Advertisement
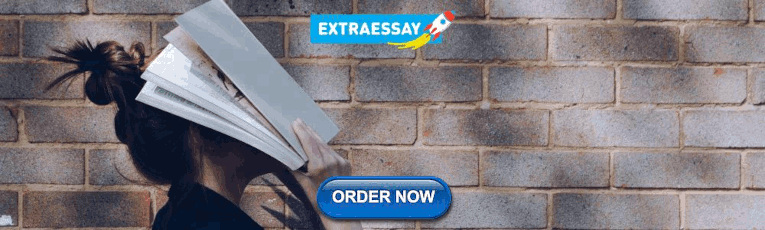
Simplified Single-Stage Planetary Gearbox and Rolling Element Bearings Dynamic Analysis Using Lagrange’s Theorem and Comparison of Vulnerable Frequencies of Vibration
- Original Paper
- Published: 25 August 2021
- Volume 10 , pages 211–223, ( 2022 )
Cite this article
- Imthiyas Manarikkal 1 ,
- Faris Elasha 1 ,
- Arnaldo Delli-Carri 1 ,
- Dina Shona Laila 1 &
- David Mba 2
291 Accesses
Explore all metrics
Introduction
Planetary gearboxes are widely used in the aerospace, automotive and renewable energy sectors. These gearboxes are the critical component in a system's reliability; hence, monitoring this component is essential.
To study the vibration characteristics, a novel dynamic modelling approach using Lagrange's equations is presented in this paper. Newtonian equations of motion have been developed and solved using Lagrange's theorem, and modal analysis has been performed to estimate the dynamic characteristics of a single-stage planetary gearbox. The torsional stiffness and time-varying mesh stiffness have been considered in this paper for dynamic modelling. This study applies the perturbation method to solve the conditions of the system. The significant resonance frequencies of the individual components in the gearbox have been identified using coordinates based on eigenvectors. The finite-element analysis results have been considered for validation purposes and compared to the numerical model. The frequency range of the gearbox components' resonances and dynamic characteristics have been obtained from the FEA.
Conclusions
The study shows that Lagrange's dynamic modes match with modes obtained from the finite-element modal analysis. In addition, the resonance frequencies produced by the sun and planet gears and the bearings are detected. Therefore, the results show a positive potential in gearbox fault diagnostics and characteristics frequencies studies for individual components in the gearbox.
This is a preview of subscription content, log in via an institution to check access.
Access this article
Price includes VAT (Russian Federation)
Instant access to the full article PDF.
Rent this article via DeepDyve
Institutional subscriptions
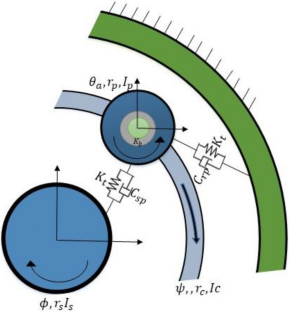
Similar content being viewed by others
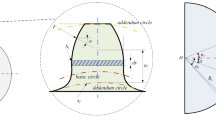
Dynamic characteristics and experimental verification of planetary gear-motor coupling system under unsteady and non-ideal states
Kongliang Zhang, Hongkun Li, … Wei Xiang
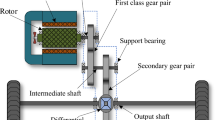
Electromechanical coupling dynamic characteristics of electric drive system for electric vehicle
Shuaishuai Ge, Longhui Qiu, … Minghui Hu
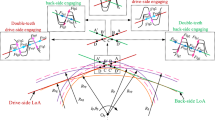
Multi-status mating mechanism and incomplete bifurcation of an internal gear system with time-variant parameters and temperature
Xiao-jing Ma, Jian-fei Shi & Li Zhang
Availability of Data and Codes
Available up on request.
Elasha F, Mba D, Togneri M, Masters I, Teixeira JA (2017) A hybrid prognostic methodology for tidal turbine gearboxes. Renewable Energy 114:1051–1061
Article Google Scholar
Deign J (2019) Gearbox faults to plummet on small part upgrades, predictive tools | reuters events | renewables. http://newenergyupdate.com/wind-energy-update/gearbox-faults-plummet-small-part-upgrades-predictive-tools . Accessed 24 May 2021
Frith J (2015) Gcube report on wind turbine gearbox downtime. http://www.maritimejournal.com/news101/insurance,-legal-andfinance/gcube-report-on-wind-turbine-gearbox-downtime . Accessed 11 Apr 2018
He S, Jia Q, Chen G, Sun H (2015) Modeling and dynamic analysis of planetary gear transmission joints with backlash. Int J Control Autom 8(2):153–162
Wang Z, Cheng Y, Mei G, Zhang W, Huang G, Yin Z (2019) Torsional vibration analysis of the gear transmission system of high-speed trains with wheel defects. Proc Instit Mech Eng Part F 234(2):123–133
Zhang L, Wang Y, Wu K, Sheng R (2017) Three-dimensional modeling and structured vibration modes of two-stage helical planetary gears used in cranes. Shock Vib 2017:1–18
Google Scholar
Jayaswal P, Wadhwani AK, Mulchandani KB (2008) Machine fault signature analysis. Int J rotat Mach 2008:1–10
Park J, Ha JM, Oh H, Youn BD, Choi J-H, Kim NH (2016) Model-based fault diagnosis of a planetary gear: a novel approach using transmission error. IEEE Trans Reliab 65(4):1830–1841
Inalpolat M, Kahraman A (2008) Dynamic modelling of planetary gears of automatic transmissions. Proc Instit Mech Eng Part K 222(3):229–242
Yang Z, Ahmat M, Geni M (2015) Dynamics numerical simulation of planetary gear system for wind turbine gearbox. Indian J Eng Mater Sci 22:71–76
Chaari F, Fakhfakh T, Haddar M (2009) Analytical modelling of spur gear tooth crack and influence on gearmesh stiffness. European Journal of Mechanics 28(3):461–468
Mohammed OD, Rantatalo M (2016) Dynamic response and time-frequency analysis for gear tooth crack detection. Mech Syst Signal Process 66–67:612–624
Mohammed OD, Rantatalo M, Aidanpää J-O (2015) Dynamic modelling of a one-stage spur gear system and vibration-based tooth crack detection analysis. Mech Syst Signal Process 54–55:293–305
Wu S, Zuo MJ, Parey A (2008) Simulation of spur gear dynamics and estimation of fault growth. J Sound Vib 317(3–5):608–624
Li G, Liang X, Li F (2018) Model-based analysis and fault diagnosis of a compound planetary gear set with damaged sun gear. J Mech Sci Technol 32(7):3081–3096
Howard I, Jia S, Wang J (2001) The dynamic modelling of a spur gear in mesh including friction and a crack. Mech Syst Signal Process 15(5):831–853
Kim W, Lee JY, Chung J (2012) Dynamic analysis for a planetary gear with time-varying pressure angles and contact ratios. J Sound Vib 331(4):883–901
Manarikkal I, Elasha F, Laila D, Mba D (2019) Dynamic modelling of planetary gearboxes with cracked tooth using vibrational analysis. Springer, Cham. https://doi.org/10.1007/978-3-030-11220-2_25
Book Google Scholar
Cooley CG, Parker RG (2014) A review of planetary and epicyclic gear dynamics and vibrations research. Appl Mech Rev. https://doi.org/10.1115/1.4027812
Xiao ZM, Qin DT (2011) Dynamic modeling of multi-stage planetary gears coupled with bearings in housing. Appl Mech Mater 86:30–34
Babu R, Kumar N, Gupta K, Gautam K, Sri D (2019) Significance of modal analysis to detect and diagnose misalignment fault in turbine rotor. https://www.researchgate.net/publication/324819731 . Accessed 6 Dec 2019
Kiekbusch T, Sappok D, Sauer B, Howard I (2011) Calculation of the combined torsional mesh stiffness of spur gears with two- and three-dimensional parametrical FE models. Strojniški Vestnik-J Mech Eng 57(11):810–818
Lin J, Parker RG (2001) Mesh Stiffness Variation Instabilities in Two-Stage Gear Systems. J Vib Acoust 124(1):68–76
Guo Y, Parker RG (2012) Dynamic analysis of planetary gears with bearing clearance. J Comput Nonlinear Dyn. https://doi.org/10.1115/1.4005929
Liang X, Zuo MJ, Pandey M (2014) Analytically evaluating the influence of crack on the mesh stiffness of a planetary gear set. Mech Mach Theory 76:20–38
Liu J, Wang C, Wu W (2020) Research on meshing stiffness and vibration response of pitting fault gears with different degrees. Int J Rotat Mach 2020:1–7
Ross Gearbox Ltd (2013) Product line overview planetary gear reducers 125–3000 KN M. https://www.rossi.com/sites/default/files/Catalog_EP_Product_Line_Overview_Edition_October_2013_en_0_0.pdf . Accessed 24 May 2021
Ahn T (2001) ASME B&PV CODE: SEC VIII, DIV. 1 + alteration and repair. https://www.nrc.gov/docs/ML0401/ML040150682.pdf . Accessed 13 Apr 2020
Fuller DD (1984) Theory and practice of lubrication for engineers. Wiley-Interscience, New York
Abu-Zeid MA, Abdel-Rahman SM (2013) Bearing problems’ effects on the dynamic performance of pumping stations. Alex Eng J 52(3):241–248
Moshrefzadeh A, Fasana A (2017) Planetary gearbox with localised bearings and gears faults: simulation and time/frequency analysis. Meccanica 52(15):3759–3779
Manarikkal I, Elasha F, Mba D (2021) Diagnostics and prognostics of planetary gearbox using CWT auto regression (AR) and K-means algorithm. App Acous 184:108314. https://doi.org/10.1016/j.apacoust.2021.108314
Download references
This is a non-funded project.
Author information
Authors and affiliations.
Future Transport and Cities Research Centre, Coventry University, Coventry, UK
Imthiyas Manarikkal, Faris Elasha, Arnaldo Delli-Carri & Dina Shona Laila
Department of Technology, De Montford University, Leicester, UK
You can also search for this author in PubMed Google Scholar
Contributions
IM performed the gearbox mathematical model and analyzed the data. FE analyzed the significance of characteristics frequencies of individual systems. AD verified the deviation of Modal analysis obtained from FE and Mathematical model. DL and DM verified the mathematical model accuracy and simplicity.
Corresponding author
Correspondence to Imthiyas Manarikkal .
Ethics declarations
Conflict of interest.
The authors declare that they have no competing interests.
Ethical Considerations and Consent to Participate
Not applicable.
Consent for Publication
Consent for publication is received from director of studies and institution.
Additional information
Publisher's note.
Springer Nature remains neutral with regard to jurisdictional claims in published maps and institutional affiliations.
Rights and permissions
Reprints and permissions
About this article
Manarikkal, I., Elasha, F., Delli-Carri, A. et al. Simplified Single-Stage Planetary Gearbox and Rolling Element Bearings Dynamic Analysis Using Lagrange’s Theorem and Comparison of Vulnerable Frequencies of Vibration. J. Vib. Eng. Technol. 10 , 211–223 (2022). https://doi.org/10.1007/s42417-021-00372-0
Download citation
Received : 12 February 2021
Revised : 15 July 2021
Accepted : 16 August 2021
Published : 25 August 2021
Issue Date : January 2022
DOI : https://doi.org/10.1007/s42417-021-00372-0
Share this article
Anyone you share the following link with will be able to read this content:
Sorry, a shareable link is not currently available for this article.
Provided by the Springer Nature SharedIt content-sharing initiative
- Modal analysis
- Find a journal
- Publish with us
- Track your research

An official website of the United States government
The .gov means it’s official. Federal government websites often end in .gov or .mil. Before sharing sensitive information, make sure you’re on a federal government site.
The site is secure. The https:// ensures that you are connecting to the official website and that any information you provide is encrypted and transmitted securely.
- Publications
- Account settings
Preview improvements coming to the PMC website in October 2024. Learn More or Try it out now .
- Advanced Search
- Journal List
- Scientific Reports

Research on fault diagnosis method of planetary gearbox based on dynamic simulation and deep transfer learning
Meng-meng song.
1 College of Information, Mechanical and Electrical Engineering, Ningde Normal University, Ningde, China
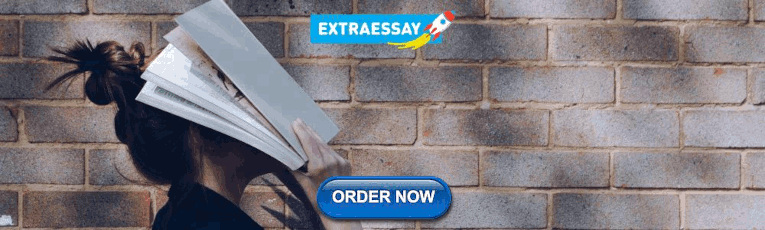
Zi-Cheng Xiong
2 College of Mechanical Engineering and Automation, Fuzhou University, Fuzhou, China
Jian-Hua Zhong
Shun-gen xiao, yao-hong tang, associated data.
The datasets generated and/or analysed during the current study are not publicly available due the data also forms part of an ongoing study, but are available from the corresponding author on reasonable request.
To address the issue of not having enough labeled fault data for planetary gearboxes in actual production, this research develops a simulation data-driven deep transfer learning fault diagnosis method that applies fault diagnosis knowledge from a dynamic simulation model to an actual planetary gearbox. Massive amounts of different fault simulation data are collected by creating a dynamic simulation model of a planetary gearbox. A fresh deep transfer learning network model is built by fusing one-dimensional convolutional neural networks, attention mechanisms, and domain adaptation methods. The network model is used to learn domain invariant features from simulated data, thereby enabling fault diagnosis on real data. The fault diagnosis experiment is verified by using the Drivetrain Diagnostics Simulator test bench. The validity of the proposed means is evaluated by comparing the diagnostic accuracy of various means on various diagnostic tasks.
Introduction
Planetary gearbox (PG) is a critical part of rotating machines, in virtue of its merit of great carrying capacity, small volume, and high driving efficiency, it has been more and more extensively applied in mechanical transmission systems of wind power, aviation, lifting and transportation, and other industries 1 . However, the working environment of PG is usually very harsh, such as often changes in the working environment, heavy loads, bad weather, and many other factors, making it easy to fail. Once the PG fails, it may cause mechanical equipment downtime. Health monitoring and fault diagnosis of PG can keep the mechanical equipment running safely and stably, and reduce possible losses caused by faults.
The conventional fault diagnosis means comprises three steps: data collection, feature extraction, and fault classification 2 . First, the data during the operation of the mechanical equipment is collected by sensors, and then the features are manually picked up from the collected data. Finally, the extracted features are used to train machine learning models such as SVM 3 , KNN 4 , RF 5 , and ANN 6 for failure prediction. However, manual feature extraction has obvious disadvantages. It requires a lot of manpower and some professional basic knowledge.
Deep learning (DL) is a major breakthrough in artificial intelligence in recent decades. It can automatically pick out representative features from raw data, and accurately build nonlinear mapping relationships of different health status features, which greatly surmounts the deficiencies of traditional fault diagnosis means. DL models such as CNN 7 , AE 8 , DBN 9 , and RNN 10 have been diffusely researched and utilized in the fault diagnosis field.
When making use of DL means for fault diagnosis, the following two assumptions usually need to be satisfied: (1) sufficient labeled data is available; (2) the feature distribution in the training data and the test data are identical. However, in actual production, majority of data are gathered when the machines is running healthy 11 , so it is very hard to obtain comprehensive and extensive labeled failure data.
In view of the scarcity of fault data, dynamic simulation analysis may be a good solution. The dynamic simulation analysis and diagnostic means frequently begins with the creation of a dynamic model of machines to simulate dynamic response of machines under multiple conditions. Then, the dynamic response may be used to do diagnostics 12 . Xiao et al. 13 – 15 revealed the dynamic response of mechanical system under the influence of rub impact fault by establishing a dynamic model. Han et al. 16 built a revised lumped parameter dynamic model, which revealed the fluctuation of the dynamic load distribution coefficient when gear crack faults were considered. Chen et al. 17 developed an analytical model for meshing stiffness calculation using the potential energy principle and uniform bending Timoshenko beam theory, and demonstrated the effect law of flexible ring gear and tooth root cracking fault on dynamic response. Park et al. 18 designed a lumped parameter model of planetary gear dynamics to extract transmission error signal, and applied the rotation error signal to fault diagnosis. Fan et al. 19 built a dynamic model of the planetary gear train with planetary carrier crack fault, and demonstrated a correlation between vibration characteristics and planetary carrier crack conditions. Duan et al. 20 used a system-level rigid-flexible coupled model consisting of shells, gears, shafts and bearings to detect the crack evolution-induced vibration, and acquired relevant spectral characteristics, statistical indicators and instantaneous energy of the crack evolution-induced vibration.
By constructing a dynamic model of the mechanical equipment to be diagnosed and solving it numerically, the simulation data of various working conditions and common fault types can be obtained easily, which solves the problem of insufficient real faults. However, limited by the complexity of the simulation model and calculation, the simulation data obtained are often too ideal, lacking noise in the actual environment, which is significantly different from the real data. Therefore, the fault diagnosis model built by the simulation data set will not attain a better precision on the real data set. Transfer learning (TL) is a useful way to settle the matter of data discrepancy.
The purpose of TL is to adapt acquired from one or more tasks (source domain) to other associated but distinct tasks (target domain). It is inspired by the human ability to reuse knowledge from previous tasks to new ones without having to start from the beginning. He et al. 21 proposed an enhanced depth transmission automatic encoder to address the issue of inadequate bearing vibration data with marks, which initialized the target model through the source model's trained parameter. Then only just a small subset of target training samples are used to fine-tune the target model to fit the features of the remaining target test samples. Li et al. 22 put forward a joint attention feature transfer network model. The established feature transfer module transfers the generalized representation obtained from the class with more samples to the class with few samples, thus expanding its feature space and solving the matter of data imbalance. Zhu et al. 23 calculated the domain loss between the source domain and the target domain through the linear combination of multiple Gaussian kernels, which enhanced the adaptive ability of the diagnostic model. Wan et al. 24 put forward a TL means combining sensitive feature selection and sparse automatic encoder, which reduces the interference of insensitive information in the original signal and raises the diagnostic precision of the model under complex operating conditions. Han et al. 25 extended the marginal distribution adaptation to joint distribution adaptation, allowing the suggested network to employ the identification structure linked to the labeled data in the source domain to adapt to the conditional distribution of the unlabeled target data and ensure higher precise distribution matching. Zheng et al. 26 put forward a normalized recursive dynamic adaptive network, which can estimate the relative weight of marginal and conditional distributions dynamically and quantitatively. Deng et al. 27 put forward a sample weighted joint adversarial network that takes use of the classification information to improve the joint domain adaptability of adversarial learning. Han et al. 28 proposed a new framework to address the issue of transfer diagnosis for sparse target data. Individual domain adaptation is performed on the source domain data and target data under the same work conditions to reduce distribution differences and avoid the negative transfer.
Aiming at the scarcity of marked fault data in practical fault diagnosis, taking PG as the research object, a fault diagnosis means based on dynamic simulation and deep transfer learning (DTL) is put forward in this paper. By building the dynamic simulation model of the PG, sufficient fault simulation data can be gathered. On this basis, the time-domain graphs (TDG) and frequency-domain graphs (FDG) of the simulation data are evaluated to confirm the validity of established models. In addition, the influence law of various simulation step sizes on simulation data is explored. A fresh DTL network model is built. The network model is composed of three parts: one-dimensional convolution neural network, attention mechanism, and domain adaptive method. Through the network model, the fault diagnosis knowledge contained in a simulation data is used in a real PG’s fault diagnosis, and the influence law of simulation data with distinct parameters on the diagnosis precision is explored.
The remaining of this article is arranged as below: “ Theoretical background ” outlines the relevant theories. “ Proposed method ” details the suggested means. “ Experiment and analysis ” studies a practical case. “ Conclusions ” summarizes this paper.
Theoretical background
Dynamics theory based on adams.
In ADAMS, the Cartesian coordinates of the rigid body's centroid and the Euler angles representing its orientation are used as generalized coordinates, hence, q = [ x , y , z , ψ , θ , φ ] T , Eq. ( 1 ) is obtained by the energy form of Lagrange equations of the first kind.
Here, T denotes the kinetic energy indicated in the generalized coordinate of the system, q j denotes the generalized coordinate, Q j represents the generalized force in the direction of q j , and the last term of the equation represents the constraint reaction in the direction of q j .
Introducing generalized momentum p j
The dynamic differential–algebraic equations of the system are established by integrating the constraint equations.
Here, Φ is the constraint function, Φ q is the Jacobian matrix of the constraint equation, H is the coordinate transformation matrix of the external force, λ is the Lagrange multiplier, and F represents the external force on the system.
There are three different integration schemes in ADAMS to reduce the order of dynamic differential–algebraic equations.
I3 integral format:
SI2 integral format:
SI1 integral format:
Transfer learning
First, various TL-related symbols are given to properly indicate the issues that need to be resolved. Given the source domain D s rc = { ( x i , y i ) } i = 1 n src with n src labeled samples and the target domain D tar = { ( x i ) } i = 1 n tar with n tar unlabeled samples, where X = { ( x i ) } i = 1 n denotes the feature space and Y = { ( y i ) } i = 1 n denotes the label space. Let P ( x ) and Q ( y|x ) represent marginal and conditional probability distribution respectively. The goal of TL is to use D src diagnostic knowledge with D tar in the case of X src = X tar , Y src = Y tar , P src ( x src ) ≠ P tar ( x tar ), Q src ( y src |x src ) ≠ Q tar ( y tar |x tar ).
Joint distribution adaptation
The joint distribution adaptation (JDA) method was come up with Long et al. 29 , and its goal is to find a transformation A to make the distance between P src (A T x src ) and P tar (A T x tar ) as close as possible, and at the same time, make the distance between the transformed conditional probability distribution Q src (y src |A T x src ) and Q tar (y tar |A T x tar ) also as close as possible.
The approach is broken down into two stages. First, the maximum mean discrepancy (MMD) would be utilized to judge the disparity between P src (x src ) and P tar (x tar ) . The distance between the sample means of D src sample and D tar sample can be demonstrated that
where tr(·) stands for a matrix's trace, X denotes the input data matrix, M 0 represents the MMD matrix and Eq. ( 8 ) shows the computation equation.
Then Q src (y src |x src ) and Q tar (y tar |x tar ) are adapted, and the distance between the conditional probability distributions can be written as
where D src ( c ) represents the source domain data with category c , D tar ( c ) represents the target domain data with category c , M 0 represents the MMD matrix involving class labels and its calculation formula is shown in Eq. ( 10 ).
Combining Eqs. ( 7 ) and ( 9 ), a general optimization objective is obtained
When Eq. ( 11 ) is the smallest, the JDA distance is the smallest, that is, the disparity between D src sample and D tar sample is the smallest.
Attention mechanism
Attention mechanism is derived from the studies of human vision. When mankind recognizes things, they often focus more on important information and ignore irrelevant information. Different features contribute differently to the final classification results throughout the training phase of a DL model. The attention method allows DL model to concentrate on features that have a significant impacts on classification precision and disregard those that do not matter, improving classification performance. CBAM put forward by woo et al. 30 , is an attention mechanism module that integrates space and channel. The method first assigns various weights to various channels of the input feature through the channel attention module. The weight given by important channels is larger and that given by unimportant channels is smaller. Then, through the spatial attention module, different weights are given to different regions of the input features. The overall calculation process is shown in Eqs. ( 12 ) and ( 13 ).
Among them, C represents the input feature map, C’ represents the feature map processed by the channel attention module, C’’ represent the output feature map, M c and M s denote the channel and spatial attention modules, respectively, and represents the element by element multiplication.
Proposed method
In this paper, a fresh fault diagnosis means for PG is put forward based on dynamic simulation and DTL. This method mainly includes three parts: Building dynamic simulation models of PG, acquiring and analyzing the simulation data and real data, and fault diagnosis of PG based on TL. Figure Figure1 1 shows the general framework of the means.

The framework of fault diagnosis means based on dynamic simulation and DTL.
Building dynamic simulation models of PG
In the light of the sizes of the actual PG related parts, use the 3D modeling software to build a 3D model, and import the 3D model into ADAMS to build a dynamic simulation model.
Acquiring and analyzing the simulation data and real data
Through the dynamic simulation model, the dynamic response of the actual PG under various fault conditions is simulated, and sufficient simulation data are obtained. By analyzing the TDG and FDG of the fault simulation data, the simulation model's validity is confirmed. By comparing the TDG and FDG of different simulation data, the influence law of simulation step size on simulation data is explored; Collect a small amount of real data from the actual PG.
Fault diagnosis of PG based on TL
The proposed DTL network model is comprised of three modules: feature extractor, health classifier, and domain adaptation. The model's framework is shown in Fig. Fig.2. 2 . The feature extractor is applied to extract high-level abstract features of data. Figure Figure3 3 shows the structure of the multi-scale feature extraction layer, which extracts features in parallel using three convolution kernel branches of various scales to obtain features of various scales. By stacking the features of these three scales, a rich multi-scale feature set is formed, and Table Table1 1 displays the feature extractor's network parameters. In the attention mechanism layer, CBAM is applied to enhance important features and suppress irrelevant features; The health status classifier is applied to distinguish health conditions of D src features. The domain adaptation part adopts joint distribution adaptation (JDA) to execute domain adaptation on D src features and D tar features.

The framework of DTL network model.

Multi-scale feature extraction layer.
Model network parameters.
Two optimization objectives must be met during model training:
- Minimize the classification loss L c of the feature extractor on D src data, L c is calculated by the cross-entropy loss function. Following is the calculating formula: L c = - ∑ i = 1 N y true i log y pred i 14 where y true represents the D src data’s true label, y pred represents the D src data’s predicted label.
- Minimize the domain loss L d , which is calculated by JDA.
By combining the classification loss L c and the domain loss L d , the overall optimization target of the model can be obtained:
in which, λ Represents the penalty factor. By minimizing L , the features learned by the feature extractor can have small domain differences and become domain invariant features, and health condition classifier can accurately classify these features. Take the simulation samples as D src and the unlabeled real samples as D tar into the DTL network model, implement fault diagnosis of actual PG, and explore influence laws of simulation data of different parameters on the diagnosis results.
Experiment and analysis
Establishment of the dynamic simulation model of pg.
This paper takes the PG in Drivetrain Diagnostics Simulator (DDS) test bench designed by SpectraQuest company of America as the research target, Fig. 4 is the structural drawing of DDS laboratory bench, which is comprised of variable speed drive motor, torque sensor and encoder, PG, parallel shaft gearbox and magnetic brake. Table Table2 2 demonstrates the fundamental parameters of the PG.

Physical drawing of DDS test bench.
Parameters of the PG.
In the light of the parameters demonstrated in Table Table2, 2 , the three-dimensional model of PG is built and assembled. In order to make the model more clearly reflect the dynamic characteristics, a number of parts that have seldom impact on the simulation results are simplified in the modeling process.
Save the 3D model as x_t format and import it into ADAMS. Considering that the rigid body model ignores the elastic deformation of the parts and cannot accurately transmit the vibration signal, the sun gear is constructed flexible. Import the 3D model of the sun gear into ANSYS, build and export a mode neutral file, replace the rigid body sun gear in ADAMS with this file, and obtain the rigid-flexible coupled model of the PG.
By replacing flexible body sun gears in the rigid-flexible coupled model, the PG simulation models of the sun gear under different health conditions are obtained. Figure 5 shows the physical drawing and flexible body model of the cracked and the missing tooth sun gear.

The physical drawing and flexible body model of the cracked and the missing tooth sun gear.
In line with the actual transmission relationship between the parts, add the constraints shown in Table Table3. 3 . Since the gear pair can only transmit the speed between the gears and cannot reflect the change of the meshing force between the gears, contact forces are added between the sun gear and the planetary gears and between the planetary gears and the ring gear to replace gear pairs.
Constraints.
The PG model designed in this paper takes the input shaft as the input, the planetary carrier as the output, and the ring gear is fixed. Therefore, a 30 Hz rotation frequency is added to the input shaft as the speed drive, and the corresponding speed is 10,800°/s. In order to prevent the speed mutation of the input shaft, resulting in infinite acceleration, the step function is used to gradually increase the speed from 0 to 10,800°/s within 0.01 s. Figure 6 demonstrates the completed rigid-flexible coupling model of the PG.

Rigid-flexible coupling model of the PG.
Acquisition and analysis of simulation data
According to the calculation formula of the transmission ratio of the epicyclic gear train, the calculation formula of the planet carrier rotation frequency f c , planetary gear train meshing frequency f m , and sun gear local fault characteristic frequency f g can be deduced
Here Z s stands for the amount of teeth of the sun gear, Z r stands for the amount of teeth of the ring gear, f s denotes the rotation frequency of the sun gear, and N denotes the amount of planetary gears. Substitute Z s = 28, Z r = 100, f s = 30 Hz, N = 4 into Eqs. ( 14 )–( 16 ) to obtain f c = 6.5625 Hz, f m = 656.25 Hz, f g = 93.75 Hz.
When the sun gear's faulty gear teeth mesh with the planetary gear teeth, the lubricating oil film between the sliding contact surfaces of the gear teeth will be ruptured, resulting in an impact phenomenon. The impact will bring about the amplitude modulation and frequency modulation effect on the meshing vibration, and the vibration signal at the meshing point can be described by the amplitude modulation and frequency modulation process. If the integer multiples of meshing frequency kf m are used as the carrier frequency, the sidebands will appear at kf m + mf g , and the spacing between the sidebands is f g 31 .
In ADAMS, set the simulation time to 0.5 s, select WSTIFF as the integration solver, and I3 as the integration format. The simulation step size will affect the results of the simulation calculation. If the step length is set too big, the calculation accuracy will be low, and if the step length is set too little, the computing time will be long. Simulate on the basis of the three groups of parameters shown in Table Table4 4 to explore the influence law of simulation step size on simulation data.
Simulation parameters.
The models used in the simulation are all rigid-flexible coupled models with missing teeth sun gear. After the end of the simulation, angular acceleration on the planet carrier is exported as simulation data. Figure 7 demonstrates TDG and FDG of various simulation data.

TDG and FDG of different simulation step sizes of rigid-flexible coupling model.
As can be aware of the TDG in Fig. 7 that the smaller the simulation step size is, the more obvious the periodic shock phenomenon appears in the TDG, and the time interval between shocks is the same as the theoretical value 1/ f g = 0.01067 s; As can be aware of the FDG of different simulation step size have obvious peaks at f m = 656.25 Hz and its integer multiples. The smaller the simulation step size is, the more obvious sidebands appear around meshing frequency f m and its integer multiple in the FDG, and the spacing between the sidebands are all f g = 93.75 Hz.
Figure 8 demonstrates the TDG and FDG of the pure rigid body model. The sun gear in the model is missing tooth fault, and the simulation step size is 8.6806 × 10 −6 s. As can be aware of Fig. 8 , the TDG of the rigid body model has no periodic impact, and the FDG has obvious peaks at the meshing frequency and its frequency doubling, but there are no sidebands.

TDG and FDG of the pure rigid body model.
Based on the above comparative analysis, it can be found out that the impact characteristics in the TDG and the fault frequency characteristics in the FDG of the simulation data are consistent with the theory, which certifies the rationality of the dynamic simulation model. Furthermore, contrasted with the pure rigid body model, the rigid-flexible coupling model can better reflect the fault characteristics of the planetary gear train, and the smaller the simulation step size, the clearer the periodic impact in the TDG and the sidebands in the FDG.
Data description
The real data are the vibration signals acquired from PG of the DDS test bench. When collecting the signal, variable speed drive motor’s rotation frequency is 30 Hz, and there are four different currents on the magnetic brake: 0 A, 0.4 A, 0.8 A, and 1.2 A (different loads can be applied to the output shaft by adjusting the magnetic brake's current). The sun gear contains three health conditions: sun gear cracks, sun gear missing teeth, sun gear normal, and sampling frequency is 12.8 kHz.
The input shaft rotation frequency of the simulation model is 30 Hz and there is no load. After the end of the simulation, angular acceleration on the planet carrier is obtained as simulation data.
The simulation data and real data are overlapping sampling to obtain simulation samples and real samples. 2048 points are sampled every 100 points, that is, 2048 data points per sample. Table Table5 5 displays the number of samples.
Data set description.
Analysis of influence law of simulation parameters
There are five different integration solvers in Adams: GSTIFF, WSTIFF, HHT, Newmark, HASTIFF, and three integration formats: I3, SI1, SI2. The characteristics of various solution means are demonstrated in Table Table6. 6 . Take the simulation data of various solution means as D src and the real data set B as D tar for TL. The diagnosis precision is demonstrated in Fig. 9 . As can be aware of Fig. 9 , when the integration solver selects WSTIFF and the integration format selects I3, the diagnosis accuracy is the highest.
Characteristics of the different solution means in ADAMS.

Diagnostic accuracy of simulation data for different solution methods.
Classification results and comparison
The validity of the proposed means is proved by five TL tasks of A → B, A → C, A → D, A → E, and B → E. In the first four TL tasks, the training dataset consists of 6000 labeled simulated samples and 3000 unlabeled real samples, and the leftover 600 real samples are used for testing. In TL task B→E, The training dataset consisted of 3000 labeled B dataset samples and 3000 unlabeled E dataset samples, and the remaining 600 E dataset samples were used for testing. CNN, DeepCoral 32 , DDC 33 , DANN 34 are selected for comparative experiments. In order to compare the accuracy of various means more reasonably, all the above means use the same CNN structure and parameters as the means proposed in this paper.
Figure 10 displays the result from several diagnostic means. In the four transfer learning tasks, the proposed means obtains the maximum accuracy, demonstrating its effectiveness; Additionally, the accuracy of transfer between real data under various working conditions reaches 100%, which is higher than the accuracy of the transfer from simulation data to real data. The reason is that there is a significant discrepancy between simulation data and real data, while the difference between real data under different working conditions is relatively small. This causes transfer between simulation data and real data to be more challenging and to have poorer accuracy. In order to intuitively show the difference between different data, the normal distribution histograms of the simulated tooth missing tooth fault data with load of 0 A, the real tooth missing tooth fault data with load of 0 A and the real tooth missing tooth fault data with load of 1.2 A are drawn, respectively. It can also be seen from Fig. 11 that the disparities between the simulation data and the real data is far greater than the disparities between the real data under different working conditions.

Diagnostic precision of various means on various transfer tasks.

Normal distribution histograms.
Conclusions
To achieve accurate fault diagnosis of PG when marked fault data is insufficient, a fault diagnosis means based on dynamic simulation and DTL is put forward in this paper. The dynamic simulation model of the PG was built by using ADAMS, and the simulation data of various health conditions were obtained. By analyzing the fault simulation data, it is found that the rigid-flexible coupling model can better mirror the fault characteristics of the planetary gear train, and the smaller the simulation step size is, the clearer the periodic impact in TDG and the sidebands in FDG are. By fusing one-dimensional convolutional neural network, attention mechanism, and domain adaptation method, a novel DTL network model is built, and the network model is used to apply diagnostic knowledge from simulation data to real PG’s fault diagnosis. The fault diagnosis experiment was verified by using DDS test bench. By contrasting the diagnosis results of the simulation data with various parameters, it was found that when the integration solver of the simulation data was WSTIFF, the integration format was I3, and the sampling frequency was the same as the real data, the diagnostic accuracy was the highest. The validity of the proposed means is verified by contrasting the diagnostic precision of various means on various transfer tasks.
Acknowledgements
This paper was supported by the following research projects: the Natural Science Foundation of Fujian Province (Grants no. 2022J011223 and no. 2020J01432), the Key Technology Innovation Project of Fujian Province (Grant no. 2022G02030) , Youth and Middle-aged Science and Technology Project of Ningde Normal University (no. 2022ZQ102), Innovation Team of Ningde Normal University (no. 2020T02), and Engineering Research Center of Mindong Aquatic Product Deep-Processing, Fujian Province University. These supports are gratefully acknowledged.
Author contributions
J.-H.Z. and S.-G.X. conceived and designed the study. M.-M.S. and Y.-H.T. contributed to data acquisition and analysis. Z.-C.X. were responsible for the model building. M.-M.S. and Z.-C.X. contributed to writing of original manuscript. J.-H.Z. and S.-G.X. were responsible for revising and reviewing. All authors reviewed the manuscript.
Data availability
Competing interests.
The authors declare no competing interests.
Publisher's note
Springer Nature remains neutral with regard to jurisdictional claims in published maps and institutional affiliations.
Contributor Information
Jian-Hua Zhong, Email: nc.ude.uzf@gnohzHj .
Shun-Gen Xiao, Email: moc.361@220negnuhsoaix .
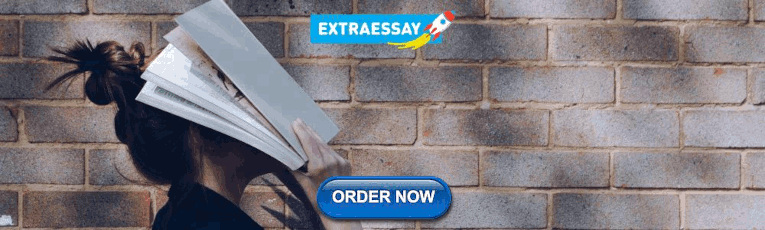
IMAGES
VIDEO
COMMENTS
Abstract and Figures. This paper focusses on the designing and optimizing of a planetary gearbox for an FSAE formula electric car. The conventional arrangement of single or double stage reduction ...
1. Introduction. Epicyclic or planetary gear (PLG) trains are of great significance for diverse drive technologies not only for hybrid vehicles but also for ships or aerospace [1], [2], [3].While the basic concepts of hybrid propulsion are more than 120 years old [4], recent research promises dramatic innovation in energy resources [5] and thus will push this concept anew.
Abstract. The main purpose of this paper is to propose a new graphical method for a planetary gear train (PGT) analysis. This is a fully graphical method that determines the rotational speeds of all transmission components, as well as the torque, power flow, power losses, and efficiency of any PGT.
To achieve reduced costs and energy conservation, this paper proposes non-standard helical spur gear reducer with one gear pair (having reduction ratio 19.25) to be the gear reducer for electric ...
With the increase in the axial misalignment quantities from 0 to 0.05 mm, the load-sharing coefficient of the planetary gear transmission system with rigid ring gear is increased from 1.03 to 1.046 and the load-sharing coefficient of the planetary gear transmission system with flexible ring gear is increased from 1.015 to 1.02.
The common lumped-parameter dynamic model of the helical planetary gear is shown in Fig. 2.For the integrity of the model description, the carrier and ring are also included in Fig. 2.There are three types of coordinate systems: (1) the static coordinate system OXYZ, (2) moving coordinate system oxyz rotating with the carrier, and (3) moving coordinate system o pn x pn y pn z pn (n = 1…
on the planetary gear dynamics (Gu and Velex, 2013). Chen and Shao performed a dynamic simulation of a planetary gear with tooth root crack in a ring gear (Chen and Shao, 2013). In the paper by Zhang et al. (2016), the dynamic modelling and vibration characteristics of a two-stage closed-form planetary gear train is shown (Zhang et al., 2016).
Introduction Planetary gearboxes are widely used in the aerospace, automotive and renewable energy sectors. These gearboxes are the critical component in a system's reliability; hence, monitoring this component is essential. Methods To study the vibration characteristics, a novel dynamic modelling approach using Lagrange's equations is presented in this paper. Newtonian equations of motion ...
Planetary gear drives are combination of three main links: a sun toothed wheel , a carrier that hosts gears and a annular gear. ... This research paper aims the fulfilment of the design approach in a generalized sense keeping in mind the required necessary parameters for planetary gear system. ...
Planetary gear systems have many advantages over other gear systems. Previous studies on its dynamic characteristics mostly used Earth as the reference system, which is inconsistent with the actual working conditions of many planetary gear systems, such as aircraft maneuvering, vehicle movement changes, etc. By analyzing representative research papers, this study summarizes the lumped ...
In this paper, we have reviewed the research papers mainly dealing with the designing and analysis of a planetary gearbox. Planetary Gear System is an assembly of gears where one or more planet gears revolve around a gear placed at centre named Sun gear and a ring gear encircles the whole system. It is capable of achieving high reduction ratios ...
Because planetary gear sets contain many components and have complex transmission structures, their dynamic models are difficult to be established. Besides, the planet gears rotate around not only their own centers, but also the center of the sun gear, and the time-varying distances from gear meshing points to the fixed transducers add the complexity of vibration response. Most of the dynamic ...
In this paper, an analysis model of planetary gearbox housing is constructed based on finite element method/boundary element method (FEM/BEM). Its vibration and acoustic radiation characteristics are investigated. Firstly, the finite element model is established using ABAQUS. The main factors affecting its dynamic characteristics are observed through modal analysis.
A flexible pin mechanism considered in the paper consists of a planet gear, a sleeve (integrated with a hydraulic bearing) and a pin. the section view of a stage of planetary gear set with the flexible pin mechanism, designed by ITRI. There are totally eight planets in the planetary gear set.
The architecture of the gearbox allowed a high gear ratio within a small form factor, and a bearingless construction with a very low number of moving parts. Layout of the Electromechanical ...
Nandeppagoudar et al. [15] redesigned a three-stage planetary gearbox by converting it into a two-stage, on the basis of space and load restrictions for reducing the weight of the machine tools. The efficacy of the modified planetary gearbox was judged by comparing the analytical and numerical results using Power Gear software.
This paper introduces a new type of high reduction bearingless gearbox which is based on a gearbox with double-row planetary pinion, and can achieve high reduction ratios in a single composite stage. We discuss the practical issues which limit the applicability of the existing double row gearbox, and motivate our innovations.
In this paper, the gear-bearing-box rigid-flexible coupling dynamics model of planetary gearbox is established, and the dynamic response of the transmission system under normal and fault conditions in stationary operation is obtained by solving the model. ... the subsequent research on the extraction method of fault characteristics under ...
This paper studies the kinematic behavior of this type of coupling by extensive literature research about hybrid and electric vehicles, transmission gearbox, power-split control systems, and ...
To address the issue of not having enough labeled fault data for planetary gearboxes in actual production, this research develops a simulation data-driven deep transfer learning fault diagnosis method that applies fault diagnosis knowledge from a dynamic simulation model to an actual planetary gearbox. ... This paper was supported by the ...
Planetary Gearbox . Opposite Case . Schmidt's Method . Low Pass. A new method to process the vibration signal acquired by an accelerometer placed in a planetary gearbox housing is proposed, which is useful to detect potential faults. The method is based on the phenomenological model and consists of the projection of the healthy vibration ...
Abstract and Figures. Goal of this research was to develop and manufacture planetary gearbox prototype using rapid prototyping technology (additive manufacturing). Developed prototype was used to ...
The fault diagnosis technology is used to monitor the working condition of the gearbox. C. Li et al. 18 employed the deep random forest fusion technique to improve the fault diagnosis performance by installing the acoustic emission and accelerometer sensor on the gearbox. Multi-fault detection technology was adopted by W. Teng et al. 19 to detect the gearbox in the wind turbine by vibration ...