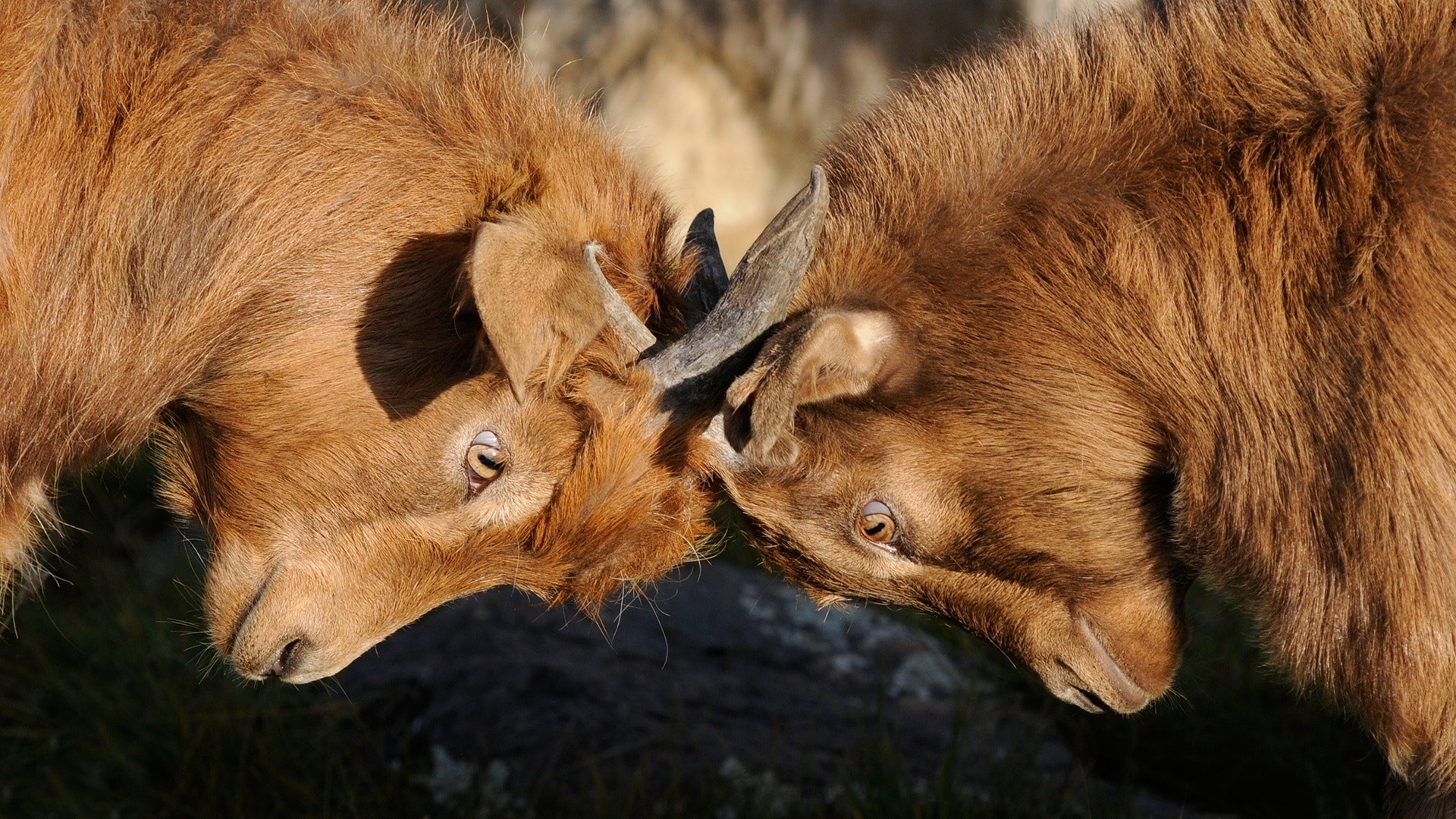
June 11, 2020 | How to's
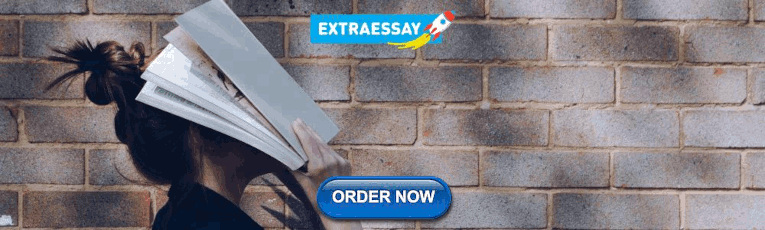
Problem Statement vs Hypothesis: which is more important for experimentation?
by Sadie Neve
When it comes to experimentation and conversion rate optimisation (CRO), we often see people relying too heavily on their instincts, abandoning logic and data in favour of their gut feelings. But really, nothing in experimentation is certain until tested. This realisation automatically makes you question everything you want to change about your website. This means experimentation should be approached like a scientific experiment that follows three core steps; identify a problem, form a hypothesis, and test that hypothesis.
But when it comes to experimentation, should you value the problem statement over the hypothesis? Or vice versa?
Which is more important: the problem statement or hypothesis?
At CreativeCX, we actually place equal importance on the problem statement and the hypothesis. This ensures that we consider both the customer problem that needs to be solved, as well as the business objectives.
All too often, we see companies either neglect the problem statement and hypothesis entirely or favour one over the other.
But weakness in either of these elements can seriously hinder the success of your experimentation programme.
So how can you structure both statements in such a way that you get the most out of your experiment?
The problem statement
First of all, what is the problem statement? A problem statement is a concise description of an issue that needs to be addressed or improved upon. In the case of digital products and services, this should be related to a problem that the customer has.
In any experiment, the problem statement should always come first. Without a problem, you have no real reason to conduct the experiment or understanding on what to conduct an experiment on.
The problem statement guides the strategic direction of your experiment while ensuring that you are always focusing on the customer.
How do we identify customer problems?
The data and research that you undertake will help you identify customer problems, either for your current customers or for your target audience. Identifying the pain points of your customer’s online behaviour should ideally come from multiple sources of data and research. This enables you to triangulate insights so that you can build a more complete picture of the problem, whilst also gaining an understanding of the magnitude of the issue. When starting your research process, you’ll probably find yourself having more questions than answers at the beginning. That’s fine; in fact, it’s normal at this early stage of the experiment.
Rather than letting this put you off, it is better to dig deeper, ask more questions and achieve a greater understanding of the customer problem before trying to find a solution. A greater understanding of the problem and how it’s affecting your customers will lead to better solutions and a higher win rate with your experiments. With this in mind, it is wise to collaborate with other teams within your business – preferably members of your CX and UX departments – who may be able to share relevant customer insights that they have discovered through their own research.
Once you have sufficient data, it is likely you will start to identify problem themes, which will help you understand the wider issues your customers are facing. This is where we start to create a clear problem statement.
How do you craft a clear problem statement?
A clear problem statement should help you identify what the problem is and the data that backs up your claim. At CreativeCX, we organise each problem statement as follows:
We believe [state the problem identified] because [state the supporting data].
Let’s demonstrate with an example. We work with an e-commerce company that sells women’s loungewear. Through our research, we discovered the following two pieces of data:
Usability testing showed users were moving back and forth between the product details page and the basket page to edit their selected size. Website data showed only 2% of customers engage with the “size guide” text link on the product details page. Based on this analysis, we have inferred a problem: users are struggling to understand which size they should choose. Through this, we are able to make the following problem statement:
We believe that users are struggling to understand which size they should choose because our data shows that users are editing their selected size multiple times on the basket and product details page and only 2% of customers engage with the sizing guide.
Can you see how much better this statement is compared to the following:
We think we have an issue with users understanding which size would fit them best.
Here are our top three questions we suggest you keep in mind when writing a problem statement:
Is my problem statement focused on my customers? Is my problem statement clear and precise? What data do I have to back up this problem? As you can see in the examples above, our first example answers all three questions while the second statement falls short on questions two and three.
Whilst your problem statement identifies the problem you hope to solve, the hypothesis helps you decide on how you will try to solve it.
The hypothesis statement
The hypothesis: you’ve probably come across this word years ago in a science class, and its meaning remains the same even in this context. Essentially, the hypothesis statement is a prediction for what you think will happen if you take a certain type of action to resolve a problem.
The hypothesis usually identifies what is going to be changed and the action’s potential outcome, as well as why you think the change will have that particular result.
Creating a hypothesis is a key part of any quality experiment and shouldn’t be rushed. Rushing over this critical step could mean that you miss out on key actions or insights further down the line.
Similar to the problem statement, the hypothesis should be precisely constructed. Having a vague hypothesis may actually be a sign that your problem statement isn’t as clear as you originally thought. An unclear problem statement or hypothesis could, in turn, result in your proposed solutions not having the desired or expected results.
How do you write a clear hypothesis?
There are many ways to write a strong hypothesis. At CreativeCX, we structure ours using the following formula:
By [state experiment change], we believe [user behaviour change], solving [state problem]. We expect to see [expected results].
Now, some may say this will create a hypothesis that is too lengthy. However, this structure clearly incorporates three key elements of an experiment: the problem we are trying to solve, the specific execution, and the expected result. More importantly, it strikes a balance between focusing on the business goals you want to achieve and optimising your customers’ online experience.
Let’s go back to our previous example. There might be multiple solutions to solving this sizing problem, all of which would require a different hypothesis. However, our problem statement has allowed us to identify that we need to increase user awareness of the sizing guide on the product page.
We have identified this as a top priority, so our hypothesis would be as follows:
By increasing link prominence for the sizing guide, we believe more customers will interact with the link, solving sizing uncertainty. We expect to see an increase in customers engaging with the size guide, as well as an increase in customers progressing from the basket to checkout.
Again, whilst this is lengthy, it is also precise. It clearly defines the experiment’s aim with both the business and its customers in mind.
Compare this to the following:
Making the sizing guide link larger will improve our profits.
Here are our top three questions to bear in mind when you’re writing a hypothesis:
Is my hypothesis a statement or a testable question? Is it clear and precise? Is my hypothesis human-friendly and keeping the customer in mind? As you can see, whilst our first example considered all three questions, the second is relatively vague and doesn’t relate to the customer at all.
What do I do once I have written a problem statement and hypothesis?
With your concise problem statement and hypothesis, you should have a great foundation for your experiment. The next step looks at designing your experiment, not in terms of actual visual designs, but what solutions you will be testing in a hope to validate your hypothesis and gain as much learnings on your customers as possible.
Look out for our future blog around how best to design your experiments and be creative with your potential variations.
If you have any questions about topics that have been covered in this blog or you’d like help with your experimentation or CRO programme, please don’t hesitate to reach out to us.
Interested in working with Creative CX?
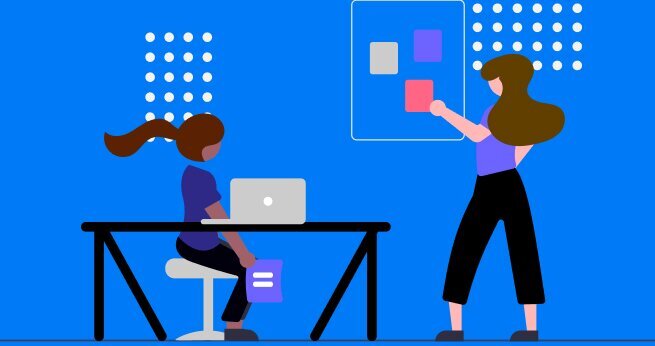
Aarhus University logo
AU Studypedia
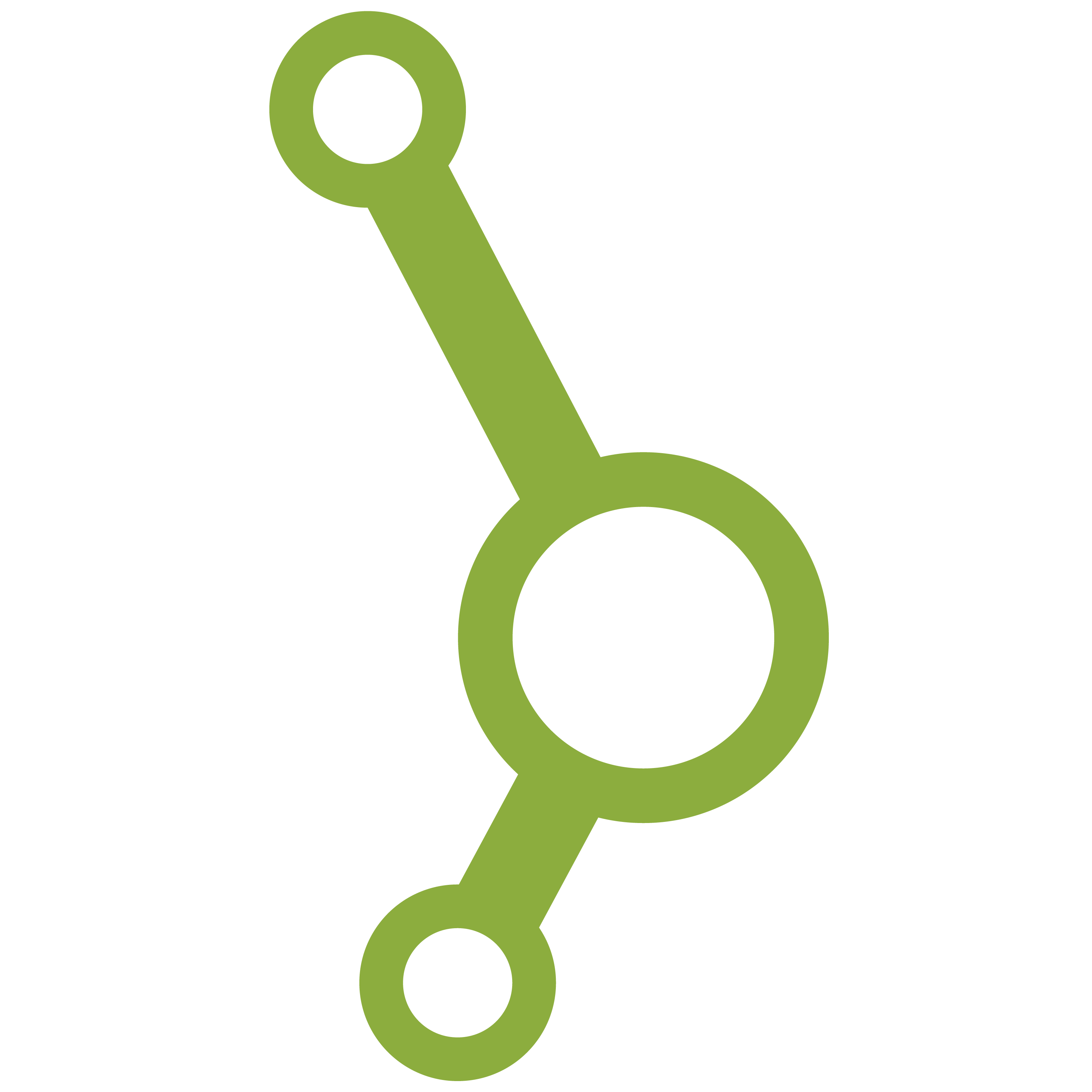
Problem statement and hypothesis
A problem statement may need to be re-worked throughout the process .
The academic problem that you are investigating in your assignment can either take the form of a problem statement, i.e. a question that you want to answer, or it can be a hypothesis that you wish to reject or confirm. How you formulate the problem influences the task you are embarking on. Problem statements as well as hypotheses must be relevant to your area of study, and you must be able to carry out the investigation using the resources and methods available to you.
Note that a problem statement or a hypothesis often changes during the writing process. Sometimes you have to change the focus of the problem statement/hypothesis, and sometimes you only have to change a single word. The amount of changes required depends on your study programme and the assignment at hand. Therefore, you should always ask your teacher or supervisor for advice.
Problem statement
A problem statement usually consists of one question to be addressed in your assignment and to be answered in your conclusion. It can include 2-5 sub-questions. A problem statement can take different forms, but generally:
It uses accurate wording, for example technical terms
It relates specifically to your project, describing what you want to study (object) and how you want to study it (theories and methods)
It not only introduces a description of the problem (what is the problem?) but also encourages explanation, reflection and discussion of the problem (how and why does the problem exist?)
The problem statement as a guiding tool
The problem statement can be a useful tool to guide you through your work process. Whether you are collecting empirical data, searching for literature or reading, always keep your problem statement in mind. This will help you narrow down your searches and your reading, and help you focus on what is relevant in order to answer the question in your problem statement.
You should also be prepared to revise your problem statement as you go along. For example if you discover a more relevant or interesting question when you start working on the investigation. Always discuss with your teacher or supervisor if you want to make radical changes to your problem statement, and thereby to your assignment.
Working on your problem statement
The problem statement sets the framework for your assignment .
Your problem statement asks the question that will be answered in the conclusion. The actual assignment - between the problem statement and the conclusion - addresses your main question. There must be a clear link between the problem statement and the conclusion.
A problem statement must comply with certain specific requirements
Your problem statement has to meet a number of formal requirements, but there are other elements that you need to consider as well. For example: Is your language clear and unambiguous, and is your topic relevant and interesting?
Checklist for the problem statement
Checklist for the problem statement .
Use the points in the checklist below to assure the quality of your problem statement. Tick off each of the points that your problem statement complies with. Continue to work on your problem statement until it complies with most or all of the items on the list. This will help you make sure that your problem statement is satisfactory.
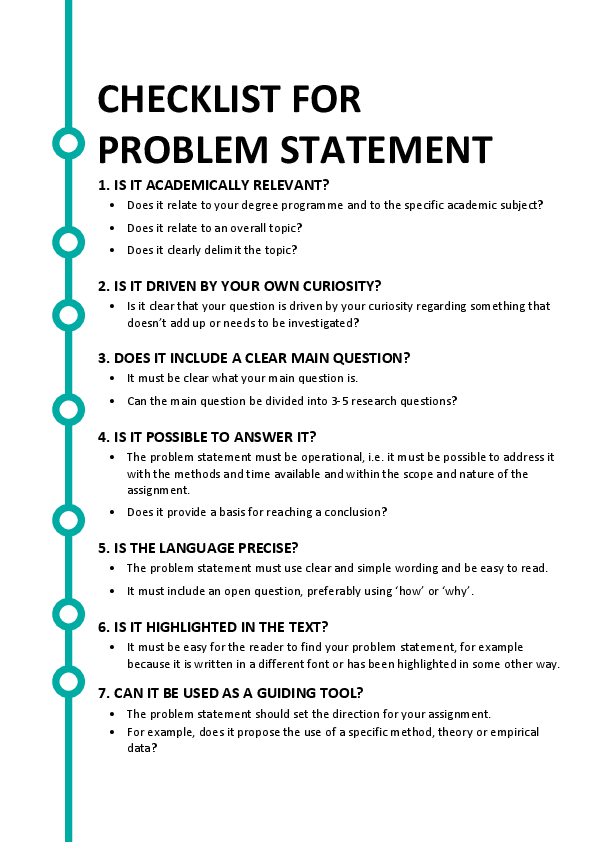
The checklist has been prepared by the editorial team in collaboration with Susanne Højlund, associate professor at the School of Culture and Society - Department of Anthropology, Aarhus University.
A hypothesis is a theoretical, hypothetical explanation that can be tested. It usually takes the form of a causal relationship or a causal explanation. You can also consider the hypothesis as a preliminary response to a research question or a problem statement. A hypothesis can be expressed in different ways, but generally, the following applies:
The hypothesis is theoretical and builds on existing knowledge and general principles.
The hypothesis can be tested through a study or an experiment.
The hypothesis can either be confirmed or rejected.
Testing a hypothesis
Your hypothesis can include a prediction of the results of your study based on a logical explanation. Your study will then show whether your hypothesis and your prediction appear to be correct or not. In other words, a good hypothesis is a hypothesis that you can test through a study or an experiment.
A good hypothesis is theoretical and is based on existing knowledge, general principles and previous research within a similar academic problem area. It can also be a good idea to consider proposing several hypotheses.
In science, it is generally believed that a hypothesis can turn out to be wrong, but that it can never be conclusively proven to be true. Consequently, your study or experiment should be designed so that it attempts to reject or falsify your hypothesis. If you fail to reject the hypothesis, it is more likely to be "correct".
Inspiration from assignments by other students
Get a list of thesis titles from your field of study, and draw inspiration from other students’ problem statements.
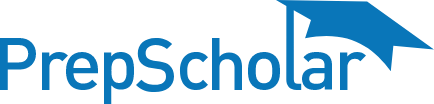
Choose Your Test
Sat / act prep online guides and tips, what is a hypothesis and how do i write one.
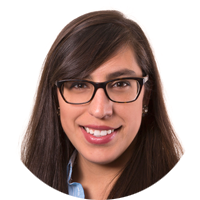
General Education
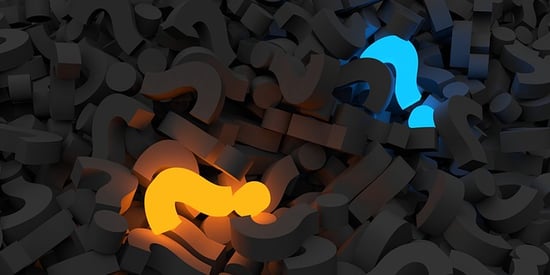
Think about something strange and unexplainable in your life. Maybe you get a headache right before it rains, or maybe you think your favorite sports team wins when you wear a certain color. If you wanted to see whether these are just coincidences or scientific fact, you would form a hypothesis, then create an experiment to see whether that hypothesis is true or not.
But what is a hypothesis, anyway? If you’re not sure about what a hypothesis is--or how to test for one!--you’re in the right place. This article will teach you everything you need to know about hypotheses, including:
- Defining the term “hypothesis”
- Providing hypothesis examples
- Giving you tips for how to write your own hypothesis
So let’s get started!
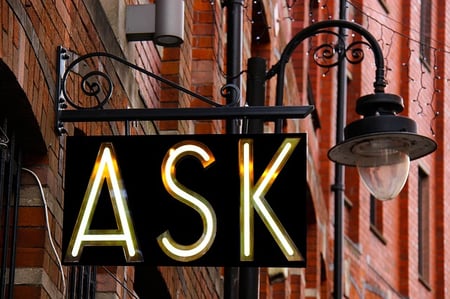
What Is a Hypothesis?
Merriam Webster defines a hypothesis as “an assumption or concession made for the sake of argument.” In other words, a hypothesis is an educated guess . Scientists make a reasonable assumption--or a hypothesis--then design an experiment to test whether it’s true or not. Keep in mind that in science, a hypothesis should be testable. You have to be able to design an experiment that tests your hypothesis in order for it to be valid.
As you could assume from that statement, it’s easy to make a bad hypothesis. But when you’re holding an experiment, it’s even more important that your guesses be good...after all, you’re spending time (and maybe money!) to figure out more about your observation. That’s why we refer to a hypothesis as an educated guess--good hypotheses are based on existing data and research to make them as sound as possible.
Hypotheses are one part of what’s called the scientific method . Every (good) experiment or study is based in the scientific method. The scientific method gives order and structure to experiments and ensures that interference from scientists or outside influences does not skew the results. It’s important that you understand the concepts of the scientific method before holding your own experiment. Though it may vary among scientists, the scientific method is generally made up of six steps (in order):
- Observation
- Asking questions
- Forming a hypothesis
- Analyze the data
- Communicate your results
You’ll notice that the hypothesis comes pretty early on when conducting an experiment. That’s because experiments work best when they’re trying to answer one specific question. And you can’t conduct an experiment until you know what you’re trying to prove!
Independent and Dependent Variables
After doing your research, you’re ready for another important step in forming your hypothesis: identifying variables. Variables are basically any factor that could influence the outcome of your experiment . Variables have to be measurable and related to the topic being studied.
There are two types of variables: independent variables and dependent variables. I ndependent variables remain constant . For example, age is an independent variable; it will stay the same, and researchers can look at different ages to see if it has an effect on the dependent variable.
Speaking of dependent variables... dependent variables are subject to the influence of the independent variable , meaning that they are not constant. Let’s say you want to test whether a person’s age affects how much sleep they need. In that case, the independent variable is age (like we mentioned above), and the dependent variable is how much sleep a person gets.
Variables will be crucial in writing your hypothesis. You need to be able to identify which variable is which, as both the independent and dependent variables will be written into your hypothesis. For instance, in a study about exercise, the independent variable might be the speed at which the respondents walk for thirty minutes, and the dependent variable would be their heart rate. In your study and in your hypothesis, you’re trying to understand the relationship between the two variables.
Elements of a Good Hypothesis
The best hypotheses start by asking the right questions . For instance, if you’ve observed that the grass is greener when it rains twice a week, you could ask what kind of grass it is, what elevation it’s at, and if the grass across the street responds to rain in the same way. Any of these questions could become the backbone of experiments to test why the grass gets greener when it rains fairly frequently.
As you’re asking more questions about your first observation, make sure you’re also making more observations . If it doesn’t rain for two weeks and the grass still looks green, that’s an important observation that could influence your hypothesis. You'll continue observing all throughout your experiment, but until the hypothesis is finalized, every observation should be noted.
Finally, you should consult secondary research before writing your hypothesis . Secondary research is comprised of results found and published by other people. You can usually find this information online or at your library. Additionally, m ake sure the research you find is credible and related to your topic. If you’re studying the correlation between rain and grass growth, it would help you to research rain patterns over the past twenty years for your county, published by a local agricultural association. You should also research the types of grass common in your area, the type of grass in your lawn, and whether anyone else has conducted experiments about your hypothesis. Also be sure you’re checking the quality of your research . Research done by a middle school student about what minerals can be found in rainwater would be less useful than an article published by a local university.
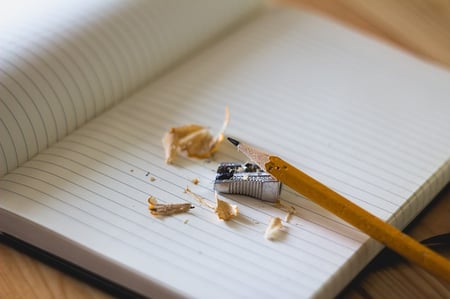
Writing Your Hypothesis
Once you’ve considered all of the factors above, you’re ready to start writing your hypothesis. Hypotheses usually take a certain form when they’re written out in a research report.
When you boil down your hypothesis statement, you are writing down your best guess and not the question at hand . This means that your statement should be written as if it is fact already, even though you are simply testing it.
The reason for this is that, after you have completed your study, you'll either accept or reject your if-then or your null hypothesis. All hypothesis testing examples should be measurable and able to be confirmed or denied. You cannot confirm a question, only a statement!
In fact, you come up with hypothesis examples all the time! For instance, when you guess on the outcome of a basketball game, you don’t say, “Will the Miami Heat beat the Boston Celtics?” but instead, “I think the Miami Heat will beat the Boston Celtics.” You state it as if it is already true, even if it turns out you’re wrong. You do the same thing when writing your hypothesis.
Additionally, keep in mind that hypotheses can range from very specific to very broad. These hypotheses can be specific, but if your hypothesis testing examples involve a broad range of causes and effects, your hypothesis can also be broad.
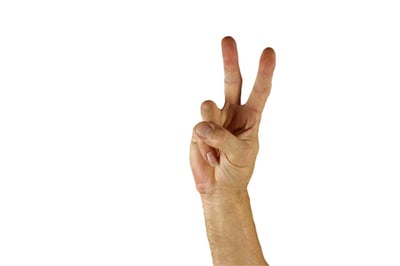
The Two Types of Hypotheses
Now that you understand what goes into a hypothesis, it’s time to look more closely at the two most common types of hypothesis: the if-then hypothesis and the null hypothesis.
#1: If-Then Hypotheses
First of all, if-then hypotheses typically follow this formula:
If ____ happens, then ____ will happen.
The goal of this type of hypothesis is to test the causal relationship between the independent and dependent variable. It’s fairly simple, and each hypothesis can vary in how detailed it can be. We create if-then hypotheses all the time with our daily predictions. Here are some examples of hypotheses that use an if-then structure from daily life:
- If I get enough sleep, I’ll be able to get more work done tomorrow.
- If the bus is on time, I can make it to my friend’s birthday party.
- If I study every night this week, I’ll get a better grade on my exam.
In each of these situations, you’re making a guess on how an independent variable (sleep, time, or studying) will affect a dependent variable (the amount of work you can do, making it to a party on time, or getting better grades).
You may still be asking, “What is an example of a hypothesis used in scientific research?” Take one of the hypothesis examples from a real-world study on whether using technology before bed affects children’s sleep patterns. The hypothesis read s:
“We hypothesized that increased hours of tablet- and phone-based screen time at bedtime would be inversely correlated with sleep quality and child attention.”
It might not look like it, but this is an if-then statement. The researchers basically said, “If children have more screen usage at bedtime, then their quality of sleep and attention will be worse.” The sleep quality and attention are the dependent variables and the screen usage is the independent variable. (Usually, the independent variable comes after the “if” and the dependent variable comes after the “then,” as it is the independent variable that affects the dependent variable.) This is an excellent example of how flexible hypothesis statements can be, as long as the general idea of “if-then” and the independent and dependent variables are present.
#2: Null Hypotheses
Your if-then hypothesis is not the only one needed to complete a successful experiment, however. You also need a null hypothesis to test it against. In its most basic form, the null hypothesis is the opposite of your if-then hypothesis . When you write your null hypothesis, you are writing a hypothesis that suggests that your guess is not true, and that the independent and dependent variables have no relationship .
One null hypothesis for the cell phone and sleep study from the last section might say:
“If children have more screen usage at bedtime, their quality of sleep and attention will not be worse.”
In this case, this is a null hypothesis because it’s asking the opposite of the original thesis!
Conversely, if your if-then hypothesis suggests that your two variables have no relationship, then your null hypothesis would suggest that there is one. So, pretend that there is a study that is asking the question, “Does the amount of followers on Instagram influence how long people spend on the app?” The independent variable is the amount of followers, and the dependent variable is the time spent. But if you, as the researcher, don’t think there is a relationship between the number of followers and time spent, you might write an if-then hypothesis that reads:
“If people have many followers on Instagram, they will not spend more time on the app than people who have less.”
In this case, the if-then suggests there isn’t a relationship between the variables. In that case, one of the null hypothesis examples might say:
“If people have many followers on Instagram, they will spend more time on the app than people who have less.”
You then test both the if-then and the null hypothesis to gauge if there is a relationship between the variables, and if so, how much of a relationship.
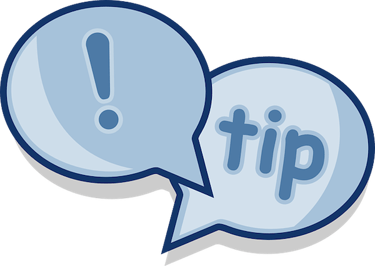
4 Tips to Write the Best Hypothesis
If you’re going to take the time to hold an experiment, whether in school or by yourself, you’re also going to want to take the time to make sure your hypothesis is a good one. The best hypotheses have four major elements in common: plausibility, defined concepts, observability, and general explanation.
#1: Plausibility
At first glance, this quality of a hypothesis might seem obvious. When your hypothesis is plausible, that means it’s possible given what we know about science and general common sense. However, improbable hypotheses are more common than you might think.
Imagine you’re studying weight gain and television watching habits. If you hypothesize that people who watch more than twenty hours of television a week will gain two hundred pounds or more over the course of a year, this might be improbable (though it’s potentially possible). Consequently, c ommon sense can tell us the results of the study before the study even begins.
Improbable hypotheses generally go against science, as well. Take this hypothesis example:
“If a person smokes one cigarette a day, then they will have lungs just as healthy as the average person’s.”
This hypothesis is obviously untrue, as studies have shown again and again that cigarettes negatively affect lung health. You must be careful that your hypotheses do not reflect your own personal opinion more than they do scientifically-supported findings. This plausibility points to the necessity of research before the hypothesis is written to make sure that your hypothesis has not already been disproven.
#2: Defined Concepts
The more advanced you are in your studies, the more likely that the terms you’re using in your hypothesis are specific to a limited set of knowledge. One of the hypothesis testing examples might include the readability of printed text in newspapers, where you might use words like “kerning” and “x-height.” Unless your readers have a background in graphic design, it’s likely that they won’t know what you mean by these terms. Thus, it’s important to either write what they mean in the hypothesis itself or in the report before the hypothesis.
Here’s what we mean. Which of the following sentences makes more sense to the common person?
If the kerning is greater than average, more words will be read per minute.
If the space between letters is greater than average, more words will be read per minute.
For people reading your report that are not experts in typography, simply adding a few more words will be helpful in clarifying exactly what the experiment is all about. It’s always a good idea to make your research and findings as accessible as possible.
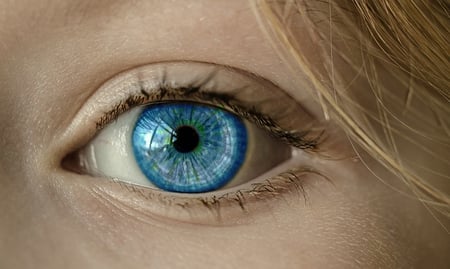
Good hypotheses ensure that you can observe the results.
#3: Observability
In order to measure the truth or falsity of your hypothesis, you must be able to see your variables and the way they interact. For instance, if your hypothesis is that the flight patterns of satellites affect the strength of certain television signals, yet you don’t have a telescope to view the satellites or a television to monitor the signal strength, you cannot properly observe your hypothesis and thus cannot continue your study.
Some variables may seem easy to observe, but if you do not have a system of measurement in place, you cannot observe your hypothesis properly. Here’s an example: if you’re experimenting on the effect of healthy food on overall happiness, but you don’t have a way to monitor and measure what “overall happiness” means, your results will not reflect the truth. Monitoring how often someone smiles for a whole day is not reasonably observable, but having the participants state how happy they feel on a scale of one to ten is more observable.
In writing your hypothesis, always keep in mind how you'll execute the experiment.
#4: Generalizability
Perhaps you’d like to study what color your best friend wears the most often by observing and documenting the colors she wears each day of the week. This might be fun information for her and you to know, but beyond you two, there aren’t many people who could benefit from this experiment. When you start an experiment, you should note how generalizable your findings may be if they are confirmed. Generalizability is basically how common a particular phenomenon is to other people’s everyday life.
Let’s say you’re asking a question about the health benefits of eating an apple for one day only, you need to realize that the experiment may be too specific to be helpful. It does not help to explain a phenomenon that many people experience. If you find yourself with too specific of a hypothesis, go back to asking the big question: what is it that you want to know, and what do you think will happen between your two variables?
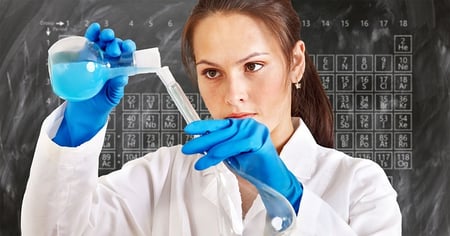
Hypothesis Testing Examples
We know it can be hard to write a good hypothesis unless you’ve seen some good hypothesis examples. We’ve included four hypothesis examples based on some made-up experiments. Use these as templates or launch pads for coming up with your own hypotheses.
Experiment #1: Students Studying Outside (Writing a Hypothesis)
You are a student at PrepScholar University. When you walk around campus, you notice that, when the temperature is above 60 degrees, more students study in the quad. You want to know when your fellow students are more likely to study outside. With this information, how do you make the best hypothesis possible?
You must remember to make additional observations and do secondary research before writing your hypothesis. In doing so, you notice that no one studies outside when it’s 75 degrees and raining, so this should be included in your experiment. Also, studies done on the topic beforehand suggested that students are more likely to study in temperatures less than 85 degrees. With this in mind, you feel confident that you can identify your variables and write your hypotheses:
If-then: “If the temperature in Fahrenheit is less than 60 degrees, significantly fewer students will study outside.”
Null: “If the temperature in Fahrenheit is less than 60 degrees, the same number of students will study outside as when it is more than 60 degrees.”
These hypotheses are plausible, as the temperatures are reasonably within the bounds of what is possible. The number of people in the quad is also easily observable. It is also not a phenomenon specific to only one person or at one time, but instead can explain a phenomenon for a broader group of people.
To complete this experiment, you pick the month of October to observe the quad. Every day (except on the days where it’s raining)from 3 to 4 PM, when most classes have released for the day, you observe how many people are on the quad. You measure how many people come and how many leave. You also write down the temperature on the hour.
After writing down all of your observations and putting them on a graph, you find that the most students study on the quad when it is 70 degrees outside, and that the number of students drops a lot once the temperature reaches 60 degrees or below. In this case, your research report would state that you accept or “failed to reject” your first hypothesis with your findings.
Experiment #2: The Cupcake Store (Forming a Simple Experiment)
Let’s say that you work at a bakery. You specialize in cupcakes, and you make only two colors of frosting: yellow and purple. You want to know what kind of customers are more likely to buy what kind of cupcake, so you set up an experiment. Your independent variable is the customer’s gender, and the dependent variable is the color of the frosting. What is an example of a hypothesis that might answer the question of this study?
Here’s what your hypotheses might look like:
If-then: “If customers’ gender is female, then they will buy more yellow cupcakes than purple cupcakes.”
Null: “If customers’ gender is female, then they will be just as likely to buy purple cupcakes as yellow cupcakes.”
This is a pretty simple experiment! It passes the test of plausibility (there could easily be a difference), defined concepts (there’s nothing complicated about cupcakes!), observability (both color and gender can be easily observed), and general explanation ( this would potentially help you make better business decisions ).
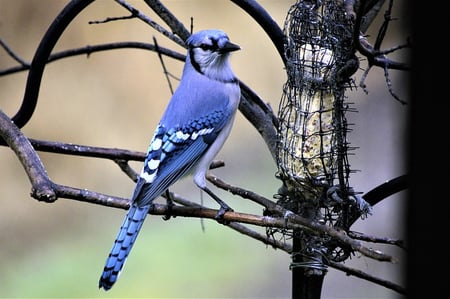
Experiment #3: Backyard Bird Feeders (Integrating Multiple Variables and Rejecting the If-Then Hypothesis)
While watching your backyard bird feeder, you realized that different birds come on the days when you change the types of seeds. You decide that you want to see more cardinals in your backyard, so you decide to see what type of food they like the best and set up an experiment.
However, one morning, you notice that, while some cardinals are present, blue jays are eating out of your backyard feeder filled with millet. You decide that, of all of the other birds, you would like to see the blue jays the least. This means you'll have more than one variable in your hypothesis. Your new hypotheses might look like this:
If-then: “If sunflower seeds are placed in the bird feeders, then more cardinals will come than blue jays. If millet is placed in the bird feeders, then more blue jays will come than cardinals.”
Null: “If either sunflower seeds or millet are placed in the bird, equal numbers of cardinals and blue jays will come.”
Through simple observation, you actually find that cardinals come as often as blue jays when sunflower seeds or millet is in the bird feeder. In this case, you would reject your “if-then” hypothesis and “fail to reject” your null hypothesis . You cannot accept your first hypothesis, because it’s clearly not true. Instead you found that there was actually no relation between your different variables. Consequently, you would need to run more experiments with different variables to see if the new variables impact the results.
Experiment #4: In-Class Survey (Including an Alternative Hypothesis)
You’re about to give a speech in one of your classes about the importance of paying attention. You want to take this opportunity to test a hypothesis you’ve had for a while:
If-then: If students sit in the first two rows of the classroom, then they will listen better than students who do not.
Null: If students sit in the first two rows of the classroom, then they will not listen better or worse than students who do not.
You give your speech and then ask your teacher if you can hand out a short survey to the class. On the survey, you’ve included questions about some of the topics you talked about. When you get back the results, you’re surprised to see that not only do the students in the first two rows not pay better attention, but they also scored worse than students in other parts of the classroom! Here, both your if-then and your null hypotheses are not representative of your findings. What do you do?
This is when you reject both your if-then and null hypotheses and instead create an alternative hypothesis . This type of hypothesis is used in the rare circumstance that neither of your hypotheses is able to capture your findings . Now you can use what you’ve learned to draft new hypotheses and test again!
Key Takeaways: Hypothesis Writing
The more comfortable you become with writing hypotheses, the better they will become. The structure of hypotheses is flexible and may need to be changed depending on what topic you are studying. The most important thing to remember is the purpose of your hypothesis and the difference between the if-then and the null . From there, in forming your hypothesis, you should constantly be asking questions, making observations, doing secondary research, and considering your variables. After you have written your hypothesis, be sure to edit it so that it is plausible, clearly defined, observable, and helpful in explaining a general phenomenon.
Writing a hypothesis is something that everyone, from elementary school children competing in a science fair to professional scientists in a lab, needs to know how to do. Hypotheses are vital in experiments and in properly executing the scientific method . When done correctly, hypotheses will set up your studies for success and help you to understand the world a little better, one experiment at a time.
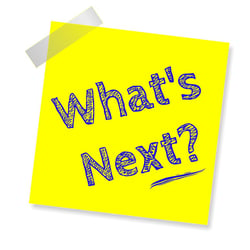
What’s Next?
If you’re studying for the science portion of the ACT, there’s definitely a lot you need to know. We’ve got the tools to help, though! Start by checking out our ultimate study guide for the ACT Science subject test. Once you read through that, be sure to download our recommended ACT Science practice tests , since they’re one of the most foolproof ways to improve your score. (And don’t forget to check out our expert guide book , too.)
If you love science and want to major in a scientific field, you should start preparing in high school . Here are the science classes you should take to set yourself up for success.
If you’re trying to think of science experiments you can do for class (or for a science fair!), here’s a list of 37 awesome science experiments you can do at home
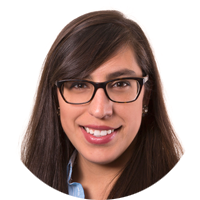
Ashley Sufflé Robinson has a Ph.D. in 19th Century English Literature. As a content writer for PrepScholar, Ashley is passionate about giving college-bound students the in-depth information they need to get into the school of their dreams.
Student and Parent Forum
Our new student and parent forum, at ExpertHub.PrepScholar.com , allow you to interact with your peers and the PrepScholar staff. See how other students and parents are navigating high school, college, and the college admissions process. Ask questions; get answers.

Ask a Question Below
Have any questions about this article or other topics? Ask below and we'll reply!
Improve With Our Famous Guides
- For All Students
The 5 Strategies You Must Be Using to Improve 160+ SAT Points
How to Get a Perfect 1600, by a Perfect Scorer
Series: How to Get 800 on Each SAT Section:
Score 800 on SAT Math
Score 800 on SAT Reading
Score 800 on SAT Writing
Series: How to Get to 600 on Each SAT Section:
Score 600 on SAT Math
Score 600 on SAT Reading
Score 600 on SAT Writing
Free Complete Official SAT Practice Tests
What SAT Target Score Should You Be Aiming For?
15 Strategies to Improve Your SAT Essay
The 5 Strategies You Must Be Using to Improve 4+ ACT Points
How to Get a Perfect 36 ACT, by a Perfect Scorer
Series: How to Get 36 on Each ACT Section:
36 on ACT English
36 on ACT Math
36 on ACT Reading
36 on ACT Science
Series: How to Get to 24 on Each ACT Section:
24 on ACT English
24 on ACT Math
24 on ACT Reading
24 on ACT Science
What ACT target score should you be aiming for?
ACT Vocabulary You Must Know
ACT Writing: 15 Tips to Raise Your Essay Score
How to Get Into Harvard and the Ivy League
How to Get a Perfect 4.0 GPA
How to Write an Amazing College Essay
What Exactly Are Colleges Looking For?
Is the ACT easier than the SAT? A Comprehensive Guide
Should you retake your SAT or ACT?
When should you take the SAT or ACT?
Stay Informed

Get the latest articles and test prep tips!
Looking for Graduate School Test Prep?
Check out our top-rated graduate blogs here:
GRE Online Prep Blog
GMAT Online Prep Blog
TOEFL Online Prep Blog
Holly R. "I am absolutely overjoyed and cannot thank you enough for helping me!”
Have a language expert improve your writing
Run a free plagiarism check in 10 minutes, automatically generate references for free.
- Knowledge Base
- Methodology
- How to Write a Strong Hypothesis | Guide & Examples
How to Write a Strong Hypothesis | Guide & Examples
Published on 6 May 2022 by Shona McCombes .
A hypothesis is a statement that can be tested by scientific research. If you want to test a relationship between two or more variables, you need to write hypotheses before you start your experiment or data collection.
Table of contents
What is a hypothesis, developing a hypothesis (with example), hypothesis examples, frequently asked questions about writing hypotheses.
A hypothesis states your predictions about what your research will find. It is a tentative answer to your research question that has not yet been tested. For some research projects, you might have to write several hypotheses that address different aspects of your research question.
A hypothesis is not just a guess – it should be based on existing theories and knowledge. It also has to be testable, which means you can support or refute it through scientific research methods (such as experiments, observations, and statistical analysis of data).
Variables in hypotheses
Hypotheses propose a relationship between two or more variables . An independent variable is something the researcher changes or controls. A dependent variable is something the researcher observes and measures.
In this example, the independent variable is exposure to the sun – the assumed cause . The dependent variable is the level of happiness – the assumed effect .
Prevent plagiarism, run a free check.
Step 1: ask a question.
Writing a hypothesis begins with a research question that you want to answer. The question should be focused, specific, and researchable within the constraints of your project.
Step 2: Do some preliminary research
Your initial answer to the question should be based on what is already known about the topic. Look for theories and previous studies to help you form educated assumptions about what your research will find.
At this stage, you might construct a conceptual framework to identify which variables you will study and what you think the relationships are between them. Sometimes, you’ll have to operationalise more complex constructs.
Step 3: Formulate your hypothesis
Now you should have some idea of what you expect to find. Write your initial answer to the question in a clear, concise sentence.
Step 4: Refine your hypothesis
You need to make sure your hypothesis is specific and testable. There are various ways of phrasing a hypothesis, but all the terms you use should have clear definitions, and the hypothesis should contain:
- The relevant variables
- The specific group being studied
- The predicted outcome of the experiment or analysis
Step 5: Phrase your hypothesis in three ways
To identify the variables, you can write a simple prediction in if … then form. The first part of the sentence states the independent variable and the second part states the dependent variable.
In academic research, hypotheses are more commonly phrased in terms of correlations or effects, where you directly state the predicted relationship between variables.
If you are comparing two groups, the hypothesis can state what difference you expect to find between them.
Step 6. Write a null hypothesis
If your research involves statistical hypothesis testing , you will also have to write a null hypothesis. The null hypothesis is the default position that there is no association between the variables. The null hypothesis is written as H 0 , while the alternative hypothesis is H 1 or H a .
Hypothesis testing is a formal procedure for investigating our ideas about the world using statistics. It is used by scientists to test specific predictions, called hypotheses , by calculating how likely it is that a pattern or relationship between variables could have arisen by chance.
A hypothesis is not just a guess. It should be based on existing theories and knowledge. It also has to be testable, which means you can support or refute it through scientific research methods (such as experiments, observations, and statistical analysis of data).
A research hypothesis is your proposed answer to your research question. The research hypothesis usually includes an explanation (‘ x affects y because …’).
A statistical hypothesis, on the other hand, is a mathematical statement about a population parameter. Statistical hypotheses always come in pairs: the null and alternative hypotheses. In a well-designed study , the statistical hypotheses correspond logically to the research hypothesis.
Cite this Scribbr article
If you want to cite this source, you can copy and paste the citation or click the ‘Cite this Scribbr article’ button to automatically add the citation to our free Reference Generator.
McCombes, S. (2022, May 06). How to Write a Strong Hypothesis | Guide & Examples. Scribbr. Retrieved 15 April 2024, from https://www.scribbr.co.uk/research-methods/hypothesis-writing/
Is this article helpful?
Shona McCombes
Other students also liked, operationalisation | a guide with examples, pros & cons, what is a conceptual framework | tips & examples, a quick guide to experimental design | 5 steps & examples.
Have a language expert improve your writing
Run a free plagiarism check in 10 minutes, generate accurate citations for free.
- Knowledge Base
Hypothesis Testing | A Step-by-Step Guide with Easy Examples
Published on November 8, 2019 by Rebecca Bevans . Revised on June 22, 2023.
Hypothesis testing is a formal procedure for investigating our ideas about the world using statistics . It is most often used by scientists to test specific predictions, called hypotheses, that arise from theories.
There are 5 main steps in hypothesis testing:
- State your research hypothesis as a null hypothesis and alternate hypothesis (H o ) and (H a or H 1 ).
- Collect data in a way designed to test the hypothesis.
- Perform an appropriate statistical test .
- Decide whether to reject or fail to reject your null hypothesis.
- Present the findings in your results and discussion section.
Though the specific details might vary, the procedure you will use when testing a hypothesis will always follow some version of these steps.
Table of contents
Step 1: state your null and alternate hypothesis, step 2: collect data, step 3: perform a statistical test, step 4: decide whether to reject or fail to reject your null hypothesis, step 5: present your findings, other interesting articles, frequently asked questions about hypothesis testing.
After developing your initial research hypothesis (the prediction that you want to investigate), it is important to restate it as a null (H o ) and alternate (H a ) hypothesis so that you can test it mathematically.
The alternate hypothesis is usually your initial hypothesis that predicts a relationship between variables. The null hypothesis is a prediction of no relationship between the variables you are interested in.
- H 0 : Men are, on average, not taller than women. H a : Men are, on average, taller than women.
Prevent plagiarism. Run a free check.
For a statistical test to be valid , it is important to perform sampling and collect data in a way that is designed to test your hypothesis. If your data are not representative, then you cannot make statistical inferences about the population you are interested in.
There are a variety of statistical tests available, but they are all based on the comparison of within-group variance (how spread out the data is within a category) versus between-group variance (how different the categories are from one another).
If the between-group variance is large enough that there is little or no overlap between groups, then your statistical test will reflect that by showing a low p -value . This means it is unlikely that the differences between these groups came about by chance.
Alternatively, if there is high within-group variance and low between-group variance, then your statistical test will reflect that with a high p -value. This means it is likely that any difference you measure between groups is due to chance.
Your choice of statistical test will be based on the type of variables and the level of measurement of your collected data .
- an estimate of the difference in average height between the two groups.
- a p -value showing how likely you are to see this difference if the null hypothesis of no difference is true.
Based on the outcome of your statistical test, you will have to decide whether to reject or fail to reject your null hypothesis.
In most cases you will use the p -value generated by your statistical test to guide your decision. And in most cases, your predetermined level of significance for rejecting the null hypothesis will be 0.05 – that is, when there is a less than 5% chance that you would see these results if the null hypothesis were true.
In some cases, researchers choose a more conservative level of significance, such as 0.01 (1%). This minimizes the risk of incorrectly rejecting the null hypothesis ( Type I error ).
The results of hypothesis testing will be presented in the results and discussion sections of your research paper , dissertation or thesis .
In the results section you should give a brief summary of the data and a summary of the results of your statistical test (for example, the estimated difference between group means and associated p -value). In the discussion , you can discuss whether your initial hypothesis was supported by your results or not.
In the formal language of hypothesis testing, we talk about rejecting or failing to reject the null hypothesis. You will probably be asked to do this in your statistics assignments.
However, when presenting research results in academic papers we rarely talk this way. Instead, we go back to our alternate hypothesis (in this case, the hypothesis that men are on average taller than women) and state whether the result of our test did or did not support the alternate hypothesis.
If your null hypothesis was rejected, this result is interpreted as “supported the alternate hypothesis.”
These are superficial differences; you can see that they mean the same thing.
You might notice that we don’t say that we reject or fail to reject the alternate hypothesis . This is because hypothesis testing is not designed to prove or disprove anything. It is only designed to test whether a pattern we measure could have arisen spuriously, or by chance.
If we reject the null hypothesis based on our research (i.e., we find that it is unlikely that the pattern arose by chance), then we can say our test lends support to our hypothesis . But if the pattern does not pass our decision rule, meaning that it could have arisen by chance, then we say the test is inconsistent with our hypothesis .
If you want to know more about statistics , methodology , or research bias , make sure to check out some of our other articles with explanations and examples.
- Normal distribution
- Descriptive statistics
- Measures of central tendency
- Correlation coefficient
Methodology
- Cluster sampling
- Stratified sampling
- Types of interviews
- Cohort study
- Thematic analysis
Research bias
- Implicit bias
- Cognitive bias
- Survivorship bias
- Availability heuristic
- Nonresponse bias
- Regression to the mean
Hypothesis testing is a formal procedure for investigating our ideas about the world using statistics. It is used by scientists to test specific predictions, called hypotheses , by calculating how likely it is that a pattern or relationship between variables could have arisen by chance.
A hypothesis states your predictions about what your research will find. It is a tentative answer to your research question that has not yet been tested. For some research projects, you might have to write several hypotheses that address different aspects of your research question.
A hypothesis is not just a guess — it should be based on existing theories and knowledge. It also has to be testable, which means you can support or refute it through scientific research methods (such as experiments, observations and statistical analysis of data).
Null and alternative hypotheses are used in statistical hypothesis testing . The null hypothesis of a test always predicts no effect or no relationship between variables, while the alternative hypothesis states your research prediction of an effect or relationship.
Cite this Scribbr article
If you want to cite this source, you can copy and paste the citation or click the “Cite this Scribbr article” button to automatically add the citation to our free Citation Generator.
Bevans, R. (2023, June 22). Hypothesis Testing | A Step-by-Step Guide with Easy Examples. Scribbr. Retrieved April 15, 2024, from https://www.scribbr.com/statistics/hypothesis-testing/
Is this article helpful?
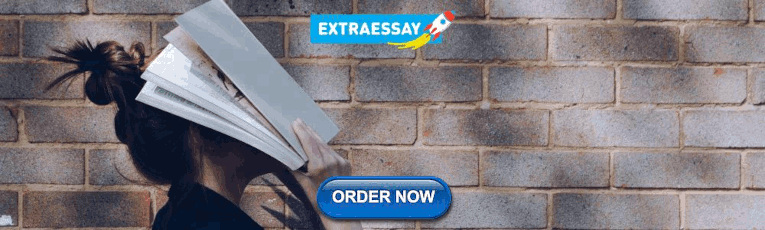
Rebecca Bevans
Other students also liked, choosing the right statistical test | types & examples, understanding p values | definition and examples, what is your plagiarism score.
- Resources Home 🏠
- Try SciSpace Copilot
- Search research papers
- Add Copilot Extension
- Try AI Detector
- Try Paraphraser
- Try Citation Generator
- April Papers
- June Papers
- July Papers

How to Write a Statement of the Problem in Research

Table of Contents
The problem statement is a foundation of academic research writing , providing a precise representation of an existing gap or issue in a particular field of study.
Crafting a sharp and focused problem statement lays the groundwork for your research project.
- It highlights the research's significance .
- Emphasizes its potential to influence the broader academic community.
- Represents the initial step for you to make a meaningful contribution to your discipline.
Therefore, in this article, we will discuss what is a statement of the problem in research and how to craft a compelling research problem statement.
What is a research problem statement?
A research problem statement is a concise, clear, and specific articulation of a gap in current knowledge that your research aims to bridge. It not only sets forth the scope and direction of your research but also establishes its relevance and significance.
Your problem statement in your research paper aims to:
- Define the gap : Clearly identify and articulate a specific gap or issue in the existing knowledge.
- Provide direction : Serve as a roadmap, guiding the course of your research and ensuring you remain focused.
- Establish relevance : Highlight the importance and significance of the problem in the context of your field or the broader world.
- Guide inquiry : Formulate the research questions or hypotheses you'll explore.
- Communicate intent : Succinctly convey the core purpose of your research to stakeholders, peers, and any audience.
- Set boundaries : Clearly define the scope of your research to ensure it's focused and achievable.
When should you write a problem statement in research?
Initiate your research by crafting a clear problem statement. This should be done before any data collection or analysis, serving as a foundational anchor that clearly identifies the specific issue you aim to address.
By establishing this early on, you shape the direction of your research, ensuring it targets a genuine knowledge gap.
Furthermore, an effective and a concise statement of the problem in research attracts collaborators, funders, and supporters, resonating with its clarity and purpose. Remember, as your research unfolds, the statement might evolve, reflecting new insights and staying pertinent.
But how do you distinguish between a well-crafted problem statement and one that falls short?
Effective vs. ineffective research problem statements
Imagine a scenario where medical researchers aim to tackle a new strain of virus. Their effective problem statement wouldn't merely state the existence of the virus. Instead, it would delve into the specifics — the regions most affected, the demographics most vulnerable, and the current limitations in medical interventions.
Whereas an ineffective research problem statement is vague, overly broad, or ambiguous, failing to provide a clear direction for the research. It may not be rooted in existing literature, might lack clarity on its significance, or could be framed in a way that makes the research objectives unachievable or irrelevant.
To understand it better, let's consider the topic of “Remote work and employee productivity.”
Effective problem statement
“Over the past decade, there has been a 70% increase in organizations adopting remote work policies. While some studies suggest remote work enhances employee productivity, others indicate potential declines due to distractions at home.
However, there’s a lack of comprehensive research examining the specific factors in a remote environment that influence productivity. This study aims to identify and analyze these factors, providing organizations with actionable insights to optimize remote work policies.”
Why is this statement of a problem in research effective?
- Specificity : The statement provides a clear percentage to highlight the rise in remote work.
- Context : It acknowledges existing research and the conflicting findings.
- Clear gap identification : It points out the lack of comprehensive research on specific factors affecting productivity in remote work.
- Purpose : The statement concludes with a clear aim for the research.
Ineffective problem statement
"People are working from home a lot now, especially since there are so many internet tools. Some say it's good; others say it's not that great. This research will just look into the whole work-from-home thing and see what's up."
Why is this statement of a problem in research ineffective?
- Informal language : Phrases like "what's up" and "the whole work-from-home thing" are not suitable for academic writing.
- Vagueness : The statement doesn't provide any specific data or context about the rise of remote work.
- Lack of clear focus : It's unclear what aspect of remote work the research will address.
- Ambiguous purpose : The statement doesn't specify the research's objectives or expected outcomes.
After gaining an understanding of what an effective research problem statement looks like, let's dive deeper into how to write one.
How to write a problem statement in research?
Drafting your research problem statement at the onset of your research journey ensures that your research remains anchored. That means by defining and articulating the main issue or challenge you intend to address at the very beginning of your research process; you provide a clear focus and direction for the entire study.
Here's a detailed guide to how you can write an effective statement of the problem in research.
Identify the research area : Before addressing a specific problem, you need to know the broader domain or field of your study. This helps in contextualizing your research and ensuring it aligns with existing academic disciplines.
Example: If you're curious about the effects of digital technology on human behavior, your broader research area might be Digital Sociology or Media Studies.
Conduct preliminary literature review : Familiarize yourself with existing research related to your topic. This will help you understand what's already known and, more importantly, identify gaps or unresolved questions in the existing knowledge. This step also ensures you're advancing upon existing work rather than replicating it.
Example: Upon reviewing literature on digital technology and behavior, you find many studies on social media's impact on youth but fewer on its effects on the elderly.
Read how to conduct an effective literature review .
Define the specific problem : After thoroughly reviewing the literature, pinpoint a particular issue that your research will address. Ensure that this chosen issue is not only of substantial importance in its field but also realistically approachable given your resources and expertise. To define it precisely, you might consider:
- Highlighting discrepancies or contradictions in existing literature.
- Emphasizing the real-world implications of this gap.
- Assessing the feasibility of exploring this issue within your means and timeframe.
Example: You decide to investigate how digital technology, especially social media, affects the mental well-being of the elderly, given the limited research in this area.
Articulate clearly and concisely : Your problem statement should be straightforward and devoid of jargon. It needs to convey the essence of your research issue in a manner that's understandable to both experts and non-experts.
Example: " The impact of social media on the mental well-being of elderly individuals remains underexplored, despite the growing adoption of digital technology in this age group. "
Highlight the significance : Explain why your chosen research problem matters. This could be due to its real-world implications, its potential to fill a knowledge gap or its relevance to current events or trends.
Example: As the elderly population grows and becomes more digitally connected, understanding the psychological effects of social media on this demographic could inform digital literacy programs and mental health interventions.
Ensure feasibility : Your research problem should be something you can realistically study, given your resources, timeframe, and expertise. It's essential to ensure that you can gather data, conduct experiments, or access necessary materials or participants.
Example: You plan to survey elderly individuals in local community centers about their social media usage and perceived mental well-being, ensuring you have the means to reach this demographic.
Seek feedback : Discuss your preliminary problem statement with peers, mentors, or experts in the field. They can provide insights, point out potential pitfalls, or suggest refinements.
Example: After discussing with a gerontologist, you decide to also consider the role of digital training in moderating the effects of social media on the elderly.
Refine and Revise : Based on feedback and further reflection, revise and improve your problem statement. This iterative process ensures clarity, relevance, and precision.
Example: Your refined statement reads: Despite the increasing digital connectivity of the elderly, the effects of social media on their mental well-being, especially in the context of digital training, remain underexplored.
By following these detailed steps, you can craft a research problem statement that is both compelling and academically rigorous.
Having explored the details of crafting a research problem statement, it's crucial to distinguish it from another fundamental element in academic research: the thesis statement.
Difference between a thesis statement and a problem statement
While both terms are central to research, a thesis statement presents your primary claim or argument, whereas a problem statement describes the specific issue your research aims to address.
Think of the thesis statement as the conclusion you're driving towards, while the problem statement identifies a specific gap in current knowledge.
For instance, a problem statement might highlight the rising mental health issues among teenagers, while the thesis statement could propose that increased screen time is a significant contributor.
Refer to the comparison table between what is a thesis and a problem statement in the research below:
Common mistakes to avoid in writing statement of the problem in research
Mistakes in the research problem statement can lead to a domino effect, causing misalignment in research objectives, wasted resources, and even inconclusive or irrelevant results.
Recognizing and avoiding these pitfalls not only strengthens the foundation of your research but also ensures that your efforts concede impactful insights.
Here's a detailed exploration of frequent subjective, qualitative, quantitative and measurable mistakes and how you can sidestep them.
Being too broad or too narrow
A problem statement that's too broad can lack focus, making it challenging to derive specific research questions or objectives. Conversely, a statement that's too narrow might limit the scope of your research or make it too trivial.
Example of mistake: "Studying the effects of diet on health" is too broad, while "Studying the effects of eating green apples at 3 pm on heart health" is overly narrow.
You can refine the scope based on preliminary research. The correct way to write this problem statement will be "Studying the effects of a high-fiber diet on heart health in adults over 50." This statement is neither too broad nor too narrow, and it provides a clear direction for the research.
Using unnecessary jargon or technical language
While academic writing often involves academic terms, overloading your problem statement with jargon can alienate readers and obscure the actual problem.
Example of Mistake: "Examining the diurnal variations in macronutrient ingestion vis-à-vis metabolic homeostasis."
To ensure it’s not complicated, you can simplify and clarify. "Examining how daily changes in nutrient intake affect metabolic balance" conveys the same idea more accessible.
Not emphasizing the "Why" of the problem
It's not enough to state a problem; you must also convey its significance. Why does this problem matter? What are the implications of not addressing it?
Example of Mistake: "Many students are not engaging with online learning platforms."
You can proceed with the approach of highlighting the significance here. "Many students are not engaging with online learning platforms, leading to decreased academic performance and widening educational disparities."
Circular reasoning and lack of relevance
Your problem statement should be grounded in existing research or observed phenomena. Avoid statements that assume what they set out to prove or lack a clear basis in current knowledge.
Example of Mistake: "We need to study X because not enough research has been done on X."
Instead, try grounding your statement based on already-known facts. "While several studies have explored Y, the specific impact of X remains unclear, necessitating further research."
Being overly ambitious
While it's commendable to aim high, your problem statement should reflect a challenge that's achievable within your means, timeframe, and resources.
Example of Mistake: "This research will solve world hunger."
Here, you need to be realistic and focused. "This research aims to develop sustainable agricultural techniques to increase crop yields in arid regions."
By being mindful of these common mistakes, you can craft a problem statement that is clear, relevant and sets a solid foundation for your research.
Over-reliance on outdated data
Using data that is no longer relevant can mislead the direction of your research. It's essential to ensure that the statistics or findings you reference are current and pertinent to the present scenario.
Example of Mistake: "According to a 1995 study, only 5% of the population uses the internet for daily tasks."
You always cross-check the dates and relevance of the data you're using. For a contemporary study on internet usage, you'd want to reference more recent statistics.
Not specifying the sample size or demographic
A problem statement should be clear about the population or sample size being studied, especially when making generalizations or claims.
Example of Mistake: "People prefer online shopping to in-store shopping."
Here, you would benefit from specifying the demographic or sample size when presenting data to avoid overgeneralization. " In a survey of 1,000 urban residents aged 18-35, 70% expressed a preference for online shopping over in-store shopping. "
Ignoring conflicting data
Cherry-picking data that supports your hypothesis while ignoring conflicting data can lead to a biased problem statement.
Example of Mistake: "Research shows that all students benefit from online learning."
You’ve to ensure a balanced view by considering all relevant data, even if it contradicts your hypothesis. " While many studies highlight the advantages of online learning for students, some research points to challenges such as decreased motivation and lack of face-to-face interaction. "
Making unsubstantiated predictions
Projecting future trends without solid data can weaken the credibility of your problem statement.
Example of Mistake: "The demand for electric cars will increase by 500% in the next year."
Base your predictions on current trends and reliable data sources, avoiding hyperbolic or unsupported claims. " With the current growth rate and recent advancements in battery technology, there's potential for a significant rise in the demand for electric cars. "
Wrapping Up
A well-crafted problem statement ensures that your research is focused, relevant, and contributes meaningfully to the broader academic community.
However, the consequences of an incorrect or poorly constructed problem statement can be severe. It can lead to misdirected research efforts, wasted resources, compromised credibility, and even ethical concerns. Such pitfalls underscore the importance of dedicating time and effort to craft a precise and impactful problem statement.
So, as you start your research journey , remember that a well-defined problem statement is not just a starting point; it guides your entire research journey, ensuring clarity, relevance, and meaningful contributions to your field.
Frequently Asked Questions
A problem statement is a clear, concise and specific articulation of a gap in current knowledge that your research aims to bridge.
The Problem Statement should highlight existing gaps in current knowledge and also the significance of the research. It should also include the research question and purpose of the research.
Clear articulation of the problem and establishing relevance; Working thesis (methods to solve the problem); Purpose and scope of study — are the 3 parts of the problem statement.
While the statement of the problem articulates and delineates a particular research problem, Objectives designates the aims, purpose and strategies to address the particular problem.
Here’s an example — “The study aims to identify and analyze the specific factors that impact employee productivity, providing organizations with actionable insights to optimize remote work policies.”
You might also like

Consensus GPT vs. SciSpace GPT: Choose the Best GPT for Research
Literature Review and Theoretical Framework: Understanding the Differences

Types of Essays in Academic Writing - Quick Guide (2024)
If you're seeing this message, it means we're having trouble loading external resources on our website.
If you're behind a web filter, please make sure that the domains *.kastatic.org and *.kasandbox.org are unblocked.
To log in and use all the features of Khan Academy, please enable JavaScript in your browser.
Biology library
Course: biology library > unit 1, the scientific method.
- Controlled experiments
- The scientific method and experimental design
Introduction
- Make an observation.
- Ask a question.
- Form a hypothesis , or testable explanation.
- Make a prediction based on the hypothesis.
- Test the prediction.
- Iterate: use the results to make new hypotheses or predictions.
Scientific method example: Failure to toast
1. make an observation..
- Observation: the toaster won't toast.
2. Ask a question.
- Question: Why won't my toaster toast?
3. Propose a hypothesis.
- Hypothesis: Maybe the outlet is broken.
4. Make predictions.
- Prediction: If I plug the toaster into a different outlet, then it will toast the bread.
5. Test the predictions.
- Test of prediction: Plug the toaster into a different outlet and try again.
- If the toaster does toast, then the hypothesis is supported—likely correct.
- If the toaster doesn't toast, then the hypothesis is not supported—likely wrong.
Logical possibility
Practical possibility, building a body of evidence, 6. iterate..
- Iteration time!
- If the hypothesis was supported, we might do additional tests to confirm it, or revise it to be more specific. For instance, we might investigate why the outlet is broken.
- If the hypothesis was not supported, we would come up with a new hypothesis. For instance, the next hypothesis might be that there's a broken wire in the toaster.
Want to join the conversation?
- Upvote Button navigates to signup page
- Downvote Button navigates to signup page
- Flag Button navigates to signup page

Research Hypothesis In Psychology: Types, & Examples
Saul Mcleod, PhD
Editor-in-Chief for Simply Psychology
BSc (Hons) Psychology, MRes, PhD, University of Manchester
Saul Mcleod, PhD., is a qualified psychology teacher with over 18 years of experience in further and higher education. He has been published in peer-reviewed journals, including the Journal of Clinical Psychology.
Learn about our Editorial Process
Olivia Guy-Evans, MSc
Associate Editor for Simply Psychology
BSc (Hons) Psychology, MSc Psychology of Education
Olivia Guy-Evans is a writer and associate editor for Simply Psychology. She has previously worked in healthcare and educational sectors.
On This Page:
A research hypothesis, in its plural form “hypotheses,” is a specific, testable prediction about the anticipated results of a study, established at its outset. It is a key component of the scientific method .
Hypotheses connect theory to data and guide the research process towards expanding scientific understanding
Some key points about hypotheses:
- A hypothesis expresses an expected pattern or relationship. It connects the variables under investigation.
- It is stated in clear, precise terms before any data collection or analysis occurs. This makes the hypothesis testable.
- A hypothesis must be falsifiable. It should be possible, even if unlikely in practice, to collect data that disconfirms rather than supports the hypothesis.
- Hypotheses guide research. Scientists design studies to explicitly evaluate hypotheses about how nature works.
- For a hypothesis to be valid, it must be testable against empirical evidence. The evidence can then confirm or disprove the testable predictions.
- Hypotheses are informed by background knowledge and observation, but go beyond what is already known to propose an explanation of how or why something occurs.
Predictions typically arise from a thorough knowledge of the research literature, curiosity about real-world problems or implications, and integrating this to advance theory. They build on existing literature while providing new insight.
Types of Research Hypotheses
Alternative hypothesis.
The research hypothesis is often called the alternative or experimental hypothesis in experimental research.
It typically suggests a potential relationship between two key variables: the independent variable, which the researcher manipulates, and the dependent variable, which is measured based on those changes.
The alternative hypothesis states a relationship exists between the two variables being studied (one variable affects the other).
A hypothesis is a testable statement or prediction about the relationship between two or more variables. It is a key component of the scientific method. Some key points about hypotheses:
- Important hypotheses lead to predictions that can be tested empirically. The evidence can then confirm or disprove the testable predictions.
In summary, a hypothesis is a precise, testable statement of what researchers expect to happen in a study and why. Hypotheses connect theory to data and guide the research process towards expanding scientific understanding.
An experimental hypothesis predicts what change(s) will occur in the dependent variable when the independent variable is manipulated.
It states that the results are not due to chance and are significant in supporting the theory being investigated.
The alternative hypothesis can be directional, indicating a specific direction of the effect, or non-directional, suggesting a difference without specifying its nature. It’s what researchers aim to support or demonstrate through their study.
Null Hypothesis
The null hypothesis states no relationship exists between the two variables being studied (one variable does not affect the other). There will be no changes in the dependent variable due to manipulating the independent variable.
It states results are due to chance and are not significant in supporting the idea being investigated.
The null hypothesis, positing no effect or relationship, is a foundational contrast to the research hypothesis in scientific inquiry. It establishes a baseline for statistical testing, promoting objectivity by initiating research from a neutral stance.
Many statistical methods are tailored to test the null hypothesis, determining the likelihood of observed results if no true effect exists.
This dual-hypothesis approach provides clarity, ensuring that research intentions are explicit, and fosters consistency across scientific studies, enhancing the standardization and interpretability of research outcomes.
Nondirectional Hypothesis
A non-directional hypothesis, also known as a two-tailed hypothesis, predicts that there is a difference or relationship between two variables but does not specify the direction of this relationship.
It merely indicates that a change or effect will occur without predicting which group will have higher or lower values.
For example, “There is a difference in performance between Group A and Group B” is a non-directional hypothesis.
Directional Hypothesis
A directional (one-tailed) hypothesis predicts the nature of the effect of the independent variable on the dependent variable. It predicts in which direction the change will take place. (i.e., greater, smaller, less, more)
It specifies whether one variable is greater, lesser, or different from another, rather than just indicating that there’s a difference without specifying its nature.
For example, “Exercise increases weight loss” is a directional hypothesis.
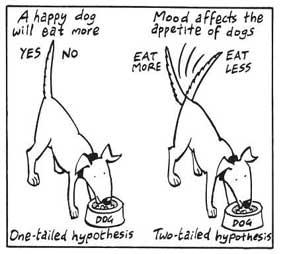
Falsifiability
The Falsification Principle, proposed by Karl Popper , is a way of demarcating science from non-science. It suggests that for a theory or hypothesis to be considered scientific, it must be testable and irrefutable.
Falsifiability emphasizes that scientific claims shouldn’t just be confirmable but should also have the potential to be proven wrong.
It means that there should exist some potential evidence or experiment that could prove the proposition false.
However many confirming instances exist for a theory, it only takes one counter observation to falsify it. For example, the hypothesis that “all swans are white,” can be falsified by observing a black swan.
For Popper, science should attempt to disprove a theory rather than attempt to continually provide evidence to support a research hypothesis.
Can a Hypothesis be Proven?
Hypotheses make probabilistic predictions. They state the expected outcome if a particular relationship exists. However, a study result supporting a hypothesis does not definitively prove it is true.
All studies have limitations. There may be unknown confounding factors or issues that limit the certainty of conclusions. Additional studies may yield different results.
In science, hypotheses can realistically only be supported with some degree of confidence, not proven. The process of science is to incrementally accumulate evidence for and against hypothesized relationships in an ongoing pursuit of better models and explanations that best fit the empirical data. But hypotheses remain open to revision and rejection if that is where the evidence leads.
- Disproving a hypothesis is definitive. Solid disconfirmatory evidence will falsify a hypothesis and require altering or discarding it based on the evidence.
- However, confirming evidence is always open to revision. Other explanations may account for the same results, and additional or contradictory evidence may emerge over time.
We can never 100% prove the alternative hypothesis. Instead, we see if we can disprove, or reject the null hypothesis.
If we reject the null hypothesis, this doesn’t mean that our alternative hypothesis is correct but does support the alternative/experimental hypothesis.
Upon analysis of the results, an alternative hypothesis can be rejected or supported, but it can never be proven to be correct. We must avoid any reference to results proving a theory as this implies 100% certainty, and there is always a chance that evidence may exist which could refute a theory.
How to Write a Hypothesis
- Identify variables . The researcher manipulates the independent variable and the dependent variable is the measured outcome.
- Operationalized the variables being investigated . Operationalization of a hypothesis refers to the process of making the variables physically measurable or testable, e.g. if you are about to study aggression, you might count the number of punches given by participants.
- Decide on a direction for your prediction . If there is evidence in the literature to support a specific effect of the independent variable on the dependent variable, write a directional (one-tailed) hypothesis. If there are limited or ambiguous findings in the literature regarding the effect of the independent variable on the dependent variable, write a non-directional (two-tailed) hypothesis.
- Make it Testable : Ensure your hypothesis can be tested through experimentation or observation. It should be possible to prove it false (principle of falsifiability).
- Clear & concise language . A strong hypothesis is concise (typically one to two sentences long), and formulated using clear and straightforward language, ensuring it’s easily understood and testable.
Consider a hypothesis many teachers might subscribe to: students work better on Monday morning than on Friday afternoon (IV=Day, DV= Standard of work).
Now, if we decide to study this by giving the same group of students a lesson on a Monday morning and a Friday afternoon and then measuring their immediate recall of the material covered in each session, we would end up with the following:
- The alternative hypothesis states that students will recall significantly more information on a Monday morning than on a Friday afternoon.
- The null hypothesis states that there will be no significant difference in the amount recalled on a Monday morning compared to a Friday afternoon. Any difference will be due to chance or confounding factors.
More Examples
- Memory : Participants exposed to classical music during study sessions will recall more items from a list than those who studied in silence.
- Social Psychology : Individuals who frequently engage in social media use will report higher levels of perceived social isolation compared to those who use it infrequently.
- Developmental Psychology : Children who engage in regular imaginative play have better problem-solving skills than those who don’t.
- Clinical Psychology : Cognitive-behavioral therapy will be more effective in reducing symptoms of anxiety over a 6-month period compared to traditional talk therapy.
- Cognitive Psychology : Individuals who multitask between various electronic devices will have shorter attention spans on focused tasks than those who single-task.
- Health Psychology : Patients who practice mindfulness meditation will experience lower levels of chronic pain compared to those who don’t meditate.
- Organizational Psychology : Employees in open-plan offices will report higher levels of stress than those in private offices.
- Behavioral Psychology : Rats rewarded with food after pressing a lever will press it more frequently than rats who receive no reward.

Go on to Assignment 1: Try Your Hand at Writing Hypotheses or Go back to Understanding Hypotheses
E-mail M. Dereshiwsky at [email protected] Call M. Dereshiwsky at (520) 523-1892
User Preferences
Content preview.
Arcu felis bibendum ut tristique et egestas quis:
- Ut enim ad minim veniam, quis nostrud exercitation ullamco laboris
- Duis aute irure dolor in reprehenderit in voluptate
- Excepteur sint occaecat cupidatat non proident
Keyboard Shortcuts
5.2 - writing hypotheses.
The first step in conducting a hypothesis test is to write the hypothesis statements that are going to be tested. For each test you will have a null hypothesis (\(H_0\)) and an alternative hypothesis (\(H_a\)).
When writing hypotheses there are three things that we need to know: (1) the parameter that we are testing (2) the direction of the test (non-directional, right-tailed or left-tailed), and (3) the value of the hypothesized parameter.
- At this point we can write hypotheses for a single mean (\(\mu\)), paired means(\(\mu_d\)), a single proportion (\(p\)), the difference between two independent means (\(\mu_1-\mu_2\)), the difference between two proportions (\(p_1-p_2\)), a simple linear regression slope (\(\beta\)), and a correlation (\(\rho\)).
- The research question will give us the information necessary to determine if the test is two-tailed (e.g., "different from," "not equal to"), right-tailed (e.g., "greater than," "more than"), or left-tailed (e.g., "less than," "fewer than").
- The research question will also give us the hypothesized parameter value. This is the number that goes in the hypothesis statements (i.e., \(\mu_0\) and \(p_0\)). For the difference between two groups, regression, and correlation, this value is typically 0.
Hypotheses are always written in terms of population parameters (e.g., \(p\) and \(\mu\)). The tables below display all of the possible hypotheses for the parameters that we have learned thus far. Note that the null hypothesis always includes the equality (i.e., =).
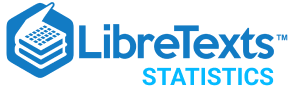
- school Campus Bookshelves
- menu_book Bookshelves
- perm_media Learning Objects
- login Login
- how_to_reg Request Instructor Account
- hub Instructor Commons
- Download Page (PDF)
- Download Full Book (PDF)
- Periodic Table
- Physics Constants
- Scientific Calculator
- Reference & Cite
- Tools expand_more
- Readability
selected template will load here
This action is not available.
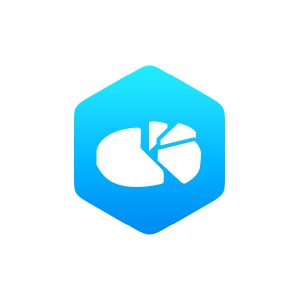
4.4: Hypothesis Testing
- Last updated
- Save as PDF
- Page ID 283
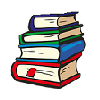
- David Diez, Christopher Barr, & Mine Çetinkaya-Rundel
- OpenIntro Statistics
Is the typical US runner getting faster or slower over time? We consider this question in the context of the Cherry Blossom Run, comparing runners in 2006 and 2012. Technological advances in shoes, training, and diet might suggest runners would be faster in 2012. An opposing viewpoint might say that with the average body mass index on the rise, people tend to run slower. In fact, all of these components might be influencing run time.
In addition to considering run times in this section, we consider a topic near and dear to most students: sleep. A recent study found that college students average about 7 hours of sleep per night.15 However, researchers at a rural college are interested in showing that their students sleep longer than seven hours on average. We investigate this topic in Section 4.3.4.
Hypothesis Testing Framework
The average time for all runners who finished the Cherry Blossom Run in 2006 was 93.29 minutes (93 minutes and about 17 seconds). We want to determine if the run10Samp data set provides strong evidence that the participants in 2012 were faster or slower than those runners in 2006, versus the other possibility that there has been no change. 16 We simplify these three options into two competing hypotheses :
- H 0 : The average 10 mile run time was the same for 2006 and 2012.
- H A : The average 10 mile run time for 2012 was different than that of 2006.
We call H 0 the null hypothesis and H A the alternative hypothesis.
Null and alternative hypotheses
- The null hypothesis (H 0 ) often represents either a skeptical perspective or a claim to be tested.
- The alternative hypothesis (H A ) represents an alternative claim under consideration and is often represented by a range of possible parameter values.
15 theloquitur.com/?p=1161
16 While we could answer this question by examining the entire population data (run10), we only consider the sample data (run10Samp), which is more realistic since we rarely have access to population data.
The null hypothesis often represents a skeptical position or a perspective of no difference. The alternative hypothesis often represents a new perspective, such as the possibility that there has been a change.
Hypothesis testing framework
The skeptic will not reject the null hypothesis (H 0 ), unless the evidence in favor of the alternative hypothesis (H A ) is so strong that she rejects H 0 in favor of H A .
The hypothesis testing framework is a very general tool, and we often use it without a second thought. If a person makes a somewhat unbelievable claim, we are initially skeptical. However, if there is sufficient evidence that supports the claim, we set aside our skepticism and reject the null hypothesis in favor of the alternative. The hallmarks of hypothesis testing are also found in the US court system.
Exercise \(\PageIndex{1}\)
A US court considers two possible claims about a defendant: she is either innocent or guilty. If we set these claims up in a hypothesis framework, which would be the null hypothesis and which the alternative? 17
Jurors examine the evidence to see whether it convincingly shows a defendant is guilty. Even if the jurors leave unconvinced of guilt beyond a reasonable doubt, this does not mean they believe the defendant is innocent. This is also the case with hypothesis testing: even if we fail to reject the null hypothesis, we typically do not accept the null hypothesis as true. Failing to find strong evidence for the alternative hypothesis is not equivalent to accepting the null hypothesis.
17 H 0 : The average cost is $650 per month, \(\mu\) = $650.
In the example with the Cherry Blossom Run, the null hypothesis represents no difference in the average time from 2006 to 2012. The alternative hypothesis represents something new or more interesting: there was a difference, either an increase or a decrease. These hypotheses can be described in mathematical notation using \(\mu_{12}\) as the average run time for 2012:
- H 0 : \(\mu_{12} = 93.29\)
- H A : \(\mu_{12} \ne 93.29\)
where 93.29 minutes (93 minutes and about 17 seconds) is the average 10 mile time for all runners in the 2006 Cherry Blossom Run. Using this mathematical notation, the hypotheses can now be evaluated using statistical tools. We call 93.29 the null value since it represents the value of the parameter if the null hypothesis is true. We will use the run10Samp data set to evaluate the hypothesis test.
Testing Hypotheses using Confidence Intervals
We can start the evaluation of the hypothesis setup by comparing 2006 and 2012 run times using a point estimate from the 2012 sample: \(\bar {x}_{12} = 95.61\) minutes. This estimate suggests the average time is actually longer than the 2006 time, 93.29 minutes. However, to evaluate whether this provides strong evidence that there has been a change, we must consider the uncertainty associated with \(\bar {x}_{12}\).
1 6 The jury considers whether the evidence is so convincing (strong) that there is no reasonable doubt regarding the person's guilt; in such a case, the jury rejects innocence (the null hypothesis) and concludes the defendant is guilty (alternative hypothesis).
We learned in Section 4.1 that there is fluctuation from one sample to another, and it is very unlikely that the sample mean will be exactly equal to our parameter; we should not expect \(\bar {x}_{12}\) to exactly equal \(\mu_{12}\). Given that \(\bar {x}_{12} = 95.61\), it might still be possible that the population average in 2012 has remained unchanged from 2006. The difference between \(\bar {x}_{12}\) and 93.29 could be due to sampling variation, i.e. the variability associated with the point estimate when we take a random sample.
In Section 4.2, confidence intervals were introduced as a way to find a range of plausible values for the population mean. Based on run10Samp, a 95% confidence interval for the 2012 population mean, \(\mu_{12}\), was calculated as
\[(92.45, 98.77)\]
Because the 2006 mean, 93.29, falls in the range of plausible values, we cannot say the null hypothesis is implausible. That is, we failed to reject the null hypothesis, H 0 .
Double negatives can sometimes be used in statistics
In many statistical explanations, we use double negatives. For instance, we might say that the null hypothesis is not implausible or we failed to reject the null hypothesis. Double negatives are used to communicate that while we are not rejecting a position, we are also not saying it is correct.
Example \(\PageIndex{1}\)
Next consider whether there is strong evidence that the average age of runners has changed from 2006 to 2012 in the Cherry Blossom Run. In 2006, the average age was 36.13 years, and in the 2012 run10Samp data set, the average was 35.05 years with a standard deviation of 8.97 years for 100 runners.
First, set up the hypotheses:
- H 0 : The average age of runners has not changed from 2006 to 2012, \(\mu_{age} = 36.13.\)
- H A : The average age of runners has changed from 2006 to 2012, \(\mu _{age} 6 \ne 36.13.\)
We have previously veri ed conditions for this data set. The normal model may be applied to \(\bar {y}\) and the estimate of SE should be very accurate. Using the sample mean and standard error, we can construct a 95% con dence interval for \(\mu _{age}\) to determine if there is sufficient evidence to reject H 0 :
\[\bar{y} \pm 1.96 \times \dfrac {s}{\sqrt {100}} \rightarrow 35.05 \pm 1.96 \times 0.90 \rightarrow (33.29, 36.81)\]
This confidence interval contains the null value, 36.13. Because 36.13 is not implausible, we cannot reject the null hypothesis. We have not found strong evidence that the average age is different than 36.13 years.
Exercise \(\PageIndex{2}\)
Colleges frequently provide estimates of student expenses such as housing. A consultant hired by a community college claimed that the average student housing expense was $650 per month. What are the null and alternative hypotheses to test whether this claim is accurate? 18
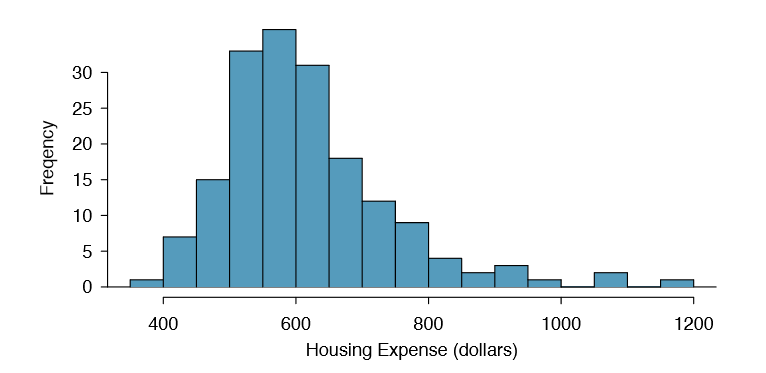
H A : The average cost is different than $650 per month, \(\mu \ne\) $650.
18 Applying the normal model requires that certain conditions are met. Because the data are a simple random sample and the sample (presumably) represents no more than 10% of all students at the college, the observations are independent. The sample size is also sufficiently large (n = 75) and the data exhibit only moderate skew. Thus, the normal model may be applied to the sample mean.
Exercise \(\PageIndex{3}\)
The community college decides to collect data to evaluate the $650 per month claim. They take a random sample of 75 students at their school and obtain the data represented in Figure 4.11. Can we apply the normal model to the sample mean?
If the court makes a Type 1 Error, this means the defendant is innocent (H 0 true) but wrongly convicted. A Type 2 Error means the court failed to reject H 0 (i.e. failed to convict the person) when she was in fact guilty (H A true).
Example \(\PageIndex{2}\)
The sample mean for student housing is $611.63 and the sample standard deviation is $132.85. Construct a 95% confidence interval for the population mean and evaluate the hypotheses of Exercise 4.22.
The standard error associated with the mean may be estimated using the sample standard deviation divided by the square root of the sample size. Recall that n = 75 students were sampled.
\[ SE = \dfrac {s}{\sqrt {n}} = \dfrac {132.85}{\sqrt {75}} = 15.34\]
You showed in Exercise 4.23 that the normal model may be applied to the sample mean. This ensures a 95% confidence interval may be accurately constructed:
\[\bar {x} \pm z*SE \rightarrow 611.63 \pm 1.96 \times 15.34 \times (581.56, 641.70)\]
Because the null value $650 is not in the confidence interval, a true mean of $650 is implausible and we reject the null hypothesis. The data provide statistically significant evidence that the actual average housing expense is less than $650 per month.
Decision Errors
Hypothesis tests are not flawless. Just think of the court system: innocent people are sometimes wrongly convicted and the guilty sometimes walk free. Similarly, we can make a wrong decision in statistical hypothesis tests. However, the difference is that we have the tools necessary to quantify how often we make such errors.
There are two competing hypotheses: the null and the alternative. In a hypothesis test, we make a statement about which one might be true, but we might choose incorrectly. There are four possible scenarios in a hypothesis test, which are summarized in Table 4.12.
A Type 1 Error is rejecting the null hypothesis when H0 is actually true. A Type 2 Error is failing to reject the null hypothesis when the alternative is actually true.
Exercise 4.25
In a US court, the defendant is either innocent (H 0 ) or guilty (H A ). What does a Type 1 Error represent in this context? What does a Type 2 Error represent? Table 4.12 may be useful.
To lower the Type 1 Error rate, we might raise our standard for conviction from "beyond a reasonable doubt" to "beyond a conceivable doubt" so fewer people would be wrongly convicted. However, this would also make it more difficult to convict the people who are actually guilty, so we would make more Type 2 Errors.
Exercise 4.26
How could we reduce the Type 1 Error rate in US courts? What influence would this have on the Type 2 Error rate?
To lower the Type 2 Error rate, we want to convict more guilty people. We could lower the standards for conviction from "beyond a reasonable doubt" to "beyond a little doubt". Lowering the bar for guilt will also result in more wrongful convictions, raising the Type 1 Error rate.
Exercise 4.27
How could we reduce the Type 2 Error rate in US courts? What influence would this have on the Type 1 Error rate?
A skeptic would have no reason to believe that sleep patterns at this school are different than the sleep patterns at another school.
Exercises 4.25-4.27 provide an important lesson:
If we reduce how often we make one type of error, we generally make more of the other type.
Hypothesis testing is built around rejecting or failing to reject the null hypothesis. That is, we do not reject H 0 unless we have strong evidence. But what precisely does strong evidence mean? As a general rule of thumb, for those cases where the null hypothesis is actually true, we do not want to incorrectly reject H 0 more than 5% of the time. This corresponds to a significance level of 0.05. We often write the significance level using \(\alpha\) (the Greek letter alpha): \(\alpha = 0.05.\) We discuss the appropriateness of different significance levels in Section 4.3.6.
If we use a 95% confidence interval to test a hypothesis where the null hypothesis is true, we will make an error whenever the point estimate is at least 1.96 standard errors away from the population parameter. This happens about 5% of the time (2.5% in each tail). Similarly, using a 99% con dence interval to evaluate a hypothesis is equivalent to a significance level of \(\alpha = 0.01\).
A confidence interval is, in one sense, simplistic in the world of hypothesis tests. Consider the following two scenarios:
- The null value (the parameter value under the null hypothesis) is in the 95% confidence interval but just barely, so we would not reject H 0 . However, we might like to somehow say, quantitatively, that it was a close decision.
- The null value is very far outside of the interval, so we reject H 0 . However, we want to communicate that, not only did we reject the null hypothesis, but it wasn't even close. Such a case is depicted in Figure 4.13.
In Section 4.3.4, we introduce a tool called the p-value that will be helpful in these cases. The p-value method also extends to hypothesis tests where con dence intervals cannot be easily constructed or applied.
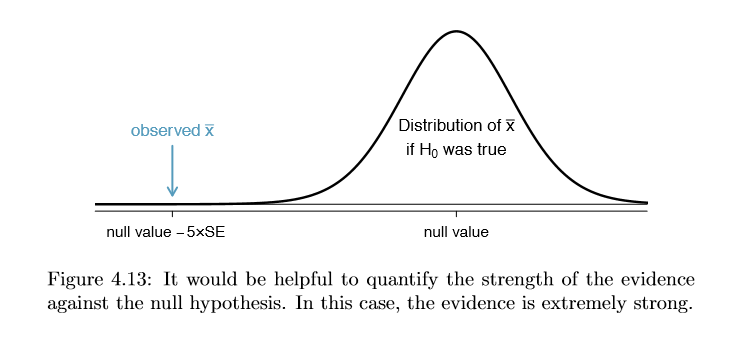
Formal Testing using p-Values
The p-value is a way of quantifying the strength of the evidence against the null hypothesis and in favor of the alternative. Formally the p-value is a conditional probability.
definition: p-value
The p-value is the probability of observing data at least as favorable to the alternative hypothesis as our current data set, if the null hypothesis is true. We typically use a summary statistic of the data, in this chapter the sample mean, to help compute the p-value and evaluate the hypotheses.
A poll by the National Sleep Foundation found that college students average about 7 hours of sleep per night. Researchers at a rural school are interested in showing that students at their school sleep longer than seven hours on average, and they would like to demonstrate this using a sample of students. What would be an appropriate skeptical position for this research?
This is entirely based on the interests of the researchers. Had they been only interested in the opposite case - showing that their students were actually averaging fewer than seven hours of sleep but not interested in showing more than 7 hours - then our setup would have set the alternative as \(\mu < 7\).
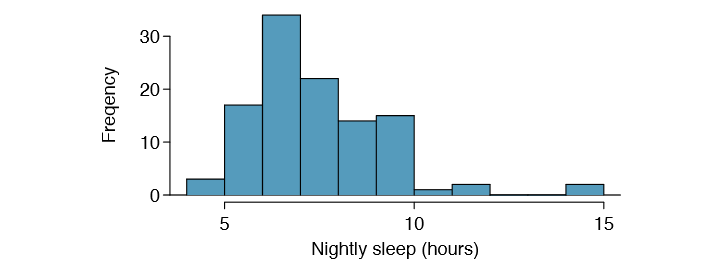
We can set up the null hypothesis for this test as a skeptical perspective: the students at this school average 7 hours of sleep per night. The alternative hypothesis takes a new form reflecting the interests of the research: the students average more than 7 hours of sleep. We can write these hypotheses as
- H 0 : \(\mu\) = 7.
- H A : \(\mu\) > 7.
Using \(\mu\) > 7 as the alternative is an example of a one-sided hypothesis test. In this investigation, there is no apparent interest in learning whether the mean is less than 7 hours. (The standard error can be estimated from the sample standard deviation and the sample size: \(SE_{\bar {x}} = \dfrac {s_x}{\sqrt {n}} = \dfrac {1.75}{\sqrt {110}} = 0.17\)). Earlier we encountered a two-sided hypothesis where we looked for any clear difference, greater than or less than the null value.
Always use a two-sided test unless it was made clear prior to data collection that the test should be one-sided. Switching a two-sided test to a one-sided test after observing the data is dangerous because it can inflate the Type 1 Error rate.
TIP: One-sided and two-sided tests
If the researchers are only interested in showing an increase or a decrease, but not both, use a one-sided test. If the researchers would be interested in any difference from the null value - an increase or decrease - then the test should be two-sided.
TIP: Always write the null hypothesis as an equality
We will find it most useful if we always list the null hypothesis as an equality (e.g. \(\mu\) = 7) while the alternative always uses an inequality (e.g. \(\mu \ne 7, \mu > 7, or \mu < 7)\).
The researchers at the rural school conducted a simple random sample of n = 110 students on campus. They found that these students averaged 7.42 hours of sleep and the standard deviation of the amount of sleep for the students was 1.75 hours. A histogram of the sample is shown in Figure 4.14.
Before we can use a normal model for the sample mean or compute the standard error of the sample mean, we must verify conditions. (1) Because this is a simple random sample from less than 10% of the student body, the observations are independent. (2) The sample size in the sleep study is sufficiently large since it is greater than 30. (3) The data show moderate skew in Figure 4.14 and the presence of a couple of outliers. This skew and the outliers (which are not too extreme) are acceptable for a sample size of n = 110. With these conditions veri ed, the normal model can be safely applied to \(\bar {x}\) and the estimated standard error will be very accurate.
What is the standard deviation associated with \(\bar {x}\)? That is, estimate the standard error of \(\bar {x}\). 25
The hypothesis test will be evaluated using a significance level of \(\alpha = 0.05\). We want to consider the data under the scenario that the null hypothesis is true. In this case, the sample mean is from a distribution that is nearly normal and has mean 7 and standard deviation of about 0.17. Such a distribution is shown in Figure 4.15.
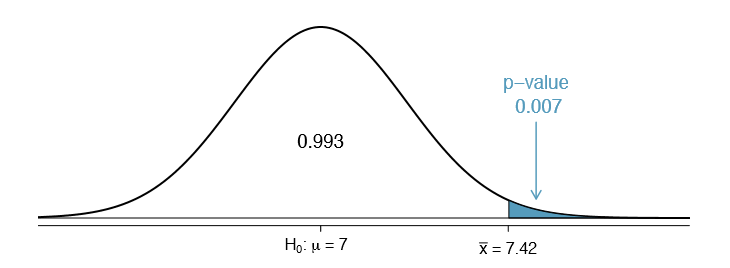
The shaded tail in Figure 4.15 represents the chance of observing such a large mean, conditional on the null hypothesis being true. That is, the shaded tail represents the p-value. We shade all means larger than our sample mean, \(\bar {x} = 7.42\), because they are more favorable to the alternative hypothesis than the observed mean.
We compute the p-value by finding the tail area of this normal distribution, which we learned to do in Section 3.1. First compute the Z score of the sample mean, \(\bar {x} = 7.42\):
\[Z = \dfrac {\bar {x} - \text {null value}}{SE_{\bar {x}}} = \dfrac {7.42 - 7}{0.17} = 2.47\]
Using the normal probability table, the lower unshaded area is found to be 0.993. Thus the shaded area is 1 - 0.993 = 0.007. If the null hypothesis is true, the probability of observing such a large sample mean for a sample of 110 students is only 0.007. That is, if the null hypothesis is true, we would not often see such a large mean.
We evaluate the hypotheses by comparing the p-value to the significance level. Because the p-value is less than the significance level \((p-value = 0.007 < 0.05 = \alpha)\), we reject the null hypothesis. What we observed is so unusual with respect to the null hypothesis that it casts serious doubt on H 0 and provides strong evidence favoring H A .
p-value as a tool in hypothesis testing
The p-value quantifies how strongly the data favor H A over H 0 . A small p-value (usually < 0.05) corresponds to sufficient evidence to reject H 0 in favor of H A .
TIP: It is useful to First draw a picture to find the p-value
It is useful to draw a picture of the distribution of \(\bar {x}\) as though H 0 was true (i.e. \(\mu\) equals the null value), and shade the region (or regions) of sample means that are at least as favorable to the alternative hypothesis. These shaded regions represent the p-value.
The ideas below review the process of evaluating hypothesis tests with p-values:
- The null hypothesis represents a skeptic's position or a position of no difference. We reject this position only if the evidence strongly favors H A .
- A small p-value means that if the null hypothesis is true, there is a low probability of seeing a point estimate at least as extreme as the one we saw. We interpret this as strong evidence in favor of the alternative.
- We reject the null hypothesis if the p-value is smaller than the significance level, \(\alpha\), which is usually 0.05. Otherwise, we fail to reject H 0 .
- We should always state the conclusion of the hypothesis test in plain language so non-statisticians can also understand the results.
The p-value is constructed in such a way that we can directly compare it to the significance level ( \(\alpha\)) to determine whether or not to reject H 0 . This method ensures that the Type 1 Error rate does not exceed the significance level standard.
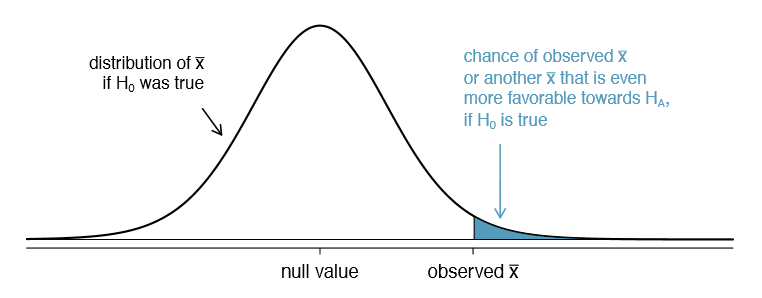
If the null hypothesis is true, how often should the p-value be less than 0.05?
About 5% of the time. If the null hypothesis is true, then the data only has a 5% chance of being in the 5% of data most favorable to H A .
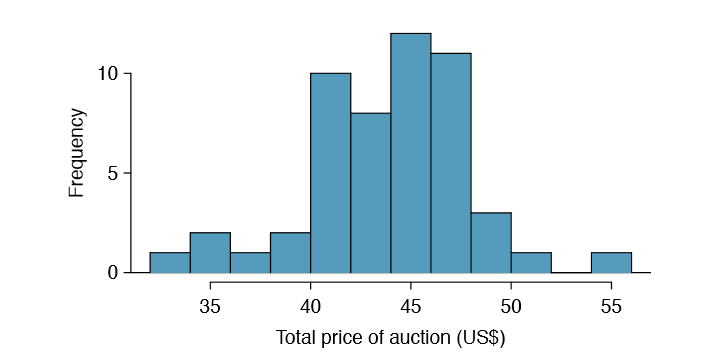
Exercise 4.31
Suppose we had used a significance level of 0.01 in the sleep study. Would the evidence have been strong enough to reject the null hypothesis? (The p-value was 0.007.) What if the significance level was \(\alpha = 0.001\)? 27
27 We reject the null hypothesis whenever p-value < \(\alpha\). Thus, we would still reject the null hypothesis if \(\alpha = 0.01\) but not if the significance level had been \(\alpha = 0.001\).
Exercise 4.32
Ebay might be interested in showing that buyers on its site tend to pay less than they would for the corresponding new item on Amazon. We'll research this topic for one particular product: a video game called Mario Kart for the Nintendo Wii. During early October 2009, Amazon sold this game for $46.99. Set up an appropriate (one-sided!) hypothesis test to check the claim that Ebay buyers pay less during auctions at this same time. 28
28 The skeptic would say the average is the same on Ebay, and we are interested in showing the average price is lower.
Exercise 4.33
During early October, 2009, 52 Ebay auctions were recorded for Mario Kart.29 The total prices for the auctions are presented using a histogram in Figure 4.17, and we may like to apply the normal model to the sample mean. Check the three conditions required for applying the normal model: (1) independence, (2) at least 30 observations, and (3) the data are not strongly skewed. 30
30 (1) The independence condition is unclear. We will make the assumption that the observations are independent, which we should report with any nal results. (2) The sample size is sufficiently large: \(n = 52 \ge 30\). (3) The data distribution is not strongly skewed; it is approximately symmetric.
H 0 : The average auction price on Ebay is equal to (or more than) the price on Amazon. We write only the equality in the statistical notation: \(\mu_{ebay} = 46.99\).
H A : The average price on Ebay is less than the price on Amazon, \(\mu _{ebay} < 46.99\).
29 These data were collected by OpenIntro staff.
Example 4.34
The average sale price of the 52 Ebay auctions for Wii Mario Kart was $44.17 with a standard deviation of $4.15. Does this provide sufficient evidence to reject the null hypothesis in Exercise 4.32? Use a significance level of \(\alpha = 0.01\).
The hypotheses were set up and the conditions were checked in Exercises 4.32 and 4.33. The next step is to find the standard error of the sample mean and produce a sketch to help find the p-value.
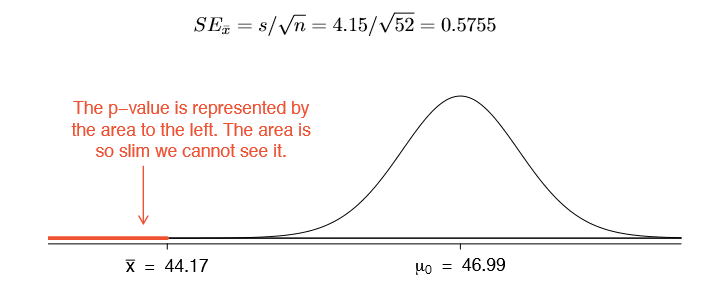
Because the alternative hypothesis says we are looking for a smaller mean, we shade the lower tail. We find this shaded area by using the Z score and normal probability table: \(Z = \dfrac {44.17 \times 46.99}{0.5755} = -4.90\), which has area less than 0.0002. The area is so small we cannot really see it on the picture. This lower tail area corresponds to the p-value.
Because the p-value is so small - specifically, smaller than = 0.01 - this provides sufficiently strong evidence to reject the null hypothesis in favor of the alternative. The data provide statistically signi cant evidence that the average price on Ebay is lower than Amazon's asking price.
Two-sided hypothesis testing with p-values
We now consider how to compute a p-value for a two-sided test. In one-sided tests, we shade the single tail in the direction of the alternative hypothesis. For example, when the alternative had the form \(\mu\) > 7, then the p-value was represented by the upper tail (Figure 4.16). When the alternative was \(\mu\) < 46.99, the p-value was the lower tail (Exercise 4.32). In a two-sided test, we shade two tails since evidence in either direction is favorable to H A .
Exercise 4.35 Earlier we talked about a research group investigating whether the students at their school slept longer than 7 hours each night. Let's consider a second group of researchers who want to evaluate whether the students at their college differ from the norm of 7 hours. Write the null and alternative hypotheses for this investigation. 31
Example 4.36 The second college randomly samples 72 students and nds a mean of \(\bar {x} = 6.83\) hours and a standard deviation of s = 1.8 hours. Does this provide strong evidence against H 0 in Exercise 4.35? Use a significance level of \(\alpha = 0.05\).
First, we must verify assumptions. (1) A simple random sample of less than 10% of the student body means the observations are independent. (2) The sample size is 72, which is greater than 30. (3) Based on the earlier distribution and what we already know about college student sleep habits, the distribution is probably not strongly skewed.
Next we can compute the standard error \((SE_{\bar {x}} = \dfrac {s}{\sqrt {n}} = 0.21)\) of the estimate and create a picture to represent the p-value, shown in Figure 4.18. Both tails are shaded.
31 Because the researchers are interested in any difference, they should use a two-sided setup: H 0 : \(\mu\) = 7, H A : \(\mu \ne 7.\)
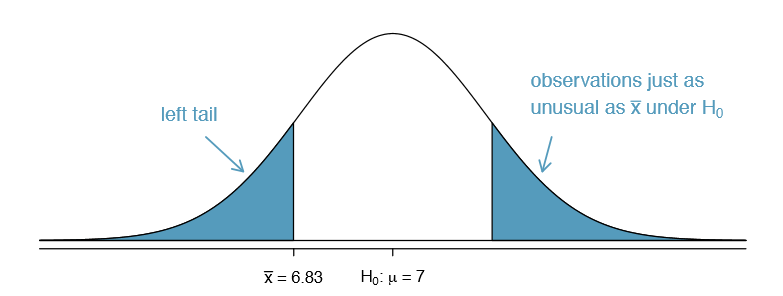
An estimate of 7.17 or more provides at least as strong of evidence against the null hypothesis and in favor of the alternative as the observed estimate, \(\bar {x} = 6.83\).
We can calculate the tail areas by rst nding the lower tail corresponding to \(\bar {x}\):
\[Z = \dfrac {6.83 - 7.00}{0.21} = -0.81 \xrightarrow {table} \text {left tail} = 0.2090\]
Because the normal model is symmetric, the right tail will have the same area as the left tail. The p-value is found as the sum of the two shaded tails:
\[ \text {p-value} = \text {left tail} + \text {right tail} = 2 \times \text {(left tail)} = 0.4180\]
This p-value is relatively large (larger than \(\mu\)= 0.05), so we should not reject H 0 . That is, if H 0 is true, it would not be very unusual to see a sample mean this far from 7 hours simply due to sampling variation. Thus, we do not have sufficient evidence to conclude that the mean is different than 7 hours.
Example 4.37 It is never okay to change two-sided tests to one-sided tests after observing the data. In this example we explore the consequences of ignoring this advice. Using \(\alpha = 0.05\), we show that freely switching from two-sided tests to onesided tests will cause us to make twice as many Type 1 Errors as intended.
Suppose the sample mean was larger than the null value, \(\mu_0\) (e.g. \(\mu_0\) would represent 7 if H 0 : \(\mu\) = 7). Then if we can ip to a one-sided test, we would use H A : \(\mu > \mu_0\). Now if we obtain any observation with a Z score greater than 1.65, we would reject H 0 . If the null hypothesis is true, we incorrectly reject the null hypothesis about 5% of the time when the sample mean is above the null value, as shown in Figure 4.19.
Suppose the sample mean was smaller than the null value. Then if we change to a one-sided test, we would use H A : \(\mu < \mu_0\). If \(\bar {x}\) had a Z score smaller than -1.65, we would reject H 0 . If the null hypothesis is true, then we would observe such a case about 5% of the time.
By examining these two scenarios, we can determine that we will make a Type 1 Error 5% + 5% = 10% of the time if we are allowed to swap to the "best" one-sided test for the data. This is twice the error rate we prescribed with our significance level: \(\alpha = 0.05\) (!).
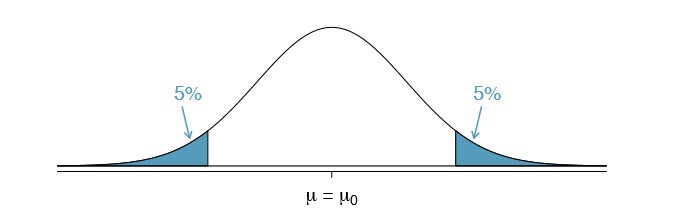
Caution: One-sided hypotheses are allowed only before seeing data
After observing data, it is tempting to turn a two-sided test into a one-sided test. Avoid this temptation. Hypotheses must be set up before observing the data. If they are not, the test must be two-sided.
Choosing a Significance Level
Choosing a significance level for a test is important in many contexts, and the traditional level is 0.05. However, it is often helpful to adjust the significance level based on the application. We may select a level that is smaller or larger than 0.05 depending on the consequences of any conclusions reached from the test.
- If making a Type 1 Error is dangerous or especially costly, we should choose a small significance level (e.g. 0.01). Under this scenario we want to be very cautious about rejecting the null hypothesis, so we demand very strong evidence favoring H A before we would reject H 0 .
- If a Type 2 Error is relatively more dangerous or much more costly than a Type 1 Error, then we should choose a higher significance level (e.g. 0.10). Here we want to be cautious about failing to reject H 0 when the null is actually false. We will discuss this particular case in greater detail in Section 4.6.
Significance levels should reflect consequences of errors
The significance level selected for a test should reflect the consequences associated with Type 1 and Type 2 Errors.
Example 4.38
A car manufacturer is considering a higher quality but more expensive supplier for window parts in its vehicles. They sample a number of parts from their current supplier and also parts from the new supplier. They decide that if the high quality parts will last more than 12% longer, it makes nancial sense to switch to this more expensive supplier. Is there good reason to modify the significance level in such a hypothesis test?
The null hypothesis is that the more expensive parts last no more than 12% longer while the alternative is that they do last more than 12% longer. This decision is just one of the many regular factors that have a marginal impact on the car and company. A significancelevel of 0.05 seems reasonable since neither a Type 1 or Type 2 error should be dangerous or (relatively) much more expensive.
Example 4.39
The same car manufacturer is considering a slightly more expensive supplier for parts related to safety, not windows. If the durability of these safety components is shown to be better than the current supplier, they will switch manufacturers. Is there good reason to modify the significance level in such an evaluation?
The null hypothesis would be that the suppliers' parts are equally reliable. Because safety is involved, the car company should be eager to switch to the slightly more expensive manufacturer (reject H 0 ) even if the evidence of increased safety is only moderately strong. A slightly larger significance level, such as \(\mu = 0.10\), might be appropriate.
Exercise 4.40
A part inside of a machine is very expensive to replace. However, the machine usually functions properly even if this part is broken, so the part is replaced only if we are extremely certain it is broken based on a series of measurements. Identify appropriate hypotheses for this test (in plain language) and suggest an appropriate significance level. 32

Crime Analysis for Problem Solvers in 60 Small Steps
Step 20: formulate hypotheses.
Whenever we confront some new and perplexing crime pattern we form hypotheses about its causes, often based on incomplete information. Experience and theory are good sources of hypotheses. You should (1) clearly state your hypotheses, (2) not be wedded to them, and (3) use data to objectively test them. Expect all hypotheses to be altered or discarded once relevant data have been examined because no hypothesis is completely right. For this reason it is often best to test multiple conflicting hypotheses.
A set of hypotheses is a roadmap for analysis. Hypotheses suggest types of data to collect, how this data should be analyzed, and how to interpret analysis results. If you were investigating drinking-related assaults in bars you might begin with the question, "How many bars are problem locations?" Based on the 80-20 rule (Step 18), you would state the hypothesis that some bars will have many fights, but most will have few or none. You would then test this hypothesis by listing the licensed drinking places and counting the number of assault reports at each over the last 12 months.
If your hypothesis was supported, you might ask the question, "What is different about the bars with many fights compared to the bars with few assaults?" The concept of risky facilities (Step 28) would help you form a set of three hypotheses:
- Risky bars have more customers.
- Risky bars have features that attract assaulters.
- Bar staff in risky bars either fail to control behaviors, or provoke fights.
You can test these hypotheses by gathering data on the number of customers at high- and low-risk bars, analyzing the number and rate of assaults per customer, observing the interactions of people at troublesome and trouble-free bars, and interviewing staff and customers.
If your first hypothesis was contradicted by the data, and you found that there was no great difference in numbers of assaults across drinking establishments, then you might ask the question, why are so many bars troublesome? This suggests another hypothesis: It's a perception problem; the city has about as many bar assaults as other comparable cities. This hypothesis suggests that you will need data from comparable cities.
If, after you collected the relevant data, you found that your city has an abnormally high number of problem bars, you might ask the question, "What is common to most bars in the city that produces a large number of assaults?" One hypothesis is that it is the way liquor licenses are dispensed and bars regulated. Another hypothesis is that there is something about the nature of bar customers in your city. Testing each would require you to collect relevant data and assess the validity of the hypothesis.
Notice how the questions and hypotheses structure the analysis. Test results - positive or negative - reveal new, more specific questions. The objective is to start with broad questions and hypotheses and, through a pruning process, come to a set of highly focused questions that point to possible responses.
Hypotheses suggest the type of data to collect. In the bar assaults example, the test of each hypothesis requires specific data. Sometimes the same data can test multiple hypotheses (as is the case with choosing among the three alternative explanations for risky bars). Often a variety of data is required to select among alternative hypotheses (as is the case with the last set of hypotheses). The more specific your hypotheses, the more focused your data collection will be. This is why it is more important to have a clear hypothesis you personally dislike, than an unclear hypothesis you approve of, or worse, no hypothesis at all.
Paralysis by Analysis
The lack of explicit hypotheses can lead to "paralysis by analysis," collecting too much data, conducting too much analysis, and not coming to any useful conclusion.
Hypotheses can help direct the analysis of data. Every clear hypothesis suggests a pattern of data that you should be able to observe, if the hypothesis is correct. In the example above, the hypotheses derived from the concept of risky facilities can be tested using a simple analytical procedure. If a bar is a crime generator, then you should see a high number of assaults, a high number of customers, but a low assault rate (see Step 17). Failure to find this pattern suggests the hypothesis is wrong. So it is important to have a clear idea of what you should observe if your hypothesis is correct, and what you should observe if your hypothesis is wrong (see third column of the table). If you cannot do this, then this is an indicator that your hypothesis may be too vague.
Hypotheses help interpret the analysis results. Let's assume that the analysis of bar fights showed that a few bars had most fights, and observations of the high- and low-risk bars indicated that the security staff of the risky bars provoked fights. This immediately suggests a possible avenue for intervention. In short, the validity of a hypothesis must make a difference. That is, if the hypothesis is true you will take a different decision than if it is false. If you will make the same decision regardless of the test results, then the hypothesis and its test are irrelevant.
In summary, hypotheses are important for guiding analysis. To formulate hypotheses you need to ask important questions, then create simple and direct speculative answers to these questions. These answers are your hypotheses. These speculations must be bold enough that they could be wrong, and there must be a way of showing whether they are right or wrong. If possible, create competing hypotheses.
Hypothesis formation is a useful group exercise, as it allows participants with contrary views to put their perspectives on the table in a way that allows clear and objective tests. In this way, participants contributing invalid hypotheses make substantial contributions to the analysis of the problem. If each hypothesis is linked to a potential solution, the test of these hypotheses simultaneously directs attention to feasible responses and rules out ineffective approaches.
Questions, Hypotheses, and Tests
- Acknowledgements
Module: Inference for One Proportion
Hypothesis testing (1 of 5), given a claim about a population, construct an appropriate set of hypotheses to test and properly interpret p values and type i / ii errors., learning objectives.
- When testing a claim, distinguish among situations involving one population mean, one population proportion, two population means, or two population proportions.
- Given a claim about a population, determine null and alternative hypotheses.
Introduction
In inference, we use a sample to draw a conclusion about a population. Two types of inference are the focus of our work in this course:
- Estimate a population parameter with a confidence interval.
- Test a claim about a population parameter with a hypothesis test.
We can also use samples from two populations to compare those populations. In this situation, the two types of inference focus on differences in the parameters.
- Estimate a difference in population parameters with a confidence interval.
- Test a claim about a difference in population parameters with a hypothesis test.
In “Estimating a Population Proportion,” we learned to estimate a population proportion using a confidence interval. For example, we estimated the proportion of all Tallahassee Community College students who are female and the proportion of all American adults who used the Internet to obtain medical information in the previous month. We will revisit confidence intervals in future modules.
Now we look more carefully at how to test a claim with a hypothesis test. Statistical investigations begin with research questions. We begin our discussion of hypothesis tests with research questions that require us to test a claim. Later we look at how a claim becomes a hypothesis.
Research Questions about Testing Claims
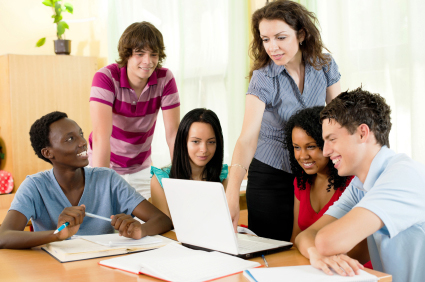
Let’s revisit some of the research questions from examples in the module Types of Statistical Studies and Producing Data that involve testing a claim.
Is the average course load for community college students less than 12 semester hours? This question contains a claim about a population mean. The question contains information about the population, the variable, and the parameter. The population is all community college students. The variable is course load in semester hours . It is quantitative, so the parameter is a mean. The claim is, “The mean course load for all community college students is less than 12 semester hours.”
Do the majority of community college students qualify for federal student loans? This question contains a claim about a population proportion and information about the population, the variable, and the parameter. The population is all community college students. The variable is Qualify for federal student loan (yes or no). It is categorical, so the parameter is a proportion. The claim is, “The proportion of community college students who qualify is greater than 0.5” (a majority means more than half, or 0.5).
In community colleges, do female students and male students have different mean GPAs? This question contains a claim that compares two population means. Again, we see information about the populations, the variable, and the parameters. The two populations are female community college students and male community college students. The variable is GPA . It is quantitative, so the parameters are means. The claim is, “The mean GPA for female community college students is different from the mean GPA for male community college students.” Notice that the claim compares the two population means, but there is no claim about the numeric value of either mean.
Are college athletes more likely than nonathletes to receive academic advising? This question contains a claim that compares two population proportions: college athletes and college students who are not athletes. The variable is Receive academic advising (yes or no). The variable is categorical, so the parameters are proportions. The claim is, “The proportion of all college athletes who receive academic advising is greater than the proportion of all nonathletes in college who receive academic advising.” Notice that the claim compares two population proportions, but there is no claim about the numeric value of either proportion.
In the case of testing a claim about a single population parameter, we compare it to a numeric value. In the case of testing a claim about two population parameters, we compare them to each other.
Learn By Doing
Identify the type of claim in each research question below.
Next Steps: Forming Hypotheses
We already know that in inference we use a sample to draw a conclusion about a population. If the research question contains a claim about the population, we translate the claim into two related hypotheses.
The null hypothesis is a hypothesis about the value of the parameter. The null hypothesis relates to our work in Linking Probability to Statistical Inference where we drew a conclusion about a population parameter on the basis of the sampling distribution. We started with an assumption about the value of the parameter, then used a simulation to simulate the selection of random samples from a population with this parameter value. Or we used the parameter value in a mathematical model to describe the center and spread of the sampling distribution. The null hypothesis gives the value of the parameter that we will use to create the sampling distribution. In this way, the null hypothesis states what we assume to be true about the population.
The alternative hypothesis usually reflects the claim in the research question about the value of the parameter. The alternative hypothesis says the parameter is “greater than” or “less than” or “not equal to” the value we assume to true in the null hypothesis.
Stating Hypotheses
Here are the hypotheses for the research questions from the previous example. The null hypothesis is abbreviated H 0 . The alternative hypothesis is abbreviated H a .
Is the average course load for community college students less than 12 semester hours?
- H 0 : The mean course load for community college students is equal to 12 semester hours.
- H a : The mean course load for community college students is less than 12 semester hours.
Do the majority of community college students qualify for federal student loans?
- H 0 : The proportion of community college students who qualify for federal student loans is 0.5.
- H a : The proportion of community college students who qualify for federal student loans is greater than 0.5.
When the research question contains a claim that compares two populations, the null hypothesis states that the parameters are equal. We will see in Modules 9 and 10 that we translate the null hypothesis into a statement about “no difference” in parameter values. We revisit this idea in more depth later.
In community colleges, do female students and male students have different mean GPAs?
- H 0 : In community colleges, female and male students have the same mean GPAs.
- H a : In community colleges, female and male students have different mean GPAs.
Are college athletes more likely than nonathletes to receive academic advising?
- H 0 : In colleges, the proportion of athletes who receive academic advising is equal to the proportion of nonathletes who receive academic advising.
- H a : In colleges, the proportion of athletes who receive academic advising is greater than the proportion of nonathletes who receive academic advising.
Here are some general observations about null and alternative hypotheses.
- The hypotheses are competing claims about the parameter or about the comparison of parameters.
- Both hypotheses are statements about the same population parameter or same two population parameters.
- The null hypothesis contains an equal sign.
- The alternative hypothesis is always an inequality statement. It contains a “less than” or a “greater than” or a “not equal to” symbol.
- In a statistical investigation, we determine the research question, and thus the hypotheses, before we collect data.
The process of forming hypotheses, collecting data, and using the data to draw a conclusion about the hypotheses is called hypothesis testing .
- Concepts in Statistics. Provided by : Open Learning Initiative. Located at : http://oli.cmu.edu . License : CC BY: Attribution
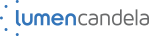
Privacy Policy
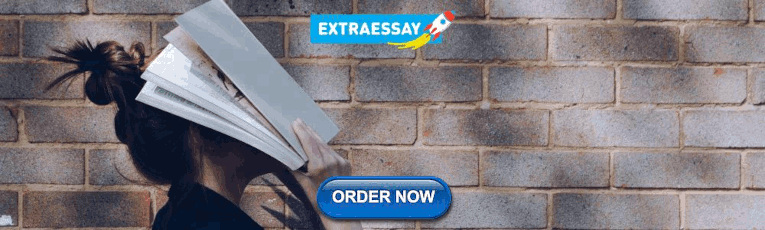
IMAGES
VIDEO
COMMENTS
At CreativeCX, we actually place equal importance on the problem statement and the hypothesis. This ensures that we consider both the customer problem that needs to be solved, as well as the business objectives. All too often, we see companies either neglect the problem statement and hypothesis entirely or favour one over the other.
3. Simple hypothesis. A simple hypothesis is a statement made to reflect the relation between exactly two variables. One independent and one dependent. Consider the example, "Smoking is a prominent cause of lung cancer." The dependent variable, lung cancer, is dependent on the independent variable, smoking. 4.
Problem statement example. Step 1: Contextualize the problem. A family-owned shoe manufacturer has been in business in New England for several generations, employing thousands of local workers in a variety of roles, from assembly to supply-chain to customer service and retail.
A hypothesis is a theoretical, hypothetical explanation that can be tested. It usually takes the form of a causal relationship or a causal explanation. You can also consider the hypothesis as a preliminary response to a research question or a problem statement. A hypothesis can be expressed in different ways, but generally, the following applies:
5. Phrase your hypothesis in three ways. To identify the variables, you can write a simple prediction in if…then form. The first part of the sentence states the independent variable and the second part states the dependent variable. If a first-year student starts attending more lectures, then their exam scores will improve.
2 Complex hypothesis. A complex hypothesis suggests the relationship between more than two variables, for example, two independents and one dependent, or vice versa. Examples: People who both (1) eat a lot of fatty foods and (2) have a family history of health problems are more likely to develop heart diseases.
Merriam Webster defines a hypothesis as "an assumption or concession made for the sake of argument.". In other words, a hypothesis is an educated guess. Scientists make a reasonable assumption--or a hypothesis--then design an experiment to test whether it's true or not.
Step 5: Phrase your hypothesis in three ways. To identify the variables, you can write a simple prediction in if … then form. The first part of the sentence states the independent variable and the second part states the dependent variable. If a first-year student starts attending more lectures, then their exam scores will improve.
Table of contents. Step 1: State your null and alternate hypothesis. Step 2: Collect data. Step 3: Perform a statistical test. Step 4: Decide whether to reject or fail to reject your null hypothesis. Step 5: Present your findings. Other interesting articles. Frequently asked questions about hypothesis testing.
Your problem statement in your research paper aims to: Define the gap: Clearly identify and articulate a specific gap or issue in the existing knowledge. Provide direction: Serve as a roadmap, guiding the course of your research and ensuring you remain focused. Establish relevance: Highlight the importance and significance of the problem in the ...
At the core of biology and other sciences lies a problem-solving approach called the scientific method. The scientific method has five basic steps, plus one feedback step: Make an observation. Ask a question. Form a hypothesis, or testable explanation. Make a prediction based on the hypothesis. Test the prediction.
Examples. A research hypothesis, in its plural form "hypotheses," is a specific, testable prediction about the anticipated results of a study, established at its outset. It is a key component of the scientific method. Hypotheses connect theory to data and guide the research process towards expanding scientific understanding.
Lesson3-2-1. EDR610 : The Class : Variables/Hypotheses : Hypotheses : Lesson3-2-1. Understanding Hypotheses Just to give us an indication of "where we've been & where we're headed ... !" We've seen that the "heart & soul" of research is the research question/problem statement; And in addition, last time we took a look at some key components of ...
Test Statistic: z = x¯¯¯ −μo σ/ n−−√ z = x ¯ − μ o σ / n since it is calculated as part of the testing of the hypothesis. Definition 7.1.4 7.1. 4. p - value: probability that the test statistic will take on more extreme values than the observed test statistic, given that the null hypothesis is true.
Key takeaways. A problem statement is a succinct explanation of a problem or concern that a research study seeks to address. A problem statement aims to offer a detailed method for problem solving and provides recommendations on how the problem can be avoided in the future. The aim of a research study is the rationale behind the study.
Countless hypotheses have been developed and tested throughout the history of science.Several examples include the idea that living organisms develop from nonliving matter, which formed the basis of spontaneous generation, a hypothesis that ultimately was disproved (first in 1668, with the experiments of Italian physician Francesco Redi, and later in 1859, with the experiments of French ...
5.2 - Writing Hypotheses. The first step in conducting a hypothesis test is to write the hypothesis statements that are going to be tested. For each test you will have a null hypothesis ( H 0) and an alternative hypothesis ( H a ). Null Hypothesis. The statement that there is not a difference in the population (s), denoted as H 0.
Example 4.4.1 4.4. 1. Next consider whether there is strong evidence that the average age of runners has changed from 2006 to 2012 in the Cherry Blossom Run. In 2006, the average age was 36.13 years, and in the 2012 run10Samp data set, the average was 35.05 years with a standard deviation of 8.97 years for 100 runners.
According to this literature, a research problem will often be followed by (at least) one research hypothesis. The problem is considered broader than its hypothesis in the sense that the problem has several possible solutions/answers, while the hypothesis corresponds to one or some of the possible solutions.
Glossary. Step 20: Formulate hypothesesWhenever we confront some new and perplexing crime pattern we form hypotheses about its causes, often based on incomplete information. Experience and theory are good sources of hypotheses. You should (1) clearly state your hypotheses, (2) not be wedded to them, and (3) use data to objectively test them.
The null hypothesis gives the value of the parameter that we will use to create the sampling distribution. In this way, the null hypothesis states what we assume to be true about the population. The alternative hypothesis usually reflects the claim in the research question about the value of the parameter. The alternative hypothesis says the ...
2.2.7 Formulating Research Problem If the topic, problem or issue has been defined, this can then be reported as a particular research problem, that is, taken from just a statement about a difficult situation to a clearly described researchable problem that describes the issues you are trying to solve.
The hypothesis/problem statement has been provided. NB For this research, learners have been provided with a formulated hypothesis/ a geographical problem statement. In this case learners will be assessed on their general presentation of the research paper (layout of the paper) and cover page.