Loading metrics
Open Access
Peer-reviewed
Research Article
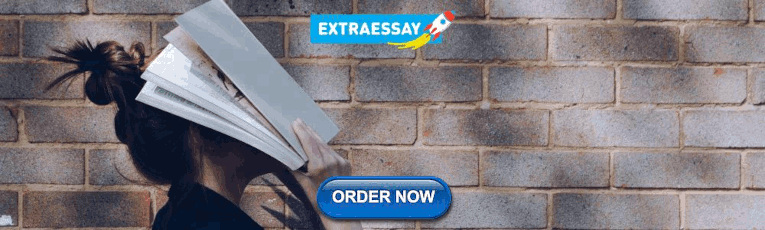
The population genetics of human disease: The case of recessive, lethal mutations
Roles Conceptualization, Data curation, Formal analysis, Investigation, Validation, Visualization, Writing – original draft
* E-mail: [email protected]
Affiliations Department of Biological Sciences, Columbia University, New York, NY, United States of America, CAPES Foundation, Ministry of Education of Brazil, Brasília, DF, Brazil

Roles Conceptualization, Formal analysis, Investigation, Methodology, Validation, Writing – original draft
Affiliation Howard Hughes Medical Institution, Stanford University, Stanford, CA, United States of America
Roles Data curation
Affiliation Department of Systems Biology, Columbia University, New York, NY, United States of America
Affiliation Universidade Federal de Santa Maria, Santa Maria, RS, Brazil
Roles Software, Writing – review & editing
Affiliation Department of Biological Sciences, Columbia University, New York, NY, United States of America
Roles Data curation, Resources, Writing – review & editing
Current address: Freenome, South San Francisco, CA, United States of America
Affiliation Counsyl, 180 Kimball Way, South San Francisco, CA, United States of America
Roles Conceptualization, Data curation, Funding acquisition, Methodology, Project administration, Supervision, Writing – review & editing
¶ ‡ These authors co-supervised this work.
Affiliations Department of Biological Sciences, Columbia University, New York, NY, United States of America, New York Genome Center, New York, NY, United States of America
Roles Conceptualization, Data curation, Funding acquisition, Investigation, Methodology, Project administration, Resources, Supervision, Validation, Writing – original draft
Affiliations Department of Biological Sciences, Columbia University, New York, NY, United States of America, Department of Systems Biology, Columbia University, New York, NY, United States of America
- Carlos Eduardo G. Amorim,
- Ziyue Gao,
- Zachary Baker,
- José Francisco Diesel,
- Yuval B. Simons,
- Imran S. Haque,
- Joseph Pickrell,
- Molly Przeworski
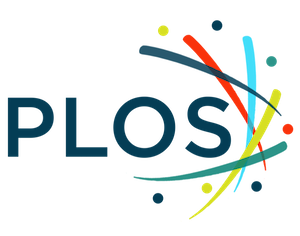
- Published: September 28, 2017
- https://doi.org/10.1371/journal.pgen.1006915
- See the preprint
- Reader Comments
2 Jul 2018: Amorim CEG, Gao Z, Baker Z, Diesel JF, Simons YB, et al. (2018) Correction: The population genetics of human disease: The case of recessive, lethal mutations. PLOS Genetics 14(7): e1007499. https://doi.org/10.1371/journal.pgen.1007499 View correction
Do the frequencies of disease mutations in human populations reflect a simple balance between mutation and purifying selection? What other factors shape the prevalence of disease mutations? To begin to answer these questions, we focused on one of the simplest cases: recessive mutations that alone cause lethal diseases or complete sterility. To this end, we generated a hand-curated set of 417 Mendelian mutations in 32 genes reported to cause a recessive, lethal Mendelian disease. We then considered analytic models of mutation-selection balance in infinite and finite populations of constant sizes and simulations of purifying selection in a more realistic demographic setting, and tested how well these models fit allele frequencies estimated from 33,370 individuals of European ancestry. In doing so, we distinguished between CpG transitions, which occur at a substantially elevated rate, and three other mutation types. Intriguingly, the observed frequency for CpG transitions is slightly higher than expectation but close, whereas the frequencies observed for the three other mutation types are an order of magnitude higher than expected, with a bigger deviation from expectation seen for less mutable types. This discrepancy is even larger when subtle fitness effects in heterozygotes or lethal compound heterozygotes are taken into account. In principle, higher than expected frequencies of disease mutations could be due to widespread errors in reporting causal variants, compensation by other mutations, or balancing selection. It is unclear why these factors would have a greater impact on disease mutations that occur at lower rates, however. We argue instead that the unexpectedly high frequency of disease mutations and the relationship to the mutation rate likely reflect an ascertainment bias: of all the mutations that cause recessive lethal diseases, those that by chance have reached higher frequencies are more likely to have been identified and thus to have been included in this study. Beyond the specific application, this study highlights the parameters likely to be important in shaping the frequencies of Mendelian disease alleles.
Author summary
What determines the frequencies of disease mutations in human populations? To begin to answer this question, we focus on one of the simplest cases: mutations that cause completely recessive, lethal Mendelian diseases. We first review theory about what to expect from mutation and selection in a population of finite size and generate predictions based on simulations using a plausible demographic scenario of recent human evolution. For a highly mutable type of mutation, transitions at CpG sites, we find that the predictions are close to the observed frequencies of recessive lethal disease mutations. For less mutable types, however, predictions substantially under-estimate the observed frequency. We discuss possible explanations for the discrepancy and point to a complication that, to our knowledge, is not widely appreciated: that there exists ascertainment bias in disease mutation discovery. Specifically, we suggest that alleles that have been identified to date are likely the ones that by chance have reached higher frequencies and are thus more likely to have been mapped. More generally, our study highlights the factors that influence the frequencies of Mendelian disease alleles.
Citation: Amorim CEG, Gao Z, Baker Z, Diesel JF, Simons YB, Haque IS, et al. (2017) The population genetics of human disease: The case of recessive, lethal mutations. PLoS Genet 13(9): e1006915. https://doi.org/10.1371/journal.pgen.1006915
Editor: Philipp W. Messer, Cornell University, UNITED STATES
Received: December 4, 2016; Accepted: July 9, 2017; Published: September 28, 2017
Copyright: © 2017 Amorim et al. This is an open access article distributed under the terms of the Creative Commons Attribution License , which permits unrestricted use, distribution, and reproduction in any medium, provided the original author and source are credited.
Data Availability: All relevant data are within the paper and its Supporting Information files and code from gitHub ( https://github.com/cegamorim/PopGenHumDisease ; https://github.com/sellalab/ForwardSimulator ).
Funding: CEGA was partially funded by a Science Without Borders fellowship from CAPES foundation (BEX 8279/11-0) and Conselho Nacional de Desenvolvimento Científico e Tecnológico (PDE 201145/2015-4), Brazil. ZG was partially supported by a postdoctoral fellowship funded by Stanford Center for Computational, Evolutionary and Human Genomics. JFD was funded by a Science Without Borders fellowship from CAPES foundation (88888.038761/2013-00). YBS was supported by NIH grant GM115889. The work was partially supported by a Research Initiative in Science and Engineering grant from Columbia University and NIGMS grants (GM121372) to JKP and MP. The computing in this project was supported by two National Institutes of Health instrumentation grants (S10OD012351 and S10OD021764) received by the Department of Systems Biology at Columbia University. The funders had no role in study design, data collection and analysis, decision to publish, or preparation of the manuscript.
Competing interests: The authors have declared that no competing interests exist.
Introduction
New disease mutations arise in heterozygotes and either drift to higher frequencies or are rapidly purged from the population, depending on the strength of selection and the demographic history of the population [ 1 – 6 ]. Elucidating the relative contributions of mutation, natural selection and genetic drift will help to understand why disease alleles persist in humans. Answers to these questions are also of practical importance, in informing how genetic variation data can be used to identify additional disease mutations [ 7 ].
In this regard, rare, Mendelian diseases, which are caused by single highly penetrant and deleterious alleles, are perhaps most amenable to investigation. A simple model for the persistence of mutations that lead to Mendelian diseases is that their frequencies reflect an equilibrium between their introduction by mutation and elimination by purifying selection, i.e., that they should be found at “mutation-selection balance” [ 4 ]. In finite populations, random drift leads to stochastic changes in the frequency of any mutation, so demographic history, in addition to mutation and natural selection, plays an important role in shaping the frequency distribution of deleterious mutations [ 3 ].
Another factor that may be important in determining the frequencies of highly penetrant disease mutations is genetic interactions. The mutation-selection balance model has been extended to scenarios with more than one disease allele, as is often seen for Mendelian diseases [ 8 , 9 ]. When compound heterozygotes have the same fitness as homozygotes for the disease allele (i.e., there is no complementation), the combined frequency of all disease alleles can be modeled similarly as the bi-allelic case, with the mutation rate given by the sum of the mutation rate to each disease allele [ 8 ]. In other cases, a disease mutation may be rescued by another mutation in the same gene [ 10 – 12 ] or by a modifier locus elsewhere in the genome that modulates the severity of the disease symptoms or the penetrance of the disease allele (e.g. [ 13 – 15 ]).
For a subset of disease alleles that are recessive, an alternative model for their persistence in the population is that there is an advantage to carrying one copy but a disadvantage to carrying two or none, such that the alleles persist due to overdominance, a form of balancing selection. Well known examples include sickle cell anemia, thalassemia and G6PD deficiency in populations living where malaria exerts strong selection pressures [ 16 ]. The importance of overdominance in maintaining the high frequency of disease mutations is unknown beyond these specific cases.
To this end, we compiled genetic information for a set of 417 mutations reported to cause fatal, recessive Mendelian diseases and estimated the frequencies of the disease-causing alleles from large exome datasets. We then compared these data to the expected frequencies of deleterious alleles based on models of mutation-selection balance in order to evaluate the effects of mutation rates and other factors in influencing these frequencies.
Mendelian recessive disease allele set
We relied on two datasets, one that describes 173 autosomal recessive diseases [ 19 ] and another from a genetic testing laboratory (Counsyl [ 20 ]; < https://www.counsyl.com/ >) that includes 110 recessive diseases of clinical interest. From these lists, we obtained a set of 44 “recessive lethal” diseases associated with 45 genes ( S1 Table ), requiring that at least one of the following conditions is met: (i) in the absence of treatment, the affected individuals die of the disease before reproductive age, (ii) reproduction is completely impaired in patients of both sexes, (iii) the phenotype includes severe mental retardation that in practice precludes reproduction, or (iv) the phenotype includes severely compromised physical development, again precluding reproduction.
Based on clinical genetics datasets and the medical literature (see Methods for details), we were able to confirm that 417 Single Nucleotide Variants (SNVs) in 32 (of the 44) genes had been reported with compelling evidence of association to the severe form of the corresponding disease and an early-onset, as well as no indication of effects in heterozygote carriers ( S2 Table ). By this approach, we obtained a set of mutations for which, at least in principle, there is no heterozygote effect, i.e., for which the dominance coefficient h = 0 in a model with relative fitness of 1 for the homozygote for the reference allele, 1- hs for the heterozygote, and 1- s for the homozygote for the deleterious allele, and the selective coefficient s is 1.
A large subset of these mutations (29.3%) consists of transitions at CpG sites (henceforth CpGti), which occur at a highly elevated rates (~17-fold higher on average) compared to other mutation types, namely CpG transversions, and non-CpG transitions and transversions [ 18 ]. This proportion is in agreement with previous estimates for a smaller set of disease genes [ 21 ] and for DMD [ 22 ].
Empirical distribution of allele frequencies of disease mutations in Europe
Allele frequency data for the 417 variants were obtained from the Exome Aggregation Consortium (ExAC) for 60,706 individuals, of whom 33,370 are non-Finnish Europeans [ 23 ]. Out of the 417 variants associated with putative recessive lethal diseases, three were found homozygous in at least one individual in this dataset (rs35269064, p.Arg108Leu in ASS1 ; rs28933375, p.Asn252Ser in PRF1 ; and rs113857788, p.Gln1352His in CFTR ). Available data quality information for these variants does not suggest genotype calling artifacts ( S2 Table ). Since these diseases have severe symptoms that lead to early death without treatment and these ExAC individuals are healthy (i.e., do not manifest severe Mendelian diseases) [ 23 ], the reported mutations are likely errors in pathogenicity classification or cases of incomplete penetrance (see a similar observation for CFTR and DHCR7 in [ 24 ]). We therefore excluded them from our analyses. In addition to the mutations present in homozygotes, we also filtered out sites that had lower coverage in ExAC (see Methods ), resulting in a final dataset of 385 variants in 32 genes ( S2 Table ).
Genotypes for a subset (91) of these mutations were also available for a larger sample size (76,314 individuals with self-reported European ancestry) generated by the company Counsyl ( S3 Table ). A comparison of the allele frequencies in this larger dataset to that of ExAC suggests that the allele frequencies for individual variants are concordant between the two datasets (Pearson’s correlation coefficient of 0.79, S1 Fig ) and that the overall distributions do not differ appreciably (Kolmogorov–Smirnov test, p-value = 0.23). Thus, both data sets appear to reflect the general distribution of these disease alleles in Europeans. In what follows, we focused on ExAC, which includes a greater number of disease mutations.
Models of mutation-selection balance
To generate expectations for the frequencies of these disease mutations under mutation-selection balance, we considered models of infinite and finite populations of constant size [ 3 ] and conducted forward simulations using a plausible demographic model for African and European populations [ 25 ] (see Methods for details). In all these models, there is a wild-type allele (A) and a deleterious allele (a, which could also represent a class of distinct deleterious alleles with the same fitness effect) at each site, such that the relative fitness of individuals of genotypes AA, Aa, or aa is given respectively by:
- w Aa = 1- hs ;
- w aa = 1- s ;
The mutation rate from A to a is u; we assume that there are no back mutations.
We note that Nei [ 3 ] assumed a Wright-Fisher model, in which there is no distinction between census and the effective population sizes. However, when the two differ, it is the effective population size that governs the dynamics of deleterious alleles, so the N in the analytical results in fact represents the effective population size. In humans, the mutation rate at each bp is very small (on the order of 10 −8 [ 18 ]) and the effective population size not that large, even recently [ 27 , 28 ], so the second approximation should apply when considering each single site independently.
Comparing mutation-selection balance models
Although an infinite population size has often been assumed when modeling deleterious allele frequencies (e.g. [ 5 , 29 – 32 ]), predictions under this assumption can differ markedly from what is expected from models of finite population sizes, assuming plausible parameter values for humans. For example, the long-term estimate of the effective population size from total polymorphism levels is ~20,000 individuals (assuming a mutation rate of 1.2 x 10 −8 per bp per generation [ 18 ] and diversity levels of 0.1% [ 33 ]). In this case and considering a mutation rate of 1.5 x 10 −8 for exons (which have a higher mutation rate than the rest of the genome, because of their base composition [ 34 ]), the average deleterious allele frequency in the model of finite population size is ~23-fold lower than that in the infinite population model ( Fig 1 ).
- PPT PowerPoint slide
- PNG larger image
- TIFF original image
The blue bar denotes the expected allele frequency under an infinite population size, the green bar the mean under a finite constant population, and the red bar the mean under a plausible demographic model for European populations; for this last case, the entire distribution across 100,000 simulations is shown in the grey histogram. All models assume s = 1 and h = 0, i.e., fully recessive, lethal mutations. For the finite constant population size model, we present the mean frequency for a population size of 20,000 (see S2A Fig for other choices). Population allele frequencies ( q ) were transformed to log10( q ), and those q = 0 were set to 10 −7 for visual purposes, but indicated as “0” on the X-axis. The density of the distribution is plotted on a log-scale on the Y-axis. The mutation rate u was set to 1.5 x 10 −8 per bp per generation in all models.
https://doi.org/10.1371/journal.pgen.1006915.g001
Because the human population size has not been constant and changes in the population size can affect the frequencies of deleterious alleles in the population (e.g. [ 2 , 35 ]), we also simulated the population dynamics of disease alleles under a plausible demographic model for European populations based on Tennessen et al. [ 25 ]. The original model assumes a genome-wide mutation rate of 2.36 x 10 −8 per bp per generation, when current, more direct estimates are approximately two-fold smaller [ 18 , 34 , 36 ]. We therefore rescaled the demographic parameters of the Tennessen et al. model, based on a mutation rate of 1.2 x 10 −8 [ 18 ] (see Methods ). Assuming a mutation rate of 1.5 x 10 −8 per bp (as recently estimated for exons [ 34 ]), the mean allele frequency of a lethal, recessive disease allele obtained from this model was 7.10 x 10 −6 , ~1.33-fold higher than expected for a constant population size model with N e = 20,000 ( Fig 1 ). The mean frequency seen in simulations instead matches the expectation for a constant population size of 35,651 individuals (see Methods and S2A Fig ). Increasing the effective population size in a constant size model is not enough to capture the dynamics of disease alleles appropriately, however. For example, if simulation results obtained under the Tennessen et al. [ 25 ] demographic model are compared to those for simulations of a constant population size of N e = 35,651, the mean allele frequencies match, but the distributions of allele frequencies are significantly different (Kolmogorov-Smirnov test, p-value < 10 −15 ; S2B and S2C Fig ). These findings thus confirm the importance of incorporating demographic history into models for understanding the population dynamics of disease alleles [ 5 , 37 , 38 ]. In what follows, we therefore tested the fit of the more realistic demographic model [ 25 ] (and variants of it) to the observed allele frequencies.
Comparing empirical and expected distributions of disease allele frequencies
The mutation rate from wild-type allele to disease allele, u , is a critical parameter in predicting the frequencies of a deleterious allele [ 4 , 39 ]. To model disease alleles, we considered four mutation types separately, with the goal of capturing most of the fine-scale heterogeneity in mutation rates [ 27 , 36 , 40 , 41 ]: transitions in methylated CpG sites (CpGti) and three less mutable types, namely transversions in CpG sites (CpGtv) and transitions and transversions outside a CpG site (nonCpGti and nonCpGtv, respectively). In order to control for the methylation status of CpG sites, we excluded 12 CpGti that occurred in CpG islands, which tend not to be methylated and thus are likely to have a lower mutation rate [ 36 ] (following Moorjani et al. [ 42 ]). To allow for heterogeneity in mutation rates within each one of these four classes considered, we modeled the within-class variation in mutation rates according to a lognormal distribution (see details in Methods and [ 27 ]).
For each mutation type, we then compared the mean allele frequency obtained from simulations to what is observed in ExAC, running 100,000 replicates. To this end, we matched simulations to the empirical data with regard to the number of individuals sampled and number of mutations observed of each mutation type and focused the analysis on the largest sample of the same common ancestry, namely Non-Finnish Europeans ( n = 33,370) ( Fig 2A ). We found significant differences between empirical and expected mean frequencies for nonCpGtv (30-fold higher on average; two-tailed p-value < 1 x 10 −4 ; see Methods for details) and nonCpGti (15-fold higher on average, two-tailed p-value < 1 x 10 −4 ), but only marginally so for CpGtv (5-fold higher on average, two-tailed p-value = 0.08). The mean frequency for CpGti is also somewhat higher than expected, but insignificantly so (1.17-fold higher on average, two-tailed p-value = 0.59). Intriguingly, the discrepancy between observed and expected frequencies becomes smaller as the mutation rate increases ( Fig 2B ).
(A) Shown are the expected and observed mean sample frequencies of disease mutations for four different mutation types. The title of the panel indicates the mutation type, followed by K , the total number of mutations of that type considered in this study, with the p-value for the difference between observed and expected mean frequencies given below. Distributions in grey are the mean sample allele frequencies across K mutations based on 100,000 simulations, and rely on a plausible demographic model for European populations [ 25 ] (see Methods ). Blue bars represent the observed mean frequencies of the four mutation types, estimated from 33,370 individuals of European ancestry from ExAC. (B) Fold increase in the observed mean allele frequency in relation to the expected, as a function of the mutation rate u (on a log-scale), for each of the four mutation classes.
https://doi.org/10.1371/journal.pgen.1006915.g002
Two additional factors that we have not included in our model should further decrease the predicted frequencies of disease alleles. Given that frequencies in ExAC are already unexpectedly high, these factors would only exacerbate the discrepancy between observed and expected frequencies of deleterious alleles. First, we have ignored the effects of compound heterozygosity, the case in which combinations of two distinct pathogenic alleles in the same gene lead to lethality. This phenomenon is known to be common [ 43 ], and indeed, in the 320 cases in which we were able to obtain this information, 58.44% were initially identified in compound heterozygotes. In the presence of compound heterozygosity, each deleterious mutation will be selected against not only when present in two copies within the same individual, but also in the presence of lethal mutations at other sites. Since the purging effect of selection against compound heterozygotes was not modeled in simulations, we would predict the frequency of a deleterious mutation to be even lower than shown (e.g., in Fig 2A ).
In order to model the effect of compound heterozygosity in our simulations, we re-ran our simulations, but focusing on a gene rather than a single site and so considering the sum of frequencies of all known recessive lethal alleles within a gene. In these simulations, we used the same set-up as in the site level analysis, except for the mutation rate, U , which is now the sum of the mutation rates u j at each site j that is known to cause a severe and early onset form of the disease [ 8 ] ( S2 Table ; see Methods for details). This approach does not consider the contribution of other mutations in the genes that cause the mild and/or late onset forms of the disease, and implicitly assumes that all combinations of known recessive lethal alleles of the same gene have the same fitness effect as homozygotes. Comparing observed frequencies of disease alleles for each gene to predictions generated by simulation, about a fourth of the 27 genes for which we implemented the gene-level analysis (see Methods ) differ from the expected distribution at the 5% level, with a clear overall trend for observed frequencies to be above expectation ( S4 Table ; Fig 3 ; Fisher’s combined probability test p-value = 6 x 10 −8 ).
The expectation (grey) is based on 1000 simulations, assuming no fitness decrease in heterozygotes, but allowing for compound heterozygosity (see Methods for details). The sum of allele frequencies of known recessive lethal disease mutations in each gene (purple bars) was obtained from ExAC considering 33,370 European individuals. Genes are ordered according to the two-tailed p-value ( S4 Table ; see Methods ). Genes are bolded when they differ significantly from expectation (at the 5% level). Violin plots show the distribution of the log 10 combined allele frequency of all segregating alleles obtained from simulations and boxes represent the fraction of simulations in which no deleterious allele was observed in the simulated sample at present time.
https://doi.org/10.1371/journal.pgen.1006915.g003
This finding is even more surprising than it may seem, because we are far from knowing the complete mutation target for each gene, i.e., all the sites at which mutations could cause the disease. If there are additional, undiscovered sites in the gene at which mutations are fatal when carried in combination with a known recessive lethal mutation, the purging effect of purifying selection on the known mutations will be under-estimated in our simulations, leading us to over-estimate the expected frequencies of the known mutations in simulations. Therefore, our predictions are, if anything, an over-estimate of the expected allele frequency, and the discrepancy between predicted and the observed is likely even larger than what we found.
The other factor that we did not consider in simulations but would reduce the expected allele frequencies is a subtle fitness decrease in heterozygotes, as has been documented in Drosophila for example [ 44 ]. To evaluate potential fitness effects in heterozygotes when none had been documented in humans, we considered the phenotypic consequences of orthologous gene knockouts in mouse. We were able to retrieve information on phenotypes for both homozygote and heterozygote mice for only eight out of the 32 genes, namely ASS1 , CFTR , DHCR7 , NPC1 , POLG , PRF1 , SLC22A5 , and SMPD1 . For all eight, homozygote knockout mice presented similar phenotypes as affected humans, and heterozygotes showed a milder but detectable phenotype ( S5 Table ). The magnitude of the heterozygote effect of these mutations in humans is unclear, but the finding with knockout mice makes it plausible that there exists a very small fitness decrease in heterozygotes in humans as well, potentially not enough to have been recognized in clinical investigations but enough to have a marked impact on the allele frequencies of the disease mutations. Indeed, even if the fitness effect in heterozygotes were as small as h = 1%, a 79% decrease in the mean allele frequency of the disease allele is expected relative to the case with complete recessivity ( h = 0) ( S3 Fig ).
To investigate the population genetics of human disease, we focused on mutations that cause Mendelian, recessive disorders that lead to early death or completely impaired reproduction. We sought to understand to what extent the frequencies of these mutations fit the expectation based on a simple balance between the input of mutations and the purging by purifying selection, as well as how other mechanisms might affect these frequencies. Many studies implicitly or explicitly compare known disease allele frequencies to expectations from mutation-selection balance [ 5 , 29 – 32 ]. In this study, we tested whether known recessive lethal disease alleles as a class fit these expectations, and found that, under a sensible demographic model for European population history with purifying selection only in homozygotes, the expectations fit the observed disease allele frequencies poorly: the mean empirical frequencies of disease alleles are substantially above expectation for all mutation types (although not significantly so for CpGti), and the fold increase in observed mean allele frequency in relation to the expectation decreases with increased mutation rate ( Fig 2 ). Furthermore, including possible effects of compound heterozygosity and subtle fitness decrease in heterozygotes will only exacerbate the discrepancy.
In principle, higher than expected disease allele frequencies could be explained by at least six (non-mutually exclusive) factors: (i) widespread errors in reporting the causal variants; (ii) misspecification of the demographic model, (iii) misspecification of the mutation rate; (iv) reproductive compensation; (v) overdominance of disease alleles; and (vi) low penetrance of disease mutations. Because widespread mis-annotation of the causal variants in disease mutation databases had previously been reported [ 23 , 45 , 46 ], we tried to minimize the effect of such errors on our analyses by filtering out any case that lacked compelling evidence of association with a recessive lethal disease, reducing our initial set of 769 mutations to 385 in which we had greater confidence (see Methods for details).
We also explored the effects of having misspecified recent demographic history or the mutation rate. Based on very large samples, it has been estimated that population growth in Europe was stronger than what we considered in our simulations [ 47 , 48 ]. When we considered higher growth rates, such that the current effective population size is up to 10-fold larger than that of the rescaled Tennessen model, we observed an increase in the expected frequency of recessive disease alleles and a larger number of segregating sites ( S4 Fig , columns A-E). However, the impact of larger growth rate is insufficient to explain the observed discrepancy: the allele frequencies observed in ExAC are still on average an order of magnitude larger than expected based on a model with a 10-fold larger current effective population size than the one initially considered [ 25 ] ( S4 Fig ). In turn, population substructure within Europe would only increase the number of homozygotes relative to what was modeled in our simulations (through the Wahlund effect [ 49 ]) and expose more recessive alleles to selection, thus decreasing the expected allele frequencies and exacerbating the discrepancy that we report.
To explore the effects of error in the mutation rate, we considered a 50% higher mean mutation rate than what has been estimated for exons [ 34 ], beyond what seems plausible based on current estimates on human mutation rates [ 42 ]. Except for the mean mutation rate (now set to 2.25 x 10 −8 ), all other parameters used for these simulations (i.e. the variance in mutation rate across simulations, the demographic model [ 25 ], absence of selective effect in heterozygotes, and selection coefficient) were kept the same as the ones used for generating S4 Fig , column A. The observed mean frequency remains significantly above what those predicted and qualitative conclusions are unchanged ( S4 Fig , column F).
Another factor to consider is that for disease phenotypes that are lethal very early on in life, there may be partial or complete reproductive compensation (e.g. [ 50 ]). This phenomenon would decrease the fitness effects of the recessive lethal mutations and could therefore lead to an increase in the allele frequency in data relative to what we predict for a selection coefficient of 1. There are no reasons, however, for this phenomenon to correlate with the mutation rate, as seen in Fig 2B .
The other two factors, overdominance and low penetrance, are likely explanations for a subset of cases. For instance, CFTR , the gene in which some mutations lead to cystic fibrosis, is the farthest above expectation (p-value < 0.004; Fig 3 ). It was long noted that there is an unusually high frequency of the CFTR deletion ΔF508 in Europeans, which led to speculation that disease alleles of this gene may be subject to over-dominance ([ 51 , 52 ], but see [ 53 ]). Regardless, it is known that disease mutations in this gene can complement one another [ 10 , 11 ] and that modifier loci in other genes also influence their penetrance [ 11 , 14 ]. Consistent with variable penetrance, Chen et al. [ 24 ] identified three purportedly healthy individuals carrying two copies of disease mutations in this gene. Similarly, DHCR7 , the gene associated with the Smith-Lemli-Opitz syndrome, is somewhat above expectation in our analysis (p-value = 0.056; Fig 3 ) and healthy individuals were found to be homozygous carriers of putatively lethal disease alleles in other studies [ 24 ]. These observations make it plausible that, in a subset of cases (particularly for CFTR ), the high frequency of deleterious mutations associated with recessive, lethal diseases are due to genetic interactions that modify the penetrance of certain recessive disease mutations. It is hard to assess the importance of this phenomenon in driving the general pattern that we observe, but two factors argue against it being a sufficient explanation for our findings at the level of single sites. First, when we removed 130 mutations in CFTR and 12 in DHCR7 , the two genes that were outliers at the gene level ( Fig 3 ; S4 Table ) and for which there is evidence of incomplete penetrance [ 24 ], the discrepancy between observed and expected allele frequencies is barely impacted ( S5 Fig ). Moreover, there is no obvious reason why the degree of incomplete penetrance would vary systematically with the mutation rate of a site, as observed ( Fig 2B ).
Instead, it seems plausible that there is an ascertainment bias in disease allele discovery and mutation identification [ 52 , 54 , 55 ]. Unlike missense or protein-truncating variants, Mendelian disease mutations cannot be annotated based solely on DNA sequences, and their identification requires reliable diagnosis of affected individuals (usually in more than one pedigree) followed by mapping of the underlying gene/mutation. Therefore, those mutations that have been identified to date are likely the ones that are segregating at higher frequencies in the population. Moreover, mutation-selection balance models predict that the frequency of a deleterious mutation should correlate with the mutation rate. Together, these considerations suggest that disease variants of a highly mutable class, such as CpGti, are more likely to have been mapped and that the mean frequency of mapped mutations will tend to be only slightly above all disease mutations in that class. In contrast, less mutable disease mutations are less likely to have been discovered to date, and the mean frequency of the subset of mutations that have been identified may tend to be far above that of all mutations in that class.
To quantify these effects, we modeled the ascertainment of disease mutations both analytically and in simulations. A large proportion of recessive Mendelian disease mutations were identified in inbred populations, likely because inbreeding leads to an excess of homozygotes compared to expected under random mating, increasing the probability that a recessive mutation would be discovered as causing a disease. Therefore, we modeled ascertainment in disease discovery in human populations with a plausible degree of inbreeding (see Methods ). As expected, we found that for a given mutation type, the probability of ascertainment increases with the sample size of the putative disease ascertainment study ( n a ) and the average inbreeding coefficient of the population under study ( F a ); in addition, the average allele frequency of mutations that have been identified is always higher than that of all existing mutations, and the discrepancy decreases as the ascertainment probability increases ( Table 1 ). Furthermore, comparison across different mutation types reveals that a higher mutation rate increases the probability of disease mutations being ascertained ( Table 1 and S6 Fig ) and decreases the magnitude of bias in the estimated allele frequency relative to the mutation class as a whole ( Table 1 ). In summary, among all the possible aforementioned explanations for the observed discrepancy between empirical and expected mean allele frequencies, the ascertainment bias hypothesis is the only one that also explains why it is more pronounced for less mutable mutation types ( Fig 2B ).
For a similar result derived from analytical modeling, see S6 Fig . Parameters for this step of the simulation correspond to plausible scenarios for human populations with widespread inbreeding (e.g., F a = 1/16 corresponds to offspring of first-cousin marriage). The last column in the bottom panel shows the fold increase of the mean allele frequency observed in ExAC in relation to simulations based on the Tennessen et al. [ 25 ] demographic model (see Methods ). Mutation rates u per bp, per generation were obtained from a large human pedigree study [ 18 ].
https://doi.org/10.1371/journal.pgen.1006915.t001
One implication of this hypothesis is that there are numerous sites at which mutations cause recessive lethal diseases yet to be discovered, particularly at non-CpG sites. More generally, this ascertainment bias complicates the interpretation of observed allele frequencies in terms of the selection pressures acting on disease alleles. Beyond this specific point, our study illustrates how the large sample sizes now made available to researchers in the context of projects like ExAC [ 23 ] can be used not only for direct discovery of disease variants, but also to test why disease alleles are segregating in the population and to understand at what frequencies we might expect to find them in the future.
Disease allele set
In order to identify single nucleotide variants within the 42 genes associated with lethal, recessive Mendelian diseases ( S1 Table ), we initially relied on the ClinVar dataset [ 56 ] (accessed on June 3 rd , 2015). We filtered out any variant that is an indel or a more complex copy number variant or that is ever classified as benign or likely benign in ClinVar (whether or not it is also classified as pathogenic or likely pathogenic). By this approach, we obtained 769 SNVs described as pathogenic or likely pathogenic. For each one of these variants, we searched the literature for evidence that it is exclusively associated to the lethal and early onset form of the disease and was never reported as causing the mild and/or late-onset form of the disease. We considered effects in the absence of medical treatment, as we were interested in the selection pressures acting on the alleles over evolutionary time scales rather than in the last one or two generations, i.e., the period over which treatment became available for some of diseases considered. To evaluate the impact of treatment, we decreased s from 1 to 0 (i.e., we assumed a complete absence of selective effects due to treatment) in the last three generations and compared the mean allele frequencies across 100,000 simulations implemented with or without this readjustment in selection coefficient. Because of the stochastic nature of the simulations, we repeated this pairwise comparison 10 times in order to get a range of expected increase in allele frequencies. We observed only a minor increase in the mean allele frequency (2.6% at most) across the 10 replicates. This simulation procedure corresponds to a scenario in which there is an extremely effective treatment for all diseases for the past three generations, which is an overestimate of the effect and length of treatment for the disease set considered.
Variants with mention of incomplete penetrance (i.e. for which homozygotes were not always affected) or with known effects in heterozygote carriers were removed from the analysis. This process yielded 417 SNVs in 32 genes associated with distinct Mendelian recessive lethal disorders ( S2 Table ). Although these mutations were purportedly associated with completely recessive diseases, we sought to examine whether there would be possible, unreported effects in heterozygous carriers. To this end, we used the Mouse Genome Database (MGD) [ 57 ] (accessed July 29 th , 2015) and were able to retrieve information for both homozygote and heterozygote mice for eight out of the 32 genes (all of which with a homologue in mice) ( S5 Table ).
In addition to the information provided by ClinVar for each one of these variants, we considered the immediate sequence context of each SNV, to tailor the mutation rate estimate accordingly [ 18 ]. To do so, we used an in-house Python script and the human genome reference sequence hg19 from UCSC (< http://hgdownload.soe.ucsc.edu/goldenPath/hg19/chromosomes/ >).
Genetic datasets
The Exome Aggregation Consortium (ExAC) [ 23 ] was accessed on August 9 th , 2016. The data consist of genotype frequencies for 60,706 individuals, assigned after Principal Component Analysis to one of seven population labels: African (n = 5,203), East Asian (n = 4,327), Finnish (n = 3,307), Latino (n = 5,789), Non-Finnish European (n = 33,370), South Asian (n = 8,256) and “other” (n = 454) [ 23 ]. We focused our analyses on those individuals of Non-Finnish European descent, because they constitute the largest sample size from a single ancestry group. We note that, some diseases mutations, for instance, those in ASPA , HEXA and SMPD1 , are known to be especially prevalent in Ashkenazi Jewish populations, which could potentially bias our results if Ashkenazi Jewish individuals constituted a great portion of the sample we considered. However, this sample includes only ~2,000 (~6%) Ashkenazi individuals (Dr. Daniel MacArthur, personal communication).
From the initial 417 mutations, we filtered out three that were homozygous in at least one individual in ExAC and 29 that had lower coverage, i.e., fewer than 80% of the individuals were sequenced to at least 15x. This approach left us with a set of 385 mutations with a minimum coverage of 27x per sample and an average coverage of 69x per sample ( S2 Table ). For 248 sites with non-zero sample frequencies, ExAC reported the number of non-Finnish European individuals that were sequenced, which was on average 32,881 individuals [ 23 ]. For the remaining 137 sites, we did not have this information. Nonetheless, the coverage across all samples is reported and does not differ significantly between the two sets of sites (by a Kolmogorov-Smirnov test, p-value = 0.90; S7 Fig ). We therefore assumed that mean number of individuals covered for all sites was 32,881 and used this number to obtain sample frequencies from simulations, as explained below.
A second genetic dataset was obtained from Counsyl (< https://www.counsyl.com/ >). Counsyl is a commercial genetic screening laboratory that offers, among other products, the “Family Prep Screen”, a genetic screening test intended to detect carrier status for up to 110 recessive Mendelian diseases in couples that are planning to have a child [ 20 ]. A subset of 294,000 of its customers was surveyed by genotyping or sequencing for “routine carrier screening”. This subset excludes individuals with indications for testing because of known personal or family history of Mendelian diseases, infertility, and consanguinity. It therefore represents a more random (with regard to the presence of disease alleles), population-based survey. For these individuals, we had details on self-reported ancestry (14 distinct ethnic/ancestry/geographic groups) and the allele frequencies for 98 mutations that match those that passed our variant selection criteria described above, of which 91 are also sequenced to high coverage in the ExAC database ( S2 Table ). We focused our analysis of this dataset on the 76,314 individuals of self-reported Northern or Southern European ancestry.
Simulating the evolution of disease alleles with population size change
We modeled the frequency of a deleterious allele in human populations by forward simulations based on a crude but plausible demographic model for human populations from Africa and Europe, inferred from exome data for African-Americans and European-Americans [ 25 ]. To this end, we used a program described in [ 1 ]. In brief, the demographic scenario consists of an Out-of-Africa demographic model, with changes in population size throughout the population history, including a severe bottleneck in Europeans following the split from the African population and a rapid, recent population growth in both populations [ 25 ]. As in Simons et al. [ 1 ], we simulated genetic drift and two-way gene flow between Africans and Europeans in recent history.
The original demographic model was inferred using a mutation rate u of 2.36 x 10 −8 per bp per generation [ 25 , 58 ]. More recent estimates, based on direct resequencing of human pedigrees, instead point to mutation rates about 50% smaller than that [ 18 , 34 , 36 ]. To incorporate what is believed to be a more accurate mutation rate estimate, we rescaled all demographic and time parameters in the original Tennessen et al. [ 25 ] model by a factor of 1.97, based on the difference between the mutation rate considered in the original study and that of Kong et al. [ 18 ] (which is similar to that found in other studies [ 48 ]). We refer to this model as the rescaled Tennessen model and rely on it throughout.
Negative selection acting on a single bi-allelic site was modeled as in the analytic models. Allele frequencies follow a Wright-Fisher sampling scheme in each generation according to these viabilities, with migration rate and population sizes varying according to the demographic scenario considered. Whenever a demographic event (e.g., growth) altered the number of individuals and the resulting number was not an integer, we rounded it to the nearest integer, as in Simons et al. [ 1 ]. A burn-in period of 10 Ne generations with constant population size Ne = 14,328 individuals was implemented in order to ensure an equilibrium distribution of segregating alleles at the onset of demographic changes in Africa, 11,643 generations ago.
In contrast to Simons et al. [ 1 ], our simulations always start with the ancestral allele A fixed and mutation occurs exclusively from this allele to the deleterious one (a), i.e., a mutation occurs with mean probability u per gamete, per generation, and there is no back-mutation. However, recurrent mutations at a site are allowed, as in Simons et al. [ 1 ].
For each mutation type, we then proceeded as follows:
- We ran two million simulations, thus obtaining the distribution of deleterious allele frequencies expected for the European population.
- We sampled K allele frequencies from the two million simulations implemented for each mutation type, where K is the number of identified mutations of that type. Sample allele frequencies were simulated from these population frequencies by Poisson sampling, so to match ExAC’s number of chromosomes.
- We repeated step (2) 100,000 times, thus obtaining a distribution for the mean allele frequency across K mutations.
To assess the significance of the deviation between observed and expected mean, we obtained a two-tailed p-value, defined as 2 x ( r +1)/(100000+1), where r is the number of simulated allele frequencies that were greater or equal to that of the empirical mean [ 60 ], for each mutation type separately.
A well-known source of heterogeneity in mutation rate within the CpGti class is methylation status, with a high transition rate seen only at methylated CpGs [ 21 ]. In our analyses, we tried to control for the methylation status of CpG sites by excluding sites located in CpG islands (CGIs), which tend to not be methylated [ 42 ]. The CGI annotation for hg19 was obtained from UCSC Genome Browser (track “Unmasked CpG”; < http://hgdownload.soe.ucsc.edu/goldenPath/hg19/database/cpgIslandExtUnmasked.txt.gz >, accessed in June 6th, 2016). BEDTools [ 61 ] was used to exclude those CpG sites located in CGIs. We note that the CpGti estimate from [ 18 ] includes CGIs, and in that sense the average mutation rate that we are using for CpGti may be a very slight underestimate of the mean rate for transitions at methylated CpG sites.
Unless otherwise noted, the expectation assumes fully recessive, lethal alleles with complete penetrance. Notably, by calculating the expected frequency one site at a time, we are ignoring possible interaction between genes (i.e., effects of the genetic background) and among different mutations within a gene (i.e., compound heterozygotes). These assumptions are relaxed in two ways. In one analysis ( S3 Fig ), we considered a very low selective effect in heterozygous individuals ( h = 1%), reasoning that such an effect could plausibly go undetected in medical examinations and yet would nonetheless impact the frequency of the disease allele. Second, when considering the gene-level analysis ( Fig 3 ), we implicitly allowed for compound heterozygosity between any pair of known lethal mutations [ 8 ]. For this analysis, we ran 1000 simulations for a total mutation rate U per gene that was calculated accounting for the heterogeneity and uncertainty in the mutation rates estimates as follows: (i) For j sites in a gene known to cause a recessive lethal disease and that passed our filtering criteria ( S2 Table ), we drew a mutation rate u j from the lognormal distribution, as described above; (ii) We then took the sum of u j as the total mutation rate U; (iii) We then ran one replicate with U as the mutation parameter, and other parameters as specified for site level analysis. Because the mutational target size considered in simulations is only comprised of those sites at which mutations are known to cause a lethal recessive disease, it is almost certainly an underestimate of the true mutation rate—potentially by a lot. We note further that by this approach, we are assuming that compound heterozygotes formed by any two lethal alleles have fitness zero, i.e., that they are identical in their effects to homozygotes for any of the lethal alleles. Moreover, we are implicitly ignoring the possibility of complementation, which is (somewhat) justified by our focus on mutations with severe effects and complete penetrance (but see Discussion ). Since we were interested in understanding the effect of compound heterozygosity, for this analysis, we did not consider the five genes in which only one mutation passed our filters ( BCS1L , FKTN , LAMA3 , PLA3G6 , and TCIRG1 ).
Modeling the effect of the ascertainment bias in disease discovery
To calculate the probability of ascertaining a recessive, lethal mutation, we assumed that all currently known disease mutations were identified in a putative ascertainment study of sample size n a in a population with an inbreeding coefficient of F a . Under this model, we can estimate P asc , the probability of ascertaining a disease mutation, as following:
We also demonstrate the relationship between the probability of ascertainment and mutation rate using simulations of ascertainment bias implemented according to the following steps:
- For each of the four mutation types considered, we generated 10 6 allele frequencies q from the results of the simulations based on a realistic demographic model [ 25 ].
- We generated n a independent diploid genotypes, given the allele frequencies from step 1 and an inbreeding coefficient F a . We ran this step for a range of n a and F a values ( Table 1 ).
- With a given combination of n a and F a values, we identified the cases (out of the 10 6 observations from step 1) where at least one homozygote individual was observed in step 2. These cases correspond to disease mutations that were ascertained; the reasoning being that given complete penetrance, a recessive disease mutation can only be identified if there is at least one affected individual in the studied population. With this step, we calculated the probability of ascertainment by taking the fraction of cases that satisfy the criteria above.
- Finally, for each one of the 10 6 simulations from step 1, we generated a sample allele frequency of the disease mutation, matching ExAC’s sample size (i.e., considering 2n = 65,762 chromosomes). We can then compare q u , the unbiased average allele frequency of all disease mutations, to q a , the mean frequency of the subset of cases ascertained in step 3, i.e., those cases for which at least one homozygote individual is observed.
These simulations were meant to illustrate the likely impact of ascertainment bias, rather than to precisely describe the disease mutation identification process or to quantify the expected effect. Notably, we performed these simulations for single sites, so the criteria for ascertainment in step 3 did not include the possibility of compound heterozygotes, despite the fact that an estimated 58.4% of the disease mutations included in our study were initially identified in compound heterozygotes. However, this simulation framework could readily be extended in this direction and it would not change our qualitative conclusion.
Supporting information
S1 table. list of lethal, recessive mendelian diseases considered in this study..
https://doi.org/10.1371/journal.pgen.1006915.s001
S2 Table. Information on 417 mutations associated with the severe form of lethal, recessive Mendelian diseases.
https://doi.org/10.1371/journal.pgen.1006915.s002
S3 Table. Information on 91 mutations associated with the severe form of lethal, recessive Mendelian diseases in Counsyl and ExAC databases.
https://doi.org/10.1371/journal.pgen.1006915.s003
S4 Table. P-values for each gene estimated by simulation, under a model of mutation-selection balance with a plausible demographic history.
https://doi.org/10.1371/journal.pgen.1006915.s004
S5 Table. Phenotypic effect of mouse knock-outs (see main text).
https://doi.org/10.1371/journal.pgen.1006915.s005
S1 Fig. Comparison of the empirical allele frequencies of recessive, lethal disease mutations in individuals of European ancestry from two large exome studies.
Shown are the allele frequencies for 91 variants associated with lethal, recessive diseases, as estimated from 33,370 individuals of non-Finnish, European ancestry in the Exome Aggregation Consortium (ExAC) database [ 23 ] and 76,314 European-ancestry individuals from a genetic testing laboratory (Counsyl [ 20 ]) (see Methods ). Points lie on the dashed blue line if the allele frequencies in Counsyl and ExAC are the same.
https://doi.org/10.1371/journal.pgen.1006915.s006
S2 Fig. Comparisons of mutation-selection balance models with constant versus changing population sizes.
(A) Population mean allele frequency as a function of effective population size, under a model of constant population size. The X-axis range corresponds to the range of effective population size over time estimated in [ 25 ]. The red bar indicates the value of a constant population size at which the mean allele frequency is the same as in simulations, for an average mutation rate of 1.5 x10 -8 per bp per generation [ 34 ]. (B-C) The allele frequency distribution (in grey) is presented for 2 x 10 6 simulations based on (B) the complex demographic scenario inferred by Tennessen et al. [ 25 ] for the evolution of European populations based on simulations (see Methods ) and of (C) the finite, constant size population model, with N set to 35,651 individuals to match the mean allele frequency with (B). Both models assume complete lethality ( s = 1) and recessivity ( h = 0).
https://doi.org/10.1371/journal.pgen.1006915.s007
S3 Fig. The impact on disease allele frequencies of a small fitness effect in heterozygotes ( h = 0.01).
Shown in each case is the distribution of the deleterious allele frequencies in the population, generated from 100,000 simulations. Means are represented by red vertical bars. For visualization, an allele frequency of q = 0 is set to 0.5 x 10 −6 . When a small fitness effect in heterozygotes is considered in the simulations, the mean allele frequency decreases by 79% relative to no effect. The two distributions differ significantly by a Kolmogorov-Smirnov test (p-value < 10 −15 ). The mutation rate u was set to 1.5 x 10 −8 per bp per generation, reflective of the mean mutation rate for exons [ 34 ].
https://doi.org/10.1371/journal.pgen.1006915.s008
S4 Fig. Effect of varying the end population size and the average mutation rate on the sample allele frequency of recessive, lethal mutations.
Tennessen et al. [ 25 ] inferred the present effective population size of Europeans to be 512,000 individuals based on a mutation rate of 2.36 x 10 −8 per bp per generation. We rescaled the parameters of this model based on a lower mutation rate estimate of 1.2 x 10 −8 [ 18 ] and show the expected distribution of sample allele frequencies of recessive, lethal mutations in column A. We further considered the effect of larger population sizes at present (2-, 4-, and 10-fold increase, denoted by columns B, C and D respectively), keeping other rescaled demographic parameters the same as in A. We also included a model (E) where rapid growth begins immediately after the out-of-Africa bottleneck, representing a more extreme scenario of population growth in comparison to the two-stage and more gradual scenario proposed by Tennessen et al. (2012). For A-E, we drew the mutation rate M from a lognormal distribution with parameters set as in Eq 8 , with u = 1.5 x 10 -8 (as implemented for Fig 2 ; see Methods ). Model F considers a larger u (2.25 x 10 −8 , i.e., a 1.5-fold increase from A-E), with all other parameters (e.g., variance in mutation rates across simulations, the demographic model) the same as in column A. The observed sample allele frequency distribution of 385 disease mutations in ExAC is shown in white. Violin plots show the density distribution of the log 10 allele frequencies for variants that were segregating in these samples, whereas boxes indicate the proportion of sites for which the deleterious mutation was not observed segregating in the sample. All distributions differ significantly from one another (i.e., all p-values are < 10 −15 by a Kolmogorov-Smirnov test).
https://doi.org/10.1371/journal.pgen.1006915.s009
S5 Fig. Expected distribution and the observed mean allele frequencies of recessive, lethal disease mutations (excluding mutations in CFTR and DHCR7 ).
As in Fig 2 , the four panels correspond to four different mutation types. The title of the panel indicates the mutation type, followed by K , the total number of mutations of that type, with p-values for the difference between observed and expected mean frequencies below. Distributions in grey are for 100,000 observations of the expected mean sample allele frequencies across K mutations, and were obtained from simulations based on a plausible demographic model for European populations [ 25 ] (see Methods ). Blue bars represent the observed values estimated from 33,370 individuals of European ancestry from ExAC. As opposed to in Fig 2 , here, we did not include mutations present in two genes ( CFTR and DHCR7 ) that were outliers in the gene-level analysis ( Fig 3 ) and were reported elsewhere to be carried by healthy homozygous individuals [ 24 ].
https://doi.org/10.1371/journal.pgen.1006915.s010
S6 Fig. The probability P asc of a mutation being ascertained, given its population allele frequency q , the sample size n a of the putative ascertainment study and the inbreeding coefficient F a in the population in which the ascertainment study was conducted.
In each case, we let only one parameter ( q , n a or F a ) vary, while fixing the others at q = 7.10 x 10 −6 (corresponding to the mean allele frequency from simulations), n a = 10,000, and F a = 1/16 (corresponding to marriage between first cousins, a plausible scenario for a population with widespread inbreeding).
https://doi.org/10.1371/journal.pgen.1006915.s011
S7 Fig. Depth of coverage for 385 mutations in ExAC known to cause lethal, Mendelian diseases.
Box plots show the mean (black bar) and the lower and upper quartiles for (A) the 248 sites with non-zero sample frequencies in ExAC, for which the number of sequenced non-Finnish European individuals was reported ( n = 32,881) and (B) the 137 sites for which we did not have this information. Since distributions of depth of coverage are similar between the two sets (by a Kolmogorov–Smirnov test, p-value = 0.90), we assumed that 32,881 individuals were sequenced at all sites, and used this number to subsample simulations to match the sample size of the ExAC data.
https://doi.org/10.1371/journal.pgen.1006915.s012
Acknowledgments
We thank Daniel MacArthur for his help with the ExAC data, Ellen Leffler for providing her Python script (available at https://github.com/cegamorim/PopGenHumDisease ), as well as members of the Pickrell, Przeworski and Sella labs, Aravinda Chakravarti, Brian Charlesworth, Damien Labuda and four anonymous reviewers for helpful discussions and comments on an earlier version of the manuscript. All codes and data to generate the figures in R [ 62 ] and the script used to get the sequence context of each mutation are available at https://github.com/cegamorim/PopGenHumDisease . The code to run the simulations is available at https://github.com/sellalab/ForwardSimulator . Allele frequencies and other information for the disease mutations employed in the analyses are in S2 and S3 Tables.
- View Article
- PubMed/NCBI
- Google Scholar
- 4. Gillespie JH (2004) Population Genetics: A Concise Guide. Baltimore, MD: Johns Hopkins University Press.
- 9. Crow JF, Kimura M (1970) Introduction to Population Genetics Theory New York: Harper & Row Publishers. 591 p.
- 62. R Core Team (2015) R: A Language and Environment for Statistical Computing. Vienna, Austria.
Genetics of neurodegenerative diseases: an overview
Affiliations.
- 1 Institute of Clinical Medicine, University of Oslo, Oslo, Norway; UCL Institute of Neurology, National Hospital for Neurology and Neurosurgery, London, United Kingdom.
- 2 UCL Institute of Neurology, National Hospital for Neurology and Neurosurgery, London, United Kingdom; Center for Neurology and Hertie Institute for Clinical Brain Research, Eberhard-Karls-University, Tübingen, Germany.
- 3 UCL Institute of Neurology, National Hospital for Neurology and Neurosurgery, London, United Kingdom. Electronic address: [email protected].
- PMID: 28987179
- DOI: 10.1016/B978-0-12-802395-2.00022-5
Genetic factors are central to the etiology of neurodegeneration, both as monogenic causes of heritable disease and as modifiers of susceptibility to complex, sporadic disorders. Over the last two decades, the identification of disease genes and risk loci has led to some of the greatest advances in medicine and invaluable insights into pathogenic mechanisms and disease pathways. Large-scale research efforts, novel study designs, and advances in methodology are rapidly expanding our understanding of the genome and the genetic architecture of neurodegenerative disease. Here, we review major developments in the field to date, highlighting overarching historic trends and general insights. Monogenic neurodegenerative diseases are discussed from the perspectives of both rare Mendelian forms of common disorders, such as Alzheimer disease and Parkinson disease, and heterogeneous heritable conditions, including ataxias and spastic paraplegias. Next, we summarize the experiences from investigations of complex neurodegenerative disorders, including genomewide association studies. In the final section, we reflect upon the limitations of current findings and outline important future directions. Genetics plays an essential role in translational research, ultimately aiming to develop novel disease-modifying therapies for neurodegenerative disorders. We anticipate that individual genetic profiling will also be increasingly relevant in a clinical context, with implications for patient care in line with the proposed ideal of personalized medicine.
Keywords: Alzheimer's diseases; Genetics; Parkinson's disease; genome-wide association study (GWAS); neurodegeneration.
Copyright © 2017 Elsevier B.V. All rights reserved.
Publication types
- Genetic Predisposition to Disease*
- Genome-Wide Association Study
- Neurodegenerative Diseases / genetics*
- Neurodegenerative Diseases / physiopathology
Grants and funding
- G0802760/MRC_/Medical Research Council/United Kingdom
- G1001253/MRC_/Medical Research Council/United Kingdom
- G108/638/MRC_/Medical Research Council/United Kingdom
- MR/J004758/1/MRC_/Medical Research Council/United Kingdom
EDITORIAL article
Editorial: the genetics and epigenetics of mental health.
- 1 Instituto de Pesquisa Pelé Pequeno Príncipe, Curitiba, Brazil
- 2 Faculdades Pequeno Príncipe, Curitiba, Brazil
- 3 Department of Genetics, Federal University of Parana, Post-graduation Program in Genetics, Curitiba, Brazil
- 4 Translational Research in Respiratory Medicine, Hospital Universitari Arnau de Vilanova-Santa Maria, Biomedical Research Institute of Lleida (IRBLleida), Lleida, Spain
- 5 CIBER of Respiratory Diseases (CIBERES), Institute of Health Carlos III, Madrid, Spain
Editorial on the Research Topic The genetics and epigenetics of mental health
Mental health conditions cover a broad spectrum of disturbances, including neurological and substance use disorders, suicide risk, and associated psychosocial, cognitive, and intellectual disabilities (WHO, 2022). Despite a substantial amount of evidence, the interaction of genetic variants, epigenetic mechanisms, and environmental risk factors involved in mental health is poorly understood. Through distinct perspectives and different experimental approaches, the genetics and epigenetics of mental health were addressed in seven relevant articles included in this Research Topic, briefly summarized below.
Stress has severe consequences on the epigenome, but the timing of its occurrence, as well as the intensity and number of events, are critical for the severity of mental health symptoms. In particular, Serpeloni et al. demonstrated that stress generated in the form of intimate partner violence (IPV) during and/or after pregnancy impacts the offspring’s epigenome, shaping its resilience. They observed that individuals exposed to maternal IPV after birth presented psychiatric issues similar to their mothers, with different outcomes if the exposure to maternal IPV occurred both prenatally and postnatally. Prenatal IPV was associated with differential methylation in CpG sites in the genes encoding the glucocorticoid receptor ( NR3C1 ) and its repressor FKBP51 ( FKBP5 ), associated with the ability to terminate hormonal stress responses. Also considering early-life experiences and data from 2008 to 2016 of the Health and Retirement Study, Shin et al. concluded that early life experiences and relationships have a significant influence, attenuating or exacerbating the risk of suffering from mental health problems among individuals with a higher polygenic risk score predisposing to autism.
Environmental and developmental factors are also strongly linked to obsessive-compulsive disorder (OCD). They may explain the apparent discrepancy between the relatively high heritability scores and the inconsistent results found in genetic association studies, owing to their impact on gene expression and regulation. Based on this, Deng et al. stratified OCD patients by the age of disease onset. The findings revealed associations between the early onset and variants of genes whose products play a role in neural development, corroborating the age-associated genetic heterogeneity of OCD.
Further exploring environmental and genetic etiological clues, Li et al. used genome-wide association study (GWAS) data to calculate polygenic risk scores for salivary and tongue dorsum microbiomes associated with anxiety and depression. Additionally, causal relationships between the oral microbiome, anxiety, and depression were detected through Mendelian randomization, unraveling potential pathogenic mechanisms and interventional targets. Constructing a similar line of evidence, Becerra et al. found associations between the epigenetic regulation of inflammatory processes, the composition of gut microbiome, and modified Rosenberg self-esteem scores in samples from the Native Hawaiian and other Pacific Islander (NHPI) populations, which present a high prevalence and mortality from chronic and immunometabolic diseases, as well as mental health problems. This warrants further investigation into the relationship of microbiota to brain activity and mental health.
There is a lot of debate regarding suicidal behavior and its relationship with psychiatric disorders, but the extent to which they share the same genetic architecture is unknown. This Research Topic was investigated by Kootbodien et al. through the use of genomic structural equation modeling and Mendelian randomization with a large genomic dataset. The authors observed a strong genetic correlation between suicidal ideation, attempts, and self-harm, as well as a moderate to strong genetic correlation between suicidal behavioral traits and a range of psychiatric disorders, most notably major depressive disorder, involving pathways related to developmental biology, signal transduction, and RNA degradation. In conclusion, the study provided evidence of a shared etiology between suicidal behavior and psychiatric disorders, with overlapping pathophysiological pathways.
Malekpour et al. , in their investigation of psychogenic non-epileptic seizures (PNES), also uncovered shared pathways with psychiatric conditions. PNES, the most prevalent non-epileptic disorder among patients referring to epilepsy centers, carries a mortality rate akin to drug-resistant epilepsy. Employing a systems biology approach, the authors pinpointed several key components influencing the disease pathogenesis network. These include brain-derived neurotrophic factor (BDNF), cortisol, norepinephrine, proopiomelanocortin (POMC), neuropeptide Y (NPY), the growth hormone receptor signaling pathway, phosphatidylinositol 3-kinase (PI3K)/protein kinase B (AKT) signaling, and the neurotrophin signaling pathway.
In general, these studies have some limitations: small sample sizes, leading to low statistical power in some cases, environmental confounding factors (such as diet and physical activity), which were not considered in the microbiome studies, incomplete phenotype descriptions, and partial coverages of human genetic diversity. Childhood adversities and adult comorbidities are among the variables that were not controlled for as possible causes of the investigated psychiatric and neurological disorders, and some results still claim for functional studies to be validated. Thus, the findings brought more elaborated questions, each of which shed some light on knowledge gaps that remain very difficult to fill. How do early-life epigenetic processes regulate our mental health resilience and disease resistance? What is the role of the microbiome in this process and how do genetic variants influence its composition? How does the impact of all these elements shape the resistance of human populations to psychiatric and neurological diseases and, most importantly, translate into public health measures in the future? We hope to engage more researchers in the pursuit of these answers.
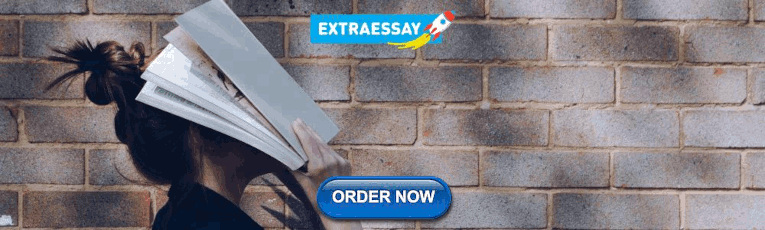
Author contributions
GCK: Conceptualization, Data curation, Writing–original draft, Writing–review and editing. ABWB: Writing–original draft, Writing–review and editing. ADST: Conceptualization, Data curation, Writing–original draft, Writing–review and editing.
The author(s) declare that financial support was received for the research, authorship, and/or publication of this article. This research was funded by Conselho Nacional de Desenvolvimento Científico e Tecnológico (CNPq) and Empresa Brasileira de Serviços Hospitalares (Ebserh) grant numbers 423317/2021-0 and 313741/2021-2 (8520137521584230), Research for the United Health SUS System (PPSUS-MS), CNPq, Fundação Araucária and SESA-PR, Protocol N°: SUS2020131000106. ABWB receives CNPq research productivity scholarships (protocols 313741/2021). ADST receives financial support from Instituto de Salud Carlos III (Miguel Servet, 2023: CP23/00095), co-funded by Fondo Social Europeo Plus (FSE+).
Conflict of interest
The authors declare that the research was conducted in the absence of any commercial or financial relationships that could be construed as a potential conflict of interest.
Publisher’s note
All claims expressed in this article are solely those of the authors and do not necessarily represent those of their affiliated organizations, or those of the publisher, the editors and the reviewers. Any product that may be evaluated in this article, or claim that may be made by its manufacturer, is not guaranteed or endorsed by the publisher.
Keywords: methylation, GWAS-genome-wide association study, microbiome & dysbiosis, poligenic risk score, neurological conditions, epigenome, genome
Citation: Kretzschmar GC, Boldt ABW and Targa ADS (2024) Editorial: The genetics and epigenetics of mental health. Front. Genet. 15:1402495. doi: 10.3389/fgene.2024.1402495
Received: 17 March 2024; Accepted: 26 March 2024; Published: 09 April 2024.
Edited and reviewed by:
Copyright © 2024 Kretzschmar, Boldt and Targa. This is an open-access article distributed under the terms of the Creative Commons Attribution License (CC BY). The use, distribution or reproduction in other forums is permitted, provided the original author(s) and the copyright owner(s) are credited and that the original publication in this journal is cited, in accordance with accepted academic practice. No use, distribution or reproduction is permitted which does not comply with these terms.
*Correspondence: Gabriela Canalli Kretzschmar, [email protected] ; Angelica Beate Winter Boldt, [email protected] ; Adriano D. S. Targa, [email protected]
Disclaimer: All claims expressed in this article are solely those of the authors and do not necessarily represent those of their affiliated organizations, or those of the publisher, the editors and the reviewers. Any product that may be evaluated in this article or claim that may be made by its manufacturer is not guaranteed or endorsed by the publisher.
- Introduction to Genomics
- Educational Resources
- Policy Issues in Genomics
- The Human Genome Project
- Funding Opportunities
- Funded Programs & Projects
- Division and Program Directors
- Scientific Program Analysts
- Contact by Research Area
- News & Events
- Research Areas
- Research investigators
- Research Projects
- Clinical Research
- Data Tools & Resources
- Genomics & Medicine
- Family Health History
- For Patients & Families
- For Health Professionals
- Jobs at NHGRI
- Training at NHGRI
- Funding for Research Training
- Professional Development Programs
- NHGRI Culture
- Social Media
- Broadcast Media
- Image Gallery
- Press Resources
- Organization
- NHGRI Director
- Mission & Vision
- Policies & Guidance
- Institute Advisors
- Strategic Vision
- Leadership Initiatives
- Diversity, Equity, and Inclusion
- Partner with NHGRI
- Staff Search
Genetic Disorders
Many human diseases have a genetic component. Some of these conditions are under investigation by researchers at or associated with the National Human Genome Research Institute (NHGRI).
A genetic disorder is a disease caused in whole or in part by a change in the DNA sequence away from the normal sequence. Genetic disorders can be caused by a mutation in one gene (monogenic disorder), by mutations in multiple genes (multifactorial inheritance disorder), by a combination of gene mutations and environmental factors, or by damage to chromosomes (changes in the number or structure of entire chromosomes, the structures that carry genes). As we unlock the secrets of the human genome (the complete set of human genes), we are learning that nearly all diseases have a genetic component. Some diseases are caused by mutations that are inherited from the parents and are present in an individual at birth, like sickle cell disease. Other diseases are caused by acquired mutations in a gene or group of genes that occur during a person's life. Such mutations are not inherited from a parent, but occur either randomly or due to some environmental exposure (such as cigarette smoke). These include many cancers, as well as some forms of neurofibromatosis.
List of Genetic Disorders
This list of genetic, orphan and rare diseases is provided for informational purposes only and is by no means comprehensive.
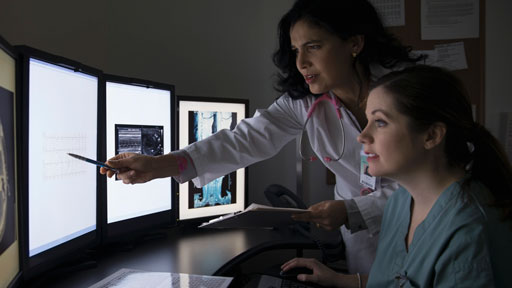
Featured Content
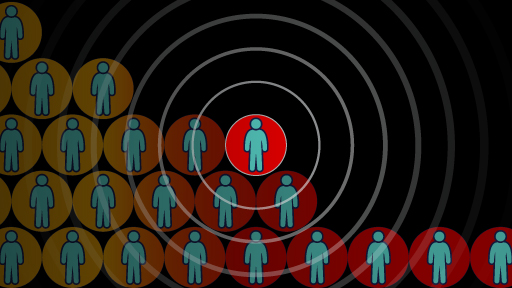
Last updated: May 18, 2018
- Uncovering the secret of long-lived stem cells
- Headed to a festival or outdoor event soon? Here is what you should know
- Healthcare on wheels: Using mobile clinics to reach children all over Houston
Research method finds new use in diagnosis of genetic disorders
- How the hand muscles have evolved
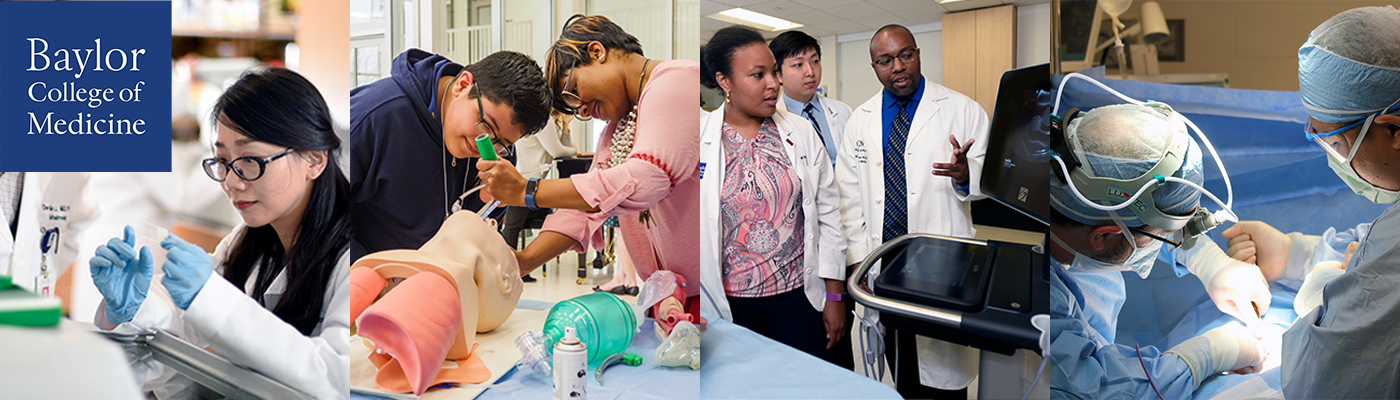
Researchers at Baylor College of Medicine have tested the feasibility of using human cell transdifferentiation with RNA sequencing to facilitate diagnoses of Mendelian disorders. The approach generated an overall diagnostic yield of 25.4% in a cohort of Undiagnosed Diseases Network cases. The findings are published in the American Journal of Human Genetics .
RNA sequencing, which reads the transcriptome or gene expression in a cell, is often needed to support a genetic diagnosis obtained through exome sequencing or whole genome sequencing. However, the effectiveness of RNA sequencing is limited by expression of disease-associated genes in clinically accessible tissues like blood or skin fibroblasts, cells that contribute to the formation of connective tissue.
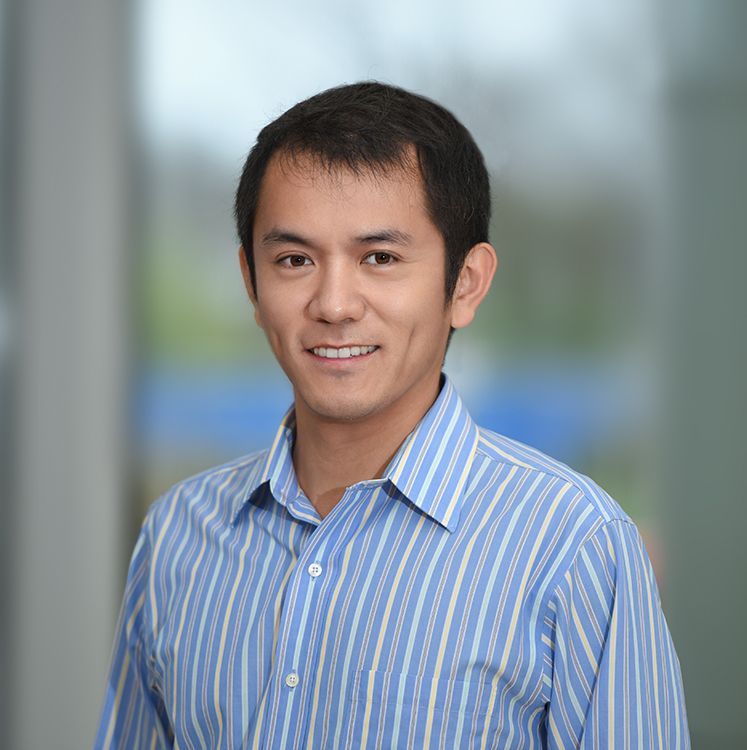
“This is especially problematic in neurological diseases because the gene causing the disorder may not be expressed in blood and skin cells,” said corresponding author Dr. Pengfei Liu , associate professor of molecular and human genetics and director of the ACGME-accredited Laboratory Genetics and Genomics Fellowship Program at Baylor.
Research method helps overcome obstacles to genetic diagnoses
To overcome this challenge, researchers led by first author Dr. Shenglan Li , staff scientist in Liu’s lab at Baylor, converted fibroblasts obtained in skin biopsies to neurons in a process called transdifferentiation. “This method activates neuron-specific gene expression and increases the probability that we can accurately characterize disease-causing mutations in these cells,” Li said.
For clinical validation, researchers generated these induced neurons for a cohort of 71 individuals with neurological characteristics in the Undiagnosed Diseases Network. RNA sequencing of the induced neurons led to a diagnosis in 18 individuals (25.4%); five of those cases could not have been diagnosed with RNA sequencing of fibroblasts alone.
This study shows that fibroblast-to-neuron transdifferentiation followed by RNA sequencing is a simple, low-cost and reproducible approach with a reasonable turnaround time, making it feasible for clinical implementation,” Liu said. “This new testing method represents a paradigm shift in laboratory genetics, moving from the traditional DNA-centric approach to one that focuses on the patient’s cells.”
“It’s exciting to apply this well-established technique, which previously has been used to study mechanisms of neurodegenerative diseases, for a new use in genetic diagnostics,” Li said.
Other authors of this work include Sen Zhao, Jefferson C. Sinson, Aleksandar Bajic, Jill A. Rosenfeld, Matthew B. Neeley, Mezthly Pena, Kim C. Worley, Lindsay C. Burrage, Monika Weisz-Hubshman, Shamika Ketkar, William J. Craigen, Gary D. Clark, Seema Lalani, Carlos A. Bacino, Keren Machol, Hsiao-Tuan Chao, Lorraine Potocki, Lisa Emrick, Jennifer Sheppard, My T.T. Nguyen, Anahita Khoramnia, Paula Patricia Hernandez, Sandesh C. S. Nagamani, Zhandong Liu, Christine M. Eng and Brendan Lee. They are affiliated with one or more of the following institutions: Baylor College of Medicine, Jan and Dan Duncan Neurological Research Institute at Texas Children’s Hospital, Texas Children’s Hospital, McNair Medical Institute at the Robert and Janice McNair Foundation and Baylor Genetics.
The research was supported by the National Institutes of Health Common Fund (U01HG007709 and U01HG007942), the National Human Genome Research Institute (R35HG011311) and the BCM Intellectual and Developmental Disabilities Research Center funded by the Eunice Kennedy Shriver National Institute of Child Health & Human Development (P50HD103555). For a full list of funding sources, see the publication .
By Molly Chiu
Follow From the Labs on X @BCMFromtheLabs and Instagram !
Receive From the Labs via email
Enter your email address to subscribe to this blog and receive notifications of new posts by email.
Email Address
Share this:
- Click to share on Facebook (Opens in new window)
- Click to share on Twitter (Opens in new window)
- Click to share on LinkedIn (Opens in new window)
- Click to email a link to a friend (Opens in new window)
You May Also Like
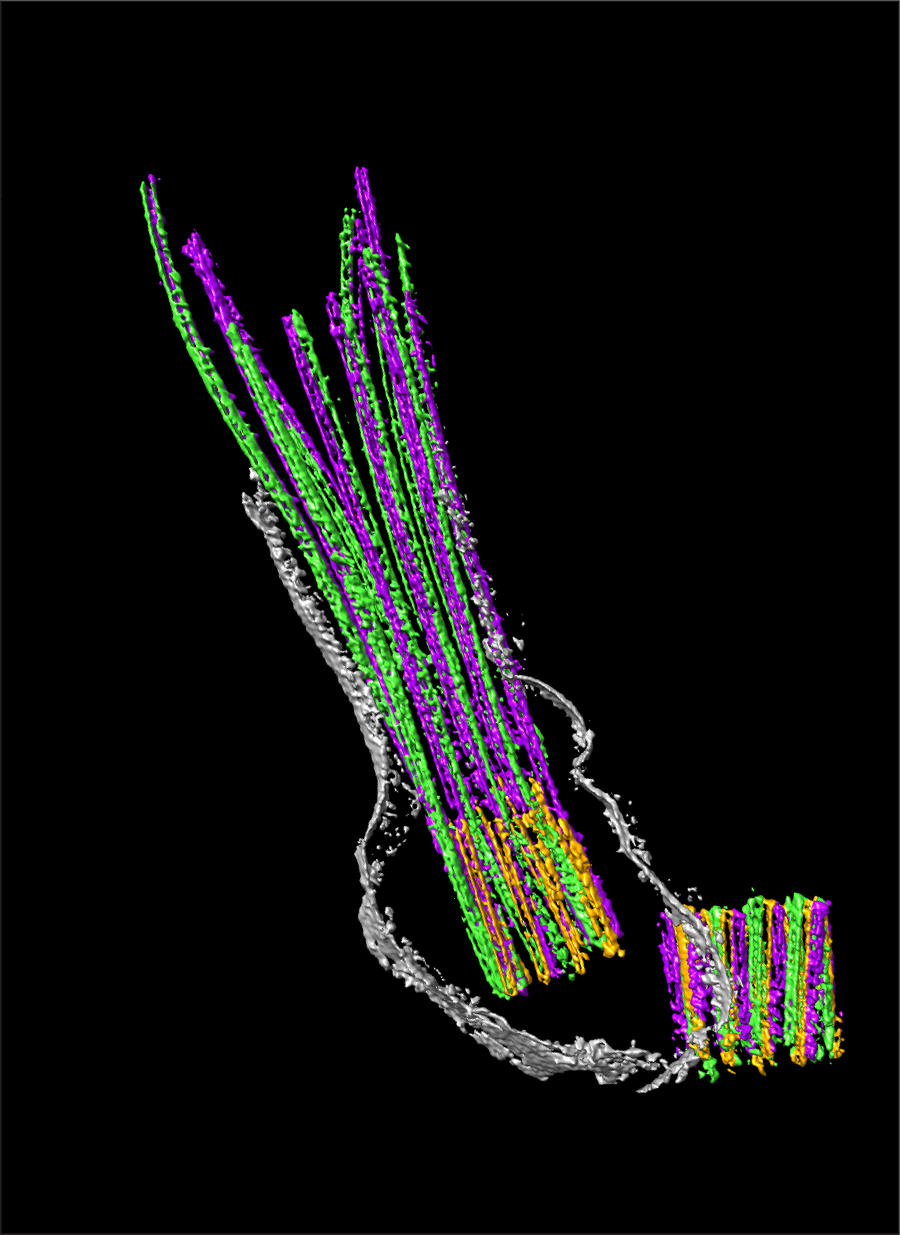
Revealing unique features of the ‘antennae’ on light-sensing neurons
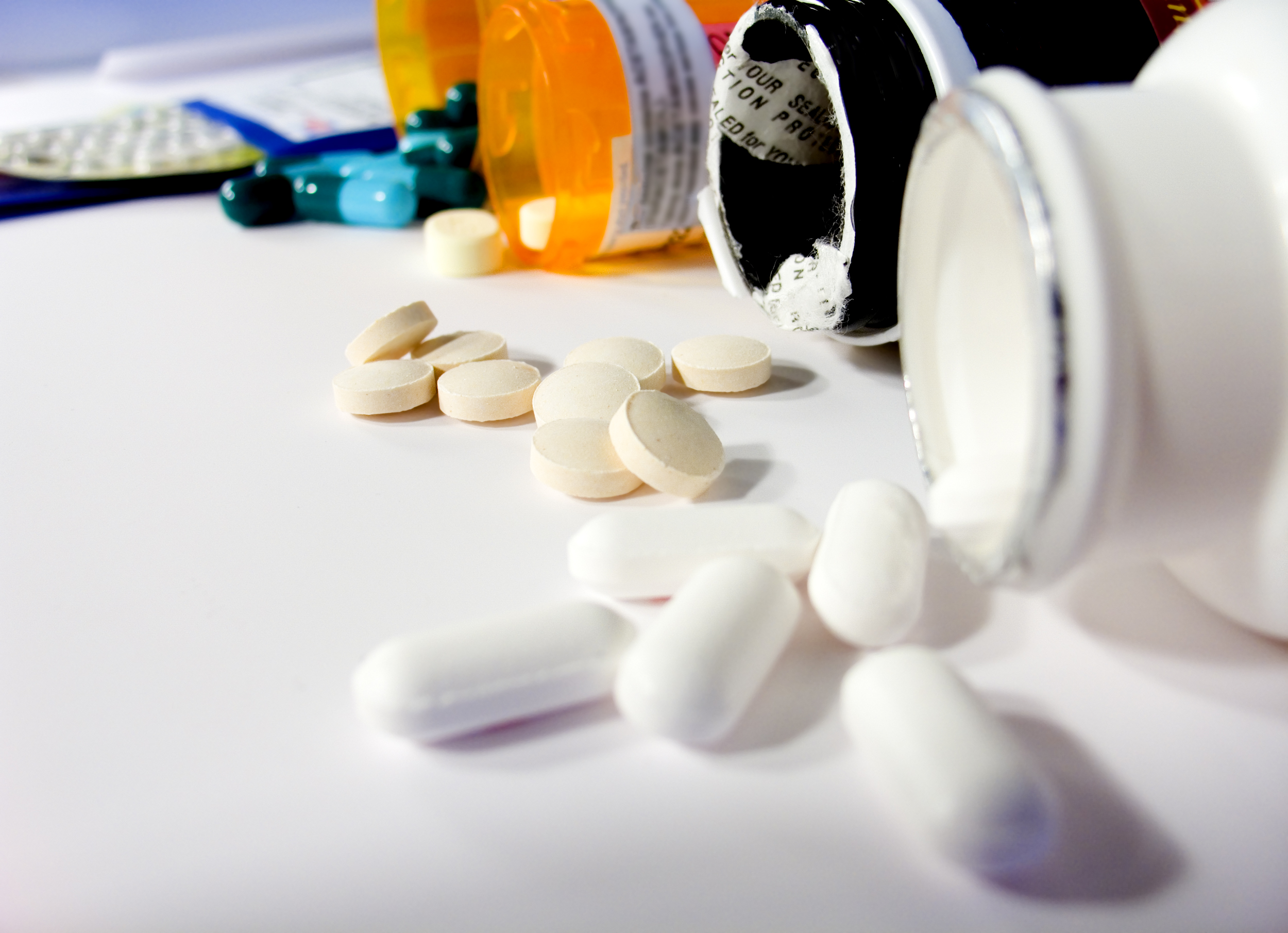
Study shows many people use non-prescription antibiotics
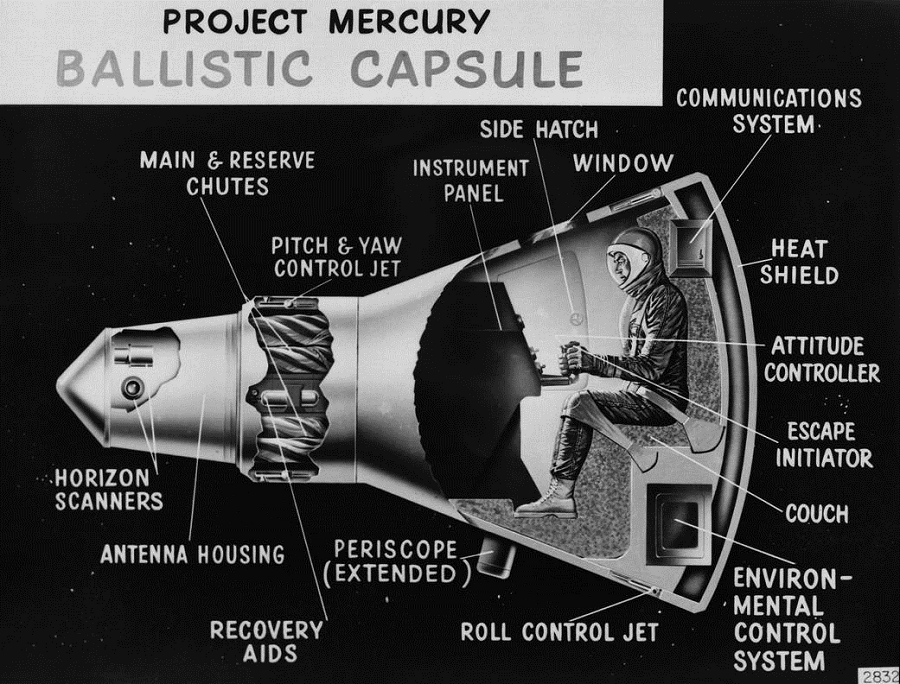
What the first American astronauts taught us about living in space
Leave a reply cancel reply.
Your email address will not be published. Required fields are marked *
Notify me of follow-up comments by email.
Notify me of new posts by email.
Thank you for visiting nature.com. You are using a browser version with limited support for CSS. To obtain the best experience, we recommend you use a more up to date browser (or turn off compatibility mode in Internet Explorer). In the meantime, to ensure continued support, we are displaying the site without styles and JavaScript.
- View all journals
- Explore content
- About the journal
- Publish with us
- Sign up for alerts
- Published: 14 March 2022
Rare diseases, common challenges
Nature Genetics volume 54 , page 215 ( 2022 ) Cite this article
11k Accesses
9 Citations
9 Altmetric
Metrics details
- Clinical genetics
- Medical research
The genetics community has a particularly important part to play in accelerating rare disease research and contributing to improving diagnosis and treatment. Innovations in sequencing technology and machine learning approaches have positively affected diagnostic success, but more coordinated efforts are needed to move towards effective therapies or even cures for these important, and sometimes overlooked, class of diseases.
Rare Disease Day was recently held on 28 February 2022, which aimed to raise awareness and promote advocacy for rare disease research. Globally, there are more than 300 million people living with rare diseases and there are no approved therapies for over 90% of these disorders. Because around 80% of rare diseases have a genetic basis, recent advances in genomic sequencing technologies and molecular gene therapies have enhanced diagnosis and expanded treatments. To ensure that these advances are benefitting as many patients as possible and doing so in an equitable manner, unified efforts that span different stakeholders across rare disease communities should be supported.
In this issue of Nature Genetics , Halley and colleagues present a Comment that calls for an integrated approach for rare disease research in the United States. The authors argue that rare diseases are an important public health issue that should be given commensurate attention for their collective effects on individual patients, disease communities and healthcare systems. As such, the approach to rare disease research needs to broader for maximum benefits to a greater number of patients. The authors call for integrated approaches to research infrastructure that would minimize barriers to making connections, whether biological, therapeutic or societal, within and between rare diseases.
The authors highlight that rare disease research is currently very siloed and often organized around single disorders. Although efforts such as the Rare Disease Clinical Research Network have taken a broader approach, overall, there is limited coordination across rare disease research networks. The single-disorder focus creates challenges for jointly combining efforts, sharing data, assessing outcomes and capturing knowledge that could be relevant across diseases. A more integrated structure with appropriate support for researchers to coordinate across rare diseases would minimize redundant efforts and increase efficiency, potentially accelerating development and the implementation of successful therapies.
Importantly, no recommendations intended to promote rare disease research can ignore equity; indeed, ensuring fair practices in funding and equitable benefits of research outcomes must be a central focus of any research initiatives into rare diseases. It is challenging to achieve greater parity across rare diseases within the current research infrastructure, as analyzing how outcomes vary within or across rare diseases in different populations or socioeconomic groups is not straightforward. A more integrated approach to rare disease research will enable the assessment of how various factors (such as income level, insurance status, or racism in health care) affect participation in rare disease research or access to its benefits.
Altogether, the authors advocate for moving towards a more coordinated approach to rare disease research that would enable analysis of the similarities and differences across diseases in terms of etiology, treatment and outcomes. Although this article is specifically focused on the United States, the authors also recognize existing international efforts, such as the Global Genes and Genetic Alliance and the International Rare Disease Research Consortium that are leading the way in facilitating coordinated research efforts and data sharing.
We are excited by new technical advances in rare disease genetics research that apply the latest technologies to improve diagnosis. As an example, also in this issue of Nature Genetics , Hsieh and colleagues report a tool that uses deep convolutional neural networks to aid in diagnosing ultra-rare disorders based on facial morphology. GestaltMatcher defines a Clinical Face Phenotype Space based on over 17,000 photographs of patients representing more than 1,100 rare disorders. An advantage of using this method is that patients who share the same genetic diagnosis can be matched, even in cases when the disorder is not part of the training set. This helps with the clinical diagnosis of both known and new phenotypes. The concept of matching patients with rare disease is also conveyed on our cover, with actual matches forming the shape of a human face.
Rare disease research encompasses passionate individuals who span different sectors of interest: clinicians, patients, genetic counselors, biologists, technicians, advocates, funders and educators. We hope that the common challenges facing rare disease research can be combatted through enhanced coordination and cooperation across research communities, with the goal of accelerating diagnosis, maximizing therapeutic benefits and reducing inefficiencies.
Rights and permissions
Reprints and permissions
About this article
Cite this article.
Rare diseases, common challenges. Nat Genet 54 , 215 (2022). https://doi.org/10.1038/s41588-022-01037-8
Download citation
Published : 14 March 2022
Issue Date : March 2022
DOI : https://doi.org/10.1038/s41588-022-01037-8
Share this article
Anyone you share the following link with will be able to read this content:
Sorry, a shareable link is not currently available for this article.
Provided by the Springer Nature SharedIt content-sharing initiative
This article is cited by
Landscape analysis of available european data sources amenable for machine learning and recommendations on usability for rare diseases screening.
- Ralitsa Raycheva
- Kostadin Kostadinov
- Rumen Stefanov
Orphanet Journal of Rare Diseases (2024)
Revealing myopathy spectrum: integrating transcriptional and clinical features of human skeletal muscles with varying health conditions
- Huahua Zhong
- Veronica Sian
Communications Biology (2024)
Highly efficient capture approach for the identification of diverse inherited retinal disorders
- Hsiao-Jung Kao
- Ting-Yi Lin
- Shun-Ping Huang
npj Genomic Medicine (2024)
Quick links
- Explore articles by subject
- Guide to authors
- Editorial policies
Sign up for the Nature Briefing newsletter — what matters in science, free to your inbox daily.

University of Utah Hospital
General questions.
- Billing & Insurance
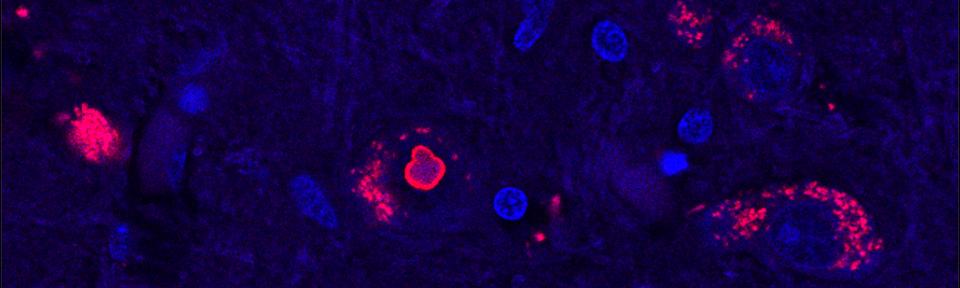
- Health Care Home
After 25 Years, Researchers Uncover Genetic Cause of Rare Neurological Disease
Media contact:.
Sophia Friesen Manager, Science Communications, University of Utah Health Email: [email protected]
Some families call it a trial of faith. Others just call it a curse. The progressive neurological disease known as spinocerebellar ataxia 4 (SCA4) is a rare condition, but its effects on patients and their families can be severe. For most people, the first sign is difficulty walking and balancing, which gets worse as time progresses. The symptoms usually start in a person’s forties or fifties but can begin as early as the late teens. There is no known cure. And, until now, there was no known cause. Now, after 25 years of uncertainty, a multinational study led by Stefan Pulst, M.D., Dr. med., professor and chair of neurology, and K. Pattie Figueroa, a project manager in neurology, both in the Spencer Fox Eccles School of Medicine at University of Utah, has conclusively identified the genetic difference that causes SCA4, bringing answers to families and opening the door to future treatments. Their results are published in the peer-reviewed journal Nature Genetics.

Solving a Genetic Enigma
SCA4’s pattern of inheritance had long made it clear that the disease was genetic, and previous research had located the gene responsible to a specific region of one chromosome. But that region proved extraordinarily difficult for researchers to analyze: full of repeated segments that look like parts of other chromosomes, and with an unusual chemical makeup that makes most genetic tests fail. To pinpoint the change that causes SCA4, Figueroa and Pulst, along with the rest of the research team, used a recently developed advanced sequencing technology. By comparing DNA from affected and unaffected people from several Utah families, they found that in SCA4 patients, a section in a gene called ZFHX3 is much longer than it should be, containing an extra-long string of repetitive DNA. Isolated human cells that have the extra-long version of ZFHX3 show signs of being sick—they don’t seem able to recycle proteins as well as they should, and some of them contain clumps of stuck-together protein. “This mutation is a toxic expanded repeat and we think that it actually jams up how a cell deals with unfolded or misfolded proteins,” says Pulst, the last author on the study. Healthy cells need to constantly break down non-functional proteins. Using cells from SCA4 patients, the group showed that the SCA4-causing mutation gums up the works of cells’ protein-recycling machinery in a way that could poison nerve cells.

Hope for the Future
Intriguingly, something similar seems to be happening in another form of ataxia, SCA2, which also interferes with protein recycling. The researchers are currently testing a potential therapy for SCA2 in clinical trials, and the similarities between the two conditions raise the possibility that the treatment might benefit patients with SCA4 as well. Finding the genetic change that leads to SCA4 is essential to develop better treatments, Pulst says. “The only step to really improve the life of patients with inherited disease is to find out what the primary cause is. We now can attack the effects of this mutation potentially at multiple levels.” But while treatments will take a long time to develop, simply knowing the cause of the disease can be incredibly valuable for families affected by SCA4, says Figueroa, the first author on the study. People in affected families can learn whether they have the disease-causing genetic change or not, which can help inform life decisions such as family planning. “They can come and get tested and they can have an answer, for better or for worse,” Figueroa says. The researchers emphasize that their discoveries would not have been possible without the generosity of SCA4 patients and their families, whose sharing of family records and biological samples allowed them to compare the DNA of affected and unaffected individuals. “Different branches of the family opened up not just their homes but their history to us,” Figueroa says. Family records were complete enough that the researchers were able to trace the origins of the disease in Utah back through history to a pioneer couple who moved to Salt Lake Valley in the 1840s. Since meeting so many families with the disease, studying SCA4 has become a personal quest, Figueroa adds. “I’ve been working on SCA4 directly since 2010 when the first family approached me, and once you go to their homes and get to know them, they’re no longer the number on the DNA vial. These are people you see every day… You can’t walk away. This is not just science. This is somebody’s life.” # # # This research was published in Nature Genetics as “GGC expansion in ZFHX3 causes SCA4 and impairs autophagy.” This work was performed in collaboration with researchers from University of Tübingen, University of Lübeck and Kiel University, University Hospital Hamburg-Eppendorf, and Veterans Administration Medical Center, Albany, NY. The study was supported by the National Institute of Neurological Disorders and Stroke of the National Institutes of Health under award number R35127253 and the DFG-funded INST 37/1049-1.
- research news

Using AI to improve diagnosis of rare genetic disorders
Diagnosing rare Mendelian disorders is a labor-intensive task, even for experienced geneticists. Investigators at Baylor College of Medicine are trying to make the process more efficient using artificial intelligence. The team developed a machine learning system called AI-MARRVEL (AIM) to help prioritize potentially causative variants for Mendelian disorders. The study is published today in NEJM AI .
Researchers from the Baylor Genetics clinical diagnostic laboratory noted that AIM's module can contribute to predictions independent of clinical knowledge of the gene of interest, helping to advance the discovery of novel disease mechanisms. "The diagnostic rate for rare genetic disorders is only about 30%, and on average, it is six years from the time of symptom onset to diagnosis. There is an urgent need for new approaches to enhance the speed and accuracy of diagnosis," said co-corresponding author Dr. Pengfei Liu, associate professor of molecular and human genetics and associate clinical director at Baylor Genetics.
AIM is trained using a public database of known variants and genetic analysis called Model organism Aggregated Resources for Rare Variant ExpLoration (MARRVEL) previously developed by the Baylor team. The MARRVEL database includes more than 3.5 million variants from thousands of diagnosed cases. Researchers provide AIM with patients' exome sequence data and symptoms, and AIM provides a ranking of the most likely gene candidates causing the rare disease.
Researchers compared AIM's results to other algorithms used in recent benchmark papers. They tested the models using three data cohorts with established diagnoses from Baylor Genetics, the National Institutes of Health-funded Undiagnosed Diseases Network (UDN) and the Deciphering Developmental Disorders (DDD) project. AIM consistently ranked diagnosed genes as the No. 1 candidate in twice as many cases than all other benchmark methods using these real-world data sets.
"We trained AIM to mimic the way humans make decisions, and the machine can do it much faster, more efficiently and at a lower cost. This method has effectively doubled the rate of accurate diagnosis," said co-corresponding author Dr. Zhandong Liu, associate professor of pediatrics -- neurology at Baylor and investigator at the Jan and Dan Duncan Neurological Research Institute (NRI) at Texas Children's Hospital.
AIM also offers new hope for rare disease cases that have remained unsolved for years. Hundreds of novel disease-causing variants that may be key to solving these cold cases are reported every year; however, determining which cases warrant reanalysis is challenging because of the high volume of cases. The researchers tested AIM's clinical exome reanalysis on a dataset of UDN and DDD cases and found that it was able to correctly identify 57% of diagnosable cases.
"We can make the reanalysis process much more efficient by using AIM to identify a high-confidence set of potentially solvable cases and pushing those cases for manual review," Zhandong Liu said. "We anticipate that this tool can recover an unprecedented number of cases that were not previously thought to be diagnosable."
Researchers also tested AIM's potential for discovery of novel gene candidates that have not been linked to a disease. AIM correctly predicted two newly reported disease genes as top candidates in two UDN cases.
"AIM is a major step forward in using AI to diagnose rare diseases. It narrows the differential genetic diagnoses down to a few genes and has the potential to guide the discovery of previously unknown disorders," said co-corresponding author Dr. Hugo Bellen, Distinguished Service Professor in molecular and human genetics at Baylor and chair in neurogenetics at the Duncan NRI.
"When combined with the deep expertise of our certified clinical lab directors, highly curated datasets and scalable automated technology, we are seeing the impact of augmented intelligence to provide comprehensive genetic insights at scale, even for the most vulnerable patient populations and complex conditions," said senior author Dr. Fan Xia, associate professor of molecular and human genetics at Baylor and vice president of clinical genomics at Baylor Genetics. "By applying real-world training data from a Baylor Genetics cohort without any inclusion criteria, AIM has shown superior accuracy. Baylor Genetics is aiming to develop the next generation of diagnostic intelligence and bring this to clinical practice."
Other authors of this work include Dongxue Mao, Chaozhong Liu, Linhua Wang, Rami AI-Ouran, Cole Deisseroth, Sasidhar Pasupuleti, Seon Young Kim, Lucian Li, Jill A.Rosenfeld, Linyan Meng, Lindsay C. Burrage, Michael Wangler, Shinya Yamamoto, Michael Santana, Victor Perez, Priyank Shukla, Christine Eng, Brendan Lee and Bo Yuan. They are affiliated with one or more of the following institutions: Baylor College of Medicine, Jan and Dan Duncan Neurological Research Institute at Texas Children's Hospital, Al Hussein Technical University, Baylor Genetics and the Human Genome Sequencing Center at Baylor.
This work was supported by the Chang Zuckerberg Initiative and the National Institute of Neurological Disorders and Stroke (3U2CNS132415).
- Diseases and Conditions
- Parkinson's Research
- Personalized Medicine
- Computers and Internet
- Computer Modeling
- Neural Interfaces
- Personality disorder
- Computer vision
- Psychopathology
- Toxic shock syndrome
- Artificial intelligence
- Computational neuroscience
- Nutrition and pregnancy
Story Source:
Materials provided by Baylor College of Medicine . Note: Content may be edited for style and length.
Journal Reference :
- Dongxue Mao, Chaozhong Liu, Linhua Wang, Rami AI-Ouran, Cole Deisseroth, Sasidhar Pasupuleti, Seon Young Kim, Lucian Li, Jill A. Rosenfeld, Linyan Meng, Lindsay C. Burrage, Michael F. Wangler, Shinya Yamamoto, Michael Santana, Victor Perez, Priyank Shukla, Christine M. Eng, Brendan Lee, Bo Yuan, Fan Xia, Hugo J. Bellen, Pengfei Liu, Zhandong Liu. AI-MARRVEL — A Knowledge-Driven AI System for Diagnosing Mendelian Disorders . NEJM AI , 2024; 1 (5) DOI: 10.1056/AIoa2300009
Cite This Page :
Explore More
- Genetic Signals Linked to Blood Pressure
- Double-Fangs of Adolescence Saber-Toothed Cats
- Microarray Patches for Vaccinating Children
- Virus to Save Billions of Gallons of Wastewater
- Weather Report On Planet 280 Light-Years Away
- Trotting Robots and Animal Gait Transitions
- Where Have All the Fireflies Gone?
- Cardio-Fitness Cuts Death and Disease by 20%
- Reusable Super-Adhesive from Smart Materials
- Long Snouts Protect Foxes Diving Into Snow
Trending Topics
Strange & offbeat.
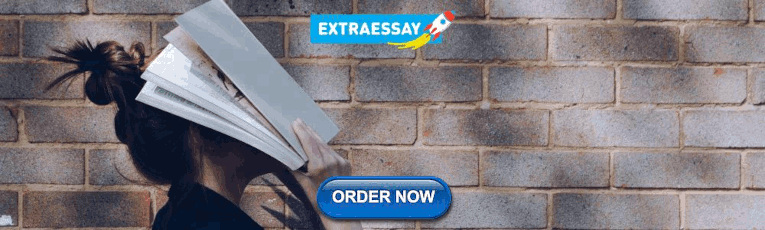
IMAGES
VIDEO
COMMENTS
Essays Biochem. 2018 Dec 3; 62(5): 643-723. ... With so many genetic disorders, it is impossible to include more than a few examples within this review, to illustrate the principles. ... The focus here will be on human disease, although much of the research that defines our understanding comes from the study of animal models that share ...
Abstract. Rare genetic diseases are the result of a continuous forward genetic screen that nature is conducting on humans. Here, we present epistemological and systems biology arguments highlighting the importance of studying these rare genetic diseases. We contend that the expanding catalog of mutations in ∼4,000 genes, which cause ∼6,500 ...
Investigating expressed RNA variants that are related to disease severity in SARS-CoV-2-infected patients with mild-to-severe disease, Egyptian Journal of Medical Human Genetics, 23, 1, (2022 ...
DYRK1A gene linked to heart defects in Down syndrome. A study shows that congenital heart defects in Down syndrome are in part caused by increased dosage of the DYRK1A gene, which lies on ...
This paper used large-scale genetic data to demonstrate the clinical potential of the polygenic scores that can be constructed for many common diseases, emphasizing that, in some situations, the ...
Essays Biochem. 2018 Dec 2;62(5):643-723. doi: 10.1042/EBC20170053. Print 2018 Dec 3. Authors Maria ... contribute to disease processes. This review explores the genetic basis of human disease, including single gene disorders, chromosomal imbalances, epigenetics, cancer and complex disorders, and considers how our understanding and ...
Interpreting the effects of genetic variants is key to understanding individual susceptibility to disease and designing personalized therapeutic approaches. Modern experimental technologies are ...
A primary goal of human genetics is to identify DNA sequence variants that influence biomedical traits, particularly those related to the onset and progression of human disease. Over the past 25 years, progress in realizing this objective has been transformed by advances in technology, foundational genomic resources and analytical tools, and by ...
Introduction. New disease mutations arise in heterozygotes and either drift to higher frequencies or are rapidly purged from the population, depending on the strength of selection and the demographic history of the population [1-6].Elucidating the relative contributions of mutation, natural selection and genetic drift will help to understand why disease alleles persist in humans.
This review explores. the genetic basis of human disease, including single gene disorders, chromosomal imbal-. ances, epigenetics, cancer and complex disorders, and considers how our understanding ...
Genetics plays an essential role in translational research, ultimately aiming to develop novel disease-modifying therapies for neurodegenerative disorders. We anticipate that individual genetic profiling will also be increasingly relevant in a clinical context, with implications for patient care in line with the proposed ideal of personalized ...
Genes & Diseases is a journal for molecular and translational medicine. The journal primarily focuses on publishing investigations on the molecular bases and experimental therapeutics of human diseases. Publication formats include full length research article, review article, …. View full aims & scope. $2500.
Feature papers represent the most advanced research with significant potential for high impact in the field. ... in genome data and methodology have accelerated the identification of candidate genes and associated variants in human genetic diseases. ... NRXN1 and its binding partner neuroligin have been associated with deficits in cognition ...
Rare diseases (RD) affect a small number of people compared to the general population and are mostly genetic in origin. The first clinical signs often appear at birth or in childhood, and patients ...
There are more than 5,000 recognized genetic diseases in humans, and Dr. Nelson believes there are likely 2,000 more to solve. It's unrealistic to expect to develop 7,000 new traditional medicines targeting each of these diseases, as "that's more drugs than exist currently across all treatments ever developed," he says.
Genetic disorders are of different types i.e. single-gene. disorders, chromosomal disorders, complex disorder s. This paper intends to be as an introductory paper for the project "Human genetic ...
Editorial. Human Genetic Diseases. Hao Deng, 1Peter Riederer,2Han-Xiang Deng,3Weidong Le,4Wei Xiong,5and Yi Guo6,7. 1 Center for Experimental Medicine and Department of Neurology, e ird Xiangya ...
The year 2022 will be important in the development of diagnostics and treatments for rare genetic diseases in prenatal, pediatric, and adult individuals. This perspective did not do justice to the breadth of clinical decision support tools, implementation projects, or legislative coverage decisions that are underway.
There is a lot of debate regarding suicidal behavior and its relationship with psychiatric disorders, but the extent to which they share the same genetic architecture is unknown. This Research Topic was investigated by Kootbodien et al. through the use of genomic structural equation modeling and Mendelian randomization with a large genomic ...
In this paper, we focus on prenatal genetic testing in Germany ... in addition to research on views of genetic testing more broadly, ... PGT is the lesser evil and if it's restricted to the most serious genetic diseases and it's done in a controlled way with individualized genetic counseling for the parents and so on, all the conditions that ...
Earlier research used many genetic variants, considered as a group, to assess disease risk. However, these risk scores were traditionally derived from those of European descent. The researchers compared three risk scores, a traditional score based only on those of European descent and two others developed by including people of different ...
A genetic disorder is a disease caused in whole or in part by a change in the DNA sequence away from the normal sequence. Genetic disorders can be caused by a mutation in one gene (monogenic disorder), by mutations in multiple genes (multifactorial inheritance disorder), by a combination of gene mutations and environmental factors, or by damage to chromosomes (changes in the number or ...
FROM THE LABS: Research method finds new use in diagnosis of genetic disorders ... McNair Medical Institute at the Robert and Janice McNair Foundation and Baylor Genetics. The research was supported by the National Institutes of Health Common Fund (U01HG007709 and U01HG007942), the National Human Genome Research Institute (R35HG011311) and the ...
The genetics community has a particularly important part to play in accelerating rare disease research and contributing to improving diagnosis and treatment. Innovations in sequencing technology ...
Spinocerebellar ataxia 4 is a severe progressive movement disease that can begin as early as the late teens. Now, a multinational research team led by University of Utah researchers has conclusively identified the genetic difference that causes the disease, bringing answers to families and opening the door to future treatments.
The Burden of Mendelian Disease. In aggregate, clinically recognized Mendelian phenotypes compose a substantial fraction (∼0.4% of live births) of known human diseases, and if all congenital anomalies are included, ∼8% of live births have a genetic disorder recognizable by early adulthood. 27 This translates to approximately eight million children born worldwide each year with a "serious ...
The study combined previously published genetic data from the UK Biobank, a large-scale biomedical database and research resource containing genetic and health information from half a million UK ...
Diagnosing rare Mendelian disorders is a labor-intensive task, even for experienced geneticists. Investigators at Baylor College of Medicine are trying to make the process more efficient using ...
With data from more than 350,000 people and information on their genetics, education, socioeconomic status and disease history, this study had strong methodology, said Dr. Aladdin Shadyab ...