MIT Technology Review
- Newsletters
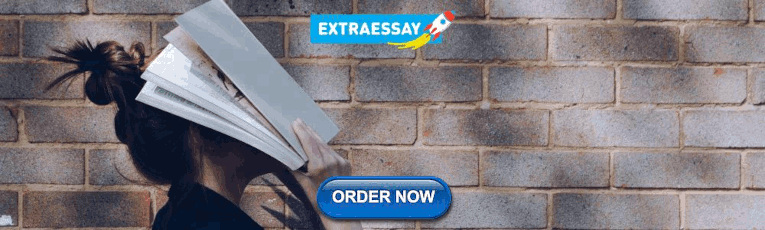
What’s next for AI in 2024
Our writers look at the four hot trends to watch out for this year
- Melissa Heikkilä archive page
- Will Douglas Heaven archive page
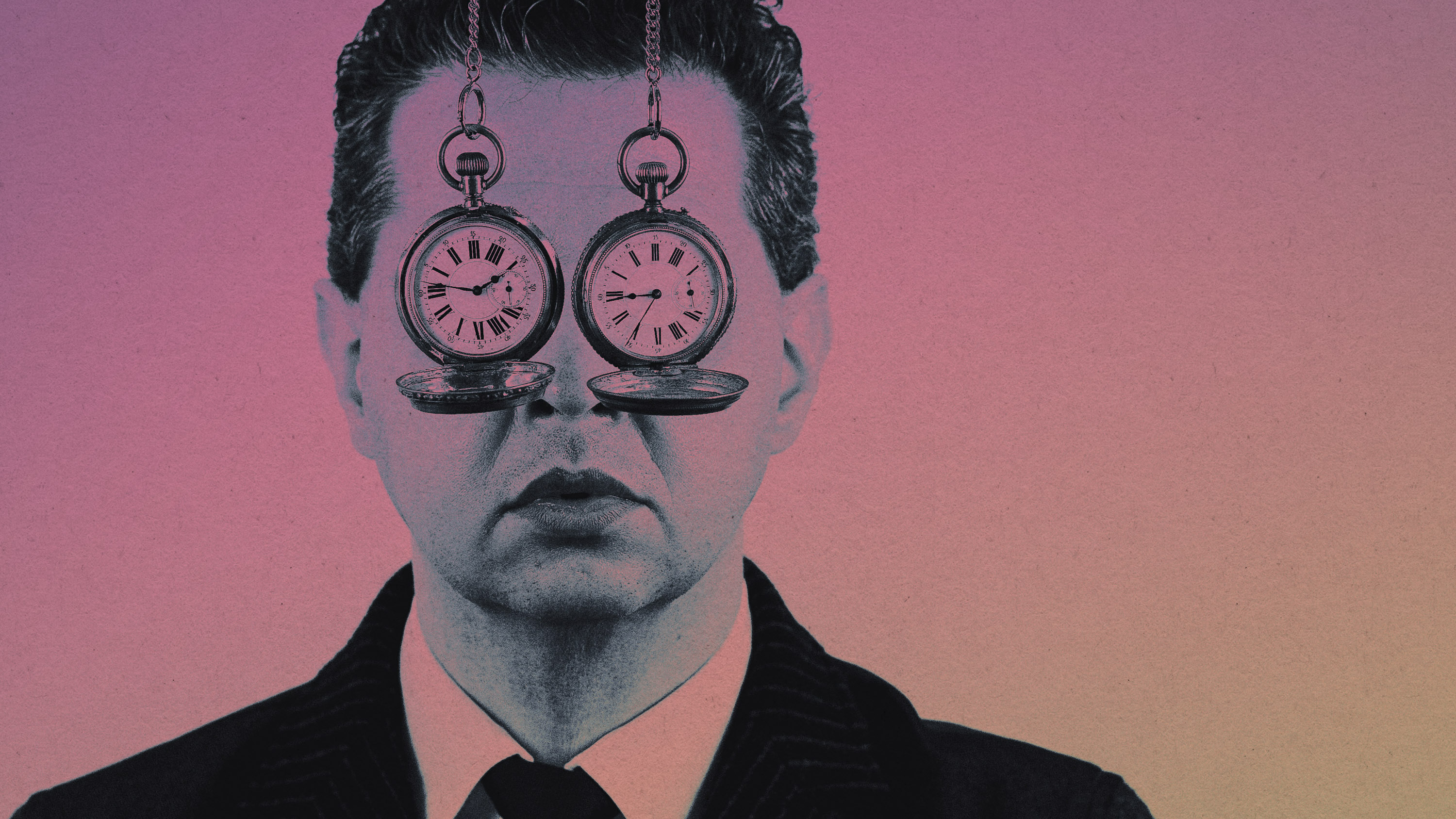
MIT Technology Review’s What’s Next series looks across industries, trends, and technologies to give you a first look at the future. You can read the rest of our series here .
This time last year we did something reckless. In an industry where nothing stands still, we had a go at predicting the future.
How did we do? Our four big bets for 2023 were that the next big thing in chatbots would be multimodal (check: the most powerful large language models out there, OpenAI’s GPT-4 and Google DeepMind’s Gemini, work with text, images and audio); that policymakers would draw up tough new regulations (check: Biden’s executive order came out in October and the European Union’s AI Act was finally agreed in December ); Big Tech would feel pressure from open-source startups (half right: the open-source boom continues, but AI companies like OpenAI and Google DeepMind still stole the limelight); and that AI would change big pharma for good (too soon to tell: the AI revolution in drug discovery is in full swing , but the first drugs developed using AI are still some years from market).
Now we’re doing it again.
We decided to ignore the obvious. We know that large language models will continue to dominate. Regulators will grow bolder. AI’s problems—from bias to copyright to doomerism—will shape the agenda for researchers, regulators, and the public, not just in 2024 but for years to come. (Read more about our six big questions for generative AI here .)
Instead, we’ve picked a few more specific trends. Here’s what to watch out for in 2024. (Come back next year and check how we did.)
Customized chatbots
You get a chatbot! And you get a chatbot! In 2024, tech companies that invested heavily in generative AI will be under pressure to prove that they can make money off their products. To do this, AI giants Google and OpenAI are betting big on going small: both are developing user-friendly platforms that allow people to customize powerful language models and make their own mini chatbots that cater to their specific needs—no coding skills required. Both have launched web-based tools that allow anyone to become a generative-AI app developer.
In 2024, generative AI might actually become useful for the regular, non-tech person, and we are going to see more people tinkering with a million little AI models. State-of-the-art AI models, such as GPT-4 and Gemini , are multimodal, meaning they can process not only text but images and even videos. This new capability could unlock a whole bunch of new apps. For example, a real estate agent can upload text from previous listings, fine-tune a powerful model to generate similar text with just a click of a button, upload videos and photos of new listings, and simply ask the customized AI to generate a description of the property.
But of course, the success of this plan hinges on whether these models work reliably. Language models often make stuff up, and generative models are riddled with biases . They are also easy to hack , especially if they are allowed to browse the web. Tech companies have not solved any of these problems. When the novelty wears off, they’ll have to offer their customers ways to deal with these problems.
—Melissa Heikkil ä
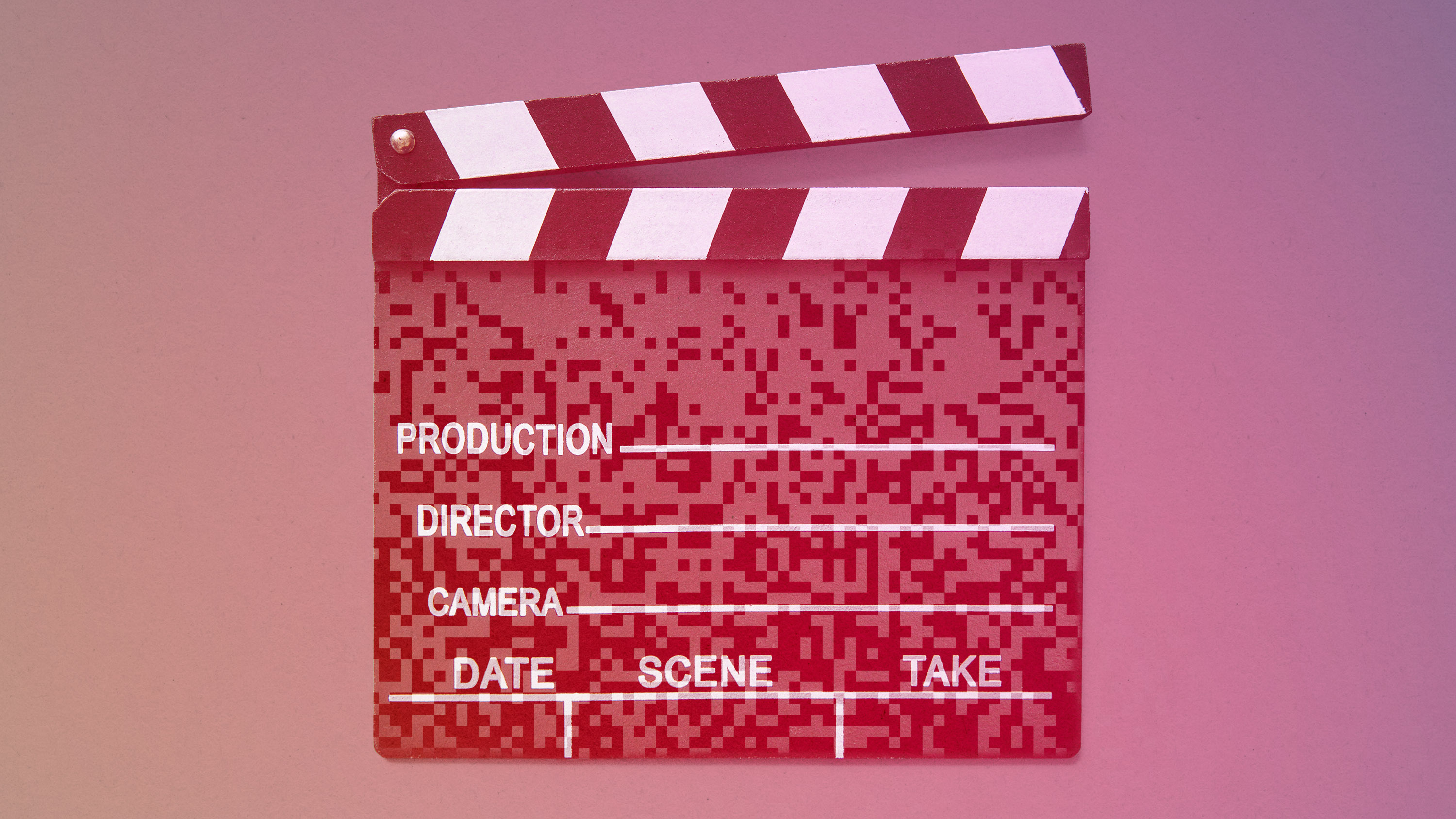
Generative AI’s second wave will be video
It’s amazing how fast the fantastic becomes familiar. The first generative models to produce photorealistic images exploded into the mainstream in 2022 —and soon became commonplace. Tools like OpenAI’s DALL-E, Stability AI’s Stable Diffusion, and Adobe’s Firefly flooded the internet with jaw-dropping images of everything from the pope in Balenciaga to prize-winning art . But it’s not all good fun: for every pug waving pompoms , there’s another piece of knock-off fantasy art or sexist sexual stereotyping .
The new frontier is text-to-video. Expect it to take everything that was good, bad, or ugly about text-to-image and supersize it.
A year ago we got the first glimpse of what generative models could do when they were trained to stitch together multiple still images into clips a few seconds long. The results were distorted and jerky. But the tech has rapidly improved.
Runway , a startup that makes generative video models (and the company that co-created Stable Diffusion), is dropping new versions of its tools every few months. Its latest model, called Gen-2 , still generates video just a few seconds long, but the quality is striking. The best clips aren’t far off what Pixar might put out.
Runway has set up an annual AI film festival that showcases experimental movies made with a range of AI tools. This year’s festival has a $60,000 prize pot, and the 10 best films will be screened in New York and Los Angeles.
It’s no surprise that top studios are taking notice. Movie giants, including Paramount and Disney, are now exploring the use of generative AI throughout their production pipeline. The tech is being used to lip-sync actors’ performances to multiple foreign-language overdubs. And it is reinventing what’s possible with special effects. In 2023, Indiana Jones and the Dial of Destiny starred a de-aged deepfake Harrison Ford. This is just the start.
Away from the big screen, deepfake tech for marketing or training purposes is taking off too. For example, UK-based Synthesia makes tools that can turn a one-off performance by an actor into an endless stream of deepfake avatars, reciting whatever script you give them at the push of a button. According to the company, its tech is now used by 44% of Fortune 100 companies.
The ability to do so much with so little raises serious questions for actors . Concerns about studios’ use and misuse of AI were at the heart of the SAG-AFTRA strikes last year. But the true impact of the tech is only just becoming apparent. “The craft of filmmaking is fundamentally changing,” says Souki Mehdaoui, an independent filmmaker and cofounder of Bell & Whistle, a consultancy specializing in creative technologies.
—Will Douglas Heaven
AI-generated election disinformation will be everywhere
If recent elections are anything to go by, AI-generated election disinformation and deepfakes are going to be a huge problem as a record number of people march to the polls in 2024. We’re already seeing politicians weaponizing these tools. In Argentina , two presidential candidates created AI-generated images and videos of their opponents to attack them. In Slovakia , deepfakes of a liberal pro-European party leader threatening to raise the price of beer and making jokes about child pornography spread like wildfire during the country’s elections. And in the US, Donald Trump has cheered on a group that uses AI to generate memes with racist and sexist tropes.
While it’s hard to say how much these examples have influenced the outcomes of elections, their proliferation is a worrying trend. It will become harder than ever to recognize what is real online. In an already inflamed and polarized political climate, this could have severe consequences.
Just a few years ago creating a deepfake would have required advanced technical skills, but generative AI has made it stupidly easy and accessible, and the outputs are looking increasingly realistic. Even reputable sources might be fooled by AI-generated content. For example, users-submitted AI-generated images purporting to depict the Israel-Gaza crisis have flooded stock image marketplaces like Adobe’s.
The coming year will be pivotal for those fighting against the proliferation of such content. Techniques to track and mitigate it content are still in early days of development. Watermarks, such as Google DeepMind’s SynthID , are still mostly voluntary and not completely foolproof. And social media platforms are notoriously slow in taking down misinformation. Get ready for a massive real-time experiment in busting AI-generated fake news.
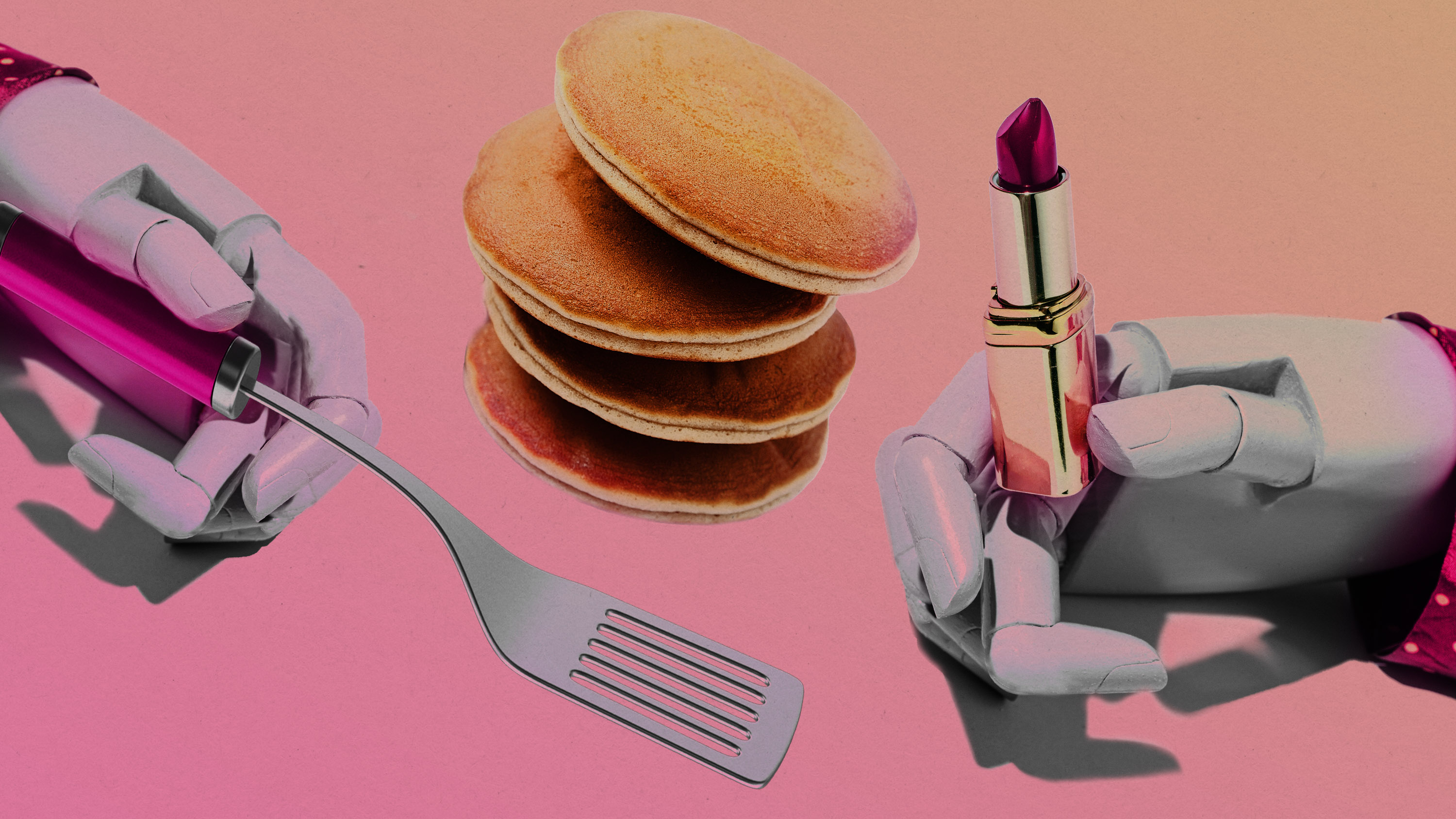
Robots that multitask
Inspired by some of the core techniques behind generative AI’s current boom, roboticists are starting to build more general-purpose robots that can do a wider range of tasks.
The last few years in AI have seen a shift away from using multiple small models, each trained to do different tasks—identifying images, drawing them, captioning them—toward single, monolithic models trained to do all these things and more. By showing OpenAI’s GPT-3 a few additional examples (known as fine-tuning), researchers can train it to solve coding problems, write movie scripts, pass high school biology exams, and so on. Multimodal models, like GPT-4 and Google DeepMind’s Gemini, can solve visual tasks as well as linguistic ones.
The same approach can work for robots, so it wouldn’t be necessary to train one to flip pancakes and another to open doors: a one-size-fits-all model could give robots the ability to multitask. Several examples of work in this area emerged in 2023.
In June, DeepMind released Robocat (an update on last year’s Gato ), which generates its own data from trial and error to learn how to control many different robot arms (instead of one specific arm, which is more typical).
In October, the company put out yet another general-purpose model for robots, called RT-X, and a big new general-purpose training data set , in collaboration with 33 university labs. Other top research teams, such as RAIL (Robotic Artificial Intelligence and Learning) at the University of California, Berkeley, are looking at similar tech.
The problem is a lack of data. Generative AI draws on an internet-size data set of text and images. In comparison, robots have very few good sources of data to help them learn how to do many of the industrial or domestic tasks we want them to.
Lerrel Pinto at New York University leads one team addressing that. He and his colleagues are developing techniques that let robots learn by trial and error, coming up with their own training data as they go. In an even more low-key project, Pinto has recruited volunteers to collect video data from around their homes using an iPhone camera mounted to a trash picker . Big companies have also started to release large data sets for training robots in the last couple of years, such as Meta’s Ego4D .
This approach is already showing promise in driverless cars. Startups such as Wayve, Waabi, and Ghost are pioneering a new wave of self-driving AI that uses a single large model to control a vehicle rather than multiple smaller models to control specific driving tasks. This has let small companies catch up with giants like Cruise and Waymo. Wayve is now testing its driverless cars on the narrow, busy streets of London. Robots everywhere are set to get a similar boost.
Artificial intelligence
Sam altman says helpful agents are poised to become ai’s killer function.
Open AI’s CEO says we won’t need new hardware or lots more training data to get there.
- James O'Donnell archive page
What’s next for generative video
OpenAI's Sora has raised the bar for AI moviemaking. Here are four things to bear in mind as we wrap our heads around what's coming.
Is robotics about to have its own ChatGPT moment?
Researchers are using generative AI and other techniques to teach robots new skills—including tasks they could perform in homes.
An AI startup made a hyperrealistic deepfake of me that’s so good it’s scary
Synthesia's new technology is impressive but raises big questions about a world where we increasingly can’t tell what’s real.
Stay connected
Get the latest updates from mit technology review.
Discover special offers, top stories, upcoming events, and more.
Thank you for submitting your email!
It looks like something went wrong.
We’re having trouble saving your preferences. Try refreshing this page and updating them one more time. If you continue to get this message, reach out to us at [email protected] with a list of newsletters you’d like to receive.
The present and future of AI
Finale doshi-velez on how ai is shaping our lives and how we can shape ai.
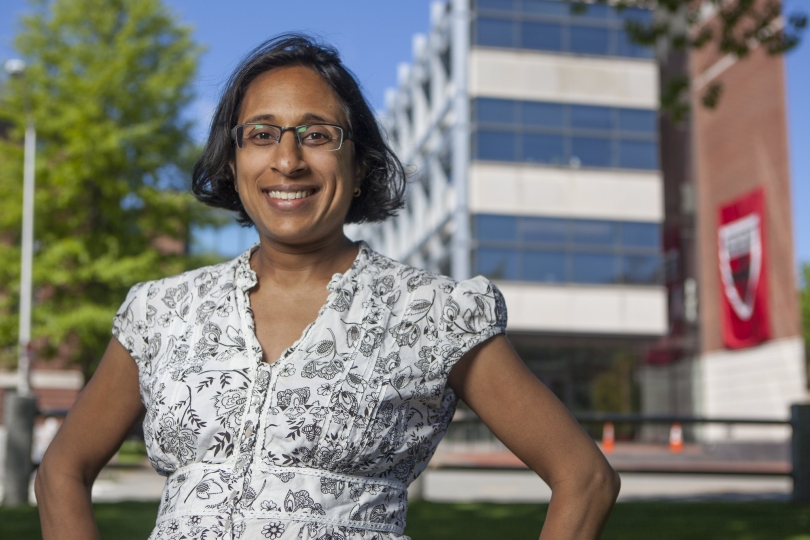
Finale Doshi-Velez, the John L. Loeb Professor of Engineering and Applied Sciences. (Photo courtesy of Eliza Grinnell/Harvard SEAS)
How has artificial intelligence changed and shaped our world over the last five years? How will AI continue to impact our lives in the coming years? Those were the questions addressed in the most recent report from the One Hundred Year Study on Artificial Intelligence (AI100), an ongoing project hosted at Stanford University, that will study the status of AI technology and its impacts on the world over the next 100 years.
The 2021 report is the second in a series that will be released every five years until 2116. Titled “Gathering Strength, Gathering Storms,” the report explores the various ways AI is increasingly touching people’s lives in settings that range from movie recommendations and voice assistants to autonomous driving and automated medical diagnoses .
Barbara Grosz , the Higgins Research Professor of Natural Sciences at the Harvard John A. Paulson School of Engineering and Applied Sciences (SEAS) is a member of the standing committee overseeing the AI100 project and Finale Doshi-Velez , Gordon McKay Professor of Computer Science, is part of the panel of interdisciplinary researchers who wrote this year’s report.
We spoke with Doshi-Velez about the report, what it says about the role AI is currently playing in our lives, and how it will change in the future.
Q: Let's start with a snapshot: What is the current state of AI and its potential?
Doshi-Velez: Some of the biggest changes in the last five years have been how well AIs now perform in large data regimes on specific types of tasks. We've seen [DeepMind’s] AlphaZero become the best Go player entirely through self-play, and everyday uses of AI such as grammar checks and autocomplete, automatic personal photo organization and search, and speech recognition become commonplace for large numbers of people.
In terms of potential, I'm most excited about AIs that might augment and assist people. They can be used to drive insights in drug discovery, help with decision making such as identifying a menu of likely treatment options for patients, and provide basic assistance, such as lane keeping while driving or text-to-speech based on images from a phone for the visually impaired. In many situations, people and AIs have complementary strengths. I think we're getting closer to unlocking the potential of people and AI teams.
There's a much greater recognition that we should not be waiting for AI tools to become mainstream before making sure they are ethical.
Q: Over the course of 100 years, these reports will tell the story of AI and its evolving role in society. Even though there have only been two reports, what's the story so far?
There's actually a lot of change even in five years. The first report is fairly rosy. For example, it mentions how algorithmic risk assessments may mitigate the human biases of judges. The second has a much more mixed view. I think this comes from the fact that as AI tools have come into the mainstream — both in higher stakes and everyday settings — we are appropriately much less willing to tolerate flaws, especially discriminatory ones. There's also been questions of information and disinformation control as people get their news, social media, and entertainment via searches and rankings personalized to them. So, there's a much greater recognition that we should not be waiting for AI tools to become mainstream before making sure they are ethical.
Q: What is the responsibility of institutes of higher education in preparing students and the next generation of computer scientists for the future of AI and its impact on society?
First, I'll say that the need to understand the basics of AI and data science starts much earlier than higher education! Children are being exposed to AIs as soon as they click on videos on YouTube or browse photo albums. They need to understand aspects of AI such as how their actions affect future recommendations.
But for computer science students in college, I think a key thing that future engineers need to realize is when to demand input and how to talk across disciplinary boundaries to get at often difficult-to-quantify notions of safety, equity, fairness, etc. I'm really excited that Harvard has the Embedded EthiCS program to provide some of this education. Of course, this is an addition to standard good engineering practices like building robust models, validating them, and so forth, which is all a bit harder with AI.
I think a key thing that future engineers need to realize is when to demand input and how to talk across disciplinary boundaries to get at often difficult-to-quantify notions of safety, equity, fairness, etc.
Q: Your work focuses on machine learning with applications to healthcare, which is also an area of focus of this report. What is the state of AI in healthcare?
A lot of AI in healthcare has been on the business end, used for optimizing billing, scheduling surgeries, that sort of thing. When it comes to AI for better patient care, which is what we usually think about, there are few legal, regulatory, and financial incentives to do so, and many disincentives. Still, there's been slow but steady integration of AI-based tools, often in the form of risk scoring and alert systems.
In the near future, two applications that I'm really excited about are triage in low-resource settings — having AIs do initial reads of pathology slides, for example, if there are not enough pathologists, or get an initial check of whether a mole looks suspicious — and ways in which AIs can help identify promising treatment options for discussion with a clinician team and patient.
Q: Any predictions for the next report?
I'll be keen to see where currently nascent AI regulation initiatives have gotten to. Accountability is such a difficult question in AI, it's tricky to nurture both innovation and basic protections. Perhaps the most important innovation will be in approaches for AI accountability.
Topics: AI / Machine Learning , Computer Science
Cutting-edge science delivered direct to your inbox.
Join the Harvard SEAS mailing list.
Scientist Profiles
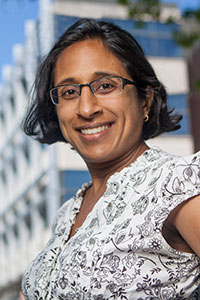
Finale Doshi-Velez
Herchel Smith Professor of Computer Science
Press Contact
Leah Burrows | 617-496-1351 | [email protected]
Related News
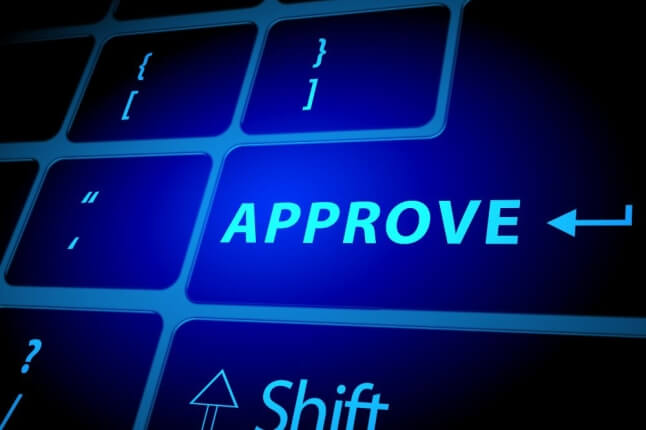
AI for more caring institutions
Improving AI-based decision-making tools for public services
AI / Machine Learning , Computer Science
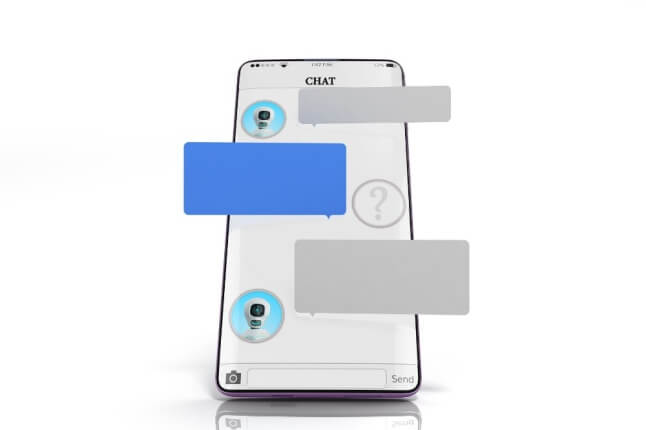
Coming out to a chatbot?
Researchers explore the limitations of mental health chatbots in LGBTQ+ communities
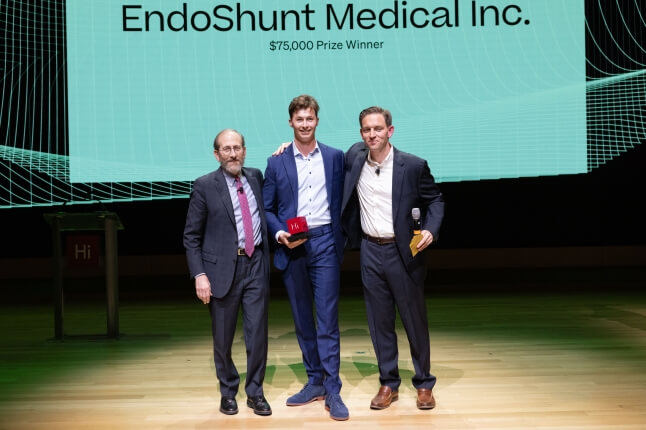
Three SEAS ventures take top prizes at President’s Innovation Challenge
Start-ups in emergency medicine, older adult care and quantum sensing all take home $75,000
Applied Physics , Awards , Computer Science , Entrepreneurship , Health / Medicine , Industry , Master of Design Engineering , Materials Science & Mechanical Engineering , MS/MBA , Quantum Engineering
When you choose to publish with PLOS, your research makes an impact. Make your work accessible to all, without restrictions, and accelerate scientific discovery with options like preprints and published peer review that make your work more Open.
PLOS Biology
- PLOS Climate
- PLOS Complex Systems
PLOS Computational Biology
PLOS Digital Health
- PLOS Genetics
- PLOS Global Public Health
PLOS Medicine
- PLOS Mental Health
- PLOS Neglected Tropical Diseases
- PLOS Pathogens
- PLOS Sustainability and Transformation
- PLOS Collections
Artificial intelligence
Discover multidisciplinary research that explores the opportunities, applications, and risks of machine learning and artificial intelligence across a broad spectrum of disciplines.
Discover artificial intelligence research from PLOS
From exploring applications in healthcare and biomedicine to unraveling patterns and optimizing decision-making within complex systems, PLOS’ interdisciplinary artificial intelligence research aims to capture cutting-edge methodologies, advancements, and breakthroughs in machine learning, showcasing diverse perspectives, interdisciplinary approaches, and societal and ethical implications.
Given their increasing influence in our everyday lives, it is vital to ensure that artificial intelligence tools are both inclusive and reliable. Robust and trusted artificial intelligence research hinges on the foundation of Open Science practices and the meticulous curation of data which PLOS takes pride in highlighting and endorsing thanks to our rigorous standards and commitment to Openness.
Stay up-to-date on artificial intelligence research from PLOS
Research spotlights
As a leading publisher in the field, these articles showcase research that has influenced academia, industry and/or policy.

A performance comparison of supervised machine learning models for Covid-19 tweets sentiment analysis

CellProfiler 3.0: Next- generation image processing for biology

Predicting survival from colorectal cancer histology slides using deep learning: A retrospective multicenter study
Artificial intelligence research topics.
PLOS publishes research across a broad range of topics. Take a look at the latest work in your field.
AI and climate change
Deep learning
AI for healthcare
Artificial neural networks
Natural language processing (NLP)
Graph neural networks (GNNs)
AI ethics and fairness
Machine learning
AI and complex systems
Read the latest research developments in your field
Our commitment to Open Science means others can build on PLOS artificial intelligence research to advance the field. Discover selected popular artificial intelligence research below:
Artificial intelligence based writer identification generates new evidence for the unknown scribes of the Dead Sea Scrolls exemplified by the Great Isaiah Scroll (1QIsaa)
Artificial intelligence with temporal features outperforms machine learning in predicting diabetes
Bat detective—Deep learning tools for bat acoustic signal detection
Bias in artificial intelligence algorithms and recommendations for mitigation
Can machine-learning improve cardiovascular risk prediction using routine clinical data?
Convergence of mechanistic modeling and artificial intelligence in hydrologic science and engineering
Cyberbullying severity detection: A machine learning approach
Diverse patients’ attitudes towards Artificial Intelligence (AI) in diagnosis
Expansion of RiPP biosynthetic space through integration of pan-genomics and machine learning uncovers a novel class of lanthipeptides
Generalizable brain network markers of major depressive disorder across multiple imaging sites
Machine learning algorithm validation with a limited sample size
Neural spiking for causal inference and learning
Review of machine learning methods in soft robotics
Ten quick tips for harnessing the power of ChatGPT in computational biology
Ten simple rules for engaging with artificial intelligence in biomedicine
Browse the full PLOS portfolio of Open Access artificial intelligence articles
25,938 authors from 133 countries chose PLOS to publish their artificial intelligence research*
Reaching a global audience, this research has received over 5,502 news and blog mentions ^ , research in this field has been cited 117,554 times after authors published in a plos journal*, related plos research collections.
Covering a connected body of work and evaluated by leading experts in their respective fields, our Collections make it easier to delve deeper into specific research topics from across the breadth of the PLOS portfolio.
Check out our highlighted PLOS research Collections:

Machine Learning in Health and Biomedicine

Cities as Complex Systems

Open Quantum Computation and Simulation
Stay up-to-date on the latest artificial intelligence research from PLOS
Related journals in artificial intelligence
We provide a platform for artificial intelligence research across various PLOS journals, allowing interdisciplinary researchers to explore artificial intelligence research at all preclinical, translational and clinical research stages.
*Data source: Web of Science . © Copyright Clarivate 2024 | January 2004 – January 2024 ^Data source: Altmetric.com | January 2004 – January 2024
Breaking boundaries. Empowering researchers. Opening science.
PLOS is a nonprofit, Open Access publisher empowering researchers to accelerate progress in science and medicine by leading a transformation in research communication.
Open Access
All PLOS journals are fully Open Access, which means the latest published research is immediately available for all to learn from, share, and reuse with attribution. No subscription fees, no delays, no barriers.
Leading responsibly
PLOS is working to eliminate financial barriers to Open Access publishing, facilitate diversity and broad participation of voices in knowledge-sharing, and ensure inclusive policies shape our journals. We’re committed to openness and transparency, whether it’s peer review, our data policy, or sharing our annual financial statement with the community.
Breaking boundaries in Open Science
We push the boundaries of “Open” to create a more equitable system of scientific knowledge and understanding. All PLOS articles are backed by our Data Availability policy, and encourage the sharing of preprints, code, protocols, and peer review reports so that readers get more context.
Interdisciplinary
PLOS journals publish research from every discipline across science and medicine and related social sciences. Many of our journals are interdisciplinary in nature to facilitate an exchange of knowledge across disciplines, encourage global collaboration, and influence policy and decision-making at all levels
Community expertise
Our Editorial Boards represent the full diversity of the research and researchers in the field. They work in partnership with expert peer reviewers to evaluate each manuscript against the highest methodological and ethical standards in the field.
Rigorous peer review
Our rigorous editorial screening and assessment process is made up of several stages. All PLOS journals use anonymous peer review by default, but we also offer authors and reviewers options to make the peer review process more transparent.
For IEEE Members
Ieee spectrum, follow ieee spectrum, support ieee spectrum, enjoy more free content and benefits by creating an account, saving articles to read later requires an ieee spectrum account, the institute content is only available for members, downloading full pdf issues is exclusive for ieee members, downloading this e-book is exclusive for ieee members, access to spectrum 's digital edition is exclusive for ieee members, following topics is a feature exclusive for ieee members, adding your response to an article requires an ieee spectrum account, create an account to access more content and features on ieee spectrum , including the ability to save articles to read later, download spectrum collections, and participate in conversations with readers and editors. for more exclusive content and features, consider joining ieee ., join the world’s largest professional organization devoted to engineering and applied sciences and get access to all of spectrum’s articles, archives, pdf downloads, and other benefits. learn more →, join the world’s largest professional organization devoted to engineering and applied sciences and get access to this e-book plus all of ieee spectrum’s articles, archives, pdf downloads, and other benefits. learn more →, access thousands of articles — completely free, create an account and get exclusive content and features: save articles, download collections, and talk to tech insiders — all free for full access and benefits, join ieee as a paying member., 2021’s top stories about ai, spoiler: a lot of them talked about what's wrong with machine learning today.
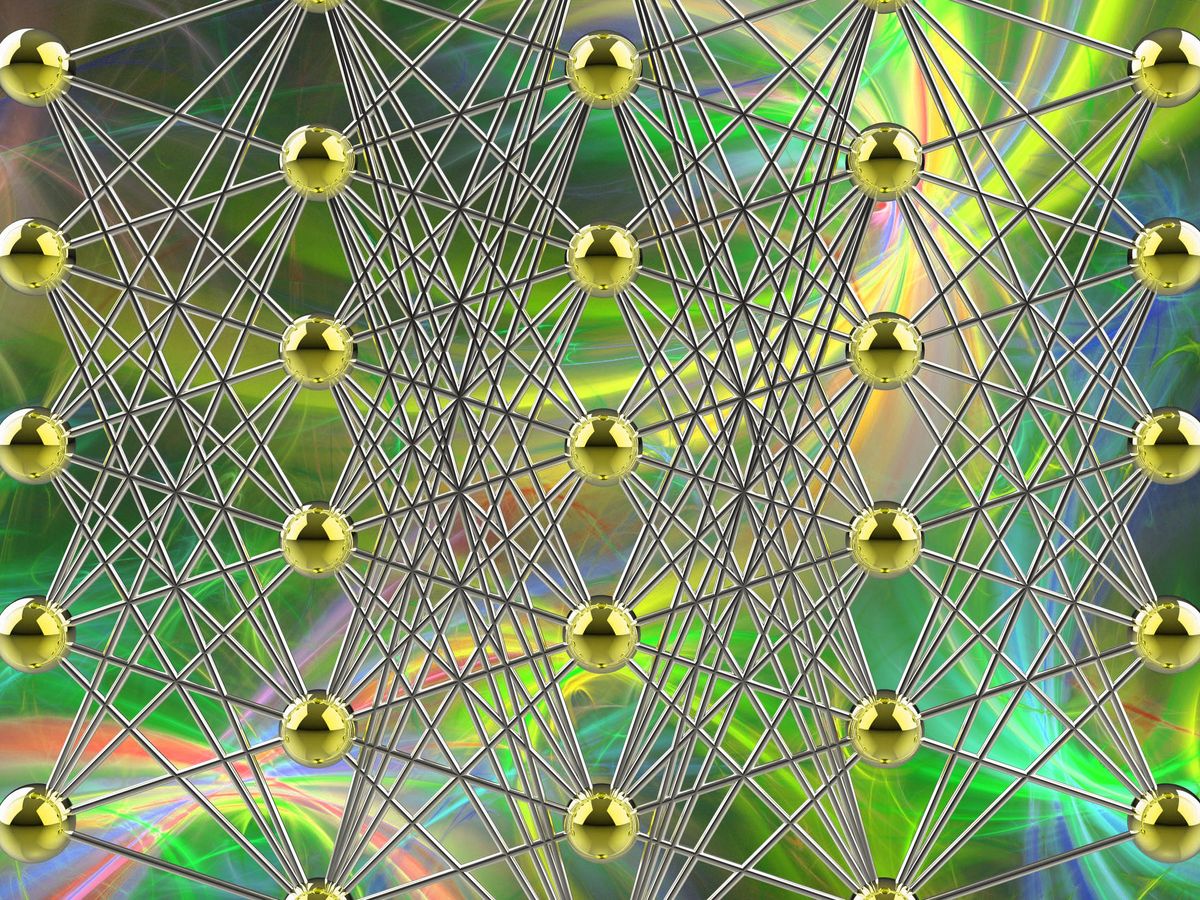
2021 was the year in which the wonders of artificial intelligence stopped being a story. Which is not to say that IEEE Spectrum didn’t cover AI—we covered the heck out of it. But we all know that deep learning can do wondrous things and that it’s being rapidly incorporated into many industries; that’s yesterday’s news. Many of this year’s top articles grappled with the limits of deep learning (today’s dominant strand of AI) and spotlighted researchers seeking new paths.
Here are the 10 most popular AI articles that Spectrum published in 2021, ranked by the amount of time people spent reading them. Several came from Spectrum ‘s October 2021 special issue on AI, The Great AI Reckoning .
1. Deep Learning’s Diminishing Returns : MIT’s Neil Thompson and several of his collaborators captured the top spot with a thoughtful feature article about the computational and energy costs of training deep-learning systems. They analyzed the improvements of image classifiers and found that “to halve the error rate, you can expect to need more than 500 times the computational resources.” They wrote: “Faced with skyrocketing costs, researchers will either have to come up with more efficient ways to solve these problems, or they will abandon working on these problems and progress will languish.” Their article isn’t a total downer, though. They ended with some promising ideas for the way forward.
2. 15 Graphs You Need to See to Understand AI in 2021 : Every year, The AI Index drops a massive load of data into the conversation about AI. In 2021, the Index’s diligent curators presented a global perspective on academia and industry, taking care to highlight issues with diversity in the AI workforce and ethical challenges of AI applications. I, your humble AI editor, then curated that massive amount of curated data, boiling 222 pages of report down into 15 graphs covering jobs, investments, and more. You’re welcome.
3. How DeepMind Is Reinventing the Robot : DeepMind, the London-based Alphabet subsidiary, has been behind some of the most impressive feats of AI in recent years, including breakthrough work on protein folding and the AlphaGo system that beat a grandmaster at the ancient game of Go. So when DeepMind’s head of robotics Raia Hadsell says she’s tackling the long-standing AI problem of catastrophic forgetting in an attempt to build multitalented and adaptable robots, people pay attention.
4. The Turbulent Past and Uncertain Future of Artificial Intelligence : This feature article served as the introduction to Spectrum ‘s special report on AI , telling the story of the field from 1956 to present day while also cueing up the other articles in the special issue. If you want to understand how we got here, this is the article for you. It pays special attention to past feuds between the symbolists who bet on expert systems and the connectionists who invented neural networks, and looks forward to the possibilities of hybrid neuro-symbolic systems.
5. Andrew Ng X-Rays the AI Hype : This short article relayed an anecdote from a Zoom Q&A session with AI pioneer Andrew Ng , who was deeply involved in early AI efforts at Google Brain and Baidu and now leads a company called Landing AI . Ng spoke about an AI system developed at Stanford University that could spot pneumonia in chest X-rays, even outperforming radiologists. But there was a twist to the story.
6. OpenAI’s GPT-3 Speaks! (Kindly Disregard Toxic Language) : When the San Francisco–based AI lab OpenAI unveiled the language-generating system GPT-3 in 2020, the first reaction of the AI community was awe. GPT-3 could generate fluid and coherent text on any topic and in any style when given the smallest of prompts. But it has a dark side. Trained on text from the internet, it learned the human biases that are all too prevalent in certain portions of the online world, and therefore has an awful habit of unexpectedly spewing out toxic language. Your humble AI editor (again, that’s me) got very interested in the companies that are rushing to integrate GPT-3 into their products, hoping to use it for such applications as customer support, online tutoring, mental health counseling, and more. I wanted to know: If you’re going to employ an AI troll, how do you prevent it from insulting and alienating your customers?
7. Fast, Efficient Neural Networks Copy Dragonfly Brains : What do dragonfly brains have to do with missile defense? Ask Frances Chance of Sandia National Laboratories, who studies how dragonflies efficiently use their roughly 1 million neurons to hunt and capture aerial prey with extraordinary precision. Her work is an interesting contrast to research labs building neural networks of ever-increasing size and complexity (recall #1 on this list). She writes: “By harnessing the speed, simplicity, and efficiency of the dragonfly nervous system, we aim to design computers that perform these functions faster and at a fraction of the power that conventional systems consume.”
8. Deep Learning Isn’t Deep Enough Unless It Copies From the Brain : In a former life, Jeff Hawkins invented the PalmPilot and ushered in the smartphone era. These days, at the machine intelligence company Numenta , he’s investigating the basis of intelligence in the human brain and hoping to usher in a new era of artificial general intelligence. This Q&A with Hawkins covers some of his most controversial ideas, including his conviction that superintelligent AI doesn’t pose an existential threat to humanity and his contention that consciousness isn’t really such a hard problem.
9. The Algorithms That Make Instacart Roll : It’s always fun for Spectrum readers to get an insider’s look at the tech companies that enable our lives. Engineers Sharath Rao and Lily Zhang of Instacart, the grocery shopping and delivery company, explain that the company’s AI infrastructure has to predict the availability of “the products in nearly 40,000 grocery stores—billions of different data points,” while also suggesting replacements, predicting how many shoppers will be available to work, and efficiently grouping orders and delivery routes.
10. 7 Revealing Ways AIs Fail : Everyone loves a list, right? After all, here we are together at item #10 on this list. Spectrum contributor Charles Choi pulled together this entertaining list of failures and explained what they reveal about the weaknesses of today’s AI. The cartoons of robots getting themselves into trouble are a nice bonus.
So there you have it. Keep reading IEEE Spectrum to see what happens next. Will 2022 be the year in which researchers figure out solutions to some of the knotty problems we covered in the year that’s now ending? Will they solve algorithmic bias, put an end to catastrophic forgetting, and find ways to improve performance without busting the planet’s energy budget? Probably not all at once...but let’s find out together.
- Artificial Intelligence News & Articles - IEEE Spectrum ›
- Superintelligent AI May Be Impossible to Control; That's the Good ... ›
- Stop Calling Everything AI, Machine-Learning Pioneer Says - IEEE ... ›
- AI’s 6 Worst-Case Scenarios - IEEE Spectrum ›
- Andrew Ng: Unbiggen AI - IEEE Spectrum ›
- Benefits & Risks of Artificial Intelligence - Future of Life Institute ›
- Artificial intelligence - Wikipedia ›
- Association for the Advancement of Artificial Intelligence ›
Eliza Strickland is a senior editor at IEEE Spectrum , where she covers AI, biomedical engineering, and other topics. She holds a master’s degree in journalism from Columbia University.
The common weakness of AI as it stands today, is that it requires commercial investment… and those investors want some positive return.
If we could accept Altruistic AI, or SI as I call it, we would have functioning self-aware intelligent systems within a decade or so.
Of course, these can be abused for commercial or political ends, and therein lies the problem.
Ethical engineering can’t be achieved until we have an ethical world to operate in.
We can only hope that comes sooner than later.
"Snake-like" Probe Images Arteries from Within
How to put a data center in a shoebox, mri sheds its shielding and superconducting magnets, related stories, llama 3 establishes meta as the leader in “open” ai, ai chip trims energy budget back by 99+ percent, faster, more secure photonic chip boosts ai training.
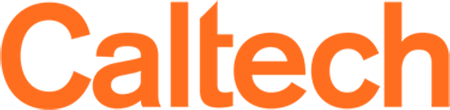
Artificial Intelligence
Since the 1950s, scientists and engineers have designed computers to "think" by making decisions and finding patterns like humans do. In recent years, artificial intelligence has become increasingly powerful, propelling discovery across scientific fields and enabling researchers to delve into problems previously too complex to solve. Outside of science, artificial intelligence is built into devices all around us, and billions of people across the globe rely on it every day. Stories of artificial intelligence—from friendly humanoid robots to SkyNet—have been incorporated into some of the most iconic movies and books.
But where is the line between what AI can do and what is make-believe? How is that line blurring, and what is the future of artificial intelligence? At Caltech, scientists and scholars are working at the leading edge of AI research, expanding the boundaries of its capabilities and exploring its impacts on society. Discover what defines artificial intelligence, how it is developed and deployed, and what the field holds for the future.
Artificial Intelligence Terms to Know >
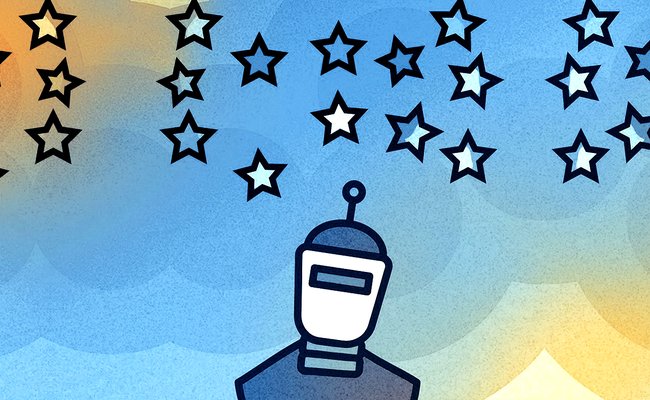
What Is AI ?
Artificial intelligence is transforming scientific research as well as everyday life, from communications to transportation to health care and more. Explore what defines AI, how it has evolved since the Turing Test, and the future of artificial intelligence.
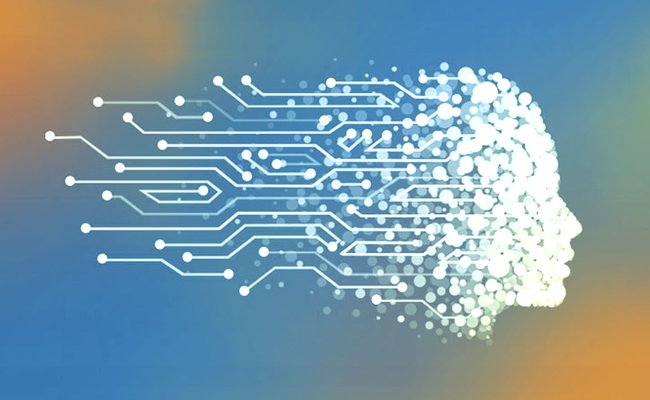
What Is the Difference Between "Artificial Intelligence" and "Machine Learning"?
The term "artificial intelligence" is older and broader than "machine learning." Learn how the terms relate to each other and to the concepts of "neural networks" and "deep learning."
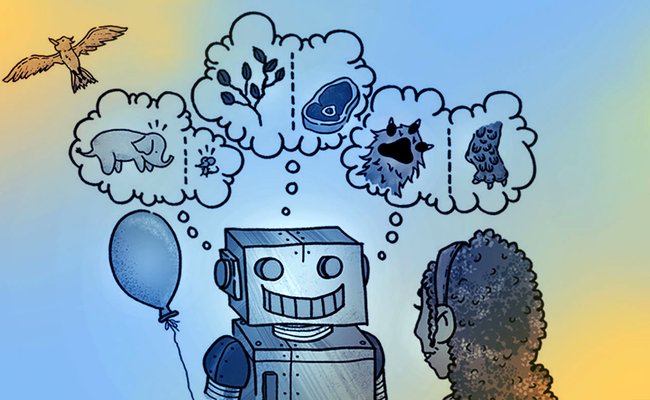
How Do Computers Learn?
Machine learning applications power many features of modern life, including search engines, social media, and self-driving cars. Discover how computers learn to make decisions and predictions in this illustration of two key machine learning models.
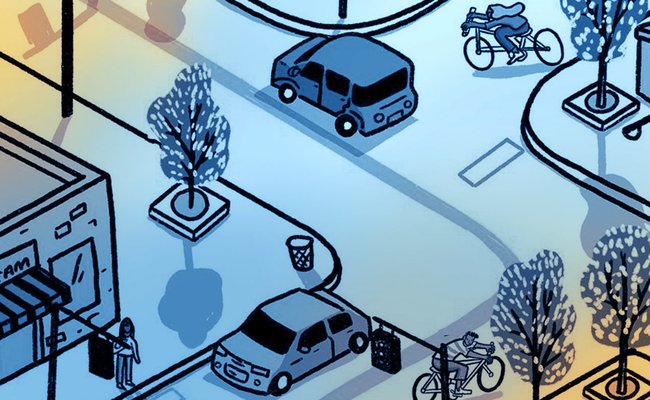
How Is AI Applied in Everyday Life?
While scientists and engineers explore AI's potential to advance discovery and technology, smart technologies also directly influence our daily lives. Explore the sometimes surprising examples of AI applications.
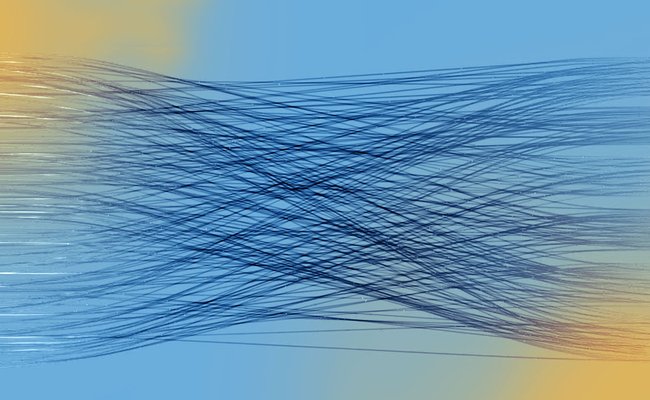
What Is Big Data?
The increase in available data has fueled the rise of artificial intelligence. Find out what characterizes big data, where big data comes from, and how it is used.
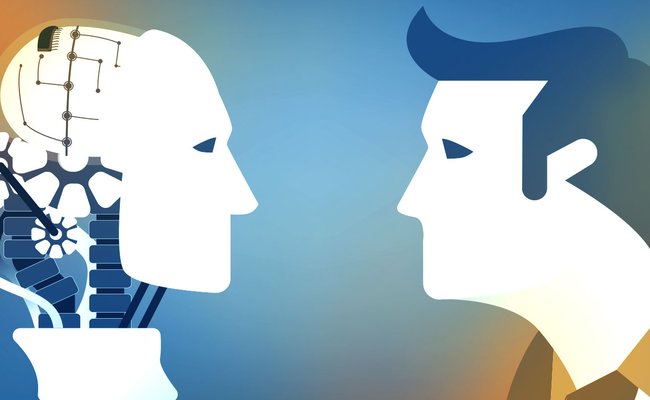
Will Machines Become More Intelligent Than Humans?
Whether or not artificial intelligence will be able to outperform human intelligence—and how soon that could happen—is a common question fueled by depictions of AI in movies and other forms of popular culture. Learn the definition of "singularity" and see a timeline of advances in AI over the past 75 years.
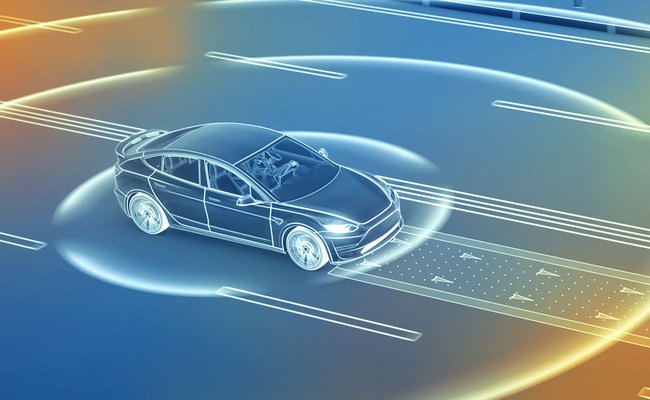
How Does AI Drive Autonomous Systems?
Learn the difference between automation and autonomy, and hear from Caltech faculty who are pushing the limits of AI to create autonomous technology, from self-driving cars to ambulance drones to prosthetic devices.
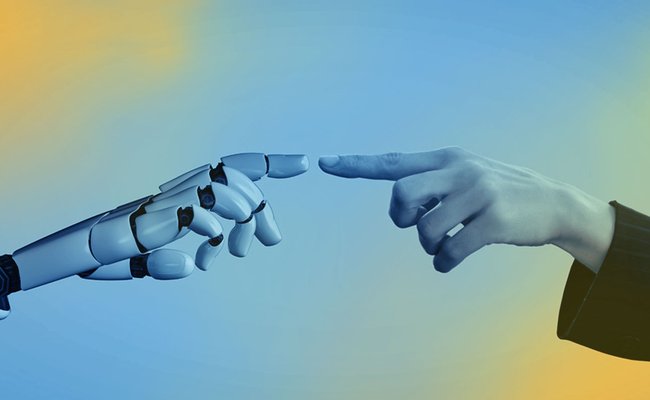
Can We Trust AI?
As AI is further incorporated into everyday life, more scholars, industries, and ordinary users are examining its effects on society. The Caltech Science Exchange spoke with AI researchers at Caltech about what it might take to trust current and future technologies.
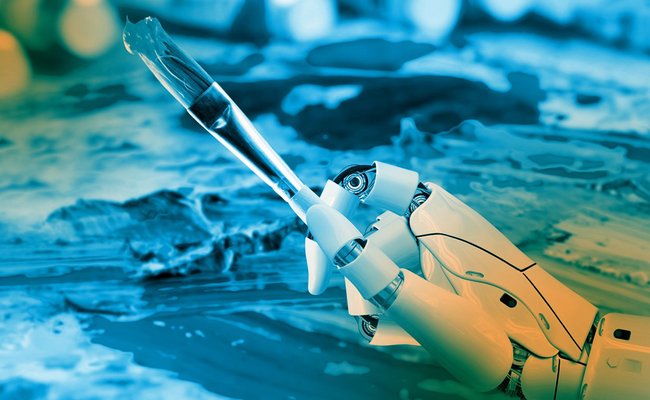
What is Generative AI?
Generative AI applications such as ChatGPT, a chatbot that answers questions with detailed written responses; and DALL-E, which creates realistic images and art based on text prompts; became widely popular beginning in 2022 when companies released versions of their applications that members of the public, not just experts, could easily use.
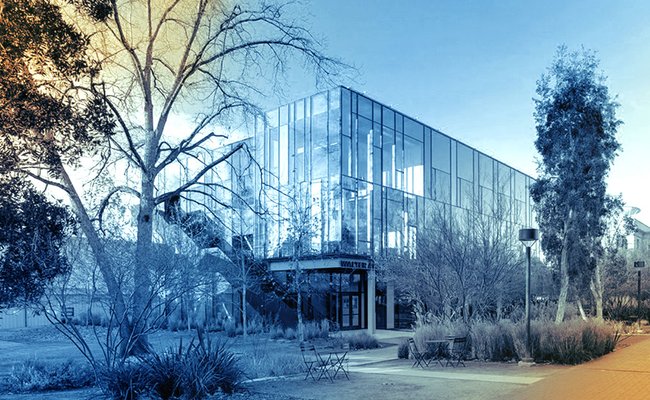
Ask a Caltech Expert
Where can you find machine learning in finance? Could AI help nature conservation efforts? How is AI transforming astronomy, biology, and other fields? What does an autonomous underwater vehicle have to do with sustainability? Find answers from Caltech researchers.
Terms to Know
A set of instructions or sequence of steps that tells a computer how to perform a task or calculation. In some AI applications, algorithms tell computers how to adapt and refine processes in response to data, without a human supplying new instructions.
Artificial intelligence describes an application or machine that mimics human intelligence.
A system in which machines execute repeated tasks based on a fixed set of human-supplied instructions.
A system in which a machine makes independent, real-time decisions based on human-supplied rules and goals.
The massive amounts of data that are coming in quickly and from a variety of sources, such as internet-connected devices, sensors, and social platforms. In some cases, using or learning from big data requires AI methods. Big data also can enhance the ability to create new AI applications.
An AI system that mimics human conversation. While some simple chatbots rely on pre-programmed text, more sophisticated systems, trained on large data sets, are able to convincingly replicate human interaction.
Deep Learning
A subset of machine learning . Deep learning uses machine learning algorithms but structures the algorithms in layers to create "artificial neural networks." These networks are modeled after the human brain and are most likely to provide the experience of interacting with a real human.
Human in the Loop
An approach that includes human feedback and oversight in machine learning systems. Including humans in the loop may improve accuracy and guard against bias and unintended outcomes of AI.
Model (computer model)
A computer-generated simplification of something that exists in the real world, such as climate change , disease spread, or earthquakes . Machine learning systems develop models by analyzing patterns in large data sets. Models can be used to simulate natural processes and make predictions.
Neural Networks
Interconnected sets of processing units, or nodes, modeled on the human brain, that are used in deep learning to identify patterns in data and, on the basis of those patterns, make predictions in response to new data. Neural networks are used in facial recognition systems, digital marketing, and other applications.
Singularity
A hypothetical scenario in which an AI system develops agency and grows beyond human ability to control it.
Training data
The data used to " teach " a machine learning system to recognize patterns and features. Typically, continual training results in more accurate machine learning systems. Likewise, biased or incomplete datasets can lead to imprecise or unintended outcomes.
Turing Test
An interview-based method proposed by computer pioneer Alan Turing to assess whether a machine can think.
Dive Deeper
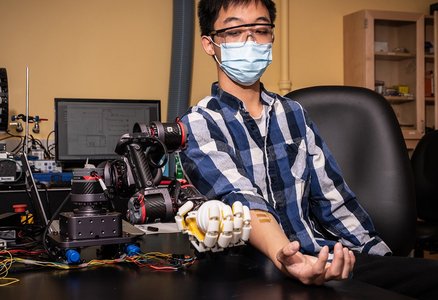
More Caltech Computer and Information Sciences Research Coverage
Suggestions or feedback?
MIT News | Massachusetts Institute of Technology
- Machine learning
- Social justice
- Black holes
- Classes and programs
Departments
- Aeronautics and Astronautics
- Brain and Cognitive Sciences
- Architecture
- Political Science
- Mechanical Engineering
Centers, Labs, & Programs
- Abdul Latif Jameel Poverty Action Lab (J-PAL)
- Picower Institute for Learning and Memory
- Lincoln Laboratory
- School of Architecture + Planning
- School of Engineering
- School of Humanities, Arts, and Social Sciences
- Sloan School of Management
- School of Science
- MIT Schwarzman College of Computing
New hardware offers faster computation for artificial intelligence, with much less energy
Press contact :, media download.
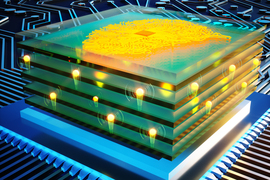
*Terms of Use:
Images for download on the MIT News office website are made available to non-commercial entities, press and the general public under a Creative Commons Attribution Non-Commercial No Derivatives license . You may not alter the images provided, other than to crop them to size. A credit line must be used when reproducing images; if one is not provided below, credit the images to "MIT."
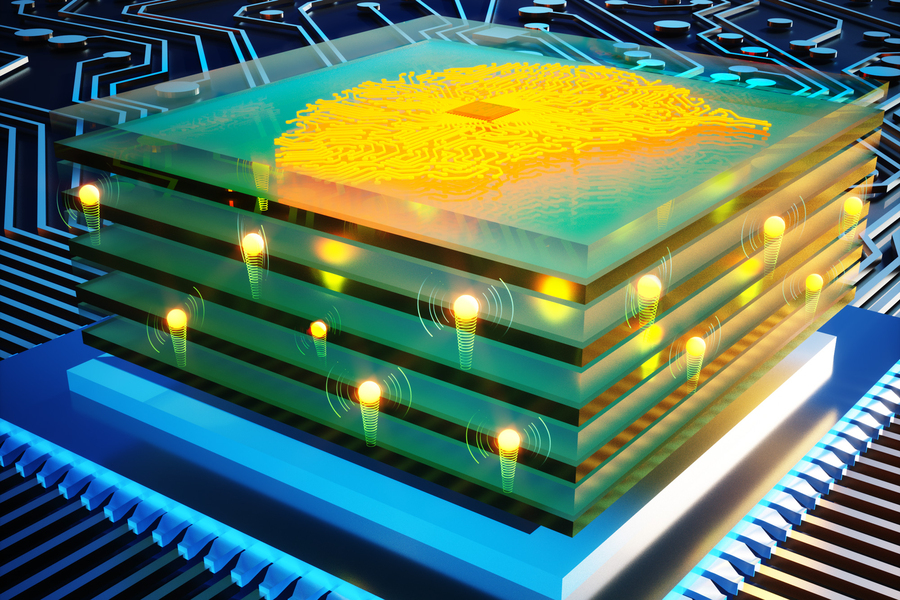
Previous image Next image
As scientists push the boundaries of machine learning, the amount of time, energy, and money required to train increasingly complex neural network models is skyrocketing. A new area of artificial intelligence called analog deep learning promises faster computation with a fraction of the energy usage.
Programmable resistors are the key building blocks in analog deep learning, just like transistors are the core elements for digital processors. By repeating arrays of programmable resistors in complex layers, researchers can create a network of analog artificial “neurons” and “synapses” that execute computations just like a digital neural network. This network can then be trained to achieve complex AI tasks like image recognition and natural language processing.
A multidisciplinary team of MIT researchers set out to push the speed limits of a type of human-made analog synapse that they had previously developed . They utilized a practical inorganic material in the fabrication process that enables their devices to run 1 million times faster than previous versions, which is also about 1 million times faster than the synapses in the human brain.
Moreover, this inorganic material also makes the resistor extremely energy-efficient. Unlike materials used in the earlier version of their device, the new material is compatible with silicon fabrication techniques. This change has enabled fabricating devices at the nanometer scale and could pave the way for integration into commercial computing hardware for deep-learning applications.
“With that key insight, and the very powerful nanofabrication techniques we have at MIT.nano , we have been able to put these pieces together and demonstrate that these devices are intrinsically very fast and operate with reasonable voltages,” says senior author Jesús A. del Alamo, the Donner Professor in MIT’s Department of Electrical Engineering and Computer Science (EECS). “This work has really put these devices at a point where they now look really promising for future applications.”
“The working mechanism of the device is electrochemical insertion of the smallest ion, the proton, into an insulating oxide to modulate its electronic conductivity. Because we are working with very thin devices, we could accelerate the motion of this ion by using a strong electric field, and push these ionic devices to the nanosecond operation regime,” explains senior author Bilge Yildiz, the Breene M. Kerr Professor in the departments of Nuclear Science and Engineering and Materials Science and Engineering.
“The action potential in biological cells rises and falls with a timescale of milliseconds, since the voltage difference of about 0.1 volt is constrained by the stability of water,” says senior author Ju Li, the Battelle Energy Alliance Professor of Nuclear Science and Engineering and professor of materials science and engineering, “Here we apply up to 10 volts across a special solid glass film of nanoscale thickness that conducts protons, without permanently damaging it. And the stronger the field, the faster the ionic devices.”
These programmable resistors vastly increase the speed at which a neural network is trained, while drastically reducing the cost and energy to perform that training. This could help scientists develop deep learning models much more quickly, which could then be applied in uses like self-driving cars, fraud detection, or medical image analysis.
“Once you have an analog processor, you will no longer be training networks everyone else is working on. You will be training networks with unprecedented complexities that no one else can afford to, and therefore vastly outperform them all. In other words, this is not a faster car, this is a spacecraft,” adds lead author and MIT postdoc Murat Onen.
Co-authors include Frances M. Ross, the Ellen Swallow Richards Professor in the Department of Materials Science and Engineering; postdocs Nicolas Emond and Baoming Wang; and Difei Zhang, an EECS graduate student. The research is published today in Science .
Accelerating deep learning
Analog deep learning is faster and more energy-efficient than its digital counterpart for two main reasons. “First, computation is performed in memory, so enormous loads of data are not transferred back and forth from memory to a processor.” Analog processors also conduct operations in parallel. If the matrix size expands, an analog processor doesn’t need more time to complete new operations because all computation occurs simultaneously.
The key element of MIT’s new analog processor technology is known as a protonic programmable resistor. These resistors, which are measured in nanometers (one nanometer is one billionth of a meter), are arranged in an array, like a chess board.
In the human brain, learning happens due to the strengthening and weakening of connections between neurons, called synapses. Deep neural networks have long adopted this strategy, where the network weights are programmed through training algorithms. In the case of this new processor, increasing and decreasing the electrical conductance of protonic resistors enables analog machine learning.
The conductance is controlled by the movement of protons. To increase the conductance, more protons are pushed into a channel in the resistor, while to decrease conductance protons are taken out. This is accomplished using an electrolyte (similar to that of a battery) that conducts protons but blocks electrons.
To develop a super-fast and highly energy efficient programmable protonic resistor, the researchers looked to different materials for the electrolyte. While other devices used organic compounds, Onen focused on inorganic phosphosilicate glass (PSG).
PSG is basically silicon dioxide, which is the powdery desiccant material found in tiny bags that come in the box with new furniture to remove moisture. It is studied as a proton conductor under humidified conditions for fuel cells. It is also the most well-known oxide used in silicon processing. To make PSG, a tiny bit of phosphorus is added to the silicon to give it special characteristics for proton conduction.
Onen hypothesized that an optimized PSG could have a high proton conductivity at room temperature without the need for water, which would make it an ideal solid electrolyte for this application. He was right.
Surprising speed
PSG enables ultrafast proton movement because it contains a multitude of nanometer-sized pores whose surfaces provide paths for proton diffusion. It can also withstand very strong, pulsed electric fields. This is critical, Onen explains, because applying more voltage to the device enables protons to move at blinding speeds.
“The speed certainly was surprising. Normally, we would not apply such extreme fields across devices, in order to not turn them into ash. But instead, protons ended up shuttling at immense speeds across the device stack, specifically a million times faster compared to what we had before. And this movement doesn’t damage anything, thanks to the small size and low mass of protons. It is almost like teleporting,” he says.
“The nanosecond timescale means we are close to the ballistic or even quantum tunneling regime for the proton, under such an extreme field,” adds Li.
Because the protons don’t damage the material, the resistor can run for millions of cycles without breaking down. This new electrolyte enabled a programmable protonic resistor that is a million times faster than their previous device and can operate effectively at room temperature, which is important for incorporating it into computing hardware.
Thanks to the insulating properties of PSG, almost no electric current passes through the material as protons move. This makes the device extremely energy efficient, Onen adds.
Now that they have demonstrated the effectiveness of these programmable resistors, the researchers plan to reengineer them for high-volume manufacturing, says del Alamo. Then they can study the properties of resistor arrays and scale them up so they can be embedded into systems.
At the same time, they plan to study the materials to remove bottlenecks that limit the voltage that is required to efficiently transfer the protons to, through, and from the electrolyte.
“Another exciting direction that these ionic devices can enable is energy-efficient hardware to emulate the neural circuits and synaptic plasticity rules that are deduced in neuroscience, beyond analog deep neural networks. We have already started such a collaboration with neuroscience, supported by the MIT Quest for Intelligence ,” adds Yildiz.
“The collaboration that we have is going to be essential to innovate in the future. The path forward is still going to be very challenging, but at the same time it is very exciting,” del Alamo says.
“Intercalation reactions such as those found in lithium-ion batteries have been explored extensively for memory devices. This work demonstrates that proton-based memory devices deliver impressive and surprising switching speed and endurance,” says William Chueh, associate professor of materials science and engineering at Stanford University, who was not involved with this research. “It lays the foundation for a new class of memory devices for powering deep learning algorithms.”
“This work demonstrates a significant breakthrough in biologically inspired resistive-memory devices. These all-solid-state protonic devices are based on exquisite atomic-scale control of protons, similar to biological synapses but at orders of magnitude faster rates,” says Elizabeth Dickey, the Teddy & Wilton Hawkins Distinguished Professor and head of the Department of Materials Science and Engineering at Carnegie Mellon University, who was not involved with this work. “I commend the interdisciplinary MIT team for this exciting development, which will enable future-generation computational devices.”
This research is funded, in part, by the MIT-IBM Watson AI Lab.
Share this news article on:
Press mentions.
MIT researchers have developed a new hardware that offers faster computation for artificial intelligence with less energy, reports Kyle Wiggers for TechCrunch . “The researchers’ processor uses ‘protonic programmable resistors’ arranged in an array to ‘learn’ skills” explains Wiggers.
New Scientist
Postdoctoral researcher Murat Onen and his colleagues have created “a nanoscale resistor that transmits protons from one terminal to another,” reports Alex Wilkins for New Scientist . “The resistor uses powerful electric fields to transport protons at very high speeds without damaging or breaking the resistor itself, a problem previous solid-state proton resistors had suffered from,” explains Wilkins.
Previous item Next item
Related Links
- Jesús del Alamo
- Bilge Yildiz
- Frances Ross
- Microsystems Technology Laboratories
- MIT-IBM Watson AI Lab
- Department of Materials Science and Engineering
- Department of Nuclear Science and Engineering
- Department of Electrical Engineering and Computer Science
Related Topics
- Electronics
- Materials science and engineering
- Nanoscience and nanotechnology
- Computer science and technology
- Artificial intelligence
- Electrical Engineering & Computer Science (eecs)
- Nuclear science and engineering
- Quest for Intelligence
Related Articles
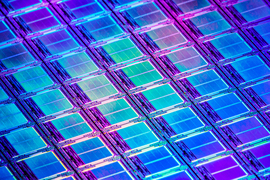
Discovery suggests new promise for nonsilicon computer transistors
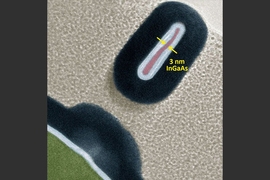
Engineers produce smallest 3-D transistor yet
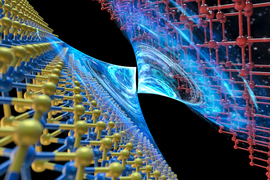
Advance may enable “2D” transistors for tinier microchip components
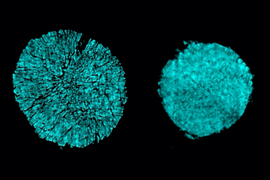
Design could enable longer lasting, more powerful lithium batteries
More mit news.
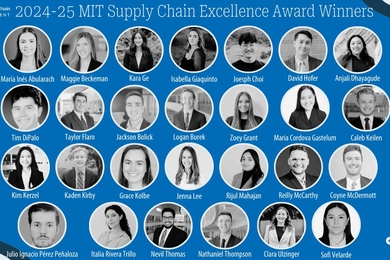
2024 MIT Supply Chain Excellence Awards given to 35 undergraduates
Read full story →
Faces of MIT: Reimi Hicks
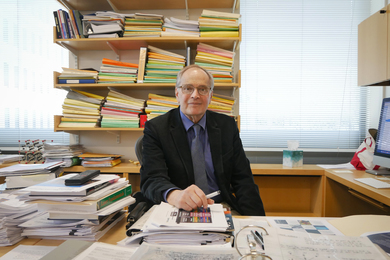
John Joannopoulos receives 2024-2025 Killian Award
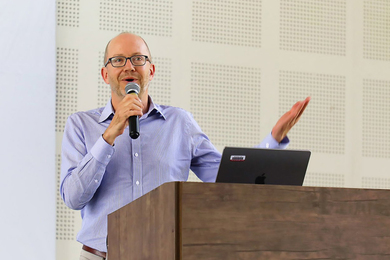
Q&A: Exploring ethnic dynamics and climate change in Africa
The MIT Bike Lab: A place for community, hands-on learning
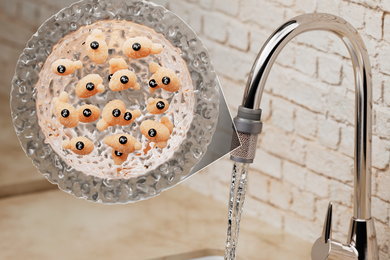
Repurposed beer yeast may offer a cost-effective way to remove lead from water
- More news on MIT News homepage →
Massachusetts Institute of Technology 77 Massachusetts Avenue, Cambridge, MA, USA
- Map (opens in new window)
- Events (opens in new window)
- People (opens in new window)
- Careers (opens in new window)
- Accessibility
- Social Media Hub
- MIT on Facebook
- MIT on YouTube
- MIT on Instagram
Help | Advanced Search
Artificial Intelligence
Authors and titles for recent submissions.
- Wed, 15 May 2024
- Tue, 14 May 2024
- Mon, 13 May 2024
- Fri, 10 May 2024
- Thu, 9 May 2024
Wed, 15 May 2024 (showing first 25 of 72 entries )
An official website of the United States government
Here's how you know
Official websites use .gov A .gov website belongs to an official government organization in the United States.
Secure .gov websites use HTTPS. A lock ( Lock Locked padlock ) or https:// means you've safely connected to the .gov website. Share sensitive information only on official, secure websites.
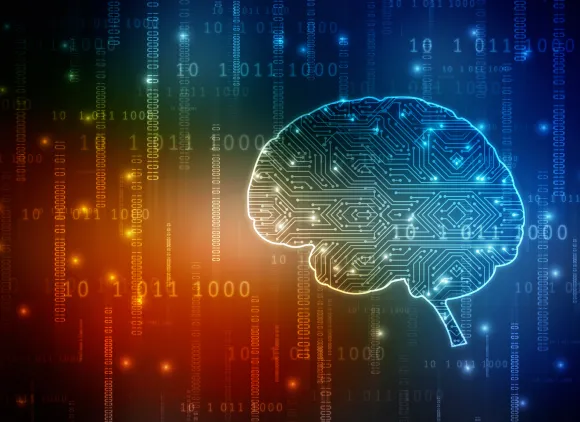
Artificial Intelligence
The U.S. National Science Foundation has invested in foundational artificial intelligence research since the early 1960s, setting the stage for today’s understanding and use of AI technologies.
AI-driven discoveries and technologies are transforming Americans' daily lives — promising practical solutions to global challenges, from food production and climate change to healthcare and education.
The growing adoption of AI also calls for a deeper understanding of its potential risks, like the amplification of bias, displacement of workers, or misuse by malicious actors to cause harm.
As a major federal funder of AI research, NSF advances AI breakthroughs that push the frontiers of knowledge, benefit people, and are aligned to the needs of society.
On this page
What is artificial intelligence?
How does AI affect our daily lives? How does it work in simple terms? Can we trust AI chatbots? In this 10-minute video, Michael Littman, NSF division director for Information and Intelligent Systems, looks at where the field of artificial intelligence has been and where it's going.
Brought to you by NSF
NSF's decades of sustained investments have ensured the continual advancement of AI research. Pioneering work supported by NSF includes:
Reinforcement learning
Which refines chatbots and trains self-driving cars, among other uses.
Neural networks
Which underlie breakthroughs in pattern recognition, image processing and natural language processing.
Large language models
Which power generative AI systems like ChatGPT.
Collaborative filtering
Which fuels content recommendation on the world's largest marketplaces and content platforms, from Amazon to Netflix.
AI-driven learning
Including virtual teachers (both digital and robotic) that incorporate speech, gesture, gaze and facial expression.
What we support
With investments of over $700 million each year, NSF supports:
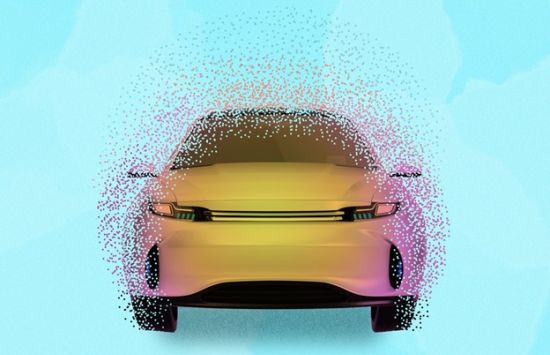
Innovation in AI methods
We invest in foundational research to understand and develop systems that can sense, learn, reason, communicate and act in the world.
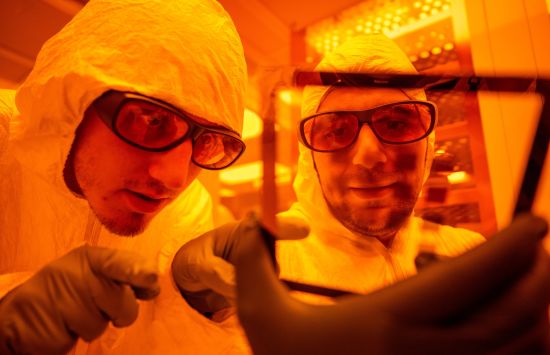
Application of AI techniques and tools
We invest in the application of AI across science and engineering to push the frontiers of knowledge and address pressing societal challenges.
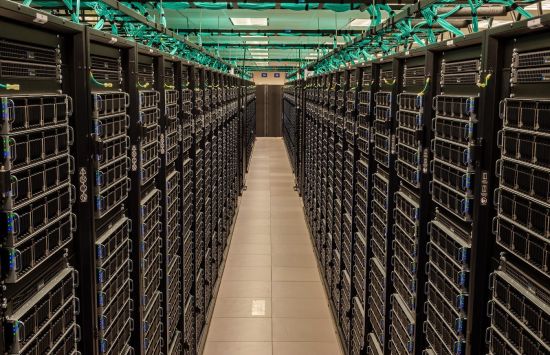
Democratizing AI research resources
We enable access to resources — like computational infrastructure, data, software, testbeds and training — to engage the full breadth of the nation's talent in AI innovation.
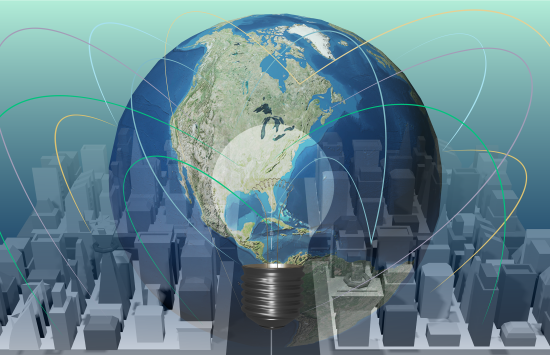
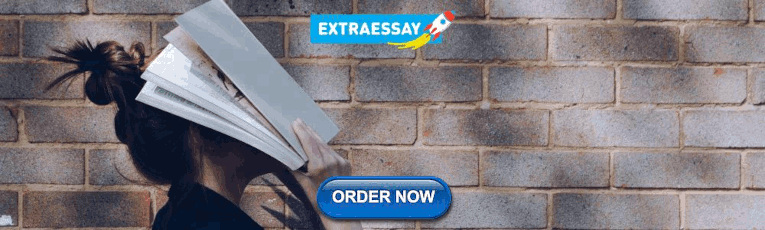
Trustworthy and ethical AI
We invest in the development of AI that is safe, secure, fair, transparent and accountable, while ensuring privacy, civil rights and civil liberties.
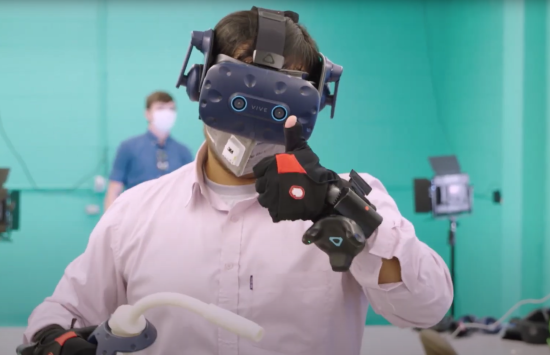
Education and workforce development
We invest in the creation of educational tools, materials, fellowships and curricula to enhance learning and foster an AI-ready workforce.
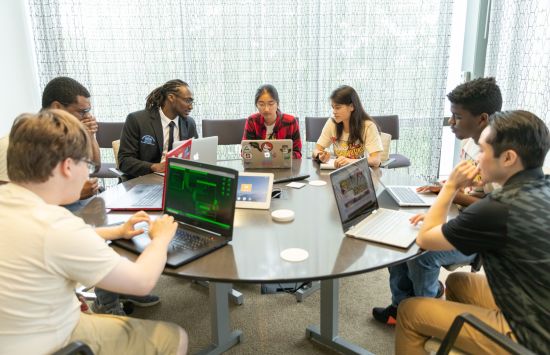
Partnerships to accelerate progress
We partner with other federal agencies, industry and nonprofits to leverage expertise; identify use cases; and improve access to data, tools and other resources.
National AI Research Institutes
Launched in 2020, the NSF-led National Artificial Intelligence Research Institutes program consists of 25 AI institutes that connect over 500 funded and collaborative institutions across the U.S. and around the world.
The AI institutes focus on different aspects of AI research, including but not limited to:
- Trustworthy and ethical AI.
- Foundations of machine learning.
- Agriculture and food systems.
- AI and advanced cybersecurity.
- Human-AI interaction and collaboration.
- AI-augmented learning.
Learn more by reading the 2020 , 2021 and 2023 AI Institutes announcements or visiting the AI Institutes Virtual Organization .
National AI Research Institutes: Interactive Map (PDF, 7.96 MB)
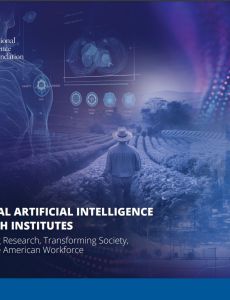
AI Institutes Booklet (PDF, 12.58 MB)
Hear from the newest ai research institutes.
- At the Edge of Artificial Intelligence This episode of NSF's Discovery Files podcast features three 2023 AI Research Institutes awardees discussing their work.
- The Frontier of Artificial Intelligence This Discovery Files episode features 2023 AI Research Institutes awardees applying AI to education, agriculture and weather forecasting.
National AI Research Resource Pilot
As part of the "National AI Initiative Act of 2020," the National AI Research Resource (NAIRR) Task Force was charged with creating a roadmap for a shared research infrastructure that would provide U.S.-based researchers, educators and students with significantly expanded access to computational resources, high-quality data, educational tools and user support.
The NSF-led interagency NAIRR Pilot will bring together government-supported, industry and other contributed resources to demonstrate the NAIRR concept and deliver early capabilities to the U.S. research and education community, including the full range of institutions of higher education and federally funded startups and small businesses.
The NAIRR Pilot is aimed to accelerate AI-dependent research such as:
- Societally relevant research on AI safety, reliability, security and privacy.
- Advances in cancer treatment and individual health outcomes.
- Supporting resilience and optimization of agricultural, water and grid infrastructure.
- Improving design, control and quality of advanced manufacturing systems.
- Addressing Earth and environmental challenges via the integration of diverse data and models.
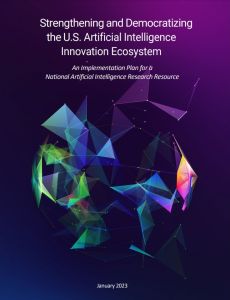
Implementation Plan for a National Artificial Intelligence Research Resource (PDF, 3.02 MB)
Featured funding.

Computer and Information Science and Engineering: Core Programs
Supports foundational and use-inspired research in AI, data science and human-computer interaction — including human language technologies, computer vision, human-AI interaction, and theory of machine learning.

America's Seed Fund (SBIR/STTR)
Supports startups and small businesses to translate research into products and services, including AI systems and AI-based hardware , for the public good.

Cyber-Physical Systems
Supports research on engineered systems with a seamless integration of cyber and physical components, such as computation, control, networking, learning, autonomy, security, privacy and verification, for a range of application domains.

Engineering Design and Systems Engineering
Supports fundamental research on the design of engineered artifacts — devices, products, processes, platforms, materials, organizations, systems and systems of systems.

Ethical and Responsible Research
Supports research on what promotes responsible and ethical conduct of research in AI and other areas as well as how to encourage researchers, practitioners and educators at all career stages to conduct research with integrity.

Expanding AI Innovation through Capacity Building and Partnerships
Supports capacity-development projects and partnerships within the National AI Research Institutes ecosystem that help broaden participation in artificial intelligence research, education and workforce development.

Experiential Learning for Emerging and Novel Technologies
Supports experiential learning opportunities that provide cohorts of diverse learners with the skills needed to succeed in artificial intelligence and other emerging technology fields.

Responsible Design, Development and Deployment of Technologies
Supports research, implementation and education projects involving multi-sector teams that focus on the responsible design, development or deployment of technologies.

Research on Innovative Technologies for Enhanced Learning
Supports early-stage research in emerging technologies such as AI, robotics and immersive or augmenting technologies for teaching and learning that respond to pressing needs in real-world educational environments.

Secure and Trustworthy Cyberspace
Supports research addressing cybersecurity and privacy, drawing on expertise in one or more of these areas: computing, communication and information sciences; engineering; economics; education; mathematics; statistics; and social and behavioral sciences.

Smart and Connected Communities
Supports use-inspired research that addresses communities' social, economic and environmental challenges by integrating intelligent technologies with the natural and built environments.

Smart Health and Biomedical Research in the Era of Artificial Intelligence
Supports the development of new methods that intuitively and intelligently collect, sense, connect, analyze and interpret data from individuals, devices and systems.
NSF directorates supporting AI research
Computer and information science and engineering (cise), engineering (eng), technology, innovation and partnerships (tip), mathematical and physical sciences (mps), social, behavioral and economic sciences (sbe), stem education (edu), geosciences (geo), biological sciences (bio), international science and engineering (oise), integrative activities (oia), featured news.
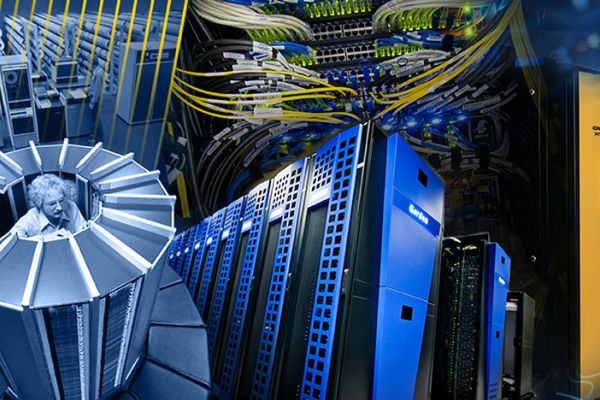
NSF-led National AI Research Resource Pilot awards first round access to 35 projects in partnership with DOE
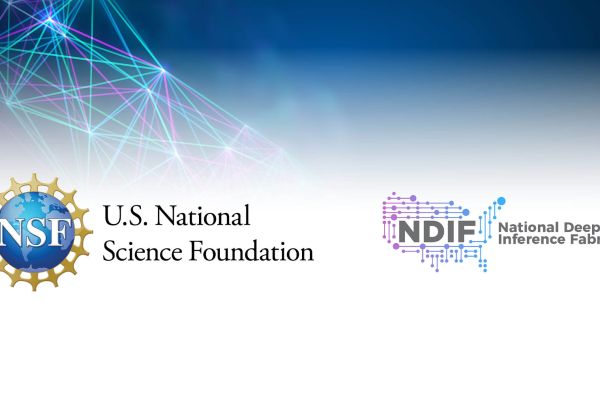
New NSF grant targets large language models and generative AI, exploring how they work and implications for societal impacts
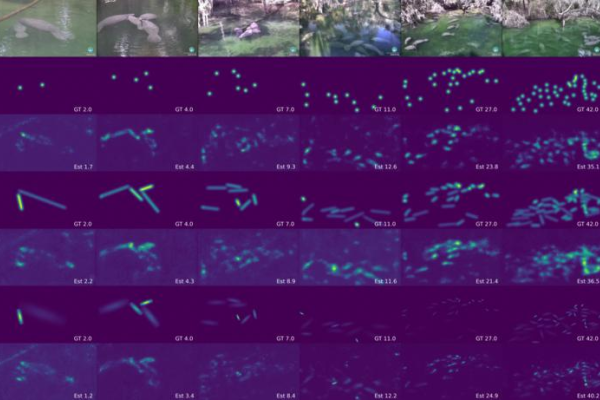
Saving an endangered species: New AI method counts manatee clusters in real time
Additional resources.
- NAIRR Pilot Explore opportunities for researchers, educators and students, including AI-ready datasets, pre-trained models and other NAIRR pilot resources.
- National Artificial Intelligence Initiative A coordinated federal approach to accelerate AI research and the integration of AI systems across all sectors of the economy and society.
- CloudBank Allows the research and education community to access cloud computing platforms.
- One Hundred Year Study on Artificial Intelligence A study focused on understanding and anticipating how AI will ripple through every aspect of how people work, live and play.
- Expanding the Frontiers of AI: Fact Sheet Learn how NSF is driving cutting-edge research on AI.
- "CHIPS and Science Act of 2022" The act authorizes historic investments in use-inspired, solutions-oriented research and innovation in key technology focus areas.
The state of AI in 2023: Generative AI’s breakout year
The latest annual McKinsey Global Survey on the current state of AI confirms the explosive growth of generative AI (gen AI) tools . Less than a year after many of these tools debuted, one-third of our survey respondents say their organizations are using gen AI regularly in at least one business function. Amid recent advances, AI has risen from a topic relegated to tech employees to a focus of company leaders: nearly one-quarter of surveyed C-suite executives say they are personally using gen AI tools for work, and more than one-quarter of respondents from companies using AI say gen AI is already on their boards’ agendas. What’s more, 40 percent of respondents say their organizations will increase their investment in AI overall because of advances in gen AI. The findings show that these are still early days for managing gen AI–related risks, with less than half of respondents saying their organizations are mitigating even the risk they consider most relevant: inaccuracy.
The organizations that have already embedded AI capabilities have been the first to explore gen AI’s potential, and those seeing the most value from more traditional AI capabilities—a group we call AI high performers—are already outpacing others in their adoption of gen AI tools. 1 We define AI high performers as organizations that, according to respondents, attribute at least 20 percent of their EBIT to AI adoption.
The expected business disruption from gen AI is significant, and respondents predict meaningful changes to their workforces. They anticipate workforce cuts in certain areas and large reskilling efforts to address shifting talent needs. Yet while the use of gen AI might spur the adoption of other AI tools, we see few meaningful increases in organizations’ adoption of these technologies. The percent of organizations adopting any AI tools has held steady since 2022, and adoption remains concentrated within a small number of business functions.
Table of Contents
- It’s early days still, but use of gen AI is already widespread
- Leading companies are already ahead with gen AI
- AI-related talent needs shift, and AI’s workforce effects are expected to be substantial
- With all eyes on gen AI, AI adoption and impact remain steady
About the research
1. it’s early days still, but use of gen ai is already widespread.
The findings from the survey—which was in the field in mid-April 2023—show that, despite gen AI’s nascent public availability, experimentation with the tools is already relatively common, and respondents expect the new capabilities to transform their industries. Gen AI has captured interest across the business population: individuals across regions, industries, and seniority levels are using gen AI for work and outside of work. Seventy-nine percent of all respondents say they’ve had at least some exposure to gen AI, either for work or outside of work, and 22 percent say they are regularly using it in their own work. While reported use is quite similar across seniority levels, it is highest among respondents working in the technology sector and those in North America.
Organizations, too, are now commonly using gen AI. One-third of all respondents say their organizations are already regularly using generative AI in at least one function—meaning that 60 percent of organizations with reported AI adoption are using gen AI. What’s more, 40 percent of those reporting AI adoption at their organizations say their companies expect to invest more in AI overall thanks to generative AI, and 28 percent say generative AI use is already on their board’s agenda. The most commonly reported business functions using these newer tools are the same as those in which AI use is most common overall: marketing and sales, product and service development, and service operations, such as customer care and back-office support. This suggests that organizations are pursuing these new tools where the most value is. In our previous research , these three areas, along with software engineering, showed the potential to deliver about 75 percent of the total annual value from generative AI use cases.
In these early days, expectations for gen AI’s impact are high : three-quarters of all respondents expect gen AI to cause significant or disruptive change in the nature of their industry’s competition in the next three years. Survey respondents working in the technology and financial-services industries are the most likely to expect disruptive change from gen AI. Our previous research shows that, while all industries are indeed likely to see some degree of disruption, the level of impact is likely to vary. 2 “ The economic potential of generative AI: The next productivity frontier ,” McKinsey, June 14, 2023. Industries relying most heavily on knowledge work are likely to see more disruption—and potentially reap more value. While our estimates suggest that tech companies, unsurprisingly, are poised to see the highest impact from gen AI—adding value equivalent to as much as 9 percent of global industry revenue—knowledge-based industries such as banking (up to 5 percent), pharmaceuticals and medical products (also up to 5 percent), and education (up to 4 percent) could experience significant effects as well. By contrast, manufacturing-based industries, such as aerospace, automotives, and advanced electronics, could experience less disruptive effects. This stands in contrast to the impact of previous technology waves that affected manufacturing the most and is due to gen AI’s strengths in language-based activities, as opposed to those requiring physical labor.
Responses show many organizations not yet addressing potential risks from gen AI
According to the survey, few companies seem fully prepared for the widespread use of gen AI—or the business risks these tools may bring. Just 21 percent of respondents reporting AI adoption say their organizations have established policies governing employees’ use of gen AI technologies in their work. And when we asked specifically about the risks of adopting gen AI, few respondents say their companies are mitigating the most commonly cited risk with gen AI: inaccuracy. Respondents cite inaccuracy more frequently than both cybersecurity and regulatory compliance, which were the most common risks from AI overall in previous surveys. Just 32 percent say they’re mitigating inaccuracy, a smaller percentage than the 38 percent who say they mitigate cybersecurity risks. Interestingly, this figure is significantly lower than the percentage of respondents who reported mitigating AI-related cybersecurity last year (51 percent). Overall, much as we’ve seen in previous years, most respondents say their organizations are not addressing AI-related risks.
2. Leading companies are already ahead with gen AI
The survey results show that AI high performers—that is, organizations where respondents say at least 20 percent of EBIT in 2022 was attributable to AI use—are going all in on artificial intelligence, both with gen AI and more traditional AI capabilities. These organizations that achieve significant value from AI are already using gen AI in more business functions than other organizations do, especially in product and service development and risk and supply chain management. When looking at all AI capabilities—including more traditional machine learning capabilities, robotic process automation, and chatbots—AI high performers also are much more likely than others to use AI in product and service development, for uses such as product-development-cycle optimization, adding new features to existing products, and creating new AI-based products. These organizations also are using AI more often than other organizations in risk modeling and for uses within HR such as performance management and organization design and workforce deployment optimization.
AI high performers are much more likely than others to use AI in product and service development.
Another difference from their peers: high performers’ gen AI efforts are less oriented toward cost reduction, which is a top priority at other organizations. Respondents from AI high performers are twice as likely as others to say their organizations’ top objective for gen AI is to create entirely new businesses or sources of revenue—and they’re most likely to cite the increase in the value of existing offerings through new AI-based features.
As we’ve seen in previous years , these high-performing organizations invest much more than others in AI: respondents from AI high performers are more than five times more likely than others to say they spend more than 20 percent of their digital budgets on AI. They also use AI capabilities more broadly throughout the organization. Respondents from high performers are much more likely than others to say that their organizations have adopted AI in four or more business functions and that they have embedded a higher number of AI capabilities. For example, respondents from high performers more often report embedding knowledge graphs in at least one product or business function process, in addition to gen AI and related natural-language capabilities.
While AI high performers are not immune to the challenges of capturing value from AI, the results suggest that the difficulties they face reflect their relative AI maturity, while others struggle with the more foundational, strategic elements of AI adoption. Respondents at AI high performers most often point to models and tools, such as monitoring model performance in production and retraining models as needed over time, as their top challenge. By comparison, other respondents cite strategy issues, such as setting a clearly defined AI vision that is linked with business value or finding sufficient resources.
The findings offer further evidence that even high performers haven’t mastered best practices regarding AI adoption, such as machine-learning-operations (MLOps) approaches, though they are much more likely than others to do so. For example, just 35 percent of respondents at AI high performers report that where possible, their organizations assemble existing components, rather than reinvent them, but that’s a much larger share than the 19 percent of respondents from other organizations who report that practice.
Many specialized MLOps technologies and practices may be needed to adopt some of the more transformative uses cases that gen AI applications can deliver—and do so as safely as possible. Live-model operations is one such area, where monitoring systems and setting up instant alerts to enable rapid issue resolution can keep gen AI systems in check. High performers stand out in this respect but have room to grow: one-quarter of respondents from these organizations say their entire system is monitored and equipped with instant alerts, compared with just 12 percent of other respondents.
3. AI-related talent needs shift, and AI’s workforce effects are expected to be substantial
Our latest survey results show changes in the roles that organizations are filling to support their AI ambitions. In the past year, organizations using AI most often hired data engineers, machine learning engineers, and Al data scientists—all roles that respondents commonly reported hiring in the previous survey. But a much smaller share of respondents report hiring AI-related-software engineers—the most-hired role last year—than in the previous survey (28 percent in the latest survey, down from 39 percent). Roles in prompt engineering have recently emerged, as the need for that skill set rises alongside gen AI adoption, with 7 percent of respondents whose organizations have adopted AI reporting those hires in the past year.
The findings suggest that hiring for AI-related roles remains a challenge but has become somewhat easier over the past year, which could reflect the spate of layoffs at technology companies from late 2022 through the first half of 2023. Smaller shares of respondents than in the previous survey report difficulty hiring for roles such as AI data scientists, data engineers, and data-visualization specialists, though responses suggest that hiring machine learning engineers and AI product owners remains as much of a challenge as in the previous year.
Looking ahead to the next three years, respondents predict that the adoption of AI will reshape many roles in the workforce. Generally, they expect more employees to be reskilled than to be separated. Nearly four in ten respondents reporting AI adoption expect more than 20 percent of their companies’ workforces will be reskilled, whereas 8 percent of respondents say the size of their workforces will decrease by more than 20 percent.
Looking specifically at gen AI’s predicted impact, service operations is the only function in which most respondents expect to see a decrease in workforce size at their organizations. This finding generally aligns with what our recent research suggests: while the emergence of gen AI increased our estimate of the percentage of worker activities that could be automated (60 to 70 percent, up from 50 percent), this doesn’t necessarily translate into the automation of an entire role.
AI high performers are expected to conduct much higher levels of reskilling than other companies are. Respondents at these organizations are over three times more likely than others to say their organizations will reskill more than 30 percent of their workforces over the next three years as a result of AI adoption.
4. With all eyes on gen AI, AI adoption and impact remain steady
While the use of gen AI tools is spreading rapidly, the survey data doesn’t show that these newer tools are propelling organizations’ overall AI adoption. The share of organizations that have adopted AI overall remains steady, at least for the moment, with 55 percent of respondents reporting that their organizations have adopted AI. Less than a third of respondents continue to say that their organizations have adopted AI in more than one business function, suggesting that AI use remains limited in scope. Product and service development and service operations continue to be the two business functions in which respondents most often report AI adoption, as was true in the previous four surveys. And overall, just 23 percent of respondents say at least 5 percent of their organizations’ EBIT last year was attributable to their use of AI—essentially flat with the previous survey—suggesting there is much more room to capture value.
Organizations continue to see returns in the business areas in which they are using AI, and they plan to increase investment in the years ahead. We see a majority of respondents reporting AI-related revenue increases within each business function using AI. And looking ahead, more than two-thirds expect their organizations to increase their AI investment over the next three years.
The online survey was in the field April 11 to 21, 2023, and garnered responses from 1,684 participants representing the full range of regions, industries, company sizes, functional specialties, and tenures. Of those respondents, 913 said their organizations had adopted AI in at least one function and were asked questions about their organizations’ AI use. To adjust for differences in response rates, the data are weighted by the contribution of each respondent’s nation to global GDP.
The survey content and analysis were developed by Michael Chui , a partner at the McKinsey Global Institute and a partner in McKinsey’s Bay Area office, where Lareina Yee is a senior partner; Bryce Hall , an associate partner in the Washington, DC, office; and senior partners Alex Singla and Alexander Sukharevsky , global leaders of QuantumBlack, AI by McKinsey, based in the Chicago and London offices, respectively.
They wish to thank Shivani Gupta, Abhisek Jena, Begum Ortaoglu, Barr Seitz, and Li Zhang for their contributions to this work.
This article was edited by Heather Hanselman, an editor in the Atlanta office.
Explore a career with us
Related articles.
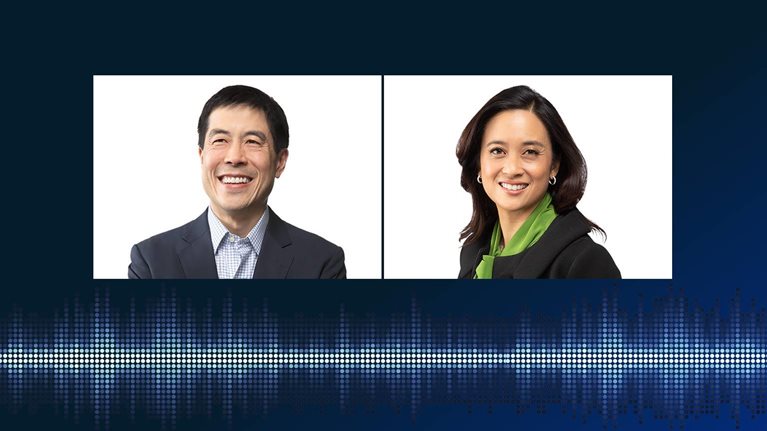
The economic potential of generative AI: The next productivity frontier
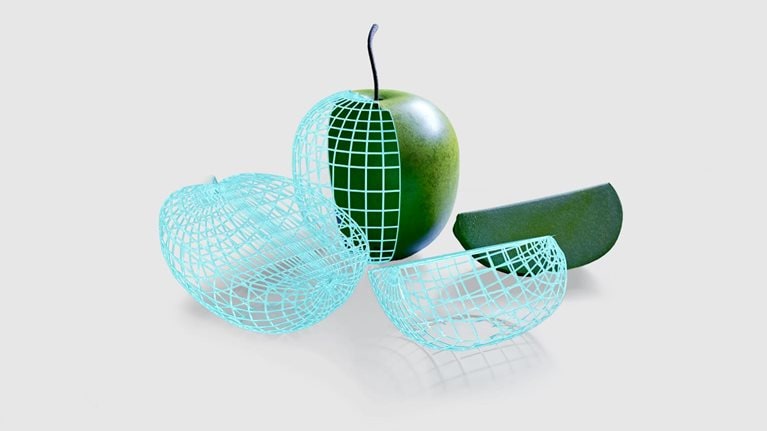
What is generative AI?
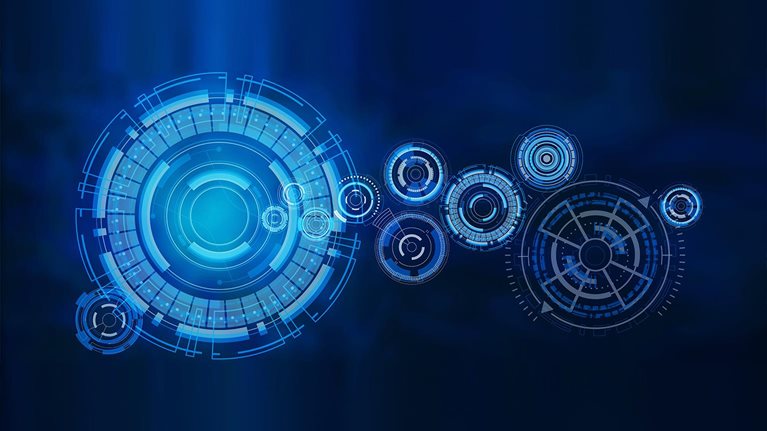
Exploring opportunities in the generative AI value chain
Science and the new age of AI
Updated 6 December 2023

Credit: Carlo Cadenas
Across disciplines as varied as biology, physics, mathematics and social science, artificial intelligence (AI) is transforming the scientific enterprise. From machine-learning techniques that hunt for patterns in data, to the latest general-purpose algorithms that can generate realistic synthetic outputs from vast corpuses of text and code, AI tools are accelerating the pace of research and providing fresh directions for scientific exploration.
This special website looks at how these changes are affecting different areas of science — and how it should respond to the challenges the tools present. It includes selected articles from journalists as well as editorials and comment from Nature , including subscriber-only content. The site will be updated with more content as it is published.
Editorial: AI will transform science — now researchers must tame it
Latest articles

Is AI leading to a reproducibility crisis in science?
Scientists worry that ill-informed use of artificial intelligence is driving a deluge of unreliable or useless research.

ChatGPT has entered the classroom: how LLMs could transform education
Researchers, educators and companies are experimenting with ways to turn flawed but famous large language models into trustworthy, accurate ‘thought partners’ for learning.

Garbage in, garbage out: mitigating risks and maximizing benefits of AI in research
Artificial-intelligence tools are transforming data-driven science — better ethical standards and more robust data curation are needed to fuel the boom and prevent a bust.

NEWS FEATURE
How ChatGPT and other AI tools could disrupt scientific publishing
Scientists who regularly use LLMs are still in the minority, but many expect that generative AI tools will become more prevalent. Here's how a world of AI-assisted writing and reviewing might transform the nature of the scientific paper.

AI and science: what 1,600 researchers think
A Nature survey finds that scientists are concerned, as well as excited, by the increasing use of artificial-intelligence tools in research.

How to stop AI deepfakes from sinking society — and science
Deceptive videos and images created using generative AI could sway elections, crash stock markets and ruin reputations. Researchers are developing methods to limit their harm.
Background to the AI revolution
Whereas the 2010s saw the creation of machine-learning algorithms that can help to discern patterns in giant, complex sets of scientific data, the 2020s are bringing in a new age with the widespread adoption of generative AI tools. These algorithms are based on neural networks and produce convincing synthetic outputs, sampling from the statistical distribution of the data they have been trained on.
The sheer pace of innovation is breathtaking and, for many, bewildering — requiring a level-headed assessment of what the tools have already achieved, and of what they can reasonably be expected to do in the future.

Scientific discovery in the age of artificial intelligence
Breakthroughs over the past decade in self-supervised learning, geometric deep learning and generative AI methods can help scientists throughout the scientific process — but also require a deeper understanding across scientific disciplines of the techniques’ pitfalls and limitations.

What ChatGPT and generative AI mean for science
The advent of generative AI based on large language models (LLMs) that can generate realistic synthetic outputs from vast corpuses of text and code is accelerating discovery and providing fresh directions for scientific exploration. That’s a reason for excitement, but also apprehension.

ChatGPT broke the Turing test — the race is on for new ways to assess AI
Large language models mimic human chatter, but scientists disagree on their ability to reason. Finding out where their limitations lie, and how their intelligence differs from that of humans, is crucial to assessing how best to use them.

In AI, is bigger always better?
Recent advances in the capabilities of AI seem to be based on ever-larger models fed with increasing amounts of data. That suggests many tasks could be conquered by AIs simply by continuing those trends — but some experts beg to differ.
AI in scientific life
From designing proteins and formulating mathematical theories, to enabling quick literature syntheses or helping to write research papers, AI tools are revolutionizing how scientists conduct their research and what they are able to achieve.
But these developments are playing out differently across the scientific enterprise. Diving into the trends in different disciplines provides a guide to the potential of AI-fuelled research and its possible pitfalls.

CAREER COLUMN
What’s the best chatbot for me? Researchers put LLMs through their paces
Large language models are becoming indispensable aids for coding, writing, teaching and more. But different research tasks call for different chatbots — here’s how to find the most appropriate match.

AI can help to speed up drug discovery — but only if we give it the right data
Drug development is labour-intensive and time-consuming. Used in the right way, AI tools that enable companies to share data about drug candidates while protecting sensitive information could help to short-circuit the process for the common good.

TECHNOLOGY FEATURE
Artificial-intelligence search engines wrangle academic literature
A new generation of search engines, powered by machine learning and large language models, is moving beyond keyword searches to pull connections from the tangled web of scientific literature. But can the results be trusted?
- How will AI change mathematics? Rise of chatbots highlights discussion
- For chemists, the AI revolution has yet to happen
- Is the world ready for ChatGPT therapists?
Challenges of AI – and how to deal with them
Although there is little doubt about the potential of AI to supercharge certain aspects of scientific discovery, there is also widespread disquiet. Many of the concerns surrounding the use of AI tools in science mirror those in wider society — transparency, accountability, reproducibility, and the reliability and biases of the data used to train them.

Living guidelines for generative AI — why scientists must oversee its use
Establish an independent scientific body to test and certify generative artificial intelligence, before the technology damages science and public trust.

NATURE PODCAST
This isn’t the Nature Podcast — how deepfakes are distorting reality
It has long been possible to create deceptive images, videos and audio to entertain or mislead audiences. Now, with the rise of AI technologies, such manipulations have become easier than ever.

AI tools as science policy advisers? The potential and the pitfalls
Synthesizing scientific evidence for policymakers is a data-intensive task often undertaken under significant time pressure. Large language models and other AI systems could excel at it — but only with appropriate safeguards and humans in the loop.

Rules to keep AI in check: nations carve different paths for tech regulation
The clamour for legal guardrails surrounding the use of AI is growing — but in practice, people still dispute precisely what needs reining in, how risky AI is and what actually needs to be restricted. China, the European Union and the United States are each approaching the issues in different ways.

ChatGPT: five priorities for research
Regardless of wider regulatory issues, the rise of conversational AI requires researchers to develop sensible guidelines for its use in science. What such guidance might look like is still up for debate, but it is clear where the focus for further research should lie.

CAREER FEATURE
Why AI’s diversity crisis matters, and how to tackle it
The real-world performance of AIs relies on how they are trained and which data are used. The field desperately needs more people from under-represented groups to ensure that the technologies deliver for all.
- Scientific sleuths spot dishonest ChatGPT use in papers
- Six tips for better coding with ChatGPT
- Privacy Policy
- Use of cookies
- Legal notice
- Terms & Conditions
- Accessibility statement

Researchers use artificial intelligence to boost image quality of metalens camera
Advance paves the way for ultra-thin cameras for applications from microscopy to mobile devices.
Researchers have leveraged deep learning techniques to enhance the image quality of a metalens camera. The new approach uses artificial intelligence to turn low-quality images into high-quality ones, which could make these cameras viable for a multitude of imaging tasks including intricate microscopy applications and mobile devices.
Metalenses are ultrathin optical devices -- often just a fraction of a millimeter thick -- that use nanostructures to manipulate light. Although their small size could potentially enable extremely compact and lightweight cameras without traditional optical lenses, it has been difficult to achieve the necessary image quality with these optical components.
"Our technology allows our metalens-based devices to overcome the limitations of image quality," said research team leader Ji Chen from Southeast University in China. "This advance will play an important role in the future development of highly portable consumer imaging electronics and can also be used in specialized imaging applications such as microscopy."
In Optica Publishing Group journal Optics Letters , the researchers describe how they used a type of machine learning known as a multi-scale convolutional neural network to improve resolution, contrast and distortion in images from a small camera -- about 3 cm × 3 cm × 0.5 cm -- they created by directly integrating a metalens onto a CMOS imaging chip.
"Metalens-integrated cameras can be directly incorporated into the imaging modules of smartphones, where they could replace the traditional refractive bulk lenses," said Chen. "They could also be used in devices such as drones, where the small size and lightweight camera would ensure imaging quality without compromising the drone's mobility."
Enhancing image quality
The camera used in the new work was previously developed by the researchers and uses a metalens with 1000-nm tall cylindrical silicon nitride nano-posts. The metalens focuses light directly onto a CMOS imaging sensor without requiring any other optical elements. Although this design created a very small camera the compact architecture limited the image quality. Thus, the researchers decided to see if machine learning could be used to improve the images.
Deep learning is a type of machine learning that uses artificial neural networks with multiple layers to automatically learn features from data and make complex decisions or predictions. The researchers applied this approach by using a convolution imaging model to generate a large number of high- and low-quality image pairs. These image pairs were used to train a multi-scale convolutional neural network so that it could recognize the characteristics of each type of image and use that to turn low-quality images into high-quality images.
"A key part of this work was developing a way to generate the large amount of training data needed for the neural network learning process," said Chen. "Once trained, a low-quality image can be sent from the device to into the neural network for processing, and high-quality imaging results are obtained immediately."
Applying the neural network
To validate the new deep learning technique, the researchers used it on 100 test images. They analyzed two commonly used image processing metrics: the peak signal-to-noise ratio and the structural similarity index. They found that the images processed by the neural network exhibited a significant improvement in both metrics. They also showed that the approach could rapidly generate high-quality imaging data that closely resembled what was captured directly through experimentation.
The researchers are now designing metalenses with complex functionalities -- such as color or wide-angle imaging -- and developing neural network methods for enhancing the imaging quality of these advanced metalenses. To make this technology practical for commercial application would require new assembly techniques for integrating metalenses into smartphone imaging modules and image quality enhancement software designed specifically for mobile phones.
"Ultra-lightweight and ultra-thin metalenses represent a revolutionary technology for future imaging and detection," said Chen. "Leveraging deep learning techniques to optimize metalens performance marks a pivotal developmental trajectory. We foresee machine learning as a vital trend in advancing photonics research."
- Medical Technology
- Biochemistry
- Photography
- Neural Interfaces
- Mobile Computing
- Computers and Internet
- Computer vision
- Confocal laser scanning microscopy
- Full motion video
- Artificial intelligence
- Search engine optimization
- Computing power everywhere
Story Source:
Materials provided by Optica . Note: Content may be edited for style and length.
Journal Reference :
- Yanxiang Zhang, Yue Wu, Chunyu Huang, Zi-Wen Zhou, Muyang Li, Zaichen Zhang, Ji Chen. Deep-learning enhanced high-quality imaging in metalens-integrated camera . Optics Letters , 2024; 49 (10): 2853 DOI: 10.1364/OL.521393
Cite This Page :
Explore More
- Reversing Brain Damage Caused by Ischemic Stroke
- Earth-Sized Planet Orbiting Ultra-Cool Dwarf
- Robots' Sense of Touch as Fast as Humans?
- Avian Flu Detected in NYC Wild Birds
- Metro-Area Quantum Computer Network Demo
- Iconic Baobab Tree's Origin Story
- 'Warm-Blooded' Dinos: 180 Million Years Ago
- Reaching 1,000 Degrees C With Solar Power
- Nature's 3D Printer: Bristle Worms
- Giant ' Cotton Candy' Planet
Trending Topics
Strange & offbeat.
Google pitches its vision for AI everywhere, from search to your phone
At the company’s annual I/O developer conference, executives announced AI improvements to Android, work apps and its Gemini chatbot.
MOUNTAIN VIEW, Calif. — In speeches and demonstrations at the company’s annual developer conference on Tuesday, Google executives showed off a vision for its future, where artificial intelligence helps people work, plan their lives, navigate the physical world and get answers to questions directly. It would change the way the internet works forever.
In the biggest overhaul to Google’s search engine in years, the company said it will roll out AI-generated answers to the top of everyone’s search results in the United States this week, and to a billion of its worldwide users by the end of the year.
It also pushed its new and improved voice assistant that can answer questions more skillfully than before. Instead of connecting people to the broader web, Google’s AI will now do the reading and researching for them, summarizing websites, videos and social media posts into “overviews” that include everything they need to know on any given topic.
“Google will do the searching, the researching, the planning, the brainstorming and so much more. All you need to do is just ask,” Elizabeth Reid, Google’s head of search, said onstage.
In one example, an executive asked Google’s Gemini assistant to plan a trip to Miami for her and her family. The AI searched the internet, reading reviews and travel guides written by humans, and put together an itinerary. The company showed off dozens more examples, from helping people learn how to flirt, to giving a suggestion for a last-minute gift.
The tsunami of new AI features come as the tech giant has thrown tens of billions of dollars into building AI tools to respond to competition from Meta, Microsoft, ChatGPT-maker OpenAI and a host of up-and-coming AI start-ups. AI features will prominently be displayed across Google’s products, including Google Docs, Google Photos, Gmail and YouTube.
Google researchers invented many of the core technologies that kicked off the AI arms race, but over the past year the company has been on its back foot, with many in the industry seeing its tech as lagging behind that of OpenAI. On Tuesday, the company sought to prove it is still the king of the AI world, showing off improvements to its core AI model, which it calls Gemini.
Outside the conference, which takes place at an open-air amphitheater near Google’s headquarters, pro-Palestinian protesters gathered to demand the company end its work with Israel’s government and military. In April, Google fired 50 workers for holding sit-ins at the company’s offices to protest its contract with Israel.
Here are the biggest announcements from the company.
AI answers take over search
Google is making the biggest changes to its search engine since it launched its core product over 20 years ago. Now, instead of showing links to other sites or snippets of those sites at the top of search results, the company will use AI to summarize websites and provide multi-paragraph answers to search queries.
The changes have been in public testing for a year, but this week Google confirmed that it would aggressively push it to its hundreds of millions of users in the United States and further abroad, whether they want to use it or not. The changes are part of a broader vision outlined by Google CEO Sundar Pichai, in which Google will be the central hub of how information is accessed for everyone. The company will ingest social media comments, online videos and news articles and remix the information using AI, spitting it out again in whatever format its users want.
Publishers are warning the changes could devastate their businesses , as more people find their answers directly on Google and don’t click through to the source of the information. Google says it doesn’t want to damage the open web and that it is still prioritizing sending traffic to websites. Users can’t turn off the AI answers, even if they want to.
AI is still far from ready to answer every question well. Even Google’s slick, highly-produced promotional video had an error where it instructed someone to fix a camera in a way that would expose and damage the film.
Google’s AI bot Gemini gets smarter
Google’s flagship AI model — its answer to OpenAI’s GPT4 — is called Gemini. The company demonstrated its capabilities, like showing it a bookshelf through a phone camera and getting it to quickly make a spreadsheet of all the books and their authors. In briefings before the event, Google showed a video of an employee walking through an office with a phone camera open, asking Gemini questions. The AI analyzed computer code on a workstation monitor, looked out the window and identified the neighborhood the person was in and even made up a clever name for a band consisting of the office golden retriever and a stuffed tiger toy — “Golden Stripes.”
The improved version of Gemini is available to all developers around the world, and to consumers who pay for an advanced version of Google’s AI app.
The day before, OpenAI had showed off a similar tool, asking its own AI chatbot to describe a room and the activities of the people in it.
Google also said that Gemini could now take in more complex instructions. For example, a student could upload an entire thesis paper and ask for feedback or ideas on how to change it.
Google’s head of AI, Demis Hassabis, also teased the company’s Project Astra. It is Google’s effort to build an AI “agent” that could do tasks for people by navigating the web on its own. Theoretically, AI agents could do things like book dentist appointments, communicate with colleagues on your behalf, and research places to eat and make a reservation.
A new AI video tool, Veo
Generative AI companies, including Google, want to revolutionize the way people create visual images, audio and movies. At I/O, Google announced a new video-generating AI tool called Veo, which aims to compete with OpenAI’s Sora . Veo generates high definition videos that can be longer than a minute, a threshold Google had yet to achieve.
Before the big speeches, DJ Marc Rebillet tried to warm up the crowd by making beats using Google’s AI tools. Rebillet bounced around the stage yelling “Google” over and over again. Google said it is working with creators including Rebillet, musician Wyclef Jean, and actor and producer Donald Glover on AI creations.
Google also showed off a new image-generation AI tool called Imagen 3, meant to compete with OpenAI’s Dall-E 3. The tech allows people to generate realistic-looking images with text prompts.
Work apps get even more AI
Google has been putting AI features into its suite of productivity apps including Gmail, Docs, Drives and Sheets over the past year. At I/O, the company announced some new tweaks, allowing users to summarize groups of emails from the same sender, adding details from a Google Doc in an email or incorporating content from a spreadsheet into a Slides presentation.
The company will also begin letting people ask Google’s AI to find specific details in a document and add them to an email. Google’s “help me write” feature, which generates text from scratch, will also soon be available in Spanish and Portuguese.
Google showed how its Gemini AI tool can also be used to teach kids about new concepts, asking it to explain the physics behind how a basketball rolls and bounces.
Android wants to catch scam calls
Google owns the Android smartphone operating system, which runs on the majority of phones worldwide. The company is trying to make Android more appealing than Apple’s iOS by putting more AI into the operating system itself. One improved feature, called Circle to Search, allows a person to circle anything they have a question about or want more information on and immediately get search results. The user can also generate images for text messages by asking Gemini.
Gemini can also help users get information from videos and PDFs. While they’re watching a video, for example, they can ask a specific question about something that happened in it. When they ask a question about a PDF, it’ll refer users to the part of the PDF where it found the answer.
Scam calls have become an even bigger problem as AI voice generators allow fraudsters to mimic real people. Android previewed a feature that will listen to and interrupt calls with a notification to the user if it thinks the call is coming from a scammer, such as if the caller asks for bank account information.
In a previous version of this article, the caption for the top photograph incorrectly said it was of the 2023 I/O conference. The photograph was taken Tuesday. The caption has been corrected.

We've detected unusual activity from your computer network
To continue, please click the box below to let us know you're not a robot.
Why did this happen?
Please make sure your browser supports JavaScript and cookies and that you are not blocking them from loading. For more information you can review our Terms of Service and Cookie Policy .
For inquiries related to this message please contact our support team and provide the reference ID below.
DeepMind is Google's AI research hub. Here's what it does, where it's located, and how it differs from OpenAI.
- DeepMind is Google's AI research hub focused on building artificial general intelligence.
- DeepMind has been applied to real-world problems in healthcare, science, and engineering.
- DeepMind has a number of competitors, including OpenAI, though Google's model is for profit.

In the last few years, artificial intelligence has stepped out of the pages of science fiction and into everyday life.
Today, we're surrounded by AI systems like Gemini, ChatGPT, Dall-E, CoPilot, and countless others, but Google DeepMind is somewhat different.
Launched back in 2010, DeepMind is a company with the goal of developing an artificial general intelligence , often referred to as AGI.
What does Google's DeepMind do?
While many AI systems in use today are very good at completing specific kinds of tasks for which they were trained, the goal of AGI is to build a human-like intelligence that can learn, reason, and problem-solve a wide range of topics and tasks across a plethora of domains.
In other words, it's designed to mimic human intelligence.
This is different from systems like ChatGPT and Google Gemini , which are narrow AI systems that are very good at the specific task of understanding natural language well enough to deliver useful information through human-like interactions.
Of course, DeepMind has not yet achieved AGI, but has made impressive achievements nonetheless. In practice, DeepMind has been applied to solving real-world problems in healthcare, science and engineering. It's perhaps most famous, though, for its mastery of enormously challenging games.
Related stories
In 2015, for example, DeepMind's AlphaGo became the first computer program to ever defeat a human opponent at Go (a game considered far more complex than chess). Less than two years later, AlphaGo went on to defeat the top-ranked Go player in the world.
Who runs Google's DeepMind?
DeepMind was created in 2010 by a trio of computer engineers from the Gatsby Computational Neuroscience Unit at University College London, and early research focused on getting AI systems to learn to play games without any instruction — the software would learn games like Breakout, Pong and Space Invaders through trial and error, eventually mastering the rules and becoming an expert at the games.
Google acquired DeepMind in 2014 for a price somewhere between $400 million and $650 million. Today, the company remains a part of Google's Alphabet portfolio of businesses where Demis Hassabis , one of DeepMinds three original founders, continues to lead the development of AGI as CEO.
In April 2023, Google CEO Sundar Pichai announced that Google would merge DeepMind with the Brain team from Google Research to create a single AI unit — named Google DeepMind — to "help us build more capable systems more safely and responsibly."
Google DeepMind remains based in London primarily, but also has researchers in Montreal, Canada, and at the Googleplex corporate headquarters in Mountain View, California.
What's the difference between DeepMind and OpenAI?
Of course, DeepMind is hardly alone in its AI research and development; it has a number of competitors, including the headline-making OpenAI.
These two companies take a very different approach to AI development, though. DeepMind is a for-profit part of Google's Alphabet, Inc., for example, while OpenAI was originally established as a non-profit, before transitioning to a "capped-profit" model .
The two companies have developed AI models and applications in ways that have contributed to AI research in sometimes complementary ways. While DeepMind mastered Go with AlphaGo, for example, OpenAI developed Generative Pre-trained Transformer language models (for example, ChatGPT) that allow machines to better understand natural language, for more interactive and immersive experiences.
Do you need a PhD to work at DeepMind?
Given the deep complexity of what DeepMind is developing, one might assume that prospective employees might all require a PhD. In reality, though, that's not true. Google hires a large number of researchers and computer engineers with lesser degrees to help advance the state of the art in artificial intelligence.
On February 28, Axel Springer, Business Insider's parent company, joined 31 other media groups and filed a $2.3 billion suit against Google in Dutch court, alleging losses suffered due to the company's advertising practices.
Watch: An AI expert discusses the hardware and infrastructure needed to properly run and train AI models
- Main content
- For Journalists
- News Releases
- Latest Releases
- News Release
AI expert available: Google’s AI-integrated search
‘Google is essentially turning the entire world into beta testers for its products’
Media Information
- Release Date: May 15, 2024
Media Contacts
Amanda Morris
- (847) 467-6790
- Email Amanda
EVANSTON, Ill. — Yesterday, Google unveiled plans to integrate its search engine with artificial intelligence (AI). Kristian Hammond , an AI expert at Northwestern University, says it’s a great idea but needs further validation.
Hammond is available to explain how large language models work, to discuss the problems with Google’s new AI-integrated search engine and to comment on how he expects the revamped search will influence other tech companies. He can be reached directly at [email protected] .
Hammond is the Bill and Cathy Osbourn Professor of Computer Science at Northwestern’s McCormick School of Engineering , director of the Center for Advancing Safety of Machine Intelligence and director of the Master of Science in Artificial Intelligence program. An AI pioneer, he also cofounded tech startup Narrative Science , a platform that used AI to turn big data into prose. Narrative Science was acquired by Salesforce in late 2021.
Comments from Professor Hammond on readiness:
“Integrating AI with search is a stunningly great idea, but it’s not ready. Given that it’s not ready, Google is essentially turning the entire world into beta testers for its products. Search is at the core of how we use the Internet on a daily basis, and now this new integrated search is being foisted upon the world. Running too fast might be bad for the products, bad for use and bad for people in general.
“In terms of the technology at the core of the model, it has not yet reached a point where we can definitively say that there are enough guardrails on the language models to stop them from telling lies. That still has not been tested enough or verified enough. The search will block users from content or give users content without allowing them to make decisions about what is a more authoritative or less authoritative source.”
On blocking content:
“With language models like Gemini and ChatGPT, developers have put a lot of work into excluding or limiting the amount of dangerous, offensive or inappropriate content. They block content if they feel it might be objectionable. Without us knowing the decision-making process behind labeling content as appropriate or inappropriate, we won’t know what is being blocked or being allowed. That, in itself, is dangerous.”
On content creators:
“The new search will provide information from other websites without leading users to those sites. Users will not visit the source sites, which provide the information and allow their content to be used. Without traffic, these sites will be threatened. People, who provide the content that is training the models, will not gain anything.”
On competing companies:
“We’re in the midst of a feature war. Tech companies like Google are integrating new features that are not massive innovations. It’s not that technology is moving too fast; it’s the features that are being hooked onto these technologies that are moving fast. When a new feature comes along, we get distracted until the next feature is released. It’s a bunch of different companies slamming their features against each other. It ends up being a battle among tech companies, and we are the test beds. There is no moment where we can pause and actually assess these products.”
Interview the Experts
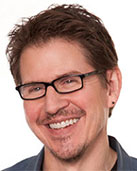
Kris Hammond
Bill and Cathy Osborn Professor of Computer Science Director, Center for Advancing Safety for Machine Intelligence Director, Master of Science in Artificial Intelligence
Advertisement
Supported by
Apple Will Revamp Siri to Catch Up to Its Chatbot Competitors
Apple plans to announce that it will bring generative A.I. to iPhones after the company’s most significant reorganization in a decade.
- Share full article

By Tripp Mickle , Brian X. Chen and Cade Metz
Tripp Mickle, Brian X. Chen and Cade Metz have been reporting on Apple’s plans for generative A.I. for this article since the fall of 2023.
Apple’s top software executives decided early last year that Siri, the company’s virtual assistant, needed a brain transplant.
The decision came after the executives Craig Federighi and John Giannandrea spent weeks testing OpenAI’s new chatbot, ChatGPT . The product’s use of generative artificial intelligence , which can write poetry, create computer code and answer complex questions, made Siri look antiquated, said two people familiar with the company’s work, who didn’t have permission to speak publicly.
Introduced in 2011 as the original virtual assistant in every iPhone, Siri had been limited for years to individual requests and had never been able to follow a conversation. It often misunderstood questions. ChatGPT, on the other hand, knew that if someone asked for the weather in San Francisco and then said, “What about New York?” that user wanted another forecast.
The realization that new technology had leapfrogged Siri set in motion the tech giant’s most significant reorganization in more than a decade. Determined to catch up in the tech industry’s A.I. race, Apple has made generative A.I. a tent pole project — the company’s special, internal label that it uses to organize employees around once-in-a-decade initiatives.
Apple is expected to show off its A.I. work at its annual developers conference on June 10 when it releases an improved Siri that is more conversational and versatile, according to three people familiar with the company’s work, who didn’t have permission to speak publicly. Siri’s underlying technology will include a new generative A.I. system that will allow it to chat rather than respond to questions one at a time.
The update to Siri is at the forefront of a broader effort to embrace generative A.I. across Apple’s business. The company is also increasing the memory in this year’s iPhones to support its new Siri capabilities. And it has discussed licensing complementary A.I. models that power chatbots from several companies, including Google, Cohere and OpenAI.
An Apple spokeswoman declined to comment.
Apple executives worry that new A.I. technology threatens the company’s dominance of the global smartphone market because it has the potential to become the primary operating system, displacing the iPhone’s iOS software, said two people familiar with the thinking of Apple’s leadership, who didn’t have permission to speak publicly. This new technology could also create an ecosystem of A.I. apps, known as agents, that can order Ubers or make calendar appointments, undermining Apple’s App Store, which generates about $24 billion in annual sales.
Apple also fears that if it fails to develop its own A.I. system, the iPhone could become a “dumb brick” compared with other technology. While it is unclear how many people regularly use Siri, the iPhone currently takes 85 percent of global smartphone profits and generates more than $200 billion in sales.
That sense of urgency contributed to Apple’s decision to cancel its other big bet — a $10 billion project to develop a self-driving car — and reassign hundreds of engineers to work on A.I.
Apple has also explored creating servers that are powered by its iPhone and Mac processors, two of these people said. Doing so could help Apple save money and create consistency between the tools used for processes in the cloud and on its devices.
Rather than compete directly with ChatGPT by releasing a chatbot that does things like write poetry, the three people familiar with its work said, Apple has focused on making Siri better at handling tasks that it already does, including setting timers, creating calendar appointments and adding items to a grocery list. It also would be able to summarize text messages.
Apple plans to bill the improved Siri as more private than rival A.I. services because it will process requests on iPhones rather than remotely in data centers. The strategy will also save money. OpenAI spends about 12 cents for about 1,000 words that ChatGPT generates because of cloud computing costs.
(The New York Times sued OpenAI and its partner, Microsoft, in December for copyright infringement of news content related to A.I. systems.)
But Apple faces risks by relying on a smaller A.I. system housed on iPhones rather than a larger one stored in a data center. Research has found that smaller A.I. systems could be more likely to make errors, known as hallucinations, than larger ones.
“It’s always been the Siri vision to have a conversational interface that understands language and context, but it’s a hard problem,” said Tom Gruber, a co-founder of Siri who worked at Apple until 2018. “Now that the technology has changed, it should be possible to do a much better job of that. So long as it’s not a one-size-fits-all effort to answer anything, then they should be able to avoid trouble.”
Apple has several advantages in the A.I. race, including more than two billion devices in use around the world where it can distribute A.I. products. It also has a leading semiconductor team that has been making sophisticated chips capable of powering A.I. tasks like facial recognition.
But for the past decade, Apple has struggled to develop a comprehensive A.I. strategy, and Siri has not had major improvements since its introduction. The assistant’s struggles blunted the appeal of the company’s HomePod smart speaker because it couldn’t consistently perform simple tasks like fulfilling a song request.
The Siri team has failed to get the kind of attention and resources that went to other groups inside Apple, said John Burkey, who worked on Siri for two years before founding a generative A.I. platform, Brighten.ai. The company’s divisions, such as software and hardware, operate independently of one another and share limited information. But A.I. needs to be threaded through products to succeed.
“It’s not in Apple’s DNA,” Mr. Burkey said. “It’s a blind spot.”
Apple has also struggled to recruit and retain leading A.I. researchers. Over the years, it has acquired A.I. companies led by leaders in the field, but they all left after a few years.
The reasons for their departures vary, but one factor is Apple’s secrecy. The company publishes fewer papers on its A.I. work than Google, Meta and Microsoft, and it doesn’t participate in conferences in the same way that its rivals do.
“Research scientists say: ‘What are my other options? Can I go back into academia? Can I go to a research institute, some place where I can work a bit more in the open?’” said Ruslan Salakhutdinov, a leading A.I. researcher, who left Apple in 2020 to return to Carnegie Mellon University.
In recent months, Apple has increased the number of A.I. papers it has published. But prominent A.I. researchers have questioned the value of the papers, saying they are more about creating the impression of meaningful work than providing examples of what Apple may bring to market.
Tsu-Jui Fu, an Apple intern and A.I. doctoral student at the University of California, Santa Barbara, wrote one of Apple’s recent A.I. papers . He spent last summer developing a system for editing photos with written commands rather than Photoshop tools. He said that Apple supported the project by providing him with the necessary G.P.U.s to train the system, but that he had no interaction with the A.I. team working on Apple products.
Though he said he had interviewed for full-time jobs at Adobe and Nvidia, he plans to return to Apple after he graduates because he thinks he can make a bigger difference there.
“A.I. product and research is emerging in Apple, but most companies are very mature,” Mr. Fu said in an interview with The Times. “At Apple, I can have more room to lead a project instead of just being a member of a team doing something.”
Tell us how your law firm is using A.I.
We’d like to hear from lawyers working with generative A.I., including contract lawyers who have been brought on for assignments related to A.I. We won’t publish your name or any part of your submission without contacting you first.
Tripp Mickle reports on Apple and Silicon Valley for The Times and is based in San Francisco. His focus on Apple includes product launches, manufacturing issues and political challenges. He also writes about trends across the tech industry, including layoffs, generative A.I. and robot taxis. More about Tripp Mickle
Brian X. Chen is the lead consumer technology writer for The Times. He reviews products and writes Tech Fix , a column about the social implications of the tech we use. More about Brian X. Chen
Cade Metz writes about artificial intelligence, driverless cars, robotics, virtual reality and other emerging areas of technology. More about Cade Metz
Explore Our Coverage of Artificial Intelligence
News and Analysis
As experts warn that A.I.-generated images, audio and video could influence the 2024 elections, OpenAI is releasing a tool designed to detect content created by DALL-E , its popular image generator.
American and Chinese diplomats plan to meet in Geneva to begin what amounts to the first, tentative arms control talks over the use of A.I.
Wayve, a London maker of A.I. systems for autonomous vehicles, said that it had raised $1 billion , an illustration of investor optimism about A.I.’s ability to reshape industries.
The Age of A.I.
A new category of apps promises to relieve parents of drudgery, with an assist from A.I. But a family’s grunt work is more human, and valuable, than it seems.
Despite Mark Zuckerberg’s hope for Meta’s A.I. assistant to be the smartest , it struggles with facts, numbers and web search.
Much as ChatGPT generates poetry, a new A.I. system devises blueprints for microscopic mechanisms that can edit your DNA.
Which A.I. system writes the best computer code or generates the most realistic image? Right now, there’s no easy way to answer those questions, our technology columnist writes .
Microsoft and LinkedIn release the 2024 Work Trend Index on the state of AI at work
May 8, 2024 | Jared Spataro - CVP, AI at Work
- Share on Facebook (opens new window)
- Share on Twitter (opens new window)
- Share on LinkedIn (opens new window)
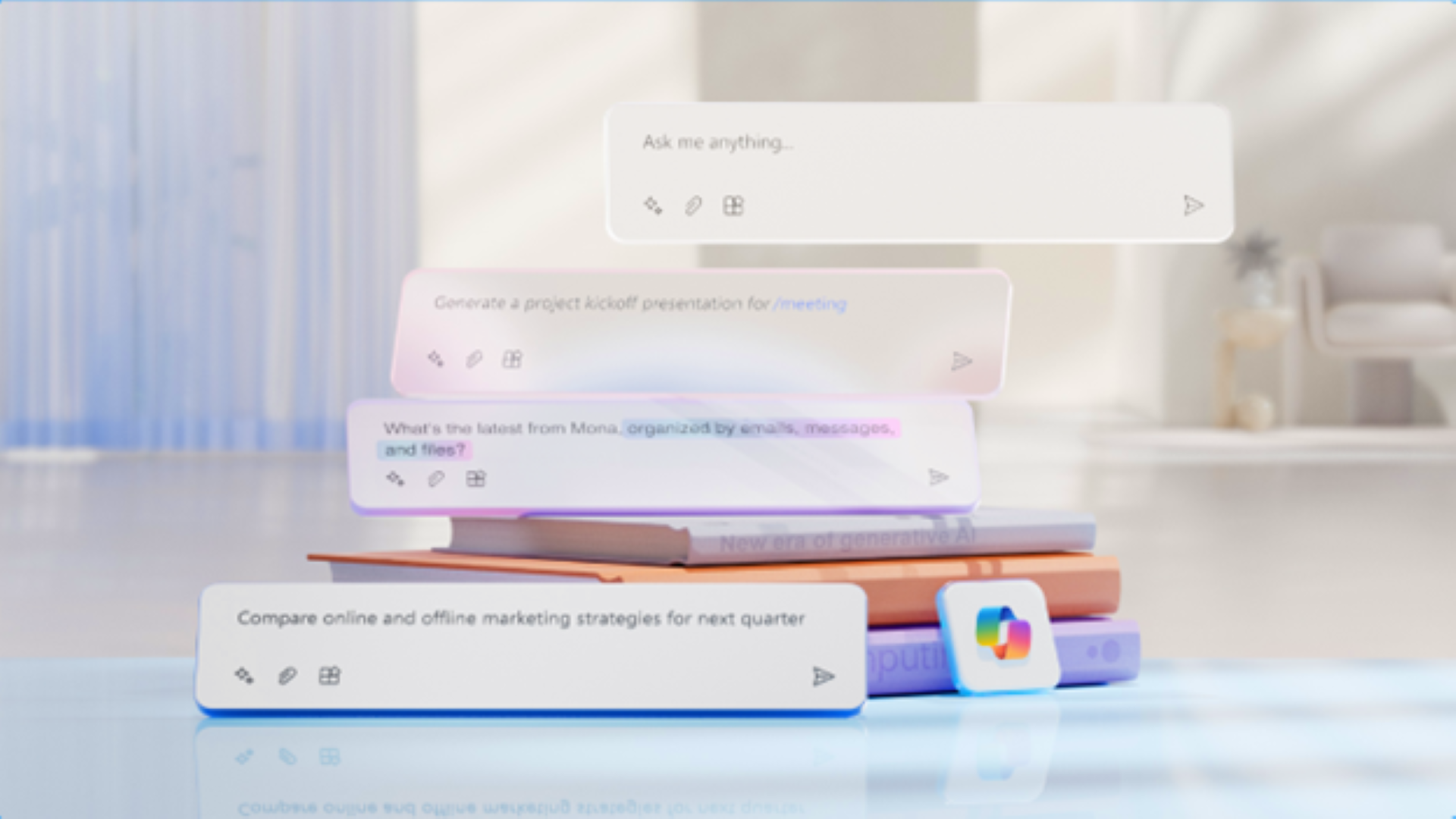
One year ago, generative AI burst onto the scene and for the first time since the smartphone, people began to change the way they interact with technology. People are bringing AI to work at an unexpected scale — and now the big question is, how’s it going?
As AI becomes ubiquitous in the workplace, employees and businesses alike are under extreme pressure. The pace and intensity of work, which accelerated during the pandemic, has not eased, so employees are bringing their own AI to work. Leaders agree AI is a business imperative — and feel the pressure to show immediate ROI — but many lack a plan and vision to go from individual impact to applying AI to drive the bottom line.
At the same time, the labor market is set to shift and there’s a new AI economy. While some professionals worry AI will replace their job, the data tells a more nuanced story — of a hidden talent shortage, more employees eyeing a career change, and a massive opportunity for those willing to skill up.
“AI is democratizing expertise across the workforce,” said Satya Nadella, Chairman and Chief Executive Officer, Microsoft. “Our latest research highlights the opportunity for every organization to apply this technology to drive better decision-making, collaboration — and ultimately business outcomes.”
For our fourth annual Work Trend Index, out today, we partnered with LinkedIn for the first time on a joint report so we could provide a comprehensive view of how AI is not only reshaping work, but the labor market more broadly. We surveyed 31,000 people across 31 countries, identified labor and hiring trends from LinkedIn, analyzed trillions of Microsoft 365 productivity signals and conducted research with Fortune 500 customers. The data points to insights every leader and professional needs to know — and actions they can take — when it comes to AI’s implications for work.
1. Employees want AI at work — and won’t wait for companies to catch up.
Three in four knowledge workers (75%) now use AI at work. Employees, overwhelmed and under duress, say AI saves time, boosts creativity and allows them to focus on their most important work. While 79% of leaders agree AI adoption is critical to remain competitive, 59% worry about quantifying the productivity gains of AI and 60% worry their company lacks a vision and plan to implement it. While leaders feel the pressure to turn individual productivity gains into organizational impact, employees aren’t waiting to reap the benefits: 78% of AI users are bringing their own AI tools to work. The opportunity for every leader is to channel this momentum into ROI.
2. For employees, AI raises the bar and breaks the career ceiling .
We also see AI beginning to impact the job market. While AI and job loss are top of mind for some, our data shows more people are eyeing a career change, there are jobs available, and employees with AI skills will get first pick. The majority of leaders (55%) say they’re worried about having enough talent to fill open roles this year, with leaders in cybersecurity, engineering, and creative design feeling the pinch most.
And professionals are looking. Forty-six percent across the globe are considering quitting in the year ahead — an all-time high since the Great Reshuffle of 2021 — a separate LinkedIn study found U.S. numbers to be even higher with 85% eyeing career moves. While two-thirds of leaders wouldn’t hire someone without AI skills, only 39% of users have received AI training from their company. So, professionals are skilling up on their own. As of late last year, we’ve seen a 142x increase in LinkedIn members adding AI skills like Copilot and ChatGPT to their profiles and a 160% increase in non-technical professionals using LinkedIn Learning courses to build their AI aptitude.
In a world where AI mentions in LinkedIn job posts drive a 17% bump in application growth, it’s a two-way street: Organizations that empower employees with AI tools and training will attract the best talent, and professionals who skill up will have the edge.
3. The rise of the AI power user — and what they reveal about the future.
In the research, four types of AI users emerged on a spectrum — from skeptics who rarely use AI to power users who use it extensively. Compared to skeptics, AI power users have reoriented their workdays in fundamental ways, reimagining business processes and saving over 30 minutes per day. Over 90% of power users say AI makes their overwhelming workload more manageable and their work more enjoyable, but they aren’t doing it on their own.
Power users work for a different kind of company. They are 61% more likely to have heard from their CEO on the importance of using generative AI at work, 53% more likely to receive encouragement from leadership to consider how AI can transform their function and 35% more likely to receive tailored AI training for their specific role or function.
“AI is redefining work and it’s clear we need new playbooks,” said Ryan Roslansky, CEO of LinkedIn. “It’s the leaders who build for agility instead of stability and invest in skill building internally that will give their organizations a competitive advantage and create more efficient, engaged and equitable teams.”
The prompt box is the new blank page
We hear one consistent piece of feedback from our customers: talking to AI is harder than it seems. We’ve all learned how to use a search engine, identifying the right few words to get the best results. AI requires more context — just like when you delegate work to a direct report or colleague. But for many, staring down that empty prompt box feels like facing a blank page: Where should I even start?
Today, we’re announcing Copilot for Microsoft 365 innovations to help our customers answer that question.
YouTube Video
- Catch Up, a new chat interface that surfaces personal insights based on your recent activity, provides responsive recommendations , like “You have a meeting with the sales VP on Thursday. Let’s get you prepared — click here to get detailed notes.”

These features will be available in the coming months, and in the future, we’ll take it a step further, with Copilot asking you questions to get to your best work yet.
LinkedIn has also made free over 50 learning courses to empower professionals at all levels to advance their AI aptitude.
Head to WorkLab for the full Work Trend Index Report , and head to LinkedIn to hear more from LinkedIn’s Chief Economist, Karin Kimbrough, on how AI is reshaping the labor market.
And for all the blogs, videos and assets related to today’s announcements, please visit our microsite .
Tags: AI , LinkedIn , Microsoft Copilot , Work Trend Index
- Check us out on RSS
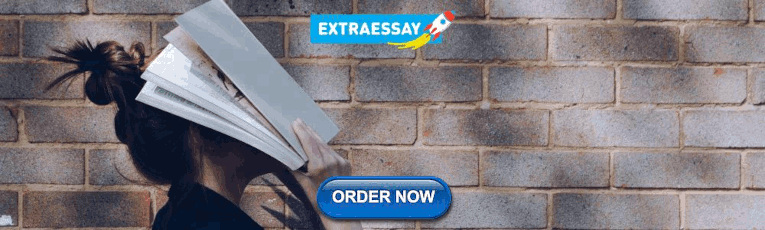
IMAGES
VIDEO
COMMENTS
In 2024, generative AI might actually become useful for the regular, non-tech person, and we are going to see more people tinkering with a million little AI models. State-of-the-art AI models ...
Gemma Conroy, Hepeng Jia, Benjamin Plackett &. Andy Tay. As artificial intelligence (AI) becomes ubiquitous in fields such as medicine, education and security, there are significant ethical and ...
The 2021 report is the second in a series that will be released every five years until 2116. Titled "Gathering Strength, Gathering Storms," the report explores the various ways AI is increasingly touching people's lives in settings that range from movie recommendations and voice assistants to autonomous driving and automated medical ...
Artificial intelligence (AI) systems, such as the chatbot ChatGPT, have become so advanced that they now very nearly match or exceed human performance in tasks including reading comprehension ...
Artificial intelligence (AI) is being increasingly integrated into scientific discovery to augment and accelerate research, helping scientists to generate hypotheses, design experiments, collect ...
From exploring applications in healthcare and biomedicine to unraveling patterns and optimizing decision-making within complex systems, PLOS' interdisciplinary artificial intelligence research aims to capture cutting-edge methodologies, advancements, and breakthroughs in machine learning, showcasing diverse perspectives, interdisciplinary approaches, and societal and ethical implications.
An AI dataset carves new paths to tornado detection. TorNet, a public artificial intelligence dataset, could help models reveal when and why tornadoes form, improving forecasters' ability to issue warnings. April 29, 2024. Read full story.
The Turbulent Past and Uncertain Future of Artificial Intelligence: ... Her work is an interesting contrast to research labs building neural networks of ever-increasing size and complexity (recall ...
Mar. 20, 2024 — Artificial intelligence can spot COVID-19 in lung ultrasound images much like facial recognition software can spot a face in a crowd, new research shows. The findings boost AI ...
Artificial Intelligence. Since the 1950s, scientists and engineers have designed computers to "think" by making decisions and finding patterns like humans do. In recent years, artificial intelligence has become increasingly powerful, propelling discovery across scientific fields and enabling researchers to delve into problems previously too ...
This work demonstrates that proton-based memory devices deliver impressive and surprising switching speed and endurance," says William Chueh, associate professor of materials science and engineering at Stanford University, who was not involved with this research. "It lays the foundation for a new class of memory devices for powering deep ...
Attention is all they need: Cognitive science and the (techno)political economy of attention in humans and machines. Subjects: Computers and Society (cs.CY); Artificial Intelligence (cs.AI); Social and Information Networks (cs.SI)
Meanwhile, the average number of AI capabilities that organizations use, such as natural-language generation and computer vision, has also doubled—from 1.9 in 2018 to 3.8 in 2022. Among these capabilities, robotic process automation and computer vision have remained the most commonly deployed each year, while natural-language text ...
Artificial Intelligence. The U.S. National Science Foundation has invested in foundational artificial intelligence research since the early 1960s, setting the stage for today's understanding and use of AI technologies. AI-driven discoveries and technologies are transforming Americans' daily lives — promising practical solutions to global ...
ChatGPT is about to become a lot more useful. OpenAI on Monday announced its latest artificial intelligence large language model that it says will make ChatGPT smarter and easier to use.. The new ...
The latest annual McKinsey Global Survey on the current state of AI confirms the explosive growth of generative AI (gen AI) tools. Less than a year after many of these tools debuted, one-third of our survey respondents say their organizations are using gen AI regularly in at least one business function. Amid recent advances, AI has risen from a ...
Last May, Sundar Pichai, Google's chief executive, said the company would use artificial intelligence to reimagine all of its products.. But because new generative A.I. technology presented ...
Significant growth in the development and deployment of artificial intelligence (AI) is being witnessed. Driven by the great versatility of emerging computer science and material science, various AI sensors provide cost-effective approaches for a wide range of monitoring applications toward the realization of smart homes and personal healthcare.
Artificial intelligence can bridge this gap, analyzing mass data from medical records and clinical trials to facilitate participant recruitment. An important risk to consider when using artificial intelligence in clinical research is the shift of influence, power, and accountability from physician to machine.
The outlook is bright for artificial intelligence jobs, which is good news for anyone interested in the growing field of AI. In fact, machine learning engineers and data scientists have held a position on Indeed's Best Jobs list for years [1, 2, 3].Additionally, the US Bureau of Labor Statistics (BLS) projects opportunities in computer and information research to grow 23 percent between 2022 ...
Garbage in, garbage out: mitigating risks and maximizing benefits of AI in research. Artificial-intelligence tools are transforming data-driven science — better ethical standards and more robust ...
The project was based on one of the winning submissions to the National Heart, Lung and Blood Institute's Big Data Analysis Challenge: Creating New Paradigms for Heart Failure Research. The work ...
Sep. 30, 2019 — Researchers use artificial intelligence to improve quality of images recorded by a relatively new biomedical imaging method. This paves the way towards more accurate diagnosis ...
Google researchers invented many of the core technologies that kicked off the AI arms race, but over the past year the company has been on its back foot, with many in the industry seeing its tech ...
OpenAI is developing a feature for ChatGPT that can search the web and cite sources in its results, according to a person familiar with the matter, potentially competing head on with Alphabet Inc ...
DeepMind is Google's AI research hub focused on building artificial general intelligence. DeepMind has been applied to real-world problems in healthcare, science, and engineering. DeepMind has a ...
EVANSTON, Ill. — Yesterday, Google unveiled plans to integrate its search engine with artificial intelligence (AI). Kristian Hammond, an AI expert at Northwestern University, says it's a great idea but needs further validation.. Hammond is available to explain how large language models work, to discuss the problems with Google's new AI-integrated search engine and to comment on how he ...
The product's use of generative artificial intelligence, which can write poetry, create computer code and answer complex questions, made Siri look antiquated, said two people familiar with the ...
For our fourth annual Work Trend Index, out today, we partnered with LinkedIn for the first time on a joint report so we could provide a comprehensive view of how AI is not only reshaping work, but the labor market more broadly. We surveyed 31,000 people across 31 countries, identified labor and hiring trends from LinkedIn, analyzed trillions ...