- Random article
- Teaching guide
- Privacy & cookies
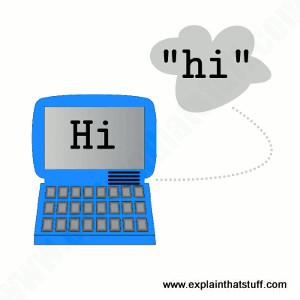
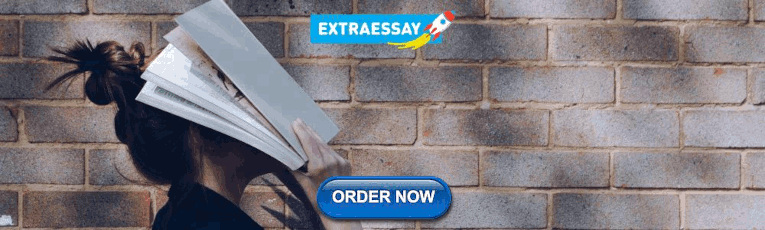
What is speech synthesis?
How does speech synthesis work.
Artwork: Context matters: A speech synthesizer needs some understanding of what it's reading.
Artwork: Concatenative versus formant speech synthesis. Left: A concatenative synthesizer builds up speech from pre-stored fragments; the words it speaks are limited rearrangements of those sounds. Right: Like a music synthesizer, a formant synthesizer uses frequency generators to generate any kind of sound.
Articulatory
What are speech synthesizers used for.
Photo: Will humans still speak to one another in the future? All sorts of public announcements are now made by recorded or synthesized computer-controlled voices, but there are plenty of areas where even the smartest machines would fear to tread. Imagine a computer trying to commentate on a fast-moving sports event, such as a rodeo, for example. Even if it could watch and correctly interpret the action, and even if it had all the right words to speak, could it really convey the right kind of emotion? Photo by Carol M. Highsmith, courtesy of Gates Frontiers Fund Wyoming Collection within the Carol M. Highsmith Archive, Library of Congress , Prints and Photographs Division.
Who invented speech synthesis?
Artwork: Speak & Spell—An iconic, electronic toy from Texas Instruments that introduced a whole generation of children to speech synthesis in the late 1970s. It was built around the TI TMC0281 chip.
Anna (c. ~2005)
Olivia (c. ~2020).
If you liked this article...
Find out more, on this website.
- Voice recognition software
Technical papers
Current research, notes and references ↑ pre-processing in described in more detail in "chapter 7: speech synthesis from textual or conceptual input" of speech synthesis and recognition by wendy holmes, taylor & francis, 2002, p.93ff. ↑ for more on concatenative synthesis, see chapter 14 ("synthesis by concatenation and signal-process modification") of text-to-speech synthesis by paul taylor. cambridge university press, 2009, p.412ff. ↑ for a much more detailed explanation of the difference between formant, concatenative, and articulatory synthesis, see chapter 2 ("low-lever synthesizers: current status") of developments in speech synthesis by mark tatham, katherine morton, wiley, 2005, p.23–37. please do not copy our articles onto blogs and other websites articles from this website are registered at the us copyright office. copying or otherwise using registered works without permission, removing this or other copyright notices, and/or infringing related rights could make you liable to severe civil or criminal penalties. text copyright © chris woodford 2011, 2021. all rights reserved. full copyright notice and terms of use . follow us, rate this page, tell your friends, cite this page, more to explore on our website....
- Get the book
- Send feedback

- There are no suggestions because the search field is empty.
What is Text-to-Speech (TTS): Initial Speech Synthesis Explained
Sep 28, 2021 1:08:38 pm.
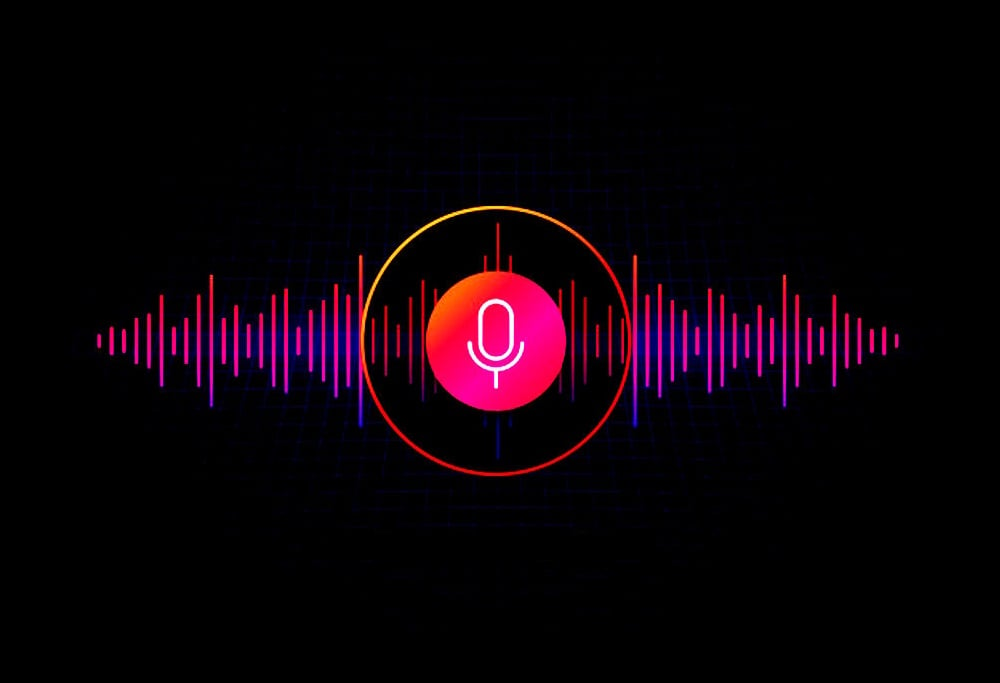
Today, speech synthesis technologies are in demand more than ever. Businesses, film studios, game producers, and video bloggers use speech synthesis to speed up and reduce the cost of content production as well as improve the customer experience.
Let's start our immersion in speech technologies by understanding how text-to-speech technology (TTS) works.
What is TTS speech synthesis?
TTS is a computer simulation of human speech from a textual representation using machine learning methods. Typically, speech synthesis is used by developers to create voice robots, such as IVR (Interactive Voice Response).
TTS saves a business time and money as it generates sound automatically, thus saving the company from having to manually record (and rewrite) audio files.
You can have any text read aloud in a voice that is as close to natural as possible, thanks to TTS synthesis. To make TTS synthesized speech sound natural, the painstaking process of honing its timbre, smoothness, placement of accents and pauses, intonation, and other areas is a long and unavoidable burden.
There are two ways developers can go about getting it done:
Concatenative - gluing together fragments of recorded audio. This synthesized speech is of high quality but requires a lot of data for machine learning.
Parametric - building a probabilistic model that selects the acoustic properties of a sound signal for a given text. Using this approach, one can synthesize a speech that is virtually indistinguishable from a real human.
What is text-to-speech technology?
To convert text to speech, the ML system must perform the following:
- Convert text to words
Firstly, the ML algorithm must convert text into a readable format. The challenge here is that the text contains not only words but numbers, abbreviations, dates, etc.
These must be translated and written in words. The algorithm then divides the text into distinct phrases, which the system then reads with the appropriate intonation. While doing that, the program follows the punctuation and stable structures in the text.
- Complete phonetic transcription
Each sentence can be pronounced differently depending on the meaning and emotional tone. To understand the right pronunciation, the system uses built-in dictionaries.
If the required word is missing, the algorithm creates the transcription using general academic rules. The algorithm also checks on the recordings of the speakers and determines which parts of the words they accentuate.
The system then calculates how many 25 millisecond fragments are in the compiled transcription. This is known as phoneme processing.
A phoneme is the minimum unit of a language’s sound structure.
The system describes each piece with different parameters: which phoneme it is a part of, the place it occupies in it, which syllable this phoneme belongs to, and so on. After that, the system recreates the appropriate intonation using data from the phrases and sentences.
- Convert transcription to speech
Finally, the system uses an acoustic model to read the processed text. The ML algorithm establishes the connection between phonemes and sounds, giving them accurate intonations.
The system uses a sound wave generator to create a vocal sound. The frequency characteristics of phrases obtained from the acoustic model are eventually loaded into the sound wave generator.
Industry TTS applications
In general, there are three most common areas to apply TTS voice conversions for your business or content production. They are:
- Voice notifications and reminders. This allows for the delivery of any information to your customers all over the world with a phone call. The good news is that the messages are delivered in the customers' native languages.
- Listening to the written content. You can hear the synthesized voice reading your favorite book, email, or website content. This is very important for people with limited reading and writing abilities, or for those who prefer listening over reading.
- Localization. It might be costly to hire employees who can speak multiple customer languages if you operate internationally. TTS allows for practically instant vocalization from English (or other languages) to any foreign language. This is considering that you use a proper translation service.
With these three in mind, you can imagine the full-scale application that covers almost any industry that you operate in with customers and that may lack personalized language experience.
Speech to speech (STS) voice synthesis helps where TTS falls short
We have extensively covered STS technology in previous blog posts. Learn more on how the deepfake tech that powers STS conversion works and some of the most disrupting applications like AI-powered dubbing or voice cloning in marketing and branding .
In short, speech synthesis powered by AI allows for covering critical use cases where you use speech (not text) as a source to generate speech in another voice.
With speech-to-speech voice cloning technology , you can make yourself sound like anyone you can imagine. Like here, where our pal Grant speaks in Barack Obama’s voice .
For those of you who want to discover more, check our FAQ page to find answers to questions about speech-to-speech voice conversion .
So why choose STS over the TTS tech? Here are just a couple of reasons:
- For obvious reasons, STS allows you to do what is impossible with TTS. Like synthesizing iconic voices of the past or saving time and money on ADR for movie production .
- STS voice cloning allows you to achieve speech of a more colorful emotional palette. The generated voice will be absolutely indistinguishable from the target voice.
- STS technology allows for the scaling of content production for those celebrities who want but can't spend time working simultaneously on several projects.
How do I find out more about speech-to-speech voice synthesis?
Try Respeecher . We have a long history of successful collaborations with Hollywood studios, video game developers, businesses, and even YouTubers for their virtual projects.
We are always willing to help ambitious projects or businesses get the most out of STS technology. Drop us a line to get a demo customized just for you.
Share this post
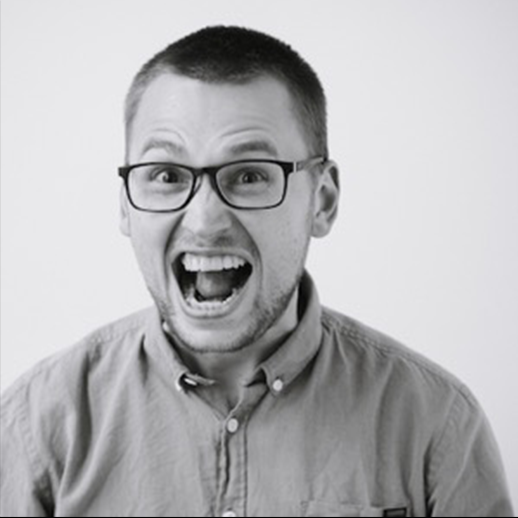
Alex Serdiuk
CEO and Co-founder
Alex founded Respeecher with Dmytro Bielievtsov and Grant Reaber in 2018. Since then the team has been focused on high-fidelity voice cloning. Alex is in charge of Business Development and Strategy. Respeecher technology is already applied in Feature films and TV projects, Video Games, Animation studios, Localization, media agencies, Healthcare, and other areas.
Stay relevant in a constantly evolving industry.
Get the monthly newsletter keeping thousands of sound professionals in the loop.
Related Articles
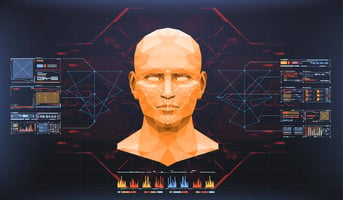
Text-to-Speech AI Voice Generator: Creating a Human-like Voice
Mar 15, 2022 1:36:00 PM
The latest technologies in voice synthesis and recognition are constantly disrupting the...
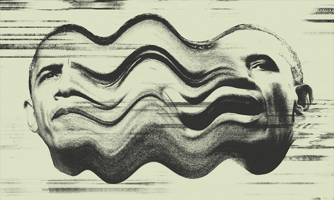
Speech Synthesis Is No More a Villain than Photoshop Was 10+ Years Ago
Sep 14, 2021 12:00:00 PM
Modern technologies deliver many benefits, completely transforming many areas of our...
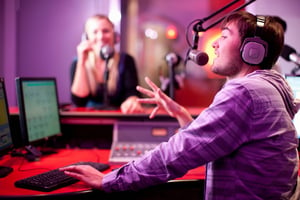
AI Voices and the Future of Speech-Based Applications
Jan 26, 2022 9:23:00 AM
While the pandemic slowed down the development of businesses and entire industries, it...
- Productivity
The Ultimate Guide to Speech Synthesis
Table of contents.
Speech synthesis is an intriguing area of artificial intelligence (AI) that’s been extensively developed by major tech corporations like Microsoft, Amazon, and Google Cloud. It employs deep learning algorithms, machine learning, and natural language processing (NLP) to convert written text into spoken words.
Basics of Speech Synthesis
Speech synthesis, also known as text-to-speech (TTS), involves the automatic production of human speech. This technology is widely used in various applications such as real-time transcription services, automated voice response systems, and assistive technology for the visually impaired. The pronunciation of words, including “robot,” is achieved by breaking down words into basic sound units or phonemes and stringing them together.
Three Stages of Speech Synthesis
Speech synthesizers go through three primary stages: Text Analysis, Prosodic Analysis, and Speech Generation.
- Text Analysis : The text to be synthesized is analyzed and parsed into phonemes, the smallest units of sound. Segmentation of the sentence into words and words into phonemes happens in this stage.
- Prosodic Analysis : The intonation, stress patterns, and rhythm of the speech are determined. The synthesizer uses these elements to generate human-like speech.
- Speech Generation : Using rules and patterns, the synthesizer forms sounds based on the phonemes and prosodic information. Concatenative and unit selection synthesizers are the two main types of speech generation. Concatenative synthesizers use pre-recorded speech segments, while unit selection synthesizers select the best unit from a large speech database.
Most Realistic TTS and Best TTS for Android
While many TTS systems produce high quality and realistic speech, Google’s TTS, part of the Google Cloud service, and Amazon’s Alexa stand out. These systems leverage machine learning and deep learning algorithms, creating seamless and almost indistinguishable-from-human speech. The best TTS engine for Android smartphones is Google’s Text-to-Speech, with a wide range of languages and high-quality voices.
Best Python Library for Text to Speech
For Python developers, the gTTS (Google Text-to-Speech) library stands out due to its simplicity and quality. It interfaces with Google Translate’s text-to-speech API, providing an easy-to-use, high-quality solution.
Speech Recognition and Text-to-Speech
While speech synthesis converts text into speech, speech recognition does the opposite. Automatic Speech Recognition (ASR) technology, like IBM’s Watson or Apple’s Siri, transcribes human speech into text. This forms the basis of voice assistants and real-time transcription services.
Pronunciation of the word “Robot”
The pronunciation of the word “robot” varies slightly depending on the speaker’s accent, but the standard American English pronunciation is /ˈroʊ.bɒt/. Here is a breakdown:
- The first syllable, “ro”, is pronounced like ‘row’ in rowing a boat.
- The second syllable, “bot”, is pronounced like ‘bot’ in ‘bottom’, but without the ‘om’ part.
Example of a Text-to-Speech Program
Google Text-to-Speech is a prominent example of a text-to-speech program. It converts written text into spoken words and is widely used in various Google services and products like Google Translate, Google Assistant, and Android devices.
Best TTS Engine for Android
The best TTS engine for Android devices is Google Text-to-Speech. It supports multiple languages, has a variety of voices to choose from, and is natively integrated with Android, providing a seamless user experience.
Difference Between Concatenative and Unit Selection Synthesizers
Concatenative and unit selection are two main techniques employed in the speech generation stage of a speech synthesizer.
- Concatenative Synthesizers : They work by stitching together pre-recorded samples of human speech. The recorded speech is divided into small pieces, each representing a phoneme or a group of phonemes. When a new speech is synthesized, the appropriate pieces are selected and concatenated together to form the final speech.
- Unit Selection Synthesizers : This approach also relies on a large database of recorded speech but uses a more sophisticated selection process to choose the best matching unit of speech for each segment of the text. The goal is to reduce the amount of ‘stitching’ required, thus producing more natural-sounding speech. It considers factors like prosody, phonetic context, and even speaker emotion while selecting the units.
Top 8 Speech Synthesis Software or Apps
- Google Text-to-Speech : A versatile TTS software integrated into Android. It supports different languages and provides high-quality voices.
- Amazon Polly : An AWS service that uses advanced deep learning technologies to synthesize speech that sounds like a human voice.
- Microsoft Azure Text to Speech : A robust TTS system with neural network capabilities providing natural-sounding speech.
- IBM Watson Text to Speech : Leverages AI to produce speech with human-like intonation.
- Apple’s Siri : Siri isn’t only a voice assistant but also provides high-quality TTS in several languages.
- iSpeech : A comprehensive TTS platform supporting various formats, including WAV.
- TextAloud 4 : A TTS software for Windows, offering conversion of text from various formats to speech.
- NaturalReader : An online TTS service with a range of natural-sounding voices.
- Previous Understanding Veed: Terms of Service, Commercial Rights, and Safe Usage
- Next How to Avoid Voice AI Scams
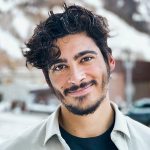
Cliff Weitzman
Cliff Weitzman is a dyslexia advocate and the CEO and founder of Speechify, the #1 text-to-speech app in the world, totaling over 100,000 5-star reviews and ranking first place in the App Store for the News & Magazines category. In 2017, Weitzman was named to the Forbes 30 under 30 list for his work making the internet more accessible to people with learning disabilities. Cliff Weitzman has been featured in EdSurge, Inc., PC Mag, Entrepreneur, Mashable, among other leading outlets.
Recent Blogs
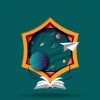
Is Text to Speech HSA Eligible?
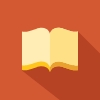
Can You Use an HSA for Speech Therapy?
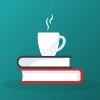
Surprising HSA-Eligible Items

Ultimate guide to ElevenLabs
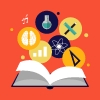
Voice changer for Discord
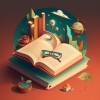
How to download YouTube audio
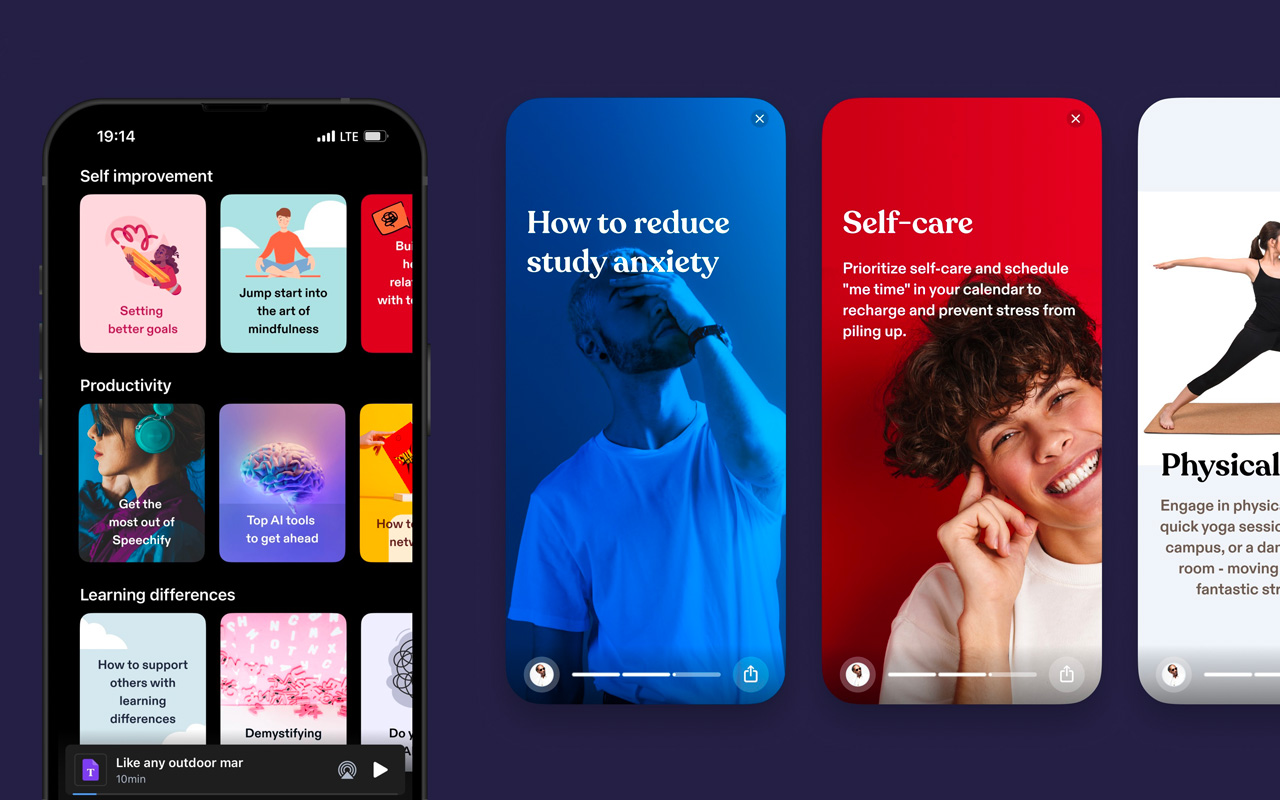
Speechify 3.0 is the Best Text to Speech App Yet.
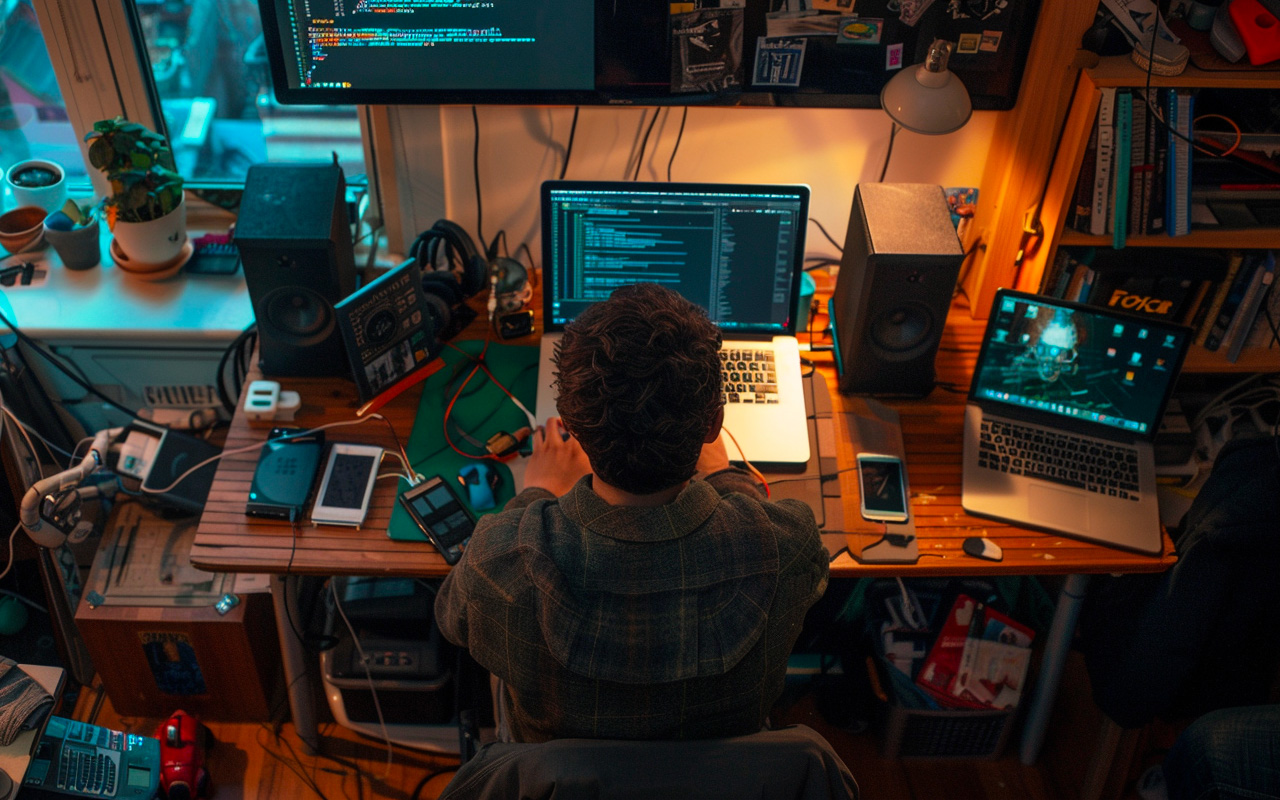
Voice API: Everything You Need to Know
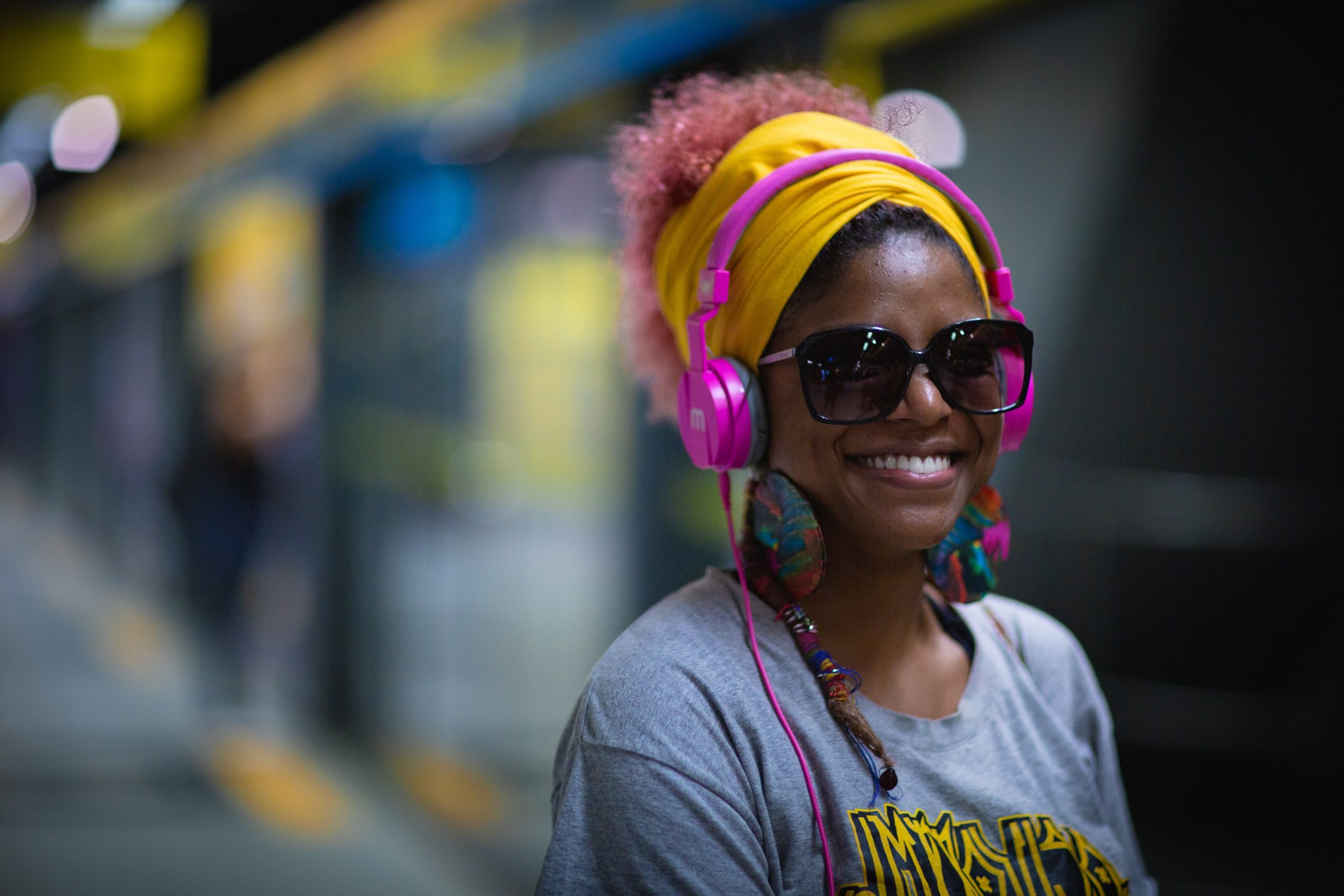
Best text to speech generator apps
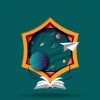
The best AI tools other than ChatGPT

Top voice over marketplaces reviewed
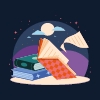
Speechify Studio vs. Descript
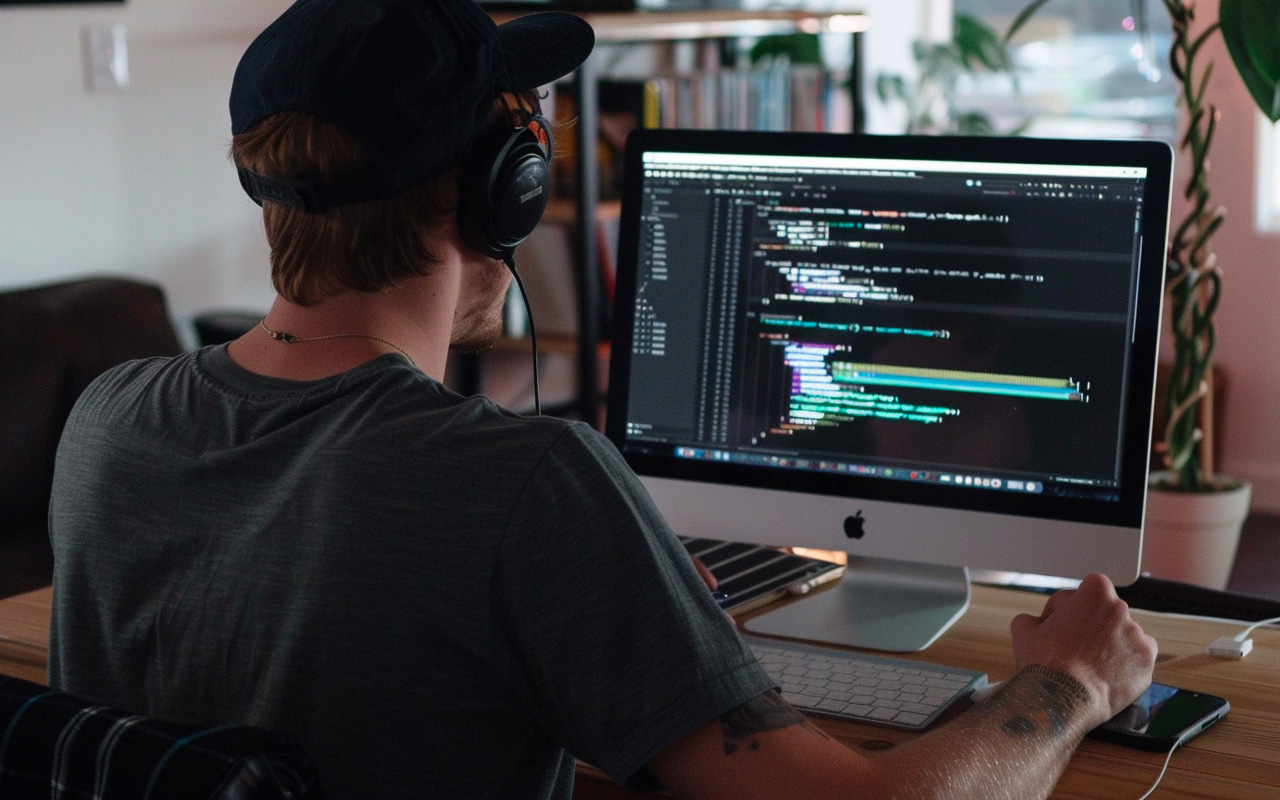
Everything to Know About Google Cloud Text to Speech API
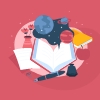
Source of Joe Biden deepfake revealed after election interference
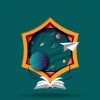
How to listen to scientific papers
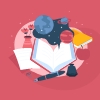
How to add music to CapCut

What is CapCut?
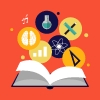
VEED vs. InVideo
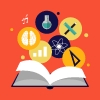
Speechify Studio vs. Kapwing
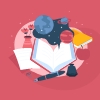
Voices.com vs. Voice123
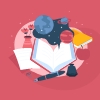
Voices.com vs. Fiverr Voice Over
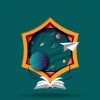
Fiverr voice overs vs. Speechify Voice Over Studio
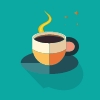
Voices.com vs. Speechify Voice Over Studio
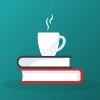
Voice123 vs. Speechify Voice Over Studio
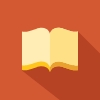
Voice123 vs. Fiverr voice overs
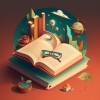
HeyGen vs. Synthesia
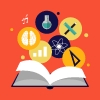
Hour One vs. Synthesia
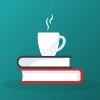
HeyGen vs. Hour One
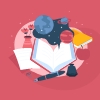
Speechify makes Google’s Favorite Chrome Extensions of 2023 list
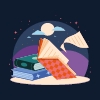
How to Add a Voice Over to Vimeo Video: A Comprehensive Guide
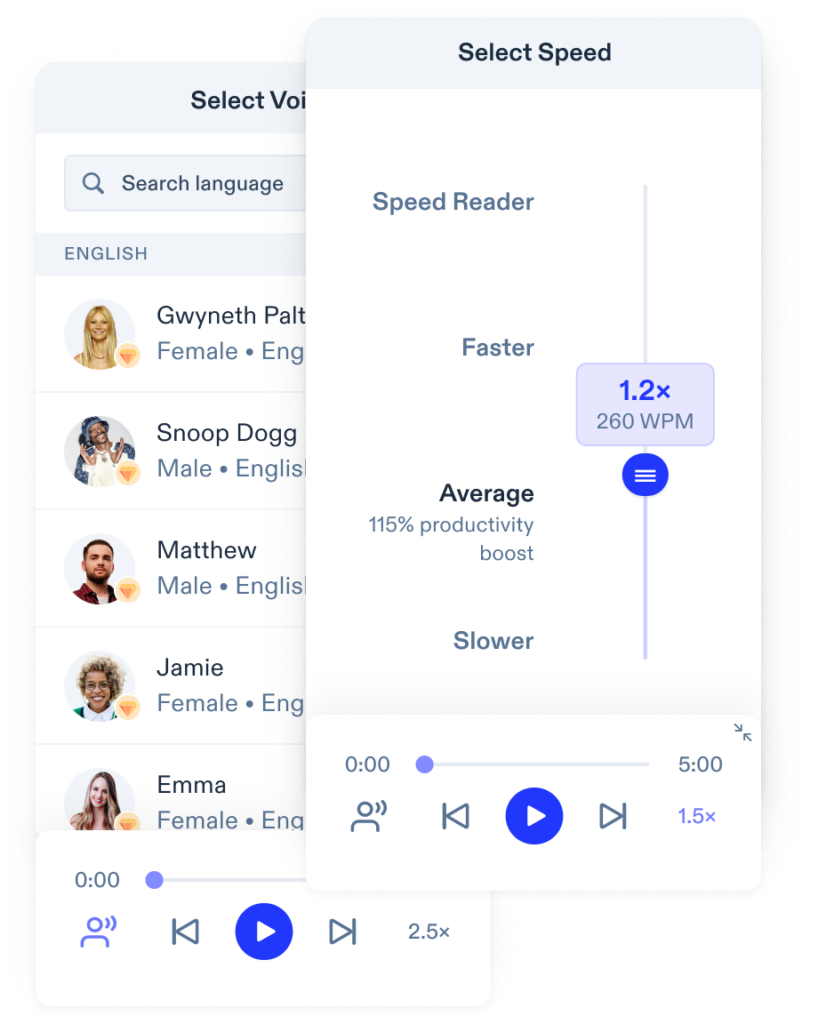
Speechify text to speech helps you save time
Popular blogs.
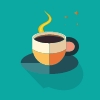
The Best Celebrity Voice Generators in 2024
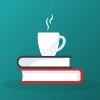
YouTube Text to Speech: Elevating Your Video Content with Speechify
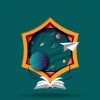
The 7 best alternatives to Synthesia.io

Everything you need to know about text to speech on TikTok
The 10 best text-to-speech apps for android, how to convert a pdf to speech, the top girl voice changers, how to use siri text to speech, obama text to speech.
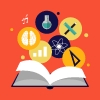
Robot Voice Generators: The Futuristic Frontier of Audio Creation
Pdf read aloud: free & paid options, alternatives to fakeyou text to speech.
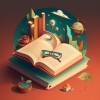
All About Deepfake Voices
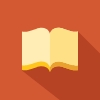
TikTok voice generator

Only available on iPhone and iPad
To access our catalog of 100,000+ audiobooks, you need to use an iOS device.
Coming to Android soon...
Join the waitlist
Enter your email and we will notify you as soon as Speechify Audiobooks is available for you.
You’ve been added to the waitlist. We will notify you as soon as Speechify Audiobooks is available for you.
- Subject List
- Take a Tour
- For Authors
- Subscriber Services
- Publications
- African American Studies
- African Studies
- American Literature
- Anthropology
- Architecture Planning and Preservation
- Art History
- Atlantic History
- Biblical Studies
- British and Irish Literature
- Childhood Studies
- Chinese Studies
- Cinema and Media Studies
- Communication
- Criminology
- Environmental Science
- Evolutionary Biology
- International Law
- International Relations
- Islamic Studies
- Jewish Studies
- Latin American Studies
- Latino Studies
Linguistics
- Literary and Critical Theory
- Medieval Studies
- Military History
- Political Science
- Public Health
- Renaissance and Reformation
- Social Work
- Urban Studies
- Victorian Literature
- Browse All Subjects
How to Subscribe
- Free Trials
In This Article Expand or collapse the "in this article" section Speech Synthesis
Introduction, textbooks, edited collections, surveys, and introductions.
- Journals and Conferences
- Formant Synthesis
- Concatenative Synthesis Based on Diphones
- Text Processing, Pronunciation Dictionaries, and Letter-to-Sound
- Fundamental Frequency Estimation
- Representing Prosody
- Predicting Prosody
- Mainstream Unit Selection
- Trainable Unit Selection and Hybrid Methods
- Source-Filter Signal Processing: Linear Prediction
- Abstracting away from the Vocal Tract Filter: The Spectral Envelope
- Avoiding Explicit Source-Filter Separation
- Statistical Parametric Speech Synthesis
- Subjective Tests
- Objective Measures
Related Articles Expand or collapse the "related articles" section about
About related articles close popup.
Lorem Ipsum Sit Dolor Amet
Vestibulum ante ipsum primis in faucibus orci luctus et ultrices posuere cubilia Curae; Aliquam ligula odio, euismod ut aliquam et, vestibulum nec risus. Nulla viverra, arcu et iaculis consequat, justo diam ornare tellus, semper ultrices tellus nunc eu tellus.
- Acoustic Phonetics
- Computational Linguistics
- Machine Translation
- Speech Perception
- Speech Production
- Voice and Voice Quality
Other Subject Areas
Forthcoming articles expand or collapse the "forthcoming articles" section.
- Cognitive Grammar
- Edward Sapir
- Teaching Pragmatics
- Find more forthcoming articles...
- Export Citations
- Share This Facebook LinkedIn Twitter
Speech Synthesis by Simon King LAST REVIEWED: 15 November 2022 LAST MODIFIED: 25 February 2016 DOI: 10.1093/obo/9780199772810-0024
Speech synthesis has a long history, going back to early attempts to generate speech- or singing-like sounds from musical instruments. But in the modern age, the field has been driven by one key application: Text-to-Speech (TTS), which means generating speech from text input. Almost universally, this complex problem is divided into two parts. The first problem is the linguistic processing of the text, and this happens in the front end of the system. The problem is hard because text clearly does not contain all the information necessary for reading out loud. So, just as human talkers use their knowledge and experience when reading out loud, machines must also bring additional information to bear on the problem; examples include rules regarding how to expand abbreviations into standard words, or a pronunciation dictionary that converts spelled forms into spoken forms. Many of the techniques currently used for this part of the problem were developed in the 1990s and have only advanced very slowly since then. In general, techniques used in the front end are designed to be applicable to almost any language, although the exact rules or model parameters will depend on the language in question. The output of the front end is a linguistic specification that contains information such as the phoneme sequence and the positions of prosodic phrase breaks. In contrast, the second part of the problem, which is to take the linguistic specification and generate a corresponding synthetic speech waveform, has received a great deal of attention and is where almost all of the exciting work has happened since around 2000. There is far more recent material available on the waveform generation part of the text-to-speech problem than there is on the text processing part. There are two main paradigms currently in use for waveform generation, both of which apply to any language. In concatenative synthesis, small snippets of prerecorded speech are carefully chosen from an inventory and rearranged to construct novel utterances. In statistical parametric synthesis, the waveform is converted into two sets of speech parameters: one set captures the vocal tract frequency response (or spectral envelope) and the other set represents the sound source, such as the fundamental frequency and the amount of aperiodic energy. Statistical models are learned from annotated training data and can then be used to generate the speech parameters for novel utterances, given the linguistic specification from the front end. A vocoder is used to convert those speech parameters back to an audible speech waveform.
Steady progress in synthesis since around 1990, and the especially rapid progress in the early 21st century, is a challenge for textbooks. Taylor 2009 provides the most up-to-date entry point to this field and is an excellent starting point for students at all levels. For a wider-ranging textbook that also provides coverage of Natural Language Processing and Automatic Speech Recognition, Jurafsky and Martin 2009 is also excellent. For those without an electrical engineering background, the chapter by Ellis giving “An Introduction to Signal Processing for Speech” in Hardcastle, et al. 2010 is essential background reading, since most other texts are aimed at readers with some previous knowledge of signal processing. Most of the advances in the field since around 2000 have been in the statistical parametric paradigm. No current textbook covers this subject in sufficient depth. King 2011 gives a short and simple introduction to some of the main concepts, and Taylor 2009 contains one relatively brief chapter. For more technical depth, it is necessary to venture beyond textbooks, and the tutorial article Tokuda, et al. 2013 is the best place to start, followed by the more technical article Zen, et al. 2009 . Some older books, such as Dutoit 1997 , still contain relevant material, especially in their treatment of the text processing part of the problem. Sproat’s comment that “text-analysis has not received anything like half the attention of the synthesis community” (p. 73) in his introduction to text processing in van Santen, et al. 1997 is still true, and Yarowsky’s chapter on homograph disambiguation in the same volume still represents a standard solution to that particular problem. Similarly, the modular system architecture described by Sproat and Olive in that volume is still the standard way of configuring a text-to-speech system.
Dutoit, Thierry. 1997. An introduction to text-to-speech synthesis . Norwell, MA: Kluwer Academic.
DOI: 10.1007/978-94-011-5730-8
Starting to get dated, but still contains useful material.
Hardcastle, W. J., J. Laver, and F. E. Gibbon. 2010. The handbook of phonetic sciences . Blackwell Handbooks in Linguistics. Oxford: Wiley-Blackwell.
DOI: 10.1002/9781444317251
A wealth of information, one highlight being the excellent chapter by Ellis introducing speech signal processing to readers with minimal technical background. The chapter on speech synthesis is too dated. Other titles in this series are worth consulting, such as the one on speech perception.
Jurafsky, D., and J. H. Martin. 2009. Speech and language processing: An introduction to natural language processing, computational linguistics, and speech recognition . 2d ed. Upper Saddle River, NJ: Prentice Hall.
A complete course in speech and language processing, very widely used for teaching at advanced undergraduate and graduate levels. The authors have a free online video lecture course covering the Natural Language Processing parts. A third edition of the book is expected.
King, S. 2011. An introduction to statistical parametric speech synthesis. Sadhana 36.5: 837–852.
DOI: 10.1007/s12046-011-0048-y
A gentle and nontechnical introduction to this topic, designed to be accessible to readers from any background. Should be read before attempting the more advanced material.
Taylor, P. 2009. Text-to-speech synthesis . Cambridge, UK: Cambridge Univ. Press.
DOI: 10.1017/CBO9780511816338
The most comprehensive and authoritative textbook ever written on the subject. The content is still up-to-date and highly relevant. Of course, developments since 2009—such as advanced techniques for HMM-based synthesis and the resurgence of Neural Networks—are not covered.
Tokuda, K., Y. Nankaku, T. Toda, H. Zen, J. Yamagishi, and K. Oura. 2013. Speech synthesis based on Hidden Markov Models. Proceedings of the IEEE 101.5: 1234–1252.
DOI: 10.1109/JPROC.2013.2251852
A tutorial article covering the main concepts of statistical parametric speech synthesis using Hidden Markov Models. Also touches on singing synthesis and controllable models.
van Santen, J. P. H., R. W. Sproat, J. P. Oliver, and J. Hirschberg, eds. 1997. Progress in speech synthesis . New York: Springer.
Covering most aspects of text-to-speech, but now dated. Material that remains relevant: Yarowsky on homograph disambiguation; Sproat’s introduction to the Linguistic Analysis section; Campbell and Black’s inclusion of prosody in the unit selection target cost, to minimize the need for subsequent signal processing (implementation details no longer relevant).
Zen, H., K. Tokuda, and A. W. Black. 2009. Statistical parametric speech synthesis. Speech Communication 51.11: 1039–1064.
DOI: 10.1016/j.specom.2009.04.004
Written before the resurgence of neural networks, this is an authoritative and technical introduction to HMM-based statistical parametric speech synthesis.
back to top
Users without a subscription are not able to see the full content on this page. Please subscribe or login .
Oxford Bibliographies Online is available by subscription and perpetual access to institutions. For more information or to contact an Oxford Sales Representative click here .
- About Linguistics »
- Meet the Editorial Board »
- Acceptability Judgments
- Acquisition, Second Language, and Bilingualism, Psycholin...
- Adpositions
- African Linguistics
- Afroasiatic Languages
- Algonquian Linguistics
- Altaic Languages
- Ambiguity, Lexical
- Analogy in Language and Linguistics
- Animal Communication
- Applicatives
- Applied Linguistics, Critical
- Arawak Languages
- Argument Structure
- Artificial Languages
- Australian Languages
- Austronesian Linguistics
- Auxiliaries
- Balkans, The Languages of the
- Baudouin de Courtenay, Jan
- Berber Languages and Linguistics
- Bilingualism and Multilingualism
- Biology of Language
- Borrowing, Structural
- Caddoan Languages
- Caucasian Languages
- Celtic Languages
- Celtic Mutations
- Chomsky, Noam
- Chumashan Languages
- Classifiers
- Clauses, Relative
- Clinical Linguistics
- Cognitive Linguistics
- Colonial Place Names
- Comparative Reconstruction in Linguistics
- Comparative-Historical Linguistics
- Complementation
- Complexity, Linguistic
- Compositionality
- Compounding
- Conditionals
- Conjunctions
- Connectionism
- Consonant Epenthesis
- Constructions, Verb-Particle
- Contrastive Analysis in Linguistics
- Conversation Analysis
- Conversation, Maxims of
- Conversational Implicature
- Cooperative Principle
- Coordination
- Creoles, Grammatical Categories in
- Critical Periods
- Cross-Language Speech Perception and Production
- Cyberpragmatics
- Default Semantics
- Definiteness
- Dementia and Language
- Dene (Athabaskan) Languages
- Dené-Yeniseian Hypothesis, The
- Dependencies
- Dependencies, Long Distance
- Derivational Morphology
- Determiners
- Dialectology
- Distinctive Features
- Dravidian Languages
- Endangered Languages
- English as a Lingua Franca
- English, Early Modern
- English, Old
- Eskimo-Aleut
- Euphemisms and Dysphemisms
- Evidentials
- Exemplar-Based Models in Linguistics
- Existential
- Existential Wh-Constructions
- Experimental Linguistics
- Fieldwork, Sociolinguistic
- Finite State Languages
- First Language Attrition
- Formulaic Language
- Francoprovençal
- French Grammars
- Gabelentz, Georg von der
- Genealogical Classification
- Generative Syntax
- Genetics and Language
- Grammar, Categorial
- Grammar, Construction
- Grammar, Descriptive
- Grammar, Functional Discourse
- Grammars, Phrase Structure
- Grammaticalization
- Harris, Zellig
- Heritage Languages
- History of Linguistics
- History of the English Language
- Hmong-Mien Languages
- Hokan Languages
- Humor in Language
- Hungarian Vowel Harmony
- Idiom and Phraseology
- Imperatives
- Indefiniteness
- Indo-European Etymology
- Inflected Infinitives
- Information Structure
- Interface Between Phonology and Phonetics
- Interjections
- Iroquoian Languages
- Isolates, Language
- Jakobson, Roman
- Japanese Word Accent
- Jones, Daniel
- Juncture and Boundary
- Khoisan Languages
- Kiowa-Tanoan Languages
- Kra-Dai Languages
- Labov, William
- Language Acquisition
- Language and Law
- Language Contact
- Language Documentation
- Language, Embodiment and
- Language for Specific Purposes/Specialized Communication
- Language, Gender, and Sexuality
- Language Geography
- Language Ideologies and Language Attitudes
- Language in Autism Spectrum Disorders
- Language Nests
- Language Revitalization
- Language Shift
- Language Standardization
- Language, Synesthesia and
- Languages of Africa
- Languages of the Americas, Indigenous
- Languages of the World
- Learnability
- Lexical Access, Cognitive Mechanisms for
- Lexical Semantics
- Lexical-Functional Grammar
- Lexicography
- Lexicography, Bilingual
- Linguistic Accommodation
- Linguistic Anthropology
- Linguistic Areas
- Linguistic Landscapes
- Linguistic Prescriptivism
- Linguistic Profiling and Language-Based Discrimination
- Linguistic Relativity
- Linguistics, Educational
- Listening, Second Language
- Literature and Linguistics
- Maintenance, Language
- Mande Languages
- Mass-Count Distinction
- Mathematical Linguistics
- Mayan Languages
- Mental Health Disorders, Language in
- Mental Lexicon, The
- Mesoamerican Languages
- Minority Languages
- Mixed Languages
- Mixe-Zoquean Languages
- Modification
- Mon-Khmer Languages
- Morphological Change
- Morphology, Blending in
- Morphology, Subtractive
- Munda Languages
- Muskogean Languages
- Nasals and Nasalization
- Niger-Congo Languages
- Non-Pama-Nyungan Languages
- Northeast Caucasian Languages
- Oceanic Languages
- Papuan Languages
- Penutian Languages
- Philosophy of Language
- Phonetics, Acoustic
- Phonetics, Articulatory
- Phonological Research, Psycholinguistic Methodology in
- Phonology, Computational
- Phonology, Early Child
- Policy and Planning, Language
- Politeness in Language
- Positive Discourse Analysis
- Possessives, Acquisition of
- Pragmatics, Acquisition of
- Pragmatics, Cognitive
- Pragmatics, Computational
- Pragmatics, Cross-Cultural
- Pragmatics, Developmental
- Pragmatics, Experimental
- Pragmatics, Game Theory in
- Pragmatics, Historical
- Pragmatics, Institutional
- Pragmatics, Second Language
- Prague Linguistic Circle, The
- Presupposition
- Psycholinguistics
- Quechuan and Aymaran Languages
- Reading, Second-Language
- Reciprocals
- Reduplication
- Reflexives and Reflexivity
- Register and Register Variation
- Relevance Theory
- Representation and Processing of Multi-Word Expressions in...
- Salish Languages
- Sapir, Edward
- Saussure, Ferdinand de
- Second Language Acquisition, Anaphora Resolution in
- Semantic Maps
- Semantic Roles
- Semantic-Pragmatic Change
- Semantics, Cognitive
- Sentence Processing in Monolingual and Bilingual Speakers
- Sign Language Linguistics
- Sociolinguistics
- Sociolinguistics, Variationist
- Sociopragmatics
- Sound Change
- South American Indian Languages
- Specific Language Impairment
- Speech, Deceptive
- Speech Synthesis
- Switch-Reference
- Syntactic Change
- Syntactic Knowledge, Children’s Acquisition of
- Tense, Aspect, and Mood
- Text Mining
- Tone Sandhi
- Transcription
- Transitivity and Voice
- Translanguaging
- Translation
- Trubetzkoy, Nikolai
- Tucanoan Languages
- Tupian Languages
- Usage-Based Linguistics
- Uto-Aztecan Languages
- Valency Theory
- Verbs, Serial
- Vocabulary, Second Language
- Vowel Harmony
- Whitney, William Dwight
- Word Classes
- Word Formation in Japanese
- Word Recognition, Spoken
- Word Recognition, Visual
- Word Stress
- Writing, Second Language
- Writing Systems
- Zapotecan Languages
- Privacy Policy
- Cookie Policy
- Legal Notice
- Accessibility
Powered by:
- [66.249.64.20|185.148.24.167]
- 185.148.24.167
- Enroll & Pay
- Prospective Students
- Current Students
- Degree Programs
What is Speech Synthesis?
Speech synthesis, or text-to-speech, is a category of software or hardware that converts text to artificial speech. A text-to-speech system is one that reads text aloud through the computer's sound card or other speech synthesis device. Text that is selected for reading is analyzed by the software, restructured to a phonetic system, and read aloud. The computer looks at each word, calculates its pronunciation then says the word in its context (Cavanaugh, 2003).
How can speech synthesis help your students?
Speech synthesis has a wide range of components that can aid in the reading process. It assists in word decoding for improved reading comprehension (Montali & Lewandowski, 1996). The software gives voice to difficult words with which students struggle by reading either scanned-in documents or imported files (such as eBooks). In word processing, it will read back students' typed text for them to hear what they have written and then make revisions. The software provides a range in options for student control such as tone, pitch, speed of speech, and even gender of speaker. Highlighting features allow the student to highlight a word or passage as it is being read.
Who can benefit from speech synthesis?
According to O'Neill (1999), there are a wide range of users who may benefit from this software, including:
- Students with a reading, learning, and/or attention disorder
- Students who are struggling with reading
- Students who speak English as a second language
- Students with low vision or certain mobility problems
What are some speech synthesis programs?
eReader by CAST
The CAST eReader has the ability to read content from the Internet, word processing files, scanned-in text or typed-in text, and further enhances that text by adding spoken voice, visual highlighting, document navigation, page navigation, type and talk capabilities. eReader is available in both Macintosh and Windows versions.
40 Harvard Mills Square, Suite 3 Wakefield, MA 01880-3233 Tel: 781-245-2212 Fax: 781-245-5212 TTY: 781-245-9320 E-mail: [email protected]
ReadPlease 2003 This free software can be used as a simple word processor that reads what is typed.
ReadPlease ReadingBar ReadingBar (a toolbar for Internet Explorer) allows users to do much more than they were able to before: have web pages read aloud, create MP3 sound files, magnify web pages, make text-only versions of any web page, dictionary look-up, and even translate web pages to and from other languages. ReadingBar is not limited to reading and recording web pages - it is just as good at reading and recording text you see on your screen from any application. ReadingBar is often used to proofread documents and even to learn other languages.
ReadPlease Corporation 121 Cherry Ridge Road Thunder Bay, ON, Canada - P7G 1A7 Phone: 807-474-7702 Fax: 807-768-1285
Read & Write v.6 Software that provides both text reading and work processing support. Features include: speech, spell checking, homophones support, word prediction, dictionary, word wizard, and teacher's toolkit.
textHELP! Systems Ltd. Enkalon Business Centre, 25 Randalstown Road, Antrim Co. Antrim BT41 4LJ N. Ireland [email protected]
Kurweil 3000 Offers a variety of reading tools to assist students with reading difficulties. Tools include: dual highlighting, tools for decoding, study skills, and writing, test taking capabilities, web access and online books, human sounding speech, bilingual and foreign language benefits, and network access and monitoring.
Kurzweil Educational Systems, Inc. 14 Crosby Drive Bedford, MA 01730-1402 From the USA or Canada: 800-894-5374 From all other countries: 781-276-0600
Max's Sandbox In MaxWrite (the Word interface), students type and then hear "Petey" the parrot read their words. In addition, it is easy to add the student's voice to the document (if you have a microphone for your computer). It is a powerful tool for documenting student writing and reading and could even be used in creating a portfolio of student language skills. In addition, MaxWrite has more than 300 clip art images for students to use, or you can easily have students access your own collection of images (scans, digital photos, or clip art). Student work can be printed to the printer you designate and saved to the folder you determine (even network folders).
Publisher: eWord Development
Where can you find more information about speech synthesis?
Research Articles
MacArthur, Charles A. (1998). Word processing with
speech synthesis and word prediction: Effects on the
Descriptive Articles
Center for Applied Special Technology (CAST) Founded in 1984 as the Center for Applied Special Technology, CAST is a not-for-profit organization whose mission is to expand educational opportunities for individuals with disabilities through the development and innovative uses of technology. CAST advances Universal Design for Learning (UDL), producing innovative concepts, educational methods, and effective, inclusive learning technologies based on theoretical and applied research. To achieve this goal, CAST:
- Conducts applied research in UDL,
- Develops and releases products that expand opportunities for learning through UDL,
- Disseminates UDL concepts through public and professional channels.
LD Online LD OnLine is a collaboration between public broadcasting and the learning disabilities community. The site offers a wide range of articles and links to information on assistive technology such as speech synthesis.

What is Speech Synthesis? A Detailed Guide
Aug 24, 2022 13 mins read
Have you ever wondered how those little voice-enabled devices like Amazon’s Alexa or Google Home work? The answer is speech synthesis! Speech synthesis is the artificial production of human speech that sounds almost like a human voice and is more precise with pitch, speech, and tone. Automation and AI-based system designed for this purpose is called a text-to-speech synthesizer and can be implemented in software or hardware.
The people in the business are fully into audio technology to automate management tasks, internal business operations, and product promotions. The super quality and cheaper audio technology are taking everyone with awe and amazement. If you’re a product marketer or content strategist, you might be wondering how you can use text-to-speech synthesis to your advantage.
Speech Synthesis for Translations of Different Languages
One of the benefits of using text to speech in translation is that it can help improve translation accuracy . It is because the synthesized speech can be controlled more precisely than human speech, making it easier to produce an accurate rendition of the original text. It saves you ample time while saving you the labor of manual work that may have a chance of being error-prone. The speech synthesis translator does not need to spend time recording themselves speaking the translated text. It can be a significant time-saving for long or complex texts.
If you’re looking for a way to improve your translation work, consider using TTS synthesis software. It can help you produce more accurate translations and save you time in the process!
If you’re considering using a text-to-speech tool for translation work, there are a few things to keep in mind:
- Choosing a high-quality speech synthesizer is essential to avoid potential errors in the synthesis process.
- You’ll need to create a script for the synthesizer that includes all the necessary pronunciations for the words and phrases in the text.
- You’ll need to test the synthesized speech to ensure it sounds natural and intelligible.
Text to Speech Synthesis for Visually Impaired People
With speech synthesis, you can not only convert text into spoken words but also control how the words are spoken. This means you can change the pitch, speed, and tone of voice. TTS is used in many applications, websites, audio newspapers, and audio blogs .
They are great for helping people who are blind or have low vision or for people who want to listen to a book instead of reading it.
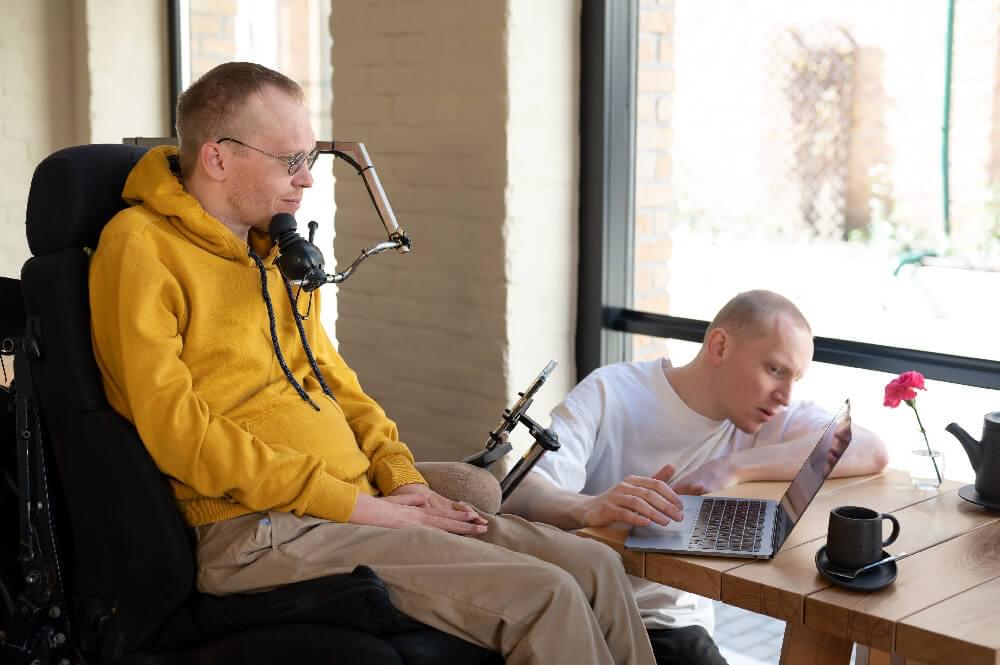
Text to Speech Synthesis for Video Content Creation
With speech synthesis, you can create engaging videos that sound natural and are easy to understand. Let’s face it; not everyone is a great speaker. But with speech synthesis, anyone can create videos that sound professional and are easy to follow.
All you need to do is type out your script. Then, the program will convert your text into spoken words . You can preview the audio to make sure it sounds like you want it to. Then, just record your video and add the audio file.
It’s that simple! With speech synthesis, anyone can create high-quality videos that sound great and are easy to understand. So if you’re looking for a way to take your YouTube channel, Instagram, or TikTok account to the next level, give speech-to-text tools a try! Boost your TikTok views with engaging audio content produced effortlessly through these innovative tools.
What Uses Does Speech Synthesis Have?
The text-to-speech tool has come a long way since its early days in the 1950s. It is now used in various applications, from helping those with speech impairments to creating realistic-sounding computer-generated characters in movies, video games, podcasts, and audio blogs.
Here are some of the most common uses for text-to-speech today:
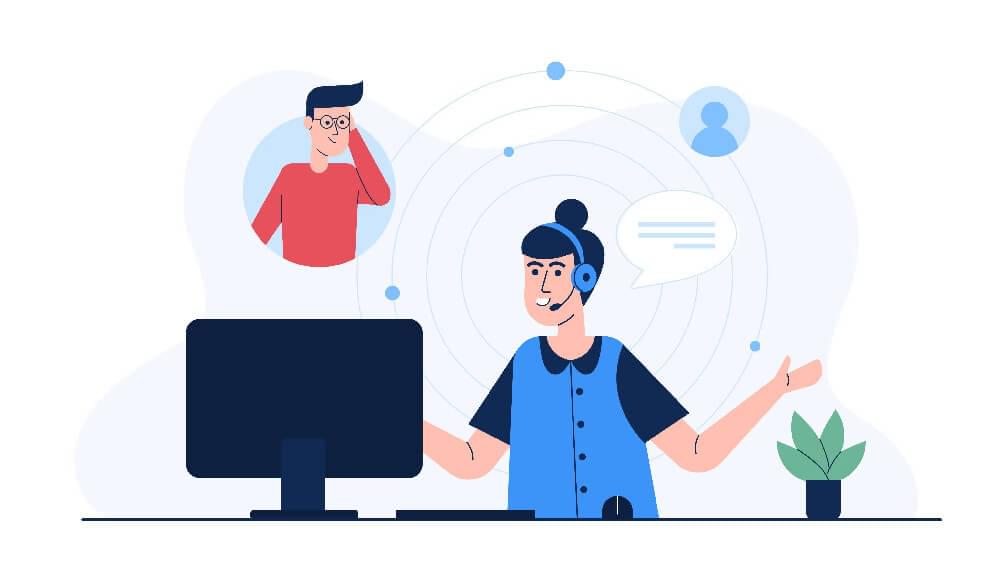
1. Assistive Technology for Those with Speech Impairments
One of the most important uses of TTS is to help those with speech impairments. Various assistive technologies, including text-to-speech (TTS) software, communication aids, and mobile apps, use speech synthesis to convert text into speech.
People with a wide range of speech impairments, including those with dysarthria (a motor speech disorder), mutism (an inability to speak), and aphasia (a language disorder), use audio tools. Nonverbal people with difficulty speaking due to temporary conditions, such as laryngitis, use TTS software.
It includes screen readers read aloud text from websites and other digital documents. Moreover, it includes navigational aids that help people with visual impairments get around.
2. Helping People with Speech Impairments Communicate
People with difficulty speaking due to a stroke or other condition can also benefit from speech synthesis. This can be a lifesaver for people who have trouble speaking but still want to be able to communicate with loved ones. Several apps and devices use this technology to help people communicate.
3. Navigation and Voice Commands—Enhancing GPS Navigation with Spoken Directions
Navigation systems and voice-activated assistants like Siri and Google Assistant are prime examples of TTS software. They convert text-based directions into speech, making it easier for drivers to stay focused on the road. The voice assistants offer voice commands for various tasks, such as sending a text message or setting a reminder. This technology benefits people unfamiliar with an area or who have trouble reading maps.
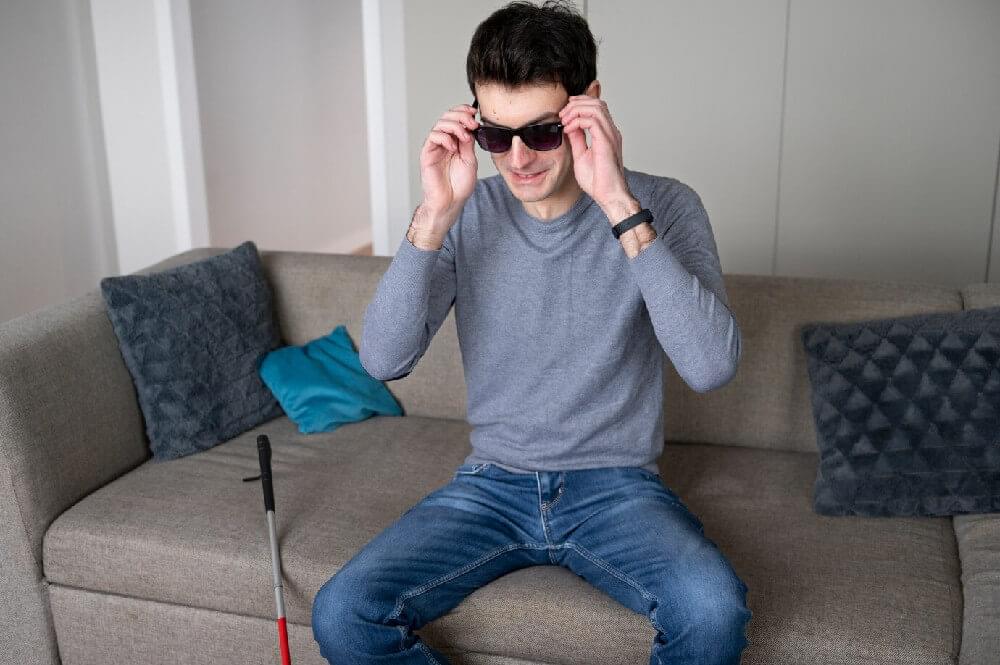
4. Educational Materials
Speech synthesizers are great to help in preparing educational materials , such as audiobooks, audio blogs and language-learning materials. Some visual learners or those who prefer to listen to material rather than read it. Now educational content creators can create materials for those with reading impairments, such as dyslexia .
After the pandemic, and so many educational programs sent online, you must give your students audio learning material to hear it out on the go. For some people, listening to material helps them focus, understand and memorize things better instead of just reading it.
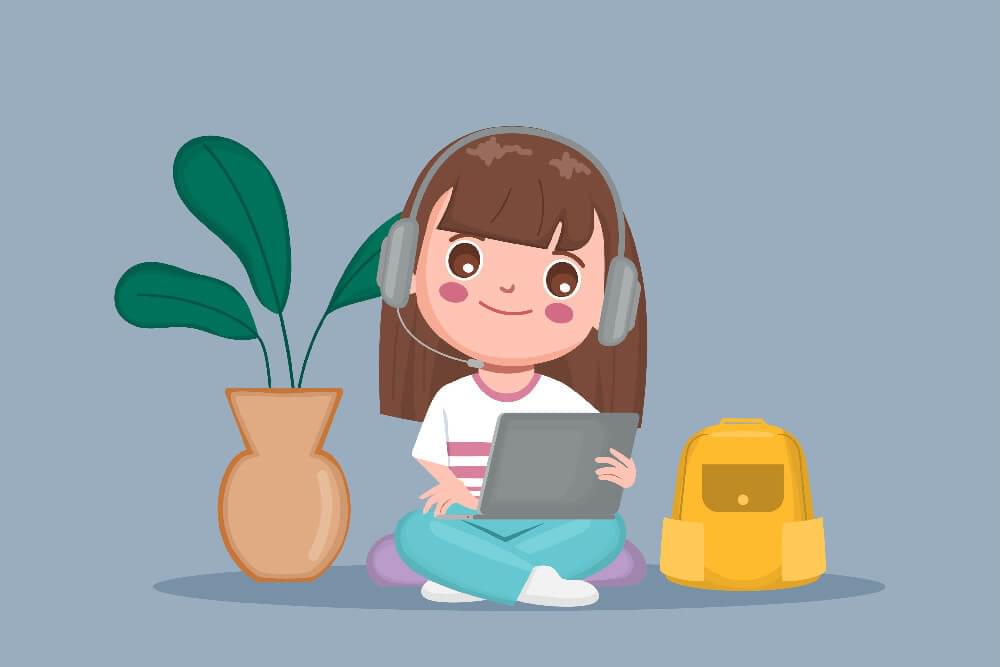
5. Text-to-Speech for Language Learning
Another great use for text-to-speech is for language learning. Hearing the words spoken aloud can be a lot easier to learn how to pronounce them and remember their meaning. Several apps and software programs use text-to-speech to help people learn new languages.
6. Audio Books
Another widespread use for speech synthesis is in audiobooks. It allows people to listen to books instead of reading them. It can be great for commuters or anyone who wants to be able to multitask while they consume content .
7. Accessibility Features in Electronic Devices
Many electronic devices, such as smartphones, tablets, and computers, now have built-in accessibility features that use speech synthesis. These features are helpful for people with visual impairments or other disabilities that make it difficult to use traditional interfaces. For example, Apple’s iPhone has a built-in screen reader called VoiceOver that uses TTS to speak the names of icons and other elements on the screen.
8. Entertainment Applications
Various entertainment applications, such as video games and movies, use speech synthesizers. In video games, they help create realistic-sounding character dialogue. In movies, adding special effects, such as when a character’s voice is artificially generated or altered. It allows developers to create unique voices for their characters without having to hire actors to provide the voices. It can save time and money and allow for more creative freedom.
These are just some of the many uses for speech synthesis today. As the technology continues to develop, we can expect to see even more innovative and exciting applications for this fascinating technology.
9. Making Videos More Engaging with Lip Sync
Lip sync is a speech synthesizer often used in videos and animations. It allows the audio to match the movement of the lips, making it appear as though the character is speaking the words. Hence, they are used for both educational and entertainment purposes.
Related: Text to Speech and Branding: How Voice Technology Enhance your Brand?
10. Generating Speech from Text in Real-Time
Several tools also use text-to-speech synthesis to generate speech from the text, like live captioning or real-time translation. Audio technology is becoming increasingly important as we move towards a more globalized world.
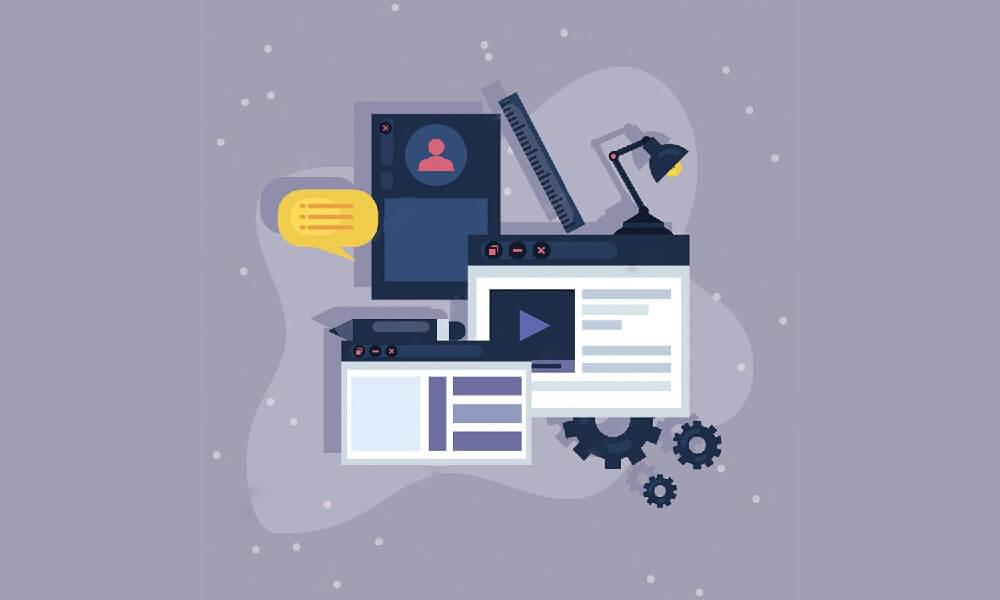
How to Choose and Integrate Speech Synthesis?
With the increasing use of speech synthesizer systems, choosing and integrating the right system for a particular application is necessary. This can be difficult as many factors to consider, such as price, quality, performance, accuracy, portability, and platform support. This article will discuss some important factors to consider when choosing and integrating a speech synthesizer system.
- The quality of a speech synthesizer means its similarity to the human voice and its ability to be understood clearly. Speech synthesis systems were first developed to aid the blind by providing a means of communicating with the outside world. The first systems were based on rule-based methods and simple concatenative synthesis . Over time, however, the quality of text-to-audio tools has improved dramatically. They are now used in various applications, including text-to-speech systems for the visually impaired, voice response systems for telephone services, children’s toys, and computer game characters.
- Another important factor to consider is the accuracy of the synthetic speech . The accuracy of synthetic speech means its ability to pronounce words and phrases correctly. Many text-to-audio tools use rule-based methods to generate synthetic speech, resulting in errors if the rules are not correctly applied. To avoid these errors, choosing a system that uses high-quality algorithms and has been tuned for the specific application is important.
- The performance of a speech synthesis system is another important factor to consider. The performance of synthetic speech means its ability to generate synthetic speech in real-time. Many TTS use pre-recorded speech units concatenated together to create synthetic speech. This can result in delays if the units are not properly aligned or if the system does not have enough resources to generate the synthetic speech in real-time. To avoid these delays, choosing a system that uses high-quality algorithms and has been tuned for the specific application is essential.
- The portability of a speech synthesis system is another essential factor to consider. The portability of synthetic speech means its ability to run on different platforms and devices. Many text-to-audio tools are designed for specific platforms and devices, limiting their portability. To avoid these limitations, choosing a system designed for portability and tested on different platforms and devices is important.
- The price of a speech synthesis system is another essential factor to consider. The price of synthetic speech is often judged by its quality and accuracy. Many text-to-audio tools are costly, so choosing a system that offers high quality and accuracy at a reasonable price is important.
The Bottom Line With technology
With the unstoppable revolution of technology, audio technology is about to bring the boom and multidimensional benefits for the people in business. You must use audio technology today to upgrade your game in the digital world.

Improve accessibility and drive user engagement with WebsiteVoice text-to-speech tool
Our solution, websitevoice.
Add voice to your website by using WebsiteVoice for free.
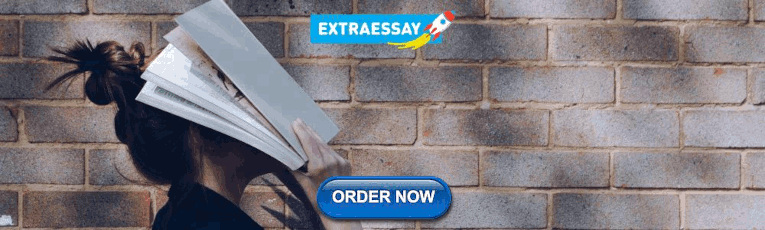
Share this post
Top articles.

Why Your Website Needs a Text-To-Speech Auto Reader?

9 Tips to Make a WordPress Website More Readable

11 Best WordPress Audio Player Plugins of 2022

10 Assistive Technology Tools to Help People with Disabilities in 2022 and Beyond

How to Make Your Website Accessible? Tips and Techniques for Website Creators
Most read from voice technology tutorials
22 apps for kids with reading issues.
Aug 10, 2021 18 mins read
What is an AI Audiobook Narration?
Jun 21, 2023 16 mins read
How AI Can Help in Creating Podcast?
Jan 18, 2024 13 mins read

We're a group of avid readers and podcast listeners who realized that sometimes it's difficult to read up on our favourite blogs, newsmedia and articles online when we're busy commuting, working, driving, doing chorse, and having our eyes and hands busy.
And so asked ourselves: wouldn't it be great if we can listen to these websites like a podcast, instead of reading? Thenext question also came up: how do people with learning disabilities and visual impairment are able to process information that are online in text?
Thus we created WebsiteVoice. The text-to-speech solution for bloggers and web content creators to allow their audience to tune in to theircontent for better user engagement, accessibility and growing more subscribers for their website.
VOICE CLONING: A MULTI-SPEAKER TEXT-TO-SPEECH SYNTHESIS APPROACH BASED ON TRANSFER LEARNING
Deep learning models are becoming predominant in many fields of machine learning. Text-to-Speech (TTS), the process of synthesizing artificial speech from text, is no exception. To this end, a deep neural network is usually trained using a corpus of several hours of recorded speech from a single speaker. Trying to produce the voice of a speaker other than the one learned is expensive and requires large effort since it is necessary to record a new dataset and retrain the model. This is the main reason why the TTS models are usually single speaker. The proposed approach has the goal to overcome these limitations trying to obtain a system which is able to model a multi-speaker acoustic space. This allows the generation of speech audio similar to the voice of different target speakers, even if they were not observed during the training phase.
Index Terms — text-to-speech, deep learning, multi-speaker speech synthesis, speaker embedding, transfer learning
1 Introduction
Text-to-Speech (TTS) synthesis, the process of generating natural speech from text, remains a challenging task despite decades of investigation. Nowadays there are several TTS systems able to get impressive results in terms of synthesis of natural voices very close to human ones. Unfortunately, many of these systems learn to synthesize text only with a single voice. The goal of this work is to build a TTS system which can generate in a data efficient manner natural speech for a wide variety of speakers, not necessarily seen during the training phase. The activity that allows the creation of this type of models is called Voice Cloning and has many applications, such as restoring the ability to communicate naturally to users who have lost their voice or customizing digital assistants such as Siri.
Over time, there has been a significant interest in end-to-end TTS models trained directly from text-audio pairs; Tacotron 2 [ 1 ] used WaveNet [ 2 ] as a vocoder to invert spectrograms generated by sequence-to-sequence with attention [ 3 ] model architecture that encodes text and decodes spectrograms, obtaining a naturalness close to the human one. It only supported a single speaker. Gibiansky et al. [ 4 ] proposed a multi-speaker variation of Tacotron able to learn a low-dimensional speaker embedding for each training speaker. Deep Voice 3 [ 5 ] introduced a fully convolutional encoder-decoder architecture which supports thousands of speakers from LibriSpeech [ 6 ] . However, these systems only support synthesis of voices seen during training since they learn a fixed set of speaker embeddings. Voiceloop [ 7 ] proposed a novel architecture which can generate speech from voices unseen during training but requires tens of minutes of speech and transcripts of the target speaker. In recent extensions, only a few seconds of speech per speaker can be used to generate new speech in that speaker’s voice. Nachmani et al. [ 8 ] for example, extended Voiceloop to utilize a target speaker encoding network to predict speaker embedding directly from a spectrogram. This network is jointly trained with the synthesis network to ensure that embeddings predicted from utterances by the same speaker are closer than embeddings computed from different speakers. Jia et al. [ 9 ] proposed a speaker encoder model similar to [ 8 ] , except that they used a network independently-trained exploring transfer learning from a pre-trained speaker verification model towards the synthesis model.
This work is similar to [ 9 ] however introduces different architectures and uses a new transfer learning technique still based on a pre-trained speaker verification model but exploiting utterance embeddings rather than speaker embeddings. In addition, we use a different strategy to condition the speech synthesis with the voice of speakers not observed before and compared several neural architectures for the speaker encoder model. The paper is organized as follows: Section 2 describes the model architecture and its formal definition; Section 3 reports experiments and results done to evaluate the proposed solution; finally conclusions are reported in Section 4 .
2 Model Architecture
Following [ 9 ] , the proposed system consists of three components: a speaker encoder , which computes a fixed-dimensional embedding vector from a few seconds of reference speech of a target speaker; a synthesizer , which predicts a mel spetrogram from an input text and an embedding vector; a neural vocoder , which infers time-domain waveforms from the mel spectrograms generated by the synthesizer. At inference time, the speaker encoder takes as input a short reference utterance of the target speaker and generates, according to its internal learned speaker characteristics space, an embedding vector. The synthesizer takes as input a phoneme (or grapheme) sequence and generates a mel spectrogram, conditioned by the speaker encoder embedding vector. Finally the vocoder takes the output of the synthesizer and generates the speech waveform. This is illustrated in Figure 1 .
2.1 Problem Definition
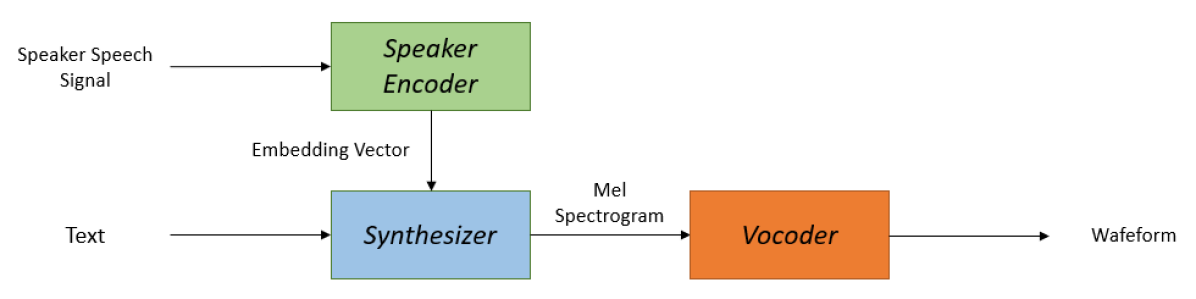
Consider a dataset of N speakers each of which has M utterances in the time-domain. Let’s denote the j-th utterance of the i-th speaker as u ij while the feature extraced from the j-th utterance of the i-th speaker as x ij ( 1 ≤ i ≤ N 1 𝑖 𝑁 1\leq i\leq N and 1 ≤ j ≤ M 1 𝑗 𝑀 1\leq j\leq M ). We chose as feature vector x ij the mel spectrogram.
The speaker encoder ℰ ℰ \mathcal{E} has the task to produce meaningful embedding vectors that should characterize the voices of the speakers. It computes the embedding vector e ij corresponding to the utterance u ij as:
where w E represents the encoder model parameters. Let’s define it utterance embedding . In addition to defining embedding at the utterance level, we can also define the speaker embedding :
In [ 9 ] , the synthesizer S 𝑆 S predicts x ij given c ij and t ij , the transcript of the utterance u ij :
where w S represents the synthesizer model parameters. In our approach, we propose to use the utterance embedding rather than the speaker embedding:
We will motivate this choice in Paragraph 2.4 .
Finally, the vocoder 𝒱 𝒱 \mathcal{V} generates u ij given x ^ i j subscript ^ x 𝑖 𝑗 \hat{\mathbf{\textbf{x}}}_{ij} . So we have:
where w V represents the vocoder model parameters.
This system could be trained in an end-to-end mode trying to optimize the following objective function:
where L V is a loss function in the time-domain. However, it requires to train the three models using the same dataset, moreover, the convergence of the combined model could be hard to reach. To overcome this drawback, the synthesizer can be trained independently to directly predict the mel spectrogram x ij of a target utterance u ij trying to optimize the following objective function:
where L S is a loss function in the time-frequency domain. It is necessary to have a pre-trained speaker encoder model available to compute the utterance embedding e ij .
The vocoder can be trained either directly on the mel spectrograms predicted by the synthesizer or on the groundtruth mel spectrograms:
where L V is a loss function in the time-domain. In the second case, a pre-trained synthesizer model is needed.
If the definition of the objective function was quite simple for both the synthesizer and the vocoder, unfortunately this is not the case for the speaker encoder. The encoder does not have labels to be trained on because its task is only to create the space of characteristics necessary to create the embedding vectors. The Generalized End-to-End (GE2E) [ 10 ] loss brings a solution to this problem and it allows the training of the speaker encoder independently. Consequently, we can define the following objective function:
where S represents a similarity matrix and L G is the GE2E loss function.
2.2 Speaker Encoder
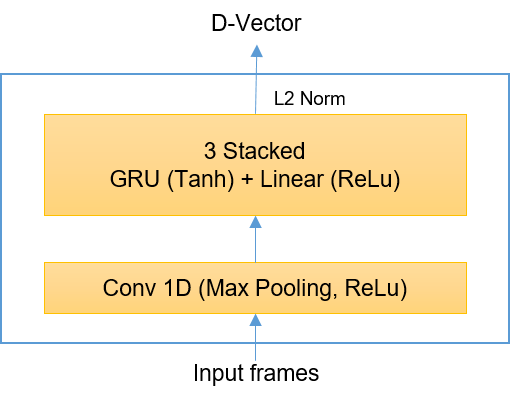
The speaker encoder must be able to produce an embedding vector that meaningfully represents speaker characteristics in the transformed space starting from a target speaker’s utterance. Furthermore, the model should identify these characteristics using a short speech signal, regardless of its phonetic content and background noise. This can be achieved by training a neural network model on a text-independent speaker verification task that tries to optimize the GE2E loss so that embeddings of utterances from the same speaker have high cosine similarity, while those of utterances from different speakers are far apart in the embedding space.
The network maps a sequence of mel spectrogram frames to a fixed-dimensional embedding vector, known as d-vector [ 11 , 12 ] . Input mel spectrograms are fed to a network consisting of one Conv1D [ 13 ] layer of 512 units followed by a stack of 3 GRU [ 14 ] layers of 512 units, each followed by a linear projection of 256 dimension. Following [ 9 ] , the final embedding dimension is 256 and it is created by L2-normalizing the output of the top layer at the final frame. This is shown in Figure 2 . We noticed that this architecture was the best among the various tried and tested, as we will see in Section 3 .
During the training phase, all the utterances are split into partial utterances that are 1.6 seconds long (160 frames). Also at inference time, the input utterance is split into segments of 1.6 seconds with 50% overlap and the model processes each segment individually. Following [ 9 , 10 ] , the final utterance-wise d-vector is generated by L2 normalizing the window-wise d-vectors and taking the element-wise average.
2.3 Synthesizer and Vocoder
The synthesizer component of the system is a sequence-to-sequence model with attention [ 1 , 3 ] which is trained on pairs of text derived token sequences and audio derived mel spectrogram sequences. Furthermore, the network is trained in a transfer learning configuration (see Paragraph 2.4 ), using an independently-trained speaker encoder to extract embedding vectors useful to condition the outcomes of this component. In view of reproducibility, the adopted vocoder component of the system is a Pytorch github implementation 1 1 1 https://github.com/fatchord/WaveRNN of the neural vocoder WaveRNN [ 15 ] . This model is not directly conditioned on the output of the speaker encoder but just on the input mel spectrogram. The multi-speaker vocoder is simply trained by using data from many speakers (see Section 3 ).
2.4 Transfer Learning Modality
The conditioning of the synthesizer via speaker encoder is the fundamental part that makes the system multi-speaker: the embedding vectors computed by the speaker encoder allow the conditioning of the mel spectrograms generated by the synthesizer so that they can incorporate the new speaker voice. In [ 9 ] , the embedding vectors are speaker embeddings obtained by Equation 2 . We used the utterance embeddings computed by Equation 1 . In fact, at inference time only one utterance of the target speaker is fed to the speaker encoder which therefore produces a single utterance-level d-vector. Thus, in this case, it is not possible to create an embedding at the speaker level since the average operation cannot be applied. This implies that only utterance embeddings can be used during the inference phase. In addition, an average mechanism could cause some loss in terms of accuracy. This is due to larger variations in pitch and voice quality often occurring in utterances of the same speaker while utterances have lower intra-variation. Following [ 9 ] , the embedding vectors computed by the speaker encoder are concatenated only with the synthesizer encoder output in order to condition the synthesis. However, we experimented with a new concatenation technique: first we passed the embedding through a single linear layer and then we applied the concatenation between the output of this layer and the synthesizer encoder one. The goal was to exploit the weights of the linear layer to make the embedding vector more meaningful, since the layer was trained together with the synthesizer. We noticed that this method achieved good convergence of training and was about 75% times faster than the former vector concatenation.
3 Experiments and Results
We used different publicly available datasets to train and evaluate the components of the system. For the speaker encoder, different neural network architectures were tested. Each of them was trained using a combination of three public sets: LibriTTS [ 16 ] train-other and dev-other; VoxCeleb [ 17 ] dev and VoxCeleb2 [ 18 ] dev. In this way, we obtained a number of speakers equal to 8,381 and a number of utterances equal to 1,419,192, not necessarily all clean and noiseless. Furthermore, transcripts were not required. The models were trained using Adam [ 19 ] as optimizer with an initial learning rate equal to 0.001. Moreover, we experimented with different learning rate decay strategies.
During the evaluation phase, we used a combination of the corresponding test sets of the training ones, obtaining a number of speakers equal to 191 and a number of utterances equal to 45,132. Both training and test sets have been sampled at 16 kHz and input mel spectrograms were computed from 25ms STFT analysis windows with a 10ms step and passed through a 40-channel mel-scale filterbank.
We separately trained the synthesizer and the vocoder using the same training set given by the combination of the two “clean” sets of LibriTTS, obtaining a number of speakers equal to 1,151, a number of utterances equal to 149,736 and a total number of hours equal to 245,14 of 22.05 kHz audio. We trained the synthesizer using the L1 loss [ 20 ] and Adam as optimizer. Moreover, the input texts were converted into phoneme sequences and target mel spectrogram features are computed on 50 ms signal windows, shifted by 12.5 ms and passed through an 80-channel mel-scale filterbank. The vocoder was trained using groundtruth waveforms rather than the synthesizer outputs.
3.1 Baseline System
We choose as baseline for our work the Corentin Jemine’s real-time voice cloning system [ 21 ] , a public re-implementation of the Google system [ 9 ] available on github 2 2 2 https://github.com/CorentinJ/Real-Time-Voice-Cloning . This system is composed out of three components: a recurrent speaker encoder consisting of 3 LSTM [ 22 ] layers and a final linear layer, each of which has 256 units; a sequence-to-sequence with attention synthesizer based on [ 1 ] and WaveRNN [ 15 ] as vocoder.
3.2 Speaker Encoder: Proposed System
To evaluate all the speaker encoder models and choose the best one, the Speaker Verification Equal Error Rate (SV-EER) was estimated by pairing each test utterance with each enrollment speaker. The models implemented are:
rec_conv network : 5 Conv1D layers, 1 GRU layer and a final linear layer;
rec_conv_2 network : 3 Conv1D layers, 2 GRU layers each followed by a linear projection layer;
gru network : 3 GRU layers each followed by a linear projection layer;
advanced_gru network : 1 Conv1D layer and 3 GRU layers each followed by a linear projection layer (Figure 2 );
lstm network : 1 Conv1D layer and 3 LSTM [ 22 ] layers each followed by a linear projection layer.
All layers have 512 units except the linear ones which have 256. Moreover, dropout rate of 0.2 was used after all the layers except before the first and after the last. All the models were trained using a batch size of 64 speakers and 10 utterances for each speaker. The results obtained are shown in Table 1 .
Name Step Time Train Loss SV-EER LR Decay rec_conv 0.33s 0.36 0.073 Reduce on Plateau rec_conv_2 0.45s 0.49 0.075 Reduce on Plateau gru 1,45s 0.33 0.054 Every 100,000 step advanced_gru 0.86s 0.14 0.040 Exponential lstm 1.08s 0.17 0.052 Exponential
We designed the advanced gru network trying to combine the advantages of convolutional and gru networks. In fact, looking at the table, this architecture was much faster than the gru network during training, and obtained the best SV-EER on the test set. Figure 3 illustrates the projection in a two-dimensional space of the utterance embeddings computed by the advanced gru network on the basis of 6 utterances extracted from 12 speakers of the test set. In Figure 4 , the 12 speakers are 6 men and 6 women. The projections were made using UMAP [ 23 ] . Both the figures show that the model has created a space of internal features that is robust regarding the speakers, creating well-formed clusters of speakers based on their utterances and nicely separating male speakers from female ones.
The SV-EER obtained on the test set from the speaker encoder model of the proposed system is 0.040 vs the baseline one which is 0.049.
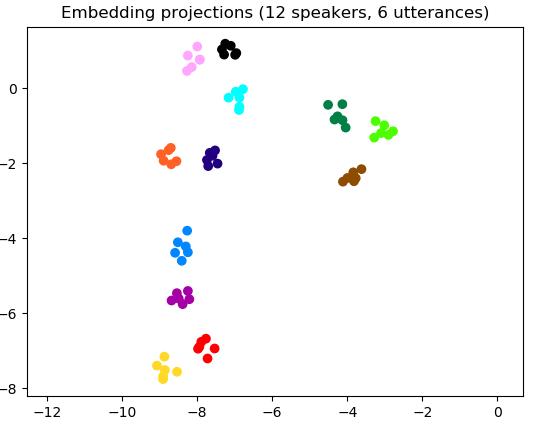
3.3 Similarity Evaluation
To assess how similar the waveforms generated by the system were from the original ones, we transformed the audio signals produced into utterance embeddings (using the speaker encoder advanced gru network) and then projected them in a two-dimensional space together with the utterance embeddings computed on the basis of the groundtruth audio. As test speakers, we randomly choose eight target speakers: four speakers (two male and two female) were extracted from the test-set-clean of LibriTTS [ 16 ] , three (two male and one female) from VCTK [ 24 ] and finally a female proprietary voice. For each speaker we randomly extracted 10 utterances and compared them with the utterances generated by the system calculating the cosine similarity. The speakers averaged values of cosine similarity between the generated and groundtruth utterance embeddings range from 0.56 to 0.76. Figure 5 shows that synthesized utterances tend to lie close to real speech from the same speaker in the embedding space.
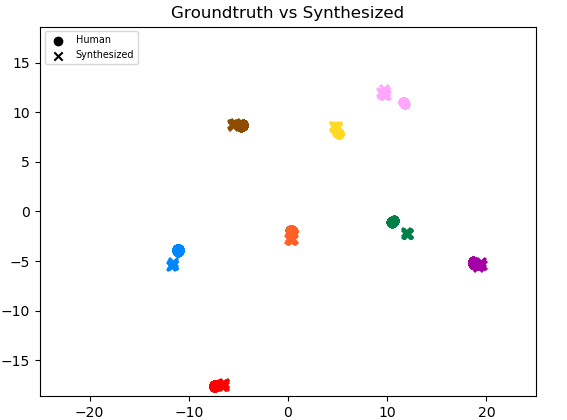
3.4 Subjective Evaluation
Finally, we evaluated how the generated utterances were, subjectively speaking, similar in terms of speech timbre to the original ones. To do this, we gathered Mean Similarity Scores (MSS) based on a 5 points mean opinion score scale, where 1 stands for “very different” and 5 for “very similar”. Ten utterances of the proprietary female voice were cloned using both the proposed and the baseline system and then 12 subjects, most of them TTS experts, were asked to listen to the 20 samples, randomly mixed, and rate them. Participants were also provided with an original utterance as reference. The question asked was: “How do you rate the similarity of these samples with respect to the reference audio? Try to focus on vocal timbre and not on content, intonation or acoustic quality of the audio”. The results obtained are shown in Table 2 . Although not conclusive, this experiment highlights a subjective evidence of the goodness of the proposed approach, despite the significant variance of both systems: this is largely due to the low number of test participants.
System MSS baseline 2.59 ± 1.03 plus-or-minus 2.59 1.03 2.59\pm 1.03 proposed 3.17 ± 0.97 plus-or-minus 3.17 0.97 3.17\pm 0.97
4 Conclusions
In this work, our goal was to build a Voice Cloning system which could generate natural speech for a variety of target speakers in a data efficient manner. Our system combines an independently trained speaker encoder network with a sequence-to-sequence with attention architecture and a neural vocoder model. Using a transfer learning technique from a speaker-discriminative encoder model based on utterance embeddings rather than speaker embeddings, the synthesizer and the vocoder are able to generate good quality speech also for speakers not observed before. Despite the experiments showed a reasonable similarity with real speech and improvements over the baseline, the proposed system does not fully reach human-level naturalness in contrast to the single speaker results from [ 1 ] . Additionally, the system is not able to reproduce the speaker prosody of the target audio. These are consequences of the additional difficulty of generating speech for a variety of speakers given significantly less data per speaker unlike when training a model on a single speaker.
5 Acknowledgements
The authors thank Roberto Esposito, Corentin Jemine, Quan Wang, Ignacio Lopez Moreno, Skjalg Lepsøy, Alessandro Garbo and Jürgen Van de Walle for their helpful discussions and feedback.
- [1] J. Shen, R. Pang, R. J. Weiss, M. Schuster, N. Jaitly, Z. Yang, Z. Chen, Y. Zhang, Y. Wang, R. Skerrv-Ryan, R. A. Saurous, Y. Agiomvrgiannakis, and Y. Wu, “Natural tts synthesis by conditioning wavenet on mel spectrogram predictions,” in 2018 IEEE International Conference on Acoustics, Speech and Signal Processing (ICASSP) , 2018, pp. 4779–4783.
- [2] Aäron van den Oord, Sander Dieleman, Heiga Zen, Karen Simonyan, Oriol Vinyals, Alex Graves, Nal Kalchbrenner, Andrew W. Senior, and Koray Kavukcuoglu, “Wavenet: A generative model for raw audio,” CoRR , vol. abs/1609.03499, 2016.
- [3] Dzmitry Bahdanau, Kyunghyun Cho, and Yoshua Bengio, “Neural machine translation by jointly learning to align and translate,” CoRR , vol. abs/1409.0473, 2015.
- [4] Andrew Gibiansky, Sercan Arik, Gregory Diamos, John Miller, Kainan Peng, Wei Ping, Jonathan Raiman, and Yanqi Zhou, “Deep voice 2: Multi-speaker neural text-to-speech,” in Advances in Neural Information Processing Systems 30 , I. Guyon, U. V. Luxburg, S. Bengio, H. Wallach, R. Fergus, S. Vishwanathan, and R. Garnett, Eds., pp. 2962–2970. Curran Associates, Inc., 2017.
- [5] Wei Ping, Kainan Peng, Andrew Gibiansky, Sercan O. Arik, Ajay Kannan, Sharan Narang, Jonathan Raiman, and John Miller, “Deep voice 3: 2000-speaker neural text-to-speech,” in International Conference on Learning Representations , 2018.
- [6] V. Panayotov, G. Chen, D. Povey, and S. Khudanpur, “Librispeech: An asr corpus based on public domain audio books,” in 2015 IEEE International Conference on Acoustics, Speech and Signal Processing (ICASSP) , 2015, pp. 5206–5210.
- [7] Yaniv Taigman, Lior Wolf, Adam Polyak, and Eliya Nachmani, “Voiceloop: Voice fitting and synthesis via a phonological loop,” in International Conference on Learning Representations , 2018.
- [8] Eliya Nachmani, Adam Polyak, Yaniv Taigman, and Lior Wolf, “Fitting new speakers based on a short untranscribed sample,” CoRR , vol. abs/1802.06984, 2018.
- [9] Ye Jia, Yu Zhang, Ron J. Weiss, Quan Wang, Jonathan Shen, Fei Ren, Zhifeng Chen, Patrick Nguyen, Ruoming Pang, Ignacio Lopez-Moreno, and Yonghui Wu, “Transfer learning from speaker verification to multispeaker text-to-speech synthesis,” CoRR , vol. abs/1806.04558, 2018.
- [10] Lipeng Wan, Qi shan Wang, Alan Papir, and Ignacio Lopez-Moreno, “Generalized end-to-end loss for speaker verification,” 2018 IEEE International Conference on Acoustics, Speech and Signal Processing (ICASSP) , pp. 4879–4883, 2018.
- [11] Georg Heigold, Ignacio Moreno, Samy Bengio, and Noam Shazeer, “End-to-end text-dependent speaker verification,” 2016 IEEE International Conference on Acoustics, Speech and Signal Processing (ICASSP) , pp. 5115–5119, 2016.
- [12] Ehsan Variani, Xin Lei, Erik McDermott, Ignacio Lopez Moreno, and Javier Gonzalez-Dominguez, “Deep neural networks for small footprint text-dependent speaker verification,” in Proc. ICASSP , 2014.
- [13] Serkan Kiranyaz, Onur Avci, Osama Abdeljaber, Turker Ince, Moncef Gabbouj, and Daniel J. Inman, “1d convolutional neural networks and applications: A survey,” ArXiv , vol. abs/1905.03554, 2019.
- [14] Junyoung Chung, Caglar Gulcehre, Kyunghyun Cho, and Yoshua Bengio, “Empirical evaluation of gated recurrent neural networks on sequence modeling,” in NIPS 2014 Workshop on Deep Learning, December 2014 , 2014.
- [15] Nal Kalchbrenner, Erich Elsen, Karen Simonyan, Seb Noury, Norman Casagrande, Edward Lockhart, Florian Stimberg, Aäron van den Oord, Sander Dieleman, and Koray Kavukcuoglu, “Efficient neural audio synthesis,” in ICML , 2018.
- [16] Heiga Zen, Viet Dang, Rob Clark, Yu Zhang, Ron J. Weiss, Ye Jia, Zhifeng Chen, and Yonghui Wu, “Libritts: A corpus derived from librispeech for text-to-speech,” in INTERSPEECH , 2019.
- [17] Arsha Nagrani, Joon Son Chung, and Andrew Zisserman, “Voxceleb: A large-scale speaker identification dataset,” in INTERSPEECH , 2017.
- [18] Joon Son Chung, Arsha Nagrani, and Andrew Zisserman, “Voxceleb2: Deep speaker recognition,” in INTERSPEECH , 2018.
- [19] Diederik Kingma and Jimmy Ba, “Adam: A method for stochastic optimization,” International Conference on Learning Representations , 12 2014.
- [20] Katarzyna Janocha and Wojciech Czarnecki, “On loss functions for deep neural networks in classification,” ArXiv , vol. abs/1702.05659, 2017.
- [21] Corentin Jemine, “Master thesis: Automatic multispeaker voice cloning,” 2019, Unpublished master’s thesis, Université de Liège, Liège, Belgique.
- [22] Klaus Greff, Rupesh K. Srivastava, Jan Koutnik, Bas R. Steunebrink, and Jurgen Schmidhuber, “Lstm: A search space odyssey,” IEEE Transactions on Neural Networks and Learning Systems , vol. 28, no. 10, pp. 2222–2232, Oct 2017.
- [23] Leland McInnes and John Healy, “Umap: Uniform manifold approximation and projection for dimension reduction,” ArXiv , vol. abs/1802.03426, 2018.
- [24] Christophe Veaux, Junichi Yamagishi, and Kirsten MacDonald, “Cstr vctk corpus: English multi-speaker corpus for cstr voice cloning toolkit,” 2018.
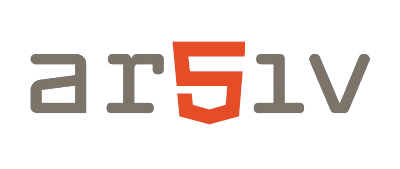
Subscribe to the PwC Newsletter
Join the community, edit method, add a method collection.
- TEXT-TO-SPEECH MODELS
Remove a collection
- TEXT-TO-SPEECH MODELS -
Add A Method Component
- BATCH NORMALIZATION
- CONVOLUTION
- LINEAR LAYER
- LOCATION SENSITIVE ATTENTION
- MIXTURE OF LOGISTIC DISTRIBUTIONS
Remove a method component
- BATCH NORMALIZATION -
- CONVOLUTION -
- LINEAR LAYER -
- LOCATION SENSITIVE ATTENTION -
- MIXTURE OF LOGISTIC DISTRIBUTIONS -
Tacotron 2 is a neural network architecture for speech synthesis directly from text. It consists of two components:
- a recurrent sequence-to-sequence feature prediction network with attention which predicts a sequence of mel spectrogram frames from an input character sequence
- a modified version of WaveNet which generates time-domain waveform samples conditioned on the predicted mel spectrogram frames
In contrast to the original Tacotron , Tacotron 2 uses simpler building blocks, using vanilla LSTM and convolutional layers in the encoder and decoder instead of CBHG stacks and GRU recurrent layers. Tacotron 2 does not use a “reduction factor”, i.e., each decoder step corresponds to a single spectrogram frame. Location-sensitive attention is used instead of additive attention .

Usage Over Time
Categories edit add remove.
Features of the Implementation of Real-Time Text-to-Speech Systems With Data Variability
Ieee account.
- Change Username/Password
- Update Address
Purchase Details
- Payment Options
- Order History
- View Purchased Documents
Profile Information
- Communications Preferences
- Profession and Education
- Technical Interests
- US & Canada: +1 800 678 4333
- Worldwide: +1 732 981 0060
- Contact & Support
- About IEEE Xplore
- Accessibility
- Terms of Use
- Nondiscrimination Policy
- Privacy & Opting Out of Cookies
A not-for-profit organization, IEEE is the world's largest technical professional organization dedicated to advancing technology for the benefit of humanity. © Copyright 2024 IEEE - All rights reserved. Use of this web site signifies your agreement to the terms and conditions.
Tacotron: Towards End-to-End Speech Synthesis
Research areas.
Speech Processing
Learn more about how we conduct our research
We maintain a portfolio of research projects, providing individuals and teams the freedom to emphasize specific types of work.
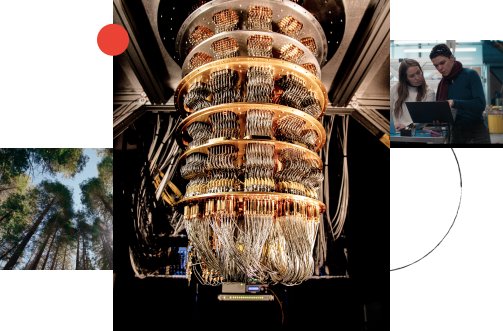
- Search for: Toggle Search
The Building Blocks of AI: Decoding the Role and Significance of Foundation Models
Editor’s note: This post is part of the AI Decoded series , which demystifies AI by making the technology more accessible, and which showcases new hardware, software, tools and accelerations for RTX PC users.
Skyscrapers start with strong foundations. The same goes for apps powered by AI.
A foundation model is an AI neural network trained on immense amounts of raw data, generally with unsupervised learning .
It’s a type of artificial intelligence model trained to understand and generate human-like language. Imagine giving a computer a huge library of books to read and learn from, so it can understand the context and meaning behind words and sentences, just like a human does.
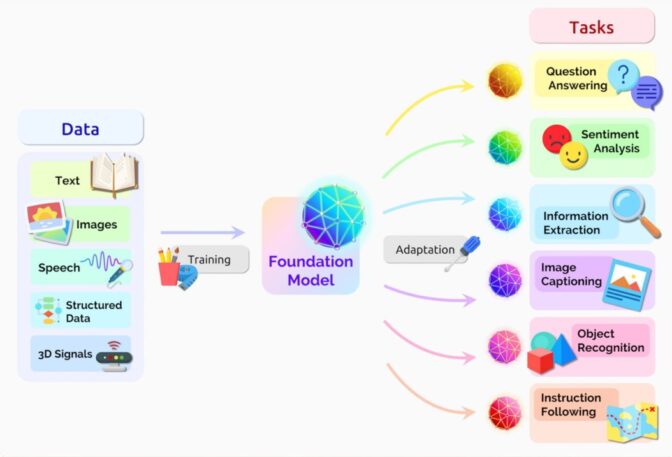
A foundation model’s deep knowledge base and ability to communicate in natural language make it useful for a broad range of applications, including text generation and summarization, copilot production and computer code analysis, image and video creation, and audio transcription and speech synthesis.
ChatGPT, one of the most notable generative AI applications, is a chatbot built with OpenAI’s GPT foundation model. Now in its fourth version, GPT-4 is a large multimodal model that can ingest text or images and generate text or image responses.
Online apps built on foundation models typically access the models from a data center. But many of these models, and the applications they power, can now run locally on PCs and workstations with NVIDIA GeForce and NVIDIA RTX GPUs.
Foundation Model Uses
Foundation models can perform a variety of functions, including:
- Language processing: understanding and generating text
- Code generation: analyzing and debugging computer code in many programming languages
- Visual processing: analyzing and generating images
- Speech: generating text to speech and transcribing speech to text
They can be used as is or with further refinement. Rather than training an entirely new AI model for each generative AI application — a costly and time-consuming endeavor — users commonly fine-tune foundation models for specialized use cases.
Pretrained foundation models are remarkably capable, thanks to prompts and data-retrieval techniques like retrieval-augmented generation , or RAG. Foundation models also excel at transfer learning , which means they can be trained to perform a second task related to their original purpose.
For example, a general-purpose large language model (LLM) designed to converse with humans can be further trained to act as a customer service chatbot capable of answering inquiries using a corporate knowledge base.
Enterprises across industries are fine-tuning foundation models to get the best performance from their AI applications.
Types of Foundation Models
More than 100 foundation models are in use — a number that continues to grow. LLMs and image generators are the two most popular types of foundation models. And many of them are free for anyone to try — on any hardware — in the NVIDIA API Catalog .
LLMs are models that understand natural language and can respond to queries. Google’s Gemma is one example; it excels at text comprehension, transformation and code generation. When asked about the astronomer Cornelius Gemma, it shared that his “contributions to celestial navigation and astronomy significantly impacted scientific progress.” It also provided information on his key achievements, legacy and other facts.
Extending the collaboration of the Gemma models , accelerated with the NVIDIA TensorRT-LLM on RTX GPUs, Google’s CodeGemma brings powerful yet lightweight coding capabilities to the community. CodeGemma models are available as 7B and 2B pretrained variants that specialize in code completion and code generation tasks.
MistralAI’s Mistral LLM can follow instructions, complete requests and generate creative text. In fact, it helped brainstorm the headline for this blog, including the requirement that it use a variation of the series’ name “AI Decoded,” and it assisted in writing the definition of a foundation model.
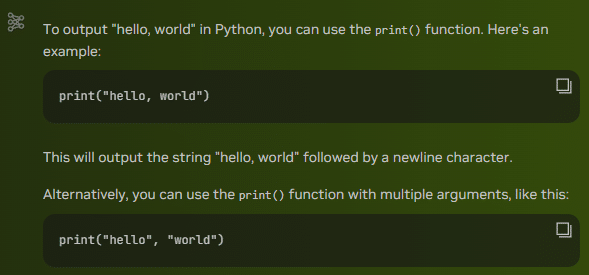
Meta’s Llama 2 is a cutting-edge LLM that generates text and code in response to prompts.
Mistral and Llama 2 are available in the NVIDIA ChatRTX tech demo, running on RTX PCs and workstations. ChatRTX lets users personalize these foundation models by connecting them to personal content — such as documents, doctors’ notes and other data — through RAG. It’s accelerated by TensorRT-LLM for quick, contextually relevant answers. And because it runs locally, results are fast and secure.
Image generators like StabilityAI’s Stable Diffusion XL and SDXL Turbo let users generate images and stunning, realistic visuals. StabilityAI’s video generator, Stable Video Diffusion , uses a generative diffusion model to synthesize video sequences with a single image as a conditioning frame.
Multimodal foundation models can simultaneously process more than one type of data — such as text and images — to generate more sophisticated outputs.
A multimodal model that works with both text and images could let users upload an image and ask questions about it. These types of models are quickly working their way into real-world applications like customer service, where they can serve as faster, more user-friendly versions of traditional manuals.
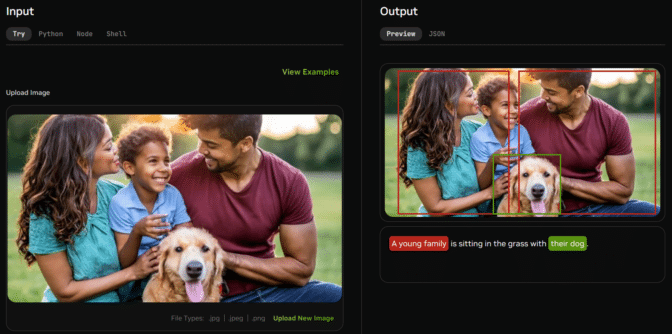
Kosmos 2 is Microsoft’s groundbreaking multimodal model designed to understand and reason about visual elements in images.
Think Globally, Run AI Models Locally
GeForce RTX and NVIDIA RTX GPUs can run foundation models locally.
The results are fast and secure. Rather than relying on cloud-based services, users can harness apps like ChatRTX to process sensitive data on their local PC without sharing the data with a third party or needing an internet connection.
Users can choose from a rapidly growing catalog of open foundation models to download and run on their own hardware. This lowers costs compared with using cloud-based apps and APIs, and it eliminates latency and network connectivity issues. Generative AI is transforming gaming, videoconferencing and interactive experiences of all kinds. Make sense of what’s new and what’s next by subscribing to the AI Decoded newsletter .
NVIDIA websites use cookies to deliver and improve the website experience. See our cookie policy for further details on how we use cookies and how to change your cookie settings.
Share on Mastodon
Multi speaker text-to-speech synthesis using generalized end-to-end loss function
- Published: 13 January 2024
Cite this article
- Owais Nazir 1 ,
- Aruna Malik ORCID: orcid.org/0000-0003-1136-6828 1 ,
- Samayveer Singh 1 &
- Al-Sakib Khan Pathan 2
174 Accesses
Explore all metrics
Multi-speaker text-to-speech synthesis involves generating unique speech patterns for individual speakers based on reference waveforms and input sequences of graphemes or phonemes. Various deep neural networks are trained for this task using a large amount of speech data recorded from a specific speaker to generate audio in their voice. The model requires a large dataset to retrain itself and learn about a new speaker not seen during training. This process is expensive in terms of time and resources. Thus, a key requirement of such techniques is to reduce time and resource consumption. In this paper, a multi-speaker text-to-speech synthesis using a generalized end-to-end loss function is developed, capable of generating speech in real-time for a given speech reference from a user and a text string as input. This method considers the speaker’s characteristics in the generated speech using the speech reference of their voice. The proposed method also assesses the effect on spontaneity and fluency in the generated language, corresponding to the speaker encoder, using the mean opinion score (MOS). However, a speaker encoder is trained with varying hours of the audio dataset, and it observes the effect on the produced speech. Furthermore, an extensive analysis is performed on the impact of the training dataset on the speaker encoder, corresponding to the generated speech, and various speaker encoder models for the speaker verification task. Based on loss function and Equal Error Rate (EER), advanced GRU is selected for generalized end-to-end loss function. The speaker verification regression test represents that the projected prototype can generate language, which the regression algorithm is able to distinguish into two sets: male and female while second test shows the above technique is able to distinguish speaker embeddings separately in clusters showing each speaker is uniquely identified. In terms of results, our proposed model achieved a MOS of 4.02 when trained on ‘Train Clean 100’, 3.74 on ‘Train-clean-360’, and 3.25 on ‘Train-clean-500’. The MOS test juxtaposes our method with prior models, demonstrating its superior performance. Conclusively, a cross-similarity matrix offers a visual representation of the similarity and disparity between utterances, underscoring the model’s robustness and efficacy.
This is a preview of subscription content, log in via an institution to check access.
Access this article
Price includes VAT (Russian Federation)
Instant access to the full article PDF.
Rent this article via DeepDyve
Institutional subscriptions
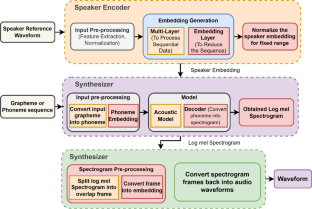
Similar content being viewed by others
Exploring Effective Speech Representation via ASR for High-Quality End-to-End Multispeaker TTS
Deep Variational Metric Learning for Transfer of Expressivity in Multispeaker Text to Speech
Pre-training Techniques for Improving Text-to-Speech Synthesis by Automatic Speech Recognition Based Data Enhancement
Data availability.
If you are interested in obtaining the data, please contact Owais Nazir at [email protected].
Zen H, Nose T, Yamagishi J, Sako S, Masuko T, Black AW, Tokuda K (2007) The HMM-based speech synthesis system (HTS) version 2.0. SSW 6:294–299
Google Scholar
Van den Oord A, Kalchbrenner N, Espeholt L, Vinyals O, Graves A (2016) Conditional image generation with pixelcnn decoders. Adv Neural Inf Process Syst 29:1–9. ArXiv, abs/1606.05328
Van Den Oord A, Kalchbrenner N, Kavukcuoglu K (2016) Pixel recurrent neural networks. In: International Conference on Machine Learning, MLR, pp. 1747–1756
Wang Y, Skerry-Ryan RJ, Stanton D, Wu Y, Weiss RJ, Jaitly N, Yang Z, Xiao Y, Chen Z, Bengio S, Le Q, Agiomyrgiannakis Y, Clark Y, Saurous RA, Saurous RA (2017) Tacotron: Towards end-to-end speech synthesis. arXiv preprint arXiv:1703.10135
Griffin D, Lim J (1984) Signal estimation from modified short-time Fourier transform. IEEE Trans Acoust Speech Signal Process 32(2):236–243
Article Google Scholar
Shen J, Pang R, Weiss RJ, Schuster M, Jaitly N, Yang Z, Chen Z, Zhang Y, Wang Y, Skerry-Ryan RJ, Saurous RA, Agiomyrgiannakis Y, Wu Y (2018) Natural tts synthesis by conditioning wavenet on mel spectrogram predictions. 2018 IEEE International Conference on Acoustics, Speech and Signal Processing (ICASSP), IEEE
Gibiansky A, Arik S, Diamos G, Miller J, Peng K, Ping W, ..., Zhou Y (2017) Deep voice 2: Multi-speaker neural text-to-speech. Adv Neural Inf Process Syst 30
Ping W, Peng K, Gibiansky A, Arik SÖ, Kannan A, Narang S, Raiman J, Miller J (2017) Deep Voice 3: 2000-Speaker Neural Text-to-Speech. https://arxiv.org/abs/1710.07654
Arik SO, Chrzanowski M, Coates A, Diamos G, Gibiansky A, Kang Y, Li X, Miller J, Ng A, Raiman J, Sengupta S, Shoeybi M (2017) Deep voice: Real-time neural text-to-speech. International Conference on Machine Learning, PMLR
Panayotov V et al (2015) Librispeech: an asr corpus based on public domain audio books. 2015 IEEE International Conference on Acoustics, Speech and Signal Processing (ICASSP), IEEE
Taigman Y, Wolf L, Polyak A, Nachmani E (2017) Voiceloop: voice fitting and synthesis via a phonological loop. arXiv preprint arXiv:1707.06588
Nachmani E et al (2018) Fitting new speakers based on a short untranscribed sample. International Conference on Machine Learning, PMLR
Jia Y, Zhang Y, Weiss RJ, Wang Q, Shen J, Ren F, Chen Z, Nguyen P, Pang R, Moreno IL, Wu Y (2018) Transfer learning from speaker verification to multispeaker text-to-speech synthesis. arXiv Preprint arXiv :180604558
Nazir O, Malik A (2021) Deep learning end to end speech synthesis: a review. 2021 2nd International Conference on Secure Cyber Computing and Communications (ICSCCC), pp 66–71. https://doi.org/10.1109/ICSCCC51823.2021.9478125
Oord AVD, Dieleman S, Zen H, Simonyan K, Vinyals O, Graves A, Kalchbrenner N, Senior A, Kavukcuoglu K (2016) Wavenet: a generative model for raw audio. arXiv Preprint arXiv :160903499
Kalchbrenner N, Oord A, Simonyan K, Danihelka I, Vinyals O, Graves A, Kavukcuoglu K (2017) Video pixel networks. In: International Conference on Machine Learning (pp. 1771–1779). PMLR
Oord A, Li Y, Babuschkin I, Simonyan K, Vinyals O, Kavukcuoglu K, Driessche G, Lockhart E, Cobo L, Stimberg F, Casagrande N (2018) Parallel wavenet: Fast high-fidelity speech synthesis. In: International Conference on Machine Learning, PMLR pp. 3918–3926
Zhang YJ, Pan S, He L, Ling ZH (2019) Learning latent representations for style control and transfer in end-to-end speech synthesis. In: ICASSP 2019–2019 IEEE International Conference on Acoustics, Speech and Signal Processing (ICASSP), IEEE, pp. 6945–6949
Kingma DP, Welling M (2013) Auto-encoding variational bayes. arXiv Preprint arXiv :13126114
Vainer J, Dušek O (2020) Speedyspeech: efficient neural speech synthesis. arXiv preprint arXiv:2008.03802
Wan L, Wang Q, Papir A, Moreno IL (2018) Generalized end-to-end loss for speaker verification. 2018 IEEE International Conference on Acoustics, Speech and Signal Processing (ICASSP), IEEE
Kuznetsova A, Sivaraman A, Kim M (2022) The potential of neural Speech synthesis-based Data Augmentation for Personalized Speech Enhancement. arXiv preprint arXiv:2211.07493
Resna S, Rajan R (2022) Multi-voice singing synthesis from lyrics. Circuits Syst Signal Process 1–15
Heigold G et al (2016) End-to-end text-dependent speaker verification. 2016 IEEE International Conference on Acoustics, Speech and Signal Processing (ICASSP), IEEE
Variani E, Lei X, McDermott E, Moreno IL, Dominguez JG (2014) Deep neural networks for small footprint text-dependent speaker verification. 2014 IEEE International Conference on Acoustics, Speech and Signal Processing (ICASSP) IEEE,
Kiranyaz S, Avci O, Abdeljaber O, Ince T, Gabbouj M, Inman DJ (2021) 1D convolutional neural networks and applications: a survey. Mech Syst Signal Process 151:107398
Chung J, Gulcehre C, Cho K, Bengio Y (2014) Empirical evaluation of gated recurrent neural networks on sequence modeling. arXiv Preprint arXiv :14123555
Nagrani A, Chung JS, Zisserman A (2017) Voxceleb: a large-scale speaker identification dataset. arXiv Preprint arXiv :170608612
Chung JS, Nagrani A, Zisserman A (2018) Voxceleb2: Deep speaker recognition. arXiv preprint arXiv:1806.05622
Kingma DP, Ba J (2014) Adam: A method for stochastic optimization. arXiv Preprint arXiv :14126980
McInnes L, Healy J, Melville J (2018) Umap: uniform manifold approximation and projection for dimension reduction. arXiv preprint arXiv:1802.03426
Wang Y, Skerry-Ryan R, Stanton D, Wu Y, Weiss RJ, Jaitly N, Yang Z, Xiao Y, Chen Z, Bengio S, Le Q, Agiomyrgiannakis Y, Clark R, Saurous RA (2017) Tacotron Towards end-to-end speech synthesis. In: Interspeech 2017, 18th Annual Conference of the International Speech Communication Association, Stockholm, Sweden, August 20–24, 2017, ISCA, pp 4006–4010. https://doi.org/10.21437/interspeech.2017-1452
Shen J, Pang R, Weiss R, Schuster M, Jaitly N, Yang Z, Chen Z, Zhang Y, Wang Y, Skerry-Ryan R, Saurous R, Agiomyrgiannakis Y, Wu Y (2018) Natural tts synthesis by conditioning wavenet on mel spectrogram predictions. 2018 IEEE International Conference on Acoustics, Speech and Signal Processing (ICASSP), pp 4779– 4783. https://doi.org/10.1109/icassp.2018.8461368
Chen L, Ren J, Chen P et al (2022) Limited text speech synthesis with electroglottograph based on Bi-LSTM and modified Tacotron-2. Appl Intell 52:15193–15209. https://doi.org/10.1007/s10489-021-03075-x
Download references
Author information
Authors and affiliations.
Department of Computer Science & Engineering, Dr B R Ambedkar National Institute of Technology Jalandhar, Jalandhar, Punjab, India
Owais Nazir, Aruna Malik & Samayveer Singh
Department of Computer Science and Engineering, United International University, Dhaka, Bangladesh
Al-Sakib Khan Pathan
You can also search for this author in PubMed Google Scholar
Corresponding author
Correspondence to Aruna Malik .
Ethics declarations
Conflict of interest.
The authors affirm that they do not have any conflicts of interest.
Additional information
Publisher’s note.
Springer Nature remains neutral with regard to jurisdictional claims in published maps and institutional affiliations.
Rights and permissions
Springer Nature or its licensor (e.g. a society or other partner) holds exclusive rights to this article under a publishing agreement with the author(s) or other rightsholder(s); author self-archiving of the accepted manuscript version of this article is solely governed by the terms of such publishing agreement and applicable law.
Reprints and permissions
About this article
Nazir, O., Malik, A., Singh, S. et al. Multi speaker text-to-speech synthesis using generalized end-to-end loss function. Multimed Tools Appl (2024). https://doi.org/10.1007/s11042-024-18121-2
Download citation
Received : 05 July 2023
Revised : 01 November 2023
Accepted : 01 January 2024
Published : 13 January 2024
DOI : https://doi.org/10.1007/s11042-024-18121-2
Share this article
Anyone you share the following link with will be able to read this content:
Sorry, a shareable link is not currently available for this article.
Provided by the Springer Nature SharedIt content-sharing initiative
- Loss function
- Multi-speaker
- Speech reference
- Text-to-speech
- Find a journal
- Publish with us
- Track your research
Thank you for visiting nature.com. You are using a browser version with limited support for CSS. To obtain the best experience, we recommend you use a more up to date browser (or turn off compatibility mode in Internet Explorer). In the meantime, to ensure continued support, we are displaying the site without styles and JavaScript.
- View all journals
- My Account Login
- Explore content
- About the journal
- Publish with us
- Sign up for alerts
- Open access
- Published: 08 April 2024
A neural speech decoding framework leveraging deep learning and speech synthesis
- Xupeng Chen 1 na1 ,
- Ran Wang 1 na1 ,
- Amirhossein Khalilian-Gourtani ORCID: orcid.org/0000-0003-1376-9583 2 ,
- Leyao Yu 2 , 3 ,
- Patricia Dugan 2 ,
- Daniel Friedman 2 ,
- Werner Doyle 4 ,
- Orrin Devinsky 2 ,
- Yao Wang ORCID: orcid.org/0000-0003-3199-3802 1 , 3 na2 &
- Adeen Flinker ORCID: orcid.org/0000-0003-1247-1283 2 , 3 na2
Nature Machine Intelligence ( 2024 ) Cite this article
236 Accesses
75 Altmetric
Metrics details
- Neural decoding
A preprint version of the article is available at bioRxiv.
Decoding human speech from neural signals is essential for brain–computer interface (BCI) technologies that aim to restore speech in populations with neurological deficits. However, it remains a highly challenging task, compounded by the scarce availability of neural signals with corresponding speech, data complexity and high dimensionality. Here we present a novel deep learning-based neural speech decoding framework that includes an ECoG decoder that translates electrocorticographic (ECoG) signals from the cortex into interpretable speech parameters and a novel differentiable speech synthesizer that maps speech parameters to spectrograms. We have developed a companion speech-to-speech auto-encoder consisting of a speech encoder and the same speech synthesizer to generate reference speech parameters to facilitate the ECoG decoder training. This framework generates natural-sounding speech and is highly reproducible across a cohort of 48 participants. Our experimental results show that our models can decode speech with high correlation, even when limited to only causal operations, which is necessary for adoption by real-time neural prostheses. Finally, we successfully decode speech in participants with either left or right hemisphere coverage, which could lead to speech prostheses in patients with deficits resulting from left hemisphere damage.
Similar content being viewed by others
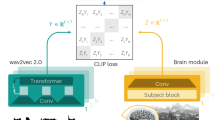
Decoding speech perception from non-invasive brain recordings
Alexandre Défossez, Charlotte Caucheteux, … Jean-Rémi King
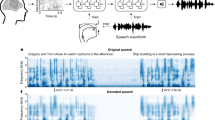
Speech synthesis from neural decoding of spoken sentences
Gopala K. Anumanchipalli, Josh Chartier & Edward F. Chang
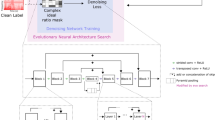
Restoring speech intelligibility for hearing aid users with deep learning
Peter Udo Diehl, Yosef Singer, … Veit M. Hofmann
Speech loss due to neurological deficits is a severe disability that limits both work life and social life. Advances in machine learning and brain–computer interface (BCI) systems have pushed the envelope in the development of neural speech prostheses to enable people with speech loss to communicate 1 , 2 , 3 , 4 , 5 . An effective modality for acquiring data to develop such decoders involves electrocorticographic (ECoG) recordings obtained in patients undergoing epilepsy surgery 4 , 5 , 6 , 7 , 8 , 9 , 10 . Implanted electrodes in patients with epilepsy provide a rare opportunity to collect cortical data during speech with high spatial and temporal resolution, and such approaches have produced promising results in speech decoding 4 , 5 , 8 , 9 , 10 , 11 .
Two challenges are inherent to successfully carrying out speech decoding from neural signals. First, the data to train personalized neural-to-speech decoding models are limited in duration, and deep learning models require extensive training data. Second, speech production varies in rate, intonation, pitch and so on, even within a single speaker producing the same word, complicating the underlying model representation 12 , 13 . These challenges have led to diverse speech decoding approaches with a range of model architectures. Currently, public code to test and replicate findings across research groups is limited in availability.
Earlier approaches to decoding and synthesizing speech spectrograms from neural signals focused on linear models. These approaches achieved a Pearson correlation coefficient (PCC) of ~0.6 or lower, but with simple model architectures that are easy to interpret and do not require large training datasets 14 , 15 , 16 . Recent research has focused on deep neural networks leveraging convolutional 8 , 9 and recurrent 5 , 10 , 17 network architectures. These approaches vary across two major dimensions: the intermediate latent representation used to model speech and the speech quality produced after synthesis. For example, cortical activity has been decoded into an articulatory movement space, which is then transformed into speech, providing robust decoding performance but with a non-natural synthetic voice reconstruction 17 . Conversely, some approaches have produced naturalistic reconstruction leveraging wavenet vocoders 8 , generative adversarial networks (GAN) 11 and unit selection 18 , but achieve limited accuracy. A recent study in one implanted patient 19 provided both robust accuracies and a naturalistic speech waveform by leveraging quantized HuBERT features 20 as an intermediate representation space and a pretrained speech synthesizer that converts the HuBERT features into speech. However, HuBERT features do not carry speaker-dependent acoustic information and can only be used to generate a generic speaker’s voice, so they require a separate model to translate the generic voice to a specific patient’s voice. Furthermore, this study and most previous approaches have employed non-causal architectures, which may limit real-time applications, which typically require causal operations.
To address these issues, in this Article we present a novel ECoG-to-speech framework with a low-dimensional intermediate representation guided by subject-specific pre-training using speech signal only (Fig. 1 ). Our framework consists of an ECoG decoder that maps the ECoG signals to interpretable acoustic speech parameters (for example, pitch, voicing and formant frequencies), as well as a speech synthesizer that translates the speech parameters to a spectrogram. The speech synthesizer is differentiable, enabling us to minimize the spectrogram reconstruction error during training of the ECoG decoder. The low-dimensional latent space, together with guidance on the latent representation generated by a pre-trained speech encoder, overcomes data scarcity issues. Our publicly available framework produces naturalistic speech that highly resembles the speaker’s own voice, and the ECoG decoder can be realized with different deep learning model architectures and using different causality directions. We report this framework with multiple deep architectures (convolutional, recurrent and transformer) as the ECoG decoder, and apply it to 48 neurosurgical patients. Our framework performs with high accuracy across the models, with the best performance obtained by the convolutional (ResNet) architecture (PCC of 0.806 between the original and decoded spectrograms). Our framework can achieve high accuracy using only causal processing and relatively low spatial sampling on the cortex. We also show comparable speech decoding from grid implants on the left and right hemispheres, providing a proof of concept for neural prosthetics in patients suffering from expressive aphasia (with damage limited to the left hemisphere), although such an approach must be tested in patients with damage to the left hemisphere. Finally, we provide a publicly available neural decoding pipeline ( https://github.com/flinkerlab/neural_speech_decoding ) that offers flexibility in ECoG decoding architectures to push forward research across the speech science and prostheses communities.
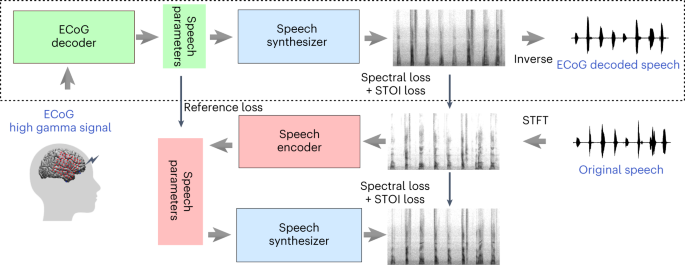
The upper part shows the ECoG-to-speech decoding pipeline. The ECoG decoder generates time-varying speech parameters from ECoG signals. The speech synthesizer generates spectrograms from the speech parameters. A separate spectrogram inversion algorithm converts the spectrograms to speech waveforms. The lower part shows the speech-to-speech auto-encoder, which generates the guidance for the speech parameters to be produced by the ECoG decoder during its training. The speech encoder maps an input spectrogram to the speech parameters, which are then fed to the same speech synthesizer to reproduce the spectrogram. The speech encoder and a few learnable subject-specific parameters in the speech synthesizer are pre-trained using speech signals only. Only the upper part is needed to decode the speech from ECoG signals once the pipeline is trained.
ECoG-to-speech decoding framework
Our ECoG-to-speech framework consists of an ECoG decoder and a speech synthesizer (shown in the upper part of Fig. 1 ). The neural signals are fed into an ECoG decoder, which generates speech parameters, followed by a speech synthesizer, which translates the parameters into spectrograms (which are then converted to a waveform by the Griffin–Lim algorithm 21 ). The training of our framework comprises two steps. We first use semi-supervised learning on the speech signals alone. An auto-encoder, shown in the lower part of Fig. 1 , is trained so that the speech encoder derives speech parameters from a given spectrogram, while the speech synthesizer (used here as the decoder) reproduces the spectrogram from the speech parameters. Our speech synthesizer is fully differentiable and generates speech through a weighted combination of voiced and unvoiced speech components generated from input time series of speech parameters, including pitch, formant frequencies, loudness and so on. The speech synthesizer has only a few subject-specific parameters, which are learned as part of the auto-encoder training (more details are provided in the Methods Speech synthesizer section). Currently, our speech encoder and speech synthesizer are subject-specific and can be trained using any speech signal of a participant, not just those with corresponding ECoG signals.
In the next step, we train the ECoG decoder in a supervised manner based on ground-truth spectrograms (using measures of spectrogram difference and short-time objective intelligibility, STOI 8 , 22 ), as well as guidance for the speech parameters generated by the pre-trained speech encoder (that is, reference loss between speech parameters). By limiting the number of speech parameters (18 at each time step; Methods section Summary of speech parameters ) and using the reference loss, the ECoG decoder can be trained with limited corresponding ECoG and speech data. Furthermore, because our speech synthesizer is differentiable, we can back-propagate the spectral loss (differences between the original and decoded spectrograms) to update the ECoG decoder. We provide multiple ECoG decoder architectures to choose from, including 3D ResNet 23 , 3D Swin Transformer 24 and LSTM 25 . Importantly, unlike many methods in the literature, we employ ECoG decoders that can operate in a causal manner, which is necessary for real-time speech generation from neural signals. Note that, once the ECoG decoder and speech synthesizer are trained, they can be used for ECoG-to-speech decoding without using the speech encoder.
Data collection
We employed our speech decoding framework across N = 48 participants who consented to complete a series of speech tasks (Methods section Experiments design). These participants, as part of their clinical care, were undergoing treatment for refractory epilepsy with implanted electrodes. During the hospital stay, we acquired synchronized neural and acoustic speech data. ECoG data were obtained from five participants with hybrid-density (HB) sampling (clinical-research grid) and 43 participants with low-density (LD) sampling (standard clinical grid), who took part in five speech tasks: auditory repetition (AR), auditory naming (AN), sentence completion (SC), word reading (WR) and picture naming (PN). These tasks were designed to elicit the same set of spoken words across tasks while varying the stimulus modality. We provided 50 repeated unique words (400 total trials per participant), all of which were analysed locked to the onset of speech production. We trained a model for each participant using 80% of available data for that participant and evaluated the model on the remaining 20% of data (with the exception of the more stringent word-level cross-validation).
Speech decoding performance and causality
We first aimed to directly compare the decoding performance across different architectures, including those that have been employed in the neural speech decoding literature (recurrent and convolutional) and transformer-based models. Although any decoder architecture could be used for the ECoG decoder in our framework, employing the same speech encoder guidance and speech synthesizer, we focused on three representative models for convolution (ResNet), recurrent (LSTM) and transformer (Swin) architectures. Note that any of these models can be configured to use temporally non-causal or causal operations. Our results show that ResNet outperformed the other models, providing the highest PCC across N = 48 participants (mean PCC = 0.806 and 0.797 for non-causal and causal, respectively), closely followed by Swin (mean PCC = 0.792 and 0.798 for non-causal and causal, respectively) (Fig. 2a ). We found the same when evaluating the three models using STOI+ (ref. 26 ), as shown in Supplementary Fig. 1a . The causality of machine learning models for speech production has important implications for BCI applications. A causal model only uses past and current neural signals to generate speech, whereas non-causal models use past, present and future neural signals. Previous reports have typically employed non-causal models 5 , 8 , 10 , 17 , which can use neural signals related to the auditory and speech feedback that is unavailable in real-time applications. Optimally, only the causal direction should be employed. We thus compared the performance of the same models with non-causal and causal temporal operations. Figure 2a compares the decoding results of causal and non-causal versions of our models. The causal ResNet model (PCC = 0.797) achieved a performance comparable to that of the non-causal model (PCC = 0.806), with no significant differences between the two (Wilcoxon two-sided signed-rank test P = 0.093). The same was true for the causal Swin model (PCC = 0.798) and its non-causal (PCC = 0.792) counterpart (Wilcoxon two-sided signed-rank test P = 0.196). In contrast, the performance of the causal LSTM model (PCC = 0.712) was significantly inferior to that of its non-causal (PCC = 0.745) version (Wilcoxon two-sided signed-rank test P = 0.009). Furthermore, the LSTM model showed consistently lower performance than ResNet and Swin. However, we did not find significant differences between the causal ResNet and causal Swin performances (Wilcoxon two-sided signed-rank test P = 0.587). Because the ResNet and Swin models had the highest performance and were on par with each other and their causal counterparts, we chose to focus further analyses on these causal models, which we believe are best suited for prosthetic applications.
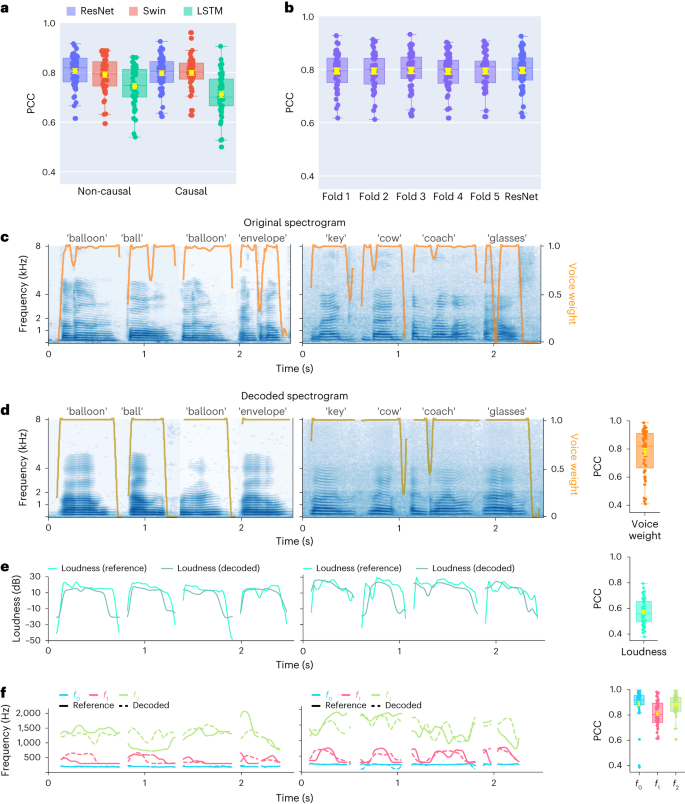
a , Performances of ResNet, Swin and LSTM models with non-causal and causal operations. The PCC between the original and decoded spectrograms is evaluated on the held-out testing set and shown for each participant. Each data point corresponds to a participant’s average PCC across testing trials. b , A stringent cross-validation showing the performance of the causal ResNet model on unseen words during training from five folds; we ensured that the training and validation sets in each fold did not overlap in unique words. The performance across all five validation folds was comparable to our trial-based validation, denoted for comparison as ResNet (identical to the ResNet causal model in a ). c – f , Examples of decoded spectrograms and speech parameters from the causal ResNet model for eight words (from two participants) and the PCC values for the decoded and reference speech parameters across all participants. Spectrograms of the original ( c ) and decoded ( d ) speech are shown, with orange curves overlaid representing the reference voice weight learned by the speech encoder ( c ) and the decoded voice weight from the ECoG decoder ( d ). The PCC between the decoded and reference voice weights is shown on the right across all participants. e , Decoded and reference loudness parameters for the eight words, and the PCC values of the decoded loudness parameters across participants (boxplot on the right). f , Decoded (dashed) and reference (solid) parameters for pitch ( f 0 ) and the first two formants ( f 1 and f 2 ) are shown for the eight words, as well as the PCC values across participants (box plots to the right). All box plots depict the median (horizontal line inside the box), 25th and 75th percentiles (box) and 25th or 75th percentiles ± 1.5 × interquartile range (whiskers) across all participants ( N = 48). Yellow error bars denote the mean ± s.e.m. across participants.
Source data
To ensure our framework can generalize well to unseen words, we added a more stringent word-level cross-validation in which random (ten unique) words were entirely held out during training (including both pre-training of the speech encoder and speech synthesizer and training of the ECoG decoder). This ensured that different trials from the same word could not appear in both the training and testing sets. The results shown in Fig. 2b demonstrate that performance on the held-out words is comparable to our standard trial-based held-out approach (Fig. 2a , ‘ResNet’). It is encouraging that the model can decode unseen validation words well, regardless of which words were held out during training.
Next, we show the performance of the ResNet causal decoder on the level of single words across two representative participants (LD grids). The decoded spectrograms accurately preserve the spectro-temporal structure of the original speech (Fig. 2c,d ). We also compare the decoded speech parameters with the reference parameters. For each parameter, we calculated the PCC between the decoded time series and the reference sequence, showing average PCC values of 0.781 (voice weight, Fig. 2d ), 0.571 (loudness, Fig. 2e ), 0.889 (pitch f 0 , Fig. 2f ), 0.812 (first formant f 1 , Fig. 2f ) and 0.883 (second formant f 2 , Fig. 2f ). Accurate reconstruction of the speech parameters, especially the pitch, voice weight and first two formants, is essential for accurate speech decoding and naturalistic reconstruction that mimics a participant’s voice. We also provide a non-causal version of Fig. 2 in Supplementary Fig. 2 . The fact that both non-causal and causal models can yield reasonable decoding results is encouraging.
Left-hemisphere versus right-hemisphere decoding
Most speech decoding studies have focused on the language- and speech-dominant left hemisphere 27 . However, little is known about decoding speech representations from the right hemisphere. To this end, we compared left- versus right-hemisphere decoding performance across our participants to establish the feasibility of a right-hemisphere speech prosthetic. For both our ResNet and Swin decoders, we found robust speech decoding from the right hemisphere (ResNet PCC = 0.790, Swin PCC = 0.798) that was not significantly different from that of the left (Fig. 3a , ResNet independent t -test, P = 0.623; Swin independent t -test, P = 0.968). A similar conclusion held when evaluating STOI+ (Supplementary Fig. 1b , ResNet independent t -test, P = 0.166; Swin independent t -test, P = 0.114). Although these results suggest that it may be feasible to use neural signals in the right hemisphere to decode speech for patients who suffer damage to the left hemisphere and are unable to speak 28 , it remains unknown whether intact left-hemisphere cortex is necessary to allow for speech decoding from the right hemisphere until tested in such patients.
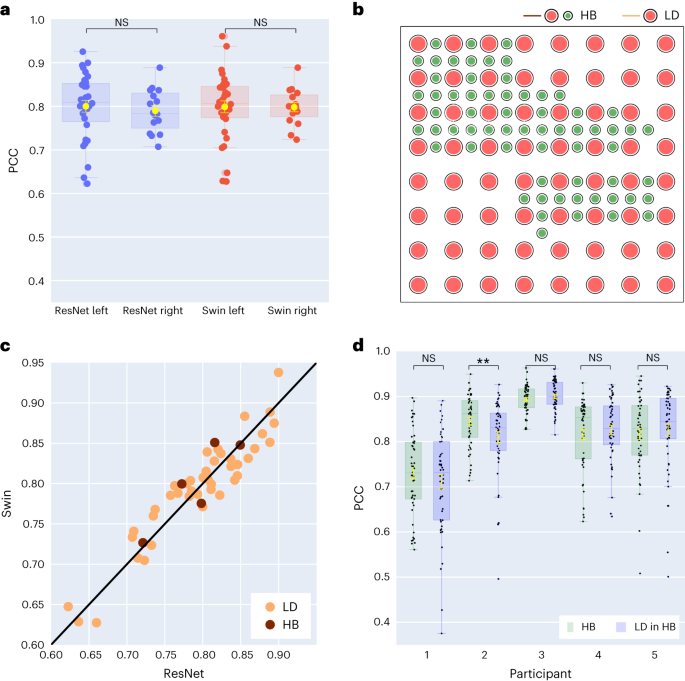
a , Comparison between left- and right-hemisphere participants using causal models. No statistically significant differences (ResNet independent t -test, P = 0.623; Swin Wilcoxon independent t -test, P = 0.968) in PCC values exist between left- ( N = 32) and right- ( N = 16) hemisphere participants. b , An example hybrid-density ECoG array with a total of 128 electrodes. The 64 electrodes marked in red correspond to a LD placement. The remaining 64 green electrodes, combined with red electrodes, reflect HB placement. c , Comparison between causal ResNet and causal Swin models for the same participant across participants with HB ( N = 5) or LD ( N = 43) ECoG grids. The two models show similar decoding performances from the HB and LD grids. d , Decoding PCC values across 50 test trials by the ResNet model for HB ( N = 5) participants when all electrodes are used versus when only LD-in-HB electrodes ( N = 5) are considered. There are no statistically significant differences for four out of five participants (Wilcoxon two-sided signed-rank test, P = 0.114, 0.003, 0.0773, 0.472 and 0.605, respectively). All box plots depict the median (horizontal line inside box), 25th and 75th percentiles (box) and 25th or 75th percentiles ± 1.5 × interquartile range (whiskers). Yellow error bars denote mean ± s.e.m. Distributions were compared with each other as indicated, using the Wilcoxon two-sided signed-rank test and independent t -test. ** P < 0.01; NS, not significant.
Effect of electrode density
Next, we assessed the impact of electrode sampling density on speech decoding, as many previous reports use higher-density grids (0.4 mm) with more closely spaced contacts than typical clinical grids (1 cm). Five participants consented to hybrid grids (Fig. 3b , HB), which typically had LD electrode sampling but with additional electrodes interleaved. The HB grids provided a decoding performance similar to clinical LD grids in terms of PCC values (Fig. 3c ), with a slight advantage in STOI+, as shown in Supplementary Fig. 3b . To ascertain whether the additional spatial sampling indeed provides improved speech decoding, we compared models that decode speech based on all the hybrid electrodes versus only the LD electrodes in participants with HB grids (comparable to our other LD participants). Our findings (Fig. 3d ) suggest that the decoding results were not significantly different from each other (with the exception of participant 2) in terms of PCC and STOI+ (Supplementary Fig. 3c ). Together, these results suggest that our models can learn speech representations well from both high and low spatial sampling of the cortex, with the exciting finding of robust speech decoding from the right hemisphere.
Contribution analysis
Finally, we investigated which cortical regions contribute to decoding to provide insight for the targeted implantation of future prosthetics, especially on the right hemisphere, which has not yet been investigated. We used an occlusion approach to quantify the contributions of different cortical sites to speech decoding. If a region is involved in decoding, occluding the neural signal in the corresponding electrode (that is, setting the signal to zero) will reduce the accuracy (PCC) of the speech reconstructed on testing data (Methods section Contribution analysis ). We thus measured each region’s contribution by decoding the reduction in the PCC when the corresponding electrode was occluded. We analysed all electrodes and participants with causal and non-causal versions of the ResNet and Swin decoders. The results in Fig. 4 show similar contributions for the ResNet and Swin models (Supplementary Figs. 8 and 9 describe the noise-level contribution). The non-causal models show enhanced auditory cortex contributions compared with the causal models, implicating auditory feedback in decoding, and underlying the importance of employing only causal models during speech decoding because neural feedback signals are not available for real-time decoding applications. Furthermore, across the causal models, both the right and left hemispheres show similar contributions across the sensorimotor cortex, especially on the ventral portion, suggesting the potential feasibility of right-hemisphere neural prosthetics.
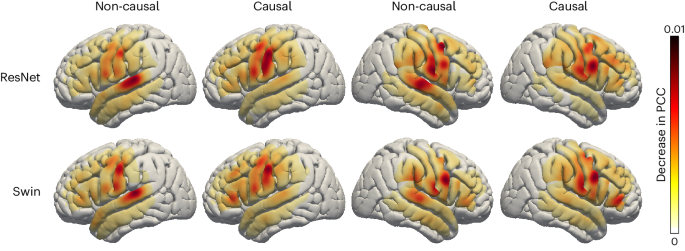
Visualization of the contribution of each cortical location to the decoding result achieved by both causal and non-causal decoding models through an occlusion analysis. The contribution of each electrode region in each participant is projected onto the standardized Montreal Neurological Institute (MNI) brain anatomical map and then averaged over all participants. Each subplot shows the causal or non-causal contribution of different cortical locations (red indicates a higher contribution; yellow indicates a lower contribution). For visualization purposes, we normalized the contribution of each electrode location by the local grid density, because there were multiple participants with non-uniform density.
Our novel pipeline can decode speech from neural signals by leveraging interchangeable architectures for the ECoG decoder and a novel differentiable speech synthesizer (Fig. 5 ). Our training process relies on estimating guidance speech parameters from the participants’ speech using a pre-trained speech encoder (Fig. 6a ). This strategy enabled us to train ECoG decoders with limited corresponding speech and neural data, which can produce natural-sounding speech when paired with our speech synthesizer. Our approach was highly reproducible across participants ( N = 48), providing evidence for successful causal decoding with convolutional (ResNet; Fig. 6c ) and transformer (Swin; Fig. 6d ) architectures, both of which outperformed the recurrent architecture (LSTM; Fig. 6e ). Our framework can successfully decode from both high and low spatial sampling with high levels of decoding performance. Finally, we provide potential evidence for robust speech decoding from the right hemisphere as well as the spatial contribution of cortical structures to decoding across the hemispheres.
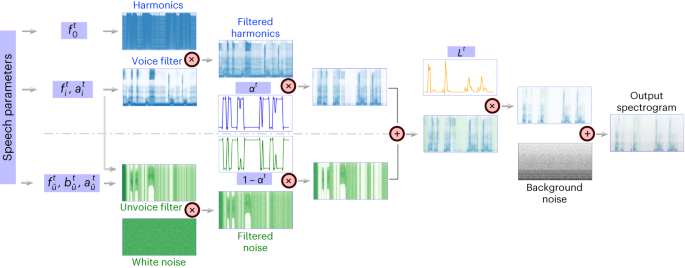
Our speech synthesizer generates the spectrogram at time t by combining a voiced component and an unvoiced component based on a set of speech parameters at t . The upper part represents the voice pathway, which generates the voiced component by passing a harmonic excitation with fundamental frequency \({f}_{0}^{\;t}\) through a voice filter (which is the sum of six formant filters, each specified by formant frequency \({f}_{i}^{\;t}\) and amplitude \({a}_{i}^{t}\) ). The lower part describes the noise pathway, which synthesizes the unvoiced sound by passing white noise through an unvoice filter (consisting of a broadband filter defined by centre frequency \({f}_{\hat{u}}^{\;t}\) , bandwidth \({b}_{\hat{u}}^{t}\) and amplitude \({a}_{\hat{u}}^{t}\) , and the same six formant filters used for the voice filter). The two components are next mixed with voice weight α t and unvoice weight 1 − α t , respectively, and then amplified by loudness L t . A background noise (defined by a stationary spectrogram B ( f )) is finally added to generate the output spectrogram. There are a total of 18 speech parameters at any time t , indicated in purple boxes.
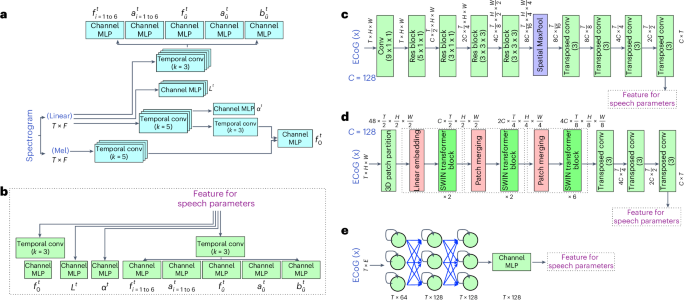
a , The speech encoder architecture. We input a spectrogram into a network of temporal convolution layers and channel MLPs that produce speech parameters. b , c , The ECoG decoder ( c ) using the 3D ResNet architecture. We first use several temporal and spatial convolutional layers with residual connections and spatiotemporal pooling to generate downsampled latent features, and then use corresponding transposed temporal convolutional layers to upsample the features to the original temporal dimension. We then apply temporal convolution layers and channel MLPs to map the features to speech parameters, as shown in b . The non-causal version uses non-causal temporal convolution in each layer, whereas the causal version uses causal convolution. d , The ECoG decoder using the 3D Swin architecture. We use three or four stages of 3D Swin blocks with spatial-temporal attention (three blocks for LD and four blocks for HB) to extract the features from the ECoG signal. We then use the transposed versions of temporal convolution layers as in c to upsample the features. The resulting features are mapped to the speech parameters using the same structure as shown in b . Non-causal versions apply temporal attention to past, present and future tokens, whereas the causal version applies temporal attention only to past and present tokens. e , The ECoG decoder using LSTM layers. We use three LSTM layers and one layer of channel MLP to generate features. We then reuse the prediction layers in b to generate the corresponding speech parameters. The non-causal version employs bidirectional LSTM in each layer, whereas the causal version uses unidirectional LSTM.
Our decoding pipeline showed robust speech decoding across participants, leading to PCC values within the range 0.62–0.92 (Fig. 2a ; causal ResNet mean 0.797, median 0.805) between the decoded and ground-truth speech across several architectures. We attribute our stable training and accurate decoding to the carefully designed components of our pipeline (for example, the speech synthesizer and speech parameter guidance) and the multiple improvements ( Methods sections Speech synthesizer , ECoG decoder and Model training ) over our previous approach on the subset of participants with hybrid-density grids 29 . Previous reports have investigated speech- or text-decoding using linear models 14 , 15 , 30 , transitional probability 4 , 31 , recurrent neural networks 5 , 10 , 17 , 19 , convolutional neural networks 8 , 29 and other hybrid or selection approaches 9 , 16 , 18 , 32 , 33 . Overall, our results are similar to (or better than) many previous reports (54% of our participants showed higher than 0.8 for the decoding PCC; Fig. 3c ). However, a direct comparison is complicated by multiple factors. Previous reports vary in terms of the reported performance metrics, as well as the stimuli decoded (for example, continuous speech versus single words) and the cortical sampling (that is, high versus low density, depth electrodes compared with surface grids). Our publicly available pipeline, which can be used across multiple neural network architectures and tested on various performance metrics, can facilitate the research community to conduct more direct comparisons while still adhering to a high accuracy of speech decoding.
The temporal causality of decoding operations, critical for real-time BCI applications, has not been considered by most previous studies. Many of these non-causal models relied on auditory (and somatosensory) feedback signals. Our analyses show that non-causal models rely on a robust contribution from the superior temporal gyrus (STG), which is mostly eliminated using a causal model (Fig. 4 ). We believe that non-causal models would show limited generalizability to real-time BCI applications due to their over-reliance on feedback signals, which may be absent (if no delay is allowed) or incorrect (if a short latency is allowed during real-time decoding). Some approaches used imagined speech, which avoids feedback during training 16 , or showed generalizability to mimed production lacking auditory feedback 17 , 19 . However, most reports still employ non-causal models, which cannot rule out feedback during training and inference. Indeed, our contribution maps show robust auditory cortex recruitment for the non-causal ResNet and Swin models (Fig. 4 , in contrast to their causal counterparts, which decode based on more frontal regions. Furthermore, the recurrent neural networks that are widely used in the literature 5 , 19 are typically bidirectional, producing non-causal behaviours and longer latencies for prediction during real-time applications. Unidirectional causal results are typically not reported. The recurrent network we tested performed the worst when trained with one direction (Fig. 2a , causal LSTM). Although our current focus was not real-time decoding, we were able to synthesize speech from neural signals with a delay of under 50 ms (Supplementary Table 1 ), which provides minimal auditory delay interference and allows for normal speech production 34 , 35 . Our data suggest that causal convolutional and transformer models can perform on par with their non-causal counterparts and recruit more relevant cortical structures for real-time decoding.
In our study we have leveraged an intermediate speech parameter space together with a novel differentiable speech synthesizer to decode subject-specific naturalistic speech (Fig. 1 . Previous reports used varying approaches to model speech, including an intermediate kinematic space 17 , an acoustically relevant intermediate space using HuBERT features 19 derived from a self-supervised speech masked prediction task 20 , an intermediate random vector (that is, GAN) 11 or direct spectrogram representations 8 , 17 , 36 , 37 . Our choice of speech parameters as the intermediate representation allowed us to decode subject-specific acoustics. Our intermediate acoustic representation led to significantly more accurate speech decoding than directly mapping ECoG to the speech spectrogram 38 , and than mapping ECoG to a random vector, which is then fed to a GAN-based speech synthesizer 11 (Supplementary Fig. 10 ). Unlike the kinematic representation, our acoustic intermediate representation using speech parameters and the associated speech synthesizer enables our decoding pipeline to produce natural-sounding speech that preserves subject-specific characteristics, which would be lost with the kinematic representation.
Our speech synthesizer is motivated by classical vocoder models for speech production (generating speech by passing an excitation source, harmonic or noise, through a filter 39 , 40 and is fully differentiable, facilitating the training of the ECoG decoder using spectral losses through backpropagation. Furthermore, the guidance speech parameters needed for training the ECoG decoder can be obtained using a speech encoder that can be pre-trained without requiring neural data. Thus, it could be trained using older speech recordings or a proxy speaker chosen by the patient in the case of patients without the ability to speak. Training the ECoG decoder using such guidance, however, would require us to revise our current training strategy to overcome the challenge of misalignment between neural signals and speech signals, which is a scope of our future work. Additionally, the low-dimensional acoustic space and pre-trained speech encoder (for generating the guidance) using speech signals only alleviate the limited data challenge in training the ECoG-to-speech decoder and provide a highly interpretable latent space. Finally, our decoding pipeline is generalizable to unseen words (Fig. 2b ). This provides an advantage compared to the pattern-matching approaches 18 that produce subject-specific utterances but with limited generalizability.
Many earlier studies employed high-density electrode coverage over the cortex, providing many distinct neural signals 5 , 10 , 17 , 30 , 37 . One question we directly addressed was whether higher-density coverage improves decoding. Surprisingly, we found a high decoding performance in terms of spectrogram PCC with both low-density and higher (hybrid) density grid coverages (Fig. 3c ). Furthermore, comparing the decoding performance obtained using all electrodes in our hybrid-density participants versus using only the low-density electrodes in the same participants revealed that the decoding did not differ significantly (albeit for one participant; Fig. 3d ). We attribute these results to the ability of our ECoG decoder to extract speech parameters from neural signals as long as there is sufficient perisylvian coverage, even in low-density participants.
A striking result was the robust decoding from right hemisphere cortical structures as well as the clear contribution of the right perisylvian cortex. Our results are consistent with the idea that syllable-level speech information is represented bilaterally 41 . However, our findings suggest that speech information is well-represented in the right hemisphere. Our decoding results could directly lead to speech prostheses for patients who suffer from expressive aphasia or apraxia of speech. Some previous studies have shown limited right-hemisphere decoding of vowels 42 and sentences 43 . However, the results were mostly mixed with left-hemisphere signals. Although our decoding results provide evidence for a robust representation of speech in the right hemisphere, it is important to note that these regions are likely not critical for speech, as evidenced by the few studies that have probed both hemispheres using electrical stimulation mapping 44 , 45 . Furthermore, it is unclear whether the right hemisphere would contain sufficient information for speech decoding if the left hemisphere were damaged. It would be necessary to collect right-hemisphere neural data from left-hemisphere-damaged patients to verify we can still achieve acceptable speech decoding. However, we believe that right-hemisphere decoding is still an exciting avenue as a clinical target for patients who are unable to speak due to left-hemisphere cortical damage.
There are several limitations in our study. First, our decoding pipeline requires speech training data paired with ECoG recordings, which may not exist for paralysed patients. This could be mitigated by using neural recordings during imagined or mimed speech and the corresponding older speech recordings of the patient or speech by a proxy speaker chosen by the patient. As discussed earlier, we would need to revise our training strategy to overcome the temporal misalignment between the neural signal and the speech signal. Second, our ECoG decoder models (3D ResNet and 3D Swin) assume a grid-based electrode sampling, which may not be the case. Future work should develop model architectures that are capable of handling non-grid data, such as strips and depth electrodes (stereo intracranial electroencephalogram (sEEG)). Importantly, such decoders could replace our current grid-based ECoG decoders while still being trained using our overall pipeline. Finally, our focus in this study was on word-level decoding limited to a vocabulary of 50 words, which may not be directly comparable to sentence-level decoding. Specifically, two recent studies have provided robust speech decoding in a few chronic patients implanted with intracranial ECoG 19 or a Utah array 46 that leveraged a large amount of data available in one patient in each study. It is noteworthy that these studies use a range of approaches in constraining their neural predictions. Metzger and colleagues employed a pre-trained large transformer model leveraging directional attention to provide the guidance HuBERT features for their ECoG decoder. In contrast, Willet and colleagues decoded at the level of phonemes and used transition probability models at both phoneme and word levels to constrain decoding. Our study is much more limited in terms of data. However, we were able to achieve good decoding results across a large cohort of patients through the use of a compact acoustic representation (rather than learnt contextual information). We expect that our approach can help improve generalizability for chronically implanted patients.
To summarize, our neural decoding approach, capable of decoding natural-sounding speech from 48 participants, provides the following major contributions. First, our proposed intermediate representation uses explicit speech parameters and a novel differentiable speech synthesizer, which enables interpretable and acoustically accurate speech decoding. Second, we directly consider the causality of the ECoG decoder, providing strong support for causal decoding, which is essential for real-time BCI applications. Third, our promising decoding results using low sampling density and right-hemisphere electrodes shed light on future neural prosthetic devices using low-density grids and in patients with damage to the left hemisphere. Last, but not least, we have made our decoding framework open to the community with documentation ( https://github.com/flinkerlab/neural_speech_decoding ), and we trust that this open platform will help propel the field forward, supporting reproducible science.
Experiments design
We collected neural data from 48 native English-speaking participants (26 female, 22 male) with refractory epilepsy who had ECoG subdural electrode grids implanted at NYU Langone Hospital. Five participants underwent HB sampling, and 43 LD sampling. The ECoG array was implanted on the left hemisphere for 32 participants and on the right for 16. The Institutional Review Board of NYU Grossman School of Medicine approved all experimental procedures. After consulting with the clinical-care provider, a research team member obtained written and oral consent from each participant. Each participant performed five tasks 47 to produce target words in response to auditory or visual stimuli. The tasks were auditory repetition (AR, repeating auditory words), auditory naming (AN, naming a word based on an auditory definition), sentence completion (SC, completing the last word of an auditory sentence), visual reading (VR, reading aloud written words) and picture naming (PN, naming a word based on a colour drawing).
For each task, we used the exact 50 target words with different stimulus modalities (auditory, visual and so on). Each word appeared once in the AN and SC tasks and twice in the others. The five tasks involved 400 trials, with corresponding word production and ECoG recording for each participant. The average duration of the produced speech in each trial was 500 ms.
Data collection and preprocessing
The study recorded ECoG signals from the perisylvian cortex (including STG, inferior frontal gyrus (IFG), pre-central and postcentral gyri) of 48 participants while they performed five speech tasks. A microphone recorded the subjects’ speech and was synchronized to the clinical Neuroworks Quantum Amplifier (Natus Biomedical), which captured ECoG signals. The ECoG array consisted of 64 standard 8 × 8 macro contacts (10-mm spacing) for 43 participants with low-density sampling. For five participants with hybrid-density sampling, the ECoG array also included 64 additional interspersed smaller electrodes (1 mm) between the macro contacts (providing 10-mm centre-to-centre spacing between macro contacts and 5-mm centre-to-centre spacing between micro/macro contacts; PMT Corporation) (Fig. 3b ). This Food and Drug Administration (FDA)-approved array was manufactured for this study. A research team member informed participants that the additional contacts were for research purposes during consent. Clinical care solely determined the placement location across participants (32 left hemispheres; 16 right hemispheres). The decoding models were trained separately for each participant using all trials except ten randomly selected ones from each task, leading to 350 trials for training and 50 for testing. The reported results are for testing data only.
We sampled ECoG signals from each electrode at 2,048 Hz and downsampled them to 512 Hz before processing. Electrodes with artefacts (for example, line noise, poor contact with the cortex, high-amplitude shifts) were rejected. The electrodes with interictal and epileptiform activity were also excluded from the analysis. The mean of a common average reference (across all remaining valid electrodes and time) was subtracted from each individual electrode. After the subtraction, a Hilbert transform extracted the envelope of the high gamma (70–150 Hz) component from the raw signal, which was then downsampled to 125 Hz. A reference signal was obtained by extracting a silent period of 250 ms before each trial’s stimulus period within the training set and averaging the signals over these silent periods. Each electrode’s signal was normalized to the reference mean and variance (that is, z -score). The data-preprocessing pipeline was coded in MATLAB and Python. For participants with noisy speech recordings, we applied spectral gating to remove stationary noise from the speech using an open-source tool 48 . We ruled out the possibility that our neural data suffer from a recently reported acoustic contamination (Supplementary Fig. 5 ) by following published approaches 49 .
To pre-train the auto-encoder, including the speech encoder and speech synthesizer, unlike our previous work in ref. 29 , which completely relied on unsupervised training, we provided supervision for some speech parameters to improve their estimation accuracy further. Specifically, we used the Praat method 50 to estimate the pitch and four formant frequencies ( \({f}_{ {{{\rm{i}}}} = {1}\,{{{\rm{to}}}}\,4}^{t}\) , in hertz) from the speech waveform. The estimated pitch and formant frequency were resampled to 125 Hz, the same as the ECoG signal and spectrogram sampling frequency. The mean square error between these speech parameters generated by the speech encoder and those estimated by the Praat method was used as a supervised reference loss, in addition to the unsupervised spectrogram reconstruction and STOI losses, making the training of the auto-encoder semi-supervised.
Speech synthesizer
Our speech synthesizer was inspired by the traditional speech vocoder, which generates speech by switching between voiced and unvoiced content, each generated by filtering a specific excitation signal. Instead of switching between the two components, we use a soft mix of the two components, making the speech synthesizer differentiable. This enables us to train the ECoG decoder and the speech encoder end-to-end by minimizing the spectrogram reconstruction loss with backpropagation. Our speech synthesizer can generate a spectrogram from a compact set of speech parameters, enabling training of the ECoG decoder with limited data. As shown in Fig. 5 , the synthesizer takes dynamic speech parameters as input and contains two pathways. The voice pathway applies a set of formant filters (each specified by the centre frequency \({f}_{i}^{\;t}\) , bandwidth \({b}_{i}^{t}\) that is dependent on \({f}_{i}^{\;t}\) , and amplitude \({a}_{i}^{t}\) ) to the harmonic excitation (with pitch frequency f 0 ) and generates the voiced component, V t ( f ), for each time step t and frequency f . The noise pathway filters the input white noise with an unvoice filter (consisting of a broadband filter defined by centre frequency \({f}_{\hat{u}}^{\;t}\) , bandwidth \({b}_{\hat{u}}^{t}\) and amplitude \({a}_{\hat{u}}^{t}\) and the same six formant filters used for the voice filter) and produces the unvoiced content, U t ( f ). The synthesizer combines the two components with a voice weight α t ∈ [0, 1] to obtain the combined spectrogram \({\widetilde{S}}^{t}{(\;f\;)}\) as
Factor α t acts as a soft switch for the gradient to flow back through the synthesizer. The final speech spectrogram is given by
where L t is the loudness modulation and B ( f ) the background noise. We describe the various components in more detail in the following.
Formant filters in the voice pathway
We use multiple formant filters in the voice pathway to model formants that represent vowels and nasal information. The formant filters capture the resonance in the vocal tract, which can help recover a speaker’s timbre characteristics and generate natural-sounding speech. We assume the filter for each formant is time-varying and can be derived from a prototype filter G i ( f ), which achieves maximum at a centre frequency \({f}_{i}^{{{\;{\rm{proto}}}}}\) and has a half-power bandwidth \({b}_{i}^{{{{\rm{proto}}}}}\) . The prototype filters have learnable parameters and will be discussed later. The actual formant filter at any time is written as a shifted and scaled version of G i ( f ). Specifically, at time t , given an amplitude \({\left({a}_{i}^{t}\right)}\) , centre frequency \({\left(\;{f}_{i}^{\;t}\right)}\) and bandwidth \({\left({b}_{i}^{t}\right)}\) , the frequency-domain representation of the i th formant filter is
where f max is half of the speech sampling frequency, which in our case is 8,000 Hz.
Rather than letting the bandwidth parameters \({b}_{i}^{t}\) be independent variables, based on the empirically observed relationships between \({b}_{i}^{t}\) and the centre frequencies \({f}_{i}^{\;t}\) , we set
The threshold frequency f θ , slope a and baseline bandwidth b 0 are three parameters that are learned during the auto-encoder training, shared among all six formant filters. This parameterization helps to reduce the number of speech parameters to be estimated at every time sample, making the representation space more compact.
Finally the filter for the voice pathway with N formant filters is given by \({F}_{{{{\rm{v}}}}}^{\;t}{(\;f\;)}={\mathop{\sum }\nolimits_{i = 1}^{N}{F}_{i}^{\;t}(\;f\;)}\) . Previous studies have shown that two formants ( N = 2) are enough for intelligible reconstruction 51 , but we use N = 6 for more accurate synthesis in our experiments.
Unvoice filters
We construct the unvoice filter by adding a single broadband filter \({F}_{\hat{u}}^{\;t}{(\;f\;)}\) to the formant filters for each time step t . The broadband filter \({F}_{\hat{u}}^{\;t}{(\;f\;)}\) has the same form as equation ( 1 ) but has its own learned prototype filter \({G}_{\hat{u}}{(f)}\) . The speech parameters corresponding to the broadband filter include \({\left({\alpha }_{\hat{u}}^{t},\,{f}_{\hat{u}}^{\;t},\,{b}_{\hat{u}}^{t}\right)}\) . We do not impose a relationship between the centre frequency \({f}_{\hat{u}}^{\;t}\) and the bandwidth \({b}_{\hat{u}}^{t}\) . This allows more flexibility in shaping the broadband unvoice filter. However, we constrain \({b}_{\hat{u}}^{t}\) to be larger than 2,000 Hz to capture the wide spectral range of obstruent phonemes. Instead of using only the broadband filter, we also retain the N formant filters in the voice pathway \({F}_{i}^{\;t}\) for the noise pathway. This is based on the observation that humans perceive consonants such as /p/ and /d/ not only by their initial bursts but also by their subsequent formant transitions until the next vowel 52 . We use identical formant filter parameters to encode these transitions. The overall unvoice filter is \({F}_{{{{\rm{u}}}}}^{\;t}{(\;f\;)}={F}_{\hat{u}}^{\;t}(\;f\;)+\mathop{\sum }\nolimits_{i = 1}^{N}{F}_{i}^{\;t}{(\;f\;)}\) .
Voice excitation
We use the voice filter in the voice pathway to modulate the harmonic excitation. Following ref. 53 , we define the harmonic excitation as \({h}^{t}={\mathop{\sum }\nolimits_{k = 1}^{K}{h}_{k}^{t}}\) , where K = 80 is the number of harmonics.
The value of the k th resonance at time step t is \({h}_{k}^{t}={\sin (2\uppi k{\phi }^{t})}\) with \({\phi }^{t}={\mathop{\sum }\nolimits_{\tau = 0}^{t}{f}_{0}^{\;\tau }}\) , where \({f}_{0}^{\;\tau }\) is the fundamental frequency at time τ . The spectrogram of h t forms the harmonic excitation in the frequency domain H t ( f ), and the voice excitation is \({V}^{\;t}{(\;f\;)}={F}_{{{{\rm{v}}}}}^{t}{(\;f\;)}{H}^{\;t}{(\;f\;)}\) .
Noise excitation
The noise pathway models consonant sounds (plosives and fricatives). It is generated by passing a stationary Gaussian white noise excitation through the unvoice filter. We first generate the noise signal n ( t ) in the time domain by sampling from the Gaussian process \({{{\mathcal{N}}}}{(0,\,1)}\) and then obtain its spectrogram N t ( f ). The spectrogram of the unvoiced component is \({U}^{\;t}{(\;f\;)}={F}_{u}^{\;t}{(\;f\;)}{N}^{\;t}{(\;f\;)}\) .
Summary of speech parameters
The synthesizer generates the voiced component at time t by driving a harmonic excitation with pitch frequency \({f}_{0}^{\;t}\) through N formant filters in the voice pathway, each described by two parameters ( \({f}_{ i}^{\;t},\,{a}_{ i}^{t}\) ). The unvoiced component is generated by filtering a white noise through the unvoice filter consisting of an additional broadband filter with three parameters ( \({f}_{\hat{u}}^{\;t},\,{b}_{\hat{u}}^{t},\,{a}_{\hat{u}}^{t}\) ). The two components are mixed based on the voice weight α t and further amplified by the loudness value L t . In total, the synthesizer input includes 18 speech parameters at each time step.
Unlike the differentiable digital signal processing (DDSP) in ref. 53 , we do not directly assign amplitudes to the K harmonics. Instead, the amplitude in our model depends on the formant filters, which has two benefits:
The representation space is more compact. DDSP requires 80 amplitude parameters \({a}_{k}^{t}\) for each of the 80 harmonic components \({f}_{k}^{\;t}\) ( k = 1, 2, …, 80) at each time step. In contrast, our synthesizer only needs a total of 18 parameters.
The representation is more disentangled. For human speech, the vocal tract shape (affecting the formant filters) is largely independent of the vocal cord tension (which determines the pitch). Modelling these two separately leads to a disentangled representation.
In contrast, DDSP specifies the amplitude for each harmonic component directly resulting in entanglement and redundancy between these amplitudes. Furthermore, it remains uncertain whether the amplitudes \({a}_{k}^{t}\) could be effectively controlled and encoded by the brain. In our approach, we explicitly model the formant filters and fundamental frequency, which possess clear physical interpretations and are likely to be directly controlled by the brain. Our representation also enables a more robust and direct estimation of the pitch.
Speaker-specific synthesizer parameters
Prototype filters.
Instead of using a predetermined prototype formant filter shape, for example, a standard Gaussian function, we learn a speaker-dependent prototype filter for each formant to allow more expressive and flexible formant filter shapes. We define the prototype filter G i ( f ) of the i th formant as a piecewise linear function, linearly interpolated from g i [ m ], m = 1, …, M , with the amplitudes of the filter at M being uniformly sampled frequencies in the range [0, f max ]. We constrain g i [ m ] to increase and then decrease monotonically so that G i ( f ) is unimodal and has a single peak value of 1. Given g i [ m ], m = 1, …, M , we can determine the peak frequency \({f}_{i}^{\;{{{\rm{proto}}}}}\) and the half-power bandwidth \({b}_{i}^{{{{\rm{proto}}}}}\) of G i ( f ).
The prototype parameters g i [ m ], m = 1, …, M of each formant filter are time-invariant and are determined during the auto-encoder training. Compared with ref. 29 , we increase M from 20 to 80 to enable more expressive formant filters, essential for synthesizing male speakers’ voices.
We similarly learn a prototype filter for the broadband filter G û ( f ) for the unvoiced component, which is specified by M parameters g û ( m ).
Background noise
The recorded sound typically contains background noise. We assume that the background noise is stationary and has a specific frequency distribution, depending on the speech recording environment. This frequency distribution B ( f ) is described by K parameters, where K is the number of frequency bins ( K = 256 for females and 512 for males). The K parameters are also learned during auto-encoder training. The background noise is added to the mixed speech components to generate the final speech spectrogram.
To summarize, our speech synthesizer has the following learnable parameters: the M = 80 prototype filter parameters for each of the N = 6 formant filters and the broadband filters (totalling M ( N + 1) = 560), the three parameters f θ , a and b 0 relating the centre frequency and bandwidth for the formant filters (totalling 18), and K parameters for the background noise (256 for female and 512 for male). The total number of parameters for female speakers is 834, and that for male speakers is 1,090. Note that these parameters are speaker-dependent but time-independent, and they can be learned together with the speech encoder during the training of the speech-to-speech auto-encoder, using the speaker’s speech only.
Speech encoder
The speech encoder extracts a set of (18) speech parameters at each time point from a given spectrogram, which are then fed to the speech synthesizer to reproduce the spectrogram.
We use a simple network architecture for the speech encoder, with temporal convolutional layers and multilayer perceptron (MLP) across channels at the same time point, as shown in Fig. 6a . We encode pitch \({f}_{0}^{\;t}\) by combining features generated from linear and Mel-scale spectrograms. The other 17 speech parameters are derived by applying temporal convolutional layers and channel MLP to the linear-scale spectrogram. To generate formant filter centre frequencies \({f}_{i = 1\,{{{\rm{to}}}}\,6}^{\;t}\) , broadband unvoice filter frequency \({f}_{\hat{u}}^{\;t}\) and pitch \({f}_{0}^{\;t}\) , we use sigmoid activation at the end of the corresponding channel MLP to map the output to [0, 1], and then de-normalize it to real values by scaling [0, 1] to predefined [ f min , f max ]. The [ f min , f max ] values for each frequency parameter are chosen based on previous studies 54 , 55 , 56 , 57 . Our compact speech parameter space facilitates stable and easy training of our speech encoder. Models were coded using PyTorch version 1.21.1 in Python.
ECoG decoder
In this section we present the design details of three ECoG decoders: the 3D ResNet ECoG decoder, the 3D Swin transformer ECoG decoder and the LSTM ECoG decoder. The models were coded using PyTorch version 1.21.1 in Python.
3D ResNet ECoG decoder
This decoder adopts the ResNet architecture 23 for the feature extraction backbone of the decoder. Figure 6c illustrates the feature extraction part. The model views the ECoG input as 3D tensors with spatiotemporal dimensions. In the first layer, we apply only temporal convolution to the signal from each electrode, because the ECoG signal exhibits more temporal than spatial correlations. In the subsequent parts of the decoder, we have four residual blocks that extract spatiotemporal features using 3D convolution. After downsampling the electrode dimension to 1 × 1 and the temporal dimension to T /16, we use several transposed Conv layers to upsample the features to the original temporal size T . Figure 6b shows how to generate the different speech parameters from the resulting features using different temporal convolution and channel MLP layers. The temporal convolution operation can be causal (that is, using only past and current samples as input) or non-causal (that is, using past, current and future samples), leading to causal and non-causal models.
3D Swin Transformer ECoG decoder
Swin Transformer 24 employs the window and shift window methods to enable self-attention of small patches within each window. This reduces the computational complexity and introduces the inductive bias of locality. Because our ECoG input data have three dimensions, we extend Swin Transformer to three dimensions to enable local self-attention in both temporal and spatial dimensions among 3D patches. The local attention within each window gradually becomes global attention as the model merges neighbouring patches in deeper transformer stages.
Figure 6d illustrates the overall architecture of the proposed 3D Swin Transformer. The input ECoG signal has a size of T × H × W , where T is the number of frames and H × W is the number of electrodes at each frame. We treat each 3D patch of size 2 × 2 × 2 as a token in the 3D Swin Transformer. The 3D patch partitioning layer produces \({\frac{T}{2}\times \frac{H}{2}\times \frac{W}{2}}\) 3D tokens, each with a 48-dimensional feature. A linear embedding layer then projects the features of each token to a higher dimension C (=128).
The 3D Swin Transformer comprises three stages with two, two and six layers, respectively, for LD participants and four stages with two, two, six and two layers for HB participants. It performs 2 × 2 × 2 spatial and temporal downsampling in the patch-merging layer of each stage. The patch-merging layer concatenates the features of each group of 2 × 2 × 2 temporally and spatially adjacent tokens. It applies a linear layer to project the concatenated features to one-quarter of their original dimension after merging. In the 3D Swin Transformer block, we replace the multi-head self-attention (MSA) module in the original Swin Transformer with the 3D shifted window multi-head self-attention module. It adapts the other components to 3D operations as well. A Swin Transformer block consists of a 3D shifted window-based MSA module followed by a feedforward network (FFN), a two-layer MLP. Layer normalization is applied before each MSA module and FFN, and a residual connection is applied after each module.
Consider a stage with T × H × W input tokens. If the 3D window size is P × M × M , we partition the input into \({\lceil \frac{T}{P}\rceil \times \lceil \frac{H}{M}\rceil \times \lceil \frac{W}{M}\rceil}\) non-overlapping 3D windows evenly. We choose P = 16, M = 2. We perform the multi-head self-attention within each 3D window. However, this design lacks connection across adjacent windows, which may limit the representation power of the architecture. Therefore, we extend the shifted 2D window mechanism of the Swin Transformer to shifted 3D windows. In the second layer of the stage, we shift the window by \(\left({\frac{P}{2},\,\frac{M}{2},\,\frac{M}{2}}\right)\) tokens along the temporal, height and width axes from the previous layer. This creates cross-window connections for the self-attention module. This shifted 3D window design enables the interaction of electrodes with longer spatial and temporal distances by connecting neighbouring tokens in non-overlapping 3D windows in the previous layer.
The temporal attention in the self-attention operation can be constrained to be causal (that is, each token only attends to tokens temporally before it) or non-causal (that is, each token can attend to tokens temporally before or after it), leading to the causal and non-causal models, respectively.
LSTM decoder
The decoder uses the LSTM architecture 25 for the feature extraction in Fig. 6e . Each LSTM cell is composed of a set of gates that control the flow of information: the input gate, the forget gate and the output gate. The input gate regulates the entry of new data into the cell state, the forget gate decides what information is discarded from the cell state, and the output gate determines what information is transferred to the next hidden state and can be output from the cell.
In the LSTM architecture, the ECoG input would be processed through these cells sequentially. For each time step T , the LSTM would take the current input x t and the previous hidden state h t − 1 and would produce a new hidden state h t and output y t . This process allows the LSTM to maintain information over time and is particularly useful for tasks such as speech and neural signal processing, where temporal dependencies are critical. Here we use three layers of LSTM and one linear layer to generate features to map to speech parameters. Unlike 3D ResNet and 3D Swin, we keep the temporal dimension unchanged across all layers.
Model training
Training of the speech encoder and speech synthesizer.
As described earlier, we pre-train the speech encoder and the learnable parameters in the speech synthesizer to perform a speech-to-speech auto-encoding task. We use multiple loss terms for the training. The modified multi-scale spectral (MSS) loss is inspired by ref. 53 and is defined as
Here, S t ( f ) denotes the ground-truth spectrogram and \({\widehat{S}}^{t}{(\;f\;)}\) the reconstructed spectrogram in the linear scale, \({S}_{{{{\rm{mel}}}}}^{t}{(\;f\;)}\) and \({\widehat{S}}_{{{{\rm{mel}}}}}^{t}{(\;f\;)}\) are the corresponding spectrograms in the Mel-frequency scale. We sample the frequency range [0, 8,000 Hz] with K = 256 bins for female participants. For male participants, we set K = 512 because they have lower f 0 , and it is better to have a higher resolution in frequency.
To improve the intelligibility of the reconstructed speech, we also introduce the STOI loss by implementing the STOI+ metric 26 , which is a variation of the original STOI metric 8 , 22 . STOI+ 26 discards the normalization and clipping step in STOI and has been shown to perform best among intelligibility evaluation metrics. First, a one-third octave band analysis 22 is performed by grouping Discrete Fourier transform (DFT) bins into 15 one-third octave bands with the lowest centre frequency set equal to 150 Hz and the highest centre frequency equal to ~4.3 kHz. Let \({\hat{x}(k,\,m)}\) denote the k th DFT bin of the m th frame of the ground-truth speech. The norm of the j th one-third octave band, referred to as a time-frequency (TF) unit, is then defined as
where k 1 ( j ) and k 2 ( j ) denote the one-third octave band edges rounded to the nearest DFT bin. The TF representation of the processed speech \({\hat{y}}\) is obtained similarly and denoted by Y j ( m ). We then extract the short-time temporal envelopes in each band and frame, denoted X j , m and Y j , m , where \({X}_{j,\,m}={\left[{X}_{j}{(m-N+1)},\,{X}_{j}{(m-N+2)},\,\ldots ,\,{X}_{j}{(m)}\right]}^{\rm{T}}\) , with N = 30. The STOI+ metric is the average of the PCC d j , m between X j , m and Y j , m , overall j and m (ref. 26 ):
We use the negative of the STOI+ metric as the STOI loss:
where J and M are the total numbers of frequency bins ( J = 15) and frames, respectively. Note that L STOI is differentiable with respect to \({\widehat{S}}^{t}{(\;f\;)}\) , and thus can be used to update the model parameters generating the predicted spectrogram \({\widehat{S}}^{t}{(\;f\;)}\) .
To further improve the accuracy for estimating the pitch \({\widetilde{f}}_{0}^{\;t}\) and formant frequencies \({\widetilde{f}}_{{{{\rm{i}}}} = {1}\,{{{\rm{to}}}}\,4}^{\;t}\) , we add supervisions to them using the formant frequencies extracted by the Praat method 50 . The supervision loss is defined as
where the weights β i are chosen to be β 1 = 0.1, β 2 = 0.06, β 3 = 0.03 and β 4 = 0.02, based on empirical trials. The overall training loss is defined as
where the weighting parameters λ i are empirically optimized to be λ 1 = 1.2 and λ 2 = 0.1 through testing the performances on three hybrid-density participants with different parameter choices.
Training of the ECoG decoder
With the reference speech parameters generated by the speech encoder and the target speech spectrograms as ground truth, the ECoG decoder is trained to match these targets. Let us denote the decoded speech parameters as \({\widetilde{C}}_{j}^{\;t}\) , and their references as \({C}_{j}^{\;t}\) , where j enumerates all speech parameters fed to the speech synthesizer. We define the reference loss as
where weighting parameters λ j are chosen as follows: voice weight λ α = 1.8, loudness λ L = 1.5, pitch \({\lambda }_{{f}_{0}}={0.4}\) , formant frequencies \({\lambda }_{{f}_{1}}={3},\,{\lambda }_{{f}_{2}}={1.8},\,{\lambda }_{{f}_{3}}={1.2},\,{\lambda }_{{f}_{4}}={0.9},\,{\lambda }_{{f}_{5}}={0.6},\,{\lambda }_{{f}_{6}}={0.3}\) , formant amplitudes \({\lambda }_{{a}_{1}}={4},\,{\lambda }_{{a}_{2}}={2.4},\,{\lambda }_{{a}_{3}}={1.2},\,{\lambda }_{{a}_{4}}={0.9},\,{\lambda }_{{a}_{5}}={0.6},\,{\lambda }_{{a}_{6}}={0.3}\) , broadband filter frequency \({\lambda }_{{f}_{\hat{u}}}={10}\) , amplitude \({\lambda }_{{a}_{\hat{u}}}={4}\) , bandwidth \({\lambda }_{{b}_{\hat{u}}}={4}\) . Similar to speech-to-speech auto-encoding, we add supervision loss for pitch and formant frequencies derived by the Praat method and use the MSS and STOI loss to measure the difference between the reconstructed spectrograms and the ground-truth spectrogram. The overall training loss for the ECoG decoder is
where weighting parameters λ i are empirically optimized to be λ 1 = 1.2, λ 2 = 0.1 and λ 3 = 1, through the same parameter search process as described for training the speech encoder.
We use the Adam optimizer 58 with hyper-parameters lr = 10 −3 , β 1 = 0.9 and β 2 = 0.999 to train both the auto-encoder (including the speech encoder and speech synthesizer) and the ECoG decoder. We train a separate set of models for each participant. As mentioned earlier, we randomly selected 50 out of 400 trials per participant as the test data and used the rest for training.
Evaluation metrics
In this Article, we use the PCC between the decoded spectrogram and the actual speech spectrogram to evaluate the objective quality of the decoded speech, similar to refs. 8 , 18 , 59 .
We also use STOI+ 26 , as described in Methods section Training of the ECoG decoder to measure the intelligibility of the decoded speech. The STOI+ value ranges from −1 to 1 and has been reported to have a monotonic relationship with speech intelligibility.
Contribution analysis with the occlusion method
To measure the contribution of the cortex region under each electrode to the decoding performance, we adopted an occlusion-based method that calculates the change in the PCC between the decoded and the ground-truth spectrograms when an electrode signal is occluded (that is, set to zeros), as in ref. 29 . This method enables us to reveal the critical brain regions for speech production. We used the following notations: S t ( f ), the ground-truth spectrogram; \({\hat{{{{{S}}}}}}^{t}{(\;f\;)}\) , the decoded spectrogram with ‘intact’ input (that is, all ECoG signals are used); \({\hat{{{{{S}}}}}}_{i}^{t}{(\;f\;)}\) , the decoded spectrogram with the i th ECoG electrode signal occluded; r ( ⋅ , ⋅ ), correlation coefficient between two signals. The contribution of i th electrode for a particular participant is defined as
where Mean{ ⋅ } denotes averaging across all testing trials of the participant.
We generate the contribution map on the standardized Montreal Neurological Institute (MNI) brain anatomical map by diffusing the contribution of each electrode of each participant (with a corresponding location in the MNI coordinate) into the adjacent area within the same anatomical region using a Gaussian kernel and then averaging the resulting map from all participants. To account for the non-uniform density of the electrodes in different regions and across the participants, we normalize the sum of the diffused contribution from all the electrodes at each brain location by the total number of electrodes in the region across all participants.
We estimate the noise level for the contribution map to assess the significance of our contribution analysis. To derive the noise level, we train a shuffled model for each participant by randomly pairing the mismatched speech segment and ECoG segment in the training set. We derive the average contribution map from the shuffled models for all participants using the same occlusion analysis as described earlier. The resulting contribution map is used as the noise level. Contribution levels below the noise levels at corresponding cortex locations are assigned a value of 0 (white) in Fig. 4 .
Reporting summary
Further information on research design is available in the Nature Portfolio Reporting Summary linked to this Article.
Data availability
The data of one participant who consented to the release of the neural and audio data are publicly available through Mendeley Data at https://data.mendeley.com/datasets/fp4bv9gtwk/2 (ref. 60 ). Although all participants consented to share their data for research purposes, not all participants agreed to share their audio publicly. Given the sensitive nature of audio speech data we will share data with researchers that directly contact the corresponding author and provide documentation that the data will be strictly used for research purposes and will comply with the terms of our study IRB. Source data are provided with this paper.
Code availability
The code is available at https://github.com/flinkerlab/neural_speech_decoding ( https://doi.org/10.5281/zenodo.10719428 ) 61 .
Schultz, T. et al. Biosignal-based spoken communication: a survey. IEEE / ACM Trans. Audio Speech Lang. Process. 25 , 2257–2271 (2017).
Google Scholar
Miller, K. J., Hermes, D. & Staff, N. P. The current state of electrocorticography-based brain-computer interfaces. Neurosurg. Focus 49 , E2 (2020).
Article Google Scholar
Luo, S., Rabbani, Q. & Crone, N. E. Brain-computer interface: applications to speech decoding and synthesis to augment communication. Neurotherapeutics 19 , 263–273 (2022).
Moses, D. A., Leonard, M. K., Makin, J. G. & Chang, E. F. Real-time decoding of question-and-answer speech dialogue using human cortical activity. Nat. Commun. 10 , 3096 (2019).
Moses, D. A. et al. Neuroprosthesis for decoding speech in a paralyzed person with anarthria. N. Engl. J. Med. 385 , 217–227 (2021).
Herff, C. & Schultz, T. Automatic speech recognition from neural signals: a focused review. Front. Neurosci. 10 , 429 (2016).
Rabbani, Q., Milsap, G. & Crone, N. E. The potential for a speech brain-computer interface using chronic electrocorticography. Neurotherapeutics 16 , 144–165 (2019).
Angrick, M. et al. Speech synthesis from ECoG using densely connected 3D convolutional neural networks. J. Neural Eng. 16 , 036019 (2019).
Sun, P., Anumanchipalli, G. K. & Chang, E. F. Brain2Char: a deep architecture for decoding text from brain recordings. J. Neural Eng. 17 , 066015 (2020).
Makin, J. G., Moses, D. A. & Chang, E. F. Machine translation of cortical activity to text with an encoder–decoder framework. Nat. Neurosci. 23 , 575–582 (2020).
Wang, R. et al. Stimulus speech decoding from human cortex with generative adversarial network transfer learning. In Proc. 2020 IEEE 17th International Symposium on Biomedical Imaging ( ISBI ) (ed. Amini, A.) 390–394 (IEEE, 2020).
Zelinka, P., Sigmund, M. & Schimmel, J. Impact of vocal effort variability on automatic speech recognition. Speech Commun. 54 , 732–742 (2012).
Benzeghiba, M. et al. Automatic speech recognition and speech variability: a review. Speech Commun. 49 , 763–786 (2007).
Martin, S. et al. Decoding spectrotemporal features of overt and covert speech from the human cortex. Front. Neuroeng. 7 , 14 (2014).
Herff, C. et al. Towards direct speech synthesis from ECoG: a pilot study. In Proc. 2016 38th Annual International Conference of the IEEE Engineering in Medicine and Biology Society ( EMBC ) (ed. Patton, J.) 1540–1543 (IEEE, 2016).
Angrick, M. et al. Real-time synthesis of imagined speech processes from minimally invasive recordings of neural activity. Commun. Biol 4 , 1055 (2021).
Anumanchipalli, G. K., Chartier, J. & Chang, E. F. Speech synthesis from neural decoding of spoken sentences. Nature 568 , 493–498 (2019).
Herff, C. et al. Generating natural, intelligible speech from brain activity in motor, premotor and inferior frontal cortices. Front. Neurosci. 13 , 1267 (2019).
Metzger, S. L. et al. A high-performance neuroprosthesis for speech decoding and avatar control. Nature 620 , 1037–1046 (2023).
Hsu, W.-N. et al. Hubert: self-supervised speech representation learning by masked prediction of hidden units. IEEE/ACM Trans. Audio Speech Lang. Process. 29 , 3451–3460 (2021).
Griffin, D. & Lim, J. Signal estimation from modified short-time fourier transform. IEEE Trans. Acoustics Speech Signal Process. 32 , 236–243 (1984).
Taal, C. H., Hendriks, R. C., Heusdens, R. & Jensen, J. A short-time objective intelligibility measure for time-frequency weighted noisy speech. In Proc. 2010 IEEE International Conference on Acoustics, Speech and Signal Processing (ed. Douglas, S.) 4214–4217 (IEEE, 2010).
He, K., Zhang, X., Ren, S. & Sun, J. Deep residual learning for image recognition. In Proc. 2016 IEEE Conference on Computer Vision and Pattern Recognition ( CVPR ) (ed. Bajcsy, R.) 770–778 (IEEE, 2016).
Liu, Z. et al. Swin Transformer: hierarchical vision transformer using shifted windows. In Proc. 2021 IEEE / CVF International Conference on Computer Vision ( ICCV ) (ed. Dickinson, S.) 9992–10002 (IEEE, 2021).
Hochreiter, S. & Schmidhuber, J. Long short-term memory. Neural Comput. 9 , 1735–1780 (1997).
Graetzer, S. & Hopkins, C. Intelligibility prediction for speech mixed with white Gaussian noise at low signal-to-noise ratios. J. Acoust. Soc. Am. 149 , 1346–1362 (2021).
Hickok, G. & Poeppel, D. The cortical organization of speech processing. Nat. Rev. Neurosci. 8 , 393–402 (2007).
Trupe, L. A. et al. Chronic apraxia of speech and Broca’s area. Stroke 44 , 740–744 (2013).
Wang, R. et al. Distributed feedforward and feedback cortical processing supports human speech production. Proc. Natl Acad. Sci. USA 120 , e2300255120 (2023).
Mugler, E. M. et al. Differential representation ofÿ articulatory gestures and phonemes in precentral and inferior frontal gyri. J. Neurosci. 38 , 9803–9813 (2018).
Herff, C. et al. Brain-to-text: decoding spoken phrases from phone representations in the brain. Front. Neurosci. 9 , 217 (2015).
Kohler, J. et al. Synthesizing speech from intracranial depth electrodes using an encoder-decoder framework. Neurons Behav. Data Anal. Theory https://doi.org/10.51628/001c.57524 (2022).
Angrick, M. et al. Towards closed-loop speech synthesis from stereotactic EEG: a unit selection approach. In Proc. 2022 IEEE International Conference on Acoustics , Speech and Signal Processing ( ICASSP ) (ed. Li, H.) 1296–1300 (IEEE, 2022).
Ozker, M., Doyle, W., Devinsky, O. & Flinker, A. A cortical network processes auditory error signals during human speech production to maintain fluency. PLoS Biol. 20 , e3001493 (2022).
Stuart, A., Kalinowski, J., Rastatter, M. P. & Lynch, K. Effect of delayed auditory feedback on normal speakers at two speech rates. J. Acoust. Soc. Am. 111 , 2237–2241 (2002).
Verwoert, M. et al. Dataset of speech production in intracranial electroencephalography. Sci. Data 9 , 434 (2022).
Berezutskaya, J. et al. Direct speech reconstruction from sensorimotor brain activity with optimized deep learning models. J. Neural Eng. 20 , 056010 (2023).
Wang, R., Wang, Y. & Flinker, A. Reconstructing speech stimuli from human auditory cortex activity using a WaveNet approach. In Proc. 2018 IEEE Signal Processing in Medicine and Biology Symposium ( SPMB ) (ed. Picone, J.) 1–6 (IEEE, 2018).
Flanagan, J. L. Speech Analysis Synthesis and Perception Vol. 3 (Springer, 2013).
Serra, X. & Smith, J. Spectral modeling synthesis: a sound analysis/synthesis system based on a deterministic plus stochastic decomposition. Comput. Music J. 14 , 12–24 (1990).
Cogan, G. B. et al. Sensory–motor transformations for speech occur bilaterally. Nature 507 , 94–98 (2014).
Ibayashi, K. et al. Decoding speech with integrated hybrid signals recorded from the human ventral motor cortex. Front. Neurosci. 12 , 221 (2018).
Soroush, P. Z. et al. The nested hierarchy of overt, mouthed and imagined speech activity evident in intracranial recordings. NeuroImage 269 , 119913 (2023).
Tate, M. C., Herbet, G., Moritz-Gasser, S., Tate, J. E. & Duffau, H. Probabilistic map of critical functional regions of the human cerebral cortex: Broca’s area revisited. Brain 137 , 2773–2782 (2014).
Long, M. A. et al. Functional segregation of cortical regions underlying speech timing and articulation. Neuron 89 , 1187–1193 (2016).
Willett, F. R. et al. A high-performance speech neuroprosthesis. Nature 620 , 1031–1036 (2023).
Shum, J. et al. Neural correlates of sign language production revealed by electrocorticography. Neurology 95 , e2880–e2889 (2020).
Sainburg, T., Thielk, M. & Gentner, T. Q. Finding, visualizing and quantifying latent structure across diverse animal vocal repertoires. PLoS Comput. Biol. 16 , e1008228 (2020).
Roussel, P. et al. Observation and assessment of acoustic contamination of electrophysiological brain signals during speech production and sound perception. J. Neural Eng. 17 , 056028 (2020).
Boersma, P. & Van Heuven, V. Speak and unSpeak with PRAAT. Glot Int. 5 , 341–347 (2001).
Chang, E. F., Raygor, K. P. & Berger, M. S. Contemporary model of language organization: an overview for neurosurgeons. J. Neurosurgery 122 , 250–261 (2015).
Jiang, J., Chen, M. & Alwan, A. On the perception of voicing in syllable-initial plosives in noise. J. Acoust. Soc. Am. 119 , 1092–1105 (2006).
Engel, J., Hantrakul, L., Gu, C. & Roberts, A. DDSP: differentiable digital signal processing. In Proc. 8th International Conference on Learning Representations https://openreview.net/forum?id=B1x1ma4tDr (Open.Review.net, 2020).
Flanagan, J. L. A difference limen for vowel formant frequency. J. Acoust. Soc. Am. 27 , 613–617 (1955).
Schafer, R. W. & Rabiner, L. R. System for automatic formant analysis of voiced speech. J. Acoust. Soc. Am. 47 , 634–648 (1970).
Fitch, J. L. & Holbrook, A. Modal vocal fundamental frequency of young adults. Arch. Otolaryngol. 92 , 379–382 (1970).
Stevens, S. S. & Volkmann, J. The relation of pitch to frequency: a revised scale. Am. J. Psychol. 53 , 329–353 (1940).
Kingma, D. P. & Ba, J. Adam: a method for stochastic optimization. In Proc. 3rd International Conference on Learning Representations (eds Bengio, Y. & LeCun, Y.) http://arxiv.org/abs/1412.6980 (arXiv, 2015).
Angrick, M. et al. Interpretation of convolutional neural networks for speech spectrogram regression from intracranial recordings. Neurocomputing 342 , 145–151 (2019).
Chen, X. ECoG_HB_02. Mendeley data, V2 (Mendeley, 2024); https://doi.org/10.17632/fp4bv9gtwk.2
Chen, X. & Wang, R. Neural speech decoding 1.0 (Zenodo, 2024); https://doi.org/10.5281/zenodo.10719428
Download references
Acknowledgements
This Work was supported by the National Science Foundation under grants IIS-1912286 and 2309057 (Y.W. and A.F.) and National Institute of Health grants R01NS109367, R01NS115929 and R01DC018805 (A.F.).
Author information
These authors contributed equally: Xupeng Chen, Ran Wang.
These authors jointly supervised this work: Yao Wang, Adeen Flinker.
Authors and Affiliations
Electrical and Computer Engineering Department, New York University, Brooklyn, NY, USA
Xupeng Chen, Ran Wang & Yao Wang
Neurology Department, New York University, Manhattan, NY, USA
Amirhossein Khalilian-Gourtani, Leyao Yu, Patricia Dugan, Daniel Friedman, Orrin Devinsky & Adeen Flinker
Biomedical Engineering Department, New York University, Brooklyn, NY, USA
Leyao Yu, Yao Wang & Adeen Flinker
Neurosurgery Department, New York University, Manhattan, NY, USA
Werner Doyle
You can also search for this author in PubMed Google Scholar
Contributions
Y.W. and A.F. supervised the research. X.C., R.W., Y.W. and A.F. conceived research. X.C., R.W., A.K.-G., L.Y., P.D., D.F., W.D., O.D. and A.F. performed research. X.C., R.W., Y.W. and A.F. contributed new reagents/analytic tools. X.C., R.W., A.K.-G., L.Y. and A.F. analysed data. P.D. and D.F. provided clinical care. W.D. provided neurosurgical clinical care. O.D. assisted with patient care and consent. X.C., Y.W. and A.F. wrote the paper.
Corresponding author
Correspondence to Adeen Flinker .
Ethics declarations
Competing interests.
The authors declare no competing interests.
Peer review
Peer review information.
Nature Machine Intelligence thanks the anonymous reviewer(s) for their contribution to the peer review of this work.
Additional information
Publisher’s note Springer Nature remains neutral with regard to jurisdictional claims in published maps and institutional affiliations.
Supplementary information
Supplementary information.
Supplementary Figs. 1–10, Table 1 and audio files list.
Reporting Summary
Supplementary audio 1.
Example original and decoded audios for eight words.
Supplementary Audio 2
Example original and decoded words from low density participants.
Supplementary Audio 3
Example original and decoded words from hybrid density participants.
Supplementary Audio 4
Example original and decoded words from left hemisphere low density participants.
Supplementary Audio 5
Example original and decoded words from right hemisphere low density participants.
Source Data Fig. 2
Data for Fig, 2a,b,d,e,f.
Source Data Fig. 3
Data for Fig, 3a,c,d.
Rights and permissions
Open Access This article is licensed under a Creative Commons Attribution 4.0 International License, which permits use, sharing, adaptation, distribution and reproduction in any medium or format, as long as you give appropriate credit to the original author(s) and the source, provide a link to the Creative Commons licence, and indicate if changes were made. The images or other third party material in this article are included in the article’s Creative Commons licence, unless indicated otherwise in a credit line to the material. If material is not included in the article’s Creative Commons licence and your intended use is not permitted by statutory regulation or exceeds the permitted use, you will need to obtain permission directly from the copyright holder. To view a copy of this licence, visit http://creativecommons.org/licenses/by/4.0/ .
Reprints and permissions
About this article
Cite this article.
Chen, X., Wang, R., Khalilian-Gourtani, A. et al. A neural speech decoding framework leveraging deep learning and speech synthesis. Nat Mach Intell (2024). https://doi.org/10.1038/s42256-024-00824-8
Download citation
Received : 29 July 2023
Accepted : 08 March 2024
Published : 08 April 2024
DOI : https://doi.org/10.1038/s42256-024-00824-8
Share this article
Anyone you share the following link with will be able to read this content:
Sorry, a shareable link is not currently available for this article.
Provided by the Springer Nature SharedIt content-sharing initiative
Quick links
- Explore articles by subject
- Guide to authors
- Editorial policies
Sign up for the Nature Briefing newsletter — what matters in science, free to your inbox daily.

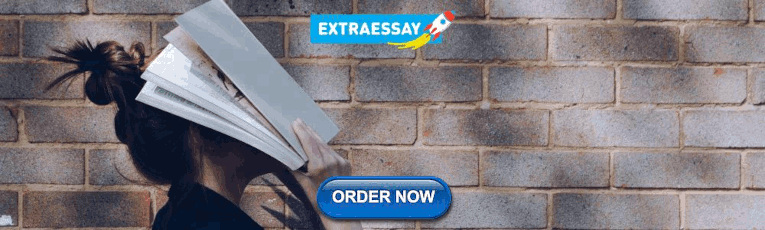
IMAGES
VIDEO
COMMENTS
Speech synthesis is the artificial production of human speech.A computer system used for this purpose is called a speech synthesizer, and can be implemented in software or hardware products. A text-to-speech (TTS) system converts normal language text into speech; other systems render symbolic linguistic representations like phonetic transcriptions into speech.
What is speech synthesis? Computers do their jobs in three distinct stages called input (where you feed information in, often with a keyboard or mouse), processing (where the computer responds to your input, say, by adding up some numbers you typed in or enhancing the colors on a photo you scanned), and output (where you get to see how the computer has processed your input, typically on a ...
Concatenative synthesis mostly relies on concatenation of separated pieces of speech stored in the database [4]. Concatenative synthesis can generate high intelligibility speech close to original voice but it requires huge amount of data to match all possible combination of speech units, but on the other hand, such systems generate
TTS is a computer simulation of human speech from a textual representation using machine learning methods. Typically, speech synthesis is used by developers to create voice robots, such as IVR (Interactive Voice Response). TTS saves a business time and money as it generates sound automatically, thus saving the company from having to manually ...
Speech synthesis, also known as text-to-speech (TTS), involves the automatic production of human speech. This technology is widely used in various applications such as real-time transcription services, automated voice response systems, and assistive technology for the visually impaired. The pronunciation of words, including "robot," is ...
The general architecture of a text-to-speech synthesis system, consisting of two components, one being concerned with text analysis (in green), the other with speech signal generation (in blue) Full size image. In the following sections, we will describe the text analysis (Fig. 4) and speech synthesis components (Fig. 5) in more detail.
Speech synthesis has a long history, going back to early attempts to generate speech- or singing-like sounds from musical instruments. But in the modern age, the field has been driven by one key application: Text-to-Speech (TTS), which means generating speech from text input. Almost universally, this complex problem is divided into two parts.
Text-to-Speech Synthesis provides a complete, end-to-end account of the process of generating speech by computer. Giving an in-depth explanation of all aspects of current speech synthesis technology, it assumes no specialised prior knowledge. Introductory chapters on linguistics, phonetics, signal processing and speech signals lay the ...
Text-to-speech synthesis (TTS) is a technology of converting written text into speech. In some parts of this book, it is simply referred to as "speech synthesis" without explicitly indicating what the input is. ... Notice that the broader definition of the term includes some of the technically simpler processes such as recording ...
Text-to-speech (TTS) aims to synthesize intelligible and natural speech based on the given text. It is a hot topic in language, speech, and machine learning research and has broad applications in industry. This book introduces neural network-based TTS in the era of deep learning, aiming to provide a good understanding of neural TTS, current ...
Text-to-speech (TTS) is a type of assistive technology that reads digital text aloud. It's sometimes called "read aloud" technology. With a click of a button or the touch of a finger, TTS can take words on a computer or other digital device and convert them into audio. TTS is very helpful for kids who struggle with reading.
As a working definition, we will take intonation synthesis to be the generation of an F0 contour from higher-level linguistic information. 17 - Further issues; ... We now turn to unit-selection synthesis which is the dominant synthesis technique in text-to-speech today. Unit selection is the natural extension of second-generation concatenative ...
Introduction. Speech synthesis (or alternatively text-to-speech synthesis) means automatically converting natural language text into speech.Speech synthesis has many potential applications. For example, it can be used as an aid to people with disabilities (see Challenges for the Future), for generating the output of spoken dialogue systems (Lemon et al., 2006; Georgila et al., 2010), for ...
Speech synthesis, or text-to-speech, is a category of software or hardware that converts text to artificial speech. A text-to-speech system is one that reads text aloud through the computer's sound card or other speech synthesis device. Text that is selected for reading is analyzed by the software, restructured to a phonetic system, and read aloud.
Text-to-Speech Synthesis. Text-to-Speech Synthesis provides a complete, end-to-end account of the process of generating speech by computer. Giving an in-depth explanation of all aspects of current speech synthesis technology, it assumes no specialized prior knowledge. Introductory chapters on linguistics, phonetics, signal processing and speech ...
Speech synthesis is artificial simulation of human speech with by a computer or other device. The counterpart of the voice recognition, speech synthesis is mostly used for translating text information into audio information and in applications such as voice-enabled services and mobile applications. Apart from this, it is also used in assistive ...
Speech synthesis is the artificial production of human speech that sounds almost like a human voice and is more precise with pitch, speech, and tone. Automation and AI-based system designed for this purpose is called a text-to-speech synthesizer and can be implemented in software or hardware.
Text-to-Speech (TTS) synthesis, the process of generating natural speech from text, remains a challenging task despite decades of investigation. Nowadays there are several TTS systems able to get impressive results in terms of synthesis of natural voices very close to human ones. ... If the definition of the objective function was quite simple ...
Text -To-Speech Synthesis is a. Technology that prov ides a means of converting written text fr om a descr iptive form to a spoken language that is easily. understandable by the end user ...
Tacotron 2 is a neural network architecture for speech synthesis directly from text. It consists of two components: a recurrent sequence-to-sequence feature prediction network with attention which predicts a sequence of mel spectrogram frames from an input character sequence a modified version of WaveNet which generates time-domain waveform samples conditioned on the predicted mel spectrogram ...
Neural speech synthesis, or text-to-speech (TTS), aims to transform a signal from the text domain to the speech domain. While developing TTS architectures that train and test on the same set of speakers has seen significant improvements, out-of-domain speaker performance still faces enormous limitations. Domain adaptation on a new set of speakers can be achieved by fine-tuning the whole model ...
This paper discusses the theoretical aspects of real-time text-to-speech synthesis systems and the implementation of such systems based on deep learning models. Such systems consist of two essential components: a text-tospeech generator responsible for generating the audio content of speech and creating mel-spectrograms, and a vocoder that synthesizes speech based on voice data. In this work ...
Abstract. In this chapter, we will address the quality experienced when listening to speech which is synthesized by state-of-the-art synthesis systems which generate artificial speech from text. Such systems are used, e.g., in information and navigation systems, but also for generating audiobooks. We describe both, auditory evaluation methods ...
A text-to-speech synthesis system typically consists of multiple stages, such as a text analysis frontend, an acoustic model and an audio synthesis module. Building these components often requires extensive domain expertise and may contain brittle design choices. In this paper, we present Tacotron, an end-to-end generative text-to-speech model ...
In fact, it helped brainstorm the headline for this blog, including the requirement that it use a variation of the series' name "AI Decoded," and it assisted in writing the definition of a foundation model. Hello, world, indeed. Meta's Llama 2 is a cutting-edge LLM that generates text and code in response to prompts.
Multi-speaker text-to-speech synthesis involves generating unique speech patterns for individual speakers based on reference waveforms and input sequences of graphemes or phonemes. Various deep neural networks are trained for this task using a large amount of speech data recorded from a specific speaker to generate audio in their voice. The model requires a large dataset to retrain itself and ...
View a PDF of the paper titled RALL-E: Robust Codec Language Modeling with Chain-of-Thought Prompting for Text-to-Speech Synthesis, by Detai Xin and 10 other authors. View PDF HTML (experimental) Abstract: We present RALL-E, a robust language modeling method for text-to-speech (TTS) synthesis. While previous work based on large language models ...
The core idea behind RALL-E is chain-of-thought (CoT) prompting, which decomposes the task into simpler steps to enhance the robustness of LLM-based TTS. To accomplish this idea, RALL-E first predicts prosody features (pitch and duration) of the input text and uses them as intermediate conditions to predict speech tokens in a CoT style.
Discrete speech tokens have been more and more popular in multiple speech processing fields, including automatic speech recognition (ASR), text-to-speech (TTS) and singing voice synthesis (SVS). In this paper, we describe the systems developed by the SJTU X-LANCE group for the TTS (acoustic + vocoder), SVS, and ASR tracks in the Interspeech 2024 Speech Processing Using Discrete Speech Unit ...
Decoding human speech from neural signals is essential for brain-computer interface (BCI) technologies that aim to restore speech in populations with neurological deficits. However, it remains a ...