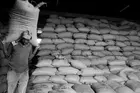
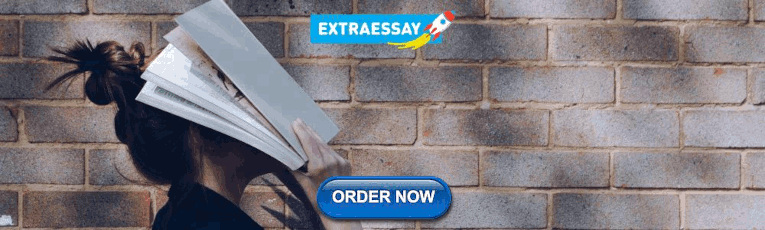
Top 40 Most Popular Case Studies of 2017
We generated a list of the 40 most popular Yale School of Management case studies in 2017 by combining data from our publishers, Google analytics, and other measures of interest and adoption. In compiling the list, we gave additional weight to usage outside Yale
We generated a list of the 40 most popular Yale School of Management case studies in 2017 by combining data from our publishers, Google analytics, and other measures of interest and adoption. In compiling the list, we gave additional weight to usage outside Yale.
Case topics represented on the list vary widely, but a number are drawn from the case team’s focus on healthcare, asset management, and sustainability. The cases also draw on Yale’s continued emphasis on corporate governance, ethics, and the role of business in state and society. Of note, nearly half of the most popular cases feature a woman as either the main protagonist or, in the case of raw cases where multiple characters take the place of a single protagonist, a major leader within the focal organization. While nearly a fourth of the cases were written in the past year, some of the most popular, including Cadbury and Design at Mayo, date from the early years of our program over a decade ago. Nearly two-thirds of the most popular cases were “raw” cases - Yale’s novel, web-based template which allows for a combination of text, documents, spreadsheets, and videos in a single case website.
Read on to learn more about the top 10 most popular cases followed by a complete list of the top 40 cases of 2017. A selection of the top 40 cases are available for purchase through our online store .
#1 - Coffee 2016
Faculty Supervision: Todd Cort
Coffee 2016 asks students to consider the coffee supply chain and generate ideas for what can be done to equalize returns across various stakeholders. The case draws a parallel between coffee and wine. Both beverages encourage connoisseurship, but only wine growers reap a premium for their efforts to ensure quality. The case describes the history of coffee production across the world, the rise of the “third wave” of coffee consumption in the developed world, the efforts of the Illy Company to help coffee growers, and the differences between “fair” trade and direct trade. Faculty have found the case provides a wide canvas to discuss supply chain issues, examine marketing practices, and encourage creative solutions to business problems.
#2 - AXA: Creating New Corporate Responsibility Metrics
Faculty Supervision: Todd Cort and David Bach
The case describes AXA’s corporate responsibility (CR) function. The company, a global leader in insurance and asset management, had distinguished itself in CR since formally establishing a CR unit in 2008. As the case opens, AXA’s CR unit is being moved from the marketing function to the strategy group occasioning a thorough review as to how CR should fit into AXA’s operations and strategy. Students are asked to identify CR issues of particular concern to the company, examine how addressing these issues would add value to the company, and then create metrics that would capture a business unit’s success or failure in addressing the concerns.
#3 - IBM Corporate Service Corps
Faculty Supervision: David Bach in cooperation with University of Ghana Business School and EGADE
The case considers IBM’s Corporate Service Corps (CSC), a program that had become the largest pro bono consulting program in the world. The case describes the program’s triple-benefit: leadership training to the brightest young IBMers, brand recognition for IBM in emerging markets, and community improvement in the areas served by IBM’s host organizations. As the program entered its second decade in 2016, students are asked to consider how the program can be improved. The case allows faculty to lead a discussion about training, marketing in emerging economies, and various ways of providing social benefit. The case highlights the synergies as well as trade-offs between pursuing these triple benefits.
#4 - Cadbury: An Ethical Company Struggles to Insure the Integrity of Its Supply Chain
Faculty Supervision: Ira Millstein
The case describes revelations that the production of cocoa in the Côte d’Ivoire involved child slave labor. These stories hit Cadbury especially hard. Cadbury's culture had been deeply rooted in the religious traditions of the company's founders, and the organization had paid close attention to the welfare of its workers and its sourcing practices. The US Congress was considering legislation that would allow chocolate grown on certified plantations to be labeled “slave labor free,” painting the rest of the industry in a bad light. Chocolate producers had asked for time to rectify the situation, but the extension they negotiated was running out. Students are asked whether Cadbury should join with the industry to lobby for more time? What else could Cadbury do to ensure its supply chain was ethically managed?
#5 - 360 State Real Options
Faculty Supervision: Matthew Spiegel
In 2010 developer Bruce Becker (SOM ‘85) completed 360 State Street, a major new construction project in downtown New Haven. Just west of the apartment building, a 6,000-square-foot pocket of land from the original parcel remained undeveloped. Becker had a number of alternatives to consider in regards to the site. He also had no obligation to build. He could bide his time. But Becker worried about losing out on rents should he wait too long. Students are asked under what set of circumstances and at what time would it be most advantageous to proceed?
#6 - Design at Mayo
Faculty Supervision: Rodrigo Canales and William Drentell
The case describes how the Mayo Clinic, one of the most prominent hospitals in the world, engaged designers and built a research institute, the Center for Innovation (CFI), to study the processes of healthcare provision. The case documents the many incremental innovations the designers were able to implement and the way designers learned to interact with physicians and vice-versa.
In 2010 there were questions about how the CFI would achieve its stated aspiration of “transformational change” in the healthcare field. Students are asked what would a major change in health care delivery look like? How should the CFI's impact be measured? Were the center's structure and processes appropriate for transformational change? Faculty have found this a great case to discuss institutional obstacles to innovation, the importance of culture in organizational change efforts, and the differences in types of innovation.
This case is freely available to the public.
#7 - Ant Financial
Faculty Supervision: K. Sudhir in cooperation with Renmin University of China School of Business
In 2015, Ant Financial’s MYbank (an offshoot of Jack Ma’s Alibaba company) was looking to extend services to rural areas in China by providing small loans to farmers. Microloans have always been costly for financial institutions to offer to the unbanked (though important in development) but MYbank believed that fintech innovations such as using the internet to communicate with loan applicants and judge their credit worthiness would make the program sustainable. Students are asked whether MYbank could operate the program at scale? Would its big data and technical analysis provide an accurate measure of credit risk for loans to small customers? Could MYbank rely on its new credit-scoring system to reduce operating costs to make the program sustainable?
#8 - Business Leadership in South Africa’s 1994 Reforms
Faculty Supervision: Ian Shapiro
This case examines the role of business in South Africa's historic transition away from apartheid to popular sovereignty. The case provides a previously untold oral history of this key moment in world history, presenting extensive video interviews with business leaders who spearheaded behind-the-scenes negotiations between the African National Congress and the government. Faculty teaching the case have used the material to push students to consider business’s role in a divided society and ask: What factors led business leaders to act to push the country's future away from isolation toward a "high road" of participating in an increasingly globalized economy? What techniques and narratives did they use to keep the two sides talking and resolve the political impasse? And, if business leadership played an important role in the events in South Africa, could they take a similar role elsewhere?
#9 - Shake Shack IPO
Faculty Supervision: Jake Thomas and Geert Rouwenhorst
From an art project in a New York City park, Shake Shack developed a devoted fan base that greeted new Shake Shack locations with cheers and long lines. When Shake Shack went public on January 30, 2015, investors displayed a similar enthusiasm. Opening day investors bid up the $21 per share offering price by 118% to reach $45.90 at closing bell. By the end of May, investors were paying $92.86 per share. Students are asked if this price represented a realistic valuation of the enterprise and if not, what was Shake Shack truly worth? The case provides extensive information on Shake Shack’s marketing, competitors, operations and financials, allowing instructors to weave a wide variety of factors into a valuation of the company.
#10 - Searching for a Search Fund Structure
Faculty Supervision: AJ Wasserstein
This case considers how young entrepreneurs structure search funds to find businesses to take over. The case describes an MBA student who meets with a number of successful search fund entrepreneurs who have taken alternative routes to raising funds. The case considers the issues of partnering, soliciting funds vs. self-funding a search, and joining an incubator. The case provides a platform from which to discuss the pros and cons of various search fund structures.
40 Most Popular Case Studies of 2017
Click on the case title to learn more about the dilemma. A selection of our most popular cases are available for purchase via our online store .
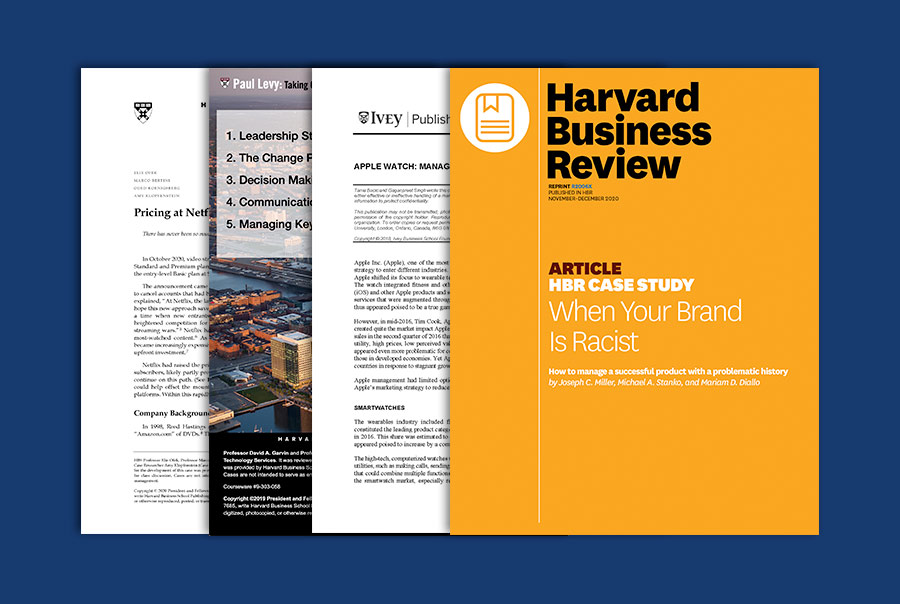
Prepare your students to navigate business challenges by immersing them in real-world scenarios.
Transform business education
Bring excitement into your classroom with engaging case discussions and introduce students to the challenge and fun of making important decisions.
Illustrate business concepts
Help students learn by doing with over 50,000+ cases featuring real-world business scenarios spanning across multiple areas of business.
Encourage new ways of thinking
Student build confidence and critical thinking skills while learning to express their ideas and convince others, setting them up for success in the real world.
Explore Different Types of Cases
Find cases that meet your particular needs.
New! Quick Cases
Quickly immerse students in focused and engaging business dilemmas. No student prep time required.
Traditional cases from HBS and 50+ leading business schools.
Multimedia Cases
Cases that keep students engaged with video, audio, and interactive components.
Search Cases in Your Discipline
Select a discipline and start browsing available cases.
- Business & Government Relations
- Business Ethics
- Entrepreneurship
- General Management
- Human Resource Management
- Information Technology
- International Business
- Negotiation
- Operations Management
- Organizational Behavior
- Service Management
- Social Enterprise
Case Teaching Seminar
Register now for our Teaching with Cases Seminar at Harvard Business School, held June 21 - 22 . Learn how to lead case discussions like a pro and earn a certificate from Harvard Business Publishing.
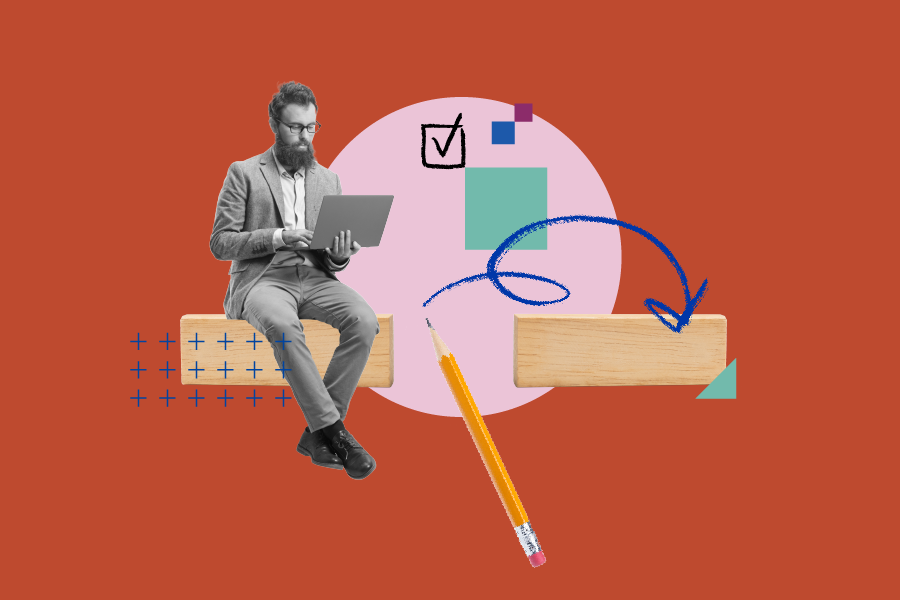
Fundamentals of Case Teaching
Our new, self-paced, online course guides you through the fundamentals for leading successful case discussions at any course level.
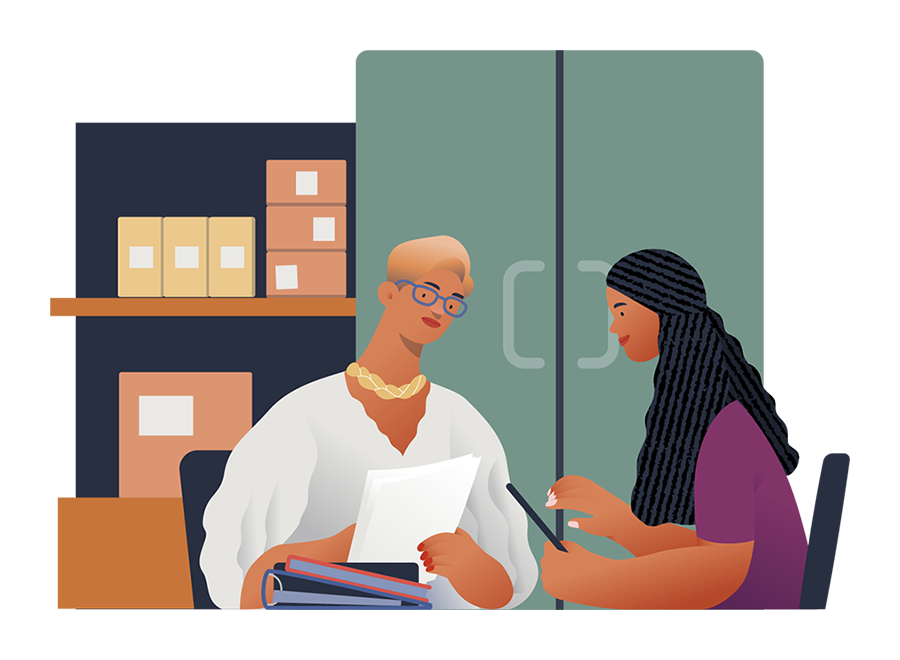
Case Companion: Build Students’ Confidence in Case Analysis
Case Companion is an engaging and interactive introduction to case study analysis that is ideal for undergraduates or any student new to learning with cases.
Discover Trending Cases
Stay up to date on cases from leading business schools.
Discover new ideas for your courses
Course Explorer lets you browse learning materials by topic, curated by our editors, partners, and faculty from leading business schools.
Teach with Cases
Explore resources designed to help you bring the case method into your classroom.
Inspiring Minds Articles on Case Teaching
Insights from leading educators about teaching with the case method.
Book: Teaching with Cases: A Practical Guide
A book featuring practical advice for instructors on managing class discussion to maximize learning.
Webinar: How ChatGPT and Other AI Tools Can Maximize the Learning Potential of Your Case-Based Classes
Register now.
Supplements: Inside the Case
Teaching tips and insights from case authors.
Guide: Teaching Cases Online
A guide for experienced educators who are new to online case teaching.
Educator Training: Selecting Cases to Use in Your Classes
Find the right materials to achieve your learning goals.
Educator Training: Teaching with Cases
Key strategies and practical advice for engaging students using the case method.
Frequently Asked Questions
What support can I offer my students around analyzing cases and preparing for discussion?
Case discussions can be a big departure from the norm for students who are used to lecture-based classes. The Case Analysis Coach is an interactive tutorial on reading and analyzing a case study. The Case Study Handbook covers key skills students need to read, understand, discuss and write about cases. The Case Study Handbook is also available as individual chapters to help your students focus on specific skills.
How can I transfer my in-person case teaching plan to an online environment?
The case method can be used in an online environment without sacrificing its benefits. We have compiled a few resources to help you create transformative online learning experiences with the case method. Learn how HBS brought the case method online in this podcast , gather some quick guidance from the article " How to Teach Any Case Online ", review the Teaching Cases Online Guide for a deep dive, and check out our Teaching Online Resources Page for more insights and inspiration.
After 35 years as an academic, I have come to the conclusion that there is a magic in the way Harvard cases are written. Cases go from specific to general, to show students that business situations are amenable to hard headed analysis that then generalize to larger theoretical insights. The students love it! Akshay Rao Professor, General Mills Chair in Marketing at the University of Minnesota
We use cookies to understand how you use our site and to improve your experience, including personalizing content. Learn More . By continuing to use our site, you accept our use of cookies and revised Privacy Policy .
- Business Essentials
- Leadership & Management
- Credential of Leadership, Impact, and Management in Business (CLIMB)
- Entrepreneurship & Innovation
- Digital Transformation
- Finance & Accounting
- Business in Society
- For Organizations
- Support Portal
- Media Coverage
- Founding Donors
- Leadership Team
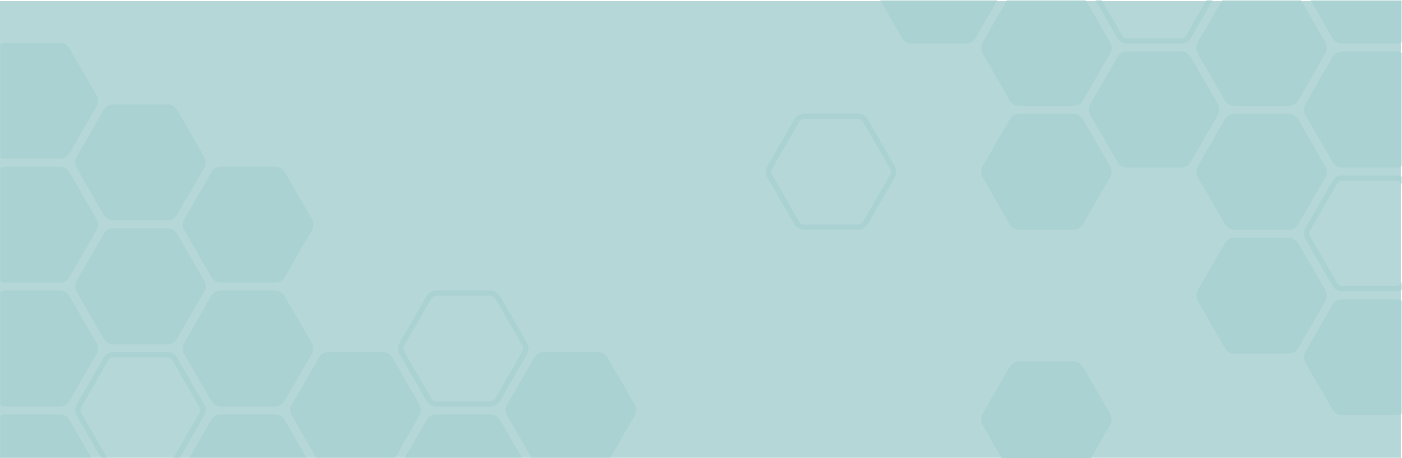
- Harvard Business School →
- HBS Online →
- Business Insights →
Business Insights
Harvard Business School Online's Business Insights Blog provides the career insights you need to achieve your goals and gain confidence in your business skills.
- Career Development
- Communication
- Decision-Making
- Earning Your MBA
- Negotiation
- News & Events
- Productivity
- Staff Spotlight
- Student Profiles
- Work-Life Balance
- AI Essentials for Business
- Alternative Investments
- Business Analytics
- Business Strategy
- Business and Climate Change
- Design Thinking and Innovation
- Digital Marketing Strategy
- Disruptive Strategy
- Economics for Managers
- Entrepreneurship Essentials
- Financial Accounting
- Global Business
- Launching Tech Ventures
- Leadership Principles
- Leadership, Ethics, and Corporate Accountability
- Leading with Finance
- Management Essentials
- Negotiation Mastery
- Organizational Leadership
- Power and Influence for Positive Impact
- Strategy Execution
- Sustainable Business Strategy
- Sustainable Investing
- Winning with Digital Platforms
5 Benefits of Learning Through the Case Study Method
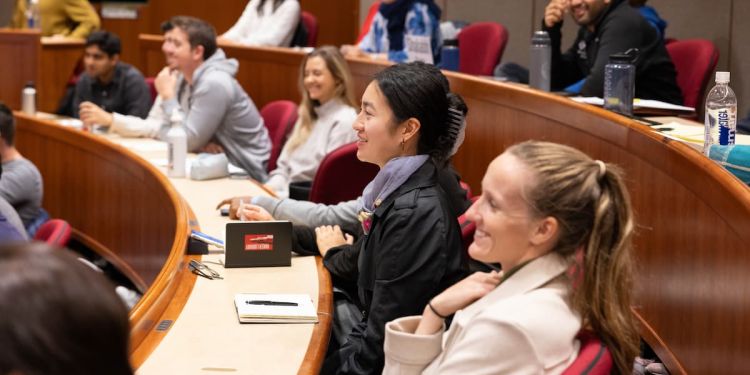
- 28 Nov 2023
While several factors make HBS Online unique —including a global Community and real-world outcomes —active learning through the case study method rises to the top.
In a 2023 City Square Associates survey, 74 percent of HBS Online learners who also took a course from another provider said HBS Online’s case method and real-world examples were better by comparison.
Here’s a primer on the case method, five benefits you could gain, and how to experience it for yourself.
Access your free e-book today.
What Is the Harvard Business School Case Study Method?
The case study method , or case method , is a learning technique in which you’re presented with a real-world business challenge and asked how you’d solve it. After working through it yourself and with peers, you’re told how the scenario played out.
HBS pioneered the case method in 1922. Shortly before, in 1921, the first case was written.
“How do you go into an ambiguous situation and get to the bottom of it?” says HBS Professor Jan Rivkin, former senior associate dean and chair of HBS's master of business administration (MBA) program, in a video about the case method . “That skill—the skill of figuring out a course of inquiry to choose a course of action—that skill is as relevant today as it was in 1921.”
Originally developed for the in-person MBA classroom, HBS Online adapted the case method into an engaging, interactive online learning experience in 2014.
In HBS Online courses , you learn about each case from the business professional who experienced it. After reviewing their videos, you’re prompted to take their perspective and explain how you’d handle their situation.
You then get to read peers’ responses, “star” them, and comment to further the discussion. Afterward, you learn how the professional handled it and their key takeaways.
HBS Online’s adaptation of the case method incorporates the famed HBS “cold call,” in which you’re called on at random to make a decision without time to prepare.
“Learning came to life!” said Sheneka Balogun , chief administration officer and chief of staff at LeMoyne-Owen College, of her experience taking the Credential of Readiness (CORe) program . “The videos from the professors, the interactive cold calls where you were randomly selected to participate, and the case studies that enhanced and often captured the essence of objectives and learning goals were all embedded in each module. This made learning fun, engaging, and student-friendly.”
If you’re considering taking a course that leverages the case study method, here are five benefits you could experience.
5 Benefits of Learning Through Case Studies
1. take new perspectives.
The case method prompts you to consider a scenario from another person’s perspective. To work through the situation and come up with a solution, you must consider their circumstances, limitations, risk tolerance, stakeholders, resources, and potential consequences to assess how to respond.
Taking on new perspectives not only can help you navigate your own challenges but also others’. Putting yourself in someone else’s situation to understand their motivations and needs can go a long way when collaborating with stakeholders.
2. Hone Your Decision-Making Skills
Another skill you can build is the ability to make decisions effectively . The case study method forces you to use limited information to decide how to handle a problem—just like in the real world.
Throughout your career, you’ll need to make difficult decisions with incomplete or imperfect information—and sometimes, you won’t feel qualified to do so. Learning through the case method allows you to practice this skill in a low-stakes environment. When facing a real challenge, you’ll be better prepared to think quickly, collaborate with others, and present and defend your solution.
3. Become More Open-Minded
As you collaborate with peers on responses, it becomes clear that not everyone solves problems the same way. Exposing yourself to various approaches and perspectives can help you become a more open-minded professional.
When you’re part of a diverse group of learners from around the world, your experiences, cultures, and backgrounds contribute to a range of opinions on each case.
On the HBS Online course platform, you’re prompted to view and comment on others’ responses, and discussion is encouraged. This practice of considering others’ perspectives can make you more receptive in your career.
“You’d be surprised at how much you can learn from your peers,” said Ratnaditya Jonnalagadda , a software engineer who took CORe.
In addition to interacting with peers in the course platform, Jonnalagadda was part of the HBS Online Community , where he networked with other professionals and continued discussions sparked by course content.
“You get to understand your peers better, and students share examples of businesses implementing a concept from a module you just learned,” Jonnalagadda said. “It’s a very good way to cement the concepts in one's mind.”
4. Enhance Your Curiosity
One byproduct of taking on different perspectives is that it enables you to picture yourself in various roles, industries, and business functions.
“Each case offers an opportunity for students to see what resonates with them, what excites them, what bores them, which role they could imagine inhabiting in their careers,” says former HBS Dean Nitin Nohria in the Harvard Business Review . “Cases stimulate curiosity about the range of opportunities in the world and the many ways that students can make a difference as leaders.”
Through the case method, you can “try on” roles you may not have considered and feel more prepared to change or advance your career .
5. Build Your Self-Confidence
Finally, learning through the case study method can build your confidence. Each time you assume a business leader’s perspective, aim to solve a new challenge, and express and defend your opinions and decisions to peers, you prepare to do the same in your career.
According to a 2022 City Square Associates survey , 84 percent of HBS Online learners report feeling more confident making business decisions after taking a course.
“Self-confidence is difficult to teach or coach, but the case study method seems to instill it in people,” Nohria says in the Harvard Business Review . “There may well be other ways of learning these meta-skills, such as the repeated experience gained through practice or guidance from a gifted coach. However, under the direction of a masterful teacher, the case method can engage students and help them develop powerful meta-skills like no other form of teaching.”

How to Experience the Case Study Method
If the case method seems like a good fit for your learning style, experience it for yourself by taking an HBS Online course. Offerings span seven subject areas, including:
- Business essentials
- Leadership and management
- Entrepreneurship and innovation
- Finance and accounting
- Business in society
No matter which course or credential program you choose, you’ll examine case studies from real business professionals, work through their challenges alongside peers, and gain valuable insights to apply to your career.
Are you interested in discovering how HBS Online can help advance your career? Explore our course catalog and download our free guide —complete with interactive workbook sections—to determine if online learning is right for you and which course to take.

About the Author
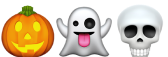
- Onsite training
3,000,000+ delegates
15,000+ clients
1,000+ locations
- KnowledgePass
- Log a ticket
01344203999 Available 24/7
Business Management Case Study: A Complete Breakdown
Gain a comprehensive understanding of the "Business Management Case Study" as we break down the concept from start to finish. Discover the incredible journeys of companies like Apple Inc., Tesla and Netflix as they navigate innovation, global expansion, and transformation. This detailed analysis will provide insights into the dynamic world of business management.

Exclusive 40% OFF
Training Outcomes Within Your Budget!
We ensure quality, budget-alignment, and timely delivery by our expert instructors.
Share this Resource
- PMP® Certification Training Course
- Introduction to Management
- Introduction to Managing People
- CAPM® Certification Training Course
- Business Management Training Course
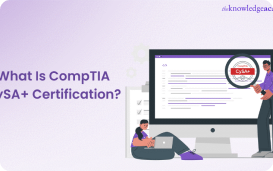
Case studies play a pivotal role in understanding real-world challenges, strategies, and outcomes in the ever-evolving field of Business Management. This blog dives into the intricacies of a compelling Business Management Case Study, dissecting its components to extract valuable insights for aspiring managers, entrepreneurs, and students alike. Learn the study behind some of the most significant Business Management Case Studies & how an online business degree can help you learn more in this article.
Table of Contents
1) What is Business Management?
2) Case Studies in Business Management
a) Apple Inc. Innovation
b) Tesla’s EV revolution
c) Amazon retailer to e-commerce giant
d) McDonald’s global expansion
e) Netflix’s transformation
3) Conclusion
What is Business Management?
Business Management refers to the set of activities, strategies, and practices employed to oversee and coordinate an organisation's operations, resources, and personnel to achieve specific goals and objectives. It encompasses a wide range of responsibilities to ensure an organisation's efficient and effective functioning across various functional areas.
Try our Business Case Training Course today and start your career!
Case Studies in Business Management
Here are some of the notable case studies in the field of Business Management that have garnered attention due to their complexity, innovative strategies, and significant impact on their respective industries:
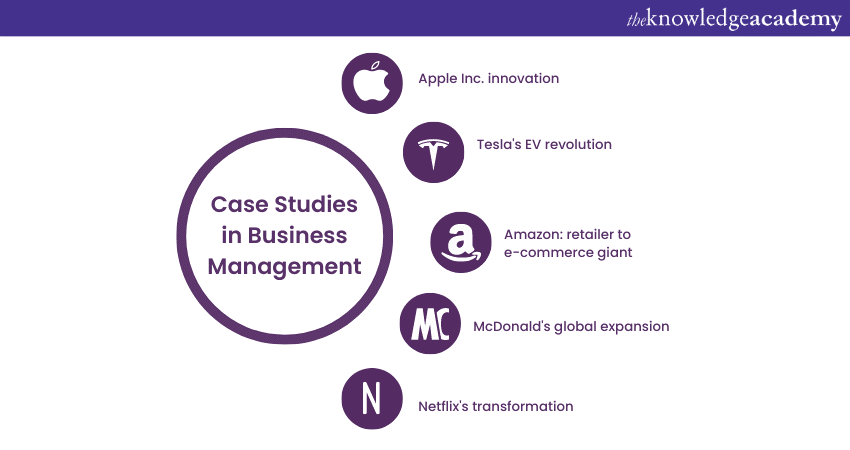
Apple Inc. innovation
a) Background: Apple Inc. is a global technology giant noted for its innovative products and design-driven approach. In the early 2000s, Apple faced intense competition and declining market share. The company needed to reinvent itself to remain relevant and competitive.
b) Problem statement: Apple's challenge was revitalising its product line and regaining market leadership while navigating a rapidly changing technological world.
c) Analysis of the situation: The Case Study dives into Apple's design thinking and customer-centric innovation to develop products that seamlessly blend form and function. The company's focus on user experience, ecosystem integration, and attention to detail set it apart from its competitors.
d) Proposed solutions: Apple's strategy involved launching breakthrough products like the iPod, iPhone, and iPad that redefined their respective markets. The company also invested heavily in creating a robust ecosystem through iTunes and the App Store.
e) Chosen strategy: Apple's commitment to user-centred design and innovation became the cornerstone of its success. The strategy encompassed cutting-edge technology, minimalist design, and exceptional user experience.
f) Implementation process: Apple's implementation involved rigorous research and development, collaboration among various teams, and meticulous attention to detail. The company also established a loyal customer base through iconic product launches and marketing campaigns.
g) Results and outcomes: Apple's strategy paid off immensely, leading to a resurgence in its market share, revenue, and brand value. The company's products became cultural touchstones, and its ecosystem approach set new standards for the technology industry.
Tesla’s EV revolution
a) Background: Tesla, led by Elon Musk, aimed to disrupt the traditional automotive industry by introducing electric vehicles (EVs) that combined sustainability, performance, and cutting-edge technology.
b) Problem statement: Tesla faced challenges related to the production, scalability, and market acceptance of electric vehicles in an industry dominated by internal combustion engine vehicles.
c) Analysis of the situation: This Case Study examines Tesla's unique approach, which combines innovation in electric powertrains, battery technology, and software. The company also adopted a direct-to-consumer sales model, bypassing traditional dealership networks.
d) Proposed solutions: Tesla's solutions included building a network of Supercharger stations, developing advanced autonomous driving technology, and leveraging over-the-air software updates to improve vehicle performance and features.
e) Chosen strategy: Tesla focused on high-quality engineering, creating a luxury brand image for EVs, and promoting a community of passionate supporters. The company also bet on long-term sustainability and energy innovation beyond just manufacturing cars.
f) Implementation process: Tesla faced production challenges, supply chain issues, and scepticism from traditional automakers. The company's determination to continuously refine its vehicles and technology resulted in incremental improvements and increased consumer interest.
g) Results and outcomes: Tesla's innovative approach catapulted it into the forefront of the EV market. The Model S, Model 3, Model X, and Model Y gained popularity for their performance, range, and technology. Tesla's market capitalisation surged, and the company played a significant part in changing the perception of electric vehicles.
Amazon retailer to e-commerce giant
a) Background: Amazon started as an online bookstore in the 1990s and quickly expanded its offerings to become the world's largest online retailer. However, its journey was riddled with challenges and risks.
b) Problem statement: Amazon faced difficulties in achieving profitability due to its aggressive expansion, heavy investments, and price competition. The company needed to find a way to sustain its growth and solidify its position in the e-commerce market.
c) Analysis of the situation: This Case Study explores Amazon's unique business model, which prioritises customer satisfaction, convenience, and diversification. The company continuously experimented with new ideas, services, and technologies.
d) Proposed solutions: Amazon's solutions included the introduction of Amazon Prime, the Kindle e-reader, and the development of its third-party seller marketplace. These initiatives aimed to enhance customer loyalty, expand product offerings, and increase revenue streams.
e) Chosen strategy: Amazon's strategy revolved around long-term thinking, customer obsession, and a willingness to invest heavily in innovation and infrastructure, even at the expense of short-term profits.
f) Implementation process: Amazon's implementation involved building a vast network of fulfilment centres, investing in advanced technology for logistics and supply chain management, and expanding its services beyond e-commerce into cloud computing (Amazon Web Services) and entertainment (Amazon Prime Video).
g) Results and outcomes: Amazon's strategy paid off as it transformed from an online bookstore to an e-commerce behemoth. The company not only achieved profitability but also diversified into various sectors, making Jeff Bezos the richest person in the world for a time.
McDonald’s global expansion
a) Background: McDonald's is one of the world's largest and most recognisable fast-food chains. The Case Study focuses on the company's global expansion strategy and challenges in adapting to diverse cultural preferences and market conditions.
b) Problem statement: McDonald's challenge was maintaining its brand identity while tailoring its menu offerings and marketing strategies to suit different countries' preferences and cultural norms.
c) Analysis: The Case Study analyses McDonald's localisation efforts, menu adaptations, and marketing campaigns in different countries. It explores how the company balances standardisation with customisation to appeal to local tastes.
d) Solutions and outcomes: McDonald's successfully combines global branding with localized strategies, resulting in sustained growth and customer loyalty in various markets. The Case Study demonstrates the importance of understanding cultural nuances in international business.
Netflix’s evolution
a) Background: Netflix started as a DVD rental-by-mail service and became a leading global streaming platform. The Case Study explores Netflix's strategic evolution, content production, and influence on the entertainment industry.
b) Problem statement: Netflix's challenge was transitioning from a traditional DVD rental business to a digital streaming service while competing with established cable networks and other streaming platforms.
c) Analysis: The Case Study analyses Netflix's shift to online streaming, its investment in original content production, and its use of data analytics to personalise user experiences and content recommendations.
d) Solutions and outcomes: Netflix's strategic pivot and focus on content quality and user experience contributed to its dominance in the streaming market. The Case Study illustrates how embracing digital disruption and customer-centric strategies can drive success.
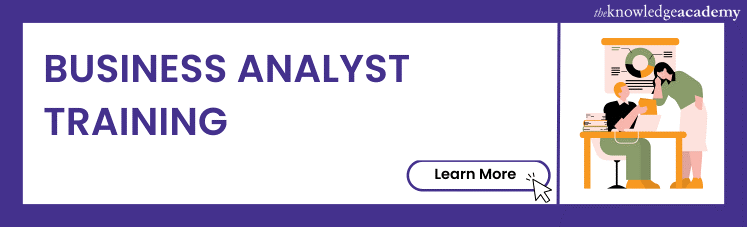
Conclusion
These case studies offer valuable insights into different facets of Business Management, including innovation, strategic decision-making, customer-centric approaches, and market disruption. Analysing these cases provides aspiring managers and entrepreneurs with real-world examples of how effective strategies, risk-taking, and adaptability can lead to remarkable success in the dynamic business world.
Try our Business Analyst Training today for a rewarding career!
Frequently Asked Questions
Upcoming business skills resources batches & dates.
Fri 31st May 2024
Fri 26th Jul 2024
Fri 27th Sep 2024
Fri 29th Nov 2024
Get A Quote
WHO WILL BE FUNDING THE COURSE?
My employer
By submitting your details you agree to be contacted in order to respond to your enquiry
- Business Analysis
- Lean Six Sigma Certification
Share this course
Our biggest spring sale.

We cannot process your enquiry without contacting you, please tick to confirm your consent to us for contacting you about your enquiry.
By submitting your details you agree to be contacted in order to respond to your enquiry.
We may not have the course you’re looking for. If you enquire or give us a call on 01344203999 and speak to our training experts, we may still be able to help with your training requirements.
Or select from our popular topics
- ITIL® Certification
- Scrum Certification
- Change Management Certification
- Business Analysis Courses
- Microsoft Azure Certification
- Microsoft Excel Courses
- Microsoft Project
- Explore more courses
Press esc to close
Fill out your contact details below and our training experts will be in touch.
Fill out your contact details below
Thank you for your enquiry!
One of our training experts will be in touch shortly to go over your training requirements.
Back to Course Information
Fill out your contact details below so we can get in touch with you regarding your training requirements.
* WHO WILL BE FUNDING THE COURSE?
Preferred Contact Method
No preference
Back to course information
Fill out your training details below
Fill out your training details below so we have a better idea of what your training requirements are.
HOW MANY DELEGATES NEED TRAINING?
HOW DO YOU WANT THE COURSE DELIVERED?
Online Instructor-led
Online Self-paced
WHEN WOULD YOU LIKE TO TAKE THIS COURSE?
Next 2 - 4 months
WHAT IS YOUR REASON FOR ENQUIRING?
Looking for some information
Looking for a discount
I want to book but have questions
One of our training experts will be in touch shortly to go overy your training requirements.
Your privacy & cookies!
Like many websites we use cookies. We care about your data and experience, so to give you the best possible experience using our site, we store a very limited amount of your data. Continuing to use this site or clicking “Accept & close” means that you agree to our use of cookies. Learn more about our privacy policy and cookie policy cookie policy .
We use cookies that are essential for our site to work. Please visit our cookie policy for more information. To accept all cookies click 'Accept & close'.
How to write a case study — examples, templates, and tools

It’s a marketer’s job to communicate the effectiveness of a product or service to potential and current customers to convince them to buy and keep business moving. One of the best methods for doing this is to share success stories that are relatable to prospects and customers based on their pain points, experiences, and overall needs.
That’s where case studies come in. Case studies are an essential part of a content marketing plan. These in-depth stories of customer experiences are some of the most effective at demonstrating the value of a product or service. Yet many marketers don’t use them, whether because of their regimented formats or the process of customer involvement and approval.
A case study is a powerful tool for showcasing your hard work and the success your customer achieved. But writing a great case study can be difficult if you’ve never done it before or if it’s been a while. This guide will show you how to write an effective case study and provide real-world examples and templates that will keep readers engaged and support your business.
In this article, you’ll learn:
What is a case study?
How to write a case study, case study templates, case study examples, case study tools.
A case study is the detailed story of a customer’s experience with a product or service that demonstrates their success and often includes measurable outcomes. Case studies are used in a range of fields and for various reasons, from business to academic research. They’re especially impactful in marketing as brands work to convince and convert consumers with relatable, real-world stories of actual customer experiences.
The best case studies tell the story of a customer’s success, including the steps they took, the results they achieved, and the support they received from a brand along the way. To write a great case study, you need to:
- Celebrate the customer and make them — not a product or service — the star of the story.
- Craft the story with specific audiences or target segments in mind so that the story of one customer will be viewed as relatable and actionable for another customer.
- Write copy that is easy to read and engaging so that readers will gain the insights and messages intended.
- Follow a standardized format that includes all of the essentials a potential customer would find interesting and useful.
- Support all of the claims for success made in the story with data in the forms of hard numbers and customer statements.
Case studies are a type of review but more in depth, aiming to show — rather than just tell — the positive experiences that customers have with a brand. Notably, 89% of consumers read reviews before deciding to buy, and 79% view case study content as part of their purchasing process. When it comes to B2B sales, 52% of buyers rank case studies as an important part of their evaluation process.
Telling a brand story through the experience of a tried-and-true customer matters. The story is relatable to potential new customers as they imagine themselves in the shoes of the company or individual featured in the case study. Showcasing previous customers can help new ones see themselves engaging with your brand in the ways that are most meaningful to them.
Besides sharing the perspective of another customer, case studies stand out from other content marketing forms because they are based on evidence. Whether pulling from client testimonials or data-driven results, case studies tend to have more impact on new business because the story contains information that is both objective (data) and subjective (customer experience) — and the brand doesn’t sound too self-promotional.

Case studies are unique in that there’s a fairly standardized format for telling a customer’s story. But that doesn’t mean there isn’t room for creativity. It’s all about making sure that teams are clear on the goals for the case study — along with strategies for supporting content and channels — and understanding how the story fits within the framework of the company’s overall marketing goals.
Here are the basic steps to writing a good case study.
1. Identify your goal
Start by defining exactly who your case study will be designed to help. Case studies are about specific instances where a company works with a customer to achieve a goal. Identify which customers are likely to have these goals, as well as other needs the story should cover to appeal to them.
The answer is often found in one of the buyer personas that have been constructed as part of your larger marketing strategy. This can include anything from new leads generated by the marketing team to long-term customers that are being pressed for cross-sell opportunities. In all of these cases, demonstrating value through a relatable customer success story can be part of the solution to conversion.
2. Choose your client or subject
Who you highlight matters. Case studies tie brands together that might otherwise not cross paths. A writer will want to ensure that the highlighted customer aligns with their own company’s brand identity and offerings. Look for a customer with positive name recognition who has had great success with a product or service and is willing to be an advocate.
The client should also match up with the identified target audience. Whichever company or individual is selected should be a reflection of other potential customers who can see themselves in similar circumstances, having the same problems and possible solutions.
Some of the most compelling case studies feature customers who:
- Switch from one product or service to another while naming competitors that missed the mark.
- Experience measurable results that are relatable to others in a specific industry.
- Represent well-known brands and recognizable names that are likely to compel action.
- Advocate for a product or service as a champion and are well-versed in its advantages.
Whoever or whatever customer is selected, marketers must ensure they have the permission of the company involved before getting started. Some brands have strict review and approval procedures for any official marketing or promotional materials that include their name. Acquiring those approvals in advance will prevent any miscommunication or wasted effort if there is an issue with their legal or compliance teams.
3. Conduct research and compile data
Substantiating the claims made in a case study — either by the marketing team or customers themselves — adds validity to the story. To do this, include data and feedback from the client that defines what success looks like. This can be anything from demonstrating return on investment (ROI) to a specific metric the customer was striving to improve. Case studies should prove how an outcome was achieved and show tangible results that indicate to the customer that your solution is the right one.
This step could also include customer interviews. Make sure that the people being interviewed are key stakeholders in the purchase decision or deployment and use of the product or service that is being highlighted. Content writers should work off a set list of questions prepared in advance. It can be helpful to share these with the interviewees beforehand so they have time to consider and craft their responses. One of the best interview tactics to keep in mind is to ask questions where yes and no are not natural answers. This way, your subject will provide more open-ended responses that produce more meaningful content.
4. Choose the right format
There are a number of different ways to format a case study. Depending on what you hope to achieve, one style will be better than another. However, there are some common elements to include, such as:
- An engaging headline
- A subject and customer introduction
- The unique challenge or challenges the customer faced
- The solution the customer used to solve the problem
- The results achieved
- Data and statistics to back up claims of success
- A strong call to action (CTA) to engage with the vendor
It’s also important to note that while case studies are traditionally written as stories, they don’t have to be in a written format. Some companies choose to get more creative with their case studies and produce multimedia content, depending on their audience and objectives. Case study formats can include traditional print stories, interactive web or social content, data-heavy infographics, professionally shot videos, podcasts, and more.
5. Write your case study
We’ll go into more detail later about how exactly to write a case study, including templates and examples. Generally speaking, though, there are a few things to keep in mind when writing your case study.
- Be clear and concise. Readers want to get to the point of the story quickly and easily, and they’ll be looking to see themselves reflected in the story right from the start.
- Provide a big picture. Always make sure to explain who the client is, their goals, and how they achieved success in a short introduction to engage the reader.
- Construct a clear narrative. Stick to the story from the perspective of the customer and what they needed to solve instead of just listing product features or benefits.
- Leverage graphics. Incorporating infographics, charts, and sidebars can be a more engaging and eye-catching way to share key statistics and data in readable ways.
- Offer the right amount of detail. Most case studies are one or two pages with clear sections that a reader can skim to find the information most important to them.
- Include data to support claims. Show real results — both facts and figures and customer quotes — to demonstrate credibility and prove the solution works.
6. Promote your story
Marketers have a number of options for distribution of a freshly minted case study. Many brands choose to publish case studies on their website and post them on social media. This can help support SEO and organic content strategies while also boosting company credibility and trust as visitors see that other businesses have used the product or service.
Marketers are always looking for quality content they can use for lead generation. Consider offering a case study as gated content behind a form on a landing page or as an offer in an email message. One great way to do this is to summarize the content and tease the full story available for download after the user takes an action.
Sales teams can also leverage case studies, so be sure they are aware that the assets exist once they’re published. Especially when it comes to larger B2B sales, companies often ask for examples of similar customer challenges that have been solved.
Now that you’ve learned a bit about case studies and what they should include, you may be wondering how to start creating great customer story content. Here are a couple of templates you can use to structure your case study.
Template 1 — Challenge-solution-result format
- Start with an engaging title. This should be fewer than 70 characters long for SEO best practices. One of the best ways to approach the title is to include the customer’s name and a hint at the challenge they overcame in the end.
- Create an introduction. Lead with an explanation as to who the customer is, the need they had, and the opportunity they found with a specific product or solution. Writers can also suggest the success the customer experienced with the solution they chose.
- Present the challenge. This should be several paragraphs long and explain the problem the customer faced and the issues they were trying to solve. Details should tie into the company’s products and services naturally. This section needs to be the most relatable to the reader so they can picture themselves in a similar situation.
- Share the solution. Explain which product or service offered was the ideal fit for the customer and why. Feel free to delve into their experience setting up, purchasing, and onboarding the solution.
- Explain the results. Demonstrate the impact of the solution they chose by backing up their positive experience with data. Fill in with customer quotes and tangible, measurable results that show the effect of their choice.
- Ask for action. Include a CTA at the end of the case study that invites readers to reach out for more information, try a demo, or learn more — to nurture them further in the marketing pipeline. What you ask of the reader should tie directly into the goals that were established for the case study in the first place.
Template 2 — Data-driven format
- Start with an engaging title. Be sure to include a statistic or data point in the first 70 characters. Again, it’s best to include the customer’s name as part of the title.
- Create an overview. Share the customer’s background and a short version of the challenge they faced. Present the reason a particular product or service was chosen, and feel free to include quotes from the customer about their selection process.
- Present data point 1. Isolate the first metric that the customer used to define success and explain how the product or solution helped to achieve this goal. Provide data points and quotes to substantiate the claim that success was achieved.
- Present data point 2. Isolate the second metric that the customer used to define success and explain what the product or solution did to achieve this goal. Provide data points and quotes to substantiate the claim that success was achieved.
- Present data point 3. Isolate the final metric that the customer used to define success and explain what the product or solution did to achieve this goal. Provide data points and quotes to substantiate the claim that success was achieved.
- Summarize the results. Reiterate the fact that the customer was able to achieve success thanks to a specific product or service. Include quotes and statements that reflect customer satisfaction and suggest they plan to continue using the solution.
- Ask for action. Include a CTA at the end of the case study that asks readers to reach out for more information, try a demo, or learn more — to further nurture them in the marketing pipeline. Again, remember that this is where marketers can look to convert their content into action with the customer.
While templates are helpful, seeing a case study in action can also be a great way to learn. Here are some examples of how Adobe customers have experienced success.
Juniper Networks
One example is the Adobe and Juniper Networks case study , which puts the reader in the customer’s shoes. The beginning of the story quickly orients the reader so that they know exactly who the article is about and what they were trying to achieve. Solutions are outlined in a way that shows Adobe Experience Manager is the best choice and a natural fit for the customer. Along the way, quotes from the client are incorporated to help add validity to the statements. The results in the case study are conveyed with clear evidence of scale and volume using tangible data.

The story of Lenovo’s journey with Adobe is one that spans years of planning, implementation, and rollout. The Lenovo case study does a great job of consolidating all of this into a relatable journey that other enterprise organizations can see themselves taking, despite the project size. This case study also features descriptive headers and compelling visual elements that engage the reader and strengthen the content.
Tata Consulting
When it comes to using data to show customer results, this case study does an excellent job of conveying details and numbers in an easy-to-digest manner. Bullet points at the start break up the content while also helping the reader understand exactly what the case study will be about. Tata Consulting used Adobe to deliver elevated, engaging content experiences for a large telecommunications client of its own — an objective that’s relatable for a lot of companies.
Case studies are a vital tool for any marketing team as they enable you to demonstrate the value of your company’s products and services to others. They help marketers do their job and add credibility to a brand trying to promote its solutions by using the experiences and stories of real customers.
When you’re ready to get started with a case study:
- Think about a few goals you’d like to accomplish with your content.
- Make a list of successful clients that would be strong candidates for a case study.
- Reach out to the client to get their approval and conduct an interview.
- Gather the data to present an engaging and effective customer story.
Adobe can help
There are several Adobe products that can help you craft compelling case studies. Adobe Experience Platform helps you collect data and deliver great customer experiences across every channel. Once you’ve created your case studies, Experience Platform will help you deliver the right information to the right customer at the right time for maximum impact.
To learn more, watch the Adobe Experience Platform story .
Keep in mind that the best case studies are backed by data. That’s where Adobe Real-Time Customer Data Platform and Adobe Analytics come into play. With Real-Time CDP, you can gather the data you need to build a great case study and target specific customers to deliver the content to the right audience at the perfect moment.
Watch the Real-Time CDP overview video to learn more.
Finally, Adobe Analytics turns real-time data into real-time insights. It helps your business collect and synthesize data from multiple platforms to make more informed decisions and create the best case study possible.
Request a demo to learn more about Adobe Analytics.
https://business.adobe.com/blog/perspectives/b2b-ecommerce-10-case-studies-inspire-you
https://business.adobe.com/blog/basics/business-case
https://business.adobe.com/blog/basics/what-is-real-time-analytics

Top 20 Project Management Case Studies [With Examples]
![case study for manager Top 20 Project Management Case Studies [With Examples]](https://www.upgrad.com/__khugblog-next/image/?url=https%3A%2F%2Fd14b9ctw0m6fid.cloudfront.net%2Fugblog%2Fwp-content%2Fuploads%2F2019%2F07%2FBlog_FI_July_upGrads-Knowledge-base.png&w=1920&q=75)
Project management case study analyses showcase and compare real-life project management processes and systems scenarios. These studies shed light on the common challenges that project managers encounter on a daily basis. This helps project managers develop effective strategies, overcome obstacles, and achieve successful results.
By leveraging project management case studies , organisations can optimise their operations by providing insights into the most effective approaches. With effective implementation of these case studies, strategies, and methodologies, ensuring successful project completion is achievable.
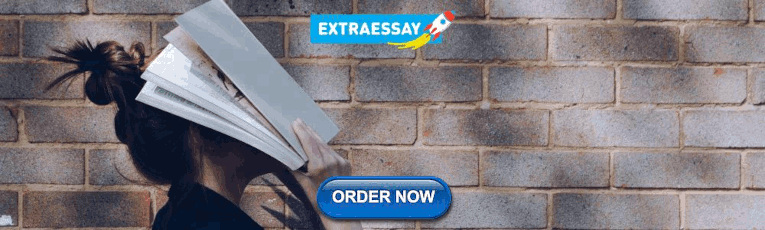
Criteria for Selection of Top 20 Case Studies
The top 20 case studies are selected based on significance, impact, challenges, project management strategies, and overall success. They provide diverse insights and lessons for project managers and organisations.
1. The Sydney Opera House Project
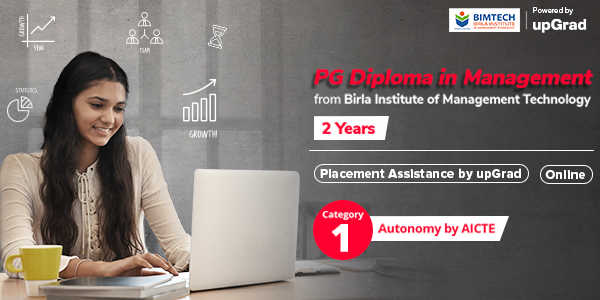
The Sydney Opera House Project is an iconic example of project management case studies as it faced multiple challenges during its construction phase. Despite facing leadership changes, budget overruns, and design failures, the project persevered and was completed in 1973, a decade later than planned. The Opera House stands as a symbol of perseverance and successful project management in the face of humankind.
2. The Airbus A380 Project
The Airbus A380 Project is a project management case study showcasing the challenges encountered during developing and producing the world’s largest commercial aircraft. The project experienced massive delays and impacted costs of more than $6 billion, with several issues arising from the manufacturing and delivery process, outsourcing, and project coordination.
However, the Airbus A380 was successfully launched through carefully planned project management strategies, delivering a world-class aircraft that met customer expectations.
3. The Panama Canal Expansion Project
The Panama Canal Expansion Project serves as a compelling case study, illustrating the management’s encounters in expanding the capacity of the Panama Canal. The project included multiple stakeholders, technological innovations, environmental concerns, and safety challenges.
4. The Boston Central Artery/Tunnel Project
The Boston Central Artery/Tunnel Project serves as a project management case study of a large-scale underground tunnel construction project. It successfully addressed traffic congestion and was completed in 2007. The project was completed in 2007, with numerous hurdles delaying progress like complexity, technology failure, ballooning budgets, media scrutiny, etc.
5. The London 2012 Olympics Project
The London 2012 Olympics Project stands as a successful project management case study, showcasing the management of a large-scale international sporting event. This project involved the construction of a new sports infrastructure, event logistics and security concerns. The project was successfully accomplished, delivering a world-class event that captivated the audience.
6. The Hoover Dam Bypass Project
The Hoover Dam Bypass Project was a construction project in the United States of America that intended to alleviate traffic from the Hoover Dam by building a new bridge. Completed in 2010, the bridge spans across the Colorado River, connecting Arizona and Nevada and offers a safer and more efficient route for motorists.
7. The Golden Gate Bridge Seismic Retrofit Project
The Golden Gate Bridge Seismic Retrofit Project is a case study example constructed in San Francisco, California. Its objective was to enhance the bridge’s resilience against earthquakes and aftershocks. Completed in 2012, the project included the installation of shock absorbers and other seismic upgrades to ensure the bridge’s safety and functionality in the event of a major earthquake.
8. The Hong Kong-Zhuhai-Macau Bridge Project
The Hong Kong-Zhuhai-Macau Bridge Project is a massive case study that intends to connect Hong Kong, Zhuhai and Macau with a bridge-tunnel system of 55 kilometres. Completed in 2018, the project required massive funds, investments and innovative engineering solutions, providing a new transport link and boosting regional connectivity.
Check out our free courses and upskill yourself.
9. The Panama Papers Investigation Project
The Panama Papers Investigation Project is a global case study of journalistic investigations into offshore tax havens. It involved leaked documents from Mossack Fonseca, a Panamanian law firm. Coordinated by the International Consortium of Investigative Journalists, the project resulted in major political and financial repercussions worldwide, garnering widespread media attention.
10. The Apple iPhone Development Project
The Apple iPhone Development Project started in 2004, aiming to create a groundbreaking mobile device. In 2007, the iPhone transformed the industry with its innovative touchscreen interface, sleek design, and advanced features. This project involved significant research, development, marketing, and supply chain management investments.
Learn Management Courses from the World’s top Universities. Earn Masters, Executive PGP, or Advanced Certificate Programs to fast-track your career.
11. The Ford Pinto Design and Launch Project
The Ford Pinto Design and Launch Project was a developmental project intended to create an affordable, fuel-efficient subcompact car. Launched in 1971, because of its fuel tank design, it became infamous for safety issues. The project was rigged for ethical and safety concerns, lawsuits, and recalls.
12. The Deepwater Horizon Oil Spill Response Project
The Deepwater Horizon Oil Spill Response Project was a response to the largest oil spill in US history, caused by an offshore drilling rig explosion in 2010. This crisis response project utilised a waterfall project management approach, where the project team followed a pattern of planning, executing, monitoring, and closing phases.
13. The NASA Challenger Space Shuttle Disaster Project
The NASA Challenger Disaster Project was a tragic space exploration mission in 1986, resulting in the loss of all seven crew members. Extensive investigations revealed design and safety flaws as the cause. This disaster prompted NASA to address decision-making processes and improve safety cultures.
14. The Three Gorges Dam Project
The Three Gorges Dam Project was a large-scale infrastructure project developed in China that aimed to build the world’s largest hydroelectric dam on the Yangtze River. Completed in 2012, it encountered environmental, social, and engineering challenges. The dam currently offers power generation, flood control, and improved navigation, but it has also resulted in ecological and cultural consequences.
15. The Big Dig Project in Boston
The Big Dig Project was a transportation infrastructure project in Boston, Massachusetts, intended to replace an old elevated highway with a newer tunnel system. Completed in 2007, it serves as one of the most complex and costly construction endeavours in US history. Despite facing many delays, cost overruns and engineering challenges, the project successfully improved traffic flow and urban aesthetics but also resulted in accidents, lawsuits, and financial burdens.
Our Top Management Programs & Articles
16. The Uber Disruptive Business Model Project
The Uber Disruptive Business Model Project was a startup that introduced a new ride business model that disrupted the taxi-cab industry by connecting riders with drivers via a mobile app. Launched in 2010, this project required innovative technology, marketing and regulatory strategies and faced legal actions and ethical challenges related to labour, safety, and competition. Uber has since then dominated the market with its ride-sharing business plan.
17. The Netflix Original Content Development Project
The Netflix Original Content Development Project was an initiative created to launch its original content for its platform. This launch by the online streaming giant in 2012 was a huge success for the company. The project required huge investments in content creation, distribution and marketing and resulted in award-winning shows and films that redefined the entire entertainment industry’s business model.
18. The Tesla Electric Car Project
The Tesla Electric Car Project was a revolutionary project that aimed to compete for its electric vehicles with gasoline-powered vehicles. The project required a strong project management plan that incorporated innovation, sustainability, and stakeholder engagement, resulting in the successful launch of the Tesla Roadster in 2008 and subsequent models. Tesla has one-handedly revolutionised the entire automobile industry on its own.
19. The Johnson & Johnson Tylenol Crisis Management Project:
The Johnson & Johnson Tylenol Crisis Management Project was a case study in crisis management in 1982. The project required quick and effective decision-making skills, stakeholder communication, and ethical leadership in response to the tampering of Tylenol capsules that led to deaths.
20. The Airbnb Online Marketplace Platform Project
The Airbnb Online Marketplace Platform Project was a startup that created an online platform which connected travellers with hosts offering short-term rental accommodations in flights. The project required innovative technology, user experience design and stakeholder management. Airbnb’s success has led to the disruption of the hospitality industry and inspired many other project case study examples of sharing economy platforms.
Explore our Popular Management Courses
Future developments in project management.
Future developments in project management include all the insights on the increased use of artificial intelligence, agile methodologies, hybrid project management approaches, and emphasis on sustainability and social responsibility, along with many more developing ideas that will address the evolving market innovations.
Key Takeaways from the Case Studies
The project management case study examples illustrate real-life examples and the importance of project management in achieving project success. The cases show the use of innovative technologies, tools, techniques, stakeholder engagement, crisis management, and agile methodologies.
Project Management also highlights the role of ethical leadership and social responsibility in project management. To learn more and more about case studies, upGrad, India’s leading education platform, has offered an Advanced General Management Program from IMT Ghaziabad that will equip you with in-demand management skills to keep up with the changing trends!

Keerthi Shivakumar
Something went wrong
Our Trending Management Courses
- PG Programme in Management - Duration 11 Months
- Post Graduate Diploma in Management - Duration 2 Years
Management Skills to Master
- Consumer Behavior Courses
- Supply Chain Management Courses
- Financial Analysis Courses
- Introduction to FinTech Courses
- Introduction to HR Analytics Courses
- Fundamentals of Communication Courses
- Art of Effective Communication Courses
- Introduction to Research Methodology Courses
- Business Communication Courses
- Mastering Sales Technique Courses
- Fundamentals of Journalism Courses
- Economics Masterclass Online Courses
- Microeconomics Online Courses
Our Popular Management Course
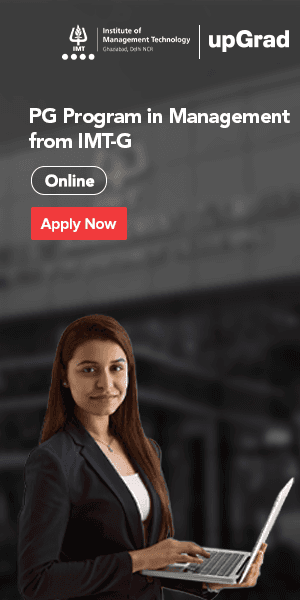
Frequently Asked Questions (FAQs)
Project Management is extensive planning, executing, monitoring and closing of a project before its deadline. Project management ensures accuracy and efficiency across all organs of a project, right from its inception to its completion.
Project Management case studies are real-life examples of projects to put an insight into all the tools, techniques and methodologies it provides.
The role of a project manager is to ensure that all day-to-day responsibilities are being met by the resources deployed in a certain project. They have the authority to manage as well as lead the functioning members as well.
Related Programs View All
Certification
16 Hrs Live Expert-Led Sessions
14 Case Studies, 3 Mock Tests
View Program
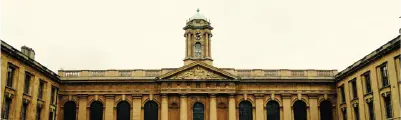
Master's Degree
Dual Credentials
16+ Hrs Expert-Led Sessions
5 Simulation Exams, 8 Mock Tests
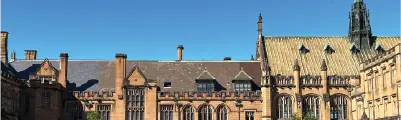
Job Assistance
32 Hrs Live Expert-Led Training
Earn 32 PDUs and SEUs
24 Hrs Live Expert-Led Training
Earn 24 PDUs and 24 SEUs
36 Hrs Live Expert-Led Training
Premium 2000+ Question Bank
88 Hours On-Demand Learning
100% Exam-Pass Guarantee
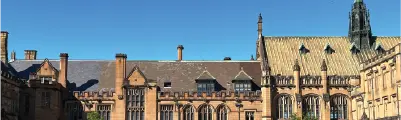
Complimentary On-Demand Course
Training by Top-Notch SPCs
1 Year SAFe® Community Membership
16 Hrs Live Expert-Led Training
Earn 16 PDUs and 16 SEUs
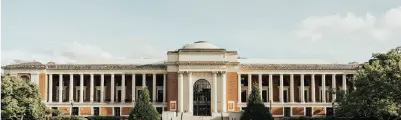
3 Day Leadership Summit in Dubai
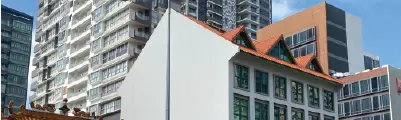
AACSB accredited
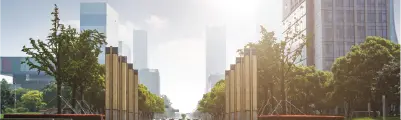
Ivy League School
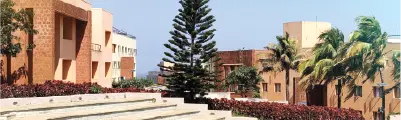
Executive PG Program
Offline Campus Experience
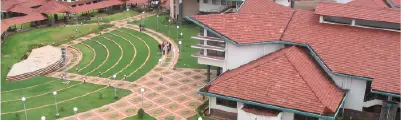
EQUIS & AMBA Accredited
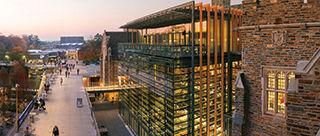
PG Certification
6-10.5 Months
2500+ Students Enrolled
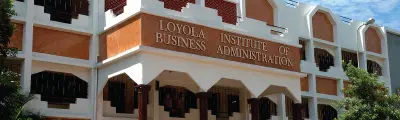
Executive Coaching
Simulations, 5 Mock Tests
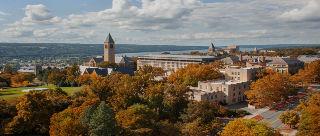
Instructor Led Model
16-Hrs Live Expert-Led Sessions
Earn 16 SEUs and 16 PDUs
16+ Hrs Expert-Led Training
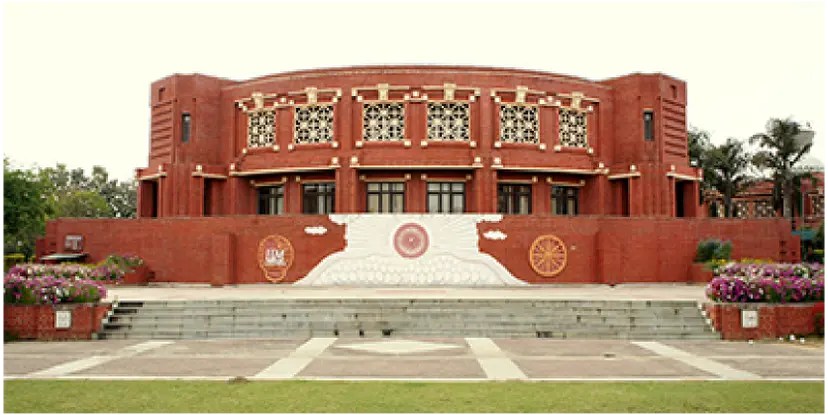
AMBA, AACSB & NIRF Accreditation
24+ Hrs Expert-Led Sessions
Simulation Exams, 24 PDUs
35 Hrs Live Expert-Led Training
35 PD Hrs, 35 CDUs & 35 PDUs
24-Hrs Live Expert-Led Sessions
Activities and Case Studies
8+ Hrs Expert-Led Sessions
Interactive Sessions, Activities
36+ Expert-Led Training
5 Simulation Exams, Projects
21 Hrs Live Expert-Led Training
Earn 21 CDUs and 21 PDUs
2-Day Live Expert-led Training
Simulations, 4 Mock Tests
16 Hours of Instructor-Led Sessions
Simulation Exams and Mock Tests
Earn 16 PDUs and 16 SEUs
24-Hrs Live Expert-Led Training
Earn 24 SEUs and 24 PDUs
Explore Free Courses
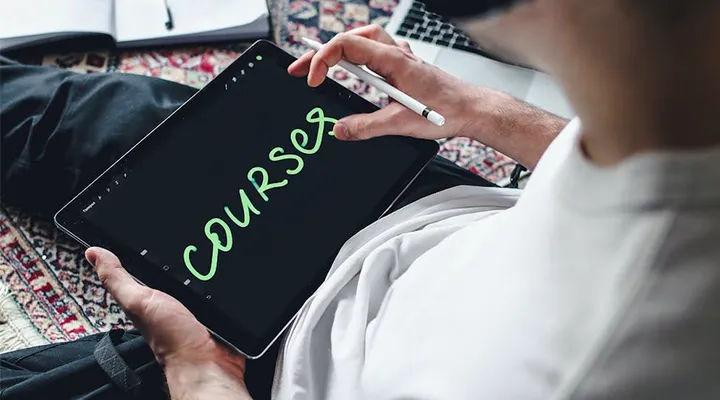
Learn more about the education system, top universities, entrance tests, course information, and employment opportunities in Canada through this course.
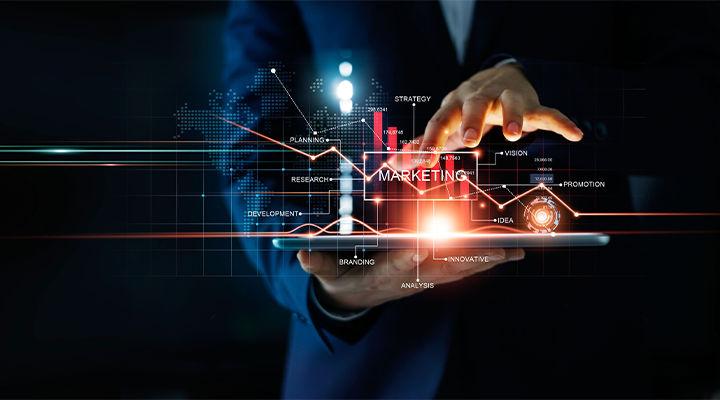
Advance your career in the field of marketing with Industry relevant free courses
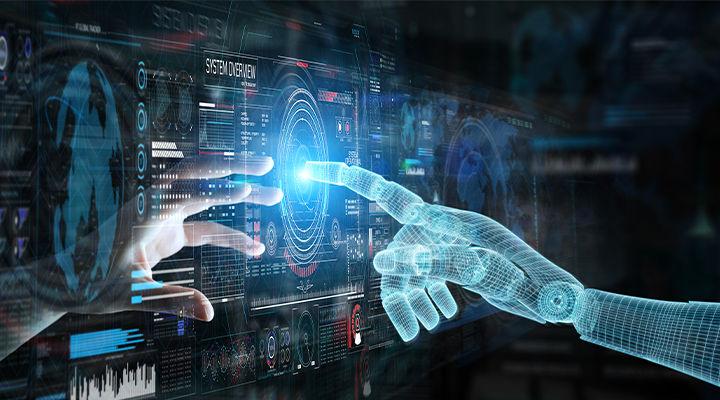
Build your foundation in one of the hottest industry of the 21st century
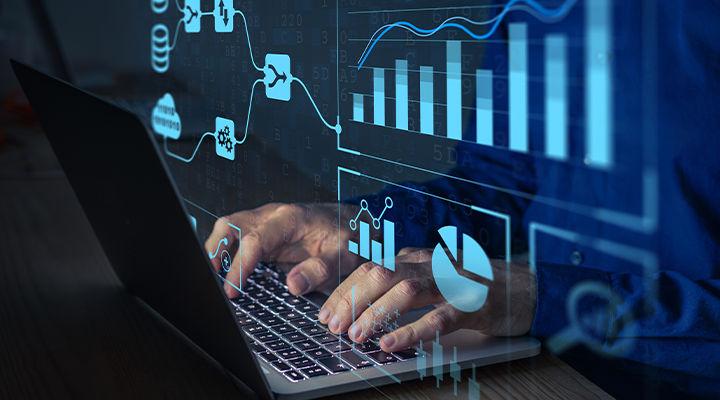
Master industry-relevant skills that are required to become a leader and drive organizational success
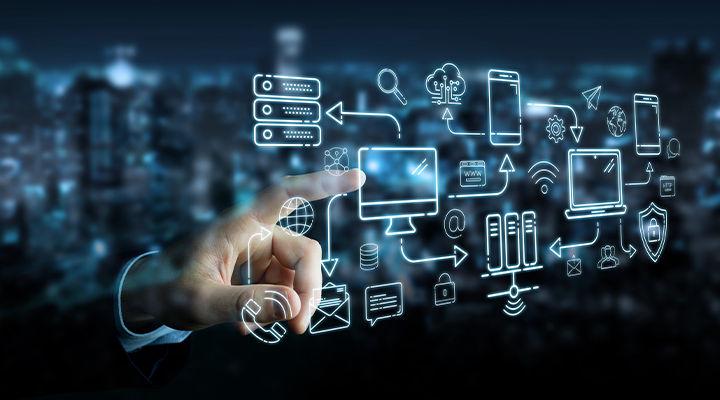
Build essential technical skills to move forward in your career in these evolving times
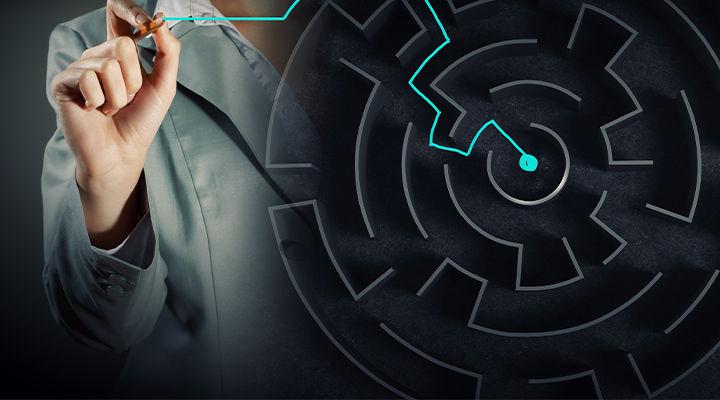
Get insights from industry leaders and career counselors and learn how to stay ahead in your career
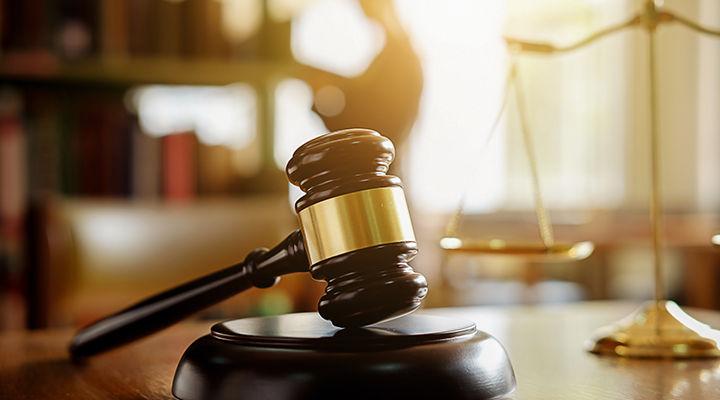
Kickstart your career in law by building a solid foundation with these relevant free courses.

Stay ahead of the curve and upskill yourself on Generative AI and ChatGPT
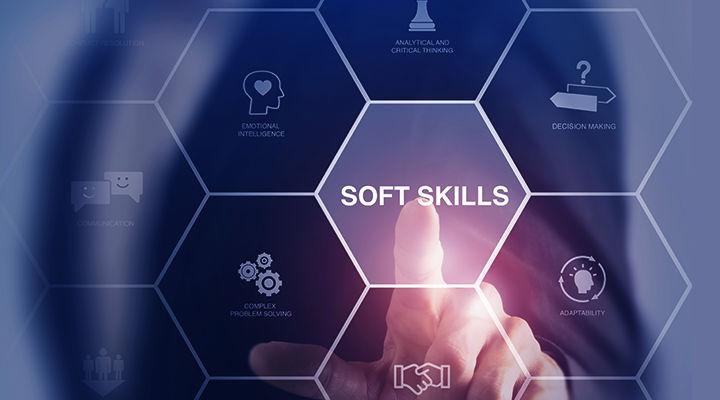
Build your confidence by learning essential soft skills to help you become an Industry ready professional.
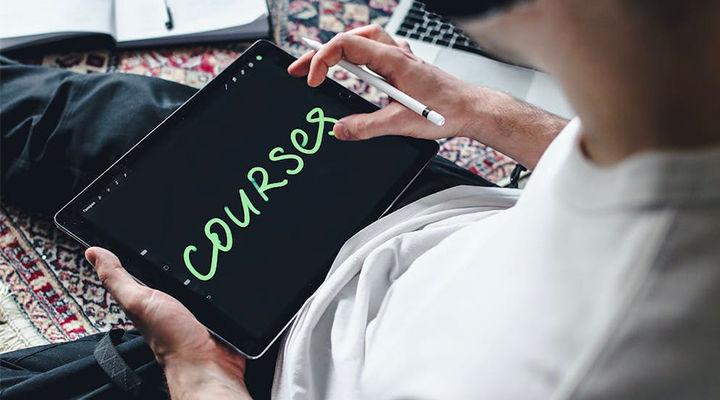
Learn more about the education system, top universities, entrance tests, course information, and employment opportunities in USA through this course.
Suggested Blogs
![case study for manager Salary after BBA: BBA Salaries in India [For Freshers & Experienced in 2023]](https://www.upgrad.com/__khugblog-next/image/?url=https%3A%2F%2Fd14b9ctw0m6fid.cloudfront.net%2Fugblog%2Fwp-content%2Fuploads%2F2021%2F04%2F1976-1.png&w=3840&q=75)
by Kamal Jacob
05 Mar 2024
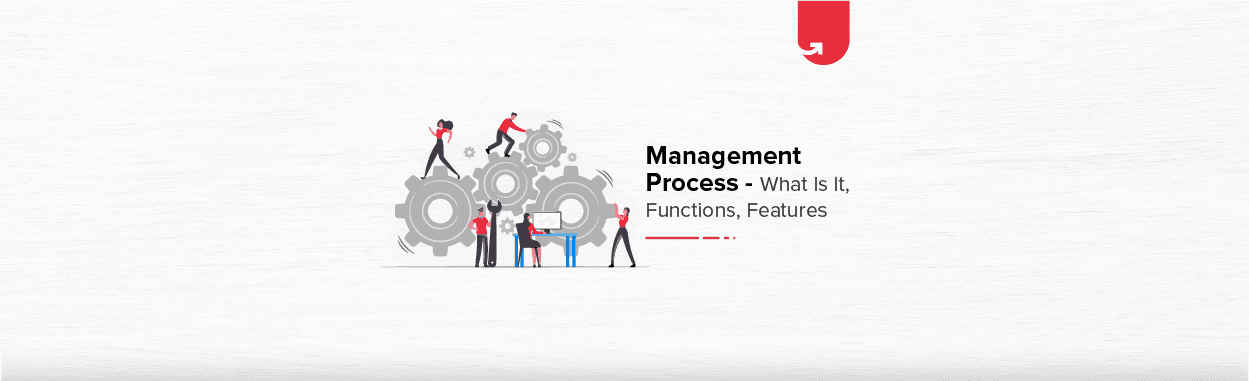
19 Feb 2024
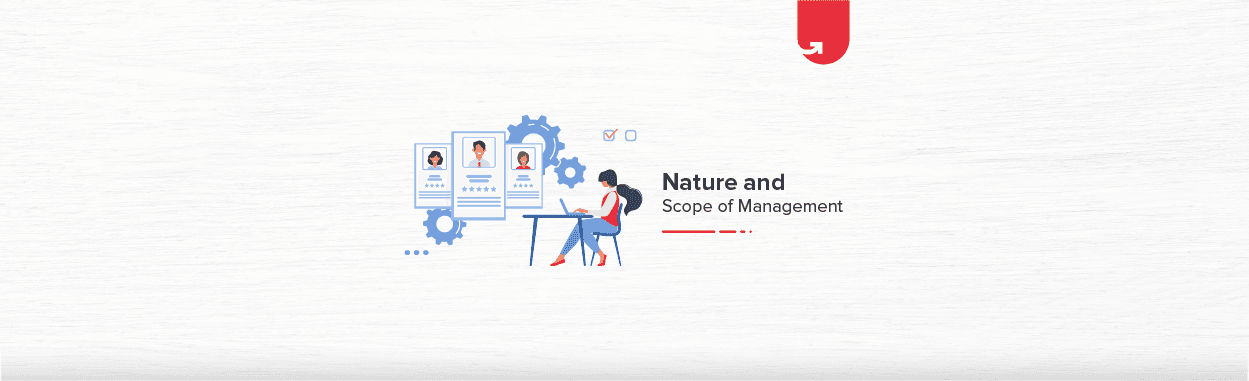
by Nitin Gurmukhani
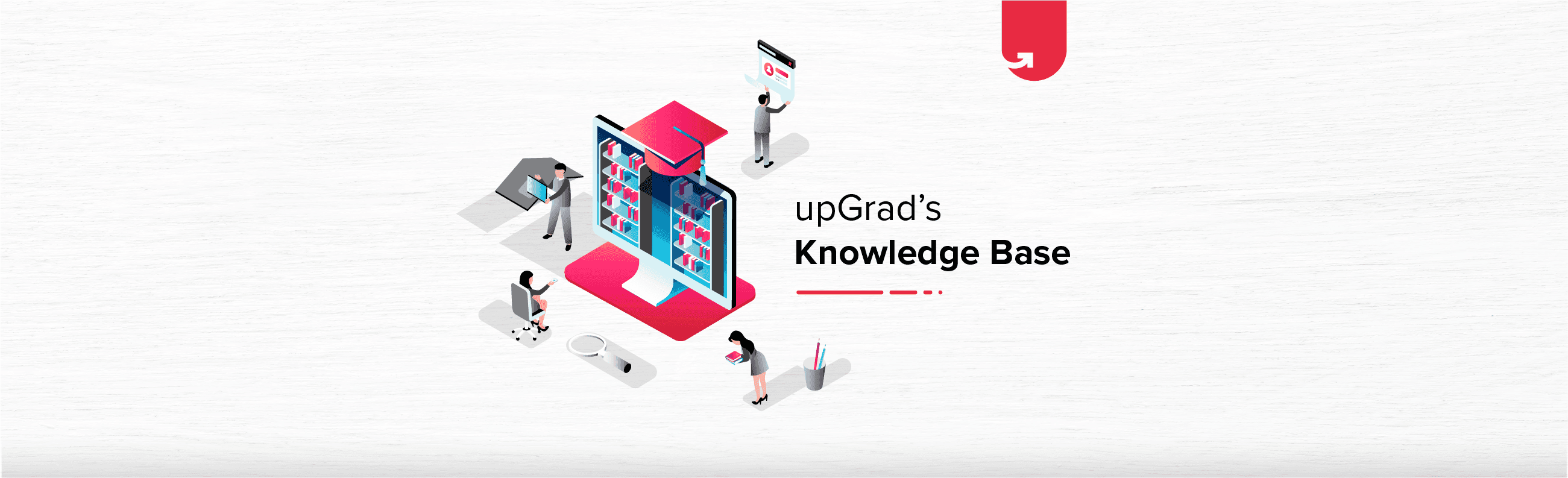
by Keerthi Shivakumar
23 Jan 2024
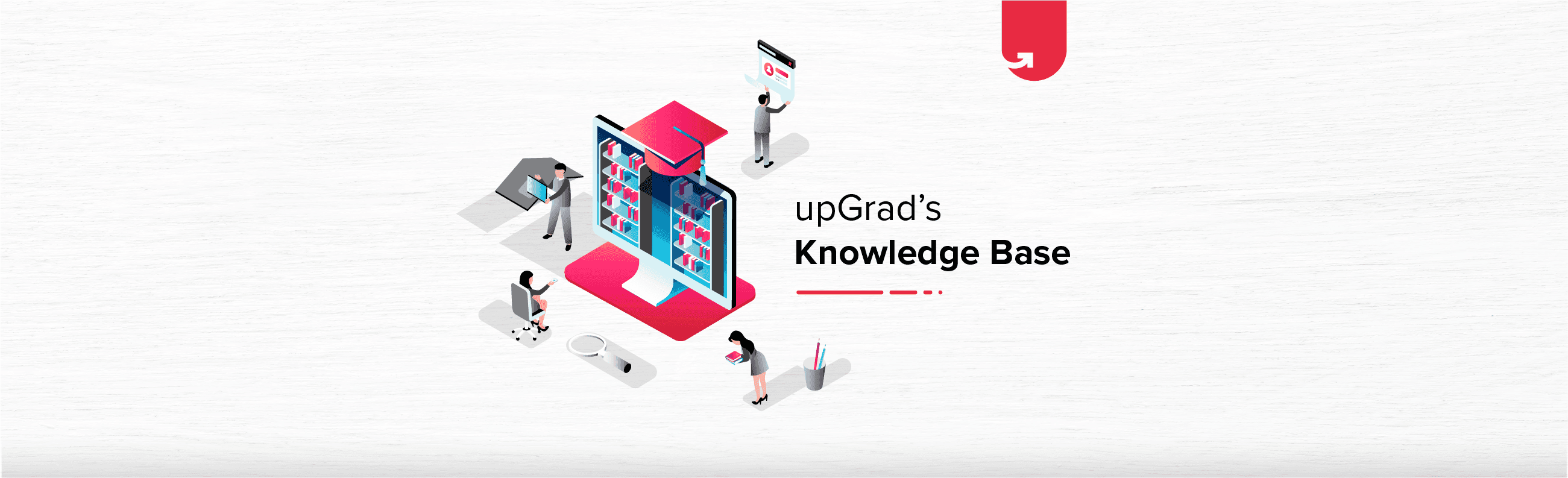
24 Sep 2023
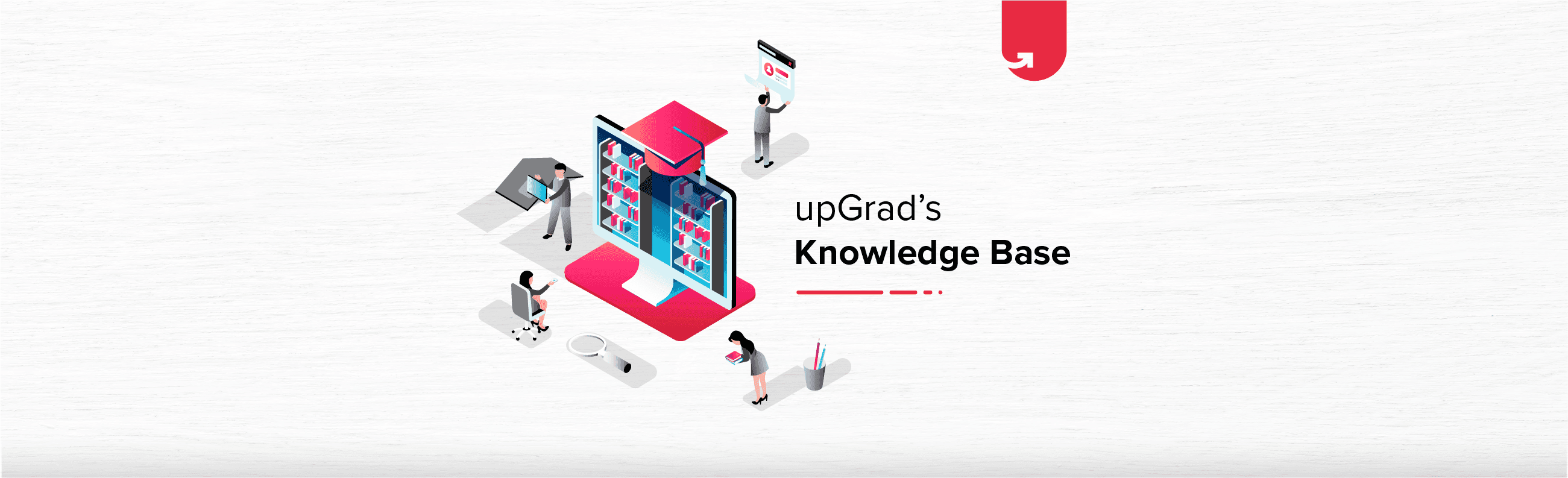
15 Sep 2023
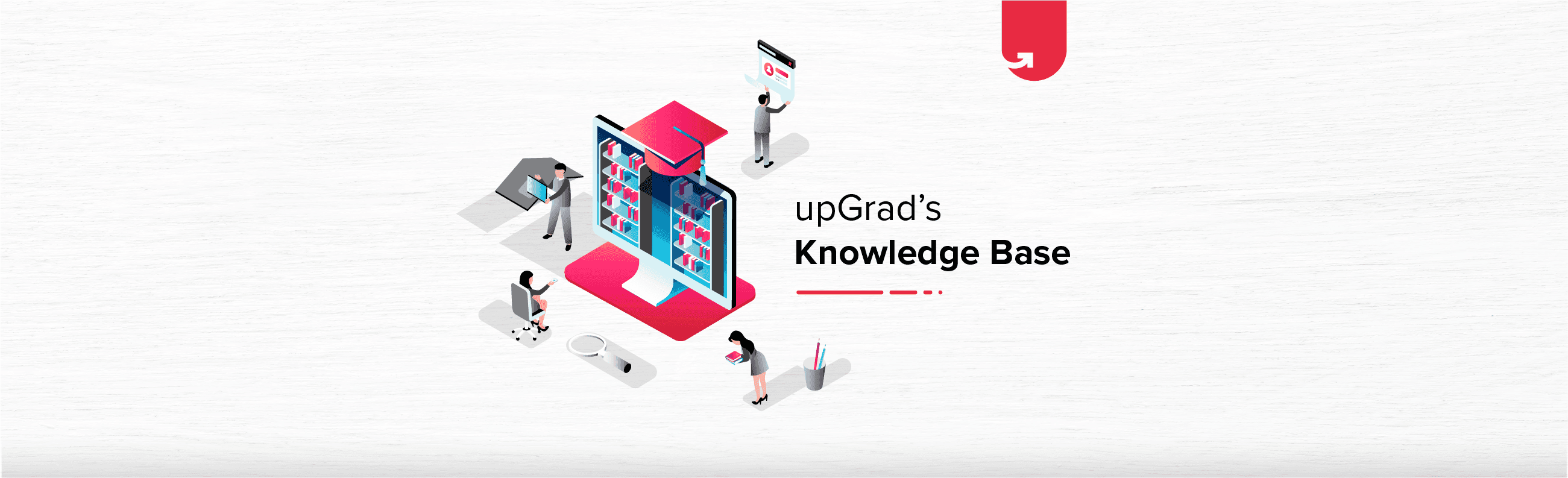
14 Sep 2023
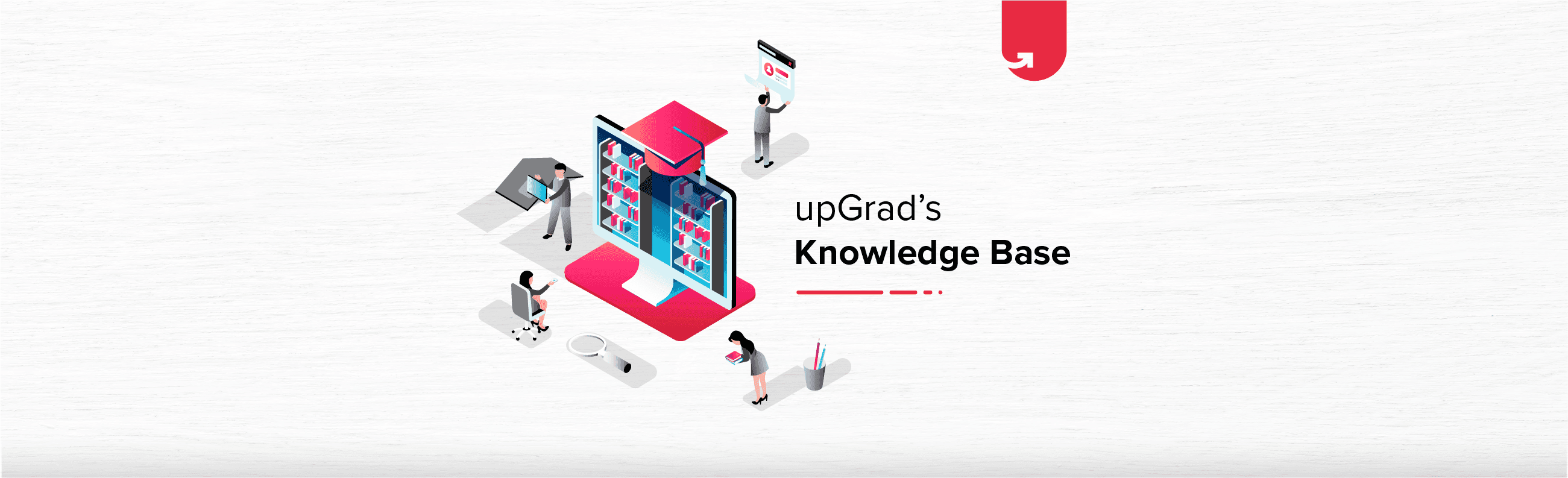
04 Sep 2023
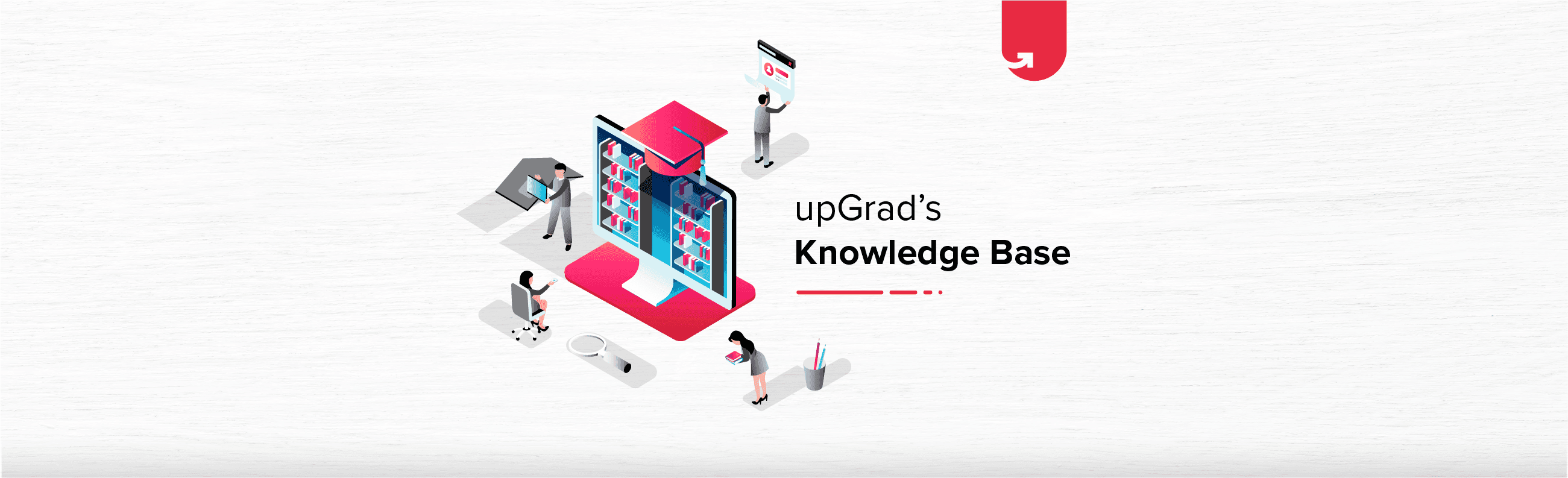
24 Aug 2023
- Schedule an Appointment
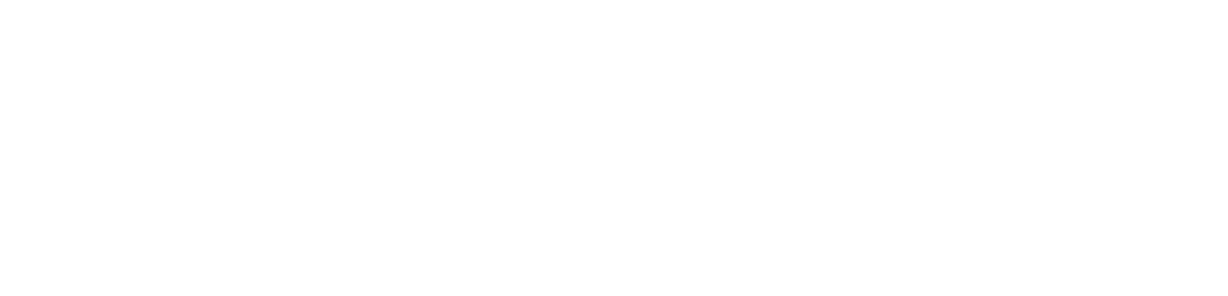
- Undergraduate Students in AS&E and SMFA
- Graduate Students in AS&E and SMFA
- Faculty & Staff
- Parents and Families
- What is a Career Community?
- Reflect, Discover & Explore Multiple Interests
- Arts, Communications & Media
- Education, Nonprofit & Social Impact
- Engineering, Technology & Physical Sciences
- Finance, Consulting, Entrepreneurship & Business
- Government, International Affairs & Law
- Healthcare, Life Sciences & the Environment
- Exploring Your Interests, Careers & Majors
- Writing Resumes & Cover Letters
- Finding an Internship
- Finding Jobs & Fellowships
- Preparing for Interviews
- Applying to Graduate & Professional School
- First Generation
- International Students
- Black, Indigenous & People of Color
- Students with Disabilities
- Students with Undocumented Status
- Women & Gender
- For Employers
- Contact & Location
- Career Fellows
- Career Services by School
Case Interview: Complete Prep Guide
- Share This: Share Case Interview: Complete Prep Guide on Facebook Share Case Interview: Complete Prep Guide on LinkedIn Share Case Interview: Complete Prep Guide on X
Welcome to our preparation tips for case interviews! Whether you are just curious about case interviews or are planning to apply for consulting internships or full-time jobs, these tips and resources will help you feel more prepared and confident.
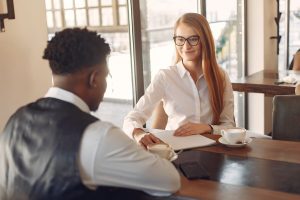
A case interview is a role playing exercise in which an employer assesses how logically and persuasively you can present a case. Rather than seeing if you get the “correct” answer, the objective is to evaluate your thought process. ( Adapted with permission from Case In Point: Complete Case Interview Preparation by Marc Cosentino).
Case interviews are very commonly used in the interview process for consulting firms and companies in similar industries. In the case interview, you will typically be given a business problem and then asked to solve it in a structured way. Learning this structure takes preparation and practice. You can learn more and practice using the resources listed below.
Why are Case Interviews Used?
Case interviews allow employers to test and evaluate the following skills:
- Analytical skills and logical ability to solve problems
- Structure and thought process
- Ability to ask for relevant data/information
- Tolerance for ambiguity and data overload
- Poise and communication skills under pressure and in front of a client
How can I prepare for Case Interviews?
1.) Read Management Consulted’s “Case Interview: Complete Prep Guide (2024)”
Management Consulted is a FREE resource for Tufts students : case and consulting resources such as 500 sample cases, Case Interview Bootcamp, Market Sizing Drills, Math Drills, case videos, consulting firm directory, and more
2.) Review additional resources:
- Case in Point – This book, by Marc Cosentino, is a comprehensive guide that walks you through the case interview process from beginning to end. This guide has helped many students over the years and can serve as an excellent foundation for how to approach business problems
- Casequestions.com – The companion website to Marc Cosentino’s book listed above offers preparation for case interviews, along with links to top 50 consulting firms
- Management Consulting Case Interviews: Cracking The Case – tips for case interviews from the other side of the table, from Argopoint, a Boston management consulting firm specializing in legal department consulting for Fortune 500 companies
- Preplounge.com – Free case preparation access for to up to 6 practice interviews with peers, selected cases, and video case solutions
- RocketBlocks – Features consulting preparation such as drills and coaching
- Practice sample online cases on consulting firm websites such as McKinsey , BCG , Bain , Deloitte and more!
3.) Schedule a mock case interview appointment with Karen Dankers or Kathy Spillane , our advisors for the Finance, Consulting, Entrepreneurship, and Business Career Community.
4.) PRACTICE PRACTICE PRACTICE cases out loud on your own (yes, that can feel odd) or preferably, with another person. See #2 and #3 above for resources and ideas to find partners to practice live cases
5.) Enjoy and have fun solving business problems!

- Browse All Articles
- Newsletter Sign-Up
ManagerialRoles →
No results found in working knowledge.
- Were any results found in one of the other content buckets on the left?
- Try removing some search filters.
- Use different search filters.
Top 10 Project Management Case Studies with Examples 2024
1. nasa's mars exploration rover: innovative project management in space exploration., 2. apple's iphone development: delivering revolutionary products with precision., 3. tesla's gigafactory construction: exemplary project execution in renewable energy., 4. netflix's content expansion: agile management in the entertainment industry., 5. amazon's prime air drone delivery: pioneering logistics project management., 6. google's waymo self-driving cars: cutting-edge technology meets project efficiency., 7. mcdonald's digital transformation: adaptive project management in fast food., 8. ikea's sustainable store design: eco-friendly project implementation in retail., 9. unicef's vaccine distribution: humanitarian project management at scale., 10. spacex's starlink satellite network: revolutionizing global connectivity with project prowess., discover more stories.
- Discounts and promotions
- Delivery and payment
Cart is empty!
Case study definition
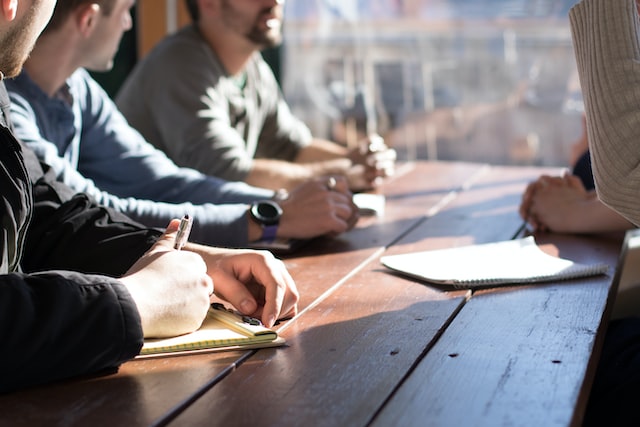
Case study, a term which some of you may know from the "Case Study of Vanitas" anime and manga, is a thorough examination of a particular subject, such as a person, group, location, occasion, establishment, phenomena, etc. They are most frequently utilized in research of business, medicine, education and social behaviour. There are a different types of case studies that researchers might use:
• Collective case studies
• Descriptive case studies
• Explanatory case studies
• Exploratory case studies
• Instrumental case studies
• Intrinsic case studies
Case studies are usually much more sophisticated and professional than regular essays and courseworks, as they require a lot of verified data, are research-oriented and not necessarily designed to be read by the general public.
How to write a case study?
It very much depends on the topic of your case study, as a medical case study and a coffee business case study have completely different sources, outlines, target demographics, etc. But just for this example, let's outline a coffee roaster case study. Firstly, it's likely going to be a problem-solving case study, like most in the business and economics field are. Here are some tips for these types of case studies:
• Your case scenario should be precisely defined in terms of your unique assessment criteria.
• Determine the primary issues by analyzing the scenario. Think about how they connect to the main ideas and theories in your piece.
• Find and investigate any theories or methods that might be relevant to your case.
• Keep your audience in mind. Exactly who are your stakeholder(s)? If writing a case study on coffee roasters, it's probably gonna be suppliers, landlords, investors, customers, etc.
• Indicate the best solution(s) and how they should be implemented. Make sure your suggestions are grounded in pertinent theories and useful resources, as well as being realistic, practical, and attainable.
• Carefully proofread your case study. Keep in mind these four principles when editing: clarity, honesty, reality and relevance.
Are there any online services that could write a case study for me?
Luckily, there are!
We completely understand and have been ourselves in a position, where we couldn't wrap our head around how to write an effective and useful case study, but don't fear - our service is here.
We are a group that specializes in writing all kinds of case studies and other projects for academic customers and business clients who require assistance with its creation. We require our writers to have a degree in your topic and carefully interview them before they can join our team, as we try to ensure quality above all. We cover a great range of topics, offer perfect quality work, always deliver on time and aim to leave our customers completely satisfied with what they ordered.
The ordering process is fully online, and it goes as follows:
• Select the topic and the deadline of your case study.
• Provide us with any details, requirements, statements that should be emphasized or particular parts of the writing process you struggle with.
• Leave the email address, where your completed order will be sent to.
• Select your payment type, sit back and relax!
With lots of experience on the market, professionally degreed writers, online 24/7 customer support and incredibly low prices, you won't find a service offering a better deal than ours.

- SUGGESTED TOPICS
- The Magazine
- Newsletters
- Managing Yourself
- Managing Teams
- Work-life Balance
- The Big Idea
- Data & Visuals
- Reading Lists
- Case Selections
- HBR Learning
- Topic Feeds
- Account Settings
- Email Preferences
Research: Negotiating Is Unlikely to Jeopardize Your Job Offer
- Einav Hart,
- Julia Bear,
- Zhiying (Bella) Ren

A series of seven studies found that candidates have more power than they assume.
Job seekers worry about negotiating an offer for many reasons, including the worst-case scenario that the offer will be rescinded. Across a series of seven studies, researchers found that these fears are consistently exaggerated: Candidates think they are much more likely to jeopardize a deal than managers report they are. This fear can lead candidates to avoid negotiating altogether. The authors explore two reasons driving this fear and offer research-backed advice on how anxious candidates can approach job negotiations.
Imagine that you just received a job offer for a position you are excited about. Now what? You might consider negotiating for a higher salary, job flexibility, or other benefits , but you’re apprehensive. You can’t help thinking: What if I don’t get what I ask for? Or, in the worst-case scenario, what if the hiring manager decides to withdraw the offer?

- Einav Hart is an assistant professor of management at George Mason University’s Costello College of Business, and a visiting scholar at the Wharton School. Her research interests include conflict management, negotiations, and organizational behavior.
- Julia Bear is a professor of organizational behavior at the College of Business at Stony Brook University (SUNY). Her research interests include the influence of gender on negotiation, as well as understanding gender gaps in organizations more broadly.
- Zhiying (Bella) Ren is a doctoral student at the Wharton School of the University of Pennsylvania. Her research focuses on conversational dynamics in organizations and negotiations.
Partner Center
Manage consent
Details cookies, important margin product information.
CFDs are complex instruments and come with a high risk of losing money rapidly due to leverage. 65% of retail investor accounts lose money when trading CFDs with this provider. You should consider whether you understand how CFDs, FX or any of our other products work and whether you can afford to take the high risk of losing your money.
Cookie policy
Our websites use cookies to offer you a better browsing experience by enabling, optimising, and analysing site operations, as well as to provide personalised ad content and allow you to connect to social media. By choosing “Accept all” you consent to the use of cookies and the related processing of personal data. Select “Manage consent” to manage your consent preferences. You can change your preferences or retract your consent at any time via the cookie policy page. Please view our cookie policy and our privacy policy .
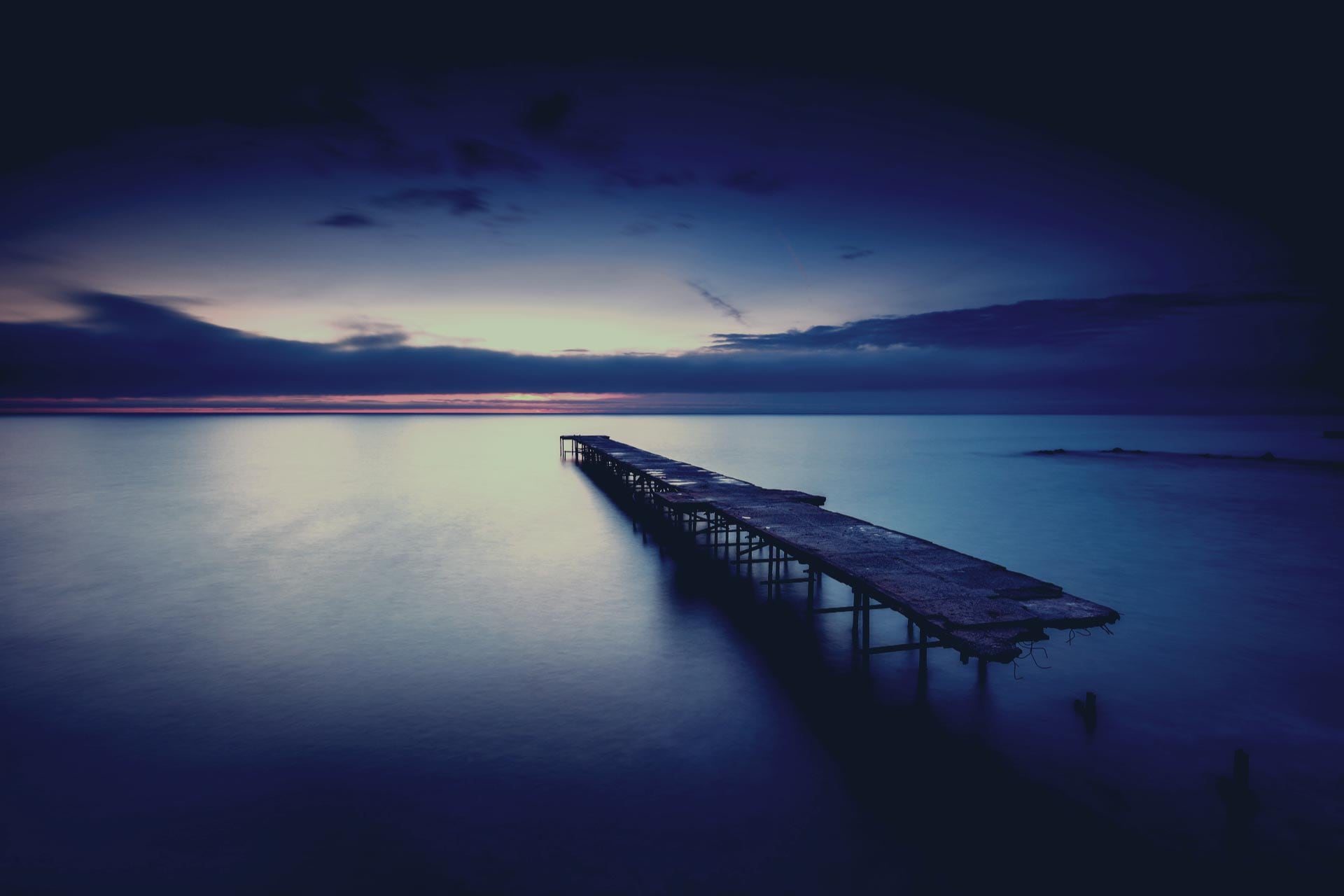
Case study: Smartly reducing your investment while maintaining market exposure
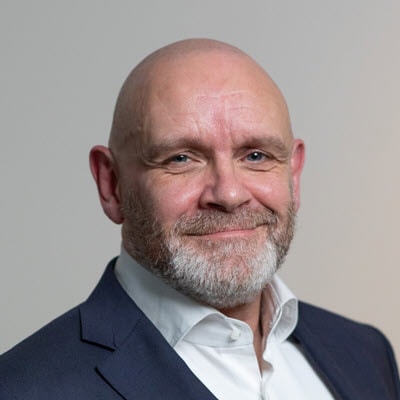
Koen Hoorelbeke
Options Strategist
Summary: This case study illustrates how Alex strategically employs long-term call options to both realize profits and maintain exposure to NVIDIA stock. By adjusting his holdings through these options, he secures gains while keeping potential for future growth, showcasing effective risk management and investment foresight.
Introduction:
In the dynamic world of investing, the ability to adapt strategies to changing market conditions is crucial for maximizing returns while managing risks. For buy-and-hold investors like Alex, who have seen substantial gains in certain stocks, the challenge often lies in realizing profits without losing potential future growth. This case study explores how strategic use of long-term call options can provide an innovative solution to this dilemma, allowing investors to secure gains and maintain market exposure simultaneously.
Important note : the strategies and examples provided in this article are purely for educational purposes. They are intended to assist in shaping your thought process and should not be replicated or implemented without careful consideration. Every investor or trader must conduct their own due diligence and take into account their unique financial situation, risk tolerance, and investment objectives before making any decisions. Remember, investing in the stock market carries risk, and it's crucial to make informed decisions.
Background:
Meet Alex, an investor with a portfolio valued at $153,319, showing a profit of $44,534 from an initial investment of $108,785. His holdings include various stocks, but a significant portion of his profit comes from his investment in NVIDIA Corporation (nvda), which currently constitutes 55% of his portfolio's total value.
Alex's NVIDIA shares have appreciated significantly, and he's looking to realize some of these gains. However, he wants to maintain his market exposure to NVIDIA due to its potential for further growth.
Solution: Using a long-term call option:
To achieve his goals, Alex decides to buy a long-term call option on NVIDIA. This option will allow him to buy NVIDIA shares at a set price of $850 each anytime until the option expires in June 2025, regardless of how high the stock price goes. This option costs him $20,300 for one contract, which covers 100 shares.
Portfolio overview:
Here is a breakdown of Alex's current portfolio before any transactions:
- NVIDIA (nvda) : 100 shares at a buy price of $496, now valued at $847.2 each, totaling $84,720.
- Other holdings : Includes stocks like PayPal (pypl), Nike (nke), and Palantir (pltr), with various performances and allocations within the portfolio.
Financial mechanics simplified:
- Current stock position : Alex holds 100 shares of NVIDIA.
- Option purchase : The call option has a cost of $20,300 and provides similar market exposure to owning approximately 66 shares of NVIDIA.
How many shares can Alex sell?
By purchasing the call option, Alex can sell about 66 shares of NVIDIA without reducing his effective market exposure to NVIDIA's future price movements. This is because the option helps maintain a similar level of investment influence as the shares he plans to sell. Specifically, the call option has a delta of 0.66, which means that one contract of the option (covering 100 shares) effectively corresponds to the exposure of owning 66 shares of the stock (0.66 * 100 = 66).
- Reduced direct investment : Alex can reduce his direct exposure by selling 66 shares, which would secure approximately $56,000 (66 shares × $847.20/share). After accounting for the cost of the option ($20,300), the net amount secured is about $35,700. This allows him to use these funds for other investment opportunities or to diversify his portfolio further.
- Maintained market exposure : The long-term option ensures that Alex still benefits from potential price increases in NVIDIA's stock.
- Flexibility and security : This strategy allows Alex to lock in profits while keeping the flexibility to participate in future growth, providing a balanced approach to managing his successful investment.
While using long-term options can offer significant advantages, there are inherent risks to consider:
- Premium cost : The initial cost of the option ($20,300) is a sunk cost, meaning it is not recoverable if the option expires worthless. This represents a fixed loss if NVIDIA's stock price does not perform as expected.
- Volatility and time decay : Options are sensitive to changes in market volatility and lose value over time as they approach expiration — a phenomenon known as time decay. If NVIDIA's stock price remains below the strike price as the expiration date nears, the value of the option could decrease significantly.
Conclusion:
This approach allows Alex to capitalize on his gains in a high-performing stock while strategically maintaining his position for future growth. By using a long-term call option, Alex smartly adjusts his portfolio to reduce risk and secure profits, demonstrating a prudent method of portfolio management in a rising market. However, it's essential for Alex to consider the risks associated with options trading and monitor his investments accordingly.
Latest Market Insights
Global Market Quick Take: Asia – May 15, 2024
UK labor data and Huw Pill's comments are not enough for a bond bull rally
Technical Update - Alibaba rebound in jeopardy after earnings miss. Check out key share price levels here
Technical Update - EURCHF likely to test key resistance shortly. USDCHF range bound for some time but be ready for break out
COT: Crude longs slump; grain purchases surge
Global Market Quick Take: Europe – 14 May 2024
Unlocking the basics of options webinar - re-watch with questions and answers from participants
AUD: Tailwinds to stay despite Budget impact
Quarterly Outlook 2024 Q2
2024: The wasted year
Macro: It’s all about elections and keeping status quo
Markets are driven by election optimism, overshadowing growing debt and liquidity concerns. The 2024 elections loom large, but economic fundamentals and debt issues warrant cautious investment.
FX: The rate cut race shifts into high gear
As US economic slowdown hints at a shift away from exceptionalism, USD faces downside with looming Fed cuts. AUD and NZD set to outperform as their rate cuts lag. JPY gains on carry unwind bets and BOJ pivot.
Equities: The AI and obesity rally is defying gravity
Amid AI and obesity drug excitement, equities see varied prospects: neutral on overvalued US stocks, negative on Japan due to JPY risks, positive on Europe. European defence stocks gain appeal.
Fixed income: Keep calm, seize the moment
With the economic slowdown, quality assets will gain favour, especially sovereign bonds up to 5 years. Central banks' potential rate cuts in Q2 suggest extending duration, despite policy and inflation concerns.
Commodities: Is the correction over?
Commodities poised for rebound. The "Year of the Metal" boosts gold and silver, copper awaits rate cuts. Grains may recover, natural gas stabilises. Gold targets $2,300-$2,500/oz, copper's breakout could signal growth.
You can access both of our platforms from a single Saxo account. Preview platform Open Account
Disclaimer The Saxo Bank Group entities each provide execution-only service and access to Analysis permitting a person to view and/or use content available on or via the website. This content is not intended to and does not change or expand on the execution-only service. Such access and use are at all times subject to (i) The Terms of Use; (ii) Full Disclaimer; (iii) The Risk Warning; (iv) the Rules of Engagement and (v) Notices applying to Saxo News & Research and/or its content in addition (where relevant) to the terms governing the use of hyperlinks on the website of a member of the Saxo Bank Group by which access to Saxo News & Research is gained. Such content is therefore provided as no more than information. In particular no advice is intended to be provided or to be relied on as provided nor endorsed by any Saxo Bank Group entity; nor is it to be construed as solicitation or an incentive provided to subscribe for or sell or purchase any financial instrument. All trading or investments you make must be pursuant to your own unprompted and informed self-directed decision. As such no Saxo Bank Group entity will have or be liable for any losses that you may sustain as a result of any investment decision made in reliance on information which is available on Saxo News & Research or as a result of the use of the Saxo News & Research. Orders given and trades effected are deemed intended to be given or effected for the account of the customer with the Saxo Bank Group entity operating in the jurisdiction in which the customer resides and/or with whom the customer opened and maintains his/her trading account. Saxo News & Research does not contain (and should not be construed as containing) financial, investment, tax or trading advice or advice of any sort offered, recommended or endorsed by Saxo Bank Group and should not be construed as a record of our trading prices, or as an offer, incentive or solicitation for the subscription, sale or purchase in any financial instrument. To the extent that any content is construed as investment research, you must note and accept that the content was not intended to and has not been prepared in accordance with legal requirements designed to promote the independence of investment research and as such, would be considered as a marketing communication under relevant laws.
Please read our disclaimers: Notification on Non-Independent Investment Research (https://www.home.saxo/legal/niird/notification) Full disclaimer (https://www.home.saxo/legal/disclaimer/saxo-disclaimer) Full disclaimer (https://www.home.saxo/legal/saxoselect-disclaimer/disclaimer)
Your browser cannot display this website correctly.
Our website is optimised to be browsed by a system running iOS 9.X and on desktop IE 10 or newer. If you are using an older system or browser, the website may look strange. To improve your experience on our site, please update your browser or system.
Machine Learning and image analysis towards improved energy management in Industry 4.0: a practical case study on quality control
- Original Article
- Open access
- Published: 13 May 2024
- Volume 17 , article number 48 , ( 2024 )
Cite this article
You have full access to this open access article
- Mattia Casini 1 ,
- Paolo De Angelis 1 ,
- Marco Porrati 2 ,
- Paolo Vigo 1 ,
- Matteo Fasano 1 ,
- Eliodoro Chiavazzo 1 &
- Luca Bergamasco ORCID: orcid.org/0000-0001-6130-9544 1
With the advent of Industry 4.0, Artificial Intelligence (AI) has created a favorable environment for the digitalization of manufacturing and processing, helping industries to automate and optimize operations. In this work, we focus on a practical case study of a brake caliper quality control operation, which is usually accomplished by human inspection and requires a dedicated handling system, with a slow production rate and thus inefficient energy usage. We report on a developed Machine Learning (ML) methodology, based on Deep Convolutional Neural Networks (D-CNNs), to automatically extract information from images, to automate the process. A complete workflow has been developed on the target industrial test case. In order to find the best compromise between accuracy and computational demand of the model, several D-CNNs architectures have been tested. The results show that, a judicious choice of the ML model with a proper training, allows a fast and accurate quality control; thus, the proposed workflow could be implemented for an ML-powered version of the considered problem. This would eventually enable a better management of the available resources, in terms of time consumption and energy usage.
Avoid common mistakes on your manuscript.
Introduction
An efficient use of energy resources in industry is key for a sustainable future (Bilgen, 2014 ; Ocampo-Martinez et al., 2019 ). The advent of Industry 4.0, and of Artificial Intelligence, have created a favorable context for the digitalisation of manufacturing processes. In this view, Machine Learning (ML) techniques have the potential for assisting industries in a better and smart usage of the available data, helping to automate and improve operations (Narciso & Martins, 2020 ; Mazzei & Ramjattan, 2022 ). For example, ML tools can be used to analyze sensor data from industrial equipment for predictive maintenance (Carvalho et al., 2019 ; Dalzochio et al., 2020 ), which allows identification of potential failures in advance, and thus to a better planning of maintenance operations with reduced downtime. Similarly, energy consumption optimization (Shen et al., 2020 ; Qin et al., 2020 ) can be achieved via ML-enabled analysis of available consumption data, with consequent adjustments of the operating parameters, schedules, or configurations to minimize energy consumption while maintaining an optimal production efficiency. Energy consumption forecast (Liu et al., 2019 ; Zhang et al., 2018 ) can also be improved, especially in industrial plants relying on renewable energy sources (Bologna et al., 2020 ; Ismail et al., 2021 ), by analysis of historical data on weather patterns and forecast, to optimize the usage of energy resources, avoid energy peaks, and leverage alternative energy sources or storage systems (Li & Zheng, 2016 ; Ribezzo et al., 2022 ; Fasano et al., 2019 ; Trezza et al., 2022 ; Mishra et al., 2023 ). Finally, ML tools can also serve for fault or anomaly detection (Angelopoulos et al., 2019 ; Md et al., 2022 ), which allows prompt corrective actions to optimize energy usage and prevent energy inefficiencies. Within this context, ML techniques for image analysis (Casini et al., 2024 ) are also gaining increasing interest (Chen et al., 2023 ), for their application to e.g. materials design and optimization (Choudhury, 2021 ), quality control (Badmos et al., 2020 ), process monitoring (Ho et al., 2021 ), or detection of machine failures by converting time series data from sensors to 2D images (Wen et al., 2017 ).
Incorporating digitalisation and ML techniques into Industry 4.0 has led to significant energy savings (Maggiore et al., 2021 ; Nota et al., 2020 ). Projects adopting these technologies can achieve an average of 15% to 25% improvement in energy efficiency in the processes where they were implemented (Arana-Landín et al., 2023 ). For instance, in predictive maintenance, ML can reduce energy consumption by optimizing the operation of machinery (Agrawal et al., 2023 ; Pan et al., 2024 ). In process optimization, ML algorithms can improve energy efficiency by 10-20% by analyzing and adjusting machine operations for optimal performance, thereby reducing unnecessary energy usage (Leong et al., 2020 ). Furthermore, the implementation of ML algorithms for optimal control can lead to energy savings of 30%, because these systems can make real-time adjustments to production lines, ensuring that machines operate at peak energy efficiency (Rahul & Chiddarwar, 2023 ).
In automotive manufacturing, ML-driven quality control can lead to energy savings by reducing the need for redoing parts or running inefficient production cycles (Vater et al., 2019 ). In high-volume production environments such as consumer electronics, novel computer-based vision models for automated detection and classification of damaged packages from intact packages can speed up operations and reduce waste (Shahin et al., 2023 ). In heavy industries like steel or chemical manufacturing, ML can optimize the energy consumption of large machinery. By predicting the optimal operating conditions and maintenance schedules, these systems can save energy costs (Mypati et al., 2023 ). Compressed air is one of the most energy-intensive processes in manufacturing. ML can optimize the performance of these systems, potentially leading to energy savings by continuously monitoring and adjusting the air compressors for peak efficiency, avoiding energy losses due to leaks or inefficient operation (Benedetti et al., 2019 ). ML can also contribute to reducing energy consumption and minimizing incorrectly produced parts in polymer processing enterprises (Willenbacher et al., 2021 ).
Here we focus on a practical industrial case study of brake caliper processing. In detail, we focus on the quality control operation, which is typically accomplished by human visual inspection and requires a dedicated handling system. This eventually implies a slower production rate, and inefficient energy usage. We thus propose the integration of an ML-based system to automatically perform the quality control operation, without the need for a dedicated handling system and thus reduced operation time. To this, we rely on ML tools able to analyze and extract information from images, that is, deep convolutional neural networks, D-CNNs (Alzubaidi et al., 2021 ; Chai et al., 2021 ).
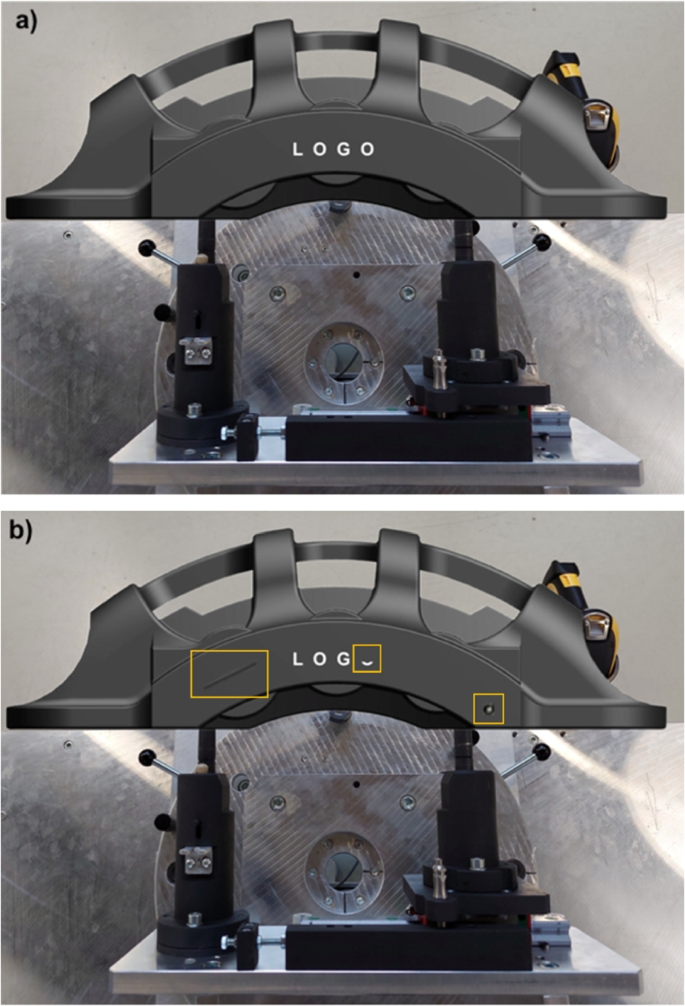
Sample 3D model (GrabCAD ) of the considered brake caliper: (a) part without defects, and (b) part with three sample defects, namely a scratch, a partially missing letter in the logo, and a circular painting defect (shown by the yellow squares, from left to right respectively)
A complete workflow for the purpose has been developed and tested on a real industrial test case. This includes: a dedicated pre-processing of the brake caliper images, their labelling and analysis using two dedicated D-CNN architectures (one for background removal, and one for defect identification), post-processing and analysis of the neural network output. Several different D-CNN architectures have been tested, in order to find the best model in terms of accuracy and computational demand. The results show that, a judicious choice of the ML model with a proper training, allows to obtain fast and accurate recognition of possible defects. The best-performing models, indeed, reach over 98% accuracy on the target criteria for quality control, and take only few seconds to analyze each image. These results make the proposed workflow compliant with the typical industrial expectations; therefore, in perspective, it could be implemented for an ML-powered version of the considered industrial problem. This would eventually allow to achieve better performance of the manufacturing process and, ultimately, a better management of the available resources in terms of time consumption and energy expense.
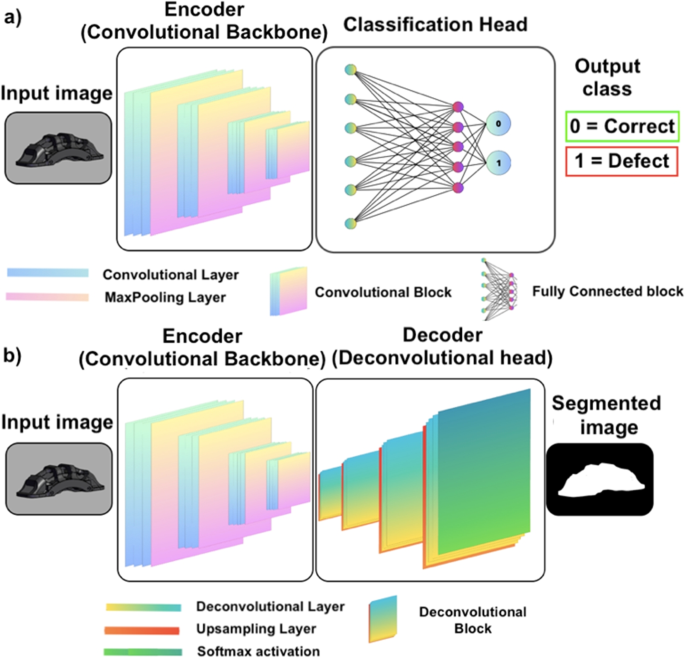
Different neural network architectures: convolutional encoder (a) and encoder-decoder (b)
The industrial quality control process that we target is the visual inspection of manufactured components, to verify the absence of possible defects. Due to industrial confidentiality reasons, a representative open-source 3D geometry (GrabCAD ) of the considered parts, similar to the original one, is shown in Fig. 1 . For illustrative purposes, the clean geometry without defects (Fig. 1 (a)) is compared to the geometry with three possible sample defects, namely: a scratch on the surface of the brake caliper, a partially missing letter in the logo, and a circular painting defect (highlighted by the yellow squares, from left to right respectively, in Fig. 1 (b)). Note that, one or multiple defects may be present on the geometry, and that other types of defects may also be considered.
Within the industrial production line, this quality control is typically time consuming, and requires a dedicated handling system with the associated slow production rate and energy inefficiencies. Thus, we developed a methodology to achieve an ML-powered version of the control process. The method relies on data analysis and, in particular, on information extraction from images of the brake calipers via Deep Convolutional Neural Networks, D-CNNs (Alzubaidi et al., 2021 ). The designed workflow for defect recognition is implemented in the following two steps: 1) removal of the background from the image of the caliper, in order to reduce noise and irrelevant features in the image, ultimately rendering the algorithms more flexible with respect to the background environment; 2) analysis of the geometry of the caliper to identify the different possible defects. These two serial steps are accomplished via two different and dedicated neural networks, whose architecture is discussed in the next section.
Convolutional Neural Networks (CNNs) pertain to a particular class of deep neural networks for information extraction from images. The feature extraction is accomplished via convolution operations; thus, the algorithms receive an image as an input, analyze it across several (deep) neural layers to identify target features, and provide the obtained information as an output (Casini et al., 2024 ). Regarding this latter output, different formats can be retrieved based on the considered architecture of the neural network. For a numerical data output, such as that required to obtain a classification of the content of an image (Bhatt et al., 2021 ), e.g. correct or defective caliper in our case, a typical layout of the network involving a convolutional backbone, and a fully-connected network can be adopted (see Fig. 2 (a)). On the other hand, if the required output is still an image, a more complex architecture with a convolutional backbone (encoder) and a deconvolutional head (decoder) can be used (see Fig. 2 (b)).
As previously introduced, our workflow targets the analysis of the brake calipers in a two-step procedure: first, the removal of the background from the input image (e.g. Fig. 1 ); second, the geometry of the caliper is analyzed and the part is classified as acceptable or not depending on the absence or presence of any defect, respectively. Thus, in the first step of the procedure, a dedicated encoder-decoder network (Minaee et al., 2021 ) is adopted to classify the pixels in the input image as brake or background. The output of this model will then be a new version of the input image, where the background pixels are blacked. This helps the algorithms in the subsequent analysis to achieve a better performance, and to avoid bias due to possible different environments in the input image. In the second step of the workflow, a dedicated encoder architecture is adopted. Here, the previous background-filtered image is fed to the convolutional network, and the geometry of the caliper is analyzed to spot possible defects and thus classify the part as acceptable or not. In this work, both deep learning models are supervised , that is, the algorithms are trained with the help of human-labeled data (LeCun et al., 2015 ). Particularly, the first algorithm for background removal is fed with the original image as well as with a ground truth (i.e. a binary image, also called mask , consisting of black and white pixels) which instructs the algorithm to learn which pixels pertain to the brake and which to the background. This latter task is usually called semantic segmentation in Machine Learning and Deep Learning (Géron, 2022 ). Analogously, the second algorithm is fed with the original image (without the background) along with an associated mask, which serves the neural networks with proper instructions to identify possible defects on the target geometry. The required pre-processing of the input images, as well as their use for training and validation of the developed algorithms, are explained in the next sections.
Image pre-processing
Machine Learning approaches rely on data analysis; thus, the quality of the final results is well known to depend strongly on the amount and quality of the available data for training of the algorithms (Banko & Brill, 2001 ; Chen et al., 2021 ). In our case, the input images should be well-representative for the target analysis and include adequate variability of the possible features to allow the neural networks to produce the correct output. In this view, the original images should include, e.g., different possible backgrounds, a different viewing angle of the considered geometry and a different light exposure (as local light reflections may affect the color of the geometry and thus the analysis). The creation of such a proper dataset for specific cases is not always straightforward; in our case, for example, it would imply a systematic acquisition of a large set of images in many different conditions. This would require, in turn, disposing of all the possible target defects on the real parts, and of an automatic acquisition system, e.g., a robotic arm with an integrated camera. Given that, in our case, the initial dataset could not be generated on real parts, we have chosen to generate a well-balanced dataset of images in silico , that is, based on image renderings of the real geometry. The key idea was that, if the rendered geometry is sufficiently close to a real photograph, the algorithms may be instructed on artificially-generated images and then tested on a few real ones. This approach, if properly automatized, clearly allows to easily produce a large amount of images in all the different conditions required for the analysis.
In a first step, starting from the CAD file of the brake calipers, we worked manually using the open-source software Blender (Blender ), to modify the material properties and achieve a realistic rendering. After that, defects were generated by means of Boolean (subtraction) operations between the geometry of the brake caliper and ad-hoc geometries for each defect. Fine tuning on the generated defects has allowed for a realistic representation of the different defects. Once the results were satisfactory, we developed an automated Python code for the procedures, to generate the renderings in different conditions. The Python code allows to: load a given CAD geometry, change the material properties, set different viewing angles for the geometry, add different types of defects (with given size, rotation and location on the geometry of the brake caliper), add a custom background, change the lighting conditions, render the scene and save it as an image.
In order to make the dataset as varied as possible, we introduced three light sources into the rendering environment: a diffuse natural lighting to simulate daylight conditions, and two additional artificial lights. The intensity of each light source and the viewing angle were then made vary randomly, to mimic different daylight conditions and illuminations of the object. This procedure was designed to provide different situations akin to real use, and to make the model invariant to lighting conditions and camera position. Moreover, to provide additional flexibility to the model, the training dataset of images was virtually expanded using data augmentation (Mumuni & Mumuni, 2022 ), where saturation, brightness and contrast were made randomly vary during training operations. This procedure has allowed to consistently increase the number and variety of the images in the training dataset.
The developed automated pre-processing steps easily allows for batch generation of thousands of different images to be used for training of the neural networks. This possibility is key for proper training of the neural networks, as the variability of the input images allows the models to learn all the possible features and details that may change during real operating conditions.
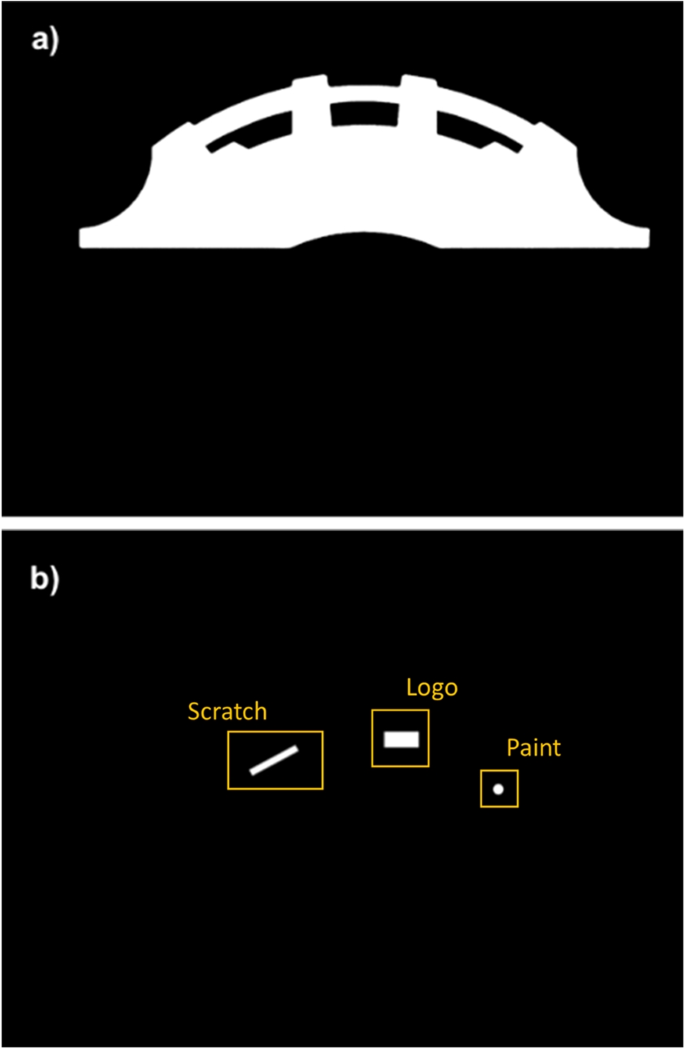
Examples of the ground truth for the two target tasks: background removal (a) and defects recognition (b)
The first tests using such virtual database have shown that, although the generated images were very similar to real photographs, the models were not able to properly recognize the target features in the real images. Thus, in a tentative to get closer to a proper set of real images, we decided to adopt a hybrid dataset, where the virtually generated images were mixed with the available few real ones. However, given that some possible defects were missing in the real images, we also decided to manipulate the images to introduce virtual defects on real images. The obtained dataset finally included more than 4,000 images, where 90% was rendered, and 10% was obtained from real images. To avoid possible bias in the training dataset, defects were present in 50% of the cases in both the rendered and real image sets. Thus, in the overall dataset, the real original images with no defects were 5% of the total.
Along with the code for the rendering and manipulation of the images, dedicated Python routines were developed to generate the corresponding data labelling for the supervised training of the networks, namely the image masks. Particularly, two masks were generated for each input image: one for the background removal operation, and one for the defect identification. In both cases, the masks consist of a binary (i.e. black and white) image where all the pixels of a target feature (i.e. the geometry or defect) are assigned unitary values (white); whereas, all the remaining pixels are blacked (zero values). An example of these masks in relation to the geometry in Fig. 1 is shown in Fig. 3 .
All the generated images were then down-sampled, that is, their resolution was reduced to avoid unnecessary large computational times and (RAM) memory usage while maintaining the required level of detail for training of the neural networks. Finally, the input images and the related masks were split into a mosaic of smaller tiles, to achieve a suitable size for feeding the images to the neural networks with even more reduced requirements on the RAM memory. All the tiles were processed, and the whole image reconstructed at the end of the process to visualize the overall final results.
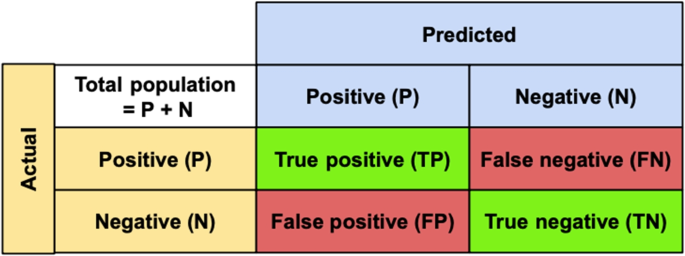
Confusion matrix for accuracy assessment of the neural networks models
Choice of the model
Within the scope of the present application, a wide range of possibly suitable models is available (Chen et al., 2021 ). In general, the choice of the best model for a given problem should be made on a case-by-case basis, considering an acceptable compromise between the achievable accuracy and computational complexity/cost. Too simple models can indeed be very fast in the response yet have a reduced accuracy. On the other hand, more complex models can generally provide more accurate results, although typically requiring larger amounts of data for training, and thus longer computational times and energy expense. Hence, testing has the crucial role to allow identification of the best trade-off between these two extreme cases. A benchmark for model accuracy can generally be defined in terms of a confusion matrix, where the model response is summarized into the following possibilities: True Positives (TP), True Negatives (TN), False Positives (FP) and False Negatives (FN). This concept can be summarized as shown in Fig. 4 . For the background removal, Positive (P) stands for pixels belonging to the brake caliper, while Negative (N) for background pixels. For the defect identification model, Positive (P) stands for non-defective geometry, whereas Negative (N) stands for defective geometries. With respect to these two cases, the True/False statements stand for correct or incorrect identification, respectively. The model accuracy can be therefore assessed as Géron ( 2022 )
Based on this metrics, the accuracy for different models can then be evaluated on a given dataset, where typically 80% of the data is used for training and the remaining 20% for validation. For the defect recognition stage, the following models were tested: VGG-16 (Simonyan & Zisserman, 2014 ), ResNet50, ResNet101, ResNet152 (He et al., 2016 ), Inception V1 (Szegedy et al., 2015 ), Inception V4 and InceptionResNet V2 (Szegedy et al., 2017 ). Details on the assessment procedure for the different models are provided in the Supplementary Information file. For the background removal stage, the DeepLabV3 \(+\) (Chen et al., 2018 ) model was chosen as the first option, and no additional models were tested as it directly provided satisfactory results in terms of accuracy and processing time. This gives preliminary indication that, from the point of view of the task complexity of the problem, the defect identification stage can be more demanding with respect to the background removal operation for the case study at hand. Besides the assessment of the accuracy according to, e.g., the metrics discussed above, additional information can be generally collected, such as too low accuracy (indicating insufficient amount of training data), possible bias of the models on the data (indicating a non-well balanced training dataset), or other specific issues related to missing representative data in the training dataset (Géron, 2022 ). This information helps both to correctly shape the training dataset, and to gather useful indications for the fine tuning of the model after its choice has been made.
Background removal
An initial bias of the model for background removal arose on the color of the original target geometry (red color). The model was indeed identifying possible red spots on the background as part of the target geometry as an unwanted output. To improve the model flexibility, and thus its accuracy on the identification of the background, the training dataset was expanded using data augmentation (Géron, 2022 ). This technique allows to artificially increase the size of the training dataset by applying various transformations to the available images, with the goal to improve the performance and generalization ability of the models. This approach typically involves applying geometric and/or color transformations to the original images; in our case, to account for different viewing angles of the geometry, different light exposures, and different color reflections and shadowing effects. These improvements of the training dataset proved to be effective on the performance for the background removal operation, with a validation accuracy finally ranging above 99% and model response time around 1-2 seconds. An example of the output of this operation for the geometry in Fig. 1 is shown in Fig. 5 .
While the results obtained were satisfactory for the original (red) color of the calipers, we decided to test the model ability to be applied on brake calipers of other colors as well. To this, the model was trained and tested on a grayscale version of the images of the calipers, which allows to completely remove any possible bias of the model on a specific color. In this case, the validation accuracy of the model was still obtained to range above 99%; thus, this approach was found to be particularly interesting to make the model suitable for background removal operation even on images including calipers of different colors.
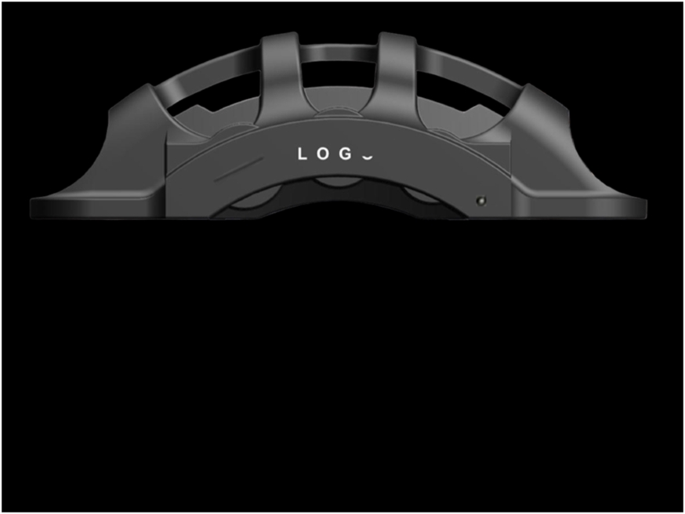
Target geometry after background removal
Defect recognition
An overview of the performance of the tested models for the defect recognition operation on the original geometry of the caliper is reported in Table 1 (see also the Supplementary Information file for more details on the assessment of different models). The results report on the achieved validation accuracy ( \(A_v\) ) and on the number of parameters ( \(N_p\) ), with this latter being the total number of parameters that can be trained for each model (Géron, 2022 ) to determine the output. Here, this quantity is adopted as an indicator of the complexity of each model.
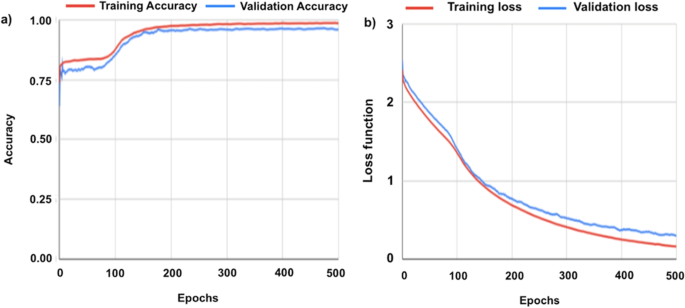
Accuracy (a) and loss function (b) curves for the Resnet101 model during training
As the results in Table 1 show, the VGG-16 model was quite unprecise for our dataset, eventually showing underfitting (Géron, 2022 ). Thus, we decided to opt for the Resnet and Inception families of models. Both these families of models have demonstrated to be suitable for handling our dataset, with slightly less accurate results being provided by the Resnet50 and InceptionV1. The best results were obtained using Resnet101 and InceptionV4, with very high final accuracy and fast processing time (in the order \(\sim \) 1 second). Finally, Resnet152 and InceptionResnetV2 models proved to be slightly too complex or slower for our case; they indeed provided excellent results but taking longer response times (in the order of \(\sim \) 3-5 seconds). The response time is indeed affected by the complexity ( \(N_p\) ) of the model itself, and by the hardware used. In our work, GPUs were used for training and testing all the models, and the hardware conditions were kept the same for all models.
Based on the results obtained, ResNet101 model was chosen as the best solution for our application, in terms of accuracy and reduced complexity. After fine-tuning operations, the accuracy that we obtained with this model reached nearly 99%, both in the validation and test datasets. This latter includes target real images, that the models have never seen before; thus, it can be used for testing of the ability of the models to generalize the information learnt during the training/validation phase.
The trend in the accuracy increase and loss function decrease during training of the Resnet101 model on the original geometry are shown in Fig. 6 (a) and (b), respectively. Particularly, the loss function quantifies the error between the predicted output during training of the model and the actual target values in the dataset. In our case, the loss function is computed using the cross-entropy function and the Adam optimiser (Géron, 2022 ). The error is expected to reduce during the training, which eventually leads to more accurate predictions of the model on previously-unseen data. The combination of accuracy and loss function trends, along with other control parameters, is typically used and monitored to evaluate the training process, and avoid e.g. under- or over-fitting problems (Géron, 2022 ). As Fig. 6 (a) shows, the accuracy experiences a sudden step increase during the very first training phase (epochs, that is, the number of times the complete database is repeatedly scrutinized by the model during its training (Géron, 2022 )). The accuracy then increases in a smooth fashion with the epochs, until an asymptotic value is reached both for training and validation accuracy. These trends in the two accuracy curves can generally be associated with a proper training; indeed, being the two curves close to each other may be interpreted as an absence of under-fitting problems. On the other hand, Fig. 6 (b) shows that the loss function curves are close to each other, with a monotonically-decreasing trend. This can be interpreted as an absence of over-fitting problems, and thus of proper training of the model.
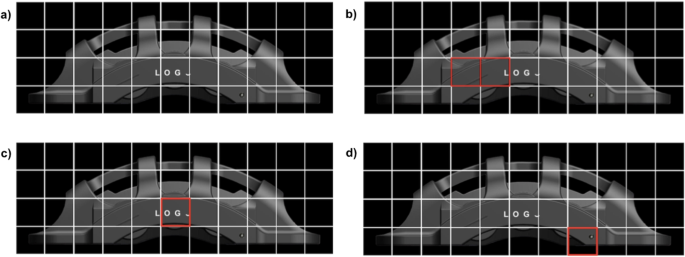
Final results of the analysis on the defect identification: (a) considered input geometry, (b), (c) and (d) identification of a scratch on the surface, partially missing logo, and painting defect respectively (highlighted in the red frames)
Finally, an example output of the overall analysis is shown in Fig. 7 , where the considered input geometry is shown (a), along with the identification of the defects (b), (c) and (d) obtained from the developed protocol. Note that, here the different defects have been separated in several figures for illustrative purposes; however, the analysis yields the identification of defects on one single image. In this work, a binary classification was performed on the considered brake calipers, where the output of the models allows to discriminate between defective or non-defective components based on the presence or absence of any of the considered defects. Note that, fine tuning of this discrimination is ultimately with the user’s requirements. Indeed, the model output yields as the probability (from 0 to 100%) of the possible presence of defects; thus, the discrimination between a defective or non-defective part is ultimately with the user’s choice of the acceptance threshold for the considered part (50% in our case). Therefore, stricter or looser criteria can be readily adopted. Eventually, for particularly complex cases, multiple models may also be used concurrently for the same task, and the final output defined based on a cross-comparison of the results from different models. As a last remark on the proposed procedure, note that here we adopted a binary classification based on the presence or absence of any defect; however, further classification of the different defects could also be implemented, to distinguish among different types of defects (multi-class classification) on the brake calipers.
Energy saving
Illustrative scenarios.
Given that the proposed tools have not yet been implemented and tested within a real industrial production line, we analyze here three perspective scenarios to provide a practical example of the potential for energy savings in an industrial context. To this, we consider three scenarios, which compare traditional human-based control operations and a quality control system enhanced by the proposed Machine Learning (ML) tools. Specifically, here we analyze a generic brake caliper assembly line formed by 14 stations, as outlined in Table 1 in the work by Burduk and Górnicka ( 2017 ). This assembly line features a critical inspection station dedicated to defect detection, around which we construct three distinct scenarios to evaluate the efficacy of traditional human-based control operations versus a quality control system augmented by the proposed ML-based tools, namely:
First Scenario (S1): Human-Based Inspection. The traditional approach involves a human operator responsible for the inspection tasks.
Second Scenario (S2): Hybrid Inspection. This scenario introduces a hybrid inspection system where our proposed ML-based automatic detection tool assists the human inspector. The ML tool analyzes the brake calipers and alerts the human inspector only when it encounters difficulties in identifying defects, specifically when the probability of a defect being present or absent falls below a certain threshold. This collaborative approach aims to combine the precision of ML algorithms with the experience of human inspectors, and can be seen as a possible transition scenario between the human-based and a fully-automated quality control operation.
Third Scenario (S3): Fully Automated Inspection. In the final scenario, we conceive a completely automated defect inspection station powered exclusively by our ML-based detection system. This setup eliminates the need for human intervention, relying entirely on the capabilities of the ML tools to identify defects.
For simplicity, we assume that all the stations are aligned in series without buffers, minimizing unnecessary complications in our estimations. To quantify the beneficial effects of implementing ML-based quality control, we adopt the Overall Equipment Effectiveness (OEE) as the primary metric for the analysis. OEE is a comprehensive measure derived from the product of three critical factors, as outlined by Nota et al. ( 2020 ): Availability (the ratio of operating time with respect to planned production time); Performance (the ratio of actual output with respect to the theoretical maximum output); and Quality (the ratio of the good units with respect to the total units produced). In this section, we will discuss the details of how we calculate each of these factors for the various scenarios.
To calculate Availability ( A ), we consider an 8-hour work shift ( \(t_{shift}\) ) with 30 minutes of breaks ( \(t_{break}\) ) during which we assume production stop (except for the fully automated scenario), and 30 minutes of scheduled downtime ( \(t_{sched}\) ) required for machine cleaning and startup procedures. For unscheduled downtime ( \(t_{unsched}\) ), primarily due to machine breakdowns, we assume an average breakdown probability ( \(\rho _{down}\) ) of 5% for each machine, with an average repair time of one hour per incident ( \(t_{down}\) ). Based on these assumptions, since the Availability represents the ratio of run time ( \(t_{run}\) ) to production time ( \(t_{pt}\) ), it can be calculated using the following formula:
with the unscheduled downtime being computed as follows:
where N is the number of machines in the production line and \(1-\left( 1-\rho _{down}\right) ^{N}\) represents the probability that at least one machine breaks during the work shift. For the sake of simplicity, the \(t_{down}\) is assumed constant regardless of the number of failures.
Table 2 presents the numerical values used to calculate Availability in the three scenarios. In the second scenario, we can observe that integrating the automated station leads to a decrease in the first factor of the OEE analysis, which can be attributed to the additional station for automated quality-control (and the related potential failure). This ultimately increases the estimation of the unscheduled downtime. In the third scenario, the detrimental effect of the additional station compensates the beneficial effect of the automated quality control on reducing the need for pauses during operator breaks; thus, the Availability for the third scenario yields as substantially equivalent to the first one (baseline).
The second factor of OEE, Performance ( P ), assesses the operational efficiency of production equipment relative to its maximum designed speed ( \(t_{line}\) ). This evaluation includes accounting for reductions in cycle speed and minor stoppages, collectively termed as speed losses . These losses are challenging to measure in advance, as performance is typically measured using historical data from the production line. For this analysis, we are constrained to hypothesize a reasonable estimate of 60 seconds of time lost to speed losses ( \(t_{losses}\) ) for each work cycle. Although this assumption may appear strong, it will become evident later that, within the context of this analysis – particularly regarding the impact of automated inspection on energy savings – the Performance (like the Availability) is only marginally influenced by introducing an automated inspection station. To account for the effect of automated inspection on the assembly line speed, we keep the time required by the other 13 stations ( \(t^*_{line}\) ) constant while varying the time allocated for visual inspection ( \(t_{inspect}\) ). According to Burduk and Górnicka ( 2017 ), the total operation time of the production line, excluding inspection, is 1263 seconds, with manual visual inspection taking 38 seconds. For the fully automated third scenario, we assume an inspection time of 5 seconds, which encloses the photo collection, pre-processing, ML-analysis, and post-processing steps. In the second scenario, instead, we add an additional time to the pure automatic case to consider the cases when the confidence of the ML model falls below 90%. We assume this happens once in every 10 inspections, which is a conservative estimate, higher than that we observed during model testing. This results in adding 10% of the human inspection time to the fully automated time. Thus, when \(t_{losses}\) are known, Performance can be expressed as follows:
The calculated values for Performance are presented in Table 3 , and we can note that the modification in inspection time has a negligible impact on this factor since it does not affect the speed loss or, at least to our knowledge, there is no clear evidence to suggest that the introduction of a new inspection station would alter these losses. Moreover, given the specific linear layout of the considered production line, the inspection time change has only a marginal effect on enhancing the production speed. However, this approach could potentially bias our scenario towards always favouring automation. To evaluate this hypothesis, a sensitivity analysis which explores scenarios where the production line operates at a faster pace will be discussed in the next subsection.
The last factor, Quality ( Q ), quantifies the ratio of compliant products out of the total products manufactured, effectively filtering out items that fail to meet the quality standards due to defects. Given the objective of our automated algorithm, we anticipate this factor of the OEE to be significantly enhanced by implementing the ML-based automated inspection station. To estimate it, we assume a constant defect probability for the production line ( \(\rho _{def}\) ) at 5%. Consequently, the number of defective products ( \(N_{def}\) ) during the work shift is calculated as \(N_{unit} \cdot \rho _{def}\) , where \(N_{unit}\) represents the average number of units (brake calipers) assembled on the production line, defined as:
To quantify defective units identified, we consider the inspection accuracy ( \(\rho _{acc}\) ), where for human visual inspection, the typical accuracy is 80% (Sundaram & Zeid, 2023 ), and for the ML-based station, we use the accuracy of our best model, i.e., 99%. Additionally, we account for the probability of the station mistakenly identifying a caliper as with a defect even if it is defect-free, i.e., the false negative rate ( \(\rho _{FN}\) ), defined as
In the absence of any reasonable evidence to justify a bias on one mistake over others, we assume a uniform distribution for both human and automated inspections regarding error preference, i.e. we set \(\rho ^{H}_{FN} = \rho ^{ML}_{FN} = \rho _{FN} = 50\%\) . Thus, the number of final compliant goods ( \(N_{goods}\) ), i.e., the calipers that are identified as quality-compliant, can be calculated as:
where \(N_{detect}\) is the total number of detected defective units, comprising TN (true negatives, i.e. correctly identified defective calipers) and FN (false negatives, i.e. calipers mistakenly identified as defect-free). The Quality factor can then be computed as:
Table 4 summarizes the Quality factor calculation, showcasing the substantial improvement brought by the ML-based inspection station due to its higher accuracy compared to human operators.
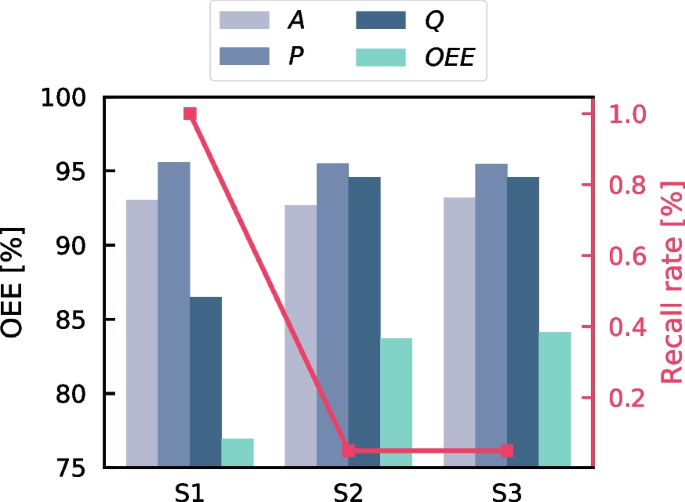
Overall Equipment Effectiveness (OEE) analysis for three scenarios (S1: Human-Based Inspection, S2: Hybrid Inspection, S3: Fully Automated Inspection). The height of the bars represents the percentage of the three factors A : Availability, P : Performance, and Q : Quality, which can be interpreted from the left axis. The green bars indicate the OEE value, derived from the product of these three factors. The red line shows the recall rate, i.e. the probability that a defective product is rejected by the client, with values displayed on the right red axis
Finally, we can determine the Overall Equipment Effectiveness by multiplying the three factors previously computed. Additionally, we can estimate the recall rate ( \(\rho _{R}\) ), which reflects the rate at which a customer might reject products. This is derived from the difference between the total number of defective units, \(N_{def}\) , and the number of units correctly identified as defective, TN , indicating the potential for defective brake calipers that may bypass the inspection process. In Fig. 8 we summarize the outcomes of the three scenarios. It is crucial to note that the scenarios incorporating the automated defect detector, S2 and S3, significantly enhance the Overall Equipment Effectiveness, primarily through substantial improvements in the Quality factor. Among these, the fully automated inspection scenario, S3, emerges as a slightly superior option, thanks to its additional benefit in removing the breaks and increasing the speed of the line. However, given the different assumptions required for this OEE study, we shall interpret these results as illustrative, and considering them primarily as comparative with the baseline scenario only. To analyze the sensitivity of the outlined scenarios on the adopted assumptions, we investigate the influence of the line speed and human accuracy on the results in the next subsection.
Sensitivity analysis
The scenarios described previously are illustrative and based on several simplifying hypotheses. One of such hypotheses is that the production chain layout operates entirely in series, with each station awaiting the arrival of the workpiece from the preceding station, resulting in a relatively slow production rate (1263 seconds). This setup can be quite different from reality, where slower operations can be accelerated by installing additional machines in parallel to balance the workload and enhance productivity. Moreover, we utilized a literature value of 80% for the accuracy of the human visual inspector operator, as reported by Sundaram and Zeid ( 2023 ). However, this accuracy can vary significantly due to factors such as the experience of the inspector and the defect type.
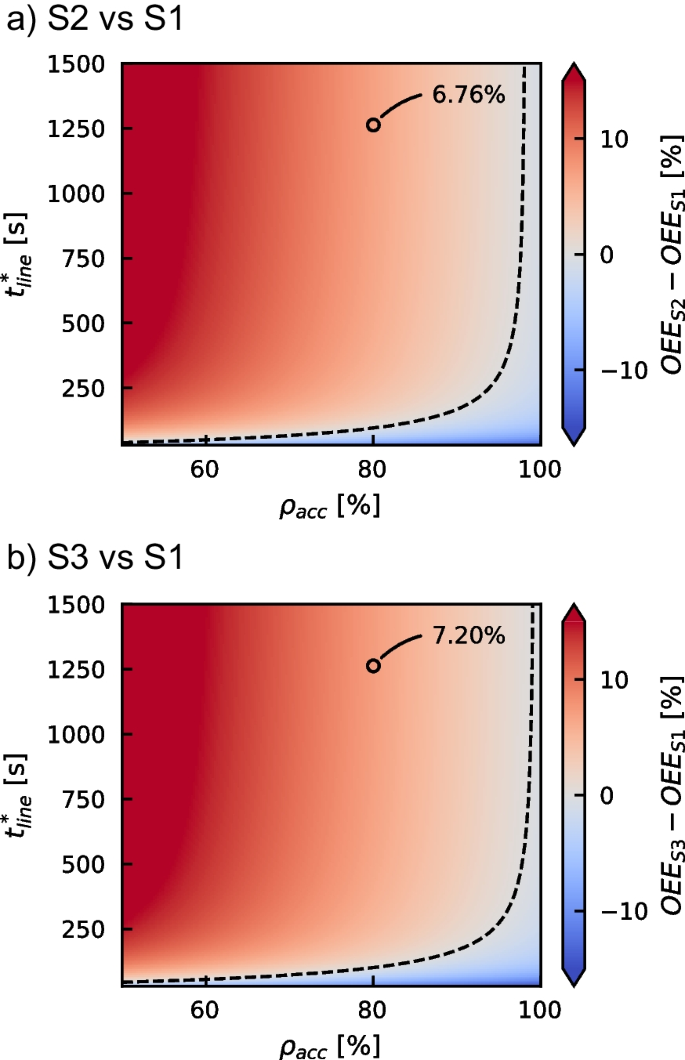
Effect of assembly time for stations (excluding visual inspection), \(t^*_{line}\) , and human inspection accuracy, \(\rho _{acc}\) , on the OEE analysis. (a) The subplot shows the difference between the scenario S2 (Hybrid Inspection) and the baseline scenario S1 (Human Inspection), while subplot (b) displays the difference between scenario S3 (Fully Automated Inspection) and the baseline. The maps indicate in red the values of \(t^*_{line}\) and \(\rho _{acc}\) where the integration of automated inspection stations can significantly improve OEE, and in blue where it may lower the score. The dashed lines denote the breakeven points, and the circled points pinpoint the values of the scenarios used in the “Illustrative scenario” Subsection.
A sensitivity analysis on these two factors was conducted to address these variations. The assembly time of the stations (excluding visual inspection), \(t^*_{line}\) , was varied from 60 s to 1500 s, and the human inspection accuracy, \(\rho _{acc}\) , ranged from 50% (akin to a random guesser) to 100% (representing an ideal visual inspector); meanwhile, the other variables were kept fixed.
The comparison of the OEE enhancement for the two scenarios employing ML-based inspection against the baseline scenario is displayed in the two maps in Fig. 9 . As the figure shows, due to the high accuracy and rapid response of the proposed automated inspection station, the area representing regions where the process may benefit energy savings in the assembly lines (indicated in red shades) is significantly larger than the areas where its introduction could degrade performance (indicated in blue shades). However, it can be also observed that the automated inspection could be superfluous or even detrimental in those scenarios where human accuracy and assembly speed are very high, indicating an already highly accurate workflow. In these cases, and particularly for very fast production lines, short times for quality control can be expected to be key (beyond accuracy) for the optimization.
Finally, it is important to remark that the blue region (areas below the dashed break-even lines) might expand if the accuracy of the neural networks for defect detection is lower when implemented in an real production line. This indicates the necessity for new rounds of active learning and an augment of the ratio of real images in the database, to eventually enhance the performance of the ML model.
Conclusions
Industrial quality control processes on manufactured parts are typically achieved by human visual inspection. This usually requires a dedicated handling system, and generally results in a slower production rate, with the associated non-optimal use of the energy resources. Based on a practical test case for quality control on brake caliper manufacturing, in this work we have reported on a developed workflow for integration of Machine Learning methods to automatize the process. The proposed approach relies on image analysis via Deep Convolutional Neural Networks. These models allow to efficiently extract information from images, thus possibly representing a valuable alternative to human inspection.
The proposed workflow relies on a two-step procedure on the images of the brake calipers: first, the background is removed from the image; second, the geometry is inspected to identify possible defects. These two steps are accomplished thanks to two dedicated neural network models, an encoder-decoder and an encoder network, respectively. Training of these neural networks typically requires a large number of representative images for the problem. Given that, one such database is not always readily available, we have presented and discussed an alternative methodology for the generation of the input database using 3D renderings. While integration of the database with real photographs was required for optimal results, this approach has allowed fast and flexible generation of a large base of representative images. The pre-processing steps required for data feeding to the neural networks and their training has been also discussed.
Several models have been tested and evaluated, and the best one for the considered case identified. The obtained accuracy for defect identification reaches \(\sim \) 99% of the tested cases. Moreover, the response of the models is fast (in the order of few seconds) on each image, which makes them compliant with the most typical industrial expectations.
In order to provide a practical example of possible energy savings when implementing the proposed ML-based methodology for quality control, we have analyzed three perspective industrial scenarios: a baseline scenario, where quality control tasks are performed by a human inspector; a hybrid scenario, where the proposed ML automatic detection tool assists the human inspector; a fully-automated scenario, where we envision a completely automated defect inspection. The results show that the proposed tools may help increasing the Overall Equipment Effectiveness up to \(\sim \) 10% with respect to the considered baseline scenario. However, a sensitivity analysis on the speed of the production line and on the accuracy of the human inspector has also shown that the automated inspection could be superfluous or even detrimental in those cases where human accuracy and assembly speed are very high. In these cases, reducing the time required for quality control can be expected to be the major controlling parameter (beyond accuracy) for optimization.
Overall the results show that, with a proper tuning, these models may represent a valuable resource for integration into production lines, with positive outcomes on the overall effectiveness, and thus ultimately leading to a better use of the energy resources. To this, while the practical implementation of the proposed tools can be expected to require contained investments (e.g. a portable camera, a dedicated workstation and an operator with proper training), in field tests on a real industrial line would be required to confirm the potential of the proposed technology.
Agrawal, R., Majumdar, A., Kumar, A., & Luthra, S. (2023). Integration of artificial intelligence in sustainable manufacturing: Current status and future opportunities. Operations Management Research, 1–22.
Alzubaidi, L., Zhang, J., Humaidi, A. J., Al-Dujaili, A., Duan, Y., Al-Shamma, O., Santamaría, J., Fadhel, M. A., Al-Amidie, M., & Farhan, L. (2021). Review of deep learning: Concepts, cnn architectures, challenges, applications, future directions. Journal of big Data, 8 , 1–74.
Article Google Scholar
Angelopoulos, A., Michailidis, E. T., Nomikos, N., Trakadas, P., Hatziefremidis, A., Voliotis, S., & Zahariadis, T. (2019). Tackling faults in the industry 4.0 era-a survey of machine—learning solutions and key aspects. Sensors, 20 (1), 109.
Arana-Landín, G., Uriarte-Gallastegi, N., Landeta-Manzano, B., & Laskurain-Iturbe, I. (2023). The contribution of lean management—industry 4.0 technologies to improving energy efficiency. Energies, 16 (5), 2124.
Badmos, O., Kopp, A., Bernthaler, T., & Schneider, G. (2020). Image-based defect detection in lithium-ion battery electrode using convolutional neural networks. Journal of Intelligent Manufacturing, 31 , 885–897. https://doi.org/10.1007/s10845-019-01484-x
Banko, M., & Brill, E. (2001). Scaling to very very large corpora for natural language disambiguation. In Proceedings of the 39th annual meeting of the association for computational linguistics (pp. 26–33).
Benedetti, M., Bonfà, F., Introna, V., Santolamazza, A., & Ubertini, S. (2019). Real time energy performance control for industrial compressed air systems: Methodology and applications. Energies, 12 (20), 3935.
Bhatt, D., Patel, C., Talsania, H., Patel, J., Vaghela, R., Pandya, S., Modi, K., & Ghayvat, H. (2021). Cnn variants for computer vision: History, architecture, application, challenges and future scope. Electronics, 10 (20), 2470.
Bilgen, S. (2014). Structure and environmental impact of global energy consumption. Renewable and Sustainable Energy Reviews, 38 , 890–902.
Blender. (2023). Open-source software. https://www.blender.org/ . Accessed 18 Apr 2023.
Bologna, A., Fasano, M., Bergamasco, L., Morciano, M., Bersani, F., Asinari, P., Meucci, L., & Chiavazzo, E. (2020). Techno-economic analysis of a solar thermal plant for large-scale water pasteurization. Applied Sciences, 10 (14), 4771.
Burduk, A., & Górnicka, D. (2017). Reduction of waste through reorganization of the component shipment logistics. Research in Logistics & Production, 7 (2), 77–90. https://doi.org/10.21008/j.2083-4950.2017.7.2.2
Carvalho, T. P., Soares, F. A., Vita, R., Francisco, R., d. P., Basto, J. P., & Alcalá, S. G. (2019). A systematic literature review of machine learning methods applied to predictive maintenance. Computers & Industrial Engineering, 137 , 106024.
Casini, M., De Angelis, P., Chiavazzo, E., & Bergamasco, L. (2024). Current trends on the use of deep learning methods for image analysis in energy applications. Energy and AI, 15 , 100330. https://doi.org/10.1016/j.egyai.2023.100330
Chai, J., Zeng, H., Li, A., & Ngai, E. W. (2021). Deep learning in computer vision: A critical review of emerging techniques and application scenarios. Machine Learning with Applications, 6 , 100134.
Chen, L. C., Zhu, Y., Papandreou, G., Schroff, F., Adam, H. (2018). Encoder-decoder with atrous separable convolution for semantic image segmentation. In Proceedings of the European conference on computer vision (ECCV) (pp. 801–818).
Chen, L., Li, S., Bai, Q., Yang, J., Jiang, S., & Miao, Y. (2021). Review of image classification algorithms based on convolutional neural networks. Remote Sensing, 13 (22), 4712.
Chen, T., Sampath, V., May, M. C., Shan, S., Jorg, O. J., Aguilar Martín, J. J., Stamer, F., Fantoni, G., Tosello, G., & Calaon, M. (2023). Machine learning in manufacturing towards industry 4.0: From ‘for now’to ‘four-know’. Applied Sciences, 13 (3), 1903. https://doi.org/10.3390/app13031903
Choudhury, A. (2021). The role of machine learning algorithms in materials science: A state of art review on industry 4.0. Archives of Computational Methods in Engineering, 28 (5), 3361–3381. https://doi.org/10.1007/s11831-020-09503-4
Dalzochio, J., Kunst, R., Pignaton, E., Binotto, A., Sanyal, S., Favilla, J., & Barbosa, J. (2020). Machine learning and reasoning for predictive maintenance in industry 4.0: Current status and challenges. Computers in Industry, 123 , 103298.
Fasano, M., Bergamasco, L., Lombardo, A., Zanini, M., Chiavazzo, E., & Asinari, P. (2019). Water/ethanol and 13x zeolite pairs for long-term thermal energy storage at ambient pressure. Frontiers in Energy Research, 7 , 148.
Géron, A. (2022). Hands-on machine learning with Scikit-Learn, Keras, and TensorFlow . O’Reilly Media, Inc.
GrabCAD. (2023). Brake caliper 3D model by Mitulkumar Sakariya from the GrabCAD free library (non-commercial public use). https://grabcad.com/library/brake-caliper-19 . Accessed 18 Apr 2023.
He, K., Zhang, X., Ren, S., & Sun, J. (2016). Deep residual learning for image recognition. In Proceedings of the IEEE conference on computer vision and pattern recognition (pp. 770–778).
Ho, S., Zhang, W., Young, W., Buchholz, M., Al Jufout, S., Dajani, K., Bian, L., & Mozumdar, M. (2021). Dlam: Deep learning based real-time porosity prediction for additive manufacturing using thermal images of the melt pool. IEEE Access, 9 , 115100–115114. https://doi.org/10.1109/ACCESS.2021.3105362
Ismail, M. I., Yunus, N. A., & Hashim, H. (2021). Integration of solar heating systems for low-temperature heat demand in food processing industry-a review. Renewable and Sustainable Energy Reviews, 147 , 111192.
LeCun, Y., Bengio, Y., & Hinton, G. (2015). Deep learning. Nature, 521 (7553), 436–444.
Leong, W. D., Teng, S. Y., How, B. S., Ngan, S. L., Abd Rahman, A., Tan, C. P., Ponnambalam, S., & Lam, H. L. (2020). Enhancing the adaptability: Lean and green strategy towards the industry revolution 4.0. Journal of cleaner production, 273 , 122870.
Liu, Z., Wang, X., Zhang, Q., & Huang, C. (2019). Empirical mode decomposition based hybrid ensemble model for electrical energy consumption forecasting of the cement grinding process. Measurement, 138 , 314–324.
Li, G., & Zheng, X. (2016). Thermal energy storage system integration forms for a sustainable future. Renewable and Sustainable Energy Reviews, 62 , 736–757.
Maggiore, S., Realini, A., Zagano, C., & Bazzocchi, F. (2021). Energy efficiency in industry 4.0: Assessing the potential of industry 4.0 to achieve 2030 decarbonisation targets. International Journal of Energy Production and Management, 6 (4), 371–381.
Mazzei, D., & Ramjattan, R. (2022). Machine learning for industry 4.0: A systematic review using deep learning-based topic modelling. Sensors, 22 (22), 8641.
Md, A. Q., Jha, K., Haneef, S., Sivaraman, A. K., & Tee, K. F. (2022). A review on data-driven quality prediction in the production process with machine learning for industry 4.0. Processes, 10 (10), 1966. https://doi.org/10.3390/pr10101966
Minaee, S., Boykov, Y., Porikli, F., Plaza, A., Kehtarnavaz, N., & Terzopoulos, D. (2021). Image segmentation using deep learning: A survey. IEEE transactions on pattern analysis and machine intelligence, 44 (7), 3523–3542.
Google Scholar
Mishra, S., Srivastava, R., Muhammad, A., Amit, A., Chiavazzo, E., Fasano, M., & Asinari, P. (2023). The impact of physicochemical features of carbon electrodes on the capacitive performance of supercapacitors: a machine learning approach. Scientific Reports, 13 (1), 6494. https://doi.org/10.1038/s41598-023-33524-1
Mumuni, A., & Mumuni, F. (2022). Data augmentation: A comprehensive survey of modern approaches. Array, 16 , 100258. https://doi.org/10.1016/j.array.2022.100258
Mypati, O., Mukherjee, A., Mishra, D., Pal, S. K., Chakrabarti, P. P., & Pal, A. (2023). A critical review on applications of artificial intelligence in manufacturing. Artificial Intelligence Review, 56 (Suppl 1), 661–768.
Narciso, D. A., & Martins, F. (2020). Application of machine learning tools for energy efficiency in industry: A review. Energy Reports, 6 , 1181–1199.
Nota, G., Nota, F. D., Peluso, D., & Toro Lazo, A. (2020). Energy efficiency in industry 4.0: The case of batch production processes. Sustainability, 12 (16), 6631. https://doi.org/10.3390/su12166631
Ocampo-Martinez, C., et al. (2019). Energy efficiency in discrete-manufacturing systems: Insights, trends, and control strategies. Journal of Manufacturing Systems, 52 , 131–145.
Pan, Y., Hao, L., He, J., Ding, K., Yu, Q., & Wang, Y. (2024). Deep convolutional neural network based on self-distillation for tool wear recognition. Engineering Applications of Artificial Intelligence, 132 , 107851.
Qin, J., Liu, Y., Grosvenor, R., Lacan, F., & Jiang, Z. (2020). Deep learning-driven particle swarm optimisation for additive manufacturing energy optimisation. Journal of Cleaner Production, 245 , 118702.
Rahul, M., & Chiddarwar, S. S. (2023). Integrating virtual twin and deep neural networks for efficient and energy-aware robotic deburring in industry 4.0. International Journal of Precision Engineering and Manufacturing, 24 (9), 1517–1534.
Ribezzo, A., Falciani, G., Bergamasco, L., Fasano, M., & Chiavazzo, E. (2022). An overview on the use of additives and preparation procedure in phase change materials for thermal energy storage with a focus on long term applications. Journal of Energy Storage, 53 , 105140.
Shahin, M., Chen, F. F., Hosseinzadeh, A., Bouzary, H., & Shahin, A. (2023). Waste reduction via image classification algorithms: Beyond the human eye with an ai-based vision. International Journal of Production Research, 1–19.
Shen, F., Zhao, L., Du, W., Zhong, W., & Qian, F. (2020). Large-scale industrial energy systems optimization under uncertainty: A data-driven robust optimization approach. Applied Energy, 259 , 114199.
Simonyan, K., & Zisserman, A. (2014). Very deep convolutional networks for large-scale image recognition. arXiv preprint arXiv:1409.1556 .
Sundaram, S., & Zeid, A. (2023). Artificial Intelligence-Based Smart Quality Inspection for Manufacturing. Micromachines, 14 (3), 570. https://doi.org/10.3390/mi14030570
Szegedy, C., Ioffe, S., Vanhoucke, V., & Alemi, A. (2017). Inception-v4, inception-resnet and the impact of residual connections on learning. In Proceedings of the AAAI conference on artificial intelligence (vol. 31).
Szegedy, C., Liu, W., Jia, Y., Sermanet, P., Reed, S., Anguelov, D., Erhan, D., Vanhoucke, V., & Rabinovich, A. (2015). Going deeper with convolutions. In Proceedings of the IEEE conference on computer vision and pattern recognition (pp. 1–9).
Trezza, G., Bergamasco, L., Fasano, M., & Chiavazzo, E. (2022). Minimal crystallographic descriptors of sorption properties in hypothetical mofs and role in sequential learning optimization. npj Computational Materials, 8 (1), 123. https://doi.org/10.1038/s41524-022-00806-7
Vater, J., Schamberger, P., Knoll, A., & Winkle, D. (2019). Fault classification and correction based on convolutional neural networks exemplified by laser welding of hairpin windings. In 2019 9th International Electric Drives Production Conference (EDPC) (pp. 1–8). IEEE.
Wen, L., Li, X., Gao, L., & Zhang, Y. (2017). A new convolutional neural network-based data-driven fault diagnosis method. IEEE Transactions on Industrial Electronics, 65 (7), 5990–5998. https://doi.org/10.1109/TIE.2017.2774777
Willenbacher, M., Scholten, J., & Wohlgemuth, V. (2021). Machine learning for optimization of energy and plastic consumption in the production of thermoplastic parts in sme. Sustainability, 13 (12), 6800.
Zhang, X. H., Zhu, Q. X., He, Y. L., & Xu, Y. (2018). Energy modeling using an effective latent variable based functional link learning machine. Energy, 162 , 883–891.
Download references
Acknowledgements
This work has been supported by GEFIT S.p.a.
Open access funding provided by Politecnico di Torino within the CRUI-CARE Agreement.
Author information
Authors and affiliations.
Department of Energy, Politecnico di Torino, Turin, Italy
Mattia Casini, Paolo De Angelis, Paolo Vigo, Matteo Fasano, Eliodoro Chiavazzo & Luca Bergamasco
R &D Department, GEFIT S.p.a., Alessandria, Italy
Marco Porrati
You can also search for this author in PubMed Google Scholar
Corresponding author
Correspondence to Luca Bergamasco .
Ethics declarations
Conflict of interest statement.
The authors declare no competing interests.
Additional information
Publisher's note.
Springer Nature remains neutral with regard to jurisdictional claims in published maps and institutional affiliations.
Supplementary Information
Below is the link to the electronic supplementary material.
Supplementary file 1 (pdf 354 KB)
Rights and permissions.
Open Access This article is licensed under a Creative Commons Attribution 4.0 International License, which permits use, sharing, adaptation, distribution and reproduction in any medium or format, as long as you give appropriate credit to the original author(s) and the source, provide a link to the Creative Commons licence, and indicate if changes were made. The images or other third party material in this article are included in the article’s Creative Commons licence, unless indicated otherwise in a credit line to the material. If material is not included in the article’s Creative Commons licence and your intended use is not permitted by statutory regulation or exceeds the permitted use, you will need to obtain permission directly from the copyright holder. To view a copy of this licence, visit http://creativecommons.org/licenses/by/4.0/ .
Reprints and permissions
About this article
Casini, M., De Angelis, P., Porrati, M. et al. Machine Learning and image analysis towards improved energy management in Industry 4.0: a practical case study on quality control. Energy Efficiency 17 , 48 (2024). https://doi.org/10.1007/s12053-024-10228-7
Download citation
Received : 22 July 2023
Accepted : 28 April 2024
Published : 13 May 2024
DOI : https://doi.org/10.1007/s12053-024-10228-7
Share this article
Anyone you share the following link with will be able to read this content:
Sorry, a shareable link is not currently available for this article.
Provided by the Springer Nature SharedIt content-sharing initiative
- Industry 4.0
- Energy management
- Artificial intelligence
- Machine learning
- Deep learning
- Convolutional neural networks
- Computer vision
- Find a journal
- Publish with us
- Track your research
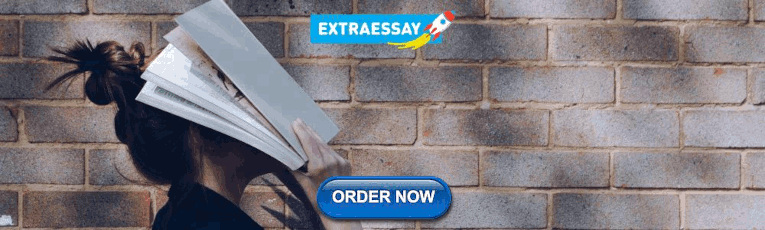
IMAGES
VIDEO
COMMENTS
Fifty four percent of raw case users came from outside the U.S.. The Yale School of Management (SOM) case study directory pages received over 160K page views from 177 countries with approximately a third originating in India followed by the U.S. and the Philippines. Twenty-six of the cases in the list are raw cases.
Professor Ashley Whillans and her co-author Hawken Lord (MBA 2023) discuss Serhant's time management techniques and consider the lessons we can all learn about making time our most valuable commodity in the case, "Ryan Serhant: Time Management for Repeatable Success.". 08 Aug 2023. Research & Ideas.
Case Study Research & Development (CRDT) | December 19, 2017. We generated a list of the 40 most popular Yale School of Management case studies in 2017 by combining data from our publishers, Google analytics, and other measures of interest and adoption. In compiling the list, we gave additional weight to usage outside Yale.
The case examines the tools a manager can use to keep her project on track and manage conflict and tension as Adobe prepares to launch Creative Suite... Published: September 24, 2008
But Coca-Cola saved its business by rereleasing the original formulation of Coke under the name Coca-Cola Classic. That, in and of itself, is an excellent case study in business management. However, the more contemporary story is how Coca-Cola has changed its business plan so that no one drink—not even Coke—can heavily influence the company ...
The Case Analysis Coach is an interactive tutorial on reading and analyzing a case study. The Case Study Handbook covers key skills students need to read, understand, discuss and write about cases. The Case Study Handbook is also available as individual chapters to help your students focus on specific skills.
What the Case Study Method Really Teaches. Summary. It's been 100 years since Harvard Business School began using the case study method. Beyond teaching specific subject matter, the case study ...
Change Management. New research on change management from Harvard Business School faculty on issues including how to plan for opportunities, how to effect change in the workplace, and case studies on how business leaders managed the economic crisis. Page 1 of 66 Results →. 12 Dec 2023.
Management Style. New research on management style from Harvard Business School faculty on issues including why dominating leaders can be poor team leaders, the benefits of 'no surprises management', and why introverts are the best leaders for proactive employees. Page 1 of 15 Results. 26 Apr 2024. HBS Case.
Case Library Control Panel. Welcome to the Case Library, Management Consulted's repository of over 600 cases, organized by firm, difficulty, and subject matter. Right now, you're looking at the Limited Case Library, a free version that lets users see one whole case and preview another. If you should have access to the whole course, but are ...
Through the case method, you can "try on" roles you may not have considered and feel more prepared to change or advance your career. 5. Build Your Self-Confidence. Finally, learning through the case study method can build your confidence. Each time you assume a business leader's perspective, aim to solve a new challenge, and express and ...
Business Management Case Study: A Complete Breakdown Eliza Taylor 14 September 2023. Gain a comprehensive understanding of the "Business Management Case Study" as we break down the concept from start to finish. Discover the incredible journeys of companies like Apple Inc., Tesla and Netflix as they navigate innovation, global expansion, and ...
Case study examples. Case studies are proven marketing strategies in a wide variety of B2B industries. Here are just a few examples of a case study: Amazon Web Services, Inc. provides companies with cloud computing platforms and APIs on a metered, pay-as-you-go basis. This case study example illustrates the benefits Thomson Reuters experienced ...
Management case studies provide valuable insights into real-world challenges and solutions faced by businesses. In both of these case studies, proactive and strategic management actions were taken to address the issues and improve the overall performance of the organizations. The key takeaways include the importance of effective communication,
Case study formats can include traditional print stories, interactive web or social content, data-heavy infographics, professionally shot videos, podcasts, and more. 5. Write your case study ... Solutions are outlined in a way that shows Adobe Experience Manager is the best choice and a natural fit for the customer. Along the way, quotes from ...
The Opera House stands as a symbol of perseverance and successful project management in the face of humankind. 2. The Airbus A380 Project. The Airbus A380 Project is a project management case study showcasing the challenges encountered during developing and producing the world's largest commercial aircraft.
Revised on November 20, 2023. A case study is a detailed study of a specific subject, such as a person, group, place, event, organization, or phenomenon. Case studies are commonly used in social, educational, clinical, and business research. A case study research design usually involves qualitative methods, but quantitative methods are ...
How can I prepare for Case Interviews? 1.) Read Management Consulted's "Case Interview: Complete Prep Guide (2024)" Management Consulted is a FREE resource for Tufts students: case and consulting resources such as 500 sample cases, Case Interview Bootcamp, Market Sizing Drills, Math Drills, case videos, consulting firm directory, and more ...
The case management process. Case management is a collaborative process in which a case manager works with clients to ensure they obtain the proper health care in the most cost-effective manner. This is what the process typically looks like: 1. Screening: The case manager reviews a client's medical records, medical history, and current ...
In choosing between persuasion and authority the manager makes a cost-benefit trade-off. This paper studies that trade-off, focusing in particular on conflicts that originate in open disagreement. Key concepts include: Persuasion and authority can be both substitutes and complements. In particular, authority and persuasion are substitutes when ...
Explore top project management case studies of 2024, from Mars exploration to self-driving cars, showcasing innovation and success across industries. Top 10 Project Management Case Studies with Examples 2024. 1. NASA's Mars Exploration Rover: Innovative project management in space exploration.
The ordering process is fully online, and it goes as follows: • Select the topic and the deadline of your case study. • Provide us with any details, requirements, statements that should be emphasized or particular parts of the writing process you struggle with. • Leave the email address, where your completed order will be sent to.
Sandeep Kumar Panda, Vaibhav Mishra, R. Balamurali, and Ahmed A. Elngar (Eds.), Artificial Intelligence and Machine Learning in Business Management: Concepts, C...
Summary. Job seekers worry about negotiating an offer for many reasons, including the worst-case scenario that the offer will be rescinded. Across a series of seven studies, researchers found that ...
This case study illustrates how Alex strategically employs long-term call options to both realize profits and maintain exposure to NVIDIA stock. By adjusting his holdings through these options, he secures gains while keeping potential for future growth, showcasing effective risk management and investment foresight.
With the advent of Industry 4.0, Artificial Intelligence (AI) has created a favorable environment for the digitalization of manufacturing and processing, helping industries to automate and optimize operations. In this work, we focus on a practical case study of a brake caliper quality control operation, which is usually accomplished by human inspection and requires a dedicated handling system ...
This study uses the Social-Ecological-Technological Systems (SETS) framework to examine the urban disaster management strategies of Toyama City, Japan. The recent seismic shocks on the Noto Peninsula highlight the importance of implementing efficient disaster management. Toyama City has incorporated the United Nations' Sustainable Development Goals into its statutory planning frameworks ...