Body Mass Index and Self-Perceived Weight: Are They Associated with Sexual and Relationship Health?
Add to collection, downloadable content.
- March 19, 2019
- Affiliation: Gillings School of Global Public Health, Department of Maternal and Child Health
- This dissertation explores associations between body mass index (BMI) and self-perceived weight during adolescence and two health outcomes during young adulthood: 1) testing positive for one or more of three sexually transmitted diseases (STD) (Chlamydia trachomatis, Neisseria gonorrhoeae, and Trichomonas vaginalis) and 2) reporting intimate partner violence (IPV) victimization. Both papers use National Longitudinal Study of Adolescent Health (Add Health) data from Waves 1, 2, and 3. In the first paper, logistic regression models examined associations between overweight BMI, self-perceived overweight, correct overweight perceptions, and misperceived overweight during adolescence and testing positive for one or more STDs (Chlamydia trachomatis, Neisseria gonorrhoeae, and Trichomonas vaginalis) during young adulthood as determined by urine testing. In unadjusted and adjusted models, adolescent overweight BMI and self-perceived overweight were not associated with young adult STD status among either gender. Adolescent correctly perceived overweight was associated with young adult STD status among males when pooled by race, and among non-Hispanic Black males in unadjusted models. Associations were no longer statistically significant when sociodemographic variables were included in models. Correctly perceived overweight and misperceived overweight were not significantly associated with STD status among females. Future research should explore the associations of interest in this paper with different adolescent body image measures and a wider variety of STD outcomes to determine if associations exist. In the second paper, logistic regression models examined the effects of adolescent overweight BMI and self-perceived overweight on the odds of experiencing IPV victimization during young adulthood. Overweight BMI and self-perceived overweight during adolescence were not significantly associated with IPV victimization during young adulthood among males. Among females, when pooled by adolescent BMI and race, adolescent overweight BMI was associated with increased odds of IPV victimization in the fully adjusted model. When analyses were stratified by race and adolescent BMI, neither adolescent weight concept was significantly associated with IPV victimization among females. Consistent with previous research, longer relationship duration, cohabitation, non-Hispanic Black race, and child abuse were risk factors for young adulthood IPV victimization. Overall, this dissertation contributes to the literature by exploring the effects of adolescent BMI and body image on understudied outcomes.
- Public health
- https://doi.org/10.17615/24wt-kc37
- Dissertation
- In Copyright
- Halpern, Carolyn
- Doctor of Philosophy
- University of North Carolina at Chapel Hill
This work has no parents.
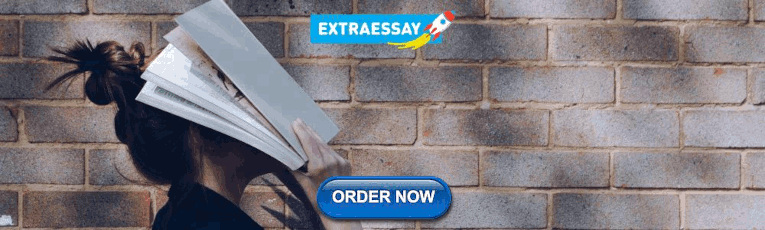
Select type of work
Master's papers.
Deposit your masters paper, project or other capstone work. Theses will be sent to the CDR automatically via ProQuest and do not need to be deposited.
Scholarly Articles and Book Chapters
Deposit a peer-reviewed article or book chapter. If you would like to deposit a poster, presentation, conference paper or white paper, use the “Scholarly Works” deposit form.
Undergraduate Honors Theses
Deposit your senior honors thesis.
Scholarly Journal, Newsletter or Book
Deposit a complete issue of a scholarly journal, newsletter or book. If you would like to deposit an article or book chapter, use the “Scholarly Articles and Book Chapters” deposit option.
Deposit your dataset. Datasets may be associated with an article or deposited separately.
Deposit your 3D objects, audio, images or video.
Poster, Presentation, Protocol or Paper
Deposit scholarly works such as posters, presentations, research protocols, conference papers or white papers. If you would like to deposit a peer-reviewed article or book chapter, use the “Scholarly Articles and Book Chapters” deposit option.
- Open access
- Published: 21 March 2024
Prevalence and risk factors of obesity among undergraduate student population in Ghana: an evaluation study of body composition indices
- Christian Obirikorang 1 , 2 ,
- Evans Asamoah Adu 1 , 2 ,
- Enoch Odame Anto 3 , 4 ,
- Anthony Afum-Adjei Awuah 1 , 2 ,
- Angela Nana Bosowah Fynn 2 ,
- George Osei-Somuah 3 ,
- Patience Nyarkoa Ansong 5 ,
- Alexander Owusu Boakye 1 , 2 ,
- Ivy Ofori-Boadu 3 ,
- Yaa Obirikorang 5 ,
- Austin Gideon Adobasom-Anane 2 ,
- Eric NY Nyarko 6 &
- Lois Balmer 4
BMC Public Health volume 24 , Article number: 877 ( 2024 ) Cite this article
431 Accesses
Metrics details
Obesity is a classified risk factor for several of the world’s leading causes of death. In this study, we combined information contained in body mass index (BMI), total percentage body fat (TPBF) and relative fat mass (RFM) to estimate obesity prevalence and examine the risk factors associated with obesity.
The study recruited 1027 undergraduate students aged between 16 and 25 years using a cross-sectional study design and two-stage stratified random sampling between January and April 2019 from the Kwame Nkrumah University of Science and Technology, Kumasi, Ghana. Demographic, lifestyle, and family history of chronic disease data, were collected using a structured questionnaire. Bioelectrical impedance, along with height, weight, age, and gender, were used to estimate BMI and TPBF. The RFM was calculated using a published equation. The TPBF and RFM ranges were evaluated based on standard BMI thresholds and an informative combined obesity prevalence estimated in a Bayesian framework. Multiple logistic regression analysis was used to evaluate potential risk factors of overweight/obesity.
Concordance between BMI, TPBF and RFM for obesity classification was 84% among female and 82.9% among male students. The Bayesian analysis revealed a combined prevalence means of obesity of 9.4% (95%CI: 6.9-12.2%) among female students and 6.7% (95%CI:4.3-9.5%) among male students. The odds of obesity were increased between 1.8 and 2.5 for females depending on the classification index. A significant increasing trend of obesity was observed with university-level. A family history of obesity was associated with a high estimate of general, central, and high TPBF.
Using multiple adiposity indicators conjointly in a Bayesian framework offers a greater power to examine obesity prevalence. We have applied this and reported high obesity prevalence, especially among female students. University level and family history of obesity were key determinants for obesity among the student population.
Peer Review reports
Introduction
Obesity is a classified risk factor for several of the world’s leading causes of death including cardiovascular diseases, diabetes, and various types of cancers [ 1 ]. It represents the 5th and 6th major level two public health problem among women and men, respectively, leading to the toll of death and disability worldwide [ 2 ]. Obesity stands out among the top leading causes of attributable disability-adjusted life years (DALYs) this is due to the rate of exposure increasing by more than 0.5% per year [ 3 ]. The prevalence of obesity has increased in pandemic dimensions over the past 50 years [ 4 ] with 650 million adults, 340 million adolescents and 39 million children classified as obese [ 5 ]. As the obesity pandemic continues, estimates indicate that approximately 167 million adults and children will become less healthy due to being overweight or obese by 2025 [ 4 ]. Especially in developing countries, the possible implications of obesity on current and future population health and healthcare spending are likely to be enormous [ 6 ].
According to existing nationwide data, the prevalence of being overweight and obese is estimated at 25.4% and 17.1%, respectively [ 7 ]. Among the adult Ghanaian population, obesity is higher in women than men and mimics the level of urbanization [ 7 ]. A meta-analysis involving 29,160 Ghanaian children (≤ 19 years) across sixteen studies reported 8.6% obesity and 10.7% overweight [ 8 ]. There exists a significant number of studies that quantify the burden of obesity in Ghana with a special focus on the general adult population [ 9 , 10 , 11 , 12 , 13 , 14 ], women [ 15 , 16 , 17 , 18 , 19 , 20 , 21 ], school-aged children [ 22 , 23 , 24 ], adolescents [ 25 , 26 , 27 ], healthcare workers [ 28 , 29 , 30 , 31 , 32 ], civil servants [ 33 , 34 , 35 , 36 , 37 , 38 ] and commercial workers [ 39 ]. However, knowledge and data about the experiences of being overweight and obese among young Ghanaian adults are inadequate. Among the few existing studies in Ghana [ 20 , 40 , 41 , 42 ], there is an inconclusive estimate of those who are overweight/obese (4.2-39.3%) among the young adult population. This is due to population non-representativeness, that is, varying lifestyle habits and health-related behaviours of these age groups.
Among undergraduate students, which mainly represent the young adult population group, poor lifestyle habits, including decreased quality of diet and physical activity, sedentary lifestyle, alcohol use and smoking, as well as decreased quality sleep, are associated with obesity [ 42 , 43 , 44 ]. Also, the concurrence of altered eating behaviours (emotional eating, uncontrolled eating, and restrained eating), depression and poor sleep are estimated to be high among undergraduate students, mainly females [ 45 ]. These are fundamental factors driving the obesity epidemic [ 1 ]. Thus, exploring obesity experiences using representative sampling among undergraduate students will allow for the acquisition of information related to young Ghanaian adults. This knowledge will go a long way in informing strategies to combat the obesity epidemic and hopefully, related medical conditions among university students and the general young adult population.
We have mainly relied on the routine use of the body mass index (BMI) as an obesity measure. However, BMI has a limitation in differentiating between body composition and body fat distribution [ 46 , 47 , 48 , 49 ]. Alternative measures, including the bioelectrical impedance analysis (BIA) and BIA-derived body fat indices [ 50 ], like the body adiposity index [ 51 ] and relative fat mass (RFM) [ 52 ], have been proposed. These measures claim to adjust the limitations of BMI and alternatively represent cost-effective indices to appropriately identify individuals with accuracy close to that of underwater weighing [ 53 ] and dual-energy X-ray absorptiometry [ 54 ]. In particular, RFM and total percentage body fat (TPBF) have been validated as being a more accurate measure compared to BMI to estimate whole-body fat percentage, in addition to improving body fat-defined obesity misclassification among different population groups [ 55 ].
In this study, we have combined information contained in body mass index (BMI), TPBF and relative fat mass (RFM) to estimate an informative obesity prevalence. Because there is no single universally accepted measure of adiposity and each index has its drawbacks, we performed an evaluation analysis of waist-to-height derived RFM, corresponding to central fatness and TPBF corresponding to overall adiposity based on the routinely used weight-to-height derived BMI thresholds. By using data from the evaluation analysis assessing concordance and the estimate of measurement properties of TPBF and RFM with BMI, we combined this classification in a Bayesian framework. Thus, we reported an informative obesity prevalence corresponding to central and general adiposity, with much power. Our governing hypothesis was that a combined estimate of obesity in a Bayesian framework does not offer a more representative estimate than commonly used BMI, RFM and TPBF in isolation.
Because it is common in population surveys to have one or multiple measures investigating the same condition, the Bayesian framework has been useful in drawing inferences on disease prevalence and measurement properties while adjusting for the possibility of conditional dependence between several disease measures [ 56 , 57 , 58 ]. In practice, two aspects exist, that can be used to estimate uncertainty and improve the accuracy of population estimate of prevalence. The first is to use the prior information from existing studies, while the second requires the integration of multiple population-based measures into one estimate [ 57 , 58 ]. In our case, we employed the second approach for this study.
Methodology
This was a cross-sectional study undertaken at the Kwame Nkrumah University of Science and Technology (KNUST), Kumasi, between August 2018 and July 2019. All students provided written informed consent for their participation in the study. Ethical approval with reference ID (CHRPE/AP/030/19) was obtained from the Committee on Human Research, Publications and Ethics (CHRPE), School of Medicine and Dentistry, Kwame Nkrumah University of Science & Technology.
Sample design
A two-stage stratified random sampling was used to select 1027 first to fourth-year undergraduate students aged 16–25 years. These students were selected to cover the six Colleges in KNUST including the College of Science (CoS), Art and Built Environment (CABE), Humanities and Social Sciences (CoHSS), Health Sciences (CoHS), Engineering (CoE), and Agric and Natural Resources (CANR). Students who were feverish, bodybuilders or highly trained athletes, and students with osteoporosis or oedema (swelling in the body) were excluded.
The targeted population consisted of undergraduate students’ population from 1st to 4th year of their academic level, across the six colleges of KNUST. The operational definition of a student’s year directly depended on the recruitment dates between January and April 2019. This period represents the second semester where first-year students have spent at least one complete semester in the college. We used a two-stage stratified cluster survey design. The study population and sampling consisted of the entire student population at KNUST (43,757) during the 2018/2019 academic year. Considering the low probability of sampling 5th and 6th -year students, available only for health sciences, were excluded from the sample. The first stage of clustering involved censoring all colleges with probabilities relative to the number of departments. From each selected college, a fixed number of departments was sampled. Eight students (two students, male and female, from levels 1st, 2nd, 3 rd, and 4th year) are sampled at random at the department level.
Sample size
The required sample size to assess overweight/obesity prevalence among students was calculated assuming p = 0.18 [ 41 ], level of acceptable precision d = 0.05 (or ± 5%) at 95% CI corresponds to 227 which relate to 28 departments of 8 students in each. Using analysis of data from a previous study [ 41 ] within the same KNUST student population, the design effect was estimated at 1.9 for overweight/obesity. This was based on 6 clusters with an average of 50 students per cluster (n = 300) and an intra-cluster coefficient of 0.1. Considering these figures and while assuming a college response rate of 90% and individual students response rate at 85%, the actual sample size was estimated to be 83 departments of 8 students each (n = 664) with male and female students having equal proportion of being sampled. In the end, we recruited 1027 participants to increase the power of our estimate. Table 1 illustrates the minimum possible sampling expectations.
The equation for sample size calculation:
\(n=(z^2 \times p(1-p\left)\right(DEEF\left)\right)/\left(\right(j\left)\right(k\left)\right(l\left)\right(d^2\left)\right)\) [ 59 ].
Z was taken at 1.96, j = is the expected response rate as a proportion (0.85 × 0.9); k is the average department size (n = 8). The proportion of the student population accounted for by the targeted of interest (l) was set at 0.8. DEFF is the design effect.
Data collection and anthropometric measurement
A structured questionnaire was used to collect data on socio-demographic characteristics, lifestyle risk factors and family history of obesity, diabetes and hypertension (Table 2 ). socio-demographic data included age, sex, year of study, and college. Lifestyle data included alcohol intake, smoking and exercise history. Height was measured with a portable height rod Stadiometer with students in a straight posture, feet placed together and flat on the ground. Waist circumference (WC) was measured using a tape measure at the point of the umbilicus and maximum gluteal protrusion. Each participant was asked to stand straight on the main unit of the OMRON BF511 Clinically Validated Full Body Composition Monitor with 8 high-precision sensors for hand-to-foot measurement (OMRON HEALTHCARE Co., Ltd.), looking straight, barefooted and with arms horizontally raised holding a display unit, extended at a 90° angle for weight, body fat mass (BFM) and TPBF estimation. The machine conforms to EN60601-1-2:2015 Electro Magnetic Compatibility (EMC) standard and uses the bioelectrical impedance, along with height, weight, age and gender information to generate results based on the OMRON’s data of body composition [ 60 ]. The Omron Full Body Sensor Body Composition Monitor and Scale estimates the TPBF by the Bioelectrical Impedance Method. The instrument sends an extremely weak electrical current of 50 kHz and less than 500 µA through the participant’s body to determine the amount of water in each tissue. The instrument takes measurements from both hands and feet to reduce the influence of water movement on body composition results. The output of the OMRON BF511 monitor included TPBF, relative visceral fat content, body mass index (BMI), and skeletal muscle. We included the TPBF and BMI output together with RFM for the analysis. RFM was calculated from WC and height:
Obesity was defined based on BMI thresholds for overweight (≥ 25 Kg/m 2 ), and obesity (≥ 30 Kg/m 2 ) according to the World Health Organization’s criteria [ 1 ].
Statistical analyses
Patient characteristics were stratified by primary clusters (colleges). Counts and corresponding percentages were used to describe categorical variables and compared using the Chi-square test. Mean and standard deviations were used to describe continuous variables if they followed the Gaussian normal distribution. Median and interquartile ranges were used if otherwise distributed. Either the One-way analysis of variance or Kruskal-Willi’s test was used to compare continuous variables among primary clusters. Multiple comparison analysis with Bonferroni correction was performed when the probability value was < 0.05. Sex-stratified prevalence estimates for obesity were determined according to BMI thresholds and the corresponding TPBF and RFM thresholds for age. The Passing and Bablok regression analysis was used to evaluate the measurement agreement and possible systematic bias for TPBF and RFM against BMI [ 61 ]. The diagnostic accuracy of TPBF and RFM was estimated based on the optimal cut-off, sensitivity, and specificity analysis, considering the area under the curve (AUC) estimated with the receiver-operating characteristic curves (ROC) analysis. We integrated the results from TPBF and RFM for obesity definition based on BMI threshold in a Bayesian framework, to report a combined obesity prevalence. Twenty chains were used to sample 50,000 samples per chain (25,000 warmups and 25,000 post warmups). Posterior densities were estimated using the Hamiltonian Monte Carlo (HMC) method. Summaries of posterior distributions including the mean and 95% credible interval were used to interpret the results. Multiple logistic regression analysis was used to evaluate potential risk factors of overweight/obesity. A two-sided p -value of 0.05 was considered statistically significant. Statistical analyses were performed using R version 4.3.0 (2023-04-21 ucrt) and MedCalc software Bvba, version 18.9.1.
Table 2 displays the characteristics of the study participants by sampling strata. There was a significant over-representation of female students at CoHSS and underrepresentation at CABE and CoE (p-value < 0.001). The proportion of students that consume alcohol was comparatively low in CoHS (p-value = 0.039). Compared with CANR and CoHSS, a significant proportion of students from CoHS (31.2%), CoE and CoS (18.4% each) never engaged in regular exercise (p-value < 0.001). We observed significant variance in TPBF% measurements compared across the colleges (p-value < 0.001).
Prevalence of being overweight and Obese based on standard BMI thresholds
Using BMI ≥ 25.0 Kg/m 2 , approximately 31% and 15% of female and male students were classified as overweight/obese. However, only 2.4% of male students and 8.0% of female students were classified as obese using BMI ≥ 30.0 Kg/m 2 (Table 3 ). We observed a trend towards increased overweight/obesity with age. Among students < 20 years, 26.3% and 13.6% female and male, respectively, were classified as being overweight and/or obese. Among ≥ 20 years female and male students, 34.5% and 15.5% were classified as overweight and/or obese.
Evaluation of RFM and BAI-derived TPBF based on BMI
Passing and Bablok regression analysis are shown in Table S1 . Here we emphasised the interpretation based on the observed random difference. The null assumption was that the observed random differences within ± 1.96 residual standard deviation (RSD) > 10%. Linearity between the variables was evaluated based on the custom test for linearity probability value (Table S1 , Figure S1 ). Concordance was observed between TPBF and BMI (RSD = 1.60, ± 1.96 = -3.14 to 3.14) compared with RFM (RSD = 2.64, ± 1.96 = -5.17 to 5.17) among male students. Similarly, among female students, TPBF demonstrated good agreement with BMI (RSD = 2.23, ± 1.96 = -4.37 to 4.37) compared to RFM (RSD = 2.98, ± 1.96 = -5.83 to 5.83). The linearity test revealed a significant deviation from linearity between TPBF and BMI (p-value < 0.01) and between BMI and RFM (p-value > 0.05). The ROC curve analysis (Fig. 1 ) identified TPBF threshold values of > 20.3% and > 20.8% and was associated with high information values for defining being overweight (BMI > 29.9 Kg/m 2 ) among male students: 15–19 years (AUC = 0.941, sensitivity = 100.0%, specificity = 87.8%) and 20–25 years (AUC = 0.942, sensitivity = 94.4%, specificity = 89.6%), respectively. Also, TPBF threshold values for defining obesity (BMI ≥ 30.0 Kg/m 2 ) among 15–19 years and 20–25 years female students were > 35.4% and > 35.2%, respectively (Fig. 1 ).
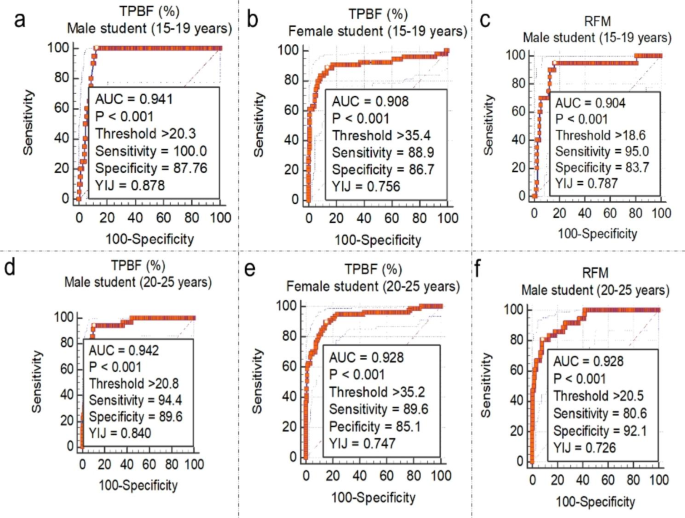
Threshold of TPBF and RFM estimates corresponding to WHO-defined BMI thresholds for overweight among male and female students
An optimal threshold value for TPBF > 26.2% (AUC = 0.981, sensitivity = 100.0%, specificity = 95.2%) for males (15–19 years) and > 24.4% (AUC = 0.938, sensitivity = 80.0%, specificity = 92.0%) had exceptional diagnostic accuracy for obesity (BMI ≥ 29.9 Kg/m 2 ) among male students (Fig. 2 ). The TPBF threshold of > 35.4% and > 35.2 was optimal for defining overweight female students 15–19 years and 20–25 years, respectively. TPBF values > 41.8% and 44.3% were optimal for defining obesity among female students 15–19 years and 20–25 years.
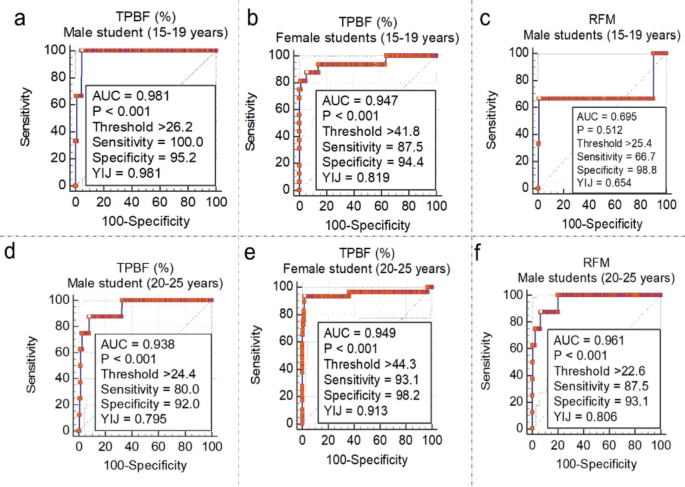
Threshold of TPBF and RFM estimates corresponding to WHO-defined BMI thresholds for obesity among male and female students
The ROC curve analysis of RFM for defining overweightness revealed an optimal cut-off of > 18.6 and > 20.5 among 15–19 years and 20–25 years male students, respectively (Fig. 1 c and f). RFM thresholds for defining obesity were > 25.4 and > 22.6 among male students 15–19 years and 20–25 years respectively (Fig. 2 a and f). Among female students (Fig. 3 a and b), RFM thresholds of > 20.7 and > 20.9 were associated with high information values for defining overweight among 15–19 years and 20 − 15 years, respectively. Moreover, obesity definition thresholds were > 24.5 and > 25.2, respectively among female students 15–19 years and 20 − 15 years (Fig. 3 c and d).
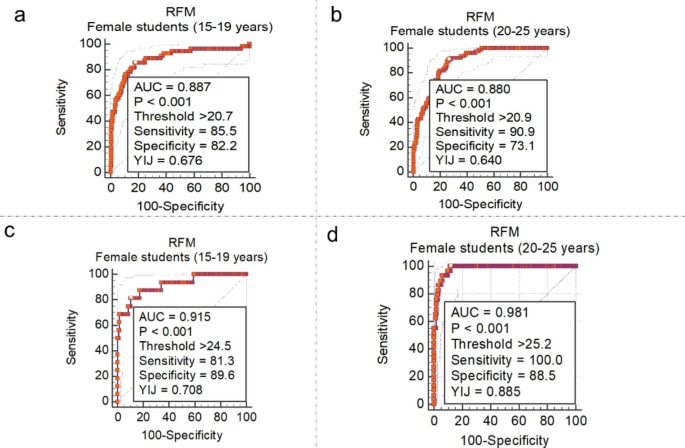
Threshold of RFM estimates corresponding to WHO-defined BMI thresholds for overweight and obesity among female students
The concordance between BMI, TPBF and RFM for obesity classification was 84% (95% lower limit = 82.0%) among female students and 82.9% (95% lower limit = 80.5%) among male students. The findings of the posterior predictive checks using the simulated data are presented in Figure S3 . The results of the Bayesian analysis suggest that the combined prevalence mean of overweight/obesity for TPBF and RFM were 33.8% (95%CI: 29.2-38.6%) among female students and 17.0% (95%CI: 13.1-21.3%) among male students. The combined prevalence mean of obesity for TPBF and RFM was 9.4% (95%CI: 6.9-12.2%) among female students and 6.7% (95%CI: 4.3-9.5%) among female students (Fig. 4 ).
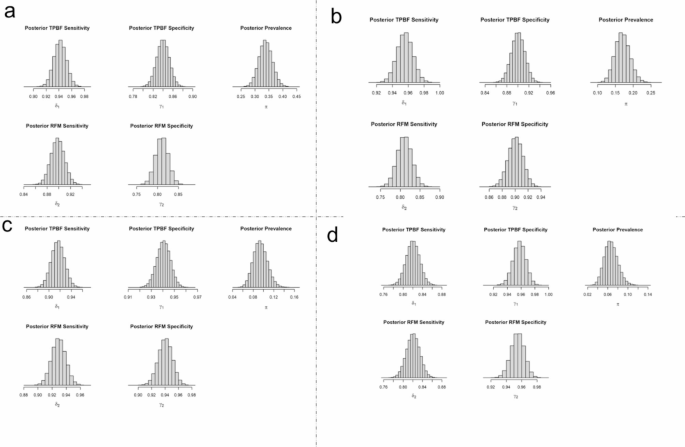
Marginal posterior density for the prevalence of obesity using combined data from TBPF and RFM. Note: π represents posterior prevalence using both TPBF and RFM data, δ1 represents sensitivity for TPBF data, γ1 represents specificity for TPBF data, δ2 represents sensitivity for RFM data, and γ2 represents specificity for RFM data
Factors associated with overweight/obesity among students
From the Multiple Logistic Regression analysis sex and family history of education were found to be consistent factors associated with general and central adiposity and percentage body fat distribution. The odds of being overweight were increased between 1.8 and 2.5, for women, depending on the classification criteria (Table 4 ). Family history of obesity was associated with increased odds of general obesity (OR = 3.48, 95%CI: 2.04–5.91), central obesity (OR = 1.98, 95%CI: 1.18–3.30) and high percentage body fat distribution (OR = 2.36, 95%CI: 1.42–3.94). Compared with first years students, the odds of central obesity and high percentage body fat were increased among third year students: OR = 2.77(1.59–4.82) and OR = 1.79(1.05–3.08), and fourth year students: OR = 3.26(1.76–6.04) and OR = 2.34(1.29–4.23), respectively.
This study sought to investigate the prevalence and risk factors of obesity among undergraduate students using multiple adiposity indices in a Byersian framework. In general the prevalence of being overweight/obese in this age group of young adults was high: 33.8% among female students and 17.0% among male students. Significant association were found between being overweight/obese and potential factors including sex, family history of obesity and university level.
Using the combined informative estimate, we observed an obesity prevalence of 6.7% among male students and 9.4% among female students. More generally, 17.0% of male students and 33.8% of female students were classified as having weight status corresponding to abnormal central and general adiposity as well as high body fat accumulation. These estimates are within the obesity prevalence range of 1.7–19.0% as estimated by a previous study in the same population group using different anthropometric indices [ 41 ]. Among the university student population in Botswana [ 43 ] and Ghana [ 44 ], similar estimates of overweight and/or obesity prevalence have been reported. In a larger study representing university students from 22 countries [ 62 ], 14.1% and 5.2% of female students and 18.9% and 5.8% of male students were reported to be overweight and obese, respectively. These data highlight the significant burden of obesity among undergraduate university students, which has a potential future health impact. In line with the current 16.2%, tertiary enrolment rate in Ghana [ 63 ], the current estimate of obesity reflects a significant national obesity problem among the young adult population with significant future health consequences.
We believe our estimate may be a true reflection of the obesity burden among student populations. First, TPBF and RFM contain high information values for obesity and fat distribution classification. Second, RFM is less accurate than BMI in lean individuals [ 64 ] whiles BAI-derived TPBF is less accurate than BMI in obese individuals [ 65 ]. Thus, combining these measures in a population estimation of obesity would provide a value informed by a broader distribution of obesity and fat distribution among the population group. Third, we relied on prior information from the concordance between both RFM and TPBF with standard BMI thresholds and a previous study from the same population [ 41 ]. Finally, the method adapted for the estimation of the combined prevalence of obesity has been successfully applied elsewhere [ 66 ]. Because this approach could be more flexible and adaptable, there is a need to test its performance in other settings with other related adiposity estimates.
An important observation of concern was the proportion of overweight/obese female students, which was higher compared with male students. This observation is consistent in several other studies [ 40 , 41 , 43 , 62 ], suggesting an increased risk for weight gain in young women and the critical need for interventions to prevent obesity and the host of associated adverse health outcomes. The evidence has been confirmed in several nationally representative surveys, where greater increases in weight are observed in young women aged 18 to 35 years compared with those seen in older women [ 67 ]. In a study among young Ghanain women aged between 15 and 24 years [ 20 ], overweight/obesity increased by 49% between 1993 and 2014 and projected a future prevalence of 35% by the year 2040. Fat deposition in women usually begins with the onset of puberty and continues unless consciously controlled [ 68 ]. Some studies has reported that female transition from adolescence to adulthood is associated with certain obesogenic dietary and physical activity behaviours to satisfy a historic valorization of large body size as a function of beauty, sexual attraction, prosperity, health and prestige [ 20 , 69 , 70 ].
In a prospective analysis of mother-daughter dyads and father-son dyads, the study reported a large and concerning increase in obesity rates over two generations of young adults, especially females [ 71 ]. These findings indicate that young adulthood represents periods of crucial importance regarding the establishment of life-long lifestyle habits and skills to control obesity. Studies have attributed this to a lack of knowledge and skills around food and nutrition, depression, anxiety, stress, satiety, neural responses, and possibly sleep patterns and premenstrual cravings [ 42 , 43 , 44 , 67 , 72 ]. Thus, there is the need to study these factors and their relationship with obesity among undergraduate students in Ghana, which can benefit future interventions.
We observed a trend in increasing obesity prevalence with academic level such that third- and fourth-year students had significantly increased obesity prevalence than first- and second-year students. Similar findings have been observed in other related studies [ 41 , 73 , 74 ] but not all [ 75 ]. This relationship may suggest the role of other factors of obesity associated with progressive academic level, which was not the focus of this study. We recommend future research to focus on changing lifestyle and eating habits of students related to progressive academic level. We replicated the association between obesity and family history and increased risk of obesity prevalence. This finding contributes to the evidence that genetics play an important role in the onset of obesity and the severity of obesity [ 76 , 77 ]. In several studies, sendentary life has been strsongly associated with being overweight/obese [ 11 , 17 , 27 , 28 ]. However, we did not find a significant association between being overweight/obese and students engagement in regular physical activity.
We would like to acknowledge some limitations of this study. First, Bayesian modelling is reliance on prior information, in our case we used the prior prevalence, concordance and diagnostic estimates obtained from the linked data and previous studies within the same population. As such, our analyses are limited by the accuracy of standard BMI thresholds for classifying obesity. Second, female students were over-represented in the dataset, which could bias the estimation of obesity prevalence. Although, we considered this in the analysis by reporting sex-specific prevalence estimates. Also, the generalizability of our estimate may be limited as data were obtained from only one tertiary institution in Ghana. This approach is significant to fill a gap in the current lack of consensus on the appropriate adiposity index and serves as the opportunity to unique data linkage and novel analytical techniques to improve obesity surveillance.
As different adiposity indices become increasingly available, multiple indicators used in combination may offer a greater power to examine obesity prevalence. We have demonstrated this by integrating central adiposity and percentage body fat criteria relative to standard BMI thresholds in a Bayesian framework and reported high obesity prevalence, especially among female students. We also demonstrated that obesity prevalence increases with university level and among students with a family history of obesity. The study suggests that the prevalence of being overweight or obese is expected to increase in the coming years, leading to several health issues. It emphasizes the requirement for public health efforts and interventions at a national level to control the problem and its associated costs and co-morbidities. Furthermore, interventions against obesity should be customized to target the socio-demographic disparities highlighted in the study.
Data Availability
The datasets and codes used and/or analysed during the current study are within the manuscript, and available at the GitHub repository ( https://github.com/EvansKCCR/obesity_among_students ).
Abbreviations
Relative fat mass
Total percentage of body fat
Body mass index
Bioelectrical impedance analysis
Kwame Nkrumah University of Science and Technology
Design effect
Receiver operative characteristics curve
Area under the curve
Hamiltonian Monte Carlo
Residual standard deviation
Obesity. and overweight. In: Department of Sustainable Development and Healthy Environments World Health Organization; 2021.
Murray CJL, Aravkin AY, Zheng P, Abbafati C, Abbas KM, Abbasi-Kangevari M, Abd-Allah F, Abdelalim A, Abdollahi M, Abdollahpour I et al. Global burden of 87 risk factors in 204 countries and territories, 1990–2019:a systematic analysis for the global burden of Disease Study 2019. The Lancet. 2020;396(10258):1223–49.
Murray CJL, Abbafati C, Abbas KM, Abbasi M, Abbasi-Kangevari M, Abd-Allah F, Abdollahi M, Abedi P, Abedi A, Abolhassani H, et al. Five insights from the global burden of Disease Study 2019. The Lancet. 2020;396(10258):1135–59.
Article Google Scholar
Blüher M. Obesity: global epidemiology and pathogenesis. Nat Reviews Endocrinol. 2019;15(5):288–98.
World Obesity Day. 2022 – Accelerating action to stop obesity. In.: World Health Organization; 2022.
Nugent R. Chronic Diseases in developing countries: health and economic burdens. Ann N Y Acad Sci. 2008;1136(1):70–9.
Article PubMed Google Scholar
Ofori-Asenso R, Agyeman AA, Laar A, Boateng D. Overweight and obesity epidemic in Ghana—a systematic review and meta-analysis. BMC Public Health. 2016;16(1):1–18.
Akowuah PK, Kobia-Acquah EJJoN. Metabolism: Childhood obesity and overweight in Ghana: a systematic review and meta-analysis. Journal of Nutrition Metabolism 2020, 2020.
Amegah A, Lumor S, Vidogo FJAJF. Agriculture, Nutrition, Development: prevalence and determinants of overweight and obesity in adult residents of Cape Coast, Ghana: a hospital-based study. Afr J Food Agric Nutr Dev 2011, 11(3).
Anderson AK. Prevalence of anemia, overweight/obesity, and undiagnosed Hypertension and Diabetes among residents of selected communities in Ghana. Int J Chronic Dis 2017, 2017.
Boateng GO, Adams EA, Odei Boateng M, Luginaah IN, Taabazuing M-M. Obesity and the burden of health risks among the elderly in Ghana: a population study. PLoS ONE. 2017;12(11):e0186947.
Article PubMed PubMed Central Google Scholar
Nyakotey DA, Ananga AS, Apprey C. Assessing physical activity, nutrient intake and obesity in middle-aged adults in Akuse, Lower Manya Krobo, Ghana. J Health Res. 2022;36(2):199–208.
Obirikorang C, Osakunor DNM, Anto EO, Amponsah SO, Adarkwa OK. Obesity and cardio-metabolic risk factors in an urban and rural population in the Ashanti Region-Ghana: a comparative cross-sectional study. PLoS ONE. 2015;10(6):e0129494.
William K. Obesity and cardiovascular risk factors in a Pentecostal population in Kumasi-Ghana. J Med Sci. 2008;8(8):682–90.
Agbeko MP, Akwasi K-K, Andrews DA, Gifty OB. Predictors of overweight and obesity among women in Ghana. Open Obes J 2013, 5(1).
Asosega KA, Adebanji AO, Abdul IW. Spatial analysis of the prevalence of obesity and overweight among women in Ghana. BMJ open. 2021;11(1):e041659.
Asosega KA, Aidoo EN, Adebanji AO, Owusu-Dabo E. Examining the risk factors for overweight and obesity among women in Ghana: A multilevel perspective. Heliyon 2023.
Marquis GS, Colecraft EK, Bannerman B, Thompson-Colón T. Overweight and obesity among women in a predominantly rural district of Ghana. The FASEB Journal 2016, 30:667.669-667.669.
Tuoyire DA. Television exposure and overweight/obesity among women in Ghana. BMC Obes. 2018;5(1):1–10.
Tuoyire DA. Overweight/obesity among 15-to 24-year-old women in Ghana: 21-year trend, future projections and socio-demographic correlates. J Biosoc Sci. 2021;53(6):839–55.
Tuoyire DA, Kumi-Kyereme A, Doku DT. Socio-demographic trends in overweight and obesity among parous and nulliparous women in Ghana. BMC Obes. 2016;3(1):1–14.
Adom T, De Villiers A, Puoane T, Kengne AP. Prevalence and correlates of overweight and obesity among school children in an urban district in Ghana. BMC Obes. 2019;6:1–11.
Aryeetey R, Lartey A, Marquis GS, Nti H, Colecraft E, Brown P. Prevalence and predictors of overweight and obesity among school-aged children in urban Ghana. BMC Obes. 2017;4:1–8.
Mogre V, Gaa PK, Abukari RNS. Overweight, obesity and thinness and associated factors among school-aged children (5–14 years) in Tamale, Northern Ghana. Eur Sci J 2013, 9(20).
Akoto S, Tandoh MA, Nsiah K, Asamoah-Boakye O, Annaful VT. Lifestyle habits, macronutrient intake, and obesity prevalence among adolescents in rural-periurban community senior high schools in the Ho municipality of Ghana. Front Nutr 2022, 9.
Peltzer K, Pengpid S. Overweight and obesity and associated factors among school-aged adolescents in Ghana and Uganda. Int J Environ Res Public Health. 2011;8(10):3859–70.
Amoh I, Appiah-Brempong E. Prevalence and risk factors of obesity among senior high school students in the Adansi North district of Ghana. Int J Community Med Public Health. 2017;4(10):3762–9.
Aryee P, Helegbe G, Baah B, Sarfo-Asante R, Quist-Therson R. Prevalence and risk factors for overweight and obesity among nurses in the tamale metropolis of Ghana. J Med Biomedical Sci. 2013;2(4):13–23.
Duodu C, Awuni TK, Attito P, Zotor FB. Assessment of overweight and obesity prevalence among practicing nurses and midwives in the Hohoe Municipality of the Volta Region, Ghana. Sci J Public Health. 2015;3(6):842–51.
Obirikorang Y, Obirikorang C, Enoch O, Acheampong E, Tuboseiyefah P, Miriam A, Emmanuella N. Prevalence and risk factors of obesity among practicing nurses at three selected hospitals in Kumasi Metropolis, Ghana. J Med Biomedical Sci. 2016;5(3):45–55.
Amo-Mensah P, Vanotoo L, Davies-Teye B. Prevalence of overweight and Obesity among Health Personnel in an urban Ghana: a descriptive cross-sectional study, 2017. Value in Health. 2017;20(9):A544.
Abubakar MB, Uthman YA, Ibrahim KG. Prevalence of overweight and obesity among health-care workers in Ghana: a systematic review. Nigerian J Experimental Clin Biosci. 2021;9(1):47.
Addo J, Smeeth L, Leon D. Obesity in urban civil servants in Ghana: association with pre-adult wealth and adult socio-economic status. Public Health. 2009;123(5):365–70.
Article CAS PubMed Google Scholar
Addo P. Prevalence of obesity and overweight and Associated factors among Financial Institution workers in Accra Metropolis, Ghana: a Case Control Study. Value in Health. 2016;19(7):A579.
Addo PN, Nyarko KM, Sackey SO, Akweongo P, Sarfo B. Prevalence of obesity and overweight and associated factors among financial institution workers in Accra Metropolis, Ghana: a cross sectional study. BMC Res Notes. 2015;8(1):1–8.
Atuahene M, Ganle JK, Adjuik M, Atuahene NF, Kampitib GB. Overweight and obesity prevalence among public servants in Nadowli district, Ghana, and associated risk factors: a cross-sectional study. BMC Obes. 2017;4(1):1–8.
Kumah DB. Prevalence of overweight and obesity among staff of a Tertiary Institution in Kumasi, Ghana. EC Nutr. 2016;5:1277–81.
Google Scholar
Nuertey BD, Alhassan AI, Nuertey AD, Mensah IA, Adongo V, Kabutey C, Addai J, Biritwum RB. Prevalence of obesity and overweight and its associated factors among registered pensioners in Ghana; a cross sectional studies. BMC Obes. 2017;4(1):1–12.
Anto EO, Owiredu W, Adua E, Obirikorang C, Fondjo LA, Annani-Akollor ME, Acheampong E, Asamoah EA, Roberts P, Wang W. Prevalence and lifestyle-related risk factors of obesity and unrecognized Hypertension among bus drivers in Ghana. Heliyon. 2020;6(1):e03147.
Mogre V, Nyaba R, Aleyira S. Lifestyle risk factors of general and abdominal obesity in students of the school of medicine and health science of the University of Development Studies, Tamale, Ghana. International Scholarly Research Notices 2014, 2014.
Obirikorang C, Anto EO, Addai P, Obirikorang Y, Acheampong E. Prevalence and risks factors of overweight/obesity among undergraduate students: an institutional based cross-sectional study, Ghana. J Med Biomedical Sci. 2017;6(1):24–34.
Agyei A, Yorke E, Boima V. Prevalence of overweight and obesity and its relation to Diet and physical activity among medical students in Accra, Ghana. Afr J Health Sci. 2022;35(2):99–113.
Tapera R, Merapelo MT, Tumoyagae T, Maswabi TM, Erick P, Letsholo B, Mbongwe B. The prevalence and factors associated with overweight and obesity among University of Botswana students. Cogent Med. 2017;4(1):1357249.
Mogre V, Nyaba R, Aleyira S. Lifestyle risk factors of general and abdominal obesity in students of the school of medicine and health science of the University of Development Studies, Tamale, Ghana. ISRN obesity 2014, 2014.
Amoako M, Amoah-Agyei F, Du C, Fenton JI, Tucker RM. Emotional Eating among Ghanaian University Students: Associations with Physical and Mental Health Measures. 2023, 15(6):1526.
Dolan CM, Kraemer H, Browner W, Ensrud K, Kelsey JL. Associations between body composition, anthropometry, and mortality in women aged 65 years and older. Am J Public Health. 2007;97(5):913–8.
Kontogianni MD, Panagiotakos DB, Skopouli FN. Does body mass index reflect adequately the body fat content in perimenopausal women? Maturitas. 2005;51(3):307–13.
Obirikorang C, Obirikorang Y, Acheampong E, Anto EO, Toboh E, Asamoah EA, Amakwaa B, Batu EN, Brenya P. Association of wrist circumference and waist-to-height ratio with cardiometabolic risk factors among type II diabetics in a Ghanaian population. Journal of diabetes research 2018, 2018.
Frankenfield DC, Rowe WA, Cooney RN, Smith JS, Becker D. Limits of body mass index to detect obesity and predict body composition. Nutrition. 2001;17(1):26–30.
Sun SS, Chumlea WC, Heymsfield SB, Lukaski HC, Schoeller D, Friedl K, Kuczmarski RJ, Flegal KM, Johnson CL, Hubbard VS. Development of bioelectrical impedance analysis prediction equations for body composition with the use of a multicomponent model for use in epidemiologic surveys. Am J Clin Nutr. 2003;77(2):331–40.
Freedman DS, Thornton JC, Pi-Sunyer FX, Heymsfield SB, Wang J, Pierson RN Jr, Blanck HM, Gallagher D. The body adiposity index (hip circumference ÷ height1. 5) is not a more accurate measure of adiposity than is BMI, waist circumference, or hip circumference. Obesity. 2012;20(12):2438–44.
Senkus KE, Crowe-White KM, Locher JL, Ard JD. Relative fat mass assessment estimates changes in adiposity among female older adults with obesity after a 12-month exercise and diet intervention. Ann Med. 2022;54(1):1160–6.
Article CAS PubMed PubMed Central Google Scholar
Brodowicz GR, Mansfield RA, McClung MR, Althoff SA. Measurement of body composition in the elderly: dual energy x-ray absorptiometry, underwater weighing, bioelectrical impedance analysis, and anthropometry. Gerontology. 1994;40(6):332–9.
Bi Y, Wang R. Application of DEXA to body composition measurement. Sports Sci Res. 2008;29(1):17.
Woolcott OO, Bergman RN. Relative fat mass (RFM) as a new estimator of whole-body fat percentage a cross-sectional study in American adult individuals. Sci Rep. 2018;8(1):10980.
Joseph L, Gyorkos TW, Coupal L. Bayesian estimation of Disease Prevalence and the parameters of diagnostic tests in the absence of a Gold Standard. Am J Epidemiol. 1995;141(3):263–72.
Erkanli A, Soyer R, Stangl D. Hierarchical bayesian analysis for Prevalence Estimation. Case studies in bayesian statistics: 1997// 1997; New York. NY: Springer New York; 1997. pp. 325–46.
Chapter Google Scholar
Speybroeck N, Devleesschauwer B, Joseph L, Berkvens DJI. Misclassification errors in prevalence estimation: bayesian handling with care. 2013, 58:791–5.
Arya R, Antonisamy B, Kumar SJTIJP. Sample size estimation in prevalence studies. 2012, 79:1482–8.
Manual OI. Full body Sensor Body Composition Monitor and Scale Model HBF–510. China: Omron Healthcare 2008.
Passing H, Bablok. A new biometrical procedure for testing the equality of measurements from two different analytical methods. Application of linear regression procedures for method comparison studies in clinical chemistry, part I. J Clin Chem Clin Biochem Z fur Klinische Chemie Und Klinische Biochemie. 1983;21(11):709–20.
CAS Google Scholar
Peltzer K, Pengpid S, Samuels TA, Özcan NK, Mantilla C, Rahamefy OH, Wong ML, Gasparishvili A. Prevalence of overweight/obesity and its associated factors among university students from 22 countries. Int J Environ Res Public Health. 2014;11(7):7425–41.
Ghana - Gross. enrolment ratio in tertiary education [ https://knoema.com/atlas/Ghana/topics/Education/Tertiary-Education/Gross-enrolment-ratio-in-tertiary-education ].
Woolcott OO, Bergman RN. Relative Fat Mass as an estimator of whole-body fat percentage among children and adolescents: a cross-sectional study using NHANES. Sci Rep. 2019;9(1):15279.
Li YC, Li CI, Lin WY, Liu CS, Hsu HS, Lee CC, Chen FN, Li TC, Lin CC. Percentage of body fat assessment using bioelectrical impedance analysis and dual-energy X-ray absorptiometry in a weight loss program for obese or overweight Chinese adults. PLoS ONE. 2013;8(4):e58272.
Edwards J, Pananos AD, Thind A, Stranges S, Chiu M, Anderson KK. A bayesian approach to estimating the population prevalence of mood and anxiety disorders using multiple measures. Epidemiol Psychiatric Sci. 2021;30:e4.
Pegington M, French DP, Harvie MN. Why young women gain weight: a narrative review of influencing factors and possible solutions. 2020, 21(5):e13002.
Ersoy C, Imamoglu S. Comparison of the obesity risk and related factors in employed and unemployed (housewife) premenopausal urban women. Diabetes Res Clin Pract. 2006;72(2):190–6.
Cohen E, Boetsch G, Palstra FP, Pasquet P. Social valorisation of stoutness as a determinant of obesity in the context of nutritional transition in Cameroon: the Bamiléké case. Social science & medicine ( 1982 ) 2013, 96:24–32.
Jokela M, Elovainio M, Kivimäki M. Lower fertility associated with obesity and underweight: the US National Longitudinal Survey of Youth. Am J Clin Nutr. 2008;88(4):886–93.
Alati R, Betts KS, Williams GM, Najman JM, Zalbahar N, Mamun A. Generational increase in obesity among young women: a prospective analysis of mother–daughter dyads. Int J Obes. 2016;40(1):176–80.
Article CAS Google Scholar
Eating Patterns of Young Women (18–25 y) with Overweight and Obesity: A Preliminary Investigation. 2023, 15(7):1652.
Elham AA, Anas A, Ikram K, Jayakumary M. Obesity among university students: a cross-sectional study in Ajman, UAE. 2015.
Ghazawy ER, Mahfouz EM, Rahman A-E, Ahmed T. Emam SAJMJoMR: Obesity/Overweight among University students. Minia Egypt. 2022;33(3):30–6.
Boukrim M, Obtel M, Lahlou L, Razine R. University students’ perceptions and factors contributing to obesity and overweigh in Southern of Morocco. Afr Health Sci. 2021;21(2):942–50.
Corica D, Aversa T, Valenzise M, Messina MF, Alibrandi A, De Luca F. Wasniewska MJFie: Does family history of obesity, cardiovascular, and metabolic diseases influence onset and severity of childhood obesity? 2018, 9:187.
van der Sande MA, Walraven GE, Milligan PJ, Banya WA, Ceesay SM, Nyan OA. McAdam KPJBotWho: family history: an opportunity for early interventions and improved control of Hypertension, obesity and Diabetes. 2001, 79(4):321–8.
Download references
Acknowledgements
We would like to thank the students of KNUST who dedicated their time and participation to this study. We also express our gratitude to the KNUST Counselling unit and the University Hospital for their support during the data collection.
This research did not receive any specific grant from funding agencies in the public, commercial, or not-for-profit sectors.
Author information
Authors and affiliations.
Department of Molecular Medicine, School of Medical Science, Kwame Nkrumah University of Science and Technology (KNUST), Kumasi, Ghana
Christian Obirikorang, Evans Asamoah Adu, Anthony Afum-Adjei Awuah & Alexander Owusu Boakye
Kumasi Centre for Collaborative Research, Kumasi, Ghana
Christian Obirikorang, Evans Asamoah Adu, Anthony Afum-Adjei Awuah, Angela Nana Bosowah Fynn, Alexander Owusu Boakye & Austin Gideon Adobasom-Anane
Department of Medical Diagnostics, Faculty of Allied Health Sciences, Kwame Nkrumah University of Science and Technology, Kumasi, Ghana
Enoch Odame Anto, George Osei-Somuah & Ivy Ofori-Boadu
Centre for Precision Health, School of Medical and Health Sciences, Edith Cowan University, Western Australia, Australia
Enoch Odame Anto & Lois Balmer
Department of Nursing, Faculty of Health Sciences, Garden City University College, Kumasi, Ghana
Patience Nyarkoa Ansong & Yaa Obirikorang
Department of Chemical Pathology, University of Ghana Medical School, University of Ghana, Accra, Ghana
Eric NY Nyarko
You can also search for this author in PubMed Google Scholar
Contributions
CO, LB, and AAA conceptualized, designed the study, and edited the manuscript. EAA, EOA and AOB analyzed, interpreted the data, and drafted the paper. ANB, GOS and PNA assisted in data acquisition and measurement. YO, IOB, ENYN and AGA contributed to writing and editing the manuscript. All authors have read and approved the final version before submission.
Corresponding author
Correspondence to Christian Obirikorang .
Ethics declarations
Ethical approval and consent to participate.
Ethical approval with reference ID (CHRPE/AP/030/19) was obtained from the Committee on Human Research, Publications and Ethics (CHRPE), School of Medicine and Dentistry, Kwame Nkrumah University of Science & Technology. Written informed consent was obtained from participants 18 years and above and assent from minors (< 18 years) who opted to participate in the study. Additionally, informed consent to participate was taken from the legal guardians of minor participants. All methods were performed following the guidelines and regulations of the Helsinki Declaration.
Consent for publication
Not applicable.
Competing interests
The authors declare no competing interests.
Additional information
Publisher’s note.
Springer Nature remains neutral with regard to jurisdictional claims in published maps and institutional affiliations.
Electronic supplementary material
Below is the link to the electronic supplementary material.
Supplementary Material 1
Rights and permissions.
Open Access This article is licensed under a Creative Commons Attribution 4.0 International License, which permits use, sharing, adaptation, distribution and reproduction in any medium or format, as long as you give appropriate credit to the original author(s) and the source, provide a link to the Creative Commons licence, and indicate if changes were made. The images or other third party material in this article are included in the article’s Creative Commons licence, unless indicated otherwise in a credit line to the material. If material is not included in the article’s Creative Commons licence and your intended use is not permitted by statutory regulation or exceeds the permitted use, you will need to obtain permission directly from the copyright holder. To view a copy of this licence, visit http://creativecommons.org/licenses/by/4.0/ . The Creative Commons Public Domain Dedication waiver ( http://creativecommons.org/publicdomain/zero/1.0/ ) applies to the data made available in this article, unless otherwise stated in a credit line to the data.
Reprints and permissions
About this article
Cite this article.
Obirikorang, C., Adu, E.A., Anto, E.O. et al. Prevalence and risk factors of obesity among undergraduate student population in Ghana: an evaluation study of body composition indices. BMC Public Health 24 , 877 (2024). https://doi.org/10.1186/s12889-023-17175-5
Download citation
Received : 26 June 2023
Accepted : 07 November 2023
Published : 21 March 2024
DOI : https://doi.org/10.1186/s12889-023-17175-5
Share this article
Anyone you share the following link with will be able to read this content:
Sorry, a shareable link is not currently available for this article.
Provided by the Springer Nature SharedIt content-sharing initiative
- Body composition
- Undergraduate students
- Risk factors
BMC Public Health
ISSN: 1471-2458
- Submission enquiries: [email protected]
- General enquiries: [email protected]
Body mass index trajectories and mortality risk in Japan using a population-based prospective cohort study: the Japan Public Health Center-based Prospective Study
Affiliations.
- 1 School of Human Evolution and Social Change, Arizona State University, Tempe, AZ, USA.
- 2 Lee Kong Chian School of Medicine, Nanyang Technological University, Singapore, Singapore.
- 3 Department of Global Health Policy, Graduate School of Medicine, The University of Tokyo, Tokyo, Japan.
- 4 Department of Psychiatry, Yale University, New Haven, CT, USA.
- 5 Department of Epidemiology and Biostatistics, Indiana University School of Public Health-Bloomington, Bloomington, IN, USA.
- 6 Department of Pediatrics, Indiana University School of Medicine-Indianapolis, Indianapolis, IN, USA.
- 7 Division of Cohort Research, National Cancer Center Institute for Cancer Control, Tokyo, Japan.
- 8 Division of Prevention, National Cancer Center Institute for Cancer Control, Tokyo, Japan.
- PMID: 37878816
- PMCID: PMC10859135
- DOI: 10.1093/ije/dyad145
Background: Recent studies have found that long-term changes in weight during adulthood are associated with a high risk of mortality. The objective of this study was to characterize body mass index (BMI) trajectories during adulthood and to examine the association between BMI trajectories and risk of death in the Japanese population.
Methods: The data were extracted from Japan Public Health Center-based Prospective Study-a population-based prospective cohort study in Japan with participants aged 40-69 years followed over 20 years. The participants were categorized into multiple BMI trajectory groups using the latent class growth model. The Cox proportional-hazards model was conducted using all-cause mortality and cause-specific mortality as outcomes and the identified BMI trajectory groups as a predictor. In total, 65 520 participants were included in the analysis.
Results: Six BMI trajectory groups were identified: underweight stable (Group 1), low-to-high normal (Group 2), high-to-low normal (Group 3), normal to overweight (Group 4), overweight to normal (Group 5) and normal to obese (Group 6). Our Cox models showed a higher hazard (risk) of all-cause mortality among participants in the BMI-declining groups [Group 3, adjusted hazard ratio (aHR): 1.10, 95% CI: 1.05-1.16; Group 5, aHR: 1.16, 95% CI: 1.08-1.26], underweight stable group (Group 1, aHR: 1.27, 95% CI: 1.21-1.33) and normal to obese group (Group 6, aHR: 1.22, 95% CI: 1.13-1.33) than Group 2 (low-to-high normal BMI trajectory).
Conclusions: Stable underweight and weight loss were associated with a high risk of mortality, both of which were uniquely observed in a Japanese population.
Keywords: Japan Public Health Center-based Prospective Study; body mass index trajectory; latent class growth model.
© The Author(s) 2023. Published by Oxford University Press on behalf of the International Epidemiological Association.
- Body Mass Index
- Japan / epidemiology
- Obesity / complications
- Overweight* / epidemiology
- Prospective Studies
- Public Health
- Risk Factors
- Thinness* / complications
- Weight Loss
Grants and funding
- 23-A-31/National Cancer Center Research and Development Fund
- Grant-in-Aid for Cancer Research from the Ministry of Health, Labour and Welfare of Japan
- KAKENHI 18K18146/Japan Society for the Promotion of Science
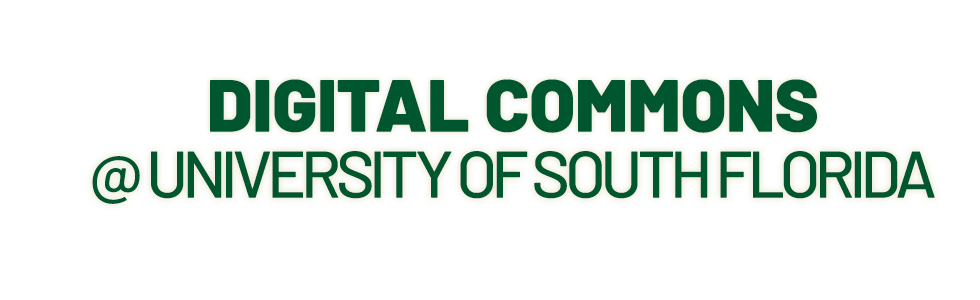
- USF Research
- USF Libraries
Digital Commons @ USF > Office of Graduate Studies > USF Graduate Theses and Dissertations > USF Tampa Theses and Dissertations > 4660
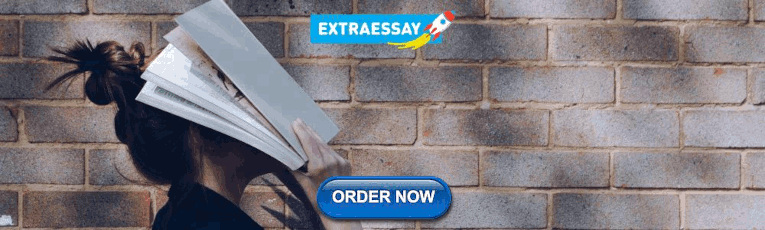
USF Tampa Graduate Theses and Dissertations
The relationship between socioeconomic status and body mass index on vitamin d levels in african american women with and without diabetes living in areas with abundant sunshine.
Shani Vann Davis , University of South Florida Follow
Graduation Year
Document type.
Dissertation
Degree Granting Department
Major professor.
Maureen Groer
African American, Obesity, Socioeconomic Status, Vitamin D
OBJECTIVE: To examine the relationships between socioeconomic status (SES), body mass index (BMI), and vitamin D levels in African American (AA) women living in areas with abundant sunshine; and to explore if diabetes moderates these relationships.
SIGNIFICANCE: More AA's live in poverty, and experience obesity, diabetes, and chronic disease compared to other groups. Eighty percent of AA women are overweight or obese, and rates of type 2 diabetes is highest in this group. Minority race, obesity, and diabetes increase risks for low vitamin D, and are associated with p
DESIGN AND METHOD: A cross-sectional descriptive research design was used to examine the specified relationships. Data from 611 non-pregnant AA women ≥ age 20 from the National Health and Nutrition Examination Survey (NHANES) cycles 2003 - 2006 were studied. SES was measured as poverty to income ratio (PIR), education level, and annual household income. Mean ± SD for BMI was 31 ± 8, and 14ng/ml ± 7ng/ml for vitamin D level. Only 8% of the sample had diabetes (n = 49). One hundred-eighty lived in areas with abundant sunshine.
RESULTS: BMI independently predicted the vitamin D level without regard for SES, or geographical locale. Vitamin D supplement use emerged as an independent predictor of vitamin D on covariate analysis. SES did not explain significant variation in the vitamin D level. A moderating influence of diabetes could not be determined.
CONCLUSIONS: BMI inversely predicts vitamin D level independent of geographic locale in AA women. Ethno/cultural measures to reduce BMI should be standard in caring for AA women which may affect vitamin D level and/or reduce morbidity and mortality in this group. Persons with low vitamin D suffer with more adverse health outcomes, and future research should examine if vitamin D deficiency accelerates risks for poor health outcomes where BMI is high.
Scholar Commons Citation
Davis, Shani Vann, "The Relationship Between Socioeconomic Status and Body Mass Index on Vitamin D Levels in African American Women with and without Diabetes Living in Areas with Abundant Sunshine" (2013). USF Tampa Graduate Theses and Dissertations. https://digitalcommons.usf.edu/etd/4660
Since September 04, 2013
Included in
Nursing Commons , Public Health Commons
Advanced Search
- Email Notifications and RSS
- All Collections
- USF Faculty Publications
- Open Access Journals
- Conferences and Events
- Theses and Dissertations
- Textbooks Collection
Useful Links
- USF Office of Graduate Studies
- Rights Information
- SelectedWorks
- Submit Research
Home | About | Help | My Account | Accessibility Statement | Language and Diversity Statements
Privacy Copyright
Body Image: Relationhsip to Attachment, Body Mass Index and Dietary Practices among College Students
Journal title, journal issn, volume title.
Body image or satisfaction with physical appearance has been established as an important aspect of self-worth and mental health across the life span. It is related to self-esteem, sexuality, family relationships and identity. Given the fact that physical appearance is a multifaceted structural concept that depends, not only on inner-biological, but also a psychological and socio-cultural components, the purpose of this study was to examine variables that are related to and influenced by satisfaction with physical appearance. Body mass index (BMI), eating disturbances, attachment (to mother, to father and to peers), global self-worth, parental control, peer influence and pressure regarding eating and media influence were examined in relation satisfaction with physical appearance. College students in a large southeastern university (195 males and 340 females) completed two subscales of Harter's Self-Perception Scale for College Students. Each subject self-reported his/her weight and height and these were used calculate weight/height ratio known as the body mass index. Participants also reported on attachment (to mother, to father and to peers) using the Inventory of Parent and Peer attachment scales (Armsden & Greenberg, 1987), Peer Influence Scale (Mukai, 1993) and the Media Influence scale which was developed for this project.
Differences between male and female perceptions of physical appearance in relationship to BMI were found: Among women, higher BMIs were associated with lower scores on perceptions of physical appearance (r = -. 429, p £ .001), whereas for males BMIs were not related to satisfaction with physical appearance. For both males and females, satisfaction with physical appearance was significantly and negatively (r = -.258, p £ .01) associated with media influence. Media influence was related to higher scores on the EAT 26 scale that measured disturbed eating attitudes and behaviors (r = .307, p £ .01). Females were affected by this association more so than were males. However, males appeared to not to be immune to such influence. Peer influence and peer pressure was another influential factor for both gender groups and it was associated with high eating disturbance scores (r = .369, p £ .01 for peer influence, and r = .413, p £ .01 for peer pressure). Attachment variables were associated with satisfaction of physical appearance and global self-worth in a different manner for adolescent females and males. For males, satisfaction with physical appearance was positively related to attachment to mother (r = .135, p £ .05) and father (r = .170, p £ .05) and negatively associated with maternal control (r = -. 246, p £ . 001). For females, only attachment to mother (r = .082, p £ .05) was positively associated satisfaction with physical appearance.
While there were many significant bivariate correlational findings, there were few significant coefficients in a regression analyses, presumably because of the high intercorrelations between the predictor variables. For females, BMI was the best predictor of satisfaction with physical appearance, whereas for males, the feeling of global self-worth was the strongest variable in predicting satisfaction with physical appearance.
Satisfaction with physical appearance is an essential part of global self-worth and is constructed differently by males and females. For females, high BMI was negatively related to satisfaction with physical appearance as well as global self-worth. On the other hand, for males neither global self-worth nor perceptions of physical appearance were affected by high BMIs. More research is needed to understand the complexity of influences on satisfaction with physical appearance as well as construction of global self-worth and its domains for both sexes.
Description
Persistent link, collections.
Influence of body mass index on health complains and life satisfaction
- Open access
- Published: 01 December 2023
- Volume 33 , pages 705–719, ( 2024 )
Cite this article
You have full access to this open access article
- Claudia Prieto-Latorre ORCID: orcid.org/0000-0002-6510-3057 1 ,
- Luis Alejandro Lopez-Agudo ORCID: orcid.org/0000-0002-0906-3206 1 &
- Oscar David Marcenaro-Gutierrez ORCID: orcid.org/0000-0003-0939-5064 1
921 Accesses
1 Altmetric
Explore all metrics
This research work investigates the influence of children’s weight status on well-being and school context in a sample of Spanish adolescences.
The Spanish records from the 2013–14 Health Behaviour in School-Aged Children Survey are used, which gathers 9,565 adolescences aged 11, 13 and 15. Studies do not usually address the endogeneity of body mass index when analysing their effect on life satisfaction and health complaints, thus resulting in biased estimates. Considering the endogeneity of body mass index, we use the frequency of alcohol consumption as an instrumental variable in order to obtain consistent estimates of its influence.
The two-stage least squares estimation shows that children’s body mass index has a significant negative influence on health complaints and it conditions the way children relate to each other at school. Likewise, results report significant influence on children’s subjective well-being and their self-assessment of general health.
Conclusions
The results of this study provide compelling evidence that BMI plays a crucial role in shaping adolescents’ well-being and their interactions with peers at school. These findings underscore the importance of addressing childhood overweight and promoting healthy body mass index levels. Furthermore, the study highlights the need for targeted policy interventions to combat the social stigma associated with being overweight, fostering a more inclusive and supportive school environment for all students.
Similar content being viewed by others
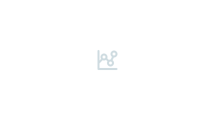
Health-related quality of life in children and adolescents with overweight and obesity: results from the German KIGGS survey
Lara Meixner, Caroline Cohrdes, … Gert B. M. Mensink
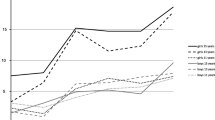
Dieting, body weight concerns and health: trends and associations in Swedish schoolchildren
Christina Berg & Christel Larsson
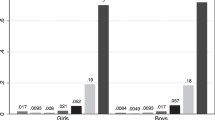
The Impact of Bodyweight on Life Satisfaction among School-Aged Children: Are the Mechanisms Gender-Based?
Victor Iturra & Mauricio Sarrias
Avoid common mistakes on your manuscript.
Introduction
Beyond health concerns, maintaining an optimal weight according to one’s height is crucial for both cognitive and non-cognitive development, as well as for various aspects of life, including social relationships [ 1 , 2 ]. This is important through life, and it becomes particularly prominent during adolescence, a period marked by strong stigmatization of heavier body sizes and its impact on social acceptance and friendships [ 3 ]. This study aims to explore the influence of body mass index (BMI) on health complaints and psychosocial relationships of Spanish teenagers following a causal methodological approach.
Children with excess weight experience poorer physical health. In this regard, childhood obesity is associated with the development of a number of medical complications, such as type 2 diabetes, hypertension, sleep apnea or cholesterol disorders [ 4 ]. All these health issues manifest as musculoskeletal, neurological and gastrointestinal pains [ 5 ] and lead to higher healthcare expenses compared to children with a healthy weight [ 6 ].
In addition to adverse effects on health status, being overweight is associated with lower life satisfaction [ 7 ]. Firstly, somatic complaints reduce the quality of life of children who are overweight [ 8 ]. Secondly, overweight children are also more likely to suffer from depression and anxiety disorders [ 9 ], which are also negatively associated with life satisfaction [ 10 ]. A third channel mediating the relationship between life satisfaction and overweight is social bias. Research indicates that overweight children are more frequently targets of bullying due to factors like social marginalization, lower self-esteem and body dissatisfaction, among others [ 11 , 12 , 13 , 14 ]. In fact, adolescents themselves perceive weight status as one of the main reasons to be bullied by their peers [ 15 ], which underscores the prevalence of weight-based stigma among teenagers [ 16 ]. This discomfort in the learning environment may explain why overweight children are more likely to be absent from school and tend to have a lower academic performance compared to their normal-weight peers [ 17 , 18 , 19 ]. Due to the associated risks of excess weight and its escalating prevalence, childhood overweight and obesity are considered as a worldwide epidemic in modern society [ 20 ].
The percentage of children with excess weight is particularly alarming in Spain, where 40.6% of children Footnote 1 fall into the overweight or obese category. This figure surpassed that of neighbouring countries, with rates of 16.5% in France, 29.6% in Portugal and 29.8% in Italy. Specifically, Spain is ranked third among the European countries with more overweight children [ 21 ]. This is surprising, given that Spain encompasses the Mediterranean diet, argued as one of the healthiest [ 22 ]. In this context, it is especially interesting to deepen the understanding of the consequences of childhood weight on perceived health (psychosomatic complaints) and school life in Spain. Therefore, our first aim is to provide new evidence on this issue. Specifically, we use the data from the “Health Behaviour in School-aged Children” for Spain—2014 (the last survey publicly available); using this dataset, we investigate how body mass index conditions students’ perceived health and school life, including violence, bullying issues and peer support.
Yet, despite the widespread interest in the consequences of weight status, relatively few works have tried to mitigate the endogenous part of weight status [ 23 ]. In this regard, children’s weight status can be endogenous since it is associated with socioeconomic conditions and individual’s surroundings [ 24 ] and these variables are determinants of some outcomes explored in this study, such as life satisfaction. Moreover, the dependencies explored herein could suffer from reverse causality problems. Let us give an example: Do children feel low because they are overweight, or could it be that feeling low predisposes them to gain weight? We found a limited number of studies which have tried to control the biases produced by such confounding problems of weight status on diverse outcomes. Previous research focusing on children's development have used the weight of a biological relative [ 25 , 26 , 27 , 28 ], genetic markers [ 28 ], child’s height [ 29 ] and past weight status of child [ 28 , 30 ] as instrument for child's weight.
In light of these methodological considerations, the evidence provided herein contributes to the existing literature in two significant ways. First, our research implements an instrumental variable procedure, using as instrument the frequency of alcohol consumption. While previous literature has frequently addressed the association between body mass index or overweight/obesity status, few studies have gone a step further and try to get close to a causal effect. The instrumental variable approach allows us to obtain consistent estimates of the influence of body mass index. Second, we provide new empirical evidence about the influence of body mass index on health complaints and school-related factors in Spain. Although some research studies have explored the consequences of weight status in the Spanish case (see [ 31 , 32 ], to the best of our knowledge, there are no previous large-scale studies which have systematically investigated the link between psychosomatic complaints and the body mass index in this country. Footnote 2
This research is based on the Spanish records from the 2013–14 Health Behaviour in School-Aged Children Survey (HBSC). The HBSC is a collaborative study coordinated and sponsored by the World Health Organization (WHO), carried out each 4 years. The goal is to collect information about adolescents’ health. According to the WHO, “health is a state of complete physical, mental and social well-being and not merely the absence of disease or infirmity”. Footnote 3 Following this definition, they collect data related to physical health, well-being, and social environment at home and at school, food and diet, substance use and physical activity. The survey consisted of an online questionnaire completed by adolescences aged 11, 13 and 15 years old in the classroom setting. To obtain a representative sample of the Spanish population of these ages, participants were recruited using a multi-stage random sampling stratified by conglomerates, taking into account the age, the Autonomous Community and the ownership of the school (public or private) [ 34 ]. The Spanish data were collected between March and December 2014.
The number of students participating in Spain was 11,136. To be eligible for the present research, students must have answered the questions related to both height and weight. In particular, around 86% of the sample answer both questions; this left a total of 9,565 students for consideration in the current analysis. Table A1 (Appendix) shows the descriptive statistics of the variables employed in the research. We have analysed if there are any differences between the sample used and the sample excluded by performing a test of mean differences and we have identified significant differences in the higher frequency of feeling psychosomatic complaints, high levels of life satisfaction, high participation in fights and bullying outcomes, and in the set of variables which measure the socioeconomic status (Table A1, Appendix). This should be taken into account when interpreting the results. Besides that, missing answers in the dependent variables may reduce the sample.
Three sets of outcomes are explored in this study: subjective health complaints and life satisfaction, fighting and bullying, and peers’ support. Table A2 (Appendix) summarises the outcome variables—the exact wording of the questions can be seen at Table A1 (Appendix).
Methodology
The main variable of interest in this research is the body mass index (BMI henceforth). This variable is a measure used to classify the population into severe thinness, thinness, normal weight, overweight and obesity, and it is derived from the relationship between height and weight:
Both weight and height are self-reported. We intend to identify empirically the effect of body mass index on health and school outcomes. To begin, we estimate the following ordered probit model:
where \({O}_{i}^{*}\) is a latent outcome variable, further \({O}_{i}^{*}=k\) if \({d}_{k-1}<{O}_{i}^{*}<{d}_{k}\) . The different outcomes are:
Psychosocial complaints: headache, stomach-ache, backache, feeling nervous, difficulties in sleeping and feeling dizzy, with \(k=\mathrm{0,1},\mathrm{2,3},4\) (0 = rarely or never, 1 = about every month, 2 = about every week, 3 = more than once/week, 4 = about every day).
Self-rated general health with \(k=\mathrm{0,1},\mathrm{2,3}\) (0 = poor, 1 = fair, 2 = good, 3 = excellent).
Subjective life satisfaction with \(k= \mathrm{0,1},\mathrm{2,3},\dots , 10\) (0 = worst possible life, 10 = best possible life).
Times of physical fight with \(k=0, 1, 2, \mathrm{3,4}\) (0 = none, 1 = 1 time, 2 = 2 times, 3 = 3 times, 4 = 4 times or more).
Bullied others, been bullied, bullying others, cyberbullied by messages, cyberbullied by pictures with \(k=\mathrm{0,1},\mathrm{2,3},4\) (0 = haven’t, 1 = once or twice, 2 = 2–3 times per month, 3 = once a week, 4 = several times a week).
Friends try to help, can count on friends, have friends to share joys and sorrows, can talk about problems with friends with \(k=\mathrm{1,2},3,\dots ,7\) (1 = very strongly disagree; 7 = very strongly agree).
The explanatory variables can be classified as:
The study-specific variable is the body mass index, represented by BMI.
Demographic characteristics. The vector of covariates \(({X}_{i})\) comprises the students’ demographic characteristics (sex and immigrant status).
Additional control variables. \({S}_{i}\) is a vector with detailed information about the socioeconomic and cultural status: parental occupation (classified from low to high), household possessions (family car, own bedrooms, number of computers, number of bathrooms, dishwasher in home, family holidays), students’ perceptions of family well-off and the practice of physical activity (frequency of doing vigorous physical activity and the hours of exercise per week).
A summary of the set of control variables can be found in Table A3 (Appendix). Since there are observations with missing socioeconomic and cultural characteristics, we replace those missing values with “0” and we add an indicator variable (i.e. missing flag variable). By doing so, we can maximize the number of observations in the sample; \({u}_{i}\) is the idiosyncratic error term.
The estimated \(\beta\) coefficient captures the influence of one point increase of student’s BMI on the outcome of interest, controlling for student’s characteristics. However, this estimation may suffer from bias due to the unobserved factors that may be correlated with BMI and the outcomes under studied. For instance, greater fast food intakes, which lead to gain weight, have been found to be associated with lower life satisfaction [ 35 ]. Besides that, around 50% of body mass index variation is due to individual choices and environment, which suggests that BMI is not exogenous [ 36 ]. To solve this, we employ the instrumental variable (IV) methodology to try to obtain the causal effect of BMI on the set of health and school outcomes. The IV approach has been used by many authors to solve the endogeneity problem of BMI (for instance [ 37 , 38 , 39 ]).
We attempt to address the potential endogeneity of BMI by using the frequency of alcohol consumption as instrumental variable ( \({Z}_{i}\) ). In particular, the question that students’ answer in the HBSC survey is: “On how many days (if any) have you drink alcohol in the last 30 days?”, with seven possible answers (never, 1–2 days, 3–5 days, 6–9 days, 10–19 days, 20–29 days and 30 days or more). This categorical variable has been recoded as a set of binary variables.
To ensure the proper application of this methodology, the instrument must satisfy a number of conditions. In Appendix B, we detail the relevant properties as well as their application to this context.
Due to the categorical nature of the outcomes, we do not directly apply the two-stage least squares (2SLS) estimation to fit the data. Instead, the model is estimated using a conditional (recursive) mixed process estimator. The base model is composed of two equations:
where \({Z}_{i}\) is the frequency of alcohol consumption, \({\varepsilon }_{i}\) and \({\omega }_{i}\) are idiosyncratic random error terms for each equation. Equation ( 3 ) would be some kind of first stage in 2SLS, while Eq. ( 2 ) would be reduced form. The dependent variable in Eq. ( 2 ) is an ordered outcome and it is estimated using ordered probit model. The dependent variable in Eq. ( 3 ) is continuous and the model is estimated using Ordinary Least Squares (OLS). The inference using this structural equation model exploits the presence of an instrumental variable through its inclusion only in Eq. ( 3 ), which is correlated with the outcome only through its effect on the BMI. This is implemented using the Stata command cmp [ 40 ], which uses a canned routine to assure that standard errors are correctly estimated.
First, we estimate the influence of BMI on health outcomes, bullying and peer support assuming that BMI is exogenous—Eq. ( 1 ) in the methodology section. Second, we implement an instrumental variables (IV) estimator that addresses the concern about the endogeneity of BMI—Eqs. ( 2 and 3 ) in the methodology section. The full set of estimates is presented in the Appendix (Tables A4, A5 and A6). Given that probit coefficients have not a meaningful interpretation far from the sign, we have computed average marginal effects to know how large and important differences are. Tables 1 , 2 , 3 , 4 , 5 and 6 show average marginal effects for the key variable (BMI). Each category of the dependent variables requires a separate estimation, which will be denoted by k. For instance, “headache” will present \(k=0, 1, 2, 3, 4\) marginal effects estimations. Tables also report the standard errors, which can be used to calculate the confidence intervals at a specific significance level:
where \(\widehat{\beta }\) is the estimated average coefficient; \(\alpha\) is the significance level; \(SE\) is the standard error; and \({z}_{1-\frac{\alpha }{2}}\) is the percentile of the normal distribution.
Tables also incorporate p -values and significance levels denoted by asterisks to facilitate the interpretation of the results (*** for 1%, ** for 5%, and * for 10%).
Health and life satisfaction
To begin, Tables 1 and 2 model the influence of BMI on health complaints, self-assessment of health and life satisfaction, controlling for socioeconomic and demographic factors. First, we assume that BMI is exogenous (Table 1 ). In this case, we identified that BMI is positively associated with health complaints but negatively associated with self-assessment of health and life satisfaction.
Then, we account for the endogeneity of BMI (Table 2 ). The direction of the association between BMI and the outcomes does not change, but coefficient estimates of BMI are considerably higher for IV than non-IV. For instance, one-point increase in the BMI raises the likelihood of having headaches more than once a week by 1.7% (average marginal effects for k = 3). The likelihood of reporting other psychosocial complaints more than once a week is very similar. With one-point increase of BMI, the likelihood of having backache increases by 1%, feeling nervous by 1.1%, having difficulties in sleeping by 1%, feeling dizzy by 1.2% and stomachache by 1.5%.
Regarding self-assessment of general health, IV specification points towards a significant and negative influence of BMI. In particular, students are 8.2 points less likely to say that their health is excellent with one-unit increase of BMI. Similarly, BMI is negatively associated with students’ subjective well-being. One-point increase of BMI decreases the likelihood of having “best possible life” by 7.7%.
Lastly, Eq. ( 3 ) models students’ BMI conditional on alcohol consumption and socioeconomic and demographic variables. As observed, the frequency of alcohol consumption is significantly associated with BMI. Just having drunk alcohol during the last 30 days once or twice—which could be considered a small frequency compared to the reference category (not having drunk)—seems to be positively associated with BMI. Particularly, it increases BMI by 1 point. This result holds for all the specifications.
Fighting and bullying
Tables 3 and 4 show the influence of BMI on fighting and bullying indicators, adjusted for socioeconomic and demographic characteristics. In Table 3 , the association between BMI and the variables under investigation is explored using ordered probit estimations. According to these models, it seems that BMI does not influence students’ participation in fights nor does the likelihood of suffering bullying. We only found a significant positive association (although weak) of BMI in the probability of bullying other peers.
However, when instrumenting the BMI (Table 4 ), its influence turns significant. As shown in Eq. ( 2 ) (IV approach), one-point increase of BMI increases the likelihood of participating in fights 4 times or more during the last 12 months by 4.2% (average marginal effects for k = 4). In the same way, having a higher BMI is positively associated with perpetrating bullying against others. The average marginal effect of BMI is equal to 2.4% increase in the likelihood of bullying other peers more than once a week ( k = 4).
Regarding experiences of being bullied, results show that an increase of BMI leads to lower probability of being a victim of bullying. In particular, the increase of BMI is associated with 1.9% likelihood of not being a victim of bullying ( k = 0). Conversely, when it comes to cyberbullying, BMI is positively associated. Specifically, one-point increase of BMI is associated with 1.4% higher likelihood of being cyberbullied by messages several times a week (e.g., instant messages, wall postings, emails and text messages, or created a website) ( k = 4) and 1.8% of being photographed without permission and the pictures posted online.
It is worth noting that the general bullying question seems related to bullying happening in school, considering the definition provided in the HBSC questionnaire. Footnote 4 Thus, higher BMI prevents students to be bullied in person (at school), but increase the likelihood of being bullied online. To the same extent, the greater physical build associated with higher BMI increases the probability of bullying other peers at school and being involved in physical fights.
Social context: peers’ support
Tables 5 and 6 report the influence of BMI on peers’ support, using as indicators: friends’ help, trust in friends, share joys and sorrows with friends and can talk with friends about problems. In the first equation (BMI exogenous, Table 5 ), it seems that BMI is significantly and positively associated with all the measures of peers’ support.
However, considering BMI as exogenous may overestimate the influence of BMI on peers’ support. When modelling BMI, the association between BMI and the questions related with having friends to share joys and sorrows and talk about problems disappears (Table 6 ). Nevertheless, the association of BMI with the statements related to friends’ help and trust in friends remains negative and significant. In particular, results show that the likelihood of strongly agreeing ( k = 7) with receiving friends’ help drops by 4% as a result of one-point increase of BMI. Similarly, it is also less likely that students strongly agree with the statement related to counting on friends when increasing BMI (average marginal effect of -2.9%).
Discussion and conclusions
In this research, we have explored the association of BMI with perceived health, bullying and social support at school. In order to undertake this study, we have employed the Spanish records from the 2013–14 Health Behaviour in School-Aged Children Survey . With the aim of mitigating the endogeneity of BMI, we have employed an IV strategy, using alcohol consumption as instrumental variable. Other important strengths of the study are the large sample size and the array of socioeconomic and demographic controls.
The empirical evidence confirms that BMI conditions the daily life of Spanish students. Firstly, we found that BMI is associated with more psychosomatic complaints, such as backaches, headaches and sleeping disorders. This positive association between BMI and psychosomatic complaints had been described in other countries like Finland [ 41 ], Germany [ 42 ] or Sweden [ 43 ]. In addition to this, an increase of BMI is associated with lower life satisfaction; this effect is within the trend observed in western countries, in which excess of weight is regarded negatively, and hence reduces subjective well-being [ 44 ].
Secondly, BMI increases the frequency of participating in physical fights and bullying other students at school. Conversely to the previous studies for Spain [ 31 , 32 ], we have found that BMI is not significantly associated with the risk of bullying victimization at school (face-to-face bullying). However, our results note that BMI is significantly associated with the bullying developed in the online realm (cyberbullying). This entails that overweight students suffer more verbal than physical abuse [ 45 ]. According to Kowalski et al. [ 46 ], the perceived anonymity of the aggressor and the higher accessibility are adverse effects of online bullying compared to face-to-face bullying, which justify its expansion.
The specific organization of a school day in Spain further illuminates the notable association of bullying victimization online and its absence during regular school hours. In Spain, the majority of schools follow a condensed schedule, with only morning sessions [ 47 ]. Consequently, the opportunities for in-person interactions out of the classroom are limited to the school break which is short, while they persist or even increase out-of-school, given the time that children spend connected to the Internet. In fact, Spanish adolescents spend most of their free time using the mobile phone, tablet or computers [ 48 ]. On average, children between 9 and 16 years old connect to the Internet more than 3 h a day in Spain, mainly for activities related to communication and entertainment [ 49 ].
Lastly, results show that the increase of BMI makes more difficult to feel peer support. Specifically, a weight gain reduces the chances of feeling friend’s help and counting on friends. The social isolation experienced by overweight students might be based on the weight stigma , which acts as a foundation for social disapproval: “overweight or obesity are negatively stereotyped as being lazy, lacking willpower and self-discipline, unmotivated to improve their health” (p. 402, [ 50 ]. An underlying driver of the lower social support might be the constant exposure to unrealistic beauty standards prevalent on the Internet. In particular, Calado et al. [ 51 ] highlight a significant association between exposure kinds of media topics related to body image—such as dieting, fashion, and fitness—and body dissatisfaction, which affects more to female than male.
Policy interventions resulting from these findings may be driven in different ways. On the one hand, they should be focused on helping children to achieve a healthy BMI. As it happens in other countries, such as the United States, health assessment at school can be a useful tool to inform parents and children about healthy weight ranges. In Spain, given that medical check-ups are available through the National Health System, there is no health assessment at school. However, we find it relevant to develop this action at school, since it ensures to reach the target group.
On the other hand, the high prevalence of overweight among Spanish youth may be attributed to their lifestyle. Research by Grosso and Galvano [ 52 ] demonstrated a low adherence to the Mediterranean diet among children and adolescents in Spain and a decreasing trend in this adherence. This relationship was mediated by various social and demographic factors; notably, individuals from socioeconomically advantaged backgrounds tended to exhibit higher adherence to the diet. In this context, a second recommendation is to implement nutrition education programs at school to promote healthy eating habits from a young age, paying special attention to low-income schools.
In addition, this study alerts to higher discrimination as a result of the increase of BMI, particularly in online environments. Consequently, policy actions should be targeted at dealing with the social stigma of overweight. Cultivating a culture of non-discrimination within school is a challenge that involves the entire educational community. In the classroom, there are various approaches, like discussing and identifying weight stereotypes, designing mechanisms to monitor incidents of discrimination or involving students in peer mediation activities. Regarding the role of parents, they should pay particular attention to the time that children spend on the Internet using social networks which, together with an inadequate use, may foster cyberbullying. In this sense, enhancing parents’ awareness of children’s use of social networks can help prevent cyberbullying. A practical approach to monitoring online activity is to use parental control apps.
Some limitations apply to the present study. First of all, the use of IV tries to deal with the endogeneity problem of BMI, but there might be other unobservable variables that we cannot control for. For instance, prior research indicates a significant correlation between adolescent life satisfaction and family structure [ 53 ], a relationship that we currently lack information on. Secondly, it is worth noting that all measures were self-reported. While this approach is advisable for some variables, for others the results should be interpreted with caution. For example, when it comes to assessing bullying comprehensively, anonymous self-reports tend to be the most reliable, as school personnel and classmates may not always be aware of every instance [ 54 ]. However, when it comes to self-reporting weight and height, there can be a risk of misclassification. Previous investigations indicate a pattern of underreporting for weight, along with over-reporting for height, which leads to a lower BMI and tends to underestimate the prevalence of overweight and obesity [ 55 ].
Further research that incorporates objective measurements of height and weight is needed to investigate the impact of BMI on experiences of bullying and peer support in a more accurate way. Additionally, it is important to note that the majority of studies examining the relationship between BMI and the school context are correlational. While this research takes a step towards establishing causality, more causal statements are needed. To better address these questions, the implementation of longitudinal assessments throughout the school period would be particularly valuable.
Data availability
The database used in this research is available through the next link: https://hbsc.org/data/ .
Data retrieved from Global Obesity Observatory. Access link: https://data.worldobesity.org/tables/prevalence-of-child-overweight-including-obesity-3/
Drosopoulou et al. [ 33 ] conducted a correlational study which analysed the relationship between psychosocial health and body mass index including a sample of 1,492 Spanish adolescences. The sample size was quite smaller than the one used in this study (n = 11,136) and they did not try to approach a causal effect.
WHO (1946). Constitution of the World Health Organization. https://www.who.int/about/governance/ constitution.
The introduction to bullying questions in the HBSC questionnaire (2013-14) indicates: “We say a student is being bullied when another student, or a group of students, say or do nasty and unpleasant things to him or her. It is also bullying when a student is teased repeatedly in a way he or she does not like or when he or she is deliberately left out of things. But it is not bullying when two students of about the same strength or power argue or fight. It is also not bullying when a student is teased in a friendly and playful way”.
Florin, T. A., Shults, J., & Stettler, N. (2011). Perception of overweight is associated with poor academic performance in US adolescents. Journal of School Health, 81 (11), 663–670. https://doi.org/10.1111/j.1746-1561.2011.00642.x
Article PubMed Google Scholar
Lin, Y. C., Latner, J. D., Fung, X. C., & Lin, C. Y. (2018). Poor health and experiences of being bullied in adolescents: Self-perceived overweight and frustration with appearance matter. Obesity, 26 (2), 397–404. https://doi.org/10.1002/oby.22041
Crosnoe, R., Frank, K., & Mueller, A. S. (2008). Gender, body size and social relations in American high schools. Social Forces, 86 (3), 1189–1216. https://doi.org/10.1353/sof.0.0004
Article Google Scholar
Barlow, S. E., & Dietz, W. H. (2002). Management of child and adolescent obesity: Summary and recommendations based on reports from pediatricians, pediatric nurse practitioners, and registered dietitians. Pediatrics, 110 (1), 236–238. https://doi.org/10.1542/peds.110.S1.236
Pinhas-Hamiel, O., Frumin, K., Gabis, L., Mazor-Aronovich, K., Modan-Moses, D., Reichman, B., & Lerner-Geva, L. (2008). Headaches in overweight children and adolescents referred to a tertiary-care center in Israel. Obesity, 16 (3), 659–663. https://doi.org/10.1038/oby.2007.88
Skinner, A. C., Mayer, M. L., Flower, K., & Weinberger, M. (2008). Health status and health care expenditures in a nationally representative sample: How do overweight and healthy-weight children compare? Pediatrics, 121 (2), e269–e277. https://doi.org/10.1542/peds.2007-0874
Lebacq, T., Dujeu, M., Méroc, E., Moreau, N., Pedroni, C., Godin, I., & Castetbon, K. (2019). Perceived social support from teachers and classmates does not moderate the inverse association between body mass index and health-related quality of life in adolescents. Quality of Life Research, 28 , 895–905. https://doi.org/10.1007/s11136-018-2079-x
Hassan, H., Paulis, W. D., Bindels, P. J., Koes, B. W., & van Middelkoop, M. (2021). Somatic complaints as a mediator in the association between body mass index and quality of life in children and adolescents. BMC Family Practice, 22 (1), 1–9. https://doi.org/10.1186/s12875-021-01562-1
Article CAS Google Scholar
BeLue, R., Francis, L. A., & Colaco, B. (2009). Mental health problems and overweight in a nationally representative sample of adolescents: Effects of race and ethnicity. Pediatrics, 123 (2), 697–702. https://doi.org/10.1542/peds.2008-0687
Zhao, G., Ford, E. S., Dhingra, S., Li, C., Strine, T. W., & Mokdad, A. H. (2009). Depression and anxiety among US adults: Associations with body mass index. International Journal of Obesity, 33 (2), 257–266. https://doi.org/10.1038/ijo.2008.268
Article CAS PubMed Google Scholar
Moradi, M., Mozaffari, H., Askari, M., & Azadbakht, L. (2021). Association between overweight/obesity with depression, anxiety, low self-esteem, and body dissatisfaction in children and adolescents: A systematic review and meta-analysis of observational studies. Critical Reviews in Food Science and Nutrition, 62 (2), 555–570. https://doi.org/10.1080/10408398.2020.1823813
Strauss, R. S., & Pollack, H. A. (2003). Social marginalization of overweight children. Archives of Pediatrics & Adolescent Medicine, 157 (8), 746–752. https://doi.org/10.1001/archpedi.157.8.746
Thompson, I., Hong, J. S., Lee, J. M., Prys, N. A., Morgan, J. T., & Udo-Inyang, I. (2020). A review of the empirical research on weight-based bullying and peer victimisation published between 2006 and 2016. Educational Review, 72 (1), 88–110. https://doi.org/10.1080/00131911.2018.1483894
Van Geel, M., Vedder, P., & Tanilon, J. (2014). Are overweight and obese youths more often bullied by their peers? A meta-analysis on the relation between weight status and bullying. International Journal of Obesity, 38 (10), 1263–1267. https://doi.org/10.1038/ijo.2014.117
Puhl, R. M., Luedicke, J., & Heuer, C. (2011). Weight-based victimization toward overweight adolescents: Observations and reactions of peers. Journal of School Health, 81 (11), 696–703. https://doi.org/10.1111/j.1746-1561.2011.00646.x
Krukowski, R. A., Smith West, D., Philyaw Perez, A., Bursac, Z., Phillips, M. M., & Raczynski, J. M. (2009). Overweight children, weight-based teasing and academic performance. International Journal of Pediatric Obesity, 4 (4), 274–280. https://doi.org/10.3109/17477160902846203
An, R., Yan, H., Shi, X., & Yang, Y. (2017). Childhood obesity and school absenteeism: A systematic review and meta-analysis. Obesity Reviews, 18 (12), 1412–1424. https://doi.org/10.1111/obr.12599
Averett, S. L., & Stifel, D. C. (2010). Race and gender differences in the cognitive effects of childhood overweight. Applied Economics Letters, 17 (17), 1673–1679. https://doi.org/10.1080/13504850903251256
Sabia, J. J., & Rees, D. I. (2015). Body weight, mental health capital, and academic achievement. Review of Economics of the Household, 13 (3), 653–684. https://doi.org/10.1007/s11150-014-9272-7
Peralta, M., Ramos, M., Lipert, A., Martins, J., & Marques, A. (2018). Prevalence and trends of overweight and obesity in older adults from 10 European countries from 2005 to 2013. Scandinavian Journal of Public Health, 46 (5), 522–529. https://doi.org/10.1177/1403494818764810
WHO (2022). WHO European regional obesity report 2022 . World health organization: Regional office for Europe. Retrieved from https://www.who.int/europe/publications/i/item/9789289057738
Sofi, F., Macchi, C., Abbate, R., Gensini, G. F., & Casini, A. (2014). Mediterranean diet and health status: An updated meta-analysis and a proposal for a literature-based adherence score. Public Health Nutrition, 17 (12), 2769–2782. https://doi.org/10.1017/S1368980013003169
Segal, A. B., Huerta, M. C., Aurino, E., & Sassi, F. (2021). The impact of childhood obesity on human capital in high-income countries: A systematic review. Obesity Reviews, 22 (1), e13104. https://doi.org/10.1111/obr.13104
Gibbs, B. G., & Forste, R. (2014). Socioeconomic status, infant feeding practices and early childhood obesity. Pediatric Obesity, 9 (2), 135–146. https://doi.org/10.1111/j.2047-6310.2013.00155.x
Iturra, V., & Sarrias, M. (2022). The impact of bodyweight on life satisfaction among school-aged children: Are the mechanisms gender-based? Child Indicators Research . https://doi.org/10.1007/s12187-022-09973-x
Sabia, J. J. (2007). The effect of body weight on adolescent academic performance. Southern Economic Journal, 73 (4), 871–900. https://doi.org/10.1002/j.2325-8012.2007.tb00809.x
Sarrias, M., & Blanco, A. (2022). Bodyweight and human capital development: Assessing the impact of obesity on socioemotional skills during childhood in Chile. Economics & Human Biology, 47 , 101190. https://doi.org/10.1016/j.ehb.2022.101190
Scholder, S. V. H. K., Smith, G. D., Lawlor, D. A., Propper, C., & Windmeijer, F. (2012). The effect of fat mass on educational attainment: Examining the sensitivity to different identification strategies. Economics & Human Biology, 10 (4), 405–418. https://doi.org/10.1016/j.ehb.2012.04.015
Katsaiti, M. S. (2012). Obesity and happiness. Applied Economics, 44 (31), 4101–4114. https://doi.org/10.1080/00036846.2011.587779
Kaestner, R., & Grossman, M. (2009). Effects of weight on children’s educational achievement. Economics of Education Review, 28 (6), 651–661. https://doi.org/10.1016/j.econedurev.2009.03.002
Garcia-Continente, X., Pérez-Giménez, A., Espelt, A., & Nebot Adell, M. (2013). Bullying among schoolchildren: Differences between victims and aggressors. Gaceta Sanitaria, 27 , 350–354. https://doi.org/10.1016/j.gaceta.2012.12.012
Lopez-Agudo, L. A., & Marcenaro-Gutierrez, O. D. (2021). The relationship between overweight and academic performance, life satisfaction and school life. Food Policy, 101 , 102077. https://doi.org/10.1016/j.foodpol.2021.102077
Drosopoulou, G., Sergentanis, T. N., Mastorakos, G., Vlachopapadopoulou, E., Michalacos, S., Tzavara, C., Bacopoulou, F., Psaltopoulou, T., & Tsitsika, A. (2021). Psychosocial health of adolescents in relation to underweight, overweight/obese status: The EU NET ADB survey. European Journal of Public Health, 31 (2), 379–384. https://doi.org/10.1093/eurpub/ckaa189
Ministerio de Sanidad, Servicios Sociales e Igualdad (2016). Informe técnico de los resultados obtenidos por el Estudio Health Behaviour in School-aged Children (HBSC) 2014 en España . Retrieved from: https://www.sanidad.gob.es/profesionales/saludPublica/prevPromocion/promocion/saludJovenes/estudioHBSC/docs/HBSC2014/HBSC2014_InformeTecnico.pdf
Ford, P. A., Jaceldo-Siegl, K., Lee, J. W., Youngberg, W., & Tonstad, S. (2013). Intake of Mediterranean foods associated with positive affect and low negative affect. Journal of Psychosomatic Research, 74 (2), 142–148. https://doi.org/10.1016/j.jpsychores.2012.11.002
Comuzzie, A. G., & Allison, D. B. (1998). The search for human obesity genes. Science, 280 (5368), 1374–1377. https://doi.org/10.1126/science.280.5368.1374
Article ADS CAS PubMed Google Scholar
Asirvatham, J., Nayga, R. M., Jr., & Thomsen, M. R. (2014). Peer-effects in obesity among public elementary school children: A grade-level analysis. Applied Economic Perspectives and Policy, 36 (3), 438–459. https://doi.org/10.1093/aepp/ppu011
Cawley, J. (2004). The impact of obesity on wages. Journal of Human Resources, 39 (2), 451–474. https://doi.org/10.3368/jhr.XXXIX.2.451
Shi, H., & Li, C. (2018). Does weight status affect academic performance? Evidence from Australian children. Applied Economics, 50 (29), 3156–3170. https://doi.org/10.1080/00036846.2017.1418077
Article MathSciNet Google Scholar
Roodman, D. (2011). Fitting fully observed recursive mixed-process models with cmp. The Stata Journal, 11 (2), 159–206. https://doi.org/10.1177/1536867X1101100202
Honkinen, P. L. K., Suominen, S. B., Välimaa, R. S., Helenius, H. Y., & Rautava, P. T. (2005). Factors associated with perceived health among 12-year-old school children. Relevance of physical exercise and sense of coherence. Scandinavian Journal of Public Health, 33 (1), 35–41. https://doi.org/10.1080/14034940410028307
Krul, M., van der Wouden, J. C., Schellevis, F. G., van Suijlekom-Smit, L. W., & Koes, B. W. (2009). Musculoskeletal problems in overweight and obese children. The Annals of Family Medicine, 7 (4), 352–356. https://doi.org/10.1370/afm.1005
Brooks, S. J., Feldman, I., Schiöth, H. B., & Titova, O. E. (2021). Important gender differences in psychosomatic and school-related complaints in relation to adolescent weight status. Scientific Reports, 11 (1), 1–9. https://doi.org/10.1038/s41598-021-93761-0
Sato, K. (2021). Unhappy and happy obesity: A comparative study on the United States and China. Journal of Happiness Studies, 22 (3), 1259–1285. https://doi.org/10.1007/s10902-020-00272-2
Wang, J., Iannotti, R. J., & Luk, J. W. (2010). Bullying victimization among underweight and overweight US youth: Differential associations for boys and girls. Journal of Adolescent Health, 47 (1), 99–101. https://doi.org/10.1016/j.jadohealth.2009.12.007
Kowalski, R. M., Giumetti, G. W., Schroeder, A. N., & Lattanner, M. R. (2014). Bullying in the digital age: A critical review and meta-analysis of cyberbullying research among youth. Psychological Bulletin, 140 (4), 1073. https://doi.org/10.1037/a0035618
Kamette, F. (2011). Organisation of school time in the European Union. European Issues, 212 , 1–6.
Google Scholar
UNICEF (2021). Impacto de la tecnología en la adolescencia. Relaciones, riesgos y oportunidades. UNICEF España: Universidad de Santiago de Compostela y Consejo General de Colegios Profesionales de Ingeniería en Informática. Retrieved from https://www.unicef.es/sites/unicef.es/files/comunicacion/Informe_estatal_impacto-tecnologia-adolescencia.pdf
Smahel, D., Machackova, H., Mascheroni, G., Dedkova, L., Staksrud, E., Ólafsson, K., Livingstone, S., & Hasebrink, U. (2020). EU kids online 2020: Survey results from 19 countries. EU Kids Online . https://doi.org/10.21953/lse.47fdeqj01ofo
Puhl, R. M., & Lessard, L. M. (2020). Weight stigma in youth: Prevalence, consequences, and considerations for clinical practice. Current Obesity Reports, 9 (4), 402–411. https://doi.org/10.1007/s13679-020-00408-8
Calado, M., Lameiras, M., Sepulveda, A. R., Rodriguez, Y., & Carrera, M. V. (2011). The association between exposure to mass media and body dissatisfaction among Spanish adolescents. Women’s Health Issues, 21 (5), 390–399. https://doi.org/10.1016/j.whi.2011.02.013
Grosso, G., & Galvano, F. (2016). Mediterranean diet adherence in children and adolescents in southern European countries. NFS Journal, 3 , 13–19. https://doi.org/10.1016/j.nfs.2016.02.004
Antaramian, S. P., Huebner, E. S., & Valois, R. F. (2008). Adolescent life satisfaction. Applied Psychology, 57 , 112–126. https://doi.org/10.1111/j.1464-0597.2008.00357.x
Lacey, A., & Cornell, D. (2013). The impact of teasing and bullying on schoolwide academic performance. Journal of Applied School Psychology, 29 (3), 262–283. https://doi.org/10.1080/15377903.2013.806883
Gorber, S. C., Tremblay, M., Moher, D., & Gorber, B. (2007). A comparison of direct vs. self-report measures for assessing height, weight and body mass index: A systematic review. Obesity Reviews, 8 (4), 307–326. https://doi.org/10.1111/j.1467-789X.2007.00347.x
Angrist, J. D., & Pischke, J. S. (2009). Mostly harmless econometrics: An empiricist’s companion . Princeton University Press.
Book Google Scholar
Kleiner, K. D., Gold, M. S., Frostpineda, K., Lenzbrunsman, B., Perri, M. G., & Jacobs, W. S. (2004). Body mass index and alcohol use. Journal of Addictive Diseases, 23 (3), 105–118. https://doi.org/10.1300/J069v23n03_08
Stock, J. H., & Yogo, M. (2005). Testing for weak instruments in linear IV regression. In D. W. K. Andrews & J. H. Stock (Eds.), Identification and inference for econometric models: Essays in honor of Thomas Rothenberg (pp. 80–108). Cambridge University Press.
Chapter Google Scholar
Swanson, S. A., & Hernán, M. A. (2018). The challenging interpretation of instrumental variable estimates under monotonicity. International Journal of Epidemiology, 47 (4), 1289–1297. https://doi.org/10.1093/ije/dyx038
Traversy, G., & Chaput, J. P. (2015). Alcohol consumption and obesity: An update. Current Obesity Reports, 4 (1), 122–130. https://doi.org/10.1007/s13679-014-0129-4
Article PubMed PubMed Central Google Scholar
Wooldridge, J. M. (2010). Econometric analysis of cross section and panel data . MIT press.
Download references
Acknowledgements
This work has been partly supported by Ministerio de Ciencia e Innovación (under Research Project PID2020-119471RB-I00), the Andalusian Regional Government (SEJ-645), the Fundación Ramón Areces (under Research Project B1-2022_23) and Universidad de Málaga/CBUA (funding for open access charge).
Funding for open access publishing: Universidad de Málaga/CBUA. This study was funded by Ministerio de Ciencia e Innovación (under Research Project PID2020-119471RB-I00), Junta de Andalucía (under Research Project SEJ-645), Fundación Ramón Areces (under Research Project B1-2022_23) and Universidad de Málaga /CBUA (funding for open access charge).
Author information
Authors and affiliations.
Departamento de Economía Aplicada (Estadística y Econometría), Facultad de Ciencias Económicas y Empresariales, Universidad de Málaga, Plaza de El Ejido s/n, 29013, Málaga, Spain
Claudia Prieto-Latorre, Luis Alejandro Lopez-Agudo & Oscar David Marcenaro-Gutierrez
You can also search for this author in PubMed Google Scholar
Corresponding author
Correspondence to Claudia Prieto-Latorre .
Ethics declarations
Conflict of interest.
All authors declare that they have no conflict of interest.
Additional information
Publisher's note.
Springer Nature remains neutral with regard to jurisdictional claims in published maps and institutional affiliations.
Supplementary Information
Below is the link to the electronic supplementary material.
Supplementary file1 (DOCX 103 kb)
Supplementary file2 (docx 17 kb), rights and permissions.
Open Access This article is licensed under a Creative Commons Attribution 4.0 International License, which permits use, sharing, adaptation, distribution and reproduction in any medium or format, as long as you give appropriate credit to the original author(s) and the source, provide a link to the Creative Commons licence, and indicate if changes were made. The images or other third party material in this article are included in the article's Creative Commons licence, unless indicated otherwise in a credit line to the material. If material is not included in the article's Creative Commons licence and your intended use is not permitted by statutory regulation or exceeds the permitted use, you will need to obtain permission directly from the copyright holder. To view a copy of this licence, visit http://creativecommons.org/licenses/by/4.0/ .
Reprints and permissions
About this article
Prieto-Latorre, C., Lopez-Agudo, L.A. & Marcenaro-Gutierrez, O.D. Influence of body mass index on health complains and life satisfaction. Qual Life Res 33 , 705–719 (2024). https://doi.org/10.1007/s11136-023-03557-0
Download citation
Accepted : 03 November 2023
Published : 01 December 2023
Issue Date : March 2024
DOI : https://doi.org/10.1007/s11136-023-03557-0
Share this article
Anyone you share the following link with will be able to read this content:
Sorry, a shareable link is not currently available for this article.
Provided by the Springer Nature SharedIt content-sharing initiative
- Body mass index
- Instrumental variable
- Psychosomatic complaints
- Life satisfaction
JEL Classification
- Find a journal
- Publish with us
- Track your research
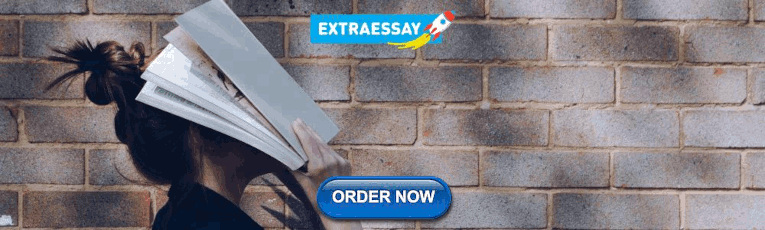
IMAGES
VIDEO
COMMENTS
Dissertation Advisor: Dr. Edward Giovannucci Donghoon Lee Predicted Lean Body Mass and Fat Mass: Novel Insights into Obesity, Chronic Disease, and Mortality Research Abstract Body mass index (BMI) is widely used measure of overall adiposity in epidemiological studies. However, BMI has a critical limitation that it cannot distinguish different ...
Background: We assessed whether body mass index (BMI) affects social and socio-economic outcomes. Methods: We used Mendelian randomization (MR), non-linear MR and non-genetic and MR within-sibling analyses, to estimate relationships of BMI with six socio-economic and four social outcomes in 378 244 people of European ancestry in UK Biobank.
personal factors, behavioral factors, and body mass index; and between behavioral and environmental factors. Positive social change implications include the U.S. Army using these findings to promote healthy lifestyles, reduce stress, and increase physical fitness among soldiers to achieve higher self-efficacy and a lower body mass index.
On Body Mass Index Analysis from Human Visual Appearance Min Jiang [email protected] Follow this and additional works at: https://researchrepository.wvu.edu/etd Recommended Citation Jiang, Min, "On Body Mass Index Analysis from Human Visual Appearance" (2020). Graduate Theses, Dissertations, and Problem Reports. 7633.
Overweight and obesity can be defined as possessing abnormal excessive body fat accumulation that can cause harm to one's health (Lavie et al., 2017). Body mass index (BMI) is a measure which is commonly used in the estimation and monitoring of overweight and obesity in the population and it is expressed mathematically as (BMI = Kg/m²).
ASSESSMENT AND PREDICTION OF BODY MASS INDEX (BMI) DISTRIBUTIONS AMONG ADULT POPULATIONS . IN MEXICO, COLOMBIA, AND PERU, 1988-2014 . by Goro Yamada . A dissertation submitted to Johns Hopkins University in conformity with the requirements for the degree of Doctor of Philosophy . Baltimore, Maryland . October, 2016
INTRODUCTION. Recent estimates show that more than 1 in 3 adults aged 60y and over in England and Scotland are obese (Body mass index or BMI, >30 kg/m 2) [], and similar rates have been found for other European countries and the US [2,3].On average, body weight increases steadily from mid-adulthood to the age of 65-70y [4-6], accompanied by ageing-related changes in body composition, such as ...
The aim of this study was to measure the association between body mass index (BMI) and satisfaction with work life. This study included participants from an international multicenter field‐test study of BODY‐Q scales. Recruitment took place at hospitals in Denmark, The Netherlands and USA between June 2019 and January 2020.
Dissertations UMSL Graduate Works 7-24-2014 Structural and cognitive correlates of body mass index in healthy older adults ... Obesity, commonly measured with body mass index (BMI), is associated with numerous deleterious health conditions and has been identified as a potential
According to the World Health Organization, obesity is one of the greatest public-health challenges of the 21st century. Body weight is also known to affect individuals' self-esteem and interpersonal relationships, including romantic ones. We estimate the "utility-maximizing" Body Mass Index (BMI) and calculate the implied monetary value of changes in both individual and spousal BMI ...
Maintaining a healthy caloric intake and expenditure balance is challenging. The preliminary study examined (a) the associations of Body Mass Index (BMI), body image, perceived stress, mental health, dietary habits, and exercise participation among 310 female college nursing students and (b) the differences in these factors among BMI subgroups. The cutoffs for underweight, normal weight ...
Siahpush et al. (2013) observed that there were marked inequities in body mass index (BMI) levels across ethnic groups. In the case of North Carolina, 36.3% and 29.6% of the individuals living in North Carolina were overweight (BMI = 25.0-29.9 kg/m2) and obese (BMI > 30 kg/m2) respectively (Hoban, 2012). In addition, obesity rates were higher ...
This dissertation explores associations between body mass index (BMI) and self-perceived weight during adolescence and two health outcomes during young adulthood: 1) testing positive for one or more of three sexually transmitted diseases (STD) (Chlamydia trachomatis, Neisseria gonorrhoeae, and Trichomonas vaginalis) and 2) reporting intimate ...
adult obesity rate (body mass index ≥30) of 17.2% and a combined overweight/obesity prevalence (body mass index ≥25) of 53.6% (Levi, et al. 2011). Body mass index is a measure of weight status calculated from weight and height (kg/m2). By 2010, Michigan's obesity rate rose to 30.5% and the combined overweight/obesity prevalence
Of 4123 participants, 2515 completed the BODY-Q Work Life scale. BMI groups showed significant difference in work life satisfaction (p < .0001). The Work Life scale mean score was 77.6 for the normal BMI group, 78.5 for the overweight group and 75.0, 68.9 and 63.8 for Class 1, 2 and 3 obesity, respectively.
Obesity is a classified risk factor for several of the world's leading causes of death. In this study, we combined information contained in body mass index (BMI), total percentage body fat (TPBF) and relative fat mass (RFM) to estimate obesity prevalence and examine the risk factors associated with obesity. The study recruited 1027 undergraduate students aged between 16 and 25 years using a ...
Background: Recent studies have found that long-term changes in weight during adulthood are associated with a high risk of mortality. The objective of this study was to characterize body mass index (BMI) trajectories during adulthood and to examine the association between BMI trajectories and risk of death in the Japanese population.
Economics ETDs Electronic Theses and Dissertations Fall 12-2018 Essays on the Factors that Contribute to Body Mass Index Veronica Salinas University of New Mexico Follow this and additional works at:https://digitalrepository.unm.edu/econ_etds Part of theHealth Economics Commons, and theLabor Economics Commons
OBJECTIVE: To examine the relationships between socioeconomic status (SES), body mass index (BMI), and vitamin D levels in African American (AA) women living in areas with abundant sunshine; and to explore if diabetes moderates these relationships. SIGNIFICANCE: More AA's live in poverty, and experience obesity, diabetes, and chronic disease compared to other groups. Eighty percent of AA women ...
A logistic regression model using secondary data from the Behavioral Risk Factor Surveillance System revealed a significant association in income levels, house hold size, dietary intake, physical activity, and increased BMI levels in African American women. African American women were significantly more likely to be obese (r = 0.93 p > 0.05 ...
Body image or satisfaction with physical appearance has been established as an important aspect of self-worth and mental health across the life span. It is related to self-esteem, sexuality, family relationships and identity. Given the fact that physical appearance is a multifaceted structural concept that depends, not only on inner-biological, but also a psychological and socio-cultural ...
Purpose This research work investigates the influence of children's weight status on well-being and school context in a sample of Spanish adolescences. Methods The Spanish records from the 2013-14 Health Behaviour in School-Aged Children Survey are used, which gathers 9,565 adolescences aged 11, 13 and 15. Studies do not usually address the endogeneity of body mass index when analysing ...
This Dissertation is brought to you for free and open access by the Walden Dissertations and Doctoral Studies Collection at ScholarWorks. It has been ... Disease Control (CDC) began keeping records on body mass index (BMI) statistics on the population in 1985; by 2012, all 50 states had an average obesity rate of more than
Theses & Dissertations 2023 Relationship between crown like structures and body mass index in breast cancer Sophie Wanjiru Irungu Aga Khan University ... multiple measures of obesity, body mass index (BMI) is the most easily obtained and the most commonly measured. In most studies of European ancestry, a BMI of 30kg/m