Finding better solutions for strategic business challenges
- Training & Education
Rotterdam School of Management, Erasmus University (RSM ) has announced a new two-day course set to teach managers to think like strategy consultants. ‘Strategic Problem-Solving’ will teach participants how to apply rigorous problem-solving methods in order to make and execute strategy.
RSM is the international business school of the Erasmus University Rotterdam. The institution offers a variety of business undergraduate and postgraduate programmes taught mostly in English.
The two-day programma ' Strategic Problem-Solving ' is mainly aimed at professionals outside of the consulting industry, the class is intended to boost participants’ problem-solving skills and their effectiveness in finding solutions to strategic business problems.
According to RSM, those in attendance will learn a variety of best practice methods and techniques as developed by top consulting firms such as McKinsey & Company and Boston Consulting Group .
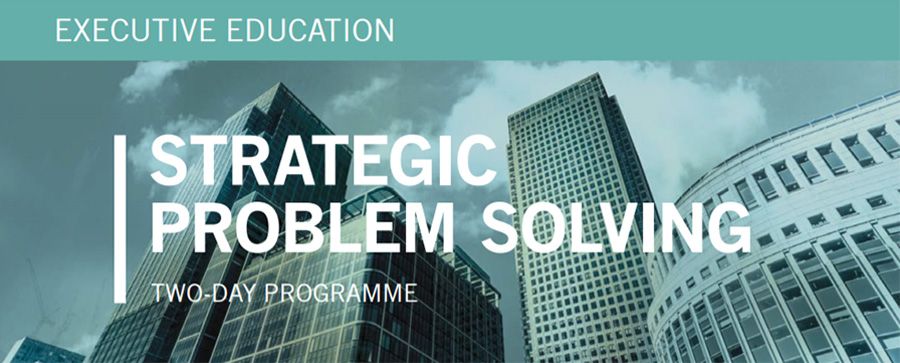
While the course is designed to help businesses think more like strategy consultants, however, that is not to say it would not also benefit consultants themselves. The event is also very relevant for internal consultants and external consultants seeking to gain more strategic skills for new or more senior roles at their respective firms.
Running again in December 2019 and April 2020, the programme will enable participants to gain more confidence as their ‘toolbox’ of available solutions for business problems grows. It will also teach them how to use these methods and techniques, their limitations and common pitfalls, and allow them to practice their newly gained skills via case discussions, small group exercises, and presentations.
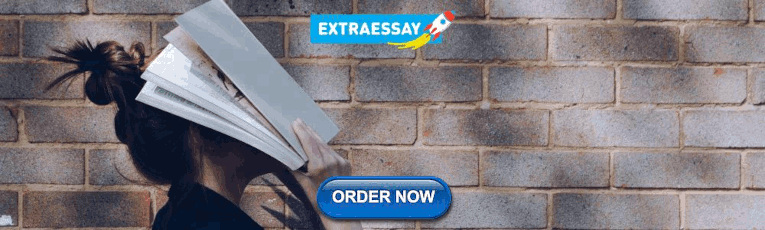
Strategy experts
The event is overseen by Marc Baaij and James Parker. Marc Baaij is an associate professor of strategic management at RSM, and specialises in methods and techniques for strategy development and execution, having previously worked for Boston Consulting Group as a manager of research and strategy consultant, and at IBM in sales and marketing positions. James Parker meanwhile is a Partner at Parma Consulting Group, and has more than 30 years of experience in consulting and managing professional organisations, having also worked for McKinsey & Company in North America, the UK and the Netherlands.
Experiences
Speaking on an earlier run of the course, Anne-Marie Hendriks, Senior Sales and Business Development Manager at Philips, said, “The course exceeded my expectations, and will change something in the way I do my work. The teacher was knowledgeable with heaps of experience, and I also liked that he used a lot of real examples to explain concepts. I walked away from the programme with several practical tools on how to approach problems, and with a good dose of energy.”
Yolanda Barreros Gutiérrez, Partner, Barreros & Gutierrez Consulting, added, “The senior level of all of the participants allowed us to move quickly from the theory to the practical application of key strategic tools. During the problem-solving workshops in small groups, we used both theoretical and real-world case studies that provided an excellent means of practicing the new approach to identifying and framing problems correctly for different strategic challenges.”
Other programmes
RSM offers a variety of programmes relevant for professionals in the professional services industry. The programme Foundations of Strategy aims to help organisations understand the main strategic tools and skills needed to maintain a competitive advantage.
The Make Strategy Work programme for senior managers focuses on strategy execution, helping attendees understand their role in making strategy work for an organisation, and to know when to amend strategy in light of changing circumstances.
Related links
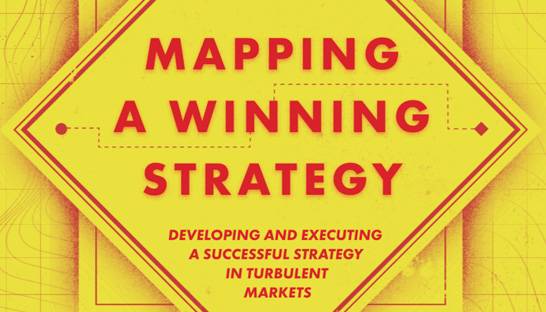
Subscribe to our newsletter.
Management news.
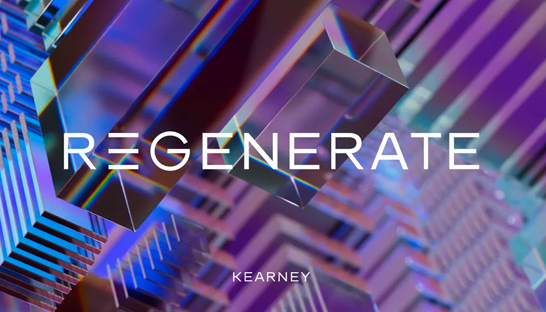
Strategy news
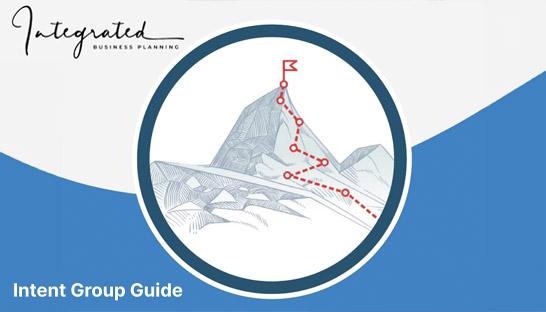
Training & Education news
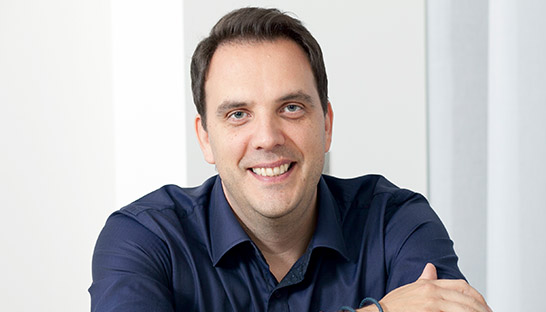
- News archive
- Consulting.us
- Consulting.ca
- Consultancy.uk
- Consultancy.in
- Consultancy.nl
- Consultancy.co.za
- Consultancy.eu
- Consultancy.asia
- Consultancy-me.com
- Consultancy.lat
- Consultancy.africa
- Consultancy.com.au
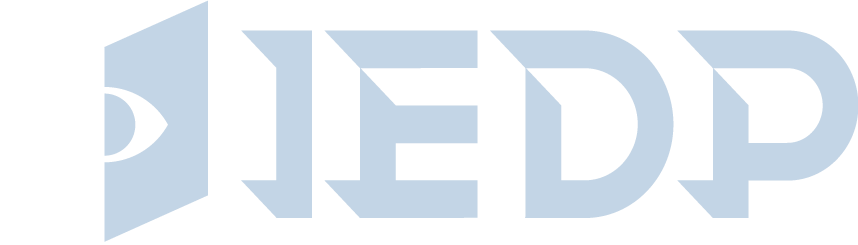
- Executive Education Index
- Program Finder
- Masters for Executives
- Developing Leaders
- Developing Leaders Quarterly
- IMPACT STORIES
- FROM THE MAGAZINE
- BOOK REVIEWS
- Managing people
- Organizational
- Customer Focus
- Articles by topic
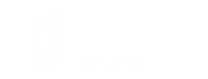
The Strategy Consultants' Approach to Problem Solving
Course dates:, language of instruction:.
Improve your problem solving skills during this two-day programme. This programme will boost your effectiveness in finding solutions to strategic business problems. You will learn a variety of best practice methods and techniques as developed by top global management consulting firms such as McKinsey & Company and BCG . In two days you will learn how to identify a problem, how to handle the planning, communication, and the successful implementation of your solution.
Before the start of the programme, you will be asked to identify a strategic issue you face in your work. Together with faculty and peers, you will work on defining an approach to solving it.
During the programme you will:
- gain more confidence as your ‘toolbox’ of available solutions for business problems grows
- learn the business problem solving methods and techniques used by top management consulting firms
- understand how to use these methods and techniques, its limitations and common pitfalls
- practise your newly gained skills via case discussions, small group exercises, and presentations.
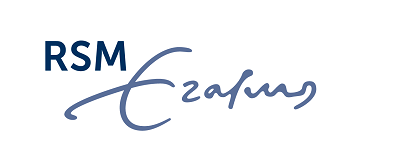
RSM - Rotterdam School of Management
Rotterdam School of Management Erasmus University Burgemeester Oudlaan 50 3062 PA Rotterdam The Netherlands
http://www.rsm.nl/executive-education/
ARTICLES YOU MIGHT LIKE
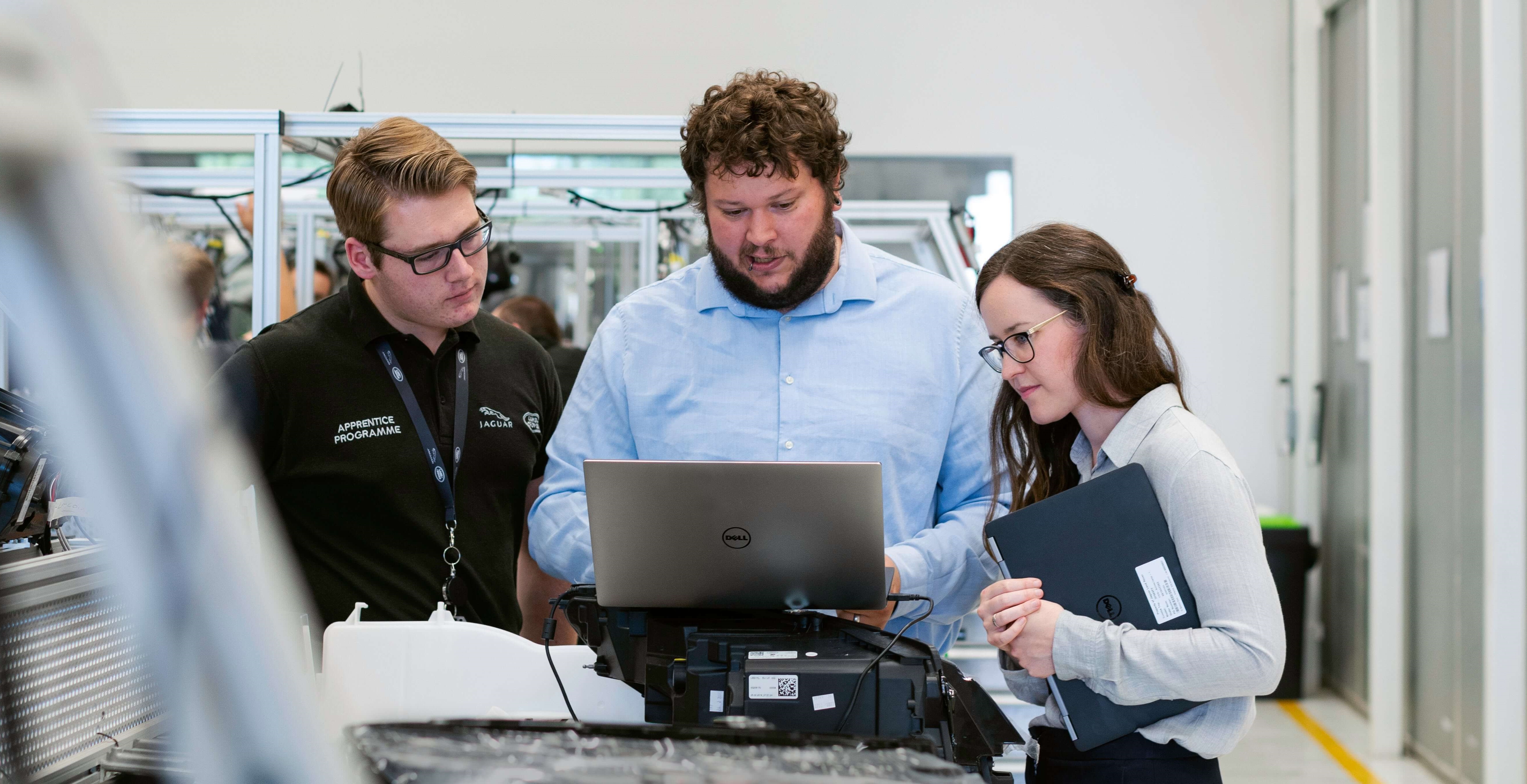
New study reveals how perceptions of competence versus interpersonal skills can be inaccurately judged when choosing team members
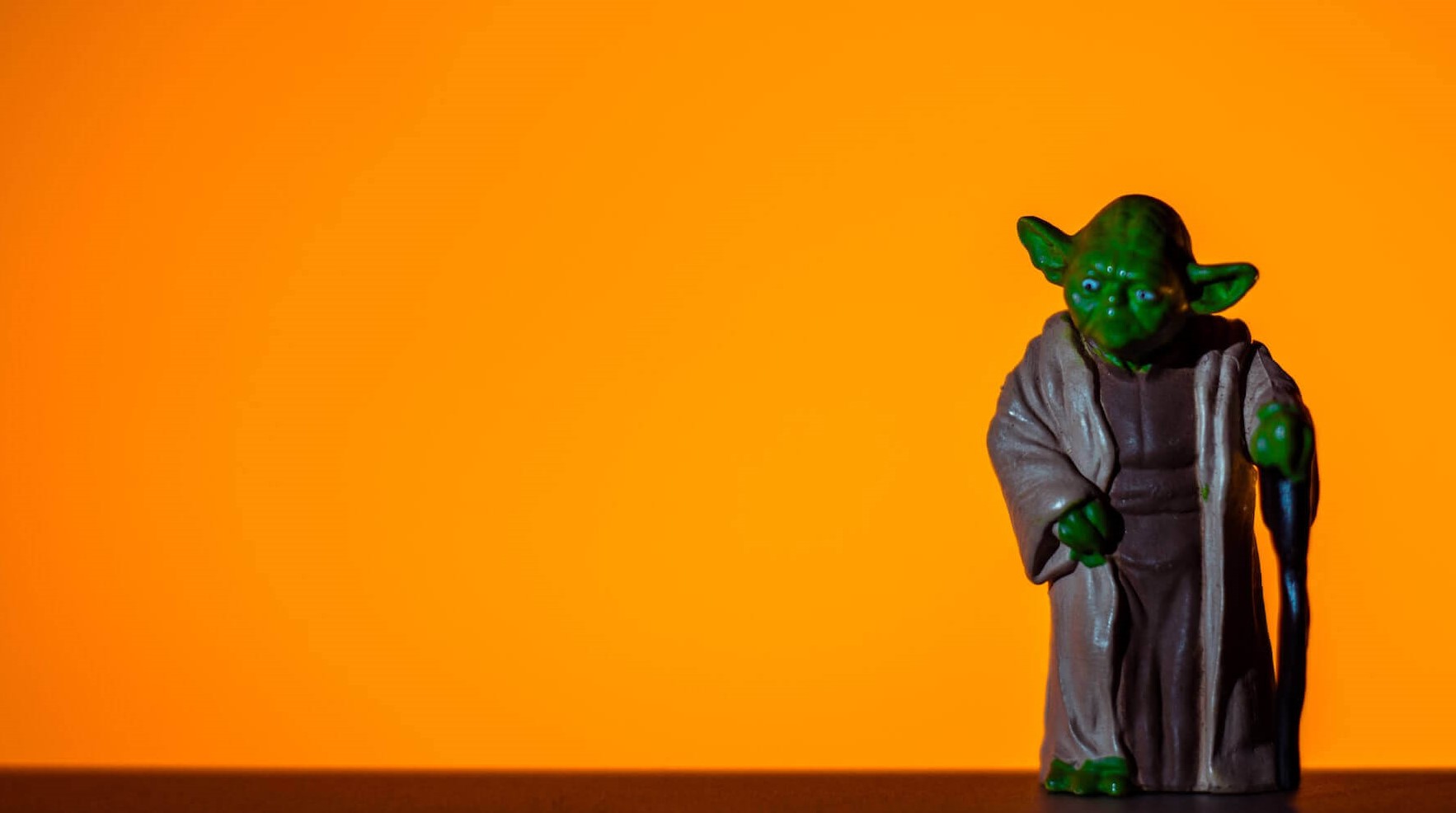
MORE FROM THIS PROVIDER
- ALL PROGRAMS BY THIS PROVIDER >
All the best analysis, articles, resources, and upcoming programs - to your inbox
DEVELOPING LEADERS QUARTERLY MAGAZINE AND WEEKLY BRIEFING EMAILS
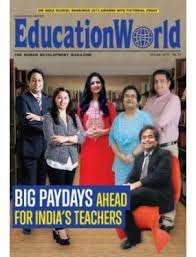
Developing Leaders Magazine: The Essential Leadership Quarterly
For information on print subscriptions sign up below and check the relevant box
The Executive Briefing and Upcoming Programs
All the latest analysis, stories and resources from IEDP.com – as well as invitations to webinars and live events, and a look at the best upcoming programs – direct to your inbox.

- privacy policy
- cookie policy
- accessibility
IEDP © 2016 All rights reserved.
How to master the seven-step problem-solving process
In this episode of the McKinsey Podcast , Simon London speaks with Charles Conn, CEO of venture-capital firm Oxford Sciences Innovation, and McKinsey senior partner Hugo Sarrazin about the complexities of different problem-solving strategies.
Podcast transcript
Simon London: Hello, and welcome to this episode of the McKinsey Podcast , with me, Simon London. What’s the number-one skill you need to succeed professionally? Salesmanship, perhaps? Or a facility with statistics? Or maybe the ability to communicate crisply and clearly? Many would argue that at the very top of the list comes problem solving: that is, the ability to think through and come up with an optimal course of action to address any complex challenge—in business, in public policy, or indeed in life.
Looked at this way, it’s no surprise that McKinsey takes problem solving very seriously, testing for it during the recruiting process and then honing it, in McKinsey consultants, through immersion in a structured seven-step method. To discuss the art of problem solving, I sat down in California with McKinsey senior partner Hugo Sarrazin and also with Charles Conn. Charles is a former McKinsey partner, entrepreneur, executive, and coauthor of the book Bulletproof Problem Solving: The One Skill That Changes Everything [John Wiley & Sons, 2018].
Charles and Hugo, welcome to the podcast. Thank you for being here.
Hugo Sarrazin: Our pleasure.
Charles Conn: It’s terrific to be here.
Simon London: Problem solving is a really interesting piece of terminology. It could mean so many different things. I have a son who’s a teenage climber. They talk about solving problems. Climbing is problem solving. Charles, when you talk about problem solving, what are you talking about?
Charles Conn: For me, problem solving is the answer to the question “What should I do?” It’s interesting when there’s uncertainty and complexity, and when it’s meaningful because there are consequences. Your son’s climbing is a perfect example. There are consequences, and it’s complicated, and there’s uncertainty—can he make that grab? I think we can apply that same frame almost at any level. You can think about questions like “What town would I like to live in?” or “Should I put solar panels on my roof?”
You might think that’s a funny thing to apply problem solving to, but in my mind it’s not fundamentally different from business problem solving, which answers the question “What should my strategy be?” Or problem solving at the policy level: “How do we combat climate change?” “Should I support the local school bond?” I think these are all part and parcel of the same type of question, “What should I do?”
I’m a big fan of structured problem solving. By following steps, we can more clearly understand what problem it is we’re solving, what are the components of the problem that we’re solving, which components are the most important ones for us to pay attention to, which analytic techniques we should apply to those, and how we can synthesize what we’ve learned back into a compelling story. That’s all it is, at its heart.
I think sometimes when people think about seven steps, they assume that there’s a rigidity to this. That’s not it at all. It’s actually to give you the scope for creativity, which often doesn’t exist when your problem solving is muddled.
Simon London: You were just talking about the seven-step process. That’s what’s written down in the book, but it’s a very McKinsey process as well. Without getting too deep into the weeds, let’s go through the steps, one by one. You were just talking about problem definition as being a particularly important thing to get right first. That’s the first step. Hugo, tell us about that.
Hugo Sarrazin: It is surprising how often people jump past this step and make a bunch of assumptions. The most powerful thing is to step back and ask the basic questions—“What are we trying to solve? What are the constraints that exist? What are the dependencies?” Let’s make those explicit and really push the thinking and defining. At McKinsey, we spend an enormous amount of time in writing that little statement, and the statement, if you’re a logic purist, is great. You debate. “Is it an ‘or’? Is it an ‘and’? What’s the action verb?” Because all these specific words help you get to the heart of what matters.
Want to subscribe to The McKinsey Podcast ?
Simon London: So this is a concise problem statement.
Hugo Sarrazin: Yeah. It’s not like “Can we grow in Japan?” That’s interesting, but it is “What, specifically, are we trying to uncover in the growth of a product in Japan? Or a segment in Japan? Or a channel in Japan?” When you spend an enormous amount of time, in the first meeting of the different stakeholders, debating this and having different people put forward what they think the problem definition is, you realize that people have completely different views of why they’re here. That, to me, is the most important step.
Charles Conn: I would agree with that. For me, the problem context is critical. When we understand “What are the forces acting upon your decision maker? How quickly is the answer needed? With what precision is the answer needed? Are there areas that are off limits or areas where we would particularly like to find our solution? Is the decision maker open to exploring other areas?” then you not only become more efficient, and move toward what we call the critical path in problem solving, but you also make it so much more likely that you’re not going to waste your time or your decision maker’s time.
How often do especially bright young people run off with half of the idea about what the problem is and start collecting data and start building models—only to discover that they’ve really gone off half-cocked.
Hugo Sarrazin: Yeah.
Charles Conn: And in the wrong direction.
Simon London: OK. So step one—and there is a real art and a structure to it—is define the problem. Step two, Charles?
Charles Conn: My favorite step is step two, which is to use logic trees to disaggregate the problem. Every problem we’re solving has some complexity and some uncertainty in it. The only way that we can really get our team working on the problem is to take the problem apart into logical pieces.
What we find, of course, is that the way to disaggregate the problem often gives you an insight into the answer to the problem quite quickly. I love to do two or three different cuts at it, each one giving a bit of a different insight into what might be going wrong. By doing sensible disaggregations, using logic trees, we can figure out which parts of the problem we should be looking at, and we can assign those different parts to team members.
Simon London: What’s a good example of a logic tree on a sort of ratable problem?
Charles Conn: Maybe the easiest one is the classic profit tree. Almost in every business that I would take a look at, I would start with a profit or return-on-assets tree. In its simplest form, you have the components of revenue, which are price and quantity, and the components of cost, which are cost and quantity. Each of those can be broken out. Cost can be broken into variable cost and fixed cost. The components of price can be broken into what your pricing scheme is. That simple tree often provides insight into what’s going on in a business or what the difference is between that business and the competitors.
If we add the leg, which is “What’s the asset base or investment element?”—so profit divided by assets—then we can ask the question “Is the business using its investments sensibly?” whether that’s in stores or in manufacturing or in transportation assets. I hope we can see just how simple this is, even though we’re describing it in words.
When I went to work with Gordon Moore at the Moore Foundation, the problem that he asked us to look at was “How can we save Pacific salmon?” Now, that sounds like an impossible question, but it was amenable to precisely the same type of disaggregation and allowed us to organize what became a 15-year effort to improve the likelihood of good outcomes for Pacific salmon.
Simon London: Now, is there a danger that your logic tree can be impossibly large? This, I think, brings us onto the third step in the process, which is that you have to prioritize.
Charles Conn: Absolutely. The third step, which we also emphasize, along with good problem definition, is rigorous prioritization—we ask the questions “How important is this lever or this branch of the tree in the overall outcome that we seek to achieve? How much can I move that lever?” Obviously, we try and focus our efforts on ones that have a big impact on the problem and the ones that we have the ability to change. With salmon, ocean conditions turned out to be a big lever, but not one that we could adjust. We focused our attention on fish habitats and fish-harvesting practices, which were big levers that we could affect.
People spend a lot of time arguing about branches that are either not important or that none of us can change. We see it in the public square. When we deal with questions at the policy level—“Should you support the death penalty?” “How do we affect climate change?” “How can we uncover the causes and address homelessness?”—it’s even more important that we’re focusing on levers that are big and movable.
Would you like to learn more about our Strategy & Corporate Finance Practice ?
Simon London: Let’s move swiftly on to step four. You’ve defined your problem, you disaggregate it, you prioritize where you want to analyze—what you want to really look at hard. Then you got to the work plan. Now, what does that mean in practice?
Hugo Sarrazin: Depending on what you’ve prioritized, there are many things you could do. It could be breaking the work among the team members so that people have a clear piece of the work to do. It could be defining the specific analyses that need to get done and executed, and being clear on time lines. There’s always a level-one answer, there’s a level-two answer, there’s a level-three answer. Without being too flippant, I can solve any problem during a good dinner with wine. It won’t have a whole lot of backing.
Simon London: Not going to have a lot of depth to it.
Hugo Sarrazin: No, but it may be useful as a starting point. If the stakes are not that high, that could be OK. If it’s really high stakes, you may need level three and have the whole model validated in three different ways. You need to find a work plan that reflects the level of precision, the time frame you have, and the stakeholders you need to bring along in the exercise.
Charles Conn: I love the way you’ve described that, because, again, some people think of problem solving as a linear thing, but of course what’s critical is that it’s iterative. As you say, you can solve the problem in one day or even one hour.
Charles Conn: We encourage our teams everywhere to do that. We call it the one-day answer or the one-hour answer. In work planning, we’re always iterating. Every time you see a 50-page work plan that stretches out to three months, you know it’s wrong. It will be outmoded very quickly by that learning process that you described. Iterative problem solving is a critical part of this. Sometimes, people think work planning sounds dull, but it isn’t. It’s how we know what’s expected of us and when we need to deliver it and how we’re progressing toward the answer. It’s also the place where we can deal with biases. Bias is a feature of every human decision-making process. If we design our team interactions intelligently, we can avoid the worst sort of biases.
Simon London: Here we’re talking about cognitive biases primarily, right? It’s not that I’m biased against you because of your accent or something. These are the cognitive biases that behavioral sciences have shown we all carry around, things like anchoring, overoptimism—these kinds of things.
Both: Yeah.
Charles Conn: Availability bias is the one that I’m always alert to. You think you’ve seen the problem before, and therefore what’s available is your previous conception of it—and we have to be most careful about that. In any human setting, we also have to be careful about biases that are based on hierarchies, sometimes called sunflower bias. I’m sure, Hugo, with your teams, you make sure that the youngest team members speak first. Not the oldest team members, because it’s easy for people to look at who’s senior and alter their own creative approaches.
Hugo Sarrazin: It’s helpful, at that moment—if someone is asserting a point of view—to ask the question “This was true in what context?” You’re trying to apply something that worked in one context to a different one. That can be deadly if the context has changed, and that’s why organizations struggle to change. You promote all these people because they did something that worked well in the past, and then there’s a disruption in the industry, and they keep doing what got them promoted even though the context has changed.
Simon London: Right. Right.
Hugo Sarrazin: So it’s the same thing in problem solving.
Charles Conn: And it’s why diversity in our teams is so important. It’s one of the best things about the world that we’re in now. We’re likely to have people from different socioeconomic, ethnic, and national backgrounds, each of whom sees problems from a slightly different perspective. It is therefore much more likely that the team will uncover a truly creative and clever approach to problem solving.
Simon London: Let’s move on to step five. You’ve done your work plan. Now you’ve actually got to do the analysis. The thing that strikes me here is that the range of tools that we have at our disposal now, of course, is just huge, particularly with advances in computation, advanced analytics. There’s so many things that you can apply here. Just talk about the analysis stage. How do you pick the right tools?
Charles Conn: For me, the most important thing is that we start with simple heuristics and explanatory statistics before we go off and use the big-gun tools. We need to understand the shape and scope of our problem before we start applying these massive and complex analytical approaches.
Simon London: Would you agree with that?
Hugo Sarrazin: I agree. I think there are so many wonderful heuristics. You need to start there before you go deep into the modeling exercise. There’s an interesting dynamic that’s happening, though. In some cases, for some types of problems, it is even better to set yourself up to maximize your learning. Your problem-solving methodology is test and learn, test and learn, test and learn, and iterate. That is a heuristic in itself, the A/B testing that is used in many parts of the world. So that’s a problem-solving methodology. It’s nothing different. It just uses technology and feedback loops in a fast way. The other one is exploratory data analysis. When you’re dealing with a large-scale problem, and there’s so much data, I can get to the heuristics that Charles was talking about through very clever visualization of data.
You test with your data. You need to set up an environment to do so, but don’t get caught up in neural-network modeling immediately. You’re testing, you’re checking—“Is the data right? Is it sound? Does it make sense?”—before you launch too far.
Simon London: You do hear these ideas—that if you have a big enough data set and enough algorithms, they’re going to find things that you just wouldn’t have spotted, find solutions that maybe you wouldn’t have thought of. Does machine learning sort of revolutionize the problem-solving process? Or are these actually just other tools in the toolbox for structured problem solving?
Charles Conn: It can be revolutionary. There are some areas in which the pattern recognition of large data sets and good algorithms can help us see things that we otherwise couldn’t see. But I do think it’s terribly important we don’t think that this particular technique is a substitute for superb problem solving, starting with good problem definition. Many people use machine learning without understanding algorithms that themselves can have biases built into them. Just as 20 years ago, when we were doing statistical analysis, we knew that we needed good model definition, we still need a good understanding of our algorithms and really good problem definition before we launch off into big data sets and unknown algorithms.
Simon London: Step six. You’ve done your analysis.
Charles Conn: I take six and seven together, and this is the place where young problem solvers often make a mistake. They’ve got their analysis, and they assume that’s the answer, and of course it isn’t the answer. The ability to synthesize the pieces that came out of the analysis and begin to weave those into a story that helps people answer the question “What should I do?” This is back to where we started. If we can’t synthesize, and we can’t tell a story, then our decision maker can’t find the answer to “What should I do?”
Simon London: But, again, these final steps are about motivating people to action, right?
Charles Conn: Yeah.
Simon London: I am slightly torn about the nomenclature of problem solving because it’s on paper, right? Until you motivate people to action, you actually haven’t solved anything.
Charles Conn: I love this question because I think decision-making theory, without a bias to action, is a waste of time. Everything in how I approach this is to help people take action that makes the world better.
Simon London: Hence, these are absolutely critical steps. If you don’t do this well, you’ve just got a bunch of analysis.
Charles Conn: We end up in exactly the same place where we started, which is people speaking across each other, past each other in the public square, rather than actually working together, shoulder to shoulder, to crack these important problems.
Simon London: In the real world, we have a lot of uncertainty—arguably, increasing uncertainty. How do good problem solvers deal with that?
Hugo Sarrazin: At every step of the process. In the problem definition, when you’re defining the context, you need to understand those sources of uncertainty and whether they’re important or not important. It becomes important in the definition of the tree.
You need to think carefully about the branches of the tree that are more certain and less certain as you define them. They don’t have equal weight just because they’ve got equal space on the page. Then, when you’re prioritizing, your prioritization approach may put more emphasis on things that have low probability but huge impact—or, vice versa, may put a lot of priority on things that are very likely and, hopefully, have a reasonable impact. You can introduce that along the way. When you come back to the synthesis, you just need to be nuanced about what you’re understanding, the likelihood.
Often, people lack humility in the way they make their recommendations: “This is the answer.” They’re very precise, and I think we would all be well-served to say, “This is a likely answer under the following sets of conditions” and then make the level of uncertainty clearer, if that is appropriate. It doesn’t mean you’re always in the gray zone; it doesn’t mean you don’t have a point of view. It just means that you can be explicit about the certainty of your answer when you make that recommendation.
Simon London: So it sounds like there is an underlying principle: “Acknowledge and embrace the uncertainty. Don’t pretend that it isn’t there. Be very clear about what the uncertainties are up front, and then build that into every step of the process.”
Hugo Sarrazin: Every step of the process.
Simon London: Yeah. We have just walked through a particular structured methodology for problem solving. But, of course, this is not the only structured methodology for problem solving. One that is also very well-known is design thinking, which comes at things very differently. So, Hugo, I know you have worked with a lot of designers. Just give us a very quick summary. Design thinking—what is it, and how does it relate?
Hugo Sarrazin: It starts with an incredible amount of empathy for the user and uses that to define the problem. It does pause and go out in the wild and spend an enormous amount of time seeing how people interact with objects, seeing the experience they’re getting, seeing the pain points or joy—and uses that to infer and define the problem.
Simon London: Problem definition, but out in the world.
Hugo Sarrazin: With an enormous amount of empathy. There’s a huge emphasis on empathy. Traditional, more classic problem solving is you define the problem based on an understanding of the situation. This one almost presupposes that we don’t know the problem until we go see it. The second thing is you need to come up with multiple scenarios or answers or ideas or concepts, and there’s a lot of divergent thinking initially. That’s slightly different, versus the prioritization, but not for long. Eventually, you need to kind of say, “OK, I’m going to converge again.” Then you go and you bring things back to the customer and get feedback and iterate. Then you rinse and repeat, rinse and repeat. There’s a lot of tactile building, along the way, of prototypes and things like that. It’s very iterative.
Simon London: So, Charles, are these complements or are these alternatives?
Charles Conn: I think they’re entirely complementary, and I think Hugo’s description is perfect. When we do problem definition well in classic problem solving, we are demonstrating the kind of empathy, at the very beginning of our problem, that design thinking asks us to approach. When we ideate—and that’s very similar to the disaggregation, prioritization, and work-planning steps—we do precisely the same thing, and often we use contrasting teams, so that we do have divergent thinking. The best teams allow divergent thinking to bump them off whatever their initial biases in problem solving are. For me, design thinking gives us a constant reminder of creativity, empathy, and the tactile nature of problem solving, but it’s absolutely complementary, not alternative.
Simon London: I think, in a world of cross-functional teams, an interesting question is do people with design-thinking backgrounds really work well together with classical problem solvers? How do you make that chemistry happen?
Hugo Sarrazin: Yeah, it is not easy when people have spent an enormous amount of time seeped in design thinking or user-centric design, whichever word you want to use. If the person who’s applying classic problem-solving methodology is very rigid and mechanical in the way they’re doing it, there could be an enormous amount of tension. If there’s not clarity in the role and not clarity in the process, I think having the two together can be, sometimes, problematic.
The second thing that happens often is that the artifacts the two methodologies try to gravitate toward can be different. Classic problem solving often gravitates toward a model; design thinking migrates toward a prototype. Rather than writing a big deck with all my supporting evidence, they’ll bring an example, a thing, and that feels different. Then you spend your time differently to achieve those two end products, so that’s another source of friction.
Now, I still think it can be an incredibly powerful thing to have the two—if there are the right people with the right mind-set, if there is a team that is explicit about the roles, if we’re clear about the kind of outcomes we are attempting to bring forward. There’s an enormous amount of collaborativeness and respect.
Simon London: But they have to respect each other’s methodology and be prepared to flex, maybe, a little bit, in how this process is going to work.
Hugo Sarrazin: Absolutely.
Simon London: The other area where, it strikes me, there could be a little bit of a different sort of friction is this whole concept of the day-one answer, which is what we were just talking about in classical problem solving. Now, you know that this is probably not going to be your final answer, but that’s how you begin to structure the problem. Whereas I would imagine your design thinkers—no, they’re going off to do their ethnographic research and get out into the field, potentially for a long time, before they come back with at least an initial hypothesis.

Want better strategies? Become a bulletproof problem solver
Hugo Sarrazin: That is a great callout, and that’s another difference. Designers typically will like to soak into the situation and avoid converging too quickly. There’s optionality and exploring different options. There’s a strong belief that keeps the solution space wide enough that you can come up with more radical ideas. If there’s a large design team or many designers on the team, and you come on Friday and say, “What’s our week-one answer?” they’re going to struggle. They’re not going to be comfortable, naturally, to give that answer. It doesn’t mean they don’t have an answer; it’s just not where they are in their thinking process.
Simon London: I think we are, sadly, out of time for today. But Charles and Hugo, thank you so much.
Charles Conn: It was a pleasure to be here, Simon.
Hugo Sarrazin: It was a pleasure. Thank you.
Simon London: And thanks, as always, to you, our listeners, for tuning into this episode of the McKinsey Podcast . If you want to learn more about problem solving, you can find the book, Bulletproof Problem Solving: The One Skill That Changes Everything , online or order it through your local bookstore. To learn more about McKinsey, you can of course find us at McKinsey.com.
Charles Conn is CEO of Oxford Sciences Innovation and an alumnus of McKinsey’s Sydney office. Hugo Sarrazin is a senior partner in the Silicon Valley office, where Simon London, a member of McKinsey Publishing, is also based.
Explore a career with us
Related articles.
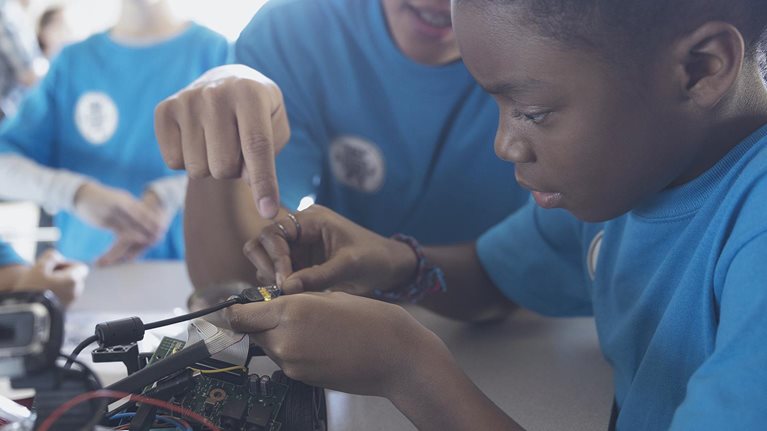
Strategy to beat the odds
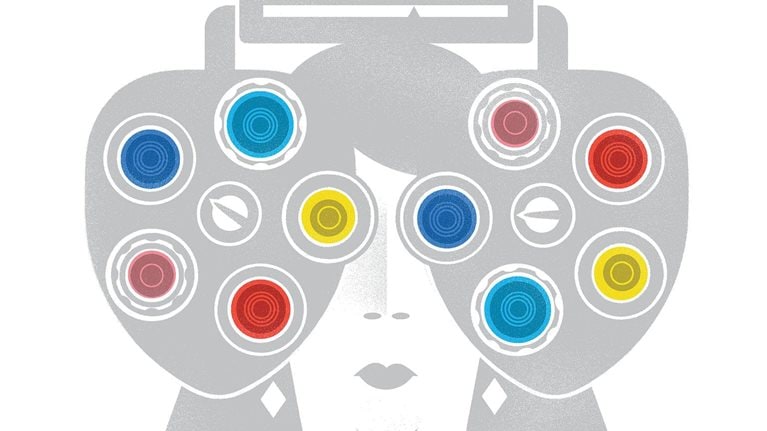
Five routes to more innovative problem solving
- Click to set high contrast
- Leadership team
- Advisory board
- Our history
- RSM Faculty Council
- Honorary doctorates
- Accreditations
- Positive Change
- Institutional partners
- Corporate partners
- Meet our employees
- Open positions
- Admission requirements
- Tuition fees
- Scholarships
- Open online courses
- Bachelor Bedrijfskunde (Dutch)
- Bachelor International Business Administration
- MSc programmes
- Premaster programmes
- Executive Masters
- International Full-time MBA
- Executive MBA
- Global Executive MBA
- Cologne-Rotterdam MBA
- Programmes for individuals
- Programmes for organisations
- Part-time PhD programme
- Full-time PhD programme
- Career Centre
- International Office
- Study Advice
- Examination board
- Faculty directory
- RSM Discovery
- Business-Society Management
- Marketing Management
- Organisation and Personnel Management
- Strategic Management and Entrepreneurship
- Technology and Operations Management
- Corporate Communication Centre
- Erasmus Centre for Leadership
- Partnerships Resource Centre
- Erasmus Centre for Data Analytics
- Erasmus Centre for Entrepreneurship
- Erasmus Initiative: Dynamics of Inclusive Prosperity
- Case Development Centre
- Erasmus Centre for Women and Organisations
- Institutions for Collective Action
- Erasmus Platform for Sustainable Value Creation
- Erasmus Centre for Study and Career Success
- Future students
- Current students
- Exchange students in & out
- Professionals
- Recruiters and organisations
- Researchers
- Journalists & media
- Future employees
Students explore structured problem-solving with Maersk Management Consulting
Date Friday, 12 June 2020
- View all news articles
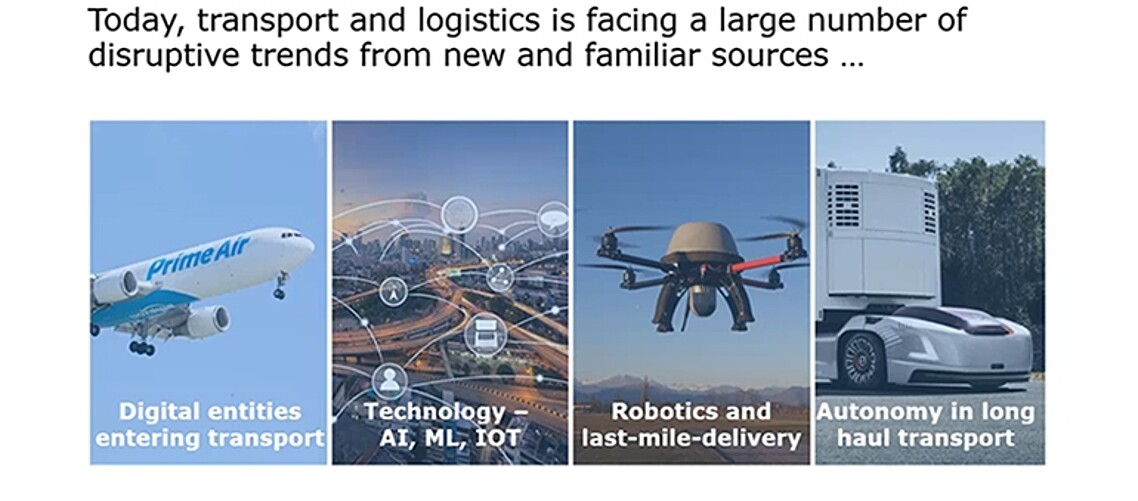
Master students from Rotterdam School of Management, Erasmus University (RSM) attended a ‘structured thinking and problem-solving’ webinar in May 2020. The online seminar was co-organised by Maersk Management Consulting (MMC) and CEMS Club Rotterdam . The students focused on structured thinking and powerful communication tools, followed by a structured problem-solving workshop, and a case study on transport logistics in Nigeria.
Around 30 business students from several master programmes were welcomed by Sergey Podolskiy , project leader at MMC and Max Weilguny , consultant at MMC and RSM alumnus. MMC offers a wide range of management consulting services to all business units within the Maersk Group – a corporate partner in the CEMS Global Alliance network – with a focus on strategy, organisation, transformation, and change management.
Powerful communication through coaching
After learning about MMC’s role in the Maersk Group and the shipping industry, there was an introduction to the concept of structured thinking, followed by advice on how to use it to create powerful communication.
The students were encouraged to test their structured thinking skills by rewriting examples of poorly formulated information. Coached by MMC consultants, the students applied their new knowledge in setting their own SMART goals.
Solutions for case in Nigeria
Students were then confronted with the MECE principal, a grouping principle to separate a set of items into subsets that are mutually exclusive and collectively exhaustive. They immediately applied this in a case study based on an actual MMC project about scenario planning for APM Terminals Nigeria . This case included a complex set of factors that affect the potential solutions. The RSM students could only reach the outcome by applying the newly learned knowledge and structuring the problem accurately.
The case was concluded using the rich experience with similar problems of the MMC consultants, which not only helped the students to evaluate their solutions but made their experience even more enriching.
The webinar – which would have been an on-campus event if it wasn’t for the Covid-19 crisis – was concluded with a Q&A session during which students asked questions about the topic, potentially starting their career at MMC, and consulting industry tips.
CEMS corporate partnership
The CEMS Global Alliance is a group of 32 prestigious business schools, and around 65 multinationals and NGOs around the world. Its global master programme recruits only the most internationally minded and talented students. RSM is one of these 32 top-ranked CEMS business schools, and offers the unique MSc International Management / CEMS programme. There are many benefits for organisations to become an RSM CEMS corporate partner , including recruitment of top talent, business projects and internships, knowledge-sharing and employer branding.
More information
Rotterdam School of Management, Erasmus University (RSM) is one of Europe’s top-ranked business schools. RSM provides ground-breaking research and education furthering excellence in all aspects of management and is based in the international port city of Rotterdam – a vital nexus of business, logistics and trade. RSM’s primary focus is on developing business leaders with international careers who can become a force for positive change by carrying their innovative mindset into a sustainable future. Our first-class range of bachelor, master, MBA, PhD and executive programmes encourage them to become critical, creative, caring and collaborative thinkers and doers. www.rsm.nl
For more information about RSM or this release, please contact Erika Harriford-McLaren, communications manager for RSM, on +31 10 408 2877 or by email at [email protected] .
Share current page as Facebook post
Share current page as Twitter post
Share current page as LinkedIn post
Share current page as e-mail message
Share current page as WhatsApp message
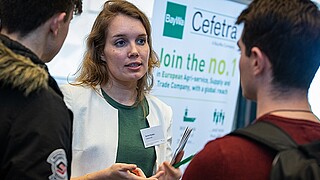
Companies search for ‘leaders of tomorrow’ at internship fair
Sixteen companies presented themselves to RSM’s business students at the Bachelor Internship Fair.
Wednesday, 8 May 2019
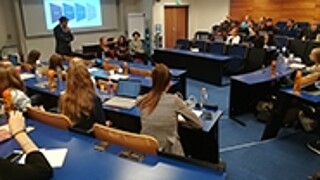
CEMS career event: “Be open to the unexpected”
Recruiters and alumni shared first-hand experiences and tips to get started on the labour market.
Thursday, 17 October 2019
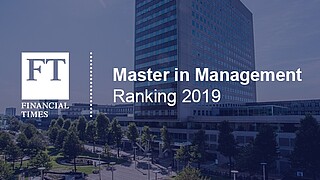
RSM climbs two places in FT Master in Management ranking
Good all-round performance consolidates RSM’s position well within the top 10.
Monday, 28 October 2019
RSM uses cookies to measure website statistics, enable social media sharing and for marketing purposes. By clicking accept cookies or by continuing to use this website, you are giving consent for us to set cookies when visiting this website. See our cookie policy for more information about cookies and how to adjust your cookie settings.
- Deutschland
- Asia, Australia & New Zealand
- Europe, Middle East & Africa
- United States & Canada
- Latinoamérica
How to become a strategic problem solver in 5 steps
A version of this tutorial originally appeared in the free Primer app .
Problem solving is an underrated talent. It helps you make clear decisions during turbulent, overwhelming times, and many experts identify it as a vital soft skill for job seekers post-pandemic .
To develop your problem-solving acumen, use a structured approach that focuses on the why, what, and how of your issue. Let’s break it down by asking five questions that will serve as a step-by-step guide.
Question 1: Why should I care about this problem?
Write a problem statement, which is a few short sentences describing an issue you’re facing that also affects your audience. Use specific, quantifiable details. Instead of, “We lose money each year due to quality issues,” a better statement would be, “In 2019, we lost $1 million due to quality errors. That’s 5% of our overall revenue.”
You don’t want to define a problem so big that you lack resources to solve it or get so specific that you miss the chance to have a greater impact. Also, avoid prematurely adding in a solution, like “We need to hire a quality assurance consultant.” Solutions come later.
Question 2: What does success look like?
Set an objective that defines what you want to achieve and key results to know when you’ve achieved it. Your objective should focus on a goal, not a solution. “Make our website an intuitive and enjoyable experience for visitors,” is an objective, but “Hire 5 UX designers,” is a solution. Then define measurable key results so you know for sure when you’ve achieved your goal. “Get increased click-throughs,” is vague, but you’ll know when you “Increase click-throughs by 30%.”
Question 3: How might I solve this problem?
Now it’s time to brainstorm potential solutions. Start by finding out as much as you can about your problem. Find out if there’s available research on problems like yours, and look to “parallel worlds” outside your industry where a similar issue has been addressed before.
If you’re holding a group brainstorm, choose one person as the moderator, and others as timekeeper and notetaker. Then brainstorm as many solutions as possible that might address your problem and help you reach your objective and key results. Tell everyone to be expansive in their thinking: Come up with both big and small ideas without self-editing.
Once you have a list of potential solutions, put them in a solution tree to help organize your goals and how you’ll get there (your strategy). Start by writing down your objective. For the sake of an easy example, let’s say your objective is “Increase product revenue.” Create branches from each objective that list solutions you brainstormed, like “Sell more of the same products,” and “Start selling new products.”
Solution tree
Think with google, share this page.
Question 4: How should I actually solve the problem?
A prioritization map helps you winnow your ideas to decide in which ones you should invest effort. Make a graph with a vertical axis labeled “impact” and a horizontal axis labeled “effort.” Then plot out all your potential solutions on the graph.
Prioritization map
High-impact and high-effort solutions will likely be the main focus of your work. Ones that are high impact and low effort are quick wins, and good for building stakeholder confidence. Low-impact and low-effort solutions should be deprioritized and only focused on when you have some free time. Low-impact and high-effort solutions are probably not worth your time.
Question 5: How can I take action?
Make a simple plan to solve your problem, avoiding unnecessary detail. You can use an action tracker, which can be a spreadsheet that breaks down who does what and when. In the first column, put all the actions that make up your problem-solving steps. In the second column, note who is responsible for each action and when it should be done.
Action tracker
Discuss and agree on task ownership and due dates with the people responsible so that everyone is on the same page. Share your action tracker with the group and regularly review it together to make sure due dates are met.
Remember that no matter how well you plan, things will change and your plan will have to change too. Be prepared to modify it so you can stay on track and achieve your goals.
Problem solving doesn’t need to be complicated. By following the right steps, you can avoid jumping into solution mode too quickly. Just think of the structure “why, what, and how,” and you’ll start to see your problems in a new way.
Others are viewing
Marketers who view this are also viewing
Why human metrics are just as important as business metrics
3 ways brands can take action today to fight racial inequity, what design thinking can teach us about teamwork in a world on pause, the ai handbook: resources and tools for marketers, 4 marketing principles to live by from the brand boss at calvin klein & pvh, how ai helps video marketers move at the speed of culture, eddie kennedy, others are viewing looking for something else, complete login.
To explore this content and receive communications from Google, please sign in with an existing Google account.
You're visiting our United States & Canada website.
Based on your location, we recommend you check out this version of the page instead:
Strategic Problem-Solving: A State of the Art
Ieee account.
- Change Username/Password
- Update Address
Purchase Details
- Payment Options
- Order History
- View Purchased Documents
Profile Information
- Communications Preferences
- Profession and Education
- Technical Interests
- US & Canada: +1 800 678 4333
- Worldwide: +1 732 981 0060
- Contact & Support
- About IEEE Xplore
- Accessibility
- Terms of Use
- Nondiscrimination Policy
- Privacy & Opting Out of Cookies
A not-for-profit organization, IEEE is the world's largest technical professional organization dedicated to advancing technology for the benefit of humanity. © Copyright 2024 IEEE - All rights reserved. Use of this web site signifies your agreement to the terms and conditions.
High Contrast
- Asia Pacific
- Latin America
- North America
- Afghanistan
- Bosnia and Herzegovina
- Cayman Islands
- Channel Islands
- Czech Republic
- Dominican Republic
- El Salvador
- Equatorial Guinea
- Hong Kong SAR, China
- Ireland (Republic of)
- Ivory Coast
- Macedonia (Republic of North)
- Netherlands
- New Zealand
- Philippines
- Puerto Rico
- Sao Tome & Principe
- Saudi Arabia
- South Africa
- Switzerland
- United Kingdom
- News releases
- RSM in the news
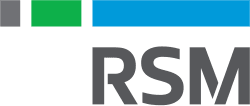
- AI, analytics and cloud services
- Audit and assurance
- Business operations and strategy
- Business tax
- Consulting services
- Family office services
- Financial management
- Global business services
- Managed services
- Mergers and acquisitions
- Private client
- Risk, fraud and cybersecurity
- See all services and capabilities
Strategic technology alliances
- Sage Intacct
- CorporateSight
- FamilySight
- PartnerSight
Featured topics
- 2024 economy and business opportunity
- Generative AI
- Middle market economics
- Environmental, social and governance
- Supply chain
Real Economy publications
- The Real Economy
- The Real Economy Industry Outlooks
- RSM US Middle Market Business Index
- The Real Economy Blog
- Construction
- Consumer goods
- Financial services
- Food and beverage
- Health care
- Life sciences
- Manufacturing
- Nonprofit and education
- Private equity
- Professional services
- Real estate
- Technology companies
- See all industry insights
- Business strategy and operations
- Family office
- Private client services
- Financial reporting resources
- Tax regulatory resources
Platform user insights and resources
- RSM Technology Blog
- Diversity and inclusion
- Middle market focus
- Our global approach
- Our strategy
- RSM alumni connection
- RSM Impact report
- RSM Classic experience
- RSM US Alliance
Experience RSM
- Your career at RSM
- Student opportunities
- Experienced professionals
- Executive careers
- Life at RSM
- Rewards and benefits
Spotlight on culture
Work with us.
- Careers in assurance
- Careers in consulting
- Careers in operations
- Careers in tax
- Our team in India
- Our team in El Salvador
- Apply for open roles
Popular Searches
Asset Management
Health Care
Partnersite
Your Recently Viewed Pages
Lorem ipsum
Dolor sit amet
Consectetur adipising
Solver enterprise CPM implementation and optimization
Manage performance better with the solver suite and rsm, consolidate data, budget, forecast and report for quicker, better decisions..
The Solver enterprise CPM platform is a powerful tool for budgeting, forecasting, reporting, self-service analytics and more. Its modern web interface makes it simple and intuitive for users, whether your deployment is in the cloud or on-premises.
Solver features a data warehouse to consolidate company information. This ensures that data is timely and accurate, and breaks down information silos that hamper fully informed decisions. With more access and insight, your team can collaborate on everything such as strategy, forecasts, budgets, reports and analysis. Flexible dashboards deliver timely information where it’s needed, when it’s needed.
As with most powerful, flexible business systems, the success of your project depends on who you work with to configure, implement and deploy your system. Ensure that your implementation partner has both the technology and business chops to handle your situation effectively. RSM brings both facets to your engagement, plus expert accounting, tax and industry advisors. You’ll rest assured.
Why RSM should implement your Solver suite
You’ve chosen to invest in an excellent CPM suite. The same level of excellence should be part of making it work for your organization.
As the US part of a global team of business advisors, you’ll find the technology, business and industry specialists you need. Wherever you are, whatever your requirements, we are there.
Your project rolls out with our proven discovery, design and implementation processes. This is not on-the-fly, let-me-read-the-instruction-manual deployment. You get seasoned professionals. More importantly, you get confidenceAdd accordion body text
Related solutions

THE POWER OF BEING UNDERSTOOD
ASSURANCE | TAX | CONSULTING
- Technologies
- RSM US client portals
- Cybersecurity
RSM US LLP is a limited liability partnership and the U.S. member firm of RSM International, a global network of independent assurance, tax and consulting firms. The member firms of RSM International collaborate to provide services to global clients, but are separate and distinct legal entities that cannot obligate each other. Each member firm is responsible only for its own acts and omissions, and not those of any other party. Visit rsmus.com/about for more information regarding RSM US LLP and RSM International.
©2024 RSM US LLP. All rights reserved.
- Terms of Use
- Do Not Sell or Share My Personal Information (California)
Advertisement
A Comprehensive Review on RSM-Coupled Optimization Techniques and Its Applications
- Review article
- Published: 23 June 2023
- Volume 30 , pages 4831–4853, ( 2023 )
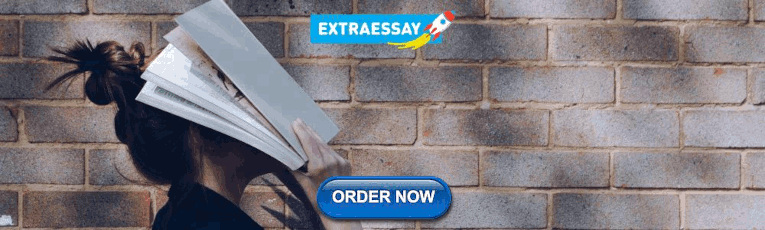
Cite this article
- Anto Susaimanickam 1 ,
- Premalatha Manickam 1 &
- Amalanathan Arputhasamy Joseph 2
416 Accesses
Explore all metrics
This review article provides a comprehensive analysis of the optimization techniques used in a wide range of engineering applications. The comparison of various approaches such as Response surface methodology (RSM), Genetic algorithm (GA) and Artificial neural network (ANN) towards optimization problems is widely elaborated. The factors that affect the optimization using various techniques are addressed along with the safety precautions to be followed in a sequential manner to achieve a better optimization model. Furthermore, the coupling of two distinct algorithms (RSM-GA, ANN-GA) are explained and this hybrid approach provides a better localizing of the optimal point with a higher accuracy.
This is a preview of subscription content, log in via an institution to check access.
Access this article
Price includes VAT (Russian Federation)
Instant access to the full article PDF.
Rent this article via DeepDyve
Institutional subscriptions
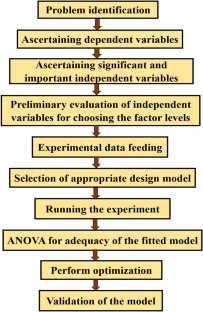
Similar content being viewed by others
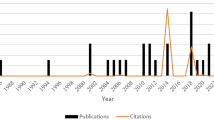
A hybrid DMAIC framework for integrating response surface methodology and multi-objective optimization methods
Aneirson Francisco da Silva, Kaio Max Aranda, … Rafael de Carvalho Miranda
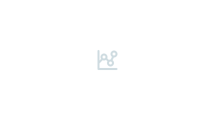
A new approach to solve multi-response statistical optimization problems using neural network, genetic algorithm, and goal attainment methods
Seyed Hamid Reza Pasandideh, Seyed Taghi Akhavan Niaki & Seyed Mahdi Atyabi
Dynamic Response Surface Method Combined with Genetic Algorithm to Optimize Extraction Process Problem
Data availability.
The datasets generated during the current study are available from the corresponding author on reasonable request.
Onwubolu GC, Babu BV (2004) New optimization techniques in engineering. Springer Berlin Heidelberg, Berlin
Book MATH Google Scholar
Pistikopoulos EN et al (2021) Process systems engineering—the generation next? Comput Chem Eng 147:107252. https://doi.org/10.1016/j.compchemeng.2021.107252
Article Google Scholar
Strawderman WE (2002) Practical optimization methods with mathematica (R) applications, optimization foundations and applications. J Am Stat Assoc 97(457):366–366. https://doi.org/10.1198/jasa.2002.s467
Sun S, Cao Z, Zhu H, Zhao J (2020) A survey of optimization methods from a machine learning perspective. IEEE Trans Cybern 50(8):3668–3681. https://doi.org/10.1109/TCYB.2019.2950779
Spedicato E, Xia Z, Zhang L (2008) ABS algorithms for optimization. Encycl Optim. https://doi.org/10.1007/978-0-387-74759-0_2
Article MATH Google Scholar
Arora RK (2016) Optimization: algorithms and applications. Choice Rev Online. https://doi.org/10.5860/choice.195857
Wilson DR, Martinez TR (2003) The general inefficiency of batch training for gradient descent learning. Neural Netw 16(10):1429–1451. https://doi.org/10.1016/S0893-6080(03)00138-2
Sergeyev YD, Kvasov DE, Mukhametzhanov MS (2018) On the efficiency of nature-inspired metaheuristics in expensive global optimization with limited budget. Sci Rep 8(1):1–9. https://doi.org/10.1038/s41598-017-18940-4
Yaqoob I et al (2016) Big data: from beginning to future. Int J Inf Manag 36(6):1231–1247. https://doi.org/10.1016/j.ijinfomgt.2016.07.009
Dean A, Voss D, Draguljić D (2017) Response surface methodology. Springer, Cham, pp 565–614
Google Scholar
Wong WK, Ming CI (2019) A review on metaheuristic algorithms: recent trends, benchmarking and applications. Int Conf Smart Comput Commun ICSCC 2019:1–5. https://doi.org/10.1109/ICSCC.2019.8843624
Dillen W, Lombaert G, Schevenels M (2021) Performance assessment of metaheuristic algorithms for structural optimization taking into account the influence of algorithmic control parameters. Front Built Environ 7(March):1–16. https://doi.org/10.3389/fbuil.2021.618851
Agrawal P, Abutarboush HF, Ganesh T, Mohamed AW (2021) Metaheuristic algorithms on feature selection: a survey of one decade of research (2009–2019). IEEE Access 9:26766–26791. https://doi.org/10.1109/ACCESS.2021.3056407
Said GAEA, Mahmoud AM (2014) A comparative study of meta-heuristic algorithms for solving quadratic assignment problem. Int J Adv Comput Sci Appl 5(1):1–6
Abdel-Basset M, Abdel-Fatah L, Sangaiah AK (2018) Chapter 10—Metaheuristic algorithms: a comprehensive review. Computational intelligence for multimedia big data on the cloud with engineering applications. Elsevier, Amsterdam, pp 185–231
Chapter Google Scholar
Zhang G, Pan L, Neri F, Gong M, Leporati A (2017) Metaheuristic optimization: algorithmic design and applications. J Optim 2017:2–4
MathSciNet MATH Google Scholar
Hussain K, Najib M, Salleh M, Cheng S, Shi Y (2019) Metaheuristic research: a comprehensive survey. Artif Intell Rev 52(4):2191–2233. https://doi.org/10.1007/s10462-017-9605-z
Slowik A, Kwasnicka H (2020) Evolutionary algorithms and their applications to engineering problems. Neural Comput Appl 32(16):12363–12379. https://doi.org/10.1007/s00521-020-04832-8
Jangid S, Puri R (2019) Evolutionary algorithms: a critical review and its future prospects. Int J Trend Res Dev 1:7–9
Chakraborty A, Kar AK (2017) Swarm intelligence: a review of algorithms swarm intelligence: a review of algorithms. Model Optim Sci Technol. https://doi.org/10.1007/978-3-319-50920-4
Brezocnik L, Fister JI, Podgorelec V (2018) Swarm intelligence algorithms for feature selection. Appl Sci. https://doi.org/10.3390/app8091521
Singh A, Kumar A (2021) Applications of nature-inspired meta-heuristic algorithms: a survey applications of nature-inspired meta-heuristic algorithms: a survey Avjeet Singh* and Anoj Kumar. Int J Adv Intell Paradig. https://doi.org/10.1504/IJAIP.2021.10027703
Priyadarshini J, Premalatha M, Cep R, Jayasudha M, Kalita K (2023) Analyzing physics-inspired metaheuristic algorithms in feature selection with K-nearest-neighbor. Appl Sci 13:906
Biswas A, Mishra KK, Tiwari S, Misra AK (2013) Physics-inspired optimization algorithms: a survey. J Optim 2013:1
Beheshti Z, Mariyam S, Shamsuddin H (2013) A review of population-based meta-heuristic algorithm. Int J Adv Soft Comput Appl 5(1):1–35
Rai R, Das A, Ray S, Gopal K (2022) Human—inspired optimization algorithms: theoretical foundations, algorithms, open—research issues and application for multi—level thresholding. Springer, Netherlands
Mingyi Zhang Y, Zhang Y (2013) The human-inspired algorithm: a hybrid nature-inspired approach to optimizing continuous functions with constraints. J Comput Intell Electron Syst 2(1):80–87
Kumar V (2022) A state-of-the-art review of heuristic and metaheuristic optimization techniques for the management of water resources. Water supply 22(4):3702–3728. https://doi.org/10.2166/ws.2022.010
Article MathSciNet Google Scholar
Bhosale V, Shastri SS, Khandare A (2017) A review of genetic algorithm used for optimizing scheduling of resource constraint construction projects. Int Res J Eng Technol 1:2869–2872
Sioshansi R, Conejo AJ (2017) Optimization in engineering, 1st edn. Springer International Publishing, Cham
Rao SS (2019) Engineering optimization: theory and practice. John Wiley & Sons
Book Google Scholar
Chen Wu Y, Wen Feng J (2018) Development and application of artificial neural network. Wirel Pers Commun 102(2):1645–1656. https://doi.org/10.1007/s11277-017-5224-x
Ilin R, Kozma R, Werbos PJ (2008) Beyond feedforward models trained by backpropagation: a practical training tool for a more efficient universal approximator. IEEE Trans Neural Networks 19(6):929–937. https://doi.org/10.1109/TNN.2008.2000396
Apicella A, Donnarumma F, Isgrò F, Prevete R (2021) A survey on modern trainable activation functions. Neural Netw 138:14–32. https://doi.org/10.1016/j.neunet.2021.01.026
Bhattacharya SS, Garlapati VK, Banerjee R (2011) Optimization of laccase production using response surface methodology coupled with differential evolution. N Biotechnol 28(1):31–39. https://doi.org/10.1016/j.nbt.2010.06.001
Sarker IH (2021) Deep learning: a comprehensive overview on techniques, taxonomy, applications and research directions. SN Comput Sci 2(6):1–20. https://doi.org/10.1007/s42979-021-00815-1
Jha AK, Sit N (2021) Comparison of response surface methodology (RSM) and artificial neural network (ANN) modelling for supercritical fluid extraction of phytochemicals from Terminalia chebula pulp and optimization using RSM coupled with desirability function (DF) and genetic. Ind Crops Prod 170:113769. https://doi.org/10.1016/j.indcrop.2021.113769
Deshwal S, Kumar A, Chhabra D (2020) Exercising hybrid statistical tools GA-RSM, GA-ANN and GA-ANFIS to optimize FDM process parameters for tensile strength improvement. CIRP J Manuf Sci Technol 31:189–199. https://doi.org/10.1016/j.cirpj.2020.05.009
Kumari M, Gupta SK (2019) Response surface methodological (RSM) approach for optimizing the removal of trihalomethanes (THMs) and its precursor’s by surfactant modified magnetic nanoadsorbents (sMNP)—an endeavor to diminish probable cancer risk. Sci Rep 9(1):1–11. https://doi.org/10.1038/s41598-019-54902-8
Sarabia LA, Ortiz MC (2009) Response surface methodology. Comprehensive chemometrics. Elsevier, Amsterdam, pp 345–390
M. C. Fu, Handbook of simulation optimization , vol. 216. 2015.
Khuri S, Mukhopadhyay AI (2010) Response surface methodology. Wiley Interdiscip Rev Comput Stat 2(2):128–149
Draper DK, Lin NR (1996) Response surface designs. Design Analy Exp 13:343–375
Article MathSciNet MATH Google Scholar
Dean D, Voss A, Draguljić D, Dean D, Voss A, Draguljić D (2017) Response surface methodology. Des Anal Exp. https://doi.org/10.1007/978-3-319-52250-0_16
Sarabia LA, Ortiz MC (2009) Response surface methodology. Compr Chemom 1(2):345–390. https://doi.org/10.1016/B978-044452701-1.00083-1
Myers S, Vining RH, Giovannitti-Jensen GG, Myers A (1992) Variance dispersion properties of second-order response surface designs. J Qual Technol 24(1):1–11
Hadiyat MA, Sopha BM, Wibowo BS (2022) Response surface methodology using observational data: a systematic literature review. Appl Sci. https://doi.org/10.3390/app122010663
Hanrahan D, Zhu G, Gibani J, Patil DG (2005) Chemometrics and statistics|experimental design. Encycl Anal Sci 8:13
Ismail M, Author C (2013) Alternative approach to fitting first-order model to the response surface methodology. Pakistan J Commer Soc Sci 7(1):157–165
Lamidi I, Olaleye S, Bankole N, Obalola Y, Aribike A, Adigun I (2022) Applications of response surface methodology (RSM) in product design, development, and process optimization. Response Surf Methodol Res Adv Appl. https://doi.org/10.5772/intechopen.106763
Alrweili H, Georgiou S, Stylianou S (2020) A new class of second-order response surface designs. IEEE Access 8:115123–115132. https://doi.org/10.1109/ACCESS.2020.3001621
Gunawan A (2014) Institutional knowledge at Singapore management university second order-response surface model for the automated parameter tuning problem second order-response surface model for the automated parameter tuning problem. IEEE Int Conf Ind Eng Eng Manag 2014:652–656
Saini N (2017) Review of selection methods in genetic algorithms. Int J Eng Comput Sci 6(12):23261–23263. https://doi.org/10.18535/ijecs/v6i12.04
Dasgupta T, Pillai NS, Rubin DB (2015) Causal inference from 2 K factorial designs by using potential outcomes. J R Stat Soc Ser B 77(4):727–753
Branson Z, Dasgupta T, Rubin DB (2016) Improving covariate balance in 2k factorial designs via rerandomization with an application to a New York city department of education high school study. Ann Appl Stat 10(4):1958–1976. https://doi.org/10.1214/16-AOAS959
Kandananond K (2013) Applying 2k factorial design to assess the performance of ANN and SVM methods for forecasting stationary and non-stationary time series. Procedia Comput Sci 22:60–69. https://doi.org/10.1016/j.procs.2013.09.081
Tablets EMI (2021) Application of Plackett–Burman design of experiments in the identification of main factors’ in the formulation of dabigatran etexilate mesylate immediate-release tablets. Int J Pharm Sci Res 12(12):6587–6592. https://doi.org/10.13040/IJPSR.0975-8232.12(12).6587-92
Ekpenyong MG, Antai SP, Asitok D, Ekpo BO (2017) Plackett–Burman design and response surface optimization of medium trace nutrients for glycolipopeptide biosurfactant production. Iran Biomed J 21(4):249–260. https://doi.org/10.18869/acadpub.ibj.21.4.249
Chaudhari SR (2020) Application of Plackett–Burman and central composite designs for screening and optimization of factor influencing the chromatographic conditions of HPTLC method for quantification of efonidipine hydrochloride. J Anal Sci Technol 11(48):1–13
Peele A, Krupanidhi S, Reddy ER, Indira M, Bobby N (2018) Plackett–Burman design for screening of process components and their effects on production of lactase by newly isolated Bacillus sp. VUVD101 strain from Dairy effluent. Beni-Suef Univ J Basic Appl Sci 7(4):543–546. https://doi.org/10.1016/j.bjbas.2018.06.006
Patel MB, Shaikh F, Patel V, Surti NI (2017) Application of simplex centroid design in formulation and optimization of floating matrix tablets of metformin. J Appl Pharm Sci 7(4):23–30. https://doi.org/10.7324/JAPS.2017.70403
Bahramparvar M, Tehrani MM (2015) Application of simplex-centroid mixture design to optimize stabilizer combinations for ice cream manufacture. J Food Sci Technol 52(3):1480–1488. https://doi.org/10.1007/s13197-013-1133-5
Article R, Reji M, Kumar R (2023) Response surface methodology (RSM): an overview to analyze multivariate data. Indian J Microbiol Res 9(4):241–248
Phanphet S (2021) Application of full factorial design for optimization of production process by turning machine. J Tianjin Univ Sci Technol ISSN 54(08):35–55. https://doi.org/10.17605/OSF.IO/3TESD
Al Sadi J (2018) Designing experiments: 3 level full factorial design and variation of processing parameters methods for polymer colors. Adv Sci Technol Eng Syst J 3(5):109–115
Salihu MM, Nwaosu CS (2021) Discrimination between 2k and 3k factorial designs using optimality based criterion Murtala Muhammad Salihu and Chigozie Sylvester Nwaosu. African Sch J pure Appl Sci 22(9):79–94
Aggarwal ML, Kaul R (1999) Hidden projection properties of some optimal designs. Stat Probab Lett 43(1):87–92. https://doi.org/10.1016/S0167-7152(98)00249-1
Kasina MM, Joseph K, John M (2020) Application of central composite design to optimize spawns propagation. Open J Optim 9:47–70. https://doi.org/10.4236/ojop.2020.93005
Sadhukhan B, Mondal NK, Chattoraj S (2016) ScienceDirect optimisation using central composite design (CCD) and the desirability function for sorption of methylene blue from aqueous solution onto Lemna major. Karbala Int J Mod Sci 2(3):145–155. https://doi.org/10.1016/j.kijoms.2016.03.005
Bayuo J, Abdullai M, Kenneth A, Pelig B (2020) Optimization using central composite design (CCD) of response surface methodology (RSM) for biosorption of hexavalent chromium from aqueous media. Appl Water Sci 10(6):1–12. https://doi.org/10.1007/s13201-020-01213-3
Hassan H, Adam SK, Alias E, Mohd M, Meor R, Affandi M (2021) Central composite design for formulation and optimization of solid lipid nanoparticles to enhance oral bioavailability of acyclovir. Molecules 26(5432):1–19
Alam P et al (2022) Box–Behnken design ( BBD ) application for optimization of chromatographic conditions in RP-HPLC method development for the estimation of thymoquinone in Nigella sativa seed powder. Processes 10:1082
Yadav P, Rastogi V, Verma A (2020) Application of Box–Behnken design and desirability function in the development and optimization of self-nanoemulsifying drug delivery system for enhanced dissolution of ezetimibe. Futur J Pharm Sci 6(1):1–20
Darvishmotevalli M, Zarei A, Moradnia M, Noorisepehr M, Mohammadi H (2019) Optimization of saline wastewater treatment using electrochemical oxidation process: Prediction by RSM method. MethodsX 6:1101–1113. https://doi.org/10.1016/j.mex.2019.03.015
Singh Pali H, Sharma A, Kumar N, Singh Y (2021) Biodiesel yield and properties optimization from Kusum oil by RSM. Fuel 291:120218. https://doi.org/10.1016/j.fuel.2021.120218
Behera SK, Meena H, Chakraborty S, Meikap BC (2018) Application of response surface methodology (RSM) for optimization of leaching parameters for ash reduction from low-grade coal. Int J Min Sci Technol 28(4):621–629. https://doi.org/10.1016/j.ijmst.2018.04.014
Hatami M (2017) Nanoparticles migration around the heated cylinder during the RSM optimization of a wavy-wall enclosure. Adv Powder Technol 28(3):890–899. https://doi.org/10.1016/j.apt.2016.12.015
Raj RA, Murugesan S (2022) Optimization of dielectric properties of pongamia pinnata methyl ester for power transformers using response surface methodology. IEEE Trans Dielectr Electr Insul 29(5):1931–1939. https://doi.org/10.1109/TDEI.2022.3190257
Kinnear KE (1994) A perspective on the work in this book. In: Kinnear KE (ed) Advances in genetic programming. MIT Press, pp 3–17
Carr J (2014) An introduction to genetic algorithms. Senior Project 1(40):7
Forrest S (1996) Genetic algorithms. ACM Comput Surv. https://doi.org/10.1145/234313.234350
McCall J (2005) Genetic algorithms for modelling and optimisation. J Comput Appl Math 184(1):205–222. https://doi.org/10.1016/j.cam.2004.07.034
Lambert-torres G, Martins HG, Coutinho MP, Silva LEB, Matsunaga FM, Carminati RA (2009) Genetic algorithm to system restoration. World Congress Electron Electric Eng. https://doi.org/10.13140/RG.2.1.4926.2482
Lima AR, de Mattos Neto PSG, Silva DA, Ferreira TAE (2016) Tests with different fitness functions for tuning of artificial neural networks with genetic algorithms. X Congresso Brasileiro de Inteligˆencia Computacional. 1(1):1–8. https://doi.org/10.21528/cbic2011-32.5
Kour H, Sharma P, Abrol P (2015) Analysis of fitness function in genetic algorithms. J Sci Tech Adv 1(3):87–89
Mandal S, Anderson TA, Turek JS, Gottschlich J, Zhou S, Muzahid A (2021) Learning fitness functions for machine programming. Proc Mach Learn Syst 1:139–155
Petridis V, Kazarlis S, Bakirtzis A (1998) Varying fitness functions in genetic algorithm constrained optimization: The cutting stock and unit commitment problems. IEEE Trans Syst Man Cybern Part B Cybern 28(5):629–640. https://doi.org/10.1109/3477.718514
Avdeenko TV, Serdyukov KE, Tsydenov ZB (2021) Formulation and research of new fitness function in the genetic algorithm for maximum code coverage. Procedia Comput Sci 186:713–720. https://doi.org/10.1016/j.procs.2021.04.194
Der Yang M, Yang YF, Su TC, Huang KS (2014) An efficient fitness function in genetic algorithm classifier for landuse recognition on satellite images. Sci World J. https://doi.org/10.1155/2014/264512
Chakraborty B (2002) Genetic algorithm with fuzzy fitness function for feature selection. IEEE Int Symp Ind Electron 1:315–319. https://doi.org/10.1109/isie.2002.1026085
Büche D, Schraudolph NN, Koumoutsakos P (2005) Accelerating evolutionary algorithms with Gaussian process fitness function models. IEEE Trans Syst Man Cybern Part C Appl Rev 35(2):183–194. https://doi.org/10.1109/TSMCC.2004.841917
Katoch S, Chauhan SS, Kumar V (2021) A review on genetic algorithm: past, present, and future. Multimed Tools Appl. https://doi.org/10.1007/s11042-020-10139-6
Haq EU, Ahmad I, Hussain A, Almanjahie IM (2019) A novel selection approach for genetic algorithms for global optimization of multimodal continuous functions. Comput Intell Neurosci. https://doi.org/10.1155/2019/8640218
Shukla A, Pandey HM, Mehrotra D (2015) Comparative review of selection techniques in genetic algorithm. Int Conf Futur Trends Comput Anal Knowl Manag. https://doi.org/10.1109/ABLAZE.2015.7154916
Jebari K, Madiafi M (2013) Selection methods for genetic algorithms. Int J Emerg Sci 3(4):333–344
Minetti G, Salto C, Alfonso H (1999) A study of performance of stochastic universal sampling versus proportional selection on genetic algorithms, I work. Investig en Ciencias la Comput 1:9–12
Pencheva T, Atanassov K, Shannon A (2009) Modelling of a stochastic universal sampling selection operator in genetic algorithms using generalized nets. Tenth Int Work Gen Nets 2009:1–7
Orong MY, Sison AM, Hernandez AA (2018) Mitigating vulnerabilities through forecasting and crime trend analysis. Eng Bus Soc Sci. https://doi.org/10.1109/ICBIR.2018.8391166
Hancock PJB (2019) Selection methods for evolutionary algorithms. Practical handbook of genetic algorithms. CRC Press, Boca Raton, pp 67–92
Champlin R, Champlin R (2018) Selection methods of genetic algorithms selection methods of genetic algorithms. Digit Commons Comput Sci 8:1
Kiran CA, Xaxa D (2015) Comparative study on various selection methods in genetic algorithm. Int J Soft Comput Artif Intell 8(3):96–103
Jannoud I, Jaradat Y, Masoud MZ, Manasrah A, Alia M (2022) The role of genetic algorithm selection operators in extending wsn stability period: a comparative study. Electron 11(1):1–16. https://doi.org/10.3390/electronics11010028
Umbarkar AJ, Sheth PD (2015) Crossover operators in genetic algorithms: a review. ICTACT J Soft Comput 06(01):1083–1092. https://doi.org/10.21917/ijsc.2015.0150
Y. Kaya, M. Uyar, and R. Tek, “A novel crossover operator for genetic algorithms: ring crossover,” arXiv Prepr. , pp. 1–4, 2011.
Anand E, Panneerselvam R (2016) A study of crossover operators for genetic algorithm and proposal of a new crossover operator to solve open shop scheduling problem. Am J Ind Bus Manag 06(06):774–789. https://doi.org/10.4236/ajibm.2016.66071
Kora P, Yadlapalli P (2017) Crossover operators in genetic algorithms: a review. Int J Comput Appl 162(10):34–36. https://doi.org/10.5120/ijca2017913370
Magalhães-Mendes J (2013) A comparative study of crossover operators for genetic algorithms to solve the job shop scheduling problem. WSEAS Trans Comput 12(4):164–173
Kumar VS, Panneerselvam R (2017) A study of crossover operators for genetic algorithms to solve VRP and its variants and new sinusoidal motion crossover operator. Int J Comput Intell Res 13(7):1717–1733
Hassanat A, Almohammadi K, Alkafaween E, Abunawas E, Hammouri A, Prasath VBS (2019) Choosing mutation and crossover ratios for genetic algorithms-a review with a new dynamic approach. Information. https://doi.org/10.3390/info10120390
O. Abdoun, J. Abouchabaka, and C. Tajani, “Analyzing the performance of mutation operators to solve the travelling salesman problem,” arXiv Prepr , 2012.
Lim SM, Sultan ABM, Sulaiman MN, Mustapha A, Leong KY (2017) Crossover and mutation operators of genetic algorithms. Int J Mach Learn Comput 7(1):9–12. https://doi.org/10.18178/ijmlc.2017.7.1.611
Nazeri Z, Khanli LM (2014) An insertion mutation operator for solving project scheduling problem. Iran Conf Intell Syst ICIS 2014:1–4. https://doi.org/10.1109/IranianCIS.2014.6802537
Soni N, Kumar T (2014) Study of various mutation operators in genetic algorithms. Int J Comput Sci Inf Technol 5(3):4519–4521
MathSciNet Google Scholar
Liu C, Kroll A (2016) Performance impact of mutation operators of a subpopulation-based genetic algorithm for multi-robot task allocation problems. Springerplus. https://doi.org/10.1186/s40064-016-3027-2
Deep K, Mebrahtu H (2011) Combined mutation operators of genetic algorithm for the travelling salesman problem Kusum. Int J Comb Optim Probl Inform 2(3):1–23
Sutton AM, Whitley LD (2014) Fitness probability distribution of bit-flip mutation. Evol Comput 23(2):217–248. https://doi.org/10.1162/EVCO
De Falco I, Della Cioppa A, Tarantino E (2002) Mutation-based genetic algorithm: performance evaluation. Appl Soft Comput 1(4):285–299. https://doi.org/10.1016/S1568-4946(02)00021-2
S. Sarmady, “An Investigation on Genetic Algorithm Parameters,” 2007.
Kumar R, Memoria M, Chandel A (2020) Performance analysis of proposed mutation operator of genetic algorithm under scheduling problem. Proc Int Conf Intell Eng Manag ICIEM 2020:193–197. https://doi.org/10.1109/ICIEM48762.2020.9160215
W et al (2020) Metadata of the chapter that will be visualized in OnlineFirst. Itib. https://doi.org/10.1007/978-3-642-03503-6
Contras D, Matei O (2016) Translation of the mutation operator from genetic algorithms to evolutionary ontologies. Int J Adv Comput Sci Appl 7(1):3–8. https://doi.org/10.14569/ijacsa.2016.070186
Bajpai P, Kumar M (2010) Genetic algorithm–an approach to solve global optimization problems. Indian J Comput Sci Eng 1(3):199–206
Elsayed SM, Sarker RA, Essam DL (2014) A new genetic algorithm for solving optimization problems. Eng Appl Artif Intell 27:57–69. https://doi.org/10.1016/j.engappai.2013.09.013
Jin Y-F, Yin Z-Y, Shen S-L, Hicher P-Y (2016) Selection of sand models and identification of parameters using an enhanced genetic algorithm. Int J Numer Anal Methods Geomech 40(8):1219–1240. https://doi.org/10.1002/nag.2487
Marcovecchio MG, Aguirre PA, Scenna NJ (2005) Global optimal design of reverse osmosis networks for seawater desalination: modeling and algorithm. Desalination 184(1–3):259–271. https://doi.org/10.1016/j.desal.2005.03.056
Al-Obaidi MA, Li JP, Kara-Zaïtri C, Mujtaba IM (2017) Optimisation of reverse osmosis based wastewater treatment system for the removal of chlorophenol using genetic algorithms. Chem Eng J 316:91–100. https://doi.org/10.1016/j.cej.2016.12.096
Ho SH, Chen CY, Lee DJ, Chang JS (2011) Perspectives on microalgal CO 2 -emission mitigation systems—a review. Biotechnol Adv 29(2):189–198. https://doi.org/10.1016/j.biotechadv.2010.11.001
Azari A, Tavakoli H, Barkdoll BD, Haddad OB (2020) Predictive model of algal biofuel production based on experimental data. Algal Res 47:101843. https://doi.org/10.1016/j.algal.2020.101843
Al-Turjman F, Abujubbeh M (2019) IoT-enabled smart grid via SM: an overview. Futur Gener Comput Syst 96:579–590. https://doi.org/10.1016/j.future.2019.02.012
Arabali A, Ghofrani M, Etezadi-Amoli M, Fadali MS, Baghzouz Y (2013) Genetic-algorithm-based optimization approach for energy management. IEEE Trans Power Deliv 28(1):162–170. https://doi.org/10.1109/TPWRD.2012.2219598
Rosa JPS, Guerra DJD, Horta NCG, Martins RMF, Lourenço NCC (2020) Overview of artificial neural networks. Appl Sci Technol 1:21–44. https://doi.org/10.1007/978-3-030-35743-6_3
Babu MS, Imai T, Sarathi R (2021) Classification of aged epoxy micro-nanocomposites through PCA- and ANN-Adopted LIBS analysis. IEEE Trans Plasma Sci 49(3):1088–1096. https://doi.org/10.1109/TPS.2021.3061410
Raghavendra U, Fujita H, Bhandary SV, Gudigar A, Tan JH, Acharya UR (2018) Deep convolution neural network for accurate diagnosis of glaucoma using digital fundus images. Inf Sci 441:41–49. https://doi.org/10.1016/j.ins.2018.01.051
Ghimire S, Deo RC, Downs NJ, Raj N (2019) Global solar radiation prediction by ANN integrated with European centre for medium range weather forecast fields in solar rich cities of Queensland Australia. J Clean Prod 216:288–310. https://doi.org/10.1016/j.jclepro.2019.01.158
Ertuğrul ÖF (2018) A novel type of activation function in artificial neural networks: trained activation function. Neural Netw 99:148–157. https://doi.org/10.1016/j.neunet.2018.01.007
Montesinos López OA, Montesinos López A, Crossa J (2022) Multivariate statistical machine learning methods for genomic prediction. Springer, Cham
Jain AK, Mao J, Mohiuddin KM (1996) Artificial neural networks: a tutorial. Computer (Long Beach Calif) 29(3):31–44. https://doi.org/10.1109/2.485891
Feng J, Lu S (2019) Performance analysis of various activation functions in artificial neural networks. J Phys Conf Ser 1237(2):111–122. https://doi.org/10.1088/1742-6596/1237/2/022030
Sharma S, Sharma S, Athaiya A (2020) Activation functions in neural networks. Int J Eng Appl Sci Technol 04(12):310–316. https://doi.org/10.33564/ijeast.2020.v04i12.054
Szandała T (2021) Review and comparison of commonly used activation functions for deep neural networks. Bio-inspired neurocomputing. Springer, Singapore, pp 203–224
Bai Y, Zhang H, Hao Y (2009) The performance of the backpropagation algorithm with varying slope of the activation function. Chaos Solitons Fractals 40(1):69–77. https://doi.org/10.1016/j.chaos.2007.07.033
Kayri M (2016) Predictive abilities of Bayesian regularization and Levenberg–Marquardt algorithms in artificial neural networks: a comparative empirical study on social data. Math Comput Appl. https://doi.org/10.3390/mca21020020
Roy RB et al (2021) A comparative performance analysis of ANN algorithms for MPPT energy harvesting in solar PV system. IEEE Access 9:102137–102152. https://doi.org/10.1109/ACCESS.2021.3096864
Namasudra S, Dhamodharavadhani S, Rathipriya R (2023) Nonlinear neural network based forecasting model for predicting COVID-19 cases. Neural Process Lett 55(1):171–191. https://doi.org/10.1007/s11063-021-10495-w
Smith JS, Member S, Wu B, Member S, Wilamowski BM, Fellow L (2019) Neural network training with Levenberg–Marquardt and adaptable weight compression. IEEE Trans Neural Netw Learning Syst 30(2):580–587
Guanabara E, Ltda K, Guanabara E, Ltda K (2022) Artificial intelligence and machine learning for EDGE computing. Elsevier. https://doi.org/10.1016/C2020-0-01569-0
A. Ranganathan, “The Levenberg-Marquardt Algorithm 3 LM as a blend of Gradient descent and Gauss-Newton itera,” Internet httpexcelsior cs ucsb educoursescs290ipdfL MA pdf , 142.June, pp. 1–5, 2004, [Online]. Available: http://twiki.cis.rit.edu/twiki/pub/Main/AdvancedDipTeamB/the-levenberg-marquardt-algorithm.pdf
Burden F, Winkler D (2008) Bayesian regularization of neural networks. Angew Chem Int Ed 6(11):951–952. https://doi.org/10.1007/978-1-60327-101-1_3
Imam A, Salami BA, Oyehan TA (2021) Predicting the compressive strength of a quaternary blend concrete using Bayesian regularized neural network. J Struct Integr Maint 6(4):237–246. https://doi.org/10.1080/24705314.2021.1892572
Yang B et al (2021) Parameter extraction of PEMFC via Bayesian regularization neural network based meta-heuristic algorithms. Energy 228:120592. https://doi.org/10.1016/j.energy.2021.120592
Liu CS, Atluri SN (2011) An iterative method using an optimal descent vector, for solving an Ill-conditioned system Bx = b, better and faster than the conjugate gradient method. C Comput Model Eng Sci 80(3–4):275–298
Møller MF (1993) A scaled conjugate gradient algorithm for fast supervised learning. Neural Netw 6(4):525–533. https://doi.org/10.1016/S0893-6080(05)80056-5
Khan TA, Alam M, Shahid Z (2019) Comparative performance analysis of Levenberg–Marquardt, Bayesian regularization and scaled conjugate gradient for the prediction of flash floods. J Inform Commun Technol Robot Appl 1:52–58
Selvamuthu D, Kumar V, Mishra A (2019) Indian stock market prediction using artificial neural networks on tick data. Financ Innov. https://doi.org/10.1186/s40854-019-0131-7
Amalanathan AJ, Vasa NJ, Harid N, Griffiths H, Sarathi R (2021) Classification of thermal ageing impact of ester fluid-impregnated pressboard material adopting LIBS. High Volt 6(4):655–664. https://doi.org/10.1049/hve2.12092
Amizhtan SK et al (2022) Experimental study and ann analysis of rheological behavior of mineral oil-based SiO 2 nanofluids. IEEE Trans Dielectr Electr Insul 29(3):956–964. https://doi.org/10.1109/TDEI.2022.3173514
Vu HL, Bolingbroke D, Ng KTW, Fallah B (2019) Assessment of waste characteristics and their impact on GIS vehicle collection route optimization using ANN waste forecasts. Waste Manag 88(2019):118–130. https://doi.org/10.1016/j.wasman.2019.03.037
Banerjee P, Sau S, Das P, Mukhopadhayay A (2015) Optimization and modelling of synthetic azo dye wastewater treatment using graphene oxide nanoplatelets: characterization toxicity evaluation and optimization using artificial neural network. Ecotoxicol Environ Saf 119:47–57. https://doi.org/10.1016/j.ecoenv.2015.04.022
Ghosal S, Chaki S (2010) Estimation and optimization of depth of penetration in hybrid CO 2 LASER-MIG welding using ANN-optimization hybrid model. Int J Adv Manuf Technol 47(9–12):1149–1157. https://doi.org/10.1007/s00170-009-2234-1
Ranade R, Alqahtani S, Farooq A, Echekki T (2019) An ANN based hybrid chemistry framework for complex fuels. Fuel 241:625–636. https://doi.org/10.1016/j.fuel.2018.12.082
Tian W, Liao Z, Zhang J (2017) An optimization of artificial neural network model for predicting chlorophyll dynamics. Ecol Modell 364:42–52. https://doi.org/10.1016/j.ecolmodel.2017.09.013
Garg A, Jain S (2020) Process parameter optimization of biodiesel production from algal oil by response surface methodology and artificial neural networks. Fuel 277:118254. https://doi.org/10.1016/j.fuel.2020.118254
Ortiz F, Simpson JR, Pignatiello JJ, Heredia-Langner A (2004) A genetic algorithm approach to multiple-response optimization. J Qual Technol 36(4):432–450. https://doi.org/10.1080/00224065.2004.11980289
Álvarez MJ, Ilzarbe L, Viles E, Tanco M (2009) The use of genetic algorithms in response surface methodology. Qual Technol Quant Manag 6(3):295–307. https://doi.org/10.1080/16843703.2009.11673201
Khoo LP, Chen CH (2001) Integration of response surface methodology with genetic algorithms. Int J Adv Manuf Technol 18(7):483–489. https://doi.org/10.1007/s0017010180483
Mustefa Beyan S, Venkatesa Prabhu S, Mumecha TK, Gemeda MT (2021) Production of alkaline proteases using Aspergillus sp. isolated from Injera: RSM-GA based process optimization and enzyme kinetics aspect. Curr Microbiol 78(5):1823–1834. https://doi.org/10.1007/s00284-021-02446-4
Sabry I, El-Zathry NE, Gadallah N, Abdel Ghafaar M (2022) Implementation of hybrid RSM-GA optimization techniques in underwater friction stir welding. J Phys Conf Ser. https://doi.org/10.1088/1742-6596/2299/1/012014
Hasanien HM, Muyeen SM (2013) A Taguchi approach for optimum design of proportional-integral controllers in cascaded control scheme. IEEE Trans Power Syst 28(2):1636–1644. https://doi.org/10.1109/TPWRS.2012.2224385
Ajala SO, Alexander ML (2020) Multi-objective optimization studies of microalgae dewatering by utilizing bio-based alkali: a case study of response surface methodology (RSM) and genetic algorithm (GA). SN Appl Sci 2(3):1–20. https://doi.org/10.1007/s42452-020-2097-5
Obi CC, Nwabanne JT, Igwegbe CA, Ohale PE, Okpala COR (2022) Multi-characteristic optimization and modeling analysis of electrocoagulation treatment of abattoir wastewater using iron electrode pairs. J Water Process Eng 49:103136. https://doi.org/10.1016/j.jwpe.2022.103136
Rhee JH, Il Kim S, Lee KM, Kim MK, Lim YM (2021) Optimization of position and number of hotspot detectors using artificial neural network and genetic algorithm to estimate material levels inside a silo. Sensors. https://doi.org/10.3390/s21134427
Chen Z, Lin X, Xiong C, Chen N (2020) Modeling the relationship of precipitation and water level using grid precipitation products with a neural network model. Remote Sens. https://doi.org/10.3390/rs12071096
Chiroma H et al (2017) Neural networks optimization through genetic algorithm searches: a review. Appl Math Inf Sci 11(6):1543–1564. https://doi.org/10.18576/amis/110602
Ferentinos KP (2005) Biological engineering applications of feedforward neural networks designed and parameterized by genetic algorithms. Neural Netw 18(7):934–950. https://doi.org/10.1016/j.neunet.2005.03.010
Ahmadi MA, Shadizadeh SR (2013) Retracted article: intelligent approach for prediction of minimum miscible pressure by evolving genetic algorithm and neural network. Neural Comput Appl 23(2):569. https://doi.org/10.1007/s00521-012-0984-4
Oreski S, Oreski D, Oreski G (2012) Hybrid system with genetic algorithm and artificial neural networks and its application to retail credit risk assessment. Expert Syst Appl 39(16):12605–12617. https://doi.org/10.1016/j.eswa.2012.05.023
Dong P, Liao X, Chen Z, Chu H (2019) An improved method for predicting CO 2 minimum miscibility pressure based on artificial neural network. Adv Geo-Energy Res 3(4):355–364. https://doi.org/10.26804/ager.2019.04.02
Ghodousian A, Babalhavaeji A (2018) An efficient genetic algorithm for solving nonlinear optimization problems defined with fuzzy relational equations and max-Lukasiewicz composition. Appl Soft Comput J 69:475–492. https://doi.org/10.1016/j.asoc.2018.04.029
Bahrami S, Doulati Ardejani F, Baafi E (2016) Application of artificial neural network coupled with genetic algorithm and simulated annealing to solve groundwater inflow problem to an advancing open pit mine. J Hydrol 536:471–484. https://doi.org/10.1016/j.jhydrol.2016.03.002
Taghavi M, Gharehghani A, Nejad FB, Mirsalim M (2019) Developing a model to predict the start of combustion in HCCI engine using ANN-GA approach. Energy Convers Manag 195:57–69. https://doi.org/10.1016/j.enconman.2019.05.015
Suresh MVJJ, Reddy KS, Kolar AK (2011) ANN-GA based optimization of a high ash coal-fired supercritical power plant. Appl Energy 88(12):4867–4873. https://doi.org/10.1016/j.apenergy.2011.06.029
Dwiputranto TH, Setiawan NA, Adji TB (2021) “DGA-based early transformer fault detection using rough set theory classifier. Int Conf Adv Mechatron Intell Manuf Ind Autom. https://doi.org/10.1109/ICAMIMIA54022.2021.9807816
Bülbül MA, Harirchian E, Işık MF, Aghakouchaki Hosseini SE, Işık E (2022) A hybrid ANN-GA model for an automated rapid vulnerability assessment of existing RC buildings. Appl Sci. https://doi.org/10.3390/app12105138
Smaali A et al (2022) Degradation of azithromycin from aqueous solution using chlorine-ferrous-oxidation: ANN-GA modeling and Daphnia magna biotoxicity test assessment. Environ Res 214(P3):114026. https://doi.org/10.1016/j.envres.2022.114026
Karimi H, Yousefi F (2012) Application of artificial neural network-genetic algorithm (ANN-GA) to correlation of density in nanofluids. Fluid Phase Equilib 336:79–83. https://doi.org/10.1016/j.fluid.2012.08.019
Download references
The authors did not receive any specific grant from funding agencies in public or non-profit sector.
Author information
Authors and affiliations.
Department of Energy and Environment, National Institute of Technology, Tiruchirappalli, Tamil Nadu, 620015, India
Anto Susaimanickam & Premalatha Manickam
Department of High Voltage Engineering, University of Applied Sciences, 02763, Zittau, Germany
Amalanathan Arputhasamy Joseph
You can also search for this author in PubMed Google Scholar
Contributions
AS-Conceptualization, methodology, writing original draft; PM-Review and Supervision; AAJ-Conceptualization, methodology, writing original draft. All the authors have read and agreed to the published version of the manuscript.
Corresponding author
Correspondence to Amalanathan Arputhasamy Joseph .
Ethics declarations
Conflict of interest.
The authors declare no conflict of interest.
Ethical Approval
The authors of the manuscript have fulfilled with all the ethical standards necessary for the publication of the journal.
Replication of Results
No results are presented.
Additional information
Publisher's note.
Springer Nature remains neutral with regard to jurisdictional claims in published maps and institutional affiliations.
Rights and permissions
Springer Nature or its licensor (e.g. a society or other partner) holds exclusive rights to this article under a publishing agreement with the author(s) or other rightsholder(s); author self-archiving of the accepted manuscript version of this article is solely governed by the terms of such publishing agreement and applicable law.
Reprints and permissions
About this article
Susaimanickam, A., Manickam, P. & Joseph, A.A. A Comprehensive Review on RSM-Coupled Optimization Techniques and Its Applications. Arch Computat Methods Eng 30 , 4831–4853 (2023). https://doi.org/10.1007/s11831-023-09963-4
Download citation
Received : 03 March 2023
Accepted : 08 June 2023
Published : 23 June 2023
Issue Date : November 2023
DOI : https://doi.org/10.1007/s11831-023-09963-4
Share this article
Anyone you share the following link with will be able to read this content:
Sorry, a shareable link is not currently available for this article.
Provided by the Springer Nature SharedIt content-sharing initiative
- Find a journal
- Publish with us
- Track your research
CEO INTERVIEW
The RSM Virtual Academy promotes problem solving amongst its rising stars
Rsm international ceo jean stephens discusses the merits of the network's new development programme with zoya malik.
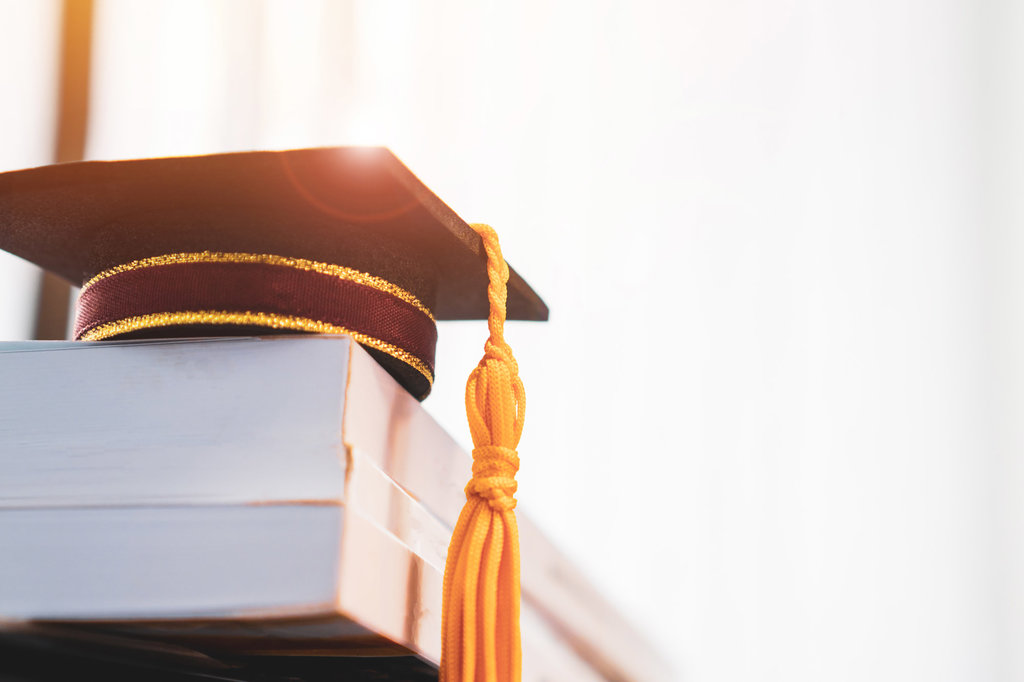
Zoya Malik: What is the objective of the RSM Virtual Academy?
Jean Stephens: First and foremost, an inherent part of our culture is our commitment to invest in the people of RSM, as they in turn will invest and share their knowledge in serving their clients to deliver The Power of Being Understood.
The RSM Academy was designed specifically for the rising stars within the RSM network to come together and take part in multi-cultural, multi-disciplined teams with the aim of collaborating on a proposal which offers a solution to an international client’s cross-border issue. Participants undergo a process which involves a challenge with problem-solving practical modules, alongside relevant tutorials by leading experts.
By working in collaborative teams, the aim is to develop a broader understanding of how our colleagues and clients think and work in different cultures and markets around the world, which will help to strengthen both the quality of our service delivery and client experience. There is a lot of power in working together and problem-solving in teams.
Jean Stephens, CEO, RSM International
ZM: Why is this a good time to launch?
JS: The RSM Academy has been a highly effective and long-standing programme and with the current situation with restricted travel, we saw this as the perfect time to launch the RSM Academy virtually to many more people globally.
The pandemic may have caused business disruption, but it has also accelerated the pace of digital transformation and been a catalyst for change. This was the perfect opportunity to innovate, adapt and connect in a meaningful way to an even greater number of rising stars within our global community.
Covid has forced us to reconsider many of our business practices, particularly in the context of collaboration, learning and sharing. This has enabled us to be more inclusive as to who can participate or contribute towards RSM’s global events and initiatives.
ZM: What investment has been made to launch the RSM Virtual Academy?
JS: The RSM Academy was originally designed as a week-long, face-to-face event, so moving this virtually required a dedicated project team to tailor the programme to a virtual environment.
The project team designed the programme using learnings from the in-person academy in addition to working with the Roffey Park Institute, a leading international, research-led learning and development organisation. We tailored it to the remote environment to make it an immersive virtual experience.
From a member firm point of view, their investment is in empowering their representatives to devote some 35 hours over a two-week period for the academy, in addition to pre-academy e-learning assignments which were designed to equip them with core information prior to the event.
ZM: What are the criteria for candidate selection?
JS: We were overwhelmed with interest, which resulted in just short of 300 candidates this year.
The individuals selected were high performers at senior manager, director or partner levels, and they needed to have a good understanding of serving clients. Importantly, the nominated individuals needed to be actively involved in international engagements, or proactively planning to do so.
After being nominated by managing partners, candidates were assessed by their level of experience – on average somewhere between eight and 10 years.
ZM: Who is the target audience and where globally will you receive most applications for courses?
JS: Ultimately, the aim of the Virtual RSM Academy is to coach and empower RSM professionals to deliver high-quality services and an exceptional client experience for their internationally active clients.
Another key objective is to develop and strengthen connections amongst professionals the world over which also contributes to a sense of connection to RSM internationally.
Although the Virtual RSM Academy was designed ultimately as a global programme, participants were selected to take part in regional programmes, working and competing as a team towards a global finale.
The regional programmes were designed carefully to ensure there was a cultural mix which also included representatives from different sized economies and different disciplines, while working around time-zones. Therefore, delegates from North America were split across two of the regional programmes.
This year saw delegates from 17 member firms from the Africa and Middle East group. The Asia-Pacific and North America group was represented by 15 member firms. From the Europe and North America regions there were participants from 27 countries. Finally, in the Latin and North America group there were participants from 21 countries in the virtual academy.
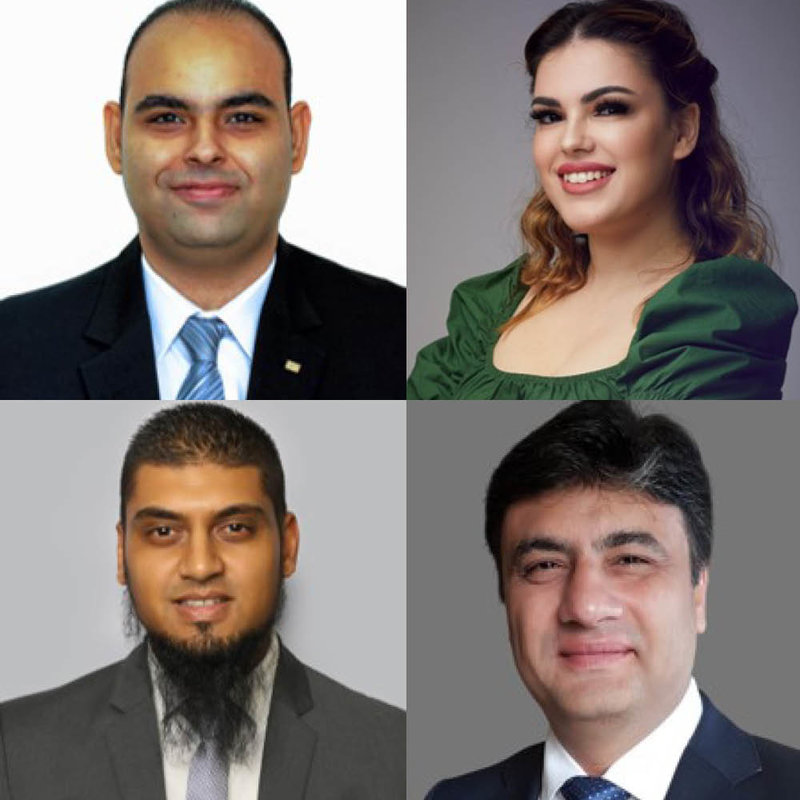
The winning team members (clockwise from top left): Eslam Ahmed (Eg ypt), Eugénia Pião (Mozambique), Ozeyr Ahmed (South Africa), Rahul Hariyani (UAE)
ZM: What training modules are being rolled out
JS: Prior to attending, delegates were asked to complete a series of e-learning modules to deepen their understanding of the RSM network. The pre-work included e-learning about RSM’s history as well as about the Network’s global strategy.
During the programme, the events were divided into two sections, learning modules and collaborative team sessions. Topics included in these modules were digital and innovation, behavioural science, technical service insights, branding, and diversity and inclusion – all tailored to enable delegates to prepare for the specific fictitious client proposal.
The pre-work simulated in the context of the virtual event was reflective of the experience a cross-border team might have when collaborating on a proposal for an international client and was supported by a significant number of tools for global business development which were available in RSM's global e-learning platform.
ZM: What is the duration of training?
JS: The e-learning pre-work took place throughout August, while the regional events lasted between two and three hours per day over 10 working days in September.
The winners from each regional event were then tasked to refine their pitches and take part in the global final, which was an hour and a half long. All the teams competing in the final were winners in their own right – however, the winning team was announced at the global finale, which took place on Thursday, 7 October.
All sessions were aimed to balance the busy schedules of our team members in serving clients with enabling the teams to collaborate towards their final goal of delivering their final presentation to the global client board.
ZM: How does the RSM Virtual Academy compete with other training courses? What differentiation does it offer?
JS: Over the last 14 years, our case study-led training simulation has helped RSM professionals work effectively as cross-cultural and cross-national teams to identify and solve unique client issues. These skills are invaluable to anyone who works in multi-disciplinary teams on cross-border work in what has been an increasingly globalised world.
The RSM Academy participants leave the experience equipped with critical insight around operating in cross-border teams, a deeper understanding of relationship building and working with different cultures and an enhanced knowledge of RSM’s global resources, common tools for business development and new digital innovations impacting our profession.
Due to the virtual nature of this year’s event, there was an even greater sense of connection with RSM as a global organisation, as the event genuinely extended across borders - far more so than via the 60 people face-to-face-event of previous years.
Now that the RSM Academy events have finished, all the delegates have been transferred to the RSM Academy Alumni Community to connect with the other 600-plus academy alumni from prior years. This ensures the networks and connections developed can be maintained.
ZM: What is the cost of training for the candidate?’
JS: There were no financial costs for participating candidates. Participation is completely free, although it is important to acknowledge that for member firms the cost translates directly to the investment of their employees’ time in taking part in the events.
ZM: How many candidates signed up, and when did the first pass through?
JS: There were hundreds of nominations globally, and the Virtual RSM Academy saw just short of 300 successful nominees take part.
The four regional winning teams came together for a special announcement on 4 October in which they received news of a twist in the challenge. This required them to move fast to refine their pitches to reflect the news from the ‘client’. Final presentations were made on 6 October with a team from Africa and MENA being ‘appointed’ by the fictitious client board of directors as the overall winning team, which was announced on 7 October.
Beyond the investment in developing those who took part, a key objective of the 2021 Virtual RSM Academy is to build on the bonds formed to strengthen connections. This year’s participants will be joining the wider global RSM Academy Alumni Community in an event on 18 November, in subsequent quarterly events and will stay connected on LinkedIn and have access to further training on our e-learning platform.
BACK TO TOP
Open Access is an initiative that aims to make scientific research freely available to all. To date our community has made over 100 million downloads. It’s based on principles of collaboration, unobstructed discovery, and, most importantly, scientific progression. As PhD students, we found it difficult to access the research we needed, so we decided to create a new Open Access publisher that levels the playing field for scientists across the world. How? By making research easy to access, and puts the academic needs of the researchers before the business interests of publishers.
We are a community of more than 103,000 authors and editors from 3,291 institutions spanning 160 countries, including Nobel Prize winners and some of the world’s most-cited researchers. Publishing on IntechOpen allows authors to earn citations and find new collaborators, meaning more people see your work not only from your own field of study, but from other related fields too.
Brief introduction to this section that descibes Open Access especially from an IntechOpen perspective
Want to get in touch? Contact our London head office or media team here
Our team is growing all the time, so we’re always on the lookout for smart people who want to help us reshape the world of scientific publishing.
Home > Books > Response Surface Methodology - Research Advances and Applications
Applications of Response Surface Methodology (RSM) in Product Design, Development, and Process Optimization
Submitted: 21 June 2022 Reviewed: 26 July 2022 Published: 16 September 2022
DOI: 10.5772/intechopen.106763
Cite this chapter
There are two ways to cite this chapter:
From the Edited Volume
Response Surface Methodology - Research Advances and Applications
Edited by Palanikumar Kayarogannam
To purchase hard copies of this book, please contact the representative in India: CBS Publishers & Distributors Pvt. Ltd. www.cbspd.com | [email protected]
Chapter metrics overview
882 Chapter Downloads
Impact of this chapter
Total Chapter Downloads on intechopen.com

Total Chapter Views on intechopen.com
In this review chapter, the authors presented a systematic exposition to the concept of Response Surface Methodology (RSM) for applications by Scientists, Engineers, Technologists and Industries. (RSM) is an empirical model which employs the use of mathematical and statistical techniques in relating input variables otherwise known as factors to the response. RSM became very useful due to the fact that other methods available such as the theoretical model could be very cumbersome to use, time-consuming, inefficient, error prone and unreliable. In order to draw meaningful conclusions and findings, an experiment is required. In an effort to obtain an objective conclusion (between the factors and the response), an experimenter needs to plan and design the experiments, and analyze the results. An approximation of the response in relation to the variables is otherwise known as RSM. This chapter reviews RSM concept for easy understanding and adoption by researchers. In section 2.0, the various terminologies used in RSM were defined. In section 3.0, RSM design types were highlighted and RSM research phases exposed in section 4.0. Section 8.0 gave some scenario applications of RSM in various fields and section 9.0 defined the RSM research cycle process. General applications and conclusions stated.
- response surface methodology
- optimization
- RSM applications
- product design
Author Information
Sheriff lamidi *.
- Lagos State University of Science and Technology (Formerly Lagos State Polytechnic), Lagos, Nigeria
Nurudeen Olaleye
Yakub bankole, aishat obalola, emmanuella aribike, idris adigun.
*Address all correspondence to: [email protected]
1. Introduction
Experimentation, Data collection, Data processing, and Analysis of data are very basic and essential to Scientists, Engineers, Technologists, and Manufacturing Industries to design, develop, improve and validate their products, processes, and operations. Response surface methodology (RSM) which is available in MINITAB and other proprietary software is a collection of both statistical and mathematical techniques useful for developing, improving, and optimizing processes [ 1 ]. RSM is known to play a pivotal role in new product design and development as well as in improving existing ones. With response surface methodology we can determine the optimum factor needed to produce the best result. RSM is a critical and very robust tool for data manipulation and analysis of research data to obtain a quality result or an improvement [ 1 ]. RSM could be applied by an industry that desires to manufacture a component (from Al-Si Alloy material) with minimum surface roughness by combining three controllable variables (cutting speed, feed rate, and, depth of cut). Because of this, the Design of Experiments (DOE) could be used to carry out the study of the effect of the three machining variables (cutting speed, feed rate, and depth of cut) on the surface roughness (Ra) of Al-Si alloy [ 2 ]. With the use of response surface methodology (RSM), a mathematical prediction model of the surface roughness would be developed in terms of cutting speed, feed rate, and depth of cut. The effects of the three process parameters on both Ra can then be investigated by using the response surface methodology (RSM). The above approach can be adopted by any industry, scientist, or researcher in getting better results (response) from several variables otherwise known as factors. RSM helps to reduce the noise in an experiment, thereby ensuring optimization. Many researchers have conducted researches on the application of RSM or other DOE concept in which the results of their findings have been used to develop a predictive model in several fields such as; tool life modeling, surface roughness prediction, for monitoring and functionality or health condition of electronic devices also for the surface roughness of Inconel using full factorial design of experiment among other areas of applications [ 2 , 3 , 4 ]. The RSM looks into an adequate approximation relationship between input and output variables and determines the best operating circumstances for a system under study or a portion of the factor field that complies with the operating requirements or conditions [ 3 , 5 , 6 ].
Response surface methodology can be better referred to as a collection of statistical and mathematical techniques employed for product design and improvement, process development and improvement as well as process optimization. It has major applications in the design, development, and, formulation of new products as well as in improving existing product design. RSM is a robust tool for the design of experiments, analysis of experimental data, and process optimization. In RSM, the response is determined by the variables and the aim is to optimize the response [ 1 , 7 , 8 ]. There are two primary experimental designs used in response surface methodology: Box-Behnken designs (BBD) and central composite designs (CCD) [ 8 , 9 ]. Recently, optimization studies have also used central composite rotatable design (CCRD) and face central composite design (FCCD) [ 8 , 10 , 11 , 12 , 13 , 14 ].
Wong [ 15 ] employed RSM concept to carry out reliability analysis of soil slopes. Tandjiria et al. [ 16 ] used response surface method for reliability analysis of laterally loaded piles. Sivakumar Babu and Amit Srivastava [ 17 ] presented a study on the analysis of allowable bearing pressures on shallow foundation using response surface method and showed that a comparative study of the results of the analysis from conventional solution and numerical analysis in terms of reliability indices enables rational choice of allowable loads. For better understanding of the RSM concept in our daily life experiences as described in Figure 1 . Take for example we have two variables (humidity and temperature) and we want to see the effects of these variables on human comfort. We can name these independent variables temperature and humidity, X1 and X2 and the response which is human comfort can be named Y. Response Surface Methodology is useful in this case for the modeling and optimization of the situation above in which the response of interest (human comfort) is influenced by the variables (humidity and temperature). In this model example, our objective is to optimize this response The visual representation of the above is otherwise known as Response Surface Methodology (RSM) or response surface modeling. To find the levels of temperature (X1) and pressure (X2) for maximum human comfort (y) in the above process.

Response surface for humidity and Temperature on human comfort.
ϵ is referred to as the error term inherent in the system
1.1 The concept of RSM
The concept of Response Surface Methodology can be used to establish an approximate explicit functional relationship between input random variables and output response through regression analysis and probabilistic analysis can be performed [ 15 ]. RSM involves a combination of metamodeling (i.e., regression) and sequential procedures (iterative optimization). Response Surface Methodology (RSM) is a collection of mathematical and statistical techniques useful for the modeling and analysis of problems. By careful design of experiments, the objective is to optimize a response (output variable) that is influenced by several independent variables (input variables). A collection of mathematical and statistical methods called Response Surface Methodology (RSM) can be used to simulate and analyze issues. The goal of meticulous experiment design is to maximize a response (output variable) that is affected by a number of independent variables (input variables). The motivation behind this work is the applicability of the concept of RSM to many areas of scientific research, engineering and manufacturing industries.
1.2 Objective of this present study
identify the sensitive parameter that provides the greatest influence on the response.
easily take decision that will impact positively the product design and process optimization.
ensure reliability, acceptability and profitability of the product developed and/or optimized condition.
2. Some useful terminologies
Factors are input variables/parameters that potentially affect the response. It can be controllable or uncontrollable, and quantitative and qualitative.
Response is a dependent variable. It is the desired results obtained from combining the interaction of independent variables.
Experiment is a series of tests, called runs, in which changes are made in the input variables to identify the reasons for changes in the output response .
Experimenter is a person experimenting for research purposes.
Treatment is a combination of one or more factors.
Levels are the values a factor can take on
Effect simply means how much a main factor or interaction between two or more factors influences the response.
One design point = one treatment
Points are typically coded to more practical values.
example. 1 factor with 2 levels – levels coded as (−1) for low level and (+1) for high level
Design Space is the range of values over which factors are to be varied or adjusted [ 18 , 19 ].
Response Surface is the unknown or experimental purpose. It is the mean response at any given level of the factors in the design space.
RSM Design Types; The summary of the various types of design available in response surface methodology is presented in Figure 2 according to [ 18 ].

Available Designs in RSM source MINITAB 20.
(ii) Central Composite Design (CCD) (2 to 10 continuous factors)
(ii) Box-Behnken Design (3,4,5,6,7,9 or 10 continuous factors)
Residual plots
Effect plots
3. RSM research phases
Use a simulation model e.g., Minitab to fit a linear regression model to the data points in the workspace and, find a better solution from the linear regression model.
Repeat the above process until the slope of the linear response surface obtained from the linear regression model is approximately zero.
Fit a nonlinear quadratic regression.
Lastly find the optimum of this equation.
4. Getting access to response surface methodology (RSM)?
MINITAB, STATISTICA, DESIGN EXPERT, etc. are software tools that can be used for experimental design and analyze data. RSM is one of the techniques that have been programmed in this software. Among all, MINITAB is highly rated when it comes to the design of experiments using response surface methodology. Minitab is a proprietary software tool, a computer program applied in statistical studies, developed in 1972. Its interface is similar to Microsoft Excel or Calc of OpenOffice, used in universities and companies, it has specific functions focused on process management and analysis of the Six Sigma suite. Minitab offers Quality Control tools, Experiment Planning (DOE) e.g., RSM, Reliability Analysis, and General Statistics [ 18 , 20 ]. Figure 3 shows the navigation process in Minitab 18 to access response surface methodology interface ( Table 1 ).

Diagram showing the navigation of RSM with MINITAB software.
Some examples of Factors and Response in RSM.
5. Advantages of RSM
Seamless statistical analysis
Optimization of manufacturing system, process, or product.
Experimental layout and design.
Interaction of variables is easily presented with clear curves and other visual aids.
Good visualization of responses or results with the use of surface plots, graphs, etc.
Associated empirical mathematical models.
6. Some scenarios of RSM Applications
6.1 a scenario of rsm in a manufacturing process.
In a quest to manufacture a component during CNC turning operation. Proper selection of process parameters or variables (cutting speed, feed rate, and depth of cut) for optimal surface quality (Response) must be achieved. This requires a more methodical approach by using experimental methods and mathematical and statistical models. The design of experiments will play a pivotal role in this regard. This will require considerable knowledge and experience of the designer to design experiments and analyze data. Note that the traditional design-of-experiment (DOE) technique requires a large number of samples to be produced. To increase machining process efficiency, strategies for optimizing machining parameters using experimental methodologies as well as mathematical and statistical models have developed significantly over time. A full factorial approach may be required to look into all potential combinations to build an approximation model that can describe interactions between design variables in this CNC turning operation. An experimental approach known as a factorial experiment involves varying design variables simultaneously rather than one at a time. It is necessary to define the lower and upper bounds for each of the n design variables in the optimization problem. Then, at various levels, the permitted range is discounted. If just the lower and upper bounds (two levels) of each variable are defined. The experimental design is referred to as 2n full factorial if each variable is defined at just the upper and lower boundaries (two levels). Second-order models can be fitted using factorial designs. When a first-order model exhibits a lack of fit as a result of the interaction between variables and surface curvature, a second-order model can considerably enhance the optimization procedure. The goal of a meticulous experiment design is to optimize the response. (Surface quality of the machined part) which is influenced by several independent input variables (cutting speed, feed rate, and depth of cut).
6.2 A scenario of RSM in the energy industry
Due to the limited availability of high-grade coal for energy production, low-grade coal can be employed. High ash levels and high moisture content are characteristics of low-grade coal. With the use of the response surface methodology, the operational parameters were optimized to generate clean coal as effectively as possible. The impact of three independent variables, including hydrofluoric acid (HF) concentration (10–20 percent by volume), temperature (60–100 o C), and time (90–180 min), for ash reduction from the low-grade coal, was explored to attain this coal optimization target. By utilizing the central composite design (CCD) method, a quadratic model was presented to correlate the independent variables for maximal ash reduction at the ideal process condition. In comparison to time and temperature, the study finds that HF concentration was the most efficient parameter for ash reduction [ 16 ].
6.3 A scenario of RSM in extraction optimization
In order to maximize the extraction process of oil from leaves, fruits etc., it is important to optimize the extraction parameters so as to get the best yield. RSM concept has been used more often in recent years to optimize various oil extractions from plant sources [ 17 , 21 ].
6.4 A scenario of RSM in drinking water treatment process
Both trihalomethanes (THMs) and Natural Organic Matter (NOM) has been characterized with cancer risk in drinking water According to [ 22 ]. The concept of RSM was used for the development of water treatment technologies and optimization of process variables in order to reduce THMs and NOM level of concentration in drinking water. A model was developed to control the process. The developed models can be effectively used to remove both THMs and NOM from drinking water.
6.5 A scenario of RSM in construction industry
The construction industry is a very germane industry in the technological advancement of any nation. The level of research-based construction has been improved lately. A study on the analysis of allowable bearing pressures on shallow foundation using response surface method was conducted and showed that a comparative study of the results of the analysis from conventional solution and numerical analysis in terms of reliability indices enables rational choice of allowable loads [ 15 ].
6.6 A scenario of RSM in product development
The effect of oven parameters such as air velocity, time, temperature etc. on formulations (sugar, water, fats, flavors, etc.,) of the quality of baked food product can the analyzed with the application of response surface methodology [ 23 ]. RSM model is a powerful tool to optimize the product quality (volume of baked product, crust and crumb color, bake loss among others). The data collected through RSM can further be used to obtain the variability of the response(s) with tested parameters [ 23 ]. In this scenario, the results of the optimization obtained is otherwise referred to as quality product.
RSM cycle processes is shown in Figure 4 .

RSM Research Cycle Process.
What data is to be collected?
How to measure it?
How does the data relate to processing performances and experimental objectives?
It is time-consuming.
Can handle one factor over time (OVAT) or one factor at a time (OFAT).
Interaction between two or more variables cannot be interpreted.
It is otherwise known as Design of Experiment (DOE)
Apply the factorial concept
It makes use of modeling to predict the behaviors of process variables e.g., RSM
The process variables could be explained through interaction plots and graphs
Saves time and improves efficiency.
An experiment is designed based on the decisions during the designing or data collection stage. The experimental design clearly states the number of experiments and how the experiment will be carried out [ 20 , 26 , 27 ].
Conducting Experiment. The next step after the experimental design is to experiment with the exact research parameters and, in the order, defined by the layout for easy statistical validity. The person experimenting is called an experimenter.
Analyzing the Results. The primary focus in this analysis stage is to obtain useful information from the experiment conducted and ascertain the level of quality or improvement recorded. In this stage, the results obtained from the experiment conducted are analyzed. The analysis of the results is targeted toward specific conclusions. Since we have several samples tested in each experimental run, different analysis techniques can be selected.
Graphical Analysis. One of the powerful features of RM is the ability to present results or responses using visual aids for easy interpretation or understanding.
Confirmation of results/Ask questions relating to the research objective. A test can be carried out to ascertain if the actual performance of the product in-service condition matches the improvement stated in the results. The test here helps to determine the research gap ( Figure 5 ).

RSM Flow chart.
7. General applications of RSM
RSM found its major application in the industry to model and design a product. Also, to optimize the manufacturing process.
RSM is capable of Data analysis, prediction, product design, and optimization [ 28 , 29 ].
RSM can predict the relationship or interaction that exists between the values of some measurable response variable(s) and those of a set of experimental factors presumed to affect the response(s).
RSM is capable to predict the response value at various process conditions.
Application of RSM can be used to screen independent variables in order to determine most significant main effect of factors among several potential ones.
With RSM a non-identified interaction effect could be determined.
With RSM application for optimization, one can easily identify the best factor(s), process interaction effect, that produce the response that brings the optimized condition. This is the real deal in parameter optimization.
RSM enhances product quality, product life span and increase productivity.
Application of response surface methodology exposes the best type of design: CCD or BBD needed to achieve optimization.
RSM can predict the relationship or interaction that exists between the values of some measurable response variables and those of a set of experimental factors presumed to affect the response.
Power visual aids such as plots graphs in 2D or 3D for easy understanding of the results.
8. Visualizing results in RSM
RSM uses a variety of surface visualization techniques according to Figures 6 – 11 to visually assess how factors affect the response. When a regression model is fitted as a result of interactions between two or more predictors, visualization better communicates the experimental results or responses. Effects plots, contour plots, residual plots, surface plots, etc. are a few examples of graphical visualization tools also known as response surface plots. These plots aid in determining the process conditions and desired response Values [ 18 , 19 , 27 , 29 , 30 , 31 , 32 , 33 , 34 , 35 ].

Figure showing standardized effect.

Central composite design.

Main effect plot in RSM.

Interaction plot explored from RSM software.

Residual plot for optimized point.

Some response surface plots for visualization of RSM results.
9. Conclusions
In this chapter, the authors provided a detailed approach for the understanding and implementing Response Surface Methodology (RSM) for the various professionals or researchers who may be involved in the application of Response Surface Methodology. In an attempt to design a product or to optimize an existing process there are several methods that can be adopted. RSM has many advantages when compared to classical methods. It requires fewer runs of experiments to understand the effects of all the factors and the optimum combination of all factor input. RSM requires less time, removes trial by error and ensure high quality results. Having presented in this chapter the huge applications of RSM in various fields of research, it can be concluded that RSM is a great research tool for product design, development and process optimization. The chapter coverage is detailed enough to give the basic insight of RSM even to a novice hearing about RSM for the very first time. However, the chapter does not cover all the information required for mastery of the RSM concept.
Acknowledgments
The authors sincerely appreciates IntechOpen with the opportunity provided to publish this chapter review in the main book; Response Surface Methodology-Research Advances and Applications.
We also give kudos to the critical peer review process.
Notes/thanks/other declarations
I hereby testify to the good work by Intechopen by way of contributing to research across all the fields from different part of the world. Indeed, there is no better way for the advancement of research than you are doing. Please, keep it up.
- 1. Raymond H, Montgomery DC, Anderson-Cook CM. Process and Product Optimization Using Designed Experiments. Third ed. New Jersey: John Wiley & Sons, Inc; 2009
- 2. Kasali Aderinmoye A, Sheriff Babatunde L. Effect of dry cutting system on surface finish in turning operation of Al-Si alloy. Journal of Multidisciplinary Engineering Science and Technology. 2021; 8 :10
- 3. Hascalik A, Caydas U. Optimization of turning parameters for surface roughness and tool life based on the Taguchi method. International Journal Advance Manufacturing Technology. 2008; 38 :896-903
- 4. Mead R, Pike DJ. A review of response surface methodology from biometric viewpoint. A literature survey. Biometrics. 1975; 31 :803-851
- 5. Hill WJ, Hunter WG. A review of response surface methodology: A literature survey. Technometrics. 1966; 4 :571-590
- 6. Peasura P. Application of response surface methodology for modeling of postweld heat treatment process in a pressure vessel steel ASTM A516 Grade 70. The Scientific World Journal. 2015
- 7. Farooq Z, Rehman S, Abid M. Application of response surface methodology to optimize composite flour for the production and enhanced storability of leavened flat bread (Naan). Journal of Food Processing and Preservation. 2013; 37 :939-945
- 8. Pishgar-Komleh SH, Keyhani A, Msm R, Jafari A. Application of response surface methodology for optimization of Picker-Husker harvesting losses in corn seed. Iranica Journal of Energy and Environment. 2012; 3 (2):134-142
- 9. Montgomery DC. Design and Analysis of Experiment. Fifth. ed. New York, USA: Wiley, Inc.; 1997
- 10. Aydar AY. Utilization of response surface methodology in optimization of extraction of plant materials. Open Access. 2018
- 11. Koç B, Kaymak-Ertekin F. Response surface methodology and food processing applications. Gıda. 2009; 7 :1-8
- 12. Prakash Maran J, Mekala V, Manikandan S. Modeling and optimization of ultrasound-assisted extraction of polysaccharide from Cucurbita moschata. Carbohydrate Polymers. 2013; 92 :2018-2026
- 13. Şahin S, Samli R, Tan AS, Barba FJ, Chemat F, Cravotto G, et al. Solvent-free microwave-assisted extraction of polyphenols from olive tree leaves: Antioxidant and antimicrobial properties. Molecules. 2017; 7 :1054
- 14. Wang J, Sun B, Cao Y, Tian Y, Li X. Optimisation of ultrasound-assisted extraction of phenolic compounds from wheat bran. Food Chemistry. 2008; 106 :804-810
- 15. Wong F. Slope stability and response surface method. Journal of Geotechnical and Geo-environmental Engineering ASCE. 1985; 111 :32-53
- 16. Tandjiria VI, Teh CI, Low BK. Reliability analysis of laterally loaded piles using response surface methods. Structural Safety. 2000; 22 :335-355
- 17. Sivakumar BG, Srivastava A. Response Surface Methodology (RSM) in the Reliability Analysis of Geotechnical Systems. In: Proceedings of 12 th International conference of International Association for Computer Methods and Advances in Geomechanics. Goa India; 2008
- 18. Wan Nor Nadyaini Wan Omar. Design of Experiment (DOE) and Response Surface Methodology (RSM). N29 Faculty of Chemical Engineering; 2015
- 19. Minitab’s 20 Help Section and DOE Navigation.
- 20. Introduction to Design of Experiment [Internet]. 2019. Available from: www.theopeneducator.com
- 21. Behera SK, Meena H, Chakraborty S, Meikap BC. Application of response surface methodology (RSM) for optimization of leaching parameters for ash reduction from low-grade coal. International Journal of Mining Science and Technology. 2018; 28 :621-629
- 22. Tian Y, Xu Z, Zheng B, Martin LY. Optimization of ultrasonic-assisted extraction of pomegranate (Punica granatum L.) seed oil. Ultrasonics Sonochemistry. 2013; 20 :202-208
- 23. Samaram S, Mirhosseini H, Tan CP, Ghazali HM, Bordbar S, Serjouie A. Optimisation of ultrasound-assisted extraction of oil from papaya seed by response surface methodology: Oil recovery, radical scavenging antioxidant activity, and oxidation stability. Food Chemistry. 2015; 172 :7-17
- 24. Cornell J. How to Apply Response Surface Methodology. American Society for Quality Control. USA: Statistic Division; 1990
- 25. Brown SR, Melemend LE. Experimental Design and Analysis Quantitative Application in the Social Science. California: Sage Publication; 1990. p. 74
- 26. Fractional factorial design [Internet]. Available from: http://en.wikipedia.org/wiki/Fractional_factorial_design#Resolution .
- 27. Raliasoft DOE Design of Experiment techniques [Internet]. 1992. Available from: www.help.synthesisplatform.net
- 28. Neto A, Oliveira S. A Software Tools Catalogue to Support the Statistical Process Control on the Software Context. In: Proceedings of the 14th International Conference on Evaluation of Novel Approaches to Software Engineering. 2019. pp. 510-517
- 29. Paul G. Mathews Design of Experiments with MINITAB. Milwaukee, Wisconsin: ASQ Quality Press;
- 30. Kumari M, Gupta SK. Response surface methodological (RSM) approach for optimizing the removal of trihalomethanes (THMs) and its precursor’s by surfactant modified magnetic nanoadsorbents (sMNP) – An endeavour to diminish probable cancer risk. Scientific Reports. 2019; 9 :18339
- 31. Dogan IS, Okut H. As a Tool Response Surface Methodology (RSM) in a New Product Development. In: International congress on Information Technology in Agriculture, Food and Environmental. Izmir, Turkey: Ege University; 2003
- 32. Russell V. Surface Plots in the RSM Package Updated to version 2.00. Iowa: Department of Statistics, The University of Iowa; 2012
- 33. Box GEP, Hunter WG, Hunter JS. Statistics for Experimenters. An Introduction to Design, Data Analysis, and Model Building. 2nd ed. New York: John Wiley & Sons; 2005
- 34. Noelle M.. How to Use MINITAB, Design of Experiments, 2014.
- 35. Khan RM. Problem-solving and Data Analysis Using Minitab. A Clear and Easy Guide to Six Sigma Methodology. 1st ed. West Sussex: Wiley; 2013
© 2022 The Author(s). Licensee IntechOpen. This chapter is distributed under the terms of the Creative Commons Attribution 3.0 License , which permits unrestricted use, distribution, and reproduction in any medium, provided the original work is properly cited.
Continue reading from the same book
Response surface methodology.
Edited by Palanikumar Kayaroganam
Published: 29 March 2023
By Nam-Ky Nguyen, John J. Borkowski and Mai Phuong Vu...
119 downloads
By Asif Ahmad, Shahnawaz Alam and Meenu Sharma
105 downloads
By Aysun Sagbas
166 downloads
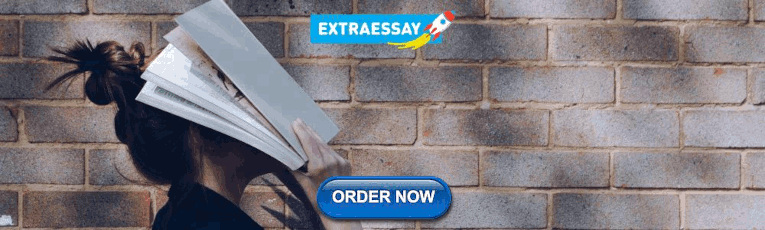
IMAGES
VIDEO
COMMENTS
RSM's business strategy advisors are passionate about innovating to drive business growth. We take an impartial look at operating models across all business functions to identify the steps necessary to automate and enhance your business operations for growth and profitability. With a focus on future-proofing your business, we incorporate ...
The four pillars of our strategy are: People: Drive an unrivaled, inclusive culture and talent experience. Clients: Deliver rich, personalized experiences through market-leading insights. Technology: Champion and embrace future-focused agile, digital and global delivery models. Solutions: Win with industry and compelling global products and ...
The MSc in Strategic Management is a 12-month programme. In the autumn semester, you'll delve into core courses (22 EC), followed by master electives (18 EC) in the spring. You also have the option to choose an elective from another MSc programme. Over the course of the year, you'll work on your master thesis project (20 EC).
Our commitment extends beyond problem-solving; we view challenges as gateways to opportunities. Leveraging data-driven insights from industry best practices, we offer end-to-end solutions to help your organization seize untapped potential. As a first-choice advisor to the middle market, we stand ready to guide and support you on your M&A journey.
Regardless of the maturity of your data strategy, RSM's experienced advisors have the knowledge and perspective to address your data challenges and successfully guide you through the data analytics life cycle. ... The information you already possess is growing and likely holds the key to solving many of your most complex business problems ...
The Excellence Academy is designed to align with RSM's strategic pillar of driving an unrivaled, inclusive culture and talent experience. ... Strengthen your technical and soft skills through collaborative real-world simulated exercises related to ethics and problem-solving. ... RSM US LLP is a limited liability partnership and the U.S ...
A force for positive change. Management education can be an instrument of positive change. RSM's solid academic roots enable us to provide people and their teams and organisations with new skills and perspectives for professional development. Our courses teach the skills and knowledge that are needed in business and management right now.
The two-day programma 'Strategic Problem-Solving ' is mainly aimed at professionals outside of the consulting industry, the class is intended to boost participants' problem-solving skills and their effectiveness in finding solutions to strategic business problems. According to RSM, those in attendance will learn a variety of best practice ...
Improve your problem solving skills during this two-day programme. This programme will boost your effectiveness in finding solutions to strategic business problems. ... Before the start of the programme, you will be asked to identify a strategic issue you face in your work. Together with faculty and peers, you will work on defining an approach ...
Make innovation happen in your organisation using design thinking. Connect and empathise with your customers' unmet needs and desires. Continuously challenge assumptions using design thinking tools and techniques. Enhance your ability to define, interpret and reframe a problem. Generate and choose solutions using a range of techniques.
In this episode of the McKinsey Podcast, Simon London speaks with Charles Conn, CEO of venture-capital firm Oxford Sciences Innovation, and McKinsey senior partner Hugo Sarrazin about the complexities of different problem-solving strategies.. Podcast transcript. Simon London: Hello, and welcome to this episode of the McKinsey Podcast, with me, Simon London.
Programmes for individuals. Improve your business skills with a short programme (1-16 days). We offer programmes in various business areas for any stage of your career. For example General Management, Finance and Accounting and Strategy and Innovation. We provide you with fresh insights, highly practical tools and the right knowledge based on ...
Master students from Rotterdam School of Management, Erasmus University (RSM) attended a 'structured thinking and problem-solving' webinar in May 2020. The online seminar was co-organised by Maersk Management Consulting (MMC) and CEMS Club Rotterdam. The students focused on structured thinking and powerful communication tools, followed by a ...
6 Monitor and evaluate the results. The sixth and final step of the strategic problem solving framework is to monitor and evaluate the results of the solution. This means measuring, analyzing, and ...
A version of this tutorial originally appeared in the free Primer app.. Problem solving is an underrated talent. It helps you make clear decisions during turbulent, overwhelming times, and many experts identify it as a vital soft skill for job seekers post-pandemic.. To develop your problem-solving acumen, use a structured approach that focuses on the why, what, and how of your issue.
The model explains common patterns of managerial reasoning and decision making, including many documented 'biases' and simplifying heuristics, and points the way to new effects and novel empirical investigations of problem solving‐oriented thinking in strategic management and types of generic strategies, driven by predictions about the ...
Strategic problem-solving is a relevant skill in business, widely used to identify problems and find inherent solutions to stop, avoid, or mitigate such problems. The literature revolving around problem-solving has been enriched over the years with several theories and approaches. Problem-solving is critical in helping maintain a successful business and looking at problems as objectively and ...
The problems involved in the real time applications often involve multiple responses where GA can be well adapted with RSM model in finding the near feasible solutions. Thus, this hybrid combination of RSM and GA provides a better optimization strategy in solving issues for problems involving large number of input variables and output responses.
Consolidate data, budget, forecast and report for quicker, better decisions. The Solver enterprise CPM platform is a powerful tool for budgeting, forecasting, reporting, self-service analytics and more. Its modern web interface makes it simple and intuitive for users, whether your deployment is in the cloud or on-premises.
Strategy based on human's problem-solving intelligence, potential to comprehend, rationale, acquiring knowledge, ability to grasp and withhold ideas, supervisory and mana-gerial powers etc. were set as basic inputs to build Human inspired optimization algorithms (HIOA). Many algorithms have been developed recently by acquiring the latest trends
JS: The RSM Academy was originally designed as a week-long, face-to-face event, so moving this virtually required a dedicated project team to tailor the programme to a virtual environment. The project team designed the programme using learnings from the in-person academy in addition to working with the Roffey Park Institute, a leading international, research-led learning and development ...
In this review chapter, the authors presented a systematic exposition to the concept of Response Surface Methodology (RSM) for applications by Scientists, Engineers, Technologists and Industries. (RSM) is an empirical model which employs the use of mathematical and statistical techniques in relating input variables otherwise known as factors to the response. RSM became very useful due to the ...
In the latest edition of the Army War College journal Parameters, Andrew Carr argues that the rising level of complexity in the world necessitates a change in how we define strategy away from a math problem of ends + ways + means and instead look to strategy as problem-solving.1 Carr's idea is that as the amount of complexity in a situation increases, the strategy should be less focused on ...
Under strategy as problem-solving, the strategist's primary contribution is the diagnosis of complex problems. Diagnosis attempts to frame, map, and probe a complex problem to identify the level of order, dispositions, and pathways for potential resolution. The word itself emerges from the Greek diagignōskein, meaning "to distinguish ...