Breast Cancer Metastasis: Mechanisms and Therapeutic Implications
Affiliations.
- 1 Department of Medical and Biological Sciences, The Catholic University of Korea, Bucheon 14662, Korea.
- 2 Department of Biotechnology, The Catholic University of Korea, Bucheon 14662, Korea.
- PMID: 35743249
- PMCID: PMC9224686
- DOI: 10.3390/ijms23126806
Breast cancer is the most common malignancy in women worldwide. Metastasis is the leading cause of high mortality in most cancers. Although predicting the early stage of breast cancer before metastasis can increase the survival rate, breast cancer is often discovered or diagnosed after metastasis has occurred. In general, breast cancer has a poor prognosis because it starts as a local disease and can spread to lymph nodes or distant organs, contributing to a significant impediment in breast cancer treatment. Metastatic breast cancer cells acquire aggressive characteristics from the tumor microenvironment (TME) through several mechanisms including epithelial-mesenchymal transition (EMT) and epigenetic regulation. Therefore, understanding the nature and mechanism of breast cancer metastasis can facilitate the development of targeted therapeutics focused on metastasis. This review discusses the mechanisms leading to metastasis and the current therapies to improve the early diagnosis and prognosis in patients with metastatic breast cancer.
Keywords: EMT; breast cancer; metastasis; tumor microenvironment.
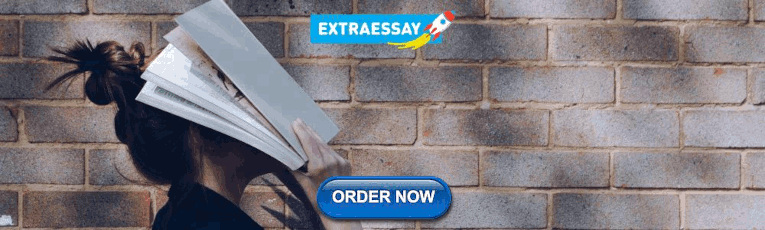
Publication types
- Breast Neoplasms* / genetics
- Epigenesis, Genetic
- Epithelial-Mesenchymal Transition
- Lymph Nodes / pathology
- Neoplasm Metastasis / pathology
- Neoplasms, Second Primary* / pathology
- Tumor Microenvironment
Grants and funding
- M2022B002600003/Korea Ministry of Education
- IT’S ADVANCING OUR UNDERSTANDING OF BREAST CANCER
- IT’S SAVING LIVES, IMPROVING OUTCOMES
- IT’S LEADING TO PREVENTION & A CURE
- RESEARCH IS THE REASON STORIES
- Our Approach
- The Ground We’ve Gained
- Areas of Focus
- Meet Our Researchers
- Collaborative Initiatives
- Start Your Fundraiser
- Make a planned gift
- Game for BCRF
- Other Ways to Give
- Become a Partner
- Find an event
- Our History
- Board of Directors
- Scientific Advisors
- Corporate Partners
- Affiliate Organizations
- Major Donors
- Blog: The Progress Report
- Podcasts: Investigating Breast Cancer
- Video Series: Behind the Breakthroughs
- Stories: Research is the reason
- BCRF Publications
- Research is the reason
Metastatic Breast Cancer Research: An Area of Focus for BCRF
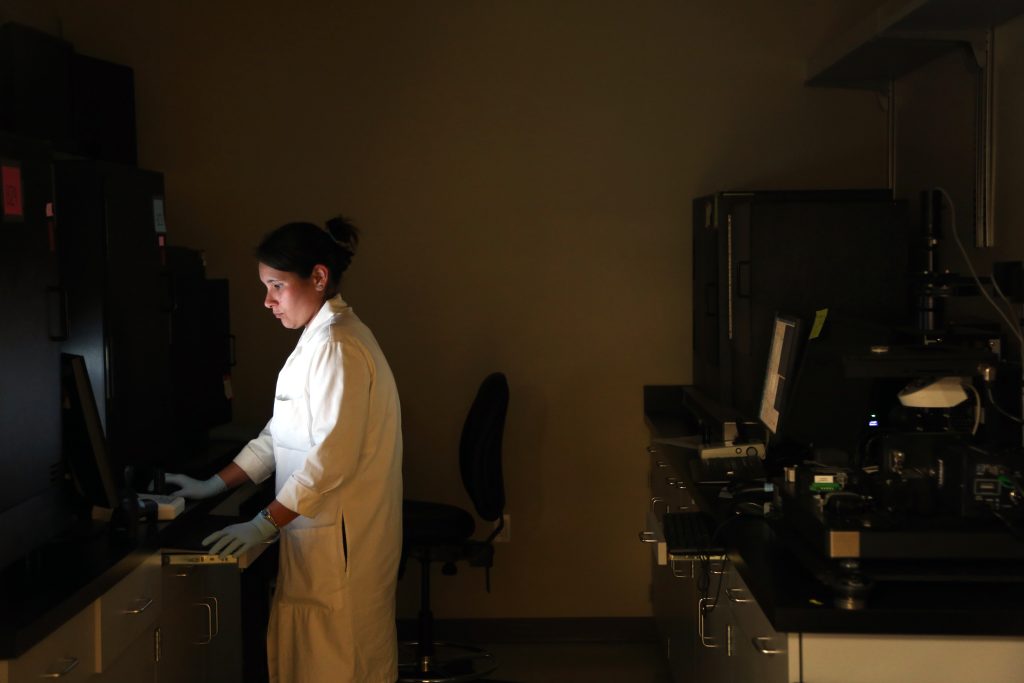
BCRF is the largest private funder of metastatic breast cancer research in the world
Metastatic breast cancer (MBC), also referred to as stage 4 or advanced breast cancer, is incurable and responsible for virtually all breast cancer related deaths, leading to about 43,000 deaths in the U.S. this year.
While we’ve made significant strides in understanding and treating primary breast cancer, we still have much to learn about metastatic breast cancer. Progress has been slow in identifying better treatments and ways to prevent metastasis.
RELATED: Metastatic Breast Cancer Symptoms, Treatments, and More to Know
Metastatic breast cancer research is a major focus for BCRF. In our 2023-24 investment year , BCRF is the largest private funder of stage 4 breast cancer research in the world and is funding 83 projects dedicated to this form of the disease. This investment totals $22.3 million.
These funded projects span every aspect of metastasis including:
- Understanding the intricacies of the events that lead to cancer cell invasion at the local tissue
- How cancer cells survive in the circulation and then grow at new sites
- Identifying new markers to predict the risk of metastasis or new targets for drug development
- Clinical trials testing new treatments for metastatic breast cancer
Read more about BCRF’s annual investment in metastatic breast cancer research here .
In addition to these projects, BCRF has supported the establishment and research of the Evelyn H. Lauder Founders’ Fund for metastatic breast cancer research.
The Evelyn H. Lauder Founder’s Fund
In 2013, under the direction of Co-Scientific Director Dr. Larry Norton and former Chairman of the Scientific Advisory Board Dr. Clifford Hudis, BCRF established a dedicated fund to support research to specifically address the persistent challenges of breast cancer metastasis. This fund now known as the Evelyn H. Lauder Founder’s Fund, has dedicated $31 million to date to support research that will accelerate discoveries to improve outcomes for people living with metastatic breast cancer and ultimately, prevent metastases altogether.
The centerpiece of the Fund are the AURORA projects, based in Europe and the United States. Inspired by The Cancer Genome Atlas (TCGA)—which compiled the landmark genomic database of primary tumors—AURORA is building the world’s largest multi-disciplinary repository of matched primary and metastatic tumor data. Deep analysis of metastatic tumor DNA, RNA, and proteins are providing new insights into the processes and mechanisms of stage 4 cancer. This invaluable resource can be mined to fuel future research and help provide answers to questions we haven’t yet formulated.
AURORA EU is based in Brussels and conducted by the Breast International Group (BIG) under the oversight of Dr. Martine Piccart. The objective of AURORA EU is to conduct precise molecular analyses of primary and stage 4 breast cancer samples to better understand the evolution of metastasis and the mechanisms of drug resistance that allow tumors to grow and spread. The program involves 60 hospitals across 11 countries throughout Europe and has already yielded new insights into metastatic disease .
AURORA US shares AURORA EU’s goals. In addition to enrolling new patients with metastatic disease, the U.S. study will more rapidly advance metastasis research by studying tumors from previously treated patients with known outcomes. The U.S. study harnesses the model clinical trials program, TBCRC , which involves 18 clinical sites and more than 100 investigators, and published its first findings in 2022 . Ongoing analysis of patient samples will help determine the features that cause metastasis and/or resistance to treatment.
Read more about the Founder’s Fund here .
Ancillary studies
While the AURORA studies are the flagship of the Evelyn H. Lauder Founder’s Fund additional projects augment the efforts of the AURORA groups to help accelerate discoveries. Ancillary projects have studied breast cancers that have metastasized to the brain; built cutting-edge lab models of metastatic tumor growth and treatment response; and leveraged the power of the internet to build a massive online registry of patient-reported metastatic tumor and clinical data.
- Metastatic Breast Cancer Alliance
BCRF is a founding member of the Metastatic Breast Cancer Alliance , a coalition of 50 nonprofits, patients, pharmaceutical companies, and biotech that work together to improve the lives and outcomes for those living with stage 4 breast cancer through education, awareness and the advancement of research. BCRF serves as the administrative home of the MBC Alliance, and BCRF staff are engaged in Alliance initiatives to advance its mission.
- Our Commitment to Metastatic Breast Cancer Research
- Metastatic Breast Cancer: Symptoms, Treatment, Research
- More BCRF-Curated Resources for People with Metastatic Breast Cancer
- Dr. Martine Piccart Discusses AURORA EU on the BCRF Podcast
Get The Latest
Connect with us.
Please remember BCRF in your will planning. Learn More
Breast Cancer Research Foundation 28 West 44th Street, Suite 609, New York, NY 10036
General Office: 646-497-2600 | Toll Free: 1-866-346-3228 [email protected] | BCRF is a 501 (c)(3) | EIN: 13-3727250
- Privacy Policy
- Find a Doctor
- Appointments and Second Opinions
- Find a Location
- Patient Portals
Metastatic Breast Cancer Clinical Trials and Research
Short placeholder heading

New Patient Appointments
2023-2024 EMBRACE Metastatic Breast Cancer Forum Series
Join us for the EMBRACE Metastatic Breast Cancer Virtual Forum Series, a series of free online educational programs for patients, families, and loved ones. This series begins in Fall 2023 and will go through Spring 2024.
Information and registration
View recordings from the 2022-2023 forum
2022-2023 EMBRACE Metastatic Breast Cancer Forum Series
The 2022-2023 EMBRACE Metastatic Breast Cancer Forum Series of online educational programs for patients, families, and loved ones was held from October 2023 to May 2024. We hope you join us for the next series!

Because we recognize that metastatic breast cancer is not just a single disease, we personalize clinical trials to each patient's type of cancer and diagnosis, matching patients with the trial that is the most compelling and relevant for their kind of breast cancer. We are conducting multiple clinical trials to study the effect of new targeted drugs, particularly in patients with metastatic disease. Much of the research work performed at Dana-Farber has resulted in treatment breakthroughs and new standards of care for patients with metastatic disease.
See our full list of clinical trials for metastatic breast cancer .
Should You Participate in a Clinical Trial?
Participating in a clinical trial is a very personal decision, and a choice that is completely yours to make. If it feels right to you, there are several good reasons to participate:
- Clinical trials are how we make progress against cancer.
- Over the past decade, thanks to participating patients, a number of new drugs have been approved for treatment of metastatic breast cancer.
- Taking part in a clinical trial can offer you new treatment options.
- By participating in a clinical trial, you contribute to knowledge that can help future patients.
Learn more about clinical trials and whether participating in a clinical trial is right for you.
Clinical Trials for Metastatic Breast Cancer
- Trial #18-516: Phase II trial of GDC-0084 in combination with trastuzumab for patients with HER2-positive breast cancer brain metastases Principal investigator: Jose Pablo Leone, MD
- Trial #20-683: ZN-A-1041 combinations for patients with HER2-positive breast cancer with or without brain metastases Principal investigator: Nancy U. Lin, MD
- Trial #21-531: Sacituzumab govitecan for patients with HER2-negative brain metastases Principal investigator: Nancy U. Lin, MD
- Trial #21-137: OP-1250 for patients with metastatic, ER-positive breast cancer Principal investigator: Nancy U. Lin, MD
- Trial #16-305: Whole-brain radiation vs. stereotactic radiation for patients with 5-20 brain metastases Principal investigator: Ayal Aizer, MD, MHS
- Trial #19-066: Screening MRI of the brain in metastatic breast cancer or inflammatory breast cancer Principal investigator: Ayal Aizer, MD, MHS
Ask your provider if any of these clinical trials may be right for you.
EMBRACE Study
Ending Metastatic Breast Cancer for Everyone (EMBRACE) is a research study conducted at Dana-Farber Cancer Institute. This study began recruiting patients in 2009, with the aim of learning more about the biology of advanced breast cancer, as well as the treatment experiences of patients living with it.
Each patient's progress is tracked by a study coordinator, and the information collected is used to help doctors understand more about the long-term journey of the metastatic breast cancer patients in this group. Investigators hope the study will bring them closer to finding a cure for this disease.
The study also sponsors a metastatic breast cancer forum every year, as well as webcasts of topics relevant to patients with metastatic disease.
For more information on EMBRACE, please email [email protected] .
Research Advances in Metastatic Breast Cancer
Research is particularly vital when it comes to cancer that has spread beyond the breast (metastatic). One hallmark of the Susan F. Smith Center for Women's Cancers is the integration of research and patient care, and the equal investment in both. At a basic level, scientists seek to understand the basic biology of how and why breast cancer can spread, and at a clinical level, clinician/scientists test potential therapies in patients. More than 30 clinical trials are now underway for women with metastatic breast cancer.
Research conducted through the Specialized Program of Research Excellence (SPORE) encompasses several metastatic breast cancer projects. This prestigious five-year grant funded by the National Cancer Institute includes projects aimed at identifying drug "targets" on breast cancer cells.
Clinical Trials for Metastatic Breast Cancer: What You Should Know
Nancy Lin, MD, and Filipa Lynce, MD, experts in breast cancer research and treatment at Dana-Farber, discuss considerations for patients considering a clinical trial for metastatic breast cancer.
Here are some examples of our current metastatic breast cancer research advances.
Clinical Trials
- Sara Tolaney MD, MPH , Erica Mayer, MD , and their colleagues have launched a series of clinical trials of drugs designed to stimulate the immune system to attack metastatic breast cancer. Trials of immunotherapy approaches are currently underway for all three major breast cancer subtypes (ER-positive, HER2-positive, and triple-negative).
- ER-positive breast cancer is typically treated with a combination of hormonal therapy with a targeted treatment, such as a CDK4/6 inhibitor (e.g. Ibrance, Kisqali, or Verzenio). Now, Sara Tolaney, MD, MPH , and Erica Mayer, MD, MPH , are leading clinical trials to test new ways to overcome resistance to CDK4/6 inhibitors, including combinations with immunotherapy or other targeted medications.
- A phase 2 clinical trial led by Rachel Freedman, MD, MPH , and Nancy Lin, MD , found that the combination of two oral medications — neratinib (Nerlynx) and capecitabine (Xeloda) — can be effective in patients with HER2-positive breast cancer that has spread to the brain. This is the first systemic treatment to gain recognition by the National Comprehensive Cancer Network (NCCN) guidelines for treatment of patients with brain metastases. Multiple trials are currently underway to test additional treatment approaches for cancer that has spread to the brain.
Basic Research
- Hormonal therapy, which deprives ER-positive breast cancers of estrogen, is part of after-surgery therapy for early ER-positive breast cancer and is often successful at preventing return of the disease. However, ER-positive breast cancer can recur many years later, often at a site far from the original tumor. Researchers led by Myles Brown, MD , have discovered that breast tumor cells can carry an abnormal "receptor" for estrogen, potentially allowing them to grow even when estrogen is absent. Researchers hope to use this finding to guide the development of new drug agents.
- Brown and his colleague Rinath Jeselsohn, MD , are studying a particularly promising class of drugs for metastatic, ER-positive breast cancers. Known as selective estrogen receptor degraders (or SERDs), they clamp onto the estrogen receptor and cause it to crumble, so the tumor cells no longer respond to estrogen.
- Other research is focusing on the molecular makeup of breast tumors — the presence of genetically distinct cells within a single tumor. Tumors with the most "heterogeneity" — the greatest diversity of cell types within them — are often more likely to resist treatment and to metastasize. Researchers led by Kornelia Polyak, MD, PhD , have discovered that the fastest-proliferating cells within a tumor aren't necessarily the main drivers of tumor growth, suggesting that efforts to develop new drugs should focus on targeting the actual driver cells.
- Up to 50 percent of HER2-positive breast cancers spread to the brain, but scientists have known little about how the process occurs. Recently a team of researchers including Nikhil Wagle, MD, Eric Winer, MD, Ian Krop, MD, PhD, Jean Zhao, PhD , Nancy Lin, MD, and Shom Goel, MD, PhD, found that approximately 12 percent of such tumors contain mutations that may explain why they don't respond well to the target drug trastuzumab, also known as Herceptin. Researchers in Zhao's lab have implanted patients' metastatic tissue into animal research models, making it possible to test various drugs to see which may be most effective against the disease.
New Research in Metastatic Breast Cancer
Harold J. Burstein, MD, PhD, provides an overview of the latest research in metastatic breast cancer at the San Antonio Breast Cancer Symposium in December 2019.
Skip to Content
- Conquer Cancer
- ASCO Journals
- f Cancer.net on Facebook
- t Cancer.net on Twitter
- q Cancer.net on YouTube
- g Cancer.net on Google
Types of Cancer
- Navigating Cancer Care
- Coping With Cancer
- Research and Advocacy
- Survivorship
Breast Cancer - Metastatic: Latest Research
ON THIS PAGE: You will read about the scientific research being done to learn more about this type of cancer and how to treat it. Use the menu to see other pages.
Doctors are working to learn more about metastatic breast cancer, including ways to prevent it, how to best treat it, and how to provide the best care to people diagnosed with this disease. The following areas of research may include new options for patients through clinical trials. Always talk with your doctor about the best diagnostic and treatment options for you.
Understanding the metastatic breast cancer process and finding ways to prevent and stop it.
Developing ways to best evaluate the genes and proteins contributing to cancer growth in each patient and each metastatic breast cancer to determine the best treatment options.
Testing new drugs and treatment combinations, such as new chemotherapies, hormonal treatments, and targeted therapies.
Studying the use of immunotherapy, which boosts the body’s natural defenses to fight cancer.
Determining the best ways to give hormonal therapy, and how to overcome tumor resistance to hormonal therapy.
Determining if surgical removal of the primary breast tumor can improve survival rates.
Testing various drugs for effectiveness against HER2-positive breast cancer that metastasizes to the brain.
Identifying new and effective treatments for triple-negative breast cancer.
Finding ways to check how well treatments are working against metastatic breast cancer and predict survival rates.
Learning more about the social and emotional factors that may affect patients’ treatment plans and quality of life.
Finding better ways of reducing symptoms and side effects of current metastatic breast cancer treatments to improve comfort and quality of life for patients and their caregivers.
Looking for More About the Latest Research?
If you would like more information about the latest areas of research in metastatic breast cancer, explore these related items that take you outside of this guide:
To find clinical trials specific to your diagnosis, talk with your doctor or search online clinical trial databases .
Visit the Cancer.Net Blog to review news and information about metastatic breast cancer, including research announced at recent scientific meetings or in the American Society of Clinical Oncology's (ASCO's) peer-reviewed journals.
Listen to podcasts from an ASCO expert discussing metastatic breast cancer research from 2021 and 2020 scientific meetings.
Get updates from Cancer.Net delivered right to your inbox. Subscribe to the Inside Cancer.Net email newsletter.
Visit the website of Conquer Cancer, the ASCO Foundation , to find out how to help support cancer research. Please note that this link takes you to a different ASCO website.
The next section in this guide is Palliative and Supportive Care . It describes how the symptoms and side effects of metastatic breast cancer and its treatment can be managed. Use the menu to choose a different section to read in this guide.
Breast Cancer - Metastatic Guide
Cancer.Net Guide Breast Cancer - Metastatic
- Introduction
- Risk Factors
- Symptoms and Signs
- Types of Treatment
- About Clinical Trials
- Latest Research
- Palliative and Supportive Care
- Coping with Treatment
- Living with Metastatic Breast Cancer
- Questions to Ask the Health Care Team
- Additional Resources
View All Pages
Timely. Trusted. Compassionate.
Comprehensive information for people with cancer, families, and caregivers, from the American Society of Clinical Oncology (ASCO), the voice of the world's oncology professionals.
Find a Cancer Doctor
metastatic breast cancer Recently Published Documents
Total documents.
- Latest Documents
- Most Cited Documents
- Contributed Authors
- Related Sources
- Related Keywords
Real-World Evidence of Trastuzumab, Pertuzumab, and Docetaxel Combination as a First-Line Treatment for Korean Patients with HER2-Positive Metastatic Breast Cancer
Survival in women with de novo metastatic breast cancer: a comparison of real-world evidence from a publicly-funded canadian province and the united states by insurance status.
Metastatic breast cancer (MBC) patient outcomes may vary according to distinct health care payers and different countries. We compared 291 Alberta (AB), Canada and 9429 US patients < 65 with de novo MBC diagnosed from 2010 through 2014. Data were extracted from the provincial Breast Data Mart and from the National Cancer Institute’s SEER program. US patients were divided by insurance status (US privately insured, US Medicaid or US uninsured). Kaplan-Meier and log-rank analyses were used to assess differences in OS and hazard ratios (HR) were estimated using Cox models. Multivariate models were adjusted for age, surgical status, and biomarker profile. No difference in OS was noted between AB and US patients (HR = 0.92 (0.77–1.10), p = 0.365). Median OS was not reached for the US privately insured and AB groups, and was 11 months and 8 months for the US Medicaid and US uninsured groups, respectively. The 3-year OS rates were comparable between US privately insured and AB groups (53.28% (51.95–54.59) and 55.54% (49.49–61.16), respectively). Both groups had improved survival (p < 0.001) relative to the US Medicaid and US uninsured groups [39.32% (37.25–41.37) and 40.53% (36.20–44.81)]. Our study suggests that a universal health care system is not inferior to a private insurance-based model for de novo MBC.
Assessment of Role of Platelet Aggregation in Metastatic Breast Cancer Patients
Background: To assess role of platelet aggregation in metastatic breast cancer patients.Methods:40 cases (Group I) of metastatic breast cancer patients and equal number of healthy control (Group II) subjects were included. Platelet aggregation studies in vitro using ADP and Thrombin were performed using an optical aggregometer. Detection of platelet aggregation was done by Chrono log series 490 dual and four channel optical aggregometer systems.Results:There were 4 subjects in group I and 12 in group II having ADP <60, 26 subjects in group I and 28 in group II with ADP 61-72 and 10 subjects in group I with ADP >72. Low thrombin <58 was seen in 8 in group II, normal thrombin between 61-72 was seen among 11 in group I and 32 in group II and high thrombin >82 among 29 in group I respectively. Amongst patients with normal platelet count, 14 patients had platelet aggregation with ADP in the normal range and 4 patients had platelet aggregation with ADP in the lower range. In patients with high platelet count, 12 showed aggregation in the normal range, and 10 patients showed aggregation in the higher range which was statistically significant (P< 0.05) (Table III, Graph II).Conclusion: Platelet aggregation has an important part to play in the tumor metastasis of breast cancer patients.
Transforming Passive into Active: Multimodal Pheophytin‐Based Carbon Dots Customize Protein Corona to Target Metastatic Breast Cancer
Aiming at a tailored cure for erbb2-positive metastatic breast cancer, multiplatform analysis of primary and metastatic breast tumors from the aurora us network identifies microenvironment and epigenetics drivers of metastasis.
Abstract Patients with metastatic breast cancer (MBC) typically have short survival times and their successful treatment represents one of most challenging aspects of patient care. This poor prognostic behavior is in part due to molecular features including increased tumor cell clonal heterogeneity, multiple drug resistance mechanisms, and alterations of the tumor microenvironment. The AURORA US Metastasis Project was established with the goal to identify molecular features specifically associated with metastasis. We therefore collected and molecularly characterized specimens from 55 metastatic breast cancer (BC) patients representing 51 primary cancers and 102 metastases. The 153 unique tumors were assayed using RNAseq, tumor/germline DNA exomes and low pass whole genome sequencing, and global DNA methylation microarrays. We found intrinsic molecular subtype differences between primary tumors and their matched metastases to be rare in triple negative breast cancer (TNBC)/Basal-like subtype tumors. Conversely, tumor subtype changes were relatively frequent in estrogen receptor positive (ER+) cancers where ~30% of Luminal A cases switched to Luminal B or HER2-enriched (HER2E) subtypes. Clonal evolution studies identified changes in expression subtype coincident with DNA clonality shifts, especially involving HER2 amplification and/or the HER2E expression subtype. We further found evidence for ER-mediated downregulation of genes involved in cell-cell adhesion in metastases. Microenvironment differences varied according to tumor subtype where ER+/Luminal metastases had lower fibroblast and endothelial cell content, while TNBC/Basal-like metastases showed a dramatic decrease in B cells and T cells. In 17% of metastatic tumors, we identified DNA hypermethylation and/or focal DNA deletions near HLA-A that were associated with its’ significantly reduced expression, and with lower immune cell infiltrates. We also identified low immune cell features in brain and liver metastases when compared to other metastatic sites, even within the same patient. These findings have direct implications for the treatment of metastatic breast cancer patients with immune- and HER2-targeting therapies and suggest potential novel therapeutic avenues for the improvement of outcomes for some patients with MBC.
Preliminary Clinical Validation of a Filtration-Based CTC Assay for Tumor Burden and HER2 Status Monitoring in Metastatic Breast Cancer
<b><i>Background:</i></b> Bearing multidimensional tumor-relevant information ranging from genomic alterations to proteomic makeup, circulating tumor cells (CTCs) constitute a promising material for liquid biopsy. The clinical validity of CTCs has been most extensively studied in metastatic breast cancer (MBC). The Cellsearch assay is currently the most widely used, while alternative strategies are pursued. A filtration-based microfluidic device has been described for CTC enrichment, but its clinical relevance remains unknown. <b><i>Methods:</i></b> In this preliminary study, we prospectively enrolled 47 MBC patients and evaluated the performance of the abovementioned CTC assay for tumor burden monitoring and human epidermal growth factor receptor 2 (HER2) status determination. <b><i>Results:</i></b> At baseline, 51.1% patients (24/47) were CTC positive. CTC count and positivity were also significantly higher in samples that accompanied poorer radiographic response evaluations. Serial blood draws suggested that CTC count enabled more accurate monitoring of tumor burden than serum markers carcinoembryonic antigen and cancer antigen 15-3. Also, in contrast to previous reports, CTC-HER2 status was moderately consistent with tumor-HER2 status. CTC-HER2 status assessment was further supported by <i>HER2</i> copy number measurements in select samples. <b><i>Conclusion:</i></b> The preliminary results from this study suggest promise for the interrogated CDC assay in several aspects, including sensitive CTC detection, accurate disease status reflection, and HER2 status determination. More studies are warranted to validate these findings and further characterize the value of CTC assay.
Outcome in patients of hormone receptor (HR) positive (Her 2) negative metastatic breast cancer treated with palbociclib – A real-world experience
Objectives: We present real-world outcome with the use of palbociclib in patients with HR-positive Her2-negative breast cancer treated at single center in India. Material and Methods: We conducted a medical audit of consecutive patients with HR-positive Her2-negative metastatic breast cancer, who were treated with palbociclib at our center between November 2016 and May 2020. Palbociclib was commenced at a dose of 125 mg orally once daily and a schedule of 21 days on therapy followed by 7 days off therapy was followed. Survival analysis included the Kaplan–Meier method using Statistical Package for the Social Sciences software (Version 26). HRs were calculated using Cox proportional hazard regression models and 95% confidence intervals (CIs) for the incidence estimates. Results: A total of 67 female patients were commenced on treatment with palbociclib between November 2016 and May 2020. The median age was 55 years (range 29–78 years). A total of 51 (76%) of these patients were postmenopausal and the remaining 16 were premenopausal. Baseline metastatic disease involved one organ/site in 23 (34%), two organs/sites in 32 (48%), three or more in 12 (18%). Bony metastasis alone was seen in 17 (25%) patients, visceral alone in 30 (45%), and the remaining 20 had both bony and visceral metastases. For these 67 patients, palbociclib was commenced as 1st line systemic therapy in 24 (36%) cases. Amongst the remaining 43 cases, it was 2nd line in 21 (31%); 3rd line and beyond in 22 (33%). Median PFS was 16.1 months (95% CI: 9.6–22.8) and median OS was 20.7 months (95% CI: 14.1–27.3). Median PFS for palbociclib use in first line was 18.7 months (95% CI: 4.6–32.9) while in subsequent lines, it was 13.8 months (95% CI: 9.8–17.9; log-rank P = 0.228). Median OS in patients who received palbociclib in first line was 23.2 months (95 % CI 20.1–26.3) and for those why received it in subsequent lines was 16.3 months (95 % CI: 12.5–20.1; P = 0.069). In total population, best response on imaging was CR in 11 (16%) cases (06 in 1st line setting and 05 in subsequent line setting); PR in 33 (49%); SD in 03; and progressive disease in 20. Median PFS with bone only metastasis: 20.9 months (95 % CI: 5.9–36.0), while with visceral metastasis 16.1 months (95% CI: 9.8–22.5; P = 0.537). Median OS with bone only metastasis: 22.7 months (95% CI: 17.8–27.5), while with visceral metastasis, it was 18.5 months (95% CI: 13.6–23.4; P = 0.314). Conclusion: Palbociclib is a useful addition in the management of HR +ve Her2 –ve breast cancer patients. Its benefit is confirmed in our real-world setting, both in the first and subsequent lines of therapy and the data are on similar lines as the global real-world data on palbociclib effectiveness.
Impact of body mass index on the efficacy of aromatase inhibitors in patients with metastatic breast cancer
Response monitoring in metastatic breast cancer: a comparison of survival times between fdg-pet/ct and ce-ct, export citation format, share document.
ORIGINAL RESEARCH article
Research trends and prospects on brain metastasis from breast cancer: a bibliometric analysis.
- 1 School of Traditional Chinese Medicine, Southern Medical University, Guangzhou, Guangdong, China
- 2 School of Chinese Medicine, Hong Kong Baptist University, Hong Kong, Hong Kong SAR, China
- 3 Department of Oncology, Affiliated Dongguan People’s Hospital, Southern Medical University, Dongguan, Guangdong, China
Introduction: Brain metastasis is the terminal event of breast cancer with poor prognoses. Therefore, this article aimed to provide an updated summary on the development, hotspots, and research trends of brain metastasis from breast cancer based on bibliometric analysis.
Method: Publications on breast cancer with brain metastasis retrieved from the Web of Science Core Collection. CiteSpace, VOSviewer, and other online bibliometric analysis platforms were used to analyze and visualize the result.
Result: In totality, 693 researchers from 3,623 institutions across 74 counties and regions published a total of 2,790 papers in 607 journals. There was a noticeable increase in publications in 2006. The United States was the dominant country with the most publications followed by China. University Texas MD Anderson Cancer Center was the most productive institution, while Dana Farber Cancer Institution was the most cited. Journal of Neuro-Oncology published the most papers, while Journal of Clinical Oncology ranked first based on cocited analysis. Nancy U. Lin was the most productive and cited author with high influence. There was a focus on basic research, clinical trials, local therapy, treatment optimization, and epidemiological studies regarding brain metastases from breast cancer. References focused on pathogenesis, prevention, treatment, and prognosis were cited most frequently, among which the clinical trial of novel treatment attracted most attention from researchers. Reference citation burst detection suggested that new therapies such as the novel tyrosine kinase inhibitor and antibody–drug conjugate may lead the research trends in the future.
Conclusion: High-income countries contributed more to the field of breast cancer with brain metastasis, while developing countries like China developed quickly. Furthermore, the success of novel therapies in recent years may lead to the new era of treatment of breast cancer with brain metastasis in the future.
1 Background
Breast cancer has been the most common malignancy in women. There has been a slight increase in breast cancer incidence rates since 2004 ( 1 ), with new cases in women reaching 281,550 in 2021, accounting for 30% of all female cancers ( 2 ). Although with a relatively high survival rate that reaches 90%, the mortality of breast cancer still ranked second in female cancer, approximately 90% of which are associated with complications from recurrent or metastatic diseases ( 3 ). Unfortunately, even patients with early-stage breast cancer will develop distant metastasis, which accounts for one-third of all cancer cases ( 4 ).
The development of breast cancer brain metastasis is regarded as a late event with a worse prognosis compared to metastasis to other organs. Different subtypes of breast cancer vary in the rate of brain metastasis. Triple-negative breast cancer [Triple-negative: hormone receptor (HR)–negative/human epidermal growth factor receptor 2 (HER2)–negative] and HER2+ breast cancer (HR-negative/HER2-positive) have a higher likelihood of developing brain metastasis, with rates of 25%–27% and 11%–20%, respectively. Both luminal A and luminal B subtypes have a lower risk of brain metastasis (l8%–15% and 11%, respectively) ( 5 – 7 ).
Despite the attention of researchers on brain metastasis from breast cancer, there has not yet been a bibliometric analysis reviewing the research output on publications concerning the topic. Herein, we utilized some bibliometric analysis tools to explore the frontiers and hotspots of studies on brain metastasis from breast cancer. Bibliometric analysis employs citation count as an assessment to measure of research quality ( 8 ). As a quantitative method, bibliometric analysis helps to trace the research profiles of different countries, institutions, and researchers that promoted the scientific production, behavior, and development in the related fields ( 9 , 10 ). Bibliometric reviews on breast cancer have covered several topics, focusing on a variety of different treatments including nanomedicine ( 9 ), immunotherapy ( 11 ), the application of pan-cancer studies in treatment ( 12 ), and radiotherapy ( 13 ).
In this study, we used CiteSpace and VOSviewer to analyze papers related to breast cancer brain metastasis and summarized the research findings. We examined the evolution and development of research hotspots in the breast cancer brain metastasis from 2006 to 2022, identifying new hotspots and topics. The aim of this study is to contribute new insights and ideas to research of breast cancer brain metastasis in the future.
2 Material and methods
2.1 data collection.
Articles related to brain metastasis from breast cancer were retrieved from the Science Citation Index Expanded (SCIE) of Web of Science Core Collection, which were all published during 1 January 1992 to 7 October 2022. Search strategy was based on the advanced search option with the following strategy: TS = (“breast cancer” OR “breast carcinoma”) AND TS = (“brain metastas*” OR “cerebral metastas*” OR “intracranial metastas*” OR “central nervous system metastas*” OR “secondary brain tumor”). The full records and cited references of the data were extracted and downloaded in a plain text file and a tab-delimited text file, consisting of publication year, authorship, title, abstract, author keywords, citation count, reference, journal title, institution, and country. Only literature written in English was contained in processes of search and downloading, which were completed within 1 day on 8 October 2022 to avoid errors caused by frequent database updates. A total of 3,096 publications were contained in the first set of data (year: 1992–2022). It may be because the data network was so complex that some functions of CiteSpace [version 6.1.R3 (64-bit)] ran very slowly. Therefore, we limited the time from 1 January 2006 to 7 October 2022 with the same strategy of search and selection mentioned earlier. A total of 2,790 publications were contained in the second set of data (year: 2006–2022). Only the annual number of publications was conducted based on the first set of data. Other analyses including country, institution, author, reference, and keyword were based on the second set of data. Occupying 89% of all the 3,096 publications in recent two decades, the analysis of the second set of data cover provided a snapshot of recent research in brain metastasis from breast cancer. Figure 1 showed the process of publication selection. Furthermore, since this study did not include any animals or experiments, ethical consent was not required.
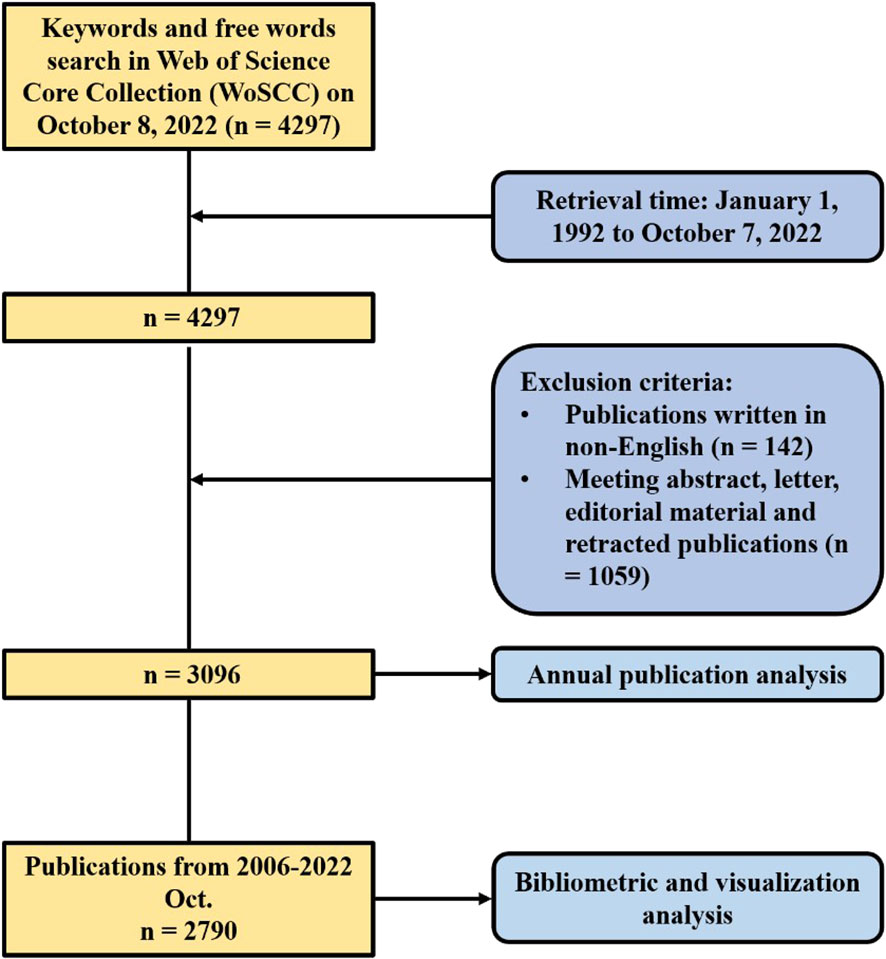
Figure 1 Flowchart of the process of publication selection.
2.2 Data analysis and visualization
Two online platforms and three software were used to analyze and visualize publications related to breast cancer brain metastasis. Datawrapper ( https://www.datawrapper.de/ ) was used to draw the map of the regional distribution of publications and BIBLIOMETRIC ( https://bibliometric.com/ ) was used to analyze the annual publications of the most productive countries as well as the collaboration between different countries. Microsoft Excel 2019 was used to analyze the annual distribution of publications on brain metastasis from breast cancer.
CiteSpace is a visualization and analyzation software designed by Prof. Chaomei Chen. It is used to discover the collaborative network and critical and pivotal points in the scientific literature of a specific topic. The analysis is based on the theory of co-occurrence and cocitation. When two publications are cited together by another publication, there is a cocitation relationship between the two publications. Burst detection is another practical function of CiteSpace to discover emerging words or references by analyzing the change of frequency of their citation or occurrence in a short time. We used CiteSpace to draw a matrix network of authors and cocited authors We also detected references with strong citation burst to find the influential references in the related field. Moreover, we detected keywords with strong occurrence burst and drew a timeline of keyword clusters, which concluded the hotspot development in different year and provided insight on the future trends in the related region. The strength of nodes was calculated of cosine, and pathfinder was used to detect the most representative network.
VOSviewer is another useful software to visualize the network map of scientific papers. We used bibliographic coupling analysis and cocitation analysis to visualize institutions and journals. We also used cooccurrence analysis to draw a cluster network of keywords. To avoid the repeat caused by expression difference, we appended the thesaurus so that the software could recognize terms like “metastasis” and “metastases” as the same term. Additionally, this study did not include any animal or experiments; thus, ethical issues were not required.
3.1 Publication output of research on breast cancer brain metastasis
Web of Science Core Collection yielded a total of 3,096 publications related to breast cancer brain metastasis in the recent three decades. As demonstrated in Figure 2 , from 1992 to 2005, the annual output rose slightly with fluctuation. In 2006, the annual number of publications was increased sharply to 57, which almost doubled that of last year. Since then, the number of publications increased substantially in general year by year and peaked in 2020 with a total of 286 publications. For this reason, we used the data from 2006 to 2022 in the follow-up analysis.
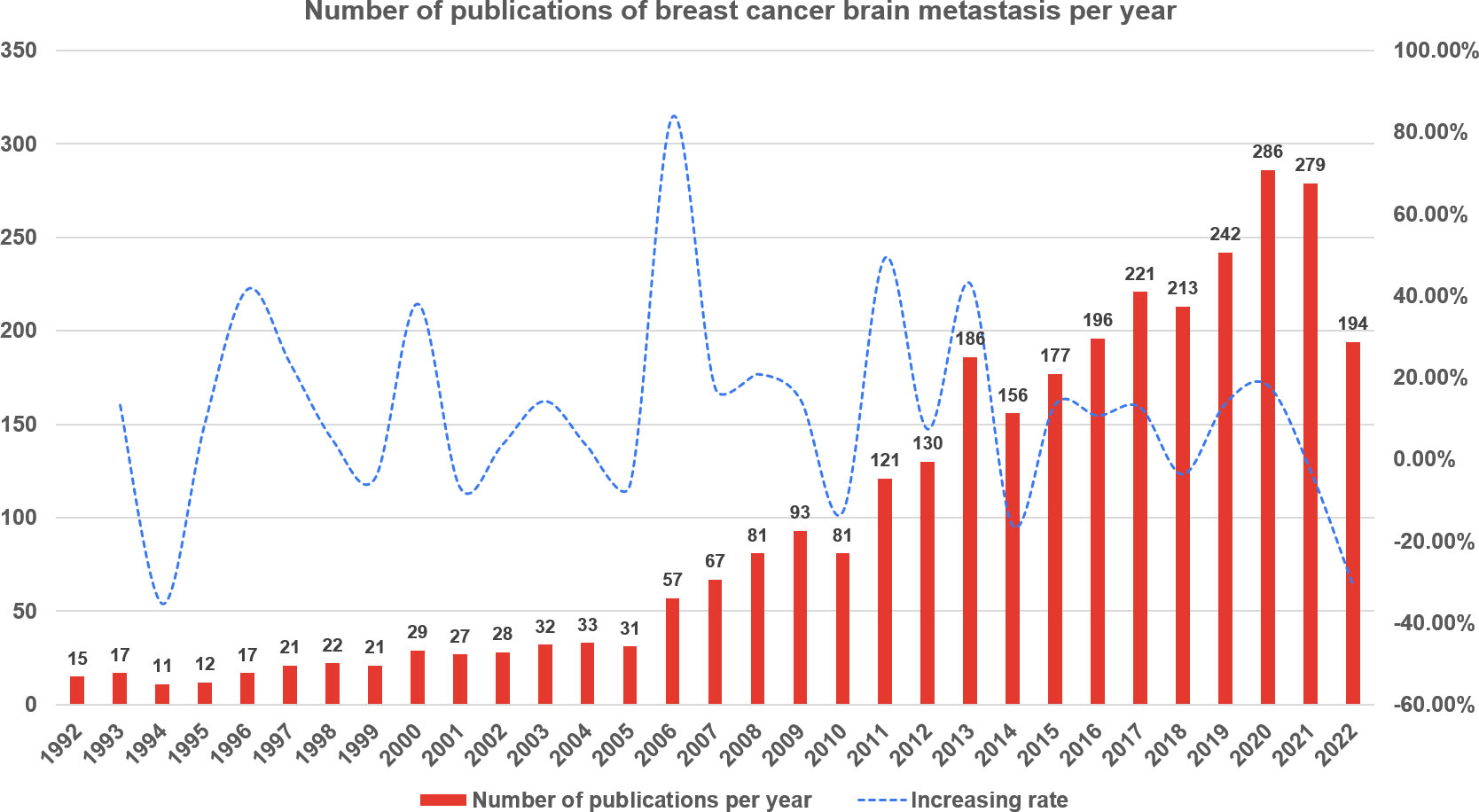
Figure 2 The annual number of publications of breast cancer brain metastasis from 1 January 1992 to 7 October 2022.
3.2 Profile of countries and institutions
A total of 74 countries or regions contributed to the studies on breast cancer brain metastasis. As shown in Figure 3 , the United States was ranked the first and China second and Germany third based on the number of publications. It is worth mentioning that China showed an emerging boost, especially in the recent decade, but the average citation stayed limited ( Table 1 ). Regarding research collaboration, the United States had the broadest range of academic collaborations with countries all around the world ( Figure 4 ).
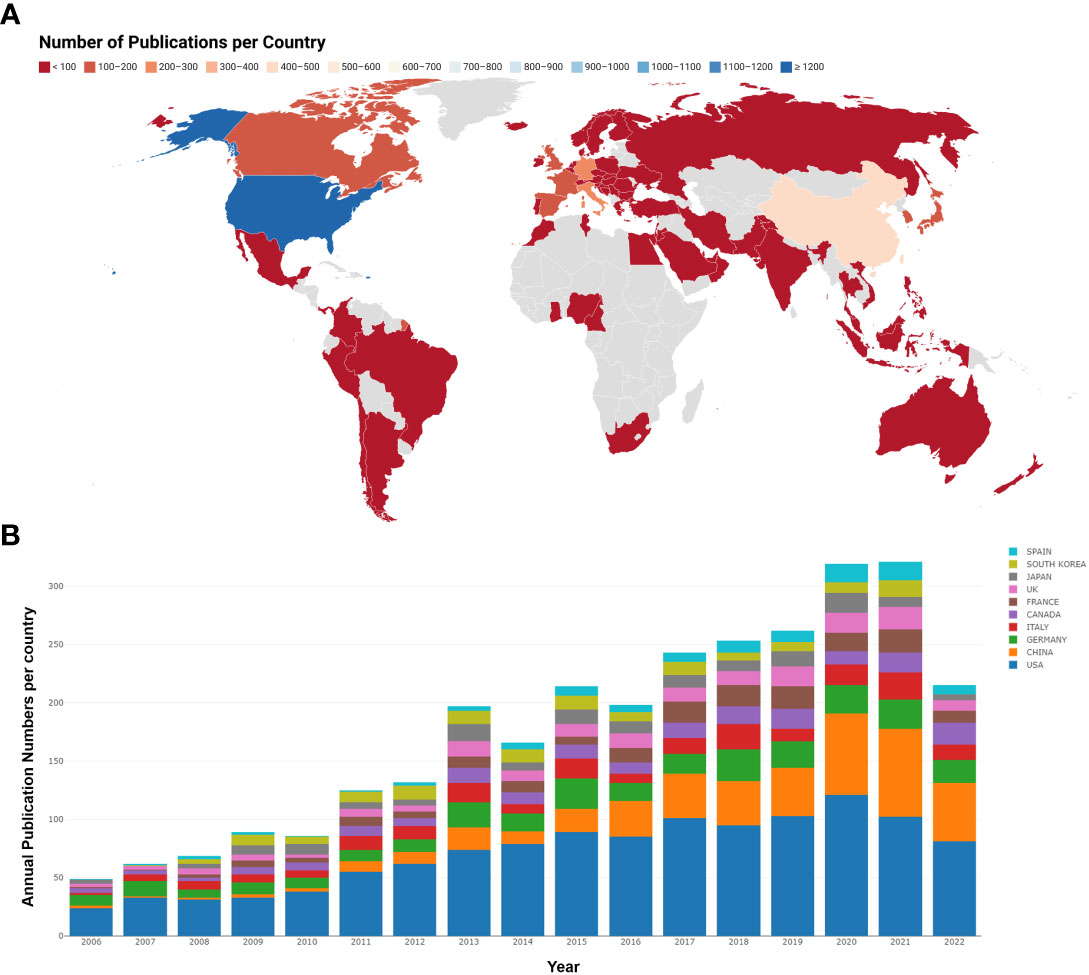
Figure 3 Analysis of country based on the number of publications. (A) The number of publications per country. Countries with less publications were colored red, while countries with most publications were colored blue. Countries without publications in the related region were colored gray. (B) The annual publication number and trend of the 10 most productive countries. Different countries were represented by different colors, and the height of each color blot reflected the number of publications of a specific country in a specific year.
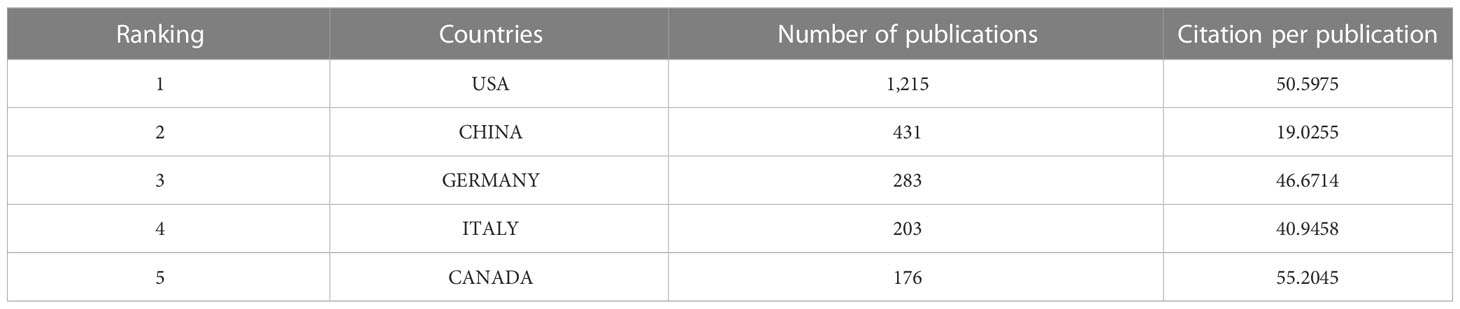
Table 1 Five most productive countries.
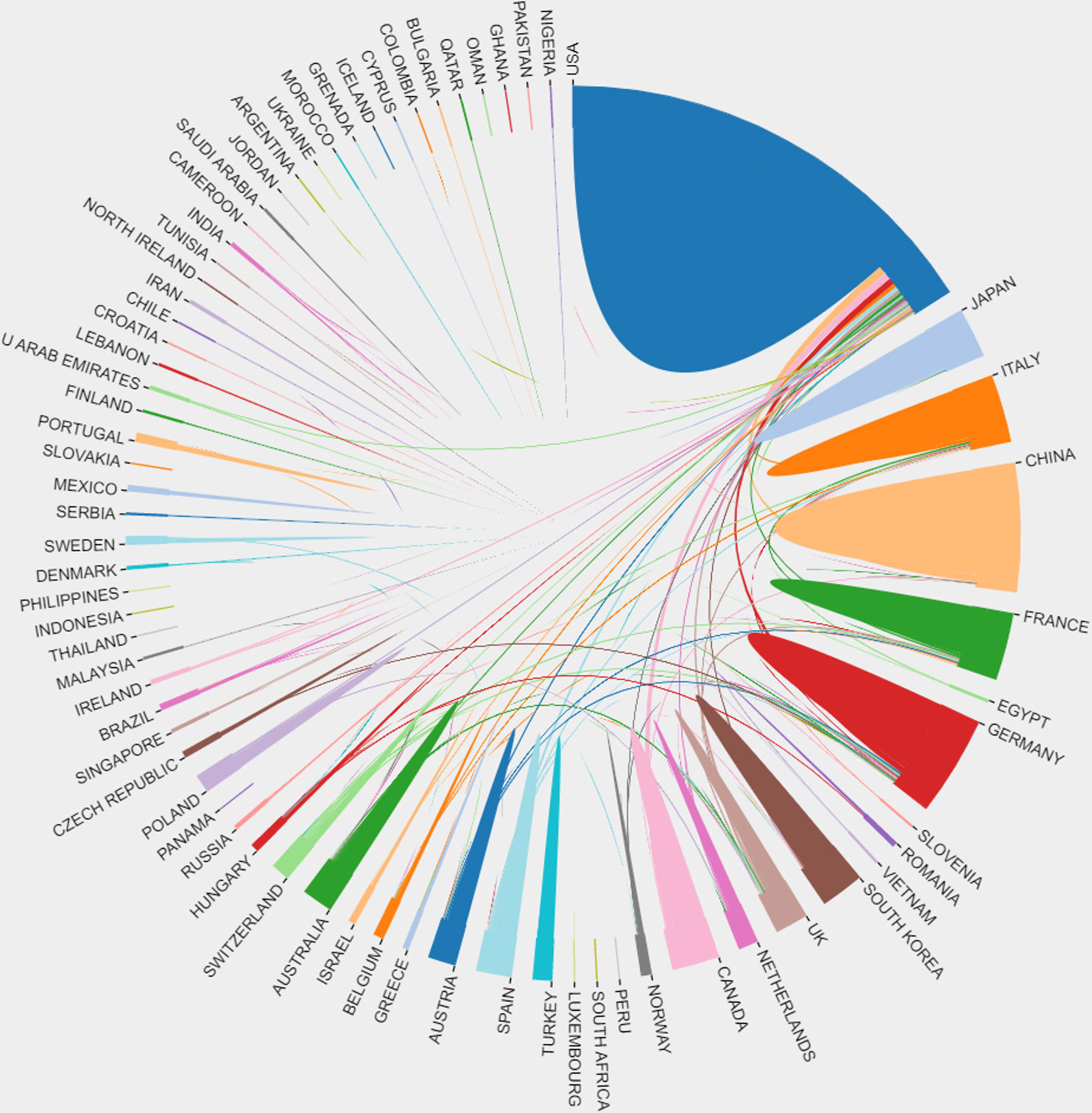
Figure 4 The connection among different countries in brain metastasis from breast cancer. Each country was represented by a fragment on the outer part of the circle. The area of the fragment is proportional to the number of publications of the correspondent country and the size of the arc to the strength of cooperation between two countries.
A total of 3,623 institutions participated in the studies of breast cancer brain metastasis, and 404 of them, with at least five publications, are shown in Figure 5 . All of the 404 institutions were divided into seven clusters based on the co-occurrence analysis. Clusters in deep blue, green, and orange, represented by the University Texas MD Anderson Cancer Center, Dana Farber Cancer Institution and University of California, San Francisco, respectively, were the institutions mainly from America. Institutions in the red cluster were mainly from Europe and America. The yellow cluster mainly consisted of institutions from China, and the purple one consisted of institutions from other East Asia countries. The light-blue cluster mainly consisted of institutions from France. When comparing Figures 5A, B , institutions in blue, green, and orange clusters were the most productive ones. Furthermore, as listed in Table 2 , nine of the most productive institutions were located in America. The University Texas MD Anderson Cancer Center was the most productive institution with a total of 131 publications, while the Dana Farber Cancer Institution was the most influential institution with the highest citation per publication and has the strongest link strength with other institutions.
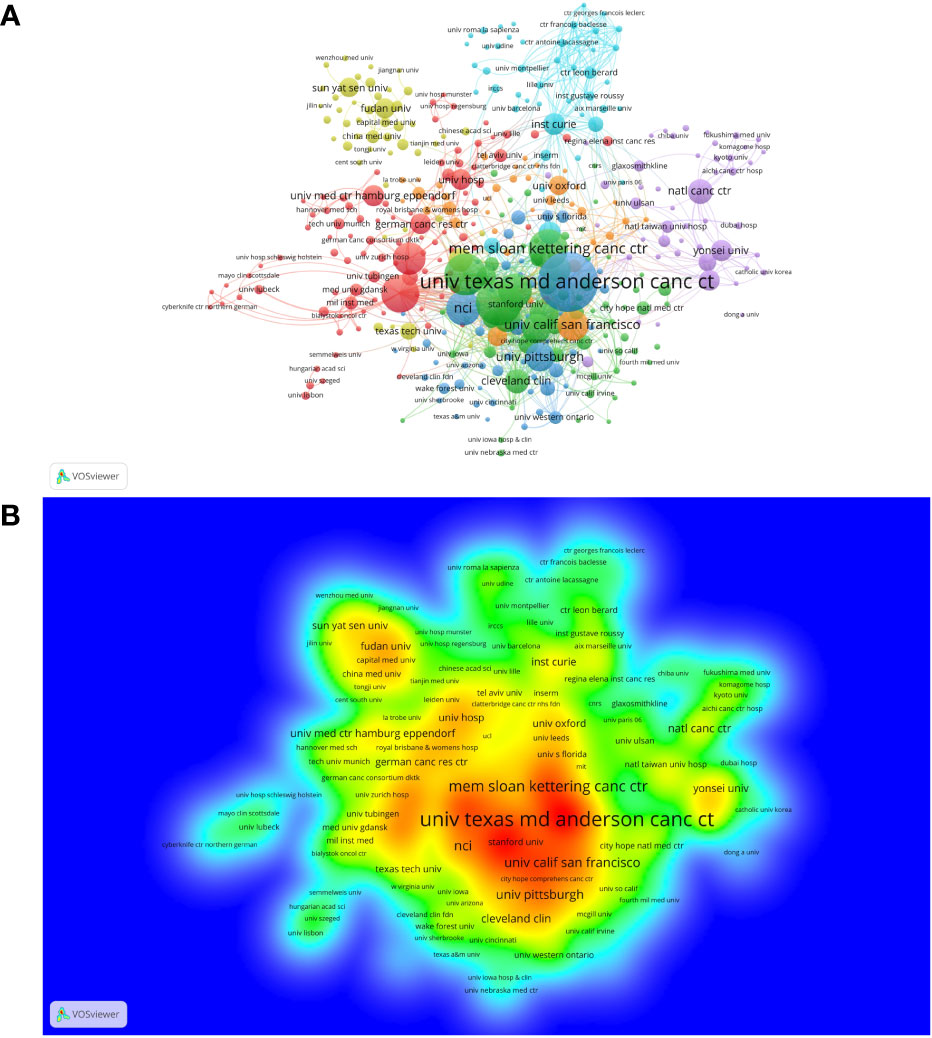
Figure 5 The co-occurrence cluster and production density of institutions. (A) cluster network analysis of institutions with at least five publications. The size of the node is proportional to the number of publications of a specific institution. The bigger the node, the more productive the institution. When two institutions occurred in the same publication for three times, there is a link between them. The width of the link represents the strength of co-occurrence between two institutions. Nodes and links in the same color belong to the same cluster, meaning the connections between these institutions are more closer according to the VOSviewer analysis. (B) The density map of institutions based on the number of publications. The labels of institutions in red area are the most productive institutions, while labels in the blue area are the less productive ones. From: VOSviewer.
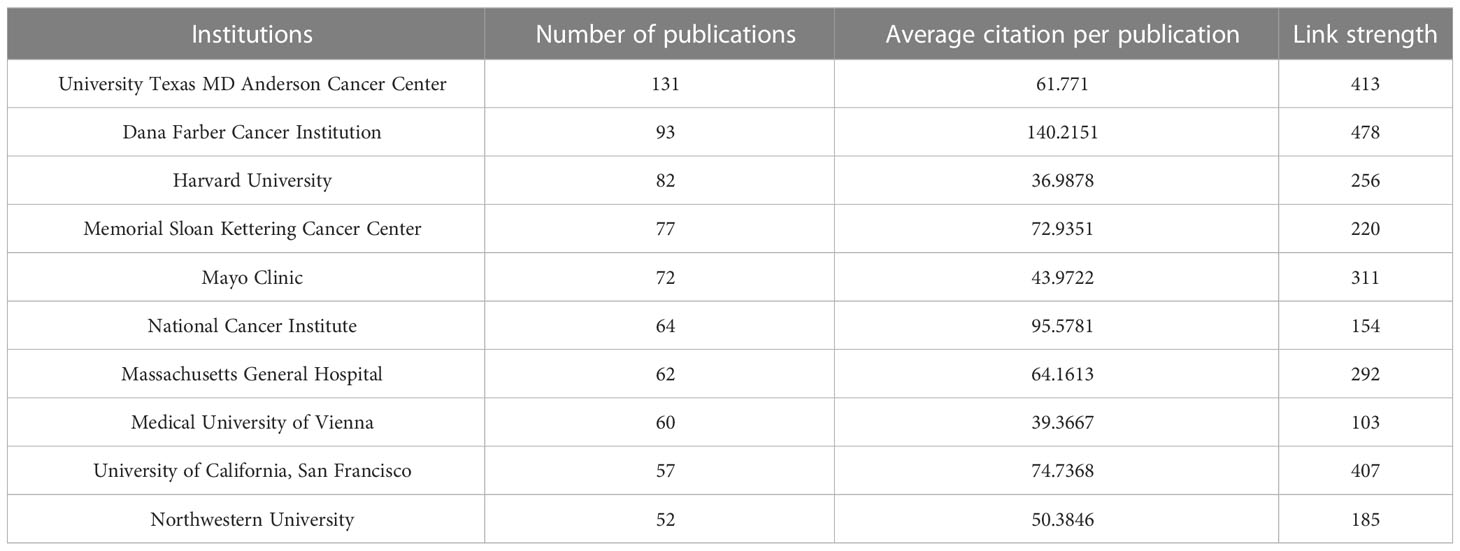
Table 2 10 most productive and cited institutions.
3.3 Profile of journals, cocited journals
VOSviewer was used to conduct the bibliographic coupling analysis and cocitation analysis of journals. A total of 2,790 papers were published in 607 journals, and 6,628 journals were cited in the references. Table 3 demonstrated the leading journals in the related research based on publication and citation. Journal of Neuro-Oncology published the most papers (n = 111), Breast Cancer Research and Treatment second (n = 82), and Cancers third (n = 68). Cocitation analysis is an effective method to discover the most influential journals in a specific topic. In the region of brain metastasis from breast cancer, Journal of Clinical Oncology ranked first with 9,861 citations, followed by Clinical Cancer Research with 4,491 citations and Cancer Research with 4257 citations. Clinical Cancer Research was the only journal both in top 10 based on production and citation, with the highest impact factor (IF = 13.801) in the list of most productive journals, indicating its special position in the related region.
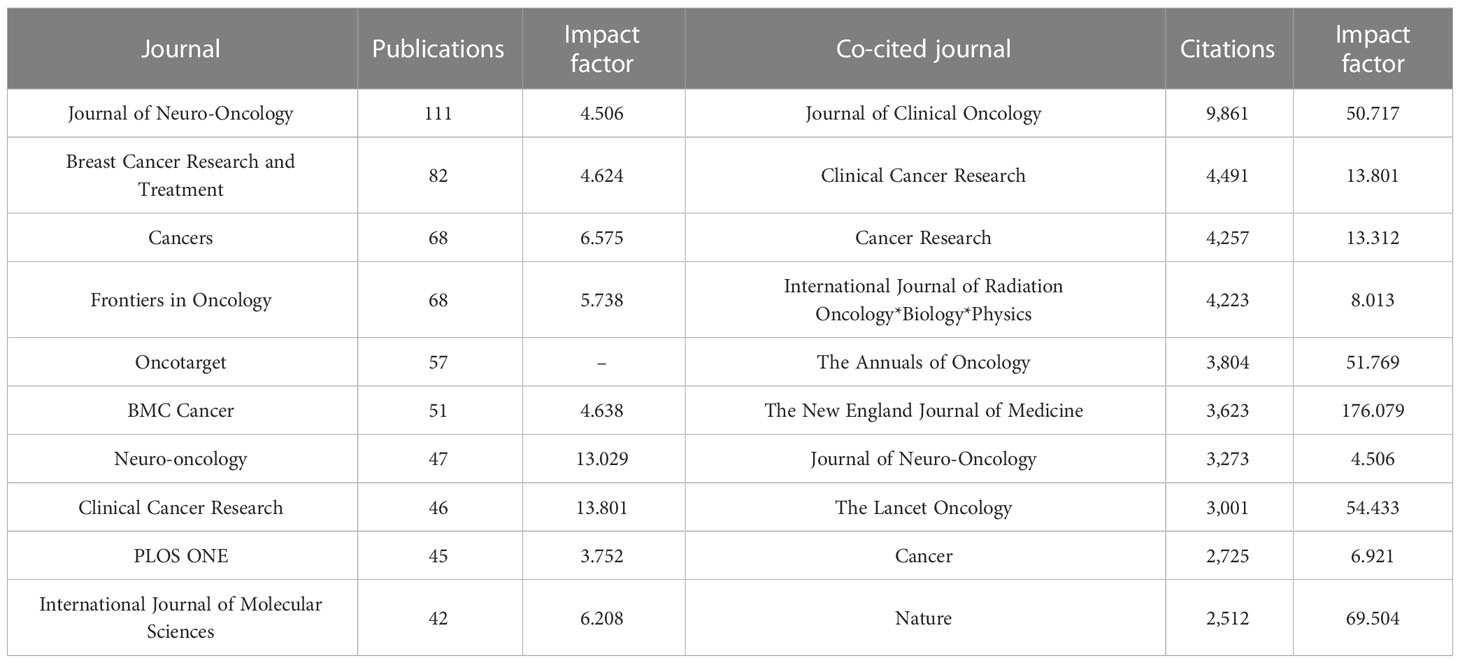
Table 3 10 most productive and most cited journals.
3.4 Profile of authors and cocited authors
CiteSpace was also used to visualize the network of authors based on co-occurrence analysis and cocited analysis. In total, 693 authors contributed to the development of research on breast cancer brain metastasis and 994 authors collaborated in the research. The top 20 authors or cocited authors in every year were selected and visualized in the network. The sizes of the nodes are proportional to the number of publications of the author shown in Figure 6A and the citation in Figure 6B . Links are thicker between two closely collaborated authors. As shown in Figure 6 , Lin NU, Kim S, Lee J, Berghoff A, and Preusser M were the top five authors based on the number of publications. As shown in Table 4 , Lin NU was active in this field all over the years with 65 publications in total. In addition, Lin NU was the most cited author based on cocited analysis with 1,000 citations. Sperduto PW ranked second with 468 citations, Pestalozzi BC ranked third with 326 citations, Patchell RA ranked fourth with 315 citations, and Bachelot T ranked fifth with 288 citations. Centrality is used to assess the relationship of one node with other nodes. When the centrality is over 0.1, the node is considered as a landmark node connected closely with other nodes in a network. The centrality of 10 most productive authors all subceeded 0.1. The centrality of 7 authors among the 10 with most citations exceeded 0.1, of which LIN NU (1.24) was the highest. These authors were the leading researchers in the field of brain metastasis from breast cancer and served as a linking bridge with other researchers.
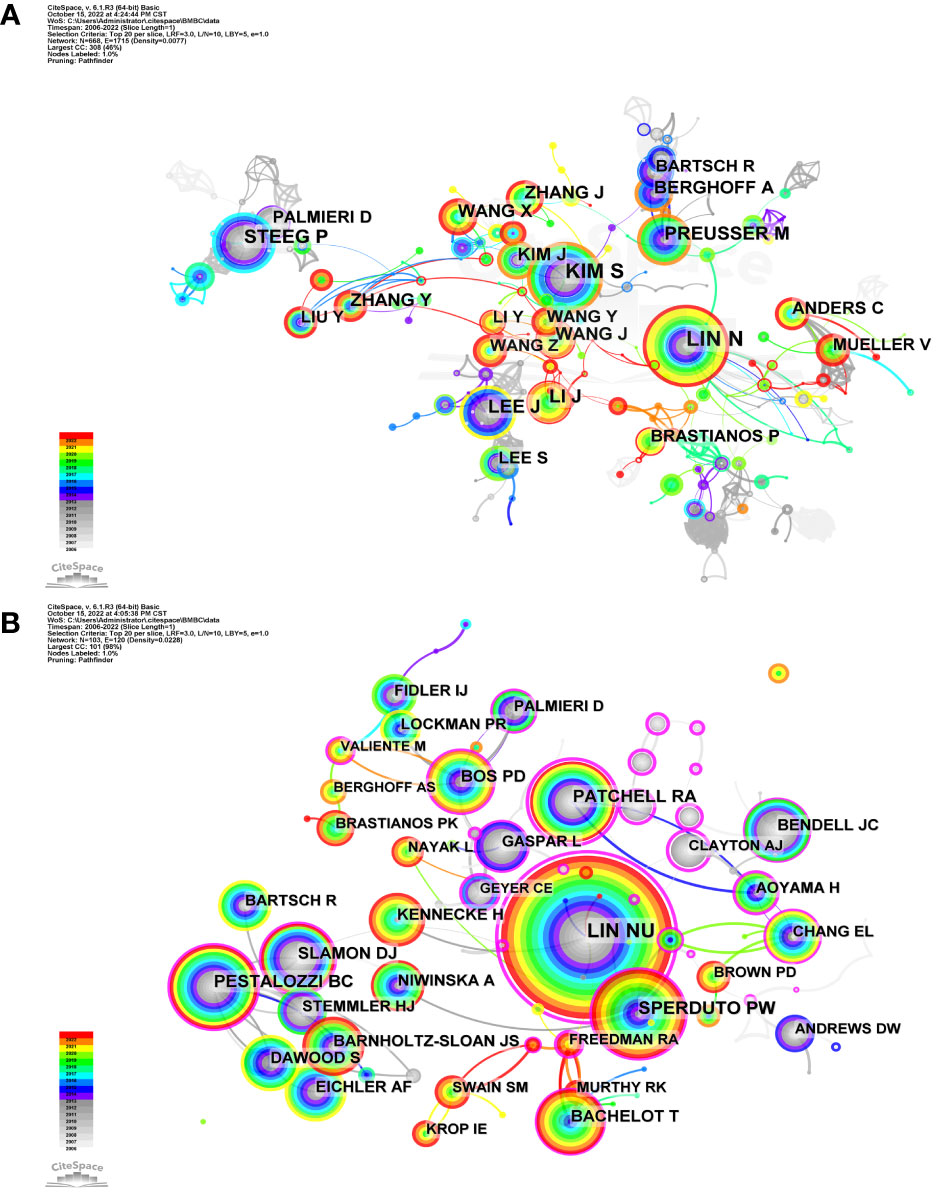
Figure 6 Network of authors and cocited authors on breast cancer brain metastasis. Each tree-ring node represents an author. (A) Author network based on cooccurrence analysis. The size of node is proportional to the research output of the author. The color of the tree-ring node represents the publication history of the author. Red tree-ring nodes represent the author who published papers in the recent 2 years, while gray ones represent authors who were productive for a decade. Colorful nodes represent authors with continuous research output. (B) Cocited author network based on cocited analysis. The larger the node, the more times the author is cited. Nodes in warmer colors represent authors cited more recently, while nodes in cooler colors represent the opposite.
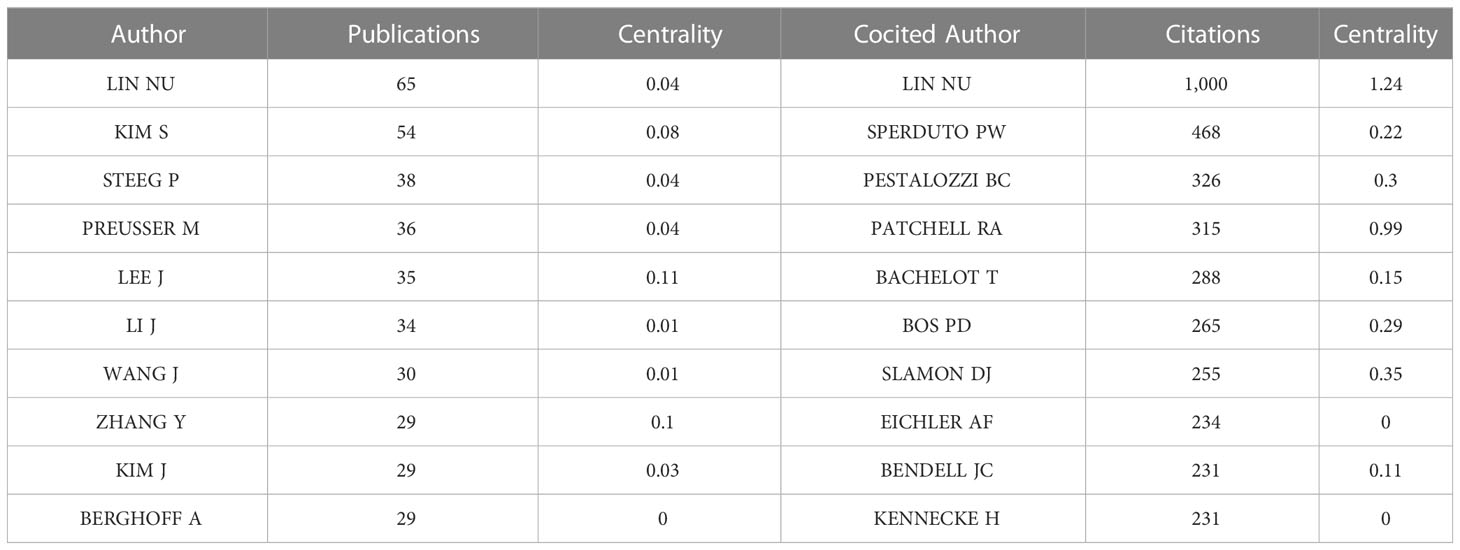
Table 4 Top 10 of the most productive authors and cocited authors.
3.5 Keywords of breast cancer brain metastasis
VOSviewer and CiteSpace were used to analyze keywords from different perspectives to provide an overlook of development and trend in the related region. After merging keywords repeated by expression difference, VOSviewer concluded 4,189 keywords and 598 of them occurred more than five times, which were divided into five clusters ( Figure 7 ). The red cluster was the largest one with 210 keywords, containing expression, in-vivo, angiogenesis, microenvironment , and endothelial growth-factor . The green cluster ranked second in size with 201 keywords including phase II trial, chemotherapy, efficacy, open-label , and lapatinib plus capecitabine . The blue cluster ranked third in size with 97 keywords including radiation-therapy, stereotactic radiosurgery, prognostic-factors, quality of life, graded prognostic assessment , and management . The yellow cluster contained 96 keywords including solid tumors, blood-brain barrier, cerebrospinal-fluid, her2-positive breast cancer, drug-delivery , and acquired-resistance . Purple cluster contained 89 keywords including survival, risk, subtype, diagnosis , and recurrence . Accordingly, keywords were roughly clustered into five categories as follows: basic study, clinical trial of new therapy, local therapy, treatment optimization, and epidemiology.
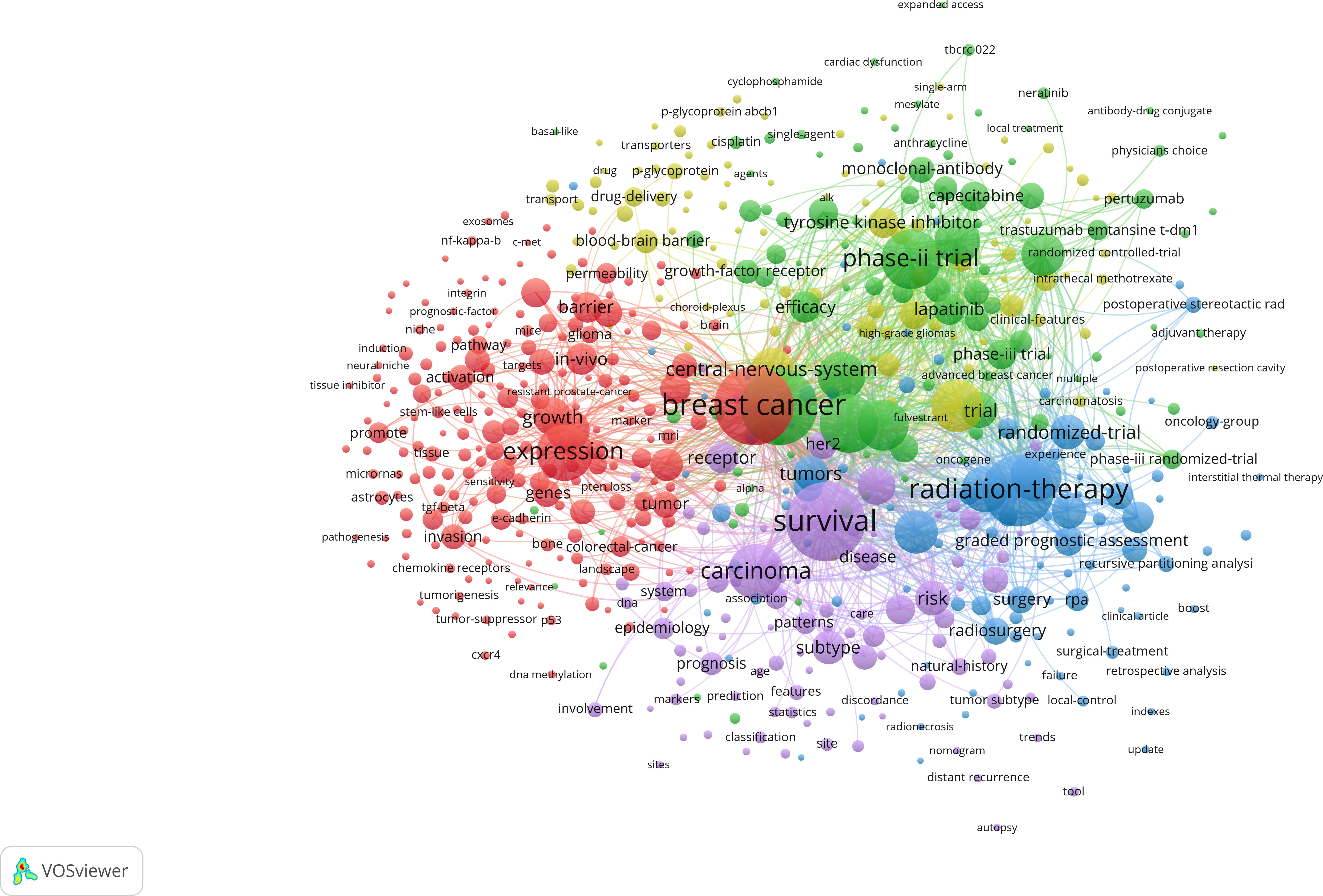
Figure 7 Co-occurrence analysis of keywords during 2006–2022. Lines between two keywords mean the two words coexisted for more than 10 times. The bigger the node, the more frequent it appears. The stronger the line, the more co-occurrences happen. At least 50 keywords are contained in one cluster. Additionally, keywords connected more closely are divided into the same cluster with the same color. From: VOSviewer.
We also used CiteSpace to perform cluster analysis based on the log likelihood ratio (LLR) and drew a timeline of the development of the hotspots in the related region ( Figure 8 ). The top 10% of keywords in a year were analyzed, which yielded a network of 253 nodes with 305 links. A cluster with the modularity of 0.8114 and the weighted mean silhouette of 0.9291 was yielded, which meant that the result was highly convincing with a significant cluster structure. There were 15 clusters including first-line treatment, epidermal growth factor receptor (EGFR) expression, pyrotinib-based therapy, the prognostic index, and other clusters, demonstrating the development of research focus on breast cancer brain metastasis.
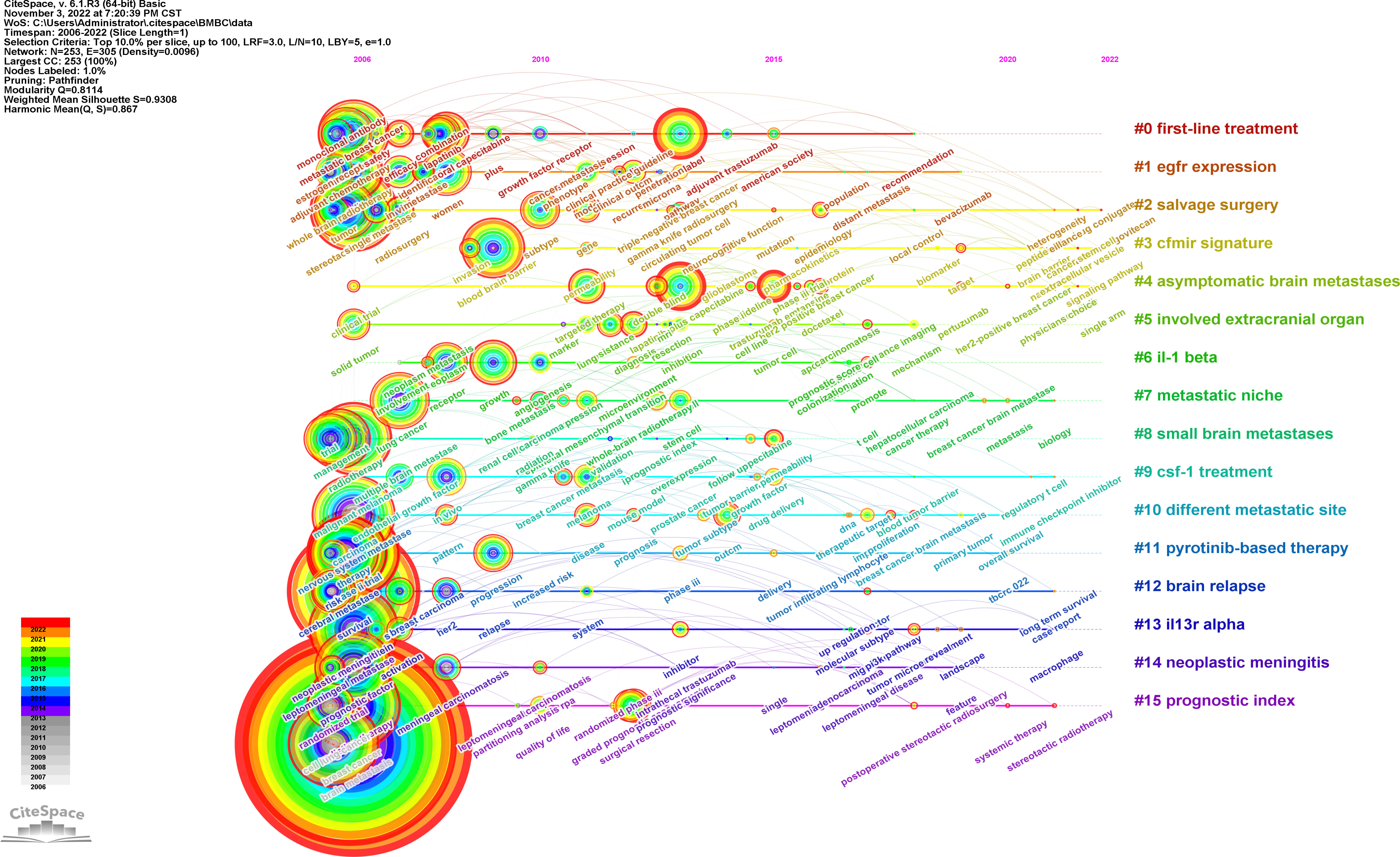
Figure 8 Cluster timeline of keywords of breast cancer brain metastasis. Each tree-ring node represents a keyword. The size of the node is proportional to the time of the occurrence of the keyword. The color of the tree-ring node represents the occurrence history of the keyword. The label of clusters was produced by LLR analysis, and the colors represent different years.
3.6 Cocited references
CiteSpace was used to visualize the network of references of records between 2006 and 2022. The top 5% of the most cited references each year were contained in the cocited analysis, and the network was refined by the pathfinder pruning method. As shown in Figure 9 , 930 references were detected and 10 of the most cocited publications are listed in Table 5 . Seven out of the top 10 co-cited publications were clinical trials, namely, five randomized controlled trials, one population-based cohort, and one retrospective cohort. The other most cocited publications included one genome wide association study (GWAS) study, one basic study, and one review. The most cited publication was “Lapatinib plus capecitabine in patients with previously untreated brain metastases from HER2-positive metastatic breast cancer (LANDSCAPE): a single-group phase 2 study ( 14 )” by Bachelot T et al., which was cited 160 times. It is worth mentioning that the sources of these publications strongly overlapped with the list of the most cited journals, which confirmed the importance of these references and journals in the studies of breast cancer brain metastasis.
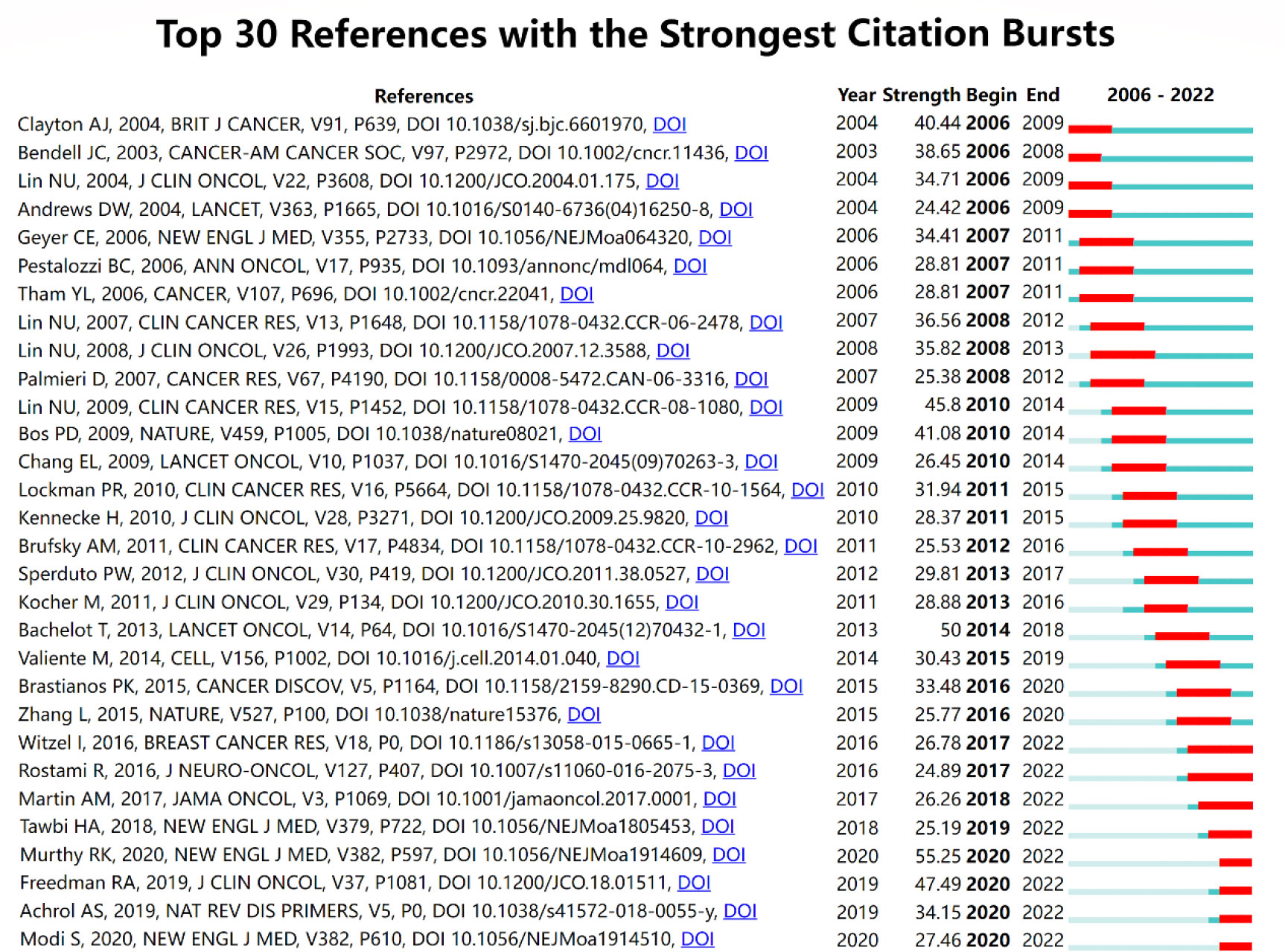
Figure 9 Top 30 references with the strongest citation bursts. The blue line reflects the duration of the citation of the paper. The red segment reflects the duration of the burst.
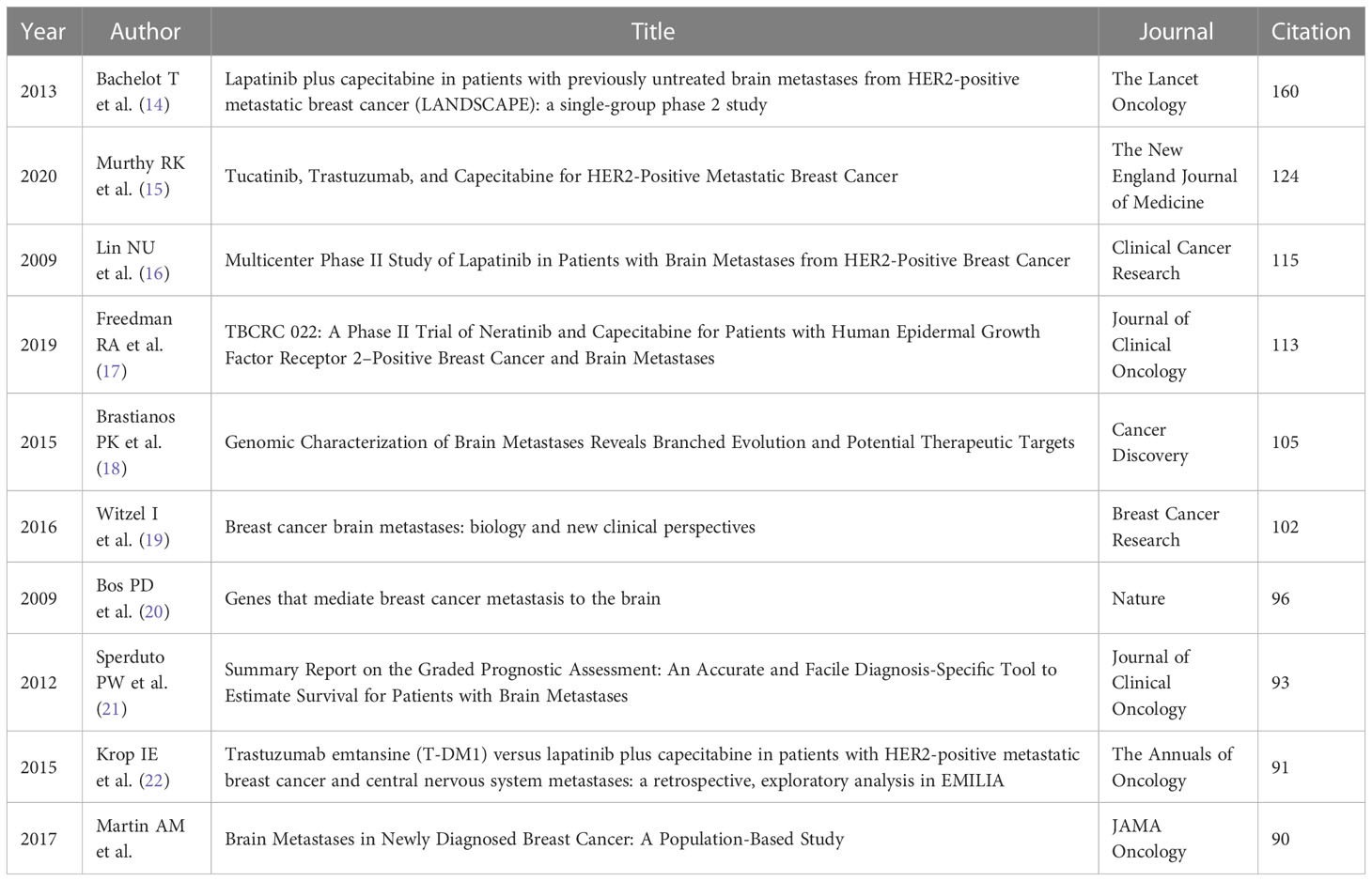
Table 5 Top 10 cocited references.
Burst detection is an effective method to find out the hotspots in different times and conclude the developmental route of a specific field supported by CiteSpace. The publication of the phase II trial of tucatinib, trastuzumab, and capecitabine for HER2-positive metastatic breast cancer ( 15 ) gained the most attention from researchers in the related field (burst strength: 55.25). The publications of another two clinical trials focused on the effects of neratinib plus capecitabine ( 17 ) and lapatinib ( 16 ) separately was ranked the second (burst strength: 47.49) and third (burst strength: 45.8), respectively. The recent publication that most appealed researchers’ attention was “Trastuzumab Deruxtecan in Previously Treated HER2-Positive Breast Cancer ( 23 )”, whose citation can be dated from 2020 with an increasing trend.
4 Discussion
4.1 general trends.
With reference from the number of publications, the publication trend of the breast cancer brain metastasis research field can be divided into two stages. From 1992 to 2005, the publications increased slowly with fluctuation. It may be due to the following two reasons. The first one is that radiotherapy was the mainstream of treatment for brain metastasis and the controversial effect of chemotherapy ( 24 ) limited the development of clinical trials. The second one is that patients with brain metastasis were always excluded from clinical trials in this period and medical oncologists were reluctant to leave the advanced patients with brain metastasis without other therapy but just the target therapy of clinical trial, which make it difficult to have enough number of patients and assess the efficacy of chemotherapy ( 25 ). In 2005, several randomized trials have confirmed the efficacy of chemotherapy in treating brain metastasis ( 24 , 26 – 28 ) and more clinical trials were ongoing, especially those focused on trastuzumab ( 28 ), which may lead to the publication boosted in 2006. From 2006 onward, the number of publications in breast cancer brain metastasis increased gradually.
In this study, we summarized the research collaboration in different dimensions as well as research focus and hotspots to understand the development and trend of breast cancer brain metastasis. A total of 693 researchers from 3,623 institutions in 74 counties and regions attributed 2,790 publications in 607 journals, indicating that breast cancer brain metastasis attracted wide attention from researchers all over the world. The United States was the leading country in the related field based on the analysis of institutions and authors’ publications and citations. Moreover, the institutions in the United States exhibited a high degree of collaboration with research institutions from all around the world, especially those from Europe. On the other hand, China had emerged as the most promising country in the last decade. Although the academic influence and research collaboration are still limited, researchers and institutions in China demonstrate diligent effort to promote the development of studies on breast cancer brain metastasis. Furthermore, regionally limited collaborations were observed based on institution analysis. Spatial isolation caused by the COVID-19 pandemic has increased the development of online meetings, which serve as a means to break the spatial boundaries between countries and accelerate the research development significantly.
Journal and cocited journal analysis listed the most productive and influential journals in the related field. The 10 top productive journals covered both basic study and clinical study, while half of the top 10 cited journals focused on clinical research, which corresponded to the result of the cocited analysis of references. It implies that clinical research has an important clinical significance in breast cancer brain metastasis. In our study, we list the 10 most productive authors and 10 most frequently cited authors. These authors contributed to the foundation of the related region. The most productive and cited author was Nancy U. Lin, who contributed 65 publications in the field. She has led and participated in numerous clinical trials on breast cancer brain metastases ( 16 , 29 , 30 ) and has participated in the development of guidelines in this field ( 31 , 32 ). In addition, Nancy U. Lin was the one with highest centrality in top 10 cocited authors, which showed her great influence in the related field.
Cocitation analysis is used to evaluate the relevance between papers, and papers with higher cocitation were considered as the milestones of a specific region. Analyzing the publications cocited most frequently helped to set up the basic knowledge and research focus of breast cancer brain metastasis. The cocited paper ranked first, second, third, fourth, and ninth based on citations were all phase 2 trials of different therapies for patients with brain metastasis from HER-2 positive breast cancer. As mentioned before, HER2-positive is the breast cancer subtype that most frequently develops brain metastasis when compared to other subtypes. Former studies found that the active therapy of HER-2 positive breast cancer, trastuzumab, seemed to increase the risk of brain metastasis. Therefore, new therapy was innovated to solve these clinical problems, including lapatinib plus capecitabine, tucatinib plus trastuzumab and capecitabine, lapatinib, neratinib plus capecitabine, and trastuzumab emtansine (T-DM1). “Lapatinib plus capecitabine in patients with previously untreated brain metastases from HER2-positive metastatic breast cancer (LANDSCAPE): a single-group phase 2 study” published by Bachelot T et al. in The Lancet Oncology was the most cited paper (160 citations). This study has confirmed the efficacy of lapatinib plus capecitabine as the first-line treatment of HER-2-positive breast cancer brain metastasis ( 14 ). The second cited paper was “Tucatinib, Trastuzumab, and Capecitabine for HER2-Positive Metastatic Breast Cancer” published by Murthy RK et al. in The New England Journal of Medicine in 2020. The novel HER-2 inhibitor, tucatinib, demonstrated inspiringly active efficacy in patients with brain metastasis from HER2-positive breast cancer. Progression-free survival in the first year was 24.9% in the tucatinib-combination group and 0% in the placebo-combination group ( 15 ). The fourth paper was “TBCRC 022: A Phase II Trial of Neratinib and Capecitabine for Patients with Human Epidermal Growth Factor Receptor 2–Positive Breast Cancer and Brain Metastases” published by Freedman RA et al., which confirmed the efficacy of neratinib plus capecitabine against refractory HER2-positive breast cancer brain metastasis ( 17 ). The ninth paper confirmed the capacity of T-DM1 to lengthen the overall survival of patients with HER2-positvie breast cancer brain metastasis when compared with lapatinib plus capecitabine treatment. The fifth cited paper was “Genomic Characterization of Brain Metastases Reveals Branched Evolution and Potential Therapeutic Targets” published by Brastianos PK et al., which clarified the value of sequence of primary biopsies by detecting alterations in the assistance of the prediction of distant metastasis including brain metastasis ( 18 ). The eighth cited paper was an evaluation of different graded prognostic assessment (GPA) indices via multi-institutional retrospective analysis ( 21 ). The seventh cited paper was the only basic study in the 10 most cited papers, which anchored COX2, HBEGF, and ST6GALNAC5 as the key genes mediating breast cancer brain metastasis ( 20 ). These references can be divided into categories: pathogenesis, prevention, treatment, and prognosis of breast cancer brain metastasis, among which treatment was the most dominant area.
4.2 Focus and hotspots
Keyword analysis was employed to identify the trends of development and research hotspots in the related fields. The co-occurrence analysis of VOSviewer divided the keywords into five clusters. We divided the five clusters into the following five categories: basic study, clinical trial, local therapy, treatment optimization, and epidemiology. Keywords with high frequency highlighted the focus within the branch category. In the red cluster, expression, cell, in vivo , and growth were the most common keywords, which were the fundamental elements of basic studies. The green cluster contained keywords such as phase II trial, trastuzumab, efficacy, open-label , and lapatinib plus capecitabine . The LANDSCAPE trial set the lapatinib plus capecitabine as the first-line therapy, and more clinical trials were registered and conducted. The combination of target therapy and chemotherapy was the mainstream in clinical trials, which complemented each other clinically ( 33 – 36 ). Local therapy was represented by the blue cluster, which included keywords such as radiation-therapy, stereotactic radiosurgery, surgical resection, prognostic-factors, management , and graded prognostic assessment . Local therapies remained the cornerstone of treatment for patients with brain metastasis from breast cancer. These therapies include surgical resection, stereotactic radiotherapy, and whole brain radiotherapy. The approach chosen for treatment is individualized based on the extent and characteristics of the brain metastasis ( 37 , 38 ). For patients with endocrine-resistant breast cancer who develop brain metastasis and are resistant to most chemotherapies, surgical tumor resection and stereotactic radiotherapy have demonstrated efficacy in improving overall survival and reducing symptoms associated with brain metastasis, which remains crucial in optimizing outcomes for this population ( 39 ). Keywords in the yellow cluster were mainly related to treatment optimization including nanoparticles and focused ultrasound , which were used in preclinical trials, and the multidisciplinary crosstalk seemed to improve the efficacy of treatment at present ( 40 , 41 ). The purple cluster was mainly related to epidemiology with keywords such as survival, risk, subtype, diagnosis , and recurrence . The development of research trends reflected by keywords were sorted out from the cluster timeline.
Burst detection was an effective method to discover the hotspots and identify potential future developmental trends. In this study, we found the references with citation burst. The burst of four references began in 2020, and the burst is still ongoing, including three clinical trials and a review. The first clinical trial was the phase II trial of tucatinib, trastuzumab, and capecitabine for HER2-positive metastatic breast cancer ( 15 ), earning the most attention of researchers in the related field (burst strength: 55.25) within 2 years. As mentioned earlier, tucatinib demonstrated apparent positive efficacy, especially in patients with brain metastasis, which was a milestone of the treatment of breast cancer brain metastasis with a tyrosine kinase inhibitor (TKI). Another clinical trial burst in 2020 was “TBCRC 022: A Phase II Trial of Neratinib and Capecitabine for Patients With Human Epidermal Growth Factor Receptor 2–Positive Breast Cancer and Brain Metastases,” which provided new possibility to the treatment of refractory HER2-positive breast cancer brain metastases ( 17 ). The third clinical trial burst recently was “Trastuzumab Deruxtecan in Previously Treated HER2-Positive Breast Cancer,” A novel antibody–drug conjugate (ADC), trastuzumab deruxtecan, showed durable antitumor activity in patients with HER2-positive metastatic breast cancer ( 23 ). The outstanding success of treating HER2-positive breast cancer and other solid tumors may represent a new era of tumor treatment ( 42 ). The last reference burst in 2 years was a review that concluded the molecular mechanisms and clinical therapies of brain metastasis ( 43 ), which provided an overview of the current understanding of brain metastasis from preclinical and clinical perspectives.
In conclusion, our analysis of keywords and citation bursts provides insight into trends and hotspots in research on brain metastasis from breast cancer. The success of novel therapies, such as TKIs and ADCs, may represent a new era of treatment in patients suffering brain metastasis from breast cancer. Our findings suggest that future research in this region may focus on these novel therapies and optimizing treatment approaches for brain metastasis.
5 Strengths and limitations
We summarized the developmental route of the research on breast cancer brain metastasis from January 2006 to October 2022. However, there are also some limitations in our bibliometric study. First, the analysis was based on publications retrieved from the SCIE of Web of Science Core Collection, which did not have papers indexed by other databases such as Scopus and Google. Second, a reference to a document can be either confirmatory or contradictory, which would result in bias in the number of citations. Third, searching based on title, abstracts, and keywords means that some of the manuscripts that involved breast cancer brain metastasis might not be included.
6 Conclusion
In conclusion, there has been a general increase in the annual number of publications on breast cancer brain metastasis from 2006 to 2022. The main findings are as follows:
a. Institutions from all over the world participated in the study of breast cancer brain metastasis. The United States was ranked first in both the number of publications and institutions. The University Texas MD Anderson Cancer Center was the most productive institution, while the Dana Farber Cancer Institution was the most cited. However, the research collaboration between countries and institutions was regionally limited.
b. The Journal of Neuro-Oncology published the most papers (n = 111), while the Journal of Clinical Oncology ranked first with 9,861 citations based on cocited analysis.
c. Nancy U. Lin was the most productive and cited author with high influence in the field.
d. Research on breast cancer brain metastasis was focused on the basic study, clinical trial, local therapy, treatment optimization, and epidemiology.
e. The most cited references were focused on pathogenesis, prevention, treatment, and prognosis, among which treatment attracted the most attention. New therapies developed rapidly in the recent 3 years, and the treatment of brain breast cancer metastasis got a breakthrough with novel TKI and ADC-based therapies, which may be the mainstream treatment in the future.
Data availability statement
The original contributions presented in the study are included in the article/supplementary material. Further inquiries can be directed to the corresponding authors.
Author contributions
S-QW and YL were responsible for the conception and design of the research. Y-TY, X-HZ, L-QC and HK contributed to the data collection and filter. S-QW and JZ participated in writing the manuscript. X-SZ revised this manuscript critically for intellectual content. This research is administrative supported by Y-FW and Y-YL. All authors contributed to the article and approved the submitted version.
This work was supported by the National Natural Science Foundation of China ( 81873205, 81803877, 82104629, 81904037), the Natural Science Foundation of Guangdong Province, China (2020B1515120063) and Postdoctoral Science Foundation of China (NO. 2022M711536).
Conflict of interest
The authors declare that the research was conducted in the absence of any commercial or financial relationships that could be construed as a potential conflict of interest.
Publisher’s note
All claims expressed in this article are solely those of the authors and do not necessarily represent those of their affiliated organizations, or those of the publisher, the editors and the reviewers. Any product that may be evaluated in this article, or claim that may be made by its manufacturer, is not guaranteed or endorsed by the publisher.
Abbreviations
TKI, tyrosine kinase inhibitor; T-DM1, trastuzumab emtansine; ADCs, antibody–drug conjugates.
1. Siegel. RL, Miller. KD, Jemal A. A: Cancer statistics, 2020. CA: Cancer J Clin (2020) 70(1):7–30. doi: 10.3322/caac.21590
PubMed Abstract | CrossRef Full Text | Google Scholar
2. Siegel. RL, Miller. KD, Fuchs. HE, Jemal A. A: Cancer statistics, 2021. CA: Cancer J Clin (2021) 71(1):7–33. doi: 10.3322/caac.21654
3. Chaffer CL, Weinberg RA. A perspective on cancer cell metastasis. Science (2011) 331(6024):1559–64. doi: 10.1126/science.1203543
4. Chen W, Hoffmann AD, Liu H, Liu X. Organotropism: New insights into molecular mechanisms of breast cancer metastasis. NPJ Precis Oncol (2018) 2(1):4. doi: 10.1038/s41698-018-0047-0
5. Persson E, Gregersson P, Gustafsson A, Fitzpatrick P, Rhost S, A. Stahlberg and G. Landberg: Patient-derived scaffolds influence secretion profiles in cancer cells mirroring clinical features and breast cancer subtypes. Cell Commun Signal (2021) 19(1):66. doi: 10.1186/s12964-021-00746-7
6. Saraf A, Grubb CS, Hwang ME, Tai CH, Wu CC, Jani A, et al. Breast cancer subtype and stage are prognostic of time from breast cancer diagnosis to brain metastasis development. J Neurooncol (2017) 134(2):453–63. doi: 10.1007/s11060-017-2549-y
7. Hosonaga M, Saya H, Arima Y. Molecular and cellular mechanisms underlying brain metastasis of breast cancer. Cancer Metastasis Rev (2020) 39(3):711–20. doi: 10.1007/s10555-020-09881-y
8. Asghar I, Cang S, Yu H. Assistive technology for people with dementia: An overview and bibliometric study. Health Info Libr J (2017) 34(1):5–19. doi: 10.1111/hir.12173
9. Teles R, Moralles HF, Cominetti MR. Global trends in nanomedicine research on triple negative breast cancer: A bibliometric analysis. Int J Nanomed (2018) 13:2321–36. doi: 10.2147/IJN.S164355
CrossRef Full Text | Google Scholar
10. McElroy T, Allen AR. A bibliometric review of publications on oxidative stress and chemobrain: 1990-2019. Antioxidants (Basel) (2020) 9(5):439. doi: 10.3390/antiox9050439
11. Ahn SK, Hwang JW. Global trends in immunotherapy research on breast cancer over the past 10 years. J Oncol (2020) 2020:4708394. doi: 10.1155/2020/4708394
12. Zhang X, Lai H, Zhang F, Wang Y, Zhang L, Yang N, et al. Visualization and analysis in the field of pan-cancer studies and its application in breast cancer treatment. Front Med (Lausanne) (2021) 8:635035. doi: 10.3389/fmed.2021.635035
13. Leite E, Moraes FY, Marta GN, Taunk NK, Vieira M, Hanna SA, et al. Trial sponsorship and self-reported conflicts of interest in breast cancer radiation therapy: An analysis of prospective clinical trials. Breast (2017) 33:29–33. doi: 10.1016/j.breast.2017.02.016
14. Bachelot T, Romieu G, Campone M, Dieras V, Cropet C, Dalenc F, et al. Lapatinib plus capecitabine in patients with previously untreated brain metastases from HER2-positive metastatic breast cancer (LANDSCAPE): A single-group phase 2 study. Lancet Oncol (2013) 14(1):64–71. doi: 10.1016/S1470-2045(12)70432-1
15. Murthy RK, Loi S, Okines A, Paplomata E, Hamilton E, Hurvitz SA, et al. Tucatinib, trastuzumab, and capecitabine for HER2-positive metastatic breast cancer. N Engl J Med (2020) 382(7):597–609. doi: 10.1056/NEJMoa1914609
16. Lin NU, Dieras V, Paul D, Lossignol D, Christodoulou C, Stemmler HJ, et al. Multicenter phase II study of lapatinib in patients with brain metastases from HER2-positive breast cancer. Clin Cancer Res (2009) 15(4):1452–9. doi: 10.1158/1078-0432.CCR-08-1080
17. Freedman RA, Gelman RS, Anders CK, Melisko ME, Parsons HA, Cropp AM, et al. TBCRC 022: A phase II trial of neratinib and capecitabine for patients with human epidermal growth factor receptor 2-positive breast cancer and brain metastases. J Clin Oncol (2019) 37(13):1081–9. doi: 10.1200/JCO.18.01511
18. Brastianos PK, Carter SL, Santagata S, Cahill DP, Taylor-Weiner A, Jones RT, et al. Genomic characterization of brain metastases reveals branched evolution and potential therapeutic targets. Cancer Discovery (2015) 5(11):1164–77. doi: 10.1158/2159-8290.CD-15-0369
19. Witzel I, Oliveira-Ferrer L, Pantel K, Muller V, Wikman H. Breast cancer brain metastases: biology and new clinical perspectives. Breast Cancer Res (2016) 18(1):8. doi: 10.1186/s13058-015-0665-1
20. Bos PD, Zhang XH, Nadal C, Shu W, Gomis RR, Nguyen DX, et al. Genes that mediate breast cancer metastasis to the brain. Nature (2009) 459(7249):1005–9. doi: 10.1038/nature08021
21. Sperduto PW, Kased N, Roberge D, Xu Z, Shanley R, Luo X, et al. Summary report on the graded prognostic assessment: An accurate and facile diagnosis-specific tool to estimate survival for patients with brain metastases. J Clin Oncol (2012) 30(4):419–25. doi: 10.1200/JCO.2011.38.0527
22. Krop IE, Lin NU, Blackwell K, Guardino E, Huober J, Lu M, et al. Trastuzumab emtansine (T-DM1) versus lapatinib plus capecitabine in patients with HER2-positive metastatic breast cancer and central nervous system metastases: A retrospective, exploratory analysis in EMILIA. Ann Oncol (2015) 26(1):113–9. doi: 10.1093/annonc/mdu486
23. Modi S, Saura C, Yamashita T, Park YH, Kim SB, Tamura K, et al. Trastuzumab deruxtecan in previously treated HER2-positive breast cancer. N Engl J Med (2020) 382(7):610–21. doi: 10.1056/NEJMoa1914510
24. Burstein HJ, Lieberman G, Slamon DJ, Winer EP, Klein P. Isolated central nervous system metastases in patients with HER2-overexpressing advanced breast cancer treated with first-line trastuzumab-based therapy. Ann Oncol (2005) 16(11):1772–7. doi: 10.1093/annonc/mdi371
25. Verger E, Gil M, Yaya R, Vinolas N, Villa S, Pujol T, et al. Temozolomide and concomitant whole brain radiotherapy in patients with brain metastases: A phase II randomized trial. Int J Radiat Oncol Biol Phys (2005) 61(1):185–91. doi: 10.1016/j.ijrobp.2004.04.061
26. Marty M, Cognetti F, Maraninchi D, Snyder R, Mauriac L, Tubiana-Hulin M, et al. Randomized phase II trial of the efficacy and safety of trastuzumab combined with docetaxel in patients with human epidermal growth factor receptor 2-positive metastatic breast cancer administered as first-line treatment: The M77001 study group. J Clin Oncol (2005) 23(19):4265–74. doi: 10.1200/JCO.2005.04.173
27. Christodoulou C, Bafaloukos D, Linardou H, Aravantinos G, Bamias A, Carina M, et al. Temozolomide (TMZ) combined with cisplatin (CDDP) in patients with brain metastases from solid tumors: a Hellenic cooperative oncology group (HeCOG) phase II study. J Neurooncol (2005) 71(1):61–5. doi: 10.1007/s11060-004-9176-0
28. Romond EH, Perez EA, Bryant J, Suman VJ, Geyer CJ, Davidson NE, et al. Trastuzumab plus adjuvant chemotherapy for operable HER2-positive breast cancer. N Engl J Med (2005) 353(16):1673–84. doi: 10.1056/NEJMoa052122
29. Lin NU, Claus E, Sohl J, Razzak AR, Arnaout A, Winer EP. Sites of distant recurrence and clinical outcomes in patients with metastatic triple-negative breast cancer: high incidence of central nervous system metastases. Cancer-Am. Cancer Soc (2008) 113(10):2638–45. doi: 10.1002/cncr.23930
30. Lin NU, Carey LA, Liu MC, Younger J, Come SE, Ewend M, et al. Phase II trial of lapatinib for brain metastases in patients with human epidermal growth factor receptor 2-positive breast cancer. J Clin Oncol (2008) 26(12):1993–9. doi: 10.1200/JCO.2007.12.3588
31. Ramakrishna N, Temin S, Chandarlapaty S, Crews JR, Davidson NE, Esteva FJ, et al. Recommendations on disease management for patients with advanced human epidermal growth factor receptor 2-positive breast cancer and brain metastases: American society of clinical oncology clinical practice guideline. J Clin Oncol (2014) 32(19):2100–8. doi: 10.1200/JCO.2013.54.0955
32. Ramakrishna N, Anders CK, Lin NU, Morikawa A, Temin S, Chandarlapaty S, et al. Management of advanced human epidermal growth factor receptor 2-positive breast cancer and brain metastases: ASCO guideline update. J Clin Oncol (2022) 40(23):2636–55. doi: 10.1200/JCO.22.00520
33. Ma F, Ouyang Q, Li W, Jiang Z, Tong Z, Liu Y, et al. Pyrotinib or lapatinib combined with capecitabine in HER2-positive metastatic breast cancer with prior taxanes, anthracyclines, and/or trastuzumab: A randomized, phase II study. J Clin Oncol (2019) 37(29):2610–9. doi: 10.1200/JCO.19.00108
34. Yan M, Ouyang Q, Sun T, Niu L, Yang J, Li L, et al. Pyrotinib plus capecitabine for patients with human epidermal growth factor receptor 2-positive breast cancer and brain metastases (PERMEATE): A multicentre, single-arm, two-cohort, phase 2 trial. Lancet Oncol (2022) 23(3):353–61. doi: 10.1016/S1470-2045(21)00716-6
35. Xu B, Yan M, Ma F, Hu X, Feng J, Ouyang Q, et al. Pyrotinib plus capecitabine versus lapatinib plus capecitabine for the treatment of HER2-positive metastatic breast cancer (PHOEBE): A multicentre, open-label, randomised, controlled, phase 3 trial. Lancet Oncol (2021) 22(3):351–60. doi: 10.1016/S1470-2045(20)30702-6
36. Bachelot T, Ciruelos E, Schneeweiss A, Puglisi F, Peretz-Yablonski T, Bondarenko I, et al. Preliminary safety and efficacy of first-line pertuzumab combined with trastuzumab and taxane therapy for HER2-positive locally recurrent or metastatic breast cancer (PERUSE). Ann Oncol (2019) 30(5):766–73. doi: 10.1093/annonc/mdz061
37. Corti C, Antonarelli G, Criscitiello C, Lin NU, Carey LA, Cortes J, et al. Targeting brain metastases in breast cancer. Cancer Treat Rev (2022) 103:102324. doi: 10.1016/j.ctrv.2021.102324
38. Lucke-Wold B, Scott K. A brief overview of neurosurgical management for breast cancer metastasis. SF J Med Oncol Cancer (2020) 1(1):1001.
PubMed Abstract | Google Scholar
39. Willman M, Willman J, Lucke-Wold B. Endocrine resistant breast cancer: Brain metastasis. Explor Target Antitumor Ther (2022) 3(2):240–51. doi: 10.37349/etat.2022.00081
40. Carney CP, Pandey N, Kapur A, Woodworth GF, Winkles JA, Kim AJ. Harnessing nanomedicine for enhanced immunotherapy for breast cancer brain metastases. Drug Deliv Transl Res (2021) 11(6):2344–70. doi: 10.1007/s13346-021-01039-9
41. Park EJ, Zhang YZ, Vykhodtseva N, McDannold N. Ultrasound-mediated blood-brain/blood-tumor barrier disruption improves outcomes with trastuzumab in a breast cancer brain metastasis model. J Control Release (2012) 163(3):277–84. doi: 10.1016/j.jconrel.2012.09.007
42. Indini A, Rijavec E, Grossi F. Trastuzumab deruxtecan: Changing the destiny of HER2 expressing solid tumors. Int J Mol Sci (2021) 22(9):4774. doi: 10.3390/ijms22094774
43. Achrol AS, Rennert RC, Anders C, Soffietti R, Ahluwalia MS, Nayak L, et al. Brain metastases. Nat Rev Dis Primers (2019) 5(1):5. doi: 10.1038/s41572-018-0055-y
Keywords: breast cancer, brain metastasis, bibliometric analysis, research trend, TKI (tyrosine kinase inhibitor), ADC (antibody-drug conjugate)
Citation: Wu S-q, Liu Y, Zhou J, You Y-t, Zhou X-h, Chen L-q, Kwan HY, Zhao X-s, Wu Y-f and Liu Y-y (2023) Research trends and prospects on brain metastasis from breast cancer: A bibliometric analysis. Front. Oncol. 13:1091249. doi: 10.3389/fonc.2023.1091249
Received: 21 November 2022; Accepted: 13 March 2023; Published: 05 April 2023.
Reviewed by:
Copyright © 2023 Wu, Liu, Zhou, You, Zhou, Chen, Kwan, Zhao, Wu and Liu. This is an open-access article distributed under the terms of the Creative Commons Attribution License (CC BY) . The use, distribution or reproduction in other forums is permitted, provided the original author(s) and the copyright owner(s) are credited and that the original publication in this journal is cited, in accordance with accepted academic practice. No use, distribution or reproduction is permitted which does not comply with these terms.
*Correspondence: Xiao-shan Zhao, [email protected] ; Yi-fen Wu, [email protected] ; Yan-yan Liu, [email protected]
† These authors have contributed equally to this work
Disclaimer: All claims expressed in this article are solely those of the authors and do not necessarily represent those of their affiliated organizations, or those of the publisher, the editors and the reviewers. Any product that may be evaluated in this article or claim that may be made by its manufacturer is not guaranteed or endorsed by the publisher.
Survivorship concerns among individuals diagnosed with metastatic cancer: Findings from the Cancer Experience Registry
- Published: 09 April 2024
Cite this article
- Rachelle S. Brick 1 ,
- Lisa Gallicchio 1 ,
- Michelle A. Mollica 1 ,
- Alexandra K. Zaleta 2 ,
- Emily S. Tonorezos 1 ,
- Paul B. Jacobsen 1 ,
- Kathleen M. Castro 1 &
- Melissa F. Miller ORCID: orcid.org/0000-0001-7099-7799 3
142 Accesses
10 Altmetric
Explore all metrics
Introduction
Individuals with metastatic cancer experience many medical, physical, and emotional challenges due to changing medical regimens, oscillating disease states, and side effects. The purpose of this study was to describe the type and prevalence of survivorship concerns reported by individuals with metastatic cancer, and their associations with cancer diagnosis, treatment, and socio-demographic variables.
This study utilized data from the Cancer Support Community’s Cancer Experience Registry. Individuals were included if they self-reported a solid tumor metastatic cancer and completed CancerSupportSource, which evaluates five domains of concerns (emotional well-being, symptom burden, body image/healthy lifestyle, healthcare team communication, and relationships/intimacy). Multivariable linear regression examined associations between independent predictors of each survivorship concern domain.
Of the 403 included participants, individuals reported a metastatic diagnosis of breast (43%), colorectal (20%), prostate (7%), lung (7%), gynecologic cancer (6%) and other. Nearly all (96%) reported at least one survivorship concern, with the most prevalent concern about cancer progression or recurrence. Survivorship concerns were higher across multiple domains for individuals unemployed due to disability. Individuals who were less than five years since diagnosis reported higher concerns related to emotional well-being, symptom burden, and healthcare communication compared to those more than five years since diagnosis.
Individuals with metastatic cancer experience a variety of moderate-to-severe survivorship concerns that warrant additional investigation.
Implications for cancer survivors
As the population of individuals with metastatic cancer lives longer, future research must investigate solutions to address modifiable factors associated with survivorship concerns, such as unemployment due to disability.
This is a preview of subscription content, log in via an institution to check access.
Access this article
Price includes VAT (Russian Federation)
Instant access to the full article PDF.
Rent this article via DeepDyve
Institutional subscriptions
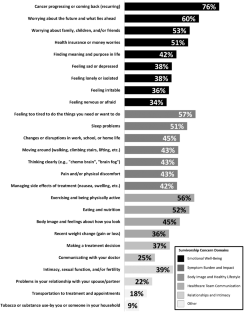
Similar content being viewed by others
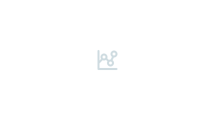
Patient-reported outcomes in cancer survivors: a population-wide cross-sectional study
Michael Jefford, Andrew C Ward, … Jim Bishop
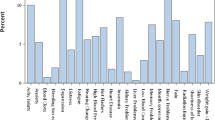
Self-reported symptoms among cancer survivors in the Women’s Health Initiative (WHI) Life and Longevity after Cancer (LILAC) cohort
Brittany M. Bernardo, Michael L. Pennell, … Electra D. Paskett
The use of a patient-reported outcome questionnaire to assess cancer survivorship concerns and psychosocial outcomes among recent survivors
Steven C. Palmer, Carrie T. Stricker, … Linda A. Jacobs
Data availability
The datasets analyzed during the current study are available from the corresponding author on reasonable request.
Gallicchio L, Devasia TP, Tonorezos E, Mollica MA, Mariotto A. Estimation of the number of individuals living with metastatic Cancer in the United States. J Natl Cancer Inst. 2022;114(11):1476–83. https://doi.org/10.1093/jnci/djac158 .
Article PubMed PubMed Central Google Scholar
Mollica MA, Smith AW, Tonorezos E, et al. Survivorship for individuals living with Advanced and metastatic cancers: National Cancer Institute Meeting Report. J Natl Cancer Inst Apr. 2022;11(4):489–95. https://doi.org/10.1093/jnci/djab223 .
Article Google Scholar
Tometich DB, Hyland KA, Soliman H, Jim HSL, Oswald L. Living with metastatic Cancer: a Roadmap for Future Research. Cancers. 2020;12(12). https://doi.org/10.3390/cancers12123684 .
Lai-Kwon J, Heynemann S, Hart NH, et al. Evolving Landscape of Metastatic Cancer Survivorship: reconsidering Clinical Care, Policy, and Research priorities for the modern era. J Clin Oncol Jun. 2023;20(18):3304–10. https://doi.org/10.1200/jco.22.02212 .
Moghaddam N, Coxon H, Nabarro S, Hardy B, Cox K. Unmet care needs in people living with advanced cancer: a systematic review. Support Care Cancer. 2016;08(8):3609–22. https://doi.org/10.1007/s00520-016-3221-3 . /01 2016.
Rimmer B, Crowe L, Todd A, Sharp L. Assessing unmet needs in advanced cancer patients: a systematic review of the development, content, and quality of available instruments. J Cancer Surviv. 2022;2022/10/01(5):960–75. https://doi.org/10.1007/s11764-021-01088-6 .
North AS, Carson L, Sharp L, Patterson J, Hamilton DW. The unmet needs of patients with advanced incurable head and neck cancer and their carers: a systematic review and meta-ethnography of qualitative data. Eur J Cancer Care. 2021/07/01 2021;30(4):e13474. https://doi.org/10.1111/ecc.13474 .
Hart NH, Crawford-Williams F, Crichton M, et al. Unmet supportive care needs of people with advanced cancer and their caregivers: a systematic scoping review. Crit Rev Oncol/Hematol. 2022. https://doi.org/10.1016/j.critrevonc.2022.103728 . /08/01/ 2022;176:103728.
Article PubMed Google Scholar
Wang T, Molassiotis A, Chung BPM, Tan JY. Unmet care needs of advanced cancer patients and their informal caregivers: a systematic review. BMC Palliat Care Jul. 2018;23(1):96. https://doi.org/10.1186/s12904-018-0346-9 .
Golant M, Zaleta AK, Ash-Lee S et al. ‘The Engaged Patient: The Cancer Support Community’s Comprehensive Model of Psychosocial Programs, Services, and Research’, in William Breitbart, and others, editors, Psycho-Oncology, 4 edn (2021; online edn, Oxford Academic, 1 Jan. 2021), https://doi.org/10.1093/med/9780190097653.003.0050 .
United States Department of Agriculture (USDA). 2013 Rural-Urban Continuum Codes. USDA Economic Research Service. 2016. https://www.ers.usda.gov/data-products/rural-urban-continuum-codes/documentation/ .
Zaleta AK, McManus S, Fortune EE et al. CancerSupportSource®-15+: development and evaluation of a short form of a distress screening program for cancer patients and survivors. Support Care Cancer. 2021/08/01 2021;29(8):4413–21. https://doi.org/10.1007/s00520-021-05988-2 .
Buzaglo JS, Zaleta AK, McManus S, Golant M, Miller MF. CancerSupportSource®: validation of a revised multi-dimensional distress screening program for cancer patients and survivors. Support Care Cancer. 2020/01/01 2020;28(1):55–64. https://doi.org/10.1007/s00520-019-04753-w .
Miller MF, Mullins CD, Onukwugha E, Golant M, Buzaglo JS. Discriminatory power of a 25-item distress screening tool: a cross-sectional survey of 251 cancer survivors. Qual Life Res. 2014;2014/12/01(10):2855–63. https://doi.org/10.1007/s11136-014-0742-4 .
Wang X, Wang N, Zhong L, et al. Prognostic value of depression and anxiety on breast cancer recurrence and mortality: a systematic review and meta-analysis of 282,203 patients. Mol Psychiatry Dec. 2020;25(12):3186–97. https://doi.org/10.1038/s41380-020-00865-6 .
Mosher CE, Johnson C, Dickler M, Norton L, Massie MJ, DuHamel K. Living with Metastatic Breast Cancer: A Qualitative Analysis of Physical, Psychological, and Social Sequelae. The Breast Journal. 2013/05/01 2013;19(3):285–292. https://doi.org/10.1111/tbj.12107 .
McClelland SI, Holland KJ, Griggs JJ. Quality of life and metastatic breast cancer: the role of body image, disease site, and time since diagnosis. Quality of Life Research. 2015/12/01 2015;24(12):2939–2943. https://doi.org/10.1007/s11136-015-1034-3 .
Ginter AC. The day you lose your hope is the day you start to die: quality of life measured by young women with metastatic breast cancer. J Psychosocial Oncol. 2020;2020/07/03(4):418–34. https://doi.org/10.1080/07347332.2020.1715523 .
Melhem SJ, Nabhani-Gebara S, Kayyali R. Latency of breast cancer stigma during survivorship and its influencing factors: a qualitative study. Original Research. Frontiers in Oncology. 2023-March-14. 2023;13. https://doi.org/10.3389/fonc.2023.1075298 .
LeBlanc TW, Temel JS, Helft PR. How much time do I have? Communicating prognosis in the era of exceptional responders. Am Soc Clin Oncol Educat Book. 2018/05/23 2018;(38):787–94. https://doi.org/10.1200/EDBK_201211 .
Duggan KJ, Wiltshire J, Strutt R, et al. Palliative care and psychosocial care in metastatic non-small cell lung cancer: factors affecting utilisation of services and impact on patient survival. Support Care Cancer. 2019;2019/03/01(3):911–9. https://doi.org/10.1007/s00520-018-4379-7 .
Ecclestone C, Chow R, Pulenzas N et al. Quality of life and symptom burden in patients with metastatic breast cancer. Support Care Cancer. 2016/09/01 2016;24(9):4035–4043. doi:10.1007/s00520-016-3217-z.
Hird A, Wong J, Zhang L et al. Exploration of symptoms clusters within cancer patients with brain metastases using the Spitzer Quality of Life Index. Support Care Cancer. 2010/03/01 2010;18(3):335–342. https://doi.org/10.1007/s00520-009-0657-8 .
Cardoso F, Wilking N, Bernardini R et al. A multi-stakeholder approach in optimising patients’ needs in the benefit assessment process of new metastatic breast cancer treatments. The Breast. 2020/08/01/ 2020;52:78–87. doi: https://doi.org/10.1016/j.breast.2020.04.011.
Schandelmaier S, Conen K, von Elm E et al. Planning and reporting of quality-of-life outcomes in cancer trials. Annals Oncol. 2015/09/01/ 2015;26(9):1966–1973. doi: https://doi.org/10.1093/annonc/mdv283.
Keane D, Phillips G, Mitchell N, Connolly RM, Hegarty J. Improving quality of life and symptom experience in patients with metastatic breast cancer: a systematic review of supportive care interventions. Psycho-oncology. 2023/08/01 2023;32(8):1192–207. https://doi.org/10.1002/pon.6183 .
Beaton R, Pagdin-Friesen W, Robertson C, Vigar C, Watson H, Harris SR. Effects of Exercise Intervention on Persons with Metastatic Cancer: A Systematic Review. Physiotherapy Canada. 2009/07/01 2009;61(3):141–153. https://doi.org/10.3138/physio.61.3.141 .
Barnes O, Wilson RL, Gonzalo-Encabo P, et al. The Effect of Exercise and Nutritional interventions on body composition in patients with Advanced or Metastatic Cancer: a systematic review. Nutrients. 2022;14(10). https://doi.org/10.3390/nu14102110 .
Gallicchio L, Tonorezos E, de Moor JS, et al. Evidence gaps in Cancer Survivorship Care: a Report from the 2019 National Cancer Institute Cancer Survivorship Workshop. J Natl Cancer Inst. 2021;113(9):1136–42. https://doi.org/10.1093/jnci/djab049 .
Mollica MA, Tesauro G, Tonorezos ES, Jacobsen PB, Smith AW, Gallicchio L. Current state of funded National Institutes of Health grants focused on individuals living with advanced and metastatic cancers: a portfolio analysis. J Cancer Surv. 2021/06/01 2021;15(3):370–4. https://doi.org/10.1007/s11764-021-01008-8 .
Mollica MA, Smith AW, Tonorezos E, et al. Survivorship for individuals living with Advanced and metastatic cancers: National Cancer Institute Meeting Report. J Natl Cancer Inst. 2022;114(4):489–95. https://doi.org/10.1093/jnci/djab223 .
de Moor JS, Alfano CM, Kent EE, et al. Recommendations for Research and practice to Improve Work outcomes among Cancer survivors. J Natl Cancer Inst. 2018;110(10):1041–7. https://doi.org/10.1093/jnci/djy154 .
Guo Y-J, Tang J, Li J-M, Zhu L-L, Xu J-S. Exploration of interventions to enhance return-to-work for cancer patients: a scoping review. Clin Rehabil. 2021;35(12):1674–93. https://doi.org/10.1177/02692155211021706 . 2021/12/01.
Tamminga SJ, Boer AGEMd, Verbeek JHAM, Frings-Dresen MHW. Return-to-work interventions integrated into cancer care: a systematic review. Occup Environ Med. 2010;67(9):639. https://doi.org/10.1136/oem.2009.050070 .
Article CAS PubMed Google Scholar
Lyons KD, Newman RM, Sullivan M, Pergolotti M, Braveman B, Cheville AL. Employment Concerns and Associated Impairments of Women Living With Advanced Breast Cancer. Arch Rehabil Res Clin Transl. 2019/06/01/ 2019;1(1):100004. https://doi.org/10.1016/j.arrct.2019.100004 .
Download references
Acknowledgements
The article was prepared as part of some of the authors’ (MAM, LG, ET, KC) official duties as employees of the US Federal Government. The findings and conclusions in this report are those of the authors and do not necessarily represent the official position of the National Cancer Institute. PBJ worked on this project during his tenure in the Division of Cancer Control and Population Sciences, National Cancer Institute. He is now retired from federal service.
Funding for the Cancer Experience Registry was provided by Astellas Pharma, Bristol Myers Squibb, Genentech, Geron, GSK, Janssen Oncology, Merck, Novartis, Pfizer, Pharmacyclics, Seagen, Taiho Oncology, Takeda Oncology, AbbVie, Amgen, AstraZeneca, Bristol-Myers Squibb, Celgene, Eli Lilly and Company, EMD Serono, Genentech (a member of the Roche Group), GlaxoSmithKline, Janssen Pharmaceuticals, Jazz Pharmaceuticals, Novartis, Pfizer, Pharmacyclics, Seagen, and Takeda Pharmaceutical.
Author information
Authors and affiliations.
Division of Cancer Control and Population Sciences, National Cancer Institute, 9609 Medical Center Drive, Rockville, MD, 20850, USA
Rachelle S. Brick, Lisa Gallicchio, Michelle A. Mollica, Emily S. Tonorezos, Paul B. Jacobsen & Kathleen M. Castro
Department of Research, CancerCare, 275 Seventh Avenue, New York, NY, 10001, USA
Alexandra K. Zaleta
Research and Training Institute, Cancer Support Community, 5614 Connecticut Avenue NW, Suite 280, Washington, D.C, 20015, USA
Melissa F. Miller
You can also search for this author in PubMed Google Scholar
Contributions
M.A.M, A.Z., L.G., K.C., R.B., E.T., P.J., M.M. were responsible for research conceptualization; M.M. conducted the analyses; R.B., M.A.M, L.G., and M.M. wrote the main manuscript text in collaboration with all authors; All authors reviewed the study design, results, and the manuscript.
Corresponding author
Correspondence to Melissa F. Miller .
Ethics declarations
Ethical approval.
Ethical and Independent Review Services (E&I, Independence, MO) served as the IRB of record (Study #23044). All procedures were in accordance with the ethical standards of the institutional research committee for studies involving human participants and with the 1964 Helsinki declaration and its later amendments or comparable ethical standards. Informed consent was obtained from all participants.
Competing interests
Dr. Zaleta reports conducting research in the past two years funded by: Astellas Pharma, Boston Scientific Foundation, Novartis, and Seagen; funding was received by Cancer Support Community. Dr. Miller reports institutional research grants from Astellas Pharma, BeiGene, Bristol Myers Squibb, Genentech, Geron, Gilead Sciences, GSK, Janssen Oncology, Merck, Novartis, Pfizer, Seagen, Taiho Oncology, and Takeda Oncology.
Additional information
Publisher’s note.
Springer Nature remains neutral with regard to jurisdictional claims in published maps and institutional affiliations.
Electronic supplementary material
Below is the link to the electronic supplementary material.
Supplementary Material 1
Rights and permissions.
Springer Nature or its licensor (e.g. a society or other partner) holds exclusive rights to this article under a publishing agreement with the author(s) or other rightsholder(s); author self-archiving of the accepted manuscript version of this article is solely governed by the terms of such publishing agreement and applicable law.
Reprints and permissions
About this article
Brick, R.S., Gallicchio, L., Mollica, M.A. et al. Survivorship concerns among individuals diagnosed with metastatic cancer: Findings from the Cancer Experience Registry. J Cancer Surviv (2024). https://doi.org/10.1007/s11764-024-01573-8
Download citation
Received : 05 January 2024
Accepted : 17 March 2024
Published : 09 April 2024
DOI : https://doi.org/10.1007/s11764-024-01573-8
Share this article
Anyone you share the following link with will be able to read this content:
Sorry, a shareable link is not currently available for this article.
Provided by the Springer Nature SharedIt content-sharing initiative
- Unmet needs
- Find a journal
- Publish with us
- Track your research
Thank you for visiting nature.com. You are using a browser version with limited support for CSS. To obtain the best experience, we recommend you use a more up to date browser (or turn off compatibility mode in Internet Explorer). In the meantime, to ensure continued support, we are displaying the site without styles and JavaScript.
- View all journals
- My Account Login
- Explore content
- About the journal
- Publish with us
- Sign up for alerts
- Open access
- Published: 29 August 2019
Deep Learning to Improve Breast Cancer Detection on Screening Mammography
- Li Shen ORCID: orcid.org/0000-0002-5190-2851 1 ,
- Laurie R. Margolies 2 ,
- Joseph H. Rothstein 3 ,
- Eugene Fluder 4 ,
- Russell McBride ORCID: orcid.org/0000-0002-3890-529X 5 &
- Weiva Sieh 3
Scientific Reports volume 9 , Article number: 12495 ( 2019 ) Cite this article
107k Accesses
491 Citations
75 Altmetric
Metrics details
- Computational science
- Computer science
- Predictive markers
The rapid development of deep learning, a family of machine learning techniques, has spurred much interest in its application to medical imaging problems. Here, we develop a deep learning algorithm that can accurately detect breast cancer on screening mammograms using an “end-to-end” training approach that efficiently leverages training datasets with either complete clinical annotation or only the cancer status (label) of the whole image. In this approach, lesion annotations are required only in the initial training stage, and subsequent stages require only image-level labels, eliminating the reliance on rarely available lesion annotations. Our all convolutional network method for classifying screening mammograms attained excellent performance in comparison with previous methods. On an independent test set of digitized film mammograms from the Digital Database for Screening Mammography (CBIS-DDSM), the best single model achieved a per-image AUC of 0.88, and four-model averaging improved the AUC to 0.91 (sensitivity: 86.1%, specificity: 80.1%). On an independent test set of full-field digital mammography (FFDM) images from the INbreast database, the best single model achieved a per-image AUC of 0.95, and four-model averaging improved the AUC to 0.98 (sensitivity: 86.7%, specificity: 96.1%). We also demonstrate that a whole image classifier trained using our end-to-end approach on the CBIS-DDSM digitized film mammograms can be transferred to INbreast FFDM images using only a subset of the INbreast data for fine-tuning and without further reliance on the availability of lesion annotations. These findings show that automatic deep learning methods can be readily trained to attain high accuracy on heterogeneous mammography platforms, and hold tremendous promise for improving clinical tools to reduce false positive and false negative screening mammography results. Code and model available at: https://github.com/lishen/end2end-all-conv .
Similar content being viewed by others
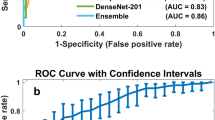
A convolutional deep learning model for improving mammographic breast-microcalcification diagnosis
Daesung Kang, Hye Mi Gweon, … Eun Ju Son
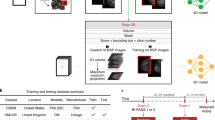
Robust breast cancer detection in mammography and digital breast tomosynthesis using an annotation-efficient deep learning approach
William Lotter, Abdul Rahman Diab, … A. Gregory Sorensen
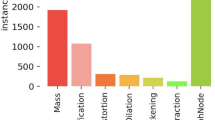
Dual view deep learning for enhanced breast cancer screening using mammography
Samuel Rahimeto Kebede, Fraol Gelana Waldamichael, … Zelalem Chimdesa Merga
Introduction
The rapid advancement of machine learning and especially deep learning continues to fuel the medical imaging community’s interest in applying these techniques to improve the accuracy of cancer screening. Breast cancer is the second leading cause of cancer deaths among U.S. women 1 and screening mammography has been found to reduce mortality 2 . Despite the benefits, screening mammography is associated with a high risk of false positives as well as false negatives. The average sensitivity of digital screening mammography in the U.S. is 86.9% and the average specificity is 88.9% 3 . To help radiologists improve the predictive accuracy of screening mammography, computer-assisted detection and diagnosis (CAD) software 4 have been developed and in clinical use since the 1990s. Unfortunately, data suggested that early commercial CAD systems had not led to significant improvement in performance 5 , 6 , 7 and progress stagnated for more than a decade since they were introduced. With the remarkable success of deep learning in visual object recognition and detection, and many other domains 8 , there is much interest in developing deep learning tools to assist radiologists and improve the accuracy of screening mammography 9 , 10 , 11 , 12 , 13 , 14 . Recent studies 15 , 16 have shown that a deep learning based CAD system performed as well as radiologists in standalone mode and improved the radiologists’ performance in support mode.
Detection of subclinical breast cancer on screening mammography is challenging as an image classification task because the tumors themselves occupy only a small portion of the image of the entire breast. For example, a full-field digital mammography (FFDM) image is typically 4000 × 3000 pixels while a potentially cancerous region of interest (ROI) can be as small as 100 × 100 pixels. For this reason, many studies 13 , 17 , 18 , 19 , 20 , 21 have limited their focus to the classification of annotated lesions. Although classifying manually annotated ROIs is an important first step, a fully automated software system must be able to operate on the entire mammogram to provide additional information beyond the known lesions and augment clinical interpretations. If ROI annotations were widely available in mammography databases then established object detection and classification methods such as the region-based convolutional neural network (R-CNN) 22 and its variants 23 , 24 , 25 could be readily applied. However, approaches that require ROI annotations 14 , 26 , 27 , 28 , 29 often cannot be transferred to large mammography databases that lack ROI annotations, which are laborious and costly to assemble. Indeed, few public mammography databases are fully annotated 30 . Other studies 9 , 10 have attempted to train neural networks using whole mammograms without relying on any annotations. However, it is hard to know if such networks were able to locate the clinically significant lesions and base predictions on the corresponding portions of the mammograms. It is well known that deep learning requires large training datasets to be most effective. Thus, it is essential to leverage both the few fully annotated datasets, as well as larger datasets labeled with only the cancer status of each image to improve the accuracy of breast cancer classification algorithms.
Pre-training is a promising method to address the problem of training a classifier when the ideal large and complete training datasets are not available. For example, Hinton et al . 31 used layer-wise pre-training to initialize the weight parameters of a deep belief net (DBN) with three hidden layers and then fine-tuned it for classification. They found that pre-training improved the training speed as well as the accuracy of handwritten digit recognition. Another popular training method is to first train a deep learning model on a large database such as the ImageNet 32 and then fine-tune the model for another task. Although the specific task may not be related to the initial training dataset, the model’s weight parameters are already initialized for recognizing primitive features, such as edges, corners and textures, which can be readily used for a different task. This often saves training time and improves the model’s performance 33 .
In this study, we propose an “end-to-end” approach in which a model to classify local image patches is pre-trained using a fully annotated dataset with ROI information. The patch classifier’s weight parameters are then used to initialize the weight parameters of the whole image classifier, which can be further fine-tuned using datasets without ROI annotations. We used a large public digitized film mammography database with thousands of images to develop the patch and whole image classifiers, and then transferred the whole image classifiers to a smaller public FFDM database with hundreds of images. We evaluated various network designs for constructing the patch and whole image classifiers to attain the best performance. The pipeline required to build a whole image classifier is presented here, as well as the pros and cons of different training strategies.
Converting a classifier from recognizing patches to whole images
To perform classification or segmentation on large complex images, a common strategy involves the use of a classifier in sliding window fashion to recognize local patches on an image to generate a grid of probabilistic outputs. This is followed by another process to summarize the patch classifier’s outputs to give the final classification or segmentation result. Such methods have been used to detect metastatic breast cancer using whole slide images of sentinel lymph node biopsies 34 and to segment neuronal membranes in microscopic images 35 . However, this strategy requires two steps that each needs to be optimized separately. Here, we propose a method to combine the two steps into a single step for training on the whole images (Fig. 1 ). Assume we have an input patch \(X\in {{\rm{IR}}}^{{\rm{p}}\times {\rm{q}}}\) and a patch classifier which is a function f so that \(f(X)\in {{\rm{IR}}}^{{\rm{c}}}\) , where the function’s output satisfies f ( X ) i ∈ [0, 1] and \({{\rm{\Sigma }}}_{i=1}^{c}f{(X)}_{i}=1\) and c is the number of classes of the patches. Here, c = 5 and the classes are: benign calcification, malignant calcification, benign mass, malignant mass and background for each patch from a mammogram. Assume the input patch is extracted from an image \(M\in {{\rm{IR}}}^{{\rm{r}}\times {\rm{s}}}\) where p ≪ r and q ≪ s . If the function f represents a convolutional neural network (CNN), then f can be applied to M without changing the network parameters so that \(f(M)\in {{\rm{IR}}}^{{\rm{u}}\times {\rm{v}}\times {\rm{c}}}\) , where u > 1 and v > 1 depend on the image size and the stride of the patch classifier. This is possible because of the weight sharing and locality properties of a CNN 36 . If the function f represents a different class of neural networks, such as the multilayer perceptron (MLP), then this becomes infeasible because a MLP requires the input to be fixed. Therefore, after changing the input from X to M , we have a u × v grid of probabilistic outputs of c classes (referred to as “heatmap”) instead of a single output of c classes. Hence the heatmap has a size of u × v × c . More layers can then be added on top of the heatmap to transform the outputs and connect with the final classification output of the image. Adding a convolutional layer on top of the patch classifier’s outputs turns the entire patch classifier into a filter and enlarges its receptive field. For example, if the patch classifier has a receptive field of 224 × 224 with a stride = 32, adding a 3 × 3 convolutional layer on top of it increases each side of the receptive field to 224 + (3 − 1) × 32 = 228. Thus, the top layers effectively use the patch classifier to “scan” the whole image, looking for cues of cancerous lesions and extracting higher level features that can finally be used for whole image classification. Using function g to represent the top layers, the whole image classification function can be written as \(h(M)=g(f(M))\in {{\rm{IR}}}^{{\rm{d}}}\) , where d is the number of classes of the whole image. Typically, d = 2 represents the two classes we want to predict: malignant and nonmalignant (benign or normal).
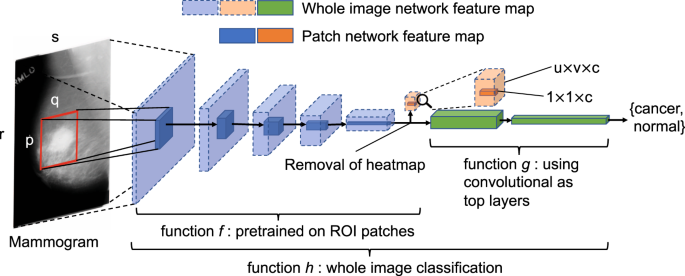
Converting a patch classifier to an end-to-end trainable whole image classifier using an all convolutional design. The function f was first trained on patches and then refined on whole images. We evaluated whether removing the heatmap improved information flow from the bottom layers of the patch classifier to the top convolutional layers in the whole image classifier. The magnifying glass shows an enlarged version of the heatmap. This figure is best viewed in color.
The function h accepts whole images as input and produces labels at the whole image level. Therefore, it is end-to-end trainable, providing two advantages over the two-step approach. First, the entire network can be jointly trained, avoiding sub-optimal solutions from each step; Second, the trained network can be transferred to another dataset without explicit reliance on ROI annotations. Large mammography databases with ROI annotations are rare and expensive to obtain. The largest public database with ROI annotations for digitized film mammograms – DDSM 37 – contains several thousand images with pixel-level annotations, which can be exploited to train a patch classifier f . Once the patch classifier is converted into a whole image classifier h , it can be fine-tuned on other databases using only image-level labels. This approach allows us to significantly reduce the requirement for ROI annotations, and has many applications in medical imaging in addition to breast cancer detection on screening mammograms.
Network design
A modern CNN is typically constructed by stacking convolutional layers on top of the input, followed by one or more fully connected (FC) layers to join with the classification output. Max pooling layers are often used amid convolutional layers to improve translational invariance and to reduce feature map size. In this study, two popular CNN structures are compared: the VGG network 38 and the residual network (Resnet) 39 . Consecutive network layers can be naturally grouped into “blocks” so that the feature map size is reduced (typically by a factor of 2) either at the beginning or at the end of a block but stays the same elsewhere in the block. For example, a “VGG block” is a stack of several 3 × 3 convolutional layers with the same depth followed by a 2 × 2 max pooling layer that reduces the feature map size by a factor of 2. Although other filter sizes can be used, 3 × 3 convolution and 2 × 2 max pooling are widely used, and employed throughout this study unless otherwise stated. Therefore, a VGG block can be represented by the pattern of N × K , where N represents the depth of each convolutional layer and K represents the number of convolutional layers. A “Resnet block” uses stride = 2 in the first convolutional layer instead of 2 × 2 max pooling to reduce feature map size at the beginning of the block, followed by the stacking of several convolutional layers. We use the “bottleneck design 39 ” which consists of repeated units of three convolutional layers that have filter sizes of 1 × 1, 3 × 3 and 1 × 1, respectively. A key feature of the Resnet block is that a shortcut is made between the two ends of each unit so that the features are directly carried over and therefore each unit can focus on learning the “residual” information 39 . Batch normalization (BN) is used in every convolutional layer in the Resnet, which is known to speedup convergence and also has a regularization effect 40 . A Resnet block can be represented by the pattern of [ L − M − N ] × K , where L , M and N represent the depths of the three convolutional layers in a unit and K represents the number of units. Here, the 16-layer VGG network (VGG16) and the 50-layer Resnet (Resnet50) are used as patch classifiers. The original design of the VGG16 38 consisted of five VGG blocks followed by two FC layers. To be consistent with the Resnet50, we replaced the two FC layers with a global average pooling layer which calculates the average activation of each feature map for the output of the last VGG block. For example, if the output of the last VGG block has a size of 7 × 7 × 512 (height × width × channel), after the global average pooling layer the output becomes 512. This output is then connected to the classification output with a FC layer.
A straightforward approach to construct a whole image classifier from a patch classifier involves flattening the heatmap and connecting it to the image’s classification output using FC layers. To increase the model’s translational invariance to the patch classifier’s output, a max pooling layer can be used after the heatmap. Further, a shortcut can be made between the heatmap and the output to make the training easier. The heatmap results directly from the patch classifier’s output which uses the softmax activation:
However, the softmax activation diminishes gradients for large inputs, which may impede gradient flow when it is used in an intermediate layer. Therefore, the rectified linear units (ReLU) can be used instead:
In the following, when we refer to the heatmap in a whole image classifier, the activation is always assumed to be ReLU unless otherwise stated.
We further propose to use convolutional layers as top layers, which preserve spatial information. Two blocks of convolutional layers (VGG or Resnet) can be added on top of the patch classifier layers, followed by a global average pooling layer and then the image’s classification output (Fig. 1 ). Therefore, this design creates an “all convolutional” network for whole image classification. As Fig. 1 shows, the heatmap abruptly reduces the depth of the feature map between the patch classifier layers and the top layers, which may cause information loss in the whole image classification. Therefore, we also evaluated the results when the heatmap is removed entirely from the whole image classifier to allow the top layers to fully utilize the features extracted from the patch classifier.
Computational environment
All experiments in this study were carried out on a Linux workstation equipped with an NVIDIA 8 GB Quadro M4000 GPU card.
Developing patch and whole image classifiers on CBIS-DDSM
Setup and processing of the dataset.
The DDSM 37 contains digitized film mammograms in a lossless-JPEG format that is now obsolete. We used a later version of the database called CBIS-DDSM 41 which contains images that are converted into the standard DICOM format. The dataset which consisted of 2478 mammography images from 1249 women was downloaded from the CBIS-DDSM website, and included both craniocaudal (CC) and mediolateral oblique (MLO) views for most of the exams. Each view was treated as a separate image in this study. We randomly split the CBIS-DDSM dataset 85:15 at the patient level to create independent training and test sets. The training data was further split 90:10 to create an independent validation set. The splits were done in a stratified fashion to maintain the same proportion of cancer cases in the training, validation and test sets. The total numbers of images in the training, validation and testing sets were: 1903, 199 and 376, respectively.
The CBIS-DDSM database contains the pixel-level annotations for the ROIs and their pathologically confirmed labels: benign or malignant. It further labels each ROI as a calcification or mass. Most mammograms contained only one ROI. All mammograms were converted into PNG format and downsized to 1152 × 896 using interpolation; no image cropping was performed. The downsizing was motivated by the limitation of GPU memory size. Two patch datasets were created by sampling image patches from ROIs and background regions. All patches had the same size of 224 × 224, which were large enough to cover most of the ROIs annotated. The first dataset (S1) consisted of sets of patches in which one was centered on the ROI and one is a random background patch from the same image. The second dataset (S10) consisted of 10 patches randomly sampled from around each ROI, with a minimum overlapping ratio of 0.9 with the ROI and inclusion of some background, to more completely capture the potentially informative region; and an equal number of background patches from the same image. All patches were classified into one of the five categories: background, malignant mass, benign mass, malignant calcification and benign calcification.
Network training
Training a whole image classifier was achieved in two steps. The first step was to train a patch classifier. We compared the networks with pre-trained weights using the ImageNet 32 database to those with randomly initialized weights. In a pre-trained network, the bottom layers represent primitive features that tend to be preserved across different tasks, whereas the top layers represent higher-order features that are more related to specific tasks and require further training. Using the same learning rate for all layers may destroy the features that were learned in the bottom layers. To prevent this, a 3-stage training strategy was employed in which the parameter learning is frozen for all but the final layer and progressively unfrozen from the top to the bottom layers, while simultaneously decreasing the learning rate. The 3-stage training strategy on the S10 patch set was as follows:
Set learning rate to 10 −3 and train the last layer for 3 epochs.
Set learning rate to 10 −4 , unfreeze the top layers and train for 10 epochs, where the top layer number is set to 46 for Resnet50 and 11 for VGG16.
Set learning rate to 10 −5 , unfreeze all layers and train for 37 epochs for a total of 50 epochs.
In the above, an epoch was defined as a sweep through the training set. For the S1 patch dataset, the total number of epochs was increased to 200 because it was much smaller and less redundant than the S10 patch dataset. For randomly initialized networks a constant learning rate of 10 −3 was used. Adam 42 was used as the optimizer and the batch size was set to be 32. The sample weights were adjusted within each batch to balance the five classes.
The second step was to train a whole image classifier converted from the patch classifier (Fig. 1 ). A 2-stage training strategy was employed to first train the newly added top layers (i.e. function g ) and then train all layers (i.e. function h ) with a reduced learning rate, which was as follows:
Set learning rate to 10 −4 , weight decay to 0.001 and train the newly added top layers for 30 epochs.
Set learning rate to 10 −5 , weight decay to 0.01 and train all layers for 20 epochs for a total of 50 epochs.
We found that the VGG-based image classifiers showed sign of continuing improvement towards the end of the 50 epochs, while the Resnet-based image classifiers had already converged. To be fair for the VGG-based image classifiers, we continued to train them with 200 additional epochs. Due to GPU memory limits, a batch size of 2 was used.
The average gray scale value of the whole image training set was subtracted from both patch and whole image datasets in training. No other preprocessing was applied. To improve the generalization of final models, data augmentation was performed using the following random transformations: horizontal and vertical flips, rotation in [−25, 25] degrees, zoom in [0.8, 1.2] ratio and intensity shift in [−20, 20] pixel values.
Development of patch classifiers
Table 1 shows the accuracy of the classification of image patches into 5 classes using Resnet50 and VGG16 in the CBIS-DDSM test set. A bootstrapping method with 3000 runs was used to derive the 95% confidence intervals for patch classification accuracy. The S10 set was more difficult to classify than the S1 set because it contained patches sampled from around ROIs, rather than centered on the ROI, that were more difficult to distinguish from background regions. On the S1 set, both randomly initialized and pre-trained Resnet50 classifiers achieved similar accuracy but the pre-trained network converged after half as many epochs as the randomly initialized one. On the S10 set, the pre-trained Resnet50 outperformed the randomly initialized one by a large margin, achieving an accuracy [95% confidence interval (CI)] of 0.89 [0.88, 0.90]. These results showed that pre-training can greatly help network convergence and performance. Therefore, pre-trained networks were used for the rest of the study. The accuracy of the pre-trained VGG16 (0.84 [0.83, 0.85]) on the S10 set was lower than that of the pre-trained Resnet50.
To further characterize performance, confusion matrix analyses were conducted on the Resnet50 and VGG16 patch classifiers in the S10 test set (Fig. 2 ). For both patch classifiers, all five classes were predicted into the correct categories with the highest probability. The background class was easiest, and malignant calcifications hardest to classify. Malignant calcifications were most likely to be misclassified as benign calcification, followed by malignant mass. Benign calcifications were most likely to be misclassified as background, followed by malignant calcification. Malignant masses were most likely to be misclassified as benign masses, while benign masses were most likely to be misclassified as malignant masses or background, depending on the patch classifier.
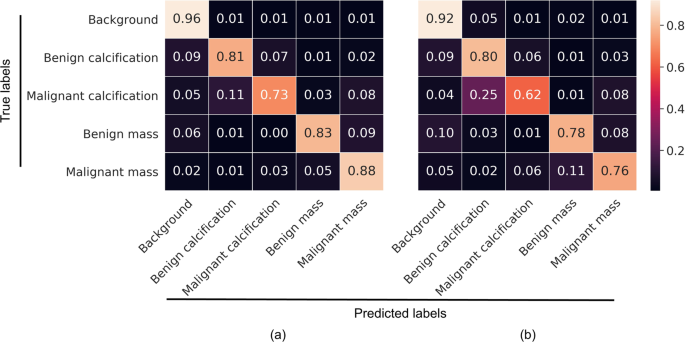
Confusion matrix analysis of 5-class patch classification for Resnet50 ( a ) and VGG16 ( b ) in the S10 test set. The matrices are normalized so that each row sums to one. This figure is best viewed in color.
Converting patch to whole image classifiers
Using pre-trained Resnet50 and VGG16 patch classifiers, we tested several different configurations for the top layers of the whole image classifiers. We also evaluated removal of the heatmap and adding two Resnet or VGG blocks on top of the patch classifier layers, followed by a global average pooling layer and the classification output. Model performance was assessed by computing the per-image AUCs on the independent test set.
Resnet-based networks: To evaluate whether the patch classifiers trained on the S1 and S10 datasets are equally useful for whole image classification, the Resnet50 patch classifiers were used. In the original design of the Resnet50 39 , L ≡ M , N is four times L and K is 3 or more; the L of the current block is also double of the L of the previous block. However, we found this design to exceed our GPU memory limit when it is used for the top layers of the whole image classifier. In the initial experiments, we used instead the same configuration of [512 − 512 − 2048] × 1 for two Resnet blocks on top of the patch classifier. A bootstrapping method with 3000 runs was used to derive 95% confidence intervals for AUCs and AUC differences. The whole image classifier trained using the S10 set (mean AUC = 0.85) performed much better than that trained using the S1 set (mean AUC = 0.63) (Table 2 ), despite its poorer patch classification accuracy (Table 1 ). The S10 dataset contains more information about the ROIs as well as their adjacent regions and other background regions on the image than the S1 dataset, which allows a patch classifier to extract more features that can be important for whole image classification. To test this hypothesis, we created another patch set (referred to as S1g) with one patch each from the ROI and background but a large patch size of 448 × 448 to include the surrounding area. The patch classification accuracy in S1g was much lower than that in S10 (Table 1 ). However, the image classification accuracy was similar for models trained on S1g and S10 (Table 2 ) with an estimated AUC difference [95% confidence interval] of −0.023 [−0.061, 0.016] supporting the hypothesis that the background regions contain useful information. For the rest of the study, only patch classifiers trained on the S10 dataset were used. Varying the configuration by using two Resnet blocks of [512 − 512 − 1024] × 2 yielded a mean AUC of 0.86, while reducing the depths and K of the two Resnet blocks to: [256 − 256 − 256] × 1 and [128 − 128 − 128] × 1 did not significantly decrease the AUC (Table 2 ). This result showed that the depths of the Resnet blocks were relatively uncorrelated with the performance of the whole image classifiers.
VGG-based networks: We tested whole image classifiers using VGG16 as the patch classifier and VGG blocks as the top layers. BN was used for the VGG blocks on the top except for the VGG16 patch classifier because it is a pre-trained network which cannot be modified. The VGG-based whole image classifiers performed similarly to the Resnet-based ones but took longer to achieve the same performance level (Table 3 ). In contrast to the Resnet, using more convolutional layers and higher depths in VGG blocks led to poorer performance: using two VGG blocks of 256 × 1 and 128 × 1 (mean AUC = 0.85) performed better than two VGG blocks of 512 × 3 (mean AUC = 0.81) with an AUC difference of 0.041 [0.011, 0.071]. Reducing the depths further to 128 and 64 did not further improve the AUC. This result illustrates that controlling model complexity (i.e., #layers and depths) is important for achieving good performance with the VGG-based networks, which are more likely to overfit.
Hybrid networks: We also created two “hybrid” networks by adding the VGG top layers that performed the best (two VGG blocks of 256 × 1 and 128 × 1) on top of the Resnet50 patch classifier; and the Resnet top layers that performed the best (two Resnet blocks of the same configuration of [512 − 512 − 1024] × 2) on top of the VGG16 patch classifier. The two hybrid networks achieved mean AUCs of 0.87 and 0.85, respectively, and were among the best performing models (Tables 2 and 3 ).
Augmented prediction and model averaging: Augmented prediction was implemented by horizontally and vertically flipping an image to obtain four images and taking an average of the four images’ scores. This technique increased the AUC (referred to as A-AUC) for each model by 0.01–0.03 (Tables 2 and 3 ), although only some of the models showed significant increase based on the 95% confidence intervals of AUC differences (Table S1 ). The four best performing models were combined into an ensemble model by taking the average of their augmented prediction scores. Two of the four best models used Resnet50 and VGG16 as patch classifiers and Resnet and VGG blocks as top layers, respectively (referred to as Resnet-Resnet and VGG-VGG); and the remaining two were hybrid models (referred to as Resnet-VGG and VGG-Resnet). Figure 3a shows the Receiver Operating Characteristic (ROC) curves of the four best models and the ensemble model, which yielded an AUC of 0.91. Because the clinical significance of a false negative (FN) is higher than that of a false positive (FP), a clinically useful system should not have significantly lower sensitivity than the current standard of care. Therefore, we evaluated model performance using a sensitivity of 86% as a benchmark based upon the estimated average for U.S. radiologists 3 , and determined the model specificity to be 80.1% at a similar sensitivity of 86.1%.
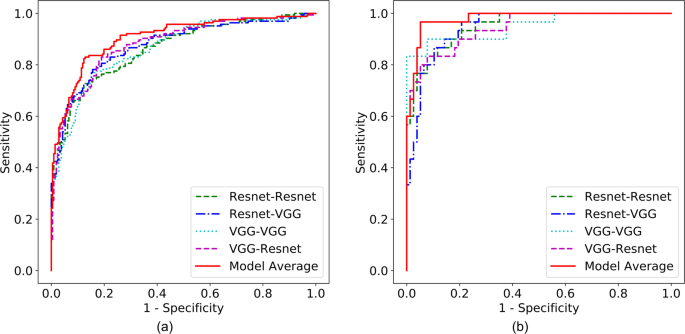
ROC curves for the four best individual models and ensemble model on the CBIS-DDSM ( a ) and INbreast ( b ) test sets. This figure is best viewed in color.
Saliency map and error analysis: Saliency maps were created using the Resnet-VGG model (Fig. 4a–c ), which showed the gradients of the input image with respect to the cancer class output. We used the guided back-propagation approach 43 that calculates only positive gradients for positive activations. A saliency map illustrates which area of the input image is considered to be responsible for the cancer prediction by a whole image classifier. Figure 4a shows the saliency map of a true positive (TP) image where the identified area is in or close to the malignant ROI. This shows that the image classifier was able to correctly locate the cancerous region on which its decision was based. Figure 4b shows a typical FP image where the identified region is located in a benign ROI that resembles a malignant ROI. Figure 4c shows a typical FN image where the malignant ROI is difficult to discern and no response passes the low cutoff.
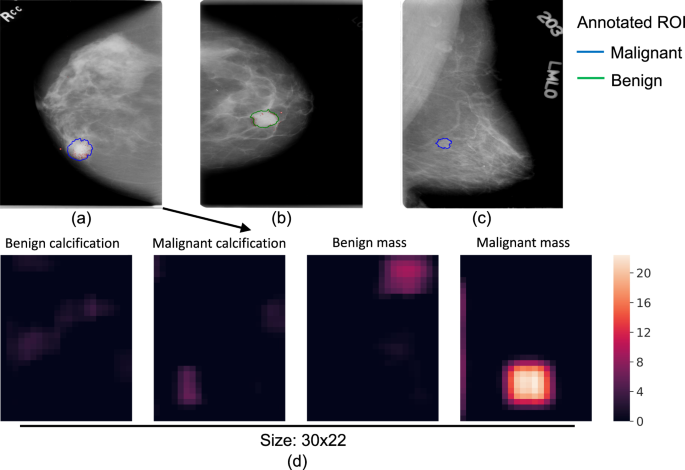
Saliency maps of TP ( a ), FP ( b ) and FN ( c ) image classifications. The outlines represent the regions of interest annotated by the radiologist, and biopsy-confirmed to contain either malignant (blue) or benign (green) tissue. The red dots represent the gradients of the input image with respect to the cancer class output. The gradients were rescaled to be within [0, 1] and a low cutoff of 0.06 was used to remove background noise. Heatmaps ( d ) of the four non-background classes for input image ( a ). The colors of the heatmaps represent the activation values after ReLU. This figure is best viewed in color.
Combining the CC and MLO views for prediction: Combining the CC and MLO views may increase performance because each view can contain unique information. After removing the samples where only a single view was available, 90% of the test set remained for analysis of both views from each of 169 breasts. We used a simple approach of taking the average score of the two views. A breast-level bootstrapping method was used (3000 runs) to compare two-view vs. single-view AUCs for the four best models above: Resnet-Resnet, Resnet-VGG, VGG-VGG and VGG-Resnet. The mean AUC differences were 0.030 [0.018, 0.042], 0.027 [0.016, 0.037], 0.040 [0.028, 0.051] and 0.048 [0.032, 0.064], respectively. Thus, using two views when available significantly increased the AUCs in comparison to single views for all the models tested here.
Max-pooling, shortcut and FC layers: We tested an alternative design by using the heatmap followed by a max-pooling and two FC layers, including a shortcut between the heatmap and the classification output. The Resnet50 and VGG16 patch classifiers were used. The FC layer sizes were chosen to gradually reduce the layer outputs. When the pooling size increased from 1 × 1 (i.e. no pooling) to 5 × 5, the AUCs did not show significant changes with the exception of pooling size 1 × 1 for the Resnet50 patch classifier, in which case the AUC was significantly lower than the others (Tables 2 and 3 ). The best mean AUC for these models was 0.74, falling short of the performance of the all convolutional models.
Evaluation of the heatmap in all convolutional networks: To test our hypothesis that the heatmap can cause information loss in the whole image classification network, we inserted a heatmap in the Resnet-based whole image classifier with two [512 − 512 − 1024] × 2 blocks as top layers. The heatmap inserted was a 1 × 1 convolutional layer that reduces the number of filters from the previous convolutional layer (2048) to 5, which corresponds to the 5 classes of the patch classifier. To facilitate the back-propagation of gradients, ReLU was used to replace the softmax activation in the heatmap. Figure 4d shows an example heatmap that provides a rough segmentation of the input image; the top layers then use the segmentation to classify the whole image. This model achieved a mean AUC of 0.80 (Table 2 ), which was significantly lower than that of the same classifier without the heatmap with an AUC difference of −0.050 [−0.088, −0.012]. To exclude the possibility that the top layers were overfit due to the shallow depth of the heatmap, another model with reduced complexity using two Resnet blocks of [64 − 64 − 256] × 2 and [128 − 128 − 512] × 2 was tested, which achieved a similar mean AUC of 0.81 (AUC difference of −0.044 [−0.075, −0.012]). These results indicated that removing the heatmap was beneficial to the whole image classification networks.
Comparison to prior two-step approach: Finally, for comparison we tested a previously reported approach 34 that used a probability cutoff to binarize the heatmap into a binary image that represents each pixel as background (0) or ROI (1). This was repeated for each of the 4 foreground classes. We then extracted regional features (such as area, major axis length and mean intensity) from the ROIs of the binary images and trained a random forest classifier (#trees = 500, max depth = 9, min samples split = 300) on the regional features. The Resnet50 patch classifier was used and the softmax activation was used in the heatmap to obtain the probabilities for the 5 classes. Four cutoffs—0.3, 0.5, 0.7 and 0.9 were used to binarize the heatmaps and the regional features were combined. This approach achieved an AUC of 0.73, and was inferior to the all convolutional models.
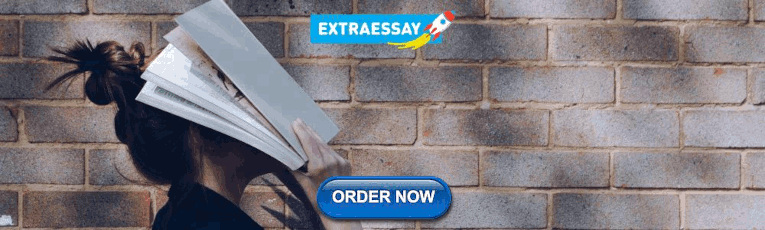
Transfer learning for whole image classification on INbreast
The INbreast 30 dataset is a public database containing more recently acquired FFDM images. These images have different intensity profiles compared with digitized film mammograms from the CBIS-DDSM, as illustrated by example images from the two databases (Fig. 5 ). Therefore, INbreast provides an excellent opportunity to test the transferability of a whole image classifier across mammography platforms. The INbreast database contains 115 patients and 410 mammograms including both CC and MLO views. We analyzed each view separately like above. The INbreast database includes radiologists’ BI-RADS 44 assessment categories which are defined as follows: 0, incomplete exam; 1, no findings; 2, benign; 3, probably benign; 4, suspicious; 5, highly suggestive of malignancy; and 6, known biopsy-proven cancer. Because the database lacks reliable pathological confirmation of malignancy, we assigned all images with BI-RADS 1 and 2 as negative; BI-RADS 4, 5 and 6 as positive; and excluded 12 patients and 23 images with BI-RADS 3 since this assessment is typically not given at screening. We split the dataset 70:30 into training and test sets at the patient-level while maintaining the same ratio of positive and negative images. The total numbers of images in the training and test sets were 280 from 72 women and 107 from 31 women, respectively. We used the same processing steps on the INbreast images as for the CBIS-DDSM images.
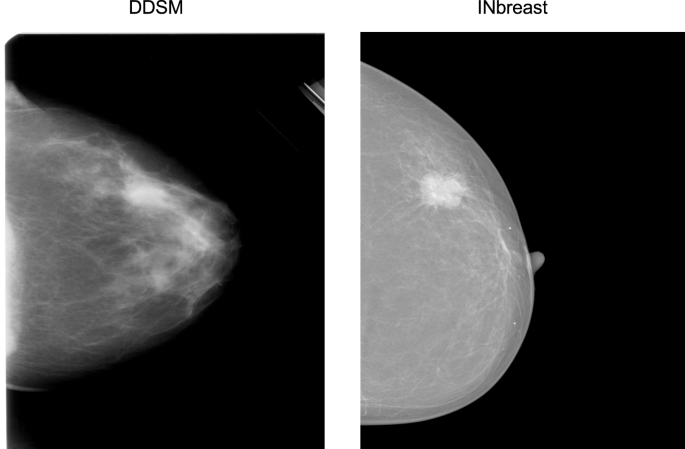
Representative examples of a digitized film mammogram from CBIS-DDSM and a digital mammogram from INbreast.
Effectiveness and efficiency of transfer learning
Although the INbreast database contains ROI annotations, they were ignored to test the transferability of the whole image classifier across different mammography platforms and databases. The four best performing models (See Tables 2 and 3 ) were directly fine-tuned on the INbreast training set and evaluated by computing per-image AUCs on the test set. Adam 42 was used as the optimizer and the learning rate was set at 10 −5 . The number of epochs was set at 200 and the weight decay at 0.01. All four models achieved an AUC of 0.95 (Table 4 ). The ensemble model based on averaging the four best models improved the AUC to 0.98 with a corresponding sensitivity of 86.7% and specificity of 96.1% (Fig. 3b ).
We also sought to determine the minimum amount of data required to fine-tune a whole image classifier to a satisfactory level of performance, to guide future studies in minimizing the resource intensive process of obtaining labels. Training subsets with 20, 30, 40, 50 and 60 patients were sampled for fine-tuning and model performance was evaluated using the same test set (Table 4 ). With as few as 20 patients or 79 images, the four models already attained AUCs between 0.87 and 0.92. The AUCs quickly approached the maximum as the training subset size increased. These results suggest that the intensive part of learning is to recognize the shapes and textures of the benign and malignant ROIs and normal tissues, and that adjusting to different intensity profiles found in different mammography datasets may require much less data. Importantly, these results clearly demonstrate that the end-to-end training approach can be successfully used to fine-tune a whole image classifier using additional small training sets with image-level labels, greatly reducing the burden of training set construction for multiple different mammography platforms.
This study shows that accurate classification of screening mammograms can be achieved with a deep learning model trained in an end-to-end fashion that relies on clinical ROI annotations only in the initial stage. Once the whole image classifier is built, it can be fine-tuned using additional datasets that lack ROI annotations, even if the pixel intensity distributions differ as is often the case for datasets assembled from heterogeneous mammography platforms. These findings indicate that deep learning algorithms can improve upon classic commercial CAD systems, such as iCAD SecondLook 1.4 and R2 ImageChecker Cenova 1.0, that are not deep learning based and have been reported to attain an average AUC of 0.72 6 . Our all convolutional networks trained using an end-to-end approach have highly competitive performance and are more generalizable across different mammography platforms compared with previous deep learning methods that have achieved AUCs in the range of 0.65–0.97 on the DDSM and INbreast databases, as well as in-house datasets 12 . Two recent studies reported that a new commercial CAD system, Transpara 1.4.0, attained an AUC of 0.89 when used to support radiologists 16 and 0.84 in standalone mode 15 . This commercial CAD used CNNs trained using the lesion annotations from 9000 mammograms with cancer to generate scores at the patch level; the scores for all detected regions were then combined into a score at the examination level. To our knowledge, the commercial CAD cannot easily be fine-tuned on different mammography datasets without lesion annotations. Our approach has the advantage of requiring only image-level labels for fine-tuning once the whole image classifier is built to facilitate scaling to larger datasets and transferring to new mammography systems as they rapidly evolve.
Two recent studies 45 , 46 developed deep learning based methods for breast cancer classification using film and digital mammograms, which were end-to-end trainable. Both studies used multi-instance learning (MIL) and modified the whole image classifier cost functions to satisfy the MIL criterion. In contrast to our approach, neither study utilized ROI annotations to train the patch classifiers first and the AUCs were lower than reported in this study. We found that the quality of the patch classifiers is critical to the accuracy of the whole image classifiers. This was supported by two lines of evidence. First, the whole image classifier based on the S10 patch set performed far better than the one based on the S1 patch set because the S10 patch set contained more information about the background than the S1 patch set. Second, it took much longer for the VGG16-based whole image classifiers to achieve the same performance as the Resnet50-based classifiers because the VGG16 was less accurate than Resnet50 in patch classification.
We also found that the accuracy of whole image classification was improved by sampling more or larger patches to include neighboring regions around the ROI and additional background regions. However, the computational burden increases linearly with the number or size of patches sampled and the performance gain may quickly diminish. Using larger patches can decrease the signal-to-noise ratio, as indicated by the lower patch classification accuracy using the S1g vs. S10 patch sets. Using larger patches also requires higher GPU memory, which may limit network choices. The saliency map analysis showed that our whole image networks were able to correctly identify the ROIs and use the information therein to predict cancer. It also showed that classification errors typically occurred in difficult cases, such as benign lesions with malignant features, or malignant lesions that were difficult to distinguish from background. Further research is needed to investigate how to sample local patches more efficiently, perhaps by augmenting the training data with difficult cases and focusing on the patches that are more likely to be misclassified. This could help overcome the computational burden of training more accurate classifiers.
Although the VGG-based image classifiers were more prone to overfitting and required longer training, the performance of VGG-based and Resnet-based image classifiers was comparable. The fact that the ensemble model performed better than any of the individual models also suggests that the VGG-based and Resnet-based classifiers can complement each other. Moreover, the VGG16 (without the two FC layers), with 15 million weight parameters, is a much smaller network than the Resnet50, with 24 million weight parameters. Having fewer parameters reduces memory requirements and training time per epoch, which is important when computational resources are limited. The Resnet is a more recently developed deep learning method, which is enhanced by shortcuts and batch normalization, both techniques that may help the network train faster and generalize better. The same techniques can be used in the VGG-based networks as well in future work, which may improve the VGG-based classifiers.
This study had some limitations. Mammograms were downsized to fit the available GPU (8 GB). As more GPU memory becomes available, future studies will be able to train models using larger image sizes, or retain the original image resolution without the need for downsizing. Retaining the full resolution of modern digital mammography images will provide finer details of the ROIs and likely improve performance. Although the CBIS-DDSM dataset included pathological confirmation of all cancer diagnoses, the INbreast dataset did not. Therefore, we used the radiologists’ BI-RADS assessments to assign labels to the images in the INbreast dataset, which has the limitation of reproducing radiologists’ impressions instead of discovering new characteristics of malignant lesions. It would be of interest in future work to include interval breast cancers that were missed by radiologists, to help train algorithms to detect more subtle signs of malignancy that may not be visually apparent. Finally, the CBIS-DDSM and INbreast datasets were not nationally representative samples and performance metrics in these datasets are not directly comparable to national estimates of radiologists’ sensitivity and specificity. Future direct comparisons between algorithms and radiologists will be facilitated by public sharing of the code and greater availability of representative benchmarking datasets.
In conclusion, our study demonstrates that deep learning models trained in an end-to-end fashion can be highly accurate and potentially readily transferable across diverse mammography platforms. Deep learning methods have enormous potential to further improve the accuracy of breast cancer detection on screening mammography as the available training datasets and computational resources expand. Our approach may assist future development of superior CAD systems that could be used to help prioritize the most suspicious cases to be read by a radiologist, or as an automatic second reader after making an initial independent interpretation. Our end-to-end approach can also be applied to other medical imaging problems where ROI annotations are scarce.
Data Availability
A preprint version of this article is available at: https://arxiv.org/abs/1708.09427 .
American Cancer Society. How Common Is Breast Cancer? https://www.cancer.org/cancer/breast-cancer/about/howcommon-is-breast-cancer.html (2018).
Oeffinger, K. C. et al . Breast Cancer Screening for Women at Average Risk: 2015 Guideline Update From the American Cancer Society. JAMA 314 , 1599–1614 (2015).
Article CAS Google Scholar
Lehman, C. D. et al . National Performance Benchmarks for Modern Screening Digital Mammography: Update from the Breast Cancer Surveillance Consortium. Radiol. 283 , 49–58 (2016).
Article Google Scholar
Elter, M. & Horsch, A. CADx of mammographic masses and clustered microcalcifications: A review. Med. Phys. 36 , 2052–2068 (2009).
Fenton, J. J. et al . Influence of Computer-Aided Detection on Performance of Screening Mammography. New Engl. J. Medicine 356 , 1399–1409 (2007).
Cole, E. B. et al . Impact of Computer-Aided Detection Systems on Radiologist Accuracy With Digital Mammography. Am. J. Roentgenol. 203 , 909–916 (2014).
Lehman, C. D. et al . Diagnostic Accuracy of Digital Screening Mammography With and Without Computer-Aided Detection. JAMA Intern. Medicine 175 , 1828–1837 (2015).
LeCun, Y., Bengio, Y. & Hinton, G. Deep learning. Nat. 521 , 436–444 (2015).
Article ADS CAS Google Scholar
Aboutalib, S. S. et al . Deep Learning to Distinguish Recalled but Benign Mammography Images in Breast Cancer Screening. Clin . Cancer Res . (2018).
Kim, E.-K. et al . Applying Data-driven Imaging Biomarker in Mammography for Breast Cancer Screening: Preliminary Study. Sci. Reports 8 , 2762 (2018).
Article ADS Google Scholar
Hamidinekoo, A., Denton, E., Rampun, A., Honnor, K. & Zwiggelaar, R. Deep learning in mammography and breast histology, an overview and future trends. Med. Image Analysis 47 , 45–67 (2018).
Burt, J. R. et al . Deep learning beyond cats and dogs: Recent advances in diagnosing breast cancer with deep neural networks. The Br . J . Radiol . 20170545 (2018).
Kooi, T. et al . Large scale deep learning for computer aided detection of mammographic lesions. Med. Image Analysis 35 , 303–312 (2017).
Agarwal, R., Diaz, O., Lladó, X., Yap, M. H. & Martí, R. Automatic mass detection in mammograms using deep convolutional neural networks. J. Med. Imaging 6 , 031409 (2019).
Rodriguez-Ruiz, A. et al . Stand-Alone Artificial Intelligence for Breast Cancer Detection in Mammography: Comparison With 101 Radiologists. JNCI: J . Natl . Cancer Inst (2019).
Rodríguez-Ruiz, A. et al . Detection of Breast Cancer with Mammography: Effect of an Artificial Intelligence Support System. Radiol. 290 , 305–314 (2018).
Jamieson, A. R., Drukker, K. & Giger, M. L. Breast image feature learning with adaptive deconvolutional networks. Proc. SPIE 8315, (6–13 (2012).
Google Scholar
Arevalo, J., González, F. A., Ramos-Pollán, R., Oliveira, J. L. & Lopez, M. A. G. Convolutional neural networks for mammography mass lesion classification. In 2015 37th Annual International Conference of the IEEE Engineering in Medicine and Biology Society ( EMBC ), 797–800, https://doi.org/10.1109/EMBC.2015.7318482 (2015).
Arevalo, J., González, F. A., Ramos-Pollán, R., Oliveira, J. L. & Guevara Lopez, M. A. Representation learning for mammography mass lesion classification with convolutional neural networks. Comput. Methods Programs Biomed. 127 , 248–257 (2016).
Lévy, D. & Jain, A. Breast Mass Classification from Mammograms using Deep Convolutional Neural Networks. arXiv preprint arXiv:1612 . 00542 (2016).
Dhungel, N., Carneiro, G. & Bradley, A. P. The Automated Learning of Deep Features for Breast Mass Classification from Mammograms. In Ourselin, S., Joskowicz, L., Sabuncu, M. R., Unal, G. & Wells, W. (eds) Medical Image Computing and Computer-Assisted Intervention – MICCAI 2016: 19th International Conference , Athens , Greece , October 17 – 21 , 2016 , Proceedings , Part II , 106–114 (Springer International Publishing, Cham, 2016).
Girshick, R., Donahue, J., Darrell, T. & Malik, J. Rich Feature Hierarchies for Accurate Object Detection and Semantic Segmentation. In Proceedings of the 2014 IEEE Conference on Computer Vision and Pattern Recognition , CVPR’ 14, 580–587 (IEEE Computer Society, Washington, DC, USA, 2014).
Girshick, R. Fast r-cnn. In Proceedings of the IEEE International Conference on Computer Vision , 1440–1448 (2015).
Ren, S., He, K., Girshick, R. & Sun, J. Faster R-CNN: Towards real-time object detection with region proposal networks. In Advances in Neural Information Processing Systems , 91–99 (2015).
Dai, J., Li, Y., He, K. & Sun, J. R-FCN: Object Detection via Region-based Fully Convolutional Networks. arXiv:1605 . 06409 [ cs ], 1605.06409 (2016).
Dhungel, N., Carneiro, G. & Bradley, A. P. Automated Mass Detection in Mammograms Using Cascaded Deep Learning and Random Forests. In 2015 International Conference on Digital Image Computing: Techniques and Applications ( DICTA ), 1–8, https://doi.org/10.1109/DICTA.2015.7371234 (2015).
Ertosun, M. G. & Rubin, D. L. Probabilistic visual search for masses within mammography images using deep learning. In 2015 IEEE International Conference on Bioinformatics and Biomedicine ( BIBM ), 1310–1315, https://doi.org/10.1109/BIBM.2015.7359868 (2015).
Akselrod-Ballin, A. et al . A Region Based Convolutional Network for Tumor Detection and Classification in Breast Mammography. In Carneiro, G. et al . (eds) Deep Learning and Data Labeling for Medical Applications: First International Workshop , LABELS 2016 , and Second International Workshop , DLMIA 2016 , Held in Conjunction with MICCAI 2016 , Athens , Greece , October 21 , 2016 , Proceedings , 197–205 (Springer International Publishing, Cham, 2016).
Ribli, D., Horváth, A., Unger, Z., Pollner, P. & Csabai, I. Detecting and classifying lesions in mammograms with Deep Learning. arXiv:1707 . 08401 [ cs ], 1707.08401 (2017).
Moreira, I. C. et al . INbreast: Toward a Full-field Digital Mammographic Database. Acad. Radiol. 19 , 236–248 (2012).
Hinton, G. E., Osindero, S. & Teh, Y.-W. A fast learning algorithm for deep belief nets. Neural Comput. 18 , 1527–1554 (2006).
Article MathSciNet Google Scholar
Russakovsky, O. et al . ImageNet Large Scale Visual Recognition Challenge. Int. J. Comput. Vis. 115 , 211–252 (2015).
Oquab, M., Bottou, L., Laptev, I. & Sivic, J. Learning and Transferring Mid-Level Image Representations using Convolutional Neural Networks. In Proceedings of the IEEE Conference on Computer Vision and Pattern Recognition , 1717–1724 (2014).
Wang, D., Khosla, A., Gargeya, R., Irshad, H. & Beck, A. H. Deep Learning for Identifying Metastatic Breast Cancer. arXiv:1606 . 05718 [ cs , q-bio ], 1606.05718 (2016).
Ciresan, D., Giusti, A., Gambardella, L. M. & Schmidhuber, J. Deep Neural Networks Segment Neuronal Membranes in Electron Microscopy Images. In Pereira, F., Burges, C. J. C., Bottou, L. & Weinberger, K. Q. (eds) Advances in Neural Information Processing Systems 25 , 2843–2851 (Curran Associates, Inc., 2012).
LeCun, Y. et al . Convolutional networks for images, speech, and time series. The handbook brain theory neural networks 3361 , 1995 (1995).
Heath, M., Bowyer, K., Kopans, D., Moore, R. & Kegelmeyer, W. The Digital Database for Screening Mammography. In Yaffe, M. (ed.) Proceedings of the Fifth International Workshop on Digital Mammography , 212–218 (Medical Physics Publishing, 2001).
Simonyan, K. & Zisserman, A. Very Deep Convolutional Networks for Large-Scale Image Recognition. arXiv:1409 . 1556 [ cs ], 1409.1556 (2014).
He, K., Zhang, X., Ren, S. & Sun, J. Deep Residual Learning for Image Recognition. arXiv:1512 . 03385 [ cs ], 1512.03385 (2015).
Ioffe, S. & Szegedy, C. Batch Normalization: Accelerating Deep Network Training by Reducing Internal Covariate Shift. arXiv:1502 . 03167 [ cs ], 1502.03167 (2015).
Lee, R. S., Gimenez, F., Hoogi, A. & Rubin, D. Curated Breast Imaging Subset of DDSM. The Cancer Imaging Arch (2016).
Kingma, D. P. & Ba, J. Adam: A Method for Stochastic Optimization. arXiv:1412 . 6980 [ cs ], 1412.6980 (2014).
Springenberg, J. T., Dosovitskiy, A., Brox, T. & Riedmiller, M. Striving for Simplicity: The All Convolutional Net. arXiv:1412 . 6806 [ cs ], 1412.6806 (2014).
D’Orsi, C., Sickles, E., Mendelson, E. & Morris, E. ACR BI-RADS R Atlas , Breast Imaging Reporting and Data System , 5th edn (American College of Radiology, Reston, Va, 2013).
Zhu, W., Lou, Q., Vang, Y. S. & Xie, X. Deep Multi-instance Networks with Sparse Label Assignment for Whole Mammogram Classification. arXiv:1705 . 08550 [ cs ], 1705.08550 (2017).
Choukroun, Y. et al . Mammogram Classification and Abnormality Detection from Nonlocal Labels using Deep Multiple Instance Neural Network. In Eurographics Workshop on Visual Computing for Biology and Medicine (2017).
Download references
Acknowledgements
This work was partially supported by the Friedman Brain Institute, the Tisch Cancer Institute (NIH P30CA196521) and a Clinical and Translational Science Award (NIH UL1TR001433). The funders had no role in study design, data collection and analysis, decision to publish, or preparation of the manuscript. This work was supported in part through the computational resources and staff expertise provided by the Department of Scientific Computing at the Icahn School of Medicine at Mount Sinai.
Author information
Authors and affiliations.
Icahn School of Medicine at Mount Sinai (ISMMS), Department of Neuroscience, New York, 10029, USA
ISMMS, Department of Diagnostic, Molecular, and Interventional Radiology, New York, 10029, USA
Laurie R. Margolies
ISMMS, Department of Population Health Science and Policy and Department of Genetics and Genomic Sciences, New York, 10029, USA
Joseph H. Rothstein & Weiva Sieh
ISMMS, Department of Scientific Computing, New York, 10029, USA
Eugene Fluder
ISMMS, Department of Pathology, New York, 10029, USA
Russell McBride
You can also search for this author in PubMed Google Scholar
Contributions
L.S. developed the algorithm, conceived and conducted the experiments and analyzed the results. L.R.M., J.R., R.M. and W.S. analyzed the results. E.F. helped with the computational resources. All authors contributed to writing and reviewing the manuscript.
Corresponding author
Correspondence to Li Shen .
Ethics declarations
Competing interests.
The authors declare no competing interests.
Additional information
Publisher’s note: Springer Nature remains neutral with regard to jurisdictional claims in published maps and institutional affiliations.
Supplementary information
Rights and permissions.
Open Access This article is licensed under a Creative Commons Attribution 4.0 International License, which permits use, sharing, adaptation, distribution and reproduction in any medium or format, as long as you give appropriate credit to the original author(s) and the source, provide a link to the Creative Commons license, and indicate if changes were made. The images or other third party material in this article are included in the article’s Creative Commons license, unless indicated otherwise in a credit line to the material. If material is not included in the article’s Creative Commons license and your intended use is not permitted by statutory regulation or exceeds the permitted use, you will need to obtain permission directly from the copyright holder. To view a copy of this license, visit http://creativecommons.org/licenses/by/4.0/ .
Reprints and permissions
About this article
Cite this article.
Shen, L., Margolies, L.R., Rothstein, J.H. et al. Deep Learning to Improve Breast Cancer Detection on Screening Mammography. Sci Rep 9 , 12495 (2019). https://doi.org/10.1038/s41598-019-48995-4
Download citation
Received : 14 May 2019
Accepted : 07 August 2019
Published : 29 August 2019
DOI : https://doi.org/10.1038/s41598-019-48995-4
Share this article
Anyone you share the following link with will be able to read this content:
Sorry, a shareable link is not currently available for this article.
Provided by the Springer Nature SharedIt content-sharing initiative
This article is cited by
Are better ai algorithms for breast cancer detection also better at predicting risk a paired case–control study.
- Ruggiero Santeramo
- Celeste Damiani
- Adam R. Brentnall
Breast Cancer Research (2024)
- Samuel Rahimeto Kebede
- Fraol Gelana Waldamichael
- Zelalem Chimdesa Merga
Scientific Reports (2024)
Deep learning system for true- and pseudo-invasion in colorectal polyps
- Charles Ling
Machine vision-based detections of transparent chemical vessels toward the safe automation of material synthesis
- Leslie Ching Ow Tiong
- Hyuk Jun Yoo
- Donghun Kim
npj Computational Materials (2024)
Robustness of Deep Networks for Mammography: Replication Across Public Datasets
- Osvaldo M. Velarde
- Clarissa Lin
- Lucas C. Parra
Journal of Imaging Informatics in Medicine (2024)
By submitting a comment you agree to abide by our Terms and Community Guidelines . If you find something abusive or that does not comply with our terms or guidelines please flag it as inappropriate.
Quick links
- Explore articles by subject
- Guide to authors
- Editorial policies
Sign up for the Nature Briefing: AI and Robotics newsletter — what matters in AI and robotics research, free to your inbox weekly.

Israeli researchers reach bone metastasis breakthrough for breast cancer patients
T el Aviv University (TAU) researchers have developed a new therapeutic strategy based on existing medications to inhibit bone metastasis in breast cancer patients. When a patient has this malignancy and it isn’t stopped from spreading, most often – in 75% of cases – it spreads to the bone, destroying its tissues and causing fractures and a great deal of pain.
Using both an animal model and tissue samples from patients in Israel and the US , the researchers showed that a combination of drugs already available on the market can hinder bone metastasis and improve survival. Based on their findings, they predict that in the future, the treatment will be applied to human patients with breast cancer as well as other types of cancer.
In this groundbreaking study, the researchers looked for a novel way to inhibit the progression of bone metastasis. The study was led by Prof. Neta Erez and Dr. Lea Monteran at Erez’s Laboratory for Tumor Biology at the pathology department of the university’s Faculty of Medical and Health Sciences. The paper has just been published in the journal Cancer Discovery under the title “Clofazimine Reduces Side Effects from Dual Immune Checkpoint Blockade.”
With today’s technologies, such as MRI and CT imaging, a diagnosis of bone metastasis mostly occurs when the disease has already gone too far and the patient can no longer be cured.
“A tumor is more than a collection of cancer cells. Just like healthy tissues, a tumor is a whole ecosystem consisting of reciprocal interactions between different cell types, including cells of the immune system, connective tissues, blood vessels, and more.
Cancer cells often ‘corrupt’ normal cells, causing them to ‘collaborate’ with the tumor and support the growth of cancer cells.
Blocking the communication channels between cancer cells and healthy cells at an early stage can hinder the growth of cancer cells in the bones. To achieve this, the early stages of the process must be investigated,” Erez explained.
Novel approach to combatting bone metastasis
To understand the process of bone metastasis, the researchers compared three types of bones from model mice – healthy, early-stage metastasis, and advanced metastasis. They found that when bone metastasis begins, T cells from the immune system arrive on the scene and penetrate the metastases but are unable to destroy them.
Next, the researchers discovered that another type of immune cell inhibits the killing activity of T cells, and they identified the proteins responsible for this effect. To neutralize these inhibitory proteins and reactivate the T-cells, they created a novel therapeutic combination that had never before been tried – a drug that counters the activity of the immune-inhibiting cells along with an antibody that activates T cells. This combination was administered to model mice, and the results were encouraging. The bone metastases were reduced, and survival was significantly improved compared to untreated model mice, the authors wrote.
At the final stage of the study, the TAU research team collaborated with Tel Aviv Sourasky Medical Center, Sheba Medical Center at Tel Hashomer, and the Baylor College of Medicine in Texas. They examined tissue samples from bone metastases taken from patients with breast and other types of cancer and found that the immune cells inhibiting T cells express the same proteins as those found in the animal model.
“Our findings suggest that the combined treatment – attacking the cells that inhibit T cells while activating the T cells – can be effective for treating bone metastasis resulting from breast cancer as well as other types of cancer,” Erez concluded. “The great advantage of our strategy is that both drugs are already available on the market, so the process of obtaining permits to use them against bone metastasis in humans can be relatively short. At the same time, clinical trials are needed to verify the effectiveness of the new therapeutic strategy.”
- Open access
- Published: 11 April 2024
METTL3 recruiting M2-type immunosuppressed macrophages by targeting m6A-SNAIL-CXCL2 axis to promote colorectal cancer pulmonary metastasis
- Peng Ouyang 1 na1 ,
- Kang Li 1 na1 ,
- Wei Xu 1 na1 ,
- Caiyun Chen 1 ,
- Yangdong Shi 1 ,
- Yao Tian 1 ,
- Jin Gong 1 &
- Zhen Bao 1
Journal of Experimental & Clinical Cancer Research volume 43 , Article number: 111 ( 2024 ) Cite this article
225 Accesses
1 Altmetric
Metrics details
The regulatory role of N6-methyladenosine (m6A) modification in the onset and progression of cancer has garnered increasing attention in recent years. However, the specific role of m6A modification in pulmonary metastasis of colorectal cancer remains unclear.
This study identified differential m6A gene expression between primary colorectal cancer and its pulmonary metastases using transcriptome sequencing and immunohistochemistry. We investigated the biological function of METTL3 gene both in vitro and in vivo using assays such as CCK-8, colony formation, wound healing, EDU, transwell, and apoptosis, along with a BALB/c nude mouse model. The regulatory mechanisms of METTL3 in colorectal cancer pulmonary metastasis were studied using methods like methylated RNA immunoprecipitation quantitative reverse transcription PCR, RNA stability analysis, luciferase reporter gene assay, Enzyme-Linked Immunosorbent Assay, and quantitative reverse transcription PCR.
The study revealed high expression of METTL3 and YTHDF1 in the tumors of patients with pulmonary metastasis of colorectal cancer. METTL3 promotes epithelial-mesenchymal transition in colorectal cancer by m6A modification of SNAIL mRNA, where SNAIL enhances the secretion of CXCL2 through the NF-κB pathway. Additionally, colorectal cancer cells expressing METTL3 recruit M2-type macrophages by secreting CXCL2.
METTL3 facilitates pulmonary metastasis of colorectal cancer by targeting the m6A-Snail-CXCL2 axis to recruit M2-type immunosuppressive macrophages. This finding offers new research directions and potential therapeutic targets for colorectal cancer treatment.
In patients with colorectal cancer (CRC), cancer metastasis is the primary cause of cancer-related deaths, with the liver and lungs being the most common target organs for metastasis [ 1 , 2 ]. While the majority of research has traditionally focused on liver metastases, colorectal cancer pulmonary metastasis (CRPM) has received relatively less attention. This gap in research is particularly significant given that the lungs are the second most common site of metastasis for CRC. Epidemiological studies estimate that pulmonary metastases occur in approximately 10–18% of rectal cancer patients and 5–6% of colon cancer patients [ 3 ]. The mechanisms of CRPM are multifaceted, encompassing intracellular factors such as genetic and epigenetic abnormalities, tumor cell heterogeneity, and the process of epithelial-mesenchymal transition (EMT). Furthermore, the influence of the tumor microenvironment cannot be underestimated [ 4 ]. Given its significance in cancer progression and treatment, in-depth research on CRPM is imperative. This not only contributes to a better understanding of the metastatic mechanisms in CRC but is also crucial for the development of novel therapeutic strategies.
In the formation process of CRPM, the EMT plays a crucial role [ 3 ]. EMT is a critical mechanism in the process of tumor initiation and development, and its activation is typically regulated by transcription factors that induce EMT. These factors include Snail family transcriptional repressor 1 (SNAIL) protein, Zinc finger E-box-binding homeobox 1 (ZEB) protein, and Twist family bHLH transcription factor 1 (TWIST1) protein [ 5 , 6 , 7 , 8 ]. This activation process leads to a series of changes in cells, including the loss of cell apical-basal polarity, disruption of cell–cell junctions, degradation of the basement membrane, and remodeling of the extracellular matrix (ECM) [ 9 , 10 ]. These changes transform epithelial cells from their original cobblestone-like appearance into spindle-shaped mesenchymal cells [ 11 ]. At the molecular level, the characteristics of EMT include a decrease in epithelial markers such as E-cadherin and an increase in mesenchymal markers such as vimentin, N-cadherin, and fibronectin [ 12 ]. Clinically, these changes enhance the mobility and invasive capacity of tumor cells, thereby promoting the distant metastasis of colorectal cancer cells [ 13 ]. Therefore, in-depth research into the EMT process is crucial for understanding the progression mechanisms of colorectal cancer and developing effective personalized treatment strategies.
Epigenetic abnormalities play a crucial role in the occurrence of CRPM [ 3 ]. Indeed, N6-methyladenosine (m6A) modification is one of the most common forms of epigenetic modifications found in messenger RNA (mRNA) [ 14 , 15 ]. Recent research has indicated that m6A plays a crucial role in various aspects of mRNA, including splicing, nuclear export, translation, and stability. Its significance extends to the development of many human diseases, including cancer [ 16 , 17 ]. This modification is controlled by a group of specialized enzymes, including "writers" that add methyl groups and "erasers" that remove methyl groups [ 18 ]. In this context, the 'writers' complex comprises enzymes such as methyltransferase-like 3 (METTL3) and methyltransferase-like 14 (METTL14), which are responsible for transferring methyl groups onto RNA [ 19 , 20 , 21 , 22 ]. The demethylation of m6A is facilitated by enzymes such as obesity-related protein (FTO) and alkB homologue 5 (ALKBH5) [ 23 , 24 ]. These modifications are recognized and interpreted by a group of proteins known as 'readers,' which include YTH domain family proteins and insulin-like growth factor 2 mRNA-binding proteins (IGF2BP) [ 25 , 26 ]. These intricate interactions influence the behavior and function of RNA, thereby playing crucial roles in the development of CRPM.
During the development of tumors, m6A often exerts its influence on the expression of key genes associated with EMT, either directly or indirectly, leading to the promotion of tumor cell EMT and facilitating their progression and metastasis. [ 27 , 28 , 29 , 30 ]. The relationship between m6A and EMT in cancer cells has been extensively studied, but its role in CRPM remains unclear. In our study, we conducted transcriptome sequencing, quantitative PCR (qPCR), Western blotting (WB), and immunohistochemical (IHC) analysis on clinical specimens of colorectal primary tumors and their lung metastatic tumors. Additionally, we integrated data from The Cancer Genome Atlas (TCGA) database and observed an increased expression of m6A-related genes, such as METTL3 and YTHDF1. These observations prompted us to further explore the potential link between these changes and colorectal cancer lung metastasis. Through in vivo and in vitro experiments, we found that METTL3 could enhance the expression of SNAIL protein through m6A modification, thereby promoting EMT in tumor cells. Furthermore, we observed that tumor cells overexpressing SNAIL could promote the secretion of CXCL2 by activating the NF-κB pathway. Secreted CXCL2, upon binding to CXCR2 on the surface of M2-type macrophages, facilitated the infiltration of these immune cells into the tumor center. These findings provide a new perspective on understanding the molecular mechanisms underlying colorectal cancer lung metastasis and may have significant implications for the development of novel therapeutic strategies.
Materials and methods
Specimens, cell culture and treatment.
The transcriptome sequencing data for CRC in this study were sourced from The Cancer Genome Atlas (TCGA) data portal (accessible at https://portal.gdc.cancer.gov/ ). Chromatin immunoprecipitation (ChIP) sequencing data were obtained from the Gene Expression Omnibus (GEO) database (see https://www.ncbi.nlm.nih.gov/gds/ ). In situ carcinoma and lung metastatic carcinoma tissue specimens from colorectal cancer lung metastasis patients were provided by the First Affiliated Hospital of Jinan University. Collection of all specimens received approval from the ethics committee of the First Affiliated Hospital of Jinan University, and written informed consent was obtained from the patients before collection.
CRC cell lines used in this study, including RKO and SW480, as well as the human monocytic cell line THP-1, were all obtained from the American Type Culture Collection (ATCC, Manassas, USA) (details available at https://www.atcc.org/ ). THP-1 cells were treated with 100 ng/ml phorbol 12-myristate 13-acetate (PMA, Sigma, Darmstadt, Germany) for 48 h to induce their differentiation into mature macrophages. Subsequently, the cells were incubated for over 48 h under conditions containing 20 ng/ml interleukin-4 (IL-4, Sigma, Darmstadt, Germany) and 20 ng/ml interleukin-13 (IL-13, Sigma, Darmstadt, Germany) to promote their polarization towards the M2 phenotype. All cell lines were cultured following the guidelines recommended by ATCC.
Production of lentiviruses
The lentiviral transfection vectors used in this study were obtained from GenePharma (Shanghai, China). Experimental groups included sh-METTL3 (METTL3 knockdown group), sh-YTHDF1 (YTHDF1 knockdown group), sh-SNAIL (SNAIL knockdown group), and Ov-SNAIL (SNAIL overexpression group), along with their corresponding negative control group sh-NC. To establish stable colorectal cancer cell lines, cell selection was performed using 400 μg/ml of neomycin.
Wound-healing assays
In this experiment, cells were initially seeded in 6-well plates. When the cells reached approximately 80% confluence, sterile 200 μl pipette tips were gently used to create scratches in the cell monolayer. Subsequently, these treated cells were incubated for an additional 48 h in serum-free culture medium. The healing process of cell injuries was observed using an inverted microscope produced by Olympus Corporation, Japan. Finally, quantitative analysis of the wound area was performed using ImageJ software to assess cell migration and healing ability.
Transwell invasion assay
In our cell invasion experiments, we utilized Transwell chambers and Matrigel from Corning, USA. Initially, a layer of extracellular matrix gel was coated in the upper chamber of the Transwell, and medium containing 20% FBS was added to the lower chamber. Once the gel had completely solidified, we added 200 µL of medium containing 1 × 10^5 cells to the upper chamber. Subsequently, the cells were incubated at 37 °C with 5% CO2 for 48 h. After the incubation period, we fixed the cells in the upper chamber with 4% paraformaldehyde and then stained them with 1% crystal violet. Finally, we observed the cells that had invaded from the upper chamber to the lower chamber using an inverted microscope produced by Olympus Corporation, Japan, and conducted quantitative analysis using ImageJ software.
Cell counting kit-8 (CCK-8) and EDU assay
In the CCK-8 experiment, cells were evenly distributed in a 96-well plate and cultured for 1, 2, 3, 4, and 5 days. Subsequently, 10 µL of CCK-8 solution was added to each well. To assess cell viability, the optical density (OD450) of each well was measured using a microplate reader from Thermo Fisher, USA.
For the EDU experiment, cell suspensions were evenly distributed in a 96-well plate to achieve a cell count of 1 × 10^4 cells per well. The culture dishes were then incubated overnight in a CO2 incubator to allow the cells to adhere to the surface. After a 2-h incubation with 10 µM EDU (Beyotime, Shanghai, China), the culture medium was removed, and 1 mL of fixation solution (Beyotime, Shanghai, China) was added to each well. The fixation solution was left at room temperature for 15 min and then removed. The cells were subsequently washed three times with 1 mL of washing solution. After removing the washing solution, 1 mL of Triton X-100 (Beyotime, Shanghai, China) was added to each well and incubated at room temperature for 10–15 min. Next, the Click reaction solution (Beyotime, Shanghai, China) was added and incubated in the dark at room temperature for 30 min. Finally, Hoechst 33,342 (Beyotime, Shanghai, China) was used for nuclear staining. Cell counting and photography were performed using a fluorescence inverted microscope, and quantitative analysis was conducted using ImageJ software.
Clone formation assays
To collect cells during the logarithmic growth phase, a cell suspension was prepared using standard digestion and centrifugation methods. After cell counting, the cells were seeded into a 6-well plate at a concentration of 500 cells per well. Once the cells reached the 6th generation, culturing was stopped. The culture medium was discarded, and cells were washed twice with PBS solution. Subsequently, the cells were fixed in methanol for 15 min and stained with 1% crystal violet solution for 10 min. After capturing photographs with a camera, quantitative analysis was performed using ImageJ software.
Apoptosis assays
In the apoptosis experiment, the detection of cell apoptosis was performed using the FITC Annexin V Cell Apoptosis Detection Kit (provided by BD Biosciences). The experimental steps were as follows: After 48 h of shRNA viral infection, the cells were seeded into 6 cm culture dishes. Cells were then cultured in medium containing 1% fetal bovine serum for 5 days. Following this, cells were digested using trypsin and mixed with the culture supernatant. After digestion was completed, cells were centrifuged, and the cell pellet was washed with PBS buffer to remove trypsin. Subsequently, the cells were resuspended and subjected to Annexin V/PI double staining. Finally, flow cytometry analysis of the samples was performed using the BD LSRII flow cytometer (BD Biosciences). Throughout the entire experiment, precautions should be taken to avoid exposure to light, and strict adherence to laboratory safety regulations is essential.
M2-type macrophage migration assay
M2-type macrophage (1 × 10^5 cells per well) were plated in the upper compartment of a transwell with a pore size of 0.8 mm, in 100 mL of 1640 medium. The lower compartment was filled with 600 mL of conditioned medium collected from RKO or SW480 cells, excluding fetal bovine serum. Following a 4-h incubation period, the migrated cells in the lower chamber were quantified.
RNA stability
Add Act-D to the cell culture medium at a concentration of 5 µg/mL when the cells have reached a confluence of 70–80%. Collect cell samples at different time points after Act-D treatment. Use TRIzol reagent (Invitrogen) to extract total RNA from the collected samples. Quantify the RNA and assess its purity using a NanoDrop spectrophotometer. Convert the extracted total RNA into cDNA using a reverse transcription reagent kit (Vazyme, Nanjing, China) following the manufacturer's instructions. Perform quantitative reverse transcription-polymerase chain reaction (qRT-PCR) analysis to detect the mRNA levels of specific genes (please specify the genes of interest). Normalize the data using GAPDH as the reference gene. Calculate the relative expression levels using the 2^-ΔΔCT method. Create line graphs illustrating the change in RNA levels over time to reflect RNA stability. Ensure that you follow the manufacturer's protocols for reagent usage and experimental procedures.
Western blotting and immunohistochemistry
Cell and tissue samples were processed using RIPA buffer from Servicebio Technology, Wuhan, China. Protein extraction was carried out following the instructions provided in the radio-immunoprecipitation assay (RIPA) kit. Protein concentrations were quantified using the bicinchoninic acid (BCA) assay kit also provided by Servicebio Technology, Wuhan, China. The protein samples were subsequently separated by electrophoresis and transferred onto membranes. After washing the membranes three times with tris-buffered saline containing Tween (TBST), they were incubated with the primary antibody overnight at 4 °C. Following incubation, the membranes were washed again and incubated with the secondary antibody. Finally, protein bands were visualized using the enhanced chemiluminescence (ECL) assay kit provided by Servicebio Technology, Wuhan, China.
Immunohistochemistry and immunofluorescence as previously described [ 31 ]. In our study, immunohistochemistry was conducted using a range of primary antibodies with the following detailed information: 1. METTL3, Vimentin, E-Cadherin, YTHDF1, YTHDF2, SNAIL, and ARG1 antibodies were all obtained from Abcam (Cambridge, UK), each with the following product codes: METTL3 (ab195352), Vimentin (ab92547), E-Cadherin (ab40772), YTHDF1 (ab252346), SNAIL (ab180714), and CD163 (ab316218). 2. P65, Phospho-P65, GAPDH, and Tubulin antibodies were sourced from Proteintech Group (Chicago, USA) and were identified by the specific product codes: P65 (80,979–1-RR), Phospho-P65 (82,335–1-RR), GAPDH (10,494–1-AP), and Tubulin (11,224–1-AP). 3. Goat anti-rabbit IgG was purchased from Biosharp Life Sciences (Beijing, China) and had the product code BL052A. The quantification of all proteins and determination of average optical density were performed using Image J software.
Luciferase reporter assays
The dual-luciferase reporter gene assay was conducted using the Dual-Luciferase® Reporter Gene Assay System provided by Promega Corporation. To standardize transfection efficiency, a co-transfection approach with the pRL-TK reporter gene (also supplied by Promega) was employed. Relative luciferase activity was calculated based on the activity of Renilla luciferase.
mRNA-sequencing and methylated RNA immunoprecipitation quantitative reverse transcription polymerase chain reaction (MeRIP qRT-PCR)
Total RNA was extracted from colorectal cancer cell lines using the TRIzol reagent provided by Invitrogen Corporation (Carlsbad, CA, USA). Subsequently, mRNA sequencing and analysis were carried out by the HaploX Genomics Center in Shenzhen, China.
For RNA immunoprecipitation (MeRIP) analysis of m6A modification, we initially subjected the RNA to fragmentation using the riboMIP™ m6A Transcriptome Analysis Kit (Ribobio, Guangzhou, China) and then performed immunoprecipitation with m6A antibodies. The RNA isolated after immunoprecipitation was analyzed using qRT-PCR to investigate the m6A modification status of RNA.
RNA Extraction and quantitative reverse transcription polymerase chain reaction (qRT-PCR)
Total RNA was extracted using TRIzol (Invitrogen, Carlsbad, USA), while cytoplasmic and nuclear RNA were extracted using cytoplasmic & nuclear RNA purification (Amyjet Scientific, Wuhan, China). qRT-PCR was performed following the protocol (Vazyme, Nanjing, China).
The cell culture medium was transferred to a sterile centrifuge tube and centrifuged at 1000 × g for 10 min at 4 °C. Aliquots of the supernatant were then dispensed into EP tubes for further use. The CXCL2 protein was detected using the manufacturer's protocols for the CXCL2 ELISA kit (Solarbio, Beijing, China).
Animal studies
The experiments conducted in this study received ethical approval from the Ethics Committee of South China Agricultural University. Female nude mice aged 4 to 5 weeks were procured from the Animal Experiment Center of Southern Medical University for the experimental procedures. During the experimentation, a total of 10 nude mice were randomly divided into two groups, each consisting of 5 mice (n = 5). they were subcutaneous injected with 2 × 10^6 RKO/SW480 cells stably silencing METTL3 or control cells. Additionally, six nude mice were randomly assigned to two groups (n = 3), and they were intravenously injected with 2 × 10^6 RKO/SW480 cells stably silencing METTL3 or control cells. These nude mice were housed under standard laboratory conditions with access to ample food and water. Tumor size and volume were monitored weekly throughout the study. Before sample collection, humane euthanasia was administered to all mice using a 2% pentobarbital sodium solution at a dose of 150 mg/kg. After a 2-week interval, mice from the subcutaneous tumor group were euthanized due to tumor development. Similarly, mice from the intravenous tumor group were euthanized at the 4-week mark. The tumors were documented through photographic imaging and weighed. Subsequently, all collected tumor specimens were stored either in liquid nitrogen or fixed using a 4% formaldehyde solution for subsequent analysis.
Statistical analysis
In this study, all statistical analyses were performed using SPSS 19.0 software and R language (version 4.2.1). Graphs and data visualization were carried out using GraphPad Prism 9.5 software. We defined a p -value of less than 0.05 as indicating statistically significant differences.
METTL3 and YTHDF1 are highly expressed in CRPM
To assess the expression profiles of m6A WERs (writers, erasers, and readers) in colon adenocarcinoma (COAD), this study analyzed data from The Cancer Genome Atlas (TCGA) database. The analysis results revealed that several m6A WERs exhibited abnormal expression in CRC (Fig. 1 a). To further investigate the status of these differentially expressed genes in colorectal cancer in situ and lung metastatic cancer, we conducted sequencing on both types of cancer samples. The sequencing results indicated a significant increase in the expression of METTL3, wilms tumor 1 associated protein (WTAP), METTL14, and YTHDF1 in lung metastatic cancer. It's noteworthy that METTL14 did not show expression differences in the TCGA database (Fig. 1 b).
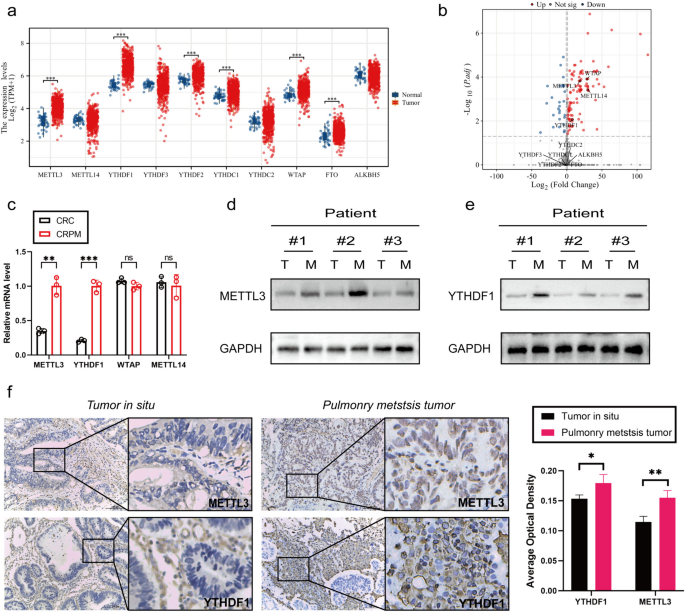
METTL3 and YTHDF1 are highly expressed in CRPM. a Expression profile of WERs in COAD based on TCGA database. b Volcano plot of differential gene sequencing WERs in colorectal cancer in situ and lung metastatic cancer. c qPCR Analysis of METTL3, WTAP, METTL14, and YTHDF1 Gene Expression in colorectal In Situ Carcinoma and Pulmonary Metastatic Carcinoma. d-e Western blotting analysis of METTL3 and YTHDF1 protein expression in colorectal in situ carcinoma and pulmonary metastatic carcinoma. T: colorectal In Situ Carcinoma. M: colorectal pulmonary metastatic carcinoma. f Immunohistochemical Analysis of METTL3 and YTHDF1 Protein Expression in colorectal In Situ Carcinoma and Pulmonary Metastatic Carcinoma. * P < 0.05, ** P < 0.01, *** P < 0.001, **** P < 0.0001
To further validate these findings, we conducted qPCR analysis on colorectal cancer in situ and lung metastatic cancer samples. The qPCR results demonstrated that the expression of METTL3 and YTHDF1 was significantly higher in colorectal cancer lung metastases compared to in situ cancer, while WTAP expression showed no significant difference (Fig. 1 c). Additionally, through WB and IHC experiments, we further confirmed the high expression of METTL3 and YTHDF1 in colorectal cancer lung metastases (Fig. 1 d-f).
Silencing the METTL3 gene inhibited the proliferation, invasion, and migration abilities of CRC cells while promoting apoptosis
In the initial stages of this study, we successfully silenced the METTL3 gene in RKO and SW480 cell lines using lentiviral infection technology. To assess the silencing effect, we performed Western blot analysis (Fig. 2 g). Subsequent cell proliferation experiments, including CCK-8, EDU staining, and plate colony assays, showed that after METTL3 knockdown, the OD450 values significantly decreased, the number of EDU-positive cells and cell colony formation were reduced (Fig. 2 c-e). These results indicate that downregulation of METTL3 weakened the proliferation ability of RKO and SW480 cells. We further investigated the impact of METTL3 on apoptosis in CRC cells. Through Annexin V-APC/PI staining experiments, we observed a significant increase in cell apoptosis rate after METTL3 inhibition (Fig. 2 f). Additionally, in vivo experiments using a nude mouse model showed that silencing METTL3 significantly reduced the size of subcutaneous tumors (Fig. 3 l). In wound healing and Transwell experiments, we found that METTL3 knockdown in CRC cell lines resulted in weakened migration and invasion abilities (Fig. 2 a-b). Another in vivo experiment also confirmed that inhibiting METTL3 significantly reduced the number of lung metastases in nude mice (Fig. 3 b). To assess whether cells underwent EMT, we conducted Western blot analysis, which revealed that after METTL3 knockdown, the expression of VIM and Snail proteins significantly decreased, while E-cadherin protein expression significantly increased (Fig. 2 g). Immunohistochemistry on tumor specimens from in vivo experiments also suggested that in the low-expression METTL3 group, both subcutaneous tumors and lung metastases showed significantly reduced expression of VIM and SNAIL proteins (Fig. 3 c-d).
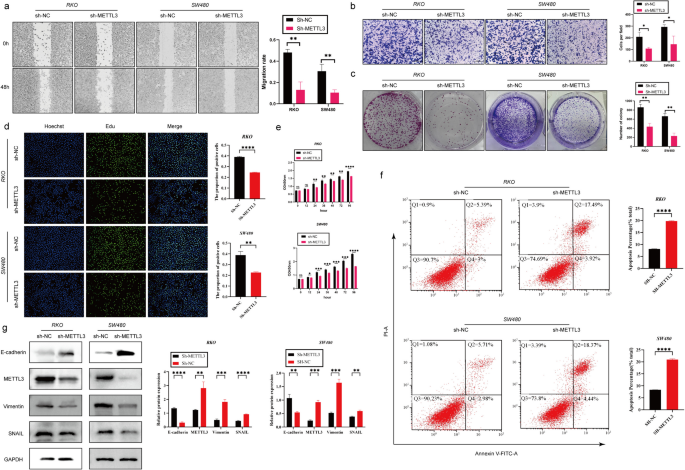
Silencing the METTL3 gene inhibited the proliferation, invasion, and migration abilities of CRC cells while promoting apoptosis. a Representative images and quantitative analysis of CRC cell migration based on wound healing assay. b Decreased expression of METTL3 restrained CRC cell invasion ability based on transwell assay. c The colony formation ability of CRC cells silencing METTL3 or not was measured by colony formation assay. d EDU assay in CRC cells was performed the to measure the proliferation level. e CCK-8 assay was applied to measure the proliferation level of CRC cells after transfected with sh-METTL3 or not. f Flow cytometry analysis the proportion of apoptosis. g Western blotting analysis was applied to measure the protein expression level of E-cadherin, METTL3, SNAIL and Vimentin. GAPDH was used as a loading control. * P < 0.05, ** P < 0.01, *** P < 0.001, **** P < 0.0001
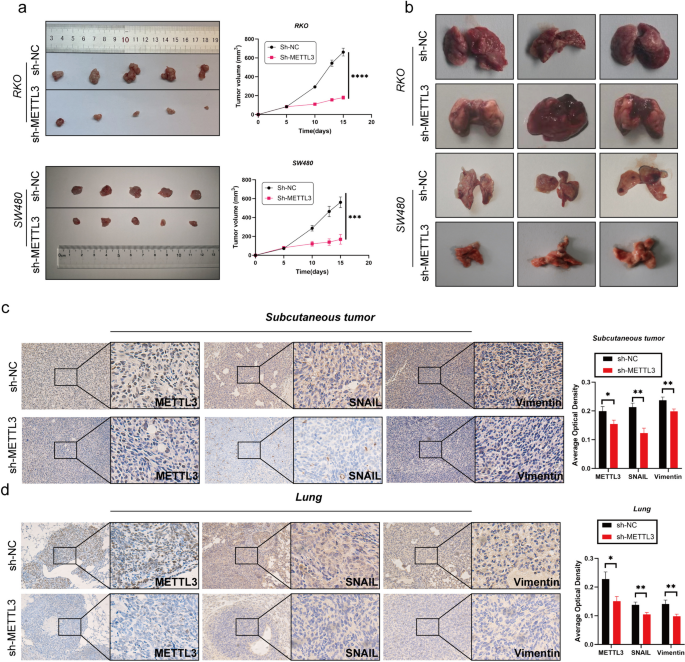
Decreased expression of METTL3 inhibits tumor growth in vivo. a Comparison of subcutaneous tumor size in nude mice after injecting RKO/SW480 CRC cells stably transfected with Sh-NC/Sh-METTL3. b The tail vein-lung metastasis model. Cells were injected into the tail vein to produce tumor cell lung metastasis. c-d IHC analysis of METTL3, SNAIL and Vimentin for tissues of subcutaneous tumor and lung metastasis tumor. * P < 0.05, ** P < 0.01, *** P < 0.001, **** P < 0.0001
METTL3 regulates tumor EMT process via m6A-Snail
According to previous studies, SNAIL plays a crucial role in the tumor microenvironment [ 31 ]. Furthermore, existing research suggests a mutual regulatory relationship between METTL3 and Snail in various types of cancer [ 28 ]. Based on this background, our study aimed to investigate whether METTL3 in colorectal cancer (CRC) exhibits a similar regulatory relationship with Snail. Our hypothesis is that METTL3 controls epithelial-mesenchymal transition (EMT) in tumors through the m6A-Snail pathway. To validate this hypothesis, we conducted Snail overexpression experiments (ov-Snail) in sh-NC (control group) and sh-METTL3 (METTL3 silenced group) cells. Western blot analysis showed that silencing METTL3 attenuated Snail expression, and the EMT process could be reversed, while overexpressing Snail could restore EMT (Fig. 4 a). This finding strongly supports the hypothesis that METTL3 regulates the EMT process through Snail. Additionally, we conducted SNAIL overexpression experiments in sh-METTL3 RKO and SW480 cell lines. The results showed that SNAIL overexpression reversed the inhibitory effect of silenced METTL3 on the proliferation, migration and invasion of CRC cells and its promotion of apoptosis (Fig. 4 b-g).
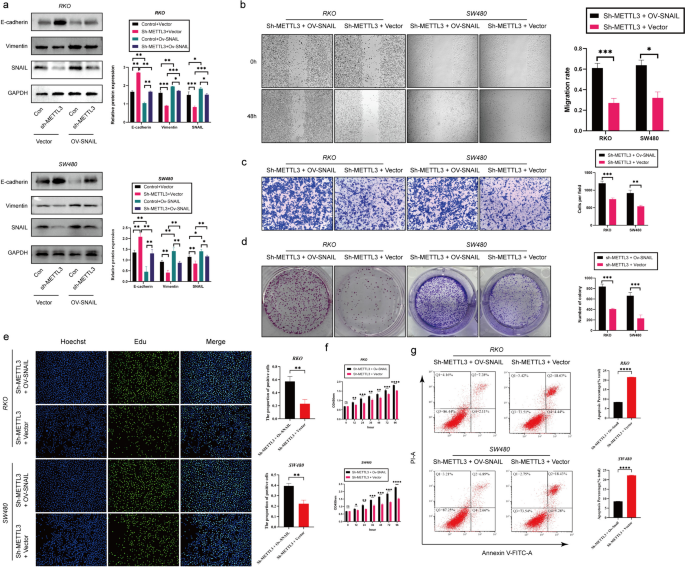
METTL3 regulates tumor process via Snail. a Western blotting analysis was applied to measure the protein expression level of E-cadherin, METTL3, SNAIL and Vimentin. GAPDH was used as a loading control. b Representative images and quantitative analysis of CRC cell migration based on wound healing assay. c Increased expression of SNAIL in sh-METTL3 CRC cell promoted CRC cell invasion ability based on transwell assay. d The colony formation ability of CRC cells overexpressing SNAIL or not was measured by colony formation assay in sh-METTL3 CRC cell. e EDU assay in CRC cells was performed the to measure the proliferation level. f CCK-8 assay was applied to measure the proliferation level of CRC cells after transfected with ov-SNAIL or not in sh-METTL3 CRC cell. g Flow cytometry analysis the proportion of apoptosis. * P < 0.05, ** P < 0.01, *** P < 0.001, **** P < 0.0001
To further explore how METTL3 regulates Snail, we first observed that silencing METTL3 led to a decrease in m6A modification levels on SNAIL mRNA using m6A qPCR technology (Fig. 5 a). Subsequently, to investigate the impact of m6A modification on SNAIL RNA, we detected the expression levels of SNAIL precursor mRNA (pre-mRNA) and mature mRNA in sh-METTL3-treated cells. The results showed that both pre-mRNA and mature mRNA levels of SNAI1 were significantly higher in the sh-METTL3 group than in the control group (Fig. 5 b). Furthermore, subcellular localization analysis of SNAI1 mRNA in sh-METTL3 and sh-NC cells revealed no significant differences between the two groups (Fig. 5 c).
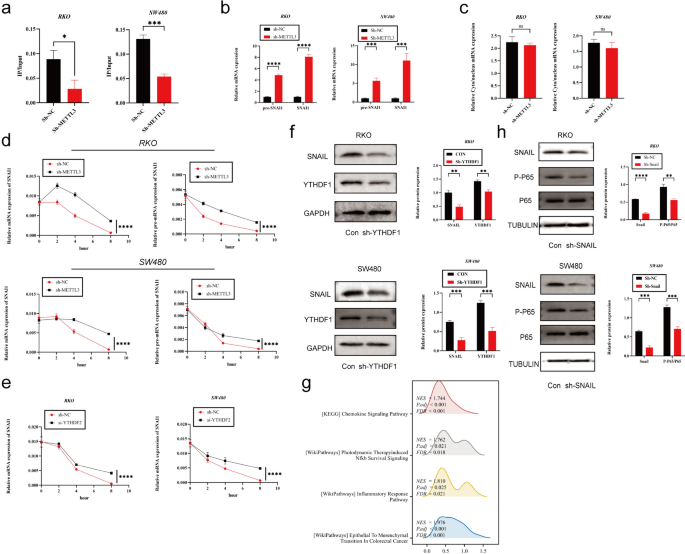
METTL3 regulates tumor EMT process via m6A-Snail. a The MeRIP qRT-PCR analysis of SNAIL mRNA in sh-NC and sh-METTL3 CRC cells. b Precursor and mature mRNA of SNAI1 in sh-NC and sh-METTL3 CRC cells. c The relative levels of the nuclear versus cytoplasmic SNAI1 mRNA in sh-NC and sh-METTL3 CRC cells. d sh-NC and sh-METTL3 CRC cells were pretreated with Act-D for 90 min, then precursor (right) or mature (left) SNAIL mRNA were analyzed at indicated times. e sh-NC and sh-YTHDF2 CRC cells were pretreated with Act-D for 90 min, then mature SNAI1 mRNA were analyzed at indicated times. f Western blotting analysis was applied to measure the protein expression level of SNAIL and YTHDF1. GAPDH was used as a loading control. g GSEA analysis of SNAIL high-expression and low-expression groups utilizing TCGA COAD data. h Western blotting analysis was applied to measure the protein expression level of SNAIL, P65 and P-P65. Tubulin was used as a loading control. * P < 0.05, ** P < 0.01, *** P < 0.001, **** P < 0.0001
To assess the stability of SNAI1 pre-mRNA and mature mRNA, we used Act-D to inhibit new RNA transcription. The experimental results showed that the half-life of pre-mRNA and mature mRNA in the sh-METTL3 group was significantly longer than that in the control group (Fig. 5 d). Given that YTHDF2 is known to mediate the degradation of m6A-modified mRNA, we hypothesized that the observed phenomenon might be related to YTHDF2-mediated mRNA degradation. Therefore, we silenced YTHDF2 in colorectal cancer cell lines and observed an increase in the stability of SNAIL mRNA (Fig. 5 e).
However, we also found some contradictory phenomena. Despite the increased expression of SNAIL mRNA in the sh-METTL3 group, previous research indicated a decrease in SNAIL protein expression in this group. We speculated that this difference might be due to the influence of m6A on SNAIL mRNA translation efficiency. Therefore, we investigated YTHDF1, which serves as a "reader" of m6A and can recognize m6A-modified mRNA, enhancing its translation [ 32 ]. To further explore this mechanism, we conducted experiments with si-YTHDF1 in colorectal cancer cell lines. The results showed that knocking down YTHDF1 significantly reduced SNAIL protein expression (Fig. 5 f).
SNAIL induces CXCL2 expression via the NF-κΒ pathway
Our previous research has revealed a regulatory relationship between SNAIL and CXCL2, but the specific regulatory mechanism remains unclear. In previous sequencing analyses, we noticed significant changes in the NF-κB pathway in the context of lung metastasis cancer (CRPM) compared to colorectal cancer (CRC). Furthermore, through gene set enrichment analysis (GSEA) of CRC data from the TCGA database, we found that in samples with high SNAIL expression, the NF-κB pathway and chemokine signaling pathway were activated, accompanied by epithelial-mesenchymal transition (EMT) (Fig. 5 g). Based on these preliminary findings, we proposed a hypothesis: Snail may regulate the expression of CXCL2 through the NF-κB pathway. To validate this hypothesis, we conducted silencing experiments using sh-Snail in SW480 and RKO cell lines and observed a significant decrease in phosphorylated P65 (Phospho-P65) levels (Fig. 5 h). Additionally, we treated control group cells with the NF-κB inhibitor BAY11-7082 and found that CXCL2 expression also significantly decreased (Fig. 6 c). Furthermore, we analyzed CHIP-seq data (GSE61198) from the Gene Expression Omnibus (GEO) database and found that Snail could bind to the transcription start site (TSS) upstream of CXCL2 (Fig. 6 a). To further explore the direct regulatory relationship between SNAIL and CXCL2, we constructed luciferase reporter gene vectors for different lengths of the CXCL2 promoter (pGL-CXCL2-1606 bp, 942 bp, and 457 bp, respectively). The experimental results showed that silencing Snail significantly reduced the activity of the CXCL2-1606 bp promoter, while it had no significant effect on the activity of the CXCL2-942 bp and CXCL2-457 bp promoters (Fig. 6 b).
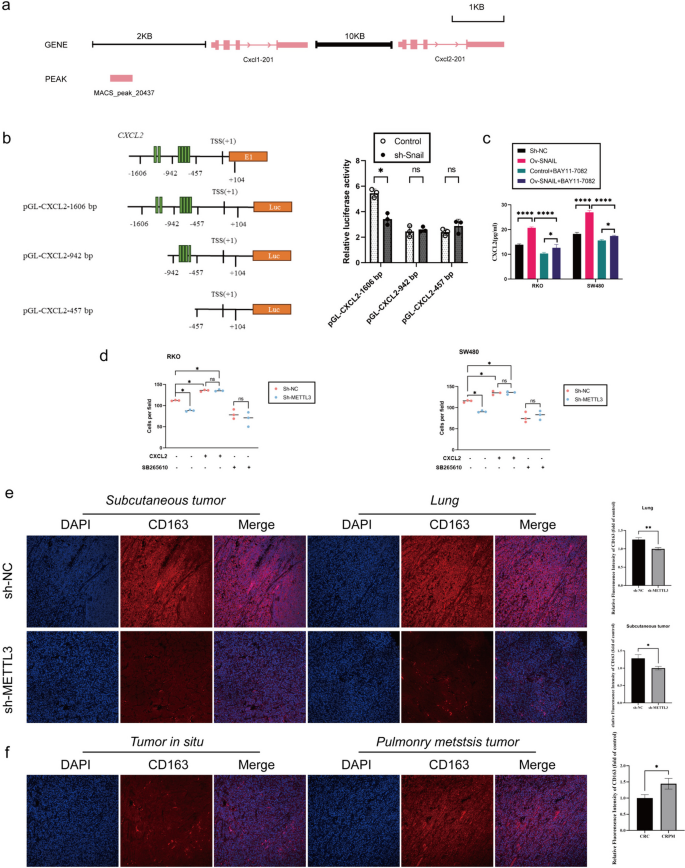
METTL3-expressing CRC Cells Recruits M2-type macrophage Via Secreting CXCL2. a Chromatin immunoprecipitation sequence data show that Snail might directly bind to Cxcl2 proximal promoters. b (Left) Schematic representation of CXCL2 promoter organization, and the luciferase reporter constructs pGL-CXCL2 (1606 bp: − 1606 to + 104 bp, 942 bp: − 942 to + 104 bp, 457 bp: − 457 to + 104 bp). TSS: transcriptional start site, E1: exon1, and Luc: luciferase. The green bars indicate E-boxes (CANNTG), which are the binding sites of Snail. (Right) Relative luciferase activities are shown. c ELISA to analyze the expression of CXCL2 in sh-NC/sh-SNAIL RKO cells (left), and the expression of CXCL2 in sh-NC/sh-SNAIL RKO cells (right), treated with or without BAY11-7082 (NF-κB inhibitor) at 10 μM for 24 h. d M2-type macrophage were seeded in the top chamber of the transwell containing 100 mL 1640 medium with or without CXCR2 inhibitor (SB265610, 10 mM). On the other hand, the bottom chamber contained 600 mL of CRC cell conditioned medium (no fetal bovine serum) with or without recombinant CXCL2 protein (1 ng/mL). After 4-h incubation, cells that have completely migrated to the bottom chamber were counted. e Immunofluorescence analysis of CD163 protein expression in colorectal in nude mice specimen. f Immunofluorescence analysis of CD163 protein expression in colorectal in situ carcinoma and pulmonary metastatic carcinoma. * P < 0.05, ** P < 0.01, *** P < 0.001, **** P < 0.0001
METTL3-expressing CRC cells recruits M2-type macrophage via secreting CXCL2
Immunohistochemical analysis of subcutaneous tumors and lung metastatic tumors in mice from the sh-METTL3 and sh-NC groups indicated that silencing METTL3 significantly reduced the expression of the M2 macrophage marker CD163 protein (Fig. 6 e). Similarly, in clinical samples, the protein expression of the M2 macrophage marker CD163 was significantly higher in lung metastatic tumors compared to primary tumors (Fig. 6 f). Next, we evaluated the role of the METTL3-CXCL2 axis in the chemotaxis of M2 macrophages in an in vitro M2 macrophage migration assay. METTL3 knockout significantly reduced the migration of M2 macrophages toward conditioned media derived from RKO and SW480 cells. The addition of recombinant CXCL2 protein rescued M2 macrophage migration in METTL3 knockout cells. On the other hand, blocking the CXCL2 receptor CXCR2 eliminated the difference in mediating M2 macrophage migration between the control and METTL3 knockout conditioned media (Fig. 6 d).
METTL3, as a core component of the multifaceted m6A methyltransferase complex (MTC), has been reported to play critical roles in various cancer types [ 33 , 34 , 35 , 36 , 37 , 38 ]. While alternative views have been proposed by other studies [ 39 , 40 , 41 , 42 , 43 ], our research findings reveal a significant oncogenic role for METTL3 in the process of tumorigenesis. Previous research has suggested that in certain cancers, METTL3 may function as a tumor suppressor gene. Given the controversies surrounding the roles of m6A modification and METTL3 in different cancer types, our study underscores the potential involvement of METTL3 in colorectal cancer. Our findings demonstrate that METTL3 promotes proliferation, invasion, migration, and inhibits apoptosis in colorectal cancer, highlighting its oncogenic potential. This further emphasizes the widespread impact of METTL3 and m6A methylation in cancer development and precision therapy.
In the field of oncology, epithelial-mesenchymal transition transcription factors (EMT-TFs) such as SNAIL play pivotal roles. SNAIL suppresses the expression of E-cadherin by binding to the e-boxes in the CDH1 promoter and recruiting the multisubunit repressor complex, a crucial process in tumor cells [ 44 , 45 ]. Aberrant Snail expression is closely associated with EMT, which, in turn, is linked to the invasion, migration, metastasis, settlement, and growth capabilities of tumors, ultimately promoting the formation of CRPM [ 46 ]. In the field of colorectal cancer research, prior investigations have already elucidated the regulatory relationship between METTL3 and SNAIL [ 47 ]. However, these studies were limited to in vitro research, leaving unanswered the question of whether METTL3 continues to exert a similar influence in the context of colorectal cancer lung metastasis. Consequently, our study is focused on elucidating the m6A modification of SNAIL mRNA by METTL3 and its subsequent ramifications in this specific context. We found that m6A modification enhances the degradation of SNAIL mRNA, which may be related to YTHDF2 specifically recognizing m6A-modified SNAIL mRNA and promoting its degradation [ 48 ]. However, this finding contradicts our experimental results, where m6A modification of SNAIL is associated with increased protein expression, raising our concern. Additionally, through sequencing and clinical sample testing, we observed elevated expression levels of METTL3 and YTHDF1 in samples of colorectal cancer lung metastasis. YTHDF1, another m6A "reader," has been found to recognize m6A-modified mRNA and enhance the translation of its targets [ 32 ]. To validate the role of YTHDF1, we silenced YTHDF1, and the results showed a significant reduction in SNAIL protein expression, suggesting that m6A methylation regulation of SNAIL is a dynamic process involving multiple factors that require further discussion. Furthermore, our research has also revealed the role of SNAIL in regulating the tumor microenvironment [ 31 ]. SNAIL not only recruits M2 macrophages to infiltrate the tumor center by promoting the secretion of CXCL2 but also indirectly regulates the expression of CXCL2 through the NF-κB pathway and directly modulates CXCL2 expression by binding to its promoter region. Moreover, colorectal cancer cells expressing METTL3 recruit M2 macrophages by secreting CXCL2, a finding confirmed through in vitro experiments and in vivo experiments with METTL3-silenced colorectal cancer cells. These findings provide a new perspective for clinical treatment, such as disrupting this malignant cycle using CXCR2 inhibitors.
In summary, our study aimed to elucidate the role and mechanism of RNA m6A methyltransferase METTL3 in CRPM. The results indicate that METTL3 plays a critical role in promoting the development of CRPM by targeting the m6A-Snail-CXCL2 axis, which is involved in recruiting M2 immunosuppressive macrophages. It is worth noting that we also observed a significant reduction in tumor migration upon inhibiting METTL3, suggesting that therapeutic strategies targeting METTL3 may be an effective approach for treating CRPM.
Availability of data and materials
The analyzed data sets generated during the present study are available from the corresponding author on reasonable request.
Abbreviations
AlkB homologue 5
American Type Culture Collection
Chromatin immunoprecipitation
Colon adenocarcinoma
Colorectal cancer
Colorectal cancer pulmonary metastasis
Extracellular matrix
Enzyme linked immunosorbent assay
Epithelial-mesenchymal transition
Fat mass and obesity-related protein
Gene Expression Omnibus
Heterogeneous nuclear ribonucleoprotein
Insulin-like growth factor 2 mRNA-binding proteins
Immunohistochemistry
Methyltransferase-like 3
Methylated RNA immunoprecipitation quantitative reverse transcription polymerase chain reaction
Messenger RNA
N6-methyladenosine
Phorbol 12-myristate 13-acetate
Quantitative reverse transcription PCR
Snail family transcriptional repressor 1
The Cancer Genome Atlas
Twist family bHLH transcription factor 1
Western blotting
Writers, erasers, and readers
Wilms tumor 1 associated protein
Zinc finger E-box-binding homeobox 1
Biller LH, Schrag D. Diagnosis and treatment of metastatic colorectal cancer: a review. JAMA-J AM MED ASSOC. 2021;325(7):669–85.
Article CAS Google Scholar
Zhou H, Liu Z, Wang Y, Wen X, Amador EH, Yuan L, Ran X, Xiong L, Ran Y, Chen W, et al. Colorectal liver metastasis: molecular mechanism and interventional therapy. Signal Transduct Tar. 2022;7(1):70.
Article Google Scholar
Chandra R, Karalis JD, Liu C, Murimwa GZ, Voth PJ, Heid CA, Reznik SI, Huang E, Minna JD, Brekken RA. The colorectal cancer tumor microenvironment and its impact on liver and lung metastasis. Cancers. 2021;13(24):6206.
Shin AE, Giancotti FG, Rustgi AK. Metastatic colorectal cancer: mechanisms and emerging therapeutics. Trends Pharmacol Sci. 2023;44(4):222–36.
Article CAS PubMed PubMed Central Google Scholar
Verstappe J, Berx G. A role for partial epithelial-to-mesenchymal transition in enabling stemness in homeostasis and cancer. Semin Cancer Biol. 2023;90:15–28.
Article CAS PubMed Google Scholar
Pastushenko I, Blanpain C. EMT Transition States during Tumor Progression and Metastasis. Trends Cell Biol. 2019;29(3):212–26.
Goossens S, Vandamme N, Van Vlierberghe P, Berx G. EMT transcription factors in cancer development re-evaluated: Beyond EMT and MET. BBA-REV Cancer. 2017;1868(2):584–91.
CAS Google Scholar
Wang Y, Shi J, Chai K, Ying X, Zhou BP. The role of snail in EMT and tumorigenesis. Curr Cancer Drug Tar. 2013;13(9):963–72.
Bakir B, Chiarella AM, Pitarresi JR, Rustgi AK. EMT, MET, plasticity, and tumor metastasis. Trends Cell Biol. 2020;30(10):764–76.
Article PubMed PubMed Central Google Scholar
Lu W, Kang Y. Epithelial-mesenchymal plasticity in cancer progression and metastasis. Dev Cell. 2019;49(3):361–74.
Huang Y, Hong W, Wei X. The molecular mechanisms and therapeutic strategies of EMT in tumor progression and metastasis. J Hematol Oncol. 2022;15(1):129.
Dongre A, Weinberg RA. New insights into the mechanisms of epithelial-mesenchymal transition and implications for cancer. Nat Rev Mol Cell Bio. 2019;20(2):69–84.
Vu T, Datta PK. Regulation of EMT in colorectal cancer: a culprit in metastasis. Cancers. 2017;9(12):171.
Wiener D, Schwartz S. The epitranscriptome beyond m(6)A. Nat Rev Genet. 2021;22(2):119–31.
He L, Li H, Wu A, Peng Y, Shu G, Yin G. Functions of N6-methyladenosine and its role in cancer. Mol Cancer. 2019;18(1):176.
He PC, He C. m(6) A RNA methylation: from mechanisms to therapeutic potential. EMBO J. 2021;40(3):e105977.
Wang T, Kong S, Tao M, Ju S. The potential role of RNA N6-methyladenosine in cancer progression. Mol Cancer. 2020;19(1):88.
Fang Z, Mei W, Qu C, Lu J, Shang L, Cao F, Li F. Role of m6A writers, erasers and readers in cancer. Exp Hematol Oncol. 2022;11(1):45.
Deng LJ, Deng WQ, Fan SR, Chen MF, Qi M, Lyu WY, Qi Q, Tiwari AK, Chen JX, Zhang DM, et al. m6A modification: recent advances, anticancer targeted drug discovery and beyond. Mol Cancer. 2022;21(1):52.
Jiang X, Liu B, Nie Z, Duan L, Xiong Q, Jin Z, Yang C, Chen Y. The role of m6A modification in the biological functions and diseases. Signal Transduct Tar. 2021;6(1):74.
Zhang Y, Chen W, Zheng X, Guo Y, Cao J, Zhang Y, Wen S, Gao W, Wu Y. Regulatory role and mechanism of m(6)A RNA modification in human metabolic diseases. Mol Ther-Oncolytics. 2021;22:52–63.
Oerum S, Meynier V, Catala M, Tisné C. A comprehensive review of m6A/m6Am RNA methyltransferase structures. Nucleic Acids Res. 2021;49(13):7239–55.
Zhao Y, Shi Y, Shen H, Xie W. m(6)A-binding proteins: the emerging crucial performers in epigenetics. J Hematol Oncol. 2020;13(1):35.
Azzam SK, Alsafar H, Sajini AA. FTO m6A demethylase in obesity and cancer: implications and underlying molecular mechanisms. Int J Mol Sci. 2022;23(7):3800.
An Y, Duan H. The role of m6A RNA methylation in cancer metabolism. Mol Cancer. 2022;21(1):14.
Sun T, Wu R, Ming L. The role of m6A RNA methylation in cancer. Biomed Pharmacother. 2019;112:108613.
Yue B, Song C, Yang L, Cui R, Cheng X, Zhang Z, Zhao G. METTL3-mediated N6-methyladenosine modification is critical for epithelial-mesenchymal transition and metastasis of gastric cancer. Mol Cancer. 2019;18(1):142.
Lin X, Chai G, Wu Y, Li J, Chen F, Liu J, Luo G, Tauler J, Du J, Lin S, et al. RNA m(6)A methylation regulates the epithelial mesenchymal transition of cancer cells and translation of Snail. Nat Commun. 2019;10(1):2065.
Chen H, Pan Y, Zhou Q, Liang C, Wong CC, Zhou Y, Huang D, Liu W, Zhai J, Gou H, et al. METTL3 inhibits antitumor immunity by targeting m(6)A-BHLHE41-CXCL1/CXCR2 axis to promote colorectal cancer. Gastroenterology. 2022;163(4):891–907.
Li T, Hu PS, Zuo Z, Lin JF, Li X, Wu QN, Chen ZH, Zeng ZL, Wang F, Zheng J, et al. METTL3 facilitates tumor progression via an m(6)A-IGF2BP2-dependent mechanism in colorectal carcinoma. Mol Cancer. 2019;18(1):112.
Bao Z, Zeng W, Zhang D, Wang L, Deng X, Lai J, Li J, Gong J, Xiang G. SNAIL induces EMT and lung metastasis of tumours secreting CXCL2 to promote the invasion of M2-type immunosuppressed macrophages in colorectal cancer. Int J Biol Sci. 2022;18(7):2867–81.
Wang X, Zhao BS, Roundtree IA, Lu Z, Han D, Ma H, Weng X, Chen K, Shi H, He C. N(6)-methyladenosine modulates messenger RNA translation efficiency. Cell. 2015;161(6):1388–99.
Vu LP, Pickering BF, Cheng Y, Zaccara S, Nguyen D, Minuesa G, Chou T, Chow A, Saletore Y, MacKay M, et al. The N(6)-methyladenosine (m(6)A)-forming enzyme METTL3 controls myeloid differentiation of normal hematopoietic and leukemia cells. Nat Med. 2017;23(11):1369–76.
Barbieri I, Tzelepis K, Pandolfini L, Shi J, Millán-Zambrano G, Robson SC, Aspris D, Migliori V, Bannister AJ, Han N, et al. Promoter-bound METTL3 maintains myeloid leukaemia by m(6)A-dependent translation control. Nature. 2017;552(7683):126–31.
Wang H, Xu B, Shi J. N6-methyladenosine METTL3 promotes the breast cancer progression via targeting Bcl-2. Gene. 2020;722:144076.
Chen M, Wei L, Law CT, Tsang FH, Shen J, Cheng CL, Tsang LH, Ho DW, Chiu DK, Lee JM, et al. RNA N6-methyladenosine methyltransferase-like 3 promotes liver cancer progression through YTHDF2-dependent posttranscriptional silencing of SOCS2. Hepatology. 2018;67(6):2254–70.
Zuo X, Chen Z, Gao W, Zhang Y, Wang J, Wang J, Cao M, Cai J, Wu J, Wang X. M6A-mediated upregulation of LINC00958 increases lipogenesis and acts as a nanotherapeutic target in hepatocellular carcinoma. J Hematol Oncol. 2020;13(1):5.
Xu H, Wang H, Zhao W, Fu S, Li Y, Ni W, Xin Y, Li W, Yang C, Bai Y, et al. SUMO1 modification of methyltransferase-like 3 promotes tumor progression via regulating Snail mRNA homeostasis in hepatocellular carcinoma. Theranostics. 2020;10(13):5671–86.
Cui Q, Shi H, Ye P, Li L, Qu Q, Sun G, Sun G, Lu Z, Huang Y, Yang CG, et al. m(6)A RNA methylation regulates the self-renewal and tumorigenesis of glioblastoma stem cells. Cell Rep. 2017;18(11):2622–34.
Liu J, Eckert MA, Harada BT, Liu SM, Lu Z, Yu K, Tienda SM, Chryplewicz A, Zhu AC, Yang Y, et al. m(6)A mRNA methylation regulates AKT activity to promote the proliferation and tumorigenicity of endometrial cancer. Nat Cell Biol. 2018;20(9):1074–83.
Jia R, Chai P, Wang S, Sun B, Xu Y, Yang Y, Ge S, Jia R, Yang YG, Fan X. m(6)A modification suppresses ocular melanoma through modulating HINT2 mRNA translation. Mol Cancer. 2019;18(1):161.
Deng R, Cheng Y, Ye S, Zhang J, Huang R, Li P, Liu H, Deng Q, Wu X, Lan P, et al. m(6)A methyltransferase METTL3 suppresses colorectal cancer proliferation and migration through p38/ERK pathways. Oncotargets Ther. 2019;12:4391–402.
Zhao S, Liu J, Nanga P, Liu Y, Cicek AE, Knoblauch N, He C, Stephens M, He X. Detailed modeling of positive selection improves detection of cancer driver genes. Nat Commun. 2019;10(1):3399.
Batlle E, Sancho E, Francí C, Domínguez D, Monfar M, Baulida J, García DHA. The transcription factor snail is a repressor of E-cadherin gene expression in epithelial tumour cells. Nat Cell Biol. 2000;2(2):84–9.
Cano A, Pérez-Moreno MA, Rodrigo I, Locascio A, Blanco MJ, Del BM, Portillo F, Nieto MA. The transcription factor snail controls epithelial-mesenchymal transitions by repressing E-cadherin expression. Nat Cell Biol. 2000;2(2):76–83.
Brabletz S, Schuhwerk H, Brabletz T, Stemmler MP. Dynamic EMT: a multi-tool for tumor progression. Embo J. 2021;40(18):e108647.
Wen J, Zhang G, Meng Y, Zhang L, Jiang M, Yu Z. RNA m(6)A methyltransferase METTL3 promotes colorectal cancer cell proliferation and invasion by regulating snail expression. Oncol Lett. 2021;22(4):711.
Wang X, Lu Z, Gomez A, Hon GC, Yue Y, Han D, Fu Y, Parisien M, Dai Q, Jia G, et al. N6-methyladenosine-dependent regulation of messenger RNA stability. Nature. 2014;505(7481):117–20.
Article PubMed Google Scholar
Download references
This study was supported by the Fundamental Research Funds for the Central Universities (21623305,21623409), Guangdong Medical Science and Technology Research Fund Project(A2023398), Guangzhou Science and Technology Plan Basic and Applied Basic Research Funding Project(2024A04J3707) and Guangzhou Science and Technology Plan City-School Joint Funding Project (202201020304).
Author information
Peng Ouyang, Kang Li and Wei Xu contributed equally to this work.
Authors and Affiliations
Department of General Surgery, The First Affiliated Hospital of Jinan University, Guangzhou, 510632, Guangdong, China
Peng Ouyang, Kang Li, Wei Xu, Caiyun Chen, Yangdong Shi, Yao Tian, Jin Gong & Zhen Bao
You can also search for this author in PubMed Google Scholar
Contributions
POY: As the first author, POY was responsible for the primary study design, experimental procedures, data collection and analysis, as well as the writing and revision of the manuscript. KL (co-first author): KL collaborated with the first author in study design, experimental procedures, participated in data analysis, and contributed to the writing of the manuscript. WX (co-first author): WX worked in collaboration with the first author on experimental design and data analysis, making significant contributions to the writing of the manuscript. CYC, YDS, YT: These three authors were involved in some experimental procedures, contributed to the collection and preliminary analysis of experimental data. JG (co-corresponding author): Jin Gong, as a co-corresponding author, participated in guiding the research, contributed to the writing, and provided final review to ensure the quality and accuracy of the study. ZB (corresponding author): As the corresponding author, Zhen Bao was responsible for overseeing the entire research project, obtaining funding, and overseeing the final review and submission of the manuscript. All authors read and approved the final manuscript.
Corresponding authors
Correspondence to Jin Gong or Zhen Bao .
Ethics declarations
Ethics approval and consent to participate.
All experiments were performed in compliance with the relevant regulations, and all patients provided written informed consent. Besides, the animal studies were approved by the Animal Ethics Committee of South China Agricultural University (2023d045). The experiments followed the Guidelines for the Care and Use of Laboratory Animals issued by the Chinese Council on Animal Research.
Consent for publication
All the authors agree to the content of the paper.
Competing interests
The authors declare no competing interests.
Additional information
Publisher’s note.
Springer Nature remains neutral with regard to jurisdictional claims in published maps and institutional affiliations.
Rights and permissions
Open Access This article is licensed under a Creative Commons Attribution 4.0 International License, which permits use, sharing, adaptation, distribution and reproduction in any medium or format, as long as you give appropriate credit to the original author(s) and the source, provide a link to the Creative Commons licence, and indicate if changes were made. The images or other third party material in this article are included in the article's Creative Commons licence, unless indicated otherwise in a credit line to the material. If material is not included in the article's Creative Commons licence and your intended use is not permitted by statutory regulation or exceeds the permitted use, you will need to obtain permission directly from the copyright holder. To view a copy of this licence, visit http://creativecommons.org/licenses/by/4.0/ . The Creative Commons Public Domain Dedication waiver ( http://creativecommons.org/publicdomain/zero/1.0/ ) applies to the data made available in this article, unless otherwise stated in a credit line to the data.
Reprints and permissions
About this article
Cite this article.
Ouyang, P., Li, K., Xu, W. et al. METTL3 recruiting M2-type immunosuppressed macrophages by targeting m6A-SNAIL-CXCL2 axis to promote colorectal cancer pulmonary metastasis. J Exp Clin Cancer Res 43 , 111 (2024). https://doi.org/10.1186/s13046-024-03035-6
Download citation
Received : 20 December 2023
Accepted : 29 March 2024
Published : 11 April 2024
DOI : https://doi.org/10.1186/s13046-024-03035-6
Share this article
Anyone you share the following link with will be able to read this content:
Sorry, a shareable link is not currently available for this article.
Provided by the Springer Nature SharedIt content-sharing initiative
- N6-Methyladenosine
- Epithelial–mesenchymal transition
- Colorectal cancer pulmonary metastases
- M2-type macrophages
Journal of Experimental & Clinical Cancer Research
ISSN: 1756-9966
- Submission enquiries: Access here and click Contact Us
- General enquiries: [email protected]
- Alzheimer's disease & dementia
- Arthritis & Rheumatism
- Attention deficit disorders
- Autism spectrum disorders
- Biomedical technology
- Diseases, Conditions, Syndromes
- Endocrinology & Metabolism
- Gastroenterology
- Gerontology & Geriatrics
- Health informatics
- Inflammatory disorders
- Medical economics
- Medical research
- Medications
- Neuroscience
- Obstetrics & gynaecology
- Oncology & Cancer
- Ophthalmology
- Overweight & Obesity
- Parkinson's & Movement disorders
- Psychology & Psychiatry
- Radiology & Imaging
- Sleep disorders
- Sports medicine & Kinesiology
- Vaccination
- Breast cancer
- Cardiovascular disease
- Chronic obstructive pulmonary disease
- Colon cancer
- Coronary artery disease
- Heart attack
- Heart disease
- High blood pressure
- Kidney disease
- Lung cancer
- Multiple sclerosis
- Myocardial infarction
- Ovarian cancer
- Post traumatic stress disorder
- Rheumatoid arthritis
- Schizophrenia
- Skin cancer
- Type 2 diabetes
- Full List »
share this!
April 16, 2024
This article has been reviewed according to Science X's editorial process and policies . Editors have highlighted the following attributes while ensuring the content's credibility:
fact-checked
peer-reviewed publication
trusted source
New insights could unlock immunotherapy for rare, deadly eye cancer
by University of Pittsburgh
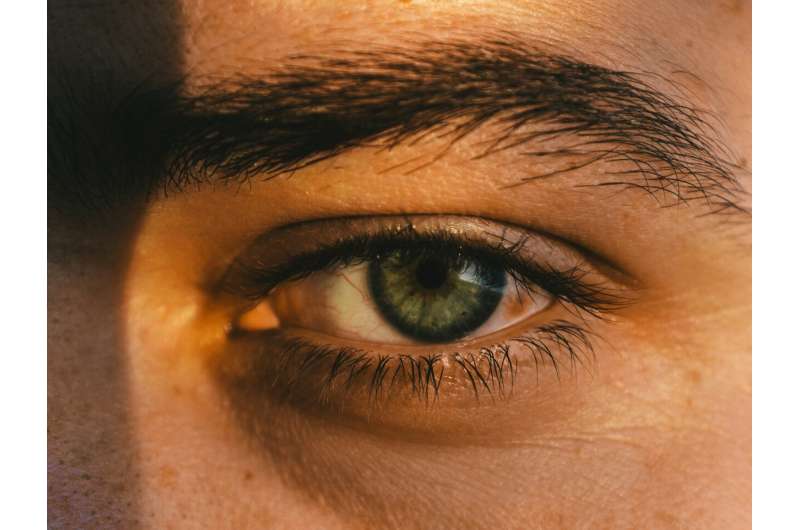
New research from the University of Pittsburgh explains why metastatic uveal melanoma is resistant to conventional immunotherapies and how adoptive therapy, which involves growing a patient's T cells outside the body before reinfusing them, can successfully treat this rare and aggressive cancer.
In a paper published in Nature Communications , the Pitt researchers also explain how they developed a new clinical tool that predicts which patients will respond to adoptive therapy . The work is helping improve personalized therapies and avoid futile treatments for metastatic uveal melanoma .
"The dogma was that uveal melanoma is a 'cold' cancer, meaning that T cells can't get into these tumors," said senior author Udai Kammula, M.D., associate professor of surgery at Pitt and director of the Solid Tumor Cell Therapy Program at UPMC Hillman Cancer Center.
"We show that T cells are in fact infiltrating metastases and they're getting activated, but they're just sitting there in a dormant state because something in the tumor is suppressing them. Adoptive therapy allows us to rescue these cells from the suppressive tumor microenvironment and successfully treat some patients."
Uveal melanoma originates in the uveal tract of the eye but has a tendency to aggressively spread throughout the body, often to the liver. When metastasis occurs, this cancer is very difficult to treat and the prognosis for patients is almost always grim.
"Cutaneous melanoma, which affects the skin, is the poster child of immunotherapy. It responds incredibly well to immune checkpoint inhibitor drugs," said Kammula. "None of these conventional immunotherapies work for uveal melanoma, but we hadn't known why—until now."
In a previous Lancet Oncology study, Kammula and his team used adoptive therapy to surgically extract metastatic tumors from 19 uveal melanoma patients and grow T cells from these tumors in the laboratory. When they infused the cells back, 35% of patients had either partial or complete regression of their cancer, evidence against the assumption that cancer-fighting cells called tumor-infiltrating lymphocytes (TILs) aren't found in uveal melanoma. But it was still a mystery why immune checkpoint inhibitors, which rev up the activity of these T cells, are ineffective in treating this disease.
Kammula saw an opportunity to answer this question using a unique resource that he and his team have been building for the last decade: the largest known repository of uveal melanoma samples, corresponding tissues and clinical information.
When the researchers analyzed 100 metastases from 84 patients, they found that over half of these tumors were chock-full of T cells. Next, they performed single cell RNA sequencing to measure gene expression in almost 100,000 cells from six metastases. They found that the TILs in some of these tumors were activated and capable of attacking tumor cells in a dish, but they weren't proliferating to high numbers in the tumor.
"We found that TILs from metastatic uveal melanoma have the potential to attack the tumor, but something in the tumor microenvironment is shutting them down, so they're in a dormant, or quiescent, state," explained Kammula. "By liberating these cells from the suppressive environment and growing them in the lab, we can rescue their tumor-fighting capacity when infused back into the patient."
But TIL therapy doesn't work for everyone, as the researchers found in their earlier study. To predict which patients will respond and which will not, Kammula and lead author Shravan Leonard-Murali, M.D., a post-doctoral fellow in the lab, developed a clinical tool called Uveal Melanoma Immunogenic Score (UMIS), a holistic measure of the tumor that reflects the activity of more than 2,000 genes expressed by tumor cells, immune cells and other cells that form the tumor microenvironment. UMIS ranged from 0.114 to 0.347 across 100 metastases, with higher values indicating tumors with more potent TILs.
When the researchers looked at patients who received adoptive therapy in the earlier study, they found that patients with higher UMIS scores had better tumor regression, suggesting that this biomarker could predict which patients are likely to respond.
They also found that patients with metastases scoring above 0.246 had significantly improved progression-free survival and overall survival than those with UMIS below this cutoff.
"If a patient's UMIS level is below this threshold, we think that adoptive therapy is not appropriate. Using a biopsy to calculate a patient's UMIS could help avoid futile therapies and unnecessarily subjecting patients to invasive operations," said Kammula.
"But the immune system is not static. UMIS offers a window into the tumor that could also help us find the optimal time to treat a patient with adoptive therapy, like picking a fruit when it's at its ripest."
Kammula is now evaluating the score prospectively in an ongoing TIL therapy clinical trial at Pitt for patients with metastatic uveal melanoma.
He and his team are also taking what they've learned from uveal melanoma to tackle other difficult-to-treat tumors such as pancreatic cancer , and they are developing a pan-cancer version of UMIS that will predict how well a patient with any type of cancer is likely to respond to adoptive therapy.
Explore further
Feedback to editors

Good blood pressure control could prevent fibroids
33 minutes ago

Study suggests the brain's reward system works to make others happy, not just ourselves

Researchers discover cause of rare congenital lung malformations

An effective drug delivery system for next-generation treatments to hitch a ride in cancer cells

Study on rats shows a junk food diet can cause long-term damage to adolescent brains

Mouse study finds small extracellular vesicles from young blood extend lifespan and restore physiological functions

From opioid overdose to treatment initiation: Outcomes associated with peer support in emergency departments

Oral contraceptive use may reduce muscle-tendon injuries

Study suggests adolescent stress may raise risk of postpartum depression in adults
2 hours ago

Scientists identify cell vulnerability 'fingerprint' related to Parkinson's, Lewy body dementia
Related stories.

Determinants of overall survival in metastatic uveal melanoma identified
Jul 13, 2023

Clinical trial: New combination treatment for eye melanoma increased patient survival
Aug 27, 2021

Immune networks in tumors found to prime responses to personalized immunotherapy
Feb 6, 2024

Researchers identify promising drug combination for melanoma
Dec 3, 2020

New approach combines oncolytic virotherapy and adoptive T cell therapy for cancer treatment
Feb 12, 2024

New cell-based immunotherapy offered for melanoma
Feb 24, 2024
Recommended for you

Asthma in children: Researchers envision novel drug to reduce the risk of the disease
9 hours ago

Many people with breast cancer 'systematically left behind' due to inaction on inequities and hidden suffering
19 hours ago

New way found to treat early relapse in leukemia
Apr 15, 2024

Epilepsy drug prevents brain tumors in mice with neurofibromatosis type 1
23 hours ago

Targeted liver cancer treatment kills cancer cells and could cut chemo side effects
Let us know if there is a problem with our content.
Use this form if you have come across a typo, inaccuracy or would like to send an edit request for the content on this page. For general inquiries, please use our contact form . For general feedback, use the public comments section below (please adhere to guidelines ).
Please select the most appropriate category to facilitate processing of your request
Thank you for taking time to provide your feedback to the editors.
Your feedback is important to us. However, we do not guarantee individual replies due to the high volume of messages.
E-mail the story
Your email address is used only to let the recipient know who sent the email. Neither your address nor the recipient's address will be used for any other purpose. The information you enter will appear in your e-mail message and is not retained by Medical Xpress in any form.
Newsletter sign up
Get weekly and/or daily updates delivered to your inbox. You can unsubscribe at any time and we'll never share your details to third parties.
More information Privacy policy
Donate and enjoy an ad-free experience
We keep our content available to everyone. Consider supporting Science X's mission by getting a premium account.
E-mail newsletter
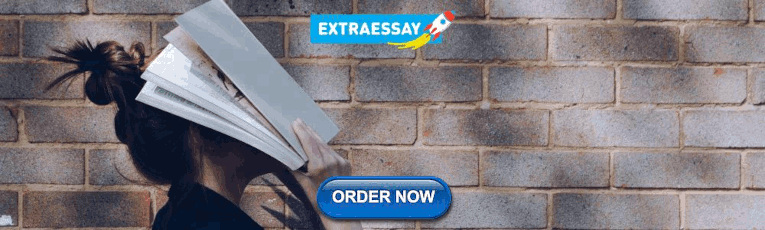
IMAGES
VIDEO
COMMENTS
Therefore, understanding the nature and mechanism of breast cancer metastasis can facilitate the development of targeted therapeutics focused on metastasis. This review discusses the mechanisms leading to metastasis and the current therapies to improve the early diagnosis and prognosis in patients with metastatic breast cancer. Keywords: EMT ...
The Aurora US Metastatic Breast Cancer Project is funded by the Breast Cancer Research Foundation (grant ID ELFF-14-002) through the Evelyn H. Lauder Founder's Fund for Metastatic Breast Cancer ...
Approximately 4% of women in the United States will develop metastatic breast cancer, which has a 10-year survival rate of less than 15%. 1-3 A major inhibitory factor toward improving these outcomes is an inability to detect metastatic disease prior to macroscopic secondary tumor formation, at which point millions of tumor cells have colonized ...
People with metastatic breast cancer without overexpression of the receptor HER2, which promotes cancer-cell growth, and who had received no more than one line of chemotherapy were selected. A ...
Cancer is an uncontrolled growth of cells in the body that can rapidly spread to any organ and 90% of cancer patients die from metastasis 1,2.Numerous types of cancer exist, but lung cancer ...
In our 2023-24 investment year, BCRF is the largest private funder of stage 4 breast cancer research in the world and is funding 83 projects dedicated to this form of the disease. This investment totals $22.3 million. These funded projects span every aspect of metastasis including: Understanding the intricacies of the events that lead to cancer ...
Breast cancer research has always generated important, pioneering ideas about metastasis evolution which then shaped thinking in other solid tumor types as well. Based on the observation that spread to locoregional lymph nodes was associated with poor outcomes in breast cancer, William Halsted developed his hypothesis of orderly sequential progression in the late 19th century.
Feature papers represent the most advanced research with significant potential for high impact in the field. A Feature Paper should be a substantial original Article that involves several techniques or approaches, provides an outlook for future research directions and describes possible research applications. ... Metastatic breast cancer is ...
A collection of articles on metastatic breast cancer. Articles (20 in this collection) Transcriptomic characterization of the histopathological growth patterns in breast cancer liver metastases Authors (first, second and last of 26) ... Content type: Research Paper Open Access; Published: 27 April 2023; Pages: 205 - 216; Sufentanil combined ...
Research Advances in Metastatic Breast Cancer. Research is particularly vital when it comes to cancer that has spread beyond the breast (metastatic). One hallmark of the Susan F. Smith Center for Women's Cancers is the integration of research and patient care, and the equal investment in both. At a basic level, scientists seek to understand the ...
To find clinical trials specific to your diagnosis, talk with your doctor or search online clinical trial databases. Visit the Cancer.Net Blog to review news and information about metastatic breast cancer, including research announced at recent scientific meetings or in the American Society of Clinical Oncology's (ASCO's) peer-reviewed journals.
Mechanisms of resistance to cancer therapy. Metastatic cancer is ultimately resistant to virtually all systemic therapies and continues to kill more than 10 million people per year around the world. This suggests a common mechanism for cancer resistan... Submission status. Closed.
Metastatic breast cancer (MBC) patient outcomes may vary according to distinct health care payers and different countries. We compared 291 Alberta (AB), Canada and 9429 US patients < 65 with de novo MBC diagnosed from 2010 through 2014. Data were extracted from the provincial Breast Data Mart and from the National Cancer Institute's SEER program.
The targeted therapy Trodelvy improved overall survival by 3.2 months in people diagnosed with metastatic, hormone receptor-positive, HER2-negative breast cancer that was previously treated with hormonal therapy, a CDK4/6 inhibitor, and at least two lines of chemotherapy. Sep 13, 2022 | Diagnosis, Metastatic Breast Cancer and Targeted Therapy.
The work of the Lancet Breast Cancer Commission highlights crucial groups, such as those living with metastatic breast cancer, and identifies how the hidden costs of breast cancer and associated suffering are considerable, varied, and have far-reaching effects. The Commission offers a forward-looking and optimistic road map for how the health ...
Research Paper Metastasis patterns and prognosis in breast cancer patients aged ≥ 80 years: a SEER database analysis Youming Han1,2, ... National Clinical Research Center for Cancer, Cancer Hospital & Shenzhen Hospital, Chinese Academy of Medical Sciences and PeKing Union Medical College, Shenzhen, 518116, China. ...
The Breast Cancer Research Foundation's recent investment in metastatic breast cancer research 21 aims to improve patients' quality of life and understand risk factors for metastasis, but does ...
Journal of Neuro-Oncology published the most papers (n = 111), Breast Cancer Research and Treatment second (n = 82), and Cancers third (n = 68). Cocitation analysis is an effective method to discover the most influential journals in a specific topic. ... Research on breast cancer brain metastasis was focused on the basic study, clinical trial ...
Key Takeaways. Clinical trials are the major way to improve existing therapies in metastatic breast cancer. In a clinical trial you may receive great medical care with a new, effective drug that ...
Introduction Individuals with metastatic cancer experience many medical, physical, and emotional challenges due to changing medical regimens, oscillating disease states, and side effects. The purpose of this study was to describe the type and prevalence of survivorship concerns reported by individuals with metastatic cancer, and their associations with cancer diagnosis, treatment, and socio ...
The research suggests that drugs targeting this altered cancer cell metabolism could boost treatments for metastatic triple-negative breast cancer. "Our research suggests triple-negative breast ...
1. Set learning rate to 10 −3 and train the last layer for 3 epochs. 2. Set learning rate to 10 −4, unfreeze the top layers and train for 10 epochs, where the top layer number is set to 46 for ...
T el Aviv University (TAU) researchers have developed a new therapeutic strategy based on existing medications to inhibit bone metastasis in breast cancer patients. When a patient has this ...
In patients with colorectal cancer (CRC), cancer metastasis is the primary cause of cancer-related deaths, with the liver and lungs being the most common target organs for metastasis [1, 2].While the majority of research has traditionally focused on liver metastases, colorectal cancer pulmonary metastasis (CRPM) has received relatively less attention.
New research from the University of Pittsburgh explains why metastatic uveal melanoma is resistant to conventional immunotherapies and how adoptive therapy, which involves growing a patient's T ...