- Open access
- Published: 12 March 2020
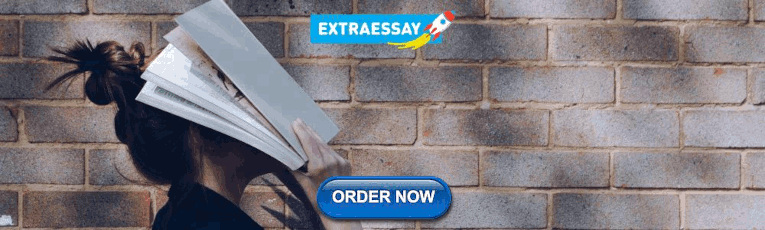
Current landscape and influence of big data on finance
- Md. Morshadul Hasan ORCID: orcid.org/0000-0001-9857-9265 1 ,
- József Popp ORCID: orcid.org/0000-0003-0848-4591 2 &
- Judit Oláh ORCID: orcid.org/0000-0003-2247-1711 2
Journal of Big Data volume 7 , Article number: 21 ( 2020 ) Cite this article
57k Accesses
86 Citations
32 Altmetric
Metrics details
Big data is one of the most recent business and technical issues in the age of technology. Hundreds of millions of events occur every day. The financial field is deeply involved in the calculation of big data events. As a result, hundreds of millions of financial transactions occur in the financial world each day. Therefore, financial practitioners and analysts consider it an emerging issue of the data management and analytics of different financial products and services. Also, big data has significant impacts on financial products and services. Therefore, identifying the financial issues where big data has a significant influence is also an important issue to explore with the influences. Based on these concepts, the objective of this paper was to show the current landscape of finance dealing with big data, and also to show how big data influences different financial sectors, more specifically, its impact on financial markets, financial institutions, and the relationship with internet finance, financial management, internet credit service companies, fraud detection, risk analysis, financial application management, and so on. The connection between big data and financial-related components will be revealed in an exploratory literature review of secondary data sources. Since big data in the financial field is an extremely new concept, future research directions will be pointed out at the end of this study.
Introduction
In the age of technological innovation, various types of data are available with the advance of information technologies, and data is seen as one of the most valuable commodities in managing automation systems [ 13 , 68 ]. In this sense, financial markets and technological evolution have become related to every human activity in the past few decades. Big data technology has become an integral part of the financial services industry and will continue to drive future innovation [ 12 ]. Financial innovations are also considered the fastest emerging issues in financial services. More specifically, they cover a variety of financial businesses such as online peer-to-peer lending, crowd-funding platforms, SME finance, wealth management and asset management platforms, trading management, crypto-currency, money/remittance transfer, mobile payments platforms, and so on. All of these services create thousands of pieces of data every day. Therefore, managing this data is also considered the most important factor in these services. Any damage to the data can cause serious problems for that specific financial industry. Nowadays, financial analysts use external and alternative data to make better investment decisions. In addition, financial industries use big data through different predictive analyses and monitor various spending patterns to develop large decision-making models. In this way, the industries can decide which financial products to offer [ 29 , 48 ]. Millions of data are transmitted among financial companies. That is why big data is receiving more attention in the financial services arena, where information affects important success and production factors. It has been playing increasingly important roles in consolidating our understanding of financial markets [ 71 ]. In any case, the financial industry is using trillions of pieces of data constantly in everyday decisions [ 22 ]. It plays an important role in changing the financial services sector, particularly in trade and investment, tax reform, fraud detection and investigation, risk analysis, and automation [ 37 ]. In addition, it has changed the financial industry by overcoming different challenges and gaining valuable insights to improve customer satisfaction and the overall banking experience [ 45 ]. Razin [ 65 ] pointed out that big data is also changing finance in five ways: creating transparency, analyzing risk, algorithmic trading, leveraging consumer data and transforming culture. Also, big data has a significant influence in economic analysis and economic modeling [ 16 , 21 ].
In this study, the views of different researchers, academics, and others related to big data and finance activities have been collected and analysed. This study not only attempts to test the existing theory but also to gain an in-depth understanding of the research from the qualitative data. However, research on big data in financial services is not as extensive as other financial areas. Few studies have precisely addressed big data in different financial research contexts. Though some studies have done these for some particular topics, the extensive views of big data in financial services haven’t done before with proper explanation of the influence and opportunity of big data on finance. Therefore, the need to identify the finance areas where big data has a significant influence is addressed. Also, the research related to big data and financial issues is extremely new. Therefore, this study presents the emerging issues of finance where big data has a significant influence, which has never been published yet by other researchers. That is why this research explores the influence of big data on financial services and this is the novelty of this study.
This paper seeks to explore the current landscape of big data in financial services. Particularly this study highlights the influence of big data on internet banking, financial markets, and financial service management. This study also presents a framework, which will facilitate the way how big data influence on finance. Some other services relating to finance are also highlighted here to specify the extended area of big data in financial services. These are the contribution of this study in the existing literatures.
This result of the study contribute to the existing literature which will help readers and researchers who are working on this topic and all target readers will obtain an integrated concept of big data in finance from this study. Furthermore, this research is also important for researchers who are working on this topic. The issue of big data has been explored here from different financing perspectives to provide a clear understanding for readers. Therefore, this study aims to outline the current state of big data technology in financial services. More importantly, an attempt has been made to focus on big data finance activities by concentrating on its impact on the finance sector from different dimensions.
Literature review
The concept of big data in finance has taken from the previous literatures, where some studies have been published by some good academic journals. At present, most of the areas of business are linked to big data. It has significant influence on various perspectives of business such as business process management, human resources management, R&D management [ 8 , 63 ], business analytics [ 19 , 26 , 42 , 59 , 63 ], B2B business process, marketing, and sales [ 30 , 39 , 53 , 58 ], industrial manufacturing process [ 7 , 15 , 40 ], enterprise’s operational performance measurement [ 20 , 69 , 81 ], policy making [ 2 ], supply chain management, decision, and performance [ 4 , 38 , 64 ], and so other business arenas.
Particularly, Rabhi et al. [ 63 ] mentioned big data as a significant factor of business process management& HR process to support the decision making. This study also talked about three sophisticated types of analytics techniques such as descriptive analytics, predictive analytics, and prescriptive analytics in order to improve the traditional data analytics process. Duan and Xiong [ 19 ], Grover and Kar [ 26 ], Ji et al. [ 42 ], and Pappas et al. [ 59 ] also explored the significance of big data in business analytics. Big data helps to solve business problems and data management through system infrastructure, which includes any technique to capture, store, transfer, and process data. Duan and Xiong [ 19 ] found that top-performing organizations use analytics as opposed to intuition almost five times more than do the lower performers. Business analytics and business strategy must be closely linked together to gain better analytics-driven insights. Grover and Kar [ 26 ] mentioned about firms, like Apple, Facebook, Google, Amazon, and eBay, that regularly use digitized transaction data such as storing the transaction time, purchase quantities, product prices, and customer credentials on regular basis to estimate the condition of their market for improving their business operations [ 61 , 76 ]. Holland et al. [ 39 ] showed the theoretical and empirical contributions of big data in business. This study inferred that B2B relationships from consumer search patterns, which used to evaluate and measure the online performance of competitors in the US airline market. Moreover, big data also help to foster B2B sales with customer data analytics. The use of customer’s big datasets significantly improve sales growth (monetary performance outcomes), and enhances the customer relationship performance (non-monetary performance outcomes) [ 30 ]. It also relates to market innovation with diversified opportunities.
Big data and its analytics and applications work as indicators of organizations’ ability to innovate to respond to market opportunities [ 78 ]. Also, big data impact on industrial manufacturing process to gain competitive advantages. After analyzing a case study of two company, Belhadi et al. [ 7 ] stated ‘NAPC aims for a qualitative leap with digital and big - data analytics to enable industrial teams to develop or even duplicate models of turnkey factories in Africa’. This study also identified an Overall framework of BDA capabilities in manufacturing process , and mentioned some values of Big Data Analytics for manufacturing process, such as enhancing transparency, improving performance, supporting decision-making and increasing knowledge. Also, Cui et al. [ 15 ] mentioned four most frequently big data applications (Monitoring, prediction, ICT framework, and data analytics) used in manufacturing. These are essential to realize the smart manufacturing process. Shamim et al. [ 69 ] argued that employee ambidexterity is important because employees’ big data management capabilities and ambidexterity are crucial for EMMNEs to manage the demands of global users. Also big data appeared as a frontier of the opportunity in improving firm performance. Yadegaridehkordi et al. [ 81 ] hypothesized that big data adoption has positive effect on firm performance. That study also mentioned that the policy makers, governments, and businesses can take well-informed decisions in adopting big data. According to Hofmann [ 38 ], velocity, variety, and volume significantly influence on supply chain management. For example, at first, velocity offers the biggest opportunity to intensification the efficiency of the processes in the supply chain. Next to this, variety supports different types of data volume in the supply chains is mostly new. After that, the volume is also a bigger interest for the multistage supply chains than to two-staged supply chains. Raman et al. [ 64 ] provided a new model, Supply Chain Operations Reference (SCOR), by incorporating SCM with big data. This model exposes the adoption of big data technology adds significant value as well as creates financial gain for the industry. This model is apt for the evaluation of the financial performance of supply chains. Also it works as a practical decision support means for examining competing decision alternatives along the chain as well as environmental assessment. Lamba and Singh [ 50 ] focused on decision making aspect of supply chain process and mentioned that data-driven decision-making is gaining noteworthy importance in managing logistics activities, process improvement, cost optimization, and better inventory management. Sahal et al. [ 67 ] and Xu and Duan [ 80 ] showed the relation of cyber physical systems and stream processing platform for Industry 4.0. Big data and IoT are considering as much influential forces for the era of Industry 4.0. These are also helping to achieve the two most important goals of Industry 4.0 applications (to increase productivity while reducing production cost & to maximum uptime throughout the production chain). Belhadi et al. [ 7 ] identified manufacturing process challenges, such as quality & process control (Q&PC), energy & environment efficiency (E&EE), proactive diagnosis and maintenance (PD&M), and safety & risk analysis (S&RA). Hofmann [ 38 ] also mentioned that one of the greatest challenges in the field of big data is to find new ways for storing and processing the different types of data. In addition, Duan and Xiong [ 19 ] mentioned that big data encompass more unstructured data such as text, graph, and time-series data compared to structured data for both data storage techniques and data analytics techniques. Zhao et al. [ 86 ] identified two major challenges for integrating both internal and external data for big data analytics. These are connecting datasets across the data sources, and selecting relevant data for analysis. Huang et al. [ 40 ] raised four challenges, first, the accuracy and applicability of the small data-based PSM paradigms is one kind of challenge; second, the traditional static-oriented PSM paradigms difficult to adapt to the dynamic changes of complex production systems; third, it is urgent to carry out research that focuses on forecasting-based PSM paradigms; and fourth, the determining the causal relationship quickly, economically and effectively is difficult, which affects safety predictions and safety decision-making.
The above discussion based on different area of business. Whatever, some studies (such as [ 6 , 11 , 14 , 22 , 23 , 41 , 45 , 54 , 68 , 71 , 73 , 75 , 83 , 85 ] focused different perspectives of financial services. Still, the contribution on this area is not expanded. Based on those researches, the current trends of big data in finance have specified in finding section.
Methodology
The purpose of this study is to locate academic research focusing on the related studies of big data and finance. To accomplish this research, secondary data sources were used to collect related data [ 31 , 32 , 34 ]. To collect secondary data, the study used the electronic database Scopus, the web of science, and Google scholar [ 33 ]. The keywords of this study are big data finance, finance and big data, big data and the stock market, big data in banking, big data management, and big data and FinTech. The search mainly focused only on academic and peer-reviewed journals, but in some cases, the researcher studied some articles on the Internet which were not published in academic and peer-reviewed journals. Sometimes, information from search engines helps understand the topic. The research area of big data has already been explored but data on big data in finance is not so extensive; this is why we did not limit the search to a certain time period because a time limitation may reduce the scope of the area of this research. Here, a structured and systematic data collection process was followed. Figure 1 presents the structured and systematic data collection process of this study. Certain renowned publishers, for example, Elsevier, Springer, Taylor & Francis, Wiley, Emerald, and Sage, among others, were prioritized when collecting the data for this study [ 35 , 36 ].
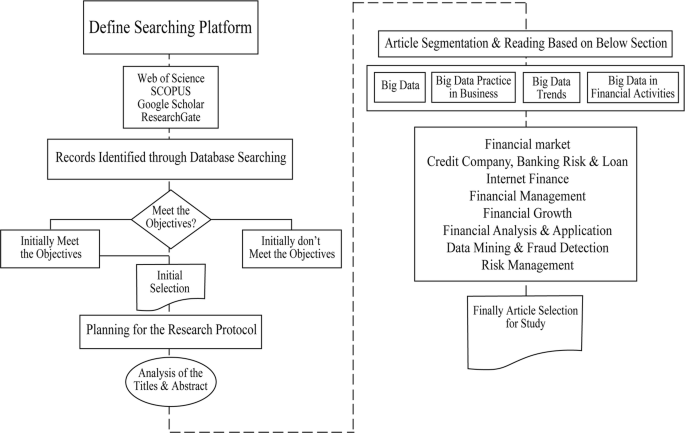
Systematic framework of the research structure. (Source: Author’s illustration)
The number of related articles collected from those databases is only 180. Following this, the collected articles were screened and a shortlist was created, featuring only 100 articles. Finally, data was used from 86 articles, of which 34 articles were directly related to ‘ Big data in Finance’ . Table 1 presents the list of those journals which will help to contribute to future research.
This literature study suggests that some major factors are related to big data and finance. In this context, it has been found that these specific factors also have a deep relationship with big data, such as financial markets, banking risk and lending, internet finance, financial management, financial growth, financial analysis and application, data mining and fraud detection, risk management, and other financial practices. Table 2 describes the focuses within the literature on the financial sector relating to big data.
Theoretical framework
After studying the literature, this study has found that big data is mostly linked to financial market, Internet finance. Credit Service Company, financial service management, financial applications and so forth. Mainly data relates with four types of financial industry such as financial market, online marketplace, lending company, and bank. These companies produce billions of data each day from their daily transaction, user account, data updating, accounts modification, and so other activities. Those companies process the billions of data and take the help to predict the preference of each consumer given his/her previous activities, and the level of credit risk for each user. Based on those data, financial institutions help in taking decisions [ 84 ]. However, different financial companies processing big data and getting help for verification and collection, credit risk prediction, and fraud detection. As the billions of data are producing from heterogeneous sources, missing data is a big concern as well as data quality and data reliability is also significant matter. Whatever, the concept of role of financial big data has taken form [ 71 ], where that study mention the sources of financial market information include the information assembled from stock market data (e.g., stock prices, stock trading volume, interest rates, and so on), social media (e.g., Facebook, twitter, newspapers, advertising, television, and so on). These data has significant roles in financial market such as predicting the market return, forecasting market volatility, valuing market position, identifying excess trading volume, analyzing the market risk, movement of the stock, option pricing, algorithmic trading, idiosyncratic volatility, and so on. Based on these discussions, a theoretical framework is illustrated in Fig. 2 .
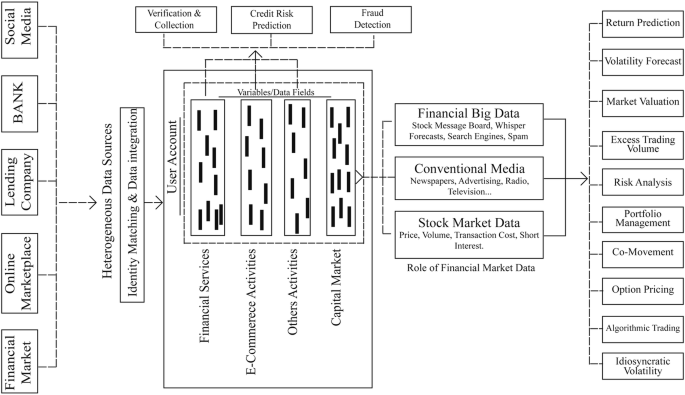
Theoretical framework of big data in financial services. Source: Author’s explanation. (This concept of this framework has been taken from Shen and Chen [ 71 ] and Zhang et al. [ 85 ])
Results and discussion
Massive data and increasingly sophisticated technologies are changing the way industries operate and compete. The financial world is also operating with these big data sets. It has not only influenced many fields of science and society, but has had an important impact on the finance industry [ 6 , 13 , 23 , 41 , 45 , 54 , 62 , 68 , 71 , 72 , 73 , 82 , 85 ]. After reviewing the literature, this study found some financial areas directly linked to big data, such as financial markets, internet credit service-companies and internet finance, financial management, analysis, and applications, credit banking risk analysis, risk management, and so forth. These areas are divided here into three groups; first, big data implications for financial markets and the financial growth of companies; second, big data implications for internet finance and value creation in internet credit-service companies; and third, big data in financial management, risk management, financial analysis, and applications. The discussion of big data in these specified financial areas is the contribution made by this study. Also, these are regarded as emerging landscape of big data in finance in this study.
Big data implications on financial markets
Financial markets always seek technological innovation for different activities, especially technological innovations that are always positively accepted, and which have a great impact on financial markets, and which have truly transforming effects on them. Shen and Chen [ 71 ] explain that the efficiency of financial markets is mostly attributed to the amount of information and its diffusion process. In this sense, social media undoubtedly plays a crucial role in financial markets. In this sense, it is considered one of the most influential forces acting on them. It generates millions of pieces of information every day in financial markets globally [ 9 ]. Big data mainly influences financial markets through return predictions, volatility forecasts, market valuations, excess trading volumes, risk analyses, portfolio management, index performance, co-movement, option pricing, idiosyncratic volatility, and algorithmic trading.
Shen and Chen [ 71 ] focus on the medium effect of big data on the financial market. This effect has two elements, effects on the efficient market hypothesis, and effects on market dynamics. The effect on the efficient market hypothesis refers to the number of times certain stock names are mentioned, the extracted sentiment from the content, and the search frequency of different keywords. Yahoo Finance is a common example of the effect on the efficient market hypothesis. On the other hand, the effect of financial big data usually relies on certain financial theories. Bollen et al. [ 9 ] emphasize that it also helps in sentiment analysis in financial markets, which represents the familiar machine learning technique with big datasets.
In another prospect, Begenau et al. [ 6 ] explore the assumption that big data strangely benefits big firms because of their extended economic activity and longer firm history. Even large firms typically produce more data compared to small firms. Big data also relates corporate finance in different ways such as attracting more financial analysis, as well as reducing equity uncertainty, cutting a firm’s cost of capital, and the costs of investors forecasting related to a financial decision. It cuts the cost of capital as investors process more data to enable large firms to grow larger. In pervasive and transformative information technology, financial markets can process more data, earnings statements, macro announcements, export market demand data, competitors’ performance metrics, and predictions of future returns. By predicting future returns, investors can reduce uncertainty about investment outcomes. In this sense Begenau et al. [ 6 ] stated that “More data processing lowers uncertainty, which reduces risk premia and the cost of capital, making investments more attractive.”.
Big data implications on internet finance and value creation at an internet credit service company
Technological advancements have caused a revolutionary transformation in financial services; especially the way banks and FinTech enterprises provide their services. Thinking about the influence of big data on the financial sector and its services, the process can be highlighted as a modern upgrade to financial access. In particular, online transactions, banking applications, and internet banking produce millions of pieces of data in a single day. Therefore, managing these millions of data is a subject to important [ 46 ]. Because managing these internet financing services has major impacts on financial markets [ 57 ]. Here, Zhang et al. [ 85 ] and Xie et al. [ 79 ] focus on data volume, service variety, information protection, and predictive correctness to show the relationship between information technologies and e-commerce and finance. Big data improves the efficiency of risk-based pricing and risk management while significantly alleviating information asymmetry problems. Also, it helps to verify and collect the data, predict credit risk status, and detect fraud [ 24 , 25 , 56 ]. Jin et al. [ 44 ], [ 47 ], Peji [ 60 ], and Hajizadeh et al. [ 28 ] identified that data mining technology plays vital roles in risk managing and fraud detection.
Big data also has a significant impact on Internet credit service companies. The first impact is to be able to assess more borrowers, even those without a good financial status. Big data also plays a vital role in credit rating bureaus. For example, the two public credit bureaus in China only have 0.3 billion individual’s ‘financial records. For other people, they at most have identity and demographic information (such as ID, name, age, marriage status, and education level), and it is not plausible to obtain reliable credit risk predictions using traditional models. This situation significantly limits financial institutions from approaching new consumers [ 85 ]. In this case, big data benefits by giving the opportunity for unlimited data access. In order to deal with credit risk effectively, financial systems take advantage of transparent information mechanisms. Big data can influence the market-based credit system of both enterprises and individuals by integrating the advantages of cloud computing and information technology. Cloud computing is another motivating factor; by using this cloud computing and big data services, mobile internet technology has opened a crystal price formation process in non-internet-based traditional financial transactions. Besides providing information to both the lenders and borrowers, it creates a positive relationship between the regulatory bodies of both banking and securities sectors. If a company has a large data set from different sources, it leads to multi-dimensional variables. However, managing these big datasets is difficult; sometimes if these datasets are not managed appropriately they may even seem a burden rather than an advantage. In this sense, the concept of data mining technology described in Hajizadeh et al. [ 28 ] to manage a huge volume of data regarding financial markets can contribute to reducing these difficulties. Managing the huge sets of data, the FinTech companies can process their information reliably, efficiently, effectively, and at a comparatively lower cost than the traditional financial institutions. They can analyze and provide services to more customers at greater depth. In addition, they can benefit from the analysis and prediction of systemic financial risks [ 82 ]. However, one critical issue is that individuals or small companies may not be able to afford to access big data directly. In this case, they can take advantage of big data through different information companies such as professional consulting companies, relevant government agencies, relevant private agencies, and so forth.
Big data in managing financial services
Big data is an emerging issue in almost all areas of business. Especially in finance, it effects with a variety of facility, such as financial management, risk management, financial analysis, and managing the data of financial applications. Big data is expressively changing the business models of financial companies and financial management. Also, it is considered a fascinating area nowadays. In this fascinating area, scientists and experts are trying to propose novel finance business models by considering big data methods, particularly, methods for risk control, financial market analysis, creating new finance sentiment indexes from social networks, and setting up information-based tools in different creative ways [ 58 ]. Sun et al. [ 73 ] mentioned the 4 V features of big data. These are volume (large data scale), variety (different data formats), velocity (real-time data streaming), and veracity (data uncertainty). These characteristics comprise different challenges for management, analytics, finance, and different applications. These challenges consist of organizing and managing the financial sector in effective and efficient ways, finding novel business models and handling traditional financial issues. The traditional financial issues are defined as high-frequency trading, credit risk, sentiments, financial analysis, financial regulation, risk management, and so on [ 73 ].
Every financial company receives billions of pieces of data every day but they do not use all of them in one moment. The data helps firms analyze their risk, which is considered the most influential factor affecting their profit maximization. Cerchiello and Giudici [ 11 ] specified systemic risk modelling as one of the most important areas of financial risk management. It mainly, emphasizes the estimation of the interrelationships between financial institutions. It also helps to control both the operational and integrated risk. Choi and Lambert [ 13 ] stated that ‘Big data are becoming more important for risk analysis’. It influences risk management by enhancing the quality of models, especially using the application and behavior scorecards. It also elaborates and interprets the risk analysis information comparatively faster than traditional systems. In addition, it also helps in detecting fraud [ 25 , 56 ] by reducing manual efforts by relating internal as well as external data in issues such as money laundering, credit card fraud, and so on. It also helps in enhancing computational efficiency, handling data storage, creating a visualization toolbox, and developing a sanity-check toolbox by enabling risk analysts to make initial data checks and develop a market-risk-specific remediation plan. Campbell-verduyn et al. [ 10 ] state “Finance is a technology of control, a point illustrated by the use of financial documents, data, models and measures in management, ownership claims, planning, accountability, and resource allocation” .
Moreover, big data techniques help to measure credit banking risk in home equity loans. Every day millions of financial operations lead to growth in companies’ databases. Managing these big databases sometimes creates problems. To resolve those problems, an automatic evaluation of credit status and risk measurements is necessary within a reasonable period of time [ 62 ]. Nowadays, bankers are facing problems in measuring the risks of credit and managing their financial databases. Big data practices are applied to manage financial databases in order to segment different risk groups. Also big data is very helpful for banks to comply with both the legal and the regulatory requirements in the credit risk and integrity risk domains [ 12 ]. A large dataset always needs to be managed with big data techniques to provide faster and unbiased estimators. Financial institutions benefit from improved and accurate credit risk evaluation. This helps to reduce the risks for financial companies in predicting a client’s loan repayment ability. In this way, more and more people get access to credit loans and at the same time banks reduce their credit risks [ 62 ].
Big data and other financial issues
One of the largest data platforms is the Internet, which is clearly playing ever-increasing roles in both the financial markets and personal finance. Information from the Internet always matters. Tumarkin and Whitelaw [ 77 ] examine the relationship between Internet message board activity and abnormal stock returns and trading volume. The study found that abnormal message activity of the stock of the Internet sector changes investors’ opinions in correlation with abnormal industry-adjusted returns, as well as causing trading volume to become abnormally high, since the Internet is the most common channel for information dissemination to investors. As a result, investors are always seeking information from the Internet and other sources. This information is mostly obtained by searching on different search engines. Drake et al. [ 18 ] found that abnormal information searches on search engines increase about two weeks prior to the earnings announcement. This study also suggests that information diffusion is not instantaneous with the release of the earnings information, but rather is spread over a period surrounding the announcement. One more significant correlation identified in this study is that information demand is positively associated with media attention and news, but negatively associated with investor distraction. Dimpfl and Jank [ 17 ] specified that search queries help predict future volatility, and their volatility will exceed the information contained in the lag volatility itself, and the volatility of the search volume will have an impact on volatility, which will last a considerable period of time. Jin et al. [ 43 ] identified that micro blogging also has a significant influence on changing the information environment, which in turn influences changes in stock market behavior.
Conclusions
Big data, machine learning, AI, and the cloud computing are fueling the finance industry toward digitalization. Large companies are embracing these technologies to implement digital transformation, bolster profit and loss, and meet consumer demand. While most companies are storing new and valuable data, the question is the implication and influence of these stored data in finance industry. In this prospect, every financial service is technologically innovative and treats data as blood circulation. Therefore, the findings of this study are reasonable to conclude that big data has revolutionized finance industry mainly with the real time stock market insights by changing trade and investments, fraud detection and prevention, and accurate risk analysis by machine learning process. These services are influencing by increasing revenue and customer satisfaction, speeding up manual processes, improving path to purchase, streamlined workflow and reliable system processing, analyze financial performance, and control growth. Despite these revolutionary service transmissions, several critical issues of big data exist in the finance world. Privacy and protection of data is one the biggest critical issue of big data services. As well as data quality of data and regulatory requirements also considered as significant issues. Even though every financial products and services are fully dependent on data and producing data in every second, still the research on big data and finance hasn’t reached its peak stage. In this perspectives, the discussion of this study reasonable to settle the future research directions. In future, varied research efforts will be important for financial data management systems to address technical challenges in order to realize the promised benefits of big data; in particular, the challenges of managing large data sets should be explored by researchers and financial analysts in order to drive transformative solutions. The common problem is that the larger the industry, the larger the database; therefore, it is important to emphasize the importance of managing large data sets for large companies compared to small firms. Managing such large data sets is expensive, and in some cases very difficult to access. In most cases, individuals or small companies do not have direct access to big data. Therefore, future research may focus on the creation of smooth access for small firms to large data sets. Also, the focus should be on exploring the impact of big data on financial products and services, and financial markets. Research is also essential into the security risks of big data in financial services. In addition, there is a need to expand the formal and integrated process of implementing big data strategies in financial institutions. In particular, the impact of big data on the stock market should continue to be explored. Finally, the emerging issues of big data in finance discussed in this study should be empirically emphasized in future research.
Availability of data and materials
Our data will be available on request.
Abbreviations
Small and medium enterprise
Research & Development
Human resource
Business to Business
Big data analytics
Supply chain management
Internet of things
Production safety management
Financial Technology
Andreasen MM, Christensen JHE, Rudebusch GD. Term structure analysis with big data: one-step estimation using bond prices. J Econom. 2019;212(1):26–46. https://doi.org/10.1016/j.jeconom.2019.04.019 .
Article MathSciNet MATH Google Scholar
Aragona B, Rosa R De. Big data in policy making. Math Popul Stud. 2018;00(00):1–7. https://doi.org/10.1080/08898480.2017.1418113 .
Article Google Scholar
Baak MA, van Hensbergen S. How big data can strengthen banking risk surveillance. Compact, 15–19. https://www.compact.nl/en/articles/how-big-data-can-strengthen-banking-risk-surveillance/ (2015).
Bag S, Wood LC, Xu L, Dhamija P, Kayikci Y. Big data analytics as an operational excellence approach to enhance sustainable supply chain performance. Resour Conserv Recycl. 2020;153:104559. https://doi.org/10.1016/j.resconrec.2019.104559 .
Barr MS, Koziara B, Flood MD, Hero A, Jagadish HV. Big data in finance: highlights from the big data in finance conference hosted at the University of Michigan October 27–28, 2016. SSRN Electron J. 2018. https://doi.org/10.2139/ssrn.3131226 .
Begenau J, Farboodi M, Veldkamp L. Big data in finance and the growth of large firms. J Monet Econ. 2018;97:71–87. https://doi.org/10.1016/j.jmoneco.2018.05.013 .
Belhadi A, Zkik K, Cherrafi A, Yusof SM, El fezazi S. Understanding big data analytics for manufacturing processes: insights from literature review and multiple case studies. Comput Ind Eng. 2019;137:106099. https://doi.org/10.1016/j.cie.2019.106099 .
Blackburn M, Alexander J, Legan JD, Klabjan D. Big data and the future of R&D management: the rise of big data and big data analytics will have significant implications for R&D and innovation management in the next decade. Res Technol Manag. 2017;60(5):43–51. https://doi.org/10.1080/08956308.2017.1348135 .
Bollen J, Mao H, Zeng X. Twitter mood predicts the stock market. J Comput Sci. 2011;2(1):1–8. https://doi.org/10.1016/j.jocs.2010.12.007 .
Campbell-verduyn M, Goguen M, Porter T. Big data and algorithmic governance: the case of financial practices. New Polit Econ. 2017;22(2):1–18. https://doi.org/10.1080/13563467.2016.1216533 .
Cerchiello P, Giudici P. Big data analysis for financial risk management. J Big Data. 2016;3(1):18. https://doi.org/10.1186/s40537-016-0053-4 .
Chen M. How the financial services industry is winning with big data. https://mapr.com/blog/how-financial-services-industry-is-winning-with-big-data/ (2018).
Choi T, Lambert JH. Advances in risk analysis with big data. Risk Anal 2017; 37(8). https://doi.org/10.1111/risa.12859 .
Corporation O. Big data in financial services and banking (Oracle Enterprise Architecture White Paper, Issue February). http://www.oracle.com/us/technologies/big-data/big-data-in-financial-services-wp-2415760.pdf (2015).
Cui Y, Kara S, Chan KC. Manufacturing big data ecosystem: a systematic literature review. Robot Comput Integr Manuf. 2020;62:101861. https://doi.org/10.1016/j.rcim.2019.101861 .
Diebold FX, Ghysels E, Mykland P, Zhang L. Big data in dynamic predictive econometric modeling. J Econ. 2019;212:1–3. https://doi.org/10.1016/j.jeconom.2019.04.017 .
Dimpfl T, Jank S. Can internet search queries help to predict stock market volatility? Eur Financ Manag. 2016;22(2):171–92. https://doi.org/10.1111/eufm.12058 .
Drake MS, Roulstone DT, Thornock JR. Investor information demand: evidence from Google Searches around earnings announcements. J Account Res. 2012;50(4):1001–40. https://doi.org/10.1111/j.1475-679X.2012.00443.x .
Duan L, Xiong Y. Big data analytics and business analytics. J Manag Anal. 2015;2(1):1–21. https://doi.org/10.1080/23270012.2015.1020891 .
Dubey R, Gunasekaran A, Childe SJ, Bryde DJ, Giannakis M, Foropon C, Roubaud D, Hazen BT. Big data analytics and artificial intelligence pathway to operational performance under the effects of entrepreneurial orientation and environmental dynamism: a study of manufacturing organisations. Int J Prod Econ. 2019. https://doi.org/10.1016/j.ijpe.2019.107599 .
Einav L, Levin J. The data revolution and economic analysis. Innov Policy Econ. 2014;14(1):1–24. https://doi.org/10.1086/674019 .
Ewen J. How big data is changing the finance industry. https://www.tamoco.com/blog/big-data-finance-industry-analytics/ (2019).
Fanning K, Grant R. Big data: implications for financial managers. J Corp Account Finance. 2013. https://doi.org/10.1002/jcaf.21872 .
Glancy FH, Yadav SB. A computational model for fi nancial reporting fraud detection. Decis Support Syst. 2011;50(3):595–601. https://doi.org/10.1016/j.dss.2010.08.010 .
Gray GL, Debreceny RS. A taxonomy to guide research on the application of data mining to fraud detection in financial statement audits. Int J Account Inform Sys. 2014. https://doi.org/10.1016/j.accinf.2014.05.006 .
Grover P, Kar AK. Big data analytics: a review on theoretical contributions and tools used in literature. Global J Flex Sys Manag. 2017;18(3):203–29. https://doi.org/10.1007/s40171-017-0159-3 .
Hagenau M, Liebmann M, Neumann D. Automated news reading: stock price prediction based on financial news using context-capturing features. Decis Support Syst. 2013;55(3):685–97. https://doi.org/10.1016/j.dss.2013.02.006 .
Hajizadeh E, Ardakani HD, Shahrabi J. Application of data mining techniques in stock markets: a survey. J Econ Int Finance. 2010;2(7):109–18.
Google Scholar
Hale G, Lopez JA. Monitoring banking system connectedness with big data. J Econ. 2019;212(1):203–20. https://doi.org/10.1016/j.jeconom.2019.04.027 .
Article MATH Google Scholar
Hallikainen H, Savimäki E, Laukkanen T. Fostering B2B sales with customer big data analytics. Ind Mark Manage. 2019. https://doi.org/10.1016/j.indmarman.2019.12.005 .
Hasan MM, Mahmud A. Risks management of ready-made garments industry in Bangladesh. Int Res J Bus Stud. 2017;10(1):1–13. https://doi.org/10.21632/irjbs.10.1.1-13 .
Hasan MM, Mahmud A, Islam MS. Deadly incidents in Bangladeshi apparel industry and illustrating the causes and effects of these incidents. J Finance Account. 2017;5(5):193–9. https://doi.org/10.11648/j.jfa.20170505.13 .
Hasan MM, Nekmahmud M, Yajuan L, Patwary MA. Green business value chain: a systematic review. Sustain Prod Consum. 2019;20:326–39. https://doi.org/10.1016/J.SPC.2019.08.003 .
Hasan MM, Parven T, Khan S, Mahmud A, Yajuan L. Trends and impacts of different barriers on Bangladeshi RMG Industry’s sustainable development. Int Res J Bus Stud. 2018;11(3):245–60. https://doi.org/10.21632/irjbs.11.3.245-260 .
Hasan MM, Yajuan L, Khan S. Promoting China’s inclusive finance through digital financial services. Global Bus Rev. 2020. https://doi.org/10.1177/0972150919895348 .
Hasan MM, Yajuan L, Mahmud A. Regional development of China’s inclusive finance through financial technology. SAGE Open. 2020. https://doi.org/10.1177/2158244019901252 .
Hill C. Where big data is taking the financial industry: trends in 2018. Big data made simple. https://bigdata-madesimple.com/where-big-data-is-taking-the-financial-industry-trends-in-2018/ (2018).
Hofmann E. Big data and supply chain decisions: the impact of volume, variety and velocity properties on the bullwhip effect. Int J Prod Res. 2017;55(17):5108–26. https://doi.org/10.1080/00207543.2015.1061222 .
Holland CP, Thornton SC, Naudé P. B2B analytics in the airline market: harnessing the power of consumer big data. Ind Mark Manage. 2019. https://doi.org/10.1016/j.indmarman.2019.11.002 .
Huang L, Wu C, Wang B. Challenges, opportunities and paradigm of applying big data to production safety management: from a theoretical perspective. J Clean Prod. 2019;231:592–9. https://doi.org/10.1016/j.jclepro.2019.05.245 .
Hussain K, Prieto E. Big data in the finance and insurance sectors. In: Cavanillas JM, Curry E, Wahlster W, editors. New horizons for a data-driven economy: a roadmap for usage and exploitation of big data in Europe. SpringerOpen: Cham; 2016. p. 2019–223. https://doi.org/10.1007/978-3-319-21569-3 .
Chapter Google Scholar
Ji W, Yin S, Wang L. A big data analytics based machining optimisation approach. J Intell Manuf. 2019;30(3):1483–95. https://doi.org/10.1007/s10845-018-1440-9 .
Jin X, Shen D, Zhang W. Has microblogging changed stock market behavior? Evidence from China. Physica A. 2016;452:151–6. https://doi.org/10.1016/j.physa.2016.02.052 .
Jin M, Wang Y, Zeng Y. Application of data mining technology in financial risk. Wireless Pers Commun. 2018. https://doi.org/10.1007/s11277-018-5402-5 .
Joshi N. How big data can transform the finance industry. BBN Times. https://www.bbntimes.com/en/technology/big-data-is-transforming-the-finance-industry .
Kh R. How big data can play an essential role in Fintech Evolutionno title. Smart Dala Collective. https://www.smartdatacollective.com/fintech-big-data-play-role-financial-evolution/ (2018).
Khadjeh Nassirtoussi A, Aghabozorgi S, Ying Wah T, Ngo DCL. Text mining for market prediction: a systematic review. Expert Syst Appl. 2014;41(16):7653–70. https://doi.org/10.1016/j.eswa.2014.06.009 .
Khan F. Big data in financial services. https://medium.com/datadriveninvestor/big-data-in-financial-services-d62fd130d1f6 (2018).
Kshetri N. Big data’s role in expanding access to financial services in China. Int J Inf Manage. 2016;36(3):297–308. https://doi.org/10.1016/j.ijinfomgt.2015.11.014 .
Lamba K, Singh SP. Big data in operations and supply chain management: current trends and future perspectives. Prod Plan Control. 2017;28(11–12):877–90. https://doi.org/10.1080/09537287.2017.1336787 .
Lien D. Business Finance and Enterprise Management in the era of big data: an introduction. North Am J Econ Finance. 2017;39:143–4. https://doi.org/10.1016/j.najef.2016.10.002 .
Liu S, Shao B, Gao Y, Hu S, Li Y, Zhou W. Game theoretic approach of a novel decision policy for customers based on big data. Electron Commer Res. 2018;18(2):225–40. https://doi.org/10.1007/s10660-017-9259-6 .
Liu Y, Soroka A, Han L, Jian J, Tang M. Cloud-based big data analytics for customer insight-driven design innovation in SMEs. Int J Inf Manage. 2019. https://doi.org/10.1016/j.ijinfomgt.2019.11.002 .
Mohamed TS. How big data does impact finance. Aksaray: Aksaray University; 2019.
Mulla J, Van Vliet B. FinQL: a query language for big data in finance. SSRN Electron J. 2015. https://doi.org/10.2139/ssrn.2685769 .
Ngai EWT, Hu Y, Wong YH, Chen Y, Sun X. The application of data mining techniques in financial fraud detection: a classification framework and an academic review of literature. Decis Support Syst. 2011;50(3):559–69. https://doi.org/10.1016/j.dss.2010.08.006 .
Niu S. Prevention and supervision of internet financial risk in the context of big data. Revista de La Facultad de Ingeniería. 2017;32(11):721–6.
Oracle. (2012) Financial services data management: big Data technology in financial services (Issue June).
Pappas IO, Mikalef P, Giannakos MN, Krogstie J, Lekakos G. Big data and business analytics ecosystems: paving the way towards digital transformation and sustainable societies. IseB. 2018;16(3):479–91. https://doi.org/10.1007/s10257-018-0377-z .
Peji M. Text mining for big data analysis in financial sector: a literature review. Sustainability. 2019. https://doi.org/10.3390/su11051277 .
Pousttchi K, Hufenbach Y. Engineering the value network of the customer interface and marketing in the data-Rich retail environment. Int J Electron Commer. 2015. https://doi.org/10.2753/JEC1086-4415180401 .
Pérez-Martín A, Pérez-Torregrosa A, Vaca M. Big Data techniques to measure credit banking risk in home equity loans. J Bus Res. 2018. https://doi.org/10.1016/j.jbusres.2018.02.008 .
Rabhi L, Falih N, Afraites A, Bouikhalene B. Big data approach and its applications in various fields: review. Proc Comput Sci. 2019;155(2018):599–605. https://doi.org/10.1016/j.procs.2019.08.084 .
Raman S, Patwa N, Niranjan I, Ranjan U, Moorthy K, Mehta A. Impact of big data on supply chain management. Int J Logist Res App. 2018;21(6):579–96. https://doi.org/10.1080/13675567.2018.1459523 .
Razin E. Big buzz about big data: 5 ways big data is changing finance. Forbes. https://www.forbes.com/sites/elyrazin/2015/12/03/big-buzz-about-big-data-5-ways-big-data-is-changing-finance/#1d055654376a (2019).
Retail banks and big data: big data as the key to better risk management. In: The Economist Intelligence Unit. https://eiuperspectives.economist.com/sites/default/files/RetailBanksandBigData.pdf (2014).
Sahal R, Breslin JG, Ali MI. Big data and stream processing platforms for Industry 4.0 requirements mapping for a predictive maintenance use case. J Manuf Sys. 2020;54:138–51. https://doi.org/10.1016/j.jmsy.2019.11.004 .
Schiff A, McCaffrey M. Redesigning digital finance for big data. SSRN Electron J. 2017. https://doi.org/10.2139/ssrn.2967122 .
Shamim S, Zeng J, Shafi Choksy U, Shariq SM. Connecting big data management capabilities with employee ambidexterity in Chinese multinational enterprises through the mediation of big data value creation at the employee level. Int Bus Rev. 2019. https://doi.org/10.1016/j.ibusrev.2019.101604 .
Shen Y (n.d.). Study on internet financial risk early warning based on big data analysis. 1919–1922.
Shen D, Chen S. Big data finance and financial markets. In: Computational social sciences (pp. 235–248). https://doi.org/10.1007/978-3-319-95465-3_12235 (2018).
Shen Y, Shen M, Chen Q. Measurement of the new economy in China: big data approach. China Econ J. 2016;9(3):304–16. https://doi.org/10.1080/17538963.2016.1211384 .
Sun Y, Shi Y, Zhang Z. Finance big data: management, analysis, and applications. Int J Electron Commer. 2019;23(1):9–11. https://doi.org/10.1080/10864415.2018.1512270 .
Sun W, Zhao Y, Sun L. Big data analytics for venture capital application: towards innovation performance improvement. Int J Inf Manage. 2018. https://doi.org/10.1016/j.ijinfomgt.2018.11.017 .
Tang Y, Xiong JJ, Luo Y, Zhang Y, Tang Y. How do the global stock markets Influence one another? Evidence from finance big data and granger causality directed network. Int J Electron Commer. 2019;23(1):85–109. https://doi.org/10.1080/10864415.2018.1512283 .
Thackeray R, Neiger BL, Hanson CL, Mckenzie JF. Enhancing promotional strategies within social marketing programs: use of Web 2.0 social media. Health Promot Pract. 2008. https://doi.org/10.1177/1524839908325335 .
Tumarkin R, Whitelaw RF. News or noise? Internet postings and stock prices. Financ Anal J. 2001;57(3):41–51. https://doi.org/10.2469/faj.v57.n3.2449 .
Wright LT, Robin R, Stone M, Aravopoulou DE. Adoption of big data technology for innovation in B2B marketing. J Business-to-Business Mark. 2019;00(00):1–13. https://doi.org/10.1080/1051712X.2019.1611082 .
Xie P, Zou C, Liu H. The fundamentals of internet finance and its policy implications in China. China Econ J. 2016;9(3):240–52. https://doi.org/10.1080/17538963.2016.1210366 .
Xu L Da, Duan L. Big data for cyber physical systems in industry 4.0: a survey. Enterp Inf Syst. 2019;13(2):148–69. https://doi.org/10.1080/17517575.2018.1442934 .
Article MathSciNet Google Scholar
Yadegaridehkordi E, Nilashi M, Shuib L, Nasir MH, Asadi M, Samad S, Awang NF. The impact of big data on firm performance in hotel industry. Electron Commer Res Appl. 2020;40:100921. https://doi.org/10.1016/j.elerap.2019.100921 .
Yang D, Chen P, Shi F, Wen C. Internet finance: its uncertain legal foundations and the role of big data in its development. Emerg Mark Finance Trade. 2017. https://doi.org/10.1080/1540496X.2016.1278528 .
Yu S, Guo S. Big data in finance. Big data concepts, theories, and application. Cham: Springer International Publishing; 2016. p. 391–412. https://doi.org/10.1007/978-3-319-27763-9 .
Yu ZH, Zhao CL, Guo SX(2017). Research on enterprise credit system under the background of big data. In: 3rd International conference on education and social development (ICESD 2017), ICESD, 903–906. https://doi.org/10.2991/wrarm-17.2017.77 .
Zhang S, Xiong W, Ni W, Li X. Value of big data to finance: observations on an internet credit Service Company in China. Financial Innov. 2015. https://doi.org/10.1186/s40854-015-0017-2 .
Zhao JL, Fan S, Hu D. Business challenges and research directions of management analytics in the big data era. J Manag Anal. 2014;1(3):169–74. https://doi.org/10.1080/23270012.2014.968643 .
Download references
Acknowledgements
All the authors are acknowledged to the reviewers who made significant comments on the review stage.
The project is funded under the program of the Minister of Science and Higher Education titled “Regional Initiative of Excellence in 2019-2022, project number 018/RID/2018/19, the amount of funding PLN 10 788 423 16”.
Author information
Authors and affiliations.
School of Finance, Nanjing Audit University, Nanjing, 211815, China
Md. Morshadul Hasan
WSB University, Cieplaka 1c, 41-300, Dabrowa Górnicza, Poland
József Popp & Judit Oláh
You can also search for this author in PubMed Google Scholar
Contributions
All the authors have the equal contribution on this paper. All authors read and approved the final manuscript.
Corresponding author
Correspondence to József Popp .
Ethics declarations
Competing interests.
There is no competing interests.
Additional information
Publisher's note.
Springer Nature remains neutral with regard to jurisdictional claims in published maps and institutional affiliations.
Rights and permissions
Open Access This article is licensed under a Creative Commons Attribution 4.0 International License, which permits use, sharing, adaptation, distribution and reproduction in any medium or format, as long as you give appropriate credit to the original author(s) and the source, provide a link to the Creative Commons licence, and indicate if changes were made. The images or other third party material in this article are included in the article's Creative Commons licence, unless indicated otherwise in a credit line to the material. If material is not included in the article's Creative Commons licence and your intended use is not permitted by statutory regulation or exceeds the permitted use, you will need to obtain permission directly from the copyright holder. To view a copy of this licence, visit http://creativecommons.org/licenses/by/4.0/ .
Reprints and permissions
About this article
Cite this article.
Hasan, M.M., Popp, J. & Oláh, J. Current landscape and influence of big data on finance. J Big Data 7 , 21 (2020). https://doi.org/10.1186/s40537-020-00291-z
Download citation
Received : 31 August 2019
Accepted : 17 February 2020
Published : 12 March 2020
DOI : https://doi.org/10.1186/s40537-020-00291-z
Share this article
Anyone you share the following link with will be able to read this content:
Sorry, a shareable link is not currently available for this article.
Provided by the Springer Nature SharedIt content-sharing initiative
- Big data finance
- Big data in financial services
- Big data in risk management
- Data management
Help | Advanced Search
Computer Science > Distributed, Parallel, and Cluster Computing
Title: analysis of distributed algorithms for big-data.
Abstract: The parallel and distributed processing are becoming de facto industry standard, and a large part of the current research is targeted on how to make computing scalable and distributed, dynamically, without allocating the resources on permanent basis. The present article focuses on the study and performance of distributed and parallel algorithms their file systems, to achieve scalability at local level (OpenMP platform), and at global level where computing and file systems are distributed. Various applications, algorithms,file systems have been used to demonstrate the areas, and their performance studies have been presented. The systems and applications chosen here are of open-source nature, due to their wider applicability.
Submission history
Access paper:.
- HTML (experimental)
- Other Formats

References & Citations
- Google Scholar
- Semantic Scholar
BibTeX formatted citation

Bibliographic and Citation Tools
Code, data and media associated with this article, recommenders and search tools.
- Institution
arXivLabs: experimental projects with community collaborators
arXivLabs is a framework that allows collaborators to develop and share new arXiv features directly on our website.
Both individuals and organizations that work with arXivLabs have embraced and accepted our values of openness, community, excellence, and user data privacy. arXiv is committed to these values and only works with partners that adhere to them.
Have an idea for a project that will add value for arXiv's community? Learn more about arXivLabs .
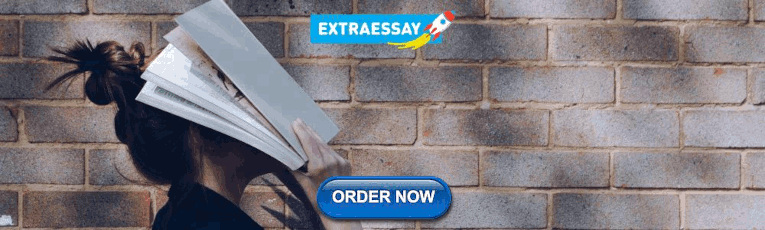
Big Data: Current Challenges and Future Scope
Ieee account.
- Change Username/Password
- Update Address
Purchase Details
- Payment Options
- Order History
- View Purchased Documents
Profile Information
- Communications Preferences
- Profession and Education
- Technical Interests
- US & Canada: +1 800 678 4333
- Worldwide: +1 732 981 0060
- Contact & Support
- About IEEE Xplore
- Accessibility
- Terms of Use
- Nondiscrimination Policy
- Privacy & Opting Out of Cookies
A not-for-profit organization, IEEE is the world's largest technical professional organization dedicated to advancing technology for the benefit of humanity. © Copyright 2024 IEEE - All rights reserved. Use of this web site signifies your agreement to the terms and conditions.
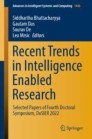
Doctoral Symposium on Intelligence Enabled Research
DoSIER 2022: Recent Trends in Intelligence Enabled Research pp 221–233 Cite as
Applications of Big Data in Various Fields: A Survey
- Sukhendu S. Mondal 18 ,
- Somen Mondal 18 &
- Sudip Kumar Adhikari ORCID: orcid.org/0000-0001-5174-397X 18
- Conference paper
- First Online: 23 June 2023
83 Accesses
Part of the book series: Advances in Intelligent Systems and Computing ((AISC,volume 1446))
A large volume of data is produced from the digital transformation with the extensive use of Internet and global communication system. Big data denotes this extensive heave of data which cannot be managed by traditional data handling methods and techniques. This data is generated in every few milliseconds in the form of structured, semi-structured, and unstructured data. Big data analytics are extensively used in enterprise which plays an important role in various fields of application. This paper presents applications of big data in various fields such as healthcare systems, social media data, e-commerce applications, agriculture application, smart city application, and intelligent transport system. The paper also tries to focus on the characteristics, storage technology of using big data in these applications. This survey provides a clear view of the state-of-the-art research areas on big data technologies and its applications in recent past.
This is a preview of subscription content, log in via an institution .
Buying options
- Available as PDF
- Read on any device
- Instant download
- Own it forever
- Available as EPUB and PDF
- Compact, lightweight edition
- Dispatched in 3 to 5 business days
- Free shipping worldwide - see info
Tax calculation will be finalised at checkout
Purchases are for personal use only
Singh, N., Lai, K.H., Vejvar, M., Cheng, T.C.E.: Big data technology: challenges, prospects, and realities. IEEE Eng. Manage. Rev. 47 (1), 58–66 (2019)
Article Google Scholar
Imran, S., Mahmood, T., Morshed, A., Sellis T.: Big data analytics in healthcare-a systematic literature review and roadmap for practical implementation, IEEE/CAA J. Automatica Sinica 8 (1), (2021)
Google Scholar
Tsai, C.W., Lai, C.F., Chao, H.C., Vasilakos, A.V.: Big data analytics: a survey. J. Big Data 21 (2015)
Rabhi, L., Falih, N., Afraites, A., Bouikhalene, B: Big data approach and its applications in various fields: review. Procedia Comput. Sci. 155 , 599–605 (2019)
Diebold, F.X.: Big data’ dynamic factor models for macroeconomic measurement and forecasting. In: Advances in Economics and Econometrics, Eighth World Congress of the Econometric Society, pp. 115–122 (2000)
Laney, D.: 3D data management: Controlling data volume, velocity, and variety, META Group, Tech. Rep., Feb. (2001)
Demchenko, Y., Ngo, C., Membrey, P.: Architecture framework and components for the big data ecosystem Draft Version 0.2, System and Network Engineering, SNE technical report SNE-UVA-2013–02, Sept (2013)
Harrison, G: Next Generation Databases: NoSQL, NewSQL, and Big Data. Apress (2015)
Wu, X., Kadambi, S., Kandhare, D., Ploetz, A.: Seven NoSQL Databases in a Week: Get Up and Running with the Fundamentals and Functionalities of Seven of the Most Popular NoSQL Databases Kindle. Packt Publishing, USA (2018)
Marz, N., Warren, J.: Big Data: Principles and Best Practices of Scalable Realtime Data Systems, USA. Manning Publications, Greenwich (2015)
Tudorica, B. G. and Bucur, C.: A comparison between several NoSQL databases with comments and notes. In: Proceeding RoEduNet International Conference 10th Edition: Networking in Education and Research. Iasi, Romania (2011)
Thusoo, A., Sarma, J.S., Jain, N., Shao, Z., Chakka, P., Zhang, N., Antony, S., Liu, H., Murthy, R.: Hive—A petabyte scale data warehouse using Hadoop. In: Proceeding of the IEEE 26th International Conference Data Engineering, pp. 996–1005. Long Beach, USA (2010)
Ercan, M. and Lane, M.: An evaluation of the suitability of NoSQL databases for distributed EHR systems. In: Proceeding 25th Australasian Conferences Information Systems. Auckland, New Zealand (2014)
Lee, B., Jeong, E.: A design of a patient-customized healthcare system based on the Hadoop with text mining (PHSHT) for an efficient disease management and prediction. Int. J. Softw. Eng. Appl. 8 (8), 131–150 (2014)
Yang, C.T., Liu, J.C., Hsu, W.H., Lu, H.W., Chu, W.C.C.: Implementation of data transform method into NoSQL database for healthcare data. In: Proceeding International Conference Parallel and Distributed Computing, pp. 198–205. Applications and Technologies, Taipei, China (2013)
Park, Y., Shankar, M, Park, B.H., Ghosh, J.: Graph databases for large-scale healthcare systems: a framework for efficient data management and data services. In: Proceeding of the IEEE 30th International Conference Data Engineering Workshops. Chicago, USA, (2014)
Štufi, M., Bacic, B., Stoimenov, L.: Big data analytics and processing platform in Czech republic healthcare. Appl. Sci. 10 (5), 1705 (2020)
Gopinath, M. P., Tamilzharasi, G.S., Aarthy, S. L. and Mohanasundram, R: An analysis and performance evaluation of NoSQL databases for efficient data management in e-health clouds. Int. J. Pure Appl. Math. 117 (21), 177–197 (2017)
Chen, K.L., Lee, H.: The impact of big data on the healthcare information systems, in transactions of the. In: International Conference Health Information Technology Advancement (2013)
Thorlby, R., Jorgensen, S., Siegel, B., Ayanian, J.Z.: How health care organizations are using data on patients’ race and ethnicity to improve quality of care. Milbank Quart. 89 (2), 226–255 (2011)
Zillner, S., Lasierra, N., Faix, W., Neururer, S.: User needs and requirements analysis for big data healthcare applications. Stud. Health Technol. Inform. 205 , 657–661 (2014)
Boinepelli, H.: Applications of big data, in Big Data. In: Primer, A. (Ed.) Springer, New Delhi, India, pp. 161–179 (2015)
Hood, L., Lovejoy, J.C., Price, N.D.: Integrating big data and actionable health coaching to optimize wellness. BMC Med. 13 (1), 4 (2015)
Rahman, M.S., Reza, H.: A systematic review towards big data analytics in social media. Big Data Min. Anal. 5 (3), 228–244 (2022)
Hou, Q., Han, M., Cai, Z.: Survey on data analysis in social media: a practical application aspect. Big Data Min. Anal. 3 (4), 259–279 (2020)
Dhawan, V., Zanini, N.: Big data and social media analytics. Res. Matt. Cambridge Assess. Publ. 18 , 36–41 (2014)
Ghani, N.A., Hamid, S., Targio Hashem, I.A, Ahmed, E.: Social media big data analytics: a survey. Comput. Hum. Behav. 101 , 417–428 (2019)
Ayele, W.Y., Juell-Skielse, G.: Social media analytics and internet of things: Survey. In: Proceeding 1st International Conference on Internet of Things and Machine Learning, pp. 1–11. Liverpool, UK (2017)
Alrumiah, S.S., Hadwan, M.: Implementing big data analytics in E-commerce: vendor and customer view. IEEE Access 9 , 37281–37286 (2021)
Akter, S., Wamba, S.F.: Big data analytics in E-commerce: a systematic review and agenda for future research. Electron. Market. 26 (2), 173–194 (2016)
Moorthi, K., Srihari, K., Karthik, S.: A survey on impact of big data in E-commerce. Int. J. Pure Appl. Math. 116 (21), 183–188 (2017)
Feng, P.: Big data analysis of E-commerce based on the internet of things. In: 2019 International Conference on Intelligent Transportation, Big Data and Smart City (ICITBS), pp. 345–347 (2019)
Bhat, S.A., Huang, N.F.: Big data and AI revolution in precision agriculture: survey and challenges. IEEE Access 9 , 110209–110222 (2021)
Bermeo-Almeida, O., Cardenas-Rodriguez, M., Samaniego-Cobo, T., Ferruzola-Gómez, E., Cabezas-Cabezas, R., Bazán-Vera, W.: Blockchain in agriculture: a systematic literature review. In: Proceeding International Conference Technology Innovations, pp. 44–56. Springer, Cham, Switzerland (2018)
Lokhande, S.A.: Effective use of big data in precision agriculture. In: 2021 International Conference on Emerging Smart Computing and Informatics (ESCI), pp. 312–316 (2021)
Jedlička, K., Charvát, K.: Visualisation of Big Data in Agriculture and Rural Development, 2018 IST-Africa Week Conference (IST-Africa), pp. 1–8 (2018)
Spandana Vaishnavi, A, Ashish, A, Sai-Pranavi, N., Amulya, S.: Big Data Analytics Based Smart Agriculture. In: 2021 6th International Conference on Communication and Electronics Systems (ICCES), pp. 534–537 (2021)
Kumar, M., Nagar, M.: Big data analytics in agriculture and distribution channel. In: 2017 International Conference on Computing Methodologies and Communication (ICCMC), pp. 384–387 (2017)
Talebkhah, M., Sali, A., Marjani, M., Gordan, M., Hashim, S.J., Rokhani, F.Z.: IoT and big data applications in smart cities: recent advances challenges, and critical issues. IEEE Access 9 , 55465–55484 (2021)
Alshawish, R.A., Alfagih, S.A.M., Musbah, M.S.: Big data applications in smart cities. 2016 International Conference on Engineering & MIS (ICEMIS), pp. 1–7 (2016)
Ismail, A.: Utilizing big data analytics as a solution for smart cities. In: 2016 3rd MEC International Conference on Big Data and Smart City (ICBDSC), pp. 1–5 (2016)
Costa, C., Santos, M.Y.: BASIS: A big data architecture for smart cities. 2016 SAI Comput. Conf. (SAI), pp. 1247–1256 (2016)
Manjunatha, Annappa, B.: Real time big data analytics in smart city applications. In: 2018 International Conference on Communication, Computing and Internet of Things (IC3IoT), pp. 279–284 (2018)
Rathore, M.M., Ahmad, A. Paul, A.: IoT-based smart city development using big data analytical approach. In: 2016 IEEE International Conference on Automatica (ICA-ACCA), pp. 1–8 (2016)
Zhu, L., Yu, F.R., Wang, Y., Ning, B., Tang, T.: Big data analytics in intelligent transportation systems: a survey. In: IEEE Transactions on Intelligent Transportation Systems, vol. 20, no. 1, pp. 383–398 (2019)
Guido, G., Rogano, D., Vitale, A., Astarita, V. and Festa, D.: Big data for public transportation: A DSS framework. In: 2017 5th IEEE International Conference on Models and Technologies for Intelligent Transportation Systems (MT-ITS) (2017)
Download references
Author information
Authors and affiliations.
Cooch Behar Government Engineering College, Cooch Behar, West Bengal, India
Sukhendu S. Mondal, Somen Mondal & Sudip Kumar Adhikari
You can also search for this author in PubMed Google Scholar
Corresponding author
Correspondence to Sudip Kumar Adhikari .
Editor information
Editors and affiliations.
Rajnagar Mahavidyalaya, Birbhum, India
Siddhartha Bhattacharyya
Cooch Behar Government Engineering College, Cooch Behar, India
Algebra University College, Zagreb, Croatia
Rights and permissions
Reprints and permissions
Copyright information
© 2023 The Author(s), under exclusive license to Springer Nature Singapore Pte Ltd.
About this paper
Cite this paper.
Mondal, S.S., Mondal, S., Adhikari, S.K. (2023). Applications of Big Data in Various Fields: A Survey. In: Bhattacharyya, S., Das, G., De, S., Mrsic, L. (eds) Recent Trends in Intelligence Enabled Research. DoSIER 2022. Advances in Intelligent Systems and Computing, vol 1446. Springer, Singapore. https://doi.org/10.1007/978-981-99-1472-2_19
Download citation
DOI : https://doi.org/10.1007/978-981-99-1472-2_19
Published : 23 June 2023
Publisher Name : Springer, Singapore
Print ISBN : 978-981-99-1471-5
Online ISBN : 978-981-99-1472-2
eBook Packages : Intelligent Technologies and Robotics Intelligent Technologies and Robotics (R0)
Share this paper
Anyone you share the following link with will be able to read this content:
Sorry, a shareable link is not currently available for this article.
Provided by the Springer Nature SharedIt content-sharing initiative
- Publish with us
Policies and ethics
- Find a journal
- Track your research

An official website of the United States government
The .gov means it’s official. Federal government websites often end in .gov or .mil. Before sharing sensitive information, make sure you’re on a federal government site.
The site is secure. The https:// ensures that you are connecting to the official website and that any information you provide is encrypted and transmitted securely.
- Publications
- Account settings
Preview improvements coming to the PMC website in October 2024. Learn More or Try it out now .
- Advanced Search
- Journal List
- Springer Nature - PMC COVID-19 Collection

The use of Big Data Analytics in healthcare
Kornelia batko.
1 Department of Business Informatics, University of Economics in Katowice, Katowice, Poland
Andrzej Ślęzak
2 Department of Biomedical Processes and Systems, Institute of Health and Nutrition Sciences, Częstochowa University of Technology, Częstochowa, Poland
Associated Data
The datasets for this study are available on request to the corresponding author.
The introduction of Big Data Analytics (BDA) in healthcare will allow to use new technologies both in treatment of patients and health management. The paper aims at analyzing the possibilities of using Big Data Analytics in healthcare. The research is based on a critical analysis of the literature, as well as the presentation of selected results of direct research on the use of Big Data Analytics in medical facilities. The direct research was carried out based on research questionnaire and conducted on a sample of 217 medical facilities in Poland. Literature studies have shown that the use of Big Data Analytics can bring many benefits to medical facilities, while direct research has shown that medical facilities in Poland are moving towards data-based healthcare because they use structured and unstructured data, reach for analytics in the administrative, business and clinical area. The research positively confirmed that medical facilities are working on both structural data and unstructured data. The following kinds and sources of data can be distinguished: from databases, transaction data, unstructured content of emails and documents, data from devices and sensors. However, the use of data from social media is lower as in their activity they reach for analytics, not only in the administrative and business but also in the clinical area. It clearly shows that the decisions made in medical facilities are highly data-driven. The results of the study confirm what has been analyzed in the literature that medical facilities are moving towards data-based healthcare, together with its benefits.
Introduction
The main contribution of this paper is to present an analytical overview of using structured and unstructured data (Big Data) analytics in medical facilities in Poland. Medical facilities use both structured and unstructured data in their practice. Structured data has a predetermined schema, it is extensive, freeform, and comes in variety of forms [ 27 ]. In contrast, unstructured data, referred to as Big Data (BD), does not fit into the typical data processing format. Big Data is a massive amount of data sets that cannot be stored, processed, or analyzed using traditional tools. It remains stored but not analyzed. Due to the lack of a well-defined schema, it is difficult to search and analyze such data and, therefore, it requires a specific technology and method to transform it into value [ 20 , 68 ]. Integrating data stored in both structured and unstructured formats can add significant value to an organization [ 27 ]. Organizations must approach unstructured data in a different way. Therefore, the potential is seen in Big Data Analytics (BDA). Big Data Analytics are techniques and tools used to analyze and extract information from Big Data. The results of Big Data analysis can be used to predict the future. They also help in creating trends about the past. When it comes to healthcare, it allows to analyze large datasets from thousands of patients, identifying clusters and correlation between datasets, as well as developing predictive models using data mining techniques [ 60 ].
This paper is the first study to consolidate and characterize the use of Big Data from different perspectives. The first part consists of a brief literature review of studies on Big Data (BD) and Big Data Analytics (BDA), while the second part presents results of direct research aimed at diagnosing the use of big data analyses in medical facilities in Poland.
Healthcare is a complex system with varied stakeholders: patients, doctors, hospitals, pharmaceutical companies and healthcare decision-makers. This sector is also limited by strict rules and regulations. However, worldwide one may observe a departure from the traditional doctor-patient approach. The doctor becomes a partner and the patient is involved in the therapeutic process [ 14 ]. Healthcare is no longer focused solely on the treatment of patients. The priority for decision-makers should be to promote proper health attitudes and prevent diseases that can be avoided [ 81 ]. This became visible and important especially during the Covid-19 pandemic [ 44 ].
The next challenges that healthcare will have to face is the growing number of elderly people and a decline in fertility. Fertility rates in the country are found below the reproductive minimum necessary to keep the population stable [ 10 ]. The reflection of both effects, namely the increase in age and lower fertility rates, are demographic load indicators, which is constantly growing. Forecasts show that providing healthcare in the form it is provided today will become impossible in the next 20 years [ 70 ]. It is especially visible now during the Covid-19 pandemic when healthcare faced quite a challenge related to the analysis of huge data amounts and the need to identify trends and predict the spread of the coronavirus. The pandemic showed it even more that patients should have access to information about their health condition, the possibility of digital analysis of this data and access to reliable medical support online. Health monitoring and cooperation with doctors in order to prevent diseases can actually revolutionize the healthcare system. One of the most important aspects of the change necessary in healthcare is putting the patient in the center of the system.
Technology is not enough to achieve these goals. Therefore, changes should be made not only at the technological level but also in the management and design of complete healthcare processes and what is more, they should affect the business models of service providers. The use of Big Data Analytics is becoming more and more common in enterprises [ 17 , 54 ]. However, medical enterprises still cannot keep up with the information needs of patients, clinicians, administrators and the creator’s policy. The adoption of a Big Data approach would allow the implementation of personalized and precise medicine based on personalized information, delivered in real time and tailored to individual patients.
To achieve this goal, it is necessary to implement systems that will be able to learn quickly about the data generated by people within clinical care and everyday life. This will enable data-driven decision making, receiving better personalized predictions about prognosis and responses to treatments; a deeper understanding of the complex factors and their interactions that influence health at the patient level, the health system and society, enhanced approaches to detecting safety problems with drugs and devices, as well as more effective methods of comparing prevention, diagnostic, and treatment options [ 40 ].
In the literature, there is a lot of research showing what opportunities can be offered to companies by big data analysis and what data can be analyzed. However, there are few studies showing how data analysis in the area of healthcare is performed, what data is used by medical facilities and what analyses and in which areas they carry out. This paper aims to fill this gap by presenting the results of research carried out in medical facilities in Poland. The goal is to analyze the possibilities of using Big Data Analytics in healthcare, especially in Polish conditions. In particular, the paper is aimed at determining what data is processed by medical facilities in Poland, what analyses they perform and in what areas, and how they assess their analytical maturity. In order to achieve this goal, a critical analysis of the literature was performed, and the direct research was based on a research questionnaire conducted on a sample of 217 medical facilities in Poland. It was hypothesized that medical facilities in Poland are working on both structured and unstructured data and moving towards data-based healthcare and its benefits. Examining the maturity of healthcare facilities in the use of Big Data and Big Data Analytics is crucial in determining the potential future benefits that the healthcare sector can gain from Big Data Analytics. There is also a pressing need to predicate whether, in the coming years, healthcare will be able to cope with the threats and challenges it faces.
This paper is divided into eight parts. The first is the introduction which provides background and the general problem statement of this research. In the second part, this paper discusses considerations on use of Big Data and Big Data Analytics in Healthcare, and then, in the third part, it moves on to challenges and potential benefits of using Big Data Analytics in healthcare. The next part involves the explanation of the proposed method. The result of direct research and discussion are presented in the fifth part, while the following part of the paper is the conclusion. The seventh part of the paper presents practical implications. The final section of the paper provides limitations and directions for future research.
Considerations on use Big Data and Big Data Analytics in the healthcare
In recent years one can observe a constantly increasing demand for solutions offering effective analytical tools. This trend is also noticeable in the analysis of large volumes of data (Big Data, BD). Organizations are looking for ways to use the power of Big Data to improve their decision making, competitive advantage or business performance [ 7 , 54 ]. Big Data is considered to offer potential solutions to public and private organizations, however, still not much is known about the outcome of the practical use of Big Data in different types of organizations [ 24 ].
As already mentioned, in recent years, healthcare management worldwide has been changed from a disease-centered model to a patient-centered model, even in value-based healthcare delivery model [ 68 ]. In order to meet the requirements of this model and provide effective patient-centered care, it is necessary to manage and analyze healthcare Big Data.
The issue often raised when it comes to the use of data in healthcare is the appropriate use of Big Data. Healthcare has always generated huge amounts of data and nowadays, the introduction of electronic medical records, as well as the huge amount of data sent by various types of sensors or generated by patients in social media causes data streams to constantly grow. Also, the medical industry generates significant amounts of data, including clinical records, medical images, genomic data and health behaviors. Proper use of the data will allow healthcare organizations to support clinical decision-making, disease surveillance, and public health management. The challenge posed by clinical data processing involves not only the quantity of data but also the difficulty in processing it.
In the literature one can find many different definitions of Big Data. This concept has evolved in recent years, however, it is still not clearly understood. Nevertheless, despite the range and differences in definitions, Big Data can be treated as a: large amount of digital data, large data sets, tool, technology or phenomenon (cultural or technological.
Big Data can be considered as massive and continually generated digital datasets that are produced via interactions with online technologies [ 53 ]. Big Data can be defined as datasets that are of such large sizes that they pose challenges in traditional storage and analysis techniques [ 28 ]. A similar opinion about Big Data was presented by Ohlhorst who sees Big Data as extremely large data sets, possible neither to manage nor to analyze with traditional data processing tools [ 57 ]. In his opinion, the bigger the data set, the more difficult it is to gain any value from it.
In turn, Knapp perceived Big Data as tools, processes and procedures that allow an organization to create, manipulate and manage very large data sets and storage facilities [ 38 ]. From this point of view, Big Data is identified as a tool to gather information from different databases and processes, allowing users to manage large amounts of data.
Similar perception of the term ‘Big Data’ is shown by Carter. According to him, Big Data technologies refer to a new generation of technologies and architectures, designed to economically extract value from very large volumes of a wide variety of data by enabling high velocity capture, discovery and/or analysis [ 13 ].
Jordan combines these two approaches by identifying Big Data as a complex system, as it needs data bases for data to be stored in, programs and tools to be managed, as well as expertise and personnel able to retrieve useful information and visualization to be understood [ 37 ].
Following the definition of Laney for Big Data, it can be state that: it is large amount of data generated in very fast motion and it contains a lot of content [ 43 ]. Such data comes from unstructured sources, such as stream of clicks on the web, social networks (Twitter, blogs, Facebook), video recordings from the shops, recording of calls in a call center, real time information from various kinds of sensors, RFID, GPS devices, mobile phones and other devices that identify and monitor something [ 8 ]. Big Data is a powerful digital data silo, raw, collected with all sorts of sources, unstructured and difficult, or even impossible, to analyze using conventional techniques used so far to relational databases.
While describing Big Data, it cannot be overlooked that the term refers more to a phenomenon than to specific technology. Therefore, instead of defining this phenomenon, trying to describe them, more authors are describing Big Data by giving them characteristics included a collection of V’s related to its nature [ 2 , 3 , 23 , 25 , 58 ]:
- Volume (refers to the amount of data and is one of the biggest challenges in Big Data Analytics),
- Velocity (speed with which new data is generated, the challenge is to be able to manage data effectively and in real time),
- Variety (heterogeneity of data, many different types of healthcare data, the challenge is to derive insights by looking at all available heterogenous data in a holistic manner),
- Variability (inconsistency of data, the challenge is to correct the interpretation of data that can vary significantly depending on the context),
- Veracity (how trustworthy the data is, quality of the data),
- Visualization (ability to interpret data and resulting insights, challenging for Big Data due to its other features as described above).
- Value (the goal of Big Data Analytics is to discover the hidden knowledge from huge amounts of data).
Big Data is defined as an information asset with high volume, velocity, and variety, which requires specific technology and method for its transformation into value [ 21 , 77 ]. Big Data is also a collection of information about high-volume, high volatility or high diversity, requiring new forms of processing in order to support decision-making, discovering new phenomena and process optimization [ 5 , 7 ]. Big Data is too large for traditional data-processing systems and software tools to capture, store, manage and analyze, therefore it requires new technologies [ 28 , 50 , 61 ] to manage (capture, aggregate, process) its volume, velocity and variety [ 9 ].
Undoubtedly, Big Data differs from the data sources used so far by organizations. Therefore, organizations must approach this type of unstructured data in a different way. First of all, organizations must start to see data as flows and not stocks—this entails the need to implement the so-called streaming analytics [ 48 ]. The mentioned features make it necessary to use new IT tools that allow the fullest use of new data [ 58 ]. The Big Data idea, inseparable from the huge increase in data available to various organizations or individuals, creates opportunities for access to valuable analyses, conclusions and enables making more accurate decisions [ 6 , 11 , 59 ].
The Big Data concept is constantly evolving and currently it does not focus on huge amounts of data, but rather on the process of creating value from this data [ 52 ]. Big Data is collected from various sources that have different data properties and are processed by different organizational units, resulting in creation of a Big Data chain [ 36 ]. The aim of the organizations is to manage, process and analyze Big Data. In the healthcare sector, Big Data streams consist of various types of data, namely [ 8 , 51 ]:
- clinical data, i.e. data obtained from electronic medical records, data from hospital information systems, image centers, laboratories, pharmacies and other organizations providing health services, patient generated health data, physician’s free-text notes, genomic data, physiological monitoring data [ 4 ],
- biometric data provided from various types of devices that monitor weight, pressure, glucose level, etc.,
- financial data, constituting a full record of economic operations reflecting the conducted activity,
- data from scientific research activities, i.e. results of research, including drug research, design of medical devices and new methods of treatment,
- data provided by patients, including description of preferences, level of satisfaction, information from systems for self-monitoring of their activity: exercises, sleep, meals consumed, etc.
- data from social media.
These data are provided not only by patients but also by organizations and institutions, as well as by various types of monitoring devices, sensors or instruments [ 16 ]. Data that has been generated so far in the healthcare sector is stored in both paper and digital form. Thus, the essence and the specificity of the process of Big Data analyses means that organizations need to face new technological and organizational challenges [ 67 ]. The healthcare sector has always generated huge amounts of data and this is connected, among others, with the need to store medical records of patients. However, the problem with Big Data in healthcare is not limited to an overwhelming volume but also an unprecedented diversity in terms of types, data formats and speed with which it should be analyzed in order to provide the necessary information on an ongoing basis [ 3 ]. It is also difficult to apply traditional tools and methods for management of unstructured data [ 67 ]. Due to the diversity and quantity of data sources that are growing all the time, advanced analytical tools and technologies, as well as Big Data analysis methods which can meet and exceed the possibilities of managing healthcare data, are needed [ 3 , 68 ].
Therefore, the potential is seen in Big Data analyses, especially in the aspect of improving the quality of medical care, saving lives or reducing costs [ 30 ]. Extracting from this tangle of given association rules, patterns and trends will allow health service providers and other stakeholders in the healthcare sector to offer more accurate and more insightful diagnoses of patients, personalized treatment, monitoring of the patients, preventive medicine, support of medical research and health population, as well as better quality of medical services and patient care while, at the same time, the ability to reduce costs (Fig. 1 ).

Healthcare Big Data Analytics applications
(Source: Own elaboration)
The main challenge with Big Data is how to handle such a large amount of information and use it to make data-driven decisions in plenty of areas [ 64 ]. In the context of healthcare data, another major challenge is to adjust big data storage, analysis, presentation of analysis results and inference basing on them in a clinical setting. Data analytics systems implemented in healthcare are designed to describe, integrate and present complex data in an appropriate way so that it can be understood better (Fig. 2 ). This would improve the efficiency of acquiring, storing, analyzing and visualizing big data from healthcare [ 71 ].

Process of Big Data Analytics
The result of data processing with the use of Big Data Analytics is appropriate data storytelling which may contribute to making decisions with both lower risk and data support. This, in turn, can benefit healthcare stakeholders. To take advantage of the potential massive amounts of data in healthcare and to ensure that the right intervention to the right patient is properly timed, personalized, and potentially beneficial to all components of the healthcare system such as the payer, patient, and management, analytics of large datasets must connect communities involved in data analytics and healthcare informatics [ 49 ]. Big Data Analytics can provide insight into clinical data and thus facilitate informed decision-making about the diagnosis and treatment of patients, prevention of diseases or others. Big Data Analytics can also improve the efficiency of healthcare organizations by realizing the data potential [ 3 , 62 ].
Big Data Analytics in medicine and healthcare refers to the integration and analysis of a large amount of complex heterogeneous data, such as various omics (genomics, epigenomics, transcriptomics, proteomics, metabolomics, interactomics, pharmacogenetics, deasomics), biomedical data, talemedicine data (sensors, medical equipment data) and electronic health records data [ 46 , 65 ].
When analyzing the phenomenon of Big Data in the healthcare sector, it should be noted that it can be considered from the point of view of three areas: epidemiological, clinical and business.
From a clinical point of view, the Big Data analysis aims to improve the health and condition of patients, enable long-term predictions about their health status and implementation of appropriate therapeutic procedures. Ultimately, the use of data analysis in medicine is to allow the adaptation of therapy to a specific patient, that is personalized medicine (precision, personalized medicine).
From an epidemiological point of view, it is desirable to obtain an accurate prognosis of morbidity in order to implement preventive programs in advance.
In the business context, Big Data analysis may enable offering personalized packages of commercial services or determining the probability of individual disease and infection occurrence. It is worth noting that Big Data means not only the collection and processing of data but, most of all, the inference and visualization of data necessary to obtain specific business benefits.
In order to introduce new management methods and new solutions in terms of effectiveness and transparency, it becomes necessary to make data more accessible, digital, searchable, as well as analyzed and visualized.
Erickson and Rothberg state that the information and data do not reveal their full value until insights are drawn from them. Data becomes useful when it enhances decision making and decision making is enhanced only when analytical techniques are used and an element of human interaction is applied [ 22 ].
Thus, healthcare has experienced much progress in usage and analysis of data. A large-scale digitalization and transparency in this sector is a key statement of almost all countries governments policies. For centuries, the treatment of patients was based on the judgment of doctors who made treatment decisions. In recent years, however, Evidence-Based Medicine has become more and more important as a result of it being related to the systematic analysis of clinical data and decision-making treatment based on the best available information [ 42 ]. In the healthcare sector, Big Data Analytics is expected to improve the quality of life and reduce operational costs [ 72 , 82 ]. Big Data Analytics enables organizations to improve and increase their understanding of the information contained in data. It also helps identify data that provides insightful insights for current as well as future decisions [ 28 ].
Big Data Analytics refers to technologies that are grounded mostly in data mining: text mining, web mining, process mining, audio and video analytics, statistical analysis, network analytics, social media analytics and web analytics [ 16 , 25 , 31 ]. Different data mining techniques can be applied on heterogeneous healthcare data sets, such as: anomaly detection, clustering, classification, association rules as well as summarization and visualization of those Big Data sets [ 65 ]. Modern data analytics techniques explore and leverage unique data characteristics even from high-speed data streams and sensor data [ 15 , 16 , 31 , 55 ]. Big Data can be used, for example, for better diagnosis in the context of comprehensive patient data, disease prevention and telemedicine (in particular when using real-time alerts for immediate care), monitoring patients at home, preventing unnecessary hospital visits, integrating medical imaging for a wider diagnosis, creating predictive analytics, reducing fraud and improving data security, better strategic planning and increasing patients’ involvement in their own health.
Big Data Analytics in healthcare can be divided into [ 33 , 73 , 74 ]:
- descriptive analytics in healthcare is used to understand past and current healthcare decisions, converting data into useful information for understanding and analyzing healthcare decisions, outcomes and quality, as well as making informed decisions [ 33 ]. It can be used to create reports (i.e. about patients’ hospitalizations, physicians’ performance, utilization management), visualization, customized reports, drill down tables, or running queries on the basis of historical data.
- predictive analytics operates on past performance in an effort to predict the future by examining historical or summarized health data, detecting patterns of relationships in these data, and then extrapolating these relationships to forecast. It can be used to i.e. predict the response of different patient groups to different drugs (dosages) or reactions (clinical trials), anticipate risk and find relationships in health data and detect hidden patterns [ 62 ]. In this way, it is possible to predict the epidemic spread, anticipate service contracts and plan healthcare resources. Predictive analytics is used in proper diagnosis and for appropriate treatments to be given to patients suffering from certain diseases [ 39 ].
- prescriptive analytics—occurs when health problems involve too many choices or alternatives. It uses health and medical knowledge in addition to data or information. Prescriptive analytics is used in many areas of healthcare, including drug prescriptions and treatment alternatives. Personalized medicine and evidence-based medicine are both supported by prescriptive analytics.
- discovery analytics—utilizes knowledge about knowledge to discover new “inventions” like drugs (drug discovery), previously unknown diseases and medical conditions, alternative treatments, etc.
Although the models and tools used in descriptive, predictive, prescriptive, and discovery analytics are different, many applications involve all four of them [ 62 ]. Big Data Analytics in healthcare can help enable personalized medicine by identifying optimal patient-specific treatments. This can influence the improvement of life standards, reduce waste of healthcare resources and save costs of healthcare [ 56 , 63 , 71 ]. The introduction of large data analysis gives new analytical possibilities in terms of scope, flexibility and visualization. Techniques such as data mining (computational pattern discovery process in large data sets) facilitate inductive reasoning and analysis of exploratory data, enabling scientists to identify data patterns that are independent of specific hypotheses. As a result, predictive analysis and real-time analysis becomes possible, making it easier for medical staff to start early treatments and reduce potential morbidity and mortality. In addition, document analysis, statistical modeling, discovering patterns and topics in document collections and data in the EHR, as well as an inductive approach can help identify and discover relationships between health phenomena.
Advanced analytical techniques can be used for a large amount of existing (but not yet analytical) data on patient health and related medical data to achieve a better understanding of the information and results obtained, as well as to design optimal clinical pathways [ 62 ]. Big Data Analytics in healthcare integrates analysis of several scientific areas such as bioinformatics, medical imaging, sensor informatics, medical informatics and health informatics [ 65 ]. Big Data Analytics in healthcare allows to analyze large datasets from thousands of patients, identifying clusters and correlation between datasets, as well as developing predictive models using data mining techniques [ 65 ]. Discussing all the techniques used for Big Data Analytics goes beyond the scope of a single article [ 25 ].
The success of Big Data analysis and its accuracy depend heavily on the tools and techniques used to analyze the ability to provide reliable, up-to-date and meaningful information to various stakeholders [ 12 ]. It is believed that the implementation of big data analytics by healthcare organizations could bring many benefits in the upcoming years, including lowering health care costs, better diagnosis and prediction of diseases and their spread, improving patient care and developing protocols to prevent re-hospitalization, optimizing staff, optimizing equipment, forecasting the need for hospital beds, operating rooms, treatments, and improving the drug supply chain [ 71 ].
Challenges and potential benefits of using Big Data Analytics in healthcare
Modern analytics gives possibilities not only to have insight in historical data, but also to have information necessary to generate insight into what may happen in the future. Even when it comes to prediction of evidence-based actions. The emphasis on reform has prompted payers and suppliers to pursue data analysis to reduce risk, detect fraud, improve efficiency and save lives. Everyone—payers, providers, even patients—are focusing on doing more with fewer resources. Thus, some areas in which enhanced data and analytics can yield the greatest results include various healthcare stakeholders (Table (Table1 1 ).
The use of analytics by various healthcare stakeholders
Source: own elaboration on the basis of [ 19 , 20 ]
Healthcare organizations see the opportunity to grow through investments in Big Data Analytics. In recent years, by collecting medical data of patients, converting them into Big Data and applying appropriate algorithms, reliable information has been generated that helps patients, physicians and stakeholders in the health sector to identify values and opportunities [ 31 ]. It is worth noting that there are many changes and challenges in the structure of the healthcare sector. Digitization and effective use of Big Data in healthcare can bring benefits to every stakeholder in this sector. A single doctor would benefit the same as the entire healthcare system. Potential opportunities to achieve benefits and effects from Big Data in healthcare can be divided into four groups [ 8 ]:
- assessment of diagnoses made by doctors and the manner of treatment of diseases indicated by them based on the decision support system working on Big Data collections,
- detection of more effective, from a medical point of view, and more cost-effective ways to diagnose and treat patients,
- analysis of large volumes of data to reach practical information useful for identifying needs, introducing new health services, preventing and overcoming crises,
- prediction of the incidence of diseases,
- detecting trends that lead to an improvement in health and lifestyle of the society,
- analysis of the human genome for the introduction of personalized treatment.
- doctors’ comparison of current medical cases to cases from the past for better diagnosis and treatment adjustment,
- detection of diseases at earlier stages when they can be more easily and quickly cured,
- detecting epidemiological risks and improving control of pathogenic spots and reaction rates,
- identification of patients who are predicted to have the highest risk of specific, life-threatening diseases by collating data on the history of the most common diseases, in healing people with reports entering insurance companies,
- health management of each patient individually (personalized medicine) and health management of the whole society,
- capturing and analyzing large amounts of data from hospitals and homes in real time, life monitoring devices to monitor safety and predict adverse events,
- analysis of patient profiles to identify people for whom prevention should be applied, lifestyle change or preventive care approach,
- the ability to predict the occurrence of specific diseases or worsening of patients’ results,
- predicting disease progression and its determinants, estimating the risk of complications,
- detecting drug interactions and their side effects.
- supporting work on new drugs and clinical trials thanks to the possibility of analyzing “all data” instead of selecting a test sample,
- the ability to identify patients with specific, biological features that will take part in specialized clinical trials,
- selecting a group of patients for which the tested drug is likely to have the desired effect and no side effects,
- using modeling and predictive analysis to design better drugs and devices.
- reduction of costs and counteracting abuse and counseling practices,
- faster and more effective identification of incorrect or unauthorized financial operations in order to prevent abuse and eliminate errors,
- increase in profitability by detecting patients generating high costs or identifying doctors whose work, procedures and treatment methods cost the most and offering them solutions that reduce the amount of money spent,
- identification of unnecessary medical activities and procedures, e.g. duplicate tests.
According to research conducted by Wang, Kung and Byrd, Big Data Analytics benefits can be classified into five categories: IT infrastructure benefits (reducing system redundancy, avoiding unnecessary IT costs, transferring data quickly among healthcare IT systems, better use of healthcare systems, processing standardization among various healthcare IT systems, reducing IT maintenance costs regarding data storage), operational benefits (improving the quality and accuracy of clinical decisions, processing a large number of health records in seconds, reducing the time of patient travel, immediate access to clinical data to analyze, shortening the time of diagnostic test, reductions in surgery-related hospitalizations, exploring inconceivable new research avenues), organizational benefits (detecting interoperability problems much more quickly than traditional manual methods, improving cross-functional communication and collaboration among administrative staffs, researchers, clinicians and IT staffs, enabling data sharing with other institutions and adding new services, content sources and research partners), managerial benefits (gaining quick insights about changing healthcare trends in the market, providing members of the board and heads of department with sound decision-support information on the daily clinical setting, optimizing business growth-related decisions) and strategic benefits (providing a big picture view of treatment delivery for meeting future need, creating high competitive healthcare services) [ 73 ].
The above specification does not constitute a full list of potential areas of use of Big Data Analysis in healthcare because the possibilities of using analysis are practically unlimited. In addition, advanced analytical tools allow to analyze data from all possible sources and conduct cross-analyses to provide better data insights [ 26 ]. For example, a cross-analysis can refer to a combination of patient characteristics, as well as costs and care results that can help identify the best, in medical terms, and the most cost-effective treatment or treatments and this may allow a better adjustment of the service provider’s offer [ 62 ].
In turn, the analysis of patient profiles (e.g. segmentation and predictive modeling) allows identification of people who should be subject to prophylaxis, prevention or should change their lifestyle [ 8 ]. Shortened list of benefits for Big Data Analytics in healthcare is presented in paper [ 3 ] and consists of: better performance, day-to-day guides, detection of diseases in early stages, making predictive analytics, cost effectiveness, Evidence Based Medicine and effectiveness in patient treatment.
Summarizing, healthcare big data represents a huge potential for the transformation of healthcare: improvement of patients’ results, prediction of outbreaks of epidemics, valuable insights, avoidance of preventable diseases, reduction of the cost of healthcare delivery and improvement of the quality of life in general [ 1 ]. Big Data also generates many challenges such as difficulties in data capture, data storage, data analysis and data visualization [ 15 ]. The main challenges are connected with the issues of: data structure (Big Data should be user-friendly, transparent, and menu-driven but it is fragmented, dispersed, rarely standardized and difficult to aggregate and analyze), security (data security, privacy and sensitivity of healthcare data, there are significant concerns related to confidentiality), data standardization (data is stored in formats that are not compatible with all applications and technologies), storage and transfers (especially costs associated with securing, storing, and transferring unstructured data), managerial skills, such as data governance, lack of appropriate analytical skills and problems with Real-Time Analytics (health care is to be able to utilize Big Data in real time) [ 4 , 34 , 41 ].
The research is based on a critical analysis of the literature, as well as the presentation of selected results of direct research on the use of Big Data Analytics in medical facilities in Poland.
Presented research results are part of a larger questionnaire form on Big Data Analytics. The direct research was based on an interview questionnaire which contained 100 questions with 5-point Likert scale (1—strongly disagree, 2—I rather disagree, 3—I do not agree, nor disagree, 4—I rather agree, 5—I definitely agree) and 4 metrics questions. The study was conducted in December 2018 on a sample of 217 medical facilities (110 private, 107 public). The research was conducted by a specialized market research agency: Center for Research and Expertise of the University of Economics in Katowice.
When it comes to direct research, the selected entities included entities financed from public sources—the National Health Fund (23.5%), and entities operating commercially (11.5%). In the surveyed group of entities, more than a half (64.9%) are hybrid financed, both from public and commercial sources. The diversity of the research sample also applies to the size of the entities, defined by the number of employees. Taking into account proportions of the surveyed entities, it should be noted that in the sector structure, medium-sized (10–50 employees—34% of the sample) and large (51–250 employees—27%) entities dominate. The research was of all-Poland nature, and the entities included in the research sample come from all of the voivodships. The largest group were entities from Łódzkie (32%), Śląskie (18%) and Mazowieckie (18%) voivodships, as these voivodships have the largest number of medical institutions. Other regions of the country were represented by single units. The selection of the research sample was random—layered. As part of medical facilities database, groups of private and public medical facilities have been identified and the ones to which the questionnaire was targeted were drawn from each of these groups. The analyses were performed using the GNU PSPP 0.10.2 software.
The aim of the study was to determine whether medical facilities in Poland use Big Data Analytics and if so, in which areas. Characteristics of the research sample is presented in Table Table2 2 .
Characteristics of the research sample
The research is non-exhaustive due to the incomplete and uneven regional distribution of the samples, overrepresented in three voivodeships (Łódzkie, Mazowieckie and Śląskie). The size of the research sample (217 entities) allows the authors of the paper to formulate specific conclusions on the use of Big Data in the process of its management.
For the purpose of this paper, the following research hypotheses were formulated: (1) medical facilities in Poland are working on both structured and unstructured data (2) medical facilities in Poland are moving towards data-based healthcare and its benefits.
The paper poses the following research questions and statements that coincide with the selected questions from the research questionnaire:
- From what sources do medical facilities obtain data? What types of data are used by the particular organization, whether structured or unstructured, and to what extent?
- From what sources do medical facilities obtain data?
- In which area organizations are using data and analytical systems (clinical or business)?
- Is data analytics performed based on historical data or are predictive analyses also performed?
- Determining whether administrative and medical staff receive complete, accurate and reliable data in a timely manner?
- Determining whether real-time analyses are performed to support the particular organization’s activities.
Results and discussion
On the basis of the literature analysis and research study, a set of questions and statements related to the researched area was formulated. The results from the surveys show that medical facilities use a variety of data sources in their operations. These sources are both structured and unstructured data (Table (Table3 3 ).
Type of data sources used in medical facility (%)
1—strongly disagree, 2—I disagree, 3—I agree or disagree, 4—I rather agree, 5—I strongly agree
According to the data provided by the respondents, considering the first statement made in the questionnaire, almost half of the medical institutions (47.58%) agreed that they rather collect and use structured data (e.g. databases and data warehouses, reports to external entities) and 10.57% entirely agree with this statement. As much as 23.35% of representatives of medical institutions stated “I agree or disagree”. Other medical facilities do not collect and use structured data (7.93%) and 6.17% strongly disagree with the first statement. Also, the median calculated based on the obtained results (median: 4), proves that medical facilities in Poland collect and use structured data (Table (Table4 4 ).
Collection and use of data determined by the size of medical facility (number of employees)
In turn, 28.19% of the medical institutions agreed that they rather collect and use unstructured data and as much as 9.25% entirely agree with this statement. The number of representatives of medical institutions that stated “I agree or disagree” was 27.31%. Other medical facilities do not collect and use structured data (17.18%) and 13.66% strongly disagree with the first statement. In the case of unstructured data the median is 3, which means that the collection and use of this type of data by medical facilities in Poland is lower.
In the further part of the analysis, it was checked whether the size of the medical facility and form of ownership have an impact on whether it analyzes unstructured data (Tables (Tables4 4 and and5). 5 ). In order to find this out, correlation coefficients were calculated.
Collection and use of data determined by the form of ownership of medical facility
Based on the calculations, it can be concluded that there is a small statistically monotonic correlation between the size of the medical facility and its collection and use of structured data (p < 0.001; τ = 0.16). This means that the use of structured data is slightly increasing in larger medical facilities. The size of the medical facility is more important according to use of unstructured data (p < 0.001; τ = 0.23) (Table (Table4 4 .).
To determine whether the form of medical facility ownership affects data collection, the Mann–Whitney U test was used. The calculations show that the form of ownership does not affect what data the organization collects and uses (Table (Table5 5 ).
Detailed information on the sources of from which medical facilities collect and use data is presented in the Table Table6 6 .
Data sources used in medical facility
1—we do not use at all, 5—we use extensively
The questionnaire results show that medical facilities are especially using information published in databases, reports to external units and transaction data, but they also use unstructured data from e-mails, medical devices, sensors, phone calls, audio and video data (Table (Table6). 6 ). Data from social media, RFID and geolocation data are used to a small extent. Similar findings are concluded in the literature studies.
From the analysis of the answers given by the respondents, more than half of the medical facilities have integrated hospital system (HIS) implemented. As much as 43.61% use integrated hospital system and 16.30% use it extensively (Table (Table7). 7 ). 19.38% of exanimated medical facilities do not use it at all. Moreover, most of the examined medical facilities (34.80% use it, 32.16% use extensively) conduct medical documentation in an electronic form, which gives an opportunity to use data analytics. Only 4.85% of medical facilities don’t use it at all.
The use of HIS and electronic documentation in medical facilities (%)
Other problems that needed to be investigated were: whether medical facilities in Poland use data analytics? If so, in what form and in what areas? (Table (Table8). 8 ). The analysis of answers given by the respondents about the potential of data analytics in medical facilities shows that a similar number of medical facilities use data analytics in administration and business (31.72% agreed with the statement no. 5 and 12.33% strongly agreed) as in the clinical area (33.04% agreed with the statement no. 6 and 12.33% strongly agreed). When considering decision-making issues, 35.24% agree with the statement "the organization uses data and analytical systems to support business decisions” and 8.37% of respondents strongly agree. Almost 40.09% agree with the statement that “the organization uses data and analytical systems to support clinical decisions (in the field of diagnostics and therapy)” and 15.42% of respondents strongly agree. Exanimated medical facilities use in their activity analytics based both on historical data (33.48% agree with statement 7 and 12.78% strongly agree) and predictive analytics (33.04% agrees with the statement number 8 and 15.86% strongly agree). Detailed results are presented in Table Table8 8 .
Conditions of using Big Data Analytics in medical facilities (%)
Medical facilities focus on development in the field of data processing, as they confirm that they conduct analytical planning processes systematically and analyze new opportunities for strategic use of analytics in business and clinical activities (38.33% rather agree and 10.57% strongly agree with this statement). The situation is different with real-time data analysis, here, the situation is not so optimistic. Only 28.19% rather agree and 14.10% strongly agree with the statement that real-time analyses are performed to support an organization’s activities.
When considering whether a facility’s performance in the clinical area depends on the form of ownership, it can be concluded that taking the average and the Mann–Whitney U test depends. A higher degree of use of analyses in the clinical area can be observed in public institutions.
Whether a medical facility performs a descriptive or predictive analysis do not depend on the form of ownership (p > 0.05). It can be concluded that when analyzing the mean and median, they are higher in public facilities, than in private ones. What is more, the Mann–Whitney U test shows that these variables are dependent from each other (p < 0.05) (Table (Table9 9 ).
Conditions of using Big Data Analytics in medical facilities determined by the form of ownership of medical facility
When considering whether a facility’s performance in the clinical area depends on its size, it can be concluded that taking the Kendall’s Tau (τ) it depends (p < 0.001; τ = 0.22), and the correlation is weak but statistically important. This means that the use of data and analytical systems to support clinical decisions (in the field of diagnostics and therapy) increases with the increase of size of the medical facility. A similar relationship, but even less powerful, can be found in the use of descriptive and predictive analyses (Table (Table10 10 ).
Conditions of using Big Data Analytics in medical facilities determined by the size of medical facility (number of employees)
Considering the results of research in the area of analytical maturity of medical facilities, 8.81% of medical facilities stated that they are at the first level of maturity, i.e. an organization has developed analytical skills and does not perform analyses. As much as 13.66% of medical facilities confirmed that they have poor analytical skills, while 38.33% of the medical facility has located itself at level 3, meaning that “there is a lot to do in analytics”. On the other hand, 28.19% believe that analytical capabilities are well developed and 6.61% stated that analytics are at the highest level and the analytical capabilities are very well developed. Detailed data is presented in Table Table11. 11 . Average amounts to 3.11 and Median to 3.
Analytical maturity of examined medical facilities (%)
The results of the research have enabled the formulation of following conclusions. Medical facilities in Poland are working on both structured and unstructured data. This data comes from databases, transactions, unstructured content of emails and documents, devices and sensors. However, the use of data from social media is smaller. In their activity, they reach for analytics in the administrative and business, as well as in the clinical area. Also, the decisions made are largely data-driven.
In summary, analysis of the literature that the benefits that medical facilities can get using Big Data Analytics in their activities relate primarily to patients, physicians and medical facilities. It can be confirmed that: patients will be better informed, will receive treatments that will work for them, will have prescribed medications that work for them and not be given unnecessary medications [ 78 ]. Physician roles will likely change to more of a consultant than decision maker. They will advise, warn, and help individual patients and have more time to form positive and lasting relationships with their patients in order to help people. Medical facilities will see changes as well, for example in fewer unnecessary hospitalizations, resulting initially in less revenue, but after the market adjusts, also the accomplishment [ 78 ]. The use of Big Data Analytics can literally revolutionize the way healthcare is practiced for better health and disease reduction.
The analysis of the latest data reveals that data analytics increase the accuracy of diagnoses. Physicians can use predictive algorithms to help them make more accurate diagnoses [ 45 ]. Moreover, it could be helpful in preventive medicine and public health because with early intervention, many diseases can be prevented or ameliorated [ 29 ]. Predictive analytics also allows to identify risk factors for a given patient, and with this knowledge patients will be able to change their lives what, in turn, may contribute to the fact that population disease patterns may dramatically change, resulting in savings in medical costs. Moreover, personalized medicine is the best solution for an individual patient seeking treatment. It can help doctors decide the exact treatments for those individuals. Better diagnoses and more targeted treatments will naturally lead to increases in good outcomes and fewer resources used, including doctors’ time.
The quantitative analysis of the research carried out and presented in this article made it possible to determine whether medical facilities in Poland use Big Data Analytics and if so, in which areas. Thanks to the results obtained it was possible to formulate the following conclusions. Medical facilities are working on both structured and unstructured data, which comes from databases, transactions, unstructured content of emails and documents, devices and sensors. According to analytics, they reach for analytics in the administrative and business, as well as in the clinical area. It clearly showed that the decisions made are largely data-driven. The results of the study confirm what has been analyzed in the literature. Medical facilities are moving towards data-based healthcare and its benefits.
In conclusion, Big Data Analytics has the potential for positive impact and global implications in healthcare. Future research on the use of Big Data in medical facilities will concern the definition of strategies adopted by medical facilities to promote and implement such solutions, as well as the benefits they gain from the use of Big Data analysis and how the perspectives in this area are seen.
Practical implications
This work sought to narrow the gap that exists in analyzing the possibility of using Big Data Analytics in healthcare. Showing how medical facilities in Poland are doing in this respect is an element that is part of global research carried out in this area, including [ 29 , 32 , 60 ].
Limitations and future directions
The research described in this article does not fully exhaust the questions related to the use of Big Data Analytics in Polish healthcare facilities. Only some of the dimensions characterizing the use of data by medical facilities in Poland have been examined. In order to get the full picture, it would be necessary to examine the results of using structured and unstructured data analytics in healthcare. Future research may examine the benefits that medical institutions achieve as a result of the analysis of structured and unstructured data in the clinical and management areas and what limitations they encounter in these areas. For this purpose, it is planned to conduct in-depth interviews with chosen medical facilities in Poland. These facilities could give additional data for empirical analyses based more on their suggestions. Further research should also include medical institutions from beyond the borders of Poland, enabling international comparative analyses.
Future research in the healthcare field has virtually endless possibilities. These regard the use of Big Data Analytics to diagnose specific conditions [ 47 , 66 , 69 , 76 ], propose an approach that can be used in other healthcare applications and create mechanisms to identify “patients like me” [ 75 , 80 ]. Big Data Analytics could also be used for studies related to the spread of pandemics, the efficacy of covid treatment [ 18 , 79 ], or psychology and psychiatry studies, e.g. emotion recognition [ 35 ].
Acknowledgements
We would like to thank those who have touched our science paths.
Authors’ contributions
KB proposed the concept of research and its design. The manuscript was prepared by KB with the consultation of AŚ. AŚ reviewed the manuscript for getting its fine shape. KB prepared the manuscript in the contexts such as definition of intellectual content, literature search, data acquisition, data analysis, and so on. AŚ obtained research funding. Both authors read and approved the final manuscript.
This research was fully funded as statutory activity—subsidy of Ministry of Science and Higher Education granted for Technical University of Czestochowa on maintaining research potential in 2018. Research Number: BS/PB–622/3020/2014/P. Publication fee for the paper was financed by the University of Economics in Katowice.
Availability of data and materials
Declarations.
Not applicable.
The author declares no conflict of interest.
Publisher's Note
Springer Nature remains neutral with regard to jurisdictional claims in published maps and institutional affiliations.
Contributor Information
Kornelia Batko, Email: [email protected] .
Andrzej Ślęzak, Email: moc.liamg@25kazelsa .
Read our research on: Gun Policy | International Conflict | Election 2024
Regions & Countries
9 facts about americans and marijuana.
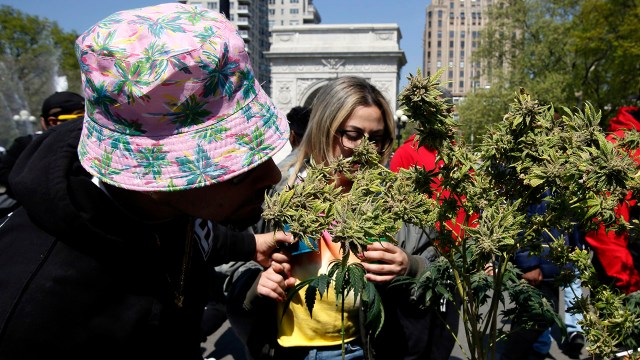
The use and possession of marijuana is illegal under U.S. federal law, but about three-quarters of states have legalized the drug for medical or recreational purposes. The changing legal landscape has coincided with a decades-long rise in public support for legalization, which a majority of Americans now favor.
Here are nine facts about Americans’ views of and experiences with marijuana, based on Pew Research Center surveys and other sources.
As more states legalize marijuana, Pew Research Center looked at Americans’ opinions on legalization and how these views have changed over time.
Data comes from surveys by the Center, Gallup , and the 2022 National Survey on Drug Use and Health from the U.S. Substance Abuse and Mental Health Services Administration. Information about the jurisdictions where marijuana is legal at the state level comes from the National Organization for the Reform of Marijuana Laws .
More information about the Center surveys cited in the analysis, including the questions asked and their methodologies, can be found at the links in the text.
Around nine-in-ten Americans say marijuana should be legal for medical or recreational use, according to a January 2024 Pew Research Center survey . An overwhelming majority of U.S. adults (88%) say either that marijuana should be legal for medical use only (32%) or that it should be legal for medical and recreational use (57%). Just 11% say the drug should not be legal in any form. These views have held relatively steady over the past five years.
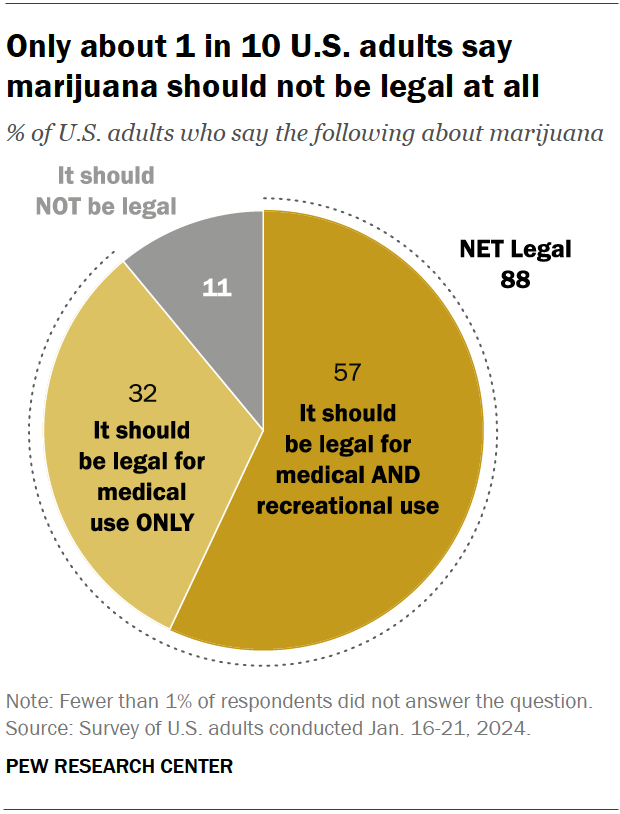
Views on marijuana legalization differ widely by age, political party, and race and ethnicity, the January survey shows.
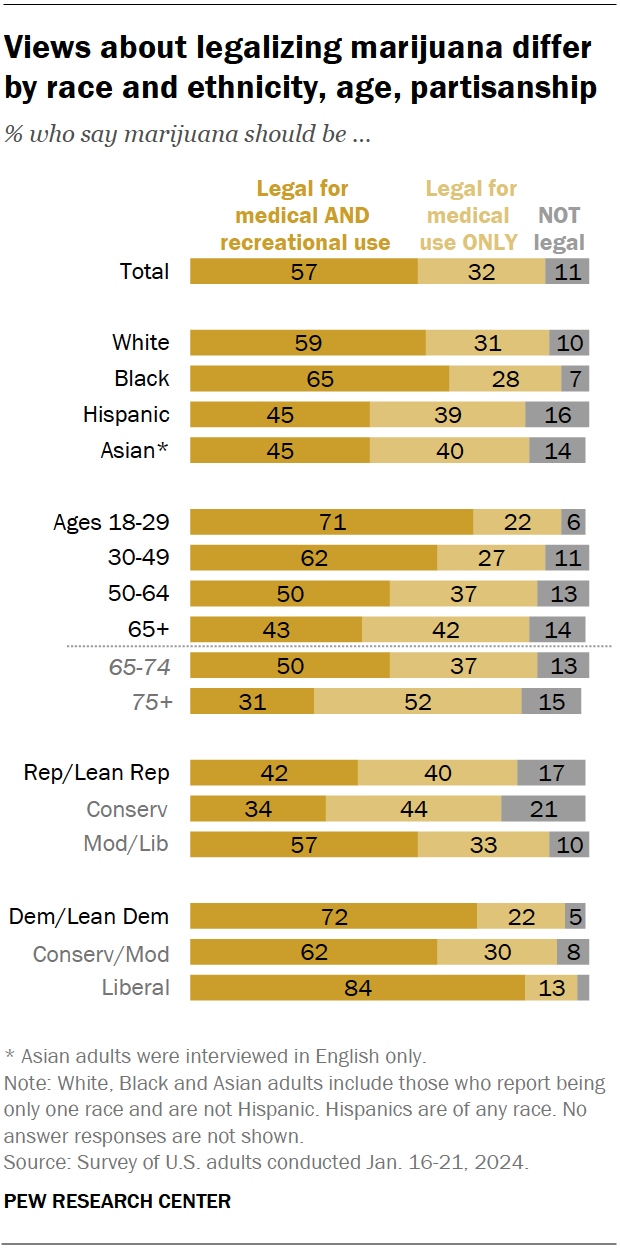
While small shares across demographic groups say marijuana should not be legal at all, those least likely to favor it for both medical and recreational use include:
- Older adults: 31% of adults ages 75 and older support marijuana legalization for medical and recreational purposes, compared with half of those ages 65 to 74, the next youngest age category. By contrast, 71% of adults under 30 support legalization for both uses.
- Republicans and GOP-leaning independents: 42% of Republicans favor legalizing marijuana for both uses, compared with 72% of Democrats and Democratic leaners. Ideological differences exist as well: Within both parties, those who are more conservative are less likely to support legalization.
- Hispanic and Asian Americans: 45% in each group support legalizing the drug for medical and recreational use. Larger shares of Black (65%) and White (59%) adults hold this view.
Support for marijuana legalization has increased dramatically over the last two decades. In addition to asking specifically about medical and recreational use of the drug, both the Center and Gallup have asked Americans about legalizing marijuana use in a general way. Gallup asked this question most recently, in 2023. That year, 70% of adults expressed support for legalization, more than double the share who said they favored it in 2000.
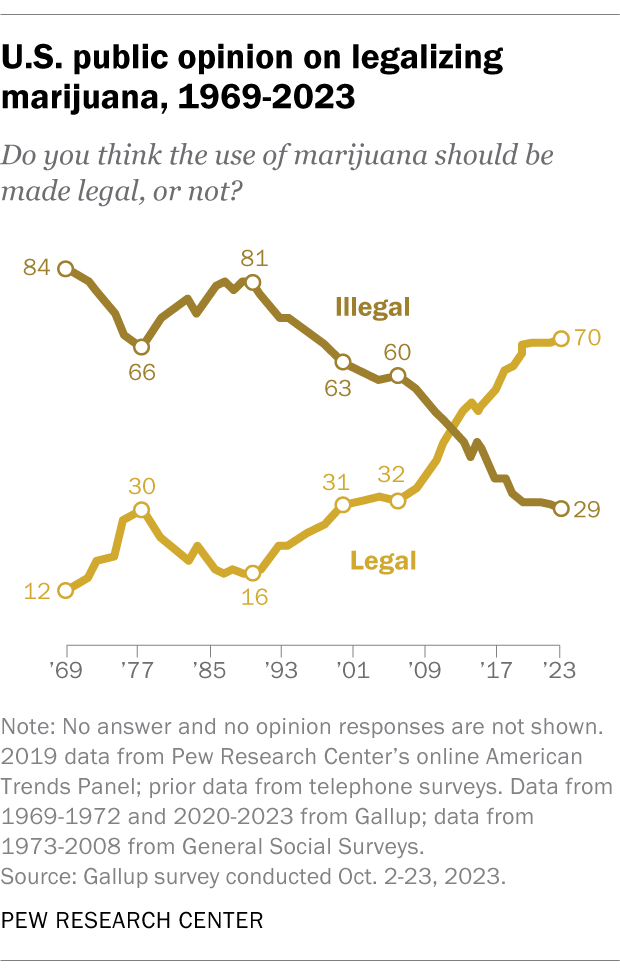
Half of U.S. adults (50.3%) say they have ever used marijuana, according to the 2022 National Survey on Drug Use and Health . That is a smaller share than the 84.1% who say they have ever consumed alcohol and the 64.8% who have ever used tobacco products or vaped nicotine.
While many Americans say they have used marijuana in their lifetime, far fewer are current users, according to the same survey. In 2022, 23.0% of adults said they had used the drug in the past year, while 15.9% said they had used it in the past month.
While many Americans say legalizing recreational marijuana has economic and criminal justice benefits, views on these and other impacts vary, the Center’s January survey shows.
- Economic benefits: About half of adults (52%) say that legalizing recreational marijuana is good for local economies, while 17% say it is bad. Another 29% say it has no impact.
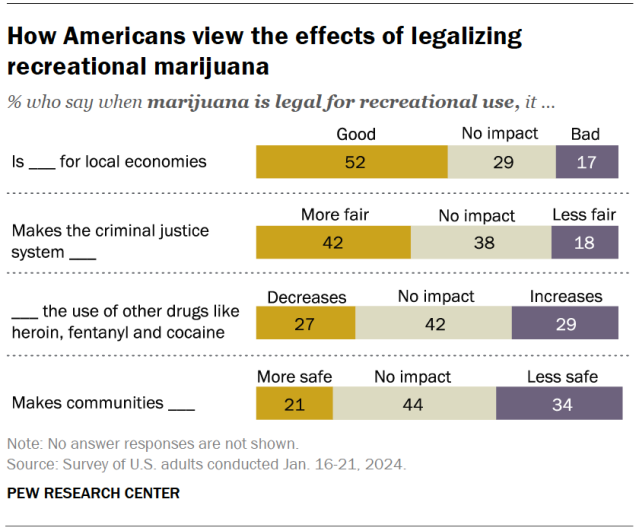
- Criminal justice system fairness: 42% of Americans say legalizing marijuana for recreational use makes the criminal justice system fairer, compared with 18% who say it makes the system less fair. About four-in-ten (38%) say it has no impact.
- Use of other drugs: 27% say this policy decreases the use of other drugs like heroin, fentanyl and cocaine, and 29% say it increases it. But the largest share (42%) say it has no effect on other drug use.
- Community safety: 21% say recreational legalization makes communities safer and 34% say it makes them less safe. Another 44% say it doesn’t impact safety.
Democrats and adults under 50 are more likely than Republicans and those in older age groups to say legalizing marijuana has positive impacts in each of these areas.
Most Americans support easing penalties for people with marijuana convictions, an October 2021 Center survey found . Two-thirds of adults say they favor releasing people from prison who are being held for marijuana-related offenses only, including 41% who strongly favor this. And 61% support removing or expunging marijuana-related offenses from people’s criminal records.
Younger adults, Democrats and Black Americans are especially likely to support these changes. For instance, 74% of Black adults favor releasing people from prison who are being held only for marijuana-related offenses, and just as many favor removing or expunging marijuana-related offenses from criminal records.
Twenty-four states and the District of Columbia have legalized small amounts of marijuana for both medical and recreational use as of March 2024, according to the National Organization for the Reform of Marijuana Laws (NORML), an advocacy group that tracks state-level legislation on the issue. Another 14 states have legalized the drug for medical use only.

Of the remaining 12 states, all allow limited access to products such as CBD oil that contain little to no THC – the main psychoactive substance in cannabis. And 26 states overall have at least partially decriminalized recreational marijuana use , as has the District of Columbia.
In addition to 24 states and D.C., the U.S. Virgin Islands , Guam and the Northern Mariana Islands have legalized marijuana for medical and recreational use.
More than half of Americans (54%) live in a state where both recreational and medical marijuana are legal, and 74% live in a state where it’s legal either for both purposes or medical use only, according to a February Center analysis of data from the Census Bureau and other outside sources. This analysis looked at state-level legislation in all 50 states and the District of Columbia.
In 2012, Colorado and Washington became the first states to pass legislation legalizing recreational marijuana.
About eight-in-ten Americans (79%) live in a county with at least one cannabis dispensary, according to the February analysis. There are nearly 15,000 marijuana dispensaries nationwide, and 76% are in states (including D.C.) where recreational use is legal. Another 23% are in medical marijuana-only states, and 1% are in states that have made legal allowances for low-percentage THC or CBD-only products.
The states with the largest number of dispensaries include California, Oklahoma, Florida, Colorado and Michigan.
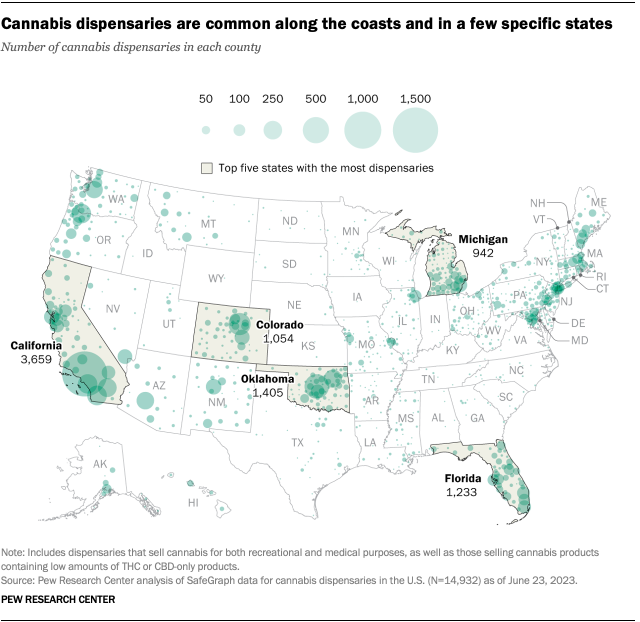
Note: This is an update of a post originally published April 26, 2021, and updated April 13, 2023.
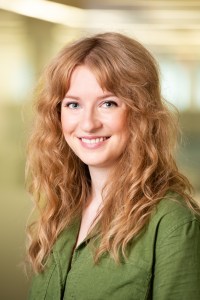
Sign up for our weekly newsletter
Fresh data delivered Saturday mornings
Americans overwhelmingly say marijuana should be legal for medical or recreational use
Religious americans are less likely to endorse legal marijuana for recreational use, four-in-ten u.s. drug arrests in 2018 were for marijuana offenses – mostly possession, two-thirds of americans support marijuana legalization, most popular.
About Pew Research Center Pew Research Center is a nonpartisan fact tank that informs the public about the issues, attitudes and trends shaping the world. It conducts public opinion polling, demographic research, media content analysis and other empirical social science research. Pew Research Center does not take policy positions. It is a subsidiary of The Pew Charitable Trusts .
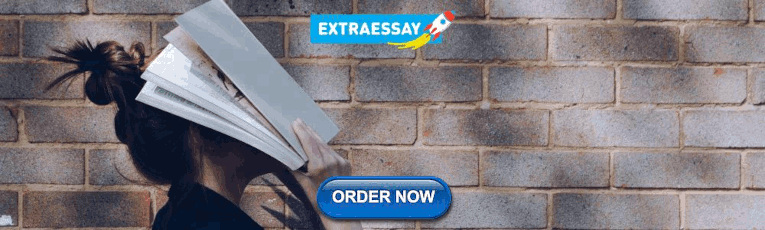
IMAGES
VIDEO
COMMENTS
Big data is one of the most recent business and technical issues in the age of technology. Hundreds of millions of events occur every day. The financial field is deeply involved in the calculation of big data events. As a result, hundreds of millions of financial transactions occur in the financial world each day. Therefore, financial practitioners and analysts consider it an emerging issue of ...
About the journal. The journal aims to promote and communicate advances in big data research by providing a fast and high quality forum for researchers, practitioners and policy makers from the very many different communities working on, and with, this topic. The journal will accept papers on foundational aspects in dealing with big data, as ...
The phrase "Big Data" refers to the vast and ever-increasing volumes of data that might overwhelm an organization (Ur Rehman et al., 2016).It gathers massive, broad, and multi-format data streams from disparate and independent data sources (X. Wu et al., 2014).Big Data is believed to have five properties, which are known as the five V's: volume, velocity, variety, veracity, and valence ...
However, the literature lacks a systematic view of the current state of big data and data analytics in public policy, and there are identifiable research gaps (Desouza & Jacob, 2017). ... This approach has been used in several research papers to form the basis for research agenda building (Suominen et al., 2019; Yuan et al., 2015).
There are three types of Articles in Press: Journal pre-proofs: versions of an article that have undergone enhancements after acceptance, such as the addition of a cover page and metadata, and formatting for readability, but are not yet definitive versions of record. These versions will undergo additional copyediting, typesetting and review ...
Prior research observed several issues related to big data accumulated in healthcare, such as data quality (Sabharwal, Gupta, and Thirunavukkarasu Citation 2016) and data quantity (Gopal et al. Citation 2019). However, there is a lack of research into the types of problems that may occur during data accumulation processes in healthcare and how ...
In this study, big data studies (01/2015-6/2018) are reviewed and several highly cited papers are identified, which indicates a growing interest in the area of big data. The papers and proceedings from international peer-reviewed journals and ranked conferences were reviewed.
by analysing 988 papers in relevant area [40]. Analyses of 406 big data papers, published in 2011, using the co-word occurrence technique, revealed key research themes in this area. Although these studies have provided valuable insights, there is no comprehensive study to show the research trends of big data based on term co-occurrence.
In this descriptive review, we highlight the roles of big data, the changing research paradigm, and easy access to research participation via the Internet fueled by the need for quick answers. Universally, data volume has increased, with the collection rate doubling every 40 months, ever since the 1980s. 4 The big data age, starting in 2002 ...
Big data collection means collecting large volumes of data to have insight into better business decisions and greater customer satisfaction. Securing big data is difficult not just because of the large amount of data it handles, but also because of the continuous streaming of data, multiple types of data, and cloud-based data storage. Additionally, traditional security and privacy methods are ...
1. Past, Present, and Future of Business Analytics and Big Data Research Seen Through the Lens of the European Conference on Information Systems. Business analytics summarises all methods, processes, technologies, applications, skills, and organisational structures necessary to analyse past or current data to manage and plan business performance.
View a PDF of the paper titled Analysis of Distributed Algorithms for Big-data, by Rajendra Purohit and 2 other authors View PDF HTML (experimental) Abstract: The parallel and distributed processing are becoming de facto industry standard, and a large part of the current research is targeted on how to make computing scalable and distributed ...
With over 4.57 billion people using the Internet in 2020, the amount of data being generated has exceeded 2.5 quintillion bytes per day. This rapid increase in the generation of data has pushed the applications of big data to new heights; one of which is cybersecurity. The paper aims to introduce a thorough survey on the use of big data analytics in building, improving, or defying ...
Big Data encompasses huge amounts of raw material which influence multitude of research fields as well as different industries performance such as business, marketing, social network analysis, educational systems, healthcare, IoT, meteorology, fraud detection. It aimed to uncover hidden trends and has prompted a development from a model-driven perspective to a data-driven approach. Among ...
This was also consistent with the view that most big data science research has focused on the technical capabilities required for data science and has overlooked the topic of ... Some of the primary studies identified in the current study can be used as seed papers in a future execution of the procedure. Second, conducting a multivocal ...
Fig 1: 8V's of Big data Courtesy: Elena. Having understood the 8V's of big data, let us look into details of research problems to be addressed. General big data research topics [3] are in the lines of: Scalability — Scalable Architectures for parallel data processing; Real-time big data analytics — Stream data processing of text, image ...
Big data analytics plays an important role in modern world. In this paper, we have briefly explained the big data characteristics and storage technology by exploring different relevant research papers. Moreover, we have also presented a detailed review of the application of big data in various fields.
With feature analysis of transportation big data, a quality assessment is conducted by three-dimensional metric sets, which is considered as a significant factor of trust measurement. ... provides an outlook for future research directions and describes possible research applications. Feature papers are submitted upon individual invitation or ...
4. Analyzing Big Data. Another important research direction is to address the privacy and the security issues in analyzing big data. Especially, recent developments in machine learning techniques have created important novel applications in many fields ranging from health care to social networking while creating important privacy challenges.
Stock market data coverage from CNN. View US markets, world markets, after hours trading, quotes, and other important stock market activity.
It also helps identify data that provides insightful insights for current as well as future decisions ... For the purpose of this paper, the following research hypotheses were formulated: (1) medical facilities in Poland are working on both structured and unstructured data (2) medical facilities in Poland are moving towards data-based ...
Around nine-in-ten Americans say marijuana should be legal for medical or recreational use, according to a January 2024 Pew Research Center survey.An overwhelming majority of U.S. adults (88%) say either that marijuana should be legal for medical use only (32%) or that it should be legal for medical and recreational use (57%).Just 11% say the drug should not be legal in any form.