Our websites may use cookies to personalize and enhance your experience. By continuing without changing your cookie settings, you agree to this collection. For more information, please see our University Websites Privacy Notice .
Neag School of Education
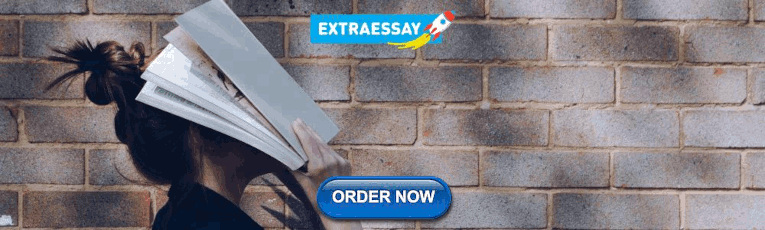
Educational Research Basics by Del Siegle
Qualitative research paradigm.
I am amazed how often we hear qualitative researchers applying their standards to quantitative research or quantitative researchers applying their standards to qualitative research. Each functions within different assumptions. Finding fault with one approach with the standards of another does little to promote understanding. Each approach should be judges on its theoretical basis.
The Assumptions of Qualitative Designs
- Qualitative researchers are concerned primarily with process , rather than outcomes or products.
- Qualitative researchers are interested in meaning: how people make sense of their lives, experiences, and their structures of the world.
- The qualitative researcher is the primary instrument for data collection and analysis. Data are mediated through this human instrument, rather than through inventories, questionnaires, or machines.
- Qualitative research involves fieldwork . The researcher physically goes to the people, setting, site, or institution to observe or record behavior in its natural setting.
- Qualitative research is descriptive in that the researcher is interested in process, meaning, and understanding gained through words or pictures.
- The process of qualitative research is inductive in that the researcher builds abstractions, concepts, hypotheses, and theories from details.
…..Merriam, S. B. (1988). Case study research in education: A qualitative approach. San Francisco, CA: Jossey-Bass.
….. Creswell, J. W. (1994). Research design: Qualitative & quantitative approaches . Thousand Oaks, CA: Sage Publications.
Arguments Supporting Qualitative Inquiry
- Human behavior is significantly influenced by the setting in which it occurs; thus one must study that behavior in situations. The physical setting (e.g., schedules, space, pay, and rewards) and the internalized notions of norms, traditions, roles, and values are crucial contextual variables. Research must be conducted in the setting where all the contextual variables are operating.
- Past researchers have not been able to derive meaning…from experimental research.
- The research techniques themselves, in experimental research, [can]…affect the findings. The lab, the questionnaire, and so on, [can]…become artifacts. Subjects [can become]…either suspicious and wary, or they [can become]…aware of what the researchers want and try to please them. Additionally, subjects sometimes do not know their feelings, interactions, and behaviors, so they cannot articulate them to respond to a questionnaire.
- One cannot understand human behavior without understanding the framework within which subjects interpret their thoughts, feelings, and actions. Researchers need to understand the framework. In fact, the “objective ” scientist, by coding and standardizing, may destroy valuable data while imposing her world on the subjects.
- Field study research can explore the processes and meanings of events.
…..Marshall, C., & Rossman, G. (1980). Designing qualitative research . Newbury Park, CA: Sage.
Predispositions of Quantitative and Qualitative Modes of Inquiry
Although some social science researchers (Lincoln & Guba, 1985; Schwandt, 1989) perceive qualitative and quantitative approaches as incompatible, others (Patton, 1990; Reichardt & Cook, 1979) believe that the skilled researcher can successfully combine approaches. The argument usually becomes muddled because one party argues from the underlying philosophical nature of each paradigm, and the other focuses on the apparent compatibility of the research methods, enjoying the rewards of both numbers and words. Because the positivist and the interpretivist paradigms rest on different assumptions about the nature of the world, they require different instruments and procedures to find the type of data desired. This does not mean, however, that the positivist never uses interviews nor that the interpretivist never uses a survey. They may, but such methods are supplementary, not dominant….Different approaches allow us to know and understand different things about the world….Nonetheless, people tend to adhere to the methodology that is most consonant with their socialized worldview. (p. 9)
….. Glesne, C., & Peshkin, A. (1992). Becoming qualitative researchers: An introduction. White Plains, NY: Longman.
Contrasting Positivist and Naturalist Axioms (Beliefs and Assumptions)
….. Lincoln, Y. S., & Guba, E. G. (1985). Naturalistic inquiry . Newbury Park, CA: Sage Publications.
….. Spradley, J. P. (1979). The ethnographic interview. Fort Worth, TX: Harcourt Brace Jovanovich College Publishers.
Five popular types of Qualitative Research are
- Ethnography
- Phenomenological
- Grounded Theory
Del Siegle, Ph.D [email protected] www.delsiegle.info

Qualitative Research
- Introduction
- Definition & Overview
- Common Paradigms
- Research Traditions
- Analytical Approaches
- Analytical Methods
- Rigor & Evaluative Criteria
- Mixed-Methods Research
Common Paradigms in Qualitative Research
Interpretivist Paradigm
- RWJF | Qualitative Research Guidelines Project : Interpretivist Paradigm
Positivist Paradigm
- RWJF | Qualitative Research Guidelines Project : Positivist Paradigm
Critical/Subtle Realist Paradigm
- RWJF | Qualitative Research Guidelines Project : Critical or Subtle Realist Paradigm
Critical Theory Paradigm
- RWJF | Qualitative Research Guidelines Project : Critical Theoretical Paradigm
Feminist Paradigm
- RWJF | Qualitative Research Guidelines Project : Feminist Paradigms
- << Previous: Definition & Overview
- Next: Research Traditions >>
- Last Updated: May 1, 2024 1:12 PM
- URL: https://msmu.libguides.com/qualitative-research
COE LIBRARY Chalon Campus
Charles Willard Coe Library 12001 Chalon Road Los Angeles, CA 90049 310.954.4370
McCARTHY LIBRARY Doheny Campus
J. Thomas McCarthy Library 10 Chester Place Los Angeles, CA 90007 213.477.2750
Jobs at the Libraries
Information Technology Resources
Institution Complaint Process
Maps & Directions
Privacy Policy
Paradigms in Qualitative Research
- First Online: 01 December 2017
Cite this chapter
- Bartosz Sławecki 4
3714 Accesses
5 Citations
The aim of the chapter is to raise novice researchers’ awareness of the significance of philosophical assumptions for their practical activity. The text presents the basic terms connected with the methodology of social sciences. The entire discussion is centered on the issue of paradigms. Various approaches within the framework of basic philosophical assumptions are discussed—concerning the nature of social reality (ontologies), the nature of scientific cognition (epistemologies), and practical ways of conducting social research (methodologies). An important element of the text is the presentation of two classifications of paradigms in social sciences with particular consideration given to qualitative research.
This work was supported by the Polish National Science Center grant 2013/11/D/HS4/03878
This is a preview of subscription content, log in via an institution to check access.
Access this chapter
- Available as PDF
- Read on any device
- Instant download
- Own it forever
- Available as EPUB and PDF
- Compact, lightweight edition
- Dispatched in 3 to 5 business days
- Free shipping worldwide - see info
- Durable hardcover edition
Tax calculation will be finalised at checkout
Purchases are for personal use only
Institutional subscriptions
Benton, T., & Craib, I. (2010). Philosophy of Social Science: The Philosophical Foundations of Social Thought (2nd ed.). Houndsmill/Basingstoke/New York: Palgrave.
Google Scholar
Burrell, G., & Morgan, G. (1985). Sociological Paradigms and Organisational Analysis: Elements of the Sociology of Corporate Life . Farnham: Routledge.
Denzin, N. K., & Lincoln, Y. S. (Eds.). (2005). The SAGE Handbook of Qualitative Research (3rd ed.). Thousand Oaks: Sage.
Giddens, A. (1993). New Rules of Sociological Method: A Positive Critique of Interpretative Sociologies . Stanford: Stanford University Press.
Guba, E. G., & Lincoln, Y. S. (2005). Paradigmatic Controversies, Contradictions, and Emerging Confluences. In N. K. Denzin & Y. S. Lincoln (Eds.), The SAGE Handbook of Qualitative Research (3rd ed., pp. 191–215). Thousand Oaks: Sage.
Heller, M. (2011). Philosophy in Science . Berlin: Springer.
Book Google Scholar
Heron, J. (1996). Co-Operative Inquiry: Research into the Human Condition . London: Sage.
Hetmański, M. (2008). Epistemology—Old Dilemmas and New Perspectives. Dialogue and Universalism, 18 (7/8), 11–28.
Article Google Scholar
Kostera, M. (1996). Postmodernizm w zarządzaniu [Postmodernism in Management]. Warsaw: PWE.
Kostera, M. (2007). Organisational Ethnography: Methods and Inspirations . Lund: Studentlitteratur.
Kuhn, T. S. (1970). The Structure of Scientific Revolutions (2nd ed., enl). Chicago: University of Chicago Press.
McCloskey, D. (1983). The Rhetoric of Economics. Journal of Economic Literature, 21 (2), 481–517.
Miles, M. B., & Huberman, A. M. (1994). Qualitative Data Analysis: An Expanded Sourcebook . Thousand Oaks: Sage.
Morgan, G. (1980). Paradigms, Metaphors, and Puzzle Solving in Organization Theory. Administrative Science Quarterly, 25 (4), 605–622.
Morgan, G. (1981). The Schismatic Metaphor and Its Implications for Organizational Analysis. Organization Studies, 2 (1), 23–44.
Morgan, G. (1983). More on Metaphor: Why We Cannot Control Tropes in Administrative Science. Administrative Science Quarterly, 28 (4), 601–607.
Morgan, G. (2006). Images of Organization (Updated ed.). Thousand Oaks: Sage.
Schutz, A. (1972). Collected Papers I . M. Natanson (Ed.) (T. 11). Dordrecht: Springer Netherlands.
Silverman, D. (2005). Doing Qualitative Research: A Practical Handbook . London: Sage.
Sintonen, M., Wolenski, J., & Niiniluoto, I. (2004). Handbook of Epistemology . Dordrecht: Kluwer Academic Publishers.
Woleński, J. (2004). The History of Epistemology. In I. Niiniluoto, M. Sintonen, & J. Woleński (Eds.), Handbook of Epistemology (pp. 3–54). Dordrecht: Springer Netherlands.
Download references
Author information
Authors and affiliations.
Poznań University of Economics and Business, Poznań, Poland
Bartosz Sławecki
You can also search for this author in PubMed Google Scholar
Editor information
Editors and affiliations.
Teesside University Business School, Teesside University, Middlesbrough, United Kingdom
Malgorzata Ciesielska
Akademia Leona Koźmińskiego, Warszawa, Poland
Dariusz Jemielniak
Rights and permissions
Reprints and permissions
Copyright information
© 2018 The Author(s)
About this chapter
Sławecki, B. (2018). Paradigms in Qualitative Research. In: Ciesielska, M., Jemielniak, D. (eds) Qualitative Methodologies in Organization Studies. Palgrave Macmillan, Cham. https://doi.org/10.1007/978-3-319-65217-7_2
Download citation
DOI : https://doi.org/10.1007/978-3-319-65217-7_2
Published : 01 December 2017
Publisher Name : Palgrave Macmillan, Cham
Print ISBN : 978-3-319-65216-0
Online ISBN : 978-3-319-65217-7
eBook Packages : Business and Management Business and Management (R0)
Share this chapter
Anyone you share the following link with will be able to read this content:
Sorry, a shareable link is not currently available for this article.
Provided by the Springer Nature SharedIt content-sharing initiative
- Publish with us
Policies and ethics
- Find a journal
- Track your research
Qualitative Research : Definition
Qualitative research is the naturalistic study of social meanings and processes, using interviews, observations, and the analysis of texts and images. In contrast to quantitative researchers, whose statistical methods enable broad generalizations about populations (for example, comparisons of the percentages of U.S. demographic groups who vote in particular ways), qualitative researchers use in-depth studies of the social world to analyze how and why groups think and act in particular ways (for instance, case studies of the experiences that shape political views).
Events and Workshops
- Introduction to NVivo Have you just collected your data and wondered what to do next? Come join us for an introductory session on utilizing NVivo to support your analytical process. This session will only cover features of the software and how to import your records. Please feel free to attend any of the following sessions below: April 25th, 2024 12:30 pm - 1:45 pm Green Library - SVA Conference Room 125 May 9th, 2024 12:30 pm - 1:45 pm Green Library - SVA Conference Room 125 May 30th, 2024 12:30 pm - 1:45 pm Green Library - SVA Conference Room 125
- Next: Choose an approach >>
- Choose an approach
- Find studies
- Learn methods
- Get software
- Get data for secondary analysis
- Network with researchers

- Last Updated: Apr 2, 2024 10:41 AM
- URL: https://guides.library.stanford.edu/qualitative_research
- Link to facebook
- Link to linkedin
- Link to twitter
- Link to youtube
- Writing Tips
Research Paradigms: Explanation and Examples
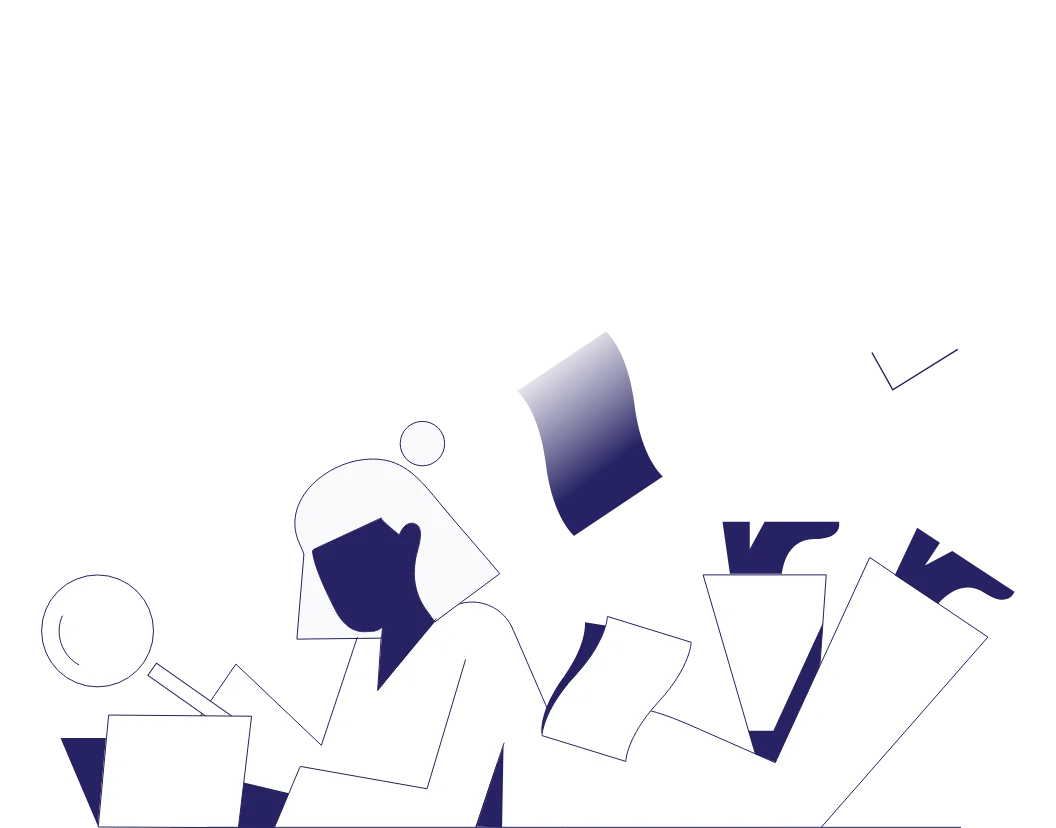
4-minute read
- 1st March 2022
Are you planning a research project? If so, you’ll need a research paradigm. But what exactly is a research paradigm, and why is it important? This blog post will cover the following:
● The definition of a research paradigm
● Why research paradigms are important
● Common examples of research paradigms
● Merging research paradigms
● Expert editing and proofreading
Read on to find out more or learn about research paradigms in the video below!
The Definition of a Research Paradigm
A research paradigm is a philosophical framework that your research is based on. It offers a pattern of beliefs and understandings from which the theories and practices of your research project operate.
A research paradigm consists of ontology, epistemology, and research methodology .
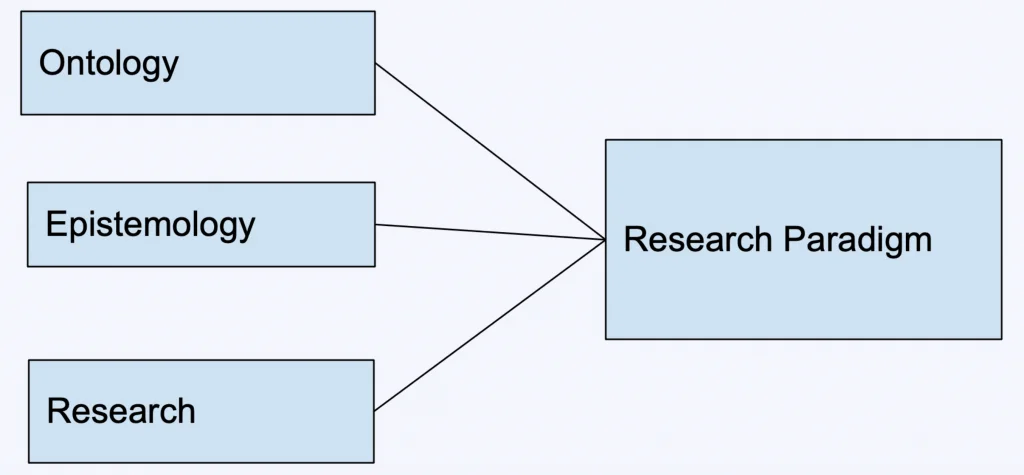
● Ontology answers the question: “What is reality?” That is, does a single reality exist within your research? An example of an ontological question would be: “Does God exist?” There are two possible realities (or ontologies) in response to this question: “Yes, God exists,” or “No, God does not exist.”
● Epistemology is the study of knowledge. It answers the question: “How is it possible to know reality?” Epistemology incorporates the validity, parameters, and methods of acquiring knowledge. An example of an epistemological question would be: “How is it possible to know whether God exists or not?”
● Research Methodology answers the question: “How do we go about discovering the answer or reality?” This includes the process of data collection and analysis. Research methodology should outline how you conduct your research and demonstrate that the findings are valid.
Find this useful?
Subscribe to our newsletter and get writing tips from our editors straight to your inbox.
Together, ontology and epistemology comprise research philosophy.
Research philosophy combined with research methodology comprises a research paradigm.

Why Are Research Paradigms Important?
Research paradigms are important because they form the philosophical basis of a research project. Research paradigms influence how different schools of learning (such as the sciences versus the humanities) undertake their research. Once a research philosophy has been determined, an appropriate methodology can be chosen.
Furthermore, a knowledge of the philosophical foundation of your research will increase its quality and improve your performance in any analysis you may have to undergo!
Common Examples of Research Paradigms
1. Positivism
Positivists believe that there’s a single reality that’s possible to measure and understand. Because of this, they’re most likely to use quantitative methods in their research. Typically, positivists propose a hypothesis that can be proved or disproved using statistical data analysis. Positivism tends to investigate the existence of a relationship between two variables rather than the reason behind it.
2. Constructivism
Constructivists believe that there’s no single reality or truth, but rather multiple realities. They devote themselves to understanding and interpreting the meaning attached to an action. For this reason, constructivists tend to use qualitative research methods , such as interviews or case studies, which focus on providing different perspectives. Constructivism aims to provide the answer to “why.” For example, asking “Why do 25% of the employees of an organization regularly arrive late to work?” rather than merely establishing the relationship between two variables (e.g., time of arrival at work and availability of nearby parking).
3. Pragmatists
Pragmatists believe that reality is continually interpreted and renegotiated against the backdrop of new and unpredictable situations. Because of this, the philosophy they apply in research depends on the research question itself. Pragmatists often combine positivist and constructivist principles in the same research project, using both qualitative and quantitative methods to investigate different components of a research problem. They believe that the optimal research methods are those that most successfully answer the research question.
Merging Research Paradigms
While most social science research operates from either a positivist (experimental) or constructivist paradigm, it’s possible to combine both, as the field of psychology often does. Quantitative and qualitative methodology are frequently used together in psychology, illustrating the subject’s footing in multiple research paradigms (positivist and constructivist).
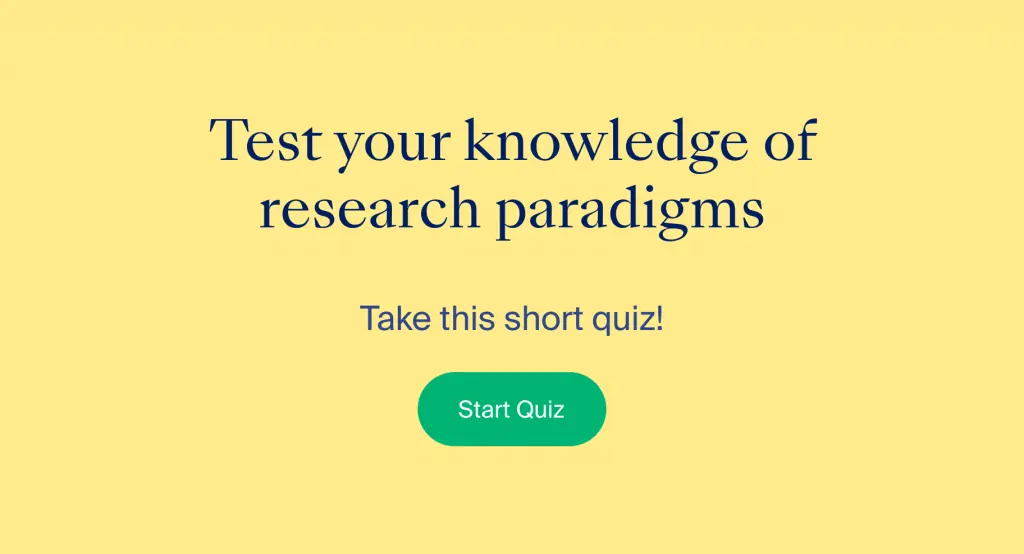
Expert Editing and Proofreading
If you’re writing a research proposal or paper , you’ll want to ensure that your writing is error-free, fluent, and precise. Although re-reading your own work is valuable, it can be very helpful to get another opinion on your writing. We offer a free trial of proofreading and editing services when you submit your first document. Find out more today!
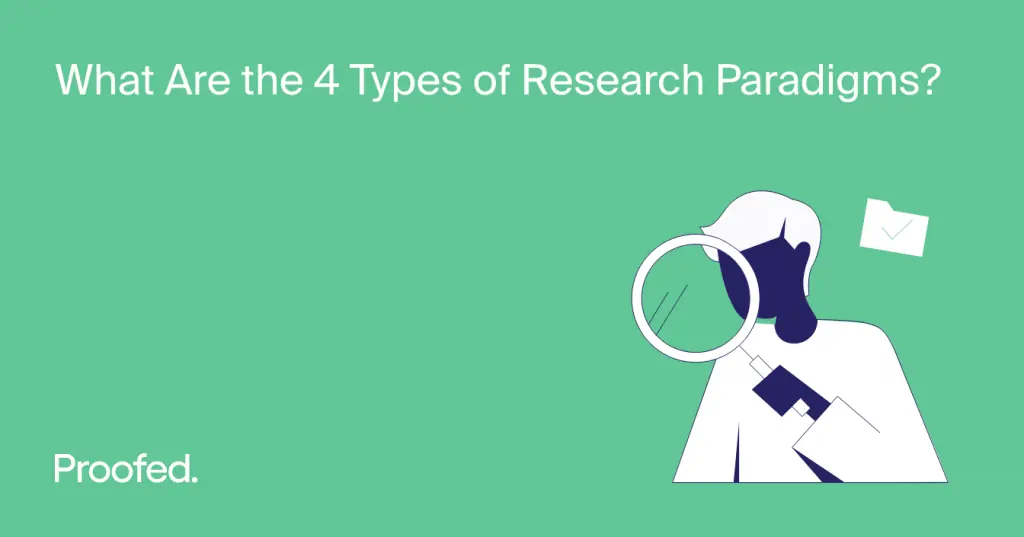
Share this article:
Post A New Comment
Got content that needs a quick turnaround? Let us polish your work. Explore our editorial business services.
3-minute read
How to Insert a Text Box in a Google Doc
Google Docs is a powerful collaborative tool, and mastering its features can significantly enhance your...
2-minute read
How to Cite the CDC in APA
If you’re writing about health issues, you might need to reference the Centers for Disease...
5-minute read
Six Product Description Generator Tools for Your Product Copy
Introduction If you’re involved with ecommerce, you’re likely familiar with the often painstaking process of...
What Is a Content Editor?
Are you interested in learning more about the role of a content editor and the...
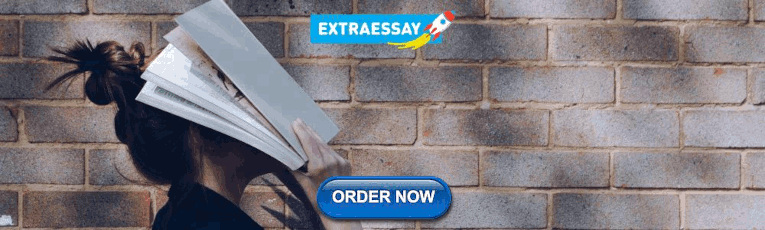
The Benefits of Using an Online Proofreading Service
Proofreading is important to ensure your writing is clear and concise for your readers. Whether...
6 Online AI Presentation Maker Tools
Creating presentations can be time-consuming and frustrating. Trying to construct a visually appealing and informative...
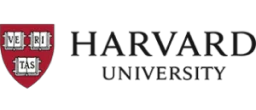
Make sure your writing is the best it can be with our expert English proofreading and editing.

An official website of the United States government
The .gov means it's official. Federal government websites often end in .gov or .mil. Before sharing sensitive information, make sure you're on a federal government site.
The site is secure. The https:// ensures that you are connecting to the official website and that any information you provide is encrypted and transmitted securely.
- Publications
- Account settings
- Browse Titles
NCBI Bookshelf. A service of the National Library of Medicine, National Institutes of Health.
StatPearls [Internet]. Treasure Island (FL): StatPearls Publishing; 2024 Jan-.

StatPearls [Internet].
Qualitative study.
Steven Tenny ; Janelle M. Brannan ; Grace D. Brannan .
Affiliations
Last Update: September 18, 2022 .
- Introduction
Qualitative research is a type of research that explores and provides deeper insights into real-world problems. [1] Instead of collecting numerical data points or intervene or introduce treatments just like in quantitative research, qualitative research helps generate hypotheses as well as further investigate and understand quantitative data. Qualitative research gathers participants' experiences, perceptions, and behavior. It answers the hows and whys instead of how many or how much. It could be structured as a stand-alone study, purely relying on qualitative data or it could be part of mixed-methods research that combines qualitative and quantitative data. This review introduces the readers to some basic concepts, definitions, terminology, and application of qualitative research.
Qualitative research at its core, ask open-ended questions whose answers are not easily put into numbers such as ‘how’ and ‘why’. [2] Due to the open-ended nature of the research questions at hand, qualitative research design is often not linear in the same way quantitative design is. [2] One of the strengths of qualitative research is its ability to explain processes and patterns of human behavior that can be difficult to quantify. [3] Phenomena such as experiences, attitudes, and behaviors can be difficult to accurately capture quantitatively, whereas a qualitative approach allows participants themselves to explain how, why, or what they were thinking, feeling, and experiencing at a certain time or during an event of interest. Quantifying qualitative data certainly is possible, but at its core, qualitative data is looking for themes and patterns that can be difficult to quantify and it is important to ensure that the context and narrative of qualitative work are not lost by trying to quantify something that is not meant to be quantified.
However, while qualitative research is sometimes placed in opposition to quantitative research, where they are necessarily opposites and therefore ‘compete’ against each other and the philosophical paradigms associated with each, qualitative and quantitative work are not necessarily opposites nor are they incompatible. [4] While qualitative and quantitative approaches are different, they are not necessarily opposites, and they are certainly not mutually exclusive. For instance, qualitative research can help expand and deepen understanding of data or results obtained from quantitative analysis. For example, say a quantitative analysis has determined that there is a correlation between length of stay and level of patient satisfaction, but why does this correlation exist? This dual-focus scenario shows one way in which qualitative and quantitative research could be integrated together.
Examples of Qualitative Research Approaches
Ethnography
Ethnography as a research design has its origins in social and cultural anthropology, and involves the researcher being directly immersed in the participant’s environment. [2] Through this immersion, the ethnographer can use a variety of data collection techniques with the aim of being able to produce a comprehensive account of the social phenomena that occurred during the research period. [2] That is to say, the researcher’s aim with ethnography is to immerse themselves into the research population and come out of it with accounts of actions, behaviors, events, etc. through the eyes of someone involved in the population. Direct involvement of the researcher with the target population is one benefit of ethnographic research because it can then be possible to find data that is otherwise very difficult to extract and record.
Grounded Theory
Grounded Theory is the “generation of a theoretical model through the experience of observing a study population and developing a comparative analysis of their speech and behavior.” [5] As opposed to quantitative research which is deductive and tests or verifies an existing theory, grounded theory research is inductive and therefore lends itself to research that is aiming to study social interactions or experiences. [3] [2] In essence, Grounded Theory’s goal is to explain for example how and why an event occurs or how and why people might behave a certain way. Through observing the population, a researcher using the Grounded Theory approach can then develop a theory to explain the phenomena of interest.
Phenomenology
Phenomenology is defined as the “study of the meaning of phenomena or the study of the particular”. [5] At first glance, it might seem that Grounded Theory and Phenomenology are quite similar, but upon careful examination, the differences can be seen. At its core, phenomenology looks to investigate experiences from the perspective of the individual. [2] Phenomenology is essentially looking into the ‘lived experiences’ of the participants and aims to examine how and why participants behaved a certain way, from their perspective . Herein lies one of the main differences between Grounded Theory and Phenomenology. Grounded Theory aims to develop a theory for social phenomena through an examination of various data sources whereas Phenomenology focuses on describing and explaining an event or phenomena from the perspective of those who have experienced it.
Narrative Research
One of qualitative research’s strengths lies in its ability to tell a story, often from the perspective of those directly involved in it. Reporting on qualitative research involves including details and descriptions of the setting involved and quotes from participants. This detail is called ‘thick’ or ‘rich’ description and is a strength of qualitative research. Narrative research is rife with the possibilities of ‘thick’ description as this approach weaves together a sequence of events, usually from just one or two individuals, in the hopes of creating a cohesive story, or narrative. [2] While it might seem like a waste of time to focus on such a specific, individual level, understanding one or two people’s narratives for an event or phenomenon can help to inform researchers about the influences that helped shape that narrative. The tension or conflict of differing narratives can be “opportunities for innovation”. [2]
Research Paradigm
Research paradigms are the assumptions, norms, and standards that underpin different approaches to research. Essentially, research paradigms are the ‘worldview’ that inform research. [4] It is valuable for researchers, both qualitative and quantitative, to understand what paradigm they are working within because understanding the theoretical basis of research paradigms allows researchers to understand the strengths and weaknesses of the approach being used and adjust accordingly. Different paradigms have different ontology and epistemologies . Ontology is defined as the "assumptions about the nature of reality” whereas epistemology is defined as the “assumptions about the nature of knowledge” that inform the work researchers do. [2] It is important to understand the ontological and epistemological foundations of the research paradigm researchers are working within to allow for a full understanding of the approach being used and the assumptions that underpin the approach as a whole. Further, it is crucial that researchers understand their own ontological and epistemological assumptions about the world in general because their assumptions about the world will necessarily impact how they interact with research. A discussion of the research paradigm is not complete without describing positivist, postpositivist, and constructivist philosophies.
Positivist vs Postpositivist
To further understand qualitative research, we need to discuss positivist and postpositivist frameworks. Positivism is a philosophy that the scientific method can and should be applied to social as well as natural sciences. [4] Essentially, positivist thinking insists that the social sciences should use natural science methods in its research which stems from positivist ontology that there is an objective reality that exists that is fully independent of our perception of the world as individuals. Quantitative research is rooted in positivist philosophy, which can be seen in the value it places on concepts such as causality, generalizability, and replicability.
Conversely, postpositivists argue that social reality can never be one hundred percent explained but it could be approximated. [4] Indeed, qualitative researchers have been insisting that there are “fundamental limits to the extent to which the methods and procedures of the natural sciences could be applied to the social world” and therefore postpositivist philosophy is often associated with qualitative research. [4] An example of positivist versus postpositivist values in research might be that positivist philosophies value hypothesis-testing, whereas postpositivist philosophies value the ability to formulate a substantive theory.
Constructivist
Constructivism is a subcategory of postpositivism. Most researchers invested in postpositivist research are constructivist as well, meaning they think there is no objective external reality that exists but rather that reality is constructed. Constructivism is a theoretical lens that emphasizes the dynamic nature of our world. “Constructivism contends that individuals’ views are directly influenced by their experiences, and it is these individual experiences and views that shape their perspective of reality”. [6] Essentially, Constructivist thought focuses on how ‘reality’ is not a fixed certainty and experiences, interactions, and backgrounds give people a unique view of the world. Constructivism contends, unlike in positivist views, that there is not necessarily an ‘objective’ reality we all experience. This is the ‘relativist’ ontological view that reality and the world we live in are dynamic and socially constructed. Therefore, qualitative scientific knowledge can be inductive as well as deductive.” [4]
So why is it important to understand the differences in assumptions that different philosophies and approaches to research have? Fundamentally, the assumptions underpinning the research tools a researcher selects provide an overall base for the assumptions the rest of the research will have and can even change the role of the researcher themselves. [2] For example, is the researcher an ‘objective’ observer such as in positivist quantitative work? Or is the researcher an active participant in the research itself, as in postpositivist qualitative work? Understanding the philosophical base of the research undertaken allows researchers to fully understand the implications of their work and their role within the research, as well as reflect on their own positionality and bias as it pertains to the research they are conducting.
Data Sampling
The better the sample represents the intended study population, the more likely the researcher is to encompass the varying factors at play. The following are examples of participant sampling and selection: [7]
- Purposive sampling- selection based on the researcher’s rationale in terms of being the most informative.
- Criterion sampling-selection based on pre-identified factors.
- Convenience sampling- selection based on availability.
- Snowball sampling- the selection is by referral from other participants or people who know potential participants.
- Extreme case sampling- targeted selection of rare cases.
- Typical case sampling-selection based on regular or average participants.
Data Collection and Analysis
Qualitative research uses several techniques including interviews, focus groups, and observation. [1] [2] [3] Interviews may be unstructured, with open-ended questions on a topic and the interviewer adapts to the responses. Structured interviews have a predetermined number of questions that every participant is asked. It is usually one on one and is appropriate for sensitive topics or topics needing an in-depth exploration. Focus groups are often held with 8-12 target participants and are used when group dynamics and collective views on a topic are desired. Researchers can be a participant-observer to share the experiences of the subject or a non-participant or detached observer.
While quantitative research design prescribes a controlled environment for data collection, qualitative data collection may be in a central location or in the environment of the participants, depending on the study goals and design. Qualitative research could amount to a large amount of data. Data is transcribed which may then be coded manually or with the use of Computer Assisted Qualitative Data Analysis Software or CAQDAS such as ATLAS.ti or NVivo. [8] [9] [10]
After the coding process, qualitative research results could be in various formats. It could be a synthesis and interpretation presented with excerpts from the data. [11] Results also could be in the form of themes and theory or model development.
Dissemination
To standardize and facilitate the dissemination of qualitative research outcomes, the healthcare team can use two reporting standards. The Consolidated Criteria for Reporting Qualitative Research or COREQ is a 32-item checklist for interviews and focus groups. [12] The Standards for Reporting Qualitative Research (SRQR) is a checklist covering a wider range of qualitative research. [13]
Examples of Application
Many times a research question will start with qualitative research. The qualitative research will help generate the research hypothesis which can be tested with quantitative methods. After the data is collected and analyzed with quantitative methods, a set of qualitative methods can be used to dive deeper into the data for a better understanding of what the numbers truly mean and their implications. The qualitative methods can then help clarify the quantitative data and also help refine the hypothesis for future research. Furthermore, with qualitative research researchers can explore subjects that are poorly studied with quantitative methods. These include opinions, individual's actions, and social science research.
A good qualitative study design starts with a goal or objective. This should be clearly defined or stated. The target population needs to be specified. A method for obtaining information from the study population must be carefully detailed to ensure there are no omissions of part of the target population. A proper collection method should be selected which will help obtain the desired information without overly limiting the collected data because many times, the information sought is not well compartmentalized or obtained. Finally, the design should ensure adequate methods for analyzing the data. An example may help better clarify some of the various aspects of qualitative research.
A researcher wants to decrease the number of teenagers who smoke in their community. The researcher could begin by asking current teen smokers why they started smoking through structured or unstructured interviews (qualitative research). The researcher can also get together a group of current teenage smokers and conduct a focus group to help brainstorm factors that may have prevented them from starting to smoke (qualitative research).
In this example, the researcher has used qualitative research methods (interviews and focus groups) to generate a list of ideas of both why teens start to smoke as well as factors that may have prevented them from starting to smoke. Next, the researcher compiles this data. The research found that, hypothetically, peer pressure, health issues, cost, being considered “cool,” and rebellious behavior all might increase or decrease the likelihood of teens starting to smoke.
The researcher creates a survey asking teen participants to rank how important each of the above factors is in either starting smoking (for current smokers) or not smoking (for current non-smokers). This survey provides specific numbers (ranked importance of each factor) and is thus a quantitative research tool.
The researcher can use the results of the survey to focus efforts on the one or two highest-ranked factors. Let us say the researcher found that health was the major factor that keeps teens from starting to smoke, and peer pressure was the major factor that contributed to teens to start smoking. The researcher can go back to qualitative research methods to dive deeper into each of these for more information. The researcher wants to focus on how to keep teens from starting to smoke, so they focus on the peer pressure aspect.
The researcher can conduct interviews and/or focus groups (qualitative research) about what types and forms of peer pressure are commonly encountered, where the peer pressure comes from, and where smoking first starts. The researcher hypothetically finds that peer pressure often occurs after school at the local teen hangouts, mostly the local park. The researcher also hypothetically finds that peer pressure comes from older, current smokers who provide the cigarettes.
The researcher could further explore this observation made at the local teen hangouts (qualitative research) and take notes regarding who is smoking, who is not, and what observable factors are at play for peer pressure of smoking. The researcher finds a local park where many local teenagers hang out and see that a shady, overgrown area of the park is where the smokers tend to hang out. The researcher notes the smoking teenagers buy their cigarettes from a local convenience store adjacent to the park where the clerk does not check identification before selling cigarettes. These observations fall under qualitative research.
If the researcher returns to the park and counts how many individuals smoke in each region of the park, this numerical data would be quantitative research. Based on the researcher's efforts thus far, they conclude that local teen smoking and teenagers who start to smoke may decrease if there are fewer overgrown areas of the park and the local convenience store does not sell cigarettes to underage individuals.
The researcher could try to have the parks department reassess the shady areas to make them less conducive to the smokers or identify how to limit the sales of cigarettes to underage individuals by the convenience store. The researcher would then cycle back to qualitative methods of asking at-risk population their perceptions of the changes, what factors are still at play, as well as quantitative research that includes teen smoking rates in the community, the incidence of new teen smokers, among others. [14] [15]
Qualitative research functions as a standalone research design or in combination with quantitative research to enhance our understanding of the world. Qualitative research uses techniques including structured and unstructured interviews, focus groups, and participant observation to not only help generate hypotheses which can be more rigorously tested with quantitative research but also to help researchers delve deeper into the quantitative research numbers, understand what they mean, and understand what the implications are. Qualitative research provides researchers with a way to understand what is going on, especially when things are not easily categorized. [16]
- Issues of Concern
As discussed in the sections above, quantitative and qualitative work differ in many different ways, including the criteria for evaluating them. There are four well-established criteria for evaluating quantitative data: internal validity, external validity, reliability, and objectivity. The correlating concepts in qualitative research are credibility, transferability, dependability, and confirmability. [4] [11] The corresponding quantitative and qualitative concepts can be seen below, with the quantitative concept is on the left, and the qualitative concept is on the right:
- Internal validity--- Credibility
- External validity---Transferability
- Reliability---Dependability
- Objectivity---Confirmability
In conducting qualitative research, ensuring these concepts are satisfied and well thought out can mitigate potential issues from arising. For example, just as a researcher will ensure that their quantitative study is internally valid so should qualitative researchers ensure that their work has credibility.
Indicators such as triangulation and peer examination can help evaluate the credibility of qualitative work.
- Triangulation: Triangulation involves using multiple methods of data collection to increase the likelihood of getting a reliable and accurate result. In our above magic example, the result would be more reliable by also interviewing the magician, back-stage hand, and the person who "vanished." In qualitative research, triangulation can include using telephone surveys, in-person surveys, focus groups, and interviews as well as surveying an adequate cross-section of the target demographic.
- Peer examination: Results can be reviewed by a peer to ensure the data is consistent with the findings.
‘Thick’ or ‘rich’ description can be used to evaluate the transferability of qualitative research whereas using an indicator such as an audit trail might help with evaluating the dependability and confirmability.
- Thick or rich description is a detailed and thorough description of details, the setting, and quotes from participants in the research. [5] Thick descriptions will include a detailed explanation of how the study was carried out. Thick descriptions are detailed enough to allow readers to draw conclusions and interpret the data themselves, which can help with transferability and replicability.
- Audit trail: An audit trail provides a documented set of steps of how the participants were selected and the data was collected. The original records of information should also be kept (e.g., surveys, notes, recordings).
One issue of concern that qualitative researchers should take into consideration is observation bias. Here are a few examples:
- Hawthorne effect: The Hawthorne effect is the change in participant behavior when they know they are being observed. If a researcher was wanting to identify factors that contribute to employee theft and tells the employees they are going to watch them to see what factors affect employee theft, one would suspect employee behavior would change when they know they are being watched.
- Observer-expectancy effect: Some participants change their behavior or responses to satisfy the researcher's desired effect. This happens in an unconscious manner for the participant so it is important to eliminate or limit transmitting the researcher's views.
- Artificial scenario effect: Some qualitative research occurs in artificial scenarios and/or with preset goals. In such situations, the information may not be accurate because of the artificial nature of the scenario. The preset goals may limit the qualitative information obtained.
- Clinical Significance
Qualitative research by itself or combined with quantitative research helps healthcare providers understand patients and the impact and challenges of the care they deliver. Qualitative research provides an opportunity to generate and refine hypotheses and delve deeper into the data generated by quantitative research. Qualitative research does not exist as an island apart from quantitative research, but as an integral part of research methods to be used for the understanding of the world around us. [17]
- Enhancing Healthcare Team Outcomes
Qualitative research is important for all members of the health care team as all are affected by qualitative research. Qualitative research may help develop a theory or a model for health research that can be further explored by quantitative research. Much of the qualitative research data acquisition is completed by numerous team members including social works, scientists, nurses, etc. Within each area of the medical field, there is copious ongoing qualitative research including physician-patient interactions, nursing-patient interactions, patient-environment interactions, health care team function, patient information delivery, etc.
- Review Questions
- Access free multiple choice questions on this topic.
- Comment on this article.
Disclosure: Steven Tenny declares no relevant financial relationships with ineligible companies.
Disclosure: Janelle Brannan declares no relevant financial relationships with ineligible companies.
Disclosure: Grace Brannan declares no relevant financial relationships with ineligible companies.
This book is distributed under the terms of the Creative Commons Attribution-NonCommercial-NoDerivatives 4.0 International (CC BY-NC-ND 4.0) ( http://creativecommons.org/licenses/by-nc-nd/4.0/ ), which permits others to distribute the work, provided that the article is not altered or used commercially. You are not required to obtain permission to distribute this article, provided that you credit the author and journal.
- Cite this Page Tenny S, Brannan JM, Brannan GD. Qualitative Study. [Updated 2022 Sep 18]. In: StatPearls [Internet]. Treasure Island (FL): StatPearls Publishing; 2024 Jan-.
In this Page
Bulk download.
- Bulk download StatPearls data from FTP
Related information
- PMC PubMed Central citations
- PubMed Links to PubMed
Similar articles in PubMed
- Suicidal Ideation. [StatPearls. 2024] Suicidal Ideation. Harmer B, Lee S, Duong TVH, Saadabadi A. StatPearls. 2024 Jan
- Folic acid supplementation and malaria susceptibility and severity among people taking antifolate antimalarial drugs in endemic areas. [Cochrane Database Syst Rev. 2022] Folic acid supplementation and malaria susceptibility and severity among people taking antifolate antimalarial drugs in endemic areas. Crider K, Williams J, Qi YP, Gutman J, Yeung L, Mai C, Finkelstain J, Mehta S, Pons-Duran C, Menéndez C, et al. Cochrane Database Syst Rev. 2022 Feb 1; 2(2022). Epub 2022 Feb 1.
- Macromolecular crowding: chemistry and physics meet biology (Ascona, Switzerland, 10-14 June 2012). [Phys Biol. 2013] Macromolecular crowding: chemistry and physics meet biology (Ascona, Switzerland, 10-14 June 2012). Foffi G, Pastore A, Piazza F, Temussi PA. Phys Biol. 2013 Aug; 10(4):040301. Epub 2013 Aug 2.
- Review Evidence Brief: The Effectiveness Of Mandatory Computer-Based Trainings On Government Ethics, Workplace Harassment, Or Privacy And Information Security-Related Topics [ 2014] Review Evidence Brief: The Effectiveness Of Mandatory Computer-Based Trainings On Government Ethics, Workplace Harassment, Or Privacy And Information Security-Related Topics Peterson K, McCleery E. 2014 May
- Review Public sector reforms and their impact on the level of corruption: A systematic review. [Campbell Syst Rev. 2021] Review Public sector reforms and their impact on the level of corruption: A systematic review. Mugellini G, Della Bella S, Colagrossi M, Isenring GL, Killias M. Campbell Syst Rev. 2021 Jun; 17(2):e1173. Epub 2021 May 24.
Recent Activity
- Qualitative Study - StatPearls Qualitative Study - StatPearls
Your browsing activity is empty.
Activity recording is turned off.
Turn recording back on
Connect with NLM
National Library of Medicine 8600 Rockville Pike Bethesda, MD 20894
Web Policies FOIA HHS Vulnerability Disclosure
Help Accessibility Careers
Thank you for visiting nature.com. You are using a browser version with limited support for CSS. To obtain the best experience, we recommend you use a more up to date browser (or turn off compatibility mode in Internet Explorer). In the meantime, to ensure continued support, we are displaying the site without styles and JavaScript.
- View all journals
- My Account Login
- Explore content
- About the journal
- Publish with us
- Sign up for alerts
- Review Article
- Open access
- Published: 01 May 2024
Paradigm shift required for translational research on the brain
- Jong Hyuk Yoon ORCID: orcid.org/0000-0002-4090-3832 1 na1 ,
- Dongha Lee 2 na1 ,
- Chany Lee 2 na1 ,
- Eunji Cho ORCID: orcid.org/0000-0002-9589-3643 1 na1 ,
- Seulah Lee 1 ,
- Amaury Cazenave-Gassiot ORCID: orcid.org/0000-0002-3050-634X 3 , 4 ,
- Kipom Kim 5 ,
- Sehyun Chae 6 ,
- Edward A. Dennis ORCID: orcid.org/0000-0003-3738-3140 7 &
- Pann-Ghill Suh 8
Experimental & Molecular Medicine ( 2024 ) Cite this article
Metrics details
- Medical research
- Neuroscience
Biomedical research on the brain has led to many discoveries and developments, such as understanding human consciousness and the mind and overcoming brain diseases. However, historical biomedical research on the brain has unique characteristics that differ from those of conventional biomedical research. For example, there are different scientific interpretations due to the high complexity of the brain and insufficient intercommunication between researchers of different disciplines owing to the limited conceptual and technical overlap of distinct backgrounds. Therefore, the development of biomedical research on the brain has been slower than that in other areas. Brain biomedical research has recently undergone a paradigm shift, and conducting patient-centered, large-scale brain biomedical research has become possible using emerging high-throughput analysis tools. Neuroimaging, multiomics, and artificial intelligence technology are the main drivers of this new approach, foreshadowing dramatic advances in translational research. In addition, emerging interdisciplinary cooperative studies provide insights into how unresolved questions in biomedicine can be addressed. This review presents the in-depth aspects of conventional biomedical research and discusses the future of biomedical research on the brain.
Introduction
Biomedical research has recently led to the successful management of several diseases, contributed to human health, and advanced the global healthcare agenda. Notably, biomedical research has successfully controlled diverse medical crises, including smallpox, pneumonia, and appendicitis 1 . Furthermore, mortality was reduced by approximately 48% from 1930 to the end of 1970, which is estimated to have resulted from active biomedical research 2 .
The field of biomedical research in the brain is still a budding research area with many unsolved questions 3 first posed by ancient theoretical and religious concepts and now addressed by modern empirical research 4 . However, unlike diseases that have been solved with the advancement of modern science, the causes of most brain disorders remain unclear. This could be due to several specific limitations that are unique to conventional brain research, such as complex brain anatomy, difficulties obtaining human brain samples, the large gap between animal and human studies, and ethical limitations, especially in psychiatric disorders 5 , 6 , 7 . Approximately 100 years ago, physicist Emerson Pugh said, “If the human brain were so simple that we could understand it, we would be so simple that we could not.” Nevertheless, brain research has rapidly advanced over the past few decades in various fields, such as medical science, pharmacology, biology, and engineering 8 . Research in each discipline is maturing rapidly in terms of biomedical applications and development 9 . Notably, as the demand for high-performance tools has increased 10 , 11 , new high-performance materials 12 , 13 and devices have been developed 14 , 15 . Moreover, cumulative databases have become invaluable references for all studies involving the identification of genomes, proteomes, and small molecules 16 , 17 . Recently, the integration and analysis of cumulative large-scale databases has become a tractable approach for understanding the brain connectome 18 , 19 .
The paradigm shift in translational research is a top-down method that preanalyzes patient-centered data and conducts data-based basic research and clinical/utilization research to overcome the limitations of conventional translational research. Collecting and analyzing patient-centered big data involves acquiring the maximum amount of data through various high-throughput analysis tools, enabling us to collect and merge data and derive meaningful results. Interdisciplinary collaboration leads to a comprehensive understanding 20 and can provide new insights. Consequently, interdisciplinary collaborative research produces better results with synergistic benefits 21 , 22 . On this basis, interdisciplinary collaborative brain research can become a powerful framework for overcoming brain diseases through new approaches to translational research. Therefore, this review presents the in-depth aspects of conventional biomedical research and discusses the future of biomedical brain research.
History of brain research
The interest in human brain research can be traced back to ancient times. The oldest known surgical text dates back to circa 1600 BCE in Egypt. It describes the restriction of head movement to treat trauma caused by a weapon, infection prevention methods, and bleeding control methods 23 . The first understanding of cranial structures, brain surface structures, and cerebrospinal fluid and 48 trauma cases were also recorded. Egyptians were believed to be knowledgeable about brain trauma and its importance. By the Renaissance, the anatomical diagram of the human brain was almost complete 24 . Since then, biomedical brain research has progressed to identifying brain structures, functions, and mechanisms. Therefore, empirical biomedical brain research has evolved according to time trends. In 1791, Luigi Galvani observed convulsions in frogs’ legs when they were in contact with a metal dissecting knife. This experiment was the first to show that an electric current can flow among living muscles, nerves, and cells and has become the basis for the development of electrophysiology 25 .
Camillo Golgi developed Golgi staining in the 1860s, and Ramon Cajal used it to reveal that neurons are the units that constitute the brain and are connected in a network. The discovery of neurons, the basic elements of the brain, in the early 20th century became the cornerstone of full-scale empirical brain research. Since then, many studies have investigated bioelectrical phenomena such as membrane potential, electrocardiography, electroencephalography, neuronal cell function, chemical transmission, nerve fibers, ion-transport mechanisms of nerve cell membranes, and cerebral function and information processing in the cerebral and visual cortex.
In the middle of the 20th century, empirical and detailed research was conducted on the behavior of neurons. This research elucidated the brain’s anatomical structure, but its information processing was poorly understood. It was later revealed that dozens of chemicals transmit information between neurons. In 1936, Henry Hallett Dale and Otto Loewi discovered and proved that acetylcholine was a chemical transporter of nerve impulses, and they won the Nobel Prize for their discovery 26 . In 1963, ion-based mechanisms associated with excitation and inhibition were discovered in the central and peripheral parts of the cell membrane, termed “action potentials” by John Carew Eccles, Alan Lloyd Hodgkin, and Andrew Fielding Huxley 27 .
The invention of the microscope led to the explosive development of biological research, including brain research. After Ernst Abbe’s diffraction limit (λ/2, where λ is the wavelength of light) was published in 1873, Stefan W. Hell developed stimulated emission depletion microscopy, which overcame the resolution limit of conventional optical microscopes using a laser excitation beam 28 . Since then, researchers have been able to observe objects <0.2 µm, enabling the understanding of viruses, interactions between individual proteins in cells, and even smaller molecules 29 . Since the early 2000s, superresolution fluorescence microscopy, especially stochastic optical reconstruction microscopy developed in 2006, has been used to randomly turn on and off fluorescent molecules to separate molecules on the time axis, overcoming the resolution limit and obtaining a high resolution of approximately 20 nm 30 . Furthermore, advances in molecular optics technology, including optical devices and sensors, have led to the development of nanoscopic techniques, enabling molecular and structural imaging of synapses and contributing to the study of physiological functions 31 , 32 . Since the brain is a large neural network, identifying the functions of each neuron is crucial. However, identifying the entire connected network and understanding its interactions are also critical 33 . Therefore, the working scale of research has moved from the microscale to the mesoscale 34 . Additionally, tissue transparency technology, which was first described in 2013, allows the internal structure of a given tissue to be observed in three dimensions after removing the lipid from the tissue and making it transparent, enabling observation of the entire brain 35 . Brain imaging at various scales has accelerated the study of neural circuits 36 , 37 , 38 . One of the most vital advancements in brain research has been the development of measurement equipment that can measure brain function externally and analyze the brain’s electrical signals. Since then, noninvasive brain imaging techniques such as in vivo and magnetic resonance imaging (MRI) have been developed. In 1973, Laterbur developed an imaging technique based on nuclear magnetic resonance technology; the technique was named nuclear magnetic resonance imaging and is now commonly referred to as MRI 39 . MRI enables noninvasive examination; provides information on the chemical structure of substances within a short examination duration; is useful for diagnosing diseases of the nervous system, such as the brain or spinal cord, which cannot be observed using radiographic scanning; and has great clinical value. The combination of MRI with positron emission tomography (PET), which displays biochemical images in three dimensions using positron-emitting radioisotope labels, results in a system capable of ultrasensitive molecular and high-resolution functional imaging, which is invaluable for understanding the physiology and function of the brain.
Conventional biomedical research
Basic information.
Conventional biomedical research includes the following procedures: observation/result analysis, target discovery, and research for basis/application. In conventional biomedical research, advances in medical diagnostics and treatment depend on a comprehensive understanding of epidemiological findings or pathophysiological processes 40 . Gathering data from studies, evaluations, and interpretations involving humans completes the framework of conventional biomedical research, which has contributed greatly to maintaining human health. In observation/result analysis, researchers use biotechnology to observe biological and pathological differences between disease and normal states 41 , design appropriate experiments to confirm these differences in a controlled system 42 , and subsequently evaluate the experimental results using reliable biotechnology and analyze the results using an appropriate statistical approach 43 . In the target discovery step, the analysis provides targets (specifically, molecular targets) 44 . In the next step, a technology for regulating the discovered targets is developed, the mode of action (basic and necessary data on the target regulatory action) 45 , 46 is investigated, and the therapeutic potential for diseases is investigated by expanding the range from the cellular level to the entire organism level 47 . Notably, a human-level clinical trial involves multiple stages in which safety and effectiveness are closely examined 48 . This process alone is a long and complex research stage that requires approximately 10 years 49 . New drugs for several diseases are being developed through conventional biomedical research. A summarized history of these drugs is presented in the Supplementary Information, including Supplementary Table 1 . We selected some of the major causes of global mortality, as ranked by the World Health Organization between 2019 and 2020, and the representative diseases that have significantly impacted human history 50 .
Considering the latest accomplishments, conventional biomedical research seems to have advantages and disadvantages. It has provided more accurate and detailed knowledge in specific and detailed research fields 51 . Furthermore, in-depth development of research using animal models has been achieved. However, there are some limitations to the conventional approach. First, in most studies, academic scientists with relatively limited clinical knowledge select animal and human participants for observational analyses; therefore, the selection criteria and results remain suboptimal 52 . Furthermore, there may be bias in classifying and comparing healthy participants and those with specific diseases in medical research due to the relative lack of clinical knowledge. Selection bias could lead to shortfalls in knowledge acquisition. Second, interpreting the effects of various drug candidates in preclinical disease animal models for reasons such as the low genetic concordance rate with humans is difficult. The results of preclinical animal studies are often insufficient to directly predict or alleviate human diseases 53 , 54 . However, prioritizing preclinical animal models is still a reasonable consideration, and several researchers depend heavily on animal models. Third, until recently, most results have been based on short-term cellular and animal experiments; however, these findings likely differ from findings in humans due to various factors. Limitations exist in predicting effectiveness and toxicity in humans because of deficiencies in experimental design strategies and biased species variance; however, animal studies are still performed. Fourth, existing biomedical studies evaluating hypotheses in clinical trials have been conducted on several patients. These hypothesis-driven studies have limitations in investigating heterogeneous and multifactorial diseases, and actual human clinical samples are difficult to collect. Therefore, in unbiased large-scale collection and clinical data analysis, it is challenging to identify patterns and generate actionable predictions regarding disease progression. Moreover, even though the new drug candidates developed through conventional biomedical research undergo numerous expensive tests, only <10% of the compounds have been approved with sufficient efficacy and adequate toxicity results to meet the predictive value of preclinical studies 52 . Table 1 summarizes the advantages and disadvantages of conventional biomedical research.
Conventional biomedical research on the brain
Neurodegenerative diseases.
Using conventional biomedical research, the observation and exploration of clinical symptoms led to the discovery of several neurodegenerative brain diseases, such as Alzheimer’s disease (AD), Parkinson’s disease (PD), multiple sclerosis 55 , 56 , 57 , and various systemic diseases. These brain diseases have unique characteristics that can be used to distinguish them from other diseases 58 , 59 , 60 . For example, in 1892, Paul Blocq and Georges Marinesco discovered senile plaques for the first time in the brain of a patient who died from epilepsy 58 . Furthermore, senile plaques were observed in patients with dementia, and in 1910, they were named “Alzheimer’s disease” by a physician named Alois Alzheimer, who observed them along with significant shrinkage of the hippocampus and neurofibrillary tangle 59 . With advancements in technology, the abnormal accumulation of amyloid plaques and tau proteins has become known as a pathological hallmark of AD. Therefore, numerous studies have focused on developing amyloid- and tau-related treatments. However, most of these treatments have failed in clinical trials because the exact causes of amyloid and tau accumulation are still unknown 61 . For AD, 76% of agents in phase III trials in 2016 were disease-modifying therapies, including amyloid- and tau-targeted agents, whereas by 2022, only 29% of the agents in phase III trials were disease-modifying therapies 62 , 63 . Additionally, AD is reportedly associated with mitochondrial dysfunction, oxidative stress, and neuroinflammation 64 , 65 , and many studies on the development of treatments for these aspects have been conducted, but the success rate is still extremely low.
Treatments for neurodegenerative diseases such as AD, which have poorly understood underlying mechanisms, are difficult to develop using a conventional biomedical research approach. Therefore, the current treatment for neurodegenerative diseases relies only on clinically observed pathological symptoms and is aimed merely at symptom improvement. For instance, the widespread loss of cholinergic neurons and overactivation of N -methyl-D-aspartate (NMDA) receptors in the brains of patients with AD have been identified, and cholinesterase inhibitors and NMDA receptor antagonists are commonly used to alleviate AD symptoms 66 . In patients with PD, the loss of dopaminergic neurons in the midbrain, dopamine precursors, dopamine agonists, and L-3,4-dihydroxyphenylalanine decarboxylase inhibitors have been identified, and catechol-O-methyl transferase (COMT) inhibitors are used to relieve PD symptoms 67 . Current treatments for neurodegenerative diseases provide symptomatic relief, but there is no conclusive evidence that they can fundamentally cure the disease. Additionally, because neurodegenerative diseases are gradually progressive, patients are on medication for prolonged durations, leading to the need for increased dosages and various side effects 68 . Therefore, novel treatments that differ from the currently used conventional methods should be developed. Table 2 summarizes the clinically approved drugs for treating neurodegenerative diseases 69 , 70 , 71 , 72 , 73 .
Psychiatric disorders
Mental illnesses have been described since ancient times; however, any true understanding of their nature was impossible, as they were considered a supernatural phenomenon caused by displeased gods, eclipses, curses, or sin 71 . As with most brain diseases, experience-based treatments and exploration of the molecular mechanisms involved in psychiatric disorders occurred almost simultaneously.
Psychiatric disorders began to be established from a somatogenic perspective in the 19th century 74 . Chlorpromazine (CPZ) was originally synthesized as a possible potentiator of general anesthesia and was accidentally developed as an antipsychotic drug 75 . Henri Laborit, a French surgeon, used cocktail lytique to prevent surgical shock and observed that when 50–100 mg of CPZ was injected intravenously, it prevented shock and induced relaxation and sedation without loss of consciousness 76 . Since then, CPZ has been significantly effective in relieving hallucinations, delusions, and disorganized thought in patients with schizophrenia 77 . Since the development of CPZ, many drugs, including thioridazine, haloperidol, and pimozide, with similar effects have been synthesized, but their molecular mechanism was unknown. Then, it was discovered that these drugs bind to dopamine receptors 78 . Therefore, because clomipramine, a tricyclic antidepressant, was found to be effective in reducing obsessive symptoms, the serotonin hypothesis for obsessive-compulsive disorder was proposed 79 , 80 . A role of gamma-aminobutyric acid (GABA) in mood disorders was proposed based on the clinical observation that valproic acid was effective in patients with bipolar disorders 81 . Psychiatric disorders are estimated to account for 13% of the global burden of disease, surpassing cardiovascular diseases and cancer 82 . However, accurate mechanisms have not yet been discovered, and only a few patients receive basic treatment 83 . Translational research can reveal novel treatments; however, it requires a coordinated effort at the disciplinary and national levels.
Other brain diseases
Attention deficit hyperactivity disorder (ADHD) is a neurodevelopmental disorder that affects 5% of children globally 84 . Its exact cause is unknown, but many risk factors, including norepinephrine and dopamine imbalances, have been identified 85 . The most common and effective medications to regulate norepinephrine are psychostimulants, including methylphenidate and dexamphetamine 85 , 86 . These treatments are effective; however, they have many side effects, such as decreased appetite, behavioral rebound, irritability, sleep problems, and tic exacerbation 87 , 88 . Epilepsy is one of the most common neurological disorders, affecting approximately 65 million people globally 89 , 90 . The exact cause of epilepsy is unknown; however, it is closely associated with neurodegeneration, ADHD, and stroke 91 , 92 , 93 . There have been significant advances in epilepsy treatment, including calcium ion channel and GABA transporter modulators, over the past few decades; however, one-third of patients are still fighting the disease, even with the currently available medications 94 . As described above, there are different types of brain diseases, including neurodegenerative, psychiatric, and neurodevelopmental disorders, but their treatments overlap and are limited. This is because the exact etiologies are unknown, and in most cases, the cause has been identified as an imbalance of neurotransmitters, including dopamine and serotonin. Therefore, a paradigm shift is needed in translational brain research. Brain diseases are more complex than systemic diseases. Consequently, special attention should be given to interdisciplinary efforts that provide a comprehensive view of the entire brain. Table 3 summarizes the available drugs for psychiatric disorders and other brain diseases, excluding treatments that are also used for various psychiatric diseases 84 , 95 , 96 , 97 , 98 , 99 , 100 , 101 , 102 , 103 .
Emerging technologies
Neuroimaging.
Neuroimaging is a noninvasive technique that is used to scan brain structures or functions in humans and animals at the macro level. There are various promising tools for brain imaging: MRI, functional MRI (fMRI), PET, electroencephalography (EEG), and magnetoencephalography. The simultaneous provision of different types of important information, such as structural, functional, and molecular information and temporal changes, makes neuroimaging an emerging high-throughput analysis toolkit.
MRI uses nuclear magnetic resonance to create images of brain structures 39 , whereas fMRI uses blood oxygenation level-dependent contrast to observe the degree and area of brain activation in humans 10 . PET observes changes in metabolic processes and blood flow by measuring positrons emitted by radioactive tracers 11 . EEG measures the brain’s electrical activity mainly generated by nerve cells 104 , whereas magnetoencephalography measures the magnetic field change derived from the brain’s electrical activity 105 .
In the history of neuroimaging, blood flow changes have been associated with brain function 106 . This has underpinned the significant progress in functional brain imaging with the development of fMRI and PET over the past 30 years 107 . When the brain structures are damaged, brain function can be disrupted. This is because the neural system is substantially flexible and organizes neural networks through regional interactions. Conversely, it is locally rigid and maintains the specificity of neural responses to brain functions that are specialized to separate regions. These properties underlie the principles describing the brain’s functional organization: segregation and integration 108 . Functional segregation was defined as “localizationism” by Franz-Joseph Gall (1758–1828), Johann Spurzheim (1776–1832), and Paul Pierre Broca (1824–1880), who stated that a function is specialized to a particular anatomical region. Therefore, injuries or lesions in that region can cause loss of function.
Conversely, according to Marie Jean Pierre Flourens (1794–1867), Kurt Goldstein (1878–1965), and Karl Lashley (1890–1958), functional integration implies networks of interactions among specialized regions. The loss of intact connections in a network causes functional loss. Deficient global integration or local segregation is associated with functional brain organization. Therefore, the functional manifestations of local brain regions have been increasingly used to understand brain diseases. Consequently, recent neuroimaging methods can be used as a translational approach in neuroscience to investigate how brain structures and functions are linked to genetic variations and disease manifestations.
Combining basic neuroscience, neuroimaging, and clinical applications to develop diagnostic methods for brain diseases has recently emerged as an intermediary approach in neuroscience. Therefore, a better understanding of the symptoms of brain disease is becoming possible 109 . Notably, numerous neuroimaging studies have attempted to diagnose neurovegetative diseases such as AD, PD, amyotrophic lateral sclerosis, and chronic traumatic encephalopathy. Recent advances in neuroimaging techniques and data analysis methods that provide the means to test the underlying organization of brain structure (MRI), function (fMRI), and metabolism (PET) from the microscopic to the macroscopic level have enabled this research. Therefore, brain injuries and neurodegenerative brain diseases can be linked through translational neuroimaging, which may provide new insights for biomedical research. For example, traumatic brain injury (TBI) studies using animal models are becoming increasingly important to match neuroimaging findings in humans with pathophysiological results in animals 110 . The idiosyncratic features of human TBI, such as heterogeneity, severity, temporal pathophysiology, and different brain systems, challenge the clinical application of mild TBI in animal models 111 . Nevertheless, translational neuroimaging results may explain the similarities and differences between humans and animals in terms of the effects of TBI 112 . In particular, resting-state fMRI in humans and mice demonstrated dynamic functional changes in mild TBI, wherein deficits and recovery occur over time 112 , 113 . Therefore, neuroimaging plays an important role in translational neuroscience because it is the cornerstone of in vivo measurements. However, its use for the collection and utilization of other types of data is limited.
The high cost of using neuroimaging methods such as MRI and PET can make translational neuroscience based on neuroimaging difficult; therefore, using a large database led by neuroimaging consortia, such as the UK Biobank ( n = 35,735), the Human Connectome Project ( n = 1200), and the Alzheimer’s Disease Neuroimaging Initiative ( n > 1800), is increasingly essential 114 . The more neuroimaging data that are shared, the more studies can progress by sharing thousands of individuals’ MRI, fMRI, and PET data for basic neuroscience, neuroimaging, and clinical studies. A large amount of data has been used to derive reliable results in replicability and longitudinal studies 115 , 116 .
Therefore, recent neuroimaging studies have attempted to combine multimodal 117 , 118 , 119 , 120 , and multispecies 121 , 122 data to interpret brain structural and functional changes based on the underlying mechanism 123 . The advantages of multimodal neuroimaging include high spatiotemporal resolution, improved data quality, and understanding of the anatomical basis of functional activity. However, the disadvantages are different resolutions, data complexities, and sample sizes 123 , 124 , 125 . Nevertheless, whether neuroimaging results are consistent with the results derived from different data types remains unclear due to the current lack of mining of multimodal data.
Technological advances in high-throughput platforms for omics-based analysis, including genomics, transcriptomics, proteomics, metabolomics, and lipidomics, have greatly contributed to understanding human health, medicine, and diseases 126 . Genomics and transcriptomics identify genetic variants and multifactorial targets associated with diseases; however, predicting the biological effects of individual variants is difficult due to epigenetic, transcriptional, and posttranslational modifications. Proteomics quantifies protein abundances and posttranslational modifications such as glycosylation, phosphorylation, and ubiquitination 127 . With improved mass spectrometry-based methods, thousands of proteins in a patient’s tissues or body fluids can be identified simultaneously. Therefore, proteomics can provide comprehensive information about actual protein functions and cellular processes associated with disease pathogenesis. However, compared with genomics, proteomics still has insufficient coverage at the genome level due to several technical issues (such as ionization efficiency for poorly responding peptides) 128 . Moreover, there are weak correlations between each type of omics data (transcripts versus proteins), mostly reflecting reactive processes, such as cellular half-life, RNA/protein degradation, splicing, and posttranslational modifications 129 . Since the etiology of most diseases involves multiple factors, it is impossible to focus on one factor, and diagnosis and treatment are difficult 128 . Therefore, comprehensive technology integration is required to identify disease-related factors. The integrative approach combines individual omics data sequentially or simultaneously to understand molecular/intermolecular interactions 130 . Metabolomics, especially lipidomics, uses mass spectrometric techniques similar to those of proteomics but analyses the products of metabolism, in which enormous numbers of metabolites vary with disease state 131 , 132 , 133 , 134 , 135 , 136 .
To date, multiomics approaches have been applied to cancer biology. In cancer diagnosis and treatment alone, multilevel information, such as mutation, fusion gene, RNA, and protein level expression changes, is needed 137 , 138 . Omics analyses have helped to elucidate key mechanisms involved in cancer onset, treatment resistance, and the risk for recurrence. Notably, integrative multiomics analyses have provided more comprehensive molecular signatures to identify cancer subtypes 139 , 140 . Recent advances in omics analysis and data archiving and processing have provided reliable data 141 . There have been global efforts to obtain new molecular information by which to treat and diagnose cancers by reproducibly obtaining and integrating omics information 142 . The Cancer Genome Atlas (TCGA) of the United States National Cancer Institute (NCI) provides multiomics datasets (including genomic, transcriptomic, epigenomic, proteomic, and phosphoproteomic data) from >20,000 patients across 33 cancer types to aid in the discovery of molecular signatures to diagnose, treat, and prevent cancer 143 . The International Cancer Genome Consortium (ICGC), a genomics and informatics consortium that started in 2007, began the 25 K project for genome analysis of 25,000 primary untreated cancers 144 . Analysis of whole-genome cancer (Pancancer Analysis of Whole Genomes project) started in 2013, and > 3000 eligible whole-cancer genomes of several cancer types are currently being analyzed 144 , 145 . The ICGC aims to accelerate genomic oncology research (Accelerating Research in Genomic Oncology project), where key clinical queries and patient clinical data drive the inquisition of cancer genomes. Clinical trials provide a unique resource of multiomics data from patients with cancer to accelerate the discovery of new therapies 146 . The Clinical Proteomic Tumor Analysis Consortium was formed and centered on the U.S. NCI by applying proteomics technology 147 . Tumor Analysis Consortium databases contain all the clinical information of patients with cancer 147 , 148 . Notably, all proteomics-based technologies, such as sample preparation, peptide generation, chemical labeling, mass spectrometry, and data processing, are optimized and shared 149 . The Tumor Analysis Consortium project has created and provided novel proteomic results for cancer biomarkers and targets 150 , 151 . Projects to discover new mechanisms and target molecules through multiomics analysis of diverse diseases are being actively undertaken as joint research between countries 151 .
Analysis of trace molecules in single cells is becoming the aim for identifying causes of diseases. Due to efforts such as the development of single-cell isolation methods and the improvement of cell resolution, the omics layer can be analyzed integrally at the single-cell level 152 , 153 . Additionally, multiomics technology development has greatly increased our understanding of the critical pathways that influence complex cell physiology and secondary metabolite production 154 . Multiomics involves comparing and interpreting vast amounts of experimental data and performing customized statistical analysis; therefore, it is time-consuming, requires professional manpower, and imposes a considerable overall economic burden. However, the diversification of analysis, interpretation, and visualization of multiomics data to overcome these limitations has led to improvements in methods, devices, and processes 130 , 155 .
Multiomics also involves several computational techniques for the integrative analysis of multiomics datasets. An unsupervised model-based method, multiomics factor analysis, integrates multiple datasets and finds principal sources of variability 156 . iCluster, capable of identifying genomic features that mostly influence biological variation, uses joint latent variable models to characterize molecular subtypes 157 . It also successfully synthesizes the complexity of multiomics data through machine learning (ML), deep learning (DL), and network-based feature extraction and transformation method development 158 , 159 . Multiomics advances are revolutionary; therefore, incorporating truly integrated multiomics analysis can rapidly advance precision medicine. Efforts are ongoing to develop an analytical infrastructure to effectively create, analyze, and annotate multiomics data 160 .
Artificial intelligence
The data obtained from basic and clinical research for translational studies are heterogeneous and large in scale 161 , 162 . These data span from the microscale to the macroscale, include human and animal data, and include molecular, cellular, regional, whole-brain, behavioral, and even textual information 163 , 164 . Therefore, innovative methods may be required to integrate and process these complex data.
Notably, various artificial intelligence (AI) types have been suggested since John McCarthy coined the term “artificial intelligence” in 1956 165 , 166 . In particular, ML, which enables learning from experience, has various applications, including classification, prediction, and generalization through supervised or unsupervised routes 167 . In the medical field, it has been successfully applied to determine the prognosis and diagnosis of diseases 166 , 168 . However, the performance of conventional ML significantly depends on the feature selection and extraction process, which DL does not require 169 . Recently, DL, a type of ML, has grown exponentially, supported by the development of various algorithms, big data, and hardware such as graphic processing units 170 , 171 , 172 . DL involves a kind of neural network with multiple and deep layers; however, it can learn from raw data, features on hidden layers, and results 173 .
Thus far, DL has successfully performed neuroscience analysis, including analyses of DNA/RNA sequences, metabolomic data, proteomic data, microscopy images, and MRI data 174 , 175 , 176 , 177 , 178 , 179 , 180 . For example, DL has been successfully applied to drug discovery, which is a costly and time-consuming process. DL can identify drug targets, biomarkers, and druggability 181 . Moreover, DL can be used to confirm drug-target interactions and drug‒drug combinations 182 , 183 . DL is a high-throughput tool because the large chemical and protein space makes it difficult for conventional methods to search for and identify the characteristics of any appreciable fraction of all possible combinations 182 , 183 . Generative DL techniques have recently emerged and have been applied in neuroscience. Since the generative adversarial network was introduced in 2014 184 , many generative adversarial network variants have been developed to classify diseases and for disease progression modeling and synthetic data generation 185 . Generative adversarial networks can produce plausible data. Therefore, a small amount of data for EEG, MRI, and multimodal neuroimages were augmented 186 , 187 , 188 , and missing multiomics data could be handled by generative DL 189 , 190 .
The next step for translational research involves developing methods to handle heterogeneous and multimodal data, a problem of algorithms, hardware, and computational systems. Nevertheless, we expect progress to be rapid and on a large scale with promising outcomes. Emerging high-throughput analysis tools, including neuroimaging, multiomics, and AI, can help researchers conduct large-scale biomedical research that induces a paradigm shift for conventional research. Figure 1 presents a streamlined workflow for new biomedical research using high-throughput analysis tools.
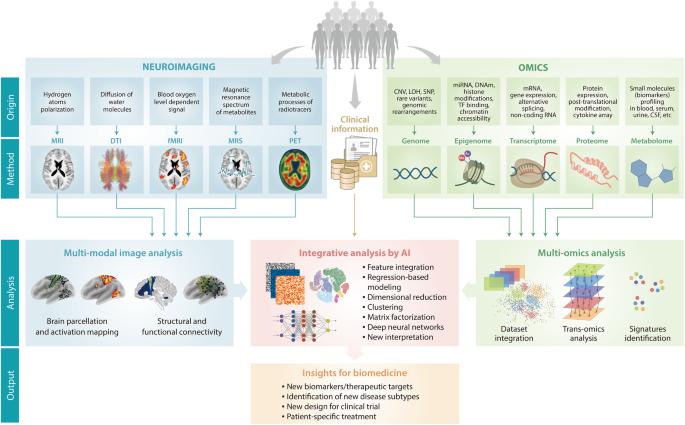
Neuroimaging, including MRI, DTI, MRS, and PET, provides multimodal imaging information. Multiomics, including genomics, proteomics, and metabolomics, provides molecular information. By linking with clinical information, AI-based integrative data analysis using multimodal neuroimaging and multiomics offers valuable new insights into biomedical brain research, such as new biomarkers, disease subtypes, and treatments. MRI magnetic resonance imaging, AI artificial intelligence, PET positron emission tomography, DTI diffusion tensor imaging, MRS magnetic resonance spectroscopy, SNP single-nucleotide polymorphism, CNV copy number variation, LOH loss of heterozygosity, fMRI functional MRI.
New directions in translational research
With conventional research methods that proceed from basic to clinical, we can fully understand living organisms; however, these methods have limitations in overcoming diseases 191 . Furthermore, academic fields have been self-focused and fragmented due to relatively slow technological advances in scientific fields, including intercommunication methods 141 . However, academic research fields are maturing and achieving success in applications based on robust fundamental backgrounds, such as electronic engineering. Based on this maturity of academic research fields and intercommunication tools, the current era is truly of smart communication.
A new era is coming for biomedical research, representing a paradigm shift 192 . We have presented emerging high-throughput analysis tools such as neuroimaging, multiomics and AI technology 193 , 194 , 195 . These tools enable patient-centered and large-scale biomedical research 196 and can ultimately drive a new approach. In new translational research, various high-throughput analyses of patients, clinical data utilization, personalized diagnostics, and treatment through the discovery of target molecules by multiomics are involved. Furthermore, the emergence of interdisciplinary cooperative studies involving basic science and engineering will provide insight into how unresolved questions in biomedicine can be solved 197 , 198 . Interdisciplinary collaborative studies could include core capability enhancement by securing large-scale data, development of analysis technology, and application of AI technology to data integration and interpretation.
Figure 2 presents a scheme of new translational research combining interdisciplinary collaborative research. It starts with multilayered patient-centered research and includes patient data acquisition steps on micro- and macroscales 163 , 164 . Since this step requires multilayered analysis with high reproducibility and reliability, high-throughput analysis tools such as multiomics and imaging and corresponding clinical data should be employed 141 . In this step, multilayered diverse information determines the next step: interdisciplinary collaborative research. AI-based data integration and interpretation are used to discover molecular and signaling signatures before the next step 143 . Subsequently, the discovered information is put into the interdisciplinary collaborative research cycle (Fig. 2 ), which includes interdisciplinary research fields such as biology, basic sciences, and technology. Each research field takes the discovered information from AI-based analysis and draws its interpretation from its point of view. Excluding biology, each of these interpretations would be unique because multilayered and patient-centered data constitute a new data type and a previously unknown principle in each research field. These findings will provide new hypotheses and insights into diseases. Therefore, subsequent experimental approaches to validate new hypotheses are needed. This step would reveal new technology questions, thereby involving the technology field. The technology field has developed new technologies and provides new data for functional research against the new hypotheses. In this cycle, each research field communicates with the others to draw more rational and advanced conclusions in their field and harmoniously across diverse disciplines. This activity promotes the theoretical advancement of each discipline and new approaches, theories, and technologies. The new technologies for diagnostics and treatments derived from this step are then applied to clinical research. This step is not a conventional clinical trial but rather the stage for testing new technologies using a group of patients with no ethical concerns, similar to the test bed scale 198 . From the clinical research step, valuable information is acquired from human specimens 199 . The acquired information is fed back to the interdisciplinary collaborative research cycle to improve their conclusions. The information is also fed back to the multilayered patient-centered research step to help improve analytical methods and availability determination.
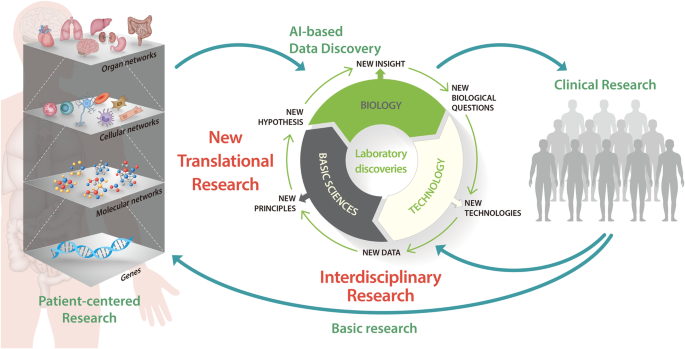
New directions in translational research start with multilayered patient-centered research using high-throughput analysis tools such as multiomics and imaging with high reproducibility and reliability. AI-based data integration and interpretation reveal new molecular and signaling signatures. Subsequently, the discovered information is inputted into the interdisciplinary collaborative research cycle. The relevant conclusions from an interdisciplinary collaboration provide new technologies for diagnoses and treatments. Then, new technologies are applied to clinical research using patient groups. The obtained information is fed back to the cycle to improve the conclusions. AI artificial intelligence.
As an example, when PD is considered in this scheme, the first step can consist of the data analysis of patients with PD (Supplementary Fig. 1 ). To acquire molecular information, proteogenomics, metabolomics, and transcriptomics of biological sources, including cerebrospinal fluid, blood, and urine, are applied 200 , 201 , 202 . Neuroimaging tools such as MRI, PET, or single-photon emission computed tomography are used to obtain macroscale information 116 . Subsequently, the corresponding clinical information of patients with PD is collected. After the fundamental analysis for data validation, this multilayered information is put into an AI-based in-depth analysis for big data integration. This step discovers well-suited and/or new target molecule and signal module candidates. Next, the results are compared and verified with previously well-known information, such as dopamine pathway-related contents 143 . In interdisciplinary collaborative research, the integrated information, including images and molecular and signal signatures, is used as new data for each research field. New hypotheses and insights from each research field will appear using the data, and preliminary interdisciplinary research on new PD targets will be conducted. The development of technology for PD diagnosis and treatment will also commence. New molecular and/or imaging targets will be discovered; therefore, the technology field can provide previously unknown diagnostic and treatment methods for PD, such as noninvasive diagnosis technology and target molecule regulation-based treatment using deep brain stimulation technology 203 , 204 . Members of the interdisciplinary cycle could correct their hypotheses and develop technologies through active intercommunication and discussion. Next, these valuable outcomes are applied to clinical research. The new findings and technologies associated with PD will be applied to limited human samples and interpreted: the technologies for precise PD diagnostics/treatment and noninvasive deep brain stimulation-based PD treatment can be tested. After analyzing the clinical research results, a new research design for in-depth basic and interdisciplinary research will be devised and executed. The acquired information is fed back to the interdisciplinary collaborative research cycle to revise the PD hypothesis and calibrate preliminary technologies, including diagnostics and deep brain stimulation.
Recent psychiatric studies have identified the necessity and feasibility of the new translational research that we suggest here. Notably, all multiomics, neuroimaging, and AI methods were not integrated; however, there were some uses of partial integration for clinical neuroscience. For example, multimodal neuroimaging (MRI and PET) and machine learning have been integrated to predict psychiatric disorders and neurodegenerative diseases 205 , 206 , 207 . PET and DL (convolutional neural network) have been integrated to differentiate patients with AD 208 . Another study reported the integration of EEG and machine learning algorithms to detect predementia AD 209 . In other studies, combining multimodal neuroimaging and multiomics was used to link neuroimaging markers with biomarkers of neurodegeneration, indicating a greater genetic risk for AD. For example, neuroimaging markers in patients with AD correlated with neurodegeneration biomarkers, such as GFAP and Ptau 181 and 217 210 . Diverse combinations of neuroimaging and multiomics have been used to classify patients with PD using DL 211 and to predict patients with PD using network analysis-based proteomics 212 . Furthermore, some studies have integrated transcriptomic and neuroimaging brain models 213 and neuroimaging-based connectomics to predict neurodegenerative processes 214 . These attempts may bridge the gap between genomics and neuroimaging and find biomarkers for treating neurodegenerative diseases 193 . These studies align with the new direction we suggest, including interdisciplinary research concepts of biology; however, in basic sciences and technology, partial integration may not address several limitations derived from conventional brain research. Therefore, considering brain structures, functions, and genes across individuals through the lens of integrated multiomics, neuroimaging, and AI information may be increasingly crucial for understanding the underlying mechanisms of brain diseases, including neurodegenerative and psychiatric disorders, and for developing treatments 215 . Thus, new translational research may be able to solve difficult problems in brain biomedical research.
In conclusion, conventional biomedical research has made numerous contributions 198 . However, its limitations are apparent, especially in biomedical brain research. To date, theories and technologies have rapidly developed and matured. Therefore, a new direction in translational research combined with the application of new technologies and interdisciplinary collaborative research will inevitably overcome the limitations of conventional approaches for brain research.
Furuse, Y. Analysis of research intensity on infectious disease by disease burden reveals which infectious diseases are neglected by researchers. Proc. Natl. Acad. Sci. USA 116 , 478–483 (2019).
Article CAS PubMed Google Scholar
Vehorn, C. L., Landefeld, J. S. & Wagner, D. P. Measuring the contribution of biomedical research to the production of health. Res. Policy 11 , 3–113 (1982).
Article Google Scholar
Adolphs, R. The unsolved problems of neuroscience. Trends Cogn. Sci. 19 , 173–175 (2015).
Article PubMed PubMed Central Google Scholar
Lee, S. K. The history of neuroscience 1: ancient neuroscience. Epilia 1 , 4–10 (2019).
Google Scholar
Tognoli, E. & Kelso, J. A. Enlarging the scope: grasping brain complexity. Front. Syst. Neurosci. 8 , 122 (2014).
Premack, D. Human and animal cognition: continuity and discontinuity. Proc. Natl. Acad. Sci. USA 104 , 13861–13867 (2007).
Article CAS PubMed PubMed Central Google Scholar
Jain, S., Kuppili, P. P., Pattanayak, R. D. & Sagar, R. Ethics in psychiatric research: issues and recommendations. Indian J. Psychol. Med. 39 , 558–565 (2017).
Amunts, K. & Lippert, T. T. Brain research challenges supercomputing. Science 374 , 1054–1055 (2021).
López-López, E., Bajorath, J. & Medina-Franco, J. L. Informatics for chemistry, biology, and biomedical sciences. J. Chem. Inf. Model. 61 , 26–35 (2021).
Article PubMed Google Scholar
Ogawa, S. et al. Intrinsic signal changes accompanying sensory stimulation: functional brain mapping with magnetic resonance imaging. Proc. Natl. Acad. Sci. USA 89 , 5951–5955 (1992).
Phelps, M. E., Hoffman, E. J., Mullani, N. A. & Ter-Pogossian, M. M. Application of annihilation coincidence detection to transaxial reconstruction tomography. J. Nucl. Med. 16 , 210–224 (1975).
CAS PubMed Google Scholar
Yang, J. & Yang, Y. W. Metal–organic frameworks for biomedical applications. Small 16 , e1906846 (2020).
Duo, Y. et al. Borophene-based biomedical applications: status and future challenges. Coord. Chem. Rev. 427 , 213549 (2021).
Article CAS Google Scholar
Kisiala, A., Kambhampati, S., Stock, N. L., Aoki, M. & Emery, R. J. N. Quantification of cytokinins using high-resolution accurate-mass Orbitrap mass spectrometry and parallel reaction monitoring (PRM). Anal. Chem. 91 , 15049–15056 (2019).
Melfi, M. T. et al. Data processing for fennel protein characterization by Fourier transform ion cyclotron resonance mass spectrometry (FT-ICR-MS). Data Brief. 35 , 106960 (2021).
Schweppe, D. K. et al. Full-featured, real-time database searching platform enables fast and accurate multiplexed quantitative proteomics. J. Proteome Res. 19 , 2026–2034 (2020).
Perez-Riverol, Y. et al. The PRIDE database resources in 2022: a hub for mass spectrometry-based proteomics evidences. Nucleic Acids Res. 50 , D543–D552 (2022).
Bassett, D. S. & Gazzaniga, M. S. Understanding complexity in the human brain. Trends Cogn. Sci. 15 , 200–209 (2011).
Poldrack, R. A. & Farah, M. J. M. J. Progress and challenges in probing the human brain. Nature 526 , 371–379 (2015).
Chen, S., Arsenault, C., Gingras, Y. & Larivière, V. Exploring the interdisciplinary evolution of a discipline: the case of biochemistry and molecular biology. Scientometrics 102 , 1307–1323 (2015).
Wen, J., Wang, W., Kozak, M., Liu, X. & Hou, H. Many brains are better than one: the importance of interdisciplinary studies on COVID-19 in and beyond tourism. Tour. Recreat. Res. 46 , 310–313 (2021).
Bloem, B. R. et al. Integrated and patient-centred management of Parkinson’s disease: a network model for reshaping chronic neurological care. Lancet Neurol. 19 , 623–634 (2020).
Breasted, J. H. The Edwin Smith Surgical Papyrus: published in facsimile and hieroglyphic transliteration with translation and commentary in two volumes. JAMA 96 , 1534 (1931).
Simeone, F. A. Andreas Vesalius: anatomist, surgeon, count palatine, and pilgrim. Am. J. Surg. 147 , 432–440 (1984).
Piccolino, M. Animal electricity and the birth of electrophysiology: the legacy of Luigi Galvani. Brain Res. Bull. 46 , 381–407 (1998).
Fishman, M. C. Sir Henry Hallett Dale and acetylcholine story. Yale J. Biol. Med. 45 , 104–118 (1972).
CAS PubMed PubMed Central Google Scholar
Eccles, J. C. Ionic mechanism of postsynaptic inhibition. Science 145 , 1140–1147 (1964).
Hell, S. W. & Wichmann, J. Breaking the diffraction resolution limit by stimulated emission: stimulated-emission-depletion fluorescence microscopy. Opt. Lett. 19 , 780–782 (1994).
Chojnacki, J. et al. Maturation-dependent HIV-1 surface protein redistribution revealed by fluorescence nanoscopy. Science 338 , 524–528 (2012).
Rust, M. J., Bates, M. & Zhuang, X. Sub-diffraction-limit imaging by stochastic optical reconstruction microscopy (STORM). Nat. Methods 3 , 793–795 (2006).
Sahl, S. J., Hell, S. W. & Jakobs, S. Fluorescence nanoscopy in cell biology. Nat. Rev. Mol. Cell Biol. 18 , 685–701 (2017).
Sidenstein, S. C. et al. Multicolour multilevel STED nanoscopy of actin/spectrin organization at synapses. Sci. Rep. 6 , 26725 (2016).
Ning, K. et al. Classifying Alzheimer’s disease with brain imaging and genetic data using a neural network framework. Neurobiol. Aging 68 , 151–158 (2018).
Tian, W. et al. Meso-structure segmentation of concrete CT image based on mask and regional convolution neural network. Mater. Des . 208 , 109919 (2021).
Chung, K. et al. Structural and molecular interrogation of intact biological systems. Nature 497 , 332–337 (2013).
Chen, J. L., Andermann, M. L., Keck, T., Xu, N. L. & Ziv, Y. Imaging neuronal populations in behaving rodents: paradigms for studying neural circuits underlying behavior in the mammalian cortex. J. Neurosci. 33 , 17631–17640 (2013).
Lutz, A., Brefczynski-Lewis, J., Johnstone, T. & Davidson, R. J. Regulation of the neural circuitry of emotion by compassion meditation: effects of meditative expertise. PLoS One 3 , e1897 (2008).
Marzluff, J. M., Miyaoka, R., Minoshima, S. & Cross, D. J. Brain imaging reveals neuronal circuitry underlying the crow’s perception of human faces. Proc. Natl. Acad. Sci. USA 109 , 15912–15917 (2012).
Lauterbur, P. C. Image formation by induced local interactions: examples employing nuclear magnetic resonance. Nature 242 , 190–191 (1973).
Bilezikian, J. P. et al. Hypoparathyroidism in the adult: epidemiology, diagnosis, pathophysiology, target‐organ involvement, treatment, and challenges for future research. J. Bone Miner. Res. 26 , 2317–2337 (2011).
Ten Kate, M. et al. Atrophy subtypes in prodromal Alzheimer’s disease are associated with cognitive decline. Brain 141 , 3443–3456 (2018).
Maes, E. et al. Designing biomedical proteomics experiments: state-of-the-art and future perspectives. Expert Rev. Proteom. 13 , 495–511 (2016).
Mishra, P. et al. Descriptive statistics and normality tests for statistical data. Ann. Card. Anaesth. 22 , 67–72 (2019).
Wong, M. et al. Whole genome, transcriptome and methylome profiling enhances actionable target discovery in high-risk pediatric cancer. Nat. Med. 26 , 1742–1753 (2020).
Trapotsi, M. A., Barrett, I., Engkvist, O. & Bender, A. In Target Discovery and Validation: Methods and Strategies for Drug Discovery (ed Plowright, A. T.) Ch. 11 78 , 323–363, (Wiley, 2019).
Davis, R. L. Mechanism of action and target identification: a matter of timing in drug discovery. Iscience 23 , 101487 (2020).
Chepelev, N. L. et al. Integrating toxicogenomics into human health risk assessment: lessons learned from the benzo[a]pyrene case study. Crit. Rev. Toxicol. 45 , 44–52 (2015).
National Academies of Sciences, Engineering, and Medicine; Health and Medicine Division; Board on Health Sciences Policy; & Committee on the Clinical Utility of Treating Patients with Compounded Bioidentical Hormone Replacement Therapy. The clinical utility of compounded bioidentical hormone therapy: a review of safety, effectiveness, and use (eds Jackson, L. M., Parker, R. M. & Mattison, D. R.) (National Academies Press, 2020).
Brody, T. Clinical trials: study design, endpoints and biomarkers, drug safety, and FDA and ICH guidelines (Elsevier, 2012).
World Health Organization. The top 10 causes of death https://www.who.int/news-room/fact-sheets/detail/the-top-10-causes-of-death#:~:text=The%20top%20global%20causes%20of,birth%20asphyxia%20and%20birth%20trauma%2C (2020).
Foster, J. G., Rzhetsky, A. & Evans, J. A. Tradition and innovation in scientists’ research strategies. Am. Sociol. Rev. 80 , 875–908 (2015).
Li, D., Azoulay, P. & Sampat, B. N. The applied value of public investments in biomedical research. Science 356 , 78–81 (2017).
Leenaars, C. H. C. et al. Animal to human translation: a systematic scoping review of reported concordance rates. J. Transl. Med. 17 , 223 (2019).
Olson, H. et al. Concordance of the toxicity of pharmaceuticals in humans and in animals. Regul. Toxicol. Pharmacol. 32 , 56–67 (2000).
Yang, H. D., Kim, D. H., Lee, S. B. & Young, L. D. History of Alzheimer’s disease. Dement. Neurocogn. Disord. 15 , 115–121 (2016).
Goetz, C. G. The history of Parkinson’s disease: early clinical descriptions and neurological therapies. Cold Spring Harb. Perspect. Med. 1 , a008862 (2011).
McKee, M. Book of the month. Priceless https://doi.org/10.1177/014107680509800615 (2005).
Buda, O., Arsene, D., Ceausu, M., Dermengiu, D. & Curca, G. C. Georges Marinesco and the early research in neuropathology. Neurology 72 , 88–91 (2009).
Goedert, M. Oskar Fischer and the study of dementia. Brain 132 , 1102–1111 (2009).
Möckl, L., Lamb, D. C. & Bräuchle, C. Super‐resolved fluorescence microscopy: Nobel Prize in Chemistry 2014 for Eric Betzig, Stefan Hell, and William E. Moerner. Angew. Chem. Int. Ed. Engl. 53 , 13972–13977 (2014).
Asher, S. & Priefer, R. Alzheimer’s disease failed clinical trials. Life Sci. 306 , 120861 (2022).
Cummings, J., Morstorf, T. & Lee, G. Alzheimer’s drug-development pipeline: 2016. Alzheimers Dement. (N. Y) 2 , 222–232 (2016).
Cummings, J. et al. Alzheimer’s disease drug development pipeline: 2022. Alzheimers Dement. (N. Y) 8 , e12295 (2022).
Wang, W., Zhao, F., Ma, X., Perry, G. & Zhu, X. Mitochondria dysfunction in the pathogenesis of Alzheimer’s disease: recent advances. Mol. Neurodegener. 15 , 30 (2020).
Leng, F. & Edison, P. Neuroinflammation and microglial activation in Alzheimer disease: where do we go from here? Nat. Rev. Neurol. 17 , 157–172 (2021).
Liu, J., Chang, L., Song, Y., Li, H. & Wu, Y. The role of NMDA receptors in Alzheimer’s disease. Front. Neurosci. 13 , 43 (2019).
Bloem, B. R., Okun, M. S. & Klein, C. Parkinson’s disease. Lancet 397 , 2284–2303 (2021).
Mathur, S., Gawas, C., Ahmad, I. Z., Wani, M. & Tabassum, H. Neurodegenerative disorders: assessing the impact of natural vs drug‐induced treatment options. Aging Med. (Milton) 6 , 82–97 (2023).
Cummings, J. L. et al. High‐dose donepezil (23 mg/day) for the treatment of moderate and severe Alzheimer’s disease: drug profile and clinical guidelines. CNS Neurosci. Ther. 19 , 294–301 (2013).
Orr, M. E., Sullivan, A. C. & Frost, B. A brief overview of tauopathy: causes, consequences, and therapeutic strategies. Trends Pharmacol. Sci. 38 , 637–648 (2017).
Horstink, M. et al. Review of the therapeutic management of Parkinson’s disease. Report of a joint task force of the European Federation of Neurological Societies (EFNS) and the Movement Disorder Society‐European Section (MDS‐ES). Part II: late (complicated) Parkinson’s disease. Eur. J. Neurol. 13 , 1186–1202 (2006).
Rascol, O., Lozano, A., Stern, M. & Poewe, W. Milestones in Parkinson’s disease therapeutics. Mov. Disord. 26 , 1072–1082 (2011).
Bar-Or, A., Pachner, A., Menguy-Vacheron, F., Kaplan, J. & Wiendl, H. Teriflunomide and its mechanism of action in multiple sclerosis. Drugs 74 , 659–674 (2014).
Eugena, K. G. PSY 142—Abnormal Psychology (Hostos Community College, 2018).
Ban, T. A. Fifty years chlorpromazine: a historical perspective. Neuropsychiatr. Dis. Treat. 3 , 495–500 (2007).
Laborit, H., Huguenard, P. & Alluaume, R. [A new vegetative stabilizer; 4560 R.P.]. Presse Med. (1893) 60 , 206–208 (1952).
Rosenbloom, M. Chlorpromazine and the psychopharmacologic revolution. JAMA 287 , 1860–1861 (2002).
Seeman, P. & Lee, T. Antipsychotic drugs: direct correlation between clinical potency and presynaptic action on dopamine neurons. Science 188 , 1217–1219 (1975).
Baumgarten, H. G. & Grozdanovic, Z. Role of serotonin in obsessive-compulsive disorder. Br. J. Psychiatry Suppl . 35 , 13–20 (1998).
McTavish, D. & Benfield, P. Clomipramine. An overview of its pharmacological properties and a review of its therapeutic use in obsessive compulsive disorder and panic disorder. Drugs 39 , 136–153 (1990).
Petty, F. GABA and mood disorders: a brief review and hypothesis. J. Affect. Disord. 34 , 275–281 (1995).
Fineberg, N. A. et al. The size, burden and cost of disorders of the brain in the UK. J. Psychopharmacol. 27 , 761–770 (2013).
World Health Organization. WHO guidelines Approved by the guidelines review committee (World Health Organization, 2009).
Faraone, S. V. et al. Attention-deficit/hyperactivity disorder. Nat. Rev. Dis. Prim. 1 , 15020 (2015).
Briars, L. & Todd, T. A review of pharmacological management of attention-deficit/hyperactivity disorder. J. Pediatr. Pharmacol. Ther. 21 , 192–206 (2016).
PubMed PubMed Central Google Scholar
Carrey, N. J., Wiggins, D. M. & Milin, R. P. Pharmacological treatment of psychiatric disorders in children and adolescents: focus on guidelines for the primary care practitioner. Drugs 51 , 750–759 (1996).
Charach, A., Ickowicz, A. & Schachar, R. Stimulant treatment over five years: adherence, effectiveness, and adverse effects. J. Am. Acad. Child Adolesc. Psychiatry 43 , 559–567 (2004).
Groth, M. et al. Critical analysis of an e-learning and interactive teaching module with respect to the interpretation of emergency computed tomography of the brain. RoFo 190 , 334–340 (2018).
Kanner, A. M. & Bicchi, M. M. Antiseizure medications for adults with epilepsy: a review. JAMA 327 , 1269–1281 (2022).
Rho, J. M. & White, H. S. H. S. Brief history of anti-seizure drug development. Epilepsia Open 3 , 114–119 (2018).
Cano, A. et al. Epilepsy in neurodegenerative diseases: related drugs and molecular pathways. Pharmaceuticals (Basel) 14 , 1057 (2021).
Fan, H. C., Chiang, K. L., Chang, K. H., Chen, C. M. & Tsai, J. D. Epilepsy and attention deficit hyperactivity disorder: connection, chance, and challenges. Int. J. Mol. Sci . 24 , 5270 (2023).
Galovic, M. et al. Seizures and epilepsy after stroke: epidemiology, biomarkers and management. Drugs Aging 38 , 285–299 (2021).
Perucca, E. The pharmacological treatment of epilepsy: recent advances and future perspectives. Acta Epileptol. 3 , 22 (2021).
Lally, J. & MacCabe, J. H. Antipsychotic medication in schizophrenia: a review. Br. Med. Bull. 114 , 169–179 (2015).
Marder, S. R. & Cannon, T. D. Schizophrenia. N. Engl. J. Med. 381 , 1753–1761 (2019).
Pittenger, C. & Bloch, M. H. Pharmacological treatment of obsessive-compulsive disorder. Psychiatr. Clin. North Am. 37 , 375–391 (2014).
Fineberg, N. & Brown, A. Pharmacotherapy for obsessive—compulsive disorder. Adv. Psychiatr. Treat. 17 , 419–434 (2011).
Carvalho, A. F., Firth, J. & Vieta, E. Bipolar disorder. N. Engl. J. Med. 383 , 58–66 (2020).
Tolou-Ghamari, Z., Zare, M., Habibabadi, J. M. & Najafi, M. R. A quick review of carbamazepine pharmacokinetics in epilepsy from 1953 to 2012. J. Res. Med. Sci. 18 , S81–S85 (2013).
Xr, A. Therapeutic class overview attention deficit/hyperactivity disorder (ADHD) agents and stimulants. https://www.semanticscholar.org/paper/Therapeutic-Class-Overview-Attention-Deficit-(ADHD)-Xr/ac77b5e861caecd907909f91133abc46b31dc933?utm_source=direct_link (2014).
Potnis, V. V., Albhar, K. G., Nanaware, P. A. & Pote, V. S. A review on epilepsy and its management. J. Drug Deliv. Ther. 10 , 273–279 (2020).
Richter, L. et al. Diazepam-bound GABAA receptor models identify new benzodiazepine binding-site ligands. Nat. Chem. Biol. 8 , 455–464 (2012).
Berger, H. About human brain waves. Über das elektroenkephalogramm des menschen. Arch. f. Psychiatr. 87 , 527–570 (1929).
Cohen, D. Magnetoencephalography: evidence of magnetic fields produced by alpha-rhythm currents. Science 161 , 784–786 (1968).
Roy, C. S. & Sherrington, C. S. On the regulation of the blood-supply of the brain. J. Physiol. 11 , 85–158.117 (1890).
Raichle, M. E. A brief history of human brain mapping. Trends Neurosci. 32 , 118–126 (2009).
Deco, G., Tononi, G., Boly, M. & Kringelbach, M. L. Rethinking segregation and integration: contributions of whole-brain modelling. Nat. Rev. Neurosci. 16 , 430–439 (2015).
Marková, I. S. Translational neuroscience and psychiatry: a conceptual analysis. J. Eval. Clin. Pract. 24 , 791–796 (2018).
Wiegand, T. L. T. et al. Translational neuroimaging in mild traumatic brain injury. J. Neurosci. Res. 100 , 1201–1217 (2022).
Bruce, E. D. et al. Neuroimaging and traumatic brain injury: state of the field and voids in translational knowledge. Mol. Cell. Neurosci. 66 , 103–113 (2015).
Boroda, E. et al. Network topology changes in chronic mild traumatic brain injury (mTBI). NeuroImage Clin. 31 , 102691 (2021).
To, X. V. & Nasrallah, F. A. A road map of brain recovery in a mouse model of concussion: insights from neuroimaging. Acta Neuropathol. Commun. 9 , 1–20 (2021).
Sudlow, C. et al. UK Biobank: an open access resource for identifying the causes of a wide range of complex diseases of middle and old age. PLOS Med. 12 , e1001779 (2015).
Bethlehem, R. A. I. et al. Brain charts for the human lifespan. Nature 604 , 525–533 (2022).
Marek, S. et al. Reproducible brain-wide association studies require thousands of individuals. Nature 603 , 654–660 (2022).
Sauter, A. W., Wehrl, H. F., Kolb, A., Judenhofer, M. S. & Pichler, B. J. Combined PET/MRI: one step further in multimodality imaging. Trends Mol. Med. 16 , 508–515 (2010).
Douaud, G. et al. Anatomically related grey and white matter abnormalities in adolescent-onset schizophrenia. Brain 130 , 2375–2386 (2007).
Watkins, K. E., Smith, S. M., Davis, S. & Howell, P. Structural and functional abnormalities of the motor system in developmental stuttering. Brain 131 , 50–59 (2008).
Mars, R. B. & Palomero-Gallagher, N. Towards multi-modal, multi-species brain atlases: part one. Brain Struct. Funct. 228 , 1041–1044 (2023).
Milham, M. P. et al. An open resource for non-human primate imaging. Neuron 100 , 61–74.e2 (2018).
Suarez, L. E. et al. A connectomics-based taxonomy of mammals. eLife 11 , e78635 (2022).
Uludağ, K. & Roebroeck, A. A. General overview on the merits of multimodal neuroimaging data fusion. Neuroimage 102 , 3–10 (2014).
Zhang, Y. D. et al. Advances in multimodal data fusion in neuroimaging: overview, challenges, and novel orientation. Inf. Fusion 64 , 149–187 (2020).
Tulay, E. E., Metin, B., Tarhan, N. & Arıkan, M. K. Multimodal neuroimaging: basic concepts and classification of neuropsychiatric diseases. Clin. EEG Neurosci. 50 , 20–33 (2019).
Krasny, L. & Huang, P. H. Data-independent acquisition mass spectrometry (DIA-MS) for proteomic applications in oncology. Mol. Omics 17 , 29–42 (2021).
Mann, M. & Jensen, O. N. Proteomic analysis of post-translational modifications. Nat. Biotechnol. 21 , 255–261 (2003).
Hasin, Y., Seldin, M. & Lusis, A. Multi-omics approaches to disease. Genome Biol. 18 , 83 (2017).
Vogel, C. & Marcotte, E. M. Insights into the regulation of protein abundance from proteomic and transcriptomic analyses. Nat. Rev. Genet. 13 , 227–232 (2012).
Bersanelli, M. et al. Methods for the integration of multi-omics data: mathematical aspects. BMC Bioinforma. 17 , 15 (2016).
Burla, B. et al. Ms-based lipidomics of human blood plasma: a community-initiated position paper to develop accepted guidelines1. J. Lipid Res. 59 , 2001–2017 (2018).
O’Donnell, V. B., Dennis, E. A., Wakelam, M. J. O. & Subramaniam, S. LIPID MAPS: serving the next generation of lipid researchers with tools, resources, data, and training. Sci. Signal. 12 , eaaw2964 (2019).
Watrous, J. D. et al. Directed non-targeted mass spectrometry and chemical networking for discovery of eicosanoids and related oxylipins. Cell Chem. Biol. 26 , 433–442.e4 (2019).
Leier, H. C. et al. A global lipid map defines a network essential for Zika virus replication. Nat. Commun. 11 , 3652 (2020).
Tkachev, A. et al. Lipid alteration signature in the blood plasma of individuals with schizophrenia, depression, and bipolar disorder. JAMA Psychiatry 80 , 250–259 (2023).
Yoon, J. H. et al. Brain lipidomics: from functional landscape to clinical significance. Sci. Adv. 8 , eadc9317 (2022).
Chakraborty, S., Hosen, M. I., Ahmed, M. & Shekhar, H. U. Onco-multi-OMICS approach: a new frontier in cancer research. BioMed. Res. Int. 2018 , 9836256 (2018).
Vasaikar, S. V., Straub, P., Wang, J. & Zhang, B. LinkedOmics: analyzing multi-omics data within and across 32 cancer types. Nucleic Acids Res. 46 , D956–D963 (2018).
Mun, D. G. et al. Proteogenomic characterization of human early-onset gastric cancer. Cancer Cell 35 , 111–124.e10 (2019).
Zhang, H. et al. Integrated proteogenomic characterization of human high-grade serous ovarian cancer. Cell 166 , 755–765 (2016).
Reska, D. et al. Integration of solutions and services for multi-omics data analysis towards personalized medicine. Biocybern. Biomed. Eng. 41 , 1646–1663 (2021).
ICGC/TCGA Pan-Cancer Analysis of Whole Genomes Consortium. Pan-cancer analysis of whole genomes. Nature 578 , 82–93 (2020).
Cancer Genome Atlas Research Network. et al. The Cancer Genome Atlas Pan-Cancer analysis project. Nat. Genet. 45 , 1113–1120 (2013).
Article PubMed Central Google Scholar
Lathrop, M. et al. International network of cancer genome projects (The International Cancer Genome Consortium). Nat. Dig. 464 , 993–998 (2010).
Stein, L. D., Knoppers, B. M., Campbell, P., Getz, G. & Korbel, J. O. Data analysis: create a cloud commons. Nature 523 , 149–151 (2015).
Zhang, J. et al. The International Cancer Genome Consortium Data Portal. Nat. Biotechnol. 37 , 367–369 (2019).
Ellis, M. J. et al. Connecting genomic alterations to cancer biology with proteomics: the NCI Clinical Proteomic Tumor Analysis Consortium. Cancer Discov. 3 , 1108–1112 (2013).
Whiteaker, J. R. et al. CPTAC Assay Portal: a repository of targeted proteomic assays. Nat. Methods 11 , 703–704 (2014).
Mertins, P. et al. Reproducible workflow for multiplexed deep-scale proteome and phosphoproteome analysis of tumor tissues by liquid chromatography–mass spectrometry. Nat. Protoc. 13 , 1632–1661 (2018).
Weiskirchen, S., Weiper, K., Tolba, R. H. & Weiskirchen, R. All you can feed: some comments on production of mouse diets used in biomedical research with special emphasis on non-alcoholic fatty liver disease research. Nutrients 12 , 163 (2020).
Wu, P. et al. Integration and analysis of CPTAC proteomics data in the context of cancer genomics in the cBioPortal*. Mol. Cell. Proteom. 18 , 1893–1898 (2019).
Hu, Y. et al. Single cell multi-omics technology: methodology and application. Front. Cell Dev. Biol. 6 , 28 (2018).
Chappell, L., Russell, A. J. C. & Voet, T. Single-cell (multi)omics technologies. Annu. Rev. Genom. Hum. Genet. 19 , 15–41 (2018).
Palazzotto, E. & Weber, T. Omics and multi-omics approaches to study the biosynthesis of secondary metabolites in microorganisms. Curr. Opin. Microbiol. 45 , 109–116 (2018).
Ma, A., McDermaid, A., Xu, J., Chang, Y. & Ma, Q. Integrative methods and practical challenges for single-cell multi-omics. Trends Biotechnol. 38 , 1007–1022 (2020).
Argelaguet, R. et al. Multi‐Omics Factor Analysis-a framework for unsupervised integration of multi‐omics data sets. Mol. Syst. Biol. 14 , e8124 (2018).
Shen, R., Olshen, A. B. & Ladanyi, M. Integrative clustering of multiple genomic data types using a joint latent variable model with application to breast and lung cancer subtype analysis. Bioinformatics 25 , 2906–2912 (2009).
Nicora, G., Vitali, F., Dagliati, A., Geifman, N. & Bellazzi, R. Integrated multi-omics analyses in oncology: a review of machine learning methods and tools. Front. Oncol. 10 , 1030 (2020).
Zhou, G., Li, S. & Xia, J. Network-based approaches for multi-omics integration. Methods Mol. Biol. 2104 , 469–487 (2020).
Olivier, M., Asmis, R., Hawkins, G. A., Howard, T. D. & Cox, L. A. The need for multi-omics biomarker signatures in precision medicine. Int. J. Mol. Sci. 20 , 4781 (2019).
Chute, C. G. et al. Some experiences and opportunities for big data in translational research. Genet. Med. 15 , 802–809 (2013).
Onitsuka, T. et al. Trends in big data analyses by multicenter collaborative translational research in psychiatry. Psychiatry Clin. Neurosci. 76 , 1–14 (2022).
Dunn, W. Jr, Burgun, A., Krebs, M. O. & Rance, B. Exploring and visualizing multidimensional data in translational research platforms. Brief. Bioinform. 18 , 1044–1056 (2017).
van den Heuvel, M. P. & Yeo, B. T. T. A spotlight on bridging microscale and macroscale human brain architecture. Neuron 93 , 1248–1251 (2017).
Almeida, S. A. Jr & Guner, S. Review of artificial neural networks and a new feed-forward network for anchorage analysis in cracked concrete. Int. Concr. Abstr. Portal 350 , 54–68 (2021).
Kaul, V., Enslin, S. & Gross, S. A. History of artificial intelligence in medicine. Gastrointest. Endosc. 92 , 807–812 (2020).
Jordan, M. I. & Mitchell, T. M. T. M. Machine learning: trends, perspectives, and prospects. Science 349 , 255–260 (2015).
Rajkomar, A., Dean, J. & Kohane, I. Machine learning in medicine. N. Engl. J. Med. 380 , 1347–1358 (2019).
Khalid, S., Khalil, T. & Nasreen, S. A survey of feature selection and feature extraction techniques in machine learning. IEEE 372–378 (2014).
Nogales, A., García-Tejedor, Á. J., Monge, D., Vara, J. S. & Antón, C. A survey of deep learning models in medical therapeutic areas. Artif. Intell. Med. 112 , 102020 (2021).
Saxe, A., Nelli, S. & Summerfield, C. If deep learning is the answer, what is the question? Nat. Rev. Neurosci. 22 , 5–67 (2021).
Yang, G. R. & Molano-Mazón, M. Towards the next generation of recurrent network models for cognitive neuroscience. Curr. Opin. Neurobiol. 70 , 182–192 (2021).
Bengio, Y., Goodfellow, I. & Courville, A. Deep learning (The MIT Press, 2016)
Komoroski, B. et al. Dapagliflozin, a novel SGLT2 inhibitor, induces dose-dependent glucosuria in healthy subjects. Clin. Pharmacol. Ther. 85 , 520–526 (2009).
Anderson, S. L. & Marrs, J. C. Dapagliflozin for the treatment of type 2 diabetes. Ann. Pharmacother. 46 , 590–598 (2012).
Agholi, M., Hatam, G. R. & Motazedian, M. H. Microsporidia and coccidia as causes of persistence diarrhea among liver transplant children: incidence rate and species/genotypes. Pediatr. Infect. Dis. J. 32 , 185–187 (2013).
Anderson, S. L. Dapagliflozin efficacy and safety: a perspective review. Ther. Adv. Drug Saf. 5 , 242–254 (2014).
Azam, A., Peerzada, M. N. & Ahmad, K. Parasitic diarrheal disease: drug development and targets. Front. Microbiol. 6 , 1183 (2015).
Miyamoto, S. et al. Transformation to small-cell lung cancer as a mechanism of acquired resistance to crizotinib and alectinib. Jpn. J. Clin. Oncol. 46 , 170–173 (2016).
PubMed Google Scholar
Dandona, P. Minimizing glycemic fluctuations in patients with Type 2 diabetes: approaches and importance. Diabetes Technol. Ther. 19 , 498–506 (2017).
Vamathevan, J. et al. Applications of machine learning in drug discovery and development. Nat. Rev. Drug Discov. 18 , 463–477 (2019).
Lu, C. et al. Systemic evolutionary chemical space exploration for drug discovery. J. Cheminform. 14 , 19 (2022).
Rifaioglu, A. S. et al. Recent applications of deep learning and machine intelligence on in silico drug discovery: methods, tools and databases. Brief. Bioinform. 20 , 1878–1912 (2019).
Goodfellow, I. et al. Generative adversarial nets. Adv. Neural Inf. Process. Syst . 27 , 2672–2680 (2014).
Wang, R. et al. Applications of generative adversarial networks in neuroimaging and clinical neuroscience. Neuroimage 269 , 119898 (2023).
Habashi, A. G., Azab, A. M., Eldawlatly, S. & Aly, G. M. Generative adversarial networks in EEG analysis: an overview. J. Neuroeng. Rehabil. 20 , 40 (2023).
Yu, W. et al. Tensorizing GAN with high-order pooling for Alzheimer’s disease assessment. IEEE Trans. Neural Netw. Learn. Syst. 33 , 4945–4959 (2022).
Lin, W. et al. Bidirectional mapping of brain MRI and PET with 3D reversible GAN for the diagnosis of Alzheimer’s disease. Front. Neurosci. 15 , 646013 (2021).
Ahmed, K. T., Sun, J., Cheng, S., Yong, J. & Zhang, W. Multi-omics data integration by generative adversarial network. Bioinformatics 38 , 179–186 (2021).
Ai, X., Smith, M. C. & Feltus, F. A. Generative adversarial networks applied to gene expression analysis: an interdisciplinary perspective. Comp. Syst. Oncol. 3 , e1050 (2023).
Khot, U. N. et al. Prevalence of conventional risk factors in patients with coronary heart disease. JAMA 290 , 898–904 (2003).
Payne, M. & Emma Reith-Hall, E. (eds) The Routledge Handbook of Social Work Theory 1st edn (ed Carey, M.) Ch. 6 (Taylor and Francis Group, 2019).
Ressler, K. J. & Williams, L. M. Big data in psychiatry: multiomics, neuroimaging, computational modeling, and digital phenotyping. Neuropsychopharmacology 46 , 1–2 (2021).
Tan, M. S., Cheah, P. L., Chin, A. V., Looi, L. M. & Chang, S. W. A review on omics-based biomarkers discovery for Alzheimer’s disease from the bioinformatics perspectives: statistical approach vs machine learning approach. Comput. Biol. Med. 139 , 104947 (2021).
Termine, A. et al. Multi-layer picture of neurodegenerative diseases: lessons from the use of big data through artificial intelligence. J. Pers. Med. 11 , 280 (2021).
Macarron, R. et al. Impact of high-throughput screening in biomedical research. Nat. Rev. Drug Discov. 10 , 188–195 (2011).
Manouchehri, E., Ghavami, V., Larki, M., Saeidi, M. & Latifnejad Roudsari, R. Domestic violence experienced by women with multiple sclerosis: a study from the North-East of Iran. BMC Women’s Health 22 , 321 (2022).
Rahman, M. M. et al. Biomedical research in developing countries: opportunities, methods, and challenges. Indian J. Gastroenterol. 39 , 292–302 (2020).
Sun, J. et al. Isolation of infectious SARS-CoV-2 from urine of a COVID-19 patient. Emerg. Microbes Infect. 9 , 991–993 (2020).
Kaiser, S. et al. Parkinson’s disease causality and heterogeneity: a proteogenomic view. medRxiv (2022).
Kurvits, L. et al. Transcriptomic profiles in Parkinson’s disease. Exp. Biol. Med. (Maywood) 246 , 584–595 (2021).
Li, X., Fan, X., Yang, H. & Liu, Y. Review of metabolomics-based biomarker research for Parkinson’s disease. Mol. Neurobiol. 59 , 1041–1057 (2022).
Boutet, A. et al. Predicting optimal deep brain stimulation parameters for Parkinson’s disease using functional MRI and machine learning. Nat. Commun. 12 , 3043 (2021).
Elkouzi, A., Vedam-Mai, V., Eisinger, R. S. & Okun, M. S. Emerging therapies in Parkinson disease—repurposed drugs and new approaches. Nat. Rev. Neurol. 15 , 204–223 (2019).
Chen, J., Guo, C., Lu, M. & Ding, S. Unifying diagnosis identification and prediction method embedding the disease ontology structure from electronic medical records. Front. Public Health 9 , 793801 (2021).
Myszczynska, M. A. et al. Applications of machine learning to diagnosis and treatment of neurodegenerative diseases. Nat. Rev. Neurol. 16 , 440–456 (2020).
Park, S. W. et al. Machine learning application for classification of Alzheimer’s disease stages using 18F-flortaucipir positron emission tomography. Biomed. Eng. OnLine 22 , 40 (2023).
Simfukwe, C., Lee, R. & Youn, Y. C., Alzheimer’s Disease and Related Dementias in Zambia (ADDIZ) Group. Classification of Abeta State from brain amyloid PET images using machine learning algorithm. Dement. Neurocogn. Disord. 22 , 61–68 (2023).
Kim, N. H. et al. PET-validated EEG-machine learning algorithm predicts brain amyloid pathology in pre-dementia Alzheimer’s disease. Sci. Rep. 13 , 10299 (2023).
Brickman, A. M. et al. Correlation of plasma and neuroimaging biomarkers in Alzheimer’s disease. Ann. Clin. Transl. Neurol. 9 , 756–761 (2022).
Chan, Y. H., Wang, C., Soh, W. K. & Rajapakse, J. C. Combining neuroimaging and omics datasets for disease classification using graph neural networks. Front. Neurosci. 16 , 866666 (2022).
Rayaprolu, S. et al. Systems-based proteomics to resolve the biology of Alzheimer’s disease beyond amyloid and tau. Neuropsychopharmacology 46 , 98–115 (2021).
Adewale, Q., Khan, A. F., Carbonell, F., Iturria-Medina, Y. & Alzheimer’s Disease Neuroimaging Initiative. Integrated transcriptomic and neuroimaging brain model decodes biological mechanisms in aging and Alzheimer’s disease. eLife 10 , e62589 (2021).
Vogel, J. W. et al. Connectome-based modelling of neurodegenerative diseases: towards precision medicine and mechanistic insight. Nat. Rev. Neurosci. 24 , 620–639 (2023).
Guo, X. et al. Inter-individual heterogeneity of functional brain networks in children with autism spectrum disorder. Mol. Autism 13 , 52 (2022).
Download references
The Korea Brain Research Institute (KBRI) basic research program through the KBRI funded by the Ministry of Science and ICT supported this research (23-BR-02-03 and 23-BR-04-02).
Author information
These authors contributed equally: Jong Hyuk Yoon, Dongha Lee, Chany Lee, Eunji Cho.
Authors and Affiliations
Neurodegenerative Diseases Research Group, Korea Brain Research Institute, Daegu, 41062, Republic of Korea
Jong Hyuk Yoon, Eunji Cho & Seulah Lee
Cognitive Science Research Group, Korea Brain Research Institute, Daegu, 41062, Republic of Korea
Dongha Lee & Chany Lee
Department of Biochemistry and Precision Medicine Translational Research Program, Yong Loo Lin School of Medicine, National University of Singapore, Singapore, 119077, Singapore
Amaury Cazenave-Gassiot
Singapore Lipidomics Incubator (SLING), Life Sciences Institute, National University of Singapore, Singapore, 117456, Singapore
Research Strategy Office, Korea Brain Research Institute, Daegu, 41062, Republic of Korea
Neurovascular Unit Research Group, Korean Brain Research Institute, Daegu, 41062, Republic of Korea
Sehyun Chae
Department of Pharmacology and Department of Chemistry and Biochemistry, University of California, San Diego, La Jolla, CA, 92093-0601, USA
Edward A. Dennis
Korea Brain Research Institute, Daegu, 41062, Republic of Korea
Pann-Ghill Suh
You can also search for this author in PubMed Google Scholar
Corresponding author
Correspondence to Jong Hyuk Yoon .
Ethics declarations
Competing interests.
The authors declare no competing interests.
Additional information
Publisher’s note Springer Nature remains neutral with regard to jurisdictional claims in published maps and institutional affiliations.
Supplementary information
Supplementary information, rights and permissions.
Open Access This article is licensed under a Creative Commons Attribution 4.0 International License, which permits use, sharing, adaptation, distribution and reproduction in any medium or format, as long as you give appropriate credit to the original author(s) and the source, provide a link to the Creative Commons licence, and indicate if changes were made. The images or other third party material in this article are included in the article’s Creative Commons licence, unless indicated otherwise in a credit line to the material. If material is not included in the article’s Creative Commons licence and your intended use is not permitted by statutory regulation or exceeds the permitted use, you will need to obtain permission directly from the copyright holder. To view a copy of this licence, visit http://creativecommons.org/licenses/by/4.0/ .
Reprints and permissions
About this article
Cite this article.
Yoon, J.H., Lee, D., Lee, C. et al. Paradigm shift required for translational research on the brain. Exp Mol Med (2024). https://doi.org/10.1038/s12276-024-01218-x
Download citation
Received : 13 October 2023
Revised : 07 February 2024
Accepted : 20 February 2024
Published : 01 May 2024
DOI : https://doi.org/10.1038/s12276-024-01218-x
Share this article
Anyone you share the following link with will be able to read this content:
Sorry, a shareable link is not currently available for this article.
Provided by the Springer Nature SharedIt content-sharing initiative
Quick links
- Explore articles by subject
- Guide to authors
- Editorial policies

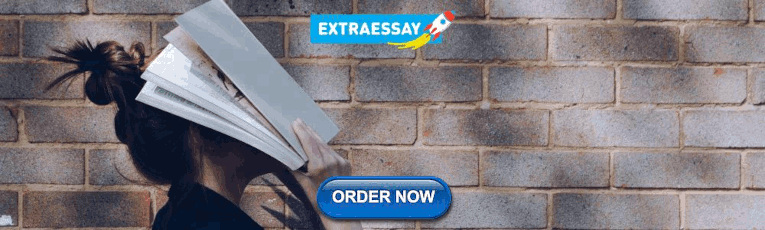
IMAGES
VIDEO
COMMENTS
The qualitative researcher is the primary instrument for data collection and analysis. Data are mediated through this human instrument, rather than through inventories, questionnaires, or machines. Qualitative research involves fieldwork. The researcher physically goes to the people, setting, site, or institution to observe or record behavior ...
Qualitative research involves collecting and analyzing non-numerical data (e.g., text, video, or audio) to understand concepts, opinions, or experiences. It can be used to gather in-depth insights into a problem or generate new ideas for research. Qualitative research is the opposite of quantitative research, which involves collecting and ...
Researchers using this paradigm are more often than not aiming to create a more just, egalitarian society in which individual and collective freedoms are secure. Both quantitative and qualitative methods can be used with this paradigm. 4. Constructivist Research Paradigm.
This review presents an investigative assessment of the pivotal features in qualitative research that can permit the readers to pass judgment on its quality and to condemn it as good research when objectively and adequately utilized. ... Qualitative Research: Interpretive Paradigms. All qualitative researchers follow highly abstract principles ...
COE LIBRARY Chalon Campus. Charles Willard Coe Library 12001 Chalon Road Los Angeles, CA 90049 310.954.4370
Unfortunately, qualitative research is commonly misunderstood. In this chapter, we describe and explain the misconceptions surrounding qualitative research enterprise, why researchers need to care about when using qualitative research, the characteristics of qualitative research, and review the paradigms in qualitative research.
Abstract. This paper aims to provide an overview of the use and assessment of qualitative research methods in the health sciences. Qualitative research can be defined as the study of the nature of phenomena and is especially appropriate for answering questions of why something is (not) observed, assessing complex multi-component interventions ...
In qualitative research, the researcher is the main data collection instrument. The researcher examines why events occur, what happens, and what those events mean to the participants studied. 1, 2. Qualitative research starts from a fundamentally different set of beliefs—or paradigms—than those that underpin quantitative research.
Theory and Methodology. Good research follows from a reasonable starting point, a theoretical concept or perspective. Quantitative research uses a positivist perspective in which evidence is objectively and systematically obtained to prove a causal model or hypothesis; what works is the focus. 3 Alternatively, qualitative approaches focus on how and why something works, to build understanding ...
Another example of a classification of paradigms in social sciences, with particular consideration given to qualitative research, is the study of Egon Guba and Yvonna Lincoln published in The SAGE Handbook of Qualitative Research. It presents the authors' proposal for five basic research orientations—updated compared to the first one dating ...
Qualitative research is the naturalistic study of social meanings and processes, using interviews, observations, and the analysis of texts and images. In contrast to quantitative researchers, whose statistical methods enable broad generalizations about populations (for example, comparisons of the percentages of U.S. demographic groups who vote in particular ways), qualitative researchers use ...
While many books and articles guide various qualitative research methods and analyses, there is currently no concise resource that explains and differentiates among the most common qualitative approaches. We believe novice qualitative researchers, students planning the design of a qualitative study or taking an introductory qualitative research course, and faculty teaching such courses can ...
There are at least three primary applications of theory in qualitative research: (1) theory of research paradigm and method (Glesne, 2011), (2) theory building as a result of data collection (Jaccard & Jacoby, 2010), and (3) theory as a framework to guide the study (Anfara & Mertz, 2015). Differentiation and clarification between these ...
The research paradigm is the framework into which the theories and practices of your discipline fit to create the research plan. This foundation guides all areas of your research plan, including the aim of the study, research question, instruments or measurements used, and analysis methods. Most research paradigms are based on one of two model ...
Research paradigms are essential to producing rigorous research (Brown & Dueñas, 2019).They represent a researcher's beliefs and understandings of reality, knowledge, and action (Crotty, 2020; Guba & Lincoln, 1994).In qualitative research, a wide variety of paradigms exist and qualitative researchers select paradigms which are theoretically aligned with their views of how power relates to ...
Purpose Traditional approaches in qualitative research have adopted one research paradigm linked to an established typology. This paper addresses the unconventional application of two research ...
Similar recommendations are found in Wagner et al.'s systematic review, which identified several studies that recommended that "students should be exposed to philosophy of science and epistemological debates related to qualitative research" (Citation 2019, p. 12), and that "paradigms linked to qualitative research be introduced in the first year and sustained throughout a curriculum ...
A qualitative research methodology was adopted for the study through the grounded theory approach. It utilized grounded theory as a research design and interpretive paradigm to guide the researcher.
Qualitative research is multimethod in focus, involving an interpretative, naturalistic approach to its subject matter. This means that qualitative researchers study things in their natural settings, attempting to make sense of, or interpret, phenomena in terms of the meanings people bring to them.
Common Examples of Research Paradigms. 1. Positivism. Positivists believe that there's a single reality that's possible to measure and understand. Because of this, they're most likely to use quantitative methods in their research. Typically, positivists propose a hypothesis that can be proved or disproved using statistical data analysis.
Defining qualitative research. "The goal of qualitative research is the development of concepts which help to understand social phenomena in natural (rather than experimental) settings, giving due emphasis to the meanings, experiences, and views of all the participants" (Pope & Mays, 1995: 44) 'The aim of such research is to investigate ...
This paper principally describes the link between the research paradigm (constructivism) and the philosophical basis (ontology, epistemology and methodology) of a qualitative study. This article begins with an overview of qualitative study which consists of its meanings and features followed by the definition of research paradigm.
Qualitative research is a type of research that explores and provides deeper insights into real-world problems.[1] Instead of collecting numerical data points or intervene or introduce treatments just like in quantitative research, qualitative research helps generate hypotheses as well as further investigate and understand quantitative data. Qualitative research gathers participants ...
Interpretivism is a research paradigm that is associated with qualitative research. A tradition of Interpretivism can be linked to the ethnographies of non-western peoples in the 19th century and ...
The paradigm shift in translational research is a top-down method that preanalyzes patient-centered data and conducts data-based basic research and clinical/utilization research to overcome the ...