- Share full article
Advertisement
Supported by
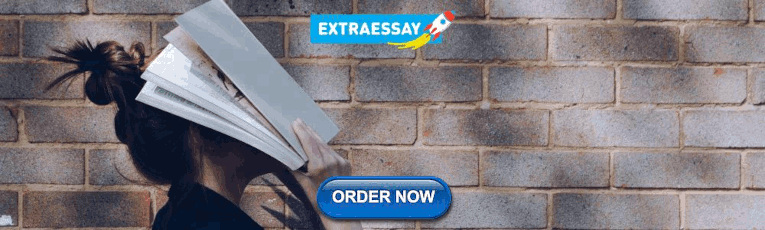
Stocks Soar in India, Luring Investors at Home and Abroad
The second-most populous country is enjoying some of the world’s strongest stock market returns. Pro-growth policies and gnawing questions about China have helped.

By Matt Phillips and Emily Schmall
Until the pandemic, India’s stock market was like another world that Dilip Kumar never had a reason to visit. But like so many other people around the world who were stuck at home, he began to see it as the place to be.
Mr. Kumar, a proposal administrator at an engineering company in New Delhi, set up a free stock trading account through Zerodha, India’s largest online brokerage firm, and plowed some of his savings into Indian Railways as well as a clothing retailer and a cinema chain.
“I invested in all the things I was using daily,” he said. Since then, he’s gotten “a big return in quick time” — more than doubling his money in a little over a year.
Plenty of others want in on the action.
India’s booming stock market is drawing both local novices and global investors to shares of the financial, industrial and technology companies that dominate its listings. The MSCI India index is up about 30 percent this year — nearly twice the return of the global index — while India’s benchmark 30-share S&P BSE Sensex is up roughly 25 percent. Both have notched a seemingly relentless string of record highs, soaring on factors including simple demographics, governmental and fiscal policy and geopolitical changes.
The enthusiasm is clear from the initial public offering this week for the parent company of the digital payments platform Paytm. The company hit its target of raising $2.5 billion — making the offering the biggest in the country’s history and valuing the company at more than $20 billion. The offering underscored the momentum of the financial and tech sectors in a country with a predominantly young population embracing digital start-ups.
At the same time, the government of Prime Minister Narendra Modi is trying to make India more self-reliant, a boon to domestic businesses offering everyday goods and services, while trying to bring more citizens — and their money — into the formal economy. And this spring, the Indian central bank embarked on a bond-buying program that’s a smaller version of the sort that has lifted stocks around the world.
Combine those factors and it’s a recipe for a retail investor boom: According to the Securities and Exchange Board of India, new securities-holding accounts have risen to an all-time high.
“There is pent-up demand among the upper middle class, who have been rushing to the market,” said Jiban Mukhopadhyay, a corporate economics professor emeritus at the S.P. Jain Institute of Management and Research.
Their confidence has been buoyed by the huge stakes that institutional investors overseas are taking in companies that have gone public this year. Abu Dhabi’s sovereign wealth fund, the Texas teachers’ pension fund and the University of Cambridge have invested a total of more than $1 billion in Paytm.
One reason: Foreign investors have lately soured on China, long the destination for those seeking high-flying returns, as growth there slows and a powerful central government cracks down on big tech companies.
“India really stands out this year, with China decelerating,” said Todd McClone, a portfolio manager at William Blair’s Emerging Markets Growth Fund. His fund sharply cut its allocation to China, moving much of that money into Indian stocks including the conglomerate Reliance Industries, the paint manufacturer Asian Paints and the specialty chemical company SRF.
“With accelerating growth, lots of good companies and all the demographics that stand behind it, I think it gave people a lot of confidence to come back to that market,” he said.
It remains to be seen how sustainable the rally will be. Emerging markets like India can often be at the mercy of decisions made by investors on the other side of the globe. Oil prices are surging, which is a particular challenge for India, a major importer.
Economists also point to an uneven recovery from the pandemic that has pushed many Indians back into poverty. The economy plunged 21 percent during India’s first lockdown, the small and midsize businesses that employ most of India’s work force continue to falter, and the government is spending billions of dollars to mop up banks’ growing number of bad loans.
But investors remain optimistic: Wall Street analysts expect Indian companies to increase their earnings more than 22 percent over the next 12 months — calculated in dollars — a faster pace of growth than benchmark indexes in either China or the United States.
“Stock prices follow earnings, and Indian corporates have the strongest fundamental momentum ,” said Brian Freiwald, an emerging-market portfolio manager at Putnam Investments in Boston.
Part of the reason for the Indian market’s rapid ascent can be traced to 2016 and a policy of demonetization. Meant to tamp down money laundering, the policy banned the most widely circulated currency notes and wiped out the savings of families and small businesses overnight. But it also bolstered companies like Paytm, a sector that benefited further as the pandemic disrupted face-to-face transactions.
Adding to the momentum are market-friendly measures delivered by Indian policymakers. In February, Mr. Modi’s government proposed a budget that called for more spending on health care and infrastructure. Then, two months later, the Reserve Bank of India began the same kind of quantitative easing programs that the Federal Reserve and other central banks instituted to support their domestic economies. Though it started its bond-buying program more than a year after the Fed’s began, India enjoyed a similar stock-market response: Shares took off.
For global investors, it was a stark contrast to what was happening in China, which had already enjoyed a rapid recovery from its pandemic shutdowns. Chinese policymakers began withdrawing some of their support for the economy early this year. Growth began to slow — it was down to just 4.9 percent in the third quarter — putting pressure on debt-laden firms that rely on continuously fast growth to pay their creditors. At the same time, the Chinese government, under the increasingly centralized power of President Xi Jinping, has begun to rein in some of the country’s most prominent tech companies.
It has been an unappealing backdrop for investors, and Chinese markets have posted some of the worst returns in the world this year.
“ India tends to do well when there’s an issue in China,” said Divya Mathur, an emerging-market portfolio manager at the money management firm Martin Currie in Edinburgh.
As rapid as the Indian market’s gains have been, they remain fragile, experts said.
Emerging markets like India can whipsaw as global investors who poured in money can pull it out quickly, particularly when central banks raise interest rates and attract investor capital. India was slammed by such a situation in 2013: When the Federal Reserve began to step back from low-interest rate policies after the 2008 financial crisis, investors pulled their money from India. Its currency, the rupee, plunged to a new low against the dollar and pushed the country to the brink of a financial crisis.
There are also fundamental demographic challenges ahead. The young people who have helped speed the country’s embrace of new technologies will put pressure on the government to keep up the rapid economic expansion. Over a quarter of India’s population — more than 360 million people — are under the age of 15, according to the World Bank.
“As this young population comes of age, can India provide enough job opportunities?” asked Ajay Krishnan, a portfolio manager who specializes in emerging markets at Wasatch Global Investors in Salt Lake City.
The pandemic also remains a threat: Roughly a quarter of India’s population is fully vaccinated, leaving it vulnerable to another surge in cases that could cause more economic damage and push more citizens into poverty.
Mr. Mukhopadhyay, the economics professor, said those dynamics are a sign that market returns aren’t an indicator of broader prosperity.
“The Indian stock market behaves like a pampered kid,” he said. “It has hardly any relationship with the movement of the economy.”
Sameer Yasir contributed reporting.
Matt Phillips covers financial markets. Before joining The New York Times in 2018, he was editor in chief at Vice Money and a founding staffer at Quartz, a business and economics website. He also spent seven years at The Wall Street Journal, where he covered stock and bond markets. More about Matt Phillips
Emily Schmall is a South Asia correspondent based in New Delhi. More about Emily Schmall
- Work & Careers
- Life & Arts
Become an FT subscriber
Try unlimited access Only $1 for 4 weeks
Then $75 per month. Complete digital access to quality FT journalism on any device. Cancel anytime during your trial.
- Global news & analysis
- Expert opinion
- Special features
- FirstFT newsletter
- Videos & Podcasts
- Android & iOS app
- FT Edit app
- 10 gift articles per month
Explore more offers.
Standard digital.
- FT Digital Edition
Premium Digital
Print + premium digital, weekend print + standard digital, weekend print + premium digital.
Today's FT newspaper for easy reading on any device. This does not include ft.com or FT App access.
- 10 additional gift articles per month
- Global news & analysis
- Exclusive FT analysis
- Videos & Podcasts
- FT App on Android & iOS
- Everything in Standard Digital
- Premium newsletters
- Weekday Print Edition
- FT Weekend Print delivery
- Everything in Premium Digital
Essential digital access to quality FT journalism on any device. Pay a year upfront and save 20%.
- Everything in Print
Complete digital access to quality FT journalism with expert analysis from industry leaders. Pay a year upfront and save 20%.
Terms & Conditions apply
Explore our full range of subscriptions.
Why the ft.
See why over a million readers pay to read the Financial Times.
International Edition
- Search Search Please fill out this field.
The BSE and NSE
Trading and settlement, market indexes, market regulation.
- Investing in India's Markets
The Bottom Line
- International Markets
Indian Stock Market: Exchanges and Indexes
:max_bytes(150000):strip_icc():format(webp)/Top100FinancialAdvisors_MargueritaCheng_2-54d1bcc14e3042818cb44df9456919f2.jpg)
With its massive population and bustling economy, India is an engine of growth. On Jan. 22, 2024, its stock market capitalization surpassed Hong Kong’s for the first time. According to data compiled by Bloomberg, the value of shares listed on Indian exchanges reached $4.33 trillion, compared to $4.29 trillion for Hong Kong.
Key Takeaways
- India has two primary stock markets, the Bombay Stock Exchange (BSE) and the National Stock Exchange (NSE).
- The BSE is India's oldest stock exchange.
- India's exchanges are regulated by the Securities Exchange Board of India (SEBI).
- The two prominent Indian market indexes are Sensex and Nifty.
Most of the trading in the Indian stock market takes place on its two stock exchanges: the Bombay Stock Exchange (BSE) and the National Stock Exchange (NSE). The BSE was established in 1875. The NSE was founded in 1992 and started trading in 1994. Both exchanges follow the same trading mechanism, trading hours, and settlement process.
As of Jan. 30, 2024, the BSE had 5,315 listed firms, whereas the rival NSE had 2,266 as of Dec. 31, 2023. Almost all the significant firms of India are listed on both exchanges. The BSE is the older stock market, and the NSE is the largest in volume.
Trading on both exchanges is through an open electronic limit order book where order matching is done by the trading computer. There are no market makers , and the entire process is order-driven by investors matched with the best limit orders. Buyers and sellers remain anonymous.
An order-driven market brings more transparency by displaying all buy and sell orders in the trading system . Institutional investors can use the direct market access (DMA) option, using trading terminals provided by brokers for placing orders directly into the stock market trading system.
Equity spot markets follow a T+1 rolling settlement , with any trade on Monday getting settled by Tuesday. All trading is conducted between 9:15 a.m. and 3:30 p.m., Indian Standard Time (+ 5.5 hours GMT), Monday through Friday. Delivery of shares must be made in dematerialized form. Each exchange has its own clearing house, which assumes all settlement risk by serving as a central counterparty.
The two prominent Indian market indexes are Sensex and Nifty. Sensex is the oldest market index for equities; it includes shares of 30 firms listed on the BSE. It was created in 1986 and provides time series data from 1979 as the base year.
The Standard and Poor's CNX Nifty includes 50 shares listed on the NSE. It was created in 1996.
The development, regulation, and supervision of India's stock market rests with the Securities and Exchange Board of India (SEBI), formed in 1992 as an independent authority. Since then, SEBI has consistently tried to lay down market rules in line with the best market practices. It enjoys vast powers of imposing penalties on market participants in case of a breach.
Investing in India's Markets
India permitted outside investments in the 1990s. Foreign investments are classified into two categories: foreign direct investment (FDI) and foreign portfolio investment (FPI). All investments in which an investor takes part in the day-to-day management and operations of the company are treated as FDI, whereas investments in shares without any control over management and operations are treated as FPI.
To make portfolio investments in India, one must be a foreign institutional investor (FII) or one of the sub-accounts of one of the registered FIIs. Both registrations are granted by the market regulator, SEBI. Foreign institutional investors mainly consist of mutual funds , pension funds, endowments, sovereign wealth funds, insurance companies, banks, and asset management companies. FIIs can also invest in unlisted securities outside stock exchanges, subject to the approval of the price by the Reserve Bank of India .
India has the fifth-largest economy in the world by GDP, with a 2023 GDP of $3.7 trillion.
Restrictions and Investment Ceilings
The government of India prescribes the FDI limit, and different ceilings have been prescribed for different sectors. The maximum limit for portfolio investment in a particular listed firm is decided by the FDI limit prescribed for the sector to which the firm belongs. However, there are two additional restrictions on portfolio investment.
According to SEBI, an FII can invest up to 10% of the equity of any one company, subject to the 24% limit on overall investments. The 24% limit may be raised to 30% for individual companies that have received shareholder approval to do so. FIIs are allowed to invest 100% of their portfolios in debt securities.
Investments for Foreign Entities
Foreign entities and individuals can gain exposure to Indian stocks through institutional investors. Investments can be made through some of the offshore instruments, like participatory notes (PNs), depositary receipts, such as American depositary receipts (ADRs) and global depositary receipts (GDRs), exchange-traded funds (ETFs), and exchange-traded notes (ETNs).
Participatory notes representing underlying Indian stocks can be issued offshore by FIIs, only to regulated entities, but small investors can invest in American depositary receipts representing the underlying stocks of some of the well-known Indian firms, listed on the New York Stock Exchange and Nasdaq. ADRs are denominated in dollars and subject to the regulations of the U.S. Securities and Exchange Commission (SEC).
Retail investors can invest in ETFs and ETNs , based on Indian stocks. India-focused ETFs mostly make investments in indexes made up of Indian stocks. Most of the equities included in the index are listed on the NYSE and Nasdaq. Two ETFs based on Indian stocks include the iShares MSCI India ETF ( INDA ) and the Wisdom-Tree India Earnings Fund ( EPI ).
What Is the Main Stock Market in India?
The main stock market in India is the Bombay Stock Exchange (BSE) which has 5,315 listed firms.
What Is the Largest Company on the Indian Stock Market?
The largest company on the Bombay Stock Exchange (BSE) is Reliance Industries with a market cap of over $229 billion as of Jan. 30, 2024.
Can Americans Invest in the Indian Stock Market?
Yes, Americans can invest in the Indian stock market. There are a few ways of doing so, such as investing in exchange-traded funds (ETFs) or purchasing American depository receipts (ADRs) of the company.
Most of the trading in the Indian stock market takes place in the Bombay Stock Exchange (BSE) and the National Stock Exchange (NSE). As of 2024, India ranked as the fourth largest market in the world. The two dominant Indian market indexes are Sensex and Nifty.
Bloomberg. " India Tops Hong Kong as World’s Fourth-Largest Stock Market ."
BSE. " History and Milestones ."
NSE. " History & Milestones ."
BSE. " All India Market Capitalization ."
NSE. " All Companies Based on Market Capitalisation ."
NSE. " Trading System ."
NSE. " Settlement Cycle ."
BSE. " Compulsory Rolling Settlement ."
BSE. " Session Timings ."
NSE. " Exchange Communications. Market Timings ."
Pib.gov.in. " BSE Sensex Is Now 25 ."
NSE. " Nifty50 ."
Securities and Exchange Board of India. " About SEBI ."
International Monetary Fund. " UNCTAD Press Release: FDI Flows to India Expanded in the 1990s ."
Securities and Exchange Board of India. " Foreign Institutional Investment ."
World Population Review. " Countries By GDP ."
Companiesmarketcap.com. " Reliance Industries ."
BSE. " Top 100 Companies by Market Capitalization ."
:max_bytes(150000):strip_icc():format(webp)/investing5-5bfc2b8e46e0fb0026016f0e.jpg)
- Terms of Service
- Editorial Policy
- Privacy Policy
- Your Privacy Choices
- Open access
- Published: 11 July 2019
Stock market and macroeconomic variables: new evidence from India
- R. Gopinathan 1 &
- S. Raja Sethu Durai 2
Financial Innovation volume 5 , Article number: 29 ( 2019 ) Cite this article
17k Accesses
23 Citations
Metrics details
Understanding the relationship between macroeconomic variables and the stock market is important because macroeconomic variables have a systematic effect on stock market returns. This study uses monthly data from India for the period from April 1994 to July 2018 to examine the long-run relationship between the stock market and macroeconomic variables. The empirical findings suggest that standard cointegration tests fail to identify any relationship among these variables. However, a transformation that extracts the actual functional relationship between these variables using the alternating conditional expectations algorithm of (J Am Stat Assoc 80:580–598, 1985) identifies strong evidence of cointegration and indicates nonlinearity in the long-run relationship. Further, the continuous partial wavelet coherency model identifies strong coherency at a lower frequency for the transformed variables, establishing the fact that the long-run relationship between stock prices and macroeconomic variables in India is nonlinear and time-varying. This evidence has far-reaching implications for understanding the dynamic relationships between the stock market and macroeconomic variables.
Introduction
Understanding the relationship between macroeconomic variables and the stock market is important because macroeconomic variables have a systematic effect on stock market returns. Economic forces affect discount rates, and through this mechanism, macroeconomic variables become part of the risk factors in equity markets [Chen et al. 1986 ]. Arbitrage pricing theory assumes that financial stocks can be influenced by the behavior of macroeconomic fundamentals; there are many channels for the relationships between the stock market and key macroeconomic variables. For example, Friedman (1988) advocated the wealth and substitution effects to measure the relationship between the stock market and money. Moreover, three separate hypotheses have been proposed to explain the theoretical relationship between the stock market and the exchange rate: Frenkel’s (1976) asset market hypothesis, Dornbusch and Fischer’s ( 1980 ) goods market hypothesis, and Frankel’s (1983) portfolio balance hypothesis.
Studies have shown that the choice of the financial and macroeconomic variables that influence the stock market is intriguing and puzzling, and have attempted to explain the anomalous relationship through different hypotheses [Fama ( 1981 ), Geske and Richard ( 1983 ), Ram and Spencer ( 1983 ), Fama ( 1990 ), Schwert ( 1990 ), Cochrane ( 1991 ) and Lee ( 1992 )]. Fama ( 1981 ) explained the anomalous negative correlation between inflation and real stock returns by proposing a proxy hypothesis, where the anomalous negative correlation is the result of the negative relationship between inflation and real output and the strong positive relationship between real output and real stock returns. Ram and Spencer ( 1983 ) found evidence of a positive relationship between inflation and real output, consistent with the Phillips curve hypothesis.
While many researchers provide empirical support for linear long-run relationships between stock markets and macroeconomic variables, studies like Mukherjee and Naka ( 1995 ), Cheung and Ng ( 1998 ), Binswanger ( 2004 ), Nasseh and Strauss ( 2000 ), Wongbangpo and Sharma ( 2002 ), Kizys and Pierdzioch ( 2009 ), Bekhet and Matar ( 2013 ), Inci and Lee ( 2014 ) and Lawala et al. ( 2018 ) employed Engle and Granger’s ( 1987 ) methodology to infer causal relationships and Granger’s ( 1988 ) and Johansen’s ( 1991 ) methodology for cointegration between these variables. Most of the earlier empirical studies implicitly assumed that the relationships between the stock market and macroeconomic variables are linear in nature and time-invariant. In their cointegration analyses, most of these studies failed to test the stability of the parameters over time or the asymmetric long-run relationships among these variables. Hansen and Johansen ( 1999 ) and Johansen et al. ( 2000 ) emphasized that the results of cointegration analysis are highly sensitive to sample selection and have potential parameter instability over time.
In this regard, McMillian ( 2005 ) estimated the time-varying relationships between the stock market and other macroeconomic variables for U.S. data using Johansen’s ( 1991 ) cointegration test in a rolling and recursive sample window and suggested that the long-run relationships between these variables are time varying. The main drawback of implementing a rolling or recursive cointegration is that the variables under consideration should have the same time series properties for all sample windows; if these properties change for a given subsample of windows, then the interpretations from the analysis are biased. On the other hand, a good number of recent studies have examined the nonlinear relationships between key macroeconomic variables such as output, inflation, and exchange rates, and found evidence in favor of the asymmetric behavior of these variables over a period. Studies like Falk ( 1986 ), Ramsey and Rothman ( 1996 ), and Bradley and Jansen ( 1997 ) all support various forms of nonlinear adjustment among key macroeconomic variables. In line with these findings, studies by Boucher ( 2007 ) and Kizys and Pierdzioch ( 2009 ) highlight that the relationships between the stock market and macroeconomic variables are asymmetric and these relationships will change in the long run.
The nonlinearities in financial markets are explained by many studies, such as Dumas ( 1992 ), Brock and LeBaron ( 1996 ), Sarantis ( 2001 ), Shleifer and Robert ( 2003 ), and Humpe and Macmillan ( 2014 ). These studies all identify the interaction between informed and noise traders, suggesting speculators are the main source for the market’s nonlinear behavior.
From an empirical standpoint, the functional form of any relationship should be appropriate before performing any analysis. When the relationship is nonlinear, a linear analysis may lead to misleading conclusions, with the potential of inferences that there is no relationship between the variables under consideration. Although some studies highlight the possible nonlinear relationships between the stock market and macroeconomic fundamentals, the issue has received little comprehensive attention in the empirical literature. A few studies, like Kanas ( 2003 , 2005 ), Zhou ( 2010 ), and Tang and Zhou ( 2013 ), have documented nonlinear relationships for different variables, and explored the alternating conditional expectations (ACE) algorithm developed by Breiman and Friedman ( 1985 ) to identify the correct functional form and transform the variables to be used in the empirical analysis. The results from these studies show that the advantage of using the ACE algorithm is its ability to identify the exact functional form of the nonlinear relationship. Once the correct functional form of the variables has been identified, further investigation can proceed.
Recent advances in time-series econometrics have produced methodologies for analyzing the relationships between variables in a frequency domain, where the actual relationship might vary at different frequencies. For example, some studies of India have used frequency domains and wavelet analysis. Durai and Bhaduri ( 2009 ) examined the stock market and economic activity using discrete wavelets to decompose the time series of the variables into different frequencies; the results suggest that the variables are connected in different ways at different frequency levels. Tiwari et al. ( 2013 ) used the continuous wavelet transformation (CWT) developed by Conraria and Soares ( 2011 ). The advantage of the CWT over discrete wavelet transformation (DWT) is the identification of the correlation between variables in different frequencies over time scales. Even though the CWT has a major disadvantage it can be used only for two variables the concepts of wavelet multiple coherencies and partial wavelet coherency to some extent compensate, allowing the CWT to move beyond bivariate analysis.
The purpose of the present study is to extend the existing nonlinear and time-varying empirical literature to clarify the relationship between stock prices and key macroeconomic fundamentals in the Indian context. The study’s main contribution is twofold. First, the analysis of nonlinearity in the relationships between the stock market and other key macroeconomic variables for Indian data provides a developing country perspective. Second, using continuous wavelets to understand the coherency between two variables in the frequency domain for different time scales provides a better understanding than implementation of a rolling or recursive cointegration.
The remainder of the paper is organized as follows. Section 2 describes the nonlinear cointegration approach using an ACE algorithm and provides a brief outline of the CWT methodology used in the study. Section 3 describes the data, and in section 4, the results of the empirical estimation are reported. Finally, section 5 draws conclusions based on the study’s empirical findings.
Nonlinear and time-varying relationships
The conventional cointegration tests of Engel and Granger (1987) and Johansen ( 1991 ) implicitly assume linearity and time invariance. The inferences drawn from these tests may be misleading if the relationships are nonlinear and time-varying. Following McMillian (2005), this study uses a rolling cointegration test for stock prices and other macroeconomic variables. In the rolling framework, we fix the size of a rolling sample window, Footnote 1 and move the sample window by adding one observation to the end and removing the first one. For each rolling sample window, we conduct conventional augmented Dickey-Fuller (ADF) unit root tests to determine the order of integration for each of the variables used in every rolling window. If the test ensures all variables are integrated of order one in all rolling windows, then we can move to implement the conventional Johansen ( 1991 ) cointegration test and the trace statistics can be observed and scaled by their 5% critical values. The null hypothesis of no cointegration can be rejected at a 5% level for the specified sub-sample period if the value of the scaled test statistic is greater than one.
The ACE algorithm and nonlinear cointegration
The ace algorithm.
Granger and Hallman ( 1991 ) and Meese and Rose ( 1991 ) analyzed the non-parametric ACE algorithm Footnote 2 developed by Breiman and Friedman ( 1985 ) for raw variables to obtain nonlinear transformations of their respective variables and used a causality test for the transformed variables to infer a nonlinear causal relationship. The ACE method converts the original variables into transformed variables, ensuring that there is maximum correlation between the variables with the highest R-squared; ACE procedures adopt extremely weak distributional assumptions and can handle a wide variety of nonlinear transformations of the data by utilizing flexible data smoothing techniques. Thus, any test on these transformed variables can be viewed as evidence for nonlinearity.
Assume a linear regression model contains k independent variables, namely x 1 t , x 2 t , … ., and x kt , and a dependent variable y t :
Where, δ 0 , δ (i = 1,2,…,k) are the regression coefficients to be estimated, and ε t is an error term. Thus eq. ( 1 ) assumes that y t, the dependent variable, is a linear function of k independent variables. An ACE algorithm using the regression model in eq. ( 1 ) can be written as.
Where f is a function of the dependent variable y, and n i is a function of the independent variables x i (i = 1,2,…,k). Equation ( 2 ) shows f (·), n 1 (·), n 2 (·) …, and nk (·) is the optimal transformation to be estimated. The ACE regression normalizes the coefficients to unity.
The ACE algorithm initially starts by defining arbitrary determinate mean zero transformations, f (y t ), n 1 (x 1t ), n 2 (x 2t ),. ., and n k (x kt ). To obtain the optimal transformation, the estimated model ensuring the maximum correlation among the variables and highest R-square from a regression as specified in eq. ( 2 ). Under the constraint of E [ f (y t )] 2 = 1, this is equivalent to minimizing the expected mean squared error of the regression. The expected mean squared error is given by
Minimization of u 2 with respect to n i (x i ) (i = 1,2,…,k) and f (yt) is carried out through a series of single-function minimizations, resulting in the following equations:
With \( \left\Vert \bullet \right\Vert \equiv {\left[E{\left(\bullet \right)}^2\right]}^{\frac{1}{2}} \)
The algorithm involves two basic mathematical operations: conditional expectations and iterative minimization; thus, the transformation is referred to as alternating conditional expectations. Using eq. ( 4 ) to transform all variables, one variable is fixed and the transformation of the variable in question is estimated using a nonparametric data smoothing technique; the algorithm then continues to the next variable. For each variable, the iterations continue until the mean squared error of eq. ( 3 ) has been minimized. Breiman and Friedman ( 1985 ) show that the ACE algorithm provides transformations such that f (y t ), n 1 (x 1t ), n 2 (x 2t ), …., and n k (x kt ) converge asymptotically to the true functional forms of the optimal transformations. The distinction of these transformations is that ACE does not treat the explanatory variables as fixed, but instead treats the variables as drawn from a joint distribution. After the estimation of n i (x it ) (i = 1,2,…,k), f (y t ) is estimated, conditioned on these estimates, according to eq. ( 5 ). Alternating between Eqs. ( 4 , 5 ), the ACE method iterates until eq. ( 3 ) is minimized. The transformations of n i *(x i ) (i = 1,2,…,k) and f * (y) that achieve minimization are the optimal transformations.
Nonlinear Cointegration
As argued in studies like Granger ( 1991 ), Granger and Hallman ( 1991 ), Meese and Rose ( 1991 ), Kanas ( 2003 , 2005 ) and Tang and Zhou ( 2013 ), nonlinear cointegration can be characterized from the linear cointegration of the ACE transformed variables. There are two steps involved in this procedure. First, as highlighted by Granger and Hallman ( 1991 ), in converting all original variables into ACE transformed variables, we have to ensure that the transformed variables do not deviate from the time-series properties of the original variables. Second, the usual Johansen ( 1991 ) type cointegration test is implemented for the transformed variables. This nonlinear cointegration can also be used in a rolling framework to examine time-varying nonlinearity in the relationship. If the transformed variables deviate from the time series properties of the original variables for the entire sample period or any subsample period in a rolling window, we cannot implement the usual cointegration test to capture the time varying aspect of the relationship. In that case, we propose using the continuous wavelet transform methodology to understand the relationship at different frequencies over time.
Continuous wavelet transform
CWT is a better alternative model for understanding the time-varying and nonlinearity of the relationships between stock prices and other macroeconomic variables. Wavelet coherency is used to determine the coherency between two variables for different frequencies over time, and partial wavelet coherency is used to determine the coherency between two variables conditional upon other variables for different frequencies over time. Following Conraria and Soares ( 2011 ), the methodology of deriving the CWT is as follows. The set of square integrables and the space of finite energy functions is denoted by L 2 (R) The minimum criteria to impose on a function ψ (t) ∈ L 2 (R) The wavelets begin with a mother (admissible or analyzing) wavelet, which consists of a technical portion of the admissibility condition.
Cψ denotes the constant of the admissibility or analyzing constant.
The purpose of the wavelet is to provide the time frequency of localization; the wavelet localized function gives both the time and frequency domains.
ψ denotes wiggles up and down in the time axis; the CWT starts with a mother wavelet ψ, and a family ψ τ,s of “wavelet daughters” can be obtained by simply scaling and translating ψ:
where s denotes a scaling or dilation factor that controls the width of the wavelet and τ denotes the translation parameter, which controls the location of the wavelet. Wavelet scaling indicates stretching if (| s | > 1) or (| s | < 1), whereas translating it means shifting its position in time. Given a time series, x (t) ∈ L 2 (R), the CWT of wavelet ψ is a function of two variables, W x:ψ (τ, s).
The time domain wavelet is given by τ , while the frequency domain is given by s . The wavelet transforms the time and frequency domains by mapping the original series into a function of τ and s ; the wavelet provides concurrent information on the time and frequency domains. The procedures of wavelet and Fourier transformations are somewhat similar. However, the Fourier transformation differs from the wavelet in that it has no time localization parameter. Moreover, we have two functions, cosine and sine, instead of a wavelet function. CWT may also be represented in the frequency as
To develop wavelet coherency, we need two more derivations: the cross-wavelet transform (XWT) and cross wavelet power (XWP). The cross wavelet transform of two times series, x(t) and y(t), was introduced by Hudgins et al. ( 1993 ) and defined as
where W x and W y represent the wavelet transforms of x and y, respectively. The cross-wavelet power provides a quantified indication of the similarity of power between two time series at each time and frequency and is defined as
Complex wavelet coherency
The set of two time series x(t) and y(t) describes their complex wavelet coherency:
The Wavelet Coherency is denoted as follows
W x and W y are the wavelet transforms of x and y, respectively, S denotes a smoothing operator in both time and scale; without smoothing, coherency would be identical across all scales and times. The partial wavelet coherency of x 1 and x j (2 ≤ j ≤ p) are denoted as follows:
r 1 jqj is measured as the absolute value
The Squared partial wavelet coherency of x i , j
Where φ denote the p x p matrix of all the smoothed cross-wavelet spectra S ij .
Wavelet coherency is the relationship to the product of the spectrum of each series, which can be the local correlation between the time and frequency domains in the two time series. This concept definition nearly replicates the traditional correlation coefficient. Wavelet coherence provides localized correlation coefficients in time and frequency space. When no correlation measures zero coherencies between the two time series and wavelet coherency is equal to one, it shows a strong correlation between the time and frequency domains. The statistical significance of estimated wavelet coherency can be explained through Monte Carlo simulation methods. There are no positive and negative co-movements between the time series for wavelet coherency. The information on positive and negative co-movements can distinguish the lead-lag relationships between two time series through the phase of the wavelets.
Following Conaria and Soares ( 2011 ), we can define the complex partial wavelet coherency between x and y after controlling z as follows
C xy and R xy are complex wavelet coherency and wavelet coherency, respectively, as defined in Eqs. ( 12 , 13 ). The partial wavelet coherency between x and y given z can be defined by taking the absolute value of the denominator in eq. ( 13 ). We may write the expression for partial wavelet coherency in terms of wavelet coherency as
The empirical analysis is conducted using monthly data from April 1994 to July 2018, a total of 292 observations. Monthly data of the Bombay Stock Exchange (BSE) Sensex Index, Index of Industrial Production (IIP), Wholesale Price Index (WPI), Broad Money (M3), and Exchange Rate are used. The choice of the sample is determined by the fact that a consistent new series of data is available for the sample period; it also reflects the post-liberalization period. The IIP is used as a proxy for output, while the WPI is used as a measure of inflation, and Rupees per U.S. dollar is used as a measure of the exchange rate. The data are collected from the following sources: the BSE Sensex Index is collected from their website ( www.bseindia.com ), and other macroeconomic variables are collected from the Handbook of Statistics on Indian Economy published by the Reserve Bank of India (RBI). All variables are seasonally adjusted, and subsequent analysis is performed on the natural logarithms Footnote 3 of these series.
Empirical results
To analyze the long-run relationships between the stock market and other key macroeconomic variables, it is necessary to perform the conventional Engle and Granger ( 1987 ) cointegration and Johansen ( 1991 ) tests. Before considering possible cointegration between these series, their orders of integration are examined using unit root tests. The standard ADF, Phillips Perron, and Kwaitkowski et al. (1992) (KPSS) unit root tests are used, and the results are presented in Table 1 . The test results show that all series are non-stationary at levels and stationary at first differences.
First, we used the conventional cointegration tests of Engle and Granger ( 1987 ) and Phillips and Ouliaris ( 1990 ) to examine the cointegration between stock prices and other macroeconomic variables. In these two tests, the null hypothesis is no cointegration, so if the calculated value of the EG and Pz statistics are significant, the null of no cointegration is rejected, indicating there is cointegration among the variables. For both these tests, the optimal lag length is determined by the Akaike information criterion, and the results are presented in Table 2 .
The results from Table 2 indicate both tests fail to reject the null of no cointegration between the stock market and the macroeconomic variables, showing there is no long-run relationship between these variables. To corroborate this, we used the Johansen ( 1991 ) cointegration test for the same set of variables and the results are presented in Table 3 .
The results indicate that the test statistics of trace and maximum Eigen statistics are both above the critical values at the 5% significance level. Hence, we reject the null of no cointegration relationship between these variables, which means a cointegrating relationship exists between the stock market and macroeconomic variables. The Engle and Granger ( 1987 ) cointegration and Phillips and Ouliaris ( 1990 ) tests fail to reject the null of no cointegration relationship between the stock market and other macroeconomic variables; however, the Johansen ( 1991 ) cointegration test shows the presence of a long-run relationship between these variables. Inferences drawn from conventional and Johansen ( 1991 ) cointegration tests depict a contrasting result for the relationship between the stock market and other macroeconomic variables. Gonzalo and Lee ( 1998 ) established the pitfalls in Johansen type cointegration tests and highlighted that validation by the Engle-Granger cointegration test is needed to avoid the drawbacks. Since the Engle and Granger cointegration test does not validate the Johansen test results, such contrary evidence leads to further investigation of these relationships.
The scatter plots
To understand these contradictory results from the two sets of cointegration tests, we need to understand the exact relationship between stock prices and each of these macroeconomic variables. A simple scatter plot of stock prices with each of these variables is depicted in Figs. 1 , 2 , 3 , 4 .
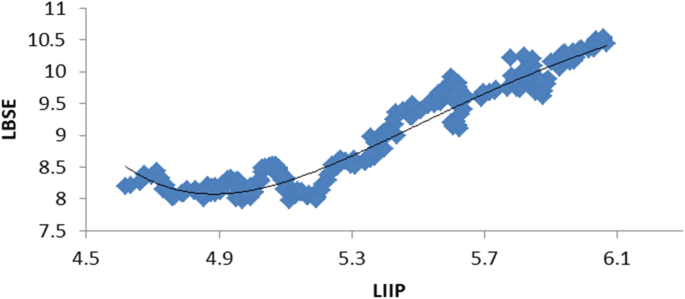
Scatter Plot of LBSE and LIIP
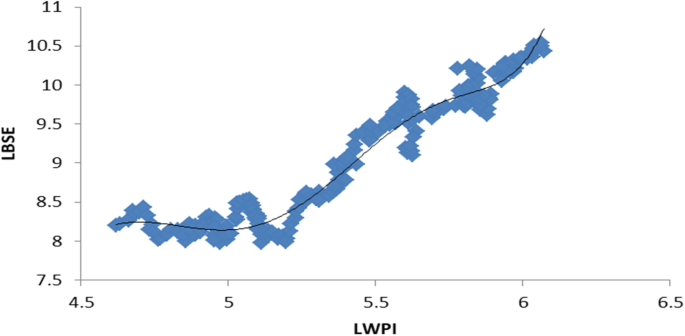
Scatter Plot of LBSE and LWPI
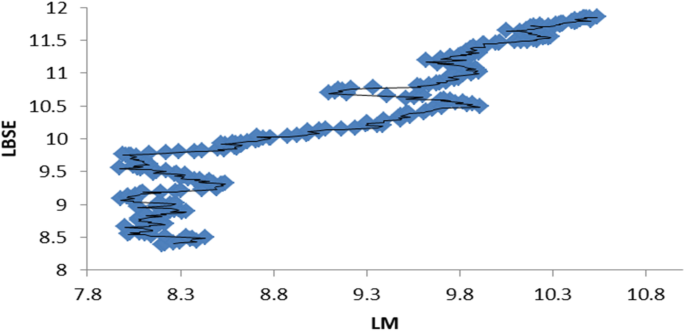
Scatter Plot of LBSE and LM
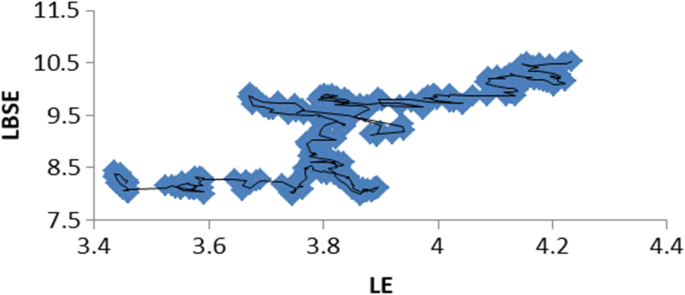
Scatter Plot of LBSE and LE
In all scatter plots, the vertical axis measures stock prices (LBSE) and all corresponding horizontal axes measure other macroeconomic variables (LIIP, LWPI, LM, and LE). These scatter plots show the nonlinear relationships between the stock market and other macroeconomic variables; moreover, the figures also show the trend lines fitted to the data. The fitted trend line for the LBSE and LIIP stands as a polynomial of order 6, whereas the trend line for LBSE and LWPI is a polynomial of order 5. For the remaining two variables, LM and LE, the trend line exhibits two and three period moving averages, respectively. Evidence from these scatter plots shows that the relationships between the stock market and other macroeconomic variables are nonlinear. This evidence restricts any linear analysis between stock prices and other macroeconomic variables in India.
To capture the nonlinearity, we employed ACE, Breiman and Friedman’s ( 1985 ) algorithm, to convert the original variables into transformed variables Footnote 4 and test their long-run cointegration relationship. As explained, the ACE transformed variables should possess the same time series properties as the original variables, so before performing cointegration analysis, this warrants ensuring that all the transformed series retain their respective time series properties. Standard ADF, Phillips Perron, and KPSS unit root tests are used for the transformed variables to examine their stationarity. We also performed the Zivot-Andrews unit-root test Footnote 5 on the transformed series and found evidence in favor of structural breaks among the variables, which supports time variation in the relationships.
The test results in Table 4 show that all series are non-stationary at levels and stationary at first differences. Since these transformed variables possess the same properties as their original counterparts, we can now use both the conventional [Engle and Granger 1987 ; Phillips and Ouliaris 1990 , and Johansen 1991 ] cointegration tests to examine the nonlinear cointegration. The results of these tests are presented in Tables 5 and 6 .
The results from both conventional tests reject the null of no cointegration between stock prices and other macroeconomic variables, and accept that there is a long-run relationship between the transformed variables at conventional levels of significance. The Johansen ( 1991 ) test results also reject the null of no cointegration relationship between these transformed variables, which indicates a cointegrating relationship exists between the stock market and macroeconomic variables. Altogether, this empirical evidence suggests that long-run cointegration exists between the ACE transformed variables, which leads to the inference that there is a nonlinear cointegration between stock prices and other macroeconomic variables for the full sample period. The key implication from this evidence is that when there is a nonlinear relationship, a linear combination of the variables may lead to deceptive inferences.
Further, regarding the possible sensitivity of the results to sample selection and parameter instability in the relationship between stock prices and other macroeconomic variables as highlighted by McMillan ( 2005 ), this study also examines the time variation in the long-run relationship between these variables using a rolling cointegration technique. Before considering the rolling cointegration test, we verify the time series properties of these variables in each subsample. In particular, the variables involved should be non-stationary in every subsample (rolling window); otherwise, the inferences might be spurious because the test statistics will be biased. Thus, we first employ rolling ADF unit root tests to check for stationarity for all series under consideration. The rolling window size is fixed at 60 observations (5 years); each time, the window is renewed by adding one observation to the end and removing the beginning observation. Thus, the first rolling window will have a sample of the first 1 to 60 observations, the second will include 2 to 61, then 3 to 62 and so on through the last observation. The test results are presented in Figs. 5 and 6 .
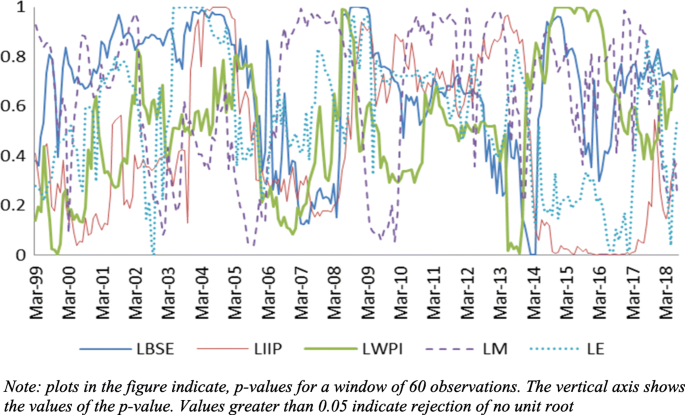
Rolling Unit Root Test for original variables
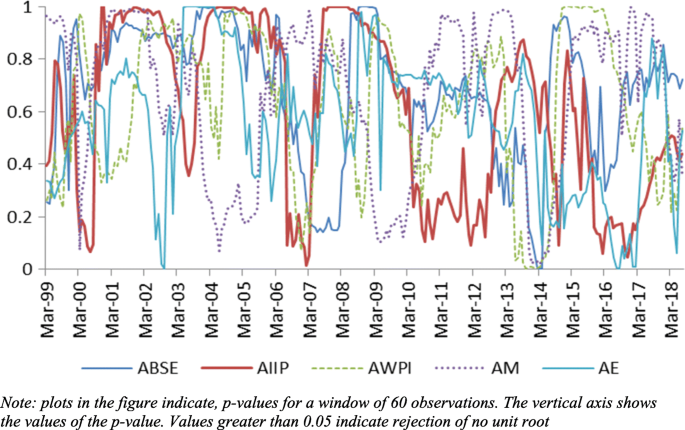
Rolling Unit Root Test for ACE Transformed Variables
Figure 5 clearly shows that for all the original variables (LBSE, LIIP, LWPI, LM, and LE), the null hypothesis of non-stationarity can be rejected in some sample windows, indicating that the variables change their time series properties. Similarly, Fig. 6 shows the same results for all the transformed variables Footnote 6 (ABSE, AIIP, AWPI, AM, and AE); in some windows variables are nonstationary, while in other windows, they are stationary at the levels. Hence, we cannot implement a rolling cointegration test to identify the time-varying relationship.
To circumvent this problem, following Conraria and Soares ( 2011 ), we use CWT for both the actual and transformed variables to understand the time variation in this relationship. In this study, we concentrate only on partial wavelet coherency to determine the coherency between two variables conditional upon other variables for different frequencies over time. The main objective is to understand the relationship between stock prices and output, retaining all other variables as control variables.
In CWT, the frequencies are split into low and high frequency, with low frequency measuring the long-run coherency between the variables and the high frequency measuring the short-run coherency. Coherency is differentiated using color codes ranging from blue to red; high coherency is indicated by the red color and the level of significance is identified with black and grey borders. Figure 7 provides the wavelet partial coherency for actual data on stock prices and output after controlling for other macroeconomic variables like inflation, money supply, and the exchange rate. The figure indicates that without transformation, there is no significant partial coherency in the low frequency, which measures the long-run partial correlation between the variables. At the high-frequency level, for some points between 2002 and 2005, there is statistically significant evidence for short-run partial coherency.
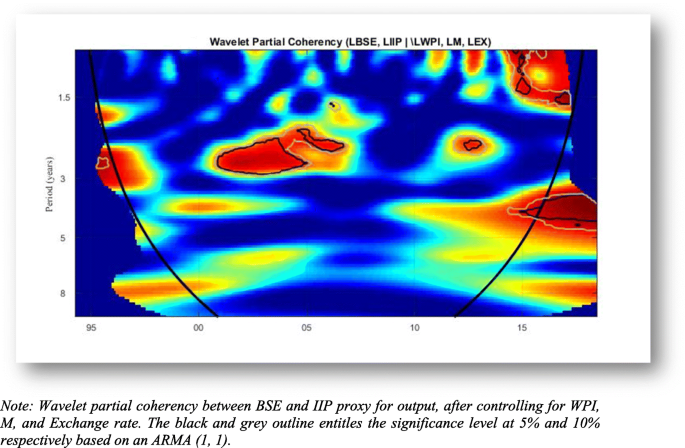
Wavelet Partial Coherency for Actual Variables
In contrast to the actual variables, Fig. 8 depicts the partial wavelet coherency for the ACE transformed variables. Like the actual data, we have analyzed the transformed stock prices and output after controlling for other transformed macroeconomic variables like inflation, money supply, and the exchange rate. The figure clearly shows a strong and significant partial coherency at a high frequency, which indicates the long-run relationship over time. The interesting inference from this analysis is that unless we identify the exact functional form of the variables, it is highly misleading to derive any plausible conclusions about their relationships. The relationships between the stock market and macroeconomic variables are nonlinearly related; if we linearly analyze and interpret them, it may result in a biased conclusion.
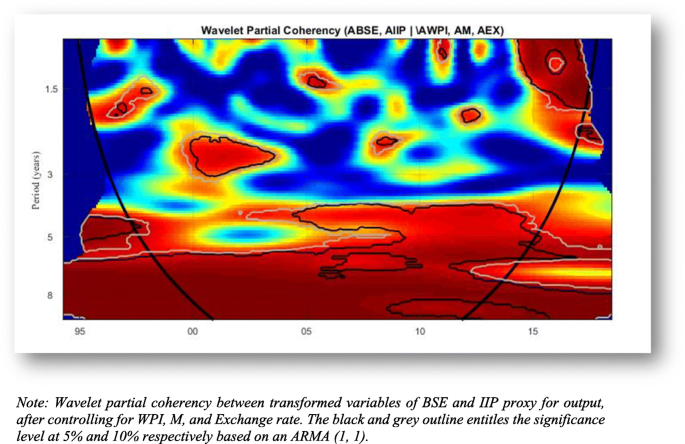
Wavelet Partial Coherency for Transformed Variables
This study aimed at understanding the time-varying, nonlinear relationships between stock prices and other key macroeconomic variables using monthly data from India for the period of April 1994 to July 2018. After checking the time series properties of the variables, different cointegration tests are implemented to understand their long-run relationships. The conventional Engle and Granger ( 1987 ) and Phillips and Ouliaris ( 1990 ) tests show that there is no relationship between stock prices and other macroeconomic variables. However, the Johansen cointegration test shows that long-run relationships exist between these variables. These two contrasting results warranted further analysis. To investigate this issue, we plot simple scatter plots that indicate these variables are not linearly related, but exhibit nonlinearity.
To address the nonlinear relationships between stock prices and other macroeconomic variables, we used the ACE algorithm of Breiman and Friedman ( 1985 ). ACE algorithms identify and extract the function of these relationships by converting the original variables into ACE transformed series, which provides the functional relationships between the variables. As highlighted by Granger and Hallman ( 1991 ), nonlinear cointegration can be characterized by implementing a cointegration test for these ACE transformed variables. To do this, we must ensure that the transformed variables do not deviate from the time series properties of the original variables. All transformed series are integrated of order one at levels for the full sample period. The conventional Engle-Granger, Phillips-Ouliaris, and Johansen cointegration tests show that there is long-run cointegration between the ACE transformed variables, which indicates that a nonlinear cointegration exists between stock prices and other key macroeconomic variables for India.
To test the time-varying aspect of this relationship, we first resort to a rolling cointegration test, but the rolling unit root test for both the actual data and transformed data show changes in the behavior of time series properties in some subsample periods (rolling windows). Hence, we cannot proceed to analyze the cointegration test to identify the relationships between stock prices and other macroeconomic variables. To understand the time variation, we used the CWT method for both actual and transformed variables, and the results identify wavelet partial coherency only for the transformed variables, not for the actual variables. The inferences drawn from the CWT highlight that stock prices and other macroeconomic variables are related nonlinearly in India.
The evidence presented in this study has far-reaching implications for policymakers, researchers, and investors. Understanding the effects of macroeconomic variables on the stock market is imperative; proper comprehension of the risk and opportunities associated with macroeconomic variables may help investors make appropriate decisions based on policy reactions and help them manage the systematic risk associated with the stock market. The evidence of nonlinearity indicates the presence in the market of noise traders, arbitrage traders, and speculators and should be supported with further empirical evidence. Hence, the findings show that understanding the nonlinear relationships is an integral part of identifying the causes of different fluctuations in economic activity. As such, this study alerts financial professionals and investors to consider nonlinear empirical consistencies when modeling the stock market and macroeconomic variables.
We used different window size for testing rolling unit root and cointegration.
All the ACE transformations is derived using the package of “ace pack” in R
Variables are named LBSE (log of BSE), LIIP (log of IIP), LWPI (log of WPI), LM (log of M3) and LE (log of Exchange rate)
Once variables are ACE Transformed then named as ABSE, AIIP, AWPI, AM and AE.
Results are available upon request.
For each rolling window the ACE transformation is derived separately.
Abbreviations
Alternating Conditional Expectation transformed Bombay Stock Exchange Sensex Index
Alternating Conditional Expectation
Augmented Dickey-Fuller
Alternating Conditional Expectation transformed Exchange rate
Alternating Conditional Expectation transformed Index of Industrial Production
Alternating Conditional Expectation transformed Broad Money
Arbitrage pricing theory
Alternating Conditional Expectation transformed Whole Sale Price Index
Bombay Stock Exchange Sensex Index
- Continuous wavelet transformation
Index of Industrial Production
Kwiatkowski, Phillip, Schmidt and Shin
Log of Bombay Stock Exchange Sensex Index
Log of Exchange rate
Log of Index of Industrial Production
Log of Broad Money
log of Whole Sale Price Index
Broad Money
Reserve Bank of India
Whole Sale Price Index
Bekhet AH, Matar A (2013) Co-integration and causality analysis between stock market prices and their determinates in Jordan. Econ Model 35:508–514
Article Google Scholar
Binswanger M (2004) How do stock prices respond to fundamental shocks? Financ Res Lett 1:90–99
Boucher C (2007) Asymmetric adjustment of stock prices to their fundamental value and the predictability of US stock returns. Econ Lett 95:339–347
Bradley M, Jansen D (1997) Nonlinear business cycle dynamics: cross-country evidence on the persistence of aggregate shocks. Econ Inq 35:495–509
Breiman L, Friedman JH (1985) Estimating transformations for multiple regression and correlation. J Am Stat Assoc 80:580–598
Brock W, LeBaron B (1996) A dynamic structural model for stock return volatility and trading volume. Rev Econ Stat 78(1):94–110
Chen NF, Roll R, Ross S (1986) Economic forces and the stock market. J Bus 59:383–403
Cheung WY, Ng LK (1998) International evidence on the stock market and aggregate economic activity, vol 5, pp 281–296
Google Scholar
Cochrane JH (1991) Production-bases asset pricing and the link between stock return and economic fluctuations. J Financ 46:209–238
Conraria LA, Soares MJ (2011) The continuous wavelet transform: a primer, Working Paper Series NIPE WP 16/ 2011
Dornbusch R, Fischer S (1980) Exchange rates and current account. Am Econ Rev 70:960–971
Dumas B (1992) Dynamic equilibrium and the real exchange rate in a spatially separated world. Rev Financ Stud 5(2):153–180
Durai SRS, Bhaduri S (2009) Stock prices, inflation and output: evidence from wavelet analysis. Econ Model 26:1089–1092
Engle R, Granger C (1987) Co-integration and error correction representation estimation and testing. Econometrica 55:251–267
Falk B (1986) Further evidence on the asymmetric behavior of economic time series over the business cycle. J Polit Econ 94:1096–1109
Fama EF (1981) Stock returns, real activity, inflation and money. Am Econ Rev 71:545–565
Fama EF (1990) Stock returns, expected returns, and real activity. J Financ 45:1089–1108
Geske R, Roll R (1983) The fiscal and monetary linkage between stock returns and inflation. J Financ 38:1–33
Gonzalo J, Lee T-H (1998) Pitfalls in testing for long run relationships. J Econ 86:129–154
Granger C (1988) Some recent development in a concept of causality. J Econ 39:199–211
Granger CWJ (1991) Some recent generalizations of cointegration and the analysis of long-run relationships, in Long-Run Economic Relationships Oxford University Press, pp 277–287
Granger CWJ, Hallman JJ (1991) Long-memory series with attractors. Oxf Bull Econ Stat 53:11–26
Hansen H, Johansen S (1999) Some tests for parameter constancy in the cointegrating VAR. Econ J 2:25–52
Hudgins L, Friehe CA, Mayer MM (1993) Wavelet Transforms and Atmospheric Turbulence. Phys Rev Lett 71(20):3279–3283
Humpe A, Macmillan P (2014) Non-linear predictability of Stock market returns: comparative evidence from Japan and the US. Invest Manag Financ Innov 11(SSRN):4
Inci AC, Lee BS (2014) Dynamic relations between stock returns and exchange rate changes. Eur Financ Manag 20(1):71–106
Johansen S (1991) Estimation and hypothesis testing of cointegration vectors in Gaussian vector autoregressive models. Econometrica 59:1551–1580
Johansen S, Mosconi R, Nielsen B (2000) Cointegration analysis in the presence of structural brakes in the deterministic trend. Econ J 3:216–249
Kanas A (2003) Non-linear Cointegration between Stock prices and dividends. Appl Econ Lett 10(7):401–405
Kanas A (2005) Nonlinearity in the stock price-dividend relation. J Int Money Financ 24:583–606
Kizys R, Pierdzioch C (2009) Changes in the international comovement of stock returns and asymmetric macroeconomic shocks. J Int Financ Mark Inst Money 19(2):289–305
Lawala AI, Somoye OR, Babajide AA, Nwanjia TI (2018) The effect of fiscal and monetary policies interaction on stock market performance: evidence from Nigeria. Future Bus J 4(1):16–33
Lee BS (1992) Causal relations among Stock returns, interest rates, real activity, and inflation. J Financ 47(4):1591–1603
MacKinnon JG (1996) Numerical distribution functions for unit root and cointegration tests. J Appl Econ 11(6):601–618
McMillan D (2005) Time variation in the cointegrating relationship between stock prices and economic activity. Int Rev Appl Econ 19:359–368
Meese RA, Rose AK (1991) An empirical assessment of nonlinearities in models of exchange rate determination. Rev Econ Stud 58:603–619
Mukherjee TK, Naka A (1995) Dynamic relations between macroeconomic variables and the Japanese stock market: an application of a vector error-correction model. J Financ Res 18:223–237
Nasseh A, Strauss J (2000) Stock prices and domestic and international macroeconomic activity: a cointegration approach. Q Rev Econ Finance 40:229–245
Phillips PCB, Ouliaris S (1990) Asymptotic properties of residual-based tests for cointegration. Econometrica 58:165–193
Ram R, Spencer DE (1983) Stock returns, real activity, inflation, and money: comment. Am Econ Rev 73:463–470
Ramsey JB, Rothman P (1996) Time irreversibility and business cycle asymmetry. J Money Credit Bank 28:1–21
Sarantis N (2001) Nonlinearities, cyclical behaviour and predictability in stock markets: international evidence. Int J Forecast 17(3):459–482
Schwert WG (1990) Stock return and real activity: a century of evidence. J Financ 4:1237–1257
Shleifer A, Robert WV (2003) Stock market driven acquisitions. J Financ Econ 70(3):295–311
Tang X, Zhou J (2013) Nonlinear relationship between the real exchange rate and economic fundamentals: evidence from China and Korea. J Int Money Financ 32:304–323
Tiwari AK, Dar AB, Bhanja N (2013) Oil price and exchange rates: a wavelet-based analysis for India. Econ Model 31:414–422
Wongbangpo P, Sharma S (2002) Stock market and macroeconomic fundamental dynamic interactions: ASEAN-5 countries. J Asian Econ 13(1):27–51
Zhou J (2010) Testing for Cointegration between house prices and economic fundamentals. Real Estate Econ 4:599–632
Download references
Acknowledgments
We thank the Editor of this Journal for all the help he extended and three anonymous referees for their useful comments and suggestions for the improvement of this paper.
The datasets used in the study were collected from BSE Sensex Index www.bseindia.com and Reverse Bank of India www.rbi.org.in
This study was not funded by any agencies, and we ensure that we have not received any grant to do this study.
Author information
Authors and affiliations.
School of Economics, Shri Mata Vaishno Devi University, Katra, Jammu & Kashmir, 182320, India
R. Gopinathan
School of Economics, University of Hyderabad, Hyderabad, Telangana, 500046, India
S. Raja Sethu Durai
You can also search for this author in PubMed Google Scholar
Contributions
R. Gopinathan is presently an Assistant Professor in the School of Economics at Shri Mata Vaishno Devi University, India. S. Raja Sethu Durai is presently an Associate Professor in the School of Economics at University of Hyderabad, India. Both authors read and approved the final manuscript.
Corresponding author
Correspondence to R. Gopinathan .
Ethics declarations
Ethics approval and consent to participate.
This article does not contain any studies with human participants or animals performed by any of the authors.
Consent for publication
Both authors are willing to permit Financial Innovation to publish our article.
Competing interests
The authors declare that they have no competing interests.
Additional information
Publisher’s note.
Springer Nature remains neutral with regard to jurisdictional claims in published maps and institutional affiliations.
Rights and permissions
Open Access This article is distributed under the terms of the Creative Commons Attribution 4.0 International License ( http://creativecommons.org/licenses/by/4.0/ ), which permits unrestricted use, distribution, and reproduction in any medium, provided you give appropriate credit to the original author(s) and the source, provide a link to the Creative Commons license, and indicate if changes were made.
Reprints and permissions
About this article
Cite this article.
Gopinathan, R., Durai, S.R.S. Stock market and macroeconomic variables: new evidence from India. Financ Innov 5 , 29 (2019). https://doi.org/10.1186/s40854-019-0145-1
Download citation
Received : 13 July 2018
Accepted : 17 June 2019
Published : 11 July 2019
DOI : https://doi.org/10.1186/s40854-019-0145-1
Share this article
Anyone you share the following link with will be able to read this content:
Sorry, a shareable link is not currently available for this article.
Provided by the Springer Nature SharedIt content-sharing initiative
- Stock prices
- Nonlinear cointegration
- Alternating conditional expectations
- Option Chain
- Daily Reports
- Press Releases
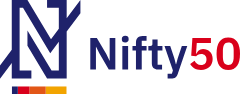
02-May-2024 10:20
03-May-2024 | 83.4275
02-May-2024 10:19
Lac Crs 403.04 | Tn $ 4.83
30-Apr-2024
- A+ | Reset | A-
- High Contrast | Reset
- Mutual Fund
You will be redirected to another link to complete the login

- View All Indices
- 22,670.55 65.70 (0.29%)
- Open 22,567.85
- High 22,680.35
- Low 22,567.85
- Advances - 31
- Declines - 19
- Unchanged - 0
TOP 5 STOCKS - NIFTY 50

Market Statistics
Stocks Traded
No. of Stocks at 52-Week High
No. of Stocks at 52-Week Low

No. of Stocks in Upper Circuit
No. of Stocks in Lower Circuit
- 65,631.90 731.40 (1.13%)
- Open 65,149.45
- High 65,689.15
- Low 65,090.80
- Advances - 35
- Declines - 15
TOP 5 STOCKS - NIFTY NEXT 50
- 11,100.10 40.35 (0.36%)
- Open 11,125.80
- High 11,153.75
- Low 11,090.65
- Advances - 15
- Declines - 9
- Unchanged - 1
TOP 5 STOCKS - NIFTY MIDCAP SELECT
- 49,416.55 19.80 (0.04%)
- Open 49,262.00
- High 49,529.35
- Low 49,221.45
- Advances - 7
- Declines - 4
TOP 5 STOCKS - NIFTY BANK
- 21,931.40 90.25 (0.41%)
- Open 21,785.45
- High 21,967.90
- Low 21,773.85
- Advances - 14
- Declines - 6
TOP 5 STOCKS - NIFTY FINANCIAL SERVICES
- with respect to Prev. Close
- with respect to adjusted price on ex-date for Dividend, Bonus, Rights & Face Value Split.
- Most Active Contracts are displayed in the descending order of Volume/Value
- In case of Option Contracts “Value” displayed is “Premium Turnover”
- Volume and Open Interest are displayed in “Contracts”
- % change is calculated with respect to “Prev. Close”
- For the Order Quotation for each Cross currency contract, refer to link
- Premium Turnover for Cross Currency pairs is calculated by using latest available Reference Rate - FBIL
Currency Spot Rates
As on 30-Apr-2024 13:30:00 IST
Interest Rates
As on May 02, 2024 10:20:36 IST
Commodity Spot * Rates
As on 30-APR-2024 IST

- Derivative to cash ratio =
- Turnover of Currency Futures and Currency options include turnover of both Non Cross Currency pairs and Cross Currency pairs.
- Currency Turnover is calculated by using latest available Reference rate-FBIL.
Complaints & Reporting
File a complaint.
(Against Stockbrokers and Listed Companies with respect to your Trades / Shareholding)
Investor Support
Toll Free : 1800 266 0050
Tel No : (022) 2659 8100 - 144
Report a tip or Suspicious activity
(Assured Returns, SMS Tips, Market Abuse, Connectivity issues, Services, etc.)
Call Us : 1800 266 0050
SMS : +91 829 183 3676
Email : [email protected]

NSE IFSC-SGX Connect Inaugurated by Hon’ble PM of India Shri Narendra Modi
Shri Ashishkumar Chauhan, Managing Director & CEO, NSE presented a memento to Hon'ble Prime Minister Narendra Modi at the launch of NSE IFSC-SGX Connect.
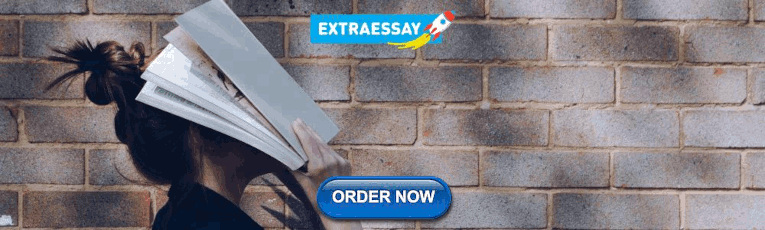
NSE Annual Highlights - 2023
Take a journey through our achievements and milestones. Here's to a year of growth, resilience, and excellence!
Don't fall for false promises of assured returns!
Any promise of assured/guaranteed returns in the securities market is illegal! Don't fall for unsolicited advice promising exorbitant returns.
Inspection & Compliance
April 30, 2024.
NSE/INSP/61851
NSE/COMP/61850
Surveillance & Investigation
NSE/SURV/61848
Securities Lending & Borrowing Scheme
NCL/CMPT/61847
Debt Segment
NCL/CC-SETLMNT/61846
NSE/CML/61845
NCL/CMPL/61844
Primary Market Segment
NSE/IPO/61843
NSE/INVG/61842
Futures & Options
NCL/CMPT/61841
NSE/INVG/61840
NSE/INVG/61839
Surveillance
Clarification by Eldeco Housing And Industries Limited Clarification by Lux Industries Limited Clarification by Lux Industries Limited Clarification by Star Cement Limited
29-Apr-2024
NSE Listing
Press Release dated April 29, 2024
Clarification by DCM Shriram Limited Clarification by Eldeco Housing And Industries Limited Clarification by Harsha Engineers International Limited Clarification by Kirloskar Industries Limited Clarification by Lux Industries Limited News about Orient Cement Limited Clarification by Viviana Power Tech Limited Clarification by WESTLIFE FOODWORLD LIMITED
NSE CLEARING
Market-wide Position Limit BIOCON
26-Apr-2024
Clarification by Arham Technologies Limited Clarification by Baweja Studios Limited Clarification by Bombay Metrics Supply Chain Limited Clarification by DCM Shriram Limited Clarification by Destiny Logistics & Infra Limited Clarification by Drone Destination Limited Clarification by Emami Realty Limited Clarification by Godfrey Phillips India Limited Clarification by Harsha Engineers International Limited Clarification by Hitachi Energy India Limited Clarification by Jyothy Labs Limited Clarification by Kirloskar Industries Limited Clarification by Konstelec Engineers Limited Clarification by Mic Electronics Limited News about Orient Cement Limited Clarification by Pashupati Cotspin Limited Clarification by Presstonic Engineering Limited Clarification by Pritika Engineering Components Limited Clarification by Swaraj Suiting Limited Clarification by TAC Infosec Limited Clarification by Vimta Labs Limited Clarification by WESTLIFE FOODWORLD LIMITED Clarification by Wealth First Portfolio Managers Limited Clarification by Zim Laboratories Limited

14-Mar-2024
Corporate Communications
National Stock Exchange (NSE) Celebrates First 5 Listings on NSE-SSE

11-Mar-2024
Joint Press Release on Caution to Investors

01-Mar-2024
NSE registered investor base crosses 9 crore (90 million) unique investors (unique PANs) and 16.9 crore (169 million) total accounts

29-Feb-2024
National Stock Exchange (NSE) Celebrates the Second Listing on Social Stock Exchange Segment by Ekalavya Foundation

28-Feb-2024
Replacements in indices w.e.f. March 28, 2024

Latest UPDATES
Select indices.
Choose upto 5 indices that you would like to view the market trend for.
Broad Market Indices
- NIFTY NEXT 50
- NIFTY MIDCAP 50
- NIFTY MIDCAP 100
- NIFTY MIDCAP 150
- NIFTY SMALLCAP 50
- NIFTY SMALLCAP 100
- NIFTY SMALLCAP 250
- NIFTY MIDSMALLCAP 400
- NIFTY500 MULTICAP 50:25:25
- NIFTY LARGEMIDCAP 250
- NIFTY MIDCAP SELECT
- NIFTY TOTAL MARKET
- NIFTY MICROCAP 250
Sectoral Indices
- NIFTY ENERGY
- NIFTY FINANCIAL SERVICES
- NIFTY FINANCIAL SERVICES 25/50
- NIFTY MEDIA
- NIFTY METAL
- NIFTY PHARMA
- NIFTY PSU BANK
- NIFTY REALTY
- NIFTY PRIVATE BANK
- NIFTY HEALTHCARE INDEX
- NIFTY CONSUMER DURABLES
- NIFTY OIL & GAS
- NIFTY MIDSMALL HEALTHCARE
Strategy Indices
- NIFTY DIVIDEND OPPORTUNITIES 50
- NIFTY50 VALUE 20
- NIFTY100 QUALITY 30
- NIFTY50 EQUAL WEIGHT
- NIFTY100 EQUAL WEIGHT
- NIFTY100 LOW VOLATILITY 30
- NIFTY ALPHA 50
- NIFTY200 QUALITY 30
- NIFTY ALPHA LOW-VOLATILITY 30
- NIFTY200 MOMENTUM 30
- NIFTY MIDCAP150 QUALITY 50
- NIFTY200 ALPHA 30
- NIFTY MIDCAP150 MOMENTUM 50
Thematic Indices
- NIFTY COMMODITIES
- NIFTY INDIA CONSUMPTION
- NIFTY INFRASTRUCTURE
- NIFTY GROWTH SECTORS 15
- NIFTY SERVICES SECTOR
- NIFTY100 LIQUID 15
- NIFTY MIDCAP LIQUID 15
- NIFTY INDIA DIGITAL
- NIFTY100 ESG
- NIFTY INDIA MANUFACTURING
- NIFTY INDIA CORPORATE GROUP INDEX - TATA GROUP 25% CAP
- NIFTY500 MULTICAP INDIA MANUFACTURING 50:30:20
- NIFTY500 MULTICAP INFRASTRUCTURE 50:30:20
Fixed Income Indices
- Securities in F&O
- Permitted to Trade
Quick Links
Quick links, for investors, for corporates, for members, old website, quick links for investors.
Dashboard for end of day reports download, quick market snapshot and important announcements.
Market Snapshot
Volume (Lakhs)
Value (Lakhs)
FFM .Cap (Lakhs)
Quick Links for Corporates
Dashboard for tracking corporate filings
Latest Corporate Filings
Showing 0 of 5 selected companies, latest circulars, quick links for members, members message area.
Exchange has published Member Help Guide and new FAQs for Access to Markets. Visit the link: https://www.nseindia.com/trade/all-member-faqs
Contact for Support
Analysis and prediction of Indian stock market: a machine-learning approach
- CASE STUDIES
- Published: 01 July 2023
- Volume 14 , pages 1567–1585, ( 2023 )
Cite this article
- Shilpa Srivastava ORCID: orcid.org/0000-0002-8566-1646 1 ,
- Millie Pant 2 &
- Varuna Gupta 1
537 Accesses
2 Citations
1 Altmetric
Explore all metrics
Prediction of financial stock market is a challenging task because of its volatile and non- linear nature. The presence of different factors like psychological, sentimental state, rational or irrational behaviour of investors make the stock market more dynamic. With the inculcation of algorithms based on artificial intelligence, deep learning algorithms, the prediction of movement of financial stock market is revolutionized in the recent years. The purpose of using these algorithms is to help the investors for taking decisions related to the Stock Pricing. A model has been proposed to predict the direction of movement of Indian stock market in the near future. This model makes use of historical Indian stock data of companies in nifty 50 since they came existence along with some financial and social indicators like financial news and tweets related to stocks. After pre-processing and normalization various machine learning algorithms like LSTM, support vector machines, KNearest neighbour, random forest, gradient boosting regressor are applied on this time series data to produce better accuracy and to minimize the RMSE error. This model has the ability to reduce major losses to the investors who invest in stock market. The social indicators will give an insight for predicting the direction of stock market. The LSTM network will make use of historical closing prices, tweets and trading volume.
This is a preview of subscription content, log in via an institution to check access.
Access this article
Price includes VAT (Russian Federation)
Instant access to the full article PDF.
Rent this article via DeepDyve
Institutional subscriptions
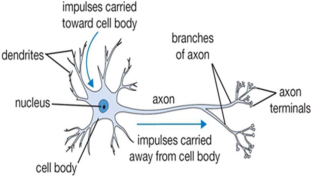
Similar content being viewed by others
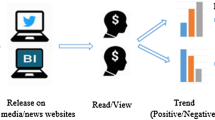
Stock market prediction using machine learning classifiers and social media, news
Financial Forecasting of Stock Market Using Sentiment Analysis and Data Analytics
A Comparative Study for Analysis and Prediction of Stock Market Movement
Abbasimehr H, Paki R (2022) Improving time series forecasting using LSTM and attention models. J Ambient Intell Human Comput 13:673–691. https://doi.org/10.1007/s12652-020-02761-x
Article Google Scholar
Alghieth M, Yang Y, Chiclana F (2016) Development of a genetic programming-based GA methodology for the prediction of short-to-medium-term stock markets. IEEE Congress on evolutionary computation (CEC) , Vancouver, BC. pp 2381–2388. https://doi.org/10.1109/CEC.2016.7744083
Altinbas H, Biskin OT (2015) Selecting macroeconomic influencers on stock markets by using feature selection algorithms. Procedia Econ Financ 30:22–29
Atkins A, Niranjan M, Gerding E (2018) Financial news predicts stock market volatility better than close price. J Financ Data Sci 4(2):120–137
Barak S, Arjmand A, Ortobelli S (2017) Fusion of multiple diverse predictors in stock market. Inf Fusion 36:90–102
Chatzis SP, Siakoulis V, Petropoulos A, Stavroulakis E, Vlachogiannakis N (2018) Forecasting stock market crisis events using deep and statistical machine learning techniques. Expert Syst Appl 112:353–371
Chauhan P, Sharma N, Sikka G (2021) The emergence of social media data and sentiment analysis in election prediction. J Ambient Intell Human Comput 12:2601–2627. https://doi.org/10.1007/s12652-020-02423-y
Chen Y, Hao Y (2017) A feature weighted support vector machine and K-nearest neighbor algorithm for stock market indices prediction. Expert Syst Appl 80:340–355
Chen W, Zhang H, Mehlawat MK, Jia L (2021) Mean–variance portfolio optimization using machine learning-based stock price prediction. Appl Soft Computi 100:106943
Chong E, Han C, Park FC (2017) Deep learning networks for stock market analysis and prediction: methodology, data representations, and case studies. Expert Syst Appli 83:187–205
de Araújo RA, Oliveira ALI, Meira S (2015) A hybrid model for high-frequency stock market forecasting. Expert Syst Appli 42(8):4081–4096
Devi KN, Bhaskaran VM, Kumar GP (2015) Cuckoo optimized SVM for stock market prediction. In: International conference on innovations in information, embedded and communication systems (ICIIECS), Coimbatore. pp 1–5
Ding S, Cui T, Xiong X et al (2020) Forecasting stock market return with nonlinearity: a genetic programming approach. J Ambient Intell Human Comput 11:4927–4939. https://doi.org/10.1007/s12652-020-01762-0
Ding X, Zhang Y, Liu T, Duan J (2015) Deep learning for event-driven stock prediction. In: Proceedings of the twenty-fourth international joint conference on artificial intelligence (IJCAI 2015). pp 2327–2333
Gao G, Bu Z, Liu L, Cao J, Wu Z (2015) A survival analysis method for stock market prediction. In: International conference on behavioral, economic and socio-cultural computing (BESC), Nanjing. pp 116–122
Ghanavati M, Wong RK, Chen F, Wang Y, Fong S (2016) A generic service framework for stock market prediction. In: 2016 IEEE international conference on services computing (SCC), San Francisco, CA. pp 283–290. https://doi.org/10.1109/SCC.2016.44
Golmaryami M, Behzadi M, Ahmadzadeh M (2015) A hybrid method based on neural networks and a meta-heuristic bat algorithm for stock price prediction. In: 2nd International conference on knowledge-based engineering and innovation (KBEI), Tehran. pp 269–275
Goykhman M, Teimouri A (2018) Machine learning in sentiment reconstruction of the simulated stock market. Phys A Stat Mech Appl 492:1729–1740
Gunduz H, Cataltepe Z, Yaslan Y (2017) Stock market direction prediction using deep neural networks. In: 25th Signal processing and communications applications conference (SIU), Antalya. pp 1–4
Gupta A, Dhingra B (2012) Stock market prediction using hidden Markov models. In: Students conference on engineering and systems, Allahabad, Uttar Pradesh. pp 1–4
Henrique BM, Sobreiro VA, Kimura H (2018) Stock price prediction using support vector regression on daily and up to the minute prices. J Financ Data Scie 4(3):183–201
https://towardsdatascience.com/how-not-to-predict-stock-prices-with-lstms-a51f564ccbca
https://towardsdatascience.com/sentiment-analysis-for-stock-price-prediction-in-python-bed40c65d178
Ismail MS, SalmiM MN, Ismail M, Razak FA, Alias MA (2020) Predicting next day direction of stock price movement using machine learning methods with persistent homology: evidence from Kuala Lumpur stock exchange. Appl Soft Computi 93:106422
Izzah A, Sari YA, Widyastuti R, Cinderatama TA (2017) Mobile app for stock prediction using improved multiple linear regression. In: International conference on sustainable information engineering and technology (SIET), Malang. pp 150–154
HS Karthik, VA Nishanth, J Manikandan (2016) Stock market prediction using optimum threshold based relevance vector machines. In: 22nd Annual international conference on advanced computing and communication (ADCOM), Bangalore. pp 21–26. https://doi.org/10.1109/ADCOM.2016.13
Khang PQ, Kaczmarczyk K, Tutak P, Golec P, Kuziak K, Depczyński R, Hernes M, Rot A (2021) Machine learning for liquidity prediction on Vietnamese stock market. Procedia Comput Sci 192:3590–3597. https://doi.org/10.1016/j.procs.2021.09.132
Kraus M, Feuerriegel S (2017) Decision support from financial disclosures with deep neural networks and transfer learning. Decis Supp Syst 104:38–48. https://doi.org/10.1016/j.dss.2017.10.001
Kumar MR, Venkatesh J, Rahman AMJMZ (2021) Data mining and machine learning in retail business: developing efficiencies for better customer retention. J Ambient Intell Human Comput. https://doi.org/10.1007/s12652-020-02711-7
Labiad B, Berrado A, Benabbou L (2016) Machine learning techniques for short term stock movements classification for Moroccan stock exchange. In: 11th International conference on intelligent systems: theories and applications (SITA), Mohammedia. pp 1–6. https://doi.org/10.1109/SITA.2016.7772259
Lee TK, Cho JH, Kwon DS, Sohn SY (2019) Global stock market investment strategies based on financial network indicators using machine learning techniques. Expert Syst Appli 117:228–242
Lee HC, Lee YH, Lu YC, Wang YC (2020) States of psychological anchors and price behavior of Japanese yen futures. N Am J Econ Financ. https://doi.org/10.1016/j.najef.2018.10.016
Leippold M, Wang Q, Zhou W (2021) Machine learning in the Chinese stock market. J Financ Econ. https://doi.org/10.1016/j.jfineco.2021.08.017
Li A, Wu J, Liu Z (2018) Market manipulation detection based on classification methods. Procedia Comput Sci 122:788–795
Li Y, Wang F, Sun R, Li R (2016a) A novel model for stock market forecasting. In: 9th International congress on image and signal processing, biomedical engineering and informatics (CISP-BMEI), Datong. pp 1995–1999. https://doi.org/10.1109/CISP-BMEI.2016.7853046
Li Q, Zhou B, Liu Q (2016b) Can twitter posts predict stock behavior?: A study of stock market with twitter social emotion. In: 2016b IEEE international conference on cloud computing and big data analysis (ICCCBDA), Chengdu. pp 359–364. https://doi.org/10.1109/ICCCBDA.2016.7529584
Liu Q, Wang C, Zhang P, Zheng K (2021) Detecting stock market manipulation via machine learning: Evidence from China Securities Regulatory Commission punishment cases. Int Revi Financ Anal 78. https://doi.org/10.1016/j.irfa.2021.101887 .
Luo B, Chen Y, Jiang W (2016) Stock market forecasting algorithm based on improved neural network. In: Eighth international conference on measuring technology and mechatronics automation (ICMTMA), Macau. pp 628–631
Maji G, Mondal D, Dey N et al (2021) Stock prediction and mutual fund portfolio management using curve fitting techniques. J Ambient Intell Human Comput 12:9521–9534. https://doi.org/10.1007/s12652-020-02693-6
Malagrino LS, Roman NT, Monteiro AM (2018) Forecasting stock market index daily direction: a Bayesian network approach. Expert Syst Appl 105:11–22
Mankar T, Hotchandani T, Madhwani M, Chidrawar A, Lifna CS (2018) Stock market prediction based on social sentiments using machine learning. In: International conference on smart city and emerging technology (ICSCET), Mumbai. pp 1–3
Mithani F, Machchhar S, Jasdanwala F (2016) A modified BPN approach for stock market prediction.In: IEEE International Conference on Computational Intelligence and Computing Research (ICCIC), Chennai. pp 1–4. https://doi.org/10.1109/ICCIC.2016.7919718
Murali P, Revathy R, Balamurali S et al (2020) Integration of RNN with GARCH refined by whale optimization algorithm for yield forecasting: a hybrid machine learning approach. J Ambient Intell Human Comput. https://doi.org/10.1007/s12652-020-01922-2
Nayak RK, Mishra D, Rath AK (2015) A naïve SVM-KNN based stock market trend reversal analysis for Indian benchmark indices. Appl Soft Comput 35:670–680
Nayak A, Pai MMM, Pai RM (2016) Prediction models for Indian stock market. Procedia Comput Sci 89:441–449
Nivetha RY, Dhaya C (2017) Developing a prediction model for stock analysis. In: International conference on technical advancements in computers and communications (ICTACC), Melmaurvathur. pp 1–3
Olaniyan R, Stamate D, Ouarbya L, Logofatu D (2015) Sentiment and stock market volatility predictive modelling—a hybrid approach. In: IEEE international conference on data science and advanced analytics (DSAA), Paris. pp 1–10
Oliveira N, Cortez P, Areal N (2016) Stock market sentiment lexicon acquisition using microblogging data and statistical measures. Decis Supp Syst 85:62–73
Oliveira N, Cortez P, Areal N (2017) The impact of microblogging data for stock market prediction: using Twitter to predict returns, volatility, trading volume and survey sentiment indices. Expert Syst Appl 73:125–144
Paniagua DC, Cubillos C, Vicari R, Urra E (2015) Decision-making system for stock exchange market using artificial emotions. Expert Syst Appli 42(20):7070–7083. https://doi.org/10.1016/j.eswa.2015.05.004
Patel J, Shah S, Thakkar P, Kotecha K (2015) Predicting stock market index using fusion of machine learning techniques. Expert Syst Appl 42(4):2162–2172
Patel HR, Parikh SM, Darji DN (2016) Prediction model for stock market using news based different classification, regression and statistical techniques: (PMSMN). In: International conference on ICT in business industry and government (ICTBIG), Indore. pp 1–5. https://doi.org/10.1109/ICTBIG.2016.7892636 .
Peng D (2019) Analysis of investor sentiment and stock market volatility trend based on big data strategy. In: International conference on robots and intelligent system (ICRIS), Haikou, China. pp 269–272
Qasem M, Thulasiram R, Thulasiram P (2015) Twitter sentiment classification using machine learning techniques for stock markets. In: International conference on advances in computing, communications and informatics (ICACCI), Kochi. pp 834–840
Rajput VS, Dubey SM (2016) Stock market sentiment analysis based on machine learning.In: 2nd International conference on next generation computing technologies (NGCT), Dehradun. pp 506–510. https://doi.org/10.1109/NGCT.2016.7877468
Rao Y, Zhong X, Lu S (2016) Social network-based stock correlation analysis and prediction. In: 2016 International conference on identification, information and knowledge in the internet of things (IIKI), Beijing. pp 573–576. https://doi.org/10.1109/IIKI.2016.102
Renault T (2017) Intraday online investor sentiment and return patterns in the U.S. stock market. J Bank Financ 84:25–40
Shah D, Isah H, Zulkernine F (2018) Predicting the effects of news sentiments on the stock market. In: IEEE international conference on big data (big data), Seattle, WA, USA. pp 4705–4708
Sharma C, Banerjee K (2015) A study of correlations in the stock market. Phys A Stat Mech Appl 432:321–330
Sharma A, Bhuriya D, Singh U (2017) Survey of stock market prediction using machine learning approach. In: International conference of electronics, communication and aerospace technology (ICECA), Coimbatore. pp 506–509
Singh P, Thakral A (2017) Stock market: statistical analysis of its indexes and its constituents. In: International conference on smart technologies for smart nation (SmartTechCon), Bangalore. pp 962–966
Soni D, Agarwal S, Agarwal T, Arora P, K Gupta (2018) Optimised prediction model for stock market trend analysis. In: Eleventh international conference on contemporary computing (IC3), Noida. pp 1–3
Stock price prediction using LSTM (Long Short-Term Memory) - DataScienceCentral.com- https://www.datasciencecentral.com/stock-price-prediction-using-lstm-long-short-term-memory/#:~:text=LSTM%20is%20an%20appropriate%20algorithm%20to%20make%20prediction,the%20dataset%20has%20a%20huge%20amount%20of%20data
Sun A, Lachanski M, Fabozzi FJ (2016) Trade the tweet: Social media text mining and sparse matrix factorization for stock market prediction. Int Rev Financ Anal 48:272–281
Umadevi KS, Gaonka A, Kulkarni R and Kannan RJ (2018) Analysis of stock market using streaming data framework. In: International conference on advances in computing, communications and informatics (ICACCI), Bangalore. pp 1388–1390
Wang Y, Wang Y (2016) Using social media mining technology to assist in price prediction of stock market.In: IEEE international conference on big data analysis (ICBDA), Hangzhou. pp 1–4. https://doi.org/10.1109/ICBDA.2016.7509794
Waqar M, Dawood H, Guo P, Shahnawaz MB, Ghazanfar MA (2017) Prediction of stock market by principal component analysis. In: 13th International conference on computational intelligence and security (CIS), Hong Kong. pp 599–602
Weng B, Lu L, Wang X, Megahed FM, Martinez W (2018) Predicting short-term stock prices using ensemble methods and online data sources. Expert Syst Appli 112:258–273
Weng W, Liu Y, Wang S, Lei K (2016) A multiclass classification model for stock news based on structured data. In: 2016 Sixth international conference on information science and technology (ICIST), Dalian. pp 72–78. https://doi.org/10.1109/ICIST.2016.7483388 .
Yin L, Zhang N, He L, Fang W (2016) A study of relationship between investor sentiment and stock price based on text mining. In: 2016 International conference on identification, information and knowledge in the internet of things (IIKI), Beijing. pp 536–539
Zhao S, Tong Y, Liu X, Tan S (2016) Correlating Twitter with the stock market through non-Gaussian SVAR . In: Eighth international conference on advanced computational intelligence (ICACI), Chiang Mai. pp 257–264. https://doi.org/10.1109/ICACI.2016.7449835
Download references
The authors did not receive support from any organization for the submitted work.
Author information
Authors and affiliations.
Christ (Deemed to be University), Delhi NCR, Ghaziabad, India
Shilpa Srivastava & Varuna Gupta
IIT Roorkee, Roorkee, India
Millie Pant
You can also search for this author in PubMed Google Scholar
Corresponding author
Correspondence to Shilpa Srivastava .
Ethics declarations
Conflict of interest.
There is no conflict of interest.
Additional information
Publisher's note.
Springer Nature remains neutral with regard to jurisdictional claims in published maps and institutional affiliations.
Rights and permissions
Springer Nature or its licensor (e.g. a society or other partner) holds exclusive rights to this article under a publishing agreement with the author(s) or other rightsholder(s); author self-archiving of the accepted manuscript version of this article is solely governed by the terms of such publishing agreement and applicable law.
Reprints and permissions
About this article
Srivastava, S., Pant, M. & Gupta, V. Analysis and prediction of Indian stock market: a machine-learning approach. Int J Syst Assur Eng Manag 14 , 1567–1585 (2023). https://doi.org/10.1007/s13198-023-01934-z
Download citation
Received : 03 October 2021
Revised : 07 February 2023
Accepted : 24 April 2023
Published : 01 July 2023
Issue Date : August 2023
DOI : https://doi.org/10.1007/s13198-023-01934-z
Share this article
Anyone you share the following link with will be able to read this content:
Sorry, a shareable link is not currently available for this article.
Provided by the Springer Nature SharedIt content-sharing initiative
- Stock market prediction
- Sentiment analysis
- RMSE error (root mean square error)
- Support vector machine
- Artificial intelligence
- Random forest
- Gradient boosting regressor
- Machine learning
- KNearest neighbour
- Normalization
- Find a journal
- Publish with us
- Track your research
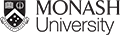
File(s) not publicly available
Essays on the indian stock market, campus location, year of award, department, school or centre, degree type, usage metrics.
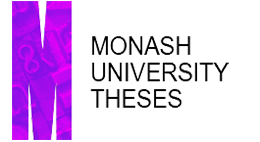
- Education News
- Learning with TOI News
Everything you ever needed to know about stock market and investing
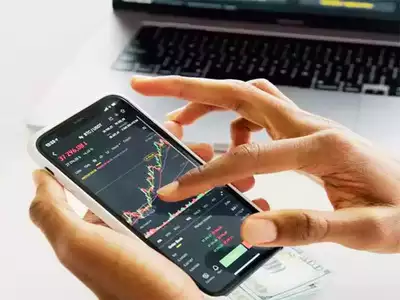
Visual Stories
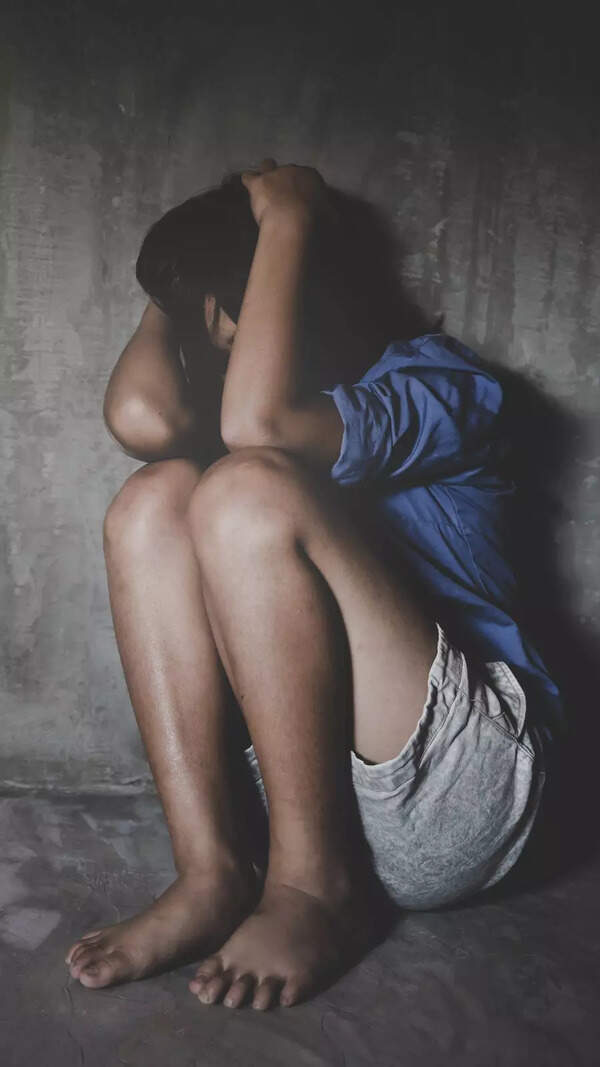
ESSAY SAUCE
FOR STUDENTS : ALL THE INGREDIENTS OF A GOOD ESSAY
Essay: Indian stock market
Essay details and download:.
- Subject area(s): Finance essays
- Reading time: 2 minutes
- Price: Free download
- Published: 30 November 2015*
- File format: Text
- Words: 531 (approx)
- Number of pages: 3 (approx)
Text preview of this essay:
This page of the essay has 531 words. Download the full version above.
The market in which shares of publicly held companies are issued and traded either through exchanges or over-the-counter markets, is known as the equity market or stock market. The stock market is one of the most important components of a free-market economy, as it provides companies with access to capital in exchange for giving investors a part of ownership in the company. The share market makes possible to its investors to grow their small initial sums of money into large ones, and to become wealthy without taking the risk of starting a business as their own or making the sacrifices that often accompany a high-paying career. A stock exchange is a place or organization for the trade of shares of listed companies. A stock exchange is a form of exchange which provides services for stock brokers and traders to buy or sell stocks, bonds, and other securities. Stock exchanges also provide facilities for issue and redemption of securities and other financial instruments, and capital events including the payment of income and dividends. Securities traded on a stock exchange include stock issued by listed companies, unit trusts, derivatives, pooled investment products and bonds. To be able to trade a security on a certain stock exchange, it must be listed there. Usually, there is a central location at least for record keeping, but trade is increasingly less linked to such a physical place, as modern markets are electronic networks, which gives them advantages of increased speed and reduced cost of transactions. Trade on an exchange is by members only. There is usually no compulsion to issue stock via the stock exchange itself, nor must stock be subsequently traded on the exchange. Such trading is said to be off exchange or over-the-counter. This is the usual way that derivatives and bonds are traded. Increasingly, stock exchanges are part of a global market for securities. In recent years, various other trading venues, such as electronic communication networks, alternative trading systems and “dark pools” have taken much of the trading activity away from traditional stock exchanges. ORIGIN OF INDIAN STOCK MARKET BSE and NSE are the leading stock exchanges in India. Apart from these two stock exchanges there are another 22 stock exchanges are also trading shares in India. But BSE and NSE established themselves as leaders in stock exchanges. There are about 80 percent of equity volume are traded in these two exchanges. BOMBAY STOCK EXCHANGE(BSE) Bombay Stock Exchange is the oldest stock exchange in Asia. It is popularly known as BSE. It was established as ‘The Native Share and Stock Broker’s Association’ in 1875. . It is the world’s 5th most active in terms of number of transactions handled through its electronic trading system. There are over 6000 stocks are listed in BSE having the market capitalization around 968000 crores BSE is the 1st in India and second in the world to obtain an ISO 9001:2000 certifications. It is also the first exchange in the country and second in the world to receive Information Security Management System. BSE’s normal trading session are from 9:15am to 3:30pm on all days of week except Saturdays Sundays and holidays declared by the Exchange in advance. BSE automated its system in1995.
...(download the rest of the essay above)
About this essay:
If you use part of this page in your own work, you need to provide a citation, as follows:
Essay Sauce, Indian stock market . Available from:<https://www.essaysauce.com/finance-essays/essay-indian-stock-market/> [Accessed 02-05-24].
These Finance essays have been submitted to us by students in order to help you with your studies.
* This essay may have been previously published on Essay.uk.com at an earlier date.
Essay Categories:
- Accounting essays
- Architecture essays
- Business essays
- Computer science essays
- Criminology essays
- Economics essays
- Education essays
- Engineering essays
- English language essays
- Environmental studies essays
- Essay examples
- Finance essays
- Geography essays
- Health essays
- History essays
- Hospitality and tourism essays
- Human rights essays
- Information technology essays
- International relations
- Leadership essays
- Linguistics essays
- Literature essays
- Management essays
- Marketing essays
- Mathematics essays
- Media essays
- Medicine essays
- Military essays
- Miscellaneous essays
- Music Essays
- Nursing essays
- Philosophy essays
- Photography and arts essays
- Politics essays
- Project management essays
- Psychology essays
- Religious studies and theology essays
- Sample essays
- Science essays
- Social work essays
- Sociology essays
- Sports essays
- Types of essay
- Zoology essays

Call us @ 08069405205

Search Here

- An Introduction to the CSE Exam
- Personality Test
- Annual Calendar by UPSC-2024
- Common Myths about the Exam
- About Insights IAS
- Our Mission, Vision & Values
- Director's Desk
- Meet Our Team
- Our Branches
- Careers at Insights IAS
- Daily Current Affairs+PIB Summary
- Insights into Editorials
- Insta Revision Modules for Prelims
- Current Affairs Quiz
- Static Quiz
- Current Affairs RTM
- Insta-DART(CSAT)
- Insta 75 Days Revision Tests for Prelims 2024
- Secure (Mains Answer writing)
- Secure Synopsis
- Ethics Case Studies
- Insta Ethics
- Weekly Essay Challenge
- Insta Revision Modules-Mains
- Insta 75 Days Revision Tests for Mains
- Secure (Archive)
- Anthropology
- Law Optional
- Kannada Literature
- Public Administration
- English Literature
- Medical Science
- Mathematics
- Commerce & Accountancy
- Monthly Magazine: CURRENT AFFAIRS 30
- Content for Mains Enrichment (CME)
- InstaMaps: Important Places in News
- Weekly CA Magazine
- The PRIME Magazine
- Insta Revision Modules-Prelims
- Insta-DART(CSAT) Quiz
- Insta 75 days Revision Tests for Prelims 2022
- Insights SECURE(Mains Answer Writing)
- Interview Transcripts
- Previous Years' Question Papers-Prelims
- Answer Keys for Prelims PYQs
- Solve Prelims PYQs
- Previous Years' Question Papers-Mains
- UPSC CSE Syllabus
- Toppers from Insights IAS
- Testimonials
- Felicitation
- UPSC Results
- Indian Heritage & Culture
- Ancient Indian History
- Medieval Indian History
- Modern Indian History
- World History
- World Geography
- Indian Geography
- Indian Society
- Social Justice
- International Relations
- Agriculture
- Environment & Ecology
- Disaster Management
- Science & Technology
- Security Issues
- Ethics, Integrity and Aptitude

- Indian Heritage & Culture
- Enivornment & Ecology
- How to Study Art & Culture?
- What is Art and Culture? What is the difference between the two?
- Indus Civilization
- Evolution of rock-cut architecture in India
- Important rock-cut caves
- The contribution of Pallavas to Rock-cut architecture
- Comparision of art form found at Ellora and Mahabalipuram
- Buddhist Architecture
- Early Temples in India
- Basic form of Hindu temple
- Dravida style of temple architecture
- Nagara Style or North India Temple style
- Vesara style of temple architecture
- Characteristic features of Indo-Islamic form of architecture
- Styles of Islamic architecture in the Indian subcontinent
- Types of buildings in Islamic architecture in the Indian subcontinent
- Evolution of this form of architecture during the medieval period
- Modern Architecture
- Post-Independence architecture
- Indus Civilization Sculpture
- Bharhut Sculptures
- Sanchi Sculptures
- Gandhara School of Sculpture
- Mathura School of Sculpture
- Amaravati School of Sculpture
- Gupta Sculpture
- Medieval School of Sculpture
- Modern Indian Sculpture
- Pre Historic Painting
- Mural Paintings & Cave Paintings
- Pala School
- Mughal Paintings
- Bundi School of Painting
- Malwa School
- Mewar School
- Basohli School
- Kangra School
- Decanni School of Painting
- Madhubani Paintings or Mithila paintings
- Pattachitra
- Kalighat Painting
- Modern Indian Paintings
- Personalities Associated to Paintings
- Christianity
- Zoroastrianism
- Six Schools of Philosophy
- Lokayata / Charvaka
- Hindustani Music
- Carnatic Music
- Folk Music Tradition
- Modern Music
- Personalities associated with Music
- Bharatanatyam
- Mohiniattam
- Folk Dances
- Modern Dance in India
- Sanskrit Theatre
- Folk Theatre
- Modern Theatre
- Personalities associated with Theatre
- History of Puppetry
- String Puppetry
- Shadow Puppetry
- Rod Puppetry
- Glove Puppetry
- Indian Cinema and Circus
- Shankaracharya
- Ramanujacharya (1017-1137AD)
- Madhvacharya
- Vallabhacharya
- Kabir (1440-1510 AD)
- Guru Nanak (1469-1538 AD)
- Chaitanya Mahaprabhu
- Shankar Dev
- Purandaradasa
- Samard Ramdas
- Classical Languages
- Scheduled Languages
- Literature in Ancient India
- Buddhist and Jain Literature
- Tamil (Sangam) Literature
- Malayalam Literature
- Telugu Literature
- Medieval Literature
- Modern Literature
- Important characteristics of Fairs and Festivals of India
- Some of the major festivals that are celebrated in India
- Art & Crafts
- Ancient Science & Technology
- Medieval Science & Technology
- Famous Personalities in Science & Technology
- Tangible Cultural Heritage
- Intangible Cultural Heritage
- Cultural Heritage Sites
- Natural Heritage Sites
- Important Institutions
- Important programmes related to promotion and preservation of Indian heritage
- Ochre Colored Pottery (OCP)
- Black and Red Ware (BRW)
- Painted Grey-Ware (PGW)
- Northern Black Polished Ware (NBPW)
- Origin of Martial arts in India
- Various forms of Martial arts in India
- Branches of economics
- Different types of sectors in an economy
- Different types of economic systems
- Difference between economic growth vs economic development
- The concept of demand and supply in an economy
- Factors of production
- National Income
- Importance of quantifying economic growth
- Various types of economic indicators or concepts related to measuring economic growth
- Output Method
- Expenditure Method
- Income method
- Determinants of National Income
- Issues associated with National Income accounting in India
- Possible solutions to Issues with National Accounting
- Indian economy at the time of independence: Basic characteristics
- Basic characteristics of the Indian economy in present times
- Features of Indian Economy
- Role of Agriculture in India
- Growth of Services sector in India
- Status of Human Resource in India
- Status of Natural Resource in India
- Challenges with Indian Economy
- Overall Solutions to achieve a New India by 2022
- Historical evolution of planning in India
- Functions of Planning Commission
- Framework of planning under the commission
- Growth of Indian economy under the various-five year plans of the Planning commission
- Analysis of the working of Planning commission
- Framework of planning under the AYOG
- Performance of the AYOG since its inception
- Issues plaguing the AYOG and measures to resolve these issues
- Definition of a resource
- Types of resources
- Need for mobilizing resource in an economy
- Sources of resource mobilization in India
- Role of Fiscal Policy in Resource Mobilization
- Role of Tax in Resource Mobilization
- Role of Capital/ Financial Markets in Resource Mobilization
- Role of Banking/ Banks in Resource Mobilization
- Issues faced in resource mobilization in recent times
- Steps to be taken to address issues resource mobilization
- Definition of inclusive growth
- Salient Features of Inclusive Growth
- Elements of Inclusive growth
- Need for Inclusion
- Reasons for Inequality in India
- Dimensions of Inclusive Growth
- Measuring Inclusive Growth
- Experience of Indian in ushering in Inclusive growth since independence
- Measures taken by India since independence to ensure inclusive growth in the economy
- Reasons why India has not been able to achieve inclusive growth in the economy
- Rural economy based growth
- Sustainable and inclusive agricultural growth
- Holistic approach to combat poverty in India
- Social sector development
- Role of public and private partnership in this regard
- Balanced regional growth
- Industrial growth and its role in ushering inclusive growth
- Definition of budget
- Types of budget
- Various important terminologies associated with budget
- Budgeting process in India
- Some recent reforms introduced in the budgeting process
- Issues associated with budgeting process in India
- Measures required to address issues related to Budgeting
- Definition, types and objectives of Fiscal policy
- Important concepts/terms related to Government revenue and expenditure
- Types of deficit
- Methods of financing deficits in India
- Changes in FRBMA policies since its introduction
- Analysis of implementation of FRBMA
- Recommendations made with regards to FRBMA policies
- Function of Reserve Bank of India (RBI)
- Definition of monetary policy
- Quantitative tools of monetary policy of RBI
- Qualitative tools of Monetary policy of RBI
- Major monetary policy reforms introduced in recent times
- An objective analysis on the efficacy of monetary policy in India
- Recommendations made by various committees and economists to improve monetary policy framework in India
- Amendments introduced in RBI act over the years
- Issues associated with RBI functioning
- Measures to address the issues plaguing RBI
- Definition of Inflation
- Types of Inflation
- Factors causing Inflation
- Indicators used to measure Inflation
- Effect of Inflation in Indian economy
- Inflation Targeting in India
- Pros and cons of Inflation targeting in India
- Various other methods of combating Inflation
- Government steps to control inflation
- Present trend of Inflation in the economy
- Definition of Taxation
- Objectives behind Taxation
- Types of taxes
- Direct taxes in India
- Indirect taxes in India
- Major taxation related reforms introduced in recent times
- Trend of tax collection in India
- Issues associated with taxation system in India
- Measures suggested/recommendations made to address issues associated with taxation system in India
- Historical evolution of banking system in India
- Functions of banks in an economy
- Scheduled banks
- Non-scheduled banks
- Nationalization of banks
- Narasimhan committee recommendation on Banking sector
- Banking reforms introduced in India in recent times
- Issues associated with banking system in India
- Way Forward : Fifth Generation Banking
- Definition of Money Market and Capital Market
- Importance of Money Market and Capital Market for an Economy
- Money Market Instruments
- Regulatory framework of Indian money market
- Money Market Reforms
- Issues / Challenges related to Money Market in India
- Gilt-Edged Market
- The Industrial Securities Market
Historical evolution of stock market in India
- Importance of stock exchanges
- Various stock exchanges in India
- Regulatory framework of Capital Market in India
- Capital Market Reforms
- Recommendations for Further Improving Capital Market in India
- Definition of investment
- Importance of investment for an economy
- Classification of investment
- Factors affecting investment
- Public investment model
- Private investment model
- Public private partnership model
- Issues associated with PPP model
- Reforms undertaken to better PPP model in recent times
- Harrod-Domar model
- Solow Swan model
- Feldman- Mahalanobis model
- Rao- Manmohan model
- Relationship between infrastructure and economic development
- Transport system in India’s economic development
- Growth in Indian railways
- Roads and road transport system in India
- Water transport in India
- Civil Aviation in India
- Communication Infrastructure in India
- Urban infrastructure
- Infrastructural reforms taken in the above sectors
- An objective analysis of the present infrastructure in the country
- Measures to further improve infrastructure in the country
- Concept of social sector and social infrastructure
- Development of Education In India
- Health and Family Welfare and The Development of Health Infrastructure
- Indian agriculture at the time of independence
- Land reforms
- Indian agriculture under the five year plans
- Food security in India
- Organization of agricultural credit in India
- Agriculture Marketing and Warehousing
- Agricultural labour
- Agriculture and Food processing industries
- Growth trajectory
- Issues plaguing Indian agriculture
- Programmes and policies introduced in India in recent times to address the issues
- Some more measures or recommendations made by the committee to improve agricultural growth in India
- Indian industry at the eve of independence
- Industrial growth under five year planning
- Major Industrial policies introduced in India since independence
- Basic characteristics of Indian industries in the present times
- Measures taken in recent times to increase the industrial growth in the country
- Issues plaguing industrial growth in India despite the measures
- Suggestions and recommendations given by various committees to increase the industrial growth in the country
- Present foreign trade of India
- Foreign trade policies
- Impact of globalization on Indian economy
- India’s balance of payments
- GATT, WTO and India’s foreign trade
- IMF and World Bank
- Recent reforms taken by Indian in this domain
- Definition of Poverty
- Classification of Poverty
- Poverty in India at the eve of Independence
- Growth of Industry in India
- Poverty Alleviation Programmes since Independence
- Impact of LPG reform on reducing poverty in India
- Reasons why poverty still exists in India
- Actions required to address the above issues
- Definition of Unemployment
- Types of unemployment
- Measurement of Unemployment in India
- Unemployment Trends in India
- Causes of unemployment in India
- Programmes, policies and measures taken to address Unemployment in India since Independence
Home » Economy » Indian financial system-II: Money and capital market in India » Stock exchanges in India » Historical evolution of stock market in India
- Corporate shares started being traded in the 1830s in Bombay with the stock of Bank and Cotton presses
- The simple and informal beginnings of stock exchanges in India take one back to the 1850s when 22 stockbrokers began trading opposite the Town Hall of Bombay under a banyan tree
- The shift continued taking place as the number of brokers increased, finally settling in 1874 at what is known as Dalal Street
- This as yet informal group known as the Native Share and Stockbrokers Association organized themselves as the Bombay Stock Exchange (BSE) in 1875
- The BSE was followed by the Ahmedabad Stock Exchange in 1894 which focused on trading in shares of textile mills
- The Calcutta Stock Exchange began operations in 1908 and began trading shares of plantations and jute mills
- The Madras Stock Exchange followed, being set up in 1920
- It was at this time, the Securities and Exchange Board of India(SEBI) was born in 1988 as a non-statutory body, which was further given statutory status in 1992
- The need for another stock exchange large enough to compete with BSE and need for transparency in stock market, gave birth to the National Stock Exchange(NSE)
- After the country gained independence, 23 stock exchanges were added apart from the BSE
- Calcutta Stock Exchange Ltd.
- Magadh Stock Exchange Ltd
- Metropolitan Stock Exchange of India Ltd
- India International Exchange (India INX)
- NSE IFSC Ltd

- Our Mission, Vision & Values
- Director’s Desk
- Commerce & Accountancy
- Previous Years’ Question Papers-Prelims
- Previous Years’ Question Papers-Mains
- Environment & Ecology
- Science & Technology
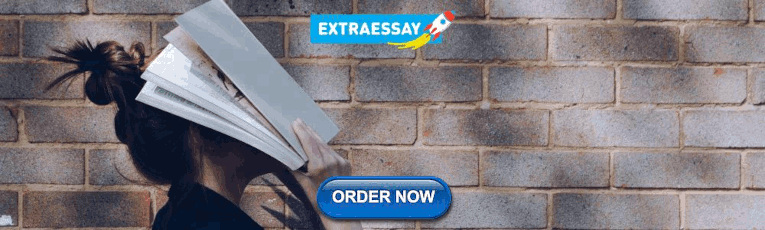
IMAGES
VIDEO
COMMENTS
India's booming stock market is drawing both local novices and global investors to shares of the financial, industrial and technology companies that dominate its listings. The MSCI India index ...
India's booming stock market. Investors are buying into a growth story. Policymakers must now ensure it plays out. The Bombay Stock Exchange building in Mumbai. The bullish narrative for stocks ...
Most of the trading in the Indian stock market takes place in the Bombay Stock Exchange (BSE) and the National Stock Exchange (NSE). As of 2024, India ranked as the fourth largest market in the world.
Furthermore, the share of percentage of stock market in India's GDP/GNI has been rising in the recent years and has been significantly high when compared on a global scale (Table 1). The researchers in the present study aim to better understand the behavior of stock markets in India and represent the decisions and policies in such a way that it ...
The study investigates the relationship between the Indian stock market performance (BSE. Sensex), Market capitalization and six macroeconomic variables, namely, index FIIs, consumer. price Index ...
Investors could assess the impact of market conditions on the stock market. India being a young and emerging economy, it captures the interest of many researchers and academicians. The interaction of these variables has significant inferences for policymakers and regulators, as sustaining the macroeconomic stability has always been a daunting ...
The onset of the COVID-19 pandemic and lockdown announcements by governments have created uncertainty in business operations globally. For the first time, a health shock has impacted the stock markets forcefully. India, one of the major emerging markets, has witnessed a massive fall of around 40% in its major stock indices' value. Therefore, we examined the short-term impact of the pandemic ...
The BSE (Bombay Stock Exchange) of India moves in tandem with other stock exchanges in the world (S&P 500, NASDAQ Composite, FTSE Of London Stock Exchange). REFERENCES 1. Chaitanya, C., 2015. Comparative analysis of international stock markets.IRACST-International Journal of Commerce, Business and Management (IJCBM), 4(1), pp.996-998. 2.
Understanding the relationship between macroeconomic variables and the stock market is important because macroeconomic variables have a systematic effect on stock market returns. This study uses monthly data from India for the period from April 1994 to July 2018 to examine the long-run relationship between the stock market and macroeconomic variables. The empirical findings suggest that ...
NSE India (National Stock Exchange of India Ltd) - LIVE Share/Stock Market Updates Today. Get all latest share market news, live charts, analysis, ipo, stock/share tips, indices, equity, currency and commodity market, derivatives, finance, budget, mutual fund, bond and corporate announcements more on NSEindia.com.
3 Lee et al. (2019) Global stock market investment strategies based on nancial network indicators using machine learn-ing technique 4 Peng (2019) Analysis of investor sentiment and stock market volatility trend based on big data strategy 5 Shah et al. (2018) Predicting the eects of news sentiments on the stock market
Odisha, India. Email: [email protected]. Abstract: This study explores the relationship between macroeconomic variables. and stock market return. The yearly data of six macroeconomic ...
The stock market is witnessing heightened activities and is increasingly gaining importance. In the current context of globalization and the subsequent integration of the global markets this paper ...
The Impact of Stock Market on the Indian Economy. The rise and fall in stock prices tend to influence numerous economic factors, including consumption and business investment. Moreover, just as how the stock market affects the economy, several conditions also impact the stock market. Listed down is the role of stock market in Indian economy-.
Essays on the Indian stock market. Cite Share. thesis. posted on 2021-07-08, 04:40 authored by Vinod Mishra. ... National Stock Exchange (India) National Stock Exchange (India) History. Stock exchanges India Investment analysis India Mathematical models Random walks (Mathematics) ...
A longish bull run that added to investor cheer over Christmas week 2023 took the most cited index of Indian stock market activity, the Sensex, to a record level of 77,240 on the last trading day of the year. But the 18 per cent increase, over the nine months starting April, that explains that level only partly captures the frenzy in the market.
Representational. The stock market is a dynamic financial platform where individuals and entities come together to buy and sell stocks, which represent ownership in publicly traded companies ...
Abstract. The development of stock markets in the financial sector has been important. The expansion of stock markets has compelled various researchers to analyze its relationship with the ...
This page of the essay has 531 words. Download the full version above. The market in which shares of publicly held companies are issued and traded either through exchanges or over-the-counter markets, is known as the equity market or stock market. The stock market is one of the most important components of a free-market economy, as it provides ...
The simple and informal beginnings of stock exchanges in India take one back to the 1850s when 22 stockbrokers began trading opposite the Town Hall of Bombay under a banyan tree. The shift continued taking place as the number of brokers increased, finally settling in 1874 at what is known as Dalal Street. This as yet informal group known as the ...
A stock exchange, also called a securities exchange or. bourse is the name given to the facility for engaging in buying and selling of shares of. stock or bonds or other financial instruments. For ...
02 June 2023. 7 min read. Elections in India have far-reaching consequences, affecting not only the political landscape but also the stock market and economy. From Dalal Street to the common labourer, everyone experiences a sense of unease during the election period. But just how much influence does a ruling party have on the future of India's ...
Recent Trends in Multi-Disciplinary Resea rch, Vol-1, 2022. Abstract: The lure of creating huge money in a short time has always attracted. investors into investing money in stock markets. However ...