
- SUGGESTED TOPICS
- The Magazine
- Newsletters
- Managing Yourself
- Managing Teams
- Work-life Balance
- The Big Idea
- Data & Visuals
- Reading Lists
- Case Selections
- HBR Learning
- Topic Feeds
- Account Settings
- Email Preferences
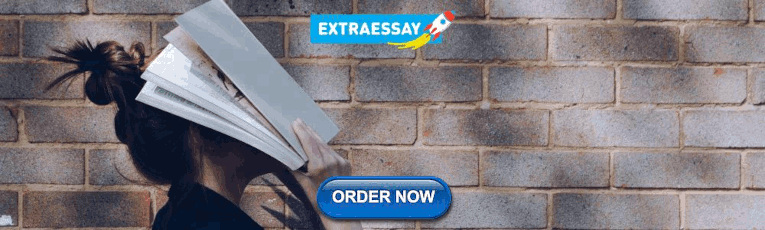
Research: How Bias Against Women Persists in Female-Dominated Workplaces
- Amber L. Stephenson,
- Leanne M. Dzubinski

A look inside the ongoing barriers women face in law, health care, faith-based nonprofits, and higher education.
New research examines gender bias within four industries with more female than male workers — law, higher education, faith-based nonprofits, and health care. Having balanced or even greater numbers of women in an organization is not, by itself, changing women’s experiences of bias. Bias is built into the system and continues to operate even when more women than men are present. Leaders can use these findings to create gender-equitable practices and environments which reduce bias. First, replace competition with cooperation. Second, measure success by goals, not by time spent in the office or online. Third, implement equitable reward structures, and provide remote and flexible work with autonomy. Finally, increase transparency in decision making.
It’s been thought that once industries achieve gender balance, bias will decrease and gender gaps will close. Sometimes called the “ add women and stir ” approach, people tend to think that having more women present is all that’s needed to promote change. But simply adding women into a workplace does not change the organizational structures and systems that benefit men more than women . Our new research (to be published in a forthcoming issue of Personnel Review ) shows gender bias is still prevalent in gender-balanced and female-dominated industries.

- Amy Diehl , PhD is chief information officer at Wilson College and a gender equity researcher and speaker. She is coauthor of Glass Walls: Shattering the Six Gender Bias Barriers Still Holding Women Back at Work (Rowman & Littlefield). Find her on LinkedIn at Amy-Diehl , Twitter @amydiehl , and visit her website at amy-diehl.com
- AS Amber L. Stephenson , PhD is an associate professor of management and director of healthcare management programs in the David D. Reh School of Business at Clarkson University. Her research focuses on the healthcare workforce, how professional identity influences attitudes and behaviors, and how women leaders experience gender bias.
- LD Leanne M. Dzubinski , PhD is acting dean of the Cook School of Intercultural Studies and associate professor of intercultural education at Biola University, and a prominent researcher on women in leadership. She is coauthor of Glass Walls: Shattering the Six Gender Bias Barriers Still Holding Women Back at Work (Rowman & Littlefield).
Partner Center
- Twitter Icon
- Facebook Icon
- Reddit Icon
- LinkedIn Icon
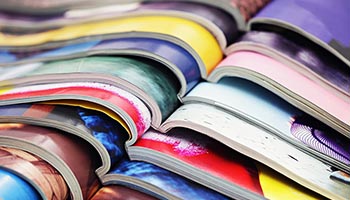
A case study of gender bias in science reporting
An analysis reveals a persistent gender disparity in the sources quoted in Nature ’s news and feature articles, although the gap is shrinking.
Gender disparities in science have attracted a lot of attention in the last decade or so, but the biases against women in media coverage of science haven’t received nearly as much focus. A recent study of Nature ’s news and feature articles sheds light on how often women are quoted in science news. The study finds that women continue to be quoted less often than men in the high-profile journal, although the gap seems to be narrowing.
Conducted by Natalie Davidson and Casey Greene of the University of Colorado School of Medicine, the study , posted in June on bioRxiv, analyzed more than 22 000 journalist-written news and feature articles that were published in the front half of Nature from 2005 to 2020. The researchers used software to approximately identify the genders and ethnicities of authors and sources. The software had a few limitations: It had a slight male bias, didn’t include a nonbinary gender assignment, and couldn’t identify all names. The researchers compared the software-assessed demographics of sources quoted in Nature ’s news section with that of the authors of the more than 13 000 research papers published in the back half of Nature during that period.
Davidson and Greene found a significant decrease in the proportion of men quoted in Nature ’s pages over those 15 years. The analysis found that in 2005, 87% of quotes were deemed to have come from men, whereas male researchers were the first authors of 73% of research papers in the study sample. By 2020 the likelihood that quotes came from men was down to about 69%. Articles about career-related topics were the only ones to achieve gender parity, the study found.
Although Nature publishes research from several disciplines with different proportions of female participation, the new study doesn’t distinguish the results by field or topic. Research papers published in Nature also may not be representative of the gender balance in the academic community or of research overall, Davidson says.
To account for that possible bias, the researchers selected another random sample of 36 000 research papers published over the same period in other journals run by publishing giant Springer Nature, which owns Nature . When compared with that data set, the estimated proportion of men quoted in Nature news and feature articles in 2020 (69%) is higher than the percentage of male first authors of the sample papers (about 63%) but lower than the rate of male last authors (76%). In contrast, the male quotation rate is lower than the rates of male first and last authors of Nature manuscripts, which are 74% and 80%, respectively. Davidson says that Nature research papers are more likely to be male-dominated (in first and last authors) than those published in other Springer Nature journals.
In an editorial published in response to the analysis, Nature acknowledges that its journalists need to work harder to eliminate biases, noting that the new analysis has shown that software can be used to recognize such trends. The editorial also mentions that Nature has been collecting data on gender diversity in its commissioned content for the last five years.
Gender bias in Physics Today
Nature is not alone in grappling with gender bias in science journalism. In an attempt to approximately replicate the Nature study’s methodology, Physics Today recorded the people quoted in 2005 and 2020 in two staff-written sections of the printed magazine: Search & Discovery, which covers new scientific research, and Issues & Events, which focuses on science policy and matters of interest to the physical sciences community.
In 2005, about 89% of quotes in those Physics Today articles were from male sources, a rate slightly more skewed than Nature ’s 87% in the same year. In 2020 the male quotation proportion was down to 74%, compared with Nature ’s 69%. Unlike in the Nature study, Physics Today did not examine the gender breakdown of the authors of journal articles in the physical sciences.
Although Physics Today is doing better than in the past, clearly there is still a lot more work to do. Physics Today ’s editors have been reaching out to more diverse sources in recent years and will continue to make those efforts a priority.
— Physics Today editors
Outside researchers say the new research, though not yet peer reviewed, is solid. Luke Holman, an evolutionary biologist at Edinburgh Napier University in the UK, says the new study has “novel, high-quality, and transparent methodology.” Holman co-authored a 2018 study that found that at the current rate of change, it would take 16 years for female researchers—averaged across scientific disciplines—to catch up with men and produce the same number of papers. In physics the gender gap would take 258 years to disappear.
Although Holman likes the new study, he notes that it doesn’t mention how many different people are quoted in each article in the sample, how many quotes are from the same sources, and how much page space is given to each source.
“It’s a really good thing that more female scientists are being quoted, even though things like this don’t normally directly contribute to tenure decisions,” says Barton Hamilton, an economist at Washington University in St Louis who has written about the gender gap in National Institutes of Health grant applications . “It’s very important that the faculty being quoted are representative of the faculty that are doing the work.”
Other analyses have also shown that women are being quoted more often in science news than in the past. For example, the World Association for Christian Communication released the latest quinquennial report on 14 July as part of the nongovernmental organization’s Global Media Monitoring Project. The report investigated, among other things, the extent to which women are quoted in the news media. Of all the news topics, women feature most often as sources and subjects in science and health news, says study editor Sarah Macharia, a consultant in gender, media, and international policy based in Toronto.
In 1995, women were 27% of the subjects and sources in science and health stories across different types of media; that representation increased to 35% by 2015. But Macharia says that as science and health news has grown as a percentage of all news coverage during the COVID-19 pandemic, the proportion of women as subjects and sources has decreased to 30%. “So as that topic has come to the limelight so dramatically and interest in the news has grown, women have been displaced from that space,” Macharia says.
Davidson and Greene’s study also compared quotation rates to rates of first or last authorship for scientists with various name origins in manuscripts published in Nature and other Springer Nature journals. They reported severe under-quotation relative to their rates of authorship for scientists with names originating in East Asia, and overrepresentation of scientists with English, Irish, Scottish, or Welsh names.
Deborah Blum, a science writer and director of the Knight Science Journalism program at MIT, explains that the reporter is responsible for identifying who did what in the studies they report on and for finding commenters from diverse backgrounds who did not work on the studies. “And that’s not just journalists trying to be politically correct,” she says. “When you have a diversity of scientists, the science is smarter. If you bring that to your reporting, your story is smarter too.”
But choosing diverse sources is not always easy or straightforward. Journalists often have to produce stories under tight deadlines and are sometimes limited to experts in a particular geographical location or time zone.
Holman sympathizes with the work that goes into finding sources but says, “If you have the opportunity to quote two equally qualified people and one of them is from an underrepresented part of the world or is a woman,” it’s best to quote that person.
Editor’s note: Dalmeet Singh Chawla regularly writes news pieces for Nature but had no involvement with the study. Madison Brewer performed the research for the Physics Today case study described in the box.

- Online ISSN 1945-0699
- Print ISSN 0031-9228
- For Researchers
- For Librarians
- For Advertisers
- Our Publishing Partners
- Physics Today
- Conference Proceedings
- Special Topics
pubs.aip.org
- Privacy Policy
- Terms of Use
Connect with AIP Publishing
This feature is available to subscribers only.
Sign In or Create an Account
- Reference Manager
- Simple TEXT file
People also looked at
Brief research report article, sex and gender bias in covid-19 clinical case reports.
- 1 Department of Medical Social Sciences, Feinberg School of Medicine, Northwestern University, Chicago, IL, United States
- 2 University of Illinois at Urbana-Champaign, Champaign, IL, United States
- 3 Division of Biostatistics, Department of Preventive Medicine, Feinberg School of Medicine, Northwestern University, Chicago, IL, United States
Clinical case reports circulate relevant information regarding disease presentation and describe treatment protocols, particularly for novel conditions. In the early months of the Covid-19 pandemic, case reports provided key insights into the pathophysiology and sequelae associated with Covid-19 infection and described treatment mechanisms and outcomes. However, case reports are often subject to selection bias due to their singular nature. To better understand how selection biases may have influenced Covid-19-releated case reports, we conducted a bibliometric analysis of Covid-19-releated case reports published in high impact journals from January 1 to June 1, 2020. Case reports were coded for patient sex, country of institutional affiliation, physiological system, and first and last author gender. Of 494 total case reports, 45% ( n = 221) of patients were male, 30% ( n = 146) were female, and 25% ( n = 124) included both sexes. Ratios of male-only to female-only case reports varied by physiological system. The majority of case reports had male first (61%, n = 302) and last (70%, n = 340) authors. Case reports with male last authors were more likely to describe male patients [ X 2 (2, n = 465) = 6.6, p = 0.037], while case reports with female last authors were more likely to include patients of both sexes [OR = 1.918 (95% CI = 1.163–3.16)]. Despite a limited sample size, these data reflect emerging research on sex-differences in the physiological presentation and impact of Covid-19 and parallel large-scale trends in authorship patterns. Ultimately, this work highlights potential biases in the dissemination of clinical information via case reports and underscores the inextricable influences of sex and gender biases within biomedicine.
Introduction
Longstanding sex and gender biases impact many facets of the biomedical research enterprise including research practices ( 1 , 2 ), clinical care ( 3 , 4 ), and workforce development ( 5 – 7 ). The persistent overrepresentation of males as research subjects, scientists, and physicians has informed our understanding of health and disease, oftentimes to the detriment of women, transgender, and gender non-binary, or non-conforming individuals.
Clinical case reports serve as an important educational tool to disseminate pertinent information regarding disease or disorder presentation, diagnosis, treatment, and prognosis ( 8 ). During the initial months of the Covid-19 pandemic, case reports provided key insights into Covid-19 pathophysiology, sequelae, and treatment and in certain circumstances, served as primary evidence for clinical decision-making.
Case report subjects are often selected semi-retrospectively for their novelty or educational benefit. As a result, singular case reports are inherently prone to selection bias. In contrast to clinical research studies which have predefined study populations and stringent inclusion or exclusion criteria, the decision to select a case report subject may lie solely with a member of the patient's care team. However, it is reasonable to expect that if case studies were compiled for a particular disease or disorder, they would closely mirror the respective patient population. In 2017, Allotey and colleagues ( 9 ) identified a significant male bias in case reports published in high-impact medical journals, which suggests that inherent biases may play a larger role than anticipated in case report selection and publication. We hypothesized that female patients may be underrepresented in Covid-19 research and clinical care due to sex differences in Covid-19 disease or due to gender biases. To determine whether Covid-19-related case reports were, in fact, subject to sex or gender biases, we characterized 494 Covid-19-related case reports published between January 1, 2020, and July 1, 2020, from 103 journals by patient sex, physiological system, country of institutional affiliation and first and last author gender.
Citation data for 1,817 articles classified as case reports were downloaded on July 1, 2020, from LitCovid, a categorical database of Covid-19 literature from PubMed ( 10 , 11 ). The LitCovid database identifies relevant articles using the National Center for Biotechnology Information's E-Utilities tool which is then further refined and categorized by machine learning and manual creation ( 11 ). Case reports were further refined by additional inclusion and exclusion criteria ( Figure 1 ). Journal impact factors [(IF), 2019 Journal Citation Reports Science Edition , Clarivate Analytics] were available for 1,466 (81%) case reports, and only those with an IF of 5 or above ( n = 498, 27%) were considered medium-to-high visibility and selected for inclusion in the study and further review. Four articles were excluded because they did not reference patients, resulting in a final sample of 494 articles. Two of the authors (ASV, AO) manually and independently screened and coded case reports for patient sex, physiological system, author first names, and country of institutional affiliation. Patient sex was determined by the use of descriptive terms such as male/female, man/woman, or inferred by the use of he/she pronouns. Only one article (0.2%) included transgender patients and did not report biological sex or gender identity. The country of institutional affiliation was determined by the institutional location of the corresponding author if the article was authored by a multinational cohort. These data were cross-checked, and the coding agreement was almost perfect for a representative subset of 55 articles (Cohen's kappa = 0.97, p < 0.001). The first and last author's gender were inferred using the name-to-gender assignment algorithm Gender API ( https://gender-api.com/ ). Gender API was selected due to its low rate of inaccuracies (7.9%) or non-classifications (3%) ( 12 ). Articles authored by an unspecified group or without full first names listed were coded as unknown.
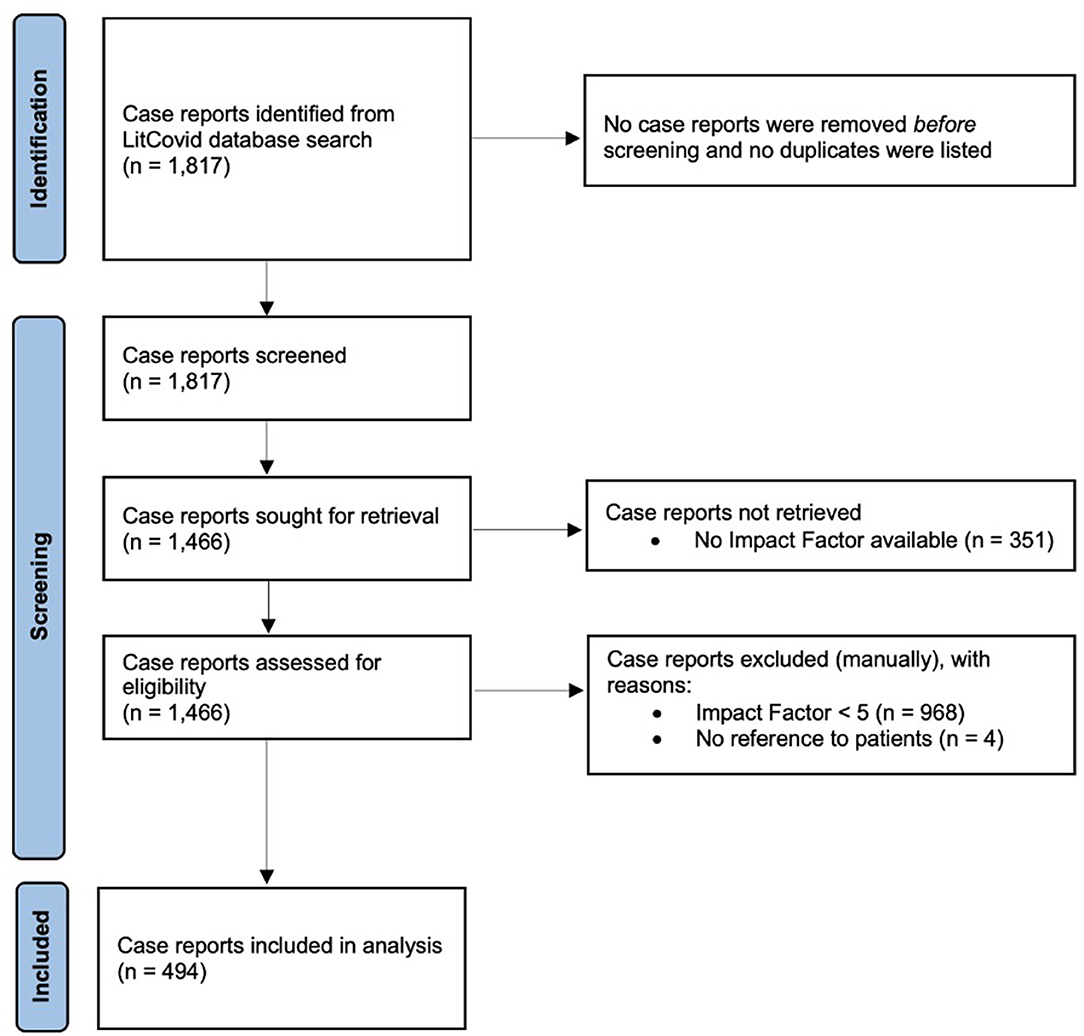
Figure 1 . Study flow diagram of the identification and screening of eligible Covid-19-related case reports retrieved from the PubMed LitCovid database.
Chi-Square tests and multinomial logistic regression models were used to examine the association between author gender and patient sex. Chi-Square tests were also used to compare patient sex by physiological system and country of institutional affiliation. Results from the multinomial logistic regression models are summarized by odds ratios (OR) and 95% confidence intervals (CI). P -values < 0.05 were considered significant.
Descriptions of patient sex or author gender follow American Psychological Association reporting standards where male/female terminology is used as descriptive adjectives when appropriate or when specifically referring to biological sex. The terms men and women are commonly used as nouns to describe groups of people.
Of the 494 case reports analyzed, the majority were related to respiratory, multi-systemic, dermatologic, hematologic, or neurologic systems ( Table 1 ). Of the patients described in the 494 case reports, forty-five % ( n = 221) were male and 30% ( n = 146) were female ( Figure 2 ). Patients of both sexes were included in 25% ( n = 124) of case reports and 0.6% ( n = 3) failed to report patient sex ( Figure 1 ). The ratio of articles reporting on male-only vs. female-only patients was highest in renal (11:1), hepatic (3.5:1), respiratory (2.3:1), multi-systemic (2.2:1), and cardiovascular (2.2:1) systems. Reproductive reports were almost exclusively female (95%, n = 20).
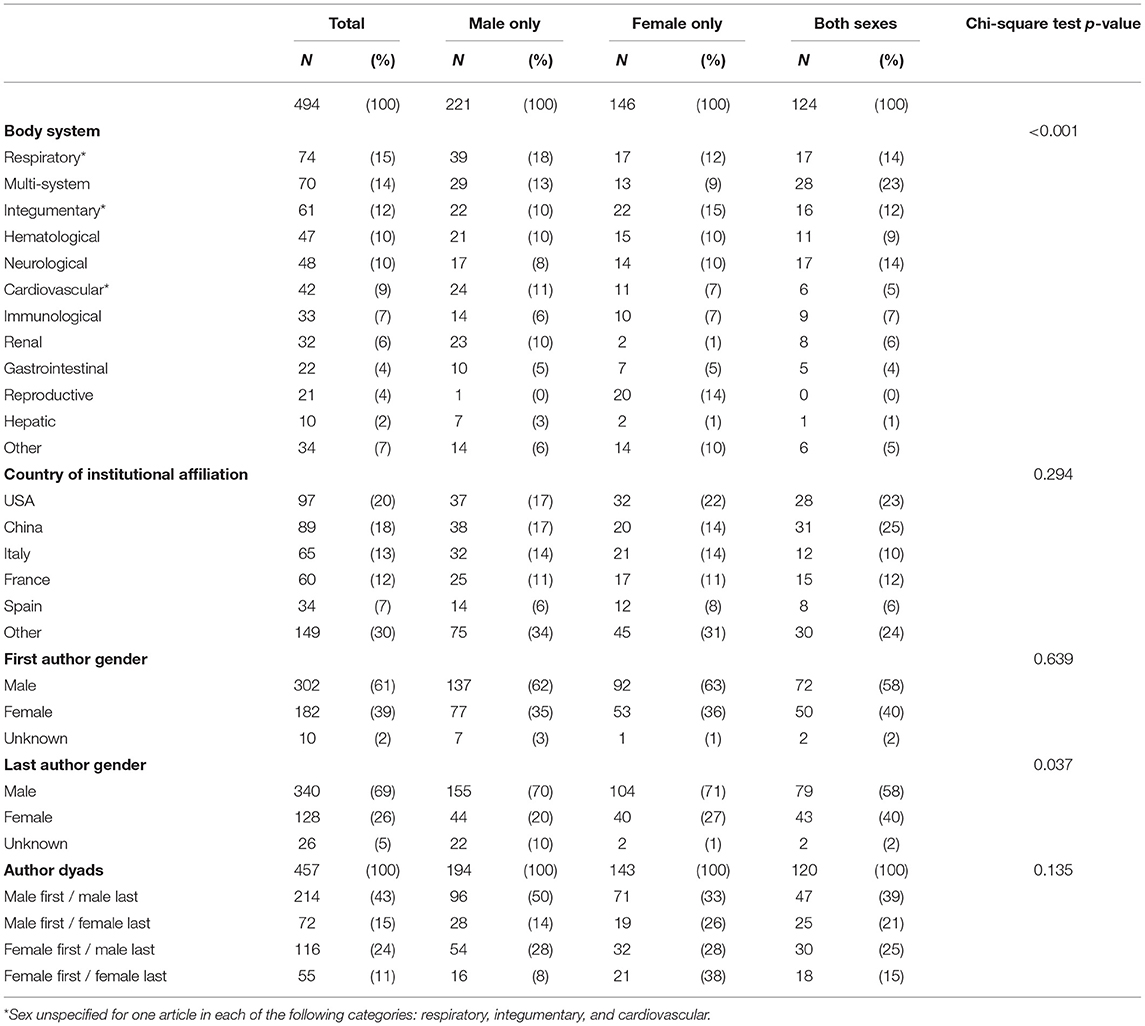
Table 1 . Case study characteristics and article metadata.
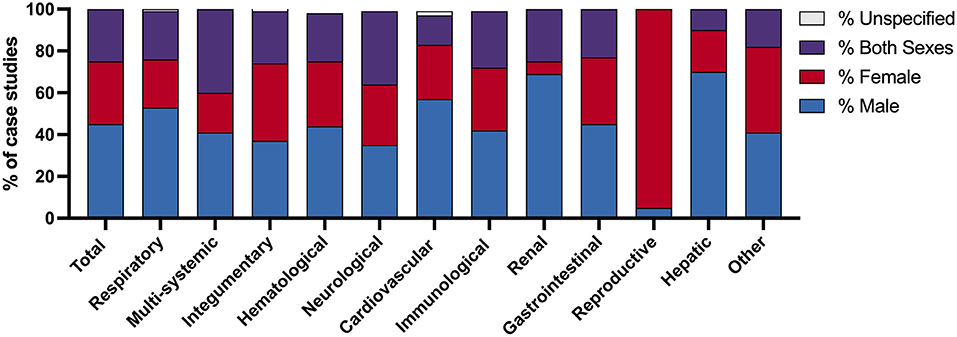
Figure 2 . Comparison of Covid-19 case studies by patient sex and physiologic body system. The percentage of Covid-19 case studies which describe patient sex as male, female, both sexes, and unspecified. Data are presented by the category of case study, coded by physiologic body system, as well as the sum of all case studies evaluated.
Case reports were primarily authored by groups with institutional affiliations in the United States (20%, n = 97), China (18%, n = 89), Italy (13%, n = 65), France (12%, n = 60), and Spain (7%, n = 34). The majority of case reports had male first (61%, n = 302) and last (70%, n = 340) authors, with 43% ( n = 214) of all reports having male first and last author dyads. The last author's gender is associated with the sex of the case report patient ( Table 1 ). Case reports with male last authors are more likely to include male-only patients ( p = 0.037) compared to female last authors. Female last authors are more likely to include patients of both sexes [OR = 1.918 (95% CI = 1.163–3.16)] in unadjusted and adjusted models [OR = 1.774 (95% CI = 1.055–2.984)] which control for impact factor, country, and physiological system.
While male bias in case reports has been previously reported ( 9 ), this is the first study to examine this in Covid-19-related case studies. The overrepresentation of male patients in Covid-19 case reports may be reflective of sex differences in disease prevalence, severity, and immune response ( 13 , 14 ). Likewise, sex and gender differences in the presence of contributing comorbidities may also influence Covid-19 disease severity and treatment outcomes ( 15 ). The high ratio of male-to-female case reports in the renal category parallels clinical data which suggests that male sex risk factor for Covid-19-related acute kidney injury ( 15 , 16 ). In comparison, the high female-to-male ratio observed in the reproductive category can be attributed to pregnancy-related case reports. Overall, the differences in patient sex ratios across physiological categories may provide insight into Covid-19 disease mechanisms. Yet, it is important to note that these data fail to fully capture the sociocultural influences on Covid-19 testing, case identification, and access to care which may differ based on gender, race, ethnicity, socioeconomic status, and geographic location as case reports typically originate from a hospital-based setting.
Gender disparities in authorship are common within the biomedical sciences ( 17 , 18 ) and have been documented for case reports ( 19 ). In a large-scale bibliometric analysis of over 20,000 case reports, Hsiehchen and colleagues ( 19 ) found that 36% of first authors and 25% of last authors are women. The data presented here are similar with female authors comprising 39% and 26% of first and last authors, respectively. Of interest, is the unique influence of the Covid-19 pandemic on gender authorship patterns. Early in the pandemic, several groups reported that women were publishing less to biomedical preprint servers compared to the same period in 2019 ( 20 , 21 ). Meanwhile, others found that women were underrepresented as first authors on Covid-19-related research studies ( 22 , 23 ). The case reports analyzed here were authored during the first 6 months of the pandemic yet reflect pre-pandemic authorship trends. This suggests that authorship trends should not solely be used as a metric for assessing the impact of Covid-19 on research productivity and more long-term, holistic evaluations of the biomedical enterprise are warranted. In depth analyses which evaluate other metrics of productivity such as grant submission and award patterns and hiring, retention, and promotion rates, at discipline- or specialty-specific levels and the availability and/or accessibility of institutional support structures would provide added insight into the impact of Covid-19 on the biomedical workforce.
Lastly, emerging evidence suggests that author gender may also influence how data are analyzed and presented ( 24 , 25 ). Prior work by Sugimoto and colleagues found that women are more likely to report and analyze data by sex ( 25 ). Here, we find that female authors are more likely to include patients of both sexes within case reports. These data suggest that female authors may be more likely to find inherent value in including clinical data derived from both sexes in case reports. Alternatively, they may be more keenly aware of, and actively seek to address sex- and gender biases in biomedicine through inclusivity. On the contrary, case reports with male last authors were more likely to include male-only patients. As last authorship generally confers seniority and intellectual leadership, these data suggest that sex or gender biases held by the senior author, whether implicit or explicit, may influence the selection of case report patients and reporting outcomes. The male-bias observed in Covid-19 case reports may be reflective of the patient population, as men who are diagnosed with Covid-19 are more likely to require hospitalization and critical care ( 26 ), however it does not fully explain the authorial differences in case report selection. The fact that female authors are more likely to include patients of both sexes is of interest and warrants further examination particularly in disease areas which are predominantly sex-specific.
This study is not without limitations. First, the sample size of case reports analyzed was limited due to stringent inclusion criteria related to journal impact factor and date of publication; although it is important to note that this sample size remains a significant and representative subset of the original sample of case reports. Journal impact factors of 5 and above were selected to represent case reports likely to be of medium-to-high visibility within the biomedical community. However, we recognize that journal impact factors are variable across biomedical disciplines and medical specialties and serve only as one metric to assess the quality, impact, and visibility of an article. As a result, case reports published in journals related to obstetrics and gynecology and reproductive health were likely omitted due to traditionally lower impact factors. The inclusion of case reports from women's health-related journals may have made the data appear more balanced and less suggestive of a sex-bias. Yet, by excluding these articles the data more broadly reflects sex and gender biases that exist outside of sex-specific fields of medicine, although we recognize that obstetric, gynecologic, and reproductive care is provided to those who identify across the gender spectrum.
In addition, these data were collected from the first 6 months of the Covid-19 pandemic, during which time the diagnosis, treatment, and understanding of the disease were rapidly evolving. We therefore cannot quantify the potential biases associated with clinical care that occurred later in the pandemic. The in silico tools to assign author gender also present another limitation as these are currently limited to gender binary options (male, female, or unknown) and therefore exclude or misrepresent the identity of those who are gender non-binary, non-conforming, two-spirit, or third gender. Moreover, some case reports did not explicitly define patient's sex or gender. For coding purposes, patient sex for these was inferred through the use of terms such as man/woman, male/female, or descriptive he/she pronouns, and there may be instances where patient sex and gender identity do not correspond. Often the terms “sex” and “gender” were used interchangeably within case reports, making it difficult to separate patient's biological sex from their gender identity. The distinctions of both biological sex and gender should be noted in case reports, as gender is a contributing social determinant of health.
The associations between author gender and patient sex suggests that sex or gender biases are contributing factors which impact patient reporting. The coordinated efforts of clinicians, reviewers, editors, and publishers are required to ensure a balanced representation of the relevant patient population. Gender has been widely recognized as a social determinant of health and as such gender biases can contribute to gender-based health disparities. Diversification of the biomedical workforce appears to be critical, but rate-limiting factor, in reducing sex- and gender biases that permeate biomedicine. As more gender-diverse perspectives are included in the selection, writing, reviewing, and publishing of case reports, their subsequent quality, and educational value are likely to improve. Acknowledging and actively addressing biases may further a better understanding of the influences of sex and gender on health and disease, ultimately minimizing health disparities.
Data Availability Statement
The raw data supporting the conclusions of this article will be made available by the authors, without undue reservation.
Author Contributions
NW: full access to all the data in the study and takes responsibility for the integrity of the data and the accuracy of the data analysis, obtained funding, administrative, technical, or material support, supervision, concept, and design. AS-V, AO, and NW: drafting of the manuscript. CY, LM, and NW: statistical analysis. All authors: acquisition, analysis, or interpretation of data and critical revision of the manuscript for important intellectual content.
This work was supported by a Women's Health Access Matters Grant to NW. The funders had no role in the design and conduct of the study; collection, management, analysis, and interpretation of the data; preparation, review, or approval of the manuscript; and decision to submit the manuscript for publication.
Conflict of Interest
The authors declare that the research was conducted in the absence of any commercial or financial relationships that could be construed as a potential conflict of interest.
Publisher's Note
All claims expressed in this article are solely those of the authors and do not necessarily represent those of their affiliated organizations, or those of the publisher, the editors and the reviewers. Any product that may be evaluated in this article, or claim that may be made by its manufacturer, is not guaranteed or endorsed by the publisher.
1. Woitowich NC, Beery A, Woodruff T. A 10-year follow-up study of sex inclusion in the biological sciences. eLife . (2020) 9:e56344. Available online at: https://www.ncbi.nlm.nih.gov/pmc/articles/PMC7282816/ (accessed May 18, 2021). doi: 10.7554/eLife.56344
PubMed Abstract | CrossRef Full Text | Google Scholar
2. Shansky RM, Murphy AZ. Considering sex as a biological variable will require a global shift in science culture. Nat Neurosci. (2021) 24:457–64. doi: 10.1038/s41593-021-00806-8
3. Alcalde-Rubio L, Hernández-Aguado I, Parker LA, Bueno-Vergara E, Chilet-Rosell E. Gender disparities in clinical practice: are there any solutions? Scoping review of interventions to overcome or reduce gender bias in clinical practice. Int J Equity Health. (2020) 19:166. doi: 10.1186/s12939-020-01283-4
4. Hay K, McDougal L, Percival V, Henry S, Klugman J, Wurie H, et al. Disrupting gender norms in health systems: making the case for change. Lancet Lond Engl. (2019) 393:2535–49. doi: 10.1016/S0140-6736(19)30648-8
5. Huang J, Gates AJ, Sinatra R, Barabási A-L. Historical comparison of gender inequality in scientific careers across countries and disciplines. Proc Natl Acad Sci . (2020) 117:4609–16. doi: 10.1073/pnas.1914221117
6. Butkus R, Serchen J, Moyer DV, Bornstein SS, Hingle ST. Achieving gender equity in physician compensation and career advancement: a position paper of the american college of physicians. Ann Intern Med. (2018) 168:721–3. doi: 10.7326/M17-3438
7. Silver JK, Bean AC, Slocum C, Poorman JA, Tenforde A, Blauwet CA. Physician workforce disparities and patient care: a narrative review. Health Equity. (2019) 3:360–77. doi: 10.1089/heq.2019.0040
8. Nissen T, Wynn R. The clinical case report: a review of its merits and limitations. BMC Res Notes. (2014) 7:264. doi: 10.1186/1756-0500-7-264
9. Allotey P, Allotey-Reidpath C, Reidpath DD. Gender bias in clinical case reports: a cross-sectional study of the “big five” medical journals. PLoS ONE. (2017) 12:e0177386. doi: 10.1371/journal.pone.0177386
10. Chen Q, Allot A, Lu Z. Keep up with the latest coronavirus research. Nature. (2020) 579:193. doi: 10.1038/d41586-020-00694-1
11. Chen Q, Allot A, Lu Z. LitCovid: an open database of COVID-19 literature. Nucleic Acids Res. (2021) 49:D1534–40. doi: 10.1093/nar/gkaa952
12. Santamaría L, Mihaljević H. Comparison and benchmark of name-to-gender inference services. PeerJ Comput Sci . (2018) 4:e156. doi: 10.7717/peerj-cs.156
13. Scully EP, Haverfield J, Ursin RL, Tannenbaum C, Klein SL. Considering how biological sex impacts immune responses and COVID-19 outcomes. Nat Rev Immunol. (2020) 20:442–7. doi: 10.1038/s41577-020-0348-8
14. Takahashi T, Iwasaki A. Sex differences in immune responses. Science. (2021) 371:347–8. doi: 10.1126/science.abe7199
15. Vahidy FS, Pan AP, Ahnstedt H, Munshi Y, Choi HA, Tiruneh Y. Sex differences in susceptibility, severity, and outcomes of coronavirus disease 2019: Cross-sectional analysis from a diverse US metropolitan area. PLoS ONE. (2021) 16:e0245556. doi: 10.1371/journal.pone.0245556
16. Zahid U, Ramachandran P, Spitalewitz S, Alasadi L, Chakraborti A, Azhar M. Acute Kidney Injury in COVID-19 Patients: an inner city hospital experience and policy implications. Am J Nephrol. (2020) 51:786–96. doi: 10.1159/000511160
17. Filardo G, da Graca B, Sass DM, Pollock BD, Smith EB, et al. Trends and comparison of female first authorship in high impact medical journals: observational study (1994-2014). BMJ . (2016) 352:i847. doi: 10.1136/bmj.i847
18. Kleijn de, Jayabalasingham M, Falk-Krzesinski B, Collins H, Kupier-Hoyng T, Cingolani LI, et al. The Researcher Journey Through a Gender Lens: An Examination of Research Participation, Career Progression and Perceptions Across the Globe. Elsevier (2020). Available online at: http://www.elsevier.com/gender-report (accessed May 19, 2021).
19. Hsiehchen D, Hsieh A, Espinoza M. Prevalence of female authors in case reports published in the medical literature. JAMA Netw Open. (2019) 2:e195000. doi: 10.1001/jamanetworkopen.2019.5000
20. Vincent-Lamarre P, Sugimoto C, Larivière V. The Decline Of Women's Research Production During The Coronavirus Pandemic. Nature Index (2020). Available online at: https://www.natureindex.com/news-blog/decline-women-scientist-research-publishing-production-coronavirus-pandemic (accessed May 19, 2021).
21. Wehner MR, Li Y, Nead KT. Comparison of the proportions of female and male corresponding authors in preprint research repositories before and during the COVID-19 pandemic. JAMA Netw Open . (2020) 3:1–4. doi: 10.1001/jamanetworkopen.2020.20335
22. Andersen JP, Nielsen MW, Simone NL, Lewiss RE, Jagsi R. COVID-19 medical papers have fewer women first authors than expected. eLife. (2020) 9:e58807. doi: 10.7554/eLife.58807
23. Lerchenmüller C, Schmallenbach L, Jena AB, Lerchenmueller MJ. Longitudinal analyses of gender differences in first authorship publications related to COVID-19. BMJ Open. (2021) 11:e045176. doi: 10.1136/bmjopen-2020-045176
24. Nielsen MW, Andersen JP, Schiebinger L, Schneider JW. One and a half million medical papers reveal a link between author gender and attention to gender and sex analysis. Nat Hum Behav. (2017) 1:791–6. doi: 10.1038/s41562-017-0235-x
25. Sugimoto CR, Ahn Y-Y, Smith E, Macaluso B, Larivière V. Factors affecting sex-related reporting in medical research: a cross-disciplinary bibliometric analysis. Lancet . (2019) 393:550–9. doi: 10.1016/S0140-6736(18)32995-7
26. Gomez JMD, Du-Fay-de-Lavallaz JM, Fugar S, Sarau A, Simmons JA, Sanghani RM, et al. Sex Differences in COVID-19 Hospitalization and Mortality. J Womens Health (Larchmt). (2021) 30:646–53. doi: 10.1089/jwh.2020.8948
Keywords: COVID-19, sex bias, gender bias, case reports (publication type), bibliometrics
Citation: Salter-Volz AE, Oyasu A, Yeh C, Muhammad LN and Woitowich NC (2021) Sex and Gender Bias in Covid-19 Clinical Case Reports. Front. Glob. Womens Health 2:774033. doi: 10.3389/fgwh.2021.774033
Received: 10 September 2021; Accepted: 29 October 2021; Published: 22 November 2021.
Reviewed by:
Copyright © 2021 Salter-Volz, Oyasu, Yeh, Muhammad and Woitowich. This is an open-access article distributed under the terms of the Creative Commons Attribution License (CC BY) . The use, distribution or reproduction in other forums is permitted, provided the original author(s) and the copyright owner(s) are credited and that the original publication in this journal is cited, in accordance with accepted academic practice. No use, distribution or reproduction is permitted which does not comply with these terms.
*Correspondence: Nicole C. Woitowich, nicole.woitowich@northwestern.edu
† These authors have contributed equally to this work
This article is part of the Research Topic
Inequalities in COVID-19 Healthcare and Research Affecting Women
- Browse All Articles
- Newsletter Sign-Up
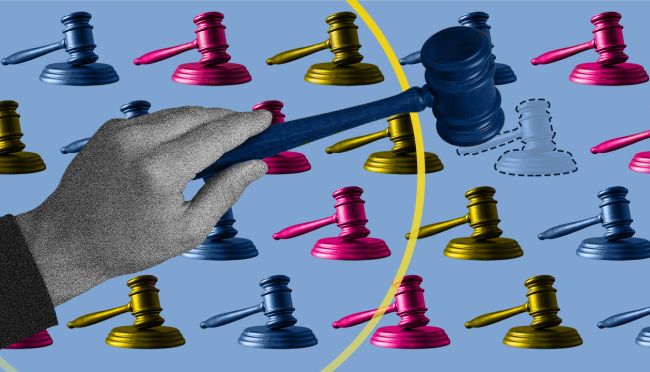
- 04 Mar 2024
- Research & Ideas
Want to Make Diversity Stick? Break the Cycle of Sameness
Whether on judicial benches or in corporate boardrooms, white men are more likely to step into roles that other white men vacate, says research by Edward Chang. But when people from historically marginalized groups land those positions, workforce diversification tends to last. Chang offers three pieces of advice for leaders striving for diversity.
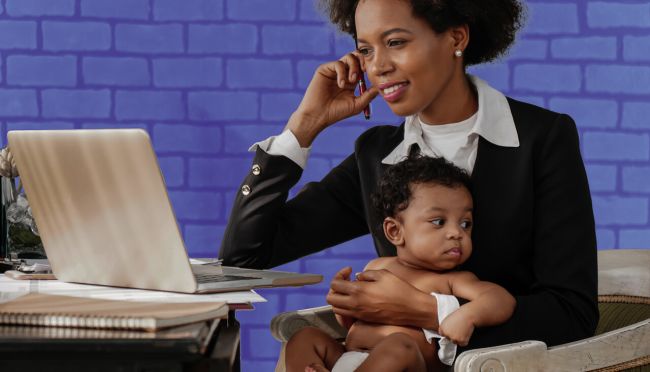
- 14 Sep 2023
Working Moms Are Mostly Thriving Again. Can We Finally Achieve Gender Parity?
The pandemic didn't destroy the workplace advancements moms had achieved. However, not all of the positive changes forced by the crisis and remote work have stuck, says research by Kathleen McGinn and Alexandra Feldberg.
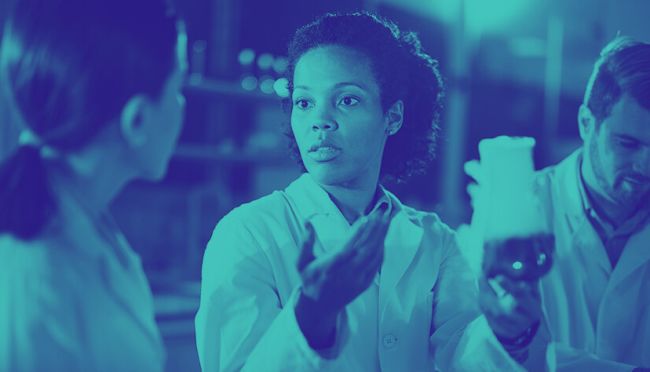
- 26 Jul 2023
STEM Needs More Women. Recruiters Often Keep Them Out
Tech companies and programs turn to recruiters to find top-notch candidates, but gender bias can creep in long before women even apply, according to research by Jacqueline Ng Lane and colleagues. She highlights several tactics to make the process more equitable.
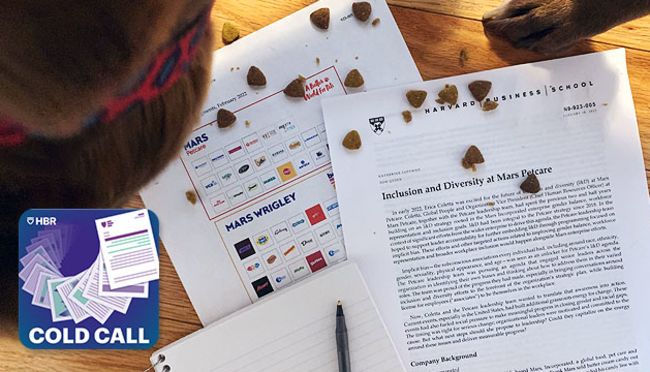
- 18 Jul 2023
- Cold Call Podcast
Diversity and Inclusion at Mars Petcare: Translating Awareness into Action
In 2020, the Mars Petcare leadership team found themselves facing critically important inclusion and diversity issues. Unprecedented protests for racial justice in the U.S. and across the globe generated demand for substantive change, and Mars Petcare's 100,000 employees across six continents were ready for visible signs of progress. How should Mars’ leadership build on their existing diversity, equity, and inclusion efforts and effectively capitalize on the new energy for change? Harvard Business School associate professor Katherine Coffman is joined by Erica Coletta, Mars Petcare’s chief people officer, and Ibtehal Fathy, global inclusion and diversity officer at Mars Inc., to discuss the case, “Inclusion and Diversity at Mars Petcare.”
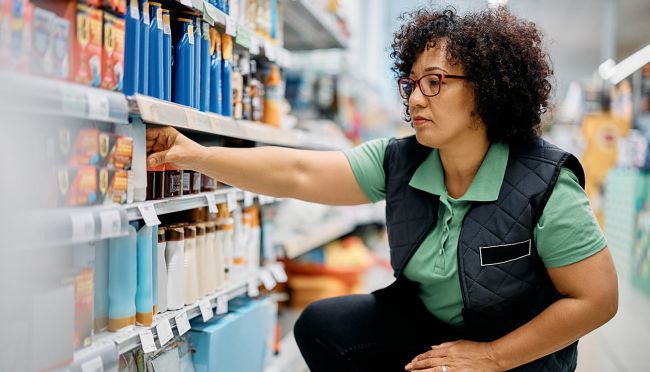
- 03 Mar 2023
When Showing Know-How Backfires for Women Managers
Women managers might think they need to roll up their sleeves and work alongside their teams to show their mettle. But research by Alexandra Feldberg shows how this strategy can work against them. How can employers provide more support?
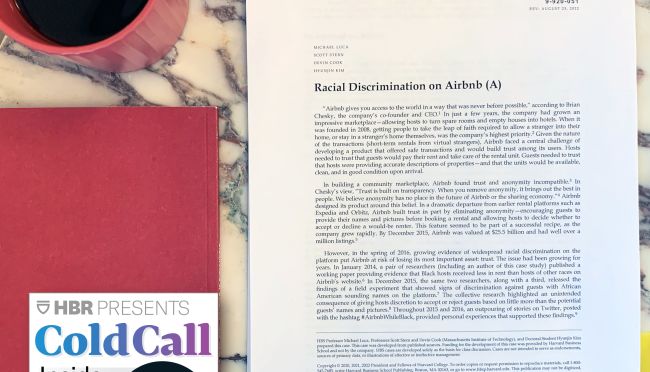
- 31 Jan 2023
Addressing Racial Discrimination on Airbnb
For years, Airbnb gave hosts extensive discretion to accept or reject a guest after seeing little more than a name and a picture, believing that eliminating anonymity was the best way for the company to build trust. However, the apartment rental platform failed to track or account for the possibility that this could facilitate discrimination. After research published by Professor Michael Luca and others provided evidence that Black hosts received less in rent than hosts of other races and showed signs of discrimination against guests with African American sounding names, the company had to decide what to do. In the case, “Racial Discrimination on Airbnb,” Luca discusses his research and explores the implication for Airbnb and other platform companies. Should they change the design of the platform to reduce discrimination? And what’s the best way to measure the success of any changes?
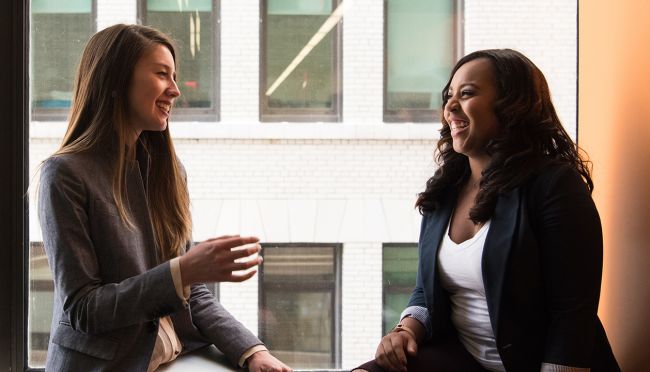
- 03 Jan 2023
Confront Workplace Inequity in 2023: Dig Deep, Build Bridges, Take Collective Action
Power dynamics tied up with race and gender underlie almost every workplace interaction, says Tina Opie. In her book Shared Sisterhood, she offers three practical steps for dismantling workplace inequities that hold back innovation.
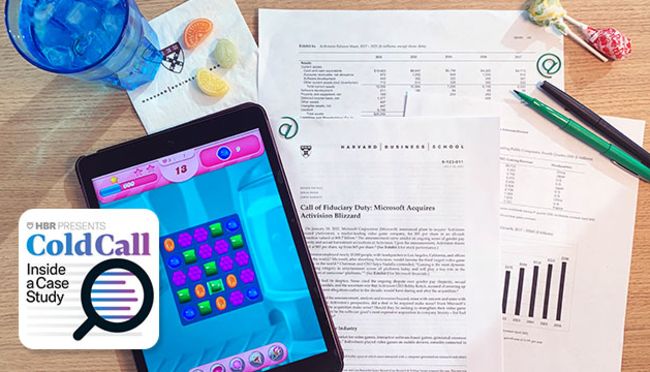
- 29 Nov 2022
How Will Gamers and Investors Respond to Microsoft’s Acquisition of Activision Blizzard?
In January 2022, Microsoft announced its acquisition of the video game company Activision Blizzard for $68.7 billion. The deal would make Microsoft the world’s third largest video game company, but it also exposes the company to several risks. First, the all-cash deal would require Microsoft to use a large portion of its cash reserves. Second, the acquisition was announced as Activision Blizzard faced gender pay disparity and sexual harassment allegations. That opened Microsoft up to potential reputational damage, employee turnover, and lost sales. Do the potential benefits of the acquisition outweigh the risks for Microsoft and its shareholders? Harvard Business School associate professor Joseph Pacelli discusses the ongoing controversies around the merger and how gamers and investors have responded in the case, “Call of Fiduciary Duty: Microsoft Acquires Activision Blizzard.”
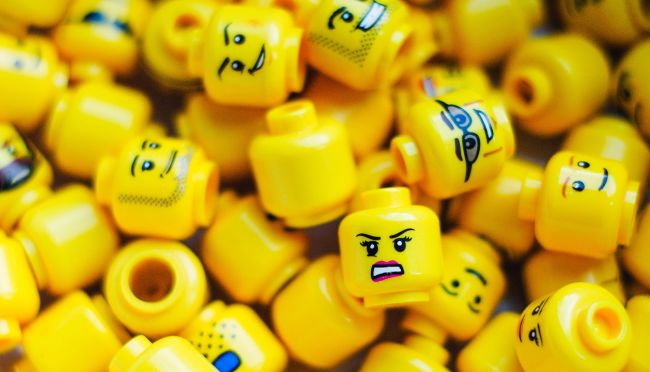
- 10 Nov 2022
Too Nice to Lead? Unpacking the Gender Stereotype That Holds Women Back
People mistakenly assume that women managers are more generous and fair when it comes to giving money, says research by Christine Exley. Could that misperception prevent companies from shrinking the gender pay gap?
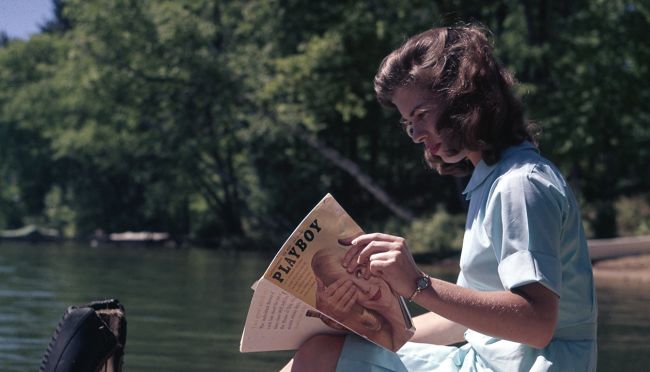
- 08 Nov 2022
How Centuries of Restrictions on Women Shed Light on Today's Abortion Debate
Going back to pre-industrial times, efforts to limit women's sexuality have had a simple motive: to keep them faithful to their spouses. Research by Anke Becker looks at the deep roots of these restrictions and their economic implications.
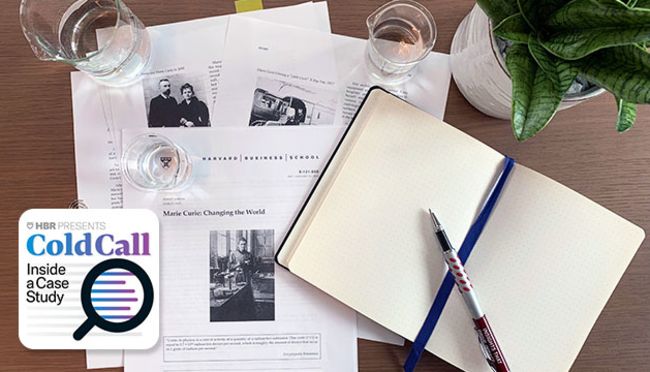
- 01 Nov 2022
Marie Curie: A Case Study in Breaking Barriers
Marie Curie, born Maria Sklodowska from a poor family in Poland, rose to the pinnacle of scientific fame in the early years of the twentieth century, winning the Nobel Prize twice in the fields of physics and chemistry. At the time women were simply not accepted in scientific fields so Curie had to overcome enormous obstacles in order to earn a doctorate at the Sorbonne and perform her pathbreaking research on radioactive materials. How did she plan her time and navigate her life choices to leave a lasting impact on the world? Professor Robert Simons discusses how Marie Curie rose to scientific fame despite poverty and gender barriers in his case, “Marie Curie: Changing the World.”
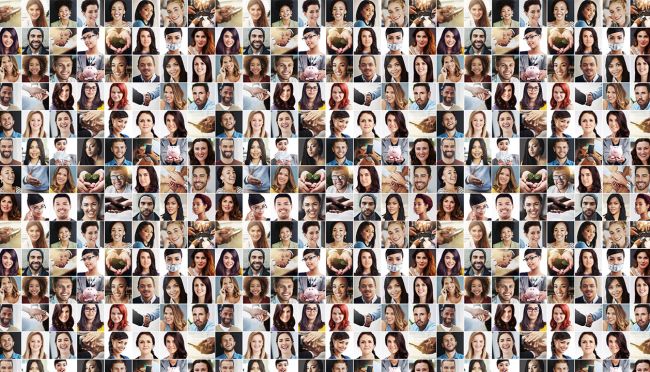
- 18 Oct 2022
When Bias Creeps into AI, Managers Can Stop It by Asking the Right Questions
Even when companies actively try to prevent it, bias can sway algorithms and skew decision-making. Ayelet Israeli and Eva Ascarza offer a new approach to make artificial intelligence more accurate.
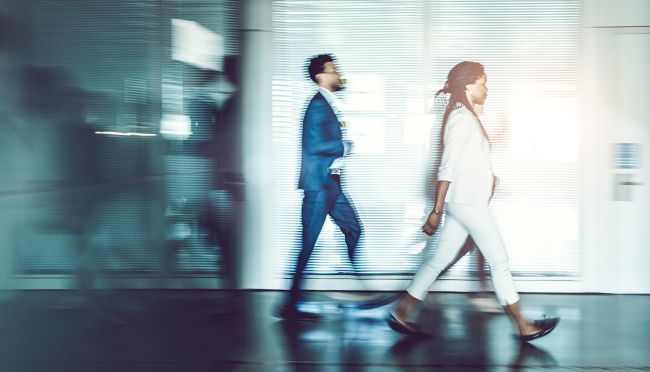
- 29 Jul 2022
Will Demand for Women Executives Finally Shrink the Gender Pay Gap?
Women in senior management have more negotiation power than they think in today's labor market, says research by Paul Healy and Boris Groysberg. Is it time for more women to seek better opportunities and bigger pay?
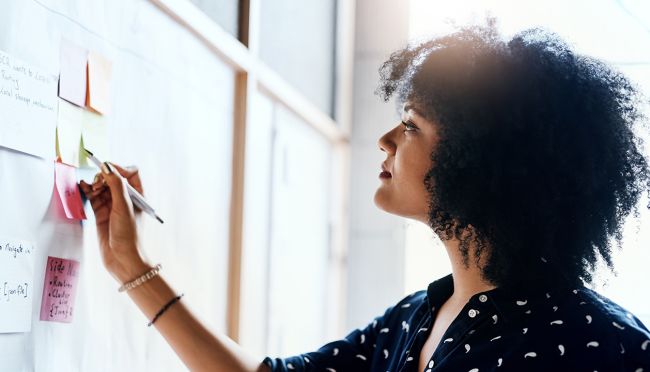
- 24 May 2022
Career Advice for Minorities and Women: Sharing Your Identity Can Open Doors
Women and people of color tend to minimize their identities in professional situations, but highlighting who they are often forces others to check their own biases. Research by Edward Chang and colleagues.
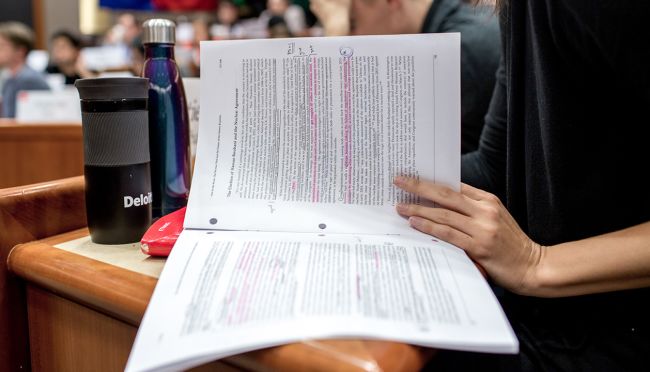
- 08 Mar 2022
Representation Matters: Building Case Studies That Empower Women Leaders
The lessons of case studies shape future business leaders, but only a fraction of these teaching tools feature women executives. Research by Colleen Ammerman and Boris Groysberg examines the gender gap in cases and its implications. Open for comment; 0 Comments.
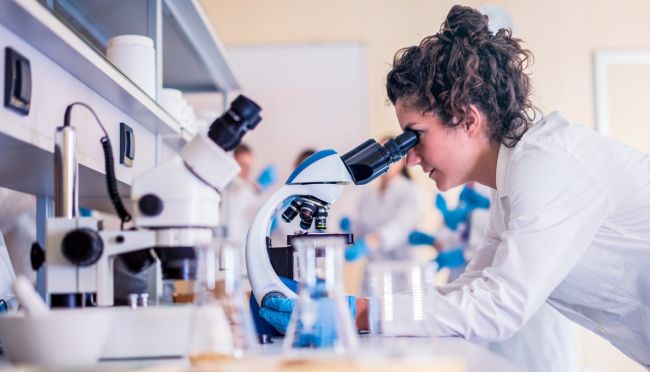
- 22 Feb 2022
Lack of Female Scientists Means Fewer Medical Treatments for Women
Women scientists are more likely to develop treatments for women, but many of their ideas never become inventions, research by Rembrand Koning says. What would it take to make innovation more equitable? Open for comment; 0 Comments.
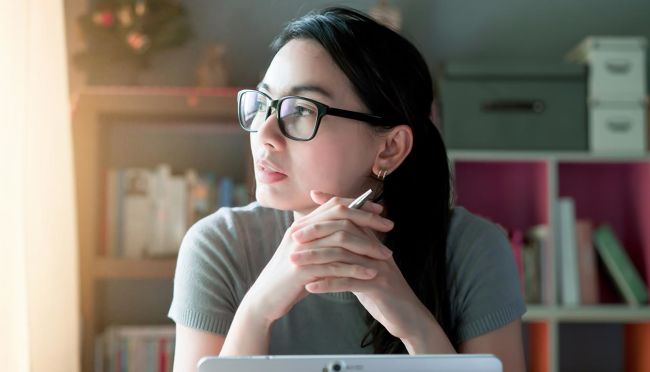
- 01 Sep 2021
How Women Can Learn from Even Biased Feedback
Gender bias often taints performance reviews, but applying three principles can help women gain meaningful insights, says Francesca Gino. Open for comment; 0 Comments.
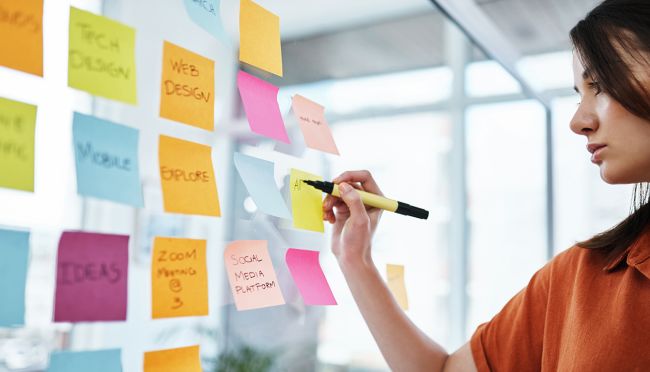
- 23 Jun 2021
One More Way the Startup World Hampers Women Entrepreneurs
Early feedback is essential to launching new products, but women entrepreneurs are more likely to receive input from men. Research by Rembrand Koning, Ramana Nanda, and Ruiqing Cao. Open for comment; 0 Comments.
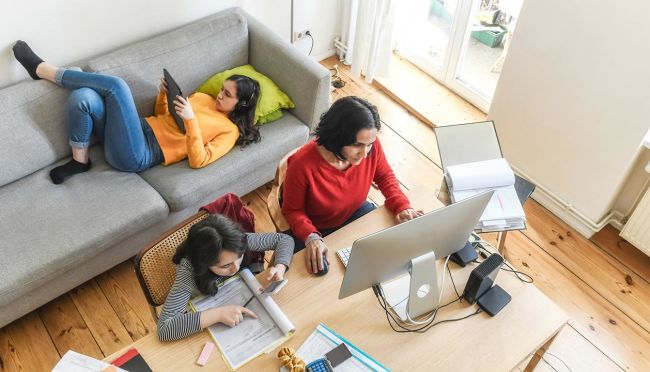
- 01 Jun 2021
- What Do You Think?
Are Employers Ready for a Flood of 'New' Talent Seeking Work?
Many people, particularly women, will be returning to the workforce as the COVID-19 pandemic wanes. What will companies need to do to harness the talent wave? asks James Heskett. Open for comment; 0 Comments.
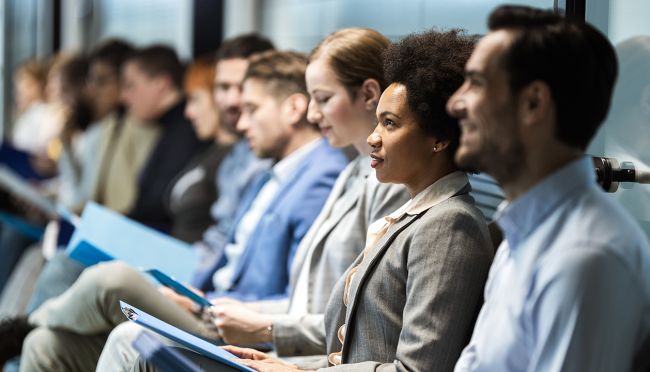
- 10 May 2021
Who Has Potential? For Many White Men, It’s Often Other White Men
Companies struggling to build diverse, inclusive workplaces need to break the cycle of “sameness” that prevents some employees from getting an equal shot at succeeding, says Robin Ely. Open for comment; 0 Comments.
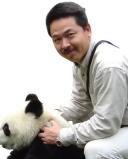
Insights From Research of Teens Unhappy With Their Gender
New studies offer critical data on teens questioning their gender..
Posted April 26, 2024 | Reviewed by Tyler Woods
- Objective information is lacking about basic demographics of adolescents unhappy with their gender.
- Two new studies reveal a sizable number of early adolescents experience dissatisfaction with their gender.
- While most teens become content with their birth gender as they grow up, some remain unsatisfied.
In the landscape of contemporary gender discourse, few topics evoke as much controversy as those concerning transgender issues. From discussions about restroom access to debates surrounding sports participation, the dialogue is fraught with polarization. These discussions often descend into heated exchanges, characterized more by accusations and counteraccusations than reasoned engagement.
Amidst the fervor and impassioned opinions, one crucial element remains conspicuously absent: concrete and objective information. Consequently, our conversations about transgender controversies frequently falter in addressing fundamental inquiries. We even don’t have basic statistics and demographics of the transgender community.
Fortunately, two recent studies have proved timely, shedding new light and offering fresh perspectives on these complex issues.
In a study published in 2021, Jen-How Kuo and Meng-Che Tsai led a team in tracking 1,806 junior high students in Taiwan from 2000 to 2009. They asked a straightforward question: “Are you satisfied with your own gender?” What they found over the nine-year period was quite telling: while the majority (86.5 percent) consistently expressed satisfaction with their genders, 7.8 percent transitioned from dissatisfaction to satisfaction, 4.8 percent shifted from satisfaction to dissatisfaction, and 0.9 percent remained consistently dissatisfied ( 1 ).
A similar study in the Netherlands, detailed in a 2024 paper by Pien Rawee, Sarah Burke, and their colleagues, queried 2,772 adolescents in their response to a similar but more nuanced statement: “I wish to be of the opposite sex .” They discovered that as many as 11 percent of early adolescents experienced gender dissatisfaction, which decreased to approximately 4 percent by the age of 25. Overall, 78 percent remained content with their genders, 19 percent transitioned from dissatisfaction to satisfaction, and 2 percent became even more dissatisfied with their birth genders as they progressed from early adolescence to young adulthood ( 2 ).
These findings hold critical significance on several fronts. First, they reveal that a sizable percentage (13.5 percent in the Taiwan study and 22 percent in the Netherlands study) of teenagers experience some level of dissatisfaction with their gender identity . However, most of them tend to resolve this uncertainty as they mature into adulthood, ultimately aligning with the gender they were assigned at birth. Nonetheless, there remains a smaller yet consistent portion of teenagers who persist in their dissatisfaction or even transition from a state of contentment to dissatisfaction with their gender identity .
“These findings indicate,” write the researchers from the Taiwan study, “that healthcare professionals should concentrate on gender non-conforming individuals at early adolescence, navigating them toward a healthy adulthood.” Clearly, they are also significant for parents, caregivers, and society at large, providing guidance on how to better care for, assist, and support adolescents, especially those prone to gender dissatisfaction.
Undoubtedly, the next crucial question revolves around identifying which adolescents will navigate out of the phase of gender uncertainty and who will persist in their dissatisfaction. While such data is currently lacking, an evidence-based approach holds the promise of transcending ideological debate to a scientific endeavor in developmental psychology.
Acknowledgments: I thank Drs. Sarah Burke and Meng-Che Tsai for double-checking the facts quoted in this essay based on their original research.
1. Kuo, J-H., Albaladejo Carrera, R., Cendra Mulyani, L., Strong, C., Lin, Y-C., Hsieh, Y-P., Tsai, M-C., and Lin, C-Y. (2021) Exploring the Interaction Effects of Gender Contentedness and Pubertal Timing on Adolescent Longitudinal Psychological and Behavioral Health Outcomes. Front. Psychiatry 12:660746.
2. Rawee, P., Rosmalen, J.G., Kalverdijk, L., and Burke, S.M. (2024) Development of Gender Non-Contentedness During Adolescence and Early Adulthood. Archives of Sexual Behavior, pp.1-13.
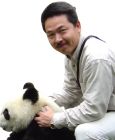
Lixing Sun, Ph.D. , is a distinguished research professor in behavior and evolution at Central Washington University.
- Find a Therapist
- Find a Treatment Center
- Find a Psychiatrist
- Find a Support Group
- Find Online Therapy
- International
- New Zealand
- South Africa
- Switzerland
- Asperger's
- Bipolar Disorder
- Chronic Pain
- Eating Disorders
- Passive Aggression
- Personality
- Goal Setting
- Positive Psychology
- Stopping Smoking
- Low Sexual Desire
- Relationships
- Child Development
- Therapy Center NEW
- Diagnosis Dictionary
- Types of Therapy
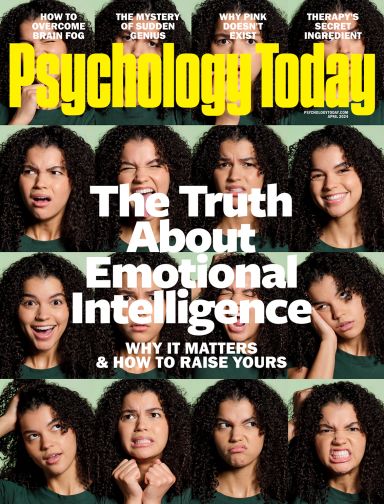
Understanding what emotional intelligence looks like and the steps needed to improve it could light a path to a more emotionally adept world.
- Emotional Intelligence
- Gaslighting
- Affective Forecasting
- Neuroscience
Assessing gender bias in machine translation: a case study with Google Translate
- Original Article
- Published: 27 March 2019
- Volume 32 , pages 6363–6381, ( 2020 )
Cite this article
- Marcelo O. R. Prates ORCID: orcid.org/0000-0002-5576-7060 1 ,
- Pedro H. Avelar 1 &
- Luís C. Lamb 1
11k Accesses
95 Citations
65 Altmetric
Explore all metrics
Recently there has been a growing concern in academia, industrial research laboratories and the mainstream commercial media about the phenomenon dubbed as machine bias , where trained statistical models—unbeknownst to their creators—grow to reflect controversial societal asymmetries, such as gender or racial bias. A significant number of Artificial Intelligence tools have recently been suggested to be harmfully biased toward some minority, with reports of racist criminal behavior predictors, Apple’s Iphone X failing to differentiate between two distinct Asian people and the now infamous case of Google photos’ mistakenly classifying black people as gorillas. Although a systematic study of such biases can be difficult, we believe that automated translation tools can be exploited through gender neutral languages to yield a window into the phenomenon of gender bias in AI. In this paper, we start with a comprehensive list of job positions from the U.S. Bureau of Labor Statistics (BLS) and used it in order to build sentences in constructions like “He/She is an Engineer” (where “Engineer” is replaced by the job position of interest) in 12 different gender neutral languages such as Hungarian, Chinese, Yoruba, and several others. We translate these sentences into English using the Google Translate API, and collect statistics about the frequency of female, male and gender neutral pronouns in the translated output. We then show that Google Translate exhibits a strong tendency toward male defaults, in particular for fields typically associated to unbalanced gender distribution or stereotypes such as STEM (Science, Technology, Engineering and Mathematics) jobs. We ran these statistics against BLS’ data for the frequency of female participation in each job position, in which we show that Google Translate fails to reproduce a real-world distribution of female workers. In summary, we provide experimental evidence that even if one does not expect in principle a 50:50 pronominal gender distribution, Google Translate yields male defaults much more frequently than what would be expected from demographic data alone. We believe that our study can shed further light on the phenomenon of machine bias and are hopeful that it will ignite a debate about the need to augment current statistical translation tools with debiasing techniques—which can already be found in the scientific literature.
This is a preview of subscription content, log in via an institution to check access.
Access this article
Price includes VAT (Russian Federation)
Instant access to the full article PDF.
Rent this article via DeepDyve
Institutional subscriptions
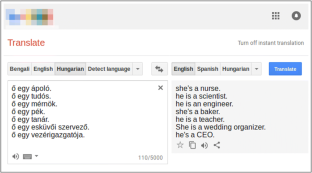
Similar content being viewed by others
Gender Bias in Machine Translation Systems
Gender and Age Bias in Commercial Machine Translation
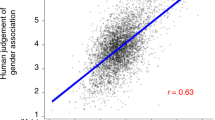
Gender stereotypes are reflected in the distributional structure of 25 languages
Angwin J, Larson J, Mattu S, Kirchner L (2016) Machine bias: there’s software used across the country to predict future criminals and it’s biased against blacks. https://www.propublica.org/article/machine-bias-risk-assessments-in-criminal-sentencing . Last visited 2017-12-17
Bahdanau D, Cho K, Bengio Y (2014) Neural machine translation by jointly learning to align and translate. arxiv:1409.0473 . Accessed 9 Mar 2019
Bellens E (2018) Google translate est sexiste. https://datanews.levif.be/ict/actualite/google-translate-est-sexiste/article-normal-889277.html?cookie_check=1549374652 . Posted 11 Sep 2018
Boitet C, Blanchon H, Seligman M, Bellynck V (2010) MT on and for the web. In: 2010 International conference on natural language processing and knowledge engineering (NLP-KE), IEEE, pp 1–10
Bolukbasi T, Chang KW, Zou JY, Saligrama V, Kalai AT (2016) Man is to computer programmer as woman is to homemaker? Debiasing word embeddings. In: Advances in neural information processing systems 29: annual conference on neural information processing systems 2016, December 5–10. Barcelona, Spain, pp 4349–4357
Boroditsky L, Schmidt LA, Phillips W (2003) Sex, syntax, and semantics. In: Getner D, Goldin-Meadow S (eds) Language in mind: advances in the study of language and thought. MIT Press, Cambridge, pp 61–79
Google Scholar
Bureau of Labor Statistics (2017) Table 11: employed persons by detailed occupation, sex, race, and Hispanic or Latino ethnicity, 2017. Labor force statistics from the current population survey, United States Department of Labor, Washington D.C
Carl M, Way A (2003) Recent advances in example-based machine translation, vol 21. Springer, Berlin
Book MATH Google Scholar
Chomsky N (2011) The golden age: a look at the original roots of artificial intelligence, cognitive science, and neuroscience (partial transcript of an interview with N. Chomsky at MIT150 Symposia: Brains, minds and machines symposium). https://chomsky.info/20110616/ . Last visited 26 Dec 2017
Clauburn T (2018) Boffins bash Google Translate for sexism. https://www.theregister.co.uk/2018/09/10/boffins_bash_google_translate_for_sexist_language/ . Posted 10 Sep 2018
Dascal M (1982) Universal language schemes in England and France, 1600–1800 comments on James Knowlson. Studia leibnitiana 14(1):98–109
Diño G (2019) He said, she said: addressing gender in neural machine translation. https://slator.com/technology/he-said-she-said-addressing-gender-in-neural-machine-translation/ . Posted 22 Jan 2019
Dryer MS, Haspelmath M (eds) (2013) WALS online. Max Planck Institute for Evolutionary Anthropology, Leipzig
Firat O, Cho K, Sankaran B, Yarman-Vural FT, Bengio Y (2017) Multi-way, multilingual neural machine translation. Comput Speech Lang 45:236–252. https://doi.org/10.1016/j.csl.2016.10.006
Article Google Scholar
Garcia M (2016) Racist in the machine: the disturbing implications of algorithmic bias. World Policy J 33(4):111–117
Google: language support for the neural machine translation model (2017). https://cloud.google.com/translate/docs/languages#languages-nmt . Last visited 19 Mar 2018
Gordin MD (2015) Scientific Babel: how science was done before and after global English. University of Chicago Press, Chicago
Book Google Scholar
Hajian S, Bonchi F, Castillo C (2016) Algorithmic bias: from discrimination discovery to fairness-aware data mining. In: Proceedings of the 22nd ACM SIGKDD international conference on knowledge discovery and data mining, ACM, pp 2125–2126
Hutchins WJ (1986) Machine translation: past, present, future. Ellis Horwood, Chichester
Johnson M, Schuster M, Le QV, Krikun M, Wu Y, Chen Z, Thorat N, Viégas FB, Wattenberg M, Corrado G, Hughes M, Dean J (2017) Google’s multilingual neural machine translation system: enabling zero-shot translation. TACL 5:339–351
Kay P, Kempton W (1984) What is the Sapir–Whorf hypothesis? Am Anthropol 86(1):65–79
Kelman S (2014) Translate community: help us improve google translate!. https://search.googleblog.com/2014/07/translate-community-help-us-improve.html . Last visited 12 Mar 2018
Kirkpatrick K (2016) Battling algorithmic bias: how do we ensure algorithms treat us fairly? Commun ACM 59(10):16–17
Knebel P (2019) Nós, os robôs e a ética dessa relação. https://www.jornaldocomercio.com/_conteudo/cadernos/empresas_e_negocios/2019/01/665222-nos-os-robos-e-a-etica-dessa-relacao.html . Posted 4 Feb 2019
Koehn P (2009) Statistical machine translation. Cambridge University Press, Cambridge
Koehn P, Hoang H, Birch A, Callison-Burch C, Federico M, Bertoldi N, Cowan B, Shen W, Moran C, Zens R, Dyer C, Bojar O, Constantin A, Herbst E (2007) Moses: open source toolkit for statistical machine translation. In: ACL 2007, Proceedings of the 45th annual meeting of the association for computational linguistics, June 23–30, 2007, Prague, Czech Republic. http://aclweb.org/anthology/P07-2045 . Accessed 9 Mar 2019
Locke WN, Booth AD (1955) Machine translation of languages: fourteen essays. Wiley, New York
MATH Google Scholar
Mills KA (2017) ’Racist’ soap dispenser refuses to help dark-skinned man wash his hands—but Twitter blames ’technology’. http://www.mirror.co.uk/news/world-news/racist-soap-dispenser-refuses-help-11004385 . Last visited 17 Dec 2017
Moss-Racusin CA, Molenda AK, Cramer CR (2015) Can evidence impact attitudes? Public reactions to evidence of gender bias in stem fields. Psychol Women Q 39(2):194–209
Norvig P (2017) On Chomsky and the two cultures of statistical learning. http://norvig.com/chomsky.html . Last visited 17 Dec 2017
Olson P (2018) The algorithm that helped Google Translate become sexist. https://www.forbes.com/sites/parmyolson/2018/02/15/the-algorithm-that-helped-google-translate-become-sexist/#1c1122c27daa . Last visited 12 Mar 2018
Papenfuss M (2017) Woman in China says colleague’s face was able to unlock her iPhone X. http://www.huffpostbrasil.com/entry/iphone-face-recognition-double_us_5a332cbce4b0ff955ad17d50 . Last visited 17 Dec 2017
Rixecker K (2018) Google Translate verstärkt sexistische vorurteile. https://t3n.de/news/google-translate-verstaerkt-sexistische-vorurteile-1109449/ . Posted 11 Sep 2018
Santacreu-Vasut E, Shoham A, Gay V (2013) Do female/male distinctions in language matter? Evidence from gender political quotas. Appl Econ Lett 20(5):495–498
Schiebinger L (2014) Scientific research must take gender into account. Nature 507(7490):9
Shankland S (2017) Google Translate now serves 200 million people daily. https://www.cnet.com/news/google-translate-now-serves-200-million-people-daily/ . Last visited 12 Mar 2018
Thompson AJ (2014) Linguistic relativity: can gendered languages predict sexist attitudes?. Linguistics Department, Montclair State University, Montclair
Wang Y, Kosinski M (2018) Deep neural networks are more accurate than humans at detecting sexual orientation from facial images. J Personal Soc Psychol 114(2):246–257
Weaver W (1955) Translation. In: Locke WN, Booth AD (eds) Machine translation of languages, vol 14. Technology Press, MIT, Cambridge, pp 15–23. http://www.mt-archive.info/Weaver-1949.pdf . Last visited 17 Dec 2017
Women’s Bureau – United States Department of Labor (2017) Traditional and nontraditional occupations. https://www.dol.gov/wb/stats/nontra_traditional_occupations.htm . Last visited 30 May 2018
Download references
Acknowledgements
This study was financed in part by the Coordenação de Aperfeiçoamento de Pessoal de Nível Superior - Brasil (CAPES) - Finance Code 001 and the Conselho Nacional de Desenvolvimento Científico e Tecnológico (CNPq).
Author information
Authors and affiliations.
Federal University of Rio Grande do Sul, Porto Alegre, Brazil
Marcelo O. R. Prates, Pedro H. Avelar & Luís C. Lamb
You can also search for this author in PubMed Google Scholar
Corresponding author
Correspondence to Marcelo O. R. Prates .
Ethics declarations
Conflict of interest.
All authors declare that they have no conflict of interest.
Additional information
Publisher's note.
Springer Nature remains neutral with regard to jurisdictional claims in published maps and institutional affiliations.
Rights and permissions
Reprints and permissions
About this article
Prates, M.O.R., Avelar, P.H. & Lamb, L.C. Assessing gender bias in machine translation: a case study with Google Translate. Neural Comput & Applic 32 , 6363–6381 (2020). https://doi.org/10.1007/s00521-019-04144-6
Download citation
Received : 19 October 2018
Accepted : 09 March 2019
Published : 27 March 2019
Issue Date : May 2020
DOI : https://doi.org/10.1007/s00521-019-04144-6
Share this article
Anyone you share the following link with will be able to read this content:
Sorry, a shareable link is not currently available for this article.
Provided by the Springer Nature SharedIt content-sharing initiative
- Machine bias
- Gender bias
- Machine learning
- Machine translation
- Find a journal
- Publish with us
- Track your research
Browse Course Material
Course info, instructors.
- Dr. Richard Fletcher
- Prof. Daniel Frey
- Dr. Mike Teodorescu
- Amit Gandhi
- Audace Nakeshimana
Departments
- Supplemental Resources
As Taught In
- Artificial Intelligence
- Curriculum and Teaching
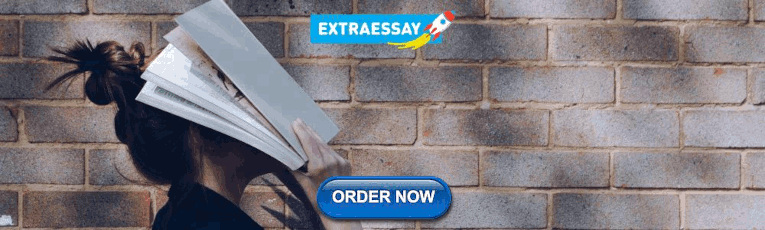
Learning Resource Types
Exploring fairness in machine learning for international development, case study with data: mitigating gender bias on the uci adult database.
- Download video
- Download transcript
Mitigating Gender Bias slides (PDF - 1.6MB)
Learning Objectives
- Explore steps and principles involved in building less-biased machine learning modules.
- Explore two classes of technique, data-based and model-based techniques for mitigating bias in machine learning.
- Apply these techniques to the UCI adult dataset.
The repository for this module can be found at Github - ML Bias Fairness .
Defining algorithmic/model bias
Bias or algorithmic bias will be defined as systematic errors in an algorithm/model that can lead to potentially unfair outcomes.
Bias can be quantified by looking at discrepancies in the model error rate for different populations.
UCI adult dataset
The UCI adult dataset is a widely cited dataset used for machine learning modeling. It includes over 48,000 data points extracted from the 1994 census data in the United States. Each data point has 15 features, including age, education, occupation, sex, race, and salary, among others.
The dataset has twice as many men as women. Additionally, the data shows income discrepancies across genders. Approximately 1 in 3 of men are reported to make over $50K, whereas only 1 in 5 women are reported to make the same amount. For high salaries, the number of data points in the male population is significantly higher than the number of data points in the female category.
Preparing data
In order to prepare the data for machine learning, we will explore different steps involved in transforming data from raw representation to appropriate numerical or categorical representation. One example is converting native country to binary, representing individuals whose native country was the US as 0 and individuals whose native country was not the US as 1. Similar representations need to be made for other attributes such as sex and salary. One-hot encoding can be used for attributes where more than two choices are possible.
Binary coding was chosen for simplicity, but this decision must be made on a case-by-case basis. Converting features like work class can be problematic if individuals from different categories have systematically different levels of income. However, not doing this can also be problematic if one category has a population that is too small to generalize from.
Illustrating gender bias
We apply the standard ML approach to the UCI adult dataset. The steps that are followed are 1) splitting the dataset into training and test data, 2) selecting model (MLPClassifier in this case), 3) fitting the model on training data, and 4) using the model to make predictions on test data. For this application, we will define the positive category to mean high income (>$50K/year) and the negative category to mean low income (<=$50K/year).
The model results show that the positive rates and true positive rates are higher for the male demographic. Additionally, the negative rate and true negative rates are higher for the female demographic. This shows consistent disparities in the error rates between the two demographics, which we will define as gender bias.
Exploring data-based debiasing techniques
We hypothesize that gender bias could come from unequal representation of male and female demographics. We attempt to re-calibrate and augment the dataset to equalize the gender representation in our training data. We will explore the following techniques and their outcomes. We will compare the results after describing each approach.
Debiasing by unawareness: we drop the gender attribute from the model so that the algorithm is unaware of an individual’s gender. Although the discrepancy in overall accuracy does not change, the positive, negative, true positive, and true negative rates are much closer for the male and female demographics.
Equalizing the number of data points: we attempt different approaches to equalizing the representation. The different equalization criteria are #male = #female, #high income male = #high income female, #high income male/#low income male = #high income female/#low income female. One of the disadvantages of equalizing the number of data points is that the dataset size is limited by the size of the smallest demographic. Equalizing the ratio can overcome this limitation.
Augment data with counterfactuals: for each data point X i with a given gender, we generate a new data point Y i that only differs with X i at the gender attribute and add it to our dataset. The gaps between male and female demographics are significantly reduced through this approach.
We see varying accuracy across different approaches on accuracy for the male and female demographics, as shown in the plot below. The counterfactual approach is shown to be the best at reducing gender bias. We see similar behavior for the positive rates and negative rates as well as the true positive and true negative rates.
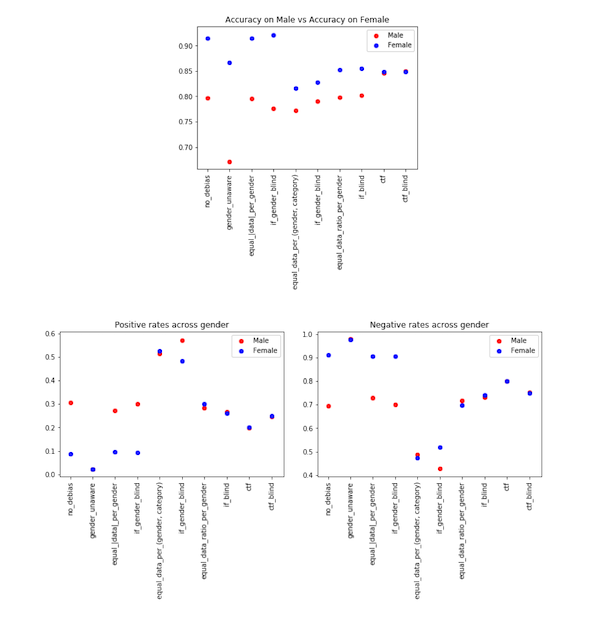
Model-based debiasing techniques
Different ML models show different levels of bias. By changing the model type and architecture, we can observe which ones will be less biased for this application. We examine single and multi-model architectures. The models that will be considered are support vector, random forest, KNN, logistic regression, and MLP classifiers. Multi-model architectures involve training a group of different models that make a final prediction based on consensus. Two approaches can be used for consensus; hard voting, where the final prediction is the majority prediction among the models and soft voting, where the final prediction is the average prediction. The following plots show the differences in overall accuracy and the discrepancies between accuracy across gender.
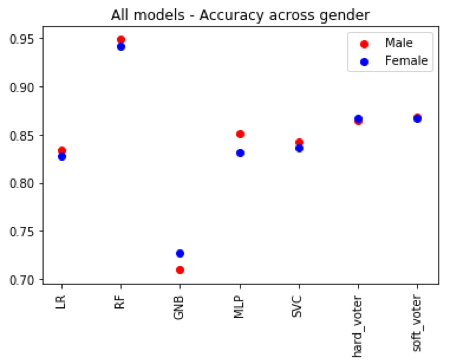
It is also important to compare the results of the models across multiple training sessions. For each model type, five instances of the model were trained and compared. Results are shown the plot below. We can see that different models have different variability in performance for different metrics of interest.
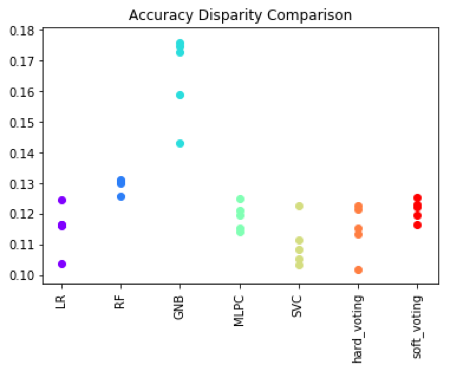
Dua, D. and Graff, C. (2019). UCI Machine Learning Repository . Irvine, CA: University of California, School of Information and Computer Science.
Bishop, Christopher M. Pattern Recognition and Machine Learning . New York: Springer 2006. ISBN: 9780387310732.
Hardt, Moritz. (2016, October 7). “ Equality of opportunity in machine learning .” Google AI Blog .
Zhong, Ziyuan. (2018, October 21). “ A tutorial on fairness in machine learning .” Toward Data Science .
Kun, Jeremy. (2015, October 19). “ One definition of algorithmic fairness: statistical parity .”
Olteanu, Alex. (2018, January 3). “ Tutorial: Learning curves for machine learning in Python .” DataQuest .
Garg, Sahaj, et al. 2018. “ Counterfactual fairness in text classification through robustness .” arXiv preprint arXiv:1809.10610.
Wikipedia contributors. (2019, September 6). “ Algorithmic bias .” In Wikipedia, The Free Encyclopedia .
Contributions
Content presented by Audace Nakeshimana (MIT).
This content was created by Audace Nakeshimana and Maryam Najafian (MIT).

You are leaving MIT OpenCourseWare
Thank you for visiting nature.com. You are using a browser version with limited support for CSS. To obtain the best experience, we recommend you use a more up to date browser (or turn off compatibility mode in Internet Explorer). In the meantime, to ensure continued support, we are displaying the site without styles and JavaScript.
- View all journals
- My Account Login
- Explore content
- About the journal
- Publish with us
- Sign up for alerts
- Open access
- Published: 26 January 2022
Gender and feminist considerations in artificial intelligence from a developing-world perspective, with India as a case study
- Shailendra Kumar ORCID: orcid.org/0000-0002-7493-5496 1 &
- Sanghamitra Choudhury ORCID: orcid.org/0000-0003-1417-1735 2 , 3 , 4 , 5 , 6
Humanities and Social Sciences Communications volume 9 , Article number: 31 ( 2022 ) Cite this article
15k Accesses
5 Citations
30 Altmetric
Metrics details
- Complex networks
- Development studies
- Information systems and information technology
- Science, technology and society
This manuscript discusses the relationship between women, technology manifestation, and likely prospects in the developing world. Using India as a case study, the manuscript outlines how Artificial Intelligence (AI) and robotics affect women’s opportunities in developing countries. Women in developing countries, notably in South Asia, are perceived as doing domestic work and are underrepresented in high-level professions. They are disproportionately underemployed and face prejudice in the workplace. The purpose of this study is to determine if the introduction of AI would exacerbate the already precarious situation of women in the developing world or if it would serve as a liberating force. While studies on the impact of AI on women have been undertaken in developed countries, there has been less research in developing countries. This manuscript attempts to fill that need.
Similar content being viewed by others
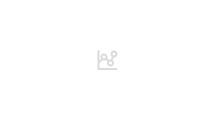
Importance and limitations of AI ethics in contemporary society
The impact of artificial intelligence on employment: the role of virtual agglomeration, towards a new generation of artificial intelligence in china, introduction.
Women in some South-Asian countries, like India, Pakistan, Bangladesh, and Afghanistan face significant hardships and problems, ranging from human trafficking to gender discrimination. Compared to their counterparts in developed countries, developing-world women encounter a biased atmosphere. Many South Asian countries have a patriarchal and male-dominated society, and their culture has a strong preference for male offspring (Kristof, 1993 ; Bhalotra et al., 2020 ; Oomman and Ganatra, 2002 ). These countries, particularly India, have experienced instances where technology has been utilized to create gender bias (Guilmoto, 2015 ). For example, there has been a great misuse of some of the techniques, of which sonography, or ultrasound , is one. The public misappropriated sonography , which was intended to determine the unborn’s health select the fetus’s gender, and perform an abortion if the fetus is female (Alkaabi et al., 2007 ; Akbulut-Yuksel and Rosenblum, 2012 ; Bowman-Smart et al., 2020 ). Many Indian states, particularly the northern states of India, have seen a significant drop in the sex ratio due to this erroneous use of technology. India’s unbalanced child sex ratio has rapidly deteriorated. In 1981, there were 962 females in the 0–6-year age group for every 1000 boys. In 1991, there were 945 girls, then 927 girls in 2001, and 918 girls at the time of the 2011 Census (Bose, 2011 ; Hu and Schlosser, 2012 ). In 1994, the Indian government passed the Pre-conception and Pre-Natal Diagnostic Techniques (Prohibition of Sex Selection) Act to halt this trend. The law made it unlawful for medical practitioners to divulge the sex of a fetus due to worries that ultrasound technology was being used to detect the sex of the unborn child and terminate the female fetus (Nidadavolu and Bracken, 2006 ; Ahankari et al., 2015 ). However, as the Indian Census data over the years demonstrates, the law did not operate as well as intended. According to public health campaigners, this is due to the Indian government’s failure to enforce the law effectively; sex determination and female feticide continue due to medical practitioners’ lack of oversight. Radiologists and gynecologists, on the other hand, argue that it’s because the law was misguided from the outset, holding the medical profession responsible for a societal problem (Tripathi, 2016 ).
In the realm of artificial intelligence, gender imbalance is a critical issue. Because of how AI systems are developed, gender bias in AI can be problematic. Algorithm developers may be unaware of their prejudices, and implicit biases and unknowingly pass their socially rooted gender prejudices onto robots. This is evident because the present trends in machine learning reinforce historical stereotypes of women, such as humility, mildness, and the need for protection. For instance, security robots are primarily male, but service and sex robots are primarily female. Another example is AI-driven risk analysis in the justice system. The algorithms may overlook the fact that women are less likely to re-offend than men, placing women at a disadvantage. Female gendering increases bots’ perceived humanity and the acceptability of AI. Consumers believe that female AI is more humane and more reliable and therefore more ready to meet users’ particular demands. Because the feminization of robots boosts their marketability, AI is attempting to humanize them by embedding feminist characteristics and striving for acceptability in a male-dominated robotic society. There’s a risk that machine learning technologies will wind up having biases encoded if women don’t make a significant contribution. Diverse teams made up of both men and women are not just better at recognizing skewed data, but they’re also more likely to spot issues that could have dire societal consequences. While women’s characteristics are highly valued in AI robots, protein-based Footnote 1 women’s jobs are in jeopardy. Presently, women hold only 22% of worldwide AI positions while men hold 78% (World Economic Forum Report, 2018 ). According to another study by wired.com, only 12% of machine learning researchers are women, which is a concerning ratio for a subject that is meant to transform society (Simonite, 2018 ). Because women make up such a small percentage of the technological workforce, technology may become the tangible incarnation of male power in the coming years. The situation in the developing world is worse, and hence a cautious approach is required to ensure that a male-dominated society in some of the developing world’s countries does not abuse AI’s power and use it to exacerbate the predicament of women who are already in a precarious situation.
This manuscript discusses the relationship between women, technology, manifestation, and probable prospects in the developing world. Taking India as a case study, the manuscript further focuses on how the ontology and epistemology perspectives used in the fields of AI, and robotics will affect the futures of women in developing countries. Women in the developing world, particularly in South Asia, are stereotyped as doing domestic work and have low representation in high-level positions. They are disproportionately underemployed and endure employment discrimination. The article will attempt to explore, whether the introduction of AI would exacerbate the already fragile position of women in South Asia or serve as a liberating force for them. While studies on the influence of AI on women have been conducted in industrialized countries, research in developing countries has been minimal. This manuscript will aim to bridge this gap.
The study tests the following hypothesis:
H01: The changing lifestyle, growing challenges, and increasing use of AI robots in daily life may not be a threat to the existing human relationship.
HA1: The changing lifestyle, growing challenges, and increasing use of AI robots in daily life may be a threat to the existing human relationship.
H02: There is no significant difference in the perception of females and males towards AI robots.
HA2: There is a significant difference in the perception of females and males towards AI robots.
H03: There is no major variation in the requirements and utilization of AI robots between men and women.
HA3: There is a considerable difference in the requirements and use of AI robots between men and women.
H04: When it comes to the gender of the robots, there is no substantial difference between male and female preferences.
HA4: When it comes to the gender of the robots, there is a substantial difference between male and female preferences.
The manuscript is divided into four sections. The first section is an introduction, followed by a literature review, methodology, results and discussion, and finally, a conclusion.
Literature review
AI’s basic assumption is that human intellect can be studied and simulated so that computers can be programmed to perform tasks that people can do (Guo, 2015 ). Alan Turing, the pioneer of AI, felt that to develop intelligent things like homo sapiens, humans ought to be considered as mechanical beings rather than as emotionally manifested super-beings so that they could be studied and replicated (Evers, 2005 ; Sanders, 2008 ). Rosalind Picard published “Affective Computing” in 1997, with the basic premise being that if we want smarter computers that interact with people more naturally, we must give them the ability to identify, interpret, and even express emotions. This approach went against popular belief, which held that pure logic was the highest type of AI (Venkatraman, 2020 ). Many human features have been transferred into non-human robots as a result of AI advancements, and expertise and knowledge will no longer be confined to only humans (Lloyd, 1985 ). Researchers like Lucy Suchman look at how agencies are now arranged at the human-machine interface and how they might be reimagined creatively and materially (Suchman, 2006 ). Weber believes that recent advancements in robotics and AI are going towards social robotics, as opposed to past achievements in robotics and AI, which can be attributed to fairly strict, law-oriented, conceding to adaptive human behavior towards machines (Weber, 2005 , p. 210). Weber goes on to say that social roboticists want to take advantage of the human predisposition to anthropomorphize machines and connect with them socially by structuring them to look like a woman, a child, or a pet (Weber, 2005 , p. 211). Reeves and Nass say humans communicate with artificial media, such as computers, in the same way, that they connect with other humans (Reeves and Naas, 1996 ). Studies further indicate that humans are increasingly relying on robots, and with the advancement of social artificially intelligent bots such as Alexa and Google Home, comme les companionship between comme les robots and humans is now becoming a reality (de Swarte et al., 2019 ; Odekerken-Schröder et al., 2020 ). It is believed that robots will not only get smarter over the next few decades, but they will also establish physical and emotional relationships with humans. This raises a fresh challenge about how people view robots intended for various types of closeness, both as friends and as possible competitors (Nordmo et al., 2020 ). According to Erika Hayasaki, artificial intelligence will be smarter than humans in the not-too-distant future. However, as technology advances, it may become increasingly racial, misogynistic, and inhospitable to women as it absorbs cultural norms from its designers and the internet (Hayasaki, 2017 ). The rapid advancement in robot and AI technology has highlighted some important problems with issues of gender bias (Bass, 2017 ; Leavy, 2018 ). There are apprehensions that algorithms will be able to target women specifically in the future (Caliskan et al., 2017 ). Given the increasing prominence of artificial intelligence in our communities, such attitudes risk leaving women stranded in many facets of life (UNESCO Report, 2020 ; Prives, 2018 ). Discrimination is no longer a purely human problem, as many decision-makers are aware that discriminating against someone based on an attribute such as sex is illegal and immoral. Therefore, they conceal their true intent behind an innocuous, constructed excuse (Santow, 2020 ). In the literature surrounding the discipline of Critical Algorithm Series, gender bias is a commonly debated topic (Pillinger, 2019 ). Donna Haraway published her famous essay titled, “A Cyborg Manifesto” in Socialist Review in 1985. In the essay, she criticized the traditional concepts of feminism as they mainly focus on identity politics. Haraway, inspires a new way of thinking about how to blur the lines between humans and machines and supports coalition-building through affinity (Pohl, 2018 ). She employs the notion of the cyborg to encourage feminists to think beyond traditional gender, feminism, and politics (Haraway, 1987 , 2006 ). She further claims that, with the help of technology, we may all promote hybrid identities, and people will forget about gender supremacy as they become more attached to their robotics (Haraway, 2008 ). To date, however, this has not been the case. In recent times, growing machine prejudice is a problem that has sparked great concern in academia, industry research laboratories, and the mainstream commercial media, where trained statistical models, unknown to their developers, have grown to reflect critical societal imbalances like gender or racial bias (Hutson, 2017 ; Hardesty, 2018 ; Nurock, 2020 ; Prates et al., 2020 ). While previous research indicates that gendered and lifelike robots are perceived as more sentient, the researchers of those studies primarily ignored the distinction between female and male bots (DiMaio, 2021 ). The study by Sylvie Borau et al. indicates that female artificial intelligence is preferred by customers because it is seen to have more good human attributes than male artificial intelligence, such as affection, understanding, and emotion (Borau et al., 2021 ). This is consistent with the literature, which suggests that male robots may appear intimidating in the home and that people trust female bots for in-home use (Carpenter, 2009 ; Niculescu et al., 2010 ). Another study indicates that when participants’ gender matched the gender of AI personal assistant Siri’s voice, participants exhibited more faith in the machine (Lee et al., 2021 ). Human–robot-interaction (HRI) researchers have undertaken various studies on gender impacts to determine if men or women are more inclined to prefer or dislike robots. According to research, males showed more positive attitudes toward interacting with social robots than females in general (Lin et al., 2012 ), and females had more negative attitudes regarding robot interactions than males in particular (Nomura and Kanda, 2003 ; Nomura et al., 2006 ). In another study, Nomura urged researchers to explore whether gendering robots for specific roles is truly required to foster human–robot interaction (Nomura, 2017 ). The investigation by R. A. Søraa demonstrates how gendering practices of humans influence mechanical species of robots, concluding that the more sapient a robot grows, the more gendered it may become (Søraa, 2017 ). “I am for more women in robotics, not for more female robots,” Martina Mara, director of the RoboPsychology R & D division at the Ars Electronica Futurelab, adds (Hieslmair, 2017 ). While there is a large body of work describing gender bias in AI robots in developed countries, little research has been done on the impact of AI robots on women in developing and undeveloped countries. This article will try to bridge the gaps in the studies.
Methodology
The overarching purpose of this exploratory project is to look at gender and feminist issues in artificial intelligence from a developing-world perspective. It also intends to depict how men and women in developing countries react to the prospect of having robot lovers, companions, and helpers in their everyday lives. A vignette experiment was conducted with 125 female and 100 male volunteers due to the lack of commercial availability of such robots. The survey was distributed online, which aided in the recruitment of volunteers. The vast majority of those who responded (76%) were university students from India. Because the study was conducted during the COVID-19 pandemic phase, the questionnaire was prepared in Google Form and distributed to participants via WhatsApp group and email. The participants ranged from 16 to 60 years old, with an average age of 27.25 (SD = 7.722) years. The vignette was created to portray a futuristic human existence as well as a variety of futuristic robots and their influence on people. The extracts from the vignette are: “At today’s science fair, Sam and Sophie, who are husband and wife, came across a variety of AI robots, including domestic robots, sex robots, doctor robots, nurse robots, engineer robots, personal assistant robots, and so on. This made them pleasantly surprised, and they were left to believe that in the future, AI robot technology will become so sophisticated that it will develop as an efficient alternative to protein-based humans. Back at home, Sophie utilizes Google Assistant and Alexa Assistant in the form of AI devices in the couple’s house to listen to music, cook food recipes, and entertain their children, while Sam uses them to get weather information, traffic information, book products online, set reminders, and so on. Mesmerized by the advancements in AI technology displayed at the science fair, Sam jokingly told Sophie that if there was a beautiful robot that could do household chores and give love and care to a partner, then the person would not need a marriage nor would he have to go through problems like break-up and divorce. Sophie disagreed, believing that the robot could not grasp or duplicate the person’s feelings and mood. Sam disagreed, claiming that a protein-based individual isn’t immune to these flaws either, because if that had been the case, human relationships would be devoid of misery, pain, violence, and breakups. Sophie thought that people need a companion but are unwilling to put up with human imperfections and that many people love the feminine traits inherent in AI robots but do not want to engage in a true relationship with real women.” After the respondents had finished reading the vignettes, they were handed the questionnaire and asked to fill it out. The questions were all delivered in English, with translation services available if necessary. Participation was entirely voluntary, and respondents were offered the option of remaining anonymous and not disclosing any further personal information such as their address, email address, or phone number. The participants gave their informed consent, and the participants’ personal information was kept private and confidential.
In this study, a varied group was subjected to demographic data sheets as well as a self-developed questionnaire. The people that have been surveyed are of different sexes, ages, educational levels, and marital statuses. The purposive (simple random) sampling technique was used to attain the objectives.
The research was conducted using a self-developed questionnaire. All the items on the scale were analyzed using IBM SPSS ® 23. Mean, standard deviation, total-item correlation, regression, and reliability analysis were performed on all the items. The questionnaire measured the items of AI using the following four scales: perspective, gender, requirements/use, and the threat of using AI. The alpha has been set to default 0.05 as a cut-off for significance.
Results and discussion
Table 1 shows a positive and significant intra-correlation between factors, such as perceptions towards AI robots, AI robot use and requirements, robot gender, and the threat from AI robots.
A regression study to identify threat factors with respect to AI robots is shown in Table 2 . The threat posed by AI robots is predicted by people’s perception of AI robots and the gender of AI robots. As a result, the hypothesis, that the changing lifestyle, growing challenges, and increasing use of AI robots in daily life may be a threat to the various existing human relationships is accepted. It could be inferred that people may be more motivated to live with AI robots due to changing lifestyles and increasing challenges and complications in human relationships (see Fig. 1 ). The majority of the respondents believe that greater use and reliance on AI robot technology will jeopardize the existence of protein-based people and lead to more prejudiced, discriminatory, and solitary societies. This is in support of the findings of the study, which show that people have an innate dread that robots will one day outwit them to the point that they will start exploiting humans (see Fig. 2 ). Moreover, the majority of respondents feel that AI may have a significant impact on gender balance in countries like India and that it may also affect gender balance in the subcontinent. This is in line with past concerns in India about the misuse of sonography technology (see Fig. 3 ).
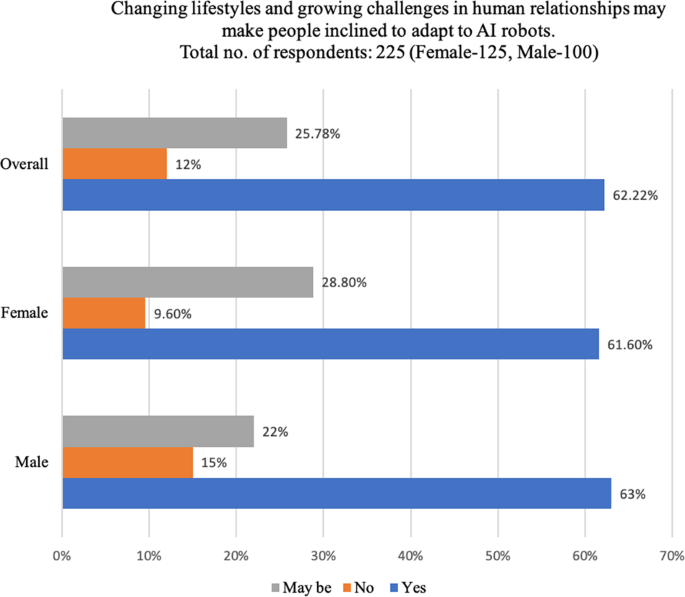
Total no. of respondents: 225 (Female: 125, Male: 100).
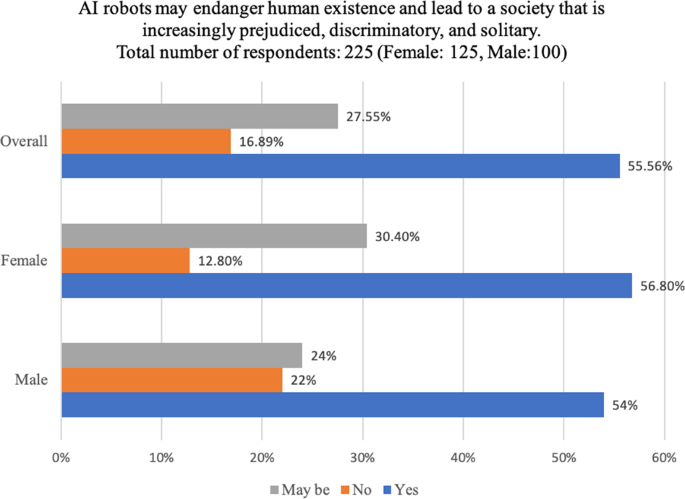
Table 3 exhibits the results of the t -test. The results portray a significant difference between male and female respondents on the perception of AI robots. Therefore, the null hypothesis is rejected and the alternate hypothesis stating that there is a significant difference in the perception of females and males towards AI robots can be accepted. The t -test further indicates that there exists no significant difference between males and females on the requirements and use of AI robots, as no significant gender difference was found on the requirements and use of AI robots by both males and females. The majority of respondents believe that living with AI robots will become a reality soon (see Fig. 4 ) and that the AI robots’ ethnic and racial appearance may have a significant impact on consumers’ AI robot purchasing preferences (see Figs. 5 and 6 ). The respondents also advocated different ethical programming for the female and male robots (see Fig. 7 ). However, as far as the use of robots is concerned, it is evident that all other categories of robots have shown roughly equal demand from both males and females, except for sex and love robots, where the gender discrepancy is more than twice, with 24% of males and 8.8% of females indicating a need for sex and love robots (see Fig. 8 ). Assistant robots, teaching robots, entertainment robots, robots that do household tasks and errands, and robots that offer care were among the most popular. Females showed the least interest in sex and love robots, while males showed the least interest in companion and friendship robots. Overall, 15.56% of respondents said they were open to having sex and love robots. This demonstrates the general concern and reluctance to engage in sexual or romantic relationships with AI computers. Females, on the other hand, appear to be more hesitant and averse to engaging in intimacy with robots than males. The key reasons for this are sentiments of envy and insecurity. The study’s findings also show that males in developing countries have a more favorable attitude toward sex robots than females. This confirms the existence of gender differences in emotional intimacy and sex preferences (Johnson, 2004 ). Sex robots have the potential to reduce or eliminate prostitution, human trafficking, and sex tourism for women (Yeoman and Mars, 2012 ) in developing countries. According to data from the Indian government’s National Crime Record Bureau (NCRB), 95% of trafficked people in India are forced into prostitution (Divya, 2020 ; Munshi, 2020 ).
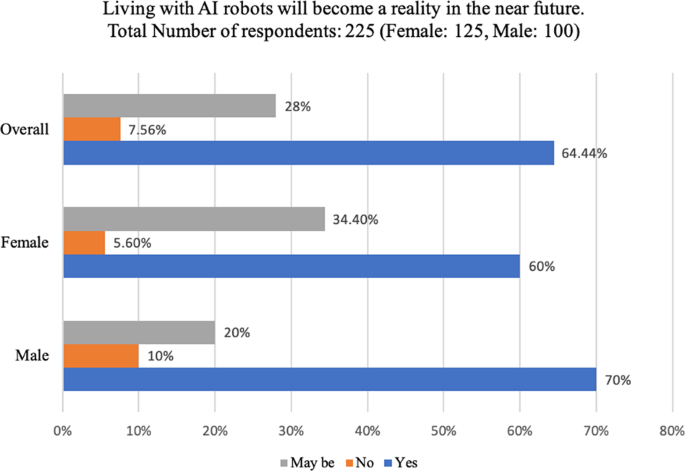
Total number of respondents:225 (Female: 125, Male: 100).
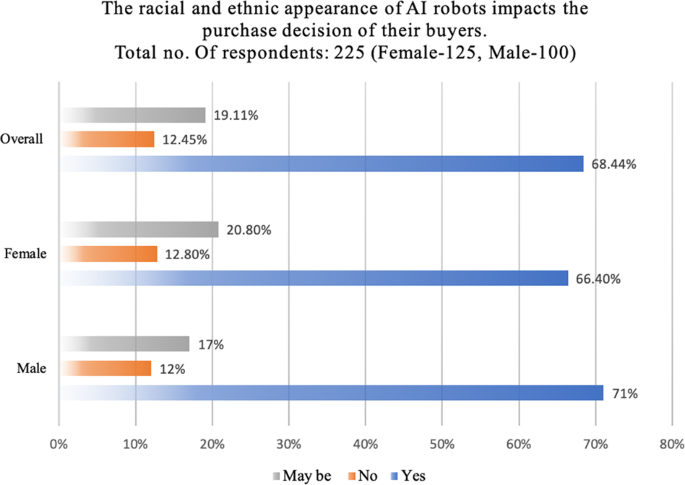
The t -test further indicates that there exists no significant difference between male and female respondents as far as the gender of the robots is concerned. However, the analysis of mean figures indicates that the majority of respondents believe that gender has a role in AI robot development and production (see Fig. 9 ), and AI will have a greater impact on both men and women (see Fig. 10 ), but when asked which gender AI will have the greatest impact on, both genders answer that AI will have the greatest impact on their gender (see Fig. 11 ). While most people disagreed with the premise that female robots are seen by them as more humane (see Fig. 12 ), they did agree that female robots are regarded differently by them (see Fig. 13 ). They further agreed that feminizing robots improves their marketability and acceptance (see Fig. 14 ), and that corporations are using feminity to humanize non-human things like AI robots (see Fig. 15 ). The responses also indicate that a majority of the respondents (55.11%) believe that the use of feminine features in robots increases the risk of females being stereotyped (see Fig. 16 ).
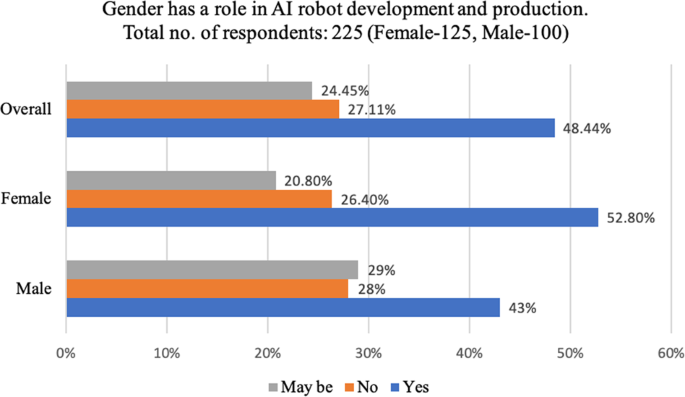
The gendering of the robots in AI is problematic and does not always lead to the desired improved acceptability, as gender makes no difference in terms of robot functionality and performance. The study contradicts the widely held belief that women in developing nations such as India are wary about living with AI robots in the near future. It also shows that both men and women feel AI will have a stronger impact on both males and females. The majority of respondents dissented that female robots are considered more commiserate than male robots, but they accede to the fact that female robots are perceived differently, even when they are robots. The majority of respondents feel that AI will have a significant impact on gender balance in nations like India just like the sonography technique that was misused for doing gender selection. However, they are in general agreement with the fact that people may be motivated to live with AI robots due to changing lifestyles and increasing challenges and complications in human relationships. Due to the rising cost of maintaining a protein-based lifestyle, humans may turn to robots to eschew the issues that come with it. The study also found people in developing countries have an innate dread that robots will one day outwit them.
Data availability
The data underpinning the study includes a dataset that has been deposited in the Harvard Dataverse repository. Please refer to Kumar, Shailendra; Choudhury, Sanghamitra, 2022, “Gender and Feminist Considerations in Artificial Intelligence from a Developing-World Perspective, with India as a Case Study”, https://doi.org/10.7910/DVN/0T3P1E , Harvard Dataverse, V1, UNF:6:oecwn9YFMHRv369S0mDW5w== [fileUNF].
The term “protein-based” has been used in the manuscript as a synonym for “humans”, with the understanding that, except for water and fat, the human body is virtually entirely made up of protein. Muscles, bones, organs, skin, and nails are all made up of protein. Muscles are made up of around 80% protein, excluding water.
Ahankari AS, Myles P, Tata LJ, Fogarty AW (2015) Banning of fetal sex determination and changes in sex ratio in India. Lancet Global Health. https://doi.org/10.1016/S2214-109X(15)00053-4
Akbulut-Yuksel M, Rosenblum D (2012) The Indian Ultrasound Paradox. IZA Discussion Paper (6273).
Alkaabi JM, Ghazal-Aswad S, Sagle M (2007) Babies as desired: ethical arguments about gender selection. Emir Med J. 25(1):1–5
Bass D (2017) Researchers combat gender and racial bias in artificial intelligence. Bloomberg 1–9. https://www.bloomberg.com/news/articles/2017-12-04/researchers-combat-gender-and-racial-bias-in-artificial-intelligence
Bhalotra S, Brulé R, Roy S (2020) Women’s inheritance rights reform and the preference for sons in India. J Dev Econ 146. https://doi.org/10.1016/j.jdeveco.2018.08.001
Borau S, Otterbring T, Laporte S, Fosso Wamba S (2021) The most human bot: female gendering increases humanness perceptions of bots and acceptance of AI. Psychol Mark 38(7):1052–1068. https://doi.org/10.1002/mar.21480
Article Google Scholar
Bose A (2011) Census of India, 2011. Econ Political Weekly
Bowman-Smart H, Savulescu J, Gyngell C, Mand C, Delatycki, MB (2020) Sex selection and non-invasive prenatal testing: a review of current practices, evidence, and ethical issues. Prenatal diagnosis. John Wiley and Sons Ltd.
Caliskan A, Bryson JJ, Narayanan A (2017) Semantics derived automatically from language corpora contain human-like biases. Science 356(6334):183–186. https://doi.org/10.1126/science.aal4230
Article ADS CAS PubMed Google Scholar
Carpenter J, Davis JM, Erwin-Stewart N, Lee TR, Bransford JD, Vye N (2009) Gender representation and humanoid robots designed for domestic use. Int J Soci Robot 1(3):261–265. https://doi.org/10.1007/s12369-009-0016-4
de Swarte T, Boufous O, Escalle P (2019) Artificial intelligence, ethics and human values: the cases of military drones and companion robots. Artif Life Robot 24(3):291–296. https://doi.org/10.1007/s10015-019-00525-1
DiMaio T (2021) Women are perceived differently from men—even when they’re robots. Acad Times. https://academictimes.com/women-are-perceived-differently-from-men-even-when-theyre-robots/
Divya A (2020) Sex workers in India on the verge of debt bondage and slavery, says a study. Indian Express. https://indianexpress.com/article/lifestyle/life-style/sex-workers-in-india-on-the-verge-of-debt-bondage-and-slavery-says-a-study-7117938/
Evers D (2005) The human being as a turing machine? The question about artificial intelligence in philosophical and theological perspectives. N Z Syst Theol Relig Philos https://doi.org/10.1515/nzst.2005.47.1.101
Guilmoto CZ (2015) Missing girls: a globalizing issue. In: Wright JD (ed.) International encyclopedia of the social & behavioral sciences, 2nd edn. Elsevier Inc., pp. 608–613. https://doi.org/10.1016/B978-0-08-097086-8.64065-5
Guo T (2015) Alan Turing: artificial intelligence as human self-knowledge. Anthropol Today 31(6):3–7. https://doi.org/10.1111/1467-8322.12209
Haraway D (1987) A manifesto for Cyborgs: science, technology, and socialist feminism in the 1980s. Aust Fem Stud2(4):1–42. https://doi.org/10.1080/08164649.1987.9961538
Haraway D (2006) A Cyborg Manifesto: science, technology, and socialist-feminism in the late 20th century. In: Weiss J, Nolan J, Hunsinger J, Trifonas P (eds) The International handbook of virtual learning environments. Springer, Dordrecht
Google Scholar
Haraway DJ (2008) When species meet. University of Minnesota Press, Minneapolis
Hardesty L (2018) Study finds gender and skin-type bias in commercial artificial-intelligence systems. MIT News 1–17. https://news.mit.edu/2018/study-finds-gender-skin-type-bias-artificial-intelligence-systems-0212 (Accessed on 12 Jun 2021)
Hayasaki E (2017) Is AI sexist?. Foreign Policy. https://foreignpolicy.com/2017/01/16/women-vs-the-machine/
Hieslmair M (2017) Martina Mara: “more women in robotics!”. Ars Electronica Blog. https://ars.electronica.art/aeblog/en/2017/03/08/women-robotics/
Hu L, Schlosser A (2012) Trends in prenatal sex selection and girls’ nutritional status in India. CESifo Econ Stud 58(2):348–372. https://doi.org/10.1093/cesifo/ifs022
Hutson M (2017) Even artificial intelligence can acquire biases against race and gender. Science. https://doi.org/10.1126/science.aal1053
Johnson HD (2004) Gender, grade, and relationship differences in emotional closeness within adolescent friendships. Adolescence 39(154):243–255
PubMed Google Scholar
Kristof ND (1993) China: ultrasound abuse in sex selection. Women’s Health J/Isis Int Latin Am Caribbean Women’s Health Netw 4:16–17
Leavy S (2018) Gender bias in artificial intelligence: The need for diversity and gender theory in machine learning. In: Proceedings—international conference on software engineering. IEEE Computer Society, pp. 14–16
Lee SK, Kavya P, Lasser SC (2021) Social interactions and relationships with an intelligent virtual agent. Int J Hum Comput Stud 150. https://doi.org/10.1016/j.ijhcs.2021.102608
Lin CH, Liu EZF, Huang YY (2012) Exploring parents’ perceptions towards educational robots: Gender and socio-economic differences. Br J Educ Technol 43(1). https://doi.org/10.1111/j.1467-8535.2011.01258.x
Lloyd D (1985) Frankenstein’s children: artificial intelligence and human value. Metaphilosophy 16(4):307–318. https://doi.org/10.1111/j.1467-9973.1985.tb00177.x
Munshi S (2020) Human trafficking hit three-year high in 2019 as maha tops list of cases followed by Delhi, Shows NCRB Data. News18 Networks. https://www.news18.com/news/india/human-trafficking-hit-three-year-high-in-2019-as-maha-tops-list-of-cases-followed-by-delhi-shows-ncrb-data-2944085.html . Accessed 15 Aug 2021
Niculescu A, Hofs D, Van Dijk B, Nijholt A (2010) How the agent’s gender influence users’ evaluation of a QA system. In: Fauzi Mohd Saman et al. (eds) Proceedings—2010 International Conference on User Science and Engineering, i-USEr 2010. pp. 16–20
Nidadavolu V, Bracken H (2006) Abortion and sex determination: conflicting messages in information materials in a District of Rajasthan, India. Reprod Health Matters 14(27):160–171. https://doi.org/10.1016/S0968-8080(06)27228-8
Article PubMed Google Scholar
Nomura T, Kanda T (2003) On proposing the concept of robot anxiety and considering measurement of it. In: Proceedings—IEEE international workshop on robot and human interactive communication. pp. 373–378
Nomura T, Kanda T, Suzuki T (2006) Experimental investigation into influence of negative attitudes toward robots on human–robot interaction. AI Soc 20(2):138–150. https://doi.org/10.1007/s00146-005-0012-7
Nomura T (2017) Robots and gender. Gend Genome 1(1):18–26. https://doi.org/10.1089/gg.2016.29002.nom
Nordmo M, Næss JØ, Husøy MF, Arnestad MN (2020) Friends, lovers or nothing: men and women differ in their perceptions of sex robots and platonic love robots. Front Psychol 11. https://doi.org/10.3389/fpsyg.2020.00355
Nurock V (2020) Can ai care? Cuad Relac Lab 38(2):217–229. https://doi.org/10.5209/CRLA.70880
Odekerken-Schröder G, Mele C, Russo-Spena T, Mahr D, Ruggiero A (2020) Mitigating loneliness with companion robots in the COVID-19 pandemic and beyond: an integrative framework and research agenda. J Serv Manag 31(6):1149–1162. https://doi.org/10.1108/JOSM-05-2020-0148
Oomman N, Ganatra BR (2002) Sex selection: the systematic elimination of girls. Reprod Health Matters 10(19):184–188. https://doi.org/10.1016/S0968-8080(02)00029-0
Pillinger A (2019) Gender and feminist aspects in robotics. GEECO Project, European Union. http://www.geecco-project.eu/fileadmin/t/geecco/FemRob_Final_plus_Deckblatt.pdf
Pohl R (2018) An analysis of Donna Haraway’s A cyborg manifesto: science, technology, and socialist-feminism in the late twentieth century. Routledge, London
Prates MOR, Avelar PH, Lamb LC (2020) Assessing gender bias in machine translation: a case study with Google Translate. Neural Comput Appl32(10):6363–6381. https://doi.org/10.1007/s00521-019-04144-6
Prives L (2018) AI for all: drawing women into the artificial intelligence field. IEE Women Eng Mag 12(2):30–32. https://doi.org/10.1109/MWIE.2018.2866890
Reeves B, Nass C (1996) The media equation: how people treat computers, television, and new media like real people and places. Cambridge University Press
Sanders, D (2008) Progress in machine intelligence. Ind Robot 35 (6). https://doi.org/10.1108/ir.2008.04935faa.002
Santow E (2020) Can artificial intelligence be trusted with our human rights? Aust Q 91(4):10–17. https://www.jstor.org/stable/26931483
Simonite T (2018) AI is the future-But where are the women?. WIRED.COM. https://www.wired.com/story/artificial-intelligence-researchers-gender-imbalance/
Søraa RA (2017) Mechanical genders: How do humans gender robots? Gend Technol Dev 21(1–2):99–115. https://doi.org/10.1080/09718524.2017.1385320
Suchman L (2006) Human-machine reconfigurations: plans and situated actions, 2nd edn. Cambridge University Press, pp. 1–314
Tripathi A (2016) Sex determination in India: Doctors tell their side of story. Scroll.in. https://scroll.in/article/805064/sex-determination-in-india-doctors-tell-their-side-of-the-story
UNESCO Report. (2020) Artificial intelligence and gender equality. Division for Gender Equality, UNESCO. https://en.unesco.org/system/files/artificial_intelligence_and_gender_equality.pdf
Venkatraman V (2020) Where logic meets emotion. Science 368(6495):1072–1072. https://doi.org/10.1126/science.abc3555
Article ADS CAS Google Scholar
Weber J (2005) Helpless machines and true loving care givers: a feminist critique of recent trends in human–robot interaction. J Inf Commun Ethics Soc. https://doi.org/10.1108/14779960580000274
World Economic Forum Report. (2018) Assessing gender gaps in artificial intelligence. https://reports.weforum.org/global-gender-gap-report-2018/assessing-gender-gaps-in-artificial-intelligence/?doing_wp_cron=1615981135.3421480655670166015625
Yeoman I, Mars M (2012) Robots, men and sex tourism. Futures 44(4):365–371. https://doi.org/10.1016/j.futures.2011.11.004
Download references
Acknowledgements
The authors are grateful to everyone who volunteered to take part in this study and helped to create this body of knowledge. Professor Lashiram Laddu Singh, Vice-Chancellor, Bodoland University, India, is to be thanked for his unwavering support and direction throughout the study’s duration. Thank you to Bishal Bhuyan and Kinnori Kashyap of Sikkim University, India for their help with data testing using SPSS software.
Author information
Authors and affiliations.
Department of Management, Sikkim Central University, Sikkim, India
- Shailendra Kumar
Department of Political Science, Bodoland State University, Kokrajhar, Assam, India
- Sanghamitra Choudhury
Department of Asian Studies, St. Antony’s College, University of Oxford, Oxford, UK
Department of History and Anthropology, Queen’s University, Belfast, Northern Ireland, UK
Hague Academy of International Law, Hague, the Netherlands
Centre for the Study of Law and Governance, Jawaharlal Nehru University, New Delhi, India
You can also search for this author in PubMed Google Scholar
Contributions
Conceptualization, SK and SC; methodology, SK and SC; software, SK; validation, SK and SC; formal analysis, SC; investigation, SK; resources, SC; data curation, SK; writing—original draft preparation, SK; writing—review and editing, SK and SC. All authors have read and agreed to the published version of the manuscript.
Corresponding author
Correspondence to Shailendra Kumar .
Ethics declarations
Competing interests.
The authors declare no competing interest.
Ethical approval
Ethical approval was obtained from Bodoland University’s Authorities and Research Ethics Committee. This study was conducted in compliance with the Charter of Fundamental Rights of the EU (2010/C 83/02), the European Union European Charter for Researchers, and the General Data Protection Regulation (GDPR).
Informed consent
Informed consent was obtained from all participants and/or their legal guardians. Every participant gave their consent before questionnaires were administered or interviews were conducted. Essentially, the online survey would get open only when the participant read, understood, and agreed with what participation in the study would entail, and each one had the option to discontinue their participation at any time.
Additional information
Publisher’s note Springer Nature remains neutral with regard to jurisdictional claims in published maps and institutional affiliations.
Rights and permissions
Open Access This article is licensed under a Creative Commons Attribution 4.0 International License, which permits use, sharing, adaptation, distribution and reproduction in any medium or format, as long as you give appropriate credit to the original author(s) and the source, provide a link to the Creative Commons license, and indicate if changes were made. The images or other third party material in this article are included in the article’s Creative Commons license, unless indicated otherwise in a credit line to the material. If material is not included in the article’s Creative Commons license and your intended use is not permitted by statutory regulation or exceeds the permitted use, you will need to obtain permission directly from the copyright holder. To view a copy of this license, visit http://creativecommons.org/licenses/by/4.0/ .
Reprints and permissions
About this article
Cite this article.
Kumar, S., Choudhury, S. Gender and feminist considerations in artificial intelligence from a developing-world perspective, with India as a case study. Humanit Soc Sci Commun 9 , 31 (2022). https://doi.org/10.1057/s41599-022-01043-5
Download citation
Received : 12 September 2021
Accepted : 10 January 2022
Published : 26 January 2022
DOI : https://doi.org/10.1057/s41599-022-01043-5
Share this article
Anyone you share the following link with will be able to read this content:
Sorry, a shareable link is not currently available for this article.
Provided by the Springer Nature SharedIt content-sharing initiative
This article is cited by
Humans, super humans, and super humanoids: debating stephen hawking’s doomsday ai forecast.
AI and Ethics (2023)
Quick links
- Explore articles by subject
- Guide to authors
- Editorial policies

McCombs School of Business
- Español ( Spanish )
Videos Concepts Unwrapped View All 36 short illustrated videos explain behavioral ethics concepts and basic ethics principles. Concepts Unwrapped: Sports Edition View All 10 short videos introduce athletes to behavioral ethics concepts. Ethics Defined (Glossary) View All 58 animated videos - 1 to 2 minutes each - define key ethics terms and concepts. Ethics in Focus View All One-of-a-kind videos highlight the ethical aspects of current and historical subjects. Giving Voice To Values View All Eight short videos present the 7 principles of values-driven leadership from Gentile's Giving Voice to Values. In It To Win View All A documentary and six short videos reveal the behavioral ethics biases in super-lobbyist Jack Abramoff's story. Scandals Illustrated View All 30 videos - one minute each - introduce newsworthy scandals with ethical insights and case studies. Video Series
Case Study UT Star Icon
Pao & Gender Bias
Ellen Pao stirred debate in the venture capital and tech industries when she filed a lawsuit against her employer on grounds of gender discrimination.
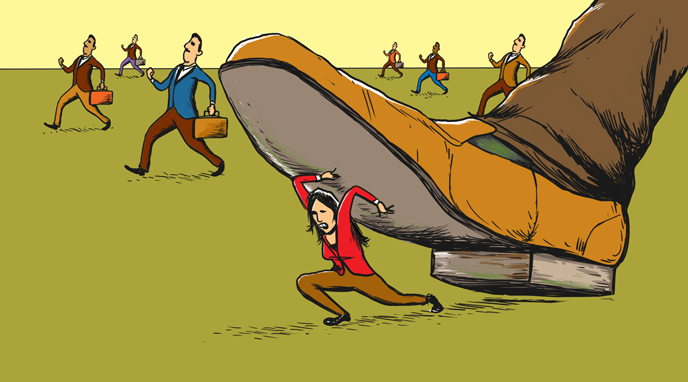
On May 10, 2012, executive Ellen Pao filed a lawsuit against her employer, Silicon Valley-based tech venture capital firm Kleiner Perkins Caufield & Byers (Kleiner Perkins), on grounds of gender discrimination. Pao began working at Kleiner Perkins in 2005. She became a junior investing partner, but after several years at the firm was passed over for a senior partner position and was eventually terminated. Pao claimed that men with similar profiles and achievements were promoted instead.
In late 2011, Pao and a coworker were asked by a senior partner to come up with ways of improving the firm’s treatment of women, but the senior partner, according to Pao, was “noncommittal.” On January 4, 2012, Pao took this issue a step further and wrote a formal memorandum to several of her superiors and the firm’s outside counsel. In the memorandum, she described harassment she had received while at the firm, claiming she had been excluded from meetings by male partners, and asserting an absence of training and policies to prevent discrimination at the firm. Pao’s memo indicated that she wished to work with the firm on improving conditions for women. She was fired on October 1, 2012. The lawsuit went to trial in February 2015.
In a testimony during the trial, Pao explained that she sued because there was no process for HR issues at the firm and believed she had exhausted all options for addressing these issues internally:
“It’s been a long journey, and I’ve tried many times to bring Kleiner Perkins to the right path. I think there should be equal opportunities for women and men to be venture capitalists. I wanted to be a VC but I wasn’t able to do so in that environment. And I think it’s important…to make those opportunities available in the future. And I wanted to make sure my story was told.”
Pao’s lawsuit made four claims against Kleiner Perkins: 1) they discriminated against Pao on the basis of gender by failing to promote her and/or terminating her employment; 2) they retaliated by failing to promote her because of conversations she had in late 2011 and/or the memo from January 4, 2012; 3) they failed to take all reasonable steps to prevent gender discrimination against her; and 4) they retaliated against her by terminating her employment because of conversations she had in late 2011 and/or the memo from January 4, 2012.
Pao’s legal team argued that men were promoted ahead of women, women who experienced sexual harassment received little support, and women’s ideas were often more quickly dismissed than men’s. Pao’s performance reviews revealed contradictory criticisms such as “too bold” and “too quiet.” Pao also accused company partner Ajit Nazre of pressuring her into an affair and subsequently retaliating against her after she ended the relationship. She said she received an inappropriate gift containing erotic imagery and was present while men at the firm were making inappropriate conversation. Further, the legal team described how Pao and other women had been left out of certain meetings and gatherings.
The defense’s case focused on Pao’s performance and character, noting that Pao received several negative performance reviews and acted entitled or resentful toward other employees and was not a team player. Evidence included evaluations, self-evaluations, meeting summaries, and messages both personal and professional. Kleiner Perkins claimed that Pao was paid more than her male counterparts, including bonuses and training. The firm also argued that Pao’s job description was mostly managerial and that limiting her involvement in investing was therefore not a form of discrimination.
The verdict was announced on March 27, 2015. The jury ruled 10 to 2 in favor of Kleiner Perkins on the first three claims, and 8 to 4 in favor of Kleiner Perkins on the fourth claim. Speaking after the trial, juror Steve Sammut said that the verdict came down to performance reviews, in which Pao’s negative criticism remained consistent each year. But he added that he wished there was some way for Kleiner Perkins to be punished for its treatment of employees, “It isn’t good. It’s like the wild, wild West.” Juror Marshalette Ramsey voted in favor of Pao, believing Pao had been discriminated against. Ramsey stated that the male junior partners who were promoted “had those same character flaws that Ellen was cited with.”
Deborah Rhode, law professor at Stanford University, said that even with this loss, Pao’s lawsuit succeeded in prompting debate about women in venture capital and tech. She stated, “This case sends a powerful signal to Silicon Valley in general and the venture capital industry in particular… Defendants who win in court sometimes lose in the world outside it.” After the verdict was announced, Pao stated that she hoped the case at least helped level the playing field for women and minorities in venture capital. She later wrote:
“I have a request for all companies: Please don’t try to silence employees who raise discrimination and harassment concerns. …I hope future cases prove me wrong and show that our community and our jurists have now developed a better understanding of how discrimination works in real life, in the tech world, in the press and in the courts.”
Pao’s case has since been credited for inspiring others facing workplace discrimination to act; similar lawsuits have been filed against companies such as Facebook, Twitter, and Microsoft.
Discussion Questions
1. At what points in this case study did Pao make the choice to voice her values? How did she voice her values in each of these instances?
2. Do you think Pao acted on her values effectively? Why or why not? Does the fact that she lost the lawsuit impact your reasoning? Explain.
3. Think through the seven pillars of GVV in relation to the case study above. Can you identify each pillar in Pao’s actions? Are there any pillars that you think Pao could have engaged more effectively? Explain.
4. If you were in Pao’s position at Kleiner Perkins, what would you have done and why? How might the pillars of GVV influence your actions? Select one of the pillars and describe how you would enact it in a situation described in the case study.
5. Based on the information in the case study, if you were a juror would you have ruled in favor of Pao or Kleiner Perkins? Why? How might your own values or biases influence your decision?
6. Have you ever worked at a job where you faced ethically questionable behavior? What did you do? In retrospect, do you wish you had done anything differently? How would you prepare for a similar situation today?
7. Have you ever witnessed or experienced discrimination in the workplace? What did you do? In retrospect, would you have done something differently? What do you think would be the ethically ideal way to handle instances of discrimination in the workplace?
Related Videos
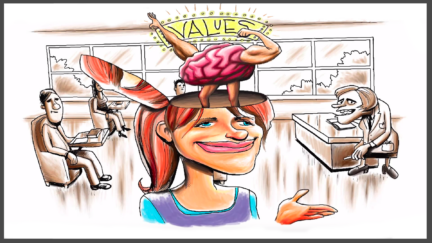
Intro to GVV
Giving Voice to Values is learning about how to act on your values effectively – not about wondering whether you could.
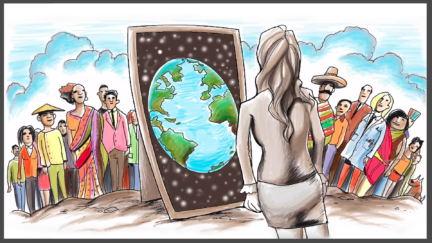
GVV Pillar 1: Values
Know and appeal to a short list of widely shared values. Don’t assume too little (or too much) commonality with the viewpoints of others.
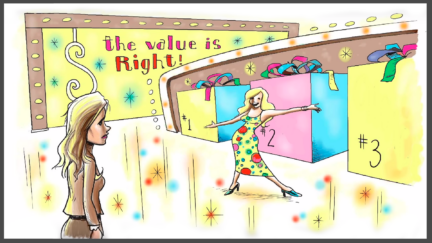
GVV Pillar 2: Choice
Believe that you have a choice about voicing your values and know what has helped – and hindered you – in the past so you can work around these factors.
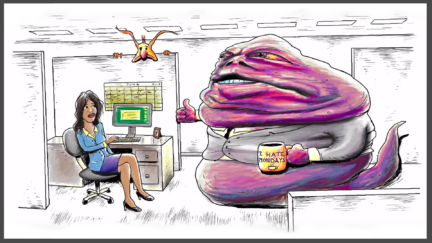
GVV Pillar 3: Normalization
Normalization means expecting values conflicts so that you approach them calmly and competently. Over-reaction can limit your choices unnecessarily.
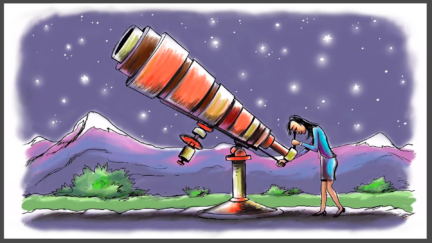
GVV Pillar 4: Purpose
Define your personal and professional purpose explicitly and broadly before conflicts arise, and appeal to this sense of purpose in others.
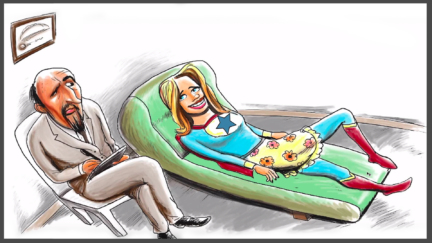
GVV Pillar 5: Self-Knowledge & Alignment
Self-knowledge and alignment means to voice and act on your values in a way that is consistent with who you are and builds on your strengths.
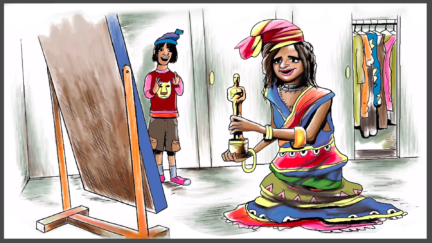
GVV Pillar 6: Voice
You are more likely to say words that you’ve pre-scripted for yourself, and more likely to “voice” your values, with scripting and practice.
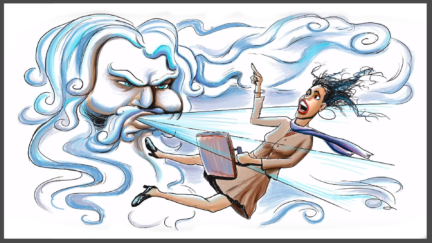
GVV Pillar 7: Reasons & Rationalizations
By anticipating the typical reasons & rationalizations given for ethically questionable behavior, you are able to identify and prepare well-reasoned responses.
Bibliography
Ellen Pao Loses Silicon Valley Bias Case Against Kleiner Perkins http://www.nytimes.com/2015/03/28/technology/ellen-pao-kleiner-perkins-case-decision.html
Kleiner Perkin Portrays Ellen Pao as Combative and Resentful in Sex Bias Trial http://www.nytimes.com/2015/03/12/technology/kleiner-perkins-portrays-ellen-pao-as-combative-and-resentful-in-sex-bias-trial.html
Ellen Pao explains why she sued: “I wanted to make sure my story was told’ http://www.businessinsider.com/ellen-pao-explains-why-she-sued-kleiner-perkins-2015-3
Ellen Pao wanted “a multimillion dollar payout,” Kleiner lawyers contend http://arstechnica.com/tech-policy/2015/03/ellen-pao-wanted-a-multimillion-dollar-payout-kleiner-lawyers-contend/
Ellen Pao asked for a $10 million payment from Kleiner Perkins as the cost of ‘not fixing problems’ http://www.businessinsider.com/ellen-pao-asked-for-10-million-2015-3
What the Jury in the Ellen Pao-Kleiner Perkins Case Needed to Decide http://www.nytimes.com/interactive/2015/03/27/technology/document-ellen-pao-kleiner-perkins-suit-verdict-form-and-jury-instructions.html
A Juror Speaks About His Vote for Kleiner Perkins but Still Wants the Firm to ‘Be Punished’ http://recode.net/2015/03/30/a-juror-speaks-about-his-vote-for-kleiner-perkins-but-still-wants-the-firm-to-be-punished/
Ellen Pao Speaks: ‘I Am Now Moving On’ http://recode.net/2015/09/10/ellen-pao-speaks-i-am-now-moving-on/
After Loss, Pao Hopes Case Leveled the Playing Field http://www.wired.com/2015/03/ellen-pao-kleiner-verdict/
Pao’s Alleged Firing Could Hurt Kleiner Perkins in Retaliation Suit http://www.wired.com/2012/10/ellen-pao-kleiner-perkins/
Gender Bias Will Soon Shine a Harsh Light on Microsoft http://www.wired.com/2015/09/microsoft-gender-lawsuit/
Stay Informed
Support our work.
Help | Advanced Search
Computer Science > Computation and Language
Title: the birth of bias: a case study on the evolution of gender bias in an english language model.
Abstract: Detecting and mitigating harmful biases in modern language models are widely recognized as crucial, open problems. In this paper, we take a step back and investigate how language models come to be biased in the first place. We use a relatively small language model, using the LSTM architecture trained on an English Wikipedia corpus. With full access to the data and to the model parameters as they change during every step while training, we can map in detail how the representation of gender develops, what patterns in the dataset drive this, and how the model's internal state relates to the bias in a downstream task (semantic textual similarity). We find that the representation of gender is dynamic and identify different phases during training. Furthermore, we show that gender information is represented increasingly locally in the input embeddings of the model and that, as a consequence, debiasing these can be effective in reducing the downstream bias. Monitoring the training dynamics, allows us to detect an asymmetry in how the female and male gender are represented in the input embeddings. This is important, as it may cause naive mitigation strategies to introduce new undesirable biases. We discuss the relevance of the findings for mitigation strategies more generally and the prospects of generalizing our methods to larger language models, the Transformer architecture, other languages and other undesirable biases.
Submission history
Access paper:.
- Other Formats

References & Citations
- Google Scholar
- Semantic Scholar
BibTeX formatted citation

Bibliographic and Citation Tools
Code, data and media associated with this article, recommenders and search tools.
- Institution
arXivLabs: experimental projects with community collaborators
arXivLabs is a framework that allows collaborators to develop and share new arXiv features directly on our website.
Both individuals and organizations that work with arXivLabs have embraced and accepted our values of openness, community, excellence, and user data privacy. arXiv is committed to these values and only works with partners that adhere to them.
Have an idea for a project that will add value for arXiv's community? Learn more about arXivLabs .
Cass Review: A victory for women, children and common sense
Dr Cass’s landmark review into gender care services in England brought us one step closer to throwing the harmful delusion that is gender ideology into the dustbin of history.

In England, a landmark independent review into gender care services for young people has exposed one of the biggest medical negligence scandals of recent times and vindicated those who have been accused of engaging in malicious “culture wars” and branded as unkind bigots for opposing unnecessary medicalisation of children.
The review, conducted by respected paediatrician Hilary Cass, found that some of the most vulnerable members of society – children presenting with “gender dysphoria” which, in many cases, masks autism, sexual abuse, trauma and confusion over same-sex attraction, among other conditions – have been let down by a lack of research and “remarkably weak” evidence on medical interventions in England’s gender care clinics.
Keep reading
Manhattan prosecutors announce retrial for film producer harvey weinstein, ‘profoundly unjust’: reactions to court overturning weinstein’s conviction, top new york court overturns harvey weinstein’s 2020 rape conviction, ten years after chibok girls kidnapping: one woman’s struggle to move on.
The National Health Service (NHS England) said it commissioned the review, published on April 10, to ensure that “children and young people who are questioning their gender identity or experiencing gender dysphoria receive a high standard of care, that meets their needs, is safe, holistic and effective.”
The review’s intentions, and thus its conclusions, are of course disputed by those who insist that anyone claiming to be transgender must be instantly affirmed – and in the case of a child, given access to puberty blockers.
Puberty blockers – hormones that stop the progress of puberty – have long been at the centre of the dispute over whether medical interventions offered to gender-questioning children are safe and fit for purpose.
In short, Cass has found that puberty blockers do indeed have side effects and negative health implications. Her inquiry concluded that there is, at best, weak evidence that these drugs are safe and beneficial to gender-questioning children, especially in the long term.
Cass told the BBC that the use of puberty blockers to “arrest puberty” started out as a clinical trial, but has been expanded to a wider group of young people before the results of that trial were available.
“It is unusual for us to give a potentially life-changing treatment to young people and not know what happens to them in adulthood, and that’s been a particular problem that we have not had the follow-up into adulthood to know what the results of this are,” she said.
To make sense of this scandal, and understand how the NHS came to offer this experimental treatment to vulnerable children without obtaining meaningful evidence for its safety and efficacy, we must examine how British institutions have been captured by “gender ideology” – the belief that an individual’s internal sense of gender, or “gender identity”, should supersede their sex in all aspects of life and under law.
In 2016, Women and Equalities Minister Maria Miller led an inquiry into transgender equality that strongly recommended that the United Kingdom legally adopt principles of gender self-declaration, which would allow any individual to decide whether they would be considered male or female according to their own “gender identity”.
Miller signed off a report advising a change to the Equality Act which would replace the protected characteristic of “gender reassignment” with “gender identity”. In so doing, she suggested that the inner feeling of “gender” should take precedence over legal sex.
Feminists complained – for us, this was a matter of protecting our hard-won sex-based rights. But Miller dismissed women’s fury about the erosion of single-sex provisions in domestic violence refuges and prisons among other aspects of life as “extraordinary” bigotry.
The feminist resistance to gender ideology and objection to harm it inflicts on women and children, however, did not start with Miller’s misguided inquiry, which ramped up institutional capture.
The first article I ever wrote on the trans issue was published in the Telegraph Magazine in November 2003. It was about those who had undergone “sex change” surgery (the popular parlance of the time).
Researching that article, I discovered that Mermaids (a charity supporting children and teenagers with “gender identity disorder”) had seen a dramatic increase in inquiries since its founding in 1995. “Sex-change” treatment, including puberty blockers, was available to children as young as 14, despite evidence (even then) from the Tavistock and Portman NHS Foundation Trust that one in four teenagers seeking “gender reassignment” would later change their minds.
Soon after, I wrote about Vancouver Rape Relief (VRR), in Canada. In August 1995, two VRR employees had asked Kimberly Nixon (a trans-identified male) to leave its counsellor training, which prepared attendees to offer face-to-face support to women traumatised as a result of male violence. The following day, Nixon filed a human rights complaint, initiating a lengthy legal battle.
The publication of this article in Guardian Weekend on January 30, 2004, headed “Gender Benders, Beware” , led directly to me being cast out by trans activists.
Invitations to prestigious events were withdrawn. I was shortlisted for awards, only to be un-shortlisted when the organisers found themselves under pressure.
Refusing to bow down to these efforts, I and a small number of other feminists continued to speak out – as did some valiant whistle-blowers. These were people working within gender clinics, horrified by the creeping normalisation of transitioning “gender-distressed” children.
In September 2017, Woman’s Place UK (WPUK) was founded by a group of feminists in response to planned new legislation by Miller, and everything changed as groups of women began to organise. Resistance to gender ideology was now driving feminist activism.
These efforts, however, did not immediately put a stop to institutional capture. There was a spike in the number of children, overwhelmingly girls, presenting with gender dysphoria and being referred to gender clinics, but this did not concern trans activists and their supporters in positions of power. More than 5,000 referrals were made to the Gender Identity Development Service (GIDS) in London in 2021-22, compared to just 500 hundred a decade earlier. Almost two-thirds of referrals in recent years were teenage girls. Still, people continued to dismiss our concerns over gender ideology and its medicalisation of children as hyperbole and bigotry.
By July 2023, the situation in schools in some parts of the country had become so urgent that concerned parents started to take matters into their own hands.
In the Brighton and Hove area, where a number of children were being allowed to “socially transition” at school, for example, concerned parents set up PSHE Brighton to assess the delivery of Personal, Social, Health, and Economic Education (PSHE) and Relationships and Sex Education (RSE) in local schools.
So far, more than 20 local families contacted them and voiced their worry that their children may be being transitioned “from the classroom to the clinic”.
One such family was 16-year-old Catherine’s*.
Catherine is autistic and, in less than two years, has gone from being a feminist and a proud lesbian to identifying as a boy. Now fixated on medical and surgical transition, she appears to have experienced rapid onset gender dysphoria after accessing material from trans-affirming charities like Mermaids online.
Her parents say she forced her school into making a social work referral by self-harming and reporting false information about her family. They explain that they had secured agreement from both Catherine’s headteacher and a private counselling service that social transition would be inappropriate for her before a full assessment of her needs had been carried out. Nevertheless, her parents say that “an unholy alliance of well-meaning teachers and social workers, misguided by potentially unlawful policies, practices, procedures, and training, have led to Catherine transitioning – first socially and then medically”.
Today, Catherine is estranged from both of her parents.
There are many, many more families going through this hell, and many children like Catherine who are exposed to experimental treatments with permanent side effects, as a result of an almost blind acceptance by individuals and institutions of gender ideology.
For years, those of us who tried to put a stop to this have been accused of being bigoted, unkind, and motivated by a dislike of trans people. For years, it has been claimed that it was not feminists and concerned parents, but ideological charities like Mermaids who were doing what is best for “trans children”. Women lost their jobs, reputations and, often, sanity for speaking up against gender ideology, and its medicalisation of vulnerable children. Thankfully, Hilary Cass has finally exposed the truth, proved that it was not us who were being “unkind” but those unnecessarily medicalising children, and brought us one step closer to throwing the harmful delusion that is gender ideology into the dustbin of history.
* Name has been changed to protect her identity
The views expressed in this article are the author’s own and do not necessarily reflect Al Jazeera’s editorial stance.
- Share full article
Advertisement
Supported by
David Brooks
The Courage to Follow the Evidence on Transgender Care

By David Brooks
Opinion Columnist
Hilary Cass is the kind of hero the world needs today. She has entered one of the most toxic debates in our culture: how the medical community should respond to the growing numbers of young people who seek gender transition through medical treatments, including puberty blockers and hormone therapies. This month, after more than three years of research, Cass, a pediatrician, produced a report , commissioned by the National Health Service in England, that is remarkable for its empathy for people on all sides of this issue, for its humility in the face of complex social trends we don’t understand and for its intellectual integrity as we try to figure out which treatments actually work to serve those patients who are in distress. With incredible courage, she shows that careful scholarship can cut through debates that have been marked by vituperation and intimidation and possibly reset them on more rational grounds.
Cass, a past president of Britain’s Royal College of Pediatrics and Child Health, is clear about the mission of her report: “This review is not about defining what it means to be trans, nor is it about undermining the validity of trans identities, challenging the right of people to express themselves or rolling back on people’s rights to health care. It is about what the health care approach should be, and how best to help the growing number of children and young people who are looking for support from the N.H.S. in relation to their gender identity.”
This issue begins with a mystery. For reasons that are not clear, the number of adolescents who have sought to medically change their sex has been skyrocketing in recent years, though the overall number remains very small. For reasons that are also not clear, adolescents who were assigned female at birth are driving this trend, whereas before the late 2000s, it was mostly adolescents who were assigned male at birth who sought these treatments.
Doctors and researchers have proposed various theories to try to explain these trends. One is that greater social acceptance of trans people has enabled people to seek these therapies. Another is that teenagers are being influenced by the popularity of searching and experimenting around identity. A third is that the rise of teen mental health issues may be contributing to gender dysphoria. In her report, Cass is skeptical of broad generalizations in the absence of clear evidence; these are individual children and adolescents who take their own routes to who they are.
Some activists and medical practitioners on the left have come to see the surge in requests for medical transitioning as a piece of the new civil rights issue of our time — offering recognition to people of all gender identities. Transition through medical interventions was embraced by providers in the United States and Europe after a pair of small Dutch studies showed that such treatment improved patients’ well-being. But a 2022 Reuters investigation found that some American clinics were quite aggressive with treatment: None of the 18 U.S. clinics that Reuters looked at performed long assessments on their patients, and some prescribed puberty blockers on the first visit.
Unfortunately, some researchers who questioned the Dutch approach were viciously attacked. This year, Sallie Baxendale, a professor of clinical neuropsychology at the University College London, published a review of studies looking at the impact of puberty blockers on brain development and concluded that “critical questions” about the therapy remain unanswered. She was immediately attacked. She recently told The Guardian, “I’ve been accused of being an anti-trans activist, and that now comes up on Google and is never going to go away.”
As Cass writes in her report, “The toxicity of the debate is exceptional.” She continues, “There are few other areas of health care where professionals are so afraid to openly discuss their views, where people are vilified on social media and where name-calling echoes the worst bullying behavior.”
Cass focused on Britain, but her description of the intellectual and political climate is just as applicable to the U.S., where brutality on the left has been matched by brutality on the right, with crude legislation that doesn’t acknowledge the well-being of the young people in question. In 24 states Republicans have passed laws banning these therapies, sometimes threatening doctors with prison time if they prescribe the treatment they think is best for their patients.
The battle lines on this issue are an extreme case, but they are not unfamiliar. On issue after issue, zealous minorities bully and intimidate the reasonable majority. Often, those who see nuance decide it’s best to just keep their heads down. The rage-filled minority rules.
Cass showed enormous courage in walking into this maelstrom. She did it in the face of practitioners who refused to cooperate and thus denied her information that could have helped inform her report. As an editorial in The BMJ puts it, “Despite encouragement from N.H.S. England,” the “necessary cooperation” was not forthcoming. “Professionals withholding data from a national inquiry seems hard to imagine, but it is what happened.”
Cass’s report does not contain even a hint of rancor, just a generous open-mindedness and empathy for all involved. Time and again in her report, she returns to the young people and the parents directly involved, on all sides of the issue. She clearly spent a lot of time meeting with them. She writes, “One of the great pleasures of the review has been getting to meet and talk to so many interesting people.”
The report’s greatest strength is its epistemic humility. Cass is continually asking, “What do we really know?” She is carefully examining the various studies — which are high quality, which are not. She is down in the academic weeds.
She notes that the quality of the research in this field is poor. The current treatments are “built on shaky foundations,” she writes in The BMJ. Practitioners have raced ahead with therapies when we don’t know what the effects will be. As Cass tells The BMJ, “I can’t think of another area of pediatric care where we give young people a potentially irreversible treatment and have no idea what happens to them in adulthood.”
She writes in her report, “The option to provide masculinizing/feminizing hormones from age 16 is available, but the review would recommend extreme caution.” She does not issue a blanket, one-size-fits-all recommendation, but her core conclusion is this: “For most young people, a medical pathway will not be the best way to manage their gender-related distress.” She realizes that this conclusion will not please many of the young people she has come to know, but this is where the evidence has taken her.
You can agree or disagree with this or that part of the report, and maybe the evidence will look different in 10 years, but I ask you to examine the integrity with which Cass did her work in such a treacherous environment.
In 1877 a British philosopher and mathematician named William Kingdon Clifford published an essay called “ The Ethics of Belief .” In it he argued that if a shipowner ignored evidence that his craft had problems and sent the ship to sea having convinced himself it was safe, then of course we would blame him if the ship went down and all aboard were lost. To have a belief is to bear responsibility, and one thus has a moral responsibility to dig arduously into the evidence, avoid ideological thinking and take into account self-serving biases. “It is wrong always, everywhere, and for anyone, to believe anything upon insufficient evidence,” Clifford wrote. A belief, he continued, is a public possession. If too many people believe things without evidence, “the danger to society is not merely that it should believe wrong things, though that is great enough; but that it should become credulous, and lose the habit of testing things and inquiring into them; for then it must sink back into savagery.”
Since the Trump years, this habit of not consulting the evidence has become the underlying crisis in so many realms. People segregate into intellectually cohesive teams, which are always dumber than intellectually diverse teams. Issues are settled by intimidation, not evidence. Our natural human tendency is to be too confident in our knowledge, too quick to ignore contrary evidence. But these days it has become acceptable to luxuriate in those epistemic shortcomings, not to struggle against them. See, for example, the modern Republican Party.
Recently it’s been encouraging to see cases in which the evidence has won out. Many universities have acknowledged that the SAT is a better predictor of college success than high school grades and have reinstated it. Some corporations have come to understand that while diversity, equity and inclusion are essential goals, the current programs often empirically fail to serve those goals and need to be reformed. I’m hoping that Hilary Cass is modeling a kind of behavior that will be replicated across academia, in the other professions and across the body politic more generally and thus save us from spiraling into an epistemological doom loop.
The Times is committed to publishing a diversity of letters to the editor. We’d like to hear what you think about this or any of our articles. Here are some tips . And here’s our email: [email protected] .
Follow the New York Times Opinion section on Facebook , Instagram , TikTok , WhatsApp , X and Threads .
David Brooks has been a columnist with The Times since 2003. He is the author, most recently, of “How to Know a Person: The Art of Seeing Others Deeply and Being Deeply Seen.” @ nytdavidbrooks
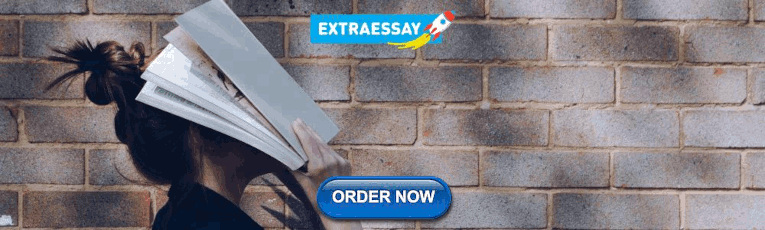
IMAGES
VIDEO
COMMENTS
Gender Bias Case Study. Despite the progress girls and women have made in school and the workplace in the past few decades, a gender gap still persists, and our research suggests that biases could be at the root of this gap. Gender bias and discrimination is surprisingly common in many schools and sometimes happens beneath school staff's radar.
Leanne M. Dzubinski. March 02, 2022. bashta/Getty Images. Summary. New research examines gender bias within four industries with more female than male workers — law, higher education, faith ...
This study has been repeatedly invoked as prima facie evidence of gender bias in grants even after controlling for productivity, for example by Kaatz et al. (2014): "Lending support to this is the classic study by Wenneras [sic] and Wold in which female applicants for a postdoctoral research fellowship needed more than twice as many ...
Abstract. Scholarly journals are often blamed for a gender gap in publication rates, but it is unclear whether peer review and editorial processes contribute to it. This article examines gender bias in peer review with data for 145 journals in various fields of research, including about 1.7 million authors and 740,000 referees.
Case reports were coded for patient sex, country of institutional affiliation, physiological system, and first and last author gender. Of 494 total case reports, 45% ( n = 221) of patients were male, 30% ( n = 146) were female, and 25% ( n = 124) included both sexes. Ratios of male-only to female-only case reports varied by physiological system.
Perceptions of gender bias ... (expert reviewers whose responsibility it is to review the quality of the retrospective impact articulated in case studies for the purposes of research evaluation ...
The study will explore in-depth case studies on four examples of both gender bias perpetuation and gender bias mitigation found in AI technologies. From these case studies, this paper will describe the existence of gender bias in algorithms, as well as how algorithms themselves could contribute to mitigating gender bias.
Gender bias in Physics Today. Nature is not alone in grappling with gender bias in science journalism. In an attempt to approximately replicate the Nature study's methodology, Physics Today recorded the people quoted in 2005 and 2020 in two staff-written sections of the printed magazine: Search & Discovery, which covers new scientific research, and Issues & Events, which focuses on science ...
One working paper study of over 500,000 physician referrals showed that women surgeons receive fewer ... Gender bias takes on different dimensions depending on other intersecting aspects of a ...
Case reports were coded for patient sex, country of institutional affiliation, physiological system, and first and last author gender. Of 494 total case reports, 45% ( n = 221) of patients were male, 30% ( n = 146) were female, and 25% ( n = 124) included both sexes. Ratios of male-only to female-only case reports varied by physiological system.
Harvard Business School Case Study: Gender Equity. Nitin Nohria, left, the dean of the Harvard Business School, acknowledging Brooke Boyarsky as a Baker scholar in May. Gretchen Ertl for The New ...
Summary. Gender bias in research influences both the selection of participants in research and perceptions about individuals' capacity to do quality research. For the selection of research participants, an androcentric, or male-focused, bias has historically led researchers to conduct their observations on males, both in biomedical studies ...
We also test whether those who believe discrimination against women in their field is no longer an issue are the most likely to express gender bias (study 2). We test these questions using samples of women and men working in the field of veterinary medicine (U.K.-based).
Gender. New research on gender in the workplace from Harvard Business School faculty on issues including "leaning-in," gender inequity, the social and economic effects of maternal employment, and gender diversity's effect on corporate financial performance. Page 1 of 122 Results →. 04 Mar 2024.
Unlike omitted variable bias—which drives discrimination in statistical models—ML discrimination is driven by changes in the model training procedure, including feature engineering and feature selection, when gender is excluded. ... Adrienne Heinrich (2022) Antidiscrimination Laws, Artificial Intelligence, and Gender Bias: A Case Study in ...
Fortunately, two recent studies have proved timely, shedding new light and offering fresh perspectives on these complex issues. In a study published in 2021, Jen-How Kuo and Meng-Che Tsai led a ...
The reporting bias which this methodology maintains creates a situation where guidelines based on the study of one sex may be generalized and applied to both. In fact, study design in the 1970s in response to sex discrimination legislation made efforts to mix gender within study groups since this was considered the best approach to equality.
Recently there has been a growing concern in academia, industrial research laboratories and the mainstream commercial media about the phenomenon dubbed as machine bias, where trained statistical models—unbeknownst to their creators—grow to reflect controversial societal asymmetries, such as gender or racial bias. A significant number of Artificial Intelligence tools have recently been ...
Illustrating gender bias We apply the standard ML approach to the UCI adult dataset. The steps that are followed are 1) splitting the dataset into training and test data, 2) selecting model (MLPClassifier in this case), 3) fitting the model on training data, and 4) using the model to make predictions on test data.
Using India as a case study, the manuscript outlines how Artificial Intelligence (AI) and robotics affect women's opportunities in developing countries. ... Hardesty L (2018) Study finds gender ...
Research has documented this bias, and gains made by women. However, much of this research examines only gender bias, ignoring other important factors such as race. In this article, we examine gender and racial bias in criminology, conceptualizing the discipline as a Bourdieusian field, characterized by hierarchically arranged positions.
Pao & Gender Bias. Ellen Pao stirred debate in the venture capital and tech industries when she filed a lawsuit against her employer on grounds of gender discrimination. On May 10, 2012, executive Ellen Pao filed a lawsuit against her employer, Silicon Valley-based tech venture capital firm Kleiner Perkins Caufield & Byers (Kleiner Perkins), on ...
Download a PDF of the paper titled The Birth of Bias: A case study on the evolution of gender bias in an English language model, by Oskar van der Wal and 3 other authors. Download PDF Abstract: Detecting and mitigating harmful biases in modern language models are widely recognized as crucial, open problems. In this paper, we take a step back ...
A photograph taken on April 10, 2024, in London, shows the entrance of the NHS Tavistock center, where the Tavistock Clinic hosted the Gender Identity Development Service (GIDS) for children until ...
Hilary Cass is the kind of hero the world needs today. She has entered one of the most toxic debates in our culture: how the medical community should respond to the growing numbers of young people ...