Innate immune suppression by SARS-CoV-2 mRNA vaccinations: The role of G-quadruplexes, exosomes, and MicroRNAs
Affiliations.
- 1 Computer Science and Artificial Intelligence Laboratory, MIT, Cambridge, MA, USA, 02139. Electronic address: [email protected].
- 2 Immersion Health, Portland, OR, 97214, USA. Electronic address: [email protected].
- 3 Research and Development, Nasco AD Biotechnology Laboratory, Department of Research and Development, Sachtouri 11, 18536, Piraeus, Greece. Electronic address: [email protected].
- 4 Truth for Health Foundation, Tucson, AZ, USA. Electronic address: [email protected].
- PMID: 35436552
- PMCID: PMC9012513
- DOI: 10.1016/j.fct.2022.113008
The mRNA SARS-CoV-2 vaccines were brought to market in response to the public health crises of Covid-19. The utilization of mRNA vaccines in the context of infectious disease has no precedent. The many alterations in the vaccine mRNA hide the mRNA from cellular defenses and promote a longer biological half-life and high production of spike protein. However, the immune response to the vaccine is very different from that to a SARS-CoV-2 infection. In this paper, we present evidence that vaccination induces a profound impairment in type I interferon signaling, which has diverse adverse consequences to human health. Immune cells that have taken up the vaccine nanoparticles release into circulation large numbers of exosomes containing spike protein along with critical microRNAs that induce a signaling response in recipient cells at distant sites. We also identify potential profound disturbances in regulatory control of protein synthesis and cancer surveillance. These disturbances potentially have a causal link to neurodegenerative disease, myocarditis, immune thrombocytopenia, Bell's palsy, liver disease, impaired adaptive immunity, impaired DNA damage response and tumorigenesis. We show evidence from the VAERS database supporting our hypothesis. We believe a comprehensive risk/benefit assessment of the mRNA vaccines questions them as positive contributors to public health.
Keywords: Cancer; Exosomes; G-quadruplexes; SARS-CoV-2 mRNA vaccines; Type I interferon Response; microRNAs.
Copyright © 2022 The Authors. Published by Elsevier Ltd.. All rights reserved.
- COVID-19 Vaccines / adverse effects
- COVID-19* / prevention & control
- Exosomes* / metabolism
- G-Quadruplexes*
- Immunity, Innate*
- MicroRNAs* / genetics
- MicroRNAs* / metabolism
- Neurodegenerative Diseases* / metabolism
- RNA, Messenger / genetics
- RNA, Messenger / metabolism
- Spike Glycoprotein, Coronavirus
- Vaccination / adverse effects
- Vaccines, Synthetic* / adverse effects
- mRNA Vaccines* / adverse effects
- COVID-19 Vaccines
- RNA, Messenger
- Vaccines, Synthetic
- mRNA Vaccines
- spike protein, SARS-CoV-2
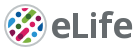
- Inside eLife
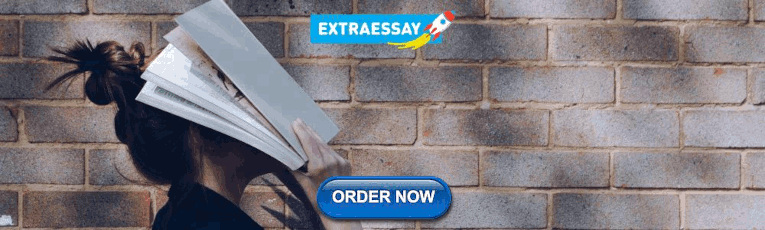
Focus Issue: Call for papers in Trained Immunity
- Open access
- Copyright information
Historically, the concept of immunological memory referred only to the ability of the adaptive immune system to develop specific responses to pathological triggers. However, this concept was challenged in 2012 by a landmark innate immune memory (also known as trained immunity) study in human and mouse experimental systems. Over the last decade, many studies built on these findings and proved that, in addition to the specific memory in adaptive immune cells, innate immune cells and structural cells can develop a non-specific memory state. Innate immune memory is a concept with evolutionary roots, as it is already present in more simple organisms such as plants, worms and insects.
The regulation of innate immune memory is based on two main pillars: metabolic reprogramming and modification of the epigenetic landscape. Numerous studies have identified triggers and regulators of trained immunity, such as certain vaccines, microbial components, metabolites, chemicals, cytokines, pathogens and diets.
Innate immune memory can substantially influence the host immune response to infections or inflammatory diseases. However, while the development of innate immune memory responses can be beneficial in the context of infectious diseases or cancer, it can also act as a double-edged sword and increase host susceptibility to infectious and inflammatory diseases. Thus, we urgently need to further expand our understanding of these host-beneficial versus host-detrimental signatures.
Currently, trained immunity therapeutics are being investigated in multiple settings, from organ transplant acceptance, to sepsis, cancer treatment, and atherosclerosis. However, even after one decade of research, there are still many questions to answer about the fundamental and clinical mechanisms of establishing innate immune memory, its risks, and how it can be used to improve health.
Aims and scope
In this eLife Focus Issue, we aim to curate Review Articles and original research articles on the history, current state, and future directions of innate immune memory studies, and overall provide a critical assessment of this new field of research. We will particularly focus on aspects that provide a better understanding of the mechanisms involving the development, regulation, maintenance and resolution of innate immune memory.
We also welcome research works on inflammatory, metabolic and epigenetic regulation of innate immune memory and its consequences in all kingdoms of life.
We hope this Focus Issue will spark new discussions and open new fields of knowledge leading to potential therapeutic interventions.
Areas of interest
We are particularly interested in research in the following areas:
- Metabolic regulation of innate immune memory
- Epigenetic mechanisms in innate immune memory
- Inflammatory signalling in trained immunity
- Targeting metabolic, epigenetic, or inflammatory signaling as therapeutic tools
- Trained immunity-based vaccines
- Evolutionary aspects of innate immune memory
- Genetics and populational aspects in trained immunity (including gender and age)
- Anti-inflammatory innate immune memory
- Modulation of trained immunity responses through lifestyle and dietary interventions
- Novel inducers of trained immunity
- Novel cell types and non-immune primary cell models of trained immunity
- Definitions of tolerance vs trained immunity vs priming
- Regulatory mechanisms in trained immunity
- Trained immunity in cancer
- Maladaptive innate immune memory
- Therapeutic applications of trained immunity-based approaches
Editors for the Focus Issue
eLife Senior Editors Carla Rothlin, Yale University, United States, and Satyajit Rath , Indian Institute of Science Education and Research (IISER), Pune, India, will oversee the curation of papers alongside Guest Editors.
eLife Senior Editors
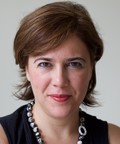
Carla Rothlin (Yale University, United States)
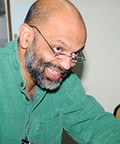
Satyajit Rath (Indian Institute of Science Education and Research (IISER), Pune, India)
Guest Editors
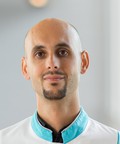
Jorge Dominguez-Andres (Radboud UMC, Netherlands)
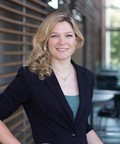
Eva Kaufmann (Queen’s University, Canada)
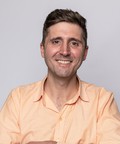
Boris Novakovic (Murdoch Children’s Research Institute, Australia)
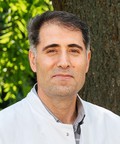
Yahya Sohrabi (University Hospital Münster, Westfälische Wilhelms-Universität, Germany)
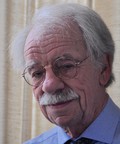
Jos van der Meer (Radboud UMC, Netherlands)
Article types
Submissions of Research Articles, Short Reports, Tools and Resources, and Research Advances are welcomed. A select number of Review Articles will be commissioned by the editors.
Details about eLife's article types are available here .
About eLife
eLife is an interdisciplinary journal committed to improving the way research is reviewed and communicated.
We publish Reviewed Preprints that combine the advantages of preprints with the scrutiny offered by peer review.
Our new publishing process
Papers submitted to eLife will now follow our new model of publishing:
- Submit your research
- Decision to review
- Consultative peer review
- Reviewed Preprint published
All papers invited for review will be published on our website as a Reviewed Preprint, accompanied by an eLife assessment and public reviews.
Reviewed Preprints will typically be online within a few weeks of the review process being completed.
Following publication of your Reviewed Preprint, it is up to you what to do next. Choose to:
- Revise and resubmit
- Declare the paper Version of Record
- Submit to another journal.
Peer review
eLife is working to improve the process of peer review so it more effectively conveys the assessment of expert reviewers to the scientific community, meaning reviews are published publicly alongside your research paper.
At the end of the peer-review process, before publication, authors have two weeks to submit a response to the assessment and reviews that can be published alongside the Reviewed Preprint.
Read more about our new process and publishing at eLife .
Submit your work
You can submit your work through eLife’s submission system .
Please include a cover letter with your submission, highlighting that the paper is for consideration for the Focus Issue on trained immunity and suggest editors from the list above.
All research articles submitted to this Focus Issue will be subject to our $2,000 publication fee , but we also offer full fee waivers where applicable. There will be no charge for Review Articles.
For more information on the submission process, read our author guide .
Submissions close on October 31, 2024 .
We welcome comments and questions from researchers as well as other journals. Please annotate publicly on the article or contact us at hello [at] elifesciences [dot] org.
For the latest in published research sign up for our bi-weekly email alerts . You can also follow eLife on Twitter , Mastodon , Facebook or LinkedIn .
Be the first to read new articles from eLife
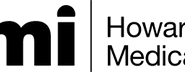

An official website of the United States government
The .gov means it’s official. Federal government websites often end in .gov or .mil. Before sharing sensitive information, make sure you’re on a federal government site.
The site is secure. The https:// ensures that you are connecting to the official website and that any information you provide is encrypted and transmitted securely.
- Publications
- Account settings
Preview improvements coming to the PMC website in October 2024. Learn More or Try it out now .
- Advanced Search
- Journal List
- Front Med (Lausanne)
Boosting the Immune System, From Science to Myth: Analysis the Infosphere With Google
Arthur cassa macedo.
1 Faculdade de Medicina, Universidade Federal de Minas Gerais, Belo Horizonte, Brazil
André Oliveira Vilela de Faria
Pietro ghezzi.
2 Brighton & Sussex Medical School, Brighton, United Kingdom
Associated Data
All datasets generated for this study are included in the manuscript and the supplementary files .
Background: The concept that one can “boost” immunity is a popular one. Although the only evidence-based approach to this is vaccination, the lay public is exposed to a wide range of information on how to boost immunity. The aim of this study was to analyze such information available on the Internet.
Methods and findings: We visited 185 webpages returned from a Google search on “boost immunity” and classified them by typology (blogs, commercial, government, no-profit, news, professional, scientific journals) and by using standard indicators of health information quality (JAMA score, HONCode). We then analyzed their content in terms of disease and “boosters” mentioned. Commercial and news websites represented one third of the results each. Of the 37 approaches to boost immunity recorded, the top ones were diet (77% of webpages), fruit (69%), vitamins (67%), antioxidants (52%), probiotics (51%), minerals (50%), and vitamin C (49%). Interestingly, vaccines ranked 27th, with only 12% of webpages mentioning them.
Conclusions: Commercial websites are an important component of the information available to the public on the topic, and thus contribute providing biased information.
Immunity is the main mechanism of host defense against infectious agents, demonstrated by the enormous success of vaccination in eradicating disease ( 1 ). The concept that vaccines are the most effective means of preventing infection is well-recognized, both by public health authorities and by the public. Daniel Davis wrote, “the public are fascinated by the connections between lifestyle choices and immunity because there may be practical implications for what it takes to be healthy” ( 2 ). There is, in particular, a public interest on increasing immune defense easily. Probably, the most popular belief is about the use of vitamin C to prevent infection, an idea that became popular after a series of scientific articles by Linus Pauling, who advocated the intake of larger amounts than those recommended at the time ( 3 , 4 ).
The popularity, among lay persons, of this concept is such that “Improving the body's immune system” is the top reason for consuming nutritional supplements ( 5 , 6 ). The “immune boosters” market includes vitamins, minerals, antioxidants, probiotics, and “functional foods” as well as other complementary and alternative medicine (CAM) approaches. A study based on the US National Health and Nutrition Examination Survey showed that over 50% of the US population reported the use of supplements ( 7 ). This has a huge commercial impact, with the global market of dietary supplements estimated around 133 billion USD ( 8 ).
An additional issue is whether the use of non-evidence based approaches to boost immunity can be considered an effective alternative to vaccination. This is a particularly important aspect at a time when vaccine hesitancy is becoming a major threat to global health, as indicated by the World Health Organization ( 9 ). A study on 9,000 US children has shown that exposure to some complementary therapies, including chiropractice and other types of alternative medicine (excluding multivitamins/multi-minerals) is associated with a lower uptake if influenza vaccine ( 10 ), although this was not observed in adults ( 11 ). Similar results were obtained in a survey of over 9,000 Australian women, with those using naturopathy or herbal medicine less likely to vaccinate against influenza ( 12 ). It is important to note that, in two of the cited studies, use of multivitamins/multiminerals was associated with a higher vaccination rate ( 10 , 12 ). However, when vaccine confidence was studied in a survey on attitudes to vaccination among 1,250 Australian adults, use of most CAM, including vitamins, was associated with lower levels of vaccination endorsement ( 13 ). It is therefore important to establish what type of information the lay public is exposed to as this is likely to be the basis of their knowledge of the topic and have important consequences on public health.
The present study aimed at obtaining a picture of the information the public is exposed to on this topic. In recent years, it became clear that we live in an online informational environment that Floridi defined as “infosphere” ( 14 ). Because most of the sources of information, including books, news outlets, governmental, and professional organizations, are now available online, we used the Google® search engine to obtain a sample of the information available on the topic, using a methodology we have successfully applied to analyze knowledge about other health-related topics ( 15 – 17 ).
The webpages returned were then analyzed in terms of type of website, whether, for instance, from commercial entities, news outlets, etc. We also analyzed the webpages returned by the search in terms of standard indicators of health information quality (HIQ). The first is the Journal of the American Medical Association (JAMA) score, a widely used HIQ tool where the transparency/trustworthiness of a webpage is assessed for the presence or absence of the following information: author, date, external references, and ownership of the website ( 18 ). We also looked for the presence of the Health On the Net (HON) code certification provided by an independent organization, the HON Foundation, based on a code of conduct comprising several criteria of quality and transparency ( 19 ).
Data Collection and Classification
We searched “boost immunity” in Google.com from Brighton, UK in November 2018. The search was done using the browser Google Chrome®, deleting cookies and browser history to limit personalization of the search results returned, although geolocalization could not be prevented as this is linked to the IP address used for the connection to the Internet. The choice of Google® as a search engine was because it has over 90% of the search engine market share ( 20 ).
We then transferred the first 200 Uniform Resource Locators (URLs) in the search engine result page (SERP) to a spreadsheet. To allow some margin in the transfer of the hyperlink we downloaded the first 204 results. Using a workflow described previously ( 15 – 17 ), we then visited each webpage and read the content. Nineteen pages were excluded because they were clearly irrelevant, not accessible (dead links) or required registration or paywall to access them. The remaining 185 webpages were then analyzed in terms of typology of website, HIQ indicators and content.
Websites were first classified according to their typology, as described in Table 1 . While all other classifications we made were based on the presence of a particular text, assigning a typology may be subjective. Therefore, this was done by two raters and disagreements were discussed until we agreed on a classification. We had a 77% agreement on the classification by typology. The inter-rater agreement gave a Cohen's kappa coefficient of 0.721 with a standard error of 0.035, and a 95% confidence interval, from 0.652 to 0.790. This strength of agreement is considered: “good.” The weighted Kappa was 0.809, considered ‘very good.’ It should be noted, however, that the agreement differed for the typologies and was as follows: blogs, 75%; commercial, 95%; excluded, 90%; government, 100%; health portals, 22%; news, 88%; no profit, 100%; professional, 100%; scientific journals, 75%. This means that the agreement was very good for all typologies but very low for health portals, suggesting that any conclusions made on the latter typology would be very weak.
Examples of websites typologies.
Indicators of Health Information Quality
Each webpage was then evaluated for standard HIQ indicators. The JAMA score was calculated assessing the presence or absence of the following information: author, date, external references, and ownership of the website ( 18 ). For each of these we assigned a score of 1, so that the total JAMA score ranged from 0 to 4. We also looked for the presence of the HONcode certification on the page ( 19 ). When deciding the presence or absence of information we applied the three-click rule, which requires the information to be present, no more than three clicks away from the webpage under analysis ( 21 ).
Content Analysis
A content analysis was then performed, recording the disease mentioned in the webpage, if any, and the type of approach mentioned in the context of boosting immunity. The list was compiled as long as the webpages were read and included supplements, dietary, and lifestyle recommendation, complementary approaches such as chiropractic or yoga, as well as medically-approved approaches such as hygiene or vaccination. Because webpages mentioning, for instance, vitamin C, could mention either eating fresh fruit or buying a vitamin C supplement or some webpages may state you should eat fruit and not supplements, we recorded the stance about supplements, whether negative or positive. Finally, we recorded whether any webpage had a negative stance about vaccines, either anti-vaccine or just vaccine-skeptical.
In some cases, we analyzed the usage of the word “supplement” or “University” in a specific set of webpages. This was done using corpus analysis. Briefly, text corpora were extracted from the webpages to be analyzed using WebBootCaT, an online tool for bootstrapping text corpora from the Internet. Then the concordance of specific words were obtained using the corpus analysis software Sketch Engine by Lexical Computing, Brno-Královo Pole, Czechia ( 22 ). Results are shown as “Key Word In Context,” i.e. a list of all the occurrences of the search word in the webpages with an equal amount of context on the left and on the right.
The spreadsheet with the raw data is available as Supplementary File 1 to allow re-analysis by different methods.
Statistical Analysis
Statistical analysis was performed with GraphPad Prism 7.04 for Windows using the tests described in the text. The number of symptoms mentioned in a specific typology of websites was compared with the rest of the search, rather than with the whole search, to avoid comparing overlapping data. To compare two groups, non-parametric tests were used: a two-tailed Mann-Whitney test for comparing two groups and a two-tailed Kruskal–Wallis test in case(s) of multiple comparisons. Frequency of typologies of websites in the first 10 results in the Google search were compared with that in the rest of the search using a two-tailed Fisher's exact test. The level of significance was set at P < 0.05. When a Fisher's test was performed in multiple comparisons, this was followed by a Benjamini–Hochberg correction set at a false discovery rate of 5%.
Ethical approval was not sought because this research did not involve human participants.
Typology of the Webpages Returned in the SERP
This first analysis looked at the composition of the SERP (185 webpages) and the top 10 webpages returned by Google in terms of typology of websites, which is essentially the details of the public or private organization who owns them. The results in Table 2 show that the two main typologies returned by Google were “commercial” and “news.” However, commercial websites were given a low visibility by Google, at least in this search, and none of them were present in the top 10 results. On the contrary, news websites ranked high, as their frequency in the top 10 was twice that in the total search. The difference in the frequency of commercial and professional websites in the top 10 webpages compared to the rest of the SERP (e.g., those in positions 11–204) was statistically significantly ( P = 0.032 by a two-tailed Fisher test for commercial websites and P = 0.014 for professional websites), while the one for news websites was not. Thus, Google gives a significantly higher visibility to professional websites wile ranking commercial websites significantly lower.
Type of websites in the SERP.
Percent of websites in each typology is shown in parenthesis (no. of webpages in the whole search, 185; in the top 10, 10) .
Standard Indicators of Health Information Quality: JAMA Score and HONcode
We then analyzed each webpage for the indication of authorship, date, ownership of the webpage, and presence of external references (the JAMA score, ranging from 0 to 4 depending on how many of these criteria were met). Figure 1 reports the JAMA score for each typology of websites. Commercial webpages scored the lowest (mean 2.0; median 2, [IQR:1, 3]; n = 60) which was significantly lower than news, blogs, and health portals (respectively, by P < 0.0001, P < 0.01, and P < 0.05 using ANOVA followed by Tukey's multiple comparison test). The JAMA score of commercial webpages was also significantly lower when compared with the rest of the SERP (all other typologies, median 3, [IQR:2, 4]; n = 125) with P < 0.0001 by a two-tailed Mann-Whitney test). Only nine webpages displayed a HONcode certification, of which five were news, four health portals, and one a no-profit. Despite being the largest typology in the SERP, none of the commercial websites displayed a HONcode certification. The conclusion from this analysis is that the information quality of commercial websites, as assessed by two tools that assess webpages' transparency and trustworthiness is lower than in other typologies of websites.

JAMA score of webpages of different typologies. Data are median, IQR, minimum, maximum. Number of webpages for each group was: commercial, 60; News, 59; Blog, 18; Professionals, 16; Health portal, 10; Non-profit organization, 9; Other, 7; Government, 3; Scientific journals, 3.
As the tools to measure HIQ described above do not take into account the scientific content of the webpage, we then visited each webpage looking at which disease conditions and approach to boost immunity they mentioned. Table 3 lists the most frequent conditions or disease processes mentioned in the webpages. We specifically recorded mention of respiratory disease, despite these largely overlap with infectious disease, mainly because of the popularity of the idea that vitamins can prevent influenza and the common cold. Infectious and respiratory disease were the most frequently mentioned in the 185 webpages.
Disease conditions mentioned in the SERP.
Total no. of webpages, 185 .
The frequency of the various approaches mentioned (with a positive or neutral stance) to “boost immunity” is shown in Figure 2 , panel A (for the whole search) and B (for the top 10 webpages). In the whole search, a healthy diet and fruit/vegetables came on top, along with vitamins. Antioxidants, probiotics, minerals, and vitamin C were also frequently mentioned, in half of the webpages or more, however, most of them referred to diet. Supplements (including vitamins) were mentioned in 36% of the webpages. A number of other approaches were mentioned by a small percentage of webpages (<10%), including chocolate, avoiding excess of medications, breastfeeding, cryotherapy, chiropractic, phytoncides, active sexual life, controlling allergies, and sauna (not shown).

Content analysis of the whole SERP (A) and the top-10 webpages (B) . Data show the percentage of webpages ( A , n = 185; B , n = 10) mentioning a specific approach in the context of boosting immunity. Only the approaches that were mentioned in at least 10% of total webpages are shown.
Interestingly, just 23 webpages (12%) mentioned vaccines (also “jab” or “shot” in British and American English, respectively) as a way of boosting immunity. Among these, there were 11 mentions of vaccines in general, 16 of influenza vaccine, two of hepatitis A, two of pneumococcus, two of shingles, one of meningococcus, and one of tetanus-diphtheria-acelluar pertussis vaccine. The prevalence of the influenza vaccine over other types of vaccines is in agreement with the focus on respiratory disease in the webpages, as described earlier ( Table 3 ).
Although the analysis of 185 webpages represents a sample of the information available online, the ranking provided by the search engine is key, as the first results presented to the viewer have a higher chance to be read. In Figure 2 , a comparison between panels A (all 185 webpages) and B (top 10 pages) highlight whether webpages mentioning specific interventions are ranked higher by Google. Diet and fruit, the two most frequently mentioned interventions are equally present in the top 10 pages. Vitamins (including vitamins in general, vitamins C, D, and A), antioxidants, oils, and minerals were mentioned less frequently or not mentioned at all (vitamins D and A, oils, minerals) in the top 10 result pages. This might reflect the fact that none of the 60 commercial webpages were present in the top 10 pages.
We also recorded whether webpages advised against specific interventions. Nineteen webpages (10%), while mentioning vitamins (six of them vitamin C), diet or probiotics, had a negative stance on the use of supplements. These “supplement-negative” webpages were mainly from professional ( n = 6), news ( n = 4), and non-profit ( n = 3) websites. The reasons behind this negative stance on supplements are various and reasons given include: vitamins can be obtained from the diet; claims are unsubstantiated; they are not effective; they add useless cost; or they can cause side effects. A sample of the use of the word “supplement” in this context using corpus analysis is provided in Supplementary File 2 .
Finally, it is important to note that none of the 185 webpages retrieved in our search had an anti-vaccine or vaccine-skeptical stance.
Commercial Bias and Newsworthiness
We asked whether commercial websites, having a financial interest, preferentially mention specific “boosts,” that is whether some of these were mentioned by commercial webpages with a higher frequency than in all other webpages in the SERP. To do so, we looked at the ratio between the frequencies of a topic in commercial webpages and compared it with the frequency of the same topic in all other webpages. Likewise, to investigate whether some of the topic were more newsworthy, we compared the frequency of the topics in news websites compared to all other websites in the SERP.
The analysis of commercial bias is shown in Figure 3A . Minerals, supplements, juices, and Echinacea were highly mentioned by commercial webpages (1.6, 2.0, 1.7, and 2.3 times more than the rest of the SERP, respectively). Conversely, vaccines were mentioned less frequently than in the rest of the SERP. The over-representation of “minerals” and “supplements” in commercial webpages was statistically significant (Fisher's test corrected for multiple comparisons using the Benjamini–Hochberg correction set at a false discovery rate of 5%).

Differential representation of approaches to boost immunity by commercial webpages (A) and news webpages (B) . Data are calculated as: 100 * (percent mention in commercial or news webpages – percent mention in the total 185 websites/percent mention in the total 185 websites. The expected value, in case of no bias should be zero. A value >0 denotes (blue) an overrepresentation (positive bias), a value <0 (red) an underrepresentation (negative bias). * Significantly over-represented by a Fisher test corrected for multiple comparison by the method of Benjamini–Hochberg with a false discovery rate set at 5%. For significance testing, the frequency a boost is mentioned by commercial or news webpage is compared with its frequency in the remaining webpages in the SERP, rather than with that in the whole SERP, to avoid comparing two groups with a significant amount of overlapping data.
In the same way, newsworthiness is shown in Figure 3B . Vaccines were mentioned 2.5-times more frequently by news webpages than the rest of the webpages, and so did “soups” (the word mostly occurred in “chicken soup”), although these differences were not statistically significant after correction for multiple comparisons.
Since many health news stories report on research studies, often relying heavily on press releases from Universities, their industrial sponsors and the academic journals they publish with ( 23 ), we counted the number of webpages mentioning the word “university.” This was done a posteriori by analyzing the corpora represented by the text of the “news” webpages downloaded automatically (see Materials and Methods) and compared with three other typologies (commercial, professional, and blog). Because not all websites allow access to robots, only a sample, although representative, of these webpages could be downloaded analyzed (68% of news webpages, 54% of commercial, 50% of professional, and 39% of blogs). The results showed that the word “university” was mentioned in 44% of news webpages compared to 19% of commercial, 25% of professional, and 14% of blogs. Full analysis of the concordance for the word “university” in news webpages shows that it was used in the context of reporting research results ( Supplementary File 3 ). In some cases, this represented a reference to a statement by an academic professional, in other cases there was a reference to a scientific publication, although the publication was not always identified. For instance three news webpages (no. 15, 21, and 188 in the Supplementary File 1 ) mention a study from the University of Vienna reporting that daily consumption of yogurt is as effective in stimulating immunity as taking probiotic pills, but we could not find a reference to that paper. These results indicates that news outlets are key in relaying results of academic research to the public.
The present study highlights a large presence of commercial websites, representing 30% of the whole SERP. It is also important to consider the ranking given by Google. In fact, many studies indicate that people often visit only the first websites presented in a SERP. In terms of typology of websites, we observed that Google tends to rank commercial websites low, as none of them appears in the top 10 hits, giving more visibility to news websites. The low raking given to commercial websites is something that we observed previously with other search queries ( 15 , 17 ). Of note, commercial webpages also ranked lowest in intrinsic (content-independent) HIQ criteria such as the JAMA score and the HONcode, which we also observed in other studies ( 15 , 16 ) and may contribute to the lower ranking.
The content analysis focused on two aspects, the disease area and the approach suggested boosting immunity. Clearly, the focus of most webpages is on infections, particularly respiratory infections (influenza and common cold), in agreement with the scientific knowledge on the main role of the immune system as a defense against pathogens. This focus on infection is confirmed by a number of approaches described (hygiene, like washing your hands) that are more about preventing an infection by different means than boosting immunity.
In terms of “boosters,” most webpages mentioned dietary advice, fruit or vegetables, and vitamins, particularly vitamin C. It should be noted, however, that when vitamins taken as supplements are suggested, the webpage would also be tagged as “supplement.” Just 36% of webpages specifically mentioned supplements (including vitamin C, flavonoids, minerals, and other agents) to boost immunity. It seems therefore, that the main message from most sources of information is that a “healthy diet,” rich in fruit and therefore vitamins, is an immune booster. One comment is that in many cases the information provided is not answering the query “boosting immunity,” as a “boost” should be something that stimulate immunity above the normal level, but rather informs on how to avoid immunodeficiency due to, for instance, malnutrition.
The high frequency with which vitamin C is mentioned is in agreement with a US survey indicating that, of all dietary supplements, vitamin C is the only one for which the most commonly reported motivation for its use (in 15% of the subjects) is “to boost immune system, prevent cold” ( 24 ). “Strengthen the immune system” is also a major reason (54% of the respondents) for the use of dietary supplements in cancer survivors ( 25 ).
It is important to note that most of the boosters mentioned are not incorporated in any medical guidelines and represent information that is around CAM. This is also true for some of the nutritional advice given (e.g., garlic, mushrooms, ginger, soups), which is either based on low-level (preclinical) scientific evidence or on traditional medicine. It should be noted, however, that the marketing of supplements is not subject to the same level of evidence-based medicine required to market a medicinal product [discussed in ( 26 )], as long as the claim is not addressing a specific disease. For instance, the European Food Standard Authority (EFSA) approved the claim that vitamin C “contributes to maintenance of the normal function of the immune system” ( 27 ).
The high frequency with which vitamin C is mentioned in the context of boosting immunity raises the question of how it became so popular in this field and in the public understanding of science ( 28 ). This does not seem to reflect clinical evidence ( 29 ) or research activity in terms of scientific publication. A search on PubMed for the term “immunity” on 31/1/2019 returned 453867 publications. Adding the term “vitamin C” returned just 306 papers (0.07%). In comparison, publications on immunity and “interferon” were 9%, immunity and “vaccine” 13.7%, immunity and “antioxidant” 2.1%, immunity and “diet” 1.5%, and immunity and “vitamin D” 0.29%. What is surprising is that vaccination, the only approved means of (literally/actively) boosting immunity against specific pathogens, ranks 27th, with only 12% of webpages mentioning it.
The highest commercial bias was around Echinacea and supplements in general. It is also not surprising, although worrisome, that these webpages, often oriented to a “natural” approach, only three out of 60 mention vaccines, although we could not identify any anti-vaccine or vaccine-skeptical webpage in the SERP. Of course natural therapies can be used as complementary, in addition to vaccination, or as an alternative to vaccination. Browne et al. suggested that vaccination compliance might be increased by appealing to features usually associated with CAM, e.g., “strengthening your natural resistance to disease” ( 13 ). Our study suggest that public health websites, educational websites, health portals, and professional organizations should specifically mention that vaccines “boost immunity” against infectious disease as otherwise their websites, and vaccines, would not be found after a search on this topic.
In conclusion, our study found that a search on boosting immunity returns a significant number (30%) of commercially-biased webpages promoting the use and sales of supplements. On the other hand, the ranking of webpages by Google gives more visibility to professional websites and news (4-times and 2-times more likely to rank in the top 10, respectively) while no commercial website was ranked high, none of them being in the top ten. In addition, none of the webpages in the search provided negative information about vaccines. Of course, while this study was performed of a sample of a reasonable size, this is the sample returned from a specific query, selected based on its popularity. Performing a more focused search on a specific supplement or disease may provide a different set of webpages. Furthermore, while the classification of most typologies was reliable, for health portals the inter-rater agreement was very poor and we could not draw any conclusion on this type of websites. Another important aspect is which language or localized version of Google is used, as a similar study on anti-vaccine website has shown major differences across different countries and languages ( 17 ). This implies that the sample uses is only representative of English language.
Data Availability
Author contributions.
PG designed the study. PG, AC, and AO collected and analyzed the data and wrote the manuscript.
Conflict of Interest Statement
The authors declare that the research was conducted in the absence of any commercial or financial relationships that could be construed as a potential conflict of interest.
Acknowledgments
We thank Zachary Clarke for critical revising the manuscript.
Supplementary Material
The Supplementary Material for this article can be found online at: https://www.frontiersin.org/articles/10.3389/fmed.2019.00165/full#supplementary-material
Supplementary File 1
List of webpages analyzed and their classification.
Supplementary File 2
Concordance of the key word “supplement” is webpages recorded as having a negative stance of supplements.
Supplementary File 3
Concordance of the key word “University” in news webpages.
Thank you for visiting nature.com. You are using a browser version with limited support for CSS. To obtain the best experience, we recommend you use a more up to date browser (or turn off compatibility mode in Internet Explorer). In the meantime, to ensure continued support, we are displaying the site without styles and JavaScript.
- View all journals
- Explore content
- About the journal
- Publish with us
- Sign up for alerts
- Published: 27 March 2024
Depleting myeloid-biased haematopoietic stem cells rejuvenates aged immunity
- Jason B. Ross ORCID: orcid.org/0000-0002-0816-1314 1 , 2 , 3 , 4 na1 ,
- Lara M. Myers 5 na1 ,
- Joseph J. Noh 1 , 2 ,
- Madison M. Collins 5 nAff8 ,
- Aaron B. Carmody 6 ,
- Ronald J. Messer 5 ,
- Erica Dhuey ORCID: orcid.org/0000-0002-5961-0722 1 , 2 ,
- Kim J. Hasenkrug ORCID: orcid.org/0000-0001-8523-4911 5 na2 &
- Irving L. Weissman ORCID: orcid.org/0000-0002-9077-7467 1 , 2 , 4 , 7 na2
Nature volume 628 , pages 162–170 ( 2024 ) Cite this article
32k Accesses
3 Citations
678 Altmetric
Metrics details
- Haematopoietic stem cells
- Stem-cell research
Ageing of the immune system is characterized by decreased lymphopoiesis and adaptive immunity, and increased inflammation and myeloid pathologies 1 , 2 . Age-related changes in populations of self-renewing haematopoietic stem cells (HSCs) are thought to underlie these phenomena 3 . During youth, HSCs with balanced output of lymphoid and myeloid cells (bal-HSCs) predominate over HSCs with myeloid-biased output (my-HSCs), thereby promoting the lymphopoiesis required for initiating adaptive immune responses, while limiting the production of myeloid cells, which can be pro-inflammatory 4 . Ageing is associated with increased proportions of my-HSCs, resulting in decreased lymphopoiesis and increased myelopoiesis 3 , 5 , 6 . Transfer of bal-HSCs results in abundant lymphoid and myeloid cells, a stable phenotype that is retained after secondary transfer; my-HSCs also retain their patterns of production after secondary transfer 5 . The origin and potential interconversion of these two subsets is still unclear. If they are separate subsets postnatally, it might be possible to reverse the ageing phenotype by eliminating my-HSCs in aged mice. Here we demonstrate that antibody-mediated depletion of my-HSCs in aged mice restores characteristic features of a more youthful immune system, including increasing common lymphocyte progenitors, naive T cells and B cells, while decreasing age-related markers of immune decline. Depletion of my-HSCs in aged mice improves primary and secondary adaptive immune responses to viral infection. These findings may have relevance to the understanding and intervention of diseases exacerbated or caused by dominance of the haematopoietic system by my-HSCs.
This is a preview of subscription content, access via your institution
Access options
Access Nature and 54 other Nature Portfolio journals
Get Nature+, our best-value online-access subscription
24,99 € / 30 days
cancel any time
Subscribe to this journal
Receive 51 print issues and online access
185,98 € per year
only 3,65 € per issue
Rent or buy this article
Prices vary by article type
Prices may be subject to local taxes which are calculated during checkout
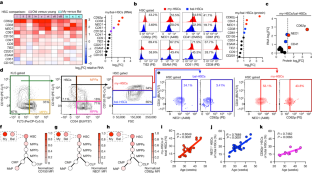
Similar content being viewed by others
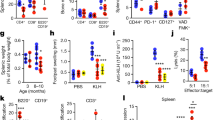
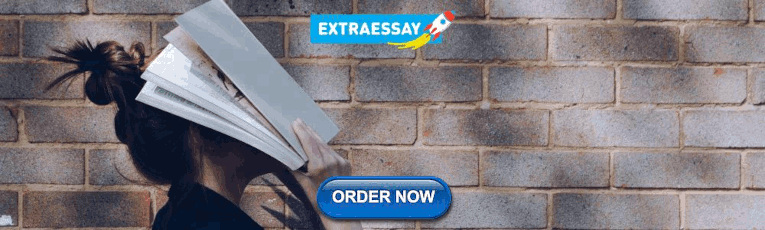
An aged immune system drives senescence and ageing of solid organs
Matthew J. Yousefzadeh, Rafael R. Flores, … Laura J. Niedernhofer
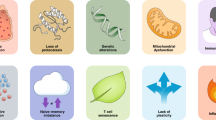
Hallmarks of T cell aging
Maria Mittelbrunn & Guido Kroemer
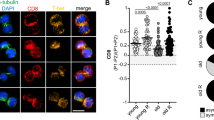
Asymmetric cell division shapes naive and virtual memory T-cell immunity during ageing
Mariana Borsa, Niculò Barandun, … Annette Oxenius
Data availability
Data for all graphical representations are provided as source data. RNA-seq data have been deposited at the GEO under accession code GSE252062 and the Sequence Read Archive (SRA) under BioProject PRJNA1054066 . The following publicly available datasets were used: GSE43729 (ref. 16 ), GSE39553 (ref. 24 ), GSE48893 (ref. 25 ), GSE109546 (ref. 26 ), GSE27686 (ref. 27 ), GSE44923 (ref. 28 ), GSE128050 (ref. 29 ), GSE47819 (ref. 30 ), GSE130504 (ref. 19 ), GSE112769 (ref. 22 ), E-MEXP-3935 (ref. 21 ), GSE32719 (ref. 3 ), GSE104406 (ref. 53 ), GSE69408 (ref. 54 ), GSE115348 (ref. 55 ), GSE107594 (ref. 104 ), GSE111410 (ref. 56 ), GSE55689 (ref. 57 ), GSE74246 (ref. 58 ), GSE132040 (ref. 108 ), GSE87633 (ref. 109 ) and GSE100428 (ref. 18 ). Source data are provided with this paper.
Morrison, S. J., Wandycz, A. M., Akashi, K., Globerson, A. & Weissman, I. L. The aging of hematopoietic stem cells. Nat. Med. 2 , 1011–1016 (1996).
Article CAS PubMed Google Scholar
Rossi, D. J., Jamieson, C. H. & Weissman, I. L. Stems cells and the pathways to aging and cancer. Cell 132 , 681–696 (2008).
Pang, W. W. et al. Human bone marrow hematopoietic stem cells are increased in frequency and myeloid-biased with age. Proc. Natl Acad. Sci. USA 108 , 20012–20017 (2011).
Article ADS CAS PubMed PubMed Central Google Scholar
Yamamoto, R. & Nakauchi, H. In vivo clonal analysis of aging hematopoietic stem cells. Mech. Ageing Dev. 192 , 111378 (2020).
Article CAS PubMed PubMed Central Google Scholar
Beerman, I. et al. Functionally distinct hematopoietic stem cells modulate hematopoietic lineage potential during aging by a mechanism of clonal expansion. Proc. Natl Acad. Sci. USA 107 , 5465–5470 (2010).
Rossi, D. J. et al. Cell intrinsic alterations underlie hematopoietic stem cell aging. Proc. Natl Acad. Sci. USA 102 , 9194–9199 (2005).
Muller-Sieburg, C. E., Cho, R. H., Karlsson, L., Huang, J. F. & Sieburg, H. B. Myeloid-biased hematopoietic stem cells have extensive self-renewal capacity but generate diminished lymphoid progeny with impaired IL-7 responsiveness. Blood 103 , 4111–4118 (2004).
Sudo, K., Ema, H., Morita, Y. & Nakauchi, H. Age-associated characteristics of murine hematopoietic stem cells. J. Exp. Med. 192 , 1273–1280 (2000).
Sieburg, H. B. et al. The hematopoietic stem compartment consists of a limited number of discrete stem cell subsets. Blood 107 , 2311–2316 (2006).
Dykstra, B. et al. Long-term propagation of distinct hematopoietic differentiation programs in vivo. Cell Stem Cell 1 , 218–229 (2007).
Dykstra, B., Olthof, S., Schreuder, J., Ritsema, M. & de Haan, G. Clonal analysis reveals multiple functional defects of aged murine hematopoietic stem cells. J. Exp. Med. 208 , 2691–2703 (2011).
Min, H., Montecino-Rodriguez, E. & Dorshkind, K. Effects of aging on the common lymphoid progenitor to pro-B cell transition. J. Immunol. 176 , 1007–1012 (2006).
Montecino-Rodriguez, E., Berent-Maoz, B. & Dorshkind, K. Causes, consequences, and reversal of immune system aging. J. Clin. Invest. 123 , 958–965 (2013).
Yang, D. & de Haan, G. Inflammation and aging of hematopoietic stem cells in their niche. Cells 10 , 1849 (2021).
Chen, J. Y. et al. Hoxb5 marks long-term haematopoietic stem cells and reveals a homogenous perivascular niche. Nature 530 , 223–227 (2016).
Beerman, I. et al. Proliferation-dependent alterations of the DNA methylation landscape underlie hematopoietic stem cell aging. Cell Stem Cell 12 , 413–425 (2013).
Gekas, C. & Graf, T. CD41 expression marks myeloid-biased adult hematopoietic stem cells and increases with age. Blood 121 , 4463–4472 (2013).
Mann, M. et al. Heterogeneous responses of hematopoietic stem cells to inflammatory stimuli are altered with age. Cell Rep. 25 , 2992–3005 (2018).
Gulati, G. S. et al. Neogenin-1 distinguishes between myeloid-biased and balanced. Proc. Natl Acad. Sci. USA 116 , 25115–25125 (2019).
Flohr Svendsen, A. et al. A comprehensive transcriptome signature of murine hematopoietic stem cell aging. Blood 138 , 439–451 (2021).
Sanjuan-Pla, A. et al. Platelet-biased stem cells reside at the apex of the haematopoietic stem-cell hierarchy. Nature 502 , 232–236 (2013).
Article ADS CAS PubMed Google Scholar
Montecino-Rodriguez, E. et al. Lymphoid-biased hematopoietic stem cells are maintained with age and efficiently generate lymphoid progeny. Stem Cell Rep. 12 , 584–596 (2019).
Article CAS Google Scholar
Zaro, B. W. et al. Proteomic analysis of young and old mouse hematopoietic stem cells and their progenitors reveals post-transcriptional regulation in stem cells. eLife 9 , e62210 (2020).
Bersenev, A. et al. Lnk deficiency partially mitigates hematopoietic stem cell aging. Aging Cell 11 , 949–959 (2012).
Flach, J. et al. Replication stress is a potent driver of functional decline in ageing haematopoietic stem cells. Nature 512 , 198–202 (2014).
Maryanovich, M. et al. Adrenergic nerve degeneration in bone marrow drives aging of the hematopoietic stem cell niche. Nat. Med. 24 , 782–791 (2018).
Norddahl, G. L. et al. Accumulating mitochondrial DNA mutations drive premature hematopoietic aging phenotypes distinct from physiological stem cell aging. Cell Stem Cell 8 , 499–510 (2011).
Wahlestedt, M. et al. An epigenetic component of hematopoietic stem cell aging amenable to reprogramming into a young state. Blood 121 , 4257–4264 (2013).
Renders, S. et al. Niche derived netrin-1 regulates hematopoietic stem cell dormancy via its receptor neogenin-1. Nat. Commun. 12 , 608 (2021).
Sun, D. et al. Epigenomic profiling of young and aged HSCs reveals concerted changes during aging that reinforce self-renewal. Cell Stem Cell 14 , 673–688 (2014).
Seita, J. et al. Gene Expression Commons: an open platform for absolute gene expression profiling. PLoS ONE 7 , e40321 (2012).
Akashi, K., Traver, D., Miyamoto, T. & Weissman, I. L. A clonogenic common myeloid progenitor that gives rise to all myeloid lineages. Nature 404 , 193–197 (2000).
Kondo, M., Weissman, I. L. & Akashi, K. Identification of clonogenic common lymphoid progenitors in mouse bone marrow. Cell 91 , 661–672 (1997).
George, B. M. et al. Antibody conditioning enables MHC-mismatched hematopoietic stem cell transplants and organ graft tolerance. Cell Stem Cell 25 , 185–192 (2019).
Czechowicz, A., Kraft, D., Weissman, I. L. & Bhattacharya, D. Efficient transplantation via antibody-based clearance of hematopoietic stem cell niches. Science 318 , 1296–1299 (2007).
Jaiswal, S. et al. CD47 is upregulated on circulating hematopoietic stem cells and leukemia cells to avoid phagocytosis. Cell 138 , 271–285 (2009).
Kuribayashi, W. et al. Limited rejuvenation of aged hematopoietic stem cells in young bone marrow niche. J. Exp. Med. 218 , e20192283 (2021).
Morrison, S. J. & Weissman, I. L. The long-term repopulating subset of hematopoietic stem cells is deterministic and isolatable by phenotype. Immunity 1 , 661–673 (1994).
Akashi, K., Kondo, M. & Weissman, I. L. Two distinct pathways of positive selection for thymocytes. Proc. Natl Acad. Sci. USA 95 , 2486–2491 (1998).
Gattinoni, L. et al. A human memory T cell subset with stem cell-like properties. Nat. Med. 17 , 1290–1297 (2011).
Elyahu, Y. et al. Aging promotes reorganization of the CD4 T cell landscape toward extreme regulatory and effector phenotypes. Sci. Adv. 5 , eaaw8330 (2019).
Hao, Y., O’Neill, P., Naradikian, M. S., Scholz, J. L. & Cancro, M. P. A B-cell subset uniquely responsive to innate stimuli accumulates in aged mice. Blood 118 , 1294–1304 (2011).
Pioli, P. D., Casero, D., Montecino-Rodriguez, E., Morrison, S. L. & Dorshkind, K. Plasma cells are obligate effectors of enhanced myelopoiesis in aging bone marrow. Immunity 51 , 351–366 (2019).
Kovtonyuk, L. V. et al. IL-1 mediates microbiome-induced inflammaging of hematopoietic stem cells in mice. Blood 139 , 44–58 (2022).
Collier, D. A. et al. Age-related immune response heterogeneity to SARS-CoV-2 vaccine BNT162b2. Nature 596 , 417–422 (2021).
Myers, L. & Hasenkrug, K. J. Retroviral immunology: lessons from a mouse model. Immunol. Res. 43 , 160–166 (2009).
Article PubMed PubMed Central Google Scholar
Dittmer, U. et al. Friend retrovirus studies reveal complex interactions between intrinsic, innate and adaptive immunity. FEMS Microbiol. Rev. 43 , 435–456 (2019).
Dittmer, U., Brooks, D. M. & Hasenkrug, K. J. Requirement for multiple lymphocyte subsets in protection by a live attenuated vaccine against retroviral infection. Nat. Med. 5 , 189–193 (1999).
Dittmer, U., Brooks, D. M. & Hasenkrug, K. J. Characterization of a live-attenuated retroviral vaccine demonstrates protection via immune mechanisms. J. Virol. 72 , 6554–6558 (1998).
Dittmer, U., Brooks, D. M. & Hasenkrug, K. J. Protection against establishment of retroviral persistence by vaccination with a live attenuated virus. J. Virol. 73 , 3753–3757 (1999).
Hasenkrug, K. J. & Dittmer, U. The role of CD4 and CD8 T cells in recovery and protection from retroviral infection: lessons from the Friend virus model. Virology 272 , 244–249 (2000).
Larochelle, A. et al. Human and rhesus macaque hematopoietic stem cells cannot be purified based only on SLAM family markers. Blood 117 , 1550–1554 (2011).
Adelman, E. R. et al. Aging human hematopoietic stem cells manifest profound epigenetic reprogramming of enhancers that may predispose to leukemia. Cancer Discov. 9 , 1080–1101 (2019).
Rundberg Nilsson, A., Soneji, S., Adolfsson, S., Bryder, D. & Pronk, C. J. Human and murine hematopoietic stem cell aging is associated with functional impairments and intrinsic megakaryocytic/erythroid bias. PLoS ONE 11 , e0158369 (2016).
Hennrich, M. L. et al. Cell-specific proteome analyses of human bone marrow reveal molecular features of age-dependent functional decline. Nat. Commun. 9 , 4004 (2018).
Article ADS PubMed PubMed Central Google Scholar
Tong, J. et al. Hematopoietic stem cell heterogeneity is linked to the initiation and therapeutic response of myeloproliferative neoplasms. Cell Stem Cell 28 , 502–513 (2021).
Woll, P. S. et al. Myelodysplastic syndromes are propagated by rare and distinct human cancer stem cells in vivo. Cancer Cell 25 , 794–808 (2014).
Corces, M. R. et al. Lineage-specific and single-cell chromatin accessibility charts human hematopoiesis and leukemia evolution. Nat. Genet. 48 , 1193–1203 (2016).
Park, C. Y., Majeti, R. & Weissman, I. L. In vivo evaluation of human hematopoiesis through xenotransplantation of purified hematopoietic stem cells from umbilical cord blood. Nat. Protoc. 3 , 1932–1940 (2008).
Bhattacharya, D. et al. Transcriptional profiling of antigen-dependent murine B cell differentiation and memory formation. J. Immunol. 179 , 6808–6819 (2007).
Luckey, C. J. et al. Memory T and memory B cells share a transcriptional program of self-renewal with long-term hematopoietic stem cells. Proc. Natl Acad. Sci. USA 103 , 3304–3309 (2006).
Saggau, C. et al. The pre-exposure SARS-CoV-2-specific T cell repertoire determines the quality of the immune response to vaccination. Immunity. 55 , 1924–1939 (2022).
Merad, M., Blish, C. A., Sallusto, F. & Iwasaki, A. The immunology and immunopathology of COVID-19. Science 375 , 1122–1127 (2022).
Jaiswal, S. & Weissman, I. L. Hematopoietic stem and progenitor cells and the inflammatory response. Ann. N. Y. Acad. Sci. 1174 , 118–121 (2009).
Hirata, Y. et al. CD150 high bone marrow Tregs maintain hematopoietic stem cell quiescence and immune privilege via adenosine. Cell Stem Cell 22 , 445–453 (2018).
Jamieson, C. H. M. & Weissman, I. L. Stem-cell aging and pathways to precancer evolution. N. Engl. J. Med. 389 , 1310–1319 (2023).
Busque, L. et al. Recurrent somatic TET2 mutations in normal elderly individuals with clonal hematopoiesis. Nat. Genet. 44 , 1179–1181 (2012).
Jan, M. et al. Clonal evolution of preleukemic hematopoietic stem cells precedes human acute myeloid leukemia. Sci. Transl. Med. 4 , 149ra118 (2012).
Jaiswal, S. & Ebert, B. L. Clonal hematopoiesis in human aging and disease. Science 366 , eaan4673 (2019).
Jaiswal, S. et al. Clonal hematopoiesis and risk of atherosclerotic cardiovascular disease. N. Engl. J. Med. 377 , 111–121 (2017).
Majeti, R. et al. Clonal expansion of stem/progenitor cells in cancer, fibrotic diseases, and atherosclerosis, and CD47 protection of pathogenic cells. Annu. Rev. Med. 73 , 307–320 (2022).
Spangrude, G. J., Heimfeld, S. & Weissman, I. L. Purification and characterization of mouse hematopoietic stem cells. Science 241 , 58–62 (1988).
Osawa, M., Hanada, K., Hamada, H. & Nakauchi, H. Long-term lymphohematopoietic reconstitution by a single CD34-low/negative hematopoietic stem cell. Science 273 , 242–245 (1996).
Smith, L. G., Weissman, I. L. & Heimfeld, S. Clonal analysis of hematopoietic stem-cell differentiation in vivo. Proc. Natl Acad. Sci. USA 88 , 2788–2792 (1991).
Yamamoto, R. et al. Large-scale clonal analysis resolves aging of the mouse hematopoietic stem cell compartment. Cell Stem Cell 22 , 600–607 (2018).
Myers, L. M. et al. A functional subset of CD8 + T cells during chronic exhaustion is defined by SIRPα expression. Nat. Commun. 10 , 794 (2019).
Chesebro, B. et al. Characterization of mouse monoclonal antibodies specific for Friend murine leukemia virus-induced erythroleukemia cells: friend-specific and FMR-specific antigens. Virology 112 , 131–144 (1981).
Marsh-Wakefield, F. M. et al. Making the most of high-dimensional cytometry data. Immunol. Cell Biol. 99 , 680–696 (2021).
Liechti, T. et al. An updated guide for the perplexed: cytometry in the high-dimensional era. Nat. Immunol. 22 , 1190–1197 (2021).
Ashhurst, T. M. et al. Integration, exploration, and analysis of high-dimensional single-cell cytometry data using Spectre. Cytometry A 101 , 237–253 (2022).
Levine, J. H. et al. Data-driven phenotypic dissection of AML reveals progenitor-like cells that correlate with prognosis. Cell 162 , 184–197 (2015).
McInnes, L., Healy, J. & Melville, J. UMAP: uniform manifold approximation and projection for dimension reduction. Preprint at arxiv.org/abs/1802.03426 (2018).
Baum, C. M., Weissman, I. L., Tsukamoto, A. S., Buckle, A. M. & Peault, B. Isolation of a candidate human hematopoietic stem-cell population. Proc. Natl Acad. Sci. USA 89 , 2804–2808 (1992).
Yiu, Y. Y. et al. CD47 blockade leads to chemokine-dependent monocyte infiltration and loss of B cells from the splenic marginal zone. J. Immunol. 208 , 1371–1377 (2022).
Brignani, S. et al. Remotely produced and axon-derived Netrin-1 instructs GABAergic neuron migration and dopaminergic substantia nigra development. Neuron 107 , 684–702 (2020).
Hadi, T. et al. Macrophage-derived netrin-1 promotes abdominal aortic aneurysm formation by activating MMP3 in vascular smooth muscle cells. Nat. Commun. 9 , 5022 (2018).
König, K. et al. The axonal guidance receptor neogenin promotes acute inflammation. PLoS ONE 7 , e32145 (2012).
Li, N. et al. Upregulation of neogenin-1 by a CREB1-BAF47 complex in vascular endothelial cells is implicated in atherogenesis. Front. Cell Dev. Biol. 10 , 803029 (2022).
Robinson, R. A. et al. Simultaneous binding of guidance cues NET1 and RGM blocks extracellular NEO1 signaling. Cell 184 , 2103–2120 (2021).
Schlegel, M. et al. Inhibition of neogenin dampens hepatic ischemia-reperfusion injury. Crit. Care Med. 42 , e610–e619 (2014).
Schlegel, M. et al. Inhibition of neogenin fosters resolution of inflammation and tissue regeneration. J. Clin. Invest. 128 , 4711–4726 (2019).
Article Google Scholar
van den Heuvel, D. M., Hellemons, A. J. & Pasterkamp, R. J. Spatiotemporal expression of repulsive guidance molecules (RGMs) and their receptor neogenin in the mouse brain. PLoS ONE 8 , e55828 (2013).
Keren, Z. et al. B-cell depletion reactivates B lymphopoiesis in the BM and rejuvenates the B lineage in aging. Blood 117 , 3104–3112 (2011).
Säwén, P. et al. Mitotic history reveals distinct stem cell populations and their contributions to hematopoiesis. Cell Rep. 14 , 2809–2818 (2016).
Boivin, G. et al. Durable and controlled depletion of neutrophils in mice. Nat. Commun. 11 , 2762 (2020).
Chhabra, A. et al. Hematopoietic stem cell transplantation in immunocompetent hosts without radiation or chemotherapy. Sci. Transl. Med. 8 , 351ra105 (2016).
Iglewicz, B. & Hoaglin, D. C. How to Detect and Handle Outliers (Asq Press, 1993).
Guide for the Care and Use of Laboratory Animals (National Research Council, 2010).
Robertson, S. J. et al. Suppression of acute anti-friend virus CD8 + T-cell responses by coinfection with lactate dehydrogenase-elevating virus. J. Virol. 82 , 408–418 (2008).
Chesebro, B., Wehrly, K. & Stimpfling, J. Host genetic control of recovery from Friend leukemia virus-induced splenomegaly: mapping of a gene within the major histocompatability complex. J. Exp. Med. 140 , 1457–1467 (1974).
Lander, M. R. & Chattopadhyay, S. K. A Mus dunni cell line that lacks sequences closely related to endogenous murine leukemia viruses and can be infected by ectropic, amphotropic, xenotropic, and mink cell focus-forming viruses. J. Virol. 52 , 695–698 (1984).
Robertson, M. N. et al. Production of monoclonal antibodies reactive with a denatured form of the Friend murine leukemia virus gp70 envelope protein: use in a focal infectivity assay, immunohistochemical studies, electron microscopy and western blotting. J. Virol. Methods 34 , 255–271 (1991).
Horton, H. et al. Optimization and validation of an 8-color intracellular cytokine staining (ICS) assay to quantify antigen-specific T cells induced by vaccination. J. Immunol. Methods 323 , 39–54 (2007).
Kumar, P. et al. HMGA2 promotes long-term engraftment and myeloerythroid differentiation of human hematopoietic stem and progenitor cells. Blood Adv. 3 , 681–691 (2019).
Mahi, N. A., Najafabadi, M. F., Pilarczyk, M., Kouril, M. & Medvedovic, M. GREIN: an interactive web platform for re-analyzing GEO RNA-seq data. Sci. Rep. 9 , 7580 (2019).
Barrett, T. et al. NCBI GEO: archive for functional genomics data sets-update. Nucleic Acids Res. 41 , D991–D995 (2013).
Edgar, R., Domrachev, M. & Lash, A. E. Gene Expression Omnibus: NCBI gene expression and hybridization array data repository. Nucleic Acids Res. 30 , 207–210 (2002).
The Tabula Muris Consortium. Single-cell transcriptomics of 20 mouse organs creates a Tabula Muris . Nature 562 , 367–372 (2018).
Article ADS CAS Google Scholar
Kadoki, M. et al. Organism-level analysis of vaccination reveals networks of protection across tissues. Cell 171 , 398–413 (2017).
Kleverov, M. et al. Phantasus: web-application for visual and interactive gene expression analysis. Preprint at bioRxiv https://doi.org/10.1101/2022.12.10.519861 (2022).
Love, M. I., Huber, W. & Anders, S. Moderated estimation of fold change and dispersion for RNA-seq data with DESeq2. Genome Biol. 15 , 550 (2014).
Ritchie, M. E. et al. limma powers differential expression analyses for RNA-sequencing and microarray studies. Nucleic Acids Res. 43 , e47 (2015).
Subramanian, A. et al. Gene set enrichment analysis: a knowledge-based approach for interpreting genome-wide expression profiles. Proc. Natl Acad. Sci. USA 102 , 15545–15550 (2005).
Liao, Y., Wang, J., Jaehnig, E. J., Shi, Z. & Zhang, B. WebGestalt 2019: gene set analysis toolkit with revamped UIs and APIs. Nucleic Acids Res. 47 , W199–W205 (2019).
Seita, J. & Weissman, I. L. Hematopoietic stem cell: self-renewal versus differentiation. Wiley Interdiscip. Rev. Syst. Biol. Med. 2 , 640–653 (2010).
Helbling, P. M. et al. Global transcriptomic profiling of the bone marrow stromal microenvironment during postnatal development, aging, and inflammation. Cell Rep. 29 , 3313–3330 (2019).
Akashi, K. & Weissman, I. L. The c-kit + maturation pathway in mouse thymic T cell development: lineages and selection. Immunity 5 , 147–161 (1996).
Loder, F. et al. B cell development in the spleen takes place in discrete steps and is determined by the quality of B cell receptor-derived signals. J. Exp. Med. 190 , 75–89 (1999).
Leins, H. et al. Aged murine hematopoietic stem cells drive aging-associated immune remodeling. Blood 132 , 565–576 (2018).
Goardon, N. et al. Coexistence of LMPP-like and GMP-like leukemia stem cells in acute myeloid leukemia. Cancer Cell 19 , 138–152 (2011).
Manz, M. G., Miyamoto, T., Akashi, K. & Weissman, I. L. Prospective isolation of human clonogenic common myeloid progenitors. Proc. Natl Acad. Sci. USA 99 , 11872–11877 (2002).
Download references
Acknowledgements
We thank the members of the Weissman and Hasenkrug laboratories for advice and discussions; A. McCarty, T. Naik, L. Quinn and T. Raveh for technical and logistical support; A. Banuelos, G. Blacker, B. George, G. Gulati, J. Liu, R. Sinha, M. Tal, N. Womack and Y. Yiu for general help, advice and experimental support; C. Carswell-Crumpton, C. Pan, J. Pasillas and the staff at the Stanford Institute for Stem Cell Biology and Regenerative Medicine FACS Core for flow cytometry assistance; H. Maecker and I. Herschmann of the Stanford Human Immune Monitoring Center (HIMC) for assistance with immunoassays; and the members of the Rocky Mountain Veterinary Branch (RMVB), especially T. Wiediger, for excellent care of the aged mice. This work was partially funded by the Intramural Research Program of the National Institute of Allergy and Infectious Diseases, National Institutes of Health, USA; the NIH/NCI Outstanding Investigator Award (R35CA220434 to I.L.W.); the NIH NIDDK (R01DK115600 to I.L.W.); the NIH NIAID (R01AI143889 to I.L.W.); and the Virginia and D.K. Ludwig Fund for Cancer Research (to I.L.W.). J.B.R. was supported by the Stanford Radiation Oncology Kaplan Research Fellowship, the RSNA Resident/Fellow Research Grant, and the Stanford Cancer Institute Fellowship Award and the Ellie Guardino Research Fund. This work was supported by the Stanford Cancer Institute, an NCI-designated Comprehensive Cancer Center. J.J.N. was supported by Stanford University Medical Scientist Training Program grant T32-GM007365 and T32-GM145402. E.D. was supported by grants from NIH NIDDK (5T32DK098132-09 and 1TL1DK139565-01). The funders had no role in study design, data collection and analysis, decision to publish or preparation of the manuscript. Some illustrations were created using BioRender.
Author information
Madison M. Collins
Present address: Department of Biological and Physical Sciences, Montana State University Billings, Billings, MT, USA
These authors contributed equally: Jason B. Ross, Lara M. Myers
These authors jointly supervised this work: Kim J. Hasenkrug, Irving L. Weissman
Authors and Affiliations
Institute for Stem Cell Biology and Regenerative Medicine, Stanford University School of Medicine, Stanford, CA, USA
Jason B. Ross, Joseph J. Noh, Erica Dhuey & Irving L. Weissman
Ludwig Center for Cancer Stem Cell Research and Medicine, Stanford University School of Medicine, Stanford, CA, USA
Department of Radiation Oncology, Stanford University School of Medicine, Stanford, CA, USA
Jason B. Ross
Stanford Cancer Institute, Stanford University School of Medicine, Stanford, CA, USA
Jason B. Ross & Irving L. Weissman
Laboratory of Persistent Viral Diseases, Rocky Mountain Laboratories, National Institutes of Allergy and Infectious Diseases, National Institutes of Health, Hamilton, MT, USA
Lara M. Myers, Madison M. Collins, Ronald J. Messer & Kim J. Hasenkrug
Research Technologies Branch, Rocky Mountain Laboratories, National Institutes of Allergy and Infectious Diseases, National Institutes of Health, Hamilton, MT, USA
Aaron B. Carmody
Department of Pathology, Stanford University School of Medicine, Stanford, CA, USA
Irving L. Weissman
You can also search for this author in PubMed Google Scholar
Contributions
J.B.R. and L.M.M. contributed equally to this work and they both have the right to be listed first in bibliographic documents. J.B.R. and L.M.M. conceived and performed experiments, analysed and interpreted all the data, and wrote the paper. J.J.N., M.M.C., A.B.C., R.J.M. and E.D. performed experiments and analysed data. L.M.M., M.M.C., A.B.C. and R.J.M. performed the Friend virus experiments. J.B.R. and E.D. designed and performed the RNA-seq and transplant experiments. I.L.W. and K.J.H. conceived experiments, supervised the research, interpreted results and wrote the paper. All of the authors reviewed, edited and approved the manuscript.
Corresponding authors
Correspondence to Kim J. Hasenkrug or Irving L. Weissman .
Ethics declarations
Competing interests.
I.L.W. is listed as an inventor on patents related to CD47 licensed to Gilead Sciences, but has no financial interests in Gilead; he is also a co-founder and equity holder of Bitterroot Bio, PHeast and 48 Bio; he is on the scientific advisory board of Appia. J.B.R. is a co-founder and equity holder of 48 Bio. I.L.W., K.J.H., J.B.R., L.M.M. and J.N.N. are listed as co-inventors on a pending patent application related to this work. The other authors declare no competing interests.
Peer review
Peer review information.
Nature thanks Jennifer Trowbridge and the other, anonymous, reviewer(s) for their contribution to the peer review of this work. Peer reviewer reports are available.
Additional information
Publisher’s note Springer Nature remains neutral with regard to jurisdictional claims in published maps and institutional affiliations.
Extended data figures and tables
Extended data fig. 1 expression of my-hsc markers in hspcs, mature cells, and tissues..
a - l , Expression of my-HSC candidate markers, Slamf1 (CD150) ( a ), Neo1 (NEO1) ( b ), Itga2b (CD41) ( c ), Selp (CD62p) ( d ), Cd38 (CD38) ( e ), Itgb3 (CD61) ( f ), Itgav (CD51) ( g ), Procr (CD201) ( h ), Tie2 ( i ), Esam ( j ), Eng (CD105) ( k ), Cd9 (CD9) ( l ), in HSC and HSPCs in normal mouse BM (top panels), and in young versus old bone marrow (bottom panels). Data and images from a – l generated and obtained directly from Gene Expression Commons 31 ; scale bars represent log2 Signal Intensity ( top ) and Gene Expression Activity ( bottom ) as defined by Gene Expression Commons 31 . m , Heatmap of relative RNA expression for CD150 ( Slamf1 ), NEO1 ( Neo1 ), CD62p ( Selp ), CD41 ( Itga2b ), CD38 ( Cd38 ), CD51 ( Itgav ), and CD61 ( Itgb3 ) in HSCs, MPPs, Progenitors, Myeloid, and Lymphoid cells. Processed data for 23 cell types were obtained directly from Gulati 19 Supplementary Table 1 . Fold-enrichment = [(average percentile of HSCs)/(average percentile of all other cell types)+100], as described in this publication. n – o , RNA expression of CD150 ( Slamf1 ), NEO1 ( Neo1 ), CD62p ( Selp ), and CD41 ( Itga2b ) in bulk mouse tissues from two independent datasets: Tabula Muris 108 ( n ) or Kadoki 109 ( o ). For n – o , Values are z-score normalized for each gene across all tissues.
Source Data
Extended Data Fig. 2 Gating strategy for total HSCs, my-HSCs, bal-HSCs, and HPCs.
a , Schematic to identify and validate my-HSC cell-surface antigens. The diagram was created using BioRender. b , Representative flow-cytometry gating of mouse BM to identify total HSC (Lin – cKIT + Sca1 + FLT3 – CD34 – CD150 + ), my-HSC (Lin – cKIT + Sca1 + FLT3 – CD34 – CD150 High ), bal-HSC (Lin – cKIT + Sca1 + FLT3 – CD34 – CD150 Low ), MPPs 115 [MPPa (Lin – cKIT + Sca1 + FLT3 – CD34 + CD150 + ), MPPb (Lin – cKIT + Sca1 + FLT3 – CD34 + CD150 – ), MPPc (Lin – cKIT + Sca1 + FLT3 + CD34 + CD150 – )], OPP (Lin – cKIT + Sca1 – ), CMP&GMP (Lin – cKIT + Sca1 – CD34 + CD41 – ), MkP (Lin – cKIT + Sca1 – CD34 + CD41 + ), MEP (Lin – cKIT + Sca1 – CD34 – CD41 + ), CLP (Lin – cKIT Lo Sca1 Lo IL7Ra + FLT3 + ). Panels are after excluding dead cells, doublets, and lineage-positive (CD3 + , or Ly-6G + /C + , or CD11b + , or CD45R + , or Ter-119 + ) cells. Used for Fig. 1b–k , Fig. 2a–f , Fig. 3b–d , Fig. 4a , Extended Data Fig. 2c–k , Extended Data Fig. 3a–l , Extended Data Fig. 4a–s , Extended Data Fig. 5c–s , Extended Data Fig. 6a Extended Data Fig. 8c–h . Illustration of Hematopoietic Stem and Progenitor Cell (HSPC) Tree Analysis. CMP is combined CMP&GMP. Gate to define my-HSC vs. bal-HSC was set as described previously 5 . c – f , Relative expression of CD41 ( c ), CD38 ( d ), CD51 ( e ), CD61 ( f ), on HSC and HSPCs. MFI values for each marker were obtained for each population and normalized from 0–1 based on the lowest to highest expression. g – h , Relative cell-surface levels ( g ) and percent-positive cells ( h ) for CD150, NEO1, CD62p, CD41, CD38, CD51, and CD61, on lineage-positive high and low cells, total HSCs, and HPCs in the BM. For cell-surface levels ( g ), MFI values for each marker were obtained for each population and normalized from 0–100 based on the lowest to highest expression. i , Percentage of total HSCs that are CD41 + (y-axis) vs. mouse age in weeks (x-axis); n = 21 mice. j , Mouse age (x-axis) vs. the frequency of total HSCs (my-HSC+bal-HSC) as a percentage of live cells in the (i) total BM (left y-axis, red ) or (ii) cKIT-enriched BM (right y-axis, blue ) in untreated mice; n = 13 mice. k , Percent-positive of my-HSCs vs. bal-HSCs for CD47 ( k , top) using independent anti-CD47 clones (MIAP301, left; MIAP410, right), and for cKIT ( k , bottom) using independent anti-cKIT clones (ACK2, left; 2B8, right). Mouse ages: 4–6 months ( b – i , k ), 3–23 months ( j ). For a – k , BM was cKIT-enriched prior to analysis. For j , total BM (non cKIT-enriched) was also examined. p -values and R values calculated with one-tailed Pearson correlation coefficient ( i – j ). n represents independent mice.
Extended Data Fig. 3 Anti-CD150 non-masking antibodies and FACS gating to isolate HSCs.
a , Schematic to identify anti-CD150 antibodies not masked by anti-CD150 antibody clone 1 (TC15), used in panels b – d ; BM cells were incubated with saturating concentrations of unlabelled anti-CD150 antibody clone 1 (TC15) and then stained with PE anti-CD150 clones 2, 3, 4 (Q38, 9D1, mShad150). b – d , Saturating concentrations of unlabelled anti-CD150 antibody clone TC15 blocks staining with PE anti-CD150 clone 4 (mShad150) ( d ), but does not block staining of PE anti-CD150 clones 2, 3 (Q38, 9D1) ( b – c ). e , Schematic to determine if anti-CD150 clones 2, 3, 4 (Q38, 9D1, mShad150) identify the same population as anti-CD150 clone 1 (TC15); used in panels f – h ; BM cells were incubated with PECy-7 anti-CD150 antibody clone 1 (TC15) and with PE anti-CD150 clones 2, 3, 4 (Q38, 9D1, mShad150). f – h , Co-staining with anti-CD150 clones 2, 3 (Q38, 9D1) identifies the same population as anti-CD150 antibody clone TC15 ( f – g ). Co-staining with anti-CD150 clone 4 (mShad150) and anti-CD150 antibody clone 1 (TC15) is mutually blocked ( h ). i , Schematic to identify anti-CD150 antibodies that are not blocked by anti-CD150 clone mShad150, used in panel j ; BM cells are incubated with saturating concentrations of unlabelled anti-CD150 antibody clone mShad150 and then stained with PE anti-CD150 clone 2 (Q38). j , Saturating concentrations of unlabelled anti-CD150 antibody clone mShad150 does not block staining of PE anti-CD150 clone 2 (Q38) ( j ). k , Schematic to determine if co-staining with anti-CD150 clone 2 (Q38) identifies the same population as anti-CD150 antibody clone mShad150; used in panel l ; BM cells are incubated with PECy-7 anti-CD150 antibody clone mShad150 and with PE anti-CD150 clone 2 (Q38). l , Co-staining with anti-CD150 clones 2 (Q38) identifies the same population as anti-CD150 antibody clone mShad150 ( l ). m – n , Representative FACS gating of mouse BM to sort total HSC (Lin – cKIT + Sca1 + FLT3 – CD34 – CD150 + ) from aged (11 months) control mice (Aged, A) or aged (11 months) mice with my-HSC depletion (Aged+Conditioning, A + C), used to sort HSCs for RNA-sequencing experiment presented in Fig. 2g, h , Extended Data Fig. 4x , and used to sort HSCs for transplant experiments presented in Fig. 2i–k , Extended Data Fig. 4t–v . My-HSC depletion was performed with anti-NEO1+anti-CD62p+anti-cKIT+CD47 and cells were collected at day 9 post-treatment; n = 3 mice (A), n = 3 mice (A + C). The diagrams ( a , e , i , k ) were created using BioRender. Mouse ages approximately: 3 months ( a – h ), 5–8 months ( i – l ), 11 months ( m – n ). For ( a – n ), BM was cKIT-enriched prior to FACS analysis or sorting. n represents independent mice.
Extended Data Fig. 4 Antibody-mediated depletion of my-HSCs in vivo.
a – d , Frequency (% live) ( a , c ) or absolute number ( b , d ) of my-HSCs and bal-HSCs ( a – b ) or of NEO1 + HSCs and NEO1 – HSCs ( c – d ) after anti-CD150 conditioning (anti-CD150, anti-CD150+anti-CD47, or anti-CD150+anti-CD47+anti-cKIT); n = 3 mice per group. e , Total HSCs (my-HSCs+bal-HSCs) as percent-live in mice receiving anti-CD47; n = 5 mice per group; P = 0.3637. f , Percentage of total HSCs that are my-HSCs in mice receiving anti-CD47; n = 5 mice per group; P = 0.6628. g , Total HSCs (my-HSCs+bal-HSCs) as a percent-live, in mice receiving anti-CD47+anti-cKIT; n = 4 mice per group; P = 0.0973. h , Percentage of total HSCs that are my-HSCs in mice receiving anti-CD47+anti-cKIT; n = 4 mice per group; P = 0.2805. i , Percentage of total HSCs that are NEO1 + HSCs in mice receiving anti-CD150 (IgG2a)+anti-CD47+anti-cKIT (e.g., protocol CD150 v2 ); n = 4 mice per group; * P = 0.0353. j – n , Frequency (% live) of CLPs ( j ), IL7Ra + cells ( k ), CMPs&GMPs ( l ), MkPs ( m ), and MEPs ( n ), after anti-CD150, anti-CD62p, or anti-NEO1 conditioning protocols. Values relative to mean of untreated control mice and log2-transformed; n = 3 mice per group (NEO1 v1 , NEO1 v2 , CD150 v1 ); n = 4 mice per group (CD62p, CD150 v2 ). o , Ratio of frequency (% live) for Lymphoid to Myeloid Progenitors (CLP)/(CMP&GMP), after anti-CD150, anti-CD62p, or anti-NEO1 antibody protocols. Values relative to mean of untreated control mice and log2-transformed; n = 3 mice per group (NEO1 v1 , NEO1 v2 , CD150 v1 ); n = 4 mice per group (CD62p, CD150 v2 ). p , Frequency (% live) of my-HSCs, bal-HSCs, NEO1 + HSCs, and NEO1 - HSCs after treatment with anti-CD150, anti-CD62p, or anti-NEO1 protocols; n = 3 mice per group (anti-CD150 v1 ); n = 4 mice per group (anti-CD62p); n = 3 control mice and n = 6 treated mice (anti-NEO1, combined 90 ug & 200 ug protocols). Values relative to mean of control mice and log2-transformed. For j – p , values for treated mice (filled circles); values for control mice (unfilled circles). q , Correlation of fraction of live cells for my-HSCs vs. NEO1 + HSCs (left), and bal-HSCs vs. NEO1 - HSCs (right), of control mice and mice receiving anti-CD150, anti-CD62p, or anti-NEO1 protocols, in cKIT-enriched BM; n = 3 mice (anti-CD150 v1 ); n = 4 mice (anti-CD62p); n = 3 control mice and n = 6 treated mice (anti-NEO1, combined 90 ug & 200 ug protocols). r , Comparison of the absolute number of cells in total (non-cKIT-enriched) BM (y-axis) vs. frequency of cells as a percent-live (in cKIT-enriched) BM (x-axis), for my-HSCs, bal-HSCs, CLP, CMP&GMP, and MkP in control mice and mice receiving anti-CD150, anti-CD150+anti-CD47, or anti-CD150+anti-CD47+anti-cKIT; n = 3 mice per condition. s , Comparison of the frequency of cells as a fraction of total (non-cKIT-enriched) BM (x-axis) vs. the frequency of cells as a fraction of cKIT-enriched BM (y-axis), for cell populations in control mice and mice receiving anti-CD150, anti-CD150+anti-CD47, or anti-CD150+anti-CD47+anti-cKIT; n = 3 mice per condition. Data for q – s log10-transformed. t – v , HSC lineage potential 8-weeks after transplantation of 100 total HSCs FACS-sorted from CD45.2 aged mice without (A) or with my-HSC depletion (A + C) into CD45.1 recipients. Donor Myeloid to Lymphoid Ratio ( t , * P = 0.0275) and Percent-Chimerism Ratio ( u , * P = 0.0340). For each recipient (y-axis), % donor cells (bottom x-axis) that are Myeloid ( red bars) or Lymphoid ( blue bars) and the Myeloid/Lymphoid log2-ratio ( red circles) (top x-axis) ( v ); n = 13 recipient mice (A), n = 14 recipient mice (A + C). w , Donor Myeloid/Lymphoid Ratio 16-weeks after transplantation of 100 total HSCs FACS-sorted from CD45.2 aged mice without (A) or with my-HSC depletion (A + C) into CD45.1 recipients; n = 12 recipient mice (A), n = 14 recipient mice (A + C); * P = 0.0232. x , Top 200 differentially expressed genes ranked by p-value for RNA-seq comparison between (A) vs. (A + C), based on Fragments Per Kilobase of transcript per Million mapped reads (FPKM); log2-transformed. Heatmap generated using Phantasus 110 (v1.21.5) with FPKM values as input and Limma 112 to define differentially expressed genes; n = 3 mice (A), n = 3 mice (A + C). Mouse ages approximately: 6-7 months ( a – d , r – s ), 7–9 months ( g – i ), 5–9 months ( j – q ), 11 months (donors, t – w ), 2 months (recipients, v ). For ( a , c , g – s ), BM was cKIT-enriched prior to analysis. For ( b , d , e – f , r – s ), total BM (non cKIT-enriched) was examined. P -values obtained by ordinary one-way ANOVA followed by one-tailed Dunnett’s multiple comparisons test with non-treated as control ( a – d ), unpaired parametric one-tailed t-test ( i - k , p , t – u ), unpaired non-parametric one-tailed t-test ( w ), or unpaired parametric two-tailed t-test ( e – h , l – o ). p -values and R values calculated with one-tailed Pearson correlation coefficient ( q – s ). CD150 v1 is rat IgG2b anti-CD150 protocol; CD150 v2 is rat IgG2a anti-CD150 protocol; NEO1 v2 includes mouse IgG2a anti-goat; α, anti-; ns, not significant. Bars indicate mean +/− s.e.m. n represents independent mice; * P < 0.05, ** P < 0.005, *** P < 0.0005; Exact P -values provided as source data.
Extended Data Fig. 5 Optimization of NEO1 depletion protocol in vitro and in vivo.
a – b , Strategy to restore balanced lineage output by depleting my-HSCs. c – h , Anti-NEO1 antibody saturation curve ( c ) determined from in vitro antibody dilution series ( d – h ). i , Schematic of in vivo saturation experiments with anti-NEO1 antibody; used in panels j – m . j – m , Dose-dependent relationship between anti-NEO1 antibody dose (0 ug, 30 ug, 90 ug, 200 ug), when combined with anti-CD47 and anti-cKIT, on the relative depletion of NEO1 + HSCs ( j ), and increase in CLPs ( k ). Optimal concentration in yellow; n = 3 mice per group. l , The increase in NEO1 – HSCs after anti-NEO1 dose escalation (0 ug, 30 ug, 90 ug, 200 ug) is correlated with the increase in CLPs. m , Impact on the ratio of Bal-HSCs/My-HSCs ( black ) and NEO1 – HSCs/NEO1 + HSCs ( blue ) as a percentage of live cells after anti-NEO1 antibody dose-escalation (0 ug, 30 ug, 90 ug, 200 ug), when combined with anti-CD47 and anti-cKIT; n = 3 per group. Values relative to mean value of control (0 ug) mice and log2-transformed. n , Schematic of double-antibody strategy to target NEO1, whereby mouse IgG2a or IgG2b monoclonal anti-goat antibodies are administered 24 h after goat anti-NEO1 93 , 94 . o – p , Schematic ( o ) to demonstrate that saturating concentrations of mouse IgG2a or IgG2b anti-goat do not reduce ability of donkey anti-goat AF488 detect goat anti-NEO1 antibody ( p ). q – s , Schematic ( q ) of experiment demonstrating that mouse anti-goat IgG2a A555 ( r ) and IgG2b PE ( s ) antibodies identify the same population as donkey anti-goat AF488 by flow-cytometry. The diagrams ( a , b , i , n , o , q ) were created using BioRender. Mouse ages approximately: 5–7 months ( c – h , n – s ), 6–9 months ( i – m ). For ( c – s ), BM was cKIT-enriched prior to analysis. For correlation p -values, one-tailed Pearson correlation coefficient (R p ), and one-tailed Spearman correlation coefficient (R s ) were calculated ( l ). p -values obtained by ordinary one-way ANOVA followed by one-tailed Dunnett’s multiple comparisons test with 0ug condition as control ( m ). MaG, mouse-anti goat; DaG, donkey anti-goat. Bars indicate mean +/− s.e.m. n represents independent mice; * P < 0.05, ** P < 0.005, *** P < 0.0005; Exact P -values provided as source data.
Extended Data Fig. 6 My-HSC depletion restores features of a youthful immune system.
a , Ratio of frequency of my-HSCs to bal-HSCs (% live) in aged (A), or aged+conditioning mice (A + C) after 8-weeks; n = 4 (A), n = 9 (A + C); P = 0.0346. b – d , Statistical significance (y-axis, -log10p) vs. fold-change (x-axis, log2-FC) of plasma protein levels at week-8 for (A)/(Y) ( b ), (A)/(A + C) ( c ), or (A)/(Y & A + C) ( d ) comparisons. Grey bar (y = 1–1.3); values above grey bar p < 0.05; n = 6 (Y, A), n = 5 (A + C). e , Overlap of top 17% of plasma proteins, ranked by statistical significance, increased in (A)/(Y) and decreased in (A + C)/(A). f , Estimated plasma concentration of CXCL5 116 at week-8; n = 6 (Y, A), n = 5 (A + C). g , Relative plasma abundance of inflammatory proteins at week-8; n = 6 (Y, A), n = 5 (A + C). Values relative to the mean for (Y) and log2-transformed. h – i , Frequency of CD11b + Ly6G/C + ( h ) and CD11b + SIRPa + ( i ) mature myeloid cells in the blood approximately 1-week after antibody-conditioning; n = 3 (A), n = 4 (A, A + C). j – k , Frequency of mature B cells (B220 + CD19 + CD43 − CD93 − IgM + IgD + ) ( j ), and progenitor B cells (B220 + CD19 − B cells) ( k ), in the BM approximately 1-week after antibody-conditioning; n = 5 (A), n = 6 (A, A + C). Thymus weight ( l ) and frequency of thymic subsets 1–8 as defined by Akashi & Weissman 117 , as % of total CD45 + cells in the thymus ( n ), 8-weeks after antibody-conditioning; representative FACS ( m ); n = 3 (Y, A), n = 9 (A + C). Populations enriched for transitional intermediate cells (areas 3&4) 117 highlighted in box ( n ). Mouse ages: Y (3–6 months); A & A + C (18–24 months). For ( a ), BM was cKIT-enriched prior to analysis. p -values obtained by unpaired parametric one-tailed t-test ( a ), ordinary one-way ANOVA followed by one-tailed Dunnett’s multiple comparisons test using Aged as control ( f ), one-way ANOVA followed by Holm multiple comparisons test ( b – d , g ), ordinary one-way ANOVA followed by two-tailed Dunnett’s multiple comparisons test using Aged as control ( h – i , l , n ), or Brown-Forsythe and Welch ANOVA tests followed by Dunnett’s T3 multiple comparisons test using Aged as control ( j – k ). Bars indicate mean +/− s.e.m. n represents independent mice; * P < 0.05, ** P < 0.005, *** P < 0.0005; Exact P -values provided as source data.
Extended Data Fig. 7 My-HSC depletion increases naïve T cells and B cells in aged mice.
a – c , Absolute numbers of ( a ) naïve (CD44 − CD62L + ); * P = 0.0430, ( b ) central memory (CM: CD44 + CD62L + ); * P = 0.0177, or ( c ) effector memory (EM: CD44 + CD62L − ); P = 0.576, T cells (CD4 & CD8), per mL of blood, approximately 8-weeks post-treatment. Values log2-transformed; n = 9 (A), n = 14 (A + C), mice pooled from 2 independent experiments. d , Absolute numbers of mature B cells (IgM + IgD + ) per mL of blood, approximately 8-weeks post-treatment; * P = 0.0498. Values log2-transformed; n = 6 (A), n = 5 (A + C). e , Absolute numbers of CD45+ cells per mL of blood, approximately 8-weeks post-treatment. Values log2-transformed; n = 3 (A), n = 9 (A + C); P = 0.069. f – g , Percentage of CM ( f ) and EM ( g ) subsets per total T cells (CD4 & CD8), 8-weeks post-treatment; n = 6 (Y, A), n = 5 (A + C). h – j , Frequency relative to Aged mice of T cell (CD4 & CD8) subsets 8-weeks after antibody treatment ( h ). Naïve, CM, and EM subsets were defined by 12-marker cluster-based analysis ( i – j ); n = 6 (Y, A), n = 5 (A + C). Values relative to mean for Aged control mice and log2-transformed. Mouse ages: Y (3–6 months); A & A + C (18–24 months). p -values obtained by unpaired parametric one-tailed t-test ( a , d ), unpaired parametric two-tailed t-test ( b – c , e ), or ordinary one-way ANOVA followed by two-tailed ( f – g ) or one-tailed ( h ) Dunnett’s multiple comparisons test using Aged as control. Bars indicate mean +/− s.e.m. n represents independent mice; * P < 0.05, ** P < 0.005, *** P < 0.0005; Exact P -values provided as source data.
Extended Data Fig. 8 Flow-cytometry gating strategy for T cells, B cells, and myeloid cells.
a – c , Gating strategy to identify: ( b ) naïve (CD44 – CD62L + ), central memory (CD44 + CD62L + ), and effector memory (CD44 + CD62L − ) T cells (combined CD4 & CD8), or ( c ) CD4 T cells that are PD1 + CD62L – or PD1 – CD62L + 41 in the blood, used for Fig. 3e,g , Extended Data Fig. 7a–c , Extended Data Fig. 7f–g . d – f , Gating strategy to identify: ( e ) mature B cells (CD19 + B220 + IgM + IgD + ) 118 , or ( f ) Aged B Cells ABCs 119 (CD19 + IgM + CD93 − CD43 − CD21/CD35 − CD23 − ) 42 in the blood, used for Fig. 3f,h , Extended Data Fig. 7d . g – i , Gating strategy to identify ( h ) progenitor B cells (B220 + CD19 − ), or ( i ) mature B cells (B220 + CD19 + CD43 − CD93 − IgM + IgD + ) in the bone-marrow, used for Extended Data Fig. 6j,k . j – l , Gating strategy to identify ( k ) CD11b + Ly6G/C + myeloid cells, or ( l ) CD11b + SIRPa + myeloid cells in the blood, used for Extended Data Fig. 6h,i .
Extended Data Fig. 9 Antibody-conditioning enhances functional immunity to infection.
a , Schematic of model to determine the impact of antibody-conditioning on functional immunity. The diagram was created using BioRender. b , Gating to identify Ter119 + cells (Ter119 + CD19 − CD3 − CD45 +/lo ) and antigen-infected cells (Ag34 + Ter119 + ) in mouse spleens, used for Extended Data Fig. 9i,j . c – h , My-HSC and NEO1 + HSC absolute numbers in total BM ( c , e ), or as percentage of total HSCs ( d , f ), with correlations ( h ), 10-weeks after anti-NEO1 v2 conditioning. Representative FACS on total HSCs ( g ). A and A + C mice received vaccination at Week 8; n = 2 (Y), n = 7 (A), n = 10 (A + C). i , Total number of Ter119 + cells per mouse spleen in mice that were Naïve, Infected, or Vaccinated & Infected with FV. Representative FACS histograms for Ter119 expression, gated on all single cells. Each row represents an independent mouse. n = 9 (Y, naïve), n = 13 (Y, FV infected), n = 13 (Y, vaccinated & FV infected), n = 6 (A, naïve), n = 10 (A, FV infected), n = 8 (A, vaccinated & FV infected), n = 5 (A + C, naïve), n = 9 (A + C, vaccinated & FV infected). Data log10-transformed. j , Total number of antigen-infected (Ag34 + Ter119 + ) cells per spleen in mice that were: Infected, or Vaccinated & Infected with FV. Representative FACS histograms for Ag34 expression, gated on Ter119+ cells. Each row represents an independent mouse; n = 13 (Y, FV infected), n = 13 (Y, vaccinated & FV infected), n = 10 (A, FV infected), n = 8 (A, vaccinated & FV infected), n = 9 (A + C, vaccinated & FV infected). Data log 10 (x + 1)-transformed. k , Correlation of Infectious virus levels (Infectious Centres; x -axis) vs. Dextramer + CD44 + cells (Percent of splenic CD8 + T cells; y -axis) in Vaccinated & FV-infected mice; n = 8 (A, vaccinated & FV-infected), n = 9 (A + C, vaccinated & FV-infected). Data log10-transformed. For i – k , data from experiments using the anti-NEO1 v1 protocol (open circles) or the anti-NEO1 v2 protocol (closed circles) were combined. Mouse ages: Y (3–6 months); A & A + C (21-22 months). For ( c – f ), BM was cKIT-enriched prior to analysis. For ( c , e , h ), total BM (non-cKIT-enriched) was also analysed to calculate total numbers of cells. p -values obtained by one-tailed unpaired parametric t-test ( c – f ), two-tailed Pearson correlation coefficient ( h , k ), or ordinary one-way ANOVA followed by Tukey’s multiple comparisons test ( i – j ). NEO1 v2 protocol is NEO1 v1 protocol (anti-NEO1+ anti-CD47+anti-cKIT)+mouse (IgG2a) anti-goat. Inf., FV infected without vaccination; Vacc. & Inf., FV infected with vaccination, Vacc. & Inf., FV infected with vaccination; LOD, limit of detection; n.s., not significant. n represents independent mice. Bars indicate mean +/− s.e.m. ( c – f ) or bars depict median ( i – j ). * P < 0.05, ** P < 0.005, *** P < 0.0005; Exact P -values provided as source data.
Extended Data Fig. 10 Mouse my-HSC markers are enriched in aged human HSCs.
a , Relative mRNA expression of CD62p ( Selp , P = 0.0243), CD41 ( Itga2b , P = 0.0001), CD61 ( Itgb 3, P = 0.0135), CD150 ( Slamf1 , P = 0.179), and NEO1 ( Neo1 , P = 0.433) in human HSCs isolated from young (age 20–26) and old (age >70) donors; data obtained from Nilsson 54 , GSE69408 . b , Relative mRNA expression of CD62p ( Selp , P = 0.0001), CD41 ( Itga2b , P = 0.0027), CD61 ( Itgb3 , P = 0.0116), and NEO1 ( Neo1 , P = 0.274) in human HSCs isolated from young (age 18–30) or old (age 65–75) donors; data obtained from Adelman 53 ( GSE104406 ). c – e , Correlation of relative mRNA expression of CD62p ( c ), CD41 ( d ), and CD61 ( e ) in human HSCs as compared to donor age; data obtained from Adelman 53 ( GSE104406 ). For a – e , values relative to mean of young samples. f , Heatmap depicting expression of candidate markers in independent datasets of human: HMGA2 + vs. HMGA2 – CD34 + cells ( e 104 ), MPN ( f 56 ) or MDS ( g 57 ) vs. normal HSCs, and Pre-AML vs. normal HSCs ( h 58 ). g – h , Representative FACS to identify human HSCs (Lin – CD34 + CD38 – CD45RA – CD90 + ) 59 , MPPs (Lin – CD34 + CD38 – CD45RA – CD90 – ) 59 , LMPPs (Lin – CD34 + CD38 – CD45RA + ) 120 , CMPs & MEPs (Lin – CD34 + CD38 + CD45RA – ) 121 , and GMPs (Lin – CD34 + CD38 + CD45RA + ) 121 in normal human BM 59 , used for Fig. 5f–i , Extended Data Fig. 10i–s . Samples are post CD34 + -enrichment. i – m , FACS of human HSCs depicting fluorescence-minus-one (FMO) control ( i ), anti-CD62p clone AK4 ( j ), anti-CD62p clone Psel.KO2.3 ( k ), anti-CD62p clone AC1.2 ( l ), and anti-CD150 ( m ); representative for n = 3 donors ( j ); n = 2 donors ( k – m ). n , Illustration of human Hematopoietic Stem and Progenitor Cell (HSPC) Tree Analysis ( n ). o – s , Relative expression of CD62p ( o ), CD150 ( p ), ESAM ( q ), and CD166 ( r ), on human HSPCs, with summary ( s ), showing percent positive HSCs, and normalized MFI for each marker on HSPCs. For o – s , FACS median fluorescent intensity (MFI) values for each marker were obtained for each population, divided by the MFI for the FMO control, and then normalized from 0–100 based on the lowest to highest expression. Red colour scale corresponds to normalized MFI values. Blue, purple, maroon scale corresponds to bins for HSC positivity (20–50%, 51–70%, and 71–100). t , Model to rejuvenate aged immune systems by depleting myeloid-biased hematopoietic stem cells. The diagram was created using BioRender. p -values obtained by unpaired parametric one-tailed t-test ( a – b ), or p -values and R values calculated with one-tailed Pearson correlation coefficient ( c – e ). MDS, myelodysplastic syndrome; MPN, myeloproliferative neoplasms. Bars indicate mean +/− s.e.m. * P < 0.05, ** P < 0.005, *** P < 0.0005; Exact P -values provided as source data.
Supplementary information
Reporting summary, peer review file, supplementary table 1.
Genes differentially expressed between HSCs isolated from control versus antibody-treated mice.
Source data
Source data fig. 1, source data fig. 2, source data fig. 3, source data fig. 4, source data fig. 5, source data extended data fig. 1, source data extended data fig. 2, source data extended data fig. 4, source data extended data fig. 5, source data extended data fig. 6, source data extended data fig. 7, source data extended data fig. 9, source data extended data fig. 10, rights and permissions.
Springer Nature or its licensor (e.g. a society or other partner) holds exclusive rights to this article under a publishing agreement with the author(s) or other rightsholder(s); author self-archiving of the accepted manuscript version of this article is solely governed by the terms of such publishing agreement and applicable law.
Reprints and permissions
About this article
Cite this article.
Ross, J.B., Myers, L.M., Noh, J.J. et al. Depleting myeloid-biased haematopoietic stem cells rejuvenates aged immunity. Nature 628 , 162–170 (2024). https://doi.org/10.1038/s41586-024-07238-x
Download citation
Received : 26 October 2022
Accepted : 26 February 2024
Published : 27 March 2024
Issue Date : 04 April 2024
DOI : https://doi.org/10.1038/s41586-024-07238-x
Share this article
Anyone you share the following link with will be able to read this content:
Sorry, a shareable link is not currently available for this article.
Provided by the Springer Nature SharedIt content-sharing initiative
This article is cited by
How to make an old immune system young again.
- Heidi Ledford
Nature (2024)
Anti-ageing antibodies revive the immune system
- Yasar Arfat T. Kasu
- Robert A. J. Signer
Restoring a youthful immune system
- Sarah Crunkhorn
Nature Reviews Drug Discovery (2024)
By submitting a comment you agree to abide by our Terms and Community Guidelines . If you find something abusive or that does not comply with our terms or guidelines please flag it as inappropriate.
Quick links
- Explore articles by subject
- Guide to authors
- Editorial policies
Sign up for the Nature Briefing newsletter — what matters in science, free to your inbox daily.

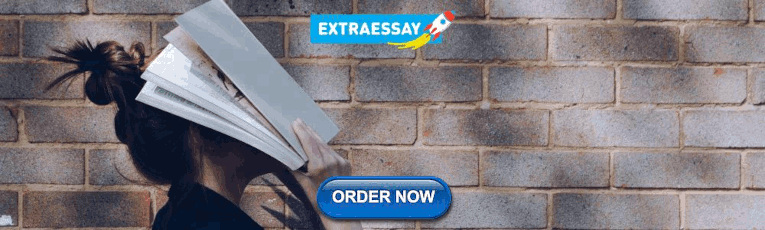
IMAGES
VIDEO
COMMENTS
The immune system is a complex network of cells, tissues and organs that work together to protect the body from harmful substances and organisms and defend against disease. The immune system can ...
In this paper we address the issue of how to think about immunity. Many immunological writings suggest a straightforward option: the view that the immune system is primarily a system of defense, which naturally invites the talk of strong immunity and strong immune response. Despite their undisputable positive role in immunology, such metaphors can also pose a risk of establishing a narrow ...
The immune system is a fascinating world of cells, soluble factors, interacting cells, and tissues, all of which are interconnected. ... Extensive research has focused on deciphering the role of ...
Essays Biochem. 2016 Oct 31; 60(3): ... Discrimination is also a key aspect of the innate immune system. This is an area of research that has grown explosively since studies at the close of the 20th Century identified families of receptors that sampled the environment for the presence of molecules associated with pathogens (called pathogen ...
Abstract. Beyond structural and chemical barriers to pathogens, the immune system has two fundamental lines of defense: innate immunity and adaptive immunity. Innate immunity is the first immunological mechanism for fighting against an intruding pathogen. It is a rapid immune response, initiated within minutes or hours after aggression, that ...
The durability of immunity resulting from SARS-CoV-2 infection and how this immunity compares with that conferred by vaccination are essential questions both at the level of an individual person ...
Immunity publishes papers that report the most important advances in immunology research. The range of subjects includes, but is not limited to, immune cell development and senescence, signal transduction, gene regulation, innate and adaptive immunity, autoimmunity, infectious disease, allergy and asthma, transplantation, and tumor immunology.
The protective effects of vaccination and prior infection against severe Covid-19 are reviewed, with proposed directions for future research, including mucosal immunity and intermittent vaccine boo...
We reviewed studies published in PubMed from inception to Sept 28, 2021, and found well conducted biological studies showing protective immunity after infection ().Furthermore, multiple epidemiological and clinical studies, including studies during the recent period of predominantly delta (B.1.617.2) variant transmission, found that the risk of repeat SARS-CoV-2 infection decreased by 80·5 ...
The mammalian immune system is a remarkable sensory system for the detection and neutralization of pathogens. History is replete with the devastating effects of plagues, and the coronavirus disease 2019 (COVID-19) pandemic is a defining global health crisis of our time. Although the development of effective vaccines has saved many lives, the ...
Innate Immunity is a peer reviewed open access journal which focuses on all aspects of innate immunity, including biologically active bacterial, viral, fungal, parasitic, and plant components, as well as relevant cells, their receptors, signalling pathways, and induced mediators.Innate Immunity is the official journal of the International Endotoxin & Innate Immunity Society (IEIIS).
Immunity publishes the most important advances in immunology research. We welcome studies that provide fundamental new immunological insights at the molecular, cellular, or whole organism level that are relevant, but not limited, to cancer, infectious disease, the nervous system, autoimmunity, allergy, mucosal immunity, and homeostasis. More.
Immune Tolerance. Tolerance is the prevention of an immune response against a particular antigen. For instance, the immune system is generally tolerant of self-antigens, so it does not usually attack the body's own cells, tissues, and organs. However, when tolerance is lost, disorders like autoimmune disease or food allergy may occur.
The protection conferred by natural immunity, vaccination, and both against symptomatic severe acute respiratory syndrome coronavirus 2 (SARS-CoV-2) infection with the BA.1 or BA.2 sublineages of t...
In this paper, we present evidence that vaccination induces a profound impairment in type I interferon signaling, which has diverse adverse consequences to human health. Immune cells that have taken up the vaccine nanoparticles release into circulation large numbers of exosomes containing spike protein along with critical microRNAs that induce ...
The immune system is a key player in maintaining physiological homeostasis and in sustaining health over disease in the human body. ... What distinguishes the paper at hand is its focus on inhalation as the only way of taking in the biogenic substances analysed. ... Very limited research has so far been carried out to address the question of ...
The research team compared the rate of SARS-CoV-2 infection, the severity of disease, hospitalization, and death in over 16s who had either vaccine-induced or natural immunity.
After a decade of research into innate immune memory, we are pleased to launch a call for papers in trained immunity addressing what we have learned and what's next for the field. Historically, the concept of immunological memory referred only to the ability of the adaptive immune system to develop specific responses to pathological triggers.
This does not seem to reflect clinical evidence or research activity in terms of scientific publication. A search on PubMed for the term "immunity" on 31/1/2019 returned 453867 publications. Adding the term "vitamin C" returned just 306 papers (0.07%).
The findings are consistent with previous a research conducted in IRAN. Citation 12. People vaccinated with any of the COVID-19 vaccines will be at risk of some side effects. In this research, we found 77 people (22%) who did not suffer from any side effect after receiving the vaccine, while 273 people (78%) suffered from different side effect.
Metrics. Ageing of the immune system is characterized by decreased lymphopoiesis and adaptive immunity, and increased inflammation and myeloid pathologies 1, 2. Age-related changes in populations ...