Household energy consumption
- Published: 29 November 2021
- Volume 24 , pages 1–5, ( 2022 )
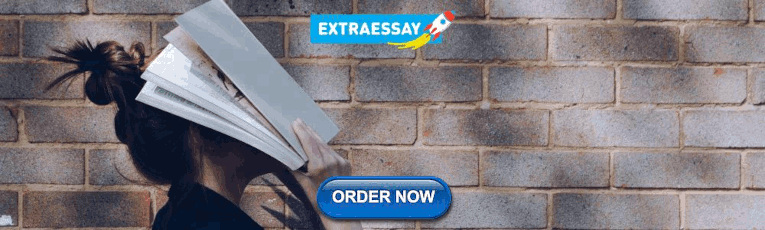
Cite this article
- Shigeru Matsumoto 1 ,
- Kenichi Mizobuchi 2 &
- Shunsuke Managi 3
6966 Accesses
8 Citations
1 Altmetric
Explore all metrics
Avoid common mistakes on your manuscript.
Energy conservation policies implemented over the last few decades have substantially improved industrial energy efficiency. In comparison, the progress of energy conservation in the residential sector has remained very slow. As a result, the share of the industrial sector in final electricity consumption decreased from 53.4% in 1973 to 42.0% in 2018, while the share of the residential sector increased from 23.1% to 26.9% (IEA 2020 ). Similarly, the industrial sector’s share of the final natural gas consumption has decreased from 54.7% to 37.0%, whereas the residential sector’s share grew from 22.7% to 29.9%. Given its increasing relative importance, countries are now strengthening energy conservation measures in the residential sector.
One of the major reasons for the delay in domestic energy conservation is the lack of basic knowledge about household energy consumption. Households employ multiple energies in their daily lives; however, it is unclear for what purpose they use each type of energy. In addition, the association between household characteristics and energy consumption is not well understood. Given the importance of energy in our daily lives, this lack of basic understanding of the energy policy design is one of the major obstacles to carbon mitigation.
Researchers examined household energy consumption using macro-level data (Espey and Espey 2004 ). However, some researchers contend that the empirical results based on macro-level data analyses that ignore household diversity are less reliable (Baker et al. 1989 ). As data for household-level energy consumption become available, analyses using microdata have become common in recent years, and the effectiveness of energy conservation measures has been assessed based on the results of such micro-level data analyses.
Although there are several residential energy conservation measures available, they can be classified into three stages. The first stage is the energy choice stage , where the policy objective is to encourage households to use energy that produces less CO 2 , preferably renewable energy. Governments attempt to influence household energy choices by changing the relative price of energy through carbon pricing schemes. Governments can reduce household energy consumption by making households choose energy-efficient products at the product purchase stage. For the last several decades, the government has attempted to design programs that effectively provide consumers with information on the energy usage of products and, in certain circumstances, has provided subsidies to consumers who purchase energy-efficient products. The total amount of energy consumed is determined by how households use the product. The objective of the conservation measures at the final energy consumption stage is to make households use the products in an energy-efficient manner. For this purpose, the government has launched various energy conservation promotion campaigns or educational programs.
This special issue includes five articles that offer useful information on the design of energy conservation measures in the residential sector.
The first two articles focus on energy savings at the product purchase stage. Even when obvious energy-saving benefits can be expected, some consumers do not purchase energy-efficient appliances. Product-choice behavior by such people cannot be fully described with a simple economic model that presupposes a rational economic man. In recent years, studies have been actively conducted to evaluate the product selection of energy-consuming products more precisely by incorporating knowledge from psychology and sociology into traditional economic models. However, most previous studies have been conducted in developed countries, mainly in Europe and the United States, and there is a lack of studies in other regions. Zainudin, Lau, and Munusami studied Malaysian consumers’ purchasing behavior of energy-consuming products based on the framework of consumer behavior science. Their empirical findings reveal that consumers’ perceived value is a major factor affecting buying intention. Their findings suggest that programs that enhance consumers’ perceptions of product values are simultaneously necessary for the market penetration of energy-efficient products.
Major electric appliances such as TVs, air conditioners, washing machines, and refrigerators are already widespread in developed countries, and households are now replacing them periodically. As the energy efficiency of products improves every year, replacing old products with new ones will lead to energy savings. Consequently, governments have encouraged the replacement of old appliances with new ones. Nevertheless, the replacement cycles of electric appliances have not been thoroughly investigated in previous studies. Wang and Matsumoto employed a duration model to develop an economic model that describes the appliance replacement cycle. Then they conducted an empirical analysis to determine how household characteristics affect the replacement cycle of refrigerators in Japan. They found that small-sized, elderly, and low-income households are reluctant to replace old appliances and contend that policies targeting such households are necessary.
Toritti’s study focused on the energy consumption stage. He observed that household electricity usage fluctuated throughout the day. On weekdays, it increases when family members wake up in the morning and then decreases after they go out during the day. It rapidly increases after family members return home and decreases after bedtime. However, electricity supply must be prepared to meet peak-hour demands, whereas additional capacity prepared for peak hours is wasted during off-peak hours. Consequently, electric power companies have aimed at equalizing electricity usage throughout the day by setting nighttime electricity charges. This peak and off-peak problem will become increasingly relevant in the use of renewable energy, which is often generated at fixed times in a day. If peak time activities can be shifted to off-peak time, it will be possible to reduce the required generation capacity and promote renewable energy more easily. However, to understand the possibility of such a time shift in household activities, we need to understand the demand-side flexibility. In his paper, Toritti developed an intrinsic flexibility index based on people’s activities during the timing of electricity demand and then analyzed the relationship between the intrinsic flexibility and spot prices in the UK wholesale electricity market.
Tanaka, Wilson, and Managi focused on the link between the energy choice stage at the initial stage and the energy consumption at the final stage. They observed that many developed countries have provided subsidies for installing solar panels in homes. In addition, some countries have adopted feed-in tariff (FIT) systems that purchase electricity generated by solar panels at a fixed price, while simultaneously raising the purchase price of electricity generated by traditional thermal power generation. Owing to such promotional programs, solar panels have rapidly become widespread in the residential sector. However, it remains unclear whether people change their electricity consumption after installing solar panels. Tanaka, Wilson, and Managi addressed this issue and examined whether the FIT system alters electricity consumption among Australian households, based on the matching method. Their empirical results reveal that Australian households increased their electricity purchases from power companies under the FIT system. This suggests that the energy consumption at the final stage is associated with the energy choice at the initial stage.
Morita, Iwata, and Arimura focused on the link between product purchases in the second stage and energy consumption in the last stage. They propose that households can reduce the unit price of obtaining energy services by purchasing energy-efficient products. If households could obtain energy services at cheaper rates, they would use more energy services than before. Therefore, some of the energy conservation benefits gained by improving energy efficiency will be lost. This concept, advocated by Jevons ( 1865 ) is known as the rebound effect and has been extensively investigated in the literature. However, previous studies on rebound effects have only examined how energy consumption has changed after the introduction of the product and have not yet investigated how product use has changed. Given that households use multiple products at home, it may be more appropriate to focus on changes in product use. Morita, Iwata, and Arimura conducted a survey to examine whether Japanese households lowered the room temperature after the installation of a new air conditioner. Their empirical results reveal that energy efficiency improvements counteract the energy-saving behaviors of the temperature setting, resulting in a rebound effect.
Although we report valuable findings for energy conservation policies in the residential sector in this special issue, we do not discuss some important aspects. All households will be affected by energy conservation policies; however, certain types of households will be affected more severely. There are economies of scale in household energy use, and small households consume more energy per capita (Ironmonger et al. 1995 ; Schröder et al. 2015 ). Therefore, compared to ordinary households, small households, such as single-parent households, have a greater energy consumption burden. It is also well known that energy expenditure increases with age (Bardazzi and Pazienza 2017 ; Inoue et al. 2021 ). Although the number of single-person households is expected to increase in Europe and East Asian countries, single-person households without sufficient retirement benefits can fall into energy poverty in the future. Further research is required to find a way to save household energy without aggravating energy poverty problems.
Household energy consumption patterns vary from region to region because they are linked to weather conditions and social customs. People at high latitudes can enjoy long, cool daylight hours in summer, albeit they require a lot more energy to heat their homes during winter. On the other hand, people in low latitudes consume less energy in winter but consume more electricity during the hot summers. People living in rural areas with weak public transportation need to use vehicles for traveling and thus consume more gasoline. On the other hand, people facing the urban heat in remote areas use air conditioners more intensively to sleep comfortably in summer. The differences in weather conditions and lifestyles should also make a difference in the demand for energy services, and thus, the type of energy services that households preferentially reduce will differ from region to region. However, current energy conservation policies in the residential sector are designed without taking into account the difference in energy saving potentials between regions. For example, the same subsidy for solar power installation is offered in areas with good sunshine and areas with poor sunshine. Further research is needed to reflect the difference in energy demand between regions in the design of carbon mitigation policies.
We intended to hold the international conference in March 2020 with financial support from the Institute of Economic Research at Aoyama Gakuin University and to invite the presented papers for this special issue. However, due to the COVID-19 pandemic, we were forced to cancel the conference at the last minute. We would like to express our gratitude to Ms. Kuramochi and Ms. Fujioka from the Institute for her invaluable support with the conference preparation. Although the workshop itself was canceled, we received many papers for this special issue. I am very pleased to have published five articles following a strict peer review at such a difficult time.
Baker P, Blundell R, Micklewright J (1989) Modelling household energy expenditures using micro-data. Econ J 99(397):720–738
Article Google Scholar
Bardazzi R, Pazienza MG (2017) Switch off the light, please! Energy use, aging population and consumption habits. Energy Econ 65:161–171
Espey JA, Espey M (2004) Turning on the lights: a meta-analysis of residential electricity demand elasticities. J Agric Appl Econ 36(1):65–81
IEA. 2020. Key world energy Statistics. https://www.iea.org/reports/key-world-energy-statistics-2020/final-consumption Accessed 17 Oct 2021
Inoue N, Matsumoto S, Mayumi K (2021) Household energy consumption pattern changes in an aging society. The case of Japan between 1989 and 2014 in retrospect. Int J Econ Policy Stud. https://doi.org/10.1007/s42495-021-00069-y
Ironmonger DS, Aitken CK, Erbas B (1995) Economies of scale in energy use in adult-only households. Energy Econ 17(4):301–310
Jevons WS (1865) 1965. The coal question, 3rd edn. Augustus M. Kelley, New York
Google Scholar
Schröder C, Rehdanz K, Narita D, Okubo T (2015) The decline in average family size and its implications for the average benefits of within-household sharing. Oxf Econ Pap 67(3):760–780
Download references
Author information
Authors and affiliations.
College of Economics, Aoyama Gakuin University, Tokyo, Japan
Shigeru Matsumoto
Faculty of Economics, Matsuyama University, Matsuyama, Japan
Kenichi Mizobuchi
Urban Institute, Kyushu University, Fukuoka, Japan
Shunsuke Managi
You can also search for this author in PubMed Google Scholar
Corresponding author
Correspondence to Shigeru Matsumoto .
Additional information
Publisher's note.
Springer Nature remains neutral with regard to jurisdictional claims in published maps and institutional affiliations.
About this article
Matsumoto, S., Mizobuchi, K. & Managi, S. Household energy consumption. Environ Econ Policy Stud 24 , 1–5 (2022). https://doi.org/10.1007/s10018-021-00331-9
Download citation
Published : 29 November 2021
Issue Date : January 2022
DOI : https://doi.org/10.1007/s10018-021-00331-9
Share this article
Anyone you share the following link with will be able to read this content:
Sorry, a shareable link is not currently available for this article.
Provided by the Springer Nature SharedIt content-sharing initiative
- Find a journal
- Publish with us
- Track your research
A Systems View Across Time and Space
- Open access
- Published: 06 July 2021
Determinants of household electricity consumption in Greece: a statistical analysis
- Dimitra Kotsila 1 &
- Persefoni Polychronidou ORCID: orcid.org/0000-0003-1517-011X 2 , 3
Journal of Innovation and Entrepreneurship volume 10 , Article number: 19 ( 2021 ) Cite this article
11k Accesses
22 Citations
Metrics details
Over the last decades, the contemporary way of living, as well as the technology development, has increased the household electricity consumption. The excessive use of electricity has a negative impact on the environment, increasing the carbon footprint and contributing to the climate change. Governments are more and more concerned about the way our societies consume energy and are committed to reduce the greenhouse emissions. As the residential sector contributes significantly to energy use, it is crucial to investigate the socio-economic parameters, dwellings’ characteristics, and climate conditions that determine the electricity consumption in households. The data of this study are collected from 1801 dwellings from all regions of Greece and two statistical models are built. Both of them conclude that the most significant determinants influencing the electricity consumption are the number of occupants, the size of the dwelling, the heating type, the heating and cooling hours, and the weather conditions.
Introduction
In the last decades due to the increased demand and the improved lifestyle, energy demand in the residential sector has increased rapidly causing the policymakers’ concern. The international climate negotiation is an opportunity for decision-makers to promote enhancement of energy efficiency and reduction of greenhouse emissions and set commitments for renewable energy consumption. The recent “2030 Energy Strategy” and the Paris climate conference (COP21) focus on the previous objectives to keep a global temperature rise this century below 2 °C. According to IEA ( 2020a ), based on the most recent data of 2018 in Greece, the CO2 emissions from fuel combustion only come from electricity and heat producers (46.8%), transport (27.4%), industry (8.1%), other energy industries (8.1%), residential (6.5%), commercial and public services (1.6%), and other (1.6%).
In the last two decades, the Greek governments have given a high priority to the environmental protection; thus, they promoted the Renewable Energy Sources (RES). The main aim concerning the RES is to be able to participate in the electricity consumption for at least 40% by the end of 2020. Specifically, under the European Renewable Energy Directive 2009/28/EC (European Parliament and Council, 2009 ) and the Law 3851/2010 (Official Government Gazette, 2018 ), Greece needs to meet the following targets for RES contribution:
20% of the final energy consumption for heating and cooling
40% of the final electricity consumption
10% of the final energy consumption in transportation
According to the most recent provided data by IEA ( 2017 ), Greece has achieved its goal in the sector of heating and cooling (58.90%), but is far from its aim in the other two sectors (22.09% and 1.43%, respectively). However, Greece has made an impressive progress in the electricity sector, thanks to the rapid growth in installed wind and solar parks and the decrease in the total electricity demand.
In 2019, RES and gas are the primary fuels of electricity production in Greece (IEA, 2020b ). In particular, the total electricity production is based on RES (35.2%), gas (35.2%), coal (22.2%), and oil (9.2%), while in 2017 the corresponding percentages were the following: RES (25.1%), gas (31%), coal (34%), and oil (10%).
Policymakers must focus on the distribution of the electricity consumption, in order to detect and improve the most inefficient sectors. In 2018, the distribution of electricity consumption per sector has been the following: commercial and public services (36%), residential (33.9%), industry (25.1%), agriculture/foster (4.6%), and transport (0.6%); it must be noted that this is similar in the last ten years (IEA, 2020c ).
In the residential sector, the final energy consumption is used to heat the space (57%), for lighting and appliances (20%), for heater heating (12%), for cooking (7%), and for space cooling (4%) (Eurostat, 2018 ).
Consumption per capita is an important indicator to observe the tendency of electricity consumption through the years, as it offers a clear view of the electricity that every individual consumes. In Greece, in 2014 the per capita electricity consumption was 5062.61 kWh/capita while in 2010 was 5333.44 kWh/capita and in 2008 was 5805.19 kWh/capita (DataBank, 2020 ). The economic crisis in Greece since 2008–2009 influenced the electricity consumption as in 2013 the average consumption fell to 5029 kWh/capita. Consumption per household is another indicator to monitor the electricity consumption in the residential sector. In 2000, the electricity per household (hh) was 3717 kWh/hh, while in 2010 it was 4023 kWh/hh (World Energy Council, 2016 ).
The increasing trend of electricity consumption is a reality. Since the residential sector is one of the most demanding sectors regarding electricity consumption, it should be studied. Unfortunately, in Greece, the socio-economic parameters that influence the electricity consumption have never been extensively examined. From the previous studies, that are outlined in the “Literature review” section, the electricity consumption is relevant with demographic data, dwelling characteristics and geographical and climate conditions. This study analyzes the aforementioned parameters in Greece and it is based on real data from 1801 customers of a Greece’s electricity provider. The variables and the methodology that are used in the current analysis are in line with the deep literature review that was conducted by Jones et al. ( 2015 ).
This paper is organized as follows: in the “Literature review” section, an overview of the literature review relevant for this study is included. A description of the regression models and the data sources is given in the “Method” section. In the “Results and discussion” section, the main results are summarized. Finally, the conclusions are presented in the “Conclusions” section.
Literature review
On the international stage, residential electricity consumption is a considerably studied subject. Relevant studies are sequenced with the oldest first and the most recent last.
Studies regarding European countries
Halicioglu ( 2007 ) examines how the energy demand in residential sector in Turkey was influenced from the price and income. The income positively influences the electricity demand and accelerates purchases of electrical goods and services. The price of electricity negatively influences the electricity demand, while the urbanization positively influences the electricity demand, as it provides greater access to electricity.
Gram-Hanssen ( 2011 ) points out that the user’s practices influence the energy consumption. He examines 8500 detached houses in Denmark and he finds that the income, the size of the house, and the presence of teenagers (13–19 years old) all have a positive effect on electricity consumption.
Wiesmann et al. ( 2011 ) examine the relationship between the per capita electricity consumption and dwelling characteristics in Portuguese consumers. They conclude that the income, the appliance ownership, and the floor area have a positive influence on per capita electricity consumption. People who live in single-family houses and/or in urban households consume more electricity than those living in a block of flats and/or rural households. People per household, dwellings per building and more heating degree-days negatively influence the consumption per capita, since in colder regions households consume less electricity per capita than those in more moderate climates.
McLoughlin et al. ( 2012 ) examine the influence of dwelling and occupant characteristics on electricity consumption of 3941 Irish dwellings. Dwelling type, number of bedrooms, age of the head of household, and electrical appliances used for water heating and cooking have a positive effect on electricity consumption.
Bedir et al. ( 2013 ) point out that in Netherlands the household size, the dwelling type, and the duration of electrical appliances’ use (such as dryers and washing machines) have significant effect on the electricity consumption. Also, in the Netherlands, Brounen et al. ( 2012 ) analyze data of 300,000 dwellings. The dwelling type affects electricity consumption as the detached and semi-detached houses consume more electricity per capita than row houses or apartments. Houses with children and especially those that have teenagers are found to have a positive effect on per capita electricity consumption. The income has positive influence whereas the number of persons in household has a negative effect on per capita electricity consumption.
Studies regarding non-European countries
Ndiaye and Gabriel ( 2011 ) analyze electricity consumption of 62 dwellings of Oshawa (Ontario, Canada). Number of residents, house status, type of fuel used to heat the pool, type of fuel used in the heating system, type of fuel used in the domestic hot water heater, type of air-conditioning, and number of air changes per hour at 50 Pa are found to have a positive effect on the electricity consumption. On the other hand, the average number of weeks that the family leaves for vacation and the existence or not of an air conditioning system have a negative effect on the electricity.
Sanquist et al. ( 2012 ) base their research on data from the Residential Energy Consumption Survey (RECS) conducted on 2005 in the USA. The air-conditioning, laundry usage, personal computers, climate zone of dwelling, and TV use significantly influence the electricity consumption. Kavousian et al. ( 2013 ) examine the residential electricity consumption of 952 US dwellings in a view of daily maximum and minimum. Daily minimum consumption is influenced by weather, location, dwelling size, and the number of refrigerators when daily maximum consumption is influenced by the use of appliances that consume a lot and the number of residents. In the summer model, the primary factor that influences the electricity consumption is the cooling degrees days.
Tewathia ( 2014 ) conducts a survey in Delhi to find the determinants of electricity consumption. The household income, the number and the usage of electrical appliances, the size of the house, the family size, time spent out of the house, and the higher educational level influence the monthly electricity consumption through all the seasons. The educational level has a negative relationship as the higher educated families tend to consume less electricity. Filippini and Pachauri ( 2004 ) analyze the electricity demand in urban Indian households. The price is inelastic in electricity demand, so the price is not an inhibiting factor in residential electricity consumption. The income, the size, the regions, and the degrees of urbanity have a significant influence into the electricity consumption. Dwellings with more residents and younger households head had the tendency to consume less electricity from those that had less elder people.
Jones et al. ( 2015 ) conduct a broad literature review to investigate the factors that influence or not the domestic electricity consumption. They study 62 factors as potential factors that determine the electricity consumption. In relation to socio-economic factors, the higher household and disposable income, the more occupants and presence of teenagers have a positive effect on electricity consumption. In relation to dwelling factors, the dwelling age, the number of rooms, the number of bedrooms, and the floor area influence the electricity consumption. Regarding appliance factors, the following ones have a positive effect: more appliances, the existence of desktop computer, television, electric oven, refrigerator, dishwasher, tumble dryer, and higher use of washing machines and tumble dryer.
Esmaeilimoakher et al. ( 2016 ) accomplish an introduction to the factors that influence the electricity consumption. Their analysis is based on a survey conducted in nine households of Perth of Western Australia. The main results are that the average annual electricity consumption per person per m 2 floor area (AAEC/P m 2 ) has a negative correlation with the number of occupants and the dwellings size.
Studies regarding Greece
The research in the field of socio-economic determinants that influence electricity consumption in Greece is poor. There are a lot of works focused on macroeconomic factors that determine energy consumption. Only the study of Sardianou ( 2007 ) provides research that includes demographic data and examines electricity conservation behavior.
Donatos and Mergos ( 1991 ) examine the residential electricity demand in Greece during the period 1961–1986. Data are collected from a public database. It is found that the electricity demand is price inelastic and income elastic. The sales of appliances, as well as the heating degrees-days, are found to have an insignificant effect on electricity demand in contrast with the number of consumers that has a significant effect.
Hondroyiannis ( 2004 ) examines the elasticity of price and income in long-run and short-run demand for residential electricity. The examined period is 1986–1999 employing monthly data. In the short-run, the electricity demand is income inelastic and independent of the price, while in the long-run period, all variables, income, price, and weighted average temperature are found to affect electricity demand.
Polemis and Dagoumas ( 2013 ) conduct a similar with Hondroyiannis ( 2004 ) research. They use cointegration techniques and the vector error correction model to observe the long-run and short-run electricity demand. The data that are taken into account are for a longer period, from 1970 to 2011. In the long-run, the electricity demand is price inelastic and income elastic, while in the short-run the relevant elasticities are inelastic.
Sardianou ( 2007 ) investigates the determinants of household energy conservation. The analysis is based on a survey that has been conducted in 586 households of five main Athens’ regions. One of the findings is that people with higher income that own their houses, and have a large family are more willing to conserve energy. Also, the number of rooms, the dwelling’s size, sex, educational level, and marital status are found that do not have a significant influence in energy conservation. However, it is found that the larger electricity expenditures negatively influence the energy conservation behaviors and the older people are more energy-intensive users than the younger ones.
All variables that have been studied in the above papers are indicated in Tables 6 and 7 in the Appendix section. Tables 6 and 7 also present which of these variables have been studied in this paper.
Model specification
The ordinary least squares (OLS) regression is used to estimate the determinants that affect the electricity consumption in Greek households. A variety of studies that examined the determinants of electricity consumption have been conducted using OLS regression (Bedir et al., 2013 ; Brounen et al., 2012 ; Filippini & Pachauri, 2004 ; Gram-Hanssen, 2011 ; Halicioglu, 2007 ; Kavousian et al., 2013 ; McLoughlin et al., 2012 ; Ndiaye & Gabriel, 2011 ; Sanquist et al., 2012 ; Sardianou, 2007 ; Wiesmann et al., 2011 ). Two different models are employed to determine the electricity consumption. More specifically, a simple OLS regression model and a log-linear regression model are used to build the aforementioned models.
Data sources and description
Data are collected from a Greek electricity provider and refer only to residential dwellings. Data for the consumption and square meters area have been provided through the actual bills. The period of actual bills is not the same between bills and differs among dwellings. Thus, in order to calculate the consumption with accuracy, the consumption per day is calculated at first. Afterwards, consumption per month is calculated and only dwellings that have consumption 2017 are selected.
Demographics and behavior data are retrieved from a questionnaire that was provided through the electricity provider’s online platform. The questionnaire was answered from every individual that covers the bill. Only dwellings that already had a monthly consumption for 2017 and have also answered all the questions are selected. The dwellings with invalid values have been excluded from the data, so the final number of dwellings that are included in the analysis is 1801. Table 1 illustrates all available variables, as well as the type and the units of the variables of both models. Table 2 illustrates the summary of statistics of the variables of both models and it shows the levels of ordinal variables and their correspondence to the converted numbers. In addition, the factor type of each variable is indicated.
In the literature review, we have found out that many studies (Donatos & Mergos, 1991 ; Kavousian et al., 2013 ; Ndiaye & Gabriel, 2011 ; Sanquist et al., 2012 ; Wiesmann et al., 2011 ) include in their models the weather factors of heating degrees-days (HDD) and cooling degrees-days (CDD). HDD and CDD is the difference, in degrees, of outside temperature and base (18.3 °C ) temperature. HDD and CDD data for each prefecture and for 2017 have been downloaded from the weather stations of National Observatory of Athens (NOA). Data have been available in the weather website ( www.meteo.gr ) and have been recorded at a daily basis (Petrou, 2018 ). The yearly HDD and CDD have been calculated per prefecture and the weather data are then connected to the consumption data based on prefecture. Units of HDD and CDD are in 1000 °C days (the values of these variables are big numbers and they should be fitted in the model as in Wiesmann et al., 2011 ).
The data preparation, manipulation, visualization, and the regression analysis is conducted using programming language R through RStudio program.
Before conducting a regression analysis, an advanced statistical analysis is conducted to observe any associations or correlation between variables. Thus, pairwise comparisons and a correlation analysis (correlation matrix can be found in Table 8 in the Appendix section) are used. The insights of the analysis show a very high negative correlation between HDD and CDD, approximately −0.809 (see Table 8 ), so HDD is removed from the model. Furthermore, a high correlation between family type and number of occupants is observed, so family type is removed from the model.
In the sample, the final independent variables along with the dummy variables count to thirty three (33). It is essential to determine whether the subset of all independent variables yields to an adequate and appropriate model. Stepwise regression is a method that attempts to find the best regression model, without examining all the possible models (Berenson et al., 2014 ). There are two approaches of stepwise regression, the “forward selection” and the “backward elimination”. Both approaches use the Akaike Information Criterion (AIC) to find the best combination of variables based on balancing the model’s complexity and accuracy. The best model is the one with minimum AIC value. The regression model is built using the forward selection, where an initial model is defined that contains only the constant and each independent variable is retained in the model only if it improves the ability of the model to predict the dependent variable.
Results and discussion
The ols regression method.
The results of the OLS regressions are presented in Table 3 . In general, the results from both models are in agreement with the literature, as it will be indicated in this section. Most of the significant variables are common in both models, but there are variables that influence one model and not the other. Τhe log-linear model (model 2) has better goodness of fit from the linear model, in terms of the R 2 . Both models explain approximately 30% of the variance in electricity consumption. Even if this value does not seem satisfactory for a regression analysis, when comparing to the literature the R 2 is within the range published. Indeed, in Sardianou ( 2007 ), R 2 is approximately 10%; in Wiesmann et al. ( 2011 ), R 2 is approximately 33%; in Bedir et al. ( 2013 ), R 2 is approximately 50%; and in most other papers presented in the literature review, the R 2 value has not been given. One basic reason for this low value is that even though we have studied thirty-three variables (most given by the electricity provider and others found by the authors), more parameters need to be studied.
Square meters area has a significant effect on the average monthly electricity consumption in both models. To be more specific, if the square meters area increases by 1m 2 then an increase of 1.389 kWh/month according to model 1 and an increase on average yearly consumption by 0.3% according to model 2 are expected. These results are in line with previous studies (Bedir et al., 2013 ; Filippini & Pachauri, 2004 ; Gram-Hanssen, 2011 ; Jones et al., 2015 ; Kavousian et al., 2013 ; Tewathia, 2014 ; Wiesmann et al., 2011 ).
Number of occupants is strongly related with the electricity consumption (because of high beta coefficient and p -value < 0.01). In both models, more occupants consume more electricity. Studies of Gram-Hanssen ( 2011 ), Jones et al. ( 2015 ), Kavousian et al. ( 2013 ), and Ndiaye and Gabriel ( 2011 ) reach the same conclusion.
Heating hours, heating type, and the presence of secondary heaters have a significant positive effect on electricity consumption in both models. That is, heating hours influence the electricity consumption regardless the heating type. On the other hand, heating type of local units significantly influences the electricity consumption. Dwellings that use local units for heating the space seem to consume more electricity. The results reveal that houses with heating type of local units use possibly electrical appliances to heat their space which has an impact on their total consumption. The presence of secondary heaters has also a significant positive effect on the electricity consumption. Those results enhance the perspective that the electrical appliances used to heat the space have an impact on electricity consumption. Jones et al. ( 2015 ) mention that there are eight studies that found a positive effect of presence of electric space heating system on electricity consumption.
Cooling hours is also related with the electricity consumption. Both models agree to the positive relationship between cooling hours and electricity consumption, as expected. CDD also is found that has a positive effect on electricity consumption in both models. The presence of air-conditioning to cool the space has a significant effect in model 2 while in model 1 it is not found that influences the electricity consumption. This result is aligned with the literature review presented by Jones et al. ( 2015 ), since they present studies with both results.
According to model 1, the dwelling type has a significant effect on electricity consumption, as the single family houses seem to consume more electricity. This result is aligned with past studies of Bedir et al. ( 2013 ), Brounen et al. ( 2012 ), McLoughlin et al. ( 2012 ), and Wiesmann et al. ( 2011 ).
Both models reveal that the age of inhabitants positively influences the electricity consumption. So, as long as the occupants that cover the bill are elderly, the more electricity is consumed. This result is aligned with and also mentioned that there are eight studies that marked the positive effect of age of head of the household on the electricity consumption.
According to model 2, dwellings with occupants that spent more time in home are found to have a positive significant effect on electricity consumption, as it is expected and it is aligned with Ndiaye and Gabriel ( 2011 ) and Tewathia ( 2014 ), respectively.
Many variables of the collected data are found that have no significant influence on the electricity consumption. Those variables are gender, educational level, house status, marital status, dwelling floor, income, light behavior, and summer holidays.
As mentioned above, most of the variables that are found significant are common in both models, but there are variables that influence one model and not the other. This happens because in model 2 a non-linear relationship exists between the independent and dependent variables.
Two-stage least squares method (2SLS)
It is possible that a two-way causation exists between variables of the above models; thus, there is the need to test for endogeneity. Variables that are tested for endogeneity are those of square meter area, number of occupants, and heating hours. Square meter area is found as significant at the level of 10%; thus, 2SLS is conducted with endogenous variable the square meters area. Number of bedrooms and number of rooms have been inserted in the model as instrumental variables. Tables 4 and 5 illustrate the results of OLS, first stage of 2SLS and second stage of 2SLS for model 1 and model 2, respectively. The coefficients of square meter area and other variables have been fitted and the significant variables are the same with the OLS method.
Conclusions
This study focuses on the investigation of the socio-economic determinants, the dwellings characteristics, and the climatic conditions that influence the household electricity consumption. Two regression models, one linear and one log-linear, are built and the results have been presented in the previous section. The two-stage last squares method (TSLS) is used to explore the two-way causation of squared meter area. Most significant variables are the number of occupants, the size of the dwelling, the heating type, the heating and cooling hours, and the weather conditions. The results of this study are aligned with the ones presented in the literature review.
When acknowledging the conclusions of this analysis, policy makers could use suitable incentives to motivate customers to reduce their electricity consumption. The variables regarding heating and cooling the space are found as significant in both models. A good practice would be for the government to motivate people to renovate their houses in order to maintain the houses’ temperature and as a consequence it could reduce the cooling and heating electricity demand. As it is shown from this study, in Greece, houses use electricity to heat their spaces and in many cases they use secondary heaters. Thus, another measure could be to give a subsidy to urge consumers to change their heating systems to more efficient and cheaper ones, such as natural gas boilers.
Another strategy that policy makers could follow is to incentivize electricity providers for the electricity per hh reduction. The utilities then could provide a discount to those households that achieve an electricity reduction year by year. Thus, both utilities and consumers will win from this strategy. The customers will have the incentive of lower cost and will try to reduce their consumption.
Simultaneously, government and utilities could enhance and promote the usage of new technology electricity appliances, such as smart appliances and appliances with A++ energy label, that consume less electricity. By large campaigns, the consumers could be informed about how they can save if they change their old wasteful appliances.
To conclude, this research shows that the dwelling and household characteristics, as well as the climatic conditions, are essential predictors in models of electricity consumption. Further research on occupants’ behavior and on presence and functions of appliances will enhance the determinants of electricity consumption in housing.
Availability of data and materials
Data of this study were collected from the database of a Greek electricity provider. The research data cannot be shared publicly, because the individual privacy could be compromised. The authors after the approval of the Greek electricity provider could provide the final data set to a third researcher.
Bedir, M., Hasselaar, E., & Itard, L. (2013). Determinants of electricity consumption in Dutch dwellings. Energy and Buildings , 58 , 194–207. https://doi.org/10.1016/j.enbuild.2012.10.016 .
Article Google Scholar
Berenson, M. L., Levine, D. M., & Szabat, K. A. (2014). Basic business statistics, student value edition , (13th ed., ). Pearson.
Brounen, D., Kok, N., & Quigley, J. M. (2012). Residential energy use and conservation: Economics and demographics. Green Building, the Economy, and Public Policy , 56 (5), 931–945.
Google Scholar
DataBank (2020). World development indicators . DataBank Available at: https://data.worldbank.org/indicator/EG.USE.ELEC.KH.PC?locations=GR (Accessed 20 Oct 2020).
Donatos, G. S., & Mergos, G. J. (1991). Residential demand for electricity: The case of Greece. Energy Economics , 13 (1), 41–47. https://doi.org/10.1016/0140-9883(91)90054-4 .
Esmaeilimoakher, P., Urmee, T., Pryor, T., & Baverstock, G. (2016). Identifying the determinants of residential electricity consumption for social housing in Perth, Western Australia. Energy and Buildings , 133 , 403–413. https://doi.org/10.1016/j.enbuild.2016.09.063 .
European Parliament and Council (2009). Directive 2009/28/EC of the European Parliament and of the Council of 23 April 2009 on the promotion of the use of energy from renewable sources and amending and subsequently repealing Directives 2001/77/EC and 2003/30/EC. Official Journal of the European Union , 52 (L140), 16–62.
Eurostat. (2018). Energy consumption in households - statistics explained , available at: http://ec.europa.eu/eurostat/statistics-explained/images/1/16/Energy_consumption_households_final.xlsx (Accessed 26 Apr 2018).
Filippini, M., & Pachauri, S. (2004). Elasticities of electricity demand in urban Indian households. Energy Policy , 32 (3), 429–436. https://doi.org/10.1016/S0301-4215(02)00314-2 .
Gram-Hanssen, K. (2011). Households’ energy use - which is the more important: efficient technologies or user practices?, Energy End-Use Efficiency Issues (EEE) , (pp. 992–999).
Halicioglu, F. (2007). Residential electricity demand dynamics in Turkey. Energy Economics , 29 (2), 199–210. https://doi.org/10.1016/j.eneco.2006.11.007 .
Hondroyiannis, G. (2004). Estimating residential demand for electricity in Greece. Energy Economics , 26 (3), 319–334. https://doi.org/10.1016/j.eneco.2004.04.001 .
International Energy Agency (2017). Energy policies of IEA Countries, Greece 2017 review , available at: https://euagenda.eu/upload/publications/untitled-110952-ea.pdf (accessed 19 October 2020).
International Energy Agency (2020a). CO2 emissions by sector, Greece 1990-2018 , available at: https://www.iea.org/data-and-statistics?country=GREECE&fuel=CO2%20emissions&indicator=CO2BySector (accessed 19 October 2020).
International Energy Agency (2020b). Electricity generation by source, Greece 1990-2019 , available at: https://www.iea.org/data-and-statistics?country=GREECE&fuel=Electricity%20and%20heat&indicator=ElecGenByFuel (Accessed 19 Oct 2020).
International Energy Agency (2020c). Electricity final consumption by sector, Greece 1990-2018 , available at: https://www.iea.org/data-and-statistics?country=GREECE&fuel=Electricity%20and%20heat&indicator=ElecConsBySector (Accessed 19 Oct 2020).
Jones, R. V., Fuertes, A., & Lomas, K. J. (2015). The socio-economic, dwelling and appliance related factors affecting electricity consumption in domestic buildings. Renewable and Sustainable Energy Reviews , 43 , 901–917. https://doi.org/10.1016/j.rser.2014.11.084 .
Kavousian, A., Rajagopal, R., & Fischer, M. (2013). Determinants of residential electricity consumption: Using smart meter data to examine the effect of climate, building characteristics, appliance stock, and occupants’ behavior. Energy , 55 , 184–194. https://doi.org/10.1016/j.energy.2013.03.086 .
McLoughlin, F., Duffy, A., & Conlon, M. (2012). Characterising domestic electricity consumption patterns by dwelling and occupant socio-economic variables: an Irish case study. Energy and Buildings , 48 , 240–248. https://doi.org/10.1016/j.enbuild.2012.01.037 .
Ndiaye, D., & Gabriel, K. (2011). Principal component analysis of the electricity consumption in residential dwellings. Energy and Buildings , 43 (2), 446–453. https://doi.org/10.1016/j.enbuild.2010.10.008 .
Official Government Gazette. (2018). Accelerating the development of renewable energy sources to deal with climate change and other regulations addressing issues under the authority of the Ministry of Environment, Energy and Climate Change, 3851/2010 , 1753–1779.
Petrou A.M. J. (2018). “meteo.gr”, meteo.gr - weather forecasts for Greece , available at: http://www.meteo.gr/ (Accessed 10 Mar 2018).
Polemis, M. L., & Dagoumas, A. S. (2013). The electricity consumption and economic growth nexus: Evidence from Greece. Energy Policy , 62 , 798–808. https://doi.org/10.1016/j.enpol.2013.06.086 .
Sanquist, T. F., Orr, H., Shui, B., & Bittner, A. C. (2012). Lifestyle factors in U.S. residential electricity consumption. Energy Policy , 42 , 354–364. https://doi.org/10.1016/j.enpol.2011.11.092 .
Sardianou, E. (2007). Estimating energy conservation patterns of Greek households. Energy Policy , 35 (7), 3778–3791. https://doi.org/10.1016/j.enpol.2007.01.020 .
Tewathia, N. (2014). Determinants of the household electricity consumption: a case study of Delhi. International Journal of Energy Economics and Policy , 4 (3), 337–348.
Wiesmann, D., Lima Azevedo, I., Ferrão, P., & Fernández, J. E. (2011). Residential electricity consumption in Portugal: findings from top-down and bottom-up models. Energy Policy , 39 (5), 2772–2779. https://doi.org/10.1016/j.enpol.2011.02.047 .
World Energy Council. (2016). Electricity use per household | Electricity Consumption Efficiency| WEC, available at: https://wec-indicators.enerdata.net/xls/household-electricity-use.xls (Accessed 26 Feb 2018).
Download references
Acknowledgements
Not applicable.
The authors declare that they have no funding for their research.
Author information
Authors and affiliations.
Master in Business Administration (MBA), Hellenic Open University, Patras, Greece
Dimitra Kotsila
Department of Economics, International Hellenic University, Serres, Greece
Persefoni Polychronidou
Hellenic Open University, Patras, Greece
You can also search for this author in PubMed Google Scholar
Contributions
The paper was jointly conceived, developed, and written by both authors. The authors read and approved the final manuscript.
Corresponding author
Correspondence to Persefoni Polychronidou .
Ethics declarations
Competing interests.
The authors declare that they have no competing interests.
Additional information
Publisher’s note.
Springer Nature remains neutral with regard to jurisdictional claims in published maps and institutional affiliations.
In the following table, the correlation matrix between types of variables is presented. Due to the varying type of variables, a compass correlation matrix is created as follows:
Continuous/discrete/ordinal pair: Pearson correlation
Continuous/discrete/categorical pair: correlation coefficient or squared root of R 2 coefficient of linear regression of integer/numeric variable over factor/categorical variable. The value lies between 0 and 1.
Categorical pair: Cramer’s V value is computed based on Chi-squared test using. The value lies between 0 and 1.
The cutoff point is set 0.6. So, the variables of Family type and HDD are omitted from the analysis.
Rights and permissions
Open Access This article is licensed under a Creative Commons Attribution 4.0 International License, which permits use, sharing, adaptation, distribution and reproduction in any medium or format, as long as you give appropriate credit to the original author(s) and the source, provide a link to the Creative Commons licence, and indicate if changes were made. The images or other third party material in this article are included in the article's Creative Commons licence, unless indicated otherwise in a credit line to the material. If material is not included in the article's Creative Commons licence and your intended use is not permitted by statutory regulation or exceeds the permitted use, you will need to obtain permission directly from the copyright holder. To view a copy of this licence, visit http://creativecommons.org/licenses/by/4.0/ .
Reprints and permissions
About this article
Cite this article.
Kotsila, D., Polychronidou, P. Determinants of household electricity consumption in Greece: a statistical analysis. J Innov Entrep 10 , 19 (2021). https://doi.org/10.1186/s13731-021-00161-9
Download citation
Received : 27 January 2020
Accepted : 04 April 2021
Published : 06 July 2021
DOI : https://doi.org/10.1186/s13731-021-00161-9
Share this article
Anyone you share the following link with will be able to read this content:
Sorry, a shareable link is not currently available for this article.
Provided by the Springer Nature SharedIt content-sharing initiative
- Electricity
- Consumption
- Determinants
- Socio-economic
- Statistical analysis
- Open access
- Published: 09 September 2020
Electricity load forecasting: a systematic review
- Isaac Kofi Nti ORCID: orcid.org/0000-0001-9257-4295 1 ,
- Moses Teimeh 2 ,
- Owusu Nyarko-Boateng ORCID: orcid.org/0000-0003-0300-2469 3 &
- Adebayo Felix Adekoya ORCID: orcid.org/0000-0002-5029-2393 3
Journal of Electrical Systems and Information Technology volume 7 , Article number: 13 ( 2020 ) Cite this article
29k Accesses
118 Citations
Metrics details
The economic growth of every nation is highly related to its electricity infrastructure, network, and availability since electricity has become the central part of everyday life in this modern world. Hence, the global demand for electricity for residential and commercial purposes has seen an incredible increase. On the other side, electricity prices keep fluctuating over the past years and not mentioning the inadequacy in electricity generation to meet global demand. As a solution to this, numerous studies aimed at estimating future electrical energy demand for residential and commercial purposes to enable electricity generators, distributors, and suppliers to plan effectively ahead and promote energy conservation among the users. Notwithstanding, load forecasting is one of the major problems facing the power industry since the inception of electric power. The current study tried to undertake a systematic and critical review of about seventy-seven (77) relevant previous works reported in academic journals over nine years (2010–2020) in electricity demand forecasting. Specifically, attention was given to the following themes: (i) The forecasting algorithms used and their fitting ability in this field, (ii) the theories and factors affecting electricity consumption and the origin of research work, (iii) the relevant accuracy and error metrics applied in electricity load forecasting, and (iv) the forecasting period. The results revealed that 90% out of the top nine models used in electricity forecasting was artificial intelligence based, with artificial neural network (ANN) representing 28%. In this scope, ANN models were primarily used for short-term electricity forecasting where electrical energy consumption patterns are complicated. Concerning the accuracy metrics used, it was observed that root-mean-square error (RMSE) (38%) was the most used error metric among electricity forecasters, followed by mean absolute percentage error MAPE (35%). The study further revealed that 50% of electricity demand forecasting was based on weather and economic parameters, 8.33% on household lifestyle, 38.33% on historical energy consumption, and 3.33% on stock indices. Finally, we recap the challenges and opportunities for further research in electricity load forecasting locally and globally.
Electricity is the pivot in upholding highly technologically advanced industrialisation in every economy [ 1 , 2 , 3 ]. Almost every activity done in this modern era hinges on electricity. The demand and usage of electric energy increase globally as the years past [ 4 ]; however, the process of generating, transmitting, and distributing electrical energy remains complicated and costly. Hence, effective grid management is an essential role in reducing the cost of energy production and increased in generating the capacity to meet the growing demand in electric energy [ 5 ].
Accordingly, effective grid management involves proper load demand planning, adequate maintenance schedule for generating, transmission and distribution lines, and efficient load distribution through the supply lines. Therefore, an accurate load forecasting will go a long way to maximise the efficiency of the planning process in the power generation industries [ 5 , 6 ]. As a means to improve the accuracy of Electrical Energy Demand (EED) forecasting, several computational and statistical techniques have been applied to enhance forecast models [ 7 ].
EED forecasting techniques can be clustered into three (3), namely correlation, extrapolation, and a combination of both. The Extrapolation techniques (Trend analysis) involve fitting trend curves to primary historical data of electrical energy demand in a way to mirror the growth trend itself [ 7 , 8 ]. Here, the future value of electricity demand is obtained from estimating the trend curve function at the preferred future point. Despite its simplicity, its results are very realistic in some instances [ 8 ].
On the other hand, correlation techniques (End-use and Economic models) involve relating the system load to several economic and demographic factors [ 7 , 8 ]. Thus, the techniques ensure that the analysts capture the association existing between load increase patterns and other measurable factors. However, the disadvantage lies in the forecasting of economic and demographic factors, which is more complicated than the load forecast itself [ 7 , 8 ]. Usually, economic and demographic factors such as population, building permits, heating, employment, ventilation, air conditioning system information, weather data, building structure, and business are used in correlation techniques [ 7 , 8 , 9 ]. Nevertheless, some researchers group EED forecasting models into two, viz. data-driven (artificial intelligence) methods (same as the extrapolation techniques) and engineering methods (same as correlation the techniques) [ 9 ]. All the same, no single method is accepted scientifically superior in all situations.
Also, proper planning and useful applications of electric load forecasting require particular “forecasting intervals,” also referred to as “lead time”. Based on the lead time, load forecasting can be grouped into four (4), namely: very short-term load forecasting (VSTLF), short-term load forecasting (STLF), medium-term load forecasting (MTLF) and long-term load forecasting (LTLF) [ 6 , 7 , 10 ]. The VSTLF is applicable in real-time control, and its predicting period is within minutes to 1 h ahead. The STLF is for making forecasting within 1 h to 7 days or month ahead [ 11 ]. It is usually used for the day-to-day operations of the utility industry, such as scheduling the generation and transmission of electric energy. The MTLF is used for forecasting of fuel purchase, maintenance, utility assessments. Its forecasting period ranges from 1 week to 1 year. While the LTLF is for making forecasting beyond a year to 20 years ahead, it is suitable for forecasting the construction of new generations, strategic planning, and changes in the electric energy supply and delivery system [ 10 ].
Notwithstanding the above-mentioned techniques and approaches available, EED forecasting is seen to be complicated and cannot easily be solved with simple mathematical formulas [ 2 ]. Also, Hong and Fan [ 12 ] pointed out that electric load forecasting has been a primary problem for the electric power industries, since the inception of the electric power. Regardless of the difficulty in electric load forecasting, the optimal and proficient economic set-up of electric power systems has continually occupied a vital position in the electric power industries [ 13 ]. This exercise permits the utility industries to examine the dynamic growth in load demand patterns to facilitate continuity planning for a better and accurate power system expansion. Consequently, inaccurate prediction leads to power shortage, which can lead to “dumsor” and unneeded development in the power system leading to unwanted expenditure [ 7 , 14 ]. Besides, a robust EED forecasting is essential in developing countries having a low rate of electrification to facilitate a way for supporting the active development of the power systems [ 15 ].
Based on the sensitive nature of electricity demand forecasting in the power industries, there is a need for researchers and professionals to identify the challenges and opportunities in this area. Besides, as argued by Moher et al. [ 16 ], systematic reviews are the established reference for generating evidence in any research field for further studies. Our partial search of literature resulted in the following [ 10 , 12 , 17 , 18 , 19 , 20 , 21 ] papers that focused on comprehensive systematic review concerning the methods, models, and several methodologies used in electric load forecasting. Hammad et al. [ 10 ] compared forty-five (45) academic papers on electric load forecasting based on inputs, outputs, time frame, the scale of the project, and value. They revealed that despite the simplicity of regression models, they are mostly useful for long-term load forecasting compared with AI-based models such as ANN, Fuzzy logic, and SVM, which are appropriate for short-term forecasting.
Similarly, Hong and Fan [ 12 ] carried out a tutorial review of probabilistic EED forecasting. The paper focused on EED forecasting methodologies, special techniques, common misunderstandings and evaluation methods. Wang et al. [ 19 ] presented a comprehensive review of factors that affects EED forecasting, such as forecast model, evaluation metric, and input parameters. The paper reported that the commonly used evaluation metrics were the mean absolute error, MAPE, and RMSE. Likewise, Kuster et al. [ 22 ] presented a systematic review of 113 studies in electricity forecasting. The paper examined the timeframe, inputs, outputs, data sample size, scale, error type as criteria for comparing models aimed at identifying which model best suited for a case or scenario.
Also, Zhou et al. [ 17 ] presented a review of electric load classification in the smart-grid environment. The paper focused on the commonly used clustering techniques and well-known evaluation methods for EED forecasting. Another study in [ 21 ] presented a review of short-term EED forecasting based on artificial intelligence. Mele [ 20 ] presented an overview of the primary machine learning techniques used for furcating short-term EED. Gonzalez-Briones et al. [ 18 ] examined the critical machine learning models for EED forecasting using a 1-year dataset of a shoe store. Panda et al. [ 23 ] presented a comprehensive review of LTLF studies examining the various techniques and approaches adopted in LTLF.
The above-discussed works of literature show that two studies [ 20 , 21 ] address a comprehensive review on STLF, [ 23 ] addresses forecasting models based on LTLF. The study in [ 24 ] was entirely dedicated to STLF. Only a fraction (10%) of above systematic review studies included STLF, MTLF and LTLF papers in their review; however, as argued in [ 10 ], the lead time (forecasting interval) is a factor that positively influences the performance of a chosen model for EED forecasting studies. Again, a high percentage of these studies [ 10 , 12 , 17 , 18 , 19 , 20 , 21 , 22 , 24 ] concentrated on the methods (models), input parameter, and timeframe. Nevertheless, Wang et al. [ 19 ] revealed that the primary factors that influence EED forecasting models are property (characteristic) parameters of the building and weather parameters include. Besides, these parameters are territorial dependant and cultural bond. Thus, the weather pattern is not the same world-wide neither do we use the same building architecture and materials globally.
Notwithstanding, a higher percentage of previous systematic review studies overlooked the origin of studies and dataset of EED forecasting paper. Also, only a few studies [ 12 , 17 , 19 ] that examined the evaluation metrics used in EED forecasting models. However, as pointed out in [ 17 ], there is no single validity index that can correctly deal with any dataset and offer better performance always.
Despite all these review studies [ 10 , 12 , 17 , 18 , 19 , 20 , 21 , 22 , 24 ] on electricity load forecasting, a comprehensive systematic review of electricity load forecasting that takes into account all possible factors, such as the forecasting load (commercial, residential and combined), the forecast model (conventional and AI), model evaluation metrics and forecasting type (STLF, MTLF, and LTLF) that influences EED forecast models is still an open gate for research. Hence, to fill in the gap, this study presents an extensive systematic review of state-of-the-art literature based on electrical energy demand forecasting. The current review is classified according to the forecasting load (commercial, residential, and combined), the forecast model (conventional, AI and hybrids), model evaluation metrics, and forecasting type (STLF, MTLF, and LTLF). The Preferred-Reporting Items for Systematic-Review and Meta-Analysis (PRISMA) flow diagram was adopted for this study based on its ability to advance the value and quality of the systematic review as compared with other guidelines [ 16 , 25 , 26 ]. The current study contributes to knowledge as follows:
A comprehensive and detailed assessment of previous state-of-the-art studies on electricity demand forecasting; based on used methods, timeframe, the train and test split of data, error, and accuracy metrics applied to forecast.
We present a concise summary of the useful characteristics of compared techniques in electric load forecasting.
We identified the challenges and opportunities for further studies in electric load forecasting.
The remaining sections of the current paper are structured as follows. “ Methodology ” section presents the methods and materials used in the current study. “ Data collection ” section presents the results and a detailed discussion of the outcomes, and “ Study framework ” section presents the summary of findings and direction for future studies.
Methodology
The current study presents a systematic review of pertinent literature on electrical energy forecasting.
Data collection
A total of eighty-one (81) state-of-the-art research works published in journals, conferences, and magazines, and student’s thesis relevant to the scope of the current study were downloaded from the internet, thus using keywords and terms which included AI, Electricity Prediction (EP), Energy Forecasting (EF), Machine Learning (ML), and combination of AI and EP, AI and EF, ML and EP, ML and EF. Each downloaded literature was then carefully studied and categorised into the two methods of electrical load forecasting data-driven (artificial intelligence) methods and engineering methods.
Study framework
According to Moher et al. [ 16 ], the quality of every systematic review is based on building protocol, which outlines the justification, hypothesis, and planned methods of the investigation. However, only a few systematic review study reports of their framework. A detailed, well-described structure for systematic reviews facilitates the understanding and evaluation of the methods adopted. Hence, the PRISMA model [ 26 ] was adopted in this study (Fig. 1 ). As shown in Fig. 1 , the PRISMA presents the flow of information from one stage to another in a systematic review of the literature and gives the total number of the research identified, excluded, and included and the reasons for inclusion and exclusions.
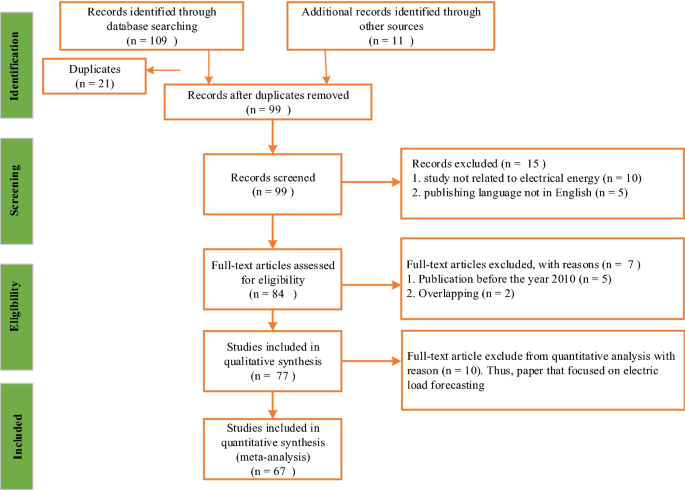
Source : Moher et al. [ 26 ]
The adopted PRISMA flow diagram.
The PRISMA flow diagram involved five (5) phases, as shown in Fig. 1 . Phase 1 consists of outlining the review scope, developing questions, and inclusion or exclusion. Phase 2 searches the literature with keywords to identify potential studies. Phase 3 includes determining the addition of a paper by screening its abstracts if it meets inclusion criteria. While phase 4 includes characterisation of paper for mapping by keywords. This review aimed to document an overview of research in the field of electric load forecasting to make way for future studies. As a result, a fifth (5) step offers an in-depth quantitative synthesis (meta-analysis) of studies included in the review.
Our search of literature retrieved one hundred and one (109) papers from online journals and eleven (11) from under sources, making one hundred and twelve (120) records in all (see Fig. 1 ). Of the 120 records, 21 were duplicates, hence, removed leaving ninety-one (99) record shortlisted for the screening stage. At the screening stage, fifteen (15) records were removed; thus, studies that were not related to electrical energy, and those that the primary publication language was not in English. Leaving eighty-four (84) records, of the 84 records, we further remove five (5) more records that were published before the year 2010, two (2) record omitted due to overlapping, this reduced eligible papers for analysis to seventy-seven (77). The 77 papers were used for the qualitative analysis. Ten (10) records that presented a review of electric load forecasting were also removed, and the remaining (67) were used for the quantitative analysis, as shown in Fig. 1 .
Results and discussion
Electrical energy consumption can be classified as residential (domestic), commercial (non-residential), or industrial. Residential or domestic refers to the home or a dwelling where people globally live from day-to-day. At the same time, commercial consumers are the business and industries that require massive supply than residential users for their businesses [ 27 ]. Selected literature was on electric load forecasting was classified into two main categories AI methods and engineering methods. However, each category was further grouped into residential and commercial or combined (residential and commercial), and the outcomes are presented. A total of seventy-seven (77) papers were eligible for the qualitative analysis, while sixty-seven (67) were included in the quantitative analysis, as already discussed above in this study.
AI methods used in electrical energy demand forecasting
This section presents the studies that were based on AI techniques.
Combined (commercial and residential)
Most works on EED forecasting sought to forecast the total load (residential and commercial) demand on the supplying authority. This section presents the selected studies that fell in this category of electric load forecasting.
Hybrid models
In a way to harness the strength in different machine learning techniques, some researchers sought to hybrid two or more ML techniques to improve the forecasting accuracy of their models.
A short-term (next-day) EED forecasting model based on the historical meteorological parameter to forecast the future load on the Greek Electric Network Grid using Support Vector Machine (SVM), ensemble XGBoost, Random Forest (RF), k-Nearest Neighbours (KNN), Neural Networks (NN) and Decision Trees (DT) was proposed by [ 28 ]. The mean absolute percentage error (MAPE) was used as a performance metric for comparison among selected models by the author. The study achieved a reduction in prediction error or + 4.74% compared with the Operator of the Electricity Market in Greece predictions. In other studies [ 29 ], long-term (10 years) EED forecasting using NN and Autoregressive Integrated Moving Average (ARIMA) was proposed to forecast the EED of Kuwait. Weather temperature and humidity, average salary, gross domestic, oil price, population, residence, passengers, currency earning rate, and economic factors like (total import and export in USD) were used as independent variables. The study concluded that NN outperformed ARIMA and weather parameters were found to be more significant than average salary, gross domestic and oil price.
A Particle Swarm Optimisation (PSO) and Differential Evolution (DE) for forecasting the Andhra Pradesh Grid using weather parameters were presented [ 6 ]. The reported concluded that better prediction accuracy was achieved with PSO and DE than the conventional time series forecast model. In another study, a Curve Fitting Algorithm (CFA) was proposed for forecasting electricity power demand for an hour/day/week/month [ 30 ]. Their study shows that future electricity demand can be effectively forecasted based on past demand. In a process to increase forecasting accuracy, historical electricity data combined with Twitter data was used as an input variable to hybrid ANN and SVM forecast model to forecast the electricity consumption in Dutch [ 14 ]. The authors compared the performance of ANN to SVM and concluded that the ANN outperformed the SVM. On the other side, the SVM performance in accuracy increases in long-term forecasting. The authors again admit that inclusion of weather data as input could not increase model performance. Similarly, in Ghana, an attempt to predict the 30-day ahead EED demand of the Bono region using a hybrid ML (MLP, SVM, and DT) based on historical weather and electricity demand was made [ 7 ]. The authors achieved 95% prediction accuracy; however, it affirms that the inclusion of household lifestyle as an input variable will improve prediction accuracy.
The hybrid of ML (SVM and RF) and time-series models Generalized Linear Model (GLM) and ARIMA forecast model was proposed for predict electricity consumption in South Africa based on the historical electricity price, load demand, and weather parameter [ 31 ]. The outcome of the study showed that the ARIMA-GLM combination performs better for long-term forecasting. Similarly, a combination of quantum search with SVM (quantum computing and the chaotic mechanics) for forecasting yearly EED in Taiwan was presented [ 32 ]. The empirical analysis revealed that the proposed model exhibits considerably enhanced forecasting performance than other SVM-based forecasting models. A hybrid of mode decomposition (EMD), PSO, and SVM model was present for forecasting short-term EED demand of the Australian electricity market [ 33 ]. Sulandari et al. [ 34 ] proposed a hybrid of ANN and Fuzzy algorithm and a recurrent formula (LRF) to predict electricity demand in Indonesian. The study results showed that the hybrid model performed well with low values of RMSE. Likewise, in [ 35 ], a hybrid of clustering technique (K-means) and ARIMA forecasting model was presented to forecast university buildings electricity demand. Paper revealed that the hybrid model outperformed the ARIMA model alone as a forecast model.
An artificial neural network (ANN)-based forecast model for short-term forecasting of Chhattisgarh State electricity demand was proposed [ 36 ], using historical weather data as input variables. The results conclude that ANN can efficiently forecast electricity demand. Likewise, an ANN model was applied to forecast the short-term electricity demand of the Iraqi National Grid [ 37 ]. The authors achieved high accuracy and a reasonable error margin. In a similar study, a DT algorithm forecast model was proposed for forecasting future EED on the Yola/Jimeta power transmission company using weather parameters. Again, a short to medium term EED forecasting using deep machine learning (ML) algorithm long short-term memory (LSTM)-based neural network enhanced with genetic algorithm (GA) for feature selection was proposed [ 9 ], to forecast France metropolitan’s electricity consumption. The mean absolute error (MAE) and root-mean-square error (RMSE) were used as the performance metrics, and the weather parameter was used in the independent variable. Their results affirm that weather parament is very useful in forecasting future electricity demand.
An enhanced Convolutional Neural Network (CNN) and enhanced SVM-based forecast model was presented for forecasting electricity price and load forecasting using [ 38 ]. Despite the enhancement made by authors, they recommended additional enhancement of classifiers to improve prediction. In a similar study, an ANN model to forecast consumer demand in North Cyprus was proposed [ 39 ]. Their results affirm the ability for ANN effective automatic modelling of electricity; however, the study concluded this could be achieved when the training and testing datasets are meaningful. A forecast model using a deep belief network using historical EED of Macedonian (2008–2014) was proposed to forecast a short-term (1 day) EED. The outcome of the forecast shows a reduction in MAPE by 8.6% by the proposed model compared with traditional techniques [ 2 ]. In Young et al. [ 40 ], an ANN with Bayesian regularisation algorithm-based model for short-term load forecasting of commercial building electricity usage was carried out. An ANN and hybrid methods to forecast electricity consumption of Turkey were proposed Aydogdu et al. [ 41 ]. The proposed model gave an average absolute prediction error of 2.25%.
Khwaja et al. [ 11 ] presented an ensemble ANN predictive model to enhance short-term electricity load forecasting. Different from existing studies, the authors combined both bagging and boosting techniques to train bagged-boosted ANNs. The study results showed that the proposed ensemble technique offered a reduction of both variance and bias compared to a bagged ANN, single ANN, and boosted ANN. Also, Ahmad et al. [ 42 ] combined Extreme Learning Machine and an enhanced Support Vector Machine to forecast short-term electricity demand. The study outcome showed that the proposed hybrid model outperformed other state-of-the-art predictive models in terms of performance and accuracy. Atef and Eltawil [ 43 ] proposed a deep-stacked LSTM forecasting model to forecast electricity demand. The paper reported that bidirectional (Bi-LSTM) networks outperformed unidirectional (Uni-LSTM) in terms of forecasting accuracy. Also, a generalised regression Neural Network (GRNN) predictive model was proposed in [ 44 ] to predict short-term electricity demand. The study results showed that a GRNN of 30 neurons offered better prediction accuracy in terms of MAPE and MAE than a GRNN of 10 neurons.
Fuzzy logic models
A Fuzzy Logic (FL)-based forecasting model for the next-day electricity demand in Albania was presented [ 45 ]. The time, the historical and forecasting value of the temperature and the previous day load (L) served as the independent variables for the forecast of the next-day consumption. The outcome of the study yielded accurate forecasting by the FL model. Motepe et al. [ 46 ] proposed an adaptive neuro-fuzzy inference system (ANFIS) model for forecasting South African electricity demand. The author concluded that adding temperature as an input parameter to the proposed model did not enhance forecast accuracy, as typically expected.
A new economy (stock indices) reflecting the STLF model for electricity demand forecasting was proposed [ 47 ]. The authors attempted to forecast the future demand for electricity based on the Taiwan Stock Exchange Capitalization Weighted Stock Index (TAIEX) data. The study revealed a significant relationship between stock market function and energy demand, which was helpful to financial analysis wanting to do reverse engineering.
Residential load forecasting
A survey in 2019 shows that EED forecasting, especially short-term load forecasting for individual (residential) electricity customers, plays an increasingly essential role in the future grid planning and operation Kong et al. [ 51 ]. Similarly, the outcome of Leahy and Lyons [ 48 ] affirms that water heating styled used by a household is even more essential than the number of electrical appliances when explaining domestic electrical energy usage. Furthermore, a study in Portugal shows that residential electricity consumption since 1990 has been increasing more rapidly than the Gross Domestic Product (GDP) per capita [ 49 ]. Therefore, studies that focused on forecasting residential EED is of necessity. However, the results revealed that eight (8) out of the sixty-seven (67) representing (11.94%) reviewed works focused on residential electricity forecasting, and a section of these studies is presented.
An hourly prediction of residential energy consumption using RF and SVM was proposed by Hedén [ 50 ], using 187 households in Austin. Error metrics are mean bias (MB), coefficient of variance (CV), and MAPE. The study achieved better forecasting accuracy compared with traditional time series models. Again, an LTSM for short-term residential load forecasting based on residential meter reading [ 51 ] and resident behaviour learning [ 52 ] was studied. The outcomes of these works showed the effectiveness of the LTSM in electricity demand forecasting.
In the same way, linear regression, RF, and SVM predictive model (data-driven) for estimation of city-scale energy use in buildings were proposed by Kontokosta and Tull [ 53 ]. The outcome of the study revealed that adequate electricity consumption in a building could be predicted using actual data from a moderately small subset of buildings. Likewise, unsupervised ML algorithms such as Self-Organising Maps (SOM), k-means, and k-medoid were used to cluster residential electricity based on their trend of electricity use within the day [ 54 ]. The study found that households and how they use electricity in the home can be categorised based on specific customer characteristics. Mcloughlin et al. [ 55 ] examined residential electricity consumption patterns in Irish based on occupant socio-economic and dwelling variables. The variable examined includes the number of bedrooms, dwelling type, age of household head, social class, household composition, and water heating.
The study outcome showed a positive association between maximum demand periods and several household appliances, especially dishwashers, electric cookers tumble dryers, with electric cook topping. The study further found that the time of use of electrical appliances was dependent on occupant characteristics, and younger occupants of a household tend to use more electricity than the older. Alike, an ARIMA, NN, and exponential smoothening forecast model were proposed for forecasting household electricity demand [ 56 ]. The authors concluded that forecasting accuracy varies considerably depending on the choice of forecasting techniques/tactic and configuration/selection of input parameters. Again, a neural network model for predicting residential building energy consumption was proposed by Biswas et al. [ 57 ]. The outcome of the study showed that models based on OWO-Newton algorithms and Levenberg–Marquardt outperformed previous literature.
Engineering and traditional electrical energy demand forecasting
This section presents the electrical energy demand forecasting study based on the traditional time-series and engineering models.
A predictive model for the prediction of medium-term (1-year) electricity consumption of general households based on the lifestyle of the household using Lasso and Group Lasso was proposed [ 58 ]. Their results showed that household lifestyle such as family composition, age, and house-type is good predictors of electricity consumption in a home. Similarly, in Nigeria, an attempt was made to estimate the electricity demand of residential users to support energy transitions using the engineering approach, such as calculating the total power consumed in a household based on the power rating of appliance and their duration of use [ 59 ]. The study concludes that the proposed system can serve policymaking in Nigeria to improve the financial sustainability of the energy supply structure. An extended Autoregressive Distributed Lag (ARDL) model was proposed to estimate residential electricity consumption per capita demand function, which depends on the GDP per capita in Algeria [ 60 ]. The study concluded that promoting financial growth among citizens of Algeria would reduce electricity consumption, since wealthier people (higher income earners) mighty use of more efficient appliances.
In Bogomolov et al. [ 61 ], authors used general public dynamics derived data from cellular network and energy consumption dataset to predict the next-day energy demand. The study could serve a model to enhance the energy meter to promote energy conservation. Likewise, the historical data of on–off times of residential appliances were used to predict the next-day electricity demand using Bayesian inference [ 62 ]. The study concluded that historical electricity consumption data only is not adequate for a decent eminence hourly forecast.
Also, an ARIMA and Holt-Winter model was proposed for forecasting the national electricity consumption of Pakistan from 1980 to 2011 [ 3 ]. The study revealed that the demand in household energy consumption would higher as compare with all other sectors. Jain et al. [ 63 ] proposed an ARIMA forecaster for forecasting electricity consumption. The proposed model achieved a MAPE of 6.63%. The authors concluded that the ARIMA model has the potential of computing in EED forecasting with other forecast techniques. Integration of three (3) forecasting model, long-range energy alternative planning (LEAP), ARIMA, and Holt-Winter, was proposed for forecasting long-term energy demand in Pakistan [ 64 ]. The study would be valid for energy supplies for accurate estimation of users’ demand in the future. The combination of Extreme Learning Machine and Multiple Regression for forecasting China’s electricity demand was proposed [ 65 ]. A quantile regression (QR) model for long-term electricity demand forecasting in South Africa within 2012 and 2015 was presented by Mokilane et al. [ 66 ]. The model was helpful to power distribution industries in the country.
An attempt was made to forecast the electricity consumption of Ghana by 2030 using ARIMA-based model. The study outcome projected that Ghana’s electricity consumption would grow from 8.5210 billion kWh in 2012 to 9.5597 billion kWh in 2030 [ 67 ]. However, in 2017, a report by the energy commission revealed that electricity consumption was 14,247 GWh [ 68 ]. A generalised additive model was adopted for forecasting medium-term electric energy demand in a South African power supply system [ 69 ]. The outcome of the study revealed a useful application of the proposed model in the power generation and distribution industries in the country. An investigation between the association of causal nexus and (environmental pollution, energy use, GDP per capita, and urbanisation) in an attempt to forecast Nigeria’s energy use by 2030 was carried out using the ARIMA and ETS models [ 70 ]. The study outcome showed better forecasting accuracy by both models, and a high rise in energy demand was observed.
Multiple regression analysis approaches for forecasting the yearly electricity demand in commercial sectors and electricity access rates in rural and urban households in some selected West African countries, which included Ghana, were carried out by Adeoye and Spataru [ 71 ]. The study showed that there is a very high variation in hourly electricity demand in the dry seasons. Their results affirm Nti et al. [ 7 ] report that the demand pattern of electricity in Ghana is highly dependent on the month of the year. In a way, one can say there is a partial agreement in these two studies. A time-series regression model for forecasting South African’s peak load demand was presented [ 72 ]. Experimental fallouts indicated that when the temperature is included as an input parameter, improvement in accuracy by the forecast model was realised. ARIMA-based predictive model for predicting both sectoral and total electrical energy consumption of Turkey for the next 15 years was proposed [ 73 ]. The study points out that the demand for electrical energy in agriculture sectors, transport, public service, residential, and commercial will keep increasing.
Similarly, partially linear additive quantile regression models for forecasting short-term electricity demand during the peak-demand periods (i.e., from 6:00 to 8:00 pm) were carried out in South African [ 74 ]. The authors found out that the use of the proposed system in power utility industries for the planning, scheduling, and dispatching of electricity activities will result in a minimal cost principally during the peak-period hours. Caro et al. [ 75 ] predicted the Spanish electricity demand using the ARIMA model. The study achieved an improvement in the short-term predictions of electricity demand with less computational time. A short-term electricity load forecasting model based on dynamic mode decomposition was proposed in [ 76 ], the proposed model showed better stability and accuracy compared with other predictive models.
Quantitative analysis of findings
The descriptive statistics of the study outcome is presented under this section with tables and charts.
Algorithms used for forecast models
As part of the aim of the review, we sought to find out the most used algorithms in electricity forecasting models. The most top nine (9) used algorithms found in the sixty-seven (67) article are presented (Fig. 2 ); this includes only algorithms that were used in more than a single paper. The study outcome revealed that 90% out of the top nine algorithms were AI-based, with ANN representing 28% of AI models used in electricity forecasting. Besides, the ANN models were primarily used for STLF where electrical energy consumption patterns are more intricate than LTLF. The traditional AFRIMA recorded 17.5% due to its efficiency in LTLF, where load fluctuations and periodicity are less critical.
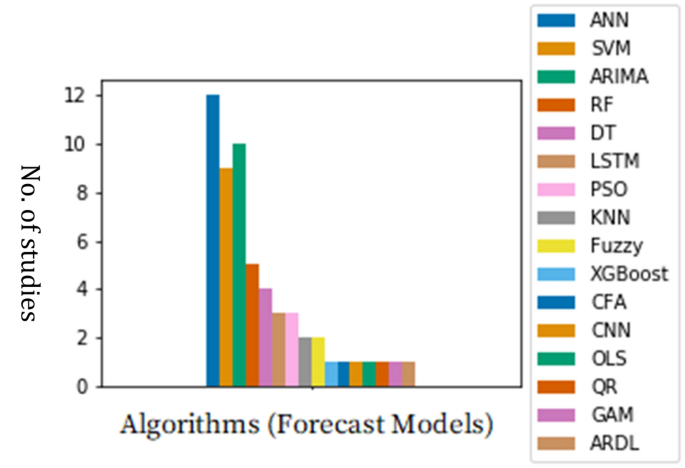
Top nine (9) most used algorithms for electricity forecasting
Additionally, a high percentage of regression models is used for LTLF prediction. The study outcome shows how AI is applied in various sectors of the economy to improve efficiency and profitability. Also, we observed that the SVM, PSO and Fuzzy are gaining more popularity in recent studies, a sign of increasing attention of researchers on these algorithms for EED forecasting.
Study origin
Table 1 shows the studies and their origin (countries). The origin of surveyed studies was examined, in order to find the linkage between the power crisis in the continent and studies on electrical energy demand forecasting. Concerning geographical coverage, it was found that a high number of studies (31.34%) were carried out in Europe, 17.91% in Africa and 19.40% in Asia. Making Africa the third-highest, however, interestingly, most of the studies in Africa were carried in South Africa (five representing 41.67%), three (3) representing 25% in Ghana, with the rest from Nigeria and other African countries. The energy crisis that hit Europe in 2008, reported by [ 77 ], can be attributed to the numerous studies in electricity forecast, as shown in Table 1 . It was further observed that 2 out of 3 studies in Ghana were based on national or regional electricity demand forecasting (one long-term, one short-term (1 month)). While the third study aimed at identifying the relationship between electricity demand and economic growth. Thus, it suggests that the limited number of studies in electricity demand forecasting by both academicians and professionals in this field might have partially contributed to the power shortages facing the nation, which in 2015 resulted in “Domsur”.
Used evaluation metrics
The performance of every forecasting model is examined based on the difference in error between the actual value \(\left( y \right)\) and the predicted value ( \(\hat{y}\) ). Several of these metrics were identified in the literature. However, we examined the most used in electrical energy demand forecasting to enable new beginners in the field of electricity demand forecasting to have a firm grip on which to apply in their study. Figure 3 shows the top four (4) most used error metrics in two or more studies. The results revealed that root-mean-square error RMSE (38%) was the most used error metric among EED forecasters, followed by mean absolute percentage error MAPE (35%). Due to the effectiveness in measure predictive model performance and their usefulness for short-term prediction, it was also observed that the MAPE is a standard primary metric as it is easy to both calculate and understand. The results affirm the findings in [ 12 , 19 , 22 ] that MAE, MAPE, and RMSE are the most commonly used evaluation metrics in EED forecasting model.
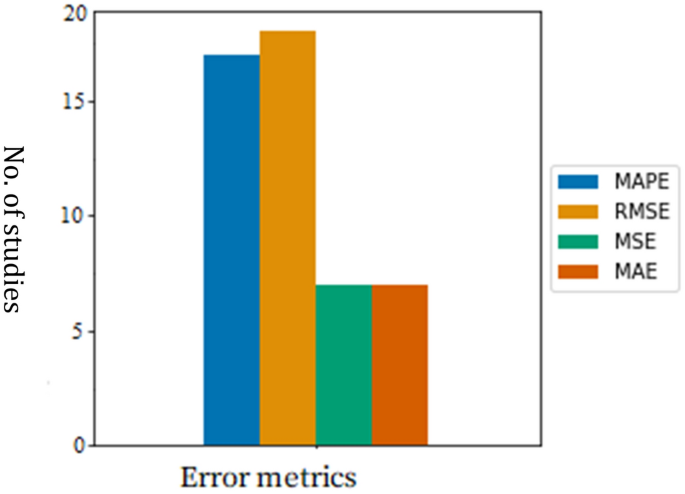
Most used error metrics in electricity load forecasting
Forecast type
Based on short-term load forecasting (STLF), medium-term load forecasting (MTLF), and long-term load forecasting (LTLF), it was observed that 80%, 15%, and 5% of the electrical energy demand forecasting were STLF, MTLF, and LTLF, respectively. The massive number of studies is based on STLF as compared with LTLF and MTLF call for further studies into the challenges associated with LTLF and MTLF electricity load forecasting. Again, 80% STLF forecasting affirms the 38% use of RMSE error metric, since it is for STLF forecasting. The results affirm the report in [ 22 ] that 43.6% of electricity forecasting are short-term prediction.
Model input parameters
The efficiency of every predictive model is believed to be partially dependent on the independent (input) variable [ 7 ]. At this level, the focus was to examine the different input variables used for electricity load forecasting. The type of independent variables (input features) used by electricity load forecasters was also examined. The current study observed that sixty (60) out of the sixty-seven (67) papers made known the input parameter to the proposed model. Table 2 presents the type of input variables and the percentage of studies that utilised it. It was observed that a high percentage (50%) of electricity demand forecasting was based on weather parameters; next was the historical electricity consumption pattern. The outcome exposed that little attention is given to household lifestyle in electricity demand forecasting. However, Nishida et al. [ 58 ] argue that residential (domestic) energy consumption differs depending on the lifestyle of the family. Family lifestyle, according to [ 56 ], cannot be undermined in electrical load forecasting. According to these studies, these factors include the life stage family composition, house type, age, home appliances possessed and their usage, family income, cultural background, social life, and lifestyle habits, which include how long to stay at home and how to spend holidays. The observations open the opportunity for further studies on the association between EED and household lifestyle.
Conclusions
The current study sought to reviewed state-of-the-art literature on electricity load forecasting to identify the challenges and opportunities for future studies. The outcome of the study revealed that electricity load forecasting is seen to be complicated for both engineers and academician and is still an ongoing area of research. The key findings are summarised as follows.
Several studies (90%) have applied AI in electrical energy demand forecasting as compared with traditional engineering and statistical method (10%) to address energy prediction problems; however, there are not enough studies benchmarking the performance of these methods.
There are few studies on EED forecasting in Africa countries (12 out of 67). Though the continent has progressive achievement in the creation of Regional Power Pools (PPP) over the last two decades, the continent still suffers from a lousy power network in most of its countries, leaving millions of people in Africa without electricity.
Temperature and rainfall as an input parameter to the EED forecasting model are seen to have a divergent view. At the same time, some sections of research recorded an improvement in accuracy and reported no improvement in accuracy when introduced and input. However, the current study attributes this to the difference in automorphic temperature globally and the different economic status among countries. An additional investigation will bring more clarity to the literature.
This study revealed that EED forecasting in the residential sector had seen little attention. On the other hand, Guo et al. [ 78 ] argue that the basic unit of electricity consumption is home.
It was observed that there had been a global increase in residential electricity demand, this according to the report in [ 49 ] can be attributed to the growing rate of buying electrical equipment and appliances of low quality due to higher living standards. However, a further probe into Soares et al. [ 49 ] assertion will bring clarity to literature because of the discrepancy in opinions in literature.
Lastly, the study revealed that there is a limited number of studies on load forecasting studies in Ghana. We, therefore, recommend rigorous researchers in this field in the country to enhance the economic growth of the country.
Our future study will focus on identifying the relationship between household lifestyle factors and electricity consumption in Ghana and predict load consumption based on identified factors since it is an area that has seen little or no attention in Ghana.
Availability of data and materials
All data generated or analysed during this study are included in this published article.
Abbreviations
- Electrical energy demand
- Artificial intelligence
Artificial neural network
Root-mean-square error
Mean absolute percentage error
Very short-term load forecasting
Short-term load forecasting
Medium-term load forecasting
Long-term load forecasting
Preferred-reporting items for systematic-review and meta-analysis
Electricity prediction
Energy forecasting
- Machine learning
Support vector machine
Random forest
k-nearest neighbours
Neural networks
Decision trees
Autoregressive integrated moving average
Particle swarm optimisation
Differential evolution
Curve fitting algorithm
Generalised linear model
Long short-term memory
Genetic algorithm
Mean absolute error
Convolutional neural network
Fuzzy logic
Adaptive neuro-fuzzy inference system
Generalised regression neural network
Taiwan stock exchange capitalisation-weighted stock index
Gross domestic product
Coefficient of variance
Self-organising maps
Autoregressive distributed lag
Long-range energy alternative planning
Quantile regression
Aung SS (2015) Electric power is the main driving force for industrialization. http://www.globalnewlightofmyanmar.com/electric-power-is-the-main-driving-force-for-industrialization/ . Accessed 2 Apr 2018
Dedinec A, Filiposka S, Dedinec A, Kocarev L (2016) Deep belief network based electricity load forecasting: an analysis of Macedonian case. Energy 115:1688–1700. https://doi.org/10.1016/j.energy.2016.07.090
Article Google Scholar
Hussain A, Rahman M, Memon JA (2016) Forecasting electricity consumption in Pakistan: the way forward. Energy Policy 90:73–80. https://doi.org/10.1016/j.enpol.2015.11.028
Jevgenijs S, Joeri deW, Kochnakyan A, Vivien F (2017) Forecasting electricity demand: an aid for practitioners. http://www.worldbank.org/energy/livewire . Accessed 15 Jun 2019
Zaman MU, Islam A, Sultana N (2018) Short term load forecasting based on internet of things (IoT). BRAC University, Dhaka
Google Scholar
Kumar CHJ, Veerakumari M (2012) Load forecasting of Andhra Pradesh grid using PSO, DE algorithms. Int J Adv Res Comput Eng Technol 1:179–184
Nti IK, Asafo-Adjei S, Agyemang M (2019) Predicting monthly electricity demand using soft-computing technique. Int Res J Eng Technol 06:1967–1973
Eeeguide.com (2014) Forecasting methodology. http://www.eeeguide.com/forecasting-methodology/ . Accessed 4 Jan 2019
Bouktif S, Fiaz A, Ouni A, Serhani MA (2018) Optimal deep learning LSTM model for electric load forecasting using feature selection and genetic algorithm: comparison with machine learning approaches †. Energies (MDPI) 11:1–20. https://doi.org/10.3390/en11071636
Hammad MA, Jereb B, Rosi B, Dragan D (2020) Methods and models for electric load forecasting: a comprehensive review. Logist Sustain Transp 11:51–76. https://doi.org/10.2478/jlst-2020-0004
Khwaja AS, Anpalagan A, Naeem M, Venkatesh B (2020) Joint bagged-boosted artificial neural networks: using ensemble machine learning to improve short-term electricity load forecasting. Electr Power Syst Res 179:106080. https://doi.org/10.1016/j.epsr.2019.106080
Hong T, Fan S (2016) Probabilistic electric load forecasting: a tutorial review. Int J Forecast 32:914–938. https://doi.org/10.1016/j.ijforecast.2015.11.011
Selvaraj KR, Sundararaj S, Ravi T (2013) Artificial neutral network based load forecasting and economic dispatch with particle swarm optimization. Int J Sci Eng Res 4:139–145
Stavast P (2014) Prediction of energy consumption using historical data and twitter. The University of Groningen, Groningen
Ouedraogo NS (2017) Modeling sustainable long-term electricity supply-demand in Africa. Appl Energy 190:1047–1067. https://doi.org/10.1016/j.apenergy.2016.12.162
Moher D, Shamseer L, Clarke M, Ghersi D, Liberati A, Petticrew M, Shekelle P, Stewart LA, Group P-P (2015) Preferred reporting items for systematic review and and explanation meta-analysis protocols (PRISMA-P) 2015: elaboration. Syst Rev 4:1–25
Zhou K, Yang S, Shen C (2013) A review of electric load classification in smart grid environment. Renew Sustain Energy Rev 24:103–110. https://doi.org/10.1016/j.rser.2013.03.023
Gonzalez-Briones A, Hernandez G, Corchado JM, Omatu S, Mohamad MS (2019) Machine learning models for electricity consumption forecasting: a review. In: 2019 2nd international conference on computer applications and information security, IEEE, pp 1–6. https://doi.org/10.1109/cais.2019.8769508
Wang Z, Li J, Zhu S, Zhao J, Deng S, Zhong S, Yin H, Li H, Qi Y, Gan Z (2019) A review of load forecasting of the distributed energy system. IOP Conf Ser Earth Environ Sci 237:042019. https://doi.org/10.1088/1755-1315/237/4/042019
Mele E (2019) A review of machine learning algorithms used for load forecasting at microgrid level. In: Sinteza 2019-International scientific conference on information technology and data related research, Singidunum University, pp 452–458. https://doi.org/10.15308/sinteza-2019-452-458
Shah RB (2019) A technological literature review on load forecasting in power system using artificial intelligence. Paripex-Indian J Res 8:14–15
Kuster C, Rezgui Y, Mourshed M (2017) Electrical load forecasting models: a critical systematic review. Sustain Cities Soc 35:257–270. https://doi.org/10.1016/j.scs.2017.08.009
Panda SK, Mohanty SN, Jagadev AK (2017) Long term electrical load forecasting: an empirical study across techniques and domains. Indian J Sci Technol 10:1–16
Jacob M, Neves C, Vukadinović-Greetham D (2020) Short term load forecasting. Forecast Assess Risk Individ Electr Peaks Math Planet Earth. https://doi.org/10.1007/978-3-030-28669-9_2
Article MATH Google Scholar
Appiahene P, Ussiph N, Missah YM (2018) Information technology impact on productivity. In: International conference on applied science and technology, Kumasi Technical University, Kumasi, Ghana, pp 122–133
Moher D, Liberati A, Tetzlaff J, Altman DG (2009) PRISMA Group, preferred reporting items for systematic reviews and meta-analyses: the PRISMA statement. Ann Intern Med 151:264–269. https://doi.org/10.7326/0003-4819-151-4-200908180-00135
Electricchoice.com (2016) Residential electricity for houses, apartments, condos, trailers, etc. https://www.electricchoice.com/residential-electricity . Accessed 10 Aug 2019
Kouroupetroglou P-N (2017) Machine learning techniques for short-term electric load forecasting. Aristotle University of Thessaloniki, Thessaloniki
Zakarya S, Abbas H, Belal M (2017) Long-term deep learning load forecasting based on social and economic factors in the Kuwait region. J Theor Appl Inf Technol 95:1524–1535
Soni A, Sharma AK (2013) Electricity load forecast for power system planning. Int Ref J Eng Sci 2:52–57
Angamuthu CR, Mukherjee A, Campion M, Salehfar H, Hansen T, Lin J, Ranganathan P (2018) A multi-stage price forecasting model for day-ahead electricity markets. Forecasting 1:3. https://doi.org/10.3390/forecast1010003
Lee CW, Lin BY (2016) Application of hybrid quantum tabu search with support vector regression (SVR) for load forecasting. Energies 9:1–16. https://doi.org/10.3390/en9110873
Hong WC, Fan GF (2019) Hybrid empirical mode decomposition with support vector regression model for short term load forecasting. Energies 12:1–16. https://doi.org/10.3390/en12061093
Sulandari W, Subanar MH, Lee PC (2020) Rodrigues, Indonesian electricity load forecasting using singular spectrum analysis, fuzzy systems and neural networks. Energy 190:116408. https://doi.org/10.1016/j.energy.2019.116408
Nepal B, Yamaha M, Yokoe A, Yamaji T (2020) Electricity load forecasting using clustering and ARIMA model for energy management in buildings. Jpn Archit Rev 3:62–76. https://doi.org/10.1002/2475-8876.12135
Ghore S, Goswami A (2015) Short term load forecasting of Chhattisgarh grid using artificial neural network. Int J Eng Dev Res 3:391–397
Kuhba H, Al-tamemi HAH (2016) Power system short-term load forecasting using artificial neural networks. Int J Eng Dev Res 4:78–87
Zahid M, Ahmed F, Javaid N, Abbasi R, Zainab KH, Javaid A, Bilal M, Akbar M, Ilahi M (2019) Electricity price and load forecasting using enhanced convolutional neural network and enhanced support vector regression in smart grids. Electronics 8:122. https://doi.org/10.3390/electronics8020122
Nwulu NI, Agboola OP (2012) Modelling and predicting electricity consumption using artificial neural networks. In: 2012 11th international conference on environmental and electrical engineering, pp 1059–1063
Young TC, Raya H, Youngdeok H, Young ML, (2016) Artificial neural network model for forecasting sub-hourly electricity usage in commercial buildings. Energy Buildings 111:184–194. https://doi.org/10.1016/j.enbuild.2015.11.045
Aydodu G, Yildiz O (2017) Forecasting the annual electricity consumption of Turkey using a hybrid model. In: 2017 25th signal processing and communication application conference, Antalya, Turkey, pp 1–4. https://doi.org/10.1109/SIU.2017.7960283
Ahmad W, Ayub N, Ali T, Irfan M, Awais M, Shiraz M, Glowacz A (2020) Towards short term electricity load forecasting using improved support vector machine and extreme learning machine. Energies 13:1–17. https://doi.org/10.3390/en13112907
Atef S, Eltawil AB (2020) Assessment of stacked unidirectional and bidirectional long short-term memory networks for electricity load forecasting. Electr Power Syst Res 187:106489. https://doi.org/10.1016/j.epsr.2020.106489
Azar AT, Khamis A, Kamal NA, Galli B (2020) Short term electricity load forecasting through machine learning. In: Hassanien A, Azar A, Gaber T, Oliva D, Tolba F (eds) International conference on artificial intelligence computer vision (AICV2020). AICV 2020. Advances in intelligent systems and computing, vol 1153, Springer, Cham. https://link.springer.com/chapter/10.1007/978-3-030-44289-7_40 . Accessed 5 July 2020
Konica JA, Hanelli L (2016) Forecasting next-day the electricity demand based on fuzzy logic method case for. J Multidiscip Eng Sci Technol 3:6172–6180
Motepe S, Hassan AN, Stopforth R (2019) South African distribution networks load forecasting using ANFIS. In: Proceedings of 2018 IEEE international conference power electronic drives energy system PEDES 2018, IEEE, Chennai, India, pp 1–6. https://doi.org/10.1109/pedes.2018.8707876
Lin CT, Der Chou L (2013) A novel economy reflecting short-term load forecasting approach. Energy Convers Manag 65:331–342. https://doi.org/10.1016/j.enconman.2012.08.001
Leahy E, Lyons S (2010) Energy use and appliance ownership in Ireland. Energy Policy 38:4265–4279. https://doi.org/10.1016/j.enpol.2010.03.056
Soares A, Gomes Á, Antunes CH (2014) Categorisation of residential electricity consumption as a basis for the assessment of the impacts of demand response actions. Renew Sustain Energy Rev 30:490–503. https://doi.org/10.1016/j.rser.2013.10.019
Hedén W (2016) Predicting hourly residential energy consumption using random forest and support vector regression an analysis of the impact of household clustering on the performance accuracy. KTH Royal Institute of Technology, Stockholm
Kong W, Dong ZY, Jia Y, Hill DJ, Xu Y, Zhang Y (2019) Short-term residential load forecasting based on LSTM recurrent neural network. IEEE Trans Smart Grid 10:841–851. https://doi.org/10.1109/TSG.2017.2753802
Kong W, Dong ZY, Hill DJ, Luo F, Xu Y (2018) Short-term residential load forecasting based on resident behaviour learning. IEEE Trans Power Syst 33:2016–2017. https://doi.org/10.1109/TPWRS.2017.2688178
Kontokosta CE, Tull C (2017) A data-driven predictive model of city-scale energy use in buildings. Appl Energy 197:303–317. https://doi.org/10.1016/j.apenergy.2017.04.005
McLoughlin F, Duffy A, Conlon M (2015) A clustering approach to domestic electricity load profile characterisation using smart metering data. Appl Energy 141:190–199. https://doi.org/10.1016/j.apenergy.2014.12.039
Mcloughlin F, Duffy A, Conlon M (2012) Characterising domestic electricity consumption patterns by dwelling and occupant socio-economic variables: an Irish case study. Energy Build 48:240–248. https://doi.org/10.1016/j.enbuild.2012.01.037
Veit A, Goebel C, Tidke R, Doblander C, Jacobsen H (2014) Household electricity demand forecasting-benchmarking state-of-the-art methods. arXiv:1603.00751 , pp 1–10
Biswas MAR, Robinson MD, Fumo N (2016) Prediction of residential building energy consumption: a neural network approach. Energy 117:84–92. https://doi.org/10.1016/j.energy.2016.10.066
Nishida K, Takeda A, Iwata S, Kiho M, Nakayama I (2017) Household energy consumption prediction by feature selection of lifestyle data. In: International conference on smart grid communication, Dresden, Germany, pp 235–240
Olaniyan K, Mclellan BC (2018) Estimating residential electricity consumption in Nigeria to support energy transitions. Sustain 10:1–22. https://doi.org/10.3390/su10051440
Bouznit M, Pablo-Romero MP (2018) Residential electricity consumption and economic growth in Algeria. Energies (MDPI). https://doi.org/10.3390/en11071656
Bogomolov A, Lepri B, Larcher R, Antonelli F, Pianesi F, Pentland A (2016) Energy consumption prediction using people dynamics derived from cellular network data. EPJ Data Sci. https://doi.org/10.1140/epjds/s13688-016-0075-3
Huber P, Gerber M, Rumsch A, Paice A (2018) Prediction of domestic appliances usage based on electrical consumption. Energy Inform 1:265–428
Jain PK, Quamer W, Pamula R (2018) Electricity consumption forecasting using time series analysis. In: Singh M, Gupta P, Tyagi V, Flusser J, Ören T (eds), Advanced computer data science ICACDS 2018. Communications in computer and information science, Springer, Singapore, Singapore. https://doi.org/10.1007/978-981-13-1813-9_33
Rehman USA, Cai Y, Fazal R, Das Walasai G, Mirjat NH (2017) An integrated modeling approach for forecasting long-term energy demand in Pakistan. Energies 10:1–23. https://doi.org/10.3390/en10111868
Liang Y, Niu D, Cao Y, Hong WC (2016) Analysis and modeling for China’s electricity demand forecasting using a hybrid method based on multiple regression and extreme learning machine: a view from carbon emission. Energies 9:1–22. https://doi.org/10.3390/en9110941
Mokilane P, Galpin J, Yadavalli VSS, Debba P, Koen R, Sibiya S (2018) Density forecasting for long-term electricity demand in South Africa using quantile regression. S Afr J Econ Manag Sci 21:1–14. https://doi.org/10.4102/sajems.v21i1.1757
Sarkodie SA (2017) Estimating Ghana’s electricity consumption by 2030: an ARIMA forecast, energy sources. Part B Econ Plan Policy 12:936–944. https://doi.org/10.1080/15567249.2017.1327993
Energy Commission, Energy Commission, Ghana 2018 Energy (Supply and Demand) Outlook for Ghana, Accra, 2018. http://www.energycom.gov.gh/planning/data-center/energy-outlook-for-ghana . Accessed 1 Feb 2020
Sigauke C (2017) Forecasting medium-term electricity demand in a South African electric power supply system. J Energy S Afr. https://doi.org/10.17159/2413-3051/2017/v28i4a2428
Asumadu-Sarkodie S, Owusu PA (2016) Forecasting Nigeria’s energy use by 2030, an econometric approach, Energy Sources. Part B Econ Plan Policy 11:990–997. https://doi.org/10.1080/15567249.2016.1217287
Adeoye O, Spataru C (2019) Modelling and forecasting hourly electricity demand in West African countries. Appl Energy 242:311–333. https://doi.org/10.1016/j.apenergy.2019.03.057
Sigauke C, Chikobvu D (2016) Peak electricity demand forecasting using time series regression models: an application to South African data. J Stat Manag Syst 19:567–586. https://doi.org/10.1080/09720510.2015.1086146
Ozturk S, Ozturk F (2018) Prediction of energy consumption of Turkey on sectoral bases by Arima model. Energy Econ Lett 5:23–30. https://doi.org/10.18488/journal.82.2018.51.23.30
Lebotsa ME, Sigauke C, Bere A, Fildes R, Boylan JE (2018) Short term electricity demand forecasting using partially linear additive quantile regression with an application to the unit commitment problem. Appl Energy 222:104–118. https://doi.org/10.1016/j.apenergy.2018.03.155
Caro E, Juan J, Cara J (2020) Periodically correlated models for short-term electricity load forecasting. Appl Math Comput 364:124642. https://doi.org/10.1016/j.amc.2019.124642
Article MathSciNet MATH Google Scholar
Kong X, Li C, Wang C, Zhang Y, Zhang J (2020) Short-term electrical load forecasting based on error correction using dynamic mode decomposition. Appl Energy 261:114368. https://doi.org/10.1016/j.apenergy.2019.114368
Auverlot D, Beeker É, Hossie G, Oriol L, Rigard-Cerison A (2014) The crisis of the european electricity system: diagnosis and possible ways forward. https://www.strategie.gouv.fr/sites/strategie.gouv.fr/files/archives/CGSP_Report_European_Electricity_System_030220141.pdf . Accessed 18 Apr 2019
Guo Z, Zhou K, Zhang C, Lu X, Chen W, Yang S (2018) Residential electricity consumption behavior: influencing factors, related theories and intervention strategies. Renew Sustain Energy Rev 81:399–412. https://doi.org/10.1016/j.rser.2017.07.046
Download references
Acknowledgements
Not applicable.
The authors declare that they did not receive any funding for this research.
Author information
Authors and affiliations.
Department of Computer Science, Sunyani Technical University, Box 206, Sunyani, Ghana
Isaac Kofi Nti
Department of Electrical and Electronic Engineering, Sunyani Technical University, Sunyani, Ghana
Moses Teimeh
Department of Computer Science and Informatics, University of Energy and Natural Resources, Sunyani, Ghana
Owusu Nyarko-Boateng & Adebayo Felix Adekoya
You can also search for this author in PubMed Google Scholar
Contributions
We, at this moment, declare that IKN and MT contributed to the design and implementation of the research. MT, IKN ON-B and AFA contributed to the analysis of the results and the writing of the manuscript. The manuscript has been read and approved by all named authors, and there are no other persons who satisfied the criteria for authorship but are not listed. All authors read and approved the final manuscript.
Corresponding author
Correspondence to Isaac Kofi Nti .
Ethics declarations
Competing interests.
The authors of the current study declare that they have no competing interests.
Additional information
Publisher's note.
Springer Nature remains neutral with regard to jurisdictional claims in published maps and institutional affiliations.
Rights and permissions
Open Access This article is licensed under a Creative Commons Attribution 4.0 International License, which permits use, sharing, adaptation, distribution and reproduction in any medium or format, as long as you give appropriate credit to the original author(s) and the source, provide a link to the Creative Commons licence, and indicate if changes were made. The images or other third party material in this article are included in the article's Creative Commons licence, unless indicated otherwise in a credit line to the material. If material is not included in the article's Creative Commons licence and your intended use is not permitted by statutory regulation or exceeds the permitted use, you will need to obtain permission directly from the copyright holder. To view a copy of this licence, visit http://creativecommons.org/licenses/by/4.0/ .
Reprints and permissions
About this article
Cite this article.
Nti, I.K., Teimeh, M., Nyarko-Boateng, O. et al. Electricity load forecasting: a systematic review. Journal of Electrical Systems and Inf Technol 7 , 13 (2020). https://doi.org/10.1186/s43067-020-00021-8
Download citation
Received : 06 April 2020
Accepted : 30 August 2020
Published : 09 September 2020
DOI : https://doi.org/10.1186/s43067-020-00021-8
Share this article
Anyone you share the following link with will be able to read this content:
Sorry, a shareable link is not currently available for this article.
Provided by the Springer Nature SharedIt content-sharing initiative
- Electricity load forecasting
- Reference Manager
- Simple TEXT file
People also looked at
Review article, research on energy consumption in household sector: a comprehensive review based on bibliometric analysis.
- 1 iSMART, Qingdao University of Technology, Qingdao, China
- 2 Faculty of Environmental Engineering, The University of Kitakyushu, Kitakyushu, Japan
Households are an important sector in carrying out human development activities, accounting for more than 30% of the total global energy consumption. The continued growth of household energy consumption (HEC) and carbon emissions is threatening economic and environmental sustainability. This review focuses on the research in the field of HEC and conducts a bibliometric analysis of research articles from the Web of Science Core Collection since 2000. The results show that: 1) HEC research has undergone rapid development since 2014, and interdisciplinary fusion and collaborative research have become dominant trends. 2) Keyword co-occurrence analysis clearly identifies the current urgent themes, including energy demand and its determinants, environmental impact factors and assessments, and energy-saving technologies and emission reduction measures. 3) The analysis of citations reveals that economic models, such as input-output models and life cycle assessment, are frequently employed in the field of HEC. Based on a summary of household energy-saving and emissions reduction work, this paper critically discusses the limitations of existing measures such as smart home technology, sustainable energy systems, and behavioral interventions. The main directions for promoting household energy-saving development in the future are identified: including improving the security and customer engagement of smart home technology, focusing on the availability and stability of sustainable energy, and paying more attention to low-income and aging households in behavioral intervention measures. One of the important obstacles facing research is how to reduce energy management efficiency and usage costs through technology and policy.
1 Introduction
Climate change and environmental impacts caused by energy consumption and carbon emissions have raised global awareness. In response to the “Paris Agreement”, many countries or regions have developed long-term policies and action plans to reduce greenhouse gas emissions. The EU introduced the “Energy Efficiency Directive”, which aims to reduce total energy consumption, reduce emissions and respond to energy poverty ( Energy Efficiency Directive, 2018 ). The Japanese government submitted its National Autonomous Contribution (NDC), stating that it will reduce greenhouse gas emissions by 26% compared to 2013 by 2030 ( Taniguchi-Matsuoka et al., 2020 ). The U.S. proposed the “CLAEN Futures Act” in the 117th Congress. It sets the goal of reducing greenhouse gas emissions from the U.S. economy by 50% by 2030 compared to 2005, and by 100% by 2050 ( CLEAN Future Act, 2021 ). As the largest developing country, China attaches great importance to addressing climate change and strengthening the implementation of the national autonomous contribution target. It has adopted “reducing CO 2 emissions per unit of GDP by 18% in 2025 compared to 2020”as a binding target in the 14th Five-Year Plan ( MEE, 2022 ). At the same time, a series of Energy Conservation and Emission Reduction Policies (ECERP) have been introduced with the aim of controlling the total amount of energy consumption, improving energy efficiency and promoting the green transformation of economic and social development ( Luo et al., 2023 ). India has a target to reduce carbon intensity of its economy to 45% by 2030 ( Amin et al., 2023 ).
Reducing building-related energy use is widely recognized as key to reaching these ambitious targets and facilitating the transition to a low-carbon and sustainable world ( Zhao et al., 2023b ). The household sector consumes large amounts of energy in the form of heating and cooling, among other things, and accounts for a significant share of greenhouse gas emissions ( Goldstein et al., 2020 ). It plays an important role in reducing carbon footprint and achieving energy sustainability. In the household sector, the widespread use of electricity is driving changes in our lifestyles. In the Kingdom of Saudi Arabia (KSA), electricity consumption in the residential sector accounts for 50% of total production ( Alqahtani and Balta-Ozkan, 2021 ); 26% of U.S. households rely on electricity as their sole energy supply ( Michelle Lewis, 2022 ). The residential sector accounts for about 24% of India’s total electricity consumption ( Ramapragada, 2022 ); which is already close to 40% in China ( CESY, 2020 ). Continued high growth in electricity demand has contributed to a rise in enthusiasm for clean, sustainable energy generation and energy independence. However, about 80% of global energy and 66% of electricity generation still originates from fossil fuels ( UNEP, 2022 ). Not only does this lead to environmental problems such as atmospheric pollution and climate warming, but for countries or regions where fossil fuels are scarce, the issue of energy supply, demand conflicts and energy security are more prominent ( Qerimi et al., 2020 ).
We are at a critical turning point in the energy transition and sustainable development, and it is urgent to achieve energy savings in the household sector. The prerequisite is a comprehensive understanding of trends in household energy consumption (HEC), quantitative analysis of environmental impacts, and actual causes so as to facilitate more sustainable measures to reduce the household carbon footprint ( Wang T et al., 2023 ). Some scholars have reviewed and summarized the research progress in the HEC.
First, the exploration of influencing factors is a topic that has been extensively covered by scholars ( Bhattacharjee and Reichard, 2011 ; Jiang et al., 2020 ). Mainly evaluated the influence of social and economic factors on household energy consumption. The behavior of family members often has a significant impact on energy consumption, equipment use decisions, etc. ( Borozan, 2018 ) integrated and external factors and individual behavioral features for multiple discussions of causes ( Hafner et al., 2019 ; Adua, 2020 ); focus on a review of factors that reduce household energy demand from a behavioral and habitual perspective ( Su et al., 2023 ). Developed a dynamic assessment model to quantify the impact of occupant behavior on household energy consumption and carbon emissions. Faced with a range of negative impacts caused by solid fuel use ( Elasu et al., 2023 ), concentrates on exploring the determinants of a clean energy transition ( Guta et al., 2022 ). Pays more attention to the barriers of energy transition for households in the middle-income and low-income countries.
Secondly, The review of quantitative methods as well as energy and carbon reduction programs was also a popular topic ( Zhang et al., 2015 ). Mainly discusses the methods for quantifying household carbon emissions, and related emission reduction measures ( Hertwich, 2005 ). Focuses on the different of the life cycle approach in sustainable consumption ( Wiedmann, 2009 ). Reviews the contribution of input-output approach (IOA) in the work on consumption and emissions accounting ( Oladokun and Odesola, 2015 ). Critically reviews modeling methods and techniques related to household energy consumption and carbon emissions ( McAndrew et al., 2021 ). Systematically examines the effectiveness of a range of household energy efficiency interventions ( Liu L et al., 2020 ). Review focused on the evaluation methods and analysis of influencing factors for HECs.
Some other studies have focused on the prospects of household energy efficiency development. The household sector, recognized as an energy-intensive and high-emission sector, has consistently garnered attention regarding the realization of energy-saving effects. Faced with the continual growth in energy consumption pressures, enhancing residential energy efficiency is regarded as a pivotal means to mitigate energy costs and reduce emissions ( Kaufmann et al., 2023 ; Zheng et al., 2024 ). Xu L et al. (2021) expressed the pioneering nature of future research in the study. Studies identified behavioral interventions, energy efficiency and energy poverty were identified as possible research frontiers. Especially with the widening scope and spatial disparities of energy poverty, scholars have initiated multidimensional explorations into its influencing factors and policy interventions. The assessment of energy efficiency levels and the projection of energy saving potentials are important prerequisites for the formulation of effective policies ( Jia and Wu, 2022 ; Stojilovska et al., 2022 ). In study of ( Calvin Nsangou et al., 2020 ), the energy demand Frontier function model was used to estimate the electricity efficiency index of households; the model was applied by ( Wang X et al., 2023 ) to evaluate a 31% energy saving potential for heating in HSCW zones in China. In addition to this, some scholars have focused on the impact of income inequality on the energy efficiency gap to explore ways to reduce energy costs for low-income households ( Chaudhuri and Huaccha, 2023 ).
There is an increasing focus on the impact of HEC. Increasing attention is being paid to accounting for household energy consumption, environmental impact assessment, and implementation of energy efficiency measures. This paper reviews the current literature related to HEC. A descriptive analysis of research trends, the main performance and contributions of countries, regions, organizations, and authors was performed in combination with bibliometric tools. Keyword cluster analysis, citation analysis, etc. , were performed in combination with co-occurrence maps.
Compared to previous review articles, the novelty of this paper lies in two aspects. Firstly, it provides a classification and comprehensive review of research methods in Household Energy consumption (HEC). These methods revolve around three aspects: environmental impact assessment, factor analysis of influences, and behavioral analysis. The advantages and disadvantages of their applications are discussed. This provides valuable information for researchers to gain a deep understanding of the development of HEC and offers targeted references for future research method selection and optimization. Another contribution of this paper is the attempt to review and critically discuss recent popular approaches to household energy conservation and emission reduction.
Furthermore, this paper summarizes important themes that have sparked widespread discussion in recent HEC research. These include key factors influencing household energy consumption, the implementation of energy-saving programs and policies, as well as the barriers faced. It also examines the development and optimization effects of smart home technologies and how residents’ consumption behavior and decision-making processes impact energy usage. This provides consumers with information and tools for energy conservation and offers guidance to utilities and governments in formulating energy plans, enabling them to make wiser decisions in sustainable energy production and utilization.
The content of the paper is following: In part 2, explained the literature search sources, databases, and analysis tools; In part 3, the results of the bibliometric analysis are described in terms of journal contribution, disciplinary contribution, literature coupling, keyword co-occurrence, and country/organizational productivity; In part 4, the currently popular research methods are summarized; In part 5, a critical discussion is presented on the aspects of energy efficiency measures that are currently of high interest.
2 Methods, data sources
2.1 methodology.
Bibliometric analysis, with its flexibility and powerful data processing capabilities, is an important tool for exploring the current stage of quantifying and analyzing a discipline or a particular research element. It can track the trends and dynamic hotspots of research topics in an objective and comprehensive manner. However, literature metrics analysis exhibits a certain degree of citation bias, where some innovative perspectives might be overlooked due to the low prominence of authors or journals. Additionally, literature databases often suffer from a time lag, impeding their ability to promptly reflect the latest developments in research. Based on this, this study will be conducted with a combination of bibliometric analysis and manual review.
Firstly, a review and statistical analysis were conducted on literature related to household energy consumption published in peer-reviewed journals over the past 20 years. A descriptive analysis was employed to characterize the current state, trends, and peer contributions. Through manual examination, duplicates and non-academic literature were excluded to ensure the validity of the analytical results. Simultaneously, recent viewpoints and research findings were introduced to discuss prevalent model approaches, dynamic focal points, and research challenges in the field.
2.2 Data sources
The bibliometric literature search of the literature database is performed on the “Web of Science Core Collection database”. The database covers many peer-reviewed, high-quality academic journals containing scientific and technical literature in more than 260 subject areas worldwide, which can provide much of the information needed for interdisciplinary development. It facilitates identification and statistical information for researchers. In addition, a multiple of data extraction formats are supported, providing a complete record of all necessary literature information that can be imported into bibliometric software tools for visual analysis.
The first step in the study was to identify the literature dataset using a keyword search. Using the literature search platform in Web of Science, the final dataset was determined by inputting keywords, date range, and other relevant criteria. The literature collection was exported in January 2023 and underwent subsequent screening processes. The keywords included “energy consumption”, “households”, and “residential”, and the time frame was set from 2000 to 2021. From the conditional search, a total of 791 open access papers were generated. Second, the search results were refined using the filtering function of web of science, and the language was set to English for ease of subsequent understanding. Among the types of literature, research papers are the main contribution to research in the field, accounting for about 85% of the total number of publications, followed by conference proceedings papers with about 13.3%, and other publications belonging to reviews as well as online publications. Since research articles usually provide more pioneering research results and have more information about authors, institutions, and citations. Therefore, only journal articles published in English are considered for further analysis in this paper, totaling 673 articles. The second step involves the article’s indicators, including title, abstract, keywords, references, authors, and organization. This data provides key information about research trends and directions in the HEC. These articles and related information are exported in text format. Finally, we need to further review the exported 673 articles. The main screening objects were withdrawn articles, duplicate articles, and articles that did not match the topic. A final literature dataset of 619 manuscripts was obtained and studied in depth using bibliometric analysis tools. Figure 1 represents the output process of the literature dataset.
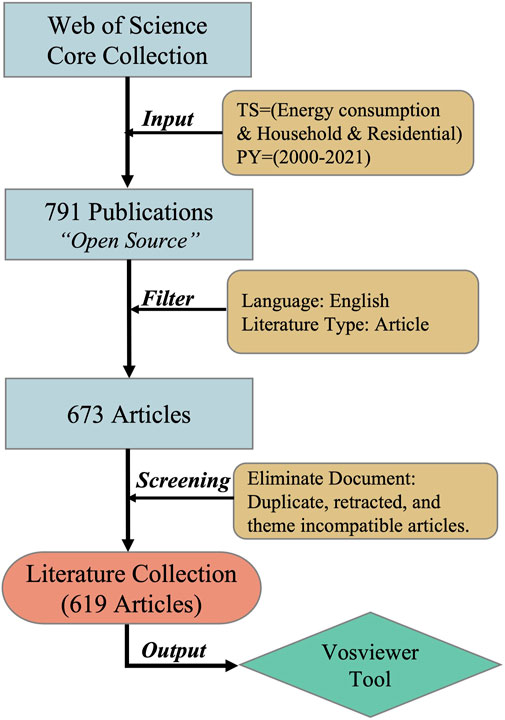
FIGURE 1 . Research flow.
2.3 Analysis tools
The selected articles have been identified, and it is necessary to use literature analysis tools for networked and visual analysis of the database. With the application and development of bibliometrics, many different types of knowledge graph tools have been derived. Among them, Citespace, HistCite, VOSViewer, Bibliometrix, etc. , are commonly used analysis tools ( Xu et al., 2023 ). Compared with other bibliometric software, VOSViewer has strong graphical presentation capabilities and is suitable for large-scale data; it can create co-authorship, bibliographic coupling and co-citation networks based on citation data, or construct keyword maps using co-occurrence data ( Hu et al., 2022 ). It can also use the thesaurus function for data processing to improve the accuracy of analysis results.
3 Results of bibliometric analysis
3.1 trends in hec research, 3.1.1 number of publications.
The statistics of article publication trends are presented in Figure 2 . The number of annual publications on household and residential energy consumption is generally on the rise. Especially since 2014, which has witnessed a period of rapid growth, with an average annual number of 76.8 articles and an average annual growth rate of 27.5%. This also indicates that energy consumption in the household sector has attracted a great deal of attention from scholars in the face of global climate action, energy depletion, and carbon emission.
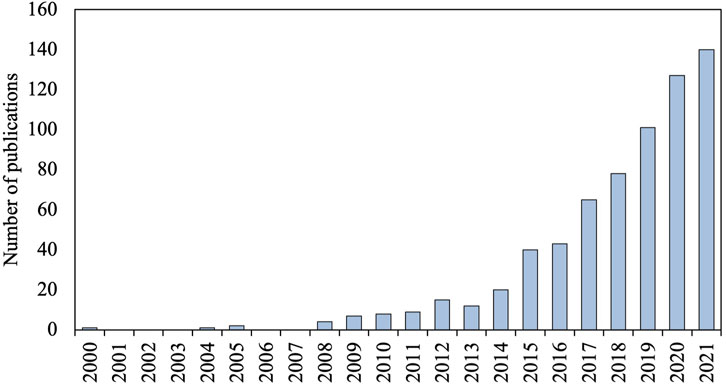
FIGURE 2 . The number of publications in HEC.
3.1.2 Discipline performance
According to the web of science, the research topics were mainly related to 65 different disciplines, and the results are detailed in Figure 3 . Interdisciplinary integration has been a common phenomenon in HEC research, with energy fuels ranking first among all disciplines, accounting for about 21.9%. This shows that studies from an energy transition perspective are a key discipline for HEC, such as the development of sustainable energy sources and how to reduce dependence on traditional energy sources. This is followed by Environmental Sciences (12.7%), Environmental Studies (12.4%), and Green and Sustainable Science Technology (8.4%); the negative impacts of HEC and how to improve environmental benefits are also gaining importance. It is worth noting that Economics (7.0%) is also one of the indispensable disciplines in HEC research. It plays an important role in improving pricing measures in the electricity sector and optimizing energy management systems. In addition, disciplines related to building technology, construction, and materials are among the major contributors to HEC. Specifically, they include Building Technology (4.8%), Engineering Civil (3.9%), Engineering Electrical Electronic (3.5%), and Engineering Chemical (2.6%). The remaining other disciplines collectively contributed 20.6%.
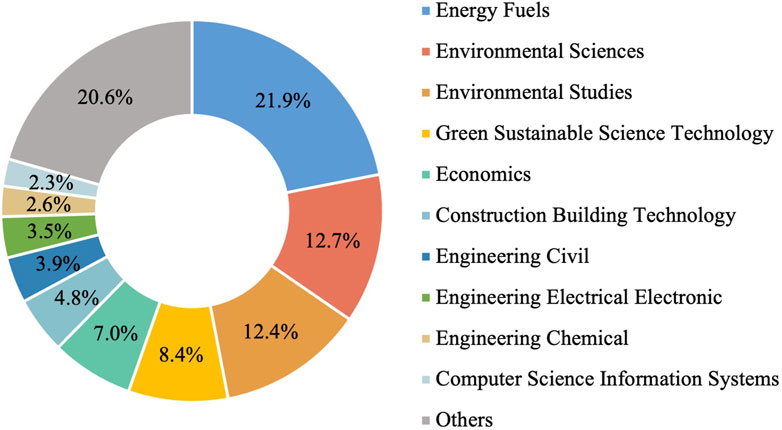
FIGURE 3 . Subject performance.
3.2 Research contribution
3.2.1 contribution of journals.
The 619 articles were published in 177 different journals, but about 66% of these journals published only one relevant article. The 10 categories of journals that contributed the most to the field are listed, showing their total publications (TP), total citations (TC), and the average number of citations (AC) for the period 2000-2021. Secondly, the impact factor (IF) of journals, which is an important indicator to evaluate the impact of articles, was also examined as one of the objects. The results are shown in Table 1 .
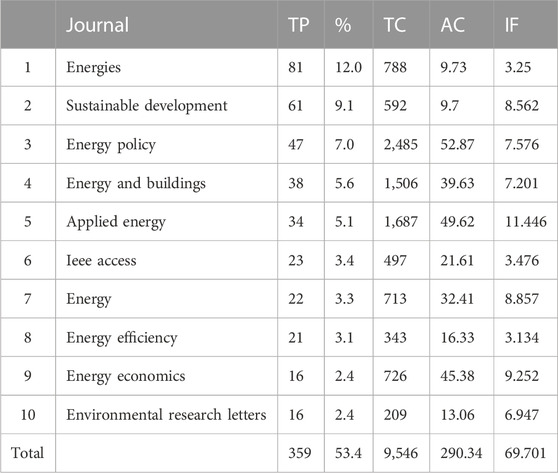
TABLE 1 . The contribution of major journals.
In terms of TP scores of journals, Energies is the most productive journal with 81 articles, but the TP, AC and IF scores are not high. This is followed by Sustainable development (61 articles) and Energy policy (47 articles). The combined TC and AC scores of the journals were considered, and Energy policy and Applied Energy were ranked first and second, respectively. This indicates that both journals contribute many high-quality articles to HEC. It is worth mentioning that Applied Energy’s IF score (11.446) is the highest score in this journal contribution review process, representing the high influence of the journal in HEC research. Energy and buildings (1,506) also have a good performance in terms of TC and AC score. Energy economics is also one of the representatives of high-quality journals. Although there are only 16 publications, the articles have relatively high TC (726) scores and AC scores (45.38) and the journal has the second highest IF score (9.252). This again confirms the high attention given to research related to energy economics. Other important journals include Sustainable development, IEEE access, Energy, Energy research and social science, Energy efficiency, Environmental research letters, Energy reports.
3.2.2 Country and region
In terms of country/region contributions, a total of 80 countries/regions published papers related to the topic of household energy consumption, with about 25.6% of countries/regions contributing only one article. The 10 countries/regions which are most active in research field are listed in Table.2 , based on the score of TP, TC and AC to articles. Joint collaborations (JC) is used to explain the strength of cooperative relationships between countries and is one of the objectives of the review. They are the United States (USA), the United Kingdom, China, Germany, Australia, Netherlands, Spain, Japan, South Korea, Canada, and Italy.
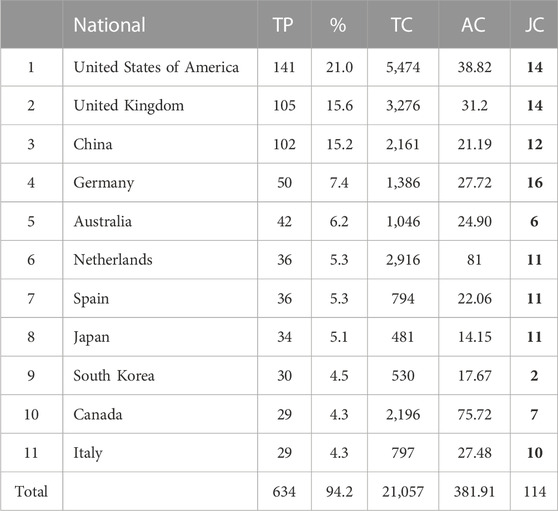
TABLE 2 . The contribution of country/region.
In view of the TP, the USA (141), the United Kingdom (105) and China (102) have the highest scores, accounting for about 51.8% of the total number of published articles. The impact of HEC has attracted high attention from carbon-emitting countries. For TC, the USA (5,474) is far ahead and has a high influence on HEC research. As one of the major countries in global energy consumption, the US has played a significant role in energy policy and technological innovation ( Subbiah et al., 2017 ). This is followed by the United Kingdom (3,276), the Netherlands (2,916). European countries are committed to promoting energy transition and enhancing energy efficiency ( De Boeck et al., 2016 ), and they have played a pivotal role in research on household energy consumption as well. China (2,161) as one of the world’s largest energy-consuming countries, is actively focusing on areas such as renewable energy and smart grids ( Gao et al., 2015 ). Although the Netherlands (81) and Canada (75.72) did not score high in TP, TC and AC scores were second only to the US and the United Kingdom, indicating that they have a strong research capacity and contribute high quality articles regarding the HEC field. In addition, most countries/regions have concentrated their publication productivity in 2017–2018.
The analysis of country/region cooperation was carried out using the full counting calculation method in VOSviewer, and the minimum threshold of published articles was set to 12, which finally filtered out 20 countries/regions that met the above conditions. Figure 4 shows the international cooperation relationships between countries/regions in the field of household energy consumption research. The countries are divided into four different color clusters based on the strength of the relationship, with the red cluster centered on the United States and containing Germany, France, and Switzerland. The green cluster is led by China and the United Kingdom, and includes Japan, Australia, Ireland, and Pakistan. The blue cluster is represented by the Netherlands and Spain, with South Korea, Denmark Sweden, Portugal, and Italy. The red cluster consists of Canada and Finland. Secondly, the circles represent the number of publications, and the connecting lines point out the cooperation relations between countries, with the thickness of each connecting line representing the intensity level of cooperation between countries.
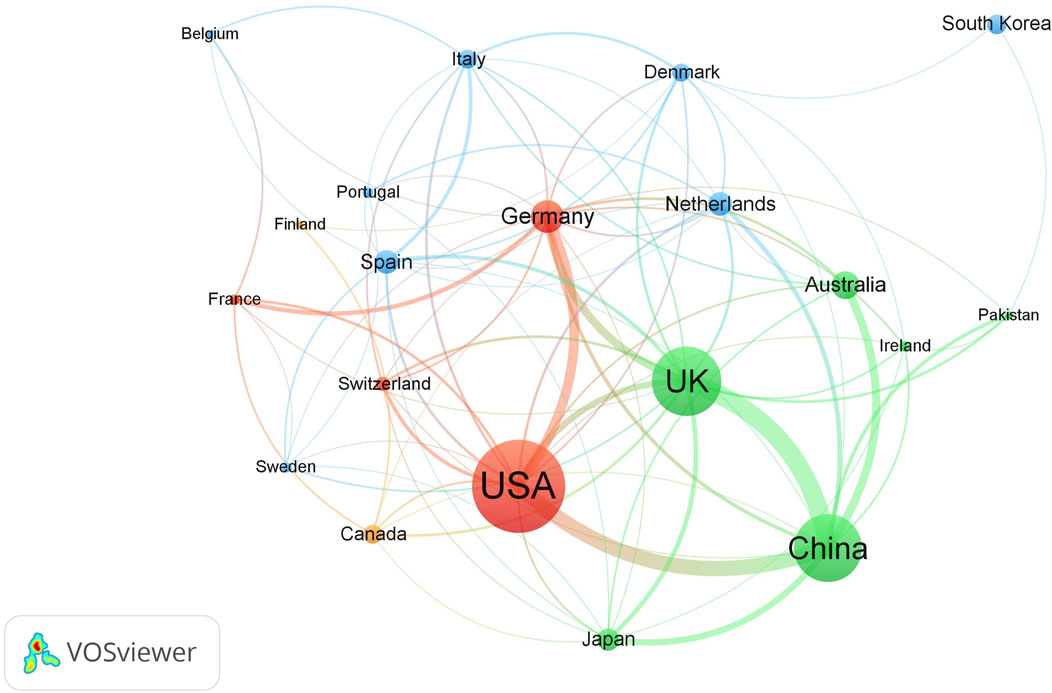
FIGURE 4 . Major Country/Region cooperation.
Germany has the densest international cooperation network among the country cooperation networks and maintains cooperation with 16 countries. Although the total number of publications in Germany is only 50, 48 of the articles are collaborative papers. Germany holds a leading position in renewable energy and energy development, and it collaborates with the United States and other European countries in joint research in this field ( Andor et al., 2021 ; Heesen and Madlener, 2021 ). The USA and the United Kingdom, as the most productive countries, maintain cooperation with 14 countries and have the strongest partnership with China. China also maintains international collaborations with 12 countries, including the USA the United Kingdom, Australia, and Japan, more than 70% of the 102 articles published are collaborative articles. The emissions and climate issues brought about by HEC have attracted global attention. International research cooperation has not only promoted the achievement of global carbon reduction goals, but also enhanced cooperation and understood among different countries.
3.2.3 Organization
A total of 871 organizations published articles on household energy consumption, with nearly 73% of them publishing only one article. The difference in the number of publications between the organizations is not significant, with the University of Oxford and Aalborg University having a relatively high number of publications, with 13 articles each. This is followed by, Delft Univ Technol, Tech Univ Denmark, Tsinghua Univ, and Univ Calif Berkeley, with 11 articles respectively. From the total number of citations of the papers, Carnegie Mellon Univ (634) was the most active, followed by Univ Calif Berkeley (517) and Univ Oxford (509). Five of the selected institutions are from the United States, reflecting the important contribution of the United States in this area of research. Two each are from China, Denmark, and the Netherlands.
By using the full counting method of VOSviewer, by setting the threshold of the minimum number of published articles to 5, a network of partnerships between organizations was obtained, and for organizations with no partnerships, the color of the circles was marked in gray. The results are shown in Figure 5 . The organizations are divided into seven clusters according to the strength of their partnerships. Blue is the largest cluster, with Univ Calif Berkeley as the core organization of the cluster and the largest number of collaborating organizations. The second largest cluster is the green cluster headed by Eindhoven Univ Technol, with 5 organizations in partnership. This is followed by the red and yellow clusters, with Trinity Coll Dublin and Univ Maryland as the cores, respectively. Meanwhile, there is a clear cross-collaboration between the blue, green, and orange clusters. In terms of the number of collaborative papers, Univ Calif Berkeley, Eindhoven Univ Technol, Hong Kong Polytech Univ, Trinity Coll Dublin, and Econ & Social Res Inst all have 8 collaborative papers and are jointly ranked first.
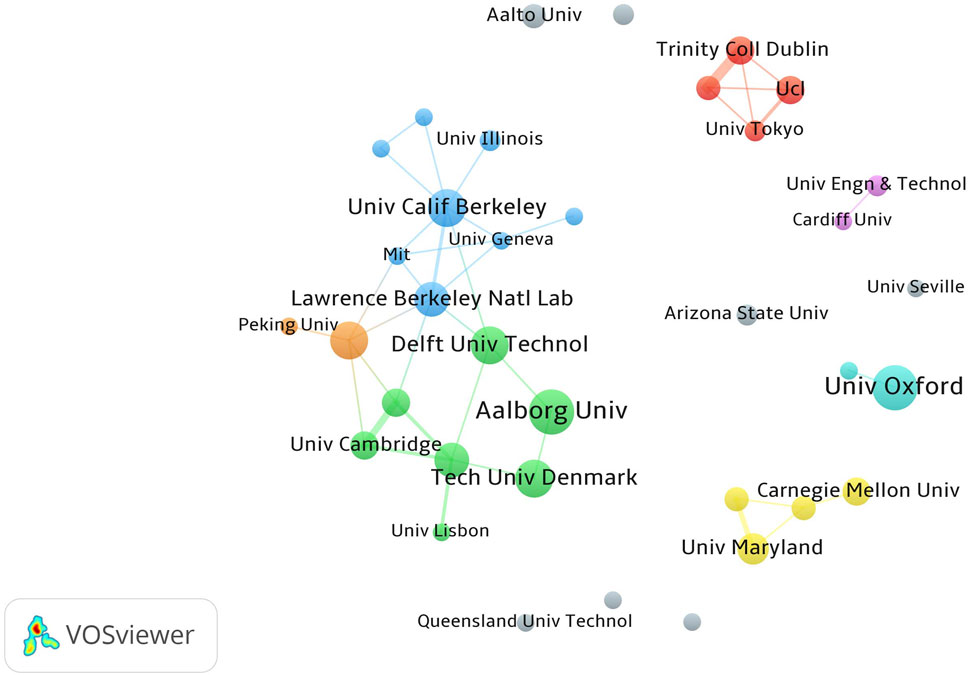
FIGURE 5 . Collaborative network of organizations.
3.2.4 Authorship
A total of 2,227 authors contributed to the study of household energy consumption, with only 167 authors having more than 3 papers. Table 3 lists the eight authors who made major contributions and the organizations to which they belonged. Also, their total number of publications, total frequency of citations, and average number of citations per article are concluded. Alberini, Anna from the University of Maryland has published 6 relevant articles with the highest total and average citation frequency, and her research has had a significant impact. Grunewald, Philipp (5 articles) from the University of Oxford is in the second. And Baik, Sung Wook from Sejong University and Ullah, Amin from Oregon State University scored relatively high in terms of the frequency of cited papers and the average number of citations.
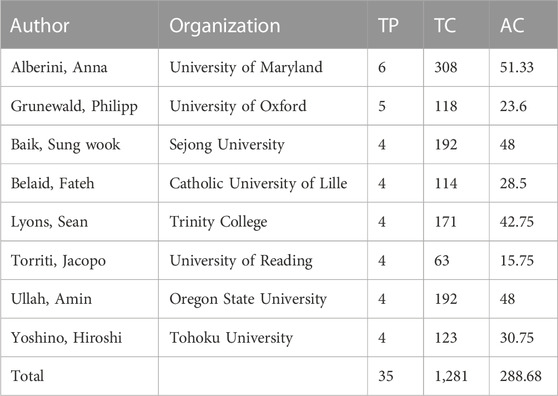
TABLE 3 . The contribution of major authors.
Figure 6 further shows the network of collaborative relationships between the authors and the strength of the links. A visual analysis of the authors’ collaboration network is performed using fractionalization in the normalization method. The most active research groups in the research are shown by using different colors, each with 1 or 2 authors at the core. In contrast to national and organizational collaborations, research groups are isolated from each other. Each circle in the figure represents an author, the size of the circle represents the number of publications by each author, and the connecting lines indicate the collaborative relationships between authors. The Blue Cluster constructed by Hanaoka, Tatsuya, Kanamori, Yuko, Masui, Yoshihiko, Xing, and Rui maintain a strong collaborative relationship. In the published articles, all four of them were collaborated. The Green Cluster, headed by Grunewald, Philipp, also formed a collaborative group. Of his five published papers, three belong to the collaborative group. The red cluster led by Alberini, Anna, although she has the largest number of papers, has only two collaborations with Torriti, Jacopo, and Filippini, Massimo. The next closest collaborations are the pink cluster with Baik, Sung wook from Sejong University and the purple cluster with Shen Guofeng, Shu Tao from Peking University.
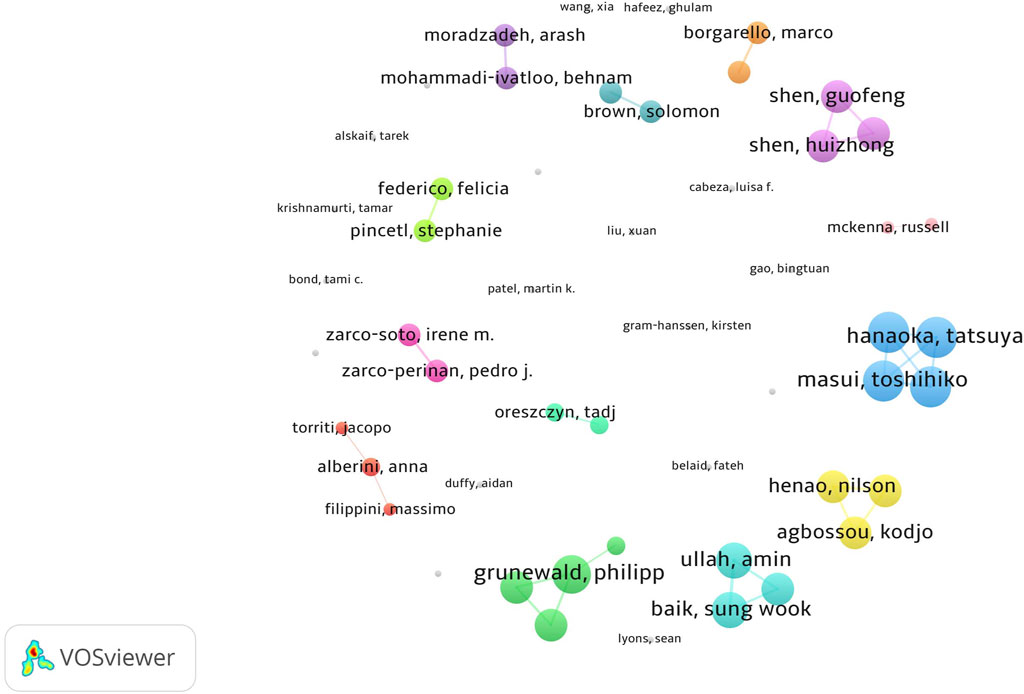
FIGURE 6 . The network map of collaborative relationships between major authors.
3.3 Theme evolution
3.3.1 keyword co-occurrence.
The co-occurrence analysis of keywords provides a clear sense of the key research content of a topic at a certain period. Using the Keywords plus function in VOSviewer, 1,231 keywords were selected from the database. To increase the accuracy of the results, the keyword dataset was processed. Firstly, we filtered words that were not relevant to the study topic, such as diffusion, refurbished, heterogeneity, etc. Secondly, we used thesaurus file function to merge and replace words with similar or same meaning, such as household and households, impact and impacts, carbon emissions, dioxide emissions, CO2 emissions, etc. To extract more accurate and valuable information, the minimum frequency of keywords was set to 8, and finally a network map containing 73 keywords was exported Figure 7 . Each circle represents a keyword, and the size of the circle is determined by the frequency of keyword occurrence, which is divided into three clusters (Cluster 1,2,3) according to the correlation strength of the keywords, and there is a close relationship between the three clusters.
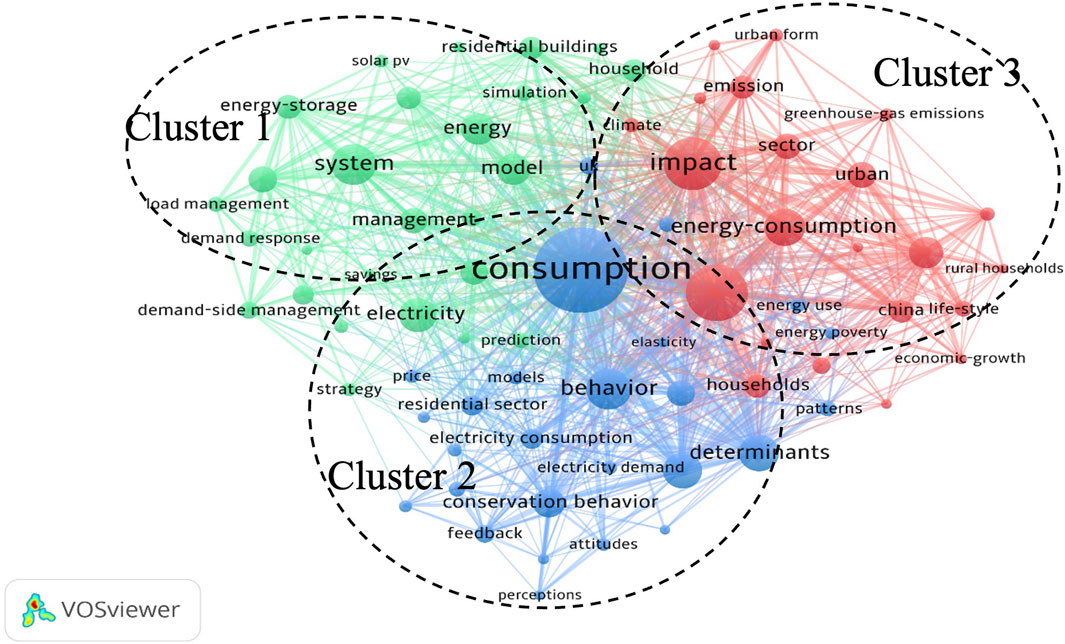
FIGURE 7 . The network map of keywords co-occurrence.
In Table 4 , list the research themes, main keywords, and their frequency of occurrence for three clusters. In the face of global concerns about climate change, HEC research has increasingly focused on sustainable energy utilization and improving energy efficiency. This includes aspects such as the utilization of renewable energy, the development of energy management systems, and technological innovations ( Abushnaf et al., 2016 ). Cluster 1 focuses on household energy efficiency and energy management systems, sustainable energy development and energy storage, and energy efficiency technology improvement and system optimization ( Bak and Yoon, 2021 ; Bataineh and Alrabee, 2018 ; Chang et al., 2018 ; Hamilton et al., 2013 ; Kumar et al., 2023 ; Xu et al., 2013 ). The development of data technology has sparked research and discussions on the potential for household electrification. Additionally, residents’ behavior and decision-making processes have emerged as popular topics, exploring how changes in residents’ energy consumption behavior can lead to reductions in energy consumption and emissions ( Das et al., 2018 ).
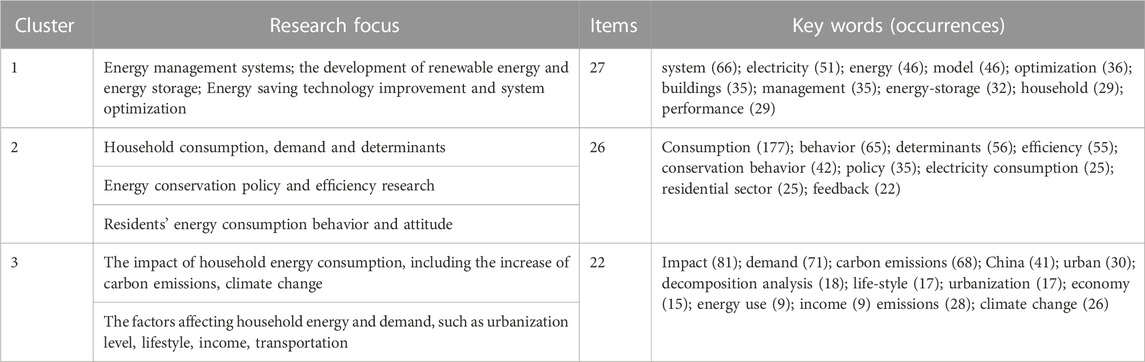
TABLE 4 . Keyword cluster and brief description.
Cluster 2 focuses on household electricity consumption, demand, and determinants; energy efficiency policies and efficiency studies; residents’ energy consumption behavior and attitudes. Energy efficiency effectiveness has become a key measure in achieving energy savings in the household sector. This includes an assessment of energy efficiency ( Hamilton et al., 2013 ), an exploration of determinants ( Adha et al., 2021 ), and a prediction of future energy savings potential ( Bataineh and Alrabee, 2018 ). The exploration of the determinants affecting energy efficiency is one of the topics that have been widely and continuously studied by scholars, which is of great significance for the development of effective policy planning and the full utilization of the economic benefits of energy ( Chang et al., 2018 ; Bak and Yoon, 2021 ). Confirmed the significant impact of dwelling characteristics, building envelope performance, etc. , on energy efficiency. Occupants’ energy behavior, rational use of equipment, and awareness of energy conservation are also factors that cannot be ignored ( Xu et al., 2013 ; Das et al., 2018 ). Second, government and local policy interventions have driven improvements in residential energy efficiency ( Jafari, 2023 ; Kumar et al., 2023 ).
Cluster 3 covers the impacts resulting from household energy consumption, including the increase in carbon emissions, climate change, etc. ( Adua et al., 2016 ); and the analysis of factors affecting household energy and demand, such as urbanization level, lifestyle, income, transportation, etc., ( Cao et al., 2019 ; Mainimo et al., 2022 ).
3.3.2 Citation analysis
Citation analysis is an activity and method that is based on the citation and referenced relationships between literature, authors, journals, and other sources. It involves studying the network or chain relationships among citations to reveal the development and connections within a field, as well as to provide insights into prospects. The more times an author or article is cited in other scholarly articles, the more influential it is in that discipline, enabling the importance of that article or researcher in any given field to be judged.
A simple method of citation analysis is to count the TC to a paper. However, the comparison of a single condition is often too abstract and causes large errors. However, the comparison of a single condition is often too abstract and causes large errors. According to the spreading pattern of academic literature, generally scientific papers reach the peak of citations within 3–5 years after publication, so recently published articles may not receive higher attention, so we will consider the average number of citations per year of the article (AC/Y) as well as the Normalized citation count of the article (NC). We analyzed the most influential articles in the field over the past 20 years, setting the minimum number of citations to 50, resulting in a total of 34 articles, which are shown in Figure 8 , where the size of the points represents the TC, and the shade of the color indicates the year of publication.
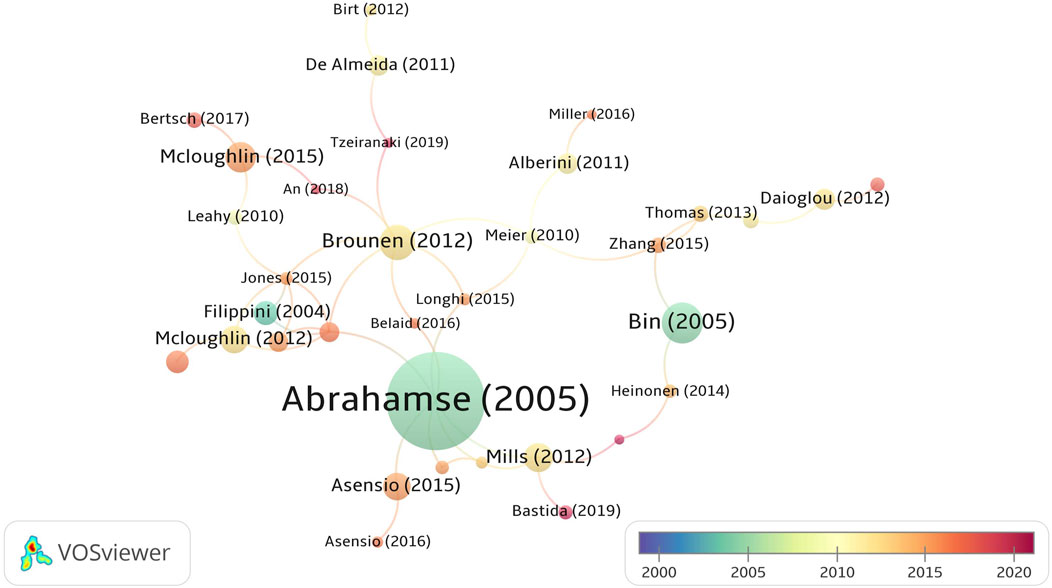
FIGURE 8 . Co-occurrence network map of citations.
Table 5 further shows the top 10 articles and their performance in terms of TC, AC/Y, and NC for the past 20 years of research in HEC. The highest combined score comes from Abrahamse et al. (2005) , reviewing research on the effectiveness of interventions to promote energy efficiency in the household. The articles by McLoughlin et al. (2015) and Asensio and Delmas (2015) ranked second and third, respectively, based on AC/Y. McLoughlin et al. (2015) extends the development of clustering methods in characterizing household electricity demand. Asensio and Delmas (2015) investigates the effectiveness of nonprice incentives in motivating energy-saving behaviour. Bin and Dowlatabadi (2005) ranked third in terms of NC, first proposed the Consumer Lifestyle Approach (CLA), which discusses the importance of consumer activities for energy conservation and emission reduction.
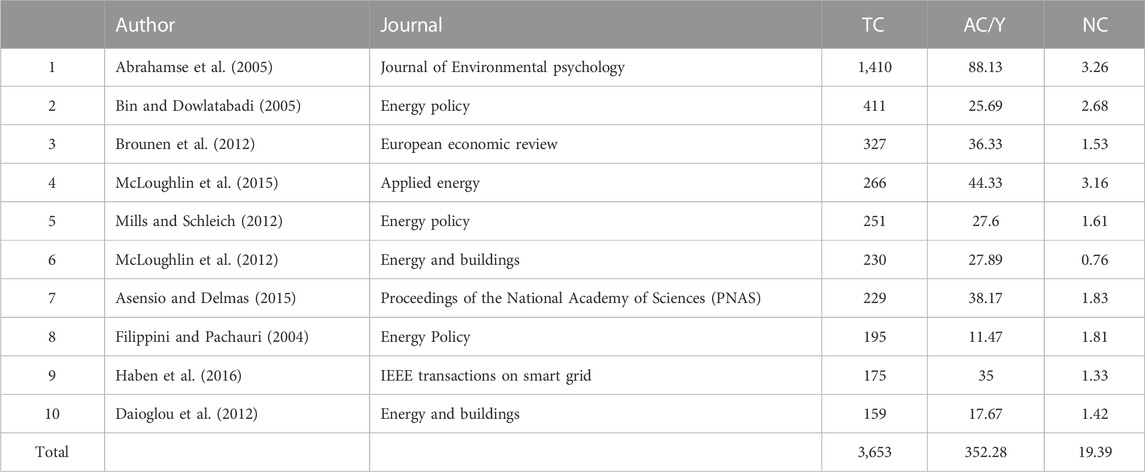
TABLE 5 . The performance of major citations.
4 Research hotspots in HEC
Household activities are attracting more attention for issues such as energy consumption and environmental impact. Research on household energy consumption (HEC) is complex and continuous, involving multiple fields and disciplines. There are variations in the research methods preferred by scholars due to different ways of collecting research data, different scales of research, and different research purposes. Firstly, a filtered search of the dataset was conducted by using the terms model, method, analysis, and quantification to identify the main popular methods and models regarding HEC. This study will discuss the popular research methods in HEC around the mainstream directions of energy use and quantification, environmental impact assessment, exploration of influencing factors. Considering that the behavior, habits, and awareness of households are closely related to changes in household activities and energy consumption, it is necessary to discuss the energy consumption behavior of households.
4.1 Environmental assessment
Environmental assessment plays an important role in the study of HEC, leading to a comprehensive understanding and management of energy use patterns, identification of potential energy waste, and the development of more effective energy management strategies for reducing negative environmental impacts ( Dioha, 2018 ; Bülbül et al., 2023 ).
4.1.1 Input-output approach
Input-output approach (IOA) is an economic model for comprehensive analysis of the quantitative dependence between inputs and outputs in economic activities. According to different measurement methods, it is divided into physical model and value model, dynamic model and static model; according to the research scope, national model, single-region model, inter-region model, sectoral model and enterprise model can be established.
In the field of household energy consumption, IOA is widely applied to the allocation and utilization of energy, as well as the assessment of potential carbon footprints and economic benefits ( Qin et al., 2022 ; Wang F et al., 2023 ). In the selected paper collection, the authors used several types of IOA to carry out their studies on energy use in the household sector. Poom and Ahas (2016) ; Tomas et al. (2020) used the environmentally extended IOA to evaluate household energy use and carbon footprint; ( Baltruszewicz et al., 2021 ; Sato and Narita, 2023 ); studied the composition and inequalities associated with household energy footprint based on the multi-regional input-output (MRIO) model. Quantifying household indirect and direct energy consumption-demand relationships using IOA is a particularly widely studied topic ( Supasa et al., 2017 ; Singh et al., 2018 ; Long et al., 2019 ). In addition, input-output models have established an important role in measuring household carbon emissions, especially in China ( Xu L et al., 2021 ; Tian et al., 2017 ; Zhang and Lei, 2017 ).
Many studies have confirmed the contribution of IOA models in promoting energy efficiency and in developing energy efficiency policies of household ( Stagnitta et al., 2020 ). However, some studies have also shown that energy efficiency improvements are instead a potential risk to retard energy efficiency effects. And IOA models also play an important role in estimating and explaining the energy rebound effect ( Thomas and Azevedo, 2013 ; Wen et al., 2018 ).
4.1.2 Life-cycle assessment
Life-cycle assessment (LCA) is a tool for evaluating the environmental impact of a product or service from production, use to disposal. It can be used to assess the overall environmental impact of a household, including buildings, equipment, water use and waste management ( García-Valiñas et al., 2023 ). LCA has been widely used in the building sector and is one of the most important tools used to assess the building performance ( Utama and Gheewala, 2009 ), energy sustainability in buildings ( Vogt Gwerder et al., 2019 ; Walzberg et al., 2019 ), building environmental impact and stress ( Vega-Azamar et al., 2017 ). Secondly, it has also been widely deployed to evaluate home energy efficiency improvements and explore improved pathways to energy conservation. Nichols and Kockelman (2014) used LCA to confirm that changes in the built environment and increases in population density can promote improvements in home energy efficiency, and ( Stephan and Crawford, 2016 ) quantified the implied energy due to changes in house size that improve the effectiveness of building energy efficiency regulations.
With the emergence of home energy management systems, LCA plays an equally important role in assessing the environmental impacts of smart buildings and home automation ( Louis et al., 2015 ; Louise and Pongracz, 2017 ).
4.2 Influencing factors analysis
Large population sizes and rapidly growing economies lead to large energy consumption and carbon emissions in the residential sector ( Xu L et al., 2021 ), which are threatening environmental sustainability. Identifying and analyzing the key drivers affecting household energy consumption (HEC) has proven to be an important step in improving energy efficiency and pursuing sustainable energy strategies ( Wang et al., 2015 ).
The analysis of factors influencing HEC typically considers two perspectives: internal and external factors within households. Internal factors mainly encompass socio-economic factors, population dynamics, and residential environmental factors ( Mashhoodi et al., 2020 ; Amber et al., 2021 ). In terms of socio-economic factors, characteristics of household members are considered a key factor discussed by many scholars. Apart from individual behavior and attitudes, factors such as household size and age composition of occupants are recognized as important determinants influencing HEC. There are studies that confirm the overburden of older households in terms of energy supply and expenditure ( Berrill et al., 2021 ), and the phenomenon of aging has been explained as a determinant of household energy use efficiency ( Pais-Magalhaes et al., 2020 ). With rapid economic development, household sizes are further shrinking, and small households or nuclear families are becoming the mainstream. This trend is expected to drive a significant increase in residential energy demand in the future ( Zeng et al., 2021 ). In addition to this, the type of residential building and the environment also have an impact on energy consumption patterns to a certain extent ( Namazkhan et al., 2020 ; Moadab et al., 2021 ).
Among the external factors, energy policies as well as climate conditions are the main perspectives discussed by scholars. The implementation of time-of-use electricity pricing plans ( Kwon et al., 2020 ) and energy-efficient appliance subsidy policies ( Nie et al., 2021 ) play a significant role in controlling electricity consumption and reducing energy costs. Climate conditions are a major driving factor in HEC and have been widely applied in estimating household energy use. In developed countries such as Europe and the United States, the number of heating and cooling degree days is an important indicator for measuring household heating and cooling demand ( Adua et al., 2016 ; Alberini et al., 2019 ). In emerging economies, Li established temperature-electricity response functions to predict the impact of climate change on electricity consumption. Additionally, ( Bezerra et al., 2021 ), developed and applied end-use models to assess the changes in HEC due to temperature and relative humidity.
Furthermore, under the global decarbonization goals, climate change has driven increased attention to the sustainable development of future residential energy and the formulation of related policies. For example, in Jordan, the implementation of this goal is reflected in the retrofitting of existing housing for improved energy efficiency ( Bataineh and Alrabee, 2018 ). In India, increasing the supply of low-income housing is considered an avenue for achieving climate mitigation ( Khosla et al., 2019 ). A number of methods or models have been used to develop the analysis of the factors affecting the drivers of household energy consumption, as shown in Table 6 .
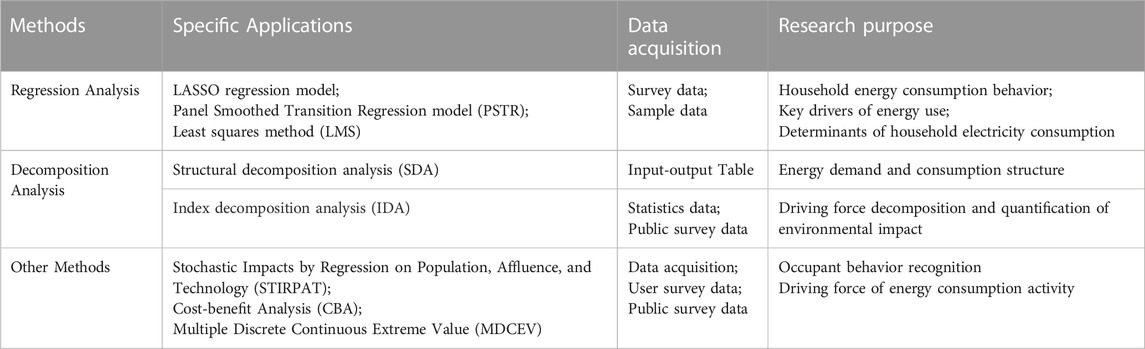
TABLE 6 . The popular methods for influencing factors.
4.2.1 Regression analysis
Economic methods are increasingly used in HEC research. It can help researchers understand household demand for energy and consumption behavior. The elastic response to energy under different economic conditions is analyzed, as well as the impact of price, income and other factors on HEC ( Guo et al., 2023 ). Regression and econometric methods are frequently used methods. It useful to deal with and analyze investigate large data sets ( Greenblatt et al., 2013 ; Copiello and Gabrielli, 2017 ). Hassen et al. (2023) used a multivariate probit model to discuss the behavioral and socio-economic factors that influence energy efficiency technologies in urban households. Shi et al. (2020) developed a LASSO regression model to identify the main drivers of direct or indirect HCEs. Belaid and Garcia (2016) conducted an empirical study on the main factors that motivate energy saving behavior in French households through econometric modeling. Zhang and Wen (2021) develop a Panel Smoothed Transition Regression (PSTR) model and explore the driving effects of urbanization level and income level on household electricity consumption according to empirical data. In addition, Least squares method (LMS) ( Besagni and Borgarello, 2019 ) and Quantile regression model ( Tilov et al., 2020 ) are frequently used to explain the effect of factors such as social-economic and household characteristics on HEC.
4.2.2 Decomposition analysis
Decomposition analysis as an analytical framework for studying the characteristics of changes in things, quantifying environmental impacts and relative contributions, has been widely used in environmental economic research. Structural decomposition analysis (SDA) and Index decomposition analysis (IDA) are the two main categories of decomposition analysis. SDA enables a more detailed description of the energy demand and consumption structure based on input-output models ( Du et al., 2018 ; Kim et al., 2015 ) which also means a higher request for data. While the IDA method is more adaptable and easier to use, the Logarithmic Mean Divisia Index (LMDI) method is the most popular ( Zhao et al., 2023a ). Most studies have used LMDI to quantitatively identify the effects of different factors on changes in energy consumption ( Roman-Collado and Jose Colinet, 2018 ; Meng et al., 2019 ; Reuter et al., 2019 ). In China, scholars paid attention to use LMDI to comprehensively analyze the differences in inter-provincial ( Li et al., 2020 ; Rong et al., 2021 ) and urban-rural ( Chen et al., 2019 ; Yang et al., 2020a ) of energy consumption and carbon emissions in the household sector. In recent years, some scholars have started to focus on the decoupling analysis of REC, Yang et al. (2020b) and Zhang and Bai (2018) combined Tapio decoupling model and LMDI method to evaluate the decoupling relationship between energy consumption and residential income. In addition, the Kaya identity is one of the main methods of decomposition analysis. Chen and Lin (2015) and Serrano et al. (2017) used the Kaya identity to evaluate the effect of economic, policy and demographic factors on the environmental impact.
4.2.3 Other method
The augmented Stochastic Impacts by Regression on Population, Affluence and Technology (STIRPAT) model is also an important method for exploring the factors influencing household energy consumption ( Ding et al., 2016 ). Investigated the spatial heterogeneity of household energy consumption and its influencing factors in China using the STIRPAT model, and the results confirmed that income level is the main factor contributing to the spatial effect. Zhou and Liu (2016) ; Bai et al. (2019) used the STIRPAT model to assess the effects of population, income, and urbanization on energy-related CO 2 . Other methods include numerical modeling, such as structural equation modeling (SEM) ( Boukarta and Berezowska-Azzag, 2018 ), Multiple Discrete Continuous Extreme Value (MDCEV) model ( Pinjari and Bhat, 2021 ), Cost-benefit analysis (CBA) ( Maljkovic et al., 2022 ).
4.3 Behavioral analysis
The consumption of energy generated by household activities is highly behavioral ( Long et al., 2019 ). The lifestyles of residents present a serious threat to energy sustainability. User behavior is considered one of the most significant factors influencing household energy consumption. As the main driver of household activities, more research focuses on exploring user consumption behavior to enhance energy efficiency and reduce environmental impact ( Eirinaki et al., 2022 ). Therefore, exploring the factors influencing households’ consumption behavior is essential to achieve energy efficiency in households. It is worth mentioning that due to the global COVID-19 pandemic in recent years, social distancing and home lockdown measures have exacerbated household energy stress, and behavioral analysis of occupants during the pandemic has generated much attention from scholars as well as policymakers ( Ludtke et al., 2021 ; Tleuken et al., 2021 ). Secondly, during the COVID-19 era, there has been a significant emphasis on energy efficiency and the willingness to purchase energy-saving home appliances ( Jamil et al., 2022 ). Due to the complexity of consumer behavior, which is also influenced by various factors, scholars have adopted different research approaches from different research perspectives.
4.3.1 Theory of planned behavior
The theory of planned behavior (TPB), which consists of the elements of attitudes, subjective norms, perceptual behavioral control, and behavioral intentions and behaviors ( Ajzen, 1991 ), is widely used in behavior-related research fields to predict, and understand human behavior ( Ajzen, 1985 ). The theory assumes that behavioral intention is the most direct factor influencing behavior, and that behavioral intention is in turn influenced by attitudes, subjective norms, and perceived behavioral control. It has been confirmed that TPB and its extended theory contribute to the explanation of household energy saving behavior and to the intervention of residential energy behavior ( Schwartz et al., 2015 ; Ru et al., 2018 ).
Since the theory is mainly based on human behavioral intentions to make judgments, the data sources for the research are mainly obtained through customer questionnaires. The use of TPB theory to explore and identify the role of consumer attitudes, subjective norms, and perceived behavioral control on building energy efficiency behaviors and intentions is commonly studied ( Liu X et al., 2020 ; Ru et al., 2018 ). Khurshid et al. (2023) based on Pakistani household data, combined Structural Equation Modeling (SEM) and TPB to gain insights into the psychological factors and emotions driving energy-saving behaviors. Tan et al. (2017) studied the indirect effects of residents’ willingness to purchase energy-efficient appliances through the extrapolation of personal moral norms in TPB. Adnan and Shahrina (2021) and Masrahi et al. (2021) based on the TPB research model, predicted consumers’ willingness to use renewable energy.
4.3.2 Consumer lifestyle approach
Bin and Dowlatabadi (2005) first proposed the Consumer Lifestyle Approach, which aims to develop a comprehensive consumer-oriented assessment framework for quantifying the environmental impacts of consumer lifestyle factors on energy use and resulting impacts. At the same time, it defines the direct and indirect impacts of consumer activities or use of products from the consumer’s perspective and is widely used to estimate trends in energy consumption and carbon footprint in the household sector. Wang and Yang (2014) used the CLA method to estimate the indirect energy consumption of households in urban and rural areas of China. Wang and Zhang (2015) analyzed the characteristics of direct and indirect household energy consumption in rural China for the period 1988-2011. As carbon emission research fever continues to rise, numerous scholars have analyzed the quantity and trends of household carbon emissions using the CLA method ( Chen et al., 2019 ; Xu et al., 2016 ). And the combination of STIRPAT model ( Jin et al., 2019 ; Wang et al., 2018 ; Wang and Yang, 2014 ) to explore the main influences of household carbon emissions has been favored by many researchers.
4.3.3 Cluster analysis
Cluster analysis is a statistical analysis method that simplifies and mines data by using different algorithms. The sample data are divided into relatively homogeneous clusters, where objects in the same cluster have high similarity and different clusters are heterogeneous from each other. Commonly used clustering algorithms include K-means, K-medoids and CLARANS ( Han et al., 2022 ). K-means algorithm is one of the relatively simple and widely used methods ( Fernandes et al., 2017 ; Trotta et al., 2020 ), its provides a new perspective for identifying occupant behavior and understanding energy use in buildings ( Yu et al., 2011 ). It has also proven to be important in improving and adjusting the usage patterns of residential air conditioning and saving energy costs ( An et al., 2018 ; Mitra et al., 2021 ). With the rise of machine learning, clustering analysis has also gained popularity and has been widely applied to household meter data in recent studies ( Roberts et al., 2019 ; Satre-Meloy et al., 2020 ), providing a basis for understanding residential electricity demand and explaining the driving forces affecting electricity consumption patterns. Cluster analysis has been beneficial in exploring residential water end-use consumption categories ( Makki et al., 2015 ), land use policies, and improving public transportation services ( Zahabi et al., 2012 ).
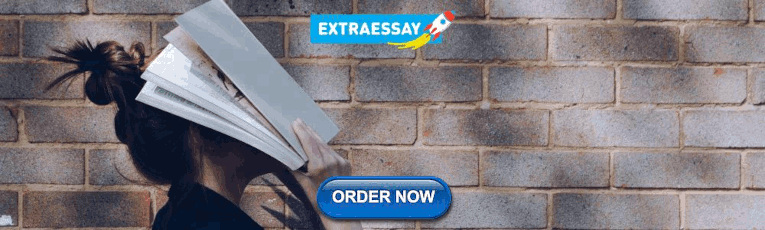
4.3.4 Other methods
With the implementation of Demand Response Programs (DRPs), Household Energy Management systems (HEMS) and its key role in achieving the Sustainable Development Goals ( Ramos et al., 2019 ). It is especially important to monitor the energy use behavior of occupants ( AlSkaif et al., 2018 ), especially in identifying the behavioral habits of electricity users ( Granell et al., 2015 ; Gajowniczek and Zabkowski, 2017 ; Khosla et al., 2019 ). Nakano and Washizu (2019) Kim et al. (2019) analyze and predict the characteristics of household electricity consumption under different behavioral patterns by using the Survey on Time Use. Sadeghianpourhamami et al. (2016) and Haben et al. (2016) based on smart meter data, conduct in-depth analysis of household behavior, and use it as a basis to build an energy load prediction model. Ouyang et al. (2009) and Wu et al. (2017) developed their studies based on the data from microdata surveys. In addition, neural network framework has been applied to analyze and understand residential electricity consumption habits and predict energy loads on the household sector ( Justo et al., 2017 ; Alhussein et al., 2020 ; Estebsari and Rajabi, 2020 ).
5 Exploration of household energy saving
The large share of the household sector in global energy consumption and the implied potential for energy savings is attracting more and more attention ( An et al., 2018 ). In response to global climate action, many countries have actively taken steps to promote sustainable development of household energy and enhance energy efficiency. The EU, for instance, has implemented energy taxation policies aimed at modifying electricity consumption through energy price adjustments. France has provided financial support, including the quelle energie premium and credits from the Family Allowance Fund, to incentivize citizens to reduce energy consumption ( Orset, 2021 ). The Chinese government has established energy efficiency standards and regulations to promote the energy efficiency of energy-consuming products ( Tao and Yu, 2011 ).
However, how to develop and implement public measures to reduce energy consumption is not just a goal for governments or utilities anymore ( Rutland and Aylett, 2008 ). For the supply side, it is effective by promoting renewable and clean energy, green energy-saving technologies to occupants ( Duan et al., 2023 ). For the demand side, how to change their own awareness and participation behavior, and flexible adoption of smart technologies and green energy is important to reduce energy costs and achieve low carbon households. Focusing on energy-saving technologies, energy transition, and behavioral interventions, it discusses the contribution of energy-saving and emission reduction measures in the household sector and the facing challenges.
5.1 Transitioning towards smart energy
The emergence of information and communication technologies has paved the way for the construction of smart cities ( Liaqat et al., 2021 ). And the development of smart cities cannot be achieved without efficient energy services. Residential customers as the main users of electrical energy, have a strong influence on electricity demand due to the randomness of their household activities and the flexibility of their appliance usage patterns ( Wang et al., 2022 ). The promotion and application of smart control systems based on energy monitoring, management, and data analysis play a crucial role in optimizing energy utilization and providing personalized recommendations ( Alsaigh et al., 2023 ; Paneru and Tarigan, 2023 ). The development and diffusion of smart home devices such as smart thermostats, smart lighting and smart appliances support the monitoring and management of energy behavior in the household ( Alhussein et al., 2020 ; Moadab et al., 2021 ), to better control energy use and improve energy efficiency. Figure 9 shows the application of a HEMS in a typical residence ( Zhao et al., 2021 ).
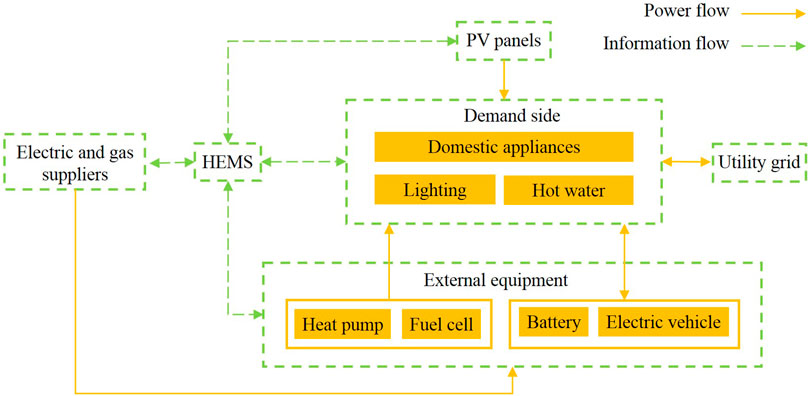
FIGURE 9 . Basic framework of HEMS used in typical household ( Zhao et al., 2021 ).
First, smart home technology allows seamless automation and control of various home systems from a single access point, such as lighting, heating, cooling, etc. , improving quality of life and convenience. In terms of energy management, it can help control electricity usage through automated systems, reducing energy bills and a smaller carbon footprint ( Louis et al., 2015 ; Pang et al., 2021 ). The data monitoring function of smart meters can effectively explore customers’ electricity consumption demand, which also supports the utility companies to optimize customer management, adjust electricity cost and policy innovation ( Gholami et al., 2021 ). Moreover, ( EL Jaouhari et al., 2019 ), proposed the possibility of smart technologies in establishing the interaction between energy, wellbeing, and health management through the study.
Although smart homes offer unlimited possibilities for future smart energy consumption in the household sector and promote the achievement of energy saving and emission reduction goals, there are still many obstacles to their promotion and popularization in households at this stage. Residential customers need to pay a high initial cost for the introduction of a smart home, which discourages many homeowners. In addition to reducing operational and service costs through technological means ( Iqbal et al., 2019 ), deepening customers’ understanding of the value for smart services ( Gonçalves and Patrício, 2022 ) and providing incentives to push the program forward. This will require more in-depth research and surveys ( Srivastava et al., 2019 ). Second, as smart home systems connect more ports, security risks are put to the test ( Komninos et al., 2014 ). The setup and maintenance of smart home technology requires a certain level of technical expertise, and customers inevitably have difficulty setting up and troubleshooting system issues. Thus, it is especially important to help customers gain a deeper understanding of the service and learn about it ( Hollebeek et al., 2019 ), and to support them in taking on new roles.
5.2 Transitioning renewable energy
The continuous growth of energy demand and the transitional dependence on fossil fuels for power generation are the main perpetrators of negative impacts such as climate change and environmental degradation. The adoption of green energy and technologies at the household level is recognized as an effective tool for achieving sustainable economic development in the future ( Eirinaki et al., 2022 ). Sustainable development goals are driving a deep energy transition among the demand side, to reduce the use of carbon energy ( Elmorshedy et al., 2021 ). The rapid growth in electricity consumption has contributed to the widespread adoption of clean and renewable energy sources ( Leitao et al., 2021 ). The use of renewable energy, mainly solar, wind and hydro power, emits almost no pollutants into the atmosphere, and produces no greenhouse gas emissions, which has the advantage of coping with climate change and effectively improves environmental benefits. Secondly, renewable energy comes from domestic sources, effectively reducing the dependence on energy imports, thus improving energy security ( Dioha, 2018 ; Khan et al., 2020 ). Solar energy plays a crucial role in renewable energy and holds significant importance for achieving energy diversification and independence. The United States has implemented solar energy policies, such as investment tax credits to incentivize the development of renewable energy ( Heng et al., 2020 ). In China, Japan, and India are gradually increasing green financing for renewable energy to support sustainable development ( Rahmani et al., 2023 ).
In the household sector, sustainable energy management measures are rapidly developing ( Heinisch et al., 2019 ; Puranen et al., 2021 ). Renewable energy systems consisting of photovoltaics, micro wind turbines, etc. , are becoming increasingly popular ( Camargo et al., 2019 ) and are playing a critical role in the energy transition ( Palm and Eriksson, 2018 ). Although the contribution of renewable energy systems in saving household energy bills as well as environmental benefits has been proven, the high cost of equipment and the stability of energy storage systems have hindered its popularity in the household sector ( Kiprop et al., 2019 ). For users, the high upfront costs and the risks to be taken are one of the main barriers ( Ellabban and Alassi, 2019 ). For national governments, overly heavy energy allowances impose a financial burden ( Hancevic et al., 2017 ). The analysis of technical, energy and economic benefits for renewable energy systems is a topic of focus for scholars ( Lazzeroni et al., 2021 ). Alqahtani and Balta-Ozkan (2021) evaluated the optimal size of PV, battery storage capacity by building a techno-economic model. Figaj et al. (2020) attempted to build a new hybrid power system to reduce the reliance on traditional energy sources for household cooling and heating, but the results of the final simulation showed that it was less economic and competitive than the conventional system due to the high cost of the system components.
In addition, as most renewable energy systems are intermittent, and require energy storage systems to provide a continuous energy supply, their energy flexibility and balance of supply are yet to be tested ( He et al., 2021 ; Li et al., 2017 ). Integrating microgrids to build a power sharing framework is one of the reliable methods available ( Quiggin et al., 2012 ). Microgrids are small systems that integrate distributed energy sources, energy storage systems, and energy conversion, and monitoring devices into one. It helps to optimize generation, distribution, and consumption ( AlSkaif et al., 2017 ). However, as energy storage and distribution systems, it is still a challenge to utilize renewable energy sources within the microgrid to generate electricity ( Bashir et al., 2018 ), optimize community energy planning, and reduce operational and investment costs ( David Mina-Casaran et al., 2021 ; Xu et al., 2022 ).
5.3 Enhancing energy-saving intentions
The molding of occupants’ behaviors toward greater energy-efficient is identified as a crucial approach in decreasing household energy consumption ( Urquizo et al., 2017 ). Especially during the recent pandemic, with increased time spent at home, some households have more frequently utilized devices such as air conditioning, water heaters, and electricity, contributing to the rise in residential energy consumption levels. Beyond the replacement of high-efficiency appliances and the adoption of energy-saving technologies, there is a growing emphasis on the shift in individual energy-saving awareness and behavior ( Bae et al., 2023 ; Conradie et al., 2023 ).
The factors influencing energy-saving behavior are diverse, including socio-economic factors, technological convenience, policy constraints, and individual awareness and attitudes ( Cvetković et al., 2021 ; Nguyen et al., 2023 ). Among them, individual awareness and attitudes are crucial for the initiation and maintenance of energy-saving behavior ( Xu Q et al., 2021 ). The balance between economic costs and benefits is a crucial prerequisite for implementing decisions related to energy-saving behavior, especially in low-income households ( Hottman and Monarch, 2020 ; Birkmann et al., 2022 ). Due to the diversity of household characteristics, there is a higher demand for the availability and convenience of energy-saving technologies, which directly impacts behavioral decisions ( Mills and Schleich, 2012 ; Adua, 2020 ). Additionally, incentives from policies and constraints from regulations can often encourage residents to adopt more energy-efficient behaviors to a certain extent ( Ren et al., 2020 ). Stringent energy efficiency standards are common in European countries to drive households to adopt more efficient heating and cooling systems ( DESNZ, 2023 ); the United States encourages the implementation of energy tax credits to encourage households to invest in energy efficiency improvements ( Adebayo and Alola, 2023 ); and Japan incentivizes households to use smart technologies to improve energy management efficiency ( Zhao et al., 2021 ).
Taking into account these factors, researchers and policymakers often implement behavior intervention policies to motivate occupants change habits, promoting the adoption of more sustainable energy practices. Behavioral interventions are usually carried out through awareness and education, information feedback, incentive measures, and social norms. Knowledge dissemination and education is a key first step in modeling eco-energy behavior ( Grodek-Szostak et al., 2021 ), usually through information campaigns, public services, or workshops. Studies have confirmed that implementing education and awareness campaigns can significantly reduce energy waste ( Song and Leng, 2020 ). Zhang J et al. (2021) evaluated the positive impact of low-carbon knowledge promotion on energy saving behavior by constructing an integrated “knowledge-intention-behavior” model.
Providing real-time feedback to households on their energy use is an incentive to reduce energy consumption ( Ruokamo et al., 2022 ). Most of the information feedback is currently done through smart grid tools, or other monitoring tools ( Kendel et al., 2017 ; Ozawa et al., 2017 ; Lazowski et al., 2018 ) combined with regression analysis, found the impact of energy feedback reports on household lifestyles, and contributed to a reduction in household electricity consumption. The economic incentives and government financial subsidy policies for reducing energy consumption, can motivate households to adopt energy efficiency measures and increase welfare ( Vuelvas and Ruiz, 2017 ; Zhang G et al., 2021 ). In the face of rapid residential electrification, increasing the share of energy-saving appliances in households is one of the effective strategies. However, emerging small appliances have not yet attracted much attention as ownership is not saturated ( Cabeza et al., 2018 ). Interventions based on social norms play an equally important role in shaping energy-efficient behavior ( Alberini and Filippini, 2018 ; Ru et al., 2018 ). Most of the mitigation measures lack heterogeneity in the consumer population, which is not substantial for low-income, elderly households ( Trotta et al., 2020 ).
6 Conclusion
The household sector is a major contributor to the increase of energy consumption and carbon emissions in a country, and the negative environmental impacts caused by its consumption activities and household behavior are seriously hindering sustainable economic and social development. This paper combines a bibliometric approach to summarize and review current work related to HEC. Analysis on the overall structure of the screened 619 papers was conducted, with descriptive statistics on research clusters and major contributors related to the topic, etc. A discussion is focused on the current mainstream research methods, relevant measures regarding household energy efficiency, and attempts to explore the values played and challenges faced by HEC in the global carbon reduction movement. This paper found that,
1. Although HEC studies have been widely conducted, less in-depth studies have been conducted on developing countries. The trend towards reducing carbon emissions and addressing climate change has sparked a global research fervor in the field of household energy efficiency and renewable energy. However, there are variations in focus across different countries and regions. Cold regions such as Canada and the United States prioritize addressing heating demand under extreme climatic conditions, while Japan concentrates on adjusting energy policies and innovating energy-efficient technologies. In developing countries like India, optimizing energy structures and traditional consumption patterns emerge as prevalent trends.
2. For accounting methods or approaches, the IOA has standardized datasets, is relatively easy to use, and is easily adaptable to other methods. But it can only output the environmental impact of the product or service itself and cannot predict the subsequent economic or social impact. Also, the modeling is highly dependent on comprehensive national or local economic data and is not applicable to all regions of the study. LCA can reflect environmental, social, and economic impacts throughout the life cycle, but data collection and practical application are time-consuming and laborious.
3. For analysis methods, TPB and CLA both focus on the influence of consumer behavior on energy consumption by establishing a structural framework. The TPB focuses more on factors such as individual awareness and perceived behavior, The CLA focuses more on the role of social and cultural factors in shaping consumer behavior. Decomposition analysis is easy to understand and highly applicable. It has a prominent position in exploring HEC drivers and can identify and quantify the impact of different factors on energy consumption and environmental change.
4. For technology, many empirical studies have demonstrated the potential of technologies such as smart homes and renewable energy systems to play a role in household energy sustainability, high operational costs, technical and system barriers, lack of customer knowledge and the risks to be taken, etc. , make diffusion extremely difficult.
Finally, this paper puts forward the future research direction of HEC. It is important to evaluate the effectiveness of energy policies and energy standards for the future development of HEC, particularly in developing countries. About household energy consumption behavior, low-income or elderly households will be the key focus. The energy consumption generated by small appliances cannot be ignored and more statistics need to be adopted to compensate. Similarly, we identified limitations in the study. The research on household energy consumption behavior is characterized by a singular data source, predominantly relying on observational data gathered through methods such as questionnaire surveys and interviews. The findings of these studies lack universality. In addition, the definition of HEC is not comprehensive, and the energy consumption of small appliances is often ignored, which hinders the accounting of HEC.
Author contributions
The manuscript was written through the contributions of all authors. TW: conceptualization, methodology, visualization, and writing–original draft. QZ: conceptualization, supervision, conceptualization, and writing–review and editing. WG: supervision, and writing–review and editing. XH: software, and visualization. All authors contributed to the article and approved the submitted version.
This work was supported by International Science and Technology Cooperation Project of the Ministry of Housing and Urban-Rural Development, China (2020-H-002) and the Kitakyushu Innovative Human Resource and Regional Development Program (JST grant code: JPMJSP2149).
Conflict of interest
The authors declare that the research was conducted in the absence of any commercial or financial relationships that could be construed as a potential conflict of interest.
Publisher’s note
All claims expressed in this article are solely those of the authors and do not necessarily represent those of their affiliated organizations, or those of the publisher, the editors and the reviewers. Any product that may be evaluated in this article, or claim that may be made by its manufacturer, is not guaranteed or endorsed by the publisher.
Abrahamse, W., Steg, L., Vlek, C., and Rothengatter, T. (2005). A review of intervention studies aimed at household energy conservation. J. Of Environ. Psychol. 25 (3), 273–291. doi:10.1016/j.jenvp.2005.08.002
CrossRef Full Text | Google Scholar
Abushnaf, J., Rassau, A., and Gornisiewicz, W. (2016). Impact on electricity use of introducing time-of-use pricing to a multi-user home energy management system. Int. Trans. Electr. Energy Syst. 26 (5), 993–1005. doi:10.1002/etep.2118
Adebayo, T. S., and Alola, A. A. (2023). Drivers of natural gas and renewable energy utilization in the USA: how about household energy efficiency-energy expenditure and retail electricity prices? Energy 283, 129022. doi:10.1016/j.energy.2023.129022
Adha, R., Hong, C.-Y., Firmansyah, M., and Paranata, A. (2021). Rebound effect with energy efficiency determinants: a two-stage analysis of residential electricity consumption in Indonesia. Sustain. Prod. Consum. 28, 556–565. doi:10.1016/j.spc.2021.06.019
Adnan, N., and Shahrina, M. N. (2021). A comprehensive approach: diffusion of environment-friendly energy technologies in residential photovoltaic markets. Sustain. Energy Technol. Assessments 46, 101289. doi:10.1016/j.seta.2021.101289
Adua, L. (2020). Reviewing the complexity of energy behavior: technologies, analytical traditions, and household energy consumption data in the United States. Energy Res. Soc. Sci. 59, 101289. doi:10.1016/j.erss.2019.101289
Adua, L., York, R., and Schuelke-Leech, B. (2016). The human dimensions of climate change: a micro-level assessment of views from the ecological modernization, political economy and human ecology perspectives. Soc. Sci. Res. 56, 26–43. doi:10.1016/j.ssresearch.2015.10.003
PubMed Abstract | CrossRef Full Text | Google Scholar
Ajzen, I. (1985). From intentions to actions: a theory of planned behavior . Berlin, Heidelberg: Springer .
Google Scholar
Ajzen, I. (1991). The theory of planned behavior. Theor. Cognitive Self-Regulation 50 (2), 179–211. doi:10.1016/0749-5978(91)90020-T
Alberini, A., and Filippini, M. (2018). Transient and persistent energy efficiency in the US residential sector: evidence from household-level data. ENERGY Effic. 11 (3), 589–601. doi:10.1007/s12053-017-9599-z
Alberini, A., Prettico, G., Shen, C., and Torriti, J. (2019). Hot weather and residential hourly electricity demand in Italy. ENERGY 177, 44–56. doi:10.1016/j.energy.2019.04.051
Alhussein, M., Aurangzeb, K., and Haider, S. (2020). Hybrid CNN-lstm model for short-term individual household load forecasting. IEEE ACCESS 8, 180544–180557. doi:10.1109/ACCESS.2020.3028281
Alqahtani, N., and Balta-Ozkan, N. (2021). Assessment of rooftop solar power generation to meet residential loads in the city of neom, Saudi Arabia. Energies 14 (13), 3805. doi:10.3390/en14133805
Alsaigh, R., Mehmood, R., and Katib, I. (2023). AI explainability and governance in smart energy systems: a review. Front. Energy Res. 11, 1071291. doi:10.3389/fenrg.2023.1071291
AlSkaif, T., Lampropoulos, I., van den Broek, M., and van Sark, W. (2018). Gamification-based framework for engagement of residential customers in energy applications. Energy Res. Soc. Sci. 44, 187–195. doi:10.1016/j.erss.2018.04.043
AlSkaif, T., Luna, A., Zapata, M., Guerrero, J., and Bellalta, B. (2017). Reputation-based joint scheduling of households appliances and storage in a microgrid with a shared battery. Energy Build. 138, 228–239. doi:10.1016/j.enbuild.2016.12.050
Amber, K. P., Ahmad, R., Farmanbar, M., Bashir, M. A., Mehmood, S., Khan, M. S., et al. (2021). Unlocking household electricity consumption in Pakistan. Buildings 11 (11), 566. doi:10.3390/buildings11110566
Amin, A., Bte Mohamed Yusoff, N. Y., Yousaf, H., Peng, S., Işık, C., Akbar, M., et al. (2023). The influence of renewable and non-renewable energy on carbon emissions in Pakistan: evidence from stochastic impacts by regression on population, affluence, and technology model. Front. Environ. Sci. 11, 1182055. doi:10.3389/fenvs.2023.1182055
An, J., Yan, D., and Hong, T. (2018). Clustering and statistical analyses of air-conditioning intensity and use patterns in residential buildings. Energy Build. 174, 214–227. doi:10.1016/j.enbuild.2018.06.035
Andor, M. A., Bernstein, D. H., and Sommer, S. (2021). Determining the efficiency of residential electricity consumption. Empir. Econ. 60 (6), 2897–2923. doi:10.1007/s00181-020-01967-4
Asensio, O., and Delmas, M. (2015). Nonprice incentives and energy conservation. Proc. Of Natl. Acad. Of Sci. Of U. S. A. 112 (6), E510–E515. doi:10.1073/pnas.1401880112
Bae, C., Lee, J. Y., Kim, D., and Chun, C. (2023). A study on the impact of residents’ energy usage behavior on heating energy consumption based on smart meter data and surveys. Energy Build. 300, 113634. doi:10.1016/j.enbuild.2023.113634
Bai, Y., Deng, X., Gibson, J., Zhao, Z., and Xu, H. (2019). How does urbanization affect residential CO2 emissions? An analysis on urban agglomerations of China. J. Of Clean. Prod. 209, 876–885. doi:10.1016/j.jclepro.2018.10.248
Bak, J., and Yoon, S. (2021). Dwelling infiltration and heating energy demand in multifamily high-rise and low-energy buildings in Korea. Renew. Sustain. Energy Rev. 148, 111284. doi:10.1016/j.rser.2021.111284
Baltruszewicz, M., Steinberger, J., Ivanova, D., Brand-Correa, L., Paavola, J., and Owen, A. (2021). Household final energy footprints in Nepal, Vietnam and Zambia: composition, inequality and links to well-being. Environ. Res. Lett. 16 (2), 025011. doi:10.1088/1748-9326/abd588
Bashir, A. A., Kasmaei, M. P., Safdarian, A., and Lehtonen, M. (2018). Matching of local load with on-site PV production in a grid-connected residential building. ENERGIES 11 (9), 2409. doi:10.3390/en11092409
Bataineh, K., and Alrabee, A. (2018). Improving the energy efficiency of the residential buildings in Jordan. Buildings 8 (7), 85. doi:10.3390/buildings8070085
Belaid, F., and Garcia, T. (2016). Understanding the spectrum of residential energy-saving behaviours: French evidence using disaggregated data. Energy Econ. 57, 204–214. doi:10.1016/j.eneco.2016.05.006
Berrill, P., Gillingham, K. T., and Hertwich, E. G. (2021). Drivers of change in US residential energy consumption and greenhouse gas emissions, 1990-2015. Environ. Res. Lett. 16 (3), 034045. doi:10.1088/1748-9326/abe325
Besagni, G., and Borgarello, M. (2019). The socio-demographic and geographical dimensions of fuel poverty in Italy. Energy Res. Soc. Sci. 49, 192–203. doi:10.1016/j.erss.2018.11.007
Bezerra, P., da Silva, F., Cruz, T., Mistry, M., Vasquez-Arroyo, E., Magalar, L., et al. (2021). Impacts of a warmer world on space cooling demand in Brazilian households. Energy Build. 234, 110696. doi:10.1016/j.enbuild.2020.110696
Bhattacharjee, S., and Reichard, G. (2011). Socio-economic factors affecting individual household energy consumption: a systematic review. Energy Sustain. 54686, 891–901. doi:10.1115/ES2011-54615
Bin, S., and Dowlatabadi, H. (2005). Consumer lifestyle approach to US energy use and the related CO2 emissions. Energy Policy 33 (2), 197–208. doi:10.1016/S0301-4215(03)00210-6
Birkmann, J., Liwenga, E., Pandey, R., Boyd, E., Djalante, R., Gemenne, F., et al. (2022). Poverty, livelihoods and sustainable development .
Borozan, D. (2018). Regional-level household energy consumption determinants: the european perspective. Renew. Sustain. Energy Rev. 90, 347–355. doi:10.1016/j.rser.2018.03.038
Boukarta, S., and Berezowska-Azzag, E. (2018). Assessing households’ gas and electricity consumption: a case study of djelfa, Algeria. Quaest. Geogr. 37 (4), 111–129. doi:10.2478/quageo-2018-0034
Brounen, D., Kok, N., and Quigley, J. M. (2012). Residential energy use and conservation: economics and demographics. Eur. Econ. Rev. 56 (5), 931–945. doi:10.1016/j.euroecorev.2012.02.007
Bülbül, H., Topal, A., Özoğlu, B., and Büyükkeklik, A. (2023). Assessment of determinants for households’ pro-environmental behaviours and direct emissions. J. Clean. Prod. 415, 137892. doi:10.1016/j.jclepro.2023.137892
Cabeza, L. F., Urge-Vorsatz, D., Urge, D., Palacios, A., and Barreneche, C. (2018). Household appliances penetration and ownership trends in residential buildings. Renew. Sustain. Energy Rev. 98, 1–8. doi:10.1016/j.rser.2018.09.006
Calvin Nsangou, J., Kenfack, J., Nzotcha, U., and Tamo, T. T. (2020). Assessment of the potential for electricity savings in households in Cameroon: a stochastic frontier approach. Energy 211, 118576. doi:10.1016/j.energy.2020.118576
Camargo, L. R., Nitsch, F., Gruber, K., Valdes, J., Wuth, J., and Dorner, W. (2019). Potential analysis of hybrid renewable energy systems for self-sufficient residential use in Germany and the Czech republic. Energies 12 (21), 4185. doi:10.3390/en12214185
Cao, Q., Kang, W., Xu, S., Sajid, M. J., and Cao, M. (2019). Estimation and decomposition analysis of carbon emissions from the entire production cycle for Chinese household consumption. J. Of Environ. Manag. 247, 525–537. doi:10.1016/j.jenvman.2019.06.044
CESY (2020). China energy statistical yearbook 2019 .
Chang, C., Zhu, N., Yang, K., and Yang, F. (2018). Data and analytics for heating energy consumption of residential buildings: the case of a severe cold climate region of China. Energy Build. 172, 104–115. doi:10.1016/j.enbuild.2018.04.037
Chaudhuri, K., and Huaccha, G. (2023). Who bears the energy cost? Local income deprivation and the household energy efficiency gap. Energy Econ. 127, 107062. doi:10.1016/j.eneco.2023.107062
Chen, C., Liu, G., Meng, F., Hao, Y., Zhang, Y., and Casazza, M. (2019). Energy consumption and carbon footprint accounting of urban and rural residents in Beijing through Consumer Lifestyle Approach. Ecol. Indic. 98, 575–586. doi:10.1016/j.ecolind.2018.11.049
Chen, Y., and Lin, S. (2015). Study on factors affecting energy-related per capita carbon dioxide emission by multi-sectoral of cities: a case study of Tianjin. Nat. Hazards 77 (2), 833–846. doi:10.1007/s11069-015-1632-y
CLEAN Future Act (2021). 117th congress . Available at: https://www.congress.gov/ .
Conradie, P., Van Hove, S., Pelka, S., Karaliopoulos, M., Anagnostopoulos, F., Brugger, H., et al. (2023). Why do people turn down the heat? Applying behavioural theories to assess reductions in space heating and energy consumption in Europe. Energy Res. Soc. Sci. 100, 103059. doi:10.1016/j.erss.2023.103059
Copiello, S., and Gabrielli, L. (2017). Analysis of building energy consumption through panel data: the role played by the economic drivers. Energy Build. 145, 130–143. doi:10.1016/j.enbuild.2017.03.053
Cvetković, D., Nešović, A., and Terzić, I. (2021). Impact of people’s behavior on the energy sustainability of the residential sector in emergency situations caused by COVID-19. Energy Build. 230, 110532. doi:10.1016/j.enbuild.2020.110532
Daioglou, V., van Ruijven, B. J., and van Vuuren, D. P. (2012). Model projections for household energy use in developing countries. Energy 37 (1), 601–615. doi:10.1016/j.energy.2011.10.044
Das, R., Richman, R., and Brown, C. (2018). Demographic determinants of Canada’s households’ adoption of energy efficiency measures: observations from the Households and Environment Survey, 2013. Energy Effic. 11 (2), 465–482. doi:10.1007/s12053-017-9578-4
David Mina-Casaran, J., Fernando Echeverry, D., Arturo Lozano, C., and Navarro-Espinosa, A. (2021). On the value of community association for microgrid development: learnings from multiple deterministic and stochastic planning designs. Appl. Sciences-Basel 11 (14), 6257. doi:10.3390/app11146257
De Boeck, L., Van Asch, S., De Bruecker, P., and Audenaert, A. (2016). Comparison of support policies for residential photovoltaic systems in the major EU markets through investment profitability. Renew. Energy 87 (1), 42–53. doi:10.1016/j.renene.2015.09.063
Desnz, D. (2023). Department for energy security & net zero: powering up Britain . London: HM. Gov .
Ding, Y., Qu, W., Niu, S., Liang, M., Qiang, W., and Hong, Z. (2016). Factors influencing the spatial difference in household energy consumption in China. Sustainability 8 (12), 1285. doi:10.3390/su8121285
Dioha, M. (2018). Modelling the impact of Nigeria household energy policies on energy consumption and CO2 emissions. Eng. Thail. 22 (6), 1–19. doi:10.4186/ej.2018.22.6.1
Du, W., Cohen, A., Shen, G., Ru, M., Shen, H., and Tao, S. (2018). Fuel use trends for boiling water in rural China (1992-2012) and environmental health implications: a national cross-sectional study. Environ. Sci. Technol. 52 (21), 12886–12894. doi:10.1021/acs.est.8b02389
Duan, M., Li, L., Liu, X., Pei, J., and Song, H. (2023). Turning awareness into behavior: meta-analysis of household residential life energy transition behavior from the dual perspective of internal driving forces and external inducing forces. Energy 279, 128072. doi:10.1016/j.energy.2023.128072
Eirinaki, M., Varlamis, I., Dahihande, J., Jaiswal, A., Pagar, A. A., and Thakare, A. (2022). Real-time recommendations for energy-efficient appliance usage in households. Front. Big Data 5, 972206. doi:10.3389/fdata.2022.972206
Elasu, J., Ntayi, J. M., Adaramola, M. S., and Buyinza, F. (2023). Drivers of household transition to clean energy fuels: a systematic review of evidence. Renew. Sustain. Energy Transition 3, 100047. doi:10.1016/j.rset.2023.100047
El Jaouhari, S., Jose Palacios-Garcia, E., Anvari-Moghaddam, A., and Bouabdallah, A. (2019). Integrated management of energy, wellbeing and health in the next generation of smart homes. Sensors 19 (3), 481. doi:10.3390/s19030481
Ellabban, O., and Alassi, A. (2019). Integrated Economic Adoption Model for residential grid-connected photovoltaic systems: an Australian case study. Energy Rep. 5, 310–326. doi:10.1016/j.egyr.2019.02.004
Elmorshedy, M. F., Elkadeem, M. R., Kotb, K. M., Taha, I. B. M., and Mazzeo, D. (2021). Optimal design and energy management of an isolated fully renewable energy system integrating batteries and supercapacitors. Energy Convers. Manag. 245, 114584. doi:10.1016/j.enconman.2021.114584
Energy Efficiency Directive (2018). European commission. Available at: https://energy.ec.europa.eu/ .
Estebsari, A., and Rajabi, R. (2020). Single residential load forecasting using deep learning and image encoding techniques. Electronics 9 (1), 68. doi:10.3390/electronics9010068
Fernandes, M., Viegas, J., Vieira, S., and Sousa, J. (2017). Segmentation of residential gas consumers using clustering analysis. Energies 10 (12), 2047. doi:10.3390/en10122047
Figaj, R., Zoladek, M., and Goryl, W. (2020). Dynamic simulation and energy economic analysis of a household hybrid ground-solar-wind system using TRNSYS software. Energies 13 (14), 3523. doi:10.3390/en13143523
Filippini, M., and Pachauri, S. (2004). Elasticities of electricity demand in urban Indian households. Energy Policy 32 (3), 429–436. doi:10.1016/S0301-4215(02)00314-2
Gajowniczek, K., and Zabkowski, T. (2017). Electricity forecasting on the individual household level enhanced based on activity patterns. PLOS ONE 12 (4), e0174098. doi:10.1371/journal.pone.0174098
Gao, B., Liu, X., Zhang, W., and Tang, Y. (2015). Autonomous household energy management based on a double cooperative game approach in the smart grid. Energies 8 (7), 7326–7343. doi:10.3390/en8077326
García-Valiñas, M., Arbués, F., and Balado-Naves, R. (2023). Assessing environmental profiles: an analysis of water consumption and waste recycling habits. J. Environ. Manag. 348, 119247. doi:10.1016/j.jenvman.2023.119247
Gholami, R., Nishant, R., and Emrouznejad, A. (2021). Modeling residential energy consumption: an application of IT-based solutions and big data analytics for sustainability. J. Glob. Inf. Manag. 29 (2), 166–193. doi:10.4018/JGIM.2021030109
Goldstein, B., Gounaridis, D., and Newell, J. (2020). The carbon footprint of household energy use in the United States. Proc. Natl. Acad. Of Sci. Of U. S. A. 117 (32), 19122–19130. doi:10.1073/pnas.1922205117
Gonçalves, L., and Patrício, L. (2022). From smart technologies to value cocreation and customer engagement with smart energy services. Energy Policy 170, 113249. doi:10.1016/j.enpol.2022.113249
Granell, R., Axon, C., and Wallom, D. (2015). Impacts of raw data temporal resolution using selected clustering methods on residential electricity load profiles. IEEE Trans. Power Syst. 30 (6), 3217–3224. doi:10.1109/TPWRS.2014.2377213
Greenblatt, J., Hopkins, A., Letschert, V., and Blasnik, M. (2013). Energy use of US residential refrigerators and freezers: function derivation based on household and climate characteristics. Energy Effic. 6 (1), 135–162. doi:10.1007/s12053-012-9158-6
Grodek-Szostak, Z., Malinowski, M., Suder, M., Kwiecien, K., Bodziacki, S., Vaverkova, M. D., et al. (2021). Energy conservation behaviors and awareness of polish, Czech and Ukrainian students: a case study. Energies 14 (18), 5599. doi:10.3390/en14185599
Guo, J., Xu, Y., Qu, Y., Wang, Y., and Wu, X. (2023). Exploring factors affecting household energy consumption in the internet era: empirical evidence from Chinese households. Energy Policy 183, 113810. doi:10.1016/j.enpol.2023.113810
Guta, D., Baumgartner, J., Jack, D., Carter, E., Shen, G., Orgill-Meyer, J., et al. (2022). A systematic review of household energy transition in low and middle income countries. Energy Res. Soc. Sci. 86, 102463. doi:10.1016/j.erss.2021.102463
Haben, S., Singleton, C., and Grindrod, P. (2016). Analysis and clustering of residential customers energy behavioral demand using smart meter data. IEEE Trans. Smart Grid 7 (1), 136–144. doi:10.1109/TSG.2015.2409786
Hafner, R. J., Elmes, D., and Read, D. (2019). Promoting behavioural change to reduce thermal energy demand in households: a review. Renew. Sustain. Energy Rev. 102, 205–214. doi:10.1016/j.rser.2018.12.004
Hamilton, I. G., Steadman, P. J., Bruhns, H., Summerfield, A. J., and Lowe, R. (2013). Energy efficiency in the British housing stock: energy demand and the homes energy efficiency database. Energy Policy 60, 462–480. doi:10.1016/j.enpol.2013.04.004
Han, J., Pei, J., and Tong, H. (2022). Data mining: concepts and techniques . Waltham, MA: Morgan kaufmann .
Hancevic, P. I., Nunez, H. M., and Rosellon, J. (2017). Distributed photovoltaic power generation: possibilities, benefits, and challenges for a widespread application in the Mexican residential sector. Energy Policy 110, 478–489. doi:10.1016/j.enpol.2017.08.046
Hassen, S., Anandarajah, G., and Seifemichael, R. (2023). Behavioral and socio-economic determinants of urban households’ investment in energy efficient technologies: evidence from Ethiopia. Front. Energy Res. 11, 1135291. doi:10.3389/fenrg.2023.1135291
He, Y., Zhou, Y., Yuan, J., Liu, Z., Wang, Z., and Zhang, G. (2021). Transformation towards a carbon-neutral residential community with hydrogen economy and advanced energy management strategies. Energy Convers. Manag. 249, 114834. doi:10.1016/j.enconman.2021.114834
Heesen, F., and Madlener, R. (2021). Revisiting heat energy consumption modeling: household production theory applied to field experimental data. Energy Policy 158, 112511. doi:10.1016/j.enpol.2021.112511
Heinisch, V., Odenberger, M., Goransson, L., and Johnsson, F. (2019). Organizing prosumers into electricity trading communities: costs to attain electricity transfer limitations and self-sufficiency goals. Int. J. Energy Res. 43 (13), 4720. doi:10.1002/er.4720
Heng, Y., Lu, C.-L., Yu, L., and Gao, Z. (2020). The heterogeneous preferences for solar energy policies among US households. Energy Policy 137, 111187. doi:10.1016/j.enpol.2019.111187
Hertwich, E. G. (2005). Life cycle approaches to sustainable consumption: a critical review. Environ. Sci. Technol. 39 (13), 4673–4684. doi:10.1021/es0497375
Hollebeek, L. D., Srivastava, R. K., and Chen, T. (2019). SD logic–informed customer engagement: integrative framework, revised fundamental propositions, and application to CRM. J. Acad. Mark. Sci. 47, 161–185. doi:10.1007/s11747-016-0494-5
Hottman, C. J., and Monarch, R. (2020). A matter of taste: estimating import price inflation across U.S. income groups. J. Int. Econ. 127, 103382. doi:10.1016/j.jinteco.2020.103382
Hu, Y., Zhang, Q., Hu, S., Xiao, G., Chen, X., Wang, J., et al. (2022). Research progress and prospects of ecosystem carbon sequestration under climate change (1992–2022). Ecol. Indic. 145, 109656. doi:10.1016/j.ecolind.2022.109656
Iqbal, M., Sajjad, M., Amin, S., Haroon, S., Liaqat, R., Khan, M., et al. (2019). Optimal scheduling of residential home appliances by considering energy storage and stochastically modelled photovoltaics in a grid exchange environment using hybrid grey wolf genetic algorithm optimizer. Appl. Sciences-Basel 9 (23), 5226. doi:10.3390/app9235226
Jafari, H. (2023). Energy storage by improving energy-efficiency of electricity home appliances under governmental supporting policies: a game-theoretic approach. J. Energy Storage 63, 106972. doi:10.1016/j.est.2023.106972
Jamil, K., Dunnan, L., Awan, F. H., Jabeen, G., Gul, R. F., Idrees, M., et al. (2022). Antecedents of consumer’s purchase intention towards energy-efficient home appliances: an agenda of energy efficiency in the post COVID-19 era. Front. Energy Res. 10, 863127. doi:10.3389/fenrg.2022.863127
Jia, W., and Wu, S. (2022). Spatial differences and influencing factors of energy poverty: evidence from provinces in China. Front. Environ. Sci. 10, 921374. doi:10.3389/fenvs.2022.921374
Jiang, L., Xue, B., Xing, R., Chen, X., Song, L., Wang, Y., et al. (2020). Rural household energy consumption of farmers and herders in the Qinghai-Tibet Plateau. Energy 192, 116649. doi:10.1016/j.energy.2019.116649
Jin, X., Li, Y., Sun, D., Zhang, J., and Zheng, J. (2019). Factors controlling urban and rural indirect carbon dioxide emissions in household consumption: a case study in beijing. Sustainability 11 (23), 6563. doi:10.3390/su11236563
Justo, D., Minussi, C., and Lotufo, A. (2017). Behavioral similarity of residential customers using a neural network based on adaptive resonance theory. Sustain. Cities Soc. 35, 483–493. doi:10.1016/j.scs.2017.08.029
Kaufmann, M., Veenman, S., Haarbosch, S., and Jansen, E. (2023). How policy instruments reproduce energy vulnerability—a qualitative study of Dutch household energy efficiency measures. Energy Res. Soc. Sci. 103, 103206. doi:10.1016/j.erss.2023.103206
Kendel, A., Lazaric, N., and Marechal, K. (2017). What do people `learn by looking’ at direct feedback on their energy consumption? Results of a field study in Southern France. Energy Policy 108, 593–605. doi:10.1016/j.enpol.2017.06.020
Khan, Z., Ullah, A., Ullah, W., Rho, S., Lee, M., and Baik, S. (2020). Electrical energy prediction in residential buildings for short-term horizons using hybrid deep learning strategy. Appl. Sciences-Basel 10 (23), 8634. doi:10.3390/app10238634
Khosla, R., Sircar, N., and Bhardwaj, A. (2019). Energy demand transitions and climate mitigation in low-income urban households in India. Environ. Res. Lett. 14 (9), 095008. doi:10.1088/1748-9326/ab3760
Khurshid, N., Shah, A., and Munir, F. (2023). Impact of socio-demographic, psychological and emotional factors on household direct and indirect electricity saving behavior: a case study of Pakistan. J. Clean. Prod. 429, 139581. doi:10.1016/j.jclepro.2023.139581
Kim, S., Jung, S., and Baek, S. (2019). A model for predicting energy usage pattern types with energy consumption information according to the behaviors of single-person households in South Korea. Sustainability 11 (1), 245. doi:10.3390/su11010245
Kim, Y.-G., Yoo, J., and Oh, W. (2015). Driving forces of rapid CO2 emissions growth: a case of Korea. Energy Policy 82, 144–155. doi:10.1016/j.enpol.2015.03.017
Kiprop, E., Matsui, K., and Maundu, N. (2019). The role of household consumers in adopting renewable energy technologies in Kenya. Environments 6 (8), 95. doi:10.3390/environments6080095
Komninos, N., Philippou, E., and Pitsillides, A. (2014). Survey in smart grid and smart home security: issues, challenges and countermeasures. IEEE Commun. Surv. Tutorials 16 (4), 1933–1954. doi:10.1109/COMST.2014.2320093
Kumar, P., Caggiano, H., Shwom, R., Felder, F. A., and Andrews, C. J. (2023). Saving from home! How income, efficiency, and curtailment behaviors shape energy consumption dynamics in US households? Energy 271, 126988. doi:10.1016/j.energy.2023.126988
Kwon, Y., Kim, T., Baek, K., and Kim, J. (2020). Multi-objective optimization of home appliances and electric vehicle considering customer’s benefits and offsite shared photovoltaic curtailment. Energies 13 (11), 2852. doi:10.3390/en13112852
Lazowski, B., Parker, P., and Rowlands, I. H. (2018). Towards a smart and sustainable residential energy culture: assessing participant feedback from a long-term smart grid pilot project. Energy Sustain. Soc. 8, 27. doi:10.1186/s13705-018-0169-9
Lazzeroni, P., Mariuzzo, I., Quercio, M., and Repetto, M. (2021). Economic, energy, and environmental analysis of PV with battery storage for Italian households. Electronics 10 (2), 146. doi:10.3390/electronics10020146
Leitao, J., Fonseca, C., Gil, P., Ribeiro, B., and Cardoso, A. (2021). A compressive receding horizon approach for smart home energy management. IEEE Access 9, 100407–100435. doi:10.1109/ACCESS.2021.3093277
Li, H., Zhao, Y., Wang, S., and Liu, Y. (2020). Spatial-temporal characteristics and drivers of the regional residential CO2 emissions in China during 2000-2017. J. Clean. Prod. 276, 124116. doi:10.1016/j.jclepro.2020.124116
Li, R., Dane, G., Finck, C., and Zeiler, W. (2017). Are building users prepared for energy flexible buildings? A large-scale survey in The Netherlands. Appl. Energy 203, 623–634. doi:10.1016/j.apenergy.2017.06.067
Liaqat, R., Sajjad, I., Waseem, M., and Alhelou, H. (2021). Appliance level energy characterization of residential electricity demand: prospects, challenges and recommendations. IEEE Access 9, 148676–148697. doi:10.1109/ACCESS.2021.3123196
Liu, L., Qu, J., Maraseni, T. N., Niu, Y., Zeng, J., Zhang, L., et al. (2020). Household CO2 emissions: current status and future perspectives. Int. J. Environ. Res. Public Health 17 (19), 7077. doi:10.3390/ijerph17197077
Liu, X., Wang, Q., Wei, H., Chi, H., Ma, Y., and Jian, I. (2020). Psychological and demographic factors affecting household energy-saving intentions: a TPB-based study in northwest China. Sustainability 12 (3), 836. doi:10.3390/su12030836
Long, Y., Yoshida, Y., Meng, J., Guan, D., Yao, L., and Zhang, H. (2019). Unequal age-based household emission and its monthly variation embodied in energy consumption—a cases study of Tokyo, Japan. Appl. Energy 247, 350–362. doi:10.1016/j.apenergy.2019.04.019
Louis, J.-N., Calo, A., Leiviskä, K., and Pongrácz, E. (2015). Environmental impacts and benefits of smart home automation: life cycle assessment of home energy management system. 8th Vienna Int. Conf. Math. Model. 48 (1), 880–885. doi:10.1016/j.ifacol.2015.05.158
Louise, J., and Pongracz, E. (2017). Life cycle impact assessment of home energy management systems (HEMS) using dynamic emissions factors for electricity in Finland. Environ. Impact Assess. Rev. 67, 109–116. doi:10.1016/j.eiar.2017.08.009
Ludtke, D., Luetkemeier, R., Schneemann, M., and Liehr, S. (2021). Increase in daily household water demand during the first wave of the covid-19 pandemic in Germany. Water 13 (3), 260. doi:10.3390/w13030260
Luo, J., Yang, J., Wan, S., Zhao, J., and Liang, J. (2023). Can energy conservation and emission reduction policies affect household carbon emissions? Evidence from China. Front. Energy Res. 11, 1138467. doi:10.3389/fenrg.2023.1138467
Mainimo, E. N., Okello, D. M., Mambo, W., and Mugonola, B. (2022). Drivers of household demand for cooking energy: a case of Central Uganda. Heliyon 8 (3), e09118. doi:10.1016/j.heliyon.2022.e09118
Makki, A., Stewart, R., Beal, C., and Panuwatwanich, K. (2015). Novel bottom-up urban water demand forecasting model: revealing the determinants, drivers and predictors of residential indoor end-use consumption. Resour. Conservation Recycl. 95, 15–37. doi:10.1016/j.resconrec.2014.11.009
Maljkovic, D., Lenz, N., and Zikovic, S. (2022). The pitfalls of shared metering: does the self-interest in district heating systems cause tragedy of the commons. Energy Res. Soc. Sci. 83, 102335. doi:10.1016/j.erss.2021.102335
Mashhoodi, B., Stead, D., and van Timmeren, A. (2020). Spatializing household energy consumption in The Netherlands: socioeconomic, urban morphology, microclimate, land surface temperature and vegetation data. Data Brief 29, 105118. doi:10.1016/j.dib.2020.105118
Masrahi, A., Wang, J., and Abudiyah, A. (2021). Factors influencing consumers’ behavioral intentions to use renewable energy in the United States residential sector. Energy Rep. 7, 7333–7344. doi:10.1016/j.egyr.2021.10.077
McAndrew, R., Mulcahy, R., Gordon, R., and Russell-Bennett, R. (2021). Household energy efficiency interventions: a systematic literature review. Energy Policy 150, 112136. doi:10.1016/j.enpol.2021.112136
McLoughlin, F., Duffy, A., and Conlon, M. (2012). Characterising domestic electricity consumption patterns by dwelling and occupant socio-economic variables: an Irish case study. Energy Build. 48, 240–248. doi:10.1016/j.enbuild.2012.01.037
McLoughlin, F., Duffy, A., and Conlon, M. (2015). A clustering approach to domestic electricity load profile characterisation using smart metering data. Appl. Energy 141, 190–199. doi:10.1016/j.apenergy.2014.12.039
MEE (2022). Progress on the implementation of China’s nationally determined contributions . Ministry of Ecology and Environment of People’s Republic of China https://www.mee.gov.cn/ .
Meng, M., Wu, S., Zhou, J., and Wang, X. (2019). What is currently driving the growth of China’s household electricity consumption? A clustering and decomposition analysis. Sustainability 11 (17), 4648. doi:10.3390/su11174648
Michelle Lewis (2022). 26% of US households use electricity as their only energy source – but there’s a caveat . https://electrek.co/ .
Mills, B., and Schleich, J. (2012). Residential energy-efficient technology adoption, energy conservation, knowledge, and attitudes: an analysis of European countries. Energy Policy 49, 616–628. doi:10.1016/j.enpol.2012.07.008
Mitra, D., Chu, Y., and Cetin, K. (2021). Cluster analysis of occupancy schedules in residential buildings in the United States. Energy Build. 236, 110791. doi:10.1016/j.enbuild.2021.110791
Moadab, N., Olsson, T., Fischl, G., and Aries, M. (2021). Smart versus conventional lighting in apartments-Electric lighting energy consumption simulation for three different households. Energy Build. 244, 111009. doi:10.1016/j.enbuild.2021.111009
Nakano, S., and Washizu, A. (2019). In which time slots can people save power? An analysis using a Japanese survey on time use. Sustainability 11 (16), 4444. doi:10.3390/su11164444
Namazkhan, M., Albers, C., and Steg, L. (2020). A decision tree method for explaining household gas consumption: the role of building characteristics, socio-demographic variables, psychological factors and household behaviour. Renew. Sustain. Energy Rev. 119, 109542. doi:10.1016/j.rser.2019.109542
Nguyen, T. T., Nguyen, D. N., and Pham, H. T. L. (2023). Survey data on energy-saving policies, energy price, crisis and household energy-saving behavior. Data Brief 51, 109646. doi:10.1016/j.dib.2023.109646
Nichols, B., and Kockelman, K. (2014). Life-cycle energy implications of different residential settings: recognizing buildings, travel, and public infrastructure. Energy Policy 68, 232–242. doi:10.1016/j.enpol.2013.12.062
Nie, H., Zhou, T., Lu, H., and Huang, S. (2021). Evaluation of the efficiency of Chinese energy-saving household appliance subsidy policy: an economic benefit perspective. Energy Policy 149, 112059. doi:10.1016/j.enpol.2020.112059
Oladokun, M. G., and Odesola, I. A. (2015). Household energy consumption and carbon emissions for sustainable cities–A critical review of modelling approaches. Int. J. Sustain. Built Environ. 4 (2), 231–247. doi:10.1016/j.ijsbe.2015.07.005
Orset, C. (2021). Is information a good policy instrument to influence the energy behaviour of households? Energy Econ. 102, 105451. doi:10.1016/j.eneco.2021.105451
Ouyang, J., Gao, L., Yan, Y., Hokao, K., and Ge, J. (2009). Effects of improved consumer behavior on energy conservation in the urban residential sector of hangzhou, China. J. Asian Archit. Build. Eng. 8 (1), 243–249. doi:10.3130/jaabe.8.243
Ozawa, A., Furusato, R., and Yoshida, Y. (2017). Tailor-made feedback to reduce residential electricity consumption: the effect of information on household lifestyle in Japan. Sustainability 9 (4), 528. doi:10.3390/su9040528
Pais-Magalhaes, V., Moutinho, V., and Robaina, M. (2020). Determinants of the household electricity consumption efficiency of an ageing population: evidence for the EU-28. Energy Rep. 6, 415–422. doi:10.1016/j.egyr.2020.11.193
Palm, J., and Eriksson, E. (2018). Residential solar electricity adoption: how households in Sweden search for and use information. Energy Sustain. Soc. 8, 14. doi:10.1186/s13705-018-0156-1
Paneru, C. P., and Tarigan, A. K. M. (2023). Reviewing the impacts of smart energy applications on energy behaviours in Norwegian households. Renew. Sustain. Energy Rev. 183, 113511. doi:10.1016/j.rser.2023.113511
Pang, Z., Chen, Y., Zhang, J., O’Neill, Z., Cheng, H., and Dong, B. (2021). How much HVAC energy could be saved from the occupant-centric smart home thermostat: a nationwide simulation study. Appl. Energy 283, 116251. doi:10.1016/j.apenergy.2020.116251
Pinjari, A., and Bhat, C. (2021). Computationally efficient forecasting procedures for Kuhn-Tucker consumer demand model systems: application to residential energy consumption analysis. J. Choice Model. 39, 100283. doi:10.1016/j.jocm.2021.100283
Poom, A., and Ahas, R. (2016). How does the environmental load of household consumption depend on residential location? Sustainability 8 (9), 799. doi:10.3390/su8090799
Puranen, P., Kosonen, A., and Ahola, J. (2021). Techno-economic viability of energy storage concepts combined with a residential solar photovoltaic system: a case study from Finland. Appl. Energy 298, 117199. doi:10.1016/j.apenergy.2021.117199
Qerimi, D., Dimitrieska, C., Vasilevska, S., and Rrecaj, A. A. (2020). Modeling of the solar thermal energy use in urban areas. Civ. Eng. Journal-Tehran 6 (7), 1349–1367. doi:10.28991/cej-2020-03091553
Qin, X., Wu, H., Zhang, X., and Wang, W. (2022). The widening wealth inequality as a contributor to increasing household carbon emissions. Front. Earth Sci. 10, 872806. doi:10.3389/feart.2022.872806
Quiggin, D., Cornell, S., Tierney, M., and Buswell, R. (2012). A simulation and optimisation study: towards a decentralised microgrid, using real world fluctuation data. Energy 41 (1), 549–559. doi:10.1016/j.energy.2012.02.007
Rahmani, A., Mashayekh, J., Aboojafari, R., and Bonyadi Naeini, A. (2023). Determinants of households’ intention for investment in renewable energy projects. Renew. Energy 205, 823–837. doi:10.1016/j.renene.2023.01.096
Ramapragada, P., Tejaswini, D., Garg, V., Mathur, J., and Gupta, R. (2022). Investigation on air conditioning load patterns and electricity consumption of typical residential buildings in tropical wet and dry climate in India. Energy Inf. 5, 61. doi:10.1186/s42162-022-00228-1
Ramos, J., Moreno, M., Delgado, M., Dominguez, S., and Cabeza, L. (2019). Potential of energy flexible buildings: evaluation of DSM strategies using building thermal mass. Energy Build. 203, 109442. doi:10.1016/j.enbuild.2019.109442
Ren, G., Sunikka-Blank, M., and Zhang, X. (2020). Young urban households in Shanghai, China: characteristics of energy use and attitudes. Sustain. Cities Soc. 60, 102174. doi:10.1016/j.scs.2020.102174
Reuter, M., Patel, M. K., and Eichhammer, W. (2019). Applying ex post index decomposition analysis to final energy consumption for evaluating European energy efficiency policies and targets. Energy Effic. 12 (5), 1329–1357. doi:10.1007/s12053-018-09772-w
Roberts, M., Haghdadi, N., Bruce, A., and MacGill, I. (2019). Characterisation of Australian apartment electricity demand and its implications for low-carbon cities. Energy 180, 242–257. doi:10.1016/j.energy.2019.04.222
Roman-Collado, R., and Jose Colinet, M. (2018). Are labour productivity and residential living standards drivers of the energy consumption changes? Energy Econ. 74, 746–756. doi:10.1016/j.eneco.2018.07.030
Rong, Y., Jia, J., Ju, M., Chen, C., Zhou, Y., and Zhong, Y. (2021). Multi-perspective analysis of household carbon dioxide emissions from direct energy consumption by the methods of logarithmic mean Divisia index and sigma convergence in Central China. Sustainability 13 (16), 9285. doi:10.3390/su13169285
Ru, X., Wang, S., and Yan, S. (2018). Exploring the effects of normative factors and perceived behavioral control on individual’s energy-saving intention: an empirical study in eastern China. Resour. Conservation Recycl. 134, 91–99. doi:10.1016/j.resconrec.2018.03.001
Ruokamo, E., Merilainen, T., Karhinen, S., Raiha, J., Suur-Uski, P., Timonen, L., et al. (2022). The effect of information nudges on energy saving: observations from a randomized field experiment in Finland. Energy Policy 161, 112731. doi:10.1016/j.enpol.2021.112731
Rutland, T., and Aylett, A. (2008). The work of policy: actor networks, governmentality, and local action on climate change in portland, Oregon. Environ. Plan. D Soc. Space 26 (4), 627–646. doi:10.1068/d6907
Sadeghianpourhamami, N., Demeester, T., Benoit, D., Strobbe, M., and Develder, C. (2016). Modeling and analysis of residential flexibility: timing of white good usage. Appl. Energy 179, 790–805. doi:10.1016/j.apenergy.2016.07.012
Sato, I., and Narita, D. (2023). Use of nested multi-regional input–output analysis for the evaluation of subnational emission reduction policies: a case of Japanese prefectures. Sustain. Prod. Consum. 37, 294–305. doi:10.1016/j.spc.2023.03.003
Satre-Meloy, A., Diakonova, M., and Grunewald, P. (2020). Cluster analysis and prediction of residential peak demand profiles using occupant activity data. Appl. Energy 260, 114246. doi:10.1016/j.apenergy.2019.114246
Schwartz, D., Bruine de Bruin, W., Fischhoff, B., and Lave, L. (2015). Advertising energy saving programs: the potential environmental cost of emphasizing monetary savings. J. Exp. Psychol. Appl. 21 (2), 158–166. doi:10.1037/xap0000042
Serrano, S., Uerge-Vorsatz, D., Barreneche, C., Palacios, A., and Cabeza, L. F. (2017). Heating and cooling energy trends and drivers in Europe. Energy 119, 425–434. doi:10.1016/j.energy.2016.12.080
Sharma, A. (2023). Economic Survey 2023: India aims 45% reduction in emission intensity of its GDP by 2030 from 2005 levels. Available at: https://www.cnbctv18.com/ .
Shi, S., Li, H., Ding, X., and Gao, X. (2020). Effects of household features on residential window opening behaviors: a multilevel logistic regression study. Build. Environ. 170, 106610. doi:10.1016/j.buildenv.2019.106610
Singh, V., Henriques, C., and Martins, A. (2018). Fostering investment on energy efficient appliances in India A multi perspective economic input-output lifecycle assessment. Energy 149, 1022–1035. doi:10.1016/j.energy.2018.01.140
Song, S., and Leng, H. (2020). Modeling the household electricity usage behavior and energy-saving management in severely cold regions. Energies 13 (21), 5581. doi:10.3390/en13215581
Srivastava, A., Van Passel, S., and Laes, E. (2019). Dissecting demand response: a quantile analysis of flexibility, household attitudes, and demographics. Energy Res. Soc. Sci. 52, 169–180. doi:10.1016/j.erss.2019.02.011
Stagnitta, R., Rocco, M., and Colombo, E. (2020). A complementary approach to traditional energy balances for assessing energy efficiency measures in final uses: the case of space heating and cooling in Argentina. Sustainability 12 (16), 6563. doi:10.3390/su12166563
Stephan, A., and Crawford, R. (2016). The relationship between house size and life cycle energy demand: implications for energy efficiency regulations for buildings. Energy 116, 1158–1171. doi:10.1016/j.energy.2016.10.038
Stojilovska, A., Guyet, R., Mahoney, K., Gouveia, J. P., Castaño-Rosa, R., Živčič, L., et al. (2022). Energy poverty and emerging debates: beyond the traditional triangle of energy poverty drivers. Energy Policy 169, 113181. doi:10.1016/j.enpol.2022.113181
Su, S., Ding, Y., Li, G., Li, X., Li, H., Skitmore, M., et al. (2023). Temporal dynamic assessment of household energy consumption and carbon emissions in China: from the perspective of occupants. Sustain. Prod. Consum. 37, 142–155. doi:10.1016/j.spc.2023.02.014
Subbiah, R., Pal, A., Nordberg, E. K., Marathe, A., and Marathe, M. V. (2017). Energy demand model for residential sector: a first principles approach. IEEE Trans. Sustain. Energy 8 (3), 1215–1224. doi:10.1109/TSTE.2017.2669990
Supasa, T., Hsiau, S., Lin, S., Wongsapai, W., and Wu, J. (2017). Household energy consumption behaviour for different demographic regions in Thailand from 2000 to 2010. Sustainability 9 (12), 2328. doi:10.3390/su9122328
Tan, C., Ooi, H., and Goh, Y. (2017). A moral extension of the theory of planned behavior to predict consumers’ purchase intention for energy-efficient household appliances in Malaysia. Energy Policy 107, 459–471. doi:10.1016/j.enpol.2017.05.027
Taniguchi-Matsuoka, A., Shimoda, Y., Sugiyama, M., Kurokawa, Y., Matoba, H., Yamasaki, T., et al. (2020). Evaluating Japan’s national greenhouse gas reduction policy using a bottom-up residential end-use energy simulation model. Appl. Energy 279, 115792. doi:10.1016/j.apenergy.2020.115792
Tao, J., and Yu, S. (2011). Implementation of energy efficiency standards of household refrigerator/freezer in China: potential environmental and economic impacts. Appl. Energy 88 (5), 1890–1905. doi:10.1016/j.apenergy.2010.11.015
Thomas, B., and Azevedo, I. (2013). Estimating direct and indirect rebound effects for US households with input-output analysis Part 1: theoretical framework. Ecol. Econ. 86, 199–210. doi:10.1016/j.ecolecon.2012.12.003
Tian, J., Lumbreras, J., Andrade, C., and Liao, H. (2017). Key sectors in carbon footprint responsibility at the city level: a case study of Beijing. Int. J. Clim. Change Strategies Manag. 9 (6), 749–776. doi:10.1108/IJCCSM-01-2017-0009
Tilov, I., Farsi, M., and Volland, B. (2020). From frugal Jane to wasteful John: a quantile regression analysis of Swiss households’ electricity demand. Energy Policy 138, 111246. doi:10.1016/j.enpol.2020.111246
Tleuken, A., Tokazhanov, G., Serikbay, A., Zhalgasbayev, K., Guney, M., Turkyilmaz, A., et al. (2021). Household water and energy consumption changes during COVID-19 pandemic lockdowns: cases of the kazakhstani cities of almaty, shymkent, and atyrau. Buildings 11 (12), 663. doi:10.3390/buildings11120663
Tomas, M., Lopez, L., and Monsalve, F. (2020). Carbon footprint, municipality size and rurality in Spain: inequality and carbon taxation. J. Clean. Prod. 266, 121798. doi:10.1016/j.jclepro.2020.121798
Trotta, G., Gram-Hanssen, K., and Jorgensen, P. (2020). Heterogeneity of electricity consumption patterns in vulnerable households. Energies 13 (18), 4713. doi:10.3390/en13184713
UNEP (2022). Renewable energy – powering a safer future . https://www.unep.org/ .
Urquizo, J., Calderon, C., and James, P. (2017). Metrics of urban morphology and their impact on energy consumption: a case study in the United Kingdom. Energy Res. Soc. Sci. 32 (SI), 193–206. doi:10.1016/j.erss.2017.03.011
Utama, A., and Gheewala, S. H. (2009). Indonesian residential high rise buildings: a life cycle energy assessment. Energy Build. 41 (11), 1263–1268. doi:10.1016/j.enbuild.2009.07.025
Vega-Azamar, R., Romero-Lopez, R., Oropeza-Garcia, N., Glaus, M., Hausler, R., and Silva-Poot, H. (2017). Emergy evaluation of dwelling operation in five housing units of Montreal island, Canada. Sustainability 9 (4), 663. doi:10.3390/su9040663
Verma, P., and Raghubanshi, A. S. (2018). Urban sustainability indicators: challenges and opportunities. Ecol. Indic. 93, 282–291. doi:10.1016/j.ecolind.2018.05.007
Vogt Gwerder, Y., Marques, P., Dias, L. C., and Freire, F. (2019). Life beyond the grid: a Life-Cycle Sustainability Assessment of household energy needs. Appl. Energy 255, 113881. doi:10.1016/j.apenergy.2019.113881
Vuelvas, J., and Ruiz, F. (2017). Rational consumer decisions in a peak time rebate program. Electr. Power Syst. Res. 143, 533–543. doi:10.1016/j.epsr.2016.11.001
Walzberg, J., Dandres, T., Merveille, N., Cheriet, M., and Samson, R. (2019). Accounting for fluctuating demand in the life cycle assessments of residential electricity consumption and demand-side management strategies. J. Clean. Prod. 240, 118251. doi:10.1016/j.jclepro.2019.118251
Wang, F., Xu, B., Si, Y., Shang, Y., Zhang, W., Cai, B., et al. (2023). Components and drivers of household water footprint inequality in China. Sustain. Prod. Consum. 43, 1–14. doi:10.1016/j.spc.2023.10.009
Wang, Q., Liu, P., Yuan, X., Cheng, X., Ma, R., Mu, R., et al. (2015). Structural evolution of household energy consumption: a China study. Sustainability 7 (4), 3919–3932. doi:10.3390/su7043919
Wang, T., Zhao, Q., Gao, W., and He, X. (2023). Subdividing end-use energy consumption based on household characteristics and climate conditions: insights from urban China. Front. Energy Res. 11, 1267975. doi:10.3389/fenrg.2023.1267975
Wang, W., and Zhang, M. (2015). Direct and indirect energy consumption of rural households in China. Nat. Hazards 79 (3), 1693–1705. doi:10.1007/s11069-015-1921-5
Wang, X., Ding, C., Zhou, M., Cai, W., Ma, X., and Yuan, J. (2023). Assessment of space heating consumption efficiency based on a household survey in the hot summer and cold winter climate zone in China. Energy 274, 127381. doi:10.1016/j.energy.2023.127381
Wang, Y., Fu, Y., Lin, H., Sun, Q., Scartezzini, J., and Wennersten, R. (2022). Uncertainty modeling of household appliance loads for smart energy management. Energy Rep. 8, 232–237. doi:10.1016/j.egyr.2021.11.097
Wang, Y., Yang, G., Dong, Y., Cheng, Y., and Shang, P. (2018). The scale, structure and influencing factors of total carbon emissions from households in 30 provinces of China-based on the extended STIRPAT model. ENERGIES 11 (5), 1125. doi:10.3390/en11051125
Wang, Z., and Yang, L. (2014). Indirect carbon emissions in household consumption: evidence from the urban and rural area in China. J. Clean. Prod. 78, 94–103. doi:10.1016/j.jclepro.2014.04.041
Wen, F., Ye, Z., Yang, H., and Li, K. (2018). Exploring the rebound effect from the perspective of household: an analysis of China’s provincial level. Energy Econ. 75, 345–356. doi:10.1016/j.eneco.2018.08.018
Wiedmann, T. (2009). A review of recent multi-region input–output models used for consumption-based emission and resource accounting. Ecol. Econ. 69 (2), 211–222. doi:10.1016/j.ecolecon.2009.08.026
Wu, J., Hou, B., Ke, R., Du, Y., Wang, C., Li, X., et al. (2017). Residential fuel choice in rural areas: field research of two counties of north China. Sustainability 9 (4), 609. doi:10.3390/su9040609
Xu, L., Geng, Y., Wu, D., Zhang, C., and Xiao, S. (2021). Carbon footprint of residents’ housing consumption and its driving forces in China. Energies 14 (13), 3890. doi:10.3390/en14133890
Xu, L., Liu, J., Pei, J., and Han, X. (2013). Building energy saving potential in Hot Summer and Cold Winter (HSCW) Zone, China—influence of building energy efficiency standards and implications. Energy Policy 57, 253–262. doi:10.1016/j.enpol.2013.01.048
Xu, Q., Hwang, B.-G., and Lu, Y. (2021). Exploring the influencing paths of behavior-driven household energy-saving intervention – household energy saving option (HESO). Sustain. Cities Soc. 71, 102951. doi:10.1016/j.scs.2021.102951
Xu, T., Gao, W., Qian, F., and Li, Y. (2022). The implementation limitation of variable renewable energies and its impacts on the public power grid. Energy 239, 121992. doi:10.1016/j.energy.2021.121992
Xu, X., Gou, X., Zhang, W., Zhao, Y., and Xu, Z. (2023). A bibliometric analysis of carbon neutrality: research hotspots and future directions. Heliyon 9 (8), e18763. doi:10.1016/j.heliyon.2023.e18763
Xu, X., Han, L., and Lv, X. (2016). Household carbon inequality in urban China, its sources and determinants. Ecol. Econ. 128, 77–86. doi:10.1016/j.ecolecon.2016.04.015
Yang, Y., Jia, J., and Chen, C. (2020a). Residential energy-related CO2 emissions in China’s less developed regions: a case study of jiangxi. Sustainability 12 (5), 2000. doi:10.3390/su12052000
Yang, Y., Jia, J., Devlin, A., Zhou, Y., Xie, D., and Ju, M. (2020b). Decoupling and decomposition analysis of residential energy consumption from economic growth during 2000-2017: a comparative study of urban and rural guangdong, China. ENERGIES 13 (17), 4461. doi:10.3390/en13174461
Yu, Z., Haghighat, F., Fung, B., Morofsky, E., and Yoshino, H. (2011). A methodology for identifying and improving occupant behavior in residential buildings. Energy 36 (11), 6596–6608. doi:10.1016/j.energy.2011.09.002
Zahabi, S., Miranda-Moreno, L., Patterson, Z., and Barla, P. (2012). Evaluating the effects of land use and strategies for parking and transit supply on mode choice of downtown commuters. J. Transp. Land Use 5 (2), 103–119. doi:10.5198/jtlu.v5i2.260
Zeng, Y., Yang, H., Wang, Z., and Li, L. (2021). Impacts of family household dynamics on residential energy demands in Hebei Province of China. GENUS 77 (1), 35. doi:10.1186/s41118-021-00148-0
Zhang, G., Zhang, C., and Nie, H. (2021). An overview of China’s energy labeling policy portfolio: China’s contribution to addressing the global goal of sustainable development. Sage Open 11 (1), 215824402098885. doi:10.1177/2158244020988858
Zhang, J., Ma, L., and Li, J. (2021). Why low-carbon publicity effect limits? The role of heterogeneous intention in reducing household energy consumption. Energies 14 (22), 7634. doi:10.3390/en14227634
Zhang, J., Zhang, L., Qin, Y., Wang, X., and Zheng, Z. (2019). Impact of residential self-selection on low-carbon behavior: evidence from zhengzhou, China. Sustainability 11 (23), 6871. doi:10.3390/su11236871
Zhang, L., and Wen, X. (2021). Nonlinear effect analysis of electricity price on household electricity consumption. Math. Problems Eng. 2021, 1–13. doi:10.1155/2021/8503158
Zhang, M., and Bai, C. (2018). Exploring the influencing factors and decoupling state of residential energy consumption in Shandong. J. Of Clean. Prod. 194, 253–262. doi:10.1016/j.jclepro.2018.05.122
Zhang, X., Luo, L., and Skitmore, M. (2015). Household carbon emission research: an analytical review of measurement, influencing factors and mitigation prospects. J. Of Clean. Prod. 103, 873–883. doi:10.1016/j.jclepro.2015.04.024
Zhang, Y., and Lei, Y. (2017). Research on the carbon emissions of Beijing residents based on the input-output model. Pol. J. Of Environ. Stud. 26 (5), 2397–2406. doi:10.15244/pjoes/69441
Zhao, Q., Gao, W., Su, Y., and Wang, T. (2023a). Carbon emissions trajectory and driving force from the construction industry with a city-scale: a case study of Hangzhou, China. Sustain. Cities Soc. 88, 104283. doi:10.1016/j.scs.2022.104283
Zhao, Q., Gao, W., Su, Y., Wang, T., and Wang, J. (2023b). How can C&D waste recycling do a carbon emission contribution for construction industry in Japan city? Energy Build. 298, 113538. doi:10.1016/j.enbuild.2023.113538
Zhao, X., Gao, W., Qian, F., and Ge, J. (2021). Electricity cost comparison of dynamic pricing model based on load forecasting in home energy management system. Energy 229, 120538. doi:10.1016/j.energy.2021.120538
Zheng, J., Dang, Y., and Assad, U. (2024). Household energy consumption, energy efficiency, and household income–Evidence from China. Appl. Energy 353, 122074. doi:10.1016/j.apenergy.2023.122074
Zhou, Y., and Liu, Y. (2016). Does population have a larger impact on carbon dioxide emissions than income? Evidence from a cross-regional panel analysis in China. Appl. Energy 180, 800–809. doi:10.1016/j.apenergy.2016.08.035
Keywords: bibliometric analysis, household energy consumption, environmental assessment, mitigation measures, household emissions reduction
Citation: Wang T, Zhao Q, Gao W and He X (2024) Research on energy consumption in household sector: a comprehensive review based on bibliometric analysis. Front. Energy Res. 11:1209290. doi: 10.3389/fenrg.2023.1209290
Received: 20 April 2023; Accepted: 13 December 2023; Published: 10 January 2024.
Reviewed by:
Copyright © 2024 Wang, Zhao, Gao and He. This is an open-access article distributed under the terms of the Creative Commons Attribution License (CC BY). The use, distribution or reproduction in other forums is permitted, provided the original author(s) and the copyright owner(s) are credited and that the original publication in this journal is cited, in accordance with accepted academic practice. No use, distribution or reproduction is permitted which does not comply with these terms.
*Correspondence: Qinfeng Zhao, [email protected]
Household Energy Consumption Prediction Using the Stationary Wavelet Transform and Transformers
Ieee account.
- Change Username/Password
- Update Address
Purchase Details
- Payment Options
- Order History
- View Purchased Documents
Profile Information
- Communications Preferences
- Profession and Education
- Technical Interests
- US & Canada: +1 800 678 4333
- Worldwide: +1 732 981 0060
- Contact & Support
- About IEEE Xplore
- Accessibility
- Terms of Use
- Nondiscrimination Policy
- Privacy & Opting Out of Cookies
A not-for-profit organization, IEEE is the world's largest technical professional organization dedicated to advancing technology for the benefit of humanity. © Copyright 2024 IEEE - All rights reserved. Use of this web site signifies your agreement to the terms and conditions.
Distribution of Market Power, Endogenous Growth, and Monetary Policy
Yumeng Gu, Sanjay R. Singh
Download PDF (1 MB)
2024-09 | March 28, 2024
We incorporate incumbent innovation in a Keynesian growth framework to generate an endogenous distribution of market power across firms. Existing firms increase markups over time through successful innovation. Entrant innovation disrupts the accumulation of market power by incumbents. Using this environment, we highlight a novel misallocation channel for monetary policy. A contractionary monetary policy shock causes an increase in markup dispersion across firms by discouraging entrant innovation relative to incumbent innovation. We characterize the circumstances when contractionary monetary policy may increase misallocation.
Article Citation
Gu, Yumeng, and Sanjay R. Singh. 2024. “Distribution of Market Power, Endogenous Growth, and Monetary Policy,” Federal Reserve Bank of San Francisco Working Paper 2024-09. Available at https://doi.org/10.24148/wp2024-09

Consumption Dynamics and Welfare Under Non-Gaussian Earnings Risk
Working Paper 2024-007A by Fatih Guvenen, Rocio Madera, and Serdar Ozkan
CORRECT ORDER OF AUTHORS: Fatih Guvenen, Serdar Ozkan, and Rocio Madera. The order of coauthors has been assigned randomly using AEA’s Author Randomization Tool. Recent empirical studies document that the distribution of earnings changes displays substantial deviations from lognormality: in particular, earnings changes are negatively skewed with extremely high kurtosis (long and thick tails), and these non-Gaussian features vary substantially both over the life cycle and with the earnings level of individuals. Furthermore, earnings changes display nonlinear (asymmetric) mean reversion. In this paper, we embed a very rich “benchmark earnings process” that captures these non-Gaussian and nonlinear features into a lifecycle consumption-saving model and study its implications for consumption dynamics, consumption insurance, and welfare. We show four main results. First, the benchmark process essentially matches the empirical lifetime earnings inequality—a first-order proxy for consumption inequality—whereas the canonical Gaussian (persistent-plus-transitory) process understates it by a factor of five to ten. Second, the welfare cost of idiosyncratic risk implied by the benchmark process is between two-to-four times higher than the canonical Gaussian one. Third, the standard method in the literature for measuring the pass-through of income shocks to consumption—can significantly overstate the degree of consumption smoothing possible under non-Gaussian shocks. Fourth, the marginal propensity to consume out of transitory income (e.g., from a stimulus check) is higher under non-Gaussian earnings risk.
Read Full Text
https://doi.org/10.20955/wp.2024.007
- Subscribe to Our Newsletter
Related Links
- Economist Pages
- JEL Classification System
- Fed In Print
SUBSCRIBE TO THE RESEARCH DIVISION NEWSLETTER
Research division.
- Legal and Privacy
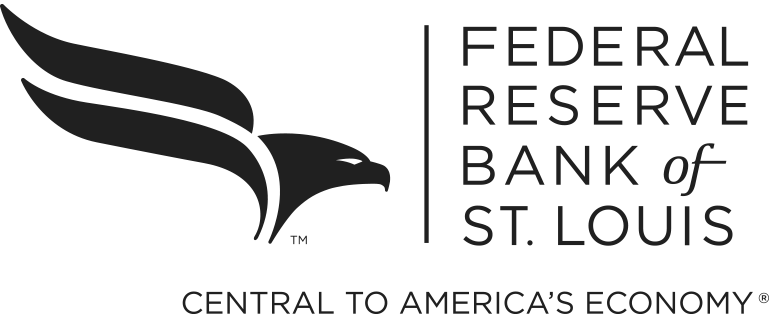
One Federal Reserve Bank Plaza St. Louis, MO 63102
Information for Visitors


The use and impact of surveillance-based technology initiatives in inpatient and acute mental health settings: A systematic review
- Find this author on Google Scholar
- Find this author on PubMed
- Search for this author on this site
- ORCID record for Jessica L. Griffiths
- For correspondence: [email protected]
- ORCID record for Katherine R. K. Saunders
- ORCID record for Una Foye
- ORCID record for Anna Greenburgh
- ORCID record for Antonio Rojas-Garcia
- ORCID record for Brynmor Lloyd-Evans
- ORCID record for Sonia Johnson
- ORCID record for Alan Simpson
- Info/History
- Supplementary material
- Preview PDF
Background: The use of surveillance technologies is becoming increasingly common in inpatient mental health settings, commonly justified as efforts to improve safety and cost-effectiveness. However, the use of these technologies has been questioned in light of limited research conducted and the sensitivities, ethical concerns and potential harms of surveillance. This systematic review aims to: 1) map how surveillance technologies have been employed in inpatient mental health settings, 2) identify any best practice guidance, 3) explore how they are experienced by patients, staff and carers, and 4) examine evidence regarding their impact. Methods: We searched five academic databases (Embase, MEDLINE, PsycInfo, PubMed and Scopus), one grey literature database (HMIC) and two pre-print servers (medRxiv and PsyArXiv) to identify relevant papers published up to 18/09/2023. We also conducted backwards and forwards citation tracking and contacted experts to identify relevant literature. Quality was assessed using the Mixed Methods Appraisal Tool. Data were synthesised using a narrative approach. Results: A total of 27 studies were identified as meeting the inclusion criteria. Included studies reported on CCTV/video monitoring (n = 13), Vision-Based Patient Monitoring and Management (VBPMM) (n = 6), Body Worn Cameras (BWCs) (n = 4), GPS electronic monitoring (n = 2) and wearable sensors (n = 2). Twelve papers (44.4%) were rated as low quality, five (18.5%) medium quality, and ten (37.0%) high quality. Five studies (18.5%) declared a conflict of interest. We identified minimal best practice guidance. Qualitative findings indicate that patient, staff and carer perceptions and experiences of surveillance technologies are mixed and complex. Quantitative findings regarding the impact of surveillance on outcomes such as self-harm, violence, aggression, care quality and cost-effectiveness were inconsistent or weak. Discussion: There is currently insufficient evidence to suggest that surveillance technologies in inpatient mental health settings are achieving the outcomes they are employed to achieve, such as improving safety and reducing costs. The studies were generally of low methodological quality, lacked lived experience involvement, and a substantial proportion (18.5%) declared conflicts of interest. Further independent coproduced research is needed to more comprehensively evaluate the impact of surveillance technologies in inpatient settings, including harms and benefits. If surveillance technologies are to be implemented, it will be important to engage all key stakeholders in the development of policies, procedures and best practice guidance to regulate their use, with a particular emphasis on prioritising the perspectives of patients.
Competing Interest Statement
AS and UF have undertaken and published research on BWCs. We have received no financial support from BWC or any other surveillance technology companies. All other authors declare no competing interests.
Clinical Protocols
https://www.crd.york.ac.uk/prospero/display_record.php?RecordID=463993
Funding Statement
This study is funded by the National Institute for Health and Care Research (NIHR) Policy Research Programme (grant no. PR-PRU-0916-22003). The views expressed are those of the author(s) and not necessarily those of the NIHR or the Department of Health and Social Care. The funders had no role in study design, data collection and analysis, decision to publish, or preparation of the manuscript. ARG was supported by the Ramon y Cajal programme (RYC2022-038556-I), funded by the Spanish Ministry of Science, Innovation and Universities.
Author Declarations
I confirm all relevant ethical guidelines have been followed, and any necessary IRB and/or ethics committee approvals have been obtained.
I confirm that all necessary patient/participant consent has been obtained and the appropriate institutional forms have been archived, and that any patient/participant/sample identifiers included were not known to anyone (e.g., hospital staff, patients or participants themselves) outside the research group so cannot be used to identify individuals.
I understand that all clinical trials and any other prospective interventional studies must be registered with an ICMJE-approved registry, such as ClinicalTrials.gov. I confirm that any such study reported in the manuscript has been registered and the trial registration ID is provided (note: if posting a prospective study registered retrospectively, please provide a statement in the trial ID field explaining why the study was not registered in advance).
I have followed all appropriate research reporting guidelines, such as any relevant EQUATOR Network research reporting checklist(s) and other pertinent material, if applicable.
Data Availability
The template data extraction form is available in Supplementary 1. MMAT quality appraisal ratings for each included study are available in Supplementary 2. All data used is publicly available in the published papers included in this review.
View the discussion thread.
Supplementary Material
Thank you for your interest in spreading the word about medRxiv.
NOTE: Your email address is requested solely to identify you as the sender of this article.

Citation Manager Formats
- EndNote (tagged)
- EndNote 8 (xml)
- RefWorks Tagged
- Ref Manager
- Tweet Widget
- Facebook Like
- Google Plus One
- Addiction Medicine (316)
- Allergy and Immunology (617)
- Anesthesia (159)
- Cardiovascular Medicine (2276)
- Dentistry and Oral Medicine (279)
- Dermatology (201)
- Emergency Medicine (370)
- Endocrinology (including Diabetes Mellitus and Metabolic Disease) (798)
- Epidemiology (11573)
- Forensic Medicine (10)
- Gastroenterology (678)
- Genetic and Genomic Medicine (3575)
- Geriatric Medicine (336)
- Health Economics (616)
- Health Informatics (2304)
- Health Policy (913)
- Health Systems and Quality Improvement (863)
- Hematology (335)
- HIV/AIDS (752)
- Infectious Diseases (except HIV/AIDS) (13149)
- Intensive Care and Critical Care Medicine (755)
- Medical Education (359)
- Medical Ethics (100)
- Nephrology (388)
- Neurology (3346)
- Nursing (191)
- Nutrition (506)
- Obstetrics and Gynecology (651)
- Occupational and Environmental Health (645)
- Oncology (1756)
- Ophthalmology (524)
- Orthopedics (209)
- Otolaryngology (284)
- Pain Medicine (223)
- Palliative Medicine (66)
- Pathology (437)
- Pediatrics (1001)
- Pharmacology and Therapeutics (422)
- Primary Care Research (406)
- Psychiatry and Clinical Psychology (3058)
- Public and Global Health (5983)
- Radiology and Imaging (1221)
- Rehabilitation Medicine and Physical Therapy (714)
- Respiratory Medicine (811)
- Rheumatology (367)
- Sexual and Reproductive Health (350)
- Sports Medicine (316)
- Surgery (386)
- Toxicology (50)
- Transplantation (170)
- Urology (142)
Thank you for visiting nature.com. You are using a browser version with limited support for CSS. To obtain the best experience, we recommend you use a more up to date browser (or turn off compatibility mode in Internet Explorer). In the meantime, to ensure continued support, we are displaying the site without styles and JavaScript.
- View all journals
- My Account Login
- Explore content
- About the journal
- Publish with us
- Sign up for alerts
- Open access
- Published: 26 March 2024
Predicting and improving complex beer flavor through machine learning
- Michiel Schreurs ORCID: orcid.org/0000-0002-9449-5619 1 , 2 , 3 na1 ,
- Supinya Piampongsant 1 , 2 , 3 na1 ,
- Miguel Roncoroni ORCID: orcid.org/0000-0001-7461-1427 1 , 2 , 3 na1 ,
- Lloyd Cool ORCID: orcid.org/0000-0001-9936-3124 1 , 2 , 3 , 4 ,
- Beatriz Herrera-Malaver ORCID: orcid.org/0000-0002-5096-9974 1 , 2 , 3 ,
- Christophe Vanderaa ORCID: orcid.org/0000-0001-7443-5427 4 ,
- Florian A. Theßeling 1 , 2 , 3 ,
- Łukasz Kreft ORCID: orcid.org/0000-0001-7620-4657 5 ,
- Alexander Botzki ORCID: orcid.org/0000-0001-6691-4233 5 ,
- Philippe Malcorps 6 ,
- Luk Daenen 6 ,
- Tom Wenseleers ORCID: orcid.org/0000-0002-1434-861X 4 &
- Kevin J. Verstrepen ORCID: orcid.org/0000-0002-3077-6219 1 , 2 , 3
Nature Communications volume 15 , Article number: 2368 ( 2024 ) Cite this article
46k Accesses
805 Altmetric
Metrics details
- Chemical engineering
- Gas chromatography
- Machine learning
- Metabolomics
- Taste receptors
The perception and appreciation of food flavor depends on many interacting chemical compounds and external factors, and therefore proves challenging to understand and predict. Here, we combine extensive chemical and sensory analyses of 250 different beers to train machine learning models that allow predicting flavor and consumer appreciation. For each beer, we measure over 200 chemical properties, perform quantitative descriptive sensory analysis with a trained tasting panel and map data from over 180,000 consumer reviews to train 10 different machine learning models. The best-performing algorithm, Gradient Boosting, yields models that significantly outperform predictions based on conventional statistics and accurately predict complex food features and consumer appreciation from chemical profiles. Model dissection allows identifying specific and unexpected compounds as drivers of beer flavor and appreciation. Adding these compounds results in variants of commercial alcoholic and non-alcoholic beers with improved consumer appreciation. Together, our study reveals how big data and machine learning uncover complex links between food chemistry, flavor and consumer perception, and lays the foundation to develop novel, tailored foods with superior flavors.
Similar content being viewed by others
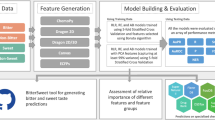
BitterSweet: Building machine learning models for predicting the bitter and sweet taste of small molecules
Rudraksh Tuwani, Somin Wadhwa & Ganesh Bagler
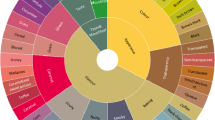
Sensory lexicon and aroma volatiles analysis of brewing malt
Xiaoxia Su, Miao Yu, … Tianyi Du
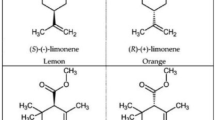
Predicting odor from molecular structure: a multi-label classification approach
Kushagra Saini & Venkatnarayan Ramanathan
Introduction
Predicting and understanding food perception and appreciation is one of the major challenges in food science. Accurate modeling of food flavor and appreciation could yield important opportunities for both producers and consumers, including quality control, product fingerprinting, counterfeit detection, spoilage detection, and the development of new products and product combinations (food pairing) 1 , 2 , 3 , 4 , 5 , 6 . Accurate models for flavor and consumer appreciation would contribute greatly to our scientific understanding of how humans perceive and appreciate flavor. Moreover, accurate predictive models would also facilitate and standardize existing food assessment methods and could supplement or replace assessments by trained and consumer tasting panels, which are variable, expensive and time-consuming 7 , 8 , 9 . Lastly, apart from providing objective, quantitative, accurate and contextual information that can help producers, models can also guide consumers in understanding their personal preferences 10 .
Despite the myriad of applications, predicting food flavor and appreciation from its chemical properties remains a largely elusive goal in sensory science, especially for complex food and beverages 11 , 12 . A key obstacle is the immense number of flavor-active chemicals underlying food flavor. Flavor compounds can vary widely in chemical structure and concentration, making them technically challenging and labor-intensive to quantify, even in the face of innovations in metabolomics, such as non-targeted metabolic fingerprinting 13 , 14 . Moreover, sensory analysis is perhaps even more complicated. Flavor perception is highly complex, resulting from hundreds of different molecules interacting at the physiochemical and sensorial level. Sensory perception is often non-linear, characterized by complex and concentration-dependent synergistic and antagonistic effects 15 , 16 , 17 , 18 , 19 , 20 , 21 that are further convoluted by the genetics, environment, culture and psychology of consumers 22 , 23 , 24 . Perceived flavor is therefore difficult to measure, with problems of sensitivity, accuracy, and reproducibility that can only be resolved by gathering sufficiently large datasets 25 . Trained tasting panels are considered the prime source of quality sensory data, but require meticulous training, are low throughput and high cost. Public databases containing consumer reviews of food products could provide a valuable alternative, especially for studying appreciation scores, which do not require formal training 25 . Public databases offer the advantage of amassing large amounts of data, increasing the statistical power to identify potential drivers of appreciation. However, public datasets suffer from biases, including a bias in the volunteers that contribute to the database, as well as confounding factors such as price, cult status and psychological conformity towards previous ratings of the product.
Classical multivariate statistics and machine learning methods have been used to predict flavor of specific compounds by, for example, linking structural properties of a compound to its potential biological activities or linking concentrations of specific compounds to sensory profiles 1 , 26 . Importantly, most previous studies focused on predicting organoleptic properties of single compounds (often based on their chemical structure) 27 , 28 , 29 , 30 , 31 , 32 , 33 , thus ignoring the fact that these compounds are present in a complex matrix in food or beverages and excluding complex interactions between compounds. Moreover, the classical statistics commonly used in sensory science 34 , 35 , 36 , 37 , 38 , 39 require a large sample size and sufficient variance amongst predictors to create accurate models. They are not fit for studying an extensive set of hundreds of interacting flavor compounds, since they are sensitive to outliers, have a high tendency to overfit and are less suited for non-linear and discontinuous relationships 40 .
In this study, we combine extensive chemical analyses and sensory data of a set of different commercial beers with machine learning approaches to develop models that predict taste, smell, mouthfeel and appreciation from compound concentrations. Beer is particularly suited to model the relationship between chemistry, flavor and appreciation. First, beer is a complex product, consisting of thousands of flavor compounds that partake in complex sensory interactions 41 , 42 , 43 . This chemical diversity arises from the raw materials (malt, yeast, hops, water and spices) and biochemical conversions during the brewing process (kilning, mashing, boiling, fermentation, maturation and aging) 44 , 45 . Second, the advent of the internet saw beer consumers embrace online review platforms, such as RateBeer (ZX Ventures, Anheuser-Busch InBev SA/NV) and BeerAdvocate (Next Glass, inc.). In this way, the beer community provides massive data sets of beer flavor and appreciation scores, creating extraordinarily large sensory databases to complement the analyses of our professional sensory panel. Specifically, we characterize over 200 chemical properties of 250 commercial beers, spread across 22 beer styles, and link these to the descriptive sensory profiling data of a 16-person in-house trained tasting panel and data acquired from over 180,000 public consumer reviews. These unique and extensive datasets enable us to train a suite of machine learning models to predict flavor and appreciation from a beer’s chemical profile. Dissection of the best-performing models allows us to pinpoint specific compounds as potential drivers of beer flavor and appreciation. Follow-up experiments confirm the importance of these compounds and ultimately allow us to significantly improve the flavor and appreciation of selected commercial beers. Together, our study represents a significant step towards understanding complex flavors and reinforces the value of machine learning to develop and refine complex foods. In this way, it represents a stepping stone for further computer-aided food engineering applications 46 .
To generate a comprehensive dataset on beer flavor, we selected 250 commercial Belgian beers across 22 different beer styles (Supplementary Fig. S1 ). Beers with ≤ 4.2% alcohol by volume (ABV) were classified as non-alcoholic and low-alcoholic. Blonds and Tripels constitute a significant portion of the dataset (12.4% and 11.2%, respectively) reflecting their presence on the Belgian beer market and the heterogeneity of beers within these styles. By contrast, lager beers are less diverse and dominated by a handful of brands. Rare styles such as Brut or Faro make up only a small fraction of the dataset (2% and 1%, respectively) because fewer of these beers are produced and because they are dominated by distinct characteristics in terms of flavor and chemical composition.
Extensive analysis identifies relationships between chemical compounds in beer
For each beer, we measured 226 different chemical properties, including common brewing parameters such as alcohol content, iso-alpha acids, pH, sugar concentration 47 , and over 200 flavor compounds (Methods, Supplementary Table S1 ). A large portion (37.2%) are terpenoids arising from hopping, responsible for herbal and fruity flavors 16 , 48 . A second major category are yeast metabolites, such as esters and alcohols, that result in fruity and solvent notes 48 , 49 , 50 . Other measured compounds are primarily derived from malt, or other microbes such as non- Saccharomyces yeasts and bacteria (‘wild flora’). Compounds that arise from spices or staling are labeled under ‘Others’. Five attributes (caloric value, total acids and total ester, hop aroma and sulfur compounds) are calculated from multiple individually measured compounds.
As a first step in identifying relationships between chemical properties, we determined correlations between the concentrations of the compounds (Fig. 1 , upper panel, Supplementary Data 1 and 2 , and Supplementary Fig. S2 . For the sake of clarity, only a subset of the measured compounds is shown in Fig. 1 ). Compounds of the same origin typically show a positive correlation, while absence of correlation hints at parameters varying independently. For example, the hop aroma compounds citronellol, and alpha-terpineol show moderate correlations with each other (Spearman’s rho=0.39 and 0.57), but not with the bittering hop component iso-alpha acids (Spearman’s rho=0.16 and −0.07). This illustrates how brewers can independently modify hop aroma and bitterness by selecting hop varieties and dosage time. If hops are added early in the boiling phase, chemical conversions increase bitterness while aromas evaporate, conversely, late addition of hops preserves aroma but limits bitterness 51 . Similarly, hop-derived iso-alpha acids show a strong anti-correlation with lactic acid and acetic acid, likely reflecting growth inhibition of lactic acid and acetic acid bacteria, or the consequent use of fewer hops in sour beer styles, such as West Flanders ales and Fruit beers, that rely on these bacteria for their distinct flavors 52 . Finally, yeast-derived esters (ethyl acetate, ethyl decanoate, ethyl hexanoate, ethyl octanoate) and alcohols (ethanol, isoamyl alcohol, isobutanol, and glycerol), correlate with Spearman coefficients above 0.5, suggesting that these secondary metabolites are correlated with the yeast genetic background and/or fermentation parameters and may be difficult to influence individually, although the choice of yeast strain may offer some control 53 .
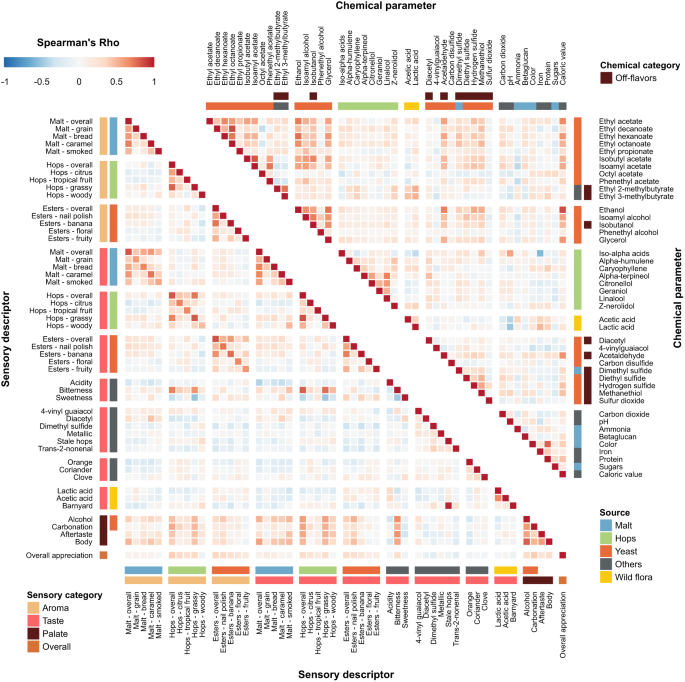
Spearman rank correlations are shown. Descriptors are grouped according to their origin (malt (blue), hops (green), yeast (red), wild flora (yellow), Others (black)), and sensory aspect (aroma, taste, palate, and overall appreciation). Please note that for the chemical compounds, for the sake of clarity, only a subset of the total number of measured compounds is shown, with an emphasis on the key compounds for each source. For more details, see the main text and Methods section. Chemical data can be found in Supplementary Data 1 , correlations between all chemical compounds are depicted in Supplementary Fig. S2 and correlation values can be found in Supplementary Data 2 . See Supplementary Data 4 for sensory panel assessments and Supplementary Data 5 for correlation values between all sensory descriptors.
Interestingly, different beer styles show distinct patterns for some flavor compounds (Supplementary Fig. S3 ). These observations agree with expectations for key beer styles, and serve as a control for our measurements. For instance, Stouts generally show high values for color (darker), while hoppy beers contain elevated levels of iso-alpha acids, compounds associated with bitter hop taste. Acetic and lactic acid are not prevalent in most beers, with notable exceptions such as Kriek, Lambic, Faro, West Flanders ales and Flanders Old Brown, which use acid-producing bacteria ( Lactobacillus and Pediococcus ) or unconventional yeast ( Brettanomyces ) 54 , 55 . Glycerol, ethanol and esters show similar distributions across all beer styles, reflecting their common origin as products of yeast metabolism during fermentation 45 , 53 . Finally, low/no-alcohol beers contain low concentrations of glycerol and esters. This is in line with the production process for most of the low/no-alcohol beers in our dataset, which are produced through limiting fermentation or by stripping away alcohol via evaporation or dialysis, with both methods having the unintended side-effect of reducing the amount of flavor compounds in the final beer 56 , 57 .
Besides expected associations, our data also reveals less trivial associations between beer styles and specific parameters. For example, geraniol and citronellol, two monoterpenoids responsible for citrus, floral and rose flavors and characteristic of Citra hops, are found in relatively high amounts in Christmas, Saison, and Brett/co-fermented beers, where they may originate from terpenoid-rich spices such as coriander seeds instead of hops 58 .
Tasting panel assessments reveal sensorial relationships in beer
To assess the sensory profile of each beer, a trained tasting panel evaluated each of the 250 beers for 50 sensory attributes, including different hop, malt and yeast flavors, off-flavors and spices. Panelists used a tasting sheet (Supplementary Data 3 ) to score the different attributes. Panel consistency was evaluated by repeating 12 samples across different sessions and performing ANOVA. In 95% of cases no significant difference was found across sessions ( p > 0.05), indicating good panel consistency (Supplementary Table S2 ).
Aroma and taste perception reported by the trained panel are often linked (Fig. 1 , bottom left panel and Supplementary Data 4 and 5 ), with high correlations between hops aroma and taste (Spearman’s rho=0.83). Bitter taste was found to correlate with hop aroma and taste in general (Spearman’s rho=0.80 and 0.69), and particularly with “grassy” noble hops (Spearman’s rho=0.75). Barnyard flavor, most often associated with sour beers, is identified together with stale hops (Spearman’s rho=0.97) that are used in these beers. Lactic and acetic acid, which often co-occur, are correlated (Spearman’s rho=0.66). Interestingly, sweetness and bitterness are anti-correlated (Spearman’s rho = −0.48), confirming the hypothesis that they mask each other 59 , 60 . Beer body is highly correlated with alcohol (Spearman’s rho = 0.79), and overall appreciation is found to correlate with multiple aspects that describe beer mouthfeel (alcohol, carbonation; Spearman’s rho= 0.32, 0.39), as well as with hop and ester aroma intensity (Spearman’s rho=0.39 and 0.35).
Similar to the chemical analyses, sensorial analyses confirmed typical features of specific beer styles (Supplementary Fig. S4 ). For example, sour beers (Faro, Flanders Old Brown, Fruit beer, Kriek, Lambic, West Flanders ale) were rated acidic, with flavors of both acetic and lactic acid. Hoppy beers were found to be bitter and showed hop-associated aromas like citrus and tropical fruit. Malt taste is most detected among scotch, stout/porters, and strong ales, while low/no-alcohol beers, which often have a reputation for being ‘worty’ (reminiscent of unfermented, sweet malt extract) appear in the middle. Unsurprisingly, hop aromas are most strongly detected among hoppy beers. Like its chemical counterpart (Supplementary Fig. S3 ), acidity shows a right-skewed distribution, with the most acidic beers being Krieks, Lambics, and West Flanders ales.
Tasting panel assessments of specific flavors correlate with chemical composition
We find that the concentrations of several chemical compounds strongly correlate with specific aroma or taste, as evaluated by the tasting panel (Fig. 2 , Supplementary Fig. S5 , Supplementary Data 6 ). In some cases, these correlations confirm expectations and serve as a useful control for data quality. For example, iso-alpha acids, the bittering compounds in hops, strongly correlate with bitterness (Spearman’s rho=0.68), while ethanol and glycerol correlate with tasters’ perceptions of alcohol and body, the mouthfeel sensation of fullness (Spearman’s rho=0.82/0.62 and 0.72/0.57 respectively) and darker color from roasted malts is a good indication of malt perception (Spearman’s rho=0.54).
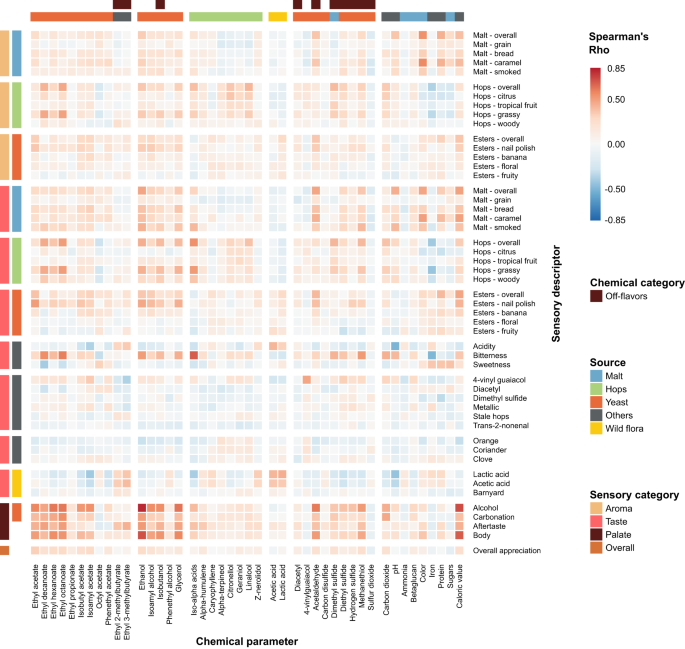
Heatmap colors indicate Spearman’s Rho. Axes are organized according to sensory categories (aroma, taste, mouthfeel, overall), chemical categories and chemical sources in beer (malt (blue), hops (green), yeast (red), wild flora (yellow), Others (black)). See Supplementary Data 6 for all correlation values.
Interestingly, for some relationships between chemical compounds and perceived flavor, correlations are weaker than expected. For example, the rose-smelling phenethyl acetate only weakly correlates with floral aroma. This hints at more complex relationships and interactions between compounds and suggests a need for a more complex model than simple correlations. Lastly, we uncovered unexpected correlations. For instance, the esters ethyl decanoate and ethyl octanoate appear to correlate slightly with hop perception and bitterness, possibly due to their fruity flavor. Iron is anti-correlated with hop aromas and bitterness, most likely because it is also anti-correlated with iso-alpha acids. This could be a sign of metal chelation of hop acids 61 , given that our analyses measure unbound hop acids and total iron content, or could result from the higher iron content in dark and Fruit beers, which typically have less hoppy and bitter flavors 62 .
Public consumer reviews complement expert panel data
To complement and expand the sensory data of our trained tasting panel, we collected 180,000 reviews of our 250 beers from the online consumer review platform RateBeer. This provided numerical scores for beer appearance, aroma, taste, palate, overall quality as well as the average overall score.
Public datasets are known to suffer from biases, such as price, cult status and psychological conformity towards previous ratings of a product. For example, prices correlate with appreciation scores for these online consumer reviews (rho=0.49, Supplementary Fig. S6 ), but not for our trained tasting panel (rho=0.19). This suggests that prices affect consumer appreciation, which has been reported in wine 63 , while blind tastings are unaffected. Moreover, we observe that some beer styles, like lagers and non-alcoholic beers, generally receive lower scores, reflecting that online reviewers are mostly beer aficionados with a preference for specialty beers over lager beers. In general, we find a modest correlation between our trained panel’s overall appreciation score and the online consumer appreciation scores (Fig. 3 , rho=0.29). Apart from the aforementioned biases in the online datasets, serving temperature, sample freshness and surroundings, which are all tightly controlled during the tasting panel sessions, can vary tremendously across online consumers and can further contribute to (among others, appreciation) differences between the two categories of tasters. Importantly, in contrast to the overall appreciation scores, for many sensory aspects the results from the professional panel correlated well with results obtained from RateBeer reviews. Correlations were highest for features that are relatively easy to recognize even for untrained tasters, like bitterness, sweetness, alcohol and malt aroma (Fig. 3 and below).
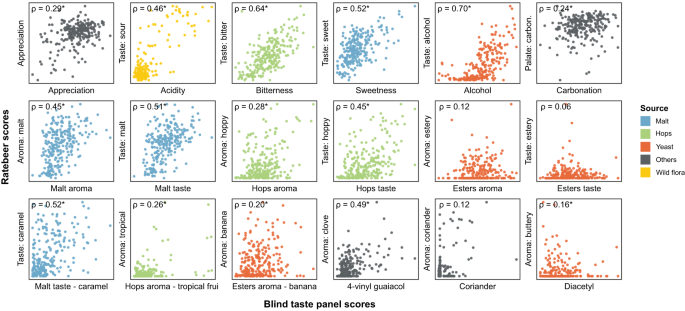
RateBeer text mining results can be found in Supplementary Data 7 . Rho values shown are Spearman correlation values, with asterisks indicating significant correlations ( p < 0.05, two-sided). All p values were smaller than 0.001, except for Esters aroma (0.0553), Esters taste (0.3275), Esters aroma—banana (0.0019), Coriander (0.0508) and Diacetyl (0.0134).
Besides collecting consumer appreciation from these online reviews, we developed automated text analysis tools to gather additional data from review texts (Supplementary Data 7 ). Processing review texts on the RateBeer database yielded comparable results to the scores given by the trained panel for many common sensory aspects, including acidity, bitterness, sweetness, alcohol, malt, and hop tastes (Fig. 3 ). This is in line with what would be expected, since these attributes require less training for accurate assessment and are less influenced by environmental factors such as temperature, serving glass and odors in the environment. Consumer reviews also correlate well with our trained panel for 4-vinyl guaiacol, a compound associated with a very characteristic aroma. By contrast, correlations for more specific aromas like ester, coriander or diacetyl are underrepresented in the online reviews, underscoring the importance of using a trained tasting panel and standardized tasting sheets with explicit factors to be scored for evaluating specific aspects of a beer. Taken together, our results suggest that public reviews are trustworthy for some, but not all, flavor features and can complement or substitute taste panel data for these sensory aspects.
Models can predict beer sensory profiles from chemical data
The rich datasets of chemical analyses, tasting panel assessments and public reviews gathered in the first part of this study provided us with a unique opportunity to develop predictive models that link chemical data to sensorial features. Given the complexity of beer flavor, basic statistical tools such as correlations or linear regression may not always be the most suitable for making accurate predictions. Instead, we applied different machine learning models that can model both simple linear and complex interactive relationships. Specifically, we constructed a set of regression models to predict (a) trained panel scores for beer flavor and quality and (b) public reviews’ appreciation scores from beer chemical profiles. We trained and tested 10 different models (Methods), 3 linear regression-based models (simple linear regression with first-order interactions (LR), lasso regression with first-order interactions (Lasso), partial least squares regressor (PLSR)), 5 decision tree models (AdaBoost regressor (ABR), extra trees (ET), gradient boosting regressor (GBR), random forest (RF) and XGBoost regressor (XGBR)), 1 support vector regression (SVR), and 1 artificial neural network (ANN) model.
To compare the performance of our machine learning models, the dataset was randomly split into a training and test set, stratified by beer style. After a model was trained on data in the training set, its performance was evaluated on its ability to predict the test dataset obtained from multi-output models (based on the coefficient of determination, see Methods). Additionally, individual-attribute models were ranked per descriptor and the average rank was calculated, as proposed by Korneva et al. 64 . Importantly, both ways of evaluating the models’ performance agreed in general. Performance of the different models varied (Table 1 ). It should be noted that all models perform better at predicting RateBeer results than results from our trained tasting panel. One reason could be that sensory data is inherently variable, and this variability is averaged out with the large number of public reviews from RateBeer. Additionally, all tree-based models perform better at predicting taste than aroma. Linear models (LR) performed particularly poorly, with negative R 2 values, due to severe overfitting (training set R 2 = 1). Overfitting is a common issue in linear models with many parameters and limited samples, especially with interaction terms further amplifying the number of parameters. L1 regularization (Lasso) successfully overcomes this overfitting, out-competing multiple tree-based models on the RateBeer dataset. Similarly, the dimensionality reduction of PLSR avoids overfitting and improves performance, to some extent. Still, tree-based models (ABR, ET, GBR, RF and XGBR) show the best performance, out-competing the linear models (LR, Lasso, PLSR) commonly used in sensory science 65 .
GBR models showed the best overall performance in predicting sensory responses from chemical information, with R 2 values up to 0.75 depending on the predicted sensory feature (Supplementary Table S4 ). The GBR models predict consumer appreciation (RateBeer) better than our trained panel’s appreciation (R 2 value of 0.67 compared to R 2 value of 0.09) (Supplementary Table S3 and Supplementary Table S4 ). ANN models showed intermediate performance, likely because neural networks typically perform best with larger datasets 66 . The SVR shows intermediate performance, mostly due to the weak predictions of specific attributes that lower the overall performance (Supplementary Table S4 ).
Model dissection identifies specific, unexpected compounds as drivers of consumer appreciation
Next, we leveraged our models to infer important contributors to sensory perception and consumer appreciation. Consumer preference is a crucial sensory aspects, because a product that shows low consumer appreciation scores often does not succeed commercially 25 . Additionally, the requirement for a large number of representative evaluators makes consumer trials one of the more costly and time-consuming aspects of product development. Hence, a model for predicting chemical drivers of overall appreciation would be a welcome addition to the available toolbox for food development and optimization.
Since GBR models on our RateBeer dataset showed the best overall performance, we focused on these models. Specifically, we used two approaches to identify important contributors. First, rankings of the most important predictors for each sensorial trait in the GBR models were obtained based on impurity-based feature importance (mean decrease in impurity). High-ranked parameters were hypothesized to be either the true causal chemical properties underlying the trait, to correlate with the actual causal properties, or to take part in sensory interactions affecting the trait 67 (Fig. 4A ). In a second approach, we used SHAP 68 to determine which parameters contributed most to the model for making predictions of consumer appreciation (Fig. 4B ). SHAP calculates parameter contributions to model predictions on a per-sample basis, which can be aggregated into an importance score.
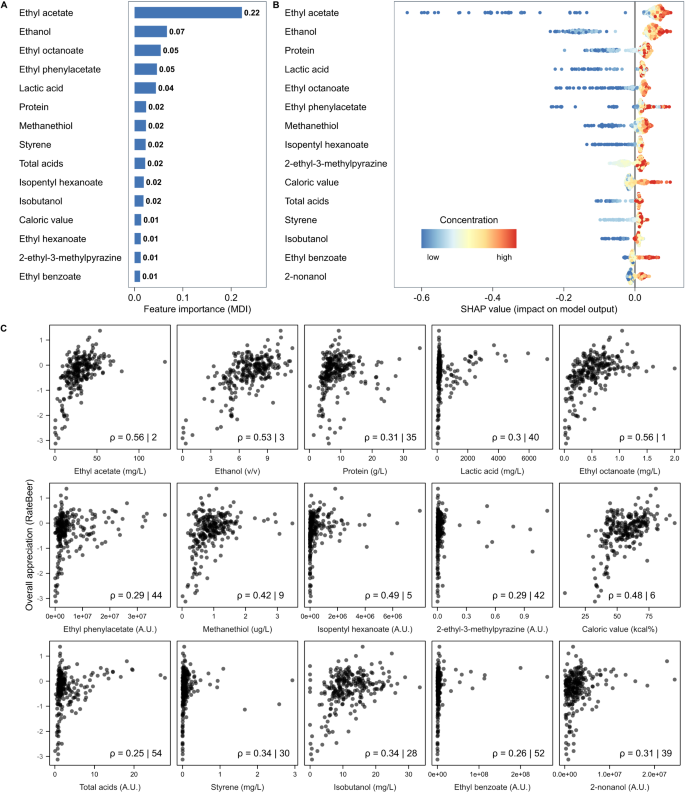
A The impurity-based feature importance (mean deviance in impurity, MDI) calculated from the Gradient Boosting Regression (GBR) model predicting RateBeer appreciation scores. The top 15 highest ranked chemical properties are shown. B SHAP summary plot for the top 15 parameters contributing to our GBR model. Each point on the graph represents a sample from our dataset. The color represents the concentration of that parameter, with bluer colors representing low values and redder colors representing higher values. Greater absolute values on the horizontal axis indicate a higher impact of the parameter on the prediction of the model. C Spearman correlations between the 15 most important chemical properties and consumer overall appreciation. Numbers indicate the Spearman Rho correlation coefficient, and the rank of this correlation compared to all other correlations. The top 15 important compounds were determined using SHAP (panel B).
Both approaches identified ethyl acetate as the most predictive parameter for beer appreciation (Fig. 4 ). Ethyl acetate is the most abundant ester in beer with a typical ‘fruity’, ‘solvent’ and ‘alcoholic’ flavor, but is often considered less important than other esters like isoamyl acetate. The second most important parameter identified by SHAP is ethanol, the most abundant beer compound after water. Apart from directly contributing to beer flavor and mouthfeel, ethanol drastically influences the physical properties of beer, dictating how easily volatile compounds escape the beer matrix to contribute to beer aroma 69 . Importantly, it should also be noted that the importance of ethanol for appreciation is likely inflated by the very low appreciation scores of non-alcoholic beers (Supplementary Fig. S4 ). Despite not often being considered a driver of beer appreciation, protein level also ranks highly in both approaches, possibly due to its effect on mouthfeel and body 70 . Lactic acid, which contributes to the tart taste of sour beers, is the fourth most important parameter identified by SHAP, possibly due to the generally high appreciation of sour beers in our dataset.
Interestingly, some of the most important predictive parameters for our model are not well-established as beer flavors or are even commonly regarded as being negative for beer quality. For example, our models identify methanethiol and ethyl phenyl acetate, an ester commonly linked to beer staling 71 , as a key factor contributing to beer appreciation. Although there is no doubt that high concentrations of these compounds are considered unpleasant, the positive effects of modest concentrations are not yet known 72 , 73 .
To compare our approach to conventional statistics, we evaluated how well the 15 most important SHAP-derived parameters correlate with consumer appreciation (Fig. 4C ). Interestingly, only 6 of the properties derived by SHAP rank amongst the top 15 most correlated parameters. For some chemical compounds, the correlations are so low that they would have likely been considered unimportant. For example, lactic acid, the fourth most important parameter, shows a bimodal distribution for appreciation, with sour beers forming a separate cluster, that is missed entirely by the Spearman correlation. Additionally, the correlation plots reveal outliers, emphasizing the need for robust analysis tools. Together, this highlights the need for alternative models, like the Gradient Boosting model, that better grasp the complexity of (beer) flavor.
Finally, to observe the relationships between these chemical properties and their predicted targets, partial dependence plots were constructed for the six most important predictors of consumer appreciation 74 , 75 , 76 (Supplementary Fig. S7 ). One-way partial dependence plots show how a change in concentration affects the predicted appreciation. These plots reveal an important limitation of our models: appreciation predictions remain constant at ever-increasing concentrations. This implies that once a threshold concentration is reached, further increasing the concentration does not affect appreciation. This is false, as it is well-documented that certain compounds become unpleasant at high concentrations, including ethyl acetate (‘nail polish’) 77 and methanethiol (‘sulfury’ and ‘rotten cabbage’) 78 . The inability of our models to grasp that flavor compounds have optimal levels, above which they become negative, is a consequence of working with commercial beer brands where (off-)flavors are rarely too high to negatively impact the product. The two-way partial dependence plots show how changing the concentration of two compounds influences predicted appreciation, visualizing their interactions (Supplementary Fig. S7 ). In our case, the top 5 parameters are dominated by additive or synergistic interactions, with high concentrations for both compounds resulting in the highest predicted appreciation.
To assess the robustness of our best-performing models and model predictions, we performed 100 iterations of the GBR, RF and ET models. In general, all iterations of the models yielded similar performance (Supplementary Fig. S8 ). Moreover, the main predictors (including the top predictors ethanol and ethyl acetate) remained virtually the same, especially for GBR and RF. For the iterations of the ET model, we did observe more variation in the top predictors, which is likely a consequence of the model’s inherent random architecture in combination with co-correlations between certain predictors. However, even in this case, several of the top predictors (ethanol and ethyl acetate) remain unchanged, although their rank in importance changes (Supplementary Fig. S8 ).
Next, we investigated if a combination of RateBeer and trained panel data into one consolidated dataset would lead to stronger models, under the hypothesis that such a model would suffer less from bias in the datasets. A GBR model was trained to predict appreciation on the combined dataset. This model underperformed compared to the RateBeer model, both in the native case and when including a dataset identifier (R 2 = 0.67, 0.26 and 0.42 respectively). For the latter, the dataset identifier is the most important feature (Supplementary Fig. S9 ), while most of the feature importance remains unchanged, with ethyl acetate and ethanol ranking highest, like in the original model trained only on RateBeer data. It seems that the large variation in the panel dataset introduces noise, weakening the models’ performances and reliability. In addition, it seems reasonable to assume that both datasets are fundamentally different, with the panel dataset obtained by blind tastings by a trained professional panel.
Lastly, we evaluated whether beer style identifiers would further enhance the model’s performance. A GBR model was trained with parameters that explicitly encoded the styles of the samples. This did not improve model performance (R2 = 0.66 with style information vs R2 = 0.67). The most important chemical features are consistent with the model trained without style information (eg. ethanol and ethyl acetate), and with the exception of the most preferred (strong ale) and least preferred (low/no-alcohol) styles, none of the styles were among the most important features (Supplementary Fig. S9 , Supplementary Table S5 and S6 ). This is likely due to a combination of style-specific chemical signatures, such as iso-alpha acids and lactic acid, that implicitly convey style information to the original models, as well as the low number of samples belonging to some styles, making it difficult for the model to learn style-specific patterns. Moreover, beer styles are not rigorously defined, with some styles overlapping in features and some beers being misattributed to a specific style, all of which leads to more noise in models that use style parameters.
Model validation
To test if our predictive models give insight into beer appreciation, we set up experiments aimed at improving existing commercial beers. We specifically selected overall appreciation as the trait to be examined because of its complexity and commercial relevance. Beer flavor comprises a complex bouquet rather than single aromas and tastes 53 . Hence, adding a single compound to the extent that a difference is noticeable may lead to an unbalanced, artificial flavor. Therefore, we evaluated the effect of combinations of compounds. Because Blond beers represent the most extensive style in our dataset, we selected a beer from this style as the starting material for these experiments (Beer 64 in Supplementary Data 1 ).
In the first set of experiments, we adjusted the concentrations of compounds that made up the most important predictors of overall appreciation (ethyl acetate, ethanol, lactic acid, ethyl phenyl acetate) together with correlated compounds (ethyl hexanoate, isoamyl acetate, glycerol), bringing them up to 95 th percentile ethanol-normalized concentrations (Methods) within the Blond group (‘Spiked’ concentration in Fig. 5A ). Compared to controls, the spiked beers were found to have significantly improved overall appreciation among trained panelists, with panelist noting increased intensity of ester flavors, sweetness, alcohol, and body fullness (Fig. 5B ). To disentangle the contribution of ethanol to these results, a second experiment was performed without the addition of ethanol. This resulted in a similar outcome, including increased perception of alcohol and overall appreciation.
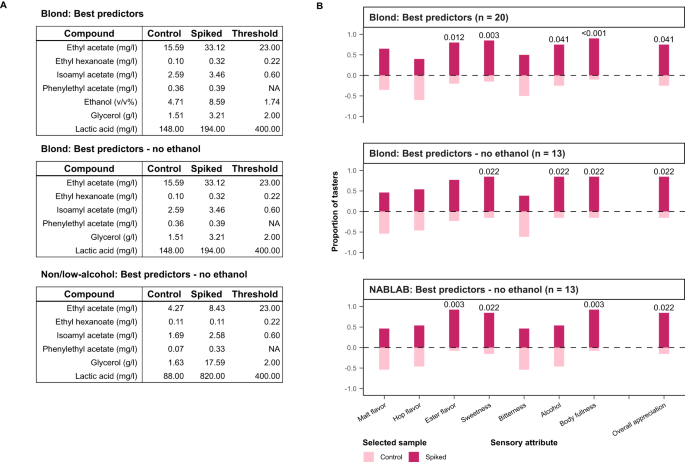
Adding the top chemical compounds, identified as best predictors of appreciation by our model, into poorly appreciated beers results in increased appreciation from our trained panel. Results of sensory tests between base beers and those spiked with compounds identified as the best predictors by the model. A Blond and Non/Low-alcohol (0.0% ABV) base beers were brought up to 95th-percentile ethanol-normalized concentrations within each style. B For each sensory attribute, tasters indicated the more intense sample and selected the sample they preferred. The numbers above the bars correspond to the p values that indicate significant changes in perceived flavor (two-sided binomial test: alpha 0.05, n = 20 or 13).
In a last experiment, we tested whether using the model’s predictions can boost the appreciation of a non-alcoholic beer (beer 223 in Supplementary Data 1 ). Again, the addition of a mixture of predicted compounds (omitting ethanol, in this case) resulted in a significant increase in appreciation, body, ester flavor and sweetness.
Predicting flavor and consumer appreciation from chemical composition is one of the ultimate goals of sensory science. A reliable, systematic and unbiased way to link chemical profiles to flavor and food appreciation would be a significant asset to the food and beverage industry. Such tools would substantially aid in quality control and recipe development, offer an efficient and cost-effective alternative to pilot studies and consumer trials and would ultimately allow food manufacturers to produce superior, tailor-made products that better meet the demands of specific consumer groups more efficiently.
A limited set of studies have previously tried, to varying degrees of success, to predict beer flavor and beer popularity based on (a limited set of) chemical compounds and flavors 79 , 80 . Current sensitive, high-throughput technologies allow measuring an unprecedented number of chemical compounds and properties in a large set of samples, yielding a dataset that can train models that help close the gaps between chemistry and flavor, even for a complex natural product like beer. To our knowledge, no previous research gathered data at this scale (250 samples, 226 chemical parameters, 50 sensory attributes and 5 consumer scores) to disentangle and validate the chemical aspects driving beer preference using various machine-learning techniques. We find that modern machine learning models outperform conventional statistical tools, such as correlations and linear models, and can successfully predict flavor appreciation from chemical composition. This could be attributed to the natural incorporation of interactions and non-linear or discontinuous effects in machine learning models, which are not easily grasped by the linear model architecture. While linear models and partial least squares regression represent the most widespread statistical approaches in sensory science, in part because they allow interpretation 65 , 81 , 82 , modern machine learning methods allow for building better predictive models while preserving the possibility to dissect and exploit the underlying patterns. Of the 10 different models we trained, tree-based models, such as our best performing GBR, showed the best overall performance in predicting sensory responses from chemical information, outcompeting artificial neural networks. This agrees with previous reports for models trained on tabular data 83 . Our results are in line with the findings of Colantonio et al. who also identified the gradient boosting architecture as performing best at predicting appreciation and flavor (of tomatoes and blueberries, in their specific study) 26 . Importantly, besides our larger experimental scale, we were able to directly confirm our models’ predictions in vivo.
Our study confirms that flavor compound concentration does not always correlate with perception, suggesting complex interactions that are often missed by more conventional statistics and simple models. Specifically, we find that tree-based algorithms may perform best in developing models that link complex food chemistry with aroma. Furthermore, we show that massive datasets of untrained consumer reviews provide a valuable source of data, that can complement or even replace trained tasting panels, especially for appreciation and basic flavors, such as sweetness and bitterness. This holds despite biases that are known to occur in such datasets, such as price or conformity bias. Moreover, GBR models predict taste better than aroma. This is likely because taste (e.g. bitterness) often directly relates to the corresponding chemical measurements (e.g., iso-alpha acids), whereas such a link is less clear for aromas, which often result from the interplay between multiple volatile compounds. We also find that our models are best at predicting acidity and alcohol, likely because there is a direct relation between the measured chemical compounds (acids and ethanol) and the corresponding perceived sensorial attribute (acidity and alcohol), and because even untrained consumers are generally able to recognize these flavors and aromas.
The predictions of our final models, trained on review data, hold even for blind tastings with small groups of trained tasters, as demonstrated by our ability to validate specific compounds as drivers of beer flavor and appreciation. Since adding a single compound to the extent of a noticeable difference may result in an unbalanced flavor profile, we specifically tested our identified key drivers as a combination of compounds. While this approach does not allow us to validate if a particular single compound would affect flavor and/or appreciation, our experiments do show that this combination of compounds increases consumer appreciation.
It is important to stress that, while it represents an important step forward, our approach still has several major limitations. A key weakness of the GBR model architecture is that amongst co-correlating variables, the largest main effect is consistently preferred for model building. As a result, co-correlating variables often have artificially low importance scores, both for impurity and SHAP-based methods, like we observed in the comparison to the more randomized Extra Trees models. This implies that chemicals identified as key drivers of a specific sensory feature by GBR might not be the true causative compounds, but rather co-correlate with the actual causative chemical. For example, the high importance of ethyl acetate could be (partially) attributed to the total ester content, ethanol or ethyl hexanoate (rho=0.77, rho=0.72 and rho=0.68), while ethyl phenylacetate could hide the importance of prenyl isobutyrate and ethyl benzoate (rho=0.77 and rho=0.76). Expanding our GBR model to include beer style as a parameter did not yield additional power or insight. This is likely due to style-specific chemical signatures, such as iso-alpha acids and lactic acid, that implicitly convey style information to the original model, as well as the smaller sample size per style, limiting the power to uncover style-specific patterns. This can be partly attributed to the curse of dimensionality, where the high number of parameters results in the models mainly incorporating single parameter effects, rather than complex interactions such as style-dependent effects 67 . A larger number of samples may overcome some of these limitations and offer more insight into style-specific effects. On the other hand, beer style is not a rigid scientific classification, and beers within one style often differ a lot, which further complicates the analysis of style as a model factor.
Our study is limited to beers from Belgian breweries. Although these beers cover a large portion of the beer styles available globally, some beer styles and consumer patterns may be missing, while other features might be overrepresented. For example, many Belgian ales exhibit yeast-driven flavor profiles, which is reflected in the chemical drivers of appreciation discovered by this study. In future work, expanding the scope to include diverse markets and beer styles could lead to the identification of even more drivers of appreciation and better models for special niche products that were not present in our beer set.
In addition to inherent limitations of GBR models, there are also some limitations associated with studying food aroma. Even if our chemical analyses measured most of the known aroma compounds, the total number of flavor compounds in complex foods like beer is still larger than the subset we were able to measure in this study. For example, hop-derived thiols, that influence flavor at very low concentrations, are notoriously difficult to measure in a high-throughput experiment. Moreover, consumer perception remains subjective and prone to biases that are difficult to avoid. It is also important to stress that the models are still immature and that more extensive datasets will be crucial for developing more complete models in the future. Besides more samples and parameters, our dataset does not include any demographic information about the tasters. Including such data could lead to better models that grasp external factors like age and culture. Another limitation is that our set of beers consists of high-quality end-products and lacks beers that are unfit for sale, which limits the current model in accurately predicting products that are appreciated very badly. Finally, while models could be readily applied in quality control, their use in sensory science and product development is restrained by their inability to discern causal relationships. Given that the models cannot distinguish compounds that genuinely drive consumer perception from those that merely correlate, validation experiments are essential to identify true causative compounds.
Despite the inherent limitations, dissection of our models enabled us to pinpoint specific molecules as potential drivers of beer aroma and consumer appreciation, including compounds that were unexpected and would not have been identified using standard approaches. Important drivers of beer appreciation uncovered by our models include protein levels, ethyl acetate, ethyl phenyl acetate and lactic acid. Currently, many brewers already use lactic acid to acidify their brewing water and ensure optimal pH for enzymatic activity during the mashing process. Our results suggest that adding lactic acid can also improve beer appreciation, although its individual effect remains to be tested. Interestingly, ethanol appears to be unnecessary to improve beer appreciation, both for blond beer and alcohol-free beer. Given the growing consumer interest in alcohol-free beer, with a predicted annual market growth of >7% 84 , it is relevant for brewers to know what compounds can further increase consumer appreciation of these beers. Hence, our model may readily provide avenues to further improve the flavor and consumer appreciation of both alcoholic and non-alcoholic beers, which is generally considered one of the key challenges for future beer production.
Whereas we see a direct implementation of our results for the development of superior alcohol-free beverages and other food products, our study can also serve as a stepping stone for the development of novel alcohol-containing beverages. We want to echo the growing body of scientific evidence for the negative effects of alcohol consumption, both on the individual level by the mutagenic, teratogenic and carcinogenic effects of ethanol 85 , 86 , as well as the burden on society caused by alcohol abuse and addiction. We encourage the use of our results for the production of healthier, tastier products, including novel and improved beverages with lower alcohol contents. Furthermore, we strongly discourage the use of these technologies to improve the appreciation or addictive properties of harmful substances.
The present work demonstrates that despite some important remaining hurdles, combining the latest developments in chemical analyses, sensory analysis and modern machine learning methods offers exciting avenues for food chemistry and engineering. Soon, these tools may provide solutions in quality control and recipe development, as well as new approaches to sensory science and flavor research.
Beer selection
250 commercial Belgian beers were selected to cover the broad diversity of beer styles and corresponding diversity in chemical composition and aroma. See Supplementary Fig. S1 .
Chemical dataset
Sample preparation.
Beers within their expiration date were purchased from commercial retailers. Samples were prepared in biological duplicates at room temperature, unless explicitly stated otherwise. Bottle pressure was measured with a manual pressure device (Steinfurth Mess-Systeme GmbH) and used to calculate CO 2 concentration. The beer was poured through two filter papers (Macherey-Nagel, 500713032 MN 713 ¼) to remove carbon dioxide and prevent spontaneous foaming. Samples were then prepared for measurements by targeted Headspace-Gas Chromatography-Flame Ionization Detector/Flame Photometric Detector (HS-GC-FID/FPD), Headspace-Solid Phase Microextraction-Gas Chromatography-Mass Spectrometry (HS-SPME-GC-MS), colorimetric analysis, enzymatic analysis, Near-Infrared (NIR) analysis, as described in the sections below. The mean values of biological duplicates are reported for each compound.
HS-GC-FID/FPD
HS-GC-FID/FPD (Shimadzu GC 2010 Plus) was used to measure higher alcohols, acetaldehyde, esters, 4-vinyl guaicol, and sulfur compounds. Each measurement comprised 5 ml of sample pipetted into a 20 ml glass vial containing 1.75 g NaCl (VWR, 27810.295). 100 µl of 2-heptanol (Sigma-Aldrich, H3003) (internal standard) solution in ethanol (Fisher Chemical, E/0650DF/C17) was added for a final concentration of 2.44 mg/L. Samples were flushed with nitrogen for 10 s, sealed with a silicone septum, stored at −80 °C and analyzed in batches of 20.
The GC was equipped with a DB-WAXetr column (length, 30 m; internal diameter, 0.32 mm; layer thickness, 0.50 µm; Agilent Technologies, Santa Clara, CA, USA) to the FID and an HP-5 column (length, 30 m; internal diameter, 0.25 mm; layer thickness, 0.25 µm; Agilent Technologies, Santa Clara, CA, USA) to the FPD. N 2 was used as the carrier gas. Samples were incubated for 20 min at 70 °C in the headspace autosampler (Flow rate, 35 cm/s; Injection volume, 1000 µL; Injection mode, split; Combi PAL autosampler, CTC analytics, Switzerland). The injector, FID and FPD temperatures were kept at 250 °C. The GC oven temperature was first held at 50 °C for 5 min and then allowed to rise to 80 °C at a rate of 5 °C/min, followed by a second ramp of 4 °C/min until 200 °C kept for 3 min and a final ramp of (4 °C/min) until 230 °C for 1 min. Results were analyzed with the GCSolution software version 2.4 (Shimadzu, Kyoto, Japan). The GC was calibrated with a 5% EtOH solution (VWR International) containing the volatiles under study (Supplementary Table S7 ).
HS-SPME-GC-MS
HS-SPME-GC-MS (Shimadzu GCMS-QP-2010 Ultra) was used to measure additional volatile compounds, mainly comprising terpenoids and esters. Samples were analyzed by HS-SPME using a triphase DVB/Carboxen/PDMS 50/30 μm SPME fiber (Supelco Co., Bellefonte, PA, USA) followed by gas chromatography (Thermo Fisher Scientific Trace 1300 series, USA) coupled to a mass spectrometer (Thermo Fisher Scientific ISQ series MS) equipped with a TriPlus RSH autosampler. 5 ml of degassed beer sample was placed in 20 ml vials containing 1.75 g NaCl (VWR, 27810.295). 5 µl internal standard mix was added, containing 2-heptanol (1 g/L) (Sigma-Aldrich, H3003), 4-fluorobenzaldehyde (1 g/L) (Sigma-Aldrich, 128376), 2,3-hexanedione (1 g/L) (Sigma-Aldrich, 144169) and guaiacol (1 g/L) (Sigma-Aldrich, W253200) in ethanol (Fisher Chemical, E/0650DF/C17). Each sample was incubated at 60 °C in the autosampler oven with constant agitation. After 5 min equilibration, the SPME fiber was exposed to the sample headspace for 30 min. The compounds trapped on the fiber were thermally desorbed in the injection port of the chromatograph by heating the fiber for 15 min at 270 °C.
The GC-MS was equipped with a low polarity RXi-5Sil MS column (length, 20 m; internal diameter, 0.18 mm; layer thickness, 0.18 µm; Restek, Bellefonte, PA, USA). Injection was performed in splitless mode at 320 °C, a split flow of 9 ml/min, a purge flow of 5 ml/min and an open valve time of 3 min. To obtain a pulsed injection, a programmed gas flow was used whereby the helium gas flow was set at 2.7 mL/min for 0.1 min, followed by a decrease in flow of 20 ml/min to the normal 0.9 mL/min. The temperature was first held at 30 °C for 3 min and then allowed to rise to 80 °C at a rate of 7 °C/min, followed by a second ramp of 2 °C/min till 125 °C and a final ramp of 8 °C/min with a final temperature of 270 °C.
Mass acquisition range was 33 to 550 amu at a scan rate of 5 scans/s. Electron impact ionization energy was 70 eV. The interface and ion source were kept at 275 °C and 250 °C, respectively. A mix of linear n-alkanes (from C7 to C40, Supelco Co.) was injected into the GC-MS under identical conditions to serve as external retention index markers. Identification and quantification of the compounds were performed using an in-house developed R script as described in Goelen et al. and Reher et al. 87 , 88 (for package information, see Supplementary Table S8 ). Briefly, chromatograms were analyzed using AMDIS (v2.71) 89 to separate overlapping peaks and obtain pure compound spectra. The NIST MS Search software (v2.0 g) in combination with the NIST2017, FFNSC3 and Adams4 libraries were used to manually identify the empirical spectra, taking into account the expected retention time. After background subtraction and correcting for retention time shifts between samples run on different days based on alkane ladders, compound elution profiles were extracted and integrated using a file with 284 target compounds of interest, which were either recovered in our identified AMDIS list of spectra or were known to occur in beer. Compound elution profiles were estimated for every peak in every chromatogram over a time-restricted window using weighted non-negative least square analysis after which peak areas were integrated 87 , 88 . Batch effect correction was performed by normalizing against the most stable internal standard compound, 4-fluorobenzaldehyde. Out of all 284 target compounds that were analyzed, 167 were visually judged to have reliable elution profiles and were used for final analysis.
Discrete photometric and enzymatic analysis
Discrete photometric and enzymatic analysis (Thermo Scientific TM Gallery TM Plus Beermaster Discrete Analyzer) was used to measure acetic acid, ammonia, beta-glucan, iso-alpha acids, color, sugars, glycerol, iron, pH, protein, and sulfite. 2 ml of sample volume was used for the analyses. Information regarding the reagents and standard solutions used for analyses and calibrations is included in Supplementary Table S7 and Supplementary Table S9 .
NIR analyses
NIR analysis (Anton Paar Alcolyzer Beer ME System) was used to measure ethanol. Measurements comprised 50 ml of sample, and a 10% EtOH solution was used for calibration.
Correlation calculations
Pairwise Spearman Rank correlations were calculated between all chemical properties.
Sensory dataset
Trained panel.
Our trained tasting panel consisted of volunteers who gave prior verbal informed consent. All compounds used for the validation experiment were of food-grade quality. The tasting sessions were approved by the Social and Societal Ethics Committee of the KU Leuven (G-2022-5677-R2(MAR)). All online reviewers agreed to the Terms and Conditions of the RateBeer website.
Sensory analysis was performed according to the American Society of Brewing Chemists (ASBC) Sensory Analysis Methods 90 . 30 volunteers were screened through a series of triangle tests. The sixteen most sensitive and consistent tasters were retained as taste panel members. The resulting panel was diverse in age [22–42, mean: 29], sex [56% male] and nationality [7 different countries]. The panel developed a consensus vocabulary to describe beer aroma, taste and mouthfeel. Panelists were trained to identify and score 50 different attributes, using a 7-point scale to rate attributes’ intensity. The scoring sheet is included as Supplementary Data 3 . Sensory assessments took place between 10–12 a.m. The beers were served in black-colored glasses. Per session, between 5 and 12 beers of the same style were tasted at 12 °C to 16 °C. Two reference beers were added to each set and indicated as ‘Reference 1 & 2’, allowing panel members to calibrate their ratings. Not all panelists were present at every tasting. Scores were scaled by standard deviation and mean-centered per taster. Values are represented as z-scores and clustered by Euclidean distance. Pairwise Spearman correlations were calculated between taste and aroma sensory attributes. Panel consistency was evaluated by repeating samples on different sessions and performing ANOVA to identify differences, using the ‘stats’ package (v4.2.2) in R (for package information, see Supplementary Table S8 ).
Online reviews from a public database
The ‘scrapy’ package in Python (v3.6) (for package information, see Supplementary Table S8 ). was used to collect 232,288 online reviews (mean=922, min=6, max=5343) from RateBeer, an online beer review database. Each review entry comprised 5 numerical scores (appearance, aroma, taste, palate and overall quality) and an optional review text. The total number of reviews per reviewer was collected separately. Numerical scores were scaled and centered per rater, and mean scores were calculated per beer.
For the review texts, the language was estimated using the packages ‘langdetect’ and ‘langid’ in Python. Reviews that were classified as English by both packages were kept. Reviewers with fewer than 100 entries overall were discarded. 181,025 reviews from >6000 reviewers from >40 countries remained. Text processing was done using the ‘nltk’ package in Python. Texts were corrected for slang and misspellings; proper nouns and rare words that are relevant to the beer context were specified and kept as-is (‘Chimay’,’Lambic’, etc.). A dictionary of semantically similar sensorial terms, for example ‘floral’ and ‘flower’, was created and collapsed together into one term. Words were stemmed and lemmatized to avoid identifying words such as ‘acid’ and ‘acidity’ as separate terms. Numbers and punctuation were removed.
Sentences from up to 50 randomly chosen reviews per beer were manually categorized according to the aspect of beer they describe (appearance, aroma, taste, palate, overall quality—not to be confused with the 5 numerical scores described above) or flagged as irrelevant if they contained no useful information. If a beer contained fewer than 50 reviews, all reviews were manually classified. This labeled data set was used to train a model that classified the rest of the sentences for all beers 91 . Sentences describing taste and aroma were extracted, and term frequency–inverse document frequency (TFIDF) was implemented to calculate enrichment scores for sensorial words per beer.
The sex of the tasting subject was not considered when building our sensory database. Instead, results from different panelists were averaged, both for our trained panel (56% male, 44% female) and the RateBeer reviews (70% male, 30% female for RateBeer as a whole).
Beer price collection and processing
Beer prices were collected from the following stores: Colruyt, Delhaize, Total Wine, BeerHawk, The Belgian Beer Shop, The Belgian Shop, and Beer of Belgium. Where applicable, prices were converted to Euros and normalized per liter. Spearman correlations were calculated between these prices and mean overall appreciation scores from RateBeer and the taste panel, respectively.
Pairwise Spearman Rank correlations were calculated between all sensory properties.
Machine learning models
Predictive modeling of sensory profiles from chemical data.
Regression models were constructed to predict (a) trained panel scores for beer flavors and quality from beer chemical profiles and (b) public reviews’ appreciation scores from beer chemical profiles. Z-scores were used to represent sensory attributes in both data sets. Chemical properties with log-normal distributions (Shapiro-Wilk test, p < 0.05 ) were log-transformed. Missing chemical measurements (0.1% of all data) were replaced with mean values per attribute. Observations from 250 beers were randomly separated into a training set (70%, 175 beers) and a test set (30%, 75 beers), stratified per beer style. Chemical measurements (p = 231) were normalized based on the training set average and standard deviation. In total, three linear regression-based models: linear regression with first-order interaction terms (LR), lasso regression with first-order interaction terms (Lasso) and partial least squares regression (PLSR); five decision tree models, Adaboost regressor (ABR), Extra Trees (ET), Gradient Boosting regressor (GBR), Random Forest (RF) and XGBoost regressor (XGBR); one support vector machine model (SVR) and one artificial neural network model (ANN) were trained. The models were implemented using the ‘scikit-learn’ package (v1.2.2) and ‘xgboost’ package (v1.7.3) in Python (v3.9.16). Models were trained, and hyperparameters optimized, using five-fold cross-validated grid search with the coefficient of determination (R 2 ) as the evaluation metric. The ANN (scikit-learn’s MLPRegressor) was optimized using Bayesian Tree-Structured Parzen Estimator optimization with the ‘Optuna’ Python package (v3.2.0). Individual models were trained per attribute, and a multi-output model was trained on all attributes simultaneously.
Model dissection
GBR was found to outperform other methods, resulting in models with the highest average R 2 values in both trained panel and public review data sets. Impurity-based rankings of the most important predictors for each predicted sensorial trait were obtained using the ‘scikit-learn’ package. To observe the relationships between these chemical properties and their predicted targets, partial dependence plots (PDP) were constructed for the six most important predictors of consumer appreciation 74 , 75 .
The ‘SHAP’ package in Python (v0.41.0) was implemented to provide an alternative ranking of predictor importance and to visualize the predictors’ effects as a function of their concentration 68 .
Validation of causal chemical properties
To validate the effects of the most important model features on predicted sensory attributes, beers were spiked with the chemical compounds identified by the models and descriptive sensory analyses were carried out according to the American Society of Brewing Chemists (ASBC) protocol 90 .
Compound spiking was done 30 min before tasting. Compounds were spiked into fresh beer bottles, that were immediately resealed and inverted three times. Fresh bottles of beer were opened for the same duration, resealed, and inverted thrice, to serve as controls. Pairs of spiked samples and controls were served simultaneously, chilled and in dark glasses as outlined in the Trained panel section above. Tasters were instructed to select the glass with the higher flavor intensity for each attribute (directional difference test 92 ) and to select the glass they prefer.
The final concentration after spiking was equal to the within-style average, after normalizing by ethanol concentration. This was done to ensure balanced flavor profiles in the final spiked beer. The same methods were applied to improve a non-alcoholic beer. Compounds were the following: ethyl acetate (Merck KGaA, W241415), ethyl hexanoate (Merck KGaA, W243906), isoamyl acetate (Merck KGaA, W205508), phenethyl acetate (Merck KGaA, W285706), ethanol (96%, Colruyt), glycerol (Merck KGaA, W252506), lactic acid (Merck KGaA, 261106).
Significant differences in preference or perceived intensity were determined by performing the two-sided binomial test on each attribute.
Reporting summary
Further information on research design is available in the Nature Portfolio Reporting Summary linked to this article.
Data availability
The data that support the findings of this work are available in the Supplementary Data files and have been deposited to Zenodo under accession code 10653704 93 . The RateBeer scores data are under restricted access, they are not publicly available as they are property of RateBeer (ZX Ventures, USA). Access can be obtained from the authors upon reasonable request and with permission of RateBeer (ZX Ventures, USA). Source data are provided with this paper.
Code availability
The code for training the machine learning models, analyzing the models, and generating the figures has been deposited to Zenodo under accession code 10653704 93 .
Tieman, D. et al. A chemical genetic roadmap to improved tomato flavor. Science 355 , 391–394 (2017).
Article ADS CAS PubMed Google Scholar
Plutowska, B. & Wardencki, W. Application of gas chromatography–olfactometry (GC–O) in analysis and quality assessment of alcoholic beverages – A review. Food Chem. 107 , 449–463 (2008).
Article CAS Google Scholar
Legin, A., Rudnitskaya, A., Seleznev, B. & Vlasov, Y. Electronic tongue for quality assessment of ethanol, vodka and eau-de-vie. Anal. Chim. Acta 534 , 129–135 (2005).
Loutfi, A., Coradeschi, S., Mani, G. K., Shankar, P. & Rayappan, J. B. B. Electronic noses for food quality: A review. J. Food Eng. 144 , 103–111 (2015).
Ahn, Y.-Y., Ahnert, S. E., Bagrow, J. P. & Barabási, A.-L. Flavor network and the principles of food pairing. Sci. Rep. 1 , 196 (2011).
Article CAS PubMed PubMed Central Google Scholar
Bartoshuk, L. M. & Klee, H. J. Better fruits and vegetables through sensory analysis. Curr. Biol. 23 , R374–R378 (2013).
Article CAS PubMed Google Scholar
Piggott, J. R. Design questions in sensory and consumer science. Food Qual. Prefer. 3293 , 217–220 (1995).
Article Google Scholar
Kermit, M. & Lengard, V. Assessing the performance of a sensory panel-panellist monitoring and tracking. J. Chemom. 19 , 154–161 (2005).
Cook, D. J., Hollowood, T. A., Linforth, R. S. T. & Taylor, A. J. Correlating instrumental measurements of texture and flavour release with human perception. Int. J. Food Sci. Technol. 40 , 631–641 (2005).
Chinchanachokchai, S., Thontirawong, P. & Chinchanachokchai, P. A tale of two recommender systems: The moderating role of consumer expertise on artificial intelligence based product recommendations. J. Retail. Consum. Serv. 61 , 1–12 (2021).
Ross, C. F. Sensory science at the human-machine interface. Trends Food Sci. Technol. 20 , 63–72 (2009).
Chambers, E. IV & Koppel, K. Associations of volatile compounds with sensory aroma and flavor: The complex nature of flavor. Molecules 18 , 4887–4905 (2013).
Pinu, F. R. Metabolomics—The new frontier in food safety and quality research. Food Res. Int. 72 , 80–81 (2015).
Danezis, G. P., Tsagkaris, A. S., Brusic, V. & Georgiou, C. A. Food authentication: state of the art and prospects. Curr. Opin. Food Sci. 10 , 22–31 (2016).
Shepherd, G. M. Smell images and the flavour system in the human brain. Nature 444 , 316–321 (2006).
Meilgaard, M. C. Prediction of flavor differences between beers from their chemical composition. J. Agric. Food Chem. 30 , 1009–1017 (1982).
Xu, L. et al. Widespread receptor-driven modulation in peripheral olfactory coding. Science 368 , eaaz5390 (2020).
Kupferschmidt, K. Following the flavor. Science 340 , 808–809 (2013).
Billesbølle, C. B. et al. Structural basis of odorant recognition by a human odorant receptor. Nature 615 , 742–749 (2023).
Article ADS PubMed PubMed Central Google Scholar
Smith, B. Perspective: Complexities of flavour. Nature 486 , S6–S6 (2012).
Pfister, P. et al. Odorant receptor inhibition is fundamental to odor encoding. Curr. Biol. 30 , 2574–2587 (2020).
Moskowitz, H. W., Kumaraiah, V., Sharma, K. N., Jacobs, H. L. & Sharma, S. D. Cross-cultural differences in simple taste preferences. Science 190 , 1217–1218 (1975).
Eriksson, N. et al. A genetic variant near olfactory receptor genes influences cilantro preference. Flavour 1 , 22 (2012).
Ferdenzi, C. et al. Variability of affective responses to odors: Culture, gender, and olfactory knowledge. Chem. Senses 38 , 175–186 (2013).
Article PubMed Google Scholar
Lawless, H. T. & Heymann, H. Sensory evaluation of food: Principles and practices. (Springer, New York, NY). https://doi.org/10.1007/978-1-4419-6488-5 (2010).
Colantonio, V. et al. Metabolomic selection for enhanced fruit flavor. Proc. Natl. Acad. Sci. 119 , e2115865119 (2022).
Fritz, F., Preissner, R. & Banerjee, P. VirtualTaste: a web server for the prediction of organoleptic properties of chemical compounds. Nucleic Acids Res 49 , W679–W684 (2021).
Tuwani, R., Wadhwa, S. & Bagler, G. BitterSweet: Building machine learning models for predicting the bitter and sweet taste of small molecules. Sci. Rep. 9 , 1–13 (2019).
Dagan-Wiener, A. et al. Bitter or not? BitterPredict, a tool for predicting taste from chemical structure. Sci. Rep. 7 , 1–13 (2017).
Pallante, L. et al. Toward a general and interpretable umami taste predictor using a multi-objective machine learning approach. Sci. Rep. 12 , 1–11 (2022).
Malavolta, M. et al. A survey on computational taste predictors. Eur. Food Res. Technol. 248 , 2215–2235 (2022).
Lee, B. K. et al. A principal odor map unifies diverse tasks in olfactory perception. Science 381 , 999–1006 (2023).
Mayhew, E. J. et al. Transport features predict if a molecule is odorous. Proc. Natl. Acad. Sci. 119 , e2116576119 (2022).
Niu, Y. et al. Sensory evaluation of the synergism among ester odorants in light aroma-type liquor by odor threshold, aroma intensity and flash GC electronic nose. Food Res. Int. 113 , 102–114 (2018).
Yu, P., Low, M. Y. & Zhou, W. Design of experiments and regression modelling in food flavour and sensory analysis: A review. Trends Food Sci. Technol. 71 , 202–215 (2018).
Oladokun, O. et al. The impact of hop bitter acid and polyphenol profiles on the perceived bitterness of beer. Food Chem. 205 , 212–220 (2016).
Linforth, R., Cabannes, M., Hewson, L., Yang, N. & Taylor, A. Effect of fat content on flavor delivery during consumption: An in vivo model. J. Agric. Food Chem. 58 , 6905–6911 (2010).
Guo, S., Na Jom, K. & Ge, Y. Influence of roasting condition on flavor profile of sunflower seeds: A flavoromics approach. Sci. Rep. 9 , 11295 (2019).
Ren, Q. et al. The changes of microbial community and flavor compound in the fermentation process of Chinese rice wine using Fagopyrum tataricum grain as feedstock. Sci. Rep. 9 , 3365 (2019).
Hastie, T., Friedman, J. & Tibshirani, R. The Elements of Statistical Learning. (Springer, New York, NY). https://doi.org/10.1007/978-0-387-21606-5 (2001).
Dietz, C., Cook, D., Huismann, M., Wilson, C. & Ford, R. The multisensory perception of hop essential oil: a review. J. Inst. Brew. 126 , 320–342 (2020).
CAS Google Scholar
Roncoroni, Miguel & Verstrepen, Kevin Joan. Belgian Beer: Tested and Tasted. (Lannoo, 2018).
Meilgaard, M. Flavor chemistry of beer: Part II: Flavor and threshold of 239 aroma volatiles. in (1975).
Bokulich, N. A. & Bamforth, C. W. The microbiology of malting and brewing. Microbiol. Mol. Biol. Rev. MMBR 77 , 157–172 (2013).
Dzialo, M. C., Park, R., Steensels, J., Lievens, B. & Verstrepen, K. J. Physiology, ecology and industrial applications of aroma formation in yeast. FEMS Microbiol. Rev. 41 , S95–S128 (2017).
Article PubMed PubMed Central Google Scholar
Datta, A. et al. Computer-aided food engineering. Nat. Food 3 , 894–904 (2022).
American Society of Brewing Chemists. Beer Methods. (American Society of Brewing Chemists, St. Paul, MN, U.S.A.).
Olaniran, A. O., Hiralal, L., Mokoena, M. P. & Pillay, B. Flavour-active volatile compounds in beer: production, regulation and control. J. Inst. Brew. 123 , 13–23 (2017).
Verstrepen, K. J. et al. Flavor-active esters: Adding fruitiness to beer. J. Biosci. Bioeng. 96 , 110–118 (2003).
Meilgaard, M. C. Flavour chemistry of beer. part I: flavour interaction between principal volatiles. Master Brew. Assoc. Am. Tech. Q 12 , 107–117 (1975).
Briggs, D. E., Boulton, C. A., Brookes, P. A. & Stevens, R. Brewing 227–254. (Woodhead Publishing). https://doi.org/10.1533/9781855739062.227 (2004).
Bossaert, S., Crauwels, S., De Rouck, G. & Lievens, B. The power of sour - A review: Old traditions, new opportunities. BrewingScience 72 , 78–88 (2019).
Google Scholar
Verstrepen, K. J. et al. Flavor active esters: Adding fruitiness to beer. J. Biosci. Bioeng. 96 , 110–118 (2003).
Snauwaert, I. et al. Microbial diversity and metabolite composition of Belgian red-brown acidic ales. Int. J. Food Microbiol. 221 , 1–11 (2016).
Spitaels, F. et al. The microbial diversity of traditional spontaneously fermented lambic beer. PLoS ONE 9 , e95384 (2014).
Blanco, C. A., Andrés-Iglesias, C. & Montero, O. Low-alcohol Beers: Flavor Compounds, Defects, and Improvement Strategies. Crit. Rev. Food Sci. Nutr. 56 , 1379–1388 (2016).
Jackowski, M. & Trusek, A. Non-Alcohol. beer Prod. – Overv. 20 , 32–38 (2018).
Takoi, K. et al. The contribution of geraniol metabolism to the citrus flavour of beer: Synergy of geraniol and β-citronellol under coexistence with excess linalool. J. Inst. Brew. 116 , 251–260 (2010).
Kroeze, J. H. & Bartoshuk, L. M. Bitterness suppression as revealed by split-tongue taste stimulation in humans. Physiol. Behav. 35 , 779–783 (1985).
Mennella, J. A. et al. A spoonful of sugar helps the medicine go down”: Bitter masking bysucrose among children and adults. Chem. Senses 40 , 17–25 (2015).
Wietstock, P., Kunz, T., Perreira, F. & Methner, F.-J. Metal chelation behavior of hop acids in buffered model systems. BrewingScience 69 , 56–63 (2016).
Sancho, D., Blanco, C. A., Caballero, I. & Pascual, A. Free iron in pale, dark and alcohol-free commercial lager beers. J. Sci. Food Agric. 91 , 1142–1147 (2011).
Rodrigues, H. & Parr, W. V. Contribution of cross-cultural studies to understanding wine appreciation: A review. Food Res. Int. 115 , 251–258 (2019).
Korneva, E. & Blockeel, H. Towards better evaluation of multi-target regression models. in ECML PKDD 2020 Workshops (eds. Koprinska, I. et al.) 353–362 (Springer International Publishing, Cham, 2020). https://doi.org/10.1007/978-3-030-65965-3_23 .
Gastón Ares. Mathematical and Statistical Methods in Food Science and Technology. (Wiley, 2013).
Grinsztajn, L., Oyallon, E. & Varoquaux, G. Why do tree-based models still outperform deep learning on tabular data? Preprint at http://arxiv.org/abs/2207.08815 (2022).
Gries, S. T. Statistics for Linguistics with R: A Practical Introduction. in Statistics for Linguistics with R (De Gruyter Mouton, 2021). https://doi.org/10.1515/9783110718256 .
Lundberg, S. M. et al. From local explanations to global understanding with explainable AI for trees. Nat. Mach. Intell. 2 , 56–67 (2020).
Ickes, C. M. & Cadwallader, K. R. Effects of ethanol on flavor perception in alcoholic beverages. Chemosens. Percept. 10 , 119–134 (2017).
Kato, M. et al. Influence of high molecular weight polypeptides on the mouthfeel of commercial beer. J. Inst. Brew. 127 , 27–40 (2021).
Wauters, R. et al. Novel Saccharomyces cerevisiae variants slow down the accumulation of staling aldehydes and improve beer shelf-life. Food Chem. 398 , 1–11 (2023).
Li, H., Jia, S. & Zhang, W. Rapid determination of low-level sulfur compounds in beer by headspace gas chromatography with a pulsed flame photometric detector. J. Am. Soc. Brew. Chem. 66 , 188–191 (2008).
Dercksen, A., Laurens, J., Torline, P., Axcell, B. C. & Rohwer, E. Quantitative analysis of volatile sulfur compounds in beer using a membrane extraction interface. J. Am. Soc. Brew. Chem. 54 , 228–233 (1996).
Molnar, C. Interpretable Machine Learning: A Guide for Making Black-Box Models Interpretable. (2020).
Zhao, Q. & Hastie, T. Causal interpretations of black-box models. J. Bus. Econ. Stat. Publ. Am. Stat. Assoc. 39 , 272–281 (2019).
Article MathSciNet Google Scholar
Hastie, T., Tibshirani, R. & Friedman, J. The Elements of Statistical Learning. (Springer, 2019).
Labrado, D. et al. Identification by NMR of key compounds present in beer distillates and residual phases after dealcoholization by vacuum distillation. J. Sci. Food Agric. 100 , 3971–3978 (2020).
Lusk, L. T., Kay, S. B., Porubcan, A. & Ryder, D. S. Key olfactory cues for beer oxidation. J. Am. Soc. Brew. Chem. 70 , 257–261 (2012).
Gonzalez Viejo, C., Torrico, D. D., Dunshea, F. R. & Fuentes, S. Development of artificial neural network models to assess beer acceptability based on sensory properties using a robotic pourer: A comparative model approach to achieve an artificial intelligence system. Beverages 5 , 33 (2019).
Gonzalez Viejo, C., Fuentes, S., Torrico, D. D., Godbole, A. & Dunshea, F. R. Chemical characterization of aromas in beer and their effect on consumers liking. Food Chem. 293 , 479–485 (2019).
Gilbert, J. L. et al. Identifying breeding priorities for blueberry flavor using biochemical, sensory, and genotype by environment analyses. PLOS ONE 10 , 1–21 (2015).
Goulet, C. et al. Role of an esterase in flavor volatile variation within the tomato clade. Proc. Natl. Acad. Sci. 109 , 19009–19014 (2012).
Article ADS CAS PubMed PubMed Central Google Scholar
Borisov, V. et al. Deep Neural Networks and Tabular Data: A Survey. IEEE Trans. Neural Netw. Learn. Syst. 1–21 https://doi.org/10.1109/TNNLS.2022.3229161 (2022).
Statista. Statista Consumer Market Outlook: Beer - Worldwide.
Seitz, H. K. & Stickel, F. Molecular mechanisms of alcoholmediated carcinogenesis. Nat. Rev. Cancer 7 , 599–612 (2007).
Voordeckers, K. et al. Ethanol exposure increases mutation rate through error-prone polymerases. Nat. Commun. 11 , 3664 (2020).
Goelen, T. et al. Bacterial phylogeny predicts volatile organic compound composition and olfactory response of an aphid parasitoid. Oikos 129 , 1415–1428 (2020).
Article ADS Google Scholar
Reher, T. et al. Evaluation of hop (Humulus lupulus) as a repellent for the management of Drosophila suzukii. Crop Prot. 124 , 104839 (2019).
Stein, S. E. An integrated method for spectrum extraction and compound identification from gas chromatography/mass spectrometry data. J. Am. Soc. Mass Spectrom. 10 , 770–781 (1999).
American Society of Brewing Chemists. Sensory Analysis Methods. (American Society of Brewing Chemists, St. Paul, MN, U.S.A., 1992).
McAuley, J., Leskovec, J. & Jurafsky, D. Learning Attitudes and Attributes from Multi-Aspect Reviews. Preprint at https://doi.org/10.48550/arXiv.1210.3926 (2012).
Meilgaard, M. C., Carr, B. T. & Carr, B. T. Sensory Evaluation Techniques. (CRC Press, Boca Raton). https://doi.org/10.1201/b16452 (2014).
Schreurs, M. et al. Data from: Predicting and improving complex beer flavor through machine learning. Zenodo https://doi.org/10.5281/zenodo.10653704 (2024).
Download references
Acknowledgements
We thank all lab members for their discussions and thank all tasting panel members for their contributions. Special thanks go out to Dr. Karin Voordeckers for her tremendous help in proofreading and improving the manuscript. M.S. was supported by a Baillet-Latour fellowship, L.C. acknowledges financial support from KU Leuven (C16/17/006), F.A.T. was supported by a PhD fellowship from FWO (1S08821N). Research in the lab of K.J.V. is supported by KU Leuven, FWO, VIB, VLAIO and the Brewing Science Serves Health Fund. Research in the lab of T.W. is supported by FWO (G.0A51.15) and KU Leuven (C16/17/006).
Author information
These authors contributed equally: Michiel Schreurs, Supinya Piampongsant, Miguel Roncoroni.
Authors and Affiliations
VIB—KU Leuven Center for Microbiology, Gaston Geenslaan 1, B-3001, Leuven, Belgium
Michiel Schreurs, Supinya Piampongsant, Miguel Roncoroni, Lloyd Cool, Beatriz Herrera-Malaver, Florian A. Theßeling & Kevin J. Verstrepen
CMPG Laboratory of Genetics and Genomics, KU Leuven, Gaston Geenslaan 1, B-3001, Leuven, Belgium
Leuven Institute for Beer Research (LIBR), Gaston Geenslaan 1, B-3001, Leuven, Belgium
Laboratory of Socioecology and Social Evolution, KU Leuven, Naamsestraat 59, B-3000, Leuven, Belgium
Lloyd Cool, Christophe Vanderaa & Tom Wenseleers
VIB Bioinformatics Core, VIB, Rijvisschestraat 120, B-9052, Ghent, Belgium
Łukasz Kreft & Alexander Botzki
AB InBev SA/NV, Brouwerijplein 1, B-3000, Leuven, Belgium
Philippe Malcorps & Luk Daenen
You can also search for this author in PubMed Google Scholar
Contributions
S.P., M.S. and K.J.V. conceived the experiments. S.P., M.S. and K.J.V. designed the experiments. S.P., M.S., M.R., B.H. and F.A.T. performed the experiments. S.P., M.S., L.C., C.V., L.K., A.B., P.M., L.D., T.W. and K.J.V. contributed analysis ideas. S.P., M.S., L.C., C.V., T.W. and K.J.V. analyzed the data. All authors contributed to writing the manuscript.
Corresponding author
Correspondence to Kevin J. Verstrepen .
Ethics declarations
Competing interests.
K.J.V. is affiliated with bar.on. The other authors declare no competing interests.
Peer review
Peer review information.
Nature Communications thanks Florian Bauer, Andrew John Macintosh and the other, anonymous, reviewer(s) for their contribution to the peer review of this work. A peer review file is available.
Additional information
Publisher’s note Springer Nature remains neutral with regard to jurisdictional claims in published maps and institutional affiliations.
Supplementary information
Supplementary information, peer review file, description of additional supplementary files, supplementary data 1, supplementary data 2, supplementary data 3, supplementary data 4, supplementary data 5, supplementary data 6, supplementary data 7, reporting summary, source data, source data, rights and permissions.
Open Access This article is licensed under a Creative Commons Attribution 4.0 International License, which permits use, sharing, adaptation, distribution and reproduction in any medium or format, as long as you give appropriate credit to the original author(s) and the source, provide a link to the Creative Commons licence, and indicate if changes were made. The images or other third party material in this article are included in the article’s Creative Commons licence, unless indicated otherwise in a credit line to the material. If material is not included in the article’s Creative Commons licence and your intended use is not permitted by statutory regulation or exceeds the permitted use, you will need to obtain permission directly from the copyright holder. To view a copy of this licence, visit http://creativecommons.org/licenses/by/4.0/ .
Reprints and permissions
About this article
Cite this article.
Schreurs, M., Piampongsant, S., Roncoroni, M. et al. Predicting and improving complex beer flavor through machine learning. Nat Commun 15 , 2368 (2024). https://doi.org/10.1038/s41467-024-46346-0
Download citation
Received : 30 October 2023
Accepted : 21 February 2024
Published : 26 March 2024
DOI : https://doi.org/10.1038/s41467-024-46346-0
Share this article
Anyone you share the following link with will be able to read this content:
Sorry, a shareable link is not currently available for this article.
Provided by the Springer Nature SharedIt content-sharing initiative
By submitting a comment you agree to abide by our Terms and Community Guidelines . If you find something abusive or that does not comply with our terms or guidelines please flag it as inappropriate.
Quick links
- Explore articles by subject
- Guide to authors
- Editorial policies
Sign up for the Nature Briefing: Translational Research newsletter — top stories in biotechnology, drug discovery and pharma.


- Vehicle Technologies Office
- About the Vehicle Technologies Office
- Technology Areas
- Technology Integration
- Reports and Publications
Office: Vehicle Technologies Office FOA number: DE-FOA-0003248 Link to apply: Apply on EERE Exchange FOA Amount: $45,800,000
Today, the Department of Energy (DOE) announced $45.8 million in new funding for projects that will advance research, development, demonstration, and deployment (RDD&D) critical to achieving net-zero greenhouse gas emissions in the transportation sector. The funding will drive innovation in equitable clean transportation and is aligned with strategies detailed in the U.S. National Blueprint for Transportation Decarbonization .
The funding is through DOE’s Office of Energy Efficiency and Renewable Energy (EERE). Topic areas in the Vehicle Technologies Office (VTO) Fiscal Year (FY) 2024 R&D funding opportunity include:
- Next-generation phosphate-based cathodes.
- Advancing the state of the art for sodium-ion batteries.
- Developing concepts for decreasing greenhouse gas emissions from off-road vehicles such as construction, agriculture, mining, and forestry vehicles.
- Developing and deploying vehicle-to-everything technologies that can lead to meaningful savings at the vehicle and transportation system level.
- Developing high-performance, domestically produced electrical steels (E-steels) for use in electrified powertrains.
- Addressing critical cybersecurity needs for smart and secure electric vehicle charging.
As part of the Biden-Harris Administration’s commitment to ensuring the benefits of a clean transportation system are shared equally, the funding seeks the participation of underserved communities and underrepresented groups. Applicants are required to describe how diversity, equity, and inclusion objectives will be incorporated into their project.
VTO provides a series of funding opportunity announcement (FOA) information session videos , which help applicants understand VTO’s FOA process and requirements. The recently released, Session 3: Tips for a Strong FOA Application, includes best practices for incorporating Diversity, Equity, Inclusion, and Accessibility in a project.
Learn more about this and other funding opportunities on VTO’s funding webpage .
Topic Areas
Topic Area 1: Next-Generation Phosphate-Based Cathodes
This topic area targets the development of phosphate-based cathode materials that surpass the performance of state-of-the-art lithium iron phosphate (LFP) cathode materials, which are currently gaining traction as an alternative low-cost solution. The primary objective of this area of interest is to develop high energy density battery cells containing phosphate-based cathodes at the material and cell level.
Topic Area 2: Na-ion Battery Seedling Projects for Electric Vehicle Applications
While shifting to alternative cathode materials like LFP can alleviate the impact of nickel and cobalt, the impact of lithium has not been adequately addressed. One alternative to lithium is sodium (Na). While there is much promise for Na-ion chemistries, key issues still limit their adoption. This objective of this topic area is to advance the state of the art for Na-ion batteries by solving key challenges for the cathode, anode, or electrolyte through the development of 1 Ah full cells utilizing cell chemistries that are significant advancements over current industry state-of-the-art Na-ion technology.
Topic Area 3: Low-GHG Concepts for Off-Road Vehicles
The objective of this topic area is to develop and validate technology concepts capable of significantly decreasing greenhouse gas emissions, energy use, harmful criteria emissions, and total cost of ownership across the entire off-road vehicle sector, including construction, agriculture, mining, forestry, ports, warehouses, etc. Concepts must demonstrate they can meet the unique requirements for off-road vehicles and gain customer acceptance.
Topic Area 4: Saving Energy with Connectivity
Research has shown that vehicle-to-everything (V2X) communications can lead to meaningful energy savings at the vehicle and transportation system level by integrating interoperable vehicle-to-vehicle (V2V), vehicle-to-infrastructure (V2I), and vehicle-to-pedestrian (V2P) communications. The objective of this topic area is to develop and deploy V2X technologies with a focus on the efficiency and convenience of the mobility ecosystem, while reducing transportation’s environmental impacts. Examples could include but are not limited to eco-driving along connected corridors, transit or freight priority, integrated corridor management, or passenger or freight trip-chaining optimization.
Topic Area 5: Domestically Produced Electrical Steels (E-Steels)
The US transportation sector is in a technology revolution where light-duty vehicles are rapidly transitioning from internal combustion engines to electrified powertrains. Although most of the vehicles are produced in the US, many of the powertrain components rely on imports and foreign supply chains. Of particular interest are traction motors and their components. The objective of this topic are is to develop E-Steels meeting properties including frequency, thickness, ductility, cost, and manufacturability.
Topic Area 6: Cybersecurity for Smart and Secure Electric Vehicle Charging
This topic area is addressing critical cybersecurity needs to address through two subtopics:
- Subtopic 6.a: Enabling Wide-scale, Cybersecure EV/EVSE Aggregation for Grid Services : To support the integration of electric vehicles (EVs) and their charging requirements with the electric grid, both government and the private sector have made significant investments in the development of smart charge management (SCM) systems and technologies for EV charging infrastructure. The objective of this subtopic area is to research, develop, and demonstrate systems, technologies, and tools necessary for the cybersecure aggregation of EVs and charging infrastructure to provide widescale, cybersecure grid services.
- Subtopic 6.b: Tools to Assess EV/EVSE/Charging System Cybersecurity Posture and Compliance with Standards and Protocols for Communications, Controls, and Monitoring : Testing and evaluation of Electric Vehicle Supply Equipment (EVSE) by DOE national laboratories has clearly indicated a lack of compliance by many vendors with certified and/or regulated EV charging standards and protocols. In addition to creating cybersecurity vulnerabilities, this non-compliance greatly inhibits interoperability, supplier-managed SCM, and right-to-repair. The objective of this subtopic is to research, develop, and validate a suite of tools and associated procedures to comprehensively assess EV/EVSE/charging system compliance with relevant standards and protocols and cybersecurity posture.
Additional Information
- Download the full funding opportunity on the EERE Exchange website.
- For FOA-specific support, contact [email protected] .
- Sign up for the Office of Energy Efficiency and Renewable Energy (EERE) funding email list to get notified of new EERE funding opportunities. Also sign up for VTO’s newsletter to stay current with the latest news.
- Watch the VTO Funding Opportunity Announcement information series webinars.
Suggestions or feedback?
MIT News | Massachusetts Institute of Technology
- Machine learning
- Social justice
- Black holes
- Classes and programs
Departments
- Aeronautics and Astronautics
- Brain and Cognitive Sciences
- Architecture
- Political Science
- Mechanical Engineering
Centers, Labs, & Programs
- Abdul Latif Jameel Poverty Action Lab (J-PAL)
- Picower Institute for Learning and Memory
- Lincoln Laboratory
- School of Architecture + Planning
- School of Engineering
- School of Humanities, Arts, and Social Sciences
- Sloan School of Management
- School of Science
- MIT Schwarzman College of Computing
Reevaluating an approach to functional brain imaging
Press contact :.
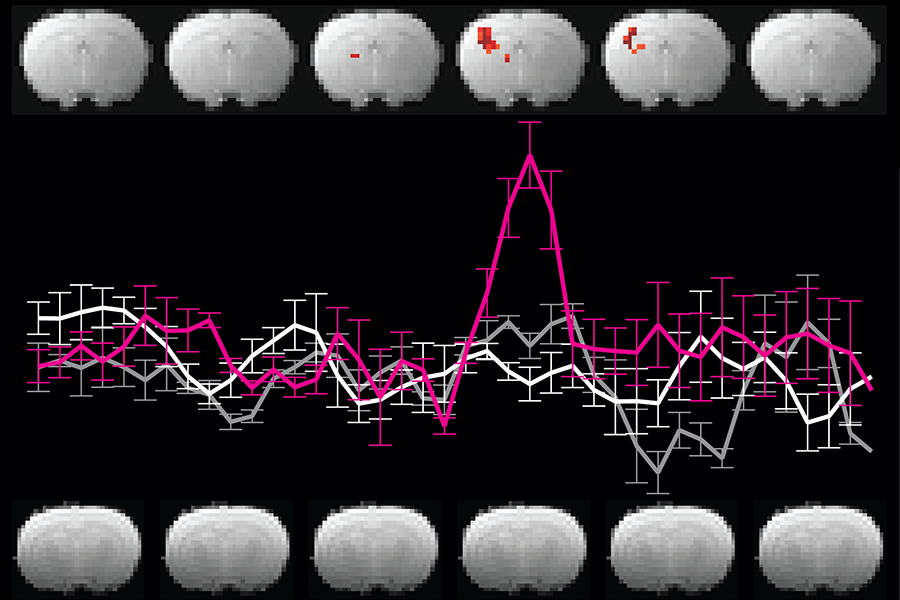
Previous image Next image
A new way of imaging the brain with magnetic resonance imaging (MRI) does not directly detect neural activity as originally reported, according to scientists at MIT’s McGovern Institute for Brain Research.
The method, first described in 2022, generated excitement within the neuroscience community as a potentially transformative approach. But a study from the lab of MIT Professor Alan Jasanoff , reported March 27 in the journal Science Advances , demonstrates that MRI signals produced by the new method are generated in large part by the imaging process itself, not neuronal activity.
Jasanoff, a professor of biological engineering, brain and cognitive sciences, and nuclear science and engineering, as well as an associate investigator of the McGovern Institute, explains that having a noninvasive means of seeing neuronal activity in the brain is a long-sought goal for neuroscientists. The functional MRI methods that researchers currently use to monitor brain activity don’t actually detect neural signaling. Instead, they use blood flow changes triggered by brain activity as a proxy. This reveals which parts of the brain are engaged during imaging, but it cannot pinpoint neural activity to precise locations, and it is too slow to truly track neurons’ rapid-fire communications.
So when a team of scientists reported in 2022 a new MRI method called DIANA, for “direct imaging of neuronal activity,” neuroscientists paid attention. The authors claimed that DIANA detected MRI signals in the brain that corresponded to the electrical signals of neurons, and that it acquired signals far faster than the methods now used for functional MRI.
“Everyone wants this,” Jasanoff says. “If we could look at the whole brain and follow its activity with millisecond precision and know that all the signals that we’re seeing have to do with cellular activity, this would be just wonderful. It could tell us all kinds of things about how the brain works and what goes wrong in disease.”
Jasanoff adds that from the initial report, it was not clear what brain changes DIANA was detecting to produce such a rapid readout of neural activity. Curious, he and his team began to experiment with the method. “We wanted to reproduce it, and we wanted to understand how it worked,” he says.
Recreating the MRI procedure reported by DIANA’s developers, postdoc Valerie Doan Phi Van imaged the brain of a rat as an electric stimulus was delivered to one paw. Phi Van says she was excited to see an MRI signal appear in the brain’s sensory cortex, exactly when and where neurons were expected to respond to the sensation on the paw. “I was able to reproduce it,” she says. “I could see the signal.”
With further tests of the system, however, her enthusiasm waned. To investigate the source of the signal, she disconnected the device used to stimulate the animal’s paw, then repeated the imaging. Again, signals showed up in the sensory processing part of the brain. But this time, there was no reason for neurons in that area to be activated. In fact, Phi Van found, the MRI produced the same kinds of signals when the animal inside the scanner was replaced with a tube of water. It was clear DIANA’s functional signals were not arising from neural activity.
Phi Van traced the source of the specious signals to the pulse program that directs DIANA’s imaging process, detailing the sequence of steps the MRI scanner uses to collect data. Embedded within DIANA’s pulse program was a trigger for the device that delivers sensory input to the animal inside the scanner. That synchronizes the two processes, so the stimulation occurs at a precise moment during data acquisition. That trigger appeared to be causing signals that DIANA’s developers had concluded indicated neural activity.
Phi Van altered the pulse program, changing the way the stimulator was triggered. Using the updated program, the MRI scanner detected no functional signal in the brain in response to the same paw stimulation that had produced a signal before. “If you take this part of the code out, then the signal will also be gone. So that means the signal we see is an artifact of the trigger,” she says.
Jasanoff and Phi Van went on to find reasons why other researchers have struggled to reproduce the results of the original DIANA report, noting that the trigger-generated signals can disappear with slight variations in the imaging process. With their postdoctoral colleague Sajal Sen, they also found evidence that cellular changes that DIANA’s developers had proposed might give rise to a functional MRI signal were not related to neuronal activity.
Jasanoff and Phi Van say it was important to share their findings with the research community, particularly as efforts continue to develop new neuroimaging methods. “If people want to try to repeat any part of the study or implement any kind of approach like this, they have to avoid falling into these pits,” Jasanoff says. He adds that they admire the authors of the original study for their ambition: “The community needs scientists who are willing to take risks to move the field ahead.”
Share this news article on:
Related links.
- Alan Jasanoff
- Jasanoff Lab
- McGovern Institute for Brain Research
- Department of Brain and Cognitive Sciences
- Department of Biological Engineering
- Department of Nuclear Science and Engineering
- https://engineering.mit.edu
Related Topics
- Neuroscience
- Magnetic resonance imaging (MRI)
- Brain and cognitive sciences
- Biological engineering
- Nuclear science and engineering
- McGovern Institute
Related Articles
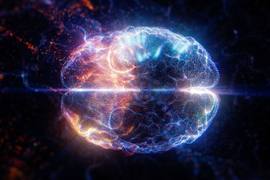
New sensor uses MRI to detect light deep in the brain
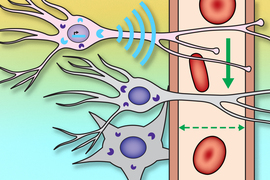
New MRI probe can reveal more of the brain’s inner workings
Previous item Next item
More MIT News
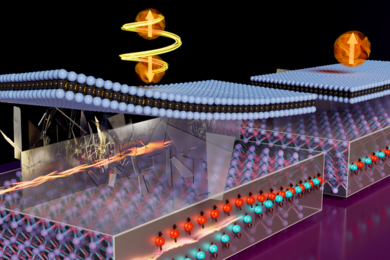
Propelling atomically layered magnets toward green computers
Read full story →
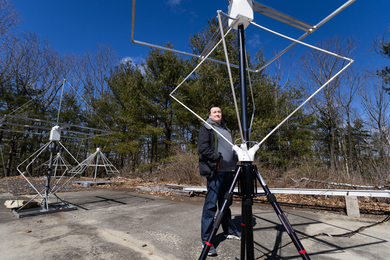
MIT Haystack scientists prepare a constellation of instruments to observe the solar eclipse’s effects
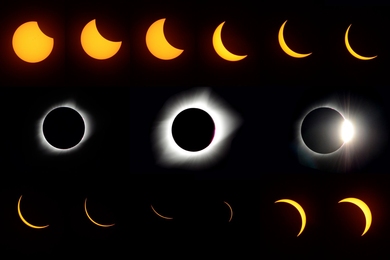
Q&A: Tips for viewing the 2024 solar eclipse
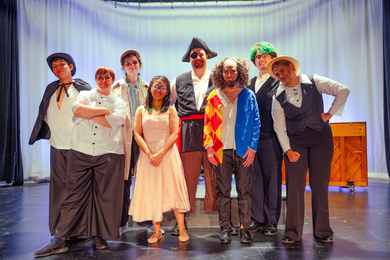
Drinking from a firehose — on stage
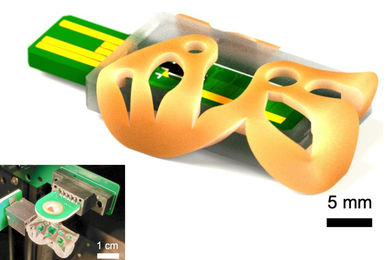
Researchers 3D print key components for a point-of-care mass spectrometer
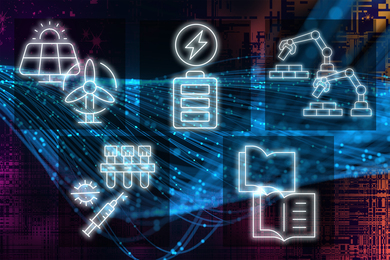
Unlocking new science with devices that control electric power
- More news on MIT News homepage →
Massachusetts Institute of Technology 77 Massachusetts Avenue, Cambridge, MA, USA
- Map (opens in new window)
- Events (opens in new window)
- People (opens in new window)
- Careers (opens in new window)
- Accessibility
- Social Media Hub
- MIT on Facebook
- MIT on YouTube
- MIT on Instagram
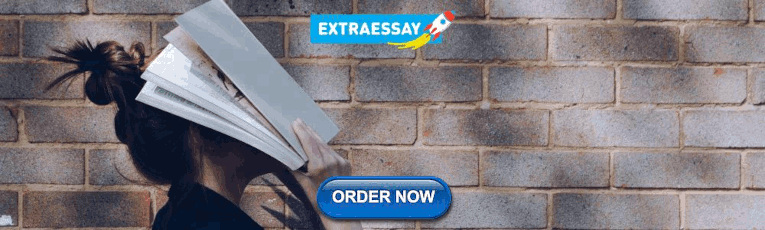
IMAGES
VIDEO
COMMENTS
Research Question: This paper presents a review of empirical methods used by authors to determine the influence of different groups of factors that influence households' electricity consumption ...
Household energy consumption accounts for almost one third of global primary energy demand and significantly affects the environment. As such, it has served as a classic and compelling theme in the literature, with a range of studies having analyzed various aspects of household consumption, including energy conservation, energy poverty, and energy efficiency. Nonetheless, overall trends and ...
1.1. Household energy consumption background. Environmental issues and social crises induce a shortage of energy all over the world. The coronavirus (COVID-19) pandemic from 2019 to 2022, in particular, resulted in a dramatic change in people's lives and activities around the world, including energy use behaviors.
However, research focusing on energy consumption, subjective well-being, and environmental-friendly energy consumption outcomes from a multi-national level perspective remain scarce. This study ...
Abstract. This study is a meta-analytic investigation of energy consumption and economic growth. It makes a literature survey on the dynamic causal relationship between broad variables of energy and economic growth for the period 1974-2021. The objective of this study is to investigate stationarity, cointegration and direction of causality ...
1. Introduction. World energy demand in a large number of contexts, including the current state-of-the-art, allowing the devastating impact of global warming on the different situations where countries and people work together to reach the Paris agreement target well below temperature 2.0 °C (Kona et al., 2018, IEA, 2017).In recent decades, the worldwide use of energy has risen exponentially ...
Feature papers represent the most advanced research with significant potential for high impact in the field. ... As energy label system is found to have a significant impact on residential electricity consumption, this paper suggests that the government may consider to promote the uses of labeling systems for more electrical appliances without ...
In comparison, the progress of energy conservation in the residential sector has remained very slow. As a result, the share of the industrial sector in final electricity consumption decreased from 53.4% in 1973 to 42.0% in 2018, while the share of the residential sector increased from 23.1% to 26.9% (IEA 2020 ).
Consumption per capita is an important indicator to observe the tendency of electricity consumption through the years, as it offers a clear view of the electricity that every individual consumes. In Greece, in 2014 the per capita electricity consumption was 5062.61 kWh/capita while in 2010 was 5333.44 kWh/capita and in 2008 was 5805.19 kWh ...
The study further revealed that 50% of electricity demand forecasting was based on weather and economic parameters, 8.33% on household lifestyle, 38.33% on historical energy consumption, and 3.33% on stock indices. Finally, we recap the challenges and opportunities for further research in electricity load forecasting locally and globally.
In this paper, non-renewable energy consumption in G20 countries is predicted by using DAGM (1,1). Results are obtained for non-renewable energy consumption prediction in G20 countries.
Households are an important sector in carrying out human development activities, accounting for more than 30% of the total global energy consumption. The continued growth of household energy consumption (HEC) and carbon emissions is threatening economic and environmental sustainability. This review focuses on the research in the field of HEC and conducts a bibliometric analysis of research ...
With the emergence of the contradictions between energy supply and demand, considerable attention has been paid to the residential household energy consumption with increasing research in this field. Based on databases of Science Citation Index Expanded and Social Sciences Citation Index, this paper applies the bibliometric method to analyze the development and evolution of this research field ...
As previously mentioned papers were excluded for a wide variety of reasons. Every paper remotely relevant to research on electricity consumption classification using smart meter data is included for further screening. After the initial search and screening of the 2099 unique articles, 552 were deemed related and relevant.
In this paper, we present a new method for forecasting power consumption. Household power consumption prediction is essential to manage and plan energy utilization. This study proposes a new technique using machine learning models based on the stationary wavelet transform (SWT) and transformers to forecast household power consumption in different resolutions. This approach works by leveraging ...
The link or the causality between energy consumption and real per capita GDP (PCGDP) plays a vital role in developing the macroeconomic policies for stabilisation purposes.In this study, an attempt has been made to find out the causality between electricity consumption and PCGDP in India. The second objective of this study is to find the direction of the causality among the variables (per ...
The total electricity end-use is of interest for the purpose of this paper, and other terms, e.g., renewable electricity generation or use (Azam et al., 2021), are not covered in this review. 2 For example, the heterogeneous Dumitrescu-Hurlin ( Dumitrescu and Hurlin, 2012 ) causality method is a procedure proposed as an extension of the Granger ...
The aim of the paper was to ascertain if renewable energy sources were sustainable and how a shift from fossil fuel-based energy sources to renewable energy sources would help reduce climate change and its impact. A qualitative research was employed by reviewing papers in the scope of the study.
Distribution of Market Power, Endogenous Growth, and Monetary Policy
limited computing power, due to the low-power nature of the system and latency constraints, us-ing a single, large, end-to-end model is infeasible: using a single LLM for this task would usually re-quire the use of a large model with long prompts for true end-to-end experiences (Wei et al.,2022). Second, consider the case when the model has to
In this paper, we embed a very rich "benchmark earnings process" that captures these non-Gaussian and nonlinear features into a lifecycle consumption-saving model and study its implications for consumption dynamics, consumption insurance, and welfare. We show four main results. First, the benchmark process essentially matches the empirical ...
Background: The use of surveillance technologies is becoming increasingly common in inpatient mental health settings, commonly justified as efforts to improve safety and cost-effectiveness. However, the use of these technologies has been questioned in light of limited research conducted and the sensitivities, ethical concerns and potential harms of surveillance. This systematic review aims to ...
In an open-access paper published March 15 in Science Advances, the team shows that the magnet ... Computer memory and processors built from magnetic materials use less energy than traditional silicon-based devices. ... This translates to something like two orders of magnitude improvement in energy efficiency." The research team is now ...
The perception and appreciation of food flavor depends on many interacting chemical compounds and external factors, and therefore proves challenging to understand and predict. Here, we combine ...
We quantitatively capture the big picture of Data Analytics research in the electricity sector, thus displaying the high-level status quo of research activity. 2. ... Most studies in our pool focus on electricity consumption. A few papers also forecast other energy sources in addition to electricity, such as petroleum [191] or natural gas ...
Office: Vehicle Technologies Office FOA number: DE-FOA-0003248 Link to apply: Apply on EERE Exchange FOA Amount: $45,800,000 Today, the Department of Energy (DOE) announced $45.8 million in new funding for projects that will advance research, development, demonstration, and deployment (RDD&D) critical to achieving net-zero greenhouse gas emissions in the transportation sector.
Recreating the MRI procedure reported by DIANA's developers, postdoc Valerie Doan Phi Van imaged the brain of a rat as an electric stimulus was delivered to one paw. Phi Van says she was excited to see an MRI signal appear in the brain's sensory cortex, exactly when and where neurons were expected to respond to the sensation on the paw.