
- Research Outputs
Original Research Article:
An article published in an academic journal can go by several names: original research, an article, a scholarly article, or a peer reviewed article. This format is an important output for many fields and disciplines. Original research articles are written by one or a number of authors who typically advance a new argument or idea to their field.
Short Reports or Letters:
Short reports or letters, sometimes also referred to as brief communications, are summaries of original research that are significantly less lengthy than academic articles. This format is often intended to quickly keep researchers and scholars abreast of current practices in a field. Short reports may also be a preview for more extensive research that is published later.
Review Articles:
A review article summarizes the state of research within a field or about a certain topic. This type of article often cites large numbers of scholars to establish a broad overview, and can inform readers about issues such as active debates in a field, noteworthy contributors or scholars, gaps in understanding, or it may predict the direction a field will go into the future.
Case Studies:
Case studies are in depth investigations of a particular person, place, group, or situation during a specified time period. The purpose of case studies is to explore and explain the underlying concepts, causal links, and impacts a case subject has in its real-life context. Case studies are common in social sciences and sciences.
Conference Presentations or Proceedings:
Conferences are organized events, usually centered on one field or topic, where researchers gather to present and discuss their work. Typically, presenters submit abstracts, or short summaries of their work, before a conference, and a group of organizers select a number of researchers who will present. Conference presentations are frequently transcribed and published in written form after they are given.
Chapter:
Books are often composed of a collection of chapters, each written by a unique author. Usually, these kinds of books are organized by theme, with each author's chapter presenting a unique argument or perspective. Books with uniquely authored chapters are often curated and organized by one or more editors, who may contribute a chapter or foreward themselves.
Often, when researchers perform their work, they will produce or work with large amounts of data, which they compile into datasets. Datasets can contain information about a wide variety of topics, from genetic code to demographic information. These datasets can then be published either independently, or as an accompaniment to another scholarly output, such as an article. Many scientific grants and journals now require researchers to publish datasets.
For some scholars, artwork is a primary research output. Scholars’ artwork can come in diverse forms and media, such as paintings, sculptures, musical performances, choreography, or literary works like poems.
Reports can come in many forms and may serve many functions. They can be authored by one or a number of people, and are frequently commissioned by government or private agencies. Some examples of reports are market reports, which analyze and predict a sector of an economy, technical reports, which can explain to researchers or clients how to complete a complex task, or white papers, which can inform or persuade an audience about a wide range of complex issues.
Digital Scholarship:
Digital scholarship is a research output that significantly incorporates or relies on digital methodologies, authoring, presentation, and presentation. Digital scholarship often complements and adds to more traditional research outputs, and may be presented in a multimedia format. Some examples include mapping projects; multimodal projects that may be composed of text, visual, and audio elements; or digital, interactive archives.
Books:
Researchers from every field and discipline produce books as a research output. Because of this, books can vary widely in content, length, form, and style, but often provide a broad overview of a topic compared to research outputs that are more limited in length, such as articles or conference proceedings. Books may be written by one or many authors, and researchers may contribute to a book in a number of ways: they could author an entire book, write a forward, or collect and organize existing works in an anthology, among others.
Interview:
Scholars may be called upon by media outlets to share their knowledge about the topic they study. Interviews can provide an opportunity for researchers to teach a more general audience about the work that they perform.
Article in a Newspaper or Magazine:
While a significant amount of researchers’ work is intended for a scholarly audience, occasionally researchers will publish in popular newspapers or magazines. Articles in these popular genres can be intended to inform a general audience of an issue in which the researcher is an expert, or they may be intended to persuade an audience about an issue.
Blog:
In addition to other scholarly outputs, many researchers also compose blogs about the work they do. Unlike books or articles, blogs are often shorter, more general, and more conversational, which makes them accessible to a wider audience. Blogs, again unlike other formats, can be published almost in real time, which can allow scholars to share current developments of their work.
- Output Types
- University of Colorado Boulder Libraries
- Research Guides
- Site: Research Strategies
- Last Updated: Oct 29, 2020 1:53 PM
- URL: https://libguides.colorado.edu/products
- Welcome to the Staff Intranet
- My Workplace
- Staff Directory
- Service Status
- Student Charter & Professional Standards
- Quick links
- Bright Red Triangle
- New to Edinburgh Napier?
- Regulations
- Academic Skills
- A-Z Resources
- ENroute: Professional Recognition Framework
- ENhance: Curriculum Enhancement Framework
- Programmes and Modules
- QAA Enhancement Themes
- Quality & Standards
- L&T ENssentials Quick Guides & Resources
- DLTE Research
- Student Interns
- Intercultural Communication
- Far From Home
- Annual Statutory Accounts
- A-Z Documents
- Finance Regulations
- Insurance Certificates
- Procurement
- Who's Who
- Staff Briefing Note on Debt Sanctions
- Operational Communications
- Who's Who in Governance & Compliance
- Governance Services
- Health & Safety
- Customer Charter
- Pay and Benefits
- HR Policy and Forms
- Working at the University
- Recruitment
- Leaving the University
- Industrial Action
- Learning Technology
- Digital Skills
- IS Policies
- Plans & Performance
- Research Cycle
- International & EU Recruitment
- International Marketing and Intelligence
- International Programmes
- Global Online
- Global Mobility
- English for Academic Purposes (EAP)
- UCAS Results Embargo
- UK Recruitment
- Visa and International Support
- Useful Documents
- Communications
- Corporate Gifts
- Development & Alumni Engagement
- NSS Staff Hub
- Planning & Performance
- Business Intelligence
- Market Intelligence
- Data Governance
- Principal & Vice-Chancellor
- University Leadership Team
- The University Chancellor
- University Strategy
- Catering, Events & Vacation Lettings
- Environmental Sustainability
- Facilities Service Desk
- Print Services
- Property and Maintenance
- Student Accommodation
- A-Z of Services
- Directorate
- Staff Documents
- Design principles
- Business Engagement
- Commercialise Your Research
- Intellectual Property
- Research Process
- Policies and Guidance
- External Projects
- Public Engagement
- Research Data
- Research Degrees
- Researcher Development
- Research Governance
- Research Induction
- Research Integrity
- Worktribe Log-in
- Worktribe RMS
- Knowledge Exchange Concordat
- Consultancy and Commercial Activity Framework
- Academic Appeals
- Academic Calendar
- Academic Integrity
- Curriculum Management
- Examinations
- Graduations
- Key Dates Calendar
- My Programme template
- Our Charter
- PASS Process Guides
- Student Centre & Campus Receptions (iPoints)
- Student Check In
- Student Decision and Status related codes
- Student Engagement Reporting
- Student Records
- Students requesting to leave
- The Student Charter
- Student Sudden Death
- Programme and Student Support (PASS)
- Timetabling
- Strategy Hub
- Careers & Skills Development
- Placements & Practice Learning
- Graduate Recruitment
- Student Ambassadors
- Confident Futures
- Disability Inclusion
- Student Funding
- Report and Support
- Keep On Track
- Student Pregnancy, Maternity, Paternity and Adoption
- Counselling
- Widening Access
- About the AUA
- Edinburgh Napier Students' Association
- Join UNISON
- Member Information & Offers
- LGPS Pensions Bulletin
- Donations made to Charity
- REF2021 - Results
- You Said, We Listened
- Outputs from Research
- Impact from Research
- REF Training and Development
- Sector Consultation
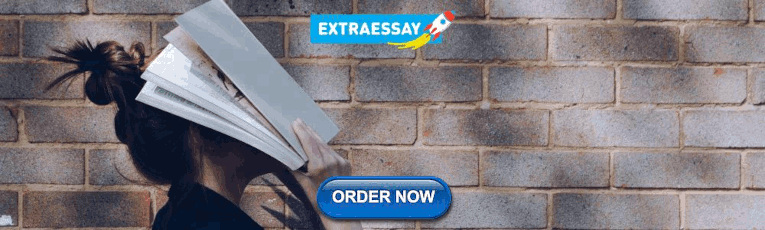
Outputs from Research
A research output is the product of research . It can take many different forms or types. See here for a full glossary of output types.
The tables below sets out the generic criteria for assessing outputs and the definitions of the starred levels, as used during the REF2021 exercise.
Definitions
'World-leading', 'internationally' and 'nationally' in this context refer to quality standards. They do not refer to the nature or geographical scope of particular subjects, nor to the locus of research, nor its place of dissemination.
Definitions of Originality, Rigour and Significance
Supplementary output criteria – understanding the thresholds:.
The 'Panel criteria' explains in more detail how the sub-panels apply the assessment criteria and interpret the thresholds:
Main Panel A: Medicine, health and life sciences Main Panel B: Physical sciences, engineering and mathematics Main Panel C: Social sciences Main Panel D: Arts and humanities
Definition of Research for the REF
1. For the purposes of the REF, research is defined as a process of investigation leading to new insights, effectively shared.
2. It includes work of direct relevance to the needs of commerce, industry, culture, society, and to the public and voluntary sectors; scholarship; the invention and generation of ideas, images, performances, artefacts including design, where these lead to new or substantially improved insights; and the use of existing knowledge in experimental development to produce new or substantially improved materials, devices, products and processes, including design and construction. It excludes routine testing and routine analysis of materials, components and processes such as for the maintenance of national standards, as distinct from the development of new analytical techniques.
It also excludes the development of teaching materials that do not embody original research.
3. It includes research that is published, disseminated or made publicly available in the form of assessable research outputs, and confidential reports
Output FAQs
Q. what is a research output.
A research output is the product of research. An underpinning principle of the REF is that all forms of research output will be assessed on a fair and equal basis. Sub-panels will not regard any particular form of output as of greater or lesser quality than another per se. You can access the full list of eligible output types here.
Q. When is the next Research Excellence Framework?
The next exercise will be REF 2029, with results published in 2029. It is therefore likely that we will make our submission towards the end of 2028, but the actual timetable hasn't been confirmed yet.
A sector-wide consultation is currently occurring to help refine the detail of the next exercise. You can learn more about the emerging REF 2029 here.
Q. Why am I being contacted now, if we don't know the final details for a future assessment?
Although we don't know all of the detail, we know that some of the core components of the previous exercise will be retained. This will include the assessment of research outputs.
To make the internal process more manageable and avoid a rush at the end of the REF cycle, we will be conducting an output review process on an annual basis, in some shape and form to spread the workload.
Furthermore, regardless of any external assessment frameworks, it is also important for us to understand the quality of research being produced at Edinburgh Napier University and to introduce support mechanisms that will enhance the quality of the research conducted. This is of benefit to the University and to you and your career development.
Q. I haven't produced any REF-eligible outputs as yet, what should I do?
We recognise that not everyone contacted this year will have produced a REF-eligible output so early on in a new REF cycle. If this is the case, you can respond with a nil return and you may be contacted again in a future annual review.
If you need additional support to help you deliver on your research objectives, please contact your line manager and/or Head of Research to discuss.
Q. I was contacted last year to identify an output, but I have not received a notification for the 2024 annual cycle, why not?
Due to administrative capacity in RIE and the lack of detail on the REF 2029 rules relating to staff and outputs, we are restricting this years' scoring activity to a manageable volume based on a set of pre-defined, targeted criteria.
An output review process will be repeated annually. If an output is not reviewed in the current year, we anticipate that it will be included in a future review process if it remains in your top selection.
Once we know more about the shape of future REF, we will adapt the annual process to meet the new eligibility criteria and aim to increase the volume of outputs being reviewed.
Q. I am unfamiliar with the REF criteria, and I do not feel well-enough equipped to provide a score or qualitative statement for my output/s, what should I do?
The output self-scoring field is optional. We appreciate that some staff may not be familiar with the criteria and are therefore unable to provide a reliable score.
The REF team has been working with Schools to develop a programme of REF awareness and output quality enhancement which aims to promote understanding of REF criteria and enable staff to score their work in future. We aim to deliver quality enhancement training in all Schools by the end of the 2023-24 academic cycle.
Please look out for further communications on this.
For those staff who do wish to provide a score and commentary, please refer specifically to the REF main panel output criteria: Main Panel A: Medicine, health and life sciences Main Panel B: Physical sciences, engineering and mathematics Main Panel C: Social sciences Main Panel D: Arts and humanities
Q. Can I refer to Journal impact factors or other metrics as a basis of Output quality?
An underpinning principle of REF is that journal impact factors or any hierarchy of journals, journal-based metrics (this includes ABS rating, journal ranking and total citations) should not be used in the assessment of outputs. No output is privileged or disadvantaged on the basis of the publisher, where it is published or the medium of its publication.
An output should be assessed on its content and contribution to advancing knowledge in its own right and in the context of the REF quality threshold criteria, irrespective of the ranking of the journal or publication outlet in which it appears.
You should refer only to the REF output quality criteria (please see definitions above) if you are adding the optional self-score and commentary field and you should not refer to any journal ranking sources.
Q. What is Open Access Policy and how does it affect my outputs?
Under current rules, to be eligible for future research assessment exercises, higher education institutions (HEIs) are required to implement processes and procedures to comply with the REF Open Access policy.
It is a requirement for all journal articles and conference proceedings with an International Standard Serial Number (ISSN), accepted for publication after 1 April 2016, to be made open access. This can be achieved by either publishing the output in an open access journal outlet or by depositing an author accepted manuscript version in the University's repository within three months of the acceptance date.
Although the current Open Access policy applies only to journal and conference proceedings with an ISSN, Edinburgh Napier University expects staff to deposit all forms of research output in the University research management system, subject to any publishers' restrictions.
You can read the University's Open Access Policy here .
Q. My Output is likely to form part of a portfolio of work (multi-component output), how do I collate and present this type of output for assessment?
The REF team will be working with relevant School research leadership teams to develop platforms to present multicomponent / portfolio submissions. In the meantime, please use the commentary section to describe how your output could form part of a multicomponent submission and provide any useful contextual information about the research question your work is addressing.
Q. How will the information I provide about my outputs be used and for what purpose?
In the 2024 output cycle, a minimum of one output identified by each identified author will be reviewed by a panel of internal and external subject experts.
The information provided will be used to enable us to report on research quality measures as identified in the University R&I strategy.
Output quality data will be recorded centrally on the University's REF module in Worktribe. Access to this data is restricted to a core team of REF staff based with the Research, Innovation and Enterprise Office and key senior leaders in the School.
The data will not be used for any other purpose, other than for monitoring REF-related preparations.
Q. Who else will be involved in reviewing my output/s?
Outputs will be reviewed by an expert panel of internal and external independent reviewers.
Q. Will I receive feedback on my Output/s?
The REF team encourages open and transparent communication relating to output review and feedback. We will be working with senior research leaders within the School to promote this.
Q. I have identified more than one Output, will all of my identified outputs be reviewed this year?
In the 2024 cycle, we are committed to reviewing at least one output from each contacted author via an internal, external and moderation review process in the 2024 cycle.
Once we know more about the shape of a future REF, we will adapt the annual process to meet the new criteria / eligibility.
Get in touch
- Report a bug
- Privacy Policy
Edinburgh Napier University is a registered Scottish charity. Registration number SC018373
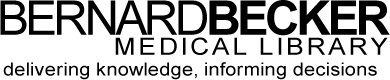
- Library Hours
- (314) 362-7080
- [email protected]
- Library Website
- Electronic Books & Journals
- Database Directory
- Catalog Home
- Library Home
Research Impact : Outputs and Activities
- Outputs and Activities
- Establishing Your Author Name and Presence
- Enhancing Your Impact
- Tracking Your Work
- Telling Your Story
- Impact Frameworks
What are Scholarly Outputs and Activities?
Scholarly/research outputs and activities represent the various outputs and activities created or executed by scholars and investigators in the course of their academic and/or research efforts.
One common output is in the form of scholarly publications which are defined by Washington University as:
". . . articles, abstracts, presentations at professional meetings and grant applications, [that] provide the main vehicle to disseminate findings, thoughts, and analysis to the scientific, academic, and lay communities. For academic activities to contribute to the advancement of knowledge, they must be published in sufficient detail and accuracy to enable others to understand and elaborate the results. For the authors of such work, successful publication improves opportunities for academic funding and promotion while enhancing scientific and scholarly achievement and repute."
Examples of activities include: editorial board memberships, leadership in professional societies, meeting organizer, consultative efforts, contributions to successful grant applications, invited talks and presentations, admininstrative roles, contribution of service to a clinical laboratory program, to name a few. For more examples of activities, see Washington University School of Medicine Appointments & Promotions Guidelines and Requirements or the "Examples of Outputs and Activities" box below. Also of interest is Table 1 in the " Research impact: We need negative metrics too " work.
Tracking your research outputs and activities is key to being able to document the impact of your research. One starting point for telling a story about your research impact is your publications. Advances in digital technology afford numerous avenues for scholars to not only disseminate research findings but also to document the diffusion of their research. The capacity to measure and report tangible outcomes can be used for a variety of purposes and tailored for various audiences ranging from the layperson, physicians, investigators, organizations, and funding agencies. Publication data can be used to craft a compelling narrative about your impact. See Quantifying the Impact of My Publications for examples of how to tell a story using publication data.
Another tip is to utilize various means of disseminating your research. See Strategies for Enhancing Research Impact for more information.
Examples of Outputs and Activities
- << Previous: Impact
- Next: Establishing Your Author Name and Presence >>
- Last Updated: Mar 12, 2024 1:26 PM
- URL: https://beckerguides.wustl.edu/impact
- Policy Library
Research Output
- All Policy and Procedure A-Z
- Policy and Procedure Categories
- Enterprise Agreement
- Current Activity
- Policy Framework
- Definitions Dictionary
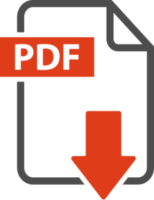
Definition overview
1 definition, 2 references, 3 definition information.
An output is an outcome of research and can take many forms. Research Outputs must meet the definition of Research.
The Excellence in Research for Australia assessment defines the following eligible research output types:
- books—authored research
- chapters in research books—authored research
- journal articles—refereed, scholarly journal
- conference publications—full paper refereed
- original creative works
- live performance of creative works
- recorded/rendered creative works
- curated or produced substantial public exhibitions and events
- research reports for an external body
Source: Australian Research Council Excellence in Research for Australia 2018 Submission Guidelines.
Complying with the law and observing Policy and Procedure is a condition of working and/or studying at the University.
* This file is available in Portable Document Format (PDF) which requires the use of Adobe Acrobat Reader. A free copy of Acrobat Reader may be obtained from Adobe. Users who are unable to access information in PDF should email [email protected] to obtain this information in an alternative format.
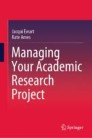
Managing Your Academic Research Project pp 101–117 Cite as
Outputs Versus Outcomes
- Jacqui Ewart 3 &
- Kate Ames 4
- First Online: 02 October 2020
549 Accesses
This chapter explores what we mean by research project deliverables—particularly the difference between outputs and outcomes. This is an increasingly important distinction to funding bodies. Research outputs, which are key performance indicators for academics, are not always the same as project outcomes. Setting expectations amongst team members and between researchers and funders is critical in the early stages of research project management, and can make the difference between whether a team is willing to work together, and/or able to be funded in an ongoing capacity. We also examine issues we can encounter when reporting for industry and government.
- Deliverables
This is a preview of subscription content, log in via an institution .
Buying options
- Available as PDF
- Read on any device
- Instant download
- Own it forever
- Available as EPUB and PDF
- Compact, lightweight edition
- Dispatched in 3 to 5 business days
- Free shipping worldwide - see info
- Durable hardcover edition
Tax calculation will be finalised at checkout
Purchases are for personal use only
Juniper, E. F. (2009). Validated questionnaires should not be modified. European Respiratory Journal , 34, 1015–1017. https://doi.org/10.1183/09031936.00110209 .
Mills-Scofield, D. (2012, November 26). It’s Not Just Semantics: Managing Outcomes Vs. Outputs. Harvard Business Review. Retrieved from https://hbr.org/2012/11/its-not-just-semantics-managing-outcomes .
Download references
Author information
Authors and affiliations.
Griffith University, Nathan, QLD, Australia
Prof. Jacqui Ewart
Central Queensland University, Brisbane, QLD, Australia
You can also search for this author in PubMed Google Scholar
Corresponding author
Correspondence to Jacqui Ewart .
Rights and permissions
Reprints and permissions
Copyright information
© 2020 Springer Nature Singapore Pte Ltd.
About this chapter
Cite this chapter.
Ewart, J., Ames, K. (2020). Outputs Versus Outcomes. In: Managing Your Academic Research Project. Springer, Singapore. https://doi.org/10.1007/978-981-15-9192-1_7
Download citation
DOI : https://doi.org/10.1007/978-981-15-9192-1_7
Published : 02 October 2020
Publisher Name : Springer, Singapore
Print ISBN : 978-981-15-9191-4
Online ISBN : 978-981-15-9192-1
eBook Packages : Education Education (R0)
Share this chapter
Anyone you share the following link with will be able to read this content:
Sorry, a shareable link is not currently available for this article.
Provided by the Springer Nature SharedIt content-sharing initiative
- Publish with us
Policies and ethics
- Find a journal
- Track your research
Imperial College London Imperial College London
Latest news.

Pollutant problems and building for NASA: News from Imperial

Water persisted in Mars’ Gale crater for longer than previously thought

Become a mentor in Imperial’s Reverse Mentoring Programme
- Research Office
- Research and Innovation
- Support for staff
Research outcomes, outputs and impact
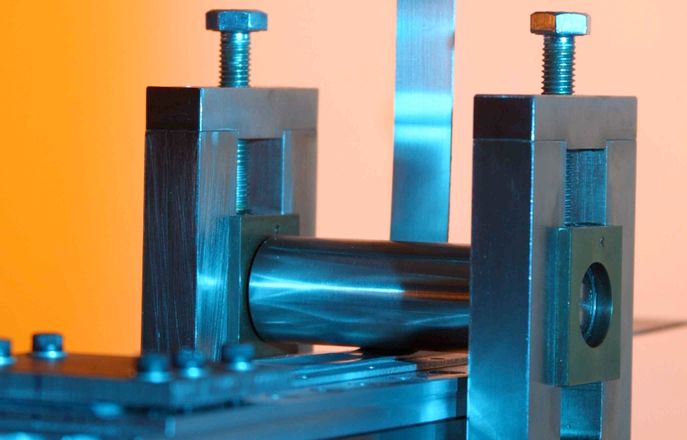
Research Impact
Impact Acceleration Accounts have helped a number of projects to expand the reach of their work and create impact.
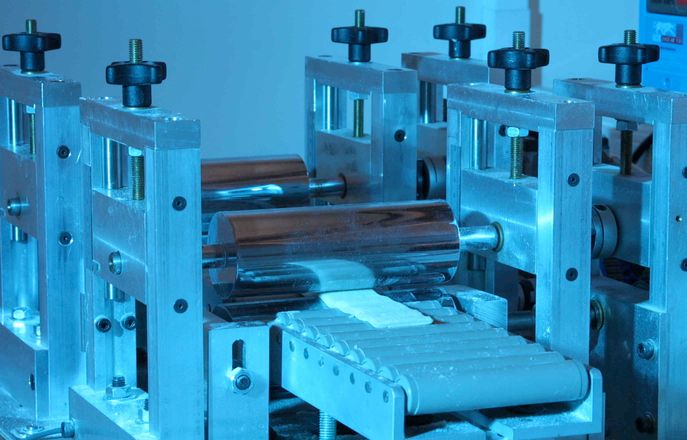
Researchfish
Researchfish is an external online system that collects research outcomes for a range of funders to help them track the impacts of their investments.
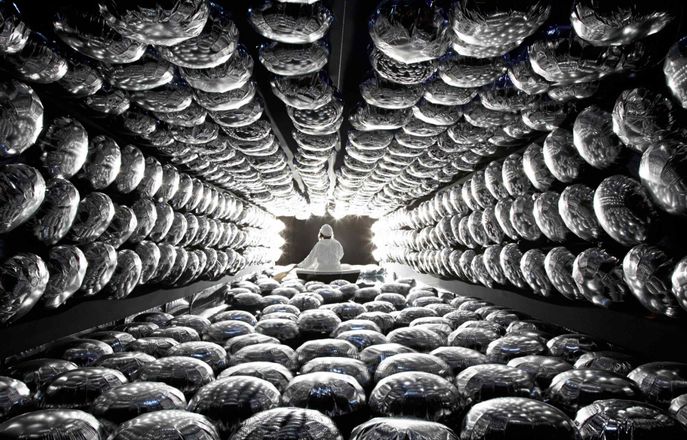
Research Data Management
College policy on the management of research data
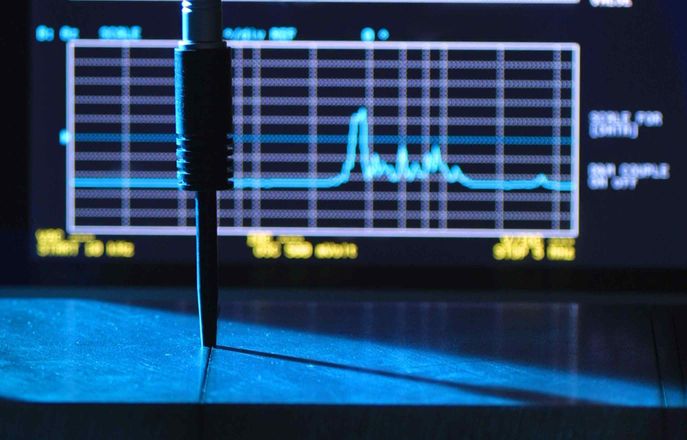
Open Access
College policy on the online availability of scholarly work (OA)
- Search Menu
- Advance articles
- Author Guidelines
- Submission Site
- Open Access
- Why Publish?
- About Research Evaluation
- Editorial Board
- Advertising and Corporate Services
- Journals Career Network
- Self-Archiving Policy
- Dispatch Dates
- Journals on Oxford Academic
- Books on Oxford Academic
Article Contents
1. introduction, 2. limitations of previous research, goals, and research questions, 3. the austrian science fund, 6. discussion, 7. conclusions.
- < Previous
Types of research output profiles: A multilevel latent class analysis of the Austrian Science Fund’s final project report data
- Article contents
- Figures & tables
- Supplementary Data
Rüdiger Mutz, Lutz Bornmann, Hans-Dieter Daniel, Types of research output profiles: A multilevel latent class analysis of the Austrian Science Fund’s final project report data, Research Evaluation , Volume 22, Issue 2, June 2013, Pages 118–133, https://doi.org/10.1093/reseval/rvs038
- Permissions Icon Permissions
Starting out from a broad concept of research output, this article looks at the question as to what research outputs can typically be expected from certain disciplines. Based on a secondary analysis of data from final project reports (ex post research evaluation) at the Austrian Science Fund (FWF), Austria’s central funding organization for basic research, the goals are (1) to find, across all scientific disciplines, types of funded research projects with similar research output profiles; and (2) to classify the scientific disciplines in homogeneous segments bottom-up according to the frequency distribution of these research output profiles. The data comprised 1,742 completed, FWF-funded research projects across 22 scientific disciplines. The multilevel latent class (LC) analysis produced four LCs or types of research output profiles: ‘Not Book’, ‘Book and Non-Reviewed Journal Article’, ‘Multiple Outputs’, and ‘Journal Article, Conference Contribution, and Career Development’. The class membership can be predicted by three covariates: project duration, requested grant sum, and project head’s age. In addition, five segments of disciplines can be distinguished: ‘Life Sciences and Medicine’, ‘Social Sciences/Arts and Humanities’, ‘Formal Sciences’, ‘Technical Sciences’, and ‘Physical Sciences’. In ‘Social Sciences/Arts and Humanities’ almost all projects are of the type ‘Book and Non-Reviewed Journal Article’, but, vice versa, not all projects of the ‘Book and Non-reviewed Journal Article’ type are in the ‘Social Sciences/Arts and Humanities’ segment. The research projects differ not only qualitatively in their output profile; they also differ quantitatively, so that projects can be ranked according to amount of output.
Research funding organizations have shown increasing interest in ex post research evaluation of the funded projects ( European Science Foundation 2011a ). For instance, the Austrian Science Fund (FWF), Austria’s central funding organization for the promotion of basic research and the subject of this article, has conducted ex post research evaluations for some years now ( Dinges 2005 ). By collecting and analysing information on the ‘progress, productivity, and quality’ ( European Science Foundation 2011b : 3) of funded projects, research funding organizations hope ‘to be able to identify gaps and opportunities, avoid duplication, encourage collaboration, and strengthen the case for research’ ( European Science Foundation 2011b : 3). As stated succinctly in the title of a 2011 working document by the European Science Foundation (ESF), a central topic in this connection is ‘The Capture and Analysis of Research Outputs’ ( European Science Foundation 2011a ). This involves the issues of what research outputs are actually important for ex post research evaluation, how they can be classified (typology) and how the data can be analysed. The ESF document provides the following definition of outputs: ‘Research outputs, as the products generated from research, include the means of evidencing, interpreting, and disseminating the findings of a research study’ ( European Science Foundation 2011a : 5).
But opinions differ on what research output categories should be included in ex post research evaluation. Without doubt, publication in a scientific journal is viewed in all scientific disciplines as the primary communication form ( European Commission 2010 ). For assessing the merits of a publication, bibliometric analyses are favoured. In the humanities and social sciences, however, the use of classical bibliometric analysis ( Glänzel 1996 ; Nederhof et al. 1989 ; Nederhof 2006 ; Van Leeuwen 2006 ) is viewed critically in the face of different forms of research outputs (e.g. monographs) and limitations of the databases ( Cronin and La Barre 2004 ; Hicks 2004 ; Archambault et al. 2006 ). For these disciplines, other forms of quantitative evaluation are under discussion ( Kousha and Thelwell 2009 ; White et al. 2009 ).
A number of authors have made a plea for extending classical biblio analysis and for broadening the concept of ‘research output’ generally ( Bourke and Butler 1996 ; Lewison 2003 ; Butler 2008 ; Huang and Chang 2008 ; Linmans 2010 ; Sarli et al. 2010 ): ‘A fair and just research evaluation should take into account the diversity of research output across disciplines and include all major forms of research publications’ ( Huang and Chang 2008 : 2018). Huang and Chang (2008) looked at an empirical analysis conducted of the publication types of all publications in the year 1998–9 across all disciplines at the University of Hong Kong and found that journal articles accounted for 90% and 99% of the total publications produced only in the disciplines medicine and physics. The other disciplines produced output in the form of very different types of written communication, such as books, book chapters, and conference and working papers. Huang and Chang’s (2008) comprehensive review of the literature on the characteristics of research output showed that especially in the humanities and social sciences, books, monographs, and book chapters are important forms of written communication.
The German Research Foundation (DFG), Germany’s central funding organization for basic research, carried out a survey in the year 2004 on the publishing strategies of researchers with regard to open access ( Deutsche Forschungsgemeinschaft 2005 ), and 1,083 DFG-funded researchers responded (response rate of 67.7%). When the researchers were asked to name their preferred form of traditional publication of their own work, they mentioned articles in scientific journals (on the average about 20 articles in 5 years). Life scientists published the largest number of journal articles (23.6 articles in 5 years) and humanities scholars and social scientists the fewest (12.7 articles in 5 years). Papers in proceedings were published far more often by engineering scholars than by researchers in other disciplines. Social scientists and humanities scholars had a greater preference for publishing their work in edited volumes and monographs than researchers in other disciplines. However, big differences in the numbers reported (e.g. number of books, number of journal articles) were found within disciplines. This study and the Huang and Chang study made it clear that not only the sciences and humanities differ greatly from other disciplines in their preferred form of written communication. There are great differences also within the natural sciences and humanities. The Expert Group on Assessment of University-Based Research set up by the European Commission came to similar conclusions ( European Commission 2010 : 26). In the opinion of the expert group, the peer-reviewed journal article is used as the primary form of written communication in all scientific disciplines. In addition, engineering scientists primarily publish in conference proceedings, whereas social scientists and humanists show a wide range of research outputs, with monographs and books as the most important forms of written communications.
The broadest concept of research output is used by the Research Council UK (RCUK) (see www.rcuk.ac.uk ), the United Kingdom’s (UK) central funding organization, and the Research Assessment Exercise (RAE) ( www.rae.ac.uk ), which in 2014 will be replaced by the new system, Research Excellence Framework (REF) (ww.ref.ac.uk). RAE and REF have the task of assessing the quality of research in higher education institutions in the UK. Whereas the RAE focuses on scientific impact, the performance measurement by the REF in addition includes societal impact—that is, any social, economic or cultural impact, or benefit beyond academia. As research output, the RAE and REF include different forms of research products (journal article, book, conference contribution, patent, software, Internet publication, and so on). The Research Outcome System (ROS) of RCUK distinguishes a total of nine categories of research outputs: publication, other research output, collaboration, communication, exploitation, recognition, staff development, further funding, and impact. The new REF is planned to extend the currently peer-supported RAE with a quantitative, indicator-based evaluation system that includes bibliometric and other quantitative methods. Butler and McAllister ( Butler and McAllister 2009 , 2011 ) spoke generally of a metric as opposed to peer review that would capture more than the classical bibliometric analysis based on journal articles does. RAE and REF are based on a research production model ( Bence and Oppenheim 2005 ) that differentiates between inputs (personnel, equipment, overheads), research generation processes, outputs (paper, articles, and so on), and utilization of research (scientific and societal impact). This kind of structuring in input, process, output, outcome/impact is also found in other frameworks for research evaluation, such as in the payback approach ( Buxton and Haney 1998 ; European Commission 2010 ; Banzi et al. 2011 ) and other national and international evaluation systems ( European Commission 2010 ).
Previous research on research outputs has had the following limitations:
As the databases for the empirical analysis, studies up to now used mainly literature databases ( Glänzel 1996 ; Nederhof et al. 1989 ) and (survey) data from researchers ( Deutsche Forschungsgemeinschaft 2005 ; Huang and Chang 2008 ). Therefore, the unit of analysis was people and not projects (European Science Foundation 2011). But the different research outputs and also inputs (e.g. human resources, funding) are tied with the research projects.
For the individual disciplines, the frequencies of certain research outputs were presented mostly in totals and separately without any closer examination of the combination of different research outputs in the form of a core profile. For example, some disciplines focus more on monographs and conference contributions and not so much on journal articles, whereas for other disciplines it is just the opposite. Beyond that, the variability of research output within a discipline, such as that found in a study conducted by the DFG ( Deutsche Forschungsgemeinschaft 2005 ), was hardly considered.
The studies often did not describe the research output comprehensively, as the RAE, REF, and RCUK do, for instance, and instead restricted the study to a specific research output category, such as journal articles. This can lead to an inadequate treatment of some disciplines. Technical sciences can be at a disadvantage, for instance, if patents are not included in the study. Moreover, mostly only selected disciplines were included in the analyses, such as social sciences and humanities, so that comparative analysis of various disciplines was not possible. But research projects in different disciplines can be very similar in the profiles of research output categories (abbreviated in the following as ‘research output profiles’).
The studies did not distinguish between quality and quantity of research outputs. For example, life sciences are similar to natural sciences in research output profiles, but life sciences have a higher volume of journal articles than the natural sciences do ( Deutsche Forschungsgemeinschaft 2005 ).
The goals of our study are:
Based on a secondary analysis of data in final project reports ( Glass 1976 ) at the FWF, Austria’s central funding organization for basic research, the goals of this study were (1) to find, across all scientific disciplines, types of funded research projects with similar research output profiles; and (2) to classify the scientific disciplines in homogeneous segments (e.g. humanities, natural sciences, engineering sciences) bottom-up according to the frequency distribution of these research output profiles. We aimed to establish the types of funded research projects using multilevel latent class analysis (MLLCA) ( Vermunt 2003 ; Kimberly and Muthén 2010 ; Mutz and Seeling 2010 ; Mutz and Daniel 2012 ).
The research questions are:
Are there any types of FWF-funded projects that have different core profiles of research outputs?
Do types of research output profiles vary across scientific disciplines? Can disciplines be clustered into segments according to the different proportions of certain types of research output profiles?
How does the probability of being in a particular type of research output profile depend on a set of project-related covariates (e.g. requested grant sum)?
Is there any additional variability within types of research output profiles that allows for a quantitative ranking of projects according to higher or lower research productivity?
The FWF is Austria’s central funding organization for the promotion of basic research. It is equally committed to all scientific disciplines. The body responsible for funding decisions at the FWF is the board of trustees, made up of 26 elected reporters and 26 alternates ( Bornmann 2012 ; Fischer and Reckling 2010 ; Mutz, Bornmann and Daniel 2012a , 2012b ; Sturn and Novak 2012 ). For each grant application, the FWF obtains at least two international expert reviews (ex ante evaluation). The number of reviewers depends on the amount of funding requested. The expert review consists (among other things) of an extensive written comment and a rating providing an overall numerical assessment of the application. At the FWF board’s decision meetings, the reporters present the written reviews and ratings of each grant application. In the period from 1999 to 2009 the approval rate of proposals was 44.2%. Since 2003, all funded projects are evaluated after completion ( Dinges 2005 ) (see www.fwf.ac.at/de/projects/evaluation-fwf.html ). The FWF surveys the FWF-funded researchers, asking them to report the outputs of their research projects using a category system that is akin to the research output system of RCUK. Additionally, referees are requested to provide a brief review giving their opinions on aspects of the final project report. They are also requested to assign a numerical rating to each aspect. The final reports were used for accountability purposes and to improve the quality of FWF’s decision procedure ( Dinges 2005 ).
The data for this study comprised 1,742 FWF-funded research projects called ‘Stand-Alone Projects’ across all fields of science (22 scientific disciplines classified into six research areas), which contributed to 60% of all FWF grants (‘Stand-Alone Projects’, ‘Special Research Programs’, ‘Awards and Prizes’, ‘Transnational Funding Activities’) and finished within a period of 9 years (2002–10). The labelling of the scientific disciplines and the research areas was adopted from the FWF ( Fischer and Reckling 2010 ). Each project head was requested to report the results of his or her research project by completing a form (final project report) containing several sections (summary for public relations; brief project report; information on project participants; attachments; collaboration with FWF).
Of the 1,742 completed FWF-funded research projects ( Table 1 ), most were in the natural sciences (31.6%), and the fewest were in the social sciences (6.0%) and technical sciences (4.5%). The finished projects (end of funding) were approved for funding in the period 1999–2010, one-third of them in 2003–4 alone. Due to still ongoing research projects, projects approved for funding in 2007–8 make up only 3.9% of the total database of 1,742 FWF-funded research projects. The average duration of the research projects was 39 months. In 84.5% of the projects, the project heads were men. The average age of the project heads was 47.
Sample description ( N = 1,742 completed FWF-funded research projects)
Note : N = frequency, per cent = column per cent, M = mean, SD = standard deviation, range = minimum and maximum.
The following six research output categories were captured in quantity and number (count data) and served as the basis for the analysis: publication (peer-reviewed journal article; non-peer-reviewed journal article, monograph, anthology, mass communication, i.e. any kind of publication in mass media, e.g. newspaper article), conference contribution (invited paper, paper, poster), award, patent, career development (diploma/degree, PhD dissertation, habilitation thesis) follow-up project (FWF funded or not). It was not differentiated between different sub-categories of the mentioned research output categories. For example, hybrid, open access and standard peer-reviewed journal articles or ongoing or terminated PhD dissertations were summarized under the respective research output category. In order to avoid problems with different publication lags, the FWF treated equally manuscripts, already published, and manuscripts, accepted for publication. The ex post evaluation approach of the FWF does not distinguish between project publications written in English and written in any other language.
Because of strongly skewed distributions, the count variables were transformed in 2-point to 5-point ordinal scale variables with at most equally sized ordinal classes, to avoid sparse classes or cells in a multivariate statistical analysis. To draw up a typology, actually, binary variables might be sufficient in which it was coded whether the particular research output category (e.g. monograph) existed (= 1) for a research project or not (= 0). However, because we wanted to differentiate a qualitative dimension (types) and a quantitative dimension (amount of output), we chose an ordinal scale with a sparse number of ordinal classes that in addition allow a quantitative assessment.
The research output variables ( Table 2 ) show a large share of zeros. The most frequently produced types of publication were reviewed journal articles (an average of five per project) and conference papers (on average nine), with a large variance across the research projects. For publication of research results, monographs are used the least (0.2 monographs per project).
Data description ( N = 1,742 FWF-funded research projects)
Note : Per cent = row per cent, M = mean of the raw data, SD = standard deviation of the raw data, Max = maximum, R 2 indicates how well an indicator is explained by the final LC model.
In a review of the literature Gonzalez-Brambila and Velosos (2007) discuss age, sex, education, and cohort effects as empirically investigated determinants of research outputs. In our study, we included the following covariates to predict research profile type membership ( Table 1 ): time period of the approval decision, time period of the project end, project duration; overall rating of the proposal, requested grant sum; gender and age of the project head. This information was taken from an ex ante evaluation of the project proposals. In the ex ante evaluation, two to three reviewers rated each proposal on a scale from 1 to 100 (ascending from poor to excellent). The mean of the overall ratings of a proposal averaged across reviewers was 89.7 (minimum: 61.7, maximum: 100).
4.2 Statistical procedure
Latent Class Analysis (LCA) in its basic structure can be defined as a statistical procedure that extracts clusters of units (latent classes (LCs)) that are homogenous with respect to the observed nominal or ordinal scale variables ( McCutcheon 1987 ). Similar to factor analysis, LCs are extracted in such a way that the correlations between the observed variables should vanish completely within each LC (local stochastic independence). LCA is favoured towards cluster analysis due to the fact that fewer pre-decisions are required than in common cluster analysis procedures (e.g. similarity measure, aggregation algorithm). Efficient algorithms for parameter estimation (maximum likelihood) are used, and a broad range of different models (LCA, IRT models, multilevel models, and more) are offered ( Magidson and Vermunt 2004 ; Vermunt and Magidson 2005a ). In a more advanced version of LCA, MLLCA, the nested data structure is additionally considered. In our study, research projects are nested within certain scientific disciplines; LCs or project types might vary between scientific disciplines. In MLLCA, not only are projects grouped according to their output profiles but also scientific disciplines will be segmented according to their different proportions of types of output profiles. In the technical framework of MLLCA, LCs represent the types of research output profile, and latent clusters (GClass) indicate the segments of disciplines. It will be presumed that a project in a certain LC behaves the same way (same research output profile) irrespective of the latent cluster to which the project belongs.
In secondary analysis the problem frequently arises that the assumption of local stochastic independence does not fully hold. For instance, career development output categories like diploma/degree and PhD dissertation are more strongly correlated with one another than with the other research output categories, so that a LCA cannot completely clarify the association between the two career development outputs. There are three possible ways to handle this problem ( Magidson and Vermunt 2004 ): First, one or more direct effects can be added that account for the residual correlations between the observed research output variables that are responsible for the violation of the local stochastic independence assumption. Second, one or more variables that are responsible for high residual correlations can be eliminated. Third, the number of latent variables (LCs, continuous latent variables) is increased. In this study we used all three strategies. After a first model run, the residuals were inspected, and a few direct effects were included in the MLLCA model. Additionally, two variables that were responsible for high residual correlations were eliminated—non-peer-reviewed journal articles and diplomas/degrees. Last but not least a MLLCA model was tested that incorporates a continuous latent variable comparable to a factor analysis. With this C-factor not only can residual correlations among the output variables be explained but also additional quantitative differences between research projects (amount of research output) can be assessed and can be taken for a ranking of projects, respectively. If, over and above, a model fits the data with the same structure (i.e. loadings of the research output variables on the factor) for all LCs as well as or better than a model with different structures in terms of different loadings of the variables in each LC, all research projects can be compared or ranked on the same scale of the latent variable.
For statistical analysis of the data we used MLLCA as implemented in the software program Latent GOLD 4.5 ( Vermunt and Magidson 2005b ). Following Bijmolt, Paas, and Vermunt (2004) , Lukočienė, Varriale, and Vermunt (2010) and Rindskopf (2006) , in a first step we calculated a simple LCA of the research outputs to obtain types of research projects with a similar research output profile. To determine the optimal number of classes (project types, segments of disciplines), information criteria were used, such as the Bayesian information criterion (BIC) or Akaike information criterion (AIC). The lower BIC or AIC the better the model fits. These information criteria penalize models for complexity (number of parameters), making it possible to make direct comparisons among models of different numbers of parameters. Results of a simulation study conducted by Lukočienė and Vermunt (2010) for MLLCA models showed that in all simulation conditions, the more advanced criteria AIC3 ( Bozdagon 1993 ) and the BIC(k) outperformed the usual BIC to identify the true number of higher-level LCs (Lukočienė, Varriale and Vermunt 2010 ). Unlike BIC, BIC(k) uses the number of groups, here the number of disciplines, in the formula for sample size n : BIC(k) =−2 * LL – df * ln(k); AIC3 = −2 * LL −3 * df, where df denotes the degrees of freedom, LL denotes the loglikelihood. In the second step , we took the hierarchical structure of data into account, calculating an MLLCA to obtain latent clusters of scientific disciplines, or segments. In a third step we fixed the number of latent clusters of the second step and again determined the number of LCs. However, Lukočienė and Vermunt’s (2010) simulation study showed that the third step results in very small improvement of 1%. We therefore abstained from applying this step.
In the last step we included covariates in the model to explain the LC membership ( Vermunt 2010 ). However, this one-step procedure has the disadvantage that by including the covariates, the model and its parameters, respectively, could change. Therefore, a three-step procedure was suggested. First, we estimated a LC model. Second, we assigned the subjects to the LCs according to their highest posterior class membership probability. Third, the LCs were regressed on a set of covariates using a multinomial regression model. However, this procedure does not take into account the uncertainty of class membership. Bolck, Croon, and Hagenaars (2004) showed that such a modelling strategy underestimates the true relationships between LCs and covariates. Recently, Vermunt (2010) developed a procedure that takes into account the uncertainty of class membership by including the classification table that cross-tabulates modal and probabilistic class assignment ( Vermunt and Magidson (2005b) as weighting matrix into the multinomial regression model. We followed this improved three-step approach. The covariates mentioned above were included for prediction of class membership ( Table 1 ).
5.1 Latent structure of research output profiles
In the first step the nested data structure (projects are nested within scientific disciplines) was ignored, and simple LC models were explored. Table 3 shows the results of fitting the models containing one to 11 LCs with and without a continuous latent C-factor, respectively. For model comparison we used the AIC3. Out of all 22 models, Model 15 with four LCs, 107 parameters, and one C-factor shows the smallest AIC3. We therefore decided on this model. With regard to our research questions, there were four types of projects with different research output profiles (qualitative dimension). Additionally, the projects differed in their productivity, i.e. the amount of outputs, represented by the continuous latent C-factor (quantitative dimension).
Fit statistics for exploratory LC models (project types)
Note : MNR = model number, NCL = number of latent classes, LL = loglikelihood, NPAR = number of parameter, AIC3 = Akaike information criterion 3. Final model grey coloured.
Figure 1 shows the four LCs or project types with different research output profiles. The 2-point to 5-point ordinal scales were re-scaled such that the numerical values varied within the range of 0–1.0 ( Vermunt and Magidson 2005b : 117). We obtained this scaling by subtracting the lowest observed value from the class-specific mean and dividing the results by the range, where the range was nothing but the difference between highest and lowest value. The advantage of this scaling is that all variables can be depicted on the same scale as the class-specific probabilities for nominal variables. It must be noted that the LC results depicted in Fig. 1 were the results of the final MLLCA model (introduced in Section 5.2 ) and not the non-nested LC model in Table 3 . However, this does not matter, because the LC models with and without nesting do not differ.
LCs of research output profiles (* = not used in the MLLCA).
The four LCs or project types with different research output profiles can be described as follows (class sizes in per cent of the total number of projects in parentheses):
Latent Class 1 ‘ Not Book ’ (37.0%): The research output profile of this research project type is quite similar to the average profile across all projects but with fewer non-reviewed journal articles, anthologies, and monographs than the average.
Latent Class 2 ‘ Book and Non-Reviewed Journal Article ’ (35.8%): this project type uses anthologies and monographs but also non-reviewed journal articles and mass communication as primary forms of written communication. Career development—such as diploma/degree, PhD dissertation and habilitation thesis—reviewed journal articles and follow-up projects score quite below the average.
Latent Class 3 ‘ Multiple Outputs ’ (17.9%): This project type generates research outputs in multiple ways with above-average outputs as peer-reviewed journal articles, non-reviewed journal articles, anthologies, monographs, conference papers, habilitation theses, PhD dissertations, diplomas/degrees, follow-up projects, but with fewer other conference contributions.
Latent Class 4 ‘ Journal Article, Conference Contribution, and Career Development ’ (9.3%): this most productive project type focuses strongly on peer-reviewed journal articles, with many published papers in combination with conference contributions (papers or other products), career development (diploma/degree, PhD dissertation, habilitation thesis), and follow-up projects, but this type uses fewer monographs as a form of written communication.
Of all the output variables, peer-reviewed journal articles and conference contributions discriminate the best between the LCs, with a discrimination index of about 0.60 ( Table 2 , last column, R 2 ).
5.2 Multilevel latent structure of research output profiles
In a multilevel latent structure model it is presumed that there is variation among the 22 scientific disciplines in the unconditional probabilities (the probabilities belonging to each LC). In an MLLCA the 22 scientific disciplines are grouped into latent clusters or segments according to their different proportion of types of research output profiles, as obtained in Section 5.1 .
Table 4 shows the results of fitting models containing one to eight latent clusters (M 1 –M 8 ), each with four LCs and with one continuous latent C-factor, respectively. With respect to BIC(k) and AIC3, a 5-GClass model will be favoured, i.e. there are five different segments of scientific disciplines with different proportions of the project types or LCs. Additionally, using the option of ‘cluster-independent C-factor’, we tested (M 9 ) whether the same loading structure can be held in all four LCs. The BIC(k) and the AIC3 improved slightly from model M 5 to the more restricted model M 9 with 122 − 89 = 33 fewer parameters than M 5 . Therefore, the assumption of a cluster-independent C-factor held, which made it possible to compare and rank all projects on the same scale. Including direct effects, such as the association between habilitation thesis and PhD dissertation, further improved the model. Only one residual (res = 3.88) was somewhat larger than the criterion of 3.84 ( Magidson and Vermunt 2004 ). To fulfil the basic model assumption of local stochastic independence, we chose model M 10 as the final model.
Fit statistics of models for variation among scientific disciplines (GClass) with four LCs and one C-factor
Note : MNR = model number, LL = loglikelihood, NPAR = number of parameters, BIC(k) = Bayesian information criterion for k clusters, AIC3 = Akaike information criterion 3.
To assess the separation between LCs, we calculated entropy-based measures, which varied between 0 and 1.0. They show how well the observed variables were able to predict the class membership (Lukočienė, Varriale and Vermunt 2010 ). For LC, the R 2 entropy amounted to 0.78, for latent clusters R 2 entropy amounted to 0.98. The separation of both the LCs and the latent clusters is therefore very large. Another model validity index is the proportion of classification error. For each project and each LC or latent cluster a posterior probability that a project belongs to the respective class can be estimated. Out of this set of probabilities the highest one indicates the LC to which a project or discipline should be assigned (modal assignment). Overall, the modal assignments can deviate from the expected assignments according to the sum of the posterior probabilities. The classification error indicates the amount of misclassification. For model M 10 the classification error was comparatively low, with 11.0% at the level of projects and 0.7% at the level of disciplines.
Based on Fig. 1 it could be supposed that the LCs do not represent a qualitative configuration but rather a quantitative dimension, in that the individual profiles run largely parallel and differ only in the level, that is, the quantity of research output. To prove this assumption the LCs were order-restricted (model M 11 ). However, the BIC(k) as well as the AIC3 of M 11 strongly increased in comparison to all other models, with the result that the assumption of a quantitative dimension behind the LCs was not very plausible.
To illustrate the meaning of these segments of scientific disciplines, Table 5 shows the distribution of the projects among the four LCs ( Fig. 1 ) of each of the five segments of disciplines (latent clusters). The last column of numbers in Table 5 indicates the size of the LCs or types of research output profiles. The last row of numbers in Table 5 indicates the proportion of disciplines that were in each discipline segment. The latent clusters or segments of scientific disciplines can be described according to the disciplines that belong to them (cluster sizes in per cent of the total number of disciplines in parentheses):
Latent Cluster 1 ‘ Life Sciences and Medicine ’ (31.6%): biology; botany; zoology; geosciences; preclinical medicine; clinical medicine; agricultural, forestry and veterinary sciences.
Latent Cluster 2 ‘ Social Sciences / Arts and Humanities ’ (31.4%): social sciences; jurisprudence; philosophy/theology; history; linguistics and literary studies; art history; other humanities fields.
Latent Cluster 3 ‘ Formal Sciences ’ (13.9%): mathematics; computer sciences; economic sciences.
Latent Cluster 4 ‘ Technical Sciences ’ (13.5%): Other natural sciences; technical sciences; psychology.
Latent Cluster 5 ‘ Physical Sciences ’ (9.6%): physics, astronomy and mechanics; chemistry.
Relative class sizes and distribution of projects among LCs (project output types) within each latent clusters (discipline segments) for M 10 (column per cent)
Note : LC size = size of the latent class, GClass size = size of the latent clusters, proportions over 0.30 (except for class sizes) are in bold face .
The remaining columns in Table 5 show the distribution of projects in each discipline segment or the probability of a project showing a specific profile type given its latent cluster membership. For instance, of all projects falling into the first GClass 84% are in LC 1 (‘Not Book’), 0% are in LC 2 (‘Book and Non-Reviewed Journal Article’), 6% are in LC 3 (‘Multiple Outputs’), and 10% are in LC 4 (‘Journal Article, Conference Contribution, and Career Development’). High proportions in a cell indicate a strong association of the corresponding segment of disciplines in the column with the corresponding type of research output profile in the row. In this respect the segment ‘Life Sciences and Medicine’ (GClass 1) was strongly associated with the ‘Not Book’ project type (LC 1) (84% of projects of this segment), but 10% of this cluster fell also in the most productive type, ‘Journal Article, Conference Contribution, and Career Development’ (LC 4). In the segment ‘Social Sciences/Arts and Humanities’ (GClass 2) almost all projects (97%) are of the second ‘Book and Non-Reviewed Journal Article’ type (LC 2). Projects of the third segment ‘Formal Sciences’ are classified about 80% in the ‘Multiple Outputs’ type, 14% also in the ‘Not Book’ type. The fourth segment, ‘Technical Sciences’, is rather heterogeneous, with over 95% of the projects of this segment in the first three project types and 37% even in the ‘Book and Non-Reviewed Journal Article’ type (LC 2). The projects of the last segment, ‘Physical Sciences’, can be divided mainly into two groups: 38% in the first project type ‘Not Book’ and 56% in the most productive project type, ‘Journal Article, Conference Contribution, and Career Development’. Overall, except for ‘Humanities’, there is no one-to-one assignment of a segment of disciplines to a special type of research output profile. Disciplines show great heterogeneity in their research output profiles.
Figure 2 shows the LC proportions for each single discipline, structured according to the latent cluster (segments of disciplines). This finding also replicated the basic findings in Table 5 at the level of single disciplines. It is of interest that the ‘Book and Non-reviewed Journal Article’ type (LC 2) played an important role not only in ‘Social Sciences/Arts and Humanities’ but also in ‘Technical Sciences’.
Estimated proportions of the four LCs of projects for each scientific discipline (stacked bars plot), classified into one of five latent clusters (1–5, separated by dashed lines).
5.3 Explaining LC membership
To explain the LC membership we conducted a modified multilevel multinomial regression model with the latent-class membership as categorical variable and the set of covariates as predictors ( Vermunt 2010 ). Beforehand, the continuous covariates time, age, duration, overall rating of a proposal (ex ante evaluation), and requested grant sum were z -transformed ( M = 0, S = 1) to facilitate the interpretation of the regression results independently of the units of the covariates ( Table 6 ).
Wald statistics are used to assess the statistical significance of a set of parameter estimates. Using Wald statistics, the restriction is tested that each estimate in a set of parameters associated with a given covariate equals zero ( Vermunt and Magidson 2005b ). A non-significant Wald statistic indicates that the respective covariate does not differ between the LCs. Additionally, we calculated a z -test for each single parameter. There are three covariates that explained the class membership with statistically significant Wald tests: project duration, requested grant sum, and the project head’s age. The overall rating of the proposal (ex ante evaluation), for instance, had no impact on the class membership. Research projects with a duration longer than the average of 39 months were more often in LC 4 (‘Journal Article, Conference Contribution, and Career Development’) than research projects with a shorter than average duration were. The higher the requested grant sum of a project, the less probable it was for the project to be in LC 2 (‘Book and Non-Reviewed Journal Article’), but the more probable it was for it to be in LC 4 (‘Journal Article, Conference Contribution, and Career Development’). Projects where the project head was older than the average age of 47 were more frequently in LC 2 (‘Book and Non-Reviewed Journal Article’), whereas projects where the project head was younger than 47 tended to be in LC 3 (‘Multiple Outputs’). Additionally, the percentage of projects in LC 4 (‘Journal Article, Conference Contribution, and Career Development’) decreased from project end year 2002 to project end 2010.
In sum, projects that belong to the ‘Book and Non-Reviewed Journal Article’ type (LC 2) tended to have rather low requested grant sums and project heads who were older than the average, whereas the most productive ‘Journal Article, Conference Contribution, and Career Development’ type was characterized by above-average requested grant sums and above-average project durations. Further, the percentage of this most productive type decreased over time (time of project end). The third type, ‘Multiple Outputs’, tended to have younger project heads.
5.4 Ranking of projects
Until now it was assumed that output profiles of research projects can be fully explained by the LC or types of output profiles into which the projects were classified. However, as Table 3 shows, projects differed not only with respect to LCs or latent cluster but also with respect to an additional quantitative dimension, a latent C-factor, referring to classical concepts of factor analysis. Unlike LCs, all output variables have positive loadings on this dimension—namely, with the same correlation or loading structure within each LC. Thus, the higher the value in any of the output variable, the higher the value of the C-factor is. Positive values in the C-factor represent productivity above average of the projects in this LC, and negative values indicate projects with less productivity with respect to projects in the same LC. In sum, the C-factor represents productivity differences of projects within each LC, similar to a Mixed-Rasch model in psychometrics ( Mutz, Borchers and Becker 2002 ; Mutz and Daniel 2007 ). This type of ranking can be used by the FWF (and other funding organizations) for comparative evaluation of the output of different projects within a certain time period.
According to the C-factor, the projects within each LC or project type could be ranked ( Fig. 3 ) from left (projects with the highest productivity) to right (projects with the lowest productivity). Additionally, Goldstein-adjusted confidence intervals are shown which makes it possible to interpret non-overlapping intervals of two projects as statistical significant differences at the 5% probability level ( Mutz and Daniel 2007 ). Roughly speaking, only the first and the last 100 projects in each LC actually showed statistically significant differences in their C-factor values.
Rankings of projects within LCs from left (largest amount of research output) to right (smallest amount of research output) and Goldstein-adjusted confidence intervals.
The aim of this study was to conduct a secondary analysis of final report data from the FWF (ex post evaluation) for the years 2002–10 (project end) and—using multilevel LCA—to build bottom-up a typology of research projects and, further, to classify scientific disciplines according to the different proportions of the types of research output profiles found. Referring to our four research questions, the results can be summarized as follows:
The 1,742 completed FWF-funded research projects available for a final report can be classified according to the research output profiles in the following four types with relatively high discrimination: 37% of all projects are in the ‘Not Book’ type, 35.8% in the ‘Book and Non-Reviewed Journal’ type, 17.9% in the ‘Multiple Outputs’ type, and 9.3% in the ‘Journal Article, Conference Contribution, and Career Development’ type, which is the most productive type in terms of number of journal articles and career-related activities. These project types represent primarily a qualitative configuration and not a quantitative dimension according to which projects can be ranked.
The 22 scientific disciplines can be divided into five segments of disciplines based on different proportions of the types of research output profiles: 31.6% of all projects can be classified in the segment ‘Life Science and Medicine’, 31.4% in ‘Social Sciences/Arts and Humanities’, 13.9% in ‘Formal Sciences’, 13.5% in ‘Technical Sciences’ and 9.6% in ‘Physical Sciences’, such as chemistry and physics. Only the ‘Social Sciences/Arts and Humanities’ segment is almost fully associated with one research output profile (‘Book and Non-Reviewed Journal Article’ type); all other segments show different proportions of the four research output profiles. Psychology and economic sciences are usually subsumed under humanities and social sciences. But the MLLCA showed that these two scientific disciplines do not belong to the segment ‘Social Sciences/Arts and Humanities’. Additionally, the fourth and most productive type of research output profile is highly represented (56%) in the fifth segment of disciplines, ‘Physical Sciences’, and with only 10% in ‘Life Science and Medicine’, contrary to the findings of the DFG ( Deutsche Forschungsgemeinschaft 2005 ) mentioned above in the introduction. ‘Life Sciences and Medicine’ is strongly associated (84%) with the ‘Not Book’ type. Projects of the third segment, ‘Formal Sciences’, are classified about 80% in the ‘Multiple Outputs’ type and 14% also in the ‘Not Book’ type. The fourth segment, ‘Technical Sciences’, is rather heterogeneous, with over 90% of the projects in this segment in the first three project types and 37% even in the ‘Book and Non-Reviewed Journal Article’ type. In the end, the findings of the Expert Group on Assessment of University-Based Research set up by the European Commission ( European Commission 2010 ) on the disciplines’ preferred forms of communication are too simple. To sum up, there are not only differences between scientific disciplines in the research output profiles; there is also great heterogeneity of research output profiles within disciplines and segments of disciplines, respectively.
Membership in a particular project type can essentially be explained by three covariates—project duration, requested grant sum, and the project head’s age. Projects that belong to the ‘Book and Non-Reviewed Journal Article’ type tend to be characterized by small requested grant sums and project heads who are older than the average, whereas the most productive type, ‘Journal Article, Conference Contribution, and Career Development’, tends to be characterized by high requested grant sums and longer than average project durations, but whose proportion decreases the more the date of the project termination approximates the year 2010. Reviewers’ overall rating of the proposal (ex ante evaluation) had no influence on latent-class membership.
Projects differ not only in the qualitative configuration of research outputs, their research output profiles, but also with respect to a quantitative dimension that makes productivity rankings of projects possible. The higher the output of a project in each of the research output variables, the higher its value on the quantitative (latent) dimension is. Only the first and the last 100 projects within each project type differed statistically significantly on this dimension.
However, there are also some limitations of our study that have to be discussed: first, the findings represent a specific picture of the research situation in one country, namely, Austria, in a 10-year period situation, and they may not necessarily apply in other countries. The quality of the research was not considered, such as through using international reference values for bibliographic indicators ( Opthof and Leydesdorff 2010 ; Bornmann and Mutz 2011 ) or through using discipline-specific quality criteria. Second, the study included only projects (in particular, ‘Stand-Alone Projects’) that were funded by the FWF. Research projects in Austria that were funded by other research funding organizations, that were not Stand-Alone Projects (40%) or that were funded by higher education institutions themselves could not be included. Further, research projects are mostly financed by mixed funding—that is, in part by grants from various research funding organizations and in part by matching funds from the relevant higher education institution (e.g. human resources), so that research output profiles cannot necessarily be explained by covariates of a single research funding organization. Third, the persons responsible for preparing a report (here, the project heads) always have a certain leeway to mention or not mention certain results of their research as results of the FWF-funded research projects in the final report (e.g. journal articles, career development). In social psychology terms, this phenomenon can be subsumed under the concept of ‘social desirability’ ( Nederhof 1985 ). Social desirability is a psychological tendency to respond in a manner that conforms to consensual standards and general expectancies in a culture. The findings of this study could thus also in part reflect different report policies in the different scientific disciplines.
Despite these limitations, we draw the following conclusions from the results:
Concept of ‘ research output ’ : If the aim is to include all disciplines in the ex post research evaluation, it is necessary to define the term ‘research output’ more broadly, as do the RCUK and the FWF, and to include—in addition to journal articles—also other output categories, such as monographs, anthologies, conference contributions, and patents, in order to treat all disciplines fairly with regard to research output.
Arts and Humanities : As has been repeatedly demanded, the arts and humanities really should be treated as an independent and relatively uniform area ( Nederhof et al. 1989 ; Nederhof 2006 ). Instead of counting only journal articles and their citations, however, it is important to include also monographs and anthologies ( Kousha and Thelwell 2009 ). Psychology and economic sciences do not belong to the segment ‘Social Sciences/Arts and Humanities’. Therefore, it is rather problematic to subsume psychology, economic sciences, social sciences, sociology, and humanities in one unique concept, ‘Social Sciences and Humanities’, as is often the case ( Archambault et al. 2006 ; Nederhof 2006 ).
Hierarchy of the sciences : A most familiar and widespread belief is that scientific disciplines can be classified as ‘hard’ sciences and ‘soft’ sciences, with physics at the top of the hierarchy, social sciences at the bottom and biology somewhere in between ( Smith et al. 2000 ). The strategy followed here made it possible to work out, bottom-up from the research outputs of funded research projects, an empirically based typology of scientific disciplines that at its heart is not hierarchically structured. The typology found reflects much more strongly the real structure of science than the top-down classification systems of sciences allow. However, the identified research output profiles do not unambiguously indicate the segment of the discipline. For instance, almost all projects in the segment ‘Social Sciences/Arts and Humanities’ are of the ‘Book and Non-Reviewed Journal Article’ type, but not all projects of the ‘Book and Non-Reviewed Journal Article’ type are in the segment ‘Social sciences/Arts and Humanities’; there is also a high proportion of ‘Book and Non-Reviewed Journal Article’ type projects in the segment ‘Technical Sciences’.
Research output profiles: Using MLLCA, research projects are not examined with regard to few arbitrarily selected project outputs; instead, the profile or combination of multiple research outputs is analysed. This should receive more attention also in ex post research evaluations of projects.
Ranking of projects : In addition, with MLLCA a qualitative dimension of different types of projects and segments of disciplines can be distinguished from a quantitative dimension that captures research productivity. In this way, projects and possibly also scientific disciplines can be ranked according to their productivity.
Selected model parameters of the regression from LCs on covariates
Note : LC = latent class, Par = parameter estimate, SE = standard error, Wald = Wald test, df = degrees of freedom.
*p < 0.05 ( z -test) **p < 0.05 (Wald test, df = 3).
Google Scholar
Google Preview
Email alerts
Citing articles via.
- Recommend to your Library
Affiliations
- Online ISSN 1471-5449
- Print ISSN 0958-2029
- Copyright © 2024 Oxford University Press
- About Oxford Academic
- Publish journals with us
- University press partners
- What we publish
- New features
- Open access
- Institutional account management
- Rights and permissions
- Get help with access
- Accessibility
- Advertising
- Media enquiries
- Oxford University Press
- Oxford Languages
- University of Oxford
Oxford University Press is a department of the University of Oxford. It furthers the University's objective of excellence in research, scholarship, and education by publishing worldwide
- Copyright © 2024 Oxford University Press
- Cookie settings
- Cookie policy
- Privacy policy
- Legal notice
This Feature Is Available To Subscribers Only
Sign In or Create an Account
This PDF is available to Subscribers Only
For full access to this pdf, sign in to an existing account, or purchase an annual subscription.
Have a language expert improve your writing
Run a free plagiarism check in 10 minutes, generate accurate citations for free.
- Knowledge Base
- Starting the research process
- How to Write a Research Proposal | Examples & Templates
How to Write a Research Proposal | Examples & Templates
Published on October 12, 2022 by Shona McCombes and Tegan George. Revised on November 21, 2023.
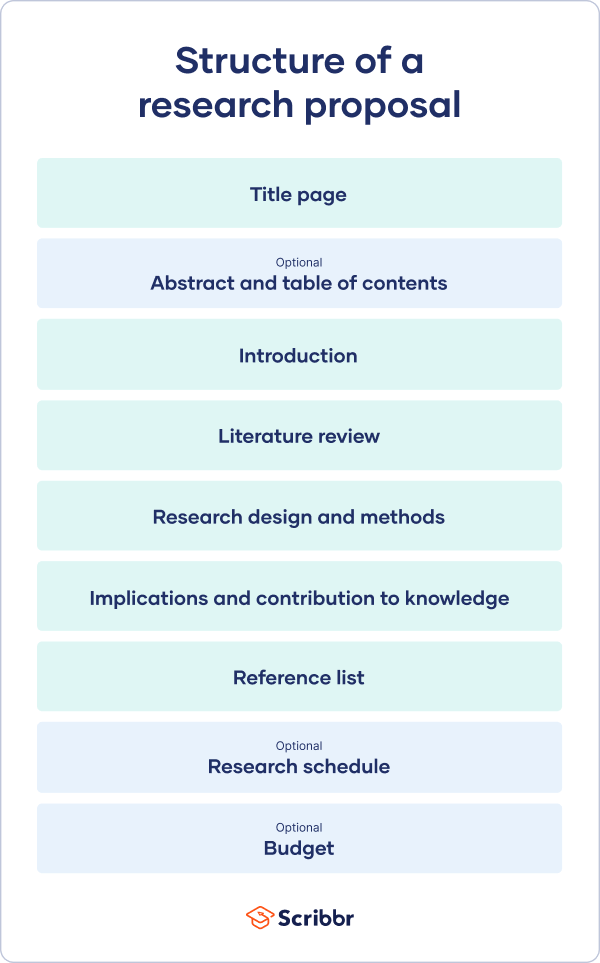
A research proposal describes what you will investigate, why it’s important, and how you will conduct your research.
The format of a research proposal varies between fields, but most proposals will contain at least these elements:
Introduction
Literature review.
- Research design
Reference list
While the sections may vary, the overall objective is always the same. A research proposal serves as a blueprint and guide for your research plan, helping you get organized and feel confident in the path forward you choose to take.
Table of contents
Research proposal purpose, research proposal examples, research design and methods, contribution to knowledge, research schedule, other interesting articles, frequently asked questions about research proposals.
Academics often have to write research proposals to get funding for their projects. As a student, you might have to write a research proposal as part of a grad school application , or prior to starting your thesis or dissertation .
In addition to helping you figure out what your research can look like, a proposal can also serve to demonstrate why your project is worth pursuing to a funder, educational institution, or supervisor.
Research proposal length
The length of a research proposal can vary quite a bit. A bachelor’s or master’s thesis proposal can be just a few pages, while proposals for PhD dissertations or research funding are usually much longer and more detailed. Your supervisor can help you determine the best length for your work.
One trick to get started is to think of your proposal’s structure as a shorter version of your thesis or dissertation , only without the results , conclusion and discussion sections.
Download our research proposal template
Receive feedback on language, structure, and formatting
Professional editors proofread and edit your paper by focusing on:
- Academic style
- Vague sentences
- Style consistency
See an example
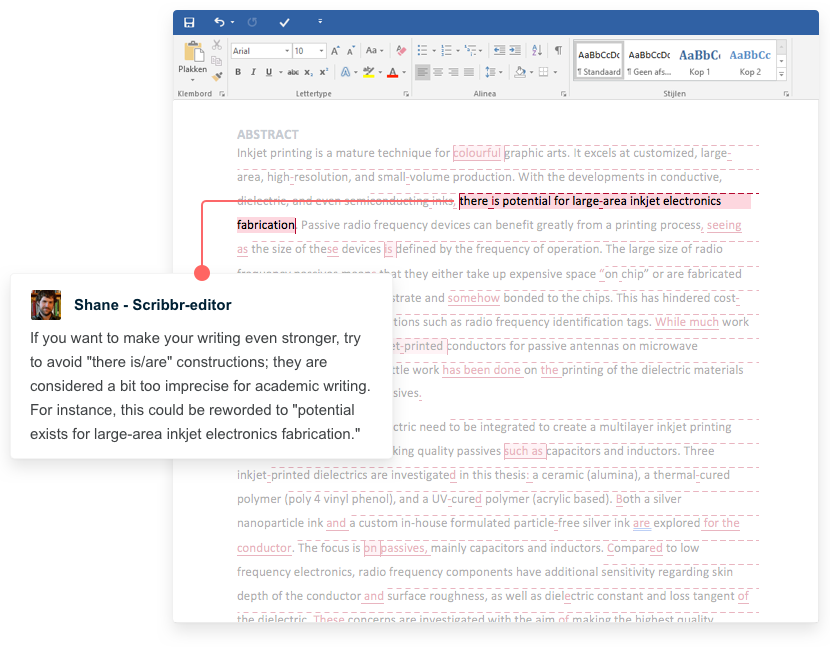
Writing a research proposal can be quite challenging, but a good starting point could be to look at some examples. We’ve included a few for you below.
- Example research proposal #1: “A Conceptual Framework for Scheduling Constraint Management”
- Example research proposal #2: “Medical Students as Mediators of Change in Tobacco Use”
Like your dissertation or thesis, the proposal will usually have a title page that includes:
- The proposed title of your project
- Your supervisor’s name
- Your institution and department
The first part of your proposal is the initial pitch for your project. Make sure it succinctly explains what you want to do and why.
Your introduction should:
- Introduce your topic
- Give necessary background and context
- Outline your problem statement and research questions
To guide your introduction , include information about:
- Who could have an interest in the topic (e.g., scientists, policymakers)
- How much is already known about the topic
- What is missing from this current knowledge
- What new insights your research will contribute
- Why you believe this research is worth doing
As you get started, it’s important to demonstrate that you’re familiar with the most important research on your topic. A strong literature review shows your reader that your project has a solid foundation in existing knowledge or theory. It also shows that you’re not simply repeating what other people have already done or said, but rather using existing research as a jumping-off point for your own.
In this section, share exactly how your project will contribute to ongoing conversations in the field by:
- Comparing and contrasting the main theories, methods, and debates
- Examining the strengths and weaknesses of different approaches
- Explaining how will you build on, challenge, or synthesize prior scholarship
Following the literature review, restate your main objectives . This brings the focus back to your own project. Next, your research design or methodology section will describe your overall approach, and the practical steps you will take to answer your research questions.
To finish your proposal on a strong note, explore the potential implications of your research for your field. Emphasize again what you aim to contribute and why it matters.
For example, your results might have implications for:
- Improving best practices
- Informing policymaking decisions
- Strengthening a theory or model
- Challenging popular or scientific beliefs
- Creating a basis for future research
Last but not least, your research proposal must include correct citations for every source you have used, compiled in a reference list . To create citations quickly and easily, you can use our free APA citation generator .
Some institutions or funders require a detailed timeline of the project, asking you to forecast what you will do at each stage and how long it may take. While not always required, be sure to check the requirements of your project.
Here’s an example schedule to help you get started. You can also download a template at the button below.
Download our research schedule template
If you are applying for research funding, chances are you will have to include a detailed budget. This shows your estimates of how much each part of your project will cost.
Make sure to check what type of costs the funding body will agree to cover. For each item, include:
- Cost : exactly how much money do you need?
- Justification : why is this cost necessary to complete the research?
- Source : how did you calculate the amount?
To determine your budget, think about:
- Travel costs : do you need to go somewhere to collect your data? How will you get there, and how much time will you need? What will you do there (e.g., interviews, archival research)?
- Materials : do you need access to any tools or technologies?
- Help : do you need to hire any research assistants for the project? What will they do, and how much will you pay them?
If you want to know more about the research process , methodology , research bias , or statistics , make sure to check out some of our other articles with explanations and examples.
Methodology
- Sampling methods
- Simple random sampling
- Stratified sampling
- Cluster sampling
- Likert scales
- Reproducibility
Statistics
- Null hypothesis
- Statistical power
- Probability distribution
- Effect size
- Poisson distribution
Research bias
- Optimism bias
- Cognitive bias
- Implicit bias
- Hawthorne effect
- Anchoring bias
- Explicit bias
Once you’ve decided on your research objectives , you need to explain them in your paper, at the end of your problem statement .
Keep your research objectives clear and concise, and use appropriate verbs to accurately convey the work that you will carry out for each one.
I will compare …
A research aim is a broad statement indicating the general purpose of your research project. It should appear in your introduction at the end of your problem statement , before your research objectives.
Research objectives are more specific than your research aim. They indicate the specific ways you’ll address the overarching aim.
A PhD, which is short for philosophiae doctor (doctor of philosophy in Latin), is the highest university degree that can be obtained. In a PhD, students spend 3–5 years writing a dissertation , which aims to make a significant, original contribution to current knowledge.
A PhD is intended to prepare students for a career as a researcher, whether that be in academia, the public sector, or the private sector.
A master’s is a 1- or 2-year graduate degree that can prepare you for a variety of careers.
All master’s involve graduate-level coursework. Some are research-intensive and intend to prepare students for further study in a PhD; these usually require their students to write a master’s thesis . Others focus on professional training for a specific career.
Critical thinking refers to the ability to evaluate information and to be aware of biases or assumptions, including your own.
Like information literacy , it involves evaluating arguments, identifying and solving problems in an objective and systematic way, and clearly communicating your ideas.
The best way to remember the difference between a research plan and a research proposal is that they have fundamentally different audiences. A research plan helps you, the researcher, organize your thoughts. On the other hand, a dissertation proposal or research proposal aims to convince others (e.g., a supervisor, a funding body, or a dissertation committee) that your research topic is relevant and worthy of being conducted.
Cite this Scribbr article
If you want to cite this source, you can copy and paste the citation or click the “Cite this Scribbr article” button to automatically add the citation to our free Citation Generator.
McCombes, S. & George, T. (2023, November 21). How to Write a Research Proposal | Examples & Templates. Scribbr. Retrieved March 23, 2024, from https://www.scribbr.com/research-process/research-proposal/
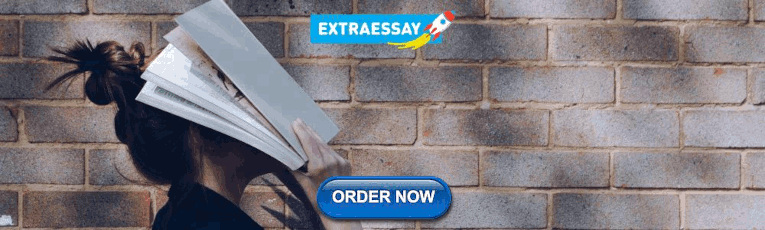
Is this article helpful?
Shona McCombes
Other students also liked, how to write a problem statement | guide & examples, writing strong research questions | criteria & examples, how to write a literature review | guide, examples, & templates, "i thought ai proofreading was useless but..".
I've been using Scribbr for years now and I know it's a service that won't disappoint. It does a good job spotting mistakes”

Library for Staff: Types of Research outputs
- Research Skills and Resources
- Information Literacy and the Library This link opens in a new window
- The Wintec Research Archive
- Copyright for Staff
- Publishing at Wintec
- Liaison Librarians
- Wintec Library Collection Policy
- Reciprocal agreement UOW & DHB
- Teaching Books
- Staff guidelines for APA assesment
- Adult and Tertiary Education
Types of Research outputs
- Last Updated: Mar 12, 2024 7:29 AM
- URL: https://libguides.wintec.ac.nz/staff

An official website of the United States government
The .gov means it’s official. Federal government websites often end in .gov or .mil. Before sharing sensitive information, make sure you’re on a federal government site.
The site is secure. The https:// ensures that you are connecting to the official website and that any information you provide is encrypted and transmitted securely.
- Publications
- Account settings
Preview improvements coming to the PMC website in October 2024. Learn More or Try it out now .
- Advanced Search
- Journal List
- Am J Pharm Educ
- v.74(8); 2010 Oct 11
Presenting and Evaluating Qualitative Research
The purpose of this paper is to help authors to think about ways to present qualitative research papers in the American Journal of Pharmaceutical Education . It also discusses methods for reviewers to assess the rigour, quality, and usefulness of qualitative research. Examples of different ways to present data from interviews, observations, and focus groups are included. The paper concludes with guidance for publishing qualitative research and a checklist for authors and reviewers.
INTRODUCTION
Policy and practice decisions, including those in education, increasingly are informed by findings from qualitative as well as quantitative research. Qualitative research is useful to policymakers because it often describes the settings in which policies will be implemented. Qualitative research is also useful to both pharmacy practitioners and pharmacy academics who are involved in researching educational issues in both universities and practice and in developing teaching and learning.
Qualitative research involves the collection, analysis, and interpretation of data that are not easily reduced to numbers. These data relate to the social world and the concepts and behaviors of people within it. Qualitative research can be found in all social sciences and in the applied fields that derive from them, for example, research in health services, nursing, and pharmacy. 1 It looks at X in terms of how X varies in different circumstances rather than how big is X or how many Xs are there? 2 Textbooks often subdivide research into qualitative and quantitative approaches, furthering the common assumption that there are fundamental differences between the 2 approaches. With pharmacy educators who have been trained in the natural and clinical sciences, there is often a tendency to embrace quantitative research, perhaps due to familiarity. A growing consensus is emerging that sees both qualitative and quantitative approaches as useful to answering research questions and understanding the world. Increasingly mixed methods research is being carried out where the researcher explicitly combines the quantitative and qualitative aspects of the study. 3 , 4
Like healthcare, education involves complex human interactions that can rarely be studied or explained in simple terms. Complex educational situations demand complex understanding; thus, the scope of educational research can be extended by the use of qualitative methods. Qualitative research can sometimes provide a better understanding of the nature of educational problems and thus add to insights into teaching and learning in a number of contexts. For example, at the University of Nottingham, we conducted in-depth interviews with pharmacists to determine their perceptions of continuing professional development and who had influenced their learning. We also have used a case study approach using observation of practice and in-depth interviews to explore physiotherapists' views of influences on their leaning in practice. We have conducted in-depth interviews with a variety of stakeholders in Malawi, Africa, to explore the issues surrounding pharmacy academic capacity building. A colleague has interviewed and conducted focus groups with students to explore cultural issues as part of a joint Nottingham-Malaysia pharmacy degree program. Another colleague has interviewed pharmacists and patients regarding their expectations before and after clinic appointments and then observed pharmacist-patient communication in clinics and assessed it using the Calgary Cambridge model in order to develop recommendations for communication skills training. 5 We have also performed documentary analysis on curriculum data to compare pharmacist and nurse supplementary prescribing courses in the United Kingdom.
It is important to choose the most appropriate methods for what is being investigated. Qualitative research is not appropriate to answer every research question and researchers need to think carefully about their objectives. Do they wish to study a particular phenomenon in depth (eg, students' perceptions of studying in a different culture)? Or are they more interested in making standardized comparisons and accounting for variance (eg, examining differences in examination grades after changing the way the content of a module is taught). Clearly a quantitative approach would be more appropriate in the last example. As with any research project, a clear research objective has to be identified to know which methods should be applied.
Types of qualitative data include:
- Audio recordings and transcripts from in-depth or semi-structured interviews
- Structured interview questionnaires containing substantial open comments including a substantial number of responses to open comment items.
- Audio recordings and transcripts from focus group sessions.
- Field notes (notes taken by the researcher while in the field [setting] being studied)
- Video recordings (eg, lecture delivery, class assignments, laboratory performance)
- Case study notes
- Documents (reports, meeting minutes, e-mails)
- Diaries, video diaries
- Observation notes
- Press clippings
- Photographs
RIGOUR IN QUALITATIVE RESEARCH
Qualitative research is often criticized as biased, small scale, anecdotal, and/or lacking rigor; however, when it is carried out properly it is unbiased, in depth, valid, reliable, credible and rigorous. In qualitative research, there needs to be a way of assessing the “extent to which claims are supported by convincing evidence.” 1 Although the terms reliability and validity traditionally have been associated with quantitative research, increasingly they are being seen as important concepts in qualitative research as well. Examining the data for reliability and validity assesses both the objectivity and credibility of the research. Validity relates to the honesty and genuineness of the research data, while reliability relates to the reproducibility and stability of the data.
The validity of research findings refers to the extent to which the findings are an accurate representation of the phenomena they are intended to represent. The reliability of a study refers to the reproducibility of the findings. Validity can be substantiated by a number of techniques including triangulation use of contradictory evidence, respondent validation, and constant comparison. Triangulation is using 2 or more methods to study the same phenomenon. Contradictory evidence, often known as deviant cases, must be sought out, examined, and accounted for in the analysis to ensure that researcher bias does not interfere with or alter their perception of the data and any insights offered. Respondent validation, which is allowing participants to read through the data and analyses and provide feedback on the researchers' interpretations of their responses, provides researchers with a method of checking for inconsistencies, challenges the researchers' assumptions, and provides them with an opportunity to re-analyze their data. The use of constant comparison means that one piece of data (for example, an interview) is compared with previous data and not considered on its own, enabling researchers to treat the data as a whole rather than fragmenting it. Constant comparison also enables the researcher to identify emerging/unanticipated themes within the research project.
STRENGTHS AND LIMITATIONS OF QUALITATIVE RESEARCH
Qualitative researchers have been criticized for overusing interviews and focus groups at the expense of other methods such as ethnography, observation, documentary analysis, case studies, and conversational analysis. Qualitative research has numerous strengths when properly conducted.
Strengths of Qualitative Research
- Issues can be examined in detail and in depth.
- Interviews are not restricted to specific questions and can be guided/redirected by the researcher in real time.
- The research framework and direction can be quickly revised as new information emerges.
- The data based on human experience that is obtained is powerful and sometimes more compelling than quantitative data.
- Subtleties and complexities about the research subjects and/or topic are discovered that are often missed by more positivistic enquiries.
- Data usually are collected from a few cases or individuals so findings cannot be generalized to a larger population. Findings can however be transferable to another setting.
Limitations of Qualitative Research
- Research quality is heavily dependent on the individual skills of the researcher and more easily influenced by the researcher's personal biases and idiosyncrasies.
- Rigor is more difficult to maintain, assess, and demonstrate.
- The volume of data makes analysis and interpretation time consuming.
- It is sometimes not as well understood and accepted as quantitative research within the scientific community
- The researcher's presence during data gathering, which is often unavoidable in qualitative research, can affect the subjects' responses.
- Issues of anonymity and confidentiality can present problems when presenting findings
- Findings can be more difficult and time consuming to characterize in a visual way.
PRESENTATION OF QUALITATIVE RESEARCH FINDINGS
The following extracts are examples of how qualitative data might be presented:
Data From an Interview.
The following is an example of how to present and discuss a quote from an interview.
The researcher should select quotes that are poignant and/or most representative of the research findings. Including large portions of an interview in a research paper is not necessary and often tedious for the reader. The setting and speakers should be established in the text at the end of the quote.
The student describes how he had used deep learning in a dispensing module. He was able to draw on learning from a previous module, “I found that while using the e learning programme I was able to apply the knowledge and skills that I had gained in last year's diseases and goals of treatment module.” (interviewee 22, male)
This is an excerpt from an article on curriculum reform that used interviews 5 :
The first question was, “Without the accreditation mandate, how much of this curriculum reform would have been attempted?” According to respondents, accreditation played a significant role in prompting the broad-based curricular change, and their comments revealed a nuanced view. Most indicated that the change would likely have occurred even without the mandate from the accreditation process: “It reflects where the profession wants to be … training a professional who wants to take on more responsibility.” However, they also commented that “if it were not mandated, it could have been a very difficult road.” Or it “would have happened, but much later.” The change would more likely have been incremental, “evolutionary,” or far more limited in its scope. “Accreditation tipped the balance” was the way one person phrased it. “Nobody got serious until the accrediting body said it would no longer accredit programs that did not change.”
Data From Observations
The following example is some data taken from observation of pharmacist patient consultations using the Calgary Cambridge guide. 6 , 7 The data are first presented and a discussion follows:
Pharmacist: We will soon be starting a stop smoking clinic. Patient: Is the interview over now? Pharmacist: No this is part of it. (Laughs) You can't tell me to bog off (sic) yet. (pause) We will be starting a stop smoking service here, Patient: Yes. Pharmacist: with one-to-one and we will be able to help you or try to help you. If you want it. In this example, the pharmacist has picked up from the patient's reaction to the stop smoking clinic that she is not receptive to advice about giving up smoking at this time; in fact she would rather end the consultation. The pharmacist draws on his prior relationship with the patient and makes use of a joke to lighten the tone. He feels his message is important enough to persevere but he presents the information in a succinct and non-pressurised way. His final comment of “If you want it” is important as this makes it clear that he is not putting any pressure on the patient to take up this offer. This extract shows that some patient cues were picked up, and appropriately dealt with, but this was not the case in all examples.
Data From Focus Groups
This excerpt from a study involving 11 focus groups illustrates how findings are presented using representative quotes from focus group participants. 8
Those pharmacists who were initially familiar with CPD endorsed the model for their peers, and suggested it had made a meaningful difference in the way they viewed their own practice. In virtually all focus groups sessions, pharmacists familiar with and supportive of the CPD paradigm had worked in collaborative practice environments such as hospital pharmacy practice. For these pharmacists, the major advantage of CPD was the linking of workplace learning with continuous education. One pharmacist stated, “It's amazing how much I have to learn every day, when I work as a pharmacist. With [the learning portfolio] it helps to show how much learning we all do, every day. It's kind of satisfying to look it over and see how much you accomplish.” Within many of the learning portfolio-sharing sessions, debates emerged regarding the true value of traditional continuing education and its outcome in changing an individual's practice. While participants appreciated the opportunity for social and professional networking inherent in some forms of traditional CE, most eventually conceded that the academic value of most CE programming was limited by the lack of a systematic process for following-up and implementing new learning in the workplace. “Well it's nice to go to these [continuing education] events, but really, I don't know how useful they are. You go, you sit, you listen, but then, well I at least forget.”
The following is an extract from a focus group (conducted by the author) with first-year pharmacy students about community placements. It illustrates how focus groups provide a chance for participants to discuss issues on which they might disagree.
Interviewer: So you are saying that you would prefer health related placements? Student 1: Not exactly so long as I could be developing my communication skill. Student 2: Yes but I still think the more health related the placement is the more I'll gain from it. Student 3: I disagree because other people related skills are useful and you may learn those from taking part in a community project like building a garden. Interviewer: So would you prefer a mixture of health and non health related community placements?
GUIDANCE FOR PUBLISHING QUALITATIVE RESEARCH
Qualitative research is becoming increasingly accepted and published in pharmacy and medical journals. Some journals and publishers have guidelines for presenting qualitative research, for example, the British Medical Journal 9 and Biomedcentral . 10 Medical Education published a useful series of articles on qualitative research. 11 Some of the important issues that should be considered by authors, reviewers and editors when publishing qualitative research are discussed below.
Introduction.
A good introduction provides a brief overview of the manuscript, including the research question and a statement justifying the research question and the reasons for using qualitative research methods. This section also should provide background information, including relevant literature from pharmacy, medicine, and other health professions, as well as literature from the field of education that addresses similar issues. Any specific educational or research terminology used in the manuscript should be defined in the introduction.
The methods section should clearly state and justify why the particular method, for example, face to face semistructured interviews, was chosen. The method should be outlined and illustrated with examples such as the interview questions, focusing exercises, observation criteria, etc. The criteria for selecting the study participants should then be explained and justified. The way in which the participants were recruited and by whom also must be stated. A brief explanation/description should be included of those who were invited to participate but chose not to. It is important to consider “fair dealing,” ie, whether the research design explicitly incorporates a wide range of different perspectives so that the viewpoint of 1 group is never presented as if it represents the sole truth about any situation. The process by which ethical and or research/institutional governance approval was obtained should be described and cited.
The study sample and the research setting should be described. Sampling differs between qualitative and quantitative studies. In quantitative survey studies, it is important to select probability samples so that statistics can be used to provide generalizations to the population from which the sample was drawn. Qualitative research necessitates having a small sample because of the detailed and intensive work required for the study. So sample sizes are not calculated using mathematical rules and probability statistics are not applied. Instead qualitative researchers should describe their sample in terms of characteristics and relevance to the wider population. Purposive sampling is common in qualitative research. Particular individuals are chosen with characteristics relevant to the study who are thought will be most informative. Purposive sampling also may be used to produce maximum variation within a sample. Participants being chosen based for example, on year of study, gender, place of work, etc. Representative samples also may be used, for example, 20 students from each of 6 schools of pharmacy. Convenience samples involve the researcher choosing those who are either most accessible or most willing to take part. This may be fine for exploratory studies; however, this form of sampling may be biased and unrepresentative of the population in question. Theoretical sampling uses insights gained from previous research to inform sample selection for a new study. The method for gaining informed consent from the participants should be described, as well as how anonymity and confidentiality of subjects were guaranteed. The method of recording, eg, audio or video recording, should be noted, along with procedures used for transcribing the data.
Data Analysis.
A description of how the data were analyzed also should be included. Was computer-aided qualitative data analysis software such as NVivo (QSR International, Cambridge, MA) used? Arrival at “data saturation” or the end of data collection should then be described and justified. A good rule when considering how much information to include is that readers should have been given enough information to be able to carry out similar research themselves.
One of the strengths of qualitative research is the recognition that data must always be understood in relation to the context of their production. 1 The analytical approach taken should be described in detail and theoretically justified in light of the research question. If the analysis was repeated by more than 1 researcher to ensure reliability or trustworthiness, this should be stated and methods of resolving any disagreements clearly described. Some researchers ask participants to check the data. If this was done, it should be fully discussed in the paper.
An adequate account of how the findings were produced should be included A description of how the themes and concepts were derived from the data also should be included. Was an inductive or deductive process used? The analysis should not be limited to just those issues that the researcher thinks are important, anticipated themes, but also consider issues that participants raised, ie, emergent themes. Qualitative researchers must be open regarding the data analysis and provide evidence of their thinking, for example, were alternative explanations for the data considered and dismissed, and if so, why were they dismissed? It also is important to present outlying or negative/deviant cases that did not fit with the central interpretation.
The interpretation should usually be grounded in interviewees or respondents' contributions and may be semi-quantified, if this is possible or appropriate, for example, “Half of the respondents said …” “The majority said …” “Three said…” Readers should be presented with data that enable them to “see what the researcher is talking about.” 1 Sufficient data should be presented to allow the reader to clearly see the relationship between the data and the interpretation of the data. Qualitative data conventionally are presented by using illustrative quotes. Quotes are “raw data” and should be compiled and analyzed, not just listed. There should be an explanation of how the quotes were chosen and how they are labeled. For example, have pseudonyms been given to each respondent or are the respondents identified using codes, and if so, how? It is important for the reader to be able to see that a range of participants have contributed to the data and that not all the quotes are drawn from 1 or 2 individuals. There is a tendency for authors to overuse quotes and for papers to be dominated by a series of long quotes with little analysis or discussion. This should be avoided.
Participants do not always state the truth and may say what they think the interviewer wishes to hear. A good qualitative researcher should not only examine what people say but also consider how they structured their responses and how they talked about the subject being discussed, for example, the person's emotions, tone, nonverbal communication, etc. If the research was triangulated with other qualitative or quantitative data, this should be discussed.
Discussion.
The findings should be presented in the context of any similar previous research and or theories. A discussion of the existing literature and how this present research contributes to the area should be included. A consideration must also be made about how transferrable the research would be to other settings. Any particular strengths and limitations of the research also should be discussed. It is common practice to include some discussion within the results section of qualitative research and follow with a concluding discussion.
The author also should reflect on their own influence on the data, including a consideration of how the researcher(s) may have introduced bias to the results. The researcher should critically examine their own influence on the design and development of the research, as well as on data collection and interpretation of the data, eg, were they an experienced teacher who researched teaching methods? If so, they should discuss how this might have influenced their interpretation of the results.
Conclusion.
The conclusion should summarize the main findings from the study and emphasize what the study adds to knowledge in the area being studied. Mays and Pope suggest the researcher ask the following 3 questions to determine whether the conclusions of a qualitative study are valid 12 : How well does this analysis explain why people behave in the way they do? How comprehensible would this explanation be to a thoughtful participant in the setting? How well does the explanation cohere with what we already know?
CHECKLIST FOR QUALITATIVE PAPERS
This paper establishes criteria for judging the quality of qualitative research. It provides guidance for authors and reviewers to prepare and review qualitative research papers for the American Journal of Pharmaceutical Education . A checklist is provided in Appendix 1 to assist both authors and reviewers of qualitative data.
ACKNOWLEDGEMENTS
Thank you to the 3 reviewers whose ideas helped me to shape this paper.
Appendix 1. Checklist for authors and reviewers of qualitative research.
Introduction
- □ Research question is clearly stated.
- □ Research question is justified and related to the existing knowledge base (empirical research, theory, policy).
- □ Any specific research or educational terminology used later in manuscript is defined.
- □ The process by which ethical and or research/institutional governance approval was obtained is described and cited.
- □ Reason for choosing particular research method is stated.
- □ Criteria for selecting study participants are explained and justified.
- □ Recruitment methods are explicitly stated.
- □ Details of who chose not to participate and why are given.
- □ Study sample and research setting used are described.
- □ Method for gaining informed consent from the participants is described.
- □ Maintenance/Preservation of subject anonymity and confidentiality is described.
- □ Method of recording data (eg, audio or video recording) and procedures for transcribing data are described.
- □ Methods are outlined and examples given (eg, interview guide).
- □ Decision to stop data collection is described and justified.
- □ Data analysis and verification are described, including by whom they were performed.
- □ Methods for identifying/extrapolating themes and concepts from the data are discussed.
- □ Sufficient data are presented to allow a reader to assess whether or not the interpretation is supported by the data.
- □ Outlying or negative/deviant cases that do not fit with the central interpretation are presented.
- □ Transferability of research findings to other settings is discussed.
- □ Findings are presented in the context of any similar previous research and social theories.
- □ Discussion often is incorporated into the results in qualitative papers.
- □ A discussion of the existing literature and how this present research contributes to the area is included.
- □ Any particular strengths and limitations of the research are discussed.
- □ Reflection of the influence of the researcher(s) on the data, including a consideration of how the researcher(s) may have introduced bias to the results is included.
Conclusions
- □ The conclusion states the main finings of the study and emphasizes what the study adds to knowledge in the subject area.

- Schools & departments

Research output
From peer-reviewed papers to book chapters, monographs and conference proceedings
There are three ways to add your research outputs to Pure. This page refers to creating records from the templates that are available in Pure.
You can also import your research outputs from an online source or from a BibTeX or RIS file .
There are a variety of research outputs and Pure has 47 sub-type templates that can be used. These are listed in the table below. Please refer to the guide for each type when adding research output records.
If you are not sure which template to use, please ask your local Pure contact for guidance. They will also be able to advise you on Open Access and/or REF-related requirements.
Please note that the research output records that you add will be validated by your College or School research administrator. Only validated research output record will be displayed on Edinburgh Research Explorer.
External Persons Affiliations
The external persons affiliations on research output records in Pure populate the research network map on the Pure Portal. If there are no external persons affiliations on a research output record, the network map on profile pages will not include that research output record and may appear empty.
Please follow the steps below to add external persons affiliations to the research output records.

Claim content
It is also possible that there is already a research output record in Pure for your research output. You can ask to be added to this existing record by claiming the record. Please use the guide below to claim content.
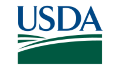
Data from polishCLR: Example input genome assemblies
[ NOTE - Data files added 2022-11-01:
- Test long reads - test.1.filtered.bam_.gz
- Test short reads R1 - testpolish_R1.fastq
- Test short reads R2 - testpolish_R2.fastq
- Chromosome 30 of H. zea - GCF_022581195.2_ilHelZeax1.1_chr30.fasta ]
In order to produce the best possible de novo , chromosome-scale genome assembly from error prone Pacific BioSciences continuous long reads (CLR) reads, we developed a publicly available, flexible and reproducible workflow that is containerized so it can be run on any conventional HPC, called polishCLR. This dataset provides example input primary contig assemblies to test and reproduce the demonstrated utility of our workflow.
The polishCLR workflow can be easily initiated from three input cases: Case 1: An unresolved primary assembly with associated contigs, the output of FALCON 2-asm: p_ctg.fasta and a_ctg.fasta Case 2: A haplotype-resolved but unpolished set, the output of FALCON-Unzip 3-unzip: all_p_ctg.fasta and all_h_ctg.fasta Case 3: A haplotype-resolved, CLR long-read, Arrow-polished set of primary and alternate contigs, the output of FALCON-Unzip 4-polish: cns_p_ctg.fasta and cns_h_ctg.fasta.
These example data are the input contigs assemblies for the pest Helicoverpa zea . These contigs are built from 49.89 Gb of raw Pacific Biosciences (PacBio) CLR data generated from a single H. zea HzStark_Cry1AcR strain male.
Adult H. zea were collected near the USDA-ARS Genetics and Sustainability Agricultural Research Unit, Starkville, MS, USA in 2011, and transported to and maintained in a colony at the USDA Southern Insect Management Unit (SIMRU), Stoneville, MS, USA as described previously. Larvae were selected on a diagnostic dose of 2.0 μg ml-1 purified Cry1Ac, and survivors used to create the strain, HzStark_Cry1AcR. HzStark_Cry1AcR was back-crossed every 5 generations to a susceptible line maintained at USDA-ARS SIMRU.
A single male pupa (homogametic, ZZ sex chromosome) from HzStark_Cry1AcR was dissected laterally into eight ~20 μg sections. High molecular weight DNA was extracted. PacBio libraries were generated from unsheared DNA using a SMRTbell Express Template Prep Kit 2.0 (Pacific Biosciences, Menlo Park, CA, USA), and 20 hour run time movies generated on a single SMRT Cell 1M v3 using the Sequel I system (Pacific Biosciences).
The raw continuous long read (CLR) subread bam files were converted to fastq format using bamtools v. 2.5.1 (Barnett et al. 2011), then used as input for the Falcon assembler (Chin et al. 2016) using the pb-assembly conda environment v. 0.0.8.1 (Pacific Biosciences; default parameters). Falcon-Unzip created primary and alternate contigs with one round of haplotype-aware polishing by Arrow (Pacific Biosciences).
Resource Title: Associated assembly contigs output from FALCON/2-asm-falcon.
File Name: a_ctg_all.fasta
Resource Title: Primary assembly contigs output from FALCON/2-asm-falcon.
File Name: p_ctg.fasta
Resource Title: Alternate haplotype assembly contigs output from FALCON Unzip 3-unzip.
File Name: all_h_ctg.fasta
Resource Title: Primary assembly contigs output from FALCON Unzip 3-unzip.
File Name: all_p_ctg.fasta
Resource Title: Alternate assembly contigs output from FALCON Unzip 4-polish.
File Name: cns_h_ctg.fasta
Resource Title: Primary assembly contigs output from FALCON Unzip 4-polish.
File Name: cns_p ctg.fasta
Resource Title: Test long reads.
File Name: test.1.filtered.bam .gz
Resource Description: For testing the pipeline, long reads that map to H. zea chromosome 30
Resource Title: Test short reads R1.
File Name: testpolish_R1.fastq
Resource Description: Short reads aligned to Chromosome 30 of H. zea
Resource Title: Test short reads R2.
File Name: testpolish_R2.fastq
Resource Description: Reverse pair (R2) short reads aligned to Chromosome 30 of H. zea
Resource Title: Chromosome 30 of H. zea.
File Name: GCF_022581195.2_ilHelZeax1.1_chr30.fasta
USDA-ARS: 5030-22000-019-00-D
Usda-ars: 0500-00093-001-00-d, data contact name, data contact email, intended use, temporal extent start date.
- Not specified
Geographic Coverage
Geographic location - description, iso topic category, national agricultural library thesaurus terms, omb bureau code.
- 005:18 - Agricultural Research Service
OMB Program Code
- 005:040 - National Research
ARS National Program Number
Pending citation, public access level, preferred dataset citation, usage metrics.
- Genomics and transcriptomics

- CBSSports.com
- Fanatics Sportsbook
- CBS Sports Home
- NCAA Tournament
- W. Tournament
- Champions League
- Motor Sports
- High School
- Horse Racing
Men's Brackets
Women's Brackets
Fantasy Baseball
Fantasy football, football pick'em, college pick'em, fantasy basketball, fantasy hockey, franchise games, 24/7 sports news network.
- CBS Sports Golazo Network
- March Madness Live
- PGA Tour on CBS
- UEFA Champions League
- UEFA Europa League
- Italian Serie A
- Watch CBS Sports Network
- TV Shows & Listings
The Early Edge
A Daily SportsLine Betting Podcast
With the First Pick
NFL Draft is coming up!
- Podcasts Home
- Eye On College Basketball
- The First Cut Golf
- NFL Pick Six
- Cover 3 College Football
- Fantasy Football Today
- Morning Kombat
- My Teams Organize / See All Teams Help Account Settings Log Out
2024 NCAA Tournament bracket predictions: March Madness expert picks, favorites to win, winners, upsets
Our experts have filled out their brackets, so check who they predict will be cutting down the nets.
We're only a few days into the 2024 NCAA Tournament but already we've ripped through dozens of games and shipped dozens of teams home as the field has steadily dwindled over the course of the first and second round. What began with 68 teams will be down to 16 by the end of the weekend, and the field – as well as our picks – will start to take some real shape.
That's mostly a good thing thus far for our experts who posted their brackets publicly, as all their title picks survived the first round and are still in the mix to win the NCAA championship. That's mostly a good thing for you, too, because if you've followed along in this space this postseason, we've been hot picking teams and done nicely arming you with knowledge in your mind to help stuff money in your pocket.
Our picks from each of our experts are in the space below to fade or follow as you wish. All of us (except one) picked a No. 1 seed to win the ship, so the ledges we went out on aren't terribly unstable, but after watching games all season we have a good feel for how things might go and have done well predicting so far how things will play out in the early rounds.
OK, let's dive into the good stuff: The brackets. ...
2024 NCAA Tournament bracket predictions
Click each bracket to enlarge.
Gary Parrish
Watching UConn become the first back-to-back national champion since Florida in 2006 and 2007 would be a blast. And let the record show that the Huskies are the betting-market favorites. So I realize picking against them might prove dumb. But, that acknowledged, I'm going to continue to do what I've been doing most of this season and put my faith in the Boilermakers. Wouldn't that be a great story -- Purdue winning the 2024 NCAA Tournament after losing to a No. 16 seed in the opening round of the 2023 NCAA Tournament? Zach Edey holding the championship trophy as a two-time National Player of the Year? Matt Painter shedding his label as the best coach yet to make a Final Four by becoming the first coach to take Purdue to the final weekend of the season since 1980? It's all such good stuff. Just getting to the Final Four will be challenging considering Tennessee, Creighton and Kansas are also in the Midwest Region. But I'm still taking the Boilermakers to make it to Arizona. And then, once they get there, I think they'll win two more games and cut nets on the second Monday in April.
Matt Norlander
A locomotive screaming down the tracks. The 31-3 reigning national champions enter this NCAA Tournament as the strongest team with the best chance to repeat of any squad since Florida in 2007. Dan Hurley's Huskies are led by All-American guard Tristen Newton (15.2 ppg, 7.0 rpg, 6.0 apg), who holds the school record for triple-doubles. In the middle is 7-foot-2 "Cling Kong," Donvan Clingan, a menace of a defender and the type of player you can't simulate in practice. The Huskies boast the nation's most efficient offense (126.6 adjusted points per 100 possessions, via KenPom.com) and overwhelm teams in a variety of ways. Sophomore Alex Karaban (39.5%) and senior Cam Spencer (44.4%) are both outstanding 3-point shooters. The Huskies have been beaten by Kansas, Seton Hall and Creighton, but all of those were road games, and there are no more road games left this season. UConn will try to become the fourth No. 1 overall seed to win the national title, joining 2007 Florida, 2012 Kentucky and 2013 Louisville.
The antagonistic side of me initially picked Purdue over UConn in the title game. But I sat and thought about it and couldn't make any reasonable case to pick any team other than UConn as champion. Of course, that doesn't guarantee the Huskies win it all and become the first repeat champs since Florida in 2007. There's a lot that can happen in the next few weeks. But they have the electric offense, the guard depth, the size down low, the shooting [takes breath] .. the passing and the pizzazz of a team that's best in the country and knows it. Every top team in this field has a high level at which they can play but no one has a top gear like UConn.
Get every pick, every play, every upset and fill out your bracket with our help! Visit SportsLine now to see which teams will make and break your bracket, and see who will cut down the nets , all from the model that nailed a whopping 20 first-round upsets by double-digit seeds.
Purdue is set for redemption after an embarrassing 2023 loss to No. 16 seed Fairleigh Dickinson in the first round. This time around, the Boilermakers are a much better 3-point shooting team and have a more favorable path than No. 1 overall seed UConn. The Huskies were the most dominant team leading up to the Big Dance the East Region bracket is filled with peril.
This is not the Purdue you have seen the last few years. Braden Smith has made a big jump from last season to this one. Fletcher Loyer is better. Lance Jones gives Purdue defense, shooting and another ball handler. And Zach Edey is better too. This is a team on a mission. This is the year they accomplish it.
Dennis Dodd
What is there not to like? The Heels won the ACC regular season. They beat Tennessee and swept Duke. RJ Davis is an elite guard and ACC Player of the Year. Hubert Davis has settled in after going to the national championship game in his first season and missing the tournament in his second. This is his best team. There will be/and always is pressure to win it all.
Armando Bacot is not as dominating as previous. Harrison Ingram (Stanford) and Cormac Ryan (Notre Dame) have been big additions in the portal. The West Region is friendly, assuming here that Alabama and Michigan State don't get in the way before the regional in L.A. An interesting regional final against Arizona looms. In the end, sometimes you go with chalk. UNC has been to the most Final Fours (21) and No. 1 seeds (18) all-time. It is tied with Kentucky for the most tournament wins ever (131). This is what the Heels do.
Chip Patterson
The selection committee set up plenty of stumbling blocks for the reigning champs, placing what I believe to be the best No. 1 seed, the best No. 2 seed (Iowa State), the best No. 3 seed (Illinois) and the best No. 4 seed (Auburn) in the Huskies bracket. And if accomplishing a historic feat like the first back-to-back title runs since 2007 is going to require that kind of epic journey, UConn has every skill and tool needed to make it back to the top of the mountain. UConn can win in all different ways, overwhelming teams with their offense in high-scoring track meets or out-executing the opponent in low-possession grinders, and it has a handful of key contributors who could each step up as needed during a title run.
Cameron Salerno
Defense wins championships. That is part of the reason why I'm picking Houston to win it all. The Cougars have the top-ranked scoring defense in the country and terrific guard play on offense to complement it. Jamal Shead is arguably the best point guard in the nation, and J'wan Roberts is an X-Factor on both ends of the floor. Houston's path to the Final Four is favorable. The Cougars weren't able to reach the Final Four in their home state last spring, but this will be the year they run the table and win their first national championship in program history.
Our Latest College Basketball Stories
Printable 2024 NCAA Women's Tournament bracket
Wajih albaroudi • 1 min read.
Winners, losers: Tennessee advances, Kansas stumbles
Cameron salerno • 7 min read.
NIT: Seton Hall, Ohio State advance to quarterfinals
Cameron salerno • 1 min read.
How to watch today's second-round NCAA women's games
Isabel gonzalez • 2 min read.
2024 NCAA Tournament schedule, scores by region
Cameron salerno • 3 min read.
2024 NCAA Tournament: How to watch live, TV tip times
Matt norlander • 3 min read.
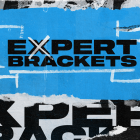
Expert brackets: Predictions for NCAA Tournament 2024
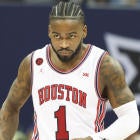
Expert picks: Today's March Madness games
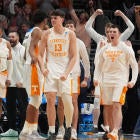
Tennessee shines; KU looks to next year
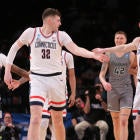
Storylines: Will No. 1s be challenged?
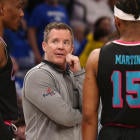
Michigan hires FAU's May to replace Howard as coach
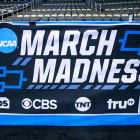
NCAA Tournament: March Madness scores, schedule
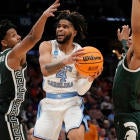
UNC punches ticket to Sweet 16 with win over Spartans
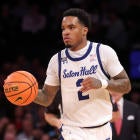
Transfer rankings: Top players in portal
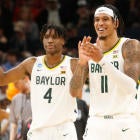
Baylor's use of freshman could work for other teams
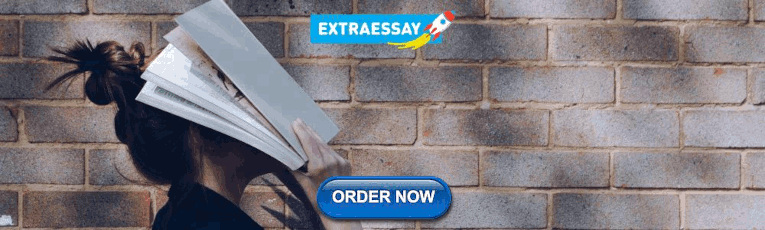
IMAGES
VIDEO
COMMENTS
Digital scholarship is a research output that significantly incorporates or relies on digital methodologies, authoring, presentation, and presentation. Digital scholarship often complements and adds to more traditional research outputs, and may be presented in a multimedia format. Some examples include mapping projects; multimodal projects that ...
Your results should always be written in the past tense. While the length of this section depends on how much data you collected and analyzed, it should be written as concisely as possible. Only include results that are directly relevant to answering your research questions. Avoid speculative or interpretative words like "appears" or ...
Originality will be understood as the extent to which the output makes an important and innovative contribution to understanding and knowledge in the field.Research outputs that demonstrate originality may do one or more of the following: produce and interpret new empirical findings or new material; engage with new and/or complex problems; develop innovative research methods, methodologies and ...
Publication data can be used to craft a compelling narrative about your impact. See Quantifying the Impact of My Publications for examples of how to tell a story using publication data. Another tip is to utilize various means of disseminating your research. See Strategies for Enhancing Research Impact for more information.
1 Definition. An output is an outcome of research and can take many forms. Research Outputs must meet the definition of Research. The Excellence in Research for Australia assessment defines the following eligible research output types: books—authored research. chapters in research books—authored research. journal articles—refereed ...
The list is vast and may include: · PhD Thesis — typically a ~40,000 word (must be <100,000 @ IC) document that will be examined at the end of your programme. · Papers — a series of disseminated documents that convey and test new ideas to the wider scientific community. These have the rigour of 'peer review' and are the 'gold ...
For example, quantifying research output as the number of publications encourages researchers to publish as many small studies as possible, whereas the interests of science might be better served by a few larger, more integrated reports (e.g., Sternberg & Sternberg 2010). Nonetheless, models for research payoffs can be helpful by clarifying the ...
Mills-Schofield argues outcomes in contrast to outputs are the "difference made by the outputs" ( 2012, n.p.). She cites examples such as better traffic flow, shorter travel times and few accidents as outcomes of a new highway, whereby the outputs are "project design and the number of highway miles built and repaired" (Mills-Scofield ...
The changing nature of research outputs has the potential to affect a wide range of organisations and people in the sector. Joined-up thinking and action could help. As the diversity of research outputs increases, we have to make choices. We can either be reactive, responding to needs and challenges as they emerge, or proactive, to help shape ...
Writing about the expected results of your study in your proposal is a good idea as it can help to establish the significance of your study. On the basis of the problems you have identified and your proposed methodology, you can describe what results can be expected from your research. It's not possible for you to predict the exact outcome of ...
INTRODUCTION. Scientific research is usually initiated by posing evidenced-based research questions which are then explicitly restated as hypotheses.1,2 The hypotheses provide directions to guide the study, solutions, explanations, and expected results.3,4 Both research questions and hypotheses are essentially formulated based on conventional theories and real-world processes, which allow the ...
Table of contents. Step 1: Write your hypotheses and plan your research design. Step 2: Collect data from a sample. Step 3: Summarize your data with descriptive statistics. Step 4: Test hypotheses or make estimates with inferential statistics.
What counts as Research? Research Funding Landscape; Non-Research; Research related policies. Intellectual Property policy (College login) Intellectual Property policy (Public access) Export Controls. Export Controls explained; Do I need an export licence? Military items; Dual-use items; End-use concerns; Exemptions; Example case studies; How ...
The following six research output categories were captured in quantity and number (count data) and served as the basis for the analysis: publication (peer-reviewed journal article; non-peer-reviewed journal article, monograph, anthology, mass communication, i.e. any kind of publication in mass media, e.g. newspaper article), conference contribution (invited paper, paper, poster), award, patent ...
Research proposal examples. Writing a research proposal can be quite challenging, but a good starting point could be to look at some examples. We've included a few for you below. Example research proposal #1: "A Conceptual Framework for Scheduling Constraint Management".
A creative research/problem-solving output in the form of design drawings, books, models, exhibitions, websites, installations or build works. This can include (but is not limited) to: fashion/textile design. graphic design. interior design. other designs. industrial design. architectural design. multimedia design.
For discovery or generative studies, an output may be a set of frameworks that can be evolved and refined based on further research. Frameworks provide us with a way of thinking about insights. Frameworks can be themes, experience maps, journey maps, user types, personas, service blueprints, illustrative design vision screens, etc.
The purpose of this paper is to help authors to think about ways to present qualitative research papers in the American Journal of Pharmaceutical Education. It also discusses methods for reviewers to assess the rigour, quality, and usefulness of qualitative research. Examples of different ways to present data from interviews, observations, and ...
There are three ways to add your research outputs to Pure. This page refers to creating records from the templates that are available in Pure. You can also import your research outputs from an online source or from a BibTeX or RIS file. There are a variety of research outputs and Pure has 47 sub-type templates that can be used.
Abstract. Purpose: A study was conducted at two merged South African higher education institutions to determine which management factors, as identified in a literature study as well as through a ...
The research projects differ not only qualitatively in their output profile; they also differ quantitatively, so that projects can be ranked according to amount of output. LCs of research output ...
The polishCLR workflow can be easily initiated from three input cases: Case 1: An unresolved primary assembly with associated contigs, the output of FALCON 2-asm: p_ctg.fasta and a_ctg.fasta Case 2: A haplotype-resolved but unpolished set, the output of FALCON-Unzip 3-unzip: all_p_ctg.fasta and all_h_ctg.fasta Case 3: A haplotype-resolved, CLR ...
The Creighton Blue Jays had a successful 2023-24 regular season, going 23-8 before falling in their first Big East Tournament game to Providence.
The antagonistic side of me initially picked Purdue over UConn in the title game. But I sat and thought about it and couldn't make any reasonable case to pick any team other than UConn as champion.