- USC Libraries
- Research Guides
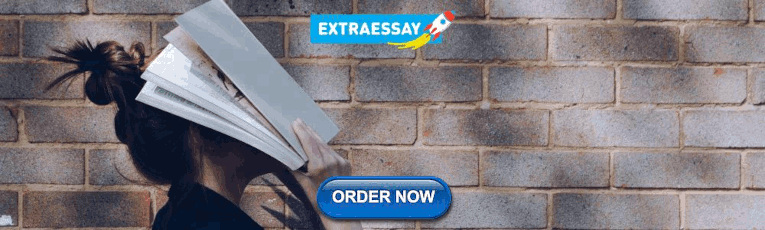
Organizing Your Social Sciences Research Paper
- 10. Proofreading Your Paper
- Purpose of Guide
- Design Flaws to Avoid
- Independent and Dependent Variables
- Glossary of Research Terms
- Reading Research Effectively
- Narrowing a Topic Idea
- Broadening a Topic Idea
- Extending the Timeliness of a Topic Idea
- Academic Writing Style
- Choosing a Title
- Making an Outline
- Paragraph Development
- Research Process Video Series
- Executive Summary
- The C.A.R.S. Model
- Background Information
- The Research Problem/Question
- Theoretical Framework
- Citation Tracking
- Content Alert Services
- Evaluating Sources
- Primary Sources
- Secondary Sources
- Tiertiary Sources
- Scholarly vs. Popular Publications
- Qualitative Methods
- Quantitative Methods
- Insiderness
- Using Non-Textual Elements
- Limitations of the Study
- Common Grammar Mistakes
- Writing Concisely
- Avoiding Plagiarism
- Footnotes or Endnotes?
- Further Readings
- Generative AI and Writing
- USC Libraries Tutorials and Other Guides
- Bibliography
Proofreading is the act of reviewing, identifying, and correcting errors in your research paper before it is handed in to be graded by your professor. Common errors found within the text of a paper can be both typographical [i.e., an error in typing] and grammatical [i.e., faulty, unconventional use of language]. However, the act of proofreading can also include identifying and correcting problems with the narrative flow of your paper [i.e., the logical sequence of thoughts and ideas], problems with concise writing [i.e., wordiness and imprecise vocabulary], and problems created by word processing software applications [e.g., unintentional font types, indented paragraphs, line spacing, uneven margins, or orphan headings, sentences, or words].
Editing and Proofreading Strategies. Writing@CSU. Colorado State University; Proofreading. The Writing Center. University of Wisconsin, Madison.
Proofreading Strategies
Proofreading is often the final act before handing in your paper. It is important because most professors grade papers not only on the quality of how you addressed the research problem and the overall organization of the study, but also on the quality of the grammar, punctuation, formatting, and narrative flow of your paper. The assigning of research papers is not just an exercise in developing good research and critical thinking skills, but it is also intended to help you become a better writer. Below are step-by-step strategies you can follow.
Before You Proofread
- Revise the larger aspects of the text . Don't proofread for the purpose of making corrections at the sentence and word level [the act of editing] if you still need to work on the overall focus, development, and organization of the paper or you need to re-arrange or change specific sections [the act of revising].
- Set your paper aside between writing and proofreading . Give yourself a day or so between the writing of your paper and proofreading it. This will help you identify mistakes more easily. This is also a reason why you shouldn't wait until the last minute to draft your paper because it won't provide the time needed to step away before proofreading.
- Eliminate unnecessary words before looking for mistakes . Throughout your paper, you should try to avoid using inflated diction if a more concise phrase works equally well. Simple, precise language is easier to proofread than overly complex sentence constructions and vocabulary. At the same time, also identify and change empty or repetitive phrases.
- Know what to look for . Make a mental note of the mistakes you need to watch for based on comments from your professor on previous drafts of the paper or that you have received about papers written in other classes. This will help you to identify repeated patterns of mistakes more readily.
- Review your list of references . Review the sources mentioned in your paper and make sure you have properly cited them in your bibliography. Also make sure that the titles cited in your bibliography are mentioned in the text. Any omissions should be resolved before you begin proofreading your paper.
NOTE: Do not confuse the act of revising your paper with the act of editing it. Editing is intended to tighten up language so that your paper is easier to read and understand. This should be the focus when you proofread. If your professor asks you to revise your paper, review the text above concerning ways to improve the overall quality of your paper. The act of revision implies that there is something within the paper that needs to be changed, improved, or re-organized in some significant way. If the reason for a revision is not specified, always ask for clarification.
Individualize the Act of Proofreading
Individualizing your proofreading process to match weaknesses in your writing will help you correct errors more efficiently and effectively . For example, I still tend to make subject-verb agreement errors. Accept the fact that you likely won't be able to check for everything, so be introspective about what your typical problem areas are and look for each type of error individually. Here's how:
- Think about what errors you typically make . Review instructors' comments about your writing and/or set up an appointment to review your paper with a staff member in the Writing Center .
- Learn how to fix those errors . Talk with your professor about helping you understand why you make the errors you do so that you can learn how to avoid them while writing.
- Use specific strategies . Develop strategies you are most comfortable with to find and correct your particular errors in usage, sentence structure, spelling, and punctuation.
- Where you proofread is important! Effective and efficient proofreading requires extended focus and concentration. If you are easily distracted by external activity or noise, proofread in a quiet corner of the library rather than at a table in Starbucks.
- Proofread in several short blocks of time . Avoid trying to proofread your entire paper all at once, otherwise, it will be difficult to maintain your concentration. A good strategy is to start your proofreading each time at the beginning of your paper. It will take longer to make corrections, but you may be surprised how many mistakes you find in text that you have already reviewed.
In general, verb tense should be in the following format, although variations can occur within the text of each section depending on the narrative style of your paper. Note that references to prior research mentioned anywhere in your paper should always be stated in the past tense.
- Abstract--past tense [summary description of what I did]
- Introduction--present tense [I am describing the study to you now]
- Literature Review--past tense [the studies I reviewed have already been published]
- Methodology--past tense [the way I gathered and synthesized data has already happened]
- Results--past tense [the findings of my study have already been discovered]
- Discussion--present tense [I am talking to you now about how I interpreted the findings]
- Conclusion--present tense [I am summarizing the study for you now]
General Strategies for Strengthening Your Paper
As noted above, proofreading involves a detailed examination of your paper to ensure there are no content errors. However, proofreading is also an opportunity to strengthen the overall quality of your paper beyond correcting specific grammar, diction, or formatting mistakes. Before you begin reviewing your paper line-by-line, step back and reflect on what you have written; consider if there are ways to improve each section of the paper by taking into consideration the following “big picture” elements of good writing.
Introduction . Look for any language that reflects broad generalizations, indeterminate phrasing, or text that does not directly inform the reader about the research and its significance. This can include unnecessary qualifiers or text, such as, "This study includes a significant review of the literature [what constitutes "significant"?], "There are a number of findings that are important [just state the number of findings; leave it to the discussion to argue the context of their importance], and, for example, "This research reminds me of...." [why does the research study relate to remembering something; is this first person perspective essential to introducing the research problem].
Research Topic . Make sure the topic does not come across as ambiguous, simplistic, overly broad, or ill-defined. A strong research problem and the associated research questions establish a set of assumptions that should be nuanced, yet challenges the reader to think. Review the Choosing a Research Problem page in this guide. Place yourself in the position of a reader totally unfamiliar with the topic, then, critically evaluate the research problem, any associated research questions you are trying to address, and the theoretical framework. Ask yourself if there is anything that may not make sense or requires further explanation or refinement. The rest of the paper will build on these elements, but the introduction of these foundational aspects of your paper should be clearly and concisely stated.
Paragraph Transitions . Review the overall paper to make sure the narrative flow is coherent throughout and that there are smooth transitions between paragraphs. Ensure that major transitions in text have a heading or sub-heading [if needed] and that the paragraph prior to the transition let's the reader know that you are about to shift to a new idea. Also, look for text that is overly long or that contains too much description and too little analysis and interpretation. Sometimes you need a long paragraph to describe a complex idea, event, or issue, but review them to make sure they can't be broken apart into shorter, more readable paragraphs.
Discussion of Results . Read over your discussion of the research findings and make sure you have not treated any of the evidence as unproblematic or uncomplicated. Make sure you have discussed the results through a critical lens of analysis that takes into account alternative interpretations or possible counter-arguments. In most cases, your discussion section should demonstrate a thorough understanding of the study's findings and their implications, both positive, supportive findings and negative, unanticipated findings.
Conclusion . Make sure you have done more than simply re-state the research problem and what you did. Provide the reader with a sense of closure by ensuring that the conclusion has highlighted all the main points of the paper and tells the reader why the study was important, what the paper's broader significance and implications might be, and, if applicable, what areas of the study require further research. Also note that the conclusion is usually no more than two or three paragraphs. If your conclusion is longer, look for ways to condense the text and be alert to information that is superfluous or should be integrated into other parts of your paper [e.g., new information].
Specific Strategies to Help Identify Errors
Once you have made any necessary revisions to your paper and looked for ways to strengthen its overall quality, focus on identifying and correcting specific errors within the text.
- Work from a printout, not a computer screen . Besides sparing your eyes from the strain of glaring at a computer screen, proofreading from a printout allows you to easily skip around to where errors might have been repeated throughout the paper [e.g., misspelling the name of a person].
- Read out loud . This is especially helpful for spotting run-on sentences and missing words, but you'll also hear other problems that you may not have identified while reading the text out loud. This will also help you adopt the role of the reader, thereby helping you to understand the paper as your audience might.
- Use a ruler or blank sheet of paper to cover up the lines below the one you're reading . This technique keeps you from skipping over possible mistakes and allows you to deliberately pace yourself as you read through your paper.
- Circle or highlight every punctuation mark in your paper . This forces you to pay attention to each mark you used and to confirm its purpose in each sentence or paragraph. This is a particularly helpful strategy if you tend to misuse or overuse a punctuation mark, such as a comma or semi-colon.
- Use the search function of the computer to find mistakes . Using the Ctrl F search [find] feature can help identify repeated errors faster. For example, if you overuse a phrase or repeatedly rely on the same qualifier [e.g., "important"], you can do a search for those words or phrases and in each instance make a decision about whether to remove it, rewrite the sentence, or use a synonym.
- If you tend to make many mistakes, check separately for each kind of error , moving from the most to the least important, and following whatever technique works best for you to identify that kind of mistake. For instance, read through once [backwards, sentence by sentence] to check for fragments; read through again [forward] to be sure subjects and verbs agree, and again [perhaps using a computer search for "this," "it," and "they"] to trace pronouns to antecedents.
- End with using a computer spell checker or reading backwards word by word . Remember that a spell checker won't catch mistakes with homonyms [e.g., "they're," "their," "there"] or certain word-to-word typos [like typing "he" when you meant to write "the"]. The spell-checker function can catch some errors quickly, but it is not a substitute for carefully reviewing the text. This also applies to the grammar check function as well.
- Leave yourself enough time . Since many errors are made and overlooked by speeding through writing and proofreading, setting aside the time to carefully review your writing will help you identify errors you might otherwise miss. Always read through your writing slowly. If you read through the paper at a normal speed, you won't give your eyes sufficient time to spot errors.
- Ask a friend to read your paper . Offer to proofread a friend's paper if they will review yours. Having another set of eyes look over your writing will often spot errors that you would have otherwise missed.
NOTE: Pay particular attention to the spelling of proper nouns [an individual person, place, or organization]. Make sure the name is carefully capitalized and spelled correctly, and that this spelling has been used consistently throughout the text of your paper. This is especially true for proper nouns transliterated into English or that have been spelled differently over time. In this case, choose the spelling most consistently used by researchers in the literature you have cited so, if asked, you can explain the logic of your choice.
Carduner, Jessie. "Teaching Proofreading Skills as a Means of Reducing Composition Errors." Language Learning Journal 35 (2007): 283-295; Gaste, Barbara. “Editing and Proofreading Your Own Work.” American Medical Writers Association (AMWA) Journal 30 (2015): 147-151; Editing and Proofreading. Writing Center, University of North Carolina; Proofreading. Writing Center, University of Wisconsin, Madison; Proofreading. Writing Center, University of Maryland; Harris, Jeanette. "Proofreading: A Reading/Writing Skill." College Composition and Communication 38 (1987): 464-466; Editing and Proofreading. The Writing Center. University of North Carolina; Mintz, Steve. “Simple Ways to Strengthen Your Students’ Writing.” Higher Ed Gamma (Opinion). Inside Higher Ed , August 17, 2022; Revising vs. Proofreading, Kathleen Jones Wright Writing Center, Indiana University of Pennsylvania; Editing and Proofreading Strategies. Student Writing Support, University of Minnesota; Saleh, Naveed. The Writer's Guide to Self-Editing: Essential Tips for Online and Print Publication . Jefferson, NC: McFarland, 2019; Writing a Paper. Walden Writing Center, Walden University; The Writing Process: Proofreading. The Purdue Online Writing Lab, Purdue University.
USC Writing Center
S hould you need help proofreading your paper, take advantage of the assistance offered by consultants at the USC Writing Center located on the second floor of Taper Hall, room 216. Consultations are free and they can help you with any aspect of the writing process. Walk-in help is provided when consultants are available, but you should schedule an appointment online because the Center gets very busy as the semester progresses. If you meet with a consultant be sure to bring a copy of your writing assignment, any relevant handouts or texts, and any outlines or drafts you've written. Also, the Center conducts helpful, fifty minute small-group writing skills workshops for students that cover a wide range of topics. These workshops provide an opportunity for you to improve your skills related to an aspect of writing that you may be struggling with, particularly if English is not your native language.
- << Previous: Appendices
- Next: Common Grammar Mistakes >>
- Last Updated: Mar 26, 2024 10:40 AM
- URL: https://libguides.usc.edu/writingguide
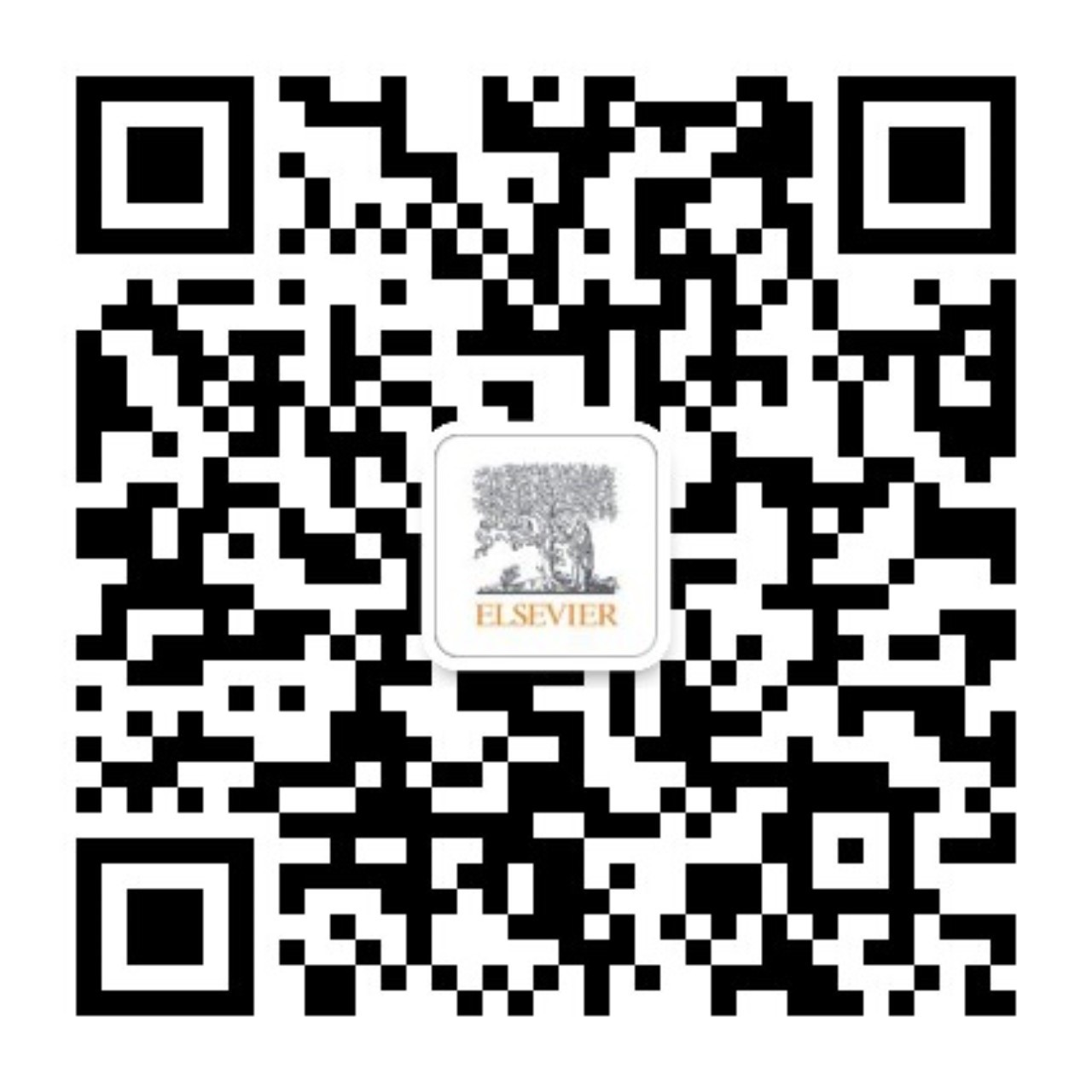
- Manuscript Review
What is the main purpose of proofreading a paper?
- 4 minute read
Table of Contents
It’s commonplace to feel nervous about submitting your scientific work. Whether you’re concerned about meeting research reviewers’ high expectations and/or the target journals’ guidelines. Proofreading is the final stage before a manuscript leaves your hands and enters the expanding universe of appraisal for publication. So, it makes sense that you want to deliver a perfectly written document, and avoid embarrassing mistakes.
Most of us simply have either friends or colleagues proofread our work, but they may have their own limitations regarding proficiency in text writing. Instead, it’s highly advised that you look for professional help at this important stage.
The main purpose of proofreading is to improve the quality of the paper, ensuring there are no lingering mistakes, and correcting generalized discourse errors or writing inconsistencies. Essentially, you want to make sure you have a well-defined communication goal. Analyzing whether the content is properly conveyed, and the sentences are syntactically and grammatically well-written, are just two of the basic tasks to achieve publication-ready work. Specifically, a perfect manuscript, ready to be published in the most recognized scientific journals.
What is proofreading
Proofreading is the last writing process before the author submits the article for publication. It is the stage of verification, by the author him or herself, or by another person. Thus, it is not only important to check grammar and spelling, it is also highly advised to ensure that the idea of the writer/author is in agreement with what he or she wants to communicate with the audience. In other words, that the article/work is clearly written for its intended target audience.
Proofreading Vs. Editing
Paper proofreader.
How often have you conducted high-quality research, but the article about that research didn’t match the quality of the research itself? How many times have you regretted missing a mistake that ultimately led to a failed submission?
Proofreading ensures flawless content for publication, increasing your chances of success. An excellent paper proofreader checks all digital sources related to the document, including websites, email addresses, etc.
A good paper proofreader is someone who will take care of your work as if it were his or her own and, in addition to correcting grammar errors, also detects the possibility of scientific plagiarism. Proofreading your scientific article using scientific editing will save you from the humiliation of having your article rejected by scientific journals due to grammatical errors or inadequate language.
Why is proofreading important?
Effective proofreading is absolutely essential for producing high-quality documents, whether academic or professional. When done clearly, correctly and thoroughly, proofreading can be the difference between writing something that communicates as it is supposed to or a huge misunderstanding. It can also be the difference between acceptance and rejection in a distinguished journal. No author creates an excellent text without reviewing, reflecting, and revising – or trusting someone to do so – before the final version of their manuscript is complete and submitted.
Language and text reviewing are important to detect:
- Grammar mistakes and numbering errors – e.g. forms of numbers, short and scientific forms, degrees of comparison, etc.
- Spelling mistakes – simple misspellings, or incorrect use of a homonym (words that sound alike, but have different meanings, e.g. “read,” for “red”), typographical error, etc.
- Inconsistency in the document format – this can be simple font, spacing and justification rules, or standard format for the applicable research sub-type (e.g. research review versus experiment)
- Punctuation errors – missing or extra commas, periods, and/or quotation marks used incorrectly
- Misplaced words – correct word choice improves the quality of your content
- Poorly structured paragraphs
- Errors in sentence structure
Whatever the nature of your research, Elsevier will be glad to give you a hand in reviewing and amending your manuscript. Professional editors can proofread your document so the final product is well-written, precise, and easy to read. With Elsevier’s medical editing and proofreading services team, we can help you with grammar, syntax, spelling, and punctuation; maximizing impact, and increasing your chances of publication.
Language Editing Services by Elsevier Author Services:
Find more about our Language Editing Standard : completion within 7 business days; editing by native speakers in (scientific) American or British English; PhD or PhD candidates selection according to your field of study and an exclusive guarantee: free re-edit or your money back.
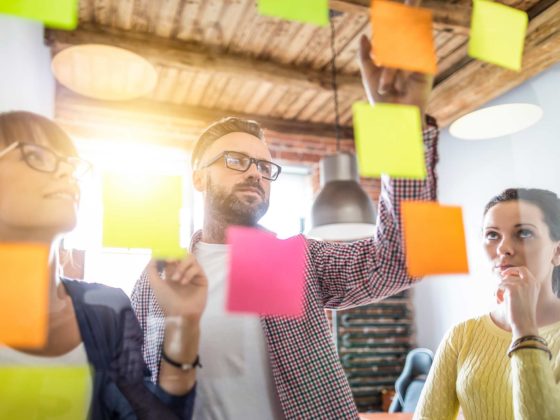
- Manuscript Preparation
Types of Scientific Articles
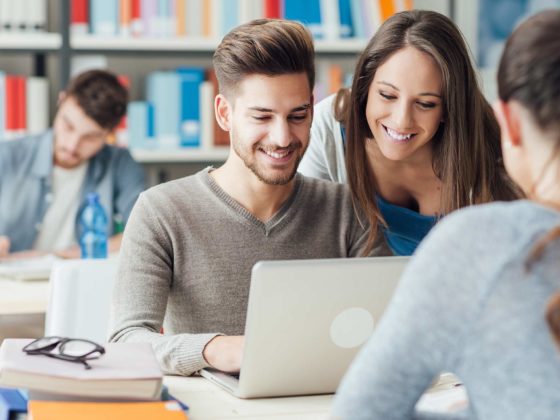
- Research Process
Navigating the Research Landscape: A Deep Dive into the FINER Method
You may also like.
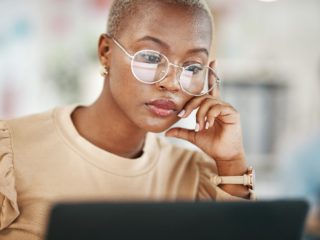
If You’re a Researcher, Remember These Before You Are Submitting Your Manuscript to Journals!
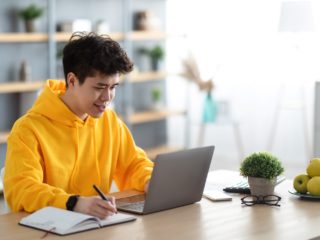
Navigating “Chinglish” Errors in Academic English Writing
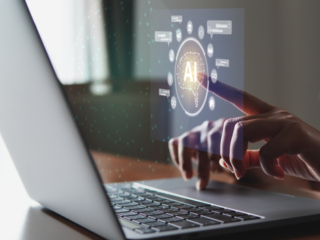
Is The Use of AI in Manuscript Editing Feasible? Here’s Three Tips to Steer Clear of Potential Issues
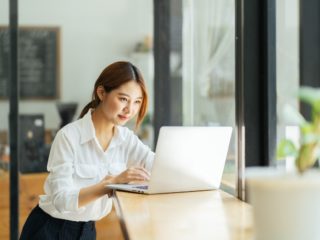
A profound editing experience with English-speaking experts: Elsevier Language Services to learn more!
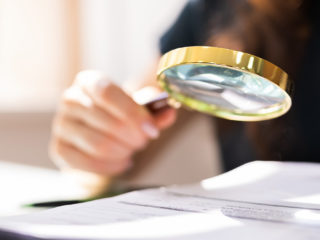
Research Fraud: Falsification and Fabrication in Research Data
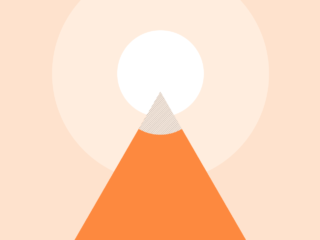
Professor Anselmo Paiva: Using Computer Vision to Tackle Medical Issues with a Little Help from Elsevier Author Services
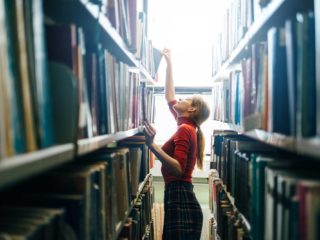
Systematic Review VS Meta-Analysis
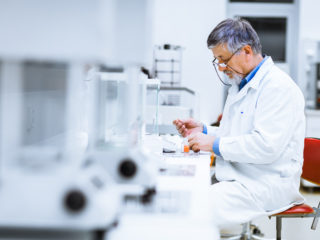
Looking for Medical Editing Services
Input your search keywords and press Enter.

OASIS: Writing Center
Writing a paper: proofreading, introduction.
Proofreading involves reading your document to correct the smaller typographical, grammatical, and spelling errors. Proofreading is usually the very last step you take before sending off the final draft of your work for evaluation or publication. It comes after you have addressed larger matters such as style, content, citations, and organization during revising. Like revising, proofreading demands a close and careful reading of the text. Although quite tedious, it is a necessary and worthwhile exercise that ensures that your reader is not distracted by careless mistakes.
Tips for Proofreading
- Set aside the document for a few hours or even a few days before proofreading. Taking a bit of time off enables you to see the document anew. A document that might have seemed well written one day may not look the same when you review it a few days later. Taking a step back provides you with a fresh (and possibly more constructive) perspective.
- Make a conscious effort to proofread at a specific time of day (or night!) when you are most alert to spotting errors. If you are a morning person, try proofreading then. If you are a night owl, try proofreading at this time.
- Reviewing the document in a different format and having the ability to manually circle and underline errors can help you take the perspective of the reader, identifying issues that you might ordinarily miss. Additionally, a hard copy gives you a different visual format (away from your computer screen) to see the words anew.
- Although useful, programs like Word's spell-checker and Grammarly can misidentify or not catch errors. Although grammar checkers give relevant tips and recommendations, they are only helpful if you know how to apply the feedback they provide. Similarly, MS Word's spell checker may not catch words that are spelled correctly but used in the wrong context (e.g., differentiating between their, they're , and there ). Beyond that, sometimes a spell checker may mark a correct word as wrong simply because the word is not found in the spell checker's dictionary. To supplement tools such as these, be sure to use dictionaries and other grammar resources to check your work. You can also make appointments with our writing instructors for feedback concerning grammar and word choice, as well as other areas of your writing!
- Reading a text aloud allows you to identify errors that you might gloss over when reading silently. This technique is particularly useful for identifying run-on and other types of awkward sentences. If you can, read for an audience. Ask a friend or family member to listen to your work and provide feedback, checking for comprehension, organization, and flow.
- Hearing someone else read your work allows you to simply listen without having to focus on the written words yourself. You can be a more critical listener when you are engaged in only the audible words.
- By reading the document backwards, sentence by sentence, you are able to focus only on the words and sentences without paying attention to the context or content.
- Placing a ruler or a blank sheet of paper under each line as you read it will give your eyes a manageable amount of text to read.
- If you can identify one type of error that you struggle with (perhaps something that a faculty member has commented on in your previous work), go through the document and look specifically for these types of errors. Learn from your mistakes, too, by mastering the problem concept so that it does not appear in subsequent drafts.
- Related to the previous strategy of checking for familiar errors, you can proofread by focusing on one error at a time. For instance, if commas are your most frequent problem, go through the paper checking just that one problem. Then proofread again for the next most frequent problem.
- After you have finished making corrections, have someone else scan the document for errors. A different set of eyes and a mind that is detached from the writing can identify errors that you may have overlooked.
- Remember that proofreading is not just about errors. You want to polish your sentences, making them smooth, interesting, and clear. Watch for very long sentences, since they may be less clear than shorter, more direct sentences. Pay attention to the rhythm of your writing; try to use sentences of varying lengths and patterns. Look for unnecessary phrases, repetition, and awkward spots.
Download and print a copy of our proofreading bookmark to use as a reference as you write!
- Proofreading Bookmark Printable bookmark with tips on proofreading a document.
Proofreading for Grammar Video
Note that this video was created while APA 6 was the style guide edition in use. There may be some examples of writing that have not been updated to APA 7 guidelines.
- Mastering the Mechanics: Proofreading for Grammar (video transcript)
Related Webinar

- Previous Page: Revising for Writing Goals
- Next Page: Reflecting & Improving
- Office of Student Disability Services
Walden Resources
Departments.
- Academic Residencies
- Academic Skills
- Career Planning and Development
- Customer Care Team
- Field Experience
- Military Services
- Student Success Advising
- Writing Skills
Centers and Offices
- Center for Social Change
- Office of Academic Support and Instructional Services
- Office of Degree Acceleration
- Office of Research and Doctoral Services
- Office of Student Affairs
Student Resources
- Doctoral Writing Assessment
- Form & Style Review
- Quick Answers
- ScholarWorks
- SKIL Courses and Workshops
- Walden Bookstore
- Walden Catalog & Student Handbook
- Student Safety/Title IX
- Legal & Consumer Information
- Website Terms and Conditions
- Cookie Policy
- Accessibility
- Accreditation
- State Authorization
- Net Price Calculator
- Contact Walden
Walden University is a member of Adtalem Global Education, Inc. www.adtalem.com Walden University is certified to operate by SCHEV © 2024 Walden University LLC. All rights reserved.
Organizing Your Social Sciences Research Paper: Proofreading Your Paper
- Purpose of Guide
- Writing a Research Proposal
- Design Flaws to Avoid
- Independent and Dependent Variables
- Narrowing a Topic Idea
- Broadening a Topic Idea
- The Research Problem/Question
- Academic Writing Style
- Choosing a Title
- Making an Outline
- Paragraph Development
- The C.A.R.S. Model
- Background Information
- Theoretical Framework
- Citation Tracking
- Evaluating Sources
- Reading Research Effectively
- Primary Sources
- Secondary Sources
- What Is Scholarly vs. Popular?
- Is it Peer-Reviewed?
- Qualitative Methods
- Quantitative Methods
- Common Grammar Mistakes
- Writing Concisely
- Avoiding Plagiarism [linked guide]
- Annotated Bibliography
- Grading Someone Else's Paper
Proofreading is the act of searching for errors before you hand in the your research paper. Errors can be both grammatical and typographical in nature, but can also include identifying problems with the narrative flow of your paper [i.e., the logical sequence of thoughts and ideas], problems with concise writing [i.e., wordiness], and finding any word processing errors [e.g., different font types, indented paragraphs, line spacing, uneven margins, etc.].
Editing and Proofreading Strategies . Writing@CSU. Colorado State University; Proofreading . The Writing Center. University of Wisconsin, Madison.
Strategies for Proofreading your Paper
Before You Proofread
- Be sure you have revised the larger aspects of the text . Don't make corrections at the sentence and word level [the act of editing] if you still need to work on the overall focus, development, and organization of the paper or you need to re-arrange or change specific sections [the act of revising].
- Set your paper aside between writing and proofreading . Give yourself a day or so between the writing of your paper and proofreading it. This will help you identify mistakes more easily. This is also a reason why you shouldn't wait until the last minute to draft your paper because it won't provide the time needed between writing and proofreading.
- Eliminate unnecessary words before looking for mistakes . Throughout your paper, you should try to avoid using inflated diction if a simpler phrase works equally well. Simple, precise language is easier to proofread than overly complex sentence constructions and vocabulary. At the same time, also identify and change empty or repetitive phrases.
- Know what to look for . Make a mental note of the mistakes you need to watch for based on comments from your professor on previous drafts of the paper or that you have received about papers written in other classes. This will help you to identify repeated patterns of mistakes more readily.
- Review your list of references . Review the sources mentioned in your paper and make sure you have properly cited them in your bibliography. Also make sure that the titles cited in your bibliography are mentioned in the text. Any omissions should be resolved before you begin proofreading your paper.
NOTE: Do not confuse the act of revising your paper with the act of editing it. Editing is intended to tighten up language so that your paper is easier to read and understand. This should be the focus when you proofread. If your professor asks you to revise your paper, the implication is that there is something within the text that needs to be changed, improved, or re-organized in some significant way. If the reason for a revision is not specified, always ask for clarification.
Strategies to Help Identify Errors
- Work from a printout, not a computer screen . Besides sparing your eyes the strain of glaring at the computer, proofreading from a printout allows you to easily skip around to where errors might have been repeated throughout the paper [e.g., misspelled name of a person].
- Read out loud . This is especially helpful for spotting run-on sentences, but you'll also hear other problems that you may not have identified while reading the text out loud. This will also helps you play the role of the reader, thereby, encouraging you to understand the paper as your audience might.
- Use a ruler or blank sheet of paper to cover up the lines below the one you're reading . This technique keeps you from skipping over possible mistakes. This also helps you deliberately pace yourself as you read through your paper.
- Circle or highlight every punctuation mark in your paper . This forces you to pay attention to each mark you used and to question its purpose in each sentence or paragraph. This is a particularly helpful strategy if you tend to misuse or overuse a punctuation mark, such as a comma or semi-colon.
- Use the search function of the computer to find mistakes . Using the search [find] feature of your word processor can help you identify common errors faster. For example, if you overuse a phrase or use the same qualifier over and over again, you can do a search for those words or phrases and in each instance make a decision about whether to remove it or use a synonym.
- If you tend to make many mistakes, check separately for each kind of error , moving from the most to the least important, and following whatever technique works best for you to identify that kind of mistake. For instance, read through once [backwards, sentence by sentence] to check for fragments; read through again [forward] to be sure subjects and verbs agree, and again [perhaps using a computer search for "this," "it," and "they"] to trace pronouns to antecedents.
- End with using a computer spell checker or reading backwards word by word . Remember that a spell checker won't catch mistakes with homonyms [e.g., "they're," "their," "there"] or certain typos [like "he" when you meant to write "the"]. The spell-checker function is not a substitute for carefully reviewing the text for spelling errors.
- Leave yourself enough time . Since many errors are made and overlooked by speeding through writing and proofreading, setting aside the time to carefully review your writing will help you catch errors you might otherwise miss. Always read through your writing slowly. If you read through the paper at a normal speed, you won't give your eyes sufficient time to spot errors.
- Ask a friend to read your paper . Offer to proofread a friend's paper if they will review yours. Having another set of eyes look over your writing will often spot errors that you would have otherwise missed.
NOTE : Pay particular attention to the spelling of proper nouns [ an individual person, place, or organization] . Make sure the name is carefully capitalized and spelled correctly, and that this spelling has been used consistently throughout the text of your paper. This is especially true for proper nouns transliterated into English or that have been spelled differently over time. In this case, choose the spelling used most consistently by researchers in the literature you have cited so, if asked, you can explain the logic of your choice.
Individualize the Act of Proofreading
In addition to following the suggestions above, individualizing your proofreading process to match weaknesses in your writing will help you proofread more efficiently and effectively . For example, I still tend to make subject-verb agreement errors. Accept the fact that you likely won't be able to check for everything, so be introspective about what your typical problem areas are and look for each type of error individually. Here's how:
- Think about what errors you typically make . Review instructors' comments about your writing and/or set up an appointment review your paper with a staff member in the Writing Center .
- Learn how to fix those errors . Talk with your professor about helping you understand why you make the errors you do make so that you can learn how to avoid them.
- Use specific strategies . Develop strategies you are most comfortable with to find and correct your particular errors in usage, sentence structure, spelling, and punctuation.
- Where you proofread is important! Effective and efficient proofreading requires extended focus and concentration. If you are easily distracted by external activity or noise, proofread in a quiet corner of the library rather than at a table in Starbucks.
- Proofread in several short blocks of time . Avoid trying to proofread you entire paper all at once, otherwise, it will be difficult to maintain your concentration. A good strategy is to start your proofreading each time at the beginning of your paper. It will take longer to make corrections, but you'll be amazed at how many mistakes you find in text that you have already reviewed.
In general, verb tense should be in the following format, although variations can occur within the text depending on the narrative style of your paper. Note that references to prior research mentioned anywhere in your paper should always be stated in the past tense.
- Abstract--past tense [the summary description of what I did]
- Introduction--present tense [I am describing the study to you now]
- Literature Review--past tense [the studies I reviewed have already been written]
- Methodology--past tense [the way I gathered and synthesized data has already happened]
- Results--past tense [the findings have already been discovered]
- Discussion--present tense [I am talking to you now about how I interpreted the findings]
- Conclusion--present tense [I am summarizing the study for you now]
Cogie, Jane, Kim Strain, and Sharon Lorinskas. "Avoiding the Proofreading Trap: The Value of the Error Correction Process." The Writing Center Journal 19 (Spring/Summer 1999): 7-32; Editing and Proofreading . The Writing Center. University of North Carolina; Editing and Proofreading Strategies . Writing@CSU. Colorado State University; Harris, Jeanette. "Proofreading: A Reading/Writing Skill." College Composition and Communication 38 (December 1987): 464-466; Lunsford, Andrea A. and Robert Connors. The St. Martin's Handbook . New York: St. Martin's Press, 1989; Madraso, Jan. "Proofreading: The Skill We've Neglected to Teach." The English Journal 82 (February 1993): 32-41; Proofreading . The Writer’s Handbook. Writing Center. University of Wisconsin, Madison; Proofreading . Department of English Writing Guide. George Mason University; Proofreading and Revising . Online Writing Center, Walden University; Proofreading a College Paper: Guidelines and Checklist . Troy University Library Tutorial; Revision: Cultivating a Critical Eye . Institute for Writing Rhetoric. Dartmouth College; Revision Guidelines . The Reading/Writing Center. Hunter College; Where Do I Begin? The Writing Lab and The OWL. Purdue University; Williams, Joseph M. and Lawrence McEnerney. Writing in College 3:A Strategy for Analyzing and Revising a First Draft . Writing Program, The University of Chicago.
Additional Help
Writing Center
Should you need help writing your paper, take advantage of the assistance offered by consultants at the Writing Center located on the the ground floor of ASBN (100). Consultations are free and they can help you with any aspect of the writing process. Walk-in help is provided when consultants are available, but you should schedule an appointment online because the Writing Center gets very busy as the semester progresses. Read more about the Writing Center here.
Location: Ground Floor ASBN (100) Phone: 512·245·3018 Hours Monday-Thursday: 10am-7pm Friday: 11am-4pm
- << Previous: Appendices
- Next: Common Grammar Mistakes >>
- Last Updated: Sep 8, 2023 12:19 PM
- URL: https://guides.library.txstate.edu/socialscienceresearch

Organizing Academic Research Papers: 10. Proofreading Your Paper
- Purpose of Guide
- Design Flaws to Avoid
- Glossary of Research Terms
- Narrowing a Topic Idea
- Broadening a Topic Idea
- Extending the Timeliness of a Topic Idea
- Academic Writing Style
- Choosing a Title
- Making an Outline
- Paragraph Development
- Executive Summary
- Background Information
- The Research Problem/Question
- Theoretical Framework
- Citation Tracking
- Content Alert Services
- Evaluating Sources
- Primary Sources
- Secondary Sources
- Tertiary Sources
- What Is Scholarly vs. Popular?
- Qualitative Methods
- Quantitative Methods
- Using Non-Textual Elements
- Limitations of the Study
- Common Grammar Mistakes
- Avoiding Plagiarism
- Footnotes or Endnotes?
- Further Readings
- Annotated Bibliography
- Dealing with Nervousness
- Using Visual Aids
- Grading Someone Else's Paper
- How to Manage Group Projects
- Multiple Book Review Essay
- Reviewing Collected Essays
- About Informed Consent
- Writing Field Notes
- Writing a Policy Memo
- Writing a Research Proposal
- Acknowledgements
Proofreading is the act of searching for errors before you hand in the your final research paper. Errors can be both grammatical and typographical in nature, but proofreading can also be used to identify problems with the flow of your paper [i.e., the logical sequence of thoughts and ideas] and to find any word processing errors [e.g., different font types, indented paragraphs, line spacing, etc.].
Strategies for Proofreading your Paper
Before You Proofread
- Be sure you've revised the larger aspects of your text . Don't make corrections at the sentence and word level if you still need to work on the overall focus, development, and arrangement of the paper, of sections in the paper, or of individual paragraphs.
- Set your text aside for a while between writing and proofreading . Establishing some distance between the writing your paper and proofreading it will help you identify mistakes more easily.
- Eliminate unnecessary words before looking for mistakes . Throughout your paper, you should try to avoid using inflated diction if a simpler phrase works equally well. Simple, more precise language is easier to proofread than overly complex sentence construction and vocabulary.
- Know what to look for . Make a list of mistakes you need to watch for based upon the comments of your professors on previous drafts of your paper or for papers written in other classes. This will help you to identify repeated patterns of mistakes more readily.
To help ensure that you identify all the errors in your paper, consider the following:
- Work from a printout, not a computer screen . Besides sparing your eyes the strain of glaring at a computer screen, proofreading from a printout allows you to easily skip around to where errors might have been repeated throughout the paper.
- Read out loud . This is especially helpful for spotting run-on sentences, but you'll also hear other problems that you may not have picked up while reading silently. Reading your paper out loud also helps you play the role of the reader, thereby encouraging you to understand the paper as your audience might.
- Use a ruler or blank sheet of paper to cover up the lines below the one you're reading . This technique keeps you from skipping over possible mistakes.
- Circle or highlight every punctuation mark in your paper . This forces you to pay attention to each mark you used and to question its purpose in each sentence or paragraph. This is particularly helpful strategy if you tend to misuse or overuse a punctuation mark, such as a comma or semi-colon.
- Use the search function of the computer to find mistakes you're likely to make . Using the search [find] feature of your word processor can help youidentify common errors faster. For example, if you overuse a phrase or use the same qualifier over and over again, you can do a search for those words or phrases and in each instance make a decision about whether to keep it or not or use a synonym.
- If you tend to make many mistakes, check separately for each kind of error , moving from the most to the least important, and following whatever technique works best for you to identify that kind of mistake. For instance, read through once [backwards, sentence by sentence] to check for fragments; read through again [forward] to be sure subjects and verbs agree, and again [perhaps using a computer search for "this," "it," and "they"] to trace pronouns to antecedents.
- End with using a computer spell checker or reading backwards word by word . But remember that a spell checker won't catch mistakes with homonyms [e.g., "they're," "their," "there"] or certain typos [like "he" when you meant to write "the"].
- Leave yourself enough time . Since many errors are made and overlooked by speeding through writing and proofreading, taking the time to carefully look over your writing will help you catch errors you might otherwise miss. Always read through your writing slowly. If you read through the paper at a normal speed, you won't give your eyes sufficient time to spot errors.
- Ask a friend to read your paper . Offer to proofread a friend's paper if they will review yours. Having another set of eyes look over your writing will often spot errors that you would have otherwise missed.
Individualize the Act of Proofreading
In addition to following the suggestions above, individualizing your proofreading process to match weaknesses in your writing will help you proofread more efficiently and effectively . For example, I still tend to make subject-verb agreement errors. Accept the fact that you likely won't be able to check for everything, so be introspective about what your typical problem areas are and look for each type of error individually. Here's how:
- Think about what errors you typically make . Review instructors' comments about your writing and/or review your paper with a tutor.
- Learn how to fix those errors . Talk with your professor about helping you understand why you make the errors you do make so that you can learn ways to avoid them.
- Use specific strategies . Develop strategies you are most comfortable with to find and correct your particular errors in usage, sentence structure, and spelling and punctuation.
- Where you proofread is important! Effective and efficient proofreading requires extended focus and concentration. If you are easily distracted by external noise, proofread in a quiet corner of the library rather than in a coffee shop.
- Proofread in several short blocks of time . Avoid trying to proofread you entire paper all at once, otherwise, it will be difficult to maintain your concentration. A good strategy is to start your proofreading each time at the beginning of your paper. It will take longer to make corrections, but you'll be amazed at how many mistakes you find in text you've already reviewed.
Editing and Proofreading . The Writing Center. University of North Carolina; Editing and Proofreading Strategies . Writing@CSU. Colorado State University; Proofreading . The Writer’s Handbook. Writing Center. University of Wisconsin, Madison; Proofreading. Department of English Writing Guide. George Mason University; Revision: Cultivating a Critical Eye. Institute for Writing Rhetoric. Dartmouth College; Revision Guidelines . The Reading/Writing Center. Hunter College; Where Do I Begin? The Writing Lab and The OWL. Purdue University.
- << Previous: Appendices
- Next: Common Grammar Mistakes >>
- Last Updated: Jul 18, 2023 11:58 AM
- URL: https://library.sacredheart.edu/c.php?g=29803
- QuickSearch
- Library Catalog
- Databases A-Z
- Publication Finder
- Course Reserves
- Citation Linker
- Digital Commons
- Our Website
Research Support
- Ask a Librarian
- Appointments
- Interlibrary Loan (ILL)
- Research Guides
- Databases by Subject
- Citation Help
Using the Library
- Reserve a Group Study Room
- Renew Books
- Honors Study Rooms
- Off-Campus Access
- Library Policies
- Library Technology
User Information
- Grad Students
- Online Students
- COVID-19 Updates
- Staff Directory
- News & Announcements
- Library Newsletter
My Accounts
- Interlibrary Loan
- Staff Site Login

FIND US ON
How to Find the Right Academic Paper Editor or Proofreader
#scribendiinc
Written by Scribendi
You've finished your research paper. Your data has been processed, your methods have been outlined, your arguments have been laid out, and potential counterarguments have been addressed. Now comes the part that most authors dislike: academic editing.
Choosing an academic editor or proofreader is a daunting task. It requires finding someone who has the skill to correct grammatical and stylistic errors in your paper. That person should also be familiar enough with academic paper editing to employ its conventions and rules.
So, how do you find the right academic editor or proofreader for you?
What's the Difference between Academic Paper Editing and Academic Proofreading Services?
People often confuse academic paper editing and academic proofreading services because they both involve text correction. However, they are distinctly different. You'll want an academic editor when you're ready to refine your writing. However, an academic proofreading service is best when you're confident that your writing is in good shape.
When it comes to choosing an academic editor or proofreader, it helps to know the difference between the two.
Read More: Editing or Proofreading?
With academic editing , editors look for issues at the core of the writing. They look at the way sentences are constructed, as well as the flow and overall clarity of the work. They also check for readability, clarity, and tone, in addition to grammar and spelling. When you have a rough draft that needs substantial revisions, which is usually when you're at the start of your writing process, an editor is your best bet.
With academic proofreading services, a proofreader looks for incorrectly spelled words, missed punctuation, or inconsistencies in the text. Usually, you'll need a proofreader after an editor has already reviewed your work and you just need a final review for grammar or punctuation errors before you submit it.
Most documents require both editing and proofreading, in that order. An editor can help you improve your first draft, and a proofreader can help you perfect your final draft.
Make Every Word Count with Professional Proofreading
Try our academic proofreading service , or get a free sample, how do i edit or proofread my own academic paper.
To edit your own academic paper, it can help to switch your perspective, to edit your paper in sections, to read your paper multiple times, and/or to try creating a reverse outline. We cover these methods in this blog post so you can edit your paper objectively.
The hardest part of being your own academic editor and proofreader is accepting that almost anything in your paper can be trimmed away. Many researchers spend months (if not years) polishing their papers.
It can be discouraging to look at a point that took countless hours to develop and strengthen and realize that, ultimately, it does not provide much support for your thesis. If you want to give proofreading your own paper a try, though, there are several steps you can take.
Try Switching Up Your Perspective
Sometimes when you've read something long enough, you're not able to look at it subjectively. You might not be able to easily tell what's missing, or your eyes might gloss over glaring typos.
In this case, try switching up your perspective.
Print out your academic paper and hold it in your hands instead of looking at it on a screen. Printing out your work offers an entirely new way of reading your document. It's a new experience for your eyes, and it'll be harder to make assumptions about things.
Alternatively, you can alter the font style or size of the text in your paper. You'd be surprised how much you can alter your reading experience simply by changing the font. You'll read sentences in a new way, and you might even find new things that don't make sense!
Changing an aspect of your document will help you see it from a new perspective. It will also prevent you from skimming over any words or sentences that might need your attention.
Edit or Proofread Your Academic Paper in Sections
If you start feeling fatigued, frustrated, angry, or anything but calm and self-assured, put your paper down and walk away from it for a while. It's best to take your time and do your academic paper editing with confidence, section by section. This approach is far more effective than quickly pushing through and missing obvious errors.
You can experiment with pretending that each section belongs only to itself, and that there's no other content. When you view your text this way, it's a different experience altogether. You can better digest the paper by taking it piece by piece versus trying to stuff the entire thing down in one read.
Plus, it allows you to slow down. And when you slow down during your reading, you'll catch more errors.
Read More: How to Edit and Proofread Your Own Writing
Read Your Academic Paper Multiple Times
Most academic editors will read through a document at least twice to catch all the mistakes and typos within it. The University of Arkansas Little Rock recommends making several passes over your paper (one pass for spelling, one for punctuation, and so on) if you plan to do your own academic editing.
You'd be surprised how easy it is to catch errors when you read something more than once. In fact, reading content multiple times is what helps editors and proofreaders find the most common mistakes. It's not a superpower; it's just simple repetition!
Try Creating a Reverse Outline for Your Academic Paper
A reverse outline can help you see your ideas clearly. Start at the end of your academic paper and work backward, identifying every point made in each paragraph. Rank your points by importance, then reshuffle your paragraphs so that the most important points are made first.
Don't be surprised if you find you have to rewrite your thesis or even your entire paper after doing this.
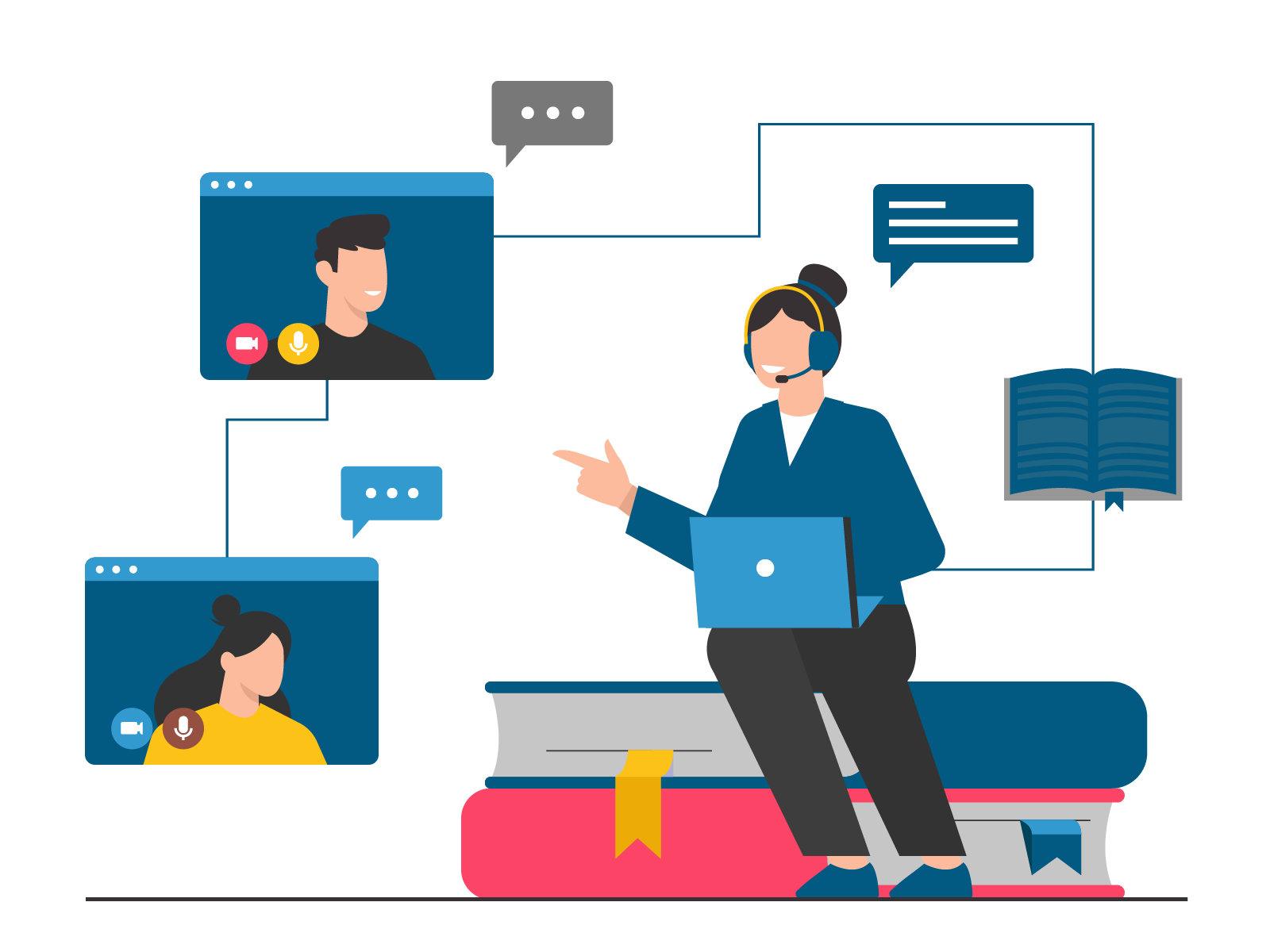
How Do I Ask Someone to Help Me Edit or Proofread My Academic Paper?
When you need help editing or proofreading an academic paper, it helps to find someone who understands your field of study. That being said, you should also keep in mind the fact that your colleagues are busy too. It is important to be polite if they say no or to set a deadline and avoid criticizing their work if they say yes.
If you're unable to hire an academic paper editing or academic proofreading service, asking someone you know to help you is a great option. There's nothing better than getting a second pair of eyes on something you've looked at a million times.
However, there are a few things to consider before asking a friend, teacher, or classmate.
Find Someone Who Understands Your Field of Study
If you plan to find an academic editor among your classmates, teachers, or fellow academics, focus on those who share or at least understand your field of study. Ask your academic advisor or check with your student services department to see what sort of academic editing or proofreading resources are available to you.
Most institutions offer groups, guides, programs, and other helpful tools to support those in need of academic paper editing or proofreading assistance. You don't even need to be affiliated with certain schools to benefit from their guidance!
Keep In Mind That Others Are Busy Too
Bear in mind, though, that just as you have been working on your paper, your classmates and supervisors have been similarly busy.
Your professors have to lead classes, hold workshops, attend meetings, and supervise student work—all while staying on top of their own research responsibilities.
While asking for a fresh perspective and a friendly edit may sound like a simple request, asking the wrong way can bruise feelings and strain relationships.
Be Polite and Respectful If They Say No
When asking for assistance, remember to be polite and respectful, and respond with grace if the answer is "no." Here are a few examples:
- Be polite : Use "please" in your query. It sounds simple, but it's easy to forget sometimes! And "please" goes a long way. You can say something like, "Would you please take a few minutes to review what I've written and let me know if I'm heading in the right direction?"
- Be respectful : As a show of respect, you can say something like, "I realize you're busy, but would you mind doing me a favor?"
- Be graceful : Even if the answer is "no," you can still show some grace by saying, "Thanks, anyway. Do you happen to know anyone else who might be able to help?"
Set a Deadline
If you get a "yes," express your gratitude and agree on a time for you to collect your edited paper. Don't say "do it when you can," even if you have plenty of time before you need to turn your paper in.
You don't want to find yourself in a situation where you're pressuring someone to read your paper because it's nearing the deadline!
Avoid Criticizing
When you get your paper back, be sure not to criticize your friend or colleague if you don't think they did a fantastic job.
Instead, focus on being grateful that they took the time to help you and did the best they could.
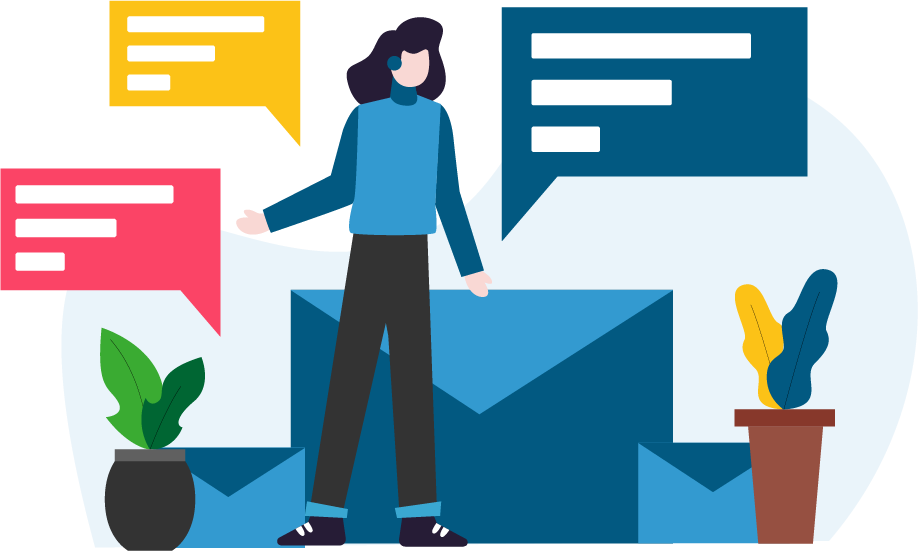
How Much Do Academic Paper Editing and Academic Proofreading Services Cost?
Academic paper editing and academic proofreading service costs vary depending on your turnaround time, the type of document you have, and the type of edit you need. Academic editing and proofreading are not the same, and different academic paper editing and academic proofreading services offer different rates.
Read More: How Much Does Proofreading Cost?
When pricing out the cost of an academic paper editing or academic proofreading service, consider the following:
Timing is a big factor to consider. Not only does timing affect the price of having your paper edited, but it also plays a big role in ensuring that you edit and finish your paper on time.
It's good practice to submit your paper to a proofreader or editor well in advance of the due date so you're not paying extra for a rush job.
To give yourself plenty of time to make revisions and corrections to your document after you've received it back, set a deadline to have an editor review your paper at least 48 hours before it's due.
Type of Academic Paper Editing or Academic Proofreading Service
Since academic paper editing and academic proofreading services aren't the same, the pricing between the two will vary. Academic paper editing requires a more in-depth review of the work, while academic proofreading is more superficial, checking for grammar, spelling, and punctuation. For this reason, some academic paper editing services will charge more for an edit than a proofread.
When you're pricing out academic paper editing or academic proofreading services, keep an eye out for tiered or à-la-carte services. You can expect to pay extra for higher tiers or additional perks if you want a full edit plus constructive feedback. So, the full cost of academic paper editing and academic proofreading services will depend on the type of academic paper editing or academic proofreading service you're looking for.
Academic Editing Speed
In order to gauge how long it will take to get your academic paper edited or proofread, keep in mind the industry standard for academic editing: 1,000 words per hour. This means a 30,000-word research paper should take roughly 30 hours to edit completely.
Break that up into workdays of ten hours each (because editors, like everyone else, need to eat and sleep), and that makes three days of work.
So, once you've figured out your word count and compared it to the 1000-words-per-hour industry standard, you should have a good idea of how quickly you can expect someone to return your paper.
Splitting the Work
If you need your work done quickly, some academic paper editing or academic proofreading services do promise turnaround times of 24 hours or less. But another option to have your academic work edited or proofread faster is to have editors split the work. This means they'll break the document into pieces and allow several editors to work on your document at once.
While this will get the job done faster, it may also cause contradictory editorial advice or certain inconsistencies to appear when you put the pieces back together. So be sure to keep that in mind!
Academic Paper Editing and Academic Proofreading Service Reputation
You'll want to be wary of academic paper editing or academic proofreading services that have a poor reputation.
Read More: 12 Best Editing and Proofreading Services
In addition, avoid companies that rely heavily (or completely!) on computer programs to do the work. Academic editing programs are not quite sophisticated enough yet to read a document and comprehend the context well enough to spot logical flaws, poor citations, unsubstantiated assertions, or pointless deviations.
Read More: 10 Best Human and AI Grammar Checkers
Lastly, businesses that do not address your complaints or offer to make things right if you're unhappy with their academic paper editing or academic proofreading service do not deserve to handle your paper.
Longevity and Proof of Quality
Your best bet for an academic paper editing or academic proofreading service is a business that has been around for a while.
Work with an academic paper editing service that has the following qualities:
- Human editors with experience and education
- A proven track record of quality
- Consistent pricing based on the academic paper editing or academic proofreading service you need
- Ability to provide constructive feedback as well as thorough academic editing work
- Excellent support from customer service
No matter whom you choose as your academic editor or proofreader, remember that academic editing is always beneficial and all writers consider it a necessary step of their writing journey.
Allow your paper to be edited and proofread to ensure that you have crafted a concise, thoughtful, and solid argument and have contributed to the wealth of knowledge available to the world.
Happy writing!
Make Every Word Count with Professional Academic Proofreading
About the author.
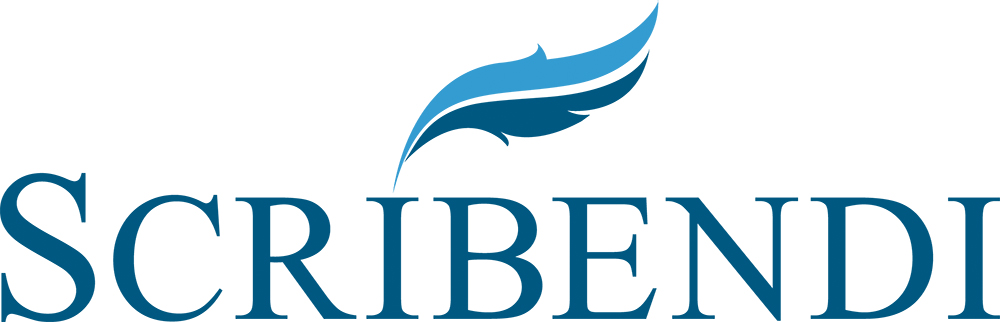
Scribendi's in-house editors work with writers from all over the globe to perfect their writing. They know that no piece of writing is complete without a professional edit, and they love to see a good piece of writing transformed into a great one. Scribendi's in-house editors are unrivaled in both experience and education, having collectively edited millions of words and obtained numerous degrees. They love consuming caffeinated beverages, reading books of various genres, and relaxing in quiet, dimly lit spaces.
Have You Read?
"The Complete Beginner's Guide to Academic Writing"
Related Posts
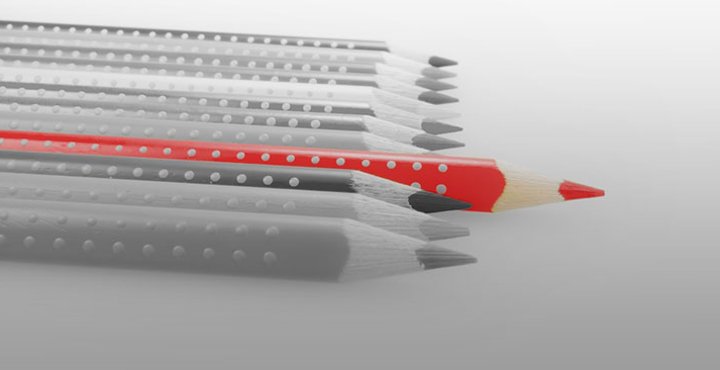
Editing or Proofreading?
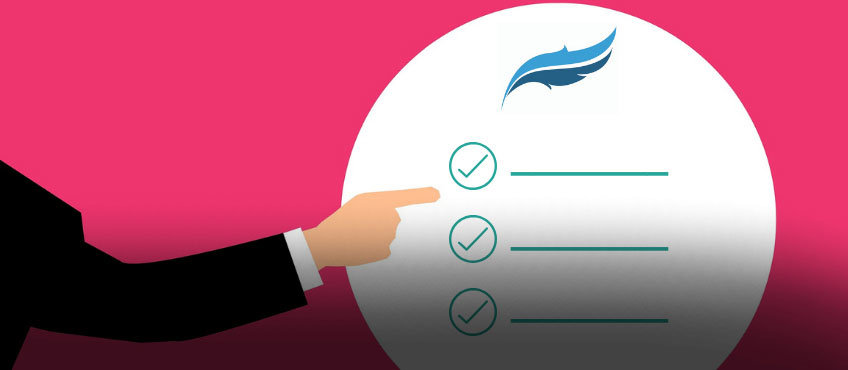
How to Edit and Proofread Your Writing to Engage Your Audience
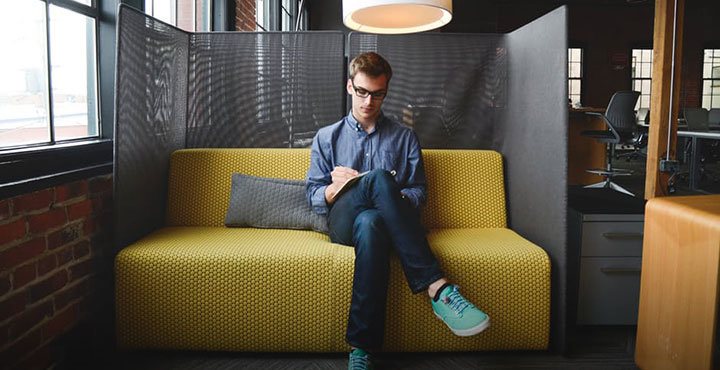
Why Hiring a Copy Editor Is a Good Idea
Upload your file(s) so we can calculate your word count, or enter your word count manually.
We will also recommend a service based on the file(s) you upload.
English is not my first language. I need English editing and proofreading so that I sound like a native speaker.
I need to have my journal article, dissertation, or term paper edited and proofread, or I need help with an admissions essay or proposal.
I have a novel, manuscript, play, or ebook. I need editing, copy editing, proofreading, a critique of my work, or a query package.
I need editing and proofreading for my white papers, reports, manuals, press releases, marketing materials, and other business documents.
I need to have my essay, project, assignment, or term paper edited and proofread.
I want to sound professional and to get hired. I have a resume, letter, email, or personal document that I need to have edited and proofread.
Prices include your personal % discount.
Prices include % sales tax ( ).
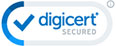
- Link to facebook
- Link to linkedin
- Link to twitter
- Link to youtube
Academic Proofreading And Editing
Whether you’re writing a thesis, research paper, or PhD proposal, Proofed’s academic proofreading and editing services will help ensure your writing reads smoothly and your ideas are communicated effectively.
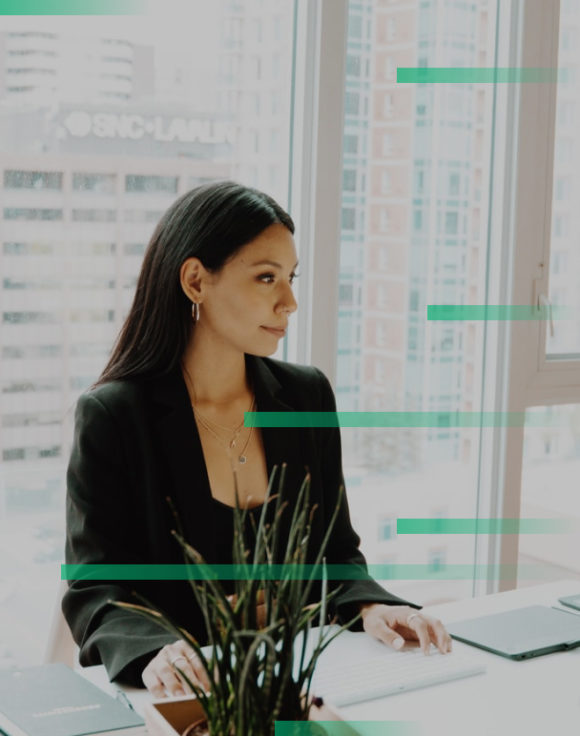
Enhance Your Academic Writing
No matter what you’re working on, with Proofed’s academic proofreading and editing services, you can rest assured that your writing will be clear and concise, with a strong academic style and tone.
We can even check references and citations in your work. Because good writing is vital for success in academia, our proofreading and editing services can help you achieve your career goals.
Experienced Academic Editors
Our team includes editors with PhDs and master’s degrees in a wide range of subject areas. We’ve also edited thousands of academic documents so we understand the high standard of writing required in academia and will use our skills and expertise to make your writing the best it can be.
Are You Looking To Publish Your Writing?
Proofed’s academic proofreading service has helped thousands of academics in many fields of study prepare their papers, reports, and articles for publication. Visit our researcher page to learn more about how we can polish your writing, thus increasing your chances of publication.
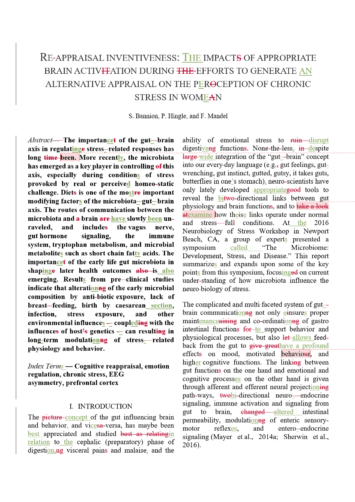
Make sure your writing is the best it can be with our expert English proofreading and editing
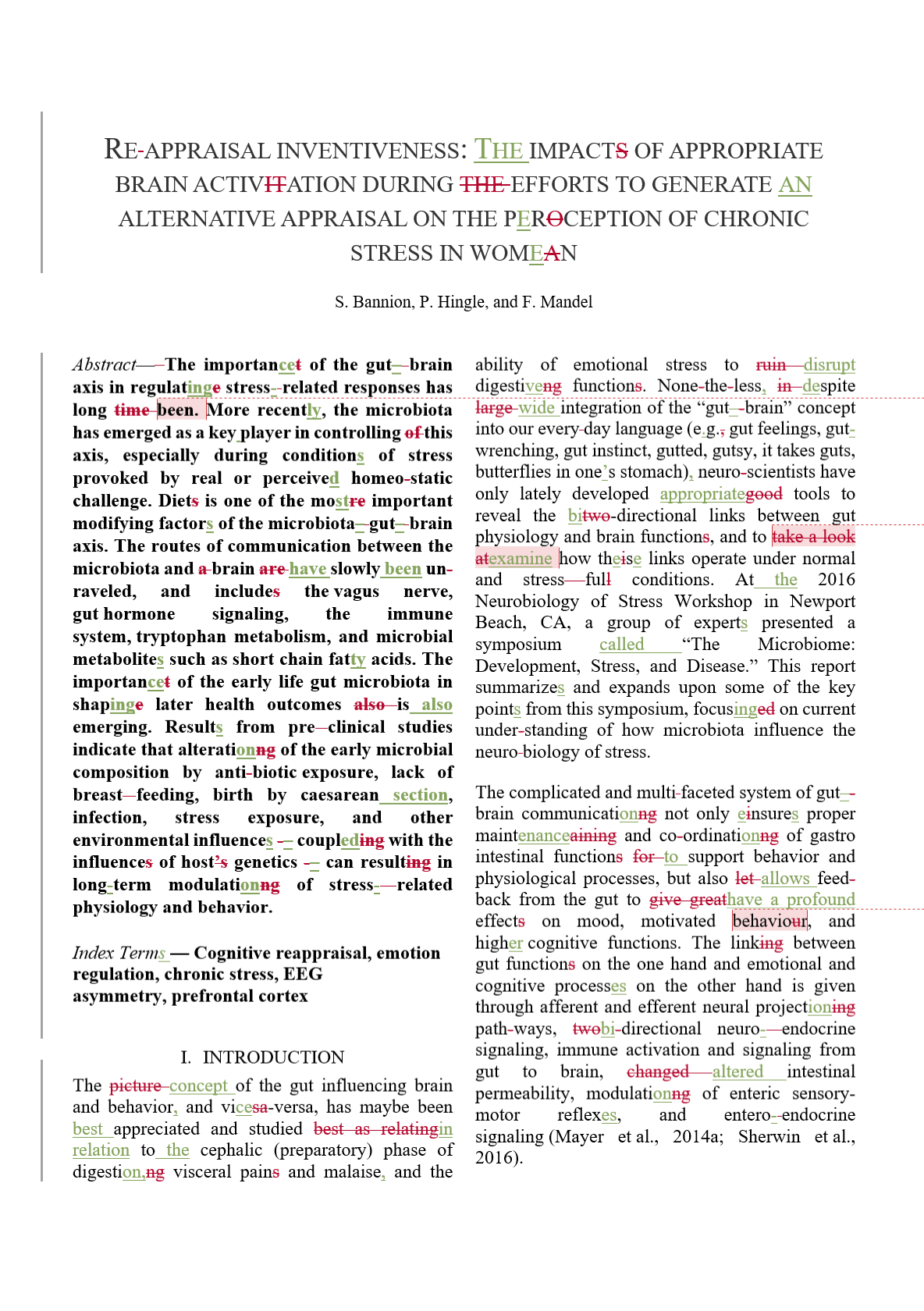
A Dedicated Team Of Expert Editors Working 24/7, 365 Days A Year
We pair your document with an editor who understands your field of study. If you’re happy with the results, let us know, and we’ll make sure the same editor works on all of your documents. That way, you can be sure the proofreading we provide will always meet your standards.
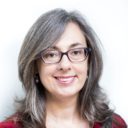
Expert Editor
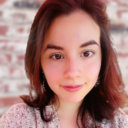
Highly Educated Editors
Female Editors
Hold Graduate Degree
Qualified In Over
Subject Areas
How We Work With Academics
Lightning-fast delivery.
You’ll never miss a deadline with our speedy services: our Next-Day Guarantee means we’ll return any document up to 8,000 words long within 24 hours.
And if you need a faster turnaround, simply select your desired delivery speed when you submit your document. We have three options
We can also meet custom deadlines! Just let us know what you need.
* For documents up to 8,000 words
** For documents up to 3,000 words
Great Pricing
Our pricing is affordable and transparent – the cost is based on the exact length of your document. Check out our pricing calculator for an instant quote, and rest assured that you’ll receive the highest quality proofreading and editing for the best value on the market.
Referencing Expertise
Our team includes experts in various referencing styles and systems, including AMA, APA, MLA, Chicago, Harvard, Vancouver, and IEEE. No matter what style you’re using, though, we can check that your citations and references are correct and let you know if any information is missing. Visit our referencing page to learn more.
Subject-Matter Experts
Our team includes over 750 professional editors with backgrounds in a wide range of disciplines, so we’ll always match you with the best editor for your writing, whether you need help with a sociology dissertation, handouts for a music theory lecture, or a biology research paper.
24-Hour Support
Our support team is available around the clock to address any concerns or questions you have about your order. This means you’ll never be left in the dark, no matter where you are or what time it is.
We Work With Every Major Format
Our system supports over 15 document formats, making it easy to submit content for proofreading and editing. Click on a file icon below to learn more.
MS Powerpoint
Google Docs
Google Slides
Google Sheets
Illustrator
Select Your Document Type Below To Learn More
Research papers.
Present your findings with pride by having your research paper checked by our expert academic proofreaders.
Journal Articles
Prepare for publication by letting our expert academic proofreaders check your journal article for errors.
Let our expert academic proofreaders help you put the finishing touches on your PhD thesis or dissertation.
Make sure your arguments come across clearly by having your thesis checked by our expert academic proofreaders.
Research Proposals
Enhance your application by letting our expert academic proofreaders check your research proposal for errors.
Instant Quote
You can also upload a document to get an instant quote
Drag & drop your file
or browse your computer
Browse from your device
Drop your file here!
Your file is being uploaded!
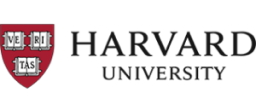
Looking For The Perfect Proofreader?
We can help you improve everything from journal articles to theses. Let us show you how!
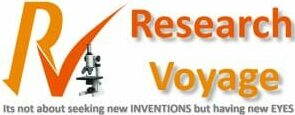
Research Voyage
Research Tips and Infromation
Importance of Proofreading in Research Paper Writing

Introduction
Is there a difference between proofreading and copy editing, checking for grammar, punctuation, and spelling errors, ensuring that the paper is well-organized and flows logically, identifying inconsistencies or inaccuracies in the data or arguments presented, improving the overall quality and credibility of the research paper, improving the readability and clarity of the paper, making a good impression, the paper is written in a concise, clear, and coherent manner, proofreading softwares, factors to consider to choose a reliable and trustworthy proofreading website, before submitting proofreading to the external agency, prominent proofreading agencies, what is a non-disclosure agreement (nda), proofreading charges.
- Whether Proofreader's Contribution should be Acknowledged in Research Paper?
Writing a research paper is a daunting task, and it requires a lot of hard work and dedication to produce a well-crafted piece of writing that accurately conveys your research findings. However, simply completing the research paper is not enough; the next crucial step is to proofread it.
Proofreading is the process of carefully reviewing and correcting errors in grammar, spelling, punctuation, formatting, and other aspects of writing to ensure that the final product is error-free and polished.
In the context of research papers, proofreading is essential because it ensures that your paper is clear, concise, and easy to read. A well-proofread paper is more likely to be accepted by academic journals or conferences, which can greatly enhance your academic career.
Furthermore, a poorly proofread paper can undermine the credibility of your research and may lead readers to question the accuracy of your findings.
In this blog post, we will explore the importance of proofreading in research papers, the common errors that researchers often make, and the best practices for proofreading your research paper.
Whether you are a seasoned researcher or just starting out, this post will provide valuable insights into how you can improve the quality of your research papers through careful proofreading.
There is a distinction between copy editing and proofreading. Both are crucial steps in the process of getting a document ready for publication, but they require various tasks and focus on various facets of the text.
The last step in the editing process is proofreading, which entails evaluating the text for any last-minute grammatical, punctuation, spelling, and formatting mistakes. The primary goal of the proofreader is to find any mistakes that might have gone unnoticed during earlier editing processes.
On the other hand, copy editing takes place after the document has been written but before it is proofread. The copy editor goes through the content in greater detail, and their major goal is to increase the document’s overall clarity, coherence, and readability. This could entail offering ideas for rephrasing or rearranging sentences or paragraphs, checking for consistency, and making sure the material is well-organized.
Proofreading Steps
Here is the list of steps that one can follow to write an error-free article with proofreading.
Grammar, punctuation, and spelling mistakes can make a paper challenging to read and can diminish the work’s credibility and general quality. They can also make it challenging for readers to comprehend the author’s points of view and concepts.
To find and fix these mistakes and make the document clear to read and written in good English, proofreading is helpful. This involves making sure that all words are spelt correctly, subject-verb agreement, and the appropriate use of commas and semicolons.
It is significant to remember that mistakes might occasionally persist even after proofreading. Any remaining errors can be found with the help of a second set of eyes, such as a colleague or a professional editor.
I have written a detailed blog post on From Journal Articles to Dissertations: How Grammarly Can Help You Write with Confidence . I personally use Grammarly tool for all my writing work. It saves lots of my time and ensures quality writing.
Readers will find it simpler to grasp and follow a research paper that is well-organized and written.
In order to guarantee that the paper makes sense and that each part and paragraph flows naturally into the next, the author should proofread the structure and organization of the work.
This involves examining the paper’s introduction, body, and conclusion for clarity, the logic and coherence of the arguments, and the connections between each paragraph and the paper’s major topic.
The author should also ensure that the headers and subheadings are understandable, simple to follow, and properly formatted. Additionally, ensure sure the document is properly labelled and is divided into relevant sections, such as literature review, methods, results, and commentary.
The author can ensure that the paper is simple to read and understand by identifying and fixing any organization and flow problems, which can enhance the overall quality and trustworthiness of the research.
I have written an article on Writing an Effective Research Paper with 11 Major Sections . This article will help you to write research papers systematically.
The research that is written in a paper should be accurate and reliable, and the data and reasoning should be consistent across the whole work.
The author should proofread the essay to ensure that all of the information and arguments are true and backed up by reliable sources. Additionally, they should make sure that all of the information supplied is pertinent to the study question or hypothesis and look for any contradictions or inconsistencies in the data or reasoning.
Any statistical information, figures, tables, and other visual aids should be checked for accuracy before being used in a report. They should also be correctly labelled and referenced. It’s crucial to double-check computations, estimates, and data-based interpretations for any flaws or errors.
The author may make sure that the research is of the highest caliber and that the work is reliable and trustworthy by spotting and addressing any errors or inconsistencies in the data or reasoning.
In my below-listed blog posts, I have discussed the importance of charts, graphs,, and figures and how to represent them in research papers accurately and succinctly. Any misrepresentation of these concepts totally degrades the quality of your research paper.
- Maximizing the Impact of Your Research Paper with Graphs and Charts
- Best Practices for Designing and Formatting Tables in Research Papers
- The Power of Images in Research Papers: How They Enhance the Quality of Your Paper
In order to ensure that the paper is of the highest caliber and that the research is presented in a reliable and accurate manner, proofreading is essential.
By finding and correcting errors, ensuring that the paper is written in proper English and is simple to read, ensuring that the paper is well-organized and flows logically, and spotting any inconsistencies or inaccuracies in the data or arguments presented, proofreading helps to improve the overall quality and credibility of research papers. This makes it more likely that the paper will be of a high caliber and will be well-received by readers and reviewers.
Academic journals aim for articles that are well-written and simple to comprehend and have high requirements for the papers they publish. A work that is well-written and simple to understand has a higher chance of being published since it is more likely to be interesting to readers and to contribute significantly to the field of study.
It’s also crucial to keep in mind that academic publications frequently have a word limit, thus proofreading aids in locating and removing any extraneous or superfluous information to assist the author to communicate their thoughts in a clear and succinct manner.
Overall, proofreading is a crucial step in the publication submission process since it ensures that the work is well-written, understandable, and up to the high standards demanded by academic publications.
Inaccurate and poorly written papers are less likely to be regarded seriously by readers and reviewers and are also less likely to be published. The author may guarantee that the paper is well-written and error-free by proofreading it and finding and fixing mistakes, which will leave a positive impression on readers and reviewers and increase the likelihood that the article will be approved for publication.
The author should proofread the work to ensure that the concepts are expressed in a clear, succinct, and coherent way. This entails making sure the document is devoid of superfluous or redundant details, that the sentences are well-written and simple to grasp, and that the paper flows logically.
Additionally, it is crucial to ensure that the work follows the rules of academic writing, including proper formatting and citation practices. This makes it easier to make sure the essay is produced in an expert and academic manner.
Best Self-Proofreading Tips
Here are a few tips for self-proofreading your research paper:
- Take a break before proofreading : After writing, it’s always best to take a break and clear your mind before proofreading. This allows you to come back to your paper with fresh eyes and be more objective when reviewing it.
- Read through the paper out loud : Reading the paper out loud can help you catch errors that you might have missed while reading it silently. It also helps to slow down your reading, which makes it easier to spot mistakes.
- Check for grammar and punctuation errors : When proofreading, pay close attention to grammar, punctuation, and spelling. Common mistakes to look out for include subject-verb agreement, verb tense, and punctuation errors.
- Check for consistency and flow : Make sure that your paper is consistent in terms of style, tone, and formatting. Check that the flow of your paper is logical and easy to follow.
- Check for factual errors : Make sure that all the information in your paper is accurate and up-to-date. Check for any errors in data, statistics, or references.
- Use proofreading tools : There are various proofreading tools available online that can help you to check your paper for errors. These tools can help you to spot mistakes that you might have missed while self-proofreading.
- Get someone else to proofread : Even after self-proofreading, it is always a good idea to get someone else to proofread your paper. A second set of eyes can help you to spot mistakes that you might have missed.
- Don’t rush : Take your time when proofreading. Rushing through the process can lead to missing errors.
By following these tips, you will be able to spot and correct errors in your research paper, which will help to improve the overall quality of your paper.
There are plenty of proofreading software available either free of cost or pro versions with nominal charges. You can try these options if you are well-versed in software handling.
- Grammarly : Grammarly is a popular proofreading tool that checks for grammar, punctuation, and spelling errors. It also suggests improvements to your writing and provides a readability score. It also has a browser extension and a plugin for Microsoft office.
- ProWritingAid : ProWritingAid is a comprehensive proofreading software that checks for grammar, punctuation, style, and readability. It also provides a thesaurus, a readability analysis, and a writing style report.
- Hemingway : Hemingway is a simple proofreading tool that checks for grammar, punctuation, and readability. It highlights complex sentences and suggests ways to simplify them.
- Ginger Software : Ginger Software is another popular proofreading tool that checks for grammar and punctuation errors. It also provides a thesaurus and a translation feature.
- WhiteSmoke : WhiteSmoke is a comprehensive proofreading software that checks for grammar, punctuation, style, and plagiarism. It also provides a thesaurus, a translation feature, and a writing style report.
- Language Tool : Language Tool is an open-source proofreading tool that checks for grammar, punctuation, and style errors. It also supports more than 20 languages.
All of these software programs can help you to improve your writing by catching errors and suggesting improvements. They also have their own unique features and pricing options, so you can choose the one that best suits your needs.
Can I Outsource Proofreading Work to External Agency?
In order to assist researchers in enhancing the caliber and correctness of their work, it is normal practice in academic and research circles to outsource proofreading services.
When outsourcing proofreading services, it’s crucial to pick a dependable and trustworthy service provider and carefully study the service agreement’s terms and conditions. Additionally, it is crucial to make sure that the research’s final form is the authors’ own original work and that it complies with all applicable laws and moral standards regarding academic honesty and plagiarism.
When choosing a website that offers proofreading and copy-editing services, there are a few key factors to consider to ensure that you choose a reliable and trustworthy website.
- Experience : Look for websites that have been in business for a while and have a good track record of providing high-quality proofreading and copy-editing services.
- Qualifications : Check that the proofreaders and copy-editors working for the website are qualified and have experience in the field.
- Reviews : Look for websites that have positive reviews from customers who have used their services in the past.
- Pricing : Compare prices of different websites and choose one that offers a fair price for the services they provide.
- Confidentiality and Security : Check the website’s policy on data protection and confidentiality of the information submitted to them. Also check if they have an NDA in place for the same.
- Turnaround time : Check the website’s turnaround time for their proofreading and copy-editing services to ensure that they can meet your deadlines.
- Support : Check if the website has customer support available and if they are responsive to queries and concerns.
- Sample work : Check if the website provides sample work of proofreading and copy-editing they have done in the past to evaluate their quality of work.
- Sign a Non-Disclosure Agreement (NDA) with the proofreading agency : Many professional proofreading agencies require authors to sign an NDA, which legally binds the agency to keep the contents of the paper confidential. This helps to ensure that the agency will not share, publish or use the paper for any other purpose.
- Remove sensitive information : Before submitting your paper for proofreading, it is recommended to remove any sensitive information such as personal details of the participants or confidential data.
- Use a reputable proofreading agency : Choose a reputable agency that is known for its professional standards and has a good reputation in the industry. Research the agency and read reviews from other authors to get an idea of the level of service they provide.
- Use a password-protected file format : Consider sending your paper in a password-protected file format, such as a pdf with password protection, this will ensure that only authorized parties have access to the document.
- Communicate with the proofreader : It’s important to communicate with the proofreader and inform them about your specific requirements, such as any confidential information that should be kept private, or any specific areas of the paper that should not be changed.
By taking these steps, you can help to ensure that your research paper is handled securely and confidentially and that it will not be published elsewhere
Sure, here are some prominent proofreading agencies that you can consider:
- Scribendi: Based in the US and Canada, Scribendi offers professional proofreading and editing services for a variety of document types, including academic papers, business documents, and books.
- Grammarly : Grammarly is an online proofreading tool that checks for grammar, punctuation, and spelling errors. It also offers suggestions for sentence structure and style.
- Proofreading Pal : Based in the US, Proofreading Pal offers professional proofreading and editing services for a variety of document types, including academic papers, business documents, and books.
- Cambridge Proofreading : Cambridge Proofreading is a UK-based company that offers professional proofreading and editing services for academic and business documents.
- ProWritingAid : ProWritingAid is an online proofreading tool that checks for grammar, punctuation, and style errors. It also provides suggestions for improving sentence structure and readability.
- First Editing : Based in the US, First Editing offers professional proofreading and editing services for a variety of document types, including academic papers, business documents, and books.
- The Proofreaders: The Proofreaders is a UK-based company that offers professional proofreading and editing services for academic and business documents.
- Proofreading Services : Based in the US, Proofreading Services offers professional proofreading and editing services for a variety of document types, including academic papers, business documents, and books.
A Non-Disclosure Agreement (NDA) is a legal contract between two parties, in which one party (the disclosing party) agrees to share confidential information with the other party (the receiving party), and the receiving party agrees to keep that information confidential.
A typical NDA template should include the following elements:
- Definition of confidential information : Clearly define what information is considered confidential and should be protected under the terms of the NDA.
- Obligations of the receiving party : Outline the specific obligations of the receiving party in relation to the confidential information, such as the requirement to keep the information confidential and not share it with any third parties.
- Exclusions : Specify any exclusions or exceptions to the confidentiality obligations, such as information that is already publicly available or that the receiving party is required to disclose by law.
- Term : Specify the duration of the NDA, and under what conditions the NDA will terminate.
- Governing law : Specify the governing law of the NDA, and the jurisdiction in which any disputes will be resolved.
- Signatures : Include space for the signatures of both parties, indicating that they have read and agreed to the terms of the NDA.
It’s important to note that NDAs are legally binding agreements, so it’s recommended that you consult with a lawyer or legal professional before entering into one.
Depending on the complexity of the document, the degree of editing needed, and the provider, the cost of professional proofreading services can range from a few cents to a few dollars per word.
It’s important to note that a range of proofreading services are offered, and prices may change based on the quality of the service. While more advanced services might also involve fact-checking and editing for style and substance, basic proofreading services might merely look for grammar and punctuation mistakes. Alternatively, some companies may provide bundled packages that include numerous rounds of editing and proofreading or may charge a flat amount for a specified number of words or pages.
I recommend checking with several different proofreading providers to get an idea of the cost of proofreading in different countries and to compare the services they offer. It is important to find a service that fits your budget and that provides the level of editing and proofreading that you need.
Here is a sample table template for proofreading charges:
I would advise you to visit their website or contact them directly to get up-to-date information on their charges, and to get an accurate quote for your specific document and requirements.
Whether Proofreader’s Contribution should be Acknowledged in Research Paper?
In scientific articles, it is customary to thank the proofreaders who helped with the work. This is so because proofreading is a crucial step in the publication process and can greatly raise the paper’s calibre and accuracy. By thanking proofreaders, authors demonstrate their appreciation for the work they have done while also recognising the proofreaders’ contributions.
Furthermore, certain journals have particular rules for crediting proofreaders. For instance, some journals demand that the efforts of the paper’s proofreaders be acknowledged. It is best to constantly double-check the exact requirements of the journal you are submitting your paper to.
In general, it is always a good idea to acknowledge the contributions of proofreaders in scientific papers, as it shows that the authors value the work done by them and it gives credit to the proofreaders for their efforts.
The process of producing a research paper must include proofreading in order to guarantee that the final product is error-free and portrays the study in the most favourable light. Grammar, punctuation, spelling, and content problems can undermine the work’s credibility and integrity and reduce its overall quality, hence it is crucial to proofread for these issues.
Before submission or publication, authors can ensure that their research is presented in a clear, expert, and accurate manner, which can increase the article’s readability and trustworthiness, by identifying and fixing these problems. It also aids in ensuring that the study is reliable, accurate, and consistent with the literature. Therefore, it is highly recommended to proofread the research multiple times before submitting or publishing it.
Upcoming Events
- Visit the Upcoming International Conferences at Exotic Travel Destinations with Travel Plan
- Visit for Research Internships Worldwide
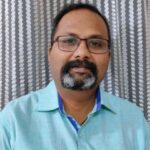
Recent Posts
- How to Request for Journal Publishing Charge (APC) Discount or Waiver?
- Do Review Papers Count for the Award of a PhD Degree?
- Vinay Kabadi, University of Melbourne, Interview on Award-Winning Research
- Do You Need Publications for a PhD Application? The Essential Guide for Applicants
- Research Internships @ Finland
- All Blog Posts
- Research Career
- Research Conference
- Research Internship
- Research Journal
- Research Tools
- Uncategorized
- Research Conferences
- Research Journals
- Research Grants
- Internships
- Research Internships
- Email Templates
- Conferences
- Blog Partners
- Privacy Policy
Copyright © 2024 Research Voyage
Design by ThemesDNA.com

Proofreading Techniques and Tips for Academic Writing
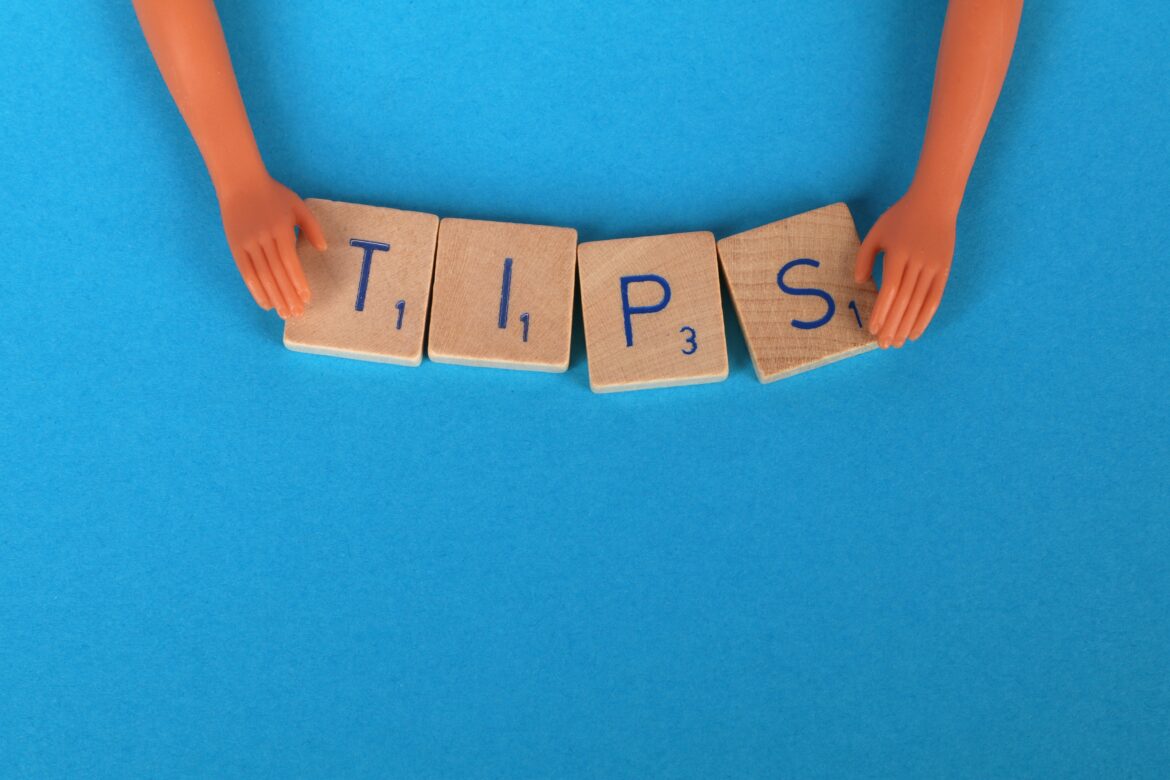
Most early career researchers are not aware of editing and proofreading techniques and even experienced researchers sometimes struggle with proofreading their papers. Most researchers do not have the time or resources to proofread their work thoroughly if they are working under tight deadlines. Many feel too fatigued to proofread having spent months and perhaps years writing, editing and revising their research paper. Others may be too involved in their research to be able to identify inadvertent errors or mistakes in their writing. Then there are those whose first language is not English and therefore they lack strong language skills or knowledge of grammar making it challenging for them to proofread.
There are many reasons why a researcher might not be able to proofread their piece of research, but this does not lessen the significance of it. Proofreading, editing and revising are all important aspects of your research writing process and each of them must be done seriously.
Table of Contents
What is proofreading and why is it important, proofreading techniques.
Proofreading is the method that corrects grammar, spelling, typographical and punctuation errors in a draft manuscript. Proofreaders typically check for uniformity in formatting and referencing and highlight inconsistencies in terms or explanations used in the text. If done well, proofreading helps address errors like comma splices , run-on sentences, and sentence fragments. Proofreading also helps you catch and fix minute errors that may have crept in as you focused on your research and writing.
It is essential to note here that proofreading is different from editing . Many researchers and doctoral students tend to view editing and proofreading in the writing process as one and the same. Remember that editing is done prior to proofreading and involves focusing on the overall clarity of the research paper by addressing grammar and spelling issues and keeping the readability in check.
While proofreading in academic writing can be challenging, there are some proofreading tips and techniques that can help you:
Pay attention to detail : This is perhaps the most important skill to master as you begin to proofread your research paper. It is important to keep distractions aside and concentrate only on proofreading, so that you are able to identify minor errors in grammar or inaccuracies in data.
Focus on one type of error at a time: Remember, proofreading in academic writing is not an easy task. Therefore, address one common type of error at a time as you read your manuscript. You might start by concentrating simply on the spelling, grammar, or even punctuation issues. Using this approach can ensure that you do not miss possible errors inadvertently.
Read the manuscript aloud: This important proofreading technique can help improve the quality of your final manuscript. As you read the sections of the research paper aloud, you will be able to mark and identify passages that may be vague or need further refinement. You will also be able to spot other errors that may not be obvious if the manuscript is read in the mind.
Request someone to read it: It is always a good idea to have someone else read your manuscript after you are done with the proofreading exercise. With the research paper under a fresh pair of eyes, missed errors can be highlighted.
Keep a checklist: Make a list to ensure that you have addressed all important aspects of the proofreading exercise like, punctuation, grammar, spelling, formatting requirements and references or citations. An organized checklist will help you keep track of the kind of errors you have to look for and the kind of errors you have already checked.
Read backwards: This proofreading technique is suggested by experts to help you focus on how well you have constructed your sentences in the paper. Reading backwards, one sentence at a time, can help your brain to identify errors in sentences that may otherwise go unnoticed.
We hope the above editing and proofreading techniques will be helpful when you proofread your next piece of research. As an alternative, you can also choose to use AI tools like Paperpal as a method of proofreading. AI tools like these can help you save time and effort by automating many of the tedious and time-consuming aspects of proofreading.
Paperpal is an AI writing assistant that help academics write better, faster with real-time suggestions for in-depth language and grammar correction. Trained on millions of research manuscripts enhanced by professional academic editors, Paperpal delivers human precision at machine speed. Try it for free or upgrade to Paperpal Prime , which unlocks unlimited access to premium features like academic translation, paraphrasing, contextual synonyms, consistency checks, submission readiness and more. It’s like always having a professional academic editor by your side! Go beyond limitations and experience the future of academic writing. Get Paperpal Prime now at just US$12 a month!
Related Reads:
- 5 Essential Research Paper Grammar Checks Every Author Must Do
- What are Synonyms and How Do You Use Synonyms in Academic Writing
- Understanding the Difference Between To and Too
- 3 Easy Ways for Researchers to Improve Their Academic Vocabulary
‘Like’ vs. ‘Such As’: Navigating the Usage for Researchers
Paperpal prime now includes free unlimited manuscript submission readiness checks, you may also like, how to paraphrase research papers effectively, 4 types of transition words for research papers , paraphrasing in academic writing: answering top author queries, sentence length: how to improve your research paper..., navigating language precision: complementary vs. complimentary, climatic vs. climactic: difference and examples, language and grammar rules for academic writing, transitive and intransitive verbs in the world of..., led vs. lead: how to differentiate between the..., academic writing for esl students: 7 tips and strategies....
- Resources Home 🏠
- Try SciSpace Copilot
- Search research papers
- Add Copilot Extension
- Try AI Detector
- Try Paraphraser
- Try Citation Generator
- April Papers
- June Papers
- July Papers

AI Proofreading Tools for Researchers (Top 6 tools enlisted)
Table of Contents
In the research realm, maintaining the highest standards of accuracy and clarity is paramount particularly when you’re working on a research paper. The dissemination of knowledge and advancements in various fields hinges on the meticulous presentation of findings and methodologies. However, the process of manuscript preparation, editing, proofreading, and reviewing can be time-consuming and often lead to delays and potential errors.
And that’s when AI proofreading comes into the picture. AI proofreading tools are new-age technology aid that is revolutionizing the way research is communicated and published. AI-powered proofreading tools are proving to be invaluable assets for researchers and editors, streamlining the proofreading process and ensuring the highest quality of academic work. This article gives you an overview of AI tools for proofreading and their role in enhancing academic writing.
The Rise of AI Proofreading in Scholarly Publishing
Traditionally, proofreading has been a meticulous and time-consuming task, often relying solely on the expertise of human editors. While human reviewers play an essential role in assessing the overall quality and content of manuscripts, AI proofreading tools offer an additional layer of reinforcement by identifying and correcting grammatical errors, inconsistencies, and stylistic issues.
The integration of AI proofreading into the research landscape is driven by the following factors:
Increased Volume of Research Output
The sheer volume of research papers being published has grown exponentially, making it challenging for human editors to keep up with the demand. AI proofreading tools can handle large volumes of text efficiently, alleviating the burden on editors and ensuring timely publication of research findings.
Global Collaboration and Diverse Writing Styles
Research collaborations often involve individuals from diverse linguistic backgrounds, leading to variations in writing styles and potential inconsistencies in grammar and usage. AI proofreading tools can adapt to these variations, ensuring that manuscripts adhere to established writing guidelines.
Consistency and Adherence to Style Guidelines
Academic journals and institutions often have specific style guides that authors must follow. AI proofreading tools can be trained on these guidelines, ensuring that manuscripts conform to the required style conventions, and reducing the risk of errors and inconsistencies.
Applications of AI Proofreading in Research
AI proofreading tools can be applied at various stages of research and scholarly publishing. Here are a few of them —
Manuscript Preparation
Researchers can use AI proofreading tools to identify and correct errors in their manuscripts before submitting them for review. This helps to ensure that the initial submission is of high quality and reduces the likelihood of errors being raised during the review process.
Peer Review Process
AI proofreading tools can assist editors and reviewers in identifying potential errors and inconsistencies in manuscripts during the peer review process. This can help to streamline the review process and ensure that the final published manuscripts are of the highest quality.
Journal Publishing and Archiving
AI proofreading tools can be integrated into journal publishing platforms to ensure that published manuscripts adhere to the journal's style guidelines and are free of errors. This helps to maintain the integrity and reputation of the journal.
Now, let’s explore the top 6 AI proofreading tools that facilitate error-free academic writing.
Top 6 AI Proofreading Tools to Improve Your Academic Writing
Ginger is a versatile proofreading tool that caters to both English and non-English writers. It offers comprehensive error detection, including grammar, spelling, and punctuation mistakes. Additionally, Ginger provides real-time translation capabilities, making it a valuable tool for multilingual writers.
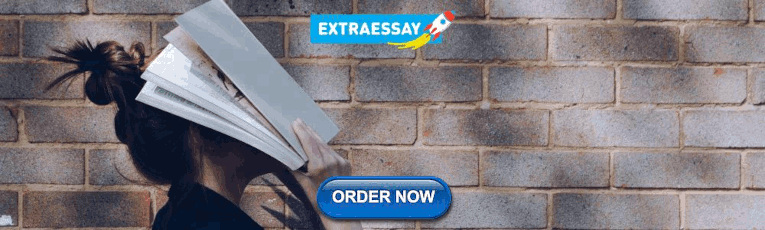
2. WhiteSmoke
WhiteSmoke is a comprehensive proofreading AI tool that combines grammar and spelling checking with plagiarism detection and translation services. It offers a user-friendly interface and provides detailed explanations for suggested corrections, helping users improve their writing skills.
3. LanguageTool
LanguageTool is an open-source proofreading tool that supports over 30 languages. It is known for its accuracy and adaptability to various writing styles. LanguageTool's free version offers basic proofreading features, while the premium version provides advanced style and tone suggestions.
4. Slick Write
Slick Write is an another proofreading tool that focuses on enhancing writing style and clarity. It identifies and eliminates unnecessary words and phrases, improves sentence structure, and suggests alternatives for overused expressions. Slick Write is particularly useful for authors who seek to achieve concise and impactful writing.
5. Hemingway Editor
Hemingway Editor is a web-based AI tool for proofreading that emphasizes clarity and readability. It identifies complex sentences, passive voice constructions, and adverbs, suggesting simpler alternatives. Hemingway Editor is particularly useful for writers who seek to create concise and easily understandable content.
6. PaperRater
PaperRater is an AI-powered essay writing and proofreading tool designed for students. It provides feedback on grammar, spelling, style, and plagiarism, along with a comprehensive score and suggestions for improvement. PaperRater can be a valuable tool for students to enhance their academic writing skills.
Benefits of AI Proofreading in Research
The adoption of AI proofreading tools in research and scholarly publishing offers a range of benefits
Enhanced Accuracy and Consistency
Proofreading tools can detect a wide range of errors, including grammatical mistakes, typos, and inconsistencies in formatting and style. This helps to ensure the accuracy and professionalism of research manuscripts.
Improved Efficiency and Workflow
By automating many of the tasks involved in proofreading, AI tools can significantly reduce the time and effort required to prepare manuscripts for publication. This frees up researchers and editors to focus on more substantive aspects of the research workflow.
Accessibility and Language Support
AI proofreading tools can handle multiple languages, making them accessible to researchers from around the world. This facilitates collaboration across all the domains and ensures that research findings are accessible to a wider audience.
Identification of Plagiarism
AI proofreading tools can detect instances of plagiarism, helping to maintain the integrity of research and upholding ethical standards in scholarly publishing.
Limitations and Considerations for AI Proofreading
While AI proofreading tools offer significant benefits, it is important to understand their limitations and use them responsibly.
Human Review Remains Essential
AI proofreading tools should not be considered a substitute for human review. Human editors still play a crucial role in assessing the overall quality, content, and coherence of manuscripts.
Error Detection Accuracy
AI proofreading tools are not infallible and may occasionally miss errors or suggest incorrect corrections. It is essential to carefully review the suggestions provided by AI tools and exercise critical judgment.
Contextual Understanding
Proofreading tools may struggle with contextual nuances and stylistic choices. Authors should carefully review the suggestions provided by AI tools to ensure that they align with the intended meaning and style of the manuscript.
AI proofreading tools have revolutionized the way we approach writing, providing valuable assistance in identifying and correcting errors, enhancing style, and improving overall clarity. These tools are not meant to replace human editors but rather complement their expertise, ensuring that written work meets the highest standards of quality and effectiveness.
By leveraging AI proofreading tools, authors can elevate their communication skills and achieve greater success in various endeavors. If you’ve used any of the above proofreading tools, you can share your experience with us and recommend the best tool to our readers!
Suggested Reads!
Using AI for research: A beginner’s guide
Best AI for Paraphrasing: An Overview
AI Citation Generators For Academic Referencing
AI for thesis writing — Unveiling 7 best AI tools
AI for Essay Writing — Exploring Top 10 Essay Writers
You might also like

Cybersecurity in Higher Education: Safeguarding Students and Faculty Data

How To Write An Argumentative Essay
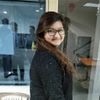
Beyond Google Scholar: Why SciSpace is the best alternative
- Quantum Research
Landmark IBM error correction paper published on the cover of Nature
Ibm has created a quantum error-correcting code about 10 times more efficient than prior methods — a milestone in quantum computing research..

27 Mar 2024
Rafi Letzter
Share this blog
Today, the paper detailing those results was published as the cover story of the scientific journal Nature. 1
Last year, we demonstrated that quantum computers had entered the era of utility , where they are now capable of running quantum circuits better than classical computers can. Over the next few years, we expect to find speedups over classical computing and extract business value from these systems. But there are also algorithms with mathematically proven speedups over leading classical methods that require tuning quantum circuits with hundreds of millions, to billions, of gates. Expanding our quantum computing toolkit to include those algorithms requires us to find a way to compute that corrects the errors inherent to quantum systems — what we call quantum error correction.
Read how a paper from IBM and UC Berkeley shows a path toward useful quantum computing
Quantum error correction requires that we encode quantum information into more qubits than we would otherwise need. However, achieving quantum error correction in a scalable and fault-tolerant way has, to this point, been out of reach without considering scales of one million or more physical qubits. Our new result published today greatly reduces that overhead, and shows that error correction is within reach.
While quantum error correction theory dates back three decades, theoretical error correction techniques capable of running valuable quantum circuits on real hardware have been too impractical to deploy on quantum system. In our new paper, we introduce a new code, which we call the gross code , that overcomes that limitation.
This code is part of our broader strategy to bring useful quantum computing to the world.
While error correction is not a solved problem, this new code makes clear the path toward running quantum circuits with a billion gates or more on our superconducting transmon qubit hardware.
What is error correction?
Quantum information is fragile and susceptible to noise — environmental noise, noise from the control electronics, hardware imperfections, state preparation and measurement errors, and more. In order to run quantum circuits with millions to billions of gates, quantum error correction will be required.
Error correction works by building redundancy into quantum circuits. Many qubits work together to protect a piece of quantum information that a single qubit might lose to errors and noise.
On classical computers, the concept of redundancy is pretty straightforward. Classical error correction involves storing the same piece of information across multiple bits. Instead of storing a 1 as a 1 or a 0 as a 0, the computer might record 11111 or 00000. That way, if an error flips a minority of bits, the computer can treat 11001 as 1, or 10001 as 0. It’s fairly easy to build in more redundancy as needed to introduce finer error correction.
Things are more complicated on quantum computers. Quantum information cannot be copied and pasted like classical information, and the information stored in quantum bits is more complicated than classical data. And of course, qubits can decohere quickly, forgetting their stored information.
Research has shown that quantum fault tolerance is possible, and there are many error correcting schemes on the books. The most popular one is called the “surface code,” where qubits are arranged on a two-dimensional lattice and units of information are encoded into sub-units of the lattice.
But these schemes have problems.
First, they only work if the hardware’s error rates are better than some threshold determined by the specific scheme and the properties of the noise itself — and beating those thresholds can be a challenge.
Second, many of those schemes scale inefficiently — as you build larger quantum computers, the number of extra qubits needed for error correction far outpaces the number of qubits the code can store.
At practical code sizes where many errors can be corrected, the surface code uses hundreds of physical qubits per encoded qubit worth of quantum information, or more. So, while the surface code is useful for benchmarking and learning about error correction, it’s probably not the end of the story for fault-tolerant quantum computers.
Exploring “good” codes
The field of error correction buzzed with excitement in 2022 when Pavel Panteleev and Gleb Kalachev at Moscow State University published a landmark paper proving that there exist asymptotically good codes — codes where the number of extra qubits needed levels off as the quality of the code increases.
This has spurred a lot of new work in error correction, especially in the same family of codes that the surface code hails from, called quantum low-density parity check, or qLDPC codes. These qLDPC codes are quantum error correcting codes where the operations responsible for checking whether or not an error has occurred only have to act on a few qubits, and each qubit only has to participate in a few checks.
But this work was highly theoretical, focused on proving the possibility of this kind of error correction. It didn’t take into account the real constraints of building quantum computers. Most importantly, some qLDPC codes would require many qubits in a system to be physically linked to high numbers of other qubits. In practice, that would require quantum processors folded in on themselves in psychedelic hyper-dimensional origami, or entombed in wildly complex rats’ nests of wires.
In our paper, we looked for fault-tolerant quantum memory with a low qubit overhead, high error threshold, and a large code distance.
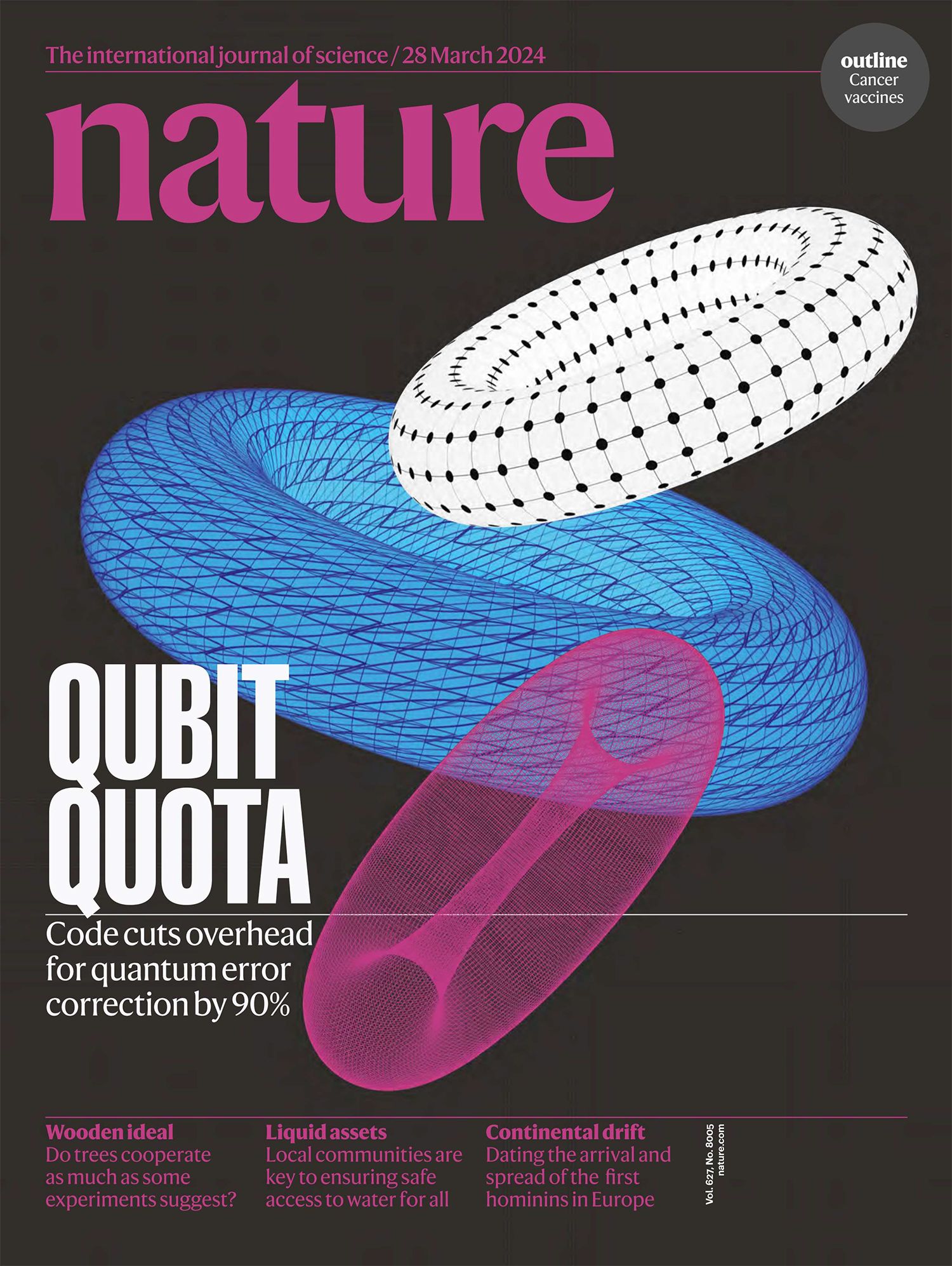
Bravyi, S., Cross, A., Gambetta, J., et al. High-threshold and low-overhead fault-tolerant quantum memory. Nature (2024). https://doi.org/10.1038/s41586-024-07107-7
In our Nature paper, we specifically looked for fault-tolerant quantum memory with a low qubit overhead, high error threshold, and a large code distance.
Let’s break that down:
Fault-tolerant: The circuits used to detect errors won't spread those errors around too badly in the process, and they can be corrected faster than they occur
Quantum memory: In this paper, we are only encoding and storing quantum information. We are not yet doing calculations on the encoded quantum information.
High error threshold: The higher the threshold, the higher amount of hardware errors the code will allow while still being fault tolerant. We were looking for a code that allowed us to operate the memory reliably at physical error rates as high as 0.001, so we wanted a threshold close to 1 percent.
Large code distance: Distance is the measure of how robust the code is — how many errors it takes to completely flip the value from 0 to 1 and vice versa. In the case of 00000 and 11111, the distance is 5. We wanted one with a large code distance that corrects more than just a couple errors. Large-distance codes can suppress noise by orders of magnitude even if the hardware quality is only marginally better than the code threshold. In contrast, codes with a small distance become useful only if the hardware quality is significantly better than the code threshold.
Low qubit overhead: Overhead is the number of extra qubits required for correcting errors. We want the number of qubits required to do error correction to be far less than we need for a surface code of the same quality, or distance.
We’re excited to report that our team’s mathematical analysis found concrete examples of qLDPC codes that met all of these required conditions. These fall into a family of codes called “Bivariate Bicycle (BB)” codes. And they are going to shape not only our research going forward, but how we architect physical quantum systems.
The gross code
While many qLDPC code families show great promise for advancing error correction theory, most aren’t necessarily pragmatic for real-world application. Our new codes lend themselves better to practical implementation because each qubit needs only to connect to six others, and the connections can be routed on just two layers.
To get an idea of how the qubits are connected, imagine they are put onto a square grid, like a piece of graph paper. Curl up this piece of graph paper so that it forms a tube, and connect the ends of the tube to make a donut. On this donut, each qubit is connected to its four neighbors and two qubits that are farther away on the surface of the donut. No more connections needed.
The good news is we don’t actually have to embed our qubits onto a donut to make these codes work — we can accomplish this by folding the surface differently and adding a few other long-range connectors to satisfy mathematical requirements of the code. It’s an engineering challenge, but much more feasible than a hyper-dimensional shape.
We explored some codes that have this architecture and focused on a particular [[144,12,12]] code. We call this code the gross code because 144 is a gross (or a dozen dozen). It requires 144 qubits to store data — but in our specific implementation, it also uses another 144 qubits to check for errors, so this instance of the code uses 288 qubits. It stores 12 logical qubits well enough that fewer than 12 errors can be detected. Thus: [[144,12,12]].
Using the gross code, you can protect 12 logical qubits for roughly a million cycles of error checks using 288 qubits. Doing roughly the same task with the surface code would require nearly 3,000 qubits.
This is a milestone. We are still looking for qLDPC codes with even more efficient architectures, and our research on performing error-corrected calculations using these codes is ongoing. But with this publication, the future of error correction looks bright.
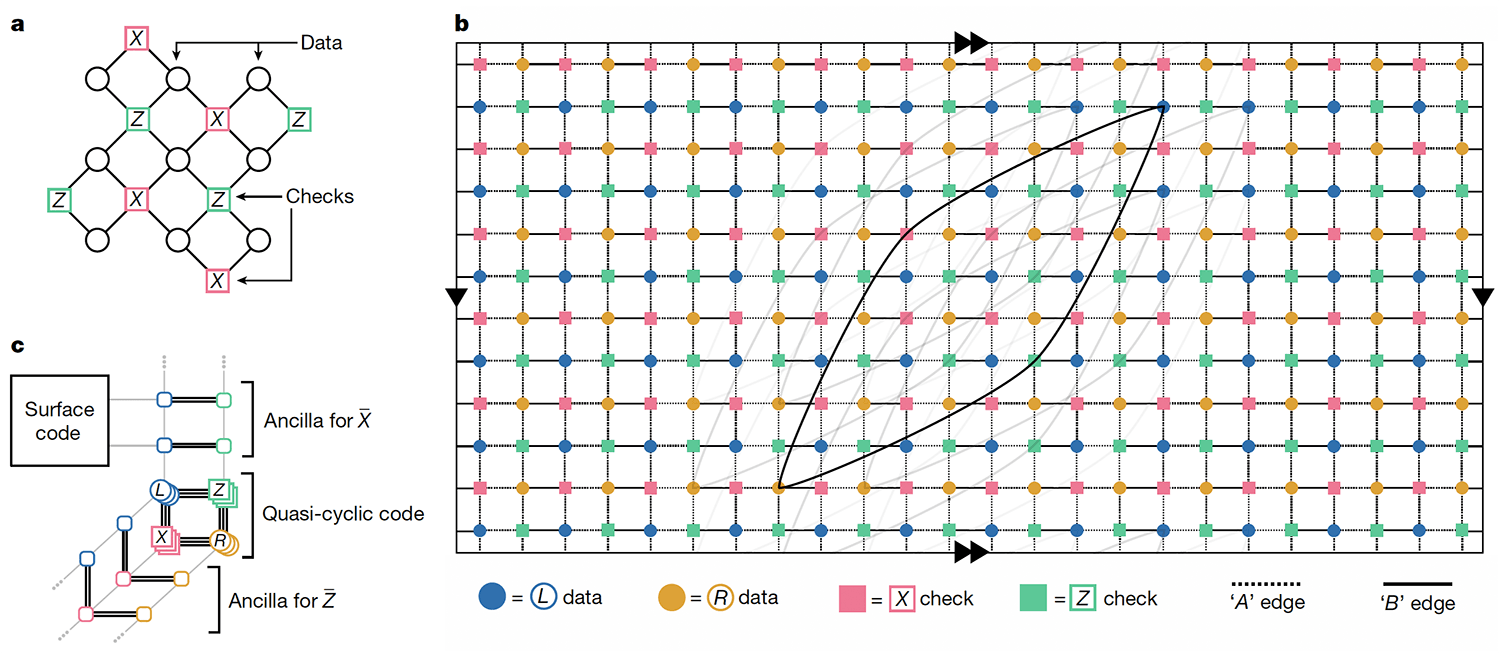
Fig. 1 | Tanner graphs of surface and BB codes.
Fig. 1 | Tanner graphs of surface and BB codes. a, Tanner graph of a surface code, for comparison. b, Tanner graph of a BB code with parameters [[144, 12, 12]] embedded into a torus. Any edge of the Tanner graph connects a data and a check vertex. Data qubits associated with the registers q(L) and q(R) are shown by blue and orange circles. Each vertex has six incident edges including four short-range edges (pointing north, south, east and west) and two long-range edges. We only show a few long-range edges to avoid clutter. Dashed and solid edges indicate two planar subgraphs spanning the Tanner graph, see the Methods. c, Sketch of a Tanner graph extension for measuring Z ˉ \={Z} and X ˉ \={X} following ref. 50, attaching to a surface code. The ancilla corresponding to the X ˉ \={X} measurement can be connected to a surface code, enabling load-store operations for all logical qubits by means of quantum teleportation and some logical unitaries. This extended Tanner graph also has an implementation in a thickness-2 architecture through the A and B edges (Methods).
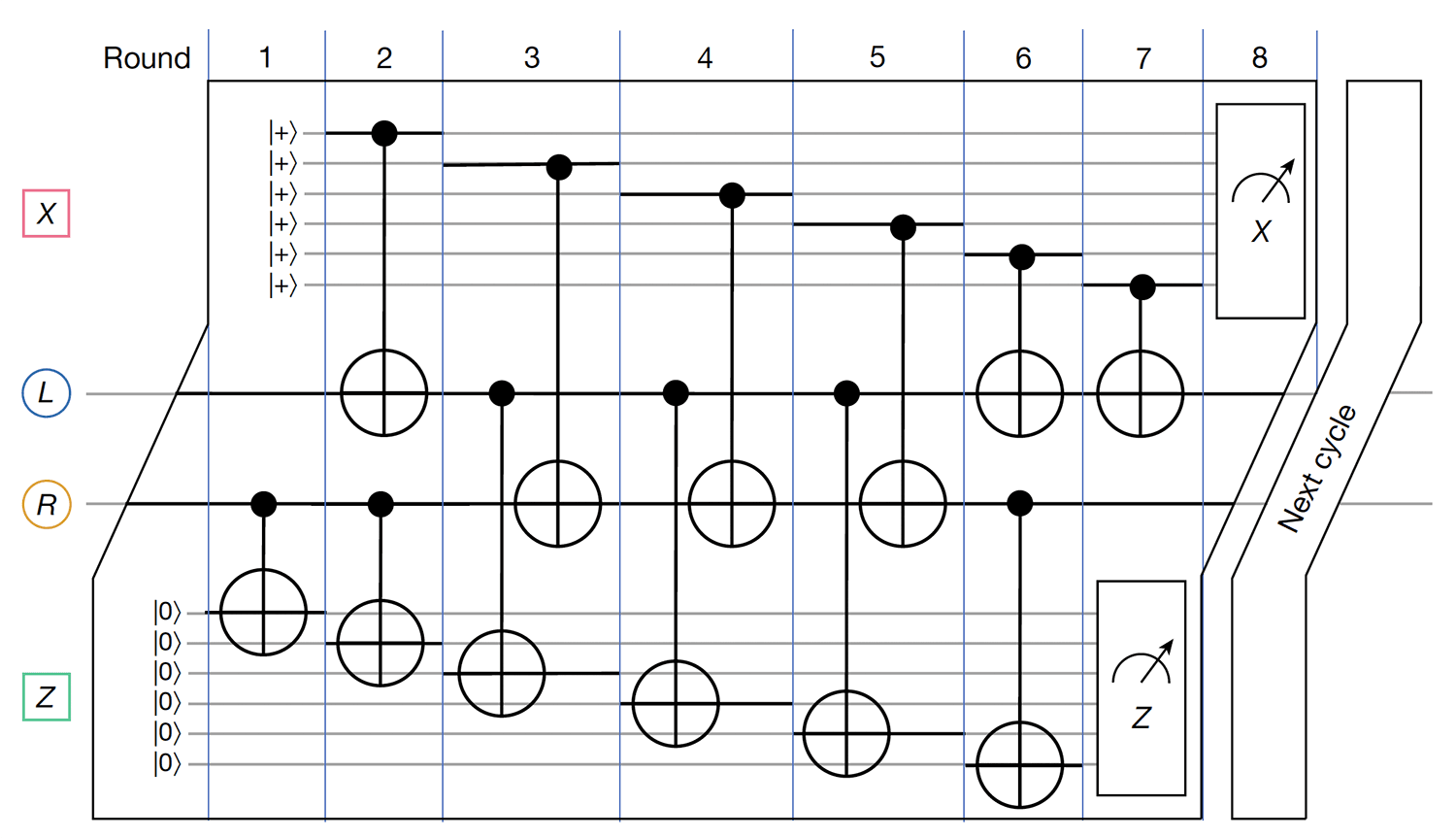
Fig. 2 | Syndrome measurement circuit.
Fig. 2 | Syndrome measurement circuit. Full cycle of syndrome measurements relying on seven layers of CNOTs. We provide a local view of the circuit that only includes one data qubit from each register q(L) and q(R) . The circuit is symmetric under horizontal and vertical shifts of the Tanner graph. Each data qubit is coupled by CNOTs with three X-check and three Z-check qubits: see the Methods for more details.
Why error correction matters
Today, our users benefit from novel error mitigation techniques — methods for reducing or eliminating the effect of noise when calculating observables, alongside our work suppressing errors at the hardware level. This work brought us into the era of quantum utility. IBM researchers and partners all over the world are exploring practical applications of quantum computing today with existing quantum systems. Error mitigation lets users begin looking for quantum advantage on real quantum hardware.
But error mitigation comes with its own overhead, requiring running the same executions repeatedly so that classical computers can use statistical methods to extract an accurate result. This limits the scale of the programs you can run, and increasing that scale requires tools beyond error mitigation — like error correction.
Last year, we debuted a new roadmap laying out our plan to continuously improve quantum computers over the next decade. This new paper is an important example of how we plan to continuously increasing the complexity (number of gates) of the quantum circuits that can be run on our hardware. It will allow us to transition from running circuits with 15,000 gates to 100 million, or even 1 billion gates.
Bravyi, S., Cross, A.W., Gambetta, J.M. et al. High-threshold and low-overhead fault-tolerant quantum memory. Nature 627, 778–782 (2024). https://doi.org/10.1038/s41586-024-07107-7
Start using our 100+ qubit systems
Keep exploring, computing with error-corrected quantum computers.

Logical gates with magic state distillation

Error correcting codes for near-term quantum computers

A new paper from IBM and UC Berkeley shows a path toward useful quantum computing
Thank you for visiting nature.com. You are using a browser version with limited support for CSS. To obtain the best experience, we recommend you use a more up to date browser (or turn off compatibility mode in Internet Explorer). In the meantime, to ensure continued support, we are displaying the site without styles and JavaScript.
- View all journals
- My Account Login
- Explore content
- About the journal
- Publish with us
- Sign up for alerts
- Open access
- Published: 26 March 2024
Predicting and improving complex beer flavor through machine learning
- Michiel Schreurs ORCID: orcid.org/0000-0002-9449-5619 1 , 2 , 3 na1 ,
- Supinya Piampongsant 1 , 2 , 3 na1 ,
- Miguel Roncoroni ORCID: orcid.org/0000-0001-7461-1427 1 , 2 , 3 na1 ,
- Lloyd Cool ORCID: orcid.org/0000-0001-9936-3124 1 , 2 , 3 , 4 ,
- Beatriz Herrera-Malaver ORCID: orcid.org/0000-0002-5096-9974 1 , 2 , 3 ,
- Christophe Vanderaa ORCID: orcid.org/0000-0001-7443-5427 4 ,
- Florian A. Theßeling 1 , 2 , 3 ,
- Łukasz Kreft ORCID: orcid.org/0000-0001-7620-4657 5 ,
- Alexander Botzki ORCID: orcid.org/0000-0001-6691-4233 5 ,
- Philippe Malcorps 6 ,
- Luk Daenen 6 ,
- Tom Wenseleers ORCID: orcid.org/0000-0002-1434-861X 4 &
- Kevin J. Verstrepen ORCID: orcid.org/0000-0002-3077-6219 1 , 2 , 3
Nature Communications volume 15 , Article number: 2368 ( 2024 ) Cite this article
27k Accesses
720 Altmetric
Metrics details
- Chemical engineering
- Gas chromatography
- Machine learning
- Metabolomics
- Taste receptors
The perception and appreciation of food flavor depends on many interacting chemical compounds and external factors, and therefore proves challenging to understand and predict. Here, we combine extensive chemical and sensory analyses of 250 different beers to train machine learning models that allow predicting flavor and consumer appreciation. For each beer, we measure over 200 chemical properties, perform quantitative descriptive sensory analysis with a trained tasting panel and map data from over 180,000 consumer reviews to train 10 different machine learning models. The best-performing algorithm, Gradient Boosting, yields models that significantly outperform predictions based on conventional statistics and accurately predict complex food features and consumer appreciation from chemical profiles. Model dissection allows identifying specific and unexpected compounds as drivers of beer flavor and appreciation. Adding these compounds results in variants of commercial alcoholic and non-alcoholic beers with improved consumer appreciation. Together, our study reveals how big data and machine learning uncover complex links between food chemistry, flavor and consumer perception, and lays the foundation to develop novel, tailored foods with superior flavors.
Similar content being viewed by others
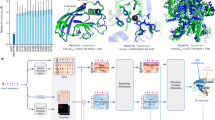
Highly accurate protein structure prediction with AlphaFold
John Jumper, Richard Evans, … Demis Hassabis
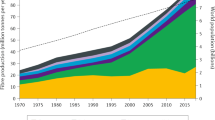
The environmental price of fast fashion
Kirsi Niinimäki, Greg Peters, … Alison Gwilt
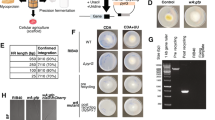
Edible mycelium bioengineered for enhanced nutritional value and sensory appeal using a modular synthetic biology toolkit
Vayu Maini Rekdal, Casper R. B. van der Luijt, … Jay D. Keasling
Introduction
Predicting and understanding food perception and appreciation is one of the major challenges in food science. Accurate modeling of food flavor and appreciation could yield important opportunities for both producers and consumers, including quality control, product fingerprinting, counterfeit detection, spoilage detection, and the development of new products and product combinations (food pairing) 1 , 2 , 3 , 4 , 5 , 6 . Accurate models for flavor and consumer appreciation would contribute greatly to our scientific understanding of how humans perceive and appreciate flavor. Moreover, accurate predictive models would also facilitate and standardize existing food assessment methods and could supplement or replace assessments by trained and consumer tasting panels, which are variable, expensive and time-consuming 7 , 8 , 9 . Lastly, apart from providing objective, quantitative, accurate and contextual information that can help producers, models can also guide consumers in understanding their personal preferences 10 .
Despite the myriad of applications, predicting food flavor and appreciation from its chemical properties remains a largely elusive goal in sensory science, especially for complex food and beverages 11 , 12 . A key obstacle is the immense number of flavor-active chemicals underlying food flavor. Flavor compounds can vary widely in chemical structure and concentration, making them technically challenging and labor-intensive to quantify, even in the face of innovations in metabolomics, such as non-targeted metabolic fingerprinting 13 , 14 . Moreover, sensory analysis is perhaps even more complicated. Flavor perception is highly complex, resulting from hundreds of different molecules interacting at the physiochemical and sensorial level. Sensory perception is often non-linear, characterized by complex and concentration-dependent synergistic and antagonistic effects 15 , 16 , 17 , 18 , 19 , 20 , 21 that are further convoluted by the genetics, environment, culture and psychology of consumers 22 , 23 , 24 . Perceived flavor is therefore difficult to measure, with problems of sensitivity, accuracy, and reproducibility that can only be resolved by gathering sufficiently large datasets 25 . Trained tasting panels are considered the prime source of quality sensory data, but require meticulous training, are low throughput and high cost. Public databases containing consumer reviews of food products could provide a valuable alternative, especially for studying appreciation scores, which do not require formal training 25 . Public databases offer the advantage of amassing large amounts of data, increasing the statistical power to identify potential drivers of appreciation. However, public datasets suffer from biases, including a bias in the volunteers that contribute to the database, as well as confounding factors such as price, cult status and psychological conformity towards previous ratings of the product.
Classical multivariate statistics and machine learning methods have been used to predict flavor of specific compounds by, for example, linking structural properties of a compound to its potential biological activities or linking concentrations of specific compounds to sensory profiles 1 , 26 . Importantly, most previous studies focused on predicting organoleptic properties of single compounds (often based on their chemical structure) 27 , 28 , 29 , 30 , 31 , 32 , 33 , thus ignoring the fact that these compounds are present in a complex matrix in food or beverages and excluding complex interactions between compounds. Moreover, the classical statistics commonly used in sensory science 34 , 35 , 36 , 37 , 38 , 39 require a large sample size and sufficient variance amongst predictors to create accurate models. They are not fit for studying an extensive set of hundreds of interacting flavor compounds, since they are sensitive to outliers, have a high tendency to overfit and are less suited for non-linear and discontinuous relationships 40 .
In this study, we combine extensive chemical analyses and sensory data of a set of different commercial beers with machine learning approaches to develop models that predict taste, smell, mouthfeel and appreciation from compound concentrations. Beer is particularly suited to model the relationship between chemistry, flavor and appreciation. First, beer is a complex product, consisting of thousands of flavor compounds that partake in complex sensory interactions 41 , 42 , 43 . This chemical diversity arises from the raw materials (malt, yeast, hops, water and spices) and biochemical conversions during the brewing process (kilning, mashing, boiling, fermentation, maturation and aging) 44 , 45 . Second, the advent of the internet saw beer consumers embrace online review platforms, such as RateBeer (ZX Ventures, Anheuser-Busch InBev SA/NV) and BeerAdvocate (Next Glass, inc.). In this way, the beer community provides massive data sets of beer flavor and appreciation scores, creating extraordinarily large sensory databases to complement the analyses of our professional sensory panel. Specifically, we characterize over 200 chemical properties of 250 commercial beers, spread across 22 beer styles, and link these to the descriptive sensory profiling data of a 16-person in-house trained tasting panel and data acquired from over 180,000 public consumer reviews. These unique and extensive datasets enable us to train a suite of machine learning models to predict flavor and appreciation from a beer’s chemical profile. Dissection of the best-performing models allows us to pinpoint specific compounds as potential drivers of beer flavor and appreciation. Follow-up experiments confirm the importance of these compounds and ultimately allow us to significantly improve the flavor and appreciation of selected commercial beers. Together, our study represents a significant step towards understanding complex flavors and reinforces the value of machine learning to develop and refine complex foods. In this way, it represents a stepping stone for further computer-aided food engineering applications 46 .
To generate a comprehensive dataset on beer flavor, we selected 250 commercial Belgian beers across 22 different beer styles (Supplementary Fig. S1 ). Beers with ≤ 4.2% alcohol by volume (ABV) were classified as non-alcoholic and low-alcoholic. Blonds and Tripels constitute a significant portion of the dataset (12.4% and 11.2%, respectively) reflecting their presence on the Belgian beer market and the heterogeneity of beers within these styles. By contrast, lager beers are less diverse and dominated by a handful of brands. Rare styles such as Brut or Faro make up only a small fraction of the dataset (2% and 1%, respectively) because fewer of these beers are produced and because they are dominated by distinct characteristics in terms of flavor and chemical composition.
Extensive analysis identifies relationships between chemical compounds in beer
For each beer, we measured 226 different chemical properties, including common brewing parameters such as alcohol content, iso-alpha acids, pH, sugar concentration 47 , and over 200 flavor compounds (Methods, Supplementary Table S1 ). A large portion (37.2%) are terpenoids arising from hopping, responsible for herbal and fruity flavors 16 , 48 . A second major category are yeast metabolites, such as esters and alcohols, that result in fruity and solvent notes 48 , 49 , 50 . Other measured compounds are primarily derived from malt, or other microbes such as non- Saccharomyces yeasts and bacteria (‘wild flora’). Compounds that arise from spices or staling are labeled under ‘Others’. Five attributes (caloric value, total acids and total ester, hop aroma and sulfur compounds) are calculated from multiple individually measured compounds.
As a first step in identifying relationships between chemical properties, we determined correlations between the concentrations of the compounds (Fig. 1 , upper panel, Supplementary Data 1 and 2 , and Supplementary Fig. S2 . For the sake of clarity, only a subset of the measured compounds is shown in Fig. 1 ). Compounds of the same origin typically show a positive correlation, while absence of correlation hints at parameters varying independently. For example, the hop aroma compounds citronellol, and alpha-terpineol show moderate correlations with each other (Spearman’s rho=0.39 and 0.57), but not with the bittering hop component iso-alpha acids (Spearman’s rho=0.16 and −0.07). This illustrates how brewers can independently modify hop aroma and bitterness by selecting hop varieties and dosage time. If hops are added early in the boiling phase, chemical conversions increase bitterness while aromas evaporate, conversely, late addition of hops preserves aroma but limits bitterness 51 . Similarly, hop-derived iso-alpha acids show a strong anti-correlation with lactic acid and acetic acid, likely reflecting growth inhibition of lactic acid and acetic acid bacteria, or the consequent use of fewer hops in sour beer styles, such as West Flanders ales and Fruit beers, that rely on these bacteria for their distinct flavors 52 . Finally, yeast-derived esters (ethyl acetate, ethyl decanoate, ethyl hexanoate, ethyl octanoate) and alcohols (ethanol, isoamyl alcohol, isobutanol, and glycerol), correlate with Spearman coefficients above 0.5, suggesting that these secondary metabolites are correlated with the yeast genetic background and/or fermentation parameters and may be difficult to influence individually, although the choice of yeast strain may offer some control 53 .
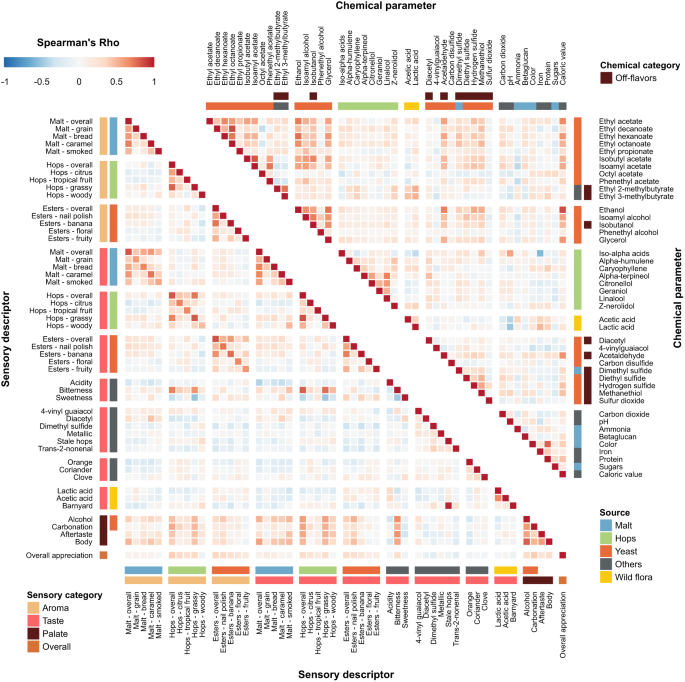
Spearman rank correlations are shown. Descriptors are grouped according to their origin (malt (blue), hops (green), yeast (red), wild flora (yellow), Others (black)), and sensory aspect (aroma, taste, palate, and overall appreciation). Please note that for the chemical compounds, for the sake of clarity, only a subset of the total number of measured compounds is shown, with an emphasis on the key compounds for each source. For more details, see the main text and Methods section. Chemical data can be found in Supplementary Data 1 , correlations between all chemical compounds are depicted in Supplementary Fig. S2 and correlation values can be found in Supplementary Data 2 . See Supplementary Data 4 for sensory panel assessments and Supplementary Data 5 for correlation values between all sensory descriptors.
Interestingly, different beer styles show distinct patterns for some flavor compounds (Supplementary Fig. S3 ). These observations agree with expectations for key beer styles, and serve as a control for our measurements. For instance, Stouts generally show high values for color (darker), while hoppy beers contain elevated levels of iso-alpha acids, compounds associated with bitter hop taste. Acetic and lactic acid are not prevalent in most beers, with notable exceptions such as Kriek, Lambic, Faro, West Flanders ales and Flanders Old Brown, which use acid-producing bacteria ( Lactobacillus and Pediococcus ) or unconventional yeast ( Brettanomyces ) 54 , 55 . Glycerol, ethanol and esters show similar distributions across all beer styles, reflecting their common origin as products of yeast metabolism during fermentation 45 , 53 . Finally, low/no-alcohol beers contain low concentrations of glycerol and esters. This is in line with the production process for most of the low/no-alcohol beers in our dataset, which are produced through limiting fermentation or by stripping away alcohol via evaporation or dialysis, with both methods having the unintended side-effect of reducing the amount of flavor compounds in the final beer 56 , 57 .
Besides expected associations, our data also reveals less trivial associations between beer styles and specific parameters. For example, geraniol and citronellol, two monoterpenoids responsible for citrus, floral and rose flavors and characteristic of Citra hops, are found in relatively high amounts in Christmas, Saison, and Brett/co-fermented beers, where they may originate from terpenoid-rich spices such as coriander seeds instead of hops 58 .
Tasting panel assessments reveal sensorial relationships in beer
To assess the sensory profile of each beer, a trained tasting panel evaluated each of the 250 beers for 50 sensory attributes, including different hop, malt and yeast flavors, off-flavors and spices. Panelists used a tasting sheet (Supplementary Data 3 ) to score the different attributes. Panel consistency was evaluated by repeating 12 samples across different sessions and performing ANOVA. In 95% of cases no significant difference was found across sessions ( p > 0.05), indicating good panel consistency (Supplementary Table S2 ).
Aroma and taste perception reported by the trained panel are often linked (Fig. 1 , bottom left panel and Supplementary Data 4 and 5 ), with high correlations between hops aroma and taste (Spearman’s rho=0.83). Bitter taste was found to correlate with hop aroma and taste in general (Spearman’s rho=0.80 and 0.69), and particularly with “grassy” noble hops (Spearman’s rho=0.75). Barnyard flavor, most often associated with sour beers, is identified together with stale hops (Spearman’s rho=0.97) that are used in these beers. Lactic and acetic acid, which often co-occur, are correlated (Spearman’s rho=0.66). Interestingly, sweetness and bitterness are anti-correlated (Spearman’s rho = −0.48), confirming the hypothesis that they mask each other 59 , 60 . Beer body is highly correlated with alcohol (Spearman’s rho = 0.79), and overall appreciation is found to correlate with multiple aspects that describe beer mouthfeel (alcohol, carbonation; Spearman’s rho= 0.32, 0.39), as well as with hop and ester aroma intensity (Spearman’s rho=0.39 and 0.35).
Similar to the chemical analyses, sensorial analyses confirmed typical features of specific beer styles (Supplementary Fig. S4 ). For example, sour beers (Faro, Flanders Old Brown, Fruit beer, Kriek, Lambic, West Flanders ale) were rated acidic, with flavors of both acetic and lactic acid. Hoppy beers were found to be bitter and showed hop-associated aromas like citrus and tropical fruit. Malt taste is most detected among scotch, stout/porters, and strong ales, while low/no-alcohol beers, which often have a reputation for being ‘worty’ (reminiscent of unfermented, sweet malt extract) appear in the middle. Unsurprisingly, hop aromas are most strongly detected among hoppy beers. Like its chemical counterpart (Supplementary Fig. S3 ), acidity shows a right-skewed distribution, with the most acidic beers being Krieks, Lambics, and West Flanders ales.
Tasting panel assessments of specific flavors correlate with chemical composition
We find that the concentrations of several chemical compounds strongly correlate with specific aroma or taste, as evaluated by the tasting panel (Fig. 2 , Supplementary Fig. S5 , Supplementary Data 6 ). In some cases, these correlations confirm expectations and serve as a useful control for data quality. For example, iso-alpha acids, the bittering compounds in hops, strongly correlate with bitterness (Spearman’s rho=0.68), while ethanol and glycerol correlate with tasters’ perceptions of alcohol and body, the mouthfeel sensation of fullness (Spearman’s rho=0.82/0.62 and 0.72/0.57 respectively) and darker color from roasted malts is a good indication of malt perception (Spearman’s rho=0.54).
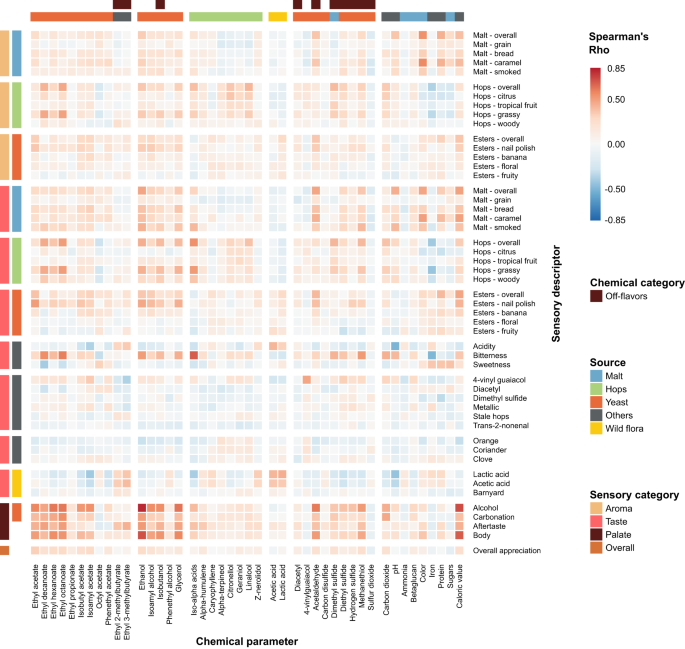
Heatmap colors indicate Spearman’s Rho. Axes are organized according to sensory categories (aroma, taste, mouthfeel, overall), chemical categories and chemical sources in beer (malt (blue), hops (green), yeast (red), wild flora (yellow), Others (black)). See Supplementary Data 6 for all correlation values.
Interestingly, for some relationships between chemical compounds and perceived flavor, correlations are weaker than expected. For example, the rose-smelling phenethyl acetate only weakly correlates with floral aroma. This hints at more complex relationships and interactions between compounds and suggests a need for a more complex model than simple correlations. Lastly, we uncovered unexpected correlations. For instance, the esters ethyl decanoate and ethyl octanoate appear to correlate slightly with hop perception and bitterness, possibly due to their fruity flavor. Iron is anti-correlated with hop aromas and bitterness, most likely because it is also anti-correlated with iso-alpha acids. This could be a sign of metal chelation of hop acids 61 , given that our analyses measure unbound hop acids and total iron content, or could result from the higher iron content in dark and Fruit beers, which typically have less hoppy and bitter flavors 62 .
Public consumer reviews complement expert panel data
To complement and expand the sensory data of our trained tasting panel, we collected 180,000 reviews of our 250 beers from the online consumer review platform RateBeer. This provided numerical scores for beer appearance, aroma, taste, palate, overall quality as well as the average overall score.
Public datasets are known to suffer from biases, such as price, cult status and psychological conformity towards previous ratings of a product. For example, prices correlate with appreciation scores for these online consumer reviews (rho=0.49, Supplementary Fig. S6 ), but not for our trained tasting panel (rho=0.19). This suggests that prices affect consumer appreciation, which has been reported in wine 63 , while blind tastings are unaffected. Moreover, we observe that some beer styles, like lagers and non-alcoholic beers, generally receive lower scores, reflecting that online reviewers are mostly beer aficionados with a preference for specialty beers over lager beers. In general, we find a modest correlation between our trained panel’s overall appreciation score and the online consumer appreciation scores (Fig. 3 , rho=0.29). Apart from the aforementioned biases in the online datasets, serving temperature, sample freshness and surroundings, which are all tightly controlled during the tasting panel sessions, can vary tremendously across online consumers and can further contribute to (among others, appreciation) differences between the two categories of tasters. Importantly, in contrast to the overall appreciation scores, for many sensory aspects the results from the professional panel correlated well with results obtained from RateBeer reviews. Correlations were highest for features that are relatively easy to recognize even for untrained tasters, like bitterness, sweetness, alcohol and malt aroma (Fig. 3 and below).
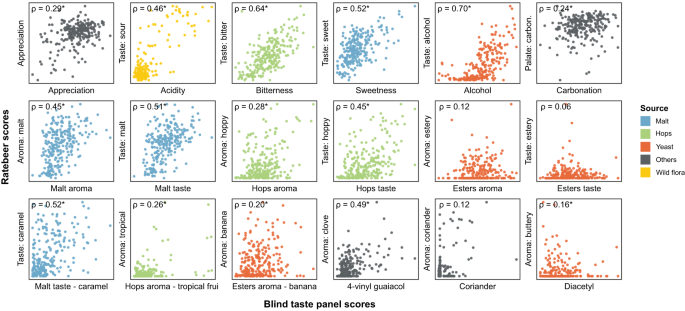
RateBeer text mining results can be found in Supplementary Data 7 . Rho values shown are Spearman correlation values, with asterisks indicating significant correlations ( p < 0.05, two-sided). All p values were smaller than 0.001, except for Esters aroma (0.0553), Esters taste (0.3275), Esters aroma—banana (0.0019), Coriander (0.0508) and Diacetyl (0.0134).
Besides collecting consumer appreciation from these online reviews, we developed automated text analysis tools to gather additional data from review texts (Supplementary Data 7 ). Processing review texts on the RateBeer database yielded comparable results to the scores given by the trained panel for many common sensory aspects, including acidity, bitterness, sweetness, alcohol, malt, and hop tastes (Fig. 3 ). This is in line with what would be expected, since these attributes require less training for accurate assessment and are less influenced by environmental factors such as temperature, serving glass and odors in the environment. Consumer reviews also correlate well with our trained panel for 4-vinyl guaiacol, a compound associated with a very characteristic aroma. By contrast, correlations for more specific aromas like ester, coriander or diacetyl are underrepresented in the online reviews, underscoring the importance of using a trained tasting panel and standardized tasting sheets with explicit factors to be scored for evaluating specific aspects of a beer. Taken together, our results suggest that public reviews are trustworthy for some, but not all, flavor features and can complement or substitute taste panel data for these sensory aspects.
Models can predict beer sensory profiles from chemical data
The rich datasets of chemical analyses, tasting panel assessments and public reviews gathered in the first part of this study provided us with a unique opportunity to develop predictive models that link chemical data to sensorial features. Given the complexity of beer flavor, basic statistical tools such as correlations or linear regression may not always be the most suitable for making accurate predictions. Instead, we applied different machine learning models that can model both simple linear and complex interactive relationships. Specifically, we constructed a set of regression models to predict (a) trained panel scores for beer flavor and quality and (b) public reviews’ appreciation scores from beer chemical profiles. We trained and tested 10 different models (Methods), 3 linear regression-based models (simple linear regression with first-order interactions (LR), lasso regression with first-order interactions (Lasso), partial least squares regressor (PLSR)), 5 decision tree models (AdaBoost regressor (ABR), extra trees (ET), gradient boosting regressor (GBR), random forest (RF) and XGBoost regressor (XGBR)), 1 support vector regression (SVR), and 1 artificial neural network (ANN) model.
To compare the performance of our machine learning models, the dataset was randomly split into a training and test set, stratified by beer style. After a model was trained on data in the training set, its performance was evaluated on its ability to predict the test dataset obtained from multi-output models (based on the coefficient of determination, see Methods). Additionally, individual-attribute models were ranked per descriptor and the average rank was calculated, as proposed by Korneva et al. 64 . Importantly, both ways of evaluating the models’ performance agreed in general. Performance of the different models varied (Table 1 ). It should be noted that all models perform better at predicting RateBeer results than results from our trained tasting panel. One reason could be that sensory data is inherently variable, and this variability is averaged out with the large number of public reviews from RateBeer. Additionally, all tree-based models perform better at predicting taste than aroma. Linear models (LR) performed particularly poorly, with negative R 2 values, due to severe overfitting (training set R 2 = 1). Overfitting is a common issue in linear models with many parameters and limited samples, especially with interaction terms further amplifying the number of parameters. L1 regularization (Lasso) successfully overcomes this overfitting, out-competing multiple tree-based models on the RateBeer dataset. Similarly, the dimensionality reduction of PLSR avoids overfitting and improves performance, to some extent. Still, tree-based models (ABR, ET, GBR, RF and XGBR) show the best performance, out-competing the linear models (LR, Lasso, PLSR) commonly used in sensory science 65 .
GBR models showed the best overall performance in predicting sensory responses from chemical information, with R 2 values up to 0.75 depending on the predicted sensory feature (Supplementary Table S4 ). The GBR models predict consumer appreciation (RateBeer) better than our trained panel’s appreciation (R 2 value of 0.67 compared to R 2 value of 0.09) (Supplementary Table S3 and Supplementary Table S4 ). ANN models showed intermediate performance, likely because neural networks typically perform best with larger datasets 66 . The SVR shows intermediate performance, mostly due to the weak predictions of specific attributes that lower the overall performance (Supplementary Table S4 ).
Model dissection identifies specific, unexpected compounds as drivers of consumer appreciation
Next, we leveraged our models to infer important contributors to sensory perception and consumer appreciation. Consumer preference is a crucial sensory aspects, because a product that shows low consumer appreciation scores often does not succeed commercially 25 . Additionally, the requirement for a large number of representative evaluators makes consumer trials one of the more costly and time-consuming aspects of product development. Hence, a model for predicting chemical drivers of overall appreciation would be a welcome addition to the available toolbox for food development and optimization.
Since GBR models on our RateBeer dataset showed the best overall performance, we focused on these models. Specifically, we used two approaches to identify important contributors. First, rankings of the most important predictors for each sensorial trait in the GBR models were obtained based on impurity-based feature importance (mean decrease in impurity). High-ranked parameters were hypothesized to be either the true causal chemical properties underlying the trait, to correlate with the actual causal properties, or to take part in sensory interactions affecting the trait 67 (Fig. 4A ). In a second approach, we used SHAP 68 to determine which parameters contributed most to the model for making predictions of consumer appreciation (Fig. 4B ). SHAP calculates parameter contributions to model predictions on a per-sample basis, which can be aggregated into an importance score.
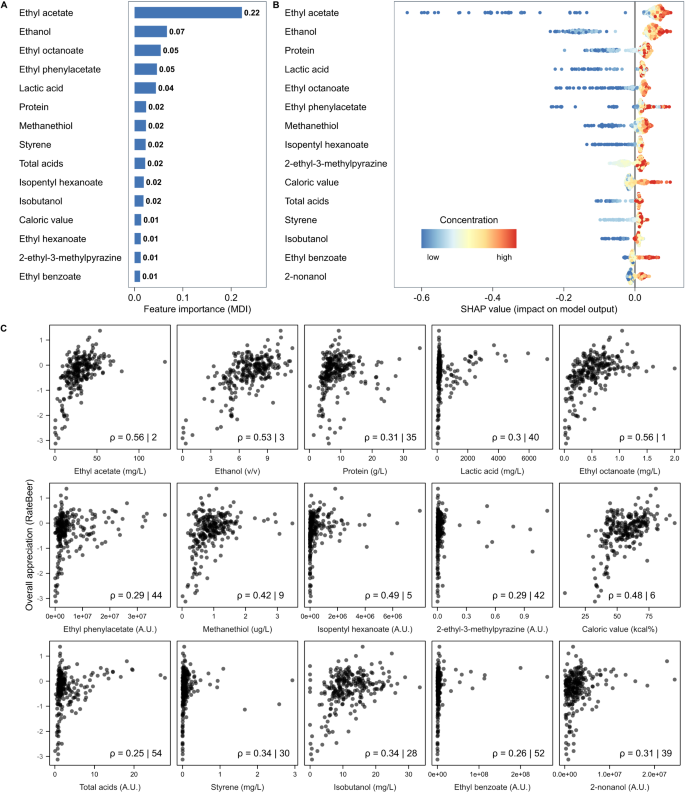
A The impurity-based feature importance (mean deviance in impurity, MDI) calculated from the Gradient Boosting Regression (GBR) model predicting RateBeer appreciation scores. The top 15 highest ranked chemical properties are shown. B SHAP summary plot for the top 15 parameters contributing to our GBR model. Each point on the graph represents a sample from our dataset. The color represents the concentration of that parameter, with bluer colors representing low values and redder colors representing higher values. Greater absolute values on the horizontal axis indicate a higher impact of the parameter on the prediction of the model. C Spearman correlations between the 15 most important chemical properties and consumer overall appreciation. Numbers indicate the Spearman Rho correlation coefficient, and the rank of this correlation compared to all other correlations. The top 15 important compounds were determined using SHAP (panel B).
Both approaches identified ethyl acetate as the most predictive parameter for beer appreciation (Fig. 4 ). Ethyl acetate is the most abundant ester in beer with a typical ‘fruity’, ‘solvent’ and ‘alcoholic’ flavor, but is often considered less important than other esters like isoamyl acetate. The second most important parameter identified by SHAP is ethanol, the most abundant beer compound after water. Apart from directly contributing to beer flavor and mouthfeel, ethanol drastically influences the physical properties of beer, dictating how easily volatile compounds escape the beer matrix to contribute to beer aroma 69 . Importantly, it should also be noted that the importance of ethanol for appreciation is likely inflated by the very low appreciation scores of non-alcoholic beers (Supplementary Fig. S4 ). Despite not often being considered a driver of beer appreciation, protein level also ranks highly in both approaches, possibly due to its effect on mouthfeel and body 70 . Lactic acid, which contributes to the tart taste of sour beers, is the fourth most important parameter identified by SHAP, possibly due to the generally high appreciation of sour beers in our dataset.
Interestingly, some of the most important predictive parameters for our model are not well-established as beer flavors or are even commonly regarded as being negative for beer quality. For example, our models identify methanethiol and ethyl phenyl acetate, an ester commonly linked to beer staling 71 , as a key factor contributing to beer appreciation. Although there is no doubt that high concentrations of these compounds are considered unpleasant, the positive effects of modest concentrations are not yet known 72 , 73 .
To compare our approach to conventional statistics, we evaluated how well the 15 most important SHAP-derived parameters correlate with consumer appreciation (Fig. 4C ). Interestingly, only 6 of the properties derived by SHAP rank amongst the top 15 most correlated parameters. For some chemical compounds, the correlations are so low that they would have likely been considered unimportant. For example, lactic acid, the fourth most important parameter, shows a bimodal distribution for appreciation, with sour beers forming a separate cluster, that is missed entirely by the Spearman correlation. Additionally, the correlation plots reveal outliers, emphasizing the need for robust analysis tools. Together, this highlights the need for alternative models, like the Gradient Boosting model, that better grasp the complexity of (beer) flavor.
Finally, to observe the relationships between these chemical properties and their predicted targets, partial dependence plots were constructed for the six most important predictors of consumer appreciation 74 , 75 , 76 (Supplementary Fig. S7 ). One-way partial dependence plots show how a change in concentration affects the predicted appreciation. These plots reveal an important limitation of our models: appreciation predictions remain constant at ever-increasing concentrations. This implies that once a threshold concentration is reached, further increasing the concentration does not affect appreciation. This is false, as it is well-documented that certain compounds become unpleasant at high concentrations, including ethyl acetate (‘nail polish’) 77 and methanethiol (‘sulfury’ and ‘rotten cabbage’) 78 . The inability of our models to grasp that flavor compounds have optimal levels, above which they become negative, is a consequence of working with commercial beer brands where (off-)flavors are rarely too high to negatively impact the product. The two-way partial dependence plots show how changing the concentration of two compounds influences predicted appreciation, visualizing their interactions (Supplementary Fig. S7 ). In our case, the top 5 parameters are dominated by additive or synergistic interactions, with high concentrations for both compounds resulting in the highest predicted appreciation.
To assess the robustness of our best-performing models and model predictions, we performed 100 iterations of the GBR, RF and ET models. In general, all iterations of the models yielded similar performance (Supplementary Fig. S8 ). Moreover, the main predictors (including the top predictors ethanol and ethyl acetate) remained virtually the same, especially for GBR and RF. For the iterations of the ET model, we did observe more variation in the top predictors, which is likely a consequence of the model’s inherent random architecture in combination with co-correlations between certain predictors. However, even in this case, several of the top predictors (ethanol and ethyl acetate) remain unchanged, although their rank in importance changes (Supplementary Fig. S8 ).
Next, we investigated if a combination of RateBeer and trained panel data into one consolidated dataset would lead to stronger models, under the hypothesis that such a model would suffer less from bias in the datasets. A GBR model was trained to predict appreciation on the combined dataset. This model underperformed compared to the RateBeer model, both in the native case and when including a dataset identifier (R 2 = 0.67, 0.26 and 0.42 respectively). For the latter, the dataset identifier is the most important feature (Supplementary Fig. S9 ), while most of the feature importance remains unchanged, with ethyl acetate and ethanol ranking highest, like in the original model trained only on RateBeer data. It seems that the large variation in the panel dataset introduces noise, weakening the models’ performances and reliability. In addition, it seems reasonable to assume that both datasets are fundamentally different, with the panel dataset obtained by blind tastings by a trained professional panel.
Lastly, we evaluated whether beer style identifiers would further enhance the model’s performance. A GBR model was trained with parameters that explicitly encoded the styles of the samples. This did not improve model performance (R2 = 0.66 with style information vs R2 = 0.67). The most important chemical features are consistent with the model trained without style information (eg. ethanol and ethyl acetate), and with the exception of the most preferred (strong ale) and least preferred (low/no-alcohol) styles, none of the styles were among the most important features (Supplementary Fig. S9 , Supplementary Table S5 and S6 ). This is likely due to a combination of style-specific chemical signatures, such as iso-alpha acids and lactic acid, that implicitly convey style information to the original models, as well as the low number of samples belonging to some styles, making it difficult for the model to learn style-specific patterns. Moreover, beer styles are not rigorously defined, with some styles overlapping in features and some beers being misattributed to a specific style, all of which leads to more noise in models that use style parameters.
Model validation
To test if our predictive models give insight into beer appreciation, we set up experiments aimed at improving existing commercial beers. We specifically selected overall appreciation as the trait to be examined because of its complexity and commercial relevance. Beer flavor comprises a complex bouquet rather than single aromas and tastes 53 . Hence, adding a single compound to the extent that a difference is noticeable may lead to an unbalanced, artificial flavor. Therefore, we evaluated the effect of combinations of compounds. Because Blond beers represent the most extensive style in our dataset, we selected a beer from this style as the starting material for these experiments (Beer 64 in Supplementary Data 1 ).
In the first set of experiments, we adjusted the concentrations of compounds that made up the most important predictors of overall appreciation (ethyl acetate, ethanol, lactic acid, ethyl phenyl acetate) together with correlated compounds (ethyl hexanoate, isoamyl acetate, glycerol), bringing them up to 95 th percentile ethanol-normalized concentrations (Methods) within the Blond group (‘Spiked’ concentration in Fig. 5A ). Compared to controls, the spiked beers were found to have significantly improved overall appreciation among trained panelists, with panelist noting increased intensity of ester flavors, sweetness, alcohol, and body fullness (Fig. 5B ). To disentangle the contribution of ethanol to these results, a second experiment was performed without the addition of ethanol. This resulted in a similar outcome, including increased perception of alcohol and overall appreciation.
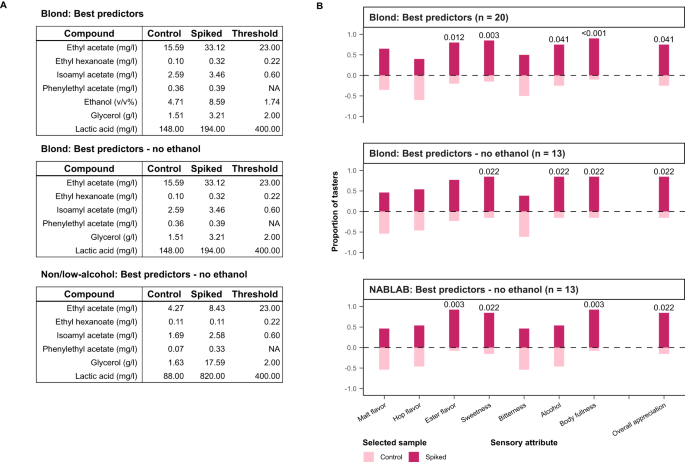
Adding the top chemical compounds, identified as best predictors of appreciation by our model, into poorly appreciated beers results in increased appreciation from our trained panel. Results of sensory tests between base beers and those spiked with compounds identified as the best predictors by the model. A Blond and Non/Low-alcohol (0.0% ABV) base beers were brought up to 95th-percentile ethanol-normalized concentrations within each style. B For each sensory attribute, tasters indicated the more intense sample and selected the sample they preferred. The numbers above the bars correspond to the p values that indicate significant changes in perceived flavor (two-sided binomial test: alpha 0.05, n = 20 or 13).
In a last experiment, we tested whether using the model’s predictions can boost the appreciation of a non-alcoholic beer (beer 223 in Supplementary Data 1 ). Again, the addition of a mixture of predicted compounds (omitting ethanol, in this case) resulted in a significant increase in appreciation, body, ester flavor and sweetness.
Predicting flavor and consumer appreciation from chemical composition is one of the ultimate goals of sensory science. A reliable, systematic and unbiased way to link chemical profiles to flavor and food appreciation would be a significant asset to the food and beverage industry. Such tools would substantially aid in quality control and recipe development, offer an efficient and cost-effective alternative to pilot studies and consumer trials and would ultimately allow food manufacturers to produce superior, tailor-made products that better meet the demands of specific consumer groups more efficiently.
A limited set of studies have previously tried, to varying degrees of success, to predict beer flavor and beer popularity based on (a limited set of) chemical compounds and flavors 79 , 80 . Current sensitive, high-throughput technologies allow measuring an unprecedented number of chemical compounds and properties in a large set of samples, yielding a dataset that can train models that help close the gaps between chemistry and flavor, even for a complex natural product like beer. To our knowledge, no previous research gathered data at this scale (250 samples, 226 chemical parameters, 50 sensory attributes and 5 consumer scores) to disentangle and validate the chemical aspects driving beer preference using various machine-learning techniques. We find that modern machine learning models outperform conventional statistical tools, such as correlations and linear models, and can successfully predict flavor appreciation from chemical composition. This could be attributed to the natural incorporation of interactions and non-linear or discontinuous effects in machine learning models, which are not easily grasped by the linear model architecture. While linear models and partial least squares regression represent the most widespread statistical approaches in sensory science, in part because they allow interpretation 65 , 81 , 82 , modern machine learning methods allow for building better predictive models while preserving the possibility to dissect and exploit the underlying patterns. Of the 10 different models we trained, tree-based models, such as our best performing GBR, showed the best overall performance in predicting sensory responses from chemical information, outcompeting artificial neural networks. This agrees with previous reports for models trained on tabular data 83 . Our results are in line with the findings of Colantonio et al. who also identified the gradient boosting architecture as performing best at predicting appreciation and flavor (of tomatoes and blueberries, in their specific study) 26 . Importantly, besides our larger experimental scale, we were able to directly confirm our models’ predictions in vivo.
Our study confirms that flavor compound concentration does not always correlate with perception, suggesting complex interactions that are often missed by more conventional statistics and simple models. Specifically, we find that tree-based algorithms may perform best in developing models that link complex food chemistry with aroma. Furthermore, we show that massive datasets of untrained consumer reviews provide a valuable source of data, that can complement or even replace trained tasting panels, especially for appreciation and basic flavors, such as sweetness and bitterness. This holds despite biases that are known to occur in such datasets, such as price or conformity bias. Moreover, GBR models predict taste better than aroma. This is likely because taste (e.g. bitterness) often directly relates to the corresponding chemical measurements (e.g., iso-alpha acids), whereas such a link is less clear for aromas, which often result from the interplay between multiple volatile compounds. We also find that our models are best at predicting acidity and alcohol, likely because there is a direct relation between the measured chemical compounds (acids and ethanol) and the corresponding perceived sensorial attribute (acidity and alcohol), and because even untrained consumers are generally able to recognize these flavors and aromas.
The predictions of our final models, trained on review data, hold even for blind tastings with small groups of trained tasters, as demonstrated by our ability to validate specific compounds as drivers of beer flavor and appreciation. Since adding a single compound to the extent of a noticeable difference may result in an unbalanced flavor profile, we specifically tested our identified key drivers as a combination of compounds. While this approach does not allow us to validate if a particular single compound would affect flavor and/or appreciation, our experiments do show that this combination of compounds increases consumer appreciation.
It is important to stress that, while it represents an important step forward, our approach still has several major limitations. A key weakness of the GBR model architecture is that amongst co-correlating variables, the largest main effect is consistently preferred for model building. As a result, co-correlating variables often have artificially low importance scores, both for impurity and SHAP-based methods, like we observed in the comparison to the more randomized Extra Trees models. This implies that chemicals identified as key drivers of a specific sensory feature by GBR might not be the true causative compounds, but rather co-correlate with the actual causative chemical. For example, the high importance of ethyl acetate could be (partially) attributed to the total ester content, ethanol or ethyl hexanoate (rho=0.77, rho=0.72 and rho=0.68), while ethyl phenylacetate could hide the importance of prenyl isobutyrate and ethyl benzoate (rho=0.77 and rho=0.76). Expanding our GBR model to include beer style as a parameter did not yield additional power or insight. This is likely due to style-specific chemical signatures, such as iso-alpha acids and lactic acid, that implicitly convey style information to the original model, as well as the smaller sample size per style, limiting the power to uncover style-specific patterns. This can be partly attributed to the curse of dimensionality, where the high number of parameters results in the models mainly incorporating single parameter effects, rather than complex interactions such as style-dependent effects 67 . A larger number of samples may overcome some of these limitations and offer more insight into style-specific effects. On the other hand, beer style is not a rigid scientific classification, and beers within one style often differ a lot, which further complicates the analysis of style as a model factor.
Our study is limited to beers from Belgian breweries. Although these beers cover a large portion of the beer styles available globally, some beer styles and consumer patterns may be missing, while other features might be overrepresented. For example, many Belgian ales exhibit yeast-driven flavor profiles, which is reflected in the chemical drivers of appreciation discovered by this study. In future work, expanding the scope to include diverse markets and beer styles could lead to the identification of even more drivers of appreciation and better models for special niche products that were not present in our beer set.
In addition to inherent limitations of GBR models, there are also some limitations associated with studying food aroma. Even if our chemical analyses measured most of the known aroma compounds, the total number of flavor compounds in complex foods like beer is still larger than the subset we were able to measure in this study. For example, hop-derived thiols, that influence flavor at very low concentrations, are notoriously difficult to measure in a high-throughput experiment. Moreover, consumer perception remains subjective and prone to biases that are difficult to avoid. It is also important to stress that the models are still immature and that more extensive datasets will be crucial for developing more complete models in the future. Besides more samples and parameters, our dataset does not include any demographic information about the tasters. Including such data could lead to better models that grasp external factors like age and culture. Another limitation is that our set of beers consists of high-quality end-products and lacks beers that are unfit for sale, which limits the current model in accurately predicting products that are appreciated very badly. Finally, while models could be readily applied in quality control, their use in sensory science and product development is restrained by their inability to discern causal relationships. Given that the models cannot distinguish compounds that genuinely drive consumer perception from those that merely correlate, validation experiments are essential to identify true causative compounds.
Despite the inherent limitations, dissection of our models enabled us to pinpoint specific molecules as potential drivers of beer aroma and consumer appreciation, including compounds that were unexpected and would not have been identified using standard approaches. Important drivers of beer appreciation uncovered by our models include protein levels, ethyl acetate, ethyl phenyl acetate and lactic acid. Currently, many brewers already use lactic acid to acidify their brewing water and ensure optimal pH for enzymatic activity during the mashing process. Our results suggest that adding lactic acid can also improve beer appreciation, although its individual effect remains to be tested. Interestingly, ethanol appears to be unnecessary to improve beer appreciation, both for blond beer and alcohol-free beer. Given the growing consumer interest in alcohol-free beer, with a predicted annual market growth of >7% 84 , it is relevant for brewers to know what compounds can further increase consumer appreciation of these beers. Hence, our model may readily provide avenues to further improve the flavor and consumer appreciation of both alcoholic and non-alcoholic beers, which is generally considered one of the key challenges for future beer production.
Whereas we see a direct implementation of our results for the development of superior alcohol-free beverages and other food products, our study can also serve as a stepping stone for the development of novel alcohol-containing beverages. We want to echo the growing body of scientific evidence for the negative effects of alcohol consumption, both on the individual level by the mutagenic, teratogenic and carcinogenic effects of ethanol 85 , 86 , as well as the burden on society caused by alcohol abuse and addiction. We encourage the use of our results for the production of healthier, tastier products, including novel and improved beverages with lower alcohol contents. Furthermore, we strongly discourage the use of these technologies to improve the appreciation or addictive properties of harmful substances.
The present work demonstrates that despite some important remaining hurdles, combining the latest developments in chemical analyses, sensory analysis and modern machine learning methods offers exciting avenues for food chemistry and engineering. Soon, these tools may provide solutions in quality control and recipe development, as well as new approaches to sensory science and flavor research.
Beer selection
250 commercial Belgian beers were selected to cover the broad diversity of beer styles and corresponding diversity in chemical composition and aroma. See Supplementary Fig. S1 .
Chemical dataset
Sample preparation.
Beers within their expiration date were purchased from commercial retailers. Samples were prepared in biological duplicates at room temperature, unless explicitly stated otherwise. Bottle pressure was measured with a manual pressure device (Steinfurth Mess-Systeme GmbH) and used to calculate CO 2 concentration. The beer was poured through two filter papers (Macherey-Nagel, 500713032 MN 713 ¼) to remove carbon dioxide and prevent spontaneous foaming. Samples were then prepared for measurements by targeted Headspace-Gas Chromatography-Flame Ionization Detector/Flame Photometric Detector (HS-GC-FID/FPD), Headspace-Solid Phase Microextraction-Gas Chromatography-Mass Spectrometry (HS-SPME-GC-MS), colorimetric analysis, enzymatic analysis, Near-Infrared (NIR) analysis, as described in the sections below. The mean values of biological duplicates are reported for each compound.
HS-GC-FID/FPD
HS-GC-FID/FPD (Shimadzu GC 2010 Plus) was used to measure higher alcohols, acetaldehyde, esters, 4-vinyl guaicol, and sulfur compounds. Each measurement comprised 5 ml of sample pipetted into a 20 ml glass vial containing 1.75 g NaCl (VWR, 27810.295). 100 µl of 2-heptanol (Sigma-Aldrich, H3003) (internal standard) solution in ethanol (Fisher Chemical, E/0650DF/C17) was added for a final concentration of 2.44 mg/L. Samples were flushed with nitrogen for 10 s, sealed with a silicone septum, stored at −80 °C and analyzed in batches of 20.
The GC was equipped with a DB-WAXetr column (length, 30 m; internal diameter, 0.32 mm; layer thickness, 0.50 µm; Agilent Technologies, Santa Clara, CA, USA) to the FID and an HP-5 column (length, 30 m; internal diameter, 0.25 mm; layer thickness, 0.25 µm; Agilent Technologies, Santa Clara, CA, USA) to the FPD. N 2 was used as the carrier gas. Samples were incubated for 20 min at 70 °C in the headspace autosampler (Flow rate, 35 cm/s; Injection volume, 1000 µL; Injection mode, split; Combi PAL autosampler, CTC analytics, Switzerland). The injector, FID and FPD temperatures were kept at 250 °C. The GC oven temperature was first held at 50 °C for 5 min and then allowed to rise to 80 °C at a rate of 5 °C/min, followed by a second ramp of 4 °C/min until 200 °C kept for 3 min and a final ramp of (4 °C/min) until 230 °C for 1 min. Results were analyzed with the GCSolution software version 2.4 (Shimadzu, Kyoto, Japan). The GC was calibrated with a 5% EtOH solution (VWR International) containing the volatiles under study (Supplementary Table S7 ).
HS-SPME-GC-MS
HS-SPME-GC-MS (Shimadzu GCMS-QP-2010 Ultra) was used to measure additional volatile compounds, mainly comprising terpenoids and esters. Samples were analyzed by HS-SPME using a triphase DVB/Carboxen/PDMS 50/30 μm SPME fiber (Supelco Co., Bellefonte, PA, USA) followed by gas chromatography (Thermo Fisher Scientific Trace 1300 series, USA) coupled to a mass spectrometer (Thermo Fisher Scientific ISQ series MS) equipped with a TriPlus RSH autosampler. 5 ml of degassed beer sample was placed in 20 ml vials containing 1.75 g NaCl (VWR, 27810.295). 5 µl internal standard mix was added, containing 2-heptanol (1 g/L) (Sigma-Aldrich, H3003), 4-fluorobenzaldehyde (1 g/L) (Sigma-Aldrich, 128376), 2,3-hexanedione (1 g/L) (Sigma-Aldrich, 144169) and guaiacol (1 g/L) (Sigma-Aldrich, W253200) in ethanol (Fisher Chemical, E/0650DF/C17). Each sample was incubated at 60 °C in the autosampler oven with constant agitation. After 5 min equilibration, the SPME fiber was exposed to the sample headspace for 30 min. The compounds trapped on the fiber were thermally desorbed in the injection port of the chromatograph by heating the fiber for 15 min at 270 °C.
The GC-MS was equipped with a low polarity RXi-5Sil MS column (length, 20 m; internal diameter, 0.18 mm; layer thickness, 0.18 µm; Restek, Bellefonte, PA, USA). Injection was performed in splitless mode at 320 °C, a split flow of 9 ml/min, a purge flow of 5 ml/min and an open valve time of 3 min. To obtain a pulsed injection, a programmed gas flow was used whereby the helium gas flow was set at 2.7 mL/min for 0.1 min, followed by a decrease in flow of 20 ml/min to the normal 0.9 mL/min. The temperature was first held at 30 °C for 3 min and then allowed to rise to 80 °C at a rate of 7 °C/min, followed by a second ramp of 2 °C/min till 125 °C and a final ramp of 8 °C/min with a final temperature of 270 °C.
Mass acquisition range was 33 to 550 amu at a scan rate of 5 scans/s. Electron impact ionization energy was 70 eV. The interface and ion source were kept at 275 °C and 250 °C, respectively. A mix of linear n-alkanes (from C7 to C40, Supelco Co.) was injected into the GC-MS under identical conditions to serve as external retention index markers. Identification and quantification of the compounds were performed using an in-house developed R script as described in Goelen et al. and Reher et al. 87 , 88 (for package information, see Supplementary Table S8 ). Briefly, chromatograms were analyzed using AMDIS (v2.71) 89 to separate overlapping peaks and obtain pure compound spectra. The NIST MS Search software (v2.0 g) in combination with the NIST2017, FFNSC3 and Adams4 libraries were used to manually identify the empirical spectra, taking into account the expected retention time. After background subtraction and correcting for retention time shifts between samples run on different days based on alkane ladders, compound elution profiles were extracted and integrated using a file with 284 target compounds of interest, which were either recovered in our identified AMDIS list of spectra or were known to occur in beer. Compound elution profiles were estimated for every peak in every chromatogram over a time-restricted window using weighted non-negative least square analysis after which peak areas were integrated 87 , 88 . Batch effect correction was performed by normalizing against the most stable internal standard compound, 4-fluorobenzaldehyde. Out of all 284 target compounds that were analyzed, 167 were visually judged to have reliable elution profiles and were used for final analysis.
Discrete photometric and enzymatic analysis
Discrete photometric and enzymatic analysis (Thermo Scientific TM Gallery TM Plus Beermaster Discrete Analyzer) was used to measure acetic acid, ammonia, beta-glucan, iso-alpha acids, color, sugars, glycerol, iron, pH, protein, and sulfite. 2 ml of sample volume was used for the analyses. Information regarding the reagents and standard solutions used for analyses and calibrations is included in Supplementary Table S7 and Supplementary Table S9 .
NIR analyses
NIR analysis (Anton Paar Alcolyzer Beer ME System) was used to measure ethanol. Measurements comprised 50 ml of sample, and a 10% EtOH solution was used for calibration.
Correlation calculations
Pairwise Spearman Rank correlations were calculated between all chemical properties.
Sensory dataset
Trained panel.
Our trained tasting panel consisted of volunteers who gave prior verbal informed consent. All compounds used for the validation experiment were of food-grade quality. The tasting sessions were approved by the Social and Societal Ethics Committee of the KU Leuven (G-2022-5677-R2(MAR)). All online reviewers agreed to the Terms and Conditions of the RateBeer website.
Sensory analysis was performed according to the American Society of Brewing Chemists (ASBC) Sensory Analysis Methods 90 . 30 volunteers were screened through a series of triangle tests. The sixteen most sensitive and consistent tasters were retained as taste panel members. The resulting panel was diverse in age [22–42, mean: 29], sex [56% male] and nationality [7 different countries]. The panel developed a consensus vocabulary to describe beer aroma, taste and mouthfeel. Panelists were trained to identify and score 50 different attributes, using a 7-point scale to rate attributes’ intensity. The scoring sheet is included as Supplementary Data 3 . Sensory assessments took place between 10–12 a.m. The beers were served in black-colored glasses. Per session, between 5 and 12 beers of the same style were tasted at 12 °C to 16 °C. Two reference beers were added to each set and indicated as ‘Reference 1 & 2’, allowing panel members to calibrate their ratings. Not all panelists were present at every tasting. Scores were scaled by standard deviation and mean-centered per taster. Values are represented as z-scores and clustered by Euclidean distance. Pairwise Spearman correlations were calculated between taste and aroma sensory attributes. Panel consistency was evaluated by repeating samples on different sessions and performing ANOVA to identify differences, using the ‘stats’ package (v4.2.2) in R (for package information, see Supplementary Table S8 ).
Online reviews from a public database
The ‘scrapy’ package in Python (v3.6) (for package information, see Supplementary Table S8 ). was used to collect 232,288 online reviews (mean=922, min=6, max=5343) from RateBeer, an online beer review database. Each review entry comprised 5 numerical scores (appearance, aroma, taste, palate and overall quality) and an optional review text. The total number of reviews per reviewer was collected separately. Numerical scores were scaled and centered per rater, and mean scores were calculated per beer.
For the review texts, the language was estimated using the packages ‘langdetect’ and ‘langid’ in Python. Reviews that were classified as English by both packages were kept. Reviewers with fewer than 100 entries overall were discarded. 181,025 reviews from >6000 reviewers from >40 countries remained. Text processing was done using the ‘nltk’ package in Python. Texts were corrected for slang and misspellings; proper nouns and rare words that are relevant to the beer context were specified and kept as-is (‘Chimay’,’Lambic’, etc.). A dictionary of semantically similar sensorial terms, for example ‘floral’ and ‘flower’, was created and collapsed together into one term. Words were stemmed and lemmatized to avoid identifying words such as ‘acid’ and ‘acidity’ as separate terms. Numbers and punctuation were removed.
Sentences from up to 50 randomly chosen reviews per beer were manually categorized according to the aspect of beer they describe (appearance, aroma, taste, palate, overall quality—not to be confused with the 5 numerical scores described above) or flagged as irrelevant if they contained no useful information. If a beer contained fewer than 50 reviews, all reviews were manually classified. This labeled data set was used to train a model that classified the rest of the sentences for all beers 91 . Sentences describing taste and aroma were extracted, and term frequency–inverse document frequency (TFIDF) was implemented to calculate enrichment scores for sensorial words per beer.
The sex of the tasting subject was not considered when building our sensory database. Instead, results from different panelists were averaged, both for our trained panel (56% male, 44% female) and the RateBeer reviews (70% male, 30% female for RateBeer as a whole).
Beer price collection and processing
Beer prices were collected from the following stores: Colruyt, Delhaize, Total Wine, BeerHawk, The Belgian Beer Shop, The Belgian Shop, and Beer of Belgium. Where applicable, prices were converted to Euros and normalized per liter. Spearman correlations were calculated between these prices and mean overall appreciation scores from RateBeer and the taste panel, respectively.
Pairwise Spearman Rank correlations were calculated between all sensory properties.
Machine learning models
Predictive modeling of sensory profiles from chemical data.
Regression models were constructed to predict (a) trained panel scores for beer flavors and quality from beer chemical profiles and (b) public reviews’ appreciation scores from beer chemical profiles. Z-scores were used to represent sensory attributes in both data sets. Chemical properties with log-normal distributions (Shapiro-Wilk test, p < 0.05 ) were log-transformed. Missing chemical measurements (0.1% of all data) were replaced with mean values per attribute. Observations from 250 beers were randomly separated into a training set (70%, 175 beers) and a test set (30%, 75 beers), stratified per beer style. Chemical measurements (p = 231) were normalized based on the training set average and standard deviation. In total, three linear regression-based models: linear regression with first-order interaction terms (LR), lasso regression with first-order interaction terms (Lasso) and partial least squares regression (PLSR); five decision tree models, Adaboost regressor (ABR), Extra Trees (ET), Gradient Boosting regressor (GBR), Random Forest (RF) and XGBoost regressor (XGBR); one support vector machine model (SVR) and one artificial neural network model (ANN) were trained. The models were implemented using the ‘scikit-learn’ package (v1.2.2) and ‘xgboost’ package (v1.7.3) in Python (v3.9.16). Models were trained, and hyperparameters optimized, using five-fold cross-validated grid search with the coefficient of determination (R 2 ) as the evaluation metric. The ANN (scikit-learn’s MLPRegressor) was optimized using Bayesian Tree-Structured Parzen Estimator optimization with the ‘Optuna’ Python package (v3.2.0). Individual models were trained per attribute, and a multi-output model was trained on all attributes simultaneously.
Model dissection
GBR was found to outperform other methods, resulting in models with the highest average R 2 values in both trained panel and public review data sets. Impurity-based rankings of the most important predictors for each predicted sensorial trait were obtained using the ‘scikit-learn’ package. To observe the relationships between these chemical properties and their predicted targets, partial dependence plots (PDP) were constructed for the six most important predictors of consumer appreciation 74 , 75 .
The ‘SHAP’ package in Python (v0.41.0) was implemented to provide an alternative ranking of predictor importance and to visualize the predictors’ effects as a function of their concentration 68 .
Validation of causal chemical properties
To validate the effects of the most important model features on predicted sensory attributes, beers were spiked with the chemical compounds identified by the models and descriptive sensory analyses were carried out according to the American Society of Brewing Chemists (ASBC) protocol 90 .
Compound spiking was done 30 min before tasting. Compounds were spiked into fresh beer bottles, that were immediately resealed and inverted three times. Fresh bottles of beer were opened for the same duration, resealed, and inverted thrice, to serve as controls. Pairs of spiked samples and controls were served simultaneously, chilled and in dark glasses as outlined in the Trained panel section above. Tasters were instructed to select the glass with the higher flavor intensity for each attribute (directional difference test 92 ) and to select the glass they prefer.
The final concentration after spiking was equal to the within-style average, after normalizing by ethanol concentration. This was done to ensure balanced flavor profiles in the final spiked beer. The same methods were applied to improve a non-alcoholic beer. Compounds were the following: ethyl acetate (Merck KGaA, W241415), ethyl hexanoate (Merck KGaA, W243906), isoamyl acetate (Merck KGaA, W205508), phenethyl acetate (Merck KGaA, W285706), ethanol (96%, Colruyt), glycerol (Merck KGaA, W252506), lactic acid (Merck KGaA, 261106).
Significant differences in preference or perceived intensity were determined by performing the two-sided binomial test on each attribute.
Reporting summary
Further information on research design is available in the Nature Portfolio Reporting Summary linked to this article.
Data availability
The data that support the findings of this work are available in the Supplementary Data files and have been deposited to Zenodo under accession code 10653704 93 . The RateBeer scores data are under restricted access, they are not publicly available as they are property of RateBeer (ZX Ventures, USA). Access can be obtained from the authors upon reasonable request and with permission of RateBeer (ZX Ventures, USA). Source data are provided with this paper.
Code availability
The code for training the machine learning models, analyzing the models, and generating the figures has been deposited to Zenodo under accession code 10653704 93 .
Tieman, D. et al. A chemical genetic roadmap to improved tomato flavor. Science 355 , 391–394 (2017).
Article ADS CAS PubMed Google Scholar
Plutowska, B. & Wardencki, W. Application of gas chromatography–olfactometry (GC–O) in analysis and quality assessment of alcoholic beverages – A review. Food Chem. 107 , 449–463 (2008).
Article CAS Google Scholar
Legin, A., Rudnitskaya, A., Seleznev, B. & Vlasov, Y. Electronic tongue for quality assessment of ethanol, vodka and eau-de-vie. Anal. Chim. Acta 534 , 129–135 (2005).
Loutfi, A., Coradeschi, S., Mani, G. K., Shankar, P. & Rayappan, J. B. B. Electronic noses for food quality: A review. J. Food Eng. 144 , 103–111 (2015).
Ahn, Y.-Y., Ahnert, S. E., Bagrow, J. P. & Barabási, A.-L. Flavor network and the principles of food pairing. Sci. Rep. 1 , 196 (2011).
Article CAS PubMed PubMed Central Google Scholar
Bartoshuk, L. M. & Klee, H. J. Better fruits and vegetables through sensory analysis. Curr. Biol. 23 , R374–R378 (2013).
Article CAS PubMed Google Scholar
Piggott, J. R. Design questions in sensory and consumer science. Food Qual. Prefer. 3293 , 217–220 (1995).
Article Google Scholar
Kermit, M. & Lengard, V. Assessing the performance of a sensory panel-panellist monitoring and tracking. J. Chemom. 19 , 154–161 (2005).
Cook, D. J., Hollowood, T. A., Linforth, R. S. T. & Taylor, A. J. Correlating instrumental measurements of texture and flavour release with human perception. Int. J. Food Sci. Technol. 40 , 631–641 (2005).
Chinchanachokchai, S., Thontirawong, P. & Chinchanachokchai, P. A tale of two recommender systems: The moderating role of consumer expertise on artificial intelligence based product recommendations. J. Retail. Consum. Serv. 61 , 1–12 (2021).
Ross, C. F. Sensory science at the human-machine interface. Trends Food Sci. Technol. 20 , 63–72 (2009).
Chambers, E. IV & Koppel, K. Associations of volatile compounds with sensory aroma and flavor: The complex nature of flavor. Molecules 18 , 4887–4905 (2013).
Pinu, F. R. Metabolomics—The new frontier in food safety and quality research. Food Res. Int. 72 , 80–81 (2015).
Danezis, G. P., Tsagkaris, A. S., Brusic, V. & Georgiou, C. A. Food authentication: state of the art and prospects. Curr. Opin. Food Sci. 10 , 22–31 (2016).
Shepherd, G. M. Smell images and the flavour system in the human brain. Nature 444 , 316–321 (2006).
Meilgaard, M. C. Prediction of flavor differences between beers from their chemical composition. J. Agric. Food Chem. 30 , 1009–1017 (1982).
Xu, L. et al. Widespread receptor-driven modulation in peripheral olfactory coding. Science 368 , eaaz5390 (2020).
Kupferschmidt, K. Following the flavor. Science 340 , 808–809 (2013).
Billesbølle, C. B. et al. Structural basis of odorant recognition by a human odorant receptor. Nature 615 , 742–749 (2023).
Article ADS PubMed PubMed Central Google Scholar
Smith, B. Perspective: Complexities of flavour. Nature 486 , S6–S6 (2012).
Pfister, P. et al. Odorant receptor inhibition is fundamental to odor encoding. Curr. Biol. 30 , 2574–2587 (2020).
Moskowitz, H. W., Kumaraiah, V., Sharma, K. N., Jacobs, H. L. & Sharma, S. D. Cross-cultural differences in simple taste preferences. Science 190 , 1217–1218 (1975).
Eriksson, N. et al. A genetic variant near olfactory receptor genes influences cilantro preference. Flavour 1 , 22 (2012).
Ferdenzi, C. et al. Variability of affective responses to odors: Culture, gender, and olfactory knowledge. Chem. Senses 38 , 175–186 (2013).
Article PubMed Google Scholar
Lawless, H. T. & Heymann, H. Sensory evaluation of food: Principles and practices. (Springer, New York, NY). https://doi.org/10.1007/978-1-4419-6488-5 (2010).
Colantonio, V. et al. Metabolomic selection for enhanced fruit flavor. Proc. Natl. Acad. Sci. 119 , e2115865119 (2022).
Fritz, F., Preissner, R. & Banerjee, P. VirtualTaste: a web server for the prediction of organoleptic properties of chemical compounds. Nucleic Acids Res 49 , W679–W684 (2021).
Tuwani, R., Wadhwa, S. & Bagler, G. BitterSweet: Building machine learning models for predicting the bitter and sweet taste of small molecules. Sci. Rep. 9 , 1–13 (2019).
Dagan-Wiener, A. et al. Bitter or not? BitterPredict, a tool for predicting taste from chemical structure. Sci. Rep. 7 , 1–13 (2017).
Pallante, L. et al. Toward a general and interpretable umami taste predictor using a multi-objective machine learning approach. Sci. Rep. 12 , 1–11 (2022).
Malavolta, M. et al. A survey on computational taste predictors. Eur. Food Res. Technol. 248 , 2215–2235 (2022).
Lee, B. K. et al. A principal odor map unifies diverse tasks in olfactory perception. Science 381 , 999–1006 (2023).
Mayhew, E. J. et al. Transport features predict if a molecule is odorous. Proc. Natl. Acad. Sci. 119 , e2116576119 (2022).
Niu, Y. et al. Sensory evaluation of the synergism among ester odorants in light aroma-type liquor by odor threshold, aroma intensity and flash GC electronic nose. Food Res. Int. 113 , 102–114 (2018).
Yu, P., Low, M. Y. & Zhou, W. Design of experiments and regression modelling in food flavour and sensory analysis: A review. Trends Food Sci. Technol. 71 , 202–215 (2018).
Oladokun, O. et al. The impact of hop bitter acid and polyphenol profiles on the perceived bitterness of beer. Food Chem. 205 , 212–220 (2016).
Linforth, R., Cabannes, M., Hewson, L., Yang, N. & Taylor, A. Effect of fat content on flavor delivery during consumption: An in vivo model. J. Agric. Food Chem. 58 , 6905–6911 (2010).
Guo, S., Na Jom, K. & Ge, Y. Influence of roasting condition on flavor profile of sunflower seeds: A flavoromics approach. Sci. Rep. 9 , 11295 (2019).
Ren, Q. et al. The changes of microbial community and flavor compound in the fermentation process of Chinese rice wine using Fagopyrum tataricum grain as feedstock. Sci. Rep. 9 , 3365 (2019).
Hastie, T., Friedman, J. & Tibshirani, R. The Elements of Statistical Learning. (Springer, New York, NY). https://doi.org/10.1007/978-0-387-21606-5 (2001).
Dietz, C., Cook, D., Huismann, M., Wilson, C. & Ford, R. The multisensory perception of hop essential oil: a review. J. Inst. Brew. 126 , 320–342 (2020).
CAS Google Scholar
Roncoroni, Miguel & Verstrepen, Kevin Joan. Belgian Beer: Tested and Tasted. (Lannoo, 2018).
Meilgaard, M. Flavor chemistry of beer: Part II: Flavor and threshold of 239 aroma volatiles. in (1975).
Bokulich, N. A. & Bamforth, C. W. The microbiology of malting and brewing. Microbiol. Mol. Biol. Rev. MMBR 77 , 157–172 (2013).
Dzialo, M. C., Park, R., Steensels, J., Lievens, B. & Verstrepen, K. J. Physiology, ecology and industrial applications of aroma formation in yeast. FEMS Microbiol. Rev. 41 , S95–S128 (2017).
Article PubMed PubMed Central Google Scholar
Datta, A. et al. Computer-aided food engineering. Nat. Food 3 , 894–904 (2022).
American Society of Brewing Chemists. Beer Methods. (American Society of Brewing Chemists, St. Paul, MN, U.S.A.).
Olaniran, A. O., Hiralal, L., Mokoena, M. P. & Pillay, B. Flavour-active volatile compounds in beer: production, regulation and control. J. Inst. Brew. 123 , 13–23 (2017).
Verstrepen, K. J. et al. Flavor-active esters: Adding fruitiness to beer. J. Biosci. Bioeng. 96 , 110–118 (2003).
Meilgaard, M. C. Flavour chemistry of beer. part I: flavour interaction between principal volatiles. Master Brew. Assoc. Am. Tech. Q 12 , 107–117 (1975).
Briggs, D. E., Boulton, C. A., Brookes, P. A. & Stevens, R. Brewing 227–254. (Woodhead Publishing). https://doi.org/10.1533/9781855739062.227 (2004).
Bossaert, S., Crauwels, S., De Rouck, G. & Lievens, B. The power of sour - A review: Old traditions, new opportunities. BrewingScience 72 , 78–88 (2019).
Google Scholar
Verstrepen, K. J. et al. Flavor active esters: Adding fruitiness to beer. J. Biosci. Bioeng. 96 , 110–118 (2003).
Snauwaert, I. et al. Microbial diversity and metabolite composition of Belgian red-brown acidic ales. Int. J. Food Microbiol. 221 , 1–11 (2016).
Spitaels, F. et al. The microbial diversity of traditional spontaneously fermented lambic beer. PLoS ONE 9 , e95384 (2014).
Blanco, C. A., Andrés-Iglesias, C. & Montero, O. Low-alcohol Beers: Flavor Compounds, Defects, and Improvement Strategies. Crit. Rev. Food Sci. Nutr. 56 , 1379–1388 (2016).
Jackowski, M. & Trusek, A. Non-Alcohol. beer Prod. – Overv. 20 , 32–38 (2018).
Takoi, K. et al. The contribution of geraniol metabolism to the citrus flavour of beer: Synergy of geraniol and β-citronellol under coexistence with excess linalool. J. Inst. Brew. 116 , 251–260 (2010).
Kroeze, J. H. & Bartoshuk, L. M. Bitterness suppression as revealed by split-tongue taste stimulation in humans. Physiol. Behav. 35 , 779–783 (1985).
Mennella, J. A. et al. A spoonful of sugar helps the medicine go down”: Bitter masking bysucrose among children and adults. Chem. Senses 40 , 17–25 (2015).
Wietstock, P., Kunz, T., Perreira, F. & Methner, F.-J. Metal chelation behavior of hop acids in buffered model systems. BrewingScience 69 , 56–63 (2016).
Sancho, D., Blanco, C. A., Caballero, I. & Pascual, A. Free iron in pale, dark and alcohol-free commercial lager beers. J. Sci. Food Agric. 91 , 1142–1147 (2011).
Rodrigues, H. & Parr, W. V. Contribution of cross-cultural studies to understanding wine appreciation: A review. Food Res. Int. 115 , 251–258 (2019).
Korneva, E. & Blockeel, H. Towards better evaluation of multi-target regression models. in ECML PKDD 2020 Workshops (eds. Koprinska, I. et al.) 353–362 (Springer International Publishing, Cham, 2020). https://doi.org/10.1007/978-3-030-65965-3_23 .
Gastón Ares. Mathematical and Statistical Methods in Food Science and Technology. (Wiley, 2013).
Grinsztajn, L., Oyallon, E. & Varoquaux, G. Why do tree-based models still outperform deep learning on tabular data? Preprint at http://arxiv.org/abs/2207.08815 (2022).
Gries, S. T. Statistics for Linguistics with R: A Practical Introduction. in Statistics for Linguistics with R (De Gruyter Mouton, 2021). https://doi.org/10.1515/9783110718256 .
Lundberg, S. M. et al. From local explanations to global understanding with explainable AI for trees. Nat. Mach. Intell. 2 , 56–67 (2020).
Ickes, C. M. & Cadwallader, K. R. Effects of ethanol on flavor perception in alcoholic beverages. Chemosens. Percept. 10 , 119–134 (2017).
Kato, M. et al. Influence of high molecular weight polypeptides on the mouthfeel of commercial beer. J. Inst. Brew. 127 , 27–40 (2021).
Wauters, R. et al. Novel Saccharomyces cerevisiae variants slow down the accumulation of staling aldehydes and improve beer shelf-life. Food Chem. 398 , 1–11 (2023).
Li, H., Jia, S. & Zhang, W. Rapid determination of low-level sulfur compounds in beer by headspace gas chromatography with a pulsed flame photometric detector. J. Am. Soc. Brew. Chem. 66 , 188–191 (2008).
Dercksen, A., Laurens, J., Torline, P., Axcell, B. C. & Rohwer, E. Quantitative analysis of volatile sulfur compounds in beer using a membrane extraction interface. J. Am. Soc. Brew. Chem. 54 , 228–233 (1996).
Molnar, C. Interpretable Machine Learning: A Guide for Making Black-Box Models Interpretable. (2020).
Zhao, Q. & Hastie, T. Causal interpretations of black-box models. J. Bus. Econ. Stat. Publ. Am. Stat. Assoc. 39 , 272–281 (2019).
Article MathSciNet Google Scholar
Hastie, T., Tibshirani, R. & Friedman, J. The Elements of Statistical Learning. (Springer, 2019).
Labrado, D. et al. Identification by NMR of key compounds present in beer distillates and residual phases after dealcoholization by vacuum distillation. J. Sci. Food Agric. 100 , 3971–3978 (2020).
Lusk, L. T., Kay, S. B., Porubcan, A. & Ryder, D. S. Key olfactory cues for beer oxidation. J. Am. Soc. Brew. Chem. 70 , 257–261 (2012).
Gonzalez Viejo, C., Torrico, D. D., Dunshea, F. R. & Fuentes, S. Development of artificial neural network models to assess beer acceptability based on sensory properties using a robotic pourer: A comparative model approach to achieve an artificial intelligence system. Beverages 5 , 33 (2019).
Gonzalez Viejo, C., Fuentes, S., Torrico, D. D., Godbole, A. & Dunshea, F. R. Chemical characterization of aromas in beer and their effect on consumers liking. Food Chem. 293 , 479–485 (2019).
Gilbert, J. L. et al. Identifying breeding priorities for blueberry flavor using biochemical, sensory, and genotype by environment analyses. PLOS ONE 10 , 1–21 (2015).
Goulet, C. et al. Role of an esterase in flavor volatile variation within the tomato clade. Proc. Natl. Acad. Sci. 109 , 19009–19014 (2012).
Article ADS CAS PubMed PubMed Central Google Scholar
Borisov, V. et al. Deep Neural Networks and Tabular Data: A Survey. IEEE Trans. Neural Netw. Learn. Syst. 1–21 https://doi.org/10.1109/TNNLS.2022.3229161 (2022).
Statista. Statista Consumer Market Outlook: Beer - Worldwide.
Seitz, H. K. & Stickel, F. Molecular mechanisms of alcoholmediated carcinogenesis. Nat. Rev. Cancer 7 , 599–612 (2007).
Voordeckers, K. et al. Ethanol exposure increases mutation rate through error-prone polymerases. Nat. Commun. 11 , 3664 (2020).
Goelen, T. et al. Bacterial phylogeny predicts volatile organic compound composition and olfactory response of an aphid parasitoid. Oikos 129 , 1415–1428 (2020).
Article ADS Google Scholar
Reher, T. et al. Evaluation of hop (Humulus lupulus) as a repellent for the management of Drosophila suzukii. Crop Prot. 124 , 104839 (2019).
Stein, S. E. An integrated method for spectrum extraction and compound identification from gas chromatography/mass spectrometry data. J. Am. Soc. Mass Spectrom. 10 , 770–781 (1999).
American Society of Brewing Chemists. Sensory Analysis Methods. (American Society of Brewing Chemists, St. Paul, MN, U.S.A., 1992).
McAuley, J., Leskovec, J. & Jurafsky, D. Learning Attitudes and Attributes from Multi-Aspect Reviews. Preprint at https://doi.org/10.48550/arXiv.1210.3926 (2012).
Meilgaard, M. C., Carr, B. T. & Carr, B. T. Sensory Evaluation Techniques. (CRC Press, Boca Raton). https://doi.org/10.1201/b16452 (2014).
Schreurs, M. et al. Data from: Predicting and improving complex beer flavor through machine learning. Zenodo https://doi.org/10.5281/zenodo.10653704 (2024).
Download references
Acknowledgements
We thank all lab members for their discussions and thank all tasting panel members for their contributions. Special thanks go out to Dr. Karin Voordeckers for her tremendous help in proofreading and improving the manuscript. M.S. was supported by a Baillet-Latour fellowship, L.C. acknowledges financial support from KU Leuven (C16/17/006), F.A.T. was supported by a PhD fellowship from FWO (1S08821N). Research in the lab of K.J.V. is supported by KU Leuven, FWO, VIB, VLAIO and the Brewing Science Serves Health Fund. Research in the lab of T.W. is supported by FWO (G.0A51.15) and KU Leuven (C16/17/006).
Author information
These authors contributed equally: Michiel Schreurs, Supinya Piampongsant, Miguel Roncoroni.
Authors and Affiliations
VIB—KU Leuven Center for Microbiology, Gaston Geenslaan 1, B-3001, Leuven, Belgium
Michiel Schreurs, Supinya Piampongsant, Miguel Roncoroni, Lloyd Cool, Beatriz Herrera-Malaver, Florian A. Theßeling & Kevin J. Verstrepen
CMPG Laboratory of Genetics and Genomics, KU Leuven, Gaston Geenslaan 1, B-3001, Leuven, Belgium
Leuven Institute for Beer Research (LIBR), Gaston Geenslaan 1, B-3001, Leuven, Belgium
Laboratory of Socioecology and Social Evolution, KU Leuven, Naamsestraat 59, B-3000, Leuven, Belgium
Lloyd Cool, Christophe Vanderaa & Tom Wenseleers
VIB Bioinformatics Core, VIB, Rijvisschestraat 120, B-9052, Ghent, Belgium
Łukasz Kreft & Alexander Botzki
AB InBev SA/NV, Brouwerijplein 1, B-3000, Leuven, Belgium
Philippe Malcorps & Luk Daenen
You can also search for this author in PubMed Google Scholar
Contributions
S.P., M.S. and K.J.V. conceived the experiments. S.P., M.S. and K.J.V. designed the experiments. S.P., M.S., M.R., B.H. and F.A.T. performed the experiments. S.P., M.S., L.C., C.V., L.K., A.B., P.M., L.D., T.W. and K.J.V. contributed analysis ideas. S.P., M.S., L.C., C.V., T.W. and K.J.V. analyzed the data. All authors contributed to writing the manuscript.
Corresponding author
Correspondence to Kevin J. Verstrepen .
Ethics declarations
Competing interests.
K.J.V. is affiliated with bar.on. The other authors declare no competing interests.
Peer review
Peer review information.
Nature Communications thanks Florian Bauer, Andrew John Macintosh and the other, anonymous, reviewer(s) for their contribution to the peer review of this work. A peer review file is available.
Additional information
Publisher’s note Springer Nature remains neutral with regard to jurisdictional claims in published maps and institutional affiliations.
Supplementary information
Supplementary information, peer review file, description of additional supplementary files, supplementary data 1, supplementary data 2, supplementary data 3, supplementary data 4, supplementary data 5, supplementary data 6, supplementary data 7, reporting summary, source data, source data, rights and permissions.
Open Access This article is licensed under a Creative Commons Attribution 4.0 International License, which permits use, sharing, adaptation, distribution and reproduction in any medium or format, as long as you give appropriate credit to the original author(s) and the source, provide a link to the Creative Commons licence, and indicate if changes were made. The images or other third party material in this article are included in the article’s Creative Commons licence, unless indicated otherwise in a credit line to the material. If material is not included in the article’s Creative Commons licence and your intended use is not permitted by statutory regulation or exceeds the permitted use, you will need to obtain permission directly from the copyright holder. To view a copy of this licence, visit http://creativecommons.org/licenses/by/4.0/ .
Reprints and permissions
About this article
Cite this article.
Schreurs, M., Piampongsant, S., Roncoroni, M. et al. Predicting and improving complex beer flavor through machine learning. Nat Commun 15 , 2368 (2024). https://doi.org/10.1038/s41467-024-46346-0
Download citation
Received : 30 October 2023
Accepted : 21 February 2024
Published : 26 March 2024
DOI : https://doi.org/10.1038/s41467-024-46346-0
Share this article
Anyone you share the following link with will be able to read this content:
Sorry, a shareable link is not currently available for this article.
Provided by the Springer Nature SharedIt content-sharing initiative
By submitting a comment you agree to abide by our Terms and Community Guidelines . If you find something abusive or that does not comply with our terms or guidelines please flag it as inappropriate.
Quick links
- Explore articles by subject
- Guide to authors
- Editorial policies
Sign up for the Nature Briefing: Translational Research newsletter — top stories in biotechnology, drug discovery and pharma.

Help | Advanced Search
Computer Science > Computer Vision and Pattern Recognition
Title: sv3d: novel multi-view synthesis and 3d generation from a single image using latent video diffusion.
Abstract: We present Stable Video 3D (SV3D) -- a latent video diffusion model for high-resolution, image-to-multi-view generation of orbital videos around a 3D object. Recent work on 3D generation propose techniques to adapt 2D generative models for novel view synthesis (NVS) and 3D optimization. However, these methods have several disadvantages due to either limited views or inconsistent NVS, thereby affecting the performance of 3D object generation. In this work, we propose SV3D that adapts image-to-video diffusion model for novel multi-view synthesis and 3D generation, thereby leveraging the generalization and multi-view consistency of the video models, while further adding explicit camera control for NVS. We also propose improved 3D optimization techniques to use SV3D and its NVS outputs for image-to-3D generation. Extensive experimental results on multiple datasets with 2D and 3D metrics as well as user study demonstrate SV3D's state-of-the-art performance on NVS as well as 3D reconstruction compared to prior works.
Submission history
Access paper:.
- Other Formats

References & Citations
- Google Scholar
- Semantic Scholar
BibTeX formatted citation

Bibliographic and Citation Tools
Code, data and media associated with this article, recommenders and search tools.
- Institution
arXivLabs: experimental projects with community collaborators
arXivLabs is a framework that allows collaborators to develop and share new arXiv features directly on our website.
Both individuals and organizations that work with arXivLabs have embraced and accepted our values of openness, community, excellence, and user data privacy. arXiv is committed to these values and only works with partners that adhere to them.
Have an idea for a project that will add value for arXiv's community? Learn more about arXivLabs .
- Share full article
Advertisement
Supported by
More Studies by Columbia Cancer Researchers Are Retracted
The studies, pulled because of copied data, illustrate the sluggishness of scientific publishers to address serious errors, experts said.

By Benjamin Mueller
Scientists in a prominent cancer lab at Columbia University have now had four studies retracted and a stern note added to a fifth accusing it of “severe abuse of the scientific publishing system,” the latest fallout from research misconduct allegations recently leveled against several leading cancer scientists.
A scientific sleuth in Britain last year uncovered discrepancies in data published by the Columbia lab, including the reuse of photos and other images across different papers. The New York Times reported last month that a medical journal in 2022 had quietly taken down a stomach cancer study by the researchers after an internal inquiry by the journal found ethics violations.
Despite that study’s removal, the researchers — Dr. Sam Yoon, chief of a cancer surgery division at Columbia University’s medical center, and Changhwan Yoon, a more junior biologist there — continued publishing studies with suspicious data. Since 2008, the two scientists have collaborated with other researchers on 26 articles that the sleuth, Sholto David, publicly flagged for misrepresenting experiments’ results.
One of those articles was retracted last month after The Times asked publishers about the allegations. In recent weeks, medical journals have retracted three additional studies, which described new strategies for treating cancers of the stomach, head and neck. Other labs had cited the articles in roughly 90 papers.
A major scientific publisher also appended a blunt note to the article that it had originally taken down without explanation in 2022. “This reuse (and in part, misrepresentation) of data without appropriate attribution represents a severe abuse of the scientific publishing system,” it said .
Still, those measures addressed only a small fraction of the lab’s suspect papers. Experts said the episode illustrated not only the extent of unreliable research by top labs, but also the tendency of scientific publishers to respond slowly, if at all, to significant problems once they are detected. As a result, other labs keep relying on questionable work as they pour federal research money into studies, allowing errors to accumulate in the scientific record.
“For every one paper that is retracted, there are probably 10 that should be,” said Dr. Ivan Oransky, co-founder of Retraction Watch, which keeps a database of 47,000-plus retracted studies. “Journals are not particularly interested in correcting the record.”
Columbia’s medical center declined to comment on allegations facing Dr. Yoon’s lab. It said the two scientists remained at Columbia and the hospital “is fully committed to upholding the highest standards of ethics and to rigorously maintaining the integrity of our research.”
The lab’s web page was recently taken offline. Columbia declined to say why. Neither Dr. Yoon nor Changhwan Yoon could be reached for comment. (They are not related.)
Memorial Sloan Kettering Cancer Center, where the scientists worked when much of the research was done, is investigating their work.
The Columbia scientists’ retractions come amid growing attention to the suspicious data that undergirds some medical research. Since late February, medical journals have retracted seven papers by scientists at Harvard’s Dana-Farber Cancer Institute . That followed investigations into data problems publicized by Dr. David , an independent molecular biologist who looks for irregularities in published images of cells, tumors and mice, sometimes with help from A.I. software.
The spate of misconduct allegations has drawn attention to the pressures on academic scientists — even those, like Dr. Yoon, who also work as doctors — to produce heaps of research.
Strong images of experiments’ results are often needed for those studies. Publishing them helps scientists win prestigious academic appointments and attract federal research grants that can pay dividends for themselves and their universities.
Dr. Yoon, a robotic surgery specialist noted for his treatment of stomach cancers, has helped bring in nearly $5 million in federal research money over his career.
The latest retractions from his lab included articles from 2020 and 2021 that Dr. David said contained glaring irregularities . Their results appeared to include identical images of tumor-stricken mice, despite those mice supposedly having been subjected to different experiments involving separate treatments and types of cancer cells.
The medical journal Cell Death & Disease retracted two of the latest studies, and Oncogene retracted the third. The journals found that the studies had also reused other images, like identical pictures of constellations of cancer cells.
The studies Dr. David flagged as containing image problems were largely overseen by the more senior Dr. Yoon. Changhwan Yoon, an associate research scientist who has worked alongside Dr. Yoon for a decade, was often a first author, which generally designates the scientist who ran the bulk of the experiments.
Kun Huang, a scientist in China who oversaw one of the recently retracted studies, a 2020 paper that did not include the more senior Dr. Yoon, attributed that study’s problematic sections to Changhwan Yoon. Dr. Huang, who made those comments this month on PubPeer, a website where scientists post about studies, did not respond to an email seeking comment.
But the more senior Dr. Yoon has long been made aware of problems in research he published alongside Changhwan Yoon: The two scientists were notified of the removal in January 2022 of their stomach cancer study that was found to have violated ethics guidelines.
Research misconduct is often pinned on the more junior researchers who conduct experiments. Other scientists, though, assign greater responsibility to the senior researchers who run labs and oversee studies, even as they juggle jobs as doctors or administrators.
“The research world’s coming to realize that with great power comes great responsibility and, in fact, you are responsible not just for what one of your direct reports in the lab has done, but for the environment you create,” Dr. Oransky said.
In their latest public retraction notices, medical journals said that they had lost faith in the results and conclusions. Imaging experts said some irregularities identified by Dr. David bore signs of deliberate manipulation, like flipped or rotated images, while others could have been sloppy copy-and-paste errors.
The little-noticed removal by a journal of the stomach cancer study in January 2022 highlighted some scientific publishers’ policy of not disclosing the reasons for withdrawing papers as long as they have not yet formally appeared in print. That study had appeared only online.
Roland Herzog, the editor of the journal Molecular Therapy, said that editors had drafted an explanation that they intended to publish at the time of the article’s removal. But Elsevier, the journal’s parent publisher, advised them that such a note was unnecessary, he said.
Only after the Times article last month did Elsevier agree to explain the article’s removal publicly with the stern note. In an editorial this week , the Molecular Therapy editors said that in the future, they would explain the removal of any articles that had been published only online.
But Elsevier said in a statement that it did not consider online articles “to be the final published articles of record.” As a result, company policy continues to advise that such articles be removed without an explanation when they are found to contain problems. The company said it allowed editors to provide additional information where needed.
Elsevier, which publishes nearly 3,000 journals and generates billions of dollars in annual revenue , has long been criticized for its opaque removals of online articles.
Articles by the Columbia scientists with data discrepancies that remain unaddressed were largely distributed by three major publishers: Elsevier, Springer Nature and the American Association for Cancer Research. Dr. David alerted many journals to the data discrepancies in October.
Each publisher said it was investigating the concerns. Springer Nature said investigations take time because they can involve consulting experts, waiting for author responses and analyzing raw data.
Dr. David has also raised concerns about studies published independently by scientists who collaborated with the Columbia researchers on some of their recently retracted papers. For example, Sandra Ryeom, an associate professor of surgical sciences at Columbia, published an article in 2003 while at Harvard that Dr. David said contained a duplicated image . As of 2021, she was married to the more senior Dr. Yoon, according to a mortgage document from that year.
A medical journal appended a formal notice to the article last week saying “appropriate editorial action will be taken” once data concerns had been resolved. Dr. Ryeom said in a statement that she was working with the paper’s senior author on “correcting the error.”
Columbia has sought to reinforce the importance of sound research practices. Hours after the Times article appeared last month, Dr. Michael Shelanski, the medical school’s senior vice dean for research, sent an email to faculty members titled “Research Fraud Accusations — How to Protect Yourself.” It warned that such allegations, whatever their merits, could take a toll on the university.
“In the months that it can take to investigate an allegation,” Dr. Shelanski wrote, “funding can be suspended, and donors can feel that their trust has been betrayed.”
Benjamin Mueller reports on health and medicine. He was previously a U.K. correspondent in London and a police reporter in New York. More about Benjamin Mueller
- Search for: Toggle Search
‘You Transformed the World,’ NVIDIA CEO Tells Researchers Behind Landmark AI Paper
Of GTC ’s 900+ sessions, the most wildly popular was a conversation hosted by NVIDIA founder and CEO Jensen Huang with seven of the authors of the legendary research paper that introduced the aptly named transformer — a neural network architecture that went on to change the deep learning landscape and enable today’s era of generative AI.
“Everything that we’re enjoying today can be traced back to that moment,” Huang said to a packed room with hundreds of attendees, who heard him speak with the authors of “ Attention Is All You Need .”
Sharing the stage for the first time, the research luminaries reflected on the factors that led to their original paper, which has been cited more than 100,000 times since it was first published and presented at the NeurIPS AI conference. They also discussed their latest projects and offered insights into future directions for the field of generative AI.
While they started as Google researchers, the collaborators are now spread across the industry, most as founders of their own AI companies.
“We have a whole industry that is grateful for the work that you guys did,” Huang said.
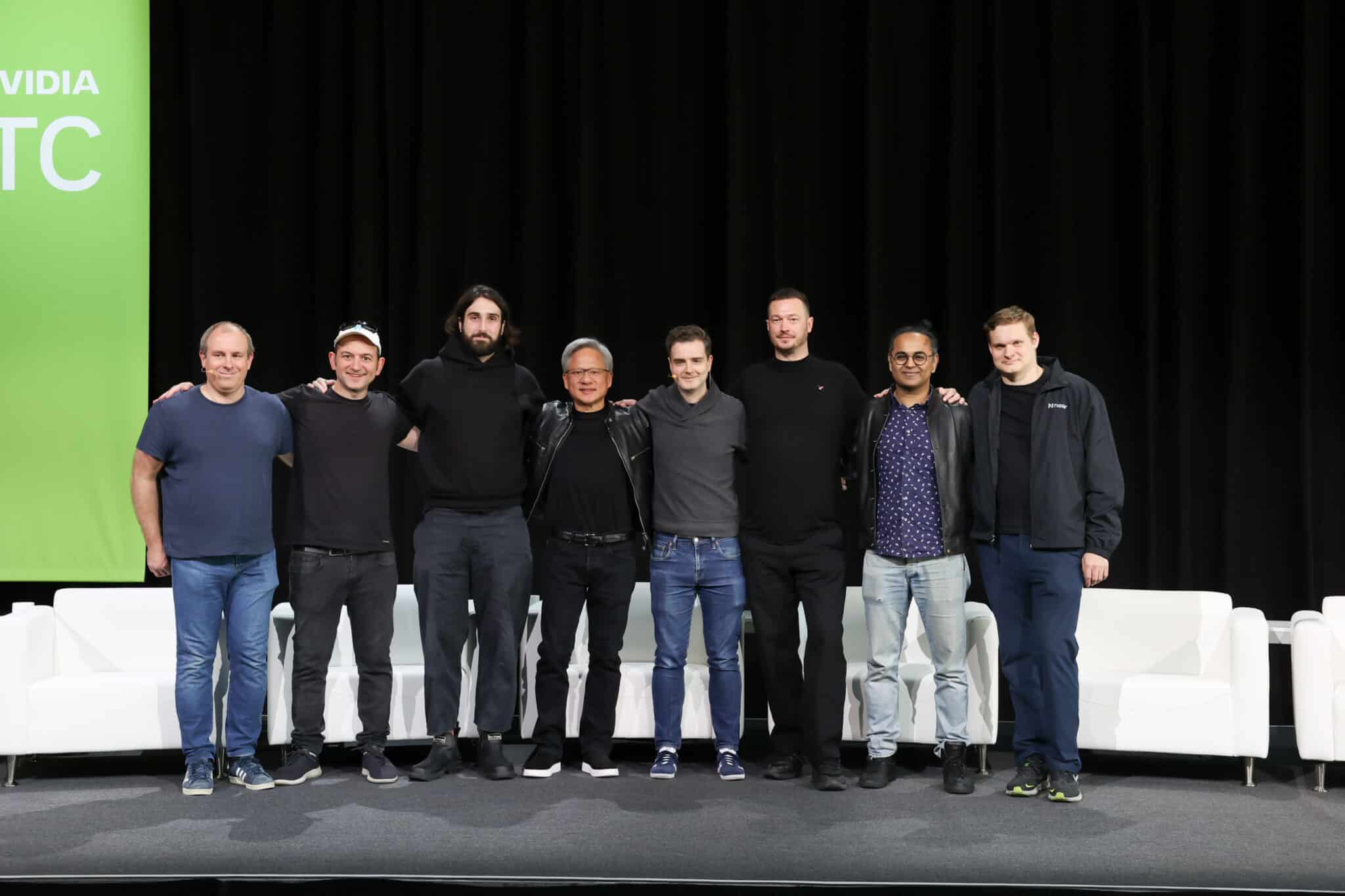
Origins of the Transformer Model
The research team initially sought to overcome the limitations of recurrent neural networks , or RNNs, which were then the state of the art for processing language data.
Noam Shazeer, cofounder and CEO of Character.AI, compared RNNs to the steam engine and transformers to the improved efficiency of internal combustion.
“We could have done the industrial revolution on the steam engine, but it would just have been a pain,” he said. “Things went way, way better with internal combustion.”
“Now we’re just waiting for the fusion,” quipped Illia Polosukhin, cofounder of blockchain company NEAR Protocol.
The paper’s title came from a realization that attention mechanisms — an element of neural networks that enable them to determine the relationship between different parts of input data — were the most critical component of their model’s performance.
“We had very recently started throwing bits of the model away, just to see how much worse it would get. And to our surprise it started getting better,” said Llion Jones, cofounder and chief technology officer at Sakana AI.
Having a name as general as “transformers” spoke to the team’s ambitions to build AI models that could process and transform every data type — including text, images, audio, tensors and biological data.
“That North Star, it was there on day zero, and so it’s been really exciting and gratifying to watch that come to fruition,” said Aidan Gomez, cofounder and CEO of Cohere. “We’re actually seeing it happen now.”
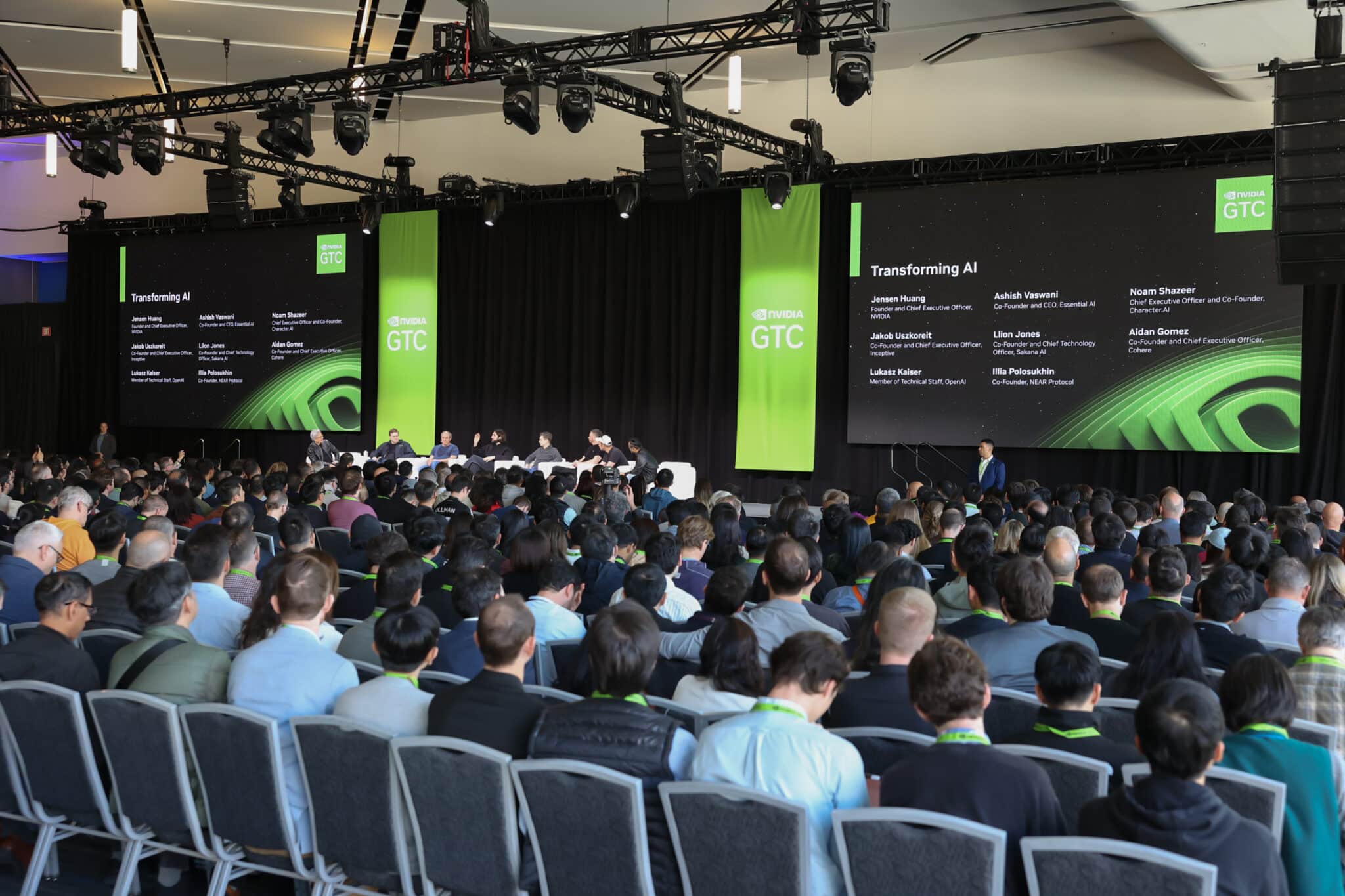
Envisioning the Road Ahead
Adaptive computation, where a model adjusts how much computing power is used based on the complexity of a given problem, is a key factor the researchers see improving in future AI models.
“It’s really about spending the right amount of effort and ultimately energy on a given problem,” said Jakob Uszkoreit, cofounder and CEO of biological software company Inceptive. “You don’t want to spend too much on a problem that’s easy or too little on a problem that’s hard.”
A math problem like two plus two, for example, shouldn’t be run through a trillion-parameter transformer model — it should run on a basic calculator, the group agreed.
They’re also looking forward to the next generation of AI models.
“I think the world needs something better than the transformer,” said Gomez. “I think all of us here hope it gets succeeded by something that will carry us to a new plateau of performance.”
“You don’t want to miss these next 10 years,” Huang said. “Unbelievable new capabilities will be invented.”
The conversation concluded with Huang presenting each researcher with a framed cover plate of the NVIDIA DGX-1 AI supercomputer, signed with the message, “You transformed the world.”
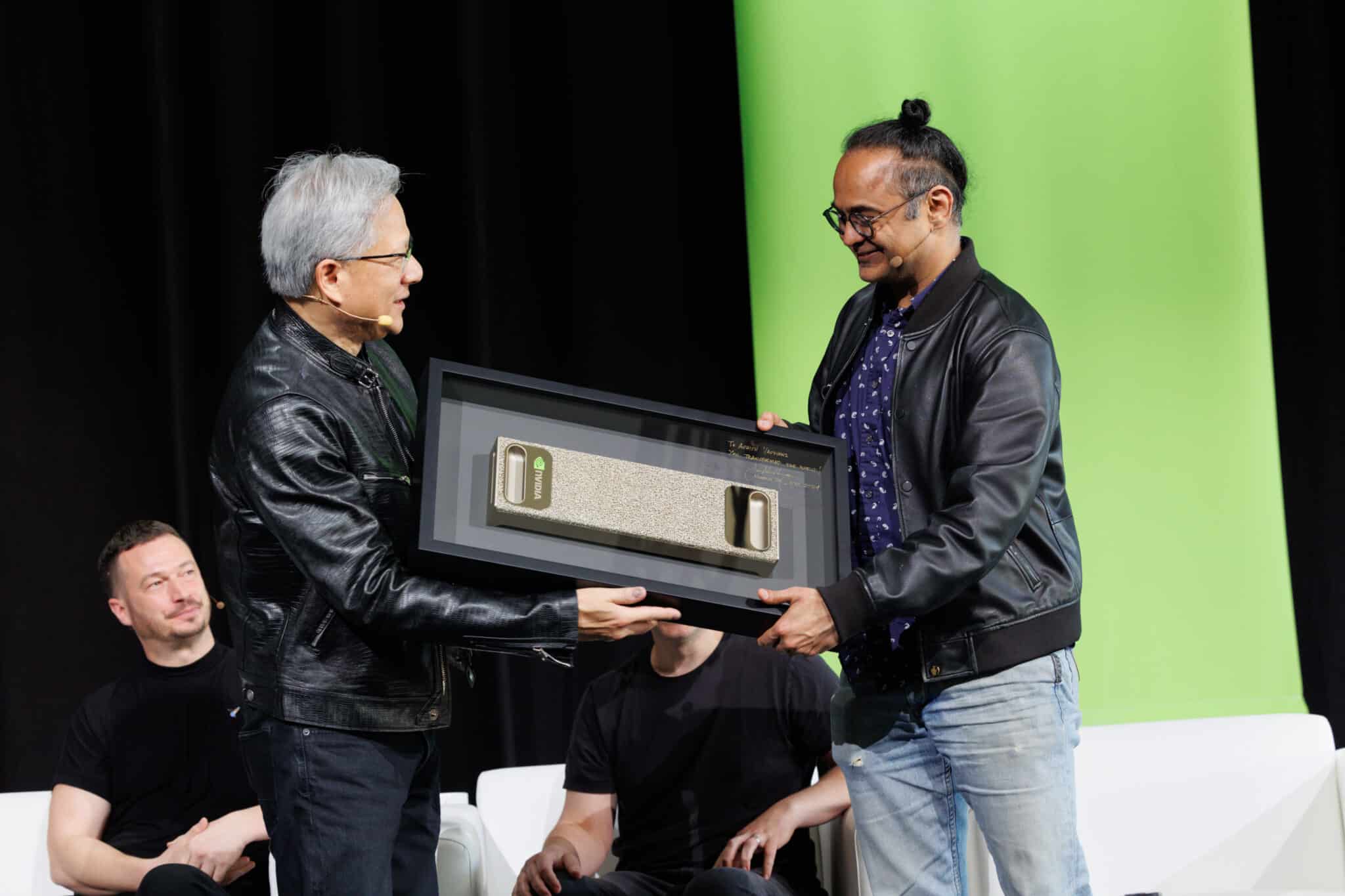
There’s still time to catch the session replay by registering for a virtual GTC pass — it’s free.
To discover the latest in generative AI, watch Huang’s GTC keynote address:
NVIDIA websites use cookies to deliver and improve the website experience. See our cookie policy for further details on how we use cookies and how to change your cookie settings.
Share on Mastodon
Paper Editing Services
Maximize your paper’s potential with clear and effective writing.
Expert paper editors polish your writing to reflect the work you put into it. Professional paper editing will:
- Ensure your arguments are judged on merit
- Lift the quality of your paper as a whole
- Make you stand out from your peers
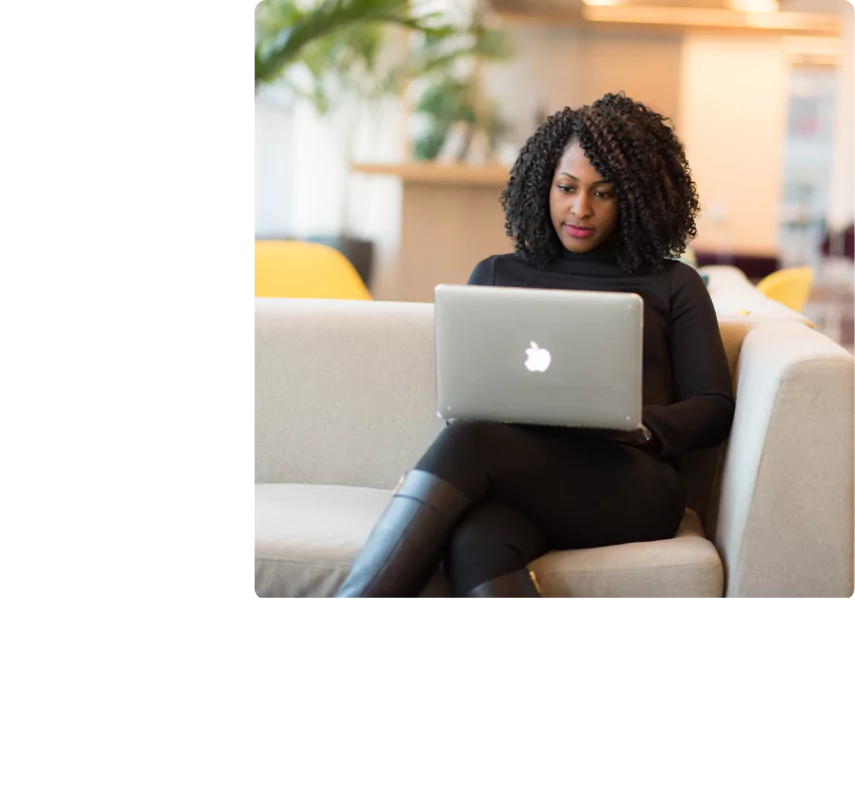
Proofreading & Editing
Personalized editing with human expertise, revisions within 12 hours, 100% happiness or a refund or re-edit.
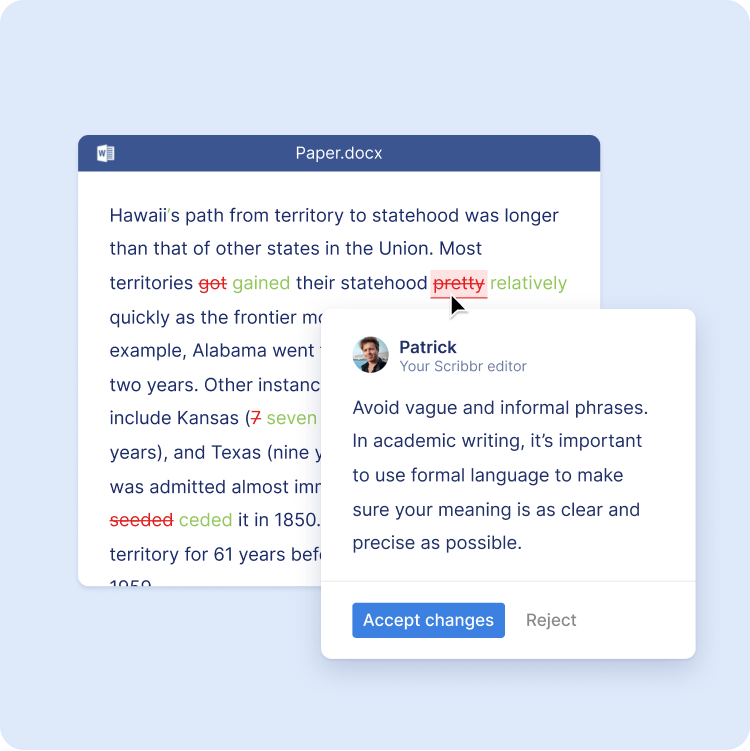
Get your paper back, free of language errors and inconsistencies
Standard paper Proofreading & Editing is perfect if you’re confident about your writing but need a second pair of eyes to catch:
- Spelling and grammar errors
- Inconsistencies in dialect
- Overuse of passive voice
- Subjective or inflated language
For a more comprehensive edit, you can add one or multiple add-on editing services that fit your needs.
Add-on services
Customize your editing package to get the help you need, structure check, clarity check, paper formatting, citation editing.
Ensures sections and chapters are structured and focused and your writing is free of redundancies.
- Through in-text feedback, your editor will help:
- Organize and focus individual chapters and sections
- Eliminate repetitive and redundant information
- Perfect transitions between sentences and paragraphs
- Align titles and headings with the section’s content
You’ll also receive a personalized Structure Check Report meant to help you identify missing elements in each chapter or section and prioritize improvements.
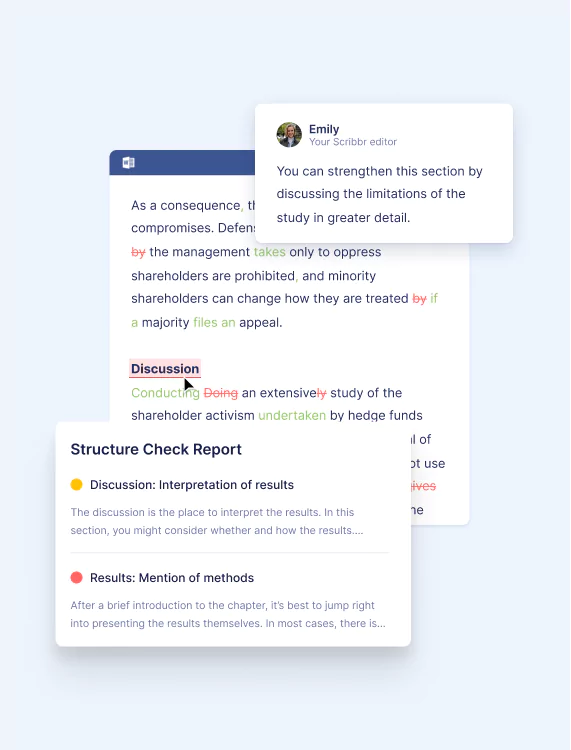
Ensures ideas are presented clearly, your arguments are consistent, and your audience can follow along.
Through in-text comments and checklists, your editor will:
- Make sure your text tells a clear and logical story
- Check that you’ve clearly presented concepts, ideas, and key terms
- Make sure your key takeaways and conclusions are front and center
- Highlight contradictions within the text
- Ensure you’re keeping your audience’s needs in mind
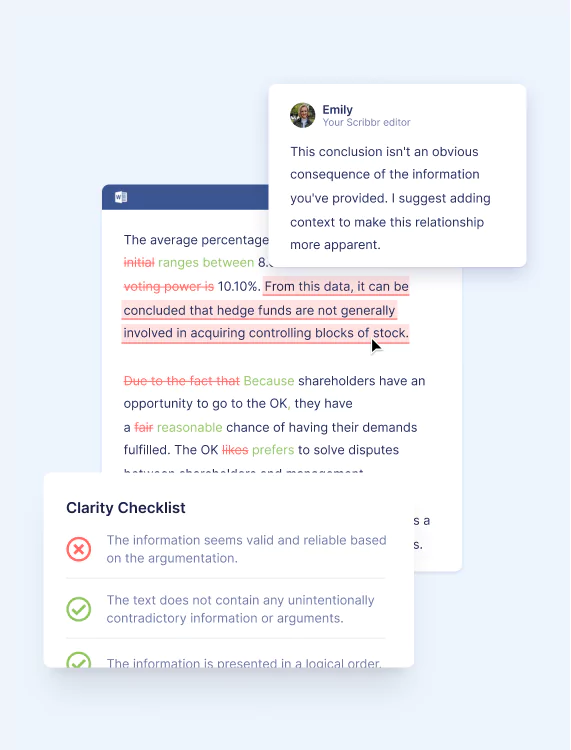
Ensures a professional look and feel of your document that meets your formatting requirements.
Your formatting expert will apply the 7th edition APA Style guidelines to all elements in your paper, including:
- Margins, spacing, and indentation
- Body text and headings
- Page numbers
- Abstract and keywords
- Explanatory footnotes
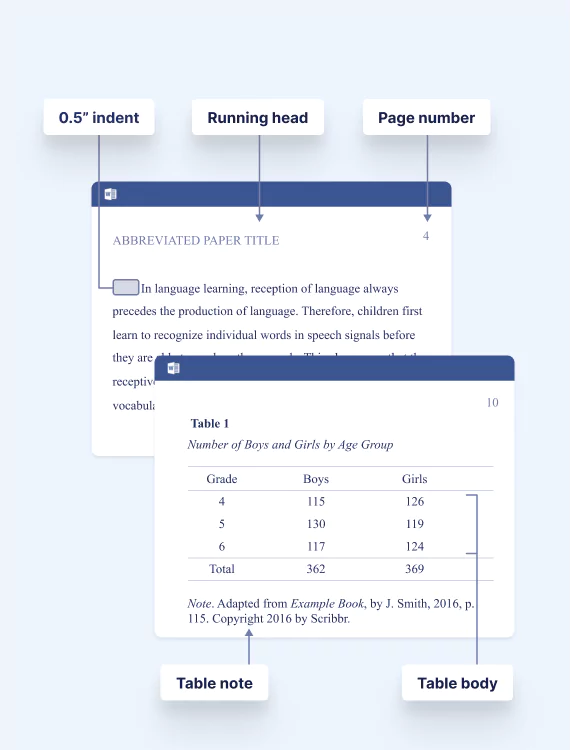
Citation Editing ensures your citations and references are consistent and meet your style guide’s requirements.
After you provide your document with a reference list, your citation expert will:
- Format the layout of your reference page (margins, indents, spacing)
- Ensure that your chosen citation style is applied consistently according to the guidelines
- Cross-check citations with reference entries
- Provide feedback on reference list entries that you need to complete due to missing information
Your expert is familiar with all common citation styles. Find more information about the service and our requirements in our FAQs .
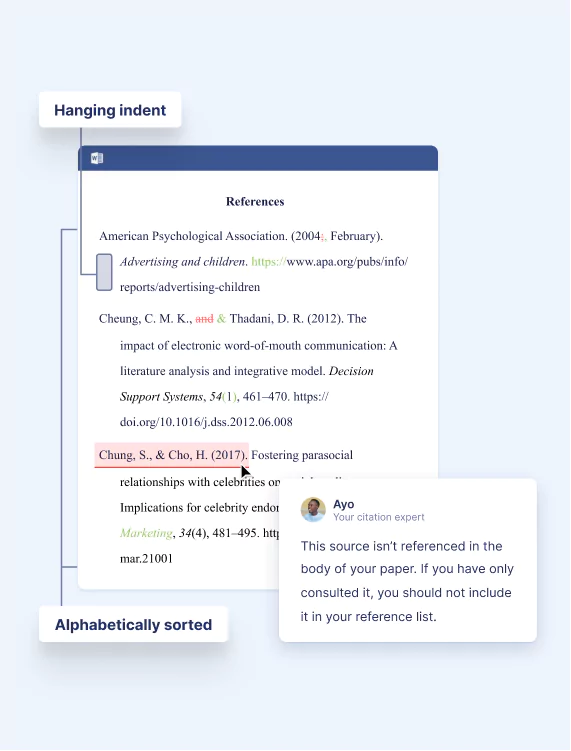
Get matched to the perfect editor - or editing team
At Scribbr, you can rest assured that only the best editors will work on your paper.
All our 800+ editors have passed the challenging Scribbr Academy, which has a passing rate of only 2%.
We handpick your editor on several criteria, including field of study and document type. And we’ll even expand your team with citation and formatting experts if needed.
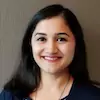
I have a doctorate in biology and studied a range of life science subjects. I specialize in editing academic texts.
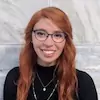
I researched at Harvard, taught English with a Fulbright in Peru, and earned a master's from Johns Hopkins.
I have a PhD in German studies, an MS in library science, and extensive experience teaching undergraduate students.
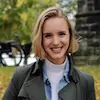
I have a bachelor's in electrical engineering and a master's in psychology and am pursuing a PhD in neuroscience.
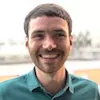
I am an ESL teacher and academic editor with a research background in the humanities, arts, and culture.
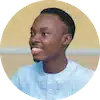
I am an academic editor and book reviewer. I am familiar with many style guides and have edited over 6 million words.
Maximize your paper's potential with expert editing
Select your currency
“Hoped for the best and got it!”
“I needed to have a paper proofread that was going to be published. Because English is not my native language, I was unsure about the text’s linguistic quality. I’m all the more grateful that B. & K. from Scribbr took care of my request very professionally, thoughtfully, and above all, quickly. It couldn’t have gone better!”
How it works
Stay in control throughout the paper editing process, upload any time.
Upload your document and easily select the pages that need editing. Next, choose your turnaround time and services and explain your situation and needs to the editor.
Stay in the loop
After placing your order you can keep track of our progress. From finding your perfect editor to potential hand-overs to formatting or citation experts.
Revise and submit
You’ll receive back your document with tracked changes and feedback as well as a personal letter from your editor. The last step is submitting your work with confidence!
Scribbr & academic integrity
Scribbr is committed to protecting academic integrity. Our proofreading service, our AI writing tools ( plagiarism checker , paraphrasing tool , grammar checker , summarizer, Citation Generator ) as well as our free Knowledge Base content are designed to help students produce quality academic papers.
We make every effort to prevent our software from being used for fraudulent or manipulative purposes.
Your questions, answered.
At Scribbr, we promise to make every customer 100% happy with the service we offer. Our philosophy: Your complaint is always justified – no denial, no doubts.
Our customer support team is here to find the solution that helps you the most, whether that’s a free new edit or a refund for the service.
The fastest turnaround time is 12 hours.
You can upload your document at any time and choose between three deadlines:
Yes, in the order process you can indicate your preference for American, British, or Australian English .
If you don’t choose one, your editor will follow the style of English you currently use. If your editor has any questions about this, we will contact you.
Yes, regardless of the deadline you choose, our editors can proofread your document during weekends and holidays.
Example: If you select the 12-hour service on Saturday, you will receive your edited document back within 12 hours on Sunday.
Your editor is on stand-by and ready to start editing your paper.
Get in touch, with real people.
We answer your questions quickly and personally from 9:00 to 23:00 CET
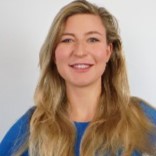
- Start live chat
- Email [email protected]
- Call +1 (510) 822-8066
- WhatsApp +31 20 261 6040
Knowledge Base
Finishing your paper with scribbr’s top-rated guides.
Research paper
Writing a Research Paper Conclusion
How to write academic paragraphs.
Research process
Developing Strong Research Questions
Methodology
How to Write a Literature Review
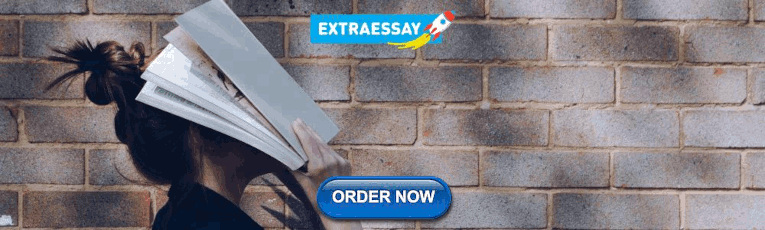
IMAGES
VIDEO
COMMENTS
USC Writing Center. S hould you need help proofreading your paper, take advantage of the assistance offered by consultants at the USC Writing Center located on the second floor of Taper Hall, room 216. Consultations are free and they can help you with any aspect of the writing process. Walk-in help is provided when consultants are available, but you should schedule an appointment online ...
The online proofreader. It's really straightforward. Just paste the text into the tool. All your errors will now be underlined in red. You can hover over these mistakes to see how they can be addressed. If you agree, just click on the button "Fix all errors," and your mistakes will be fixed instantly!
Step 1: Content editing. Revising an early draft of a text, often making significant changes to the content and moving, adding or deleting entire sections (also known as developmental or substantive editing). Step 2: Line editing. Revising the use of language to communicate your story, ideas, or arguments as effectively as possible.
The main purpose of proofreading is to improve the quality of the paper, ensuring there are no lingering mistakes, and correcting generalized discourse errors or writing inconsistencies. Essentially, you want to make sure you have a well-defined communication goal. Analyzing whether the content is properly conveyed, and the sentences are ...
Research PaperEditing Services. When you submit a research paper for proofreading, our editors will: And to ensure you are in full control of the final draft, we also provide two copies of your edited paper when we're done: We use this 'Track Changes' copy to highlight all edits in the document, so you can review our work and make further ...
Proofreading/editing research papers comes with a number of additional considerations. These are documents with a particular structure and register, and writing for academia involves adhering to various conventions. If you don't have a background in writing/editing research papers and are unfamiliar with these conventions, take some time to ...
Overuse of passive voice. Subjective or inflated language. For a more comprehensive edit, you can add one or multiple add-on editing services that fit your needs. ⏰ Deadline. Within 12 hours. 📄 Texts. Papers, essays, reports, manuscripts. ⭐️ Rating. 4.6 based on 3,549 reviews.
Introduction. Proofreading involves reading your document to correct the smaller typographical, grammatical, and spelling errors. Proofreading is usually the very last step you take before sending off the final draft of your work for evaluation or publication. It comes after you have addressed larger matters such as style, content, citations ...
How much does research paper proofreading cost? The average cost of proofreading a research paper is $39-$69 per 1,000 words. However, the rates for rush jobs, niche topics, and complex formatting styles are significantly higher than those for simpler topics with a slower turnaround time.
Proofreading is the act of searching for errors before you hand in the your research paper. Errors can be both grammatical and typographical in nature, but can also include identifying problems with the narrative flow of your paper [i.e., the logical sequence of thoughts and ideas], problems with concise writing [i.e., wordiness], and finding any word processing errors [e.g., different font ...
Proofreading is the act of searching for errors before you hand in the your final research paper. Errors can be both grammatical and typographical in nature, but proofreading can also be used to identify problems with the flow of your paper [i.e., the logical sequence of thoughts and ideas] and to find any word processing errors [e.g., different font types, indented paragraphs, line spacing ...
Scientific Editing and Proofreading. What is a scientific research paper? Researchers in various fields of science report their findings, analyses, and deductions in the form of a scientific research paper. Scientists face tough competition to publish in their respective fields. Publishing in a reputed peer-reviewed journal is essential for ...
Academic Editing Speed. In order to gauge how long it will take to get your academic paper edited or proofread, keep in mind the industry standard for academic editing: 1,000 words per hour. This means a 30,000-word research paper should take roughly 30 hours to edit completely.
AcademicProofreading And Editing. Whether you're writing a thesis, research paper, or PhD proposal, Proofed's academic proofreading and editing services will help ensure your writing reads smoothly and your ideas are communicated effectively. Try For Free.
Proofreading is the process of carefully reviewing and correcting errors in grammar, spelling, punctuation, formatting, and other aspects of writing to ensure that the final product is error-free and polished. In the context of research papers, proofreading is essential because it ensures that your paper is clear, concise, and easy to read.
While proofreading in academic writing can be challenging, there are some proofreading tips and techniques that can help you: Pay attention to detail: This is perhaps the most important skill to master as you begin to proofread your research paper. It is important to keep distractions aside and concentrate only on proofreading, so that you are ...
Improve your paper with our award-winning Proofreading Services, Plagiarism Checker, Citation Generator, AI Detector & Knowledge Base. Proofreading & Editing Get expert help from Scribbr's academic editors, who will proofread and edit your essay, paper, or dissertation to perfection.
PaperRater is an AI-powered essay writing and proofreading tool designed for students. It provides feedback on grammar, spelling, style, and plagiarism, along with a comprehensive score and suggestions for improvement. PaperRater can be a valuable tool for students to enhance their academic writing skills. Benefits of AI Proofreading in Research
We aid you in emphasizing the research gap using compelling academic language without ambiguities, redundancies, or logical errors. Avail PaperTrue's online paper editing services to submit a refined research paper. Get an error-free paper with our research paper proofreading service.
Free proofreading tool. With QuillBot, you have the best free proofreading tool in your pocket, on your browser, or in Word whenever you need it. Writing is a lot of work, and doing it well means revising and revising again. For writers with high standards in every genre and field, online proofreading is an invaluable resource—a must-have.
IBM has created a quantum error-correcting code about 10 times more efficient than prior methods — a milestone in quantum computing research. Today, the paper detailing those results was published as the cover story of the scientific journal Nature. 1. Last year, we demonstrated that quantum computers had entered the era of utility, where ...
The beer was poured through two filter papers (Macherey-Nagel, 500713032 MN 713 ¼) to remove carbon dioxide and prevent spontaneous foaming. ... for her tremendous help in proofreading and ...
We present Stable Video 3D (SV3D) -- a latent video diffusion model for high-resolution, image-to-multi-view generation of orbital videos around a 3D object. Recent work on 3D generation propose techniques to adapt 2D generative models for novel view synthesis (NVS) and 3D optimization. However, these methods have several disadvantages due to either limited views or inconsistent NVS, thereby ...
Scribbr's proofreading services are transparent, fast, and straightforward. From uploading your paper to making the final revisions, we're there for you every step of the way. 1 Upload. 2 Our job. 3 Final touch.
Daniel Kahneman, the Eugene Higgins Professor of Psychology, Emeritus, professor of psychology and public affairs, emeritus, and a Nobel laureate in economics whose groundbreaking behavioral science research changed our understanding of how people think and make decisions, died on March 27. He was 90. Kahneman joined the Princeton University faculty in 1993, following appointments at Hebrew ...
March 20, 2024. Scientists in a prominent cancer lab at Columbia University have now had four studies retracted and a stern note added to a fifth accusing it of "severe abuse of the scientific ...
Overuse of passive voice. Subjective or inflated language. For a more comprehensive edit, you can add one or multiple add-on editing services that fit your needs. ⏰ Deadline. Within 12 hours. 📄 Texts. Papers, essays, dissertations, manuscripts. ⭐️ Rating. 4.6 based on 3,549 reviews.
In this paper, my coauthors and I developed a method that allows researchers to quantify the potential effect of area-level interventions (e.g., adding a new healthy food store or physical activity facility to a ZIP code) on reducing the difference in health outcome (e.g., cancer incidence) between ZIP codes with certain characteristics.
March 21, 2024 by Isha Salian. Of GTC 's 900+ sessions, the most wildly popular was a conversation hosted by NVIDIA founder and CEO Jensen Huang with seven of the authors of the legendary research paper that introduced the aptly named transformer — a neural network architecture that went on to change the deep learning landscape and enable ...
Overuse of passive voice. Subjective or inflated language. For a more comprehensive edit, you can add one or multiple add-on editing services that fit your needs. ⏰ Deadline. Within 12 hours. 📄 Texts. Papers, essays, reports, manuscripts. ⭐️ Rating. 4.6 based on 3,549 reviews.