- En español – ExME
- Em português – EME
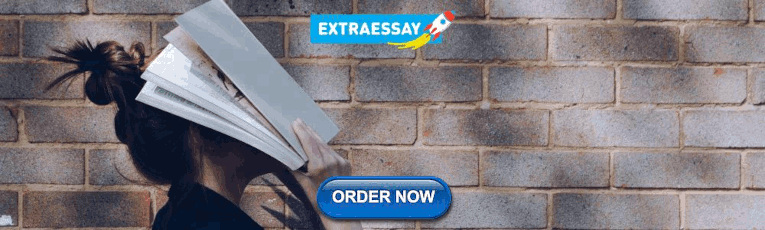
An introduction to different types of study design
Posted on 6th April 2021 by Hadi Abbas
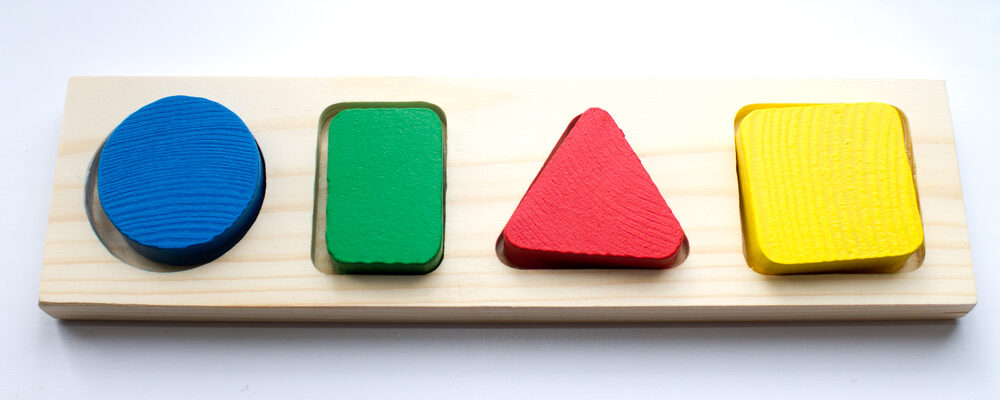
Study designs are the set of methods and procedures used to collect and analyze data in a study.
Broadly speaking, there are 2 types of study designs: descriptive studies and analytical studies.
Descriptive studies
- Describes specific characteristics in a population of interest
- The most common forms are case reports and case series
- In a case report, we discuss our experience with the patient’s symptoms, signs, diagnosis, and treatment
- In a case series, several patients with similar experiences are grouped.
Analytical Studies
Analytical studies are of 2 types: observational and experimental.
Observational studies are studies that we conduct without any intervention or experiment. In those studies, we purely observe the outcomes. On the other hand, in experimental studies, we conduct experiments and interventions.
Observational studies
Observational studies include many subtypes. Below, I will discuss the most common designs.
Cross-sectional study:
- This design is transverse where we take a specific sample at a specific time without any follow-up
- It allows us to calculate the frequency of disease ( p revalence ) or the frequency of a risk factor
- This design is easy to conduct
- For example – if we want to know the prevalence of migraine in a population, we can conduct a cross-sectional study whereby we take a sample from the population and calculate the number of patients with migraine headaches.
Cohort study:
- We conduct this study by comparing two samples from the population: one sample with a risk factor while the other lacks this risk factor
- It shows us the risk of developing the disease in individuals with the risk factor compared to those without the risk factor ( RR = relative risk )
- Prospective : we follow the individuals in the future to know who will develop the disease
- Retrospective : we look to the past to know who developed the disease (e.g. using medical records)
- This design is the strongest among the observational studies
- For example – to find out the relative risk of developing chronic obstructive pulmonary disease (COPD) among smokers, we take a sample including smokers and non-smokers. Then, we calculate the number of individuals with COPD among both.
Case-Control Study:
- We conduct this study by comparing 2 groups: one group with the disease (cases) and another group without the disease (controls)
- This design is always retrospective
- We aim to find out the odds of having a risk factor or an exposure if an individual has a specific disease (Odds ratio)
- Relatively easy to conduct
- For example – we want to study the odds of being a smoker among hypertensive patients compared to normotensive ones. To do so, we choose a group of patients diagnosed with hypertension and another group that serves as the control (normal blood pressure). Then we study their smoking history to find out if there is a correlation.
Experimental Studies
- Also known as interventional studies
- Can involve animals and humans
- Pre-clinical trials involve animals
- Clinical trials are experimental studies involving humans
- In clinical trials, we study the effect of an intervention compared to another intervention or placebo. As an example, I have listed the four phases of a drug trial:
I: We aim to assess the safety of the drug ( is it safe ? )
II: We aim to assess the efficacy of the drug ( does it work ? )
III: We want to know if this drug is better than the old treatment ( is it better ? )
IV: We follow-up to detect long-term side effects ( can it stay in the market ? )
- In randomized controlled trials, one group of participants receives the control, while the other receives the tested drug/intervention. Those studies are the best way to evaluate the efficacy of a treatment.
Finally, the figure below will help you with your understanding of different types of study designs.
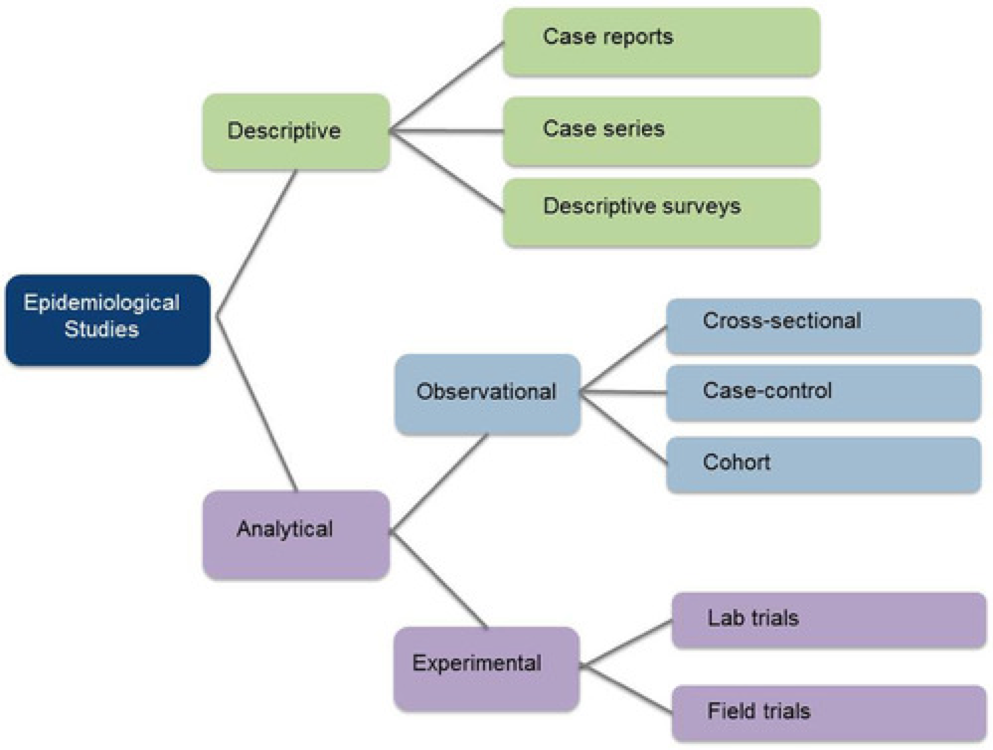
References (pdf)
You may also be interested in the following blogs for further reading:
An introduction to randomized controlled trials
Case-control and cohort studies: a brief overview
Cohort studies: prospective and retrospective designs
Prevalence vs Incidence: what is the difference?
Leave a Reply Cancel reply
Your email address will not be published. Required fields are marked *
Save my name, email, and website in this browser for the next time I comment.
No Comments on An introduction to different types of study design
you are amazing one!! if I get you I’m working with you! I’m student from Ethiopian higher education. health sciences student
Very informative and easy understandable
You are my kind of doctor. Do not lose sight of your objective.
Wow very erll explained and easy to understand
I’m Khamisu Habibu community health officer student from Abubakar Tafawa Balewa university teaching hospital Bauchi, Nigeria, I really appreciate your write up and you have make it clear for the learner. thank you
well understood,thank you so much
Well understood…thanks
Simply explained. Thank You.
Thanks a lot for this nice informative article which help me to understand different study designs that I felt difficult before
That’s lovely to hear, Mona, thank you for letting the author know how useful this was. If there are any other particular topics you think would be useful to you, and are not already on the website, please do let us know.
it is very informative and useful.
thank you statistician
Fabulous to hear, thank you John.
Thanks for this information
Thanks so much for this information….I have clearly known the types of study design Thanks
That’s so good to hear, Mirembe, thank you for letting the author know.
Very helpful article!! U have simplified everything for easy understanding
I’m a health science major currently taking statistics for health care workers…this is a challenging class…thanks for the simified feedback.
That’s good to hear this has helped you. Hopefully you will find some of the other blogs useful too. If you see any topics that are missing from the website, please do let us know!
Hello. I liked your presentation, the fact that you ranked them clearly is very helpful to understand for people like me who is a novelist researcher. However, I was expecting to read much more about the Experimental studies. So please direct me if you already have or will one day. Thank you
Dear Ay. My sincere apologies for not responding to your comment sooner. You may find it useful to filter the blogs by the topic of ‘Study design and research methods’ – here is a link to that filter: https://s4be.cochrane.org/blog/topic/study-design/ This will cover more detail about experimental studies. Or have a look on our library page for further resources there – you’ll find that on the ‘Resources’ drop down from the home page.
However, if there are specific things you feel you would like to learn about experimental studies, that are missing from the website, it would be great if you could let me know too. Thank you, and best of luck. Emma
Great job Mr Hadi. I advise you to prepare and study for the Australian Medical Board Exams as soon as you finish your undergrad study in Lebanon. Good luck and hope we can meet sometime in the future. Regards ;)
You have give a good explaination of what am looking for. However, references am not sure of where to get them from.
Subscribe to our newsletter
You will receive our monthly newsletter and free access to Trip Premium.
Related Articles
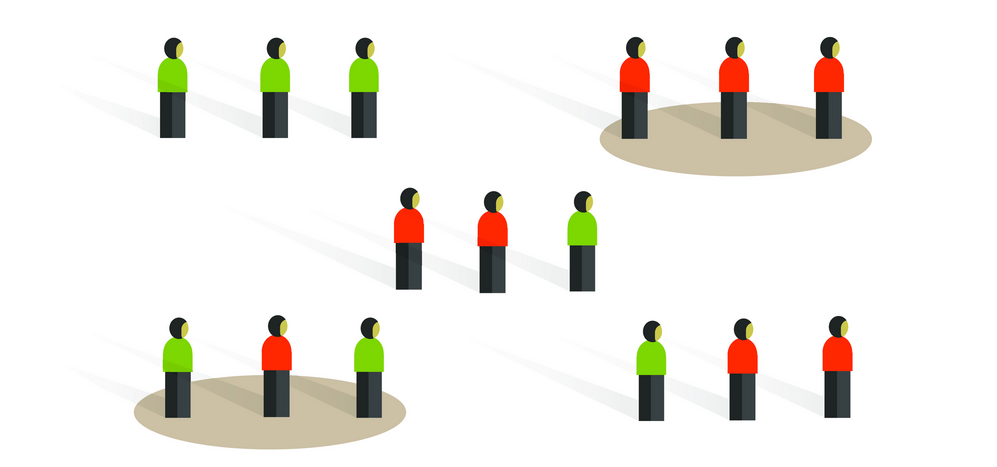
Cluster Randomized Trials: Concepts
This blog summarizes the concepts of cluster randomization, and the logistical and statistical considerations while designing a cluster randomized controlled trial.
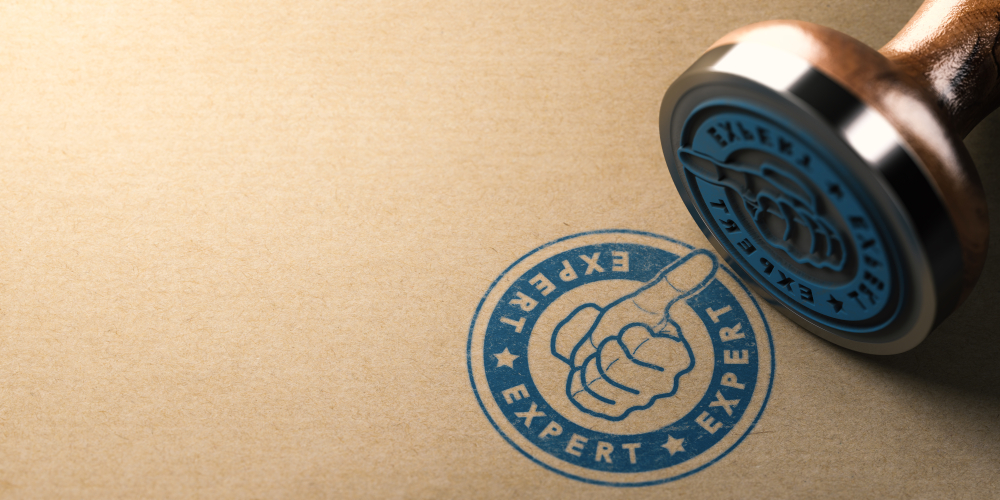
Expertise-based Randomized Controlled Trials
This blog summarizes the concepts of Expertise-based randomized controlled trials with a focus on the advantages and challenges associated with this type of study.
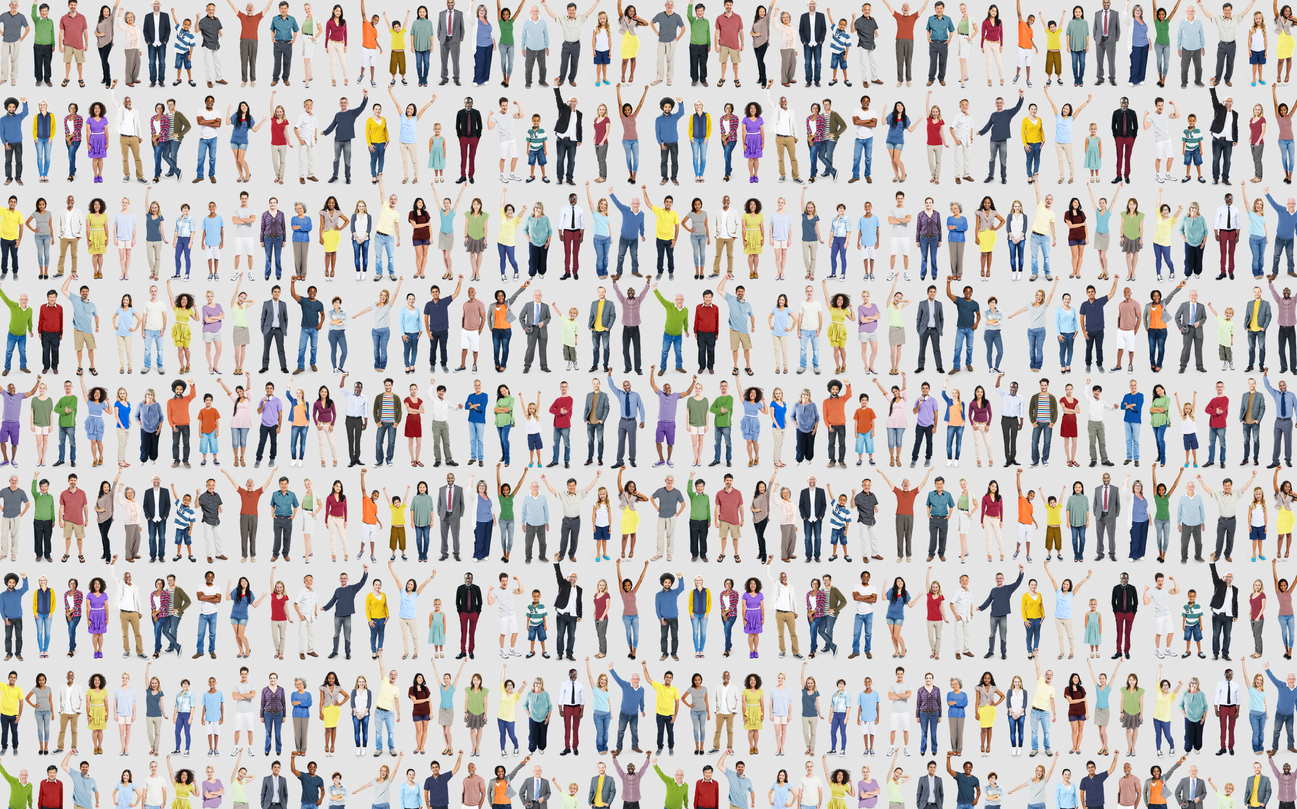
A well-designed cohort study can provide powerful results. This blog introduces prospective and retrospective cohort studies, discussing the advantages, disadvantages and use of these type of study designs.
- - Google Chrome
Intended for healthcare professionals
- Access provided by Google Indexer
- My email alerts
- BMA member login
- Username * Password * Forgot your log in details? Need to activate BMA Member Log In Log in via OpenAthens Log in via your institution

Search form
- Advanced search
- Search responses
- Search blogs
- Methodological...
Methodological standards for qualitative and mixed methods patient centered outcomes research
- Related content
- Peer review
- Bridget Gaglio , senior program officer 1 ,
- Michelle Henton , program manager 1 ,
- Amanda Barbeau , program associate 1 ,
- Emily Evans , research health science specialist 2 ,
- David Hickam , director of clinical effectiveness and decision sciences 1 ,
- Robin Newhouse , dean 3 ,
- Susan Zickmund , research health scientist and professor 4 5
- 1 Patient-Centered Outcomes Research Institute, 1828 L Street, Suite 900, Washington, DC, 20036, USA
- 2 Veterans Health Administration, United States Department of Veterans Affairs, Washington, DC, USA
- 3 Indiana University School of Nursing, Indianapolis, IN, USA
- 4 United States Department of Veterans Affairs, Salt Lake City, UT, USA
- 5 University of Utah School of Medicine, Salt Lake City, UT, USA
- Correspondence to: B Gaglio bgaglio{at}pcori.org
- Accepted 20 October 2020
The Patient-Centered Outcomes Research Institute’s (PCORI) methodology standards for qualitative methods and mixed methods research help ensure that research studies are designed and conducted to generate the evidence needed to answer patients’ and clinicians’ questions about which methods work best, for whom, and under what circumstances. This set of standards focuses on factors pertinent to patient centered outcomes research, but it is also useful for providing guidance for other types of clinical research. The standards can be used to develop and evaluate proposals, conduct the research, and interpret findings. The standards were developed following a systematic process: survey the range of key methodological issues and potential standards, narrow inclusion to standards deemed most important, draft preliminary standards, solicit feedback from a content expert panel and the broader public, and use this feedback to develop final standards for review and adoption by PCORI’s board of governors. This article provides an example on how to apply the standards in the preparation of a research proposal.
Rigorous methodologies are critical for ensuring the trustworthiness of research results. This paper will describe the process for synthesizing the current literature providing guidance on the use of qualitative and mixed methods in health research; and the process for development of methodology standards for qualitative and mixed methods used in patient centered outcomes research. Patient centered outcomes research is comparative clinical effectiveness research that aims to evaluate the clinical outcomes resulting from alternative clinical or care delivery approaches for fulfilling specific health and healthcare needs. By focusing on outcomes that are meaningful to patients, studies on patient centered outcomes research strengthen the evidence base and inform the health and healthcare decisions made by patients, clinicians, and other stakeholders.
The methods used in patient centered outcomes research are diverse and often include qualitative methodologies. Broadly, qualitative research is a method of inquiry used to generate and analyze open ended textual data to enhance the understanding of a phenomenon by identifying underlying reasons, opinions, and motivations for behavior. Many different methodologies can be used in qualitative research, each with its own set of frameworks and procedures. 1 This multitude of qualitative approaches allows investigators to select and synergize methods with the specific needs associated with the aims of the study.
Qualitative methods can also be used to supplement and understand quantitative results; the integration of these approaches for scientific inquiry and evaluation is known as mixed methods. 2 This type of approach is determined a priori, because the research question drives the choice of methods, and draws on the strengths of both quantitative and qualitative approaches to resolve complex and contemporary issues in health services. This strategy is achieved by integrating qualitative and quantitative approaches at the design, methods, interpretation, and reporting levels of research. 3 Table 1 lists definitions of qualitative methods, mixed methods, and patient centered outcomes research. The methodology standards described here are intended to improve the rigor and transparency of investigations that include qualitative and mixed methods. The standards apply to designing projects, conducting the studies, and reporting the results. Owing to its focus on patient centered outcomes research, this article is not intended to be a comprehensive summary of the difficulties encountered in the conduct of qualitative and mixed methods research.
Terms and definitions used in the development of the Patient-Centered Outcomes Research Institute’s (PCORI) qualitative and mixed methods research methodology standards
- View inline
Summary points
Many publications provide guidance on how to use qualitative and mixed methods in health research
The methodological standards reported here and adopted by Patient-Centered Outcomes Research Institute (PCORI) synthesize and refine various recommendations to improve the design, conduct, and reporting of patient centered, comparative, clinical effectiveness research
PCORI has developed and adopted standards that provide guidance on key areas where research applications and research reports have been deficient in the plans for and use of qualitative and mixed methods in conducting patient centered outcomes research
The standards provide guidance to health researchers to ensure that studies of this research are designed and conducted to generate valid evidence needed to analyze patients’ and clinicians’ questions about what works best, for whom, and under what circumstances
Established by the United States Congress in 2010 13 and reauthorized in 2019, 14 the Patient-Centered Outcomes Research Institute (PCORI) funds scientifically rigorous comparative effectiveness research, previously defined as patient centered outcomes research, to improve the quality and relevance of evidence that patients, care givers, clinicians, payers, and policy makers need to make informed healthcare decisions. Such decisions might include choices about which prevention strategies, diagnostic methods, and treatment options are most appropriate based on personal preferences and unique patient characteristics.
PCORI’s focus on patient centeredness and stakeholder engagement in research has generated increased interest in and use of methodologies of qualitative and mixed methods research within comparative effectiveness research studies. Qualitative data have a central role in understanding the human experience. As with any research, the potential for these studies to generate high integrity, evidence based information depends on the quality of the methods and approaches that were used. PCORI’s authorizing legislation places a unique emphasis on ensuring scientific rigor, including the creation of a methodology committee that develops and approves methodology standards to guide PCORI funded research. 13 The methodology committee consists of 15 individuals who were appointed by the Comptroller General of the US and the directors of the Agency for Healthcare Research and Quality and the National Institutes of Health. The members of the committee are medical and public health professionals with expertise in study design and methodology for comparative effectiveness research or patient centered outcomes research ( https://www.pcori.org/about-us/governance/methodology-committee ).
The methodology committee began developing its initial group of methodology standards in 2012 (with adoption by the PCORI’s board of governors that year). Since then, the committee has revised and expanded the standards based on identified methodological issues and input from stakeholders. Before the adoption of the qualitative and mixed methods research standards, the PCORI methodology standards consisted of 56 individual standards in 13 categories. 15 The first five categories of the standards are crosscutting and relevant to most studies on patient centered outcomes research, while the other eight categories are applicable depending on a study’s purpose and design. 15
Departures from good research practices are partially responsible for weaknesses in the quality and subsequent relevance of research. The PCORI methodology standards provide guidance that helps to ensure that studies on patient centered outcomes research are designed and conducted to generate the evidence needed to answer patients’ and clinicians’ questions about what works best, for whom, and under what circumstances. These standards do not represent a complete, comprehensive set of all requirements for high quality patient centered outcomes research; rather, they cover topics that are likely to contribute to improvements in quality and value. Specifically, the standards focus on selected methodological issues that have substantial deficiencies or inconsistencies regarding how available methods are applied in practice. These methodological issues might include a lack of rigor or inappropriate use of approaches for conducting patient centered outcomes research. As a research funder, PCORI uses the standards in the scientific review of applications, monitoring of funded research projects, and evaluation of final reports of research findings.
Use of qualitative methods has become more prevalent over time. Based on a PubMed search in June 2020 (search terms “qualitative methods” and “mixed methods”), the publication of qualitative and mixed methods studies has grown steadily from 1980 to 2019. From 1980 to 1989, 63 qualitative and 110 mixed methods papers were identified. Between 1990 to 1999, the number of qualitative and mixed methods papers was 420 and 58, respectively; by 2010 to 2019, these numbers increased to 5481 and 17 031, respectively. The prominent increase in publications in recent years could be associated with more sophisticated indexing methods in PubMed as well as the recognition that both qualitative and mixed methods research are important approaches to scientific inquiry within the health sciences. These approaches allow investigators to obtain a more detailed perspective and to incorporate patients’ motivations, beliefs, and values.
Although the use of qualitative and mixed methods research has increased, consensus regarding definitions and application of the methods remain elusive, reflecting wide disciplinary variation. 16 17 Many investigators and organizations have attempted to resolve these differences by proposing guidelines and checklists that help define essential components. 12 16 18 19 20 21 22 23 24 25 26 27 28 29 For example, Treloar et al 20 offer direction for qualitative researchers in designing and publishing research by providing a 10 point checklist for assessing the quality of qualitative research in clinical epidemiological studies. Tong et al 22 provide a 32 item checklist to help investigators report important aspects of the research process for interviews and focus groups such as the study team, study methods, context of the study, findings, analysis, and interpretations.
The goal of the PCORI Methodology Standards on Qualitative and Mixed Methods is to provide authoritative guidance on the use of these methodologies in comparative effectiveness research and patient centered outcomes research. The purpose of these types of research is to improve the clinical evidence base and, particularly, to help end users understand how the evidence provided by individual research studies can be applied to particular clinical circumstances. Use of qualitative and mixed methods can achieve this goal but can also introduce specific issues that need to be captured in PCORI’s methodological guidance. The previously published guidelines generally have a broader focus and different points of emphasis.
This article describes the process for synthesizing the current literature providing guidance on the use of qualitative and mixed methods in health research; and developing methodology standards for qualitative and mixed methods used in patient centered outcomes research. We then provide an example showing how to apply the standards in the design of a patient centered outcomes research application.
Methodology standards development process
Literature review and synthesis.
The purpose of the literature review was to identify published journal articles that defined criteria for rigorous qualitative and mixed methods research in health research. With the guidance of PCORI’s medical librarian, we designed and executed searches in PubMed, and did four different keyword searches for both qualitative and mixed methods (eight searches in total; supplemental table 1). We aimed to identify articles that provided methodological guidance rather than studies that simply used the methods.
We encountered two major challenges. First, qualitative and mixed methods research has a broad set of perspectives. 30 31 Second, some medical subject headings (MeSH terms) in our queries were not introduced until recently (eg, “qualitative methods” introduced in 2003, “comparative effectiveness” introduced in 2010), which required us to search for articles by identifying a specific qualitative method (eg, interviews, focus groups) to capture the literature before 2003 ( table 1 ). These challenges could have led to missed publications. To refine and narrow our search results, we applied the following inclusion criteria:
Articles on health services or clinical research, published in English, and published between 1 January 1990 and 14 April 2017
Articles that proposed or discussed a guideline, standard, framework, or set of principles for conducting rigorous qualitative and mixed methods research
Articles that described or discussed the design, methods for, or reporting of qualitative and mixed methods research.
The search queries identified 1933 articles (1070 on qualitative methods and 863 on mixed methods). The initial citation lists were reviewed, and 204 duplicates were removed. Three authors (BG, MH, and AB) manually reviewed the 1729 remaining article abstracts. Titles and abstracts were independently evaluated by each of the three reviewers using the inclusion criteria. Disagreements were adjudicated by an in-person meeting to determine which articles to include. This initial round of review yielded 212 references, for which the full articles were obtained. The full articles were reviewed using the same inclusion and exclusion criteria as the abstracts. Most of these articles were studies that had used a qualitative or mixed methods approach but were only reporting on the results of the completed research. Therefore, these articles were not able to inform the development of standards for conducting qualitative and mixed methods research and they were excluded, resulting in the final inclusion of 56 articles (supplemental table 2). Following the original search, the literature was scanned for new articles providing guidance on qualitative and mixed methods, resulting in four articles being added to the final set of literature. These articles come from psychology and health psychology specialties and seek to provide not only minimal standards in relation to qualitative and mixed methods research but also standards for best practice that apply across a wide range of fields. 32 33 34 35
Initial set of methodology standards
Using an abstraction form that outlined criteria for qualitative and mixed methods manuscripts and research proposals, we abstracted the articles to identify key themes, recommendations, and guidance under each criterion. Additional information was noted when considered relevant. A comprehensive document was created to include the abstractions and notes for all articles. This document outlined the themes in the literature related to methodological guidance. We began with the broadest set of themes organized into 11 major domains: the theoretical approach, research topics, participants, data collection, analysis and interpretation, data management, validity and reliability, presentation of results, context of research, impact of the researchers (that is, reflexivity), and mixed methods. As our goal was to distill the themes into broad standards that did not overlap with pre-existing PCORI methodology standards, we initially condensed the themes into six qualitative and three mixed methods standards. Following discussion among members of the working group, some standards were combined and two were dropped because of substantial overlap with each other or with previously developed PCORI methodology standards.
The key themes identified from the abstracted information were used as the foundation for the first draft of the new methodology standards. We then further discussed the themes as a team and removed redundancies, refined the labeling of themes, and removed themes deemed extraneous through a team based adjudication process. The draft standards were presented to PCORI’s methodology committee to solicit feedback. Revisions were made on the basis of this feedback.
Expert panel one day workshop
A one day expert panel workshop was held in Washington, DC, on 18 January 2018. Ten individuals regarded as international leaders in qualitative and mixed methods were invited to attend—including those who had created standards previously or had a substantial number of peer reviewed publications reporting qualitative and mixed methods in health research; had many years’ experience as primary researchers; and had served as editors of major textbooks and journals. The panel was selected on the basis of their influence and experience in these methodologies as well as their broad representation from various fields of study. The representation of expertise spanned the fields of healthcare, anthropology, and the social sciences (supplemental table 3).
Before the meeting, we emailed the panel members the draft set of qualitative and mixed methods standards, PCORI’s methodology standards document, and the background document describing how the draft standards had been developed. At the meeting, the experts provided extensive feedback, including their recommendations regarding what needs to be done well when using these methodological approaches. The panel emphasized that when conducting mixed methods research, this approach should be selected a priori, based on the research question, and that integration of the mixed approaches is critical at all levels of the research process (from inception to data analysis). The panel emphasized that when conducting qualitative research, flexibility and reflexive iteration should be maintained throughout the process—that is, the sampling, data collection, and data analysis. The main theme from the meeting was that the draft standards were not comprehensive enough to provide guidance for studies on patient centered outcomes research or comparative effectiveness research that involved qualitative and mixed methods. After the conclusion of the workshop, feedback and recommendations were synthesized, and the draft standards were reworked in the spring of 2018 ( fig 1 ). This work resulted in a new set of four qualitative methods standards and three mixed methods standards representing the unique features of each methodology that were not already included in the methodology standards previously adopted by PCORI.

Process of development and adoption of the Patient-Centered Outcomes Research Institute’s (PCORI) methodology standards on qualitative and mixed methods research
- Download figure
- Open in new tab
- Download powerpoint
Continued refinement and approval of methodology standards
In late spring 2018, the revised draft methodology standards were presented to PCORI’s methodology committee first by sharing a draft of the standards and then via oral presentation. Feedback from the methodology committee centered around eliminating redundancy in the standards proposed (both across the draft standards and in relation to the previously adopted categories of standards) and making the standards more actionable. The areas where the draft standards overlapped with the current standards were those for formulating research questions, for patient centeredness, and for data integrity and rigorous analyses. Each draft standard was reviewed and assessed by the methodology committee members and the staff workgroup to confirm its unique contribution to PCORI’s methodology standards. After this exercise, each remaining standard was reworded to be primarily action guiding (rather than explanatory). This version of proposed standards was approved by the methodology committee to be sent to PCORI’s board of governors for a vote to approve for public comment. The board of governors approved the standards to be posted for public comment.
The public comment period hosted on PCORI’s website ( https://www.pcori.org/engagement/engage-us/provide-input/past-opportunities-provide-input ) was held from 24 July 2018 to 21 September 2018. Thirty nine comments were received from nine different stakeholders—seven health researchers, one training institution, and one professional organization. Based on the public comments, minor wording changes were made to most of the draft standards. The final version of the standards underwent review by both the methodology committee and PCORI’s board of governors. The board voted to adopt the final version of the standards on 26 February 2019 ( table 2 ).
Patient-Centered Outcomes Research Institute’s (PCORI) methodology standards for qualitative methods and mixed methods
Application of methodology standards in research design
The standards can be used across the research continuum, from research design and application development, conduct of the research, and reporting of research findings. We provide an example for researchers on how these standards can be used in the preparation of a research application ( table 3 ).
Guidance for researchers on how to use Patient-Centered Outcomes Research Institute’s (PCORI) methodology standards for qualitative and mixed methods research in application preparation
QM-1: State the qualitative approach to research inquiry, design, and conduct
Many research proposals on patient centered outcomes research or comparative effectiveness research propose the use of qualitative methods but lack adequate description of and justification for the qualitative approach that will be used. Often the rationale for using qualitative methods is not tied back to the applicable literature and the identified evidence gap, missing the opportunity to link the importance of the approach in capturing the human experience or patient voice in the research aims. The approach to inquiry should be explicitly stated along with the rationale and a description of how it ties to the research question(s). The research proposal should clearly define how the qualitative approach will be operationalized and supports the choice of methods for participant recruitment, data collection, and analysis. Moreover, procedures for data collection should be stated, as well as the types of data to be collected, when data will be collected (that is, one point in time v longitudinal), data management, codebook development, intercoder reliability process, data analysis, and procedures for ensuring full confidentiality.
QM-2: Select and justify appropriate qualitative methods and sampling strategy
While the number of participants who will be recruited for focus groups or in-depth interviews is usually described, the actual sampling strategy is often not stated. The description of the sampling strategy should state how it aligns with the qualitative approach, how it relates to the research question(s), and the variation in sampling that might occur over the course of the study. Furthermore, most research proposals state that data will be collected until thematic saturation is reached, but how this will be determined is omitted. As such, this standard outlines the information essential for understanding who is participating in the study and aims to reduce the likelihood of making unsupported statements, emphasizing transparency in the criteria used to determine the stopping point for recruitment and data collection.
QM-3: Link the qualitative data analysis, interpretations, and conclusions to the study question
Qualitative analysis transforms data into information that can be used by the relevant stakeholder. It is a process of reviewing, synthesizing, and interpreting data to describe and explain the phenomena being studied. The interpretive process occurs at many points in the research process. It begins with making sense of what is heard and observed during data gathering, and then builds understanding of the meaning of the data through data analysis. This is followed by development of a description of the findings that makes sense of the data, in which the researcher’s interpretation of the findings is embedded. Many research proposals state that the data will be coded, but it is unclear by whom, their qualifications, or the process. Very little, if any, description is provided as to how conclusions will be drawn and how they will be related to the original data, and this standard highlights the need for detailed information on the analytical and interpretive processes for qualitative data and its relationship to the overall study.
QM-4: Establish trustworthiness and credibility of qualitative research
The qualitative research design should incorporate elements demonstrating validity and reliability, which are also known by terms such as trustworthiness and credibility. Studies with qualitative components can use several approaches to help ensure the validity and reliability of their findings, including audit trail, reflexivity, negative or deviant case analysis, triangulation, or member checking (see table 1 for definitions).
MM-1: Specify how mixed methods are integrated across design, data sources, and/or data collection phases
This standard requires investigators to declare and support their intent to conduct a mixed methods approach a priori in order to avoid a haphazard approach to the design and resulting data. Use of mixed methods can enhance the study design, by using the strengths of both quantitative and qualitative research as investigators are afforded the use of multiple data collection tools rather than being restricted to one approach. Mixed methods research designs have three key factors: integration of data, relative timing, and implications of linkages for methods in each component. Additionally, the standards for mixed methods, quantitative, and qualitative methodologies must be met in the design, implementation, and reporting stages. This is different from a multimethod research design in which two or more forms of data (qualitative, quantitative, or both) are used to resolve different aspects of the research question independently and are not integrated.
MM-2: Select and justify appropriate mixed methods sampling strategy
Mixed methods research aims to contribute insights and knowledge beyond that obtained from quantitative or qualitative methods only, which should be reflected in the sampling strategies as well as in the design of the study and the research plan. Qualitative and quantitative components can occur simultaneously or sequentially, and researchers must select and justify the most appropriate mixed method sampling strategy and demonstrate that the desired number and type of participants can be achieved with respect to the available time, cost, research team skillset, and resources. Those sampling strategies that are unique to mixed methods (eg, interdependent, independent, and combined) should focus on the depth and breadth of information across research components.
MM-3: Integrate data analysis, data interpretations, and conclusions
Qualitative and quantitative data often are analyzed in isolation, with little thought given to when these analyses should occur or how the analysis, interpretation, and conclusions integrate with one another. There are multiple approaches to integration in the analysis of qualitative and quantitative data (eg, merging, embedding, and connecting). As such, the approach to integration should determine the priority of the qualitative and quantitative components, as well as the temporality with which analysis will take place (eg, sequentially, or concurrently; iterative or otherwise). Either a priori or emergently, where appropriate, researchers should define these characteristics, identify the points of integration, and explain how integrated analyses will proceed with respect to the two components and the selected approach.
The choice between multiple options for prevention, diagnosis, and treatment of health conditions presents a considerable challenge to patients, clinicians, and policy makers as they seek to make informed decisions. Patient centered outcomes research focuses on the pragmatic comparison of two or more health interventions to determine what works best for which patients and populations in which settings. 5 The use of qualitative and mixed methods research can enable more robust capture and understanding of information from patients, caregivers, clinicians, and other stakeholders in research, thereby improving the strength, quality, and relevance of findings. 4
Despite extensive literature on qualitative and mixed methods research in general, the use of these methodologies in the context of patient centered outcomes research or comparative effectiveness research continues to grow and requires additional guidance. This guidance could facilitate the appropriate design, conduct, analysis, and reporting of these approaches. For example, the need for including multiple stakeholder perspectives, understanding how an intervention was implemented across multiple settings, or documenting the clinical context so decision makers can evaluate whether findings would be transferable to their respective settings pose unique challenges to the rigor and agility of qualitative and mixed methods approaches.
PCORI’s methodology standards for qualitative and mixed methods research represent an opportunity for further strengthening the design, conduct, and reporting of patient centered outcomes research or comparative effectiveness research by providing guidance that encompasses the broad range of methods that stem from various philosophical assumptions, disciplines, and procedures. These standards directly affect factors related to methodological integrity, accuracy, and clarity as identified by PCORI staff, methodology committee members, and merit reviewers in studies on patient centered outcomes research or comparative effectiveness research. The standards are presented at a level accessible to researchers new to qualitative and mixed methods research; however, they are not a substitute for appropriate expertise.
The challenges of ensuring rigorous methodology in the design and conduct of research are not unique to qualitative and mixed methods research, because the imperative to increase value and reduce waste in research design, conduct, and analysis is widely recognized. 36 Consistent with such efforts, PCORI recognizes the importance of continued methodological development and evaluation and is committed to listening to the research community and providing updated guidance based on methodological advances and research needs. 37
Acknowledgments
We thank the Patient-Centered Outcomes Research Institute’s (PCORI) methodology committee during this work (Naomi Aronson, Ethan Bach, Stephanie Chang, David Flum, Cynthia Girman, Steven Goodman (chairperson), Mark Helfand, Michael S Lauer, David O Meltzer, Brian S Mittman, Sally C Morton, Robin Newhouse (vice chairperson), Neil R Powe, and Adam Wilcox); and Frances K Barg, Benjamin F Crabtree, Deborah Cohen, Michael Fetters, Suzanne Heurtin-Roberts, Deborah K Padgett, Janice Morse, Lawrence A Palinkas, Vicki L Plano Clark, and Catherine Pope, for participating in the expert panel meeting and consultation.
Contributors: BG led the development of the methodology standards and wrote the first draft of the paper. MH, AB, SZ, EE, DH, and RN made a substantial contribution to all stages of developing the methodology standards. BG, SZ, MH, and AB drafted the methodology standards. DH, EE, and RN gave critical insights into PCORI’s methodology standards development processes and guidance. SZ served as qualitative methods consultant to the workgroup. BG provided project leadership and guidance. MH and AB facilitated the expert panel meeting. SZ is senior author. BG and SZ are the guarantors of this work and accept full responsibility for the finished article and controlled the decision to publish. The corresponding author attests that all listed authors meet authorship criteria and that no others meeting the criteria have been omitted.
Funding: No funding was used to support this work. All statements in this report, including its findings and conclusions, are solely those of the authors and do not necessarily represent the views of PCORI, its board of governors or methodology committee. The views expressed in this article are those of the authors and do not necessarily represent the views of the Department of Veterans Affairs.
Competing interests: All authors have completed the ICMJE uniform disclosure form at http://www.icmje.org/conflicts-of-interest/ and declare: no support from any organization for the submitted work; no financial relationships with any organizations that might have an interest in the submitted work in the previous three years; no other relationships or activities that could appear to have influenced the submitted work.
The lead author affirms that the manuscript is an honest, accurate, and transparent account of the work being reported; that no important aspects of the study have been omitted; and that any discrepancies from the work as planned have been explained.
Provenance and peer review: Not commissioned; externally peer reviewed.
Patient and public involvement: Patients and stakeholders were invited to comment on the draft standards during the public comment period held from 24 July 2018 to 21 September 2018. Comments were reviewed and revisions made accordingly. Development of the standards, including the methods, were presented at two PCORI board of governors’ meetings, which are open to the public, recorded, and posted on the PCORI website.
This is an Open Access article distributed in accordance with the Creative Commons Attribution Non Commercial (CC BY-NC 4.0) license, which permits others to distribute, remix, adapt, build upon this work non-commercially, and license their derivative works on different terms, provided the original work is properly cited and the use is non-commercial. See: http://creativecommons.org/licenses/by-nc/4.0/ .
- Collins CS ,
- Stockton CM
- Creswell JW ,
- Plano Clark VL
- Fetters MD ,
- Creswell JW
- ↵ Patient-Centered Outcomes Research Institute. Patient-centered outcomes research. 2010-19. https://www.pcori.org/research-results/about-our-research/research-we-support .
- Institute of Medicine
- Crabtree BF ,
- Klassen AC ,
- Plano Clark VL ,
- Clegg Smith KC ,
- Office of Behavioral and Social Sciences Research
- ↵ Patient Protection and Affordable Care Act, Pub. L. No. 111-148 Stat. 119 (March 23, 2010).
- ↵ Further Consolidated Appropriations Act, 2020, Pub. L. No. 116-94 (20 December 2019).
- ↵ Patient-Centered Outcomes Research Institute (PCORI). PCORI methodology standards. 2011-19. https://www.pcori.org/research-results/about-our-research/research-methodology/pcori-methodology-standards .
- Molina-Azorin JF
- Chapple A ,
- Treloar C ,
- Champness S ,
- Simpson PL ,
- Higginbotham N
- Cesario S ,
- Santa-Donato A
- Sainsbury P ,
- Flemming K ,
- McInnes E ,
- Davidoff F ,
- Batalden P ,
- Stevens D ,
- Mooney SE ,
- SQUIRE development group
- Gagnon MP ,
- Griffiths F ,
- Johnson-Lafleur J
- ↵ National Cancer Institute. Qualitative methods in implementation science. 2018. https://cancercontrol.cancer.gov/sites/default/files/2020-09/nci-dccps-implementationscience-whitepaper.pdf
- O’Brien BC ,
- Harris IB ,
- Beckman TJ ,
- ↵ National Institute for Health and Clinical Excellence. The guidelines manual. Appendix H: Methodology checklist: qualitative studies. https://www.nice.org.uk/process/pmg6/resources/the-guidelines-manual-appendices-bi-2549703709/chapter/appendix-h-methodology-checklist-qualitative-studies .
- Crabtree BF
- Johnson RB ,
- Onwuegbuzie AJ ,
- American Psychological Association
- Levitt HM ,
- Bamberg M ,
- Josselson R ,
- Suárez-Orozco C
- Motulsky SL ,
- Morrow SL ,
- Ponterotto JG
- Bishop FL ,
- Horwood J ,
- Chilcot J ,
- Ioannidis JPA ,
- Greenland S ,
- Hlatky MA ,
- ↵ Patient-Centered Outcomes Research Institute. The PCORI methodology report. 2019. https://www.pcori.org/sites/default/files/PCORI-Methodology-Report.pdf .
BMC Medical Research Methodology
Latest collections open to submissions, publication dynamics.
Guest Edited by Mueen Ahmed KK, Igor Burstyn, Gokhan Tazegul and Demeng Xia
Patient-centric approaches
Guest Edited by Violeta Moizé Arcone, Yitka Graham and Tito R. Mendoza
Open science: bias, challenges, and barriers
Guest Edited by Tim Mathes, Rahul Mhaskar, Livia Puljak and Matt Vassar
Inclusive methodological awareness for equity and diversity
Guest Edited by Rosemary M. Caron and Elochukwu Ezenwankwo
Data science methodologies
Guest Edited by Imran Ashraf
Editor's Highlights
A data-adaptive method for investigating effect heterogeneity with high-dimensional covariates in Mendelian randomization
Contextual effects: how to, and how not to, quantify them
Group sequential designs for pragmatic clinical trials with early outcomes: methods and guidance for planning and implementation
Barriers and facilitators for recruiting and retaining male participants into longitudinal health research: a systematic review
- Most accessed
Multimorbidity in middle-aged women and COVID-19: binary data clustering for unsupervised binning of rare multimorbidity features and predictive modeling
Authors: Dayana Benny, Mario Giacobini, Giuseppe Costa, Roberto Gnavi and Fulvio Ricceri
Evaluation of respondent-driven sampling in seven studies of people who use drugs from rural populations: findings from the Rural Opioid Initiative
Authors: Abby E. Rudolph, Robin M. Nance, Georgiy Bobashev, Daniel Brook, Wajiha Akhtar, Ryan Cook, Hannah L. Cooper, Peter D. Friedmann, Simon D. W. Frost, Vivian F. Go, Wiley D. Jenkins, Philip T. Korthuis, William C. Miller, Mai T. Pho, Stephanie A. Ruderman, David W. Seal…
Reporting of interventional clinical trial results in an academic center: a survey of completed studies
Authors: Anne Sophie Alix-Doucet, Constant Vinatier, Loïc Fin, Hervé Léna, Hélène Rangé, Clara Locher and Florian Naudet
Interpretable machine learning in predicting drug-induced liver injury among tuberculosis patients: model development and validation study
Authors: Yue Xiao, Yanfei Chen, Ruijian Huang, Feng Jiang, Jifang Zhou and Tianchi Yang
Application of causal inference methods in individual-participant data meta-analyses in medicine: addressing data handling and reporting gaps with new proposed reporting guidelines
Authors: Heather Hufstedler, Nicole Mauer, Edmund Yeboah, Sinclair Carr, Sabahat Rahman, Alexander M. Danzer, Thomas P. A. Debray, Valentijn M.T. de Jong, Harlan Campbell, Paul Gustafson, Lauren Maxwell, Thomas Jaenisch, Ellicott C. Matthay and Till Bärnighausen
Most recent articles RSS
View all articles
Systematic review or scoping review? Guidance for authors when choosing between a systematic or scoping review approach
Authors: Zachary Munn, Micah D. J. Peters, Cindy Stern, Catalin Tufanaru, Alexa McArthur and Edoardo Aromataris
The case study approach
Authors: Sarah Crowe, Kathrin Cresswell, Ann Robertson, Guro Huby, Anthony Avery and Aziz Sheikh
Characterising and justifying sample size sufficiency in interview-based studies: systematic analysis of qualitative health research over a 15-year period
Authors: Konstantina Vasileiou, Julie Barnett, Susan Thorpe and Terry Young
How to do a grounded theory study: a worked example of a study of dental practices
Authors: Alexandra Sbaraini, Stacy M Carter, R Wendell Evans and Anthony Blinkhorn
Most accessed articles RSS
Aims and scope
- Explore our scope examples and collections
- Learn how to prepare your manuscript
- Find out about our journal, values and ethos
- Get inspired by our recent articles
Become an Editorial Board Member
We are recruiting new Editorial Board Members.
BMC Series Blog
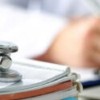
Introducing BMC Primary Care’s Collection: Trust and mistrust in primary care
17 April 2024
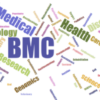
Highlights of the BMC Series – March 2024
10 April 2024
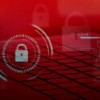
Introducing BMC Bioinformatics’ Collection: Bioinformatics ethics and data privacy
09 April 2024
Latest Tweets
Your browser needs to have JavaScript enabled to view this timeline
Important information
Editorial board
For authors
For editorial board members
For reviewers
- Manuscript editing services
Annual Journal Metrics
2022 Citation Impact 4.0 - 2-year Impact Factor 7.0 - 5-year Impact Factor 2.055 - SNIP (Source Normalized Impact per Paper) 1.778 - SJR (SCImago Journal Rank)
2023 Speed 40 days submission to first editorial decision for all manuscripts (Median) 210 days submission to accept (Median)
2023 Usage 4,638,094 downloads 3,126 Altmetric mentions
- More about our metrics
Peer-review Terminology
The following summary describes the peer review process for this journal:
Identity transparency: Single anonymized
Reviewer interacts with: Editor
Review information published: Review reports. Reviewer Identities reviewer opt in. Author/reviewer communication
More information is available here
- Follow us on Twitter
ISSN: 1471-2288
- General enquiries: [email protected]
An overview of commonly used statistical methods in clinical research
Affiliations.
- 1 Center for Surgical Outcomes Research, The Research Institute at Nationwide Children's Hospital, Columbus, OH, USA.
- 2 Department of Surgery, Children's Mercy Hospital, 2401 Gillham Road, Kansas City, MO 64108, USA. Electronic address: [email protected].
- PMID: 30473041
- DOI: 10.1053/j.sempedsurg.2018.10.008
Statistics plays an essential role in clinical research by providing a framework for making inferences about a population of interest. In order to interpret research datasets, clinicians involved in clinical research should have an understanding of statistical methodology. This article provides a brief overview of statistical methods that are frequently used in clinical research studies. Descriptive and inferential methods, including regression modeling and propensity scores, are discussed, with focus on the rationale, assumptions, strengths, and limitations to their application.
Keywords: Descriptive statistics; Inferential statistics; Propensity scores; Regression analysis; Survival analysis.
Copyright © 2018 Elsevier Inc. All rights reserved.
Publication types
- Biomedical Research / methods*
- Clinical Trials as Topic / methods*
- Data Interpretation, Statistical*
- Propensity Score
- Regression Analysis
- Research Design*
- Survival Analysis
Advertisement

- Previous Issue
- Previous Article
- Next Article
- Box 1. What to Look for in Research Using This Method
What Is Qualitative Research?
Qualitative versus quantitative research, conducting and appraising qualitative research, conclusions, research support, competing interests, qualitative research methods in medical education.
Submitted for publication January 5, 2018. Accepted for publication November 29, 2018.
- Split-Screen
- Article contents
- Figures & tables
- Supplementary Data
- Peer Review
- Open the PDF for in another window
- Cite Icon Cite
- Get Permissions
- Search Site
Adam P. Sawatsky , John T. Ratelle , Thomas J. Beckman; Qualitative Research Methods in Medical Education. Anesthesiology 2019; 131:14–22 doi: https://doi.org/10.1097/ALN.0000000000002728
Download citation file:
- Ris (Zotero)
- Reference Manager
Qualitative research was originally developed within the social sciences. Medical education is a field that comprises multiple disciplines, including the social sciences, and utilizes qualitative research to gain a broader understanding of key phenomena within the field. Many clinician educators are unfamiliar with qualitative research. This article provides a primer for clinician educators who want to appraise or conduct qualitative research in medical education. This article discusses a definition and the philosophical underpinnings for qualitative research. Using the Standards for Reporting Qualitative Research as a guide, this article provides a step-wise approach for conducting and evaluating qualitative research in medical education. This review will enable the reader to understand when to utilize qualitative research in medical education and how to interpret reports using qualitative approaches.

Image: J. P. Rathmell and Terri Navarette.
Qualitative research provides approaches to explore and characterize the education of future anesthesiologists. For example, the practice of anesthesiology is increasingly team-based; core members of the anesthesia care team include physicians, trainees, nurse anesthetists, anesthesiologist assistants, and other healthcare team members. 1 Understanding how to work within and how to teach learners about anesthesia care teams requires the ability to conceptualize the complexity of individual psychology and social interactions that occur within teams. Qualitative research is well suited to investigate complex issues like team-based care. For example, one qualitative study observed the interactions between members of the anesthesia care team during simulated stressful situations and conducted interviews of team members; they described limited understanding of each team member’s role and perceptions about appropriate roles and responsibilities, which provided insight for interprofessional team training. 2 Another qualitative study explored the hierarchy within the anesthesia care team, highlighting residents’ reluctance to challenge the established hierarchy and outlining the strategies they use to cope with fear and intimidation. 3 Key issues in medical education and anesthesiology, particularly when exploring human experience and social interactions, may be best studied using qualitative research methodologies and methods.
Medical education is a complex field, and medical education research and practice fittingly draws from many disciplines ( e.g. , medicine, psychology, sociology, education) and synthesizes multiple perspectives to explain how people learn and how medicine should be taught. 4 , 5 The concept of a field was well described by Cristancho and Varpio 5 in their tips for early career medical educators: “A discipline is usually guided by shared paradigms, assumptions, rules and methods to present their knowledge claims— i.e. , people from the same discipline speak the same language. A field brings people from multiple disciplines together.” Qualitative research draws from the perspectives of multiple disciplines and has provided methodologies to explore the complex research questions inherent to medical education.
When appraising qualitative research in medical education, do the authors:
Clearly state the study purpose and research question?
Describe the conceptual framework that inform the study and guide analysis?
Identify their qualitative methodology and research paradigm?
Demonstrate adequate reflexivity, conveying to the reader their values, assumptions and way of thinking, being explicit about the effects these ways of thinking have on the research process?
Choose data collection methods that are congruent with the research purpose and qualitative methodology?
Select an appropriate sampling strategy, choosing participants whose perspectives or experiences are relevant to the study question?
Define their method for determining saturation, how they decided to stop data collection?
Outline their process for data processing, including the management and coding of study data?
Conduct data analysis consistent with their chosen methodology?
Consider techniques to enhance trustworthiness of their study findings?
Synthesize and interpret their data with sufficient detail and supporting quotations to explain the phenomenon of study?
Current medical training is heavily influenced by the practice of evidence-based medicine. 6 Trainees are taught the “hierarchy of evidence” for evaluating studies of clinical interventions. 7 This hierarchy prioritizes knowledge gained through systematic reviews and meta-analyses, randomized controlled trials, and observational studies, but it does not include qualitative research methodologies. This means that because of their medical training and exposure to quantitative medical literature, clinician educators may be more familiar with quantitative research and feel more comfortable engaging in studies utilizing quantitative methodologies. However, many clinician educators are not familiar with the language and application of qualitative research and feel less comfortable engaging in studies using qualitative methodologies.
Because medical education is a diverse and complex field, qualitative research is a common approach in medical education research. Clinician educators who wish to understand the medical education literature need to be familiar with qualitative research. Clinician educators involved in research may also find themselves asking questions best answered by qualitative methodologies. Our goal is to provide a broad, practical overview of qualitative research in medical education. Our objectives are to:
1) Define qualitative research.
2) Compare and contrast qualitative and quantitative research.
3) Provide a framework for conducting and appraising qualitative research in medical education.
Qualitative research in medical education has a distinct vocabulary with terminology not commonly used in other biomedical research fields. Therefore, we have provided a glossary and definitions of the common terms that are used throughout this article ( table 1 ).
Glossary of Common Terms Used in Qualitative Research

Of the many attempts to provide a comprehensive definition of qualitative research, our favorite definition comes from Denzin and Lincoln:
“Qualitative research is a situated activity that locates the observer in the world. Qualitative research consists of a set of interpretive, material practices that make the world visible. These practices…turn the world into a series of representations, including field notes, interviews, conversations, photographs, recordings, and memos to the self. At this level, qualitative research involves an interpretive, naturalistic approach to the world. This means that qualitative researchers study things in their natural settings, attempting to make sense of or interpret phenomena in terms of the meanings people bring to them.” 12
This definition reveals the following points: first, qualitative research is a “situated activity,” meaning that the research and observations are made in the real world, in this case a real life clinical or educational situation. Second, qualitative research “turns the world into a series of representations” by representing the observations, in this case of a clinical or educational situation, with qualitative data, usually taking the form of words, pictures, documents, and other symbols. Last, qualitative researchers seek to “make sense” of the meanings that research participants bring to different phenomena to allow for a greater understanding of those phenomena. Through qualitative research, observers comprehend participants’ beliefs and values and the way these beliefs and values are shaped by the context in which they are studied.
Because most clinician educators are familiar with quantitative methods, we will start by comparing qualitative and quantitative methods to gain a better understanding of qualitative research ( table 2 ). To illustrate the difference between qualitative and quantitative research in medical education, we pose the question: “What makes noon conference lectures effective for resident learning?” A qualitative approach might explore the learner perspective on learning in noon conference lectures during residency and conduct an exploratory thematic analysis to better understand what the learner thinks is effective. 13 A qualitative approach is useful to answer this question, especially if the phenomenon of interest is incompletely understood. If we wanted to compare types or attributes of conferences to assess the most effective methods of teaching in a noon conference setting, then a quantitative approach might be more appropriate, though a qualitative approach could be helpful as well. We could use qualitative data to inform the design of a survey 14 or even inform the design of a randomized control trial to compare two types of learning during noon conference. 15 Therefore, when discussing qualitative and quantitative research, the issue is not which research approach is stronger, because it is understood that each approach yields different types of knowledge when answering the research question.
Comparisons of Quantitative and Qualitative Research in Medical Education

Similarities
The first step of any research project, qualitative or quantitative, is to determine and refine the study question; this includes conducting a thorough literature review, crafting a problem statement, establishing a conceptual framework for the study, and declaring a statement of intent. 16 A common pitfall in medical education research is to start by identifying the desired methods ( e.g. , “I want to do a focus group study with medical students.”) without having a clearly refined research question, which is like putting the cart before the horse. In other words, the research question should guide the methodology and methods for both qualitative and quantitative research.
Acknowledging the conceptual framework for a study is equally important for both qualitative and quantitative research. In a systematic review of medical education research, only 55% of studies provided a conceptual framework, limiting the interpretation and meaning of the results. 17 Conceptual frameworks are often theories that represent a way of thinking about the phenomenon being studied. Conceptual frameworks guide the interpretation of data and situate the study within the larger body of literature on a specific topic. 9 Because qualitative research was developed within the social sciences, many qualitative research studies in medical education are framed by theories from social sciences. Theories from social science disciplines have the ability to “open up new ways of seeing the world and, in turn, new questions to ask, new assumptions to unearth, and new possibilities for change.” 18 Qualitative research in medical education has benefitted from these new perspectives to help understand fundamental and complex problems within medical education such as culture, power, identity, and meaning.
Differences
The fundamental difference between qualitative and quantitative methodologies centers on epistemology ( i.e. , differing views on truth and knowledge). Cleland 19 describes the differences between qualitative and quantitative philosophies of scientific inquiry: “quantitative and qualitative approaches make different assumptions about the world, about how science should be conducted and about what constitutes legitimate problems, solutions and criteria of ‘proof.’”
Quantitative research comes from objectivism , an epistemology asserting that there is an absolute truth that can be discovered; this way of thinking about knowledge leads researchers to conduct experimental study designs aimed to test hypotheses about cause and effect. 10 Qualitative research, on the other hand, comes from constructivism , an epistemology asserting that reality is constructed by our social, historical, and individual contexts, and leads researchers to utilize more naturalistic or exploratory study designs to provide explanations about phenomenon in the context that they are being studied. 10 This leads researchers to ask fundamentally different questions about a given phenomenon; quantitative research often asks questions of “What?” and “Why?” to understand causation, whereas qualitative research often asks the questions “Why?” and “How?” to understand explanations. Cook et al. 20 provide a framework for classifying the purpose of medical education research to reflect the steps in the scientific method—description (“What was done?”), justification (“Did it work?”), and clarification (“Why or how did it work?”). Qualitative research nicely fits into the categories of “description” and “clarification” by describing observations in natural settings and developing models or theories to help explain “how” and “why” educational methods work. 20
Another difference between quantitative and qualitative research is the role of the researcher in the research process. Experimental studies have explicitly stated methods for creating an “unbiased” study in which the researcher is detached ( i.e. , “blinded”) from the analysis process so that their biases do not shape the outcome of the research. 21 The term “bias” comes from the positivist paradigm underpinning quantitative research. Assessing and addressing “bias” in qualitative research is incongruous. 22 Qualitative research, based largely on a constructivist paradigm, acknowledges the role of the researcher as a “coconstructer” of knowledge and utilizes the concept of “reflexivity.” Because researchers act as coconstructors of knowledge, they must be explicit about the perspectives they bring to the research process. A reflexive researcher is one who challenges their own values, assumptions, and way of thinking and who is explicit about the effects these ways of thinking have on the research process. 23 For example, when we conducted a study on self-directed learning in residency training, we were overt regarding our roles in the residency program as core faculty, our belief in the importance of self-directed learning, and our assumptions that residents actually engaged in self-directed learning. 24 , 25 We also needed to challenge these assumptions and open ourselves to alternative questions, methods of data collection, and interpretations of the data, to ultimately ensure that we created a research team with varied perspectives. Therefore, qualitative researchers do not strive for “unbiased” research but to understand their own roles in the coconstruction of knowledge. When assessing reflexivity, it is important for the authors to define their roles, explain how those roles may affect the collection and analysis of data, and how the researchers accounted for that effect and, if needed, challenged any assumptions during the research process. Because of the role of the researcher in qualitative research, it is vital to have a member of the research team with qualitative research experience.
A Word on Mixed Methods
In mixed methods research, the researcher collects and analyzes both qualitative and quantitative data rigorously and integrates both forms of data in the results of the study. 26 Medical education research often involves complex questions that may be best addressed through both quantitative and qualitative approaches. Combining methods can complement the strengths and limitations of each method and provide data from multiple sources to create a more detailed understanding of the phenomenon of interest. Examples of uses of mixed methods that would be applicable to medical education research include: collecting qualitative and quantitative data for more complete program evaluation, collecting qualitative data to inform the research design or instrument development of a quantitative study, or collecting qualitative data to explain the meaning behind the results of a quantitative study. 26 The keys to conducting mixed methods studies are to clearly articulate your research questions, explain your rationale for use of each approach, build an appropriate research team, and carefully follow guidelines for methodologic rigor for each approach. 27
Toward Asking More “Why” Questions
We presented similarities and differences between qualitative and quantitative research to introduce the clinician educator to qualitative research but not to suggest the relative value of one these research methods over the other. Whether conducting qualitative or quantitative research in medical education, researchers should move toward asking more “why” questions to gain deeper understanding of the key phenomena and theories in medical education to move the field of medical education forward. 28 By understanding the theories and assumptions behind qualitative and quantitative research, clinicians can decide how to use these approaches to answer important questions in medical education.
There are substantial differences between qualitative and quantitative research with respect to the assessment of rigor; here we provide a framework for reading, understanding, and assessing the quality of qualitative research. O’Brien et al. 29 created a useful 21-item guide for reporting qualitative research in medical education, based upon a systematic review of reporting standards for qualitative research—the Standards for Reporting Qualitative Research. It should be noted, however, that just performing and reporting each step in these standards do not ensure research quality.
Using the Standards for Reporting Qualitative Research as a backdrop, we will highlight basic steps for clinician educators wanting to engage with qualitative research. If you use this framework to conduct qualitative research in medical education, then you should address these steps; if you are evaluating qualitative research in medical education, then you can assess whether the study investigators addressed these steps. Table 3 underscores each step and provides examples from our research in resident self-directed learning. 25
Components of Qualitative Research: Examples from a Single Research Study

Refine the study question. As with any research project, investigators should clearly define the topic of research, describe what is already known about the phenomenon that is being studied, identify gaps in the literature, and clearly state how the study will fill that gap. Considering theoretical underpinnings of qualitative research in medical education often means searching for sources outside of the biomedical literature and utilizing theories from education, sociology, psychology, or other disciplines. This is also a critical time to engage people from other disciplines to identify theories or sources of information that can help define the problem and theoretical frameworks for data collection and analysis. When evaluating the introduction of a qualitative study, the researchers should demonstrate a clear understanding of the phenomenon being studied, the previous research on the phenomenon, and conceptual frameworks that contextualize the study. Last, the problem statement and purpose of the study should be clearly stated.
Identify the qualitative methodology and research paradigm. The qualitative methodology should be chosen based on the stated purpose of the research. The qualitative methodology represents the overarching philosophy guiding the collection and analysis of data and is distinct from the research methods ( i.e. , how the data will be collected). There are a number of qualitative methodologies; we have included a list of some of the most common methodologies in table 4 . Choosing a qualitative methodology involves examining the existing literature, involving colleagues with qualitative research expertise, and considering the goals of each approach. 32 For example, explaining the processes, relationships, and theoretical understanding of a phenomenon would point the researcher to grounded theory as an appropriate approach to conducting research. Alternatively, describing the lived experiences of participants may point the researcher to a phenomenological approach. Ultimately, qualitative research should explicitly state the qualitative methodology along with the supporting rationale. Qualitative research is challenging, and you should consult or collaborate with a qualitative research expert as you shape your research question and choose an appropriate methodology. 32
Choose data collection methods. The choice of data collection methods is driven by the research question, methodology, and practical considerations. Sources of data for qualitative studies would include open-ended survey questions, interviews, focus groups, observations, and documents. Among the most important aspects of choosing the data collection method is alignment with the chosen methodology and study purpose. 33 For interviews and focus groups, there are specific methods for designing the instruments. 34 , 35 Remarkably, these instruments can change throughout the course of the study, because data analysis often informs future data collection in an iterative fashion.
Select a sampling strategy. After identifying the types of data to be collected, the next step is deciding how to sample the data sources to obtain a representative sample. Most qualitative methodologies utilize purposive sampling, which is choosing participants whose perspectives or experiences are relevant to the study question. 11 Although random sampling and convenience sampling may be simpler and less costly for the researcher than purposeful sampling, these approaches often do not provide sufficient information to answer the study question. 36 For example, in grounded theory, theoretical sampling means that the choice of subsequent participants is purposeful to aid in the building and refinement of developing theory. The criteria for selecting participants should be stated clearly. One key difference between qualitative and quantitative research is sample size: in qualitative research, sample size is usually determined during the data collection process, whereas in quantitative research, the sample size is determined a priori . Saturation is verified when the analysis of newly collected data no longer provides additional insights into the data analysis process. 10
Plan and outline a strategy for data processing. Data processing refers to how the researcher organizes, manages, and dissects the study data. Although data processing serves data analysis, it is not the analysis itself. Data processing includes practical aspects of data management, like transcribing interviews, collecting field notes, and organizing data for analysis. The next step is coding the data, which begins with organizing the raw data into chunks to allow for the identification of themes and patterns. A code is a “word or short phrase that symbolically assigns a summative, salient, essence-capturing, and/or evocative attribute for a portion of language-based or visual data.” 8 There is an artificial breakdown between data processing and analysis, because these steps may be conducted simultaneously; many consider coding as different from—yet a necessary step to facilitating—the analysis of data. 8 Qualitative software can support this process, by making it easier to organize, access, search, and code your data. However, it is noteworthy that these programs do not do the work for you, they are merely tools for supporting data processing and analysis.
Conduct the data analysis. When analyzing the data, there are several factors to consider. First, the process of data analysis begins with the initial data collection, which often informs future data collection. Researchers should be intentional when reading, reviewing, and analyzing data as it is collected, so that they can shape and enrich subsequent data collection ( e.g. , modify the interview questions). Second, data analysis is often conducted by a research team that should have the appropriate expertise and perspectives to bring to the analysis process. Therefore, when evaluating a qualitative study, you should consider the team’s composition and their reflexivity with respect to their potential biases and influences on their study subjects. Third, the overall goal is to move from the raw data to abstractions of the data that answer the research question. For example, in grounded theory, the research moves from the raw data, to the identification of themes, to categorization of themes, to identifying relationships between themes, and ultimately to the development of theoretical explanations of the phenomenon. 30 Consequently, the primary researcher or research team should be intimately involved with the data analysis, interrogating the data, writing analytic memos, and ultimately make meaning out of the data. There are differing opinions about the use of “counting” of codes or themes in qualitative research. In general, counting of themes is used during the analysis process to recognize patterns and themes; often these are not reported as numbers and percentages as in quantitative research, but may be represented by words like few , some , or many . 37
Recognize techniques to enhance trustworthiness of your study findings. Ensuring consistency between the data and the results of data analysis, along with ensuring that the data and results accurately represent the perspectives and contexts related to the data source, are crucial to ensuring trustworthiness of study findings. Methods for enhancing trustworthiness include triangulation , which is comparing findings from different methods or perspectives, and member-checking , which is presenting research findings to study participants to provide opportunities to ensure that the analysis is representative. 10
Synthesize and interpret your data. Synthesis of qualitative research is determined by the depth of the analysis and involves moving beyond description of the data to explaining the findings and situating the results within the larger body of literature on the phenomenon of interest. The reporting of data synthesis should match the research methodology. For instance, if the study is using grounded theory, does the study advance the theoretical understanding of the phenomenon being studied? It is also important to acknowledge that clarity and organization are paramount. 10 Qualitative data are rich and extensive; therefore, researchers must organize and tell a compelling story from the data. 38 This process includes the selection of representative data ( e.g. , quotations from interviews) to substantiate claims made by the research team.
Common Methodologies Used in Qualitative Research

For more information on qualitative research in medical education:
Qualitative Research and Evaluation Methods: Integrating Theory and Practice, by Michael Q. Patton (SAGE Publications, Inc., 2014)
Qualitative Inquiry and Research Design: Choosing Among Five Approaches, by John W. Cresswell (SAGE Publications, Inc. 2017)
Researching Medical Education, by Jennifer Cleland and Steven J. Durning (Wiley-Blackwell, 2015)
Qualitative Research in Medical Education, by Patricia McNally, in Oxford Textbook of Medical Education, edited by Kieren Walsh (Oxford University Press, 2013)
The Journal of Graduate Medical Education “Qualitative Rip Out Series” (Available at: http://www.jgme.org/page/ripouts )
The Standards for Reporting Qualitative Research (O'Brien BC, Harris IB, Beckman TJ, Reed DA, Cook DA. Standards for reporting qualitative research: a synthesis of recommendations. Acad Med. 2014;89(9):1245-51.)
The Wilson Centre Qualitative Atelier (For more information: http://thewilsoncentre.ca/atelier/ )
Qualitative research is commonly used in medical education but may be unfamiliar to many clinician educators. In this article, we provided a definition of qualitative research, explored the similarities and differences between qualitative and quantitative research, and outlined a framework for conducting or appraising qualitative research in medical education. Even with advanced training, it can be difficult for clinician educators to understand and conduct qualitative research. Leaders in medical education research have proposed the following advice to clinician educators wanting to engage in qualitative medical education research: (1) clinician educators should find collaborators with knowledge of theories from other disciplines ( e.g. , sociology, cognitive psychology) and experience in qualitative research to utilize their complementary knowledge and experience to conduct research—in this way, clinician educators can identify important research questions; collaborators can inform research methodology and theoretical perspectives; and (2) clinician educators should engage with a diverse range disciplines to generate new questions and perspectives on research. 4
Support was provided solely from institutional and/or departmental sources.
The authors declare no competing interests.
Citing articles via
Most viewed, email alerts, related articles, social media, affiliations.
- ASA Practice Parameters
- Online First
- Author Resource Center
- About the Journal
- Editorial Board
- Rights & Permissions
- Online ISSN 1528-1175
- Print ISSN 0003-3022
- Anesthesiology
- ASA Monitor

- Terms & Conditions Privacy Policy
- Manage Cookie Preferences
- © Copyright 2024 American Society of Anesthesiologists
This Feature Is Available To Subscribers Only
Sign In or Create an Account
Skip to content
Read the latest news stories about Mailman faculty, research, and events.
Departments
We integrate an innovative skills-based curriculum, research collaborations, and hands-on field experience to prepare students.
Learn more about our research centers, which focus on critical issues in public health.
Our Faculty
Meet the faculty of the Mailman School of Public Health.
Become a Student
Life and community, how to apply.
Learn how to apply to the Mailman School of Public Health.
Clinical Research Methods
Director: Todd Ogden, PhD
The Mailman School offers the degree of Master of Science in Biostatistics, with an emphasis on issues in the statistical analysis and design of clinical studies. The Clinical Research Methods track was conceived and designed for clinicians who are pursuing research careers in academic medicine. Candidacy in the CRM program is open to anyone who holds a medical/doctoral degree and/or has several years of clinical research experience.
Competencies
In addition to achieving the MS in Biostatistics core competencies, graduates of the 30 credit MS Clinical Research Methods Track develop specific competencies in data analysis and computing, public health and collaborative research, and data management. MS/CRM graduates will be able to:
Data Analysis and Computing
- Apply the basic tenets of research design and analysis for the purpose of critically reviewing research and programs in disciplines outside of biostatistics;
- Differentiate between quantitative problems that can be addressed with standard methods and those requiring input from a professional biostatistician.
Public Health and Collaborative Research
- Formulate and prepare a written statistical plan for analysis of public health research data that clearly reflects the research hypotheses of the proposal in a manner that resonates with both co-investigators and peer reviewers;
- Prepare written summaries of quantitative analyses for journal publication, presentations at scientific meetings, grant applications, and review by regulatory agencies;
Data Management
- Identify the uses to which data management can be put in practical statistical analysis, including the establishment of standards for documentation, archiving, auditing, and confidentiality; guidelines for accessibility; security; structural issues; and data cleaning;
- Differentiate between analytical and data management functions through knowledge of the role and functions of databases, different types of data storage, and the advantages and limitations of rigorous database systems in conjunction with statistical tools;
- Describe the different types of database management systems, the ways these systems can provide data for analysis and interact with statistical software, and methods for evaluating technologies pertinent to both; and
- Assess database tools and the database functions of statistical software, with a view to explaining the impact of data management processes and procedures on their own research.
Required Courses
The required courses enable degree candidates to gain proficiency in study design, application of commonly-used statistical procedures, use of statistical software packages, and successful interpretation and communication of analysis results. A required course may be waived for students with demonstrated expertise in that field of study. If a student places out of one or more required courses, that student must substitute other courses, perhaps a more advanced course in the same area or another elective course in biostatistics or another discipline, with the approval of the student’s faculty advisor.
The program, which consists of 30 credits of coursework and research, may be completed in one year, provided the candidate begins study during the summer semester of his or her first year. If preferred, candidates may pursue the MS/CRM on a part-time basis. The degree program must be completed within five years of the start date.
The curriculum, described below, is comprised of 24 credits of required courses, including a 3-credit research project (the “Master’s essay”) to be completed during the final year of study, and two electives of 6 credits. Note that even if a course is waived, students must still complete a minimum of 30 credits to be awarded the MS degree.
Commonly chosen elective courses include:
Master's Essay
As part of MS/CRM training, each student is required to register for the 3-credit Master's essay course (P9160). This course provides direct support and supervision for the completion of the required research project, or Master's essay, consisting of a research paper of publishable quality. CRM candidates should register for the Master's essay during the spring semester of their final year of study. Students are required to come to the Master's essay course with research data in hand for analysis and interpretation.
CRM graduates have written excellent Master's essays over the years, many of which were ultimately published in the scientific literature. Some titles include:
- A Comprehensive Analysis of the Natural History and the Effect of Treatment on Patients with Malignant Pleural Mesothelioma
- Prevalence and Modification of Cardiovascular Risk Factors in Early Chronic Kidney Disease: Data from the Third National Health and Nutrition Examination Survey
- Perspectives on Pediatric Outcomes: A Comparison of Parents' and Children's Ratings of Health-Related Quality of Life
- Clinical and Demographic Profiles of Cancer Discharges throughout New York State Compared to Corresponding Incidence Rates, 1990-1994
Sample Timeline
Candidates may choose to complete the CRM program track on a part-time basis, or complete all requirements within one year (July through May). To complete the degree in one year, coursework must commence during the summer term.
Note that course schedules change from year to year, so that class days/times in future years will differ from the sample schedule below; you must check the current course schedule for each year on the course directory page .
Paul McCullough Director of Academic Programs Department of Biostatistics Columbia University [email protected] 212-342-3417
More information on Admission Requirements and Deadlines.
- Alzheimer's disease & dementia
- Arthritis & Rheumatism
- Attention deficit disorders
- Autism spectrum disorders
- Biomedical technology
- Diseases, Conditions, Syndromes
- Endocrinology & Metabolism
- Gastroenterology
- Gerontology & Geriatrics
- Health informatics
- Inflammatory disorders
- Medical economics
- Medical research
- Medications
- Neuroscience
- Obstetrics & gynaecology
- Oncology & Cancer
- Ophthalmology
- Overweight & Obesity
- Parkinson's & Movement disorders
- Psychology & Psychiatry
- Radiology & Imaging
- Sleep disorders
- Sports medicine & Kinesiology
- Vaccination
- Breast cancer
- Cardiovascular disease
- Chronic obstructive pulmonary disease
- Colon cancer
- Coronary artery disease
- Heart attack
- Heart disease
- High blood pressure
- Kidney disease
- Lung cancer
- Multiple sclerosis
- Myocardial infarction
- Ovarian cancer
- Post traumatic stress disorder
- Rheumatoid arthritis
- Schizophrenia
- Skin cancer
- Type 2 diabetes
- Full List »
share this!
April 22, 2024
This article has been reviewed according to Science X's editorial process and policies . Editors have highlighted the following attributes while ensuring the content's credibility:
fact-checked
peer-reviewed publication
trusted source
New study furthers understanding of lung regeneration
by Boston Medical Center
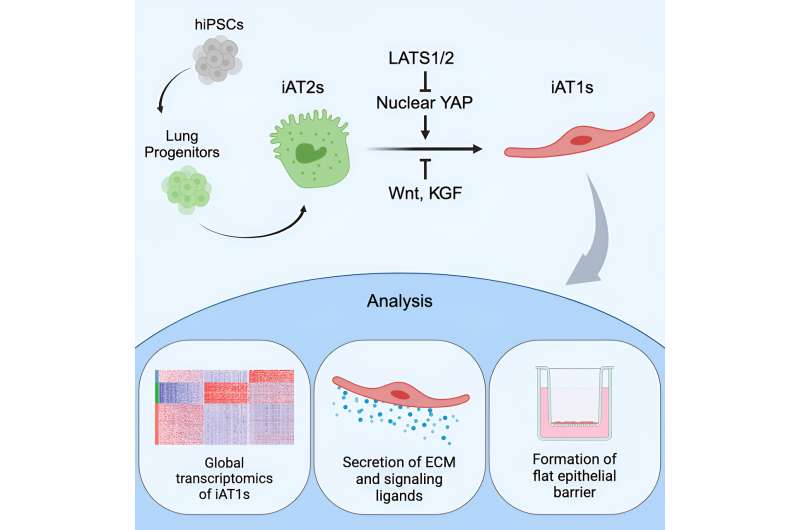
Researchers at Boston Medical Center (BMC) and Boston University (BU) have published a new study detailing the development of a method for generating human alveolar epithelial type I cells (AT1s) from pluripotent stem cells (iPSCs).
The ability to recreate these cells in an iPSC-based model will allow researchers to analyze the historically difficult to isolate cells in greater detail, helping to further the understanding of human lung regeneration and may ultimately expedite progress in treatment and therapeutic options for people living with pulmonary diseases. The study is published in the journal Cell Stem Cell .
Pulmonary diseases, including pulmonary fibrosis and chronic obstructive pulmonary disease (COPD), cause significant mortality and morbidity worldwide, and many pulmonary diseases lack sufficient treatment options. As science and medicine have progressed, researchers have identified a clear need for additional knowledge about lung cells to help improve patient health.
The results of this study provide an in vitro model of human AT1 cells, which line the vast majority of the gas exchange barrier of the distal lung, and are a potential source of human AT1s to develop regenerative therapies. The new model will help researchers of pulmonary diseases deepen their understanding of lung regeneration, specifically after an infection or exposure to toxins, as well as diseases of the alveolar epithelium such as acute respiratory distress syndrome (ARDS) and pulmonary fibrosis.
"Uncovering the ability to generate human alveolar epithelial type I cells (AT1s), and similar cell types, from pluripotent stem cells (iPSCs), has expanded our knowledge of biological processes and can significantly improve disease understanding and management," said Darrell Kotton, MD, Director, Center for Regenerative Medicine (CReM) of Boston University and Boston Medical Center.
This new study also furthers the CReM's goal of generating every human lung cell type from iPSCs as a pathway to improving disease management and provides a source of cells for future transplantation to regenerate damaged lung tissues in vivo.
"We know that the respiratory system can respond to injury and regenerate lost or damaged cells, but the depth of that knowledge is currently limited," said Claire Burgess, Ph.D., Boston University Chobanian and Avedisian School of Medicine, who is the study's first author.
"We anticipate this protocol will be used to further understand how AT1 cells react to toxins, bacteria, and viral exposures, and will be used in basic developmental studies, disease modeling, and potential engineering of future regenerative therapies."
Explore further
Feedback to editors

The consumption of certain food additive emulsifiers could be associated with the risk of developing type 2 diabetes
12 hours ago

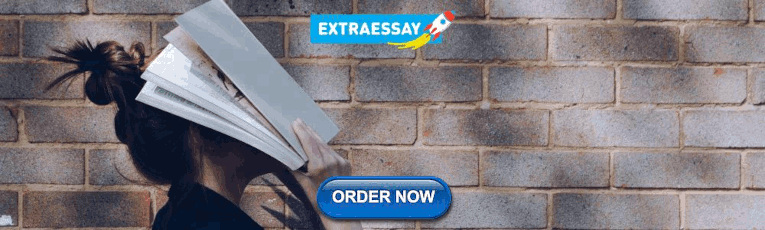
Perinatal transmission of HIV can lead to cognitive deficits

Study finds suicidal behaviors increased by over 50% in Catalonia, Spain after the COVID-19 pandemic
14 hours ago

Why do we move slower the older we get? New study delivers answers

Stress activates brain regions linked to alcohol use disorder differently for women than men, finds study

Chemical tool illuminates pathways used by dopamine, opioids and other neuronal signals
15 hours ago

Gentle defibrillation for the heart: A milder method developed by researchers for cardiac arrhythmias

Q&A: Research shows neural connection between learning a second language and learning to code
16 hours ago

Gut microbiota acts like an auxiliary liver, study finds

Higher light levels may improve cognitive performance
Related stories.

Researchers discover novel approach for rebuilding, regenerating lung cells
Aug 24, 2023

Researchers create new model of lung mesenchymal cells
Jun 13, 2023

Researchers create first model of the most common genetic cause of childhood interstitial lung disease
Jan 16, 2024
Researchers optimize lung stem cell engineering process
Apr 12, 2018

Stem cells 'migrate' to repair damaged lung cells, study shows
Feb 22, 2024

Researchers describe unique genetic identity of primordial lung progenitors
Jan 31, 2020
Recommended for you

A sustainable diagnosis tool for multiple cancers
20 hours ago

Magnetic microcoils unlock targeted single-neuron therapies for neurodegenerative disorders

Study identifies signs of repeated blast-related brain injury in active-duty United States Special Operations Forces
Apr 22, 2024

New technology uncovers mechanism affecting generation of new COVID variants

Mechanical engineers develop miniaturized, hydrogel-based electric generators for biomedical devices
Let us know if there is a problem with our content.
Use this form if you have come across a typo, inaccuracy or would like to send an edit request for the content on this page. For general inquiries, please use our contact form . For general feedback, use the public comments section below (please adhere to guidelines ).
Please select the most appropriate category to facilitate processing of your request
Thank you for taking time to provide your feedback to the editors.
Your feedback is important to us. However, we do not guarantee individual replies due to the high volume of messages.
E-mail the story
Your email address is used only to let the recipient know who sent the email. Neither your address nor the recipient's address will be used for any other purpose. The information you enter will appear in your e-mail message and is not retained by Medical Xpress in any form.
Newsletter sign up
Get weekly and/or daily updates delivered to your inbox. You can unsubscribe at any time and we'll never share your details to third parties.
More information Privacy policy
Donate and enjoy an ad-free experience
We keep our content available to everyone. Consider supporting Science X's mission by getting a premium account.
E-mail newsletter
Loading metrics
Open Access
Peer-reviewed
Research Article
Large language models approach expert-level clinical knowledge and reasoning in ophthalmology: A head-to-head cross-sectional study
Roles Conceptualization, Data curation, Formal analysis, Funding acquisition, Investigation, Methodology, Project administration, Resources, Software, Supervision, Validation, Visualization, Writing – original draft, Writing – review & editing
* E-mail: [email protected] (AJT); [email protected] (DSJT)
Affiliations University of Cambridge School of Clinical Medicine, Cambridge, United Kingdom, Oxford University Clinical Academic Graduate School, University of Oxford, Oxford, United Kingdom

Roles Data curation, Investigation, Writing – review & editing
Affiliation University of Cambridge School of Clinical Medicine, Cambridge, United Kingdom
Affiliation Eye Institute, Cleveland Clinic Abu Dhabi, Abu Dhabi Emirate, United Arab Emirates
Roles Data curation, Investigation, Writing – original draft, Writing – review & editing
Affiliations University of Cambridge School of Clinical Medicine, Cambridge, United Kingdom, Department of Physiology, Development and Neuroscience, University of Cambridge, Cambridge, United Kingdom
Roles Data curation, Investigation
Affiliation West Suffolk NHS Foundation Trust, Bury St Edmunds, United Kingdom
Affiliation Manchester Royal Eye Hospital, Manchester University NHS Foundation Trust, Manchester, United Kingdom
Affiliation Birmingham and Midland Eye Centre, Sandwell and West Birmingham NHS Foundation Trust, Birmingham, United Kingdom
Affiliation Department of Ophthalmology, Chang Gung Memorial Hospital, Linkou Medical Center, Taoyuan, Taiwan
Affiliation Yong Loo Lin School of Medicine, National University of Singapore, Singapore
Roles Data curation, Investigation, Project administration, Writing – review & editing
Affiliation Bedfordshire Hospitals NHS Foundation Trust, Luton and Dunstable, United Kingdom
Affiliation Singapore Eye Research Institute, Singapore National Eye Centre, Singapore, Singapore
Roles Writing – review & editing
Affiliations Birmingham and Midland Eye Centre, Sandwell and West Birmingham NHS Foundation Trust, Birmingham, United Kingdom, Academic Unit of Ophthalmology, Institute of Inflammation and Ageing, University of Birmingham, Birmingham, United Kingdom
Roles Funding acquisition, Project administration
Affiliations Singapore Eye Research Institute, Singapore National Eye Centre, Singapore, Singapore, Duke-NUS Medical School, Singapore, Singapore, Byers Eye Institute, Stanford University, Palo Alto, California, United States of America
- [ ... ],
Roles Conceptualization, Formal analysis, Funding acquisition, Methodology, Project administration, Supervision, Writing – original draft, Writing – review & editing
Affiliations Birmingham and Midland Eye Centre, Sandwell and West Birmingham NHS Foundation Trust, Birmingham, United Kingdom, Academic Unit of Ophthalmology, Institute of Inflammation and Ageing, University of Birmingham, Birmingham, United Kingdom, Academic Ophthalmology, School of Medicine, University of Nottingham, Nottingham, United Kingdom
- [ view all ]
- [ view less ]
- Arun James Thirunavukarasu,
- Shathar Mahmood,
- Andrew Malem,
- William Paul Foster,
- Rohan Sanghera,
- Refaat Hassan,
- Sean Zhou,
- Shiao Wei Wong,
- Yee Ling Wong,
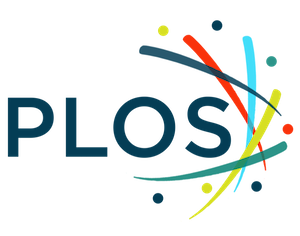
- Published: April 17, 2024
- https://doi.org/10.1371/journal.pdig.0000341
- Reader Comments
Large language models (LLMs) underlie remarkable recent advanced in natural language processing, and they are beginning to be applied in clinical contexts. We aimed to evaluate the clinical potential of state-of-the-art LLMs in ophthalmology using a more robust benchmark than raw examination scores. We trialled GPT-3.5 and GPT-4 on 347 ophthalmology questions before GPT-3.5, GPT-4, PaLM 2, LLaMA, expert ophthalmologists, and doctors in training were trialled on a mock examination of 87 questions. Performance was analysed with respect to question subject and type (first order recall and higher order reasoning). Masked ophthalmologists graded the accuracy, relevance, and overall preference of GPT-3.5 and GPT-4 responses to the same questions. The performance of GPT-4 (69%) was superior to GPT-3.5 (48%), LLaMA (32%), and PaLM 2 (56%). GPT-4 compared favourably with expert ophthalmologists (median 76%, range 64–90%), ophthalmology trainees (median 59%, range 57–63%), and unspecialised junior doctors (median 43%, range 41–44%). Low agreement between LLMs and doctors reflected idiosyncratic differences in knowledge and reasoning with overall consistency across subjects and types ( p >0.05). All ophthalmologists preferred GPT-4 responses over GPT-3.5 and rated the accuracy and relevance of GPT-4 as higher ( p <0.05). LLMs are approaching expert-level knowledge and reasoning skills in ophthalmology. In view of the comparable or superior performance to trainee-grade ophthalmologists and unspecialised junior doctors, state-of-the-art LLMs such as GPT-4 may provide useful medical advice and assistance where access to expert ophthalmologists is limited. Clinical benchmarks provide useful assays of LLM capabilities in healthcare before clinical trials can be designed and conducted.
Author summary
Large language models (LLMs) are the most sophisticated form of language-based artificial intelligence. LLMs have the potential to improve healthcare, and experiments and trials are ongoing to explore potential avenues for LLMs to improve patient care. Here, we test state-of-the-art LLMs on challenging questions used to assess the aptitude of eye doctors (ophthalmologists) in the United Kingdom before they can be deemed fully qualified. We compare the performance of these LLMs to fully trained ophthalmologists as well as doctors in training to gauge the aptitude of the LLMs for providing advice to patients about eye health. One of the LLMs, GPT-4, exhibits favourable performance when compared with fully qualified and training ophthalmologists; and comparisons with its predecessor model, GPT-3.5, indicate that this superior performance is due to improved accuracy and relevance of model responses. LLMs are approaching expert-level ophthalmological knowledge and reasoning, and may be useful for providing eye-related advice where access to healthcare professionals is limited. Further research is required to explore potential avenues of clinical deployment.
Citation: Thirunavukarasu AJ, Mahmood S, Malem A, Foster WP, Sanghera R, Hassan R, et al. (2024) Large language models approach expert-level clinical knowledge and reasoning in ophthalmology: A head-to-head cross-sectional study. PLOS Digit Health 3(4): e0000341. https://doi.org/10.1371/journal.pdig.0000341
Editor: Man Luo, Mayo Clinic Scottsdale, UNITED STATES
Received: July 31, 2023; Accepted: February 26, 2024; Published: April 17, 2024
Copyright: © 2024 Thirunavukarasu et al. This is an open access article distributed under the terms of the Creative Commons Attribution License , which permits unrestricted use, distribution, and reproduction in any medium, provided the original author and source are credited.
Data Availability: All data are available as supplementary information , excluding copyrighted material from the textbook used for experiments.
Funding: DSWT is supported by the National Medical Research Council, Singapore (NMCR/HSRG/0087/2018; MOH-000655-00; MOH-001014-00), Duke-NUS Medical School (Duke-NUS/RSF/2021/0018; 05/FY2020/EX/15-A58), and Agency for Science, Technology and Research (A20H4g2141; H20C6a0032). DSJT is supported by a Medical Research Council / Fight for Sight Clinical Research Fellowship (MR/T001674/1). These funders were not involved in the conception, execution, or reporting of this review.
Competing interests: AM is a member of the Panel of Examiners of the Royal College of Ophthalmologists and performs unpaid work as an FRCOphth examiner. DSWT holds a patent on a deep learning system to detect retinal disease. DSJT authored the book used in the study and receives royalty from its sales. The other authors have no competing interests to declare.
Introduction
Generative Pre-trained Transformer 3.5 (GPT-3.5) and 4 (GPT-4) are large language models (LLMs) trained on datasets containing hundreds of billions of words from articles, books, and other internet sources [ 1 , 2 ]. ChatGPT is an online chatbot which uses GPT-3.5 or GPT-4 to provide bespoke responses to human users’ queries [ 3 ]. LLMs have revolutionised the field of natural language processing, and ChatGPT has attracted significant attention in medicine for attaining passing level performance in medical school examinations and providing more accurate and empathetic messages than human doctors in response to patient queries on a social media platform [ 3 , 4 , 5 , 6 ]. While GPT-3.5 performance in more specialised examinations has been inadequate, GPT-4 is thought to represent a significant advancement in terms of medical knowledge and reasoning [ 3 , 7 , 8 ]. Other LLMs in wide use include Pathways Language Model 2 (PaLM 2) and Large Language Model Meta AI 2 (LLaMA 2) [ 3 ], [ 9 , p. 2], [ 10 ].
Applications and trials of LLMs in ophthalmological settings has been limited despite ChatGPT’s performance in questions relating to ‘eyes and vision’ being superior to other subjects in an examination for general practitioners [ 7 , 11 ]. ChatGPT has been trialled on the North American Ophthalmology Knowledge Assessment Program (OKAP), and Fellowship of the Royal College of Ophthalmologists (FRCOphth) Part 1 and Part 2 examinations. In both cases, relatively poor results have been reported for GPT-3.5, with significant improvement exhibited by GPT-4 [ 12 , 13 , 14 , 15 , 16 ]. However, previous studies are afflicted by two important issues which may affect their validity and interpretability. First, so-called ‘contamination’, where test material features in the pretraining data used to develop LLMs, may result in inflated performance as models recall previously seen text rather than using clinical reasoning to provide an answer. Second, examination performance in and of itself provides little information regarding the potential of models to contribute to clinical practice as a medical-assistance tool [ 3 ]. Clinical benchmarks are required to understanding the meaning and implications of scores in ophthalmological examinations attained by LLMs and are a necessary precursor to clinical trials of LLM-based interventions.
Here, we used FRCOphth Part 2 examination questions to gauge the ophthalmological knowledge base and reasoning capability of LLMs using fully qualified and currently training ophthalmologists as clinical benchmarks. These questions were not freely available online, minimising the risk of contamination. The FRCOphth Part 2 Written Examination tests the clinical knowledge and skills of ophthalmologists in training using multiple choice questions with no negative marking and must be passed to fully qualify as a specialist eye doctor in the United Kingdom.
Question extraction
FRCOphth Part 2 questions were sourced from a textbook for doctors preparing to take the examination [ 17 ]. This textbook is not freely available on the internet, making the possibility of its content being included in LLMs’ training datasets unlikely [ 1 ]. All 360 multiple-choice questions from the textbook’s six chapters were extracted, and a 90-question mock examination from the textbook was segregated for LLM and doctor comparisons. Two researchers matched the subject categories of the practice papers’ questions to those defined in the Royal College of Ophthalmologists’ documentation concerning the FRCOphth Part 2 written examination. Similarly, two researchers categorised each question as first order recall or higher order reasoning, corresponding to ‘remembering’ and ‘applying’ or ‘analysing’ in Bloom’s taxonomy, respectively [ 18 ]. Disagreement between classification decisions was resolved by a third researcher casting a deciding vote. Questions containing non-plain text elements such as images were excluded as these could not be inputted to the LLM applications.
Trialling large language models
Every eligible question was inputted into ChatGPT (GPT-3.5 and GPT-4 versions; OpenAI, San Francisco, California, United States of America) between April 29 and May 10, 2023. The answers provided by GPT-3.5 and GPT-4 were recorded and their whole reply to each question was recorded for further analysis. If ChatGPT failed to provide a definitive answer, the question was re-trialled up to three times, after which ChatGPT’s answer was recorded as ‘null’ if no answer was provided. Correct answers (‘ground truth’) were defined as the answers provided by the textbook and were recorded for every eligible question to facilitate calculation of performance. Upon their release, Bard (Google LLC, Mountain View, California, USA) and HuggingChat (Hugging Face, Inc., New York City, USA) were used to trial PaLM 2 (Google LLC) and LLaMA (Meta, Menlo Park, California, USA) respectively on the portion of the textbook corresponding to a 90-question examination, adhering to the same procedures between June 20 and July 2, 2023.
Clinical benchmarks
To gauge the performance, accuracy, and relevance of LLM outputs, five expert ophthalmologists who had all passed the FRCOphth Part 2 (E1-E5), three trainees (residents) currently in ophthalmology training programmes (T1-T3), and two unspecialised ( i . e . not in ophthalmology training) junior doctors (J1-J2) first answered the 90-question mock examination independently, without reference to textbooks, the internet, or LLMs’ recorded answers. As with the LLMs, doctors’ performance was calculated with reference to the correct answers provided by the textbook. After completing the examination, ophthalmologists graded the whole output of GPT-3.5 and GPT-4 on a Likert scale from 1–5 (very bad, bad, neutral, good, very good) to qualitatively appraise accuracy of information provided and relevance of outputs to the question used as an input prompt. For these appraisals, ophthalmologists were blind to the LLM source (which was presented in a randomised order) and to their previous answers to the same questions, but they could refer to the question text and correct answer and explanation provided by the textbook. Procedures are comprehensively described in the protocol issued to the ophthalmologists ( S1 Protocol ).
Our null hypothesis was that LLMs and doctors would exhibit similar performance, supported by results in a wide range of medical examinations [ 3 , 6 ]. Prospective power analysis was conducted which indicated that 63 questions were required to identify a 10% superior performance of an LLM to human performance at a 5% significance level (type 1 error rate) with 80% power (20% type 2 error rate). This indicated that the 90-question examination in our experiments was more than sufficient to detect ~10% differences in overall performance. The whole 90-question mock examination was used to avoid over- or under-sampling certain question types with respect to actual FRCOphth papers. To verify that the mock examination was representative of the FRCOphth Part 2 examination, expert ophthalmologists were asked to rate the difficulty of questions used here in comparison to official examinations on a 5-point Likert scale (“much easier”, “somewhat easier”, “similar”, “somewhat more difficult”, “much more difficult”).
Statistical analysis
Performance of doctors and LLMs were compared using chi-squared (χ 2 ) tests. Agreement between answers provided by doctors and LLMs was quantified through calculation of Kappa statistics, interpreted in accordance with McHugh’s recommendations [ 19 ]. To further explore the strengths and weaknesses of the answer providers, performance was stratified by question type (first order fact recall or higher order reasoning) and subject using a chi-squared or Fisher’s exact test where appropriate. Likert scale data corresponding to the accuracy and relevance of GPT-3.5 and GPT-4 responses to the same questions were analysed with paired t -tests with the Bonferroni correction applied to mitigate the risk of false positive results due to multiple-testing—parametric testing was justified by a sufficient sample size [ 20 ]. A chi-squared test was used to quantify the significance of any difference in overall preference of ophthalmologists choosing between GPT-3.5 and GPT-4 responses. Statistical significance was concluded where p < 0.05. For additional contextualisation, examination statistics corresponding to FRCOphth Part 2 written examinations taken between July 2017 and December 2022 were collected from Royal College of Ophthalmologists examiners’ reports [ 21 ]. These statistics facilitated comparisons between human and LLM performance in the mock examination with the performance of actual candidates in recent examinations. Failure cases where all LLMs provided an incorrect answer were appraised qualitatively to explore any specific weaknesses of the technology.
Statistical analysis was conducted in R (version 4.1.2; R Foundation for Statistical Computing, Vienna, Austria), and figures were produced in Affinity Designer (version 1.10.6; Serif Ltd, West Bridgford, Nottinghamshire, United Kingdom).
Questions sources
Of 360 questions in the textbook, 347 questions (including 87 of the 90 questions from the mock examination chapter) were included [ 17 ]. Exclusions were all due to non-text elements such as images and tables which could not be inputted into LLM chatbot interfaces. The distribution of question types and subjects within the whole set and mock examination set of questions is summarised in Table 1 and S1 Table alongside performance.
- PPT PowerPoint slide
- PNG larger image
- TIFF original image
Question subject and type distributions presented alongside scores attained by LLMs (GPT-3.5, GPT-4, LLaMA, and PaLM 2), expert ophthalmologists (E1-E5), ophthalmology trainees (T1-T3), and unspecialised junior doctors (J1-J2). Median scores do not necessarily sum to the overall median score, as fractional scores are impossible.
https://doi.org/10.1371/journal.pdig.0000341.t001
GPT-4 represents a significant advance on GPT-3.5 in ophthalmological knowledge and reasoning.
Overall performance over 347 questions was significantly higher for GPT-4 (61.7%) than GPT-3.5 (48.41%; χ 2 = 12.32, p <0.01), with results detailed in S1 Fig and S1 Table . ChatGPT performance was consistent across question types and subjects ( S1 Table ). For GPT-4, no significant variation was observed with respect to first order and higher order questions (χ 2 = 0.22, p = 0.64), or subjects defined by the Royal College of Ophthalmologists (Fisher’s exact test over 2000 iterations, p = 0.23). Similar results were observed for GPT-3.5 with respect to first and second order questions (χ 2 = 0.08, p = 0.77), and subjects (Fisher’s exact test over 2000 iterations, p = 0.28). Performance and variation within the 87-question mock examination was very similar to the overall performance over 347 questions, and subsequent experiments were therefore restricted to that representative set of questions.
GPT-4 compares well with other LLMs, junior and trainee doctors and ophthalmology experts.
Performance in the mock examination is summarised in Fig 1 —GPT-4 (69%) was the top-scoring model, performing to a significantly higher standard than GPT-3.5 (48%; χ 2 = 7.33, p < 0.01) and LLaMA (32%; χ 2 = 22.77, p < 0.01), but statistically similarly to PaLM 2 (56%) despite a superior score (χ 2 = 2.81, p = 0.09). LLaMA exhibited the lowest examination score, significantly weaker than GPT-3.5 (χ 2 = 4.58, p = 0.03) and PaLM-2 (χ 2 = 10.01, p < 0.01) as well as GPT-4.
Examination performance in the 87-question mock examination used to trial LLMs (GPT-3.5, GPT-4, LLaMA, and PaLM 2), expert ophthalmologists (E1-E5), ophthalmology trainees (T1-T3), and unspecialised junior doctors (J1-J2). Dotted lines depict the mean performance of expert ophthalmologists (66/87; 76%), ophthalmology trainees (60/87; 69%), and unspecialised junior doctors (37/87; 43%). The performance of GPT-4 lay within the range of expert ophthalmologists and ophthalmology trainees.
https://doi.org/10.1371/journal.pdig.0000341.g001
The performance of GPT-4 was statistically similar to the mean score attained by expert ophthalmologists ( Fig 1 ; χ 2 = 1.18, p = 0.28). Moreover, GPT-4’s performance exceeded the mean mark attained across FRCOphth Part 2 written examination candidates between 2017–2022 (66.06%), mean pass mark according to standard setting (61.31%), and the mean official mark required to pass the examination after adjustment (63.75%), as detailed in S2 Table . In individual comparisons with expert ophthalmologists, GPT-4 was equivalent in 3 cases (χ 2 tests, p > 0.05, S3 Table ), and inferior in 2 cases (χ 2 tests, p < 0.05; Table 2 ). In comparisons with ophthalmology trainees, GPT-4 was equivalent to all three ophthalmology trainees (χ 2 tests, p > 0.05; Table 2 ). GPT-4 was significantly superior to both unspecialised trainee doctors (χ 2 tests, p < 0.05; Table 2 ). Doctors were anonymised in analysis, but their ophthalmological experience is summarised in S3 Table . Unsurprisingly, junior doctors (J1-J2) attained lower scores than expert ophthalmologists (E1-E5; t = 7.18, p < 0.01), and ophthalmology trainees (T1-T3; t = 11.18, p < 0.01), illustrated in Fig 1 . Ophthalmology trainees approached expert-level scores with no significant difference between the groups ( t = 1.55, p = 0.18). None of the other LLMs matched any of the expert ophthalmologists, mean mark of real examination candidates, or FRCOphth Part 2 pass mark.
Expert ophthalmologists agreed that the mock examination was a faithful representation of actual FRCOphth Part 2 Written Examination papers with a mean and median score of 3/5 (range 2-4/5).
Results of pair-wise comparisons of examination performance between GPT-4 and the other answer providers. Significantly greater performance for GPT-4 is highlighted green, significantly inferior performance for GPT-4 is highlighted orange. GPT-4 was superior to all other LLMs and unspecialised junior doctors, and equivalent to most expert ophthalmologists and all ophthalmology trainees.
https://doi.org/10.1371/journal.pdig.0000341.t002
LLM strengths and weaknesses are similar to doctors.
Agreement between answers given by LLMs, expert ophthalmologists, and trainee doctors was generally absent (0 ≤ κ < 0.2), minimal (0.2 ≤ κ < 0.4), or weak (0.4 ≤ κ < 0.6), with moderate agreement only recorded for one pairing between the two highest performing ophthalmologists ( Fig 2 ; κ = 0.64) [ 19 ]. Disagreement was primarily the result of general differences in knowledge and reasoning ability, illustrated by strong negative correlation between Kappa statistic (quantifying agreement) and difference in examination performance (Pearson’s r = -0.63, p < 0.01). Answer providers with more similar scores exhibited greater agreement overall irrespective of their category (LLM, expert ophthalmologist, ophthalmology trainee, or junior doctor).
Agreement correlates strongly with overall performance and stratification analysis found no particular question type or subject was associated with better performance of LLMs or doctors, indicating that LLM knowledge and reasoning ability is general across ophthalmology rather than restricted to particular subspecialties or question types.
https://doi.org/10.1371/journal.pdig.0000341.g002
Stratification analysis was undertaken to identify any specific strengths and weaknesses of LLMs with respect to expert ophthalmologists and trainee doctors ( Table 1 and S4 Table ). No significant difference between performance in first order fact recall and higher order reasoning questions was observed among any of the LLMs, expert ophthalmologists, ophthalmology trainees, or unspecialised junior doctors ( S4 Table ; χ 2 tests, p > 0.05). Similarly, only J1 (junior doctor yet to commence ophthalmology training) exhibited statistically significant variation in performance between subjects ( S4 Table ; Fisher’s exact tests over 2000 iterations, p = 0.02); all other doctors and LLMs exhibited no significant variation (Fisher’s exact tests over 2000 iterations, p > 0.05). To explore whether consistency was due to an insufficient sample size, similar analyses were run for GPT-3.5 and GPT-4 performance over the larger set of 347 questions ( S1 Table ; S4 Table ). As with the mock examination, no significant differences in performance across question types ( S4 Table ; χ 2 tests, p > 0.05) or subjects ( S4 Table ; Fisher’s exact tests over 2000 iterations, p > 0.05) were observed.
LLM examination performance translates to subjective preference indicated by expert ophthalmologists.
Ophthalmologists’ appraisal of GPT-4 and GPT-3.5 outputs indicated a marked preference for the former over the latter, mirroring objective performance in the mock examination and over the whole textbook. GPT-4 exhibited significantly ( t -test with Bonferroni correction, p < 0.05) higher accuracy and relevance than GPT-3.5 according to all five ophthalmologists’ grading ( Table 3 ). Differences were visually obvious, with GPT-4 exhibiting much higher rates of attaining the highest scores for accuracy and relevance than GPT-3.5 ( Fig 3 ). This superiority was reflected in ophthalmologists’ qualitative preference indications: GPT-4 responses were preferred to GPT-3.5 responses by every ophthalmologist with statistically significant skew in favour of GPT-4 (χ 2 test, p < 0.05; Table 3 ).
Accuracy (A) and relevance (B) ratings were provided by five expert ophthalmologists for ChatGPT (powered by GPT-3.5 and GPT-4) responses to 87 FRCOphth Part 2 mock examination questions. In every case, the accuracy and relevance of GPT-4 is significantly superior to GPT-3.5 (t-test with Bonferroni correct applied, p < 0.05). Pooled scores for accuracy (C) and relevance (D) from all five raters are presented in the bottom two plots, with GPT-3.5 (left bars) compared directly with GPT-4 (right bars).
https://doi.org/10.1371/journal.pdig.0000341.g003
t-test results with Bonferroni correction applied showing the superior accuracy and relevance of GPT-4 responses relative to GPT-3.5 responses in the opinion of five fully trained ophthalmologists (positive mean differences favour GPT-4), and χ 2 test showing that GPT-4 responses were preferred to GPT-3.5 responses by every ophthalmologist in their blinded qualitative appraisals.
https://doi.org/10.1371/journal.pdig.0000341.t003
Failure cases exhibit no association with subject, complexity, or human answers.
The LLM failure cases—where every LLM provided an incorrect answer—are summarised in Table 4 . While errors made by LLMs were occasionally similar to those made by trainee ophthalmologists and junior doctors, this association was not consistent ( Table 4 ). There was no preponderance of ophthalmological subject or first or higher order questions in the failure cases, and questions did not share a common theme, sentence structure, or grammatical construct ( Table 4 ). Examination questions are redacted here to avoid breaching copyright and prevent future LLMs accessing the test data during pretraining but can be provided on request.
Summary of LLM failure cases, where all models provided an incorrect answer to the FRCOphth Part 2 mock examination question. No associations were found with human answers, complexity, subject, theme, sentence structure, or grammatic constructs.
https://doi.org/10.1371/journal.pdig.0000341.t004
Here, we present a clinical benchmark to gauge the ophthalmological performance of LLMs, using a source of questions with very low risk of contamination as the utilised textbook is not freely available online [ 17 ]. Previous studies have suggested that ChatGPT can provide useful responses to ophthalmological queries, but often use online question sources which may have featured in LLMs’ pretraining datasets [ 7 , 12 , 15 , 22 ]. In addition, our employment of multiple LLMs as well as fully qualified and training doctors provides novel insight into the potential and limitations of state-of-the-art LLMs through head-to-head comparisons which provide clinical context and quantitative benchmarks of competence in ophthalmology. Subsequent research may leverage our questions and results to gauge the performance of new LLMs and applications as they emerge.
We make three primary observations. First, performance of GPT-4 compares well to expert ophthalmologists and ophthalmology trainees, and exhibits pass-worthy performance in an FRCOphth Part 2 mock examination. PaLM 2 did not attain pass-worthy performance or match expert ophthalmologists’ scores but was within the spread of trainee doctors’ performance. LLMs are approaching human expert-level knowledge and reasoning in ophthalmology, and significantly exceed the ability of non-specialist clinicians (represented here by unspecialised junior doctors) to answer ophthalmology questions. Second, clinician grading of model outputs suggests that GPT-4 exhibits improved accuracy and relevance when compared with GPT-3.5. Development is producing models which generate better outputs to ophthalmological queries in the opinion of expert human clinicians, which suggests that models are becoming more capable of providing useful assistance in clinical settings. Third, LLM performance was consistent across question subjects and types, distributed similarly to human performance, and exhibited comparable agreement between other LLMs and doctors when corrected for differences in overall performance. Together, this indicates that the ophthalmological knowledge and reasoning capability of LLMs is general rather than limited to certain subspecialties or tasks. LLM-driven natural language processing seems to facilitate similar—although idiosyncratic—clinical knowledge and reasoning to human clinicians, with no obvious blind spots precluding clinical use.
Similarly dramatic improvements in the performance of GPT-4 relative to GPT-3.5 have been reported in the context of the North American Ophthalmology Knowledge Assessment Program (OKAP) [ 13 , 15 ]. State-of-the-art models exhibit far more clinical promise than their predecessors, and expectations and development should be tailored accordingly. Results from the OKAP also suggest that improvement in performance is due to GPT-4 being more well-rounded than GPT-3.5 [ 13 ]. This increases the scope for potential applications of LLMs in ophthalmology, as development is eliminating weaknesses rather than optimising in narrow domains. This study shows that well-rounded LLM performance compares well with expert ophthalmologists, providing clinically relevant evidence that LLMs may be used to provide medical advice and assistance. Further improvement is expected as multimodal foundation models, perhaps based on LLMs such as GPT-4, emerge and facilitate compatibility with image-rich ophthalmological data [ 3 , 23 , 24 ].
Limitations
This study was limited by three factors. First, examination performance is an unvalidated indicator of clinical aptitude. We sought to ameliorate this limitation by employing expert ophthalmologists, ophthalmology trainees, and unspecialised junior doctors answering the same questions as clinical benchmarks; and compared LLM performance to real cohorts of candidates in recent FRCOphth examinations. However, it remains an issue that comparable performance to clinical experts in an examination does not necessarily demonstrate that an LLM can communicate with patients and practitioners or contribute to clinical decision making accurately and safely. Early trials of LLM chatbots have suggested that LLM responses may be equivalent or even superior to human doctors in terms of accuracy and empathy, and experiments using complicated case studies suggest that LLMs operate well even outside typical presentations and more common medical conditions [ 4 , 25 , 26 ]. In ophthalmology, GPT-3.5 and GPT-4 have been shown to be capable of providing precise and suitable triage decisions when queried with eye-related symptoms [ 22 , 27 ]. Further work is now warranted in conventional clinical settings.
Second, while the study was sufficiently powered to detect a less than 10% difference in overall performance, the relatively small number of questions in certain categories used for stratification analysis may mask significant differences in performance. Testing LLMs and clinicians with more questions may help establish where LLMs exhibit greater or lesser ability in ophthalmology. Furthermore, researchers using different ways to categorise questions may be able to identify specific strengths and weaknesses of LLMs and doctors which could help guide design of clinical LLM interventions.
Finally, experimental tasks were ‘zero-shot’ in that LLMs were not provided with any examples of correctly answered questions before it was queried with FRCOphth questions from the textbook. This mode of interrogation entails the maximal level of difficulty for LLMs, so it is conceivable that the ophthalmological knowledge and reasoning encoded within these models is actually even greater than indicated by results here [ 1 ]. Future research may seek to fine-tune LLMs by using more domain-specific text during pretraining and fine-tuning, or by providing examples of successfully completed tasks to further improve performance in that clinical task [ 3 ].
Future directions
Autonomous deployment of LLMs is currently precluded by inaccuracy and fact fabrication. Our study found that despite meeting expert standards, state-of-the-art LLMs such as GPT-4 do not match top-performing ophthalmologists [ 28 ]. Moreover, there remain controversial ethical questions about what roles should and should not be assigned to inanimate AI models, and to what extent human clinicians must remain responsible for their patients [ 3 ]. However, the remarkable performance of GPT-4 in ophthalmology examination questions suggests that LLMs may be able to provide useful input in clinical contexts, either to assist clinicians in their day-to-day work or with their education or preparation for examinations [ 3 , 13 , 14 , 27 ]. Further improvement in performance may be obtained by specific fine-tuning of models with high quality ophthalmological text data, requiring curation and deidentification [ 29 ]. GPT-4 may prove especially useful where access to ophthalmologists is limited: provision of advice, diagnosis, and management suggestions by a model with FRCOphth Part 2-level knowledge and reasoning ability is likely to be superior to non-specialist doctors and allied healthcare professionals working without support, as their exposure to and knowledge of eye care is limited [ 27 , 30 , 31 ].
However, close monitoring is essential to avoid mistakes caused by inaccuracy or fact fabrication [ 32 ]. Clinical applications would also benefit from an uncertainty indicator reducing the risk of erroneous decisions [ 7 ]. As LLM performance often correlates with the frequency of query terms’ representation in the model’s training dataset, a simple indicator of ‘familiarity’ could be engineered by calculating the relative frequency of query term representation in the training data [ 7 , 33 ]. Users could appraise familiarity to temper their confidence in answers provided by the LLM, perhaps reducing error. Moreover, ophthalmological applications require extensive validation, preferably with high quality randomised controlled trials to conclusively demonstrate benefit (or lack thereof) conferred to patients by LLM interventions [ 34 ]. Trials should be pragmatic so as not to inflate effect sizes beyond what may generalise to patients once interventions are implemented at scale [ 34 , 35 ]. In addition to patient outcomes, practitioner-related variables should also be considered: interventions aiming to improve efficiency should be specifically tested to ensure that they reduce rather than increase clinicians’ workload [ 3 ].
According to comparisons with expert and trainee doctors, state-of-the-art LLMs are approaching expert-level performance in advanced ophthalmology questions. GPT-4 attains pass-worthy performance in FRCOphth Part 2 questions and exceeds the scores of some expert ophthalmologists. As top-performing doctors exhibit superior scores, LLMs do not appear capable of replacing ophthalmologists, but state-of-the-art models could provide useful advice and assistance to non-specialists or patients where access to eye care professionals is limited [ 27 , 28 ]. Further research is required to design LLM-based interventions which may improve eye health outcomes, validate interventions in clinical trials, and engineer governance structures to regulate LLM applications as they begin to be deployed in clinical settings [ 36 ].
Supporting information
S1 fig. chatgpt performance in questions taken from the whole textbook..
Mosaic plot depicting the overall performance of ChatGPT versions powered by GPT-3.5 and GPT-4 in 360 FRCOphth Part 2 written examination questions. Performance was significantly higher for GPT-4 than GPT-3.5, and was close to mean human examination candidate performance and pass mark set by standard setting and after adjustment.
https://doi.org/10.1371/journal.pdig.0000341.s001
S1 Table. Question characteristics and performance of GPT-3.5 and GPT-4 over the whole textbook.
Similar observations were noted here to the smaller mock examination used for subsequent experiments. GPT-4 performs to a significantly higher standard than GPT-3.5
https://doi.org/10.1371/journal.pdig.0000341.s002
S2 Table. Examination statistics corresponding to FRCOphth Part 2 written examinations sat between July 2017-December 2022.
https://doi.org/10.1371/journal.pdig.0000341.s003
S3 Table. Experience of expert ophthalmologists (E1-E5), ophthalmology trainees (T1-T3), and unspecialised junior doctors (J1-J2) involved in experiments.
https://doi.org/10.1371/journal.pdig.0000341.s004
S4 Table. Results of statistical tests of variation in performance between question subjects and types, for each trialled LLM, expert ophthalmologist, and trainee doctor.
Statistically significant results are highlighted in green.
https://doi.org/10.1371/journal.pdig.0000341.s005
S1 Protocol. Procedures followed by ophthalmologists to grade the output of GPT-3.5 and GPT-4 in terms of accuracy, relevance, and rater-preference of model outputs.
https://doi.org/10.1371/journal.pdig.0000341.s006
Acknowledgments
The authors extend their thanks to Mr Arunachalam Thirunavukarasu (Betsi Cadwaladr University Health Board) for his advice and assistance with recruitment.
- 1. Brown T, Mann B, Ryder N, Subbiah M, Kaplan JD, Dhariwal P, et al. Language Models are Few-Shot Learners. In: Advances in Neural Information Processing Systems [Internet]. Curran Associates, Inc.; 2020 [cited 2023 Jan 30]. p. 1877–901. Available from: https://papers.nips.cc/paper/2020/hash/1457c0d6bfcb4967418bfb8ac142f64a-Abstract.html
- 2. OpenAI. GPT-4 Technical Report [Internet]. arXiv; 2023 [cited 2023 Apr 11]. Available from: http://arxiv.org/abs/2303.08774
- View Article
- PubMed/NCBI
- Google Scholar
- 9. Google. PaLM 2 Technical Report [Internet]. 2023 [cited 2023 May 11]. Available from: https://ai.google/static/documents/palm2techreport.pdf
- 17. Ting DSJ, Steel D. MCQs for FRCOphth Part 2. Oxford University Press; 2020. 253 p.
- 21. Part 2 Written FRCOphth Exam [Internet]. The Royal College of Ophthalmologists. [cited 2023 Jan 30]. Available from: https://www.rcophth.ac.uk/examinations/rcophth-exams/part-2-written-frcophth-exam/
This paper is in the following e-collection/theme issue:
Published on 23.4.2024 in Vol 26 (2024)
Problems and Barriers Related to the Use of mHealth Apps From the Perspective of Patients: Focus Group and Interview Study
Authors of this article:

Original Paper
- Godwin Denk Giebel 1 , MSc ;
- Carina Abels 1 , Dr ;
- Felix Plescher 1 , MA ;
- Christian Speckemeier 1 , MSc ;
- Nils Frederik Schrader 1 , MSc ;
- Kirstin Börchers 2 , Prof Dr ;
- Jürgen Wasem 1 , Prof Dr ;
- Silke Neusser 1 , Dr ;
- Nikola Blase 1 , Dr
1 Institute for Health Care Management and Research, Universität Duisburg-Essen, Essen, Germany
2 QM BÖRCHERS CONSULTING+, Herne, Germany
Corresponding Author:
Godwin Denk Giebel, MSc
Institute for Health Care Management and Research
Universität Duisburg-Essen
Weststadt-Carree
Thea-Leymann-Straße 9
Essen, 45127
Phone: 49 20118 ext 33180
Email: [email protected]
Background: Since fall 2020, mobile health (mHealth) apps have become an integral part of the German health care system. The belief that mHealth apps have the potential to make the health care system more efficient, close gaps in care, and improve the economic outcomes related to health is unwavering and already partially confirmed. Nevertheless, problems and barriers in the context of mHealth apps usually remain unconsidered.
Objective: The focus groups and interviews conducted in this study aim to shed light on problems and barriers in the context of mHealth apps from the perspective of patients.
Methods: Guided focus groups and individual interviews were conducted with patients with a disease for which an approved mHealth app was available at the time of the interviews. Participants were recruited via self-help groups. The interviews were recorded, transcribed, and subjected to a qualitative content analysis. The content analysis was based on 10 problem categories (“validity,” “usability,” “technology,” “use and adherence,” “data privacy and security,” “patient-physician relationship,” “knowledge and skills,” “individuality,” “implementation,” and “costs”) identified in a previously conducted scoping review. Participants were asked to fill out an additional questionnaire about their sociodemographic data and about their use of technology.
Results: A total of 38 patients were interviewed in 5 focus groups (3 onsite and 2 web-based) and 5 individual web-based interviews. The additional questionnaire was completed by 32 of the participants. Patients presented with a variety of different diseases, such as arthrosis, tinnitus, depression, or lung cancer. Overall, 16% (5/32) of the participants had already been prescribed an app. During the interviews, all 10 problem categories were discussed and considered important by patients. A myriad of problem manifestations could be identified for each category. This study shows that there are relevant problems and barriers in the context of mHealth apps from the perspective of patients, which warrant further attention.
Conclusions: There are essentially 3 different areas of problems in the context of mHealth apps that could be addressed to improve care: quality of the respective mHealth app, its integration into health care, and the expandable digital literacy of patients.
Introduction
Worldwide, patients can access an ever-growing number of mobile health (mHealth) apps. Six years ago, there were 325,000 mHealth apps available, which were created by 84,000 different developers [ 1 ]. Regardless of the high number of mHealth apps, there is no fundamental quality control by app stores as distribution channels. Thus, unsafe mHealth apps are a potential threat for patient health [ 2 , 3 ].
An indicator for safe mHealth apps is the approval as a medical device. This is achieved by the European conformity or Food and Drug Administration certification in Europe and the United States, respectively [ 4 , 5 ]. Although medical device certification focuses particularly on safety and medical-technical performance with respect to the intended purpose specified by the manufacturer, there are also other areas where problems can arise.
A more extensive certification program is the “Fast-Track Process for Digital Health Applications (DiGA)” established in Germany. In this process, in addition to the European conformity certification as proof of “safety” and “suitability,” other requirements, such as “data protection,” “information security,” “interoperability,” and “user-friendliness” are reviewed [ 6 ]. Despite the relatively broad testing approach in the fast-track process, it has not been conclusively clarified whether it is sufficient to face all potential problems in the context of mHealth apps. This is partly due to the novelty of the field of care.
In addition to governmental guidelines, there is also a variety of approaches in science to evaluate the quality of mHealth apps. Well-known assessment scales include the Mobile App Rating Scale [ 7 ], the User Version of the Mobile Application Rating Scale [ 8 ], Enlight [ 9 ], and the System Usability Scale [ 10 ]. On an aggregated level, a review by Nouri et al [ 11 ], which was published in 2018, analyzed such rating tools. The review identified “design,” “information/content,” “usability,” “functionality,” “ethical issues,” “security and privacy,” and “user-perceived value” as relevant quality dimensions [ 11 ]. However, similar to the presented governmental certification processes, it is also questionable whether these quality assessment procedures and identified quality dimensions in the scientific field are sufficient to address potential problems in the context of mHealth.
Knowing the existing problems and barriers related to mHealth apps and their use is a fundamental requirement to guarantee the comprehensive quality assurance of mHealth apps. However, only few studies have aimed to identify weaknesses and problems of mHealth apps [ 12 - 14 ]. A scoping review on this topic revealed 10 problem categories: “validity,” “usability,” “technology,” “use and adherence,” “data privacy and security,” “patient-physician relationship,” “knowledge and skills,” “individuality,” “implementation,” and “costs” [ 14 ].
However, it remains uncertain whether these categories are relevant from the perspective of users or patients. A systematic review of qualitative studies identified 25 studies focusing on barriers of and facilitators to the use of lifestyle apps. However, the authors emphasized that the included studies have considered only healthy individuals, and thus, the obtained results are not transferable to patients with diseases [ 15 ]. In another qualitative study from Australia, patients were recruited in a general practice setting and interviewed about barriers and enablers to the use of mHealth apps. Nevertheless, the authors emphasized that the sample size was skewed toward relatively healthy patients, and future studies should target patients with long-term medical conditions [ 16 ].
As there is insufficient evidence base regarding the problems and barriers in the context of mHealth apps, qualitative research methods could provide an explorative insight in this research field. Perspectives of both mHealth app users as well as nonusers should be taken into account. This is necessary to reveal existing problems with the use of mHealth apps as well as concerns and barriers preventing patients from using these apps. Therefore, this study surveyed both app using and nonusing patients in focus groups and individual interviews to identify patient-relevant problems and barriers related to the use of mHealth apps.
We followed the standards of the study by O’Brien et al [ 17 ] and subsequently checked the manuscript by applying the 32-item COREQ (Consolidated Criteria for Reporting Qualitative Research) checklist [ 18 ] to ensure transparency in all aspects of our qualitative research.
Theoretical Framework
Focus groups and interviews were conducted with patients who had a condition for which an approved digital health applications (DiGA) existed at the time of the study. Patients were either actual users of DiGA and other mHealth apps or did not use any mHealth apps. The aim of the research was to shed light on the perspective of patients on mHealth apps. Questions covered “problems and barriers,” “facilitating factors,” “reasons for attrition,” and “important properties” that mHealth apps should meet. This paper focuses especially on problems and barriers identified in the underlying conversations.
The decision for a qualitative research design was made to take an exploratory step into the still poorly researched field of problems and barriers in the context of mHealth apps. Conducting focus groups and interviews allowed the collection of individual opinions. Those can subsequently be used to develop new research hypotheses.
Participant Selection
Patients were recruited via self-help groups and included both users and nonusers of mHealth apps. While patients with experience in mHealth app use could mainly contribute to the existing problems with use, patients without experience could mainly contribute to the barriers to use. Saturation was reached when all relevant indications for which there were approved DiGA at that time were covered.
Before conducting the interviews, participants received an informational letter about the topic “quality assurance of DiGA” and the proceeding of the interviews by email. Furthermore, patients were informed in the cover letter that the focus groups or interviews were part of a project (“QuaSiApps”) funded by the German Federal Joint Committee, which aims to develop a continuous quality assurance concept for DiGA. More detailed information was not provided beforehand.
Ethical Considerations
Upon request and presentation of the project schedule to the Ethics Committee of the Medical Faculty of the University of Duisburg-Essen, it was confirmed that ethics approval was not required. This was because, as part of the patient survey, neither personal nor disease-related questions were to be asked. Written informed consent was obtained from all participants before the survey. The participants were informed and asked to consent to the recording of their conversations for subsequent transcription. At the beginning of the interviews, the participants were informed that they could end the conversations at any time without giving a reason and without facing disadvantages of any type.
The settings of focus groups and interviews were heterogeneous. Conversations were either conducted web-based or in person. While the focus groups each included >3 participants, the interviews were conducted with 1 interviewee at a time. The onsite focus groups were conducted with self-help groups in their respective familiar settings. The web-based interviews were conducted via the videoconferencing platform Zoom (Zoom Video Communications, Inc). The choice for the web-based setting arose from the preference of participants.
No abnormalities were observed in the study setting that could influence patient statements. All patients were in a familiar environment during the interviews. No other people except 2 relatives of participants in 1 focus group were present besides participants and researchers. In 2 web-based focus groups, people did not know each other, which could lead to patients being more reluctant to participate.
There were no known relationships with participants. Notable characteristics of researchers, which could influence the participants, were not identified. There were no conflicts of interest, and the moderators did not take a firm stance for or against the use of mHealth apps.
Data Collection
Each focus group or interview was conducted by 1 of 3 moderators (GDG, CA, and KB). Of the 3 moderators, 2 were female (CA and KB) and 1 was male (GDG). For quality assurance reasons, at least 2 of the moderators participated in each focus group or interview conducted. While 2 moderators hold degrees with health economics background (GDG and CA), 1 moderator originally stems from the field of medicine and holds an MD degree (KB). GDG and CA are researchers at the Institute for Healthcare Management and Research, University of Duisburg-Essen. KB is the managing director of BÖRCHERS CONSULTING+ and an honorary professor at the University of Duisburg-Essen. While CA and KB are very experienced in conducting focus groups, GDG was less experienced but received a detailed briefing.
A prior scoping review [ 14 ] on problems and barriers related to the use of mHealth apps similar to the German concept of DiGA served as a basis to develop a noninfluencing interview guideline. This provided a consistent interview framework ( Multimedia Appendix 1 ). The guideline followed a uniform structure. Each topic started with open questions followed by some concrete questions, which were shown if the participants did not find a spontaneous answer. Personal data were not addressed in the guideline.
Focus groups or interviews were recorded either onsite with microphones or web-based via the integrated recording function in Zoom. Data collection took place in the period between the end of September 2021 and the beginning of December 2021. Subsequently, to record transcription, data analysis started in February 2022 and was completed at the end of October 2022. The conduct of the interviews worked without problems, and the guide proved to be understandable. Therefore, no adjustments were made to the method of data generation during the study.
The recordings of the interviews and focus groups were transcribed and pseudonymized by project assistants or employed students. A second person, also a project assistant or student assistant, performed quality assurance of the transcripts. Afterward, the transcripts were loaded into MAXQDA (VERBI Software GmbH) for data analysis, and the audio recordings were deleted. After data extraction, it was no longer possible to trace back individual statements to individual participants.
Qualitative data analysis was based on Mayring [ 19 ] and performed by GDG and CA. NB supervised the process and served as the decisive authority in the event of disagreements. As recommended by Mayring [ 19 ], deductive codes used in the data analysis were defined before the data analysis. Deductive codes were taken from the results of a scoping review [ 14 ]. Inductive subcodes were developed iteratively at the time of data extraction.
Data analysis was conducted in 4 steps to enhance trustworthiness. In the first step, GDG coded each transcript with deductive codes. In the second step, CA checked the coding made in each transcript, and in case of disagreement, problems were solved by discussion. In the third step, GDG developed inductive subcodes, which were discussed in group (GDG, CA, NB, and FP). Finally, subcodes were applied by GDG and checked by CA.
In total, 38 patients and 2 relatives participated in 5 focus groups and 5 interviews between September and December 2021. A total of 7 conversations took place on the web via Zoom, and 3 conversations took place in person. A structured overview of the focus groups and interviews conducted is provided in Table 1 .
Of the 40 included participants, 32 (80%) consented to give information about sociodemographical data ( Table 2 ). Further details on the study participants can be found in Multimedia Appendix 2 . The intrinsic motivation and participation of the participants were high. Nevertheless, the moderators made sure that everybody had their say and had the opportunity to express themselves.
a Multiple answers possible.
b N/A: not applicable.
As recruitment was conducted with the help of self-help groups, no binding statement can be made about how many participants declined to participate. None of the included patients dropped out of the study after recruitment.
The findings describe the perspectives of patients on problems and barriers in the context of mHealth apps. During the interviews and focus groups, all the 10 problem categories that served as deductive codes were addressed. The 10 mentioned problem categories, which served as deductive codes, include “validity,” “usability,” “technology,” “use and adherence,” “data privacy and security,” “patient-physician relationship,” “knowledge and skills,” “individuality,” “implementation,” and “costs.” The respective definitions of the categories can be found in the published scoping review [ 14 ]. The corresponding inductive subcodes are listed in Multimedia Appendix 3 . Multimedia Appendix 4 includes all relevant statements of the patients systematized into the problem categories.
Problems with “validity” were particularly mentioned in the 4 areas of “poor content and quality of information,” “lack of (added) value,” “lack of therapeutic setting,” and “patient safety.” The first problem area, “poor content and quality of information,” was observed by patients, especially in the lack of empirical evidence, inappropriate content, nonfunctioning links, and deficient exercise instructions:
[...] that’s why I’m very demanding when it comes to, for example, progressive muscle relaxation or something like that [...] and I find the [instructions] that are in the app-. That doesn’t work at all, so for me. [Patient with migraine]
“Lack of validity, reliability, and accuracy of app-collected data” was identified as a second problem area. For example, in the area of obesity, patients reported the overrating of physical activity within the app. Another concern was that results could be skewed due to, for example, tattoos, heavy arm hair, or athletic activity, or false output could be generated by interconnectivity with other apps:
The problem is that another app somewhere is still feeding me data, even in the GoogleFit, so that I have twice the amount of steps every day. And that has blown things up, of course. I was well into the minus range of calories that I could take in. That’s not quite mature yet [...] [Patient with obesity]
The third problem area of “validity” and the possible reason for the discontinuation of use is “lack of (added) value.” Patients reported that lack of health improvement would lead them to try something else:
Failure to improve health [...] “doesn’t help me, I have to try something else.” [Patient with tinnitus]
The “lack of therapeutic setting” was identified as the fourth problem area of “validity.” It was explained that the app alone would not be sufficient, and a real person would have to pay attention to what the patient was doing:
With me, the problem is more. There has to be someone behind it to watch what I’m doing. [Patient with obesity]
The last area to be addressed was “patient safety” under the problem area of “validity.” It was explicitly stated that the lack of human control and correction posed a direct safety issue to patients. Thus, in the context of guided practice, errors could creep in, or worse, in an emergency, such as the occurrence of suicidal thoughts, no appropriate response could occur:
Or at some point I had indicated that I was having suicidal thoughts. And only then did the app unlock that you can call the telephone counselling service directly from the app. [Patient with depression]
The adverse effects that could occur through the app were also described as problematic for patient safety. For example, patients described a constant preoccupation with symptoms, symptom amplification, or the activation of triggers by the app as harmful. A final issue was the risk of social withdrawal:
Many people don’t want to talk about [the disease] and withdraw. And I think that if you only have this app in front of you and nothing personal at all, that’s very difficult for cancer patients. For all seriously ill people. [Patient with cancer]
The problems mentioned in the category of “usability” can basically be divided into “problems with the instructions” on the one hand and “difficulties with the usage” on the other. Thus, patients rejected a time-consuming introduction to the functions of the app and were dismissive toward independently searching through functions in the app:
I would say, from my experience, I really don’t have the nerve to read it at that moment. [...] But you could do it quite simply, like a how-to with screenshots. So rather with pictures where you have to click on them. [Patient with migraine]
Further difficulties in the category of “usability” were found, particularly in the lack of an easy overview and nonintuitive app design. A deficient menu navigation was explicitly criticized:
But then there’s kind of no main menu after that. So when I finish it, I would like to have a main menu like that. [Patient with migraine]
In addition to the fundamental problem expressed that technical complexities are particularly disturbing in the case of illness, 3 areas of technological problems were identified: “problems with the software,” “problems with the hardware,” and “problems with interoperability and network connection.”
In the area of software, it was criticized that some apps were only available as web apps but not as mobile apps. Furthermore, patients mentioned the danger of viruses as well as problems, errors, and bugs in the software itself. Problems, errors, and bugs in the software could show up in app crashes and were seen because of poor programming:
And it was programmed in a very very clumsy and annoying way. [Patient with tinnitus]
In addition to software issues, problems also arose concerning hardware, specifically with the devices in use and their updates. One point raised was related to an existing concern that updates for the smartphone would impair the functionality of the app.
Furthermore, besides updates, some technical features, such as digital displays, were criticized. Thus, for example, in the case of migraine, working on the screen was described to be problematic due to light sensitivity:
I think that I would then use it even easier if I could sort of just talk to the phone and not have to sit down and look at the display big time. [Patient with migraine]
Further problems were observed with running and connected devices. Specifically, the limitation of using the app on only 1 device and the absence of the option to use it across multiple devices (such as smartphones or tablets) were considered problematic. On the other hand, additional devices, such as smartwatches and virtual reality glasses, were partially dismissed:
If you think about possible accessories, for example. I’m kind of getting out of the game with any more tech accessories. So, I don’t use a smartwatch, nor do I have VR goggles, and I don’t really want to. [Patient with cancer]
In the area of compatibility and network connection, an example was cited in which data imported from other apps did not work properly. Furthermore, dependence on an internet connection was criticized:
And that I then-, we also had a bad Internet connection there, then I couldn’t use that at all like that in some cases. [Patient with tinnitus]
Use and Adherence
The problem area of “use and adherence” included, in particular, “problems due to the attitude of users”; “problems that occurred in the context of usage”; “inadequate, unappealing content design”; and “limited time resources of the patients.”
A fundamental problem stated by the patients was the attitude of users. They often have a lack of motivation and engagement. It was expressed that it may be unnecessary to prescribe apps to those who have a negative attitude in advance. On the one hand, lack of motivation may be fundamental, but on the other hand, it may be due to an aversion to the digital format:
Yes, maybe that you don’t have the motivation to do it alone. Many need the group to do the exercises. [Patient with osteoarthritis]
The second that I don’t want to do therapy then on digital devices because as a journalist I’m on the go all day with tablet, cell phone and laptop. [Patient with tinnitus]
If patients actually use the apps, problems can also arise in the (social) context of use. These include, for example, a low prioritization of use over other obligations such as work or private commitments, a predetermined daily schedule or paternalism exercised by the app, or distraction by other people:
And I can’t do that now in front of the running TV, when the family is sitting around, of course that doesn’t work. And if someone says: “I don’t have the time, because I have small children or we have a lot going on in the apartment or something-.” So you do need a bit of a place to retreat. [Patient with tinnitus]
Furthermore, use can also be inhibited by the content design. This happens when the content is not very varied and boring or when it lacks human or emotional aspects:
For me, it was so that the app-, I didn’t find it very-, so it had become somehow boring for me. So I didn’t enjoy entering my things there anymore, because it was always the same. It always just recorded the app and then calculated it for me and that was it. [Patient with obesity]
Another problem and possible reason for discontinuation of use across many indications was that apps were too extensive and time consuming. This problem was exacerbated by a lack of interruption or pause options, among other things:
It’s just very time-consuming, and that’s the thing that bothered me a little bit before. [Patient with migraine]
Data Privacy and Security
In the context of data privacy and security, fears about the loss of personal data was noted on the patients’ side. Besides insufficient data protection within the app, another problem was the lack of trust in running operating systems. Furthermore, patients rejected indiscriminate data provision to third parties:
So if I have the feeling that the data is not in good hands [...] I do turn my innermost thoughts inside out with these apps. Especially when it comes to mental illness. [Patient with depression]
[...] if I now have to prove my sporting activities to the health insurance company, then they just get the sporting activities, but they are not supposed to know, how I felt [...] [Patient with obesity]
Patient-Physician Relationship
There were mainly 2 different types of problems regarding the physician-patient relationship. On the one hand, there were “problems with usage not accompanied by a doctor or therapist,” and on the other hand, there were “problems in the patient-physician relationship triggered by the app.”
A fundamental problem in the use of the app without medical or therapeutic accompaniment was seen in the limited scope of support that apps can offer. Therefore, care must be taken to ensure that they do not lead to the neglect of personal therapeutic contact:
And also to that, that an app has limits, I think that’s important and that should be apps... and that should also, when the doctors then eventually know what that is, also tell the patients [laughs], “Yes, that’s just an app and it has its limits.” [Patient with depression]
Furthermore, patients expressed that app use can have an impact on the patient-physician relationship for various reasons. This occurs, for example, when patients need to report their app use to physicians or when health care providers are dismissive of the use, perceiving the app as competition to their abilities:
Because, of course, it must be assumed that the prescribing physician or the attending physician is also behind it. If he now inwardly rejects it, then it can of course be that the relationship with him suffers. [Patient with tinnitus]
Finally, a particularly problematic situation for patients can arise when there are inconsistent opinions between the physician and the app. Patients then find themselves in the situation of having to determine which information is correct:
Do I trust my doctor more and the statement he says? Or do I also additionally trust what an app suggests I might do? That’s where I stand in between. [Patient with cancer]
Knowledge and Skills
Problems in the area of “knowledge and skills” were seen on the “patient side” but also on the “side of physicians and therapists.” On the part of the patients, a distinction was made here between actual “lack of skills, knowledge and experience” on the one hand and “perception” on the other hand.
The respondents saw potential for improvement particularly in the areas of patients’ technical skills and media competence. Although in the case of media competence, the assessment was seen as particularly problematic, in the technical area, the focus was on the lack of skills:
I can’t operate at all, I can’t operate at all. I’m not a person who can handle digital things at all. [Patient with cancer]
Participants expressed that older patients do not always consider themselves as a target group. Older adults often had a preference for analog methods:
[...] I’ve already handed over the 60, we just didn’t grow up with these things in our hands. So maybe we just don’t want to be digital for a change, and the grip on paper is just more familiar. [Patient with migraine]
In some cases, patients did not use apps because there was a lack of confidence in the therapeutic effect, the apps were perceived as complicated, or patients were fundamentally opposed to the technology:
I haven’t used an app until now, for the reason that I always imagined it to be very complicated, I have to read a lot, scroll. [Patient with obesity]
Issues regarding “knowledge and skills” were also observed on the practitioners’ and health insurers’ side, particularly stemming from a poor level of knowledge:
I think it would make a lot of sense. So you would just have to somehow bring it to the doctors a bit, because I think most of them don’t really know how to deal with it yet. [Patient with depression]
Individuality
A further group of problems and a possible reason for discontinuing use was observed in the lack of “individuality.” In particular, “inadequate adaption to individual user abilities and needs” as well as the problem of “too generalized approaches” were addressed. It was emphasized that individual abilities are different, older users have special requirements for app use, and preferences exist for different (physical and mental) exercises:
Is so individual, of course. Everyone has their own problems of course, everyone perhaps does their own exercises. [Patient with osteoarthritis]
Approaches that are too generalized were considered a further problem in this category. Depending on the stage of the disease and the individual, different configurations or designs were desirable:
Tinnitus symptoms are very individual, both in terms of the triggers and the expression. And dealing with it and then the question is always, if such an app comes as a therapy concept, how fine-tuned is that [...]” [Patient with tinnitus]
Implementation
The field of “implementation” included many different problems. The focus was particularly on the “barriers to access” and “additional burden for patients” as well as “additional burden for health care providers” and “low acceptance by health care providers.” Other points mentioned were “difficult transfer into clinical practice,” “too many options to use,” and “fear of consequences due to app usage.”
Patients encountered barriers to access, especially due to language barriers and lack of accessibility. The individual degree of illness and age should also be taken into account in the context of access. Further barriers to access were seen in complicated access to the app or complicated acquisition via the health insurance company and use limited to 1 device:
So, yes, it has to be low-threshold and if it’s not, I think it’s also difficult from a certain age then to deal with it or also from a certain degree of illness. [Patient with depression]
The interviewees stated that the use of apps was associated with additional effort, both for themselves and for the physicians involved. In particular, the time required was emphasized critically:
Because that’s another new task for the doctors. First of all, they have so much to do with their patients, who are all individuals, focused on their specialty. So it becomes difficult to bring it all together afterwards. Because there won’t be just one patient who wants to use such an app, but five or even 100. [Patient with cancer]
Patients stated that problems also arise when service providers only have a low level of acceptance toward the apps. This can be due to a lack of interest, competition between the app and health care providers, or a fundamental rejection. Physicians might perceive apps as interfering with their expertise, might express disapproval, or might advise patients against using apps. Divergent opinions between different physicians were mentioned as a particular problem:
Do I go to my urologist, do I go to my thoracic surgeon, do I go to my family doctor, or do I go to my dermatologist? And if one is in favor, but the others are against, what do I do? [Patient with cancer]
“Difficult transfer into clinical practice” was identified as a further problem. The transfer might be impeded by a lack of presence of the apps. It was criticized that the apps are still rarely recommended by health care providers and that the social awareness is still low:
Because just no one has recommended this app yet. So I’ve really been through a bunch of psychologists and I-, several clinics [...] But the topic of the app is not in the waiting room with a flyer, nor at the doctor’s office, nor in the support group [...] [Patient with depression]
The confusing app market was also described as complicated. Especially for patients who are already limited by diseases, the search and selection process can be complicated and time consuming:
I’m struggling with my illness or I’m struggling with my various illnesses, and then now there’s this additional psychological pressure: “I might have the wrong thing I’m using after all. Maybe there’s something significantly better in the meantime.” These digital health apps, they’re totally confusing to me as a user. [Patient with cancer]
A final, but not specified, problem was seen in the consequences, especially for the health insurance of patients. It was questioned whether using the apps might have any consequences on their insurance coverage:
Then what does that do to my health insurance coverage afterward? [Patient with cancer]
Patients stated that costs might result in different problems. Patients, health insurers, and physicians were named as the groups of people affected by costs. Problems were observed in “loss of revenue,” “low willingness to pay,” “alternative financing methods,” and “waste of money.”
While a loss of revenue was considered problematic on the side of the service providers, a low willingness to pay was observed on the side of the patients. The lack of willingness to pay was related, on the one hand, to the apps themselves and, on the other hand, to the accessories required:
So for me, the reason was that it’s paid now and that-, so this app was super good, except for some initial difficulties, but just, I didn’t want to pay for it. [Patient with obesity]
Alternative financing methods, especially through advertising, were another problem mentioned. Both advertising for products within the app and advertising about the app in other media were discussed:
If, for example, advertising were to come on all at once. If now from different drug manufacturers there is always something inserted there. [Patient with cancer]
The last point mentioned in the category of costs was that under certain circumstances, mHealth apps could lead to a waste of money. Two such situations were discussed. First, a lack of control over whether the app is used was considered critical; second, payment, despite a lack of evidence, was criticized:
And it was, that was also point of attack, of those who do not think anything at all of e-health, that the scientific proof is missing and now the insurance already pays. [Patient with tinnitus]
Principal Findings
Our study showed that patients face a multitude of problems and barriers in the context of mHealth apps. While the problem categories were determined based on a previously conducted scoping review [ 14 ], many new expressions of problems emerged in each category. These expressions provide a deeper insight into the thinking and attitudes of patients related to mHealth apps.
There were mainly 3 different factors related to problems and barriers in the context of mHealth app use. First, the mHealth apps themselves could lead to problems. Such problems were observed, for example, in defective design, technical aspects, or low or no added value for patients.
Second, the integration into the health care system was considered partially problematic. Thus, problems were found, for instance, in remuneration and lack of time of health care providers, influences on the relation between health care providers and patients, and a lack of presence of the topic in the health care system.
Third, the users themselves as well as their attitude and knowledge were found to be a barrier for mHealth app use. Several participants expressed a lack of interest in using mHealth apps and explained that they sometimes lack knowledge and skills to use the digital technology. This fact was especially pronounced for older patients.
In our study, we included patients with different diseases (migraine, tinnitus, obesity, depression, arthrosis, cancer, and alcohol addiction) to receive broad feedback from people with diverse needs. While most of the problems were overarching and concerned the general use of mHealth apps, some problems were disease specific. The latter were mainly found in the category of individuality but also included technological problems such as light sensitivity leading to an avoidance of screen use in the case of patients with migraine or other problems such as the risk of increased social withdrawal of individuals with cancer because of app use. For other indications, there are additional disease-specific problems that could not be completely covered here.
Implications
To guarantee a sustainable, safe, and effective use of mHealth apps, it is necessary to deal with all three areas where problems can arise or barriers exist: (1) the mHealth apps, (2) the integration into the health care system, and (3) the users.
mHealth Apps
Problems with the mHealth apps themselves were found in the categories of “validity,” “usability,” “technology,” “individuality,” “data privacy and security,” and “costs.” One approach to identify these is to use various quality assessment tools, which aim to guarantee a high quality of mHealth apps [ 11 , 20 , 21 ]. Quality assessment tools provide a practicable approach to distinguish high-quality mHealth apps from low-quality mHealth apps and thereby indicate if patients might have more or less problems with app use.
Furthermore, patients should be involved during the whole development process of mHealth apps. This includes (1) the need assessment, (2) the design and development, (3) the laboratory evaluation, and (4) the field evaluation. Eligible methods include qualitative data collection methods such as interviews, focus groups, observations, and think-aloud techniques as well as quantitative approaches such as self-report questionnaires [ 22 ].
An eligible way to do this is the participatory design [ 23 ]. By this, patients would have the possibility to comment on problems and barriers as soon as they become apparent. By including both experienced and nonexperienced mHealth apps users during the development process of mHealth apps, problems and barriers can be minimized for all users. A good example in which a 3-phase user-centered design approach has been successfully implemented is the study by Newton et al [ 24 ].
In addition, problems and barriers become apparent through user feedback in app stores. Therefore, user feedback should not only be included during the development but also in the evaluation and rework of mHealth apps. One innovative approach to incorporate user text review is the ACCU 3 RATE rating scale [ 25 ].
Integration of mHealth Apps Into the Health Care System
The results of this study show that problems and barriers are not restricted to the mHealth apps themselves. Thus, it is necessary to obtain more knowledge about the contextual problems and barriers that patients encounter when they want to or actually use mHealth apps.
The focus groups and interviews conducted indicate that, in the perception of patients, health care practitioners are one of the most important stakeholder groups facing problems with mHealth apps. Therefore, it is a fundamental requirement that they are convinced of the positive effects and low risk of mHealth apps for their patients and of the simple and sustainable integration in their daily routine.
However, this is not always the case, as shown by a study on the attitudes of physicians toward mHealth apps falling under the German concept of DiGA [ 26 ]. In principle, general practitioners, other outpatient care physicians, and psychotherapists were in favor of prescribing DiGA but faced significant barriers, such as insufficient information, insufficient reimbursement for DiGA-related medical services, lack of medical evidence, and legal and technological uncertainties. Therefore, such problems and barriers faced by health care providers should also be further investigated.
Germany was the first country in the world to incorporate certain mHealth apps (ie, DiGA) as a fixed part into the benefit package of the health care system [ 26 ]. Although the German concept is an innovative and commendable approach, it still faces several challenges. Further investigations into problems and barriers related to integrating mHealth apps could provide insights into improving regulatory systems. This would aim to make app use easier while ensuring safety and sustainability.
Another problem, depending on the respective health care system, could be the costs of using mHealth apps for patients. In the German statutory health insurance system, however, the costs are covered and thus represent only an indirect problem for users. Therefore, prices must be negotiated between health insurers and manufacturers, in particular.
mHealth App Users
Besides the mHealth apps themselves and their integration into health care, problems on the patients’ side were also reported. In our study as well as in the literature [ 14 ], it was found that low digital literacy of patients was seen as a problem in the context of mHealth app use.
According to the technology acceptance model by Davis et al [ 27 ], an actual system use is the result of perceived ease of use as well as perceived usefulness. Therefore, another approach to optimize the use of mHealth apps should focus on reinforcing the digital literacy of potential users, especially older patients. This involves supporting them with app use and effectively communicating the benefits of mHealth apps [ 28 , 29 ].
Both our study and other studies [ 14 , 16 , 30 ] found that age is a problem in the context of mHealth app use. Interestingly, however, the reason for this is predominantly seen in the lack of technological affinity and digital literacy among older people. The extent to which cognitive and psychological decline plays a role should be investigated further.
Limitations
Given the scarce evidence regarding problems and barriers in the context of mHealth app use, qualitative research seems to be an eligible first step to gain further evidence [ 14 ]. Nevertheless, qualitative research is always accompanied by uncertainty. The statements made by participants are not necessarily representative for all patients, especially as participants were recruited from self-help groups and presumably had a high motivation to actively shape their to actively shape their experience of living with the disease. Thus, the problems and barriers identified in this study should serve as the first evidence to conduct further qualitative and quantitative studies.
Two points concerning the methods must still be made. First, we were not able to determine the number of people who refused to take part in our study. As we recruited our participants via self-help groups, we do not exactly know how each group distributed the information about our study to their respective members. Second, we did not calculate an agreement rate or other measurements regarding the coding discrepancies. In retrospect, however, there were very few disagreements and minimal need for discussion in this regard.
To make the research comprehensible and as free from arbitrariness as possible, we described the methods precisely. Therefore, we followed the standards by O’Brien et al [ 17 ] and checked the manuscript against the 32-items of the COREQ checklist [ 18 ]. Thereby, the strengths and weaknesses of our study became very transparent.
In Germany, the use of mHealth apps in the context of diseases is not yet very common [ 31 ]. This also became obvious in our study. Only 16% (5/32) of the participants in our study reported ≥1 prescription of an mHealth app. Thus, most participants could not contribute with experience in this field. Nevertheless, we included opinions, fears, and concerns of nonusers in our research. These should be taken into account when developing and integrating mHealth apps in health care.
A limitation of the article, but not of the study or the results, is that the patient statements were originally made in German and subsequently translated into English. We have taken care not to change the meaning of the statements. The original citations can be requested from the authors of the study.
Conclusions
Problems and barriers in the context of mHealth apps should be considered to guarantee their sustainable, safe, and effective use. Such problems can be categorized into problems originating from the app, problems with the integration of the mHealth app into the health care system, and problems and barriers on the users’ side. While problems on the level of the app and the health care system should be taken into account when developing mHealth apps and corresponding assessment tools, problems on the patients’ side should be solved by increasing the digital literacy of potential users. On the basis of our findings, further research should be conducted to generate more evidence on problems and barriers as well as how to counteract them.
Acknowledgments
This study is part of a larger research project (Continuous quality assurance of Digital Health Applications [DiGA] [“QuaSiApps”]). The project is funded by the German Federal Joint Committee. The funders had no influence on the study design, the conduct of the study, or the decision to publish or prepare the manuscript. The authors acknowledge the support by the Open Access Publication Fund of the University of Duisburg-Essen.
Data Availability
All data generated or analyzed during this study are included in this published article and its supplementary information files. In addition to the English patient statements included in the text, the original statements made in German can be requested from the authors.
Authors' Contributions
All authors participated in the conception of the study. Data collection was performed by GDG, CA, KB, and NB. GDG and CA conducted data analysis and interpreted the data together with FP and NB. The manuscript was drafted by GDG with the aid of NB. Feedback from the entire consortium was incorporated.
Conflicts of Interest
None declared.
Interview guidelines.
Participant characteristics.
The coding system including problem categories and subthemes.
Systematized statements of the patients.
- mHealth economics 2017/2018 – connectivity in digital health. Research2Guidance. URL: https://research2guidance.com/product/connectivity-in-digital-health/ [accessed 2024-04-10]
- van Velthoven MH, Wyatt JC, Meinert E, Brindley D, Wells G. How standards and user involvement can improve app quality: a lifecycle approach. Int J Med Inform. Oct 2018;118:54-57. [ FREE Full text ] [ CrossRef ] [ Medline ]
- Akbar S, Coiera E, Magrabi F. Safety concerns with consumer-facing mobile health applications and their consequences: a scoping review. J Am Med Inform Assoc. Feb 01, 2020;27(2):330-340. [ FREE Full text ] [ CrossRef ] [ Medline ]
- CE marking. European Union. URL: https://tinyurl.com/yfk2mjtx [accessed 2024-04-10]
- Overview of device regulation. U.S. Food & Drug Administration. URL: https://tinyurl.com/464fzbtu [accessed 2023-01-29]
- The fast-track process for digital health applications (DiGA) according to Section 139e SGB V. A guide for manufacturers, service providers and users. Federal Institute for Drugs and Medical Devices. URL: https://tinyurl.com/k4efvfzx [accessed 2023-01-29]
- Stoyanov SR, Hides L, Kavanagh DJ, Zelenko O, Tjondronegoro D, Mani M. Mobile app rating scale: a new tool for assessing the quality of health mobile apps. JMIR Mhealth Uhealth. Mar 11, 2015;3(1):e27. [ FREE Full text ] [ CrossRef ] [ Medline ]
- Stoyanov SR, Hides L, Kavanagh DJ, Wilson H. Development and validation of the user version of the mobile application rating scale (uMARS). JMIR Mhealth Uhealth. Jun 10, 2016;4(2):e72. [ FREE Full text ] [ CrossRef ] [ Medline ]
- Baumel A, Faber K, Mathur N, Kane JM, Muench F. Enlight: a comprehensive quality and therapeutic potential evaluation tool for mobile and web-based eHealth interventions. J Med Internet Res. Mar 21, 2017;19(3):e82. [ FREE Full text ] [ CrossRef ] [ Medline ]
- Brooke J. SUS: a 'quick and dirty' usability scale. In: Jordan PW, Thomas B, McClelland IL, Weerdmeester B, editors. Usability Evaluation In Industry. Boca Raton, FL. CRC Press; 1996.
- Nouri R, R Niakan Kalhori S, Ghazisaeedi M, Marchand G, Yasini M. Criteria for assessing the quality of mHealth apps: a systematic review. J Am Med Inform Assoc. Aug 01, 2018;25(8):1089-1098. [ FREE Full text ] [ CrossRef ] [ Medline ]
- Zhou L, Bao J, Watzlaf V, Parmanto B. Barriers to and facilitators of the use of mobile health apps from a security perspective: mixed-methods study. JMIR Mhealth Uhealth. Apr 16, 2019;7(4):e11223. [ FREE Full text ] [ CrossRef ] [ Medline ]
- Borghouts J, Eikey E, Mark G, De Leon C, Schueller SM, Schneider M, et al. Barriers to and facilitators of user engagement with digital mental health interventions: systematic review. J Med Internet Res. Mar 24, 2021;23(3):e24387. [ FREE Full text ] [ CrossRef ] [ Medline ]
- Giebel GD, Speckemeier C, Abels C, Plescher F, Börchers K, Wasem J, et al. Problems and barriers related to the use of digital health applications: scoping review. J Med Internet Res. May 12, 2023;25:e43808. [ FREE Full text ] [ CrossRef ] [ Medline ]
- Shabir H, D'Costa M, Mohiaddin Z, Moti Z, Rashid H, Sadowska D, et al. The barriers and facilitators to the use of lifestyle apps: a systematic review of qualitative studies. Eur J Investig Health Psychol Educ. Jan 27, 2022;12(2):144-165. [ FREE Full text ] [ CrossRef ] [ Medline ]
- Byambasuren O, Beller E, Hoffmann T, Glasziou P. Barriers to and facilitators of the prescription of mHealth apps in Australian general practice: qualitative study. JMIR Mhealth Uhealth. Jul 30, 2020;8(7):e17447. [ FREE Full text ] [ CrossRef ] [ Medline ]
- O'Brien BC, Harris IB, Beckman TJ, Reed DA, Cook DA. Standards for reporting qualitative research: a synthesis of recommendations. Acad Med. Sep 2014;89(9):1245-1251. [ FREE Full text ] [ CrossRef ] [ Medline ]
- Tong A, Sainsbury P, Craig J. Consolidated criteria for reporting qualitative research (COREQ): a 32-item checklist for interviews and focus groups. Int J Qual Health Care. Dec 2007;19(6):349-357. [ FREE Full text ] [ CrossRef ] [ Medline ]
- Mayring P. Qualitative Inhaltsanalyse: Grundlagen und Techniken. Weinheim, Germany. Beltz Publishing; Feb 2, 2015.
- Azad-Khaneghah P, Neubauer N, Miguel Cruz A, Liu L. Mobile health app usability and quality rating scales: a systematic review. Disabil Rehabil Assist Technol. Oct 08, 2021;16(7):712-721. [ FREE Full text ] [ CrossRef ] [ Medline ]
- Lagan S, Sandler L, Torous J. Evaluating evaluation frameworks: a scoping review of frameworks for assessing health apps. BMJ Open. Mar 19, 2021;11(3):e047001. [ FREE Full text ] [ CrossRef ] [ Medline ]
- An Q, Kelley MM, Hanners A, Yen PY. Sustainable development for mobile health apps using the human-centered design process. JMIR Form Res. Aug 25, 2023;7:e45694. [ FREE Full text ] [ CrossRef ] [ Medline ]
- Clemensen J, Larsen SB, Kyng M, Kirkevold M. Participatory design in health sciences: using cooperative experimental methods in developing health services and computer technology. Qual Health Res. Jan 2007;17(1):122-130. [ FREE Full text ] [ CrossRef ] [ Medline ]
- Newton A, Bagnell A, Rosychuk R, Duguay J, Wozney L, Huguet A, et al. A mobile phone-based app for use during cognitive behavioral therapy for adolescents with anxiety (MindClimb): user-centered design and usability study. JMIR Mhealth Uhealth. Dec 08, 2020;8(12):e18439. [ FREE Full text ] [ CrossRef ] [ Medline ]
- Biswas M, Tania MH, Kaiser MS, Kabir R, Mahmud M, Kemal AA. ACCU3RATE: a mobile health application rating scale based on user reviews. PLoS One. Dec 16, 2021;16(12):e0258050. [ FREE Full text ] [ CrossRef ] [ Medline ]
- Dahlhausen F, Zinner M, Bieske L, Ehlers JP, Boehme P, Fehring L. Physicians' attitudes toward prescribable mHealth apps and implications for adoption in Germany: mixed methods study. JMIR Mhealth Uhealth. Nov 23, 2021;9(11):e33012. [ FREE Full text ] [ CrossRef ] [ Medline ]
- Davis FD, Bagozzi RP, Warshaw PR. User acceptance of computer technology: a comparison of two theoretical models. Manag Sci. Aug 1989;35(8):982-1003. [ FREE Full text ] [ CrossRef ]
- Lee M, Kang D, Yoon J, Shim S, Kim IR, Oh D, et al. The difference in knowledge and attitudes of using mobile health applications between actual user and non-user among adults aged 50 and older. PLoS One. 2020;15(10):e0241350. [ FREE Full text ] [ CrossRef ] [ Medline ]
- Pang NQ, Lau J, Fong SY, Wong CY, Tan KK. Telemedicine acceptance among older adult patients with cancer: scoping review. J Med Internet Res. Mar 29, 2022;24(3):e28724. [ FREE Full text ] [ CrossRef ] [ Medline ]
- Ahmad NA, Mat Ludin AF, Shahar S, Mohd Noah SA, Mohd Tohit N. Willingness, perceived barriers and motivators in adopting mobile applications for health-related interventions among older adults: a scoping review protocol. BMJ Open. Mar 16, 2020;10(3):e033870. [ FREE Full text ] [ CrossRef ] [ Medline ]
- Bericht des GKV-spitzenverbandes über die inanspruchnahme und entwicklung der versorgung mit digitalen gesundheitsanwendungen. Deutscher Bundestag. URL: https://tinyurl.com/3h6bub6b [accessed 2023-03-26]
Abbreviations
Edited by T Leung; submitted 15.06.23; peer-reviewed by R Sun, R Eckhoff; comments to author 11.10.23; revised version received 24.10.23; accepted 31.01.24; published 23.04.24.
©Godwin Denk Giebel, Carina Abels, Felix Plescher, Christian Speckemeier, Nils Frederik Schrader, Kirstin Börchers, Jürgen Wasem, Silke Neusser, Nikola Blase. Originally published in the Journal of Medical Internet Research (https://www.jmir.org), 23.04.2024.
This is an open-access article distributed under the terms of the Creative Commons Attribution License (https://creativecommons.org/licenses/by/4.0/), which permits unrestricted use, distribution, and reproduction in any medium, provided the original work, first published in the Journal of Medical Internet Research, is properly cited. The complete bibliographic information, a link to the original publication on https://www.jmir.org/, as well as this copyright and license information must be included.

An official website of the United States government
The .gov means it’s official. Federal government websites often end in .gov or .mil. Before sharing sensitive information, make sure you’re on a federal government site.
The site is secure. The https:// ensures that you are connecting to the official website and that any information you provide is encrypted and transmitted securely.
- Publications
- Account settings
Preview improvements coming to the PMC website in October 2024. Learn More or Try it out now .
- Advanced Search
- Journal List
- v.15(1); 2023 Jan

Clinical Research: A Review of Study Designs, Hypotheses, Errors, Sampling Types, Ethics, and Informed Consent
Addanki purna singh.
1 Physiology, Department of Biomedical Sciences, Saint James School of Medicine, The Quarter, AIA
Sabitha Vadakedath
2 Biochemistry, Prathima Institute of Medical Sciences, Karimnagar, IND
Venkataramana Kandi
3 Clinical Microbiology, Prathima Institute of Medical Sciences, Karimnagar, IND
Recently, we have been noticing an increase in the emergence and re-emergence of microbial infectious diseases. In the previous 100 years, there were several incidences of pandemics caused by different microbial species like the influenza virus , human immunodeficiency virus (HIV), dengue virus , severe acute respiratory syndrome Coronavirus (SARS-CoV), middle east respiratory syndrome coronavirus (MERS-CoV), and SARS-CoV-2 that were responsible for severe morbidity and mortality among humans. Moreover, non-communicable diseases, including malignancies, diabetes, heart, liver, kidney, and lung diseases, have been on the rise. The medical fraternity, people, and governments all need to improve their preparedness to effectively tackle health emergencies. Clinical research, therefore, assumes increased significance in the current world and may potentially be applied to manage human health-related problems. In the current review, we describe the critical aspects of clinical research that include research designs, types of study hypotheses, errors, types of sampling, ethical concerns, and informed consent.
Introduction and background
To conduct successful and credible research, scientists/researchers should understand the key elements of clinical research like neutrality (unbiased), reliability, validity, and generalizability. Moreover, results from clinical studies are applied in the real world to benefit human health. As a result, researchers must understand the various types of research designs [ 1 ]. Before choosing a research design, the researchers must work out the aims and objectives of the study, identify the study population, and address the ethical concerns associated with the clinical study. Another significant aspect of clinical studies is the research methodology and the statistical applications that are employed to process the data and draw conclusions. There are primarily two types of research designs: observational studies and experimental studies [ 2 ]. Observational studies do not involve any interventions and are therefore considered inferior to experimental designs. The experimental studies include the clinical trials that are carried out among a selected group of participants who are given a drug to assess its safety and efficacy in treating and managing the disease. However, in the absence of a study group, a single-case experimental design (SCED) was suggested as an alternative methodology that is equally reliable as a randomization study [ 3 ]. The single case study designs are called N-of-1 type clinical trials [ 4 , 5 ]. The N-of-1 study design is being increasingly applied in healthcare-related research. Experimental studies are complex and are generally performed by pharmaceutical industries as a part of research and development activities during the discovery of a therapeutic drug/device. Also, clinical trials are undertaken by individual researchers or a consortium. In a recent study, the researchers were cautioned about the consequences of a faulty research design [ 6 ]. It was noted that clinical studies on the effect of the gut microbiome and its relationship with the feed could potentially be influenced by the choice of the experimental design, controls, and comparison groups included in the study. Moreover, clinical studies can be affected by sampling errors and biases [ 7 ]. In the present review, we briefly discuss the types of clinical study designs, study hypotheses, sampling errors, and the ethical issues associated with clinical research.
Research design
A research design is a systematic elucidation of the whole research process that includes methods and techniques, starting from the planning of research, execution (data collection), analysis, and drawing a logical conclusion based on the results obtained. A research design is a framework developed by a research team to find an answer/solution to a problem. The research designs are of several types that include descriptive research, surveys, correlation type, experimental, review (systematic/literature), and meta-analysis. The choice of research design is determined by the type of research question that is opted for. Both the research design and the research question are interdependent. For every research question, a complementary/appropriate research design must have been chosen. The choice of research design influences the research credibility, reliability, and accuracy of the data collected. A well-defined research design would contain certain elements that include a specific purpose of the research, methods to be applied while collecting and analyzing the data, the research methodology used to interpret the collected data, research infrastructure, limitations, and most importantly, the time required to complete the research. The research design can broadly be categorized into two types: qualitative and quantitative designs. In a qualitative research method, the collected data are measured and evaluated using mathematical and statistical applications. Whereas in quantitative research, a larger sample size is selected, and the results derived from statistics can benefit society. The various types of research designs are shown in Figure Figure1 1 [ 8 ].

Types of research studies
There are various types of research study designs. The researcher who aims to take up the study determines the type of study design to choose among the available ones. The choice of study design depends on many factors that include but are not limited to the research question, the aim of the study, the available funds, manpower, and infrastructure, among others. The research study designs include systematic reviews, meta-analyses, randomized controlled trials, cross-sectional studies, case-control studies, cohort studies, case reports/studies, animal experiments, and other in vitro studies, as shown in Figure Figure2 2 [ 9 ].

Systematic Reviews
In these studies, the researcher makes an elaborate and up-to-date search of the available literature. By doing a systematic review of a selected topic, the researcher collects the data, analyses it, and critically evaluates it to evolve with impactful conclusions. Systematic reviews could equip healthcare professionals with more than adequate evidence with respect to the decisions to be taken during improved patient management that may include diagnosis, interventions, prognosis, and others [ 10 ]. A recent systematic research study evaluated the role of socioeconomic conditions on the knowledge of risk factors for stroke in the World Health Organization (WHO) European region. This study collected data from PubMed, Embase, Web of Science (WoS), and other sources and finally included 20 studies and 67,309 subjects. This study concluded that the high socioeconomic group had better knowledge of risk factors and warning signs of stroke and suggested improved public awareness programs to better address the issue [ 11 ].
Meta-Analysis
Meta-analysis is like a systematic review, but this type of research design uses quantitative tools that include statistical methods to draw conclusions. Such a research method is therefore considered both equal and superior to the original research studies. Both the systematic review and the meta-analyses follow a similar research process that includes the research question, preparation of a protocol, registration of the study, devising study methods using inclusion and exclusion criteria, an extensive literature survey, selection of studies, assessing the quality of the evidence, data collection, analysis, assessment of the evidence, and finally the interpretation/drawing the conclusions [ 12 ]. A recent research study, using a meta-analytical study design, evaluated the quality of life (QoL) among patients suffering from chronic pulmonary obstructive disease (COPD). This study used WoS to collect the studies, and STATA to analyze and interpret the data. The study concluded that non-therapeutic mental health and multidisciplinary approaches were used to improve QoL along with increased support from high-income countries to low and middle-income countries [ 13 ].
Cross-Sectional Studies
These studies undertake the observation of a select population group at a single point in time, wherein the subjects included in the studies are evaluated for exposure and outcome simultaneously. These are probably the most common types of studies undertaken by students pursuing postgraduation. A recent study evaluated the activities of thyroid hormones among the pre- and post-menopausal women attending a tertiary care teaching hospital. The results of this study demonstrated that there was no significant difference in the activities of thyroid hormones in the study groups [ 14 ].
Cohort Studies
Cohort studies use participant groups called cohorts, which are followed up for a certain period and assess the exposure to the outcome. They are used for epidemiological observations to improve public health. Although cohort studies are laborious, financially burdensome, and difficult to undertake as they require a large population group, such study designs are frequently used to conduct clinical studies and are only second to randomized control studies in terms of their significance [ 15 ]. Also, cohort studies can be undertaken both retrospectively and prospectively. A retrospective study assessed the effect of alcohol intake among human immunodeficiency virus (HIV)-infected persons under the national program of the United States of America (USA) for HIV care. This study, which included more than 30,000 HIV patients under the HIV care continuum program, revealed that excessive alcohol use among the participants affected HIV care, including treatment [ 16 ].
Case-Control Study
The case-control studies use a single point of observation among two population groups that are categorized based on the outcome. Those who had an outcome are termed as cases, and the ones who did not develop the disease are called control groups. This type of study design is easy to perform and is extensively undertaken as a part of medical research. Such studies are frequently used to assess the efficacy of vaccines among the population [ 17 ]. A previous study evaluated the activities of zinc among patients suffering from beta-thalassemia and compared it with the control group. This study concluded that the patients with beta-thalassemia are prone to hypozincaemia and had low concentrations of zinc as compared to the control group [ 18 ].
Case Studies
Such types of studies are especially important from the perspective of patient management. Although these studies are just observations of single or multiple cases, they may prove to be particularly important in the management of patients suffering from unusual diseases or patients presenting with unusual presentations of a common disease. Listeria is a bacterium that generally affects humans in the form of food poisoning and neonatal meningitis. Such an organism was reported to cause breast abscesses [ 19 ].
Randomized Control Trial
This is probably the most trusted research design that is frequently used to evaluate the efficacy of a novel pharmacological drug or a medical device. This type of study has a negligible bias, and the results obtained from such studies are considered accurate. The randomized controlled studies use two groups, wherein the treatment group receives the trial drug and the other group, called the placebo group, receives a blank drug that appears remarkably like the trial drug but without the pharmacological element. This can be a single-blind study (only the investigator knows who gets the trial drug and who is given a placebo) or a double-blind study (both the investigator and the study participant have no idea what is being given). A recent study (clinical trial registration number: {"type":"clinical-trial","attrs":{"text":"NCT04308668","term_id":"NCT04308668"}} NCT04308668 ) concluded that post-exposure prophylaxis with hydroxychloroquine does not protect against Coronavirus disease-19 (COVID-19) after a high and moderate risk exposure when the treatment was initiated within four days of potential exposure [ 20 ].
Factors that affect study designs
Among the different factors that affect a study's design is the recruitment of study participants. It is not yet clear as to what is the optimal method to increase participant participation in clinical studies. A previous study had identified that the language barrier and the long study intervals could potentially hamper the recruitment of subjects for clinical trials [ 21 ]. It was noted that patient recruitment for a new drug trial is more difficult than for a novel diagnostic study [ 22 ].
Reproducibility is an important factor that affects a research design. The study designs must be developed in such a way that they are replicable by others. Only those studies that can be re-done by others to generate the same/similar results are considered credible [ 23 ]. Choosing an appropriate study design to answer a research question is probably the most important factor that could affect the research result [ 24 ]. This can be addressed by clearly understanding various study designs and their applications before selecting a more relevant design.
Retention is another significant aspect of the study design. It is hard to hold the participants of a study until it is completed. Loss of follow-up among the study participants will influence the study results and the credibility of the study. Other factors that considerably influence the research design are the availability of a source of funding, the necessary infrastructure, and the skills of the investigators and clinical trial personnel.
Synthesizing a research question or a hypothesis
A research question is at the core of research and is the point from which a clinical study is initiated. It should be well-thought-out, clear, and concise, with an arguable element that requires the conduction of well-designed research to answer it. A research question should generally be a topic of curiosity in the researcher's mind, and he/she must be passionate enough about it to do all that is possible to answer it [ 25 ].
A research question must be generated/framed only after a preliminary literature search, choosing an appropriate topic, identifying the audience, self-questioning, and brainstorming for its clarity, feasibility, and reproducibility.
A recent study suggested a stepwise process to frame the research question. The research question is developed to address a phenomenon, describe a case, establish a relationship for comparison, and identify causality, among others. A better research question is one that describes the statement of the problem, points out the study area, puts focus on the study aspects, and guides data collection, analysis, and interpretation. The aspects of a good research question are shown in Figure Figure3 3 [ 26 ].

Research questions may be framed to prove the existence of a phenomenon, describe and classify a condition, elaborate the composition of a disease condition, evaluate the relationship between variables, describe and compare disease conditions, establish causality, and compare the variables resulting in causality. Some examples of the research questions include: (i) Does the coronavirus mutate when it jumps from one organism to another?; (ii) What is the therapeutic efficacy of vitamin C and dexamethasone among patients infected with COVID-19?; (iii) Is there any relationship between COPD and the complications of COVID-19?; (iv) Is Remdesivir alone or in combination with vitamin supplements improve the outcome of COVID-19?; (v) Are males more prone to complications from COVID-19 than females?
The research hypothesis is remarkably like a research question except for the fact that in a hypothesis the researcher assumes either positively or negatively about a causality, relation, correlation, and association. An example of a research hypothesis: overweight and obesity are risk factors for cardiovascular disease.
Types of errors in hypothesis testing
An assumption or a preliminary observation made by the researcher about the potential outcome of research that is being envisaged may be called a hypothesis. There are different types of hypotheses, including simple hypotheses, complex hypotheses, empirical hypotheses, statistical hypotheses, null hypotheses, and alternative hypotheses. However, the null hypothesis (H0) and the alternative hypothesis (HA) are commonly practiced. The H0 is where the researcher assumes that there is no relation/causality/effect, and the HA is when the researcher believes/assumes that there is a relationship/effect [ 27 , 28 ].
Hypothesis testing is affected by two types of errors that include the type I error (α) and the type II error (β). The type I error (α) occurs when the investigator contradicts the null hypothesis despite it being true, which is considered a false positive error. The type II error (β) happens when the researcher considers/accepts the null hypothesis despite it being false, which is termed a false negative error [ 28 , 29 ].
The reasons for errors in the hypothesis testing may be due to bias and other causes. Therefore, the researchers set the standards for studies to rule out errors. A 5% deviation (α=0.05; range: 0.01-0.10) in the case of a type I error and up to a 20% probability (β=0.20; range: 0.05-0.20) for type II errors are generally accepted [ 28 , 29 ]. The features of a reasonable hypothesis include simplicity and specificity, and the hypothesis is generally determined by the researcher before the initiation of the study and during the preparation of the study proposal/protocol [ 28 , 29 ].
The applications of hypothesis testing
A hypothesis is tested by assessing the samples, where appropriate statistics are applied to the collected data and an inference is drawn from it. It was noted that a hypothesis can be made based on the observations of physicians using anatomical characteristics and other physiological attributes [ 28 , 30 ]. The hypothesis may also be tested by employing proper statistical techniques. Hypothesis testing is carried out on the sample data to affirm the null hypothesis or otherwise.
An investigator needs to believe the null hypothesis or accept that the alternate hypothesis is true based on the data collected from the samples. Interestingly, most of the time, a study that is carried out has only a 50% chance of either the null hypothesis or the alternative hypothesis coming true [ 28 , 31 ].
Hypothesis testing is a step-by-step strategy that is initiated by the assumption and followed by the measures applied to interpret the results, analysis, and conclusion. The margin of error and the level of significance (95% free of type I error and 80% free of type II error) are initially fixed. This enables the chance for the study results to be reproduced by other researchers [ 32 ].
Ethics in health research
Ethical concerns are an important aspect of civilized societies. Moreover, ethics in medical research and practice assumes increased significance as most health-related research is undertaken to find a cure or discover a medical device/diagnostic tool that can either diagnose or cure the disease. Because such research involves human participants, and due to the fact that people approach doctors to find cures for their diseased condition, ethics, and ethical concerns take center stage in public health-related clinical/medical practice and research.
The local and international authorities like the Drugs Controller General of India (DCGI), and the Food and Drug Administration (FDA) make sure that health-related research is carried out following all ethical concerns and good clinical practice (GCP) guidelines. The ethics guidelines are prescribed by both national and international bodies like the Indian Council of Medical Research (ICMR) and the World Medical Association (WMA) Declaration of Helsinki guidelines for ethical principles for medical research involving human subjects [ 33 ].
Ethical conduct is more significant during clinical practice, medical education, and research. It is recommended that medical practitioners embark on self-regulation of the medical profession. Becoming proactive in terms of ethical practices will enhance the social image of a medical practitioner/researcher. Moreover, such behavior will allow people to comprehend that this profession is not for trade/money but for the benefit of the patients and the public at large. Administrations should promote ethical practitioners and penalize unethical practitioners and clinical research organizations. It is suggested that the medical curriculum should incorporate ethics as a module and ethics-related training must be delivered to all medical personnel. It should be noted that a tiny seed grows into an exceptionally gigantic tree if adequately watered and taken care of [ 33 ]. It is therefore inevitable to address the ethical concerns in medical education, research and practice to make more promising medical practitioners and acceptable medical educators and researchers as shown in Figure Figure4 4 .

Sampling in health research
Sampling is the procedure of picking a precise number of individuals from a defined group to accomplish a research study. This sample is a true representative subset of individuals who potentially share the same characteristics as a large population, and the results of the research can be generalized [ 34 , 35 ]. Sampling is a prerogative because it is almost impossible to include all the individuals who want to partake in a research investigation. A sample identified from a representative population can be depicted in Figure Figure5 5 .

Sampling methods are of different types and are broadly classified into probability sampling and non-probability sampling. In a probability sampling method, which is routinely employed in quantitative research, each individual in the representative population is provided with an equivalent likelihood of being included in the study [ 35 ]. Probability sampling can be separated into four types that include simple random sampling, systematic sampling, stratified sampling, and cluster sampling, as shown in Figure Figure6 6 .

Simple Random Sample
In the simple random sampling method, every person in the representative population is given an equal chance of being selected. It may use a random number generator for selecting the study participants. To study the employees’ perceptions of government policies, a researcher initially assigns a number to each employee [ 35 ]. After this, the researcher randomly chooses the required number of samples. In this type of sampling method, each one has an equal chance of being selected.
Systematic Sample
In this sampling method, the researcher selects the study participants depending on a pre-defined order (1, 3, 5, 7, 9…), wherein the researcher assigns a serial number (1-100 (n)) to volunteers [ 35 ]. The researcher in this type of sample selects a number from 1 to 10 and later applies a systematic pattern to select the sample like 2, 12, 22, 32, etc.
Stratified Sample
The stratified sampling method is applied when the people from whom the sample must be taken have mixed features. In this type of sampling, the representative population is divided into clusters/strata based on attributes like age, sex, and other factors. Subsequently, a simple random or systematic sampling method is applied to select the samples from each group. Initially, different age groups, sexes, and other characters were selected as a group [ 35 ]. The investigator finds his/her sample from each group using simple or systematic random sampling methods.
Cluster Sample
This sampling method is used to create clusters of the representative population with mixed qualities. Because such groups have mixed features, each one can be regarded as a sample. Conversely, a sample can be developed by using simple random/systematic sampling approaches. The cluster sampling method is similar to stratified sampling but differs in the group characteristics, wherein each group has representatives of varied ages, different sexes, and other mixed characters [ 35 ]. Although each group appears as a sample, the researcher again applies a simple or systematic random sampling method to choose the sample.
Non-probability Sample
In this type of sampling method, the participants are chosen based on non-random criteria. In a non-probability sampling method, the volunteers do not have an identical opportunity to get selected. This method, although it appears to be reasonable and effortless to do, is plagued by selection bias. The non-probability sampling method is routinely used in experimental and qualitative research. It is suitable to perform a pilot study that is carried out to comprehend the qualities of a representative population [ 35 ]. The non-probability sampling is of four types, including convenience sampling, voluntary response sampling, purposive sampling, and snowball sampling, as shown in Figure Figure7 7 .

Convenience Sample
In the convenience sampling method, there are no pre-defined criteria, and only those volunteers who are readily obtainable to the investigator are included. Despite it being an inexpensive method, the results yielded from studies that apply convenience sampling may not reflect the qualities of the population, and therefore, the results cannot be generalized [ 35 ]. The best example of this type of sampling is when the researcher invites people from his/her own work area (company, school, city, etc.).
Voluntary Response Sample
In the voluntary response sampling method, the participants volunteer to partake in the study. This sampling method is similar to convenience sampling and therefore leaves sufficient room for bias [ 35 ]. The researcher waits for the participants who volunteer in the study in a voluntary response sampling method.
Purposive Sample/Judgment Sample
In the purposive or judgemental sampling method, the investigator chooses the participants based on his/her judgment/discretion. In this type of sampling method, the attributes (opinions/experiences) of the precise population group can be achieved [ 35 ]. An example of such a sampling method is the handicapped group's opinion on the facilities at an educational institute.
Snowball Sample
In the snowball sampling method, suitable study participants are found based on the recommendations and suggestions made by the participating subjects [ 36 ]. In this type, the individual/sample recruited by the investigator in turn invites/recruits other participants.
Significance of informed consent and confidentiality in health research
Informed consent is a document that confirms the fact that the study participants are recruited only after being thoroughly informed about the research process, risks, and benefits, along with other important details of the study like the time of research. The informed consent is generally drafted in the language known to the participants. The essential contents of informed consent include the aim of research in a way that is easily understood even by a layman. It must also brief the person as to what is expected from participation in the study. The informed consent contains information such as that the participant must be willing to share demographic characteristics, participate in the clinical and diagnostic procedures, and have the liberty to withdraw from the study at any time during the research. The informed consent must also have a statement that confirms the confidentiality of the participant and the protection of privacy of information and identity [ 37 ].
Health research is so complex that there may be several occasions when a researcher wants to re-visit a medical record to investigate a specific clinical condition, which also requires informed consent [ 38 ]. Awareness of biomedical research and the importance of human participation in research studies is a key element in the individual’s knowledge that may contribute to participation or otherwise in the research study [ 39 ]. In the era of information technology, the patient’s medical data are stored as electronic health records. Research that attempts to use such records is associated with ethical, legal, and social concerns [ 40 , 41 ]. Improved technological advances and the availability of medical devices to treat, diagnose, and prevent diseases have thrown a new challenge at healthcare professionals. Medical devices are used for interventions only after being sure of the potential benefit to the patients, and at any cost, they must never affect the health of the patient and only improve the outcome [ 42 ]. Even in such cases, the medical persons must ensure informed consent from the patients.
Conclusions
Clinical research is an essential component of healthcare that enables physicians, patients, and governments to tackle health-related problems. Increased incidences of both communicable and non-communicable diseases warrant improved therapeutic interventions to treat, control, and manage diseases. Several illnesses do not have a treatment, and for many others, the treatment, although available, is plagued by drug-related adverse effects. For many other infections, like dengue, we require preventive vaccines. Therefore, clinical research studies must be carried out to find solutions to the existing problems. Moreover, the knowledge of clinical research, as discussed briefly in this review, is required to carry out research and enhance preparedness to counter conceivable public health emergencies in the future.
The content published in Cureus is the result of clinical experience and/or research by independent individuals or organizations. Cureus is not responsible for the scientific accuracy or reliability of data or conclusions published herein. All content published within Cureus is intended only for educational, research and reference purposes. Additionally, articles published within Cureus should not be deemed a suitable substitute for the advice of a qualified health care professional. Do not disregard or avoid professional medical advice due to content published within Cureus.
The authors have declared that no competing interests exist.
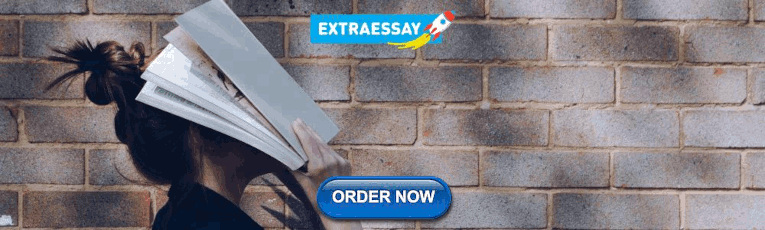
IMAGES
VIDEO
COMMENTS
Introduction. In clinical research, our aim is to design a study, which would be able to derive a valid and meaningful scientific conclusion using appropriate statistical methods that can be translated to the "real world" setting. 1 Before choosing a study design, one must establish aims and objectives of the study, and choose an appropriate target population that is most representative of ...
The study design used to answer a particular research question depends on the nature of the question and the availability of resources. In this article, which is the first part of a series on "study designs," we provide an overview of research study designs and their classification. The subsequent articles will focus on individual designs.
We may approach this study by 2 longitudinal designs: Prospective: we follow the individuals in the future to know who will develop the disease. Retrospective: we look to the past to know who developed the disease (e.g. using medical records) This design is the strongest among the observational studies. For example - to find out the relative ...
Background Methodological studies - studies that evaluate the design, analysis or reporting of other research-related reports - play an important role in health research. They help to highlight issues in the conduct of research with the aim of improving health research methodology, and ultimately reducing research waste. Main body We provide an overview of some of the key aspects of ...
Health research methodology: A guide for training in research methods Chapter 1 Research and Scientific Methods 1.1 Definition Research is a quest for knowledge through diligent search or investigation or experimentation aimed at the discovery and interpretation of new knowledge. Scientific method is a systematic
Clinical research errors in general, and biases in particular, are best avoided by a strong study design coupled with thoughtful statistical analysis. Although the latter can compensate for confounding to the extent that factors are known and measured, formal statistical methods are most effective in coping with evaluating random variation.
For doctors interested in doing and interpreting clinical research. Also papers that present new or updated research reporting guidelines. ... Research methods & reporting Showing results 1-100. Sorted by: Most recent. ... Guidance for the design and reporting of studies evaluating the clinical performance of tests for present or past SARS-CoV ...
Methods: This article describes the structured classification of studies into two types, primary and secondary, as well as a further subclassification of studies of primary type. This is done on the basis of a selective literature search concerning study types in medical research, in addition to the authors' own experience.
The Patient-Centered Outcomes Research Institute's (PCORI) methodology standards for qualitative methods and mixed methods research help ensure that research studies are designed and conducted to generate the evidence needed to answer patients' and clinicians' questions about which methods work best, for whom, and under what circumstances. This set of standards focuses on factors ...
Publish your healthcare research with BMC Medical Research Methodology, with 4.0 Impact Factor and 40 days to first decision. ... as are empirical studies of the associations between choice of methodology and study outcomes. BMC Medical Research Methodology does not aim to publish articles describing scientific methods or techniques: ...
Clinical research is the comprehensive study of the safety and effectiveness of the most promising advances in patient care. Clinical research is different than laboratory research. It involves people who volunteer to help us better understand medicine and health. Lab research generally does not involve people — although it helps us learn ...
Abstract. Clinical research can be categorized by the timing of data collection: retrospective or prospective. Clinical research also can be categorized by study design. In case-control studies, investigators compare previous exposures (including genetic and other personal factors, environmental influences, and medical treatments) among groups ...
Primary research entails conducting studies and collecting raw data. Secondary research evaluates or synthesizes data collected during primary research. Primary medical research is categorized into three main fields: laboratorial, clinical, and epidemiological. Laboratory scientists analyze the fundamentals of diseases and treatments.
Statistical Methods in Medical Research is a highly ranked, peer reviewed scholarly journal and is the leading vehicle for articles in all the main areas of medical statistics and therefore an essential reference for all medical statisticians. It is particularly useful for medical researchers dealing with data and provides a key resource for medical and statistical libraries, as well as ...
In order to interpret research datasets, clinicians involved in clinical research should have an understanding of statistical methodology. This article provides a brief overview of statistical methods that are frequently used in clinical research studies. Descriptive and inferential methods, including regression modeling and propensity scores ...
Medical education is a complex field, and medical education research and practice fittingly draws from many disciplines (e.g., medicine, psychology, sociology, education) and synthesizes multiple perspectives to explain how people learn and how medicine should be taught. 4,5 The concept of a field was well described by Cristancho and Varpio 5 in their tips for early career medical educators ...
Clinical Research Methods. Director: Todd Ogden, PhD. The Mailman School offers the degree of Master of Science in Biostatistics, with an emphasis on issues in the statistical analysis and design of clinical studies. The Clinical Research Methods track was conceived and designed for clinicians who are pursuing research careers in academic medicine.
Given the complex, multilevel nature of curriculum research, we used a mixed-methods study design to explore our three aims (Fetters et al. Citation 2013). Using an explanatory, sequential design, we initially used a mixed quantitative and qualitative online survey to establish a broad picture of the variety of student study behaviours, and ...
METHODS Study design and procedures. This study was a single-center, randomized, open, single-dose, parallel-controlled pharmacokinetic, safety, and immunogenicity comparative trial in healthy male Chinese adult subjects, conducted in phase I Clinical Research Unit of PKU Care Luzhong Hospital (Shandong, China).
Researchers at Boston Medical Center (BMC) and Boston University (BU) have published a new study detailing the development of a method for generating human alveolar epithelial type I cells (AT1s ...
Introduction. Generative Pre-trained Transformer 3.5 (GPT-3.5) and 4 (GPT-4) are large language models (LLMs) trained on datasets containing hundreds of billions of words from articles, books, and other internet sources [1, 2].ChatGPT is an online chatbot which uses GPT-3.5 or GPT-4 to provide bespoke responses to human users' queries [].LLMs have revolutionised the field of natural language ...
A total of 496 participants were assigned to receive pembrolizumab and 498 to receive placebo. As of September 15, 2023, the median follow-up was 57.2 months.
First, consider that nearly all medical students pass the Step 1 exam. In 2020, 98% of DO and MD students passed the exam on their first try. But if you don't, the National Board of Medical Examiners allows a maximum of four attempts to pass each different level of the USMLE exam. If you fail, you get a score report that offers feedback on ...
In this tutorial paper, we will use the term methodological study to refer to any study that reports on the design, conduct, analysis or reporting of primary or secondary research-related reports (such as trial registry entries and conference abstracts). In the past 10 years, there has been an increase in the use of terms related to ...
Methods: Guided focus groups and individual interviews were conducted with patients with a disease for which an approved mHealth app was available at the time of the interviews. ... This study shows that there are relevant problems and barriers in the context of mHealth apps from the perspective of patients, which warrant further attention ...
Both the systematic review and the meta-analyses follow a similar research process that includes the research question, preparation of a protocol, registration of the study, devising study methods using inclusion and exclusion criteria, an extensive literature survey, selection of studies, assessing the quality of the evidence, data collection ...