Tobacco, Nicotine, and E-Cigarettes Research Report How can we prevent tobacco use?
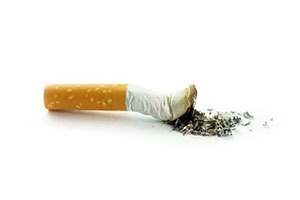
The medical consequences of tobacco use—including secondhand exposure—make tobacco control and smoking prevention crucial parts of any public health strategy. Since the first Surgeon General’s Report on Smoking and Health in 1964, states and communities have made efforts to reduce initiation of smoking, decrease exposure to smoke, and increase cessation. Researchers estimate that these tobacco control efforts are associated with averting an estimated 8 million premature deaths and extending the average life expectancy of men by 2.3 years and of women by 1.6 years. 18 But there is a long way yet to go: roughly 5.6 million adolescents under age 18 are expected to die prematurely as a result of an illness related to smoking. 13
Prevention can take the form of policy-level measures, such as increased taxation of tobacco products; stricter laws (and enforcement of laws) regulating who can purchase tobacco products; how and where they can be purchased; where and when they can be used (i.e., smoke-free policies in restaurants, bars, and other public places); and restrictions on advertising and mandatory health warnings on packages. Over 100 studies have shown that higher taxes on cigarettes, for example, produce significant reductions in smoking, especially among youth and lower-income individuals. 217 Smoke-free workplace laws and restrictions on advertising have also shown benefits. 218
Prevention can also take place at the school or community level. Merely educating potential smokers about the health risks has not proven effective. 218 Successful evidence-based interventions aim to reduce or delay initiation of smoking, alcohol use, and illicit drug use, and otherwise improve outcomes for children and teens by reducing or mitigating modifiable risk factors and bolstering protective factors. Risk factors for smoking include having family members or peers who smoke, being in a lower socioeconomic status, living in a neighborhood with high density of tobacco outlets, not participating in team sports, being exposed to smoking in movies, and being sensation-seeking. 219 Although older teens are more likely to smoke than younger teens, the earlier a person starts smoking or using any addictive substance, the more likely they are to develop an addiction. Males are also more likely to take up smoking in adolescence than females.
Some evidence-based interventions show lasting effects on reducing smoking initiation. For instance, communities utilizing the intervention-delivery system, Communities that Care (CTC) for students aged 10 to14 show sustained reduction in male cigarette initiation up to 9 years after the end of the intervention. 220

Subscribe Follow Watch
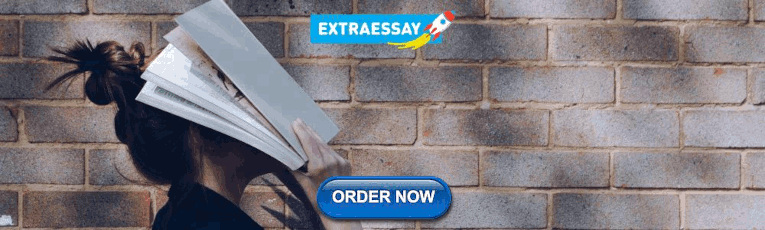
Research Priorities
Tobacco use.
The NIH supports research studying tobacco use prevention, including projects in regulatory science, addiction, tobacco control, health effects, cancer prevention, and behavioral studies.
Screening, Brief Intervention and Referral to Treatment or Prevention (SBIRT/P) for alcohol, tobacco, and other drugs (ATOD) use and misuse in adult populations that experience health disparities (R01, Clinical Trial Required) [PAR-23-270] . First available due date: February 5, 2024 (expires May 8, 2027)
Resources for Researchers
Explore databases and tools for tobacco research.
Tobacco Use Supplement to the Current Population Survey (TUS-CPS)
A survey of tobacco use that has been administered as part of the U.S. Census Bureau's Current Population Survey since 1992, including past data and statistics.
PhenX Toolkit
A catalog of recommended standard measures of phenotypes and environmental exposures for use in biomedical research. This includes common data elements for tobacco regulatory research.
Learn more about the PhenX Tobacco Regulatory Project and how these measures were identified, selected, and approved . Contact Dr. Kay Wanke , Deputy Director, TRSP/OD/NIH, for more information.
CDC Smoking & Tobacco Use Surveys
A comprehensive list of national and international surveys on tobacco use, smoking cessation, secondhand smoke exposure, and other tobacco-related topics among youth, adults, and specific populations.
Population Assessment of Tobacco and Health (PATH) Study
A national longitudinal study of tobacco use and how it affects the health of people in the United States, begun in 2013.
Interested in funding opportunities or planning to submit an application in tobacco use research? Use these tools to find what you need:
- Funding Opportunities
- Funded Projects
NIH-Funded Projects
- ClinicalTrials.gov
NIH Institutes/Centers, and Federal Partner Activities
Many NIH Institutes and Centers (ICs) actively engage in and support tobacco research and may be helpful starting points as you plan your research or if you’re exploring the latest work in a specific area. Because ICs may have several ongoing tobacco programs and projects, we encourage you to use the links provided to find the most up-to-date information, including how to contact program officials who may be able to address your specific questions.
National Institutes of Health (NIH)
- Collaborative Research on Addiction at NIH (CRAN)
Fogarty International Center (FIC)
- International Tobacco and Health Research and Capacity Building Program (TOBAC)
National Cancer Institute (NCI)
- Tobacco Control Research Branch (TCRB)
U.S. Department of Health and Human Services (HHS) Office of Disease Prevention and Health Promotion (ODPHP)
- Healthy People 2030 – Tobacco Use
U.S. Food & Drug Administration (FDA)
- Center for Tobacco Products (CTP)
National Institute on Drug Abuse (NIDA)
- Nicotine and Tobacco Research Team (NTRT)
National Institute of Dental and Craniofacial Research (NIDCR)
- Behavioral and Social Sciences Research Branch (BSSRB)
National Heart, Lung, and Blood Institute (NHLBI)
- Division of Lung Diseases (DLD)
National Institute on Environmental Health Sciences (NIEHS)
- Environmental Epigenomics and Disease Group
Office of Disease Prevention (ODP)
- Tobacco Regulatory Science Program (TRSP)
Recent NIH News
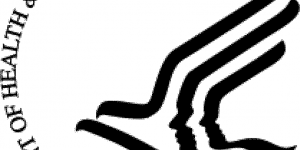
HHS Announces New Smoking Cessation Framework to Support Quitting
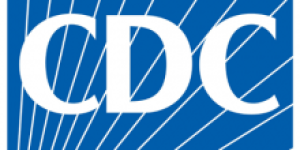
CDC’s Tips From Former Smokers® Launches New Ads to Encourage People to Quit Smoking
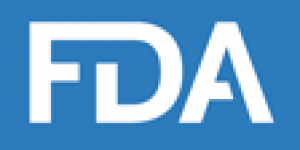
CTP Releases Five-Year Strategic Plan
- Open access
- Published: 30 July 2021
Impact of tobacco and/or nicotine products on health and functioning: a scoping review and findings from the preparatory phase of the development of a new self-report measure
- Esther F. Afolalu ORCID: orcid.org/0000-0001-8866-4765 1 ,
- Erica Spies 1 ,
- Agnes Bacso 1 ,
- Emilie Clerc 1 ,
- Linda Abetz-Webb 2 ,
- Sophie Gallot 1 &
- Christelle Chrea 1
Harm Reduction Journal volume 18 , Article number: 79 ( 2021 ) Cite this article
14k Accesses
4 Citations
10 Altmetric
Metrics details
Measuring self-reported experience of health and functioning is important for understanding the changes in the health status of individuals switching from cigarettes to less harmful tobacco and/or nicotine products (TNP) or reduced-risk products (RRP) and for supporting tobacco harm reduction strategies.
This paper presents insights from three research activities from the preparatory phase of the development of a new self-report health and functioning measure. A scoping literature review was conducted to identify the positive and negative impact of TNP use on health and functioning. Focus groups ( n = 29) on risk perception and individual interviews ( n = 40) on perceived dependence in people who use TNPs were reanalyzed in the context of health and functioning, and expert opinion was gathered from five key opinion leaders and five technical consultants.
Triangulating the findings of the review of 97 articles, qualitative input from people who use TNPs, and expert feedback helped generate a preliminary conceptual framework including health and functioning and conceptually-related domains impacted by TNP use. Domains related to the future health and functioning measurement model include physical health signs and symptoms, general physical appearance, functioning (physical, sexual, cognitive, emotional, and social), and general health perceptions.
Conclusions
This preliminary conceptual framework can inform future research on development and validation of new measures for assessment of overall health and functioning impact of TNPs from the consumers’ perspective.
As a leading cause of preventable morbidity and mortality worldwide, smoking remains a major public health problem. Compared with those who do not smoke, people who smoke are significantly more likely to develop heart diseases, lung cancer, chronic obstructive pulmonary disease (COPD), and other diseases [ 1 , 2 ]. It is well established that the best way to avoid the health risks associated with smoking is for people to never start and for those who smoke to quit [ 1 , 3 ]. Tobacco harm reduction is one way to alleviate the health risk for individuals who choose not to quit smoking [ 4 ], by providing less harmful tobacco and/or nicotine products (TNP), such as reduced-risk products (RRP) (used here to refer to products that present, are likely to present, or have the potential to present, less risk of harm to people who smoke and switch to these products versus continued smoking) or modified risk tobacco products (MRTP).
Several smokeless tobacco products and a heated tobacco product were recently authorized for marketing with modified risk claims through the United States (US) Food and Drug Administration (FDA) MRTP pathway [ 5 ]. The guidance on MRTP applications [ 6 ] specifies that health outcomes should be assessed during premarket evaluation and postmarket surveillance of modified risk TNPs such as these. These health outcomes comprise not only objective clinical and biological measures but also self-reported outcomes [ 6 , 7 ]. Studies and reports have recently started providing evidence on the health impact of new TNPs [ 8 ]. For instance, recent papers have investigated the effects of e-cigarettes and heated tobacco products on cardiopulmonary outcomes [ 9 , 10 , 11 , 12 , 13 , 14 ]. However, the papers have mainly focused on clinical measurements, such as spirometry and other lung function tests; consumer perception is rarely explored or the focus of the research. Measuring self-reported experience is important for understanding the changes in the health status of individuals switching from cigarettes to RRPs and is a key component of tobacco harm reduction strategies [ 7 ]. Self-reported ratings of RRP effectiveness or adverse events might differ from clinical measures and provide another perspective as useful as the clinicians. In addition, consumer perception of positive changes in health status, functioning and other behavioral outcomes will also subsequently influence use behaviors and switching to RRPs rather than continuing smoking.
Self-perceived health status is a complex concept to define and measure, particularly within the context of TNP use [ 15 ]. While generic health status measures, such as the Medical Outcomes Study 36-item Short-Form Health Survey (SF-36), have been used to evaluate the health status of people who smoke [ 16 , 17 ], comparisons have mainly been made between those who currently smoke, those who used to smoke, and those who never smoked [ 18 , 19 ]. Results from these studies strongly suggest that, in healthy populations, existing generic measures are not sensitive enough to detect change over time when stopping or switching from cigarettes to other TNPs, owing to high ceiling effects [ 20 ]. While a few smoking-specific quality of life measures have been developed, these measures have not been widely implemented or standardized [ 15 , 17 , 21 , 22 ], and the application of these smoking-specific measures to different TNP use across the risk continuum is scarce [ 20 ].
As part of the A ssessment of B ehavioral OU tcomes related to T obacco and Nicotine Products (ABOUT™) Toolbox initiative [ 23 ], the present project aims at developing a new self-report measure (ABOUT™— Health and Functioning ) to address the current gap and assess the impact of TNPs on health and functioning (including health status, functional status and other health-related quality of life constructs). This paper presents insights from three research activities [ 24 , 25 ] from the preparatory phase of development of the measure—that is, a scoping literature review, reanalysis of consumer focus groups/interviews, and expert opinion. These three activities serve as background research to support the development of a preliminary conceptual framework of health and functioning associated with the use of TNPs.
Scoping literature review
The purpose of the review was to address two main questions among individuals who use TNPs:
What are the positive and negative health and functioning impacts of TNP use?
What concepts are evaluated by measures used to assess the positive and negative impacts of TNP use?
Given the nature and breadth of the research questions and the number of potentially relevant publications, a scoping literature review was used as it provides a means of identifying the literature and mapping the concepts and evidence on a topic by using an informative and iterative research process [ 26 ]. The scoping review involved a PubMed search (August 2018) and application of Sciome’s rapid Evidence Mapping (rEM) [ 27 ], followed by additional manual screening and review. rEM is a proprietary methodology developed by Sciome ( https://www.sciome.com/ ) to rapidly summarize and produce a quantitative representation of the available body of scientific evidence in a particular area. The study by Lam et al. demonstrated a proof-of-concept application of the rEM methodology [ 27 ]. The PubMed search terms targeted qualitative and quantitative research among people who use TNPs (Table 1 ). This was supplemented by a second, parallel step of manually identifying relevant literature through other known sources. Table 2 describes the general inclusion and exclusion criteria that were applied to the scoping literature review.
After the initial rEM exercise, two reviewers (EC, SG) further manually screened the titles and abstracts of the articles identified through the automated rEM exercise against the inclusion and exclusion criteria. Finally, the selected publications underwent a full screening by two reviewers (VL and DF) for determining their relevance to the research questions for data extraction and one of the co-authors (LA-W) cross-checked the screening and resolved differences in opinion among the reviewers.
The World Health Organization (WHO) International Classification of Functioning, Disability and Health (ICF) [ 28 ] framework and the revised Wilson and Cleary [ 29 , 30 ] model were used as a guide to broadly inform categories for data extraction from the literature on TNP use and health and functioning. These established models enable the conceptualization and description of health status and functioning (the combination of which is often referred to as health-related quality of life) [ 31 , 32 ], and related outcomes and determinants. To complement and refine this and to ensure relevance to those who use TNPs, the data extracted from the literature was also grouped and labeled based on the contents of the literature reviewed.
The elements extracted from the selected papers were as follows:
Author, citation details, and publication type
Objectives and/or research questions
Sample type, size, and principle demographics
Type(s) of TNP and definitions of levels of consumption
Methodology, questionnaires, and statistical methods used
Main results
Results grouped in broad categories: Health Signs and Symptoms; General Health Perceptions; Quality of Life, Health-Related Quality of Life, and Functional Status; Individual Characteristics; Environmental and Social Characteristics; Biomarkers and Biological Endpoints.
Reanalysis of focus groups/in-depth interviews
The objective of the secondary analyses of existing qualitative data in people who use TNPs was to inform the drafting of the initial conceptual framework, as well as interview guides for planned concept elicitation qualitative studies to identify concepts and develop items to detect what is relevant to measure in this context. Two sets of qualitative data containing information related to health and functioning were reanalyzed and participants had consented for their data to be used in future studies. The first was from 29 focus groups (total number of participants n = 229) that were originally designed to discuss perceived risk, appeal, and intent to use TNPs [ 33 , 34 ]. The focus groups—stratified by smoking status—were conducted in the United States (US; n = 12), Japan ( n = 4), Italy ( n = 4), and the United Kingdom (UK; n = 9) between December 2012 and August 2013. The second dataset included 40 in-depth interviews conducted in North Carolina, USA, with people who use TNPs, to discuss issues centered on perceived dependence on TNPs [ 35 ]. While 21 interviewees were people who were poly-TNPs users, 19 were people who were exclusive users of one of the following types of TNPs: cigarettes ( n = 5), smokeless tobacco ( n = 5), e-cigarettes ( n = 5), or another type of TNP (pipes, waterpipes, or nicotine replacement therapy [NRT] products; n = 4). These interviews were conducted in August 2017. The demographics of both data sets are presented in Table 3 . For reanalyzing the data, an initial codebook guided by the literature review data extraction categories was developed; however, new codes were created to complement these categories based on the thematic content analysis of the transcripts. The qualitative analysis software Quirkos [ 36 ] was used for the reanalysis.
Expert panel review
An expert panel consisting of five key opinion leaders (KOL) and five technical consultants was convened in August 28, 2018, in Neuchâtel, Switzerland. The KOLs were subject matter experts in the fields of nicotine and smoking cessation ( n = 1), Patients Reported Outcomes (PRO) evaluation and scale development ( n = 3), and health economics ( n = 1). The consultants were experts on nicotine dependence ( n = 1), psychometric validation ( n = 2), market research ( n = 1), and PRO development and validation ( n = 1). The meeting followed an agenda and semi-structured discussion guide to facilitate conversations. First, the panel was presented with the principles underlying the tobacco harm reduction assessment strategy [ 4 ]. This session was followed by an open elicitation phase, during which two experienced moderators asked the panel to identify and discuss concepts related to health and functioning in people who use TNPs that different stakeholders might find important. Then, the panel was asked to review and respond to the concepts identified in the literature review and in the qualitative research reanalysis. These findings were discussed in depth to arrive at a consolidated preliminary conceptual framework. Each concept was presented, and the experts were asked to rank and agree on concepts to be included and how the concepts should be grouped by domains in the framework. In generating the framework, the project team and expert panel considered the themes and concepts identified under each of the categories from the scoping literature review, specific concepts from the secondary analyses of the qualitative data, and the expert panel meeting. The authors then synthesized and re-organized concepts emerging from the different preparatory phase activities under main health and functioning and conceptually-related domains. The participants also provided their input on the best strategies for planned qualitative studies to inform and support the development and validity of the proposed health and functioning measure.
The literature search identified 4761 articles. Figure 1 (flow diagram) depicts the results of the search and screening process. Titles and abstracts were screened by the rEM exercise until the machine learning algorithms predicted 97.7% relevant references; thus, 707 abstracts were not screened. After applying the inclusion/exclusion criteria to the remaining 4,054 abstracts, 281 were identified as part of the rEM exercise. After additional manual screening and review of the abstracts and articles against the inclusion/exclusion criteria, 90 full-text articles were included for data extraction [ 20 , 37 – 125 ]. Seven additional full-text articles were also included on the basis of a manual search [ 126 , 127 , 128 , 129 , 130 , 131 , 132 ]. Findings are summarized in Table 4 and a detailed description and data extracted from all the articles from the literature review is presented in Additional File 1 .
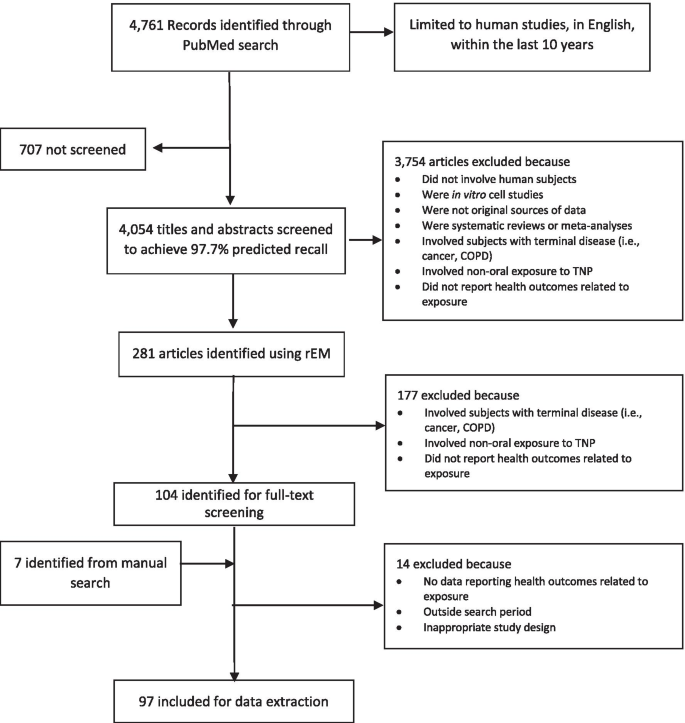
Flow diagram Sciome’s rapid Evidence Mapping (rEM) and manual screening processes of the scoping literature review
Fifty-six publications (56/97; 58%) presented data related to health signs and symptoms . These are grouped under five core areas: mental health and cognitive functioning (28/97; 29%); pain and physical trauma (6/97; 6%); respiratory, cardiovascular and inflammatory conditions (5/97; 5%); “other” health conditions , which included insomnia, liver disease, eye health, and hearing loss (5/97; 5%); and oral health (4/97; 4%). There were also eight publications related to the effects of smoking cessation on health signs and symptoms, mostly benefits of cessation but also including perceived dependence, addiction, and withdrawal symptoms (8/97; 8%). Overall, the burden and impact of cigarette smoking on both physical and mental health symptoms was negative and generally worse among people who smoke relative to those who do not smoke. On the other hand, quitting smoking was accompanied by improvements in general physical health and psychological wellbeing. However, in spite of the positive impact of quitting smoking, loss of moments of pleasure, struggle to manage stress, the social aspects of smoking, and withdrawal symptoms were seen as barriers to quitting.
The general health perceptions of various adults who use TNPs were reported in 18 of the 97 articles (18%), with 9 of them detailing the general health perceptions related to cigarettes and 9 being related to e-cigarettes and other TNPs. Perceptions were determined by questionnaires and focus groups for evaluating the health impacts, fear of diseases, harm to others and self, social impacts (both positive [e.g., inclusion and looking “cool”] and negative [e.g., stigma and exclusion]), and other reasons for taking up or considering/attempting smoking cessation.
Quality of life, health-related quality of life, and functional status was studied in 9 of the 97 included articles (9%). These studies mostly demonstrated with generic and specific QoL, HRQoL, or functional status questionnaires that cigarette smoking was associated with a worse quality of life and that smoking cessation often resulted in an improved quality of life. However, in some cases, the use of TNPs also reportedly enabled individuals to manage their levels of anxiety and improve some aspects of social engagement and functional status.
Individual, environmental and social characteristics were found to influence the decision to smoke and/or consider or attempt to quit smoking or switching to other TNPs, as reported in 8 (8%) and 11 (11%) of 97 publications, respectively. Some key characteristics and determinants of smoking behavior included low socioeconomic status, male sex, living alone, family, and close social environment, societal stigma, and local regulations.
Finally, 12 of the 97 publications (12%) were related to studies on biomarkers and biological endpoints in people who use TNPs and showed that smoking cigarettes negatively influenced cardiovascular, respiratory, oral, renal, stress, metabolic, and inflammatory-related biomarkers and physiological assessments.
The themes from this reanalysis are summarized below and organized on the basis of the narrative of the participants of their experiences.
Perceived negative impact of smoking
Other than health, the biggest and most salient reported negative impact of smoking was the perceived lack of control related to addiction and emotional health and wellbeing. Participants reported feeling that cigarette smoking was running their lives or “holding them hostage.” They reported that this perceived lack of a sense of control or willpower often led to feelings of weakness or a feeling that they were a “slave” to cigarettes. Many respondents reported smoking even when they did not necessarily want to and experiencing feelings of obsession and craving.
Perceived lack of control and addiction were also related to the activities of the participants throughout the day. People who smoke often reported altering their activities to smoke because of patterns of behavior or routine and the experienced need for a smoke. They reported that the “need for a smoke” sensation would cause them to leave work or social events early, not attend events if smoking was not allowed, interrupt what they were doing to smoke, and get up in the middle of the night.
Fear of withdrawal symptoms, with a strong emphasis on mental/emotional health, was also prominent among reported negative impacts of smoking. This fear was often reported as limiting the willingness of individuals to try to quit smoking or facilitating a return to prior smoking behavior. Individuals reported fearing the following symptoms they associated with withdrawal: mood swings and irritability, violent or aggressive behavior, inability to concentrate, anxiety, anger, and weight gain.
Perceived benefits of smoking
Several perceived benefits were identified that keep individuals smoking or using cigarettes. These included perceptions of enhanced cognitive functioning, relaxation, a way to take a break, use as a coping strategy, a social function, a weight management tool, the perception that it feels good, and being part of one’s identity. It is also important to note that the perceived benefits of smoking often outweighed the risks and the feeling of lack of control in the participant discussions. Even people who used to smoke noted they missed the relaxation and breaks they associated with smoking.
Recognition of symptoms/diseases related to smoking
Table 5 summarizes the negative symptoms and diseases related to smoking recognized by participants in both the focus groups and interviews. These were mostly related to six main body systems (cardiovascular, digestive, oral, neurological, reproductive, and respiratory).
Impacts on physical functioning
The participants noted how smoking impacts their physical functioning. In particular, they noted how their exercise capacity during running, playing sports, walking upstairs, and general physical activity was diminished. They also reported reduced stamina and endurance, decreased physical strength, and feeling tired more easily.
Effects on emotional health
The participants also described how smoking impacts their emotional health and wellbeing. People who smoke reported feelings of shame, guilt, weakness, and a lack of control or powerlessness. They also reported feelings of depression and anxiety associated with worry about health risks. Furthermore, the participants indicated that they experienced a fear of going to places where they could not smoke, being a bad role model for their children, and (in case of people who used to smoke) going back to smoking.
Positive and negative social impacts
Smoking was perceived to have both negative and positive impacts on the social lives of participants. Smoking impacted life negatively when it was not allowed in certain environments, such as in homes, at work, and in cars and airplanes. Stigma was also associated with smoking in an environment where peers and family members do not smoke, but it was also seen as a source of group identity within social networks that had a higher prevalence of smoking behaviors. Participants reported that smoking had some positive impacts on their social interaction, because it facilitated work breaks and increased communication with peers.
Reasons people decided to try to quit
Throughout the focus groups and interviews, individuals identified several reasons why they tried to quit smoking. These included: health, diagnosis of cancer (self, family, or friend), gum disease, pregnancy, hospital stay, worry that it will “kill me,” dislike of taste or odor, social reasons, change in surroundings (fewer smoking spaces), and price.
Reasons people do not like alternatives to cigarettes
The participants’ reasons for not liking alternatives to cigarettes (i.e., less harmful TNPs/RRPs) included perceptions that the alternatives did not work (i.e., the participants still had cravings and experienced withdrawal symptoms), made them feel or get ill (nausea and vomiting), were not “the same” as cigarettes in terms of the ritual, taste, or “feeling,” or were inconvenient/too big to carry.
The conclusions of the expert panel widely supported the findings of the literature review and the input from the reanalyzed focus groups and interviews. Some of the experts working in field of tobacco and nicotine provided additional insights based on their extensive experience with people who use TNPs; they highlighted the importance of the enjoyment of smoking for people who find it difficult to quit, the positive immediate benefits of quitting, and the smoking-related biomarkers that might be on a causal pathway between switching and changes in health and functioning status.
The following main areas were discussed and agreed during the meeting: (1) utility of use, referring to the perceived satisfaction and enjoyment of smoking (e.g., craving relief, weight control, and social affiliation); (2) signs and symptoms of withdrawal (e.g., anxiety, depression, and anger) and the positive immediate physical health effects of quitting smoking (e.g., better general and oral hygiene, less coughing, and improved exercise capacity); (3) functioning, including cognitive, physical, sexual, social, emotional, and role functioning; (4) worry associated with smoking and smoking-related diseases; (5) general health perceptions and quality of life; (6) association with smoking-related biomarkers that could be on the causal pathway between switching and changes in health and functioning; and (7) TNP use patterns and maintenance of switching to RRPs.
Generation of the preliminary conceptual framework
Triangulation of the findings from the literature review, qualitative input from people who use TNPs, and expert panel feedback helped generate a preliminary descriptive conceptual framework that includes the health and functioning and conceptually-related domains impacted by TNP use (Fig. 2 ).

Health and functioning conceptual framework related to tobacco and/or nicotine product use from the preparatory phase research findings
Four domains related to the future health and functioning measurement model for TNP use are indicated in grey rectangular boxes and include (moving down from proximal to distal parameters) physical health symptoms (e.g., oral and respiratory symptoms), general physical condition (e.g., appearance and hygiene), functioning (physical, sexual, cognitive, emotional, and social functioning), and general health perceptions, which will be the most distal measure of health and functioning. The preparatory phase research also identified six conceptually-related domains (in dashed rectangular boxes), which are not direct indicators of health status but might influence the impact of TNP use on health and functioning. These include attitudinal variables (worry about the health risks of using TNPs and perceived dependence/fear of withdrawal symptoms associated with quitting smoking), and utilitarian ones (perceived appeal, satisfaction, and benefits of TNP use). In addition, personal factors (e.g., sociodemographic) and environmental factors (e.g., peer/family influence, policies and regulations and sociocultural context) are also reflected in the conceptual framework as indirect indicators of health and functioning.
The framework further indicates that specific behavioral indicators (i.e., TNP use patterns over time) might influence any impact of TNP use on health and functioning. Whilst other causal and reciprocal relationships and hierarchies might exist within the domains, these are not explicitly characterized in this initial draft of the framework and will have to be tested with further empirical data. Finally, identified biomarkers of potential harm (in italics and dashed box) are also integrated in this conceptual framework as part of the conceptually-related domains, because they are on a causal pathway between TNP use and changes in health and functioning [ 133 , 134 ]. Biomarkers are not part of the measurement model that will be considered for a new self-report measure; however, because they are the most proximal parameters to health and functioning, they will be assessed independently as appropriate endpoints by objective clinical or biological analyses.
Triangulation of published literature, reanalysis of qualitative data, and expert opinion helped develop the presented preliminary conceptual framework as the foundation for a new measure to assess the impact of TNPs on self-reported health and functioning. This is essential for identifying relevant concepts and understanding what is important to measure in people who use TNPs. The findings reveal the importance of not only the perceived impacts of TNP use on physical health and physical functioning, but also on aspects of mental health and social interactions and functioning, and general perceptions of health and health-related quality of life.
For the literature review, the WHO ICF [ 28 ] and Wilson and Cleary model [ 29 , 30 ] served as useful guides to develop categories for data abstraction. The scoping literature review yielded 97 articles on TNP use and the relationship to health, perceptions of health, social and individual functioning, and quality of life. Overall, most studies had focused on the known negative effects of cigarette smoking (e.g., mental, respiratory, and oral health) and the rationale and motivation to quit smoking. The WHO ICF and Wilson and Clearly models were not always sufficient for identifying the breadth of relevant concepts, especially from the perspective of TNP use. Development of new codes for the reanalysis of existing qualitative data allowed for the development, extension, and exploration of the topic and provided valuable insights reported in the qualitative data reanalysis, such as the perceived benefits/satisfaction from cigarette smoking, and the rationale for quitting smoking or switching to an RRP. The findings show how this manner of secondary analysis can be valuable in health-related fields where the topic is broad and an existing body of knowledge can contribute by offering a different perspective [ 135 ].
The presentation of the preliminary conceptual framework from this preparatory phase is specific to TNP use and marks a slight departure from the established norms and characterization of the variables typically observed in existing generic health and functioning and health-related quality of life models, such as the WHO ICF and Wilson and Clearly models. Notably, specific hypothesized relationships and the hierarchy between domains are not explicitly characterized in the current draft of the framework. The framework provided an exploratory representation of the current findings to reflect a measurement instrument in people who use TNPs that would ideally be able to assess and demonstrate improvements in self-reported health and functioning status, stability of perceived positive aspects of using TNPs, and no worsening in key areas of physical and emotional health and functioning upon switching to RRPs. Nevertheless, the framework could still undergo further refinement to support the development and validation of a new measure and to further characterize and test the relationships and hierarchies between domains.
This work is not without limitations. For the scoping literature review, among the reviewed articles, not many reported on the use of e-cigarettes and other alternative tobacco or nicotine-delivery devices, because most studies had focused exclusively on cigarettes. It is possible that concepts associated with health and functioning that are relevant to other TNPs were not identified. This is most likely the consequence of the large number of publications related to cigarette use. Some concepts might also have been missed, given the large evidence base on health and functioning-related themes and concepts. However, this was also not a systematic literature search; a scoping review is generally broader than a systematic review in terms of the former having a less-defined research question, broader inclusion and exclusion criteria, and no systematic appraisal of study quality [ 26 ]. Nevertheless, the present scoping review methodology provides a lens on the overall evidence base, and regular updates on the search—specifically related to RRPs and novel TNPs and their health and functioning impacts—could be considered for fully understanding the evolving state of the art in this context. The reanalysis of existing qualitative data also has limitations related to data fit and completeness of preexisting data [ 136 ]. The insights collected from these reanalyzed studies were originally for a different purpose several years prior to the present research, and this might not completely and accurately reflect the objectives of the new project.
Considering the findings of the current research, the development of a health and functioning measure can continue to follow the FDA’s Guidance on PRO measures. As specified within the guideline, gaining input directly from the intended use populations through concept elicitation is a critical activity for ensuring content validity during the development of any new self-reported measure [ 137 ]. Continuous engagement with an expert panel can also support the refinement of the conceptual framework as well as the development of the draft and final measure.
The goal of this research was to identify from varied research activities key concepts and aspects of health and functioning and related changes associated with the use of TNPs. The resulting preliminary conceptual framework provides the basis for informing future research to further understand health and functioning concepts important to measure in individual who switch to RRPs and to develop a new self-report measure to assess this from the consumers’ perspective.
Availability of data and materials
All data generated or analyzed during this study are included in this published article and its supplementary information files.
Abbreviations
Assessment of Behavioral OUtcomes related to Tobacco and Nicotine Products Toolbox
Chronic obstructive pulmonary disease
Food and Drug Administration
Health-related quality of life
International Classification of Functioning, Disability and Health
- Modified risk tobacco products
Nicotine replacement therapy
Patient-Reported Outcomes
Quality of life
Reduced-risk products
Rapid Evidence Mapping
- Tobacco and/or nicotine products
United Kingdom
United States
36-Item Short-Form Health Survey
World Health Organization
U.S. Department of Health and Human Services. The health consequences of smoking: 50 years of progress. A report of the surgeon general. Atlanta, GA: U.S. Department of Health and Human Services, Centers for Disease Control and Prevention, National Center for Chronic Disease Prevention and Health Promotion, Office on Smoking and Health; 2014.
Google Scholar
Danaei G, Ding EL, Mozaffarian D, Taylor B, Rehm J, Murray CJL, et al. The preventable causes of death in the United States: comparative risk assessment of dietary, lifestyle, and metabolic risk factors. PLoS Med. 2009;6(4):e1000058.
Article PubMed PubMed Central Google Scholar
Bilano V, Gilmour S, Moffiet T, d’Espaignet ET, Stevens GA, Commar A, et al. Global trends and projections for tobacco use, 1990–2025: an analysis of smoking indicators from the WHO Comprehensive Information Systems for Tobacco Control. Lancet. 2015;385(9972):966–76.
Article PubMed Google Scholar
Zeller M, Hatsukami D. The strategic dialogue on tobacco harm reduction: a vision and blueprint for action in the US. Tob Control. 2009;18(4):324–32.
Food and Drug Administration. Modified risk orders 2020. https://www.fda.gov/tobacco-products/advertising-and-promotion/modified-risk-orders .
Food and Drug Administration. Modified risk tobacco product applications: draft guidance for industry 2012. https://www.fda.gov/regulatory-information/search-fda-guidance-documents/modified-risk-tobacco-product-applications .
Institute of Medicine. Scientific standards for studies on modified risk tobacco products. Washington, DC: The National Academies Press; 2012. p. 370.
Stratton K, Kwan LY, Eaton DL, editors. National Academies of Sciences Engineering and Medicine. Public health consequences of e-cigarettes. Washington, DC: The National Academies Press; 2018. p. 774.
Lappas AS, Tzortzi AS, Konstantinidi EM, Teloniatis SI, Tzavara CK, Gennimata SA, et al. Short-term respiratory effects of e-cigarettes in healthy individuals and smokers with asthma. Respirology. 2018;23(3):291–7.
Meo SA, Ansary MA, Barayan FR, Almusallam AS, Almehaid AM, Alarifi NS, et al. Electronic cigarettes: impact on lung function and fractional exhaled nitric oxide among healthy adults. Am J Men’s Health. 2019;13(1):1557988318806073.
Article Google Scholar
Polosa R, Cibella F, Caponnetto P, Maglia M, Prosperini U, Russo C, et al. Health impact of E-cigarettes: a prospective 3.5-year study of regular daily users who have never smoked. Sci Rep. 2017;7(1):13825.
Article PubMed PubMed Central CAS Google Scholar
Sharman A, Zhussupov B, Sharman D, Kim I, Yerenchina E. Lung function in users of a smoke-free electronic device with HeatSticks (iQOS) versus smokers of conventional cigarettes: protocol for a longitudinal cohort observational study. JMIR Res Protoc. 2018;7(11):e10006.
Skotsimara G, Antonopoulos AS, Oikonomou E, Siasos G, Ioakeimidis N, Tsalamandris S, et al. Cardiovascular effects of electronic cigarettes: a systematic review and meta-analysis. Eur J Prev Cardiol. 2019;26(11):1219–28.
Wang JB, Olgin JE, Nah G, Vittinghoff E, Cataldo JK, Pletcher MJ, et al. Cigarette and e-cigarette dual use and risk of cardiopulmonary symptoms in the Health eHeart Study. PLoS ONE. 2018;13(7):e0198681.
Ware JE Jr, Gandek B, Kulasekaran A, Guyer R. Evaluation of smoking-specific and generic quality of life measures in current and former smokers in Germany and the United States. Health Qual Life Outcomes. 2015;13:128.
Frendl DM, Ware JE Jr. Patient-reported functional health and well-being outcomes with drug therapy: a systematic review of randomized trials using the SF-36 health survey. Med Care. 2014;52(5):439–45.
Goldenberg M, Danovitch I, IsHak WW. Quality of life and smoking. Am J Addict. 2014;23(6):540–62.
Olufade AO, Shaw JW, Foster SA, Leischow SJ, Hays RD, Coons SJ. Development of the smoking cessation quality of life questionnaire. Clin Ther. 1999;21(12):2113–30.
Article CAS PubMed Google Scholar
Sarna L, Bialous SA, Cooley ME, Jun HJ, Feskanich D. Impact of smoking and smoking cessation on health-related quality of life in women in the Nurses’ Health Study. Qual Life Res. 2008;17(10):1217–27.
Kulasekaran A, Proctor C, Papadopoulou E, Shepperd CJ, Guyer R, Gandek B, et al. Preliminary evaluation of a new german translated tobacco quality of life impact tool to discriminate between healthy current and former smokers and to explore the effect of switching smokers to a reduced toxicant prototype cigarette. Nicotine Tob Res. 2015;17(12):1456–64.
Edelen MO. The PROMIS smoking assessment toolkit–background and introduction to supplement. Nicotine Tob Res. 2014;16(Suppl 3):S170–4.
Shaw JW, Coons SJ, Foster SA, Leischow SJ, Hays RD. Responsiveness of the Smoking Cessation Quality of Life (SCQoL) questionnaire. Clin Ther. 2001;23(6):957–69.
Chrea C, Acquadro C, Afolalu EF, Spies E, Salzberger T, Abetz-Webb L, et al. Developing fit-for-purpose self-report instruments for assessing consumer responses to tobacco and nicotine products: the ABOUT Toolbox initiative. F1000Res. 2018;7:1878.
Food and Drug Administration. Patient-reported outcome measures: use in medical product development to support labeling claims 2009. https://www.fda.gov/regulatory-information/search-fda-guidance-documents/patient-reported-outcome-measures-use-medical-product-development-support-labeling-claims .
Food and Drug Administration. Patient-focused drug development: methods to identify what is important to patients guidance for industry, Food and Drug Administration Staff, and Other Stakeholders 2019. https://www.fda.gov/regulatory-information/search-fda-guidance-documents/patient-focused-drug-development-methods-identify-what-important-patients-guidance-industry-food-and .
Munn Z, Peters MDJ, Stern C, Tufanaru C, McArthur A, Aromataris E. Systematic review or scoping review? Guidance for authors when choosing between a systematic or scoping review approach. BMC Med Res Methodol. 2018;18(1):143.
Lam J, Howard BE, Thayer K, Shah RR. Low-calorie sweeteners and health outcomes: a demonstration of rapid evidence mapping (rEM). Environ Int. 2019;123:451–8.
World Health Organisation. International classification of functioning, disability and health (ICF) 2001. https://www.who.int/classifications/icf/en/ .
Ferrans CE, Zerwic JJ, Wilbur JE, Larson JL. Conceptual model of health-related quality of life. J Nurs Scholarsh. 2005;37(4):336–42.
Wilson IB, Cleary PD. Linking clinical variables with health-related quality of life. A conceptual model of patient outcomes. JAMA. 1995;273(1):59–65.
Karimi M, Brazier J. Health, health-related quality of life, and quality of life: what is the difference? Pharmacoeconomics. 2016;34(7):645–9.
Moons P. Why call it health-related quality of life when you mean perceived health status? Eur J Cardiovasc Nurs. 2004;3(4):275–7.
Cano S, Chrea C, Salzberger T, Alfieri T, Emilien G, Mainy N, et al. Development and validation of a new instrument to measure perceived risks associated with the use of tobacco and nicotine-containing products. Health Qual Life Outcomes. 2018;16(1):192.
Salzberger T, Chrea C, Cano SJ, Martin M, Atkison M, Emilien G, et al. Perceived risks associated with the use of tobacco and nicotine-containing products: findings from qualitative research. Tobacco Sci Technol. 2017;50:32–42.
Chrea C, Salzberger T, Abetz-Webb L, Afolalu EF, Cano S, Rose J, et al. PRM183—development of a fit-for-purpose tobacco and nicotine products dependence instrument. Barcelona: ISPOR Europe; 2018.
Book Google Scholar
Quirkos 2.3.1 [Computer Software] 2020. https://www.quirkos.com .
Abu-Helalah MA, Alshraideh HA, Al-Serhan AA, Nesheiwat AI, Da’na M, Al-Nawafleh A. Epidemiology, attitudes and perceptions toward cigarettes and hookah smoking amongst adults in Jordan. Environ Health Prev Med. 2015;20(6):422–33.
Akturk E, Yağmur J, Açıkgöz N, Ermi N, Cansel M, Karaku Y, et al. Assessment of atrial conduction time by tissue Doppler echocardiography and P-wave dispersion in smokers. J Interv Card Electrophysiol. 2012;34(2):247–53.
Aubin HJ, Peiffer G, Stoebner-Delbarre A, Vicaut E, Jeanpetit Y, Solesse A, et al. The French Observational Cohort of Usual Smokers (FOCUS) cohort: French smokers perceptions and attitudes towards smoking cessation. BMC Public Health. 2010;10(100):1–8.
Becoña E, Vázquez MI, Míguez MC, Fernández del Río E, López-Durán A, Martínez Ú, et al. Smoking habit profile and health-related quality of life. Psicothema. 2013;25(4):421–6.
PubMed Google Scholar
Bennasar Veny M, Pericas Beltrán J, González Torrente S, Segui González P, Aguiló Pons A, Tauler RP. Self-perceived factors associated with smoking cessation among primary health care nurses: a qualitative study. Rev Latino-Am Enfermagem. 2011;19(6):1437–44.
Bommelé J, Schoenmakers TM, Kleinjan M, van Straaten B, Wits E, Snelleman M, et al. Perceived pros and cons of smoking and quitting in hard-core smokers: a focus group study. BMC Public Health. 2014;14:175–85.
Borland R, Yong HH, O’Connor RJ, Hyland A, Thompson ME. The reliability and predictive validity of the Heaviness of Smoking Index and its two components: findings from the International Tobacco Control Four Country study. Nicotine Tob Res. 2010;12(Suppl 1):S45-50.
Bot M, Vink J, Milaneschi Y, Smit JH, Kluft C, Neuteboom J, et al. Plasma cotinine levels in cigarette smokers: impact of mental health and other correlates. Eur Addict Res. 2014;20(4):183–91.
Brody AL, Olmstead RE, Abrams AL, Costello MR, Khan A, Kozman D, et al. Effect of a history of major depressive disorder on smoking-induced dopamine release. Biol Psychiatry. 2009;66(9):898–901.
Article CAS PubMed PubMed Central Google Scholar
Brook DW, Rubenstone E, Zhang C, Brook JS. Trajectories of cigarette smoking in adulthood predict insomnia among women in late mid-life. Sleep Med. 2012;13(9):1130–7.
Brotman RM, He X, Gajer P, Fadrosh D, Sharma E, Mongodin EF, et al. Association between cigarette smoking and the vaginal microbiota: a pilot study. BMC Infect Dis. 2014;14:471–82.
Brown-Johnson CG, Cataldo JK, Orozco N, Lisha NE, Hickman NJ 3rd, Prochaska JJ. Validity and reliability of the Internalized Stigma of Smoking Inventory: an exploration of shame, isolation, and discrimination in smokers with mental health diagnoses. Am J Addict. 2015;24(5):410–8.
Bush T, Hsu C, Levine MD, Magnusson B, Miles L. Weight gain and smoking: perceptions and experiences of obese quitline participants. BMC Public Health. 2014;14:1229.
Caldirola D, Cavedini P, Riva A, Di Chiaro NV, Perna G. Cigarette smoking has no pro-cognitive effect in subjects with obsessive-compulsive disorder: a preliminary study. Psychiatr Danub. 2016;28(1):86–90.
Caldirola D, Daccò S, Grassi M, Citterio A, Menotti R, Cavedini P, et al. Effects of cigarette smoking on neuropsychological performance in mood disorders: a comparison between smoking and nonsmoking inpatients. J Clin Psychiatry. 2013;74(2):e130–6.
Carpenter MJ, Gray KM. A pilot randomized study of smokeless tobacco use among smokers not interested in quitting: changes in smoking behavior and readiness to quit. Nicotine Tob Res. 2010;12(2):136–43.
Croucher R, Haque MF, Kassim S. Oral pain before and after smokeless tobacco cessation in U.K.-resident Bangladeshi women: cross-sectional analyses. Nicotine Tobacco Res. 2013;15(5):896–903.
Article CAS Google Scholar
Depp CA, Bowie CR, Mausbach BT, Wolyniec P, Thornquist MH, Luke JR, et al. Current smoking is associated with worse cognitive and adaptive functioning in serious mental illness. Acta Psychiatr Scand. 2015;131(5):333–41.
Ditre JW, Zale EL, Heckman BW, Hendricks PS. A measure of perceived pain and tobacco smoking interrelations: pilot validation of the pain and smoking inventory. Cogn Behav Ther. 2017;46(6):339–51.
Doiron M, Dupré N, Langlois M, Provencher P, Simard M. Smoking history is associated to cognitive impairment in Parkinson’s disease. Aging Ment Health. 2017;21(3):322–6.
Gonzalez A, Zvolensky MJ, Vujanovic AA, Leyro TM, Marshall EC. An evaluation of anxiety sensitivity, emotional dysregulation, and negative affectivity among daily cigarette smokers: relation to smoking motives and barriers to quitting. J Psychiatr Res. 2008;43(2):138–47.
Grogan S, Fry G, Gough B, Conner M. Smoking to stay thin or giving up to save face? Young men and women talk about appearance concerns and smoking. Br J Health Psychol. 2009;14(1):175–86.
Hawari FI, Obeidat NA, Ghonimat IM, Ayub HS, Dawahreh SS. The effect of habitual waterpipe tobacco smoking on pulmonary function and exercise capacity in young healthy males: A pilot study. Respir Med. 2017;122:71–5.
Heffernan TM, O’Neill TS, Moss M. Smoking-related prospective memory deficits in a real-world task. Drug Alcohol Depend. 2012;120(1–3):1–6.
Highland KB, McChargue DE. Stress-induced cardiovascular reactivity among African American smokers. Am J Health Behav. 2011;35(1):51–9.
Holley AL, Law EF, Tham SW, Myaing M, Noonan C, Strachan E, et al. Current smoking as a predictor of chronic musculoskeletal pain in young adult twins. J Pain. 2013;14(10):1131–9.
Ichikawa Y, Kitagawa K, Kato S, Dohi K, Hirano T, Ito M, et al. Altered coronary endothelial function in young smokers detected by magnetic resonance assessment of myocardial blood flow during the cold pressor test. Int J Cardiovasc Imaging. 2014;30:73–80.
Javed F, Abduljabbar T, Vohra F, Malmstrom H, Rahman I, Romanos GE. Comparison of periodontal parameters and self-perceived oral symptoms among cigarette smokers, individuals vaping electronic cigarettes, and never-smokers. J Periodontol. 2017;88(10):1059–65.
Johnson AL, McLeish AC. Differences in panic psychopathology between smokers with and without asthma. Psychol Health Med. 2017;22(1):110–20.
Kahler CW, Daughters SB, Leventhal AM, Rogers ML, Clark MA, Colby SM, et al. Personality, psychiatric disorders, and smoking in middle-aged adults. Nicotine Tob Res. 2009;11(7):833–41.
Karadoğan D, Önal Ö, Şahin D, Yazıcı S, Kanbay Y. Evaluation of school teachers’ sociodemographic characteristics and quality of life according to their cigarette smoking status: a cross-sectional study from eastern Black Sea region of Turkey. Tuberkuloz ve toraks. 2017;65(1):18–24.
Kim J, Gall SL, Dewey HM, Macdonell RA, Sturm JW, Thrift AG. Baseline smoking status and the long-term risk of death or nonfatal vascular event in people with stroke: a 10-year survival analysis. Stroke. 2012;43(12):3173–8.
Kim J, Lee S. Using focus group interviews to analyze the behavior of users of new types of tobacco products. J Prev Med Public Health. 2017;50(5):336–46.
Kralikova E, Novak J, West O, Kmetova A, Hajek P. Do e-cigarettes have the potential to compete with conventional cigarettes?: a survey of conventional cigarette smokers’ experiences with e-cigarettes. Chest. 2013;144(5):1609–14.
Lao XQ, Jiang CQ, Zhang WS, Adab P, Lam TH, Cheng KK, et al. Smoking, smoking cessation and inflammatory markers in older Chinese men: The Guangzhou Biobank Cohort Study. Atherosclerosis. 2009;203(1):304–10.
Lappan S, Thorne CB, Long D, Hendricks PS. Longitudinal and reciprocal relationships between psychological well-being and smoking. Nicotine Tob Res. 2018;22(1):18–23.
Article PubMed Central Google Scholar
Lynch ME, Johnson KC, Kable JA, Carroll J, Coles CD. Smoking in pregnancy and parenting stress: maternal psychological symptoms and socioeconomic status as potential mediating variables. Nicotine Tob Res. 2011;13(7):532–9.
Lyvers M, Carlopio C, Honours VB, Edwards MS. Mood, mood regulation, and frontal systems functioning in current smokers, long-term abstinent ex-smokers, and never-smokers. J Psychoactive Drugs. 2014;46(2):133–9.
Martin LM, Sayette MA. A review of the effects of nicotine on social functioning. Exp Clin Psychopharmacol. 2018;26(5):425–39.
Martinasek MP, McDermott RJ, Bryant CA. Antecedents of university students’ hookah smoking intention. Am J Health Behav. 2013;37(5):599–609.
Mattey DL, Dawson SR, Healey EL, Packham JC. Relationship between smoking and patient-reported measures of disease outcome in ankylosing spondylitis. J Rheumatol. 2011;38(12):2608–15.
McCann SJ. Subjective well-being, personality, demographic variables, and American state differences in smoking prevalence. Nicotine Tob Res. 2010;12(9):895–904.
McDonald EA, Ling PM. One of several “toys” for smoking: young adult experiences with electronic cigarettes in New York City. Tob Control. 2015;24(6):588–93.
McLeish AC, Zvolensky MJ, Del Ben KS, Burke RS. Anxiety sensitivity as a moderator of the association between smoking rate and panic-relevant symptoms among a community sample of middle-aged adult daily smokers. Am J Addict. 2009;18(1):93–9.
Melis M, Lobo SL, Ceneviz C, Ruparelia UN, Zawawi KH, Chandwani BP, et al. Effect of cigarette smoking on pain intensity of TMD patients: a pilot study. Cranio. 2010;28(3):187–92.
Memon A, Barber J, Rumsby E, Parker S, Mohebati L, de Visser RO, et al. What factors are important in smoking cessation and relapse in women from deprived communities? A qualitative study in Southeast England. Public Health. 2016;134:39–45.
Mendiondo MS, Alexander LA, Crawford T. Health profile differences for menthol and non-menthol smokers: findings from the National Health Interview Survey. Addiction. 2010;105(Suppl 1):124–40.
Miyatake N, Numata T, Nishii K, Sakano N, Suzue T, Hirao T, et al. Influence of cigarette smoking on estimated glomerular filtration rate (eGFR) in Japanese male workers. Acta Med Okayama. 2010;64:385–90.
CAS PubMed Google Scholar
Mohammadi S, Mazhari MM, Mehrparvar AH, Attarchi MS. Cigarette smoking and occupational noise-induced hearing loss. Eur J Public Health. 2009;20(4):452–5.
Mohammadnezhad M, Tsourtos G, Wilson C, Ratcliffe J, Ward P. “I have never experienced any problem with my health. So far, it hasn’t been harmful”: older Greek-Australian smokers’ views on smoking: a qualitative study. BMC Public Health. 2015;15:304–15.
Morris MC, Mielock AS, Rao U. Salivary stress biomarkers of recent nicotine use and dependence. Am J Drug Alcohol Abuse. 2016;42(6):640–8.
Muir S, Marshall B. Changes in health perceptions of male prisoners following a smoking cessation program. J Correct Health Care. 2016;22(3):247–56.
Nelson JP, Pederson LL, Lewis J. Tobacco use in the Army: illuminating patterns, practices, and options for treatment. Mil Med. 2009;174(2):162–9.
Nemeth JM, Liu ST, Klein EG, Ferketich AK, Kwan MP, Wewers ME. Factors influencing smokeless tobacco use in rural Ohio Appalachia. J Community Health. 2012;37(6):1208–17.
Nikcevic AV, Spada MM. Metacognitions about smoking: a preliminary investigation. Clin Psychol Psychother. 2010;17(6):536–42.
Nunes SO, Vargas HO, Brum J, Prado E, Vargas MM, de Castro MR, et al. A comparison of inflammatory markers in depressed and nondepressed smokers. Nord J Psychiatry. 2012;14:540–6.
CAS Google Scholar
Oh DL, Heck JE, Dresler C, Allwright S, Haglund M, Del Mazo SS, et al. Determinants of smoking initiation among women in five European countries: a cross-sectional survey. BMC Public Health. 2010;10(74):1–11.
Pasco JA, Williams LJ, Jacka FN, Ng F, Henry MJ, Nicholson GC, et al. Tobacco smoking as a risk factor for major depressive disorder: population-based study. Br J Psychiatry. 2008;193(4):322–6.
Pina JA, Namba MD, Leyrer-Jackson JM, Cabrera-Brown G, Gipson CD. Social influences on nicotine-related behaviors. Int Rev Neurobiol. 2018;140:1–32.
Poole-Di Salvo E, Liu YH, Brenner S, Weitzman M. Adult household smoking is associated with increased child emotional and behavioral problems. J Dev Behav Pediatr. 2010;31(2):107–15.
Rahman MA, Mahmood MA, Spurrier N, Rahman M, Choudhury SR, Leeder S. Why do Bangladeshi people use smokeless tobacco products? Asia-Pacific J Public Health. 2015;27(2):NP2197–209.
Rollini F, Franchi F, Cho JR, Degroat C, Bhatti M, Ferrante E, et al. Cigarette smoking and antiplatelet effects of aspirin monotherapy versus clopidogrel monotherapy in patients with atherosclerotic disease: results of a prospective pharmacodynamic study. J Cardiovasc Transl Res. 2014;7(1):53–63.
Romijnders K, van Osch L, de Vries H, Talhout R. Perceptions and reasons regarding e-cigarette use among users and non-users: a narrative literature review. Int J Environ Res Public Health. 2018;15(6):1190.
Rooke C, Cunningham-Burley S, Amos A. Smokers’ and ex-smokers’ understanding of electronic cigarettes: a qualitative study. Tob Control. 2016;25:e60–6.
Roth B, Bengtsson M, Ohlsson B. Diarrhoea is not the only symptom that needs to be treated in patients with microscopic colitis. Eur J Intern Med. 2013;24(6):573–8.
Sales MP, Oliveira MI, Mattos IM, Viana CM, Pereira ED. The impact of smoking cessation on patient quality of life. J Bras Pneumol. 2009;35(5):436–41.
Schane RE, Prochaska JJ, Glantz SA. Counseling nondaily smokers about secondhand smoke as a cessation message: a pilot randomized trial. Nicotine Tob Res. 2013;15(2):334–42.
Schnoll RA, Goren A, Annunziata K, Suaya JA. The prevalence, predictors and associated health outcomes of high nicotine dependence using three measures among US smokers. Addiction. 2013;108(11):1989–2000.
Segarra R, Zabala A, Eguíluz JI, Ojeda N, Elizagarate E, Sánchez P, et al. Cognitive performance and smoking in first-episode psychosis: the self-medication hypothesis. Eur Arch Psychiatry Clin Neurosci. 2011;261(4):241–50.
Sherratt FC, Newson L, Marcus MW, Field JK, Robinson J. Perceptions towards electronic cigarettes for smoking cessation among Stop Smoking Service users. Br J Health Psychol. 2015;21(2):421–33.
Soneji SS, Sung HY, Primack BA, Pierce JP, Sargent JD. Quantifying population-level health benefits and harms of e-cigarette use in the United States. PLoS ONE. 2018;13(3):e0193328.
Talati A, Wickramaratne PJ, Keyes KM, Hasin DS, Levin FR, Weissman MM. Smoking and psychopathology increasingly associated in recent birth cohorts. Drug Alcohol Depend. 2013;133(2):724–32.
Tan QH. Smoking spaces as enabling spaces of wellbeing. Health Place. 2013;24:173–82.
Tatullo M, Gentile S, Paduano F, Santacroce L, Marrelli M. Crosstalk between oral and general health status in e-smokers. Medicine. 2016;95(49):e5589.
Taylor G, McNeill A, Girling A, Farley A, Lindson-Hawley N, Aveyard P. Change in mental health after smoking cessation: systematic review and meta-analysis. BMJ. 2014;348:g1151-g.
Thompson TP, Greaves CJ, Ayres R, Aveyard P, Warren FC, Byng R, et al. An exploratory analysis of the smoking and physical activity outcomes from a pilot randomized controlled trial of an exercise assisted reduction to stop smoking intervention in disadvantaged groups. Nicotine Tob Res. 2016;18(3):289–97.
Torigian DA, Green-McKenzie J, Liu X, Shofer FS, Werner T, Smith CE, et al. A study of the feasibility of FDG-PET/CT to systematically detect and quantify differential metabolic effects of chronic tobacco use in organs of the whole body—a prospective pilot study. Acad Radiol. 2017;24:930–40.
Ulvik A, Ebbing M, Hustad S, Midttun Ø, Nygård O, Vollset SE, et al. Long- and short-term effects of tobacco smoking on circulating concentrations of B vitamins. Clin Chem. 2010;56(5):755–63.
Vidrine JI, Businelle MS, Cinciripini P, Li Y, Marcus MT, Waters AJ, et al. Associations of mindfulness with nicotine dependence, withdrawal, and agency. Subst Abus. 2009;30(4):318–27.
Volkman JE, DeRycke EC, Driscoll MA, Becker WC, Brandt CA, Mattocks KM, et al. Smoking status and pain intensity among OEF/OIF/OND veterans. Pain Med. 2015;16(9):1690–6.
Vujanovic AA, Marshall-Berenz EC, Beckham JC, Bernstein A, Zvolensky MJ. Posttraumatic stress symptoms and cigarette deprivation in the prediction of anxious responding among trauma-exposed smokers: a laboratory test. Nicotine Tob Res. 2010;12(11):1080–8.
Wadsworth E, Neale J, McNeill A, Hitchman SC. How and why do smokers start using e-cigarettes? Qualitative study of Vapers in London, UK. Int J Environ Res Public Health. 2016;13(7):661–74.
Article PubMed Central CAS Google Scholar
Xie X, Dijkstra AE, Vonk JM, Oudkerk M, Vliegenthart R, Groen HJ. Chronic respiratory symptoms associated with airway wall thickening measured by thin-slice low-dose CT. Am J Roentgenol. 2014;203(4):W383–90.
Yang T, Shiffman S, Rockett IR, Cui X, Cao R. Nicotine dependence among Chinese city dwellers: a population-based cross-sectional study. Nicotine Tob Res. 2011;13(7):556–64.
Yu A, Cai X, Zhang Z, Shi H, Liu D, Zhang P, et al. Effect of nicotine dependence on opioid requirements of patients after thoracic surgery. Acta Anaesthesiol Scand. 2015;59(1):115–22.
Zhang X, Kahende J, Fan AZ, Barker L, Thompson TJ, Mokdad AH, et al. Smoking and visual impairment among older adults with age-related eye diseases. Prev Chronic Dis. 2011;8(4):A84.
PubMed PubMed Central Google Scholar
Zhao L, Xu L, Lai Y, Che C, Zhou Y. Temporal changes of smoking status and motivation in Chinese patients with hepatitis B: relationship with anxiety and depression. J Clin Nurs. 2012;21(15–16):2193–201.
Zorlu N, Cropley VL, Zorlu PK, Delibas DH, Adibelli ZH, Baskin EP, et al. Effects of cigarette smoking on cortical thickness in major depressive disorder. J Psychiatr Res. 2017;84:1–8.
Miyatake N, Numata T, Nishii K, Sakano N, Suzue T, Hirao T, et al. Relation between cigarette smoking and ventilatory threshold in the Japanese. Environ Health Prev Med. 2011;16(3):185–90.
Bjørngaard JH, Gunnell D, Elvestad MB, Davey Smith G, Skorpen F, Krokan H, et al. The causal role of smoking in anxiety and depression: a Mendelian randomization analysis of the HUNT study. Psychol Med. 2012;43(4):711–9.
Chen SC, Chen HF, Peng HL, Lee LY, Chiang TY, Chiu HC. Psychometric testing of the Chinese-Version Glover-Nilsson Smoking Behavioral Questionnaire (GN-SBQ-C) for the identification of nicotine dependence in adult smokers in Taiwan. Int J Behav Med. 2017;24(2):272–9.
Doran N, Spring B, McChargue D, Pergadia M, Richmond M. Impulsivity and smoking relapse. Nicotine Tob Res. 2004;6:641–7.
Haller CS, Etter JF, Courvoisier DS. Trajectories in cigarette dependence as a function of anxiety: a multilevel analysis. Drug Alcohol Depend. 2014;139:115–20.
Jamal M, Van der Does AJW, Cuijpers P, Penninx BW. Association of smoking and nicotine dependence with severity and course of symptoms in patients with depressive or anxiety disorder. Drug Alcohol Depend. 2012;126(1–2):138–46.
Krishnan-Sarin S, Reynolds B, Duhig A, Smith A, Liss T, McFetridge A, et al. Behavioral impulsivity predicts treatment outcome in a smoking cessation program for adolescent smokers. Drug Alcohol Depend. 2007;88:79–82.
Tidey JW, Pacek LR, Koopmeiners JS, Vandrey R, Nardone N, Drobes DJ, et al. Effects of 6-week use of reduced-nicotine content cigarettes in smokers with and without elevated depressive symptoms. Nicotine Tob Res. 2017;19(Suppl 1):59–67.
Chang CM, Cheng YC, Cho TM, Mishina EV, Del Valle-Pinero AY, van Bemmel DM, et al. Biomarkers of potential harm: summary of an FDA-sponsored public workshop. Nicotine Tob Res. 2019;21(1):3–13.
Haziza C, de La Bourdonnaye G, Donelli A, Skiada D, Poux V, Weitkunat R, et al. Favorable changes in biomarkers of potential harm to reduce the adverse health effects of smoking in smokers switching to the menthol tobacco heating system 2.2 for three months (part 2). Nicotine Tob Res. 2019;22(4):549–59.
Bishop L, Kuula-Luumi A. Revisiting qualitative data reuse: a decade on. SAGE Open. 2017;7(1):1–15.
Sherif V. Evaluating preexisting qualitative research data for secondary analysis. In: Forum: qualitative social research, vol. 19, no. 2. 2018.
Patrick D, Burke L, Gwaltney C, Leidy N, Martin M, Molsen E, et al. Content validity—establishing and reporting the evidence in newly developed patient-reported outcomes (PRO) instruments for medical product evaluation: ISPOR PRO good research practices task force report: part 1—eliciting concepts for a new PRO instrument. Value Health. 2011;14(8):967–77.
Download references
Acknowledgements
We thank the team at Sciome LLC for their assistance and contribution to the literature review. We thank Vivienne Law and David Floyd for their contributions to the literature review, reanalysis of qualitative data, and assistance with review of the draft manuscript. We thank Catherine Acquadro for her review of the draft manuscript. We also thank John Ware, Jed Rose, Ashley Slagle, Donald Patrick, Karl Fagerström, Stefan Cano, and Thomas Salzberger for their input and review.
Philip Morris International is the sole source of funding and sponsor of this research.
Author information
Authors and affiliations.
PMI R&D, Philip Morris Product S.A., Quai Jeanrenaud 5, 2000, Neuchâtel, Switzerland
Esther F. Afolalu, Erica Spies, Agnes Bacso, Emilie Clerc, Sophie Gallot & Christelle Chrea
Patient-Centered Outcomes Assessments Ltd., 1 Springbank, Bollington, Macclesfield, Cheshire, SK10 5LQ, UK
Linda Abetz-Webb
You can also search for this author in PubMed Google Scholar
Contributions
EA, ES and CC performed conceptualization. EA, ES and LA-W performed methodology. EA, ES, SG, EC and LA-W were involved in the investigation. EA and ES were involved in writing—original draft. EA, EC, LA-W and CC were involved in writing—review & editing. EA performed visualization. ES and CC performed supervision. AB, EC and SG were involved in data curation. AB and EC were involved in project administration. LA-W performed formal analysis. CC was involved in funding acquisition. All authors read and approved the final manuscript.
Corresponding author
Correspondence to Esther F. Afolalu .
Ethics declarations
Ethics approval and consent to participate.
Not applicable.
Consent for publication
Competing interests.
Esther F. Afolalu, Emilie Clerc, and Christelle Chrea are employees of Philip Morris International. Agnes Bacso, Erica Spies, and Sophie Gallot completed the work during prior employment with Philip Morris International. Linda Abetz-Webb is a consultant for Philip Morris International.
Additional information
Publisher's note.
Springer Nature remains neutral with regard to jurisdictional claims in published maps and institutional affiliations.
Work completed during prior affiliation with PMI R&D: Erica Spies, Agnes Bacso, Sophie Gallot.
Supplementary Information
Additional file 1..
Summary tables of results of scoping literature review
Rights and permissions
Open Access This article is licensed under a Creative Commons Attribution 4.0 International License, which permits use, sharing, adaptation, distribution and reproduction in any medium or format, as long as you give appropriate credit to the original author(s) and the source, provide a link to the Creative Commons licence, and indicate if changes were made. The images or other third party material in this article are included in the article's Creative Commons licence, unless indicated otherwise in a credit line to the material. If material is not included in the article's Creative Commons licence and your intended use is not permitted by statutory regulation or exceeds the permitted use, you will need to obtain permission directly from the copyright holder. To view a copy of this licence, visit http://creativecommons.org/licenses/by/4.0/ . The Creative Commons Public Domain Dedication waiver ( http://creativecommons.org/publicdomain/zero/1.0/ ) applies to the data made available in this article, unless otherwise stated in a credit line to the data.
Reprints and permissions
About this article
Cite this article.
Afolalu, E.F., Spies, E., Bacso, A. et al. Impact of tobacco and/or nicotine products on health and functioning: a scoping review and findings from the preparatory phase of the development of a new self-report measure. Harm Reduct J 18 , 79 (2021). https://doi.org/10.1186/s12954-021-00526-z
Download citation
Received : 14 October 2020
Accepted : 21 July 2021
Published : 30 July 2021
DOI : https://doi.org/10.1186/s12954-021-00526-z
Share this article
Anyone you share the following link with will be able to read this content:
Sorry, a shareable link is not currently available for this article.
Provided by the Springer Nature SharedIt content-sharing initiative
- Health and functioning
- Scoping review
- Qualitative research
- Conceptual framework
Harm Reduction Journal
ISSN: 1477-7517
- Submission enquiries: [email protected]
Click through the PLOS taxonomy to find articles in your field.
For more information about PLOS Subject Areas, click here .
Loading metrics
Open Access
Peer-reviewed
Research Article
Mixed methods research in tobacco control with youth and young adults: A methodological review of current strategies
Roles Conceptualization, Data curation, Formal analysis, Funding acquisition, Investigation, Methodology, Project administration, Resources, Supervision, Validation, Visualization, Writing – original draft, Writing – review & editing
* E-mail: [email protected]
Affiliations Department of Behavioral and Community Health, School of Public Health, University of Maryland, College Park, Maryland, United States of America, Maryland Center for Health Equity, School of Public Health, University of Maryland, College Park, Maryland, United States of America

Roles Data curation, Formal analysis, Investigation, Project administration, Validation, Visualization, Writing – original draft, Writing – review & editing
Affiliation Department of Behavioral and Community Health, School of Public Health, University of Maryland, College Park, Maryland, United States of America
Roles Formal analysis, Investigation, Methodology, Validation, Visualization, Writing – original draft, Writing – review & editing
Affiliation Educational Studies, School of Education, University of Cincinnati, Cincinnati, Ohio, United States of America
Roles Conceptualization, Formal analysis, Investigation, Methodology, Resources, Supervision, Validation, Visualization, Writing – original draft, Writing – review & editing
- Craig S. Fryer,
- Elizabeth L. Seaman,
- Rachael S. Clark,
- Vicki L. Plano Clark
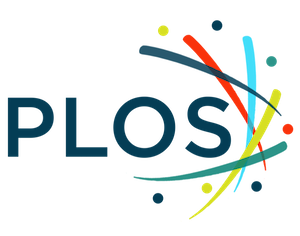
- Published: August 25, 2017
- https://doi.org/10.1371/journal.pone.0183471
- Reader Comments
Introduction
Tobacco use among young people is a complex and serious global dilemma that demands innovative and diverse research approaches. The purpose of this methodological review was to examine the current use of mixed methods research in tobacco control with youth and young adult populations and to develop practical recommendations for tobacco control researchers interested in this methodology.
Using PubMed, we searched five peer-reviewed journals that publish tobacco control empirical literature for the use of mixed methods research to study young populations, age 12–25 years. Our team analyzed the features of each article in terms of tobacco control topic, population, youth engagement strategies, and several essential elements of mixed methods research.
We identified 23 mixed methods studies published by authors from five different countries reported between 2004 and 2015. These 23 articles examined various topics that included tobacco use behavior, tobacco marketing and branding, and cessation among youth and young adults. The most common mixed methods approach was variations of the concurrent design in which the qualitative and quantitative strands were administered at the same time and given equal priority. This review documented several innovative applications of mixed methods research as well as challenges in the reporting of the complex research designs.
Conclusions
The use of mixed methods research in tobacco control has great potential for advancing the understanding of complex behavioral and sociocultural issues for all groups, especially youth and young adults.
Citation: Fryer CS, Seaman EL, Clark RS, Plano Clark VL (2017) Mixed methods research in tobacco control with youth and young adults: A methodological review of current strategies. PLoS ONE 12(8): e0183471. https://doi.org/10.1371/journal.pone.0183471
Editor: Hiroyoshi Ariga, Hokkaido Daigaku, JAPAN
Received: June 3, 2016; Accepted: July 7, 2017; Published: August 25, 2017
Copyright: © 2017 Fryer et al. This is an open access article distributed under the terms of the Creative Commons Attribution License , which permits unrestricted use, distribution, and reproduction in any medium, provided the original author and source are credited.
Data Availability: All relevant data are within the paper and its Supporting Information files.
Funding: This study was funded in part by the National Institutes of Health (NIH)/National Cancer Institute (NCI) by grant # K01CA148789: CSF Principal Investigator. The funders had no role in the study design, data collection and analysis, decision to publish, or preparation of the manuscript.
Competing interests: The authors have declared that no competing interests exist.
Tobacco use among young people is a global public health issue.[ 1 ] The majority of smokers began smoking in their youth[ 2 , 3 ] and more than 80–90% of smokers in the United States (U.S.) began smoking in their teens.[ 4 ] Currently, approximately 50% of young men and 10% of young women smoke worldwide with annual tobacco-attributable deaths projected to rise from 5 million in 2010 to more than 10 million by 2030.[ 5 ] Tobacco-caused morbidity and mortality is the most preventable disease among humans.[ 6 ] Consequently, tobacco use among young people is a global challenge demanding concerted efforts among tobacco control experts to develop effective prevention, treatment, and cessation modalities.
To address this challenge, the field of tobacco control needs research approaches that are able to address the complex trends and contexts related to tobacco use by youth and young adults. Youth tobacco use behavior in the U.S. is consistently changing with the greater availability, marketing, and promotion of a new and diverse constellation of combustible and non-combustible tobacco products, including the emergence of flavored little cigars and cigarillos, hookah, pipes, snus, dissolvables, and e-cigarettes.[ 7 ] It is well established that the tobacco industry aggressively targets youth and young adults with multifaceted tobacco product promotions.[ 8 , 9 ] Additionally, how young people intentionally change dependent behaviors is not well understood within tobacco control research.[ 10 ] For instance, nicotine dependence is based on adult models and conflicting information exists on nicotine dependence as a concept among youth[ 11 , 12 ] despite it being a significant barrier to smoking cessation.[ 13 ] Current priority tobacco control issues with young people that need to be addressed include: (a) the development of an optimal measure of novel tobacco products (i.e., small cigar and cigarillos);[ 14 ] (b) better understanding the sensory appeal (i.e., smell and sight) of flavored tobacco products;[ 14 ] (c) the escalating trend for dual, poly-tobacco, and nonconventional tobacco product use.[ 15 ]
Mixed methods research is a methodology for collecting, analyzing, and integrating both quantitative and qualitative data during the research process to gain a better understanding of complex research problems.[ 16 , 17 ] Mixed methods approaches add multiple dimensions and rigor to more traditional single-stranded research designs because of the power of integration.[ 18 ] As a result, mixed methods research can provide stronger inferences about a finding and provides the opportunity for presenting a range of divergent viewpoints regarding the phenomena under study and engaging vulnerable populations.[ 17 , 19 ] Therefore, mixed methods research designs offer novel approaches to explicate the complexity of tobacco control issues (e.g., concomitant use and nicotine dependence) among vulnerable youth and young adult smokers.
There are several indicators of the need to consider mixed methods research for addressing complex tobacco control issues. The prevalence of the use of mixed methods designs has increased in recent years, particularly in the fields of the behavioral sciences[ 20 ] and health sciences[ 21 – 24 ] with more than 40 types of mixed methods research designs reported in the literature.[ 25 , 26 ] Furthermore, the Office of Behavioral and Social Sciences Research (OBSSR) of the National Institutes of Health (NIH) commissioned a leadership team in 2010 to develop a guide for investigators regarding mixed methods research. The subsequent report, Best Practices for Mixed Methods Research in the Health Sciences ,[ 27 ] represents an important step in defining mixed methods research to the broader health sciences community. While this growth is encouraging, it is unclear how investigators are using mixed methods designs in tobacco control research to address the complex problems associated with youth and young adult populations.
Therefore, our aim was to examine and describe the use of mixed methods research in tobacco control research about youth and young adults. This methodological review contributes to understanding the adoption and use of mixed methods, which is one of five major methodological domains identified by Creswell.[ 26 , 28 ] Specifically, we addressed the following research questions: (a) What mixed methods designs are investigators using to study young people in tobacco control research ? ; (b) What substantive topics are investigators addressing when using mixed methods ? ; and (c) How are investigators engaging youth in mixed methods research designs ?
There are important audiences for this methodological review. The review has the potential to help tobacco control investigators better understand key practices involved in the design and conduct of mixed methods research and the intricacies of the varied study designs. A description of current practices can also assist funding agencies in identifying mixed methods exemplars as a way to improve evaluation of this important research approach.
Our methods build on established procedures for conducting rigorous methodological reviews and prevalence studies of the use of mixed methods research within disciplinary contexts.[ 29 – 34 ] These procedures guided our decisions about journal selection, search terms, inclusion criteria, and sampling.
A critical issue for methodological reviews is the identification of the appropriate journals for the search. While some methodological reviews have examined only one journal[ 35 ], it is most common to assess several journals.[ 34 ] Additionally, authors often focus on the leading journals within specific disciplines for the review in order to identify high-quality research applications.[ 34 ] Building upon highly-cited reviews in other fields,[ 23 , 29 , 36 ] we decided to limit our search to specific journals that publish tobacco control research to focus the scope of our review and ensure results were replicable and face validity was high. Our aim was to review the best subset of articles from which to learn; exemplars in the field. We solicited the opinions of six nationally-known tobacco research experts to determine the best journals in the field of tobacco control with interest in young populations. Combining this consultation with our expertise and years of experience working in the field of tobacco control research, five high quality, peer-reviewed journals were identified to be included for analysis: Addictive Behaviors, Health Education Research, Nicotine and Tobacco Research, Social Science and Medicine, and Tobacco Control. To ensure that we were not missing a significant source of mixed methods articles, we conducted informal searches of other high-quality, peer-reviewed journals (i.e., Addiction and Preventive Medicine), but did not locate articles that met all study inclusion criteria.
Inclusion criteria
The inclusion criteria of our methodological review encompassed three main designations: youth and young adults, tobacco control research, and mixed methods research defined as:
- Youth or Young Adults—Articles involving youth between the ages of 12–17 years and/or young adults aged 18–25 years.[ 6 ]
- Tobacco Control–Articles involving empirical research regarding any aspect of tobacco use and nicotine dependence.
- Mixed Methods Research–Articles reporting the use of research designs that collect, analyze, and integrate quantitative and qualitative data within a single study or multiple phases of a program of research[ 16 ].
The sampling phase utilized a four-step process: identification, screening, eligibility, and inclusion. An illustration of this process is presented in Fig 1 .
- PPT PowerPoint slide
- PNG larger image
- TIFF original image
https://doi.org/10.1371/journal.pone.0183471.g001
The first step was sample identification. The scientific research articles were identified through a broad, multifaceted search strategy. On October 1, 2016 we implemented our search of tobacco control empirical studies that used mixed methods research designs with young people published during January 1, 2004 to December 31, 2015. We searched the selected five journals for articles that matched our first two inclusion criteria (participant age and tobacco control) using PubMed. We chose PubMed as our database because of its comprehensiveness (5,153 journals in Index Medicus and 479 non- Index Medicus journals) and management by the National Institutes of Health’s U.S. National Library of Medicine. This search used broad subject terms, but did not include the term “mixed methods research” because mixed methods experts have cautioned against relying on this term when searching electronic databases as it may result in missing publications that combine quantitative and qualitative methods without self-identifying as mixed methods [ 34 ]. The search strategy used was: (((((((((youth or Adolescen* or "young adult")) AND ("Nicotine & tobacco research: official journal of the Society for Research on Nicotine and Tobacco"[Journal])) OR "Tobacco control"[Journal]) OR "Health education research"[Journal]) OR "Social science & medicine (1982)"[Journal]) OR "Addictive behaviors"[Journal])) AND (Tobacco OR cigarette* or Smok*[Title]). This search of titles yielded 6,924 records. Next, we applied our year range filter (2004–2015) and the results were reduced to 4,487 records. The full yield of 4,487 records was then assessed against our three inclusion criteria.
The second step was screening. Abstracts were obtained for each of the 4,487 titles. The second author (ELS) screened the entire sample by reviewing abstracts to identify all articles that potentially met the inclusion criteria for full review. Often the second and first author (CSF) met to discuss screening decisions. Letters to the editor, systematic reviews, commentaries, special communications, research briefs, and editorials were excluded. To confirm the accuracy of the screening, a randomly selected subsample of 5% (225 titles and abstracts) of the original 4,487 results was sent to the third author (RSC). RSC used the identical inclusion criteria and identified two abstracts among the 225 that warranted a full review. The two abstracts matched those identified by ELS’s review, resulting in a 100% agreement for the 225 abstracts. The screening yielded 44 abstracts of articles eligible for full review.
The third step was to determine the eligibility of all 44 abstracts by obtaining their respective full-text copies. The full articles were then examined and discussed by all four members of the research team. From this review, we excluded 21 articles that reported only qualitative or quantitative methods and thus, did not meet our inclusion criteria for mixed methods research.
The fourth step assessed the remaining articles for inclusion in the sample. Our process resulted in a final sample of 23 articles for the methodological review.
Data coding
The first author (CSF) in consultation with the senior author (VPC) developed a codebook to examine the articles based on categories used in methodological reviews of the use of mixed methods in other topical areas.[ 29 , 36 , 37 ] The codebook was comprised of names and brief descriptions highlighting seven different article features: Substantive Content, Quantitative Component, Qualitative Component, Mixed Methods Features, Youth Engagement Features, Other Issues, and Reflections. Details of the codebook, including the dataset are provided in S1 File .
Two team members (ELS and RSC) were responsible for the primary coding of all articles. Each article was given a randomly generated identification number and randomly assigned to ELS or RSC. They independently coded each assigned article using an Excel spreadsheet accessible by all team members through GoogleDocs TM including direct quotes from the articles and the coder’s interpretations of the information. After initial coding of each article was complete, the senior members (CSF AND VPC) were randomly assigned to each article, reviewed and recorded information and discussed any discrepancies with the primary coder. Final consensus was reached among the full team through discussion for each article in the dataset.
Data analysis
To characterize the current state of the use of mixed methods research with youth and young adults in tobacco control research, the studies were examined to assess research methods and designs, research participants, sampling, approaches to analysis, and the tobacco control topic or behavior investigated. Each team member was assigned a section of the data codebook to analyze and provided a summary of their findings. During weekly meetings, each team member presented their findings and the remaining three members provided critical feedback. The result was an inductive analysis process that examined how specific features of each article holistically described the mixed methods research being conducted in tobacco control with young people.
Table 1 lists the 23 articles included in this methodological review. The articles were published between 2004 and 2015. The majority (n = 16) were published between 2006 and 2011 and by scientists from the U.S.A. (n = 13) and the United Kingdom (n = 7). We also identified mixed methods studies with young people conducted in India (n = 1), Israel (n = 1), and New Zealand (n = 1). The country affiliation of the authors of these studies may have differed from the location of the research such as Nichter.[ 38 ] The number of co-authors ranged from two to nine. The preponderance of the first authors were in the behavioral sciences (n = 14) including anthropology, psychology, and public health. Others were from the fields of medicine (n = 6), marketing (n = 2), and statistics (n = 1).
https://doi.org/10.1371/journal.pone.0183471.t001
Next, we describe the substantive content, youth engagement methods including recruitment, and mixed methods research design of these 23 articles.
Substantive content
Of the 23 articles reviewed, two articles reported on setting-specific smoking prevention programs[ 45 , 58 ] while five reported on perceptions of smokers and various tobacco products.[ 42 , 43 , 48 , 50 , 51 ] Five articles focused on smoking prevalence and behavior[ 39 , 46 , 47 , 59 , 60 ] while three explored the influences of tobacco marketing and branding.[ 38 , 44 , 54 ] Three articles [ 40 , 41 , 53 ] examined aspects of smoking cessation including services, two examined the use of media literacy to prevent smoking [ 49 , 57 ], and the remaining articles focused on cigar product modification,[ 55 ] motives for and against genetic testing[ 56 ] and the reliability and validity of self-reported data.[ 52 ] Furthermore, two-thirds (n = 14) of the articles reported on the use of cigarette smoking only and one reported on e-cigarette use and cessation only among middle school, high school, and college students.[ 46 ] The authors of five articles focused on the use of cigarettes and another tobacco product such as smokeless tobacco, cigars, hookah, bidis, and cloves. One article reported on the use of cigarettes and cannabis[ 47 ] and another on cigar modification.[ 55 ]
Youth engagement through recruitment and intervention
The populations of young people represented in the 23 articles were varied and reflected the international scope of tobacco research. Given that many countries worldwide prohibit sales and restrict access to youth for tobacco products[ 38 ] as well as the increasing denormalization of the social acceptability of smoking, engaging young people in tobacco control research is challenging.[ 38 , 61 ] Therefore, we examined how the investigators engaged their participants through the phases of planning, recruitment, and retention in the mixed methods research.
We were particularly interested in recruitment strategies used in these studies. The investigators utilized both passive (e.g., flyers, email, posters) and active recruitment methods (e.g., staff of the schools or community organizations, referrals, presentations to groups) to engage young people in their research studies ( Table 1 ). A little more than two-thirds (n = 16) utilized the school setting (i.e., middle or high school and college) for recruitment. The study samples reflected the ethnic distribution of the geographic locations, yet few studies had ample numbers of young people across racial and ethnic categories to perform group comparison analyses. The majority of the research teams used traditional recruitment methods (e.g., flyers and email) and the recruitment strategies supported the diverse research designs (cross sectional or longitudinal), sampling techniques (convenient or purposeful), data collection methods (quantitative or qualitative), and populations of interests (youth or young adults).
Furthermore, several of the articles engaged youth through the development, implementation, or evaluation of tobacco control interventions. [ 41 , 43 , 45 , 58 ] In this review, investigators were strategic in how they engaged youth in their research employing participatory and culturally-sensitive approaches. The participatory approaches varied in the studies. For example, Audrey et al [ 41 ] employed a peer nomination process and counseling techniques to identify and gain peer support for a student-led smoking prevention program. The most nominated students were trained as peer supporters and charged to recruit a wide range of friendship groups within a ten week period. The team reported 87% of those invited accepted the position, were trained, and completed their duties and 86% attended all follow-up sessions. Elsey and her research team [ 43 ] examined the feasibility and acceptability of using a social norms approach in five schools among seventh graders to prevent smoking. Findings demonstrated youth had significant misperceptions between self-reported and perceived smoking among their peers in the same grade. As a result, they collaborated with a local arts organization to both engage and assist students in the development of their own social norm campaigns to correct misperceptions of peer smoking. Investigators in this review also used culturally-sensitive approaches to engage young people in their research. For example, Goenka and colleagues[ 45 ] conducted a process evaluation of a classroom-based, tobacco prevention intervention in India. The intervention addressed both smokeless and smoked forms of tobacco among sixth to ninth graders and was tailored to Indian culture in context, content, and communication. The seven-session curriculum utilized mascots ( Disha and Deepak ), posters, and the creation of skits to sustain the interests of youth. Results indicated higher levels of student and peer leader communication and higher levels of student participation in discussion. Finally, one study used a combined participatory and culturally-sensitive approach. Sorensen et al[ 58 ] investigated the use of a workplace intervention to increase knowledge about the harms of smoking among teenage smokers. The intervention developed teen advisory boards to facilitate discussions about the negative impact of smoking on health with study participants. In addition, participants were recruited from and the study was implemented in 10 grocery stores in Boston, USA. As such, the culture of the workplace setting was an important component to the study design. Contrary to the team's predictions, most teen workers preferred to quit on their own, suggesting interventions with working teens may not be maximally effective.
Use of mixed methods research
Our analysis of the articles focused on the researcher’s reports of their use of mixed methods research. Consistent with our definition of mixed methods research, we examined the quantitative and qualitative components of each study and how the authors integrated these two components. Based on this information, we classified each study in terms of one of the five major mixed methods research designs discussed by Creswell and Plano Clark.[ 16 ] This information is summarized in Table 2 and discussed in the sections that follow.
Quantitative components.
Each of the reviewed mixed methods studies included a quantitative component that reflected the researchers’ decisions about sampling, collection, and data analysis. A range of sampling strategies were used for the quantitative component of the studies including purposive sampling,[ 40 , 44 , 46 , 60 ] probability or random stratified sampling,[ 49 , 54 ] multi-stage sampling,[ 55 ] and a combination of sampling methods.[ 47 ] We concluded that the remaining 15 studies utilized convenience sampling based on the described contextual information. The sample sizes of the quantitative strands of the studies varied greatly. The smallest sample included 15 parent/child dyads[ 50 ] and the largest study enrolled 10,370 participants.[ 41 ]
https://doi.org/10.1371/journal.pone.0183471.t002
The majority of the studies utilized a survey or a questionnaire for the quantitative data collection. Some studies combined a survey or questionnaire with another quantitative component such as a video activity,[ 49 ] ranking tobacco packaging,[ 54 ] and a salivary cotinine sample.[ 41 ] Two studies used “web assessments” to collect data at more than one time point.[ 39 , 59 ] Three studies utilized validated measures such as the Beck Depression Inventory and the Teen Smoking Questionnaire.[ 50 , 51 , 53 ] Several studies used more innovative ways to collect quantitative data, such as quantifying participants’ brand attribute associations to create a perceptual map.[ 44 ]
Qualitative components.
Each of the reviewed mixed methods studies also included a qualitative component that reflected the researchers’ decisions about sampling, collection, and data analysis. In terms of sampling, 13 studies used a subset of the quantitative sample for their qualitative data collection (e.g.,[ 41 , 48 , 53 ]). The subsets were chosen from the full sample size in different ways. One study relied on participants to volunteer for the qualitative portion and bring a friend,[ 60 ] whereas two studies used nominations to select which students to include in the qualitative phase of the study.[ 41 , 49 ] Nine studies used the same sample in the qualitative and quantitative components (e.g., [ 40 , 44 , 57 ]). The sample sizes for the qualitative components ranged from 18 to 531 participants.
Several studies combined qualitative methods to examine both the opinions of the group and individuals, such as focus groups and interviews.[ 53 ] Two studies utilized more than two qualitative methods, such as combining interviews, focus groups, informal discussions and ethnographic fieldwork.[ 38 ] Some studies employed uncommon combinations–for instance focus groups with media diaries[ 49 ] or semi-structured interviews with adjective cards to describe participants’ smoking experiences.[ 39 ] Many of the studies provided little information about the specific qualitative data analysis. Six studies directly mentioned performing a thematic analysis, all with varying levels of detail.[ 38 , 40 , 44 , 55 , 56 , 60 ] One study team cited a focus group guide[ 62 ] to describe their analysis[ 42 ], while another performed a descriptive content analysis on YouTube videos and a thematic analysis on the comments associated with each video.[ 55 ]
Reasons for and value of mixing methods.
Central to the use of mixed methods is integrating the quantitative and qualitative components of a mixed methods study. This integration often starts by the researchers specifying a rationale for mixing methods. Due to the complexity that mixed methods brings to research, it is important for researchers to describe the rationale and value that the mixed methods approach brings to a study.[ 63 , 64 ] Of the 23 articles reviewed, only three explicitly stated the authors’ reason for using mixed methods.[ 41 , 49 , 57 ] Therefore, we analyzed the remaining articles to uncover the implicit rationale for each study.
Common rationales included triangulation (n = 7) and complementarity (n = 7). Triangulation involves corroborating results from the quantitative and qualitative methods to obtain more validated conclusions.[ 63 ] One study[ 47 ] used mixed methods to triangulate patterns of behavior by comparing quantitative and qualitative data to identify and understand “culturally relevant meanings” that shaped participants’ substance use behaviors. Complementarity is used when quantitative and qualitative methods examine different aspects of the same phenomenon resulting in a deeper, more complex understanding of the topic.[ 64 ] For instance, investigators[ 56 ] discovered novel information from open-ended items on a questionnaire that explained differences in quantitative and qualitative findings regarding interest in genetic testing to determine cancer risk. Some studies used the results of one method to develop or inform the use of subsequent methods.[ 64 ] Examples of development include needing quantitative results to select participants[ 53 ] or using qualitative results to develop a new measure.[ 49 ]
Timing of components.
Timing refers to the order in which the qualitative and quantitative components were used in a mixed methods study.[ 16 , 65 ] Some of the articles did not include a clear description of when the strands were administered, which made timing challenging to determine. Based upon our interpretation, many studies used concurrent timing (n = 12), which occurs when researchers administer the qualitative and quantitative components concurrently. For example, researchers[ 44 ] collected quantitative (questionnaire) and qualitative (focus groups) data within the same session.
Nine studies used sequential timing, which occurs when researchers fully implement one phase (quantitative or qualitative) before the other so the second phase depends on the results of the first phase. For example, in a study to characterize and measure media health literacy,[ 49 ] the researchers first implemented the qualitative component and then used those results to create a questionnaire utilized in the subsequent quantitative phase. Two studies used multiphase timing by combining multiple concurrent and/or sequential components.
Priority describes the relative importance of the quantitative and qualitative components for addressing the overall study purpose.[ 16 ] In a mixed methods study, the two components may have equal weight in addressing the study purpose or one method may be given more weight than the other. We assessed studies as having quantitative priority (n = 11), qualitative priority (n = 7), and equal priority (n = 5). For example, Nichter et al[ 38 ] assigned priority to the quantitative methods in their study of perceptions of tobacco use and behavior associated with use because the article contained many tables of quantitative results, but no qualitative data illustrations. Audrey and colleagues[ 41 ] demonstrated qualitative priority because they used extensive analysis of peer leader diaries and focus group data to conduct a process evaluation of a peer support and intervention program. Equal priority was illustrated by a study of self-report measures of youth smoking in which researchers used data from questionnaires and semi-structured interviews to examine inconsistencies in reliability between measures.[ 52 ]
Mixing strategies.
Mixing strategies can be classified into three broad categories: merging, connecting, and embedding.[ 16 ] Merging occurs when the qualitative and quantitative results are combined during analysis. Connecting occurs when the results or findings from one strand are used to design the data collection of another strand. Embedding occurs when a secondary qualitative or quantitative component is added to an overall quantitative or qualitative design. For example, researchers may embed a qualitative component into an experiment or a quantitative component into a case study.
Eleven studies used merging to integrate results. For example, researchers[ 40 ] described the merging of the quantitative and qualitative data sets as, “Responses to questions on quitting from the structured questionnaire were analyzed in SPSS and contrasted with what was said in the interviews” (p.183). Another form of merging occurs when one type of data is transformed into another in order to facilitate analysis and comparison. Primack, Fine, and Yang[ 57 ] illustrated this type of mixing, as the researchers collected qualitative data and transformed the qualitative results into quantitative data in order to “illuminate results of the outcome evaluation” (p. 326).
Connecting was used as a mixing strategy in nine articles. For example, one team[ 53 ] selected participants for the qualitative strand based on results from the quantitative strand. The authors described the desire to interview individuals of “both genders who wanted to give up smoking and were in three older year groups and from a range of different schools” (p. 543). Once the quantitative results were collected, the researchers purposefully selected six representative schools and all students were invited to participate in the qualitative phase of the study.
Investigators used embedding in a study of a peer-led smoking intervention.[ 41 ] The qualitative data collection occurred within the context of an experiment and included diary entries, semi-structured interviews, and focus groups that were analyzed and used to augment the quantitative data and complete the process evaluation. Two studies used a combination of mixing strategies.[ 38 , 60 ]
Overall mixed methods design.
As indicated in Table 2 , we applied the mixed methods design typology of Creswell and Plano Clark[ 16 ] and identified examples of five different mixed methods designs: concurrent, explanatory sequential, exploratory sequential, embedded, and multiphase.[ 16 ] Of note, no study reported the use of the transformative mixed methods design discussed in Creswell and Plano Clark.[ 16 ] Eleven studies used a variation of the concurrent design. The concurrent design (Quan + Qual) typically entails administering the quantitative and qualitative strands concurrently, emphasizing each strand equally, and merging the results from both strands during the final analysis and interpretation for the purposes of triangulation and complementarity.[ 16 ] In a study on self-reported smoking data, survey and focus group results were given equal weight and used for comparison to test the reliability over time and across settings.[ 52 ]
Six studies used the explanatory sequential design (Quan→Qual). This design entails collecting and analyzing quantitative and qualitative data sequentially where the qualitative phase builds on the quantitative results and is used to help explain the quantitative results.[ 16 ] In a study of perceptions of cigarette packaging,[ 54 ] the investigators used an explanatory sequential design to first measure the impact of non-branded cigarette packs and then explain why the impact occurred by providing smokers the opportunity to explain and expand upon their quantitative results.
As described in Creswell and Plano Clark[ 16 ], the remaining six studies used the exploratory sequential approach (Qual→Quan),[ 46 , 49 , 58 ] the embedded QUAN(qual) design,[ 41 ] or the multiphase design (Quan→qual) →[qual+quan].[ 38 , 60 ]
Mixed methods reporting issues.
The main issues with reporting were: i) the use of clear language and ii) the scope of the information reported. In many of the articles, the authors did not clearly distinguish the different study components of their work. Some authors made explicit choices about their language use, which facilitated understanding the study’s components. For example, one research team[ 60 ] explicitly discussed their choice of terminology to distinguish study participants based on their participation in the quantitative or qualitative study strand. They wrote, "In this paper, in order to clarify whether we are referring to individuals who were surveyed or those who were interviewed, the former will be referred to as pupils , the latter as participants " (p. 1517, italics in original).[ 60 ] The other reporting issue was the scope of information that was included in the articles. Many studies were extensive and reported only a fraction of the studies’ information in the articles. Several strategies were noted to deal with the issue of scope in these situations. Some authors described the methods of the full study but then reported only a subset of the study results, such as results for one phase[ 42 , 54 ] or one wave of data collection.[ 57 ] Other authors specifically referred to information available outside of the article to supplement the description of the study’s methods, such as reported in other publications,[ 48 ] available online,[ 45 ] or to contact the corresponding author.[ 44 ]
Population-based systematic reviews examining access to tobacco,[ 66 ] smoking initiation,[ 67 ] tobacco control interventions and social inequalities,[ 68 , 69 ] longitudinal studies and smoking cessation trials,[ 70 , 71 ] and tobacco control policies[ 72 ] have been published for more than a decade. To our knowledge, this is the first methodological review of the use of mixed methods research designs in tobacco control being employed with youth and young adults–which we consider to be novel and an important contribution to the scientific literature. Our team identified 4,487 articles in five journals and synthesized evidence from 23 published articles representing 22 mixed methods studies in this review. The total yield is less than 1% of the original sample amplifying that mixed methods research represents a small, yet important body of work within tobacco control research with youth and young adults.
In summary, studies combining qualitative and quantitative methods were successfully being implemented with young people in tobacco control research both globally and across many disciplines (see [ 45 , 47 , 51 , 54 ]). In the field, investigators addressed relevant issues facing young people across the continuum of prevention, treatment, and cessation utilizing multiple variations of five of the six major mixed methods research designs.[ 16 ]
Of the 23 studies in this methodological review, the most common approach was some variation of the concurrent design. These findings are congruent with other methodological reviews that report the increased implementation of mixed methods designed studies across many disciplines[ 22 , 23 , 73 , 74 ] and the concurrent design identified as the most commonly utilized mixed methods design.[ 29 , 75 ] Additionally, although most of the investigators utilized traditional data collection procedures (i.e., surveys, focus groups, and individual interviews), a smaller group of the articles described other combinations of data collection methods, including video activities, biological sample collection, and perceptual mapping. Plano Clark [ 73 ] found similar results in a review of federally funded, health-related grant applications, yet noted that this was not the case in a review completed two years before in family sciences.[ 29 ] This suggests that as mixed methods research designs increase in popularity and use, the more intricate features of the designs may become.
As previously mentioned, mixed methods research can provide stronger inferences about a finding and offer insights regarding the phenomena under study that could not be gleaned from the quantitative or qualitative component alone.[ 16 , 17 , 25 ] This review uncovered several exemplars of such inferences for tobacco control. For example, Turner and colleagues[ 60 ] utilized a multiphase mixed methods research design in their examination of peer group influence on school smoking rates. While their qualitative findings complemented those of the quantitative analysis regarding attitudes of smoking among non-smoking girls, the mixed methods analysis provided a deeper understanding of the mechanisms operating among these groups of girls at the two socioeconomically different schools. Specifically, the processes of selection (i.e., modeling) were operating at one school, while those of influence (i.e., coercion) were found at the other school. A different, but equally salient example of the power of mixed methods study designs was found in the work of Mair et al.[ 52 ] This investigative team utilized a concurrent mixed methods research design with longitudinal qualitative and quantitative data to assess the reliability of self-reported smoking data among young people. Once they merged their study results during data analysis and interpretation, they learned that the ‘inconsistencies’ found statistically were actually important self-characterizations young people had of themselves overtime. As a result, they concluded that the ability to identify inconsistencies was a strength of the research. More importantly, the inconsistencies were not related to the self-reported data, but investigators’ tendencies to employ analytical models that are not germane to the lived experience of the young participants.
Although a few investigators reported creative ways to engage young people in their research–utilizing the arts, peer counseling, and culturally-appropriate mascots, [ 41 , 43 , 45 ],overall our sample lacked innovative exemplars of youth engagement. In an effort to protect the health of youth and young adults, tobacco control investigators must be as effective in engaging youth in research as the tobacco industry has been in attracting young people to the multitude of new tobacco products continuously emerging on the global market. Equally important are contextually appropriate and sensitive methods to examine the feasibility, process, and outcomes of such interventions. Our sample provides examples of using mixed methods research designs to do that [ 43 , 54 , 57 ] and these techniques should be applied to develop more innovative intervention approaches such as the use of new media strategies like social media platforms (i.e., Instagram or Youtube) or smartphone applications (i.e., Smokerface or QuitStart). Furthermore, the use of existing anti-smoking programs like the truth ® #FinishIt campaign and the Fresh Empire ® music contests and events to name a few, can be novel mechanisms in tobacco control intervention designs to engage young adult interests across the continuum of planning, recruitment, and retention.
While we applaud the successful use and variety of mixed methods designed studies in this review, we found unevenness and inconsistency in the reporting of this important work in the published tobacco control empirical literature. We reflect upon three broad, pertinent issues found in our review: lack of common terminology, lack of information, and the lack of clear descriptions and explanations of procedures that impact our ability to assess the quality and rigor of these published works.[ 22 ]
Lack of common terminology
Since the early 1990s, the field of mixed methods research has developed a wealth of resources regarding the conceptualization, design, and implementation of mixed methods studies.[ 16 , 25 , 73 , 76 – 78 ] Despite such resources, the researchers of the studies examined herein often did not use common terminology in the reporting of their work.[ 22 ] Case in point, unlike methodological reviews in other disciplines,[ 73 , 74 ] very few of the authors used mixed methods terminology such as ‘mixed methods’ or self-identified their study as ‘quantitative and qualitative’ in the description of their research. As reported in the Methods section of this article, because there was no consistent language to identify mixed methods studies, we decided to implement a broad, multifaceted search strategy in a stepwise procedure. Thus, we used simple key words in our search and then assessed a larger sample of articles to find information-rich examples of mixed methods studies. The lack of clear terminology hampers the proliferation of innovative techniques and prohibits learning. By understanding the many possible mixed methods approaches, including advantages and challenges, tobacco control investigators will be able to better choose and articulate their mixed methods designs. It is imperative that investigators use common terminology so that the work can be identified, assessed, and ultimately replicated in the pursuit of scientific advancement. We recommend that authors using mixed methods approaches name their approach “mixed methods research” and that they provide a formal mixed methods design name (e.g., explanatory sequential mixed methods design) as discussed in the literature and used in this review.[ 16 ]
Lack of information
Another overarching concern was the lack of information provided to effectively ascertain the study implementation procedures. For example, some authors mentioned the setting in which recruitment took place (i.e., a school or a workplace), yet did not describe the process by which young participants were recruited to the individual qualitative or quantitative component of the study. As a result, we could not easily determine the recruitment methods utilized by investigators and reported the lack of information in our findings (See Table 1 ). Another example was the very unbalanced descriptions of procedural and analytic methods between the quantitative and qualitative components of the study. Often investigators provided in-depth reports of the quantitative methods, analyses, and results, yet qualitative methods and analysis procedures were briefly described. In several cases, the amount of information provided about the qualitative component would not be sufficient for replication. O’Cathain et al[ 22 ] found this issue in their review of mixed methods studies in health services research and offered that it was indicative of the “historical dominance of quantitative methods in health services research.” One could argue that this is also true in the field of tobacco control research. Additionally, the lack of information impacts the ability to determine not only the quality of the qualitative and quantitative components, but the rigor of the overall study and the implications of the study findings. We recommend that authors using mixed methods approaches be consistent in their description of both the qualitative and quantitative strands of their study. They must ensure that they report the same information from each phase of the mixed methods design. Moreover, investigators can prioritize journals that recognize the richness of mixed methods designs and have less restrictive manuscript word limits.
Lack of clear explanations of mixed methods procedures
The last issue is the lack of clear descriptions and explanations of mixed methods procedures. In the effort to assess the quality of mixed methods research, several investigators have created criteria to examine the rigor of the study.[ 16 , 17 , 22 , 76 ] O’Cathain et al[ 22 ] provided guidelines for Good Reporting of a Mixed Methods Study (GRAMMS). These quality criteria focus on how to clearly report seminal features of the mixed methods study design including the rationale, design, and the integration or “mixing” of the methods. The use of the major design names, explanation of the purpose or rationale of the design, as well as descriptions of the timing, priority, and integration were frequently omitted among this collection of studies hindering our ability to assess the quality of the research study. For instance, some studies’ mixing approaches involved using data from a previous study or embedding the current study within a larger parent study. Without an understanding of the how these qualitative and quantitative strands were integrated, we could not ascertain the synthesizability or quality of the research.[ 79 ] This is the central purpose of mixed methods research designs–the integration of both components.[ 18 , 80 ]Currently, mixed methods research experts refer to this issue as the “integration challenge.”[ 81 ] Specifically, although integration is required in mixed methods research to go beyond the separate quantitative and qualitative components; it is challenging to do so. We conducted this methodological review to assess studies that attempted to take on the integration challenge and thus articles that reported on only one component of their study did not meet our inclusion criteria. Moreover, investigators must fully appreciate that each mixed methods design is best suited for specific purposes/rationales. Thus, to successfully respond to the study’s purpose and research questions, one must logically and consistently implement and describe the various aspects of the study thoughtfully. Bryman[ 80 ] suggests that if investigators self-reflect on the original purpose/rationale for conducting their work, they can then use this information to conduct an integrative analysis. In essence, can researchers demonstrate that their integrated findings have enhanced their understanding of the phenomena under study beyond that of a single component of the study?[ 80 ] We recommend that tobacco control investigators become familiar with and master reporting guidelines such as GRAMMS[ 22 ] to meet the field of mixed methods research reporting and quality criteria and to effectively describe the rigor of mixed methods designed studies. The ability to articulate the important features of mixed methods research is paramount to advancing its use in tobacco control. Finally, given the word limits of journal articles, researchers should write robust descriptions of their study methods and restrict their embellishment of the introduction or background sections of their manuscripts.
It is important to note that the aforementioned issues are impacted by word and space limits set by journals and its respective publisher. These are critically important parameters that have been reported as an important barrier to publishing mixed methods research.[ 82 ] While there are tradeoffs for what should be highlighted in an article, guidelines exist for effectively reporting mixed methods research[ 22 , 23 ] to further advance the use of mixed methods designs in the field.
Limitations
A few limitations of this methodological review must be considered in the interpretation of our results. Our work builds on established procedures for conducting rigorous methodological reviews within disciplinary contexts, including the journals selected for the review. To this end, these results represent articles published in five select journals; not all journals of the scientific tobacco control empirical literature and only those published in the English language. More importantly, our work contributes to understanding the current practices of tobacco control researchers with the use of the five major mixed methods designs identified by Creswell.[ 26 , 28 ] Articles that reported on only one component (quantitative or qualitative) of a study did not meet our definition of mixed methods research and was excluded from our sample. Additionally, the results represent a specific range in time during which our search was conducted. Although our methodological review covers a 12-year span (2004–2015), we did not include articles that have been published since January 2016.
Mixed methods research designs can be of great promise in tobacco control research efforts. The central purpose of mixed methods designs is the integration of the quantitative and qualitative components. To this end, mixed methods research designs have the ability to provide findings of integrated data that are beyond the limitations of quantitative and qualitative data alone. Investigators utilizing mixed methods study designs can contribute to the complexities of youth and young adult behavior and prevention, the treatment of tobacco-caused problems such as dependence, including symptoms of withdrawal and cessation. The application of mixed methods designs can also provide the opportunity to discover new areas of research as the field grapples to address the emergence of a plethora of novel, non-conventional tobacco products specifically targeting youth and young adults such as the co-use or multiuse of tobacco products and other substances among young people. In the future, we recommend that tobacco control investigators be explicit about the study’s purpose and research design and use clear language in describing the many facets of their mixed methods approach. This would include the priority given to the qualitative or quantitative strand of the design as well the rationale for integrating or mixing the two strands. We believe our work is the beginning of a rich and fruitful discussion regarding the most meaningful use of mixed methods study designs when working with young people. Ultimately, the field of tobacco control needs more innovative research methods including innovative interventions in order to achieve the insights necessary to understand and overcome the complex issues of tobacco use among the world’s youth. Mixed methods research gives tobacco control researchers a powerful methodological strategy for taking on this challenge.
Supporting information
S1 file. study dataset..
https://doi.org/10.1371/journal.pone.0183471.s001
S2 File. PRISMA 2009 checklist.
https://doi.org/10.1371/journal.pone.0183471.s002
Acknowledgments
The authors would like to thank Ms. Joanne D’Silva for a previous review of the manuscript. Its contents are solely the responsibility of the authors and do not necessarily represent the official views of funders.
- 1. Eriksen M., Mackay J., Ross H. The Tobacco Atlas. 4th ed. New York, NY: American Cancer Society; 2014.
- View Article
- PubMed/NCBI
- Google Scholar
- 4. Results from the 2012 National Survey on Drug Use and Health (NSDUH) [Internet]. Substance Abuse and Mental Health Services Administration (SAMHSA); 2013. Available: http://media.samhsa.gov/data/NSDUH/2012SummNatFindDetTables/NationalFindings/NSDUHresults2012.pdf
- 6. The Health Consequences of Smoking—50 Years of Progress. A Report of the Surgeon General [Internet]. U.S. Department of Health and Human Services; Available: http://www.surgeongeneral.gov/library/reports/50-years-of-progress/full-report.pdf
- 7. Tobacco Use Among Middle and High School Students—United States, 2013 [Internet]. CDC; 2014. Available: http://www.cdc.gov/mmwr/preview/mmwrhtml/mm6345a2.htm
- 16. Creswell J.W., Plano Clark V.L. Designing and Conducting Mixed Methods Research. 2nd ed. Thousand Oaks, CA: Sage; 2011.
- 17. Tashakkori A., Teddlie C., editors. SAGE Handbook of Mixed Methods in Social and Behavioral Research. 2nd ed. Thousand Oaks, CA: Sage; 2010.
- 24. Curry L., Nunez-Smith M. Mixed Methods in Health Sciences Research–A Practical Primer. Los Angeles, CA: Sage; 2015.
- 25. Greene J.C. Mixed Methods in Social Inquiry. San Francisco, CA: Jossey-Bass; 2007.
- 26. Creswell J.W. Research Design: Qualitative, Quantitative, and Mixed Methods Approaches. 4th ed. Thousand Oaks, CA: Sage; 2014.
- 27. Creswell J.W., Klassen A.C., Plano Clark V.L. Best practices for mixed methods research in the health sciences [Internet]. Office of Behavioral and Social Sciences Research. National Institutes of Health; 2011. Available: http://obssr.od.nih.gov/mixed_methods_research/
- 62. Morgan D.L., Krueger R.A. The focus group kit. Thousand Oaks, CA: Sage; 1988.
- 66. Sutcliffe K., Brunton G., Twamley K., Hinds K., O’Mara- Eves A.J., Thomas J. Young people’s access to tobacco: a mixed-method systematic review [Internet]. London: EPPI-Centre, Social Science Research Unit, Institute of Education; 2011. Available: http://eprints.ioe.ac.uk/16193/1/Sutcliffe_et_al._2011._Young_people_s_access_to_tobacco.pdf
- 74. Plano Clark V.L., Wang S.C. Chapter 34: Adapting Mixed Methods Research to Multicultural Counseling. In: Ponterotto J.G., Casas J.M., Suzuki A.L., Alexander C.M., editors. Handbook of Multicultural Counseling. Thousand Oaks, CA: Sage; 2010. pp. 427–438.
- 77. Morse J.M., Niehaus L. Mixed method design, principles and procedures. Walnut Creek, CA: Left Coast Press; 2009.
- 78. Plano Clark V.L., Ivankova N.V. Mixed methods research: A guide to the field. Thousand Oaks, CA: Sage; 2015.
- 79. O’Cathain A. Chapter 21: Assessing the Quality of Mixed Methods Research: Towards a Comprehensive Framework. In: Tashakkori A., Teddlie C, editors. SAGE Handbook of Mixed Methods in Social & Behavioral Research. Thousand Oaks, CA: Sage; pp. 531–555.
Thank you for visiting nature.com. You are using a browser version with limited support for CSS. To obtain the best experience, we recommend you use a more up to date browser (or turn off compatibility mode in Internet Explorer). In the meantime, to ensure continued support, we are displaying the site without styles and JavaScript.
- View all journals
- My Account Login
- Explore content
- About the journal
- Publish with us
- Sign up for alerts
- Open access
- Published: 07 December 2022
Genetic diversity fuels gene discovery for tobacco and alcohol use
- Gretchen R. B. Saunders 1 na1 ,
- Xingyan Wang 2 na1 ,
- Fang Chen ORCID: orcid.org/0000-0003-3108-8204 2 na1 ,
- Seon-Kyeong Jang 1 na1 ,
- Mengzhen Liu ORCID: orcid.org/0000-0001-6550-6959 1 na1 ,
- Chen Wang ORCID: orcid.org/0000-0002-2727-7240 2 na1 ,
- Shuang Gao 2 ,
- Yu Jiang 3 ,
- Chachrit Khunsriraksakul 2 ,
- Jacqueline M. Otto 1 ,
- Clifton Addison 4 ,
- Masato Akiyama 5 , 6 ,
- Christine M. Albert 7 , 8 ,
- Fazil Aliev 9 ,
- Alvaro Alonso 10 ,
- Donna K. Arnett 11 ,
- Allison E. Ashley-Koch 12 , 13 ,
- Aneel A. Ashrani 14 ,
- Kathleen C. Barnes 15 , 16 ,
- R. Graham Barr 17 ,
- Traci M. Bartz 18 , 19 ,
- Diane M. Becker 20 ,
- Lawrence F. Bielak 21 ,
- Emelia J. Benjamin 22 , 23 ,
- Joshua C. Bis 18 ,
- Gyda Bjornsdottir 24 ,
- John Blangero 25 ,
- Eugene R. Bleecker 26 ,
- Jason D. Boardman 27 ,
- Eric Boerwinkle 28 ,
- Dorret I. Boomsma 29 ,
- Meher Preethi Boorgula 15 ,
- Donald W. Bowden 30 ,
- Jennifer A. Brody 18 ,
- Brian E. Cade 31 , 32 , 33 ,
- Daniel I. Chasman 8 ,
- Sameer Chavan 15 ,
- Yii-Der Ida Chen 34 ,
- Zhengming Chen 35 , 36 ,
- Iona Cheng 37 , 38 ,
- Michael H. Cho 39 , 40 ,
- Hélène Choquet 41 ,
- John W. Cole 42 , 43 ,
- Marilyn C. Cornelis 44 ,
- Francesco Cucca 45 ,
- Joanne E. Curran 25 ,
- Mariza de Andrade 46 ,
- Danielle M. Dick 9 ,
- Anna R. Docherty 47 , 48 , 49 ,
- Ravindranath Duggirala 25 ,
- Charles B. Eaton 50 ,
- Marissa A. Ehringer 51 , 52 ,
- Tõnu Esko 53 ,
- Jessica D. Faul 54 ,
- Lilian Fernandes Silva 55 ,
- Edoardo Fiorillo 56 ,
- Myriam Fornage 28 , 57 ,
- Barry I. Freedman 58 ,
- Maiken E. Gabrielsen 59 ,
- Melanie E. Garrett 12 , 13 ,
- Sina A. Gharib 18 , 60 , 61 ,
- Christian Gieger 62 ,
- Nathan Gillespie 48 ,
- David C. Glahn 63 ,
- Scott D. Gordon 64 ,
- Charles C. Gu 65 ,
- Dongfeng Gu 66 ,
- Daniel F. Gudbjartsson 24 , 67 ,
- Xiuqing Guo 68 ,
- Jeffrey Haessler 69 ,
- Michael E. Hall 70 ,
- Toomas Haller 53 ,
- Kathleen Mullan Harris 71 ,
- Jiang He 72 , 73 ,
- Pamela Herd 74 ,
- John K. Hewitt 51 , 75 ,
- Ian Hickie 76 ,
- Bertha Hidalgo 77 ,
- John E. Hokanson 78 ,
- Christian Hopfer 79 ,
- JoukeJan Hottenga 29 ,
- Lifang Hou 44 ,
- Hongyan Huang 80 , 81 ,
- Yi-Jen Hung 82 ,
- David J. Hunter 83 ,
- Kristian Hveem 59 , 84 , 85 ,
- Shih-Jen Hwang 86 ,
- Chii-Min Hwu 87 ,
- William Iacono 1 ,
- Marguerite R. Irvin 77 ,
- Yon Ho Jee 80 ,
- Eric O. Johnson 88 , 89 ,
- Yoonjung Y. Joo 44 , 90 ,
- Eric Jorgenson 91 ,
- Anne E. Justice 92 , 93 ,
- Yoichiro Kamatani 5 , 94 ,
- Robert C. Kaplan 69 , 95 ,
- Jaakko Kaprio 96 ,
- Sharon L. R. Kardia 21 ,
- Matthew C. Keller 51 , 75 ,
- Tanika N. Kelly 72 , 73 ,
- Charles Kooperberg 19 , 69 ,
- Tellervo Korhonen 96 ,
- Peter Kraft 80 , 81 ,
- Kenneth Krauter 97 ,
- Johanna Kuusisto 98 , 99 ,
- Markku Laakso 98 ,
- Jessica Lasky-Su 100 ,
- Wen-Jane Lee 101 ,
- James J. Lee 1 ,
- Daniel Levy 86 ,
- Liming Li 102 ,
- Kevin Li 103 ,
- Yuqing Li 37 ,
- Kuang Lin 35 ,
- Penelope A. Lind 104 , 105 , 106 ,
- Chunyu Liu 107 ,
- Donald M. Lloyd-Jones 108 ,
- Sharon M. Lutz 109 , 110 ,
- Jiantao Ma 86 , 111 ,
- Reedik Mägi 52 ,
- Ani Manichaikul 112 ,
- Nicholas G. Martin 64 ,
- Ravi Mathur 88 ,
- Nana Matoba 5 , 113 ,
- Patrick F. McArdle 114 ,
- Matt McGue 1 ,
- Matthew B. McQueen 115 ,
- Sarah E. Medland 104 ,
- Andres Metspalu 53 ,
- Deborah A. Meyers 26 ,
- Iona Y. Millwood 35 , 36 ,
- Braxton D. Mitchell 114 , 116 ,
- Karen L. Mohlke 117 ,
- Matthew Moll 39 , 40 ,
- May E. Montasser 114 ,
- Alanna C. Morrison 28 ,
- Antonella Mulas 56 ,
- Jonas B. Nielsen 59 , 118 ,
- Kari E. North 93 ,
- Elizabeth C. Oelsner 17 ,
- Yukinori Okada 119 , 120 , 121 , 122 ,
- Valeria Orrù 56 ,
- Nicholette D. Palmer 30 ,
- Teemu Palviainen 96 ,
- Anita Pandit 103 ,
- S. Lani Park 123 ,
- Ulrike Peters 69 , 124 ,
- Annette Peters 125 , 126 , 127 ,
- Patricia A. Peyser 21 ,
- Tinca J. C. Polderman 128 , 129 ,
- Nicholas Rafaels 15 ,
- Susan Redline 31 , 32 , 130 ,
- Robert M. Reed 131 ,
- Alex P. Reiner 69 , 124 ,
- John P. Rice 132 ,
- Stephen S. Rich 112 ,
- Nicole E. Richmond 78 ,
- Carol Roan 133 ,
- Jerome I. Rotter 68 ,
- Michael N. Rueschman 31 ,
- Valgerdur Runarsdottir 134 ,
- Nancy L. Saccone 65 , 135 ,
- David A. Schwartz 136 ,
- Aladdin H. Shadyab 137 ,
- Jingchunzi Shi 138 ,
- Suyash S. Shringarpure 138 ,
- Kamil Sicinski 133 ,
- Anne Heidi Skogholt 59 ,
- Jennifer A. Smith 21 , 54 ,
- Nicholas L. Smith 124 , 139 , 140 ,
- Nona Sotoodehnia 18 , 141 ,
- Michael C. Stallings 51 , 75 ,
- Hreinn Stefansson 24 ,
- Kari Stefansson 24 , 142 ,
- Jerry A. Stitzel 51 ,
- Xiao Sun 72 ,
- Moin Syed 1 ,
- Ruth Tal-Singer 143 ,
- Amy E. Taylor 144 , 145 , 146 ,
- Kent D. Taylor 68 ,
- Marilyn J. Telen 12 ,
- Khanh K. Thai 41 ,
- Hemant Tiwari 147 ,
- Constance Turman 80 , 81 ,
- Thorarinn Tyrfingsson 134 ,
- Tamara L. Wall 148 ,
- Robin G. Walters 35 , 36 ,
- David R. Weir 54 ,
- Scott T. Weiss 100 ,
- Wendy B. White 149 ,
- John B. Whitfield 64 ,
- Kerri L. Wiggins 150 ,
- Gonneke Willemsen 29 ,
- Cristen J. Willer 151 , 152 , 153 ,
- Bendik S. Winsvold 59 , 154 , 155 ,
- Huichun Xu 114 ,
- Lisa R. Yanek 20 ,
- Jie Yin 41 ,
- Kristin L. Young 156 ,
- Kendra A. Young 78 ,
- Bing Yu 28 ,
- Wei Zhao 21 ,
- Wei Zhou 153 , 157 ,
- Sebastian Zöllner 158 , 159 ,
- Luisa Zuccolo 144 , 146 , 160 ,
- 23andMe Research Team ,
- The Biobank Japan Project ,
- Chiara Batini 161 ,
- Andrew W. Bergen 162 , 163 ,
- Laura J. Bierut 132 ,
- Sean P. David 164 , 165 ,
- Sarah A. Gagliano Taliun 166 , 167 , 168 ,
- Dana B. Hancock 88 ,
- Bibo Jiang 2 ,
- Marcus R. Munafò 144 , 145 , 169 ,
- Thorgeir E. Thorgeirsson 24 ,
- Dajiang J. Liu ORCID: orcid.org/0000-0001-6553-858X 2 na2 &
- Scott Vrieze ORCID: orcid.org/0000-0003-3861-7930 1 na2
Nature volume 612 , pages 720–724 ( 2022 ) Cite this article
53k Accesses
66 Citations
399 Altmetric
Metrics details
- Genome-wide association studies
- Human behaviour
- Risk factors
This article has been updated
Tobacco and alcohol use are heritable behaviours associated with 15% and 5.3% of worldwide deaths, respectively, due largely to broad increased risk for disease and injury 1 , 2 , 3 , 4 . These substances are used across the globe, yet genome-wide association studies have focused largely on individuals of European ancestries 5 . Here we leveraged global genetic diversity across 3.4 million individuals from four major clines of global ancestry (approximately 21% non-European) to power the discovery and fine-mapping of genomic loci associated with tobacco and alcohol use, to inform function of these loci via ancestry-aware transcriptome-wide association studies, and to evaluate the genetic architecture and predictive power of polygenic risk within and across populations. We found that increases in sample size and genetic diversity improved locus identification and fine-mapping resolution, and that a large majority of the 3,823 associated variants (from 2,143 loci) showed consistent effect sizes across ancestry dimensions. However, polygenic risk scores developed in one ancestry performed poorly in others, highlighting the continued need to increase sample sizes of diverse ancestries to realize any potential benefit of polygenic prediction.
Similar content being viewed by others
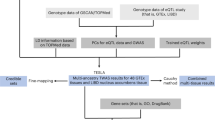
Multi-ancestry transcriptome-wide association analyses yield insights into tobacco use biology and drug repurposing
Fang Chen, Xingyan Wang, … Dajiang J. Liu
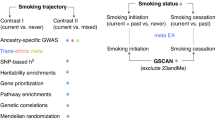
Genome-wide association study of smoking trajectory and meta-analysis of smoking status in 842,000 individuals
Ke Xu, Boyang Li, … VA Million Veteran Program
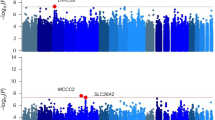
Multi-ancestry genome-wide association study of cannabis use disorder yields insight into disease biology and public health implications
Daniel F. Levey, Marco Galimberti, … Joel Gelernter
We developed a multi-ancestry meta-regression method to meta-analyse ancestrally diverse genome-wide association study (GWAS) summary statistics from 60 cohorts with 3,383,199 individuals (Supplementary Table 1 ; see Supplementary Fig. 1 for an overview of the project), representing major clines of recent human ancestry (Fig. 1a ). The meta-analytic method used here uses meta-regression to account for per study axes of genetic ancestry variation combined with a random effect to capture further unexplained heterogeneity in the effect of a given genetic variant. Although ancestry here is continuous, we also performed secondary analyses of continental groups reflecting four ancestry clines, including individuals of African (AFR; maximum n = 119,589) and American (AMR; n = 286,026) recently admixed ancestries primarily from the United States; individuals of East Asian ancestries (EAS; n = 296,438) primarily from the United States, People’s Republic of China and Japan; and individuals of European ancestries (EUR; n = 2,669,029) from the United States, Europe and Australia (see Extended Data Fig. 1 and Supplementary Note ). Smoking phenotypes were selected to represent different stages of tobacco use and addiction, including initiation, the onset of regular use, amount smoked and cessation. Measures of onset included whether an individual ever smoked regularly (smoking initiation (SmkInit); n = 3,383,199) and the age at which the individual began smoking regularly (AgeSmk; n = 728,826). Amount smoked among current and former regular smokers was measured as cigarettes smoked per day (CigDay; n = 784,353). Smoking cessation (SmkCes; n = 1,400,535) contrasted current versus former smokers. Alcohol use was widely available across most studies, measured as drinks per week (DrnkWk; n = 2,965,643).
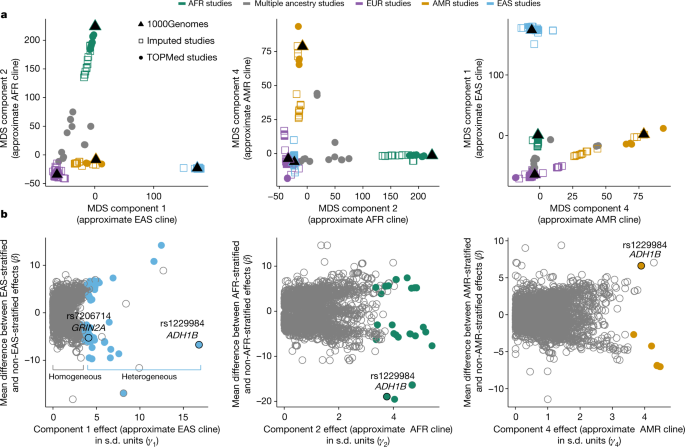
a , Ancestry compositions of contributing studies (each point is a study). Colours are coded by primary ancestry of individuals in the cohort. Studies with less than 90% of individuals assignable to a single ancestry group are shown in grey. Ancestry component 3 was a north–south EUR cline, which was omitted here as we did not conduct meta-analyses stratified by northern versus southern Europe. TOPMed, Trans-Omics for Precision Medicine. b , Extent of effect size moderation as a function of the same ancestry dimensions as shown in a . The full moderation results are in Supplementary Table 2 . Each point in b represents an independent variant with the standardized MDS component coefficient from our trans-ancestry models (that is, γ ) along the x axes, and the corresponding mean difference in effect sizes ( β ) for the ancestry-stratified meta-analysis of the given ancestry versus all other ancestries along the y axes. The grey circles indicate variants showing little to no evidence of effect size heterogeneity across ancestry, whereas the coloured circles represent variants with adequate evidence of effect size heterogeneity. The plots highlight that the majority of variants have similar effect sizes across all ancestry clines, with some potentially interesting exceptions in which the variant effects sizes differ substantially between ancestry clines.
Multi-ancestry meta-analysis
Using our multi-ancestry meta-analysis, we identified 2,143 associated loci across all phenotypes (sentinel variant P < 5 × 10 −9 ), with 3,823 independently associated variants (Extended Data Fig. 2 , Supplementary Tables 2 and 3 and Supplementary Figs. 2 and 3 ). Of these, 1,346 loci and 2,486 independent variants were associated with SmkInit, 33 loci (39 variants) with AgeSmk, 140 loci (243 variants) with CigDay, 128 loci (206 variants) with SmkCes and 496 loci (849 variants) with DrnkWk. Approximately 64% ( n = 1,364) of loci were phenotype-specific, five loci were associated with all four smoking phenotypes but not with DrnkWk, and five loci were associated with all five phenotypes. All sentinel variants within identified loci had high posterior probabilities that their effect would replicate in a sufficiently powered study according to a trans-ancestry extension of our GWAS cross-validation technique 6 . Only 17 sentinel variants (0.7%) had such posterior probabilities of less than 0.99 and were therefore removed from the counts above and from further consideration (additional details on these 17 variants are shown in Supplementary Fig. 4 ).
Inclusion of diverse ancestry may improve the discovery of new variants through a combination of increased genetic variation, larger sample sizes and improved fine-mapping due to diverse patterns of linkage disequilibrium (LD). We quantified gains in power from the use of our multi-ancestry model over a simpler ancestry-naive fixed-effects model excluding the ancestry meta-regression. Comparing the number of associated variants, we found 721 additional independent variants that were identified only by the multi-ancestry meta-regression analysis. Both sets of models were fit to the same data, such that the larger number of significantly associated variants identified with the multi-ancestry model indicates increased power from accounting for axes of genetic variation and residual heterogeneity. Included among these 721 were newly associated variants in genes related to nervous system function (for example, NRXN1 ) including glutamatergic ( GRIN2A ) neurotransmission, which is of relevance to neurocircuitry in addiction 7 , 8 .
To isolate likely causal variants, we used a fine-mapping procedure (see Supplementary Note ) that leverages variation in LD across ancestry groups to construct 90% credible intervals. We identified 597 loci (27.9%) in which the 90% credible intervals included fewer than five variants, including 192 loci (9.0%) with a single fine-mapped variant. Overall, credible intervals contained medians of 9–19 variants and median spans of 32–78 kb across phenotypes (Supplementary Table 4 ). Compared with the EUR-stratified GWAS (described in the next section), the trans-ancestry fine-mapping increased the number of 90% credible intervals containing fewer than five variants by 27.6%, and containing a single variant by 41.2%. Across all 2,143 loci, 1,330 (62.1%) loci had a reduced number of variants in the credible intervals in the multi-ancestry analysis. To determine the gain in resolution attributable to increased sample size (versus LD differences), we ‘downsampled’ the multi-ancestry analysis by removing EUR ancestry cohorts until the total sample size was approximately equal to that of the EUR-stratified analysis and regenerated fine-mapping results. Using the 1,330 loci with improved resolution in multi-ancestry analysis, we found that the credible intervals were reduced from a median of 22 variants in the EUR-stratified analysis to 12 variants in the downsampled multi-ancestry analysis, suggesting that approximately 55% of the observed improvement in fine-mapping is attributable to larger multi-ancestry sample sizes alone. These findings highlight the utility of both increased sample size and diverse ancestry in fine-mapping variants for these complex behavioural phenotypes. To characterize genes prioritized from fine-mapping, we conducted a series of functional enrichment analyses. We first selected intervals fine-mapped to fewer than five variants from the multi-ancestry results and mapped each variant to the nearest gene to identify ‘high-priority’ genes. Relative to genes mapped from variants with posterior inclusion probabilities (PIP) < 0.01, the high-priority genes were enriched across brain and nerve tissues (Extended Data Fig. 3a and Supplementary Table 5 ). Within the brain, cell-type enrichment of the high-priority genes was observed for projecting glutamatergic neurons from the cortex, hippocampus and amygdala (telencephalon excitatory projection neurons) and projection GABA neurons from medium spiny neurons of the striatum (telencephalon inhibitory projecting neurons), along with neurons in various subcortical structures such as the hypothalamus and midbrain, consistent with aspects of the mesolimbic theory of addiction 7 , 8 (Extended Data Fig. 3b ). Finally, these high-priority genes that were strongly associated with substance use were enriched in gene pathways related to neurogenesis, neuronal development, neuronal differentiation and synaptic function. The neurodevelopmental aspect of the high-priority genes could indicate a role for these genes in processes that predispose individuals to risk of substance use and/or may contribute to brain circuit rewiring during drug use.
The multi-ancestry meta-analysis method also allowed for tests of whether a variant effect size differed (that is, was moderated) by ancestry along four ancestry dimensions estimated from multidimensional scaling (MDS) of allele frequencies from each participating study (Fig. 1a ). Roughly, the first axis represents an EAS ancestry cline, the second axis an AFR cline, the third a EUR cline (north to south EUR) and the fourth an AMR cline. There was minimal evidence of effect size moderation by ancestry for most independent variants, ranging from 76.6% (187 variants) in CigDay to 85.0% (175 variants) in SmkCes. Another 7.7–18.1% showed modest evidence for moderation. Finally, roughly 3.6% of all independent variants, reflecting 136 variants from 84 distinct loci, showed strong evidence of effect size moderated by ancestry (complete results are shown in Supplementary Table 2 ). Comparisons between the variants with strong evidence for effect size moderation by ancestry and those with no evidence suggested that the identification of these 136 variants was not driven to a large extent by differences in imputation quality, LD scores or Fst (fixation index) across ancestries.
Across phenotypes, 88 of these 136 variants showed moderation by the first axis of ancestry variation (approximate EAS cline; Fig. 1b , left), 29 variants by the second axis (approximate AFR cline; Fig. 1b , middle) and 10 variants by the fourth axis (approximate AMR cline; Fig. 1b , right). Nine variants showed differences in effect size moderated by the third axis (EUR cline). Only the effect of one variant was moderated by three or more ancestry clines (EAS, AFR and AMR): rs1229984, a missense variant in the alcohol dehydrogenase gene ADH1B , which has been shown to be protective against alcohol consumption 9 . An increase on any of these clines was associated with a reduced effect size of this allele, on average. For example, if there are two people who both carry one copy of the protective T allele for this variant but are separated by 1 s.d. on MDS component 1 (EAS cline), the person with a lower value on that MDS cline would be expected to drink 0.3 fewer drinks than the person with a higher MDS value, despite the same rs1229984 genotype in ADH1B .
To further evaluate causal genes and relevant tissues through which associated variants may be operating, we applied a trans-ancestry transcriptome-wide association study (TWAS) analysis to each phenotype across 49 tissues derived from the GTEx Consortium 10 . Using a P value threshold Bonferroni-corrected for the total number of genes and tissues within a phenotype, we found 1,167 genes significantly associated with SmkInit, 21 genes with AgeSmk, 203 genes with CigDay, 188 genes with SmkCes and 504 genes with DrnkWk (resulting in 1,705 unique genes across phenotypes; Supplementary Table 6 ). For each of our five phenotypes, matrix decomposition parallel analysis 11 of the per-tissue P value correlation matrix suggested two components: one explaining 53.7–55.2% of the variance in P values, and another explaining 3.5–3.8% of the variance in P values. Similar loading patterns were observed for all phenotypes such that all tissues loaded strongly (all loadings > 0.12) on the first component, suggesting that it represents a general effect across tissues, whereas only brain tissues had strong loadings on the second component (all loadings > 0.12), indicating the importance of brain-specific gene expression effects for these tobacco and alcohol use phenotypes. Pathway enrichment analyses of the TWAS-associated genes identified 1,029 unique gene pathways across phenotypes that were broadly enriched across tissues (Supplementary Table 7 ), including many of obvious relevance to neurotransmission and neurodevelopment.
To further illustrate several variants within genes of interest, we integrated findings described above to select variants for which there was evidence of association across analytic methods and for which the availability of diverse ancestries was clearly relevant. Illustrative variants were chosen in a similar way as described for the enrichment analyses above: (1) we extracted variants from multi-ancestry fine-mapped credible intervals containing less than five variants, and (2) we cross-referenced the resulting variants with the multi-ancestry TWAS cis -expression quantitative trait loci and their significantly associated genes. We highlight five of the 52 genes that resulted from this process.
We found the nicotinic gene cluster CHRNA5–A3–B4 to be significantly associated with SmkInit 12 with a fine-mapped 90% credible interval that shrank from 53 variants in EUR-stratified results to just two variants in multi-ancestry results (rs2869055 and rs28438420; Supplementary Table 4 ). These variants are not in high LD ( r 2 = 0.31 for both variants) with the well-known variant rs16969968 in this gene cluster. By contrast, this locus was fine-mapped to two variants in high LD with rs16969968 for CigDay ( r 2 = 0.84 and 0.86), suggesting that the variants underlying this signal for smoking initiation may be distinct from those for cigarettes per day. We also found a novel association between SmkInit and CACNA1B , which encodes a voltage-gated calcium channel (Ca v 2.2) that controls neuronal neurotransmitter release and has been associated with cocaine reinstatement 13 , increased aggression and vigilance, and reduced startle and exploration 14 . CACNA1B is linked to multiple psychiatric disorders, including schizophrenia, bipolar disorder and autism spectrum disorders 15 , 16 , 17 .
CigDay was associated with variants in neurturin ( NRTN ), a type of glial cell line-derived neurotrophic factor involved in the development and survival of dopamine neurons 18 . This gene has been studied in relation to Parkinson disease for its potential to restore dopamine neurocircuitry 19 . Likewise, PAK6 was another novel gene strongly associated with CigDay in TWAS results and was fine-mapped to just three variants in the 90% credible interval. PAK6 encodes a p21-activated kinase that is highly expressed in the striatum and hippocampus, has been implicated in the migration of GABAergic interneurons 20 as well as the modulation of dopaminergic neurotransmission 21 , and is involved in locomotor activity and cognitive function 22 . PAK6 has been robustly associated with schizophrenia 23 and neurodegenerative diseases 24 , 25 , such as Parkinson disease and Alzheimer disease, further highlighting its role in synaptic changes. Finally, we found a novel association between ECE2 and DrnkWk. ECE2 is involved in cortical development 26 as well as the processing of several neuroendocrine peptides, including neurotensin and substance P 27 , and may also have a role in amyloid-β processing 28 . ECE2 also generates peptides such as BAM 12 (which shows κ -opioid receptor selectivity) and BAM 22 (which shows μ -opioid receptor selectivity), suggesting a link with pain transmission 27 .
Genetic correlation and polygenic scores
To evaluate heritability, genetic correlation and polygenic scoring, we generated ancestry-stratified GWAS meta-analysis results for each of the four continental groups: AFR, AMR, EAS and EUR (Supplementary Table 2 lists ancestry-stratified loci). Heritability and cross-phenotype genetic correlations were generally similar in sign and modest in magnitude in each ancestry (Fig. 2a and Supplementary Tables 8 and 9 ). Smoking phenotypes were moderately genetically correlated with each other (| r g | = 0.30–0.63) and with DrnkWk (| r g | = 0.16–0.27). Genetic correlations for the same phenotype between each of the largest contributing cohorts and all remaining cohorts (restricted to EUR ancestries only) were generally high for each smoking phenotype (mean r g of 0.93) and DrnkWk (mean r g of 0.72), indicating that these measures were reliable across cohorts (Supplementary Table 9 ).
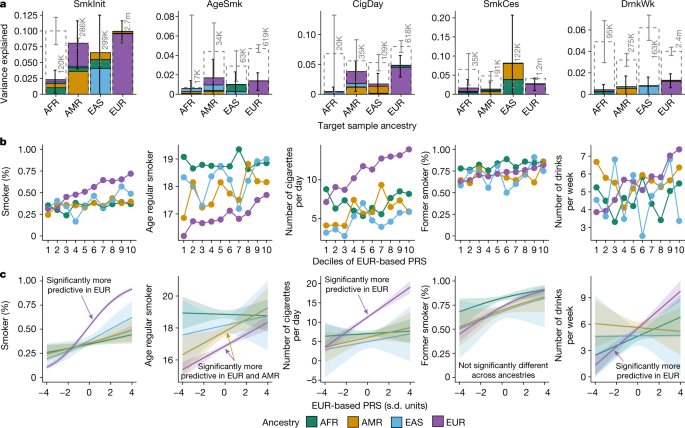
a , Incremental variance explained for each target ancestry group. The colour of the stacked bars indicates the ancestry from which the polygenic score was derived; the total height of each set of the stacked bars (and 95% confidence intervals) correspond to the total variance explained by all four ancestry-stratified scores combined. For example, in the target EUR subsample, non-EUR polygenic scores add little over and above the EUR score. Note that some comparisons are underpowered to detect differences in predictive accuracy across ancestry (see Supplementary Note ). Heritabilities, estimated by LD score regression, of each phenotype–ancestry combination are depicted by the grey dashed bar (with 95% confidence intervals) and corresponding sample sizes; these represent the maximum expected accuracy of the polygenic risk score (PRS). b , The manner in which the phenotype mean in the target sample changes as a function of the EUR PRS deciles. c , Results from an interaction model, in which each phenotype was modelled as a function of an interaction between the EUR-based PRS and target ancestry (coded as a factor with EUR ancestry as the reference and scores scaled within ancestry). The bands around each line denote the 95% confidence intervals. Significant interactions are noted with text. Using SmkInit as an example, the purple line represents the predicted proportion of regular smokers as a function of the EUR PRS in the EUR subsample of Add Health, the blue lines show the predicted proportion of regular smokers by standard deviation of the EUR PRS in the EAS subsample, and so on. In this case, the magnitude of the association between the EUR-based PRS and SmkInit (that is, the slope) was significantly greater in the EUR target ancestry than all other ancestries. Full PRS results are in Supplementary Table 12 .
To characterize the multifactorial genetic aetiology of tobacco and alcohol use, we computed genetic correlations of our EUR-stratified results with 1,141 medical, biomarker and behavioural phenotypes from the UK Biobank 29 (Supplementary Tables 10 and 11 ). An affinity propagation clustering algorithm 30 was used to aid interpretability by grouping UK Biobank phenotypes such that each of the five current phenotypes were exemplars (Supplementary Fig. 5 ). SmkInit and AgeSmk clustered together, as did SmkCes and CigDay, with all four forming a broad higher-level smoking cluster. Phenotypes with high positive genetic correlations with SmkInit included addiction to any substance, neighbourhood material deprivation, diagnosis of chronic obstructive pulmonary disease, and a negative correlation with age at first sexual intercourse (| r g | = 0.57–0.64). For AgeSmk, the largest genetic correlations were with reproductive phenotypes such as age at first birth ( r g = 0.69–0.71) and measures of years of education and attainment ( r g = 0.58–0.69). CigDay and SmkCes were most highly positively correlated with respiratory and cardiovascular diseases and cancers ( r g = 0.52–0.72), highlighting their genetic link to adverse disease outcomes. Finally, DrnkWk was most strongly correlated with problematic drinking behaviours ( r g = 0.52–0.70), indicating extensive overlap in the genetic architecture of DrnkWk and measures of alcohol use, problems and alcohol use disorder. This is consistent with previous findings of strong but imperfect genetic correlations (for example, r g = 0.8) between alcohol consumption and alcohol use disorder from large-scale GWAS 31 , 32 . We note, however, that genetic correlations can be difficult to interpret 33 , 34 as they may be affected by genetic confounding, mediation effects or sampling bias.
We used the ancestry-stratified meta-analysis results to construct ancestry-specific polygenic risk scores in Add Health 35 , an independent target sample of individuals of diverse ancestries from the United States ( n = 2,199 AFR, 1,132 AMR, 525 EAS and 6,092 EUR). To evaluate within-ancestry and across-ancestry performance of polygenic scores, we iteratively fit a multiple regression model and evaluated the incremental predictive accuracy of each ancestry-based score, over and above scores already entered into the model (that is, first including the AMR-based score, then adding the AFR-based, EAS-based and EUR-based scores one at a time to evaluate incremental prediction accuracy). EUR-based scores in EUR ancestries outperformed ancestry-matched scores in non-EUR ancestries (Fig. 2a ) and showed significantly stronger associations with most phenotypes in EUR ancestries than in non-EUR ancestries (described by decile plots and tested by modelling an interaction between the EUR-based polygenic risk score and the target sample ancestry group), consistent with expectations 36 (Fig. 2b,c ). For each ancestry and phenotype, the EUR-based score on its own outperformed the ancestry-matched score on its own (Supplementary Table 12 ). These results highlight the relative utility of current polygenic scores for EUR ancestries versus all others. In interpreting these results, however, we note that some comparisons may be underpowered to identify differences in the variance explained by polygenic scores between ancestries. Finally, EUR-based scores overpredicted tobacco and alcohol use for individuals of non-EUR ancestry and underpredicted for individuals of EUR ancestry, although this prediction bias is readily eliminated through statistical correction with genetic principal components.
Tobacco and alcohol use are heritable behaviours that can be radically affected by environmental factors, including cultural context 37 and public health policies 38 , 39 . Despite this, we found that a large majority of associated genetic variants showed homogeneous effect size estimates across diverse ancestries, suggesting that the genetic variants associated with substance use affect such individuals similarly. The limited extent of variant effect size heterogeneity, coupled with similar heritability estimates and cross-trait genetic correlations, indicates that the genetic architecture underlying substance use is not markedly different across ancestries. There are some potentially interesting exceptions of ancestrally heterogeneous effects in genes such as ADH1B and CACNA1B . By contrast, polygenic scores generally performed well in EUR ancestries but with mixed-to-limited results in other ancestries, suggesting that portability of such scores across ancestries remains challenging, even when discovery sample sizes across all ancestries are more than 100,000. Explanations for this apparent discrepancy have been proposed 40 , but more stringent and sensitive tests will be required to draw strong conclusions about such patterns of heredity.
Most individuals of EUR, AFR and AMR ancestries in the current study live in the United States and Europe and share somewhat similar environments regarding tobacco and alcohol availability and policies surrounding use of these substances, and all included individuals were adults. Further increases in genetic diversity and consideration of environmental moderators, including cultural factors, will continue to add to our understanding of the genetic architecture of both substance use and related behaviours and diseases.
Here we describe an overview of the methods used to conduct the association, fine-mapping and downstream in silico functional analysis. Additional details can be found in the Supplementary Note .
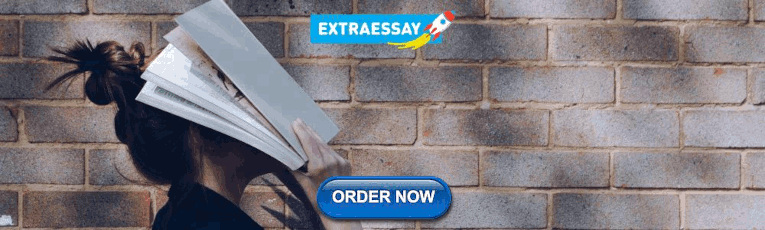
Generation of summary statistics and ancestry considerations
Except for TOPMed studies, in which the genetic data were derived from deep whole-genome sequencing, participants in all studies were genotyped on genome-wide arrays. The majority of studies imputed their genotypes to the Haplotype Reference Consortium 41 (for EUR ancestries) or 1000 Genomes 42 (Supplementary Table 1 ). GWAS summary statistics were generated in each study sample typically using RVTESTS 43 , BOLT-LMM 44 or SAIGE 45 with covariates of sex, age, age squared and genetic principal components according to an analysis plan detailed in the Supplementary Note . Studies composed primarily of closely related individuals (for example, family studies) first regressed out covariates, inverse-normalized the residuals as necessary and then tested additive variant effects under a linear mixed model with a genetic kinship matrix for all phenotypes. Some studies of unrelated individuals followed the same analysis for quasi-continuous phenotypes (AgeSmk, CigDay and DrnkWk), but estimated additive genetic effects under a logistic model for binary phenotypes (SmkInit and SmkCes).
We used terminology and acronyms from the 1000 Genomes Project 42 to describe ancestry. The majority of participating cohorts stratified their sample by ancestry before generation of summary statistics. Cohorts composed of substantial samples of multiple ancestry groups provided summary statistics stratified by ancestry, as well as results based on all individuals regardless of ancestry for use in the multi-ancestry meta-analyses. As TOPMed served multiple functions in the present study, including as an LD reference panel, we detailed the ancestry analyses and classification of TOPMed data in the Supplementary Note . For example, for both ancestry-stratified and multi-ancestry conditional analysis, we created TOPMed reference panels for estimating LD. We first created ancestry-stratified reference samples, resulting in matched ancestry reference sample sizes of n = 28,665 AFR, n = 19,737 AMR, n = 4,918 EAS and n = 51,656 EUR. To create a TOPMed-based reference sample for multi-ancestry analyses, we combined the matched ancestry individuals, resulting in a diverse ancestry reference panel ( n = 104,976) that matches the ancestry proportions of the included cohorts to estimate LD.
Extensive quality control and filtering were performed on the summary statistics from each cohort. We removed studies with a sample size of less than 100, and those with genomic control values greater than 1.1 or less than 0.9 and a sample size of less than 10,000 (per study sample size and genomic control values are listed in Supplementary Table 1 ), as well as variants with an imputation quality of less than 0.3.
Ancestry-stratified meta-analyses
Ancestry-stratified meta analyses were performed using the software package rareGWAMA (see URLs for software use). Specifically, the method aggregated weighted Z -score statistics, that is,
where Z k is the Z -score statistic in study k . The weight w k is defined by \({w}_{k}=\sqrt{{N}_{k}{p}_{k}(1-{p}_{k}){R}_{k}^{2}},\) where \({p}_{k}\) is the variant allele frequency, and \({R}_{k}^{2}\) is the imputation quality in study k . This method accounts for between-study heterogeneity in phenotype measures, imputation accuracy, allele frequencies and sample sizes.
Multi-ancestry meta-analyses
Multi-ancestry meta-analyses were performed using mixed-effects meta-regression for optimal trans-ancestry meta-analysis (MEMO) implemented in rareGWAMA (see URLs for software use). The full model is \({b}_{{jk}}={\sum }_{l=0}^{L}{C}_{{lk}}{\gamma }_{{jl}}+{e}_{{jk}}+{{\epsilon }}_{{jk}},\) where \({b}_{{jk}}\) is the genetic effect estimate for the j th variant in the k th study, and \({C}_{{lk}}\) is the l th ancestry component for the k th study. Note that we set \({C}_{0k}=1\) , so \({\gamma }_{j0}\) serves as the intercept. The regression coefficient \({\gamma }_{{jl}}\) captures the effect of the l th axis of genetic variation for the j th variant, with \({\gamma }_{j0}\) as an intercept in the model, and \({e}_{{jk}}\sim N\left(0,{\tau }^{2}\right)\) is the random effect that captures unexplained effect size heterogeneity after adjusting for genetic variation. Finally, \({{\epsilon }}_{{jk}}\sim N\left(0,{s}_{{jk}}^{2}\right)\) is the random error term, where \({s}_{{jk}}^{2}\) is the variance of the genetic effect estimate \({b}_{{jk}}\) . This method models heterogeneity of effects attributable to ancestry as well as a random effect to capture residual heterogeneity. The MEMO model contains fixed-effect, random-effect and meta-regression models as special cases. Specifically, removing the random effect \({e}_{{jk}}\) results in a regular meta-regression model, removing the covariates of genetic variation ( \({C}_{{lk}})\) , but retaining \({e}_{{jk}}\) results in a random-effect meta-analysis model, whereas removing both \({e}_{{jk}}\) and \({C}_{{lk}}\) results in a fixed-effect meta-analysis model.
Per study ancestry variation, \({C}_{{lk}}\) is calculated using MDS on the basis of allele frequency. We defined the genetic distance between two studies, that is, study k and k ′, with J variants, as \({d}_{k{k}^{{\prime} }}=\sqrt{{\sum }_{j}{\left({f}_{{jk}}-{f}_{j{k}^{{\prime} }}\right)}^{2}},\) where \({f}_{{jk}}\) and \({f}_{j{k}^{{\prime} }}\) are the allele frequency for the j th variant for study k and k ′, respectively. We fit models with 0, 1, 2, 3 and 4 MDS components and combined the results using a minimal P value approach (see Extended Data Fig. 1a for a visual representation of the first four MDS components).
To better ensure robustness, for each phenotype, we filtered variants from the meta-analytic results to variants that were present in at least three studies, had an effective sample size (sample size multiplied by imputation accuracy) to maximum sample size ratio of ≥ 0.1, and minor allele frequency (MAF) > 0.001 in the multi-ancestry and EUR-stratified meta-analysis or MAF > 0.01 for AMR-stratified, AFR-stratified and EAS-stratified meta-analysis, given the expected drop off in imputation accuracy for those ancestries. These filters reduce potential artefacts arising from sparse data or poor imputation and retain variants with reasonable statistical power.
With increasing imputation accuracy and the inclusion of variants with MAF down to 0.1% (for EUR), genome-wide significant variants were identified using a threshold of P < 5 × 10 −9 , to account for approximately 10 million independent tests. The threshold was chosen based on previous work on low-frequency variants 5 , 46 , 47 . All statistical tests are two-sided unless otherwise stated.
Robustness and replicability of signals
We applied genomic control correction for low-frequency variants (MAF < 1%) in both multi-ancestry and ancestry-stratified meta-analyses. Genomic control correction for common variants was not applied given that elevation of genomic control values is expected with high polygenicity (that is, it assumes sparsity) and very large sample sizes 48 ; such a correction may be overly conservative. To evaluate this decision, we estimated the replicability of associated loci using a trans-ancestry extension of an existing method 6 . This method, ‘RATES’, incorporates cohort-level summary statistics (single-nucleotide polymorphism (SNP) effect sizes and their corresponding standard errors), along with allele frequency-based MDS components per study to assign a posterior probability that each sentinel variant effect would replicate in a sufficiently powered study. To further evaluate robustness of our results, we estimated LD score regression (LDSC) intercepts and attenuation ratios to account for bias in the intercept test when sample sizes become extreme, as in the present case. Results were within expected limits and consistent with a limited effect of population stratification on the meta-analysis results 44 (Supplementary Table 8 ). Then, we compared the sign of SNP effect size estimates between EUR-stratified results and within-sibling GWAS results from the UK Biobank, finding sign concordance estimates of 63.4–80% across phenotypes, all of which were significantly higher than would be expected if our results were driven entirely by population stratification or cryptic relatedness and were consistent in magnitude with other large-scale association studies 49 . Finally, given reduced power in the within-sibling GWAS, we additionally compared the sign of SNP effect size estimates between EUR-stratified 23andMe summary statistics (the largest participating cohort) and EUR-stratified summary statistics with all cohorts except 23andMe, finding sign concordance estimates of 94.3–100%. See the Supplementary Note for further details on the methods and full results, including the list of excluded variants and loci.
Conditional analyses and locus definitions
We performed sequential forward selection to identify independently associated variants in each locus 50 for ancestry-stratified and multi-ancestry results. The procedure begins by including only the top association signal into a set of independently associated variants ( ϕ ) per locus. Conditional analysis is then conducted on the remaining variants, conditioning on variants in ϕ . If any of these conditional signals remained significant (that is, P < 5 × 10 −9 ), we added the top signal to the set ϕ . The process iterates until there are no remaining significantly associated variants. The method requires an external genomic reference panel to estimate LD patterns. For ancestry-stratified conditional analyses, we used ancestry-matched individuals from TOPMed to estimate LD (sample sizes given previously). For multi-ancestry conditional analyses, we used the diverse ancestry TOPMed reference panel ( n = 104,976) that matched the ancestry proportions of the included cohorts.
Loci were defined based in part on the conditional analysis, using a multi-step approach. First, consistent with previous GWAS meta-analysis 5 in EUR ancestries, we identified all 1-Mb windows surrounding sentinel variants and collapsed overlapping windows. This resulted in a total of 1,449 such windows. For each window, we then used our ancestry-aware conditional analysis 51 (described previously) with an ancestry-matched reference panel from TOPMed to enumerate all independent variants within each window. Then, for each independent variant, we defined a locus as the region including all variants in LD of r 2 > 0.1, based on the same ancestry-matched TOPMed reference panel (Supplementary Table 3 and Supplementary Fig. 3 ). Overlapping loci were then collapsed. This procedure avoids conventional definitions of a locus based on work in EUR ancestries and is tailored to the multi-ancestry data at hand.
Allelic effect size moderation
We evaluated evidence of effect size moderation by ancestry in the multi-ancestry model for each independent variant. To do so, we extended the MEMO model into a mixture model that separated variants with homogenous effects (models with only an intercept term) from those with possible heterogeneous effects (on at least one axis of genetic variation). We considered six sub-models including the null model, and the models in which the number of included components varied from 0 to 4.
where \(p({b}_{j}|{\rm{N}}{\rm{U}}{\rm{L}}{\rm{L}})\) and \(p\left({b}_{j}| M{R}_{l}\right)\) are the likelihoods of the variant j effect sizes under the null model and the meta-regression models with l axes of genetic variation, respectively; \({p}_{a}^{{\rm{NULL}}}\) and \({p}_{a}^{{\rm{ALT}}}\) are the probabilities of locus a carrying zero or at least one causal variant, respectively. The term \({q}_{{jl}}\) is the probability that the model with l axes of genetic variation best fit the data. We selected the model with the largest posterior probability for each variant as the best-fitting model to capture the genetic effect heterogeneity. Variants in which the zero component model was selected (that is, all models with at least one component were rejected) were considered to have homogeneous effects across ancestry. Among the remaining variants, we considered which one of the meta-regression models (that is, 1–4 components) best described the extent of effect heterogeneities based on the posterior probabilities for each model. In addition, we required that strongly heterogeneous variants had an MDS component effect that was significantly different from zero and were polymorphic in two or more ancestry-stratified cohorts to ease interpretation of heterogeneous effects. For example, a variant in which the model with two components best fit the data was considered at least weakly heterogeneous. If this variant also had a component two effect significantly different than zero ( \({\gamma }_{j2}\ne 0,\) from above) and was polymorphic in at least two ancestries, it was considered strongly heterogeneous.
Fine-mapping
On the basis of the selected genetic effect model (above), for each variant in a locus, we calculated the Bayes factor by \({\varLambda }_{j}={\rm{\exp }}\left[\frac{{X}_{j}-\left(T+1\right){\rm{\log }}K}{2}\right]\) , where \({X}_{j}\) denotes the chi-squared test statistic for variant j , T denotes the number of axes of genetic variation included in the best-fitting model (that is, 0–4 MDS components) and K denotes the number of studies contributing to the GWAS. Using the approximate Bayes factor, we then calculated the posterior inclusion probability for each variant as \({\pi }_{j}=\frac{{\varLambda }_{j}}{{\sum }_{i}{\varLambda }_{i}}\) , where i indexes each locus. Finally, we derived 90% credible intervals by ranking variants within a locus by their single posterior estimate and selecting variants until the cumulative posterior inclusion probability reached 0.90.
For EUR-stratified fine-mapping, we approximated the Bayes factor as above with T set to 0. Fine-mapping was conducted in EUR-stratified results, using identical loci as in multi-ancestry fine-mapping, to describe the increased resolution attributable to diverse ancestry inclusion and differences in sample size.
Functional enrichment analysis was conducted to test whether high-priority genes identified in the fine-mapping results were expressed in specific tissue types or enriched in certain cell types or gene pathways. High-priority genes were defined as those mapped from variants in credible intervals containing less than five variants. That is, for each variant in credible intervals with less than five variants, we used the UCSC genome annotation database to assign genes. We assigned intergenic variants to the nearest gene. We mapped genes from variants with PIP < 0.01 (as ‘control’ genes) in the same way. Functional enrichment was then evaluated by estimating a relative risk (as described and implemented previously 52 ), defined as the ratio of the proportion of genes mapped from variants in credible intervals with less than five variants that are in a given annotation category to the proportion of genes mapped from variants, within associated loci, with PIP < 0.01 in the same annotation category. Annotation categories were derived from GTEx tissue expression 53 , central nervous systems cell types 50 and gene pathways 54 .
TWAS were performed using a trans-ancestry method. In brief, this method fits a series of meta-regression models including the first four axes of genetic variation (MDS components), similar to that of our multi-ancestry meta-analysis model minus the random-effect term. Genetic effect estimates from these four models were then used to estimate phenotypic effects of each variant. Together, with variant weights taken from PrediXcan 55 based on 49 tissues from GTEx 10 release version 8 (which includes up to 15% of individuals of non-EUR ancestry), the phenotypic effect estimates were used to construct a single TWAS statistic for each MDS component. A minimum P value approach 56 was then applied to combine all four TWAS statistic P values. Finally, we used a Cauchy combination test 57 to combine P values across all available tissues for each gene. The final, combined P value was subjected to a Bonferroni correction for 22,121 genes in 49 tissues. We present our TWAS results based on per gene P values combined across all available tissues, resulting in a 5 (phenotype) × 22,121 (gene) matrix of P values. Pathway enrichment was also conducted using a weighted regression approach 58 with the TWAS per-tissue P values to quantify the enrichment of identified genes in each pathway.
Heritability and genetic correlations
LDSC 59 was used to estimate heritability of our five phenotypes for EAS and EUR ancestries using a standard 1-cM window size. For ancestries with more recent admixture (AFR and AMR ancestries), we used covariate-adjusted LDSC 60 for the same analyses in which in-sample LD scores were calculated using ancestry-matched TOPMed reference samples and adjusted by the first 50 principal components. For more recently admixed AFR and AMR ancestries, which tend to show longer-range LD, we used a 20-cM window size when calculating LD scores. For both LDSC and covariate-adjusted LDSC, variants were subset to HapMap3 (ref. 61 ) with MAF > 0.05, as recommended for this approach.
We calculated genetic correlations between our five phenotypes and 4,065 UK Biobank phenotypes (both restricted to EUR ancestry) using bivariate LDSC with 1000 Genomes-based pre-calculated EUR LD scores for HapMap3 variants. We excluded phenotypes with heritability Z -scores less than 3 (reflecting near-zero heritability), genetic correlations with our phenotypes less than −0.8 or greater than 0.8, to remove phenotypes approaching redundancy with our target tobacco and alcohol use measures (for example, cigarettes per day versus packs per day), and those whose genetic correlations were unable to be estimated largely due to negative heritability estimates, leaving 1,141 UK Biobank phenotypes. Affinity propagation clustering 62 , a message-passing algorithm based on exemplars that identifies their corresponding set of clusters, was then used to further interpret the pattern of genetic correlations and multifactorial nature of substance use. A Bonferroni-corrected P value threshold for 1,141 UK Biobank phenotypes was used to identify genetic correlations that were significantly different from zero.
Polygenic scoring
Polygenic risk scores were computed using LDpred for each ancestry group separately, an approach that incorporates the correlation between genetic variants to re-weight effect size estimates 63 . We used an independent prediction cohort, Add Health 35 , to validate each score. Add Health is a nationally representative sample of US adolescents enrolled in grades 7 through 12 during the 1994–1995 school year. The mean birth year of respondents was 1979 (s.d. = 1.8) and the mean age at assessment (here, wave 4) was 29.0 years (s.d. = 1.8), which is comparable, in general, to the age of participants in the 23andMe cohort but younger, on average, than those in other cohorts. Add Health is composed of individuals from the same four major ancestral groups (defined with reference to 1000 Genomes; see Supplementary Note for details) comprising our ancestry-stratified results (EUR, AFR, AMR and EAS). Phenotypic descriptive statistics are given in Supplementary Table 12 . Across the full Add Health sample, approximately 41% ever smoke regularly and reported an average of 7.3 cigarettes per day. For each polygenic score, we used only HapMap3 variants and those with MAF > 0.01. We used each Add Health ancestry group as its own LD reference panel for construction of each polygenic score, after removing related individuals, except for EAS in which we use 1000 Genomes due to the small sample size in Add Health.
Prediction accuracy of each polygenic score was estimated by taking the difference in the coefficient of determination ( R 2 ) between a base model that included only the covariates of age, sex, age × sex interaction, and the first ten genetic principal components, and a full model that additionally included the polygenic score. All scores were scaled to have a mean of zero and standard deviation of one.
URLs for software use
BCFtools, http://samtools.github.io/bcftools/ ; BOLT-LMM, https://data.broadinstitute.org/alkesgroup/BOLT-LMM/ ; cov-LDSC, https://github.com/immunogenomics/cov-ldsc ; EAGLE, https://alkesgroup.broadinstitute.org/Eagle/ ; GCTA, http://cnsgenomics.com/software/gcta/ ; IMPUTE2, https://mathgen.stats.ox.ac.uk/impute/impute_v2.html ; LDpred, https://github.com/bvilhjal/ldpred/ ; LDSC, https://github.com/bulik/ldsc/ ; MEMO (rareGWAMA), https://github.com/dajiangliu/rareGWAMA/ ; Minimac3, https://genome.sph.umich.edu/wiki/Minimac3 ; PLINK, https://www.cog-genomics.org/plink/ ; R, https://www.r-project.org/ ; RATES, https://github.com/wangc29/RATES ; RVTESTS, https://github.com/zhanxw/rvtests/ ; SAIGE, https://github.com/weizhouUMICH/SAIGE ; SHAPEIT, http://mathgen.stats.ox.ac.uk/genetics_software/shapeit/shapeit.html ; TESLA, https://github.com/funfunchen/rareGWAMA ; VCFtools, https://vcftools.github.io/index.html .
Ethical review and approval were provided by the University of Minnesota institutional review board. All human participants provided informed consent.
Reporting summary
Further information on research design is available in the Nature Portfolio Reporting Summary linked to this article.
Data availability
GWAS summary statistics can be downloaded online ( https://doi.org/10.13020/przg-dp88 ) with more information available here: https://genome.psych.umn.edu/index.php/GSCAN . We have provided association results for variants that passed quality-control filters in the multi-ancestry and ancestry-stratified results for each of the five substance use phenotypes, excluding data provided by 23andMe. Ancestry-stratified polygenic score weights based on ancestry-stratified summary statistics are also provided. 23andMe results are available directly from the company.
Code availability
All software used to perform these analyses is publicly available. Software tools used are listed in the main text and Methods.
Change history
26 january 2023.
An amendment to the underlying article code was made to enable an author name to appear correctly in PubMed.
World Health Organization. Tobacco. WHO https://www.who.int/news-room/fact-sheets/detail/tobacco (2022).
World Health Organization. Alcohol. WHO https://www.who.int/news-room/fact-sheets/detail/alcohol (2022).
World Health Organization. The top 10 causes of death. WHO https://www.who.int/news-room/fact-sheets/detail/the-top-10-causes-of-death (2020).
Griswold, M. G. et al. Alcohol use and burden for 195 countries and territories, 1990–2016: a systematic analysis for the Global Burden of Disease Study 2016. Lancet 392 , 1015–1035 (2018).
Article Google Scholar
Liu, M. et al. Association studies of up to 1.2 million individuals yield new insights into the genetic etiology of tobacco and alcohol use. Nat. Genet. 51 , 237–244 (2019).
Article CAS Google Scholar
McGuire, D. et al. Model-based assessment of replicability for genome-wide association meta-analysis. Nat. Commun. 12 , 1964 (2021).
Article ADS CAS Google Scholar
Volkow, N. D., Koob, G. F. & McLellan, A. T. Neurobiologic advances from the brain disease model of addiction. N. Engl. J. Med. 374 , 363–371 (2016).
Koob, G. F. & Volkow, N. D. Neurocircuitry of Addiction. Neuropsychopharmacology 35 , 217–238 (2010).
Bierut, L. J. et al. ADH1B is associated with alcohol dependence and alcohol consumption in populations of European and African ancestry. Mol. Psychiatry 17 , 445–450 (2012).
The GTEx Consortium. The GTEx Consortium atlas of genetic regulatory effects across human tissues. Science 369 , 1318–1330 (2020).
Horn, J. L. A rationale and test for the number of factors in factor analysis. Psychometrika 30 , 179–185 (1965).
Article CAS MATH Google Scholar
Berrettini, W. H. & Doyle, G. A. The CHRNA5–A3–B4 gene cluster in nicotine addiction. Mol. Psychiatry 17 , 856–866 (2012).
Buchta, W. C. et al. Dynamic CRMP2 regulation of CaV2.2 in the prefrontal cortex contributes to the reinstatement of cocaine seeking. Mol. Neurobiol. 57 , 346–357 (2020).
Andrade, A. et al. Genetic associations between voltage-gated calcium channels and psychiatric disorders. Int. J. Mol. Sci. 20 , 3537 (2019).
Purcell, S. M. et al. Common polygenic variation contributes to risk of schizophrenia and bipolar disorder. Nature 460 , 748–752 (2009).
Moskvina, V. et al. Gene-wide analyses of genome-wide association data sets: evidence for multiple common risk alleles for schizophrenia and bipolar disorder and for overlap in genetic risk. Mol. Psychiatry 14 , 252–260 (2009).
Liao, X. & Li, Y. Genetic associations between voltage-gated calcium channels and autism spectrum disorder: a systematic review. Mol. Brain 13 , 96 (2020).
Koskela, M. et al. Update of neurotrophic factors in neurobiology of addiction and future directions. Neurobiol. Dis. 97 , 189–200 (2017).
Domanskyi, A., Saarma, M. & Airavaara, M. Prospects of neurotrophic factors for Parkinson’s disease: comparison of protein and gene therapy. Hum. Gene Ther. 26 , 550–559 (2015).
Zhang, K., Wang, Y., Fan, T., Zeng, C. & Sun, Z. S. The p21-activated kinases in neural cytoskeletal remodeling and related neurological disorders. Protein Cell 13 , 6–25 (2020).
Civiero, L. & Greggio, E. PAKs in the brain: function and dysfunction. Biochim. Biophys. Acta 1864 , 444–453 (2018).
Nekrasova, T., Jobes, M. L., Ting, J. H., Wagner, G. C. & Minden, A. Targeted disruption of the Pak5 and Pak6 genes in mice leads to deficits in learning and locomotion. Dev. Biol. 322 , 95–108 (2008).
Landek-Salgado, M. A., Faust, T. E. & Sawa, A. Molecular substrates of schizophrenia: homeostatic signaling to connectivity. Mol. Psychiatry 21 , 10–28 (2016).
Civiero, L. et al. Leucine-rich repeat kinase 2 interacts with p21-activated kinase 6 to control neurite complexity in mammalian brain. J. Neurochem. 135 , 1242–1256 (2015).
Ma, Q.-L. et al. p21-Activated kinase-aberrant activation and translocation in Alzheimer disease pathogenesis. J. Biol. Chem. 283 , 14132–14143 (2008).
Buchsbaum, I. Y. et al. ECE2 regulates neurogenesis and neuronal migration during human cortical development. EMBO Rep. 21 , e48204 (2020).
Mzhavia, N., Pan, H., Che, F.-Y., Fricker, L. D. & Devi, L. A. Characterization of endothelin-converting enzyme-2. Implication for a role in the nonclassical processing of regulatory peptides. J. Biol. Chem. 278 , 14704–14711 (2003).
Baranello, R. J. et al. Amyloid-β protein clearance and degradation (ABCD) pathways and their role in Alzheimer’s disease. Curr. Alzheimer Res. 12 , 32–46 (2015).
Bycroft, C. et al. The UK Biobank resource with deep phenotyping and genomic data. Nature 562 , 203–209 (2018).
Bodenhofer, U., Kothmeier, A. & Hochreiter, S. APCluster: an R package for affinity propagation clustering. Bioinformatics 27 , 2463–2464 (2011).
Mallard, T. T. et al. Item-level genome-wide association study of the alcohol use disorders identification test in three population-based cohorts. Am. J. Psychiatry 179 , 58–70 (2022).
Zhou, H. et al. Genome-wide meta-analysis of problematic alcohol use in 435,563 individuals yields insights into biology and relationships with other traits. Nat. Neurosci. 23 , 809–818 (2020).
Bulik-Sullivan, B. et al. An atlas of genetic correlations across human diseases and traits. Nat. Genet. 47 , 1236–1241 (2015).
Kraft, P., Chen, H. & Lindström, S. The use of genetic correlation and Mendelian randomization studies to increase our understanding of relationships between complex traits. Curr. Epidemiol. Rep. 7 , 104–112 (2020).
Harris, K. M. et al. Cohort profile: the National Longitudinal Study of Adolescent to Adult Health (Add Health). Int. J. Epidemiol. 48 , 1415–1415k (2019).
Martin, A. R. et al. Human demographic history impacts genetic risk prediction across diverse populations. Am. J. Hum. Genet. 100 , 635–649 (2017).
Hermalin, L. The Age Prevalence of Smoking among Chinese Women: A Case of Arrested Diffusion (Population Studies Center, 2010).
Flor, L. S., Reitsma, M. B., Gupta, V., Ng, M. & Gakidou, E. The effects of tobacco control policies on global smoking prevalence. Nat. Med. 27 , 239–243 (2021).
Burton, R. et al. A rapid evidence review of the effectiveness and cost-effectiveness of alcohol control policies: an English perspective. Lancet 389 , 1558–1580 (2017).
Mathieson, I. The omnigenic model and polygenic prediction of complex traits. Am. J. Hum. Genet. 108 , 1558–1563 (2021).
McCarthy, S. et al. A reference panel of 64,976 haplotypes for genotype imputation. Nat. Genet. 48 , 1279–1283 (2016).
1000 Genomes Project Consortium et al. A global reference for human genetic variation. Nature 526 , 68–74 (2015).
Zhan, X., Hu, Y., Li, B., Abecasis, G. R. & Liu, D. J. RVTESTS: an efficient and comprehensive tool for rare variant association analysis using sequence data. Bioinformatics 32 , 1423–1426 (2016).
Loh, P.-R., Kichaev, G., Gazal, S., Schoech, A. P. & Price, A. L. Mixed-model association for biobank-scale datasets. Nat. Genet. 50 , 906–908 (2018).
Zhou, W. et al. Efficiently controlling for case–control imbalance and sample relatedness in large-scale genetic association studies. Nat. Genet. 50 , 1335–1341 (2018).
Chen, Z. & Liu, Q. A new approach to account for the correlations among single nucleotide polymorphisms in genome-wide association studies. Hum. Hered. 72 , 1–9 (2011).
Gao, X., Becker, L. C., Becker, D. M., Starmer, J. D. & Province, M. A. Avoiding the high Bonferroni penalty in genome-wide association studies. Genet. Epidemiol. 34 , 100–105 (2010).
Google Scholar
Yang, J. et al. Genomic inflation factors under polygenic inheritance. Eur. J. Hum. Genet. 19 , 807–812 (2011).
Lee, J. J. et al. Gene discovery and polygenic prediction from a genome-wide association study of educational attainment in 1.1 million individuals. Nat. Genet. 50 , 1112–1121 (2018).
Bryois, J. et al. Genetic identification of cell types underlying brain complex traits yields insights into the etiology of Parkinson’s disease. Nat. Genet. 52 , 482–493 (2020).
Jiang, Y. et al. Proper conditional analysis in the presence of missing data: application to large scale meta-analysis of tobacco use phenotypes. PLoS Genet. 14 , e1007452 (2018).
Kanai, M. et al. Insights from complex trait fine-mapping across diverse populations. Preprint at medRxiv https://doi.org/10.1101/2021.09.03.21262975 (2021).
Lonsdale, J. et al. The Genotype-Tissue Expression (GTEx) project. Nat. Genet. 45 , 580–585 (2013).
Gene Ontology Consortium. The Gene Ontology resource: enriching a GOld mine. Nucleic Acids Res. 49 , D325–D334 (2021).
Gamazon, E. R. et al. A gene-based association method for mapping traits using reference transcriptome data. Nat. Genet. 47 , 1091–1098 (2015).
Lin, D.-Y. & Tang, Z.-Z. A general framework for detecting disease associations with rare variants in sequencing studies. Am. J. Hum. Genet. 89 , 354–367 (2011).
Liu, Y. & Xie, J. Cauchy combination test: a powerful test with analytic p-value calculation under arbitrary dependency structures. J. Am. Stat. Assoc. 115 , 393–402 (2020).
Leeuw, C. A., de, Mooij, J. M., Heskes, T. & Posthuma, D. MAGMA: generalized gene-set analysis of GWAS data. PLoS Comput. Biol. 11 , e1004219 (2015).
Bulik-Sullivan, B. K. et al. LD score regression distinguishes confounding from polygenicity in genome-wide association studies. Nat. Genet. 47 , 291–295 (2015).
Luo, Y. et al. Estimating heritability and its enrichment in tissue-specific gene sets in admixed populations. Hum. Mol. Genet. 30 , 1521–1534 (2021).
CAS Google Scholar
Altshuler, D. M. et al. Integrating common and rare genetic variation in diverse human populations. Nature 467 , 52–58 (2010).
Frey, B. J. & Dueck, D. Clustering by passing messages between data points. Science 315 , 972–976 (2007).
Article ADS CAS MATH Google Scholar
Vilhjálmsson, B. J. et al. Modeling linkage disequilibrium increases accuracy of polygenic risk scores. Am. J. Hum. Genet. 97 , 576–592 (2015).
Download references
Acknowledgements
This study was designed and carried out by the GWAS and Sequencing Consortium of Alcohol and Nicotine use (GSCAN). It was conducted by using the UK Biobank Resource under application number 16651. This study was supported by funding from US National Institutes of Health awards R56HG011035, R01DA044283, R01DA042755 and U01DA041120 to S.V., and R01GM126479, R56HG011035, R03OD032630, R01HG011035 and R56HG012358 to D.J.L. G.R.B.S. was also supported by National Institutes of Health award T32DA050560. D.J.L. and X.W. were in part supported by the Penn State College of Medicine’s Biomedical Informatics and Artificial Intelligence Program in the Strategic Plan. A full list of acknowledgements is provided in the Supplementary Note .
Author information
These authors contributed equally: Gretchen R.B. Saunders, Xingyan Wang, Fang Chen, Seon-Kyeong Jang, Mengzhen Liu, Chen Wang
These authors jointly supervised this work: Dajiang J. Liu, Scott Vrieze
Authors and Affiliations
Department of Psychology, University of Minnesota, Minneapolis, MN, USA
Gretchen R. B. Saunders, Seon-Kyeong Jang, Mengzhen Liu, Jacqueline M. Otto, William Iacono, James J. Lee, Matt McGue, Moin Syed & Scott Vrieze
Department of Public Health Sciences, Penn State College of Medicine, Hershey, PA, USA
Xingyan Wang, Fang Chen, Chen Wang, Shuang Gao, Chachrit Khunsriraksakul, Bibo Jiang & Dajiang J. Liu
Department of Epidemiology & Population Health at Stanford University, Stanford, CA, USA
Jackson Heart Study (JHS) Graduate Training and Education Center (GTEC), Department of Epidemiology and Biostatistics, School of Public Health, Jackson State University, Jackson, MS, USA
Clifton Addison
Laboratory for Statistical and Translational Genetics, RIKEN Center for Integrative Medical Sciences, Yokohama, Japan
Masato Akiyama, Yoichiro Kamatani & Nana Matoba
Department of Ocular Pathology and Imaging Science, Kyushu University Graduate School of Medical Sciences, Fukuoka, Japan
- Masato Akiyama
Smidt Heart Institute, Cedars-Sinai Medical Center, Los Angeles, CA, USA
Christine M. Albert
Division of Preventive Medicine, Brigham and Women’s Hospital and Harvard Medical School, Boston, MA, USA
Christine M. Albert & Daniel I. Chasman
Department of Psychiatry, Rutgers Robert Wood Johnson Medical School, New Brunswick, NJ, USA
Fazil Aliev & Danielle M. Dick
Department of Epidemiology, Rollins School of Public Health, Emory University, Atlanta, GA, USA
Alvaro Alonso
Dean’s Office and Department of Epidemiology, College of Public Health, University of Kentucky, Lexington, KY, USA
Donna K. Arnett
Department of Medicine and Duke Comprehensive Sickle Cell Center, Duke University School of Medicine, Durham, NC, USA
Allison E. Ashley-Koch, Melanie E. Garrett & Marilyn J. Telen
Duke Molecular Physiology Institute, Duke University School of Medicine, Durham, NC, USA
Allison E. Ashley-Koch & Melanie E. Garrett
Division of Hematology, Department of Medicine, Mayo Clinic College of Medicine and Science, Rochester, MN, USA
Aneel A. Ashrani
Division of Biomedical Informatics & Personalized Medicine, University of Colorado Anschutz Medical Campus, Aurora, CO, USA
Kathleen C. Barnes, Meher Preethi Boorgula, Sameer Chavan & Nicholas Rafaels
Tempus, Chicago, IL, USA
Kathleen C. Barnes
Department of Medicine, Columbia University Medical Center, New York, NY, USA
R. Graham Barr & Elizabeth C. Oelsner
Cardiovascular Health Research Unit, Department of Medicine, University of Washington, Seattle, WA, USA
Traci M. Bartz, Joshua C. Bis, Jennifer A. Brody, Sina A. Gharib & Nona Sotoodehnia
Department of Biostatistics, University of Washington, Seattle, WA, USA
Traci M. Bartz & Charles Kooperberg
Department of Medicine, Johns Hopkins University School of Medicine, Baltimore, MD, USA
Diane M. Becker & Lisa R. Yanek
Department of Epidemiology, School of Public Health, University of Michigan, Ann Arbor, MI, USA
Lawrence F. Bielak, Sharon L. R. Kardia, Patricia A. Peyser, Jennifer A. Smith & Wei Zhao
Department of Medicine, Boston Medical Center, Boston University School of Medicine, Boston, MA, USA
Emelia J. Benjamin
Department of Epidemiology, Boston University School of Public Health, Boston, MA, USA
deCODE Genetics/Amgen, Inc., Reykjavik, Iceland
Gyda Bjornsdottir, Daniel F. Gudbjartsson, Hreinn Stefansson, Kari Stefansson & Thorgeir E. Thorgeirsson
Department of Human Genetics and South Texas Diabetes and Obesity Institute, University of Texas Rio Grande Valley School of Medicine, Brownsville, TX, USA
John Blangero, Joanne E. Curran & Ravindranath Duggirala
Department of Medicine, University of Arizona, Tucson, AZ, USA
Eugene R. Bleecker & Deborah A. Meyers
Institute of Behavioral Science, University of Colorado Boulder, Boulder, CO, USA
Jason D. Boardman
Human Genetics Center, Department of Epidemiology, Human Genetics, and Environmental Sciences, School of Public Health, The University of Texas Health Science Center at Houston, Houston, TX, USA
Eric Boerwinkle, Myriam Fornage, Alanna C. Morrison & Bing Yu
Netherlands Twin Register, Dept Biological Psychology, Vrije Universiteit Amsterdam, Amsterdam, The Netherlands
Dorret I. Boomsma, JoukeJan Hottenga & Gonneke Willemsen
Department of Biochemistry, Wake Forest School of Medicine, Winston-Salem, NC, USA
Donald W. Bowden & Nicholette D. Palmer
Division of Sleep and Circadian Disorders, Brigham and Women’s Hospital, Boston, MA, USA
Brian E. Cade, Susan Redline & Michael N. Rueschman
Division of Sleep Medicine, Harvard Medical School, Boston, MA, USA
Brian E. Cade & Susan Redline
Program in Medical and Population Genetics, Broad Institute, Cambridge, MA, USA
Brian E. Cade
Institute for Translational Genomics and Population Sciences, Department of Pediatrics, Lundquist Institute for Biomedical Innovation at Harbor-UCLA Medical Center, Torrance, CA, USA
Yii-Der Ida Chen
Clinical Trial Service Unit and Epidemiological Studies Unit, Nuffield Department of Population Health, University of Oxford, Oxford, UK
Zhengming Chen, Kuang Lin, Iona Y. Millwood & Robin G. Walters
MRC Population Health Research Unit, Nuffield Department of Population Health, University of Oxford, Oxford, UK
Zhengming Chen, Iona Y. Millwood & Robin G. Walters
Department of Epidemiology & Biostatistics, University of California, San Francisco, CA, USA
Iona Cheng & Yuqing Li
UCSF Helen Diller Family Comprehensive Cancer Center, University of California, San Francisco, CA, USA
Channing Division of Network Medicine, Department of Medicine, Brigham and Women’s Hospital, Boston, MA, USA
Michael H. Cho & Matthew Moll
Division of Pulmonary and Critical Care Medicine, Department of Medicine, Brigham and Women’s Hospital, Boston, MA, USA
Kaiser Permanente Northern California (KPNC), Division of Research, Oakland, CA, USA
Hélène Choquet, Khanh K. Thai & Jie Yin
Department of Neurology, Baltimore Veterans Affairs Medical Center, Baltimore, MD, USA
John W. Cole
Division of Vascular Neurology, Department of Neurology, University of Maryland School of Medicine, Baltimore, MD, USA
Department of Preventive Medicine, Northwestern University Feinberg School of Medicine, Chicago, IL, USA
Marilyn C. Cornelis, Lifang Hou & Yoonjung Y. Joo
University of Sassari, Sassari SS, Italy
Francesco Cucca
Division of Clinical Trials and Biostatistics, Department of Quantitative Health Sciences, Mayo Clinic College of Medicine and Science, Rochester, MN, USA
Mariza de Andrade
Department of Psychiatry, University of Utah School of Medicine, Salt Lake City, UT, USA
Anna R. Docherty
Virginia Institute for Psychiatric and Behavioral Genetics, Virginia Commonwealth University, Virginia, USA
Anna R. Docherty & Nathan Gillespie
Huntsman Mental Health Institute, Salt Lake City, UT, USA
Department of Family Medicine, Brown University, Providence, RI, USA
Charles B. Eaton
Institute for Behavioral Genetics, University of Colorado Boulder, Boulder, CO, USA
Marissa A. Ehringer, John K. Hewitt, Matthew C. Keller, Michael C. Stallings & Jerry A. Stitzel
Department of Integrative Physiology, University of Colorado Boulder, Boulder, CO, USA
Marissa A. Ehringer & Reedik Mägi
Institute of Genomics, University of Tartu, Tartu, Estonia
Tõnu Esko, Toomas Haller & Andres Metspalu
Survey Research Center, Institute for Social Research, University of Michigan, Ann Arbor, MI, USA
Jessica D. Faul, Jennifer A. Smith & David R. Weir
Institute of Clinical Medicine, Internal Medicine, University of Eastern Finland, Kuopio, Finland
Lilian Fernandes Silva
Istituto di Ricerca Genetica e Biomedica, Consiglio Nazionale delle Ricerche (CNR), Monserrato, Italy
Edoardo Fiorillo, Antonella Mulas & Valeria Orrù
Brown Foundation Institute of Molecular Medicine, McGovern Medical School, University of Texas Health Science Center at Houston, Houston, TX, USA
Myriam Fornage
Department of Internal Medicine-Section on Nephrology, Wake Forest School of Medicine, Winston-Salem, NC, USA
Barry I. Freedman
K.G. Jebsen Center for Genetic Epidemiology, Department of Public Health and Nursing, NTNU, Norwegian University of Science and Technology, Trondheim, Norway
Maiken E. Gabrielsen, Kristian Hveem, Jonas B. Nielsen, Anne Heidi Skogholt & Bendik S. Winsvold
Division of Pulmonary, Critical Care, and Sleep Medicine, Department of Medicine, University of Washington, Seattle, WA, USA
Sina A. Gharib
Center for Lung Biology, Department of Medicine, University of Washington, Seattle, WA, USA
Research Unit Molecular Epidemiology, Institute of Epidemiology, Helmholtz Zentrum München, German Research Center for Environmental Health, Neuherberg, Germany
Christian Gieger
Department of Psychiatry & Behavioral Sciences, Boston Children’s Hospital & Harvard Medical School, Boston, MA, USA
David C. Glahn
Genetic Epidemiology, QIMR Berghofer Medical Research Institute, Brisbane, Australia
Scott D. Gordon, Nicholas G. Martin & John B. Whitfield
Division of Biostatistics, Washington University School of Medicine, St. Louis, MO, USA
Charles C. Gu & Nancy L. Saccone
Department of Epidemiology and Key Laboratory of Cardiovascular Epidemiology, Fuwai Hospital, National Center for Cardiovascular Diseases, Chinese Academy of Medical Sciences and Peking Union Medical College, Beijing, China
Dongfeng Gu
School of Engineering and Natural Sciences, University of Iceland, Reykjavik, Iceland
Daniel F. Gudbjartsson
The Institute for Translational Genomics and Population Sciences, Department of Pediatrics, The Lundquist Institute for Biomedical Innovation at Harbor-UCLA Medical Center, Torrance, CA, USA
Xiuqing Guo, Jerome I. Rotter & Kent D. Taylor
Public Health Sciences Division, Fred Hutchinson Cancer Research Center, Seattle, WA, USA
Jeffrey Haessler, Robert C. Kaplan, Charles Kooperberg, Ulrike Peters & Alex P. Reiner
Department of Medicine, University of Mississippi Medical Center, Jackson, MS, USA
Michael E. Hall
Department of Sociology and the Carolina Population Center, University of North Carolina, Chapel Hill, NC, USA
Kathleen Mullan Harris
Department of Epidemiology, Tulane University, New Orleans, LA, USA
Jiang He, Tanika N. Kelly & Xiao Sun
Translational Sciences Institute, Tulane University, New Orleans, LA, USA
Jiang He & Tanika N. Kelly
McCourt School of Public Policy, Georgetown University, Washington, DC, USA
Pamela Herd
Department Of Psychology and Neuroscience, University of Colorado Boulder, Boulder, CO, USA
John K. Hewitt, Matthew C. Keller & Michael C. Stallings
Youth Mental Health & Technology Team, Brain and Mind Centre, University of Sydney, Sydney, Australia
Department of Epidemiology, School of Public Health, University of Alabama at Birmingham, Birmingham, AL, USA
Bertha Hidalgo & Marguerite R. Irvin
Department of Epidemiology, University of Colorado Anschutz Medical Campus, Aurora, CO, USA
John E. Hokanson, Nicole E. Richmond & Kendra A. Young
Department of Psychiatry, University of Colorado Anschutz Medical Center, Denver, CO, USA
Christian Hopfer
Department of Epidemiology, Harvard T.H. Chan School of Public Health, Boston, MA, USA
Hongyan Huang, Yon Ho Jee, Peter Kraft & Constance Turman
Program in Genetic Epidemiology and Statistical Genetics, Harvard T.H. Chan School of Public Health, Boston, MA, USA
Hongyan Huang, Peter Kraft & Constance Turman
Institute of Preventive Medicine, National Defense Medical Center, New Taipei City, Taiwan
Yi-Jen Hung
Nuffield Department of Population Health, University of Oxford, Oxford, UK
David J. Hunter
HUNT Research Center, Department of Public Health and Nursing, Faculty of Medicine and Health Sciences, Norwegian University of Science and Technology (NTNU), Trondheim, Norway
Kristian Hveem
Department of Research, Innovation and Education, St. Olavs Hospital, Trondheim University Hospital, Trondheim, Norway
Population Sciences Branch, National Heart, Lung, and Blood Institute, National Institutes of Health, Bethesda, MD, USA
Shih-Jen Hwang, Daniel Levy & Jiantao Ma
Section of Endocrinology and Metabolism, Department of Medicine, Taipei Veterans General Hospital, Taipei, Taiwan
Chii-Min Hwu
GenOmics, Bioinformatics, and Translational Research Center, RTI International, Research Triangle Park, NC, USA
Eric O. Johnson, Ravi Mathur & Dana B. Hancock
Fellow Program, RTI International, Research Triangle Park, NC, USA
Eric O. Johnson
Institute of Data Science, Korea University, Seoul, South Korea
Yoonjung Y. Joo
Regeneron Genetics Center, Tarrytown, NY, USA
Eric Jorgenson
Department of Population Health Sciences, Geisinger, Danville, PA, USA
Anne E. Justice
Department of Epidemiology, University of North Carolina at Chapel Hill, Chapel Hill, NC, USA
Anne E. Justice & Kari E. North
Laboratory of Complex Trait Genomics, Department of Computational Biology and Medical Sciences, Graduate School of Frontier Sciences, The University of Tokyo, Tokyo, Japan
Yoichiro Kamatani
Department of Epidemiology and Population Health, Albert Einstein College of Medicine, Bronx, NY, USA
Robert C. Kaplan
Institute for Molecular Medicine Finland - FIMM, University of Helsinki, Helsinki, Finland
Jaakko Kaprio, Tellervo Korhonen & Teemu Palviainen
Department of Molecular, Cellular and Developmental Biology, University of Colorado, Boulder, CO, USA
Kenneth Krauter
Institute of Clinical Medicine, Internal Medicine, University of Eastern Finland and Kuopio University Hospital, Kuopio, Finland
Johanna Kuusisto & Markku Laakso
Center for Medicine and Clinical Research, Kuopio University Hospital, Kuopio, Finland
Johanna Kuusisto
Brigham and Women’s Hospital, Department of Medicine, Channing Division of Network Medicine, Boston, MA, USA
Jessica Lasky-Su & Scott T. Weiss
Department of Medical Research, Taichung Veterans General Hospital, Taichung City, Taiwan
Wen-Jane Lee
Department of Epidemiology and Biostatistics, School of Public Health, Peking University Health Science Center, Beijing, China
Center for Statistical Genetics, Department of Biostatistics, University of Michigan, Ann Arbor, MI, USA
Kevin Li & Anita Pandit
Psychiatric Genetics, QIMR Berghofer Medical Research Institute, Brisbane, Australia
Penelope A. Lind & Sarah E. Medland
School of Biomedical Sciences, Faculty of Medicine, University of Queensland, Brisbane, Australia
Penelope A. Lind
School of Biomedical Sciences, Queensland University of Technology, Brisbane, Australia
Department of Biostatistics, Boston University School of Public Health, Boston, MA, USA
Departments of Preventive Medicine, Medicine, and Pediatrics, Northwestern University Feinberg School of Medicine, Chicago, IL, USA
Donald M. Lloyd-Jones
Department of Population Medicine, Harvard Pilgrim Health Care Institute, Boston, MA, USA
Sharon M. Lutz
Department of Biostatics, Harvard T.H. Chan School of Public Health, Boston, MA, USA
Division of Nutrition Epidemiology and Data Science, Friedman School of Nutrition Science and Policy, Tufts University, Boston, MA, USA
Center for Public Health Genomics, Department of Public Health Sciences, University of Virginia School of Medicine, Charlottesville, VA, USA
Ani Manichaikul & Stephen S. Rich
Department of Genetics, UNC Neuroscience Center, University of North Carolina at Chapel Hill, Chapel Hill, NC, USA
Nana Matoba
Division of Endocrinology, Diabetes and Nutrition, Department of Medicine, University of Maryland School of Medicine, Baltimore, MD, USA
Patrick F. McArdle, Braxton D. Mitchell, May E. Montasser & Huichun Xu
Department of Integrative Physiology, University of Colorado, Boulder, CO, USA
Matthew B. McQueen
Geriatrics Research and Education Clinical Center, Baltimore Veterans Administration Medical Center, Baltimore, MD, USA
Braxton D. Mitchell
Department of Genetics, University of North Carolina, Chapel Hill, NC, USA
Karen L. Mohlke
Department of Internal Medicine, Division of Cardiovascular Medicine, University of Michigan, Ann Arbor, MI, USA
Jonas B. Nielsen
Laboratory for Systems Genetics, RIKEN Center for Integrative Medical Sciences, Yokohama, Japan
Yukinori Okada
Department of Statistical Genetics, Osaka University Graduate School of Medicine, Suita, Japan
Laboratory of Statistical Immunology, Immunology Frontier Research Center (WPI-IFReC), Osaka University, Suita, Japan
Department of Genome Informatics, Graduate School of Medicine, the University of Tokyo, Tokyo, Japan
Population Sciences of the Pacific Program, University of Hawaii Cancer Center, Honolulu, HI, USA
S. Lani Park
Department of Epidemiology, University of Washington, Seattle, WA, USA
Ulrike Peters, Alex P. Reiner & Nicholas L. Smith
Institute of Epidemiology, Helmholtz Zentrum München, German Research Center for Environmental Health, Neuherberg, Germany
Annette Peters
Institute for Medical Information Processing, Biometry and Epidemiology, Ludwig Maximilians University Munich, Munich, Germany
German Centre for Cardiovascular Research, DZHK, Partner Site Munich, Munich, Germany
Department of Clinical Developmental Psychology, Vrije Universiteit, Amsterdam, The Netherlands
Tinca J. C. Polderman
Department of Child and Adolescent Psychiatry, Amsterdam UMC, Amsterdam, The Netherlands
Division of Pulmonary, Critical Care, and Sleep Medicine, Beth Israel Deaconess Medical Center, Boston, MA, USA
Susan Redline
Division of Pulmonary and Critical Care Medicine, Department of Medicine, University of Maryland School of Medicine, Baltimore, MD, USA
Robert M. Reed
Department of Psychiatry, Washington University School of Medicine, St. Louis, MO, USA
John P. Rice & Laura J. Bierut
Center for Demography of Health and Aging, University of Wisconsin-Madison, Madison, WI, USA
Carol Roan & Kamil Sicinski
SAA-National Center of Addiction Medicine, Vogur Hospital, Reykjavik, Iceland
Valgerdur Runarsdottir & Thorarinn Tyrfingsson
Department of Genetics, Washington University School of Medicine, St. Louis, MO, USA
Nancy L. Saccone
Division of Pulmonary Sciences and Critical Care Medicine; Department of Medicine and Immunology, University of Colorado, Aurora, CO, USA
David A. Schwartz
Herbert Wertheim School of Public Health and Human Longevity Science, University of California, San Diego, La Jolla, CA, USA
Aladdin H. Shadyab
23andMe, Inc, Sunnyvale, CA, USA
Jingchunzi Shi & Suyash S. Shringarpure
Kaiser Permanente Washington Health Research Institute, Kaiser Permanente Washington, Seattle, WA, USA
Nicholas L. Smith
Seattle Epidemiologic Research and Information Center, Department of Veterans Affairs Office of Research and Development, Seattle, WA, USA
Division of Cardiology, Department of Medicine, University of Washington, Seattle, WA, USA
Nona Sotoodehnia
Faculty of Medicine, University of Iceland, Reykjavik, Iceland
Kari Stefansson
COPD Foundation, Washington, DC, USA
Ruth Tal-Singer
MRC Integrative Epidemiology Unit, Population Health Sciences, University of Bristol, Bristol, UK
Amy E. Taylor, Luisa Zuccolo & Marcus R. Munafò
National Institute for Health Research Biomedical Research Centre at the University Hospitals Bristol NHS Foundation Trust and the University of Bristol, Bristol, UK
Amy E. Taylor & Marcus R. Munafò
Department of Population Health Sciences, Bristol Medical School, University of Bristol, Bristol, UK
Amy E. Taylor & Luisa Zuccolo
Department of Biostatistics, School of Public Health, University of Alabama at Birmingham, Birmingham, AL, USA
Hemant Tiwari
Department of Psychiatry, University of California San Diego, San Diego, CA, USA
Tamara L. Wall
Jackson Heart Study Undergraduate Training and Education Center, Tougaloo College, Tougaloo, MS, USA
Wendy B. White
Department of Medicine, University of Washington, Seattle, WA, USA
Kerri L. Wiggins
Department of Internal Medicine, Division of Cardiology, University of Michigan, Ann Arbor, MI, USA
Cristen J. Willer
Department of Human Genetics, University of Michigan, Ann Arbor, MI, USA
Department of Computational Medicine and Bioinformatics, University of Michigan, Ann Arbor, MI, USA
Cristen J. Willer & Wei Zhou
Department of Research and Innovation, Division of Clinical Neuroscience, Oslo University Hospital, Oslo, Norway
Bendik S. Winsvold
Department of Neurology, Oslo University Hospital, Oslo, Norway
Department of Epidemiology, Gillings School of Global Public Health, University of North Carolina at Chapel Hill, Chapel Hill, NC, USA
Kristin L. Young
Analytic and Translational Genetics Unit, Massachusetts General Hospital, Boston, MA, USA
Department of Biostatistics, University of Michigan, Ann Arbor, MI, USA
Sebastian Zöllner
Department of Psychiatry, University of Michigan, Ann Arbor, MI, USA
Health Data Science Centre, Fondazione Human Technopole, Milan, Italy
Luisa Zuccolo
Department of Population Health Sciences, University of Leicester, Leicester, UK
Chiara Batini
Oregon Research Institute, Springfield, OR, USA
Andrew W. Bergen
BioRealm, LLC, Walnut, CA, USA
Outcomes Research Network & Department of Family Medicine, NorthShore University HealthSystem, Evanston, IL, USA
Sean P. David
Department of Family Medicine, University of Chicago, Chicago, IL, USA
Department of Medicine, Université de Montréal, Montréal, Québec, Canada
Sarah A. Gagliano Taliun
Department of Neurosciences, Université de Montréal, Montréal, Québec, Canada
Research Centre, Montréal Heart Institute, Montréal, Québec, Canada
School of Psychological Science, University of Bristol, Bristol, UK
Marcus R. Munafò
You can also search for this author in PubMed Google Scholar
23andMe Research Team
- Jingchunzi Shi
- & Suyash S. Shringarpure
The Biobank Japan Project
- , Yoichiro Kamatani
- , Nana Matoba
- & Yukinori Okada
Contributions
D.J.L. and S.V. designed, led and oversaw the study. G.R.B.S and X.W. were the lead analysts for the study, and they were assisted by D.J.L., S.V., F. Chen, S.-K.J., M. Liu and C.W. Phenotype definitions were developed by L.J.B., M.C.C., J. Kaprio., E.J., D.J.L., M. McGue, M.R.M., S.V. and L.Z. Software development was carried out by X.W., D.J.L., F. Chen and C.W. Multi-ancestry meta-analyses were performed by X.W. Ancestry-stratified meta-analyses were performed by G.R.B.S. and M. Liu. Conditional analyses were performed by X.W. and G.R.B.S. Fine-mapping and allelic heterogeneity were performed by X.W. and G.R.B.S. Replicability analyses were performed by C.W., S.-K.J. and G.R.B.S. Multi-ancestry TWAS were performed by F. Chen. Heritability and genetic correlation analyses were performed by S.-K.J. Polygenic scoring analyses was performed by G.R.B.S. Bioinformatics analyses were performed and interpreted by F. Chen, S.-K.J., G.R.B.S., S.V. and J.A. Stitzel. Figures were created by M. Liu, G.R.B.S., S.-K.J. and S.V. M. Liu and S.V. coordinated among participating cohorts. M.A.E. and M.C.K. helped with data access. G.R.B.S. coordinated authorship and acknowledgement details. C.B., A.W.B., L.B., S.P.D., S.A.G.T., D.B.H., M.R.M. and T.E.T. provided helpful advice and feedback on study design and the manuscript. All authors contributed to and critically reviewed the manuscript. G.R.B.S., X.W., S.-K.J., F. Chen, C.W., D.J.L. and S.V. made major contributions to the writing and editing.
Corresponding authors
Correspondence to Dajiang J. Liu or Scott Vrieze .
Ethics declarations
Competing interests.
The spouse of N.L. Saccone is listed as an inventor on issued U.S. patent 8080371 ‘Markers of addiction’, covering the use of certain single-nucleotide polymorphisms in determining the diagnosis, prognosis and treatment of addiction. M.H.C. has received grant funding from GSK and Bayer, and speaking or consulting fees from AstraZeneca, Illumina and Genentech. R.T.-S. is a former employee and current shareholder of GSK and is currently a non-executive member of the ENA Respiratory board of directors. She reports personal fees from Teva, Immunomet, Vocalis Health and ENA Respiratory (until January 2021). D.A.S. is the founder and chief scientific officer of Eleven P15, a company focused on the early diagnosis of treatment of pulmonary fibrosis. J.B.N. and E.J. are employed by Regeneron Pharmaceuticals, Inc. The spouse of C.J.W. is employed by Regeneron Pharmaceuticals, Inc. L.J.B. is listed as an inventor on Issued U.S. Patent 8080371 ‘Markers for addiction’, covering the use of certain single-nucleotide polymorphisms in determining the diagnosis, prognosis and treatment of addiction. The 23andMe Research Team, including J.S. and S.S.S., are employees of 23andMe, Inc., and hold stock and/or stock options in 23andMe. T.E.T., D.F.G., H.S., G.B. and K. Stefansson are employees of deCODE genetics/AMGEN. M. Moll received grant support from Bayer. A.W.B. is listed as a co-inventor on a U.S. patent application ‘Biosignature discovery for substance use disorder using statistical learning’ assigned to BioRealm, LLC, and serves as a scientific advisor and consultant to BioRealm, LLC. All other authors declare no competing interests.
Peer review
Peer review information.
Nature thanks David Balding, Ditte Demontis, Eske Derks and the other, anonymous, reviewer(s) for their contribution to the peer review of this work. Peer reviewer reports are available.
Additional information
Publisher’s note Springer Nature remains neutral with regard to jurisdictional claims in published maps and institutional affiliations.
Extended data figures and tables
Extended data fig. 1 ancestry space of studies contributing to meta-analysis (panel a), versus individuals from topmed and 1000 genomes (panel b)..
The meta-regression within the MEMO model requires specification of ancestry clines. To ensure consistency in the meaning of ancestry clines across all five MEMO analyses (one for each phenotype) we created a single multidimensional scaling solution based on allele frequencies from all phenotypes in all participating cohorts. These solutions are plotted in panel a (circles correspond to TOPMed cohorts, squares are all other cohorts which used imputed microarray genotypes, and triangles are 1000 Genomes ancestry groups). Colors of points correspond to the primary assigned ancestry of each cohort (studies with < 90% of individuals coming from a single ancestry group are shown in grey). Panel b shows projection of principal components (after OADP transformation) of TOPMed individuals onto PCs of 1000 Genomes individuals, in colored triangles. Each 1000 Genomes individual is colored by their known ancestry. This PC information was used in assigning ancestry to TOPMed individuals for the purpose of reference panel creation (individuals of South Asian ancestry were not included in analyses). The PCs in panel b were reordered or reversed in some cases to align with panel a. These transformations are noted in the axis labels.
Extended Data Fig. 2 Multi-ancestry meta-analysis Manhattan plots.
Black horizontal line corresponds to P = 5 × 10 −9 , the GWAS significance threshold used for all analyses. Note that some y-axis scales are discontinuous to better illustrate variants with very small P -values (e.g., the Drinks per Week y-axis is cut at 30 with a maximum value of 307.7, denoting a P -value of 1.9 × 10 −308 ). All P -values are from two-sided statistical tests.
Extended Data Fig. 3 Tissue expression and brain cell type enrichment in high priority genes.
Panel a shows tissue expression enrichment in ‘high priority’ genes. We define high priority genes here as those located nearest to the variants in fine-mapped credible intervals containing less than five variants. These genes were compared to ‘control’ genes identified in the same way, but from variants in credible intervals with PIP < 0.01 from the trans-ancestry fine-mapping. The x-axis denotes GTEx tissue types. The y-axis represents relative risk estimates comparing high priority to control genes. Panel b shows similar relative risk comparisons with 39 brain cell types. Data are presented as relative risk values with error bars denoting bootstrapped 95% confidence intervals. Further details on estimating relative risk are included in the Supplementary Note section ‘Functional enrichment’.
Supplementary information
Supplementary information.
This file contains Supplementary Notes, Supplementary Figs. 1–5, Supplementary References, and Acknowledgements – see Contents page for details.
Reporting Summary
Supplementary tables.
Supplementary Tables 1–12.
Peer Review File
Rights and permissions.
Open Access This article is licensed under a Creative Commons Attribution 4.0 International License, which permits use, sharing, adaptation, distribution and reproduction in any medium or format, as long as you give appropriate credit to the original author(s) and the source, provide a link to the Creative Commons licence, and indicate if changes were made. The images or other third party material in this article are included in the article’s Creative Commons licence, unless indicated otherwise in a credit line to the material. If material is not included in the article’s Creative Commons licence and your intended use is not permitted by statutory regulation or exceeds the permitted use, you will need to obtain permission directly from the copyright holder. To view a copy of this licence, visit http://creativecommons.org/licenses/by/4.0/ .
Reprints and permissions
About this article
Cite this article.
Saunders, G.R.B., Wang, X., Chen, F. et al. Genetic diversity fuels gene discovery for tobacco and alcohol use. Nature 612 , 720–724 (2022). https://doi.org/10.1038/s41586-022-05477-4
Download citation
Received : 09 March 2022
Accepted : 25 October 2022
Published : 07 December 2022
Issue Date : 22 December 2022
DOI : https://doi.org/10.1038/s41586-022-05477-4
Share this article
Anyone you share the following link with will be able to read this content:
Sorry, a shareable link is not currently available for this article.
Provided by the Springer Nature SharedIt content-sharing initiative
This article is cited by
Are novel treatments for brain disorders hiding in plain sight.
- Laramie Duncan
- Karl Deisseroth
Neuropsychopharmacology (2024)
Genetic factors associated with suicidal behaviors and alcohol use disorders in an American Indian population
- David A. Gilder
- Cindy L. Ehlers
Molecular Psychiatry (2024)
Estimating the direct effects of the genetic liabilities to bipolar disorder, schizophrenia, and behavioral traits on suicide attempt using a multivariable Mendelian randomization approach
- Brenda Cabrera-Mendoza
- Necla Aydin
- Renato Polimanti
Paternal cocaine-seeking motivation defines offspring’s vulnerability to addiction by down-regulating GABAergic GABRG3 in the ventral tegmental area
Translational Psychiatry (2024)
Pleiotropy and genetically inferred causality linking multisite chronic pain to substance use disorders
- Dora Koller
- Eleni Friligkou
By submitting a comment you agree to abide by our Terms and Community Guidelines . If you find something abusive or that does not comply with our terms or guidelines please flag it as inappropriate.
Quick links
- Explore articles by subject
- Guide to authors
- Editorial policies
Sign up for the Nature Briefing newsletter — what matters in science, free to your inbox daily.

- Alzheimer's disease & dementia
- Arthritis & Rheumatism
- Attention deficit disorders
- Autism spectrum disorders
- Biomedical technology
- Diseases, Conditions, Syndromes
- Endocrinology & Metabolism
- Gastroenterology
- Gerontology & Geriatrics
- Health informatics
- Inflammatory disorders
- Medical economics
- Medical research
- Medications
- Neuroscience
- Obstetrics & gynaecology
- Oncology & Cancer
- Ophthalmology
- Overweight & Obesity
- Parkinson's & Movement disorders
- Psychology & Psychiatry
- Radiology & Imaging
- Sleep disorders
- Sports medicine & Kinesiology
- Vaccination
- Breast cancer
- Cardiovascular disease
- Chronic obstructive pulmonary disease
- Colon cancer
- Coronary artery disease
- Heart attack
- Heart disease
- High blood pressure
- Kidney disease
- Lung cancer
- Multiple sclerosis
- Myocardial infarction
- Ovarian cancer
- Post traumatic stress disorder
- Rheumatoid arthritis
- Schizophrenia
- Skin cancer
- Type 2 diabetes
- Full List »
share this!
April 3, 2024
This article has been reviewed according to Science X's editorial process and policies . Editors have highlighted the following attributes while ensuring the content's credibility:
fact-checked
peer-reviewed publication
trusted source
Study finds e-cigarette users now more likely to quit traditional cigarettes
by Oxford University Press
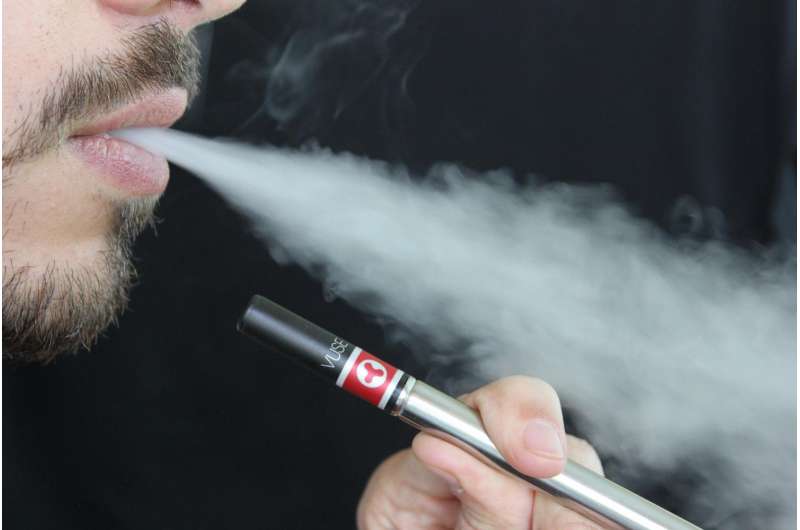
A new paper in Nicotine & Tobacco Research finds that smokers who switch to electronic cigarettes are now more likely to stop smoking regular cigarettes. In the past, smokers who began using electronic cigarettes mostly continued smoking. The paper is titled, "Divergence in cigarette discontinuation rates by use of electronic nicotine delivery systems (ENDS): Longitudinal findings from the U.S. PATH Study Waves 1-6."
Electronic nicotine delivery systems first emerged on the U.S. market in 2007. The first e-cigarettes resembled conventional cigarettes (in appearance) and used fixed low-voltage batteries. Beginning in 2016, manufacturers introduced e-liquids containing nicotine salt formulations. These new e-cigarettes became widely available. These nicotine salts are lower in pH than freebase formulations, which allows manufacturers to increase nicotine concentration while avoiding harshness and bitterness.
Past population-level research provided conflicting findings on whether vaping helps people who smoke combustible cigarettes to quit smoking. Some research suggests improved cigarette quitting-related outcomes with e-cigarette use , while other research suggests the opposite.
Inconsistent findings may be due to differences in the samples and measures considered, differences in the analytic approaches of researchers used, the rapidly changing product environment, or policy contexts.
The researchers here examined differences in real-world trends in population-level cigarette discontinuation rates from 2013 to 2021, comparing U.S. adults who smoked combustible cigarettes and used e-cigarettes with U.S. adults who smoked combustible cigarettes and did not use e-cigarettes.
Using data from among adults (ages 21+) in the Population Assessment of Tobacco and Health (PATH) Study, a national longitudinal study of tobacco use from people from all over the United States, the researchers found that between 2013 and 2016, rates of discontinuing cigarette smoking among adults in the U.S. population were statistically indistinguishable between those who used e-cigarettes and those who did not. Cigarette discontinuation rates were 15.5% for those who used e-cigarettes and 15.6% for those who did not.
But the quit rates changed in subsequent years; the researchers here found that between 2018 and 2021 only 20% of smokers who did not use e-cigarettes stopped smoking combustible cigarettes, but some 30.9% of smokers who used e-cigarettes stopped smoking combustible cigarettes.
The paper notes that the full study period spanned a time in the United States when the e-cigarette marketplace was expanding; salt-based nicotine formulations gained market share in 2016 and vaping products became available with increased nicotine yields over time.
This was also a period in which state and federal governments restricted tobacco in various ways, including increasing the tobacco-purchase age to 21 and restricting flavored e-cigarettes.
"Our findings here suggest that the times have changed when it comes to vaping and smoking cessation for adults in the US," notes study first author, Karin Kasza, an assistant professor of oncology in the Department of Health Behavior at Roswell Park Comprehensive Cancer Center in Buffalo, NY.
"While our study doesn't give the answers as to why vaping is associated with cigarette quitting in the population today when it wasn't associated with quitting years ago, design changes leading to e-cigarettes that deliver nicotine more effectively should be investigated. This work underscores the importance of using the most recent data to inform public health decisions."
Explore further
Feedback to editors

Small protein plays big role in chronic HIV infection
38 minutes ago

Vaping additives harm a vital membrane in the lungs, researchers find

Research offers insight into future understanding of MS and its treatments
43 minutes ago

Fans are not a magic bullet for beating the heat, modeling study shows
44 minutes ago

Gene editing technique shows promise for effectively curing a hereditary liver disease

Study reveals that the brain's cerebellum can shape cognition

Research details adaptation tactics of pancreatic cancer

US company withdraws ALS drug after it fails in trial
2 hours ago

Discovery of how to limit asthma attack damage could stop disease

Stool transplant shows promise for Parkinson's disease
Related stories.

E-cigarette use is rising among pregnant adolescents, study finds
Dec 20, 2023

Daily vaping dramatically ups quit rate in heavy smokers not aiming to quit
Dec 29, 2021
Are e-cigarettes with higher nicotine associated with more smoking, vaping?
Oct 23, 2017

Daily e-cigarette use may help smokers quit regular cigarettes
Jul 23, 2019

Most e-cigarette users want to quit, study finds
May 21, 2019

New study finds largest population increase among US adult ecigarette users is in younger adults
Oct 5, 2020
Recommended for you

Team moves forward in developing a vaccine for the 'zombie drug' xylazine
Apr 3, 2024

In people with opioid use disorder, telemedicine for HCV was more than twice as successful as off-site referral

Study suggests lung cancer does not decrease in line with reduced smoking
Apr 2, 2024

Reducing late-night alcohol sales curbed all violent crimes by 23% annually in a Baltimore neighborhood: Study
Apr 1, 2024

How blocking a neural receptor responsible for addiction could reduce alcohol use
Mar 29, 2024

Prescribing alcohol use disorder medications upon discharge from alcohol-related hospitalizations works
Let us know if there is a problem with our content.
Use this form if you have come across a typo, inaccuracy or would like to send an edit request for the content on this page. For general inquiries, please use our contact form . For general feedback, use the public comments section below (please adhere to guidelines ).
Please select the most appropriate category to facilitate processing of your request
Thank you for taking time to provide your feedback to the editors.
Your feedback is important to us. However, we do not guarantee individual replies due to the high volume of messages.
E-mail the story
Your email address is used only to let the recipient know who sent the email. Neither your address nor the recipient's address will be used for any other purpose. The information you enter will appear in your e-mail message and is not retained by Medical Xpress in any form.
Newsletter sign up
Get weekly and/or daily updates delivered to your inbox. You can unsubscribe at any time and we'll never share your details to third parties.
More information Privacy policy
Donate and enjoy an ad-free experience
We keep our content available to everyone. Consider supporting Science X's mission by getting a premium account.
E-mail newsletter

- News Releases
E-cigarette users now more likely to quit traditional cigarettes
Oxford University Press USA
A new paper in Nicotine & Tobacco Research , published by Oxford University Press, finds that smokers who switch to electronic cigarettes are now more likely to stop smoking regular cigarettes. In the past, smokers who began using electronic cigarettes mostly continued smoking.
Electronic nicotine delivery systems first emerged on the U.S. market in 2007. The first e-cigarettes resembled conventional cigarettes (in appearance) and used fixed low-voltage batteries. Beginning in 2016, manufacturers introduced e-liquids containing nicotine salt formulations. These new e-cigarettes became widely available. These nicotine salts are lower in pH than freebase formulations, which allow manufacturers to increase nicotine concentration while avoiding harshness and bitterness.
Past population-level research provided conflicting findings on whether vaping helps people who smoke combustible cigarettes to quit smoking. Some research suggests improved cigarette quitting-related outcomes with e-cigarette use, while other research suggests the opposite. Inconsistent findings may be due to differences in the samples and measures considered, differences in the analytic approaches of researchers used, the rapidly changing product environment, or policy contexts.
The researchers here examined differences in real-world trends in population-level cigarette discontinuation rates from 2013 to 2021, comparing U.S. adults who smoked combustible cigarettes and used e-cigarettes with U.S. adults who smoked combustible cigarettes and did not use e-cigarettes.
Using data from among adults (ages 21+) in the Population Assessment of Tobacco and Health (PATH) Study, a national longitudinal study of tobacco use from people from all over the United States, the researchers found that between 2013 and 2016, rates of discontinuing cigarette smoking among adults in the U.S. population were statistically indistinguishable between those who used e-cigarettes and those who did not. Cigarette discontinuation rates were 15.5% for those who used e-cigarettes and 15.6% for those who did not.
But the quit rates changed in subsequent years; the researchers here found that between 2018 and 2021 only 20% of smokers who did not use e-cigarettes stopped smoking combustible cigarettes, but some 30.9% of smokers who used e-cigarettes stopped smoking combustible cigarettes.
The paper notes that the full study period spanned a time in the United States when the e-cigarette marketplace was expanding; salt-based nicotine formulations gained market share in 2016 and vaping products became available with increased nicotine yields over time. This was also a period in which state and federal governments restricted tobacco in various ways, including increasing the tobacco-purchase age to 21 and restricting flavored e-cigarettes.
“Our findings here suggest that the times have changed when it comes to vaping and smoking cessation for adults in the US,” notes study first author, Karin Kasza, an assistant professor of oncology in the Department of Health Behavior at Roswell Park Comprehensive Cancer Center in Buffalo, NY. “While our study doesn’t give the answers as to why vaping is associated with cigarette quitting in the population today when it wasn’t associated with quitting years ago, design changes leading to e-cigarettes that deliver nicotine more effectively should be investigated. This work underscores the importance of using the most recent data to inform public health decisions.”
The paper, “Divergence in cigarette discontinuation rates by use of electronic nicotine delivery systems (ENDS): Longitudinal findings from the U.S. PATH Study Waves 1-6,” is available (at midnight on April 3rd) at https://doi.org/10.1093/ntr/ntae027 .
Direct correspondence to: Karin Kasza Department of Health Behavior Roswell Park Comprehensive Cancer Center 665 Elm St Buffalo, NY 14203 [email protected]
To request a copy of the study, please contact: Daniel Luzer [email protected]
Nicotine & Tobacco Research
10.1093/ntr/ntae027
Method of Research
Observational study
Subject of Research
Article title.
Divergence in cigarette discontinuation rates by use of electronic nicotine delivery systems (ENDS): Longitudinal findings from the U.S. PATH Study Waves 1-6
Article Publication Date
Coi statement.
Disclaimer: AAAS and EurekAlert! are not responsible for the accuracy of news releases posted to EurekAlert! by contributing institutions or for the use of any information through the EurekAlert system.
Help | Advanced Search
Computer Science > Computation and Language
Title: mapping the increasing use of llms in scientific papers.
Abstract: Scientific publishing lays the foundation of science by disseminating research findings, fostering collaboration, encouraging reproducibility, and ensuring that scientific knowledge is accessible, verifiable, and built upon over time. Recently, there has been immense speculation about how many people are using large language models (LLMs) like ChatGPT in their academic writing, and to what extent this tool might have an effect on global scientific practices. However, we lack a precise measure of the proportion of academic writing substantially modified or produced by LLMs. To address this gap, we conduct the first systematic, large-scale analysis across 950,965 papers published between January 2020 and February 2024 on the arXiv, bioRxiv, and Nature portfolio journals, using a population-level statistical framework to measure the prevalence of LLM-modified content over time. Our statistical estimation operates on the corpus level and is more robust than inference on individual instances. Our findings reveal a steady increase in LLM usage, with the largest and fastest growth observed in Computer Science papers (up to 17.5%). In comparison, Mathematics papers and the Nature portfolio showed the least LLM modification (up to 6.3%). Moreover, at an aggregate level, our analysis reveals that higher levels of LLM-modification are associated with papers whose first authors post preprints more frequently, papers in more crowded research areas, and papers of shorter lengths. Our findings suggests that LLMs are being broadly used in scientific writings.
Submission history
Access paper:.
- HTML (experimental)
- Other Formats

References & Citations
- Google Scholar
- Semantic Scholar
BibTeX formatted citation

Bibliographic and Citation Tools
Code, data and media associated with this article, recommenders and search tools.
- Institution
arXivLabs: experimental projects with community collaborators
arXivLabs is a framework that allows collaborators to develop and share new arXiv features directly on our website.
Both individuals and organizations that work with arXivLabs have embraced and accepted our values of openness, community, excellence, and user data privacy. arXiv is committed to these values and only works with partners that adhere to them.
Have an idea for a project that will add value for arXiv's community? Learn more about arXivLabs .

An official website of the United States government
The .gov means it’s official. Federal government websites often end in .gov or .mil. Before sharing sensitive information, make sure you’re on a federal government site.
The site is secure. The https:// ensures that you are connecting to the official website and that any information you provide is encrypted and transmitted securely.
- Publications
- Account settings
Preview improvements coming to the PMC website in October 2024. Learn More or Try it out now .
- Advanced Search
- Journal List
- HHS Author Manuscripts

Top 100 Cited Articles in Recent Tobacco Research
E. melinda mahabee-gittens.
1 Division of Emergency Medicine, Cincinnati Children’s Hospital Medical Center, Cincinnati, Ohio, USA
2 College of Medicine, University of Cincinnati, Cincinnati, Ohio, USA
Judith S. Gordon
3 Department of Family and Community Medicine, University of Arizona, Tucson, Arizona, USA
Katie F. Melink
Ashley l. merianos.
4 School of Human Services, University of Cincinnati, Cincinnati, Ohio, USA
The total citations that a peer-reviewed manuscript has is often used to measure the impact that a publication has in its respective field of study. Both the citation count and total number of publications are often used as measures of academic productivity and success. This issue has been previously investigated in the field of tobacco control research. Given the changing landscape in the field of tobacco research since 2004, we sought to re-examine this issue. The study purpose was to identify the 100 top-cited tobacco-related articles published since 2005, and to categorize and describe the fields of study represented in these articles.
We searched the Scopus library database to determine the citations of the top 100 tobacco-related articles. Information was gathered on: title, number of authors, publication year, journal name and impact factor, country of origin, article type and subject category. Articles were selected and analyzed by two independent investigators.
We identified the 100 top-cited articles published in 58 journals, led by The New England Journal of Medicine (8) and Lancet (6), between 2005 and 2014. The United States was the most common country of origin for the highly-cited articles. The top article types were observation (27%), basic science (26%), and review articles (24%). The most common article subject area was medicine (74%). A statistically significant association was found between the journal impact factor and the number of top 100 cited articles ( p = 0.03).
Conclusions
This review may be helpful to identify articles that may be contributing to the conduct of current and future tobacco research. The analysis can be used as a reference to review and evaluate the publications that are making a high impact in the field of tobacco research.
Although an imperfect measure, the total citations that a peer-reviewed manuscript has is often used to measure the impact that a publication has in its respective field of study. Manuscripts that are highly cited are often those that describe topics, experimental designs and current trends in the field. Moreover, many institutions scrutinize the total number of citations that a faculty member has received for their total collective publications as a measure of recognition in their scientific field of research. Both the citation count and total number of publications are often used as measures of academic productivity and success [ 1 - 6 ].
This issue has been previously investigated in the field of tobacco control research. In 2005, Bryne and Chapman published the results of their investigation of the most highly cited authors in tobacco control from 1980-2004 [ 4 ]. This study found that approximately 48.5% of the most highly cited authors conducted research in the field of epidemiology followed by 14.5% in the field of psychology and 14.5% in the field of medicine. This analysis also identified and analyzed the top 50 most highly cited papers. Given the changing landscape in the field of tobacco research since 2004, we sought to re-examine this issue. The goal of this study was to identify the 100 top-cited tobacco-related articles published since 2005, and to identify characteristics of those articles such as journal names, impact factors and immediacy index, author names and sex, article type, and subject areas.
We did a document search of the Scopus Library database ( www.scopus.com ) in January 2016 to obtain citations of published, peer-reviewed, tobacco-related, research articles. Scopus was used in the present study since this accurate and comprehensive database has the largest number of abstracts and citations with more than 20,500 titles from over 5,000 international publishers ( www.scopus.com ). In order to compile a comprehensive list of tobacco-related manuscripts published during 2005-2014, we searched the Scopus database for the following keywords in the title using the “OR” operator: tobacco, tobacco use, nicotine, electronic nicotine delivery system, electronic cigarette, e-cigarette, smoke exposure, secondhand smoke, secondhand smoke exposure, environmental tobacco smoke, tobacco smoke exposure, passive smoke exposure, passive smoke, or smoke. “All” document types and “English” language were selected. The articles identified by these search terms were accessed and reviewed online through the National Institutes of Health library. Electronic copies were available for all articles. The articles from the database were sorted from most-to-least citations by using the option ‘times cited’. The first 124 articles identified were reviewed using previously described methods [ 7 - 9 ]. Two independent investigators (ALM, EMMG) reviewed the articles and excluded one clinical practice guideline, one non-peer reviewed conference paper, one review article in a non-peer reviewed book, nine Centers for Disease Control and Prevention’s Morbidity and Mortality Weekly Reports [ 10 ], and four non-peer reviewed articles from the Cochrane Database of Systematic Reviews [ 11 ]. Additionally, to maintain focus on tobacco research, three articles with a sole focus on never smokers that did not report on any tobacco measures were excluded. Five articles that focused on tobacco plants, tobacco mosaic virus, smoke antimicrobials, and non-tobacco smoke-related pollution were also excluded.
Of the remaining 113 articles, the top 100 were exported into an Excel spreadsheet and reviewed. The following data were compiled: title, year of publication, number of citations, journal name, first author, last author, number of authors, country of origin, journal impact factor, and immediacy index. Subsequently, two independent investigators (ALM, JSG) reviewed the 100 articles and determined article type: original research (randomized clinical trial; both single-blind and double blind were included), observational study (which included case-control studies, cohort studies, and case series), basic science study, meta-analysis, or review article. In order to categorize manuscripts by subject area, we queried Scopus to determine “Subject Areas” which included medicine, nursing, biochemistry/genetics/molecular biology, and other areas. Since the Scopus “Subject Areas” did not include epidemiology, all articles were subcategorized into epidemiology or not epidemiology by the same two independent reviewers. Disagreements were resolved by a third investigator (EMMG). The journal impact factor and immediacy index were obtained using the ISI Web of Knowledge database [ 12 ] for the year 2014.
Using the Pearson product-moment correlation coefficient, we evaluated the strength and direction of the linear relationship between the impact factor of the journal and the number of top 100 cited articles included in the list. The statistical software package used for data analysis was SPSS version 23.0. Data are presented as means, medians, and interquartile ranges. A significance level of α = 0.05 was used for all the comparisons.
Our investigation did not require Institutional Review Board approval as routine data were used in the present study.
The number of citations for the top 100 cited articles from 2005-2014 ranged from 209 to 899 ( Table 1 ). The mean number of citations was 327.16 and the median was 272 (interquartile range: 118). The mean number of authors was 13.75 and the median was seven (interquartile range: 10.75). Most articles ( n = 79) were published in the three-year period between 2005 and 2008. The journal with the highest number of top 100 cited articles was the New England Journal of Medicine (NEJM) with eight ( Table 2 ), followed by the Lancet with six. The Journal of the American Medical Association (JAMA), Nature , and Nicotine and Tobacco Research each had four highly-cited articles. The impact factors for journals with the top 100 cited articles ranged from 1.929 to 55.873. The highest immediacy index also belonged to the journal with the highest impact factor ( NEJM ). We found a statistically significant association between journal impact factor and the number of top 100 cited articles ( p =.03).
List of 100 most cited articles on tobacco
Article citations, impact factor, and immediacy index according to journal
There were 17 different countries of origin for the highly-cited articles. The United States (U.S.) had the most articles (55). The United Kingdom published a total of nine articles. Canada and France each published five articles and Italy published four articles.
The top-cited article was “Efficacy of varenicline, an α4β2 nicotinic acetylcholine receptor partial agonist, vs placebo or sustained-release bupropion for smoking cessation: A randomized controlled trial” published in JAMA by Jorenby DE, et al. [ 13 ]. The author with the highest number of papers published was Reeves KR with five publications. There were seven authors who had four publications and these authors were Benowitz NL, Billing CB, Breslau N, Hatsukami D, Madden PAF, Williams KE, and Watsky EJ. The majority of the first authors (66%) were male.
Agreement on article type was 88%; disagreements were resolved by a third investigator. The article types were reviewed as follows: observational (27%), basic science (26%), review articles (24%), meta-analyses (12%), and RCT (11%). The top three “Subject Areas” as classified by Scopus were: medicine (74%), biochemistry/genetics/molecular biology (28%), and neuroscience (6%); 1% were in the area of psychology. We further subcategorized the articles into epidemiology versus non-epidemiology with 84% agreement; disagreements were resolved by a third investigator. A total of 35 articles were subcategorized as belonging to the “epidemiology” field.
We identified the 100 most cited tobacco-related articles in the ten-year period from 2005-2014 This bibliometric literature analysis is useful in understanding the recent areas of interest and potential impact of tobacco research. The top five articles were published in journals with impact factors that ranged from 17.81 to 55.873. It is interesting to note that only six of the articles on our list were published in the two specific tobacco journals of Nicotine and Tobacco Research and Tobacco Control , and that the first article on the list from those two journals appeared at number 30 (in Nicotine and Tobacco Research) . In 2012, another investigation identified the top 20 cited papers specifically published in Tobacco Control between 1998 and September 2011 [ 14 ]. The two papers published in Tobacco Control that were on our list were not included in this prior investigation due to search techniques. Unlike the investigation published in 2005 [ 4 ] which found that 80% of the top 50 papers were published in journals with impact factors of 2.352 and above, we found that 85% of the top 100 papers were published in journals with impact factors over 5.0. It is also worth noting that four of the top five articles were classified by Scopus as belonging in the “medicine” category. Again, unlike the investigation published in 2005, [ 4 ] epidemiology papers did not dominate this analysis but the majority of articles were in the field of medicine. However, similar to the previous investigation and other similar analyses, higher impact journals had a higher number of publications. Additionally, there was a dominance of male first authors (66%) and the majority came from authors in the U.S., which may reflect a greater availability for funding of tobacco research or a bias towards publication of studies that are conducted in the U.S.[ 4 , 8 , 15 ].
Similar to other bibliometric analyses, there are potential limitations. This analysis will disproportionately favor older published articles which have had a longer time to garner citations [ 16 , 17 ]. The use of the Scopus database is a potential shortcoming as it is a dynamic database which updates daily, thus our results may not be accurate if the same search strategy were performed today. Although Scopus is the world’s largest abstract and citation database, tobacco publications with high article metrics that were not indexed in this particular database were excluded. Thus, we may have missed important top cited tobacco articles. Further, we may have inadvertently omitted important publications by search criteria chosen. Lastly, the method of using journal citations as a measure is potentially skewed due to authors’ self-citations or due to a “snowball effect” which can result when some authors cite certain articles simply because they have been cited before but not necessarily due to their relevance to a publication [ 18 , 19 ].
However, our analysis provides information into the frequency of citations of tobacco-related research articles and the content areas of these influential publications. This review may be helpful to identify articles that may be contributing to the conduct of current and future tobacco research. The analysis can be used as a reference to review and evaluate the publications that are making a high impact in the field of tobacco research.
Acknowledgments
This study was funded by the Eunice Kennedy Shriver National Institute of Child Health and Human Development (1R01HD083354).
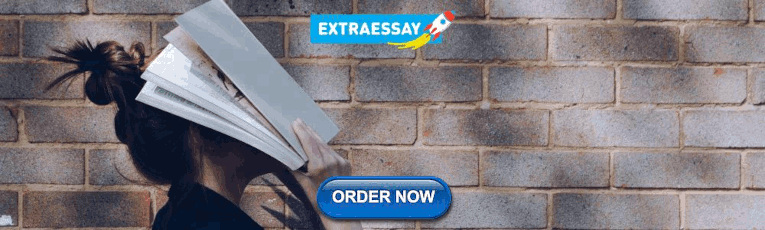
IMAGES
VIDEO
COMMENTS
Background and objectives: Despite reductions in prevalence in recent years, tobacco smoking remains one of the main preventable causes of ill-health and premature death worldwide.This paper reviews the extent and nature of harms caused by smoking, the benefits of stopping, patterns of smoking, psychological, pharmacological and social factors that contribute to uptake and maintenance of ...
Cutting-edge neuroimaging technologies have identified brain changes associated with nicotine dependence and smoking. Using functional magnetic resonance imaging (fMRI), scientists can visualize smokers' brains as they respond to cigarette-associated cues that can trigger craving and relapse. 231 Such research may lead to a biomarker for ...
For instance, recent papers have investigated the effects of e-cigarettes and heated tobacco products on cardiopulmonary outcomes [9-14]. However, the papers have mainly focused on clinical measurements, such as spirometry and other lung function tests; consumer perception is rarely explored or the focus of the research.
Decades after its ill effects on human health were first documented, tobacco smoking remains one of the major global drivers of premature death and disability. In 2017, smoking was responsible for ...
Abstract. Tobacco smoking is a major determinant of preventable morbidity and mortality worldwide. More than a billion people smoke, and without major increases in cessation, at least half will ...
In their Article in The Lancet Public Health, Marissa Reitsma and colleagues1 report their comprehensive analysis of smoking tobacco use in young people from more than 3000 tobacco surveys from 204 countries and territories around the world. The result is an invaluable overview of an epidemic that causes millions of deaths every year. Their detailed mapping of the prevalence of smoking tobacco ...
In 2014, the Nation marked the 50th anniversary of the first Surgeon General's Report on Smoking and Health. In 1964, more than 40 percent of the adult population smoked. Once the link between smoking and its medical consequences—including cancers and heart and lung diseases—became a part of the public consciousness, education efforts and public policy changes were enacted to reduce the ...
60 Monitor tobacco use and prevention policies 64 Protect people from tobacco smoke 68 Offer help to quit tobacco use 72 Warn about the dangers of tobacco use 76 Anti-tobacco mass media campaigns 80 Enforce bans on tobacco advertising, promotion and sponsorship 86 Raise taxes on tobacco 94 National tobacco control programmes
Tobacco Use Insights is an international, peer reviewed, open access journal that looks at all aspects of the health impacts of tobacco use and smoking cessation. The journal is multidisciplinary and includes research from the social, psychological, epidemiological, prevention, economic, and treatment arenas. View full journal description
We identified three outcomes with a 4-star association with smoking: COPD (72% increase in risk based on the BPRF, 0.54 ROS), lower respiratory tract infection (54%, 0.43) and pancreatic cancer ...
Nicotine & Tobacco Research aims to provide a forum for empirical findings, critical reviews, and conceptual papers on the many aspects of nicotine and tobacco, including research from the biobehavioral, neurobiological, molecular biologic, epidemiological, prevention, and treatment arenas. Find out more here.
Prevention can also take place at the school or community level. Merely educating potential smokers about the health risks has not proven effective. 218 Successful evidence-based interventions aim to reduce or delay initiation of smoking, alcohol use, and illicit drug use, and otherwise improve outcomes for children and teens by reducing or ...
A survey of tobacco use that has been administered as part of the U.S. Census Bureau's Current Population Survey since 1992, including past data and statistics. PhenX Toolkit. A catalog of recommended standard measures of phenotypes and environmental exposures for use in biomedical research. This includes common data elements for tobacco ...
The relationship between tobacco use and health stems initially from clinical observations about lung cancer, the first disease definitively linked to tobacco use. Almost 35 years ago, the Office of the Surgeon General of the United States Health Service reviewed over 7000 research papers on the topic of smoking and health, and publicly ...
While the health effects of cigarette smoking are well recognized and documented, the environmental impacts of tobacco are less appreciated and often overlooked. Here, we evaluate tobacco's global footprint across its entire supply chain, looking at resource needs, waste, and emissions of the full cradle-to-grave life cycle of cigarettes. The cultivation of 32.4 Mt of green tobacco used for ...
This descriptive paper examines differences in real-world trends in population-level cigarette discontinuation rates across 2013/14-2021, comparing U.S. adults who smoked combustible cigarettes and used ENDS with U.S. adults who smoked combustible cigarettes and did not use ENDS (regardless of other tobacco product use). Methods Participants
Tobacco farming employs millions of small-scale tobacco farmers around the globe, most of whom are out growers who rely on the tobacco industry. This paper aims to map the regions of greatest tobacco production globally (i.e., the US, Brazil, China, Indonesia, India, and Zambia) and tobacco use rates in these locations.
Measuring self-reported experience of health and functioning is important for understanding the changes in the health status of individuals switching from cigarettes to less harmful tobacco and/or nicotine products (TNP) or reduced-risk products (RRP) and for supporting tobacco harm reduction strategies. This paper presents insights from three research activities from the preparatory phase of ...
Introduction. Tobacco use among young people is a global public health issue.[] The majority of smokers began smoking in their youth[2,3] and more than 80-90% of smokers in the United States (U.S.) began smoking in their teens.[] Currently, approximately 50% of young men and 10% of young women smoke worldwide with annual tobacco-attributable deaths projected to rise from 5 million in 2010 to ...
Tobacco and alcohol use are heritable behaviours associated with 15% and 5.3% of worldwide deaths, respectively, due largely to broad increased risk for disease and injury 1,2,3,4.These substances ...
A new paper in Nicotine & Tobacco Research finds that smokers who switch to electronic cigarettes are now more likely to stop smoking regular cigarettes. In the past, smokers who began using ...
Tobacco use, in any form, can be described as a behavioral process which elicits psychological and physiologic addictive mood among users. ... There is also emerging data suggesting that e-cigarettes may facilitate smoking cessation but further research is needed to compare the effectiveness and safety of e-cigarettes compared to other nicotine ...
A new paper in Nicotine & Tobacco Research finds that smokers who switch to electronic cigarettes are now more likely to stop smoking regular cigarettes. In the past, smokers who began using ...
The research will be presented at the International Conference on Learning Representations. Finding facts. Most large language models, also called transformer models, are neural networks. Loosely based on the human brain, neural networks contain billions of interconnected nodes, or neurons, that are grouped into many layers, and which encode ...
Our findings reveal a steady increase in LLM usage, with the largest and fastest growth observed in Computer Science papers (up to 17.5%). In comparison, Mathematics papers and the Nature portfolio showed the least LLM modification (up to 6.3%). Moreover, at an aggregate level, our analysis reveals that higher levels of LLM-modification are ...
All patients should receive tobacco cessation support to improve therapeutic outcomes, but clinicians could also consider the integration of tobacco use assessment and tobacco cessation into research programmes. 37 Screening patients using paper-based systems that capture subjective tobacco use responses might be useful to encourage referrals ...
April 4, 2024 The School of Computing and Information (SCI), along with Carnegie Library of Pittsburgh (CLP), is honored to present the Association for Library Service to Children's (ALSC) 2024 Children's Literature Lecture on April 17. Bestselling and award-winning author Rita Williams-Garcia will present her paper, "A Funny Thing About Memory." "We are proud to host the Children's ...
The journal with the highest number of top 100 cited articles was the New England Journal of Medicine (NEJM) with eight ( Table 2 ), followed by the Lancet with six. The Journal of the American Medical Association (JAMA), Nature, and Nicotine and Tobacco Research each had four highly-cited articles. The impact factors for journals with the top ...