- Browse All Articles
- Newsletter Sign-Up
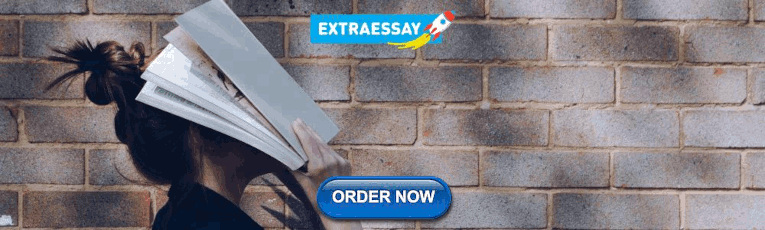
OnlineAdvertising →
No results found in working knowledge.
- Were any results found in one of the other content buckets on the left?
- Try removing some search filters.
- Use different search filters.
Online-to-offline advertisements as field experiments
- Special Issue: Article
- Lab and Field Experiments
- Open access
- Published: 18 October 2021
- Volume 73 , pages 211–242, ( 2022 )
Cite this article
You have full access to this open access article
- Akira Matsui 1 &
- Daisuke Moriwaki 2
6181 Accesses
Explore all metrics
Online advertisements have become one of today’s most widely used tools for enhancing businesses partly because of their compatibility with A/B testing. A/B testing allows sellers to find effective advertisement strategie,s such as ad creatives or segmentations. Even though several studies propose a technique to maximize the effect of an advertisement, there is insufficient comprehension of the customers’ offline shopping behavior invited by the online advertisements. Herein, we study the difference in offline behavior between customers who received online advertisements and regular customers (i.e., the customers visits the target shop voluntary), and the duration of this difference. We analyze approximately three thousand users’ offline behavior with their 23.5 million location records through 31 A/B testings. We first demonstrate the externality that customers with advertisements traverse larger areas than those without advertisements, and this spatial difference lasts several days after their shopping day. We then find a long-run effect of this externality of advertising that a certain portion of the customers invited to the offline shops revisit these shops. Finally, based on this revisit effect findings, we utilize a causal machine learning model to propose a marketing strategy to maximize the revisit ratio. Our results suggest that advertisements draw customers who have different behavior traits from regular customers. This study demonstrates that a simple analysis may underrate the effects of advertisements on businesses, and an analysis considering externality can attract potentially valuable customers.
Similar content being viewed by others
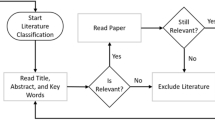
A review on customer segmentation methods for personalized customer targeting in e-commerce use cases
Miguel Alves Gomes & Tobias Meisen
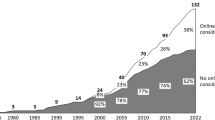
Competitive pricing on online markets: a literature review
Torsten J. Gerpott & Jan Berends
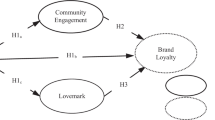
Do Social Media Marketing Activities Improve Brand Loyalty? An Empirical Study on Luxury Fashion Brands
Abdulla H. Fetais, Raed S. Algharabat, … Nripendra P. Rana
Avoid common mistakes on your manuscript.
1 Introduction
In this introduction, we describe the background of our study and propose the research questions to be answered. This section also provides the literature review.
1.1 Background and research questions
Online advertisements play an integral role in today’s World Wide Web. Regardless of whether they are for online or offline shopping, the center of interest in ad technology developments is to increase the sales of the targeted items or shops. Therefore, even post hoc analysis of the advertisements mainly focuses on these outcomes. However, to propose an effective marketing strategy, we need to disentangle what type of the customers advertisements invite to the target shop. The customers invited by the advertisement may show different shopping patterns from customers who visit the shop voluntarily. If these differences exist, an appropriate advertisement strategy for each group is not the same. Studying customer behavior during their shopping will advance understanding of what customers are induced by the advertisements and develop the additional marketing strategy to enhance the business.
Recent studies, for instance, have investigated customers’ offline shopping trajectories, who have received advertisements. The customers who received advertisements increase in-store traffic, resulting in unplanned purchases (Hui et al., 2013a , 2013b ). The field experiment in a shopping mall suggests a similar phenomenon in which location-based advertisements change customers’ shopping behavior trajectories (Ghose et al., 2019 ). These studies imply that just focusing on the traditional simple measurement may neglect the behavioral differences between regular customers and the customers who are invited to the shops by the advertisement.
Although the above literature provides insightful findings on customers’ shopping behavior, several vital issues remain unexplored. First, they only cover a limited area for shopping, such as in a grocery store (Hui et al., 2013a , 2013b ) or shopping mall (Ghose et al., 2019 ). In addition, they only focus on the trajectories of the target shop visit day, and not much is known about the long term trajectory of the customers who received advertisements. In other words, few studies have focused on the entire picture of the long-run customers’ trajectory. However, such comprehension of customers’ offline behavior is essential to maximize the effectiveness of brick-and-mortar advertising. For example, suppose a customer visits several stores when they visit the advertised shop. In that case, additional advertisements for those other stores would have a better conversion rate. In addition, analyzing long-term offline trajectories enables us to understand the type of customers who are likely to revisit the store (i.e., those who will become loyal customers). This long term analysis will enable us to maximize repeat customers through advertisements.
To fill the gaps mentioned above, we analyzed the long-term customer trajectory data from the mobile devices that consist of 4 months of customers’ location data. We first map the experimental data for Online to Offline (O2O) advertisements and the customers’ location data. Thereafter, we address the following three major research questions:
Do the customers invited by the advertisement show different shopping trajectories than regular customers on the target shop visit day?
How long does that trajectory difference last?
How likely customers will return to the same shop after the visit, and what factors are associated with that revisiting behavior?
With the answers to the third research question, we will utilize our findings to propose an effective advertising strategy that maximizes the number of revisit customers. To this end, we use an uplift model, a framework of causal machine learning, to propose an advertisement campaign strategy aiming to maximize the revisiting effect.
This paper contributes to the current state-of-the-art in three ways. First, we study the trajectory differences between the customers invited by the advertisement and regular customers who voluntarily visit the shop on a large scale. With the long-term user trajectory data, we also study the duration of the trajectory differences between the two groups. Our holistic analysis implies that the existing studies omit unignorable behavioral differences between the two groups. We also find that a certain fraction of the users invited to the physical shop revisit the same shop. Utilizing this discovery, we propose a prediction model that maximizes the revisiting effect of the advertisement.
1.2 Literature review
This subsection first reviews prior studies on the human offline trajectory mining in general and then focuses on the offline customer trajectory. Finally, we discuss the application of the mining human trajectory location-based advertisements, including O2O, and we also introduce the important marketing concept in these topics, commercial area.
1.2.1 Mining human offline trajectory
The mining of human offline trajectory has been an essential computer science topic, which focuses on analyzing the user’s physical behavior theoretically or empirically to extract useful information from this high-dimensional data. One of the most fundamental interests in this topic is to find frequent trajectory patterns (Gaffney & Smyth, 1999 ; Liu et al., 2011 ), as frequent patterns allow us to categorize users. For example, the frequent trajectory patterns are often used for clustering the users. Accordingly, the researchers study the trajectories’ semantics to generate interpretable results (Ghosh & Ghosh, 2016 ; Lee et al., 2007 ; Nanni & Pedreschi, 2006 ; Zhou et al., 2019 ). In recent years, embedding techniques such as word2vec have been used to approximate high-dimensional offline trajectories to lower dimensions (Crivellari & Beinat, 2019 , 2020 ).
There are several applications of mining offline human trajectory. The first important application is to predict the next offline trajectory of users (Monreale et al., 2009 ) because of its usefulness not only for the business but also for the public policy for human mobility (Gambs et al., 2012 ; Gao et al., 2019 ). However, the limits of predictions based on offline trajectories have been discussed in the context of social networks (Song et al., 2010 ). In social network analysis, this prediction problem is defined as edge connection prediction (Althoff et al., 2017 ; Sapiezynski et al., 2018 ), which reveals the importance of the social network. In addition, the users’ offline trajectory can predict their demographic information (Bao et al., 2016 ; Hinds & Joinson, 2018 ; Ravi et al., 2018 ; Wang et al., 2017 ; Zhong et al., 2015 ).
1.2.2 Customer offline trajectory
Marketing scientists are especially interested in a more specific human offline trajectory and customer shopping trajectory. This is because it conveys the most critical aspect of consumer behavior during their shopping, for instance, the selection of the times of purchase by the customer. From this perspective, marketing scientists have proposed models that incorporate the customer shopping trajectory, such as traditional marketing strategy models or statistical models.
We would like to emphasize that marketing researchers have mainly focused on customers’ in-store offline trajectories in the context of spatial analysis (Bronnenberg, 2005 ; Bronnenberg et al., 2005 ). For instance, some early work on customers’ in-shop trajectory study the customers’ shopping path in a super market (Larson et al., 2005 )
To understand the in-store trajectory pattern, Hui et al. deeply exploited customer behaviors. They first modeled the customer shopping path (Hui et al., 2009a , 2009b ). To investigate the advertisement and shopping trajectory, they conducted field experiments on in-store purchases with coupon (Hui et al., 2013b ) detailed analysis with video data (Hui et al., 2013a ). A more extended study by Ghose et al. ( 2019 ) conducted a large field experiment in a shopping mall to understand the effectiveness of the redeem campaign, and study the customers’ trajectory within the mall.
1.2.3 Location base advertisement and commercial area
Recently, online advertisements have used location data, including the offline trajectory of the targeted customers. This capability makes online advertisements different from traditional advertisements in several aspects (Goldfarb, 2014 ). Location information from mobile devices has been the source of most information of the customers’ trajectory. Recently, the importance of customers’ location information for online advertisements has garnered significant attention (Agarwal et al., 2011 , 2020 ; Johnson et al., 2016 ). This information is also effective for marketing strategies, such as coupons (Molitor et al., 2020 ). In addition, the most recent papers in this topic link the location advertisement to the causal inference machine learning to assess the effect of given advertisements (Kawanaka & Moriwaki, 2019 ; Moriwaki et al., 2020 ).
To distribute the location-based advertisement effectively, a typical strategy sets a commercial area for potential customers, because persons far from the target shop are unlikely to visit that shop. Traditionally, marketing researchers have been interested in market areas (Greenhut, 1952 ), and they study the evolution of the marketing area (Thelen & Woodside, 1997 ) expansion (Allaway et al., 1994 ) and their driving force (Allaway et al., 2003 ).
Overall, previous studies found the customers’ trajectory differences according to the advertisements they received. In addition, the importance of location information for online advertisements has been indicated. In particular, marketing scientists have mainly studied the first point with field experiments, and computer scientists have focused on the second point in the context of the ad technology. Our study aims to connect these two dots using A/B testing for O2O advertisements and offline customers’ trajectory data.
2 Study design and data
In this section, we report our study design and data. We first discuss our strategy to answer the three research questions introduced in Sect. 1 . Thereafter, we explain our data set, which consists of the A/B testings for the advertisements. We also introduce a glossary of terms in Table 1 to make our deliberations in this paper consistent and concise.
2.1 Study design to answer the RQs
Our three research questions ask if the difference in the motivation for visiting the shops reflect the trajectory differences. To answer these questions, we compose the A/B testing data for O2O advertisements.
As a regular A/B testing, the users in the experiment are randomly split into two groups to study the effectiveness of the O2O advertisements. One group receives the advertisements (Treatment), whereas the other group does not distribute the advertisements (Control). The primary interest of these A/B testing is the target shops that the online advertisement causes customers to visit. To understand this point, the location data is collected from the users’ mobile devices to check whether the users actually visit the target shop.
In this study, we investigate the experimental data from a different point of view, summarized in the schematic example in Fig. 1 . We leverage the situation in which the A/B testing for O2O advertisements causes motivational distinctions. The experimental data contains the users of the two groups, and this difference corresponds to the motivation to visit the shop. The control group users are supposed to visit the target shop voluntarily, because any O2O advertisement is not distributed to this group. In contrast, the treatment group can have an extrinsic motivation provided by the distributed advertisement. To understand if such motivational difference reflects their offline trajectories, we compare the trajectory between the voluntary customers (Control) and those of the invited customers (Treatment). Table 1 summarizes the terms that we introduced in this section.
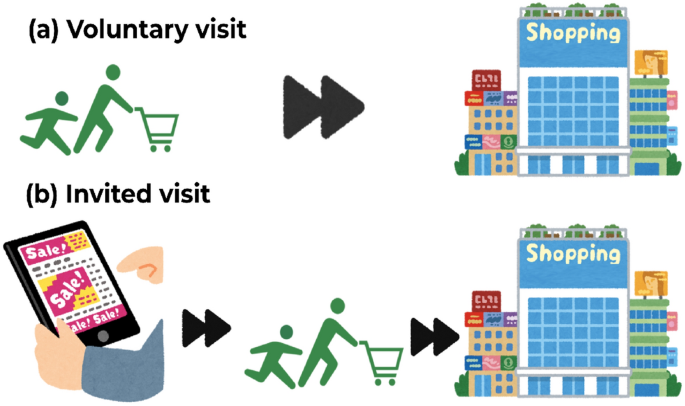
Schematic of the voluntary and invited visit. Schematic of our research design. We compare the two groups that have different motivations to visit the target shop. The users in a Voluntary visit group visits the target shop without seeing any O2O advertisement. The users in (a) are supposed to have an intrinsic motivation for shopping. On the other hand, b Invited visit group is the users that receive the O2O advertisements, and hence they are supposed to have an extrinsic motivation for shopping. In this study, we study if this motivational difference reflect the trajectory difference
2.2 Volume of the audiences and the resulting sales
We conduct our study based on the data generated from the A/B testing of the O2O advertisements conducted by CyberAgent, Inc., a Japan-based major advertising agency. They conduct nation-wide advertisement campaigns for offline shopping, aiming at inviting potential customers to a physical shop through online advertisements. Their campaign distributes online advertisements based on customer behavior.
As typical online advertisements, the advertisement campaigns use a demand-side platform (DSP) to deliver their advertisement creatives to potential customers’ mobile devices through a real-time bidding (RTB) system. During the campaigns, users’ visiting histories are used to count the number of visits to target stores. To understand the effectiveness of each campaign, the company randomly split the user pool into two groups as follows: treatment and control. Only the users in the treatment group have a chance to receive the advertisements, whereas those in the control group do not receive any treatment.
We study the multiple campaigns for multiple businesses, covering the various categories from electronic stores to super markets Footnote 1 . As we introduced in Sect. 2.1 , this study focuses on the customers who visited a target shop, resulting in the number of customers in the control group being 1440 and the number of customers in the treatment group is 1515. We also report the balance test at Appendix A.1 .
2.3 Offline behavior trajectory data
In addition to the visiting history to the target shop, we are interested in their trajectories near the target shop visit day. We collect the anonymized customer location data from their mobile devices and label them with the actual commercial place data. We trace the customers’ shopping trajectories, matching the location data from the customer with the commercial place data set. We then only label “visited” if a customer stayed for more than 10 min within a 20 m radius of a location registered in the shop data set.
For this threshold for “visited”, we use the most strict threshold in the service, which is the source of our data (the O2O advertisement product operated by CyberAgent, Inc. Footnote 2 ). In the service, they use the different thresholds depending on the advertised shop (3 min, 5 min, etc.). Those thresholds are determined based on the consultations between the advertisers and the retailers. Therefore, the thresholds used in the product is recognized as valid among the advertisers who actually pay the advertisement cost and operate the business. Our threshold, “10 min within a 20 m radius” is the most strict threshold among those thresholds. While it would be more appropriate to choose the threshold size depending on the type of the advertised shop, we decided to use the most strict threshold for research purpose. For this reason, we believe that our threshold is valid to study the customer behavior of interest.
In addition, we label the visited location with the three commercial categories as follows: shopping, food, and service. Consequently, our data set contains 23,549,902 location histories for 2955 users from January 1, 2020 to April 1, 2020. For the additional analysis, we also study the post-experiment user location data from April 1, 2020 to July 31, 2020 for the revisit analysis to be described in the subsequent section. Our mobility data is collected legally from the apps on their mobile device strictly following the privacy policy of the data provider (CyberAgent, 2021 ). On each app, the OS (i.e., iOS or Android) is designed to explicitly ask the user’s consent for mobility data usage. Our data provider obtains these mobility data from the app developers within the user consent.
2.4 Offline behavior features for the uplift model
We also compose the users’ behavior features built on the user offline trajectory data for uplift modeling to be detailed in Sect. 3.4 . To prevent the data leakage, we do not use any data for the analysis for RQ1-3 described in Sects. 2.2 and 2.3 . The demographic features are predicted values for each demographic characteristics that are provided by CyberAgent, Inc. The prediction model for demographic information is implemented using regression models and XGboost (Chen & Guestrin, 2016 ). This prediction model is not technically difficult and it is well known that the offline trajectories of the users can predict their demographic information (Bao et al., 2016 ; Hinds & Joinson, 2018 ; Ravi et al., 2018 ; Wang et al., 2017 ; Zhong et al., 2015 ). Note that this estimation results are used only for the uplift and not for our main research questions (RQ1–3). In addition, we use 96 shopping categories of the shops that the users visited which covers shopping categories from Fishing Shop to Bakery. Finally, we estimate the users’ home location and calculate the distance from their home to the target shops. We only use the data before the period of the experiments to prevent data leaking.
In this section, we describe the methods to answer the aforementioned three research questions. For RQ1 , we discuss our spatial trajectory analysis in Sect. 3.1 . Then we explain our panel regression model to study RQ2 at Sect. 3.2 . Finally, for RQ3 , we propose the method to calculate the revisit effect of the O2O advertisements at Sect. 3.3 , and the causal machine learning model to maximize the lift effect of the revisit at Sect. 3.4 .
3.1 RQ1: spatial trajectory analysis
With the compiled shopping trajectory data, we study whether the invited customers show different shopping trajectories compared with the voluntary customers. To this end, we model customers’ spatial trajectory. We first align the user location data such that we could analyze the customer behavior on the same coordinate and run a regression model to study the spatial features of each group.
3.1.1 Aligning spatial shopping trajectories from the different places
As discussed in the previous sections, the experiments target multiple offline shops and are on a nationwide scale. This makes it impossible to map the customers’ movement in one actual map as a typical geological analysis does. In other words, the raw trajectory data contains the user location history in different places across the nation, and hence, they do not allow us to summarize their trajectories as a single model. Therefore, we align the customer trajectory data into the same coordinate.
We refer to the location of the target shop for this alignment. For each customer travel point \((u_i, v_i)\) , we align it taking longitude and latitude differences as follows:
where \(u^{l}_{i}\) and \(v^{l}_{i}\) denote the latitude and longitude of the l , \(l=\{raw, ref\}\) . raw represents the raw customer’s geographical position, whereas ref represents the position of the reference points. The reference point in this study is the location of the target shop.
3.1.2 Features of aligned location data
We also note that shop categories are annotated in our location data. Here, we formally denote our aligned location data, \(G\in {\mathbb {R}}_{+}^{ U \times V \times N \times M}\) and \(g_{u, v, c, t} \in T\) . \(g_{u, v, c, t}\) represents the number of visits to a shop of category c , and its aligned latitude and longitude are ( u , v ), and t represents its experimental group (Treatment or Control).
3.1.3 Specification of spatial difference of the shopping trajectory
For RQ1 , we investigate if the customer in the treatment group shows a different spatial trajectory from the control group, modeling a spatial relationship between shop visiting behaviors and the distance from the target shop.
Because spatial analysis can easily experience difficulties, such as spatial dependency or auto-correlation among regions, we employ geographically weighted regression (GWR) (Brunsdon et al., 1998 ), a weighted regression model that incorporates spatial heterogeneity. We specify the following geographically weighted logistic regression model:
where \(y_{i}\) is a binary dependent variable that represents the dominance of either group; \(x_{i,j}\) is a vector of independent variables for area \(\left( u_{i}, v_{i}\right)\) ; \(\beta _{bw, j}\) represents that for each feature j , we use a different bandwidth bw for weighting. Dependent variables, here is whether the treatment group dominates are \(\left( u_{i}, v_{i}\right)\) . For the independent variable at position i , \(x_{i,j}\) , we use the distance from the reference points and shop category shares.
We set \(y_{i}\) as 1 when the customers in the treatment group visit a block \(\left( u_{i}, v_{i}\right)\) more frequently than the customers in the control group, and otherwise 0. Because the number of observed data points for each group is different, we normalized the data as described in Appendix A.2 .
3.2 RQ2: travel distance differences after the target shop visit day
When a customer changes their trajectory, it would be of paramount interest whether that alternation is a permanent or tentative. We study the shopping trajectory after the day when the customer visits the target shop. To trace the customer trajectory, we calculate the travel distances nearly 3 days before and after the first visit day, denoting the travel distances of user i at day s as \(d_{i,s}\) . Here, \(d_{i,s}\) represents the position from the day that customer i visits the target shop, and we focus on 3 days before and after the target shop visit day (i.e., \(s = \{j\}_{j=-3}^{3}\) ). For example, \(d_{i,s=0}\) is the travel distance of the day that user i visited the target shop. We calculate the travel distance after the visit to the shop between the control and treatment groups using the following regressions.
where \(\alpha\) denotes an intercept; \(T_{i}\) the dummy variable that takes 1 when customer i is in the treatment group and otherwise 0; \({Aft}_{s}\) becomes 1 after the shop visit (i.e., \(s > 0\) ); otherwise, 0; \(X_{i s}\) represents the control variables that incorporate customer fixed effects; \(\epsilon _{i s}\) denotes the error term.
In this model specification, \(\beta\) represents the average differences in the travel distance between the voluntary (control) and invited (treatment) group after the shop visit day. For control variables, \(X_{i s}\) , we use several sets of fixed effect variables that might affect travel distance as follows: the day of week, the day, the advertisement, and individual fixed effect. We will attempt several settings of X and discuss how it affects the estimation of the travel distance differences. For instance, having a fixed individual effect implies that we control the customer’s difference, such as their travel distance preference. Especially, when we incorporate the individual and time fixed effects, this regression model becomes the same as the framework of difference-in-difference, which is a widely used causal inference technique not only in economics (Angrist & Pischke, 2008 ; Card & Krueger, 1993 ; Lechner, 2011 ) but in a recent computer science study (Kusmierczyk & Gomez-Rodriguez, 2018 ; Li et al., 2020 ). We use robust standard errors clustered at the advertisement level or the individual level Footnote 3
3.3 RQ3: revisit effect of advertisements
We are also interested in studying the long-term effect of the advertisements, the revisiting effect. In other words, we intend to know the ratio of the customers that the advertisement makes them revisit. To study this effect, we subtract the revisit ratio in the control group from the treatment groups’ revisit ratio. Because the control group did not receive an advertisement, the subtracted revisit ratio can be interpreted as the effect of the advertisement. In addition to the simple calculation, we conduct meta-analysis to consider the advertisement differences.
3.3.1 Calculating the revisiting by the advertisement
As in Sect. 2.1 , we assume that there are two types of customers: Induced and organic customers. Induced customers are the customers induced by the advertisements. Meanwhile, there are customers who visit the target shops regardless of the advertisements, voluntary customers.
The revisit probability Pr ( RV ) can be formalized as follows: \(Pr(RV) = Pr(RV_{organic}) + Pr(RV_{induced})\) , where \(Pr(RV_{organic})\) and \(Pr(RV_{induced})\) denote the revisit ratios of the organic and induced customers, respectively Footnote 4 . With this formalization, we obtain \(Pr(RV_{induced})\) . As a definition, all of the re-visiting customers in the control group do not receive an advertisement. Therefore, we estimate \(Pr(RV_{induced})\) as Footnote 5
3.3.2 Controlling the differences among the advertisements
Although estimating Eq. 4 is straightforward, it might contain some biases, because our data set consists of the experiments for the various advertising campaigns. Differences among them can yield heterogeneous effects on revisiting, resulting in an imprecise estimation. To overcome this potential problem, we employ the model of the variance of the experiments and study the overall effect of the experiments. To this end, we conducted a meta-analysis using the Mantel–Haenszel method with random effects, which is a popular method in clinical research (Harrer et al., 2019 ). This meta-analysis method returns a weighted risk ratio assuming the variance among the experiments as random effects. To make our results comparable, we will report the odds ratio of revisiting for the invited customer (treatment) group with the voluntary (control) group by the following two methods: the direct calculation described in Eq. 4 and the meta-analysis.
3.4 Prediction model to maximize the revisit effect of O2O Ad
Finally, we propose a prediction model for high-value customers and discuss the marketing strategy using the data we observed in our experiments. In our analysis, we will find the customers who revisit the target shops after the first visit. These customers are supposed to bring more profit to the business than the customers who visit only once. Therefore, a model to predict the revisiting effect is of paramount interest for advertisers.
The most natural solution for this prediction task might be to find the factors associated with customers’ revisit behaviors. For example, logistic regression analysis will promptly find the variables that are associated with the revisiting behavior. Several marketing studies conventionally perform this type of post-experiment analysis. However, the challenge that advertisers face in reality and practice is the efficient distribution of advertisements to potential customers under the budget constraint.
3.4.1 The aim of uplift model
To attain the goal mention above, we use Uplift model that predicts the degree to which an advertisement improves the probability of revisiting.
The first term on the right-hand side of Eq. 7 is the probability that customer i revisits the target shop if it receives the advertisements, whereas the second term is the probability that customer i revisits the target shop if it does not receive the advertisements. Intuitively, Eq. 7 calculates the degree that the advertisement lifts the probability of customer i revisiting.
Our interest in this task is to sort the customers by descending order of \(\tau _{i}\) , and distribute the ad following that order to maximize the aggregated effect of \(\tau _{i}\) . In the literature of computer science, this \(\tau _{i}\) is called as lift effect and the prediction models of lift effect are called as uplift model (Gutierrez & Gérardy, 2017 ; Kawanaka & Moriwaki, 2019 ; Rzepakowski & Jaroszewicz, 2012 ; Zaniewicz & Jaroszewicz, 2013 ).
3.4.2 Learning the lift effect of O2O ad by uplift model
The apparent obstacle to learning the lift effect described in Eq. 7 is that we only observe one of the two in the data Footnote 6 . In other word, for user i who did not receive the O2O ad in the experiment, we need to study the probability of revisit “what if” she/he had received the O2O Footnote 7 .
To learn a function that predicts the \(\tau\) value for a given customer, we utilize the experimental data. With the data, we want to learn the function as follows:
where \(X_i\) denotes the feature vector of customer i discussed in Sect. 2.4 , \(G={T, C}\) represents whether a customer receives a treatment (i.e., receives an advertisement) T or not C . The first term describes the probability of customer i ’s revisiting given \(X_i\) when they are in treatment groups and the second term is the probability of revisiting even when they do not receive an advertisement.
We could learn the first and second term separately from the treatment and control group data, but it requires training two different models for a single prediction function. To avoid this cumbersome task, we use the reverted model proposed by (Jaskowski & Jaroszewicz, 2012 ), which is a transformed version of Eq. 8 ; that is, introducing the indicator Z as follows:
As (Jaskowski & Jaroszewicz, 2012 ) explains, Z takes 1 if the assignment G is ideal. In other words, we want to see the customers with the treatment revisit, and the customers without the treatment not revisiting. Note that Z takes 0 when a treated customer does not revisit, or a non-treated customer revisits. We will not gain any treatment for both cases. Under the assumption that the treatment assignment is random, we obtain Footnote 8
Therefore, we will estimate the function \(\tau (X)\) through \(P\left( Z=1 \mid X\right)\) .
3.4.3 Evaluation of the uplift model
To evaluate the uplift model, we will study the mechanism through which the advertisement distribution based on the model increases the uplift effect compared to the random advertisement distribution. Following practical conventions (Radcliffe & Surry, 2011 ; Rzepakowski & Jaroszewicz, 2012 ; Zaniewicz & Jaroszewicz, 2013 ), we use the area under the uplift curve (AUUC) for the evaluation. The AUUC describes the uplift gain when we distribute the advertisements from the users with the highest uplift scores to the users with the lowest uplift socre as follows:
where \(f_{o}(k),\ o \in \{1, 0\}\) denotes the lift effect of the k th sample in the ordered sample o . For \(o=1\) , we sort the sample based on the uplift effect prediction (descending). In contrast, we arrange the sample in a random manner when \(o=0\) .
The AUUC describes an advertisement strategy that maximizes the revisit effect based on following natural observation. First of all, it is not cost-efficient to distribute an O2O ad to the customer who is not likely to revisit the shop by the ad. Similarly, we do not need to pay attention to the customer who revisits the shop regardless of the ad. Therefore, an optimal advertisement strategy is to prioritize the customers who have a high lift (i.e., revisit effect described as \(\tau\) ), which means such customers are likely to revisit the shop because of the ad. The function \(f_{1}(k)\) describes the revisit rate when we distribute the O2O ad to the customer whose \(\tau\) is more than the kth percentile. The function \(f_{0}(k)\) , on the other hand, returns the revisit rate when we randomly distribute the O2O ad to the kth percent of the customers. Thus, \(f_{1}(k)-f_{0}(k)\) describes the gain of the revisit (rate) by the advertisements campaign in which we consider the lift effect. Consequently, we attain the maximized lift effect with \(k^{*}\) such that \(f_{1}(k)-f_{0}(k)\) is maximized.
This section reports our results that answer the three research questions proposed in the introduction section. First, we focus on the customer trajectory near the target shop during their first target shop visit ( RQ1 ). In addition to presenting the actual customers’ trajectory, we model the customers’ spatial behavior and report the model prediction to show the distinctions between the two groups. Furthermore, we trace the trajectory 3 days after the first target shop visit day and calculate the travel distances to observe the persistence of the advertisement effects ( RQ2 ). Then, we will study the revisiting effect of advertisements ( RQ3 ), comparing the revisiting ratio between the control and treatment groups. Finally, with the finding in RQ3 , we propose a counterfactual machine learning model for marketing strategy based on our analysis. We use the uplift model to predict the revisit effect and a strategy for delivering the advertisement to maximize the revisit effects.
4.1 RQ1: shopping trajectory differences
Our first research question asks; ”Do the customers invited by the advertisement show different shopping spatial trajectories on the target shop visit day?” To investigate this trajectory difference, we compare the trajectories of the voluntary visit customers (the control group) and the invited visit customers (the treatment group) during their first target visit day.
4.1.1 Customer location history near the target shop
To answer RQ1 , we study the customer location data near the target shop to compare the differences in visit points between the two groups. We first align the customer location history to map their trajectories into the same coordination as in Sect. 3.1.1 . After coordination, we focus on an area of 2km from the target shops in radius, and calculate the number of visits in each grid (111 m 2 ), as discussed in Sect. 3.1.3 . Figure 2 shows the area near the target shop with a label that represents the group that dominates a particular area. Not compelling but Fig. 2 alludes to the fact that the customers in the control group are dense near the shop and the treatment group are dominant in the outward area.
4.1.2 Customer spatial trajectory modeling
To conclude the hypothesis proposed above, we will employ a GWR to model a spatial relationship between the distance from the target shop and the dominant area. We first model a simple regression, where we only use the distance from the shop. The results of the regression in Table 2 demonstrate a positive relationship between distance and treatment group dominance. The further from the target shop a place is located, the more likely the treatment group dominates that place. These results are qualitatively the same even when we add some control variables that might affect the area preference. In the second model, we add the ratio of the shops in each category, shopping and food, and found a positive relationship between distance and treatment group dominance.
This finding becomes more evident as we observe the prediction results using the estimated models. Figure 3 depicts the prediction result, where the control group dominates the center areas, whereas the treatment group dominates the outer areas. We also found a similar result when we used the second multivariate model. Note that the aim of these prediction models is not to prediction the behavior, but to abstract the important factors from the customers’ trajectories.
These prediction results contrast the trajectories of customers with two different motivations for visiting the target shop. Voluntary visitors gather around the area near the target shops. Meanwhile, the invited customers move to wider areas. The customers who shop in a wide area would bring more benefits to the shops near the target shop. In other words, the invited customers bring a larger externality in the sense that the shops near the target shop benefit from them, even though the campaign did not advertise these nearby shops (Fig. 4 ).
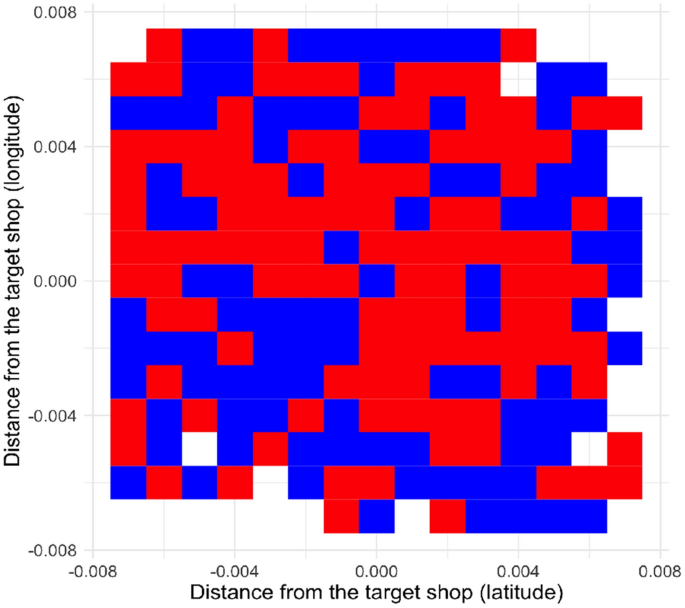
Dominant areas around the target shop. We align the target shop to the center of the plot and then split the area around the target shops by grids (the area of a square is about 111 m × 111 m). This figure shows the areas the invited customers (blue, treatment) group dominant and the voluntary customers (red, control) group dominant. We calculate which blocks are dominant following the procedure described in Sect. 3.1.3
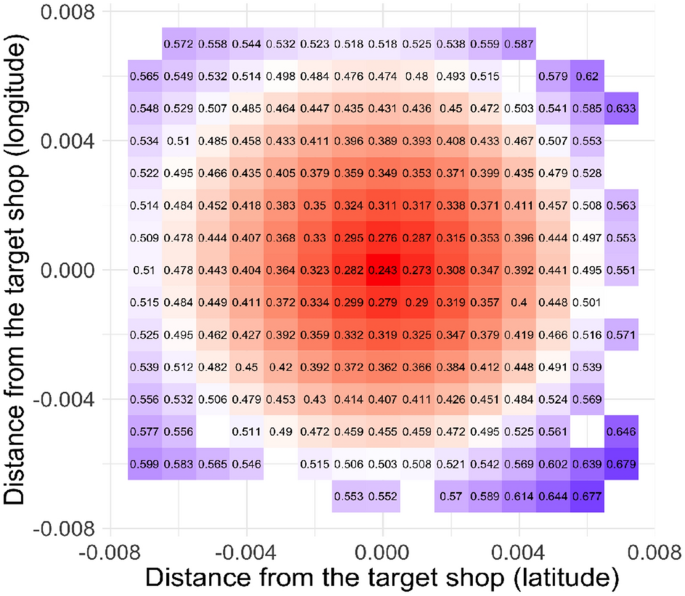
GWR prediction (simple model, Model 1). We align the target shop to the center of the plot and then split the area around the target shops by grids (the area of a square is about 111 m × 111 m). This figure describes the prediction by the estimated GWR model (Model 1 in Table 2 ). The figure shows that the model predicts that the invited customers’ dominants the outer areas (Blue), whereas the voluntary visit customer condence around the target shop (Red)
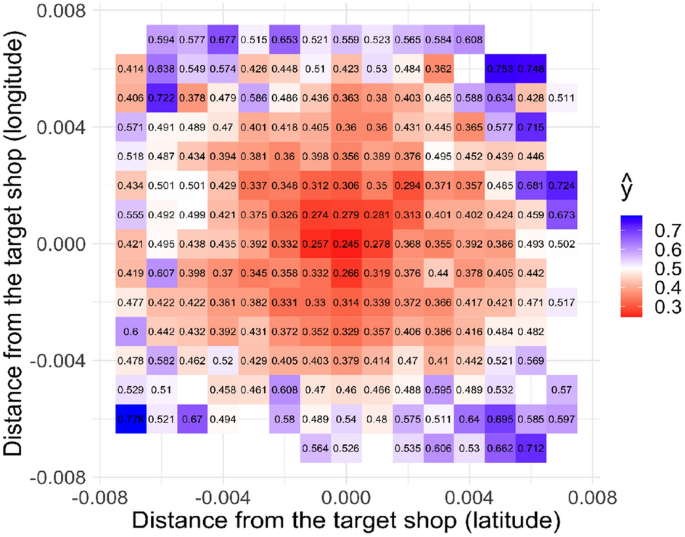
GWR prediction (multivariate model, Model 2). We align the target shop to the center of the plot and then split the area around the target shops by grids (the area of a square is about 111 m × 111 m). This figure provides the prediction by the GWR model with multiple independent variables (Model 2 in Table 2 ). Model 2 also predicts the dominance of the outer areas by the treatment groups
4.2 RQ2: persistence of trajectory difference
After RQ1 , one interesting question would be; “How long do these trajectory differences persist? ( RQ2 )”. Such an analysis will enable us to know the duration of the behavior difference between the voluntary and invited customers found in RQ1 .
To understand the persistence of the advertisement externality, we investigate the daily travel distances of the customers before and after the first target shop visit day. Because we found that the invited (treatment) group travels wider areas than the control group, the travel distances of each group should be a suitable indicator of such externality. In this analysis, we calculate the daily average travel distance for each group. There are several confounding factors that might affect their travel distance, such as advertisement differences, individual differences, and differences among days. Therefore, we control these differences by incorporating control variables, as discussed in Sect. 3.2 .
4.2.1 First look
We first calculate the travel distance difference among the two groups for each day, controlling the advertisement differences by the advertisement fixed effect. We run the regression analysis and report the coefficients of the day-dummy in Fig. 5 . The figure shows that the travel distances increase on the target shop visit day, and the distance differences remain relatively higher than the day before the visit. Even though the confidence intervals of days 1 and 2 are on the zero bound, we are not sure if these differences are robust. For this first analysis, we only control for differences among the advertisements, but each individual might respond differently. We, therefore, need to conduct a regression analysis modeling these differences to determine if this finding is robust.
4.2.2 Analysis with elaborated models
We attempt four settings of the control variables and report the results in Table 3 . The table calculates the day average travel distances 3 days after the target shop visit day Footnote 9 . Consequently, we find that the invited (treatment) group travels a longer distance even after the shop visit day. We estimate Model 1 as a baseline that only incorporates the dummy variables of the advertisements. For Model 2, we also incorporate the day-of-week dummy into the models. These first two models return similar results in the travel distance differences, suggesting that the day-of-week effect is negligible.
On the contrary, the last two models with the heterogeneity among the customers (Models 3 and 4) report smaller travel differences than the first two (Models 1 and 2), indicating that there is a certain heterogeneity among the customers and its overestimates of travel distances. While detecting the overestimation in the first look analysis, our analysis of the persistence of trajectory difference demonstrates consistent results that the treatment group travels longer distances even after the target shop visit day.
4.3 RQ3: revisit odds differences
Finally, we expand the time horizon of the analysis and ask RQ3 , studying if the customers revisit the shop within 4 months after the visit. To answer this question, we calculated the re-visitation ratio difference between the treatment and control groups, studying if a user revisits the shop within 4 months after the visit. We also present a causal machine learning model to predict the re-visitation effect of the advertisement and discuss the marketing strategy for re-visitation.
4.3.1 Revisiting ratio difference
As discussed in Sect. 3.3 , we interpret the differences among the groups in the revisit ratio as the revisits invited by the advertisements. We calculate the odds ratio of revisiting for the invited customer (treatment) group with the voluntary (control) group. Because our data consists of multiple advertisement campaigns, the effect of advertising may vary. We, therefore, present the odd ratio using Mentel–Haenszel methods to take into account the advertisement effects’ heterogeneity.
Figure 6 reports the results of the two different odds with 95% confidence intervals. In both calculations, the odd ratios are greater than 1, implying that the customers who received an advertisement are more likely to revisit than those who visit a shop voluntary. In other words, the advertisements encourage revisiting of the customers who do not revisit without the advertisements. Figure 6 also shows that the odds estimation by the meta analysis has a narrow confidence interval than the estimation using the direct method. This overestimation by the direct methods suggests that the revisit effect varies among the advertisements. However, after modifying these differences, the odd ratio is still greater than 1, implying that the advertisements increase the revisit ratio.
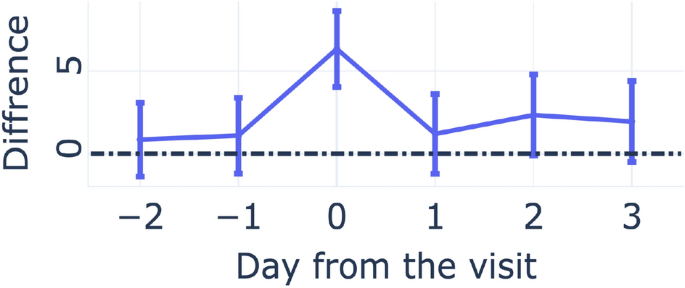
Travel distances difference (km) before and after the shop visit day. Figure 5 describes the transition of the travel distance differences (km) between the voluntary and invited customers (the error bars represent 95% confidence interval)
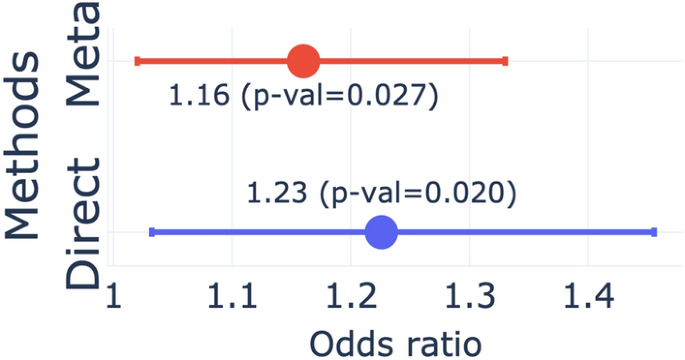
Odds ratio of revisiting. Figure 6 compares the odds ratio of revisiting for the invited customer (treatment) group with the voluntary (control) group by the two different methods: Direct and Meta analysis. Direct : The odds ratio of the whole sample. Meta : The odds ratio by the Mantel–Haenszel method that controls the differences between the advertisements
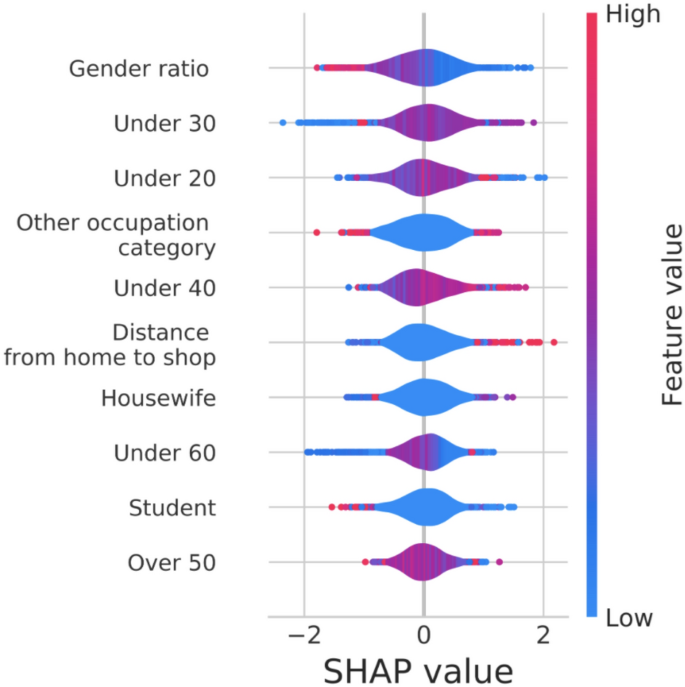
Distribution of the SHAP values of the top 10 higest mean SHAP value features. The top 10 features by the SHAP value by (Lundberg & Lee, 2017 ) for the uplift model. The descriptions of the features are in Appendix A.4 Except for Distance from home to shop , the other demographic variables represent the probability that a customer in that demographic category. For example, the high value of Other occupation category means the prediction model returns the high probability that the customer is not in the specific occupation category. The color represents the value of the feature (Reg: high, Blue: low). A high SHAP value represents a positive impact on the lift effect, and vice versa. For instance, the figure shows that the customer who lives the spot with fewer woman residents contributes to the high lift effect predictions. Overall, this SHAP value analysis suggests that younger male persons tend to have a higher lift effect
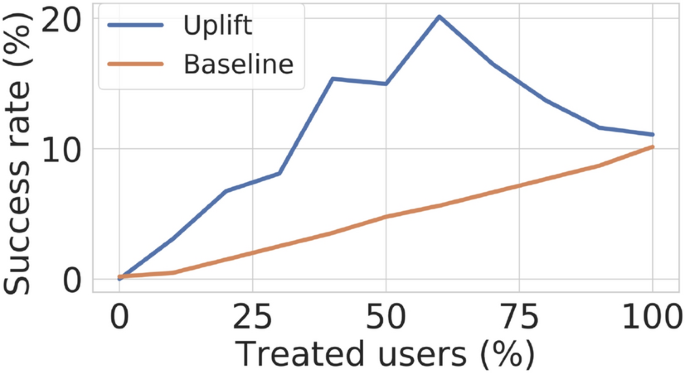
Uplift curve. Figure 8 shows the marketing strategy to maximize the lift effect of revisiting by the advertisements. The baseline describes the revisit effect when we randomly chose the users to get advertisements from the targeted user pool. The figure shows that revisiting is maximized when the customers whose lift effect is more than the 60th percentile receive the advertisements
4.4 Predicting the revisiting lift effects
We found that the advertisement encourages the customers to revisit the target shop. A natural next goal is to study the way to distribute the advertisement to maximize the revisiting effect. As we discussed in Sect. 3.4 , this goal is not achievable only without modeling the effects that the advertisement lifts (increases) the probability of revisiting a given customer. To this end, we use uplift modeling to predict the revisiting effect for each customer, and discuss a reasonable advertisement strategy. We split the data set into three and conducted feature selection optimization, train model, and predict the uplift with each subset of the data. With the trained model, we study what features are associated with the revisiting effect prediction.
To construct the uplift model, we first determine the best combination of features to maximize the uplift effects (i.e., the AUUC) from the set of features described in Sect. 2.4 . A number of candidate features for the model include customer profiles and location information. The best set of features is useful not only for improving the prediction accuracy but also in the sense that it enables us to know the features that are associated with the revisiting behavior. We conduct feature selection optimization via the state-of-the-art framework for hyper-parameter optimization, Optuna (Akiba et al., 2019 ). The feature optimization selects 60 features that consist of visit place histories, demographic information, such as age, and distance from home to the target shops.
With the features selected by the Optuna (Akiba et al., 2019 ), we use the XGBoost classifier (Chen & Guestrin, 2016 ) to estimate our uplift model and train it on the train data set. Footnote 10 To understand each feature’s contribution to the prediction of the lift effect, we utilize the SHapley Additive exPlanations (SHAP) value (Lundberg & Lee, 2017 ). The SHAP value represents the degree of the contribution of each feature on the prediction. Footnote 11 . We chose the top 10 features based on the mean absolute SHAP values. Footnote 12 A high SHAP value of a feature implies that a high value of that feature has a positive effect on a lift effect prediction and vice versa. Figure 7 reports the distribution of the SHAP values for the top 10 features, and it suggests the importance of predicted demographic information, such as age or occupation. The distribution of the SHAP values of the most three important features suggest that our uplift model predicts a high lift effect for young males. In addition, we find that location information is also important, as represented by Dist from the shop to home . The figure demonstrates that the longer distance predicts a high lift effect. This result implies that such customers wouldn’t have revisit the shop, because they are from the shop, and the O2O advertisements can cause the revisit.
With the trained uplift model, we study the optimal advertisement strategy to maximize the revisit effects. We calculate the cumulative success rate and depict it in Fig. 8 . The figure demonstrates the cumulative success rate when we distribute the advertisement to the customers based on the model’s predicted uplift score. The y -axis represents the cumulative success rate (i.e., cumulative revisit rate), and the x -axis represents the percentage of the customers who received the advertisement. The cumulative success rate achieves the peak when approximately 60% of people received the advertisement. Hence, the uplift model’s optimal strategy by this model is to distribute to the customers in the top 60% uplift scores.
5 Limitation
The A/B testings used in this study does not force customers to visit the target shops, but the customers who are willing to do so show up in our trajectory data as we could do in some field experiments. Hence, there is a possibility that the advertisement does not alter the customers’ trajectory and just picks up those who intend to change their trajectory. Therefore, it would be an oversimplification to claim that the O2O advertisements is the only source of the trajectory differences found in this study. d, as we discussed in the introduction section, the existing research continuously found that advertisements alter the behavior trajectory (Ghose et al., 2019 ; Hui et al., 2013a , 2013b ). In addition, this limitation can remain in a more rigid random control study. Even with such limitation, the body of our research manifests that the customers who received the O2O advertisements show different trajectories than the voluntary visit customers: move a wider area, travel a longer distance, and have a higher revisit ratio. We believe that our research conducts a large-scale quasi-field experiment that proposes a promising direction of behavior research in this marketing area. We also would like to mention here that our result can change according to the threshold discussed in Sect. 2.3 . To discuss an impact of the threshold on the result, we discussed the robustness check in Appendix A.3 .
6 Conclusions and discussion
In summary, our results demonstrate that the customers who are invited by the advertisement to the physical shops show different trajectories than those who visit the shop voluntarily. In our analysis, the invited customers demonstrate a more active shopping trajectory on their visit day, whereas the voluntary customers’ trajectories concentrate near the target shop. The invited customers move not just for longer distances, but also in larger areas than voluntary visit customers. These trajectory differences last even after the target shop visit day; their travel distances are approximately 6.8% longer than the voluntary visit customers. In addition, we found that the advertisements pick up customers who have higher revisit odds, implying that the invited customers are valuable for businesses. While estimating the exact economic value on businesses (e.g., sales, revenue, etc.) is out of the scope of the current paper, we can assume that the economic value to gain a marginal revisit customer is more than the price that an advertiser pay to gain a marginal visit Footnote 13 .
Based on this finding, we proposed a causal inference prediction model to devise an advertisement strategy for increasing the revisiting probability. Our model does not just predict the revisit probability for a given customer, rather than how much the advertisement will increase the number of revisits, enabling us to deliver the advertisements efficiently.
These insights provide opportunities for retailers who have their physical shops and the owners of huge commercial facilities, such as shopping malls. For example, the advertisements can invite new customers to their places, and such customers travel to more extensive areas that contribute to the business administrated by shopping malls. The customers who travel to more extensive areas would be exposed to more shopping spots, resulting in a high likelihood of committing unplanned purchases. These implications are also useful for the advertising industry. The price of advertisements conventionally relies on simple metrics of volume of the audiences and the resulting sales. Typical pricing of display advertisement depends the number of clicks a given ad earns (cost-per-click, CPC) or the number of conversions they earn (cost-per-action, CPA). However, our findings suggest some arbitrage between the values that advertisements bring and their actual price. For example, we could augment the value of O2O advertisements, demonstrating the economic value that would be brought to their marketplace.
The results here are not without limitations as discussed in the previous section, calling calls for further studies. First, while we found that O2O advertisements invited customers with a more massive externality, we cannot conclude that the advertisement itself is only the cause of such externality. Second, because we have no access to the customers’ spending history, we are not able to estimate the economic value of the externalizes. Therefore, future work will need to collect customer spending information to estimate the economic value of invited customers to the area near the target shops. As we proposed the revisit uplift model, one possible direction for further work is to propose a causal machine learning model that maximizes the causal externality effect by O2O advertisements.
The number of campaigns (i.e., experiments) with the category of the target shop; Variety store: 15; Sport good store: 6; Electronic store: 6; Housing-related store: 2; Super market: 1; Store in other category: 2.
https://www.airtrack.jp/ .
We report which cluster we used for which model in the results.
Note that \(\#\text{revisit customers}= \#\text{organic revisit customers}\) + \(\#\text{induced revisit customers}\) and, therefore,
\(\text {Pr(RV)}=\frac{ \#\text{revisit customers}}{ \#\text{first visit customers}} = \frac{ \#\text{organic revisit customers}}{ \#\text{first visit customers}} +\frac{ \#\text{induced revisit customers}}{ \#\text{first visit customers}}\)
Note that this is not the average treatment effect (ATE), because the re-visit is only defined for the population who visit the shops at least one time.
We use the term “learn” rather than “estimate” to emphasize that we study the prediction model rather than estimate the effect.
Focusing on this causal inference-like interpretation, the literature in computer science classifies uplift model into the class of causal machine learning. However, uplift model is different from other causal machine learning models popular in economics, such as causal forces in terms of its aim that estimates heterogeneity of treatment effects (HTE).
For the detail of the calculation see (Jaskowski & Jaroszewicz, 2012 )
The target shop visit day is not included.
max_depth: 10, n_estimators:100.
Online version of Molnar (2019) provides a good introduction of SHAP value.
We report the descriptions of the features and the absolute mean SHAP values of them in Appendix A.4 .
Such price is strict confidential business information. To the best of our knowledge, (Google, 2021 ) only provides an example of the price that the retailers (the advertisers) pay for their marginal gain of customer visit by O2O ad, and its average is ¥329 (Supermarket: ¥165; Convenience store: ¥123; Car dealer shop: ¥754; Department store: ¥288). In this case, the average economical value for \(x\%\) revisit rate gain can be 3.29 x yen.
Agarwal, S., Jensen, J. B., & Monte, F. (2020). Consumer mobility and the local structure of consumption industries. Working paper at National Bureau of Economic Research.
Agarwal, A., Hosanagar, K., & Smith, M. D. (2011). Location, location, location: An analysis of profitability of position in online advertising markets. Journal of Marketing Research, 48 (6), 1057–1073.
Article Google Scholar
Akiba, T., Sano, S., Yanase, T., Ohta, T., & Koyama, M. (2019). Optuna: A next-generation hyperparameter optimization framework. In Proceedings of the 25th ACM SIGKDD international conference on knowledge discovery & data mining (pp. 2623-2631).
Allaway, A. W., Berkowitz, D., & D’Souza, G. (2003). Spatial diffusion of a new loyalty program through a retail market. Journal of Retailing, 79 (3), 137–151.
Allaway, A. W., Black, W. C., Richard, M. D., & Mason, J. B. (1994). Evolution of a retail market area: An event-history model of spatial diffusion. Economic Geography, 70 (1), 23–40.
Althoff, T., Jindal, P., & Leskovec, J. (2017). Online actions with offline impact: How online social networks influence online and offline user behavior. In Proceedings of the tenth ACM international conference on web search and data mining (pp. 537–546).
Angrist, J. D., & Pischke, J. S. (2008). Mostly harmless econometrics: An empiricist’s companion . Princeton University Press.
Book Google Scholar
Bao, J., Lian, D., Zhang, F., & Yuan, N. J. (2016). Geo-social media data analytic for user modeling and location-based services. SIGSPATIAL Special, 7 (3), 11–18. https://doi.org/10.1145/2876480.2876484 .
Bronnenberg, J. (2005). Spatial models in marketing research and practice. Applied Stochastic Models in Business and Industry, 21 (4–5), 335–343.
Bronnenberg, B. J., Arora, N., Bell, D., & Thomadsen, R. (2005). Spatial models in marketing. Marketing Letters, 16 (3/4), 267–278.
Google Scholar
Brunsdon, C., Fotheringham, S., & Charlton, M. (1998). Geographically weighted regression. Journal of the Royal Statistical Society: Series D (The Statistician), 47 (3), 431–443.
Card, D., & Krueger, A. B. (1993). Minimum wages and employment: A case study of the fast food industry in new jersey and pennsylvania . Tech. rep., National Bureau of Economic Research.
Chen, T., & Guestrin, C. (2016). Xgboost: A scalable tree boosting system. In Proceedings of the 22nd acm sigkdd international conference on knowledge discovery and data mining (pp. 785–794).
Crivellari, A., & Beinat, E. (2019). From motion activity to geo-embeddings: Generating and exploring vector representations of locations, traces and visitors through large-scale mobility data. ISPRS International Journal of Geo-Information, 8 (3), 134.
Crivellari, A., & Beinat, E. (2020). Trace2trace-a feasibility study on neural machine translation applied to human motion trajectories. Sensors, 20 (12), 3503.
CyberAgent Inc. (2021). Privacy policy:ai business unit privacy policy. https://privacy-policy.cyberagent.ai/index-en.html . Last Accessed 10 May 2021.
Gaffney, S., & Smyth, P. (1999). Trajectory clustering with mixtures of regression models. In Proceedings of the fifth ACM SIGKDD international conference on knowledge discovery and data mining (pp. 63–72).
Gambs, S., Killijian, M. O., & del Prado Cortez, M. N. (2012). Next place prediction using mobility markov chains. In Proceedings of the first workshop on measurement, privacy, and mobility (pp. 1–6).
Gao, Q., Zhou, F., Trajcevski, G., Zhang, K., Zhong, T., & Zhang, F. (2019). Predicting human mobility via variational attention . The World Wide Web Conference, Association for Computing Machinery (pp. 2750–2756). New York, NY: WWW ’19. https://doi.org/10.1145/3308558.3313610
Ghose, A., Li, B., & Liu, S. (2019). Mobile targeting using customer trajectory patterns. Management Science, 65 (11), 5027–5049.
Ghosh, S., & Ghosh, S. K. (2016). Thump: Semantic analysis on trajectory traces to explore human movement pattern. In WWW ’16 companion proceedings of the 25th international conference companion on world wide web (pp. 35–36).
Goldfarb, A. (2014). What is different about online advertising? Review of Industrial Organization, 44 (2), 115–129.
Google, Inc. (2017). Visualizing the effects of online advertising on store visits. https://www.thinkwithgoogle.com/intl/ja-jp/marketing-strategies/data-and-measurement/awa-o2o2/ . Last Accessed 27 Jul 2022. ( in Japanese ).
Greenhut, M. L. (1952). The size and shape of the market area of a firm. Southern Economic Journal, 37–50.
Gutierrez, P., & Gérardy, J. Y. (2017). Causal inference and uplift modelling: A review of the literature. In International conference on predictive applications and APIs (pp. 1–13).
Harrer, M., Cuijpers, P., Furukawa, T. A., & Ebert, D. D. (2019). Doing meta-analysis in r: A hands-on guide. https://bookdown.org/MathiasHarrer/Doing_Meta_Analysis_in_R/
Hinds, J., & Joinson, A. N. (2018). What demographic attributes do our digital footprints reveal? A systematic review. PLOS ONE, 13 (11), e0207112.
Hui, S. K., Bradlow, E. T., & Fader, P. S. (2009a). Testing behavioral hypotheses using an integrated model of grocery store shopping path and purchase behavior. Journal of Consumer Research, 36 (3), 478–493.
Hui, S. K., Fader, P. S., & Bradlow, E. T. (2009b). Path data in marketing: An integrative framework and prospectus for model building. Marketing Science, 28 (2), 320–335.
Hui, S. K., Huang, Y., Suher, J., & Inman, J. J. (2013a). Deconstructing the “first moment of truth”: Understanding unplanned consideration and purchase conversion using in-store video tracking. Journal of Marketing Research, 50 (4), 445–462.
Hui, S. K., Inman, J. J., Huang, Y., & Suher, J. (2013b). The effect of in-store travel distance on unplanned spending: Applications to mobile promotion strategies. Journal of Marketing, 77 (2), 1–16. https://doi.org/10.1509/jm.11.0436 .
Jaskowski, M., & Jaroszewicz, S. (2012). Uplift modeling for clinical trial data. ICML Workshop on Clinical Data Analysis.
Johnson, G., Lewis, R. A., & Reiley, D. (2016). Location, location, location: repetition and proximity increase advertising effectiveness. Mimeo, Available at SSRN 2268215.
Kawanaka, S., & Moriwaki, D. (2019). Uplift modeling for location-based online advertising. In Proceedings of the 3rd ACM SIGSPATIAL international workshop on location-based recommendations, geosocial networks and geoadvertising (pp. 1–4).
Kusmierczyk, T., & Gomez-Rodriguez, M. (2018). On the causal effect of badges. In Proceedings of the 2018 world wide web conference (pp. 659–668).
Larson, J. S., Bradlow, E. T., & Fader, P. S. (2005). An exploratory look at supermarket shopping paths. International Journal of Research in Marketing, 22 (4), 395–414.
Lechner, M. (2011). The estimation of causal effects by difference-in-difference methods. Foundations and Trends in Econometrics .
Lee, J. G., Han, J., & Whang, K. Y. (2007). Trajectory clustering: a partition-and-group framework. In Proceedings of the 2007 ACM SIGMOD international conference on management of data (pp 593–604).
Li, A., Wang, A., Nazari, Z., Chandar, P., & Carterette, B. (2020). Do podcasts and music compete with one another? Understanding users’ audio streaming habits. In Proceedings of the web conference 2020 (pp. 1920–1931).
Liu, Y., Zhao, Y., Chen, L., Pei, J., & Han, J. (2011). Mining frequent trajectory patterns for activity monitoring using radio frequency tag arrays. IEEE Transactions on Parallel and Distributed Systems, 23 (11), 2138–2149.
Lundberg, S. M., & Lee, S. I. (2017). A unified approach to interpreting model predictions. In I. Guyon, U. V. Luxburg, S. Bengio, H. Wallach, R. Fergus, S. Vishwanathan, & R. Garnett (Eds.), Advances in neural information processing systems (vol. 30, pp. 4765–4774). Curran Associates, Inc. http://papers.nips.cc/paper/7062-a-unified-approach-to-interpreting-model-predictions.pdf
Molitor, D., Spann, M., Ghose, A., & Reichhart, P. (2020). Effectiveness of location-based advertising and the impact of interface design. Journal of Management Information Systems, 37 (2), 431–456.
Molnar, C. (2019). Interpretable machine learning: A guide for making black box models explainable. https://christophm.github.io/interpretable-ml-book
Monreale, A., Pinelli, F., Trasarti, R., & Giannotti, F. (2009). Wherenext: A location predictor on trajectory pattern mining. In Proceedings of the 15th ACM SIGKDD international conference on knowledge discovery and data mining (pp. 637–646).
Moriwaki, D., Hayakawa, Y., Munemasa, I., Saito, Y., & Matsui, A. (2020). Unbiased lift-based bidding system. arXiv preprint arXiv:200704002
Nanni, M., & Pedreschi, D. (2006). Time-focused clustering of trajectories of moving objects. Journal of Intelligent Information Systems, 27 (3), 267–289.
Radcliffe, N. J., & Surry, P. D. (2011). Real-world uplift modelling with significance-based uplift trees. White Paper TR-2011-1, Stochastic Solutions, pp. 1–33.
Ravi, A., Sangaralingam, K., & Datta, A. (2018). Predicting consumer level brand preferences using persistent mobility patterns. In 2018 IEEE international conference on big data (big data) (pp. 1986–1991).
Rzepakowski, P., & Jaroszewicz, S. (2012). Decision trees for uplift modeling with single and multiple treatments. Knowledge and Information Systems, 32 (2), 303–327.
Sapiezynski, P., Stopczynski, A., Wind, D. K., Leskovec, J., & Lehmann, S. (2018). Offline behaviors of online friends. arXiv preprint arXiv:181103153
Song, C., Qu, Z., Blumm, N., & Barabási, A. L. (2010). Limits of predictability in human mobility. Science, 327 (5968), 1018–1021.
Thelen, E. M., & Woodside, A. G. (1997). What evokes the brand or store? consumer research on accessibility theory applied to modeling primary choice. International Journal of Research in Marketing, 14 (2), 125–145.
Wang, P., Sun, F., Wang, D., Tao, J., Guan, X., & Bifet, A. (2017). Inferring demographics and social networks of mobile device users on campus from ap-trajectories. In Proceedings of the 26th international conference on world wide web companion (pp. 139–147).
Zaniewicz, L., & Jaroszewicz, S. (2013). Support vector machines for uplift modeling. In ICDMW ’13: Proceedings of the 2013 IEEE 13th international conference on data mining workshops, IEEE (pp. 131–138).
Zhong, Y., Yuan, N. J., Zhong, W., Zhang, F., & Xie, X. (2015). You are where you go: Inferring demographic attributes from location check-ins. In Proceedings of the tenth ACM international conference on web search and data mining (pp. 295–304).
Zhou, F., Yue, X., Trajcevski, G., Zhong, T., & Zhang, K. (2019). Context-aware variational trajectory encoding and human mobility inference. In The world wide web conference (pp. 3469–3475).
Download references
Author information
Authors and affiliations.
University of Southern California, Los Angeles, CA, USA
Akira Matsui
CyberAgent, Inc., Shibuya, Tokyo, Japan
Daisuke Moriwaki
You can also search for this author in PubMed Google Scholar
Corresponding author
Correspondence to Akira Matsui .
Additional information
Publisher's note.
Springer Nature remains neutral with regard to jurisdictional claims in published maps and institutional affiliations.
1.1 A.1 Balance check
While typical RCT experiments track the key attribute of the participants, such as genders or age, the ground truth of such attributes are not available in our experiments but only we can estimate them by our prediction model discussed in Sect. 2.4 . Therefore, to study if the treatment and control group are balanced, we study the odd ratio of the visit rate before they received the O2O ad, using the method used in Sect. 3.3 . The result shows that we are not able to reject the null hypothesis are not rejected (Direct: 0.0971 ( \(p= 0.240\) ); Mentel–Haensze: 1.08 ( \(p=0.304\) ), Fig. 9 ).
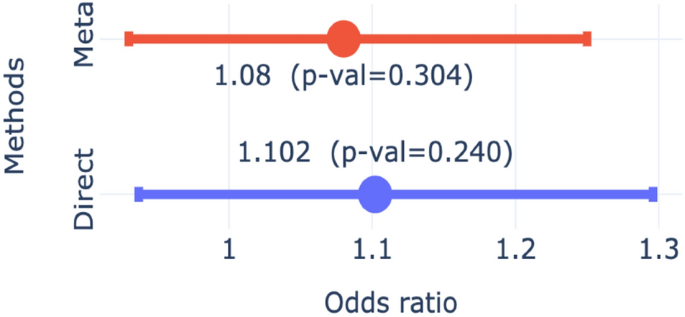
Odds ratio of visit before receiving the advertisement (before advertisement). Figure 9 compares the odds ratio of revisiting for the invited customer (treatment) group with the voluntary (control) group by the two different methods: Direct and Meta analysis. Direct : The odds ratio of the whole sample. Meta : The odds ratio by the Mantel–Haenszel method that controls the differences between the advertisements
1.2 A.2 Normalization
We set \(y_{i}\) as 1 when the customers in treatment group visit a block \(\left( u_{i}, v_{i}\right)\) more frequent than the people in the control group. To be fair, we standardize the number of visits taking considering the differences of the observed activities between Treatment and Control groups. We calculate the following value q for each user. \(q_{i}^{a}\) , for each group \(a \in \{Treatment, Control\}\) each block \(\left( u_{i}, v_{i}\right)\) .
where \(V_{i}^{a}\) is the set of customer j who are in group a . \(r_{j}\) is the value of visit of customer j calculated as
where \(n_j\) is the number of observation of the customer j movement within the area of interests; \(C^{a}\) is the set of customers in group a .
1.3 A.3 Robustness check
This subsection report a robustness check of the threshold for the “visited” label discussed in Sect. 2.3 (a customer stayed for more than 10 min within a 20 m radius). We change the threshold changing to study the threshold, where the differences between the two groups are not observed. The result does not change when we only change the radius (to 30 m radius). However, we found that the result changes when we increase the threshold for staying min. We report the results with “12.5 min within a 30 m radius”. The result shows similar results in the behavior on the shop visit day (RQ1: Table 4 and Figs. 10 , 11 , 12 ) and the travel distance differences (RQ2: Table 5 ), but we do not found that significant revisit rate differences (RQ3: Fig. 13 ). We also found that we do not find the significant result with “15 min within a 30 m radius”. While those results shows that the results become unclear when we increase the threshold, the threshold in this study is already strict as discussed in Sect. 2.3 . Therefore, we interpreted this result as an indication that the threshold used in the study was already severe.
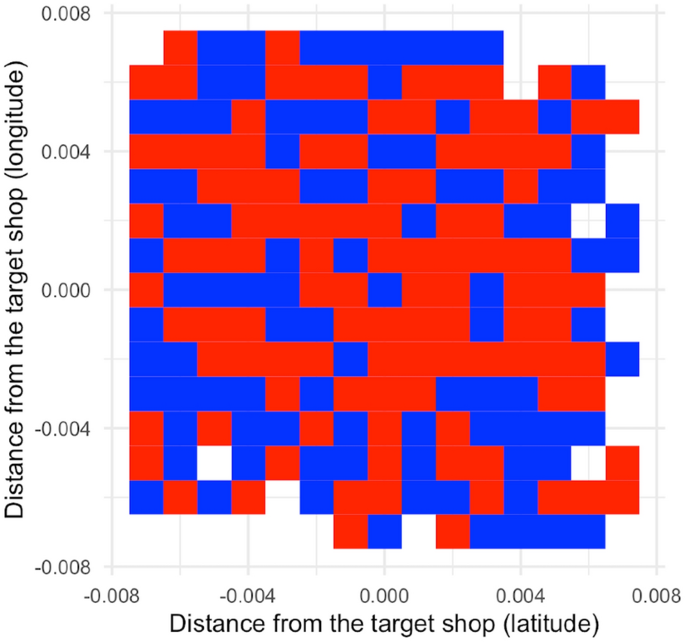
Dominant areas around the target shop. We align the target shop to the center of the plot and then split the area around the target shops by grids (the area of a square is about 111 m × 111 m). This figure shows the areas the invited customers (blue, treatment) group dominant and the voluntary customers (red, control) group dominant. We calculate which blocks are dominant following the procedure described in Sect. 3.1.3
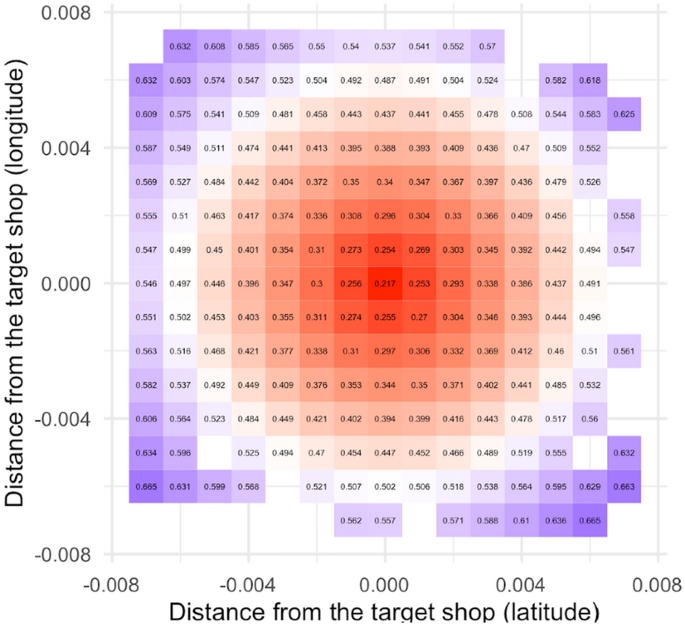
GWR prediction (simple model, Model 1: 30 m, 12.5 min threshold). We align the target shop to the center of the plot and then split the area around the target shops by grids (the area of a square is about 111 m × 111 m). This figure describes the prediction by the estimated GWR model (Model 1 in Table 4 ). The figure shows that the model predicts that the invited customers’ dominants the outer areas (Blue), whereas the voluntary visit customers condence around the target shop (Red)
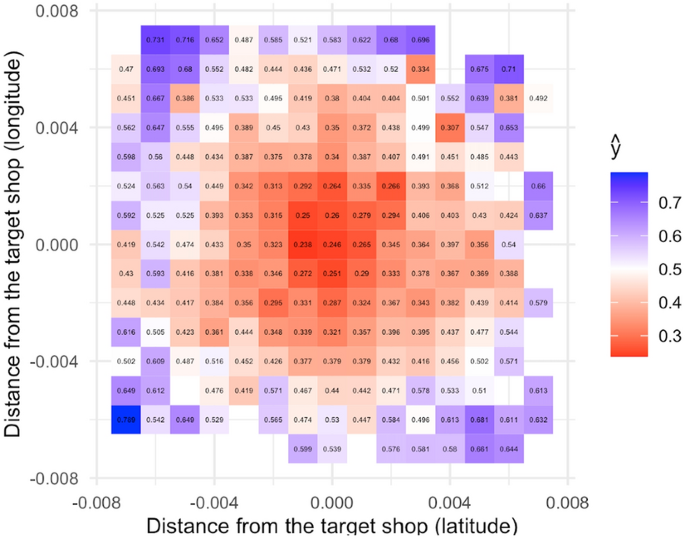
GWR prediction (multivariate model, Model 2: 30 m, 12.5 min threshold). We align the target shop to the center of the plot and then split the area around the target shops by grids (the area of a square is about 111 m × 111 m). This figure provides the prediction by the GWR model with multiple independent variables (Model 2 in Table 4 ). The second model also predicts the dominance of the outer areas by the treatment groups
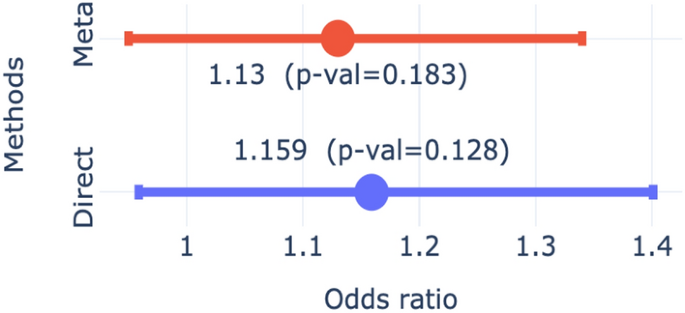
Odds ratio of revisiting (30 m, 12.5 min). Figure 13 compares the odds ratio of revisiting for the invited customer (treatment) group with the voluntary (control) group by the two different methods: Direct and Meta analysis. Direct : The odds ratio of the whole sample. Meta : The odds ratio by the Mantel–Haenszel method that controls the differences between the advertisements. ( \(n=2055\) )
1.4 A.4 The 10 features by the SHAP value for the uplift modeling
In this subsection, we will provide the details of the top 10 features by SHAP value (Lundberg & Lee, 2017 ). Table 6 reports the descriptions of the top 10 features in Fig. 7 . These features are the estimated demographic information and the distance from the user’s home to the target shops introduced in Sect. 2.4 . Except for “Distance from home to shop”, the all features the estimated probability that the user has that demographic information, such as being a student. We also present the mean values of the absolute SHAP values at Fig. 14
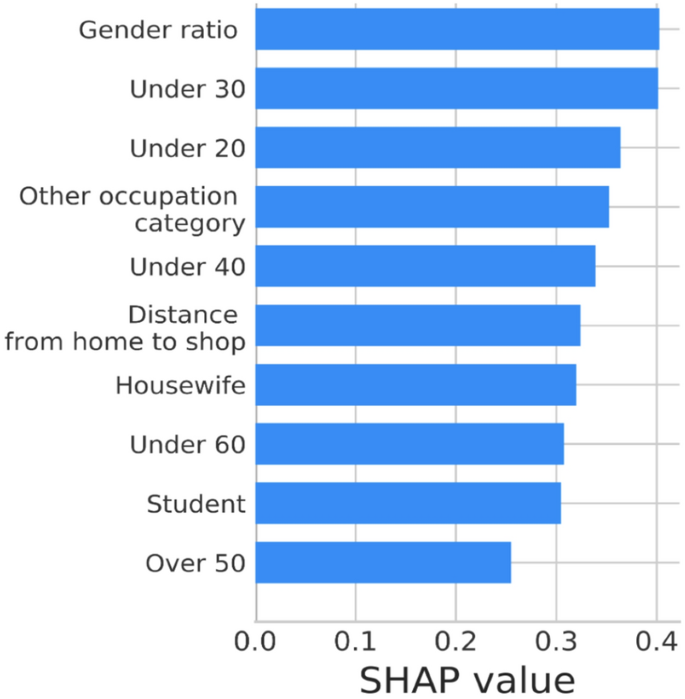
Top 10 mean absolute SHAP value features. The top 10 mean absolute SHAP value features (Lundberg & Lee, 2017 ) for the uplift model. The x axis represents the absolute mean SHAP value that stands for the importance of the features for the uplift model
Rights and permissions
Open Access This article is licensed under a Creative Commons Attribution 4.0 International License, which permits use, sharing, adaptation, distribution and reproduction in any medium or format, as long as you give appropriate credit to the original author(s) and the source, provide a link to the Creative Commons licence, and indicate if changes were made. The images or other third party material in this article are included in the article's Creative Commons licence, unless indicated otherwise in a credit line to the material. If material is not included in the article's Creative Commons licence and your intended use is not permitted by statutory regulation or exceeds the permitted use, you will need to obtain permission directly from the copyright holder. To view a copy of this licence, visit http://creativecommons.org/licenses/by/4.0/ .
Reprints and permissions
About this article
Matsui, A., Moriwaki, D. Online-to-offline advertisements as field experiments. JER 73 , 211–242 (2022). https://doi.org/10.1007/s42973-021-00101-y
Download citation
Received : 14 May 2021
Revised : 08 August 2021
Accepted : 27 September 2021
Published : 18 October 2021
Issue Date : January 2022
DOI : https://doi.org/10.1007/s42973-021-00101-y
Share this article
Anyone you share the following link with will be able to read this content:
Sorry, a shareable link is not currently available for this article.
Provided by the Springer Nature SharedIt content-sharing initiative
- Online advertisement
- Trajectory mining
- Online-to-offline advertisement
- Find a journal
- Publish with us
- Track your research
Market Research: A How-To Guide and Template
Discover the different types of market research, how to conduct your own market research, and use a free template to help you along the way.
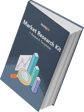
MARKET RESEARCH KIT
5 Research and Planning Templates + a Free Guide on How to Use Them in Your Market Research
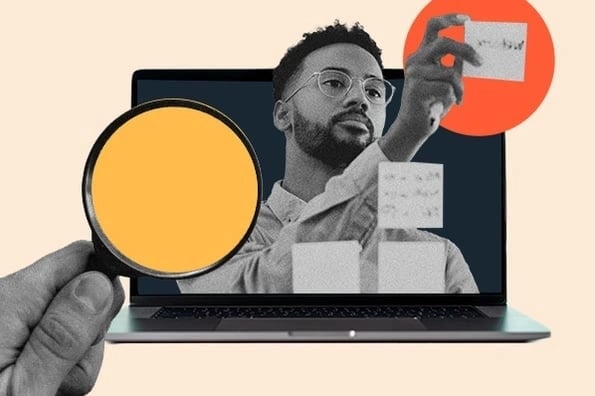
Updated: 02/21/24
Published: 02/21/24
Today's consumers have a lot of power. As a business, you must have a deep understanding of who your buyers are and what influences their purchase decisions.
Enter: Market Research.
![research project online advertising → Download Now: Market Research Templates [Free Kit]](https://no-cache.hubspot.com/cta/default/53/6ba52ce7-bb69-4b63-965b-4ea21ba905da.png)
Whether you're new to market research or not, I created this guide to help you conduct a thorough study of your market, target audience, competition, and more. Let’s dive in.
Table of Contents
What is market research?
Primary vs. secondary research, types of market research, how to do market research, market research report template, market research examples.
Market research is the process of gathering information about your target market and customers to verify the success of a new product, help your team iterate on an existing product, or understand brand perception to ensure your team is effectively communicating your company's value effectively.
Market research can answer various questions about the state of an industry. But if you ask me, it's hardly a crystal ball that marketers can rely on for insights on their customers.
Market researchers investigate several areas of the market, and it can take weeks or even months to paint an accurate picture of the business landscape.
However, researching just one of those areas can make you more intuitive to who your buyers are and how to deliver value that no other business is offering them right now.
How? Consider these two things:
- Your competitors also have experienced individuals in the industry and a customer base. It‘s very possible that your immediate resources are, in many ways, equal to those of your competition’s immediate resources. Seeking a larger sample size for answers can provide a better edge.
- Your customers don't represent the attitudes of an entire market. They represent the attitudes of the part of the market that is already drawn to your brand.
The market research services market is growing rapidly, which signifies a strong interest in market research as we enter 2024. The market is expected to grow from roughly $75 billion in 2021 to $90.79 billion in 2025 .
.png)
Free Market Research Kit
- SWOT Analysis Template
- Survey Template
- Focus Group Template
You're all set!
Click this link to access this resource at any time.
Why do market research?
Market research allows you to meet your buyer where they are.
As our world becomes louder and demands more of our attention, this proves invaluable.
By understanding your buyer's problems, pain points, and desired solutions, you can aptly craft your product or service to naturally appeal to them.
Market research also provides insight into the following:
- Where your target audience and current customers conduct their product or service research
- Which of your competitors your target audience looks to for information, options, or purchases
- What's trending in your industry and in the eyes of your buyer
- Who makes up your market and what their challenges are
- What influences purchases and conversions among your target audience
- Consumer attitudes about a particular topic, pain, product, or brand
- Whether there‘s demand for the business initiatives you’re investing in
- Unaddressed or underserved customer needs that can be flipped into selling opportunity
- Attitudes about pricing for a particular product or service
Ultimately, market research allows you to get information from a larger sample size of your target audience, eliminating bias and assumptions so that you can get to the heart of consumer attitudes.
As a result, you can make better business decisions.
To give you an idea of how extensive market research can get , consider that it can either be qualitative or quantitative in nature — depending on the studies you conduct and what you're trying to learn about your industry.
Qualitative research is concerned with public opinion, and explores how the market feels about the products currently available in that market.
Quantitative research is concerned with data, and looks for relevant trends in the information that's gathered from public records.
That said, there are two main types of market research that your business can conduct to collect actionable information on your products: primary research and secondary research.
Primary Research
Primary research is the pursuit of first-hand information about your market and the customers within your market.
It's useful when segmenting your market and establishing your buyer personas.
Primary market research tends to fall into one of two buckets:
- Exploratory Primary Research: This kind of primary market research normally takes place as a first step — before any specific research has been performed — and may involve open-ended interviews or surveys with small numbers of people.
- Specific Primary Research: This type of research often follows exploratory research. In specific research, you take a smaller or more precise segment of your audience and ask questions aimed at solving a suspected problem.
Secondary Research
Secondary research is all the data and public records you have at your disposal to draw conclusions from (e.g. trend reports, market statistics, industry content, and sales data you already have on your business).
Secondary research is particularly useful for analyzing your competitors . The main buckets your secondary market research will fall into include:
- Public Sources: These sources are your first and most-accessible layer of material when conducting secondary market research. They're often free to find and review — like government statistics (e.g., from the U.S. Census Bureau ).
- Commercial Sources: These sources often come in the form of pay-to-access market reports, consisting of industry insight compiled by a research agency like Pew , Gartner , or Forrester .
- Internal Sources: This is the market data your organization already has like average revenue per sale, customer retention rates, and other historical data that can help you draw conclusions on buyer needs.
- Focus Groups
- Product/ Service Use Research
- Observation-Based Research
- Buyer Persona Research
- Market Segmentation Research
- Pricing Research
- Competitive Analysis Research
- Customer Satisfaction and Loyalty Research
- Brand Awareness Research
- Campaign Research
1. Interviews
Interviews allow for face-to-face discussions so you can allow for a natural flow of conversation. Your interviewees can answer questions about themselves to help you design your buyer personas and shape your entire marketing strategy.
2. Focus Groups
Focus groups provide you with a handful of carefully-selected people that can test out your product and provide feedback. This type of market research can give you ideas for product differentiation.
3. Product/Service Use Research
Product or service use research offers insight into how and why your audience uses your product or service. This type of market research also gives you an idea of the product or service's usability for your target audience.
4. Observation-Based Research
Observation-based research allows you to sit back and watch the ways in which your target audience members go about using your product or service, what works well in terms of UX , and which aspects of it could be improved.
5. Buyer Persona Research
Buyer persona research gives you a realistic look at who makes up your target audience, what their challenges are, why they want your product or service, and what they need from your business or brand.
6. Market Segmentation Research
Market segmentation research allows you to categorize your target audience into different groups (or segments) based on specific and defining characteristics. This way, you can determine effective ways to meet their needs.
7. Pricing Research
Pricing research helps you define your pricing strategy . It gives you an idea of what similar products or services in your market sell for and what your target audience is willing to pay.
8. Competitive Analysis
Competitive analyses give you a deep understanding of the competition in your market and industry. You can learn about what's doing well in your industry and how you can separate yourself from the competition .
9. Customer Satisfaction and Loyalty Research
Customer satisfaction and loyalty research gives you a look into how you can get current customers to return for more business and what will motivate them to do so (e.g., loyalty programs , rewards, remarkable customer service).
10. Brand Awareness Research
Brand awareness research tells you what your target audience knows about and recognizes from your brand. It tells you about the associations people make when they think about your business.
11. Campaign Research
Campaign research entails looking into your past campaigns and analyzing their success among your target audience and current customers. The goal is to use these learnings to inform future campaigns.
- Define your buyer persona.
- Identify a persona group to engage.
- Prepare research questions for your market research participants.
- List your primary competitors.
- Summarize your findings.
1. Define your buyer persona.
You have to understand who your customers are and how customers in your industry make buying decisions.
This is where your buyer personas come in handy. Buyer personas — sometimes referred to as marketing personas — are fictional, generalized representations of your ideal customers.
Use a free tool to create a buyer persona that your entire company can use to market, sell, and serve better.
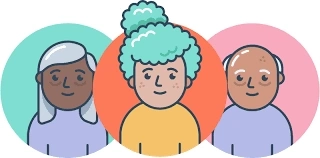
Don't forget to share this post!
Related articles.
![research project online advertising SWOT Analysis: How To Do One [With Template & Examples]](https://blog.hubspot.com/hubfs/marketingplan_20.webp)
SWOT Analysis: How To Do One [With Template & Examples]
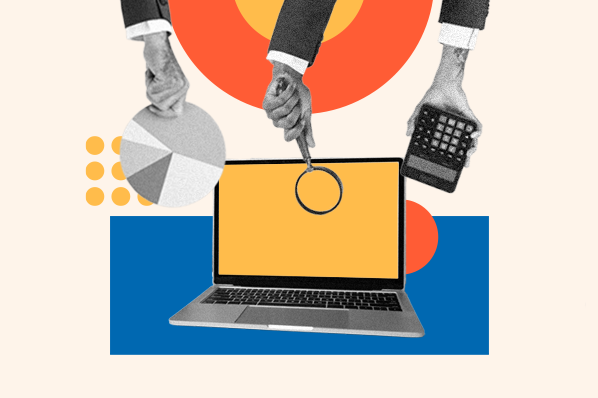
20+ Tools & Resources for Conducting Market Research
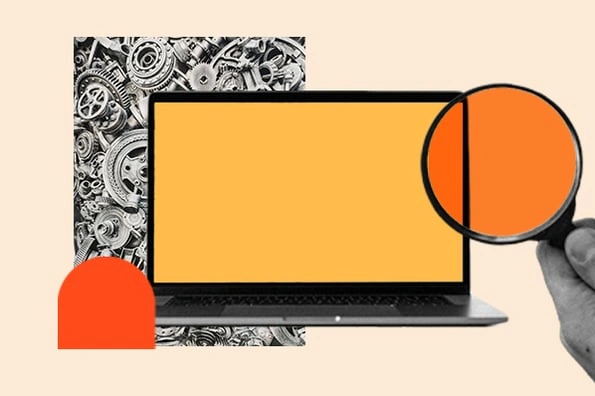
What's a Competitive Analysis & How Do You Conduct One?
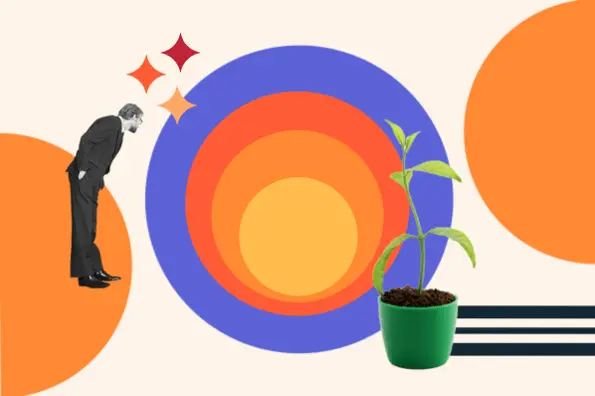
TAM SAM SOM: What Do They Mean & How Do You Calculate Them?
![research project online advertising How to Run a Competitor Analysis [Free Guide]](https://blog.hubspot.com/hubfs/Google%20Drive%20Integration/how%20to%20do%20a%20competitor%20analysis_122022.jpeg)
How to Run a Competitor Analysis [Free Guide]
![research project online advertising 5 Challenges Marketers Face in Understanding Audiences [New Data + Market Researcher Tips]](https://blog.hubspot.com/hubfs/challenges%20marketers%20face%20in%20understanding%20the%20customer%20.png)
5 Challenges Marketers Face in Understanding Audiences [New Data + Market Researcher Tips]
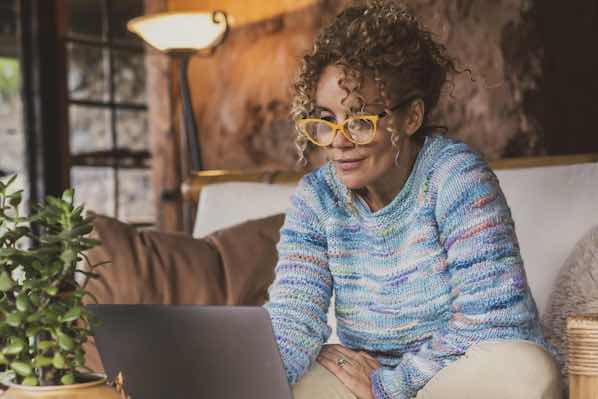
Causal Research: The Complete Guide
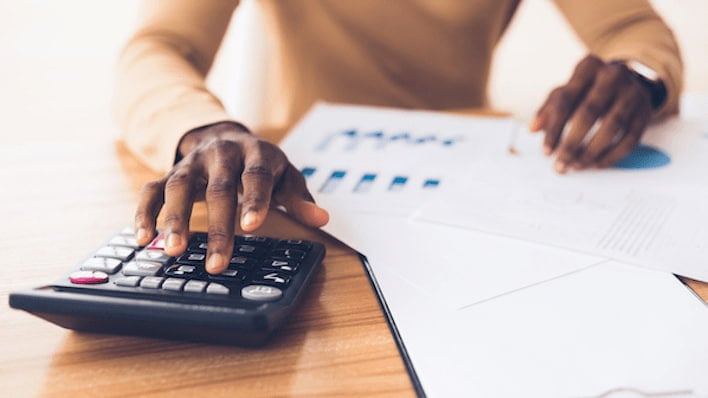
Total Addressable Market (TAM): What It Is & How You Can Calculate It
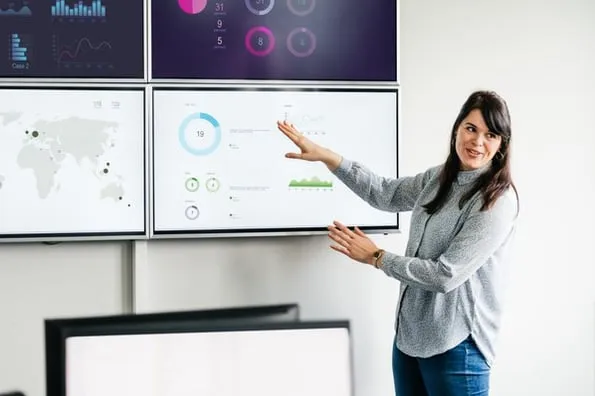
What Is Market Share & How Do You Calculate It?
![research project online advertising 3 Ways Data Privacy Changes Benefit Marketers [New Data]](https://blog.hubspot.com/hubfs/how-data-privacy-benefits-marketers_1.webp)
3 Ways Data Privacy Changes Benefit Marketers [New Data]
Free Guide & Templates to Help Your Market Research
Marketing software that helps you drive revenue, save time and resources, and measure and optimize your investments — all on one easy-to-use platform

Research Topics & Ideas: Marketing
50+ Marketing Research Topic Ideas To Fast-Track Your Project
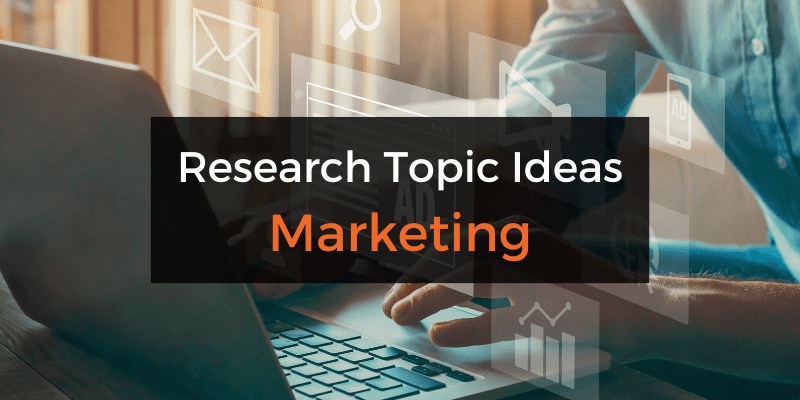
Finding and choosing a strong research topic is the critical first step when it comes to crafting a high-quality dissertation, thesis or research project. If you’ve landed on this post, chances are you’re looking for a marketing-related research topic , but aren’t sure where to start. If so, you’ve come to the right place.
Here, we’ll explore a variety of marketing-related research ideas and topic thought-starters, covering both traditional marketing (offline media) and digital marketing (including social media marketing, content marketing and the like). We’ll also look at actual dissertations and theses from marketing students to give you a view of what a well-defined research topic looks like.
NB – This is just the start…
The topic ideation and evaluation process has multiple steps . In this post, we’ll kickstart the process by sharing some research topic ideas within the marketing domain. This is the starting point, but to develop a well-defined research topic, you’ll need to identify a clear and convincing research gap , along with a well-justified plan of action to fill that gap.
If you’re new to the oftentimes perplexing world of research, or if this is your first time undertaking a formal academic research project, be sure to check out our free dissertation mini-course. In it, we cover the process of writing a dissertation or thesis from start to end. Be sure to also sign up for our free webinar that explores how to find a high-quality research topic.
Overview: Marketing-Related Topics
- How to find a research topic (video)
- Traditional (offline) marketing topics/ideas
- Digital (online) marketing topics/ideas
- Examples of actual dissertation topics
- Free Webinar : Topic Ideation 101
- Where to get extra help
How To Find A Research Topic
Before we look at specific research ideas and topics, it’s useful to first understand what the topic ideation process entails and how to go about finding and refining viable topic ideas. In the video below, we cover exactly that. So, if you’re a first-time researcher, be sure to watch this tutorial before jumping into the collection of marketing research topics we present below.
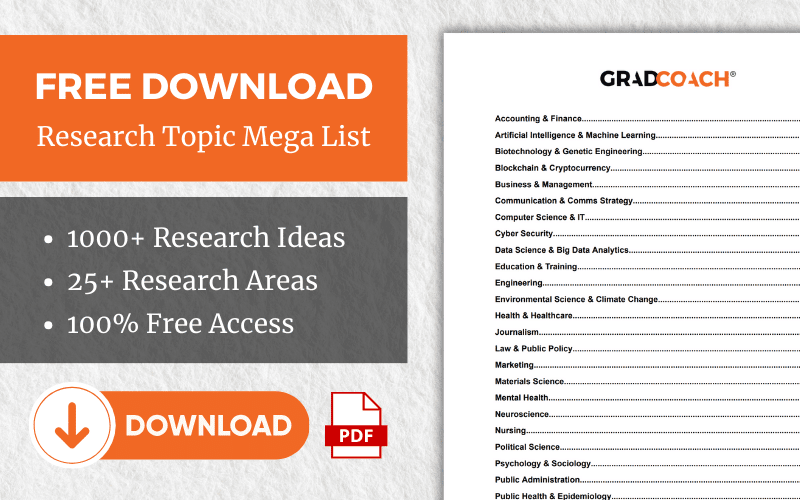
Now that you’ve got a big-picture view of the topic ideation process, we can dive into a few research ideas to get your creative juices flowing. Please note that these research topic ideas are intentionally broad and generic, so you will need to refine them a fair deal using the techniques we discussed in the video above.
To make life a little easier for you, we’ve grouped the topic ideas based on two main categories – traditional marketing and digital / online marketing . For the latter, we’ve divided it up into a few subcategories, including social media marketing, content marketing and search marketing. That said, there is naturally some overlap between topics, so keep this in mind.
Research Ideas: Traditional Marketing
- The perceived effectiveness of different types of direct mail campaigns (e.g., postcards, catalogues, brochures) among small business owners in New York
- The perception and use of traditional marketing in creating and nurturing customer loyalty and retention among UK Financial Firms.
- The impact of demographic targeting on traditional marketing performance among green businesses
- The perception of different types of sales promotion (e.g., discounts, coupons, contests) among GenZ consumers
- The continued use of celebrity endorsements in traditional marketing campaigns, post-2020.
- Exploring factors related to outdoor advertising recall in India
- Measuring the ROI of traditional marketing efforts: a review of current methods
- Comparing the effectiveness of different traditional media channels (e.g., television, radio, print) in driving engagement and conversions among established brands
- The use of public relations in traditional marketing campaigns among large insurance companies
- The role of event marketing in building brand awareness and engagement: perceptions among marketing professionals in the UK
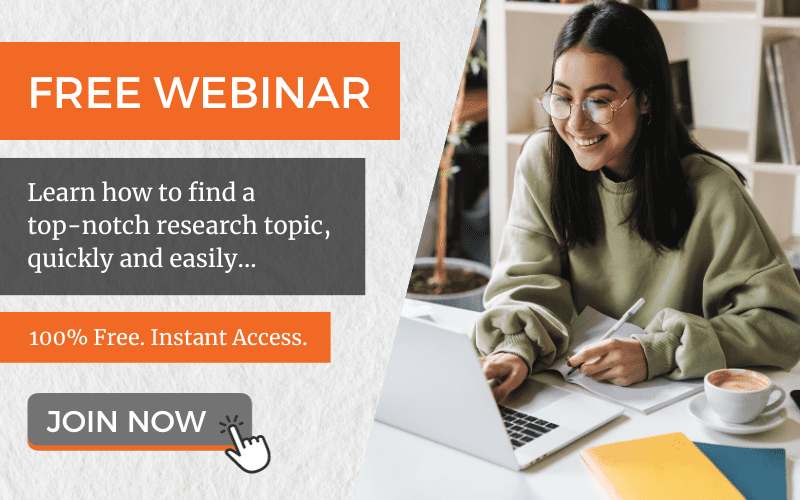
Research Ideas: Digital/Online Marketing
Social media marketing
- The use of artificial intelligence in social media marketing among online fashion companies in Sweden
- The impact of social media on public relations and media relations: a Case Study of Nandos
- The role of Twitter in crisis communication and reputation management: a discourse analysis
- The impact of social media influencer presence on brand loyalty and customer retention among Gen X.
- The use of social media for market research and consumer insights among SMEs in Kenya
- The impact of social media on consumer buying behaviour of fast food in Italy
- The challenges and opportunities of social media in global and multicultural marketing: perceptions of marketing departments in large corporations
- The relationship between social media engagement and website traffic: an analysis of fitness brands
- The effectiveness of influencer marketing on social media platforms for household cleaning products in the UK
- The perceptions and understanding of paid vs organic social media advertising among Gen Z consumers in San Francisco
Content marketing
- The use and understanding of micro-moments in creating personalized content experiences among content creators in the USA
- Comparing the effectiveness of methods of personalization of content marketing: an evaluation of best practice
- The experiences and use of SEO in content marketing strategy among small online businesses
- The use of social media influencers in content marketing campaigns: a Case Study of Hello Fresh
- Comparing different content distribution channels for different types of content: perceptions of best practices among content marketing experts
- The use of user-generated content in building brand trust and engagement among green companies in the UK
- Measuring the ROI of content marketing efforts among luxury brands
- The perception and use of Chat GPT in content creation among small online businesses
- The perceptions of interactive and immersive content formats (e.g., virtual reality, augmented reality) in marketing among consumers: an experimental study
- Comparing image and text-based content formats in driving engagement and conversions on social media: a multi-case study of NGOs
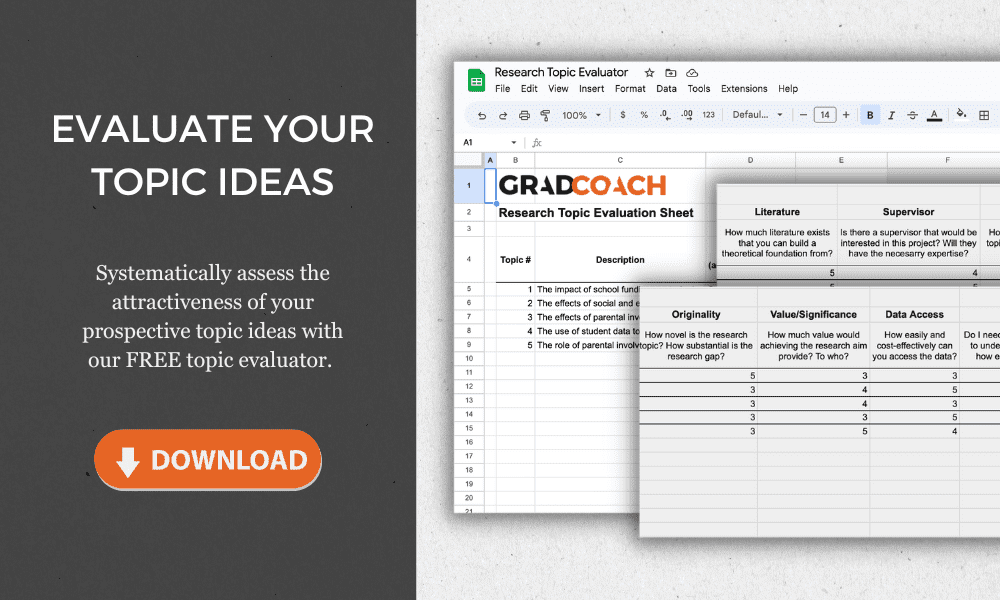
Search engine marketing
- The effectiveness of different ad formats (e.g., text ads, shopping ads, video ads) in Search Engine Marketing for tourist destinations
- The impact of search engine algorithm updates on Search Engine Marketing performance – who are the winners and losers?
- The perception and use of AI and machine learning in Search Engine Marketing strategy and bidding optimization within a UK marketing agency
- The use of voice search and its impact on Search Engine Marketing among food retailers in Switzerland
- The role of local SEO in driving brick-and-mortar sales: a case study of a local fashion outlet in France
- The impact of mobile optimization on Search Engine Marketing performance and conversion rates in Nigeria
- Comparing analytical tools for measuring ROI of Search Engine Marketing campaigns
- The effectiveness of different types of keywords and search queries in driving traffic towards restaurants in Italy
- The use of remarketing and retargeting in search engine marketing campaigns among marketers
- The understanding and use of schema markup and structured data in improving Search engine marketing performance among Start-Ups
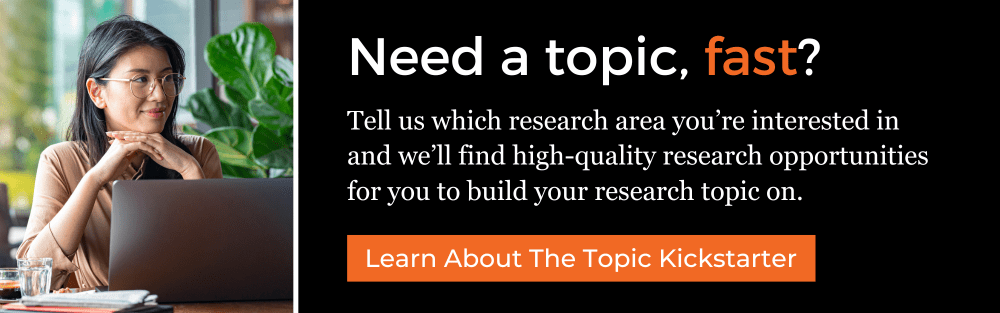
Email marketing
- The perception and use of AI and machine learning in email marketing among NGOs: a focus on personalized content and dynamic segmentation
- The effectiveness of email subject line strategies in driving open and click-through rates: an experimental study
- Comparing A/B testing and multivariate testing in improving email marketing performance: a case study
- The use of personalization on email marketing performance among craft bloggers
- The impact of automated email marketing among local online retailers on customer loyalty and retention
- The use of automation and triggered emails in increasing engagement and conversion rates for online courses
- The perception and understanding of GDPR and other data privacy regulations on email marketing among SMEs
- The role of mobile optimization in email marketing targeting Gen Z consumers
- The challenges of measuring the ROI of email marketing campaigns: perceptions of SMEs
- The effectiveness of different email formats (e.g., text-based, HTML, interactive) in driving engagement: A/B testing for a small online retailer
Marketing-Related Dissertations & Theses
While the ideas we’ve presented above are a decent starting point for finding marketing-related research topic, they are fairly generic and non-specific. So, it helps to look at actual dissertations and theses to see how this all comes together.
Below, we’ve included a selection of research projects from various marketing-related degree programs to help refine your thinking. These are actual dissertations and theses, written as part of Master’s and PhD-level programs, so they can provide some useful insight as to what a research topic looks like in practice.
- The Influences of Brand Personality, Culture, and Social Media on Iconic Brand Preferences (Whelan, 2021)
- Customer Success and the Transformation of Customer Relationships (Raines, 2022)
- The Effects of Online Incentivized Reviews on Organic Review Ratings (Jeong, 2020)
- Are You For Real? The Consumption-Driven Self-Authentication Process And Its Effects On Perceived Brand Authenticity (Kuchmaner, 2020)
- You Are What You Eat: How Food Texture And Packaging Influence Consumer Well-Being (Ning, 2020)
- Social Dialogic Listening: Connecting Marketing Activity To Strategy (Collins, 2020)
- Millennials’ Attitudes Towards Influencer Marketing And Purchase Intentions (Yu, 2019)
- Cannibalization between Two Mercedes-Benz Models and Consumer Behavior (Ma, 2020)
- Selling and Buying Aspects of Used Products That Are Brand Anthropomorphized (Kim, 2019)
- Global Identity: Conceptualization, Measurement, And Implications For Marketing Strategy (Yoruk, 2022)
- The Intersection of Organizational Frontline Marketing and a High-Tech World (Krotz, 2021)
- The Unexplored Impacts of Communication Elements in Marketing (Trinh, 2022)
- Founder Social Identity As A Predictor Of Customer And Competitor Orientation In Small And Medium-Sized Enterprises (Rutherford, 2021)
Looking at these titles, you can probably pick up that the research topics here are far more specific and narrowly-focused , compared to the generic ones presented earlier. This is an important thing to keep in mind as you develop your own research topic. That is to say, to create a top-notch research topic, you must be precise and target a specific context with specific variables of interest . In other words, you need to identify a clear, well-justified research gap.
Fast-Track Your Research Topic
If you’re still feeling a bit unsure about how to find a research topic for your marketing dissertation or thesis, check out our 1-on-1 private coaching services below.
You Might Also Like:
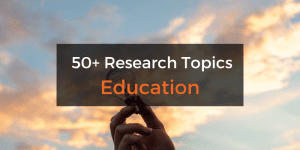
Submit a Comment Cancel reply
Your email address will not be published. Required fields are marked *
Save my name, email, and website in this browser for the next time I comment.
- Print Friendly
Learn / Blog / Article
Back to blog
How to do market research in 4 steps: a lean approach to marketing research
From pinpointing your target audience and assessing your competitive advantage, to ongoing product development and customer satisfaction efforts, market research is a practice your business can only benefit from.
Learn how to conduct quick and effective market research using a lean approach in this article full of strategies and practical examples.
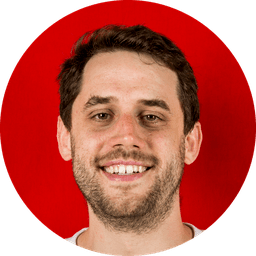
Last updated
Reading time.
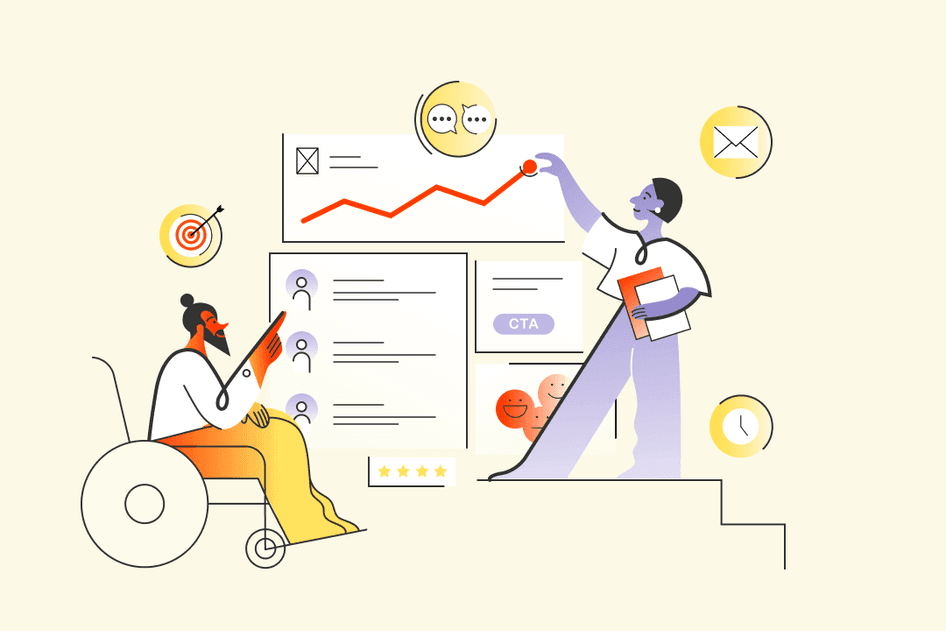
A comprehensive (and successful) business strategy is not complete without some form of market research—you can’t make informed and profitable business decisions without truly understanding your customer base and the current market trends that drive your business.
In this article, you’ll learn how to conduct quick, effective market research using an approach called 'lean market research'. It’s easier than you might think, and it can be done at any stage in a product’s lifecycle.
How to conduct lean market research in 4 steps
What is market research, why is market research so valuable, advantages of lean market research, 4 common market research methods, 5 common market research questions, market research faqs.
We’ll jump right into our 4-step approach to lean market research. To show you how it’s done in the real world, each step includes a practical example from Smallpdf , a Swiss company that used lean market research to reduce their tool’s error rate by 75% and boost their Net Promoter Score® (NPS) by 1%.
Research your market the lean way...
From on-page surveys to user interviews, Hotjar has the tools to help you scope out your market and get to know your customers—without breaking the bank.
The following four steps and practical examples will give you a solid market research plan for understanding who your users are and what they want from a company like yours.
1. Create simple user personas
A user persona is a semi-fictional character based on psychographic and demographic data from people who use websites and products similar to your own. Start by defining broad user categories, then elaborate on them later to further segment your customer base and determine your ideal customer profile .
How to get the data: use on-page or emailed surveys and interviews to understand your users and what drives them to your business.
How to do it right: whatever survey or interview questions you ask, they should answer the following questions about the customer:
Who are they?
What is their main goal?
What is their main barrier to achieving this goal?
Pitfalls to avoid:
Don’t ask too many questions! Keep it to five or less, otherwise you’ll inundate them and they’ll stop answering thoughtfully.
Don’t worry too much about typical demographic questions like age or background. Instead, focus on the role these people play (as it relates to your product) and their goals.
How Smallpdf did it: Smallpdf ran an on-page survey for a couple of weeks and received 1,000 replies. They learned that many of their users were administrative assistants, students, and teachers.
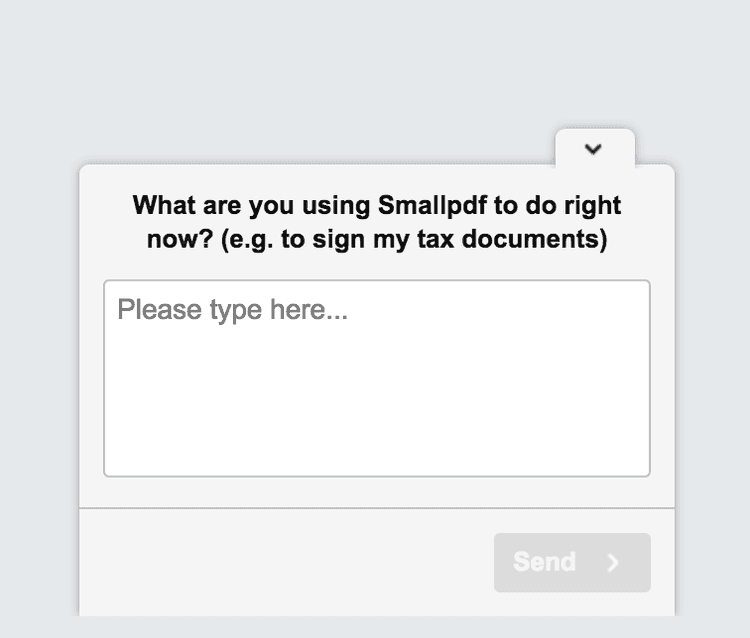
Next, they used the survey results to create simple user personas like this one for admins:
Who are they? Administrative Assistants.
What is their main goal? Creating Word documents from a scanned, hard-copy document or a PDF where the source file was lost.
What is their main barrier to achieving it? Converting a scanned PDF doc to a Word file.
💡Pro tip: Smallpdf used Hotjar Surveys to run their user persona survey. Our survey tool helped them avoid the pitfalls of guesswork and find out who their users really are, in their own words.
You can design a survey and start running it in minutes with our easy-to-use drag and drop builder. Customize your survey to fit your needs, from a sleek one-question pop-up survey to a fully branded questionnaire sent via email.
We've also created 40+ free survey templates that you can start collecting data with, including a user persona survey like the one Smallpdf used.
2. Conduct observational research
Observational research involves taking notes while watching someone use your product (or a similar product).
Overt vs. covert observation
Overt observation involves asking customers if they’ll let you watch them use your product. This method is often used for user testing and it provides a great opportunity for collecting live product or customer feedback .
Covert observation means studying users ‘in the wild’ without them knowing. This method works well if you sell a type of product that people use regularly, and it offers the purest observational data because people often behave differently when they know they’re being watched.
Tips to do it right:
Record an entry in your field notes, along with a timestamp, each time an action or event occurs.
Make note of the users' workflow, capturing the ‘what,’ ‘why,’ and ‘for whom’ of each action.
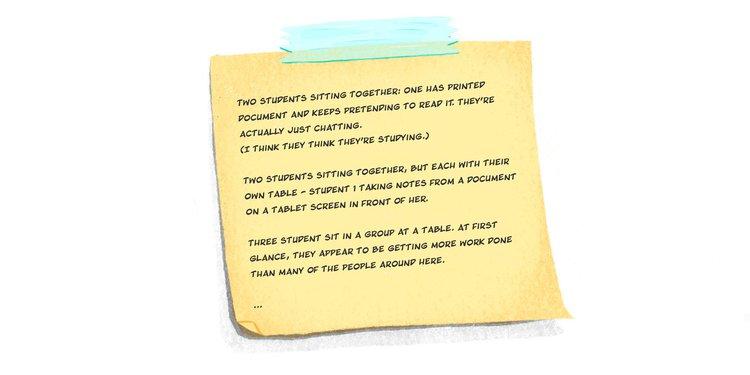
Don’t record identifiable video or audio data without consent. If recording people using your product is helpful for achieving your research goal, make sure all participants are informed and agree to the terms.
Don’t forget to explain why you’d like to observe them (for overt observation). People are more likely to cooperate if you tell them you want to improve the product.
💡Pro tip: while conducting field research out in the wild can wield rewarding results, you can also conduct observational research remotely. Hotjar Recordings is a tool that lets you capture anonymized user sessions of real people interacting with your website.
Observe how customers navigate your pages and products to gain an inside look into their user behavior . This method is great for conducting exploratory research with the purpose of identifying more specific issues to investigate further, like pain points along the customer journey and opportunities for optimizing conversion .
With Hotjar Recordings you can observe real people using your site without capturing their sensitive information
How Smallpdf did it: here’s how Smallpdf observed two different user personas both covertly and overtly.
Observing students (covert): Kristina Wagner, Principle Product Manager at Smallpdf, went to cafes and libraries at two local universities and waited until she saw students doing PDF-related activities. Then she watched and took notes from a distance. One thing that struck her was the difference between how students self-reported their activities vs. how they behaved (i.e, the self-reporting bias). Students, she found, spent hours talking, listening to music, or simply staring at a blank screen rather than working. When she did find students who were working, she recorded the task they were performing and the software they were using (if she recognized it).
Observing administrative assistants (overt): Kristina sent emails to admins explaining that she’d like to observe them at work, and she asked those who agreed to try to batch their PDF work for her observation day. While watching admins work, she learned that they frequently needed to scan documents into PDF-format and then convert those PDFs into Word docs. By observing the challenges admins faced, Smallpdf knew which products to target for improvement.
“Data is really good for discovery and validation, but there is a bit in the middle where you have to go and find the human.”
3. Conduct individual interviews
Interviews are one-on-one conversations with members of your target market. They allow you to dig deep and explore their concerns, which can lead to all sorts of revelations.
Listen more, talk less. Be curious.
Act like a journalist, not a salesperson. Rather than trying to talk your company up, ask people about their lives, their needs, their frustrations, and how a product like yours could help.
Ask "why?" so you can dig deeper. Get into the specifics and learn about their past behavior.
Record the conversation. Focus on the conversation and avoid relying solely on notes by recording the interview. There are plenty of services that will transcribe recorded conversations for a good price (including Hotjar!).
Avoid asking leading questions , which reveal bias on your part and pushes respondents to answer in a certain direction (e.g. “Have you taken advantage of the amazing new features we just released?).
Don't ask loaded questions , which sneak in an assumption which, if untrue, would make it impossible to answer honestly. For example, we can’t ask you, “What did you find most useful about this article?” without asking whether you found the article useful in the first place.
Be cautious when asking opinions about the future (or predictions of future behavior). Studies suggest that people aren’t very good at predicting their future behavior. This is due to several cognitive biases, from the misguided exceptionalism bias (we’re good at guessing what others will do, but we somehow think we’re different), to the optimism bias (which makes us see things with rose-colored glasses), to the ‘illusion of control’ (which makes us forget the role of randomness in future events).
How Smallpdf did it: Kristina explored her teacher user persona by speaking with university professors at a local graduate school. She learned that the school was mostly paperless and rarely used PDFs, so for the sake of time, she moved on to the admins.
A bit of a letdown? Sure. But this story highlights an important lesson: sometimes you follow a lead and come up short, so you have to make adjustments on the fly. Lean market research is about getting solid, actionable insights quickly so you can tweak things and see what works.
💡Pro tip: to save even more time, conduct remote interviews using an online user research service like Hotjar Engage , which automates the entire interview process, from recruitment and scheduling to hosting and recording.
You can interview your own customers or connect with people from our diverse pool of 200,000+ participants from 130+ countries and 25 industries. And no need to fret about taking meticulous notes—Engage will automatically transcribe the interview for you.
4. Analyze the data (without drowning in it)
The following techniques will help you wrap your head around the market data you collect without losing yourself in it. Remember, the point of lean market research is to find quick, actionable insights.
A flow model is a diagram that tracks the flow of information within a system. By creating a simple visual representation of how users interact with your product and each other, you can better assess their needs.
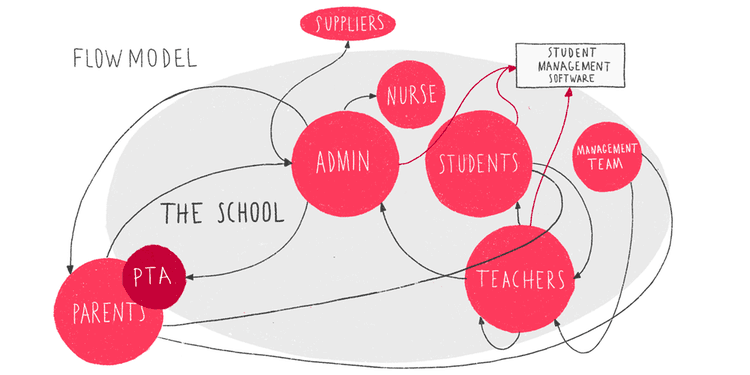
You’ll notice that admins are at the center of Smallpdf’s flow model, which represents the flow of PDF-related documents throughout a school. This flow model shows the challenges that admins face as they work to satisfy their own internal and external customers.
Affinity diagram
An affinity diagram is a way of sorting large amounts of data into groups to better understand the big picture. For example, if you ask your users about their profession, you’ll notice some general themes start to form, even though the individual responses differ. Depending on your needs, you could group them by profession, or more generally by industry.
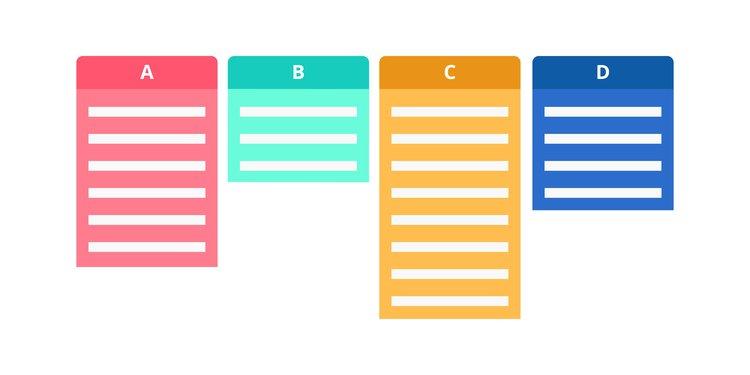
We wrote a guide about how to analyze open-ended questions to help you sort through and categorize large volumes of response data. You can also do this by hand by clipping up survey responses or interview notes and grouping them (which is what Kristina does).
“For an interview, you will have somewhere between 30 and 60 notes, and those notes are usually direct phrases. And when you literally cut them up into separate pieces of paper and group them, they should make sense by themselves.”
Pro tip: if you’re conducting an online survey with Hotjar, keep your team in the loop by sharing survey responses automatically via our Slack and Microsoft Team integrations. Reading answers as they come in lets you digest the data in pieces and can help prepare you for identifying common themes when it comes time for analysis.
Hotjar lets you easily share survey responses with your team
Customer journey map
A customer journey map is a diagram that shows the way a typical prospect becomes a paying customer. It outlines their first interaction with your brand and every step in the sales cycle, from awareness to repurchase (and hopefully advocacy).
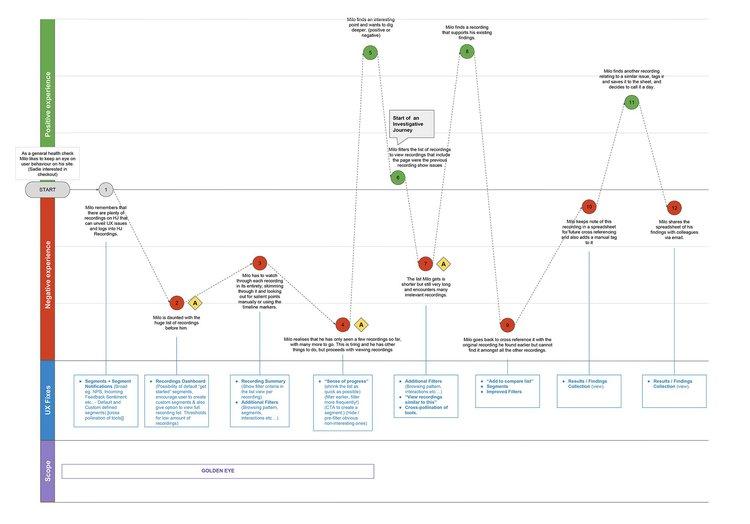
The above customer journey map , created by our team at Hotjar, shows many ways a customer might engage with our tool. Your map will be based on your own data and business model.
📚 Read more: if you’re new to customer journey maps, we wrote this step-by-step guide to creating your first customer journey map in 2 and 1/2 days with free templates you can download and start using immediately.
Next steps: from research to results
So, how do you turn market research insights into tangible business results? Let’s look at the actions Smallpdf took after conducting their lean market research: first they implemented changes, then measured the impact.
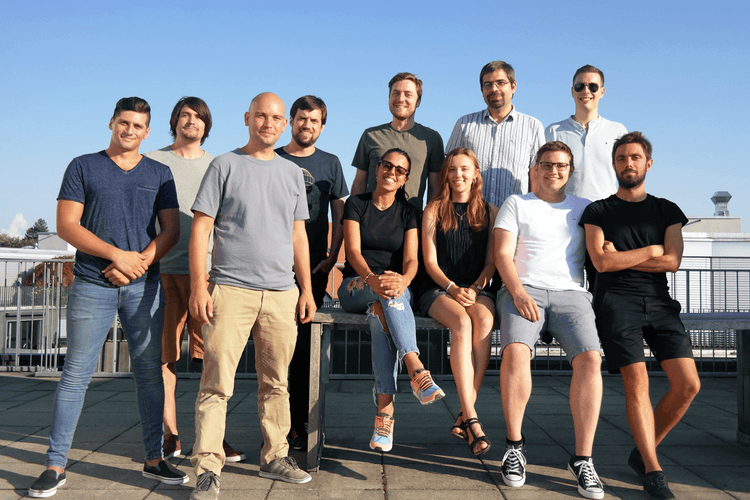
Implement changes
Based on what Smallpdf learned about the challenges that one key user segment (admins) face when trying to convert PDFs into Word files, they improved their ‘PDF to Word’ conversion tool.
We won’t go into the details here because it involves a lot of technical jargon, but they made the entire process simpler and more straightforward for users. Plus, they made it so that their system recognized when you drop a PDF file into their ‘Word to PDF’ converter instead of the ‘PDF to Word’ converter, so users wouldn’t have to redo the task when they made that mistake.
In other words: simple market segmentation for admins showed a business need that had to be accounted for, and customers are happier overall after Smallpdf implemented an informed change to their product.
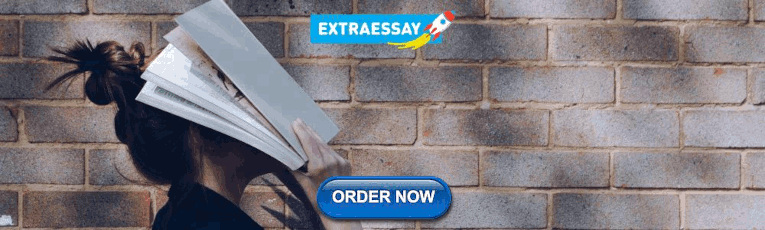
Measure results
According to the Lean UX model, product and UX changes aren’t retained unless they achieve results.
Smallpdf’s changes produced:
A 75% reduction in error rate for the ‘PDF to Word’ converter
A 1% increase in NPS
Greater confidence in the team’s marketing efforts
"With all the changes said and done, we've cut our original error rate in four, which is huge. We increased our NPS by +1%, which isn't huge, but it means that of the users who received a file, they were still slightly happier than before, even if they didn't notice that anything special happened at all.”
Subscribe to fresh and free monthly insights.
Over 50,000 people interested in UX, product, digital empathy, and beyond, receive our newsletter every month. No spam, just thoughtful perspectives from a range of experts, new approaches to remote work, and loads more valuable insights. If that floats your boat, why not become a subscriber?
I have read and accepted the message outlined here: Hotjar uses the information you provide to us to send you relevant content, updates and offers from time to time. You can unsubscribe at any time by clicking the link at the bottom of any email.
Market research (or marketing research) is any set of techniques used to gather information and better understand a company’s target market. This might include primary research on brand awareness and customer satisfaction or secondary market research on market size and competitive analysis. Businesses use this information to design better products, improve user experience, and craft a marketing strategy that attracts quality leads and improves conversion rates.
David Darmanin, one of Hotjar’s founders, launched two startups before Hotjar took off—but both companies crashed and burned. Each time, he and his team spent months trying to design an amazing new product and user experience, but they failed because they didn’t have a clear understanding of what the market demanded.
With Hotjar, they did things differently . Long story short, they conducted market research in the early stages to figure out what consumers really wanted, and the team made (and continues to make) constant improvements based on market and user research.
Without market research, it’s impossible to understand your users. Sure, you might have a general idea of who they are and what they need, but you have to dig deep if you want to win their loyalty.
Here’s why research matters:
Obsessing over your users is the only way to win. If you don’t care deeply about them, you’ll lose potential customers to someone who does.
Analytics gives you the ‘what’, while research gives you the ‘why’. Big data, user analytics , and dashboards can tell you what people do at scale, but only research can tell you what they’re thinking and why they do what they do. For example, analytics can tell you that customers leave when they reach your pricing page, but only research can explain why.
Research beats assumptions, trends, and so-called best practices. Have you ever watched your colleagues rally behind a terrible decision? Bad ideas are often the result of guesswork, emotional reasoning, death by best practices , and defaulting to the Highest Paid Person’s Opinion (HiPPO). By listening to your users and focusing on their customer experience , you’re less likely to get pulled in the wrong direction.
Research keeps you from planning in a vacuum. Your team might be amazing, but you and your colleagues simply can’t experience your product the way your customers do. Customers might use your product in a way that surprises you, and product features that seem obvious to you might confuse them. Over-planning and refusing to test your assumptions is a waste of time, money, and effort because you’ll likely need to make changes once your untested business plan gets put into practice.
Lean User Experience (UX) design is a model for continuous improvement that relies on quick, efficient research to understand customer needs and test new product features.
Lean market research can help you become more...
Efficient: it gets you closer to your customers, faster.
Cost-effective: no need to hire an expensive marketing firm to get things started.
Competitive: quick, powerful insights can place your products on the cutting edge.
As a small business or sole proprietor, conducting lean market research is an attractive option when investing in a full-blown research project might seem out of scope or budget.
There are lots of different ways you could conduct market research and collect customer data, but you don’t have to limit yourself to just one research method. Four common types of market research techniques include surveys, interviews, focus groups, and customer observation.
Which method you use may vary based on your business type: ecommerce business owners have different goals from SaaS businesses, so it’s typically prudent to mix and match these methods based on your particular goals and what you need to know.
1. Surveys: the most commonly used
Surveys are a form of qualitative research that ask respondents a short series of open- or closed-ended questions, which can be delivered as an on-screen questionnaire or via email. When we asked 2,000 Customer Experience (CX) professionals about their company’s approach to research , surveys proved to be the most commonly used market research technique.
What makes online surveys so popular?
They’re easy and inexpensive to conduct, and you can do a lot of data collection quickly. Plus, the data is pretty straightforward to analyze, even when you have to analyze open-ended questions whose answers might initially appear difficult to categorize.
We've built a number of survey templates ready and waiting for you. Grab a template and share with your customers in just a few clicks.
💡 Pro tip: you can also get started with Hotjar AI for Surveys to create a survey in mere seconds . Just enter your market research goal and watch as the AI generates a survey and populates it with relevant questions.
Once you’re ready for data analysis, the AI will prepare an automated research report that succinctly summarizes key findings, quotes, and suggested next steps.
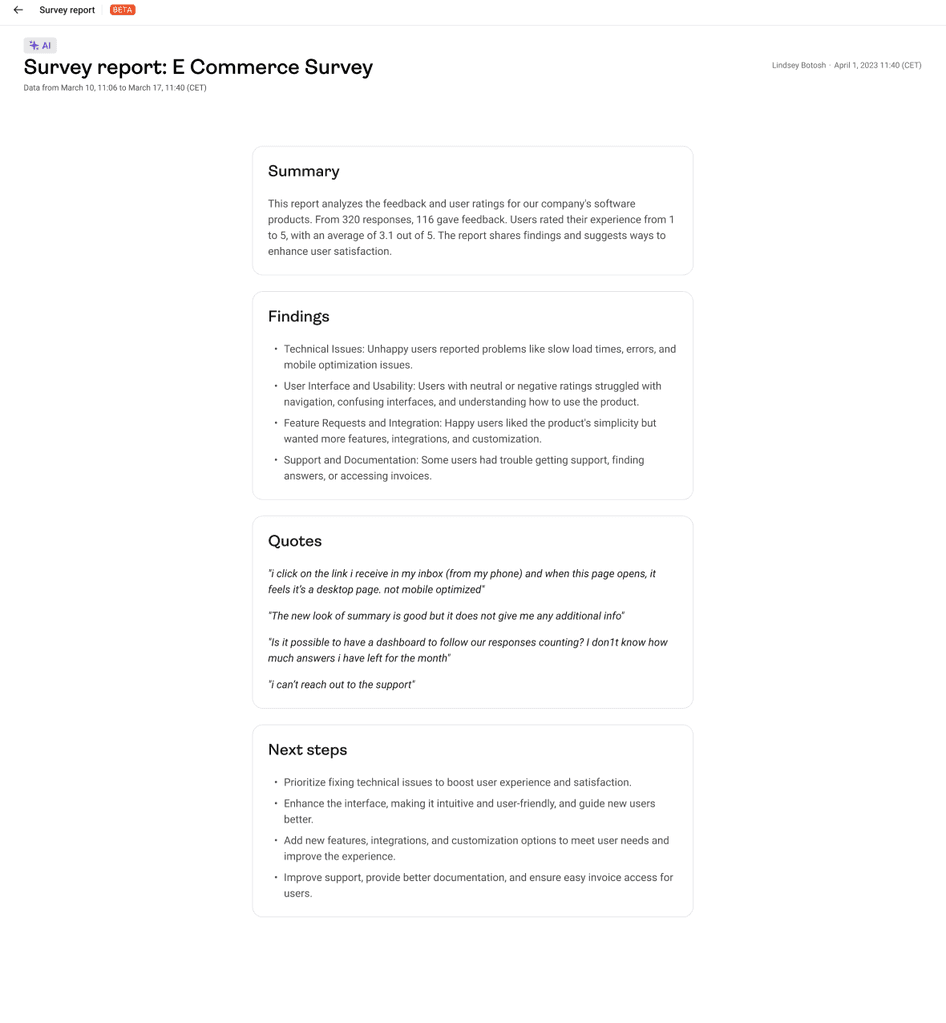
An example research report generated by Hotjar AI for Surveys
2. Interviews: the most insightful
Interviews are one-on-one conversations with members of your target market. Nothing beats a face-to-face interview for diving deep (and reading non-verbal cues), but if an in-person meeting isn’t possible, video conferencing is a solid second choice.
Regardless of how you conduct it, any type of in-depth interview will produce big benefits in understanding your target customers.
What makes interviews so insightful?
By speaking directly with an ideal customer, you’ll gain greater empathy for their experience , and you can follow insightful threads that can produce plenty of 'Aha!' moments.
3. Focus groups: the most unreliable
Focus groups bring together a carefully selected group of people who fit a company’s target market. A trained moderator leads a conversation surrounding the product, user experience, or marketing message to gain deeper insights.
What makes focus groups so unreliable?
If you’re new to market research, we wouldn’t recommend starting with focus groups. Doing it right is expensive , and if you cut corners, your research could fall victim to all kinds of errors. Dominance bias (when a forceful participant influences the group) and moderator style bias (when different moderator personalities bring about different results in the same study) are two of the many ways your focus group data could get skewed.
4. Observation: the most powerful
During a customer observation session, someone from the company takes notes while they watch an ideal user engage with their product (or a similar product from a competitor).
What makes observation so clever and powerful?
‘Fly-on-the-wall’ observation is a great alternative to focus groups. It’s not only less expensive, but you’ll see people interact with your product in a natural setting without influencing each other. The only downside is that you can’t get inside their heads, so observation still isn't a recommended replacement for customer surveys and interviews.
The following questions will help you get to know your users on a deeper level when you interview them. They’re general questions, of course, so don’t be afraid to make them your own.
1. Who are you and what do you do?
How you ask this question, and what you want to know, will vary depending on your business model (e.g. business-to-business marketing is usually more focused on someone’s profession than business-to-consumer marketing).
It’s a great question to start with, and it’ll help you understand what’s relevant about your user demographics (age, race, gender, profession, education, etc.), but it’s not the be-all-end-all of market research. The more specific questions come later.
2. What does your day look like?
This question helps you understand your users’ day-to-day life and the challenges they face. It will help you gain empathy for them, and you may stumble across something relevant to their buying habits.
3. Do you ever purchase [product/service type]?
This is a ‘yes or no’ question. A ‘yes’ will lead you to the next question.
4. What problem were you trying to solve or what goal were you trying to achieve?
This question strikes to the core of what someone’s trying to accomplish and why they might be willing to pay for your solution.
5. Take me back to the day when you first decided you needed to solve this kind of problem or achieve this goal.
This is the golden question, and it comes from Adele Revella, Founder and CEO of Buyer Persona Institute . It helps you get in the heads of your users and figure out what they were thinking the day they decided to spend money to solve a problem.
If you take your time with this question, digging deeper where it makes sense, you should be able to answer all the relevant information you need to understand their perspective.
“The only scripted question I want you to ask them is this one: take me back to the day when you first decided that you needed to solve this kind of problem or achieve this kind of a goal. Not to buy my product, that’s not the day. We want to go back to the day that when you thought it was urgent and compelling to go spend money to solve a particular problem or achieve a goal. Just tell me what happened.”
— Adele Revella , Founder/CEO at Buyer Persona Institute
Bonus question: is there anything else you’d like to tell me?
This question isn’t just a nice way to wrap it up—it might just give participants the opportunity they need to tell you something you really need to know.
That’s why Sarah Doody, author of UX Notebook , adds it to the end of her written surveys.
“I always have a last question, which is just open-ended: “Is there anything else you would like to tell me?” And sometimes, that’s where you get four paragraphs of amazing content that you would never have gotten if it was just a Net Promoter Score [survey] or something like that.”
What is the difference between qualitative and quantitative research?
Qualitative research asks questions that can’t be reduced to a number, such as, “What is your job title?” or “What did you like most about your customer service experience?”
Quantitative research asks questions that can be answered with a numeric value, such as, “What is your annual salary?” or “How was your customer service experience on a scale of 1-5?”
→ Read more about the differences between qualitative and quantitative user research .
How do I do my own market research?
You can do your own quick and effective market research by
Surveying your customers
Building user personas
Studying your users through interviews and observation
Wrapping your head around your data with tools like flow models, affinity diagrams, and customer journey maps
What is the difference between market research and user research?
Market research takes a broad look at potential customers—what problems they’re trying to solve, their buying experience, and overall demand. User research, on the other hand, is more narrowly focused on the use (and usability ) of specific products.
What are the main criticisms of market research?
Many marketing professionals are critical of market research because it can be expensive and time-consuming. It’s often easier to convince your CEO or CMO to let you do lean market research rather than something more extensive because you can do it yourself. It also gives you quick answers so you can stay ahead of the competition.
Do I need a market research firm to get reliable data?
Absolutely not! In fact, we recommend that you start small and do it yourself in the beginning. By following a lean market research strategy, you can uncover some solid insights about your clients. Then you can make changes, test them out, and see whether the results are positive. This is an excellent strategy for making quick changes and remaining competitive.
Net Promoter, Net Promoter System, Net Promoter Score, NPS, and the NPS-related emoticons are registered trademarks of Bain & Company, Inc., Fred Reichheld, and Satmetrix Systems, Inc.
Related articles
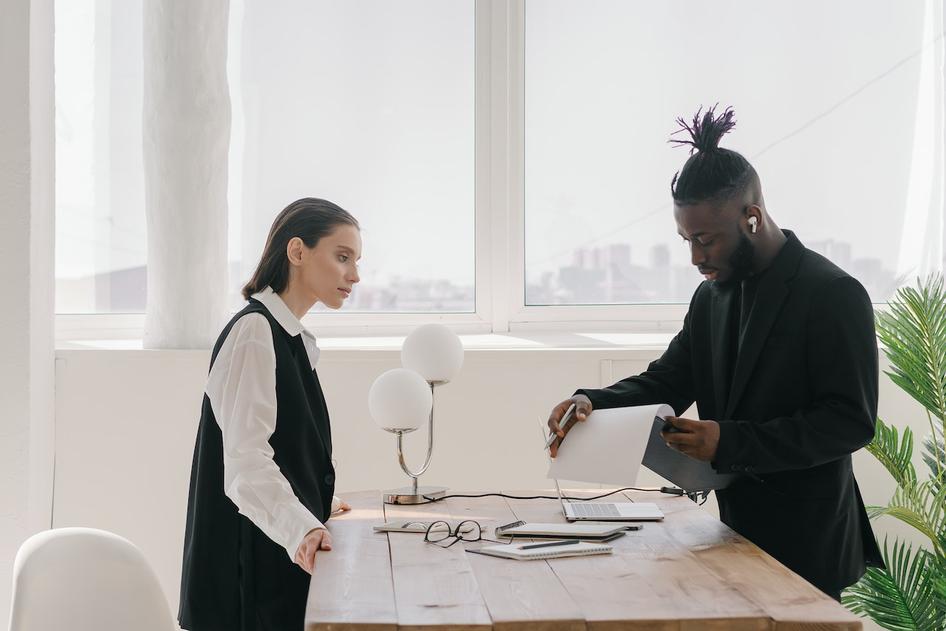
6 traits of top marketing leaders (and how to cultivate them in yourself)
Stepping into a marketing leadership role can stir up a mix of emotions: excitement, optimism, and, often, a gnawing doubt. "Do I have the right skills to truly lead and inspire?" If you've ever wrestled with these uncertainties, you're not alone.
Hotjar team
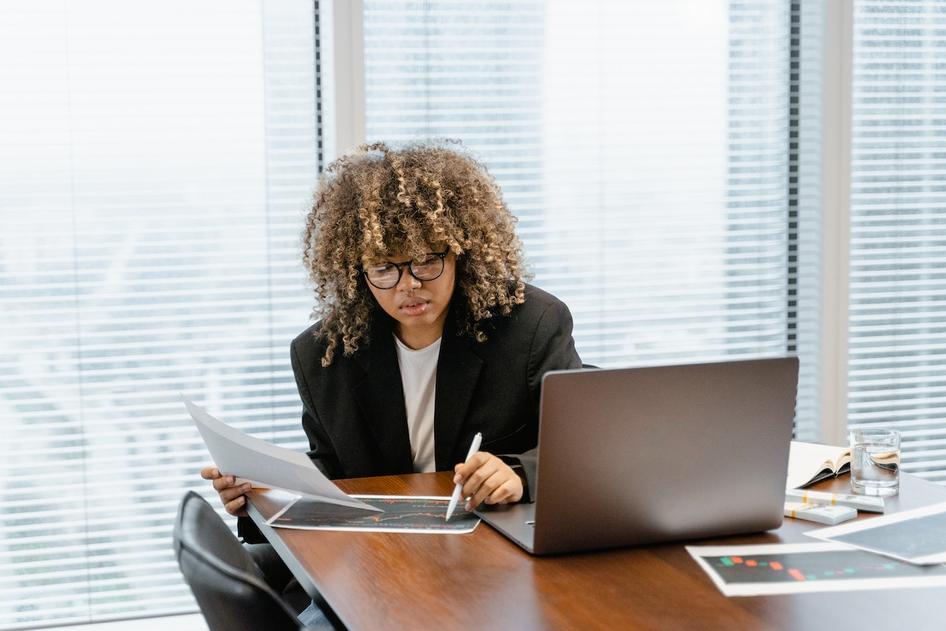
The 7 best BI tools for marketers in 2024 (and how to use them)
Whether you're sifting through campaign attribution data or reviewing performance reports from different sources, extracting meaningful business insights from vast amounts of data is an often daunting—yet critical—task many marketers face. So how do you efficiently evaluate your results and communicate key learnings?
This is where business intelligence (BI) tools come in, transforming raw data into actionable insights that drive informed, customer-centric decisions.
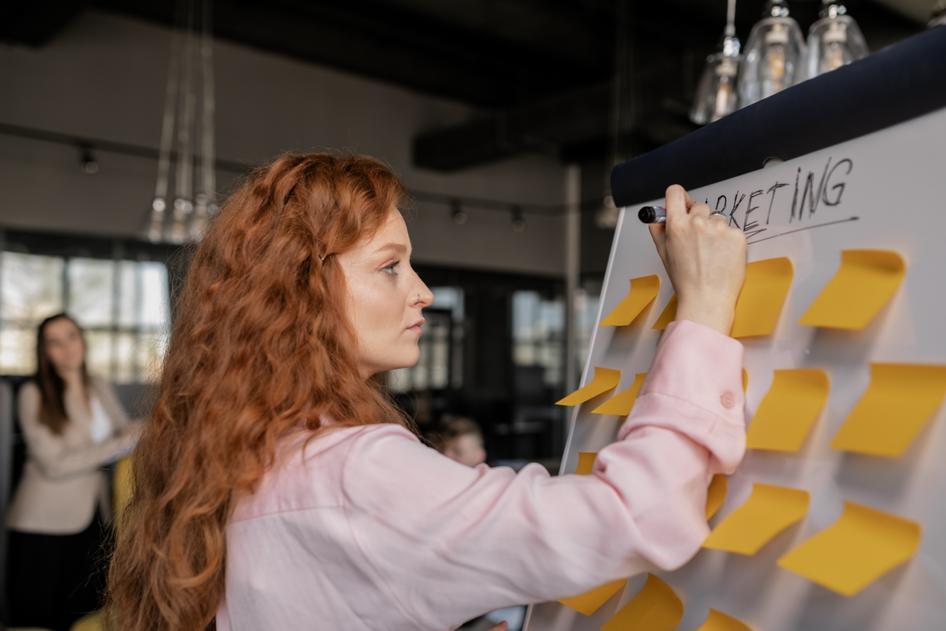
6 marketing trends that will shape the future of ecommerce in 2023
Today, marketing trends evolve at the speed of technology. Ecommerce businesses that fail to update their marketing strategies to meet consumers where they are in 2023 will be left out of the conversations that drive brand success.
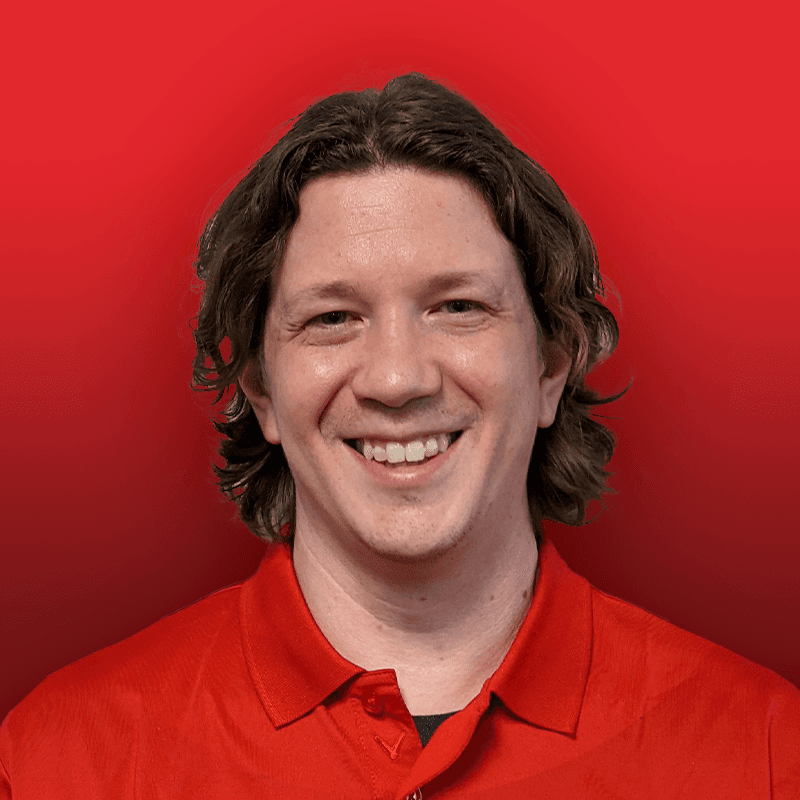
Geoff Whiting
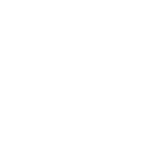
Online Market Research: What It Is and How To Do It

From building a business to buying a house, on Competitive analysis line market research is an effective tool for making sound decisions.
Oh, and it’s not just you.
Over 53% of consumers do online research routinely, with an astounding 95% reading online reviews before making a purchase.
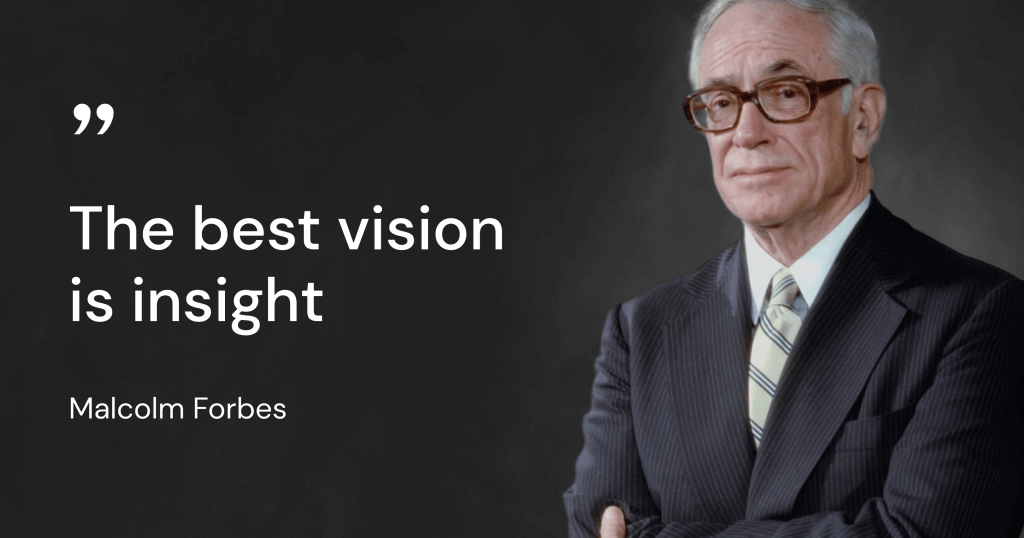
What is online market research?
Online market research takes secondary data from the internet or collects primary data online to support research or expand knowledge for a chosen topic. The information is then analyzed to inform or substantiate a theory.
Here are a few things you can do with digital market research:
- Discover new topics or content to write about
- Track consumer journeys
- See what creatives and ads perform well
- Find the best marketing channels
- Explore which markets are ripe for entry
- Analyze a target audience
- Research potential product or service improvements
- Benchmark your business
- Understand market dynamics and spot trends
- Find out what your competitors are doing and unpack their tactics
- Uncover new opportunities
Digital market research can help a company grow efficiently and effectively —supporting a business in its quest to stay relevant; and to survive and thrive in its market.
Take two, and hear first-hand from Harvard Business School Professor Rem Koning about the difference between traditional market research and state-of-the-art Digital Intelligence powered by behavioral data from Similarweb.
Online market research methods
Almost all online market research methods can be grouped into two subcategories; primary or secondary market research . Within these two core groups, you also have qualitative and quantitative research methods to consider.
Here’s a quick explainer of the core four types of online market research, including what they are and how to use them.
Primary Market Research
This is the first-hand collection of data by an organization. It gives full control over what questions are asked. The data obtained isn’t freely available online to anyone else , and the person or company conducting the research retains full ownership of the information they acquire.
This type of research wasn’t always this easy to do online. Thanks to advancements in technology and the heightened use of video conferencing in our daily lives – many types of primary research can be done remotely. Market research surveys , online focus groups, and interviews are perhaps the most widely adopted form of online primary research online.
See below for a complete list of primary research types.
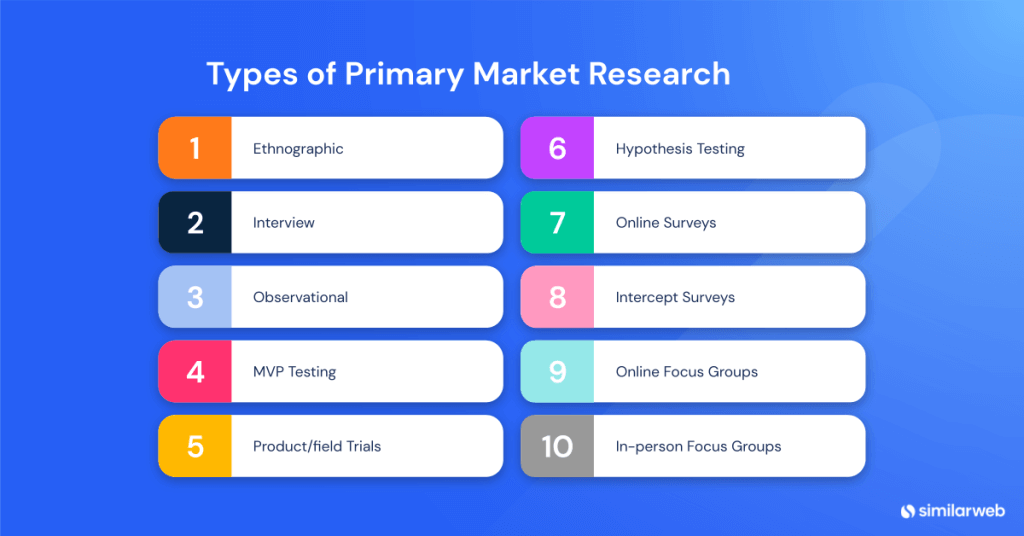
Secondary Market Research
This takes information from various sources online and repurposes it to inform or pad-out research into a chosen topic. It’s often quicker and cheaper to conduct than primary market research but offers fewer controls regarding research methodologies and customization. This data is freely available to anyone online, providing no exclusivity with the insights or information used.
This type of digital market research is also known as desk research and is a tried and tested way to gather facts or insights into a market, consumers, competitors, or products. Research reports, digital intelligence platforms, media outlets, and rival websites are some of the most relevant types of research.
But there are others 👇
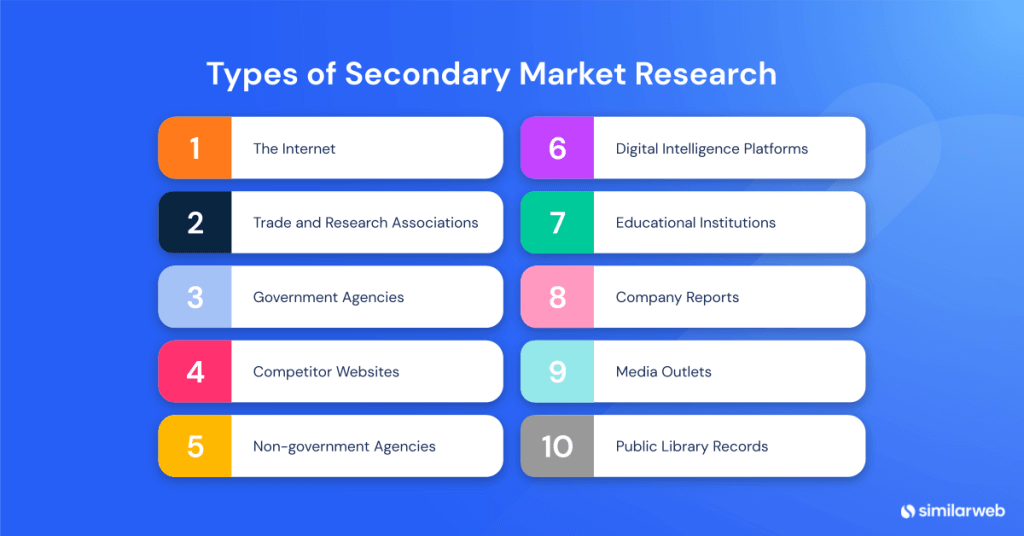
Aside from the core online market research methods, there are two other well-known research types to consider.
Qualitative Market Research
This type of digital market research looks at how people feel about a specific topic or brand . It takes more time to plan than most other forms of research, often with a smaller group of people. Let’s say you want to trial a new feature or product; you’d do this form of research pre-launch or post-trial to get under the hood and understand consumer sentiment.
As a form of primary data, it’s a highly tailored form of research that aims to give granular insights into why things are the way they are. Some of the most popular forms of qualitative research that can be done entirely online include focus groups, online forums, and interviews.
Stop Guessing, Start Analyzing
Get actionable insights for market research here
Quantitative Market Research
Quantitative market research is a numbers game. It’s a process that collects and analyzes data, dealing with primary and secondary information contained in a numerical format. The uses of quantitative market research include making predictions, trendspotting, finding patterns, and establishing averages in a market or business.
If an organization needs to do market sizing , market validation , and forecasting – they’ll usually use quantitative research to inform these activities. It can be done online when you need to gather numerical data from or about a target audience . It’s delivered in mixed formats, including raw data, charts, graphics, and tables. A common form of quantitative research that’s done online is a survey—specifically those using close-ended questions with answer options that have a numerical value. Once the values are collected, various analysis tools can be used to turn them into insights.
Read More: 98 Quantitative Market Research Questions & Examples
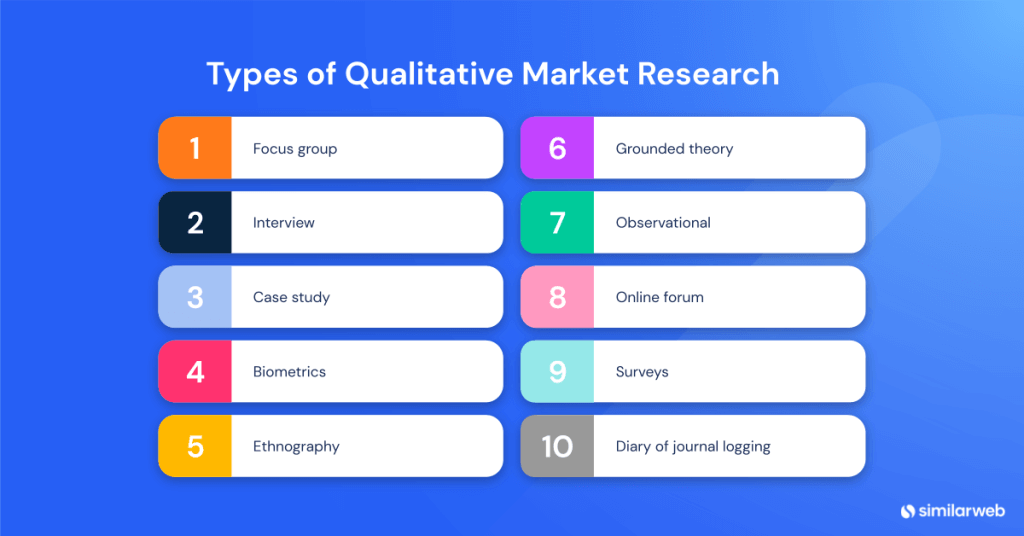
How to do market research online
As with any project, you must establish its goals, AKA your research objective. Once you have clarity on what you’re trying to achieve , you can map out how to get from point A to point B.
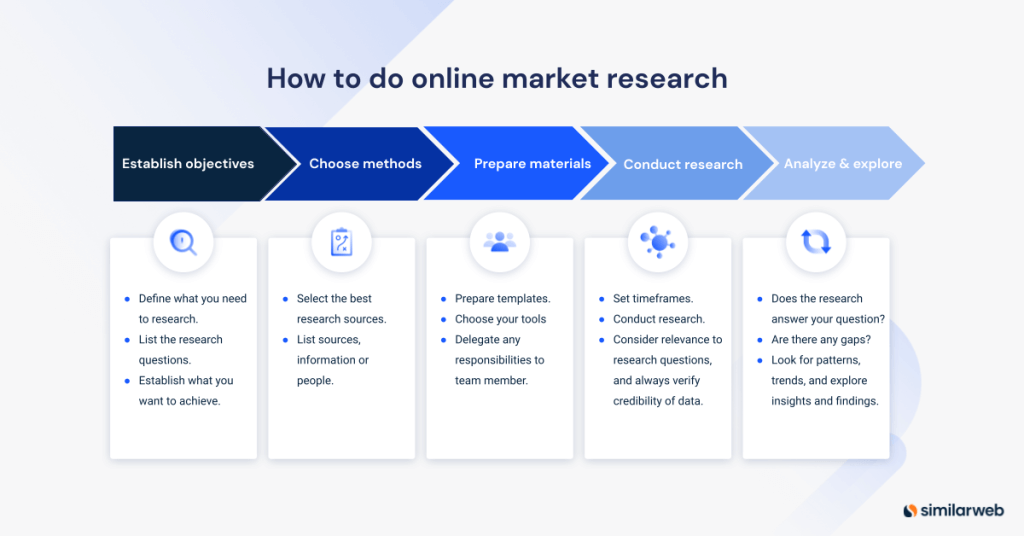
Here’s a quick explainer to guide you through the process.
1. Establish research objectives
Consider what you want to learn from your research. Are you trying to find which market could be best to enter next? Perhaps you need to find new opportunities for efficient growth . You might even want to flesh out some ideas for future enhancements to your product.
Whatever it is, write it down and make it your project goal.
2. Select the right online market research method/s
Now you know your goal, it’s time to lay out the foundation of your online market research strategy. At this point, the only thing to consider is the type of data you need and the right types of market research to use to help you get it.
There are four core types of market research to choose from.
- Primary market research
- Secondary market research (also known as desk research )
- Qualitative market research
- Quantitative market research
Not sure where to start? Read this post to learn more about the different types of market research .
3. Prepare research materials
As with most things in life, the better prepared you are, the easier the task is to complete. Online market research is no different. This essential third step in the digital research process entails laying the foundations for what’s to come. This means you need to collate any:
- Research questions
- Delegation of any responsibilities
As someone who’s written extensively about doing online market research and spoken with many thought leaders and professionals about the topic, the biggest tip I can provide is this.
Pro Tip: Take a systematic approach to documenting your findings. Record everything in a workable file in a centralized space. Having a mishmash of files, data, and assets will slow you down when it comes to the analysis phase.
Whatever type of online market research you’re doing, finding a template to use a framework can help speed things up . We’ve put together a free pack of 6 templates for you below.
4. Conduct your research
Carry out your online market research. Set a time limit, and try to stick to it. Regardless of the type of digital market research you choose, always keep your research goal in mind. If you’re using tools like Similarweb Digital Research Intelligence to do industry, company, or market research, then make sure you compare the right data sets that examine the same periods and location.
For secondary research, ensure that any reports or stats are backed up with verifiable sources. And for primary research, specifically, focus groups or surveys – be open to needing to change the line or type of questions being asked if you don’t feel confident the feedback will answer your research question adequately.
5. Analysis time
This final step in doing online market research involves summarizing your uncovered data. If you took on board the tip about using a systematic way of collecting data, this is where your foundation-setting will really start to pay off.
Once the data is summarized, you can start to analyze and uncover key insights that give you answers to your initial research questions and help bring you closer to your goals.
The market research process is ongoing and should never be viewed as a one-time thing. The more often you conduct it, the more insights and information you’ll uncover.
If you’re new to online market research, check out some free market research courses via the Similarweb Academy .
Online market research tools
Historically, market research was an arduous task that took time to do. Often, it would be done quarterly or perhaps yearly, using data that was already, to some extent, outdated.
Thankfully, times have changed.
Today, modern online market research tools deliver real-time market data and fresh insights as and when you need them.
Online Surveys
A firm favorite for companies looking to get feedback fast. Surveys can be done with or without a mailing list; and shared on a website or on socials. Typeform and Survey Monkey .
Market research intelligence platforms (like Similarweb)
Software like Similarweb can give you highly relevant, up-to-date information about any market, company, or topic. Most types of online market research benefit from using digital intelligence tools. The data is trusted, it’s dynamic, and it’s one of the quickest types of online market research you can do.
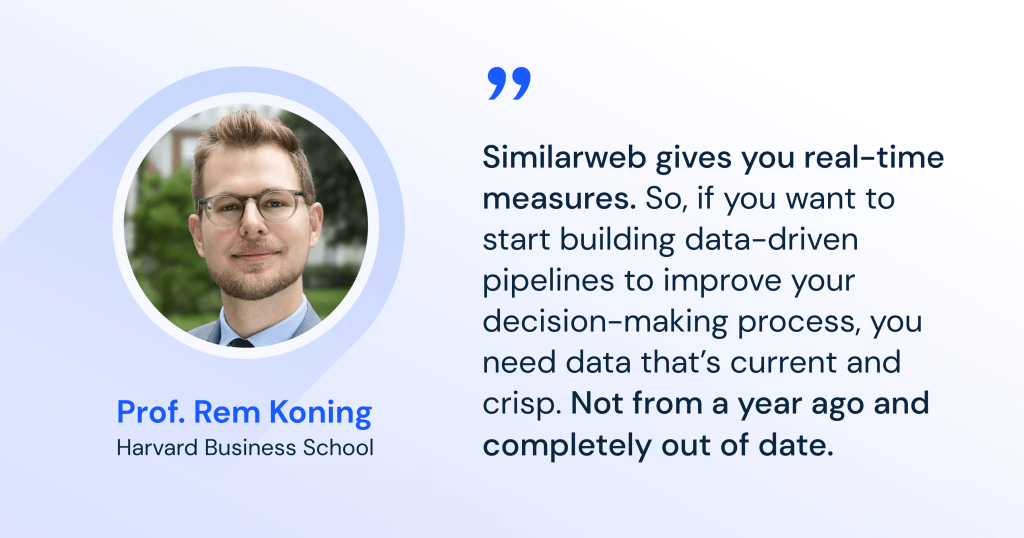
Think with Google
Great for doing research into persona development, consumer insights, and digital trends.
Answer the Public
If you’re doing online market research into a specific topic, this is a real gem of a tool. It shows
what questions people are asking online relative to your theme, and can give insights into a target audience and their relative concerns.
The Internet
I know it sounds a little elementary, but there’s a world of information available online. From trade associations to research companies that provide syndicated research reports , through to your rivals and even their social media profiles – there are plenty of insights waiting to be uncovered.
Read our dedicated blog on the topic if you want to find more online market research tools .
Similarweb for online market research
Similarweb Digital Research Intelligence is your gateway to endless online market research insights. Whether analyzing an industry or market, doing competitive research, or examining a target audience and their online behaviors, you can do this and more from within a single platform.
Competitive analysis is one of the most valuable types of online market research any firm can do. It takes a rival’s website and unpacks its successes in its basic form. When you do this systematically with your industry leaders and rising stars, you can see what channels, keywords, social platforms, ads, and content work best in your market.
Here, you can view website traffic and engagement metrics , marketing channels, competition keywords, and core audience demographic information, like age, geographics, device split, and gender. It shows the specific tactics and successes of any site.
Oh, and it’s not just for market research; you can also use Similarweb to do audience analysis , benchmarking , company research , and consumer journey tracking across the web, mobile, and app channels.
Wrapping up: online market research
Whether you’re just starting or part of an established firm, you face dilemmas that online market research can help solve . In the past, market research was timely and costly, but that’s not the case anymore. With tools like Similarweb, you have a world of information and insight at your fingertips.
It’s easier, quicker, and more resolute than ever before.
Try it out, for free, for a week – and see what you can discover about your market and the people in it.
A type of digital market research conducted online. It uses primary, secondary, quantitative, or qualitative research methods and is designed to help inform strategy or uncover more information about a topic.
What are the benefits of online market research?
You can do online market research with little-to-no professional training, it’s quick to conduct, and almost anyone can access secondary research data on the internet. Online research tools like Similarweb can significantly speed up the time it takes to complete a research project.
What is the best type of digital market research?
The best type of market research will depend on three things—your research goals, the time available, and your resources. Choosing the correct method of research will be crucial to your success. Read our guide to the different types of market research to see which is best for you.
Related Posts

From AI to Buy: The Role of Artificial Intelligence in Retail

How to Conduct a Social Media Competitor Analysis: 5 Quick Steps

Industry Research: The Data-Backed Approach
Wondering what similarweb can do for you.
Here are two ways you can get started with Similarweb today!
- 18+ Risks and Disadvantages of Technology
How to Build Your Business Identity on a Tight Budget
- Best Green Tea Brands in the world in 2020: What makes them the best?
- Global Milk brands in 2020 – What makes them successful?
- What is a Triple Net Lease? Its Advantages and Disadvantages
- Adaptation Level Phenomenon – understanding its importance
- Risk Matrix – Factors of a risk matrix and how to implement it
- Prioritization Matrix – Different types and how to use a prioritization matrix
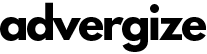
71+ Research Paper Topics & Ideas for Marketing Students
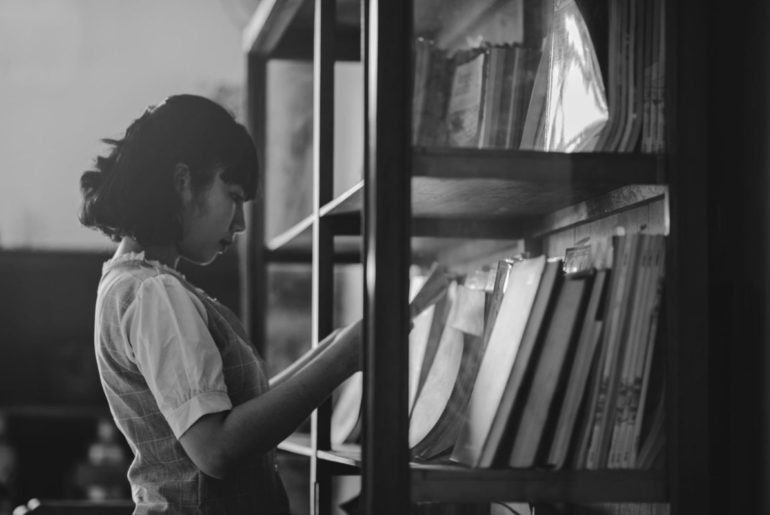
As a marketing student, you probably have access to a plethora of resources such as your college library and of course, the internet, to come up with great research paper topics.
However, the thought of writing your research paper can be daunting, especially if you’re still brainstorming and don’t know what to write about.
Just like any other piece of writing, start by keeping your audience in mind. Then, make a list of research paper topics that are more relevant to your interests, or a new under-developed field (for example; augmented reality, or people sentiments towards Artificial Intelligence), or a unique research topic that intrigues your audience.
But if you’re still struggling to pin down one out of the many research paper topics for your program, we’ll suggest a number of them for you to either choose from; or for you to take inspiration from and come up with your own.
Table of Contents
How to Choose the Best Research Paper Topics
Before we dive into the details, you’ll have to familiarize yourself with the basics. For starters, pick up a pen and paper and brainstorm different topics that you’d like to write about.
While personal interest is definitely important, we also suggest you opt for a topic that will intrigue your readers. Here are a couple of factors you ought to keep in mind while selecting a topic:
Keeping your personal interest in mind
You probably won’t be able to write a stellar research paper if you’re not interested in the topic. Sit down with your peers and advisors to discuss possible ideas. It will be easier for you to discuss different themes once you’ve written down all your ideas in one place. If you’ve decided on a specific keyword for instance “consumer behaviour”, you can look for similar research papers on the internet.
A research paper isn’t a descriptive essay which you can drag aimlessly. Your research paper needs to be based on factual data and that’s only possible if you’ve conducted thorough research. While jotting down points for your first draft, ensure your statements are supported with references or examples citing credible academicals and research work.
Don’t leave it till the last day
A lot of students tend to undermine the writing process and leave for the last few days. Bear in mind that you can’t possibly write your entire research paper overnight. In order to succeed, you’ll have to devote sufficient amount of time to research.
Also, be prepared to schedule meetings with your advisor on a regular basis as you’re bound to require help along the way. At this point, make sure you only rely on credible sources that will support your dissertation.
Examples & List of Research Paper Topics
If you’re still unable to decide a topic of your interest, here is a list of 70 unique marketing research topics that you can use as marketing project topics for your MBA, or any other marketing course:
- How do organizations use CSR ( corporate social responsibility ) to reinforce brand equity?
- What manipulation tactics do brands use to get more customers?
- How can brand image be communicated via social media marketing?
- How can social media impact the buying choices of shoppers?
- Are consumers equipped to shield themselves from direct marketing strategies?
- Determine and analyze consumer buying behavior for [product name]
- How does advertising impact consumer behaviour?
- How does family orientation impact marketing communications?
- What characteristics do buyers look for when purchasing a product online?
- How does global marketing incorporate standardization?
- What attributes do consumers look for when comparing products online?
- How do financial institutions differentiate their goods and services on the basis of social class?
- Is direct marketing really the most effective form of marketing?
- What internet marketing trends can be expected for the future?
- How to marketing strategies differ across different cultures?
- Can brand advertising impact political campaigns?
- How do brands exploit impulsive buying?
- How does loyalty cards encourage sales and boost customer loyalty?
- Can well-marketed brands get away with selling substandard quality products?
- How is globalization having on impact on consumer behaviour?
- Impact of brand image on customer loyalty
- Brand attributes that lead to an increase in customer loyalty
- Successful marketing approaches that helped break through strong market monopoly
- Impact of cause marketing on brand affinity with young mothers
- Effect of consumer promotions and discount offerings on brand equity
- The outcomes of advertising in a recession
- Influence on Social Media advertising on consumer behaviour
- Effect of TV advertising on top of mind awareness
- Understanding customer perceptions around event sponsorships
- Does corporate social responsibility translate into sales?
- Canadians perspective on being targeted with mobile ads based on their browser history
- Is direct marketing welcomed by people?
- Are customers able to differentiate between various mortgage offering by competing banks?
- Does social media influence buying behaviour
- Do people like being click baited into sponsored posts?
- Understanding the impact of celebrity endorsements on ROI for CPG brands
- Women’s sentiments around comparison advertising
- How effective is comparison advertising to build brand equity?
- Do consumers prefer purchasing routine grocery products online?
- Is earned media perceived to be as important as it appears to be?
- What makes people want to share content to their friends?
- Understanding why content goes viral
- Marketing challenges around the evolving family structures
- Are we losing the emotional value and significance of money being in a cashless society?
- Is centralized global marketing a good idea for brand health in local markets?
- How is augmented reality going to enhance marketing experiences?
- How will artificial intelligence support in making better marketing decisions?
- Is immersion marketing through virtual reality technology going to be accepted?
- What does the luxury auto buyer look for in a car?
- How to instill a desire to purchase for customers in the luxury category
- Harmful effects of advertising to kids
- Impact of in-store branding on brand salience
- Effect marketing strategies for restaurant businesses
- Habit formation and ways to integrate new products in consumer lifestyles
- Is display advertising going to die?
- Can Snapchat help small business grow?
- How do customers perceive the brand who advertise on Instagram?
- The impact of humour in advertising
- Do customers pay attention to nutrition labels?
- What triggers impulse buying behaviour
- Essentials to sky rocket a new brand to heights of awareness
- The factors that lead to customer satisfaction in young adults
- Elements that help build an emotional connection with your audience
- How do males and females differ in their buying behaviour of mobile phones
- Does language targeting help in ethnic advertising?
- Customer Perceptions: Are well known brands good in quality?
- Is radio still an effective method of advertising?
- Rural vs Urban marketing challenges to be mindful of
- Impact of internal branding on employee retention and turnover
- An in-depth analysis of political marketing in Canada
More Categories of Research Topics
Still in need of some inspiration? Here are a few research paper areas that you can explore:
- Distribution
- Consumer Behavior
- Relationship Marketing
- Brand Management
- Nonprofit Marketing
- Market Segmentation & Targeting
- Internet Marketing
- Marketing Planning & Forecasting
- Product Design & Positioning
- Direct Marketing
- Advertising
- Purchasing & Materials Management
Hopefully, these marketing thesis topics will help you come up with a few topics of your own. If you’re still confused about which area, you’d like to work with, we suggest you consult your advisor for some additional help. Good luck!
135+ Rumi Quotes on Love, Beauty & Happiness
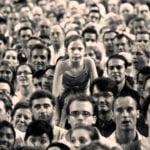
Explanation of Group Polarization with Real Life Examples
Related posts.
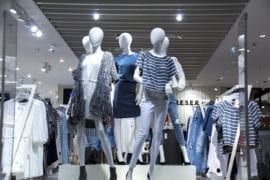
Dell Marketing Strategy – Details of the multi-million-dollar monopoly
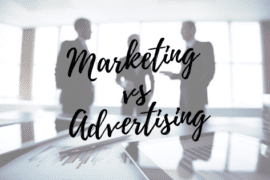
Difference between Marketing and Advertising: What’s confusing?
Write a comment cancel reply.
Save my name, email, and website in this browser for the next time I comment.
This site uses Akismet to reduce spam. Learn how your comment data is processed .
- ADVERTISING
- SOCIAL MEDIA
- ENTREPRENEURSHIP
- WEB DEVELOPMENT
- MAKE MONEY WITHOUT PAYING ANYTHING
- HOW TO MAKE QUICK MONEY
- WAYS TO MAKE MONEY BLOGGING
- BIGGEST SLOGANS GUIDE
- DIFFERENT TYPES OF MARKETING
- MARKETING MIX EXPLANATION
- TYPES OF DISTRIBUTION STRATEGIES
- AFFILIATE MARKETING FOR BEGINNERS
- LEARN TO MAKE A BLOG
- FREE PRINTABLE CALENDARS 2019
- DMCA Notice
- Privacy Policy
Type above and press Enter to search. Press Esc to cancel.
Home • Knowledge hub • Online market research: a comprehensive guide
Online market research: a comprehensive guide
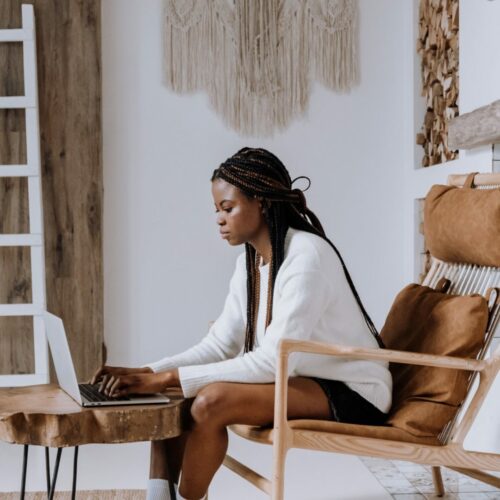
As internet penetration has increased across the world, online market research has seen rapid growth. The COVID-19 pandemic acted as a catalyst for further adoption as restrictions on face-to-face contact meant that businesses had to embrace new research approaches. The result? Many companies experiencing the advantages of online research for the first time. What’s more, the widespread adoption of tech during the pandemic – particularly amongst audiences that were traditionally easier to reach offline – means that online market research is a now a viable way of engaging more people than ever before.
In this guide we’ll:
- Explore the key advantages and disadvantages of online market research
- Explain the different methodologies available to you and when to use them
- Share our top tips for setting your project up for success, moderation and analysis
What is online market research?
As the name suggests, online market research is a type of market research where data collection takes place on the internet. In this way it differs from more traditional forms of market research where data collection takes places offline (either in person or over the phone) such as focus groups or telephone interviews.
What are the main advantages and disadvantages of online market research?
Advantages of online market research include being faster and in some cases, cheaper to conduct than face-to-face market research, meaning that you can obtain results more quickly. One of the major disadvantages of online market research is that it relies on the people you’re trying to reach having access to the internet and as such it may not be suitable for all audiences. Some online qualitative methodologies also require a degree of tech savviness, and a longer time commitment than offline approaches.
Beyond this:
- Online market research is particularly useful for global projects. If you’re looking to enter a new market and don’t have boots on the ground, online market research can help you build your understanding without having to fork out for flights and focus groups. It’s also well suited to multi-market research as you can conduct research in a number of countries in parallel to identify common trends.
- Online research can help you get closer to customers and bring them to life for stakeholders. In a focus group or a face to face interview, you only have a short amount of time to get to know respondents. In contrast, some forms of online qualitative research take place over a series of days, weeks or even months. This allows us to dig deeper and build a richer understanding of customers and their needs than we might offline. Some methodologies even allow you to set photo and videos tasks. These result in rich multimedia outputs can be used to bring customers to life for stakeholders.
- Online techniques allow for a more iterative approach to research. The longitudinal nature of some online qualitative methodologies means that it’s possible to adapt your discussion guide as the research progresses to capitalize on emerging insights. This is particularly effective for product development research. For example, in an online community, you can share initial concepts with consumers, iteratively improve them based on feedback and then put them back into the community for further testing. This approach allows you to build and refine the concepts as the research progresses.
- Online market research can be good for sensitive topics. Many online methodologies offer a greater degree of anonymity than face to face research. As such, they can be better suited to discussing difficult subjects such as illness or sex.
What type of business questions can online market research help me answer?
Online market research is extremely versatile and can be harnessed to explore a broad range of challenges, including:
- Better understanding your target market
- Product or service development
- Brand and advertising research to guide marketing strategy
- Determining customer satisfaction
What are the main methodologies used in online market research and when should I use them?
There are a number of different research tools you can use to collect data online. They include:
Online surveys
An online survey is the primary method for collecting quantitative data online. Online surveys can be completed by your customers or respondents can be sourced from an online panel (a group of people that have agreed to take part in online research). If you opt for this route you can build a representative sample and extrapolate your findings to the wider population.
When to use an online survey
- To understand trends and patterns of behavior e.g. buying habits
- To understand attitudes towards your brand versus the competition
- To compare different groups (e.g. by age, gender or market) to understand similarities or differences
- To track metrics over time e.g. brand awareness, customer satisfaction
When to avoid an online survey
- Projects where you need to qualitatively explore an issue with consumers to understand motivations and the “why” behind behaviors
- Projects where you need to co-create with consumers to iteratively improve an idea
- It can be tempting to pack your survey full of questions but you need to be mindful of time. An online survey should take a maximum of 15 – 20 minutes to complete. Any longer than this and you risk seeing respondent fatigue or a significant proportion of people dropping off before the end. This will mean that fieldwork will take longer to complete.
- Mix up the type of questions you use to keep the survey engaging for respondents
Online communities
Online communities are a qualitative technique for exploring a topic over a number of days, weeks or even months. Pre-screened respondents are invited to a secure online platform where they take part in a number of activities each day. This can involve discussing topics in groups, one on one or taking part in video, photo or audio tasks.
When to use an online community
- To deepen understanding of a target audience
- To explore content preferences and consumption
- To test audience attitude and brand perceptions
- To test new ideas with consumers – this can be anything from products and services to packaging or new marketing concepts. Online communities are particularly well suited to this. Many platforms enable consumers to mark-up concepts so you can gather in-depth feedback whilst also protecting the confidential nature of the stimulus, through functionality such watermarking or setting videos to self-destruct once they’ve been watched.
When to avoid an online community
- When you need to explore a topic with consumers on an individual level
- Do your research on the types of platforms available. Each offer different functionality so go back to your objectives to ensure you’re selecting most suitable for your project and what you want to achieve.
Digital depths
A digital depth is essentially an audio or video interview which takes places online. These tend to last about 30 – 90 minutes. A typical program might involve 15 – 20 interviews per market, although this can vary based on your business objectives.
When to use a digital depth
- To explore in-home brand or product usage or explore attitudes towards particular brands or products
- To gain B2B market insights
When to avoid a digital depth
- Projects where you require respondents to share and bounce ideas off each other
- Projects that require large sample sizes as running 100s or 1000s of depths online or offline is just not feasible
- One of the challenges levelled against online research is that it can be harder to build rapport online. To overcome this, consider pre-tasks that allow you to get to know the respondent beforehand and help them feel comfortable. This can be anything from asking them to complete a short diary task to some quickfire WhatsApp questions before you get started.
- Be aware of market differences. In markets that have traditionally favored face to face methods, using video as part of a digital depth is a must for engendering trust.
Digital ethnographies
The objective of a digital ethnography is to view a consumer’s life as it happens. Ethnography emerged as an offline discipline, but developments in technology mean that it is now possible to conduct ethnography online. From 360 cameras to smart home technology right through to the humble old smartphone, there are a number of tools you can use. And in some cases, these new approaches can uncover insights that you just would not gather when there’s a researcher in the room.
When to use digital ethnographies
- To explore in-home brand or product usage or to explore attitudes towards particular brands or products
- To identify unmet needs
- To understand cultural differences between markets
When not to use digital ethnographies
- Projects where you need respondents to bounce ideas off one another
- Not everyone will be suited to take part in digital ethnography. You need respondents who are open and willing to let you into their lives. One way of doing this is to include a casting phase as part of the project to identify the right people to participate.
Online focus groups
An online focus group is where a group of pre-screened respondents are invited to join a private online platform for a few hours. Like an in-person focus group, a moderator will guide the discussion, which can be either text or video based.
When to use an online focus group
- To test attitudes and responses to concepts and ideas at a high level
- To sense check ideas quickly with consumers
When not to use an online focus group
- To obtain detailed inputs from respondents at a one-to-one level
- If you are used to running focus groups in person, don’t automatically assume that online focus groups are the next best thing. Depending on what you’re trying to achieve, another methodology may better meet your objectives.
- If you’re running a video based online focus group make sure that you’re able to see all of the participants at once. This will help in interpreting non-verbal cues and bringing people into the conversation.
Questions to ask yourself when designing your online research approach
As you can see, there are a number of different online methodologies available to use, each with their own distinct use cases, benefits and drawbacks. To help you design the best research approach, we’d recommend asking yourself 5 key questions:
- What am I trying to achieve? Some clients come to us dead set on using a particular methodology. We’d advise against this. By starting with your business objectives and then considering which methodology best allows you to meet these, you’ll be in a much stronger position to design an effective research approach.
- Do I need to collect quantitative or qualitative data? If you’re looking to conduct research at scale, you’ll need to opt for an online survey whereas if you want to explore a topic qualitatively, there are a number of different options available.
- What depth of insight do I require? Are you sense checking an idea or do you need to explore needs in real detail? This will have implications for the methodology you choose.
- Do I need to speak to consumers one-on-one or in a group? Different methodologies and platforms facilitate a different type of engagement with respondents. Think carefully about which you require up front.
- Which markets am I exploring? Not every methodology will work in every market. There are cultural factors and connectivity issues you’ll need to consider. For instance, if you’re conducting research in India outside metros or tier 1 cities, you’ll need to make sure your approach is mobile optimized but doesn’t require too much bandwidth. Or if looking to test concepts in China, you’ll need to do so in a one-on-one setting due to the Chinese habit to moderate answers and avoid causing offence in group environments. Our guide to conducting online research in Asia , can help you consider the best approach to take in each market. Remember, if you’re running a multi-market project, you don’t need to use the same methodology in every country. Harnessing an approach that will ensure you can answer your business objectives is more important than consistency of approach.
Get regular insights
Keep up to date with the latest insights from our research as well as all our company news in our free monthly newsletter.
- First Name *
- Last Name *
- Business Email *
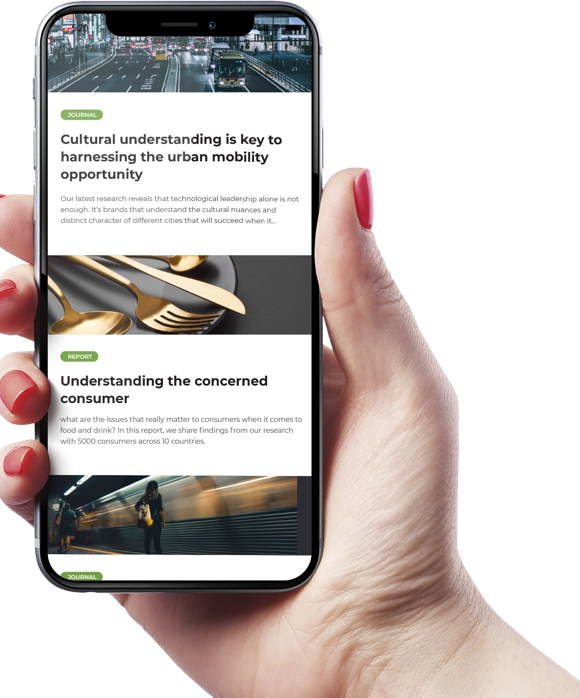
Setting your online research study up for success
So you’ve decided on the best methodology for your study. The next step is to get into the nitty gritty of setting up the project. Here there are a number of key things to consider.
- Simplicity. When you’re writing your questionnaire or discussion guide, focus on making it as easy as possible to understand. Cut out the jargon to ensure a consistent understanding amongst respondents.
- Flexibility . For online qualitative projects, it’s worth building in an element of flexibility into your discussion guide. This will allow you to delve into emerging insights during the course of the research.
- Cultural nuances. We talked about the importance of culture when selecting your methodology. It’s crucial to bear this in mind throughout the entire research project. When you’re writing a questionnaire, preparing a discussion guide or designing specific tasks, consider how cultural differences might affect participants’ responses. For instance, in Japan people tend to avoid giving negative feedback so you use a traditional 5 point Likert scale here you’ll find that most people will answer somewhere in the middle, making it hard to ascertain the trend. To get a clearer go / no-go result, you’ll want to consider a 4 point scale in this market.
- Testing and training. Some online qualitative research approaches rely on external technology platforms. Make sure you build in a comprehensive testing phase to ensure these work across different browsers and in different markets, particularly if you’re using a tool for the first time. You should also consider preparing training materials for respondents. What might seem intuitive to us as researchers, may be not be as straight forward for the person taking part in the research.
How to moderate online research
Moderation is arguably even more important online than it is offline. So what should you bear in mind?
- Don’t forget the basics. Just because the research is taking place online doesn’t mean that you can forget everything you’ve learnt about great moderation. Dress appropriately and try and mimic face-to-face interactions – even something as simple as waving hello at the start of an interview can help to build rapport and set someone at ease.
- Read the (virtual) room. Sometimes what people choose not to say can be as revealing as what they do.
- Do your homework. Earlier in this guide, we mentioned the value that pre-tasks can bring. Even a short WhatsApp exchange can be useful in helping you to understand more about a respondent and how they feel about certain topics so you can get the most out of them in the session.
- Curate the conversation. Unlike a focus group or an interview which might only last 90 minutes, online communities take place over a longer period and therefore require ongoing moderation. Moderators should think about how they curate the conversation by connecting individual respondents up to foster group discussion.
- Conduct research in the local language. If you’re running a project in another country, use local language moderators who’ll be able to understand and draw out local nuances.
Analyzing online research projects
Online market research is often considered to deliver more bang for your buck than a face-to-face approach because of the sheer amount of data you can collect. This is particularly true of online communities due to their longitudinal nature. As such, you need to carefully structure your analysis, ensuring you’re focusing on the ‘so what’ for your stakeholders.
There are also cultural considerations to take into account when it comes to analysis. You’ll need to bear in mind that consumers in different markets can answer questions differently when you’re interpreting the data. For instance, when asking about interest levels or purchase intent, the figures you see in ASEAN developing countries tend to be higher than in other markets. Even within markets, there can be regional differences to take account of. In Vietnam, for instance, there are cultural differences between consumers in the two major cities – Hanoi in the North and Ho Chi Minh in the South. Those in the North tend to favour products from well-known brands, while those in the South are more open to trying new things, and this is reflected in the data you see from these areas.
Considering harnessing online research in your own organization?
This is where we’re in our element. Find out about some of our online methodologies like online surveys or online communities or get in touch to discuss a project .
Helping brands uncover valuable insights
We’ve been working with Kadence on a couple of strategic projects, which influenced our product roadmap roll-out within the region. Their work has been exceptional in providing me the insights that I need. Senior Marketing Executive Arla Foods
Kadence’s reports give us the insight, conclusion and recommended execution needed to give us a different perspective, which provided us with an opportunity to relook at our go to market strategy in a different direction which we are now reaping the benefits from. Sales & Marketing Bridgestone
Kadence helped us not only conduct a thorough and insightful piece of research, its interpretation of the data provided many useful and unexpected good-news stories that we were able to use in our communications and interactions with government bodies. General Manager PR -Internal Communications & Government Affairs Mitsubishi
Kadence team is more like a partner to us. We have run a number of projects together and … the pro-activeness, out of the box thinking and delivering in spite of tight deadlines are some of the key reasons we always reach out to them. Vital Strategies
Kadence were an excellent partner on this project; they took time to really understand our business challenges, and developed a research approach that would tackle the exam question from all directions. The impact of the work is still being felt now, several years later. Customer Intelligence Director Wall Street Journal
Get In Touch
" (Required) " indicates required fields
Privacy Overview
6.3 Steps in a Successful Marketing Research Plan
Learning outcomes.
By the end of this section, you will be able to:
- 1 Identify and describe the steps in a marketing research plan.
- 2 Discuss the different types of data research.
- 3 Explain how data is analyzed.
- 4 Discuss the importance of effective research reports.
Define the Problem
There are seven steps to a successful marketing research project (see Figure 6.3 ). Each step will be explained as we investigate how a marketing research project is conducted.
The first step, defining the problem, is often a realization that more information is needed in order to make a data-driven decision. Problem definition is the realization that there is an issue that needs to be addressed. An entrepreneur may be interested in opening a small business but must first define the problem that is to be investigated. A marketing research problem in this example is to discover the needs of the community and also to identify a potentially successful business venture.
Many times, researchers define a research question or objectives in this first step. Objectives of this research study could include: identify a new business that would be successful in the community in question, determine the size and composition of a target market for the business venture, and collect any relevant primary and secondary data that would support such a venture. At this point, the definition of the problem may be “Why are cat owners not buying our new cat toy subscription service?”
Additionally, during this first step we would want to investigate our target population for research. This is similar to a target market, as it is the group that comprises the population of interest for the study. In order to have a successful research outcome, the researcher should start with an understanding of the problem in the current situational environment.
Develop the Research Plan
Step two is to develop the research plan. What type of research is necessary to meet the established objectives of the first step? How will this data be collected? Additionally, what is the time frame of the research and budget to consider? If you must have information in the next week, a different plan would be implemented than in a situation where several months were allowed. These are issues that a researcher should address in order to meet the needs identified.
Research is often classified as coming from one of two types of data: primary and secondary. Primary data is unique information that is collected by the specific researcher with the current project in mind. This type of research doesn’t currently exist until it is pulled together for the project. Examples of primary data collection include survey, observation, experiment, or focus group data that is gathered for the current project.
Secondary data is any research that was completed for another purpose but can be used to help inform the research process. Secondary data comes in many forms and includes census data, journal articles, previously collected survey or focus group data of related topics, and compiled company data. Secondary data may be internal, such as the company’s sales records for a previous quarter, or external, such as an industry report of all related product sales. Syndicated data , a type of external secondary data, is available through subscription services and is utilized by many marketers. As you can see in Table 6.1 , primary and secondary data features are often opposite—the positive aspects of primary data are the negative side of secondary data.
There are four research types that can be used: exploratory, descriptive, experimental, and ethnographic research designs (see Figure 6.4 ). Each type has specific formats of data that can be collected. Qualitative research can be shared through words, descriptions, and open-ended comments. Qualitative data gives context but cannot be reduced to a statistic. Qualitative data examples are categorical and include case studies, diary accounts, interviews, focus groups, and open-ended surveys. By comparison, quantitative data is data that can be reduced to number of responses. The number of responses to each answer on a multiple-choice question is quantitative data. Quantitative data is numerical and includes things like age, income, group size, and height.
Exploratory research is usually used when additional general information in desired about a topic. When in the initial steps of a new project, understanding the landscape is essential, so exploratory research helps the researcher to learn more about the general nature of the industry. Exploratory research can be collected through focus groups, interviews, and review of secondary data. When examining an exploratory research design, the best use is when your company hopes to collect data that is generally qualitative in nature. 7
For instance, if a company is considering a new service for registered users but is not quite sure how well the new service will be received or wants to gain clarity of exactly how customers may use a future service, the company can host a focus group. Focus groups and interviews will be examined later in the chapter. The insights collected during the focus group can assist the company when designing the service, help to inform promotional campaign options, and verify that the service is going to be a viable option for the company.
Descriptive research design takes a bigger step into collection of data through primary research complemented by secondary data. Descriptive research helps explain the market situation and define an “opinion, attitude, or behavior” of a group of consumers, employees, or other interested groups. 8 The most common method of deploying a descriptive research design is through the use of a survey. Several types of surveys will be defined later in this chapter. Descriptive data is quantitative in nature, meaning the data can be distilled into a statistic, such as in a table or chart.
Again, descriptive data is helpful in explaining the current situation. In the opening example of LEGO , the company wanted to describe the situation regarding children’s use of its product. In order to gather a large group of opinions, a survey was created. The data that was collected through this survey allowed the company to measure the existing perceptions of parents so that alterations could be made to future plans for the company.
Experimental research , also known as causal research , helps to define a cause-and-effect relationship between two or more factors. This type of research goes beyond a correlation to determine which feature caused the reaction. Researchers generally use some type of experimental design to determine a causal relationship. An example is A/B testing, a situation where one group of research participants, group A, is exposed to one treatment and then compared to the group B participants, who experience a different situation. An example might be showing two different television commercials to a panel of consumers and then measuring the difference in perception of the product. Another example would be to have two separate packaging options available in different markets. This research would answer the question “Does one design sell better than the other?” Comparing that to the sales in each market would be part of a causal research study. 9
The final method of collecting data is through an ethnographic design. Ethnographic research is conducted in the field by watching people interact in their natural environment. For marketing research, ethnographic designs help to identify how a product is used, what actions are included in a selection, or how the consumer interacts with the product. 10
Examples of ethnographic research would be to observe how a consumer uses a particular product, such as baking soda. Although many people buy baking soda, its uses are vast. So are they using it as a refrigerator deodorizer, a toothpaste, to polish a belt buckle, or to use in baking a cake?
Select the Data Collection Method
Data collection is the systematic gathering of information that addresses the identified problem. What is the best method to do that? Picking the right method of collecting data requires that the researcher understand the target population and the design picked in the previous step. There is no perfect method; each method has both advantages and disadvantages, so it’s essential that the researcher understand the target population of the research and the research objectives in order to pick the best option.
Sometimes the data desired is best collected by watching the actions of consumers. For instance, how many cars pass a specific billboard in a day? What website led a potential customer to the company’s website? When are consumers most likely to use the snack vending machines at work? What time of day has the highest traffic on a social media post? What is the most streamed television program this week? Observational research is the collecting of data based on actions taken by those observed. Many data observations do not require the researched individuals to participate in the data collection effort to be highly valuable. Some observation requires an individual to watch and record the activities of the target population through personal observations .
Unobtrusive observation happens when those being observed aren’t aware that they are being watched. An example of an unobtrusive observation would be to watch how shoppers interact with a new stuffed animal display by using a one-way mirror. Marketers can identify which products were handled more often while also determining which were ignored.
Other methods can use technology to collect the data instead. Instances of mechanical observation include the use of vehicle recorders, which count the number of vehicles that pass a specific location. Computers can also assess the number of shoppers who enter a store, the most popular entry point for train station commuters, or the peak time for cars to park in a parking garage.
When you want to get a more in-depth response from research participants, one method is to complete a one-on-one interview . One-on-one interviews allow the researcher to ask specific questions that match the respondent’s unique perspective as well as follow-up questions that piggyback on responses already completed. An interview allows the researcher to have a deeper understanding of the needs of the respondent, which is another strength of this type of data collection. The downside of personal interviews it that a discussion can be very time-consuming and results in only one respondent’s answers. Therefore, in order to get a large sample of respondents, the interview method may not be the most efficient method.
Taking the benefits of an interview and applying them to a small group of people is the design of a focus group . A focus group is a small number of people, usually 8 to 12, who meet the sample requirements. These individuals together are asked a series of questions where they are encouraged to build upon each other’s responses, either by agreeing or disagreeing with the other group members. Focus groups are similar to interviews in that they allow the researcher, through a moderator, to get more detailed information from a small group of potential customers (see Figure 6.5 ).
Link to Learning
Focus groups.
Focus groups are a common method for gathering insights into consumer thinking and habits. Companies will use this information to develop or shift their initiatives. The best way to understand a focus group is to watch a few examples or explanations. TED-Ed has this video that explains how focus groups work.
You might be asking when it is best to use a focus group or a survey. Learn the differences, the pros and cons of each, and the specific types of questions you ask in both situations in this article .
Preparing for a focus group is critical to success. It requires knowing the material and questions while also managing the group of people. Watch this video to learn more about how to prepare for a focus group and the types of things to be aware of.
One of the benefits of a focus group over individual interviews is that synergy can be generated when a participant builds on another’s ideas. Additionally, for the same amount of time, a researcher can hear from multiple respondents instead of just one. 11 Of course, as with every method of data collection, there are downsides to a focus group as well. Focus groups have the potential to be overwhelmed by one or two aggressive personalities, and the format can discourage more reserved individuals from speaking up. Finally, like interviews, the responses in a focus group are qualitative in nature and are difficult to distill into an easy statistic or two.
Combining a variety of questions on one instrument is called a survey or questionnaire . Collecting primary data is commonly done through surveys due to their versatility. A survey allows the researcher to ask the same set of questions of a large group of respondents. Response rates of surveys are calculated by dividing the number of surveys completed by the total number attempted. Surveys are flexible and can collect a variety of quantitative and qualitative data. Questions can include simplified yes or no questions, select all that apply, questions that are on a scale, or a variety of open-ended types of questions. There are four types of surveys (see Table 6.2 ) we will cover, each with strengths and weaknesses defined.
Let’s start off with mailed surveys —surveys that are sent to potential respondents through a mail service. Mailed surveys used to be more commonly used due to the ability to reach every household. In some instances, a mailed survey is still the best way to collect data. For example, every 10 years the United States conducts a census of its population (see Figure 6.6 ). The first step in that data collection is to send every household a survey through the US Postal Service (USPS). The benefit is that respondents can complete and return the survey at their convenience. The downside of mailed surveys are expense and timeliness of responses. A mailed survey requires postage, both when it is sent to the recipient and when it is returned. That, along with the cost of printing, paper, and both sending and return envelopes, adds up quickly. Additionally, physically mailing surveys takes time. One method of reducing cost is to send with bulk-rate postage, but that slows down the delivery of the survey. Also, because of the convenience to the respondent, completed surveys may be returned several weeks after being sent. Finally, some mailed survey data must be manually entered into the analysis software, which can cause delays or issues due to entry errors.
Phone surveys are completed during a phone conversation with the respondent. Although the traditional phone survey requires a data collector to talk with the participant, current technology allows for computer-assisted voice surveys or surveys to be completed by asking the respondent to push a specific button for each potential answer. Phone surveys are time intensive but allow the respondent to ask questions and the surveyor to request additional information or clarification on a question if warranted. Phone surveys require the respondent to complete the survey simultaneously with the collector, which is a limitation as there are restrictions for when phone calls are allowed. According to Telephone Consumer Protection Act , approved by Congress in 1991, no calls can be made prior to 8:00 a.m. or after 9:00 p.m. in the recipient’s time zone. 12 Many restrictions are outlined in this original legislation and have been added to since due to ever-changing technology.
In-person surveys are when the respondent and data collector are physically in the same location. In-person surveys allow the respondent to share specific information, ask questions of the surveyor, and follow up on previous answers. Surveys collected through this method can take place in a variety of ways: through door-to-door collection, in a public location, or at a person’s workplace. Although in-person surveys are time intensive and require more labor to collect data than some other methods, in some cases it’s the best way to collect the required data. In-person surveys conducted through a door-to-door method is the follow-up used for the census if respondents do not complete the mailed survey. One of the downsides of in-person surveys is the reluctance of potential respondents to stop their current activity and answer questions. Furthermore, people may not feel comfortable sharing private or personal information during a face-to-face conversation.
Electronic surveys are sent or collected through digital means and is an opportunity that can be added to any of the above methods as well as some new delivery options. Surveys can be sent through email, and respondents can either reply to the email or open a hyperlink to an online survey (see Figure 6.7 ). Additionally, a letter can be mailed that asks members of the survey sample to log in to a website rather than to return a mailed response. Many marketers now use links, QR codes, or electronic devices to easily connect to a survey. Digitally collected data has the benefit of being less time intensive and is often a more economical way to gather and input responses than more manual methods. A survey that could take months to collect through the mail can be completed within a week through digital means.
Design the Sample
Although you might want to include every possible person who matches your target market in your research, it’s often not a feasible option, nor is it of value. If you did decide to include everyone, you would be completing a census of the population. Getting everyone to participate would be time-consuming and highly expensive, so instead marketers use a sample , whereby a portion of the whole is included in the research. It’s similar to the samples you might receive at the grocery store or ice cream shop; it isn’t a full serving, but it does give you a good taste of what the whole would be like.
So how do you know who should be included in the sample? Researchers identify parameters for their studies, called sample frames . A sample frame for one study may be college students who live on campus; for another study, it may be retired people in Dallas, Texas, or small-business owners who have fewer than 10 employees. The individual entities within the sampling frame would be considered a sampling unit . A sampling unit is each individual respondent that would be considered as matching the sample frame established by the research. If a researcher wants businesses to participate in a study, then businesses would be the sampling unit in that case.
The number of sampling units included in the research is the sample size . Many calculations can be conducted to indicate what the correct size of the sample should be. Issues to consider are the size of the population, the confidence level that the data represents the entire population, the ease of accessing the units in the frame, and the budget allocated for the research.
There are two main categories of samples: probability and nonprobability (see Figure 6.8 ). Probability samples are those in which every member of the sample has an identified likelihood of being selected. Several probability sample methods can be utilized. One probability sampling technique is called a simple random sample , where not only does every person have an identified likelihood of being selected to be in the sample, but every person also has an equal chance of exclusion. An example of a simple random sample would be to put the names of all members of a group into a hat and simply draw out a specific number to be included. You could say a raffle would be a good example of a simple random sample.
Another probability sample type is a stratified random sample , where the population is divided into groups by category and then a random sample of each category is selected to participate. For instance, if you were conducting a study of college students from your school and wanted to make sure you had all grade levels included, you might take the names of all students and split them into different groups by grade level—freshman, sophomore, junior, and senior. Then, from those categories, you would draw names out of each of the pools, or strata.
A nonprobability sample is a situation in which each potential member of the sample has an unknown likelihood of being selected in the sample. Research findings that are from a nonprobability sample cannot be applied beyond the sample. Several examples of nonprobability sampling are available to researchers and include two that we will look at more closely: convenience sampling and judgment sampling.
The first nonprobability sampling technique is a convenience sample . Just like it sounds, a convenience sample is when the researcher finds a group through a nonscientific method by picking potential research participants in a convenient manner. An example might be to ask other students in a class you are taking to complete a survey that you are doing for a class assignment or passing out surveys at a basketball game or theater performance.
A judgment sample is a type of nonprobability sample that allows the researcher to determine if they believe the individual meets the criteria set for the sample frame to complete the research. For instance, you may be interested in researching mothers, so you sit outside a toy store and ask an individual who is carrying a baby to participate.
Collect the Data
Now that all the plans have been established, the instrument has been created, and the group of participants has been identified, it is time to start collecting data. As explained earlier in this chapter, data collection is the process of gathering information from a variety of sources that will satisfy the research objectives defined in step one. Data collection can be as simple as sending out an email with a survey link enclosed or as complex as an experiment with hundreds of consumers. The method of collection directly influences the length of this process. Conducting personal interviews or completing an experiment, as previously mentioned, can add weeks or months to the research process, whereas sending out an electronic survey may allow a researcher to collect the necessary data in a few days. 13
Analyze and Interpret the Data
Once the data has been collected, the process of analyzing it may begin. Data analysis is the distillation of the information into a more understandable and actionable format. The analysis itself can take many forms, from the use of basic statistics to a more comprehensive data visualization process. First, let’s discuss some basic statistics that can be used to represent data.
The first is the mean of quantitative data. A mean is often defined as the arithmetic average of values. The formula is:
A common use of the mean calculation is with exam scores. Say, for example, you have earned the following scores on your marketing exams: 72, 85, 68, and 77. To find the mean, you would add up the four scores for a total of 302. Then, in order to generate a mean, that number needs to be divided by the number of exam scores included, which is 4. The mean would be 302 divided by 4, for a mean test score of 75.5. Understanding the mean can help to determine, with one number, the weight of a particular value.
Another commonly used statistic is median. The median is often referred to as the middle number. To generate a median, all the numeric answers are placed in order, and the middle number is the median. Median is a common statistic when identifying the income level of a specific geographic region. 14 For instance, the median household income for Albuquerque, New Mexico, between 2015 and 2019 was $52,911. 15 In this case, there are just as many people with an income above the amount as there are below.
Mode is another statistic that is used to represent data of all types, as it can be used with quantitative or qualitative data and represents the most frequent answer. Eye color, hair color, and vehicle color can all be presented with a mode statistic. Additionally, some researchers expand on the concept of mode and present the frequency of all responses, not just identifying the most common response. Data such as this can easily be presented in a frequency graph, 16 such as the one in Figure 6.9 .
Additionally, researchers use other analyses to represent the data rather than to present the entirety of each response. For example, maybe the relationship between two values is important to understand. In this case, the researcher may share the data as a cross tabulation (see Figure 6.10 ). Below is the same data as above regarding social media use cross tabulated with gender—as you can see, the data is more descriptive when you can distinguish between the gender identifiers and how much time is spent per day on social media.
Not all data can be presented in a graphical format due to the nature of the information. Sometimes with qualitative methods of data collection, the responses cannot be distilled into a simple statistic or graph. In that case, the use of quotations, otherwise known as verbatims , can be used. These are direct statements presented by the respondents. Often you will see a verbatim statement when reading a movie or book review. The critic’s statements are used in part or in whole to represent their feelings about the newly released item.
Infographics
As they say, a picture is worth a thousand words. For this reason, research results are often shown in a graphical format in which data can be taken in quickly, called an infographic .
Check out this infographic on what components make for a good infographic. As you can see, a good infographic needs four components: data, design, a story, and the ability to share it with others. Without all four pieces, it is not as valuable a resource as it could be. The ultimate infographic is represented as the intersection of all four.
Infographics are particularly advantageous online. Refer to this infographic on why they are beneficial to use online .
Prepare the Research Report
The marketing research process concludes by sharing the generated data and makes recommendations for future actions. What starts as simple data must be interpreted into an analysis. All information gathered should be conveyed in order to make decisions for future marketing actions. One item that is often part of the final step is to discuss areas that may have been missed with the current project or any area of further study identified while completing it. Without the final step of the marketing research project, the first six steps are without value. It is only after the information is shared, through a formal presentation or report, that those recommendations can be implemented and improvements made. The first six steps are used to generate information, while the last is to initiate action. During this last step is also when an evaluation of the process is conducted. If this research were to be completed again, how would we do it differently? Did the right questions get answered with the survey questions posed to the respondents? Follow-up on some of these key questions can lead to additional research, a different study, or further analysis of data collected.
Methods of Quantifying Marketing Research
One of the ways of sharing information gained through marketing research is to quantify the research . Quantifying the research means to take a variety of data and compile into a quantity that is more easily understood. This is a simple process if you want to know how many people attended a basketball game, but if you want to quantify the number of students who made a positive comment on a questionnaire, it can be a little more complicated. Researchers have a variety of methods to collect and then share these different scores. Below are some of the most common types used in business.
Is a customer aware of a product, brand, or company? What is meant by awareness? Awareness in the context of marketing research is when a consumer is familiar with the product, brand, or company. It does not assume that the consumer has tried the product or has purchased it. Consumers are just aware. That is a measure that many businesses find valuable. There are several ways to measure awareness. For instance, the first type of awareness is unaided awareness . This type of awareness is when no prompts for a product, brand, or company are given. If you were collecting information on fast-food restaurants, you might ask a respondent to list all the fast-food restaurants that serve a chicken sandwich. Aided awareness would be providing a list of products, brands, or companies and the respondent selects from the list. For instance, if you give a respondent a list of fast-food restaurants and ask them to mark all the locations with a chicken sandwich, you are collecting data through an aided method. Collecting these answers helps a company determine how the business location compares to those of its competitors. 17
Customer Satisfaction (CSAT)
Have you ever been asked to complete a survey at the end of a purchase? Many businesses complete research on buying, returning, or other customer service processes. A customer satisfaction score , also known as CSAT, is a measure of how satisfied customers are with the product, brand, or service. A CSAT score is usually on a scale of 0 to 100 percent. 18 But what constitutes a “good” CSAT score? Although what is identified as good can vary by industry, normally anything in the range from 75 to 85 would be considered good. Of course, a number higher than 85 would be considered exceptional. 19
Customer Acquisition Cost (CAC) and Customer Effort Score (CES)
Other metrics often used are a customer acquisition cost (CAC) and customer effort score (CES). How much does it cost a company to gain customers? That’s the purpose of calculating the customer acquisition cost. To calculate the customer acquisition cost , a company would need to total all expenses that were accrued to gain new customers. This would include any advertising, public relations, social media postings, etc. When a total cost is determined, it is divided by the number of new customers gained through this campaign.
The final score to discuss is the customer effort score , also known as a CES. The CES is a “survey used to measure the ease of service experience with an organization.” 20 Companies that are easy to work with have a better CES than a company that is notorious for being difficult. An example would be to ask a consumer about the ease of making a purchase online by incorporating a one-question survey after a purchase is confirmed. If a number of responses come back negative or slightly negative, the company will realize that it needs to investigate and develop a more user-friendly process.
Knowledge Check
It’s time to check your knowledge on the concepts presented in this section. Refer to the Answer Key at the end of the book for feedback.
- Defining the problem
- Developing the research plan
- Selecting a data collection method
- Designing the sample
- you are able to send it to all households in an area
- it is inexpensive
- responses are automatically loaded into the software
- the data comes in quickly
- Primary data
- Secondary data
- Secondary and primary data
- Professional data
- It shows how respondents answered two variables in relation to each other and can help determine patterns by different groups of respondents.
- By presenting the data in the form of a picture, the information is easier for the reader to understand.
- It is an easy way to see how often one answer is selected by the respondents.
- This analysis can used to present interview or focus group data.
As an Amazon Associate we earn from qualifying purchases.
This book may not be used in the training of large language models or otherwise be ingested into large language models or generative AI offerings without OpenStax's permission.
Want to cite, share, or modify this book? This book uses the Creative Commons Attribution License and you must attribute OpenStax.
Access for free at https://openstax.org/books/principles-marketing/pages/1-unit-introduction
- Authors: Dr. Maria Gomez Albrecht, Dr. Mark Green, Linda Hoffman
- Publisher/website: OpenStax
- Book title: Principles of Marketing
- Publication date: Jan 25, 2023
- Location: Houston, Texas
- Book URL: https://openstax.org/books/principles-marketing/pages/1-unit-introduction
- Section URL: https://openstax.org/books/principles-marketing/pages/6-3-steps-in-a-successful-marketing-research-plan
© Jan 9, 2024 OpenStax. Textbook content produced by OpenStax is licensed under a Creative Commons Attribution License . The OpenStax name, OpenStax logo, OpenStax book covers, OpenStax CNX name, and OpenStax CNX logo are not subject to the Creative Commons license and may not be reproduced without the prior and express written consent of Rice University.
Education During Coronavirus
A Smithsonian magazine special report
Science | June 15, 2020
Seventy-Five Scientific Research Projects You Can Contribute to Online
From astrophysicists to entomologists, many researchers need the help of citizen scientists to sift through immense data collections
:focal(300x157:301x158)/https://tf-cmsv2-smithsonianmag-media.s3.amazonaws.com/filer/e2/ca/e2ca665f-77b7-4ba2-8cd2-46f38cbf2b60/citizen_science_mobile.png)
Rachael Lallensack
Former Assistant Editor, Science and Innovation
If you find yourself tired of streaming services, reading the news or video-chatting with friends, maybe you should consider becoming a citizen scientist. Though it’s true that many field research projects are paused , hundreds of scientists need your help sifting through wildlife camera footage and images of galaxies far, far away, or reading through diaries and field notes from the past.
Plenty of these tools are free and easy enough for children to use. You can look around for projects yourself on Smithsonian Institution’s citizen science volunteer page , National Geographic ’s list of projects and CitizenScience.gov ’s catalog of options. Zooniverse is a platform for online-exclusive projects , and Scistarter allows you to restrict your search with parameters, including projects you can do “on a walk,” “at night” or “on a lunch break.”
To save you some time, Smithsonian magazine has compiled a collection of dozens of projects you can take part in from home.
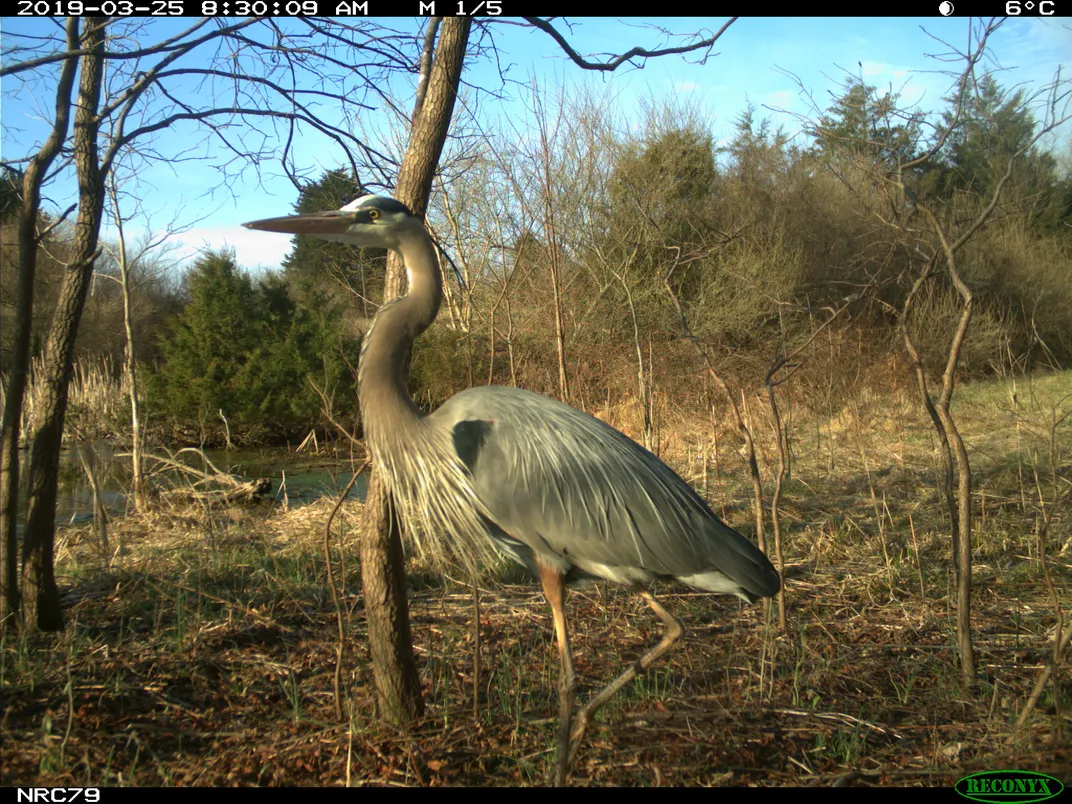
American Wildlife
If being home has given you more time to look at wildlife in your own backyard, whether you live in the city or the country, consider expanding your view, by helping scientists identify creatures photographed by camera traps. Improved battery life, motion sensors, high-resolution and small lenses have made camera traps indispensable tools for conservation.These cameras capture thousands of images that provide researchers with more data about ecosystems than ever before.
Smithsonian Conservation Biology Institute’s eMammal platform , for example, asks users to identify animals for conservation projects around the country. Currently, eMammal is being used by the Woodland Park Zoo ’s Seattle Urban Carnivore Project, which studies how coyotes, foxes, raccoons, bobcats and other animals coexist with people, and the Washington Wolverine Project, an effort to monitor wolverines in the face of climate change. Identify urban wildlife for the Chicago Wildlife Watch , or contribute to wilderness projects documenting North American biodiversity with The Wilds' Wildlife Watch in Ohio , Cedar Creek: Eyes on the Wild in Minnesota , Michigan ZoomIN , Western Montana Wildlife and Snapshot Wisconsin .
"Spend your time at home virtually exploring the Minnesota backwoods,” writes the lead researcher of the Cedar Creek: Eyes on the Wild project. “Help us understand deer dynamics, possum populations, bear behavior, and keep your eyes peeled for elusive wolves!"
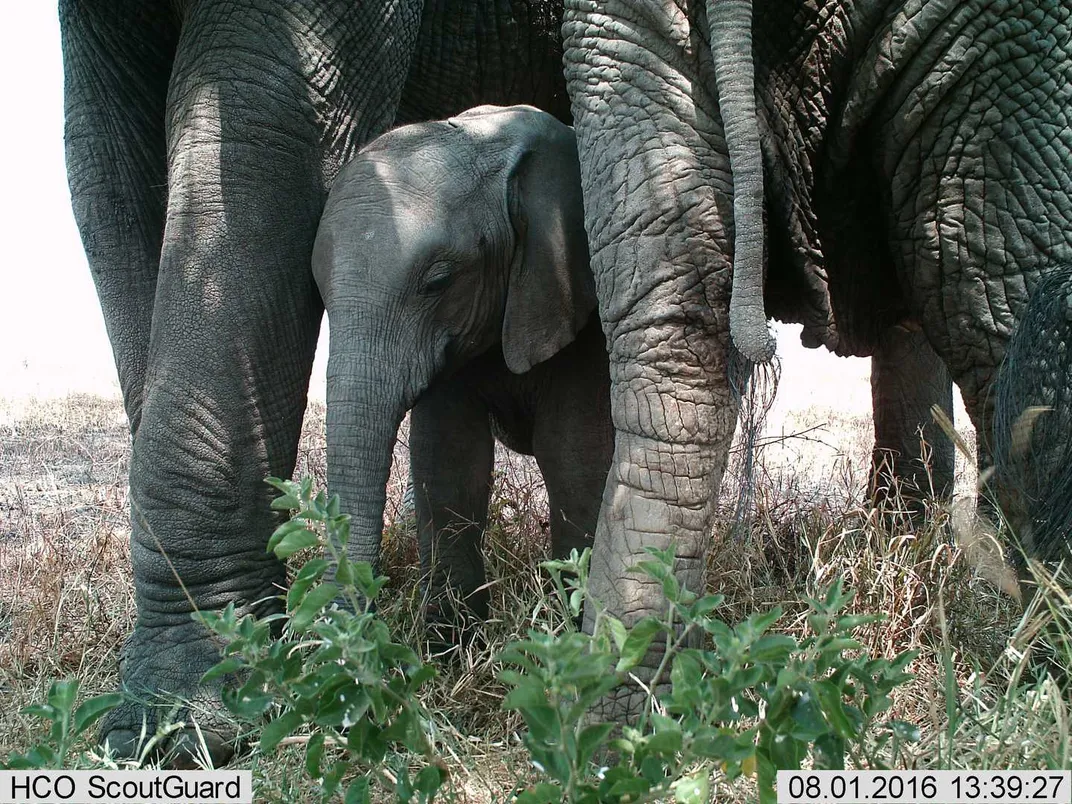
If being cooped up at home has you daydreaming about traveling, Snapshot Safari has six active animal identification projects. Try eyeing lions, leopards, cheetahs, wild dogs, elephants, giraffes, baobab trees and over 400 bird species from camera trap photos taken in South African nature reserves, including De Hoop Nature Reserve and Madikwe Game Reserve .
With South Sudan DiversityCam , researchers are using camera traps to study biodiversity in the dense tropical forests of southwestern South Sudan. Part of the Serenegeti Lion Project, Snapshot Serengeti needs the help of citizen scientists to classify millions of camera trap images of species traveling with the wildebeest migration.
Classify all kinds of monkeys with Chimp&See . Count, identify and track giraffes in northern Kenya . Watering holes host all kinds of wildlife, but that makes the locales hotspots for parasite transmission; Parasite Safari needs volunteers to help figure out which animals come in contact with each other and during what time of year.
Mount Taranaki in New Zealand is a volcanic peak rich in native vegetation, but native wildlife, like the North Island brown kiwi, whio/blue duck and seabirds, are now rare—driven out by introduced predators like wild goats, weasels, stoats, possums and rats. Estimate predator species compared to native wildlife with Taranaki Mounga by spotting species on camera trap images.
The Zoological Society of London’s (ZSL) Instant Wild app has a dozen projects showcasing live images and videos of wildlife around the world. Look for bears, wolves and lynx in Croatia ; wildcats in Costa Rica’s Osa Peninsula ; otters in Hampshire, England ; and both black and white rhinos in the Lewa-Borana landscape in Kenya.
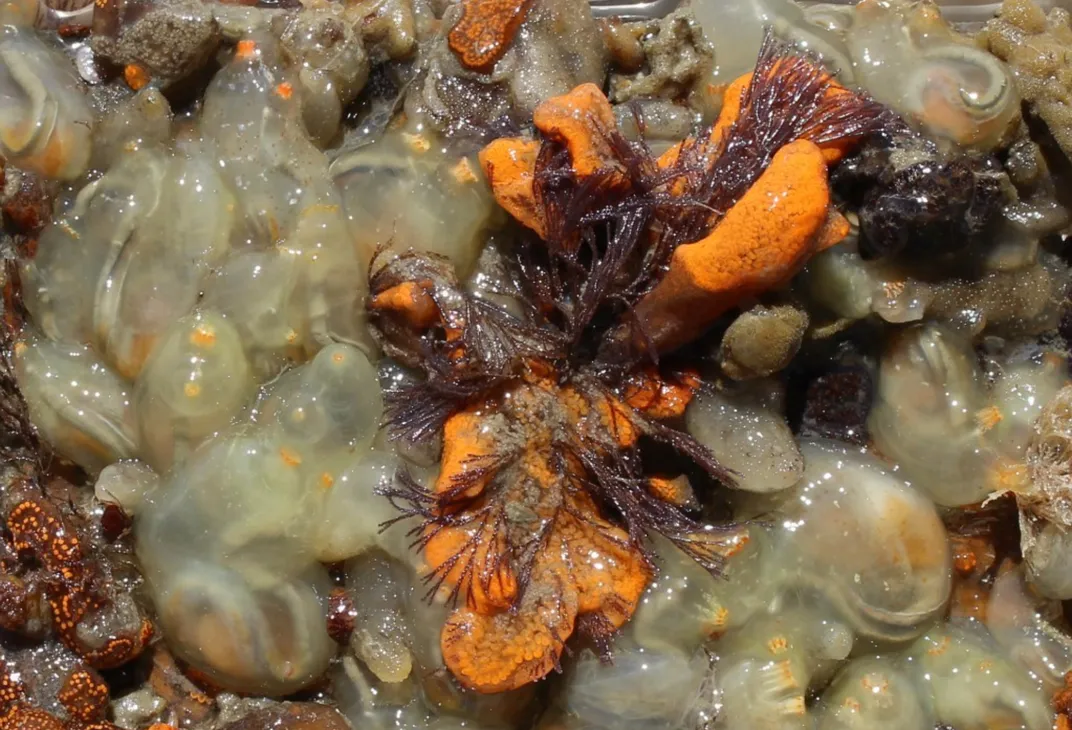
Under the Sea
Researchers use a variety of technologies to learn about marine life and inform conservation efforts. Take, for example, Beluga Bits , a research project focused on determining the sex, age and pod size of beluga whales visiting the Churchill River in northern Manitoba, Canada. With a bit of training, volunteers can learn how to differentiate between a calf, a subadult (grey) or an adult (white)—and even identify individuals using scars or unique pigmentation—in underwater videos and images. Beluga Bits uses a “ beluga boat ,” which travels around the Churchill River estuary with a camera underneath it, to capture the footage and collect GPS data about the whales’ locations.
Many of these online projects are visual, but Manatee Chat needs citizen scientists who can train their ear to decipher manatee vocalizations. Researchers are hoping to learn what calls the marine mammals make and when—with enough practice you might even be able to recognize the distinct calls of individual animals.
Several groups are using drone footage to monitor seal populations. Seals spend most of their time in the water, but come ashore to breed. One group, Seal Watch , is analyzing time-lapse photography and drone images of seals in the British territory of South Georgia in the South Atlantic. A team in Antarctica captured images of Weddell seals every ten minutes while the seals were on land in spring to have their pups. The Weddell Seal Count project aims to find out what threats—like fishing and climate change—the seals face by monitoring changes in their population size. Likewise, the Año Nuevo Island - Animal Count asks volunteers to count elephant seals, sea lions, cormorants and more species on a remote research island off the coast of California.
With Floating Forests , you’ll sift through 40 years of satellite images of the ocean surface identifying kelp forests, which are foundational for marine ecosystems, providing shelter for shrimp, fish and sea urchins. A project based in southwest England, Seagrass Explorer , is investigating the decline of seagrass beds. Researchers are using baited cameras to spot commercial fish in these habitats as well as looking out for algae to study the health of these threatened ecosystems. Search for large sponges, starfish and cold-water corals on the deep seafloor in Sweden’s first marine park with the Koster seafloor observatory project.
The Smithsonian Environmental Research Center needs your help spotting invasive species with Invader ID . Train your eye to spot groups of organisms, known as fouling communities, that live under docks and ship hulls, in an effort to clean up marine ecosystems.
If art history is more your speed, two Dutch art museums need volunteers to start “ fishing in the past ” by analyzing a collection of paintings dating from 1500 to 1700. Each painting features at least one fish, and an interdisciplinary research team of biologists and art historians wants you to identify the species of fish to make a clearer picture of the “role of ichthyology in the past.”
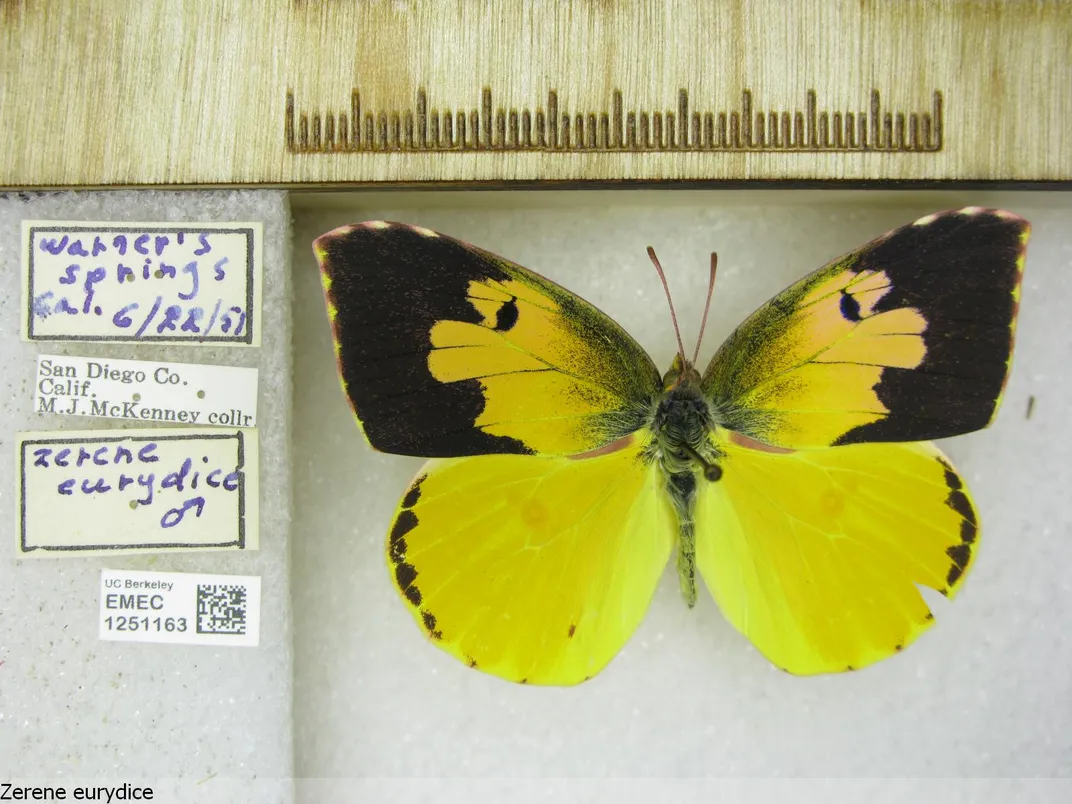
Interesting Insects
Notes from Nature is a digitization effort to make the vast resources in museums’ archives of plants and insects more accessible. Similarly, page through the University of California Berkeley’s butterfly collection on CalBug to help researchers classify these beautiful critters. The University of Michigan Museum of Zoology has already digitized about 300,000 records, but their collection exceeds 4 million bugs. You can hop in now and transcribe their grasshopper archives from the last century . Parasitic arthropods, like mosquitos and ticks, are known disease vectors; to better locate these critters, the Terrestrial Parasite Tracker project is working with 22 collections and institutions to digitize over 1.2 million specimens—and they’re 95 percent done . If you can tolerate mosquito buzzing for a prolonged period of time, the HumBug project needs volunteers to train its algorithm and develop real-time mosquito detection using acoustic monitoring devices. It’s for the greater good!
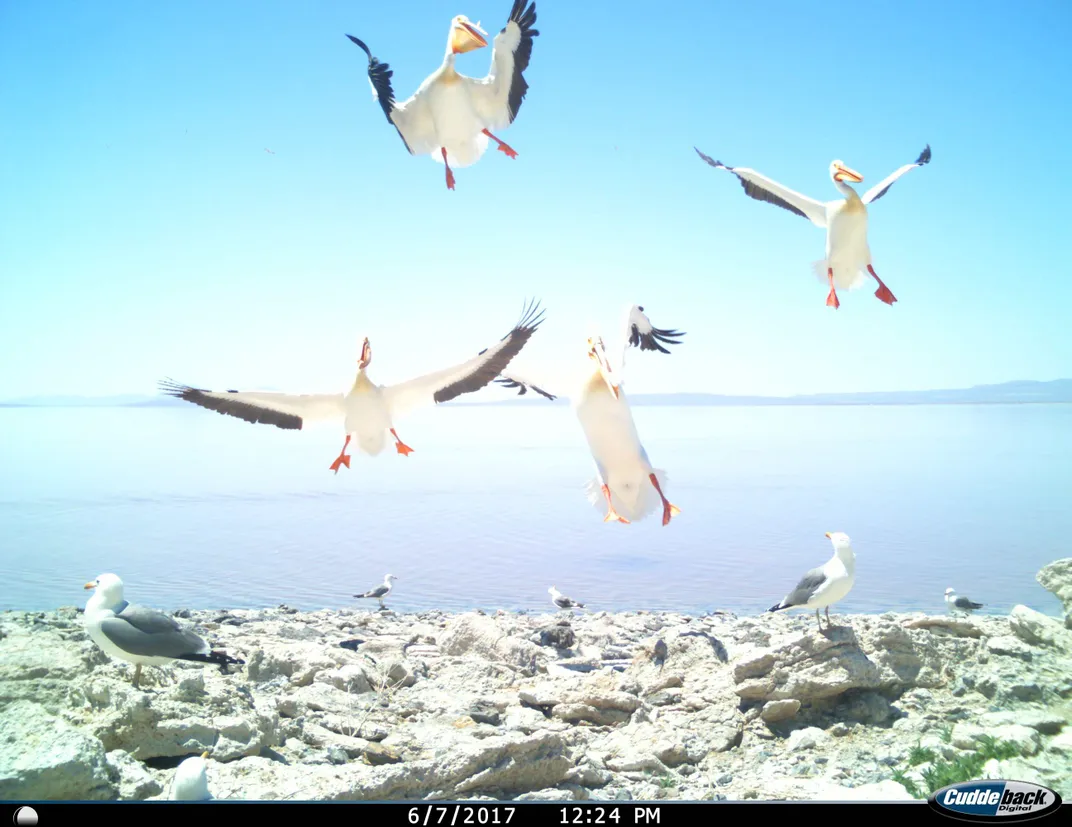
For the Birders
Birdwatching is one of the most common forms of citizen science . Seeing birds in the wilderness is certainly awe-inspiring, but you can birdwatch from your backyard or while walking down the sidewalk in big cities, too. With Cornell University’s eBird app , you can contribute to bird science at any time, anywhere. (Just be sure to remain a safe distance from wildlife—and other humans, while we social distance ). If you have safe access to outdoor space—a backyard, perhaps—Cornell also has a NestWatch program for people to report observations of bird nests. Smithsonian’s Migratory Bird Center has a similar Neighborhood Nest Watch program as well.
Birdwatching is easy enough to do from any window, if you’re sheltering at home, but in case you lack a clear view, consider these online-only projects. Nest Quest currently has a robin database that needs volunteer transcribers to digitize their nest record cards.
You can also pitch in on a variety of efforts to categorize wildlife camera images of burrowing owls , pelicans , penguins (new data coming soon!), and sea birds . Watch nest cam footage of the northern bald ibis or greylag geese on NestCams to help researchers learn about breeding behavior.
Or record the coloration of gorgeous feathers across bird species for researchers at London’s Natural History Museum with Project Plumage .
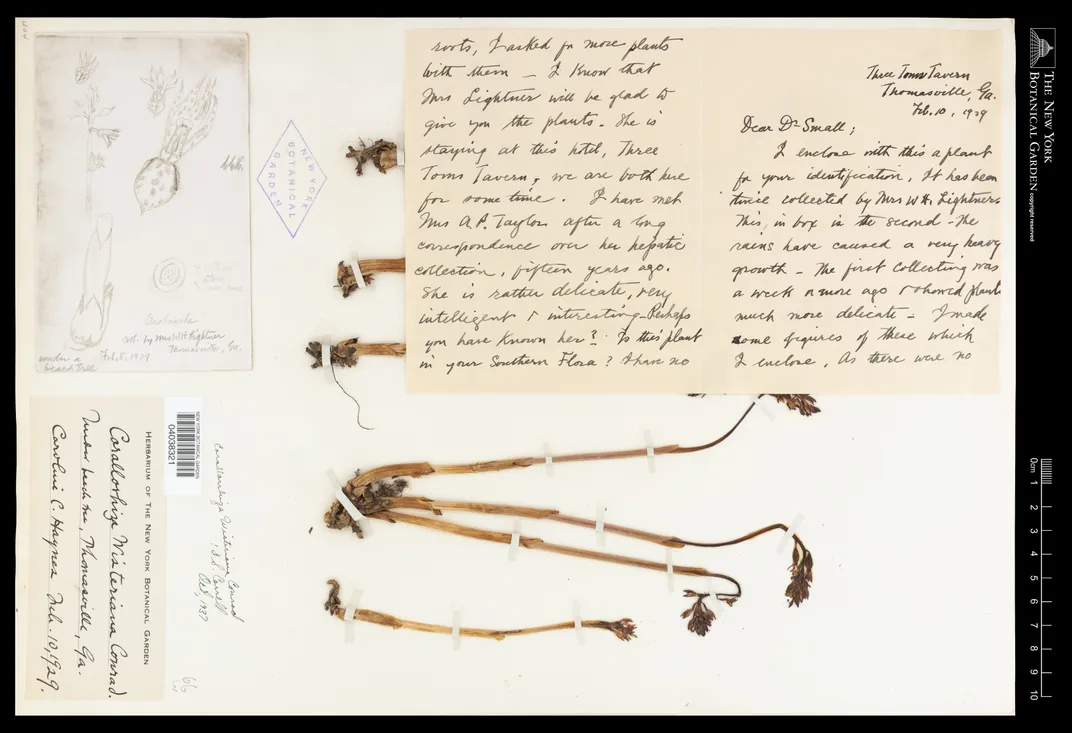
Pretty Plants
If you’re out on a walk wondering what kind of plants are around you, consider downloading Leafsnap , an electronic field guide app developed by Columbia University, the University of Maryland and the Smithsonian Institution. The app has several functions. First, it can be used to identify plants with its visual recognition software. Secondly, scientists can learn about the “ the ebb and flow of flora ” from geotagged images taken by app users.
What is older than the dinosaurs, survived three mass extinctions and still has a living relative today? Ginko trees! Researchers at Smithsonian’s National Museum of Natural History are studying ginko trees and fossils to understand millions of years of plant evolution and climate change with the Fossil Atmospheres project . Using Zooniverse, volunteers will be trained to identify and count stomata, which are holes on a leaf’s surface where carbon dioxide passes through. By counting these holes, or quantifying the stomatal index, scientists can learn how the plants adapted to changing levels of carbon dioxide. These results will inform a field experiment conducted on living trees in which a scientist is adjusting the level of carbon dioxide for different groups.
Help digitize and categorize millions of botanical specimens from natural history museums, research institutions and herbaria across the country with the Notes from Nature Project . Did you know North America is home to a variety of beautiful orchid species? Lend botanists a handby typing handwritten labels on pressed specimens or recording their geographic and historic origins for the New York Botanical Garden’s archives. Likewise, the Southeastern U.S. Biodiversity project needs assistance labeling pressed poppies, sedums, valerians, violets and more. Groups in California , Arkansas , Florida , Texas and Oklahoma all invite citizen scientists to partake in similar tasks.
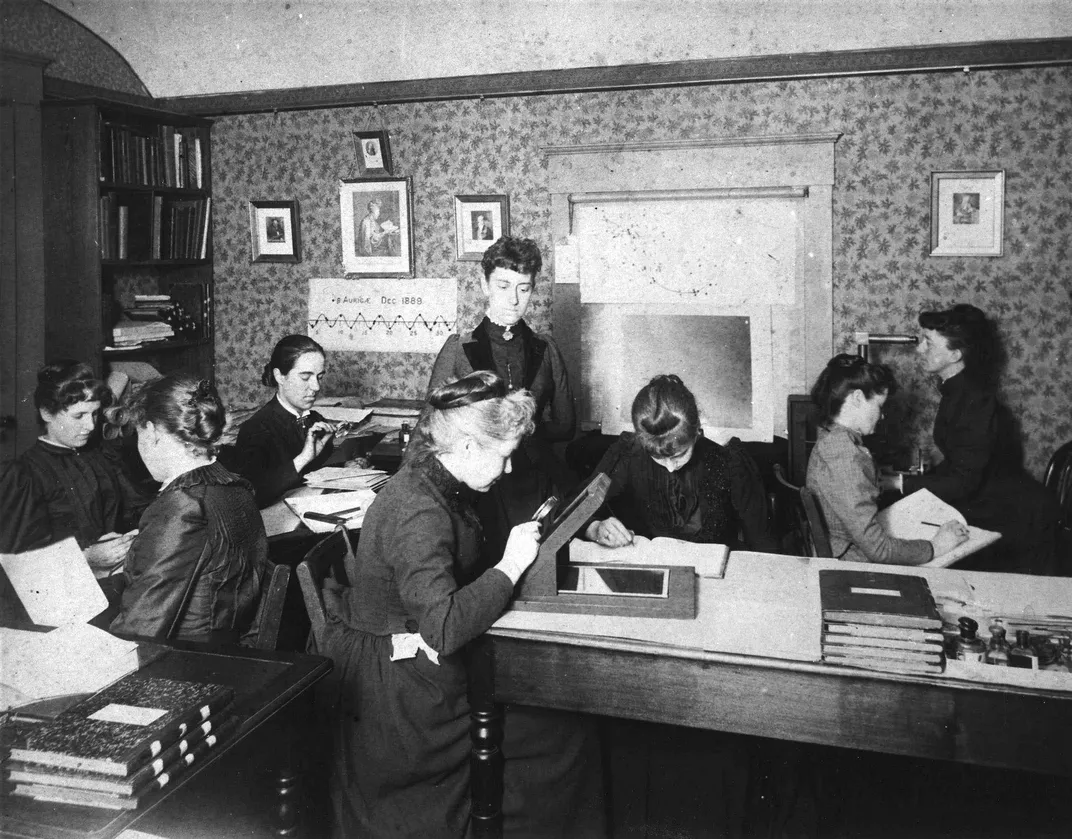
Historic Women in Astronomy
Become a transcriber for Project PHaEDRA and help researchers at the Harvard-Smithsonian Center for Astrophysics preserve the work of Harvard’s women “computers” who revolutionized astronomy in the 20th century. These women contributed more than 130 years of work documenting the night sky, cataloging stars, interpreting stellar spectra, counting galaxies, and measuring distances in space, according to the project description .
More than 2,500 notebooks need transcription on Project PhaEDRA - Star Notes . You could start with Annie Jump Cannon , for example. In 1901, Cannon designed a stellar classification system that astronomers still use today. Cecilia Payne discovered that stars are made primarily of hydrogen and helium and can be categorized by temperature. Two notebooks from Henrietta Swan Leavitt are currently in need of transcription. Leavitt, who was deaf, discovered the link between period and luminosity in Cepheid variables, or pulsating stars, which “led directly to the discovery that the Universe is expanding,” according to her bio on Star Notes .
Volunteers are also needed to transcribe some of these women computers’ notebooks that contain references to photographic glass plates . These plates were used to study space from the 1880s to the 1990s. For example, in 1890, Williamina Flemming discovered the Horsehead Nebula on one of these plates . With Star Notes, you can help bridge the gap between “modern scientific literature and 100 years of astronomical observations,” according to the project description . Star Notes also features the work of Cannon, Leavitt and Dorrit Hoffleit , who authored the fifth edition of the Bright Star Catalog, which features 9,110 of the brightest stars in the sky.
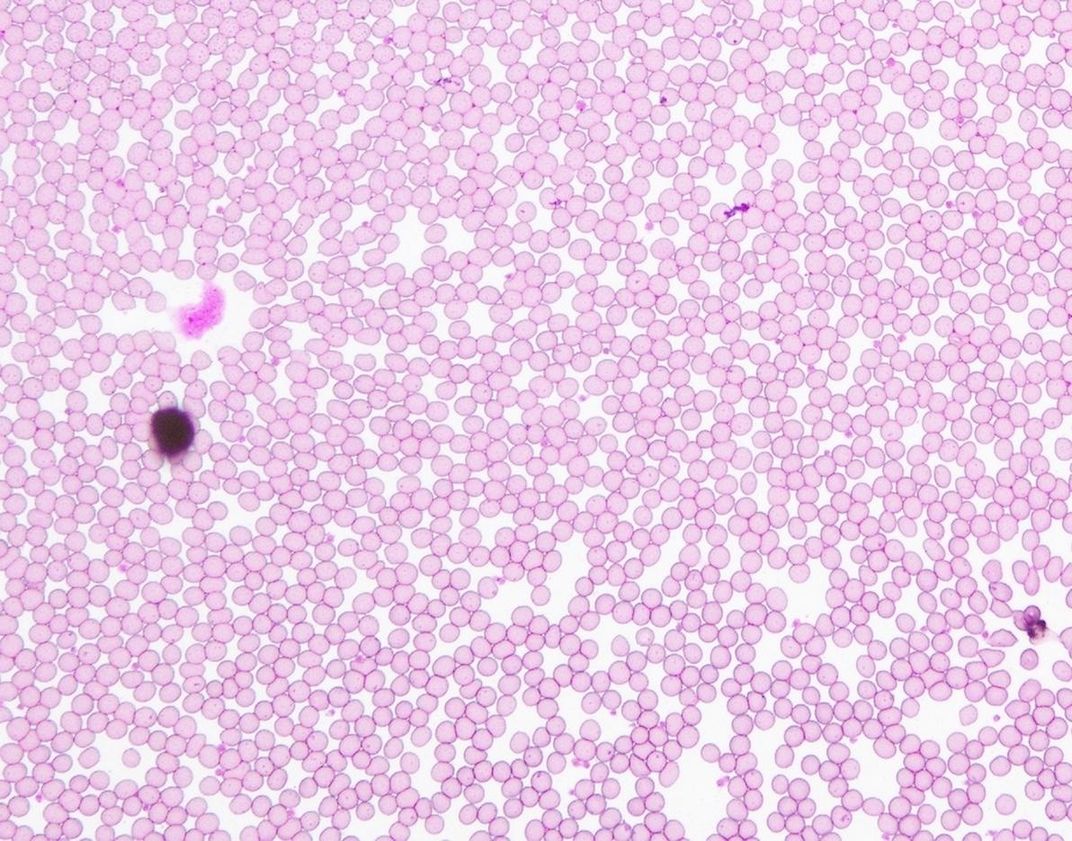
Microscopic Musings
Electron microscopes have super-high resolution and magnification powers—and now, many can process images automatically, allowing teams to collect an immense amount of data. Francis Crick Institute’s Etch A Cell - Powerhouse Hunt project trains volunteers to spot and trace each cell’s mitochondria, a process called manual segmentation. Manual segmentation is a major bottleneck to completing biological research because using computer systems to complete the work is still fraught with errors and, without enough volunteers, doing this work takes a really long time.
For the Monkey Health Explorer project, researchers studying the social behavior of rhesus monkeys on the tiny island Cayo Santiago off the southeastern coast of Puerto Rico need volunteers to analyze the monkeys’ blood samples. Doing so will help the team understand which monkeys are sick and which are healthy, and how the animals’ health influences behavioral changes.
Using the Zooniverse’s app on a phone or tablet, you can become a “ Science Scribbler ” and assist researchers studying how Huntington disease may change a cell’s organelles. The team at the United Kingdom's national synchrotron , which is essentially a giant microscope that harnesses the power of electrons, has taken highly detailed X-ray images of the cells of Huntington’s patients and needs help identifying organelles, in an effort to see how the disease changes their structure.
Oxford University’s Comprehensive Resistance Prediction for Tuberculosis: an International Consortium—or CRyPTIC Project , for short, is seeking the aid of citizen scientists to study over 20,000 TB infection samples from around the world. CRyPTIC’s citizen science platform is called Bash the Bug . On the platform, volunteers will be trained to evaluate the effectiveness of antibiotics on a given sample. Each evaluation will be checked by a scientist for accuracy and then used to train a computer program, which may one day make this process much faster and less labor intensive.
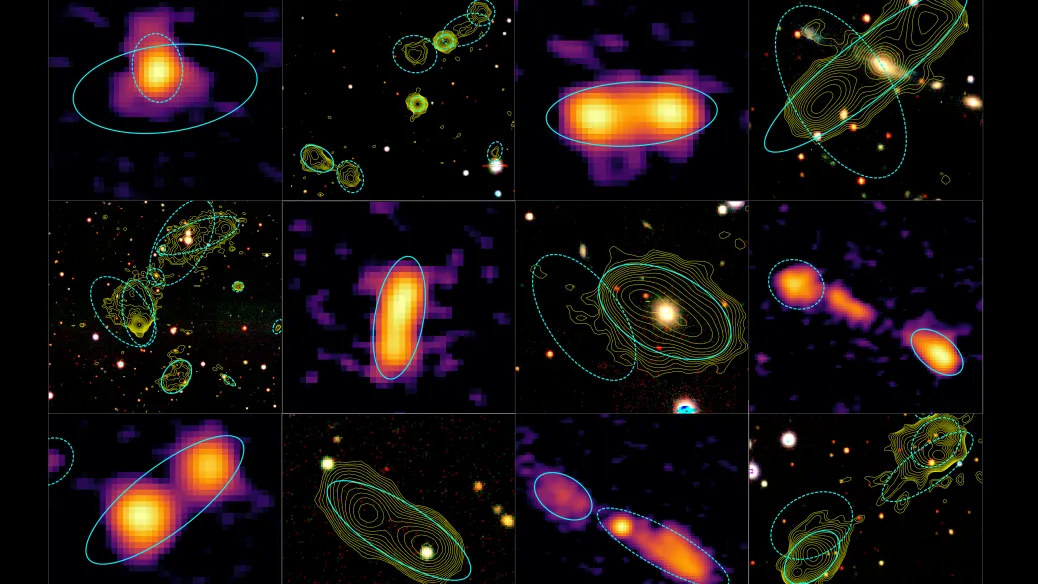
Out of This World
If you’re interested in contributing to astronomy research from the comfort and safety of your sidewalk or backyard, check out Globe at Night . The project monitors light pollution by asking users to try spotting constellations in the night sky at designated times of the year . (For example, Northern Hemisphere dwellers should look for the Bootes and Hercules constellations from June 13 through June 22 and record the visibility in Globe at Night’s app or desktop report page .)
For the amateur astrophysicists out there, the opportunities to contribute to science are vast. NASA's Wide-field Infrared Survey Explorer (WISE) mission is asking for volunteers to search for new objects at the edges of our solar system with the Backyard Worlds: Planet 9 project .
Galaxy Zoo on Zooniverse and its mobile app has operated online citizen science projects for the past decade. According to the project description, there are roughly one hundred billion galaxies in the observable universe. Surprisingly, identifying different types of galaxies by their shape is rather easy. “If you're quick, you may even be the first person to see the galaxies you're asked to classify,” the team writes.
With Radio Galaxy Zoo: LOFAR , volunteers can help identify supermassive blackholes and star-forming galaxies. Galaxy Zoo: Clump Scout asks users to look for young, “clumpy” looking galaxies, which help astronomers understand galaxy evolution.
If current events on Earth have you looking to Mars, perhaps you’d be interested in checking out Planet Four and Planet Four: Terrains —both of which task users with searching and categorizing landscape formations on Mars’ southern hemisphere. You’ll scroll through images of the Martian surface looking for terrain types informally called “spiders,” “baby spiders,” “channel networks” and “swiss cheese.”
Gravitational waves are telltale ripples in spacetime, but they are notoriously difficult to measure. With Gravity Spy , citizen scientists sift through data from Laser Interferometer Gravitational-Wave Observatory, or LIGO , detectors. When lasers beamed down 2.5-mile-long “arms” at these facilities in Livingston, Louisiana and Hanford, Washington are interrupted, a gravitational wave is detected. But the detectors are sensitive to “glitches” that, in models, look similar to the astrophysical signals scientists are looking for. Gravity Spy teaches citizen scientists how to identify fakes so researchers can get a better view of the real deal. This work will, in turn, train computer algorithms to do the same.
Similarly, the project Supernova Hunters needs volunteers to clear out the “bogus detections of supernovae,” allowing researchers to track the progression of actual supernovae. In Hubble Space Telescope images, you can search for asteroid tails with Hubble Asteroid Hunter . And with Planet Hunters TESS , which teaches users to identify planetary formations, you just “might be the first person to discover a planet around a nearby star in the Milky Way,” according to the project description.
Help astronomers refine prediction models for solar storms, which kick up dust that impacts spacecraft orbiting the sun, with Solar Stormwatch II. Thanks to the first iteration of the project, astronomers were able to publish seven papers with their findings.
With Mapping Historic Skies , identify constellations on gorgeous celestial maps of the sky covering a span of 600 years from the Adler Planetarium collection in Chicago. Similarly, help fill in the gaps of historic astronomy with Astronomy Rewind , a project that aims to “make a holistic map of images of the sky.”
Get the latest Science stories in your inbox.
/https://tf-cmsv2-smithsonianmag-media.s3.amazonaws.com/accounts/headshot/rachael.png)
Rachael Lallensack | READ MORE
Rachael Lallensack is the former assistant web editor for science and innovation at Smithsonian .
- International edition
- Australia edition
- Europe edition
Can TikToks reduce gun violence? Ask these teenagers
Project Unloaded invited students in Chicago to create online marketing campaigns – here’s what happened
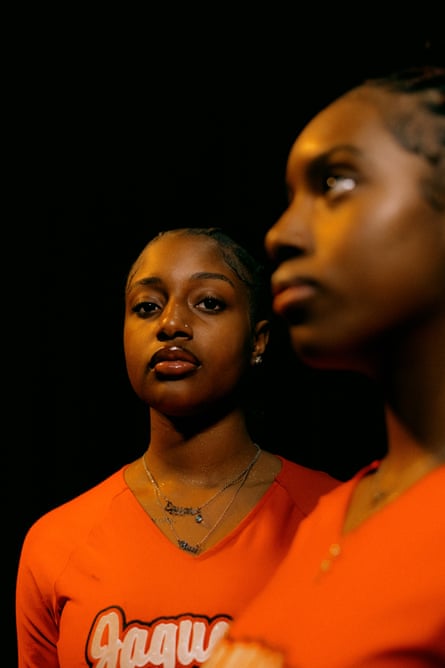
This story is a collaboration between the Guardian and the Trace , a non-profit newsroom covering guns in America. A more detailed version of the story can be found here .
Once upon a time in America, people rolled their eyes at seatbelts, four out of 10 high school students smoked cigarettes, and campers left their fires smoldering through the night. Then we changed. Not primarily because of policy reforms, but because teams of marketers crafted public health messaging campaigns in which chatty crash test dummies told us to buckle up, teens piled 1,200 body bags in front of the Philip Morris headquarters, and Smokey the Bear identified who can prevent forest fires.
Recently, big players in gun violence prevention have begun asking whether this tradition of transforming the US through advertising might be used to reform our relationship to guns.
Since its inception, the gun violence prevention movement has invested the bulk of its time and money in a top-down strategy: change gun policy in courtrooms and statehouses, the thinking goes, and the trajectory of gun violence in America will change too. But despite spending hundreds of millions of dollars on this approach and enjoying the support of most of the American public, the movement has failed to budge the metrics that matter most. Gun deaths have been climbing for decades, and in 2020, guns surpassed car accidents as the leading cause of death for young people. These losses have persuaded some gun reform advocates that it’s time to try a different strategy: go directly to the people.
For the first time, this bottom-up approach has been drawing major attention and funding in the gun violence prevention world. The Ad Council – kingmaker in the realm of public service announcements – is in various stages of campaign development with three separate gun violence prevention groups. Meanwhile, the Biden administration has been building out its own gun-safety messaging efforts focused on service members and veterans and, just last month, on red-flag laws .
But one of the most ambitious efforts to change Americans’ relationship to guns is being led by a non-profit founded two years ago, called Project Unloaded. Rather than trying to improve how existing gun owners handle their weapons, or curry public support for a specific policy, Project Unloaded is using marketing to attack the problem at the heart of gun violence: too many households own guns, and more access to guns leads to more deaths and injuries .
Project Unloaded has flooded the social media feeds of young people in a dozen cities with ads and influencer content about the threat that owning a gun poses, reaching more than 2 million teens an average of 30 times. And last summer, the group experimented with deeper in-person engagement on Chicago’s South Side, where gun violence is not an abstract problem. For six weeks last summer, 50 students from Chicago Public Schools were paid to design and implement a bespoke public-messaging campaign that several teenagers say fundamentally changed the way they saw guns – and made them question whether they want to own one.
A single statistic led to Project Unloaded’s founding: a Gallup poll found that the portion of Americans who believe that having a gun in the house makes it a safer place had jumped from 35% to 63% between 2000 and 2014.
The rise rattled Nina Vinik, the project’s founder and a civil rights attorney who has long been a player in the gun violence prevention world – not just because piles of public health research show the belief to be false, but because the falsehood has nonetheless been accepted by many people who are not part of the traditional gun-owning demographic. Women believe it. People of color believe it. Young people believe it.
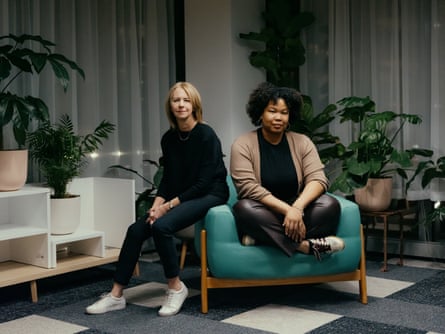
It occurred to Vinik that while the gun industry had been aggressively pushing the message that guns are the solution to an unsafe world, there’s been no major push back with the empirical truth, uncovered in study after study: in an unsafe world, owning a gun makes you more vulnerable. Having a gun in the house makes suicide attempts more deadly, shooting accidents more common and domestic disputes more dangerous.
Project Unloaded’s wager is that if enough young people know the facts, and even a portion of them choose not to arm themselves, America’s gun death trends will, at long last, reverse course.
Focusing on young people makes sense. Half of gen Zers report that they think about mass shootings at least once a week, which isn’t surprising given that most were taught how to respond to an active school shooter before they were taught to read. Yet, even more than their elders do, young people have come to believe the myth that guns provide safety: 68% of people under 34 believe a gun makes a house a safer place, compared with 61% of baby boomers.
When Vinik first learned of that contradiction, it struck her as an opportunity. Like older adults, young people have absorbed the falsehood. But it hasn’t yet been cemented into their worldview.
Project Unloaded launched its first campaign in 2022. It was dubbed Snug, short for Safer Not Using Guns and, handily, the word “guns” backwards.
The campaign’s ads featured bright colors, lo-fi beats and simple, shorthand messages: “DYK… SUICIDES R 4X HIGHER 4 KIDS W/ GUNS @ 🏠… R U SNUG?” reads their top-performing 15-second ad . They placed ads on TikTok, Snapchat and Instagram in 12 cities, and have paid influencers to talk about the #yousnug campaign. One hair care influencer styled her curls while explaining that a gun can make domestic violence disputes more deadly. An actor and comedian in New York City, sipping her morning iced coffee, talked about finding herself in an active shooter situation on the subway. “That’s why I choose to be Snug,” she concluded.
Meanwhile, Project Unloaded hired a dozen teenagers from around the US, whom they pay a stipend for providing feedback and TikToks. Vinik’s team runs all ad content by this youth council, Anvesha Guru, a 16-year-old high school senior from Wisconsin and the council’s social media chair, told me: “With the Snug ads, they were like: ‘Are these colors too bright for young people? Will they find it cringy?’”
In addition to giving advice and making content, the council has taken on its own projects. This year, they launched a teen-focused SMS text program that sends out gun-safety stats, and are developing a toolkit for student clubs. They also published an independent research project showing that during the Hollywood labor strikes, depictions of gun violence shrank significantly without affecting ratings.
Most of the teens on Project Unloaded’s youth council grew up in places where shootings are most commonly self-inflicted. But could the messaging strategy work in a place like Chicago’s South Side, one of the rare places in the US where firearm homicides significantly outnumber firearm suicides?
Last July, Project Unloaded paid 50 Chicago Public School students to create a campaign against gun violence. The teenagers, who were connected with Project Unloaded after signing up for a youth summer jobs program, were split into teams and tasked with researching public health literature on gun ownership and violence. After that, they would work with professional mentors to develop a marketing campaign informing other young people of the facts.
But within days, some students were so restive that there was talk in the district of aborting the pilot and reassigning students to other jobs. Some students felt the assignment was dumb: they could do it, but why? It’s not like anyone listened to teenagers anyway. A couple of students reasoned that gun violence was a problem caused by adults, and it was wrong to ask kids to fix it on their summer break. One said she went to bed every night wondering if she’d wake up to a call that her brother had been shot. Googling studies on gun violence felt emotionally taxing.
District officials listened and tried to affirm their concerns. The group brainstormed how to make the project feel more authentic and useful to them and their peers. By the third hour of talking things through, the students said they were feeling better. The next day they got to work.
I told this story to Selwyn Rogers, founding director of the University of Chicago’s trauma and acute care center, the place most people on the South Side go for treatment if they are shot. Rogers, who also sits on Project Unloaded’s steering committee, wasn’t surprised. The young people he speaks with have divided feelings about guns. On one hand, they greatly fear them. On the other, having a gun gives them a sense of control over their life.
Still, Rogers said, there’s a role for Project Unloaded in places like the South Side. Gun violence, he said, is neither inevitable, nor unshakable. “You could never convince a 76-year-old guy that they should give up the gun they’ve had their entire life because they’re battling depression after their wife died,” he said. Young people are different: “Kids can change,” he said.
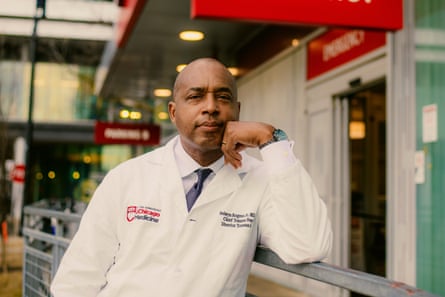
I asked everyone I spoke to for this story what they see as the closest historical analogue to these new cultural efforts on guns. Most pointed to the campaign against drunk driving, another effort of the Ad Council. In 1983, a time when “one more for the road” was still America’s general vibe, the first Drinking and Driving Can Kill a Friendship ad appeared on TV. A few years later, the ads adopted the term “designated driver”. Alcohol-fueled auto fatalities have dropped for a number of reasons; most analyses show that public health messaging has played a sizable role.
But none of the campaigns that have taken on America’s stickiest problems – drunk driving, teen smoking, seatbelts, littering – and found success are perfect parallels to gun campaigns, because guns occupy a unique place in American politics. There’s no pro-littering lobby, nor a constitutional amendment protecting Americans’ right to resist seatbelts. Crucially, these historical analogues got help from policymakers, who implemented smoking bans, drinking age increases and littering fines.
Still, Vinik says, there’s hope. Surveys indicate that at least 15% of teens repeatedly exposed to Project Unloaded’s messages shifted their view on whether owning a gun would make them safer, the group says.
Michael Anestis, the executive director of the New Jersey gun violence research center at Rutgers, has devoted much of his research in recent years to studying what makes an effective firearm suicide prevention messaging campaign. When I first spoke with him, he wasn’t familiar with Project Unloaded or the Snug campaign, but liked the premise. One of the gun violence prevention movement’s “great failures”, he said, has been allowing the gun industry’s messages to “go into a vacuum”, with no pushback.
But he wondered whether a campaign like Snug might work better if it offered an alternative, like how the drunk-driving campaign suggested designated drivers. What if, he posited, a campaign against gun violence did the same: don’t feel safe at home? Consider an alarm system. Or maybe adopt a dog. They both keep you safer than a gun would.
Even if messaging is fruitful, I asked Vinik: can America’s gun culture change without major policy wins prodding it along? The gun violence prevention movement has made policy inroads in some states, but has backslid in many others, and state borders are porous. The federal gun safety law passed in 2021 – the first of its kind in decades – is often criticized as toothless. The supreme court 2022 Bruen decision will make gun restrictions harder to pass and maintain, because it requires them to have historical precedents from the nation’s earliest era.
Vinik admitted that cultural campaigns have their limits. “There’s no messaging campaign that’s going to solve this problem on its own,” she said. Then she threw the question back: would the policy fights have been more successful if they’d happened alongside efforts to change our culture? She’s convinced the answer is yes.
Each of the three groups in Project Unloaded’s summer jobs pilot program in Chicago gave themselves a name. Best friends Arinek Shorter and Egypt Paige, both 17, were on a team that dubbed itself Peace Love Justice, or PLJ.
On the first day of their summer jobs pilot program, Olivia Brown, Project Unloaded’s program director, handed out a survey to see where the students stood on gun ownership. Twenty per cent said they didn’t plan to be gun owners, and another 19% weren’t sure. The majority said they would either probably or definitely own a gun one day.
Both Arinek and Egypt found the data showing that guns don’t make you safer persuasive, but other members were less moved. “They felt that guns will protect them from all the dangers in Chicago,” said Egypt. “But we showed them the research and it’s like, no, this is not what’s keeping you safe, this is what’s putting you in harm’s way.”
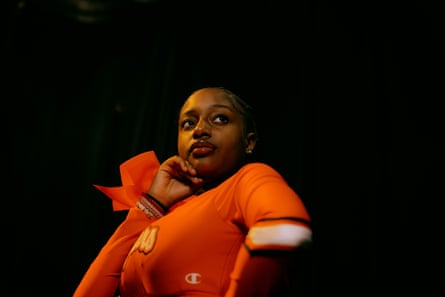
The team found themselves debating the issue over and over. Arinek understood why many of her peers disagreed with her change of heart. They were the product of an environment that lacked resources, opportunity and safety. “In our community, all we know is guns. All we know is that it’s a dog-eat-dog world. And so they’re like: ‘If they have a gun, why can’t I have a gun?’” she said.
It was in the middle of one of these debates that the team came up with the marketing slogan they would eventually use for their marketing campaign: “A lot of people kept saying: ‘Guns, they help you, they give you power, they keep you safer,’ and it was just like a click – like: ‘Guns don’t give you power!”
The phrase quickly became a refrain. “Every time somebody said they need a gun to be safe, we would hit them with: ‘Guns don’t give you power’ – and let me give you the facts and statistics why they don’t,” said Arinek, who aspires one day to be an attorney.
On the last day of the program, the three teams presented their marketing proposals to an assembly of the 500 other teens in the district’s summer jobs programs. Chicago’s mayor, Brandon Johnson, was on hand to judge; the winning proposal would be incorporated into Project Unloaded’s next major campaign, which the organization was developing specifically to share in communities with high levels of community violence.
The first group’s campaign slogan was: “Enough is enough – change the lyrics!” They proposed asking hip-hop artists and producers to create a “gun edit” of their songs that left out language glorifying gun violence. The second campaign, “Watch where you aim”, encouraged teens to aim higher than a life of gun violence.
Arinek and Egypt’s team went last. Arinek introduced their theme, and Egypt, who aims to one day be a doctor, followed with a skit debunking gun myths. Next, their group performed a spoken-word poem they had collectively written on the theme “Guns don’t give you power”.
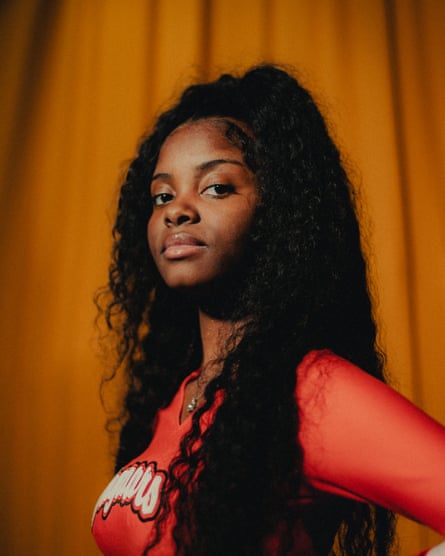
“When I see a gun, my mind automatically tells me to run,” Egypt began. “Run from the pain. Run from the shame. Run from the streets calling my name.” Arinek’s lines came later: “Fear of love. Fear of fun. Fear of letting my guard down. knowing that one gun can take my life. Fear that I might not even make it home tonight. Fear that my dreams are no longer in sight.”
When the votes were in, Peace Love Justice had won. The team erupted in cheers.
Before the students left, Vinik and Brown tried to wrangle them into taking another survey, but many of them had already headed for the doors. Of the 18 who took it, 20% had moved from “probably” or “definitely will own a gun” to “undecided/unsure”. Vinik counted that as a win.
Among the students who did not fill out the survey that day was a young man on a team called the Strikers. He had been unable to help deliver their “Watch where you aim” presentation, because he was recuperating. Not long before, he had been shot in the leg.
- Marketing & PR
- Guns and lies
- US gun control
Most viewed

An official website of the United States government
The .gov means it’s official. Federal government websites often end in .gov or .mil. Before sharing sensitive information, make sure you’re on a federal government site.
The site is secure. The https:// ensures that you are connecting to the official website and that any information you provide is encrypted and transmitted securely.
Environmental Factor
Your online source for niehs news, researchers team up with tribe, community to fight pfas with plants.
NIEHS Superfund researchers, Mi’kmaq Nation use hemp and nanomaterials to combat contaminants.
By Mali Velasco
Scientists supported by the NIEHS Superfund Research Program (SRP) together with community and tribal members are using phytoremediation to remove PFAS from a contaminated site in northern Maine. Phytoremediation is a technique that takes advantage of plants’ ability to take up and accumulate hazardous substances from the environment.
To boost the plants’ uptake of PFAS, the research team also plans to use nanoparticles made from silica, a chemical that is the main constituent of most rocks and minerals, and small carbon nanoparticles, called carbon dots .
“I hope that phytoremediation can become a feasible option for removing PFAS from soil,” said project researcher Sara Nason, Ph.D., of the Connecticut Agricultural Experiment Station (CAES). “Current options are very limited overall, and phytoremediation on its own is not effective enough to produce the results many industries are seeking.”
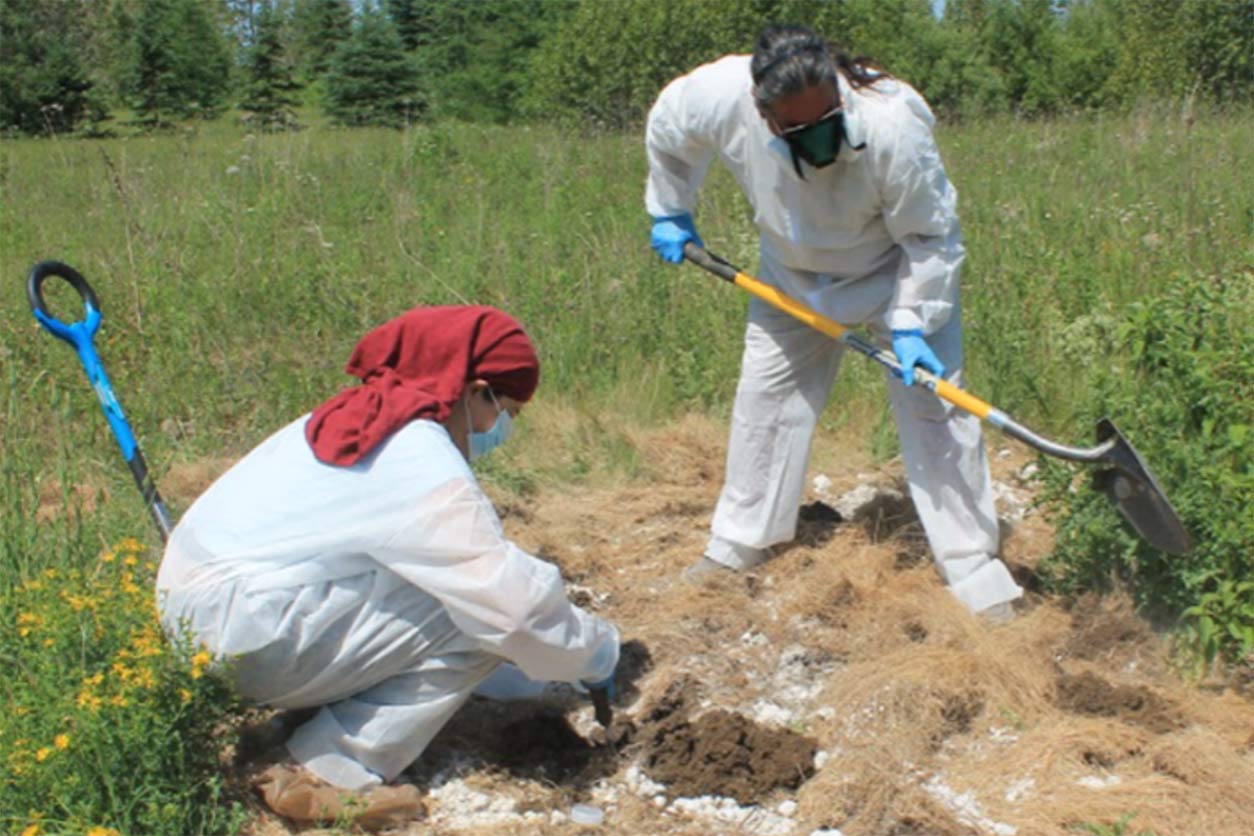
The start of a collaborative project
In 2019, members of the Mi’kmaq Nation, an Indigenous tribe of about 1,500 people, and Upland Grassroots contacted CAES, a state government research organization. The goal was to kick-start a project using fiber hemp plants to remove PFAS from contaminated water and soil on land belonging to the Aroostook Band of the Mi’kmaq Nation. The land was formerly the site of Loring Air Force Base, which had for decades been a firefighting testing area. Firefighting foams usually contain PFAS because of the chemicals’ ability to suppress fire.
The collaborators chose fiber hemp because it grows quickly, takes up large amounts of water, and is usually not grazed by livestock. In addition, parts of the plant that are less suitable for PFAS storage, such as stems, may be used by tribal members to make products such as bricks and rope.
However, hemp plants are not able to remove all PFAS from soil and water because some of the molecules stay stuck in the soil. To address this challenge, CAES teamed up with researchers at Yale University and the University of Minnesota to study how nanomaterials can improve the ability of plants to absorb PFAS. SRP began to fund this research in 2021.
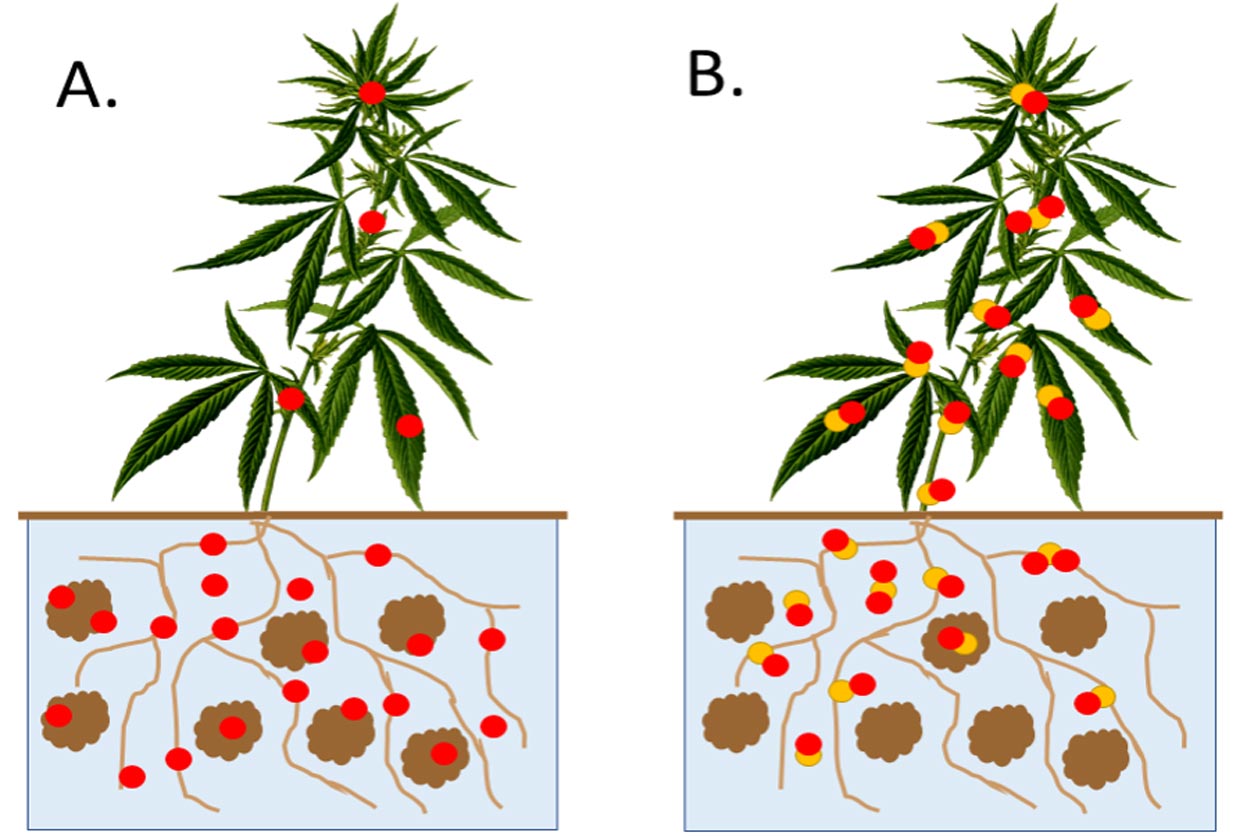
Nurturing community-based research
“I am proud that what started as a small pilot project working with Mi’kmaq community members and local organizations has grown into a large, multi-institution effort that still has ties to the community where the work started,” Nason said. “Having CAES staff funded by our SRP project has enabled us to be a stronger participant in remediation work with the tribe, while also pursuing new technologies to improve our current remediation options.”
While the nanomaterials are not yet ready for deployment in the field, work at the former Loring site has continued. CAES scientists work closely with Upland Grassroots and tribal leaders to develop plans for soil sample collection and hemp planting that allows the community to conduct most of the field research, while producing scientifically useful data.
“Listening to the needs of community researchers and designing projects around their interests and capabilities has been key to our success,” Nason said. “I hope that our work with Upland Grassroots and the Mi’kmaq Nation can be an example to other scientists doing community-based research.”
Citations : Nason SL, Thomas S, Stanley C, Silliboy S, Blumenthal M, Zhang W, Liang Y, Jones JP, Zuverza-Mena N, White JC, Haynes CL, Vasiliou V, Timko MP, Berger BW. 2024. A comprehensive trial on PFAS remediation: hemp phytoextraction and PFAS degradation in harvested plants . Env Sci Adv 3(2):304-313.
Lewis RE, Huang CH, White JC, Haynes CL. 2023. Using 19F NMR to investigate cationic carbon dot association with per- and polyfluoroalkyl substances (PFAS) . ACS Nanosci Au 3(5):408-417.
(Mali Velasco is a research and communication specialist for MDB Inc., a contractor for the NIEHS Division of Extramural Research and Training.)
Modified Iron Particles Could Improve Bioremediation of PFAS
This September 2023 NIEHS video describes how SRP-funded researchers at Princeton University are using nanoparticles to improve the ability of a type of bacteria to break down PFAS in the environment. (1:18)
Related Articles
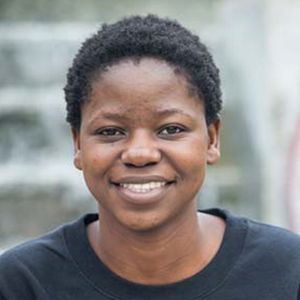
Oral histories shed light on environmental injustice in Louisiana
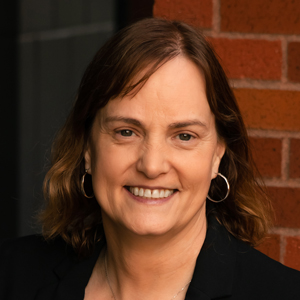
Zebrafish represent key piece to toxicology puzzle, Robyn Tanguay says
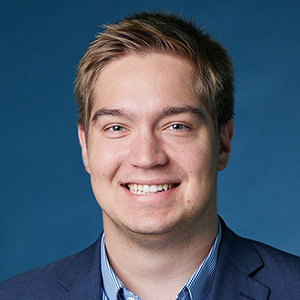
Rollie Mills wins 2023 Wetterhahn Award
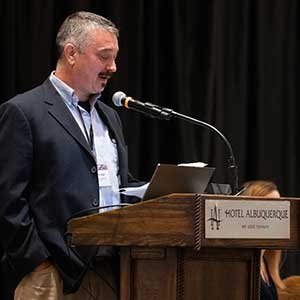
Collaboration and training key to Superfund success
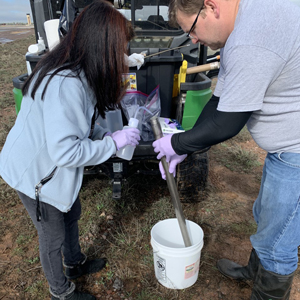
For PFAS-polluted sites, forum highlights best research practices
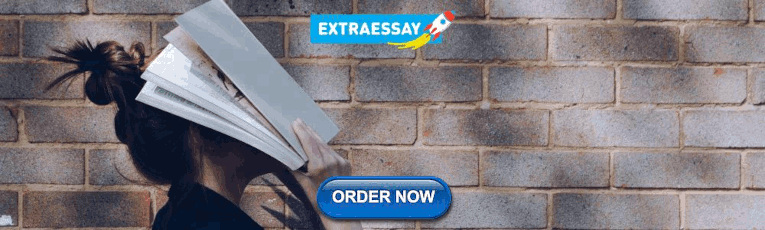
IMAGES
VIDEO
COMMENTS
The present research report is concentrating on quantifying the impact of online advertising on consumer buying behaviour, which raises the consumers' intentions towards the product and buying ...
This is due to the fact that 'consuming in shops' is changing to 'online consuming'. Companies are using different online marketing strategies to attract prospective buyers. Different tools and techniques are used to influence the purchasing decision of consumers. This case study on online marketing, research through survey and analysis ...
The amount of research related to Internet marketing has grown rapidly since the dawn of the Internet Age. A review of the literature base will help identify the topics that have been explored as well as identify topics for further research. This research project collects, synthesizes, and analyses both the research strategies (i.e., methodologies) and content (e.g., topics, focus, categories ...
Time and the Value of Data. by Ehsan Valavi, Joel Hestness, Newsha Ardalani, and Marco Iansiti. This paper studies the impact of time-dependency and data perishability on a dataset's effectiveness in creating value for a business, and shows the value of data in the search engine and advertisement businesses perishes quickly. 19 May 2020.
Online advertisements have become one of today's most widely used tools for enhancing businesses partly because of their compatibility with A/B testing. A/B testing allows sellers to find effective advertisement strategie,s such as ad creatives or segmentations. Even though several studies propose a technique to maximize the effect of an advertisement, there is insufficient comprehension of ...
SWOT Analysis Template. A SWOT (Strengths, Weaknesses, Opportunities, Threats) analysis looks at your internal strengths and weaknesses, and your external opportunities and threats within the market. A SWOT analysis highlights direct areas of opportunity your company can continue, build, focus on, and work to overcome.
Digital native advertising is a subtle form of digital advertising that is integrated closely with its context. Practitioners are increasingly assigning budgets to this advertising strategy. On the basis of 22 in-depth expert interviews with senior executives of advertising brands, publishing companies, and media agencies, this study provides ...
Finding and choosing a strong research topic is the critical first step when it comes to crafting a high-quality dissertation, thesis or research project. If you've landed on this post, chances are you're looking for a marketing-related research topic, but aren't sure where to start.If so, you've come to the right place.
Deep, ongoing market research is one of the best ways to truly understand your customers. Whether over the phone, on Skype, or over coffee, one-on-one conversations are often the most effective ways to get inside your target market's head. If you're starting market research, I recommend this as your first step.
How to conduct lean market research in 4 steps. The following four steps and practical examples will give you a solid market research plan for understanding who your users are and what they want from a company like yours. 1. Create simple user personas. A user persona is a semi-fictional character based on psychographic and demographic data ...
Integrate with 100+ apps and plug-ins to get more done. SurveyMonkey Forms. Build and customize online forms to collect info and payments. SurveyMonkey Genius. Create better surveys and spot insights quickly with built-in AI. Market Research Solutions. Purpose-built solutions for all of your market research needs. INDUSTRIES.
Software like Similarweb can give you highly relevant, up-to-date information about any market, company, or topic. Most types of online market research benefit from using digital intelligence tools. The data is trusted, it's dynamic, and it's one of the quickest types of online market research you can do.
Nonprofit Marketing. Market Segmentation & Targeting. Internet Marketing. Marketing Planning & Forecasting. Product Design & Positioning. Direct Marketing. Advertising. Purchasing & Materials Management. Hopefully, these marketing thesis topics will help you come up with a few topics of your own.
June 3, 2021 28 min read. Market research is a process of gathering, analyzing, and interpreting information about a given market. It takes into account geographic, demographic, and psychographic data about past, current, and potential customers, as well as competitive analysis to evaluate the viability of a product offer.
Online market research is extremely versatile and can be harnessed to explore a broad range of challenges, including: Better understanding your target market. Product or service development. Brand and advertising research to guide marketing strategy. Determining customer satisfaction.
Learning Outcomes. By the end of this section, you will be able to: 1 Identify and describe the steps in a marketing research plan. 2 Discuss the different types of data research. 3 Explain how data is analyzed. 4 Discuss the importance of effective research reports.
Online market research is a crucial first step in making a variety of business decisions, including: Starting a business. Creating a pricing strategy. Launching new product or service. Entering a new market. Drafting a marketing campaign. There are a whole host of benefits that make market research helpful in the above situations.
Science | June 15, 2020. Seventy-Five Scientific Research Projects You Can Contribute to Online. From astrophysicists to entomologists, many researchers need the help of citizen scientists to sift ...
1. INTRODUCTION Advertising is a way of c 0mmunicati n t0 enc urage an audience f r making purchase decisi 0n ab 0ut a pr duct r service and c nveying inf 0rmati n t0 viewers. It is c0nsidered as a vital and essential element f 0r the ec 0n mic gr wth 0f the marketers and businesses.Advertising is usually a paid f 0rm f exp 0sure r pr m0ti0n by s 0me sp ns r that
A Systematic Review. Mohammed T. Nuseir , Ghaleb A. El Refae, Ahmad Aljumah, Muhammad Alshurideh , Sarah Urabi, and Barween Al Kurdi. Abstract The aim of this study is to explore the contemporary ...
Project Unloaded invited students in Chicago to create online marketing campaigns - here's what happened High school students Egypt Paige, left, and Aeja Hood at Percy Julian high school in ...
The start of a collaborative project. In 2019, members of the Mi'kmaq Nation, an Indigenous tribe of about 1,500 people, and Upland Grassroots contacted CAES, a state government research organization. The goal was to kick-start a project using fiber hemp plants to remove PFAS from contaminated water and soil on land belonging to the Aroostook ...
Developing and Validating the Qualitative Characteristics of Children's Play Assessment System Supported by Caplan Foundation Project led by: PI: Michael Haslip, PhD This one-year project in the McNichol ECE lab is validating an assessment created to measure young children's play skill development, called the Qualitative Characteristics of Children's Play or QCCP, and building a new ...