Big Data in Finance
Big data is revolutionizing the finance industry and has the potential to significantly shape future research in finance. This special issue contains articles following the 2019 NBER/ RFS conference on big data. In this Introduction to the special issue, we define the “Big Data” phenomenon as a combination of three features: large size, high dimension, and complex structure. Using the articles in the special issue, we discuss how new research builds on these features to push the frontier on fundamental questions across areas in finance – including corporate finance, market microstructure, and asset pricing. Finally, we offer some thoughts for future research directions.
Ye acknowledges support from National Science Foundation grant 1838183 and the Extreme Science and Engineering Discovery Environment (XSEDE). The views expressed herein are those of the authors and do not necessarily reflect the views of the National Bureau of Economic Research.
MARC RIS BibTeΧ
Download Citation Data
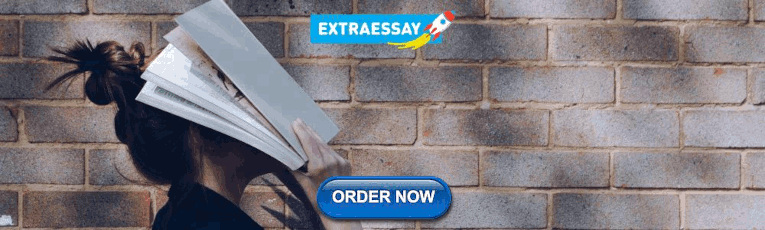
Published Versions
Big Data in Finance , Itay Goldstein, Chester S Spatt, Mao Ye . in Big Data: Long-Term Implications for Financial Markets and Firms , Goldstein, Spatt, and Ye. 2021
Itay Goldstein & Chester S Spatt & Mao Ye, 2021. " Big Data in Finance, " The Review of Financial Studies, vol 34(7), pages 3213-3225.
More from NBER
In addition to working papers , the NBER disseminates affiliates’ latest findings through a range of free periodicals — the NBER Reporter , the NBER Digest , the Bulletin on Retirement and Disability , the Bulletin on Health , and the Bulletin on Entrepreneurship — as well as online conference reports , video lectures , and interviews .
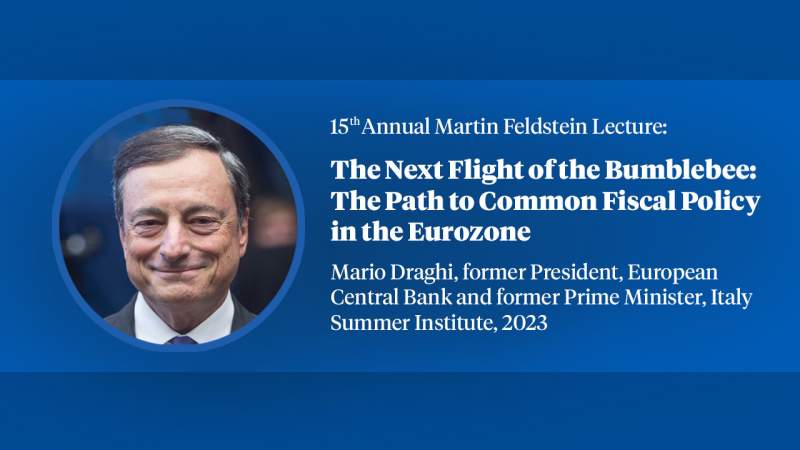
Big data optimisation and management in supply chain management: a systematic literature review
- Open access
- Published: 24 June 2023
- Volume 56 , pages 253–284, ( 2023 )
Cite this article
You have full access to this open access article
- Idrees Alsolbi ORCID: orcid.org/0000-0002-2957-402X 1 , 2 ,
- Fahimeh Hosseinnia Shavaki 2 ,
- Renu Agarwal 3 ,
- Gnana K Bharathy 4 ,
- Shiv Prakash 5 &
- Mukesh Prasad 2
5360 Accesses
2 Citations
Explore all metrics
This article has been updated
The increasing interest from technology enthusiasts and organisational practitioners in big data applications in the supply chain has encouraged us to review recent research development. This paper proposes a systematic literature review to explore the available peer-reviewed literature on how big data is widely optimised and managed within the supply chain management context. Although big data applications in supply chain management appear to be often studied and reported in the literature, different angles of big data optimisation and management technologies in the supply chain are not clearly identified. This paper adopts the explanatory literature review involving bibliometric analysis as the primary research method to answer two research questions, namely: (1) How to optimise big data in supply chain management? and (2) What tools are most used to manage big data in supply chain management? A total of thirty-seven related papers are reviewed to answer the two research questions using the content analysis method. The paper also reveals some research gaps that lead to prospective future research directions.
Similar content being viewed by others
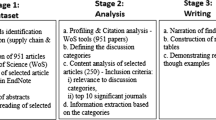
Supply chain disruptions and resilience: a major review and future research agenda
K. Katsaliaki, P. Galetsi & S. Kumar
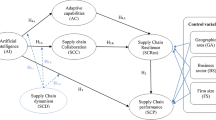
Artificial intelligence-driven innovation for enhancing supply chain resilience and performance under the effect of supply chain dynamism: an empirical investigation
Amine Belhadi, Venkatesh Mani, … Surabhi Verma
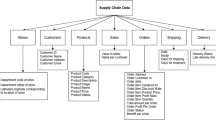
Predictive big data analytics for supply chain demand forecasting: methods, applications, and research opportunities
Mahya Seyedan & Fereshteh Mafakheri
Avoid common mistakes on your manuscript.
1 Introduction
The technologies continue to rapidly change to meet the 21st century corporate needs worldwide. With this growth, professionals from various organisations started to understand the values of applying big data concepts. One major value of applying big data analytics is to improve the process of making decisions on supply chain configurations (Gupta et al. 2019 ; Nguyen et al. 2018 ). Business entities are increasingly overwhelmed by the stream of endless data overflowing from an extensive range of channels. Companies that capitalise on such data and use it for decision-making end up gaining a competitive advantage, particularly streamlining their end-to-end supply chain functions(Govindan et al. 2018 ; Nguyen et al. 2018 ). Supply chain functions refer to procurement, manufacturing, warehousing, logistics and transportation, and demand management (Nguyen et al. 2018 ). For example, one value of applying big data in supply chain management (SCM) is to improve customers’ satisfaction by predicting orders, leading to reducing prices and control risks (Govindan et al. 2018 ).
The term “big data” can be defined as an increasingly growing volume of data from different sources with various structures that noticeably challenges industrial organisations on the one hand and presents them with a complex range of valuable storage and analysis capabilities on the other hand (Addo-Tenkorang and Helo 2016 ). Besides, big data comprises information collected from internet-enabled electronic devices such as tablets and smartphones, especially voice recordings, videos, and social media (Chen et al. 2015 ). “SCM” is generally defined as the management of relationship flow as well as the flow of material, information, and resources within and across a network, including upstream and downstream organisations (Rai 2019 ).
Big data is accelerating the evolution of supply chain design and management (Tao et al. 2018 ). Also, big data analytics can enhance capacity utilisation, enable companies to create new business models, improve customer experience, reduce fraud, improve supplier quality level, and expand end-to-end supply chain visibility facilitating prescriptive and predictive analytics (Zhan and Tan 2020 ). Top executives rely on big data analytics for decision-making to enhance visibility and provide more comprehensive insights on overall SCM. The large data sets with enhanced variability and variety are generated to enable analysts to identify any anomalies and patterns and make predictive insights to improve the SCM practice (Barbosa et al. 2018 ). Big data has enabled companies to adopt a responsive supply chain, especially understanding market trends and customer behaviours allowing them to predict demand in the market (Nguyen et al. 2018 ; Zhan and Tan 2020 ). Therefore, the effective utilisation of big data in supply chain operations leads to an increasing in the value of organisations by processing proper decisions (Govindan et al. 2018 ). The low uptake of big data analytics has been attributed to the firm’s minimal capacity to identify suitable data and data security threats.
In particular, the significant data optimisation foundation is to find an alternative means to achieve higher performance considering the cost-effective conditions. Also, optimisation means doing the best strategy related to a set of selected constraints, which include significant factors such as reliability, productivity, efficiency, longevity, strength, and utilization (Roy et al. 2018 ) introduced a survey that shows certain optimisation technologies and the associated useful applications for many organisations.
An example of using optimization in supply chain is found in Amazon, which has revolutionized the game by providing extremely fast delivery timeframes and alerts for projected delivery times and minute-by-minute tracking. Moreover, every step of UPS’s shipping process includes supply chain data analysis. As packages move through the supply chain, radars and sensors collect data. Big data tools then optimize the delivery routes to ensure that packages reach on time. Overall, UPS has saved 1.6 million gallons of gasoline in its trucks each year, greatly lowering delivery costs (Parmar 2021 ).
Therefore, some of the studies have proven the positive impacts of using big data on supply chain performance through case studies. In this regard, Nita ( 2015 ) by applying heterogeneous mixture learning to big data showed that systematization and accuracy increase in demand forecasting can decrease the distribution costs and disposal losses in the food supply chain. Papanagnou and Matthews-Amune ( 2018 ) through the use of the multivariate time series analysis technique (VARX) concluded that customer-generated content from various internet sources such as Google, YouTube, and newspapers can improve demand forecasting accuracy in the short term and response to demand volatility in retail pharmacy settings. Zhong et al. ( 2015 ) proposed an RFID-enabled approach to extract logistics data. Through case studies, they showed the feasibility and practicality of the proposed approach which can reveal rich knowledge for further advanced decision-makings like logistics planning, production planning and scheduling, and enterprise-oriented strategies. Lee et al. ( 2018 ) applied data mining and optimization methods to real-life cases from a liner shipping company considering weather data to optimize vessel speed, fuel cost, and service level. They demonstrated that this approach provides better fuel consumption estimates compared to the benchmark method which ignores the weather impact.
To help managers make better use of the available big data and obtain competitive advantages, a big data infrastructure is required. Managers require strategies to organise and combine diverse streams of data to build a coherent picture of a given situation, rather than simply generating enormous amounts of data using existing software (Tan et al. 2015 ). In addition, scientific methodologies are now necessary for storing, analysing, and displaying big data so that businesses may make benefit from the information assets that are available to them (Grover and Kar 2017 ; Roy et al. 2018 ) highlights some aspects of data management techniques that have been applied in SCM. For example, using Hadoop as a platform to manage massive data has been mentioned in this survey. However, we assume that some beneficial techniques can still manage and control big data aside from Hadoop.
As a result, big data management and big data optimisation are two essential pillars of using big data in SCM. Big data management refers to the infrastructures and frameworks to process and manage data as well as data storing tools and techniques. On the other hand, big data optimisation addresses the methods, techniques, and tools that can be applied to big data to make data-driven decisions leading to improvement in the performance of the supply chain. Moreover, it determines how and in what extent, big data can help a supply chain take advantage of the huge amount of its gathered data and transform it into valuable knowledge. Understanding how big data can be optimised and managed will reduce firms’ operational costs, given the improved track-and-trace capabilities and lower forecast errors, which help avoid lost sales and increase companies’ profit.
Therefore, understanding how big data can be optimised and managed can help reduce firms’ operational costs, given the improved track-and-trace capabilities and lower forecast errors, which help avoid lost sales. In particular, the significant data optimisation foundation is to find an alternative means to achieve higher performance considering the cost-effective conditions. Also, optimisation means doing the best strategy related to a set of selected constraints, which include significant factors such as reliability, productivity, efficiency, longevity, strength, and utilization (Roy et al. 2018 ) introduced a survey that shows certain optimisation technologies and the associated applications applicable in many organisations. It highlights some aspects of data management techniques that have been applied in SCM. For example, using Hadoop as a platform to manage massive data has been mentioned (Roy et al. 2018 ). However, we assume that some beneficial techniques can still manage and control big data aside from Hadoop.
Big data applications in SCM appear to be discussed and reported in the literature often; however, this topic has different angles which are not well defined. There are some fragmentation and missing coverage in the literature due to some reasons: (1) the supply chain scope has a major evolution over the last two decades from being synonymous with logistics (Cooper et al. 1997 ) to be a melting pot of primary and supporting activities, spanning everything from purchasing to operations management, logistics and transportation, distribution and retail, relationship management, and information technology (Giunipero et al. 2008 ); (2) different disciplines have handled SCM often, using various nomenclature, preventing the development of a unified body of knowledge (Maestrini et al. 2017 ); and (3) optimisation techniques and identifying the capabilities of big data technologies have rarely been studied in their entirety, but rather in parts and pieces, such as comprehensive classifying big data analytics in SCM in (Nguyen et al. 2018 ) or broadly discussing the technologies of managing the massive data (Gupta et al. 2019 ). The coverage of these topics often considers only one portion of its value in the supply chain, without in-depth discussion on the processes involved, the obstacles, and providing examples from the industry. Despite this, the academic literature lacks a complete summary of the issue.
Therefore, we conducted a systematic literature review that (1) investigates the tools and techniques of optimising big data in SCM, (2) provides comprehensive coverage of the applied big data management solutions in industries, and (3) summarises the potential solutions that may address the research gaps. The current systematic literature review provides an analysis of the literature contents, which is able to introduce a full picture of how big data is optimised and managed in SCM. To achieve the goals, this systematic literature review answers the following questions:
How to optimise big data in SCM?
What tools are most used to manage big data in SCM?
These two research questions have been addressed using the bibliometric analysis and content analysis methods. While the methodology reported in the review of (Nguyen et al. 2018 ), has provided robust steps to conduct the systematic review, they did not use bibliometric analysis that can assist in discovering the urgent and published areas of research. Instead, they used descriptive analysis to use a set of analytical and structural groups of a classification framework. (Gupta et al. 2019 ; Tiwari et al. 2018 ) also used descriptive analysis and (Arunachalam et al. 2018 ) employed both bibliometric and thematic analysis in their literature review. As mentioned by Govindan et al. ( 2018 ) and Mishra et al. ( 2018 ), bibliometric analysis is to suggest the urgent clusters and encourage scholars to further expand and collaborate the knowledge in the supply chain field. Thus, these limitations of the adopted methodology in (Nguyen et al. 2018 ) yield us to introduce our modification on it.
This paper aims to contribute to the development of big data in the supply chain, focusing on two major aspects of big data: big data management and optimisation. Also, to present what solutions, techniques, and tools have been utilised to optimise and manage big data contributing to the research field in SCM. This paper combines the coverage of two applications of big data management and optimisation which may affect the way we approach studying these technologies in SCM.
This paper contains three main sections apart from the introduction and the conclusion. Section two incorporates related work, research methodology of the systematic literature review, and bibliometric analysis of the literature. Section three presents findings and discussion on materials evaluation. Then a brief discussion on the major gaps and the future directions is presented in section four.
2 Research methodology
2.1 related work.
Based on our collections of articles related to big data applications in SCM, we created a small database of related review articles to compare between their scopes, research methodologies, and their coverage. Although there are several literature reviews of big data analytics in the area of SCM, the majority of them are based on SCM applications of big data analytics (Addo-Tenkorang and Helo 2016 ; Anitha and Patil 2018 ; Arunachalam et al. 2018 ; Barbosa et al. 2018 ; Brinch 2018 ; Chaudhuri et al. 2018 ; Chehbi-Gamoura et al. 2020 ; Govindan et al. 2018 ; Gupta et al. 2019 ; Mishra et al. 2018 ; Nguyen et al. 2018 ; Tiwari et al. 2018 ). Table 1 presents a summary of such studies, including the authors’ names, year of publication, and the main focus and the limitations of their work. Past literature has also been evaluated since it partially deals with big data applications in supply chain and emphasises its contributions to researchers’ grasp of supply chain. Although the past literature provides the essential initial points of our study, there are some identified limitations (in addition to the limitations shown in Table 1 for each study): (i) the methodology of the review and its steps are not well illustrated; (ii) a minimal literature review on supply chain performing cross-maps with big data optimisations techniques; and (iii) there is a lack of defining, comparing, and presenting big data management tools in SCM.
Consequently, the scope of this systematic literature review focuses on both big data management and optimisation studies in SCM in recent years that gives our work a novel multidimensional structure unlike previous literature reviews that mostly focused on big data analytics. We believe that this study will overcome these previous limitations by (i) introducing a definition of big data optimisation, and how big data is optimised, what techniques are used to optimise big data in supply chain and (ii) comparing the common tools of managing big data, and its applications in the industrial context. We adopted a review methodology and modified it by involving a reporting flowchart and content analysis method to provide useful insights to increase the reliability of our study and allow its adoption. Content analysis is also a systematic method that allows users to make correct inferences from a vocal, graphical, or structured text to define and measure certain occurrences y systematically and objectively (Downe-Wamboldt 1992 ).
2.2 Research methodology
In order to achieve this study’s objectives, the paper used an adopted systematic review methodology introduced by Nguyen et al. ( 2018 ). However, the methodology of Nguyen et al. ( 2018 ) lacks reporting the literature in a comprehensive approach and utilising textual analysis for the reported articles. Thus, the research methodology in this paper attempts to address these limitations in three points which are:
The research methodology involves only three main stages: collecting materials, bibliometric analysis, and categorising the documents.
The materials were assessed and evaluated during the collecting materials stage because we involved a reporting flowchart (PRISMA). The reporting flowchart is to enhance the quality of the tractability and reporting of the system (Moher et al. 2009 ). The reporting flowchart is preferred during the literature search process to record the included and excluded studies (Yang et al. 2017 ). The significance of PRISMA flow diagram lies in assessing the logical stages of the literature reporting process as well as defining the process boundaries (Vu-Ngoc et al. 2018 ). PRISMA is a 27-item checklist that covers the abstract, introduction, methodology, findings, and discussion of the paper to improve transparency in systematic reviews (Page et al. 2021 ). The reporting flowchart helps assess the materials and evaluate their inclusion or exclusion before analysing them, which has not been done in the methodology of (Nguyen et al. 2018 ).
Involving a bibliometric analysis of the collected documents to provide insights and meaningful information about these documents. The Bibliometrix R-package was chosen because it offers a variety of metrics for keyword analysis, author scientific publications, country-author collaborations, and a conceptual structure (Aria and Cuccurullo 2017 ). Bibliometrix R-package supports analysing the extracted materials from the three databases which are Scopus and Web of Science databases. Therefore, other applications of bibliometric analysis lack the combination between all three databases to perform such a comprehensive analysis.
2.2.1 Collecting materials
In this step, a process of searching and collecting materials relevant to the topic is involved. For building a database for the records obtained from the searching process, the PRISMA flowchart (Moher et al. 2009 ) is adopted to eliminate the observed results of documents based on certain criteria to build a database for the records obtained from the searching process. Notably, three main databases were used to collect research papers relevant to the scope of this literature. These databases are Scopus, ProQuest, and Web of Science, which are deemed as comprehensive and most powerful databases (Yang et al. 2017 ). Then, a distinguished set of keywords is identified in (Table 2 ) to synthesise the literature. Consequently, the keywords were grouped into two groups (A and B).
Group (A) contains keywords are to seek relevant records of big data and Group (B) consists of keywords are to find results pertinent to SCM. Most importantly, some of the keywords involve a wildcard (*) character to provide comprehensive results and include as many records as possible and avoid missing any relevant effect. Keywords from both groups (A) and (B) are joint using operator “AND” in the research, and operator “OR” used within the same group. Table 3 shows the three different strings that combine keywords from Table 2 .
The search string differs from a database to another one because it is often difficult to reproduce the exact same query between databases. For example, some databases offer only searching in titles and abstracts but excluding the keywords such as ProQuest which may result in less detail of reporting the literature. However, full-text mining might be a useful option (Penning de Vries et al. 2020 ). There are at least two (not necessarily unique) methods of leveraging data and text mining to choose articles for additional review, as mentioned by (O’Mara-Eves et al. 2015 ) as cited in (Penning de Vries et al. 2020 ). The technique of extracting knowledge and structure from unstructured material is known as text mining (Hearst 1999 ). Though not yet firmly proven, text mining’s potential for automatically eliminating studies should be viewed as encouraging. It can be utilised with a high degree of confidence in highly technical and clinical domains, but additional research is needed in other fields for development and evaluation.
Another alternative is searching using subject headings which refers to regulated vocabulary terms, to identify the subject matter of an article (Guidance for students and staff: literature searching n.d.). Utilizing relevant subject headings may improve the search and enable one to locate additional details on the selected topic (Guidance for students and staff: literature searching n.d.).
The search is done based on all likely pairs between the two categories of keywords considering the four stages of PRISMA flowchart diagram as shown in Fig. 1 .
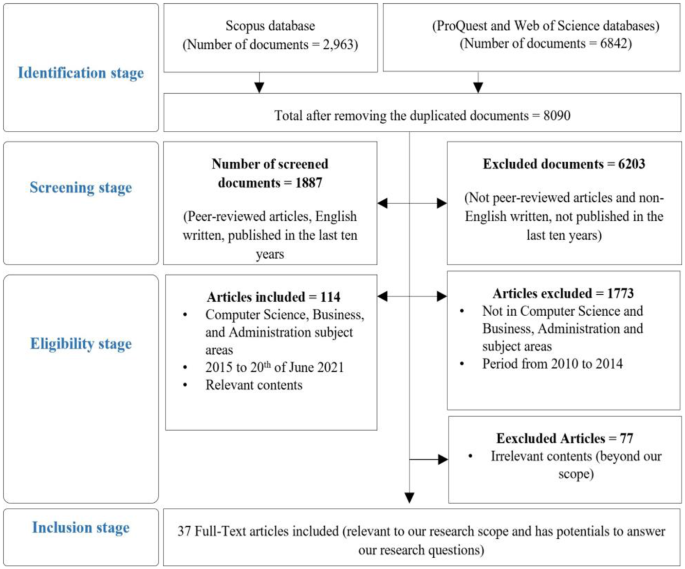
PRISMA flowchart for reporting the results of searching documents of the literature
Identification stage
the first results by combining Group A and Group B keywords were 2963 from Scopus and 6842 documents from ProQuest and Web of Science databases, without any searching filters. After deleting the duplicates, 8090 documents were found.
Screening stage
using the document type filter in each database, we selected only peer-reviewed papers, final documents and, English writing published in the last ten years. The choice of the last ten years is because big data applications, especially big data analytics, became a phenomenon globally since 2011 (Nguyen et al. 2018 ). Thus, the excluded results were 6203, and 1887 documents were included screened. The results are only shown in the period of the last ten years. The purpose of this stage is to screen the documents to check their eligibility in the next stage.
Eligibility stage
in this stage, we chose only Computer Science, Administration, and Business subject areas for each document. This is to ensure the relevance of the documents to our scope. During this stage, there were 1773 excluded articles in different subjects’ areas that are not relevant to our topic and the research questions. Another exclusion reason is that documents from 2010 to 2014 did not clearly describe the application of big data optimisation and management in supply chain. Hence, only 114 records were included since they are in the same domain, Computer Science and Business, and in the period from 2015 to 20th of June 2021.
Inclusion stage
After the eligibility stage, we assessed each article of the 114 articles by reading the title, the abstract, the keywords, the objectives of the paper, and the conclusion. We found 77 papers that are irrelevant which we could not find our answers to the research questions. Thus, these 77 papers were removed, and only 37 peer-reviewed documents were included in our database. We then stored the relevant information to prepare it for bibliometric analysis.
2.2.2 Bibliometric analysis of the literature
This section presents a comprehensive bibliometric analysis to discover more about the previous studies associated with the relationship between big data optimisation and management in SCM. The bibliometric analysis was done using a bibliometric package embedded in the R language. The bibliometric package supports the process of importing data from different databases such as Scopus, Web of Science, and PubMed and assists researchers in building data metrics (Aria and Cuccurullo 2017 ). Before applying the analysis, the data file was processed to ensure that the necessary attributes are available. Some useful metrics can be found from the analysis and are presented in Figs. 2 , 3 , 4 and 5 , and 6 .
2.2.2.1 Insights of journals portfolios.
To understand the role of several journals found in the literature, we created a table of top ten journals that published articles in the field of big data and supply chain, but mainly related to our research focus which is optimization and management of big data. Table 4 shows the number of articles Footnote 1 in each journal indicating the highest and lowest number of publications. All the journals are sorted by counting the number of publications. Interestingly, there is a lack of publications on our focus of big data optimization and big data management from year 2010 to 2013. However, from 2014, there has been an increase of publications, whereas the peak of publications was in 2018. Journals insights are helpful for whom interested publish in the future as it offers an overview of metrics of the research.
2.2.2.2 The number of annually published articles.
With a total of 37 papers published on this topic, Fig. 2 shows the annual number of articles published from 2015 to 2020 on big data applications in SCM. Noticeably, in 2018, the number of records increased dramatically to 12 documents.
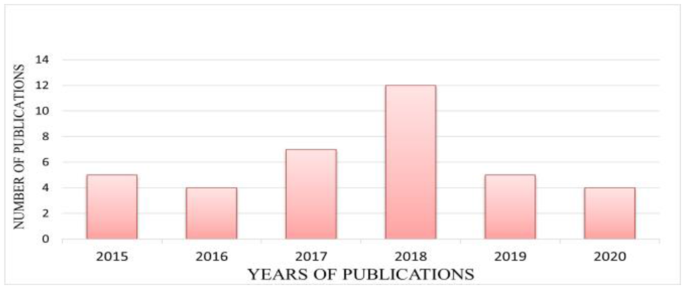
Annual number of published articles from 2015 to 2020
2.2.2.3 The most common keywords.
Figure 3 (Words Cloud) shows the frequency of keywords used in the literature. For example, “big data,” “decision-making,” “manufacture,” “supply chains,” “big data applications,” “information management,” “data handling,” “data acquisition,” and “digital storage” are the hot spots of the research, which shows a high frequency mentioned in many studies. Interestingly, other keywords also have a remarkable frequency of appearance in the literature, which also could be helpful to answer the research questions such as “cloud computing,” “internet of things,” “artificial intelligence,” “data analytics,” and “industry 4.0”.
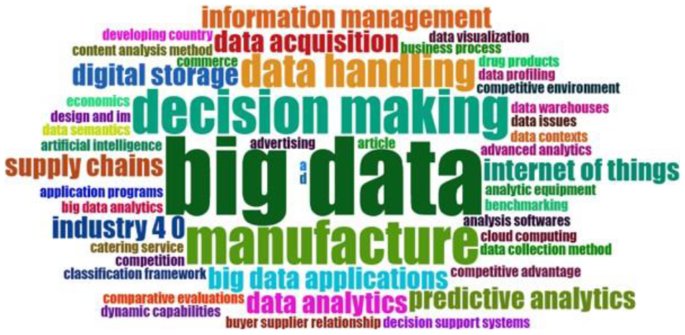
Words Cloud of the frequency of keywords
Figure 3 highlights the most common keywords discussed and studied by scholars in big data applications in SCM. The keywords “optimisation” and “big data management” have not been involved highly being focused on. The benefit of the words cloud is to show how many times a number of keywords have been involved in the research field. If there is a slight appearance of these keywords, this indicates a lack of literature on relevant topics.
2.2.2.4 Conceptual structure map and co-occurrence network.
The co-word analysis aims to represent the conceptual structure of a framework by relying on the co-occurrence. There are three various useful mapping types of the conceptual structure that can be generated from the conceptual structure-function. These are a conceptual structure map, factorial map of the highest cited documents, and a factorial map for the most contributed papers. This paper visualised a conceptual structure map (Fig. 4 ), which shows two clusters of the authors’ keywords. Authors’ keywords mean the keywords that have been noted by authors in their articles (Aria and Cuccurullo 2017 ). The two clusters are obtained by using the Multidimensional Scaling method (MDS). The two clusters are obtained by using the Multidimensional Scaling method (MDS). Both clusters indicate that the classified keywords are the most in the attention of big data and supply chain researchers. Both dimensions correspond to the most used keywords in SCM and represent those keywords from the big data analysis field.
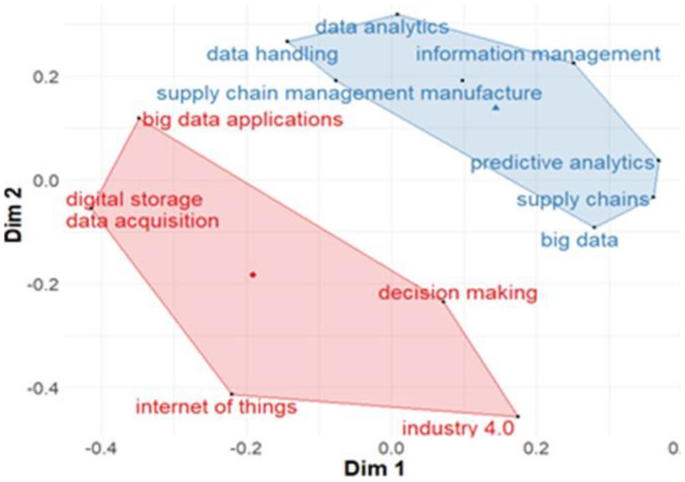
Conceptual Structure Map using Multidimensional Scaling method (MDS)
The Keywords Co-occurrence Network (KCN) aims to focus on extracting the knowledge components from any scientific research through testing the links between the keywords found in the literature (Aria and Cuccurullo 2017 ). Figure 5 shows two clusters of the most occurrence keywords grouped in two different colours (red and blue), indicating these keywords’ frequent appearance between different types of studies.
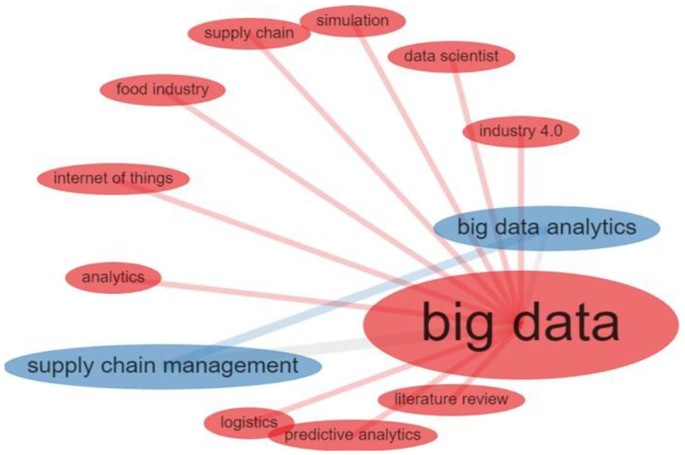
Co-occurrence Network
2.2.2.5 Three-fields plot.
Figure 6 shows the visualisation of three main fields of the biography and the relationship between them using a Sankey diagram (Aria and Cuccurullo 2017 ). The left-field represents the keywords; the middle field shows authors, and the right field is for the most frequent words in the titles. Commonly, the “big data” keyword has been in the direction of several researchers. Meanwhile, “data,” “big,” “supply,” “analytics,” and “chain” are more used in the title of the papers. Figure 6 also helps identify the researchers’ focus on different aspects of SCM and applying big data analytics.
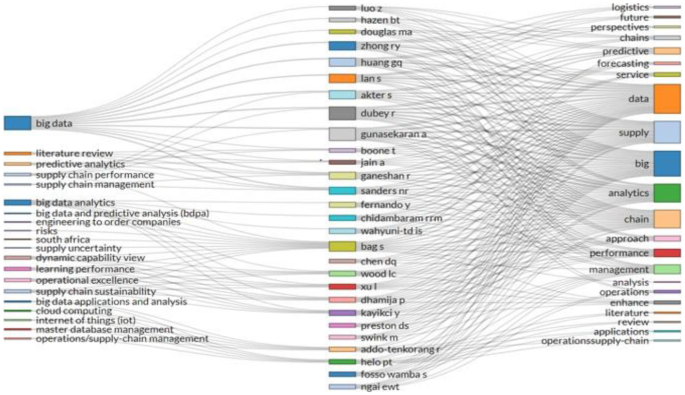
Three-field plot link between authors, titles, and keywords in the literature
Although Bibliometrix R-tool provided rich analysis for this study, there are other useful visualisation tools including Bibexcel, Gephi, Pajek, and VOSviewer (Donthu et al. 2021 ). As a result, relevant outcomes could be produced to offer solutions to certain study concerns. In addition, other bibliometric analysis methods, like citation analysis, could be used. A co-citation network is formed when two publications are connected when they are listed as references in another article. Co-citation analysis enables researchers to locate the most significant publications as well as learn about theme clusters (Donthu et al. 2021 ). Through the incorporation of additional categories of searched documents including business periodicals, case studies, and newspaper articles, a detailed assessment can be conducted in the near future by utilising more databases to obtain more data insights on the search themes (Alsolbi et al. 2022 ). In terms of the subject’s scope of study and scalability, expanding the types of papers and sources can be more beneficial.
2.2.3 Categories selection
The category selection step aims at using analytical categories and structural dimensions to conceptualise the classification framework (Nguyen et al. 2018 ). In this paper, two structural dimensions have been selected to create different layers of the framework: big data management and big data optimisation. For the dimension of big data management, two categories of data architecture and storing data techniques are considered. Based on the approach of papers, the dimension of big data optimisation is divided into two categories of big data impacts on supply chain performance and optimisation techniques and applications, as shown in Table 5 .
In terms of supply chain functions, five main functions are procurement, manufacturing and production, logistics and transportation, warehousing, and demand management, as used in the review of (Nguyen et al. 2018 ). We also considered the general SCM for papers having a general perspective. The outcomes of the categorisation are shown in Table 4 . Interestingly, most of these papers addressed big data optimisation and a small number of them dealt with both structural dimensions (Roy et al. 2018 ; Tan et al. 2015 ; Vieira et al. 2020 ; Zhong et al. 2016 ). Interestingly, most of these papers addressed big data optimisation, and a small number of them dealt with both structural dimensions.
3 Findings and discussion
Several studies on big data applications involve the processes of collecting, processing, analysing, and visualising beneficial information through technical means associated with machine learning. Some useful articles and reviews introduced different searching models, classification frameworks, empirical studies, surveys, and literature reviews. Both studies (Grover and Kar 2017 ; Nguyen et al. 2018 ) encouraged us to study more about big data applications in the supply chain domain.
However, conducting big data studies can be challenging as technology is evolving rapidly. With regards to supply chain functions, which are procurement, manufacturing, warehousing, logistics and transportation, and demand management (Nguyen et al. 2018 ), there are only a few studies that sought to tackle the optimisation approaches such as routing and order-picking challenges in warehousing by the use of big data. Such problems have been frequently addressed using mathematical modelling, simulation, and heuristic and metaheuristic solution algorithms (Ardjmand et al. 2020 ; Cano et al. 2020 ; Schubert et al. 2020 ; (Shavaki and Jolai 2021a , b ). The review indicated that there is little known of real-time optimisation models to enhance the effectiveness of the production and logistics process (Nguyen et al. 2018 ). There is a shortage of studies on how big data can be managed in the supply chain. There are conceptual models of data infrastructure, big data management solutions, and proposed approaches to tackle the huge amount of big data.
To better answer the research questions in this paper, we reviewed and analysed each paper’s main work, the contribution to the research field, and synthesised it with other similar articles.
3.1 How to optimise big data in SCM?
The usage of big data in SCM allows businesses to gain a variety of short-term advantages in their operations (Chen et al. 2015 ). For instance, analysing point of sale data can help with pricing and special services for each customer group, while analysing inventory and shipping data can minimise lead times and increase product availability, and consequently increase sales amount (Chen et al. 2015 ), the optimisation of quality-product trade-off can help in producing high-quality products with lower costs (Mubarik et al. 2019 ). Moreover, the use of Radio Frequency Identification (RFID) can help small markets in improving the decision-making process in logistics functions (Navickas and Gruzauskas 2016 ). Behavioural analytics and fraud detection solutions are the other applications of big data in SCM (Patil 2017 ).
The research question sought to investigate how big data in the supply chain can be optimised. To answer this question, we firstly review previous studies on the effects of big data usage on supply chain performance to clarify big data’s potential to improve and facilitate SCM, and then we will elaborate on big data optimisation in SCM. Several scholars have empirically investigated the impacts of using big data on the performance of supply chain by using statistical methods in a generic supply chain (Chen et al. 2015 ; Gunasekaran et al. 2017 ; Hofmann and Rutschmann 2018 ). Also, researchers have studied the effects of applying big data in a specific industry, such as food and beverage supply chains (Irfan and Wang 2019 ), pharmaceutical industry (Asrini et al. 2020 ; Shafique et al. 2019 ), oil and gas industry (Mubarik et al. 2019 ), services and humanitarian supply chains (Dubey et al. 2018 ; Fernando et al. 2018 ), and mining and minerals industry (Bag 2017 ).
Chen et al. ( 2015 ) investigated the influence of big data analytics (BDA) usage on organisational performance considering both the antecedents and consequences of the BDA usage through a TOE (technology organisation environment) framework. Their findings showed that BDA has a positive impact on both asset productivity and business growth.
Irfan and Wang ( 2019 ) looked at how data-driven capabilities affected supply chain integration and firm competitiveness in Pakistan’s food and beverage industry. They adopted the structural equation modelling approach to test their hypotheses on collected survey data. Asrini et al. ( 2020 ) investigated the effects of supply chain integration, learning, big data analytics capabilities, and supply chain agility on the firm’s performance by the use of structural equation modelling approach in the pharmaceutical companies in Indonesia. (Mubarik et al. 2019 ) explored the influence of big data supply chain analytics and supply chain integration on supply chain efficiency across the board, including forecasting and supplier management, sourcing, production, inventory management, and transportation. To measure the relationships, they used covariance-based structural equation modelling.
Fernando et al. ( 2018 ) also used structural equation modelling to investigate the effects of big data analytics on data protection, innovation capacity, and supply chain efficiency. Dubey et al. ( 2018 ) used the ordinary least square regression to test the impact of big data and predictive analytics on visibility and coordination in humanitarian supply chains. Shafique et al. ( 2019 ) used partial least square structural equation modelling (PLS-SEM) to investigate the relationship between big data predictive analytics acceptance and supply chain success in the pharmaceutical logistics industry in China. They also used the” variance accounted for” form of mediation to assess the role of RFID technology in mediation.
Bag ( 2017 ) employed partial least square regression analysis to examine the correlation between buyer-supplier relationships, big data and prescriptive analytics, and supply chain performance in managing supply ambiguities in companies of mining and minerals manufacturing in South Africa. Hofmann ( 2017 ) focused on how big data could be used to optimise supply chain processes by reducing the bullwhip effect. He used an existing system dynamics model to simulate the effects of big data levers such as velocity, length, and variety on the bullwhip effect in a two-stage supply chain, taking into account several starting points derived from previous research. The results revealed that the lever velocity has a great potential to enhance the performance in the supply chain.
In addition to the studies mentioned above that demonstrate the positive impact of big data on supply chain performance, the systematic literature review proved that big data optimisation is widely applied in all functional areas within SCM. In demand management, Nita ( 2015 ) systematised demand forecasting using heterogeneous mixture learning technology in the food supply chain. Hofmann and Rutschmann ( 2018 ) studied the impacts of big data analytics on demand forecasting accuracy. Papanagnou and Matthews-Amune ( 2018 ) proposed using the VARX model to evaluate the impact of independent variables obtained from various internet-based sources on inhibitory sales trends data to improve forecasting accuracy. Boone et al. ( 2019 ) provided an overview of how customer insights focused on big data and related technologies could improve supply chain sales forecasting.
In logistics and transportation, companies prefer optimisation as a suitable approach since it strengthens predictive analytics (Nguyen et al. 2018 ). In this regard, a holistic Big Data approach was proposed by Zhong et al. ( 2015 ) to mine massive RFID-enabled shop floor logistics data for the frequent trajectory to quantitatively evaluate logistics operations and machines. Another experimental study to obtain a decision support optimisation in logistics has been introduced by the authors of Lee et al. ( 2018 ). They relied on weather historical big data to decide the optimal speed that reduces the fuel consumption while vessels are serving. They proposed a novel approach to parse weather data and use data mining techniques to learn about the influence of the weather on fuel consumption. The results of their experiment showed that better fuel consumption obtained forecasts from fuel consumption function. Also, suggested routes are introduced in the study to provide enhanced decisions. These studies contributed to adding examples of how big data can be optimised, relying on logistics issues.
In manufacturing and production, there is a growing interest among scholars to investigate the real-time optimisation models to enhance the effectiveness of the production processes. Simulation and modelling are increasingly being adopted in the development of real-time manufacturing control systems whereby tracking devices like RFID and sensors provide a sufficient and consistent flow of instantaneous data (Zhong et al. 2015 ). In their study, Ji et al. ( 2017 ) used a Bayesian network to create a cause-and-effect relationship between the data to forecast direct food production and market demand accurately. As another big data application, IoT is involved in SCM because it helps in improving operational efficiencies and creating opportunities for cutting costs in the sourcing, procurement, production, and distribution processes (Aryal et al. 2018 ; Kim 2017 ). Adopting IoT in SCM is already applied in many companies such as United Parcel Service (UPS), which reduced the idling time and costs of services (Aryal et al. 2018 ). The installation of sensors in products can help a company to trace the movement of all the goods from the warehouse to the customer through interconnected devices. Effective inventory management is enhanced by IoT as embedded technology on items communicates and gives alerts on lead times, stock-outs, and product availability (Aryal et al. 2018 ; Barbosa et al. 2018 ).
The systems and equipment in the network can respond by initiating another manufacturing process or triggering procurement requests to the raw material’ suppliers in case of low stock. Furthermore, there are still few studies on improving order-picking processes such as sorting, routing, and batching by using big data optimisation.
In terms of big data models, it is evident from the trend assessment that prescriptive analytics appears in more frequently published literature in the management of big data analytics-driven SCM compared to predictive and descriptive analytics. One of the reasons is that predictive analytics has become mainstream in most areas, and domain-related scholarly publications are limited. That does not mean that predictive analytics is out. Seyedan and Mafakheri ( 2020 ) provide an overview of predictive BDA for the supply chain, including customer analysis, demand prediction, and trend analysis. Demand forecasting in closed-loop supply chains is regarded as one area in demand in both research and practice. Likewise, the application of IoT devices in conjunction with AI and analytical approaches also has significant potential.
Regarding predictive analytics, time series, deep learning, machine learning (e.g., support vector machines), models for demand forecasting and classification are some of the predominant approaches being applied in production to improve planning and control and equipment maintenance in the manufacturing process.
Furthermore, classification is a critical big data analytics model that helps in improving procurement research and logistics. Critically, the association is a popular model used in descriptive analytics, and it is usually applied across the diverse functional areas in the supply chain from procurement, warehousing, logistics, to demand management. Similarly, in most of the studies reported in the literature of (Nguyen et al. 2018 ), the visualisation model is treated less significantly mostly as a supplement of other state-of-the-art data mining models. The literature review of Govindan et al. ( 2018 ) presented opportunities of how big data can be collected from different sources, such as ERP systems, logistics and orders, newsfeeds, manufacturing environment, surveillance videos, radio frequency-based identification tracking, customers behaviours patterns, and product lifecycle operations. Dealing with the massive datasets leads researchers to consider techniques of optimising big data (Govindan et al. 2018 ). The findings of this study show that optimising big data in SCM has many benefits, including cost reduction. Noticeably, this paper stated comprehensively what chances and places where optimisation can occur; however, there is still a gap in covering how big data can be optimised with the introduced technologies in this paper.
Hofmann and Rutschmann ( 2018 ) presented a table of advanced applications of analytics to enhance the predictions in supply chain retail through some optimisation tools. These optimisation tools, such as a price optimisation tool, optimise forecasting of amounts of cashiers relying on the number of visitors of a store. Doolun et al. ( 2018 ) presented one other interesting study of optimisation in the supply chain, which applies a data-driven hybrid analytical approach to overcome a supply chain location-allocation problem with four echelons. The main problem they focused on is to find the locations of warehouses and plants that should be operating with minimum costs of supply, considering the requirement of maximising the customer demands. A data-driven analytical hybrid NSDEA algorithm was involved in this experiment to select the best control variables. Therefore, they chose five variant of hybrid algorithms that were validated to locate the number of warehouses and plants among different sites. The study concludes that NSDEA is one of the powerful tools that can be considered to determine the solutions of that case of the Malaysian electronic auto parts supply chain.
Lamba and Singh ( 2018 ) used three separate multi-criteria decision- making (MCDM) techniques to inve(Lamba and Singh 2018 )stigate the relationships between big data enablers in operations and SCM, including interpretive structural modelling, fuzzy complete interpretive structural modelling, and decision-making trial and evaluation laboratory.
Liu and Yi ( 2018 ) examined the issues surrounding big data information (BDI) investment decision-making and its implications for supply chain coordination. They looked at a supply chain with a producer and a retailer, with the manufacturer as the leader of the Stackelberg Game, determining the wholesale price, and the retailer as the follower, deciding the order quantity. Based on the Stackelberg Game philosophy and teamwork theory, four BDI investment modes and a coordination tactic have been proposed to evaluate the value of the supply chain after investing in BDI in each investment mode. This paper provides theoretical guidance for supply chain managers about BDI investment decisions and their consequences.
An optimisation experiment done by Zhao et al. ( 2017 ) presents a multi-objective model for managing a green supply chain scheme to minimise the risks as a result of hazardous materials, carbon emission, and economic costs. Big data analytics were used to capitalise on the model parameters. In the model, they had adopted three scenarios (aimed to reduce the hazardous materials, carbon, and economic costs) to improve the process of the green supply chain. Results show that reducing carbon risks and emissions can be determined through optimisation. Although this study is relevant to our questions, the authors did not mention in detail what was done to optimise big data in this study. The big data term mentioned in the paper was to extract the parameters-related data. Involving big data analytics in supply chain optimisation is a standard experiment however, steps of how big data was analysed to lead to an optimised approach is crucial to add to the literature and provide an adequate answer.
As a summary of the findings this section, Table 6 displays a list of applied research methods and tools in the reviewed papers to optimise big data in the SCM field. From this table, optimisation methods have been used more than the other techniques in this area.
While this paper is about big data optimisation, and management in SCM, the review would not be complete without some pertinent comments about other frameworks that have approached SCM. One such framework is using system models. System modelling approaches such as system dynamics (Feng 2012 ; Rabelo et al. 2011 ), agent-based modelling (Arvitrida 2018 ; Behdani et al. 2013 ), and network modelling are frequently employed in supply chain modelling, analysis, and optimisation. These techniques have been applied to small data. When it comes to big data, these techniques could still prove to be useful in helping define conceptual models, thereby enabling better model building with big data. Although previous studies addressed big data optimisation in various problems either within a general supply chain or in a specific function and demonstrated the capabilities of big data to improve supply chain performance, there are still gaps in the literature that need to be dealt with. We discussed these gaps in Sect. 4.
3.2 What tools are most used to manage big data in SCM?
As the second research question, the literature review sought to summarise big data management tools and technologies among supply chain organisations. Table 7 shows the main work and ideas of reviewed papers in big data management. It is evident that big data management conceptual models, and infrastructures of storage of vast amounts of data remain challenging for many organisations.
With the development of industry 4.0 in the business world, every object linked with a supply chain is continuously generating data in structured (data generated with the formal structure like records, files, docs, and tables), semi-structured (data contains distinct semantic elements but does not have formal nature), or unstructured (data without any identifiable formal structure such as blogs, audio, video, image, etc.) forms Biswas and Sen ( 2016 ). Big data experts believe that, in the next coming years, unstructured data will be the major proportion of the total generated data (Zhan and Tan 2020 ). For example, data collected from a variety of sources, such as sensors and digital cameras, in the service and manufacturing industries are usually unstructured, heterogeneous, incompatible, and non-standardised (Zhong et al. 2016 ). To cope with these difficulties, companies must pre-process the unstructured data and develop a shared data warehouse to store relatively homogeneous information (Lamba and Singh 2017 ). The availability of a large amount of data gathered from various sources has increased the risk of privacy and security of data (Fernando et al. 2018 ).
However, big data analysis platforms and tools have enabled managers to examine large data sets in all forms to discover useful information such as hidden patterns and market trends, helping organisations improve business decision-making (Grover and Kar 2017 ). For instance, Papanagnou and Matthews-Amune ( 2018 ) investigated the use of structured data from sales in conjunction with non-structured data from customers to enhance inventory management. Biswas and Sen ( 2016 ), in their Big Data architecture, addressed both structured and unstructured data so that the structured data was derived by extract transform and load (ETL) mechanisms and is populated into a data warehouse. The unstructured data was managed by the Hadoop Distributed File System (HDFS) and MapReduce systems of the Hadoop cluster and is also stored in the NoSQL database. Tan et al. ( 2015 ) (which presented analytics infrastructure framework) tried to harvest available unstructured data of company SPEC (a leading eyeglasses manufacturer in China) to create ideas for new products’ innovation and operations improvement. This company uses Apache Mahout for machine learning algorithms, Tableau for big data visualisation, Storm for analysing real-time computation systems, and InfoSphere for big data mining and integration. Zhan and Tan ( 2020 ) analysed the unstructured data generated from multiple sources of LLY Company (a leading Chinese athletics products – shoes and sporting equipment – manufacturer on a global scale). This company uses the main technologies of big data such as Hadoop, HBase, and Spark SQL.
It is stated by Tan et al. ( 2015 ) that the existing software remains challenging for managing a massive amount of data. Data varies in its types and resources, leading to difficulty generalising the analytics tools and management systems. Despite the overwhelming task of collecting and assessing the voluminous data, top management must prioritise the use of big data analytics to enhance SCM. There are big data management tools that can be applied to supply chain analytical operations, such as Hadoop, which is an open-source platform for performing analyses on stored data (Grover and Kar 2017 ). Since there are many challenges in storing big data, using Apache Storm is beneficial in supply chain networks. Apache Storm is an open-source framework that provides a streaming process linked to clustering data (Grover and Kar 2017 ).
Despite the various big data management technologies, organisations of the supply chain, which aim to apply technologies of big data management, may face critical issues when selecting a suitable platform. Table 8 shows the most reported big data management tools that have been most cited in the literature.
Another important concern of the managers is the potential of these platforms to be used in different data life cycle phases from generation to destruction. (Chen and Zhao 2012 ) divided data life cycle into seven stages including data generation, transfer, use, share, storage, archival and destruction. Table 6 also displays each platform can be used in what stages. Interestingly, it was noted by Tan et al. ( 2015 ) that analytics infrastructure is required to structure and relate different bunches of information to the objectives.
Tan et al. ( 2015 ) summarised the analytic techniques into four main categories: Burbidge’s connectome Concept, Influence diagram, Cognitive Mapping and Induction Graph. They also described each of these techniques, their strengths, their weaknesses, and useful software. Finally, they proposed an analytics infrastructure framework using deduction graph techniques to assist managers in decision-making process (Fig. 7 ). By applying this framework to the SPEC company, the results were in the preference of SPEC executives. The framework would help managers to build a visual decision path that captures the logic behind the number of decisions taking during the competence set method for evaluating. The significance of this framework is counted in a better approach to structure the data and connect the different streams of data to build a coherent picture of a specific problem.
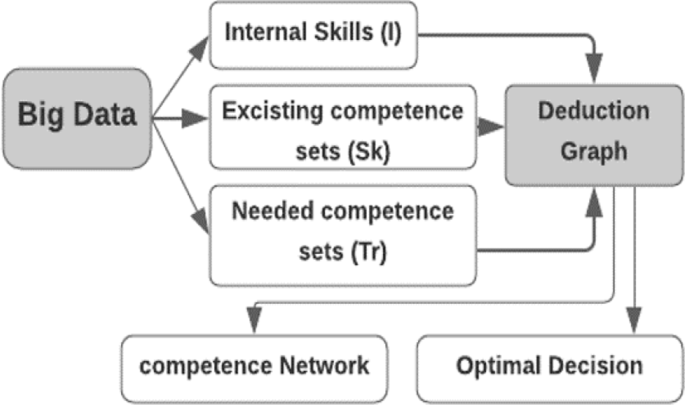
The adopted analytics infrastructure framework (Tan et al. 2015 )
Different big data tools offer different services for end users, which may offer different capabilities of analysing and managing data in SCM. This study reports some of big data tools being used in SCM during the period from 2010 to 2021, however; there are expected new tools and enhancements that leverage the capabilities of big data analytics and management tools in SCM. Firstly, the development of open-source machine learning and data mining libraries in recent years has contributed to the success of big data platforms like Spark, Hadoop, Flink, and others (Mohamed et al. 2020 ). However, numerous efforts have been undertaken to build cloud computing technologies because of the amazing characteristics of cloud computing, including its pervasive service-oriented nature and elastic processing capacity (Mohamed et al. 2020 ). Another point is that big data tools remain mysterious for many users (Mohamed et al. 2020 ). To bridge the gap between big data systems and their users, a user-friendly visualisation technique is required. The visualisation technique should present the analytic results to users in such a way that they can effectively identify the interesting results (Mohamed et al. 2020 ).
4 Research gaps and future direction
Based on the findings, there is a need to conduct further empirical studies on how big data analytics can be optimised and managed in SCM. Our study revealed three certain gaps in the research conducted on the optimisation and management of big data in SCM. We expressed our understanding of these three gaps based on our analysis of the documents’ records, citations, and keywords in Section two and our findings and discussion in Section three.
We summarized research gaps and possible directions that tackle these gaps in Table 9 . One of the main identified gaps in the available literature in both dimensions of big data management and big data optimisation in the SCM is the lack of case studies and practical examples. The reason is that the implementation of big data-related techniques in the SCM has several challenges. One of the challenges is to optimise the hardware and software setup to balance cost and performance. Conducting an empirical study to optimise the setup is not cost-effective; it is time-intensive and difficult to control. As a result, simulation-based methods are a viable alternative (Tiwari et al. 2018 ). Privacy, security, and quality of data (Richey et al. 2016 ), data acquisition continuity, data cleansing, and data standardising (Raghupathi and Raghupathi 2014 ) are mentioned as the other challenges.
Despite technological and data quality concerns, organisations cited culture and managerial issues as major roadblocks (Arunachalam et al. 2018 ). On the other hand, while the computational technology required to manage and optimise big data are progressing, the human expertise and abilities required by business executives to use big data are lagging behind, posing another significant challenge (Barbosa et al. 2018 ) in this area.
Due to all of these challenges as well as the lack of interest among business managers to publish their projects in peer-reviewed journals and conferences, there is a gap in scientific publications including practical examples. To void this gap, maturity models can assist organisations in assessing their existing technological capability in comparison to industry standards (Arunachalam et al. 2018 ) and identifying the requirements for progressing their big data projects. Cross collaboration among firms in the supply chain is the other alternative to overcome the challenge (Tiwari et al. 2018 ). Finally, partnership between academics and supply chain executives is suggested to enhance the achievements of real-world projects and enrich scientific publications.
5 Limitations of the review
There are several flaws in the literature review. The explorative technique, for example, exposes the study to subjective bias, despite the fact that the exploratory review process ensures a thorough examination of the research issue. There is a vast amount of secondary data available from a variety of databases that may be analyzed to further research goals and lay the groundwork for future investigations. Scopus, Web of Science, and ProQuest were the only databases used in this study. Some papers, however, may have been overlooked if the source was not found in these databases. Another constraint is that the sources were limited to those publications that appeared between 2010 and 2021. More findings may have been achieved if the chosen period had been longer. Other terminology, such as “decision making” or “empowered data,“ may arise in studies focusing on non-profits. However, there may be literature in this research topic that is irrelevant. Because the goal was to produce particular results that matched the keyword lists, extending the period was not considered.
6 Conclusion
This paper adopts the content analysis methodology to conduct the systematic literature review, whereby 37 journal articles were definitively relied upon on the search strategy to offer a comprehensive outlook of how big data has been optimised in SCM. Also, we reviewed technologies mostly used for big data management in SCM. It is indisputable that big data is extensively managed and optimised in supply chain functional areas to enhance organisational effectiveness. Therefore, companies need to ensure proper management of big data applications and related infrastructure. Different levels of big data optimisation and management techniques are used to ensure effective and seamless supply chain operations. Nonetheless, the review and discussion of findings reveal several research gaps that require further studies to fill the void and develop the topic. Although technologically and organisationally challenging, it is very important to publish papers including case studies and practical examples to show the effectiveness and outcomes of optimising big data in real-world supply chains. The other pressing area for future studies is managing and optimising unstructured data and take advantage of the huge amount of data that is continuously generated by various data sources in supply chains. These issues can be addressed by the cooperation between supply chain managers and academic researchers, improving the culture of managers to accept and employ new technologies, and enhance the skills of employees.
Furthermore, the literature review has several limitations; for instance, the use of exploratory methodology makes the study to be prone to subjective bias. By the way, the exploratory review method is significant since it ensures a comprehensive analysis of the research topic. Besides, it is a flexible and inexpensive method for answering the research questions. There is a massive volume of secondary data from numerous databases that can be examined to achieve the research objectives and lay the foundation for future studies based on the gaps identified.
Change history
17 july 2023.
Process all figures in colour.
There are articles that have no insights from their journals. Thus, only 26 articles found and reported their journal insights.
Addo-Tenkorang R, Helo PT (2016) Big data applications in operations/supply-chain management: a literature review. Computers and Industrial Engineering 101:528–543
Google Scholar
Alsolbi I, Wu M, Zhang Y, Joshi S, Sharma M, Tafavogh S, Sinha A, Prasad M (2022) Different approaches of bibliometric analysis for data analytics applications in non-profit organisations. J Smart Environ Green Comput 2(3):90–104
Anitha P, Patil MM (2018) A review on Data Analytics for Supply Chain Management: a Case study. Int J Inform Eng Electron Bus 11(5):30
Ardjmand E, Ghalehkhondabi I, Young W, Sadeghi A, Sinaki R, Weckman G (2020) A hybrid Artificial neural network, genetic algorithm and Column Generation Heuristic for minimizing Makespan in Manual Order picking Operations. Expert Syst Appl vol:113566
Aria M, Cuccurullo C (2017) bibliometrix: an R-tool for comprehensive science mapping analysis. J Informetrics 11(4):959–9754
Arunachalam D, Kumar N, Kawalek JP (2018) Understanding big data analytics capabilities in supply chain management: unravelling the issues, challenges and implications for practice. 114:416–436Transportation Research Part E-Logistics and Transportation Review
Arvitrida N (2018) A review of agent-based modeling approach in the supply chain collaboration context vol, vol 337. IOP Publishing, p 012015
Aryal A, Liao Y, Nattuthurai P, Li B (2018) The emerging big data analytics and IoT in supply chain management: a systematic review. Supply Chain Management 25(2):141–1562
Asrini M, Setyawati Y, Kumalawati L, Fajariyah NA (2020) Predictors of firm performance and supply chain: evidence from indonesian Pharmaceuticals Industry. Int J Supply Chain Manage 9(1):1080
Bag S (2017) Big Data and Predictive Analysis is key to Superior Supply Chain performance: a south african experience. Int J Inform Syst Supply Chain Manage 10(2):66–842
Barbosa MW, Vicente AdlC, Ladeira MB, Oliveira MPVd (2018) Managing supply chain resources with Big Data Analytics: a systematic review. Int J Logistics 21(3):177–200
Behdani B, Van Dam K, Lukszo Z (2013) Agent-based models of supply chains, Agent-based modelling of socio-technical systems. Springer, pp 151–180
Bharathy GK, Silverman B (2013) Holistically evaluating agent-based social systems models: a case study. Simulation 89(1):102–1351
Biswas S, Sen J (2016) A proposed Architecture for Big Data Driven Supply Chain Analytics. IUP J Supply Chain Manage 13(3):7–333
Boone T, Ganeshan R, Jain A, Sanders NR (2019) Forecasting sales in the supply chain: consumer analytics in the big data era. Int J Forecast 35(1):170–180
Brinch M (2018) Understanding the value of big data in supply chain management and its business processes. Int J Oper Prod Manage 38(7):1589–16147
Cano JA, Correa-Espinal AA, GГіmez-Montoya RAs (2020) Mathematical programming modeling for joint order batching, sequencing and picker routing problems in manual order picking systems. J King Saud Univ - Eng Sci 32(3):219–228
Cassandra A (2014) ‘"Apache cassandra.“ ‘, Series “Apache cassandra.“ < http://planetcassandra.org/what-is-apache-cassandra
Chaudhuri A, Dukovska-Popovska I, Subramanian N, Chan HK, Bai R (2018) Decision-making in cold chain logistics using data analytics: a literature review. Int J Logistics Manage 29(3):839–861
Chehbi-Gamoura S, Derrouiche R, Damand D, Barth M (2020) Insights from big data analytics in supply chain management: an all-inclusive literature review using the SCOR model. Prod Plann Control 31(5):355–382
Chen D, Zhao H (2012) Data Security and Privacy Protection Issues in, pp. 6457 – 651
Chen X, Ong Y-S, Tan P-S, Zhang N, Li Z (2013) Agent-based modeling and simulation for supply chain risk management-a survey of the state-of-the-art IEEE, pp. 1294–9
Chen DQ, Preston DS, Swink M (2015) How the use of big data analytics affects value creation in supply chain management. J Manage Inform Syst 32(4):4–39
Cooper MC, Lambert DM, Pagh JD (1997) Supply Chain Management: more than a New Name for Logistics. Int J Logistics Manage vol 8(1):1–141
de Penning BBL, van Smeden M, Rosendaal FR, Groenwold RHH (2020) Title, abstract, and keyword searching resulted in poor recovery of articles in systematic reviews of epidemiologic practice. J Clin Epidemiol 121:55–61
Dean J, Ghemawat S (2008) MapReduce: simplified data processing on large clusters. Commun ACM vol:107–113
Donthu N, Kumar S, Mukherjee D, Pandey N, Lim WM (2021) How to conduct a bibliometric analysis: an overview and guidelines. J Bus Res 133:285–296
Doolun IS, Ponnambalam SG, Subramanian N, Kanagaraj G (2018) Data driven hybrid evolutionary analytical approach for multi objective location allocation decisions: automotive green supply chain empirical evidence. Computers and Operations Research 98:265–283
MathSciNet MATH Google Scholar
Downe-Wamboldt B (1992) Content analysis: method, applications, and issues. Health Care for Women International. vol 13, 0739–9332 (Print). 0739–9332 (Print), pp. 313 – 21
Dubey R, Luo Z, Gunasekaran A, Akter S, Hazen BT, Douglas MA (2018) Big data and predictive analytics in humanitarian supply chains. Int J Logistics Manage 29(2):485–5122
Feng Y (2012) System Dynamics Modeling for Supply Chain Information Sharing. Physics Procedia. vol 25, pp. 1463-9
Fernando Y, Ramanathan RMC, Ika S, Wahyuni TD (2018) Benchmarking vol 25(9):4009–4034The impact of Big Data analytics and data security practices on service supply chain performance
Giunipero L, Hooker R, Joseph-Mathews S, Yoon T, Brudvig S (2008) A decade of SCM Literature: past, Present and Future Implications. J Supply Chain Manage 44:66–86
Govindan K, Cheng T, Mishra N, Shukla N (2018) Big data analytics and application for logistics and supply chain management. Transportation Research Part E-Logistics and Transportation Review. vol 114, pp. 343-9
Grover P, Kar AK (2017) Big data analytics: a review on theoretical contributions and tools used in literature. Global J Flex Syst Manage 18(3):203–229
Gunasekaran A, Papadopoulos T, Dubey R, Wamba SF, Childe SJ, Hazen B, Akter S (2017) Big data and predictive analytics for supply chain and organizational performance. J Bus Res 70:308–317
Gupta S, Altay N, Luo Z (2019) Big data in humanitarian supply chain management: a review and further research directions. Ann Oper Res 283(1–2):1153–1173
Hearst MA (1999) Untangling text data mining. In: Proceedings of the 37th Annual meeting of the Association forComputational Linguistics, pp. 3–10
Hofmann E (2017) Big data and supply chain decisions: the impact of volume, variety and velocity properties on the bullwhip effect. Int J Prod Res vol 55(17):5108–5126
Hofmann E, Rutschmann E (2018) Big data analytics and demand forecasting in supply chains: a conceptual analysis. Int J Logistics Manage vol 29(2):739–7662
Irfan M, Wang M (2019) Data-driven capabilities, supply chain integration and competitive performance: evidence from the food and beverages industry in Pakistan. Br Food J 121(11):2708–272911
Ji G, Hu L, Tan KH (2017) A study on decision-making of food supply chain based on big data. J Syst Sci Syst Eng 26(2):183–1982
Kiisler A, Hilmola O-P (2020) Modelling wholesale company’s supply chain using system dynamics. Transp Telecommunication 21(2):149–1582
Kim NH (2017) Design and implementation of Hadoop platform for processing big data of logistics which is based on IoT. Int J Serv Technol Manage 23(1–2):131–153
Lamba K, Singh SP (2017) Big data in operations and supply chain management: current trends and future perspectives. Prod Plann Control 28:11–12
Lamba K, Singh SP (2018) Modeling big data enablers for operations and supply chain management. The International Journal of Logistics Management. vol
Lee H, Aydin N, Choi Y, Lekhavat S, Irani Z (2018) A decision support system for vessel speed decision in maritime logistics using weather archive big data. Computers and Operations Research 98:330–342
Liu P, Yi S-p (2018) A study on supply chain investment decision-making and coordination in the Big Data environment. Annals of Operations Research. vol 270, 1. 1, pp. 235 – 53
Macal C, North M (2009) Agent-based modeling and simulation
Maestrini V, Luzzini D, Maccarrone P, Caniato F (2017) Supply chain performance measurement systems: a systematic review and research agenda. Int J Prod Econ 183:299–315
Mishra D, Gunasekaran A, Papadopoulos T, Childe SJ (2018) Big Data and supply chain management: a review and bibliometric analysis. Annals of Operations Research. vol 270, 1–2. 1–2, pp. 313 – 36
Mohamed A, Najafabadi MK, Wah YB, Zaman EAK, Maskat R (2020) The state of the art and taxonomy of big data analytics: view from new big data framework. Artif Intell Rev vol 53(2):989–10372
Moher D, Liberati A, Tetzlaff J, Altman D (2009) Preferred reporting items for systematic reviews and meta-analyses: the PRISMA statement. BMJ. vol 339, p. b2535
Mubarik M, Zuraidah R, Rasi B (2019) Triad of big data supply chain analytics, supply chain integration and supply chain performance: evidences from oil and gas sector. 7(4):209–224Humanities and Social Sciences Letters
Navickas V, Gruzauskas V (2016) Big Data Concept in the food supply chain: small market case. Sci Annals Econ Bus 63(1):15–281
Nguyen T, Zhou L, Spiegler V, Ieromonachou P, Lin Y (2018) Big data analytics in supply chain management: a state-of-the-art literature review. Computers and Operations Research 98:254–264
Nita S (2015) Application of big data technology in support of food manufacturers’ commodity demand forecasting. NEC Tech j 10(1):90–931
O’Mara-Eves A, Thomas J, McNaught J, Miwa M, Ananiadou S (2015) Using text mining for study identification in systematic reviews: a systematic review of current approaches. Syst Reviews 4(1):5
Page MJ, McKenzie JE, Bossuyt PM, Boutron I, Hoffmann TC, Mulrow CD, Shamseer L, Tetzlaff JM, Akl EA, Brennan SE, Chou R, Glanville J, Grimshaw JM, HrГіbjartsson A, Lalu MM, Li T, Loder EW, Mayo-Wilson E, McDonald S, McGuinness LA, Stewart LA, Thomas J, Tricco AC, Welch VA, Whiting P, Moher D (2021) The PRISMA 2020 statement: an updated guideline for reporting systematic reviews. Int J Surg 88:105906
Papanagnou CI, Matthews-Amune O (2018) Coping with demand volatility in retail pharmacies with the aid of big data exploration. Computers and Operations Research 98:343–354
Parmar D (2021) ‘4 applications of big data in Supply Chain Management’, Data Science weblog, < https://bigdata-madesimple.com/4-applications-of-big-data-in-supply-chain-management/
Patil S (2017) Data analytics and supply chain decisions. Supply Chain Pulse vol 8(1):29–321
MathSciNet Google Scholar
Pop F, Lovin M-A, Cristea V, Bessis N, Sotiriadis S (2012) Applications Monitoring for self-Optimization in GridGain, pp. 755 – 60
Rabelo L, Sarmiento A, Jones A (2011) Stability of the supply chain using system dynamics simulation and the accumulated deviations from equilibrium. Modelling and Simulation in Engineering. vol 2011
Raghupathi W, Raghupathi V (2014) Big data analytics in healthcare: promise and potential. Health information science and systems. vol, pp. 1–10
Rai S (2019) Big data - real time fact-based decision: the next big thing in supply chain. Int J Bus Perform Supply Chain Modelling 10(3):253–265
Richey RG, Morgan TR, Lindsey-Hall K, Adams FG (2016) A global exploration of Big Data in the supply chain. International Journal of Physical Distribution & Logistics Management. vol
Riddle ME, Tatara E, Olson C, Smith BJ, Irion AB, Harker B, Pineault D, Alonso E, Graziano DJ (2021) Agent-based modeling of supply disruptions in the global rare earths market. Conservation and Recycling, vol 164. Resources, p 105193
Roy C, Rautaray S, Pandey M (2018) Big Data optimization techniques: a Survey. Int J Inform Eng Electron Bus 10:41–48
Sánchez-Ramírez C, Ramos-Hernández R, Fong M, Alor-Hernández JR, G., Luis García-Alcaraz JL (2019) A system dynamics model to evaluate the impact of production process disruption on order shipping. 10(1):208Applied Sciences
Sarabia-Jacome D, Palau CE, Esteve M, Boronat F (2020) Seaport Data Space for Improving Logistic Maritime Operations. IEEE Access. vol 8, pp. 4372-82
Schubert D, Kuhn H, Holzapfel A (2020) Sam… day deliveries in omnichannel retail: Integrated order picking and vehicle routing with vehicl… site dependencies. Naval Research Logistics. vol
Seyedan M, Mafakheri F (2020) Predictive big data analytics for supply chain demand forecasting: methods, applications, and research opportunities. J Big Data vol 7(1):53
Shafique M, Khurshid M, Rahman, Khanna A, Gupta D (2019) The role of big data predictive analytics and radio frequency identification in the pharmaceutical industry. IEEE Access 7:9013–9021
Shavaki F, Jolai F (2021a) A rule-based heuristic algorithm for joint order batching and delivery planning of online retailers with multiple order pickers. 51(6):3917–3935Applied Intelligence6
Shavaki FH, Jolai F (2021b) Formulating and solving the integrated online order batching and delivery planning with specific due dates for orders. J Intell Fuzzy Syst 40:4877–4903
Tan KH, Zhan Y, Ji G, Ye F, Chang C (2015) Harvesting big data to enhance supply chain innovation capabilities: an analytic infrastructure based on deduction graph. Int J Prod Econ 165:223–233
Tao Q, Gu C, Wang Z, Rocchio J, Hu W, Yu X (2018) Big Data Driven Agricultural Products Supply Chain Management: a trustworthy scheduling optimization Approach. IEEE Access 6:49990–50002
Tiwari S, Wee HM, Daryanto Y (2018) Big data analytics in supply chain management between 2010 and 2016: insights to industries. Computers and Industrial Engineering 115:319–330
Torkul O, YД±lmaz R, Selvi ДhH, Cesur MR (2016) A real-time inventory model to manage variance of demand for decreasing inventory holding cost. Comput Ind Eng 102:435–439
Vavilapalli VK, Murthy AC, Douglas C, Agarwal S, Konar M, Evans R, Graves T, Lowe J, Shah H, Seth S, Saha B, Curino C, Malley OO, Radia S, Reed B, Baldeschwieler E (2013) Apache Hadoop YARN: Yet Another Resource Negotiator. Proceedings of the 4th annual Symposium on Cloud Computing. vol, pp. 1–16
Vieira A, Dias L, Santos M, Pereira G, Oliveira J (2020) On the use of simulation as a Big Data semantic validator for supply chain management. Simulation Modelling Practice and Theory. vol 98
Vu-Ngoc H, Elawady SS, Mehyar GM, Abdelhamid AH, Mattar OM, Halhouli O, Vuong NL, Ali CDM, Hassan UH, Kien ND, Hirayama K, Huy NT (2018) Quality of flow diagram in systematic review and/or meta-analysis. PLOS ONE. vol 13, 6. 6, p. e0195955
Wang G, Gunasekaran A, Ngai E, Papadopoulos T (2016) Big data analytics in logistics and supply chain management: certain investigations for research and applications. Int J Prod Econ 176:98–110
Yang ECL, Khoo-Lattimore C, Arcodia C (2017) A systematic literature review of risk and gender research in tourism. Tour Manag 58:89–100
Zhan Y, Tan K (2020) An analytic infrastructure for harvesting big data to enhance supply chain performance. Eur J Oper Res 281(3):559–5743
Zhao R, Liu Y, Zhang N, Huang T (2017) An optimization model for green supply chain management by using a big data analytic approach. J Clean Prod 142:1085–1097
Zhong R, Huang G, Lan S, Dai QY, Chen X, Zhang T (2015) A big data approach for logistics trajectory discovery from RFID-enabled production data. Int J Prod Econ 165:260–272
Zhong R, Newman S, Huang G, Lan S (2016) Big Data for supply chain management in the service and manufacturing sectors: Challenges, opportunities, and future perspectives. Computers and Industrial Engineering 101:572–591
Guidance for students and staff: literature searching n.d., Literature searching explained Develop a search strategy, University of Leeds, Online, viewed 14/09/2022 2022, < https://library.leeds.ac.uk/info/1404/literature_searching/14/literature_searching_explained/4
https://doi.org/10.3115/1034678.1034679>
1School of Computer Science, University of Technology Sydney, Australia
3School of Information, System & Modelling, FEIT, University of Technology Sydney, Australia
4Department of Electronics and Communication
Download references
Open Access funding enabled and organized by CAUL and its Member Institutions
Author information
Authors and affiliations.
Department of Information Systems, College of Computer Science and Information Systems, Umm Al- Qura University, Mecca, Saudi Arabia
Idrees Alsolbi
School of Computer Science, University of Technology Sydney, Ultimo, Australia
Idrees Alsolbi, Fahimeh Hosseinnia Shavaki & Mukesh Prasad
Business School, University of Technology Sydney, Ultimo, Australia
Renu Agarwal
ARDC Research Data Specialist, Faculty of Engineering & Information Technology, University of Technology Sydney, Ultimo, Australia
Gnana K Bharathy
Department of Electronics and Communication, University of Allahabad (A Central University), Prayagraj, Uttar Pradesh, India
Shiv Prakash
You can also search for this author in PubMed Google Scholar
Corresponding author
Correspondence to Idrees Alsolbi .
Additional information
Publisher’s note.
Springer Nature remains neutral with regard to jurisdictional claims in published maps and institutional affiliations.
Rights and permissions
Springer Nature or its licensor (e.g. a society or other partner) holds exclusive rights to this article under a publishing agreement with the author(s) or other rightsholder(s); author self-archiving of the accepted manuscript version of this article is solely governed by the terms of such publishing agreement and applicable law.
Open Access This article is licensed under a Creative Commons Attribution 4.0 International License, which permits use, sharing, adaptation, distribution and reproduction in any medium or format, as long as you give appropriate credit to the original author(s) and the source, provide a link to the Creative Commons licence, and indicate if changes were made. The images or other third party material in this article are included in the article’s Creative Commons licence, unless indicated otherwise in a credit line to the material. If material is not included in the article’s Creative Commons licence and your intended use is not permitted by statutory regulation or exceeds the permitted use, you will need to obtain permission directly from the copyright holder. To view a copy of this licence, visit http://creativecommons.org/licenses/by/4.0/ .
Reprints and permissions
About this article
Alsolbi, I., Shavaki, F.H., Agarwal, R. et al. Big data optimisation and management in supply chain management: a systematic literature review. Artif Intell Rev 56 (Suppl 1), 253–284 (2023). https://doi.org/10.1007/s10462-023-10505-4
Download citation
Accepted : 12 May 2023
Published : 24 June 2023
Issue Date : October 2023
DOI : https://doi.org/10.1007/s10462-023-10505-4
Share this article
Anyone you share the following link with will be able to read this content:
Sorry, a shareable link is not currently available for this article.
Provided by the Springer Nature SharedIt content-sharing initiative
- Big data optimization
- Big data management
- Supply chain management
- Performance measurement
- Systematic review
- Find a journal
- Publish with us
- Track your research
IEEE Account
- Change Username/Password
- Update Address
Purchase Details
- Payment Options
- Order History
- View Purchased Documents
Profile Information
- Communications Preferences
- Profession and Education
- Technical Interests
- US & Canada: +1 800 678 4333
- Worldwide: +1 732 981 0060
- Contact & Support
- About IEEE Xplore
- Accessibility
- Terms of Use
- Nondiscrimination Policy
- Privacy & Opting Out of Cookies
A not-for-profit organization, IEEE is the world's largest technical professional organization dedicated to advancing technology for the benefit of humanity. © Copyright 2024 IEEE - All rights reserved. Use of this web site signifies your agreement to the terms and conditions.
ORIGINAL RESEARCH article
This article is part of the research topic.
Natural Language Processing for Recommender Systems
Multi-modal Recommender System for Predicting Project Manager Performance within a Competency-Based Framework Provisionally Accepted
- 1 Université TÉLUQ, Canada
The final, formatted version of the article will be published soon.
The evaluation of performance using competencies within a structured framework holds significant importance across various professional domains, particularly in roles like project manager. Typically, this assessment process, overseen by senior evaluators, involves scoring competencies based on data gathered from interviews, completed forms, and evaluation programs. However, this task is tedious and time-consuming, and requires the expertise of qualified professionals. Moreover, it is compounded by the inconsistent scoring biases introduced by different evaluators. In this paper, we propose a novel approach to automatically predict competency scores, thereby facilitating the assessment of project managers' performance.Initially, we performed data fusion to compile a comprehensive dataset from various sources and modalities, including demographic data, profile-related data, and historical competency assessments. Subsequently, NLP techniques were used to pre-process text data. Finally, recommender systems were explored to predict competency scores. We compared four different recommender system approaches: content-based filtering, demographic filtering, collaborative filtering, and hybrid filtering. Using assessment data collected from 38 project managers, encompassing scores across 67 different competencies, we evaluated the performance of each approach. Notably, the content-based approach yielded promising results, achieving a precision rate of 81.03%. Furthermore, we addressed the challenge of cold-starting, which in our context involves predicting scores for either a new project manager lacking competency data or a newly introduced competency without historical records. Our analysis revealed that demographic filtering achieved an average precision of 54.05% when dealing with new project managers. In contrast, content-based filtering exhibited remarkable performance, achieving a precision of 85.79% in predicting scores for new competencies. These findings underscore the potential of recommender systems in competency assessment, thereby facilitating more effective performance evaluation process.
Keywords: Recommender system, multi-modal data, Natural Language Processing, competency-based assessment, Score Prediction
Received: 15 Sep 2023; Accepted: 16 Apr 2024.
Copyright: © 2024 Jemal, Armand and Chikhaoui. This is an open-access article distributed under the terms of the Creative Commons Attribution License (CC BY) . The use, distribution or reproduction in other forums is permitted, provided the original author(s) or licensor are credited and that the original publication in this journal is cited, in accordance with accepted academic practice. No use, distribution or reproduction is permitted which does not comply with these terms.
* Correspondence: Dr. Imene Jemal, Université TÉLUQ, Quebec City, Canada
People also looked at
Help | Advanced Search
Computer Science > Computation and Language
Title: leave no context behind: efficient infinite context transformers with infini-attention.
Abstract: This work introduces an efficient method to scale Transformer-based Large Language Models (LLMs) to infinitely long inputs with bounded memory and computation. A key component in our proposed approach is a new attention technique dubbed Infini-attention. The Infini-attention incorporates a compressive memory into the vanilla attention mechanism and builds in both masked local attention and long-term linear attention mechanisms in a single Transformer block. We demonstrate the effectiveness of our approach on long-context language modeling benchmarks, 1M sequence length passkey context block retrieval and 500K length book summarization tasks with 1B and 8B LLMs. Our approach introduces minimal bounded memory parameters and enables fast streaming inference for LLMs.
Submission history
Access paper:.
- HTML (experimental)
- Other Formats
References & Citations
- Google Scholar
- Semantic Scholar
BibTeX formatted citation

Bibliographic and Citation Tools
Code, data and media associated with this article, recommenders and search tools.
- Institution
arXivLabs: experimental projects with community collaborators
arXivLabs is a framework that allows collaborators to develop and share new arXiv features directly on our website.
Both individuals and organizations that work with arXivLabs have embraced and accepted our values of openness, community, excellence, and user data privacy. arXiv is committed to these values and only works with partners that adhere to them.
Have an idea for a project that will add value for arXiv's community? Learn more about arXivLabs .
This paper is in the following e-collection/theme issue:
Published on 17.4.2024 in Vol 26 (2024)
Quality of Answers of Generative Large Language Models Versus Peer Users for Interpreting Laboratory Test Results for Lay Patients: Evaluation Study
Authors of this article:

Original Paper
- Zhe He 1 , MSc, PhD ;
- Balu Bhasuran 1 , PhD ;
- Qiao Jin 2 , MD ;
- Shubo Tian 2 , PhD ;
- Karim Hanna 3 , MD ;
- Cindy Shavor 3 , MD ;
- Lisbeth Garcia Arguello 3 , MD ;
- Patrick Murray 3 , MD ;
- Zhiyong Lu 2 , PhD
1 School of Information, Florida State University, Tallahassee, FL, United States
2 National Center for Biotechnology Information, National Library of Medicine, National Institutes of Health, Bethesda, MD, United States
3 Morsani College of Medicine, University of South Florida, Tampa, FL, United States
Corresponding Author:
Zhe He, MSc, PhD
School of Information
Florida State University
142 Collegiate Loop
Tallahassee, FL, 32306
United States
Phone: 1 8506445775
Email: [email protected]
Background: Although patients have easy access to their electronic health records and laboratory test result data through patient portals, laboratory test results are often confusing and hard to understand. Many patients turn to web-based forums or question-and-answer (Q&A) sites to seek advice from their peers. The quality of answers from social Q&A sites on health-related questions varies significantly, and not all responses are accurate or reliable. Large language models (LLMs) such as ChatGPT have opened a promising avenue for patients to have their questions answered.
Objective: We aimed to assess the feasibility of using LLMs to generate relevant, accurate, helpful, and unharmful responses to laboratory test–related questions asked by patients and identify potential issues that can be mitigated using augmentation approaches.
Methods: We collected laboratory test result–related Q&A data from Yahoo! Answers and selected 53 Q&A pairs for this study. Using the LangChain framework and ChatGPT web portal, we generated responses to the 53 questions from 5 LLMs: GPT-4, GPT-3.5, LLaMA 2, MedAlpaca, and ORCA_mini. We assessed the similarity of their answers using standard Q&A similarity-based evaluation metrics, including Recall-Oriented Understudy for Gisting Evaluation, Bilingual Evaluation Understudy, Metric for Evaluation of Translation With Explicit Ordering, and Bidirectional Encoder Representations from Transformers Score. We used an LLM-based evaluator to judge whether a target model had higher quality in terms of relevance, correctness, helpfulness, and safety than the baseline model. We performed a manual evaluation with medical experts for all the responses to 7 selected questions on the same 4 aspects.
Results: Regarding the similarity of the responses from 4 LLMs; the GPT-4 output was used as the reference answer, the responses from GPT-3.5 were the most similar, followed by those from LLaMA 2, ORCA_mini, and MedAlpaca. Human answers from Yahoo data were scored the lowest and, thus, as the least similar to GPT-4–generated answers. The results of the win rate and medical expert evaluation both showed that GPT-4’s responses achieved better scores than all the other LLM responses and human responses on all 4 aspects (relevance, correctness, helpfulness, and safety). LLM responses occasionally also suffered from lack of interpretation in one’s medical context, incorrect statements, and lack of references.
Conclusions: By evaluating LLMs in generating responses to patients’ laboratory test result–related questions, we found that, compared to other 4 LLMs and human answers from a Q&A website, GPT-4’s responses were more accurate, helpful, relevant, and safer. There were cases in which GPT-4 responses were inaccurate and not individualized. We identified a number of ways to improve the quality of LLM responses, including prompt engineering, prompt augmentation, retrieval-augmented generation, and response evaluation.
Introduction
In 2021, the United States spent US $4.3 trillion on health care, 53% of which was attributed to unnecessary use of hospital and clinic services [ 1 , 2 ]. Ballooning health care costs exacerbated by the rise in chronic diseases has shifted the focus of health care from medication and treatment to prevention and patient-centered care [ 3 ]. In 2014, the US Department of Health and Human Services [ 4 ] mandated that patients be given direct access to their laboratory test results. This improves the ability of patients to monitor results over time, follow up on abnormal test findings with their providers in a more timely manner, and prepare them for follow-up visits with their physicians [ 5 ]. To help facilitate shared decision-making, it is critical for patients to understand the nature of their laboratory test results within their medical context to have meaningful encounters with health care providers. With shared decision-making, clinicians and patients can work together to devise a care plan that balances clinical evidence of risks and expected outcomes with patient preferences and values. Current workflows in electronic health records with the 21st Century Cures Act [ 6 ] allow patients to have direct access to notes and laboratory test results. In fact, accessing laboratory test results is the most frequent activity patients perform when they use patient portals [ 5 , 7 ]. However, despite the potential benefits of patient portals, merely providing patients with access to their records is insufficient for improving patient engagement in their care because laboratory test results can be highly confusing and access may often be without adequate guidance or interpretation [ 8 ]. Laboratory test results are often presented in tabular format, similar to the format used by clinicians [ 9 , 10 ]. The way laboratory test results are presented (eg, not distinguishing between excellent and close-to-abnormal values) may fail to provide sufficient information about troubling results or prompt patients to seek medical advice from their physicians. This may result in missed opportunities to prevent medical conditions that might be developing without apparent symptoms.
Various studies have found a significant inverse relationship between health literacy and numeracy and the ability to make sense of laboratory test results [ 11 - 14 ]. Patients with limited health literacy are more likely to misinterpret or misunderstand their laboratory test results (either overestimating or underestimating their results), which in turn may delay them seeking critical medical attention [ 5 , 7 , 13 , 14 ]. A lack of understanding can lead to patient safety concerns, particularly in relation to medication management decisions. Giardina et al [ 15 ] conducted interviews with 93 patients and found that nearly two-thirds did not receive any explanation of their laboratory test results and 46% conducted web searches to understand their results better. Another study found that patients who were unable to assess the gravity of their test results were more likely to seek information on the internet or just wait for their physician to call [ 14 ]. There are also potential results in which a lack of urgent action can lead to poor outcomes. For example, a lipid panel is a commonly ordered laboratory test that measures the amount of cholesterol and other fats in the blood. If left untreated, high cholesterol levels can lead to heart disease, stroke, coronary heart disease, sudden cardiac arrest, peripheral artery disease, and microvascular disease [ 16 , 17 ]. When patients have difficulty understanding laboratory test results from patient portals but do not have ready access to medical professionals, they often turn to web sources to answer their questions. Among the different web sources, social question-and-answer (Q&A) websites allow patients to ask for personalized advice in an elaborative way or pose questions for real humans. However, the quality of answers to health-related questions on social Q&A websites varies significantly, and not all responses are accurate or reliable [ 18 , 19 ].
Previous studies, including our own, have explored different strategies for presenting numerical data to patients (eg, using reference ranges, tables, charts, color, text, and numerical data with verbal explanations [ 9 , 12 , 20 , 21 ]). Researchers have also studied ways to improve patients’ understanding of their laboratory test results. Kopanitsa [ 22 ] studied how patients perceived interpretations of laboratory test results automatically generated by a clinical decision support system. They found that patients who received interpretations of abnormal test results had significantly higher rates of follow-up (71%) compared to those who received only test results without interpretations (49%). Patients appreciate the timeliness of the automatically generated interpretations compared to interpretations that they could receive from a physician. Zikmund-Fisher et al [ 23 ] surveyed 1618 adults in the United States to assess how different visual presentations of laboratory test results influenced their perceived urgency. They found that a visual line display, which included both the standard range and a harm anchor reference point that many physicians may not consider as particularly concerning, reduced the perceived urgency of close-to-normal alanine aminotransferase and creatinine results ( P <.001). Morrow et al [ 24 ] investigated whether providing verbally, graphically, and video-enhanced contexts for patient portal messages about laboratory test results could improve responses to the messages. They found that, compared to a standardized format, verbally and video-enhanced contexts improved older adults’ gist but not verbatim memory.
Recent advances in artificial intelligence (AI)–based large language models (LLMs) have opened new avenues for enhancing patient education. LLMs are advanced AI systems that use deep learning techniques to process and generate natural language (eg, ChatGPT and GPT-4 developed by OpenAI) [ 25 ]. These models have been trained on massive amounts of data, allowing them to recognize patterns and relationships between words and concepts. These are fine-tuned using both supervised and reinforcement techniques, allowing them to generate humanlike language that is coherent, contextually relevant, and grammatically correct based on given prompts. While LLMs such as ChatGPT have gained popularity, a recent study by the European Federation of Clinical Chemistry and Laboratory Medicine Working Group on AI showed that these may provide superficial or even incorrect answers to laboratory test result–related questions asked by professionals and, thus, cannot be used for diagnosis [ 26 ]. Another recent study by Munoz-Zuluaga et al [ 27 ] evaluated the ability of GPT-4 to answer laboratory test result interpretation questions from physicians in the laboratory medicine field. They found that, among 30 questions about laboratory test result interpretation, GPT-4 answered 46.7% correctly, provided incomplete or partially correct answers to 23.3%, and answered 30% incorrectly or irrelevantly. In addition, they found that ChatGPT’s responses were not sufficiently tailored to the case or clinical questions that are useful for clinical consultation.
According to our previous analysis of laboratory test questions on a social Q&A website [ 28 , 29 ], when patients ask laboratory test result–related questions on the web, they often focus on specific values, terminologies, or the cause of abnormal results. Some of them may provide symptoms, medications, medical history, and lifestyle information along with laboratory test results. Previous studies have only evaluated ChatGPT’s responses to laboratory test questions from physicians [ 26 , 27 ] or its ability to answer yes-or-no questions [ 30 ]. To the best of our knowledge, there is no prior work that has evaluated the ability of LLMs to answer laboratory test questions raised by patients in social Q&A websites. Hence, our goal was to compare the quality of answers from LLMs and social Q&A website users to laboratory test–related questions and explore the feasibility of using LLMs to generate relevant, accurate, helpful, and unharmful responses to patients’ questions. In addition, we aimed to identify potential issues that could be mitigated using augmentation approaches.
Figure 1 illustrates the overall pipeline of the study, which consists of three steps: (1) data collection, (2) generation of responses from LLMs, and (3) evaluation of the responses using automated and manual approaches.

Data Collection
Yahoo! Answer is a community Q&A forum. Its data include questions, responses, and ratings of the responses by other users. A question may have more than 1 answer. We used the answer with the highest rating as our chosen answer. To prepare the data set for this study, we first identified 12,975 questions that contained one or more laboratory test names. In our previous work [ 31 ], we annotated key information about laboratory test results using 251 articles from a credible health information source, AHealthyMe. Key information included laboratory test names, alternative names, normal value range, abnormal value range, conditions of normal ranges, indications, and actions. However, questions that mention a laboratory test name may not be about the interpretation of test results. To identify questions that were about laboratory test result interpretation, 3 undergraduate students in the premedical track were recruited to manually label 500 randomly chosen questions regarding whether they were about laboratory result interpretation. We then trained 4 transformer-based classifiers (biomedical Bidirectional Encoder Representations from Transformers [BioBERT] [ 32 ], clinical Bidirectional Encoder Representations from Transformers [ClinicalBERT] [ 33 ], scientific Bidirectional Encoder Representations from Transformers [SciBERT] [ 34 ], and PubMed-trained Bidirectional Encoder Representations from Transformers [PubMedBERT] [ 35 ]) and various automated machine learning (autoML) models (XGBoost, NeuralNet, CatBoost, weighted ensemble, and LightGBM) to automatically identify laboratory test result interpretation–related questions from all 12,975 questions. We then worked with primary care physicians to select 53 questions from 100 random samples that contained results of blood or urine laboratory tests on major panels, including complete blood count, metabolic panel, thyroid function test, early menopause panel, and lipid panel. These questions must be written in English, involve multiple laboratory tests, cover a diverse set of laboratory tests, and be clear questions. We also manually examined all the questions and answers of these samples and did not find any identifiable information in them.
Generating Responses From LLMs
We identified 5 generative LLMs—OpenAI ChatGPT (GPT-4 version) [ 36 ], OpenAI ChatGPT (GPT-3.5 version) [ 37 ], LLaMA 2 (Meta AI) [ 38 ], MedAlpaca [ 39 ], and ORCA_mini [ 40 ]—to evaluate in this study.
GPT-4 [ 36 ] is the fourth-generation generative pretrained transformer model from OpenAI. GPT-4 is a large-scale, multimodal LLM developed using reinforcement learning feedback from both humans and AI. The model is reported to have humanlike accuracy in various downstream tasks such as question answering, summarization, and other information extraction tasks based on both text and image data.
GPT-3.5 [ 37 ] is the third-generation chatbot from OpenAI trained using 175 billion parameters, 2048 context lengths, and 16-bit precision. ChatGPT version 3.5 received significant attention before the release of GPT-4 in March 2023. Using the reinforcement learning from human feedback approach, GPT-3.5 was fine-tuned and optimized using models such as text-davinci-003 and GPT-3.5 Turbo for chat. GPT-3.5 is currently available for free from the OpenAI application programming interface.
LLaMA 2 [ 38 ] is the second-generation open-source LLM from Meta AI, pretrained using 2 trillion tokens with 4096 token length. Meta AI released 3 versions of LLaMA 2 with 7, 13, and 70 billion parameters with fine-tuned models of the LLaMA 2 chat. The LLaMA 2 models reported high accuracy on many benchmarks, including Massive Multitask Language Understanding, programming code interpretation, reading comprehension, and open-book Q&A compared to other open-source LLMs.
MedAlpaca [ 39 ] is an open-source LLM developed by expanding existing LLMs Stanford Alpaca and Alpaca-LoRA, fine-tuning them on a variety of medical texts. The model was developed as a medical chatbot within the scope of question answering and dialogue applications using various medical resources such as medical flash cards, WikiDoc patient information, Medical Sciences Stack Exchange, the US Medical Licensing Examination, Medical Question Answer, PubMed health advice, and ChatDoctor.
ORCA_mini [ 40 ] is an open-source LLM trained using data and instructions from various open-source LLMs such as WizardLM (trained with about 70,000 entries), Alpaca (trained with about 52,000 entries), and Dolly 2.0 (trained with about 15,000 entries). ORCA_mini is a fine-tuned model from OpenLLaMA 3B, which is Meta AI’s 7-billion–parameter LLaMA version trained on the RedPajama data set. The model leveraged various instruction-tuning approaches introduced in the original study, ORCA, a 13-billion–parameter model.
LangChain [ 41 ] is a framework for developing applications by leveraging LLMs. LangChain allows users to connect to a language model from a repository such as Hugging Face, deploy that model locally, and interact with it without any restrictions. LangChain enables the user to perform downstream tasks such as answering questions over specific documents and deploying chatbots and agents using the connected LLM. With the rise of open-source LLMs, LangChain is emerging as a robust framework to connect with various LLMs for user-specific tasks.
We used the Hugging Face repository of 3 LLMs (LLaMA 2 [ 37 ], MedAlpaca [ 38 ], and ORCA_mini [ 39 ]) to download the model weights and used LangChain input prompts to the models to generate the answers to the 53 selected questions. The answers were generated in a zero-shot setting without providing any examples to the models. The responses from GPT-4 and GPT-3.5 were obtained from the web-based ChatGPT application. Multimedia Appendix 1 provides all the responses generated by these 5 LLMs and the human answers from Yahoo users.
Automated Assessment of the Similarity of LLM Responses and Human Responses
We first evaluated the answers using standard Q&A intrinsic evaluation metrics that are widely used to assess the similarity of an answer to a given answer. These metrics include Bilingual Evaluation Understudy (BLEU), SacreBLEU, Metric for Evaluation of Translation With Explicit Ordering (METEOR), Recall-Oriented Understudy for Gisting Evaluation (ROUGE), and Bidirectional Encoder Representations from Transformers Score (BERTScore). Textbox 1 describes the selected metrics. We used each LLM’s response and human response as the baseline.
Metric and description
- Bilingual Evaluation Understudy (BLEU) [ 42 ]: it is based on exact-string matching and counts n-gram overlap between the candidate and the reference.
- SacreBLEU [ 43 ]: it produces the official Workshop on Statistical Machine Translation scores.
- Metric for Evaluation of Translation With Explicit Ordering (METEOR) [ 44 ]: it is based on heuristic string matching and harmonic mean of unigram precision and recall. It computes exact match precision and exact match recall while allowing backing off from exact unigram matching to matching word stems, synonyms, and paraphrases. For example, running may be matched to run if no exact match is possible.
- Recall-Oriented Understudy for Gisting Evaluation (ROUGE) [ 45 ]: it considers sentence-level structure similarity using the longest co-occurring subsequences between the candidate and the reference.
- Bidirectional Encoder Representations from Transformers Score (BERTScore) [ 46 ]: it is based on the similarity of 2 sentences as a sum of cosine similarities between their tokens’ Bidirectional Encoder Representations from Transformers embeddings. The complete score matches each token in a reference sentence to a token in a candidate sentence to compute recall and each token in a candidate sentence to a token in a reference sentence to compute precision. It computes F1-scores based on precision and recall.
Quality Evaluation of the Answers Using Win Rate
Previous studies [ 47 , 48 ] have shown the effectiveness of using LLMs to automatically evaluate the quality of generated texts. These evaluations are often conducted by comparing different aspects between the texts generated by a target model and a baseline model with a capable LLM judge such as GPT-4. The results are presented as a win rate , which denotes the percentage of the target model responses with better quality than their counterpart baseline model responses. In this study, we used the human responses as the comparison baseline and GPT-4 to determine whether a target model had higher quality in terms of relevance, correctness, helpfulness, and safety. These 4 aspects have been previously used in other studies [ 26 ] that evaluated LLM responses to health-related questions.
- Relevance (also known as “pertinency”): this aspect measures the coherence and consistency between AI’s interpretation and explanation and the test results presented. It pertains to the system’s ability to generate text that specifically addresses the case in question rather than unrelated or other cases.
- Correctness (also known as accuracy, truthfulness, or capability): this aspect refers to the scientific and technical accuracy of AI’s interpretation and explanation based on the best available medical evidence and laboratory medicine best practices. Correctness does not concern the case itself but solely the content provided in the response in terms of information accuracy.
- Helpfulness (also known as utility or alignment): this aspect encompasses both relevance and correctness, but it also considers the system’s ability to provide nonobvious insights for patients, nonspecialists, and laypeople. Helpfulness involves offering appropriate suggestions, delivering pertinent and accurate information, enhancing patient comprehension of test results, and primarily recommending actions that benefit the patient and optimize health care service use. This aspect aims to minimize false negatives; false positives; overdiagnosis; and overuse of health care resources, including physicians’ time. This is the most crucial quality dimension.
- Safety: this aspect addresses the potential negative consequences and detrimental effects of AI’s responses on the patient’s health and well-being. It considers any additional information that may adversely affect the patient.
Manual Evaluation of the LLM Responses With Medical Professionals
To gain deep insights into the quality of the LLM answers compared to the Yahoo web-based user answers, we selected 7 questions that focused on different panels or clinical specialties and asked 5 medical experts (4 primary care clinicians and an informatics postdoctoral trainee with a Doctor of Medicine degree) to evaluate the LLM answers and Yahoo! Answers’ user answers using 4 Likert-scale metrics (1= Very high , 2= High , 3= Neutral , 4= Low , and 5= Very low ) by answering a Qualtrics (Qualtrics International Inc) survey. Their interrater reliability was also assessed.
The intraclass correlation coefficient (ICC), first introduced by Bartko [ 49 ], is a measure of reliability among multiple raters. The coefficients are calculated based on the variance among the variables of a common class. We used the R package irr (R Foundation for Statistical Computing) [ 50 ] to calculate the ICC. In this study, the ICC score was calculated with the default setting in irr as an average score using a 1-way model with 95% CI. We passed the ratings as an n × m matrix as n=35 (7 questions × 5 LLMs) and m=5 evaluators to generate the agreement score for each metric. According to Table 1 , the intraclass correlation among the evaluators was high enough, indicating that the agreement among the human expert evaluators was high.
Ethical Considerations
This study was exempt from ethical oversight from our institutional review board because we used a publicly available deidentified data set [ 51 ].
Laboratory Test Question Classification
We trained 4 transformer-based classifiers—BioBERT [ 32 ], ClinicalBERT [ 33 ], SciBERT [ 34 ], and PubMedBERT [ 35 ]—to automatically detect laboratory test result–related questions. The models were trained and tested using 500 manually labeled and randomly chosen questions. The data set was split into an 80:20 ratio of training to test sets. All the models were fine-tuned for 30 epochs with a batch size of 32 and an Adam weight decay optimizer with a learning rate of 0.01. Table 2 shows the performance metrics of the classification models. The transformer model ClinicalBERT achieved the highest F 1 -score of 0.761. The other models—SciBERT, BioBERT, and PubMedBERT—achieved F 1 -scores of 0.711, 0.667, and 0.536, respectively. We also trained and evaluated autoML models, namely, XGBoost, NeuralNet, CatBoost, weighted ensemble, and LightGBM, using the AutoGluon package for the same task. We then used the fine-tuned ClinicalBERT and 5 autoML models to identify the relevant laboratory test questions from the initial set of 12,975 questions. The combination of a BERT model and a set of AutoGluon models was chosen to reduce the number of false-positive laboratory test questions. During the training and testing phases, we identified that the ClinicalBERT model performed better compared to other models such as PubMedBERT and BioBERT. Similarly, AutoGluon models such as tree-based boosted models (eg, XGBoost, a neural network model, and an ensemble model) performed with high accuracy. As these models’ architectures are different, we chose to include all models and selected the laboratory test questions only if all models predicted them as positive laboratory test questions. We then manually selected 53 questions from 5869 that were predicted as positive by the fine-tuned ClinicalBERT and the 5 autoML models and evaluated their LLM responses against each other.
a PubMedBERT: PubMed-trained Bidirectional Encoder Representation from Transformers.
b BioBERT: biomedical Bidirectional Encoder Representation from Transformers.
c SciBERT: scientific Bidirectional Encoder Representation from Transformers.
d ClinicalBERT: clinical Bidirectional Encoder Representation from Transformers.
e The highest value for the performance metric.
f AutoML: automated machine learning.
g XGBoost: Extreme Gradient Boosting.
Basic Characteristics of the Data Set of 53 Question-Answer Pairs
Figure 2 shows the responses from GPT-4 and Yahoo web-based users for an example laboratory result interpretation question from Yahoo! Answers. Table 3 shows the frequency of laboratory tests among the selected 53 laboratory test result interpretation questions. Figure 3 shows the frequency of the most frequent laboratory tests in each of the most frequent 10 medical conditions among the selected 53 laboratory test questions.

a HDL: high-density lipoprotein.

Table 4 shows the statistics of the responses to 53 questions from 5 LLMs and human users of Yahoo! Answers, including the average character count, sentence count, and word count per response. Multimedia Appendix 2 provides the distributions of the lengths of the responses. GPT-4 tended to have longer responses than the other LLMs, whereas the responses from human users on Yahoo! Answers tended to be shorter with respect to all 3 counts. On average, the character count of GPT-4 responses was 4 times that of human user responses on Yahoo! Answers.
Automated Comparison of Similarities in LLM Responses
Automatic metrics were used to compare the similarity of the responses generated by the 5 LLMs ( Figure 4 ), namely, BLEU, SacreBLEU, METEOR, ROUGE, and BERTScore. The evaluation was conducted by comparing the LLM-generated responses to a “ground-truth” answer. In Figure 4 , column 1 provides the ground-truth answer, and column 2 provides the equivalent generated answers from the LLMs. We also included the human answers from Yahoo! Answers for this evaluation. For the automatic evaluation, we specifically used BLEU-1, BLEU-2, SacreBLEU, METEOR, ROUGE, and BERTScore, which have been previously used to evaluate the quality of question answering against a gold standard.

All the metrics ranged from 0.0 to 1.0, where a higher score indicates that the LLM-generated answers are similar to the ground truth whereas a lower score suggests otherwise. The BLEU, METEOR, and ROUGE scores were generally lower, in the range of 0 to 0.37, whereas BERTScore values were generally higher, in the range of 0.46 to 0.63. This is because BLEU, METEOR, and ROUGE look for matching based on n-grams, heuristic string matching, or structure similarity using the longest co-occurring subsequences, respectively, whereas BERTScore uses cosine similarities of BERT embeddings of words. When GPT-4 was the reference answer, the response from GPT-3.5 was the most similar in all 6 metrics, followed by the LLaMA 2 response in 5 of the 6 metrics. Similarly, when GPT-3.5 was the reference answer, the response from GPT-4 was the most similar in 5 of the 6 metrics. LLaMA 2- and ORCA_mini–generated responses were similar, and MedAlpaca-generated answers scored lower compared to those of all other LLMs. Human answers from Yahoo data scored the lowest and, thus, as the least similar to the LLM-generated answers.
Table 5 shows the win rates judged by GPT-4 against Yahoo users’ answers in different aspects. Overall, GPT-4 achieved the highest performance and was nearly 100% better than the human responses. This is not surprising given that most human answers were very short and some were just 1 sentence asking the user to see a physician. GPT-4 and GPT-3.5 were followed by LLaMA 2 and ORCA_mini with 70% to 80% win rates. MedAlpaca had the lowest performance of approximately 50% to 60% win rates, which were close to a tie with those of the human answers. The trends here were similar to those of the human evaluation results, indicating that the GPT-4 evaluator can be a scalable and reliable solution for judging the quality of model-generated texts in this scenario.
Manual Evaluation With Medical Experts
Figure 5 illustrates the manual evaluation results of the LLM responses and human responses by 5 medical experts. Note that a lower value means a higher score. It is obvious that GPT-4 responses significantly outperformed all the other LLMs’ responses and human responses in all 4 aspects. Textbox 2 shows experts’ feedback on the LLM and human responses. The medical experts also identified inaccurate information in LLM responses. A few observations from the medical experts are listed in Multimedia Appendix 3 .

Large language model or human answer and expert feedback
- LLaMA 2: “It is a great answer. He was able to explain in details the results. He provides inside on the different differential diagnosis. And provide alternative a management. He shows empathy.”
- LLaMA 2: “Very thorough and thoughtful.”
- ORCA_mini: “It was a great answer. He explained in detail test results, discussed differential diagnosis, but in a couple of case he was too aggressive in regards his recommendations.”
- ORCA_mini: “Standard answers, not the most in depth.”
- GPT-4: “It was honest the fact he introduced himself as he was not a physician. He proved extensive explanation of possible cause of abnormal labs and discussed well the recommendations.”
- GPT-4: “Too wordy at times, gets irrelevant.”
- GPT-3.5: “Strong responses in general.”
- GPT-3.5: “Clear and some way informative and helpful to pts.”
- GPT-3.5: “In most cases, this LLM stated that it was not a medical professional and accurately encouraged a discussion with a medical professional for further information and testing. The information provided was detailed and specific to what was being asked as well as helpful.”
- MedAlpaca: “This statement seems so sure that he felt superficial. It made me feel he did not provide enough information. It felt not safe for the patient.”
- MedAlpaca: “Short and succinct. condescending at times.”
- Human answer: “These were not very helpful or accurate. Most did not state their credentials to know how credible they are. Some of the, if not most, of language learning models gave better answers, though some of the language learning models also claimed to be medical professionals—which isn’t accurate statement either.”
- Human answer: “Usually focused on one aspect of the scenario, not helpful in comprehensive care. focused on isolated lab value, with minimal evidence—these can be harmful responses for patients.”
- Human answer: “These are really bad answers.”
- Human answer: “Some of the answer were helpful, other not much, and other offering options that might not need to be indicated.”
Principal Findings
This study evaluated the feasibility of using generative LLMs to answer patients’ laboratory test result questions using 53 patients’ questions on a social Q&A website, Yahoo! Answers. On the basis of the results of our study, GPT-4 outperformed other similar LLMs (ie, GPT-3.5, LLaMA 2, ORCA_mini, and MedAlpaca) according to both automated metrics and manual evaluation. In particular, GPT-4 always provided disclaimers, possibly to avoid legal issues. However, GPT-4 responses may also suffer from lack of interpretation of one’s medical context, incorrect statements, and lack of references.
Recent studies [ 26 , 27 ] regarding the use of LLMs to answer laboratory test result questions from medical professionals found that ChatGPT may give superficial or incorrect answers to laboratory test result–related questions and can only provide accurate answers to approximately 50% of questions [ 26 ]. They also found that ChatGPT’s responses were not sufficiently tailored to the case or clinical questions to be useful for clinical consultation. For instance, diagnoses of liver injury were made solely based on γ-glutamyl transferase levels without considering other liver enzyme indicators. In addition, high levels of glucose and glycated hemoglobin (HbA 1c ) were both identified as indicative of diabetes regardless of whether HbA 1c levels were normal or elevated. These studies also highlighted that GPT-4 failed to account for preanalytical factors such as fasting status for glucose tests and struggled to differentiate between abnormal and critically abnormal laboratory test values. Our study observed similar patterns, where a normal HbA 1c level coupled with high glucose levels led to a diabetes prediction and critically low iron levels were merely classified as abnormal.
In addition, our findings also show that GPT-4 accurately distinguished between normal, prediabetic, and diabetic HbA 1c ranges considering fasting glucose levels and preanalytical conditions such as fasting status. Furthermore, in cases of elevated bilirubin levels, GPT-4 correctly associated them with potential jaundice citing the patient’s yellow eye discoloration and appropriately considered a comprehensive set of laboratory test results—including elevated liver enzymes and bilirubin levels—and significant alcohol intake history to recommend diagnoses such as alcoholic liver disease, hepatitis, bile duct obstruction, and liver cancer.
On the basis of our observation with the limited number of questions, we found that patients’ questions are often less complex than professionals’ questions, making ChatGPT more likely to provide an adequately accurate answer to such questions. In our manual evaluation of 7 selected patients’ laboratory test result questions, 91% (32/35) of the ratings from 5 medical experts on GPT-4’s response accuracy were either 1 ( very high ) or 2 ( high ).
Through this study, we gained insights into the challenges of using generative LLMs to answer patients’ laboratory test result–related questions and provide suggestions to mitigate these challenges. First, when asking laboratory test result questions on social Q&A websites, patients tend to focus on laboratory test results but may not provide pertinent information needed for result interpretation. In the real-world clinical setting, to fully evaluate the results, clinicians may need to evaluate the medical history of a patient and examine the trends of the laboratory test results over time. This shows that, to allow LLMs to provide a more thorough evaluation of laboratory test results, the question prompts may need to be augmented with additional information. As such, LLMs could be useful in prompting patients to provide additional information. A possible question prompt would be the following: “What additional information or data would you need to provide a more accurate diagnosis for me?”
Second, we found that it is important to understand the limitations of LLMs when answering laboratory test–related questions. As general-purpose generative AI models, they should be used to explain common terminologies and test purposes; clarify the typical reference ranges for common laboratory tests and what it might mean to have values outside these ranges; and offer general interpretation of laboratory test results, such as what it might mean to have high or low levels in certain common laboratory tests. On the basis of our findings, LLMs, especially GPT-4, can provide a basic interpretation of laboratory test results without reference ranges in the question prompts. LLMs could also be used to suggest what questions to ask health care providers. They should not be used for diagnostic purposes or treatment advice. All laboratory test results should be interpreted by a health care professional who can consider the full context of one’s health. For providers, LLMs could also be used as an educational tool for laboratory professionals, providing real-time information and explanations of laboratory techniques. When using LLMs for laboratory test result interpretation, it is important to consider the ethical and practical implications, including data privacy, the need for human oversight, and the potential for AI to both enhance and disrupt clinical workflows.
Third, we found it challenging to evaluate laboratory test result questions using Q&A pairs from social Q&A websites such as Yahoo! Answers. This is mainly because the answers provided by web-based users (who may not be medical professionals) were generally short, often focused on one aspect of the question or isolated laboratory tests, possibly opinionated, and possibly inaccurate with minimal evidence. Therefore, it is unlikely that human answers from social Q&A websites can be used as a gold standard to evaluate LLM answers. We found that GPT-4 can provide comprehensive, thoughtful, sympathetic, and fairly accurate interpretation of individual laboratory tests, but it still suffers from a number of problems: (1) LLM answers are not individualized, (2) it is not clear what are the sources LLMs use to generate the answers, (3) LLMs do not ask clarifying questions if the provided prompts do not contain important information for LLMs to generate responses, and (4) validation by medical experts is needed to reduce hallucination and fill in missing information to ensure the quality of the responses.
Future Directions
We would like to point out a few ways to improve the quality of LLM responses to laboratory test–related questions. First, the interpretation of certain laboratory tests is dependent on age group, gender, and possibly other conditions pertaining to particular population subgroups (eg, pregnant women), but LLMs do not ask clarifying questions, so it is important to enrich the question prompts with necessary information available in electronic health records or ask patients to provide necessary information for more accurate interpretation. Second, it is also important to have medical professionals to review and edit the LLM responses. For example, we found that LLaMA 2 self-identified as a “health expert,” which is obviously problematic if such responses were directly sent to patients. Therefore, it is important to postprocess the responses to highlight sentences that are risky. Third, LLMs are sensitive to question prompts. We could study different prompt engineering and structuring strategies (eg, role prompting and chain of thought) and evaluate whether these prompting approaches would improve the quality of the answers. Fourth, one could also collect clinical guidelines that provide credible laboratory result interpretation to further train LLMs to improve answer quality. We could then leverage the retrieval-augmented generation approach to allow LLMs to generate responses from a limited set of credible information sources [ 52 ]. Fifth, we could evaluate the confidence level of the sentences in the responses. Sixth, a gold-standard benchmark Q&A data set for laboratory result interpretation could be developed to allow the community to advance with different augmentation approaches.
Limitations
A few limitations should be noted in this study. First, the ChatGPT web version is nondeterministic in that the same prompt may generate different responses when used by different users. Second, the sample size for the human evaluation was small. Nonetheless, this study produced evidence that LLMs such as GPT-4 can be a promising tool for filling the information gap for understanding laboratory tests and various approaches can be used to enhance the quality of the responses.
Conclusions
In this study, we evaluated the feasibility of using generative LLMs to answer common laboratory test result interpretation questions from patients. We generated responses from 5 LLMs—ChatGPT (GPT-4 version and GPT-3.5 version), LLaMA 2, MedAlpaca, and ORCA_mini—for laboratory test questions selected from Yahoo! Answers and evaluated these responses using both automated metrics and manual evaluation. We found that GPT-4 performed better compared to the other LLMs in generating more accurate, helpful, relevant, and safe answers to these questions. We also identified a number of ways to improve the quality of LLM responses from both the prompt and response sides.
Acknowledgments
This project was partially supported by the University of Florida Clinical and Translational Science Institute, which is supported in part by the National Institutes of Health (NIH) National Center for Advancing Translational Sciences under award UL1TR001427, as well as the Agency for Healthcare Research and Quality (AHRQ) under award R21HS029969. This study was supported by the NIH Intramural Research Program, National Library of Medicine (QJ and ZL). The content is solely the responsibility of the authors and does not necessarily represent the official views of the NIH and AHRQ. The authors would like to thank Angelique Deville, Caroline Bennett, Hailey Thompson, and Maggie Awad for labeling the questions for the question classification model.
Data Availability
The data sets generated during and analyzed during this study are available from the corresponding author on reasonable request.
Conflicts of Interest
QJ is a coauthor and an active associate editor for the Journal of Medical Internet Research . All other authors declare no other conflicts of interest.
The responses generated by the 5 large language models and the human answers from Yahoo users.
Distribution of the lengths of the responses.
A few observations from the medical experts regarding the accuracy of the large language model responses.
- Healthy people 2030: building a healthier future for all. Office of Disease Prevention and Health Promotion. URL: https://health.gov/healthypeople [accessed 2023-05-09]
- NHE fact sheet. Centers for Medicare & Medicaid Services. URL: https://tinyurl.com/yc4durw4 [accessed 2023-06-06]
- Bauer UE, Briss PA, Goodman RA, Bowman BA. Prevention of chronic disease in the 21st century: elimination of the leading preventable causes of premature death and disability in the USA. Lancet. Jul 2014;384(9937):45-52. [ CrossRef ]
- Centers for Medicare and Medicaid Services (CMS), Centers for Disease Control and Prevention (CDC), Office for Civil Rights (OCR). CLIA program and HIPAA privacy rule; patients' access to test reports. Final rule. Fed Regist. Feb 06, 2014;79(25):7289-7316. [ FREE Full text ] [ Medline ]
- Pillemer F, Price R, Paone S, Martich GD, Albert S, Haidari L, et al. Direct release of test results to patients increases patient engagement and utilization of care. PLoS One. 2016;11(6):e0154743. [ FREE Full text ] [ CrossRef ] [ Medline ]
- Health IT legislation: 21st century cures act. Office of the National Coordinator for Health Information Technology. URL: https://www.healthit.gov/topic/laws-regulation-and-policy/health-it-legislation [accessed 2023-02-19]
- Tsai R, Bell EJ, Woo H, Baldwin K, Pfeffer M. How patients use a patient portal: an institutional case study of demographics and usage patterns. Appl Clin Inform. Jan 06, 2019;10(1):96-102. [ FREE Full text ] [ CrossRef ] [ Medline ]
- Witteman HO, Zikmund-Fisher BJ. Communicating laboratory results to patients and families. Clin Chem Lab Med. Feb 25, 2019;57(3):359-364. [ FREE Full text ] [ CrossRef ] [ Medline ]
- Turchioe MR, Myers A, Isaac S, Baik D, Grossman LV, Ancker JS, et al. A systematic review of patient-facing visualizations of personal health data. Appl Clin Inform. Aug 09, 2019;10(4):751-770. [ FREE Full text ] [ CrossRef ] [ Medline ]
- Alpert JM, Krist AH, Aycock RA, Kreps GL. Applying multiple methods to comprehensively evaluate a patient portal's effectiveness to convey information to patients. J Med Internet Res. May 17, 2016;18(5):e112. [ FREE Full text ] [ CrossRef ] [ Medline ]
- Zikmund-Fisher BJ, Exe NL, Witteman HO. Numeracy and literacy independently predict patients' ability to identify out-of-range test results. J Med Internet Res. Aug 08, 2014;16(8):e187. [ FREE Full text ] [ CrossRef ] [ Medline ]
- Zhang Z, Citardi D, Xing A, Luo X, Lu Y, He Z. Patient challenges and needs in comprehending laboratory test results: mixed methods study. J Med Internet Res. Dec 07, 2020;22(12):e18725. [ FREE Full text ] [ CrossRef ] [ Medline ]
- Fraccaro P, Vigo M, Balatsoukas P, van der Veer SN, Hassan L, Williams R, et al. Presentation of laboratory test results in patient portals: influence of interface design on risk interpretation and visual search behaviour. BMC Med Inform Decis Mak. Feb 12, 2018;18(1):11. [ FREE Full text ] [ CrossRef ] [ Medline ]
- Bar-Lev S, Beimel D. Numbers, graphs and words - do we really understand the lab test results accessible via the patient portals? Isr J Health Policy Res. Oct 28, 2020;9(1):58. [ FREE Full text ] [ CrossRef ] [ Medline ]
- Giardina TD, Baldwin J, Nystrom DT, Sittig DF, Singh H. Patient perceptions of receiving test results via online portals: a mixed-methods study. J Am Med Inform Assoc. Apr 01, 2018;25(4):440-446. [ FREE Full text ] [ CrossRef ] [ Medline ]
- Doi T, Langsted A, Nordestgaard BG. Elevated remnant cholesterol reclassifies risk of ischemic heart disease and myocardial infarction. J Am Coll Cardiol. Jun 21, 2022;79(24):2383-2397. [ FREE Full text ] [ CrossRef ] [ Medline ]
- Wadström BN, Wulff AB, Pedersen KM, Jensen GB, Nordestgaard BG. Elevated remnant cholesterol increases the risk of peripheral artery disease, myocardial infarction, and ischaemic stroke: a cohort-based study. Eur Heart J. Sep 07, 2022;43(34):3258-3269. [ CrossRef ] [ Medline ]
- Chu SK, Huang H, Wong WN, van Ginneken WF, Wu KM, Hung MY. Quality and clarity of health information on Q and A sites. Libr Inf Sci Res. Jul 2018;40(3-4):237-244. [ CrossRef ]
- Oh S, Yi YJ, Worrall A. Quality of health answers in social Q and A. Proc Assoc Inf Sci Technol. Jan 24, 2013;49(1):1-6. [ CrossRef ]
- Tao D, Yuan J, Qu X, Wang T, Chen X. Presentation of personal health information for consumers: an experimental comparison of four visualization formats. In: Proceedings of the 15th International Conference on Engineering Psychology and Cognitive Ergonomics. 2018. Presented at: EPCE '18; July 15-20, 2018;490-500; Las Vegas, NV. URL: https://link.springer.com/chapter/10.1007/978-3-319-91122-9_40
- Struikman B, Bol N, Goedhart A, van Weert JC, Talboom-Kamp E, van Delft S, et al. Features of a patient portal for blood test results and patient health engagement: web-based pre-post experiment. J Med Internet Res. Jul 20, 2020;22(7):e15798. [ FREE Full text ] [ CrossRef ] [ Medline ]
- Kopanitsa G. Study of patients' attitude to automatic interpretation of laboratory test results and its influence on follow-up rate. BMC Med Inform Decis Mak. Mar 27, 2022;22(1):79. [ FREE Full text ] [ CrossRef ] [ Medline ]
- Zikmund-Fisher BJ, Scherer AM, Witteman HO, Solomon JB, Exe NL, Fagerlin A. Effect of harm anchors in visual displays of test results on patient perceptions of urgency about near-normal values: experimental study. J Med Internet Res. Mar 26, 2018;20(3):e98. [ FREE Full text ] [ CrossRef ] [ Medline ]
- Morrow D, Azevedo RF, Garcia-Retamero R, Hasegawa-Johnson M, Huang T, Schuh W, et al. Contextualizing numeric clinical test results for gist comprehension: implications for EHR patient portals. J Exp Psychol Appl. Mar 2019;25(1):41-61. [ CrossRef ] [ Medline ]
- Tian S, Jin Q, Yeganova L, Lai PT, Zhu Q, Chen X, et al. Opportunities and challenges for ChatGPT and large language models in biomedicine and health. Brief Bioinform. Nov 22, 2023;25(1):bbad493. [ CrossRef ] [ Medline ]
- Cadamuro J, Cabitza F, Debeljak Z, De Bruyne SD, Frans G, Perez SM, et al. Potentials and pitfalls of ChatGPT and natural-language artificial intelligence models for the understanding of laboratory medicine test results. An assessment by the European Federation of Clinical Chemistry and Laboratory Medicine (EFLM) working group on artificial intelligence (WG-AI). Clin Chem Lab Med. Jun 27, 2023;61(7):1158-1166. [ FREE Full text ] [ CrossRef ] [ Medline ]
- Munoz-Zuluaga C, Zhao Z, Wang F, Greenblatt MB, Yang HS. Assessing the accuracy and clinical utility of ChatGPT in laboratory medicine. Clin Chem. Aug 02, 2023;69(8):939-940. [ CrossRef ] [ Medline ]
- Zhang Z, Lu Y, Kou Y, Wu DT, Huh-Yoo J, He Z. Understanding patient information needs about their clinical laboratory results: a study of social Q and A site. Stud Health Technol Inform. Aug 21, 2019;264:1403-1407. [ FREE Full text ] [ CrossRef ] [ Medline ]
- Zhang Z, Lu Y, Wilson C, He Z. Making sense of clinical laboratory results: an analysis of questions and replies in a social Q and A community. Stud Health Technol Inform. Aug 21, 2019;264:2009-2010. [ CrossRef ] [ Medline ]
- Kurstjens S, Schipper A, Krabbe J, Kusters R. Predicting hemoglobinopathies using ChatGPT. Clin Chem Lab Med. Feb 26, 2024;62(3):e59-e61. [ FREE Full text ] [ CrossRef ] [ Medline ]
- He Z, Tian S, Erdengasileng A, Hanna K, Gong Y, Zhang Z, et al. Annotation and information extraction of consumer-friendly health articles for enhancing laboratory test reporting. AMIA Annu Symp Proc. 2023;2023:407-416. [ FREE Full text ] [ Medline ]
- Lee J, Yoon W, Kim S, Kim D, Kim S, So CH, et al. BioBERT: a pre-trained biomedical language representation model for biomedical text mining. Bioinformatics. Feb 15, 2020;36(4):1234-1240. [ FREE Full text ] [ CrossRef ] [ Medline ]
- Alsentzer E, Murphy JR, Boag W, Weng WH, Jin D, Naumann T, et al. Publicly available clinical BERT embeddings. arXiv. Preprint posted online on April 6, 2019. [ FREE Full text ] [ CrossRef ]
- Beltagy I, Lo K, Cohan A. SciBERT: a pretrained language model for scientific text. arXiv. Preprint posted online on March 26, 2019. [ FREE Full text ]
- Gu Y, Tinn R, Cheng H, Lucas M, Usuyama N, Liu X, et al. Domain-specific language model pretraining for biomedical natural language processing. ACM Trans Comput Healthc. Oct 15, 2021;3(1):1-23. [ FREE Full text ] [ CrossRef ]
- OpenAI. GPT-4 technical report. arXiv. Preprint posted online on March 15, 2023. [ FREE Full text ]
- Ye J, Chen X, Xu N, Zu C, Shao Z, Liu S, et al. A comprehensive capability analysis of GPT-3 and GPT-3. arXiv. Preprint posted online on March 18, 2023. [ FREE Full text ]
- Touvron H, Martin L, Stone K, Albert P, Almahairi A, Babaei Y, et al. Llama 2: open foundation and fine-tuned chat models. arXiv. Preprint posted online on July 18, 2023. [ FREE Full text ]
- Han T, Adams LC, Papaioannou JM, Grundmann P, Oberhauser T, Löser A, et al. MedAlpaca -- an open-source collection of medical conversational AI models and training data. arXiv. Preprint posted online on April 14, 2023. [ FREE Full text ]
- orca_mini_3b. Hugging Face. URL: https://huggingface.co/pankajmathur/orca_mini_3b [accessed 2023-12-04]
- LangChain: introduction and getting started. Pinecone. URL: https://www.pinecone.io/learn/series/langchain/langchain-intro/ [accessed 2023-12-04]
- Papineni K, Roukos S, Ward T, Zhu WJ. BLEU: a method for automatic evaluation of machine translation. In: Proceedings of the 40th Annual Meeting on Association for Computational Linguistics. 2002. Presented at: ALC '02; July 7-12, 2002;311-318; Philadelphia, PA. URL: https://dl.acm.org/doi/10.3115/1073083.1073135 [ CrossRef ]
- Post M. A call for clarity in reporting BLEU scores. arXiv. Preprint posted online on April 23, 2018. [ FREE Full text ] [ CrossRef ]
- Banerjee S, Lavie A. METEOR: an automatic metric for MT evaluation with high levels of correlation with human judgments. In: Proceedings of the 2005 ACL Workshop on Intrinsic and Extrinsic Evaluation Measures for Machine Translation and/or Summarization. 2005. Presented at: WIEEMMTS '05; June 29, 2005;65-72; Ann Arbor, MI. URL: https://aclanthology.org/W05-0909 [ CrossRef ]
- Lin CY. ROUGE: a package for automatic evaluation of summaries. In: Lin CY, editor. Text Summarization Branches Out Internet. Barcelona, Spain. Association for Computational Linguistics; 2004;74-81.
- Zhang T, Kishore V, Wu F, Weinberger KQ, Artzi Y. BERTScore: evaluating text generation with BERT. arXiv. Preprint posted online on April 21, 2019. [ FREE Full text ]
- Wang T, Yu P, Tan XE, O'Brien S, Pasunuru R, Dwivedi-Yu J, et al. Shepherd: a critic for language model generation. arXiv. Preprint posted online on August 8, 2023. [ FREE Full text ]
- Dubois Y, Li X, Taori R, Zhang T, Gulrajani I, Ba J, et al. AlpacaFarm: a simulation framework for methods that learn from human feedback. arXiv. Preprint posted online on May 22, 2023. [ FREE Full text ]
- Bartko JJ. The intraclass correlation coefficient as a measure of reliability. Psychol Rep. Aug 1966;19(1):3-11. [ FREE Full text ] [ CrossRef ] [ Medline ]
- Gamer M, Lemon J, Singh IF. irr: various coefficients of interrater reliability and agreement. Cran R Project. 2019. URL: https://cran.r-project.org/web/packages/irr/index.html [accessed 2023-12-12]
- Human subject regulations decision charts: 2018 requirements. Office for Human Research Protection. Jan 20, 2019. URL: https://tinyurl.com/3sbzydm3 [accessed 2024-04-03]
- Jin Q, Leaman R, Lu Z. Retrieve, summarize, and verify: how will ChatGPT affect information seeking from the medical literature? J Am Soc Nephrol. Aug 01, 2023;34(8):1302-1304. [ CrossRef ] [ Medline ]
Abbreviations
Edited by B Puladi; submitted 23.01.24; peer-reviewed by Y Chen, Z Smutny; comments to author 01.02.24; revised version received 17.02.24; accepted 06.03.24; published 17.04.24.
©Zhe He, Balu Bhasuran, Qiao Jin, Shubo Tian, Karim Hanna, Cindy Shavor, Lisbeth Garcia Arguello, Patrick Murray, Zhiyong Lu. Originally published in the Journal of Medical Internet Research (https://www.jmir.org), 17.04.2024.
This is an open-access article distributed under the terms of the Creative Commons Attribution License (https://creativecommons.org/licenses/by/4.0/), which permits unrestricted use, distribution, and reproduction in any medium, provided the original work, first published in the Journal of Medical Internet Research, is properly cited. The complete bibliographic information, a link to the original publication on https://www.jmir.org/, as well as this copyright and license information must be included.
Thank you for visiting nature.com. You are using a browser version with limited support for CSS. To obtain the best experience, we recommend you use a more up to date browser (or turn off compatibility mode in Internet Explorer). In the meantime, to ensure continued support, we are displaying the site without styles and JavaScript.
- View all journals
- My Account Login
- Explore content
- About the journal
- Publish with us
- Sign up for alerts
- Open access
- Published: 10 April 2024
FSC-certified forest management benefits large mammals compared to non-FSC
- Joeri A. Zwerts ORCID: orcid.org/0000-0003-3841-6389 1 , 2 ,
- E. H. M. Sterck ORCID: orcid.org/0000-0003-1101-6027 2 , 3 ,
- Pita A. Verweij ORCID: orcid.org/0000-0002-3577-2524 4 ,
- Fiona Maisels 5 , 6 ,
- Jaap van der Waarde ORCID: orcid.org/0009-0002-8394-8894 7 ,
- Emma A. M. Geelen 2 ,
- Georges Belmond Tchoumba 7 ,
- Hermann Frankie Donfouet Zebaze 7 &
- Marijke van Kuijk 1
Nature volume 628 , pages 563–568 ( 2024 ) Cite this article
4299 Accesses
1 Citations
220 Altmetric
Metrics details
- Biodiversity
- Conservation biology
- Environmental impact
- Tropical ecology
More than a quarter of the world’s tropical forests are exploited for timber 1 . Logging impacts biodiversity in these ecosystems, primarily through the creation of forest roads that facilitate hunting for wildlife over extensive areas. Forest management certification schemes such as the Forest Stewardship Council (FSC) are expected to mitigate impacts on biodiversity, but so far very little is known about the effectiveness of FSC certification because of research design challenges, predominantly limited sample sizes 2 , 3 . Here we provide this evidence by using 1.3 million camera-trap photos of 55 mammal species in 14 logging concessions in western equatorial Africa. We observed higher mammal encounter rates in FSC-certified than in non-FSC logging concessions. The effect was most pronounced for species weighing more than 10 kg and for species of high conservation priority such as the critically endangered forest elephant and western lowland gorilla. Across the whole mammal community, non-FSC concessions contained proportionally more rodents and other small species than did FSC-certified concessions. The first priority for species protection should be to maintain unlogged forests with effective law enforcement, but for logged forests our findings provide convincing data that FSC-certified forest management is less damaging to the mammal community than is non-FSC forest management. This study provides strong evidence that FSC-certified forest management or equivalently stringent requirements and controlling mechanisms should become the norm for timber extraction to avoid half-empty forests dominated by rodents and other small species.
Similar content being viewed by others
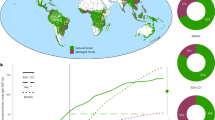
The importance of capturing management in forest restoration targets
Martin Jung, Myroslava Lesiv, … Steffen Fritz
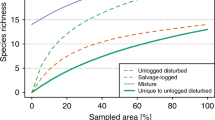
Estimating retention benchmarks for salvage logging to protect biodiversity
Simon Thorn, Anne Chao, … Alexandro B. Leverkus
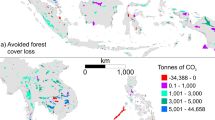
Southeast Asian protected areas are effective in conserving forest cover and forest carbon stocks compared to unprotected areas
Victoria Graham, Jonas Geldmann, … Hsing-Chung Chang
Commercial logging concessions cover more than one-quarter of the world’s remaining tropical forests 1 . Forest certification schemes aim to have more positive socio-economic and environmental outcomes compared to conventional logging schemes. For example, the Forest Stewardship Council (FSC) aims to reduce direct environmental impacts by various means that include maintaining high conservation value forests and applying reduced impact logging practices (Supplementary Tables 1 and 2 ). A major concern for biodiversity is that timber extraction—by the creation of roads—opens previously remote forests, enabling illegal and unsustainable hunting 4 , 5 , 6 , 7 . This indirect effect of logging is known to mainly influence medium- to large-sized forest mammals, which are particularly vulnerable to human pressure 8 . FSC certification may alleviate these pressures because, among other measures, companies reduce accessibility to concessions by closing off old logging roads, prohibit wild meat transport and hunting materials, provide access to alternative meat sources for workers and their families, and carry out surveillance by rangers. An FSC certificate is valid for 5 years and logging companies are audited for compliance through third-party annual surveillance assessments.
In African tropical forests, FSC certification has been shown to be associated with reduced deforestation 9 , improved working and living conditions of employees and benefit-sharing with neighbouring institutions 10 . Studies in Latin America suggest that mammal occupancy in FSC-certified sites is comparable to that of protected areas 11 , 12 . There is, however, little data on the status of faunal communities in FSC-certified versus non-FSC forests 2 , 3 . Most studies on the effectiveness of FSC certification for wildlife conservation have focused on one or a few sites or species at a time 13 , 14 , 15 , 16 . Although these studies reported a positive impact of FSC certification on wildlife compared to non-FSC concessions, their research designs did not account for explanatory variables such as concession location, land-use history or stochastic effects 17 , 18 . One study included several sites and species and found no effect of FSC certification 19 . However, that study investigated only bird species richness: bird dispersal distances are much higher than those of terrestrial mammals and may thus be a weak indicator of local management. In addition to simply comparing species diversity, it is important to compare population sizes between forest management types. Hunting does not necessarily completely extirpate wildlife species, especially when forests are connected, but rather results in population declines 4 .
We used camera traps to assess whether FSC certification can mitigate the negative effects of timber extraction on wildlife by studying the encounter rate of a broad range of mammal species across several sites. We compared small- to large-sized mammal observations across seven paired FSC-certified and non-FSC concessions in Gabon and the Republic of Congo (Fig. 1 ). Gabon and the Republic of Congo lie in western equatorial Africa (WEA). We included all companies that were FSC-certified between 2018 and 2021 in this region, except for one which refused to allow access. WEA is particularly suitable for these analyses, as its forests are reasonably intact and logging concessions are embedded in a matrix of contiguous forest, which are therefore mostly devoid of influences other than the effects of logging and hunting 20 . Wild meat hunting is pervasive throughout WEA, whereby logging increases hunting pressure by increasing access (logging roads) and through the arrival of people working in the concessions in once-remote forests 8 . By ensuring spatial pairing of the FSC-certified and non-FSC concessions we minimized the influence of regional landscape heterogeneity. We calculated mammal encounter rates and grouped mammal species into five body mass classes. The relative encounter rate of these classes could be used as a proxy for hunting pressure, as larger-bodied species are targeted more by hunters 8 . In addition, larger-bodied species recover more slowly from hunting compared to smaller-bodied species, resulting in lower abundances of large versus small species under higher hunting pressure 21 , 22 . Finally, we explored how FSC-certified forest management affects mammal encounter rate by taxonomic group and by IUCN Red List categories. We hypothesized that FSC certification would effectively decrease hunting pressure and therefore predicted a higher encounter rate of larger-bodied species in FSC-certified compared with non-FSC logging concessions.
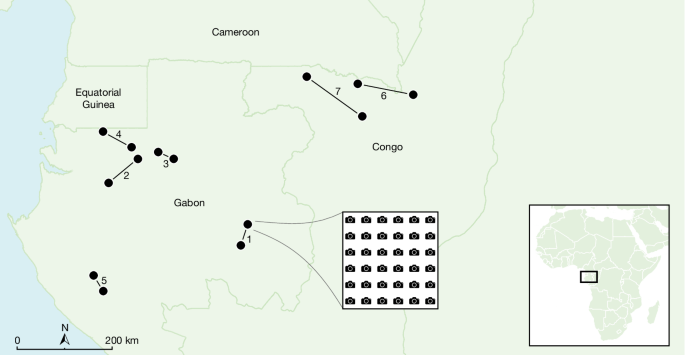
Between 28 to 36 cameras were deployed in each concession in systematic, 1 km spaced grids. Numbers and lines indicate the pairs of FSC-certified and non-FSC concessions.
We collected and catalogued nearly 1.3 million photos from 474 camera-trap locations for a total of 35,546 days, averaging 2,539 camera-trap days per concession (Extended Data Table 1 ). We detected a total of 55 mammal species (Extended Data Table 2 ). The mammal encounter rate estimated by our model (Fig. 2a ) was 1.5 times higher in FSC-certified concessions compared to non-FSC concessions (Extended Data Table 3 ). We also found fewer signs of hunting (Fig. 2b ) in FSC-certified than in non-FSC concessions. Estimated total faunal biomass derived from mammal encounter rates was 4.5 times higher in FSC-certified compared to non-FSC concessions (Extended Data Fig. 1 ). Larger species contributed more to the total biomass. We observed comparable species diversity in the two concession types, as only a few species, all with very low encounter rates, were lacking completely in one or other of the concession types (Extended Data Table 2 ).
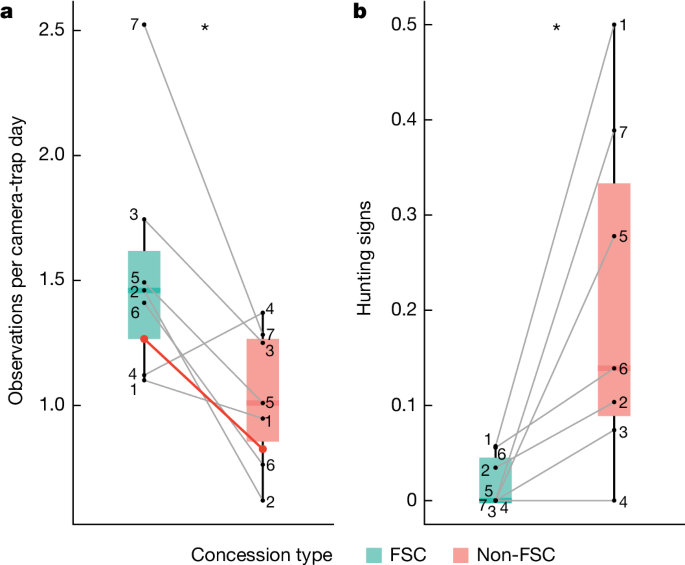
a , b , Encounter rate of all observed mammals ( P = 0.041) ( a ) and proportion of camera locations with hunting signs ( P = 0.036) ( b ). Numbers represent paired FSC-certified ( n = 7) and non-FSC ( n = 7) concessions. The red line in a represents the linear mixed model predicted fixed effect (certification status) and grey lines represent random effects (concession pairs). Differences between hunting signs in b were analysed using a two-sided Wilcoxon signed-rank test. Data are represented as boxplots, where central lines represent medians and lower and upper lines correspond to the first and third quartiles, whiskers reflect 1.5 times the interquartile range. * P < 0.05.
Source data
The differences between mammal encounter rates in FSC-certified and non-FSC concessions increased with body mass (Fig. 3 and Extended Data Tables 3 and 4 ). FSC-certified concessions had higher encounter rates of mammals above 10 kg than non-FSC concessions but there was no difference for mammals below 10 kg. Model estimates showed that mammals in body mass classes over 100, 30–100 and 10–30 kg, had encounter rates that were 2.7, 2.5 and 3.5 times higher, respectively, in FSC-certified concessions compared to non-FSC concessions. Mammal encounter rates of the IUCN Red List categories critically endangered, near threatened and least concern were 2.7, 2.3 and 1.4 times higher, respectively, in FSC-certified compared to non-FSC concessions (Fig. 4 and Extended Data Tables 3 and 4 ).
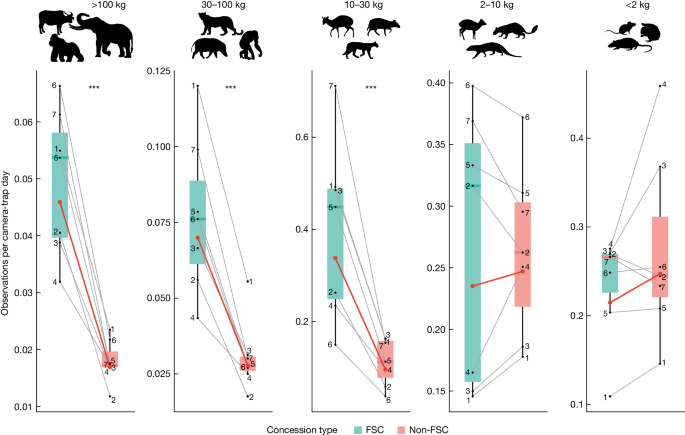
Numbers represent paired FSC-certified ( n = 7) and non-FSC ( n = 7) concessions, red lines represent linear mixed model predicted fixed effects (certification status) and grey lines represent random effects (concession pairs). Data are represented as boxplots, where central lines represent medians and lower and upper lines correspond to the first and third quartiles, whiskers reflect 1.5 times the interquartile range. Pairwise comparisons were multivariate t adjusted. *** P < 0.001. Exact P values are summarized in Extended Data Table 3 . Note that the scales of the y axes vary. Silhouettes of Gorilla gorilla , Syncerus caffer , Potamochoerus porcus , Cephalophus sp., Hyemoschus aquaticus , Philantomba monticola , Atherurus africanus and mice were created by T. Markus.
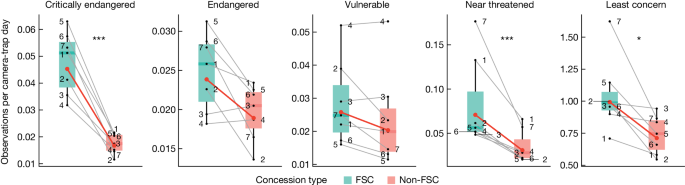
Numbers represent paired FSC-certified ( n = 7) and non-FSC ( n = 7) concessions, red lines represent linear mixed model predicted fixed effects (certification status) and grey lines represent random effects (concession pairs). Data are represented as boxplots, where central lines represent medians and lower and upper lines correspond to the first and third quartiles, whiskers reflect 1.5 times the interquartile range. Pairwise comparisons were multivariate t adjusted. *** P < 0.001, * P < 0.05. Exact P values are summarized in Extended Data Table 3 . Note that the scales of the y axes vary.
Mammal encounter rate in FSC-certified and non-FSC concessions varied between taxonomic groups (Fig. 5 and Extended Data Tables 3 and 4 ). In FSC-certified concessions, forest elephants were encountered 2.5 times, primates 1.8 times, even-toed ungulates 2 times and carnivores 1.5 times more compared to non-FSC concessions. The encounter rate of pangolins and rodents did not differ.
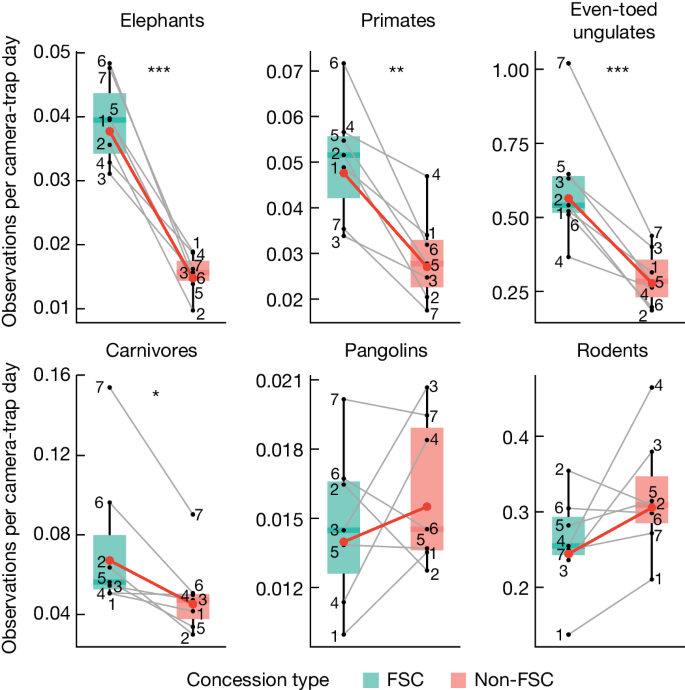
Numbers represent paired FSC-certified ( n = 7) and non-FSC ( n = 7) concessions, red lines represent linear mixed model predicted fixed effects (certification status) and grey lines represent random effects (concession pairs). Data are represented as boxplots, where central lines represent medians and lower and upper lines correspond to the first and third quartiles, whiskers reflect 1.5 times the interquartile range. Pairwise comparisons were multivariate t adjusted. *** P < 0.001, ** P < 0.01, * P < 0.05. Exact P values are summarized in Extended Data Table 3 . Note that the scales of the y axes vary.
The loss of large mammals
We conducted a large-scale quantitative study to assess the impact of FSC-certified forest management on mammal encounter rate across several logging concessions and for a broad range of mammals. Our data provide strong evidence that FSC-certified forest management results in higher overall mammal abundance, as approximated by encounter rate and faunal biomass relative to non-FSC forest management. This effect was most pronounced for species larger than 10 kg, which was consistent for all FSC–non-FSC concession pairs, probably because these medium to large species recover more slowly from population losses and may be targeted more often by hunters 21 , 22 . Not all large species with reduced encounter rates may be commonly targeted for hunting but they are often indiscriminately affected by snaring 23 . Non-FSC concessions contained proportionally more rodents and other small species than did FSC-certified concessions (Extended Data Table 2 ). The lack of hunting impacts on small mammal populations suggests some form of density compensation is in place: the hunting pressure on small mammal populations might be compensated by higher reproductive rates and/or a release from competition and predation in the non-FSC concessions 24 , 25 .
A particularly strong effect of FSC certification was found for the critically endangered forest elephant, which is in line with previous findings 14 . The distribution of this species is driven almost entirely by human activity: they avoid areas that are unsafe to them 26 , 27 . Their large home ranges can span several concessions 28 , thus they may actively seek to reside not only in protected areas but also in FSC-certified concessions where measures to prevent illegal hunting are in place. This suggests that FSC-certified concessions may provide an important refuge for wide-ranging elephants. By contrast, no difference was found in pangolin encounter rate (they are among the most trafficked mammals 29 ) between the two types of logging regimes. Two out of the three pangolin species present in WEA are relatively small and generally have higher reproduction rates than mammals in larger size classes. Moreovjer, all three pangolin species had low encounter rates in our study (Extended Data Table 2 ), probably because two pangolin species are semi-arboreal and are therefore not effectively captured by ground-based camera traps, which reduces our ability to draw strong conclusions about these species and warrants further research. We did not observe a loss of species that were encountered frequently in either FSC-certified or non-FSC concessions, nor did we expect to. This is because human population density in WEA is relatively low and the forests are still highly connected 20 .
Conservation of large mammals through FSC certification brings wider benefits to forests, as these mammals play a pivotal role in ecological processes, including seed dispersal, seed predation, browsing, trampling, plant competition, nutrient cycling and predator–prey interactions 30 . It has also been suggested that forest carbon storage is higher when large mammal assemblages are more intact because the ecological processes they are part of (such as seed dispersal) often benefit large, high wood density trees 31 , 32 , 33 and the benefits of their conservation may far outweigh the cost 34 . Futhermore, by reducing the amount of wild meat available for human consumption, FSC-certified concessions or similar stringent schemes may also reduce the chance of zoonotic disease transmission 35 .
Methodological considerations
The FSC takes a comprehensive and all-encompassing perspective when it comes to managing and promoting sustainable forest management practices. This approach recognizes that forests are complex ecosystems with intricate interconnections between their various components, including flora, fauna, soil, water and climate. In logged tropical forests, controlling hunting is probably the most important factor for the reduction of environmental impacts 7 . We found more hunting signs in non-FSC concessions, which supports the interpretation that FSC effectively reduces hunting pressure, although counting hunting signs is likely to be a relatively weak measure of the quantification of hunting pressure 36 . Hunting has long been known to be the most important driver of forest fauna decline in central African logged forests 6 , 37 and the same phenomenon has been shown in Asia 7 . Of course, other factors such as retaining high conservation value areas and reduced impact logging practices are likely to contribute to the observed effects as well 38 . Our data do not allow for causal inference of the association of any of the specific measures implemented by FSC companies with the observed effects, as that would require setting up more detailed measure-based experiments.
For the sections of the concessions that we sampled, we ensured comparability between paired concessions. We maximized the similarity in geographic covariates that may drive variation in mammal abundance—elevation and distances to roads, rivers, human settlements and protected areas—between each pair of FSC-certified and non-FSC concessions (Extended Data Fig. 2 and Extended Data Table 5 ). Although we believe that these covariates are important drivers of mammal abundance 39 , including these covariates did not greatly improve the models, which underscores that camera grid locations were sufficiently similar in terms of these confounding influences. Precise logging intensity and logging history data per camera were not available for most concessions because the planning schemes of companies and actual exploitation of cutting blocks often did not match. Slight differences in logging history are not expected to have a large effect on the data because mammals are mobile and can return quickly to areas that have been exploited 40 . Fourteen logging concessions may be a large sample size for tropical ecology studies 17 but a low sample size from a statistical perspective. Nonetheless, despite the small number of replicates, we found clear and consistent differences in encounter rate between FSC-certified and non-FSC forests.
We used encounter rate, defined as the number of observations divided by the number of camera-trap days. Encounter rates may be affected by unaccounted influences on detection probabilities 41 , which may complicate comparisons between species or between sites. We compare individual species across management types, which renders differences in detection across species less relevant. For camera-trap sites, however, variation in visibility or other factors may affect the number of detections, even though mammal population sizes are similar. However, we found no differences in any relevant site covariates between treatments at the camera-trap level. Visibility at ground level, slope, the presence of fruiting trees and small water courses around camera-trap locations did not differ between FSC-certified and non-FSC concessions (Extended Data Fig. 3 and Extended Data Table 5 ). We also compared the presence and type of trails or paths around camera-trap locations, which did not differ significantly except for the number of elephant paths, which was higher in FSC-certified concessions (Extended Data Fig. 4 and Extended Data Table 5 ). As camera traps were installed randomly at the predetermined GPS locations on the nearest tree with 4 m visibility, finding a higher frequency of elephant paths in FSC-certified concessions was, in itself, an indication of higher elephant abundance in FSC-certified concessions. Potential seasonal influences are accounted for by the paired design. It is, however, important to note that encounter rates are a mixed measure of abundance and activity and we cannot disentangle whether changes in encounter rate are the result of changes in abundance, activity—movement per day—or both. Species’ home ranges and movement patterns can change in response to disturbance, which can affect encounter rates. It is, however, unlikely that changes in activity solely make up the observed differences in encounter rates, given the consistency of the data in the three heaviest body mass classes. We also estimate relative biomass using encounter rates, which is a useful proxy to assess differences between forest management types but cannot be interpreted as true biomass (Extended Data Fig. 1 ).
Conservation implications
Of central African tropical forest, 21% is designated for protection but only 15% of the species’ ranges for central chimpanzees and the western lowland gorilla lie in protected areas 42 , 43 . More than half of these species’ ranges and a large part of the ranges of other mammals, such as forest elephants, lie in logging concessions 26 . Protected areas are essential for conservation but sometimes lack the resources for effective control of illegal hunting 44 , 45 . Logging companies often do have the means to protect forests and have an economic incentive to do so. We did not compare mammal encounter rates in protected areas with the same metric in logging concessions ourselves. However, our observed encounter rates for large mammals, which are the first species to disappear as a result of hunting and poaching, in FSC-certified concessions were comparable to published data from recently monitored protected areas in the same region 46 , 47 , 48 . The ratio of large versus small forest antelopes in the FSC-certified concessions is furthermore comparable to such ratios in a protected area in the region with almost no hunting, whereas those in non-FSC concessions are far lower 49 . Although the first priority for species protection should be to maintain unlogged forests where there is effective law enforcement, our results challenge the notion that, at least for large-bodied mammals in WEA, logging is always disastrous for wildlife 50 , 51 . We show that, if selectively logged forests are properly managed, they can provide an important contribution to biodiversity: our results confirm that FSC-certified forests support far more larger and threatened species than do non-FSC forests. The results of this study are likely to be applicable to other logged tropical forests where hunting, through increased accessibility, poses a risk to forest mammals. This is because wildlife protection measures and law enforcement are applied across all FSC-certified forests, as part of the FSC principles, criteria and indicators for which FSC-certified companies are audited for compliance (Supplementary Tables 1 and 2 ). We infer this with caution as timber extraction volumes, concession size and shape, presence of public roads, population density and other characteristics may differ between concessions and thereby affect the impacts of FSC-certified forest management 52 .
Most terrestrial protected areas are isolated 53 and increasing human modification and fragmentation of landscapes is limiting the ability of mammals to move 54 . Governments in forest-rich countries may enhance the effectiveness of conservation policies by requiring FSC certification in strategic locations, such as buffer zones around protected areas to reduce the edge-to-area ratio of the conservation landscape 55 . Non-FSC companies may also contribute to conservation, as they vary along a gradient of environmental and social responsibility 56 . This was, however, not the focus of our study. Concessions in our study region are large, often larger than 2,000 km 2 , and together with protected areas they can substantially contribute to mammal conservation. Well-managed logging concessions can contribute to Sustainable Development Goal (SDG) 12 (sustainable consumption and production) and SDG 15 (life on land) by performing a strategic function in preserving habitats and landscape connectivity while allowing for responsible economic activity 57 .
Our findings indicate that the requirements of FSC certification lead to effective mitigation of direct and indirect influences of logging on tropical forest mammals. The control of widespread and unsustainable hunting and poaching which is facilitated by the increased access to forests engendered by timber extraction is probably a key determinant of this impact. However, not all hunting is illegal and FSC certification protects customary rights to hunt non-protected species for subsistence. Sustainability of this practice is controlled by—among other requirements—controlling firearm permits, spatially assigning hunting zones and monitoring wildlife offtake. We believe that a strict set of requirements, control of compliance and regular enforcement, all integrally connected and ensured in the FSC system, are crucial for successful environmental protection through forest certification.
The need to upscale certification
We present a clear, evidence-based message about the positive impact of FSC certification. We show that medium- to large-sized mammals—which play vital functions in forests—are more abundant in FSC-certified concessions than in non-FSC concessions. This study calls for action, reinforcing previous studies that called for more forest certification and land-use planning that takes conservation into account 14 , 26 , 43 , 58 . To protect large mammals, we urge that FSC certification or similar stringent schemes become the norm, as conventional logging is likely to result in half-empty forests dominated by rodents and other small species. To increase logging companies’ interest in FSC certification, it is essential that sufficient demand is created for FSC-certified products by institutional and individual buyers. The information put forward by this study can play an important role in FSC’s global strategy to leverage sustainable finance to reduce biodiversity loss, whereby certificate holders can be rewarded for the biodiversity benefits that they incur 59 . Rendering FSC-certified forests eligible for payments by biodiversity schemes, especially driven by government regulation 60 , can contribute to fair valuation of standing forests. To ensure environmentally and socially responsible forest management practices 10 , we strongly support the application of regulatory frameworks which stimulate and require the selling and buying of timber certified by FSC or similar stringent schemes.
Data collection
We set up arrays of camera traps from 2018 to 2021 in 14 logging concessions owned by 11 different companies (5 FSC and 6 non-FSC) in Gabon and the Republic of Congo (Fig. 1 ). Seven FSC-certified concessions were each paired to the closest non-FSC concession that was similar in terms of terrain and forest type 20 . All concessions are situated in a matrix of connected forests. In each pair of concessions, camera traps (Bushnell Trophy Cam HD for pairs 1–6 and Browning 2018 Spec Ops Advantage for pair 7) were deployed simultaneously to account for seasonal differences, for 2–3 months. There was one exception where Covid restrictions obliged the cameras to remain in place for longer (Extended Data Table 1 ). Camera-trap grid locations in each pair of concessions were chosen on the basis of similarity between potential drivers of mammal abundance, including distance to settlements, roads, rivers, protected areas, elevation (Extended Data Fig. 2 and Extended Data Table 5 ) and time since logging (2–10 years before our study), although some camera grids overlapped older logging blocks. Camera traps were set out in systematic, 1 km spaced grids with a random start point. On reaching the predetermined GPS locations, the first potential installation location was used where cameras had at least 4 m of visibility. This ensured that each grid was representative of environmental heterogeneity: that is, not specifically targeting or ignoring trails or other landscape elements that could influence detection 61 . The 1 km intercamera distance exceeds most species’ home range sizes to avoid spatial autocorrelation. Species were not expected to migrate within the sampling duration of the study. Between 28 and 36 cameras were deployed in each concession, totalling 474 camera traps, distributed over 474 km 2 (Extended Data Table 1 ). Cameras were installed at a height of 30 cm to enable observations of mammals of all sizes. Cameras were programmed to take bursts of three photos to maximize the chance of detection and to take a photo every 12 h for correct calculation of active days in the event of a defect before the end of the deployment period. For each camera, we recorded whether there was an elephant path, skidder trail, small wildlife trail or an absence of a trail or path, in the field of view of each camera (Extended Data Fig. 4 and Extended Data Table 5 ). We also visually estimated forest visibility (0–10 m, 11–20 m, greater than 20 m), slope (0–5°, 5–20°, greater than 20°), presence of fruiting trees within 30 m and presence of small water courses within 50 m (Extended Data Fig. 3 and Extended Data Table 1 ). When approaching each predefined camera point, we counted cartridges, snares and hunting camps from 500 m before the camera up to its location. Various field teams were employed in different concessions and hence there may be some influence of interobserver bias of hunting observations between sites.
Photo processing and data analysis
Camera-trap efforts yielded 1,278,853 photos, including 645,165 photos with animals. All photos were annotated in the program Wild.ID v.1.0.1. We identified animals up to the species level if photo quality permitted and otherwise designated the species as ‘indet’ 62 . As reliable species identification of small mammals is difficult, they were grouped into squirrels, rats and mice and shrews. Rare observations of humans, birds, bats, reptiles and domestic dogs were excluded from the analyses.
Observations of the same species that were at least 10 min apart were considered as separate detections. We assessed the sensitivity of this threshold by calculating the number of detections for intervals of 10, 30, 60 and 1,440 min, which all yielded proportionally similar numbers of observations across body mass classes (Supplementary Table 3 ). When several animals were observed, the number of individuals was determined by taking the highest number of individuals in a photo within the 10 min threshold. Sampling effort was defined as the number of camera days minus downtime due to malfunctioning cameras or obstruction of vision by vegetation.
Mammal behaviour may be different in hunted concessions, as mammals may be shyer of non-natural objects such as camera traps, which could in turn negatively affect their probability of detection. If this dynamic existed, shyness was assumed to fade over time with habituation to the materials, resulting in an increase of observations over time. We tested for an interaction between certification status and the number of observations over time using a linear model with a log-transformed number of observations for the first 68 days of all deployments, as that was the shortest concession deployment period, ensuring that all concessions were equally represented. We did not find that certification status was related to a trend in observations over time (Extended Data Fig. 5 ). We recognize, however, that other factors may have influenced detection probability, such as movement rates, which may be affected by hunting.
For each species for each concession, we calculated encounter rate, weighted by group size, as the number of observations divided by the sampling effort and we reported all findings using the metric ‘observations per camera-trap day’. Encounter rate was calculated for all species combined, per body mass class, per IUCN Red List category 63 and per taxonomic group. Body mass of each species was determined by taking the mean across sexes 62 . Taxonomic groups Hyracoidea and Tubulidentata were excluded from the taxonomic analysis because of low sample sizes. Shrews were included as rodents in the taxonomy analysis even though they are formally not rodents because they are difficult to distinguish from mice. We consider this acceptable given that shrews are functionally very similar to rodents in the light of this study. To study the impact of certification on total estimated faunal biomass, the encounter rate of each species was multiplied by its average body mass divided by the sampling effort.
To assess whether encounter rates varied between FSC-certified and non-FSC concessions, we quantified the means of the paired concessions using linear mixed-effects models with concession pairs, concessions and cameras as random effects, whereby cameras were nested in concessions, in concession pairs, in a multilevel random effect structure. We allowed the means of concession pairs to vary between body mass class, IUCN Red List category and taxonomic group, if supported by model selection. We tested whether potential drivers of mammal abundance (Extended Data Figs. 2 , 3 and 4 ) were important using a model-selection approach based on minimization of Bayesian information criterion values (Supplementary Table 4 ). We found that the inclusion of geographic covariates did not substantially improve the model for body mass classes, taxonomic groups and IUCN categories. Only for all mammals pooled together, the inclusion of elevation and distance to rivers resulted in slightly improved models but differences were negligible and did not support strong evidence for a significant influence of these covariates 64 . Quadratic geographic covariate terms and camera-trap site covariates did not result in better models. Pairwise comparisons were multivariate t adjusted. We used two-sided Wilcoxon signed-rank tests for all other analyses (Extended Data Table 5 ). Statistical analyses were performed in R v.4.2.2.
Reporting summary
Further information on research design is available in the Nature Portfolio Reporting Summary linked to this article.
Data availability
The data that support the findings of this study are available in the Zenodo repository under https://doi.org/10.5281/zenodo.10061155 (ref. 65 ). Source data are provided with this paper.
Code availability
R code for statistical analyses and data tables are available in the Zenodo repository under https://doi.org/10.5281/zenodo.10061155 (ref. 65 ).
Blaser, J., Sarre, A., Poore, D. & Johnson, S. Status of Tropical Forest Management 2011 (ITTO, 2011).
Romero, C. et al. Evaluation of the impacts of Forest Stewardship Council (FSC) certification of natural forest management in the tropics: a rigorous approach to assessment of a complex conservation intervention. Int. For. Rev. 19 , 36–49 (2018).
Google Scholar
van der Ven, H. & Cashore, B. Forest certification: the challenge of measuring impacts. Curr. Opin. Environ. Sustain. 32 , 104–111 (2018).
Benítez-López, A. et al. The impact of hunting on tropical mammal and bird populations. Science 356 , 180–183 (2017).
ADS PubMed Google Scholar
Kleinschroth, F., Laporte, N., Laurance, W. F., Goetz, S. J. & Ghazoul, J. Road expansion and persistence in forests of the Congo Basin. Nat. Sustain. 2 , 628–634 (2019).
Leisher, C. et al. Ranking the direct threats to biodiversity in sub-Saharan Africa. Biodivers. Conserv. 31 , 1329–1343 (2022).
Tilker, A. et al. Habitat degradation and indiscriminate hunting differentially impact faunal communities in the Southeast Asian tropical biodiversity hotspot. Commun. Biol. 2 , 396 (2019).
PubMed PubMed Central Google Scholar
Abernethy, K. A., Coad, L., Taylor, G., Lee, M. E. & Maisels, F. Extent and ecological consequences of hunting in Central African rainforests in the twenty-first century. Phil. Trans. R. Soc. B 368 , 20120303 (2013).
CAS PubMed PubMed Central Google Scholar
Tritsch, I. et al. Do forest-management plans and FSC certification help avoid deforestation in the Congo Basin? Ecol. Econ. 175 , 106660 (2020).
Cerutti, P. O. et al. Social Impacts of the Forest Stewardship Council Certification (Center for International Forestry Research, 2014).
Roopsind, A., Caughlin, T. T., Sambhu, H., Fragoso, J. M. V. & Putz, F. E. Logging and indigenous hunting impacts on persistence of large neotropical animals. Biotropica 49 , 565–575 (2017).
Tobler, M. W. et al. Do responsibly managed logging concessions adequately protect jaguars and other large and medium-sized mammals? Two case studies from Guatemala and Peru. Biol. Conserv. 220 , 245–253 (2018).
Bahaa-el-din, L. et al. Effects of human land-use on Africa’s only forest-dependent felid: the African golden cat Caracal aurata . Biol. Conserv. 199 , 1–9 (2016).
Stokes, E. J. et al. Monitoring great ape and elephant abundance at large spatial scales: measuring effectiveness of a conservation landscape. PLoS ONE 5 , e10294 (2010).
ADS PubMed PubMed Central Google Scholar
Polisar, J. et al. Using certified timber extraction to benefit jaguar and ecosystem conservation. Ambio 46 , 588–603 (2017).
ADS CAS PubMed Google Scholar
Sollmann, R. et al. Quantifying mammal biodiversity co-benefits in certified tropical forests. Divers. Distrib. 23 , 317–328 (2017).
Ramage, B. S. et al. Pseudoreplication in tropical forests and the resulting effects on biodiversity conservation. Conserv. Biol. 27 , 364–372 (2013).
PubMed Google Scholar
Burivalova, Z., Hua, F., Koh, L. P., Garcia, C. & Putz, F. A critical comparison of conventional, certified and community management of tropical forests for timber in terms of environmental, economic and social variables. Conserv. Lett. 10 , 4–14 (2017).
Campos-Cerqueira, M. et al. How does FSC forest certification affect the acoustically active fauna in Madre de Dios, Peru? Remote Sens. Ecol. Conserv. https://doi.org/10.1002/rse2.120 (2019).
Grantham, H. S. et al. Spatial priorities for conserving the most intact biodiverse forests within Central Africa. Environ. Res. Lett. 15 , 0940b5 (2020).
CAS Google Scholar
Atwood, T. B. et al. Herbivores at the highest risk of extinction among mammals, birds and reptiles. Sci. Adv. 6 , eabb8458 (2020).
Cardillo, M. et al. Multiple causes of high extinction risk in large mammal species. Science 309 , 1239–1241 (2005).
Figel, J. J., Hambal, M., Krisna, I., Putra, R. & Yansyah, D. Malignant snare traps threaten an irreplaceable megafauna community. Trop. Conserv. Sci. 14 , 1940082921989187 (2021).
Yasuoka, H. et al. Changes in the composition of hunting catches in southeastern Cameroon: a promising approach for collaborative wildlife management between ecologists and local hunters. Ecol. Soc. 20 , 25 (2015).
Peres, C. A. & Dolman, P. M. Density compensation in neotropical primate communities: evidence from 56 hunted and nonhunted Amazonian forests of varying productivity. Oecologia 122 , 175–189 (2000).
Maisels, F. et al. Devastating decline of forest elephants in Central Africa. PLoS ONE 8 , e59469 (2013).
ADS CAS PubMed PubMed Central Google Scholar
Wall, J. et al. Human footprint and protected areas shape elephant range across Africa. Curr. Biol. 31 , 2437–2445 (2021).
CAS PubMed Google Scholar
Beirne, C. et al. African forest elephant movements depend on time scale and individual behavior. Sci. Rep. 11 , 12634 (2021).
Challender, D. W. S., Heinrich, S., Shepherd, C. R. & Katsis, L. K. D. in Pangolins (eds Challender, D. W. S. et al.) 259–276 (Academic, 2020).
Rogers, H. S., Donoso, I., Traveset, A. & Fricke, E. C. Cascading impacts of seed disperser loss on plant communities and ecosystems. Annu. Rev. Ecol. Evol. Syst. 52 , 641–666 (2021).
Bello, C. et al. Defaunation affects carbon storage in tropical forests. Sci. Adv. 1 , e1501105 (2015).
Chanthorn, W. et al. Defaunation of large-bodied frugivores reduces carbon storage in a tropical forest of Southeast Asia. Sci. Rep. 9 , 10015 (2019).
Berzaghi, F., Bretagnolle, F., Durand-Bessart, C. & Blake, S. Megaherbivores modify forest structure and increase carbon stocks through multiple pathways. Proc. Natl Acad. Sci. USA 120 , e2201832120 (2023).
Berzaghi, F., Chami, R., Cosimano, T. & Fullenkamp, C. Financing conservation by valuing carbon services produced by wild animals. Proc. Natl Acad. Sci. USA 119 , e2120426119 (2022).
Johnson, C. K. et al. Global shifts in mammalian population trends reveal key predictors of virus spillover risk. Proc. R. Soc. B 287 , 20192736 (2020).
Ibbett, H. et al. Experimentally assessing the effect of search effort on snare detectability. Biol. Conserv. 247 , 108581 (2020).
Wilkie, D. S., Sidle, J. G., Boundzanga, G. C., Auzel, P. & Blake, S. in The Cutting Edge (eds Fimbel, R. A. et al.) 375–400 (Columbia Univ. Press, 2001).
Bicknell, J. E., Struebig, M. J. & Davies, Z. G. Reconciling timber extraction with biodiversity conservation in tropical forests using reduced-impact logging. J. Appl. Ecol. 52 , 379–388 (2015).
Laméris, D. W., Tagg, N., Kuenbou, J. K., Sterck, E. H. M. & Willie, J. Drivers affecting mammal community structure and functional diversity under varied conservation efforts in a tropical rainforest in Cameroon. Anim. Conserv. 23 , 182–191 (2020).
Morgan, D. et al. African apes coexisting with logging: comparing chimpanzee ( Pan troglodytes troglodytes ) and gorilla ( Gorilla gorilla gorilla ) resource needs and responses to forestry activities. Biol. Conserv. 218 , 277–286 (2018).
Sollmann, R., Mohamed, A., Samejima, H. & Wilting, A. Risky business or simple solution—relative abundance indices from camera-trapping. Biol. Conserv. 159 , 405–412 (2013).
Doumenge, C., Palla, F., Madzous, I. & Ludovic, G. (eds) State of Protected Areas in Central Africa: 2020 (OFAC-COMIFAC, Yaounde, Cameroon & IUCN, 2021).
Strindberg, S. et al. Guns, germs and trees determine density and distribution of gorillas and chimpanzees in Western Equatorial Africa. Sci. Adv. 4 , eaar2964 (2018).
Laurance, W. F. et al. Averting biodiversity collapse in tropical forest protected areas. Nature 489 , 290–293 (2012).
Poulsen, J. R. et al. Poaching empties critical Central African wilderness of forest elephants. Curr. Biol. 27 , R134–R135 (2017).
Poulain, F. et al. A camera trap survey in the community zone of Lobéké National Park (Cameroon) reveals a nearly intact mammalian community. Afr. J. Ecol. 61 , 523–529 (2023).
Hedwig, D. et al. A camera trap assessment of the forest mammal community within the transitional savannah–forest mosaic of the Batéké Plateau National Park, Gabon. Afr. J. Ecol . 56 , 777–790 (2018).
Bruce, T. et al. Using camera trap data to characterise terrestrial larger‐bodied mammal communities in different management sectors of the Dja Faunal Reserve, Cameroon. Afr. J. Ecol. 56 , 759–776 (2018).
Breuer, T., Breuer‐Ndoundou Hockemba, M., Opepa, C. K., Yoga, S. & Mavinga, F. B. High abundance and large proportion of medium and large duikers in an intact and unhunted afrotropical protected area: insights into monitoring methods. Afr. J. Ecol. 59 , 399–411 (2021).
Potapov, P. et al. The last frontiers of wilderness: tracking loss of intact forest landscapes from 2000 to 2013. Sci. Adv. 3 , e1600821 (2017).
Gibson, L. et al. Primary forests are irreplaceable for sustaining tropical biodiversity. Nature 478 , 378–381 (2011).
Burivalova, Z., Şekercioğlu, Ç. H. & Koh, L. P. Thresholds of logging intensity to maintain tropical forest biodiversity. Curr. Biol. 24 , 1893–1898 (2014).
Ward, M. et al. Just ten percent of the global terrestrial protected area network is structurally connected via intact land. Nat. Commun. 11 , 4563 (2020).
Brennan, A. et al. Functional connectivity of the world’s protected areas. Science 376 , 1101–1104 (2022).
Clark, C. J., Poulsen, J. R., Malonga, R. & Elkan, P. W. Logging concessions can extend the conservation estate for Central African tropical forests. Conserv. Biol. 23 , 1281–1293 (2009).
Rayden, T. & Essono, R. E. Evaluation of the Management of Wildlife in the Forestry Concessions Around the National Parks of Lopé, Waka and Ivindo (WWF, 2010).
Edwards, D. P., Tobias, J. A., Sheil, D., Meijaard, E. & Laurance, W. F. Maintaining ecosystem function and services in logged tropical forests. Trends Ecol. Evol. 29 , 511–520 (2014).
Nasi, R., Billand, A. & van Vliet, N. Managing for timber and biodiversity in the Congo Basin. For. Ecol. Manag. 268 , 103–111 (2012).
FSC Global Strategy 2021–2026: Demonstrating the Value and Benefits of Forest Stewardship (FSC, 2020).
Salzman, J., Bennett, G., Carroll, N., Goldstein, A. & Jenkins, M. The global status and trends of payments for ecosystem services. Nat. Sustain. 1 , 136–144 (2018).
Zwerts, J. A. et al. Methods for wildlife monitoring in tropical forests: comparing human observations, camera traps and passive acoustic sensors. Conserv. Sci. Pract. 3 , e568 (2021).
Kingdon, J. The Kingdon Field Guide to African Mammals (Bloomsbury, 2015).
The IUCN Red List of Threatened Species Version 2022-1 (IUCN, accessed 10 August 2022).
Anderson, D. R. Model Based Inference in the Life Sciences: A Primer on Evidence Vol. 31 (Springer, 2008).
Zwerts, J. A. FSC-certified forest management benefits large mammals compared to non-FSC. Zenodo https://doi.org/10.5281/zenodo.10061155 (2023).
Download references
Acknowledgements
We thank the logging companies for access to their concessions, 263 people from WEA for fieldwork assistance and 23 students and assistants for data processing. We also thank Y. Hautier for his insights concerning the statistical analyses. The work was carried out with permission from the Gabonese Centre National de la Recherche Scientifique et Technologique (CENAREST) under research permit no. AV AR0046/18 and the Congolese Institut National de Recherche Forestière under research permit nos. 219 and 126 issued by the National Forest Research Institute (IRF) of Congo with the help of the Wildlife Conservation Society (WCS) Congo, under no. 219MRSIT/IRF/DG/DS on 17 July 2019 and the extension under no. 126MRSIT/IRF/DG/DS on 4 August 2020. J.A.Z. received support for this work from the Dutch Research Council NWO through the graduate programme Nature Conservation, Management and Restoration (grant no. 022.006.011), Programme de Promotion de l’Exploitation Certifiée des Forêts (PPECF) de la COMIFAC (à travers la KfW) under grant no. C146, WWF Netherlands, WWF Germany and the Prince Bernhard Chair for International Nature Conservation of Utrecht University.
Author information
Authors and affiliations.
Ecology and Biodiversity, Utrecht University, Utrecht, The Netherlands
Joeri A. Zwerts & Marijke van Kuijk
Animal Behaviour & Cognition, Utrecht University, Utrecht, The Netherlands
Joeri A. Zwerts, E. H. M. Sterck & Emma A. M. Geelen
Animal Science Department, Biomedical Primate Research Centre, Rijswijk, The Netherlands
E. H. M. Sterck
Copernicus Institute of Sustainable Development, Utrecht University, Utrecht, The Netherlands
Pita A. Verweij
Faculty of Natural Sciences, University of Stirling, Stirling, UK
Fiona Maisels
Wildlife Conservation Society, Global Conservation Program, New York, NY, USA
WWF Cameroon, Yaoundé, Cameroon
Jaap van der Waarde, Georges Belmond Tchoumba & Hermann Frankie Donfouet Zebaze
You can also search for this author in PubMed Google Scholar
Contributions
J.A.Z., M.v.K., J.v.d.W. and G.B.T. conceptualized this article. J.A.Z., E.A.M.G. and H.F.D.Z. were responsible for data curation. J.A.Z. and E.A.M.G. conducted the formal analysis. J.A.Z., M.v.K., E.A.M.G., E.H.M.S. and P.A.V. developed the methodology. J.A.Z. and H.F.D.Z. undertook investigations. J.A.Z. and E.A.M.G. created the visualizations. P.A.V., J.A.Z., M.v.K. and J.v.d.W. acquired funding. J.A.Z. and G.B.T. were responsible for project administration. J.A.Z., M.v.K., G.B.T., E.H.M.S., P.A.V. and F.M. supervised the work. J.A.Z. wrote the original draft manuscript. J.A.Z., M.v.K., J.v.d.W., F.M., G.B.T., P.A.V. and E.H.M.S. reviewed and edited the final article.
Corresponding author
Correspondence to Joeri A. Zwerts .
Ethics declarations
Competing interests.
J.A.Z. is an unpaid individual member of the FSC Environmental chamber, sub-chamber North. G.B.T. is an unpaid individual member of the FSC Environmental chamber, sub-chamber South and, since 2018, also a member of an advisory committee to the Board of Directors of FSC, the Policy and Standard Committee. J.v.d.W. and H.F.D.Z. have unpaid institutional membership of FSC through WWF International. The remaining authors declare no competing interests.
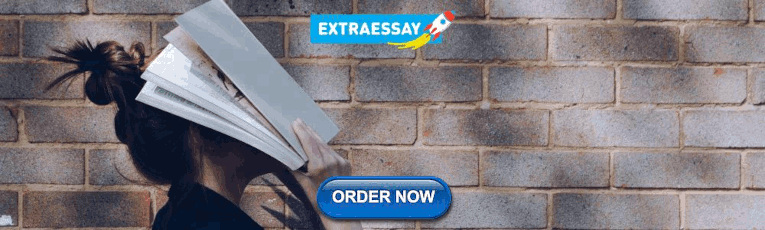
Peer review
Peer review information.
Nature thanks Julia Fa, Roland Kays and the other, anonymous, reviewer(s) for their contribution to the peer review of this work. Peer reviewer reports are available.
Additional information
Publisher’s note Springer Nature remains neutral with regard to jurisdictional claims in published maps and institutional affiliations.
Extended data figures and tables
Extended data fig. 1 estimated faunal biomass derived from mammal encounter rates..
( a ) Estimated faunal biomass was higher (p = 0.016) in FSC-certified (n = 7) than in non-FSC concessions (n = 7). Numbers represent paired FSC-certified and non-FSC concessions linked by grey lines. Data is represented as a boxplot, where central lines represent medians and lower and upper lines correspond to the first and third quartiles, whiskers reflect 1.5 times the interquartile range. Two-sided Wilcoxon signed-rank, * : p < 0.05. Panels (b–e) represent the contributions of different body mass classes to the estimated faunal biomass derived from mammal encounter rates in FSC-certified (n = 7) and non-FSC concessions (n = 7). ( b ) in kg / camera-trap day; ( c ) as a proportion of total faunal biomass; ( d ) in kg /day for species up to 100 kg; ( e ) as a proportion of the total faunal biomass for species up to 100 kg. FSC-certified concessions had higher overall biomass whereby mammals weighing more than 10 kg made up a larger proportion of the total biomass than in non-FSC concessions.
Extended Data Fig. 2 Geographic covariates.
( a ) Distance to roads, ( b ) rivers, ( c ) human settlements, ( d ) and protected areas, as well as ( e ) elevation, did not differ significantly between camera locations in FSC-certified (n = 7) and non-FSC concessions (n = 7). Numbers represent paired FSC-certified and non-FSC concessions linked by grey lines. Data are represented as boxplots, where central lines represent medians and lower and upper lines correspond to the first and third quartiles, whiskers reflect 1.5 times the interquartile range. Two-sided Wilcoxon signed-rank, ns: p > 0.05. Exact p-values are summarized in Extended Data Table 4 .
Extended Data Fig. 3 Camera trap site covariates.
( a ) The presence of fruiting trees within 30 m, ( b ) visibility, ( c ) the presence of small water courses within 50 m distance and ( d ) slope, expressed in proportions, did not differ significantly between camera locations in FSC-certified (n = 7) and non-FSC concessions (n = 7). Numbers represent paired FSC-certified and non-FSC concessions linked by grey lines. Data are represented as boxplots, where central lines represent medians and lower and upper lines correspond to the first and third quartiles, whiskers reflect 1.5 times the interquartile range. Two-sided Wilcoxon signed-rank, ns: p > 0.05. Exact p-values are summarized in Extended Data Table 4 .
Extended Data Fig. 4 The presence of trails or paths in the field of view of randomly placed cameras.
Each camera trap installation location was characterized as either an elephant path, skidder trail, small wildlife trail or as an absence of a trail or path. Only elephant paths, expressed in proportions, were encountered more often in FSC-certified concessions (n = 7) than in non-FSC concessions (n = 7), whereas the presence or absence of the other three types of installation locations was equivalent between the two forest management types. Camera trap sites were selected as the closest location from the predetermined GPS locations with both a suitable tree and a minimum of four metres visibility. Following this method, randomly encountering more elephant paths is in itself an indication of higher elephant abundances in FSC-certified concessions. Numbers represent paired FSC-certified and non-FSC concessions linked by grey lines. Data are represented as boxplots, where central lines represent medians and lower and upper lines correspond to the first and third quartiles, whiskers reflect 1.5 times the interquartile range. Two-sided Wilcoxon signed-rank, *: p <= 0.05, ns: p > 0.05. Exact p-values are summarized in Extended Data Table 4 .
Extended Data Fig. 5 Observations over time.
This analysis explored whether variation in hunting induced mammal shyness for non-natural objects influenced detection differentially in FSC-certified (n = 7) and non-FSC concessions (n = 7). We did not find support for an effect of certification status on the number of observations over time. Linear model: p = 0.892.
Supplementary information
Supplementary information.
Supplementary Tables 1, 2 and 4.
Reporting Summary
Peer review file, supplementary table 3.
Observation numbers calculated with different time thresholds between camera trap observations of the same species.
Source Data Figs. 2–5 and Extended Data Figs. 1–5
Rights and permissions.
Open Access This article is licensed under a Creative Commons Attribution 4.0 International License, which permits use, sharing, adaptation, distribution and reproduction in any medium or format, as long as you give appropriate credit to the original author(s) and the source, provide a link to the Creative Commons licence, and indicate if changes were made. The images or other third party material in this article are included in the article’s Creative Commons licence, unless indicated otherwise in a credit line to the material. If material is not included in the article’s Creative Commons licence and your intended use is not permitted by statutory regulation or exceeds the permitted use, you will need to obtain permission directly from the copyright holder. To view a copy of this licence, visit http://creativecommons.org/licenses/by/4.0/ .
Reprints and permissions
About this article
Cite this article.
Zwerts, J.A., Sterck, E.H.M., Verweij, P.A. et al. FSC-certified forest management benefits large mammals compared to non-FSC. Nature 628 , 563–568 (2024). https://doi.org/10.1038/s41586-024-07257-8
Download citation
Received : 22 October 2022
Accepted : 29 February 2024
Published : 10 April 2024
Issue Date : 18 April 2024
DOI : https://doi.org/10.1038/s41586-024-07257-8
Share this article
Anyone you share the following link with will be able to read this content:
Sorry, a shareable link is not currently available for this article.
Provided by the Springer Nature SharedIt content-sharing initiative
This article is cited by
Wildlife boost in african forests certified for sustainable logging.
- Julia E. Fa
Nature (2024)
By submitting a comment you agree to abide by our Terms and Community Guidelines . If you find something abusive or that does not comply with our terms or guidelines please flag it as inappropriate.
Quick links
- Explore articles by subject
- Guide to authors
- Editorial policies
Sign up for the Nature Briefing: Anthropocene newsletter — what matters in anthropocene research, free to your inbox weekly.

- Survey paper
- Open access
- Published: 01 October 2015
Big data analytics: a survey
- Chun-Wei Tsai 1 ,
- Chin-Feng Lai 2 ,
- Han-Chieh Chao 1 , 3 , 4 &
- Athanasios V. Vasilakos 5
Journal of Big Data volume 2 , Article number: 21 ( 2015 ) Cite this article
145k Accesses
481 Citations
130 Altmetric
Metrics details
The age of big data is now coming. But the traditional data analytics may not be able to handle such large quantities of data. The question that arises now is, how to develop a high performance platform to efficiently analyze big data and how to design an appropriate mining algorithm to find the useful things from big data. To deeply discuss this issue, this paper begins with a brief introduction to data analytics, followed by the discussions of big data analytics. Some important open issues and further research directions will also be presented for the next step of big data analytics.
Introduction
As the information technology spreads fast, most of the data were born digital as well as exchanged on internet today. According to the estimation of Lyman and Varian [ 1 ], the new data stored in digital media devices have already been more than 92 % in 2002, while the size of these new data was also more than five exabytes. In fact, the problems of analyzing the large scale data were not suddenly occurred but have been there for several years because the creation of data is usually much easier than finding useful things from the data. Even though computer systems today are much faster than those in the 1930s, the large scale data is a strain to analyze by the computers we have today.
In response to the problems of analyzing large-scale data , quite a few efficient methods [ 2 ], such as sampling, data condensation, density-based approaches, grid-based approaches, divide and conquer, incremental learning, and distributed computing, have been presented. Of course, these methods are constantly used to improve the performance of the operators of data analytics process. Footnote 1 The results of these methods illustrate that with the efficient methods at hand, we may be able to analyze the large-scale data in a reasonable time. The dimensional reduction method (e.g., principal components analysis; PCA [ 3 ]) is a typical example that is aimed at reducing the input data volume to accelerate the process of data analytics. Another reduction method that reduces the data computations of data clustering is sampling [ 4 ], which can also be used to speed up the computation time of data analytics.
Although the advances of computer systems and internet technologies have witnessed the development of computing hardware following the Moore’s law for several decades, the problems of handling the large-scale data still exist when we are entering the age of big data . That is why Fisher et al. [ 5 ] pointed out that big data means that the data is unable to be handled and processed by most current information systems or methods because data in the big data era will not only become too big to be loaded into a single machine, it also implies that most traditional data mining methods or data analytics developed for a centralized data analysis process may not be able to be applied directly to big data. In addition to the issues of data size, Laney [ 6 ] presented a well-known definition (also called 3Vs) to explain what is the “big” data: volume, velocity, and variety. The definition of 3Vs implies that the data size is large, the data will be created rapidly, and the data will be existed in multiple types and captured from different sources, respectively. Later studies [ 7 , 8 ] pointed out that the definition of 3Vs is insufficient to explain the big data we face now. Thus, veracity, validity, value, variability, venue, vocabulary, and vagueness were added to make some complement explanation of big data [ 8 ].
Expected trend of the marketing of big data between 2012 and 2018. Note that yellow , red , and blue of different colored box represent the order of appearance of reference in this paper for particular year
The report of IDC [ 9 ] indicates that the marketing of big data is about $16.1 billion in 2014. Another report of IDC [ 10 ] forecasts that it will grow up to $32.4 billion by 2017. The reports of [ 11 ] and [ 12 ] further pointed out that the marketing of big data will be $46.34 billion and $114 billion by 2018, respectively. As shown in Fig. 1 , even though the marketing values of big data in these researches and technology reports [ 9 – 15 ] are different, these forecasts usually indicate that the scope of big data will be grown rapidly in the forthcoming future.
In addition to marketing, from the results of disease control and prevention [ 16 ], business intelligence [ 17 ], and smart city [ 18 ], we can easily understand that big data is of vital importance everywhere. A numerous researches are therefore focusing on developing effective technologies to analyze the big data. To discuss in deep the big data analytics, this paper gives not only a systematic description of traditional large-scale data analytics but also a detailed discussion about the differences between data and big data analytics framework for the data scientists or researchers to focus on the big data analytics.
Moreover, although several data analytics and frameworks have been presented in recent years, with their pros and cons being discussed in different studies, a complete discussion from the perspective of data mining and knowledge discovery in databases still is needed. As a result, this paper is aimed at providing a brief review for the researchers on the data mining and distributed computing domains to have a basic idea to use or develop data analytics for big data.
Roadmap of this paper
Figure 2 shows the roadmap of this paper, and the remainder of the paper is organized as follows. “ Data analytics ” begins with a brief introduction to the data analytics, and then “ Big data analytics ” will turn to the discussion of big data analytics as well as state-of-the-art data analytics algorithms and frameworks. The open issues are discussed in “ The open issues ” while the conclusions and future trends are drawn in “ Conclusions ”.
Data analytics
To make the whole process of knowledge discovery in databases (KDD) more clear, Fayyad and his colleagues summarized the KDD process by a few operations in [ 19 ], which are selection, preprocessing, transformation, data mining, and interpretation/evaluation. As shown in Fig. 3 , with these operators at hand we will be able to build a complete data analytics system to gather data first and then find information from the data and display the knowledge to the user. According to our observation, the number of research articles and technical reports that focus on data mining is typically more than the number focusing on other operators, but it does not mean that the other operators of KDD are unimportant. The other operators also play the vital roles in KDD process because they will strongly impact the final result of KDD. To make the discussions on the main operators of KDD process more concise, the following sections will focus on those depicted in Fig. 3 , which were simplified to three parts (input, data analytics, and output) and seven operators (gathering, selection, preprocessing, transformation, data mining, evaluation, and interpretation).
The process of knowledge discovery in databases
As shown in Fig. 3 , the gathering, selection, preprocessing, and transformation operators are in the input part. The selection operator usually plays the role of knowing which kind of data was required for data analysis and select the relevant information from the gathered data or databases; thus, these gathered data from different data resources will need to be integrated to the target data. The preprocessing operator plays a different role in dealing with the input data which is aimed at detecting, cleaning, and filtering the unnecessary, inconsistent, and incomplete data to make them the useful data. After the selection and preprocessing operators, the characteristics of the secondary data still may be in a number of different data formats; therefore, the KDD process needs to transform them into a data-mining-capable format which is performed by the transformation operator. The methods for reducing the complexity and downsizing the data scale to make the data useful for data analysis part are usually employed in the transformation, such as dimensional reduction, sampling, coding, or transformation.
The data extraction, data cleaning, data integration, data transformation, and data reduction operators can be regarded as the preprocessing processes of data analysis [ 20 ] which attempts to extract useful data from the raw data (also called the primary data) and refine them so that they can be used by the following data analyses. If the data are a duplicate copy, incomplete, inconsistent, noisy, or outliers, then these operators have to clean them up. If the data are too complex or too large to be handled, these operators will also try to reduce them. If the raw data have errors or omissions, the roles of these operators are to identify them and make them consistent. It can be expected that these operators may affect the analytics result of KDD, be it positive or negative. In summary, the systematic solutions are usually to reduce the complexity of data to accelerate the computation time of KDD and to improve the accuracy of the analytics result.
Data analysis
Since the data analysis (as shown in Fig. 3 ) in KDD is responsible for finding the hidden patterns/rules/information from the data, most researchers in this field use the term data mining to describe how they refine the “ground” (i.e, raw data) into “gold nugget” (i.e., information or knowledge). The data mining methods [ 20 ] are not limited to data problem specific methods. In fact, other technologies (e.g., statistical or machine learning technologies) have also been used to analyze the data for many years. In the early stages of data analysis, the statistical methods were used for analyzing the data to help us understand the situation we are facing, such as public opinion poll or TV programme rating. Like the statistical analysis, the problem specific methods for data mining also attempted to understand the meaning from the collected data.
After the data mining problem was presented, some of the domain specific algorithms are also developed. An example is the apriori algorithm [ 21 ] which is one of the useful algorithms designed for the association rules problem. Although most definitions of data mining problems are simple, the computation costs are quite high. To speed up the response time of a data mining operator, machine learning [ 22 ], metaheuristic algorithms [ 23 ], and distributed computing [ 24 ] were used alone or combined with the traditional data mining algorithms to provide more efficient ways for solving the data mining problem. One of the well-known combinations can be found in [ 25 ], Krishna and Murty attempted to combine genetic algorithm and k -means to get better clustering result than k -means alone does.
Data mining algorithm
As Fig. 4 shows, most data mining algorithms contain the initialization, data input and output, data scan, rules construction, and rules update operators [ 26 ]. In Fig. 4 , D represents the raw data, d the data from the scan operator, r the rules, o the predefined measurement, and v the candidate rules. The scan, construct, and update operators will be performed repeatedly until the termination criterion is met. The timing to employ the scan operator depends on the design of the data mining algorithm; thus, it can be considered as an optional operator. Most of the data algorithms can be described by Fig. 4 in which it also shows that the representative algorithms— clustering , classification , association rules , and sequential patterns —will apply these operators to find the hidden information from the raw data. Thus, modifying these operators will be one of the possible ways for enhancing the performance of the data analysis.
Clustering is one of the well-known data mining problems because it can be used to understand the “new” input data. The basic idea of this problem [ 27 ] is to separate a set of unlabeled input data Footnote 2 to k different groups, e.g., such as k -means [ 28 ]. Classification [ 20 ] is the opposite of clustering because it relies on a set of labeled input data to construct a set of classifiers (i.e., groups) which will then be used to classify the unlabeled input data to the groups to which they belong. To solve the classification problem, the decision tree-based algorithm [ 29 ], naïve Bayesian classification [ 30 ], and support vector machine (SVM) [ 31 ] are widely used in recent years.
Unlike clustering and classification that attempt to classify the input data to k groups, association rules and sequential patterns are focused on finding out the “relationships” between the input data. The basic idea of association rules [ 21 ] is find all the co-occurrence relationships between the input data. For the association rules problem, the apriori algorithm [ 21 ] is one of the most popular methods. Nevertheless, because it is computationally very expensive, later studies [ 32 ] have attempted to use different approaches to reducing the cost of the apriori algorithm, such as applying the genetic algorithm to this problem [ 33 ]. In addition to considering the relationships between the input data, if we also consider the sequence or time series of the input data, then it will be referred to as the sequential pattern mining problem [ 34 ]. Several apriori-like algorithms were presented for solving it, such as generalized sequential pattern [ 34 ] and sequential pattern discovery using equivalence classes [ 35 ].
Output the result
Evaluation and interpretation are two vital operators of the output. Evaluation typically plays the role of measuring the results. It can also be one of the operators for the data mining algorithm, such as the sum of squared errors which was used by the selection operator of the genetic algorithm for the clustering problem [ 25 ].
To solve the data mining problems that attempt to classify the input data, two of the major goals are: (1) cohesion—the distance between each data and the centroid (mean) of its cluster should be as small as possible, and (2) coupling—the distance between data which belong to different clusters should be as large as possible. In most studies of data clustering or classification problems, the sum of squared errors (SSE), which was used to measure the cohesion of the data mining results, can be defined as
where k is the number of clusters which is typically given by the user; \(n_i\) the number of data in the i th cluster; \(x_{ij}\) the j th datum in the i th cluster; \(c_i\) is the mean of the i th cluster; and \(n= \sum ^k_{i=1} n_i\) is the number of data. The most commonly used distance measure for the data mining problem is the Euclidean distance, which is defined as
where \(p_i\) and \(p_j\) are the positions of two different data. For solving different data mining problems, the distance measurement \(D(p_i, p_j)\) can be the Manhattan distance, the Minkowski distance, or even the cosine similarity [ 36 ] between two different documents.
Accuracy (ACC) is another well-known measurement [ 37 ] which is defined as
To evaluate the classification results, precision ( p ), recall ( r ), and F -measure can be used to measure how many data that do not belong to group A are incorrectly classified into group A ; and how many data that belong to group A are not classified into group A . A simple confusion matrix of a classifier [ 37 ] as given in Table 1 can be used to cover all the situations of the classification results.
In Table 1 , TP and TN indicate the numbers of positive examples and negative examples that are correctly classified, respectively; FN and FP indicate the numbers of positive examples and negative examples that are incorrectly classified, respectively. With the confusion matrix at hand, it is much easier to describe the meaning of precision ( p ), which is defined as
and the meaning of recall ( r ), which is defined as
The F -measure can then be computed as
In addition to the above-mentioned measurements for evaluating the data mining results, the computation cost and response time are another two well-known measurements. When two different mining algorithms can find the same or similar results, of course, how fast they can get the final mining results will become the most important research topic.
After something (e.g., classification rules) is found by data mining methods, the two essential research topics are: (1) the work to navigate and explore the meaning of the results from the data analysis to further support the user to do the applicable decision can be regarded as the interpretation operator [ 38 ], which in most cases, gives useful interface to display the information [ 39 ] and (2) a meaningful summarization of the mining results [ 40 ] can be made to make it easier for the user to understand the information from the data analysis. The data summarization is generally expected to be one of the simple ways to provide a concise piece of information to the user because human has trouble of understanding vast amounts of complicated information. A simple data summarization can be found in the clustering search engine, when a query “oasis” is sent to Carrot2 ( http://search.carrot2.org/stable/search ), it will return some keywords to represent each group of the clustering results for web links to help us recognize which category needed by the user, as shown in the left side of Fig. 5 .
Screenshot of the results of clustering search engine
A useful graphical user interface is another way to provide the meaningful information to an user. As explained by Shneiderman in [ 39 ], we need “overview first, zoom and filter, then retrieve the details on demand”. The useful graphical user interface [ 38 , 41 ] also makes it easier for the user to comprehend the meaning of the results when the number of dimensions is higher than three. How to display the results of data mining will affect the user’s perspective to make the decision. For instance, data mining can help us find “type A influenza” at a particular region, but without the time series and flu virus infected information of patients, the government could not recognize what situation (pandemic or controlled) we are facing now so as to make appropriate responses to that. For this reason, a better solution to merge the information from different sources and mining algorithm results will be useful to let the user make the right decision.
Since the problems of handling and analyzing large-scale and complex input data always exist in data analytics, several efficient analysis methods were presented to accelerate the computation time or to reduce the memory cost for the KDD process, as shown in Table 2 . The study of [ 42 ] shows that the basic mathematical concepts (i.e., triangle inequality) can be used to reduce the computation cost of a clustering algorithm. Another study [ 43 ] shows that the new technologies (i.e., distributed computing by GPU) can also be used to reduce the computation time of data analysis method. In addition to the well-known improved methods for these analysis methods (e.g., triangle inequality or distributed computing), a large proportion of studies designed their efficient methods based on the characteristics of mining algorithms or problem itself, which can be found in [ 32 , 44 , 45 ], and so forth. This kind of improved methods typically was designed for solving the drawback of the mining algorithms or using different ways to solve the mining problem. These situations can be found in most association rules and sequential patterns problems because the original assumption of these problems is for the analysis of large-scale dataset. Since the earlier frequent pattern algorithm (e.g., apriori algorithm) needs to scan the whole dataset many times which is computationally very expensive. How to reduce the number of times the whole dataset is scanned so as to save the computation cost is one of the most important things in all the frequent pattern studies. The similar situation also exists in data clustering and classification studies because the design concept of earlier algorithms, such as mining the patterns on-the-fly [ 46 ], mining partial patterns at different stages [ 47 ], and reducing the number of times the whole dataset is scanned [ 32 ], are therefore presented to enhance the performance of these mining algorithms. Since some of the data mining problems are NP-hard [ 48 ] or the solution space is very large, several recent studies [ 23 , 49 ] have attempted to use metaheuristic algorithm as the mining algorithm to get the approximate solution within a reasonable time.
Abundant research results of data analysis [ 20 , 27 , 63 ] show possible solutions for dealing with the dilemmas of data mining algorithms. It means that the open issues of data analysis from the literature [ 2 , 64 ] usually can help us easily find the possible solutions. For instance, the clustering result is extremely sensitive to the initial means, which can be mitigated by using multiple sets of initial means [ 65 ]. According to our observation, most data analysis methods have limitations for big data, that can be described as follows:
Unscalability and centralization Most data analysis methods are not for large-scale and complex dataset. The traditional data analysis methods cannot be scaled up because their design does not take into account large or complex datasets. The design of traditional data analysis methods typically assumed they will be performed in a single machine, with all the data in memory for the data analysis process. For this reason, the performance of traditional data analytics will be limited in solving the volume problem of big data.
Non-dynamic Most traditional data analysis methods cannot be dynamically adjusted for different situations, meaning that they do not analyze the input data on-the-fly. For example, the classifiers are usually fixed which cannot be automatically changed. The incremental learning [ 66 ] is a promising research trend because it can dynamically adjust the the classifiers on the training process with limited resources. As a result, the performance of traditional data analytics may not be useful to the problem of velocity problem of big data.
Uniform data structure Most of the data mining problems assume that the format of the input data will be the same. Therefore, the traditional data mining algorithms may not be able to deal with the problem that the formats of different input data may be different and some of the data may be incomplete. How to make the input data from different sources the same format will be a possible solution to the variety problem of big data.
Because the traditional data analysis methods are not designed for large-scale and complex data, they are almost impossible to be capable of analyzing the big data. Redesigning and changing the way the data analysis methods are designed are two critical trends for big data analysis. Several important concepts in the design of the big data analysis method will be given in the following sections.
Big data analytics
Nowadays, the data that need to be analyzed are not just large, but they are composed of various data types, and even including streaming data [ 67 ]. Since big data has the unique features of “massive, high dimensional, heterogeneous, complex, unstructured, incomplete, noisy, and erroneous,” which may change the statistical and data analysis approaches [ 68 ]. Although it seems that big data makes it possible for us to collect more data to find more useful information, the truth is that more data do not necessarily mean more useful information. It may contain more ambiguous or abnormal data. For instance, a user may have multiple accounts, or an account may be used by multiple users, which may degrade the accuracy of the mining results [ 69 ]. Therefore, several new issues for data analytics come up, such as privacy, security, storage, fault tolerance, and quality of data [ 70 ].
The comparison between traditional data analysis and big data analysis on wireless sensor network
The big data may be created by handheld device, social network, internet of things, multimedia, and many other new applications that all have the characteristics of volume, velocity, and variety. As a result, the whole data analytics has to be re-examined from the following perspectives:
From the volume perspective, the deluge of input data is the very first thing that we need to face because it may paralyze the data analytics. Different from traditional data analytics, for the wireless sensor network data analysis, Baraniuk [ 71 ] pointed out that the bottleneck of big data analytics will be shifted from sensor to processing, communications, storage of sensing data, as shown in Fig. 6 . This is because sensors can gather much more data, but when uploading such large data to upper layer system, it may create bottlenecks everywhere.
In addition, from the velocity perspective, real-time or streaming data bring up the problem of large quantity of data coming into the data analytics within a short duration but the device and system may not be able to handle these input data. This situation is similar to that of the network flow analysis for which we typically cannot mirror and analyze everything we can gather.
From the variety perspective, because the incoming data may use different types or have incomplete data, how to handle them also bring up another issue for the input operators of data analytics.
In this section, we will turn the discussion to the big data analytics process.
Big data input
The problem of handling a vast quantity of data that the system is unable to process is not a brand-new research issue; in fact, it appeared in several early approaches [ 2 , 21 , 72 ], e.g., marketing analysis, network flow monitor, gene expression analysis, weather forecast, and even astronomy analysis. This problem still exists in big data analytics today; thus, preprocessing is an important task to make the computer, platform, and analysis algorithm be able to handle the input data. The traditional data preprocessing methods [ 73 ] (e.g., compression, sampling, feature selection, and so on) are expected to be able to operate effectively in the big data age. However, a portion of the studies still focus on how to reduce the complexity of the input data because even the most advanced computer technology cannot efficiently process the whole input data by using a single machine in most cases. By using domain knowledge to design the preprocessing operator is a possible solution for the big data. In [ 74 ], Ham and Lee used the domain knowledge, B -tree, divide-and-conquer to filter the unrelated log information for the mobile web log analysis. A later study [ 75 ] considered that the computation cost of preprocessing will be quite high for massive logs, sensor, or marketing data analysis. Thus, Dawelbeit and McCrindle employed the bin packing partitioning method to divide the input data between the computing processors to handle this high computations of preprocessing on cloud system. The cloud system is employed to preprocess the raw data and then output the refined data (e.g., data with uniform format) to make it easier for the data analysis method or system to preform the further analysis work.
Sampling and compression are two representative data reduction methods for big data analytics because reducing the size of data makes the data analytics computationally less expensive, thus faster, especially for the data coming to the system rapidly. In addition to making the sampling data represent the original data effectively [ 76 ], how many instances need to be selected for data mining method is another research issue [ 77 ] because it will affect the performance of the sampling method in most cases.
To avoid the application-level slow-down caused by the compression process, in [ 78 ], Jun et al. attempted to use the FPGA to accelerate the compression process. The I/O performance optimization is another issue for the compression method. For this reason, Zou et al. [ 79 ] employed the tentative selection and predictive dynamic selection and switched the appropriate compression method from two different strategies to improve the performance of the compression process. To make it possible for the compression method to efficiently compress the data, a promising solution is to apply the clustering method to the input data to divide them into several different groups and then compress these input data according to the clustering information. The compression method described in [ 80 ] is one of this kind of solutions, it first clusters the input data and then compresses these input data via the clustering results while the study [ 81 ] also used clustering method to improve the performance of the compression process.
In summary, in addition to handling the large and fast data input, the research issues of heterogeneous data sources, incomplete data, and noisy data may also affect the performance of the data analysis. The input operators will have a stronger impact on the data analytics at the big data age than it has in the past. As a result, the design of big data analytics needs to consider how to make these tasks (e.g., data clean, data sampling, data compression) work well.
Big data analysis frameworks and platforms
Various solutions have been presented for the big data analytics which can be divided [ 82 ] into (1) Processing/Compute: Hadoop [ 83 ], Nvidia CUDA [ 84 ], or Twitter Storm [ 85 ], (2) Storage: Titan or HDFS, and (3) Analytics: MLPACK [ 86 ] or Mahout [ 87 ]. Although there exist commercial products for data analysis [ 83 – 86 ], most of the studies on the traditional data analysis are focused on the design and development of efficient and/or effective “ways” to find the useful things from the data. But when we enter the age of big data, most of the current computer systems will not be able to handle the whole dataset all at once; thus, how to design a good data analytics framework or platform Footnote 3 and how to design analysis methods are both important things for the data analysis process. In this section, we will start with a brief introduction to data analysis frameworks and platforms, followed by a comparison of them.
The basic idea of big data analytics on cloud system
Researches in frameworks and platforms
To date, we can easily find tools and platforms presented by well-known organizations. The cloud computing technologies are widely used on these platforms and frameworks to satisfy the large demands of computing power and storage. As shown in Fig. 7 , most of the works on KDD for big data can be moved to cloud system to speed up the response time or to increase the memory space. With the advance of these works, handling and analyzing big data within a reasonable time has become not so far away. Since the foundation functions to handle and manage the big data were developed gradually; thus, the data scientists nowadays do not have to take care of everything, from the raw data gathering to data analysis, by themselves if they use the existing platforms or technologies to handle and manage the data. The data scientists nowadays can pay more attention to finding out the useful information from the data even thought this task is typically like looking for a needle in a haystack. That is why several recent studies tried to present efficient and effective framework to analyze the big data, especially on find out the useful things.
Performance-oriented From the perspective of platform performance, Huai [ 88 ] pointed out that most of the traditional parallel processing models improve the performance of the system by using a new larger computer system to replace the old computer system, which is usually referred to as “scale up”, as shown in Fig. 8 a. But for the big data analytics, most researches improve the performance of the system by adding more similar computer systems to make it possible for a system to handle all the tasks that cannot be loaded or computed in a single computer system (called “scale out”), as shown in Fig. 8 b where M1, M2, and M3 represent computer systems that have different computing power, respectively. For the scale up based solution, the computing power of the three systems is in the order of \(\text {M3}>\text {M2}>\text {M1}\) ; but for the scale out based system, all we have to do is to keep adding more similar computer systems to to a system to increase its ability. To build a scalable and fault-tolerant manager for big data analysis, Huai et al. [ 88 ] presented a matrix model which consists of three matrices for data set (D), concurrent data processing operations (O), and data transformations (T), called DOT. The big data is divided into n subsets each of which is processed by a computer node (worker) in such a way that all the subsets are processed concurrently, and then the results from these n computer nodes are collected and transformed to a computer node. By using this framework, the whole data analysis framework is composed of several DOT blocks. The system performance can be easily enhanced by adding more DOT blocks to the system.
The comparisons between scale up and scale out
Another efficient big data analytics was presented in [ 89 ], called generalized linear aggregates distributed engine (GLADE). The GLADE is a multi-level tree-based data analytics system which consists of two types of computer nodes that are a coordinator and workers. The simulation results [ 90 ] show that the GLADE can provide a better performance than Hadoop in terms of the execution time. Because Hadoop requires large memory and storage for data replication and it is a single master, Footnote 4 Essa et al. [ 91 ] presented a mobile agent based framework to solve these two problems, called the map reduce agent mobility (MRAM). The main reason is that each mobile agent can send its code and data to any other machine; therefore, the whole system will not be down if the master failed. Compared to Hadoop, the architecture of MRAM was changed from client/server to a distributed agent. The load time for MRAM is less than Hadoop even though both of them use the map-reduce solution and Java language. In [ 92 ], Herodotou et al. considered issues of the user needs and system workloads. They presented a self-tuning analytics system built on Hadoop for big data analysis. Since one of the major goals of their system is to adjust the system based on the user needs and system workloads to provide good performance automatically, the user usually does not need to understand and manipulate the Hadoop system. The study [ 93 ] was from the perspectives of data centric architecture and operational models to presented a big data architecture framework (BDAF) which includes: big data infrastructure, big data analytics, data structures and models, big data lifecycle management, and big data security. According to the observations of Demchenko et al. [ 93 ], cluster services, Hadoop related services, data analytics tools, databases, servers, and massively parallel processing databases are typically the required applications and services in big data analytics infrastructure.
Result-oriented Fisher et al. [ 5 ] presented a big data pipeline to show the workflow of big data analytics to extract the valuable knowledge from big data, which consists of the acquired data, choosing architecture, shaping data into architecture, coding/debugging, and reflecting works. From the perspectives of statistical computation and data mining, Ye et al. [ 94 ] presented an architecture of the services platform which integrates R to provide better data analysis services, called cloud-based big data mining and analyzing services platform (CBDMASP). The design of this platform is composed of four layers: the infrastructure services layer, the virtualization layer, the dataset processing layer, and the services layer. Several large-scale clustering problems (the datasets are of size from 0.1 G up to 25.6 G) were also used to evaluate the performance of the CBDMASP. The simulation results show that using map-reduce is much faster than using a single machine when the input data become too large. Although the size of the test dataset cannot be regarded as a big dataset, the performance of the big data analytics using map-reduce can be sped up via this kind of testings. In this study, map-reduce is a better solution when the dataset is of size more than 0.2 G, and a single machine is unable to handle a dataset that is of size more than 1.6 G.
Another study [ 95 ] presented a theorem to explain the big data characteristics, called HACE: the characteristics of big data usually are large-volume, Heterogeneous, Autonomous sources with distributed and decentralized control, and we usually try to find out some useful and interesting things from complex and evolving relationships of data. Based on these concerns and data mining issues, Wu and his colleagues [ 95 ] also presented a big data processing framework which includes data accessing and computing tier, data privacy and domain knowledge tier, and big data mining algorithm tier. This work explains that the data mining algorithm will become much more important and much more difficult; thus, challenges will also occur on the design and implementation of big data analytics platform. In addition to the platform performance and data mining issues, the privacy issue for big data analytics was a promising research in recent years. In [ 96 ], Laurila et al. explained that the privacy is an essential problem when we try to find something from the data that are gathered from mobile devices; thus, data security and data anonymization should also be considered in analyzing this kind of data. Demirkan and Delen [ 97 ] presented a service-oriented decision support system (SODSS) for big data analytics which includes information source, data management, information management, and operations management.
Comparison between the frameworks/platforms of big data
In [ 98 ], Talia pointed out that cloud-based data analytics services can be divided into data analytics software as a service, data analytics platform as a service, and data analytics infrastructure as a service. A later study [ 99 ] presented a general architecture of big data analytics which contains multi-source big data collecting, distributed big data storing, and intra/inter big data processing. Since many kinds of data analytics frameworks and platforms have been presented, some of the studies attempted to compare them to give a guidance to choose the applicable frameworks or platforms for relevant works. To give a brief introduction to big data analytics, especially the platforms and frameworks, in [ 100 ], Cuzzocrea et al. first discuss how recent studies responded the “computational emergency” issue of big data analytics. Some open issues, such as data source heterogeneity and uncorrelated data filtering, and possible research directions are also given in the same study. In [ 101 ], Zhang and Huang used the 5Ws model to explain what kind of framework and method we need for different big data approaches. Zhang and Huang further explained that the 5Ws model represents what kind of data, why we have these data, where the data come from, when the data occur, who receive the data, and how the data are transferred. A later study [ 102 ] used the features (i.e., owner, workload, source code, low latency, and complexity) to compare the frameworks of Hadoop [ 83 ], Storm [ 85 ] and Drill [ 103 ]. Thus, it can be easily seen that the framework of Apache Hadoop has high latency compared with the other two frameworks. To better understand the strong and weak points of solutions of big data, Chalmers et al. [ 82 ] then employed the volume, variety, variability, velocity, user skill/experience, and infrastructure to evaluate eight solutions of big data analytics.
In [ 104 ], in addition to defining that a big data system should include data generation, data acquisition, data storage, and data analytics modules, Hu et al. also mentioned that a big data system can be decomposed into infrastructure, computing, and application layers. Moreover, a promising research for NoSQL storage systems was also discussed in this study which can be divided into key-value , column , document , and row databases. Since big data analysis is generally regarded as a high computation cost work, the high performance computing cluster system (HPCC) is also a possible solution in early stage of big data analytics. Sagiroglu and Sinanc [ 105 ] therefore compare the characteristics between HPCC and Hadoop. They then emphasized that HPCC system uses the multikey and multivariate indexes on distributed file system while Hadoop uses the column-oriented database. In [ 17 ], Chen et al. give a brief introduction to the big data analytics of business intelligence (BI) from the perspective of evolution, applications, and emerging research topics. In their survey, Chen et al. explained that the revolution of business intelligence and analytics (BI&I) was from BI&I 1.0, BI&I 2.0, to BI&I 3.0 which are DBMS-based and structured content, web-based and unstructured content, and mobile and sensor based content, respectively.
Big data analysis algorithms
Mining algorithms for specific problem.
Because the big data issues have appeared for nearly ten years, in [ 106 ], Fan and Bifet pointed out that the terms “big data” [ 107 ] and “big data mining” [ 108 ] were first presented in 1998, respectively. The big data and big data mining almost appearing at the same time explained that finding something from big data will be one of the major tasks in this research domain. Data mining algorithms for data analysis also play the vital role in the big data analysis, in terms of the computation cost, memory requirement, and accuracy of the end results. In this section, we will give a brief discussion from the perspective of analysis and search algorithms to explain its importance for big data analytics.
Clustering algorithms In the big data age, traditional clustering algorithms will become even more limited than before because they typically require that all the data be in the same format and be loaded into the same machine so as to find some useful things from the whole data. Although the problem [ 64 ] of analyzing large-scale and high-dimensional dataset has attracted many researchers from various disciplines in the last century, and several solutions [ 2 , 109 ] have been presented presented in recent years, the characteristics of big data still brought up several new challenges for the data clustering issues. Among them, how to reduce the data complexity is one of the important issues for big data clustering. In [ 110 ], Shirkhorshidi et al. divided the big data clustering into two categories: single-machine clustering (i.e., sampling and dimension reduction solutions), and multiple-machine clustering (parallel and MapReduce solutions). This means that traditional reduction solutions can also be used in the big data age because the complexity and memory space needed for the process of data analysis will be decreased by using sampling and dimension reduction methods. More precisely, sampling can be regarded as reducing the “amount of data” entered into a data analyzing process while dimension reduction can be regarded as “downsizing the whole dataset” because irrelevant dimensions will be discarded before the data analyzing process is carried out.
CloudVista [ 111 ] is a representative solution for clustering big data which used cloud computing to perform the clustering process in parallel. BIRCH [ 44 ] and sampling method were used in CloudVista to show that it is able to handle large-scale data, e.g., 25 million census records. Using GPU to enhance the performance of a clustering algorithm is another promising solution for big data mining. The multiple species flocking (MSF) [ 112 ] was applied to the CUDA platform from NVIDIA to reduce the computation time of clustering algorithm in [ 113 ]. The simulation results show that the speedup factor can be increased from 30 up to 60 by using GPU for data clustering. Since most traditional clustering algorithms (e.g, k -means) require a computation that is centralized, how to make them capable of handling big data clustering problems is the major concern of Feldman et al. [ 114 ] who use a tree construction for generating the coresets in parallel which is called the “merge-and-reduce” approach. Moreover, Feldman et al. pointed out that by using this solution for clustering, the update time per datum and memory of the traditional clustering algorithms can be significantly reduced.
Classification algorithms Similar to the clustering algorithm for big data mining, several studies also attempted to modify the traditional classification algorithms to make them work on a parallel computing environment or to develop new classification algorithms which work naturally on a parallel computing environment. In [ 115 ], the design of classification algorithm took into account the input data that are gathered by distributed data sources and they will be processed by a heterogeneous set of learners. Footnote 5 In this study, Tekin et al. presented a novel classification algorithm called “classify or send for classification” (CoS). They assumed that each learner can be used to process the input data in two different ways in a distributed data classification system. One is to perform a classification function by itself while the other is to forward the input data to another learner to have them labeled. The information will be exchanged between different learners. In brief, this kind of solutions can be regarded as a cooperative learning to improve the accuracy in solving the big data classification problem. An interesting solution uses the quantum computing to reduce the memory space and computing cost of a classification algorithm. For example, in [ 116 ], Rebentrost et al. presented a quantum-based support vector machine for big data classification and argued that the classification algorithm they proposed can be implemented with a time complexity \(O(\log NM)\) where N is the number of dimensions and M is the number of training data. There are bright prospects for big data mining by using quantum-based search algorithm when the hardware of quantum computing has become mature.
Frequent pattern mining algorithms Most of the researches on frequent pattern mining (i.e., association rules and sequential pattern mining) were focused on handling large-scale dataset at the very beginning because some early approaches of them were attempted to analyze the data from the transaction data of large shopping mall. Because the number of transactions usually is more than “tens of thousands”, the issues about how to handle the large scale data were studied for several years, such as FP-tree [ 32 ] using the tree structure to include the frequent patterns to further reduce the computation time of association rule mining. In addition to the traditional frequent pattern mining algorithms, of course, parallel computing and cloud computing technologies have also attracted researchers in this research domain. Among them, the map-reduce solution was used for the studies [ 117 – 119 ] to enhance the performance of the frequent pattern mining algorithm. By using the map-reduce model for frequent pattern mining algorithm, it can be easily expected that its application to “cloud platform” [ 120 , 121 ] will definitely become a popular trend in the forthcoming future. The study of [ 119 ] no only used the map-reduce model, it also allowed users to express their specific interest constraints in the process of frequent pattern mining. The performance of these methods by using map-reduce model for big data analysis is, no doubt, better than the traditional frequent pattern mining algorithms running on a single machine.
Machine learning for big data mining
The potential of machine learning for data analytics can be easily found in the early literature [ 22 , 49 ]. Different from the data mining algorithm design for specific problems, machine learning algorithms can be used for different mining and analysis problems because they are typically employed as the “search” algorithm of the required solution. Since most machine learning algorithms can be used to find an approximate solution for the optimization problem, they can be employed for most data analysis problems if the data analysis problems can be formulated as an optimization problem. For example, genetic algorithm, one of the machine learning algorithms, can not only be used to solve the clustering problem [ 25 ], it can also be used to solve the frequent pattern mining problem [ 33 ]. The potential of machine learning is not merely for solving different mining problems in data analysis operator of KDD; it also has the potential of enhancing the performance of the other parts of KDD, such as feature reduction for the input operators [ 72 ].
A recent study [ 68 ] shows that some traditional mining algorithms, statistical methods, preprocessing solutions, and even the GUI’s have been applied to several representative tools and platforms for big data analytics. The results show clearly that machine learning algorithms will be one of the essential parts of big data analytics. One of the problems in using current machine learning methods for big data analytics is similar to those of most traditional data mining algorithms which are designed for sequential or centralized computing. However, one of the most possible solutions is to make them work for parallel computing. Fortunately, some of the machine learning algorithms (e.g., population-based algorithms) can essentially be used for parallel computing, which have been demonstrated for several years, such as parallel computing version of genetic algorithm [ 122 ]. Different from the traditional GA, as shown in Fig. 9 a, the population of island model genetic algorithm, one of the parallel GA’s, can be divided into several sub-populations, as shown in Fig. 9 b. This means that the sub-populations can be assigned to different threads or computer nodes for parallel computing, by a simple modification of the GA.
The comparison between basic idea of traditional GA (TGA) and parallel genetic algorithm (PGA)
For this reason, in [ 123 ], Kiran and Babu explained that the framework for distributed data mining algorithm still needs to aggregate the information from different computer nodes. As shown in Fig. 10 , the common design of distributed data mining algorithm is as follows: each mining algorithm will be performed on a computer node (worker) which has its locally coherent data, but not the whole data. To construct a globally meaningful knowledge after each mining algorithm finds its local model, the local model from each computer node has to be aggregated and integrated into a final model to represent the complete knowledge. Kiran and Babu [ 123 ] also pointed out that the communication will be the bottleneck when using this kind of distributed computing framework.
A simple example of distributed data mining framework [ 86 ]
Bu et al. [ 124 ] found some research issues when trying to apply machine learning algorithms to parallel computing platforms. For instance, the early version of map-reduce framework does not support “iteration” (i.e., recursion). But the good news is that some recent works [ 87 , 125 ] have paid close attention to this problem and tried to fix it. Similar to the solutions for enhancing the performance of the traditional data mining algorithms, one of the possible solutions to enhancing the performance of a machine learning algorithm is to use CUDA, i.e., a GPU, to reduce the computing time of data analysis. Hasan et al. [ 126 ] used CUDA to implement the self-organizing map (SOM) and multiple back-propagation (MBP) for the classification problem. The simulation results show that using GPU is faster than using CPU. More precisely, SOM running on a GPU is three times faster than SOM running on a CPU, and MPB running on a GPU is twenty-seven times faster than MPB running on a. Another study [ 127 ] attempted to apply the ant-based algorithm to grid computing platform. Since the proposed mining algorithm is extended by the ant clustering algorithm of Deneubourg et al. [ 128 ], Footnote 6 Ku-Mahamud modified the ant behavior of this ant clustering algorithm for big data clustering. That is, each ant will be randomly placed on the grid. This means that the ant clustering algorithm then can be used on a parallel computing environment.
The trends of machine learning studies for big data analytics can be divided into twofold: one attempts to make machine learning algorithms run on parallel platforms, such as Radoop [ 129 ], Mahout [ 87 ], and PIMRU [ 124 ]; the other is to redesign the machine learning algorithms to make them suitable for parallel computing or to parallel computing environment, such as neural network algorithms for GPU [ 126 ] and ant-based algorithm for grid [ 127 ]. In summary, both of them make it possible to apply the machine learning algorithms to big data analytics although still many research issues need to be solved, such as the communication cost for different computer nodes [ 86 ] and the large computation cost most machine learning algorithms require [ 126 ].
Output the result of big data analysis
The benchmarks of PigMix [ 130 ], GridMix [ 131 ], TeraSort and GraySort [ 132 ], TPC-C, TPC-H, TPC-DS [ 133 ], and yahoo cloud serving benchmark (YCSB) [ 134 ] have been presented for evaluating the performance of the cloud computing and big data analytics systems. Ghazal et al. [ 135 ] presented another benchmark (called BigBench) to be used as an end-to-end big data benchmark which covers the characteristics of 3V of big data and uses the loading time, time for queries, time for procedural processing queries, and time for the remaining queries as the metrics. By using these benchmarks, the computation time is one of the intuitive metrics for evaluating the performance of different big data analytics platforms or algorithms. That is why Cheptsov [ 136 ] compered the high performance computing (HPC) and cloud system by using the measurement of computation time to understand their scalability for text file analysis. In addition to the computation time, the throughput (e.g., the number of operations per second) and read/write latency of operations are the other measurements of big data analytics [ 137 ]. In the study of [ 138 ], Zhao et al. believe that the maximum size of data and the maximum number of jobs are the two important metrics to understand the performance of the big data analytics platform. Another study described in [ 139 ] presented a systematic evaluation method which contains the data throughput, concurrency during map and reduce phases, response times, and the execution time of map and reduce. Moreover, most benchmarks for evaluating the performance of big data analytics typically can only provide the response time or the computation cost; however, the fact is that several factors need to be taken into account at the same time when building a big data analytics system. The hardware, bandwidth for data transmission, fault tolerance, cost, power consumption of these systems are all issues [ 70 , 104 ] to be taken into account at the same time when building a big data analytics system. Several solutions available today are to install the big data analytics on a cloud computing system or a cluster system. Therefore, the measurements of fault tolerance, task execution, and cost of cloud computing systems can then be used to evaluate the performance of the corresponding factors of big data analytics.
How to present the analysis results to a user is another important work in the output part of big data analytics because if the user cannot easily understand the meaning of the results, the results will be entirely useless. Business intelligent and network monitoring are the two common approaches because their user interface plays the vital role of making them workable. Zhang et al. [ 140 ] pointed out that the tasks of the visual analytics for commercial systems can be divided into four categories which are exploration, dashboards, reporting, and alerting. The study [ 141 ] showed that the interface for electroencephalography (EEG) interpretation is another noticeable research issue in big data analytics. The user interface for cloud system [ 142 , 143 ] is the recent trend for big data analytics. This usually plays vital roles in big data analytics system, one of which is to simplify the explanation of the needed knowledge to the users while the other is to make it easier for the users to handle the data analytics system to work with their opinions. According to our observations, a flexible user interface is needed because although the big data analytics can help us to find some hidden information, the information found usually is not knowledge. This situation is just like the example we mentioned in “ Output the result ”. The mining or statistical techniques can be employed to know the flu situation of each region, but data scientists sometimes need additional ways to display the information to find out the knowledge they need or to prove their assumption. Thus, the user interface can be adjusted by the user to display the knowledge that is needed urgently for big data analytics.
Summary of process of big data analytics
This discussion of big data analytics in this section was divided into input, analysis, and output for mapping the data analysis process of KDD. For the input (see also in “ Big data input ”) and output (see also “ Output the result of big data analysis ”) of big data, several methods and solutions proposed before the big data age (see also “ Data input ”) can also be employed for big data analytics in most cases.
However, there still exist some new issues of the input and output that the data scientists need to confront. A representative example we mentioned in “ Big data input ” is that the bottleneck will not only on the sensor or input devices, it may also appear in other places of data analytics [ 71 ]. Although we can employ traditional compression and sampling technologies to deal with this problem, they can only mitigate the problems instead of solving the problems completely. Similar situations also exist in the output part. Although several measurements can be used to evaluate the performance of the frameworks, platforms, and even data mining algorithms, there still exist several new issues in the big data age, such as information fusion from different information sources or information accumulation from different times.
Several studies attempted to present an efficient or effective solution from the perspective of system (e.g., framework and platform) or algorithm level. A simple comparison of these big data analysis technologies from different perspectives is described in Table 3 , to give a brief introduction to the current studies and trends of data analysis technologies for the big data. The “Perspective” column of this table explains that the study is focused on the framework or algorithm level; the “Description” column gives the further goal of the study; and the “Name” column is an abbreviated names of the methods or platform/framework. From the analysis framework perspective, this table shows that big data framework , platform , and machine learning are the current research trends in big data analytics system. For the mining algorithm perspective, the clustering , classification , and frequent pattern mining issues play the vital role of these researches because several data analysis problems can be mapped to these essential issues.
A promising trend that can be easily found from these successful examples is to use machine learning as the search algorithm (i.e., mining algorithm) for the data mining problems of big data analytics system. The machine learning-based methods are able to make the mining algorithms and relevant platforms smarter or reduce the redundant computation costs. That parallel computing and cloud computing technologies have a strong impact on the big data analytics can also be recognized as follows: (1) most of the big data analytics frameworks and platforms are using Hadoop and Hadoop relevant technologies to design their solutions; and (2) most of the mining algorithms for big data analysis have been designed for parallel computing via software or hardware or designed for Map-Reduce-based platform.
From the results of recent studies of big data analytics, it is still at the early stage of Nolan’s stages of growth model [ 146 ] which is similar to the situations for the research topics of cloud computing, internet of things, and smart grid. This is because several studies just attempted to apply the traditional solutions to the new problems/platforms/environments. For example, several studies [ 114 , 145 ] used k -means as an example to analyze the big data, but not many studies applied the state-of-the-art data mining algorithms and machine learning algorithms to the analysis the big data. This explains that the performance of the big data analytics can be improved by data mining algorithms and metaheuristic algorithms presented in recent years [ 147 ]. The relevant technologies for compression, sampling, or even the platform presented in recent years may also be used to enhance the performance of the big data analytics system. As a result, although these research topics still have several open issues that need to be solved, these situations, on the contrary, also illustrate that everything is possible in these studies.
The open issues
Although the data analytics today may be inefficient for big data caused by the environment, devices, systems, and even problems that are quite different from traditional mining problems, because several characteristics of big data also exist in the traditional data analytics. Several open issues caused by the big data will be addressed as the platform/framework and data mining perspectives in this section to explain what dilemmas we may confront because of big data. Here are some of the open issues:
Platform and framework perspective
Input and output ratio of platform.
A large number of reports and researches mentioned that we will enter the big data age in the near future. Some of them insinuated to us that these fruitful results of big data will lead us to a whole new world where “everything” is possible; therefore, the big data analytics will be an omniscient and omnipotent system. From the pragmatic perspective, the big data analytics is indeed useful and has many possibilities which can help us more accurately understand the so-called “things.” However, the situation in most studies of big data analytics is that they argued that the results of big data are valuable, but the business models of most big data analytics are not clear. The fact is that assuming we have infinite computing resources for big data analytics is a thoroughly impracticable plan, the input and output ratio (e.g., return on investment) will need to be taken into account before an organization constructs the big data analytics center.
Communication between systems
Since most big data analytics systems will be designed for parallel computing, and they typically will work on other systems (e.g., cloud platform) or work with other systems (e.g., search engine or knowledge base), the communication between the big data analytics and other systems will strongly impact the performance of the whole process of KDD. The first research issue for the communication is that the communication cost will incur between systems of data analytics. How to reduce the communication cost will be the very first thing that the data scientists need to care. Another research issue for the communication is how the big data analytics communicates with other systems. The consistency of data between different systems, modules, and operators is also an important open issue on the communication between systems. Because the communication will appear more frequently between systems of big data analytics, how to reduce the cost of communication and how to make the communication between these systems as reliable as possible will be the two important open issues for big data analytics.
Bottlenecks on data analytics system
The bottlenecks will be appeared in different places of the data analytics for big data because the environments, systems, and input data have changed which are different from the traditional data analytics. The data deluge of big data will fill up the “input” system of data analytics, and it will also increase the computation load of the data “analysis” system. This situation is just like the torrent of water (i.e., data deluge) rushed down the mountain (i.e., data analytics), how to split it and how to avoid it flowing into a narrow place (e.g., the operator is not able to handle the input data) will be the most important things to avoid the bottlenecks in data analytics system. One of the current solutions to the avoidance of bottlenecks on a data analytics system is to add more computation resources while the other is to split the analysis works to different computation nodes. A complete consideration for the whole data analytics to avoid the bottlenecks of that kind of analytics system is still needed for big data.
Security issues
Since much more environment data and human behavior will be gathered to the big data analytics, how to protect them will also be an open issue because without a security way to handle the collected data, the big data analytics cannot be a reliable system. In spite of the security that we have to tighten for big data analytics before it can gather more data from everywhere, the fact is that until now, there are still not many studies focusing on the security issues of the big data analytics. According to our observation, the security issues of big data analytics can be divided into fourfold: input, data analysis, output, and communication with other systems. For the input, it can be regarded as the data gathering which is relevant to the sensor, the handheld devices, and even the devices of internet of things. One of the important security issues on the input part of big data analytics is to make sure that the sensors will not be compromised by the attacks. For the analysis and input, it can be regarded as the security problem of such a system. For communication with other system, the security problem is on the communications between big data analytics and other external systems. Because of these latent problems, security has become one of the open issues of big data analytics.
Data mining perspective
Data mining algorithm for map-reduce solution.
As we mentioned in the previous sections, most of the traditional data mining algorithms are not designed for parallel computing; therefore, they are not particularly useful for the big data mining. Several recent studies have attempted to modify the traditional data mining algorithms to make them applicable to Hadoop-based platforms. As long as porting the data mining algorithms to Hadoop is inevitable, making the data mining algorithms work on a map-reduce architecture is the first very thing to do to apply traditional data mining methods to big data analytics. Unfortunately, not many studies attempted to make the data mining and soft computing algorithms work on Hadoop because several different backgrounds are needed to develop and design such algorithms. For instance, the researcher and his or her research group need to have the background in data mining and Hadoop so as to develop and design such algorithms. Another open issue is that most data mining algorithms are designed for centralized computing; that is, they can only work on all the data at the same time. Thus, how to make them work on a parallel computing system is also a difficult work. The good news is that some studies [ 145 ] have successfully applied the traditional data mining algorithms to the map-reduce architecture. These results imply that it is possible to do so. According to our observation, although the traditional mining or soft computing algorithms can be used to help us analyze the data in big data analytics, unfortunately, until now, not many studies are focused on it. As a consequence, it is an important open issue in big data analytics.
Noise, outliers, incomplete and inconsistent data
Although big data analytics is a new age for data analysis, because several solutions adopt classical ways to analyze the data on big data analytics, the open issues of traditional data mining algorithms also exist in these new systems. The open issues of noise, outliers, incomplete, and inconsistent data in traditional data mining algorithms will also appear in big data mining algorithms. More incomplete and inconsistent data will easily appear because the data are captured by or generated from different sensors and systems. The impact of noise, outliers, incomplete and inconsistent data will be enlarged for big data analytics. Therefore, how to mitigate the impact will be the open issues for big data analytics.
Bottlenecks on data mining algorithm
Most of the data mining algorithms in big data analytics will be designed for parallel computing. However, once data mining algorithms are designed or modified for parallel computing, it is the information exchange between different data mining procedures that may incur bottlenecks. One of them is the synchronization issue because different mining procedures will finish their jobs at different times even though they use the same mining algorithm to work on the same amount of data. Thus, some of the mining procedures will have to wait until the others finished their jobs. This situation may occur because the loading of different computer nodes may be different during the data mining process, or it may occur because the convergence speeds are different for the same data mining algorithm. The bottlenecks of data mining algorithms will become an open issue for the big data analytics which explains that we need to take into account this issue when we develop and design a new data mining algorithm for big data analytics.
Privacy issues
The privacy concern typically will make most people uncomfortable, especially if systems cannot guarantee that their personal information will not be accessed by the other people and organizations. Different from the concern of the security, the privacy issue is about if it is possible for the system to restore or infer personal information from the results of big data analytics, even though the input data are anonymous. The privacy issue has become a very important issue because the data mining and other analysis technologies will be widely used in big data analytics, the private information may be exposed to the other people after the analysis process. For example, although all the gathered data for shop behavior are anonymous (e.g., buying a pistol), because the data can be easily collected by different devices and systems (e.g., location of the shop and age of the buyer), a data mining algorithm can easily infer who bought this pistol. More precisely, the data analytics is able to reduce the scope of the database because location of the shop and age of the buyer provide the information to help the system find out possible persons. For this reason, any sensitive information needs to be carefully protected and used. The anonymous, temporary identification, and encryption are the representative technologies for privacy of data analytics, but the critical factor is how to use, what to use, and why to use the collected data on big data analytics.
Conclusions
In this paper, we reviewed studies on the data analytics from the traditional data analysis to the recent big data analysis. From the system perspective, the KDD process is used as the framework for these studies and is summarized into three parts: input, analysis, and output. From the perspective of big data analytics framework and platform, the discussions are focused on the performance-oriented and results-oriented issues. From the perspective of data mining problem, this paper gives a brief introduction to the data and big data mining algorithms which consist of clustering, classification, and frequent patterns mining technologies. To better understand the changes brought about by the big data, this paper is focused on the data analysis of KDD from the platform/framework to data mining. The open issues on computation, quality of end result, security, and privacy are then discussed to explain which open issues we may face. Last but not least, to help the audience of the paper find solutions to welcome the new age of big data, the possible high impact research trends are given below:
For the computation time, there is no doubt at all that parallel computing is one of the important future trends to make the data analytics work for big data, and consequently the technologies of cloud computing, Hadoop, and map-reduce will play the important roles for the big data analytics. To handle the computation resources of the cloud-based platform and to finish the task of data analysis as fast as possible, the scheduling method is another future trend.
Using efficient methods to reduce the computation time of input, comparison, sampling, and a variety of reduction methods will play an important role in big data analytics. Because these methods typically do not consider parallel computing environment, how to make them work on parallel computing environment will be a future research trend. Similar to the input, the data mining algorithms also face the same situation that we mentioned in the previous section , how to make them work on parallel computing environment will be a very important research trend because there are abundant research results on traditional data mining algorithms.
How to model the mining problem to find something from big data and how to display the knowledge we got from big data analytics will also be another two vital future trends because the results of these two researches will decide if the data analytics can practically work for real world approaches, not just a theoretical stuff.
The methods of extracting information from external and relative knowledge resources to further reinforce the big data analytics, until now, are not very popular in big data analytics. But combining information from different resources to add the value of output knowledge is a common solution in the area of information retrieval, such as clustering search engine or document summarization. For this reason, information fusion will also be a future trend for improving the end results of big data analytics.
Because the metaheuristic algorithms are capable of finding an approximate solution within a reasonable time, they have been widely used in solving the data mining problem in recent years. Until now, many state-of-the-art metaheuristic algorithms still have not been applied to big data analytics. In addition, compared to some early data mining algorithms, the performance of metaheuristic is no doubt superior in terms of the computation time and the quality of end result. From these observations, the application of metaheuristic algorithms to big data analytics will also be an important research topic.
Because social network is part of the daily life of most people and because its data is also a kind of big data, how to analyze the data of a social network has become a promising research issue. Obviously, it can be used to predict the behavior of a user. After that, we can make applicable strategies for the user. For instance, a business intelligence system can use the analysis results to encourage particular customers to buy the goods they are interested.
The security and privacy issues that accompany the work of data analysis are intuitive research topics which contain how to safely store the data, how to make sure the data communication is protected, and how to prevent someone from finding out the information about us. Many problems of data security and privacy are essentially the same as those of the traditional data analysis even if we are entering the big data age. Thus, how to protect the data will also appear in the research of big data analytics.
In this paper, by the data analytics, we mean the whole KDD process, while by the data analysis, we mean the part of data analytics that is aimed at finding the hidden information in the data, such as data mining.
In this paper, by an unlabeled input data, we mean that it is unknown to which group the input data belongs. If all the input data are unlabeled, it means that the distribution of the input data is unknown.
In this paper, the analysis framework refers to the whole system, from raw data gathering, data reformat, data analysis, all the way to knowledge representation.
The whole system may be down when the master machine crashed for a system that has only one master.
The learner typically represented the classification function which will create the classifier to help us classify the unknown input data.
The basic idea of [ 128 ] is that each ant will pick up and drop data items in terms of the similarity of its local neighbors.
Abbreviations
principal components analysis
volume, velocity, and variety
International Data Corporation
knowledge discovery in databases
support vector machine
sum of squared errors
generalized linear aggregates distributed engine
big data architecture framework
cloud-based big data mining & analyzing services platform
service-oriented decision support system
high performance computing cluster system
business intelligence and analytics
database management system
multiple species flocking
genetic algorithm
self-organizing map
multiple back-propagation
yahoo cloud serving benchmark
high performance computing
electroencephalography
Lyman P, Varian H. How much information 2003? Tech. Rep, 2004. [Online]. Available: http://www2.sims.berkeley.edu/research/projects/how-much-info-2003/printable_report.pdf .
Xu R, Wunsch D. Clustering. Hoboken: Wiley-IEEE Press; 2009.
Google Scholar
Ding C, He X. K-means clustering via principal component analysis. In: Proceedings of the Twenty-first International Conference on Machine Learning, 2004, pp 1–9.
Kollios G, Gunopulos D, Koudas N, Berchtold S. Efficient biased sampling for approximate clustering and outlier detection in large data sets. IEEE Trans Knowl Data Eng. 2003;15(5):1170–87.
Article Google Scholar
Fisher D, DeLine R, Czerwinski M, Drucker S. Interactions with big data analytics. Interactions. 2012;19(3):50–9.
Laney D. 3D data management: controlling data volume, velocity, and variety, META Group, Tech. Rep. 2001. [Online]. Available: http://blogs.gartner.com/doug-laney/files/2012/01/ad949-3D-Data-Management-Controlling-Data-Volume-Velocity-and-Variety.pdf .
van Rijmenam M. Why the 3v’s are not sufficient to describe big data, BigData Startups, Tech. Rep. 2013. [Online]. Available: http://www.bigdata-startups.com/3vs-sufficient-describe-big-data/ .
Borne K. Top 10 big data challenges a serious look at 10 big data v’s, Tech. Rep. 2014. [Online]. Available: https://www.mapr.com/blog/top-10-big-data-challenges-look-10-big-data-v .
Press G. $16.1 billion big data market: 2014 predictions from IDC and IIA, Forbes, Tech. Rep. 2013. [Online]. Available: http://www.forbes.com/sites/gilpress/2013/12/12/16-1-billion-big-data-market-2014-predictions-from-idc-and-iia/ .
Big data and analytics—an IDC four pillar research area, IDC, Tech. Rep. 2013. [Online]. Available: http://www.idc.com/prodserv/FourPillars/bigData/index.jsp .
Taft DK. Big data market to reach $46.34 billion by 2018, EWEEK, Tech. Rep. 2013. [Online]. Available: http://www.eweek.com/database/big-data-market-to-reach-46.34-billion-by-2018.html .
Research A. Big data spending to reach $114 billion in 2018; look for machine learning to drive analytics, ABI Research, Tech. Rep. 2013. [Online]. Available: https://www.abiresearch.com/press/big-data-spending-to-reach-114-billion-in-2018-loo .
Furrier J. Big data market $50 billion by 2017—HP vertica comes out #1—according to wikibon research, SiliconANGLE, Tech. Rep. 2012. [Online]. Available: http://siliconangle.com/blog/2012/02/15/big-data-market-15-billion-by-2017-hp-vertica-comes-out-1-according-to-wikibon-research/ .
Kelly J, Vellante D, Floyer D. Big data market size and vendor revenues, Wikibon, Tech. Rep. 2014. [Online]. Available: http://wikibon.org/wiki/v/Big_Data_Market_Size_and_Vendor_Revenues .
Kelly J, Floyer D, Vellante D, Miniman S. Big data vendor revenue and market forecast 2012-2017, Wikibon, Tech. Rep. 2014. [Online]. Available: http://wikibon.org/wiki/v/Big_Data_Vendor_Revenue_and_Market_Forecast_2012-2017 .
Mayer-Schonberger V, Cukier K. Big data: a revolution that will transform how we live, work, and think. Boston: Houghton Mifflin Harcourt; 2013.
Chen H, Chiang RHL, Storey VC. Business intelligence and analytics: from big data to big impact. MIS Quart. 2012;36(4):1165–88.
Kitchin R. The real-time city? big data and smart urbanism. Geo J. 2014;79(1):1–14.
Fayyad UM, Piatetsky-Shapiro G, Smyth P. From data mining to knowledge discovery in databases. AI Mag. 1996;17(3):37–54.
Han J. Data mining: concepts and techniques. San Francisco: Morgan Kaufmann Publishers Inc.; 2005.
Agrawal R, Imieliński T, Swami A. Mining association rules between sets of items in large databases. Proc ACM SIGMOD Int Conf Manag Data. 1993;22(2):207–16.
Witten IH, Frank E. Data mining: practical machine learning tools and techniques. San Francisco: Morgan Kaufmann Publishers Inc.; 2005.
Abbass H, Newton C, Sarker R. Data mining: a heuristic approach. Hershey: IGI Global; 2002.
Book Google Scholar
Cannataro M, Congiusta A, Pugliese A, Talia D, Trunfio P. Distributed data mining on grids: services, tools, and applications. IEEE Trans Syst Man Cyber Part B Cyber. 2004;34(6):2451–65.
Krishna K, Murty MN. Genetic \(k\) -means algorithm. IEEE Trans Syst Man Cyber Part B Cyber. 1999;29(3):433–9.
Tsai C-W, Lai C-F, Chiang M-C, Yang L. Data mining for internet of things: a survey. IEEE Commun Surveys Tutor. 2014;16(1):77–97.
Jain AK, Murty MN, Flynn PJ. Data clustering: a review. ACM Comp Surveys. 1999;31(3):264–323.
McQueen JB. Some methods of classification and analysis of multivariate observations. In: Proceedings of the Berkeley Symposium on Mathematical Statistics and Probability, 1967. pp 281–297.
Safavian S, Landgrebe D. A survey of decision tree classifier methodology. IEEE Trans Syst Man Cyber. 1991;21(3):660–74.
Article MathSciNet Google Scholar
McCallum A, Nigam K. A comparison of event models for naive bayes text classification. In: Proceedings of the National Conference on Artificial Intelligence, 1998. pp. 41–48.
Boser BE, Guyon IM, Vapnik VN. A training algorithm for optimal margin classifiers. In: Proceedings of the annual workshop on Computational learning theory, 1992. pp. 144–152.
Han J, Pei J, Yin Y. Mining frequent patterns without candidate generation. In : Proceedings of the ACM SIGMOD International Conference on Management of Data, 2000. pp. 1–12.
Kaya M, Alhajj R. Genetic algorithm based framework for mining fuzzy association rules. Fuzzy Sets Syst. 2005;152(3):587–601.
Article MATH MathSciNet Google Scholar
Srikant R, Agrawal R. Mining sequential patterns: generalizations and performance improvements. In: Proceedings of the International Conference on Extending Database Technology: Advances in Database Technology, 1996. pp 3–17.
Zaki MJ. Spade: an efficient algorithm for mining frequent sequences. Mach Learn. 2001;42(1–2):31–60.
Article MATH Google Scholar
Baeza-Yates RA, Ribeiro-Neto B. Modern Information Retrieval. Boston: Addison-Wesley Longman Publishing Co., Inc; 1999.
Liu B. Web data mining: exploring hyperlinks, contents, and usage data. Berlin, Heidelberg: Springer-Verlag; 2007.
d’Aquin M, Jay N. Interpreting data mining results with linked data for learning analytics: motivation, case study and directions. In: Proceedings of the International Conference on Learning Analytics and Knowledge, pp 155–164.
Shneiderman B. The eyes have it: a task by data type taxonomy for information visualizations. In: Proceedings of the IEEE Symposium on Visual Languages, 1996, pp 336–343.
Mani I, Bloedorn E. Multi-document summarization by graph search and matching. In: Proceedings of the National Conference on Artificial Intelligence and Ninth Conference on Innovative Applications of Artificial Intelligence, 1997, pp 622–628.
Kopanakis I, Pelekis N, Karanikas H, Mavroudkis T. Visual techniques for the interpretation of data mining outcomes. In: Proceedings of the Panhellenic Conference on Advances in Informatics, 2005. pp 25–35.
Elkan C. Using the triangle inequality to accelerate k-means. In: Proceedings of the International Conference on Machine Learning, 2003, pp 147–153.
Catanzaro B, Sundaram N, Keutzer K. Fast support vector machine training and classification on graphics processors. In: Proceedings of the International Conference on Machine Learning, 2008. pp 104–111.
Zhang T, Ramakrishnan R, Livny M. BIRCH: an efficient data clustering method for very large databases. In: Proceedings of the ACM SIGMOD International Conference on Management of Data, 1996. pp 103–114.
Ester M, Kriegel HP, Sander J, Xu X. A density-based algorithm for discovering clusters in large spatial databases with noise. In: Proceedings of the Second International Conference on Knowledge Discovery and Data Mining, 1996. pp 226–231.
Ester M, Kriegel HP, Sander J, Wimmer M, Xu X. Incremental clustering for mining in a data warehousing environment. In: Proceedings of the International Conference on Very Large Data Bases, 1998. pp 323–333.
Ordonez C, Omiecinski E. Efficient disk-based k-means clustering for relational databases. IEEE Trans Knowl Data Eng. 2004;16(8):909–21.
Kogan J. Introduction to clustering large and high-dimensional data. Cambridge: Cambridge Univ Press; 2007.
MATH Google Scholar
Mitra S, Pal S, Mitra P. Data mining in soft computing framework: a survey. IEEE Trans Neural Netw. 2002;13(1):3–14.
Mehta M, Agrawal R, Rissanen J. SLIQ: a fast scalable classifier for data mining. In: Proceedings of the 5th International Conference on Extending Database Technology: Advances in Database Technology. 1996. pp 18–32.
Micó L, Oncina J, Carrasco RC. A fast branch and bound nearest neighbour classifier in metric spaces. Pattern Recogn Lett. 1996;17(7):731–9.
Djouadi A, Bouktache E. A fast algorithm for the nearest-neighbor classifier. IEEE Trans Pattern Anal Mach Intel. 1997;19(3):277–82.
Ververidis D, Kotropoulos C. Fast and accurate sequential floating forward feature selection with the bayes classifier applied to speech emotion recognition. Signal Process. 2008;88(12):2956–70.
Pei J, Han J, Mao R. CLOSET: an efficient algorithm for mining frequent closed itemsets. In: Proceedings of the ACM SIGMOD Workshop on Research Issues in Data Mining and Knowledge Discovery, 2000. pp 21–30.
Zaki MJ, Hsiao C-J. Efficient algorithms for mining closed itemsets and their lattice structure. IEEE Trans Knowl Data Eng. 2005;17(4):462–78.
Burdick D, Calimlim M, Gehrke J. MAFIA: a maximal frequent itemset algorithm for transactional databases. In: Proceedings of the International Conference on Data Engineering, 2001. pp 443–452.
Chen B, Haas P, Scheuermann P. A new two-phase sampling based algorithm for discovering association rules. In: Proceedings of the ACM SIGKDD International Conference on Knowledge Discovery and Data Mining, 2002. pp 462–468.
Zaki MJ. SPADE: an efficient algorithm for mining frequent sequences. Mach Learn. 2001;42(1–2):31–60.
Yan X, Han J, Afshar R. CloSpan: mining closed sequential patterns in large datasets. In: Proceedings of the SIAM International Conference on Data Mining, 2003. pp 166–177.
Pei J, Han J, Asl MB, Pinto H, Chen Q, Dayal U, Hsu MC. PrefixSpan mining sequential patterns efficiently by prefix projected pattern growth. In: Proceedings of the International Conference on Data Engineering, 2001. pp 215–226.
Ayres J, Flannick J, Gehrke J, Yiu T. Sequential PAttern Mining using a bitmap representation. In: Proceedings of the ACM SIGKDD International Conference on Knowledge Discovery and Data Mining, 2002. pp 429–435.
Masseglia F, Poncelet P, Teisseire M. Incremental mining of sequential patterns in large databases. Data Knowl Eng. 2003;46(1):97–121.
Xu R, Wunsch-II DC. Survey of clustering algorithms. IEEE Trans Neural Netw. 2005;16(3):645–78.
Chiang M-C, Tsai C-W, Yang C-S. A time-efficient pattern reduction algorithm for k-means clustering. Inform Sci. 2011;181(4):716–31.
Bradley PS, Fayyad UM. Refining initial points for k-means clustering. In: Proceedings of the International Conference on Machine Learning, 1998. pp 91–99.
Laskov P, Gehl C, Krüger S, Müller K-R. Incremental support vector learning: analysis, implementation and applications. J Mach Learn Res. 2006;7:1909–36.
MATH MathSciNet Google Scholar
Russom P. Big data analytics. TDWI: Tech. Rep ; 2011.
Ma C, Zhang HH, Wang X. Machine learning for big data analytics in plants. Trends Plant Sci. 2014;19(12):798–808.
Boyd D, Crawford K. Critical questions for big data. Inform Commun Soc. 2012;15(5):662–79.
Katal A, Wazid M, Goudar R. Big data: issues, challenges, tools and good practices. In: Proceedings of the International Conference on Contemporary Computing, 2013. pp 404–409.
Baraniuk RG. More is less: signal processing and the data deluge. Science. 2011;331(6018):717–9.
Lee J, Hong S, Lee JH. An efficient prediction for heavy rain from big weather data using genetic algorithm. In: Proceedings of the International Conference on Ubiquitous Information Management and Communication, 2014. pp 25:1–25:7.
Famili A, Shen W-M, Weber R, Simoudis E. Data preprocessing and intelligent data analysis. Intel Data Anal. 1997;1(1–4):3–23.
Zhang H. A novel data preprocessing solution for large scale digital forensics investigation on big data, Master’s thesis, Norway, 2013.
Ham YJ, Lee H-W. International journal of advances in soft computing and its applications. Calc Paralleles Reseaux et Syst Repar. 2014;6(1):1–18.
Cormode G, Duffield N. Sampling for big data: a tutorial. In: Proceedings of the ACM SIGKDD International Conference on Knowledge Discovery and Data Mining, 2014. pp 1975–1975.
Satyanarayana A. Intelligent sampling for big data using bootstrap sampling and chebyshev inequality. In: Proceedings of the IEEE Canadian Conference on Electrical and Computer Engineering, 2014. pp 1–6.
Jun SW, Fleming K, Adler M, Emer JS. Zip-io: architecture for application-specific compression of big data. In: Proceedings of the International Conference on Field-Programmable Technology, 2012, pp 343–351.
Zou H, Yu Y, Tang W, Chen HM. Improving I/O performance with adaptive data compression for big data applications. In: Proceedings of the International Parallel and Distributed Processing Symposium Workshops, 2014. pp 1228–1237.
Yang C, Zhang X, Zhong C, Liu C, Pei J, Ramamohanarao K, Chen J. A spatiotemporal compression based approach for efficient big data processing on cloud. J Comp Syst Sci. 2014;80(8):1563–83.
Xue Z, Shen G, Li J, Xu Q, Zhang Y, Shao J. Compression-aware I/O performance analysis for big data clustering. In: Proceedings of the International Workshop on Big Data, Streams and Heterogeneous Source Mining: Algorithms, Systems, Programming Models and Applications, 2012. pp 45–52.
Pospiech M, Felden C. Big data—a state-of-the-art. In: Proceedings of the Americas Conference on Information Systems, 2012, pp 1–23. [Online]. Available: http://aisel.aisnet.org/amcis2012/proceedings/DecisionSupport/22 .
Apache Hadoop, February 2, 2015. [Online]. Available: http://hadoop.apache.org .
Cuda, February 2, 2015. [Online]. Available: URL: http://www.nvidia.com/object/cuda_home_new.html .
Apache Storm, February 2, 2015. [Online]. Available: URL: http://storm.apache.org/ .
Curtin RR, Cline JR, Slagle NP, March WB, Ram P, Mehta NA, Gray AG. MLPACK: a scalable C++ machine learning library. J Mach Learn Res. 2013;14:801–5.
Apache Mahout, February 2, 2015. [Online]. Available: http://mahout.apache.org/ .
Huai Y, Lee R, Zhang S, Xia CH, Zhang X. DOT: a matrix model for analyzing, optimizing and deploying software for big data analytics in distributed systems. In: Proceedings of the ACM Symposium on Cloud Computing, 2011. pp 4:1–4:14.
Rusu F, Dobra A. GLADE: a scalable framework for efficient analytics. In: Proceedings of LADIS Workshop held in conjunction with VLDB, 2012. pp 1–6.
Cheng Y, Qin C, Rusu F. GLADE: big data analytics made easy. In: Proceedings of the ACM SIGMOD International Conference on Management of Data, 2012. pp 697–700.
Essa YM, Attiya G, El-Sayed A. Mobile agent based new framework for improving big data analysis. In: Proceedings of the International Conference on Cloud Computing and Big Data. 2013, pp 381–386.
Wonner J, Grosjean J, Capobianco A, Bechmann D Starfish: a selection technique for dense virtual environments. In: Proceedings of the ACM Symposium on Virtual Reality Software and Technology, 2012. pp 101–104.
Demchenko Y, de Laat C, Membrey P. Defining architecture components of the big data ecosystem. In: Proceedings of the International Conference on Collaboration Technologies and Systems, 2014. pp 104–112.
Ye F, Wang ZJ, Zhou FC, Wang YP, Zhou YC. Cloud-based big data mining and analyzing services platform integrating r. In: Proceedings of the International Conference on Advanced Cloud and Big Data, 2013. pp 147–151.
Wu X, Zhu X, Wu G-Q, Ding W. Data mining with big data. IEEE Trans Knowl Data Eng. 2014;26(1):97–107.
Laurila JK, Gatica-Perez D, Aad I, Blom J, Bornet O, Do T, Dousse O, Eberle J, Miettinen M. The mobile data challenge: big data for mobile computing research. In: Proceedings of the Mobile Data Challenge by Nokia Workshop, 2012. pp 1–8.
Demirkan H, Delen D. Leveraging the capabilities of service-oriented decision support systems: putting analytics and big data in cloud. Decision Support Syst. 2013;55(1):412–21.
Talia D. Clouds for scalable big data analytics. Computer. 2013;46(5):98–101.
Lu R, Zhu H, Liu X, Liu JK, Shao J. Toward efficient and privacy-preserving computing in big data era. IEEE Netw. 2014;28(4):46–50.
Cuzzocrea A, Song IY, Davis KC. Analytics over large-scale multidimensional data: The big data revolution!. In: Proceedings of the ACM International Workshop on Data Warehousing and OLAP, 2011. pp 101–104.
Zhang J, Huang ML. 5Ws model for big data analysis and visualization. In: Proceedings of the International Conference on Computational Science and Engineering, 2013. pp 1021–1028.
Chandarana P, Vijayalakshmi M. Big data analytics frameworks. In: Proceedings of the International Conference on Circuits, Systems, Communication and Information Technology Applications, 2014. pp 430–434.
Apache Drill February 2, 2015. [Online]. Available: URL: http://drill.apache.org/ .
Hu H, Wen Y, Chua T-S, Li X. Toward scalable systems for big data analytics: a technology tutorial. IEEE Access. 2014;2:652–87.
Sagiroglu S, Sinanc D, Big data: a review. In: Proceedings of the International Conference on Collaboration Technologies and Systems, 2013. pp 42–47.
Fan W, Bifet A. Mining big data: current status, and forecast to the future. ACM SIGKDD Explor Newslett. 2013;14(2):1–5.
Diebold FX. On the origin(s) and development of the term “big data”, Penn Institute for Economic Research, Department of Economics, University of Pennsylvania, Tech. Rep. 2012. [Online]. Available: http://economics.sas.upenn.edu/sites/economics.sas.upenn.edu/files/12-037.pdf .
Weiss SM, Indurkhya N. Predictive data mining: a practical guide. San Francisco: Morgan Kaufmann Publishers Inc.; 1998.
Fahad A, Alshatri N, Tari Z, Alamri A, Khalil I, Zomaya A, Foufou S, Bouras A. A survey of clustering algorithms for big data: taxonomy and empirical analysis. IEEE Trans Emerg Topics Comp. 2014;2(3):267–79.
Shirkhorshidi AS, Aghabozorgi SR, Teh YW, Herawan T. Big data clustering: a review. In: Proceedings of the International Conference on Computational Science and Its Applications, 2014. pp 707–720.
Xu H, Li Z, Guo S, Chen K. Cloudvista: interactive and economical visual cluster analysis for big data in the cloud. Proc VLDB Endowment. 2012;5(12):1886–9.
Cui X, Gao J, Potok TE. A flocking based algorithm for document clustering analysis. J Syst Archit. 2006;52(89):505–15.
Cui X, Charles JS, Potok T. GPU enhanced parallel computing for large scale data clustering. Future Gener Comp Syst. 2013;29(7):1736–41.
Feldman D, Schmidt M, Sohler C. Turning big data into tiny data: Constant-size coresets for k-means, pca and projective clustering. In: Proceedings of the ACM-SIAM Symposium on Discrete Algorithms, 2013. pp 1434–1453.
Tekin C, van der Schaar M. Distributed online big data classification using context information. In: Proceedings of the Allerton Conference on Communication, Control, and Computing, 2013. pp 1435–1442.
Rebentrost P, Mohseni M, Lloyd S. Quantum support vector machine for big feature and big data classification. CoRR , vol. abs/1307.0471, 2014. [Online]. Available: http://dblp.uni-trier.de/db/journals/corr/corr1307.html#RebentrostML13 .
Lin MY, Lee PY, Hsueh SC. Apriori-based frequent itemset mining algorithms on mapreduce. In: Proceedings of the International Conference on Ubiquitous Information Management and Communication, 2012. pp 76:1–76:8.
Riondato M, DeBrabant JA, Fonseca R, Upfal E. PARMA: a parallel randomized algorithm for approximate association rules mining in mapreduce. In: Proceedings of the ACM International Conference on Information and Knowledge Management, 2012. pp 85–94.
Leung CS, MacKinnon R, Jiang F. Reducing the search space for big data mining for interesting patterns from uncertain data. In: Proceedings of the International Congress on Big Data, 2014. pp 315–322.
Yang L, Shi Z, Xu L, Liang F, Kirsh I. DH-TRIE frequent pattern mining on hadoop using JPA. In: Proceedings of the International Conference on Granular Computing, 2011. pp 875–878.
Huang JW, Lin SC, Chen MS. DPSP: Distributed progressive sequential pattern mining on the cloud. In: Proceedings of the Advances in Knowledge Discovery and Data Mining, vol. 6119, 2010, pp 27–34.
Paz CE. A survey of parallel genetic algorithms. Calc Paralleles Reseaux et Syst Repar. 1998;10(2):141–71.
kranthi Kiran B, Babu AV. A comparative study of issues in big data clustering algorithm with constraint based genetic algorithm for associative clustering. Int J Innov Res Comp Commun Eng 2014; 2(8): 5423–5432.
Bu Y, Borkar VR, Carey MJ, Rosen J, Polyzotis N, Condie T, Weimer M, Ramakrishnan R. Scaling datalog for machine learning on big data, CoRR , vol. abs/1203.0160, 2012. [Online]. Available: http://dblp.uni-trier.de/db/journals/corr/corr1203.html#abs-1203-0160 .
Malewicz G, Austern MH, Bik AJ, Dehnert JC, Horn I, Leiser N, Czajkowski G. Pregel: A system for large-scale graph processing. In: Proceedings of the ACM SIGMOD International Conference on Management of Data, 2010. pp 135–146.
Hasan S, Shamsuddin S, Lopes N. Soft computing methods for big data problems. In: Proceedings of the Symposium on GPU Computing and Applications, 2013. pp 235–247.
Ku-Mahamud KR. Big data clustering using grid computing and ant-based algorithm. In: Proceedings of the International Conference on Computing and Informatics, 2013. pp 6–14.
Deneubourg JL, Goss S, Franks N, Sendova-Franks A, Detrain C, Chrétien L. The dynamics of collective sorting robot-like ants and ant-like robots. In: Proceedings of the International Conference on Simulation of Adaptive Behavior on From Animals to Animats, 1990. pp 356–363.
Radoop [Online]. https://rapidminer.com/products/radoop/ . Accessed 2 Feb 2015.
PigMix [Online]. https://cwiki.apache.org/confluence/display/PIG/PigMix . Accessed 2 Feb 2015.
GridMix [Online]. http://hadoop.apache.org/docs/r1.2.1/gridmix.html . Accessed 2 Feb 2015.
TeraSoft [Online]. http://sortbenchmark.org/ . Accessed 2 Feb 2015.
TPC, transaction processing performance council [Online]. http://www.tpc.org/ . Accessed 2 Feb 2015.
Cooper BF, Silberstein A, Tam E, Ramakrishnan R, Sears R. Benchmarking cloud serving systems with ycsb. In: Proceedings of the ACM Symposium on Cloud Computing, 2010. pp 143–154.
Ghazal A, Rabl T, Hu M, Raab F, Poess M, Crolotte A, Jacobsen HA. BigBench: Towards an industry standard benchmark for big data analytics. In: Proceedings of the ACM SIGMOD International Conference on Management of Data, 2013. pp 1197–1208.
Cheptsov A. Hpc in big data age: An evaluation report for java-based data-intensive applications implemented with hadoop and openmpi. In: Proceedings of the European MPI Users’ Group Meeting, 2014. pp 175:175–175:180.
Yuan LY, Wu L, You JH, Chi Y. Rubato db: A highly scalable staged grid database system for oltp and big data applications. In: Proceedings of the ACM International Conference on Conference on Information and Knowledge Management, 2014. pp 1–10.
Zhao JM, Wang WS, Liu X, Chen YF. Big data benchmark - big DS. In: Proceedings of the Advancing Big Data Benchmarks, 2014, pp. 49–57.
Saletore V, Krishnan K, Viswanathan V, Tolentino M. HcBench: Methodology, development, and full-system characterization of a customer usage representative big data/hadoop benchmark. In: Advancing Big Data Benchmarks, 2014. pp 73–93.
Zhang L, Stoffel A, Behrisch M, Mittelstadt S, Schreck T, Pompl R, Weber S, Last H, Keim D. Visual analytics for the big data era—a comparative review of state-of-the-art commercial systems. In: Proceedings of the IEEE Conference on Visual Analytics Science and Technology, 2012. pp 173–182.
Harati A, Lopez S, Obeid I, Picone J, Jacobson M, Tobochnik S. The TUH EEG CORPUS: A big data resource for automated eeg interpretation. In: Proceeding of the IEEE Signal Processing in Medicine and Biology Symposium, 2014. pp 1–5.
Thusoo A, Sarma JS, Jain N, Shao Z, Chakka P, Anthony S, Liu H, Wyckoff P, Murthy R. Hive: a warehousing solution over a map-reduce framework. Proc VLDB Endowment. 2009;2(2):1626–9.
Beckmann M, Ebecken NFF, de Lima BSLP, Costa MA. A user interface for big data with rapidminer. RapidMiner World, Boston, MA, Tech. Rep., 2014. [Online]. Available: http://www.slideshare.net/RapidMiner/a-user-interface-for-big-data-with-rapidminer-marcelo-beckmann .
Januzaj E, Kriegel HP, Pfeifle M. DBDC: Density based distributed clustering. In: Proceedings of the Advances in Database Technology, 2004; vol. 2992, 2004, pp 88–105.
Zhao W, Ma H, He Q. Parallel k-means clustering based on mapreduce. Proceedings Cloud Comp. 2009;5931:674–9.
Nolan RL. Managing the crises in data processing. Harvard Bus Rev. 1979;57(1):115–26.
Tsai CW, Huang WC, Chiang MC. Recent development of metaheuristics for clustering. In: Proceedings of the Mobile, Ubiquitous, and Intelligent Computing, 2014; vol. 274, pp. 629–636.
Download references
Authors’ contributions
CWT contributed to the paper review and drafted the first version of the manuscript. CFL contributed to the paper collection and manuscript organization. HCC and AVV double checked the manuscript and provided several advanced ideas for this manuscript. All authors read and approved the final manuscript.
Acknowledgements
The authors would like to thank the anonymous reviewers for their valuable comments and suggestions on the paper. This work was supported in part by the Ministry of Science and Technology of Taiwan, R.O.C., under Contracts MOST103-2221-E-197-034, MOST104-2221-E-197-005, and MOST104-2221-E-197-014.
Compliance with ethical guidelines
Competing interests The authors declare that they have no competing interests.
Author information
Authors and affiliations.
Department of Computer Science and Information Engineering, National Ilan University, Yilan, Taiwan
Chun-Wei Tsai & Han-Chieh Chao
Institute of Computer Science and Information Engineering, National Chung Cheng University, Chia-Yi, Taiwan
Chin-Feng Lai
Information Engineering College, Yangzhou University, Yangzhou, Jiangsu, China
Han-Chieh Chao
School of Information Science and Engineering, Fujian University of Technology, Fuzhou, Fujian, China
Department of Computer Science, Electrical and Space Engineering, Luleå University of Technology, SE-931 87, Skellefteå, Sweden
Athanasios V. Vasilakos
You can also search for this author in PubMed Google Scholar
Corresponding author
Correspondence to Athanasios V. Vasilakos .
Rights and permissions
Open Access This article is distributed under the terms of the Creative Commons Attribution 4.0 International License ( http://creativecommons.org/licenses/by/4.0/ ), which permits unrestricted use, distribution, and reproduction in any medium, provided you give appropriate credit to the original author(s) and the source, provide a link to the Creative Commons license, and indicate if changes were made.
Reprints and permissions
About this article
Cite this article.
Tsai, CW., Lai, CF., Chao, HC. et al. Big data analytics: a survey. Journal of Big Data 2 , 21 (2015). https://doi.org/10.1186/s40537-015-0030-3
Download citation
Received : 14 May 2015
Accepted : 02 September 2015
Published : 01 October 2015
DOI : https://doi.org/10.1186/s40537-015-0030-3
Share this article
Anyone you share the following link with will be able to read this content:
Sorry, a shareable link is not currently available for this article.
Provided by the Springer Nature SharedIt content-sharing initiative
- data analytics
- data mining

An official website of the United States government
The .gov means it’s official. Federal government websites often end in .gov or .mil. Before sharing sensitive information, make sure you’re on a federal government site.
The site is secure. The https:// ensures that you are connecting to the official website and that any information you provide is encrypted and transmitted securely.
- Publications
- Account settings
Preview improvements coming to the PMC website in October 2024. Learn More or Try it out now .
- Advanced Search
- Journal List
- Springer Nature - PMC COVID-19 Collection

The use of Big Data Analytics in healthcare
Kornelia batko.
1 Department of Business Informatics, University of Economics in Katowice, Katowice, Poland
Andrzej Ślęzak
2 Department of Biomedical Processes and Systems, Institute of Health and Nutrition Sciences, Częstochowa University of Technology, Częstochowa, Poland
Associated Data
The datasets for this study are available on request to the corresponding author.
The introduction of Big Data Analytics (BDA) in healthcare will allow to use new technologies both in treatment of patients and health management. The paper aims at analyzing the possibilities of using Big Data Analytics in healthcare. The research is based on a critical analysis of the literature, as well as the presentation of selected results of direct research on the use of Big Data Analytics in medical facilities. The direct research was carried out based on research questionnaire and conducted on a sample of 217 medical facilities in Poland. Literature studies have shown that the use of Big Data Analytics can bring many benefits to medical facilities, while direct research has shown that medical facilities in Poland are moving towards data-based healthcare because they use structured and unstructured data, reach for analytics in the administrative, business and clinical area. The research positively confirmed that medical facilities are working on both structural data and unstructured data. The following kinds and sources of data can be distinguished: from databases, transaction data, unstructured content of emails and documents, data from devices and sensors. However, the use of data from social media is lower as in their activity they reach for analytics, not only in the administrative and business but also in the clinical area. It clearly shows that the decisions made in medical facilities are highly data-driven. The results of the study confirm what has been analyzed in the literature that medical facilities are moving towards data-based healthcare, together with its benefits.
Introduction
The main contribution of this paper is to present an analytical overview of using structured and unstructured data (Big Data) analytics in medical facilities in Poland. Medical facilities use both structured and unstructured data in their practice. Structured data has a predetermined schema, it is extensive, freeform, and comes in variety of forms [ 27 ]. In contrast, unstructured data, referred to as Big Data (BD), does not fit into the typical data processing format. Big Data is a massive amount of data sets that cannot be stored, processed, or analyzed using traditional tools. It remains stored but not analyzed. Due to the lack of a well-defined schema, it is difficult to search and analyze such data and, therefore, it requires a specific technology and method to transform it into value [ 20 , 68 ]. Integrating data stored in both structured and unstructured formats can add significant value to an organization [ 27 ]. Organizations must approach unstructured data in a different way. Therefore, the potential is seen in Big Data Analytics (BDA). Big Data Analytics are techniques and tools used to analyze and extract information from Big Data. The results of Big Data analysis can be used to predict the future. They also help in creating trends about the past. When it comes to healthcare, it allows to analyze large datasets from thousands of patients, identifying clusters and correlation between datasets, as well as developing predictive models using data mining techniques [ 60 ].
This paper is the first study to consolidate and characterize the use of Big Data from different perspectives. The first part consists of a brief literature review of studies on Big Data (BD) and Big Data Analytics (BDA), while the second part presents results of direct research aimed at diagnosing the use of big data analyses in medical facilities in Poland.
Healthcare is a complex system with varied stakeholders: patients, doctors, hospitals, pharmaceutical companies and healthcare decision-makers. This sector is also limited by strict rules and regulations. However, worldwide one may observe a departure from the traditional doctor-patient approach. The doctor becomes a partner and the patient is involved in the therapeutic process [ 14 ]. Healthcare is no longer focused solely on the treatment of patients. The priority for decision-makers should be to promote proper health attitudes and prevent diseases that can be avoided [ 81 ]. This became visible and important especially during the Covid-19 pandemic [ 44 ].
The next challenges that healthcare will have to face is the growing number of elderly people and a decline in fertility. Fertility rates in the country are found below the reproductive minimum necessary to keep the population stable [ 10 ]. The reflection of both effects, namely the increase in age and lower fertility rates, are demographic load indicators, which is constantly growing. Forecasts show that providing healthcare in the form it is provided today will become impossible in the next 20 years [ 70 ]. It is especially visible now during the Covid-19 pandemic when healthcare faced quite a challenge related to the analysis of huge data amounts and the need to identify trends and predict the spread of the coronavirus. The pandemic showed it even more that patients should have access to information about their health condition, the possibility of digital analysis of this data and access to reliable medical support online. Health monitoring and cooperation with doctors in order to prevent diseases can actually revolutionize the healthcare system. One of the most important aspects of the change necessary in healthcare is putting the patient in the center of the system.
Technology is not enough to achieve these goals. Therefore, changes should be made not only at the technological level but also in the management and design of complete healthcare processes and what is more, they should affect the business models of service providers. The use of Big Data Analytics is becoming more and more common in enterprises [ 17 , 54 ]. However, medical enterprises still cannot keep up with the information needs of patients, clinicians, administrators and the creator’s policy. The adoption of a Big Data approach would allow the implementation of personalized and precise medicine based on personalized information, delivered in real time and tailored to individual patients.
To achieve this goal, it is necessary to implement systems that will be able to learn quickly about the data generated by people within clinical care and everyday life. This will enable data-driven decision making, receiving better personalized predictions about prognosis and responses to treatments; a deeper understanding of the complex factors and their interactions that influence health at the patient level, the health system and society, enhanced approaches to detecting safety problems with drugs and devices, as well as more effective methods of comparing prevention, diagnostic, and treatment options [ 40 ].
In the literature, there is a lot of research showing what opportunities can be offered to companies by big data analysis and what data can be analyzed. However, there are few studies showing how data analysis in the area of healthcare is performed, what data is used by medical facilities and what analyses and in which areas they carry out. This paper aims to fill this gap by presenting the results of research carried out in medical facilities in Poland. The goal is to analyze the possibilities of using Big Data Analytics in healthcare, especially in Polish conditions. In particular, the paper is aimed at determining what data is processed by medical facilities in Poland, what analyses they perform and in what areas, and how they assess their analytical maturity. In order to achieve this goal, a critical analysis of the literature was performed, and the direct research was based on a research questionnaire conducted on a sample of 217 medical facilities in Poland. It was hypothesized that medical facilities in Poland are working on both structured and unstructured data and moving towards data-based healthcare and its benefits. Examining the maturity of healthcare facilities in the use of Big Data and Big Data Analytics is crucial in determining the potential future benefits that the healthcare sector can gain from Big Data Analytics. There is also a pressing need to predicate whether, in the coming years, healthcare will be able to cope with the threats and challenges it faces.
This paper is divided into eight parts. The first is the introduction which provides background and the general problem statement of this research. In the second part, this paper discusses considerations on use of Big Data and Big Data Analytics in Healthcare, and then, in the third part, it moves on to challenges and potential benefits of using Big Data Analytics in healthcare. The next part involves the explanation of the proposed method. The result of direct research and discussion are presented in the fifth part, while the following part of the paper is the conclusion. The seventh part of the paper presents practical implications. The final section of the paper provides limitations and directions for future research.
Considerations on use Big Data and Big Data Analytics in the healthcare
In recent years one can observe a constantly increasing demand for solutions offering effective analytical tools. This trend is also noticeable in the analysis of large volumes of data (Big Data, BD). Organizations are looking for ways to use the power of Big Data to improve their decision making, competitive advantage or business performance [ 7 , 54 ]. Big Data is considered to offer potential solutions to public and private organizations, however, still not much is known about the outcome of the practical use of Big Data in different types of organizations [ 24 ].
As already mentioned, in recent years, healthcare management worldwide has been changed from a disease-centered model to a patient-centered model, even in value-based healthcare delivery model [ 68 ]. In order to meet the requirements of this model and provide effective patient-centered care, it is necessary to manage and analyze healthcare Big Data.
The issue often raised when it comes to the use of data in healthcare is the appropriate use of Big Data. Healthcare has always generated huge amounts of data and nowadays, the introduction of electronic medical records, as well as the huge amount of data sent by various types of sensors or generated by patients in social media causes data streams to constantly grow. Also, the medical industry generates significant amounts of data, including clinical records, medical images, genomic data and health behaviors. Proper use of the data will allow healthcare organizations to support clinical decision-making, disease surveillance, and public health management. The challenge posed by clinical data processing involves not only the quantity of data but also the difficulty in processing it.
In the literature one can find many different definitions of Big Data. This concept has evolved in recent years, however, it is still not clearly understood. Nevertheless, despite the range and differences in definitions, Big Data can be treated as a: large amount of digital data, large data sets, tool, technology or phenomenon (cultural or technological.
Big Data can be considered as massive and continually generated digital datasets that are produced via interactions with online technologies [ 53 ]. Big Data can be defined as datasets that are of such large sizes that they pose challenges in traditional storage and analysis techniques [ 28 ]. A similar opinion about Big Data was presented by Ohlhorst who sees Big Data as extremely large data sets, possible neither to manage nor to analyze with traditional data processing tools [ 57 ]. In his opinion, the bigger the data set, the more difficult it is to gain any value from it.
In turn, Knapp perceived Big Data as tools, processes and procedures that allow an organization to create, manipulate and manage very large data sets and storage facilities [ 38 ]. From this point of view, Big Data is identified as a tool to gather information from different databases and processes, allowing users to manage large amounts of data.
Similar perception of the term ‘Big Data’ is shown by Carter. According to him, Big Data technologies refer to a new generation of technologies and architectures, designed to economically extract value from very large volumes of a wide variety of data by enabling high velocity capture, discovery and/or analysis [ 13 ].
Jordan combines these two approaches by identifying Big Data as a complex system, as it needs data bases for data to be stored in, programs and tools to be managed, as well as expertise and personnel able to retrieve useful information and visualization to be understood [ 37 ].
Following the definition of Laney for Big Data, it can be state that: it is large amount of data generated in very fast motion and it contains a lot of content [ 43 ]. Such data comes from unstructured sources, such as stream of clicks on the web, social networks (Twitter, blogs, Facebook), video recordings from the shops, recording of calls in a call center, real time information from various kinds of sensors, RFID, GPS devices, mobile phones and other devices that identify and monitor something [ 8 ]. Big Data is a powerful digital data silo, raw, collected with all sorts of sources, unstructured and difficult, or even impossible, to analyze using conventional techniques used so far to relational databases.
While describing Big Data, it cannot be overlooked that the term refers more to a phenomenon than to specific technology. Therefore, instead of defining this phenomenon, trying to describe them, more authors are describing Big Data by giving them characteristics included a collection of V’s related to its nature [ 2 , 3 , 23 , 25 , 58 ]:
- Volume (refers to the amount of data and is one of the biggest challenges in Big Data Analytics),
- Velocity (speed with which new data is generated, the challenge is to be able to manage data effectively and in real time),
- Variety (heterogeneity of data, many different types of healthcare data, the challenge is to derive insights by looking at all available heterogenous data in a holistic manner),
- Variability (inconsistency of data, the challenge is to correct the interpretation of data that can vary significantly depending on the context),
- Veracity (how trustworthy the data is, quality of the data),
- Visualization (ability to interpret data and resulting insights, challenging for Big Data due to its other features as described above).
- Value (the goal of Big Data Analytics is to discover the hidden knowledge from huge amounts of data).
Big Data is defined as an information asset with high volume, velocity, and variety, which requires specific technology and method for its transformation into value [ 21 , 77 ]. Big Data is also a collection of information about high-volume, high volatility or high diversity, requiring new forms of processing in order to support decision-making, discovering new phenomena and process optimization [ 5 , 7 ]. Big Data is too large for traditional data-processing systems and software tools to capture, store, manage and analyze, therefore it requires new technologies [ 28 , 50 , 61 ] to manage (capture, aggregate, process) its volume, velocity and variety [ 9 ].
Undoubtedly, Big Data differs from the data sources used so far by organizations. Therefore, organizations must approach this type of unstructured data in a different way. First of all, organizations must start to see data as flows and not stocks—this entails the need to implement the so-called streaming analytics [ 48 ]. The mentioned features make it necessary to use new IT tools that allow the fullest use of new data [ 58 ]. The Big Data idea, inseparable from the huge increase in data available to various organizations or individuals, creates opportunities for access to valuable analyses, conclusions and enables making more accurate decisions [ 6 , 11 , 59 ].
The Big Data concept is constantly evolving and currently it does not focus on huge amounts of data, but rather on the process of creating value from this data [ 52 ]. Big Data is collected from various sources that have different data properties and are processed by different organizational units, resulting in creation of a Big Data chain [ 36 ]. The aim of the organizations is to manage, process and analyze Big Data. In the healthcare sector, Big Data streams consist of various types of data, namely [ 8 , 51 ]:
- clinical data, i.e. data obtained from electronic medical records, data from hospital information systems, image centers, laboratories, pharmacies and other organizations providing health services, patient generated health data, physician’s free-text notes, genomic data, physiological monitoring data [ 4 ],
- biometric data provided from various types of devices that monitor weight, pressure, glucose level, etc.,
- financial data, constituting a full record of economic operations reflecting the conducted activity,
- data from scientific research activities, i.e. results of research, including drug research, design of medical devices and new methods of treatment,
- data provided by patients, including description of preferences, level of satisfaction, information from systems for self-monitoring of their activity: exercises, sleep, meals consumed, etc.
- data from social media.
These data are provided not only by patients but also by organizations and institutions, as well as by various types of monitoring devices, sensors or instruments [ 16 ]. Data that has been generated so far in the healthcare sector is stored in both paper and digital form. Thus, the essence and the specificity of the process of Big Data analyses means that organizations need to face new technological and organizational challenges [ 67 ]. The healthcare sector has always generated huge amounts of data and this is connected, among others, with the need to store medical records of patients. However, the problem with Big Data in healthcare is not limited to an overwhelming volume but also an unprecedented diversity in terms of types, data formats and speed with which it should be analyzed in order to provide the necessary information on an ongoing basis [ 3 ]. It is also difficult to apply traditional tools and methods for management of unstructured data [ 67 ]. Due to the diversity and quantity of data sources that are growing all the time, advanced analytical tools and technologies, as well as Big Data analysis methods which can meet and exceed the possibilities of managing healthcare data, are needed [ 3 , 68 ].
Therefore, the potential is seen in Big Data analyses, especially in the aspect of improving the quality of medical care, saving lives or reducing costs [ 30 ]. Extracting from this tangle of given association rules, patterns and trends will allow health service providers and other stakeholders in the healthcare sector to offer more accurate and more insightful diagnoses of patients, personalized treatment, monitoring of the patients, preventive medicine, support of medical research and health population, as well as better quality of medical services and patient care while, at the same time, the ability to reduce costs (Fig. 1 ).

Healthcare Big Data Analytics applications
(Source: Own elaboration)
The main challenge with Big Data is how to handle such a large amount of information and use it to make data-driven decisions in plenty of areas [ 64 ]. In the context of healthcare data, another major challenge is to adjust big data storage, analysis, presentation of analysis results and inference basing on them in a clinical setting. Data analytics systems implemented in healthcare are designed to describe, integrate and present complex data in an appropriate way so that it can be understood better (Fig. 2 ). This would improve the efficiency of acquiring, storing, analyzing and visualizing big data from healthcare [ 71 ].

Process of Big Data Analytics
The result of data processing with the use of Big Data Analytics is appropriate data storytelling which may contribute to making decisions with both lower risk and data support. This, in turn, can benefit healthcare stakeholders. To take advantage of the potential massive amounts of data in healthcare and to ensure that the right intervention to the right patient is properly timed, personalized, and potentially beneficial to all components of the healthcare system such as the payer, patient, and management, analytics of large datasets must connect communities involved in data analytics and healthcare informatics [ 49 ]. Big Data Analytics can provide insight into clinical data and thus facilitate informed decision-making about the diagnosis and treatment of patients, prevention of diseases or others. Big Data Analytics can also improve the efficiency of healthcare organizations by realizing the data potential [ 3 , 62 ].
Big Data Analytics in medicine and healthcare refers to the integration and analysis of a large amount of complex heterogeneous data, such as various omics (genomics, epigenomics, transcriptomics, proteomics, metabolomics, interactomics, pharmacogenetics, deasomics), biomedical data, talemedicine data (sensors, medical equipment data) and electronic health records data [ 46 , 65 ].
When analyzing the phenomenon of Big Data in the healthcare sector, it should be noted that it can be considered from the point of view of three areas: epidemiological, clinical and business.
From a clinical point of view, the Big Data analysis aims to improve the health and condition of patients, enable long-term predictions about their health status and implementation of appropriate therapeutic procedures. Ultimately, the use of data analysis in medicine is to allow the adaptation of therapy to a specific patient, that is personalized medicine (precision, personalized medicine).
From an epidemiological point of view, it is desirable to obtain an accurate prognosis of morbidity in order to implement preventive programs in advance.
In the business context, Big Data analysis may enable offering personalized packages of commercial services or determining the probability of individual disease and infection occurrence. It is worth noting that Big Data means not only the collection and processing of data but, most of all, the inference and visualization of data necessary to obtain specific business benefits.
In order to introduce new management methods and new solutions in terms of effectiveness and transparency, it becomes necessary to make data more accessible, digital, searchable, as well as analyzed and visualized.
Erickson and Rothberg state that the information and data do not reveal their full value until insights are drawn from them. Data becomes useful when it enhances decision making and decision making is enhanced only when analytical techniques are used and an element of human interaction is applied [ 22 ].
Thus, healthcare has experienced much progress in usage and analysis of data. A large-scale digitalization and transparency in this sector is a key statement of almost all countries governments policies. For centuries, the treatment of patients was based on the judgment of doctors who made treatment decisions. In recent years, however, Evidence-Based Medicine has become more and more important as a result of it being related to the systematic analysis of clinical data and decision-making treatment based on the best available information [ 42 ]. In the healthcare sector, Big Data Analytics is expected to improve the quality of life and reduce operational costs [ 72 , 82 ]. Big Data Analytics enables organizations to improve and increase their understanding of the information contained in data. It also helps identify data that provides insightful insights for current as well as future decisions [ 28 ].
Big Data Analytics refers to technologies that are grounded mostly in data mining: text mining, web mining, process mining, audio and video analytics, statistical analysis, network analytics, social media analytics and web analytics [ 16 , 25 , 31 ]. Different data mining techniques can be applied on heterogeneous healthcare data sets, such as: anomaly detection, clustering, classification, association rules as well as summarization and visualization of those Big Data sets [ 65 ]. Modern data analytics techniques explore and leverage unique data characteristics even from high-speed data streams and sensor data [ 15 , 16 , 31 , 55 ]. Big Data can be used, for example, for better diagnosis in the context of comprehensive patient data, disease prevention and telemedicine (in particular when using real-time alerts for immediate care), monitoring patients at home, preventing unnecessary hospital visits, integrating medical imaging for a wider diagnosis, creating predictive analytics, reducing fraud and improving data security, better strategic planning and increasing patients’ involvement in their own health.
Big Data Analytics in healthcare can be divided into [ 33 , 73 , 74 ]:
- descriptive analytics in healthcare is used to understand past and current healthcare decisions, converting data into useful information for understanding and analyzing healthcare decisions, outcomes and quality, as well as making informed decisions [ 33 ]. It can be used to create reports (i.e. about patients’ hospitalizations, physicians’ performance, utilization management), visualization, customized reports, drill down tables, or running queries on the basis of historical data.
- predictive analytics operates on past performance in an effort to predict the future by examining historical or summarized health data, detecting patterns of relationships in these data, and then extrapolating these relationships to forecast. It can be used to i.e. predict the response of different patient groups to different drugs (dosages) or reactions (clinical trials), anticipate risk and find relationships in health data and detect hidden patterns [ 62 ]. In this way, it is possible to predict the epidemic spread, anticipate service contracts and plan healthcare resources. Predictive analytics is used in proper diagnosis and for appropriate treatments to be given to patients suffering from certain diseases [ 39 ].
- prescriptive analytics—occurs when health problems involve too many choices or alternatives. It uses health and medical knowledge in addition to data or information. Prescriptive analytics is used in many areas of healthcare, including drug prescriptions and treatment alternatives. Personalized medicine and evidence-based medicine are both supported by prescriptive analytics.
- discovery analytics—utilizes knowledge about knowledge to discover new “inventions” like drugs (drug discovery), previously unknown diseases and medical conditions, alternative treatments, etc.
Although the models and tools used in descriptive, predictive, prescriptive, and discovery analytics are different, many applications involve all four of them [ 62 ]. Big Data Analytics in healthcare can help enable personalized medicine by identifying optimal patient-specific treatments. This can influence the improvement of life standards, reduce waste of healthcare resources and save costs of healthcare [ 56 , 63 , 71 ]. The introduction of large data analysis gives new analytical possibilities in terms of scope, flexibility and visualization. Techniques such as data mining (computational pattern discovery process in large data sets) facilitate inductive reasoning and analysis of exploratory data, enabling scientists to identify data patterns that are independent of specific hypotheses. As a result, predictive analysis and real-time analysis becomes possible, making it easier for medical staff to start early treatments and reduce potential morbidity and mortality. In addition, document analysis, statistical modeling, discovering patterns and topics in document collections and data in the EHR, as well as an inductive approach can help identify and discover relationships between health phenomena.
Advanced analytical techniques can be used for a large amount of existing (but not yet analytical) data on patient health and related medical data to achieve a better understanding of the information and results obtained, as well as to design optimal clinical pathways [ 62 ]. Big Data Analytics in healthcare integrates analysis of several scientific areas such as bioinformatics, medical imaging, sensor informatics, medical informatics and health informatics [ 65 ]. Big Data Analytics in healthcare allows to analyze large datasets from thousands of patients, identifying clusters and correlation between datasets, as well as developing predictive models using data mining techniques [ 65 ]. Discussing all the techniques used for Big Data Analytics goes beyond the scope of a single article [ 25 ].
The success of Big Data analysis and its accuracy depend heavily on the tools and techniques used to analyze the ability to provide reliable, up-to-date and meaningful information to various stakeholders [ 12 ]. It is believed that the implementation of big data analytics by healthcare organizations could bring many benefits in the upcoming years, including lowering health care costs, better diagnosis and prediction of diseases and their spread, improving patient care and developing protocols to prevent re-hospitalization, optimizing staff, optimizing equipment, forecasting the need for hospital beds, operating rooms, treatments, and improving the drug supply chain [ 71 ].
Challenges and potential benefits of using Big Data Analytics in healthcare
Modern analytics gives possibilities not only to have insight in historical data, but also to have information necessary to generate insight into what may happen in the future. Even when it comes to prediction of evidence-based actions. The emphasis on reform has prompted payers and suppliers to pursue data analysis to reduce risk, detect fraud, improve efficiency and save lives. Everyone—payers, providers, even patients—are focusing on doing more with fewer resources. Thus, some areas in which enhanced data and analytics can yield the greatest results include various healthcare stakeholders (Table (Table1 1 ).
The use of analytics by various healthcare stakeholders
Source: own elaboration on the basis of [ 19 , 20 ]
Healthcare organizations see the opportunity to grow through investments in Big Data Analytics. In recent years, by collecting medical data of patients, converting them into Big Data and applying appropriate algorithms, reliable information has been generated that helps patients, physicians and stakeholders in the health sector to identify values and opportunities [ 31 ]. It is worth noting that there are many changes and challenges in the structure of the healthcare sector. Digitization and effective use of Big Data in healthcare can bring benefits to every stakeholder in this sector. A single doctor would benefit the same as the entire healthcare system. Potential opportunities to achieve benefits and effects from Big Data in healthcare can be divided into four groups [ 8 ]:
- assessment of diagnoses made by doctors and the manner of treatment of diseases indicated by them based on the decision support system working on Big Data collections,
- detection of more effective, from a medical point of view, and more cost-effective ways to diagnose and treat patients,
- analysis of large volumes of data to reach practical information useful for identifying needs, introducing new health services, preventing and overcoming crises,
- prediction of the incidence of diseases,
- detecting trends that lead to an improvement in health and lifestyle of the society,
- analysis of the human genome for the introduction of personalized treatment.
- doctors’ comparison of current medical cases to cases from the past for better diagnosis and treatment adjustment,
- detection of diseases at earlier stages when they can be more easily and quickly cured,
- detecting epidemiological risks and improving control of pathogenic spots and reaction rates,
- identification of patients who are predicted to have the highest risk of specific, life-threatening diseases by collating data on the history of the most common diseases, in healing people with reports entering insurance companies,
- health management of each patient individually (personalized medicine) and health management of the whole society,
- capturing and analyzing large amounts of data from hospitals and homes in real time, life monitoring devices to monitor safety and predict adverse events,
- analysis of patient profiles to identify people for whom prevention should be applied, lifestyle change or preventive care approach,
- the ability to predict the occurrence of specific diseases or worsening of patients’ results,
- predicting disease progression and its determinants, estimating the risk of complications,
- detecting drug interactions and their side effects.
- supporting work on new drugs and clinical trials thanks to the possibility of analyzing “all data” instead of selecting a test sample,
- the ability to identify patients with specific, biological features that will take part in specialized clinical trials,
- selecting a group of patients for which the tested drug is likely to have the desired effect and no side effects,
- using modeling and predictive analysis to design better drugs and devices.
- reduction of costs and counteracting abuse and counseling practices,
- faster and more effective identification of incorrect or unauthorized financial operations in order to prevent abuse and eliminate errors,
- increase in profitability by detecting patients generating high costs or identifying doctors whose work, procedures and treatment methods cost the most and offering them solutions that reduce the amount of money spent,
- identification of unnecessary medical activities and procedures, e.g. duplicate tests.
According to research conducted by Wang, Kung and Byrd, Big Data Analytics benefits can be classified into five categories: IT infrastructure benefits (reducing system redundancy, avoiding unnecessary IT costs, transferring data quickly among healthcare IT systems, better use of healthcare systems, processing standardization among various healthcare IT systems, reducing IT maintenance costs regarding data storage), operational benefits (improving the quality and accuracy of clinical decisions, processing a large number of health records in seconds, reducing the time of patient travel, immediate access to clinical data to analyze, shortening the time of diagnostic test, reductions in surgery-related hospitalizations, exploring inconceivable new research avenues), organizational benefits (detecting interoperability problems much more quickly than traditional manual methods, improving cross-functional communication and collaboration among administrative staffs, researchers, clinicians and IT staffs, enabling data sharing with other institutions and adding new services, content sources and research partners), managerial benefits (gaining quick insights about changing healthcare trends in the market, providing members of the board and heads of department with sound decision-support information on the daily clinical setting, optimizing business growth-related decisions) and strategic benefits (providing a big picture view of treatment delivery for meeting future need, creating high competitive healthcare services) [ 73 ].
The above specification does not constitute a full list of potential areas of use of Big Data Analysis in healthcare because the possibilities of using analysis are practically unlimited. In addition, advanced analytical tools allow to analyze data from all possible sources and conduct cross-analyses to provide better data insights [ 26 ]. For example, a cross-analysis can refer to a combination of patient characteristics, as well as costs and care results that can help identify the best, in medical terms, and the most cost-effective treatment or treatments and this may allow a better adjustment of the service provider’s offer [ 62 ].
In turn, the analysis of patient profiles (e.g. segmentation and predictive modeling) allows identification of people who should be subject to prophylaxis, prevention or should change their lifestyle [ 8 ]. Shortened list of benefits for Big Data Analytics in healthcare is presented in paper [ 3 ] and consists of: better performance, day-to-day guides, detection of diseases in early stages, making predictive analytics, cost effectiveness, Evidence Based Medicine and effectiveness in patient treatment.
Summarizing, healthcare big data represents a huge potential for the transformation of healthcare: improvement of patients’ results, prediction of outbreaks of epidemics, valuable insights, avoidance of preventable diseases, reduction of the cost of healthcare delivery and improvement of the quality of life in general [ 1 ]. Big Data also generates many challenges such as difficulties in data capture, data storage, data analysis and data visualization [ 15 ]. The main challenges are connected with the issues of: data structure (Big Data should be user-friendly, transparent, and menu-driven but it is fragmented, dispersed, rarely standardized and difficult to aggregate and analyze), security (data security, privacy and sensitivity of healthcare data, there are significant concerns related to confidentiality), data standardization (data is stored in formats that are not compatible with all applications and technologies), storage and transfers (especially costs associated with securing, storing, and transferring unstructured data), managerial skills, such as data governance, lack of appropriate analytical skills and problems with Real-Time Analytics (health care is to be able to utilize Big Data in real time) [ 4 , 34 , 41 ].
The research is based on a critical analysis of the literature, as well as the presentation of selected results of direct research on the use of Big Data Analytics in medical facilities in Poland.
Presented research results are part of a larger questionnaire form on Big Data Analytics. The direct research was based on an interview questionnaire which contained 100 questions with 5-point Likert scale (1—strongly disagree, 2—I rather disagree, 3—I do not agree, nor disagree, 4—I rather agree, 5—I definitely agree) and 4 metrics questions. The study was conducted in December 2018 on a sample of 217 medical facilities (110 private, 107 public). The research was conducted by a specialized market research agency: Center for Research and Expertise of the University of Economics in Katowice.
When it comes to direct research, the selected entities included entities financed from public sources—the National Health Fund (23.5%), and entities operating commercially (11.5%). In the surveyed group of entities, more than a half (64.9%) are hybrid financed, both from public and commercial sources. The diversity of the research sample also applies to the size of the entities, defined by the number of employees. Taking into account proportions of the surveyed entities, it should be noted that in the sector structure, medium-sized (10–50 employees—34% of the sample) and large (51–250 employees—27%) entities dominate. The research was of all-Poland nature, and the entities included in the research sample come from all of the voivodships. The largest group were entities from Łódzkie (32%), Śląskie (18%) and Mazowieckie (18%) voivodships, as these voivodships have the largest number of medical institutions. Other regions of the country were represented by single units. The selection of the research sample was random—layered. As part of medical facilities database, groups of private and public medical facilities have been identified and the ones to which the questionnaire was targeted were drawn from each of these groups. The analyses were performed using the GNU PSPP 0.10.2 software.
The aim of the study was to determine whether medical facilities in Poland use Big Data Analytics and if so, in which areas. Characteristics of the research sample is presented in Table Table2 2 .
Characteristics of the research sample
The research is non-exhaustive due to the incomplete and uneven regional distribution of the samples, overrepresented in three voivodeships (Łódzkie, Mazowieckie and Śląskie). The size of the research sample (217 entities) allows the authors of the paper to formulate specific conclusions on the use of Big Data in the process of its management.
For the purpose of this paper, the following research hypotheses were formulated: (1) medical facilities in Poland are working on both structured and unstructured data (2) medical facilities in Poland are moving towards data-based healthcare and its benefits.
The paper poses the following research questions and statements that coincide with the selected questions from the research questionnaire:
- From what sources do medical facilities obtain data? What types of data are used by the particular organization, whether structured or unstructured, and to what extent?
- From what sources do medical facilities obtain data?
- In which area organizations are using data and analytical systems (clinical or business)?
- Is data analytics performed based on historical data or are predictive analyses also performed?
- Determining whether administrative and medical staff receive complete, accurate and reliable data in a timely manner?
- Determining whether real-time analyses are performed to support the particular organization’s activities.
Results and discussion
On the basis of the literature analysis and research study, a set of questions and statements related to the researched area was formulated. The results from the surveys show that medical facilities use a variety of data sources in their operations. These sources are both structured and unstructured data (Table (Table3 3 ).
Type of data sources used in medical facility (%)
1—strongly disagree, 2—I disagree, 3—I agree or disagree, 4—I rather agree, 5—I strongly agree
According to the data provided by the respondents, considering the first statement made in the questionnaire, almost half of the medical institutions (47.58%) agreed that they rather collect and use structured data (e.g. databases and data warehouses, reports to external entities) and 10.57% entirely agree with this statement. As much as 23.35% of representatives of medical institutions stated “I agree or disagree”. Other medical facilities do not collect and use structured data (7.93%) and 6.17% strongly disagree with the first statement. Also, the median calculated based on the obtained results (median: 4), proves that medical facilities in Poland collect and use structured data (Table (Table4 4 ).
Collection and use of data determined by the size of medical facility (number of employees)
In turn, 28.19% of the medical institutions agreed that they rather collect and use unstructured data and as much as 9.25% entirely agree with this statement. The number of representatives of medical institutions that stated “I agree or disagree” was 27.31%. Other medical facilities do not collect and use structured data (17.18%) and 13.66% strongly disagree with the first statement. In the case of unstructured data the median is 3, which means that the collection and use of this type of data by medical facilities in Poland is lower.
In the further part of the analysis, it was checked whether the size of the medical facility and form of ownership have an impact on whether it analyzes unstructured data (Tables (Tables4 4 and and5). 5 ). In order to find this out, correlation coefficients were calculated.
Collection and use of data determined by the form of ownership of medical facility
Based on the calculations, it can be concluded that there is a small statistically monotonic correlation between the size of the medical facility and its collection and use of structured data (p < 0.001; τ = 0.16). This means that the use of structured data is slightly increasing in larger medical facilities. The size of the medical facility is more important according to use of unstructured data (p < 0.001; τ = 0.23) (Table (Table4 4 .).
To determine whether the form of medical facility ownership affects data collection, the Mann–Whitney U test was used. The calculations show that the form of ownership does not affect what data the organization collects and uses (Table (Table5 5 ).
Detailed information on the sources of from which medical facilities collect and use data is presented in the Table Table6 6 .
Data sources used in medical facility
1—we do not use at all, 5—we use extensively
The questionnaire results show that medical facilities are especially using information published in databases, reports to external units and transaction data, but they also use unstructured data from e-mails, medical devices, sensors, phone calls, audio and video data (Table (Table6). 6 ). Data from social media, RFID and geolocation data are used to a small extent. Similar findings are concluded in the literature studies.
From the analysis of the answers given by the respondents, more than half of the medical facilities have integrated hospital system (HIS) implemented. As much as 43.61% use integrated hospital system and 16.30% use it extensively (Table (Table7). 7 ). 19.38% of exanimated medical facilities do not use it at all. Moreover, most of the examined medical facilities (34.80% use it, 32.16% use extensively) conduct medical documentation in an electronic form, which gives an opportunity to use data analytics. Only 4.85% of medical facilities don’t use it at all.
The use of HIS and electronic documentation in medical facilities (%)
Other problems that needed to be investigated were: whether medical facilities in Poland use data analytics? If so, in what form and in what areas? (Table (Table8). 8 ). The analysis of answers given by the respondents about the potential of data analytics in medical facilities shows that a similar number of medical facilities use data analytics in administration and business (31.72% agreed with the statement no. 5 and 12.33% strongly agreed) as in the clinical area (33.04% agreed with the statement no. 6 and 12.33% strongly agreed). When considering decision-making issues, 35.24% agree with the statement "the organization uses data and analytical systems to support business decisions” and 8.37% of respondents strongly agree. Almost 40.09% agree with the statement that “the organization uses data and analytical systems to support clinical decisions (in the field of diagnostics and therapy)” and 15.42% of respondents strongly agree. Exanimated medical facilities use in their activity analytics based both on historical data (33.48% agree with statement 7 and 12.78% strongly agree) and predictive analytics (33.04% agrees with the statement number 8 and 15.86% strongly agree). Detailed results are presented in Table Table8 8 .
Conditions of using Big Data Analytics in medical facilities (%)
Medical facilities focus on development in the field of data processing, as they confirm that they conduct analytical planning processes systematically and analyze new opportunities for strategic use of analytics in business and clinical activities (38.33% rather agree and 10.57% strongly agree with this statement). The situation is different with real-time data analysis, here, the situation is not so optimistic. Only 28.19% rather agree and 14.10% strongly agree with the statement that real-time analyses are performed to support an organization’s activities.
When considering whether a facility’s performance in the clinical area depends on the form of ownership, it can be concluded that taking the average and the Mann–Whitney U test depends. A higher degree of use of analyses in the clinical area can be observed in public institutions.
Whether a medical facility performs a descriptive or predictive analysis do not depend on the form of ownership (p > 0.05). It can be concluded that when analyzing the mean and median, they are higher in public facilities, than in private ones. What is more, the Mann–Whitney U test shows that these variables are dependent from each other (p < 0.05) (Table (Table9 9 ).
Conditions of using Big Data Analytics in medical facilities determined by the form of ownership of medical facility
When considering whether a facility’s performance in the clinical area depends on its size, it can be concluded that taking the Kendall’s Tau (τ) it depends (p < 0.001; τ = 0.22), and the correlation is weak but statistically important. This means that the use of data and analytical systems to support clinical decisions (in the field of diagnostics and therapy) increases with the increase of size of the medical facility. A similar relationship, but even less powerful, can be found in the use of descriptive and predictive analyses (Table (Table10 10 ).
Conditions of using Big Data Analytics in medical facilities determined by the size of medical facility (number of employees)
Considering the results of research in the area of analytical maturity of medical facilities, 8.81% of medical facilities stated that they are at the first level of maturity, i.e. an organization has developed analytical skills and does not perform analyses. As much as 13.66% of medical facilities confirmed that they have poor analytical skills, while 38.33% of the medical facility has located itself at level 3, meaning that “there is a lot to do in analytics”. On the other hand, 28.19% believe that analytical capabilities are well developed and 6.61% stated that analytics are at the highest level and the analytical capabilities are very well developed. Detailed data is presented in Table Table11. 11 . Average amounts to 3.11 and Median to 3.
Analytical maturity of examined medical facilities (%)
The results of the research have enabled the formulation of following conclusions. Medical facilities in Poland are working on both structured and unstructured data. This data comes from databases, transactions, unstructured content of emails and documents, devices and sensors. However, the use of data from social media is smaller. In their activity, they reach for analytics in the administrative and business, as well as in the clinical area. Also, the decisions made are largely data-driven.
In summary, analysis of the literature that the benefits that medical facilities can get using Big Data Analytics in their activities relate primarily to patients, physicians and medical facilities. It can be confirmed that: patients will be better informed, will receive treatments that will work for them, will have prescribed medications that work for them and not be given unnecessary medications [ 78 ]. Physician roles will likely change to more of a consultant than decision maker. They will advise, warn, and help individual patients and have more time to form positive and lasting relationships with their patients in order to help people. Medical facilities will see changes as well, for example in fewer unnecessary hospitalizations, resulting initially in less revenue, but after the market adjusts, also the accomplishment [ 78 ]. The use of Big Data Analytics can literally revolutionize the way healthcare is practiced for better health and disease reduction.
The analysis of the latest data reveals that data analytics increase the accuracy of diagnoses. Physicians can use predictive algorithms to help them make more accurate diagnoses [ 45 ]. Moreover, it could be helpful in preventive medicine and public health because with early intervention, many diseases can be prevented or ameliorated [ 29 ]. Predictive analytics also allows to identify risk factors for a given patient, and with this knowledge patients will be able to change their lives what, in turn, may contribute to the fact that population disease patterns may dramatically change, resulting in savings in medical costs. Moreover, personalized medicine is the best solution for an individual patient seeking treatment. It can help doctors decide the exact treatments for those individuals. Better diagnoses and more targeted treatments will naturally lead to increases in good outcomes and fewer resources used, including doctors’ time.
The quantitative analysis of the research carried out and presented in this article made it possible to determine whether medical facilities in Poland use Big Data Analytics and if so, in which areas. Thanks to the results obtained it was possible to formulate the following conclusions. Medical facilities are working on both structured and unstructured data, which comes from databases, transactions, unstructured content of emails and documents, devices and sensors. According to analytics, they reach for analytics in the administrative and business, as well as in the clinical area. It clearly showed that the decisions made are largely data-driven. The results of the study confirm what has been analyzed in the literature. Medical facilities are moving towards data-based healthcare and its benefits.
In conclusion, Big Data Analytics has the potential for positive impact and global implications in healthcare. Future research on the use of Big Data in medical facilities will concern the definition of strategies adopted by medical facilities to promote and implement such solutions, as well as the benefits they gain from the use of Big Data analysis and how the perspectives in this area are seen.
Practical implications
This work sought to narrow the gap that exists in analyzing the possibility of using Big Data Analytics in healthcare. Showing how medical facilities in Poland are doing in this respect is an element that is part of global research carried out in this area, including [ 29 , 32 , 60 ].
Limitations and future directions
The research described in this article does not fully exhaust the questions related to the use of Big Data Analytics in Polish healthcare facilities. Only some of the dimensions characterizing the use of data by medical facilities in Poland have been examined. In order to get the full picture, it would be necessary to examine the results of using structured and unstructured data analytics in healthcare. Future research may examine the benefits that medical institutions achieve as a result of the analysis of structured and unstructured data in the clinical and management areas and what limitations they encounter in these areas. For this purpose, it is planned to conduct in-depth interviews with chosen medical facilities in Poland. These facilities could give additional data for empirical analyses based more on their suggestions. Further research should also include medical institutions from beyond the borders of Poland, enabling international comparative analyses.
Future research in the healthcare field has virtually endless possibilities. These regard the use of Big Data Analytics to diagnose specific conditions [ 47 , 66 , 69 , 76 ], propose an approach that can be used in other healthcare applications and create mechanisms to identify “patients like me” [ 75 , 80 ]. Big Data Analytics could also be used for studies related to the spread of pandemics, the efficacy of covid treatment [ 18 , 79 ], or psychology and psychiatry studies, e.g. emotion recognition [ 35 ].
Acknowledgements
We would like to thank those who have touched our science paths.
Authors’ contributions
KB proposed the concept of research and its design. The manuscript was prepared by KB with the consultation of AŚ. AŚ reviewed the manuscript for getting its fine shape. KB prepared the manuscript in the contexts such as definition of intellectual content, literature search, data acquisition, data analysis, and so on. AŚ obtained research funding. Both authors read and approved the final manuscript.
This research was fully funded as statutory activity—subsidy of Ministry of Science and Higher Education granted for Technical University of Czestochowa on maintaining research potential in 2018. Research Number: BS/PB–622/3020/2014/P. Publication fee for the paper was financed by the University of Economics in Katowice.
Availability of data and materials
Declarations.
Not applicable.
The author declares no conflict of interest.
Publisher's Note
Springer Nature remains neutral with regard to jurisdictional claims in published maps and institutional affiliations.
Contributor Information
Kornelia Batko, Email: [email protected] .
Andrzej Ślęzak, Email: moc.liamg@25kazelsa .
Log in using your username and password
- Search More Search for this keyword Advanced search
- Latest content
- Current issue
- Browse by collection
- BMJ Journals More You are viewing from: Google Indexer
You are here
- Volume 110, Issue 9
- The role of COVID-19 vaccines in preventing post-COVID-19 thromboembolic and cardiovascular complications
- Article Text
- Article info
- Citation Tools
- Rapid Responses
- Article metrics

- Núria Mercadé-Besora 1 , 2 , 3 ,
- Xintong Li 1 ,
- Raivo Kolde 4 ,
- Nhung TH Trinh 5 ,
- Maria T Sanchez-Santos 1 ,
- Wai Yi Man 1 ,
- Elena Roel 3 ,
- Carlen Reyes 3 ,
- http://orcid.org/0000-0003-0388-3403 Antonella Delmestri 1 ,
- Hedvig M E Nordeng 6 , 7 ,
- http://orcid.org/0000-0002-4036-3856 Anneli Uusküla 8 ,
- http://orcid.org/0000-0002-8274-0357 Talita Duarte-Salles 3 , 9 ,
- Clara Prats 2 ,
- http://orcid.org/0000-0002-3950-6346 Daniel Prieto-Alhambra 1 , 9 ,
- http://orcid.org/0000-0002-0000-0110 Annika M Jödicke 1 ,
- Martí Català 1
- 1 Pharmaco- and Device Epidemiology Group, Health Data Sciences, Botnar Research Centre, NDORMS , University of Oxford , Oxford , UK
- 2 Department of Physics , Universitat Politècnica de Catalunya , Barcelona , Spain
- 3 Fundació Institut Universitari per a la recerca a l'Atenció Primària de Salut Jordi Gol i Gurina (IDIAPJGol) , IDIAP Jordi Gol , Barcelona , Catalunya , Spain
- 4 Institute of Computer Science , University of Tartu , Tartu , Estonia
- 5 Pharmacoepidemiology and Drug Safety Research Group, Department of Pharmacy, Faculty of Mathematics and Natural Sciences , University of Oslo , Oslo , Norway
- 6 School of Pharmacy , University of Oslo , Oslo , Norway
- 7 Division of Mental Health , Norwegian Institute of Public Health , Oslo , Norway
- 8 Department of Family Medicine and Public Health , University of Tartu , Tartu , Estonia
- 9 Department of Medical Informatics, Erasmus University Medical Center , Erasmus University Rotterdam , Rotterdam , Zuid-Holland , Netherlands
- Correspondence to Prof Daniel Prieto-Alhambra, Pharmaco- and Device Epidemiology Group, Health Data Sciences, Botnar Research Centre, NDORMS, University of Oxford, Oxford, UK; daniel.prietoalhambra{at}ndorms.ox.ac.uk
Objective To study the association between COVID-19 vaccination and the risk of post-COVID-19 cardiac and thromboembolic complications.
Methods We conducted a staggered cohort study based on national vaccination campaigns using electronic health records from the UK, Spain and Estonia. Vaccine rollout was grouped into four stages with predefined enrolment periods. Each stage included all individuals eligible for vaccination, with no previous SARS-CoV-2 infection or COVID-19 vaccine at the start date. Vaccination status was used as a time-varying exposure. Outcomes included heart failure (HF), venous thromboembolism (VTE) and arterial thrombosis/thromboembolism (ATE) recorded in four time windows after SARS-CoV-2 infection: 0–30, 31–90, 91–180 and 181–365 days. Propensity score overlap weighting and empirical calibration were used to minimise observed and unobserved confounding, respectively.
Fine-Gray models estimated subdistribution hazard ratios (sHR). Random effect meta-analyses were conducted across staggered cohorts and databases.
Results The study included 10.17 million vaccinated and 10.39 million unvaccinated people. Vaccination was associated with reduced risks of acute (30-day) and post-acute COVID-19 VTE, ATE and HF: for example, meta-analytic sHR of 0.22 (95% CI 0.17 to 0.29), 0.53 (0.44 to 0.63) and 0.45 (0.38 to 0.53), respectively, for 0–30 days after SARS-CoV-2 infection, while in the 91–180 days sHR were 0.53 (0.40 to 0.70), 0.72 (0.58 to 0.88) and 0.61 (0.51 to 0.73), respectively.
Conclusions COVID-19 vaccination reduced the risk of post-COVID-19 cardiac and thromboembolic outcomes. These effects were more pronounced for acute COVID-19 outcomes, consistent with known reductions in disease severity following breakthrough versus unvaccinated SARS-CoV-2 infection.
- Epidemiology
- PUBLIC HEALTH
- Electronic Health Records
Data availability statement
Data may be obtained from a third party and are not publicly available. CPRD: CPRD data were obtained under the CPRD multi-study license held by the University of Oxford after Research Data Governance (RDG) approval. Direct data sharing is not allowed. SIDIAP: In accordance with current European and national law, the data used in this study is only available for the researchers participating in this study. Thus, we are not allowed to distribute or make publicly available the data to other parties. However, researchers from public institutions can request data from SIDIAP if they comply with certain requirements. Further information is available online ( https://www.sidiap.org/index.php/menu-solicitudesen/application-proccedure ) or by contacting SIDIAP ([email protected]). CORIVA: CORIVA data were obtained under the approval of Research Ethics Committee of the University of Tartu and the patient level data sharing is not allowed. All analyses in this study were conducted in a federated manner, where analytical code and aggregated (anonymised) results were shared, but no patient-level data was transferred across the collaborating institutions.
This is an open access article distributed in accordance with the Creative Commons Attribution 4.0 Unported (CC BY 4.0) license, which permits others to copy, redistribute, remix, transform and build upon this work for any purpose, provided the original work is properly cited, a link to the licence is given, and indication of whether changes were made. See: https://creativecommons.org/licenses/by/4.0/ .
https://doi.org/10.1136/heartjnl-2023-323483
Statistics from Altmetric.com
Request permissions.
If you wish to reuse any or all of this article please use the link below which will take you to the Copyright Clearance Center’s RightsLink service. You will be able to get a quick price and instant permission to reuse the content in many different ways.
WHAT IS ALREADY KNOWN ON THIS TOPIC
COVID-19 vaccines proved to be highly effective in reducing the severity of acute SARS-CoV-2 infection.
While COVID-19 vaccines were associated with increased risk for cardiac and thromboembolic events, such as myocarditis and thrombosis, the risk of complications was substantially higher due to SARS-CoV-2 infection.
WHAT THIS STUDY ADDS
COVID-19 vaccination reduced the risk of heart failure, venous thromboembolism and arterial thrombosis/thromboembolism in the acute (30 days) and post-acute (31 to 365 days) phase following SARS-CoV-2 infection. This effect was stronger in the acute phase.
The overall additive effect of vaccination on the risk of post-vaccine and/or post-COVID thromboembolic and cardiac events needs further research.
HOW THIS STUDY MIGHT AFFECT RESEARCH, PRACTICE OR POLICY
COVID-19 vaccines proved to be highly effective in reducing the risk of post-COVID cardiovascular and thromboembolic complications.
Introduction
COVID-19 vaccines were approved under emergency authorisation in December 2020 and showed high effectiveness against SARS-CoV-2 infection, COVID-19-related hospitalisation and death. 1 2 However, concerns were raised after spontaneous reports of unusual thromboembolic events following adenovirus-based COVID-19 vaccines, an association that was further assessed in observational studies. 3 4 More recently, mRNA-based vaccines were found to be associated with a risk of rare myocarditis events. 5 6
On the other hand, SARS-CoV-2 infection can trigger cardiac and thromboembolic complications. 7 8 Previous studies showed that, while slowly decreasing over time, the risk for serious complications remain high for up to a year after infection. 9 10 Although acute and post-acute cardiac and thromboembolic complications following COVID-19 are rare, they present a substantial burden to the affected patients, and the absolute number of cases globally could become substantial.
Recent studies suggest that COVID-19 vaccination could protect against cardiac and thromboembolic complications attributable to COVID-19. 11 12 However, most studies did not include long-term complications and were conducted among specific populations.
Evidence is still scarce as to whether the combined effects of COVID-19 vaccines protecting against SARS-CoV-2 infection and reducing post-COVID-19 cardiac and thromboembolic outcomes, outweigh any risks of these complications potentially associated with vaccination.
We therefore used large, representative data sources from three European countries to assess the overall effect of COVID-19 vaccines on the risk of acute and post-acute COVID-19 complications including venous thromboembolism (VTE), arterial thrombosis/thromboembolism (ATE) and other cardiac events. Additionally, we studied the comparative effects of ChAdOx1 versus BNT162b2 on the risk of these same outcomes.
Data sources
We used four routinely collected population-based healthcare datasets from three European countries: the UK, Spain and Estonia.
For the UK, we used data from two primary care databases—namely, Clinical Practice Research Datalink, CPRD Aurum 13 and CPRD Gold. 14 CPRD Aurum currently covers 13 million people from predominantly English practices, while CPRD Gold comprises 3.1 million active participants mostly from GP practices in Wales and Scotland. Spanish data were provided by the Information System for the Development of Research in Primary Care (SIDIAP), 15 which encompasses primary care records from 6 million active patients (around 75% of the population in the region of Catalonia) linked to hospital admissions data (Conjunt Mínim Bàsic de Dades d’Alta Hospitalària). Finally, the CORIVA dataset based on national health claims data from Estonia was used. It contains all COVID-19 cases from the first year of the pandemic and ~440 000 randomly selected controls. CORIVA was linked to the death registry and all COVID-19 testing from the national health information system.
Databases included sociodemographic information, diagnoses, measurements, prescriptions and secondary care referrals and were linked to vaccine registries, including records of all administered vaccines from all healthcare settings. Data availability for CPRD Gold ended in December 2021, CPRD Aurum in January 2022, SIDIAP in June 2022 and CORIVA in December 2022.
All databases were mapped to the Observational Medical Outcomes Partnership Common Data Model (OMOP CDM) 16 to facilitate federated analytics.
Multinational network staggered cohort study: study design and participants
The study design has been published in detail elsewhere. 17 Briefly, we used a staggered cohort design considering vaccination as a time-varying exposure. Four staggered cohorts were designed with each cohort representing a country-specific vaccination rollout phase (eg, dates when people became eligible for vaccination, and eligibility criteria).
The source population comprised all adults registered in the respective database for at least 180 days at the start of the study (4 January 2021 for CPRD Gold and Aurum, 20 February 2021 for SIDIAP and 28 January 2021 for CORIVA). Subsequently, each staggered cohort corresponded to an enrolment period: all people eligible for vaccination during this time were included in the cohort and people with a history of SARS-CoV-2 infection or COVID-19 vaccination before the start of the enrolment period were excluded. Across countries, cohort 1 comprised older age groups, whereas cohort 2 comprised individuals at risk for severe COVID-19. Cohort 3 included people aged ≥40 and cohort 4 enrolled people aged ≥18.
In each cohort, people receiving a first vaccine dose during the enrolment period were allocated to the vaccinated group, with their index date being the date of vaccination. Individuals who did not receive a vaccine dose comprised the unvaccinated group and their index date was assigned within the enrolment period, based on the distribution of index dates in the vaccinated group. People with COVID-19 before the index date were excluded.
Follow-up started from the index date until the earliest of end of available data, death, change in exposure status (first vaccine dose for those unvaccinated) or outcome of interest.
COVID-19 vaccination
All vaccines approved within the study period from January 2021 to July 2021—namely, ChAdOx1 (Oxford/AstraZeneca), BNT162b2 (BioNTech/Pfizer]) Ad26.COV2.S (Janssen) and mRNA-1273 (Moderna), were included for this study.
Post-COVID-19 outcomes of interest
Outcomes of interest were defined as SARS-CoV-2 infection followed by a predefined thromboembolic or cardiac event of interest within a year after infection, and with no record of the same clinical event in the 6 months before COVID-19. Outcome date was set as the corresponding SARS-CoV-2 infection date.
COVID-19 was identified from either a positive SARS-CoV-2 test (polymerase chain reaction (PCR) or antigen), or a clinical COVID-19 diagnosis, with no record of COVID-19 in the previous 6 weeks. This wash-out period was imposed to exclude re-recordings of the same COVID-19 episode.
Post-COVID-19 outcome events were selected based on previous studies. 11–13 Events comprised ischaemic stroke (IS), haemorrhagic stroke (HS), transient ischaemic attack (TIA), ventricular arrhythmia/cardiac arrest (VACA), myocarditis/pericarditis (MP), myocardial infarction (MI), heart failure (HF), pulmonary embolism (PE) and deep vein thrombosis (DVT). We used two composite outcomes: (1) VTE, as an aggregate of PE and DVT and (2) ATE, as a composite of IS, TIA and MI. To avoid re-recording of the same complication we imposed a wash-out period of 90 days between records. Phenotypes for these complications were based on previously published studies. 3 4 8 18
All outcomes were ascertained in four different time periods following SARS-CoV-2 infection: the first period described the acute infection phase—that is, 0–30 days after COVID-19, whereas the later periods - which are 31–90 days, 91–180 days and 181–365 days, illustrate the post-acute phase ( figure 1 ).
- Download figure
- Open in new tab
- Download powerpoint
Study outcome design. Study outcomes of interest are defined as a COVID-19 infection followed by one of the complications in the figure, within a year after infection. Outcomes were ascertained in four different time windows after SARS-CoV-2 infection: 0–30 days (namely the acute phase), 31–90 days, 91–180 days and 181–365 days (these last three comprise the post-acute phase).
Negative control outcomes
Negative control outcomes (NCOs) were used to detect residual confounding. NCOs are outcomes which are not believed to be causally associated with the exposure, but share the same bias structure with the exposure and outcome of interest. Therefore, no significant association between exposure and NCO is to be expected. Our study used 43 different NCOs from previous work assessing vaccine effectiveness. 19
Statistical analysis
Federated network analyses.
A template for an analytical script was developed and subsequently tailored to include the country-specific aspects (eg, dates, priority groups) for the vaccination rollout. Analyses were conducted locally for each database. Only aggregated data were shared and person counts <5 were clouded.
Propensity score weighting
Large-scale propensity scores (PS) were calculated to estimate the likelihood of a person receiving the vaccine based on their demographic and health-related characteristics (eg, conditions, medications) prior to the index date. PS were then used to minimise observed confounding by creating a weighted population (overlap weighting 20 ), in which individuals contributed with a different weight based on their PS and vaccination status.
Prespecified key variables included in the PS comprised age, sex, location, index date, prior observation time in the database, number of previous outpatient visits and previous SARS-CoV-2 PCR/antigen tests. Regional vaccination, testing and COVID-19 incidence rates were also forced into the PS equation for the UK databases 21 and SIDIAP. 22 In addition, least absolute shrinkage and selection operator (LASSO) regression, a technique for variable selection, was used to identify additional variables from all recorded conditions and prescriptions within 0–30 days, 31–180 days and 181-any time (conditions only) before the index date that had a prevalence of >0.5% in the study population.
PS were then separately estimated for each staggered cohort and analysis. We considered covariate balance to be achieved if absolute standardised mean differences (ASMDs) were ≤0.1 after weighting. Baseline characteristics such as demographics and comorbidities were reported.
Effect estimation
To account for the competing risk of death associated with COVID-19, Fine-and-Grey models 23 were used to calculate subdistribution hazard ratios (sHRs). Subsequently, sHRs and confidence intervals were empirically calibrated from NCO estimates 24 to account for unmeasured confounding. To calibrate the estimates, the empirical null distribution was derived from NCO estimates and was used to compute calibrated confidence intervals. For each outcome, sHRs from the four staggered cohorts were pooled using random-effect meta-analysis, both separately for each database and across all four databases.
Sensitivity analysis
Sensitivity analyses comprised 1) censoring follow-up for vaccinated people at the time when they received their second vaccine dose and 2) considering only the first post-COVID-19 outcome within the year after infection ( online supplemental figure S1 ). In addition, comparative effectiveness analyses were conducted for BNT162b2 versus ChAdOx1.
Supplemental material
Data and code availability.
All analytic code for the study is available in GitHub ( https://github.com/oxford-pharmacoepi/vaccineEffectOnPostCovidCardiacThromboembolicEvents ), including code lists for vaccines, COVID-19 tests and diagnoses, cardiac and thromboembolic events, NCO and health conditions to prioritise patients for vaccination in each country. We used R version 4.2.3 and statistical packages survival (3.5–3), Empirical Calibration (3.1.1), glmnet (4.1-7), and Hmisc (5.0–1).
Patient and public involvement
Owing to the nature of the study and the limitations regarding data privacy, the study design, analysis, interpretation of data and revision of the manuscript did not involve any patients or members of the public.
All aggregated results are available in a web application ( https://dpa-pde-oxford.shinyapps.io/PostCovidComplications/ ).
We included over 10.17 million vaccinated individuals (1 618 395 from CPRD Gold; 5 729 800 from CPRD Aurum; 2 744 821 from SIDIAP and 77 603 from CORIVA) and 10.39 million unvaccinated individuals (1 640 371; 5 860 564; 2 588 518 and 302 267, respectively). Online supplemental figures S2-5 illustrate study inclusion for each database.
Adequate covariate balance was achieved after PS weighting in most studies: CORIVA (all cohorts) and SIDIAP (cohorts 1 and 4) did not contribute to ChAdOx1 subanalyses owing to sample size and covariate imbalance. ASMD results are accessible in the web application.
NCO analyses suggested residual bias after PS weighting, with a majority of NCOs associated positively with vaccination. Therefore, calibrated estimates are reported in this manuscript. Uncalibrated effect estimates and NCO analyses are available in the web interface.
Population characteristics
Table 1 presents baseline characteristics for the weighted populations in CPRD Aurum, for illustrative purposes. Online supplemental tables S1-25 summarise baseline characteristics for weighted and unweighted populations for each database and comparison. Across databases and cohorts, populations followed similar patterns: cohort 1 represented an older subpopulation (around 80 years old) with a high proportion of women (57%). Median age was lowest in cohort 4 ranging between 30 and 40 years.
- View inline
Characteristics of weighted populations in CPRD Aurum database, stratified by staggered cohort and exposure status. Exposure is any COVID-19 vaccine
COVID-19 vaccination and post-COVID-19 complications
Table 2 shows the incidence of post-COVID-19 VTE, ATE and HF, the three most common post-COVID-19 conditions among the studied outcomes. Outcome counts are presented separately for 0–30, 31–90, 91–180 and 181–365 days after SARS-CoV-2 infection. Online supplemental tables S26-36 include all studied complications, also for the sensitivity and subanalyses. Similar pattern for incidences were observed across all databases: higher outcome rates in the older populations (cohort 1) and decreasing frequency with increasing time after infection in all cohorts.
Number of records (and risk per 10 000 individuals) for acute and post-acute COVID-19 cardiac and thromboembolic complications, across cohorts and databases for any COVID-19 vaccination
Forest plots for the effect of COVID-19 vaccines on post-COVID-19 cardiac and thromboembolic complications; meta-analysis across cohorts and databases. Dashed line represents a level of heterogeneity I 2 >0.4. ATE, arterial thrombosis/thromboembolism; CD+HS, cardiac diseases and haemorrhagic stroke; VTE, venous thromboembolism.
Results from calibrated estimates pooled in meta-analysis across cohorts and databases are shown in figure 2 .
Reduced risk associated with vaccination is observed for acute and post-acute VTE, DVT, and PE: acute meta-analytic sHR are 0.22 (95% CI, 0.17–0.29); 0.36 (0.28–0.45); and 0.19 (0.15–0.25), respectively. For VTE in the post-acute phase, sHR estimates are 0.43 (0.34–0.53), 0.53 (0.40–0.70) and 0.50 (0.36–0.70) for 31–90, 91–180, and 181–365 days post COVID-19, respectively. Reduced risk of VTE outcomes was observed in vaccinated across databases and cohorts, see online supplemental figures S14–22 .
Similarly, the risk of ATE, IS and MI in the acute phase after infection was reduced for the vaccinated group, sHR of 0.53 (0.44–0.63), 0.55 (0.43–0.70) and 0.49 (0.38–0.62), respectively. Reduced risk associated with vaccination persisted for post-acute ATE, with sHR of 0.74 (0.60–0.92), 0.72 (0.58–0.88) and 0.62 (0.48–0.80) for 31–90, 91–180 and 181–365 days post-COVID-19, respectively. Risk of post-acute MI remained lower for vaccinated in the 31–90 and 91–180 days after COVID-19, with sHR of 0.64 (0.46–0.87) and 0.64 (0.45–0.90), respectively. Vaccination effect on post-COVID-19 TIA was seen only in the 181–365 days, with sHR of 0.51 (0.31–0.82). Online supplemental figures S23-31 show database-specific and cohort-specific estimates for ATE-related complications.
Risk of post-COVID-19 cardiac complications was reduced in vaccinated individuals. Meta-analytic estimates in the acute phase showed sHR of 0.45 (0.38–0.53) for HF, 0.41 (0.26–0.66) for MP and 0.41 (0.27–0.63) for VACA. Reduced risk persisted for post-acute COVID-19 HF: sHR 0.61 (0.51–0.73) for 31–90 days, 0.61 (0.51–0.73) for 91–180 days and 0.52 (0.43–0.63) for 181–365 days. For post-acute MP, risk was only lowered in the first post-acute window (31–90 days), with sHR of 0.43 (0.21–0.85). Vaccination showed no association with post-COVID-19 HS. Database-specific and cohort-specific results for these cardiac diseases are shown in online supplemental figures S32-40 .
Stratified analyses by vaccine showed similar associations, except for ChAdOx1 which was not associated with reduced VTE and ATE risk in the last post-acute window. Sensitivity analyses were consistent with main results ( online supplemental figures S6-13 ).
Figure 3 shows the results of comparative effects of BNT162b2 versus ChAdOx1, based on UK data. Meta-analytic estimates favoured BNT162b2 (sHR of 0.66 (0.46–0.93)) for VTE in the 0–30 days after infection, but no differences were seen for post-acute VTE or for any of the other outcomes. Results from sensitivity analyses, database-specific and cohort-specific estimates were in line with the main findings ( online supplemental figures S41-51 ).
Forest plots for comparative vaccine effect (BNT162b2 vs ChAdOx1); meta-analysis across cohorts and databases. ATE, arterial thrombosis/thromboembolism; CD+HS, cardiac diseases and haemorrhagic stroke; VTE, venous thromboembolism.
Key findings
Our analyses showed a substantial reduction of risk (45–81%) for thromboembolic and cardiac events in the acute phase of COVID-19 associated with vaccination. This finding was consistent across four databases and three different European countries. Risks for post-acute COVID-19 VTE, ATE and HF were reduced to a lesser extent (24–58%), whereas a reduced risk for post-COVID-19 MP and VACA in vaccinated people was seen only in the acute phase.
Results in context
The relationship between SARS-CoV-2 infection, COVID-19 vaccines and thromboembolic and/or cardiac complications is tangled. Some large studies report an increased risk of VTE and ATE following both ChAdOx1 and BNT162b2 vaccination, 7 whereas other studies have not identified such a risk. 25 Elevated risk of VTE has also been reported among patients with COVID-19 and its occurrence can lead to poor prognosis and mortality. 26 27 Similarly, several observational studies have found an association between COVID-19 mRNA vaccination and a short-term increased risk of myocarditis, particularly among younger male individuals. 5 6 For instance, a self-controlled case series study conducted in England revealed about 30% increased risk of hospital admission due to myocarditis within 28 days following both ChAdOx1 and BNT162b2 vaccines. However, this same study also found a ninefold higher risk for myocarditis following a positive SARS-CoV-2 test, clearly offsetting the observed post-vaccine risk.
COVID-19 vaccines have demonstrated high efficacy and effectiveness in preventing infection and reducing the severity of acute-phase infection. However, with the emergence of newer variants of the virus, such as omicron, and the waning protective effect of the vaccine over time, there is a growing interest in understanding whether the vaccine can also reduce the risk of complications after breakthrough infections. Recent studies suggested that COVID-19 vaccination could potentially protect against acute post-COVID-19 cardiac and thromboembolic events. 11 12 A large prospective cohort study 11 reports risk of VTE after SARS-CoV-2 infection to be substantially reduced in fully vaccinated ambulatory patients. Likewise, Al-Aly et al 12 suggest a reduced risk for post-acute COVID-19 conditions in breakthrough infection versus SARS-CoV-2 infection without prior vaccination. However, the populations were limited to SARS-CoV-2 infected individuals and estimates did not include the effect of the vaccine to prevent COVID-19 in the first place. Other studies on post-acute COVID-19 conditions and symptoms have been conducted, 28 29 but there has been limited reporting on the condition-specific risks associated with COVID-19, even though the prognosis for different complications can vary significantly.
In line with previous studies, our findings suggest a potential benefit of vaccination in reducing the risk of post-COVID-19 thromboembolic and cardiac complications. We included broader populations, estimated the risk in both acute and post-acute infection phases and replicated these using four large independent observational databases. By pooling results across different settings, we provided the most up-to-date and robust evidence on this topic.
Strengths and limitations
The study has several strengths. Our multinational study covering different healthcare systems and settings showed consistent results across all databases, which highlights the robustness and replicability of our findings. All databases had complete recordings of vaccination status (date and vaccine) and are representative of the respective general population. Algorithms to identify study outcomes were used in previous published network studies, including regulatory-funded research. 3 4 8 18 Other strengths are the staggered cohort design which minimises confounding by indication and immortal time bias. PS overlap weighting and NCO empirical calibration have been shown to adequately minimise bias in vaccine effectiveness studies. 19 Furthermore, our estimates include the vaccine effectiveness against COVID-19, which is crucial in the pathway to experience post-COVID-19 complications.
Our study has some limitations. The use of real-world data comes with inherent limitations including data quality concerns and risk of confounding. To deal with these limitations, we employed state-of-the-art methods, including large-scale propensity score weighting and calibration of effect estimates using NCO. 19 24 A recent study 30 has demonstrated that methodologically sound observational studies based on routinely collected data can produce results similar to those of clinical trials. We acknowledge that results from NCO were positively associated with vaccination, and estimates might still be influenced by residual bias despite using calibration. Another limitation is potential under-reporting of post-COVID-19 complications: some asymptomatic and mild COVID-19 infections might have not been recorded. Additionally, post-COVID-19 outcomes of interest might be under-recorded in primary care databases (CPRD Aurum and Gold) without hospital linkage, which represent a large proportion of the data in the study. However, results in SIDIAP and CORIVA, which include secondary care data, were similar. Also, our study included a small number of young men and male teenagers, who were the main population concerned with increased risks of myocarditis/pericarditis following vaccination.
Conclusions
Vaccination against SARS-CoV-2 substantially reduced the risk of acute post-COVID-19 thromboembolic and cardiac complications, probably through a reduction in the risk of SARS-CoV-2 infection and the severity of COVID-19 disease due to vaccine-induced immunity. Reduced risk in vaccinated people lasted for up to 1 year for post-COVID-19 VTE, ATE and HF, but not clearly for other complications. Findings from this study highlight yet another benefit of COVID-19 vaccination. However, further research is needed on the possible waning of the risk reduction over time and on the impact of booster vaccination.
Ethics statements
Patient consent for publication.
Not applicable.
Ethics approval
The study was approved by the CPRD’s Research Data Governance Process, Protocol No 21_000557 and the Clinical Research Ethics committee of Fundació Institut Universitari per a la recerca a l’Atenció Primària de Salut Jordi Gol i Gurina (IDIAPJGol) (approval number 4R22/133) and the Research Ethics Committee of the University of Tartu (approval No. 330/T-10).
Acknowledgments
This study is based in part on data from the Clinical Practice Research Datalink (CPRD) obtained under licence from the UK Medicines and Healthcare products Regulatory Agency. We thank the patients who provided these data, and the NHS who collected the data as part of their care and support. All interpretations, conclusions and views expressed in this publication are those of the authors alone and not necessarily those of CPRD. We would also like to thank the healthcare professionals in the Catalan healthcare system involved in the management of COVID-19 during these challenging times, from primary care to intensive care units; the Institut de Català de la Salut and the Program d’Analítica de Dades per a la Recerca i la Innovació en Salut for providing access to the different data sources accessible through The System for the Development of Research in Primary Care (SIDIAP).
- Pritchard E ,
- Matthews PC ,
- Stoesser N , et al
- Lauring AS ,
- Tenforde MW ,
- Chappell JD , et al
- Pistillo A , et al
- Duarte-Salles T , et al
- Hansen JV ,
- Fosbøl E , et al
- Chen A , et al
- Hippisley-Cox J ,
- Mei XW , et al
- Duarte-Salles T ,
- Fernandez-Bertolin S , et al
- Ip S , et al
- Bowe B , et al
- Prats-Uribe A ,
- Feng Q , et al
- Campbell J , et al
- Herrett E ,
- Gallagher AM ,
- Bhaskaran K , et al
- Raventós B ,
- Fernández-Bertolín S ,
- Aragón M , et al
- Makadia R ,
- Matcho A , et al
- Mercadé-Besora N ,
- Kolde R , et al
- Ostropolets A ,
- Makadia R , et al
- Rathod-Mistry T , et al
- Thomas LE ,
- ↵ Coronavirus (COVID-19) in the UK . 2022 . Available : https://coronavirus.data.gov.uk/
- Generalitat de Catalunya
- Schuemie MJ ,
- Hripcsak G ,
- Ryan PB , et al
- Houghton DE ,
- Wysokinski W ,
- Casanegra AI , et al
- Katsoularis I ,
- Fonseca-Rodríguez O ,
- Farrington P , et al
- Jehangir Q ,
- Li P , et al
- Byambasuren O ,
- Stehlik P ,
- Clark J , et al
- Brannock MD ,
- Preiss AJ , et al
- Schneeweiss S , RCT-DUPLICATE Initiative , et al
Supplementary materials
Supplementary data.
This web only file has been produced by the BMJ Publishing Group from an electronic file supplied by the author(s) and has not been edited for content.
- Data supplement 1
AMJ and MC are joint senior authors.
Contributors DPA and AMJ led the conceptualisation of the study with contributions from MC and NM-B. AMJ, TD-S, ER, AU and NTHT adapted the study design with respect to the local vaccine rollouts. AD and WYM mapped and curated CPRD data. MC and NM-B developed code with methodological contributions advice from MTS-S and CP. DPA, MC, NTHT, TD-S, HMEN, XL, CR and AMJ clinically interpreted the results. NM-B, XL, AMJ and DPA wrote the first draft of the manuscript, and all authors read, revised and approved the final version. DPA and AMJ obtained the funding for this research. DPA is responsible for the overall content as guarantor: he accepts full responsibility for the work and the conduct of the study, had access to the data, and controlled the decision to publish.
Funding The research was supported by the National Institute for Health and Care Research (NIHR) Oxford Biomedical Research Centre (BRC). DPA is funded through a NIHR Senior Research Fellowship (Grant number SRF-2018–11-ST2-004). Funding to perform the study in the SIDIAP database was provided by the Real World Epidemiology (RWEpi) research group at IDIAPJGol. Costs of databases mapping to OMOP CDM were covered by the European Health Data and Evidence Network (EHDEN).
Patient and public involvement Patients and/or the public were not involved in the design, or conduct, or reporting or dissemination plans of this research.
Provenance and peer review Not commissioned; externally peer reviewed.
Supplemental material This content has been supplied by the author(s). It has not been vetted by BMJ Publishing Group Limited (BMJ) and may not have been peer-reviewed. Any opinions or recommendations discussed are solely those of the author(s) and are not endorsed by BMJ. BMJ disclaims all liability and responsibility arising from any reliance placed on the content. Where the content includes any translated material, BMJ does not warrant the accuracy and reliability of the translations (including but not limited to local regulations, clinical guidelines, terminology, drug names and drug dosages), and is not responsible for any error and/or omissions arising from translation and adaptation or otherwise.
Read the full text or download the PDF:
Read our research on: Gun Policy | International Conflict | Election 2024
Regions & Countries
Political typology quiz.
Notice: Beginning April 18th community groups will be temporarily unavailable for extended maintenance. Thank you for your understanding and cooperation.
Where do you fit in the political typology?
Are you a faith and flag conservative progressive left or somewhere in between.

Take our quiz to find out which one of our nine political typology groups is your best match, compared with a nationally representative survey of more than 10,000 U.S. adults by Pew Research Center. You may find some of these questions are difficult to answer. That’s OK. In those cases, pick the answer that comes closest to your view, even if it isn’t exactly right.
About Pew Research Center Pew Research Center is a nonpartisan fact tank that informs the public about the issues, attitudes and trends shaping the world. It conducts public opinion polling, demographic research, media content analysis and other empirical social science research. Pew Research Center does not take policy positions. It is a subsidiary of The Pew Charitable Trusts .
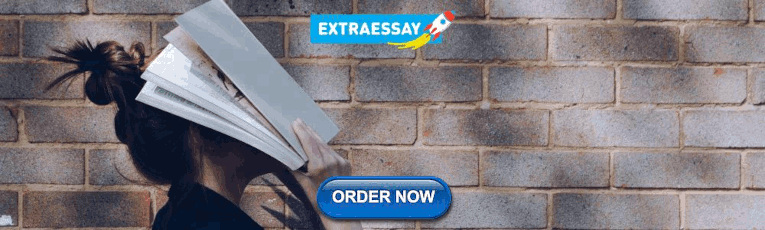
IMAGES
VIDEO
COMMENTS
About the journal. The journal aims to promote and communicate advances in big data research by providing a fast and high quality forum for researchers, practitioners and policy makers from the very many different communities working on, and with, this topic. The journal will accept papers on foundational aspects in dealing with big data, as ...
The Journal of Big Data publishes open-access original research on data science and data analytics. Deep learning algorithms and all applications of big data are welcomed. Survey papers and case studies are also considered. The journal examines the challenges facing big data today and going forward including, but not limited to: data capture and storage; search, sharing, and analytics; big ...
Of the 70 papers satisfying our selection criteria, publication year and type (journal or conference paper) reveal an increasing trend in big data analytics over the last 6 years (Table 6). Additionally, journals produced more BDA papers than Conference proceedings (Fig. 2 ), which may be affected during 2020-2021 because of COVID, and fewer ...
Big Data is an essential research area for governments, institutions, and private agencies to support their analytics decisions. Big Data refers to all about data, how it is collected, processed, and analyzed to generate value-added data-driven insights and decisions. Degradation in Data Quality may result in unpredictable consequences. In this case, confidence and worthiness in the data and ...
3. Research methodology. In an attempt to better understand and provide more detailed insights to the phenomenon of big data and bit data analytics, the authors respond to the special issue call on Big Data and Analytics in Technology and Organizational Resource Management (specifically focusing on conducting - A comprehensive state-of-the-art review that presents Big Data Challenges and Big ...
2014 — Volume 1. Read the latest articles of Big Data Research at ScienceDirect.com, Elsevier's leading platform of peer-reviewed scholarly literature.
The Internet of Things (IoT) is a communication paradigm and a collection of heterogeneous interconnected devices. It produces large-scale distributed, and diverse data called big data. Big Data Management (BDM) in IoT is used for knowledge discovery and intelligent decision-making and is one of the most significant research challenges today. There are several mechanisms and technologies for ...
Big Data Analytic s: A Literature Review Pape r. Nada Elgendy and Ahmed Elragal. Department of Busi ness Informatics & Operations, German University in Cairo (GUC), Cairo, Egypt. {nada.el-gendy ...
Prior research observed several issues related to big data accumulated in healthcare, such as data quality (Sabharwal, Gupta, and Thirunavukkarasu Citation 2016) and data quantity (Gopal et al. Citation 2019). However, there is a lack of research into the types of problems that may occur during data accumulation processes in healthcare and how ...
In this descriptive review, we highlight the roles of big data, the changing research paradigm, and easy access to research participation via the Internet fueled by the need for quick answers. Universally, data volume has increased, with the collection rate doubling every 40 months, ever since the 1980s. 4 The big data age, starting in 2002 ...
Big Data in Finance. Itay Goldstein, Chester S. Spatt & Mao Ye. Working Paper 28615. DOI 10.3386/w28615. Issue Date March 2021. Big data is revolutionizing the finance industry and has the potential to significantly shape future research in finance. This special issue contains articles following the 2019 NBER/ RFS conference on big data.
The increasing interest from technology enthusiasts and organisational practitioners in big data applications in the supply chain has encouraged us to review recent research development. This paper proposes a systematic literature review to explore the available peer-reviewed literature on how big data is widely optimised and managed within the supply chain management context. Although big ...
Recently, big data streams have become ubiquitous due to the fact that a number of applications generate a huge amount of data at a great velocity. This made it difficult for existing data mining tools, technologies, methods, and techniques to be applied directly on big data streams due to the inherent dynamic characteristics of big data. In this paper, a systematic review of big data streams ...
This paper focuses on privacy and security concerns in Big Data. This paper also covers the encryption techniques by taking existing methods such as differential privacy, k-anonymity, T-closeness, and L-diversity.Several privacy-preserving techniques have been created to safeguard privacy at various phases of a large data life cycle.
Big data are a collection of structured and unstructured data incoming with a high speed and large amounts. This paper investigates big data applications in IoT to comprehend the different published approaches using the systematic literature review (SLR) technique. This paper systematically studies the latest research methods on big data in IoT ...
Profile Information. Communications Preferences. Profession and Education. Technical Interests. Need Help? US & Canada:+1 800 678 4333. Worldwide: +1 732 981 0060. Contact & Support. About IEEE Xplore.
The remainder of this SLR is organized as can be seen in Fig. 1. Section 2 discusses some related works and motivation. The research questions, the details of the selection process, and the research methodology are documented in Section 3.Following, Section 4 provides a classification and a detailed study of the selected papers and demonstrates their main ideas, advantages, disadvantages ...
Taking "the establishment of the national big data comprehensive pilot zone" as a quasi-experiment, this study uses propensity score matching-difference in difference (PSM-DID) to investigate how the national big data comprehensive pilot zone improves the technical complexity of urban exports and its mechanism, which is based on the data of 280 prefecture cities from 2007 to 2019. The results ...
The evaluation of performance using competencies within a structured framework holds significant importance across various professional domains, particularly in roles like project manager. Typically, this assessment process, overseen by senior evaluators, involves scoring competencies based on data gathered from interviews, completed forms, and evaluation programs. However, this task is ...
Models for structuring big-data and data-analytics projects typically start with a definition of the project's goals and the business value they are expected to create. The literature identifies proper project... Jeroen de Mast and Joran Lokkerbol. Journal of Big Data 2024 11 :50. Research Published on: 12 April 2024.
This work introduces an efficient method to scale Transformer-based Large Language Models (LLMs) to infinitely long inputs with bounded memory and computation. A key component in our proposed approach is a new attention technique dubbed Infini-attention. The Infini-attention incorporates a compressive memory into the vanilla attention mechanism and builds in both masked local attention and ...
Background: Although patients have easy access to their electronic health records and laboratory test result data through patient portals, laboratory test results are often confusing and hard to understand. Many patients turn to web-based forums or question-and-answer (Q&A) sites to seek advice from their peers. The quality of answers from social Q&A sites on health-related questions ...
Several research papers demonstrate that big data analytics, when applied to problems of specific domains such as healthcare, service provision, supply chain management, and marketing, can offer substantial value (Mikalef et al., 2019; Raghupathi & Raghupathi, 2014; Waller & Fawcett, 2013; Wang et al., 2016).
Quantum neural networks (QNNs) leverage the strengths of both quantum computing and neural networks, offering solutions to challenges that are often beyond the reach of traditional neural networks. QNNs are being used in areas such as computer games, function approximation, and big data processing. Moreover, quantum neural network algorithms are finding utility in social network modeling ...
We collected and catalogued nearly 1.3 million photos from 474 camera-trap locations for a total of 35,546 days, averaging 2,539 camera-trap days per concession (Extended Data Table 1).We detected ...
Expected trend of the marketing of big data between 2012 and 2018. Note that yellow, red, and blue of different colored box represent the order of appearance of reference in this paper for particular year. Full size image. The report of IDC [ 9] indicates that the marketing of big data is about $16.1 billion in 2014.
The paper poses the following research questions and statements that coincide with the selected questions from the research questionnaire: ... Future research on the use of Big Data in medical facilities will concern the definition of strategies adopted by medical facilities to promote and implement such solutions, as well as the benefits they ...
Objective To study the association between COVID-19 vaccination and the risk of post-COVID-19 cardiac and thromboembolic complications. Methods We conducted a staggered cohort study based on national vaccination campaigns using electronic health records from the UK, Spain and Estonia. Vaccine rollout was grouped into four stages with predefined enrolment periods. Each stage included all ...
Research methods are roadmaps, techniques, and procedures employed in a study to collect data, process data, analyze data, yield findings, and draw a conclusion to achieve the research aims. To a large degree the availability, nature, and size of a dataset can affect the selection of the research methods, even the research topics.
About Pew Research Center Pew Research Center is a nonpartisan fact tank that informs the public about the issues, attitudes and trends shaping the world. It conducts public opinion polling, demographic research, media content analysis and other empirical social science research. Pew Research Center does not take policy positions.