
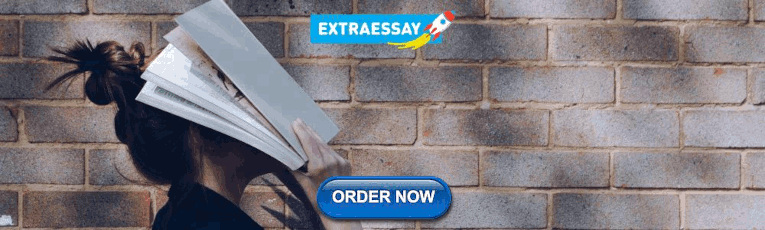
Research Paper Writing: 6. Results / Analysis
- 1. Getting Started
- 2. Abstract
- 3. Introduction
- 4. Literature Review
- 5. Methods / Materials
- 6. Results / Analysis
- 7. Discussion
- 8. Conclusion
- 9. Reference
Writing about the information
There are two sections of a research paper depending on what style is being written. The sections are usually straightforward commentary of exactly what the writer observed and found during the actual research. It is important to include only the important findings, and avoid too much information that can bury the exact meaning of the context.
The results section should aim to narrate the findings without trying to interpret or evaluate, and also provide a direction to the discussion section of the research paper. The results are reported and reveals the analysis. The analysis section is where the writer describes what was done with the data found. In order to write the analysis section it is important to know what the analysis consisted of, but does not mean data is needed. The analysis should already be performed to write the results section.
Written explanations
How should the analysis section be written?
- Should be a paragraph within the research paper
- Consider all the requirements (spacing, margins, and font)
- Should be the writer’s own explanation of the chosen problem
- Thorough evaluation of work
- Description of the weak and strong points
- Discussion of the effect and impact
- Includes criticism
How should the results section be written?
- Show the most relevant information in graphs, figures, and tables
- Include data that may be in the form of pictures, artifacts, notes, and interviews
- Clarify unclear points
- Present results with a short discussion explaining them at the end
- Include the negative results
- Provide stability, accuracy, and value
How the style is presented
Analysis section
- Includes a justification of the methods used
- Technical explanation
Results section
- Purely descriptive
- Easily explained for the targeted audience
- Data driven
Example of a Results Section
Publication Manual of the American Psychological Association Sixth Ed. 2010
- << Previous: 5. Methods / Materials
- Next: 7. Discussion >>
- Last Updated: Nov 7, 2023 7:37 AM
- URL: https://wiu.libguides.com/researchpaperwriting
- PRO Courses Guides New Tech Help Pro Expert Videos About wikiHow Pro Upgrade Sign In
- EDIT Edit this Article
- EXPLORE Tech Help Pro About Us Random Article Quizzes Request a New Article Community Dashboard This Or That Game Popular Categories Arts and Entertainment Artwork Books Movies Computers and Electronics Computers Phone Skills Technology Hacks Health Men's Health Mental Health Women's Health Relationships Dating Love Relationship Issues Hobbies and Crafts Crafts Drawing Games Education & Communication Communication Skills Personal Development Studying Personal Care and Style Fashion Hair Care Personal Hygiene Youth Personal Care School Stuff Dating All Categories Arts and Entertainment Finance and Business Home and Garden Relationship Quizzes Cars & Other Vehicles Food and Entertaining Personal Care and Style Sports and Fitness Computers and Electronics Health Pets and Animals Travel Education & Communication Hobbies and Crafts Philosophy and Religion Work World Family Life Holidays and Traditions Relationships Youth
- Browse Articles
- Learn Something New
- Quizzes Hot
- This Or That Game
- Train Your Brain
- Explore More
- Support wikiHow
- About wikiHow
- Log in / Sign up
- Finance and Business
- Business Skills
- Business Writing
How to Write an Analysis
Last Updated: April 3, 2024 Fact Checked
This article was co-authored by Christopher Taylor, PhD and by wikiHow staff writer, Megaera Lorenz, PhD . Christopher Taylor is an Adjunct Assistant Professor of English at Austin Community College in Texas. He received his PhD in English Literature and Medieval Studies from the University of Texas at Austin in 2014. There are 14 references cited in this article, which can be found at the bottom of the page. This article has been fact-checked, ensuring the accuracy of any cited facts and confirming the authority of its sources. This article has been viewed 293,668 times.
An analysis is a piece of writing that looks at some aspect of a document in detail. To write a good analysis, you’ll need to ask yourself questions that focus on how and why the document works the way it does. You can start the process by gathering information about the subject of your analysis and defining the questions your analysis will answer. Once you’ve outlined your main arguments, look for specific evidence to support them. You can then work on putting your analysis together into a coherent piece of writing.
Gathering Information and Building Your Argument

- If your analysis is supposed to answer a specific question or focus on a particular aspect of the document you are analyzing.
- If there are any length or formatting requirements for the analysis.
- The citation style your instructor wants you to use.
- On what criteria your instructor will evaluate your analysis (e.g., organization, originality, good use of references and quotations, or correct spelling and grammar).

- The title of the document (if it has one).
- The name of the creator of the document. For example, depending on the type of document you’re working with, this could be the author, artist, director, performer, or photographer.
- The form and medium of the document (e.g., “Painting, oil on canvas”).
- When and where the document was created.
- The historical and cultural context of the work.

- Who you believe the intended audience is for the advertisement.
- What rhetorical choices the author made to persuade the audience of their main point.
- What product is being advertised.
- How the poster uses images to make the product look appealing.
- Whether there is any text in the poster, and, if so, how it works together with the images to reinforce the message of the ad.
- What the purpose of the ad is or what its main point is.

- For example, if you’re analyzing an advertisement poster, you might focus on the question: “How does this poster use colors to symbolize the problem that the product is intended to fix? Does it also use color to represent the beneficial results of using the product?”

- For example, you might write, “This poster uses the color red to symbolize the pain of a headache. The blue elements in the design represent the relief brought by the product.”
- You could develop the argument further by saying, “The colors used in the text reinforce the use of colors in the graphic elements of the poster, helping the viewer make a direct connection between the words and images.”

- For example, if you’re arguing that the advertisement poster uses red to represent pain, you might point out that the figure of the headache sufferer is red, while everyone around them is blue. Another piece of evidence might be the use of red lettering for the words “HEADACHE” and “PAIN” in the text of the poster.
- You could also draw on outside evidence to support your claims. For example, you might point out that in the country where the advertisement was produced, the color red is often symbolically associated with warnings or danger.
Tip: If you’re analyzing a text, make sure to properly cite any quotations that you use to support your arguments. Put any direct quotations in quotation marks (“”) and be sure to give location information, such as the page number where the quote appears. Additionally, follow the citation requirements for the style guide assigned by your instructor or one that's commonly used for the subject matter you're writing about.
Organizing and Drafting Your Analysis

- For example, “The poster ‘Say! What a relief,’ created in 1932 by designer Dorothy Plotzky, uses contrasting colors to symbolize the pain of a headache and the relief brought by Miss Burnham’s Pep-Em-Up Pills. The red elements denote pain, while blue ones indicate soothing relief.”
Tip: Your instructor might have specific directions about which information to include in your thesis statement (e.g., the title, author, and date of the document you are analyzing). If you’re not sure how to format your thesis statement or topic sentence, don’t hesitate to ask.

- a. Background
- ii. Analysis/Explanation
- iii. Example
- iv. Analysis/Explanation
- III. Conclusion

- For example, “In the late 1920s, Kansas City schoolteacher Ethel Burnham developed a patent headache medication that quickly achieved commercial success throughout the American Midwest. The popularity of the medicine was largely due to a series of simple but eye-catching advertising posters that were created over the next decade. The poster ‘Say! What a relief,’ created in 1932 by designer Dorothy Plotzky, uses contrasting colors to symbolize the pain of a headache and the relief brought by Miss Burnham’s Pep-Em-Up Pills.”

- Make sure to include clear transitions between each argument and each paragraph. Use transitional words and phrases, such as “Furthermore,” “Additionally,” “For example,” “Likewise,” or “In contrast . . .”
- The best way to organize your arguments will vary based on the individual topic and the specific points you are trying to make. For example, in your analysis of the poster, you might start with arguments about the red visual elements and then move on to a discussion about how the red text fits in.

- For example, you might end your essay with a few sentences about how other advertisements at the time might have been influenced by Dorothy Plotzky’s use of colors.

- For example, in your discussion of the advertisement, avoid stating that you think the art is “beautiful” or that the advertisement is “boring.” Instead, focus on what the poster was supposed to accomplish and how the designer attempted to achieve those goals.
Polishing Your Analysis

- For example, if your essay currently skips around between discussions of the red and blue elements of the poster, consider reorganizing it so that you discuss all the red elements first, then focus on the blue ones.

- For example, you might look for places where you could provide additional examples to support one of your major arguments.

- For example, if you included a paragraph about Dorothy Plotzky’s previous work as a children’s book illustrator, you may want to cut it if it doesn’t somehow relate to her use of color in advertising.
- Cutting material out of your analysis may be difficult, especially if you put a lot of thought into each sentence or found the additional material really interesting. Your analysis will be stronger if you keep it concise and to the point, however.

- You may find it helpful to have someone else go over your essay and look for any mistakes you might have missed.
Tip: When you’re reading silently, it’s easy to miss typos and other small errors because your brain corrects them automatically. Reading your work out loud can make problems easier to spot.
Sample Analysis Outline and Conclusion

Expert Q&A

You Might Also Like

- ↑ https://lsa.umich.edu/sweetland/undergraduates/writing-guides/how-do-i-make-sure-i-understand-an-assignment-.html
- ↑ https://www.bucks.edu/media/bcccmedialibrary/pdf/HOWTOWRITEALITERARYANALYSISESSAY_10.15.07_001.pdf
- ↑ https://owl.purdue.edu/owl/general_writing/visual_rhetoric/analyzing_visual_documents/elements_of_analysis.html
- ↑ https://lsa.umich.edu/sweetland/undergraduates/writing-guides/how-can-i-create-stronger-analysis-.html
- ↑ https://lsa.umich.edu/sweetland/undergraduates/writing-guides/how-do-i-decide-what-i-should-argue-.html
- ↑ https://lsa.umich.edu/sweetland/undergraduates/writing-guides/how-do-i-effectively-integrate-textual-evidence-.html
- ↑ https://writingcenter.uagc.edu/writing-a-thesis
- ↑ https://owl.purdue.edu/owl/general_writing/visual_rhetoric/analyzing_visual_documents/organizing_your_analysis.html
- ↑ https://lsa.umich.edu/sweetland/undergraduates/writing-guides/how-do-i-write-an-intro--conclusion----body-paragraph.html
- ↑ http://utminers.utep.edu/omwilliamson/engl0310/Textanalysis.htm
- ↑ https://owl.purdue.edu/owl/graduate_writing/graduate_writing_topics/graduate_writing_organization_structure_new.html
- ↑ https://owl.purdue.edu/owl/general_writing/mechanics/sentence_clarity.html
- ↑ https://writingcenter.unc.edu/tips-and-tools/conciseness-handout/
- ↑ https://writingcenter.unc.edu/tips-and-tools/editing-and-proofreading/
About This Article

If you need to write an analysis, first look closely at your assignment to make sure you understand the requirements. Then, gather background information about the document you’ll be analyzing and do a close read so that you’re thoroughly familiar with the subject matter. If it’s not already specified in your assignment, come up with one or more specific question’s you’d like your analysis to answer, then outline your main arguments. Finally, gather evidence and examples to support your arguments. Read on to learn how to organize, draft, and polish your analysis! Did this summary help you? Yes No
- Send fan mail to authors
Did this article help you?

Featured Articles

Trending Articles

Watch Articles

- Terms of Use
- Privacy Policy
- Do Not Sell or Share My Info
- Not Selling Info
Get all the best how-tos!
Sign up for wikiHow's weekly email newsletter

How To Write The Results/Findings Chapter
For quantitative studies (dissertations & theses).
By: Derek Jansen (MBA) | Expert Reviewed By: Kerryn Warren (PhD) | July 2021
So, you’ve completed your quantitative data analysis and it’s time to report on your findings. But where do you start? In this post, we’ll walk you through the results chapter (also called the findings or analysis chapter), step by step, so that you can craft this section of your dissertation or thesis with confidence. If you’re looking for information regarding the results chapter for qualitative studies, you can find that here .
Overview: Quantitative Results Chapter
- What exactly the results chapter is
- What you need to include in your chapter
- How to structure the chapter
- Tips and tricks for writing a top-notch chapter
- Free results chapter template
What exactly is the results chapter?
The results chapter (also referred to as the findings or analysis chapter) is one of the most important chapters of your dissertation or thesis because it shows the reader what you’ve found in terms of the quantitative data you’ve collected. It presents the data using a clear text narrative, supported by tables, graphs and charts. In doing so, it also highlights any potential issues (such as outliers or unusual findings) you’ve come across.
But how’s that different from the discussion chapter?
Well, in the results chapter, you only present your statistical findings. Only the numbers, so to speak – no more, no less. Contrasted to this, in the discussion chapter , you interpret your findings and link them to prior research (i.e. your literature review), as well as your research objectives and research questions . In other words, the results chapter presents and describes the data, while the discussion chapter interprets the data.
Let’s look at an example.
In your results chapter, you may have a plot that shows how respondents to a survey responded: the numbers of respondents per category, for instance. You may also state whether this supports a hypothesis by using a p-value from a statistical test. But it is only in the discussion chapter where you will say why this is relevant or how it compares with the literature or the broader picture. So, in your results chapter, make sure that you don’t present anything other than the hard facts – this is not the place for subjectivity.
It’s worth mentioning that some universities prefer you to combine the results and discussion chapters. Even so, it is good practice to separate the results and discussion elements within the chapter, as this ensures your findings are fully described. Typically, though, the results and discussion chapters are split up in quantitative studies. If you’re unsure, chat with your research supervisor or chair to find out what their preference is.
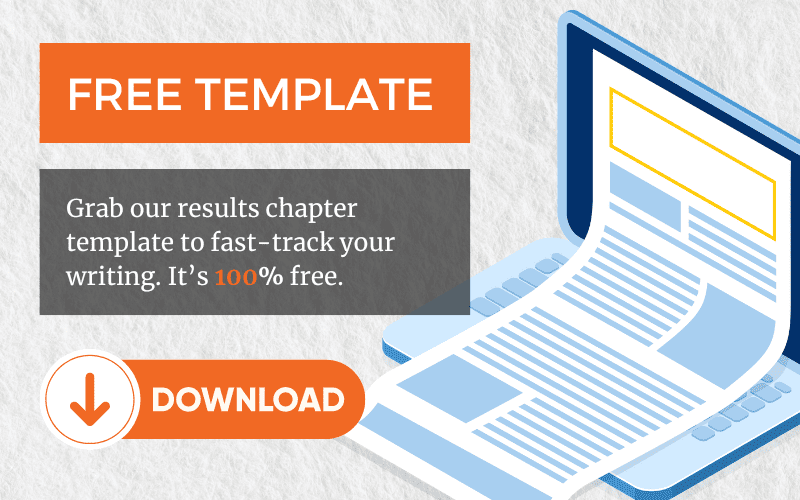
What should you include in the results chapter?
Following your analysis, it’s likely you’ll have far more data than are necessary to include in your chapter. In all likelihood, you’ll have a mountain of SPSS or R output data, and it’s your job to decide what’s most relevant. You’ll need to cut through the noise and focus on the data that matters.
This doesn’t mean that those analyses were a waste of time – on the contrary, those analyses ensure that you have a good understanding of your dataset and how to interpret it. However, that doesn’t mean your reader or examiner needs to see the 165 histograms you created! Relevance is key.
How do I decide what’s relevant?
At this point, it can be difficult to strike a balance between what is and isn’t important. But the most important thing is to ensure your results reflect and align with the purpose of your study . So, you need to revisit your research aims, objectives and research questions and use these as a litmus test for relevance. Make sure that you refer back to these constantly when writing up your chapter so that you stay on track.
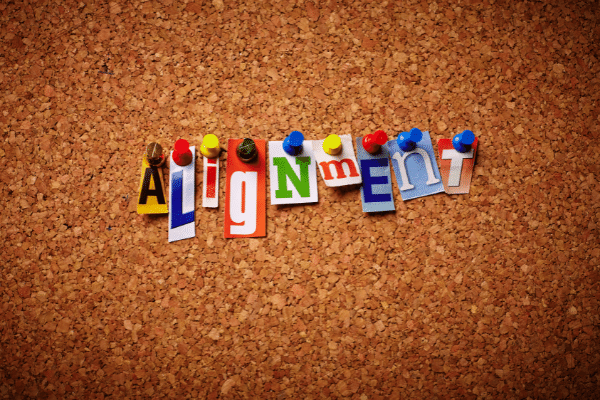
As a general guide, your results chapter will typically include the following:
- Some demographic data about your sample
- Reliability tests (if you used measurement scales)
- Descriptive statistics
- Inferential statistics (if your research objectives and questions require these)
- Hypothesis tests (again, if your research objectives and questions require these)
We’ll discuss each of these points in more detail in the next section.
Importantly, your results chapter needs to lay the foundation for your discussion chapter . This means that, in your results chapter, you need to include all the data that you will use as the basis for your interpretation in the discussion chapter.
For example, if you plan to highlight the strong relationship between Variable X and Variable Y in your discussion chapter, you need to present the respective analysis in your results chapter – perhaps a correlation or regression analysis.
Need a helping hand?
How do I write the results chapter?
There are multiple steps involved in writing up the results chapter for your quantitative research. The exact number of steps applicable to you will vary from study to study and will depend on the nature of the research aims, objectives and research questions . However, we’ll outline the generic steps below.
Step 1 – Revisit your research questions
The first step in writing your results chapter is to revisit your research objectives and research questions . These will be (or at least, should be!) the driving force behind your results and discussion chapters, so you need to review them and then ask yourself which statistical analyses and tests (from your mountain of data) would specifically help you address these . For each research objective and research question, list the specific piece (or pieces) of analysis that address it.
At this stage, it’s also useful to think about the key points that you want to raise in your discussion chapter and note these down so that you have a clear reminder of which data points and analyses you want to highlight in the results chapter. Again, list your points and then list the specific piece of analysis that addresses each point.
Next, you should draw up a rough outline of how you plan to structure your chapter . Which analyses and statistical tests will you present and in what order? We’ll discuss the “standard structure” in more detail later, but it’s worth mentioning now that it’s always useful to draw up a rough outline before you start writing (this advice applies to any chapter).
Step 2 – Craft an overview introduction
As with all chapters in your dissertation or thesis, you should start your quantitative results chapter by providing a brief overview of what you’ll do in the chapter and why . For example, you’d explain that you will start by presenting demographic data to understand the representativeness of the sample, before moving onto X, Y and Z.
This section shouldn’t be lengthy – a paragraph or two maximum. Also, it’s a good idea to weave the research questions into this section so that there’s a golden thread that runs through the document.
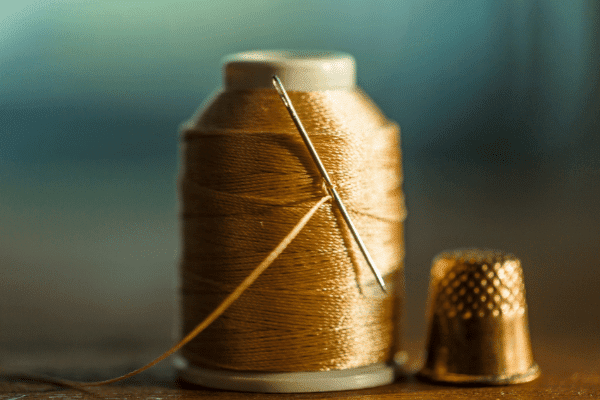
Step 3 – Present the sample demographic data
The first set of data that you’ll present is an overview of the sample demographics – in other words, the demographics of your respondents.
For example:
- What age range are they?
- How is gender distributed?
- How is ethnicity distributed?
- What areas do the participants live in?
The purpose of this is to assess how representative the sample is of the broader population. This is important for the sake of the generalisability of the results. If your sample is not representative of the population, you will not be able to generalise your findings. This is not necessarily the end of the world, but it is a limitation you’ll need to acknowledge.
Of course, to make this representativeness assessment, you’ll need to have a clear view of the demographics of the population. So, make sure that you design your survey to capture the correct demographic information that you will compare your sample to.
But what if I’m not interested in generalisability?
Well, even if your purpose is not necessarily to extrapolate your findings to the broader population, understanding your sample will allow you to interpret your findings appropriately, considering who responded. In other words, it will help you contextualise your findings . For example, if 80% of your sample was aged over 65, this may be a significant contextual factor to consider when interpreting the data. Therefore, it’s important to understand and present the demographic data.
Step 4 – Review composite measures and the data “shape”.
Before you undertake any statistical analysis, you’ll need to do some checks to ensure that your data are suitable for the analysis methods and techniques you plan to use. If you try to analyse data that doesn’t meet the assumptions of a specific statistical technique, your results will be largely meaningless. Therefore, you may need to show that the methods and techniques you’ll use are “allowed”.
Most commonly, there are two areas you need to pay attention to:
#1: Composite measures
The first is when you have multiple scale-based measures that combine to capture one construct – this is called a composite measure . For example, you may have four Likert scale-based measures that (should) all measure the same thing, but in different ways. In other words, in a survey, these four scales should all receive similar ratings. This is called “ internal consistency ”.
Internal consistency is not guaranteed though (especially if you developed the measures yourself), so you need to assess the reliability of each composite measure using a test. Typically, Cronbach’s Alpha is a common test used to assess internal consistency – i.e., to show that the items you’re combining are more or less saying the same thing. A high alpha score means that your measure is internally consistent. A low alpha score means you may need to consider scrapping one or more of the measures.
#2: Data shape
The second matter that you should address early on in your results chapter is data shape. In other words, you need to assess whether the data in your set are symmetrical (i.e. normally distributed) or not, as this will directly impact what type of analyses you can use. For many common inferential tests such as T-tests or ANOVAs (we’ll discuss these a bit later), your data needs to be normally distributed. If it’s not, you’ll need to adjust your strategy and use alternative tests.
To assess the shape of the data, you’ll usually assess a variety of descriptive statistics (such as the mean, median and skewness), which is what we’ll look at next.
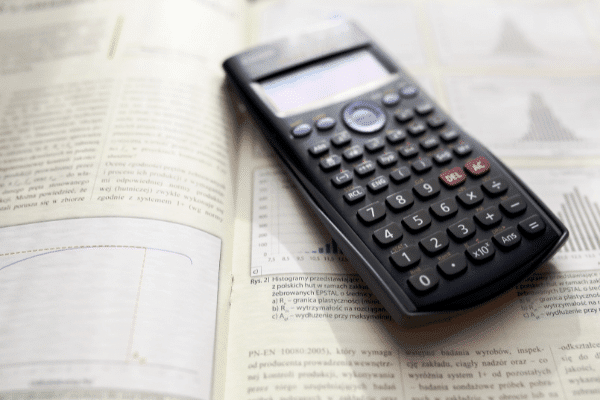
Step 5 – Present the descriptive statistics
Now that you’ve laid the foundation by discussing the representativeness of your sample, as well as the reliability of your measures and the shape of your data, you can get started with the actual statistical analysis. The first step is to present the descriptive statistics for your variables.
For scaled data, this usually includes statistics such as:
- The mean – this is simply the mathematical average of a range of numbers.
- The median – this is the midpoint in a range of numbers when the numbers are arranged in order.
- The mode – this is the most commonly repeated number in the data set.
- Standard deviation – this metric indicates how dispersed a range of numbers is. In other words, how close all the numbers are to the mean (the average).
- Skewness – this indicates how symmetrical a range of numbers is. In other words, do they tend to cluster into a smooth bell curve shape in the middle of the graph (this is called a normal or parametric distribution), or do they lean to the left or right (this is called a non-normal or non-parametric distribution).
- Kurtosis – this metric indicates whether the data are heavily or lightly-tailed, relative to the normal distribution. In other words, how peaked or flat the distribution is.
A large table that indicates all the above for multiple variables can be a very effective way to present your data economically. You can also use colour coding to help make the data more easily digestible.
For categorical data, where you show the percentage of people who chose or fit into a category, for instance, you can either just plain describe the percentages or numbers of people who responded to something or use graphs and charts (such as bar graphs and pie charts) to present your data in this section of the chapter.
When using figures, make sure that you label them simply and clearly , so that your reader can easily understand them. There’s nothing more frustrating than a graph that’s missing axis labels! Keep in mind that although you’ll be presenting charts and graphs, your text content needs to present a clear narrative that can stand on its own. In other words, don’t rely purely on your figures and tables to convey your key points: highlight the crucial trends and values in the text. Figures and tables should complement the writing, not carry it .
Depending on your research aims, objectives and research questions, you may stop your analysis at this point (i.e. descriptive statistics). However, if your study requires inferential statistics, then it’s time to deep dive into those .
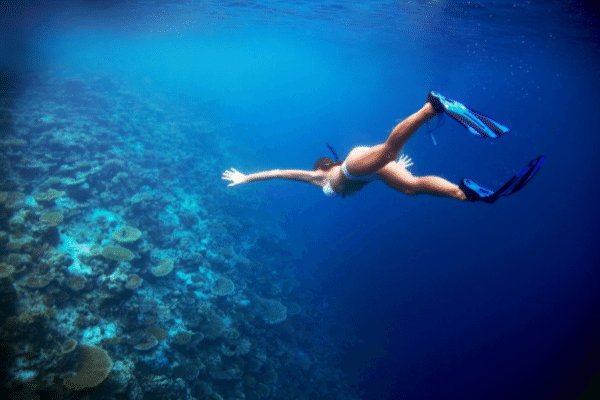
Step 6 – Present the inferential statistics
Inferential statistics are used to make generalisations about a population , whereas descriptive statistics focus purely on the sample . Inferential statistical techniques, broadly speaking, can be broken down into two groups .
First, there are those that compare measurements between groups , such as t-tests (which measure differences between two groups) and ANOVAs (which measure differences between multiple groups). Second, there are techniques that assess the relationships between variables , such as correlation analysis and regression analysis. Within each of these, some tests can be used for normally distributed (parametric) data and some tests are designed specifically for use on non-parametric data.
There are a seemingly endless number of tests that you can use to crunch your data, so it’s easy to run down a rabbit hole and end up with piles of test data. Ultimately, the most important thing is to make sure that you adopt the tests and techniques that allow you to achieve your research objectives and answer your research questions .
In this section of the results chapter, you should try to make use of figures and visual components as effectively as possible. For example, if you present a correlation table, use colour coding to highlight the significance of the correlation values, or scatterplots to visually demonstrate what the trend is. The easier you make it for your reader to digest your findings, the more effectively you’ll be able to make your arguments in the next chapter.
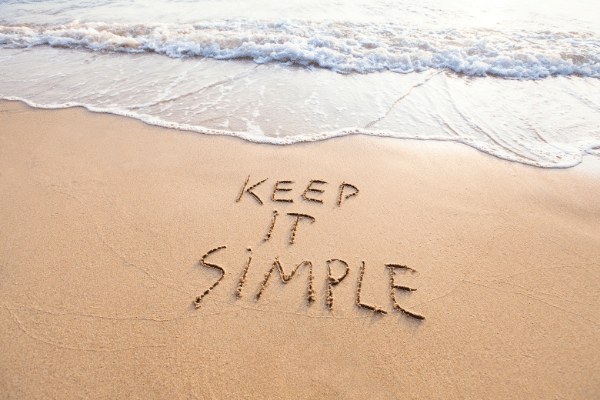
Step 7 – Test your hypotheses
If your study requires it, the next stage is hypothesis testing. A hypothesis is a statement , often indicating a difference between groups or relationship between variables, that can be supported or rejected by a statistical test. However, not all studies will involve hypotheses (again, it depends on the research objectives), so don’t feel like you “must” present and test hypotheses just because you’re undertaking quantitative research.
The basic process for hypothesis testing is as follows:
- Specify your null hypothesis (for example, “The chemical psilocybin has no effect on time perception).
- Specify your alternative hypothesis (e.g., “The chemical psilocybin has an effect on time perception)
- Set your significance level (this is usually 0.05)
- Calculate your statistics and find your p-value (e.g., p=0.01)
- Draw your conclusions (e.g., “The chemical psilocybin does have an effect on time perception”)
Finally, if the aim of your study is to develop and test a conceptual framework , this is the time to present it, following the testing of your hypotheses. While you don’t need to develop or discuss these findings further in the results chapter, indicating whether the tests (and their p-values) support or reject the hypotheses is crucial.
Step 8 – Provide a chapter summary
To wrap up your results chapter and transition to the discussion chapter, you should provide a brief summary of the key findings . “Brief” is the keyword here – much like the chapter introduction, this shouldn’t be lengthy – a paragraph or two maximum. Highlight the findings most relevant to your research objectives and research questions, and wrap it up.
Some final thoughts, tips and tricks
Now that you’ve got the essentials down, here are a few tips and tricks to make your quantitative results chapter shine:
- When writing your results chapter, report your findings in the past tense . You’re talking about what you’ve found in your data, not what you are currently looking for or trying to find.
- Structure your results chapter systematically and sequentially . If you had two experiments where findings from the one generated inputs into the other, report on them in order.
- Make your own tables and graphs rather than copying and pasting them from statistical analysis programmes like SPSS. Check out the DataIsBeautiful reddit for some inspiration.
- Once you’re done writing, review your work to make sure that you have provided enough information to answer your research questions , but also that you didn’t include superfluous information.
If you’ve got any questions about writing up the quantitative results chapter, please leave a comment below. If you’d like 1-on-1 assistance with your quantitative analysis and discussion, check out our hands-on coaching service , or book a free consultation with a friendly coach.
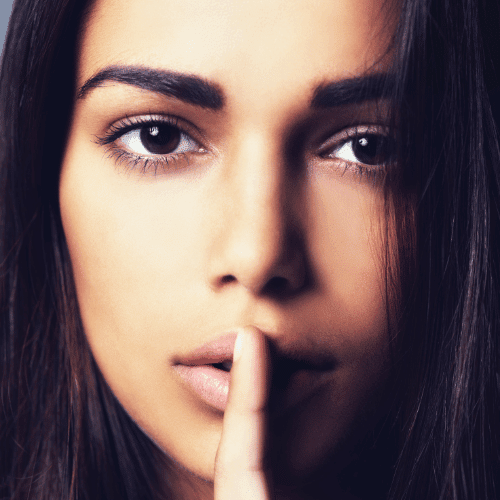
Psst... there’s more!
This post was based on one of our popular Research Bootcamps . If you're working on a research project, you'll definitely want to check this out ...
You Might Also Like:
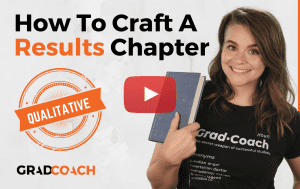
Thank you. I will try my best to write my results.
Awesome content 👏🏾
this was great explaination
Submit a Comment Cancel reply
Your email address will not be published. Required fields are marked *
Save my name, email, and website in this browser for the next time I comment.
- Print Friendly
Have a language expert improve your writing
Run a free plagiarism check in 10 minutes, automatically generate references for free.
- Knowledge Base
- Methodology
Research Design | Step-by-Step Guide with Examples
Published on 5 May 2022 by Shona McCombes . Revised on 20 March 2023.
A research design is a strategy for answering your research question using empirical data. Creating a research design means making decisions about:
- Your overall aims and approach
- The type of research design you’ll use
- Your sampling methods or criteria for selecting subjects
- Your data collection methods
- The procedures you’ll follow to collect data
- Your data analysis methods
A well-planned research design helps ensure that your methods match your research aims and that you use the right kind of analysis for your data.
Table of contents
Step 1: consider your aims and approach, step 2: choose a type of research design, step 3: identify your population and sampling method, step 4: choose your data collection methods, step 5: plan your data collection procedures, step 6: decide on your data analysis strategies, frequently asked questions.
- Introduction
Before you can start designing your research, you should already have a clear idea of the research question you want to investigate.
There are many different ways you could go about answering this question. Your research design choices should be driven by your aims and priorities – start by thinking carefully about what you want to achieve.
The first choice you need to make is whether you’ll take a qualitative or quantitative approach.
Qualitative research designs tend to be more flexible and inductive , allowing you to adjust your approach based on what you find throughout the research process.
Quantitative research designs tend to be more fixed and deductive , with variables and hypotheses clearly defined in advance of data collection.
It’s also possible to use a mixed methods design that integrates aspects of both approaches. By combining qualitative and quantitative insights, you can gain a more complete picture of the problem you’re studying and strengthen the credibility of your conclusions.
Practical and ethical considerations when designing research
As well as scientific considerations, you need to think practically when designing your research. If your research involves people or animals, you also need to consider research ethics .
- How much time do you have to collect data and write up the research?
- Will you be able to gain access to the data you need (e.g., by travelling to a specific location or contacting specific people)?
- Do you have the necessary research skills (e.g., statistical analysis or interview techniques)?
- Will you need ethical approval ?
At each stage of the research design process, make sure that your choices are practically feasible.
Prevent plagiarism, run a free check.
Within both qualitative and quantitative approaches, there are several types of research design to choose from. Each type provides a framework for the overall shape of your research.
Types of quantitative research designs
Quantitative designs can be split into four main types. Experimental and quasi-experimental designs allow you to test cause-and-effect relationships, while descriptive and correlational designs allow you to measure variables and describe relationships between them.
With descriptive and correlational designs, you can get a clear picture of characteristics, trends, and relationships as they exist in the real world. However, you can’t draw conclusions about cause and effect (because correlation doesn’t imply causation ).
Experiments are the strongest way to test cause-and-effect relationships without the risk of other variables influencing the results. However, their controlled conditions may not always reflect how things work in the real world. They’re often also more difficult and expensive to implement.
Types of qualitative research designs
Qualitative designs are less strictly defined. This approach is about gaining a rich, detailed understanding of a specific context or phenomenon, and you can often be more creative and flexible in designing your research.
The table below shows some common types of qualitative design. They often have similar approaches in terms of data collection, but focus on different aspects when analysing the data.
Your research design should clearly define who or what your research will focus on, and how you’ll go about choosing your participants or subjects.
In research, a population is the entire group that you want to draw conclusions about, while a sample is the smaller group of individuals you’ll actually collect data from.
Defining the population
A population can be made up of anything you want to study – plants, animals, organisations, texts, countries, etc. In the social sciences, it most often refers to a group of people.
For example, will you focus on people from a specific demographic, region, or background? Are you interested in people with a certain job or medical condition, or users of a particular product?
The more precisely you define your population, the easier it will be to gather a representative sample.
Sampling methods
Even with a narrowly defined population, it’s rarely possible to collect data from every individual. Instead, you’ll collect data from a sample.
To select a sample, there are two main approaches: probability sampling and non-probability sampling . The sampling method you use affects how confidently you can generalise your results to the population as a whole.
Probability sampling is the most statistically valid option, but it’s often difficult to achieve unless you’re dealing with a very small and accessible population.
For practical reasons, many studies use non-probability sampling, but it’s important to be aware of the limitations and carefully consider potential biases. You should always make an effort to gather a sample that’s as representative as possible of the population.
Case selection in qualitative research
In some types of qualitative designs, sampling may not be relevant.
For example, in an ethnography or a case study, your aim is to deeply understand a specific context, not to generalise to a population. Instead of sampling, you may simply aim to collect as much data as possible about the context you are studying.
In these types of design, you still have to carefully consider your choice of case or community. You should have a clear rationale for why this particular case is suitable for answering your research question.
For example, you might choose a case study that reveals an unusual or neglected aspect of your research problem, or you might choose several very similar or very different cases in order to compare them.
Data collection methods are ways of directly measuring variables and gathering information. They allow you to gain first-hand knowledge and original insights into your research problem.
You can choose just one data collection method, or use several methods in the same study.
Survey methods
Surveys allow you to collect data about opinions, behaviours, experiences, and characteristics by asking people directly. There are two main survey methods to choose from: questionnaires and interviews.
Observation methods
Observations allow you to collect data unobtrusively, observing characteristics, behaviours, or social interactions without relying on self-reporting.
Observations may be conducted in real time, taking notes as you observe, or you might make audiovisual recordings for later analysis. They can be qualitative or quantitative.
Other methods of data collection
There are many other ways you might collect data depending on your field and topic.
If you’re not sure which methods will work best for your research design, try reading some papers in your field to see what data collection methods they used.
Secondary data
If you don’t have the time or resources to collect data from the population you’re interested in, you can also choose to use secondary data that other researchers already collected – for example, datasets from government surveys or previous studies on your topic.
With this raw data, you can do your own analysis to answer new research questions that weren’t addressed by the original study.
Using secondary data can expand the scope of your research, as you may be able to access much larger and more varied samples than you could collect yourself.
However, it also means you don’t have any control over which variables to measure or how to measure them, so the conclusions you can draw may be limited.
As well as deciding on your methods, you need to plan exactly how you’ll use these methods to collect data that’s consistent, accurate, and unbiased.
Planning systematic procedures is especially important in quantitative research, where you need to precisely define your variables and ensure your measurements are reliable and valid.
Operationalisation
Some variables, like height or age, are easily measured. But often you’ll be dealing with more abstract concepts, like satisfaction, anxiety, or competence. Operationalisation means turning these fuzzy ideas into measurable indicators.
If you’re using observations , which events or actions will you count?
If you’re using surveys , which questions will you ask and what range of responses will be offered?
You may also choose to use or adapt existing materials designed to measure the concept you’re interested in – for example, questionnaires or inventories whose reliability and validity has already been established.
Reliability and validity
Reliability means your results can be consistently reproduced , while validity means that you’re actually measuring the concept you’re interested in.
For valid and reliable results, your measurement materials should be thoroughly researched and carefully designed. Plan your procedures to make sure you carry out the same steps in the same way for each participant.
If you’re developing a new questionnaire or other instrument to measure a specific concept, running a pilot study allows you to check its validity and reliability in advance.
Sampling procedures
As well as choosing an appropriate sampling method, you need a concrete plan for how you’ll actually contact and recruit your selected sample.
That means making decisions about things like:
- How many participants do you need for an adequate sample size?
- What inclusion and exclusion criteria will you use to identify eligible participants?
- How will you contact your sample – by mail, online, by phone, or in person?
If you’re using a probability sampling method, it’s important that everyone who is randomly selected actually participates in the study. How will you ensure a high response rate?
If you’re using a non-probability method, how will you avoid bias and ensure a representative sample?
Data management
It’s also important to create a data management plan for organising and storing your data.
Will you need to transcribe interviews or perform data entry for observations? You should anonymise and safeguard any sensitive data, and make sure it’s backed up regularly.
Keeping your data well organised will save time when it comes to analysing them. It can also help other researchers validate and add to your findings.
On their own, raw data can’t answer your research question. The last step of designing your research is planning how you’ll analyse the data.
Quantitative data analysis
In quantitative research, you’ll most likely use some form of statistical analysis . With statistics, you can summarise your sample data, make estimates, and test hypotheses.
Using descriptive statistics , you can summarise your sample data in terms of:
- The distribution of the data (e.g., the frequency of each score on a test)
- The central tendency of the data (e.g., the mean to describe the average score)
- The variability of the data (e.g., the standard deviation to describe how spread out the scores are)
The specific calculations you can do depend on the level of measurement of your variables.
Using inferential statistics , you can:
- Make estimates about the population based on your sample data.
- Test hypotheses about a relationship between variables.
Regression and correlation tests look for associations between two or more variables, while comparison tests (such as t tests and ANOVAs ) look for differences in the outcomes of different groups.
Your choice of statistical test depends on various aspects of your research design, including the types of variables you’re dealing with and the distribution of your data.
Qualitative data analysis
In qualitative research, your data will usually be very dense with information and ideas. Instead of summing it up in numbers, you’ll need to comb through the data in detail, interpret its meanings, identify patterns, and extract the parts that are most relevant to your research question.
Two of the most common approaches to doing this are thematic analysis and discourse analysis .
There are many other ways of analysing qualitative data depending on the aims of your research. To get a sense of potential approaches, try reading some qualitative research papers in your field.
A sample is a subset of individuals from a larger population. Sampling means selecting the group that you will actually collect data from in your research.
For example, if you are researching the opinions of students in your university, you could survey a sample of 100 students.
Statistical sampling allows you to test a hypothesis about the characteristics of a population. There are various sampling methods you can use to ensure that your sample is representative of the population as a whole.
Operationalisation means turning abstract conceptual ideas into measurable observations.
For example, the concept of social anxiety isn’t directly observable, but it can be operationally defined in terms of self-rating scores, behavioural avoidance of crowded places, or physical anxiety symptoms in social situations.
Before collecting data , it’s important to consider how you will operationalise the variables that you want to measure.
The research methods you use depend on the type of data you need to answer your research question .
- If you want to measure something or test a hypothesis , use quantitative methods . If you want to explore ideas, thoughts, and meanings, use qualitative methods .
- If you want to analyse a large amount of readily available data, use secondary data. If you want data specific to your purposes with control over how they are generated, collect primary data.
- If you want to establish cause-and-effect relationships between variables , use experimental methods. If you want to understand the characteristics of a research subject, use descriptive methods.
Cite this Scribbr article
If you want to cite this source, you can copy and paste the citation or click the ‘Cite this Scribbr article’ button to automatically add the citation to our free Reference Generator.
McCombes, S. (2023, March 20). Research Design | Step-by-Step Guide with Examples. Scribbr. Retrieved 14 May 2024, from https://www.scribbr.co.uk/research-methods/research-design/
Is this article helpful?
Shona McCombes
- Skip to main content
- Skip to primary sidebar
- Skip to footer
- QuestionPro

- Solutions Industries Gaming Automotive Sports and events Education Government Travel & Hospitality Financial Services Healthcare Cannabis Technology Use Case NPS+ Communities Audience Contactless surveys Mobile LivePolls Member Experience GDPR Positive People Science 360 Feedback Surveys
- Resources Blog eBooks Survey Templates Case Studies Training Help center

Home Market Research
Data Analysis in Research: Types & Methods
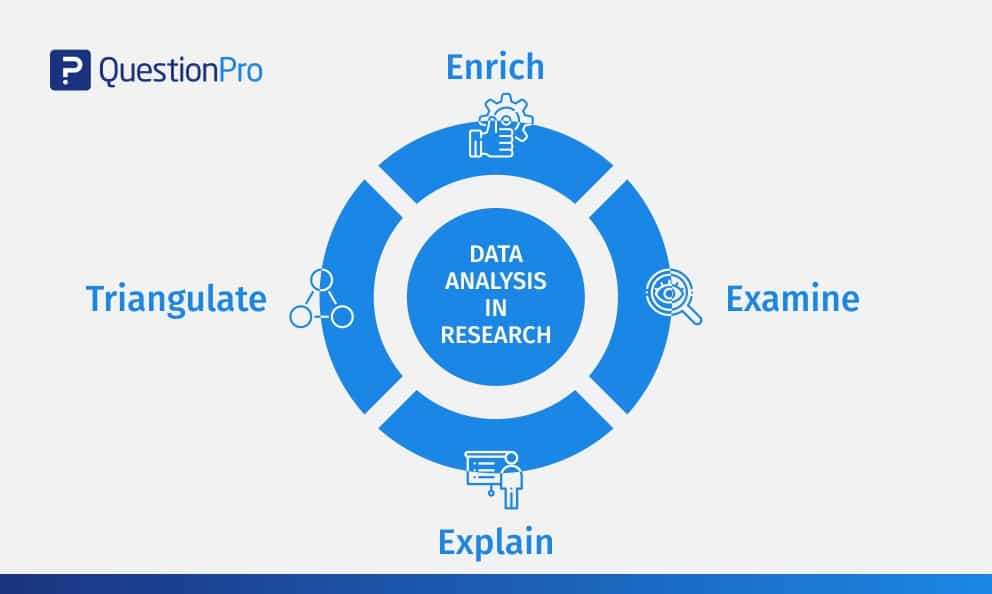
Content Index
Why analyze data in research?
Types of data in research, finding patterns in the qualitative data, methods used for data analysis in qualitative research, preparing data for analysis, methods used for data analysis in quantitative research, considerations in research data analysis, what is data analysis in research.
Definition of research in data analysis: According to LeCompte and Schensul, research data analysis is a process used by researchers to reduce data to a story and interpret it to derive insights. The data analysis process helps reduce a large chunk of data into smaller fragments, which makes sense.
Three essential things occur during the data analysis process — the first is data organization . Summarization and categorization together contribute to becoming the second known method used for data reduction. It helps find patterns and themes in the data for easy identification and linking. The third and last way is data analysis – researchers do it in both top-down and bottom-up fashion.
LEARN ABOUT: Research Process Steps
On the other hand, Marshall and Rossman describe data analysis as a messy, ambiguous, and time-consuming but creative and fascinating process through which a mass of collected data is brought to order, structure and meaning.
We can say that “the data analysis and data interpretation is a process representing the application of deductive and inductive logic to the research and data analysis.”
Researchers rely heavily on data as they have a story to tell or research problems to solve. It starts with a question, and data is nothing but an answer to that question. But, what if there is no question to ask? Well! It is possible to explore data even without a problem – we call it ‘Data Mining’, which often reveals some interesting patterns within the data that are worth exploring.
Irrelevant to the type of data researchers explore, their mission and audiences’ vision guide them to find the patterns to shape the story they want to tell. One of the essential things expected from researchers while analyzing data is to stay open and remain unbiased toward unexpected patterns, expressions, and results. Remember, sometimes, data analysis tells the most unforeseen yet exciting stories that were not expected when initiating data analysis. Therefore, rely on the data you have at hand and enjoy the journey of exploratory research.
Create a Free Account
Every kind of data has a rare quality of describing things after assigning a specific value to it. For analysis, you need to organize these values, processed and presented in a given context, to make it useful. Data can be in different forms; here are the primary data types.
- Qualitative data: When the data presented has words and descriptions, then we call it qualitative data . Although you can observe this data, it is subjective and harder to analyze data in research, especially for comparison. Example: Quality data represents everything describing taste, experience, texture, or an opinion that is considered quality data. This type of data is usually collected through focus groups, personal qualitative interviews , qualitative observation or using open-ended questions in surveys.
- Quantitative data: Any data expressed in numbers of numerical figures are called quantitative data . This type of data can be distinguished into categories, grouped, measured, calculated, or ranked. Example: questions such as age, rank, cost, length, weight, scores, etc. everything comes under this type of data. You can present such data in graphical format, charts, or apply statistical analysis methods to this data. The (Outcomes Measurement Systems) OMS questionnaires in surveys are a significant source of collecting numeric data.
- Categorical data: It is data presented in groups. However, an item included in the categorical data cannot belong to more than one group. Example: A person responding to a survey by telling his living style, marital status, smoking habit, or drinking habit comes under the categorical data. A chi-square test is a standard method used to analyze this data.
Learn More : Examples of Qualitative Data in Education
Data analysis in qualitative research
Data analysis and qualitative data research work a little differently from the numerical data as the quality data is made up of words, descriptions, images, objects, and sometimes symbols. Getting insight from such complicated information is a complicated process. Hence it is typically used for exploratory research and data analysis .
Although there are several ways to find patterns in the textual information, a word-based method is the most relied and widely used global technique for research and data analysis. Notably, the data analysis process in qualitative research is manual. Here the researchers usually read the available data and find repetitive or commonly used words.
For example, while studying data collected from African countries to understand the most pressing issues people face, researchers might find “food” and “hunger” are the most commonly used words and will highlight them for further analysis.
LEARN ABOUT: Level of Analysis
The keyword context is another widely used word-based technique. In this method, the researcher tries to understand the concept by analyzing the context in which the participants use a particular keyword.
For example , researchers conducting research and data analysis for studying the concept of ‘diabetes’ amongst respondents might analyze the context of when and how the respondent has used or referred to the word ‘diabetes.’
The scrutiny-based technique is also one of the highly recommended text analysis methods used to identify a quality data pattern. Compare and contrast is the widely used method under this technique to differentiate how a specific text is similar or different from each other.
For example: To find out the “importance of resident doctor in a company,” the collected data is divided into people who think it is necessary to hire a resident doctor and those who think it is unnecessary. Compare and contrast is the best method that can be used to analyze the polls having single-answer questions types .
Metaphors can be used to reduce the data pile and find patterns in it so that it becomes easier to connect data with theory.
Variable Partitioning is another technique used to split variables so that researchers can find more coherent descriptions and explanations from the enormous data.
LEARN ABOUT: Qualitative Research Questions and Questionnaires
There are several techniques to analyze the data in qualitative research, but here are some commonly used methods,
- Content Analysis: It is widely accepted and the most frequently employed technique for data analysis in research methodology. It can be used to analyze the documented information from text, images, and sometimes from the physical items. It depends on the research questions to predict when and where to use this method.
- Narrative Analysis: This method is used to analyze content gathered from various sources such as personal interviews, field observation, and surveys . The majority of times, stories, or opinions shared by people are focused on finding answers to the research questions.
- Discourse Analysis: Similar to narrative analysis, discourse analysis is used to analyze the interactions with people. Nevertheless, this particular method considers the social context under which or within which the communication between the researcher and respondent takes place. In addition to that, discourse analysis also focuses on the lifestyle and day-to-day environment while deriving any conclusion.
- Grounded Theory: When you want to explain why a particular phenomenon happened, then using grounded theory for analyzing quality data is the best resort. Grounded theory is applied to study data about the host of similar cases occurring in different settings. When researchers are using this method, they might alter explanations or produce new ones until they arrive at some conclusion.
LEARN ABOUT: 12 Best Tools for Researchers
Data analysis in quantitative research
The first stage in research and data analysis is to make it for the analysis so that the nominal data can be converted into something meaningful. Data preparation consists of the below phases.
Phase I: Data Validation
Data validation is done to understand if the collected data sample is per the pre-set standards, or it is a biased data sample again divided into four different stages
- Fraud: To ensure an actual human being records each response to the survey or the questionnaire
- Screening: To make sure each participant or respondent is selected or chosen in compliance with the research criteria
- Procedure: To ensure ethical standards were maintained while collecting the data sample
- Completeness: To ensure that the respondent has answered all the questions in an online survey. Else, the interviewer had asked all the questions devised in the questionnaire.
Phase II: Data Editing
More often, an extensive research data sample comes loaded with errors. Respondents sometimes fill in some fields incorrectly or sometimes skip them accidentally. Data editing is a process wherein the researchers have to confirm that the provided data is free of such errors. They need to conduct necessary checks and outlier checks to edit the raw edit and make it ready for analysis.
Phase III: Data Coding
Out of all three, this is the most critical phase of data preparation associated with grouping and assigning values to the survey responses . If a survey is completed with a 1000 sample size, the researcher will create an age bracket to distinguish the respondents based on their age. Thus, it becomes easier to analyze small data buckets rather than deal with the massive data pile.
LEARN ABOUT: Steps in Qualitative Research
After the data is prepared for analysis, researchers are open to using different research and data analysis methods to derive meaningful insights. For sure, statistical analysis plans are the most favored to analyze numerical data. In statistical analysis, distinguishing between categorical data and numerical data is essential, as categorical data involves distinct categories or labels, while numerical data consists of measurable quantities. The method is again classified into two groups. First, ‘Descriptive Statistics’ used to describe data. Second, ‘Inferential statistics’ that helps in comparing the data .
Descriptive statistics
This method is used to describe the basic features of versatile types of data in research. It presents the data in such a meaningful way that pattern in the data starts making sense. Nevertheless, the descriptive analysis does not go beyond making conclusions. The conclusions are again based on the hypothesis researchers have formulated so far. Here are a few major types of descriptive analysis methods.
Measures of Frequency
- Count, Percent, Frequency
- It is used to denote home often a particular event occurs.
- Researchers use it when they want to showcase how often a response is given.
Measures of Central Tendency
- Mean, Median, Mode
- The method is widely used to demonstrate distribution by various points.
- Researchers use this method when they want to showcase the most commonly or averagely indicated response.
Measures of Dispersion or Variation
- Range, Variance, Standard deviation
- Here the field equals high/low points.
- Variance standard deviation = difference between the observed score and mean
- It is used to identify the spread of scores by stating intervals.
- Researchers use this method to showcase data spread out. It helps them identify the depth until which the data is spread out that it directly affects the mean.
Measures of Position
- Percentile ranks, Quartile ranks
- It relies on standardized scores helping researchers to identify the relationship between different scores.
- It is often used when researchers want to compare scores with the average count.
For quantitative research use of descriptive analysis often give absolute numbers, but the in-depth analysis is never sufficient to demonstrate the rationale behind those numbers. Nevertheless, it is necessary to think of the best method for research and data analysis suiting your survey questionnaire and what story researchers want to tell. For example, the mean is the best way to demonstrate the students’ average scores in schools. It is better to rely on the descriptive statistics when the researchers intend to keep the research or outcome limited to the provided sample without generalizing it. For example, when you want to compare average voting done in two different cities, differential statistics are enough.
Descriptive analysis is also called a ‘univariate analysis’ since it is commonly used to analyze a single variable.
Inferential statistics
Inferential statistics are used to make predictions about a larger population after research and data analysis of the representing population’s collected sample. For example, you can ask some odd 100 audiences at a movie theater if they like the movie they are watching. Researchers then use inferential statistics on the collected sample to reason that about 80-90% of people like the movie.
Here are two significant areas of inferential statistics.
- Estimating parameters: It takes statistics from the sample research data and demonstrates something about the population parameter.
- Hypothesis test: I t’s about sampling research data to answer the survey research questions. For example, researchers might be interested to understand if the new shade of lipstick recently launched is good or not, or if the multivitamin capsules help children to perform better at games.
These are sophisticated analysis methods used to showcase the relationship between different variables instead of describing a single variable. It is often used when researchers want something beyond absolute numbers to understand the relationship between variables.
Here are some of the commonly used methods for data analysis in research.
- Correlation: When researchers are not conducting experimental research or quasi-experimental research wherein the researchers are interested to understand the relationship between two or more variables, they opt for correlational research methods.
- Cross-tabulation: Also called contingency tables, cross-tabulation is used to analyze the relationship between multiple variables. Suppose provided data has age and gender categories presented in rows and columns. A two-dimensional cross-tabulation helps for seamless data analysis and research by showing the number of males and females in each age category.
- Regression analysis: For understanding the strong relationship between two variables, researchers do not look beyond the primary and commonly used regression analysis method, which is also a type of predictive analysis used. In this method, you have an essential factor called the dependent variable. You also have multiple independent variables in regression analysis. You undertake efforts to find out the impact of independent variables on the dependent variable. The values of both independent and dependent variables are assumed as being ascertained in an error-free random manner.
- Frequency tables: The statistical procedure is used for testing the degree to which two or more vary or differ in an experiment. A considerable degree of variation means research findings were significant. In many contexts, ANOVA testing and variance analysis are similar.
- Analysis of variance: The statistical procedure is used for testing the degree to which two or more vary or differ in an experiment. A considerable degree of variation means research findings were significant. In many contexts, ANOVA testing and variance analysis are similar.
- Researchers must have the necessary research skills to analyze and manipulation the data , Getting trained to demonstrate a high standard of research practice. Ideally, researchers must possess more than a basic understanding of the rationale of selecting one statistical method over the other to obtain better data insights.
- Usually, research and data analytics projects differ by scientific discipline; therefore, getting statistical advice at the beginning of analysis helps design a survey questionnaire, select data collection methods , and choose samples.
LEARN ABOUT: Best Data Collection Tools
- The primary aim of data research and analysis is to derive ultimate insights that are unbiased. Any mistake in or keeping a biased mind to collect data, selecting an analysis method, or choosing audience sample il to draw a biased inference.
- Irrelevant to the sophistication used in research data and analysis is enough to rectify the poorly defined objective outcome measurements. It does not matter if the design is at fault or intentions are not clear, but lack of clarity might mislead readers, so avoid the practice.
- The motive behind data analysis in research is to present accurate and reliable data. As far as possible, avoid statistical errors, and find a way to deal with everyday challenges like outliers, missing data, data altering, data mining , or developing graphical representation.
LEARN MORE: Descriptive Research vs Correlational Research The sheer amount of data generated daily is frightening. Especially when data analysis has taken center stage. in 2018. In last year, the total data supply amounted to 2.8 trillion gigabytes. Hence, it is clear that the enterprises willing to survive in the hypercompetitive world must possess an excellent capability to analyze complex research data, derive actionable insights, and adapt to the new market needs.
LEARN ABOUT: Average Order Value
QuestionPro is an online survey platform that empowers organizations in data analysis and research and provides them a medium to collect data by creating appealing surveys.
MORE LIKE THIS
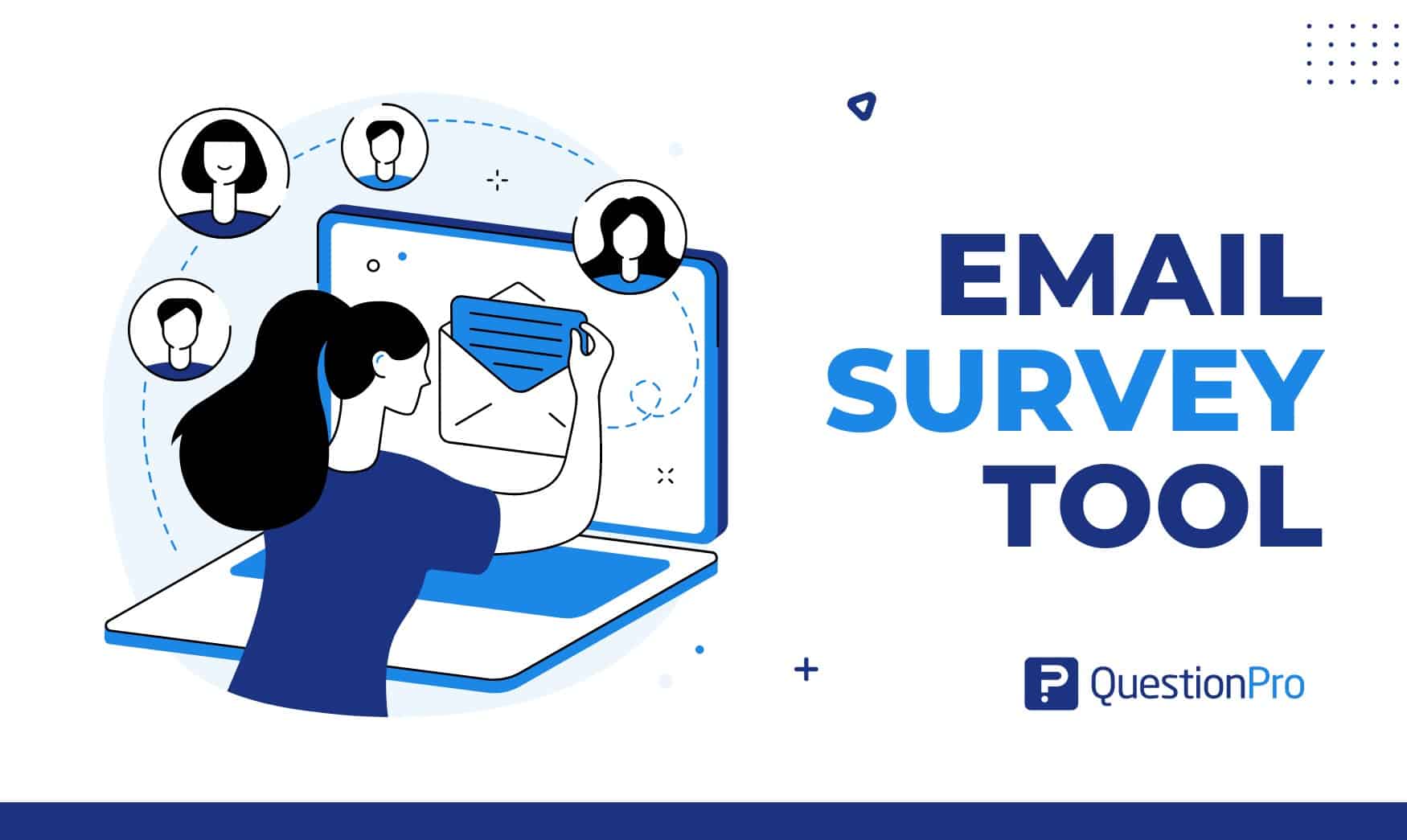
The Best Email Survey Tool to Boost Your Feedback Game
May 7, 2024
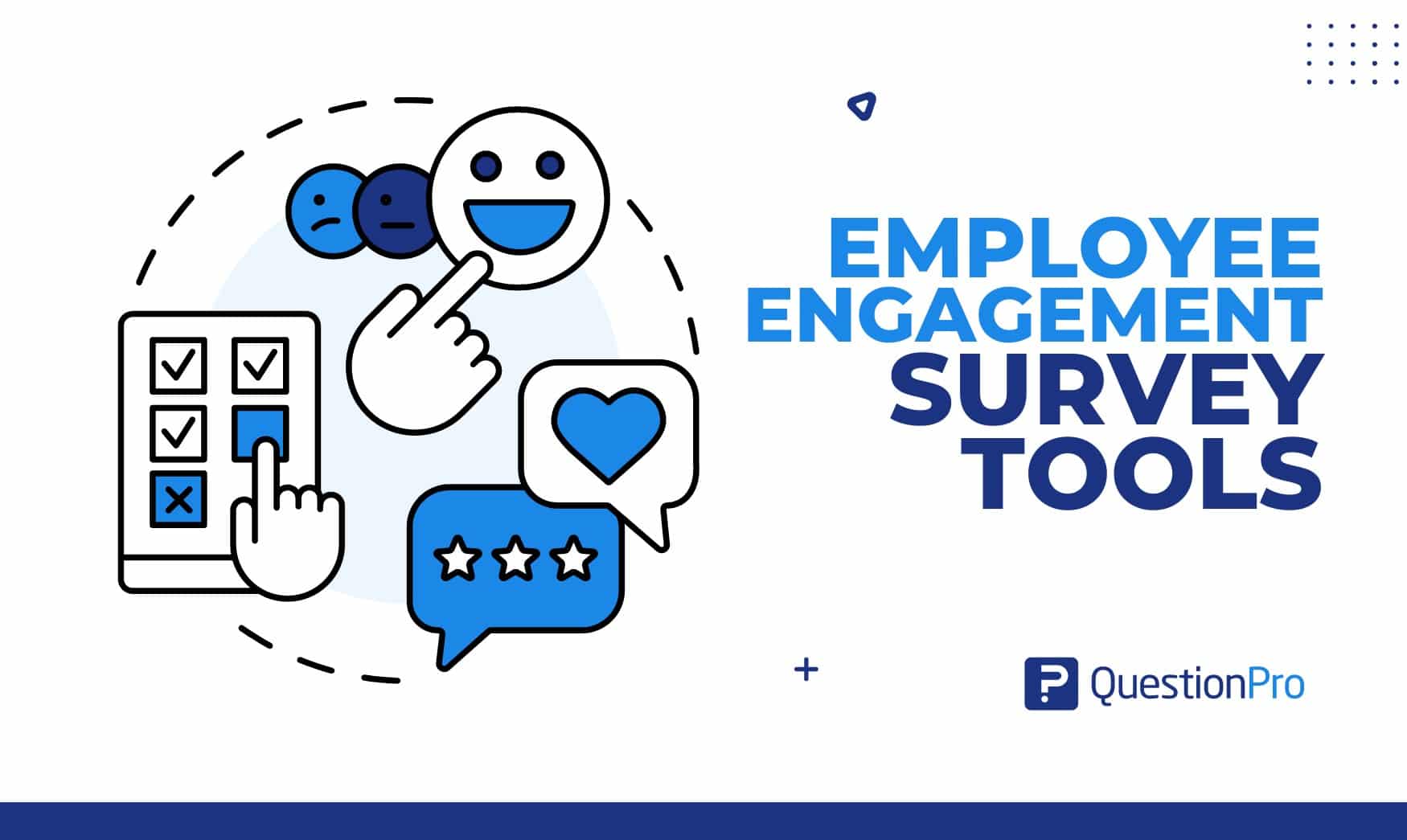
Top 10 Employee Engagement Survey Tools
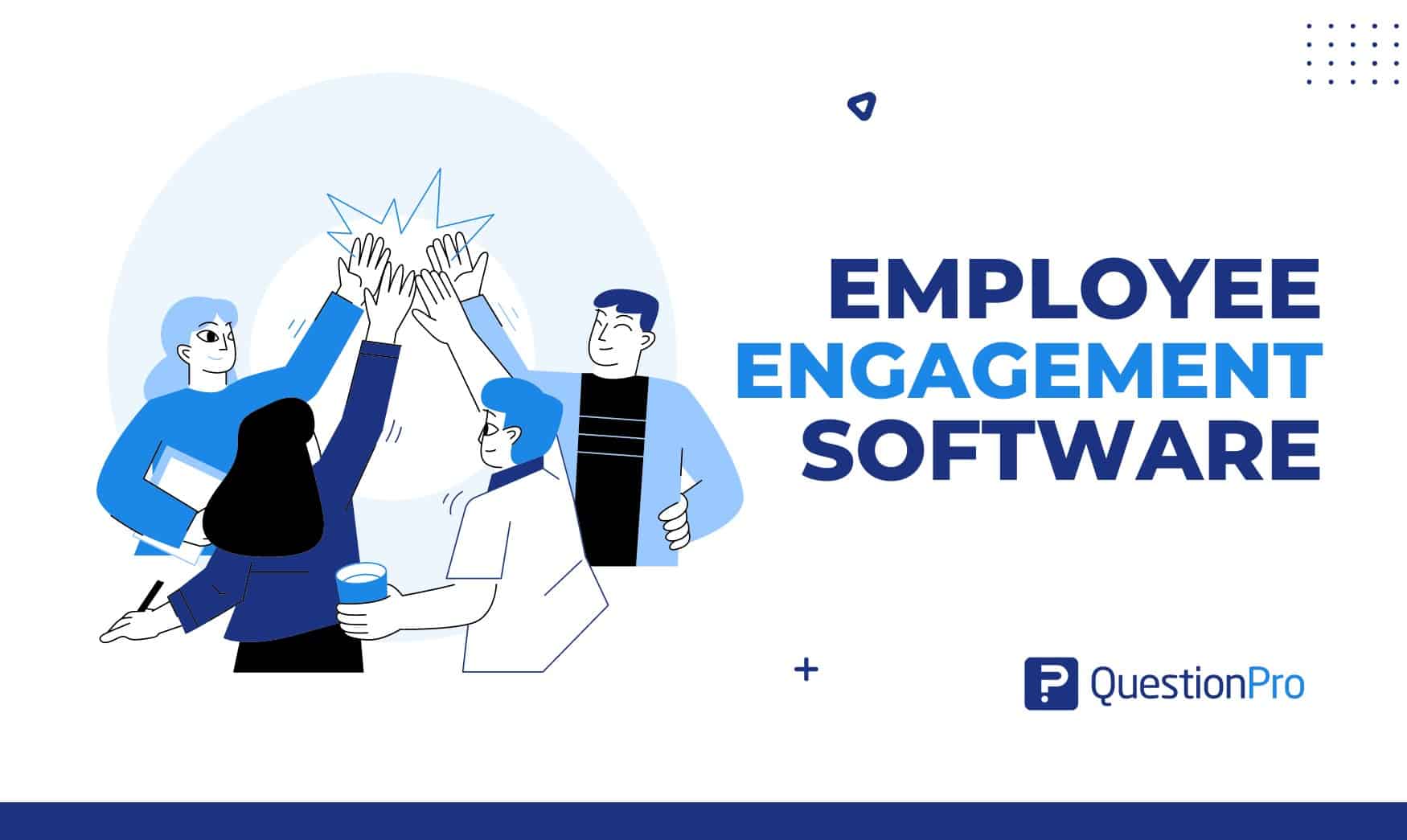
Top 20 Employee Engagement Software Solutions
May 3, 2024
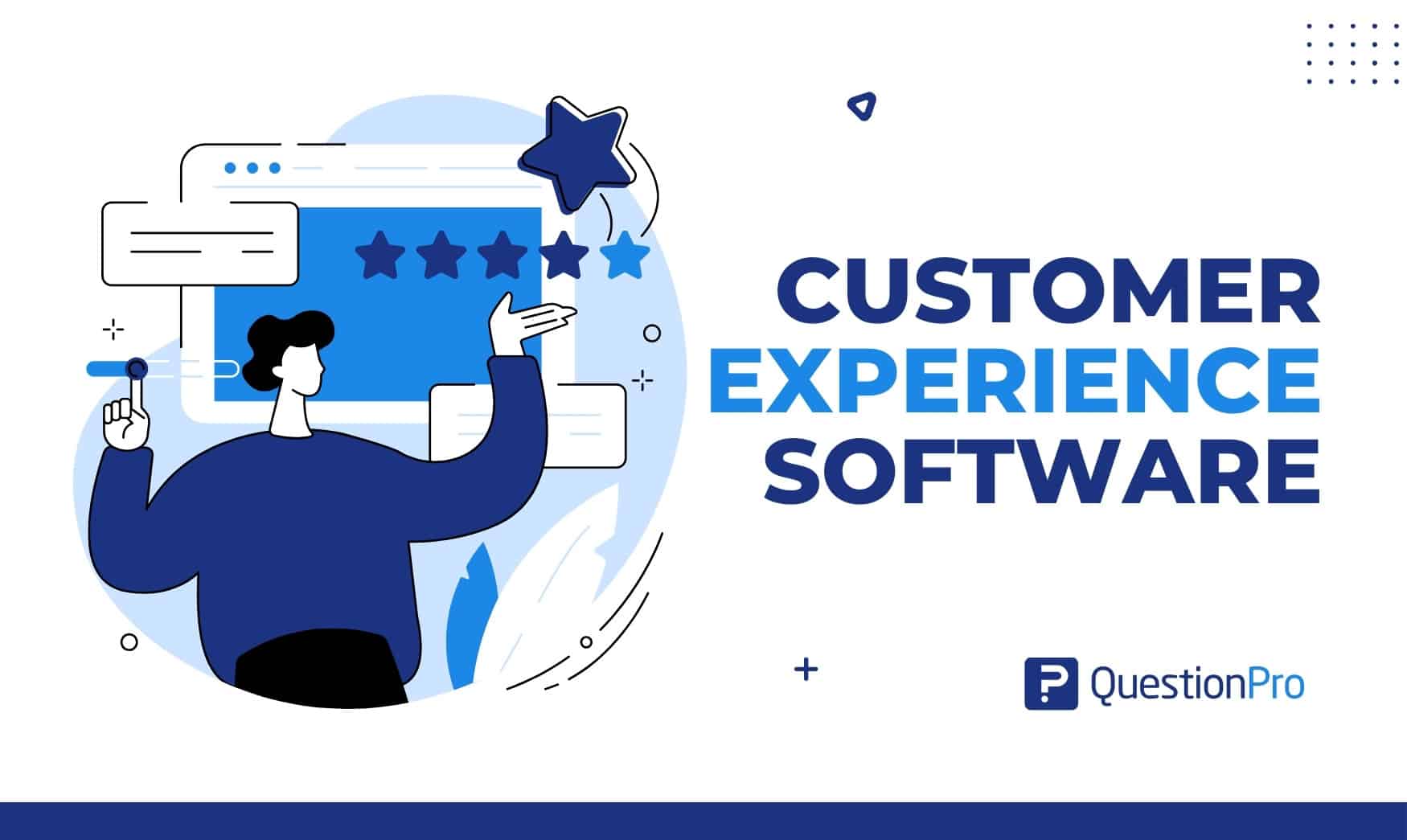
15 Best Customer Experience Software of 2024
May 2, 2024
Other categories
- Academic Research
- Artificial Intelligence
- Assessments
- Brand Awareness
- Case Studies
- Communities
- Consumer Insights
- Customer effort score
- Customer Engagement
- Customer Experience
- Customer Loyalty
- Customer Research
- Customer Satisfaction
- Employee Benefits
- Employee Engagement
- Employee Retention
- Friday Five
- General Data Protection Regulation
- Insights Hub
- Life@QuestionPro
- Market Research
- Mobile diaries
- Mobile Surveys
- New Features
- Online Communities
- Question Types
- Questionnaire
- QuestionPro Products
- Release Notes
- Research Tools and Apps
- Revenue at Risk
- Survey Templates
- Training Tips
- Uncategorized
- Video Learning Series
- What’s Coming Up
- Workforce Intelligence
Purdue Online Writing Lab Purdue OWL® College of Liberal Arts
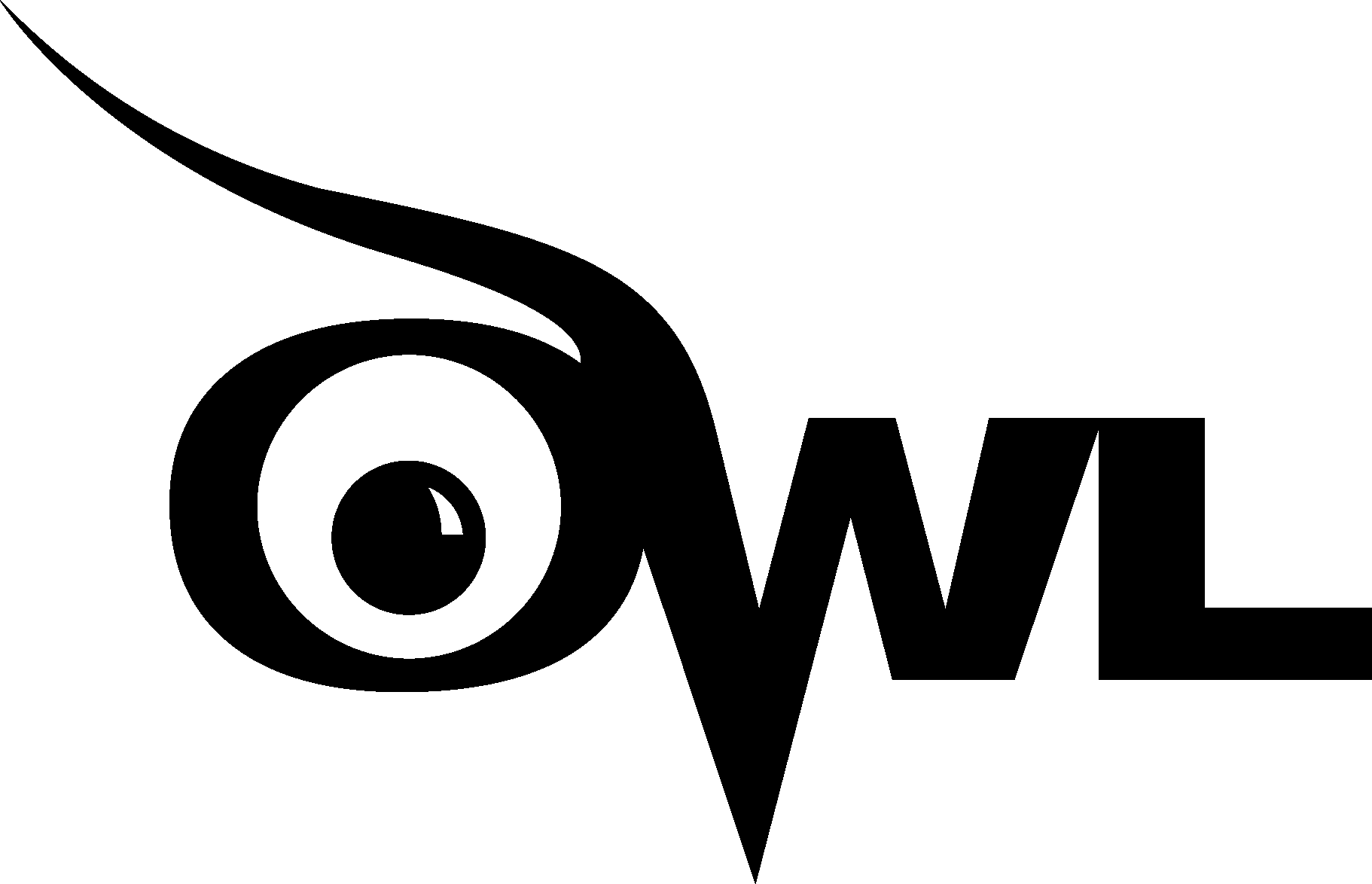
Welcome to the Purdue OWL
This page is brought to you by the OWL at Purdue University. When printing this page, you must include the entire legal notice.
Copyright ©1995-2018 by The Writing Lab & The OWL at Purdue and Purdue University. All rights reserved. This material may not be published, reproduced, broadcast, rewritten, or redistributed without permission. Use of this site constitutes acceptance of our terms and conditions of fair use.
Analysis is a type of primary research that involves finding and interpreting patterns in data, classifying those patterns, and generalizing the results. It is useful when looking at actions, events, or occurrences in different texts, media, or publications. Analysis can usually be done without considering most of the ethical issues discussed in the overview, as you are not working with people but rather publicly accessible documents. Analysis can be done on new documents or performed on raw data that you yourself have collected.
Here are several examples of analysis:
- Recording commercials on three major television networks and analyzing race and gender within the commercials to discover some conclusion.
- Analyzing the historical trends in public laws by looking at the records at a local courthouse.
- Analyzing topics of discussion in chat rooms for patterns based on gender and age.
Analysis research involves several steps:
- Finding and collecting documents.
- Specifying criteria or patterns that you are looking for.
- Analyzing documents for patterns, noting number of occurrences or other factors.
- Privacy Policy

Home » Research Methodology – Types, Examples and writing Guide
Research Methodology – Types, Examples and writing Guide
Table of Contents

Research Methodology
Definition:
Research Methodology refers to the systematic and scientific approach used to conduct research, investigate problems, and gather data and information for a specific purpose. It involves the techniques and procedures used to identify, collect , analyze , and interpret data to answer research questions or solve research problems . Moreover, They are philosophical and theoretical frameworks that guide the research process.
Structure of Research Methodology
Research methodology formats can vary depending on the specific requirements of the research project, but the following is a basic example of a structure for a research methodology section:
I. Introduction
- Provide an overview of the research problem and the need for a research methodology section
- Outline the main research questions and objectives
II. Research Design
- Explain the research design chosen and why it is appropriate for the research question(s) and objectives
- Discuss any alternative research designs considered and why they were not chosen
- Describe the research setting and participants (if applicable)
III. Data Collection Methods
- Describe the methods used to collect data (e.g., surveys, interviews, observations)
- Explain how the data collection methods were chosen and why they are appropriate for the research question(s) and objectives
- Detail any procedures or instruments used for data collection
IV. Data Analysis Methods
- Describe the methods used to analyze the data (e.g., statistical analysis, content analysis )
- Explain how the data analysis methods were chosen and why they are appropriate for the research question(s) and objectives
- Detail any procedures or software used for data analysis
V. Ethical Considerations
- Discuss any ethical issues that may arise from the research and how they were addressed
- Explain how informed consent was obtained (if applicable)
- Detail any measures taken to ensure confidentiality and anonymity
VI. Limitations
- Identify any potential limitations of the research methodology and how they may impact the results and conclusions
VII. Conclusion
- Summarize the key aspects of the research methodology section
- Explain how the research methodology addresses the research question(s) and objectives
Research Methodology Types
Types of Research Methodology are as follows:
Quantitative Research Methodology
This is a research methodology that involves the collection and analysis of numerical data using statistical methods. This type of research is often used to study cause-and-effect relationships and to make predictions.
Qualitative Research Methodology
This is a research methodology that involves the collection and analysis of non-numerical data such as words, images, and observations. This type of research is often used to explore complex phenomena, to gain an in-depth understanding of a particular topic, and to generate hypotheses.
Mixed-Methods Research Methodology
This is a research methodology that combines elements of both quantitative and qualitative research. This approach can be particularly useful for studies that aim to explore complex phenomena and to provide a more comprehensive understanding of a particular topic.
Case Study Research Methodology
This is a research methodology that involves in-depth examination of a single case or a small number of cases. Case studies are often used in psychology, sociology, and anthropology to gain a detailed understanding of a particular individual or group.
Action Research Methodology
This is a research methodology that involves a collaborative process between researchers and practitioners to identify and solve real-world problems. Action research is often used in education, healthcare, and social work.
Experimental Research Methodology
This is a research methodology that involves the manipulation of one or more independent variables to observe their effects on a dependent variable. Experimental research is often used to study cause-and-effect relationships and to make predictions.
Survey Research Methodology
This is a research methodology that involves the collection of data from a sample of individuals using questionnaires or interviews. Survey research is often used to study attitudes, opinions, and behaviors.
Grounded Theory Research Methodology
This is a research methodology that involves the development of theories based on the data collected during the research process. Grounded theory is often used in sociology and anthropology to generate theories about social phenomena.
Research Methodology Example
An Example of Research Methodology could be the following:
Research Methodology for Investigating the Effectiveness of Cognitive Behavioral Therapy in Reducing Symptoms of Depression in Adults
Introduction:
The aim of this research is to investigate the effectiveness of cognitive-behavioral therapy (CBT) in reducing symptoms of depression in adults. To achieve this objective, a randomized controlled trial (RCT) will be conducted using a mixed-methods approach.
Research Design:
The study will follow a pre-test and post-test design with two groups: an experimental group receiving CBT and a control group receiving no intervention. The study will also include a qualitative component, in which semi-structured interviews will be conducted with a subset of participants to explore their experiences of receiving CBT.
Participants:
Participants will be recruited from community mental health clinics in the local area. The sample will consist of 100 adults aged 18-65 years old who meet the diagnostic criteria for major depressive disorder. Participants will be randomly assigned to either the experimental group or the control group.
Intervention :
The experimental group will receive 12 weekly sessions of CBT, each lasting 60 minutes. The intervention will be delivered by licensed mental health professionals who have been trained in CBT. The control group will receive no intervention during the study period.
Data Collection:
Quantitative data will be collected through the use of standardized measures such as the Beck Depression Inventory-II (BDI-II) and the Generalized Anxiety Disorder-7 (GAD-7). Data will be collected at baseline, immediately after the intervention, and at a 3-month follow-up. Qualitative data will be collected through semi-structured interviews with a subset of participants from the experimental group. The interviews will be conducted at the end of the intervention period, and will explore participants’ experiences of receiving CBT.
Data Analysis:
Quantitative data will be analyzed using descriptive statistics, t-tests, and mixed-model analyses of variance (ANOVA) to assess the effectiveness of the intervention. Qualitative data will be analyzed using thematic analysis to identify common themes and patterns in participants’ experiences of receiving CBT.
Ethical Considerations:
This study will comply with ethical guidelines for research involving human subjects. Participants will provide informed consent before participating in the study, and their privacy and confidentiality will be protected throughout the study. Any adverse events or reactions will be reported and managed appropriately.
Data Management:
All data collected will be kept confidential and stored securely using password-protected databases. Identifying information will be removed from qualitative data transcripts to ensure participants’ anonymity.
Limitations:
One potential limitation of this study is that it only focuses on one type of psychotherapy, CBT, and may not generalize to other types of therapy or interventions. Another limitation is that the study will only include participants from community mental health clinics, which may not be representative of the general population.
Conclusion:
This research aims to investigate the effectiveness of CBT in reducing symptoms of depression in adults. By using a randomized controlled trial and a mixed-methods approach, the study will provide valuable insights into the mechanisms underlying the relationship between CBT and depression. The results of this study will have important implications for the development of effective treatments for depression in clinical settings.
How to Write Research Methodology
Writing a research methodology involves explaining the methods and techniques you used to conduct research, collect data, and analyze results. It’s an essential section of any research paper or thesis, as it helps readers understand the validity and reliability of your findings. Here are the steps to write a research methodology:
- Start by explaining your research question: Begin the methodology section by restating your research question and explaining why it’s important. This helps readers understand the purpose of your research and the rationale behind your methods.
- Describe your research design: Explain the overall approach you used to conduct research. This could be a qualitative or quantitative research design, experimental or non-experimental, case study or survey, etc. Discuss the advantages and limitations of the chosen design.
- Discuss your sample: Describe the participants or subjects you included in your study. Include details such as their demographics, sampling method, sample size, and any exclusion criteria used.
- Describe your data collection methods : Explain how you collected data from your participants. This could include surveys, interviews, observations, questionnaires, or experiments. Include details on how you obtained informed consent, how you administered the tools, and how you minimized the risk of bias.
- Explain your data analysis techniques: Describe the methods you used to analyze the data you collected. This could include statistical analysis, content analysis, thematic analysis, or discourse analysis. Explain how you dealt with missing data, outliers, and any other issues that arose during the analysis.
- Discuss the validity and reliability of your research : Explain how you ensured the validity and reliability of your study. This could include measures such as triangulation, member checking, peer review, or inter-coder reliability.
- Acknowledge any limitations of your research: Discuss any limitations of your study, including any potential threats to validity or generalizability. This helps readers understand the scope of your findings and how they might apply to other contexts.
- Provide a summary: End the methodology section by summarizing the methods and techniques you used to conduct your research. This provides a clear overview of your research methodology and helps readers understand the process you followed to arrive at your findings.
When to Write Research Methodology
Research methodology is typically written after the research proposal has been approved and before the actual research is conducted. It should be written prior to data collection and analysis, as it provides a clear roadmap for the research project.
The research methodology is an important section of any research paper or thesis, as it describes the methods and procedures that will be used to conduct the research. It should include details about the research design, data collection methods, data analysis techniques, and any ethical considerations.
The methodology should be written in a clear and concise manner, and it should be based on established research practices and standards. It is important to provide enough detail so that the reader can understand how the research was conducted and evaluate the validity of the results.
Applications of Research Methodology
Here are some of the applications of research methodology:
- To identify the research problem: Research methodology is used to identify the research problem, which is the first step in conducting any research.
- To design the research: Research methodology helps in designing the research by selecting the appropriate research method, research design, and sampling technique.
- To collect data: Research methodology provides a systematic approach to collect data from primary and secondary sources.
- To analyze data: Research methodology helps in analyzing the collected data using various statistical and non-statistical techniques.
- To test hypotheses: Research methodology provides a framework for testing hypotheses and drawing conclusions based on the analysis of data.
- To generalize findings: Research methodology helps in generalizing the findings of the research to the target population.
- To develop theories : Research methodology is used to develop new theories and modify existing theories based on the findings of the research.
- To evaluate programs and policies : Research methodology is used to evaluate the effectiveness of programs and policies by collecting data and analyzing it.
- To improve decision-making: Research methodology helps in making informed decisions by providing reliable and valid data.
Purpose of Research Methodology
Research methodology serves several important purposes, including:
- To guide the research process: Research methodology provides a systematic framework for conducting research. It helps researchers to plan their research, define their research questions, and select appropriate methods and techniques for collecting and analyzing data.
- To ensure research quality: Research methodology helps researchers to ensure that their research is rigorous, reliable, and valid. It provides guidelines for minimizing bias and error in data collection and analysis, and for ensuring that research findings are accurate and trustworthy.
- To replicate research: Research methodology provides a clear and detailed account of the research process, making it possible for other researchers to replicate the study and verify its findings.
- To advance knowledge: Research methodology enables researchers to generate new knowledge and to contribute to the body of knowledge in their field. It provides a means for testing hypotheses, exploring new ideas, and discovering new insights.
- To inform decision-making: Research methodology provides evidence-based information that can inform policy and decision-making in a variety of fields, including medicine, public health, education, and business.
Advantages of Research Methodology
Research methodology has several advantages that make it a valuable tool for conducting research in various fields. Here are some of the key advantages of research methodology:
- Systematic and structured approach : Research methodology provides a systematic and structured approach to conducting research, which ensures that the research is conducted in a rigorous and comprehensive manner.
- Objectivity : Research methodology aims to ensure objectivity in the research process, which means that the research findings are based on evidence and not influenced by personal bias or subjective opinions.
- Replicability : Research methodology ensures that research can be replicated by other researchers, which is essential for validating research findings and ensuring their accuracy.
- Reliability : Research methodology aims to ensure that the research findings are reliable, which means that they are consistent and can be depended upon.
- Validity : Research methodology ensures that the research findings are valid, which means that they accurately reflect the research question or hypothesis being tested.
- Efficiency : Research methodology provides a structured and efficient way of conducting research, which helps to save time and resources.
- Flexibility : Research methodology allows researchers to choose the most appropriate research methods and techniques based on the research question, data availability, and other relevant factors.
- Scope for innovation: Research methodology provides scope for innovation and creativity in designing research studies and developing new research techniques.
Research Methodology Vs Research Methods
About the author.
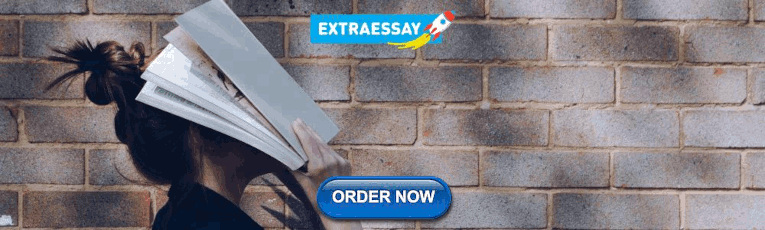
Muhammad Hassan
Researcher, Academic Writer, Web developer
You may also like

How to Cite Research Paper – All Formats and...

Data Collection – Methods Types and Examples

Delimitations in Research – Types, Examples and...

Research Paper Format – Types, Examples and...

Research Process – Steps, Examples and Tips

Research Design – Types, Methods and Examples
- Utility Menu

User Research Center
User research analysis guide, by meg mcmahon.
Analyzing the notes from a user research study is an essential part of answering the research questions that prompted the study.
During the analysis, you or your team decide what insights are generated from the notes taken about the research studies. The insights should relate to the research questions posed at the beginning of the study or point to future research.
Before Analysis
Become familiar with the study.
Read over the research questions, script, background information on the project, and any notes moderators took during interviews.
Decide on analysis type
There are two types of analysis that the URC team uses frequently, an affinity diagramming session and thematic coding.
- Affinity diagramming: this is a process in which all of the notes are separated into individual sticky notes (virtual or physical) and grouped by question or participant at first. Then the analyzers take an even number of groups and sort them individually and then together to find themes. We at the URC use Miro or Dovetail to virtually facilitate this process.
- Thematic coding: this is a process where the notes or a transcript are marked up using codes. Codes are like tags or hashtags. They are bits of metadata that you use to describe other data. After the codes are created the analyzers go through and draw insights from the coded information. We at the URC use Dovetail for this type of analysis.
Decide on who should help with analysis
Often it is a good idea to include stakeholders, as well as yourself, in the analysis process for two primary reasons. One, the stakeholders are able to be a subject matter expert on the system, process, or workflow you are evaluating and two, by participating in the analysis process they are more likely to feel ownership over the final findings and recommendations.
Analysis Basics
Keep a scratch document.
While analyzing your data, it can be helpful to have a scratch document (a document separate from the main notes document) open to the side. You can use this scratch document to:
- Write down quotes from notes that are especially powerful or meaningful.
- Write down any initial insights you have. An insight informs the research questions or furthers your research goals in some way.
Give insights specific names
Insights are created from the themes or codes created during affinity diagramming or thematic coding. Look at the connections between themes or codes, what are larger insights you can draw from them?
Give each insight a name and be as specific as possible. Insights should help your stakeholders understand their research questions.
- DO: use a strong, clear insight: Participants collaboratively search with a collection specialist as a part of the search process. This was noted to build researchers' special collections research skills.
- DON’T: use a hard to understand, non-specific insight: collaborative search
Review the data with other analyzers
After reviewing the data on your own, discuss what you think of the first themes. Why are they important? What pattern do they surface? How do they relate to the research questions?
Back up insights with quotes
Quote or paraphrase ideas directly from the participants that relate to insights add more impact in the future report.
Look for research gaps
After having insights figured out the next step is to review the insights for any research gaps. Can you answer the research questions in your research plan? If not, what additional research is needed? Or are there other themes that don’t directly relate to the research questions which point to a future follow up study?
Prioritize insights most closely related to research questions
As a user researcher your job is to answer the research questions that started the study. If there is an insight that is only tangentially related to the research questions, it is okay to prioritize insights that are more directly related to the research questions when writing the report.
Affinity diagramming
Take the time to set up session.
Affinity diagramming takes time to set up, but it is important to have every note grouped either by participant or by question.
Create top level themes and sub themes
Start to sort the notes into themes. It is okay to create multiple levels of themes if the data lends itself to this kind of grouping. This is helpful for reports to group like insights together for readability.
Themes are not insights
Themes are not insights, they are building blocks for insights. When you are grouping themes it is okay to keep them more vague than you would an insight.
Collapse themes together if necessary
Often when affinity diagramming you may find similar themes within different questions. It is okay to collapse those themes together under a unifying heading. Themes (and insights) don’t need to be based on only a single question.
Avoid creating a "group" of unrelated notes
There are times in affinity diagramming where there are notes that don’t relate easily to any other notes for the study. It is okay to have orphan notes, notes that don’t relate to any theme. If the finding is important enough, it is okay for the orphan note to be its own theme.
Thematic coding
Codes (tags) are not insights.
Codes (tags) are not insights, they are building blocks for insights. When you are adding codes to the notes or transcripts it is okay to keep them more vague than you would an insight.
Use nested coding
When coding you may need to use nested codes. For example, say you’ve created a tag labeled “Coffee”. Now, if you are talking about how people like their coffee served you may make it a two word code/tag like “Coffee Served”.
If you want to specify that someone likes the coffee “black”, you can add a third word. However, considering “black” is a word that now describes “Coffee Served”, it would be best to create a nested tag which should look something like this: “Coffee Served: Black”.
This makes the tag structure easy to use again. For example, if you have to tag a comment later where someone mentions they like the coffee with cream and sugar, it’s easy to add that as “Coffee Served: Cream & Sugar”.
Repeat codes if necessary
Your codes should inform your research questions and you should repeat codes as necessary. If someone talks about the same thing at various times throughout the interview, code each of those instances with the same code.
Blog posts by month
- October 2023 (1)
- August 2023 (2)
- July 2023 (1)
- June 2023 (1)
- May 2023 (2)

An official website of the United States government
The .gov means it’s official. Federal government websites often end in .gov or .mil. Before sharing sensitive information, make sure you’re on a federal government site.
The site is secure. The https:// ensures that you are connecting to the official website and that any information you provide is encrypted and transmitted securely.
- Publications
- Account settings
Preview improvements coming to the PMC website in October 2024. Learn More or Try it out now .
- Advanced Search
- Journal List
- BMJ Open Access

How to write statistical analysis section in medical research
Alok kumar dwivedi.
Department of Molecular and Translational Medicine, Division of Biostatistics and Epidemiology, Texas Tech University Health Sciences Center El Paso, El Paso, Texas, USA
Associated Data
jim-2022-002479supp001.pdf
Data sharing not applicable as no datasets generated and/or analyzed for this study.
Reporting of statistical analysis is essential in any clinical and translational research study. However, medical research studies sometimes report statistical analysis that is either inappropriate or insufficient to attest to the accuracy and validity of findings and conclusions. Published works involving inaccurate statistical analyses and insufficient reporting influence the conduct of future scientific studies, including meta-analyses and medical decisions. Although the biostatistical practice has been improved over the years due to the involvement of statistical reviewers and collaborators in research studies, there remain areas of improvement for transparent reporting of the statistical analysis section in a study. Evidence-based biostatistics practice throughout the research is useful for generating reliable data and translating meaningful data to meaningful interpretation and decisions in medical research. Most existing research reporting guidelines do not provide guidance for reporting methods in the statistical analysis section that helps in evaluating the quality of findings and data interpretation. In this report, we highlight the global and critical steps to be reported in the statistical analysis of grants and research articles. We provide clarity and the importance of understanding study objective types, data generation process, effect size use, evidence-based biostatistical methods use, and development of statistical models through several thematic frameworks. We also provide published examples of adherence or non-adherence to methodological standards related to each step in the statistical analysis and their implications. We believe the suggestions provided in this report can have far-reaching implications for education and strengthening the quality of statistical reporting and biostatistical practice in medical research.
Introduction
Biostatistics is the overall approach to how we realistically and feasibly execute a research idea to produce meaningful data and translate data to meaningful interpretation and decisions. In this era of evidence-based medicine and practice, basic biostatistical knowledge becomes essential for critically appraising research articles and implementing findings for better patient management, improving healthcare, and research planning. 1 However, it may not be sufficient for the proper execution and reporting of statistical analyses in studies. 2 3 Three things are required for statistical analyses, namely knowledge of the conceptual framework of variables, research design, and evidence-based applications of statistical analysis with statistical software. 4 5 The conceptual framework provides possible biological and clinical pathways between independent variables and outcomes with role specification of variables. The research design provides a protocol of study design and data generation process (DGP), whereas the evidence-based statistical analysis approach provides guidance for selecting and implementing approaches after evaluating data with the research design. 2 5 Ocaña-Riola 6 reported a substantial percentage of articles from high-impact medical journals contained errors in statistical analysis or data interpretation. These errors in statistical analyses and interpretation of results do not only impact the reliability of research findings but also influence the medical decision-making and planning and execution of other related studies. A survey of consulting biostatisticians in the USA reported that researchers frequently request biostatisticians for performing inappropriate statistical analyses and inappropriate reporting of data. 7 This implies that there is a need to enforce standardized reporting of the statistical analysis section in medical research which can also help rreviewers and investigators to improve the methodological standards of the study.
Biostatistical practice in medicine has been improving over the years due to continuous efforts in promoting awareness and involving expert services on biostatistics, epidemiology, and research design in clinical and translational research. 8–11 Despite these efforts, the quality of reporting of statistical analysis in research studies has often been suboptimal. 12 13 We noticed that none of the methods reporting documents were developed using evidence-based biostatistics (EBB) theory and practice. The EBB practice implies that the selection of statistical analysis methods for statistical analyses and the steps of results reporting and interpretation should be grounded based on the evidence generated in the scientific literature and according to the study objective type and design. 5 Previous works have not properly elucidated the importance of understanding EBB concepts and related reporting in the write-up of statistical analyses. As a result, reviewers sometimes ask to present data or execute analyses that do not match the study objective type. 14 We summarize the statistical analysis steps to be reported in the statistical analysis section based on review and thematic frameworks.
We identified articles describing statistical reporting problems in medicine using different search terms ( online supplemental table 1 ). Based on these studies, we prioritized commonly reported statistical errors in analytical strategies and developed essential components to be reported in the statistical analysis section of research grants and studies. We also clarified the purpose and the overall implication of reporting each step in statistical analyses through various examples.
Supplementary data
Although biostatistical inputs are critical for the entire research study ( online supplemental table 2 ), biostatistical consultations were mostly used for statistical analyses only 15 . Even though the conduct of statistical analysis mismatched with the study objective and DGP was identified as the major problem in articles submitted to high-impact medical journals. 16 In addition, multivariable analyses were often inappropriately conducted and reported in published studies. 17 18 In light of these statistical errors, we describe the reporting of the following components in the statistical analysis section of the study.
Step 1: specify study objective type and outcomes (overall approach)
The study objective type provides the role of important variables for a specified outcome in statistical analyses and the overall approach of the model building and model reporting steps in a study. In the statistical framework, the problems are classified into descriptive and inferential/analytical/confirmatory objectives. In the epidemiological framework, the analytical and prognostic problems are broadly classified into association, explanatory, and predictive objectives. 19 These study objectives ( figure 1 ) may be classified into six categories: (1) exploratory, (2) association, (3) causal, (4) intervention, (5) prediction and (6) clinical decision models in medical research. 20

Comparative assessments of developing and reporting of study objective types and models. Association measures include odds ratio, risk ratio, or hazard ratio. AUC, area under the curve; C, confounder; CI, confidence interval; E, exposure; HbA1C: hemoglobin A1c; M, mediator; MFT, model fit test; MST, model specification test; PI, predictive interval; R 2 , coefficient of determinant; X, independent variable; Y, outcome.
The exploratory objective type is a specific type of determinant study and is commonly known as risk factors or correlates study in medical research. In an exploratory study, all covariates are considered equally important for the outcome of interest in the study. The goal of the exploratory study is to present the results of a model which gives higher accuracy after satisfying all model-related assumptions. In the association study, the investigator identifies predefined exposures of interest for the outcome, and variables other than exposures are also important for the interpretation and considered as covariates. The goal of an association study is to present the adjusted association of exposure with outcome. 20 In the causal objective study, the investigator is interested in determining the impact of exposure(s) on outcome using the conceptual framework. In this study objective, all variables should have a predefined role (exposures, confounders, mediators, covariates, and predictors) in a conceptual framework. A study with a causal objective is known as an explanatory or a confirmatory study in medical research. The goal is to present the direct or indirect effects of exposure(s) on an outcome after assessing the model’s fitness in the conceptual framework. 19 21 The objective of an interventional study is to determine the effect of an intervention on outcomes and is often known as randomized or non-randomized clinical trials in medical research. In the intervention objective model, all variables other than the intervention are treated as nuisance variables for primary analyses. The goal is to present the direct effect of the intervention on the outcomes by eliminating biases. 22–24 In the predictive study, the goal is to determine an optimum set of variables that can predict the outcome, particularly in external settings. The clinical decision models are a special case of prognostic models in which high dimensional data at various levels are used for risk stratification, classification, and prediction. In this model, all variables are considered input features. The goal is to present a decision tool that has high accuracy in training, testing, and validation data sets. 20 25 Biostatisticians or applied researchers should properly discuss the intention of the study objective type before proceeding with statistical analyses. In addition, it would be a good idea to prepare a conceptual model framework regardless of study objective type to understand study concepts.
A study 26 showed a favorable effect of the beta-blocker intervention on survival outcome in patients with advanced human epidermal growth factor receptor (HER2)-negative breast cancer without adjusting for all the potential confounding effects (age or menopausal status and Eastern Cooperative Oncology Performance Status) in primary analyses or validation analyses or using a propensity score-adjusted analysis, which is an EBB preferred method for analyzing non-randomized studies. 27 Similarly, another study had the goal of developing a predictive model for prediction of Alzheimer’s disease progression. 28 However, this study did not internally or externally validate the performance of the model as per the requirement of a predictive objective study. In another study, 29 investigators were interested in determining an association between metabolic syndrome and hepatitis C virus. However, the authors did not clearly specify the outcome in the analysis and produced conflicting associations with different analyses. 30 Thus, the outcome should be clearly specified as per the study objective type.
Step 2: specify effect size measure according to study design (interpretation and practical value)
The study design provides information on the selection of study participants and the process of data collection conditioned on either exposure or outcome ( figure 2 ). The appropriate use of effect size measure, tabular presentation of results, and the level of evidence are mostly determined by the study design. 31 32 In cohort or clinical trial study designs, the participants are selected based on exposure status and are followed up for the development of the outcome. These study designs can provide multiple outcomes, produce incidence or incidence density, and are preferred to be analyzed with risk ratio (RR) or hazards models. In a case–control study, the selection of participants is conditioned on outcome status. This type of study can have only one outcome and is preferred to be analyzed with an odds ratio (OR) model. In a cross-sectional study design, there is no selection restriction on outcomes or exposures. All data are collected simultaneously and can be analyzed with a prevalence ratio model, which is mathematically equivalent to the RR model. 33 The reporting of effect size measure also depends on the study objective type. For example, predictive models typically require reporting of regression coefficients or weight of variables in the model instead of association measures, which are required in other objective types. There are agreements and disagreements between OR and RR measures. Due to the constancy and symmetricity properties of OR, some researchers prefer to use OR in studies with common events. Similarly, the collapsibility and interpretability properties of RR make it more appealing to use in studies with common events. 34 To avoid variable practice and interpretation issues with OR, it is recommended to use RR models in all studies except for case–control and nested case–control studies, where OR approximates RR and thus OR models should be used. Otherwise, investigators may report sufficient data to compute any ratio measure. Biostatisticians should educate investigators on the proper interpretation of ratio measures in the light of study design and their reporting. 34 35

Effect size according to study design.
Investigators sometimes either inappropriately label their study design 36 37 or report effect size measures not aligned with the study design, 38 39 leading to difficulty in results interpretation and evaluation of the level of evidence. The proper labeling of study design and the appropriate use of effect size measure have substantial implications for results interpretation, including the conduct of systematic review and meta-analysis. 40 A study 31 reviewed the frequency of reporting OR instead of RR in cohort studies and randomized clinical trials (RCTs) and found that one-third of the cohort studies used an OR model, whereas 5% of RCTs used an OR model. The majority of estimated ORs from these studies had a 20% or higher deviation from the corresponding RR.
Step 3: specify study hypothesis, reporting of p values, and interval estimates (interpretation and decision)
The clinical hypothesis provides information for evaluating formal claims specified in the study objectives, while the statistical hypothesis provides information about the population parameters/statistics being used to test the formal claims. The inference about the study hypothesis is typically measured by p value and confidence interval (CI). A smaller p value indicates that the data support against the null hypothesis. Since the p value is a conditional probability, it can never tell about the acceptance or rejection of the null hypothesis. Therefore, multiple alternative strategies of p values have been proposed to strengthen the credibility of conclusions. 41 42 Adaption of these alternative strategies is only needed in the explanatory objective studies. Although exact p values are recommended to be reported in research studies, p values do not provide any information about the effect size. Compared with p values, the CI provides a confidence range of the effect size that contains the true effect size if the study were repeated and can be used to determine whether the results are statistically significant or not. 43 Both p value and 95% CI provide complementary information and thus need to be specified in the statistical analysis section. 24 44
Researchers often test one or more comparisons or hypotheses. Accordingly, the side and the level of significance for considering results to be statistically significant may change. Furthermore, studies may include more than one primary outcome that requires an adjustment in the level of significance for multiplicity. All studies should provide the interval estimate of the effect size/regression coefficient in the primary analyses. Since the interpretation of data analysis depends on the study hypothesis, researchers are required to specify the level of significance along with the side (one-sided or two-sided) of the p value in the test for considering statistically significant results, adjustment of the level of significance due to multiple comparisons or multiplicity, and reporting of interval estimates of the effect size in the statistical analysis section. 45
A study 46 showed a significant effect of fluoxetine on relapse rates in obsessive-compulsive disorder based on a one-sided p value of 0.04. Clearly, there was no reason for using a one-sided p value as opposed to a two-sided p value. A review of the appropriate use of multiple test correction methods in multiarm clinical trials published in major medical journals in 2012 identified over 50% of the articles did not perform multiple-testing correction. 47 Similar to controlling a familywise error rate due to multiple comparisons, adjustment of the false discovery rate is also critical in studies involving multiple related outcomes. A review of RCTs for depression between 2007 and 2008 from six journals reported that only limited studies (5.8%) accounted for multiplicity in the analyses due to multiple outcomes. 48
Step 4: account for DGP in the statistical analysis (accuracy)
The study design also requires the specification of the selection of participants and outcome measurement processes in different design settings. We referred to this specific design feature as DGP. Understanding DGP helps in determining appropriate modeling of outcome distribution in statistical analyses and setting up model premises and units of analysis. 4 DGP ( figure 3 ) involves information on data generation and data measures, including the number of measurements after random selection, complex selection, consecutive selection, pragmatic selection, or systematic selection. Specifically, DGP depends on a sampling setting (participants are selected using survey sampling methods and one subject may represent multiple participants in the population), clustered setting (participants are clustered through a recruitment setting or hierarchical setting or multiple hospitals), pragmatic setting (participants are selected through mixed approaches), or systematic review setting (participants are selected from published studies). DGP also depends on the measurements of outcomes in an unpaired setting (measured on one occasion only in independent groups), paired setting (measured on more than one occasion or participants are matched on certain subject characteristics), or mixed setting (measured on more than one occasion but interested in comparing independent groups). It also involves information regarding outcomes or exposure generation processes using quantitative or categorical variables, quantitative values using labs or validated instruments, and self-reported or administered tests yielding a variety of data distributions, including individual distribution, mixed-type distribution, mixed distributions, and latent distributions. Due to different DGPs, study data may include messy or missing data, incomplete/partial measurements, time-varying measurements, surrogate measures, latent measures, imbalances, unknown confounders, instrument variables, correlated responses, various levels of clustering, qualitative data, or mixed data outcomes, competing events, individual and higher-level variables, etc. The performance of statistical analysis, appropriate estimation of standard errors of estimates and subsequently computation of p values, the generalizability of findings, and the graphical display of data rely on DGP. Accounting for DGP in the analyses requires proper communication between investigators and biostatisticians about each aspect of participant selection and data collection, including measurements, occasions of measurements, and instruments used in the research study.

Common features of the data generation process.
A study 49 compared the intake of fresh fruit and komatsuna juice with the intake of commercial vegetable juice on metabolic parameters in middle-aged men using an RCT. The study was criticized for many reasons, but primarily for incorrect statistical methods not aligned with the study DGP. 50 Similarly, another study 51 highlighted that 80% of published studies using the Korean National Health and Nutrition Examination Survey did not incorporate survey sampling structure in statistical analyses, producing biased estimates and inappropriate findings. Likewise, another study 52 highlighted the need for maintaining methodological standards while analyzing data from the National Inpatient Sample. A systematic review 53 identified that over 50% of studies did not specify whether a paired t-test or an unpaired t-test was performed in statistical analysis in the top 25% of physiology journals, indicating poor transparency in reporting of statistical analysis as per the data type. Another study 54 also highlighted the data displaying errors not aligned with DGP. As per DGP, delay in treatment initiation of patients with cancer defined from the onset of symptom to treatment initiation should be analyzed into three components: patient/primary delay, secondary delay, and tertiary delay. 55 Similarly, the number of cancerous nodes should be analyzed with count data models. 56 However, several studies did not analyze such data according to DGP. 57 58
Step 5: apply EBB methods specific to study design features and DGP (efficiency and robustness)
The continuous growth in the development of robust statistical methods for dealing with a specific problem produced various methods to analyze specific data types. Since multiple methods are available for handling a specific problem yet with varying performances, heterogeneous practices among applied researchers have been noticed. Variable practices could also be due to a lack of consensus on statistical methods in literature, unawareness, and the unavailability of standardized statistical guidelines. 2 5 59 However, it becomes sometimes difficult to differentiate whether a specific method was used due to its robustness, lack of awareness, lack of accessibility of statistical software to apply an alternative appropriate method, intention to produce expected results, or ignorance of model diagnostics. To avoid heterogeneous practices, the selection of statistical methodology and their reporting at each stage of data analysis should be conducted using methods according to EBB practice. 5 Since it is hard for applied researchers to optimally select statistical methodology at each step, we encourage investigators to involve biostatisticians at the very early stage in basic, clinical, population, translational, and database research. We also appeal to biostatisticians to develop guidelines, checklists, and educational tools to promote the concept of EBB. As an effort, we developed the statistical analysis and methods in biomedical research (SAMBR) guidelines for applied researchers to use EBB methods for data analysis. 5 The EBB practice is essential for applying recent cutting-edge robust methodologies to yield accurate and unbiased results. The efficiency of statistical methodologies depends on the assumptions and DGP. Therefore, investigators may attempt to specify the choice of specific models in the primary analysis as per the EBB.
Although details of evidence-based preferred methods are provided in the SAMBR checklists for each study design/objective, 5 we have presented a simplified version of evidence-based preferred methods for common statistical analysis ( online supplemental table 3 ). Several examples are available in the literature where inefficient methods not according to EBB practice have been used. 31 57 60
Step 6: report variable selection method in the multivariable analysis according to study objective type (unbiased)
Multivariable analysis can be used for association, prediction or classification or risk stratification, adjustment, propensity score development, and effect size estimation. 61 Some biological, clinical, behavioral, and environmental factors may directly associate or influence the relationship between exposure and outcome. Therefore, almost all health studies require multivariable analyses for accurate and unbiased interpretations of findings ( figure 1 ). Analysts should develop an adjusted model if the sample size permits. It is a misconception that the analysis of RCT does not require adjusted analysis. Analysis of RCT may require adjustment for prognostic variables. 23 The foremost step in model building is the entry of variables after finalizing the appropriate parametric or non-parametric regression model. In the exploratory model building process due to no preference of exposures, a backward automated approach after including any variables that are significant at 25% in the unadjusted analysis can be used for variable selection. 62 63 In the association model, a manual selection of covariates based on the relevance of the variables should be included in a fully adjusted model. 63 In a causal model, clinically guided methods should be used for variable selection and their adjustments. 20 In a non-randomized interventional model, efforts should be made to eliminate confounding effects through propensity score methods and the final propensity score-adjusted multivariable model may adjust any prognostic variables, while a randomized study simply should adjust any prognostic variables. 27 Maintaining the event per variable (EVR) is important to avoid overfitting in any type of modeling; therefore, screening of variables may be required in some association and explanatory studies, which may be accomplished using a backward stepwise method that needs to be clarified in the statistical analyses. 10 In a predictive study, a model with an optimum set of variables producing the highest accuracy should be used. The optimum set of variables may be screened with the random forest method or bootstrap or machine learning methods. 64 65 Different methods of variable selection and adjustments may lead to different results. The screening process of variables and their adjustments in the final multivariable model should be clearly mentioned in the statistical analysis section.
A study 66 evaluating the effect of hydroxychloroquine (HDQ) showed unfavorable events (intubation or death) in patients who received HDQ compared with those who did not (hazard ratio (HR): 2.37, 95% CI 1.84 to 3.02) in an unadjusted analysis. However, the propensity score-adjusted analyses as appropriate with the interventional objective model showed no significant association between HDQ use and unfavorable events (HR: 1.04, 95% CI 0.82 to 1.32), which was also confirmed in multivariable and other propensity score-adjusted analyses. This study clearly suggests that results interpretation should be based on a multivariable analysis only in observational studies if feasible. A recent study 10 noted that approximately 6% of multivariable analyses based on either logistic or Cox regression used an inappropriate selection method of variables in medical research. This practice was more commonly noted in studies that did not involve an expert biostatistician. Another review 61 of 316 articles from high-impact Chinese medical journals revealed that 30.7% of articles did not report the selection of variables in multivariable models. Indeed, this inappropriate practice could have been identified more commonly if classified according to the study objective type. 18 In RCTs, it is uncommon to report an adjusted analysis based on prognostic variables, even though an adjusted analysis may produce an efficient estimate compared with an unadjusted analysis. A study assessing the effect of preemptive intervention on development outcomes showed a significant effect of an intervention on reducing autism spectrum disorder symptoms. 67 However, this study was criticized by Ware 68 for not reporting non-significant results in unadjusted analyses. If possible, unadjusted estimates should also be reported in any study, particularly in RCTs. 23 68
Step 7: provide evidence for exploring effect modifiers (applicability)
Any variable that modifies the effect of exposure on the outcome is called an effect modifier or modifier or an interacting variable. Exploring the effect modifiers in multivariable analyses helps in (1) determining the applicability/generalizability of findings in the overall or specific subpopulation, (2) generating ideas for new hypotheses, (3) explaining uninterpretable findings between unadjusted and adjusted analyses, (4) guiding to present combined or separate models for each specific subpopulation, and (5) explaining heterogeneity in treatment effect. Often, investigators present adjusted stratified results according to the presence or absence of an effect modifier. If the exposure interacts with multiple variables statistically or conceptually in the model, then the stratified findings (subgroup) according to each effect modifier may be presented. Otherwise, stratified analysis substantially reduces the power of the study due to the lower sample size in each stratum and may produce significant results by inflating type I error. 69 Therefore, a multivariable analysis involving an interaction term as opposed to a stratified analysis may be presented in the presence of an effect modifier. 70 Sometimes, a quantitative variable may emerge as a potential effect modifier for exposure and an outcome relationship. In such a situation, the quantitative variable should not be categorized unless a clinically meaningful threshold is not available in the study. In fact, the practice of categorizing quantitative variables should be avoided in the analysis unless a clinically meaningful cut-off is available or a hypothesis requires for it. 71 In an exploratory objective type, any possible interaction may be obtained in a study; however, the interpretation should be guided based on clinical implications. Similarly, some objective models may have more than one exposure or intervention and the association of each exposure according to the level of other exposure should be presented through adjusted analyses as suggested in the presence of interaction effects. 70
A review of 428 articles from MEDLINE on the quality of reporting from statistical analyses of three (linear, logistic, and Cox) commonly used regression models reported that only 18.5% of the published articles provided interaction analyses, 17 even though interaction analyses can provide a lot of useful information.
Step 8: assessment of assumptions, specifically the distribution of outcome, linearity, multicollinearity, sparsity, and overfitting (reliability)
The assessment and reporting of model diagnostics are important in assessing the efficiency, validity, and usefulness of the model. Model diagnostics include satisfying model-specific assumptions and the assessment of sparsity, linearity, distribution of outcome, multicollinearity, and overfitting. 61 72 Model-specific assumptions such as normal residuals, heteroscedasticity and independence of errors in linear regression, proportionality in Cox regression, proportionality odds assumption in ordinal logistic regression, and distribution fit in other types of continuous and count models are required. In addition, sparsity should also be examined prior to selecting an appropriate model. Sparsity indicates many zero observations in the data set. 73 In the presence of sparsity, the effect size is difficult to interpret. Except for machine learning models, most of the parametric and semiparametric models require a linear relationship between independent variables and a functional form of an outcome. Linearity should be assessed using a multivariable polynomial in all model objectives. 62 Similarly, the appropriate choice of the distribution of outcome is required for model building in all study objective models. Multicollinearity assessment is also useful in all objective models. Assessment of EVR in multivariable analysis can be used to avoid the overfitting issue of a multivariable model. 18
Some review studies highlighted that 73.8%–92% of the articles published in MEDLINE had not assessed the model diagnostics of the multivariable regression models. 17 61 72 Contrary to the monotonically, linearly increasing relationship between systolic blood pressure (SBP) and mortality established using the Framingham’s study, 74 Port et al 75 reported a non-linear relationship between SBP and all-cause mortality or cardiovascular deaths by reanalysis of the Framingham’s study data set. This study identified a different threshold for treating hypertension, indicating the role of linearity assessment in multivariable models. Although a non-Gaussian distribution model may be required for modeling patient delay outcome data in cancer, 55 a study analyzed patient delay data using an ordinary linear regression model. 57 An investigation of the development of predictive models and their reporting in medical journals identified that 53% of the articles had fewer EVR than the recommended EVR, indicating over half of the published articles may have an overfitting model. 18 Another study 76 attempted to identify the anthropometric variables associated with non-insulin-dependent diabetes and found that none of the anthropometric variables were significant after adjusting for waist circumference, age, and sex, indicating the presence of collinearity. A study reported detailed sparse data problems in published studies and potential solutions. 73
Step 9: report type of primary and sensitivity analyses (consistency)
Numerous considerations and assumptions are made throughout the research processes that require assessment, evaluation, and validation. Some assumptions, executions, and errors made at the beginning of the study data collection may not be fixable 13 ; however, additional information collected during the study and data processing, including data distribution obtained at the end of the study, may facilitate additional considerations that need to be verified in the statistical analyses. Consistencies in the research findings via modifications in the outcome or exposure definition, study population, accounting for missing data, model-related assumptions, variables and their forms, and accounting for adherence to protocol in the models can be evaluated and reported in research studies using sensitivity analyses. 77 The purpose and type of supporting analyses need to be specified clearly in the statistical analyses to differentiate the main findings from the supporting findings. Sensitivity analyses are different from secondary or interim or subgroup analyses. 78 Data analyses for secondary outcomes are often referred to as secondary analyses, while data analyses of an ongoing study are called interim analyses and data analyses according to groups based on patient characteristics are known as subgroup analyses.
Almost all studies require some form of sensitivity analysis to validate the findings under different conditions. However, it is often underutilized in medical journals. Only 18%–20.3% of studies reported some forms of sensitivity analyses. 77 78 A review of nutritional trials from high-quality journals reflected that 17% of the conclusions were reported inappropriately using findings from sensitivity analyses not based on the primary/main analyses. 77
Step 10: provide methods for summarizing, displaying, and interpreting data (transparency and usability)
Data presentation includes data summary, data display, and data from statistical model analyses. The primary purpose of the data summary is to understand the distribution of outcome status and other characteristics in the total sample and by primary exposure status or outcome status. Column-wise data presentation should be preferred according to exposure status in all study designs, while row-wise data presentation for the outcome should be preferred in all study designs except for a case–control study. 24 32 Summary statistics should be used to provide maximum information on data distribution aligned with DGP and variable type. The purpose of results presentation primarily from regression analyses or statistical models is to convey results interpretation and implications of findings. The results should be presented according to the study objective type. Accordingly, the reporting of unadjusted and adjusted associations of each factor with the outcome may be preferred in the determinant objective model, while unadjusted and adjusted effects of primary exposure on the outcome may be preferred in the explanatory objective model. In prognostic models, the final predictive models may be presented in such a way that users can use models to predict an outcome. In the exploratory objective model, a final multivariable model should be reported with R 2 or area under the curve (AUC). In the association and interventional models, the assessment of internal validation is critically important through various sensitivity and validation analyses. A model with better fit indices (in terms of R 2 or AUC, Akaike information criterion, Bayesian information criterion, fit index, root mean square error) should be finalized and reported in the causal model objective study. In the predictive objective type, the model performance in terms of R 2 or AUC in training and validation data sets needs to be reported ( figure 1 ). 20 21 There are multiple purposes of data display, including data distribution using bar diagram or histogram or frequency polygons or box plots, comparisons using cluster bar diagram or scatter dot plot or stacked bar diagram or Kaplan-Meier plot, correlation or model assessment using scatter plot or scatter matrix, clustering or pattern using heatmap or line plots, the effect of predictors with fitted models using marginsplot, and comparative evaluation of effect sizes from regression models using forest plot. Although the key purpose of data display is to highlight critical issues or findings in the study, data display should essentially follow DGP and variable types and should be user-friendly. 54 79 Data interpretation heavily relies on the effect size measure along with study design and specified hypotheses. Sometimes, variables require standardization for descriptive comparison of effect sizes among exposures or interpreting small effect size, or centralization for interpreting intercept or avoiding collinearity due to interaction terms, or transformation for achieving model-related assumptions. 80 Appropriate methods of data reporting and interpretation aligned with study design, study hypothesis, and effect size measure should be specified in the statistical analysis section of research studies.
Published articles from reputed journals inappropriately summarized a categorized variable with mean and range, 81 summarized a highly skewed variable with mean and standard deviation, 57 and treated a categorized variable as a continuous variable in regression analyses. 82 Similarly, numerous examples from published studies reporting inappropriate graphical display or inappropriate interpretation of data not aligned with DGP or variable types are illustrated in a book published by Bland and Peacock. 83 84 A study used qualitative data on MRI but inappropriately presented with a Box-Whisker plot. 81 Another study reported unusually high OR for an association between high breast parenchymal enhancement and breast cancer in both premenopausal and postmenopausal women. 85 This reporting makes suspicious findings and may include sparse data bias. 86 A poor tabular presentation without proper scaling or standardization of a variable, missing CI for some variables, missing unit and sample size, and inconsistent reporting of decimal places could be easily noticed in table 4 of a published study. 29 Some published predictive models 87 do not report intercept or baseline survival estimates to use their predictive models in clinical use. Although a direct comparison of effect sizes obtained from the same model may be avoided if the units are different among variables, 35 a study had an objective to compare effect sizes across variables but the authors performed comparisons without standardization of variables or using statistical tests. 88
A sample for writing statistical analysis section in medical journals/research studies
Our primary study objective type was to develop a (select from figure 1 ) model to assess the relationship of risk factors (list critical variables or exposures) with outcomes (specify type from continuous/discrete/count/binary/polytomous/time-to-event). To address this objective, we conducted a (select from figure 2 or any other) study design to test the hypotheses of (equality or superiority or non-inferiority or equivalence or futility) or develop prediction. Accordingly, the other variables were adjusted or considered as (specify role of variables from confounders, covariates, or predictors or independent variables) as reflected in the conceptual framework. In the unadjusted or preliminary analyses as per the (select from figure 3 or any other design features) DGP, (specify EBB preferred tests from online supplemental table 3 or any other appropriate tests) were used for (specify variables and types) in unadjusted analyses. According to the EBB practice for the outcome (specify type) and DGP of (select from figure 3 or any other), we used (select from online supplemental table 1 or specify a multivariable approach) as the primary model in the multivariable analysis. We used (select from figure 1 ) variable selection method in the multivariable analysis and explored the interaction effects between (specify variables). The model diagnostics including (list all applicable, including model-related assumptions, linearity, or multicollinearity or overfitting or distribution of outcome or sparsity) were also assessed using (specify appropriate methods) respectively. In such exploration, we identified (specify diagnostic issues if any) and therefore the multivariable models were developed using (specify potential methods used to handle diagnostic issues). The other outcomes were analyzed with (list names of multivariable approaches with respective outcomes). All the models used the same procedure (or specify from figure 1 ) for variable selection, exploration of interaction effects, and model diagnostics using (specify statistical approaches) depending on the statistical models. As per the study design, hypothesis, and multivariable analysis, the results were summarized with effect size (select as appropriate or from figure 2 ) along with (specify 95% CI or other interval estimates) and considered statistically significant using (specify the side of p value or alternatives) at (specify the level of significance) due to (provide reasons for choosing a significance level). We presented unadjusted and/or adjusted estimates of primary outcome according to (list primary exposures or variables). Additional analyses were conducted for (specific reasons from step 9) using (specify methods) to validate findings obtained in the primary analyses. The data were summarized with (list summary measures and appropriate graphs from step 10), whereas the final multivariable model performance was summarized with (fit indices if applicable from step 10). We also used (list graphs) as appropriate with DGP (specify from figure 3 ) to present the critical findings or highlight (specify data issues) using (list graphs/methods) in the study. The exposures or variables were used in (specify the form of the variables) and therefore the effect or association of (list exposures or variables) on outcome should be interpreted in terms of changes in (specify interpretation unit) exposures/variables. List all other additional analyses if performed (with full details of all models in a supplementary file along with statistical codes if possible).
Concluding remarks
We highlighted 10 essential steps to be reported in the statistical analysis section of any analytical study ( figure 4 ). Adherence to minimum reporting of the steps specified in this report may enforce investigators to understand concepts and approach biostatisticians timely to apply these concepts in their study to improve the overall quality of methodological standards in grant proposals and research studies. The order of reporting information in statistical analyses specified in this report is not mandatory; however, clear reporting of analytical steps applicable to the specific study type should be mentioned somewhere in the manuscript. Since the entire approach of statistical analyses is dependent on the study objective type and EBB practice, proper execution and reporting of statistical models can be taught to the next generation of statisticians by the study objective type in statistical education courses. In fact, some disciplines ( figure 5 ) are strictly aligned with specific study objective types. Bioinformaticians are oriented in studying determinant and prognostic models toward precision medicine, while epidemiologists are oriented in studying association and causal models, particularly in population-based observational and pragmatic settings. Data scientists are heavily involved in prediction and classification models in personalized medicine. A common thing across disciplines is using biostatistical principles and computation tools to address any research question. Sometimes, one discipline expert does the part of others. 89 We strongly recommend using a team science approach that includes an epidemiologist, biostatistician, data scientist, and bioinformatician depending on the study objectives and needs. Clear reporting of data analyses as per the study objective type should be encouraged among all researchers to minimize heterogeneous practices and improve scientific quality and outcomes. In addition, we also encourage investigators to strictly follow transparent reporting and quality assessment guidelines according to the study design ( https://www.equator-network.org/ ) to improve the overall quality of the study, accordingly STROBE (Strengthening the Reporting of Observational Studies in Epidemiology) for observational studies, CONSORT (Consolidated Standards of Reporting Trials) for clinical trials, STARD (Standards for Reporting Diagnostic Accuracy Studies) for diagnostic studies, TRIPOD (Transparent Reporting of a multivariable prediction model for Individual Prognosis OR Diagnosis) for prediction modeling, and ARRIVE (Animal Research: Reporting of In Vivo Experiments) for preclinical studies. The steps provided in this document for writing the statistical analysis section is essentially different from other guidance documents, including SAMBR. 5 SAMBR provides a guidance document for selecting evidence-based preferred methods of statistical analysis according to different study designs, while this report suggests the global reporting of essential information in the statistical analysis section according to study objective type. In this guidance report, our suggestion strictly pertains to the reporting of methods in the statistical analysis section and their implications on the interpretation of results. Our document does not provide guidance on the reporting of sample size or results or statistical analysis section for meta-analysis. The examples and reviews reported in this study may be used to emphasize the concepts and related implications in medical research.

Summary of reporting steps, purpose, and evaluation measures in the statistical analysis section.

Role of interrelated disciplines according to study objective type.
Acknowledgments
The author would like to thank the reviewers for their careful review and insightful suggestions.
Contributors: AKD developed the concept and design and wrote the manuscript.
Funding: The authors have not declared a specific grant for this research from any funding agency in the public, commercial or not-for-profit sectors.
Competing interests: AKD is a Journal of Investigative Medicine Editorial Board member. No other competing interests declared.
Provenance and peer review: Commissioned; externally peer reviewed.
Supplemental material: This content has been supplied by the author(s). It has not been vetted by BMJ Publishing Group Limited (BMJ) and may not have been peer-reviewed. Any opinions or recommendations discussed are solely those of the author(s) and are not endorsed by BMJ. BMJ disclaims all liability and responsibility arising from any reliance placed on the content. Where the content includes any translated material, BMJ does not warrant the accuracy and reliability of the translations (including but not limited to local regulations, clinical guidelines, terminology, drug names and drug dosages), and is not responsible for any error and/or omissions arising from translation and adaptation or otherwise.
Data availability statement
Ethics statements, patient consent for publication.
Not required.
A strong analytical question
- speaks to a genuine dilemma presented by your sources . In other words, the question focuses on a real confusion, problem, ambiguity, or gray area, about which readers will conceivably have different reactions, opinions, or ideas.
- yields an answer that is not obvious . If you ask, "What did this author say about this topic?” there’s nothing to explore because any reader of that text would answer that question in the same way. But if you ask, “how can we reconcile point A and point B in this text,” readers will want to see how you solve that inconsistency in your essay.
- suggests an answer complex enough to require a whole essay's worth of discussion. If the question is too vague, it won't suggest a line of argument. The question should elicit reflection and argument rather than summary or description.
- can be explored using the sources you have available for the assignment , rather than by generalizations or by research beyond the scope of your assignment.
How to come up with an analytical question
One useful starting point when you’re trying to identify an analytical question is to look for points of tension in your sources, either within one source or among sources. It can be helpful to think of those points of tension as the moments where you need to stop and think before you can move forward. Here are some examples of where you may find points of tension:
- You may read a published view that doesn’t seem convincing to you, and you may want to ask a question about what’s missing or about how the evidence might be reconsidered.
- You may notice an inconsistency, gap, or ambiguity in the evidence, and you may want to explore how that changes your understanding of something.
- You may identify an unexpected wrinkle that you think deserves more attention, and you may want to ask a question about it.
- You may notice an unexpected conclusion that you think doesn’t quite add up, and you may want to ask how the authors of a source reached that conclusion.
- You may identify a controversy that you think needs to be addressed, and you may want to ask a question about how it might be resolved.
- You may notice a problem that you think has been ignored, and you may want to try to solve it or consider why it has been ignored.
- You may encounter a piece of evidence that you think warrants a closer look, and you may raise questions about it.
Once you’ve identified a point of tension and raised a question about it, you will try to answer that question in your essay. Your main idea or claim in answer to that question will be your thesis.
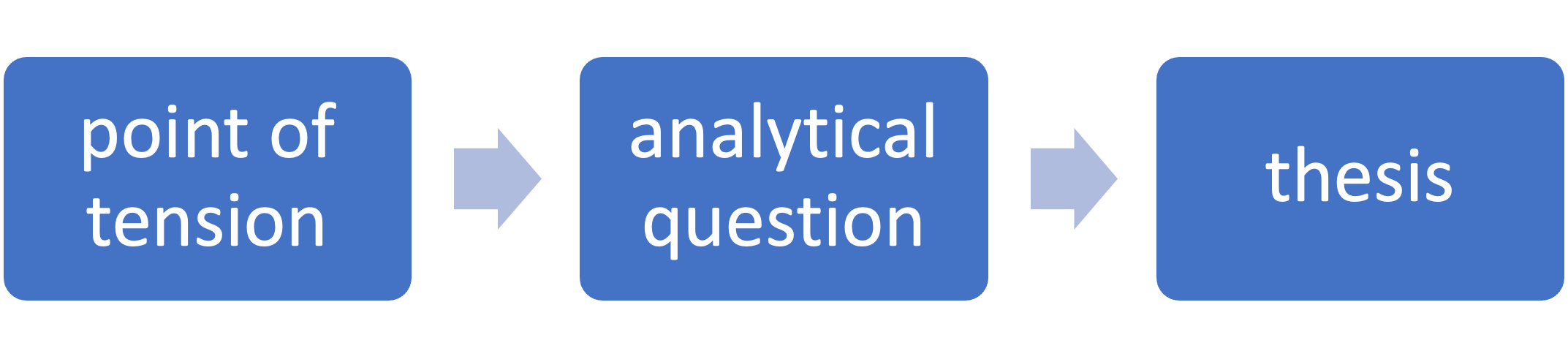
- "How" and "why" questions generally require more analysis than "who/ what/when/where” questions.
- Good analytical questions can highlight patterns/connections, or contradictions/dilemmas/problems.
- Good analytical questions establish the scope of an argument, allowing you to focus on a manageable part of a broad topic or a collection of sources.
- Good analytical questions can also address implications or consequences of your analysis.
- picture_as_pdf Asking Analytical Questions
Cookie consent
We use our own and third-party cookies to show you more relevant content based on your browsing and navigation history. Please accept or manage your cookie settings below. Here's our cookie policy
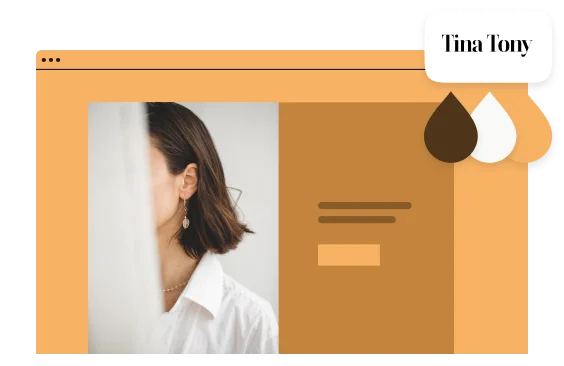
- Form Builder Signups and orders
- Survey maker Research and feedback
- Quiz Maker Trivia and product match
- Find Customers Generate more leads
- Get Feedback Discover ways to improve
- Do research Uncover trends and ideas
- Marketers Forms for marketing teams
- Product Forms for product teams
- HR Forms for HR teams
- Customer success Forms for customer success teams
- Business Forms for general business
- Form templates
- Survey templates
- Quiz templates
- Poll templates
- Order forms
- Feedback forms
- Satisfaction surveys
- Application forms
- Feedback surveys
- Evaluation forms
- Request forms
- Signup forms
- Business surveys
- Marketing surveys
- Report forms
- Customer feedback form
- Registration form
- Branding questionnaire
- 360 feedback
- Lead generation
- Contact form
- Signup sheet

- Help center Find quick answers
- Contact us Speak to someone
- Our blog Get inspired
- Our community Share and learn
- Our guides Tips and how-to
- Updates News and announcements
- Brand Our guidelines
- Partners Browse or join
- Careers Join our team
- → Opinions & Expertise
- → How to analyze survey data: Survey da...
How to analyze survey data: Survey data analysis best practices
Survey data analysis involves reviewing raw materials and transforming them into key insights. Learn how to analyze survey data and best practices here.
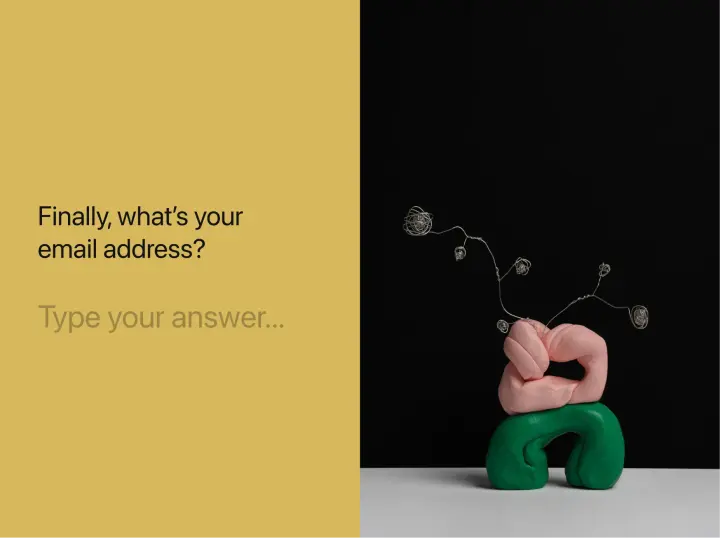
Latest posts on Opinions & Expertise
Typeform | 05.2024
Sheena Fronk | 04.2024
Lydia Kentowski | 04.2024
Norma Ventura | 04.2024
The results are in. You’ve written the questions, found the right people to ask, and got your answers back—now what?
Perfect surveys sent to insightful respondents can become entirely useless if the results aren't coherently and comprehensively analyzed..
So, don’t run and hide.
We know the phrase “survey data analysis” sounds technical and exclusive, but it’s not as scary as it seems. By following a few simple guidelines on how to analyze survey data, you can draw insights from it yourself.
What is survey data analysis?
Survey data analysis is the process of turning survey responses into compelling insights. This includes taking all of your collected data and transforming it into something actionable. Whether it’s open-ended essays, multiple-choice answers, or other questions to ask , you can take this information and uncover patterns and helpful insights.
Some survey data analysis methods include sorting data into categories and using statistical tactics to identify trends and patterns. The goal is to take these raw data responses and turn them into a clear story that answers your research questions to help you make informed decisions.
Getting started with survey data analysis
Before you get started crunching the numbers and performing a survey data analysis, there are a few pieces of information you need to gather.
First, you need to know the number of total respondents. This number will indicate how large your sample is and how much you can rely on your results. It’s always a good idea to gather people’s opinions, but if 5,000 people attended a concert and only five people answered your survey, you can’t really treat those five answers as representative of the whole group.
Secondly, you need to calculate your survey response rate . This is a straightforward percentage—calculate it by dividing the number of responses you received in total by the number of people you asked to fill out the survey. The higher your response rate and the higher your total number of respondents, the more you can trust your survey data to be representative of the sample as a whole.
How to analyze survey data
The first step when analyzing survey data is to turn your individualized responses into aggregated numbers. This sounds complicated, but really, it just means you need to do some counting.
For every question in your survey, you need to know the total number of people who answered with each response. Take a look at this example question:
By aggregating your responses, you're simply counting how many people answered a, b, c, and d, respectively. If 100 people took your survey, the aggregated results would look something like this:
In the last six months: 30
Six months to a year ago: 40
One to two years ago: 20
Over two years ago: 10
Now, if your survey was conducted through a survey host, your online survey results should be aggregated automatically, so there’ll be no need to add the numbers.
Qualitative vs. quantitative data
Once you have all of your aggregated answers, it’s time to start making some sense of the survey data.
Our brains can make sense of percentages much more quickly and easily than whole numbers. It's also far easier to compare different percentages rather than whole numbers.
Say you wrote a survey asking 5-year-olds for their favorite colors. Just saying that 67 children chose red as their favorite color means very little. However, saying that 23% of the children chose red as their favorite color, compared to 50% who chose blue, gives you a much clearer indication of the relative popularity of one color.
If you’ve asked people to write feedback or long-form answers, leave these until the end.
You don’t want the qualitative data to bias your quantitative analysis. Focus on the numbers first, and hopefully, once you have a clear idea of what the sentiment is, the qualitative answers will be able to help you understand why that might be the case.
How to cross-tabulate survey data
Cross-tabulating your data is where you can really begin to draw insights from your survey results instead of just statistics. It can help you add context to your numbers and explore how different groups of people behave or how different factors might affect a single outcome.
When you plan your survey, you'll have considered the different comparisons you'd like to make. For example, maybe you’d like to know if older people are more likely to enjoy eating olives.
Your question might be something like this:
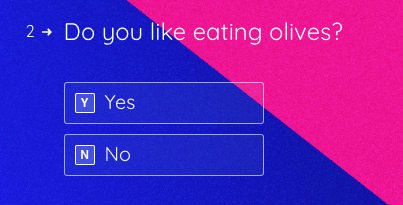
Now, in the first round of your data analysis, you might have already divided the respondents into two groups to work out the split between people who like and don't like eating olives.
So let’s say the results of this olive question were:
Like olives: 542 people (46%)
Dislike olives: 630 people (54%)
To cross-tabulate your data, you’ll need to map another variable onto this one.
We’re interested in whether tastes change with age, so let’s use that age as our second variable and ask:
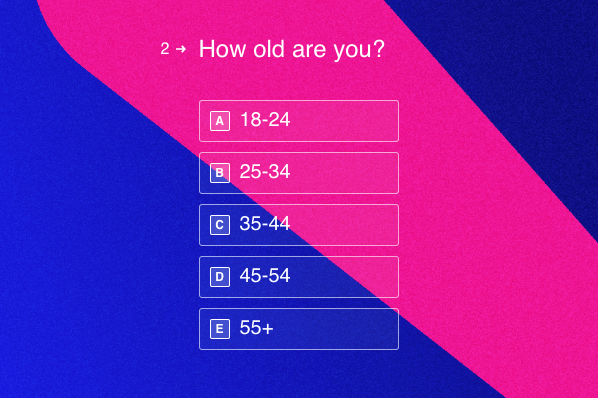
With these results, you can plug them into a Google Sheet and start to see if there are any correlations:
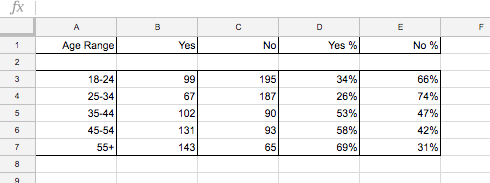
Imagine you have a client who is looking at marketing their olive brand directly to people under 35. You could ask these two questions and look at the split between olive lovers and haters just within this subgroup and see how it compares to the overall average splits.
Benchmarking survey data
Data means very little to us without context and meaning. Turning your numbers into percentages makes comparisons easier, but although proportionally, we can recognize exactly what 75% means, how can we know if that is good?
The answer is benchmarks.
Setting benchmarks is key to making sense of the data and working out what those percentages really mean.
Some of the most common benchmarking techniques involve comparisons between this survey’s results and the data from the last time the survey was collected. To do this effectively, you need to make sure that you are comparing the results of the same question from each survey .
Setting a benchmark using last year’s data is easy. You simply take the percentage splits of responses to a certain question and treat these as your starting point. Then, you can easily see if this month’s data is above or below that benchmark.
Year-over-year or month-over-month comparisons are an excellent way of tracking progress. They allow you to see whether trends are emerging or how much responses have changed in a given period. This is known as longitudinal analysis.
If this is your first time collecting data, no worries, you can still set some benchmarks. Instead of comparing your results to last month's or last year’s data, you can calculate the overall total split between responses for each question and treat this as your benchmark or baseline.
Once you begin to cross-tabulate and break your respondents down into further categories, you can compare their results to your benchmark to place their statistics in context.
If a value is higher than the average , we can say that this category is over-indexing , and if the value is lower , we can say that the category under-indexes . This gives some context to the statistics and starts letting you draw out some real insights from your survey data.
Why you need to analyze survey data
Quantitative data is extremely valuable when interpreting survey results. However, the numbers themselves are unlikely to provide a concrete answer as to why something happened or why people hold a certain opinion.
Understanding why respondents answered in the way that they did is when you can really start to address problems and make changes. This is where the real insight is born.
Sometimes, the “why” will be answered with direct questions in the survey and sometimes with multiple-choice boxes. Other times, it will be up to you as the survey analyst to determine causation, if possible. And this is where we need to be careful.
It's easy to become sucked into a trap when analyzing survey data and start to see patterns everywhere. This isn't necessarily a bad thing, as identifying a correlation between two variables is a key part of interpreting survey results. However, the danger is that we often make assumptions instead.
Assumptions about the data can be hopes or expectations, conscious or subconscious. However, realizing when we are making assumptions can help us avoid any problems further down the line and prevent us from wasting time.
Ultimately, no one wants to find out their assumptions were false after the survey analysis is complete. Similarly, you wouldn’t want a critical assumption to be false and never even realized.
Survey data analysis examples
Correlation occurs when two different variables move at the same time.
A classic example is the sale of seasonal products. During the summer, swimming pool and barbecue sales rise. When plotted on a graph, the two variables move in the same direction at the same time. However, there's no direct connection between these two variables. People buying barbecues isn't the reason the sales of swimming pools increase.
Causation, on the other hand, occurs when one factor directly causes a change in another factor.
For example, in the case of seasonal products, the weather is a key factor. As the temperature rises in the summer, so do barbecue sales. Barbecue sales here are a variable that's dependent on the weather, and there's a key link between them.
When interpreting survey results, it's easy to mistake correlation for causation. Just because two variables move at the same time, it doesn't mean that one is directly influencing the other.
This is where qualitative data comes in. If you’ve asked your respondents to fill in longer-form answers to explain why they chose a certain response, analyzing these answers can give you the insight you need to work out why.
How to report back on your survey data
When sharing your survey data analysis, remember that the story is what makes it interesting, not the numbers.
The percentages you've calculated are vital evidence for your argument, but your analysis needs a narrative to have a real impact on people's thinking.
If you can, always provide context with your statistics, either comparing them to the same survey from last year or comparing groups of people in the same year’s data. Benchmark your numbers so that your audience is immediately aware of whether what they are seeing is positive or negative.
If you are unable to provide recommended actions based on your survey data analysis, at least signpost the key areas that need attention so the relevant parties can begin to tackle the problem if necessary.
When you visualize your data, remember that while long reports can be fascinating, most people won’t read them. Whoever you are presenting to is unlikely to want to listen or read as you walk them through your survey analysis methods step-by-step, so don’t feel like you have to include every single calculation you made in your report.
Put yourself in your audience’s shoes and determine their interests and priorities. Only give them the information if it is relevant to them, they will understand it, and there's something they can do with this new information.
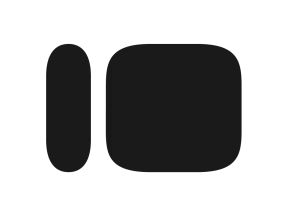
About the author
We're Typeform - a team on a mission to transform data collection by bringing you refreshingly different forms.
Liked that? Check these out:
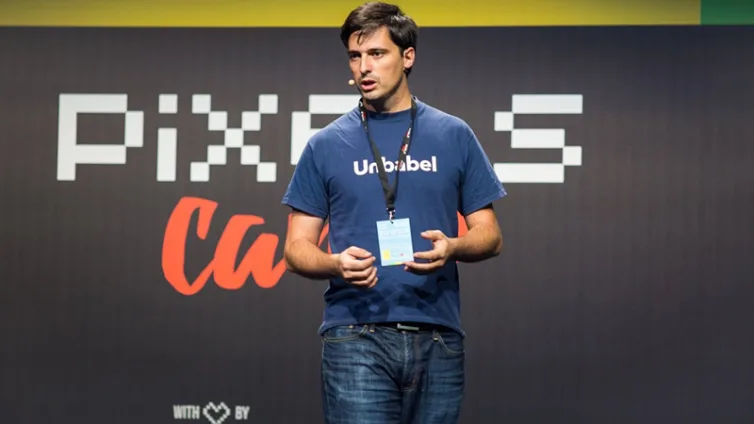
Machines or Humans: who’s cracking the language barrier?
Perfect translations to any language in an instant? We spoke with Unbabel CEO Vasco Pedro on how they do this, and the future of brain-machine interfaces.
Eric Johnson | 05.2019

Lost on a beach: an interview with designpreneur Tomas Laurinavicius
Wake up to a sunrise in Thailand. Explore new and ancient Europe. Pull papaya right off a tree in Central America. Six months here, three months there. All while working 5.5 hours a day. Behold the lifestyle of Tomas Laurinavicius.
Eric Johnson | 07.2019
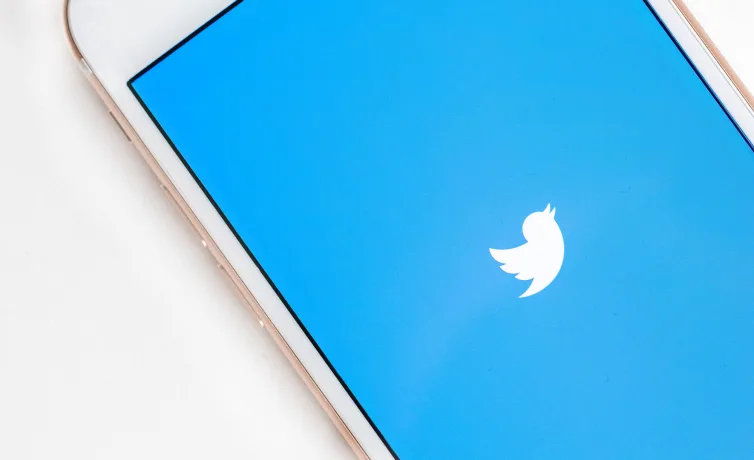
9 lessons @POTUS taught us about social media
Learn the golden rules of social media marketing—as demonstrated by Barack Obama himself.
Beatrice Murray-Nag | 05.2016
Thank you for visiting nature.com. You are using a browser version with limited support for CSS. To obtain the best experience, we recommend you use a more up to date browser (or turn off compatibility mode in Internet Explorer). In the meantime, to ensure continued support, we are displaying the site without styles and JavaScript.
- View all journals
- Explore content
- About the journal
- Publish with us
- Sign up for alerts
- Published: 06 May 2024
APOE4 homozygozity represents a distinct genetic form of Alzheimer’s disease
- Juan Fortea ORCID: orcid.org/0000-0002-1340-638X 1 , 2 , 3 na1 ,
- Jordi Pegueroles ORCID: orcid.org/0000-0002-3554-2446 1 , 2 ,
- Daniel Alcolea ORCID: orcid.org/0000-0002-3819-3245 1 , 2 ,
- Olivia Belbin ORCID: orcid.org/0000-0002-6109-6371 1 , 2 ,
- Oriol Dols-Icardo ORCID: orcid.org/0000-0003-2656-8748 1 , 2 ,
- Lídia Vaqué-Alcázar 1 , 4 ,
- Laura Videla ORCID: orcid.org/0000-0002-9748-8465 1 , 2 , 3 ,
- Juan Domingo Gispert 5 , 6 , 7 , 8 , 9 ,
- Marc Suárez-Calvet ORCID: orcid.org/0000-0002-2993-569X 5 , 6 , 7 , 8 , 9 ,
- Sterling C. Johnson ORCID: orcid.org/0000-0002-8501-545X 10 ,
- Reisa Sperling ORCID: orcid.org/0000-0003-1535-6133 11 ,
- Alexandre Bejanin ORCID: orcid.org/0000-0002-9958-0951 1 , 2 ,
- Alberto Lleó ORCID: orcid.org/0000-0002-2568-5478 1 , 2 &
- Víctor Montal ORCID: orcid.org/0000-0002-5714-9282 1 , 2 , 12 na1
Nature Medicine ( 2024 ) Cite this article
13k Accesses
4455 Altmetric
Metrics details
- Alzheimer's disease
- Predictive markers
This study aimed to evaluate the impact of APOE4 homozygosity on Alzheimer’s disease (AD) by examining its clinical, pathological and biomarker changes to see whether APOE4 homozygotes constitute a distinct, genetically determined form of AD. Data from the National Alzheimer’s Coordinating Center and five large cohorts with AD biomarkers were analyzed. The analysis included 3,297 individuals for the pathological study and 10,039 for the clinical study. Findings revealed that almost all APOE4 homozygotes exhibited AD pathology and had significantly higher levels of AD biomarkers from age 55 compared to APOE3 homozygotes. By age 65, nearly all had abnormal amyloid levels in cerebrospinal fluid, and 75% had positive amyloid scans, with the prevalence of these markers increasing with age, indicating near-full penetrance of AD biology in APOE4 homozygotes. The age of symptom onset was earlier in APOE4 homozygotes at 65.1, with a narrower 95% prediction interval than APOE3 homozygotes. The predictability of symptom onset and the sequence of biomarker changes in APOE4 homozygotes mirrored those in autosomal dominant AD and Down syndrome. However, in the dementia stage, there were no differences in amyloid or tau positron emission tomography across haplotypes, despite earlier clinical and biomarker changes. The study concludes that APOE4 homozygotes represent a genetic form of AD, suggesting the need for individualized prevention strategies, clinical trials and treatments.
This is a preview of subscription content, access via your institution
Access options
Access Nature and 54 other Nature Portfolio journals
Get Nature+, our best-value online-access subscription
24,99 € / 30 days
cancel any time
Subscribe to this journal
Receive 12 print issues and online access
195,33 € per year
only 16,28 € per issue
Buy this article
- Purchase on Springer Link
- Instant access to full article PDF
Prices may be subject to local taxes which are calculated during checkout
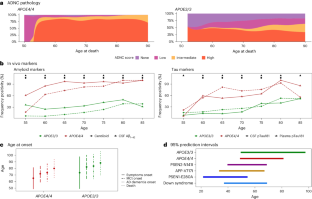
Similar content being viewed by others
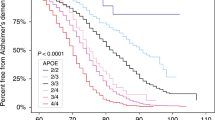
Exceptionally low likelihood of Alzheimer’s dementia in APOE2 homozygotes from a 5,000-person neuropathological study
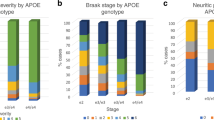
Association of APOE e2 genotype with Alzheimer’s and non-Alzheimer’s neurodegenerative pathologies
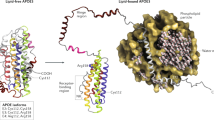
Apolipoprotein E and Alzheimer disease: pathobiology and targeting strategies
Data availability.
Access to tabular data from ADNI ( https://adni.loni.usc.edu/ ), OASIS ( https://oasis-brains.org/ ), A4 ( https://ida.loni.usc.edu/collaboration/access/appLicense.jsp ) and NACC ( https://naccdata.org/ ) can be requested online, as publicly available databases. All requests will be reviewed by each studyʼs scientific board. Concrete inquiries to access the WRAP ( https://wrap.wisc.edu/data-requests-2/ ) and ALFA + ( https://www.barcelonabeta.org/en/alfa-study/about-the-alfa-study ) cohort data can be directed to each study team for concept approval and feasibility consultation. Requests will be reviewed to verify whether the request is subject to any intellectual property.
Code availability
All statistical analyses and raw figures were generated using R (v.4.2.2). We used the open-sourced R packages of ggplot2 (v.3.4.3), dplyr (v.1.1.3), ggstream (v.0.1.0), ggpubr (v.0.6), ggstatsplot (v.0.12), Rmisc (v.1.5.1), survival (v.3.5), survminer (v.0.4.9), gtsummary (v.1.7), epitools (v.0.5) and statsExpression (v.1.5.1). Rscripts to replicate our findings can be found at https://gitlab.com/vmontalb/apoe4-asdad (ref. 32 ). For neuroimaging analyses, we used Free Surfer (v.6.0) and ANTs (v.2.4.0).
Bellenguez, C. et al. New insights into the genetic etiology of Alzheimer’s disease and related dementias. Nat. Genet. 54 , 412–436 (2022).
Article CAS PubMed PubMed Central Google Scholar
Frisoni, G. B. et al. The probabilistic model of Alzheimer disease: the amyloid hypothesis revised. Nat. Rev. Neurosci. 23 , 53–66 (2022).
Article CAS PubMed Google Scholar
Bateman R. J. et al. Clinical and biomarker changes in dominantly inherited Alzheimer’s disease. N. Engl. J. Med. 367 , 795–804 (2012).
Genin, E. et al. APOE and Alzheimer disease: a major gene with semidominant inheritance. Mol. Psychiatry 16 , 903–907 (2011).
Fortea, J. et al. Alzheimer’s disease associated with Down syndrome: a genetic form of dementia. Lancet Neurol. 20 , 930–942 (2021).
Fortea, J. et al. Clinical and biomarker changes of Alzheimer’s disease in adults with Down syndrome: a cross-sectional study. Lancet 395 , 1988–1997 (2020).
Jansen, W. J. et al. Prevalence of cerebral amyloid pathology in persons without dementia: a meta-analysis. JAMA 313 , 1924–1938 (2015).
Article PubMed PubMed Central Google Scholar
Saddiki H. et al. Age and the association between apolipoprotein E genotype and Alzheimer disease: a cerebrospinal fluid biomarker-based case-control study. PLoS Med. https://doi.org/10.1371/JOURNAL.PMED.1003289 (2020).
Jack, C. R. et al. NIA‐AA Research Framework: toward a biological definition of Alzheimer’s disease. Alzheimer’s Dement. 14 , 535–562 (2018).
Article Google Scholar
Beekly, D. L. et al. The National Alzheimer’s Coordinating Center (NACC) Database: an Alzheimer disease database. Alzheimer Dis. Assoc. Disord. 18 , 270–277 (2004).
PubMed Google Scholar
Montine, T. J. et al. National Institute on Aging–Alzheimer’s Association guidelines for the neuropathologic assessment of Alzheimer’s disease: a practical approach. Acta Neuropathol. 123 , 1–11 (2012).
Reiman, E. M. et al. Exceptionally low likelihood of Alzheimer’s dementia in APOE2 homozygotes from a 5,000-person neuropathological study. Nat. Commun. 11 , 1–11 (2020).
Iulita M. F. et al. Association of Alzheimer disease with life expectancy in people with Down syndrome. JAMA Netw. Open https://doi.org/10.1001/JAMANETWORKOPEN.2022.12910 (2022).
Corder, E. H. et al. Gene dose of apolipoprotein E type 4 allele and the risk of Alzheimer’s disease in late onset families. Science 261 , 921–923 (1993).
Fortea, J., Quiroz, Y. T. & Ryan, N. S. Lessons from Down syndrome and autosomal dominant Alzheimer’s disease. Lancet Neurol. 22 , 5–6 (2023).
Therriault, J. et al. Frequency of biologically defined Alzheimer’s disease in relation to age, sex, APOE ε4, and cognitive impairment. Neurology 96 , e975–e985 (2021).
Betthauser, T. J. et al. Multi-method investigation of factors influencing amyloid onset and impairment in three cohorts. Brain 145 , 4065–4079 (2022).
Snellman, A. et al. APOE ε4 gene dose effect on imaging and blood biomarkers of neuroinflammation and beta-amyloid in cognitively unimpaired elderly. Alzheimers Res. Ther. 15 , 71 (2023).
Ghisays, V. et al. Brain imaging measurements of fibrillar amyloid-β burden, paired helical filament tau burden, and atrophy in cognitively unimpaired persons with two, one, and no copies of the APOE ε4 allele. Alzheimers Dement. 16 , 598–609 (2020).
Mehta, R. I. & Schneider, J. A. What is ‘Alzheimer’s disease’? The neuropathological heterogeneity of clinically defined Alzheimer’s dementia. Curr. Opin. Neurol. 34 , 237–245 (2021).
van der Lee, S. J. et al. The effect of APOE and other common genetic variants on the onset of Alzheimer’s disease and dementia: a community-based cohort study. Lancet Neurol. 17 , 434–444 (2018).
Belloy, M. E., Napolioni, V. & Greicius, M. D. A quarter century of APOE and Alzheimera’s disease: progress to date and the path forward. Neuron 101 , 820–838 (2019).
Belloy, M. E. et al. APOE genotype and Alzheimer disease risk across age, sex, and population ancestry. JAMA Neurol. 80 , 1284–1294 (2023).
Jack, C. R. et al. Long-term associations between amyloid positron emission tomography, sex, apolipoprotein E and incident dementia and mortality among individuals without dementia: hazard ratios and absolute risk. Brain Commun. 4 , fcac017 (2022).
Morris, J. C. The Clinical Dementia Rating (CDR): current version and scoring rules. Neurology 43 , 2412–2414 (1993).
Weiner, M. W. et al. The Alzheimer’s Disease Neuroimaging Initiative 3: continued innovation for clinical trial improvement. Alzheimer’s Dement. 13 , 561–571 (2017).
Sperling R. A. et al. The A4 Study: stopping AD before symptoms begin? Sci. Transl. Med. https://doi.org/10.1126/scitranslmed.3007941 (2014).
Molinuevo, J. L. et al. The ALFA project: a research platform to identify early pathophysiological features of Alzheimer’s disease. Alzheimer’s Dement.: Transl. Res. Clin. Interventions 2 , 82–92 (2016).
Johnson, S. C. et al. The Wisconsin Registry for Alzheimer’s Prevention: a review of findings and current directions. Alzheimer’s Dement.: Diagnosis, Assess. Dis. Monit. 10 , 130–142 (2018).
Google Scholar
LaMontagne P. J. et al. OASIS-3: longitudinal neuroimaging, clinical and cognitive dataset for normal aging and Alzheimer disease. Preprint at MedRxiv https://doi.org/10.1101/2019.12.13.19014902 (2019).
La Joie, R. et al. Multisite study of the relationships between antemortem [ 11 C]PIB-PET Centiloid values and postmortem measures of Alzheimer’s disease neuropathology. Alzheimers Dement. 15 , 205–216 (2019).
Montal, V. APOE4-ASDAD. GitLab https://gitlab.com/vmontalb/apoe4-asdad (2024).
Download references
Acknowledgements
We acknowledge the contributions of several consortia that provided data for this study. We extend our appreciation to the NACC, the Alzheimer’s Disease Neuroimaging Initiative, The A4 Study, the ALFA Study, the Wisconsin Register for Alzheimer’s Prevention and the OASIS3 Project. Without their dedication to advancing Alzheimer’s disease research and their commitment to data sharing, this study would not have been possible. We also thank all the participants and investigators involved in these consortia for their tireless efforts and invaluable contributions to the field. We also thank the institutions that funded this study, the Fondo de Investigaciones Sanitario, Carlos III Health Institute, the Centro de Investigación Biomédica en Red sobre Enfermedades Neurodegenerativas and the Generalitat de Catalunya and La Caixa Foundation, as well as the NIH, Horizon 2020 and the Alzheimer’s Association, which was crucial for this research. Funding: National Institute on Aging. This study was supported by the Fondo de Investigaciones Sanitario, Carlos III Health Institute (INT21/00073, PI20/01473 and PI23/01786 to J.F., CP20/00038, PI22/00307 to A.B., PI22/00456 to M.S.-C., PI18/00435 to D.A., PI20/01330 to A.L.) and the Centro de Investigación Biomédica en Red sobre Enfermedades Neurodegenerativas Program 1, partly jointly funded by Fondo Europeo de Desarrollo Regional, Unión Europea, Una Manera de Hacer Europa. This work was also supported by the National Institutes of Health grants (R01 AG056850; R21 AG056974, R01 AG061566, R01 AG081394 and R61AG066543 to J.F., S10 OD025245, P30 AG062715, U54 HD090256, UL1 TR002373, P01 AG036694 and P50 AG005134 to R.S.; R01 AG027161, R01 AG021155, R01 AG037639, R01 AG054059; P50 AG033514 and P30 AG062715 to S.J.) and ADNI (U01 AG024904), the Department de Salut de la Generalitat de Catalunya, Pla Estratègic de Recerca I Innovació en Salut (SLT006/17/00119 to J.F.; SLT002/16/00408 to A.L.) and the A4 Study (R01 AG063689, U24 AG057437 to R.A.S). It was also supported by Fundación Tatiana Pérez de Guzmán el Bueno (IIBSP-DOW-2020-151 o J.F.) and Horizon 2020–Research and Innovation Framework Programme from the European Union (H2020-SC1-BHC-2018-2020 to J.F.; 948677 and 847648 to M.S.-C.). La Caixa Foundation (LCF/PR/GN17/50300004 to M.S.-C.) and EIT Digital (Grant 2021 to J.D.G.) also supported this work. The Alzheimer Association also participated in the funding of this work (AARG-22-923680 to A.B.) and A4/LEARN Study AA15-338729 to R.A.S.). O.D.-I. receives funding from the Alzheimer’s Association (AARF-22-924456) and the Jerome Lejeune Foundation postdoctoral fellowship.
Author information
These authors contributed equally: Juan Fortea, Víctor Montal.
Authors and Affiliations
Sant Pau Memory Unit, Hospital de la Santa Creu i Sant Pau - Biomedical Research Institute Sant Pau, Barcelona, Spain
Juan Fortea, Jordi Pegueroles, Daniel Alcolea, Olivia Belbin, Oriol Dols-Icardo, Lídia Vaqué-Alcázar, Laura Videla, Alexandre Bejanin, Alberto Lleó & Víctor Montal
Centro de Investigación Biomédica en Red de Enfermedades Neurodegenerativas. CIBERNED, Barcelona, Spain
Juan Fortea, Jordi Pegueroles, Daniel Alcolea, Olivia Belbin, Oriol Dols-Icardo, Laura Videla, Alexandre Bejanin, Alberto Lleó & Víctor Montal
Barcelona Down Medical Center, Fundació Catalana Síndrome de Down, Barcelona, Spain
Juan Fortea & Laura Videla
Department of Medicine, Faculty of Medicine and Health Sciences, Institute of Neurosciences, University of Barcelona, Barcelona, Spain
Lídia Vaqué-Alcázar
Barcelonaβeta Brain Research Center (BBRC), Pasqual Maragall Foundation, Barcelona, Spain
Juan Domingo Gispert & Marc Suárez-Calvet
Neurosciences Programme, IMIM - Hospital del Mar Medical Research Institute, Barcelona, Spain
Department of Medicine and Life Sciences, Universitat Pompeu Fabra, Barcelona, Spain
Centro de Investigación Biomédica en Red Bioingeniería, Biomateriales y Nanomedicina. Instituto de Salud carlos III, Madrid, Spain
Centro Nacional de Investigaciones Cardiovasculares (CNIC), Madrid, Spain
Wisconsin Alzheimer’s Disease Research Center, University of Wisconsin-Madison School of Medicine and Public Health, Madison, WI, USA
Sterling C. Johnson
Brigham and Women’s Hospital Massachusetts General Hospital, Harvard Medical School, Boston, MA, USA
Reisa Sperling
Barcelona Supercomputing Center, Barcelona, Spain
Víctor Montal
You can also search for this author in PubMed Google Scholar
Contributions
J.F. and V.M. conceptualized the research project and drafted the initial manuscript. V.M., J.P. and J.F. conducted data analysis, interpreted statistical findings and created visual representations of the data. O.B. and O.D.-I. provided valuable insights into the genetics of APOE. L.V., A.B. and L.V.-A. meticulously reviewed and edited the manuscript for clarity, accuracy and coherence. J.D.G., M.S.-C., S.J. and R.S. played pivotal roles in data acquisition and securing funding. A.L. and D.A. contributed to the study design, offering guidance and feedback on statistical analyses, and provided critical review of the paper. All authors carefully reviewed the manuscript, offering pertinent feedback that enhanced the study’s quality, and ultimately approved the final version.
Corresponding authors
Correspondence to Juan Fortea or Víctor Montal .
Ethics declarations
Competing interests.
S.C.J. has served at scientific advisory boards for ALZPath, Enigma and Roche Diagnostics. M.S.-C. has given lectures in symposia sponsored by Almirall, Eli Lilly, Novo Nordisk, Roche Diagnostics and Roche Farma, received consultancy fees (paid to the institution) from Roche Diagnostics and served on advisory boards of Roche Diagnostics and Grifols. He was granted a project and is a site investigator of a clinical trial (funded to the institution) by Roche Diagnostics. In-kind support for research (to the institution) was received from ADx Neurosciences, Alamar Biosciences, Avid Radiopharmaceuticals, Eli Lilly, Fujirebio, Janssen Research & Development and Roche Diagnostics. J.D.G. has served as consultant for Roche Diagnostics, receives research funding from Hoffmann–La Roche, Roche Diagnostics and GE Healthcare, has given lectures in symposia sponsored by Biogen, Philips Nederlands, Esteve and Life Molecular Imaging and serves on an advisory board for Prothena Biosciences. R.S. has received personal consulting fees from Abbvie, AC Immune, Acumen, Alector, Bristol Myers Squibb, Janssen, Genentech, Ionis and Vaxxinity outside the submitted work. O.B. reported receiving personal fees from Adx NeuroSciences outside the submitted work. D.A. reported receiving personal fees for advisory board services and/or speaker honoraria from Fujirebio-Europe, Roche, Nutricia, Krka Farmacéutica and Esteve, outside the submitted work. A.L. has served as a consultant or on advisory boards for Almirall, Fujirebio-Europe, Grifols, Eisai, Lilly, Novartis, Roche, Biogen and Nutricia, outside the submitted work. J.F. reported receiving personal fees for service on the advisory boards, adjudication committees or speaker honoraria from AC Immune, Adamed, Alzheon, Biogen, Eisai, Esteve, Fujirebio, Ionis, Laboratorios Carnot, Life Molecular Imaging, Lilly, Lundbeck, Perha, Roche and outside the submitted work. O.B., D.A., A.L. and J.F. report holding a patent for markers of synaptopathy in neurodegenerative disease (licensed to Adx, EPI8382175.0). The remaining authors declare no competing interests.
Peer review
Peer review information.
Nature Medicine thanks Naoyuki Sato, Yadong Huang and the other, anonymous, reviewer(s) for their contribution to the peer review of this work. Primary Handling Editor: Jerome Staal, in collaboration with the Nature Medicine team.
Additional information
Publisher’s note Springer Nature remains neutral with regard to jurisdictional claims in published maps and institutional affiliations.
Supplementary information
Supplementary information.
Supplementary Methods, Results, Bibliography, Figs. 1–7 and Tables 1–3.
Reporting Summary
Supplementary code.
This code is also available in the GitLab repository.
Rights and permissions
Springer Nature or its licensor (e.g. a society or other partner) holds exclusive rights to this article under a publishing agreement with the author(s) or other rightsholder(s); author self-archiving of the accepted manuscript version of this article is solely governed by the terms of such publishing agreement and applicable law.
Reprints and permissions
About this article
Cite this article.
Fortea, J., Pegueroles, J., Alcolea, D. et al. APOE4 homozygozity represents a distinct genetic form of Alzheimer’s disease. Nat Med (2024). https://doi.org/10.1038/s41591-024-02931-w
Download citation
Received : 03 November 2023
Accepted : 19 March 2024
Published : 06 May 2024
DOI : https://doi.org/10.1038/s41591-024-02931-w
Share this article
Anyone you share the following link with will be able to read this content:
Sorry, a shareable link is not currently available for this article.
Provided by the Springer Nature SharedIt content-sharing initiative
Quick links
- Explore articles by subject
- Guide to authors
- Editorial policies
Sign up for the Nature Briefing newsletter — what matters in science, free to your inbox daily.

BUSINESS STRATEGIES
How to create a gym business plan
- Annabelle Amery
- Sep 5, 2023
- 10 min read

When starting a business , it’s crucial to create a comprehensive and clear business plan. This applies to all types of businesses , including a gym business . A gym business plan is a strategic document that outlines the goals, objectives and strategies for starting and running a successful gym.
A gym business plan serves as a roadmap for entrepreneurs, providing guidance on how to navigate the challenges and complexities of the fitness industry. It helps entrepreneurs define their vision, mission and target market, as well as identify the resources and steps needed to achieve their goals.
Need a way to promote your gym business online? Get started with Wix’s website builder , which includes built-in marketing and scheduling tools.
Why create a gym business plan?
A gym business plan is particularly important for individuals who are new to entrepreneurship. It provides a structured approach to starting a gym by outlining the necessary steps, such as securing funding, finding a suitable location and acquiring equipment. Some of the other main advantages include being able to:
Attract funding: A well-written gym business plan can attract potential investors or lenders who may be interested in supporting your venture financially. It demonstrates your understanding of the market, your competitive advantage and your plans for profitability.
Understand resource requirements: A comprehensive gym business plan helps entrepreneurs identify the resources required to start and operate their gym successfully. This includes business registration (cost to start an LLC ), equipment, staff, gym marketing materials and other supplies necessary for day-to-day operations.
Achieve long-term success: A gym business plan provides entrepreneurs with an opportunity to thoroughly analyze their target market and competition. By conducting market research and competitive analysis, they can develop strategies to differentiate their gym from others in the industry.
Set goals: A well-crafted gym business plan helps entrepreneurs set realistic and measurable goals for their gym. These goals can include financial targets, membership growth, customer satisfaction levels and other key performance indicators.
Make better decisions: Having a clear business plan in place helps entrepreneurs make informed decisions about various aspects of their gym, such as pricing, marketing strategies and expansion plans. It serves as a reference point for evaluating options and making choices that align with the overall business strategy.
Guide your website messaging and design: A gym business plan also includes considerations for creating a business website . Today, making a website is crucial for attracting customers and promoting your gym. The business plan can outline the website's purpose, design, functionality and marketing strategies to drive traffic to the site. Be inspired by these fitness website examples .
How to write a gym business plan in 6 steps
A gym business plan is a crucial document that outlines the strategies and goals for starting and running a successful gym. It provides a roadmap for entrepreneurs, guiding them through the process of establishing and growing their fitness facility. To create an effective gym business plan, it is important to include six main parts:
Executive summary
Business and domain names
Market analysis and research
Operations plan
Marketing and advertising
Financial plan
01. Executive summary
The executive summary is a concise overview of your gym business plan. It provides an introduction to your gym, highlighting its unique selling points and summarizing the key elements of your plan. The executive summary should include a brief description of your gym, your mission statement, an overview of the target market and competitive landscape, a summary of your marketing, your advertising strategies and an outline of your financial projections (including how you plan to raise money for your business ).
Example of an executive summary for a gym:
“ABC Fitness is a state-of-the-art gym located in downtown Cityville. Our mission is to provide a welcoming and inclusive environment where individuals of all fitness levels can achieve their health and wellness goals. With top-of-the-line equipment, certified trainers and a variety of group exercise classes, we aim to be the premier fitness destination in our community.
In an increasingly health-conscious society, the demand for fitness facilities continues to grow. ABC Fitness differentiates itself by offering personalized training programs tailored to each member's specific needs. Our strategic location in the heart of the city ensures easy accessibility for our target market of urban professionals.
To attract customers, we will implement a comprehensive marketing campaign that includes online advertising, social media promotions, and partnerships with local businesses. We project steady growth in membership numbers over the next three years, resulting in increased revenue and profitability.”
02. Business and domain names
Choosing the right business name for your gym is crucial for building brand awareness and trust. It should reflect your gym's values, target market and unique selling proposition. Here are some steps to consider when deciding on a company name:
Brainstorm keywords and concepts related to fitness, health and wellness
Use a business name generator (or specifically a small business name generator ) for inspiration and to generate unique name ideas
Consider the availability of domain names associated with your chosen company name
Check the availability of the chosen domain name using domain registration websites
Be inspired: Gym business name ideas , Fitness business name ideas
Learn more about registering your business once you’ve landed on your business name and legal structure.
03. Market analysis and research
Including market analysis and research in your gym business plan is essential for understanding the competitive environment and developing a better business strategy.
Target market: Analyze the target market by identifying demographics, psychographics, and fitness preferences of potential customers. Conduct a SWOT analysis (strengths, weaknesses, opportunities and threats) to assess your gym's internal and external factors.
Competitors: Research competitors in your area to understand their strengths, weaknesses, pricing strategies and marketing efforts. Identify opportunities for differentiation by offering unique services or targeting underserved segments of the market.
04. Operations plan
The operations plan outlines the logistical aspects of your gym, including location, premises, equipment and staffing needs. Make sure to select a suitable location that is easily accessible, has ample parking and aligns with where your target market is based.
Then determine the size and layout of your gym space based on the number of equipment, exercise areas, locker rooms and amenities. Research and budget for the necessary gym equipment, including cardio machines, weightlifting equipment and fitness accessories.
Lastly outline staffing requirements by identifying the number of trainers, instructors, front desk staff and maintenance personnel needed.
05. Marketing and advertising plan
The marketing and advertising plan lays out the strategies and campaigns you will implement to promote your gym business. To do this you’ll need to first identify your target audience and tailor your marketing efforts to reach them effectively.
Then, plan out a strong brand identity by creating a logo, color scheme and visual elements that resonate with your target market. (Need help creating a logo? Check out these fitness logo ideas or Wix’s free logo maker .)
Consider using various marketing channels such as social media platforms, online advertising, local partnerships and community events. Then plan compelling content that highlights the benefits of joining your gym and showcases success stories from existing members. You can also implement referral programs or incentives to encourage current members to bring in new customers.
06. Financial plan
The financial plan is a cornerstone of any gym business plan, providing an in-depth overview of how the business will secure initial funding, manage expenses, generate revenue and outline the projected time frame for achieving profitability. This section is pivotal in demonstrating the financial viability and sustainability of the gym business to potential investors, stakeholders and lenders.
Startup costs and funding: Starting a gym business entails various upfront costs that should be carefully estimated for accurate financial planning. These costs include lease or purchase of the gym space, equipment procurement, interior design, licensing and permits, marketing, initial staffing and operational supplies. Funding for these startup costs can come from diverse sources, such as personal savings, business loans, grants, partnerships or investments.
Revenue projections: Revenue projections provide a forecast of the expected income over a specific period, usually the first few years of the gym's operation. These projections should be based on thorough market research, competitor analysis and realistic expectations.
Profit and loss projection: The profit and loss projection, also known as the income statement , offers an overview of the gym business's revenue, expenses and net profit or loss over a specific period. It provides insights into the financial health of the business and its potential profitability.
Time frame for profitability: The time frame for achieving profitability in a gym business depends on factors like membership growth, marketing strategies, operational efficiency and retention rates. Typically, gyms aim to reach profitability within the first year or two of operation
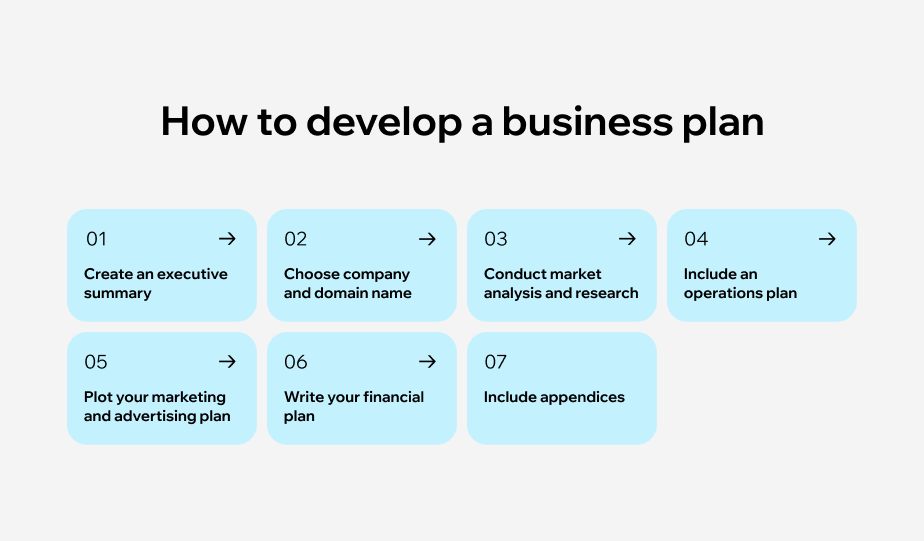
Gym business plan examples
Creating a gym business plan from scratch can be a daunting task, especially if you're new to entrepreneurship. Fortunately, there are many resources available that provide gym business plan templates to help you get started. These templates serve as a framework for organizing your ideas and ensuring that you include all the necessary components in your plan. Here are two draft business plans for hypothetical gym businesses, incorporating the main parts discussed earlier.
By using templates as a starting point, you can customize them to fit your specific vision and goals for your gym. Remember to conduct thorough research, analyze your target market and develop strategies that set your gym apart from the competition. With a well-crafted gym business plan in hand, you'll be well-equipped to start your journey towards building a successful fitness facility.
Business plan template 1: neighborhood fitness studio
The Neighborhood Fitness Studio is a boutique gym located in a residential area, catering to individuals seeking a personalized fitness experience. Our mission is to provide a welcoming and supportive environment where members can achieve their health and wellness goals. With certified trainers, state-of-the-art equipment and a variety of group exercise classes, we aim to become the go-to fitness destination in our community.
Company name and domain name
We have chosen the name "FitHub" for our neighborhood fitness studio. The domain name www.fithub.com is available and aligns well with our brand identity.
Our target market consists of local residents aged 25 to 45 who value convenience and personalized attention
Competitor analysis reveals that there is no other gym within a two-mile radius offering specialized fitness programs tailored to individual needs
We plan to differentiate ourselves by offering personalized training plans, nutrition counseling and small group classes
We have secured a 2,000 square foot space in a commercial building with ample parking for our gym
The gym will be equipped with cardio machines, free weights, strength training equipment and a designated area for group exercise classes
We will hire certified personal trainers, fitness instructors and front desk staff to ensure excellent customer service
Marketing and advertising plan
We will utilize social media platforms like Facebook and Instagram to promote our gym and engage with potential customers
Local partnerships with health food stores and wellness centers will allow us to reach a wider audience
We will offer a free trial period for new members and implement a referral program to incentivize word-of-mouth marketing
Business plan template 2: upscale fitness center
The Upscale Fitness Center is a premium gym targeting affluent individuals who prioritize luxury and exclusivity. Our mission is to provide a high-end fitness experience that combines cutting-edge equipment, personalized training and luxurious amenities. With our state-of-the-art facility, expert trainers and exclusive membership perks, we aim to become the preferred fitness destination for the elite in our city.
We have chosen the name "Elevate Fitness" for our upscale fitness center. The domain name www.elevatefitness.com is available and reflects our commitment to helping members elevate their fitness journey.
Marketing analysis and research
Our target market consists of high-income individuals aged 35 to 55 who value personalized service and are willing to invest in their health
Competitor analysis reveals that there are no other fitness centers in our city offering the same level of luxury and exclusivity
We plan to differentiate ourselves by offering VIP amenities, personalized training programs and exclusive member events
We have secured a 10,000 square foot space in a prime location, featuring high-end finishes and top-of-the-line fitness equipment
The fitness center will include dedicated areas for cardio, strength training, group exercise classes and a spa-like relaxation area
We will hire certified personal trainers, nutritionists and spa professionals to provide a comprehensive wellness experience for our members
We will leverage digital marketing strategies, such as targeted online advertising and influencer partnerships to reach our affluent target market
Exclusive membership events and collaborations with luxury brands will create buzz and attract high-profile individuals
We will offer personalized consultations and complimentary services to prospective members to showcase the unique value of our fitness center
Got your eye on other business types?
How to start an online business
How to start a consulting business
How to start a fitness business
How to start a fitness clothing line
How to start a makeup line
How to start a candle business
How to start a clothing business
How to start an online boutique
How to start a T-shirt business
How to start a jewelry business
How to start a subscription box business
How to start a beauty business
How to start a frozen food business
How to start a DJ business
How to start a flower business
How to start a plumbing business
How to start a baking business
How to start a babysitting business
How to start a virtual assistant business
How to start a car wash business
How to start a food prep business
How to start a pool cleaning business
How to start an eCommerce business
How to start a dropshipping business
How to start a farming business
How to start a rental property business
Looking to start a business in a specific state?
How to start a business in Arizona
How to start a business in South Carolina
How to start a business in Virginia
How to start a business in Michigan
How to start a business in California
How to start a business in Florida
How to start a business in Texas
How to start a business in Wisconsin
Want to create another type of business plan?
How to create a real estate business plan
How to create a flower business plan
How to create a medical supply business plan
How to create a car wash business plan
How to create a contractor business plan
How to create a DJ business plan
How to create a dog walking business plan
How to create a clothing line business plan
How to create a construction business plan
How to create a painting business plan
How to create a plumbing business plan
How to create a rental property business plan
How to create a bar business plan
How to create a photographer business plan
How to create a cleaning business plan
How to create a restaurant business plan
How to create a coffee shop business plan
Related Posts
How to write an effective nail salon business plan
How to create flower business plan
Was this article helpful?
Money blog: McDonald's changes iconic Happy Meal box; AI-powered mortgage lender cuts rates
The fast food giant has made the change to shine a light on mental health. Read this and all the latest consumer and personal finance news in the Money blog - and leave a comment or your money problem in the box below.
Tuesday 14 May 2024 21:00, UK
- Strong wage growth shrinks hope of interest rate cut
- McDonald's changes iconic Happy Meal box
- AI-powered mortgage lender cuts rates twice in a week
- Traitors winner reveals what he's spent his prize money on so far
Essential reads
- The 'fast food' trend hitting Michelin starred restaurants
- What is PIP - and what could government changes mean?
- How to make sure your car passes its MOT
- 'Loud budgeting': The money-saving trend that has nothing to do with giving up your daily coffee
- Money Problem: My workplace is bringing in new clock-in system to pay us by the minute - is this allowed?
- Best of the Money blog - an archive
Ask a question or make a comment
The Duke and Duchess of Sussex's Archewell Foundation has been labelled "delinquent" in the US for failing to submit annual records.
A letter was sent to the charity on 3 May by by California's Registry of Charities and Fundraisers, saying it has been "listed as delinquent" for "failing to submit required annual report(s) and/or renewal fees".
The letter said an organisation listed as delinquent is banned from "soliciting or disbursing charitable funds" and its registration may be "suspended or revoked".
It is understood that a physical cheque was sent by Archewell Foundation but not received, and a new one has been sent to resolve the issue.
It is believed the charity was only made aware of this when the delinquency notice was published.
Read more on this story below...
Shares of US video game retailer GameStop have soared again today, fuelled by the return of online influencer "Roaring Kitty" to social media.
Real name Keith Gill, the influencer's first online post caused shares to jump yesterday, with another surge reported today.
The retailer's shares rallied 132% in pre-market trading before falling back to about 80% up as US markets opened.
Mr Gill shared a meme and more than 10 clips from movies including The Avengers and Tombstone. Though the posts didn't mention any company names, GameStop and US cinema chain AMC were the most-traded stocks by investors yesterday and today, according to data from JP Morgan.
He is credited with helping to fuel the "meme stock" craze during the COVID pandemic, which saw GameStop shares rise more than 1,000%. They later collapsed as interest faded.
Tesco's managing director has seen his pay deal more than double to almost £10m.
That's 431 times the wage of the average £23,010 salary for a Tesco worker.
Ken Murphy received a pay packet worth £9.93m for the year to February, the supermarket's annual report revealed.
His pay deal came to £4.4m in the previous financial year.
The rise was driven by £4.91m from his performance share plan (PSP) after he helped lead the company to higher profits in the face of challenging inflation.
This PSP payment will be paid out in Tesco shares and is based on the company's performance since 2021.
It comes on top of an annual salary of £1.64m and an annual bonus of £3.38m.
The group's chief finance officer, Imran Nawaz, also saw his annual pay package more than double.
He received a total £4.95m for the year, jumping from £2.27m in the previous financial year.
The retailer was criticised for revealing a £2.83bn profit for the year to February when many customers had been impacted by rampant food and drink inflation.
Alison Platt, chairwoman of the Tesco remuneration committee, said the pay boost reflects the fact "Tesco has delivered for all of its stakeholders over the last year".
She added: "Tesco remains committed to a competitive and fair reward package for all colleagues and over the last two years we have invested more than £800m in colleague pay, as well as significantly enhancing the range of wellbeing benefits we offer."
Sony's operating profit has climbed 5% this business year - even as it forecasts lower PlayStation 5 sales.
The Japanese entertainment and electronics company said its operating profit is expected to come in at 1.28 trillion yen (£6.5bn) in the year ending March.
Sony, a major supplier of image sensors for smartphones, said its chips business is expected to book a 40% rise in operating profit on higher sales and lower costs.
At its gaming unit, revenues are expected to fall with the PlayStation 5 in its fourth year, but Sony said user engagement and cost control could drive future profitability at the business.
It predicted PlayStation 5 sales will fall to 18 million units from last year's 20.8 million.
Cheaper energy deals for new customers could potentially return in October, with the industry regulator announcing a review of their ban.
Ofgem is consulting on removing the block on acquisition-only tariffs in an attempt to encourage competition between suppliers.
The ban was introduced as a short-term measure in April 2022 to protect consumers during the energy crisis, and was due to be lifted in March next year.
Now, the regulator has said that it is the right time to consider removing it as the energy market continues to stabilise.
MoneySavingExpert Martin Lewis welcomed the consultation, saying: "We need anything possible right now to stimulate competition and bring prices down."
"In normal times, I wouldn't call for firms to be allowed to offer new customers cheaper prices than existing, yet these aren't normal times."
Melinda French Gates has left the charity she set up with her former husband, Microsoft billionaire Bill Gates, after the couple's divorce.
In a statement, she said she would step down from her position at the Bill & Melinda Gates Foundation on 7 June.
You can see her full statement below...
The foundation was created in 2000 and it is one of the most influential charitable organisations in the world.
It has spent billions working to tackle poverty and disease around the world.
Bill and Melinda Gates announced they were divorcing three years ago after being married for 27 years.
An AI-powered mortgage lender has cut rates for a second time this week.
MPowered has reduced all its two and five year fixed deals, with rates starting at 4.37% down from 4.59%.
"The swap markets are moving at pace at present, and it is important that as a responsible lender we are able to react and pass on any savings we can to borrowers," said Matt Surridge, sales director of MPowered Mortgages.
"I'm therefore really pleased we are one of the first, if not the first, to cut rates this week, having already cut rates once in the past week."
The company uses AI in its mortgage process and is a fully digital platform.
McDonald's has decided to remove the iconic smile from its Happy Meal box in a bid to teach children about their emotions.
Instead, a sheet of stickers depicting different moods will be placed inside, which children can use to express their feelings.
A QR code for a mental health hub will also be placed on the red packaging to provide its younger customers with different resources about emotional wellbeing.
The move comes as part of Mental Health Week, with research by the fast food chain finding nearly half of children feel pressure to be happy all the time.
Football legend Rio Ferdinand has teamed up with the company to support the campaign, which runs until 19 May.
The father-of-five said: "It's our job to empower our children to express themselves freely and support them every step of the way in understanding that it's okay to not be happy all the time."
The Traitors' winner Harry Clark has revealed he's only spent some of his prize money so far, and it's gone towards clearing his relatives' debts.
The 23-year-old won £95,150 after successfully convincing his fellow contestants that he was a faithful in the second season of the show.
Speaking on the TV BAFTAs red carpet, the former British Army engineer said his dad has stopped him from spending the cash and has been looking after him.
"He's got his head screwed on. He's been making sure I can get my first place," he told reporters.
"I've just given my family some dosh, just to pay off their debts and stuff like that, so they don't have to worry anymore.
"That's all I've wanted to do in my life."
Police are no longer interested in dealing with shoplifting and retailers are being forced to spend "a lot of money" on protecting themselves, the chairman of M&S has claimed.
Archie Norman said stores have resorted to installing new camera systems and employing store detectives to try to keep crime rates down.
"We get very little help from the police," he told LBC's Nick Ferrari at Breakfast.
"I think we have to accept that the police are not interested in this sort of crime any more. Whether we like it or not, that's the way it has gone."
Shoplifting is at the highest levels since records began in 2003, according to the Office for National Statistics.
It has risen by 37% since last year.
Mr Norman said thefts had surged since the pandemic, and the rising cost of living crisis was also causing problems.
"When people are hard up, or particularly when there's a growth in other forms of crime, particularly drugs-related crime, then one way of financing it is to go and steal from shops… it's understandable given what we've been through in the last couple of years, we've seen more of that," he added.
A change to the law in 2014 now means shoplifting goods worth less than £200 is only a summary offence.
This may have prompted police to pay less attention to it, and it has been on the rise since.
Home Office data also show the number of shoplifting charges has fallen in recent years.
Taking further action wasn't considered to be "in the public interest" in most cases.
Sky News has contacted the Home Office for comment.
By James Sillars , business news reporter
The prospects for a Bank of England interest rate cut are almost 50/50.
That's according to the latest financial market expectations in reaction to this morning's employment figures.
They showed the pace of wage growth remaining stubbornly high - overshooting the expectations of economists.
Strong wage growth is not what the Bank wants to see, as it fears a surge in consumer spending power driving a new wave of inflation.
There is a further set of wage data before the Bank's next rate-setting meeting on 20 June.
That may not help those seeking a cut in borrowing costs, however, as it will reflect the impact of April's big rise in the National Living Wage.
Away from the interest rate cut speculation, the FTSE 100 has opened flat for a second day.
Currys is among stocks doing well on the wider stock market.
The electricals retailer saw its shares trading almost 8% higher in early deals after it raised its annual profit outlook.
Those of Greggs, however, were down almost 1% despite a leap in sales.
The bakery to fast food chain said its performance was in line with expectations and, as such, it had no impact on its forecasts for the full year.
Wages grew by 6% in the three months to March, excluding bonuses, according to the Office for National Statistics.
This is slightly above economists' expectations - bad news for the Bank of England, which wants to see wage growth fall to help ease inflation as it weighs when to cut 16-year-high interest rates.
The Bank is watching wages closely as it looks to bring inflation back to its 2% target, and cooling earnings growth is seen as being key to paving the way for it to begin cutting rates.
In real terms - taking Consumer Prices Index inflation into account - pay rose 2.4% across the period.
In March alone, that figure was 3% - the highest level of growth since July 2021, when it hit 3.9%.
"Earnings growth in cash terms remains high, with the recent falls in the rate now levelling off while, with inflation falling, real pay growth remains at its highest level in well over two years," said ONS director of economic statistics Liz McKeown.
Meanwhile, unemployment ticked up to 4.3% from January to March, compared to 4.2% in the previous three months, December to February.
The number of job vacancies remains about pre-pandemic levels, but has been declining for 22 consecutive months, said Ms McKeown.
"With unemployment also increasing, the number of unemployed people per vacancy has continued to rise, approaching levels seen before the onset of COVID-19."
Be the first to get Breaking News
Install the Sky News app for free

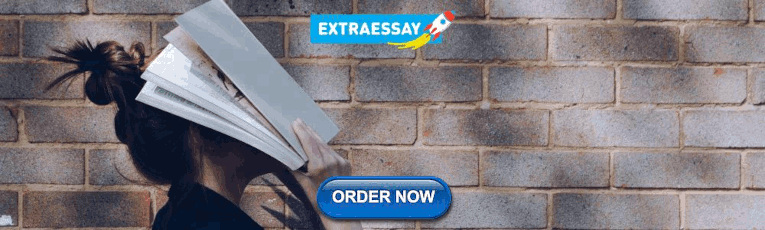
IMAGES
VIDEO
COMMENTS
Writing an analysis requires a particular structure and key components to create a compelling argument. The following steps can help you format and write your analysis: Choose your argument. Define your thesis. Write the introduction. Write the body paragraphs. Add a conclusion. 1. Choose your argument.
Create a research paper outline. Write a first draft of the research paper. Write the introduction. Write a compelling body of text. Write the conclusion. The second draft. The revision process. Research paper checklist. Free lecture slides.
The analysis shows that you can evaluate the evidence presented in the research and explain why the research could be important. Summary. The summary portion of the paper should be written with enough detail so that a reader would not have to look at the original research to understand all the main points. At the same time, the summary section ...
Step 1: Find a topic and review the literature. As we mentioned earlier, in a research paper, you, as the researcher, will try to answer a question.More specifically, that's called a research question, and it sets the direction of your entire paper. What's important to understand though is that you'll need to answer that research question with the help of high-quality sources - for ...
Step 1: Write your hypotheses and plan your research design. To collect valid data for statistical analysis, you first need to specify your hypotheses and plan out your research design. Writing statistical hypotheses. The goal of research is often to investigate a relationship between variables within a population. You start with a prediction ...
Definition: Research Paper is a written document that presents the author's original research, analysis, and interpretation of a specific topic or issue. It is typically based on Empirical Evidence, and may involve qualitative or quantitative research methods, or a combination of both. The purpose of a research paper is to contribute new ...
Communications Program. 79 John F. Kennedy Street Cambridge, Massachusetts 02138. TIPS FOR WRITING ANALYTIC RESEARCH PAPERS. • Papers require analysis, not just description. When you describe an existing situation (e.g., a policy, organization, or problem), use that description for some analytic purpose: respond to it, evaluate it according ...
The most logical way to structure quantitative results is to frame them around your research questions or hypotheses. For each question or hypothesis, share: A reminder of the type of analysis you used (e.g., a two-sample t test or simple linear regression). A more detailed description of your analysis should go in your methodology section.
The results are reported and reveals the analysis. The analysis section is where the writer describes what was done with the data found. In order to write the analysis section it is important to know what the analysis consisted of, but does not mean data is needed. The analysis should already be performed to write the results section.
2. Create an outline for your analysis. Building on your thesis and the arguments you sketched out while doing your close read of the document, create a brief outline. Make sure to include the main arguments you would like to make as well as the evidence you will use to support each argument.
A research question is what a study aims to answer after data analysis and interpretation. The answer is written in length in the discussion section of the paper. ... of the study meant to address the problem posed in the research question.1 An excellent research question clarifies the research writing while facilitating understanding of the ...
The results chapter (also referred to as the findings or analysis chapter) is one of the most important chapters of your dissertation or thesis because it shows the reader what you've found in terms of the quantitative data you've collected. It presents the data using a clear text narrative, supported by tables, graphs and charts.
Table of contents. Step 1: Consider your aims and approach. Step 2: Choose a type of research design. Step 3: Identify your population and sampling method. Step 4: Choose your data collection methods. Step 5: Plan your data collection procedures. Step 6: Decide on your data analysis strategies.
Definition of research in data analysis: According to LeCompte and Schensul, research data analysis is a process used by researchers to reduce data to a story and interpret it to derive insights. The data analysis process helps reduce a large chunk of data into smaller fragments, which makes sense. Three essential things occur during the data ...
Analysis. Analysis is a type of primary research that involves finding and interpreting patterns in data, classifying those patterns, and generalizing the results. It is useful when looking at actions, events, or occurrences in different texts, media, or publications. Analysis can usually be done without considering most of the ethical issues ...
Qualitative Research Methodology. This is a research methodology that involves the collection and analysis of non-numerical data such as words, images, and observations. This type of research is often used to explore complex phenomena, to gain an in-depth understanding of a particular topic, and to generate hypotheses.
By Meg McMahon. Analyzing the notes from a user research study is an essential part of answering the research questions that prompted the study. During the analysis, you or your team decide what insights are generated from the notes taken about the research studies. The insights should relate to the research questions posed at the beginning of ...
Abstract. Reporting of statistical analysis is essential in any clinical and translational research study. However, medical research studies sometimes report statistical analysis that is either inappropriate or insufficient to attest to the accuracy and validity of findings and conclusions. Published works involving inaccurate statistical ...
Step 4: Create a research design. The research design is a practical framework for answering your research questions. It involves making decisions about the type of data you need, the methods you'll use to collect and analyze it, and the location and timescale of your research. There are often many possible paths you can take to answering ...
The conclusion of your literature review should summarize the primary arguments highlighted in the review and position your research within the context of the literature. The primary aim of this section is to explain the necessity of your research study based on the gaps it fills in the existing literature.The conclusion should align your research study with the upcoming thesis chapters.
By writing about a source or collection of sources, you will have the chance to wrestle with some of the ideas that you are learning about in the course. Through the careful work of considering evidence and assumptions and thinking through the logic of arguments, you will begin to figure out what you think about complicated or controversial topics.
Writing the annotation - Evaluation and analysis; Writing the annotation - Reflection; Conclusion and check your understanding; Case study; Essay. How to build an essay; Example essay outlines; Taking it further; Journal article. Planning the article; Writing the article; Submitting the article; Literature review. The process of writing a ...
Some survey data analysis methods include sorting data into categories and using statistical tactics to identify trends and patterns. The goal is to take these raw data responses and turn them into a clear story that answers your research questions to help you make informed decisions.
The analysis included 3,297 individuals for the pathological study and 10,039 for the clinical study. ... Future research must prioritize the inclusion of diverse populations to elucidate the full ...
Table of contents. Step 1: Reading the text and identifying literary devices. Step 2: Coming up with a thesis. Step 3: Writing a title and introduction. Step 4: Writing the body of the essay. Step 5: Writing a conclusion. Other interesting articles.
The executive summary is a concise overview of your gym business plan. It provides an introduction to your gym, highlighting its unique selling points and summarizing the key elements of your plan. The executive summary should include a brief description of your gym, your mission statement, an overview of the target market and competitive landscape, a summary of your marketing, your ...
The suitability model I intend to follow includes collecting and gathering soil type and habitat data, along with elevation and DEM data. I will then reclassify those rasters by the value's importance/suitability to milkweed growth and use the reclassified values to conduct a weighted overlay analysis, which will provide a raster that gives the least and most suitable areas for milkweed growth.
Research proposal examples. Writing a research proposal can be quite challenging, but a good starting point could be to look at some examples. We've included a few for you below. Example research proposal #1: "A Conceptual Framework for Scheduling Constraint Management".
The fast food giant has made the change to shine a light on mental health. Read this and all the latest consumer and personal finance news in the Money blog - and leave a comment or your money ...
To analyze data collected in a statistically valid manner (e.g. from experiments, surveys, and observations). Meta-analysis. Quantitative. To statistically analyze the results of a large collection of studies. Can only be applied to studies that collected data in a statistically valid manner.