Communication
iResearchNet
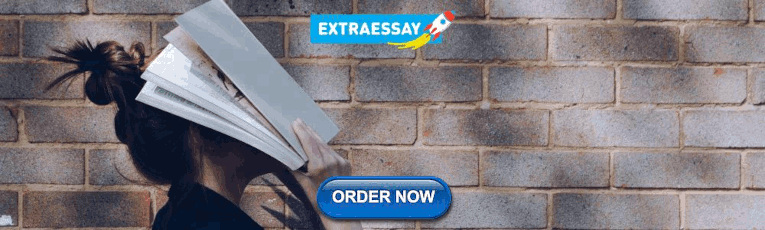
Custom Writing Services
Quantitative content analysis.
Quantitative content analysis is an empirical method used in the social sciences primarily for analyzing recorded human communication in a quantitative, systematic, and intersubjective way. This material can include newspaper articles, films, advertisements, interview transcripts, or observational protocols, for instance. Thus, a quantitative content analysis can be applied to verbal material, and also to visual material like the evening news or television entertainment. Surveys, observations, and quantitative content analysis are the main three methods of data collection in empirical communication research, with quantitative content analysis the most prominent in the field. In other disciplines like psychology or sociology quantitative content analysis is not used as widely.
Quantitative And Qualitative Content Analysis
Ole R. Holsti (1969) defines quantitative content analysis as “any technique for making inferences by objectively and systematically identifying specified characteristics of messages.” Bernhard Berelson (1952) speaks of “a research technique for the objective, systematic and quantitative description of the manifest content of communication.” There has been much debate on this classical definition of quantitative content analysis: what does the word “manifest” mean, and is it possible to analyze latent structures of human communication beyond the surface of the manifest text, i.e., the “black marks on white”? In a more practical sense, the word “manifest” should be interpreted in terms of “making it manifest.” For example, if one were to look for irony in political commentaries, the construct of “irony” is not manifest in the sense of being directly identifiable from “black marks on white.” Whether or not there is irony in a commentary has to be interpreted. Thus, before a commentary is coded, it has to be precisely determined what words, phrases, key words, or arguments should serve as indicators for the category “irony.” In other words, this “latent” aspect of communication is made manifest by its definition.
With newspaper articles, the logic of quantitative content analysis can be described as a systematic form of “newspaper reading.” Here, the reader, i.e., the person charged with coding, assigns numeric codes (taken from a category system) to certain elements of the articles (e.g., issues or politicans mentioned in articles) by following a fixed plan (written down in a codebook). Of course, one does not analyze only one but many articles, reports, or commentaries from different newspapers. In this respect, quantitative content analysis differs from qualitative content analysis. While qualitative content analysis is limited to a number of roughly 50 to 100 sample elements (e.g., newspaper reports, interview transcripts, or observational protocols), quantitative content analysis can deal with a huge number of sample elements. Qualitative content analysis works rather inductively by summarizing and classifying elements or parts of the text material and by assigning labels or categories to them. Quantitative content analysis, however, works deductively and measures quantitatively by assigning numeric codes to parts of the material to be coded – which is called coding in quantitative content analysis.
Fields Of Application
Originally, quantitative content analysis was linked to propaganda research, for instance, propaganda material in World War II. Nowadays, quantitative content analysis is applied to many different forms of human communication like print and television coverage, public relations, entertainment, advertisements, photographs, pictures, or films. For instance, subjects of analysis can include national images in media coverage about foreign affairs, the role models presented in television advertisements, the kind and quality of arguments in company press releases, or the features of actors in modern Asian film. In theoretical terms, quantitative content analysis is being applied to many different fields of research in communication science, for example, in analyzing the kind of issues covered by the media in the context of agenda-setting. Similarly, quantitative content analysis is a key method in the cultivation approach, where it is called message system analysis. In both these approaches, content analysis is combined with a survey in field design. Further applications of quantitative content analysis are in: election studies, where it is used to examine election campaigns themselves or media coverage about the candidates, parties, and campaigns; and in media coverage of or public discourses on social problems and issues like racism, social movements, or collective violence.
Purposes Of Quantitative Content Analysis
Generally speaking, there are three major purposes and thus three basic types of quantitative content analysis which follow the popular “Lasswell formula,” which asks who says what, to whom, why, to what extent, and with what effect. The first purpose is to make inferences about the antecedents of communication, for example, examining the coverage of a liberal and a conservative newspaper (who). If there is a political bias in the commentaries or in the news reports (what), the bias would be explained (why), e.g., differences between the newspapers in their editorial lines or political orientation.
The second purpose is merely to describe communication (what, to what extent). Here, different techniques can be applied (which are described below). The third purpose is to draw inferences about the effects of communication (to whom, with what effect). Agendasetting and cultivation studies are good examples for this basic type of quantitative content analysis. Yet, strictly speaking, such an inference is not possible when nothing but the message is examined. Additionally, a survey should be carried out, for instance, before a statement is made about mass media coverage influencing people’s thoughts and perceptions of the world, as in the cultivation approach. The reason for this can be explained by constructivism. From the constructivist point of view, quantitative content analysis is a reactive method, like surveys for example, since the message to be coded is not fixed in an objective sense. Several people reading the same message may interpret it differently due to their individual schemata, beliefs, and attitudes. Thus, when making inferences about message effects, one should carry out not only a quantitative content analysis but also a reception study or an effects study.
Types Of Quantitative Content Analysis
There are different types of quantitative content analysis, i.e., different techniques of describing human communication. The first focuses on frequencies, where one merely counts, e.g., the appearance of issues, persons, or arguments in newspaper coverage. Many studies using the agenda-setting approach are such simple frequency content analyses, and most rely only on media archives. Thus, they do not examine the content or text, e.g. of a newspaper article, but focus only on the headline. This is a simplified version of quantitative content analysis.
The second technique focuses on tendencies. The above-mentioned example of political bias in the commentaries of a liberal and a conservative newspaper is a good example. In another example, the analysis would not only measure the number of articles on nuclear energy, but also their viewpoint on the issue, e.g., by noting the advantages and disadvantages mentioned. If the advantages reported exceed the disadvantages, the article would be observed to be “in favor” of nuclear energy, while if the disadvantages reported exceed the advantages, it would be considered to show “disapproval” or a “negative tendency.”
The third technique does not only focus on tendencies, but also on intensities. Here, one would not just code on an ordinal scale (using, e.g., “positive,” “ambivalent,” and “negative”), but use interval measurement (e.g., “strongly positive,” “positive,” “ambivalent,” “negative,” and “strongly negative”).
Finally, there are also several techniques that meet the popular objection that quantitative content analysis “disassembles” communication. Critics of quantitative content analysis (e.g., Siegfried Kracauer) claim that quantitative content analysis cannot examine relations between elements of communication, i.e., the semantic and syntactical structures of communication. They argue, for instance, that when arguments and persons in newspaper reports are counted separately, the fact that arguments are raised by persons is neglected; or that when statements are separately coded, the fact that a statement can refer to another statement, e.g., by supporting it, is not taken into account. The latter objection may apply with reference to most agenda-setting studies, but it is not a justified objection against quantitative content analysis in general.
A good example of a technique that meets the semantic and syntactical structures of communication is the Semantische Strukturund Inhaltsanalyse (Semantic Structure and Content Analysis) developed by Werner Früh. Without going into detail, the technique considers various elements of communication as well as the relations between them; for instance, it analyzes persons and roles mentioned in newspaper articles, but it also examines time aspects like anteriority and so-called “modifications” like persons’ features or local specifications. In addition, it looks for so-called “k-relations,” such as causal, intentional, or conditional relations as well as for “R-relations” mentioned in, e.g., news reports. Thus, newspaper articles are deconstructed into micro-propositions, but the semantic structure and content analysis reconstructs all relations between these micropropositions.
Most quantitative content analyses examine text or verbal material, i.e., transcribed or recorded human communication. Studies analyzing visual material like films, television advertisements, or televised debates between presidential candidates are comparably rare. There are three major reasons for this. First, copies of newspaper articles are easier to access than copies of the evening news, for instance; in retrospective studies (which most quantitative content analyses are), especially, visual material is often no longer available. Second, visual material is more complex than verbal or text material. For example, television news not only provides information via the audio channel but also via the visual channel. Since verbal and visual information can deliver different messages, one has to code both streams of information. This is more expensive than just coding print news. Finally, coding visual material like evening news on television requires more detailed coding instructions and more complex category definitions than a codebook for analyzing newspaper coverage.
A more or less recent development in quantitative content analysis is automatic, that is, computer-assisted, content analysis, where a computer program counts keywords and searches for related words in the same paragraph, for example. Before the coding process begins, all the relevant keywords or phrases in a so-called coding dictionary – an equivalent of the codebook of a conventional content analysis – have to be listed. While there has been some progress in this technique, it will be a while before the human coder becomes redundant.
Current challenges for quantitative content analysis stem from the world wide web, where the content of a private weblog, arguments in online chat, or the pictures in an online gallery can be subject to analysis. Compared to newspaper coverage, for example, a content analysis of online communication can be quite a problem. Here, the population from which a sample is taken for analysis is not fixed but changes from day to day, or even more quickly. It is therefore important to store all relevant communication for a specific study. But even if this were possible, one seldom has a view of the complete population since the world wide web, or the Internet, as a whole is not easy to grasp. Thus most studies that analyze online communication work with samples that are more or less clearly defined.
Like any other method in the social sciences, quantitative content analysis has to meet certain standards of quantitative empirical research. The first criterion of intersubjectivity calls for transparent research. This means that all the details of a quantitative content analysis have to be described and explained so that exactly what has been done is clear. The second criterion of systematics requires that the coding rules and sampling criteria are invariantly applied to all material. The third criterion of reliability calls for the codebook to be dependable. Different coders do not always agree on coding. For example, one coder may identify an argument in a newspaper article as argument 13 from the argument list in the codebook, while another coder will choose argument 15, with the result that the numeric codes assigned to the argument in the newspaper article do not match. Yet, in other cases the two coders may agree. Using all codings of both coders, one can divide the doubled number of matching pairs (e.g., 17 identical codings) by the number of all codings of the first coder and the number of all codings of the second coder (e.g., 20 codings each), to obtain a ratio called the Holsti-formula, which is a simple reliability coefficient. In this example we would find R = 17 * 2 / (20 + 20) = 0.85. The values of all reliability coefficients range from 0 (no matching at all) to 1 (perfect matching). Another popular reliability coefficient – better known from index calculation – is Cronbach’s alpha α.
The fourth standard for a quantitative content analysis is validity. An instrument of empirical research, i.e., the codebook with reference to CA, can claim to be valid when it measures what it intends to measure. For instance, if the codebook contains a category “stereotype,” then the coders should not measure political bias or irony when applying the coding rules for this category, but code stereotypes. According to Klaus Krippendorff, there are different forms of validity. The type of validity in the example can be called face validity. Predictive validity and concurrent validity both refer to an external criterion measure for validating data obtained by a quantitative CA. Such a measure may be another quantitative content analysis or may be statistical data from governmental sources. With concurrent validity the validity test is administered at the same time as the criterion is collected. With predictive validity, scores are predicted on some criterion measure.
Content Analysis As Research Practice
The research process using quantitative content analysis comprises six steps. It usually begins with theoretical considerations, literature review, and deducing empirical hypotheses. In the second step, the sample material that is to be coded, i.e., examined with the codebook, is defined. In the third step, the coding units (e.g., articles or arguments) are described. In the fourth step, the codebook with the category system is developed and pre-tested. The actual measurement, i.e., the process of coding, represents the fifth step of a CA. The final step is data analysis and data interpretation.
Most quantitative content analysis requires multilevel sampling, for instance, analysis of campaign coverage would involve the choice of a limited number of national newspapers which represent diverse political standpoints (e.g., from liberal to conservative). On the next level the time span to be analyzed (e.g., every day in the critical phase of the election campaign) is set. On the next level, the articles to be coded are determined (e.g., all articles on the front page). Usually the sample is called the unit of analysis. The coding unit, however, is the most important unit in quantitative CA. It defines the level of measurement. For example, if the features of an article (e.g., the main issue of the article) are examined, the single article is the coding unit, but if the attributes of an argument (e.g., the issues mentioned in an argument) are examined, then the single argument is the coding unit. In the first case, 100 articles (with 5 arguments per article) will lead to 100 codes, in the second case the same number of articles will produce 500 codes. The level of coding depends on the sample size as well as on the research question. If the the study focuses on argumentation structures, the article will not be chosen as the coding unit. If political coverage in 10 newspapers in the last 50 years is the focus of the study, the argument or statement will not be chosen as a coding unit, otherwise a vast number of cases will have to be coded.
References:
- Berelson, B. (1952). Content analysis in communication research. Glencoe, IL: Free Press.
- Früh, W. (1998). Inhaltsanalyse: Theorie und Praxis, 4th edn. [Content analysis: Theory and practice]. Constance: UVK.
- Holsti, O. R. (1969). Content analysis for the social sciences and humanities. Reading, MA: AddisonWesley.
- Krippendorff, K. (2004). Content analysis: An introduction to its methodology. Beverly Hills, CA: Sage.
- Neuendorf, K. A. (2002). The content analysis guidebook. Thousand Oaks, CA: Sage.
- Popping, R. (2000). Computer-assisted text analysis. Thousand Oaks, CA: Sage.
- Riffe, D., Lacy, S., & Fico, F. G. (2005). Analyzing media messages: Using quantitative content analysis in research. Mahwah, NJ: LEA.
- Weber, R. P. (1990). Basic content analysis, 2nd edn. Newbury Park, London, and New Delhi: Sage.
Guide to Communication Research Methodologies: Quantitative, Qualitative and Rhetorical Research
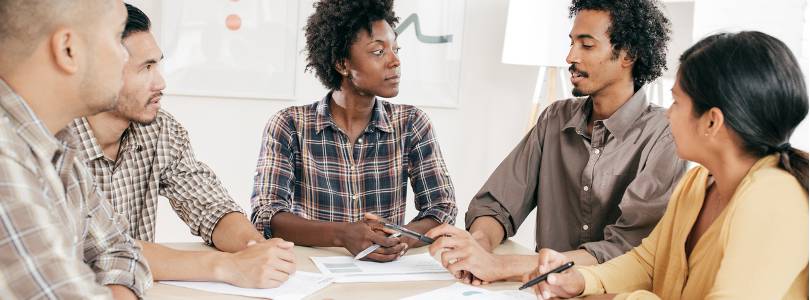
Overview of Communication
Communication research methods, quantitative research, qualitative research, rhetorical research, mixed methodology.
Students interested in earning a graduate degree in communication should have at least some interest in understanding communication theories and/or conducting communication research. As students advance from undergraduate to graduate programs, an interesting change takes place — the student is no longer just a repository for knowledge. Rather, the student is expected to learn while also creating knowledge. This new knowledge is largely generated through the development and completion of research in communication studies. Before exploring the different methodologies used to conduct communication research, it is important to have a foundational understanding of the field of communication.
Defining communication is much harder than it sounds. Indeed, scholars have argued about the topic for years, typically differing on the following topics:
- Breadth : How many behaviors and actions should or should not be considered communication.
- Intentionality : Whether the definition includes an intention to communicate.
- Success : Whether someone was able to effectively communicate a message, or merely attempted to without it being received or understood.
However, most definitions discuss five main components, which include: sender, receiver, context/environment, medium, and message. Broadly speaking, communication research examines these components, asking questions about each of them and seeking to answer those questions.
As students seek to answer their own questions, they follow an approach similar to most other researchers. This approach proceeds in five steps: conceptualize, plan and design, implement a methodology, analyze and interpret, reconceptualize.
- Conceptualize : In the conceptualization process, students develop their area of interest and determine if their specific questions and hypotheses are worth investigating. If the research has already been completed, or there is no practical reason to research the topic, students may need to find a different research topic.
- Plan and Design : During planning and design students will select their methods of evaluation and decide how they plan to define their variables in a measurable way.
- Implement a Methodology : When implementing a methodology, students collect the data and information they require. They may, for example, have decided to conduct a survey study. This is the step when they would use their survey to collect data. If students chose to conduct a rhetorical criticism, this is when they would analyze their text.
- Analyze and Interpret : As students analyze and interpret their data or evidence, they transform the raw findings into meaningful insights. If they chose to conduct interviews, this would be the point in the process where they would evaluate the results of the interviews to find meaning as it relates to the communication phenomena of interest.
- Reconceptualize : During reconceptualization, students ask how their findings speak to a larger body of research — studies related to theirs that have already been completed and research they should execute in the future to continue answering new questions.
This final step is crucial, and speaks to an important tenet of communication research: All research contributes to a better overall understanding of communication and moves the field forward by enabling the development of new theories.
In the field of communication, there are three main research methodologies: quantitative, qualitative, and rhetorical. As communication students progress in their careers, they will likely find themselves using one of these far more often than the others.
Quantitative research seeks to establish knowledge through the use of numbers and measurement. Within the overarching area of quantitative research, there are a variety of different methodologies. The most commonly used methodologies are experiments, surveys, content analysis, and meta-analysis. To better understand these research methods, you can explore the following examples:
Experiments : Experiments are an empirical form of research that enable the researcher to study communication in a controlled environment. For example, a researcher might know that there are typical responses people use when they are interrupted during a conversation. However, it might be unknown as to how frequency of interruption provokes those different responses (e.g., do communicators use different responses when interrupted once every 10 minutes versus once per minute?). An experiment would allow a researcher to create these two environments to test a hypothesis or answer a specific research question. As you can imagine, it would be very time consuming — and probably impossible — to view this and measure it in the real world. For that reason, an experiment would be perfect for this research inquiry.
Surveys : Surveys are often used to collect information from large groups of people using scales that have been tested for validity and reliability. A researcher might be curious about how a supervisor sharing personal information with his or her subordinate affects way the subordinate perceives his or her supervisor. The researcher could create a survey where respondents answer questions about a) the information their supervisors self-disclose and b) their perceptions of their supervisors. The data collected about these two variables could offer interesting insights about this communication. As you would guess, an experiment would not work in this case because the researcher needs to assess a real relationship and they need insight into the mind of the respondent.
Content Analysis : Content analysis is used to count the number of occurrences of a phenomenon within a source of media (e.g., books, magazines, commercials, movies, etc.). For example, a researcher might be interested in finding out if people of certain races are underrepresented on television. They might explore this area of research by counting the number of times people of different races appear in prime time television and comparing that to the actual proportions in society.
Meta-Analysis : In this technique, a researcher takes a collection of quantitative studies and analyzes the data as a whole to get a better understanding of a communication phenomenon. For example, a researcher might be curious about how video games affect aggression. This researcher might find that many studies have been done on the topic, sometimes with conflicting results. In their meta-analysis, they could analyze the existing statistics as a whole to get a better understanding of the relationship between the two variables.
Qualitative research is interested in exploring subjects’ perceptions and understandings as they relate to communication. Imagine two researchers who want to understand student perceptions of the basic communication course at a university. The first researcher, a quantitative researcher, might measure absences to understand student perception. The second researcher, a qualitative researcher, might interview students to find out what they like and dislike about a course. The former is based on hard numbers, while the latter is based on human experience and perception.
Qualitative researchers employ a variety of different methodologies. Some of the most popular are interviews, focus groups, and participant observation. To better understand these research methods, you can explore the following examples:
Interviews : This typically consists of a researcher having a discussion with a participant based on questions developed by the researcher. For example, a researcher might be interested in how parents exert power over the lives of their children while the children are away at college. The researcher could spend time having conversations with college students about this topic, transcribe the conversations and then seek to find themes across the different discussions.
Focus Groups : A researcher using this method gathers a group of people with intimate knowledge of a communication phenomenon. For example, if a researcher wanted to understand the experience of couples who are childless by choice, he or she might choose to run a series of focus groups. This format is helpful because it allows participants to build on one another’s experiences, remembering information they may otherwise have forgotten. Focus groups also tend to produce useful information at a higher rate than interviews. That said, some issues are too sensitive for focus groups and lend themselves better to interviews.
Participant Observation : As the name indicates, this method involves the researcher watching participants in their natural environment. In some cases, the participants may not know they are being studied, as the researcher fully immerses his or herself as a member of the environment. To illustrate participant observation, imagine a researcher curious about how humor is used in healthcare. This researcher might immerse his or herself in a long-term care facility to observe how humor is used by healthcare workers interacting with patients.
Rhetorical research (or rhetorical criticism) is a form of textual analysis wherein the researcher systematically analyzes, interprets, and critiques the persuasive power of messages within a text. This takes on many forms, but all of them involve similar steps: selecting a text, choosing a rhetorical method, analyzing the text, and writing the criticism.
To illustrate, a researcher could be interested in how mass media portrays “good degrees” to prospective college students. To understand this communication, a rhetorical researcher could take 30 articles on the topic from the last year and write a rhetorical essay about the criteria used and the core message argued by the media.
Likewise, a researcher could be interested in how women in management roles are portrayed in television. They could select a group of popular shows and analyze that as the text. This might result in a rhetorical essay about the behaviors displayed by these women and what the text says about women in management roles.
As a final example, one might be interested in how persuasion is used by the president during the White House Correspondent’s Dinner. A researcher could select several recent presidents and write a rhetorical essay about their speeches and how they employed persuasion during their delivery.
Taking a mixed methods approach results in a research study that uses two or more techniques discussed above. Often, researchers will pair two methods together in the same study examining the same phenomenon. Other times, researchers will use qualitative methods to develop quantitative research, such as a researcher who uses a focus group to discuss the validity of a survey before it is finalized.
The benefit of mixed methods is that it offers a richer picture of a communication phenomenon by gathering data and information in multiple ways. If we explore some of the earlier examples, we can see how mixed methods might result in a better understanding of the communication being studied.
Example 1 : In surveys, we discussed a researcher interested in understanding how a supervisor sharing personal information with his or her subordinate affects the way the subordinate perceives his or her supervisor. While a survey could give us some insight into this communication, we could also add interviews with subordinates. Exploring their experiences intimately could give us a better understanding of how they navigate self-disclosure in a relationship based on power differences.
Example 2 : In content analysis, we discussed measuring representation of different races during prime time television. While we can count the appearances of members of different races and compare that to the composition of the general population, that doesn’t tell us anything about their portrayal. Adding rhetorical criticism, we could talk about how underrepresented groups are portrayed in either a positive or negative light, supporting or defying commonly held stereotypes.
Example 3 : In interviews, we saw a researcher who explored how power could be exerted by parents over their college-age children who are away at school. After determining the tactics used by parents, this interview study could have a phase two. In this phase, the researcher could develop scales to measure each tactic and then use those scales to understand how the tactics affect other communication constructs. One could argue, for example, that student anxiety would increase as a parent exerts greater power over that student. A researcher could conduct a hierarchical regression to see how each power tactic effects the levels of stress experienced by a student.
As you can see, each methodology has its own merits, and they often work well together. As students advance in their study of communication, it is worthwhile to learn various research methods. This allows them to study their interests in greater depth and breadth. Ultimately, they will be able to assemble stronger research studies and answer their questions about communication more effectively.
Note : For more information about research in the field of communication, check out our Guide to Communication Research and Scholarship .
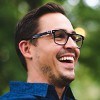
Quantitative Data Analysis: A Comprehensive Guide
By: Ofem Eteng Published: May 18, 2022
Related Articles
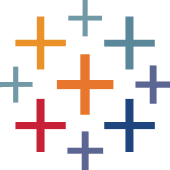
A healthcare giant successfully introduces the most effective drug dosage through rigorous statistical modeling, saving countless lives. A marketing team predicts consumer trends with uncanny accuracy, tailoring campaigns for maximum impact.
Table of Contents
These trends and dosages are not just any numbers but are a result of meticulous quantitative data analysis. Quantitative data analysis offers a robust framework for understanding complex phenomena, evaluating hypotheses, and predicting future outcomes.
In this blog, we’ll walk through the concept of quantitative data analysis, the steps required, its advantages, and the methods and techniques that are used in this analysis. Read on!
What is Quantitative Data Analysis?
Quantitative data analysis is a systematic process of examining, interpreting, and drawing meaningful conclusions from numerical data. It involves the application of statistical methods, mathematical models, and computational techniques to understand patterns, relationships, and trends within datasets.
Quantitative data analysis methods typically work with algorithms, mathematical analysis tools, and software to gain insights from the data, answering questions such as how many, how often, and how much. Data for quantitative data analysis is usually collected from close-ended surveys, questionnaires, polls, etc. The data can also be obtained from sales figures, email click-through rates, number of website visitors, and percentage revenue increase.
Quantitative Data Analysis vs Qualitative Data Analysis
When we talk about data, we directly think about the pattern, the relationship, and the connection between the datasets – analyzing the data in short. Therefore when it comes to data analysis, there are broadly two types – Quantitative Data Analysis and Qualitative Data Analysis.
Quantitative data analysis revolves around numerical data and statistics, which are suitable for functions that can be counted or measured. In contrast, qualitative data analysis includes description and subjective information – for things that can be observed but not measured.
Let us differentiate between Quantitative Data Analysis and Quantitative Data Analysis for a better understanding.
Data Preparation Steps for Quantitative Data Analysis
Quantitative data has to be gathered and cleaned before proceeding to the stage of analyzing it. Below are the steps to prepare a data before quantitative research analysis:
- Step 1: Data Collection
Before beginning the analysis process, you need data. Data can be collected through rigorous quantitative research, which includes methods such as interviews, focus groups, surveys, and questionnaires.
- Step 2: Data Cleaning
Once the data is collected, begin the data cleaning process by scanning through the entire data for duplicates, errors, and omissions. Keep a close eye for outliers (data points that are significantly different from the majority of the dataset) because they can skew your analysis results if they are not removed.
This data-cleaning process ensures data accuracy, consistency and relevancy before analysis.
- Step 3: Data Analysis and Interpretation
Now that you have collected and cleaned your data, it is now time to carry out the quantitative analysis. There are two methods of quantitative data analysis, which we will discuss in the next section.
However, if you have data from multiple sources, collecting and cleaning it can be a cumbersome task. This is where Hevo Data steps in. With Hevo, extracting, transforming, and loading data from source to destination becomes a seamless task, eliminating the need for manual coding. This not only saves valuable time but also enhances the overall efficiency of data analysis and visualization, empowering users to derive insights quickly and with precision
Hevo is the only real-time ELT No-code Data Pipeline platform that cost-effectively automates data pipelines that are flexible to your needs. With integration with 150+ Data Sources (40+ free sources), we help you not only export data from sources & load data to the destinations but also transform & enrich your data, & make it analysis-ready.
Start for free now!
Now that you are familiar with what quantitative data analysis is and how to prepare your data for analysis, the focus will shift to the purpose of this article, which is to describe the methods and techniques of quantitative data analysis.
Methods and Techniques of Quantitative Data Analysis
Quantitative data analysis employs two techniques to extract meaningful insights from datasets, broadly. The first method is descriptive statistics, which summarizes and portrays essential features of a dataset, such as mean, median, and standard deviation.
Inferential statistics, the second method, extrapolates insights and predictions from a sample dataset to make broader inferences about an entire population, such as hypothesis testing and regression analysis.
An in-depth explanation of both the methods is provided below:
- Descriptive Statistics
- Inferential Statistics
1) Descriptive Statistics
Descriptive statistics as the name implies is used to describe a dataset. It helps understand the details of your data by summarizing it and finding patterns from the specific data sample. They provide absolute numbers obtained from a sample but do not necessarily explain the rationale behind the numbers and are mostly used for analyzing single variables. The methods used in descriptive statistics include:
- Mean: This calculates the numerical average of a set of values.
- Median: This is used to get the midpoint of a set of values when the numbers are arranged in numerical order.
- Mode: This is used to find the most commonly occurring value in a dataset.
- Percentage: This is used to express how a value or group of respondents within the data relates to a larger group of respondents.
- Frequency: This indicates the number of times a value is found.
- Range: This shows the highest and lowest values in a dataset.
- Standard Deviation: This is used to indicate how dispersed a range of numbers is, meaning, it shows how close all the numbers are to the mean.
- Skewness: It indicates how symmetrical a range of numbers is, showing if they cluster into a smooth bell curve shape in the middle of the graph or if they skew towards the left or right.
2) Inferential Statistics
In quantitative analysis, the expectation is to turn raw numbers into meaningful insight using numerical values, and descriptive statistics is all about explaining details of a specific dataset using numbers, but it does not explain the motives behind the numbers; hence, a need for further analysis using inferential statistics.
Inferential statistics aim to make predictions or highlight possible outcomes from the analyzed data obtained from descriptive statistics. They are used to generalize results and make predictions between groups, show relationships that exist between multiple variables, and are used for hypothesis testing that predicts changes or differences.
There are various statistical analysis methods used within inferential statistics; a few are discussed below.
- Cross Tabulations: Cross tabulation or crosstab is used to show the relationship that exists between two variables and is often used to compare results by demographic groups. It uses a basic tabular form to draw inferences between different data sets and contains data that is mutually exclusive or has some connection with each other. Crosstabs help understand the nuances of a dataset and factors that may influence a data point.
- Regression Analysis: Regression analysis estimates the relationship between a set of variables. It shows the correlation between a dependent variable (the variable or outcome you want to measure or predict) and any number of independent variables (factors that may impact the dependent variable). Therefore, the purpose of the regression analysis is to estimate how one or more variables might affect a dependent variable to identify trends and patterns to make predictions and forecast possible future trends. There are many types of regression analysis, and the model you choose will be determined by the type of data you have for the dependent variable. The types of regression analysis include linear regression, non-linear regression, binary logistic regression, etc.
- Monte Carlo Simulation: Monte Carlo simulation, also known as the Monte Carlo method, is a computerized technique of generating models of possible outcomes and showing their probability distributions. It considers a range of possible outcomes and then tries to calculate how likely each outcome will occur. Data analysts use it to perform advanced risk analyses to help forecast future events and make decisions accordingly.
- Analysis of Variance (ANOVA): This is used to test the extent to which two or more groups differ from each other. It compares the mean of various groups and allows the analysis of multiple groups.
- Factor Analysis: A large number of variables can be reduced into a smaller number of factors using the factor analysis technique. It works on the principle that multiple separate observable variables correlate with each other because they are all associated with an underlying construct. It helps in reducing large datasets into smaller, more manageable samples.
- Cohort Analysis: Cohort analysis can be defined as a subset of behavioral analytics that operates from data taken from a given dataset. Rather than looking at all users as one unit, cohort analysis breaks down data into related groups for analysis, where these groups or cohorts usually have common characteristics or similarities within a defined period.
- MaxDiff Analysis: This is a quantitative data analysis method that is used to gauge customers’ preferences for purchase and what parameters rank higher than the others in the process.
- Cluster Analysis: Cluster analysis is a technique used to identify structures within a dataset. Cluster analysis aims to be able to sort different data points into groups that are internally similar and externally different; that is, data points within a cluster will look like each other and different from data points in other clusters.
- Time Series Analysis: This is a statistical analytic technique used to identify trends and cycles over time. It is simply the measurement of the same variables at different times, like weekly and monthly email sign-ups, to uncover trends, seasonality, and cyclic patterns. By doing this, the data analyst can forecast how variables of interest may fluctuate in the future.
- SWOT analysis: This is a quantitative data analysis method that assigns numerical values to indicate strengths, weaknesses, opportunities, and threats of an organization, product, or service to show a clearer picture of competition to foster better business strategies
How to Choose the Right Method for your Analysis?
Choosing between Descriptive Statistics or Inferential Statistics can be often confusing. You should consider the following factors before choosing the right method for your quantitative data analysis:
1. Type of Data
The first consideration in data analysis is understanding the type of data you have. Different statistical methods have specific requirements based on these data types, and using the wrong method can render results meaningless. The choice of statistical method should align with the nature and distribution of your data to ensure meaningful and accurate analysis.
2. Your Research Questions
When deciding on statistical methods, it’s crucial to align them with your specific research questions and hypotheses. The nature of your questions will influence whether descriptive statistics alone, which reveal sample attributes, are sufficient or if you need both descriptive and inferential statistics to understand group differences or relationships between variables and make population inferences.
Pros and Cons of Quantitative Data Analysis
1. Objectivity and Generalizability:
- Quantitative data analysis offers objective, numerical measurements, minimizing bias and personal interpretation.
- Results can often be generalized to larger populations, making them applicable to broader contexts.
Example: A study using quantitative data analysis to measure student test scores can objectively compare performance across different schools and demographics, leading to generalizable insights about educational strategies.
2. Precision and Efficiency:
- Statistical methods provide precise numerical results, allowing for accurate comparisons and prediction.
- Large datasets can be analyzed efficiently with the help of computer software, saving time and resources.
Example: A marketing team can use quantitative data analysis to precisely track click-through rates and conversion rates on different ad campaigns, quickly identifying the most effective strategies for maximizing customer engagement.
3. Identification of Patterns and Relationships:
- Statistical techniques reveal hidden patterns and relationships between variables that might not be apparent through observation alone.
- This can lead to new insights and understanding of complex phenomena.
Example: A medical researcher can use quantitative analysis to pinpoint correlations between lifestyle factors and disease risk, aiding in the development of prevention strategies.
1. Limited Scope:
- Quantitative analysis focuses on quantifiable aspects of a phenomenon , potentially overlooking important qualitative nuances, such as emotions, motivations, or cultural contexts.
Example: A survey measuring customer satisfaction with numerical ratings might miss key insights about the underlying reasons for their satisfaction or dissatisfaction, which could be better captured through open-ended feedback.
2. Oversimplification:
- Reducing complex phenomena to numerical data can lead to oversimplification and a loss of richness in understanding.
Example: Analyzing employee productivity solely through quantitative metrics like hours worked or tasks completed might not account for factors like creativity, collaboration, or problem-solving skills, which are crucial for overall performance.
3. Potential for Misinterpretation:
- Statistical results can be misinterpreted if not analyzed carefully and with appropriate expertise.
- The choice of statistical methods and assumptions can significantly influence results.
This blog discusses the steps, methods, and techniques of quantitative data analysis. It also gives insights into the methods of data collection, the type of data one should work with, and the pros and cons of such analysis.
Gain a better understanding of data analysis with these essential reads:
- Data Analysis and Modeling: 4 Critical Differences
- Exploratory Data Analysis Simplified 101
- 25 Best Data Analysis Tools in 2024
Carrying out successful data analysis requires prepping the data and making it analysis-ready. That is where Hevo steps in.
Want to give Hevo a try? Sign Up for a 14-day free trial and experience the feature-rich Hevo suite first hand. You may also have a look at the amazing Hevo price , which will assist you in selecting the best plan for your requirements.
Share your experience of understanding Quantitative Data Analysis in the comment section below! We would love to hear your thoughts.
Ofem is a freelance writer specializing in data-related topics, who has expertise in translating complex concepts. With a focus on data science, analytics, and emerging technologies.
No-code Data Pipeline for your Data Warehouse
- Data Analysis
- Data Warehouse
- Quantitative Data Analysis
Continue Reading

Principles of Running a High Performance Data Team
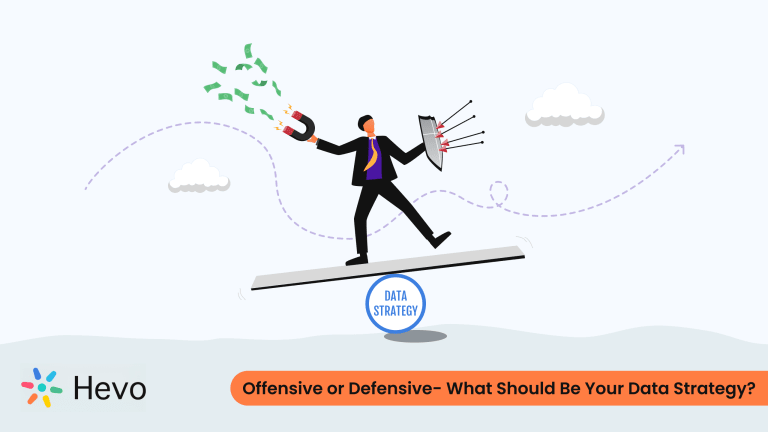
Willem Koenders
Offensive vs Defensive Data Strategy: Do You Really Need to Choose?
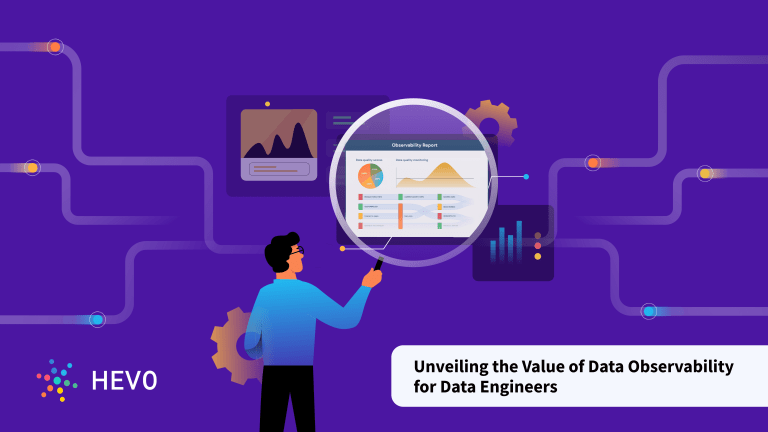
Kyle Kirwan
The Data Engineer’s Crystal Ball: How Data Observability Helps You See What’s Coming
I want to read this e-book.

Quantitative Data Analysis 101
The lingo, methods and techniques, explained simply.
By: Derek Jansen (MBA) and Kerryn Warren (PhD) | December 2020
Quantitative data analysis is one of those things that often strikes fear in students. It’s totally understandable – quantitative analysis is a complex topic, full of daunting lingo , like medians, modes, correlation and regression. Suddenly we’re all wishing we’d paid a little more attention in math class…
The good news is that while quantitative data analysis is a mammoth topic, gaining a working understanding of the basics isn’t that hard , even for those of us who avoid numbers and math . In this post, we’ll break quantitative analysis down into simple , bite-sized chunks so you can approach your research with confidence.
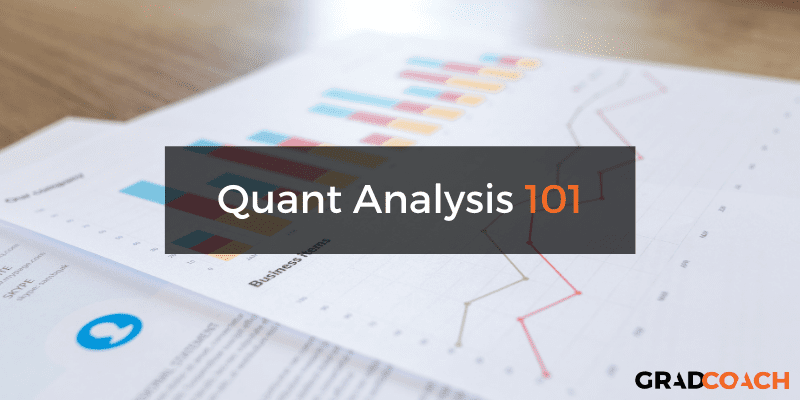
Overview: Quantitative Data Analysis 101
- What (exactly) is quantitative data analysis?
- When to use quantitative analysis
- How quantitative analysis works
The two “branches” of quantitative analysis
- Descriptive statistics 101
- Inferential statistics 101
- How to choose the right quantitative methods
- Recap & summary
What is quantitative data analysis?
Despite being a mouthful, quantitative data analysis simply means analysing data that is numbers-based – or data that can be easily “converted” into numbers without losing any meaning.
For example, category-based variables like gender, ethnicity, or native language could all be “converted” into numbers without losing meaning – for example, English could equal 1, French 2, etc.
This contrasts against qualitative data analysis, where the focus is on words, phrases and expressions that can’t be reduced to numbers. If you’re interested in learning about qualitative analysis, check out our post and video here .
What is quantitative analysis used for?
Quantitative analysis is generally used for three purposes.
- Firstly, it’s used to measure differences between groups . For example, the popularity of different clothing colours or brands.
- Secondly, it’s used to assess relationships between variables . For example, the relationship between weather temperature and voter turnout.
- And third, it’s used to test hypotheses in a scientifically rigorous way. For example, a hypothesis about the impact of a certain vaccine.
Again, this contrasts with qualitative analysis , which can be used to analyse people’s perceptions and feelings about an event or situation. In other words, things that can’t be reduced to numbers.
How does quantitative analysis work?
Well, since quantitative data analysis is all about analysing numbers , it’s no surprise that it involves statistics . Statistical analysis methods form the engine that powers quantitative analysis, and these methods can vary from pretty basic calculations (for example, averages and medians) to more sophisticated analyses (for example, correlations and regressions).
Sounds like gibberish? Don’t worry. We’ll explain all of that in this post. Importantly, you don’t need to be a statistician or math wiz to pull off a good quantitative analysis. We’ll break down all the technical mumbo jumbo in this post.
Need a helping hand?
As I mentioned, quantitative analysis is powered by statistical analysis methods . There are two main “branches” of statistical methods that are used – descriptive statistics and inferential statistics . In your research, you might only use descriptive statistics, or you might use a mix of both , depending on what you’re trying to figure out. In other words, depending on your research questions, aims and objectives . I’ll explain how to choose your methods later.
So, what are descriptive and inferential statistics?
Well, before I can explain that, we need to take a quick detour to explain some lingo. To understand the difference between these two branches of statistics, you need to understand two important words. These words are population and sample .
First up, population . In statistics, the population is the entire group of people (or animals or organisations or whatever) that you’re interested in researching. For example, if you were interested in researching Tesla owners in the US, then the population would be all Tesla owners in the US.
However, it’s extremely unlikely that you’re going to be able to interview or survey every single Tesla owner in the US. Realistically, you’ll likely only get access to a few hundred, or maybe a few thousand owners using an online survey. This smaller group of accessible people whose data you actually collect is called your sample .
So, to recap – the population is the entire group of people you’re interested in, and the sample is the subset of the population that you can actually get access to. In other words, the population is the full chocolate cake , whereas the sample is a slice of that cake.
So, why is this sample-population thing important?
Well, descriptive statistics focus on describing the sample , while inferential statistics aim to make predictions about the population, based on the findings within the sample. In other words, we use one group of statistical methods – descriptive statistics – to investigate the slice of cake, and another group of methods – inferential statistics – to draw conclusions about the entire cake. There I go with the cake analogy again…
With that out the way, let’s take a closer look at each of these branches in more detail.
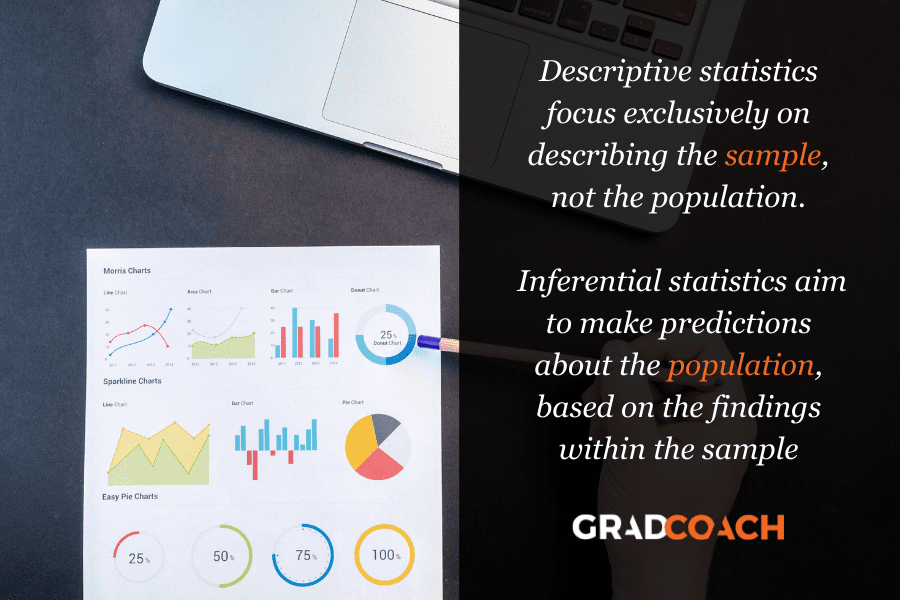
Branch 1: Descriptive Statistics
Descriptive statistics serve a simple but critically important role in your research – to describe your data set – hence the name. In other words, they help you understand the details of your sample . Unlike inferential statistics (which we’ll get to soon), descriptive statistics don’t aim to make inferences or predictions about the entire population – they’re purely interested in the details of your specific sample .
When you’re writing up your analysis, descriptive statistics are the first set of stats you’ll cover, before moving on to inferential statistics. But, that said, depending on your research objectives and research questions , they may be the only type of statistics you use. We’ll explore that a little later.
So, what kind of statistics are usually covered in this section?
Some common statistical tests used in this branch include the following:
- Mean – this is simply the mathematical average of a range of numbers.
- Median – this is the midpoint in a range of numbers when the numbers are arranged in numerical order. If the data set makes up an odd number, then the median is the number right in the middle of the set. If the data set makes up an even number, then the median is the midpoint between the two middle numbers.
- Mode – this is simply the most commonly occurring number in the data set.
- In cases where most of the numbers are quite close to the average, the standard deviation will be relatively low.
- Conversely, in cases where the numbers are scattered all over the place, the standard deviation will be relatively high.
- Skewness . As the name suggests, skewness indicates how symmetrical a range of numbers is. In other words, do they tend to cluster into a smooth bell curve shape in the middle of the graph, or do they skew to the left or right?
Feeling a bit confused? Let’s look at a practical example using a small data set.
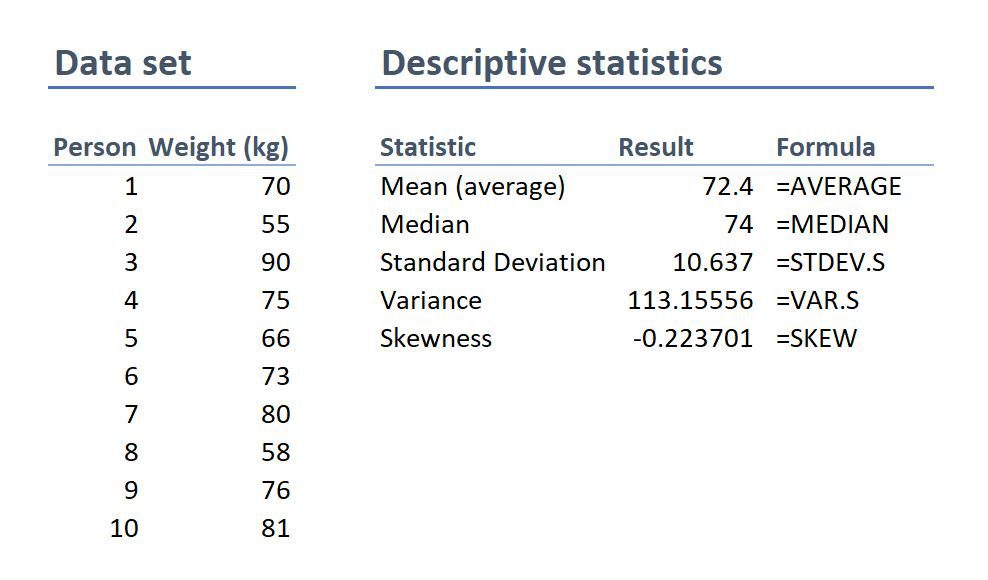
On the left-hand side is the data set. This details the bodyweight of a sample of 10 people. On the right-hand side, we have the descriptive statistics. Let’s take a look at each of them.
First, we can see that the mean weight is 72.4 kilograms. In other words, the average weight across the sample is 72.4 kilograms. Straightforward.
Next, we can see that the median is very similar to the mean (the average). This suggests that this data set has a reasonably symmetrical distribution (in other words, a relatively smooth, centred distribution of weights, clustered towards the centre).
In terms of the mode , there is no mode in this data set. This is because each number is present only once and so there cannot be a “most common number”. If there were two people who were both 65 kilograms, for example, then the mode would be 65.
Next up is the standard deviation . 10.6 indicates that there’s quite a wide spread of numbers. We can see this quite easily by looking at the numbers themselves, which range from 55 to 90, which is quite a stretch from the mean of 72.4.
And lastly, the skewness of -0.2 tells us that the data is very slightly negatively skewed. This makes sense since the mean and the median are slightly different.
As you can see, these descriptive statistics give us some useful insight into the data set. Of course, this is a very small data set (only 10 records), so we can’t read into these statistics too much. Also, keep in mind that this is not a list of all possible descriptive statistics – just the most common ones.
But why do all of these numbers matter?
While these descriptive statistics are all fairly basic, they’re important for a few reasons:
- Firstly, they help you get both a macro and micro-level view of your data. In other words, they help you understand both the big picture and the finer details.
- Secondly, they help you spot potential errors in the data – for example, if an average is way higher than you’d expect, or responses to a question are highly varied, this can act as a warning sign that you need to double-check the data.
- And lastly, these descriptive statistics help inform which inferential statistical techniques you can use, as those techniques depend on the skewness (in other words, the symmetry and normality) of the data.
Simply put, descriptive statistics are really important , even though the statistical techniques used are fairly basic. All too often at Grad Coach, we see students skimming over the descriptives in their eagerness to get to the more exciting inferential methods, and then landing up with some very flawed results.
Don’t be a sucker – give your descriptive statistics the love and attention they deserve!
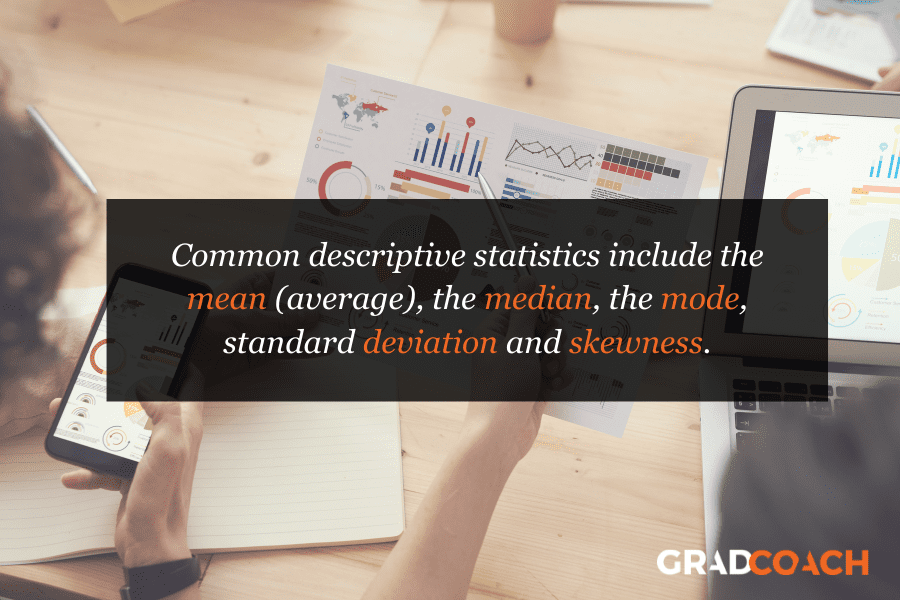
Branch 2: Inferential Statistics
As I mentioned, while descriptive statistics are all about the details of your specific data set – your sample – inferential statistics aim to make inferences about the population . In other words, you’ll use inferential statistics to make predictions about what you’d expect to find in the full population.
What kind of predictions, you ask? Well, there are two common types of predictions that researchers try to make using inferential stats:
- Firstly, predictions about differences between groups – for example, height differences between children grouped by their favourite meal or gender.
- And secondly, relationships between variables – for example, the relationship between body weight and the number of hours a week a person does yoga.
In other words, inferential statistics (when done correctly), allow you to connect the dots and make predictions about what you expect to see in the real world population, based on what you observe in your sample data. For this reason, inferential statistics are used for hypothesis testing – in other words, to test hypotheses that predict changes or differences.
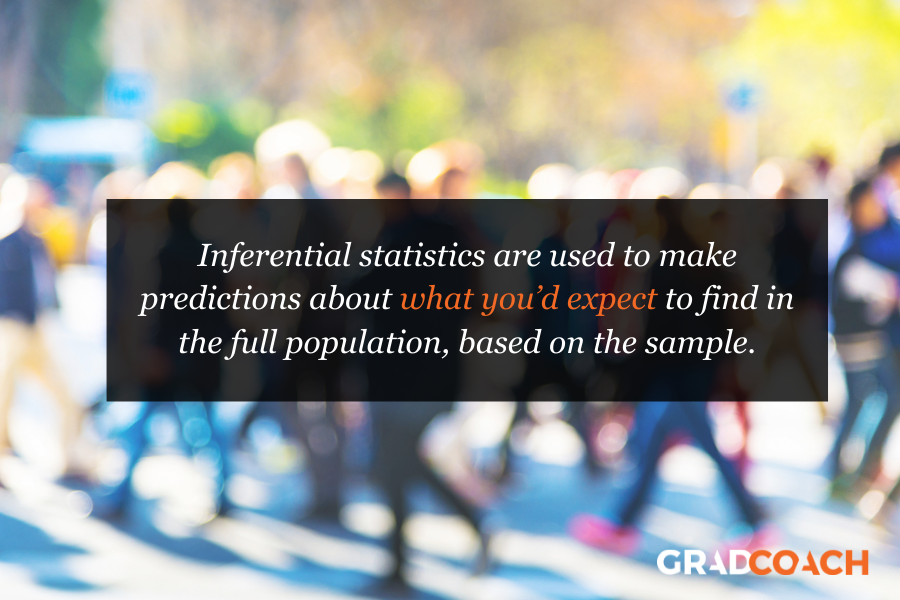
Of course, when you’re working with inferential statistics, the composition of your sample is really important. In other words, if your sample doesn’t accurately represent the population you’re researching, then your findings won’t necessarily be very useful.
For example, if your population of interest is a mix of 50% male and 50% female , but your sample is 80% male , you can’t make inferences about the population based on your sample, since it’s not representative. This area of statistics is called sampling, but we won’t go down that rabbit hole here (it’s a deep one!) – we’ll save that for another post .
What statistics are usually used in this branch?
There are many, many different statistical analysis methods within the inferential branch and it’d be impossible for us to discuss them all here. So we’ll just take a look at some of the most common inferential statistical methods so that you have a solid starting point.
First up are T-Tests . T-tests compare the means (the averages) of two groups of data to assess whether they’re statistically significantly different. In other words, do they have significantly different means, standard deviations and skewness.
This type of testing is very useful for understanding just how similar or different two groups of data are. For example, you might want to compare the mean blood pressure between two groups of people – one that has taken a new medication and one that hasn’t – to assess whether they are significantly different.
Kicking things up a level, we have ANOVA, which stands for “analysis of variance”. This test is similar to a T-test in that it compares the means of various groups, but ANOVA allows you to analyse multiple groups , not just two groups So it’s basically a t-test on steroids…
Next, we have correlation analysis . This type of analysis assesses the relationship between two variables. In other words, if one variable increases, does the other variable also increase, decrease or stay the same. For example, if the average temperature goes up, do average ice creams sales increase too? We’d expect some sort of relationship between these two variables intuitively , but correlation analysis allows us to measure that relationship scientifically .
Lastly, we have regression analysis – this is quite similar to correlation in that it assesses the relationship between variables, but it goes a step further to understand cause and effect between variables, not just whether they move together. In other words, does the one variable actually cause the other one to move, or do they just happen to move together naturally thanks to another force? Just because two variables correlate doesn’t necessarily mean that one causes the other.
Stats overload…
I hear you. To make this all a little more tangible, let’s take a look at an example of a correlation in action.
Here’s a scatter plot demonstrating the correlation (relationship) between weight and height. Intuitively, we’d expect there to be some relationship between these two variables, which is what we see in this scatter plot. In other words, the results tend to cluster together in a diagonal line from bottom left to top right.
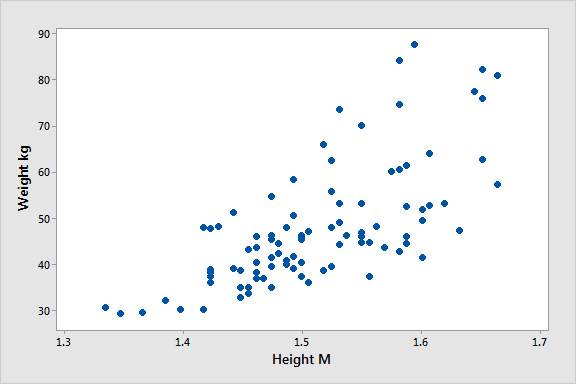
As I mentioned, these are are just a handful of inferential techniques – there are many, many more. Importantly, each statistical method has its own assumptions and limitations.
For example, some methods only work with normally distributed (parametric) data, while other methods are designed specifically for non-parametric data. And that’s exactly why descriptive statistics are so important – they’re the first step to knowing which inferential techniques you can and can’t use.
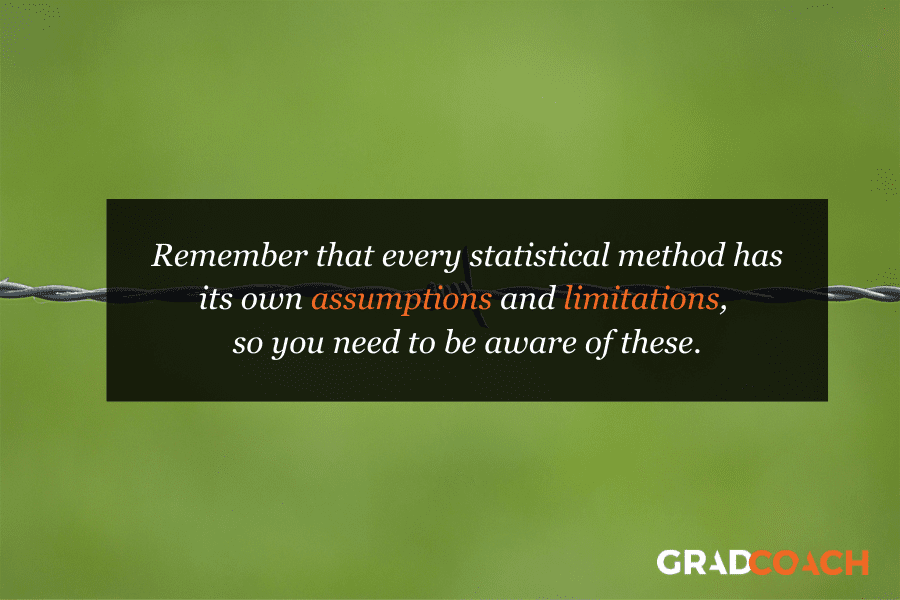
How to choose the right analysis method
To choose the right statistical methods, you need to think about two important factors :
- The type of quantitative data you have (specifically, level of measurement and the shape of the data). And,
- Your research questions and hypotheses
Let’s take a closer look at each of these.
Factor 1 – Data type
The first thing you need to consider is the type of data you’ve collected (or the type of data you will collect). By data types, I’m referring to the four levels of measurement – namely, nominal, ordinal, interval and ratio. If you’re not familiar with this lingo, check out the video below.
Why does this matter?
Well, because different statistical methods and techniques require different types of data. This is one of the “assumptions” I mentioned earlier – every method has its assumptions regarding the type of data.
For example, some techniques work with categorical data (for example, yes/no type questions, or gender or ethnicity), while others work with continuous numerical data (for example, age, weight or income) – and, of course, some work with multiple data types.
If you try to use a statistical method that doesn’t support the data type you have, your results will be largely meaningless . So, make sure that you have a clear understanding of what types of data you’ve collected (or will collect). Once you have this, you can then check which statistical methods would support your data types here .
If you haven’t collected your data yet, you can work in reverse and look at which statistical method would give you the most useful insights, and then design your data collection strategy to collect the correct data types.
Another important factor to consider is the shape of your data . Specifically, does it have a normal distribution (in other words, is it a bell-shaped curve, centred in the middle) or is it very skewed to the left or the right? Again, different statistical techniques work for different shapes of data – some are designed for symmetrical data while others are designed for skewed data.
This is another reminder of why descriptive statistics are so important – they tell you all about the shape of your data.
Factor 2: Your research questions
The next thing you need to consider is your specific research questions, as well as your hypotheses (if you have some). The nature of your research questions and research hypotheses will heavily influence which statistical methods and techniques you should use.
If you’re just interested in understanding the attributes of your sample (as opposed to the entire population), then descriptive statistics are probably all you need. For example, if you just want to assess the means (averages) and medians (centre points) of variables in a group of people.
On the other hand, if you aim to understand differences between groups or relationships between variables and to infer or predict outcomes in the population, then you’ll likely need both descriptive statistics and inferential statistics.
So, it’s really important to get very clear about your research aims and research questions, as well your hypotheses – before you start looking at which statistical techniques to use.
Never shoehorn a specific statistical technique into your research just because you like it or have some experience with it. Your choice of methods must align with all the factors we’ve covered here.
Time to recap…
You’re still with me? That’s impressive. We’ve covered a lot of ground here, so let’s recap on the key points:
- Quantitative data analysis is all about analysing number-based data (which includes categorical and numerical data) using various statistical techniques.
- The two main branches of statistics are descriptive statistics and inferential statistics . Descriptives describe your sample, whereas inferentials make predictions about what you’ll find in the population.
- Common descriptive statistical methods include mean (average), median , standard deviation and skewness .
- Common inferential statistical methods include t-tests , ANOVA , correlation and regression analysis.
- To choose the right statistical methods and techniques, you need to consider the type of data you’re working with , as well as your research questions and hypotheses.
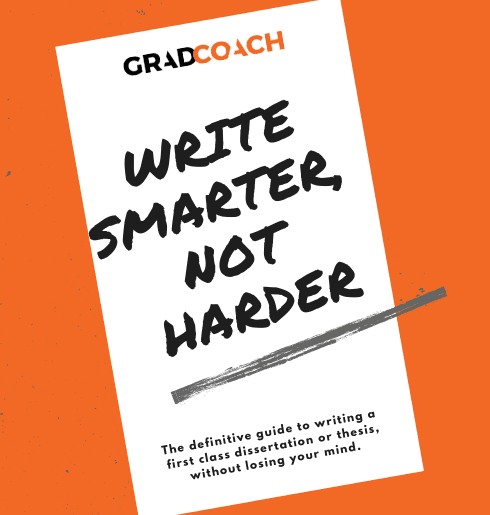
Psst… there’s more (for free)
This post is part of our dissertation mini-course, which covers everything you need to get started with your dissertation, thesis or research project.
You Might Also Like:
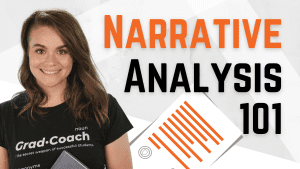
74 Comments
Hi, I have read your article. Such a brilliant post you have created.
Thank you for the feedback. Good luck with your quantitative analysis.
Thank you so much.
Thank you so much. I learnt much well. I love your summaries of the concepts. I had love you to explain how to input data using SPSS
Amazing and simple way of breaking down quantitative methods.
This is beautiful….especially for non-statisticians. I have skimmed through but I wish to read again. and please include me in other articles of the same nature when you do post. I am interested. I am sure, I could easily learn from you and get off the fear that I have had in the past. Thank you sincerely.
Send me every new information you might have.
i need every new information
Thank you for the blog. It is quite informative. Dr Peter Nemaenzhe PhD
It is wonderful. l’ve understood some of the concepts in a more compréhensive manner
Your article is so good! However, I am still a bit lost. I am doing a secondary research on Gun control in the US and increase in crime rates and I am not sure which analysis method I should use?
Based on the given learning points, this is inferential analysis, thus, use ‘t-tests, ANOVA, correlation and regression analysis’
Well explained notes. Am an MPH student and currently working on my thesis proposal, this has really helped me understand some of the things I didn’t know.
I like your page..helpful
wonderful i got my concept crystal clear. thankyou!!
This is really helpful , thank you
Thank you so much this helped
Wonderfully explained
thank u so much, it was so informative
THANKYOU, this was very informative and very helpful
This is great GRADACOACH I am not a statistician but I require more of this in my thesis
Include me in your posts.
This is so great and fully useful. I would like to thank you again and again.
Glad to read this article. I’ve read lot of articles but this article is clear on all concepts. Thanks for sharing.
Thank you so much. This is a very good foundation and intro into quantitative data analysis. Appreciate!
You have a very impressive, simple but concise explanation of data analysis for Quantitative Research here. This is a God-send link for me to appreciate research more. Thank you so much!
Avery good presentation followed by the write up. yes you simplified statistics to make sense even to a layman like me. Thank so much keep it up. The presenter did ell too. i would like more of this for Qualitative and exhaust more of the test example like the Anova.
This is a very helpful article, couldn’t have been clearer. Thank you.
Awesome and phenomenal information.Well done
The video with the accompanying article is super helpful to demystify this topic. Very well done. Thank you so much.
thank you so much, your presentation helped me a lot
I don’t know how should I express that ur article is saviour for me 🥺😍
It is well defined information and thanks for sharing. It helps me a lot in understanding the statistical data.
I gain a lot and thanks for sharing brilliant ideas, so wish to be linked on your email update.
Very helpful and clear .Thank you Gradcoach.
Thank for sharing this article, well organized and information presented are very clear.
VERY INTERESTING AND SUPPORTIVE TO NEW RESEARCHERS LIKE ME. AT LEAST SOME BASICS ABOUT QUANTITATIVE.
An outstanding, well explained and helpful article. This will help me so much with my data analysis for my research project. Thank you!
wow this has just simplified everything i was scared of how i am gonna analyse my data but thanks to you i will be able to do so
simple and constant direction to research. thanks
This is helpful
Great writing!! Comprehensive and very helpful.
Do you provide any assistance for other steps of research methodology like making research problem testing hypothesis report and thesis writing?
Thank you so much for such useful article!
Amazing article. So nicely explained. Wow
Very insightfull. Thanks
I am doing a quality improvement project to determine if the implementation of a protocol will change prescribing habits. Would this be a t-test?
The is a very helpful blog, however, I’m still not sure how to analyze my data collected. I’m doing a research on “Free Education at the University of Guyana”
tnx. fruitful blog!
So I am writing exams and would like to know how do establish which method of data analysis to use from the below research questions: I am a bit lost as to how I determine the data analysis method from the research questions.
Do female employees report higher job satisfaction than male employees with similar job descriptions across the South African telecommunications sector? – I though that maybe Chi Square could be used here. – Is there a gender difference in talented employees’ actual turnover decisions across the South African telecommunications sector? T-tests or Correlation in this one. – Is there a gender difference in the cost of actual turnover decisions across the South African telecommunications sector? T-tests or Correlation in this one. – What practical recommendations can be made to the management of South African telecommunications companies on leveraging gender to mitigate employee turnover decisions?
Your assistance will be appreciated if I could get a response as early as possible tomorrow
This was quite helpful. Thank you so much.
wow I got a lot from this article, thank you very much, keep it up
Thanks for yhe guidance. Can you send me this guidance on my email? To enable offline reading?
Thank you very much, this service is very helpful.
Every novice researcher needs to read this article as it puts things so clear and easy to follow. Its been very helpful.
Wonderful!!!! you explained everything in a way that anyone can learn. Thank you!!
I really enjoyed reading though this. Very easy to follow. Thank you
Many thanks for your useful lecture, I would be really appreciated if you could possibly share with me the PPT of presentation related to Data type?
Thank you very much for sharing, I got much from this article
This is a very informative write-up. Kindly include me in your latest posts.
Very interesting mostly for social scientists
Thank you so much, very helpfull
You’re welcome 🙂
woow, its great, its very informative and well understood because of your way of writing like teaching in front of me in simple languages.
I have been struggling to understand a lot of these concepts. Thank you for the informative piece which is written with outstanding clarity.
very informative article. Easy to understand
Beautiful read, much needed.
Always greet intro and summary. I learn so much from GradCoach
Quite informative. Simple and clear summary.
I thoroughly enjoyed reading your informative and inspiring piece. Your profound insights into this topic truly provide a better understanding of its complexity. I agree with the points you raised, especially when you delved into the specifics of the article. In my opinion, that aspect is often overlooked and deserves further attention.
Absolutely!!! Thank you
Thank you very much for this post. It made me to understand how to do my data analysis.
Submit a Comment Cancel reply
Your email address will not be published. Required fields are marked *
Save my name, email, and website in this browser for the next time I comment.
- Print Friendly
- Search Menu
- Browse content in Arts and Humanities
- Browse content in Archaeology
- Anglo-Saxon and Medieval Archaeology
- Archaeological Methodology and Techniques
- Archaeology by Region
- Archaeology of Religion
- Archaeology of Trade and Exchange
- Biblical Archaeology
- Contemporary and Public Archaeology
- Environmental Archaeology
- Historical Archaeology
- History and Theory of Archaeology
- Industrial Archaeology
- Landscape Archaeology
- Mortuary Archaeology
- Prehistoric Archaeology
- Underwater Archaeology
- Urban Archaeology
- Zooarchaeology
- Browse content in Architecture
- Architectural Structure and Design
- History of Architecture
- Residential and Domestic Buildings
- Theory of Architecture
- Browse content in Art
- Art Subjects and Themes
- History of Art
- Industrial and Commercial Art
- Theory of Art
- Biographical Studies
- Byzantine Studies
- Browse content in Classical Studies
- Classical History
- Classical Philosophy
- Classical Mythology
- Classical Literature
- Classical Reception
- Classical Art and Architecture
- Classical Oratory and Rhetoric
- Greek and Roman Epigraphy
- Greek and Roman Law
- Greek and Roman Archaeology
- Greek and Roman Papyrology
- Late Antiquity
- Religion in the Ancient World
- Digital Humanities
- Browse content in History
- Colonialism and Imperialism
- Diplomatic History
- Environmental History
- Genealogy, Heraldry, Names, and Honours
- Genocide and Ethnic Cleansing
- Historical Geography
- History by Period
- History of Agriculture
- History of Education
- History of Emotions
- History of Gender and Sexuality
- Industrial History
- Intellectual History
- International History
- Labour History
- Legal and Constitutional History
- Local and Family History
- Maritime History
- Military History
- National Liberation and Post-Colonialism
- Oral History
- Political History
- Public History
- Regional and National History
- Revolutions and Rebellions
- Slavery and Abolition of Slavery
- Social and Cultural History
- Theory, Methods, and Historiography
- Urban History
- World History
- Browse content in Language Teaching and Learning
- Language Learning (Specific Skills)
- Language Teaching Theory and Methods
- Browse content in Linguistics
- Applied Linguistics
- Cognitive Linguistics
- Computational Linguistics
- Forensic Linguistics
- Grammar, Syntax and Morphology
- Historical and Diachronic Linguistics
- History of English
- Language Acquisition
- Language Variation
- Language Families
- Language Evolution
- Language Reference
- Lexicography
- Linguistic Theories
- Linguistic Typology
- Linguistic Anthropology
- Phonetics and Phonology
- Psycholinguistics
- Sociolinguistics
- Translation and Interpretation
- Writing Systems
- Browse content in Literature
- Bibliography
- Children's Literature Studies
- Literary Studies (Asian)
- Literary Studies (European)
- Literary Studies (Eco-criticism)
- Literary Studies (Modernism)
- Literary Studies (Romanticism)
- Literary Studies (American)
- Literary Studies - World
- Literary Studies (1500 to 1800)
- Literary Studies (19th Century)
- Literary Studies (20th Century onwards)
- Literary Studies (African American Literature)
- Literary Studies (British and Irish)
- Literary Studies (Early and Medieval)
- Literary Studies (Fiction, Novelists, and Prose Writers)
- Literary Studies (Gender Studies)
- Literary Studies (Graphic Novels)
- Literary Studies (History of the Book)
- Literary Studies (Plays and Playwrights)
- Literary Studies (Poetry and Poets)
- Literary Studies (Postcolonial Literature)
- Literary Studies (Queer Studies)
- Literary Studies (Science Fiction)
- Literary Studies (Travel Literature)
- Literary Studies (War Literature)
- Literary Studies (Women's Writing)
- Literary Theory and Cultural Studies
- Mythology and Folklore
- Shakespeare Studies and Criticism
- Browse content in Media Studies
- Browse content in Music
- Applied Music
- Dance and Music
- Ethics in Music
- Ethnomusicology
- Gender and Sexuality in Music
- Medicine and Music
- Music Cultures
- Music and Religion
- Music and Culture
- Music and Media
- Music Education and Pedagogy
- Music Theory and Analysis
- Musical Scores, Lyrics, and Libretti
- Musical Structures, Styles, and Techniques
- Musicology and Music History
- Performance Practice and Studies
- Race and Ethnicity in Music
- Sound Studies
- Browse content in Performing Arts
- Browse content in Philosophy
- Aesthetics and Philosophy of Art
- Epistemology
- Feminist Philosophy
- History of Western Philosophy
- Metaphysics
- Moral Philosophy
- Non-Western Philosophy
- Philosophy of Science
- Philosophy of Action
- Philosophy of Law
- Philosophy of Religion
- Philosophy of Language
- Philosophy of Mind
- Philosophy of Perception
- Philosophy of Mathematics and Logic
- Practical Ethics
- Social and Political Philosophy
- Browse content in Religion
- Biblical Studies
- Christianity
- East Asian Religions
- History of Religion
- Judaism and Jewish Studies
- Qumran Studies
- Religion and Education
- Religion and Health
- Religion and Politics
- Religion and Science
- Religion and Law
- Religion and Art, Literature, and Music
- Religious Studies
- Browse content in Society and Culture
- Cookery, Food, and Drink
- Cultural Studies
- Customs and Traditions
- Ethical Issues and Debates
- Hobbies, Games, Arts and Crafts
- Lifestyle, Home, and Garden
- Natural world, Country Life, and Pets
- Popular Beliefs and Controversial Knowledge
- Sports and Outdoor Recreation
- Technology and Society
- Travel and Holiday
- Visual Culture
- Browse content in Law
- Arbitration
- Browse content in Company and Commercial Law
- Commercial Law
- Company Law
- Browse content in Comparative Law
- Systems of Law
- Competition Law
- Browse content in Constitutional and Administrative Law
- Government Powers
- Judicial Review
- Local Government Law
- Military and Defence Law
- Parliamentary and Legislative Practice
- Construction Law
- Contract Law
- Browse content in Criminal Law
- Criminal Procedure
- Criminal Evidence Law
- Sentencing and Punishment
- Employment and Labour Law
- Environment and Energy Law
- Browse content in Financial Law
- Banking Law
- Insolvency Law
- History of Law
- Human Rights and Immigration
- Intellectual Property Law
- Browse content in International Law
- Private International Law and Conflict of Laws
- Public International Law
- IT and Communications Law
- Jurisprudence and Philosophy of Law
- Law and Politics
- Law and Society
- Browse content in Legal System and Practice
- Courts and Procedure
- Legal Skills and Practice
- Primary Sources of Law
- Regulation of Legal Profession
- Medical and Healthcare Law
- Browse content in Policing
- Criminal Investigation and Detection
- Police and Security Services
- Police Procedure and Law
- Police Regional Planning
- Browse content in Property Law
- Personal Property Law
- Study and Revision
- Terrorism and National Security Law
- Browse content in Trusts Law
- Wills and Probate or Succession
- Browse content in Medicine and Health
- Browse content in Allied Health Professions
- Arts Therapies
- Clinical Science
- Dietetics and Nutrition
- Occupational Therapy
- Operating Department Practice
- Physiotherapy
- Radiography
- Speech and Language Therapy
- Browse content in Anaesthetics
- General Anaesthesia
- Neuroanaesthesia
- Browse content in Clinical Medicine
- Acute Medicine
- Cardiovascular Medicine
- Clinical Genetics
- Clinical Pharmacology and Therapeutics
- Dermatology
- Endocrinology and Diabetes
- Gastroenterology
- Genito-urinary Medicine
- Geriatric Medicine
- Infectious Diseases
- Medical Oncology
- Medical Toxicology
- Pain Medicine
- Palliative Medicine
- Rehabilitation Medicine
- Respiratory Medicine and Pulmonology
- Rheumatology
- Sleep Medicine
- Sports and Exercise Medicine
- Clinical Neuroscience
- Community Medical Services
- Critical Care
- Emergency Medicine
- Forensic Medicine
- Haematology
- History of Medicine
- Browse content in Medical Dentistry
- Oral and Maxillofacial Surgery
- Paediatric Dentistry
- Restorative Dentistry and Orthodontics
- Surgical Dentistry
- Medical Ethics
- Browse content in Medical Skills
- Clinical Skills
- Communication Skills
- Nursing Skills
- Surgical Skills
- Medical Statistics and Methodology
- Browse content in Neurology
- Clinical Neurophysiology
- Neuropathology
- Nursing Studies
- Browse content in Obstetrics and Gynaecology
- Gynaecology
- Occupational Medicine
- Ophthalmology
- Otolaryngology (ENT)
- Browse content in Paediatrics
- Neonatology
- Browse content in Pathology
- Chemical Pathology
- Clinical Cytogenetics and Molecular Genetics
- Histopathology
- Medical Microbiology and Virology
- Patient Education and Information
- Browse content in Pharmacology
- Psychopharmacology
- Browse content in Popular Health
- Caring for Others
- Complementary and Alternative Medicine
- Self-help and Personal Development
- Browse content in Preclinical Medicine
- Cell Biology
- Molecular Biology and Genetics
- Reproduction, Growth and Development
- Primary Care
- Professional Development in Medicine
- Browse content in Psychiatry
- Addiction Medicine
- Child and Adolescent Psychiatry
- Forensic Psychiatry
- Learning Disabilities
- Old Age Psychiatry
- Psychotherapy
- Browse content in Public Health and Epidemiology
- Epidemiology
- Public Health
- Browse content in Radiology
- Clinical Radiology
- Interventional Radiology
- Nuclear Medicine
- Radiation Oncology
- Reproductive Medicine
- Browse content in Surgery
- Cardiothoracic Surgery
- Gastro-intestinal and Colorectal Surgery
- General Surgery
- Neurosurgery
- Paediatric Surgery
- Peri-operative Care
- Plastic and Reconstructive Surgery
- Surgical Oncology
- Transplant Surgery
- Trauma and Orthopaedic Surgery
- Vascular Surgery
- Browse content in Science and Mathematics
- Browse content in Biological Sciences
- Aquatic Biology
- Biochemistry
- Bioinformatics and Computational Biology
- Developmental Biology
- Ecology and Conservation
- Evolutionary Biology
- Genetics and Genomics
- Microbiology
- Molecular and Cell Biology
- Natural History
- Plant Sciences and Forestry
- Research Methods in Life Sciences
- Structural Biology
- Systems Biology
- Zoology and Animal Sciences
- Browse content in Chemistry
- Analytical Chemistry
- Computational Chemistry
- Crystallography
- Environmental Chemistry
- Industrial Chemistry
- Inorganic Chemistry
- Materials Chemistry
- Medicinal Chemistry
- Mineralogy and Gems
- Organic Chemistry
- Physical Chemistry
- Polymer Chemistry
- Study and Communication Skills in Chemistry
- Theoretical Chemistry
- Browse content in Computer Science
- Artificial Intelligence
- Computer Architecture and Logic Design
- Game Studies
- Human-Computer Interaction
- Mathematical Theory of Computation
- Programming Languages
- Software Engineering
- Systems Analysis and Design
- Virtual Reality
- Browse content in Computing
- Business Applications
- Computer Security
- Computer Games
- Computer Networking and Communications
- Digital Lifestyle
- Graphical and Digital Media Applications
- Operating Systems
- Browse content in Earth Sciences and Geography
- Atmospheric Sciences
- Environmental Geography
- Geology and the Lithosphere
- Maps and Map-making
- Meteorology and Climatology
- Oceanography and Hydrology
- Palaeontology
- Physical Geography and Topography
- Regional Geography
- Soil Science
- Urban Geography
- Browse content in Engineering and Technology
- Agriculture and Farming
- Biological Engineering
- Civil Engineering, Surveying, and Building
- Electronics and Communications Engineering
- Energy Technology
- Engineering (General)
- Environmental Science, Engineering, and Technology
- History of Engineering and Technology
- Mechanical Engineering and Materials
- Technology of Industrial Chemistry
- Transport Technology and Trades
- Browse content in Environmental Science
- Applied Ecology (Environmental Science)
- Conservation of the Environment (Environmental Science)
- Environmental Sustainability
- Environmentalist Thought and Ideology (Environmental Science)
- Management of Land and Natural Resources (Environmental Science)
- Natural Disasters (Environmental Science)
- Nuclear Issues (Environmental Science)
- Pollution and Threats to the Environment (Environmental Science)
- Social Impact of Environmental Issues (Environmental Science)
- History of Science and Technology
- Browse content in Materials Science
- Ceramics and Glasses
- Composite Materials
- Metals, Alloying, and Corrosion
- Nanotechnology
- Browse content in Mathematics
- Applied Mathematics
- Biomathematics and Statistics
- History of Mathematics
- Mathematical Education
- Mathematical Finance
- Mathematical Analysis
- Numerical and Computational Mathematics
- Probability and Statistics
- Pure Mathematics
- Browse content in Neuroscience
- Cognition and Behavioural Neuroscience
- Development of the Nervous System
- Disorders of the Nervous System
- History of Neuroscience
- Invertebrate Neurobiology
- Molecular and Cellular Systems
- Neuroendocrinology and Autonomic Nervous System
- Neuroscientific Techniques
- Sensory and Motor Systems
- Browse content in Physics
- Astronomy and Astrophysics
- Atomic, Molecular, and Optical Physics
- Biological and Medical Physics
- Classical Mechanics
- Computational Physics
- Condensed Matter Physics
- Electromagnetism, Optics, and Acoustics
- History of Physics
- Mathematical and Statistical Physics
- Measurement Science
- Nuclear Physics
- Particles and Fields
- Plasma Physics
- Quantum Physics
- Relativity and Gravitation
- Semiconductor and Mesoscopic Physics
- Browse content in Psychology
- Affective Sciences
- Clinical Psychology
- Cognitive Neuroscience
- Cognitive Psychology
- Criminal and Forensic Psychology
- Developmental Psychology
- Educational Psychology
- Evolutionary Psychology
- Health Psychology
- History and Systems in Psychology
- Music Psychology
- Neuropsychology
- Organizational Psychology
- Psychological Assessment and Testing
- Psychology of Human-Technology Interaction
- Psychology Professional Development and Training
- Research Methods in Psychology
- Social Psychology
- Browse content in Social Sciences
- Browse content in Anthropology
- Anthropology of Religion
- Human Evolution
- Medical Anthropology
- Physical Anthropology
- Regional Anthropology
- Social and Cultural Anthropology
- Theory and Practice of Anthropology
- Browse content in Business and Management
- Business Strategy
- Business History
- Business Ethics
- Business and Government
- Business and Technology
- Business and the Environment
- Comparative Management
- Corporate Governance
- Corporate Social Responsibility
- Entrepreneurship
- Health Management
- Human Resource Management
- Industrial and Employment Relations
- Industry Studies
- Information and Communication Technologies
- International Business
- Knowledge Management
- Management and Management Techniques
- Operations Management
- Organizational Theory and Behaviour
- Pensions and Pension Management
- Public and Nonprofit Management
- Strategic Management
- Supply Chain Management
- Browse content in Criminology and Criminal Justice
- Criminal Justice
- Criminology
- Forms of Crime
- International and Comparative Criminology
- Youth Violence and Juvenile Justice
- Development Studies
- Browse content in Economics
- Agricultural, Environmental, and Natural Resource Economics
- Asian Economics
- Behavioural Finance
- Behavioural Economics and Neuroeconomics
- Econometrics and Mathematical Economics
- Economic Systems
- Economic Methodology
- Economic History
- Economic Development and Growth
- Financial Markets
- Financial Institutions and Services
- General Economics and Teaching
- Health, Education, and Welfare
- History of Economic Thought
- International Economics
- Labour and Demographic Economics
- Law and Economics
- Macroeconomics and Monetary Economics
- Microeconomics
- Public Economics
- Urban, Rural, and Regional Economics
- Welfare Economics
- Browse content in Education
- Adult Education and Continuous Learning
- Care and Counselling of Students
- Early Childhood and Elementary Education
- Educational Equipment and Technology
- Educational Strategies and Policy
- Higher and Further Education
- Organization and Management of Education
- Philosophy and Theory of Education
- Schools Studies
- Secondary Education
- Teaching of a Specific Subject
- Teaching of Specific Groups and Special Educational Needs
- Teaching Skills and Techniques
- Browse content in Environment
- Applied Ecology (Social Science)
- Climate Change
- Conservation of the Environment (Social Science)
- Environmentalist Thought and Ideology (Social Science)
- Natural Disasters (Environment)
- Social Impact of Environmental Issues (Social Science)
- Browse content in Human Geography
- Cultural Geography
- Economic Geography
- Political Geography
- Browse content in Interdisciplinary Studies
- Communication Studies
- Museums, Libraries, and Information Sciences
- Browse content in Politics
- African Politics
- Asian Politics
- Chinese Politics
- Comparative Politics
- Conflict Politics
- Elections and Electoral Studies
- Environmental Politics
- European Union
- Foreign Policy
- Gender and Politics
- Human Rights and Politics
- Indian Politics
- International Relations
- International Organization (Politics)
- International Political Economy
- Irish Politics
- Latin American Politics
- Middle Eastern Politics
- Political Methodology
- Political Communication
- Political Philosophy
- Political Sociology
- Political Theory
- Political Behaviour
- Political Economy
- Political Institutions
- Politics and Law
- Public Administration
- Public Policy
- Quantitative Political Methodology
- Regional Political Studies
- Russian Politics
- Security Studies
- State and Local Government
- UK Politics
- US Politics
- Browse content in Regional and Area Studies
- African Studies
- Asian Studies
- East Asian Studies
- Japanese Studies
- Latin American Studies
- Middle Eastern Studies
- Native American Studies
- Scottish Studies
- Browse content in Research and Information
- Research Methods
- Browse content in Social Work
- Addictions and Substance Misuse
- Adoption and Fostering
- Care of the Elderly
- Child and Adolescent Social Work
- Couple and Family Social Work
- Developmental and Physical Disabilities Social Work
- Direct Practice and Clinical Social Work
- Emergency Services
- Human Behaviour and the Social Environment
- International and Global Issues in Social Work
- Mental and Behavioural Health
- Social Justice and Human Rights
- Social Policy and Advocacy
- Social Work and Crime and Justice
- Social Work Macro Practice
- Social Work Practice Settings
- Social Work Research and Evidence-based Practice
- Welfare and Benefit Systems
- Browse content in Sociology
- Childhood Studies
- Community Development
- Comparative and Historical Sociology
- Economic Sociology
- Gender and Sexuality
- Gerontology and Ageing
- Health, Illness, and Medicine
- Marriage and the Family
- Migration Studies
- Occupations, Professions, and Work
- Organizations
- Population and Demography
- Race and Ethnicity
- Social Theory
- Social Movements and Social Change
- Social Research and Statistics
- Social Stratification, Inequality, and Mobility
- Sociology of Religion
- Sociology of Education
- Sport and Leisure
- Urban and Rural Studies
- Browse content in Warfare and Defence
- Defence Strategy, Planning, and Research
- Land Forces and Warfare
- Military Administration
- Military Life and Institutions
- Naval Forces and Warfare
- Other Warfare and Defence Issues
- Peace Studies and Conflict Resolution
- Weapons and Equipment

- < Previous chapter
- Next chapter >
7 Quantitative Data Analysis
- Published: January 2022
- Cite Icon Cite
- Permissions Icon Permissions
Many people tend to privilege evidence generated from quantitative research over, for example, qualitative research. Politicians and the public often demand “hard evidence” to support decisions. The descriptor ‘hard” often implies evidence generated by quantitative research. Although quantitative research and methods can provide good evidence, it is important for novice researchers to learn basic elements of quantitative data analysis in order to better understand and interpret their own research or that of others. This chapter discusses how to analyze and interpret quantitative data and connect data analysis to stakeholder inclusion, positionality, and ethics. The chapter provides additional resources for further exploration of quantitative data analysis.
Signed in as
Institutional accounts.
- Google Scholar Indexing
- GoogleCrawler [DO NOT DELETE]
Personal account
- Sign in with email/username & password
- Get email alerts
- Save searches
- Purchase content
- Activate your purchase/trial code
Institutional access
- Sign in with a library card Sign in with username/password Recommend to your librarian
- Institutional account management
- Get help with access
Access to content on Oxford Academic is often provided through institutional subscriptions and purchases. If you are a member of an institution with an active account, you may be able to access content in one of the following ways:
IP based access
Typically, access is provided across an institutional network to a range of IP addresses. This authentication occurs automatically, and it is not possible to sign out of an IP authenticated account.
Sign in through your institution
Choose this option to get remote access when outside your institution. Shibboleth/Open Athens technology is used to provide single sign-on between your institution’s website and Oxford Academic.
- Click Sign in through your institution.
- Select your institution from the list provided, which will take you to your institution's website to sign in.
- When on the institution site, please use the credentials provided by your institution. Do not use an Oxford Academic personal account.
- Following successful sign in, you will be returned to Oxford Academic.
If your institution is not listed or you cannot sign in to your institution’s website, please contact your librarian or administrator.
Sign in with a library card
Enter your library card number to sign in. If you cannot sign in, please contact your librarian.
Society Members
Society member access to a journal is achieved in one of the following ways:
Sign in through society site
Many societies offer single sign-on between the society website and Oxford Academic. If you see ‘Sign in through society site’ in the sign in pane within a journal:
- Click Sign in through society site.
- When on the society site, please use the credentials provided by that society. Do not use an Oxford Academic personal account.
If you do not have a society account or have forgotten your username or password, please contact your society.
Sign in using a personal account
Some societies use Oxford Academic personal accounts to provide access to their members. See below.
A personal account can be used to get email alerts, save searches, purchase content, and activate subscriptions.
Some societies use Oxford Academic personal accounts to provide access to their members.
Viewing your signed in accounts
Click the account icon in the top right to:
- View your signed in personal account and access account management features.
- View the institutional accounts that are providing access.
Signed in but can't access content
Oxford Academic is home to a wide variety of products. The institutional subscription may not cover the content that you are trying to access. If you believe you should have access to that content, please contact your librarian.
For librarians and administrators, your personal account also provides access to institutional account management. Here you will find options to view and activate subscriptions, manage institutional settings and access options, access usage statistics, and more.
Our books are available by subscription or purchase to libraries and institutions.
- About Oxford Academic
- Publish journals with us
- University press partners
- What we publish
- New features
- Open access
- Rights and permissions
- Accessibility
- Advertising
- Media enquiries
- Oxford University Press
- Oxford Languages
- University of Oxford
Oxford University Press is a department of the University of Oxford. It furthers the University's objective of excellence in research, scholarship, and education by publishing worldwide
- Copyright © 2024 Oxford University Press
- Cookie settings
- Cookie policy
- Privacy policy
- Legal notice
This Feature Is Available To Subscribers Only
Sign In or Create an Account
This PDF is available to Subscribers Only
For full access to this pdf, sign in to an existing account, or purchase an annual subscription.
Have a language expert improve your writing
Run a free plagiarism check in 10 minutes, generate accurate citations for free.
- Knowledge Base
Methodology
- What Is Quantitative Research? | Definition, Uses & Methods
What Is Quantitative Research? | Definition, Uses & Methods
Published on June 12, 2020 by Pritha Bhandari . Revised on June 22, 2023.
Quantitative research is the process of collecting and analyzing numerical data. It can be used to find patterns and averages, make predictions, test causal relationships, and generalize results to wider populations.
Quantitative research is the opposite of qualitative research , which involves collecting and analyzing non-numerical data (e.g., text, video, or audio).
Quantitative research is widely used in the natural and social sciences: biology, chemistry, psychology, economics, sociology, marketing, etc.
- What is the demographic makeup of Singapore in 2020?
- How has the average temperature changed globally over the last century?
- Does environmental pollution affect the prevalence of honey bees?
- Does working from home increase productivity for people with long commutes?
Table of contents
Quantitative research methods, quantitative data analysis, advantages of quantitative research, disadvantages of quantitative research, other interesting articles, frequently asked questions about quantitative research.
You can use quantitative research methods for descriptive, correlational or experimental research.
- In descriptive research , you simply seek an overall summary of your study variables.
- In correlational research , you investigate relationships between your study variables.
- In experimental research , you systematically examine whether there is a cause-and-effect relationship between variables.
Correlational and experimental research can both be used to formally test hypotheses , or predictions, using statistics. The results may be generalized to broader populations based on the sampling method used.
To collect quantitative data, you will often need to use operational definitions that translate abstract concepts (e.g., mood) into observable and quantifiable measures (e.g., self-ratings of feelings and energy levels).
Note that quantitative research is at risk for certain research biases , including information bias , omitted variable bias , sampling bias , or selection bias . Be sure that you’re aware of potential biases as you collect and analyze your data to prevent them from impacting your work too much.
Receive feedback on language, structure, and formatting
Professional editors proofread and edit your paper by focusing on:
- Academic style
- Vague sentences
- Style consistency
See an example
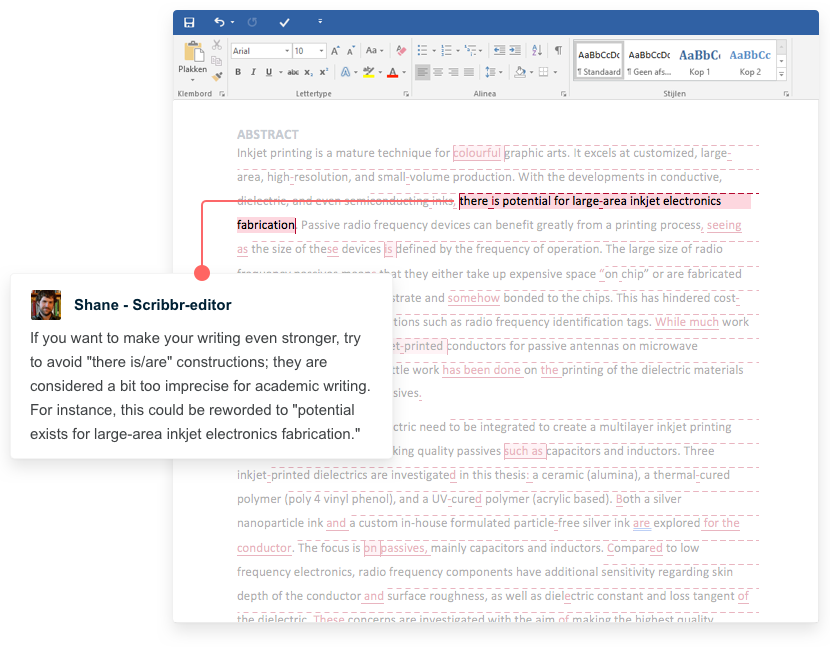
Once data is collected, you may need to process it before it can be analyzed. For example, survey and test data may need to be transformed from words to numbers. Then, you can use statistical analysis to answer your research questions .
Descriptive statistics will give you a summary of your data and include measures of averages and variability. You can also use graphs, scatter plots and frequency tables to visualize your data and check for any trends or outliers.
Using inferential statistics , you can make predictions or generalizations based on your data. You can test your hypothesis or use your sample data to estimate the population parameter .
First, you use descriptive statistics to get a summary of the data. You find the mean (average) and the mode (most frequent rating) of procrastination of the two groups, and plot the data to see if there are any outliers.
You can also assess the reliability and validity of your data collection methods to indicate how consistently and accurately your methods actually measured what you wanted them to.
Quantitative research is often used to standardize data collection and generalize findings . Strengths of this approach include:
- Replication
Repeating the study is possible because of standardized data collection protocols and tangible definitions of abstract concepts.
- Direct comparisons of results
The study can be reproduced in other cultural settings, times or with different groups of participants. Results can be compared statistically.
- Large samples
Data from large samples can be processed and analyzed using reliable and consistent procedures through quantitative data analysis.
- Hypothesis testing
Using formalized and established hypothesis testing procedures means that you have to carefully consider and report your research variables, predictions, data collection and testing methods before coming to a conclusion.
Despite the benefits of quantitative research, it is sometimes inadequate in explaining complex research topics. Its limitations include:
- Superficiality
Using precise and restrictive operational definitions may inadequately represent complex concepts. For example, the concept of mood may be represented with just a number in quantitative research, but explained with elaboration in qualitative research.
- Narrow focus
Predetermined variables and measurement procedures can mean that you ignore other relevant observations.
- Structural bias
Despite standardized procedures, structural biases can still affect quantitative research. Missing data , imprecise measurements or inappropriate sampling methods are biases that can lead to the wrong conclusions.
- Lack of context
Quantitative research often uses unnatural settings like laboratories or fails to consider historical and cultural contexts that may affect data collection and results.
If you want to know more about statistics , methodology , or research bias , make sure to check out some of our other articles with explanations and examples.
- Chi square goodness of fit test
- Degrees of freedom
- Null hypothesis
- Discourse analysis
- Control groups
- Mixed methods research
- Non-probability sampling
- Inclusion and exclusion criteria
Research bias
- Rosenthal effect
- Implicit bias
- Cognitive bias
- Selection bias
- Negativity bias
- Status quo bias
Quantitative research deals with numbers and statistics, while qualitative research deals with words and meanings.
Quantitative methods allow you to systematically measure variables and test hypotheses . Qualitative methods allow you to explore concepts and experiences in more detail.
In mixed methods research , you use both qualitative and quantitative data collection and analysis methods to answer your research question .
Data collection is the systematic process by which observations or measurements are gathered in research. It is used in many different contexts by academics, governments, businesses, and other organizations.
Operationalization means turning abstract conceptual ideas into measurable observations.
For example, the concept of social anxiety isn’t directly observable, but it can be operationally defined in terms of self-rating scores, behavioral avoidance of crowded places, or physical anxiety symptoms in social situations.
Before collecting data , it’s important to consider how you will operationalize the variables that you want to measure.
Reliability and validity are both about how well a method measures something:
- Reliability refers to the consistency of a measure (whether the results can be reproduced under the same conditions).
- Validity refers to the accuracy of a measure (whether the results really do represent what they are supposed to measure).
If you are doing experimental research, you also have to consider the internal and external validity of your experiment.
Hypothesis testing is a formal procedure for investigating our ideas about the world using statistics. It is used by scientists to test specific predictions, called hypotheses , by calculating how likely it is that a pattern or relationship between variables could have arisen by chance.
Cite this Scribbr article
If you want to cite this source, you can copy and paste the citation or click the “Cite this Scribbr article” button to automatically add the citation to our free Citation Generator.
Bhandari, P. (2023, June 22). What Is Quantitative Research? | Definition, Uses & Methods. Scribbr. Retrieved April 6, 2024, from https://www.scribbr.com/methodology/quantitative-research/
Is this article helpful?
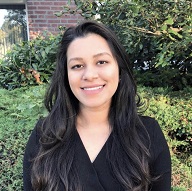
Pritha Bhandari
Other students also liked, descriptive statistics | definitions, types, examples, inferential statistics | an easy introduction & examples, what is your plagiarism score.
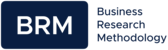
Quantitative Data Analysis
In quantitative data analysis you are expected to turn raw numbers into meaningful data through the application of rational and critical thinking. Quantitative data analysis may include the calculation of frequencies of variables and differences between variables. A quantitative approach is usually associated with finding evidence to either support or reject hypotheses you have formulated at the earlier stages of your research process .
The same figure within data set can be interpreted in many different ways; therefore it is important to apply fair and careful judgement.
For example, questionnaire findings of a research titled “A study into the impacts of informal management-employee communication on the levels of employee motivation: a case study of Agro Bravo Enterprise” may indicate that the majority 52% of respondents assess communication skills of their immediate supervisors as inadequate.
This specific piece of primary data findings needs to be critically analyzed and objectively interpreted through comparing it to other findings within the framework of the same research. For example, organizational culture of Agro Bravo Enterprise, leadership style, the levels of frequency of management-employee communications need to be taken into account during the data analysis.
Moreover, literature review findings conducted at the earlier stages of the research process need to be referred to in order to reflect the viewpoints of other authors regarding the causes of employee dissatisfaction with management communication. Also, secondary data needs to be integrated in data analysis in a logical and unbiased manner.
Let’s take another example. You are writing a dissertation exploring the impacts of foreign direct investment (FDI) on the levels of economic growth in Vietnam using correlation quantitative data analysis method . You have specified FDI and GDP as variables for your research and correlation tests produced correlation coefficient of 0.9.
In this case simply stating that there is a strong positive correlation between FDI and GDP would not suffice; you have to provide explanation about the manners in which the growth on the levels of FDI may contribute to the growth of GDP by referring to the findings of the literature review and applying your own critical and rational reasoning skills.
A set of analytical software can be used to assist with analysis of quantitative data. The following table illustrates the advantages and disadvantages of three popular quantitative data analysis software: Microsoft Excel, Microsoft Access and SPSS.
Advantages and disadvantages of popular quantitative analytical software
Quantitative data analysis with the application of statistical software consists of the following stages [1] :
- Preparing and checking the data. Input of data into computer.
- Selecting the most appropriate tables and diagrams to use according to your research objectives.
- Selecting the most appropriate statistics to describe your data.
- Selecting the most appropriate statistics to examine relationships and trends in your data.
It is important to note that while the application of various statistical software and programs are invaluable to avoid drawing charts by hand or undertake calculations manually, it is easy to use them incorrectly. In other words, quantitative data analysis is “a field where it is not at all difficult to carry out an analysis which is simply wrong, or inappropriate for your data or purposes. And the negative side of readily available specialist statistical software is that it becomes that much easier to generate elegantly presented rubbish” [2] .
Therefore, it is important for you to seek advice from your dissertation supervisor regarding statistical analyses in general and the choice and application of statistical software in particular.
My e-book, The Ultimate Guide to Writing a Dissertation in Business Studies: a step by step approach contains a detailed, yet simple explanation of quantitative data analysis methods . The e-book explains all stages of the research process starting from the selection of the research area to writing personal reflection. Important elements of dissertations such as research philosophy, research approach, research design, methods of data collection and data analysis are explained in simple words. John Dudovskiy
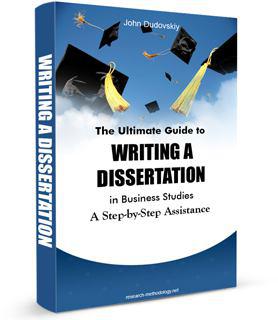
[1] Saunders, M., Lewis, P. & Thornhill, A. (2012) “Research Methods for Business Students” 6th edition, Pearson Education Limited.
[2] Robson, C. (2011) Real World Research: A Resource for Users of Social Research Methods in Applied Settings (3rd edn). Chichester: John Wiley.

An official website of the United States government
The .gov means it’s official. Federal government websites often end in .gov or .mil. Before sharing sensitive information, make sure you’re on a federal government site.
The site is secure. The https:// ensures that you are connecting to the official website and that any information you provide is encrypted and transmitted securely.
- Publications
- Account settings
Preview improvements coming to the PMC website in October 2024. Learn More or Try it out now .
- Advanced Search
- Journal List
- J Korean Med Sci
- v.37(16); 2022 Apr 25

A Practical Guide to Writing Quantitative and Qualitative Research Questions and Hypotheses in Scholarly Articles
Edward barroga.
1 Department of General Education, Graduate School of Nursing Science, St. Luke’s International University, Tokyo, Japan.
Glafera Janet Matanguihan
2 Department of Biological Sciences, Messiah University, Mechanicsburg, PA, USA.
The development of research questions and the subsequent hypotheses are prerequisites to defining the main research purpose and specific objectives of a study. Consequently, these objectives determine the study design and research outcome. The development of research questions is a process based on knowledge of current trends, cutting-edge studies, and technological advances in the research field. Excellent research questions are focused and require a comprehensive literature search and in-depth understanding of the problem being investigated. Initially, research questions may be written as descriptive questions which could be developed into inferential questions. These questions must be specific and concise to provide a clear foundation for developing hypotheses. Hypotheses are more formal predictions about the research outcomes. These specify the possible results that may or may not be expected regarding the relationship between groups. Thus, research questions and hypotheses clarify the main purpose and specific objectives of the study, which in turn dictate the design of the study, its direction, and outcome. Studies developed from good research questions and hypotheses will have trustworthy outcomes with wide-ranging social and health implications.
INTRODUCTION
Scientific research is usually initiated by posing evidenced-based research questions which are then explicitly restated as hypotheses. 1 , 2 The hypotheses provide directions to guide the study, solutions, explanations, and expected results. 3 , 4 Both research questions and hypotheses are essentially formulated based on conventional theories and real-world processes, which allow the inception of novel studies and the ethical testing of ideas. 5 , 6
It is crucial to have knowledge of both quantitative and qualitative research 2 as both types of research involve writing research questions and hypotheses. 7 However, these crucial elements of research are sometimes overlooked; if not overlooked, then framed without the forethought and meticulous attention it needs. Planning and careful consideration are needed when developing quantitative or qualitative research, particularly when conceptualizing research questions and hypotheses. 4
There is a continuing need to support researchers in the creation of innovative research questions and hypotheses, as well as for journal articles that carefully review these elements. 1 When research questions and hypotheses are not carefully thought of, unethical studies and poor outcomes usually ensue. Carefully formulated research questions and hypotheses define well-founded objectives, which in turn determine the appropriate design, course, and outcome of the study. This article then aims to discuss in detail the various aspects of crafting research questions and hypotheses, with the goal of guiding researchers as they develop their own. Examples from the authors and peer-reviewed scientific articles in the healthcare field are provided to illustrate key points.
DEFINITIONS AND RELATIONSHIP OF RESEARCH QUESTIONS AND HYPOTHESES
A research question is what a study aims to answer after data analysis and interpretation. The answer is written in length in the discussion section of the paper. Thus, the research question gives a preview of the different parts and variables of the study meant to address the problem posed in the research question. 1 An excellent research question clarifies the research writing while facilitating understanding of the research topic, objective, scope, and limitations of the study. 5
On the other hand, a research hypothesis is an educated statement of an expected outcome. This statement is based on background research and current knowledge. 8 , 9 The research hypothesis makes a specific prediction about a new phenomenon 10 or a formal statement on the expected relationship between an independent variable and a dependent variable. 3 , 11 It provides a tentative answer to the research question to be tested or explored. 4
Hypotheses employ reasoning to predict a theory-based outcome. 10 These can also be developed from theories by focusing on components of theories that have not yet been observed. 10 The validity of hypotheses is often based on the testability of the prediction made in a reproducible experiment. 8
Conversely, hypotheses can also be rephrased as research questions. Several hypotheses based on existing theories and knowledge may be needed to answer a research question. Developing ethical research questions and hypotheses creates a research design that has logical relationships among variables. These relationships serve as a solid foundation for the conduct of the study. 4 , 11 Haphazardly constructed research questions can result in poorly formulated hypotheses and improper study designs, leading to unreliable results. Thus, the formulations of relevant research questions and verifiable hypotheses are crucial when beginning research. 12
CHARACTERISTICS OF GOOD RESEARCH QUESTIONS AND HYPOTHESES
Excellent research questions are specific and focused. These integrate collective data and observations to confirm or refute the subsequent hypotheses. Well-constructed hypotheses are based on previous reports and verify the research context. These are realistic, in-depth, sufficiently complex, and reproducible. More importantly, these hypotheses can be addressed and tested. 13
There are several characteristics of well-developed hypotheses. Good hypotheses are 1) empirically testable 7 , 10 , 11 , 13 ; 2) backed by preliminary evidence 9 ; 3) testable by ethical research 7 , 9 ; 4) based on original ideas 9 ; 5) have evidenced-based logical reasoning 10 ; and 6) can be predicted. 11 Good hypotheses can infer ethical and positive implications, indicating the presence of a relationship or effect relevant to the research theme. 7 , 11 These are initially developed from a general theory and branch into specific hypotheses by deductive reasoning. In the absence of a theory to base the hypotheses, inductive reasoning based on specific observations or findings form more general hypotheses. 10
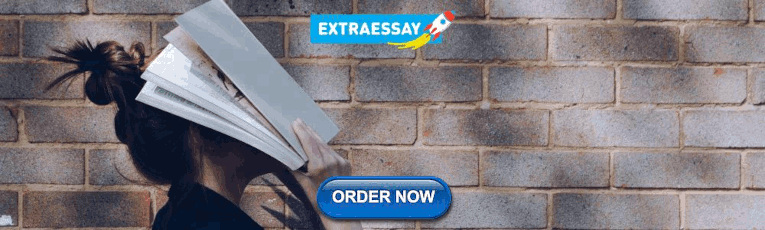
TYPES OF RESEARCH QUESTIONS AND HYPOTHESES
Research questions and hypotheses are developed according to the type of research, which can be broadly classified into quantitative and qualitative research. We provide a summary of the types of research questions and hypotheses under quantitative and qualitative research categories in Table 1 .
Research questions in quantitative research
In quantitative research, research questions inquire about the relationships among variables being investigated and are usually framed at the start of the study. These are precise and typically linked to the subject population, dependent and independent variables, and research design. 1 Research questions may also attempt to describe the behavior of a population in relation to one or more variables, or describe the characteristics of variables to be measured ( descriptive research questions ). 1 , 5 , 14 These questions may also aim to discover differences between groups within the context of an outcome variable ( comparative research questions ), 1 , 5 , 14 or elucidate trends and interactions among variables ( relationship research questions ). 1 , 5 We provide examples of descriptive, comparative, and relationship research questions in quantitative research in Table 2 .
Hypotheses in quantitative research
In quantitative research, hypotheses predict the expected relationships among variables. 15 Relationships among variables that can be predicted include 1) between a single dependent variable and a single independent variable ( simple hypothesis ) or 2) between two or more independent and dependent variables ( complex hypothesis ). 4 , 11 Hypotheses may also specify the expected direction to be followed and imply an intellectual commitment to a particular outcome ( directional hypothesis ) 4 . On the other hand, hypotheses may not predict the exact direction and are used in the absence of a theory, or when findings contradict previous studies ( non-directional hypothesis ). 4 In addition, hypotheses can 1) define interdependency between variables ( associative hypothesis ), 4 2) propose an effect on the dependent variable from manipulation of the independent variable ( causal hypothesis ), 4 3) state a negative relationship between two variables ( null hypothesis ), 4 , 11 , 15 4) replace the working hypothesis if rejected ( alternative hypothesis ), 15 explain the relationship of phenomena to possibly generate a theory ( working hypothesis ), 11 5) involve quantifiable variables that can be tested statistically ( statistical hypothesis ), 11 6) or express a relationship whose interlinks can be verified logically ( logical hypothesis ). 11 We provide examples of simple, complex, directional, non-directional, associative, causal, null, alternative, working, statistical, and logical hypotheses in quantitative research, as well as the definition of quantitative hypothesis-testing research in Table 3 .
Research questions in qualitative research
Unlike research questions in quantitative research, research questions in qualitative research are usually continuously reviewed and reformulated. The central question and associated subquestions are stated more than the hypotheses. 15 The central question broadly explores a complex set of factors surrounding the central phenomenon, aiming to present the varied perspectives of participants. 15
There are varied goals for which qualitative research questions are developed. These questions can function in several ways, such as to 1) identify and describe existing conditions ( contextual research question s); 2) describe a phenomenon ( descriptive research questions ); 3) assess the effectiveness of existing methods, protocols, theories, or procedures ( evaluation research questions ); 4) examine a phenomenon or analyze the reasons or relationships between subjects or phenomena ( explanatory research questions ); or 5) focus on unknown aspects of a particular topic ( exploratory research questions ). 5 In addition, some qualitative research questions provide new ideas for the development of theories and actions ( generative research questions ) or advance specific ideologies of a position ( ideological research questions ). 1 Other qualitative research questions may build on a body of existing literature and become working guidelines ( ethnographic research questions ). Research questions may also be broadly stated without specific reference to the existing literature or a typology of questions ( phenomenological research questions ), may be directed towards generating a theory of some process ( grounded theory questions ), or may address a description of the case and the emerging themes ( qualitative case study questions ). 15 We provide examples of contextual, descriptive, evaluation, explanatory, exploratory, generative, ideological, ethnographic, phenomenological, grounded theory, and qualitative case study research questions in qualitative research in Table 4 , and the definition of qualitative hypothesis-generating research in Table 5 .
Qualitative studies usually pose at least one central research question and several subquestions starting with How or What . These research questions use exploratory verbs such as explore or describe . These also focus on one central phenomenon of interest, and may mention the participants and research site. 15
Hypotheses in qualitative research
Hypotheses in qualitative research are stated in the form of a clear statement concerning the problem to be investigated. Unlike in quantitative research where hypotheses are usually developed to be tested, qualitative research can lead to both hypothesis-testing and hypothesis-generating outcomes. 2 When studies require both quantitative and qualitative research questions, this suggests an integrative process between both research methods wherein a single mixed-methods research question can be developed. 1
FRAMEWORKS FOR DEVELOPING RESEARCH QUESTIONS AND HYPOTHESES
Research questions followed by hypotheses should be developed before the start of the study. 1 , 12 , 14 It is crucial to develop feasible research questions on a topic that is interesting to both the researcher and the scientific community. This can be achieved by a meticulous review of previous and current studies to establish a novel topic. Specific areas are subsequently focused on to generate ethical research questions. The relevance of the research questions is evaluated in terms of clarity of the resulting data, specificity of the methodology, objectivity of the outcome, depth of the research, and impact of the study. 1 , 5 These aspects constitute the FINER criteria (i.e., Feasible, Interesting, Novel, Ethical, and Relevant). 1 Clarity and effectiveness are achieved if research questions meet the FINER criteria. In addition to the FINER criteria, Ratan et al. described focus, complexity, novelty, feasibility, and measurability for evaluating the effectiveness of research questions. 14
The PICOT and PEO frameworks are also used when developing research questions. 1 The following elements are addressed in these frameworks, PICOT: P-population/patients/problem, I-intervention or indicator being studied, C-comparison group, O-outcome of interest, and T-timeframe of the study; PEO: P-population being studied, E-exposure to preexisting conditions, and O-outcome of interest. 1 Research questions are also considered good if these meet the “FINERMAPS” framework: Feasible, Interesting, Novel, Ethical, Relevant, Manageable, Appropriate, Potential value/publishable, and Systematic. 14
As we indicated earlier, research questions and hypotheses that are not carefully formulated result in unethical studies or poor outcomes. To illustrate this, we provide some examples of ambiguous research question and hypotheses that result in unclear and weak research objectives in quantitative research ( Table 6 ) 16 and qualitative research ( Table 7 ) 17 , and how to transform these ambiguous research question(s) and hypothesis(es) into clear and good statements.
a These statements were composed for comparison and illustrative purposes only.
b These statements are direct quotes from Higashihara and Horiuchi. 16
a This statement is a direct quote from Shimoda et al. 17
The other statements were composed for comparison and illustrative purposes only.
CONSTRUCTING RESEARCH QUESTIONS AND HYPOTHESES
To construct effective research questions and hypotheses, it is very important to 1) clarify the background and 2) identify the research problem at the outset of the research, within a specific timeframe. 9 Then, 3) review or conduct preliminary research to collect all available knowledge about the possible research questions by studying theories and previous studies. 18 Afterwards, 4) construct research questions to investigate the research problem. Identify variables to be accessed from the research questions 4 and make operational definitions of constructs from the research problem and questions. Thereafter, 5) construct specific deductive or inductive predictions in the form of hypotheses. 4 Finally, 6) state the study aims . This general flow for constructing effective research questions and hypotheses prior to conducting research is shown in Fig. 1 .

Research questions are used more frequently in qualitative research than objectives or hypotheses. 3 These questions seek to discover, understand, explore or describe experiences by asking “What” or “How.” The questions are open-ended to elicit a description rather than to relate variables or compare groups. The questions are continually reviewed, reformulated, and changed during the qualitative study. 3 Research questions are also used more frequently in survey projects than hypotheses in experiments in quantitative research to compare variables and their relationships.
Hypotheses are constructed based on the variables identified and as an if-then statement, following the template, ‘If a specific action is taken, then a certain outcome is expected.’ At this stage, some ideas regarding expectations from the research to be conducted must be drawn. 18 Then, the variables to be manipulated (independent) and influenced (dependent) are defined. 4 Thereafter, the hypothesis is stated and refined, and reproducible data tailored to the hypothesis are identified, collected, and analyzed. 4 The hypotheses must be testable and specific, 18 and should describe the variables and their relationships, the specific group being studied, and the predicted research outcome. 18 Hypotheses construction involves a testable proposition to be deduced from theory, and independent and dependent variables to be separated and measured separately. 3 Therefore, good hypotheses must be based on good research questions constructed at the start of a study or trial. 12
In summary, research questions are constructed after establishing the background of the study. Hypotheses are then developed based on the research questions. Thus, it is crucial to have excellent research questions to generate superior hypotheses. In turn, these would determine the research objectives and the design of the study, and ultimately, the outcome of the research. 12 Algorithms for building research questions and hypotheses are shown in Fig. 2 for quantitative research and in Fig. 3 for qualitative research.

EXAMPLES OF RESEARCH QUESTIONS FROM PUBLISHED ARTICLES
- EXAMPLE 1. Descriptive research question (quantitative research)
- - Presents research variables to be assessed (distinct phenotypes and subphenotypes)
- “BACKGROUND: Since COVID-19 was identified, its clinical and biological heterogeneity has been recognized. Identifying COVID-19 phenotypes might help guide basic, clinical, and translational research efforts.
- RESEARCH QUESTION: Does the clinical spectrum of patients with COVID-19 contain distinct phenotypes and subphenotypes? ” 19
- EXAMPLE 2. Relationship research question (quantitative research)
- - Shows interactions between dependent variable (static postural control) and independent variable (peripheral visual field loss)
- “Background: Integration of visual, vestibular, and proprioceptive sensations contributes to postural control. People with peripheral visual field loss have serious postural instability. However, the directional specificity of postural stability and sensory reweighting caused by gradual peripheral visual field loss remain unclear.
- Research question: What are the effects of peripheral visual field loss on static postural control ?” 20
- EXAMPLE 3. Comparative research question (quantitative research)
- - Clarifies the difference among groups with an outcome variable (patients enrolled in COMPERA with moderate PH or severe PH in COPD) and another group without the outcome variable (patients with idiopathic pulmonary arterial hypertension (IPAH))
- “BACKGROUND: Pulmonary hypertension (PH) in COPD is a poorly investigated clinical condition.
- RESEARCH QUESTION: Which factors determine the outcome of PH in COPD?
- STUDY DESIGN AND METHODS: We analyzed the characteristics and outcome of patients enrolled in the Comparative, Prospective Registry of Newly Initiated Therapies for Pulmonary Hypertension (COMPERA) with moderate or severe PH in COPD as defined during the 6th PH World Symposium who received medical therapy for PH and compared them with patients with idiopathic pulmonary arterial hypertension (IPAH) .” 21
- EXAMPLE 4. Exploratory research question (qualitative research)
- - Explores areas that have not been fully investigated (perspectives of families and children who receive care in clinic-based child obesity treatment) to have a deeper understanding of the research problem
- “Problem: Interventions for children with obesity lead to only modest improvements in BMI and long-term outcomes, and data are limited on the perspectives of families of children with obesity in clinic-based treatment. This scoping review seeks to answer the question: What is known about the perspectives of families and children who receive care in clinic-based child obesity treatment? This review aims to explore the scope of perspectives reported by families of children with obesity who have received individualized outpatient clinic-based obesity treatment.” 22
- EXAMPLE 5. Relationship research question (quantitative research)
- - Defines interactions between dependent variable (use of ankle strategies) and independent variable (changes in muscle tone)
- “Background: To maintain an upright standing posture against external disturbances, the human body mainly employs two types of postural control strategies: “ankle strategy” and “hip strategy.” While it has been reported that the magnitude of the disturbance alters the use of postural control strategies, it has not been elucidated how the level of muscle tone, one of the crucial parameters of bodily function, determines the use of each strategy. We have previously confirmed using forward dynamics simulations of human musculoskeletal models that an increased muscle tone promotes the use of ankle strategies. The objective of the present study was to experimentally evaluate a hypothesis: an increased muscle tone promotes the use of ankle strategies. Research question: Do changes in the muscle tone affect the use of ankle strategies ?” 23
EXAMPLES OF HYPOTHESES IN PUBLISHED ARTICLES
- EXAMPLE 1. Working hypothesis (quantitative research)
- - A hypothesis that is initially accepted for further research to produce a feasible theory
- “As fever may have benefit in shortening the duration of viral illness, it is plausible to hypothesize that the antipyretic efficacy of ibuprofen may be hindering the benefits of a fever response when taken during the early stages of COVID-19 illness .” 24
- “In conclusion, it is plausible to hypothesize that the antipyretic efficacy of ibuprofen may be hindering the benefits of a fever response . The difference in perceived safety of these agents in COVID-19 illness could be related to the more potent efficacy to reduce fever with ibuprofen compared to acetaminophen. Compelling data on the benefit of fever warrant further research and review to determine when to treat or withhold ibuprofen for early stage fever for COVID-19 and other related viral illnesses .” 24
- EXAMPLE 2. Exploratory hypothesis (qualitative research)
- - Explores particular areas deeper to clarify subjective experience and develop a formal hypothesis potentially testable in a future quantitative approach
- “We hypothesized that when thinking about a past experience of help-seeking, a self distancing prompt would cause increased help-seeking intentions and more favorable help-seeking outcome expectations .” 25
- “Conclusion
- Although a priori hypotheses were not supported, further research is warranted as results indicate the potential for using self-distancing approaches to increasing help-seeking among some people with depressive symptomatology.” 25
- EXAMPLE 3. Hypothesis-generating research to establish a framework for hypothesis testing (qualitative research)
- “We hypothesize that compassionate care is beneficial for patients (better outcomes), healthcare systems and payers (lower costs), and healthcare providers (lower burnout). ” 26
- Compassionomics is the branch of knowledge and scientific study of the effects of compassionate healthcare. Our main hypotheses are that compassionate healthcare is beneficial for (1) patients, by improving clinical outcomes, (2) healthcare systems and payers, by supporting financial sustainability, and (3) HCPs, by lowering burnout and promoting resilience and well-being. The purpose of this paper is to establish a scientific framework for testing the hypotheses above . If these hypotheses are confirmed through rigorous research, compassionomics will belong in the science of evidence-based medicine, with major implications for all healthcare domains.” 26
- EXAMPLE 4. Statistical hypothesis (quantitative research)
- - An assumption is made about the relationship among several population characteristics ( gender differences in sociodemographic and clinical characteristics of adults with ADHD ). Validity is tested by statistical experiment or analysis ( chi-square test, Students t-test, and logistic regression analysis)
- “Our research investigated gender differences in sociodemographic and clinical characteristics of adults with ADHD in a Japanese clinical sample. Due to unique Japanese cultural ideals and expectations of women's behavior that are in opposition to ADHD symptoms, we hypothesized that women with ADHD experience more difficulties and present more dysfunctions than men . We tested the following hypotheses: first, women with ADHD have more comorbidities than men with ADHD; second, women with ADHD experience more social hardships than men, such as having less full-time employment and being more likely to be divorced.” 27
- “Statistical Analysis
- ( text omitted ) Between-gender comparisons were made using the chi-squared test for categorical variables and Students t-test for continuous variables…( text omitted ). A logistic regression analysis was performed for employment status, marital status, and comorbidity to evaluate the independent effects of gender on these dependent variables.” 27
EXAMPLES OF HYPOTHESIS AS WRITTEN IN PUBLISHED ARTICLES IN RELATION TO OTHER PARTS
- EXAMPLE 1. Background, hypotheses, and aims are provided
- “Pregnant women need skilled care during pregnancy and childbirth, but that skilled care is often delayed in some countries …( text omitted ). The focused antenatal care (FANC) model of WHO recommends that nurses provide information or counseling to all pregnant women …( text omitted ). Job aids are visual support materials that provide the right kind of information using graphics and words in a simple and yet effective manner. When nurses are not highly trained or have many work details to attend to, these job aids can serve as a content reminder for the nurses and can be used for educating their patients (Jennings, Yebadokpo, Affo, & Agbogbe, 2010) ( text omitted ). Importantly, additional evidence is needed to confirm how job aids can further improve the quality of ANC counseling by health workers in maternal care …( text omitted )” 28
- “ This has led us to hypothesize that the quality of ANC counseling would be better if supported by job aids. Consequently, a better quality of ANC counseling is expected to produce higher levels of awareness concerning the danger signs of pregnancy and a more favorable impression of the caring behavior of nurses .” 28
- “This study aimed to examine the differences in the responses of pregnant women to a job aid-supported intervention during ANC visit in terms of 1) their understanding of the danger signs of pregnancy and 2) their impression of the caring behaviors of nurses to pregnant women in rural Tanzania.” 28
- EXAMPLE 2. Background, hypotheses, and aims are provided
- “We conducted a two-arm randomized controlled trial (RCT) to evaluate and compare changes in salivary cortisol and oxytocin levels of first-time pregnant women between experimental and control groups. The women in the experimental group touched and held an infant for 30 min (experimental intervention protocol), whereas those in the control group watched a DVD movie of an infant (control intervention protocol). The primary outcome was salivary cortisol level and the secondary outcome was salivary oxytocin level.” 29
- “ We hypothesize that at 30 min after touching and holding an infant, the salivary cortisol level will significantly decrease and the salivary oxytocin level will increase in the experimental group compared with the control group .” 29
- EXAMPLE 3. Background, aim, and hypothesis are provided
- “In countries where the maternal mortality ratio remains high, antenatal education to increase Birth Preparedness and Complication Readiness (BPCR) is considered one of the top priorities [1]. BPCR includes birth plans during the antenatal period, such as the birthplace, birth attendant, transportation, health facility for complications, expenses, and birth materials, as well as family coordination to achieve such birth plans. In Tanzania, although increasing, only about half of all pregnant women attend an antenatal clinic more than four times [4]. Moreover, the information provided during antenatal care (ANC) is insufficient. In the resource-poor settings, antenatal group education is a potential approach because of the limited time for individual counseling at antenatal clinics.” 30
- “This study aimed to evaluate an antenatal group education program among pregnant women and their families with respect to birth-preparedness and maternal and infant outcomes in rural villages of Tanzania.” 30
- “ The study hypothesis was if Tanzanian pregnant women and their families received a family-oriented antenatal group education, they would (1) have a higher level of BPCR, (2) attend antenatal clinic four or more times, (3) give birth in a health facility, (4) have less complications of women at birth, and (5) have less complications and deaths of infants than those who did not receive the education .” 30
Research questions and hypotheses are crucial components to any type of research, whether quantitative or qualitative. These questions should be developed at the very beginning of the study. Excellent research questions lead to superior hypotheses, which, like a compass, set the direction of research, and can often determine the successful conduct of the study. Many research studies have floundered because the development of research questions and subsequent hypotheses was not given the thought and meticulous attention needed. The development of research questions and hypotheses is an iterative process based on extensive knowledge of the literature and insightful grasp of the knowledge gap. Focused, concise, and specific research questions provide a strong foundation for constructing hypotheses which serve as formal predictions about the research outcomes. Research questions and hypotheses are crucial elements of research that should not be overlooked. They should be carefully thought of and constructed when planning research. This avoids unethical studies and poor outcomes by defining well-founded objectives that determine the design, course, and outcome of the study.
Disclosure: The authors have no potential conflicts of interest to disclose.
Author Contributions:
- Conceptualization: Barroga E, Matanguihan GJ.
- Methodology: Barroga E, Matanguihan GJ.
- Writing - original draft: Barroga E, Matanguihan GJ.
- Writing - review & editing: Barroga E, Matanguihan GJ.
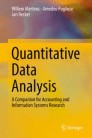
Quantitative Data Analysis
A Companion for Accounting and Information Systems Research
- Willem Mertens 0 ,
- Amedeo Pugliese 1 ,
- Jan Recker ORCID: https://orcid.org/0000-0002-2072-5792 2
QUT Business School, Queensland University of Technology, Brisbane, Australia
You can also search for this author in PubMed Google Scholar
Dept. of Economics and Management, University of Padova, Padova, Italy
School of accountancy, queensland university of technology, brisbane, australia.
- Offers a guide through the essential steps required in quantitative data analysis
- Helps in choosing the right method before starting the data collection process
- Presents statistics without the math!
- Offers numerous examples from various diciplines in accounting and information systems
- No need to invest in expensive and complex software packages
44k Accesses
22 Citations
13 Altmetric
- Table of contents
About this book
Authors and affiliations, about the authors, bibliographic information.
- Publish with us
This is a preview of subscription content, log in via an institution to check access.
Access this book
- Available as EPUB and PDF
- Read on any device
- Instant download
- Own it forever
- Compact, lightweight edition
- Dispatched in 3 to 5 business days
- Free shipping worldwide - see info
- Durable hardcover edition
Tax calculation will be finalised at checkout
Other ways to access
Licence this eBook for your library
Institutional subscriptions
Table of contents (9 chapters)
Front matter, introduction.
- Willem Mertens, Amedeo Pugliese, Jan Recker
Comparing Differences Across Groups
Assessing (innocuous) relationships, models with latent concepts and multiple relationships: structural equation modeling, nested data and multilevel models: hierarchical linear modeling, analyzing longitudinal and panel data, causality: endogeneity biases and possible remedies, how to start analyzing, test assumptions and deal with that pesky p -value, keeping track and staying sane, back matter.
- quantitative data analysis
- nested models
- quantitative data analysis method
- building data analysis skills
Willem Mertens
Amedeo Pugliese
Willem Mertens is a Postdoctoral Research Fellow at Queensland University of Technology, Brisbane, Australia, and a Research Fellow of Vlerick Business School, Belgium. His main research interests lie in the areas of innovation, positive deviance and organizational behavior in general.
Amedeo Pugliese (PhD, University of Naples, Federico II) is currently Associate Professor of Financial Accounting and Governance at the University of Padova and Colin Brain Research Fellow in Corporate Governance and Ethics at Queensland University of Technology. His research interests span across boards of directors and the role of financial information and corporate disclosure on capital markets. Specifically he is studying how information risk faced by board members and its effects on the decision-making quality and monitoring in the boardroom.
Jan Recker is Alexander-von-Humboldt Fellow and tenured Full Professor of Information Systems at Queensland University of Technology. His research focuses on process-oriented systems analysis, Green Information Systems and IT-enabled innovation. He has written a textbook on scientific research in Information Systems that is used in many doctoral programs all over the world. He is Editor-in-Chief of the Communications of the Association for Information Systems, and Associate Editor for the MIS Quarterly.
Book Title : Quantitative Data Analysis
Book Subtitle : A Companion for Accounting and Information Systems Research
Authors : Willem Mertens, Amedeo Pugliese, Jan Recker
DOI : https://doi.org/10.1007/978-3-319-42700-3
Publisher : Springer Cham
eBook Packages : Business and Management , Business and Management (R0)
Copyright Information : Springer International Publishing Switzerland 2017
Hardcover ISBN : 978-3-319-42699-0 Published: 10 October 2016
Softcover ISBN : 978-3-319-82640-0 Published: 14 June 2018
eBook ISBN : 978-3-319-42700-3 Published: 29 September 2016
Edition Number : 1
Number of Pages : X, 164
Number of Illustrations : 9 b/w illustrations, 20 illustrations in colour
Topics : Business Information Systems , Statistics for Business, Management, Economics, Finance, Insurance , Information Systems and Communication Service , Corporate Governance , Methodology of the Social Sciences
Policies and ethics
- Find a journal
- Track your research
- Privacy Policy
Buy Me a Coffee

Home » Quantitative Data – Types, Methods and Examples
Quantitative Data – Types, Methods and Examples
Table of Contents

Quantitative Data
Definition:
Quantitative data refers to numerical data that can be measured or counted. This type of data is often used in scientific research and is typically collected through methods such as surveys, experiments, and statistical analysis.
Quantitative Data Types
There are two main types of quantitative data: discrete and continuous.
- Discrete data: Discrete data refers to numerical values that can only take on specific, distinct values. This type of data is typically represented as whole numbers and cannot be broken down into smaller units. Examples of discrete data include the number of students in a class, the number of cars in a parking lot, and the number of children in a family.
- Continuous data: Continuous data refers to numerical values that can take on any value within a certain range or interval. This type of data is typically represented as decimal or fractional values and can be broken down into smaller units. Examples of continuous data include measurements of height, weight, temperature, and time.
Quantitative Data Collection Methods
There are several common methods for collecting quantitative data. Some of these methods include:
- Surveys : Surveys involve asking a set of standardized questions to a large number of people. Surveys can be conducted in person, over the phone, via email or online, and can be used to collect data on a wide range of topics.
- Experiments : Experiments involve manipulating one or more variables and observing the effects on a specific outcome. Experiments can be conducted in a controlled laboratory setting or in the real world.
- Observational studies : Observational studies involve observing and collecting data on a specific phenomenon without intervening or manipulating any variables. Observational studies can be conducted in a natural setting or in a laboratory.
- Secondary data analysis : Secondary data analysis involves using existing data that was collected for a different purpose to answer a new research question. This method can be cost-effective and efficient, but it is important to ensure that the data is appropriate for the research question being studied.
- Physiological measures: Physiological measures involve collecting data on biological or physiological processes, such as heart rate, blood pressure, or brain activity.
- Computerized tracking: Computerized tracking involves collecting data automatically from electronic sources, such as social media, online purchases, or website analytics.
Quantitative Data Analysis Methods
There are several methods for analyzing quantitative data, including:
- Descriptive statistics: Descriptive statistics are used to summarize and describe the basic features of the data, such as the mean, median, mode, standard deviation, and range.
- Inferential statistics : Inferential statistics are used to make generalizations about a population based on a sample of data. These methods include hypothesis testing, confidence intervals, and regression analysis.
- Data visualization: Data visualization involves creating charts, graphs, and other visual representations of the data to help identify patterns and trends. Common types of data visualization include histograms, scatterplots, and bar charts.
- Time series analysis: Time series analysis involves analyzing data that is collected over time to identify patterns and trends in the data.
- Multivariate analysis : Multivariate analysis involves analyzing data with multiple variables to identify relationships between the variables.
- Factor analysis : Factor analysis involves identifying underlying factors or dimensions that explain the variation in the data.
- Cluster analysis: Cluster analysis involves identifying groups or clusters of observations that are similar to each other based on multiple variables.
Quantitative Data Formats
Quantitative data can be represented in different formats, depending on the nature of the data and the purpose of the analysis. Here are some common formats:
- Tables : Tables are a common way to present quantitative data, particularly when the data involves multiple variables. Tables can be used to show the frequency or percentage of data in different categories or to display summary statistics.
- Charts and graphs: Charts and graphs are useful for visualizing quantitative data and can be used to highlight patterns and trends in the data. Some common types of charts and graphs include line charts, bar charts, scatterplots, and pie charts.
- Databases : Quantitative data can be stored in databases, which allow for easy sorting, filtering, and analysis of large amounts of data.
- Spreadsheets : Spreadsheets can be used to organize and analyze quantitative data, particularly when the data is relatively small in size. Spreadsheets allow for calculations and data manipulation, as well as the creation of charts and graphs.
- Statistical software : Statistical software, such as SPSS, R, and SAS, can be used to analyze quantitative data. These programs allow for more advanced statistical analyses and data modeling, as well as the creation of charts and graphs.
Quantitative Data Gathering Guide
Here is a basic guide for gathering quantitative data:
- Define the research question: The first step in gathering quantitative data is to clearly define the research question. This will help determine the type of data to be collected, the sample size, and the methods of data analysis.
- Choose the data collection method: Select the appropriate method for collecting data based on the research question and available resources. This could include surveys, experiments, observational studies, or other methods.
- Determine the sample size: Determine the appropriate sample size for the research question. This will depend on the level of precision needed and the variability of the population being studied.
- Develop the data collection instrument: Develop a questionnaire or survey instrument that will be used to collect the data. The instrument should be designed to gather the specific information needed to answer the research question.
- Pilot test the data collection instrument : Before collecting data from the entire sample, pilot test the instrument on a small group to identify any potential problems or issues.
- Collect the data: Collect the data from the selected sample using the chosen data collection method.
- Clean and organize the data : Organize the data into a format that can be easily analyzed. This may involve checking for missing data, outliers, or errors.
- Analyze the data: Analyze the data using appropriate statistical methods. This may involve descriptive statistics, inferential statistics, or other types of analysis.
- Interpret the results: Interpret the results of the analysis in the context of the research question. Identify any patterns, trends, or relationships in the data and draw conclusions based on the findings.
- Communicate the findings: Communicate the findings of the analysis in a clear and concise manner, using appropriate tables, graphs, and other visual aids as necessary. The results should be presented in a way that is accessible to the intended audience.
Examples of Quantitative Data
Here are some examples of quantitative data:
- Height of a person (measured in inches or centimeters)
- Weight of a person (measured in pounds or kilograms)
- Temperature (measured in Fahrenheit or Celsius)
- Age of a person (measured in years)
- Number of cars sold in a month
- Amount of rainfall in a specific area (measured in inches or millimeters)
- Number of hours worked in a week
- GPA (grade point average) of a student
- Sales figures for a product
- Time taken to complete a task.
- Distance traveled (measured in miles or kilometers)
- Speed of an object (measured in miles per hour or kilometers per hour)
- Number of people attending an event
- Price of a product (measured in dollars or other currency)
- Blood pressure (measured in millimeters of mercury)
- Amount of sugar in a food item (measured in grams)
- Test scores (measured on a numerical scale)
- Number of website visitors per day
- Stock prices (measured in dollars)
- Crime rates (measured by the number of crimes per 100,000 people)
Applications of Quantitative Data
Quantitative data has a wide range of applications across various fields, including:
- Scientific research: Quantitative data is used extensively in scientific research to test hypotheses and draw conclusions. For example, in biology, researchers might use quantitative data to measure the growth rate of cells or the effectiveness of a drug treatment.
- Business and economics: Quantitative data is used to analyze business and economic trends, forecast future performance, and make data-driven decisions. For example, a company might use quantitative data to analyze sales figures and customer demographics to determine which products are most popular among which segments of their customer base.
- Education: Quantitative data is used in education to measure student performance, evaluate teaching methods, and identify areas where improvement is needed. For example, a teacher might use quantitative data to track the progress of their students over the course of a semester and adjust their teaching methods accordingly.
- Public policy: Quantitative data is used in public policy to evaluate the effectiveness of policies and programs, identify areas where improvement is needed, and develop evidence-based solutions. For example, a government agency might use quantitative data to evaluate the impact of a social welfare program on poverty rates.
- Healthcare : Quantitative data is used in healthcare to evaluate the effectiveness of medical treatments, track the spread of diseases, and identify risk factors for various health conditions. For example, a doctor might use quantitative data to monitor the blood pressure levels of their patients over time and adjust their treatment plan accordingly.
Purpose of Quantitative Data
The purpose of quantitative data is to provide a numerical representation of a phenomenon or observation. Quantitative data is used to measure and describe the characteristics of a population or sample, and to test hypotheses and draw conclusions based on statistical analysis. Some of the key purposes of quantitative data include:
- Measuring and describing : Quantitative data is used to measure and describe the characteristics of a population or sample, such as age, income, or education level. This allows researchers to better understand the population they are studying.
- Testing hypotheses: Quantitative data is often used to test hypotheses and theories by collecting numerical data and analyzing it using statistical methods. This can help researchers determine whether there is a statistically significant relationship between variables or whether there is support for a particular theory.
- Making predictions : Quantitative data can be used to make predictions about future events or trends based on past data. This is often done through statistical modeling or time series analysis.
- Evaluating programs and policies: Quantitative data is often used to evaluate the effectiveness of programs and policies. This can help policymakers and program managers identify areas where improvements can be made and make evidence-based decisions about future programs and policies.
When to use Quantitative Data
Quantitative data is appropriate to use when you want to collect and analyze numerical data that can be measured and analyzed using statistical methods. Here are some situations where quantitative data is typically used:
- When you want to measure a characteristic or behavior : If you want to measure something like the height or weight of a population or the number of people who smoke, you would use quantitative data to collect this information.
- When you want to compare groups: If you want to compare two or more groups, such as comparing the effectiveness of two different medical treatments, you would use quantitative data to collect and analyze the data.
- When you want to test a hypothesis : If you have a hypothesis or theory that you want to test, you would use quantitative data to collect data that can be analyzed statistically to determine whether your hypothesis is supported by the data.
- When you want to make predictions: If you want to make predictions about future trends or events, such as predicting sales for a new product, you would use quantitative data to collect and analyze data from past trends to make your prediction.
- When you want to evaluate a program or policy : If you want to evaluate the effectiveness of a program or policy, you would use quantitative data to collect data about the program or policy and analyze it statistically to determine whether it has had the intended effect.
Characteristics of Quantitative Data
Quantitative data is characterized by several key features, including:
- Numerical values : Quantitative data consists of numerical values that can be measured and counted. These values are often expressed in terms of units, such as dollars, centimeters, or kilograms.
- Continuous or discrete : Quantitative data can be either continuous or discrete. Continuous data can take on any value within a certain range, while discrete data can only take on certain values.
- Objective: Quantitative data is objective, meaning that it is not influenced by personal biases or opinions. It is based on empirical evidence that can be measured and analyzed using statistical methods.
- Large sample size: Quantitative data is often collected from a large sample size in order to ensure that the results are statistically significant and representative of the population being studied.
- Statistical analysis: Quantitative data is typically analyzed using statistical methods to determine patterns, relationships, and other characteristics of the data. This allows researchers to make more objective conclusions based on empirical evidence.
- Precision : Quantitative data is often very precise, with measurements taken to multiple decimal points or significant figures. This precision allows for more accurate analysis and interpretation of the data.
Advantages of Quantitative Data
Some advantages of quantitative data are:
- Objectivity : Quantitative data is usually objective because it is based on measurable and observable variables. This means that different people who collect the same data will generally get the same results.
- Precision : Quantitative data provides precise measurements of variables. This means that it is easier to make comparisons and draw conclusions from quantitative data.
- Replicability : Since quantitative data is based on objective measurements, it is often easier to replicate research studies using the same or similar data.
- Generalizability : Quantitative data allows researchers to generalize findings to a larger population. This is because quantitative data is often collected using random sampling methods, which help to ensure that the data is representative of the population being studied.
- Statistical analysis : Quantitative data can be analyzed using statistical methods, which allows researchers to test hypotheses and draw conclusions about the relationships between variables.
- Efficiency : Quantitative data can often be collected quickly and efficiently using surveys or other standardized instruments, which makes it a cost-effective way to gather large amounts of data.
Limitations of Quantitative Data
Some Limitations of Quantitative Data are as follows:
- Limited context: Quantitative data does not provide information about the context in which the data was collected. This can make it difficult to understand the meaning behind the numbers.
- Limited depth: Quantitative data is often limited to predetermined variables and questions, which may not capture the complexity of the phenomenon being studied.
- Difficulty in capturing qualitative aspects: Quantitative data is unable to capture the subjective experiences and qualitative aspects of human behavior, such as emotions, attitudes, and motivations.
- Possibility of bias: The collection and interpretation of quantitative data can be influenced by biases, such as sampling bias, measurement bias, or researcher bias.
- Simplification of complex phenomena: Quantitative data may oversimplify complex phenomena by reducing them to numerical measurements and statistical analyses.
- Lack of flexibility: Quantitative data collection methods may not allow for changes or adaptations in the research process, which can limit the ability to respond to unexpected findings or new insights.
About the author
Muhammad Hassan
Researcher, Academic Writer, Web developer
You may also like

Primary Data – Types, Methods and Examples

Qualitative Data – Types, Methods and Examples

Research Data – Types Methods and Examples

Secondary Data – Types, Methods and Examples

Information in Research – Types and Examples
Thank you for visiting nature.com. You are using a browser version with limited support for CSS. To obtain the best experience, we recommend you use a more up to date browser (or turn off compatibility mode in Internet Explorer). In the meantime, to ensure continued support, we are displaying the site without styles and JavaScript.
- View all journals
- My Account Login
- Explore content
- About the journal
- Publish with us
- Sign up for alerts
- Open access
- Published: 05 April 2024
Quantitative analysis of the intensity distribution of optical rogue waves
- Éva Rácz ORCID: orcid.org/0000-0003-1747-5347 1 ,
- Kirill Spasibko ORCID: orcid.org/0000-0001-6667-5084 2 ,
- Mathieu Manceau 3 , 4 ,
- László Ruppert 1 ,
- Maria V. Chekhova ORCID: orcid.org/0000-0002-3399-2101 4 , 5 &
- Radim Filip ORCID: orcid.org/0000-0003-4114-6068 1
Communications Physics volume 7 , Article number: 119 ( 2024 ) Cite this article
Metrics details
- Characterization and analytical techniques
- Nonlinear optics
- Supercontinuum generation
The field of optical rogue waves is a rapidly expanding topic with a focus on explaining their emergence. To complement this research, instead of providing a microscopic model that generates extreme events, we concentrate on a general quantitative description of the observed behavior. We explore two complementary top-down approaches to estimating the exponent describing the power-law decaying distribution of optical rogue waves observed in supercontinuum generated in a single-mode fiber in the normal-dispersion regime by applying a highly fluctuating pump. The two distinct approaches provide consistent results, outperforming the standard Hill estimator. Further analysis of the distributions reveals the breakdown of power-law behavior due to pump depletion and detector saturation. Either of our methods is adaptable to analyze extreme-intensity events from arbitrary experimental data.
Similar content being viewed by others
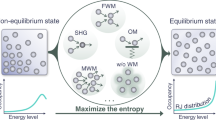
Universality of light thermalization in multimoded nonlinear optical systems
Qi Zhong, Fan O. Wu, … Demetrios N. Christodoulides
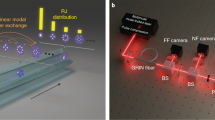
Direct observations of thermalization to a Rayleigh–Jeans distribution in multimode optical fibres
Hamed Pourbeyram, Pavel Sidorenko, … Frank Wise
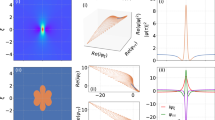
Analysis of interaction dynamics and rogue wave localization in modulation instability using data-driven dominant balance
Andrei V. Ermolaev, Mehdi Mabed, … John M. Dudley
Introduction
Once only featured in sailors’—mostly disbelieved—stories, rogue waves were first measured only relatively recently: in 1995 at an oil rig in the North Sea 1 . The concept, namely ocean waves that seem to appear out of nowhere and whose size far exceeds what is considered typical, has been observed or predicted in multiple fields where the wave analogy is applicable: Bose–Einstein condensates 2 , 3 , plasmas 4 , 5 , 6 , atmospheric rogue waves 7 , superfluids 8 . Optical rogue waves were first reported in 2007 9 . The focus of theoretical research has been on providing generating mechanisms for such extreme behavior 10 , 11 , 12 .
Although with different points of emphasis, extreme and hard-to-predict behavior has been reported and studied considerably longer in other areas of research (see, for example, Pareto’s seminal work on the distribution of wealth from 1896 13 ). Further examples include incomes 13 , 14 , insurance claims 15 , 16 , number of citations of scientific publications 17 , 18 , 19 , earthquake intensities 20 , 21 , 22 , avalanche sizes 23 , solar flares 24 , degree distributions of various social and biological networks 25 , 26 , 27 , and many more. Unsurprisingly, the mathematical background of extreme behavior has been most extensively studied with the motivation of mitigating financial risks 28 , 29 , 30 , 31 , 32 . This broader area of research is referred to as extreme value theory, and heavy-tailed distributions as well as their estimation play an important role in it.
A rogue wave is, by definition 33 , an unpredictably appearing wave with an amplitude at least twice as large than the significant wave height, with the tail of the amplitude probability density function (PDF) decaying slower than a Gaussian. While multiple sets of criteria exist 33 for using the term rogue wave, a systematic way of estimating and comparing how extreme rogue waves are is needed. The simplest estimators for heavy tails 34 , 35 , 36 build on the hypothesis that the distribution of interest is such that there exists a sharp, finite threshold beyond which the PDF decays exactly at a power-law rate. This is, of course, in most situations not true, and the basic estimators’ bias can be reduced by taking into account higher-order behavior 37 .
In comparison with more traditional uses of extreme value theory, non-linear optics 38 , 39 has clear advantages in controllability, reproducibility, and statistical significance of the generated optical extreme events. Optical experiments producing light with heavy-tailed intensity distributions allow high repetition rates and, therefore, large samples to study unstable non-linear phenomena and their sources 40 , 41 , 42 , 43 . However, obtaining the correct statistics of rogue-wave events is, even so, a non-trivial problem 44 , 45 .
The aim of the experiment under consideration 46 was to produce highly fluctuating intensities. The authors achieved this in the normal-dispersion regime with a fiber that had a relatively low non-linear refractive index, where such rogue events (without cascading 12 ) were not expected. The reason for the observed level of fluctuations is mainly the super-Poissonian pumping that was applied to the single-mode fiber, which further enhanced intensity fluctuations through nonlinear frequency conversion.
What the literature is lacking is a focus on the essential quantitative description of the measured intensities. Since the size of rogue events can exceed the median by orders of magnitude, their detection is difficult. Any physical measurement device has its limits; this is also the case for optical detectors: the detector response becomes non-linear and saturates with the increase of light power 47 . For example, for commonly used biased photodiodes, the detector response cannot be larger than the reverse bias voltage; the further increase of input light power leads to the same output. Therefore, detector saturation will almost inevitably spoil the statistics of very energetic rogue events.
Furthermore, the efficiency of an optical non-linear process strongly depends on the intensity of its pump. This is also the case for the experiment under consideration 46 , where the amount of produced photons and the efficiency of the process depend exponentially on the pump intensity. Thus, the efficiency of supercontinuum generation usually increases with the increase of pump power. However, as soon as the amount of converted pump energy becomes significant (tens of percent), the remaining pump cannot feed the process efficiently anymore; it is already depleted. In this case, increasing input pump power does not increase the output intensity 48 , 49 .
The focus of the current work is to tackle the rich phenomenology of optical rogue waves from a non-conventional angle. Instead of concentrating on their origin, our study provides a quantitative description of the measured intensities of optical rogue waves. To this aim, we explore two approaches to adapting the general statistics toolkit for tail estimation (proposed by Rácz and et al. 50 ) to the special case of rogue waves resulting from supercontinuum generation, using data collected during experiments similar to those reported by Manceau and colleagues 46 . In this reference, an infinite-mean power-law distribution for supercontinuum generation was observed for the first time. However, a detailed statistical analysis of such phenomena requires complementary approaches. Furthermore, we also describe the breakdown of the ideal behavior due to pump depletion and detector saturation.
The primary focus of this paper is on estimating the exponent related to the power-law decay of the distribution of measured intensities in the experiment 46 whose schematics are shown in Fig. 1 . Highly fluctuating super-Poissonian light was used to pump a single-mode fiber in the normal-dispersion regime (see under Experiment in Methods). As a result of these large fluctuations in the pumping light, the observed intensity fluctuations at the other end of the fiber were also quite large in comparison to coherent pumping. In effect, fluctuations in the incoming light were drastically enhanced due to the non-linear effects present in the fiber. Depending on the the orientation of the barium borate (BBO) crystals, the experiment used either non-degenerate parametric down-conversion (PDC) producing light with thermal intensity statistics (exponentially distributed) or degenerate PDC producing bright squeezed vacuum (gamma-distributed intensities).
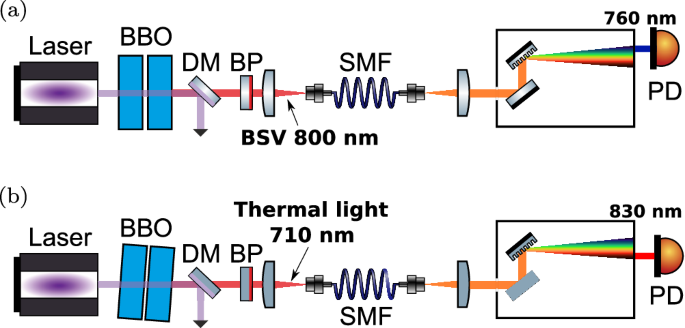
Pulsed 400 nm light is used to pump parametric down-conversion (PDC) in two cascaded barium borate (BBO) crystals. Depending on the phase-matching angle of the crystals, either ( a ) bright squeezed vacuum (BSV) is generated via degenerate PDC at 800 nm, or ( b ) thermal light is generated via non-degenerate PDC at 710 nm. These two types of light are filtered by a band-pass filter at 710 ± 5 nm for thermal light or at 800 ± 5 nm for BSV, and used to generate supercontinuum in a single-mode fiber. The resulting intensity is measured (PD) after spectrally filtering the supercontinuum by a monochromator.
The current experiment exhibits a spectral broadening in the normal-dispersion regime 51 (for further details, check under Experiment in Methods); we refer to this aspect as supercontinuum generation. While this produces less of a broadening effect than in the anomalous-dispersion case, since, to our knowledge, there is no consensus in the literature on exactly what level of broadening constitutes supercontinuum, we will go on using this term throughout the article. Furthermore, rather than spectral broadening, we focus on the amplification effect, which we attribute primarily to four-wave mixing (see Rogue wave generation in Methods). For more details regarding the experiment, see the first two subsections under Methods and the experimental study 46 .
We will discuss the results for both types of pumping and compare two different approaches to estimating the tail exponent:
First, a generalization of the Hill estimator 34 introduced in Rácz et al. 50 , which directly estimates the exponent only (Direct estimation of the tail exponent);
Second, fitting a multi-parameter model to the observations (see Tail exponent estimation as part of a model fit), where one of the parameters corresponds to the exponent of interest.
We will also explore the validity of the two approaches and quantitatively describe the intensity distribution where it is affected by both pump depletion and detector saturation (see Supplementary Note 2) .
Preliminaries
This section provides a brief summary of terminology and basic quantities; for further details, see Methods and Supplementary Note 3 . The results are mainly presented in terms of exceedance probabilities (or complementary cumulative distribution function, or tail function). For a real-valued random variable X , the exceedance probability function (EPF) is defined as the probability that the value of the variable exceeds a pre-defined threshold x :
with \({{{{{{{\bf{P}}}}}}}}\left(\cdot \right)\) denoting the probability of an event. From a finite sample, one can obtain the empirical exceedance probability (EEPF) for any limit x simply as the fraction of observations that exceed x . Empirical exceedance probabilities have multiple advantages over histograms: there is no binning involved; it is smoother; tail behavior is visually more apparent.
The Pareto (or power-law) distribution is the archetype of heavy-tailed distributions and can be given with the PDF \(f(x)={x}_{0}^{-1}{\left(x/{x}_{0}\right)}^{-\alpha -1}\) , for x 0 , α > 0, and x ≥ x 0 . The constant α is referred to as the tail exponent, and as a measure of tail heaviness, it can be generalized to a broader class of distributions referred to as Pareto-type, or regularly varying distributions. For such distributions, the exceedance probability plot is asymptotically a straight line on a doubly logarithmic scale. As Fig. 2 (yellow lines) shows, our data do not quite behave like this: power-law behavior has an upper limit, which is why alternative approaches are needed to estimate the exponent.
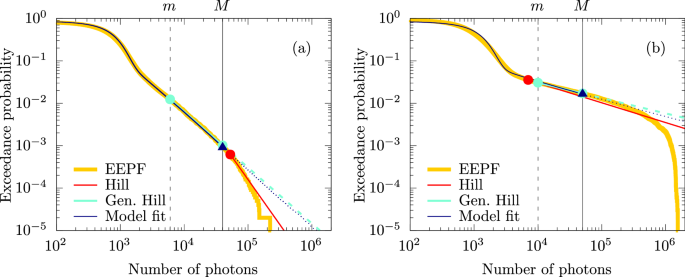
We compare different fitting approaches for ( a ) thermal, and ( b ) BSV pumping. The yellow line shows the empirical exceedance probabilities (EEPF) from an experimental data set. The red line shows the result of applying the traditional Hill estimator ( 3 ) combined with Guillou & Hall’s estimator 66 of the tail length k , the red dot marks the beginning of the line. The light blue line (and its dashed extension) shows the result of the generalized version ( 4 ) combined with a heuristic choice of the lower and upper cutoff parameters m and M , the light blue dots mark the ends of the interval. The dark blue line (and its dotted extension) show the result of fitting the model ( 5 ) to the sample; the value of the upper cutoff was the same as for the generalized Hill estimator and is marked by a dark blue triangle.
Thermal pumping
Non-degenerate parametric down-conversion produces light with thermal intensity statistics; that is, in a continuous approximation, the distribution of pumping intensities is exponential.
First, we investigate the properties of the exceedance probability function for intensities observed in supercontinuum generation experiments using a thermal source as a pump (Fig. 2 a). Experimental results show that under the investigated circumstances, the single-mode fiber produces exponential amplification, which can be explained in terms of four-wave mixing (see Rogue wave generation and the experimental work 46 ). Since the intensity distribution of the pumping light decays exponentially, after exponential amplification, the resulting intensity distribution should decay at a power-law rate. Visually, the empirical exceedance probability function (at least for the largest values) should be linear on a log-log scale. However, the dependence of the EEPF from experimental data (yellow) seems linear only in the middle section. For low values (below m ), this is due to the different types of noises, while for high values (above M ), pump depletion affects the distribution in a significant way. It is worth noting that even though we have quite large sample sizes (~10 5 ), power-law behavior manifests itself only for the top few percent of observations, so the informative or effective sample size is considerably smaller (~10 3 ).
The standard Hill estimator ( 3 ) in a realistic experiment is not the best choice of estimation method (red line) since the distribution clearly does not decay at a power-law rate for the largest values, which introduces a significant bias. A better direct estimation can be achieved after discarding the largest observations affected by saturation or pump depletion (that is, estimating the value of the tail exponent from the middle section only) by using the generalized Hill estimator from ( 4 ) (light blue line). Finally, we have also used the modeling approach (see Tail exponent estimation as part of a model fit), consisting of constructing a detailed multi-parameter model of the process and estimating its parameters simultaneously, including the one that determines the value of the tail exponent (dark blue line). We can approximate the real process very accurately already with this simple model.
Bright squeezed vacuum
If one uses bright squeezed vacuum (BSV) as a pump instead of thermal light to generate the supercontinuum, the situation remains quite similar (Fig. 2 b), but there are also a few significant differences. The difficulty comes from the fact that even though the asymptotic behavior is similar to the thermal case, the convergence to the asymptotics is slower. The Hill estimator (red line) is, as expected, biased as it includes the large, saturated values as well. But if we use the generalized Hill estimator (light blue line) with a properly chosen interval, we can obtain a reasonable estimate even in this case. Note, however, that for this specific measurement, the range within which power-law decay is a reasonable assumption is quite short, about 1%, of the data. The issue is that by using either version of the Hill estimator, one essentially estimates a gamma distribution with an exponential, which works well only asymptotically. However, the largest values are distorted as a result of pump depletion and detector saturation.
This is why the modeling approach can potentially provide more information. The fitted distribution (dark blue line) is not as close to the actual data as for a thermal source. This has a technical reason: in the thermal pumping version of the experiment, fluctuations in the mean intensity of the laser were accounted for by post-selecting the output intensity conditional on the value of the laser intensity. No such post-selection was done in the BSV case, and the model described in Tail exponent estimation as part of a model fit does not take into account fluctuations in the mean incoming intensity. Nevertheless, despite the inaccuracy close to the noise floor, the model sufficiently captures the stretching of the distribution’s tail, which we are ultimately interested in.
Method comparison
Let us highlight the sensitivities of the different methods to choosing the limit(s) of the application interval. Firstly, looking at the direct estimation methods (Fig. 3 a) using different lower limits, it becomes more evident why the value of the Hill estimator is rather incidental. For an ideal, strictly power-law distributed sample, the expectancy of the Hill estimator is constant, with its standard deviation decreasing as the number of points taken into account increases. For distributions that are only asymptotically power-law, there is an ideal tail length corresponding to a trade-off between minimizing bias and standard deviation.
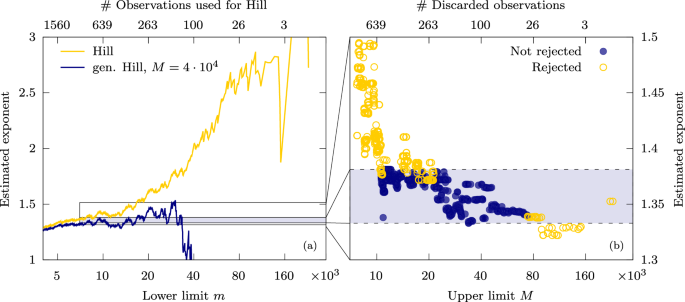
a Direct fitting methods: \({\hat{\alpha }}_{{{{{{{{\rm{H}}}}}}}}}\left[k(m)\right]\) and \({\hat{\alpha }}_{{{{{{{{\rm{gH}}}}}}}}}\left[k(m),j=100\right]\) . b Modeling approach results as a function of the upper cutoff M (all observations below M were taken into account). The blue circles indicate the estimates that were accepted at a 5% significance level by a binomial test, the estimates denoted by yellow circles were rejected (see Supplementary Note 1) .
A simple approach to estimating this ideal value is looking for a plateau in the Hill plot 52 , where the estimator’s value is stable. Unfortunately, in our case, the Hill estimator (yellow line in Fig. 3 a) presents a clear trend throughout the plotted interval as a function of m due to the bias introduced by the fact that the largest observations are clearly not Pareto-distributed. As a consequence, tail length estimators produce somewhat random values (red points in Fig. 2 ).
In contrast, throwing out the top observations really makes a difference compared to the standard Hill estimator: the generalized Hill estimator (blue line in Fig. 3 a) is much less sensitive to the choice of the lower bound, that is, it produces relatively stable estimates in a wide range of values for m . Note that compared to the traditional Hill estimator, the upper limit M is an extra parameter to choose, which makes this method somewhat more complicated. But in practice, the estimator is also relatively stable in regard of choosing that parameter too, so it is not necessary to have a very accurate estimate of the endpoints of the interval [ m , M ].
Looking at the sensitivity of the modeling approach (Fig. 3 b), we see that the estimates are even more stable with regard to the endpoint of the interval (in this case we only have an upper limit, M ). More precisely, this approach provides a similar range of estimates as the generalized Hill estimator, but in addition, we can quantify the fit of the model with the estimated parameters to the measured data, and one can reject the values which are not a good fit (yellow points). And if we only look at the remaining, accepted estimates (blue points), these range only between 1.33 and 1.38 (light blue area), which is a really narrow interval, especially comparing it to the fluctuation of the traditional Hill estimates.
Of course, there is no guarantee that the given estimators are unbiased, so even if they are stable they could give a wrong result. That is why it is generally very useful to have at least two independent estimation methods (which are biased in different ways) and check whether they provide consistent results. We can compare the results of different estimators and can conclude that the modeling approach provides estimates close to the generalized Hill estimator (see Supplementary Table 1 ). We were interested in seeing if an extended analysis that enables us to use a much larger part of the data can provide an improvement over the generalized Hill estimator, which discards both the smallest and largest observations. If we only look at the estimation of the tail exponent, the answer to this question is no; however, the results of fitting the detailed model provide an independent reference point for the generalized Hill estimator. The fact that the two approaches provide similar estimates increases the confidence in the results. So, in summary, the two approaches are not necessarily meant to compete but rather to complement each other. The advantage of the generalized Hill estimator is that it is easy to compute and is applicable in a broader range of situations as-is, without needing to know the details of the process. The modeling approach, on the other hand, gives us more insight into the specifics of the phenomenon, but at the same time, the exact model applied here is only applicable in a narrow context. This means that a new model should be worked out for the specifics of the process of interest, while the direct approach is applicable out of the box for any process that produces power-law tails.
Breakdown of power-law behavior
As Fig. 2 shows, power-law behavior eventually breaks down; using a semi-logarithmic scale instead of the original log-log (Fig. 4 ) reveals exactly how: the linear sections in the plots indicate exponential decay rather than power-law (i.e., EEPF ∝ e − λ x for some λ > 0). This behavior can be attributed to pump depletion: in the case of a fluctuating pump, the depletion manifests itself not only in the power reduction and decrease of generation efficiency but also in the change of pump statistics 46 , 53 . In the presence of pump depletion, the most energetic bursts are not as strongly amplified as weaker ones. The result is that the empirical variance of the intensities is smaller than it would be in the absence of depletion. It is possible to account for this effect in the black-box approach taken in Tail exponent estimation as part of a model fit by altering the response of the fiber (by exchanging the \({\sinh }^{2}\) function for a function that increases exponentially for small values but is only linear for large values), but if one is only interested in the stretching caused by exponential amplification, it is easier to discard the values affected by it. This is what we have done in the previous section. Here, we will also look at the description of the distribution beyond the validity of the power-law functional form.
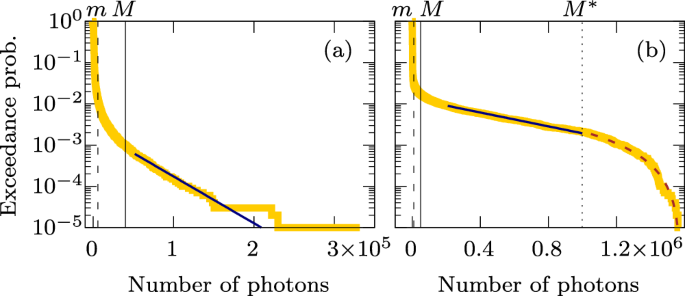
The plots show empirical exceedance probabilities on a semi-logarithmic scale; the samples are the same as shown in Fig. 2 , with ( a ) thermal, and ( b ) BSV pumping. The same values for the power-law interval [ m , M ] are indicated by vertical lines. The dark blue solid lines show exponential fits, to observations above 5 ⋅ 10 4 in the thermal case, and between 2 ⋅ 10 5 and M * = 10 6 in the BSV case, providing exponential rate parameter estimates of \({\hat{\lambda }}_{a}=2.6\cdot 1{0}^{-5}\) and \({\hat{\lambda }}_{b}=1.9\cdot 1{0}^{-6}\) , respectively. The brown dashed line shows the generalized Pareto fit of the observations beyond M * , with \({\hat{i}}_{\max }=1.58\cdot 1{0}^{6}\) and \(\hat{\gamma }=1.59\) .
Furthermore, as Fig. 4 b shows, if intensities are high enough (beyond about M * = 10 6 ), the decay becomes even faster than exponential due to detector saturation. It can be shown (see Supplementary Note 2) that combining an exponential distribution with an exponentially saturating detector results in a generalized Pareto distribution. More accurately, the EEPF is \(\propto {\left({i}_{\max }-x\right)}^{\gamma }\) . Here, \({i}_{\max }\) denotes the maximum output of the detector, and γ > 0 the related exponent.
The parameters of the exponential ( λ ) and the generalized Pareto distributions ( \({i}_{\max }\) and γ ) can be estimated in a similar fashion to the tail exponent via a conditional maximum likelihood approach (see Supplementary Note 2) . The results of the exponential fits are shown in blue in Fig. 4 , the result of the generalized Pareto fit is shown in Fig. 4 b using a brown dashed line. Note that the parameter values obtained for Fig. 4 b are in line with the direct measurement of the detector response shown in Supplementary Fig. 1 : \(\hat{\gamma }/{\hat{\lambda }}_{b}/{\hat{i}}_{\max }=0.54\) versus 0.62 in the direct measurement.
As an interesting note, we mention that the generalized Pareto distribution (EEPF \(\propto {\left(1+\xi x\right)}^{-1/\xi }\) ) includes the regular Pareto distribution ( ξ > 0), the exponential distribution ( ξ = 0), and the distribution derived for the observations beyond M * ( ξ < 0) as well, so it should be possible to introduce a decreasing ξ ( x ) function to treat the three types of behavior at the same time. Moreover, in principle, one could include these aspects in the modeling approach of estimating the tail exponent as well; however, as the tail exponent has only little effect on the few observations beyond M , doing so would not likely improve the tail exponent estimation. On the contrary, introducing the extra parameters would negatively affect the stability of the numerical optimization.
Optical rogue waves present an exciting direction of research and provide excellent means to study extreme statistical behavior in a controllable and reproducible fashion. In this study, we analyzed intensity data gathered from supercontinuum generation experiments fed by thermal light and bright squeezed vacuum. Even though optical experiments provide large amounts of data, they are highly affected by noise, pump depletion, and detector saturation. As a result, the effective sample size (observations that are not or are little affected by either issue) is much smaller than the total sample size, which makes the estimation problem non-trivial.
The rogueness of the observed intensity distributions comes from the fact that they display a power-law decay. We used two different, advanced methods to estimate the related exponent. Accurately estimating the rate of power-law decay is of both theoretical and practical importance. Knowing whether the theoretical distribution (unaffected by saturation issues) has a finite second moment or not determines whether, for example, calculating the correlation function g (2) is meaningful, and having a proper estimate of the tail exponent also helps with designing further experiments.
The first method estimates the value of the tail exponent directly and is the generalization of the well-known Hill estimator. As pump depletion and detector saturation have an effect on the largest values only, the estimation of the tail is much more precise if we discard these affected observations. This requires a modification of the Hill estimator but is otherwise quite straightforward. The second approach consists of devising a simplified physical model of the process (including noises and further limitations), and performing a maximum likelihood fit of its parameters based on the data. This approach is, of course, tailored to the specific problem at hand, and in a different context, requires setting up a different model. The largest values are discarded similarly to the first approach, which means that instead of having to add extra parameters to the model to describe pump depletion and the saturation curve of the detector, we only need to choose a limit beyond which values are discarded. Since the fit is quite good, this means we not only accurately estimated the parameters (including the tail exponent), but we also have a reasonably good grasp of the imperfections in the system. Using the two approaches in parallel is useful as well since they produce independent estimates. Consistent values indicate that our view of the process, and our implementation of the estimators are appropriate.
This dual approach is especially useful in the case of pumping with bright squeezed vacuum. We have a fundamental problem in both our estimation schemes: the direct method is better suited for an underlying exponential distribution than for gamma, while the modeling approach has problems with the fit as the physical process is more complex. Nevertheless, either method provides similar tail exponents, meaning that albeit the estimation procedures are more sensitive in the BSV case, we can still perform a consistent tail estimation.
Other than estimating the exponent of the power-law decay, we also showed how power-law behavior breaks down for the largest observations. The primary cause of this is pump depletion, turning the power-law decay into exponential. Furthermore, especially in the case of pumping with bright squeezed vacuum, high intensities beyond the detector’s linear response range were achieved, which additionally distorted the empirical distribution. We were able to provide a model to characterize this post-power-law range of observations through an exponential and a generalized Pareto distribution.
In summary, we have extensively addressed the problem of accurately describing rogue waves. In contrast to most publications related to rogue waves, we did not attempt to introduce a microscopic model to explain why our system produces extreme behavior. Instead, we applied a top-down approach: we used advanced statistical analysis of the available data, and from that, we obtained a quantitative description of the extreme behavior that also takes into account different physical imperfections of the system. Through investigating the particular case of supercontinuum generation, we provided a practical toolkit to analyze similar highly volatile processes that result in rogue waves.
In the experiment (Fig. 1 ), the radiation of a titanium-sapphire laser is frequency doubled to generate pulses at 400 nm with a 1.4 ps pulse duration and up to 200 μJ of energy per pulse. This 400 nm radiation is used to pump two cascaded 3 mm BBO crystals in a type-I collinear parametric down-conversion (PDC) scheme. Depending on the crystal orientation, we can implement two processes 54 : (i) degenerate PDC, for which the signal and idler radiations have the same central wavelength (800 nm), (ii) non-degenerate PDC, for which the signal and idler radiations have different central wavelengths (signal 710 nm, idler 916 nm). To stress the different intensity statistics of these two types of light, in the following, we will call the signal radiation of the non-degenerate PDC at 710 nm thermal light and the result of the degenerate PDC at 800 nm bright squeezed vacuum (BSV). The radiation of non-degenerate PDC has intensity fluctuations identical to those of thermal light, larger than laser light 55 , 56 . The radiation of degenerate PDC (BSV) has even stronger intensity fluctuations 46 . The energy per pulse for BSV and thermal light was a few tens of nJ. These two light sources were filtered by bandpass filters: (i) at 710 ± 5 nm for thermal light, (ii) at 800 ± 5 nm for BSV, and were subsequently used to pump a 5 m single-mode fiber (SMF) with Ge-doped silica core (Thorlabs P3-780A-FC-5 patch cable with 780HP fiber) to generate a supercontinuum centered at 710 nm for thermal light pumping, and at 800 nm for BSV pumping. The fiber had normal dispersion in the studied range of wavelengths (from 700 –900 nm) 57 , and a nonlinear refractive index n 2 around 3 × 10 −20 m 2 /W 58 , 59 .
At the output of the fiber, the supercontinuum was spectrally filtered by a monochromator with 1 nm resolution and measured by a photodetector (PD) to reduce the averaging of statistics by the detector over different wavelengths. The filtering was performed at the extreme red part of the supercontinuum (830 nm) for thermal light and the extreme blue part of the supercontinuum (760 nm) for BSV. The photodetector was calibrated to convert its output voltage signal into the number of photons per pulse, which is the data used in our analysis. The upper limit to the detector’s linear response was ~10 6 photons per pulse, with a maximum output ~2 ⋅ 10 6 photons per pulse. Note that in some measurements, the data were post-selected to avoid pump intensity fluctuations in the right polarization for PDC generation. This means that the laser power was monitored continuously, and data corresponding to the power falling outside a certain window were discarded. In other cases, no post-selection was done in order to be able to check for temporal correlations. In either case, data was collected until the sample size of 10 5 was reached. Importantly, the decrease in pump power fluctuations did not have a large effect on the distribution of measured intensities, and, therefore, the applicability of our approach.
Rogue wave generation
In optical fibers, rogue waves are typically associated with solitons appearing under anomalous dispersion. However, the measurements by Manceau et al. 46 (and also by Hammani et al. 60 in a somewhat different regime) were done at wavelengths in the normal-dispersion regime. Moreover, the nonlinear refractive index of the fiber was also relatively low. Therefore, the appearance of very high-magnitude events was unexpected. The explanation, however, is simple: the super-Poissonian statistics of the pumping PDC light played the dominant role in the appearance of rogue waves under these conditions. In other words, the amplification effect is much more prominent if the pumping light is already highly fluctuating (compared to coherent pumping).
As a simple demonstration of rogue behavior, let us look at Fig. 5 a, which shows a typical outcome of an experiment. The yellow points correspond to the top 1% of observations, the blue points to the bottom 99%. The histogram of the lower 99% is shown in Fig. 5 b. From the histogram, one might mistakenly conclude that the interesting part of the distribution ends at about 5 × 10 3 photons per pulse. For example, for an exponential sample of 10 5 observations, the expectancy of the sample maximum is only about 2.6 times larger than the 99th percentile. In contrast, for the particular measurement depicted in Fig. 5 , many observations are above this value, and the actual maximal value is about 41 times larger than the 99th percentile (i.e., an order higher than expected). This is the behavior that distinguishes these rogue waves from quieter processes, for which not much of interest happens regarding the largest observations. If one takes a look at the traditional criterion, namely an event whose magnitude exceeds twice the significant wave height, about 2% of observations in the sample shown in Fig. 5 are rogue.
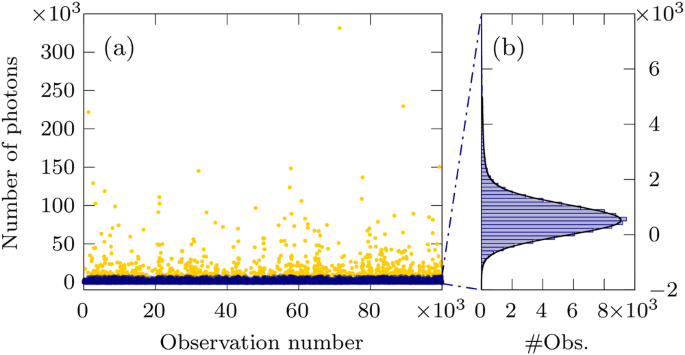
a Sample time series of the number of photons per pulse observed in an experiment. Time is shown in the number of observations; the sampling frequency was 5 kHz. The bottom 99% of photon number observations are colored blue, and the top 1% are colored yellow. b Histogram based on the blue dots in ( a ).
Regarding the origin of rogue waves, the spectral broadening in the regime of normal group velocity dispersion with picosecond pulsing is usually explained by the combination of self- and cross-phase modulation (SPM and XPM) together with the four-wave-mixing (FWM) processes 61 . Similar to Takushima et al. 62 , our spectral broadening is symmetrical with respect to pump wavelength, see Fig. 6 (see also Manceau et al. 46 , Fig. 4 depicting similar spectra), which suggests that the stimulated Raman scattering should not dominate in our case.
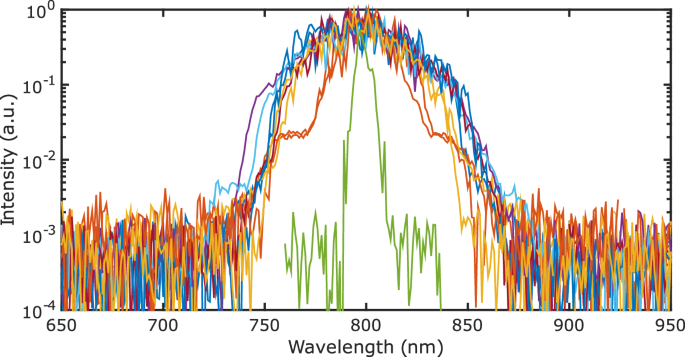
The violet, blue, yellow, orange, and red lines correspond to output spectra at a BSV pumping power of about 50 nJ; the BSV pump spectrum is shown in green. The supercontinuum spectra are symmetric with respect to pump wavelength and demonstrate high fluctuations at both edges.
We are observing rogue waves well-detuned from the pump frequency; therefore, we can use four-wave mixing to explain the amplification effect. For normal group velocity dispersion, the main question is whether it is possible to get for our detuning Ω a positive parametric gain G and the corresponding \({\sinh }^{2}G\) power dependence, respectively. Indeed, following the works of Stolen 63 and Wang 64 , the parametric gain is equal to
where P p and λ p are the pumping peak power and wavelength, A eff and β 2 are effective mode area and group velocity dispersion of the fiber, L coh = 2 π / ∣ β 2 Ω 2 ∣ is the coherence length of the interaction. Taking into account the parameters of our fiber at λ p = 800 nm, β 2 = 40 fs 2 /mm and A eff = 20 μm 2 , for the observation of FWM from BSV at 760 nm (Fig. 2 b), we obtain a positive gain for BSV energy per pulse >33 nJ. It is somewhat smaller than the mean energy used for Fig. 2 b (40 nJ), for which we are getting G around 2.1, taking into account L coh = 1.1 cm. However, the gain is much larger for more energetic pulses from the fluctuating BSV pump. Note that these estimates do not take into account the shift of the amplification band, which happens due to SPM and XPM.
In agreement with this estimate, the experiment demonstrates an exponential dependence of the output number of photons on the input pulse energy. Figure 7 shows the mean number of photons generated at 780 nm (blue points) as a function of the pulse energy of BSV pumping at 800 nm fitted by \({\sinh }^{2}(C\times {P}_{{{{{{{{\rm{p}}}}}}}}})\) function (red curve), where C is a constant. The estimate of parametric gain using Eq. ( 2 ) in this case gives a positive gain for BSV pump energies of >8 nJ per pulse, which is where we start observing some converted photons. The fit gives us the gain G = 3.4, which is close to the number estimated from Eq. ( 2 ).
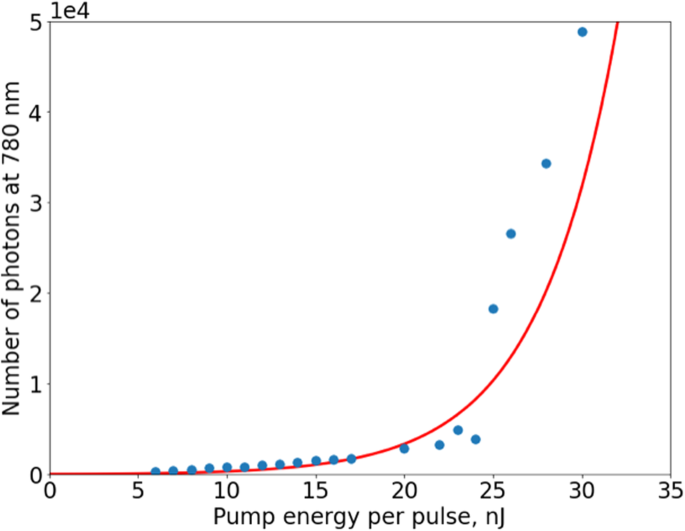
Number of photons at 780 nm as a function of BSV pump energy per pulse (blue points) together with the corresponding fit (red curve).
Direct estimation of the tail exponent
In the supercontinuum intensity data to be analyzed, power-law behavior has both a lower and an upper limit. This upper limit is not considered in the statistical literature of tail exponent estimation because, in the usual contexts, it is much harder to attain. For example, as there is a finite amount of water on Earth, flood sizes are, of course, limited; however, no recorded flood size has ever come close to that limit. In our case, an upper limit exists because the values of the largest observations are affected by detector saturation and pump depletion. In other words, even though the observed data is strictly speaking not heavy-tailed, we posit that this is due only to experimental limitations and would like to minimize their effect on exponent estimates.
We refer to the first estimation approach as direct because it estimates the value of the tail exponent directly, supposing that there is a finite interval within the range of observed values in which the density of the intensity observations shows a power-law decay. The advantages of this approach are that it is straightforward and it is also more generally applicable than the current context of supercontinuum generation (it only assumes that there is an interval where the exceedance probability function decays at a power-law rate). However, it does not take into account deviations from exact power-law behavior (e.g., which might cause some bias for BSV source); nevertheless, it is an easy-to-implement tool that, in our case, has only little bias.
The standard option for directly estimating the value of the tail exponent is the Hill estimator 34 , defined as
with x ( i ) denoting the i th largest element of the sample. Note that this formula is based on the k + 1 largest observations. However, since we have data that clearly do not decay at a power-law rate for large values, this method provides unreliable results (see Fig. 2 , red line). Even if the tail of the distribution decays asymptotically at a power-law rate, for finite samples, the problem of choosing k is non-trivial and has an extensive literature 65 , 66 , 67 , 68 . The basic approach is visual and is referred to as the Hill plot: one has to look for a range of values of k where \({\hat{\alpha }}_{{{{{{{{\rm{H}}}}}}}}}^{-1}(k)\) is flat, that is, it is insensitive to the choice of k . Note that choosing the tail length is equivalent to choosing a lower limit m beyond which observations are taken into account. That is, the tail length k can be expressed as \(k(m)=\max \{i:{x}_{(i)} \, > \, m\}\) . We prefer plotting the parameter estimate as a function of the limit m because it makes for an easy comparison of different estimators (see Fig. 3 a).
In our previous work 50 , we proposed a generalization of ( 3 ) for distributions for which power-law behavior has both a lower limit m and a finite upper limit M . With \(k=\max \{i:{x}_{(i)} > m\}\) and \(j=\max \{i:{x}_{(i)} \, > \, M\}\) , this generalized Hill estimator can be given as
That is, out of the top k + 1 observations, one discards the j largest elements, and uses the remaining k + 1 − j observations to estimate the tail exponent; note that \({\hat{\alpha }}_{{{{{{{{\rm{H}}}}}}}}}^{-1}(k)\equiv {\hat{\alpha }}_{{{{{{{{\rm{gH}}}}}}}}}^{-1}(k,0)\) .
Clearly, with no prior information on m and M , choosing their values based on the sample only is more involved than choosing the lower limit for the Hill estimator. We adapted an approach similar to the Hill plot, namely looking for an area where \({\hat{\alpha }}_{{{{{{{{\rm{gH}}}}}}}}}\) is not sensitive to the choice of [ m , M ]. This can, for example, be done by plotting the value of \({\hat{\alpha }}_{{{{{{{{\rm{gH}}}}}}}}}\) for several fixed values of M as a function of m . The likely ranges for the limits can be pinpointed by looking at the EEPF on a log-log scale. This visual approach is, of course, not feasible if one has a large number of samples to evaluate, but can be automated, for example, similarly to the heuristic algorithm proposed by Neves et al. 69 .
Tail exponent estimation as part of a model fit
The second approach, which we will refer to as the modeling approach, consists of fitting a multi-parameter model to the whole process, where the tail exponent is only one parameter out of a few.
As opposed to simulating the evolution of the non-linear Schrödinger equation, we take an opposite approach: we treat the process as a black box and fit a physically motivated functional form to the empirical distribution obtained from the measured data. This simplest model is based on four-wave mixing 46 , which we think is at the root of the amplification effect during the process. Due to the simplicity of the model, we can obtain a semi-analytic goodness of fit function, which helps with the stability and accuracy of the fit. Even though, similarly to the previously discussed direct approach, this procedure still takes a birds-eye view of the process, it also quantifies major experimental limitations. The model is the following:
I OUT denoting the measured intensity at the end of the fiber;
I IN standing for the incoming intensity with a constant mean μ (further details on incoming light statistics are in Supplementary Note 3) , which is
exponentially distributed for thermal(PDF \(\propto \exp \{-x/\mu \}\) ),
gamma-distributed for a BSV source(PDF \(\propto {x}^{-1/2}\exp \left\{-x/2\mu \right\}\) );
K : constant factor related to choosing the unit;
\({\omega }_{i} \sim {{{{{{{\mathcal{N}}}}}}}}\left(0,{\sigma }_{i}^{2}\right)\) : independent Gaussian noises;
R ( ⋅ ): detector response function.
The noise ω 1 corresponds to additive noises that affect the incoming intensity even before the light enters the fiber (due to the \({\sinh }^{2}(\cdot )\) transformation, this is essentially a multiplicative noise), whereas ω 2 is an additive detection noise. In order to avoid introducing extra parameters for the response function, we did not fit the model to the observations affected by detector saturation. This amounted to only taking into account the non-linearity of detector response about the noise floor l , through \(R(x)=\max \left\{l,x\right\}\) , and discarding the largest observations.
In order to have a better understanding of how the individual parameters affect the output intensity distribution, let us look at the asymptotic exceedance probability for the thermal case in the absence of detection noise ( σ 2 = 0) 50 :
This shows that the decay exponent is solely determined by the mean input intensity, whereas \({x}_{0}=K\cdot {e}^{{\sigma }_{1}^{2}/\mu }/4\) is a scaling factor.
This model can, of course, be further refined, but we were interested in the simplest version able to describe the observed process. This simplest version has five parameters: ϑ = ( μ , l , K , σ 1 , σ 2 ). The tail exponent of the output of this model is α = (2 μ ) −1 for the thermal, and α = (4 μ ) −1 for the BSV case.
The advantage of the model defined by ( 5 ) is that its density and distribution functions can be calculated semi-analytically. This gives us the opportunity to relatively easily perform a conditional (only observations below a pre-specified limit M are taken into account) maximum likelihood fit of its parameters. After the parameters are estimated, a simple binomial test can be performed to determine whether the fit should be rejected or not (further details of the method are discussed in Supplementary Note 1 ).
Data availability
The data that support the findings of this study are available from the corresponding author upon reasonable request.
Sunde, A. Kjempebølger i nordsjøen (Extreme waves in the North Sea). Vær & Klima, 18 , 1 (1995).
Bludov, Y. V., Konotop, V. V. & Akhmediev, N. Matter rogue waves. Phys. Rev. A 80 , 033610 (2009).
Article ADS Google Scholar
Manikandan, K., Muruganandam, P., Senthilvelan, M. & Lakshmanan, M. Manipulating matter rogue waves and breathers in Bose-Einstein condensates. Phys. Rev. E 90 , 062905 (2014).
Ruderman, M. S. Freak waves in laboratory and space plasmas. Eur. Phy. J. Spec. Top. 185 , 57–66 (2010).
Article Google Scholar
Moslem, W. M., Shukla, P. K. & Eliasson, B. Surface plasma rogue waves. Europhys. Lett. 96 , 25002 (2011).
Tsai, Y.-Y., Tsai, J.-Y. & I, L. Generation of acoustic rogue waves in dusty plasmas through three-dimensional particle focusing by distorted waveforms. Nat. Phys. 12 , 573–577 (2016).
Stenflo, L. & Marklund, M. Rogue waves in the atmosphere. J. Plasma Phy. 76 , 293–295 (2010).
Ganshin, A. N., Efimov, V. B., Kolmakov, G. V., Mezhov-Deglin, L. P. & McClintock, P. V. E. Observation of an inverse energy cascade in developed acoustic turbulence in superfluid helium. Phys. Rev. Lett. 101 , 065303 (2008).
Solli, D. R., Ropers, C., Koonath, P. & Jalali, B. Optical rogue waves. Nature 450 , 1054–1057 (2007).
Buccoliero, D., Steffensen, H., Ebendorff-Heidepriem, H., Monro, T. M. & Bang, O. Midinfrared optical rogue waves in soft glass photonic crystal fiber. Opt. Express 19 , 17973–17978 (2011).
Onorato, M., Residori, S., Bortolozzo, U., Montina, A. & Arecchi, F. Rogue waves and their generating mechanisms in different physical contexts. Phys. Rep. 528 , 47–89 (2013).
Article ADS MathSciNet Google Scholar
Hansen, R. E., Engelsholm, R. D., Petersen, C. R. & Bang, O. Numerical observation of spm rogue waves in normal dispersion cascaded supercontinuum generation. J. Opt. Soc. Am. B 38 , 2754–2764 (2021).
Pareto, V. in Cours d’économie politique professé à l’Université de Lausanne , 299–345 (F. Rouge, 1896).
Yakovenko, V. M. & Rosser, J. B. Colloquium: Statistical mechanics of money, wealth, and income. Rev. Mod. Phys. 81 , 1703–1725 (2009).
Shpilberg, D. C. The probability distribution of fire loss amount. J. Risk Insur. 44 , 103–115 (1977).
Rootzén, H. & Tajvidi, N. Extreme value statistics and wind storm losses: a case study. Scand. Actuarial J. 1 , 70–94 (1995).
Google Scholar
de Solla Price, D. J. Networks of scientific papers. Science 149 , 510–515 (1965).
Redner, S. How popular is your paper? an empirical study of the citation distribution. Eur. Phys. J. B 4 , 131–134 (1998).
Golosovsky, M. Power-law citation distributions are not scale-free. Phys. Rev. E 96 , 032306 (2017).
Gutenberg, B. & Richter, C. F. Frequency of earthquakes in California. Bull. Seismol. Soc. Am. 34 , 185–188 (1944).
Christensen, K., Danon, L., Scanlon, T. & Bak, P. Unified scaling law for earthquakes. Proc. Natil Acad. Sci. 99 , 2509–2513 (2002).
Newberry, M. G. & Savage, V. M. Self-similar processes follow a power law in discrete logarithmic space. Phys. Rev. Lett. 122 , 158303 (2019).
Birkeland, K. W. & Landry, C. C. Power-laws and snow avalanches. Geophys.Res. Lett. 29 , 49–1–49–3 (2002).
Lu, E. T. & Hamilton, R. J. Avalanches and the distribution of solar flares. Astrophys. J. 380 , L89–L92 (1991).
Pastor-Satorras, R., Vázquez, A. & Vespignani, A. Dynamical and correlation properties of the internet. Phys. Rev. Lett. 87 , 258701 (2001).
Stumpf, M. P. H. & Ingram, P. J. Probability models for degree distributions of protein interaction networks. Europhys. Lett.(EPL) 71 , 152–158 (2005).
Newman, M. Power laws, Pareto distributions and Zipf’s law. Contemp. Phy. 46 , 323–351 (2005).
Zipf, G. K. The distribution of economic power and social status. In Human Behavior and the Principle of Least Effort Ch. 11, Vol. 588, 445–516 (Addison-Wesley Press, Oxford, England, 1949).
Mandelbrot, B. The Pareto–Lévy law and the distribution of income. Int. Econ. Rev. 1 , 79–106 (1960).
Mandelbrot, B. New methods in statistical economics. J. Pol. Econ. 71 , 421–440 (1963).
Embrechts, P., Resnick, S. I. & Samorodnitsky, G. Extreme value theory as a risk management tool. N. Am. Actuar. J. 3 , 30–41 (1999).
Article MathSciNet Google Scholar
Rachev, S. (ed.) Handbook of Heavy Tailed Distributions in Finance 1st edn, vol1 (Elsevier, 2003).
Akhmediev, N. & Pelinovsky, E. Editorial – introductory remarks on “discussion & debate: rogue waves – towards a unifying concept?”. Eur. Phy. J. Spec. Top. 185 , 1–4 (2010).
Hill, B. M. A simple general approach to inference about the tail of a distribution. Ann. Statist. 3 , 1163–1174 (1975).
Pickands III, J. Statistical inference using extreme order statistics. Annal. Stat. 3 , 119–131 (1975).
Kratz, M. & Resnick, S. I. The qq-estimator and heavy tails. Commun. Stat. Stoch. Models 12 , 699–724 (1996).
Feuerverger, A. & Hall, P. Estimating a tail exponent by modelling departure from a pareto distribution. Annal. Stat. 27 , 760 – 781 (1999).
Boyd, R. W. Nonlinear Optics 3rd edn (Academic Press, Inc. USA, 2008).
Grynberg, G., Aspect, A. & Fabre, C. Introduction to Quantum Optics: From the Semi-classical Approach to Quantized Light 696 (Cambridge University Press, 2010).
Barthelemy, P., Bertolotti, J. & Wiersma, D. S. A lévy flight for light. Nature 453 , 495–498 (2008).
Mercadier, N., Guerin, W., Chevrollier, M. & Kaiser, R. Lévy flights of photons in hot atomic vapours. Nat. Phy. 5 , 602–605 (2009).
Solli, D. R., Ropers, C. & Jalali, B. Active control of rogue waves for stimulated supercontinuum generation. Phys. Rev. Lett. 101 , 233902 (2008).
Wetzel, B. et al. Random walks and random numbers from supercontinuum generation. Opt. Express 20 , 11143–11152 (2012).
Sørensen, S. T., Bang, O., Wetzel, B. & Dudley, J. M. Describing supercontinuum noise and rogue wave statistics using higher-order moments. Optics Communications 285 , 2451–2455 (2012).
Wetzel, B. et al. Real-time full bandwidth measurement of spectral noise in supercontinuum generation. Sci. Rep. 2 , 882 (2012).
Manceau, M., Spasibko, K. Y., Leuchs, G., Filip, R. & Chekhova, M. V. Indefinite-mean pareto photon distribution from amplified quantum noise. Phys. Rev. Lett. 123 , 123606 (2019).
Quimby, R. Photonics and Lasers: An Introduction 1st edn, 536 14-2 253–259 (Wiley-Interscience, 2006).
Fedotov, A. B. et al. Pump-depleting four-wave mixing in supercontinuum-generating microstructure fibers. Appl. Phys. B 77 , 313–317 (2003).
Vanholsbeeck, F., Martin-Lopez, S., González-Herráez, M. & Coen, S. The role of pump incoherence in continuous-wave supercontinuum generation. Opt. Express 13 , 6615–6625 (2005).
Rácz, É., Ruppert, L. & Filip, R. Estimation of heavy tails in optical non-linear processes. N. J. Phys. 23 , 043013 (2021).
Stolen, R. H. & Lin, C. Self-phase-modulation in silica optical fibers. Phys. Rev. A 17 , 1448–1453 (1978).
Drees, H., de Haan, L. & Resnick, S. How to make a hill plot. Annal. Stat. 28 , 254–274 (2000).
Flórez, J., Lundeen, J. S. & Chekhova, M. V. Pump depletion in parametric down-conversion with low pump energies. Opt. Lett. 45 , 4264–4267 (2020).
López-Durán, J. & Rosas-Ortiz, O. Exact solutions for vector phase-matching conditions in nonlinear uniaxial crystals. Symmetry 14 , 2272 (2022).
Walls, D. & Milburn, G. Quantum Optics Ch. 5 (pp. 73-91) (Springer Berlin Heidelberg, 2008).
Paleari, F., Andreoni, A., Zambra, G. & Bondani, M. Thermal photon statistics in spontaneous parametric downconversion. Opt. Express 12 , 2816–2824 (2004).
Coherent Corp. 780-HP Dispersion Data Howpublished https://www.coherent.com/resources/application-note/components-and-accessories/specialty-optical-fibers/780-hp-dispersion.pdf .
Kato, T., Suetsugu, Y. & Nishimura, M. Estimation of nonlinear refractive index in various silica-based glasses for optical fibers. Opt. Lett. 20 , 2279–2281 (1995).
Iakushev, S. O. et al. Formation of ultrashort triangular pulses in optical fibers. Opt. Express 22 , 29119–29134 (2014).
Hammani, K., Finot, C., Dudley, J. M. & Millot, G. Optical rogue-wave-like extreme value fluctuations in fiber raman amplifiers. Opt. Express 16 , 16467–16474 (2008).
Agrawal, G. Nonlinear Fiber Optics 5th edn, 652, 423–426 (Elsevier, 2012).
Takushima, Y., Futami, F. & Kikuchi, K. Generation of over 140-nm-wide super-continuum from a normal dispersion fiber by using a mode-locked semiconductor laser source. IEEE Photonics Technol. Lett. 10 , 1560–1562 (1998).
Stolen, R. & Bjorkholm, J. Parametric amplification and frequency conversion in optical fibers. IEEE J. Quant. Electronics 18 , 1062–1072 (1982).
Wang, L. J., Hong, C. K. & Friberg, S. R. Generation of correlated photons via four-wave mixing in optical fibres. J. Opt. B: Quantum Semiclass. Optics 3 , 346 (2001).
Drees, H. & Kaufmann, E. Selecting the optimal sample fraction in univariate extreme value estimation. Stoch. Process. Their Appl. 75 , 149 – 172 (1998).
Guillou, A. & Hall, P. A diagnostic for selecting the threshold in extreme value analysis. J. R. Stat. Soc. Ser. B (Stat. Methodol.) 63 , 293–305 (2001).
Danielsson, J., de Haan, L., Peng, L. & de Vries, C. Using a bootstrap method to choose the sample fraction in tail index estimation. J. Multivar. Anal. 76 , 226–248 (2001).
Caeiro, F. & Gomes, M. Threshold selection in extreme value analysis: methods and applications. In Extreme Value Modeling and Risk Analysis: Methods and Applications 1st edn (eds Dey, D. K. & Yan, J.) 69–86 (Taylor & Francis, New York, 2016).
Neves, M. M., Gomes, M. I., Figueiredo, F. & Gomes, D. P. Modeling extreme events: Sample fraction adaptive choice in parameter estimation. J. Stat. Theory Pract. 9 , 184–199 (2015).
Download references
Acknowledgements
R. F. acknowledges the support of project 21-13265X of the Czech Science Foundation. L. R. and É. R. acknowledge funding from the MEYS of the Czech Republic (Grant Agreement 8C22001), M. V. C. acknowledges funding from the Deutsche Forschungsgemeinschaft (grant number CH 1591/16-1). Project SPARQL has received funding from the European Union’s Horizon 2020 Research and Innovation Programme under Grant Agreement no. 731473 and 101017733 (QuantERA). L. R., É. R. and R. F. have further been supported by the European Union’s 2020 research and innovation programme (CSA - Coordination and support action, H2020-WIDESPREAD-2020-5) under grant agreement No. 951737 (NONGAUSS).
Author information
Authors and affiliations.
Department of Optics, Palacký University, 17. listopadu 1192/12, 771 46, Olomouc, Czech Republic
Éva Rácz, László Ruppert & Radim Filip
Q.ANT GmbH, Handwerkstraße 29, 70565, Stuttgart, Germany
Kirill Spasibko
Laboratoire de Physique des Lasers, Université Sorbonne Paris Nord, CNRS, UMR 7538, 93430, Villetaneuse, France
Mathieu Manceau
Max Planck Institute for the Science of Light, Staudtstraße 2, 91058, Erlangen, Germany
Mathieu Manceau & Maria V. Chekhova
Friedrich-Alexander Universität Erlangen-Nürnberg, Staudtstraße 7/B2, 91058, Erlangen, Germany
Maria V. Chekhova
You can also search for this author in PubMed Google Scholar
Contributions
K.S., M.M., and M.V.C. conceived the design and performed the experimental part of the study. É.R., L.R., and R.F. developed the theoretical methods and calculated the relevant numerical estimators. É.R., K.S., M.M., L.R., M.V.C., and R.F. analyzed and interpreted the results and contributed to the composition of the manuscript.
Corresponding author
Correspondence to Éva Rácz .
Ethics declarations
Competing interests.
The authors declare no competing interests.
Peer review
Peer review information.
Communications Physics thanks Christian Rosenberg Petersen, Dong Mao and the other, anonymous reviewer for their contribution to the peer review of this work.
Additional information
Publisher’s note Springer Nature remains neutral with regard to jurisdictional claims in published maps and institutional affiliations.
Supplementary information
Supplementary information, rights and permissions.
Open Access This article is licensed under a Creative Commons Attribution 4.0 International License, which permits use, sharing, adaptation, distribution and reproduction in any medium or format, as long as you give appropriate credit to the original author(s) and the source, provide a link to the Creative Commons licence, and indicate if changes were made. The images or other third party material in this article are included in the article’s Creative Commons licence, unless indicated otherwise in a credit line to the material. If material is not included in the article’s Creative Commons licence and your intended use is not permitted by statutory regulation or exceeds the permitted use, you will need to obtain permission directly from the copyright holder. To view a copy of this licence, visit http://creativecommons.org/licenses/by/4.0/ .
Reprints and permissions
About this article
Cite this article.
Rácz, É., Spasibko, K., Manceau, M. et al. Quantitative analysis of the intensity distribution of optical rogue waves. Commun Phys 7 , 119 (2024). https://doi.org/10.1038/s42005-024-01592-y
Download citation
Received : 28 July 2023
Accepted : 08 March 2024
Published : 05 April 2024
DOI : https://doi.org/10.1038/s42005-024-01592-y
Share this article
Anyone you share the following link with will be able to read this content:
Sorry, a shareable link is not currently available for this article.
Provided by the Springer Nature SharedIt content-sharing initiative
By submitting a comment you agree to abide by our Terms and Community Guidelines . If you find something abusive or that does not comply with our terms or guidelines please flag it as inappropriate.
Quick links
- Explore articles by subject
- Guide to authors
- Editorial policies
Sign up for the Nature Briefing newsletter — what matters in science, free to your inbox daily.

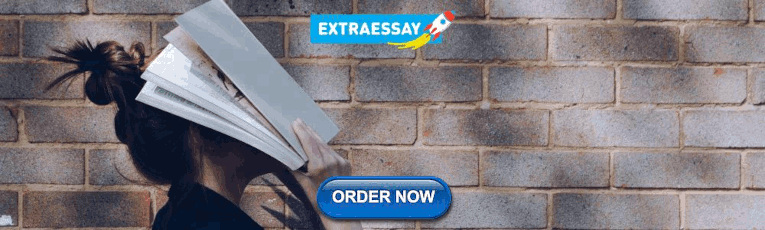
IMAGES
VIDEO
COMMENTS
Teaching quantitative data analysis is not teaching number crunching, but teaching a way of critical thinking for how to analyze the data. The goal of data analysis is to reveal the underlying patterns, trends, and relationships of a study's contextual situation. Learning data analysis is not learning how to use statistical tests to crunch ...
In theoretical terms, quantitative content analysis is being applied to many different fields of research in communication science, for example, in analyzing the kind of issues covered by the media in the context of agenda-setting. Similarly, quantitative content analysis is a key method in the cultivation approach, where it is called message ...
Quantitative communication research is typically based on a highly conventionalized approach to social science. Science and research, like all human endeavors, are subject to social pressures and normative ... proach to data analysis that Gigerenzer et al. (1990) call modern hybrid statistics. This approach involves
Quantitative methods for social justice use the "po wer of numbers" and the scientific. method for social good to bring about meaningful and measurable social change. Quantitative ...
Meta-Analysis: In this technique, a researcher takes a collection of quantitative studies and analyzes the data as a whole to get a better understanding of a communication phenomenon. For example, a researcher might be curious about how video games affect aggression.
Whether the statistical analysis was proper, the data clean, the surveyors well trained, etc., innumerable attacks can be brought against any study if the reader does not care to accept the propriety of the argument. ... Three Types of Communication Research Methods: Quantitative, Qualitative, and Participatory. In: Servaes, J. (eds) Handbook ...
Quantitative content analysis is a research method in which features of textual, visual, or aural material are systematically categorized and recorded so that they can be analyzed. Widely employed in the field of communication, it also has utility in a range of other fields. Central to content analysis is the process of coding, which involves ...
Quantitative data has to be gathered and cleaned before proceeding to the stage of analyzing it. Below are the steps to prepare a data before quantitative research analysis: Step 1: Data Collection. Before beginning the analysis process, you need data. Data can be collected through rigorous quantitative research, which includes methods such as ...
Abstract. Quantitative data analysis serves as part of an essential process of evidence-making in health and social sciences. It is adopted for any types of research question and design whether it is descriptive, explanatory, or causal. However, compared with qualitative counterpart, quantitative data analysis has less flexibility.
Quantitative data analysis is one of those things that often strikes fear in students. It's totally understandable - quantitative analysis is a complex topic, full of daunting lingo, like medians, modes, correlation and regression.Suddenly we're all wishing we'd paid a little more attention in math class…. The good news is that while quantitative data analysis is a mammoth topic ...
nominal. It is important to know w hat kind of data you are planning to collect or analyse as this w ill. affect your analysis method. A 12 step approach to quantitative data analysis. Step 1 ...
Although quantitative research and methods can provide good evidence, it is important for novice researchers to learn basic elements of quantitative data analysis in order to better understand and interpret their own research or that of others. This chapter discusses how to analyze and interpret quantitative data and connect data analysis to ...
CMM aims to bring developments in methodology, both qualitative and quantitative, to the attention of communication scholars, to provide an outlet for discussion and dissemination of methodological tools and approaches to researchers across the field, to comment on practices with suggestions for improvement in both research design and analysis, and to introduce new methods of measurement ...
Revised on June 22, 2023. Quantitative research is the process of collecting and analyzing numerical data. It can be used to find patterns and averages, make predictions, test causal relationships, and generalize results to wider populations. Quantitative research is the opposite of qualitative research, which involves collecting and analyzing ...
clinical research. research. statistical analysis. SPECIAL COMMUNICATION Basics of Research (Part 6): Quantitative Data Analysis Cheryl Bagley Thompson, PhD, RN, CS,1 Robert Schwartz, MD, MPH,2 Eric Davis, MD,3 Edward A. Panacek, MD4 1. University of Utah College of Nursing, Salt Lake City, Utah 2. Department of Emergency Medicine, Center for ...
conducting communication research is an enormous. field of study that requires very skilled and knowl-. edgeable researchers who are aware of its many. areas, approaches, designs, methods, tools ...
Quantitative data analysis may include the calculation of frequencies of variables and differences between variables. A quantitative approach is usually associated with finding evidence to either support or reject hypotheses you have formulated at the earlier stages of your research process. The same figure within data set can be interpreted in ...
quantitative research. Quantitative research remains particularly intricate to define and understand. A basic definition suggests it is an approach used to quantify problems by generating numerical data that can be transformed into usable statistics. Nonetheless, this definition remains insufficient. Kombo and Tromp offer a similar definition,
Hypothesis-testing (Quantitative hypothesis-testing research) - Quantitative research uses deductive reasoning. - This involves the formation of a hypothesis, collection of data in the investigation of the problem, analysis and use of the data from the investigation, and drawing of conclusions to validate or nullify the hypotheses.
Offers a guide through the essential steps required in quantitative data analysis; Helps in choosing the right method before starting the data collection process; Presents statistics without the math! Offers numerous examples from various diciplines in accounting and information systems; No need to invest in expensive and complex software packages
The purpose of quantitative data is to provide a numerical representation of a phenomenon or observation. Quantitative data is used to measure and describe the characteristics of a population or sample, and to test hypotheses and draw conclusions based on statistical analysis. Some of the key purposes of quantitative data include:
Drawing inspiration from previous works using 1H FID MRSI, this study quantifies metabolite concentrations at 9.4 T in the human cerebrum of a volunteer cohort and performs a respective group analysis to derive region specific metabolite concentrations. Voxel-specific corrections were performed for both water and individual metabolites, as well as used tissue specific T1-relaxation times.
Communications Physics - While a lot of research efforts have been directed towards determining the emergence mechanism of optical rogue waves, less attention has been given to characterizing the ...
The research results show that: under normal conditions, the HEP of high-speed rail train dispatchers is 9.1586×10 -5 , and under abnormal conditions, the HEP of highspeed rail train dispatchers is 2.1189×10 -3 . ... At the same time, compared with other methods, the model has certain advantages in terms of weight sensitivity, data ...