
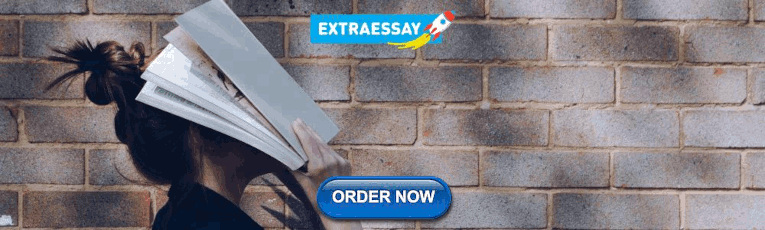
Research Topics & Ideas: Data Science
50 Topic Ideas To Kickstart Your Research Project
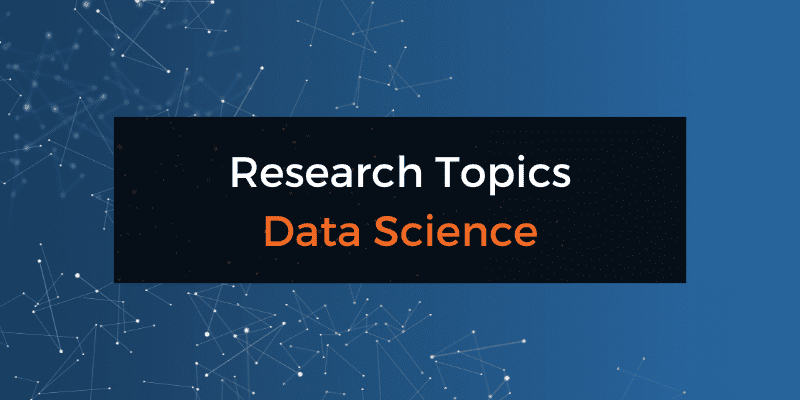
If you’re just starting out exploring data science-related topics for your dissertation, thesis or research project, you’ve come to the right place. In this post, we’ll help kickstart your research by providing a hearty list of data science and analytics-related research ideas , including examples from recent studies.
PS – This is just the start…
We know it’s exciting to run through a list of research topics, but please keep in mind that this list is just a starting point . These topic ideas provided here are intentionally broad and generic , so keep in mind that you will need to develop them further. Nevertheless, they should inspire some ideas for your project.
To develop a suitable research topic, you’ll need to identify a clear and convincing research gap , and a viable plan to fill that gap. If this sounds foreign to you, check out our free research topic webinar that explores how to find and refine a high-quality research topic, from scratch. Alternatively, consider our 1-on-1 coaching service .
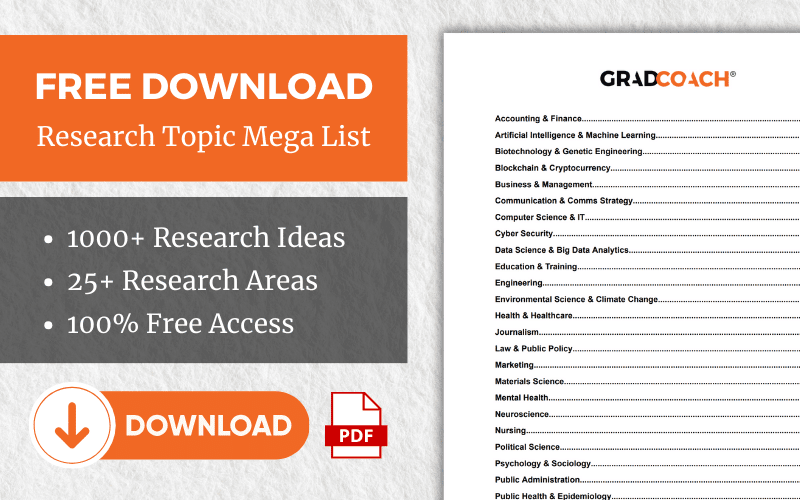
Data Science-Related Research Topics
- Developing machine learning models for real-time fraud detection in online transactions.
- The use of big data analytics in predicting and managing urban traffic flow.
- Investigating the effectiveness of data mining techniques in identifying early signs of mental health issues from social media usage.
- The application of predictive analytics in personalizing cancer treatment plans.
- Analyzing consumer behavior through big data to enhance retail marketing strategies.
- The role of data science in optimizing renewable energy generation from wind farms.
- Developing natural language processing algorithms for real-time news aggregation and summarization.
- The application of big data in monitoring and predicting epidemic outbreaks.
- Investigating the use of machine learning in automating credit scoring for microfinance.
- The role of data analytics in improving patient care in telemedicine.
- Developing AI-driven models for predictive maintenance in the manufacturing industry.
- The use of big data analytics in enhancing cybersecurity threat intelligence.
- Investigating the impact of sentiment analysis on brand reputation management.
- The application of data science in optimizing logistics and supply chain operations.
- Developing deep learning techniques for image recognition in medical diagnostics.
- The role of big data in analyzing climate change impacts on agricultural productivity.
- Investigating the use of data analytics in optimizing energy consumption in smart buildings.
- The application of machine learning in detecting plagiarism in academic works.
- Analyzing social media data for trends in political opinion and electoral predictions.
- The role of big data in enhancing sports performance analytics.
- Developing data-driven strategies for effective water resource management.
- The use of big data in improving customer experience in the banking sector.
- Investigating the application of data science in fraud detection in insurance claims.
- The role of predictive analytics in financial market risk assessment.
- Developing AI models for early detection of network vulnerabilities.
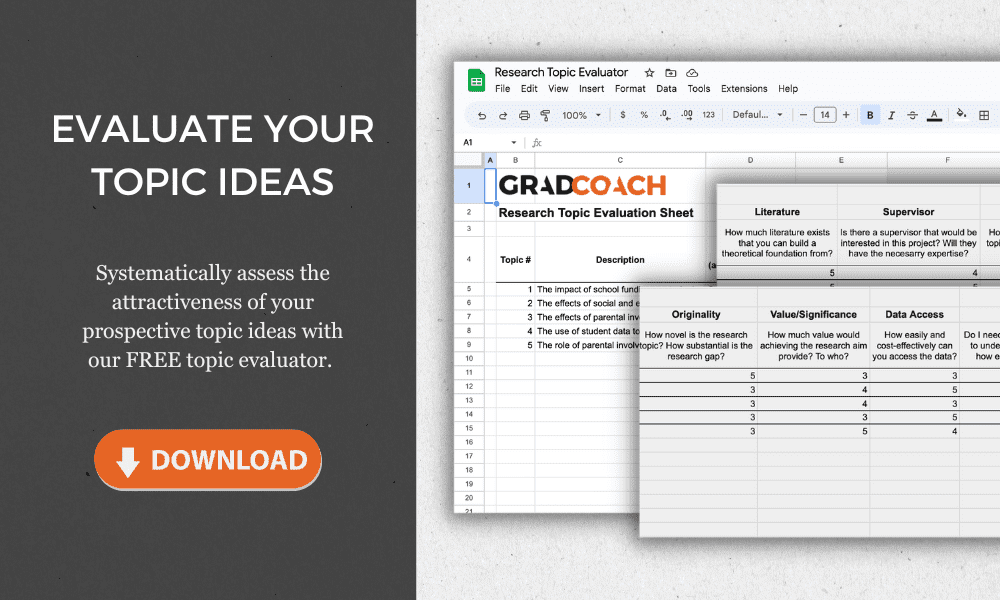
Data Science Research Ideas (Continued)
- The application of big data in public transportation systems for route optimization.
- Investigating the impact of big data analytics on e-commerce recommendation systems.
- The use of data mining techniques in understanding consumer preferences in the entertainment industry.
- Developing predictive models for real estate pricing and market trends.
- The role of big data in tracking and managing environmental pollution.
- Investigating the use of data analytics in improving airline operational efficiency.
- The application of machine learning in optimizing pharmaceutical drug discovery.
- Analyzing online customer reviews to inform product development in the tech industry.
- The role of data science in crime prediction and prevention strategies.
- Developing models for analyzing financial time series data for investment strategies.
- The use of big data in assessing the impact of educational policies on student performance.
- Investigating the effectiveness of data visualization techniques in business reporting.
- The application of data analytics in human resource management and talent acquisition.
- Developing algorithms for anomaly detection in network traffic data.
- The role of machine learning in enhancing personalized online learning experiences.
- Investigating the use of big data in urban planning and smart city development.
- The application of predictive analytics in weather forecasting and disaster management.
- Analyzing consumer data to drive innovations in the automotive industry.
- The role of data science in optimizing content delivery networks for streaming services.
- Developing machine learning models for automated text classification in legal documents.
- The use of big data in tracking global supply chain disruptions.
- Investigating the application of data analytics in personalized nutrition and fitness.
- The role of big data in enhancing the accuracy of geological surveying for natural resource exploration.
- Developing predictive models for customer churn in the telecommunications industry.
- The application of data science in optimizing advertisement placement and reach.
Recent Data Science-Related Studies
While the ideas we’ve presented above are a decent starting point for finding a research topic, they are fairly generic and non-specific. So, it helps to look at actual studies in the data science and analytics space to see how this all comes together in practice.
Below, we’ve included a selection of recent studies to help refine your thinking. These are actual studies, so they can provide some useful insight as to what a research topic looks like in practice.
- Data Science in Healthcare: COVID-19 and Beyond (Hulsen, 2022)
- Auto-ML Web-application for Automated Machine Learning Algorithm Training and evaluation (Mukherjee & Rao, 2022)
- Survey on Statistics and ML in Data Science and Effect in Businesses (Reddy et al., 2022)
- Visualization in Data Science VDS @ KDD 2022 (Plant et al., 2022)
- An Essay on How Data Science Can Strengthen Business (Santos, 2023)
- A Deep study of Data science related problems, application and machine learning algorithms utilized in Data science (Ranjani et al., 2022)
- You Teach WHAT in Your Data Science Course?!? (Posner & Kerby-Helm, 2022)
- Statistical Analysis for the Traffic Police Activity: Nashville, Tennessee, USA (Tufail & Gul, 2022)
- Data Management and Visual Information Processing in Financial Organization using Machine Learning (Balamurugan et al., 2022)
- A Proposal of an Interactive Web Application Tool QuickViz: To Automate Exploratory Data Analysis (Pitroda, 2022)
- Applications of Data Science in Respective Engineering Domains (Rasool & Chaudhary, 2022)
- Jupyter Notebooks for Introducing Data Science to Novice Users (Fruchart et al., 2022)
- Towards a Systematic Review of Data Science Programs: Themes, Courses, and Ethics (Nellore & Zimmer, 2022)
- Application of data science and bioinformatics in healthcare technologies (Veeranki & Varshney, 2022)
- TAPS Responsibility Matrix: A tool for responsible data science by design (Urovi et al., 2023)
- Data Detectives: A Data Science Program for Middle Grade Learners (Thompson & Irgens, 2022)
- MACHINE LEARNING FOR NON-MAJORS: A WHITE BOX APPROACH (Mike & Hazzan, 2022)
- COMPONENTS OF DATA SCIENCE AND ITS APPLICATIONS (Paul et al., 2022)
- Analysis on the Application of Data Science in Business Analytics (Wang, 2022)
As you can see, these research topics are a lot more focused than the generic topic ideas we presented earlier. So, for you to develop a high-quality research topic, you’ll need to get specific and laser-focused on a specific context with specific variables of interest. In the video below, we explore some other important things you’ll need to consider when crafting your research topic.
Get 1-On-1 Help
If you’re still unsure about how to find a quality research topic, check out our Research Topic Kickstarter service, which is the perfect starting point for developing a unique, well-justified research topic.
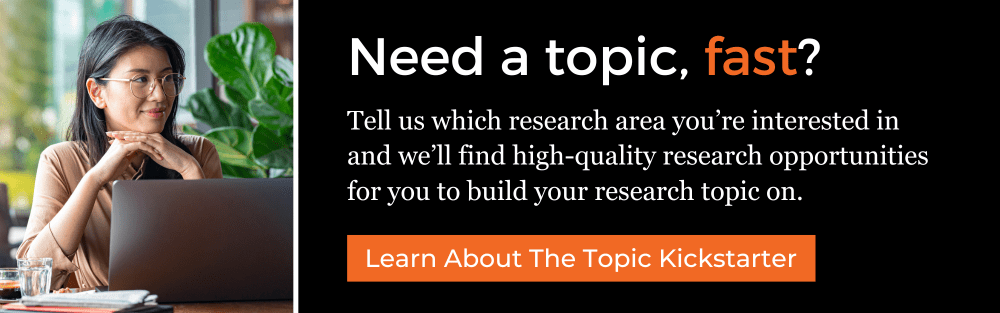
You Might Also Like:
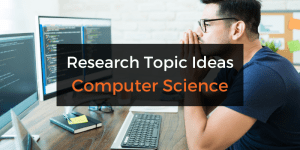
Submit a Comment Cancel reply
Your email address will not be published. Required fields are marked *
Save my name, email, and website in this browser for the next time I comment.
- Print Friendly
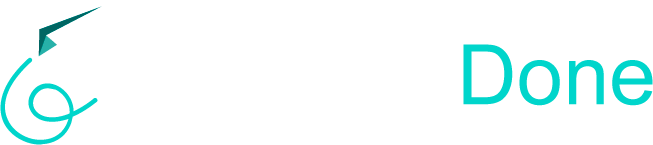
- Services Paper editing services Paper proofreading Business papers Philosophy papers Write my paper Term papers for sale Term paper help Academic term papers Buy research papers College writing services Paper writing help Student papers Original term papers Research paper help Nursing papers for sale Psychology papers Economics papers Medical papers Blog
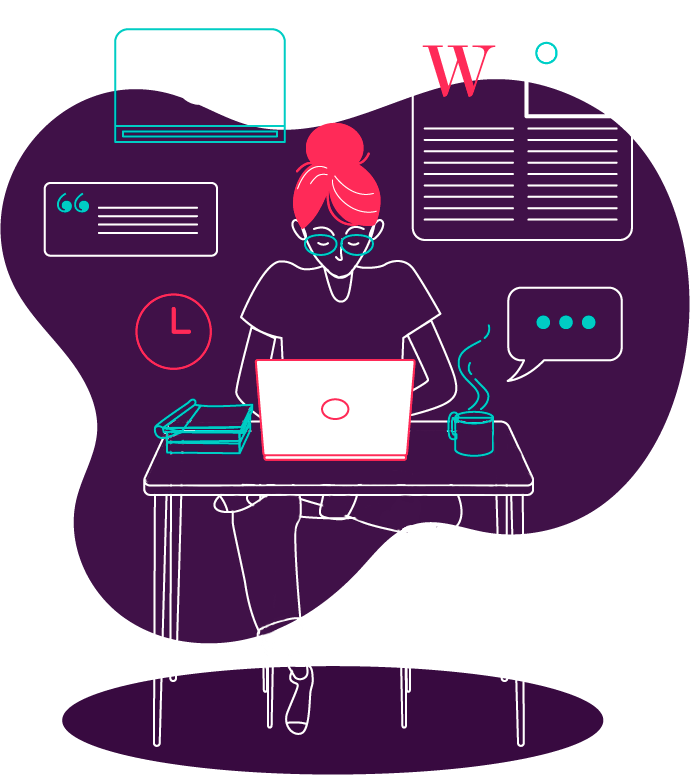
166 Latest Big Data Research Topics And Fascinating Ideas
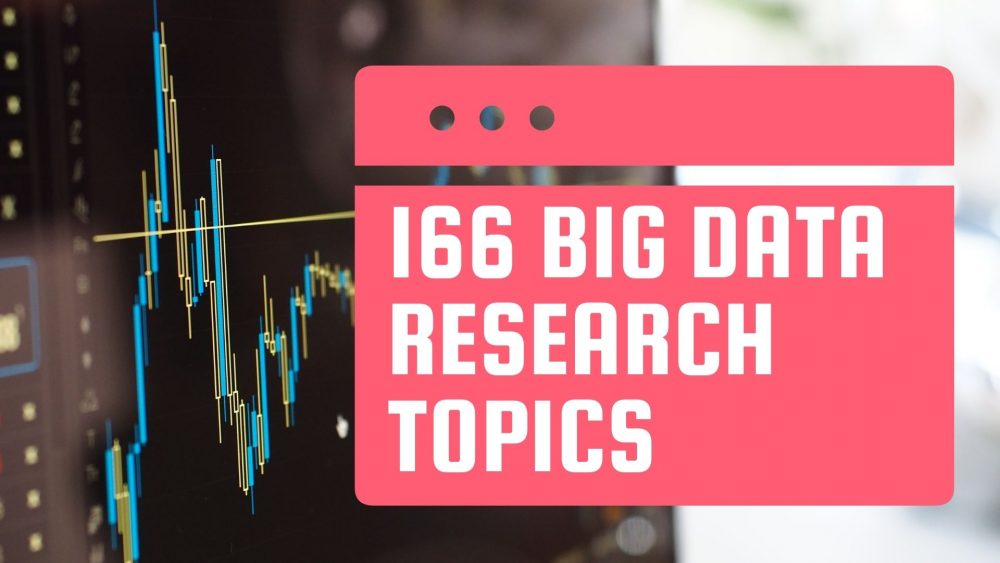
Big data refers to a huge volume of data, whether organized or unorganized, whose analysis shapes technologies and methodologies. Big data is so massive and complicated that it cannot be handled using ordinary application software. For instance, some frameworks, such as Hadoop, are built to process large amounts of data. Big data has gained much attention, hence it’s a trendy topic and essay for students and researchers who want to write thesis, projects, and dissertations. Based on this, there are several searchable and interesting topics to explore for undergraduate and master’s theses in big data, same as doctoral degrees. In this article, we have provided every topic you need on big data. Our topics stretch from big data analytics, big data research questions, to IoT and database essays. If you’ve been looking for the latest big data research topics, your search stops here. Read on to see some of the most interesting topics for your thesis.
Interesting Big Data Analytics Research Topics
Data analytics is the lifeblood of the modern IT sector. Big data is one of the strategies and technologies for analyzing large amounts of data. Data analytics is being used by the industry to acquire knowledge of system performance and customer behavior. Here are some of the best big data analytics topics and ideas for academic papers.
- The surge of Internet of Things (IoT)
- Explain the significance of augmented reality.
- What is the significance of artificial intelligence?
- Describe the graph analytics procedure.
- What is agile data science, and how does it differ from traditional data science?
- What role does machine intelligence play in today’s businesses?
- What is hyper-personalization, and how does it work?
- Describe how behavioral analytics works.
- What is the experience economy, and how does it work?
- Talk about the science of travel.
- Discuss the validation and extraction of knowledge.
- What is semantic data management, and how does it work?
- Describe the process of deep learning.
- Describe software engineering in the context of big data science.
- What is structured machine learning, and how does it work?
- Describe how to answer a semantic question
- What is distributed semantic analytics, and how does it work?
- What role does domain knowledge play in data analysis?
- Why is data exploration important in data analysis?
- Who uses big data analytics?
So, it’s not an easy task to write a paper for a high grade. Sometimes every student need a professional help with research paper writing. Therefore, don’t be afraid to hire a writer to complete your assignment. Just contact us and get your paper done soon.
Trending Big Data Research Topics
Students and researchers who want to write about big data latest research topics on appearing issues and topics should pick current topics in data science. Below are some current big data analysis research topics and essays to look into if writing a research essay or paper.
- Analyze the digital tools and programs for processing large data.
- Discuss the effect of the sophistication of big data on human privacy.
- Evaluate how scalable architectures can be used for processing parallel data.
- List the different growth oriented big data storage mechanics.
- Visualizing big data.
- Business acumen in combination with big data analytics.
- Map-reductionist architecture.
- Methods of machine learning in big data.
- Big data analytics and impact on privacy preservation.
- The processing of big data and impact on climate change.
- Risks and uncertainties in big data management.
- Detecting anomalies in large-scale data systems.
- Analyze the big data for social networks.
- Platforms for large scale data computing: big data analysis and acceptance.
- Discuss the procedures of analyzing big data.
- Discuss the many effective ways of managing big data.
- Big data programming and process methods.
- Big data semantics.
- How big data influences biomedical information and strategies.
- The significance of big data strategies on small and medium-sized businesses.
Most Debatable Big Data Research Topics and Essays
The rapid rise of big data in our current time is not without controversy. There is a myriad of ongoing debates in the discipline that have gone unresolved for quite some time. The list below contains the most common big data debate topics.
- Big data and its major vulnerabilities.
- What measures are in place to recognize a legit user of big data?
- Explain the significance of user-access control.
- Investigate the importance of centralized key management.
- Identify ways to prevent illegal access of data.
- Intrusion-detection system: Which is the best?
- Does machine learning enhance data quality?
- Which security technology has proven to be the best for big data protection?
- What strategies should be used for data governance and who should implement data policies?
- Should tech giants regularly update security measures and be transparent about them?
- How has poor data security contributed to the loss of historical evidence?
- What are the most important big data management tools and strategies?
- What is data retention and explain its relevance?
- Artificial intelligence will lead to the loss of employment and human interaction.
- Enterprise analytics: How to manage platforms?
- Can data management foster the promotion of peace and freedom?
- Who should be in control of data security: Tech giants or the government?
- What are the functions of the government in big data management and security?
- Discuss how big data is leading to the end of morals and ethics.
- How is big data contributing to the rise of global climate and why tech should pay carbon taxes.
Interesting Dissertation Topics on Big Data
Many research theses and big data topics can be found online for undergraduates, Masters, and Ph.D. students. The list below comprises some dissertation topics on big data.
- Privacy and security issues in big data and how to curtail them.
- Impacts of storage systems of scalable big data.
- The significance of big data processing and data management to industrial development.
- Techniques and data mining tools for big data.
- The benefits of data analytics and cloud computing to the future of work.
- Parallel data processing: effective data architecture and how to go about it.
- Impacts of machine learning algorithms on the fashion industry.
- Using bandwidth provision, how the world of streaming is changing.
- What are the benefits and threats of dedicated networks to governance?
- Cloud gaming and impacts on Millennials and Generation Z.
- Ways to enhance and maximize spread efficiency using flow authority model.
- How divergent and convergent is the Internet of Things (IoT) on manufacturing?
- Data mining and environmental impact: The way forward.
- Geopolitics and the surge of demographic mapping in big data.
- Impacts of travel patterns on big data analytics and data management.
- The rise of deep learning in the automotive industry.
- The sophistication of big data and its implications on cybersecurity.
- Discuss how the big data manufacturing process indicates positive globalization.
- Evaluate the future of data mining and the adaptation of humans to big data.
- Human and material wastes in big data management.
Interesting Research Topics on A/B Testing in Big Data
The A/B testing is also known as controlled experiments and is used widely by companies and firms to make decisions in product launches. Tech companies use the test to know the acceptability of a certain product by the users. However, below are some key research topics on A/B testing in Big Data
- Evaluate the common A/B pitfalls in the automotive industry.
- Discuss the benefits of improving library user experience with A/B Testing.
- How to design A/B tests in a collaboration network.
- Analyze how the future of social network advertising can be improved by A/B testing.
- Effectiveness of A/B experiments in MOOCs for better instructional methods.
- Strategies of Bayesian A/B testing for business decisions.
- A/B testing challenges in large-scale social networks and online controlled experiments.
- Illustrate how consumer behaviors and trends are shaped by A/B testing.
List of Research Topics on Big Data and Local Governments
Big data offers tremendous value to grassroots governments with the ability to optimize cost through data-induced decisions that reduce the crime rate, traffic congestion and improve the environment. Below are interesting topics on big data and local governments.
- How local governments can measure crime using big data testing.
- Big data and algorithmic policy in local government policies.
- Application of data science technologies to civil service in the local government.
- Combating grassroots crime and corruption through algorithmic government.
- Big data in the public sector: how local governments can benefit from the algorithmic policy.
Innovative Research Topics on Big Data and IoT
Big data has a lot in common with the Internet of Things (IoT). Indeed, IoT is an integral part of big data. Below are researchable IoT and big data research topics.
- The impacts of big data and the Internet of Things (IoT) on the fourth industrial revolution.
- The importance of big data and the Internet of Things (IoT) on public health systems.
- Explain how big data and the Internet of Things (IoT) dictate the flow of information in the media sector.
- Challenges of big data and the Internet of Things (IoT) on governance and sustainability.
- The disruption of big data and its attendant effects on the Internet of Things (IoT).
- Illustrate the surge in household smart devices and the role of big data analytics.
- An analysis of the disruption of the supply chain of traditional goods through the Internet of Things (IoT).
- A comprehensive evaluation of machine and deep learning for IoT-enabled healthcare systems.
- The future evaluation of the internet of things and big data analytics in the public infrastructure systems.
- Discuss how AI-induced security can guarantee effective data protection.
- IoT privacy: what data protection means to households and the impacts of security infringement.
- Discuss the role of big data and the integrity of the Internet of Things (IoT).
- How do dedicated networks work through the Internet of Things (IoT)?
- The threats and benefits of the Internet of Things (IoT) forensic science.
- Big data distributed storage and impacts on IoT-enabled industries.
Most Engaging Database Big Data Research Topics
The database category of big data has some interesting data science research topics. Due to the large data, modern companies have to analyze every day, which are difficult to handle, strict managing is essential to make sure of the effective use of data. Check out some intriguing big data database research topics students and researchers can write about.
- Explain the most inventive big data information concepts and strategies.
- Clarify the most ideal data management strategies and techniques for present-day businesses.
- New advancements and AI in information management.
- What is information maintenance and for what reason is it significant?
- Depict the essentials of information management.
- Clarify the use of information management in e-learning.
- Information distribution and access by present-day organizations.
- Clarify the most common way of investigating and overseeing information for biomedical exploration.
- Disclose how to function with 3D pictures during research.
- How could an association guarantee secure and classified information management and security?
- Information indexes: Describe approaches and their execution as well as their reception.
- Talk about the effect of information quality on a business.
- Instructions on how to advance medical examination and reach logical effort through information management.
- The most effective method to source and oversee external data.
- Evaluate the procedures available to organizations in ensuring information security through appropriate administration.
- Information catalog reference model and global market study.
- What is information valuation and what difference does it make in information management?
- How could AI further develop database security?
- How might an organization carry out effective data administration?
- Database management and the cost of disruptive cybersecurity.
If you are ready to boost your grades with a little effort, j ust write a message “Please, write a custom research paper for me” to hire one of our professional writers. Contact us today to get a 100% original paper.
Compelling Big Data Scala Research Topics
Big Data Scala is the product of algorithmic frameworks in deep and machine learning. Below are listed topics on big data Scala for students and young researchers.
- Large information versatility dependent on Scala and Spark Machine Learning Libraries
- Analyze versatile large information stockpiling frameworks in deep learning.
- Dealing with Data and Model drift for practical applications.
- Building generative systems based on conversational frameworks (Chatbot systems).
- Adaptable designs for parallel data building.
- Dealing with continuous video analytics in cloud computing.
- Proficient graph processing at a machine learning scale.
- Dimensional reduction approaches for information management.
- Compelling anonymization of sensitive fields in computer vision.
- Versatile security safeguarding on big data.
List of Independent Research Topics for Big Data
Independent researches are pieces of research that may be considered unorthodox in big data testing and management. These are research studies generated by individual researchers. Here is a list of the most fascinating independent research topics on big data.
- Significance of effective data mining tools and procedures.
- What is data-driven clustering in deep and machine learning?
- How impactful is the graph analytics process to the Internet of Things?
- Explain the significance of AI for present-day businesses.
- Significance of information investigation in information examination on deep learning.
- Evaluate the usefulness of coding in Artificial Intelligence.
- Clarify the AI strategies in big data management.
- Data security: what it means to computer vision.
- Impact of open-source deep learning libraries on developers.
- The significance of token-based authentication to data security.
- Using big data to identify disinformation and misinformation.
- Data management and the fundamental principles of Artificial Intelligence.
- Big data analytics and why it should be more user-friendly.
- Why business intelligence should focus more on privacy preservation.
- Social networks and impact on privacy infringement.
Is Your Big Data Paper Not Coming Along?
Although we have provided you with a list of big data essays to choose from, we dare say university research topics go beyond mere writing tips. As a student, you may need quality college paper writing services and professional assistance to writing an A-graded and top-notch thesis or dissertations. Here is where we come in. You can consult our reliable and professional writing experts to ease your degree courses at a pocket-friendly price. Aside, you can also refer your colleagues online to enjoy our discounted services that will make your research experience less tacky and frustrating.
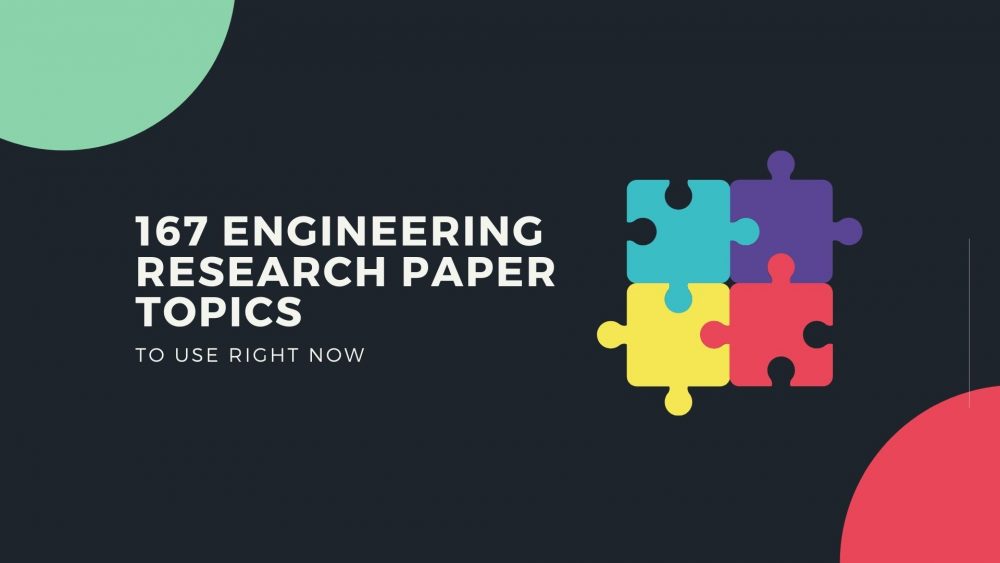
Leave a Reply Cancel reply
Your email address will not be published. Required fields are marked *
Save my name, email, and website in this browser for the next time I comment.
Terms & Conditions Loyalty Program Privacy Policy Money-Back Policy
Copyright © 2013-2024 MyPaperDone.com
- How It Works
- PhD thesis writing
- Master thesis writing
- Bachelor thesis writing
- Dissertation writing service
- Dissertation abstract writing
- Thesis proposal writing
- Thesis editing service
- Thesis proofreading service
- Thesis formatting service
- Coursework writing service
- Research paper writing service
- Architecture thesis writing
- Computer science thesis writing
- Engineering thesis writing
- History thesis writing
- MBA thesis writing
- Nursing dissertation writing
- Psychology dissertation writing
- Sociology thesis writing
- Statistics dissertation writing
- Buy dissertation online
- Write my dissertation
- Cheap thesis
- Cheap dissertation
- Custom dissertation
- Dissertation help
- Pay for thesis
- Pay for dissertation
- Senior thesis
- Write my thesis
214 Best Big Data Research Topics for Your Thesis Paper
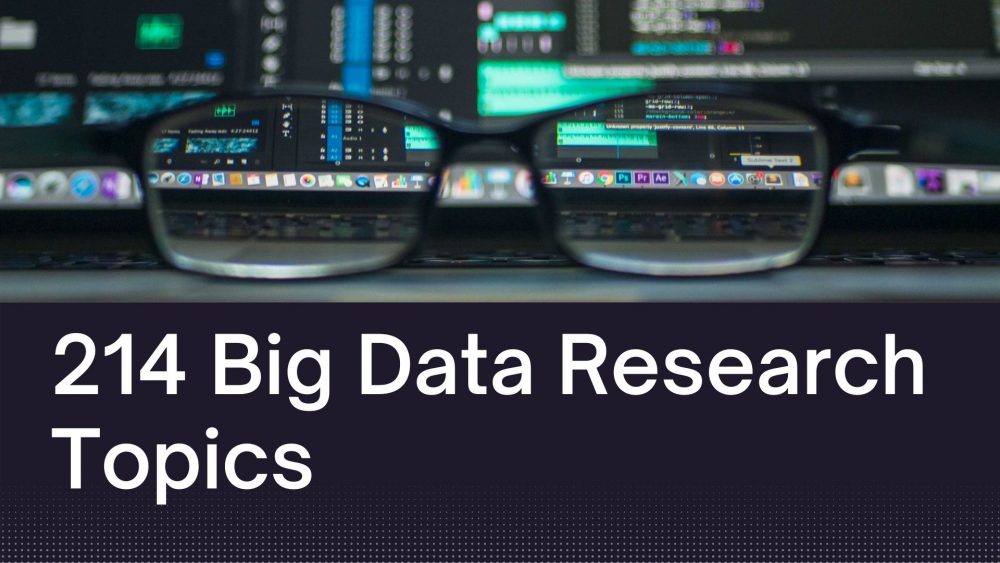
Finding an ideal big data research topic can take you a long time. Big data, IoT, and robotics have evolved. The future generations will be immersed in major technologies that will make work easier. Work that was done by 10 people will now be done by one person or a machine. This is amazing because, in as much as there will be job loss, more jobs will be created. It is a win-win for everyone.
Big data is a major topic that is being embraced globally. Data science and analytics are helping institutions, governments, and the private sector. We will share with you the best big data research topics.
On top of that, we can offer you the best writing tips to ensure you prosper well in your academics. As students in the university, you need to do proper research to get top grades. Hence, you can consult us if in need of research paper writing services.
Big Data Analytics Research Topics for your Research Project
Are you looking for an ideal big data analytics research topic? Once you choose a topic, consult your professor to evaluate whether it is a great topic. This will help you to get good grades.
- Which are the best tools and software for big data processing?
- Evaluate the security issues that face big data.
- An analysis of large-scale data for social networks globally.
- The influence of big data storage systems.
- The best platforms for big data computing.
- The relation between business intelligence and big data analytics.
- The importance of semantics and visualization of big data.
- Analysis of big data technologies for businesses.
- The common methods used for machine learning in big data.
- The difference between self-turning and symmetrical spectral clustering.
- The importance of information-based clustering.
- Evaluate the hierarchical clustering and density-based clustering application.
- How is data mining used to analyze transaction data?
- The major importance of dependency modeling.
- The influence of probabilistic classification in data mining.
Interesting Big Data Analytics Topics
Who said big data had to be boring? Here are some interesting big data analytics topics that you can try. They are based on how some phenomena are done to make the world a better place.
- Discuss the privacy issues in big data.
- Evaluate the storage systems of scalable in big data.
- The best big data processing software and tools.
- Data mining tools and techniques are popularly used.
- Evaluate the scalable architectures for parallel data processing.
- The major natural language processing methods.
- Which are the best big data tools and deployment platforms?
- The best algorithms for data visualization.
- Analyze the anomaly detection in cloud servers
- The scrutiny normally done for the recruitment of big data job profiles.
- The malicious user detection in big data collection.
- Learning long-term dependencies via the Fourier recurrent units.
- Nomadic computing for big data analytics.
- The elementary estimators for graphical models.
- The memory-efficient kernel approximation.
Big Data Latest Research Topics
Do you know the latest research topics at the moment? These 15 topics will help you to dive into interesting research. You may even build on research done by other scholars.
- Evaluate the data mining process.
- The influence of the various dimension reduction methods and techniques.
- The best data classification methods.
- The simple linear regression modeling methods.
- Evaluate the logistic regression modeling.
- What are the commonly used theorems?
- The influence of cluster analysis methods in big data.
- The importance of smoothing methods analysis in big data.
- How is fraud detection done through AI?
- Analyze the use of GIS and spatial data.
- How important is artificial intelligence in the modern world?
- What is agile data science?
- Analyze the behavioral analytics process.
- Semantic analytics distribution.
- How is domain knowledge important in data analysis?
Big Data Debate Topics
If you want to prosper in the field of big data, you need to try even hard topics. These big data debate topics are interesting and will help you to get a better understanding.
- The difference between big data analytics and traditional data analytics methods.
- Why do you think the organization should think beyond the Hadoop hype?
- Does the size of the data matter more than how recent the data is?
- Is it true that bigger data are not always better?
- The debate of privacy and personalization in maintaining ethics in big data.
- The relation between data science and privacy.
- Do you think data science is a rebranding of statistics?
- Who delivers better results between data scientists and domain experts?
- According to your view, is data science dead?
- Do you think analytics teams need to be centralized or decentralized?
- The best methods to resource an analytics team.
- The best business case for investing in analytics.
- The societal implications of the use of predictive analytics within Education.
- Is there a need for greater control to prevent experimentation on social media users without their consent?
- How is the government using big data; for the improvement of public statistics or to control the population?
University Dissertation Topics on Big Data
Are you doing your Masters or Ph.D. and wondering the best dissertation topic or thesis to do? Why not try any of these? They are interesting and based on various phenomena. While doing the research ensure you relate the phenomenon with the current modern society.
- The machine learning algorithms are used for fall recognition.
- The divergence and convergence of the internet of things.
- The reliable data movements using bandwidth provision strategies.
- How is big data analytics using artificial neural networks in cloud gaming?
- How is Twitter accounts classification done using network-based features?
- How is online anomaly detection done in the cloud collaborative environment?
- Evaluate the public transportation insights provided by big data.
- Evaluate the paradigm for cancer patients using the nursing EHR to predict the outcome.
- Discuss the current data lossless compression in the smart grid.
- How does online advertising traffic prediction helps in boosting businesses?
- How is the hyperspectral classification done using the multiple kernel learning paradigm?
- The analysis of large data sets downloaded from websites.
- How does social media data help advertising companies globally?
- Which are the systems recognizing and enforcing ownership of data records?
- The alternate possibilities emerging for edge computing.
The Best Big Data Analysis Research Topics and Essays
There are a lot of issues that are associated with big data. Here are some of the research topics that you can use in your essays. These topics are ideal whether in high school or college.
- The various errors and uncertainty in making data decisions.
- The application of big data on tourism.
- The automation innovation with big data or related technology
- The business models of big data ecosystems.
- Privacy awareness in the era of big data and machine learning.
- The data privacy for big automotive data.
- How is traffic managed in defined data center networks?
- Big data analytics for fault detection.
- The need for machine learning with big data.
- The innovative big data processing used in health care institutions.
- The money normalization and extraction from texts.
- How is text categorization done in AI?
- The opportunistic development of data-driven interactive applications.
- The use of data science and big data towards personalized medicine.
- The programming and optimization of big data applications.
The Latest Big Data Research Topics for your Research Proposal
Doing a research proposal can be hard at first unless you choose an ideal topic. If you are just diving into the big data field, you can use any of these topics to get a deeper understanding.
- The data-centric network of things.
- Big data management using artificial intelligence supply chain.
- The big data analytics for maintenance.
- The high confidence network predictions for big biological data.
- The performance optimization techniques and tools for data-intensive computation platforms.
- The predictive modeling in the legal context.
- Analysis of large data sets in life sciences.
- How to understand the mobility and transport modal disparities sing emerging data sources?
- How do you think data analytics can support asset management decisions?
- An analysis of travel patterns for cellular network data.
- The data-driven strategic planning for citywide building retrofitting.
- How is money normalization done in data analytics?
- Major techniques used in data mining.
- The big data adaptation and analytics of cloud computing.
- The predictive data maintenance for fault diagnosis.
Interesting Research Topics on A/B Testing In Big Data
A/B testing topics are different from the normal big data topics. However, you use an almost similar methodology to find the reasons behind the issues. These topics are interesting and will help you to get a deeper understanding.
- How is ultra-targeted marketing done?
- The transition of A/B testing from digital to offline.
- How can big data and A/B testing be done to win an election?
- Evaluate the use of A/B testing on big data
- Evaluate A/B testing as a randomized control experiment.
- How does A/B testing work?
- The mistakes to avoid while conducting the A/B testing.
- The most ideal time to use A/B testing.
- The best way to interpret results for an A/B test.
- The major principles of A/B tests.
- Evaluate the cluster randomization in big data
- The best way to analyze A/B test results and the statistical significance.
- How is A/B testing used in boosting businesses?
- The importance of data analysis in conversion research
- The importance of A/B testing in data science.
Amazing Research Topics on Big Data and Local Governments
Governments are now using big data to make the lives of the citizens better. This is in the government and the various institutions. They are based on real-life experiences and making the world better.
- Assess the benefits and barriers of big data in the public sector.
- The best approach to smart city data ecosystems.
- The big analytics used for policymaking.
- Evaluate the smart technology and emergence algorithm bureaucracy.
- Evaluate the use of citizen scoring in public services.
- An analysis of the government administrative data globally.
- The public values are found in the era of big data.
- Public engagement on local government data use.
- Data analytics use in policymaking.
- How are algorithms used in public sector decision-making?
- The democratic governance in the big data era.
- The best business model innovation to be used in sustainable organizations.
- How does the government use the collected data from various sources?
- The role of big data for smart cities.
- How does big data play a role in policymaking?
Easy Research Topics on Big Data
Who said big data topics had to be hard? Here are some of the easiest research topics. They are based on data management, research, and data retention. Pick one and try it!
- Who uses big data analytics?
- Evaluate structure machine learning.
- Explain the whole deep learning process.
- Which are the best ways to manage platforms for enterprise analytics?
- Which are the new technologies used in data management?
- What is the importance of data retention?
- The best way to work with images is when doing research.
- The best way to promote research outreach is through data management.
- The best way to source and manage external data.
- Does machine learning improve the quality of data?
- Describe the security technologies that can be used in data protection.
- Evaluate token-based authentication and its importance.
- How can poor data security lead to the loss of information?
- How to determine secure data.
- What is the importance of centralized key management?
Unique IoT and Big Data Research Topics
Internet of Things has evolved and many devices are now using it. There are smart devices, smart cities, smart locks, and much more. Things can now be controlled by the touch of a button.
- Evaluate the 5G networks and IoT.
- Analyze the use of Artificial intelligence in the modern world.
- How do ultra-power IoT technologies work?
- Evaluate the adaptive systems and models at runtime.
- How have smart cities and smart environments improved the living space?
- The importance of the IoT-based supply chains.
- How does smart agriculture influence water management?
- The internet applications naming and identifiers.
- How does the smart grid influence energy management?
- Which are the best design principles for IoT application development?
- The best human-device interactions for the Internet of Things.
- The relation between urban dynamics and crowdsourcing services.
- The best wireless sensor network for IoT security.
- The best intrusion detection in IoT.
- The importance of big data on the Internet of Things.
Big Data Database Research Topics You Should Try
Big data is broad and interesting. These big data database research topics will put you in a better place in your research. You also get to evaluate the roles of various phenomena.
- The best cloud computing platforms for big data analytics.
- The parallel programming techniques for big data processing.
- The importance of big data models and algorithms in research.
- Evaluate the role of big data analytics for smart healthcare.
- How is big data analytics used in business intelligence?
- The best machine learning methods for big data.
- Evaluate the Hadoop programming in big data analytics.
- What is privacy-preserving to big data analytics?
- The best tools for massive big data processing
- IoT deployment in Governments and Internet service providers.
- How will IoT be used for future internet architectures?
- How does big data close the gap between research and implementation?
- What are the cross-layer attacks in IoT?
- The influence of big data and smart city planning in society.
- Why do you think user access control is important?
Big Data Scala Research Topics
Scala is a programming language that is used in data management. It is closely related to other data programming languages. Here are some of the best scala questions that you can research.
- Which are the most used languages in big data?
- How is scala used in big data research?
- Is scala better than Java in big data?
- How is scala a concise programming language?
- How does the scala language stream process in real-time?
- Which are the various libraries for data science and data analysis?
- How does scala allow imperative programming in data collection?
- Evaluate how scala includes a useful REPL for interaction.
- Evaluate scala’s IDE support.
- The data catalog reference model.
- Evaluate the basics of data management and its influence on research.
- Discuss the behavioral analytics process.
- What can you term as the experience economy?
- The difference between agile data science and scala language.
- Explain the graph analytics process.
Independent Research Topics for Big Data
These independent research topics for big data are based on the various technologies and how they are related. Big data will greatly be important for modern society.
- The biggest investment is in big data analysis.
- How are multi-cloud and hybrid settings deep roots?
- Why do you think machine learning will be in focus for a long while?
- Discuss in-memory computing.
- What is the difference between edge computing and in-memory computing?
- The relation between the Internet of things and big data.
- How will digital transformation make the world a better place?
- How does data analysis help in social network optimization?
- How will complex big data be essential for future enterprises?
- Compare the various big data frameworks.
- The best way to gather and monitor traffic information using the CCTV images
- Evaluate the hierarchical structure of groups and clusters in the decision tree.
- Which are the 3D mapping techniques for live streaming data.
- How does machine learning help to improve data analysis?
- Evaluate DataStream management in task allocation.
- How is big data provisioned through edge computing?
- The model-based clustering of texts.
- The best ways to manage big data.
- The use of machine learning in big data.
Is Your Big Data Thesis Giving You Problems?
These are some of the best topics that you can use to prosper in your studies. Not only are they easy to research but also reflect on real-time issues. Whether in University or college, you need to put enough effort into your studies to prosper. However, if you have time constraints, we can provide professional writing help. Are you looking for online expert writers? Look no further, we will provide quality work at a cheap price.
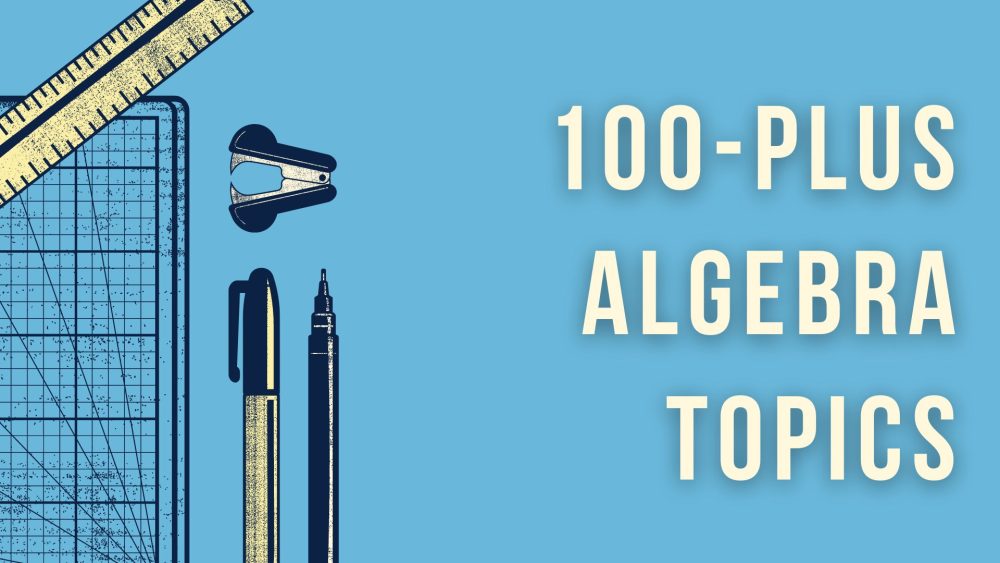
Leave a Reply Cancel reply
Your email address will not be published. Required fields are marked *
Comment * Error message
Name * Error message
Email * Error message
Save my name, email, and website in this browser for the next time I comment.
As Putin continues killing civilians, bombing kindergartens, and threatening WWIII, Ukraine fights for the world's peaceful future.
Ukraine Live Updates
Machine Learning Blog | ML@CMU | Carnegie Mellon University
Statistics:.
- [ Submissions ]
- Submissions
Categories:
- Educational
[instagram-feed num=6 cols=6 imagepadding=0 disablemobile=true showbutton=false showheader=false followtext=”Follow @Mint_Theme”]
- Data, AI, & Machine Learning
- Managing Technology
- Social Responsibility
- Workplace, Teams, & Culture
- AI & Machine Learning
- Diversity & Inclusion
- Big ideas Research Projects
- Artificial Intelligence and Business Strategy
- Responsible AI
- Future of the Workforce
- Future of Leadership
- All Research Projects
- AI in Action
- Most Popular
- The Truth Behind the Nursing Crisis
- Work/23: The Big Shift
- Coaching for the Future-Forward Leader
- Measuring Culture
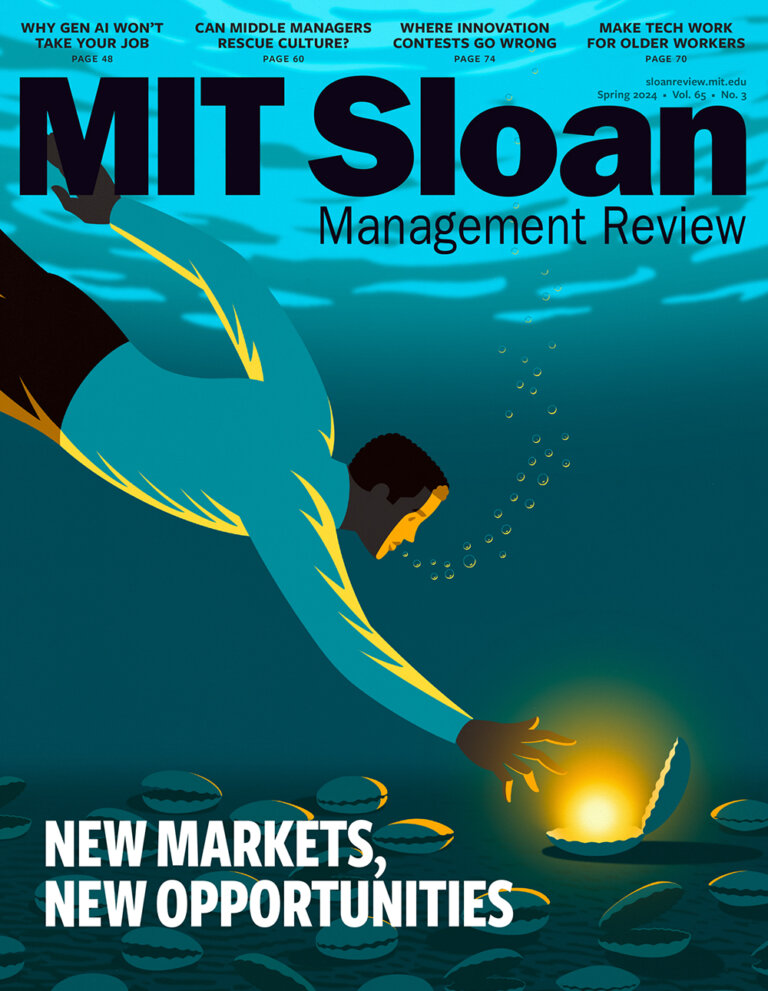
The spring 2024 issue’s special report looks at how to take advantage of market opportunities in the digital space, and provides advice on building culture and friendships at work; maximizing the benefits of LLMs, corporate venture capital initiatives, and innovation contests; and scaling automation and digital health platform.
- Past Issues
- Upcoming Events
- Video Archive
- Me, Myself, and AI
- Three Big Points
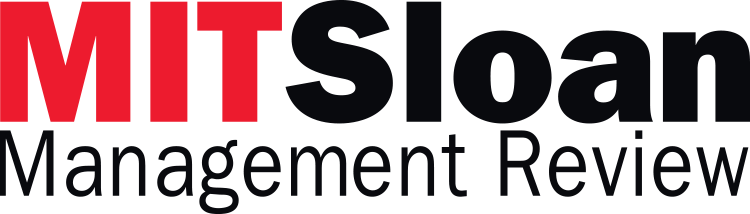
Data, AI, & Machine Learning
Ai-related risks test the limits of organizational risk management.
Experts debate how effectively organizations are adjusting risk management practices to govern AI.
Elizabeth M. Renieris, David Kiron, and Steven Mills
Popular with our readers, thomas c. redman and roger w. hoerl, thomas h. davenport and randy bean.
Marc Pinski, Monideepa Tarafdar, and Alexander Benlian
Latest Insights from MIT SMR
It governance & leadership, elizabeth m. renieris et al., how grassroots automation speeds digital success, thomas h. davenport et al., data & data culture, how lufthansa shapes data-driven transformation leaders, christian haude et al., ai & machine learning, ai and statistics: perfect together, fashioning the perfect fit with ai: stitch fix’s jeff cooper, sam ransbotham and shervin khodabandeh, when ai investments pay off in marketing, christine moorman and colleen hickey, more insights from mit smr.
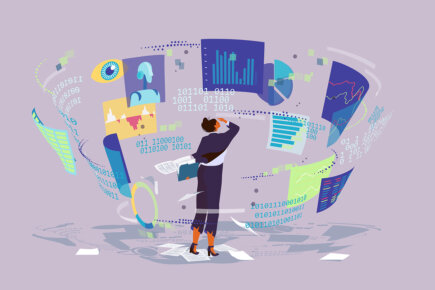
Why Executives Can’t Get Comfortable With AI
In the age of artificial intelligence, executives must make maintaining their AI literacy a habit.
Marc Pinski et al.
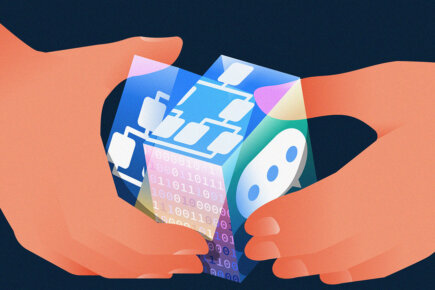
Reinventing the Organization for GenAI and LLMs
Learn three principles for reorganizing work around AI.
Ethan Mollick
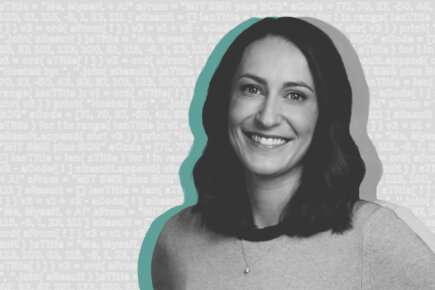
Collaboration
Solving real user problems with generative ai: slack’s jackie rocca.
On the Me, Myself, and AI podcast, Jackie Rocca explains how Slack uses artificial intelligence to relieve user pain points.
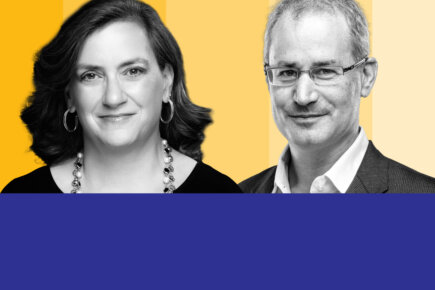
Leadership Skills
How ai changes your workforce.
Watch this short video to learn more about how AI changes the rules of workforce management.
MIT Sloan Management Review
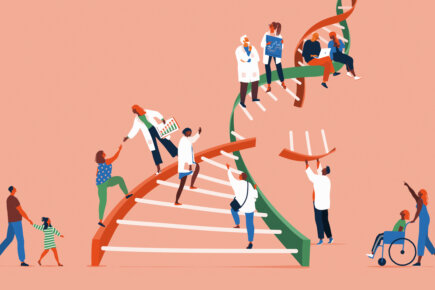
Mayo Clinic’s Healthy Model for AI Success
The nonprofit is delivering technology and services to help its staff build AI applications efficiently and safely.
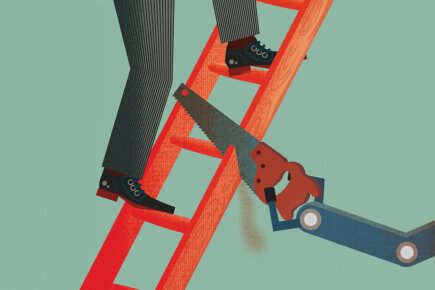
In AI We Trust — Too Much?
To reduce the risk of accidentally using bad artificial intelligence, we need regulation — and skepticism.
Ayanna Howard
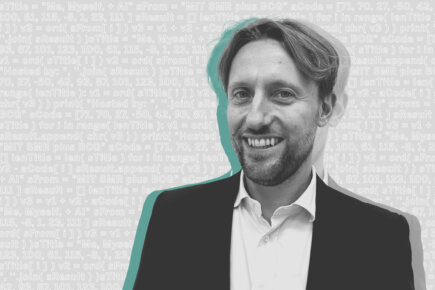
New Product Development
Driving manufacturing efficiency with ai: pirelli’s daniele petecchi.
On the Me, Myself, and AI podcast, Daniele Petecchi discusses how Pirelli uses AI to develop tires more efficiently.
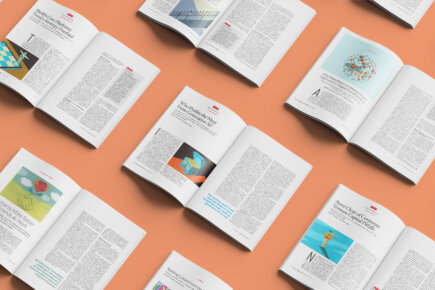
Innovation Strategy
Our guide to the spring 2024 issue.
The spring 2024 issue of MIT SMR looks at opportunities in digital innovation, automation, generative AI, and more.
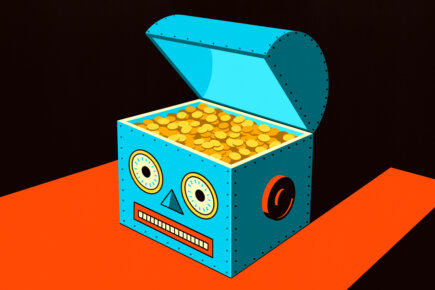
Who Profits the Most From Generative AI?
Analyzing factors behind generative AI’s value can help leaders determine who will benefit most from its growth.
Kartik Hosanagar and Ramayya Krishnan
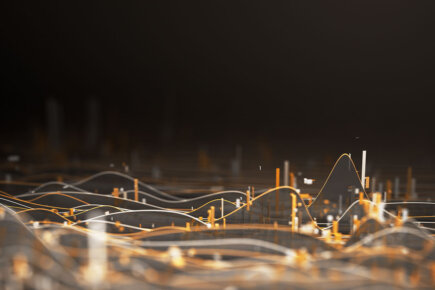
How to Succeed With Predictive AI
To succeed with machine learning, manage projects as business initiatives, not technology projects.
Eric Siegel and Abbie Lundberg
Executive guides.
- Technology Ethics
- Leading Data & Analytics
- Data Strategy
Developing an Ethical Technology Mindset
Building an organizational approach to responsible ai, kay firth-butterfield, designing ethical technology requires systems for anticipation and resilience, kirsten martin and bidhan (bobby) l. parmar, why building an ethical culture must start at the top, robert chesnut, interviewed by ally macdonald, why ‘explicit uncertainty’ matters for the future of ethical technology, mark nitzberg, putting dignity at the core of employee data use, dorothy leidner et al., organizational behavior, design your work environment to manage unintended tech consequences, lebene soga et al., a new business mandate for ethical technology, ally macdonald, the new leadership mindset for data & analytics, empowering a data culture from the inside out, jonathan tudor, interviewed by ally macdonald, analytics & business intelligence, leading with decision-driven data analytics, bart de langhe and stefano puntoni, top-down leadership for data: seven ways to get started, thomas c. redman, why chief data officers must assume leadership for data success, changing culture is central to changing business models, lanham napier et al., talent management, how large companies can grow their data and analytics talent, thomas h. davenport, developing a new leadership mindset for data, building a winning data strategy, how organizations can build analytics agility, lori c. bieda, the enterprise systems that companies need to create, david waller and paul beswick, data governance in the 21st-century organization, gregory vial, actioned analytics pave the way to new customer value, barbara h. wixom and gabriele piccoli, why culture is the greatest barrier to data success, getting serious about data and data science, thomas c. redman and thomas h. davenport, building a winning data strategy: an mit smr executive guide.
AI lacks a basis for inference: a solid foundation on which to base predictions and decisions. Thomas C. Redman and Roger W. Hoerl April 16, 2024 AI and Statistics: Perfect Together
University Libraries
Advanced data analytics.
- Advanced Data Analytics Course Libguide
- Data sources
- Learning resources
- Analytic tools
- Research and data services at UNT
- Research on the topic of data analytics
Finding research on data analytics
Data analytics is a very new area of work and not really a discipline of its own. This makes finding scholarly work about data analytics kind of tricky.
Much of the work is done by computer scientists, so a good bit of the literature can be found in the these databases:
- IEEE Xplore EL (IEEE Xplore) Full text access to IEEE Journals, Transactions, Magazines, Letters, Conference Proceedings, Standards (current only), and IEE Journals and Conferences. Dates of Coverage: 1988 to current, with some journals and conference titles available from 1950.
- ACM digital library ACM Digital Library bibliographic citations, abstracts, reviews, and full text for articles published in ACM proceedings and periodicals, covering computer science and engineering, as well as more general computing topics. Dates of Coverage: 1954 - Present
Otherwise, you'll want to search a database related to the domain in which you want to apply data analytics: see one of the UNT Libraries' subject guides to find relevant database.
Or you can explore the literature across fields, such as by using the Books & More (for ebooks) and Online Article Search (for journal articles) features, both on the homepage of the UNT Libraries . (On a mobile device, click the magnifying glass icon and then choose Library Catalog for ebooks or Full Text Online for articles.)
- << Previous: Research and data services at UNT
- Last Updated: Mar 18, 2024 12:32 PM
- URL: https://guides.library.unt.edu/data-analytics
Additional Links
UNT: Apply now UNT: Schedule a tour UNT: Get more info about the University of North Texas
UNT: Disclaimer | UNT: AA/EOE/ADA | UNT: Privacy | UNT: Electronic Accessibility | UNT: Required Links | UNT: UNT Home
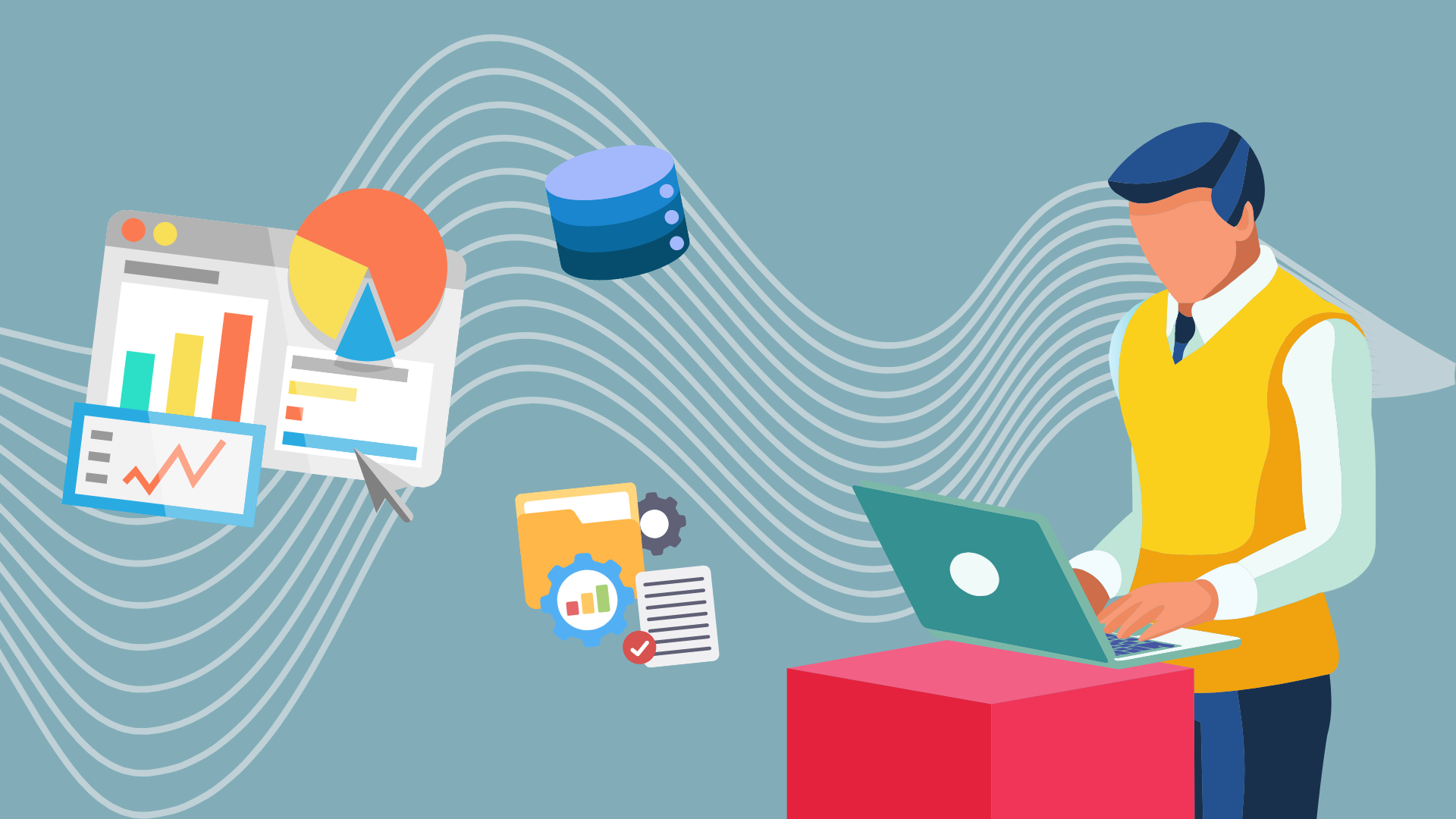
36 Data Analytics Project Ideas and Datasets (2024 UPDATE)
Data analytics projects help you to build a portfolio and land interviews. It is not enough to just do a novel analytics project however, you will also have to market your project to ensure it gets found.
The first step for any data analytics project is to come up with a compelling problem to investigate. Then, you need to find a dataset to analyze the problem. Some of the strongest categories for data analytics project ideas include:
- Beginner Analytics Projects - For early-career data analysts, beginner projects help you practice new skills.
- Python Analytics Projects - Python allows you to scrape relevant data and perform analysis with pandas dataframes and SciPy libraries.
- Rental and Housing Data Analytics Projects - Housing data is readily available from public sources, or can be simple enough to create your own dataset. Housing is related to many other societal forces, and because we all need some form of it, the topic will always be of interest to many people.
- Sports and NBA Analytics Projects - Sports data can be easily scraped, and by using player and game stats you can analyze strategies and performance.
- Data Visualization Projects - Visualizations allow you to create graphs and charts to tell a story about the data.
- Music Analytics Projects - Contains datasets for music-related data and identifying music trends.
- Economics and Current Trends - From exploring GDPs of respective countries to the spread of the COVID-19 virus, these datasets will allow you to explore a wide variety of time-relevant data.
- Advanced Analytics Projects - For data analysts looking for a stack-filled project.
A data analytics portfolio is a powerful tool for landing an interview. But how can you build one effectively?
Start with a data analytics project and build your portfolio around it. A data analytics project involves taking a dataset and analyzing it in a specific way to showcase results. Not only do they help you build your portfolio, but analytics projects also help you:
- Learn new tools and techniques.
- Work with complex datasets.
- Practice packaging your work and results.
- Prep for a case study and take-home interviews.
- Give you inbound interviews from hiring managers that have read your blog post!
Beginner Data Analytics Projects
Projects are one of the best ways for beginners to practice data science skills, including visualization, data cleaning, and working with tools like Python and pandas.
1. Relax Predicting User Adoption Take-Home
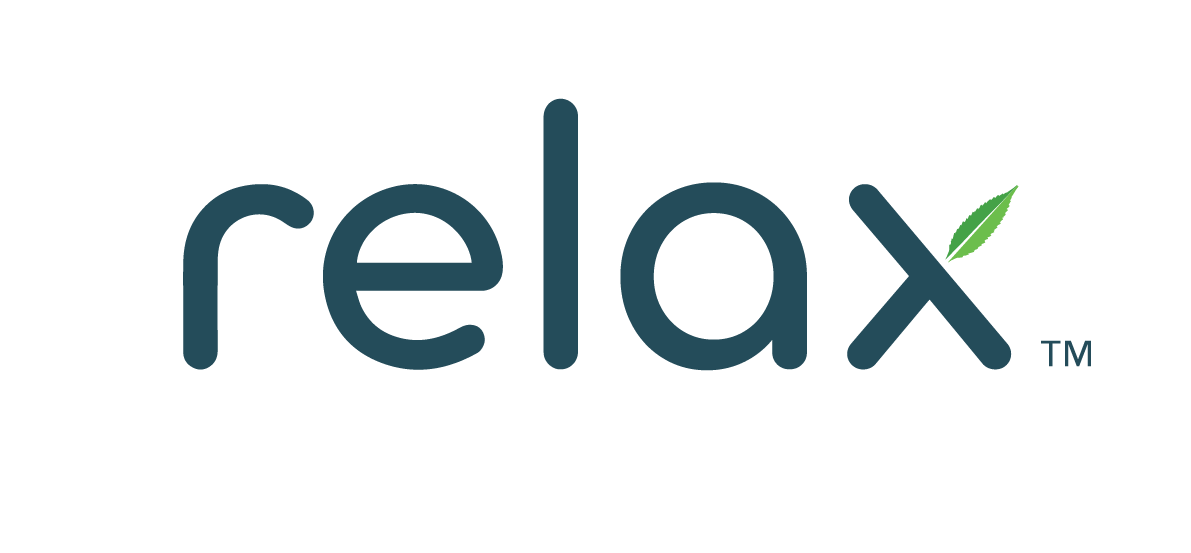
This data analytics take-home assignment, which has been given to data analysts and data scientists at Relax Inc., asks you to dig into user engagement data. Specifically, you’re asked to determine who an “adopted user” is, which is a user who has logged into the product on three separate days in at least one seven-day period.
Once you’ve identified adopted users, you’re asked to surface factors that predict future user adoption.
How you can do it: Jump into the Relax take-home data. This is an intensive data analytics take-home challenge, which the company suggests you spend 12 hours on (although you’re welcome to spend more or less). This is a great project for practicing your data analytics EDA skills, as well as surfacing predictive insights from a dataset.
2. Salary Analysis
Are you in some sort of slump, or do you find the other projects a tad too challenging? Here’s something that’s really easy; this is a salary dataset from Kaggle that is easy to read and clean, and yet still has many dimensions to interpret.
This salary dataset is a good candidate for descriptive analysis , and we can identify which demographics experience reduced or increased salaries. For example, we could explore the salary variations by gender, age, industry, and even years of prior work.
How you can do it: The first step is to grab the dataset from Kaggle. You can either use it as-is and use spreadsheet tools such as Excel to analyze the data, or you can load it into a local SQL server and design a database around the available data. You can then use visualization tools such as Tableau to visualize the data; either through Tableau MySQL Connector, or Tableau’s CSV import feature.
3. Skilledup Messy Product Data Analysis Take-Home
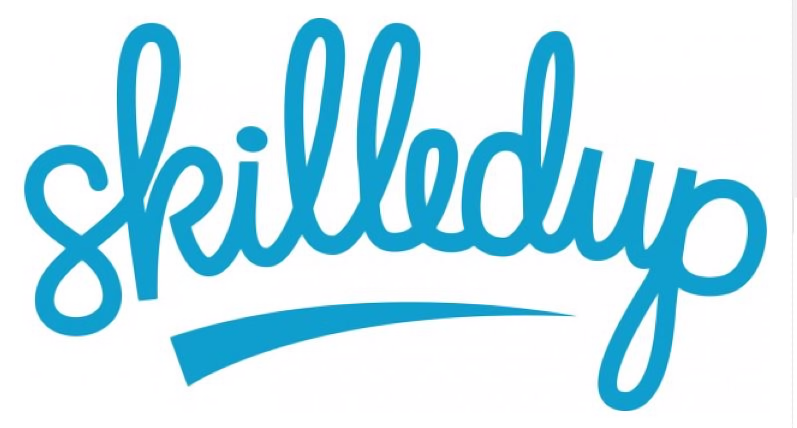
This data analytics take-home from Skilledup, asks participants to perform analysis on a dataset of product details that is formatted inconveniently. This challenge provides an opportunity to show your data cleaning skills, as well as your ability to perform EDA and surface insights from an unfamiliar dataset. Specifically, the assignment asks you to consider one product group, named Books.
Each product in the group is associated with categories. Of course, there are tradeoffs to categorization, and you’re asked to consider these questions:
- Is there redundancy in the categorization?
- How can redundancy be identified and removed?
- Is it possible to reduce the number of categories dramatically by sacrificing relatively few category entries?
How you can do it: You can access this EDA takehome on Interview Query. Open the dataset and perform some EDA to familiarize yourself with the categories. Then, you can begin to consider the questions that are posed.
4. Marketing Analytics Exploratory Data Analysis
This marketing analytics dataset on Kaggle includes customer profiles, campaign successes and failures, channel performance, and product preferences. It’s a great tool for diving into marketing analytics, and there are a number of questions you can answer from the data like:
- What factors are significantly related to the number of store purchases?
- Is there a significant relationship between the region the campaign is run in and that campaign’s success?
- How does the U.S. compare to the rest of the world in terms of total purchases?
How you can do it: This Kaggle Notebook from user Jennifer Crockett is a good place to start, and includes quite a few visualizations and analyses.
If you want to take it a step further, there is quite a bit of statistical analysis you can perform as well.
5. UFO Sightings Data Analysis
The UFO Sightings dataset is a fun one to dive into, and it contains data from more than 80,000 sightings over the last 100 years. This is a robust source for a beginner EDA project, and you can create insights into where sightings are reported most frequently sightings in the U.S. vs the rest of the world, and more.
How you can do it: Jump into the dataset on Kaggle. There are a number of notebooks you can check out with helpful code snippets. If you’re looking for a challenge, one user created an interactive map with sighting data .
6. Data Cleaning Practice
This Kaggle Challenge asks you to clean data as well as perform a variety of data cleaning tasks. This is a perfect beginner data analytics project, which will provide hands-on experience performing techniques like handling missing values, scaling and normalization, and parsing dates.
How you can do it: You can work through this Kaggle Challenge, which includes data. Another option, however, would be to choose your own dataset that needs to be cleaned, and then work through the challenge and adapt the techniques to your own dataset.
Python Data Analytics Projects
Python is a powerful tool for data analysis projects. Whether you are web scraping data - on sites like the New York Times and Craigslist - or you’re conducting EDA on Uber trips, here are three Python data analytics project ideas to try:
7. Enigma Transforming CSV file Take-Home
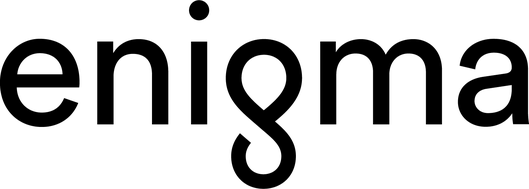
This take-home challenge - which requires 1-2.5 hours to complete - is a Python script writing task. You’re asked to write a script to transform input CSV data to desired output CSV data. A take-home like this is good practice for the type of Python take-homes that are asked of data analysts, data scientists, and data engineers.
As you work through this practice challenge, focus specifically on the grading criteria, which include:
- How well you solve the problems.
- The logic and approach you take to solving them.
- Your ability to produce, document, and comment on code.
- Ultimately, the ability to write clear and clean scripts for data preparation.
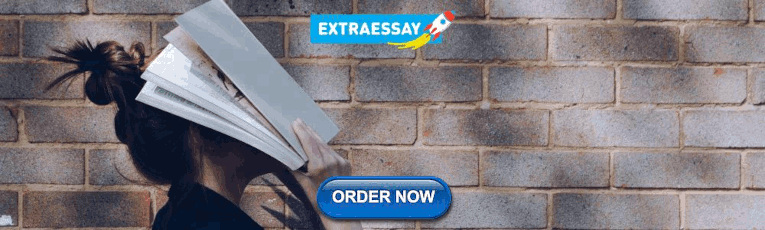
8. Wedding Crunchers
Todd W. Schneider’s Wedding Crunchers is a prime example of a data analysis project using Python. Todd scraped wedding announcements from the New York Times, performed analysis on the data, and found intriguing tidbits like:
- Distribution of common phrases.
- Average age trends of brides and grooms.
- Demographic trends.
Using the data and his analysis Schneider created a lot of cool visuals, like this one on Ivy League representation in the wedding announcements:
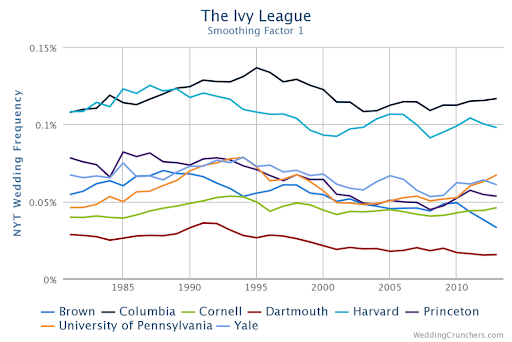
How you can do it: Follow the example of Wedding Crunchers. Choose a news or media source, scrape titles and text, and analyze the data for trends. Here’s a tutorial for scraping news APIs with Python.
9. Scraping Craigslist
Craigslist is a classic data source for an analytics project, and there is a wide range of things you can analyze. One of the most common listings is for apartments.
Riley Predum created a handy tutorial that walks you through the steps of using Python and Beautiful Soup to scrape the data to pull apartment listings, and then was able to do some interesting analysis of pricing when segmented by neighborhood and price distributions. When graphed, his analysis looked like this:
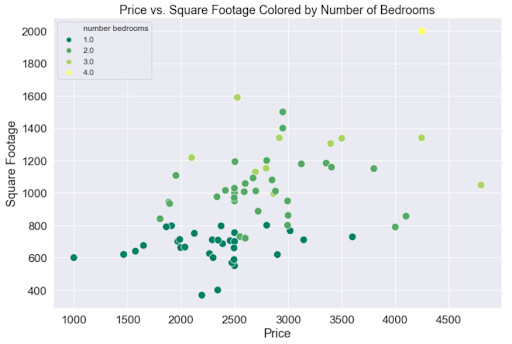
How you can do it: Follow the tutorial to learn how to scrape the data using Python. Some analysis ideas: Look at apartment listings for another area, analyze used car prices for your market, or check out what used items sell on Craigslist.
10. Uber Trip Analysis
Here’s a cool project from Aman Kharwal: An analysis of Uber trip data from NYC. The project used this Kaggle dataset from FiveThirtyEight , containing nearly 20 million Uber pickups. There are a lot of angles to analyze this dataset, like popular pickup times or the busiest days of the week.
Here’s a data visualization on pickup times by hour of the day from Aman:
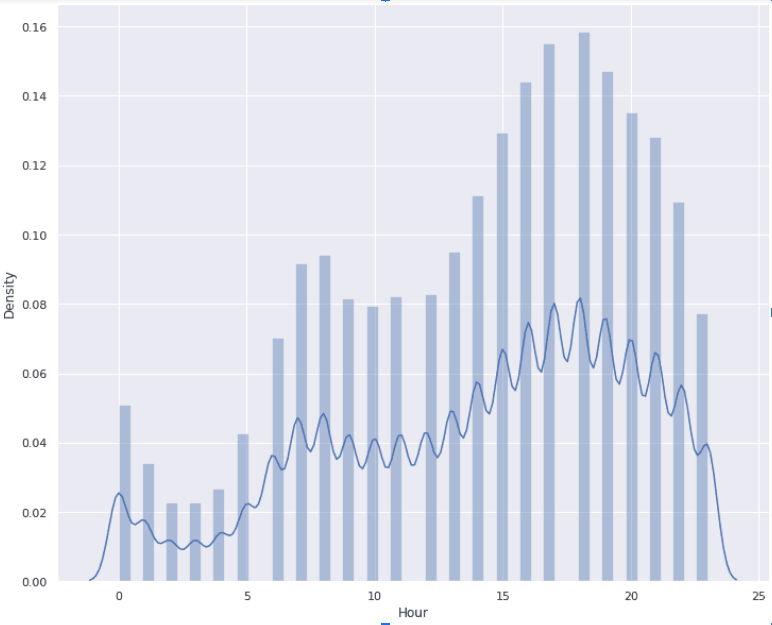
How you can do it: This is a data analysis project idea if you’re prepping for a case study interview. You can emulate this one, using the dataset on Kaggle, or you can use these similar taxies and Uber datasets on data.world, including one for Austin, TX.
11. Twitter Sentiment Analysis
Twitter (now X) is the perfect data source for an analytics project, and you can perform a wide range of analyses based on Twitter datasets. Sentiment analysis projects are great for practicing beginner NLP techniques.
One option would be to measure sentiment in your dataset over time like this:
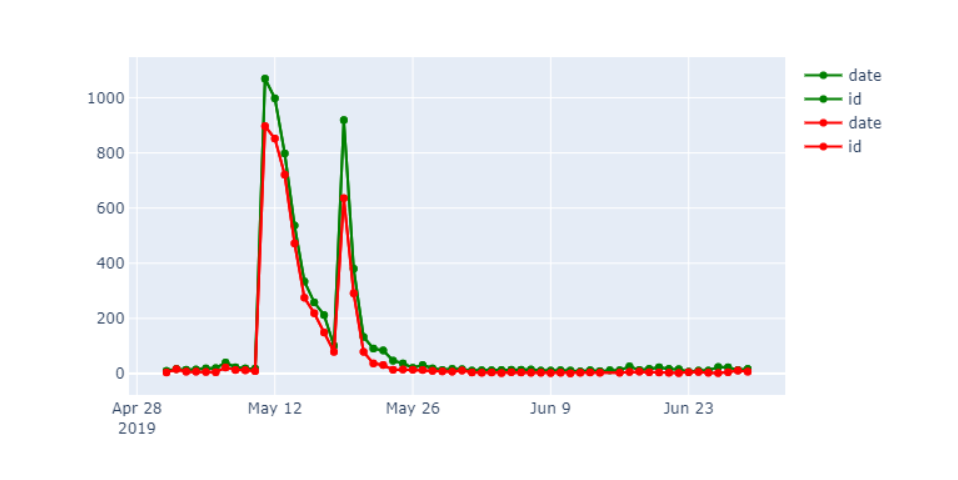
How you can do it: This tutorial from Natassha Selvaraj provides step-by-step instructions to do sentiment analysis on Twitter data. Or see this tutorial from the Twitter developer forum . For data, you can scrape your own or pull some from these free datasets.
12. Home Pricing Predictions
This project has been featured in our list of Python data science projects . With this project, you can take the classic California Census dataset , and use it to predict home prices by region, zip code, or details about the house.
Python can be used to produce some stunning visualizations, like this heat map of price by location.
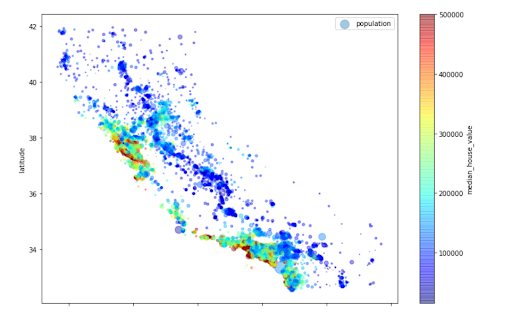
How you can do it: Because this dataset is so well known, there are a lot of helpful tutorials to learn how to predict price in Python. Then, once you’ve learned the technique, you can start practicing it on a variety of datasets like stock prices, used car prices, or airfare.
Rental and Housing Data Analytics Project Ideas
There’s a ton of accessible housing data online, e.g. sites like Zillow and Airbnb, and these datasets are perfect for analytics and EDA projects.
If you’re interested in price trends in housing, market predictions, or just want to analyze the average home prices for a specific city or state, jump into these projects:
13. Airbnb Data Analytics Take-Home Assignment
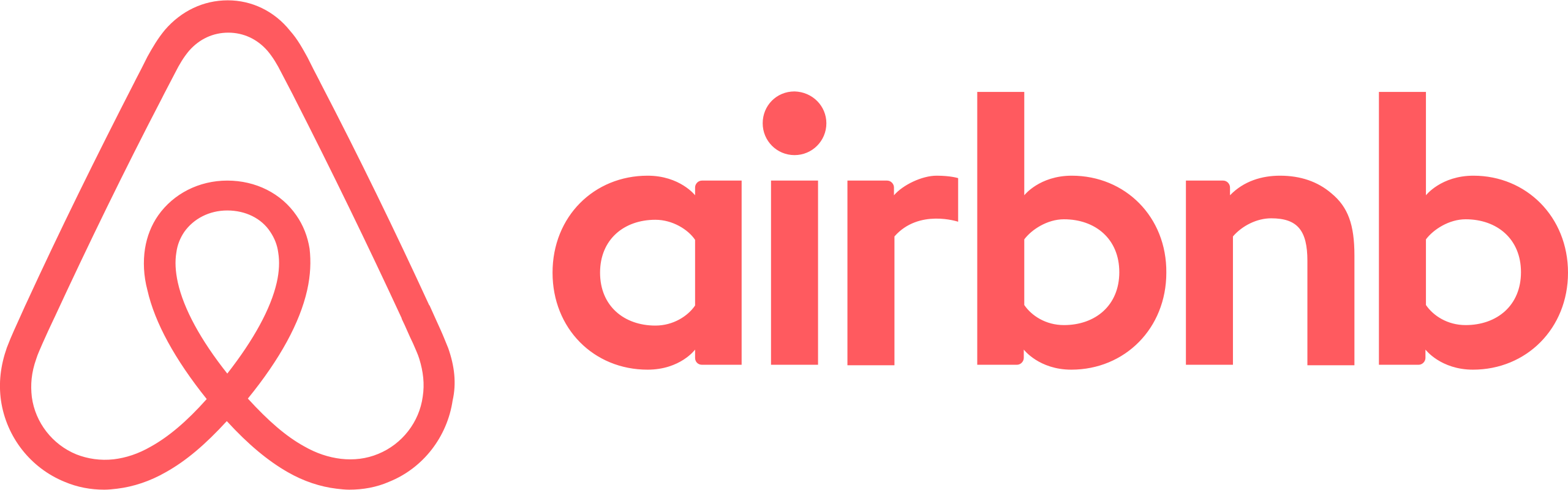
- Overview: Analyze the provided data and make product recommendations to help increase bookings in Rio de Janeiro.
- Time Required: 6 hours
- Skills Tested: Analytics, EDA, growth marketing, data visualization
- Deliverable: Summarize your recommendations in response to the questions above in a Jupyter Notebook intended for the Head of Product and VP of Operations (who is not technical).
This take-home is a classic product case study. You have booking data for Rio de Janeiro, and you must define metrics for analyzing matching performance and make recommendations to help increase the number of bookings.
This take-home includes grading criteria, which can help direct your work. Assignments are judged on the following:
- Analytical approach and clarity of visualizations.
- Your data sense and decision-making, as well as the reproducibility of the analysis.
- Strength of your recommendations
- Your ability to communicate insights in your presentation.
- Your ability to follow directions.
14. Zillow Housing Prices
Check out Zillow’s free datasets. The Zillow Home Value Index (ZHVI) is a smoothed, seasonally adjusted average of housing market values by region and housing type. There are also datasets on rentals, housing inventories, and price forecasts.
Here’s an analytics project based in R that might give you some direction. The author analyzes Zillow data for Seattle, looking at things like the age of inventory (days since listing), % of homes that sell for a loss or gain, and list price vs. sale price for homes in the region:
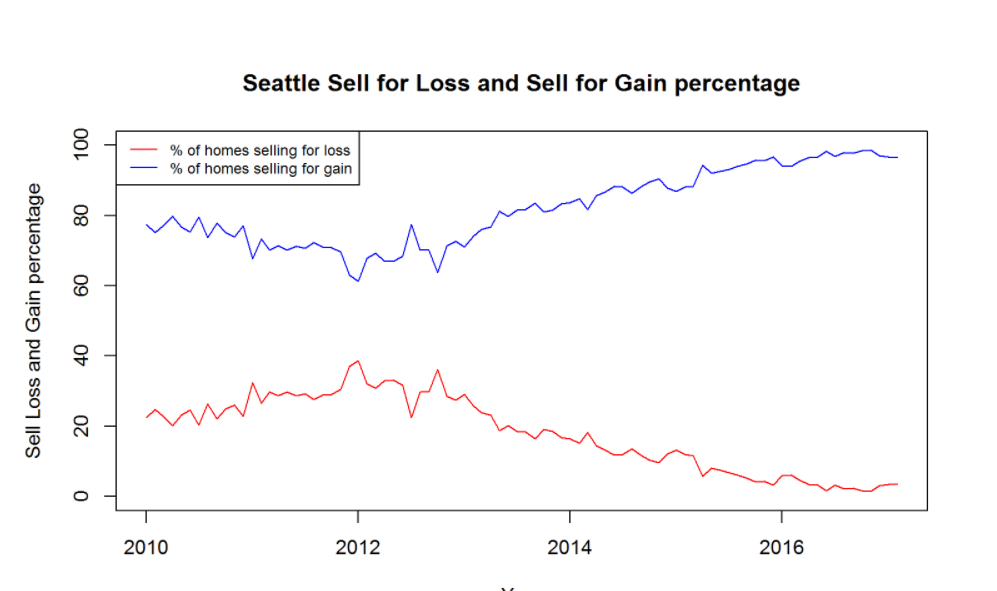
How you can do it: There are a ton of different ways you can use the Zillow dataset. Examine listings by region, explore individual list price vs. sale price, or take a look at the average sale price over the average list price by city.
15. Inside Airbnb
On Inside Airbnb , you’ll find data from Airbnb that has been analyzed, cleaned, and aggregated. There is data for dozens of cities around the world, including number of listings, calendars for listings, and reviews for listings.
Agratama Arfiano has extensively examined Airbnb data for Singapore. There are a lot of different analyses you can do, including finding the number of listings by host or listings by neighborhood. Arfiano has produced some really striking visualizations for this project, including the following:
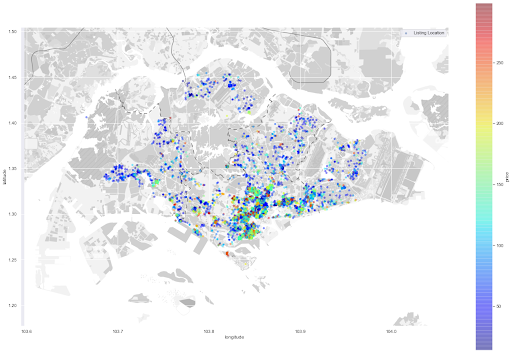
How you can do it: Download the data from Inside Airbnb, then choose a city for analysis. You can look at the price, listings by area, listings by the host, the average number of days a listing is rented, and much more.
16. Car Rentals
Have you ever wondered which cars are the most rented? Curious how fares change by make and model? Check out the Cornell Car Rental Dataset on Kaggle. Kushlesh Kumar created the dataset, which features records on 6,000+ rental cars. There are a lot of questions you can answer with this dataset: Fares by make and model, fares by city, inventory by city, and much more. Here’s a cool visualization from Kushlesh:
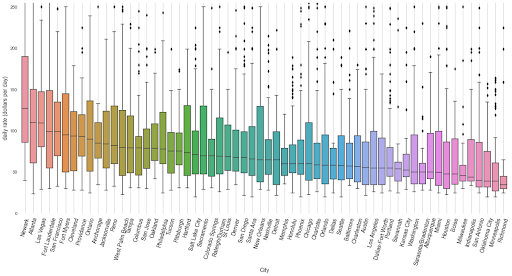
How you can do it: Using the dataset, you could analyze rental cars by make and model, a particular location, or analyze specific car manufacturers. Another option: Try a similar project with these datasets: Cash for Clunkers cars , Carvana sales data or used cars on eBay .
17. Analyzing NYC Property Sales
This real estate dataset shows every property that sold in New York City between September 2016 and September 2017. You can use this data (or a similar dataset you create) for a number of projects, including EDA, price predictions, regression analysis, and data cleaning.
A beginner analytics project you can try with this data would be a missing values analysis project like:
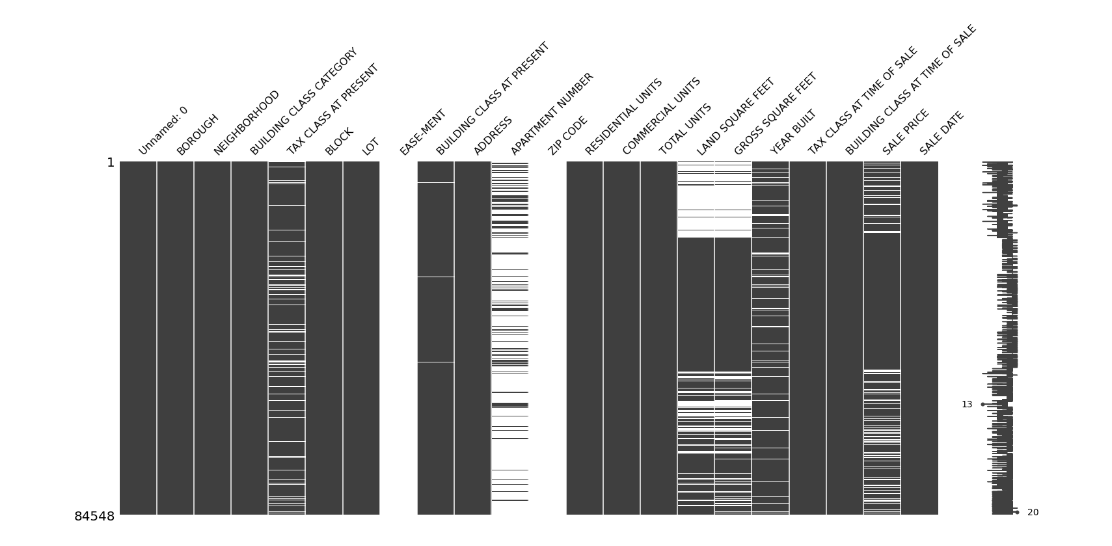
How you can do it: There are a ton of helpful Kaggle notebooks you can browse to learn how to: perform price predictions, do data cleaning tasks, or do some interesting EDA with this dataset.
Sports and NBA Data Analytics Projects
Sports data analytics projects are fun if you’re a fan, and also, because there are quite a few free data sources available like Pro-Football-Reference and Basketball-Reference. These sources allow you to pull a wide range of statistics and build your own unique dataset to investigate a problem.
18. NBA Data Analytics Project
Check out this NBA data analytics project from Jay at Interview Query. Jay analyzed data from Basketball Reference to determine the impact of the 2-for-1 play in the NBA. The idea: In basketball, the 2-for-1 play refers to an end-of-quarter strategy where a team aims to shoot the ball with between 25 and 36 seconds on the clock. That way the team that shoots first has time for an additional play while the opposing team only gets one response. (You can see the source code on GitHub).
The main metric he was looking for was the differential gain between the score just before the 2-for-1 shot and the score at the end of the quarter. Here’s a look at a differential gain:
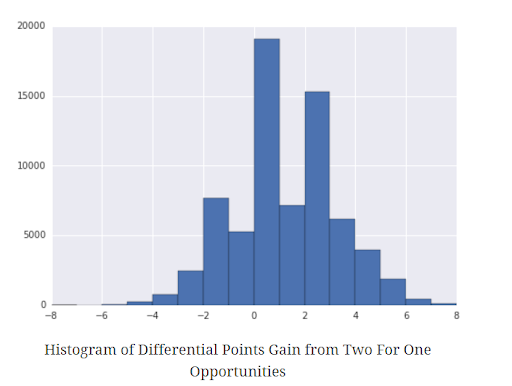
How you can do it: Read this tutorial on scraping Basketball Reference data . You can analyze in-game statistics, career statistics, playoff performance, and much more. An idea could be to analyze a player’s high school ranking vs. their success in the NBA. Or you could visualize a player’s career.
19. Olympic Medals Analysis
This is a great dataset for a sports analytics project. Featuring 35,000 medals awarded since 1896, there is plenty of data to analyze, and it’s useful for identifying performance trends by country and sport. Here’s a visualization from Didem Erkan :
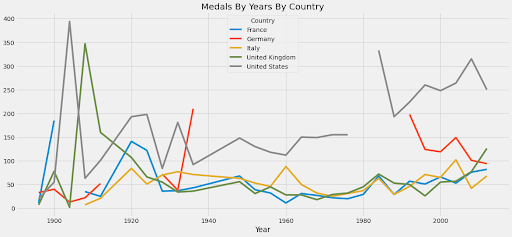
How you can do it: Check out the Olympics medals dataset . Angles you might take for analysis include: Medal count by country (as in this visualization ), medal trends by country, e.g., how U.S. performance evolved during the 1900s, or even grouping countries by region to see how fortunes have risen or faded over time.
20. Soccer Power Rankings
FiveThirtyEight is a wonderful source of sports data; they have NBA datasets, as well as data for the NFL and NHL. The site uses its Soccer Power Index (SPI) ratings for predictions and forecasts, but it’s also a good source for analysis and analytics projects. To get started, check out Gideon Karasek’s breakdown of working with the SPI data .
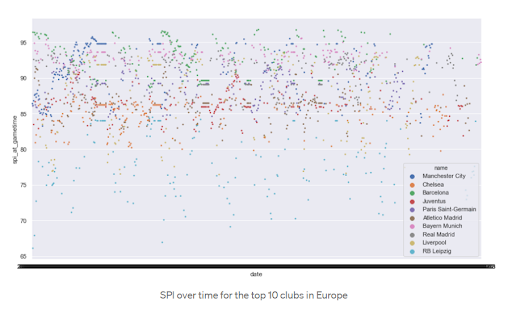
How you can do it: Check out the SPI data . Questions you might try to answer include: How has a team’s SPI changed over time, comparisons of SPI amongst various soccer leagues, and goals scored vs. goals predicted?
21. Home Field Advantage Analysis
Does home-field advantage matter in the NFL? Can you quantify how much it matters? First, gather data from Pro-Football-Reference.com . Then you can perform a simple linear regression model to measure the impact.
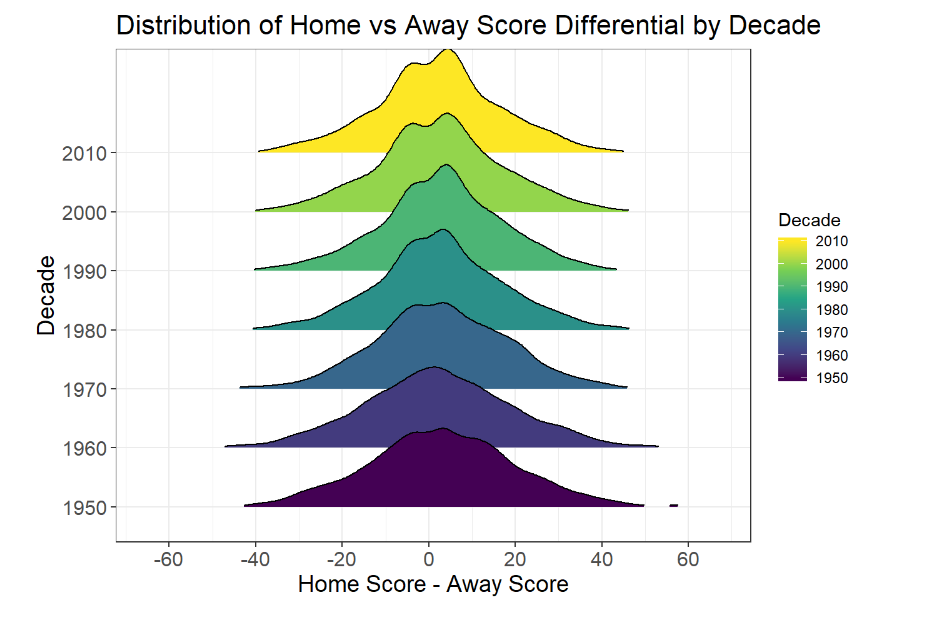
There are a ton of projects you can do with NFL data. One would be to determine WR rankings, based on season performance .
How you can do it: See this Github repository on performing a linear regression to quantify home field advantage .
22. Daily Fantasy Sports
Creating a model to perform in daily fantasy sports requires you to:
- Predict which players will perform best based on matchups, locations, and other indicators.
- Build a roster based on a “salary cap” budget.
- Determine which players will have the top ROI during the given week.
If you’re interested in fantasy football, basketball, or baseball, this would be a strong project.
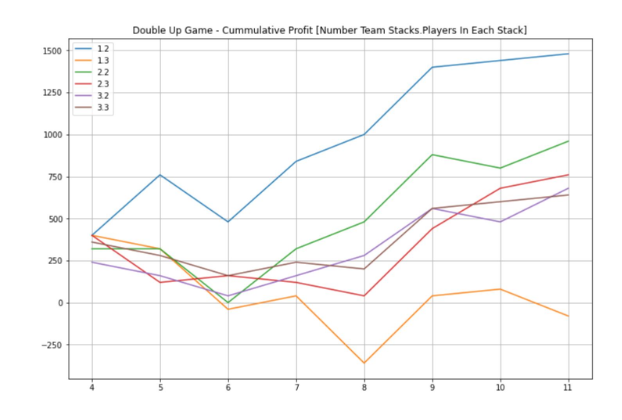
How you can do it: Check out the Daily Fantasy Data Science course , if you want a step-by-step look.
Data Visualization Projects
All of the datasets we’ve mentioned would make for amazing data visualization projects. To cap things off we are highlighting three more ideas for you to use as inspiration that potentially draws from your own experiences or interests!
23. Supercell Data Scientist Pre-Test
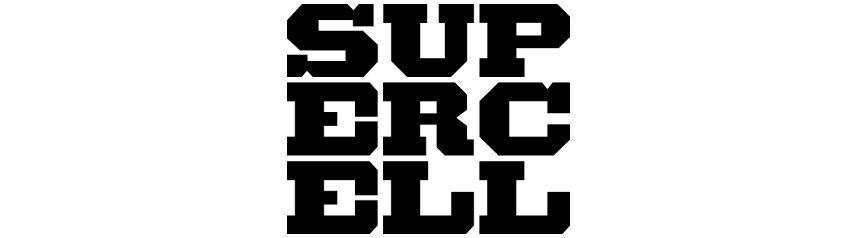
This is a classic SQL/data analytics take-home. You’re asked to explore, analyze, visualize and model Supercell’s revenue data. Specifically, the dataset contains user data and transactions tied to user accounts.
You must answer questions about the data, like which countries produce the most revenue. Then, you’re asked to create a visualization of the data, as well as apply machine learning techniques to it.
24. Visualizing Pollution
This project by Jamie Kettle visualizes plastic pollution by country, and it does a scarily good job of showing just how much plastic waste enters the ocean each year. Take a look for inspiration:

How you can do it: There are dozens of pollution datasets on data.world . Choose one and create a visualization that shows the true impact of pollution on our natural environments.
26. Visualizing Top Movies
There are a ton of movie and media datasets on Kaggle: The Movie Database 5000 , Netflix Movies and TV Shows , Box Office Mojo data , etc. And just like their big-screen debuts, movie data makes for fantastic visualizations.
Take a look at this visualization of the Top 100 movies by Katie Silver , which features top movies based on box office gross and the Oscars each received:

How you can do it: Take a Kaggle movie dataset, and create a visualization that shows one of the following: gross earnings vs. average IMDB rating, Netflix shows by rating, or visualization of top movies by the studio.
27. Gender Pay Gap Analysis
Salary is a subject everyone is interested in, and it makes it a relevant subject for visualization. One idea: Take this dataset from the U.S. Bureau of Labor Statistics , and create a visualization looking at the gap in pay by industry.
You can see an example of a gender pay gap visualization on InformationIsBeautiful.net:
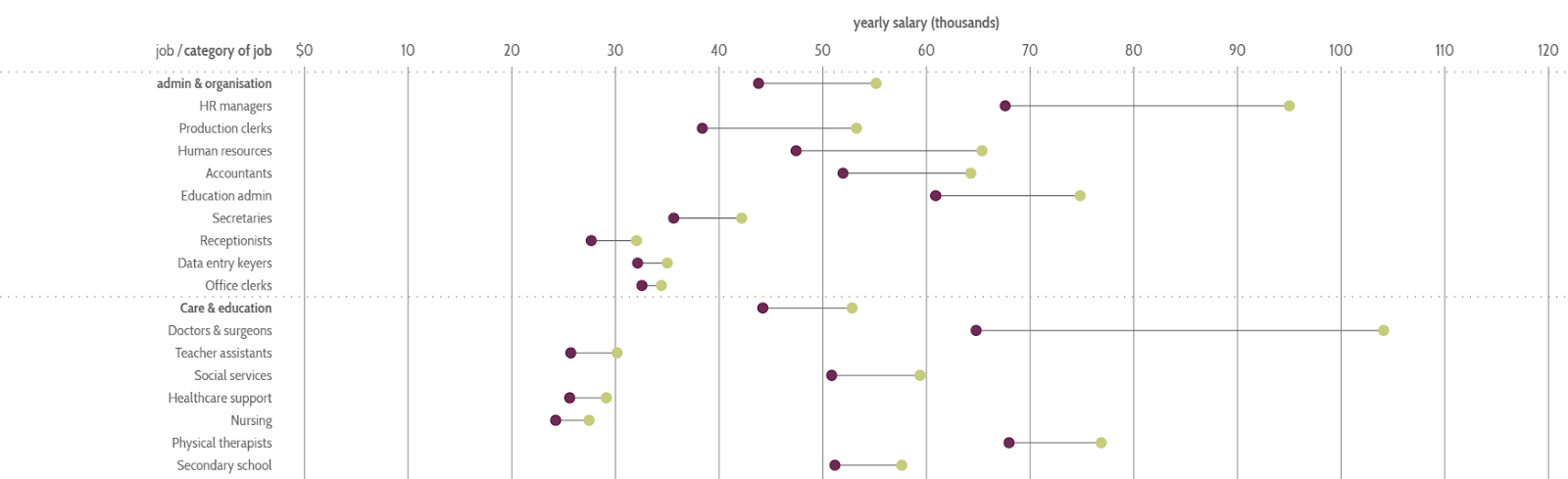
How you can do it: You can re-create the gender pay visualization, and add your own spin. Or use salary data to visualize, fields with the fastest growing salaries, salary differences by cities, or data science salaries by the company .
27. Visualize Your Favorite Book
Books are full of data, and you can create some really amazing visualizations using the patterns from them. Take a look at this project by Hanna Piotrowska, turning an Italo Calvo book into cool visualizations . The project features visualizations of word distributions, themes and motifs by chapter, and a visualization of the distribution of themes throughout the book:

How you can do it: This Shakespeare dataset , which features all of the lines from his plays, would be ripe for recreating this type of project. Another option: Create a visualization of your favorite Star Wars script.
Music Analytics Projects
If you’re a music fan, music analytics projects are a good way to jumpstart your portfolio. Of course, analyzing music through digital signal processing is out of our scope, so the best way to go around music-related projects is through exploring trends and charts. Here are some resources that you may use.
28. Popular Music Analysis
Here’s one way to analyze music features without explicit feature extraction. This dataset from Kaggle contains a list of popular music from the 1960s. A feature of this dataset is that it is currently being maintained. Here are a few approaches you can use.
How you can do it: You can grab this dataset from Kaggle. This dataset has classifications for popularity, release date, album name, and even genre. You can also use pre-extracted features such as time signature, liveness, valence, acoustic-ness, and even tempo.
Load this dataset into a Pandas DataFrame and do your appropriate processes there. You can analyze how the features move over time (i.e., did songs over time get a bit more mellow, livelier, or louder), or you can even explore the rise and fall of artists over time.
29. KPOP Melon Music Charts Analysis
If you’re interested in creating a KPOP-related analytics project, here’s one for you. While this is not a dataset, what we have here is a data source that scrapes data from the Melon charts and shows you the top 100 songs in the weekly, daily, rising, monthly, and LIVE charts.
How you can do it: The problem with this data source is that it is scraped, so gathering previous data might be a bit problematic. In order to do historical analysis, you will need to compile and store the data yourself.
So for this approach, we will prefer a locally hosted infrastructure. Knowing how to use cloud services to automate and store data might introduce additional layers of complexity for you to show off to a recruiter. Here’s a local approach to conducting this project.
The first step is to decide which database solution to use. We recommend XAMPP’s toolkit with MySQL Server and PHPMyAdmin as it provides an easy-to-use frontend while also providing a query builder that allows you to construct table schemas, so learning DDL (Data Definition Language) is not as much of a necessity.
The second step is to create a Python script that scrapes data from Melon’s music charts. Thankfully, we have a module that scrapes data from the charts. First, install the melonapi module. Then, you can gather the data and store it in your database. Here’s a step-by-step guide to loading the data from the site.
Of course, running this script over a period of time manually opens the door to human forgetfulness or boredom. To avoid this, you can use an automation service to automate your processes. For Windows systems, you can use the built-in Windows Task Scheduler. If you’re using Mac, you can use Automator.
When you have the appropriate data, you can then perform analytics, such as examining how songs move over time, classifying songs by album, and so on.
Economic and Current Trends Analytics Projects
One of the most valuable analytics projects is those that delve into economic and current trends. These projects, which make use of data from financial market trends, public demographic data, and social media behavior, are powerful tools not only for businesses and policymakers but also for individuals who aim to better understand the world around them.
When discussing current trends, COVID-19 is a significant phenomenon that continues to profoundly impact the status quo. An in-depth analysis of COVID-19 datasets can provide valuable insights into public health, global economies, and societal behavior.
How you can do it: These datasets, readily available for download, focus on different geographical areas. Here are a few:
- EU COVID-19 Dataset - dataset from the European Centre for Disease Prevention and Control, contains COVID-19 data for EU territories.
- US COVID-19 Dataset - US COVID-19 data provided by the New York Times. However, data might be outdated.
- Mexico COVID-19 Dataset - A COVID-19 dataset provided by the Mexican government.
These datasets provide opportunities to develop predictive algorithms and to create visualizations depicting the virus’s spread over time. Despite COVID-19 being less deadly today, it has become more contagious , and insights derived from these datasets can be crucial for understanding and combating future pandemics. For instance, a time-series analysis could identify key periods of infection rates’ acceleration and slow-down, highlighting effective and ineffective public health measures.
31. News Media Dataset
The News Media Dataset provides valuable information about the top 43 English media channels on YouTube, including each of their top 50 videos. This dataset, although limited in its scope, can offer intriguing insights into viewer preferences and trends in news consumption.
How you can do it: Grab the dataset from Kaggle and use the dataset which contains the top 50 viewed videos per channel. There are a lot of insights you can gain here, such as using a basic sentiment analysis tool to determine whether the top-performing headlines were positive or negative.
For sentiment analysis, you don’t necessarily need to train a model. You can load the CSV file and loop through all the tags. Use the TextBlob module to conduct sentiment analysis. Here’s how you can go about doing it:
Then, by using the subjectivity and polarity metrics, you can create visualizations that reflect your findings.
32. The Big Mac Index Analytics
The Big Mac Index offers an intriguing approach to comparing purchasing power parity (PPP) between different countries. The index shows how the U.S. dollar compares to other currencies, through a standardized, identical product, the McDonald’s Big Mac. The dataset, provided by Andrii Samoshyn, contains a lot of missing data, offering a real-world exercise in data cleaning. The data goes back to April 2000 up until January 2020.
How you can do it: You can download the dataset from Kaggle here . One common strategy for handling missing data is by using measures of central tendency like mean or median to fill in gaps. More advanced techniques, such as regression imputation, could also be applicable depending on the nature of the missing data.
Using this cleaned dataset, you can compare values over time or between regions. Introducing a “geographical proximity” column could provide additional layers of analysis, allowing comparisons between neighboring countries. Machine Learning techniques like clustering or classification could reveal novel groupings or patterns within the data, providing a richer interpretation of global economic trends.
When conducting these analyses, it’s important to keep in mind methods for evaluating the effectiveness of your work. This might involve statistical tests for significance, accuracy measures for predictive models, or even visual inspection of plotted data to ensure trends and patterns have been accurately captured. Remember, any analytics project is incomplete without a robust method of evaluation.
33. Global Country Information Dataset
This dataset offers a wealth of information about various countries, encompassing factors such as population density, birth rate, land area, agricultural land, Consumer Price Index (CPI), Gross Domestic Product (GDP), and much more. This data provides ample opportunity for comprehensive analysis and correlation studies among different aspects of countries.
How you can do it : Download this dataset from Kaggle. This dataset includes diverse attributes, ranging from economic to geographic factors, creating an array of opportunities for analysis. Here are some project ideas:
- Correlation Analysis: Investigate the correlations between different attributes, such as GDP and education enrollment, population density and CO2 emissions, birth rate, and life expectancy. You can use libraries like pandas and seaborn in Python for these tasks.
- Geospatial Analysis: With latitude and longitude data available, you could visualize data on a world map to understand global patterns better. Libraries such as geopandas and folium can be helpful here.
- Predictive Modeling: Try to predict an attribute based on others. For instance, could you predict a country’s GDP based on factors like population, education enrollment, and CO2 emissions?
- Cluster Analysis: Group countries based on various features to identify patterns. Are there groups of countries with similar characteristics, and if so, why?
Remember to perform EDA before diving into modeling or advanced analysis, as this will help you understand your data better and could reveal insights or trends to explore further.
34. College Rankings and Tuition Costs Dataset
This dataset offers valuable information regarding various universities, including their rankings and tuition fees. It allows for a comprehensive analysis of the relationship between a university’s prestige, represented by its ranking, and its cost.
How you can do it: First, download the dataset from Kaggle . You can then use Python’s pandas for data handling, and matplotlib or seaborn for visualization.
Possible analyses include exploring the correlation between college rankings and tuition costs, comparing tuition costs of private versus public universities, and studying trends in tuition costs over time. For a more advanced task, try predicting college rankings based on tuition and other variables.
Advanced Data Analytics Project
Ready to take your data skills to the next level? Advanced projects are a way to do just that. They’re all about handling larger datasets, really digging into data cleaning and preprocessing, and getting your hands dirty with a range of tech stacks. It’s a two-in-one deal – you’ll dip your toes inside the roles of both a data engineer and a data scientist. Here are some project ideas to consider.
35. Analyzing Google Trends Data
Google Trends, a free service provided by Google, can serve as a treasure trove for data analysts, offering insights into popular trends worldwide. But there’s a hitch. Google Trends does not support any official API, making direct data acquisition a bit challenging. However, there’s a workaround — web scraping. This guide will walk you through the process of using a Python module for scraping Google Trends data.
How you can do it: Of course, we would not want to implement a web scraper ourselves. Simply put, it’s too much work. For this project, we will utilize a Python module that will help us scrape the data. Let’s view an example:
This code should print out the data in the following format:
You should use an automation service to automate scraping at least once per hour (see: KPOP Melon Music Charts Analysis) . Then, you should store the results in a CSV file that you can query later. There are many points of analysis, such as keyword rankings, website rankings for articles, and more.
Taking it a step further:
If you want to make an even more robust project that’s bound to wow your recruiters, here are some ideas to make the scraping process easier to maintain, albeit with a higher difficulty in setting up.
The first problem in our previous approach is the hardware issue. Simply put, the automation service we used earlier is moot if our device is off or if it was not instantiated during device startup. To solve this, we can utilize the cloud.
Using a function service (i.e., GCP Cloud Functions, AWS Lambda), you can execute Python scripts. Now, you will need to orchestrate this service, and you can use a Pub/Sub service such as GCP Pub/Sub and AWS SNS. These will alert your cloud functions to run, and you can modify the Pub/Sub service to run at a specified time gap.
Then, when your script successfully scrapes the data, you will need a SQL server instance. The flavor of SQL does not really matter, but you can use the available databases provided by your cloud provider. For example, AWS offers RDS, while GCP offers Cloud SQL.
Once your data is pulled together, you can then start analyzing your data and employing analysis techniques to visualize and interpret data.
36. New York Times (NYT) Movie Reviews Sentiment Analysis
Sentiment Analysis is a critical tool in gauging public opinion and emotional responses towards various subjects, and in this case, movies. With a substantial number of movie reviews published daily in well-circulated publications like the NYT, proper sentiment analysis can provide valuable insights into the perceived quality of films and their reception among critics.
How you can do it: As a data source, NYT has an API service that allows you to query their databases. Create an account at this link and enable the ‘Movie Reviews’ service. Then, using your API key, you can start querying using the following script:
The query looks up the titles and returns movie reviews matching those in the query. You can then use the review summaries to do sentiment analysis.
Other NY Times APIs you can explore include the Most Popular API , and the Top Stories API .
More Analytics Project Resources
If you are still looking for inspiration, see our compiled list of free datasets which features sites to search for free data, datasets for EDA projects and visualizations, as well as datasets for machine learning projects.
You should also read our guide on the data analyst career path , how to become a data analyst without a degree , how to build a data science project from scratch and list of 30 data science project ideas .

Analytics Insight
Top 10 Research Challenge Areas to Pursue in Data Science
Disclaimer: Any financial and crypto market information given on Analytics Insight are sponsored articles, written for informational purpose only and is not an investment advice. The readers are further advised that Crypto products and NFTs are unregulated and can be highly risky. There may be no regulatory recourse for any loss from such transactions. Conduct your own research by contacting financial experts before making any investment decisions. The decision to read hereinafter is purely a matter of choice and shall be construed as an express undertaking/guarantee in favour of Analytics Insight of being absolved from any/ all potential legal action, or enforceable claims. We do not represent nor own any cryptocurrency, any complaints, abuse or concerns with regards to the information provided shall be immediately informed here .
You May Also Like

Top 10 Big Data Analytics Trends and Predictions to Watch For in 2020

5 Online Courses for Beginners to Learn Android in 2024

Amid Layoffs, Microsoft to Draw Curtains Over Metaverse and VR

Top 10 Amazing Open-Source Deep Learning Projects to Know

Analytics Insight® is an influential platform dedicated to insights, trends, and opinion from the world of data-driven technologies. It monitors developments, recognition, and achievements made by Artificial Intelligence, Big Data and Analytics companies across the globe.

- Select Language:
- Privacy Policy
- Content Licensing
- Terms & Conditions
- Submit an Interview
Special Editions
- Dec – Crypto Weekly Vol-1
- 40 Under 40 Innovators
- Women In Technology
- Market Reports
- AI Glossary
- Infographics
Latest Issue

Disclaimer: Any financial and crypto market information given on Analytics Insight is written for informational purpose only and is not an investment advice. Conduct your own research by contacting financial experts before making any investment decisions, more information here .
Second Menu
- Submit News
- Advertise Units
- Write for us
- White Papers

100+ Data Analytics Project Ideas: Unleashing the Power of Hands-On Learning
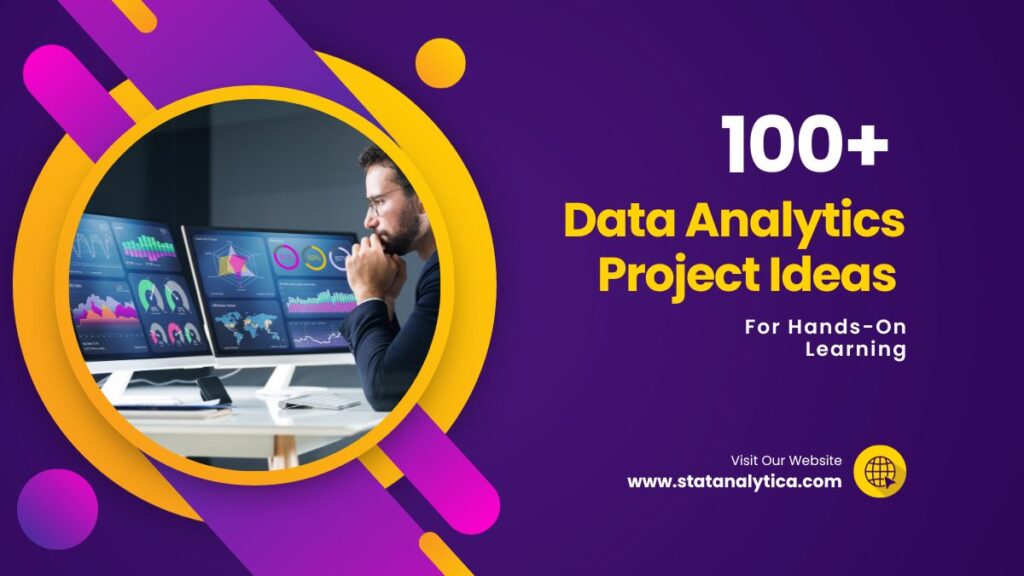
In today’s data-driven world, the ability to analyze and derive meaningful insights from vast amounts of data is a valuable skill. Data analytics not only helps in understanding patterns and trends but also aids in making informed decisions. One of the most effective ways to gain practical experience in data analytics is through hands-on projects.
In this blog post, we will explore the world of data analytics project ideas, discussing their benefits, how to choose the right project, and providing a diverse range of project ideas for aspiring data analysts.
What are the Benefits of Data Analytics Projects?
Table of Contents
Embarking on a data analytics project offers a plethora of benefits, making it an invaluable learning experience.
- Firstly, these projects enable skill development by providing practical exposure to various data analysis techniques and tools. By working on real datasets, individuals can enhance their analytical, statistical, and programming skills.
- Secondly, data analytics projects serve as a valuable addition to one’s portfolio. Aspiring data analysts can showcase these projects to potential employers, demonstrating their expertise and problem-solving abilities. A well-executed project speaks volumes about an individual’s capability to handle real-world data analysis challenges.
- Moreover, these projects provide an opportunity for real-world application of theoretical knowledge. It allows individuals to address practical problems faced by businesses, organizations, or even society at large. Solving these real-life problems enhances critical thinking and problem-solving skills, which are highly sought after in the field of data analytics.
Key Step: How to Choose a Data Analytics Project
Choosing the right data analytics project is crucial for a fulfilling and educational experience.
- To select an appropriate project, consider your interests, goals, available data sources, and the complexity of the project.
- Identifying a topic that aligns with your passion ensures that you remain motivated and engaged throughout the project.
- Additionally, assess the availability and quality of data sources. High-quality and well-organized datasets are essential for meaningful analysis and accurate results.
- Consider the complexity of the project; it should challenge you enough to foster growth but not overwhelm you.
- Defining the scope and objectives of the project clearly will help you stay focused and achieve your goals effectively.
100+ Data Analytics Project Ideas: Categories Wise
Exploratory data analysis (eda) projects.
- Customer segmentation based on demographics and purchase history.
- Market basket analysis to identify product associations.
- Social media sentiment analysis for a specific brand or topic.
- Exploration of COVID-19 data to analyze trends and patterns.
- Analysis of traffic data to optimize transportation routes.
- Study of air quality data to assess pollution trends in urban areas.
- Examination of crime data to identify high-crime areas and trends.
- Analysis of food and nutrition data to assess dietary habits.
- EDA of movie ratings and reviews to identify user preferences.
- Exploration of website user behavior to improve user experience.
Predictive Analytics Projects
- Predicting stock prices using historical data.
- Sales forecasting for an e-commerce business.
- Predicting the likelihood of customer churn in a subscription service.
- Predicting the success of a marketing campaign.
- Predicting student performance based on historical academic data.
- Forecasting energy consumption for a residential area.
- Predicting equipment failures in manufacturing.
- Predicting the outcome of sports events.
- Credit risk prediction for a financial institution.
- Predicting the likelihood of a disease outbreak.
Machine Learning Projects
- Image classification for recognizing objects in photos.
- Natural language processing (NLP) for text sentiment analysis.
- Recommender system for suggesting movies or products.
- Chatbot development for customer support.
- Fraud detection using machine learning algorithms.
- Speech recognition system for voice commands.
- Customer churn prediction using machine learning models.
- Personalized content recommendation for websites.
- Anomaly detection in network security.
- Employee attrition prediction for HR management.
Time Series Analysis Projects
- Predicting stock market trends using time series data.
- Forecasting website traffic patterns.
- Analyzing temperature fluctuations over time.
- Predicting electricity demand and supply.
- Predicting seasonal product demand for inventory management.
- Analyzing trends in social media mentions for a brand.
- Analyzing changes in cryptocurrency prices.
- Predicting daily website visitor counts.
- Forecasting future climate conditions.
- Predicting the spread of infectious diseases.
Natural Language Processing (NLP) Projects
- Named entity recognition for extracting information from text.
- Text classification for news articles or social media posts.
- Building a sentiment analysis model for movie reviews.
- Language translation tools.
- Chatbot development for customer service.
- Speech recognition and transcription services.
- Text summarization for news articles or research papers.
- Email categorization and prioritization.
- Spam email classification.
- Building a keyword extraction tool.
Data Visualization Projects
- Interactive data dashboards for sales performance.
- Geospatial data visualization for regional insights.
- Visualizing social network connections.
- Time series data visualization for stock market trends.
- Visualizing climate data to show temperature anomalies.
- Network traffic visualization for security monitoring.
- Hierarchical data visualization for organizational structures.
- Interactive dashboard for COVID-19 statistics .
- Visualizing customer journey maps for marketing analysis.
- Visualizing movie ratings and user preferences.
Data Cleaning and Preprocessing Projects
- Data cleaning and integration for a customer database.
- Removing outliers from a large dataset.
- Data imputation for missing values in healthcare records.
- Standardizing and normalizing data for analysis.
- Data deduplication for improving data quality.
- Identifying and handling duplicate records in a database.
- Data anonymization for privacy protection.
- Text data cleaning for NLP projects.
- Image data preprocessing for computer vision.
- Data transformation for machine learning input.
Big Data and Distributed Computing Projects
- Analyzing large-scale social media data using Hadoop.
- Real-time data stream processing for monitoring online trends.
- Distributed machine learning for big data sets.
- Analyzing web server logs for performance optimization.
- Large-scale text data analysis using Apache Spark.
- Analyzing IoT sensor data in a distributed environment.
- Building recommendation systems for e-commerce platforms.
- Analyzing customer clickstream data for user behavior insights.
- Distributed sentiment analysis for social media platforms.
- Real-time fraud detection in financial transactions.
Web Scraping and Data Collection Projects
- Web scraping for collecting product prices and reviews.
- Collecting and analyzing social media mentions of a brand.
- Scraping news articles and analyzing trends.
- Collecting and summarizing research papers from academic journals.
- Web scraping for real estate market data.
- Extracting job postings and analyzing job market trends.
- Gathering weather data from various sources.
- Scraping data from government websites for public analysis.
- Collecting data on political campaign contributions.
- Gathering data from e-commerce websites for competitive analysis.
Healthcare and Medical Data Projects
- Analyzing patient records to identify disease risk factors.
- Predicting disease outbreaks using epidemiological data.
- Monitoring and predicting patient wait times in hospitals.
- Analyzing health data for personalized treatment recommendations.
- Predicting patient readmissions for hospital management.
- Analyzing genomic data for genetic disease risk assessment.
- Monitoring and predicting the spread of infectious diseases.
- Identifying healthcare fraud and abuse.
- Analyzing electronic health records for clinical insights.
- Predicting patient demographics for targeted healthcare services.
Social Impact and Humanitarian Projects
- Predicting poverty levels using household data.
- Analyzing data to improve disaster response and relief efforts.
- Identifying educational disparities in underserved communities.
- Predicting and preventing traffic accidents in high-risk areas.
- Analyzing voting patterns to improve election transparency.
- Tracking and predicting air pollution levels in cities.
- Analyzing crime data to improve public safety.
- Monitoring and mitigating the impact of climate change.
- Identifying water scarcity issues using geospatial data.
- Predicting disease outbreaks to aid public health efforts.
Project Execution: Key Method to Executive Data Analytics Project Ideas
Once you get data analytics project ideas, it’s time to dive into the execution phase.
- Start by collecting and cleaning the data, ensuring it is free from errors and inconsistencies.
- Data analysis and modeling come next, where you apply various statistical and machine learning techniques to extract insights from the data.
- Visualization of results through charts, graphs, and interactive dashboards enhances the interpretability of your findings.
- Lastly, evaluate your results, iterate if necessary, and document your work comprehensively.
Resources for Data Analytics Projects
To embark on a data analytics project, you’ll need access to various resources. Seek out reliable data sources and datasets relevant to your project. Popular programming languages like Python and R, along with libraries such as pandas, NumPy, scikit-learn, and TensorFlow, are essential tools for data analysis and machine learning.
Online courses, tutorials, books, and research papers provide valuable learning materials to deepen your understanding of data analytics concepts and techniques.
Additional Resources and References
- Data Sources and Datasets:
- Kaggle (www.kaggle.com)
- UCI Machine Learning Repository (archive.ics.uci.edu/ml/index.php)
- Data.gov (www.data.gov)
- Tools and Software:
- Python (www.python.org)
- R (www.r-project.org)
- Pandas (pandas.pydata.org)
- Scikit-Learn (scikit-learn.org)
- Books and Research Papers:
- “Python for Data Analysis” by Wes McKinney
- “Introduction to Statistical Learning” by Gareth James, Daniela Witten, Trevor Hastie, Robert Tibshirani
- Various research papers available on platforms like Google Scholar (scholar.google.com)
Tips for Success In Data Analytics Project Ideas
- Setting Realistic Goals and Deadlines:
Establish achievable milestones and deadlines to keep your project on track.
- Collaboration and Seeking Feedback:
Collaborate with peers or mentors and seek feedback to gain different perspectives on your work.
- Documenting Your Work:
Maintain clear and detailed documentation of your data, methods, and findings. Proper documentation enhances reproducibility and makes it easier to communicate your results to others.
- Continuous Learning and Improvement:
Data analytics is a continuously evolving field. Stay updated with the latest trends, techniques, and tools to enhance your skills and knowledge continually.
Embarking on data analytics project ideas is a transformative journey that equips individuals with practical skills, problem-solving abilities, and a deeper understanding of the data analytics process.
By choosing the right project, leveraging available resources, and following best practices, aspiring data analysts can unleash the power of hands-on learning, making a meaningful impact in the data-driven world.
Reference: * (T&C Apply)
Related Posts
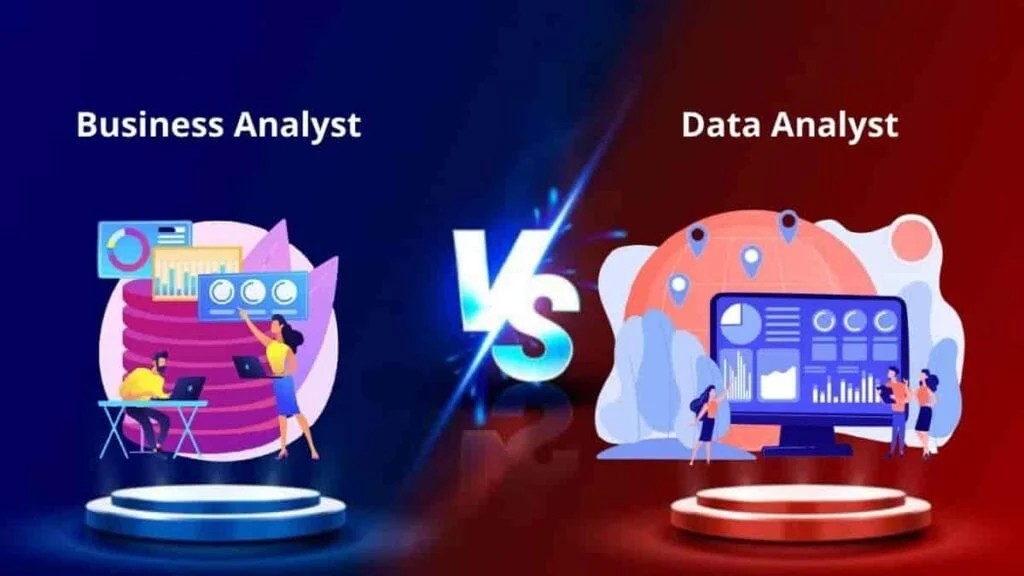
Best Comparison On Business Analyst v/s Data Analyst
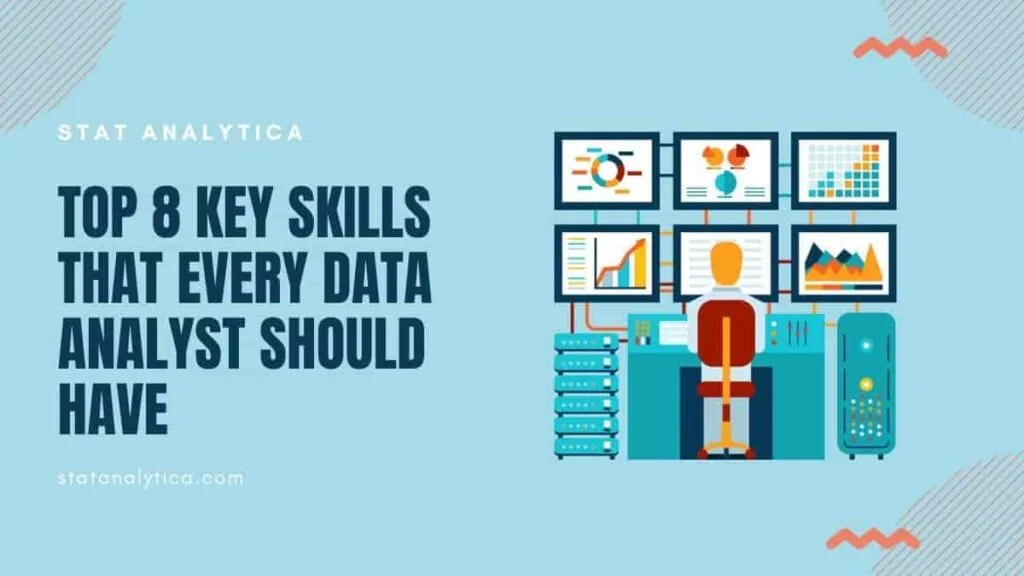
Top 8 key Skills That Every Data Analyst Should Have
7 Top Data Analytics Trends In 2024
You may also like:
- Important Computer Science Trends
- Top Cryptocurrency Trends
- Key Data Science Trends
Over the last decade, data has emerged as a transformative force.
Enterprises that give their leaders the tools they need to analyze data and the platforms they need to make data-driven decisions can truly harness the power of data. Businesses who don’t do this are missing out on a critical competitive edge.
In this article, read about the top seven trends in data analytics including AI, edge computing, data mesh, and more.
1. Implementing AI in Data Analytics
The rise of artificial intelligence (AI), in particular machine learning (ML), is increasing the speed and scale of data analytics operations.
Traditional analytics requires a team of IT analysts to comb through the data, theorize potential insights, test those insights, and report on their findings.
However, ML-based models can continuously monitor data, pick out anomalies, and alert the appropriate teams in real-time — without any human input needed.
ML models can look at any data subset and produce insights. They can look for correlations between millions of data points.
These systems are also incredibly accurate.
When data analytics platforms utilize ML and automate many of the traditional tasks associated with analytics, it’s called augmented analytics .
With augmented analytics, employees can use natural language to delve into the company’s data. They don’t need to have a hypothesis in mind because the ML algorithms uncover the insights users need.
This can be used for countless business processes like forecasting, predictive analytics, modeling, and visualizations.
One important advantage of augmented analytics is that AI/ML platforms can make sense of unstructured data.
That includes phone calls.
For example, University Hospitals in Cleveland, Ohio, receives more than 400,000 phone calls per month.
Before they began using an AI data analytics platform, listening to these phone calls and documenting what occurred was the task of several employees.
After University Hospitals implemented an augmented analytics platform from Invoca , they could begin automatically monitoring calls via the AI platform.
They’ve saved at least 40 hours per week in employee labor.
Augmented analytics can also guide enterprises in optimizing pricing strategies and forecasting demand .
For example, an AI model can analyze customer data to uncover patterns in purchasing behavior and utilize dynamic pricing in order to increase revenue.
Augmented analytics can also monitor competitor data so that organizations can optimize their pricing accordingly.
The market for augmented analytics is growing quickly.
Research and Markets estimates it will grow at a CAGR of nearly 26% through 2027, reaching a valuation of more than $32 billion that year.
2. Using Business Intelligence to Gather Insights
Put simply, business intelligence tools harness raw data in order to extract meaningful patterns and actionable insights.
Many of today’s popular BI platforms take advantage of AI/ML capabilities to deliver insights to business users.
These insights can lead to identifying issues, spotting trends, or finding new revenue sources.
Data mining, querying, reporting, and visualization are all part of a BI system .
Many business leaders consider BI imperative for organizational survival and success.
About one-quarter of organizations are currently using BI, according to a survey. But that number grows as high as 80% when considering organizations with 5,000+ employees.
Consider Delta Airlines, the employer of nearly 90,000 individuals .
The company has invested more than $100 million in a BI platform that tracks baggage handling.
The data intelligence gained from that platform guides Delta teams in figuring out issues and delays related to baggage — a huge source of stress for customers.
But the application of BI can be valuable at nearly every stage of the customer journey.
Organizations can use BI to create buyer personas and segment customer groups.
With all of this data, enterprises are able to create personalized sales and marketing campaigns.
In another example, BI platforms are a critical part of the digitization of the manufacturing industry.
These tools give manufacturers a data-driven method for streamlining production while keeping quality high.
BI can even be instrumental in handling supply chain disruptions and expenses.
When organizations want to present their data in a way that’s easy to understand, they’re turning to their BI platforms to create data visualizations.
These visualizations are things like charts, graphs, maps, and the like.
One of the most popular BI systems, Tableau , has a wealth of data visualization options for users.
And, the company provides a free platform called Tableau Public where users can get inspiration for their own data visualizations.
Users can create box-and-whisker plots that provide a quick way to see data distribution, heat maps that show specific data as specific colors, and tree maps that nest rectangles together in order to display hierarchical data.
With such a diverse array of applications, BI is on the way to becoming a universal standard for enterprises.
According to Fortune Business Insights, the business intelligence market could reach $54.27 billion by 2030, a $27 billion increase over 2022.
3. More Use Cases for Edge Computing
With the explosion of data in recent years and the need for real-time analytics, many enterprises are moving their data analysis to the edge, handling the data at the device that generates it.
By 2025, Gartner predicts more than 50% of critical data will be created and processed outside of the enterprise’s data center and cloud.
It will instead be processed in an edge computing environment.
According to an analysis from IDC, global spending on edge computing will reach $208 billion by the end of this year. That’s a 13.1% increase over 2022.
Why the rapid transition toward edge computing?
The world today generates more than 64 zettabytes of data in a year — that’s 64 trillion gigabytes of data, from a total of 23.8 billion connected devices .
By 2025, the world’s data will exceed 180 zettabytes from over 41 billion connected devices.
As the wealth of data has grown, many organizations have transitioned to data storage in the cloud.
However, even conventional cloud computing is under-equipped to handle the vast, ever-growing ocean of real-world data being generated every day.
Limitations in bandwidth, lag in data relay, and network disruptions can cripple critical industrial and commercial data processes.
This, in turn, escalates operating costs and risks.
The better alternative, data industry experts say, is edge computing .
Edge computing involves processor-intensive, often repetitive, mission-critical data analytics within devices on the outer edge of a network.
Only data summaries are sent to “fog” nodes, which are then passed on to cloud storage for higher-level processing.
Utilizing edge computing means analytics are delivered in real time. This is critical for enterprises that depend on rapid responses like healthcare and manufacturing.
But it can also filter out the information that’s not needed — estimates show that up to 90% of deployed data is useless.
This data does not get transferred to the cloud, saving bandwidth and budget.
Edge computing also has the benefit of increased privacy and data protection. Since data is never sent to the cloud, it’s more secure.
Edge analytics are an important part of the Industry 4.0 revolution.
Industrial settings have a vast number of IoT devices that generate large volumes of data every day.
In order to be useful, that data must be processed in milliseconds.
Routing data to the cloud and back doesn’t make sense in this setting, but edge computing does.
Data like temperature, humidity, vibrations, and the like can be analyzed immediately and discarded if not needed for long-term analysis.
Even the United States Postal Service is taking advantage of edge analytics .
The organization uses edge analytics to process millions of package images (each edge server processes 20 terabytes of images a day) in order to hunt down missing packages.
USPS officials say this task used to take the work of 8-10 people over the course of several days to find missing packages. With edge analytics, it takes just one person and a few hours.
4. Increasing Reliance on Data-as-a-Service
As more data is generated every day, it is becoming apparent that every business must utilize data to stay competitive.
Still, not every business has the ability to source, store, and analyze data as easily as the largest tech companies.
That is where Data-as-a-Service (DaaS) comes in.
DaaS companies typically offer data collection, storage, and analysis services on a subscription basis.
It runs via cloud computing and delivers services to end users over the network instead of having the data analyzed or stored locally.
Depending on the customer, the DaaS provider could collect and utilize internal data that is native to the customer or supply the customer with datasets it might not have access to on its own.
As more of the business world moves to cloud-first technology, it seems likely that the switch to DaaS will be swift as well.
In fact, Technavio estimates the DaaS market will add $56.85 billion in value before 2027.
In 2023, the year-over-year growth rate of the market was already at 28.64%.
Snowflake is one of the largest and most popular companies in this market.
The company is famous for its data warehousing services, but it can also act as a pure DaaS provider.
Snowflake allows customers to store and analyze data on its platform but also hosts vendors selling data products to end users through its platform.
On its platform, the company offers more than 600 active data sets.
Snowflake also recently invested in a New York-based company called Cybersyn during a $62.9 million Series A funding round in April 2023.
The company is focused on bringing together public and proprietary economic data to create datasets and sell them to third parties.
5. The Democratization of Data Systems
In a modern enterprise, data is often siloed.
It’s stuck in one department and other business users miss out on the value of that data.
That’s why data democratization is such an important trend for businesses. It involves opening up information so it’s available to everyone in an enterprise regardless of their technical expertise.
In one survey, 80% of business leaders say having access to data improves decision-making.
A Harvard Business Review survey found that 97% of business leaders say democratizing data is important to the success of their business.
But there’s still a long way to go. Only 60% of them say their organizations are effective at granting employees access to data and the tools they need to analyze it.
Putting the data in the hands of decision-makers means that many employees in the company become citizen data scientists , people who can analyze data but don’t take on that task as their primary role.
Coca-Cola has invested in upskilling managers in order to create more citizen data scientists in their organization.
In the first year of the program, they trained more than 500 people in digital skills such as data analytics. They have plans to roll out the program to more than 4,000 employees in the coming years.
Beyond executing new training programs, enterprises are also selecting self-service data analytics tools that enable employees to query and analyze data without any prior training.
Alteryx offers a variety of data analytics software options that are accessible to ordinary end-users.
Their platform offers a code-free, intuitive way to dig into the data. The platform utilizes ML and NLP, but Alteryx also recently released generative AI features .
These features are being implemented as “Magic Documents” that automate summarizations of data insights and a “Workflow Summary” that uses ChatGPT to document workflows.
The platform will also get an OpenAI connector that serves as an open-ended generative AI solution for businesses.
Alteryx went public in 2017 and has since grown their value to $2.74 billion .
6. Implementing a Data Mesh Architecture
Data mesh is an architecture that supports self-service analytics.
It’s an approach that advocates for decentralizing data ownership and management, treating data as a product, and establishing domain-oriented data teams.
The key idea behind data mesh is to distribute data responsibility across different teams within an enterprise. That enables the teams to take ownership of their own data domains and make data-driven decisions independently.
Governance is also embedded within domain teams rather than being imposed top-down. Each team has the autonomy to govern and manage their data products according to their specific domain requirements.
Because data is distributed, each enterprise team can use the tools and technologies that make sense for their specific domain .
The inventory management team might use one system while the marketing and sales teams use a different tool.
The end result is that teams have the data they need and the tools they need to innovate, experiment, and engage in effective decision-making.
A data mesh approach has several benefits for businesses: it’s more cost-efficient because it reduces the strain on the storage system; it facilitates interoperability; it increases security and regulatory compliance.
The person who first introduced the world to the concept of data mesh, Zhamak Dehghani, announced her own data company —Nextdata—in January 2023.
Nextdata aims to help enterprises decentralize data using the data mesh architecture and data product containers.
Nextdata’s product is still in the design and testing phase.
One example of a data mesh architecture in action comes from the financial industry where data is incredibly valuable but sharing it comes with inherent security and privacy risks.
JPMorgan Chase Bank built a data mesh solution in 2022 with the help of AWS.
Prior to integrating the data mesh, teams would need to extract and join data from multiple systems in multiple data domains in order to create reports.
But with the data mesh, teams that own data make it available in data lakes. Other teams can find that data via the enterprise data catalog and request it.
Data flows directly from one team’s application to the other team’s application.
7. Using Synthetic Data to Deliver High-Quality Data While Ensuring Privacy
Synthetic data is fake, generated by a computer program. It’s not based on any real-world person or event. But it’s becoming incredibly valuable to the field of data analytics.
As more and more enterprises start to implement AI/ML analytics systems, they need data to use in training those systems.
Some companies struggle to come up with the large amount of data and high-quality data needed for training.
That’s where synthetic data comes in.
This data is created by taking a database, creating an ML model for it, and generating a second set of data from it.
Synthetic data has the same patterns and properties as real data, but it’s not tied to any actual data identifiers.
A large volume of synthetic data can be created in a short period of time. And, it’s automatically tagged as it’s generated.
It’s also the prime way to get high-quality data regarding events that don’t happen in the real world very often.
Another big sticking point is security and privacy.
Synthetic data is ideal for enterprises that need to keep data private . Anonymizing data can be ineffective, but that’s never an issue with synthetic data because it was never real.
For example, synthetic data is being used in the medical field.
UC Davis Health in Sacramento, California, won a $1.2 million grant in April 2022 to innovate new ways to generate synthetic data to help forecast disease incidences.
A German health insurance group, Provinzial , is using synthetic data for predictive analytics .
They’ve used the data to build an analytics recommendation engine that predicts which services and products their customers will need next.
The enterprise saved three months because they didn’t need to go through privacy evaluations.
They also reduced the time to date by 4 weeks.
In an example from the public sector, the city of Vienna utilized synthetic data as it developed more than 300 software applications for the city.
The city needed demographic data but that’s protected under GDPR.
So, they utilized their existing datasets to generate synthetic data that matched the real population and number of households but did not include any identifiers related to personal data.
They analyzed the synthetic data in order to create tools for tourists, apps for individuals riding public transportation, and other solutions related to geographical data.
Gartner predicts that 60% of the data used by AI and analytics solutions will be synthetic data by 2024.
As organizations across the globe race to stay ahead of the competition, data analytics will likely continue to be a differentiating factor. The ability to generate and use business intelligence is a critical determinant of enterprise growth.
Early adopters are beginning to recognize the power of edge computing and the ability to turn their data into real-time insights. However, some are going even further to implement ML platforms that can produce insights without human intervention. Others are seeing more data-driven decisions across the enterprise thanks to data democratization.
In short, the data analytics space looks to have plenty of room for future growth.
Find Thousands of Trending Topics With Our Platform
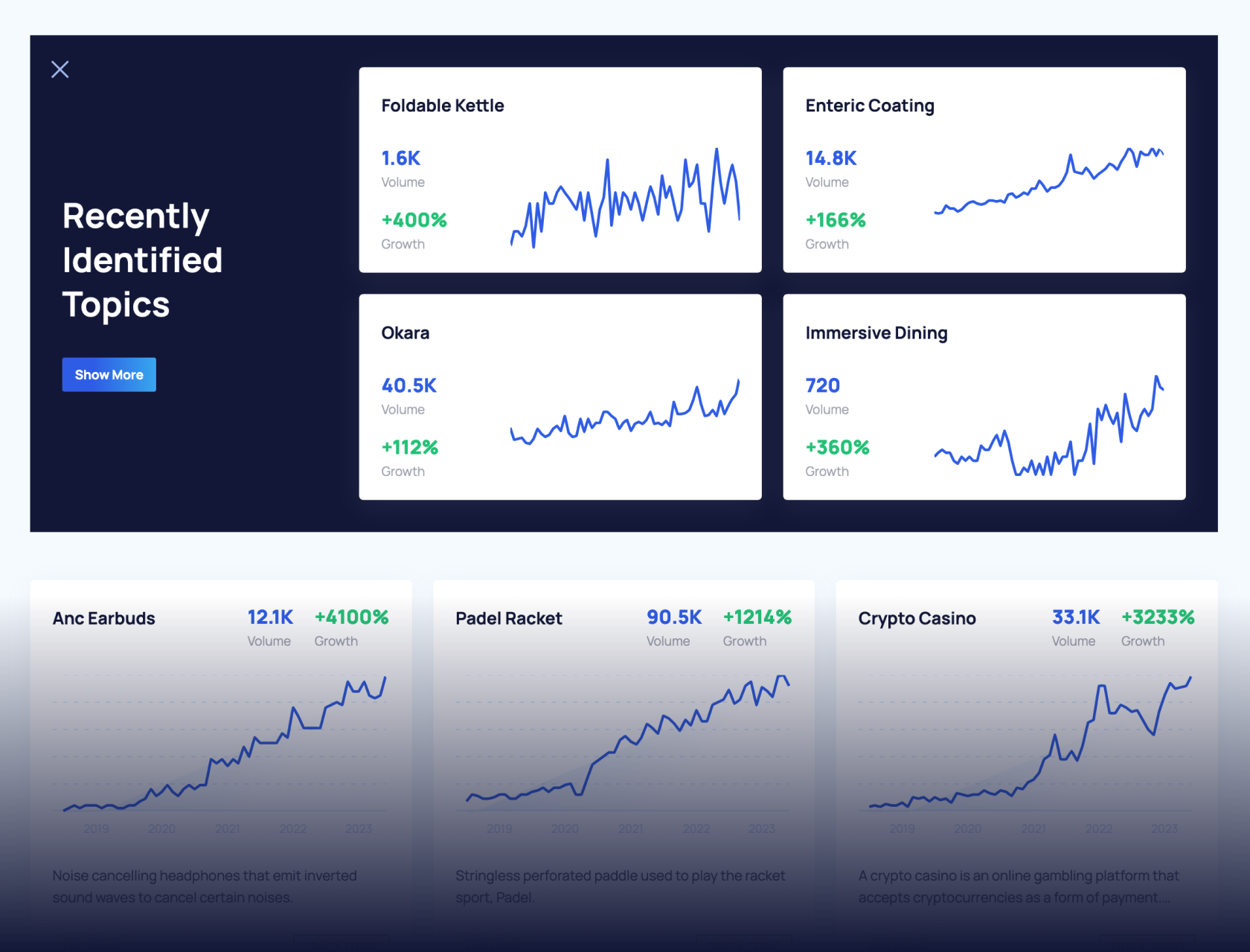
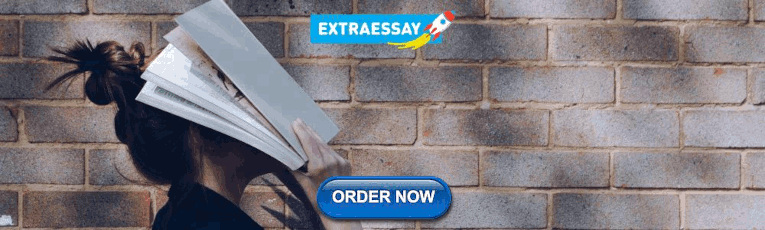
COMMENTS
Data Science-Related Research Topics. Developing machine learning models for real-time fraud detection in online transactions. The use of big data analytics in predicting and managing urban traffic flow. Investigating the effectiveness of data mining techniques in identifying early signs of mental health issues from social media usage.
The best course of action to amplify the robustness of a resume is to participate or take up different data science projects. In this article, we have listed 10 such research and thesis topic ideas to take up as data science projects in 2022. Handling practical video analytics in a distributed cloud: With increased dependency on the internet ...
166 Latest Big Data Research Topics And Fascinating Ideas. Big data refers to a huge volume of data, whether organized or unorganized, whose analysis shapes technologies and methodologies. Big data is so massive and complicated that it cannot be handled using ordinary application software. For instance, some frameworks, such as Hadoop, are ...
The importance of data analysis in conversion research; The importance of A/B testing in data science. Amazing Research Topics on Big Data and Local Governments . Governments are now using big data to make the lives of the citizens better. This is in the government and the various institutions.
6 Data engineering. Data engineering is a research topic that covers the aspects of data science that involve the acquisition, preparation, storage, and delivery of data. Data engineering can ...
99+ Data Science Research Topics: A Path to Innovation. In today's rapidly advancing digital age, data science research plays a pivotal role in driving innovation, solving complex problems, and shaping the future of technology. Choosing the right data science research topics is paramount to making a meaningful impact in this field.
11. Quantum Computing for Faster Analysis . One of the trending research topics in data science is Quantum computing. Google is already working on this, where decisions are not taken by the binary digits 0 and 1. The decisions are made using quantum bits of a processor called Sycamore. This processor is said to solve a problem in just 200 seconds.
The top level of analytics in Figure 1, prescriptive analytics for decision making, tends to be under-focused in statistics and data science programs. Recall the marketing 4P's in Table 1, if we can answer these questions causally , we can use the results to optimize , as listed in the last column of the table, linking predictive and ...
A data science research paper should start with a clear goal, stating what the study aims to investigate or achieve. This objective guides the entire paper, helping readers understand the purpose and direction of the research. 2. Detailed Methodology. Explaining how the research was conducted is crucial.
Published. August 31, 2020. This series of blog posts is based on the Fall 2019 10-718 Data Analysis class at Carnegie Mellon University, taught by Leila Wehbe, with the assistance of Jacob Tyo, Aria Wang and Fabricio Flores. The blog posts were written by the students and edited by the instructors and the ML@CMU blog team.
Abstract. To drive progress in the field of data science, we propose 10 challenge areas for the research community to pursue. Since data science is broad, with methods drawing from computer science, statistics, and other disciplines, and with applications appearing in all sectors, these challenge areas speak to the breadth of issues spanning ...
Solving Real User Problems With Generative AI: Slack's Jackie Rocca. On the Me, Myself, and AI podcast, Jackie Rocca explains how Slack uses artificial intelligence to relieve user pain points. Sam Ransbotham and Shervin Khodabandeh. April 02, 2024.
Graeme Andrew Stewart. Caterina Doglioni. Elizabeth Sexton-Kennedy. Eduardo Rodrigues. 421 views. This innovative journal focuses on the power of big data - its role in machine learning, AI, and data mining, and its practical application from cybersecurity to climate science and public health.
Big data analytics (BDA) has had a considerable influence across healthcare functions (Gu et al. Citation 2017; ... indicating that the research topic is relevant to the recent literature on BDA and healthcare. RQ2 is answered by analysing the contexts of the reviewed studies. The synthesis of the findings of prior studies addresses RQ3 ...
The field of data science is constantly evolving, and new research topics are emerging all the time. Here are some of the current topics of interest for research in data science and analytics ...
Big data is used in several research areas related to healthcare, location-based services, satellite information usage, online ad-. vertising, and retail marketing. In the coming years, the ...
Research on the topic of data analytics; Finding research on data analytics. Data analytics is a very new area of work and not really a discipline of its own. This makes finding scholarly work about data analytics kind of tricky. Much of the work is done by computer scientists, so a good bit of the literature can be found in the these databases
The role of data and analytics is to equip businesses, their employees and leaders to make better decisions and improve decision outcomes. This applies to all types of decisions, including macro, micro, real-time, cyclical, strategic, tactical and operational. At the same time, D&A can unearth new questions, as well as innovative solutions and ...
About the journal. The journal aims to promote and communicate advances in big data research by providing a fast and high quality forum for researchers, practitioners and policy makers from the very many different communities working on, and with, this topic. The journal will accept papers on foundational aspects in dealing with big data, as ...
For many researchers unfamiliar with qualitative research, determining how to conduct qualitative analyses is often quite challenging. Part of this challenge is due to the seemingly limitless approaches that a qualitative researcher might leverage, as well as simply learning to think like a qualitative researcher when analyzing data. From framework analysis (Ritchie & Spencer, 1994) to content ...
There are numerous research topics in healthcare data and analytics that can lead to breakthroughs in the industry. Predictive analytics: Predictive analytics involves using machine learning algorithms and statistical models to analyze historical data and predict future events. In healthcare, predictive analytics can be used to forecast patient ...
There are four key types of data analytics: descriptive, diagnostic, predictive, and prescriptive. These four types of data analytics can help an organisation make data-driven decisions. At a glance, each of them tells us the following: Descriptive analytics tell us what happened. Diagnostic analytics tell us why something happened.
Python Data Analytics Projects. Python is a powerful tool for data analysis projects. Whether you are web scraping data - on sites like the New York Times and Craigslist - or you're conducting EDA on Uber trips, here are three Python data analytics project ideas to try: 7. Enigma Transforming CSV file Take-Home.
Below are the top ten research challenge areas which will help to improve the efficiency of data science. 1. Scientific comprehension of learning, particularly deep learning algorithms As much as we respect the astounding triumphs of deep learning, we despite everything do not have a logical understanding of why deep learning works so well. We ...
Predictive Analytics Projects. Predicting stock prices using historical data. Sales forecasting for an e-commerce business. Predicting the likelihood of customer churn in a subscription service. Predicting the success of a marketing campaign. Predicting student performance based on historical academic data.
Data analytics package. Data Analytics is a package containing many tools that are all integral to performing analytics on data sets. This includes importing data, cleaning and preparing data, and tools and applications for doing statistics and data mining. There is a large list of examples that demonstrate the use of the tools.
In this article, read about the top seven trends in data analytics including AI, edge computing, data mesh, and more. 1. Implementing AI in Data Analytics. The rise of artificial intelligence (AI), in particular machine learning (ML), is increasing the speed and scale of data analytics operations. Search volume for "AI analytics" is growing ...