
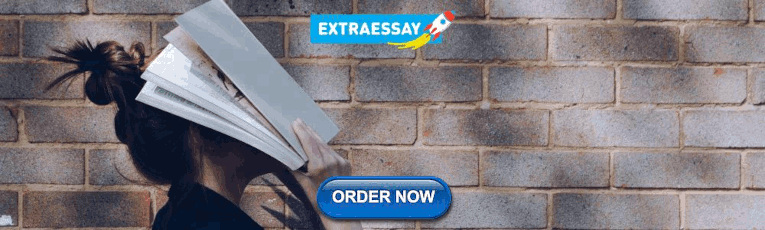
How to Create a Data Analysis Plan: A Detailed Guide
by Barche Blaise | Aug 12, 2020 | Writing

If a good research question equates to a story then, a roadmap will be very vita l for good storytelling. We advise every student/researcher to personally write his/her data analysis plan before seeking any advice. In this blog article, we will explore how to create a data analysis plan: the content and structure.
This data analysis plan serves as a roadmap to how data collected will be organised and analysed. It includes the following aspects:
- Clearly states the research objectives and hypothesis
- Identifies the dataset to be used
- Inclusion and exclusion criteria
- Clearly states the research variables
- States statistical test hypotheses and the software for statistical analysis
- Creating shell tables
1. Stating research question(s), objectives and hypotheses:
All research objectives or goals must be clearly stated. They must be Specific, Measurable, Attainable, Realistic and Time-bound (SMART). Hypotheses are theories obtained from personal experience or previous literature and they lay a foundation for the statistical methods that will be applied to extrapolate results to the entire population.
2. The dataset:
The dataset that will be used for statistical analysis must be described and important aspects of the dataset outlined. These include; owner of the dataset, how to get access to the dataset, how the dataset was checked for quality control and in what program is the dataset stored (Excel, Epi Info, SQL, Microsoft access etc.).
3. The inclusion and exclusion criteria :
They guide the aspects of the dataset that will be used for data analysis. These criteria will also guide the choice of variables included in the main analysis.
4. Variables:
Every variable collected in the study should be clearly stated. They should be presented based on the level of measurement (ordinal/nominal or ratio/interval levels), or the role the variable plays in the study (independent/predictors or dependent/outcome variables). The variable types should also be outlined. The variable type in conjunction with the research hypothesis forms the basis for selecting the appropriate statistical tests for inferential statistics. A good data analysis plan should summarize the variables as demonstrated in Figure 1 below.

5. Statistical software
There are tons of software packages for data analysis, some common examples are SPSS, Epi Info, SAS, STATA, Microsoft Excel. Include the version number, year of release and author/manufacturer. Beginners have the tendency to try different software and finally not master any. It is rather good to select one and master it because almost all statistical software have the same performance for basic and the majority of advance analysis needed for a student thesis. This is what we recommend to all our students at CRENC before they begin writing their results section .
6. Selecting the appropriate statistical method to test hypotheses
Depending on the research question, hypothesis and type of variable, several statistical methods can be used to answer the research question appropriately. This aspect of the data analysis plan outlines clearly why each statistical method will be used to test hypotheses. The level of statistical significance (p-value) which is often but not always <0.05 should also be written. Presented in figures 2a and 2b are decision trees for some common statistical tests based on the variable type and research question
A good analysis plan should clearly describe how missing data will be analysed.

7. Creating shell tables
Data analysis involves three levels of analysis; univariable, bivariable and multivariable analysis with increasing order of complexity. Shell tables should be created in anticipation for the results that will be obtained from these different levels of analysis. Read our blog article on how to present tables and figures for more details. Suppose you carry out a study to investigate the prevalence and associated factors of a certain disease “X” in a population, then the shell tables can be represented as in Tables 1, Table 2 and Table 3 below.
Table 1: Example of a shell table from univariate analysis

Table 2: Example of a shell table from bivariate analysis

Table 3: Example of a shell table from multivariate analysis

aOR = adjusted odds ratio
Now that you have learned how to create a data analysis plan, these are the takeaway points. It should clearly state the:
- Research question, objectives, and hypotheses
- Dataset to be used
- Variable types and their role
- Statistical software and statistical methods
- Shell tables for univariate, bivariate and multivariate analysis
Further readings
Creating a Data Analysis Plan: What to Consider When Choosing Statistics for a Study https://www.ncbi.nlm.nih.gov/pmc/articles/PMC4552232/pdf/cjhp-68-311.pdf
Creating an Analysis Plan: https://www.cdc.gov/globalhealth/healthprotection/fetp/training_modules/9/creating-analysis-plan_pw_final_09242013.pdf
Data Analysis Plan: https://www.statisticssolutions.com/dissertation-consulting-services/data-analysis-plan-2/
Photo created by freepik – www.freepik.com

Dr Barche is a physician and holds a Masters in Public Health. He is a senior fellow at CRENC with interests in Data Science and Data Analysis.
Post Navigation
16 comments.
Thanks. Quite informative.
Educative write-up. Thanks.
Easy to understand. Thanks Dr
Very explicit Dr. Thanks
I will always remember how you help me conceptualize and understand data science in a simple way. I can only hope that someday I’ll be in a position to repay you, my dear friend.
Plan d’analyse

This is interesting, Thanks
Very understandable and informative. Thank you..
love the figures.
Nice, and informative
This is so much educative and good for beginners, I would love to recommend that you create and share a video because some people are able to grasp when there is an instructor. Lots of love
Thank you Doctor very helpful.
Educative and clearly written. Thanks
Well said doctor,thank you.But when do you present in tables ,bars,pie chart etc?
Very informative guide!
Submit a Comment Cancel Reply
Your email address will not be published. Required fields are marked *
Notify me of follow-up comments by email.
Notify me of new posts by email.
Submit Comment
Receive updates on new courses and blog posts
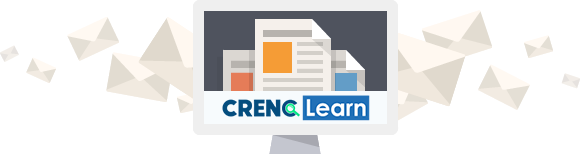
Never Miss a Thing!
Subscribe to our mailing list to receive the latest news and updates on our webinars, articles and courses.
You have Successfully Subscribed!

An official website of the United States government
The .gov means it’s official. Federal government websites often end in .gov or .mil. Before sharing sensitive information, make sure you’re on a federal government site.
The site is secure. The https:// ensures that you are connecting to the official website and that any information you provide is encrypted and transmitted securely.
- Publications
- Account settings
Preview improvements coming to the PMC website in October 2024. Learn More or Try it out now .
- Advanced Search
- Journal List
- Can J Hosp Pharm
- v.68(4); Jul-Aug 2015

Creating a Data Analysis Plan: What to Consider When Choosing Statistics for a Study
There are three kinds of lies: lies, damned lies, and statistics. – Mark Twain 1
INTRODUCTION
Statistics represent an essential part of a study because, regardless of the study design, investigators need to summarize the collected information for interpretation and presentation to others. It is therefore important for us to heed Mr Twain’s concern when creating the data analysis plan. In fact, even before data collection begins, we need to have a clear analysis plan that will guide us from the initial stages of summarizing and describing the data through to testing our hypotheses.
The purpose of this article is to help you create a data analysis plan for a quantitative study. For those interested in conducting qualitative research, previous articles in this Research Primer series have provided information on the design and analysis of such studies. 2 , 3 Information in the current article is divided into 3 main sections: an overview of terms and concepts used in data analysis, a review of common methods used to summarize study data, and a process to help identify relevant statistical tests. My intention here is to introduce the main elements of data analysis and provide a place for you to start when planning this part of your study. Biostatistical experts, textbooks, statistical software packages, and other resources can certainly add more breadth and depth to this topic when you need additional information and advice.
TERMS AND CONCEPTS USED IN DATA ANALYSIS
When analyzing information from a quantitative study, we are often dealing with numbers; therefore, it is important to begin with an understanding of the source of the numbers. Let us start with the term variable , which defines a specific item of information collected in a study. Examples of variables include age, sex or gender, ethnicity, exercise frequency, weight, treatment group, and blood glucose. Each variable will have a group of categories, which are referred to as values , to help describe the characteristic of an individual study participant. For example, the variable “sex” would have values of “male” and “female”.
Although variables can be defined or grouped in various ways, I will focus on 2 methods at this introductory stage. First, variables can be defined according to the level of measurement. The categories in a nominal variable are names, for example, male and female for the variable “sex”; white, Aboriginal, black, Latin American, South Asian, and East Asian for the variable “ethnicity”; and intervention and control for the variable “treatment group”. Nominal variables with only 2 categories are also referred to as dichotomous variables because the study group can be divided into 2 subgroups based on information in the variable. For example, a study sample can be split into 2 groups (patients receiving the intervention and controls) using the dichotomous variable “treatment group”. An ordinal variable implies that the categories can be placed in a meaningful order, as would be the case for exercise frequency (never, sometimes, often, or always). Nominal-level and ordinal-level variables are also referred to as categorical variables, because each category in the variable can be completely separated from the others. The categories for an interval variable can be placed in a meaningful order, with the interval between consecutive categories also having meaning. Age, weight, and blood glucose can be considered as interval variables, but also as ratio variables, because the ratio between values has meaning (e.g., a 15-year-old is half the age of a 30-year-old). Interval-level and ratio-level variables are also referred to as continuous variables because of the underlying continuity among categories.
As we progress through the levels of measurement from nominal to ratio variables, we gather more information about the study participant. The amount of information that a variable provides will become important in the analysis stage, because we lose information when variables are reduced or aggregated—a common practice that is not recommended. 4 For example, if age is reduced from a ratio-level variable (measured in years) to an ordinal variable (categories of < 65 and ≥ 65 years) we lose the ability to make comparisons across the entire age range and introduce error into the data analysis. 4
A second method of defining variables is to consider them as either dependent or independent. As the terms imply, the value of a dependent variable depends on the value of other variables, whereas the value of an independent variable does not rely on other variables. In addition, an investigator can influence the value of an independent variable, such as treatment-group assignment. Independent variables are also referred to as predictors because we can use information from these variables to predict the value of a dependent variable. Building on the group of variables listed in the first paragraph of this section, blood glucose could be considered a dependent variable, because its value may depend on values of the independent variables age, sex, ethnicity, exercise frequency, weight, and treatment group.
Statistics are mathematical formulae that are used to organize and interpret the information that is collected through variables. There are 2 general categories of statistics, descriptive and inferential. Descriptive statistics are used to describe the collected information, such as the range of values, their average, and the most common category. Knowledge gained from descriptive statistics helps investigators learn more about the study sample. Inferential statistics are used to make comparisons and draw conclusions from the study data. Knowledge gained from inferential statistics allows investigators to make inferences and generalize beyond their study sample to other groups.
Before we move on to specific descriptive and inferential statistics, there are 2 more definitions to review. Parametric statistics are generally used when values in an interval-level or ratio-level variable are normally distributed (i.e., the entire group of values has a bell-shaped curve when plotted by frequency). These statistics are used because we can define parameters of the data, such as the centre and width of the normally distributed curve. In contrast, interval-level and ratio-level variables with values that are not normally distributed, as well as nominal-level and ordinal-level variables, are generally analyzed using nonparametric statistics.
METHODS FOR SUMMARIZING STUDY DATA: DESCRIPTIVE STATISTICS
The first step in a data analysis plan is to describe the data collected in the study. This can be done using figures to give a visual presentation of the data and statistics to generate numeric descriptions of the data.
Selection of an appropriate figure to represent a particular set of data depends on the measurement level of the variable. Data for nominal-level and ordinal-level variables may be interpreted using a pie graph or bar graph . Both options allow us to examine the relative number of participants within each category (by reporting the percentages within each category), whereas a bar graph can also be used to examine absolute numbers. For example, we could create a pie graph to illustrate the proportions of men and women in a study sample and a bar graph to illustrate the number of people who report exercising at each level of frequency (never, sometimes, often, or always).
Interval-level and ratio-level variables may also be interpreted using a pie graph or bar graph; however, these types of variables often have too many categories for such graphs to provide meaningful information. Instead, these variables may be better interpreted using a histogram . Unlike a bar graph, which displays the frequency for each distinct category, a histogram displays the frequency within a range of continuous categories. Information from this type of figure allows us to determine whether the data are normally distributed. In addition to pie graphs, bar graphs, and histograms, many other types of figures are available for the visual representation of data. Interested readers can find additional types of figures in the books recommended in the “Further Readings” section.
Figures are also useful for visualizing comparisons between variables or between subgroups within a variable (for example, the distribution of blood glucose according to sex). Box plots are useful for summarizing information for a variable that does not follow a normal distribution. The lower and upper limits of the box identify the interquartile range (or 25th and 75th percentiles), while the midline indicates the median value (or 50th percentile). Scatter plots provide information on how the categories for one continuous variable relate to categories in a second variable; they are often helpful in the analysis of correlations.
In addition to using figures to present a visual description of the data, investigators can use statistics to provide a numeric description. Regardless of the measurement level, we can find the mode by identifying the most frequent category within a variable. When summarizing nominal-level and ordinal-level variables, the simplest method is to report the proportion of participants within each category.
The choice of the most appropriate descriptive statistic for interval-level and ratio-level variables will depend on how the values are distributed. If the values are normally distributed, we can summarize the information using the parametric statistics of mean and standard deviation. The mean is the arithmetic average of all values within the variable, and the standard deviation tells us how widely the values are dispersed around the mean. When values of interval-level and ratio-level variables are not normally distributed, or we are summarizing information from an ordinal-level variable, it may be more appropriate to use the nonparametric statistics of median and range. The first step in identifying these descriptive statistics is to arrange study participants according to the variable categories from lowest value to highest value. The range is used to report the lowest and highest values. The median or 50th percentile is located by dividing the number of participants into 2 groups, such that half (50%) of the participants have values above the median and the other half (50%) have values below the median. Similarly, the 25th percentile is the value with 25% of the participants having values below and 75% of the participants having values above, and the 75th percentile is the value with 75% of participants having values below and 25% of participants having values above. Together, the 25th and 75th percentiles define the interquartile range .
PROCESS TO IDENTIFY RELEVANT STATISTICAL TESTS: INFERENTIAL STATISTICS
One caveat about the information provided in this section: selecting the most appropriate inferential statistic for a specific study should be a combination of following these suggestions, seeking advice from experts, and discussing with your co-investigators. My intention here is to give you a place to start a conversation with your colleagues about the options available as you develop your data analysis plan.
There are 3 key questions to consider when selecting an appropriate inferential statistic for a study: What is the research question? What is the study design? and What is the level of measurement? It is important for investigators to carefully consider these questions when developing the study protocol and creating the analysis plan. The figures that accompany these questions show decision trees that will help you to narrow down the list of inferential statistics that would be relevant to a particular study. Appendix 1 provides brief definitions of the inferential statistics named in these figures. Additional information, such as the formulae for various inferential statistics, can be obtained from textbooks, statistical software packages, and biostatisticians.
What Is the Research Question?
The first step in identifying relevant inferential statistics for a study is to consider the type of research question being asked. You can find more details about the different types of research questions in a previous article in this Research Primer series that covered questions and hypotheses. 5 A relational question seeks information about the relationship among variables; in this situation, investigators will be interested in determining whether there is an association ( Figure 1 ). A causal question seeks information about the effect of an intervention on an outcome; in this situation, the investigator will be interested in determining whether there is a difference ( Figure 2 ).

Decision tree to identify inferential statistics for an association.

Decision tree to identify inferential statistics for measuring a difference.
What Is the Study Design?
When considering a question of association, investigators will be interested in measuring the relationship between variables ( Figure 1 ). A study designed to determine whether there is consensus among different raters will be measuring agreement. For example, an investigator may be interested in determining whether 2 raters, using the same assessment tool, arrive at the same score. Correlation analyses examine the strength of a relationship or connection between 2 variables, like age and blood glucose. Regression analyses also examine the strength of a relationship or connection; however, in this type of analysis, one variable is considered an outcome (or dependent variable) and the other variable is considered a predictor (or independent variable). Regression analyses often consider the influence of multiple predictors on an outcome at the same time. For example, an investigator may be interested in examining the association between a treatment and blood glucose, while also considering other factors, like age, sex, ethnicity, exercise frequency, and weight.
When considering a question of difference, investigators must first determine how many groups they will be comparing. In some cases, investigators may be interested in comparing the characteristic of one group with that of an external reference group. For example, is the mean age of study participants similar to the mean age of all people in the target group? If more than one group is involved, then investigators must also determine whether there is an underlying connection between the sets of values (or samples ) to be compared. Samples are considered independent or unpaired when the information is taken from different groups. For example, we could use an unpaired t test to compare the mean age between 2 independent samples, such as the intervention and control groups in a study. Samples are considered related or paired if the information is taken from the same group of people, for example, measurement of blood glucose at the beginning and end of a study. Because blood glucose is measured in the same people at both time points, we could use a paired t test to determine whether there has been a significant change in blood glucose.
What Is the Level of Measurement?
As described in the first section of this article, variables can be grouped according to the level of measurement (nominal, ordinal, or interval). In most cases, the independent variable in an inferential statistic will be nominal; therefore, investigators need to know the level of measurement for the dependent variable before they can select the relevant inferential statistic. Two exceptions to this consideration are correlation analyses and regression analyses ( Figure 1 ). Because a correlation analysis measures the strength of association between 2 variables, we need to consider the level of measurement for both variables. Regression analyses can consider multiple independent variables, often with a variety of measurement levels. However, for these analyses, investigators still need to consider the level of measurement for the dependent variable.
Selection of inferential statistics to test interval-level variables must include consideration of how the data are distributed. An underlying assumption for parametric tests is that the data approximate a normal distribution. When the data are not normally distributed, information derived from a parametric test may be wrong. 6 When the assumption of normality is violated (for example, when the data are skewed), then investigators should use a nonparametric test. If the data are normally distributed, then investigators can use a parametric test.
ADDITIONAL CONSIDERATIONS
What is the level of significance.
An inferential statistic is used to calculate a p value, the probability of obtaining the observed data by chance. Investigators can then compare this p value against a prespecified level of significance, which is often chosen to be 0.05. This level of significance represents a 1 in 20 chance that the observation is wrong, which is considered an acceptable level of error.
What Are the Most Commonly Used Statistics?
In 1983, Emerson and Colditz 7 reported the first review of statistics used in original research articles published in the New England Journal of Medicine . This review of statistics used in the journal was updated in 1989 and 2005, 8 and this type of analysis has been replicated in many other journals. 9 – 13 Collectively, these reviews have identified 2 important observations. First, the overall sophistication of statistical methodology used and reported in studies has grown over time, with survival analyses and multivariable regression analyses becoming much more common. The second observation is that, despite this trend, 1 in 4 articles describe no statistical methods or report only simple descriptive statistics. When inferential statistics are used, the most common are t tests, contingency table tests (for example, χ 2 test and Fisher exact test), and simple correlation and regression analyses. This information is important for educators, investigators, reviewers, and readers because it suggests that a good foundational knowledge of descriptive statistics and common inferential statistics will enable us to correctly evaluate the majority of research articles. 11 – 13 However, to fully take advantage of all research published in high-impact journals, we need to become acquainted with some of the more complex methods, such as multivariable regression analyses. 8 , 13
What Are Some Additional Resources?
As an investigator and Associate Editor with CJHP , I have often relied on the advice of colleagues to help create my own analysis plans and review the plans of others. Biostatisticians have a wealth of knowledge in the field of statistical analysis and can provide advice on the correct selection, application, and interpretation of these methods. Colleagues who have “been there and done that” with their own data analysis plans are also valuable sources of information. Identify these individuals and consult with them early and often as you develop your analysis plan.
Another important resource to consider when creating your analysis plan is textbooks. Numerous statistical textbooks are available, differing in levels of complexity and scope. The titles listed in the “Further Reading” section are just a few suggestions. I encourage interested readers to look through these and other books to find resources that best fit their needs. However, one crucial book that I highly recommend to anyone wanting to be an investigator or peer reviewer is Lang and Secic’s How to Report Statistics in Medicine (see “Further Reading”). As the title implies, this book covers a wide range of statistics used in medical research and provides numerous examples of how to correctly report the results.
CONCLUSIONS
When it comes to creating an analysis plan for your project, I recommend following the sage advice of Douglas Adams in The Hitchhiker’s Guide to the Galaxy : Don’t panic! 14 Begin with simple methods to summarize and visualize your data, then use the key questions and decision trees provided in this article to identify relevant statistical tests. Information in this article will give you and your co-investigators a place to start discussing the elements necessary for developing an analysis plan. But do not stop there! Use advice from biostatisticians and more experienced colleagues, as well as information in textbooks, to help create your analysis plan and choose the most appropriate statistics for your study. Making careful, informed decisions about the statistics to use in your study should reduce the risk of confirming Mr Twain’s concern.
Appendix 1. Glossary of statistical terms * (part 1 of 2)
- 1-way ANOVA: Uses 1 variable to define the groups for comparing means. This is similar to the Student t test when comparing the means of 2 groups.
- Kruskall–Wallis 1-way ANOVA: Nonparametric alternative for the 1-way ANOVA. Used to determine the difference in medians between 3 or more groups.
- n -way ANOVA: Uses 2 or more variables to define groups when comparing means. Also called a “between-subjects factorial ANOVA”.
- Repeated-measures ANOVA: A method for analyzing whether the means of 3 or more measures from the same group of participants are different.
- Freidman ANOVA: Nonparametric alternative for the repeated-measures ANOVA. It is often used to compare rankings and preferences that are measured 3 or more times.
- Fisher exact: Variation of chi-square that accounts for cell counts < 5.
- McNemar: Variation of chi-square that tests statistical significance of changes in 2 paired measurements of dichotomous variables.
- Cochran Q: An extension of the McNemar test that provides a method for testing for differences between 3 or more matched sets of frequencies or proportions. Often used as a measure of heterogeneity in meta-analyses.
- 1-sample: Used to determine whether the mean of a sample is significantly different from a known or hypothesized value.
- Independent-samples t test (also referred to as the Student t test): Used when the independent variable is a nominal-level variable that identifies 2 groups and the dependent variable is an interval-level variable.
- Paired: Used to compare 2 pairs of scores between 2 groups (e.g., baseline and follow-up blood pressure in the intervention and control groups).
Lang TA, Secic M. How to report statistics in medicine: annotated guidelines for authors, editors, and reviewers. 2nd ed. Philadelphia (PA): American College of Physicians; 2006.
Norman GR, Streiner DL. PDQ statistics. 3rd ed. Hamilton (ON): B.C. Decker; 2003.
Plichta SB, Kelvin E. Munro’s statistical methods for health care research . 6th ed. Philadelphia (PA): Wolters Kluwer Health/ Lippincott, Williams & Wilkins; 2013.
This article is the 12th in the CJHP Research Primer Series, an initiative of the CJHP Editorial Board and the CSHP Research Committee. The planned 2-year series is intended to appeal to relatively inexperienced researchers, with the goal of building research capacity among practising pharmacists. The articles, presenting simple but rigorous guidance to encourage and support novice researchers, are being solicited from authors with appropriate expertise.
Previous articles in this series:
- Bond CM. The research jigsaw: how to get started. Can J Hosp Pharm . 2014;67(1):28–30.
- Tully MP. Research: articulating questions, generating hypotheses, and choosing study designs. Can J Hosp Pharm . 2014;67(1):31–4.
- Loewen P. Ethical issues in pharmacy practice research: an introductory guide. Can J Hosp Pharm. 2014;67(2):133–7.
- Tsuyuki RT. Designing pharmacy practice research trials. Can J Hosp Pharm . 2014;67(3):226–9.
- Bresee LC. An introduction to developing surveys for pharmacy practice research. Can J Hosp Pharm . 2014;67(4):286–91.
- Gamble JM. An introduction to the fundamentals of cohort and case–control studies. Can J Hosp Pharm . 2014;67(5):366–72.
- Austin Z, Sutton J. Qualitative research: getting started. C an J Hosp Pharm . 2014;67(6):436–40.
- Houle S. An introduction to the fundamentals of randomized controlled trials in pharmacy research. Can J Hosp Pharm . 2014; 68(1):28–32.
- Charrois TL. Systematic reviews: What do you need to know to get started? Can J Hosp Pharm . 2014;68(2):144–8.
- Sutton J, Austin Z. Qualitative research: data collection, analysis, and management. Can J Hosp Pharm . 2014;68(3):226–31.
- Cadarette SM, Wong L. An introduction to health care administrative data. Can J Hosp Pharm. 2014;68(3):232–7.
Competing interests: None declared.
Further Reading
- Devor J, Peck R. Statistics: the exploration and analysis of data. 7th ed. Boston (MA): Brooks/Cole Cengage Learning; 2012. [ Google Scholar ]
- Lang TA, Secic M. How to report statistics in medicine: annotated guidelines for authors, editors, and reviewers. 2nd ed. Philadelphia (PA): American College of Physicians; 2006. [ Google Scholar ]
- Mendenhall W, Beaver RJ, Beaver BM. Introduction to probability and statistics. 13th ed. Belmont (CA): Brooks/Cole Cengage Learning; 2009. [ Google Scholar ]
- Norman GR, Streiner DL. PDQ statistics. 3rd ed. Hamilton (ON): B.C. Decker; 2003. [ Google Scholar ]
- Plichta SB, Kelvin E. Munro’s statistical methods for health care research. 6th ed. Philadelphia (PA): Wolters Kluwer Health/Lippincott, Williams & Wilkins; 2013. [ Google Scholar ]
- Skip to main content
- Skip to primary sidebar
- Skip to footer
- QuestionPro

- Solutions Industries Gaming Automotive Sports and events Education Government Travel & Hospitality Financial Services Healthcare Cannabis Technology Use Case NPS+ Communities Audience Contactless surveys Mobile LivePolls Member Experience GDPR Positive People Science 360 Feedback Surveys
- Resources Blog eBooks Survey Templates Case Studies Training Help center

Home Market Research
Data Analysis in Research: Types & Methods
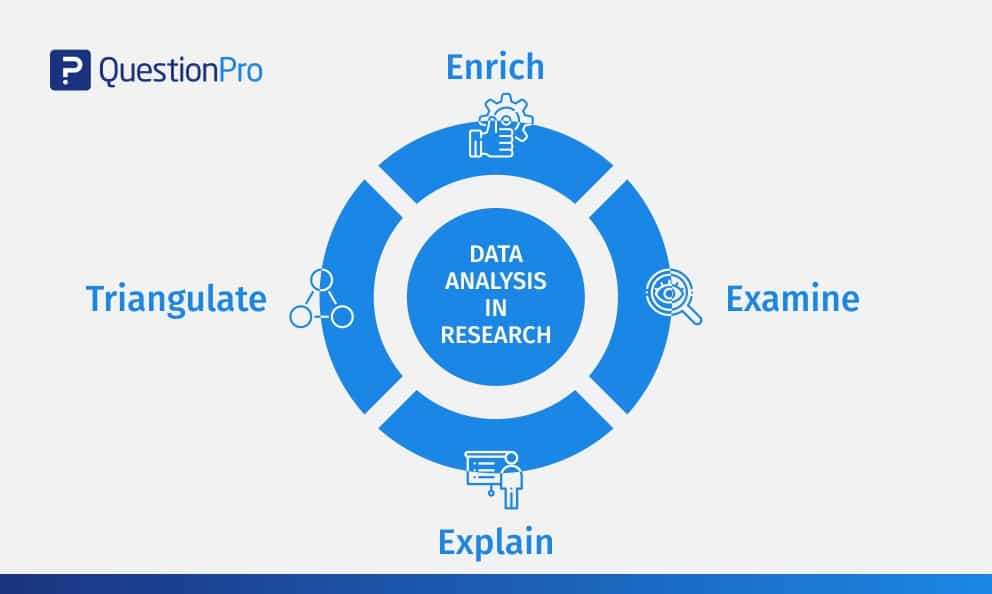
Content Index
Why analyze data in research?
Types of data in research, finding patterns in the qualitative data, methods used for data analysis in qualitative research, preparing data for analysis, methods used for data analysis in quantitative research, considerations in research data analysis, what is data analysis in research.
Definition of research in data analysis: According to LeCompte and Schensul, research data analysis is a process used by researchers to reduce data to a story and interpret it to derive insights. The data analysis process helps reduce a large chunk of data into smaller fragments, which makes sense.
Three essential things occur during the data analysis process — the first is data organization . Summarization and categorization together contribute to becoming the second known method used for data reduction. It helps find patterns and themes in the data for easy identification and linking. The third and last way is data analysis – researchers do it in both top-down and bottom-up fashion.
LEARN ABOUT: Research Process Steps
On the other hand, Marshall and Rossman describe data analysis as a messy, ambiguous, and time-consuming but creative and fascinating process through which a mass of collected data is brought to order, structure and meaning.
We can say that “the data analysis and data interpretation is a process representing the application of deductive and inductive logic to the research and data analysis.”
Researchers rely heavily on data as they have a story to tell or research problems to solve. It starts with a question, and data is nothing but an answer to that question. But, what if there is no question to ask? Well! It is possible to explore data even without a problem – we call it ‘Data Mining’, which often reveals some interesting patterns within the data that are worth exploring.
Irrelevant to the type of data researchers explore, their mission and audiences’ vision guide them to find the patterns to shape the story they want to tell. One of the essential things expected from researchers while analyzing data is to stay open and remain unbiased toward unexpected patterns, expressions, and results. Remember, sometimes, data analysis tells the most unforeseen yet exciting stories that were not expected when initiating data analysis. Therefore, rely on the data you have at hand and enjoy the journey of exploratory research.
Create a Free Account
Every kind of data has a rare quality of describing things after assigning a specific value to it. For analysis, you need to organize these values, processed and presented in a given context, to make it useful. Data can be in different forms; here are the primary data types.
- Qualitative data: When the data presented has words and descriptions, then we call it qualitative data . Although you can observe this data, it is subjective and harder to analyze data in research, especially for comparison. Example: Quality data represents everything describing taste, experience, texture, or an opinion that is considered quality data. This type of data is usually collected through focus groups, personal qualitative interviews , qualitative observation or using open-ended questions in surveys.
- Quantitative data: Any data expressed in numbers of numerical figures are called quantitative data . This type of data can be distinguished into categories, grouped, measured, calculated, or ranked. Example: questions such as age, rank, cost, length, weight, scores, etc. everything comes under this type of data. You can present such data in graphical format, charts, or apply statistical analysis methods to this data. The (Outcomes Measurement Systems) OMS questionnaires in surveys are a significant source of collecting numeric data.
- Categorical data: It is data presented in groups. However, an item included in the categorical data cannot belong to more than one group. Example: A person responding to a survey by telling his living style, marital status, smoking habit, or drinking habit comes under the categorical data. A chi-square test is a standard method used to analyze this data.
Learn More : Examples of Qualitative Data in Education
Data analysis in qualitative research
Data analysis and qualitative data research work a little differently from the numerical data as the quality data is made up of words, descriptions, images, objects, and sometimes symbols. Getting insight from such complicated information is a complicated process. Hence it is typically used for exploratory research and data analysis .
Although there are several ways to find patterns in the textual information, a word-based method is the most relied and widely used global technique for research and data analysis. Notably, the data analysis process in qualitative research is manual. Here the researchers usually read the available data and find repetitive or commonly used words.
For example, while studying data collected from African countries to understand the most pressing issues people face, researchers might find “food” and “hunger” are the most commonly used words and will highlight them for further analysis.
LEARN ABOUT: Level of Analysis
The keyword context is another widely used word-based technique. In this method, the researcher tries to understand the concept by analyzing the context in which the participants use a particular keyword.
For example , researchers conducting research and data analysis for studying the concept of ‘diabetes’ amongst respondents might analyze the context of when and how the respondent has used or referred to the word ‘diabetes.’
The scrutiny-based technique is also one of the highly recommended text analysis methods used to identify a quality data pattern. Compare and contrast is the widely used method under this technique to differentiate how a specific text is similar or different from each other.
For example: To find out the “importance of resident doctor in a company,” the collected data is divided into people who think it is necessary to hire a resident doctor and those who think it is unnecessary. Compare and contrast is the best method that can be used to analyze the polls having single-answer questions types .
Metaphors can be used to reduce the data pile and find patterns in it so that it becomes easier to connect data with theory.
Variable Partitioning is another technique used to split variables so that researchers can find more coherent descriptions and explanations from the enormous data.
LEARN ABOUT: Qualitative Research Questions and Questionnaires
There are several techniques to analyze the data in qualitative research, but here are some commonly used methods,
- Content Analysis: It is widely accepted and the most frequently employed technique for data analysis in research methodology. It can be used to analyze the documented information from text, images, and sometimes from the physical items. It depends on the research questions to predict when and where to use this method.
- Narrative Analysis: This method is used to analyze content gathered from various sources such as personal interviews, field observation, and surveys . The majority of times, stories, or opinions shared by people are focused on finding answers to the research questions.
- Discourse Analysis: Similar to narrative analysis, discourse analysis is used to analyze the interactions with people. Nevertheless, this particular method considers the social context under which or within which the communication between the researcher and respondent takes place. In addition to that, discourse analysis also focuses on the lifestyle and day-to-day environment while deriving any conclusion.
- Grounded Theory: When you want to explain why a particular phenomenon happened, then using grounded theory for analyzing quality data is the best resort. Grounded theory is applied to study data about the host of similar cases occurring in different settings. When researchers are using this method, they might alter explanations or produce new ones until they arrive at some conclusion.
LEARN ABOUT: 12 Best Tools for Researchers
Data analysis in quantitative research
The first stage in research and data analysis is to make it for the analysis so that the nominal data can be converted into something meaningful. Data preparation consists of the below phases.
Phase I: Data Validation
Data validation is done to understand if the collected data sample is per the pre-set standards, or it is a biased data sample again divided into four different stages
- Fraud: To ensure an actual human being records each response to the survey or the questionnaire
- Screening: To make sure each participant or respondent is selected or chosen in compliance with the research criteria
- Procedure: To ensure ethical standards were maintained while collecting the data sample
- Completeness: To ensure that the respondent has answered all the questions in an online survey. Else, the interviewer had asked all the questions devised in the questionnaire.
Phase II: Data Editing
More often, an extensive research data sample comes loaded with errors. Respondents sometimes fill in some fields incorrectly or sometimes skip them accidentally. Data editing is a process wherein the researchers have to confirm that the provided data is free of such errors. They need to conduct necessary checks and outlier checks to edit the raw edit and make it ready for analysis.
Phase III: Data Coding
Out of all three, this is the most critical phase of data preparation associated with grouping and assigning values to the survey responses . If a survey is completed with a 1000 sample size, the researcher will create an age bracket to distinguish the respondents based on their age. Thus, it becomes easier to analyze small data buckets rather than deal with the massive data pile.
LEARN ABOUT: Steps in Qualitative Research
After the data is prepared for analysis, researchers are open to using different research and data analysis methods to derive meaningful insights. For sure, statistical analysis plans are the most favored to analyze numerical data. In statistical analysis, distinguishing between categorical data and numerical data is essential, as categorical data involves distinct categories or labels, while numerical data consists of measurable quantities. The method is again classified into two groups. First, ‘Descriptive Statistics’ used to describe data. Second, ‘Inferential statistics’ that helps in comparing the data .
Descriptive statistics
This method is used to describe the basic features of versatile types of data in research. It presents the data in such a meaningful way that pattern in the data starts making sense. Nevertheless, the descriptive analysis does not go beyond making conclusions. The conclusions are again based on the hypothesis researchers have formulated so far. Here are a few major types of descriptive analysis methods.
Measures of Frequency
- Count, Percent, Frequency
- It is used to denote home often a particular event occurs.
- Researchers use it when they want to showcase how often a response is given.
Measures of Central Tendency
- Mean, Median, Mode
- The method is widely used to demonstrate distribution by various points.
- Researchers use this method when they want to showcase the most commonly or averagely indicated response.
Measures of Dispersion or Variation
- Range, Variance, Standard deviation
- Here the field equals high/low points.
- Variance standard deviation = difference between the observed score and mean
- It is used to identify the spread of scores by stating intervals.
- Researchers use this method to showcase data spread out. It helps them identify the depth until which the data is spread out that it directly affects the mean.
Measures of Position
- Percentile ranks, Quartile ranks
- It relies on standardized scores helping researchers to identify the relationship between different scores.
- It is often used when researchers want to compare scores with the average count.
For quantitative research use of descriptive analysis often give absolute numbers, but the in-depth analysis is never sufficient to demonstrate the rationale behind those numbers. Nevertheless, it is necessary to think of the best method for research and data analysis suiting your survey questionnaire and what story researchers want to tell. For example, the mean is the best way to demonstrate the students’ average scores in schools. It is better to rely on the descriptive statistics when the researchers intend to keep the research or outcome limited to the provided sample without generalizing it. For example, when you want to compare average voting done in two different cities, differential statistics are enough.
Descriptive analysis is also called a ‘univariate analysis’ since it is commonly used to analyze a single variable.
Inferential statistics
Inferential statistics are used to make predictions about a larger population after research and data analysis of the representing population’s collected sample. For example, you can ask some odd 100 audiences at a movie theater if they like the movie they are watching. Researchers then use inferential statistics on the collected sample to reason that about 80-90% of people like the movie.
Here are two significant areas of inferential statistics.
- Estimating parameters: It takes statistics from the sample research data and demonstrates something about the population parameter.
- Hypothesis test: I t’s about sampling research data to answer the survey research questions. For example, researchers might be interested to understand if the new shade of lipstick recently launched is good or not, or if the multivitamin capsules help children to perform better at games.
These are sophisticated analysis methods used to showcase the relationship between different variables instead of describing a single variable. It is often used when researchers want something beyond absolute numbers to understand the relationship between variables.
Here are some of the commonly used methods for data analysis in research.
- Correlation: When researchers are not conducting experimental research or quasi-experimental research wherein the researchers are interested to understand the relationship between two or more variables, they opt for correlational research methods.
- Cross-tabulation: Also called contingency tables, cross-tabulation is used to analyze the relationship between multiple variables. Suppose provided data has age and gender categories presented in rows and columns. A two-dimensional cross-tabulation helps for seamless data analysis and research by showing the number of males and females in each age category.
- Regression analysis: For understanding the strong relationship between two variables, researchers do not look beyond the primary and commonly used regression analysis method, which is also a type of predictive analysis used. In this method, you have an essential factor called the dependent variable. You also have multiple independent variables in regression analysis. You undertake efforts to find out the impact of independent variables on the dependent variable. The values of both independent and dependent variables are assumed as being ascertained in an error-free random manner.
- Frequency tables: The statistical procedure is used for testing the degree to which two or more vary or differ in an experiment. A considerable degree of variation means research findings were significant. In many contexts, ANOVA testing and variance analysis are similar.
- Analysis of variance: The statistical procedure is used for testing the degree to which two or more vary or differ in an experiment. A considerable degree of variation means research findings were significant. In many contexts, ANOVA testing and variance analysis are similar.
- Researchers must have the necessary research skills to analyze and manipulation the data , Getting trained to demonstrate a high standard of research practice. Ideally, researchers must possess more than a basic understanding of the rationale of selecting one statistical method over the other to obtain better data insights.
- Usually, research and data analytics projects differ by scientific discipline; therefore, getting statistical advice at the beginning of analysis helps design a survey questionnaire, select data collection methods, and choose samples.
LEARN ABOUT: Best Data Collection Tools
- The primary aim of data research and analysis is to derive ultimate insights that are unbiased. Any mistake in or keeping a biased mind to collect data, selecting an analysis method, or choosing audience sample il to draw a biased inference.
- Irrelevant to the sophistication used in research data and analysis is enough to rectify the poorly defined objective outcome measurements. It does not matter if the design is at fault or intentions are not clear, but lack of clarity might mislead readers, so avoid the practice.
- The motive behind data analysis in research is to present accurate and reliable data. As far as possible, avoid statistical errors, and find a way to deal with everyday challenges like outliers, missing data, data altering, data mining , or developing graphical representation.
LEARN MORE: Descriptive Research vs Correlational Research The sheer amount of data generated daily is frightening. Especially when data analysis has taken center stage. in 2018. In last year, the total data supply amounted to 2.8 trillion gigabytes. Hence, it is clear that the enterprises willing to survive in the hypercompetitive world must possess an excellent capability to analyze complex research data, derive actionable insights, and adapt to the new market needs.
LEARN ABOUT: Average Order Value
QuestionPro is an online survey platform that empowers organizations in data analysis and research and provides them a medium to collect data by creating appealing surveys.
MORE LIKE THIS
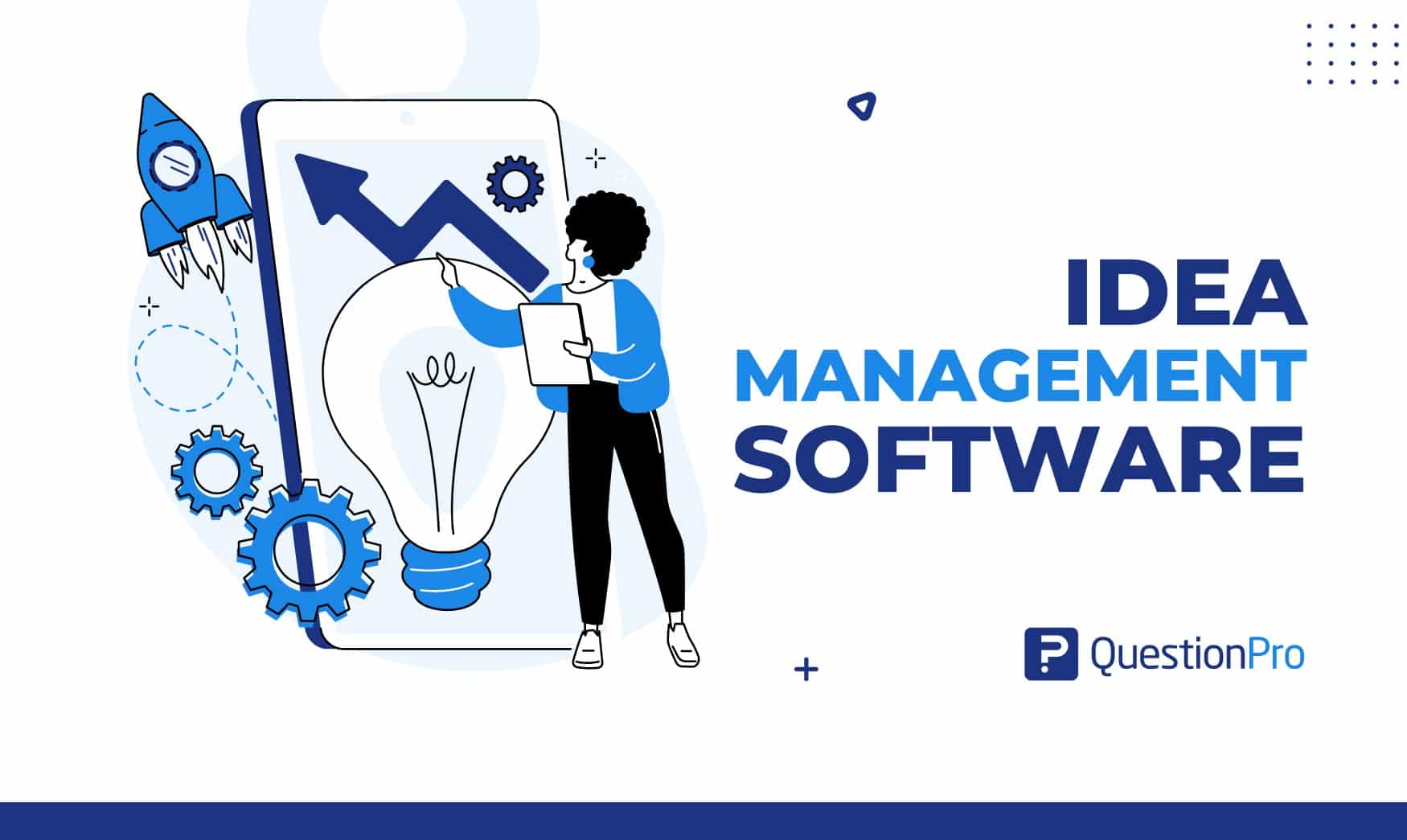
Unlocking Creativity With 10 Top Idea Management Software
Mar 23, 2024
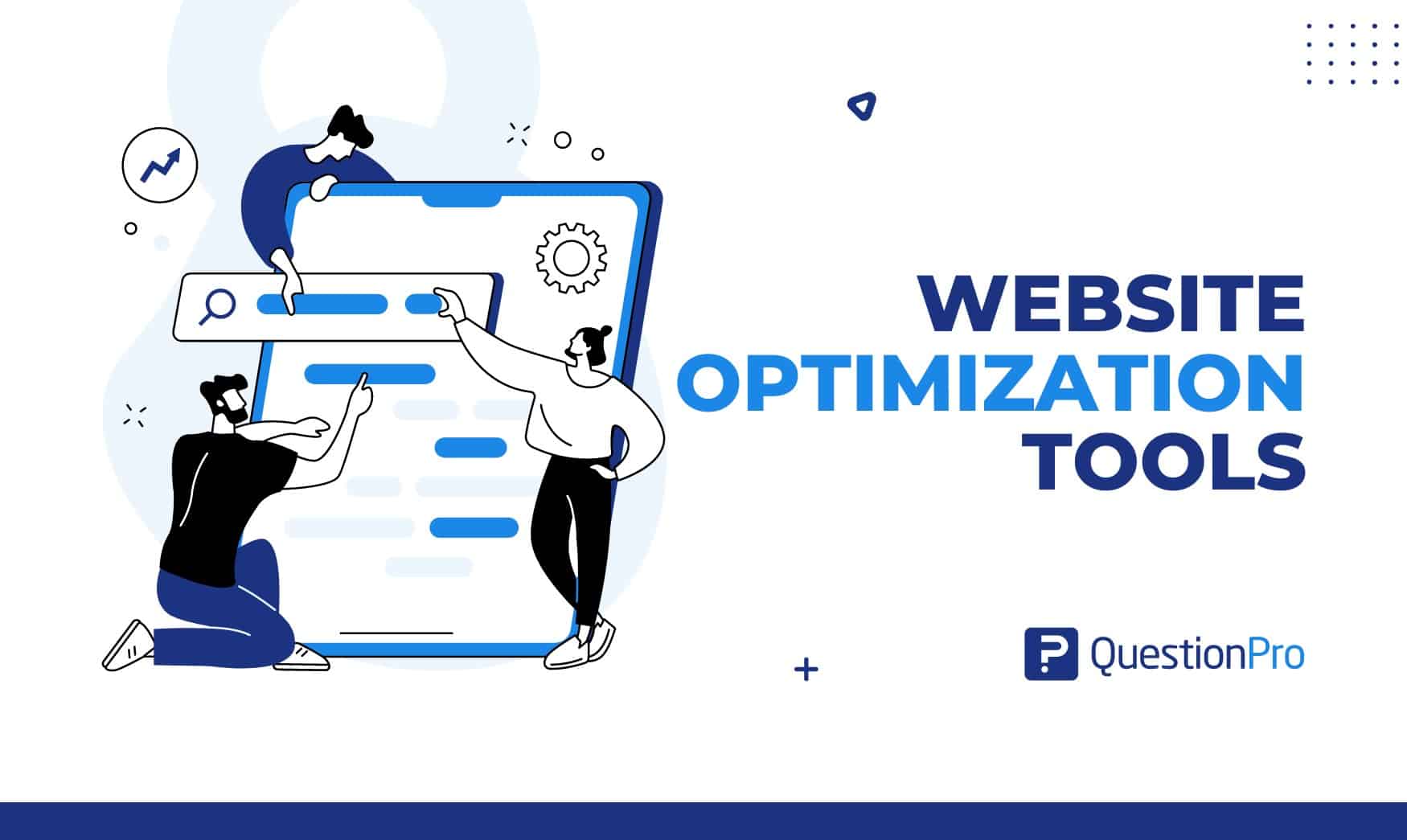
20 Best Website Optimization Tools to Improve Your Website
Mar 22, 2024
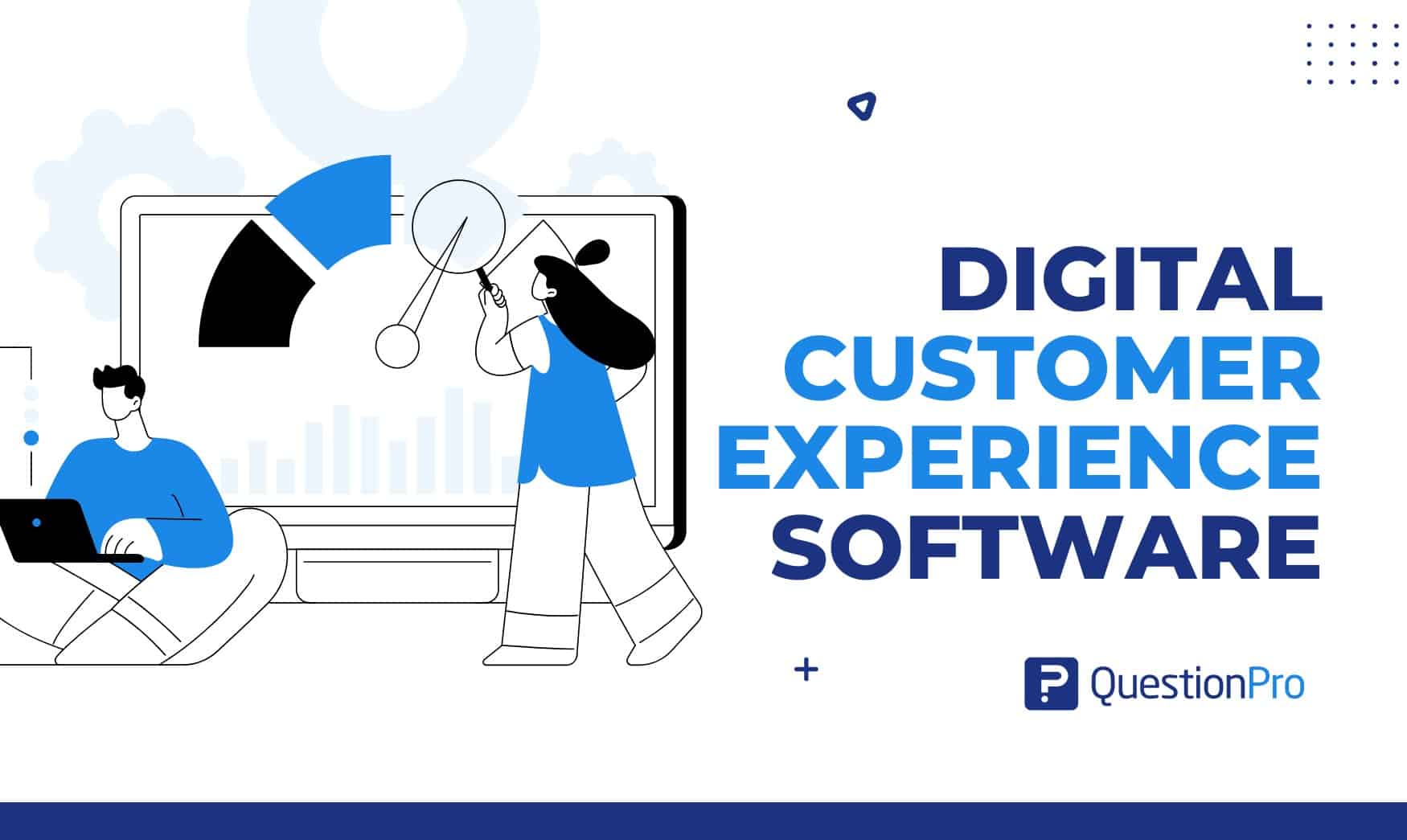
15 Best Digital Customer Experience Software of 2024
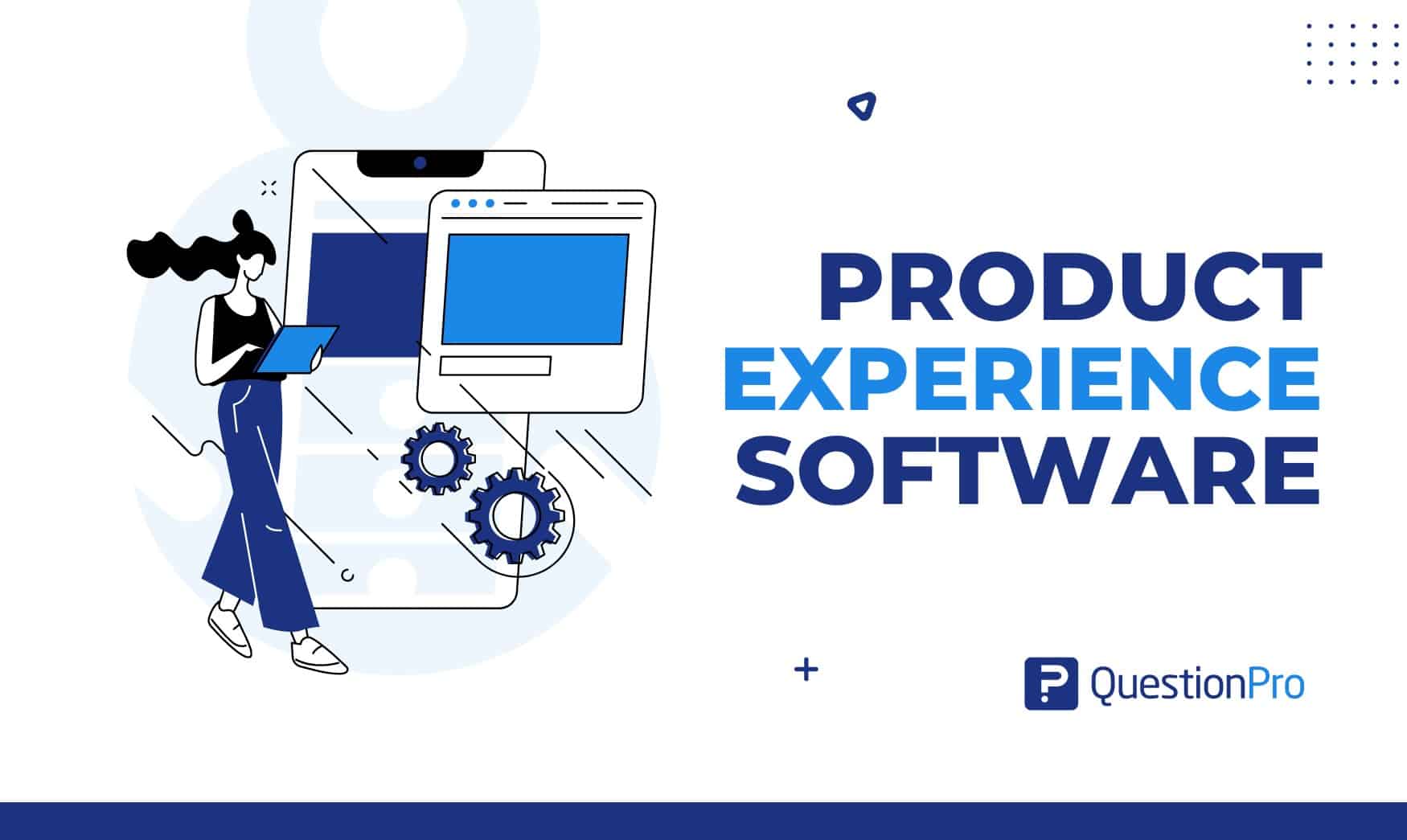
15 Best Product Experience Software of 2024
Other categories.
- Academic Research
- Artificial Intelligence
- Assessments
- Brand Awareness
- Case Studies
- Communities
- Consumer Insights
- Customer effort score
- Customer Engagement
- Customer Experience
- Customer Loyalty
- Customer Research
- Customer Satisfaction
- Employee Benefits
- Employee Engagement
- Employee Retention
- Friday Five
- General Data Protection Regulation
- Insights Hub
- Life@QuestionPro
- Market Research
- Mobile diaries
- Mobile Surveys
- New Features
- Online Communities
- Question Types
- Questionnaire
- QuestionPro Products
- Release Notes
- Research Tools and Apps
- Revenue at Risk
- Survey Templates
- Training Tips
- Uncategorized
- Video Learning Series
- What’s Coming Up
- Workforce Intelligence
Data Analysis Plan: Ultimate Guide and Examples
Learn the post survey questions you need to ask attendees for valuable feedback.
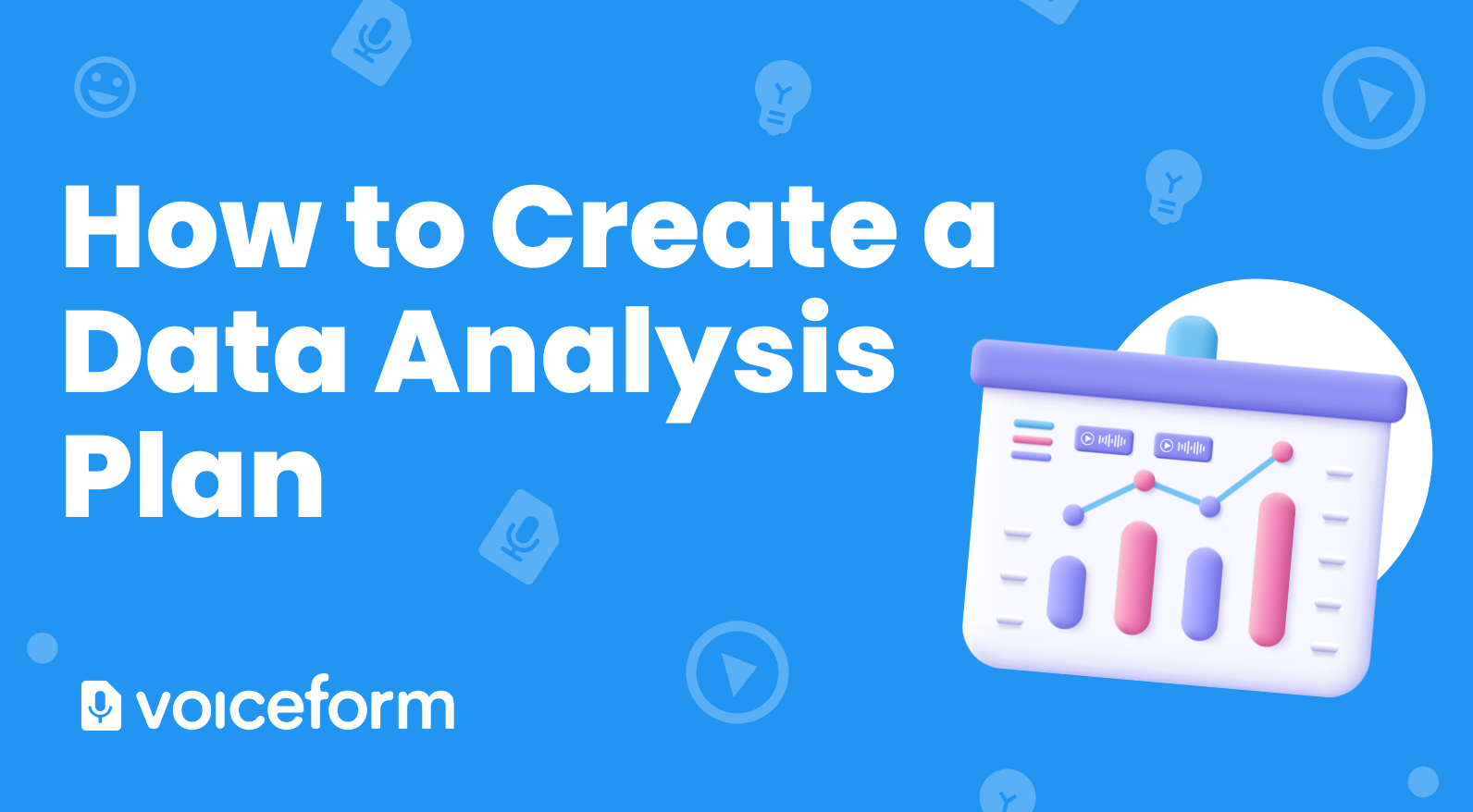
Once you get survey feedback , you might think that the job is done. The next step, however, is to analyze those results. Creating a data analysis plan will help guide you through how to analyze the data and come to logical conclusions.
So, how do you create a data analysis plan? It starts with the goals you set for your survey in the first place. This guide will help you create a data analysis plan that will effectively utilize the data your respondents provided.
What can a data analysis plan do?
Think of data analysis plans as a guide to your organization and analysis, which will help you accomplish your ultimate survey goals. A good plan will make sure that you get answers to your top questions, such as “how do customers feel about this new product?” through specific survey questions. It will also separate respondents to see how opinions among various demographics may differ.
Creating a data analysis plan
Follow these steps to create your own data analysis plan.
Review your goals
When you plan a survey, you typically have specific goals in mind. That might be measuring customer sentiment, answering an academic question, or achieving another purpose.
If you’re beta testing a new product, your survey goal might be “find out how potential customers feel about the new product.” You probably came up with several topics you wanted to address, such as:
- What is the typical experience with the product?
- Which demographics are responding most positively? How well does this match with our idea of the target market?
- Are there any specific pain points that need to be corrected before the product launches?
- Are there any features that should be added before the product launches?
Use these objectives to organize your survey data.
Evaluate the results for your top questions
Your survey questions probably included at least one or two questions that directly relate to your primary goals. For example, in the beta testing example above, your top two questions might be:
- How would you rate your overall satisfaction with the product?
- Would you consider purchasing this product?
Those questions offer a general overview of how your customers feel. Whether their sentiments are generally positive, negative, or neutral, this is the main data your company needs. The next goal is to determine why the beta testers feel the way they do.
Assign questions to specific goals
Next, you’ll organize your survey questions and responses by which research question they answer. For example, you might assign questions to the “overall satisfaction” section, like:
- How would you describe your experience with the product?
- Did you encounter any problems while using the product?
- What were your favorite/least favorite features?
- How useful was the product in achieving your goals?
Under demographics, you’d include responses to questions like:
- Education level
This helps you determine which questions and answers will answer larger questions, such as “which demographics are most likely to have had a positive experience?”
Pay special attention to demographics
Demographics are particularly important to a data analysis plan. Of course you’ll want to know what kind of experience your product testers are having with the product—but you also want to know who your target market should be. Separating responses based on demographics can be especially illuminating.
For example, you might find that users aged 25 to 45 find the product easier to use, but people over 65 find it too difficult. If you want to target the over-65 demographic, you can use that group’s survey data to refine the product before it launches.
Other demographic segregation can be helpful, too. You might find that your product is popular with people from the tech industry, who have an easier time with a user interface, while those from other industries, like education, struggle to use the tool effectively. If you’re targeting the tech industry, you may not need to make adjustments—but if it’s a technological tool designed primarily for educators, you’ll want to make appropriate changes.
Similarly, factors like location, education level, income bracket, and other demographics can help you compare experiences between the groups. Depending on your ultimate survey goals, you may want to compare multiple demographic types to get accurate insight into your results.
Consider correlation vs. causation
When creating your data analysis plan, remember to consider the difference between correlation and causation. For instance, being over 65 might correlate with a difficult user experience, but the cause of the experience might be something else entirely. You may find that your respondents over 65 are primarily from a specific educational background, or have issues reading the text in your user interface. It’s important to consider all the different data points, and how they might have an effect on the overall results.
Moving on to analysis
Once you’ve assigned survey questions to the overall research questions they’re designed to answer, you can move on to the actual data analysis. Depending on your survey tool, you may already have software that can perform quantitative and/or qualitative analysis. Choose the analysis types that suit your questions and goals, then use your analytic software to evaluate the data and create graphs or reports with your survey results.
At the end of the process, you should be able to answer your major research questions.
Power your data analysis with Voiceform
Once you have established your survey goals, Voiceform can power your data collection and analysis. Our feature-rich survey platform offers an easy-to-use interface, multi-channel survey tools, multimedia question types, and powerful analytics. We can help you create and work through a data analysis plan. Find out more about the product, and book a free demo today !
We make collecting, sharing and analyzing data a breeze
Get started for free. Get instant access to Voiceform features that get you amazing data in minutes.

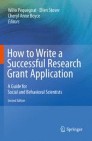
How to Write a Successful Research Grant Application pp 283–298 Cite as
Writing the Data Analysis Plan
- A. T. Panter 4
- First Online: 01 January 2010
5741 Accesses
3 Altmetric
You and your project statistician have one major goal for your data analysis plan: You need to convince all the reviewers reading your proposal that you would know what to do with your data once your project is funded and your data are in hand. The data analytic plan is a signal to the reviewers about your ability to score, describe, and thoughtfully synthesize a large number of variables into appropriately-selected quantitative models once the data are collected. Reviewers respond very well to plans with a clear elucidation of the data analysis steps – in an appropriate order, with an appropriate level of detail and reference to relevant literatures, and with statistical models and methods for that map well into your proposed aims. A successful data analysis plan produces reviews that either include no comments about the data analysis plan or better yet, compliments it for being comprehensive and logical given your aims. This chapter offers practical advice about developing and writing a compelling, “bullet-proof” data analytic plan for your grant application.
- Latent Class Analysis
- Grant Application
- Grant Proposal
- Data Analysis Plan
- Latent Transition Analysis
These keywords were added by machine and not by the authors. This process is experimental and the keywords may be updated as the learning algorithm improves.
This is a preview of subscription content, log in via an institution .
Buying options
- Available as PDF
- Read on any device
- Instant download
- Own it forever
- Available as EPUB and PDF
- Compact, lightweight edition
- Dispatched in 3 to 5 business days
- Free shipping worldwide - see info
Tax calculation will be finalised at checkout
Purchases are for personal use only
Aiken, L. S. & West, S. G. (1991). Multiple regression: testing and interpreting interactions . Newbury Park, CA: Sage.
Google Scholar
Aiken, L. S., West, S. G., & Millsap, R. E. (2008). Doctoral training in statistics, measurement, and methodology in psychology: Replication and extension of Aiken, West, Sechrest and Reno’s (1990) survey of PhD programs in North America. American Psychologist , 63 , 32–50.
Article PubMed Google Scholar
Allison, P. D. (2003). Missing data techniques for structural equation modeling. Journal of Abnormal Psychology , 112 , 545–557.
American Psychological Association (APA) Task Force to Increase the Quantitative Pipeline (2009). Report of the task force to increase the quantitative pipeline . Washington, DC: American Psychological Association.
Bauer, D. & Curran, P. J. (2004). The integration of continuous and discrete latent variables: Potential problems and promising opportunities. Psychological Methods , 9 , 3–29.
Bollen, K. A. (1989). Structural equations with latent variables . New York: Wiley.
Bollen, K. A. & Curran, P. J. (2007). Latent curve models: A structural equation modeling approach . New York: Wiley.
Cohen, J., Cohen, P., West, S. G., & Aiken, L. S. (2003). Multiple correlation/regression for the behavioral sciences (3rd ed.). Mahwah, NJ: Erlbaum.
Curran, P. J., Bauer, D. J., & Willoughby, M. T. (2004). Testing main effects and interactions in hierarchical linear growth models. Psychological Methods , 9 , 220–237.
Embretson, S. E. & Reise, S. P. (2000). Item response theory for psychologists . Mahwah, NJ: Erlbaum.
Enders, C. K. (2006). Analyzing structural equation models with missing data. In G. R. Hancock & R. O. Mueller (Eds.), Structural equation modeling: A second course (pp. 313–342). Greenwich, CT: Information Age.
Hosmer, D. & Lemeshow, S. (1989). Applied logistic regression . New York: Wiley.
Hoyle, R. H. & Panter, A. T. (1995). Writing about structural equation models. In R. H. Hoyle (Ed.), Structural equation modeling: Concepts, issues, and applications (pp. 158–176). Thousand Oaks: Sage.
Kaplan, D. & Elliott, P. R. (1997). A didactic example of multilevel structural equation modeling applicable to the study of organizations. Structural Equation Modeling , 4 , 1–23.
Article Google Scholar
Lanza, S. T., Collins, L. M., Schafer, J. L., & Flaherty, B. P. (2005). Using data augmentation to obtain standard errors and conduct hypothesis tests in latent class and latent transition analysis. Psychological Methods , 10 , 84–100.
MacKinnon, D. P. (2008). Introduction to statistical mediation analysis . Mahwah, NJ: Erlbaum.
Maxwell, S. E. (2004). The persistence of underpowered studies in psychological research: Causes, consequences, and remedies. Psychological Methods , 9 , 147–163.
McCullagh, P. & Nelder, J. (1989). Generalized linear models . London: Chapman and Hall.
McDonald, R. P. & Ho, M. R. (2002). Principles and practices in reporting structural equation modeling analyses. Psychological Methods , 7 , 64–82.
Messick, S. (1989). Validity. In R. L. Linn (Ed.), Educational measurement (3rd ed., pp. 13–103). New York: Macmillan.
Muthén, B. O. (1994). Multilevel covariance structure analysis. Sociological Methods & Research , 22 , 376–398.
Muthén, B. (2008). Latent variable hybrids: overview of old and new models. In G. R. Hancock & K. M. Samuelsen (Eds.), Advances in latent variable mixture models (pp. 1–24). Charlotte, NC: Information Age.
Muthén, B. & Masyn, K. (2004). Discrete-time survival mixture analysis. Journal of Educational and Behavioral Statistics , 30 , 27–58.
Muthén, L. K. & Muthén, B. O. (2004). Mplus, statistical analysis with latent variables: User’s guide . Los Angeles, CA: Muthén &Muthén.
Peugh, J. L. & Enders, C. K. (2004). Missing data in educational research: a review of reporting practices and suggestions for improvement. Review of Educational Research , 74 , 525–556.
Preacher, K. J., Curran, P. J., & Bauer, D. J. (2006). Computational tools for probing interaction effects in multiple linear regression, multilevel modeling, and latent curve analysis. Journal of Educational and Behavioral Statistics , 31 , 437–448.
Preacher, K. J., Curran, P. J., & Bauer, D. J. (2003, September). Probing interactions in multiple linear regression, latent curve analysis, and hierarchical linear modeling: Interactive calculation tools for establishing simple intercepts, simple slopes, and regions of significance [Computer software]. Available from http://www.quantpsy.org .
Preacher, K. J., Rucker, D. D., & Hayes, A. F. (2007). Addressing moderated mediation hypotheses: Theory, methods, and prescriptions. Multivariate Behavioral Research , 42 , 185–227.
Raudenbush, S. W. & Bryk, A. S. (2002). Hierarchical linear models: Applications and data analysis methods (2nd ed.). Thousand Oaks, CA: Sage.
Radloff, L. (1977). The CES-D scale: A self-report depression scale for research in the general population. Applied Psychological Measurement , 1 , 385–401.
Rosenberg, M. (1965). Society and the adolescent self-image . Princeton, NJ: Princeton University Press.
Schafer. J. L. & Graham, J. W. (2002). Missing data: Our view of the state of the art. Psychological Methods , 7 , 147–177.
Schumacker, R. E. (2002). Latent variable interaction modeling. Structural Equation Modeling , 9 , 40–54.
Schumacker, R. E. & Lomax, R. G. (2004). A beginner’s guide to structural equation modeling . Mahwah, NJ: Erlbaum.
Selig, J. P. & Preacher, K. J. (2008, June). Monte Carlo method for assessing mediation: An interactive tool for creating confidence intervals for indirect effects [Computer software]. Available from http://www.quantpsy.org .
Singer, J. D. & Willett, J. B. (1991). Modeling the days of our lives: Using survival analysis when designing and analyzing longitudinal studies of duration and the timing of events. Psychological Bulletin , 110 , 268–290.
Singer, J. D. & Willett, J. B. (1993). It’s about time: Using discrete-time survival analysis to study duration and the timing of events. Journal of Educational Statistics , 18 , 155–195.
Singer, J. D. & Willett, J. B. (2003). Applied longitudinal data analysis: Modeling change and event occurrence . New York: Oxford University.
Book Google Scholar
Vandenberg, R. J. & Lance, C. E. (2000). A review and synthesis of the measurement invariance literature: Suggestions, practices, and recommendations for organizational research. Organizational Research Methods , 3 , 4–69.
Wirth, R. J. & Edwards, M. C. (2007). Item factor analysis: Current approaches and future directions. Psychological Methods , 12 , 58–79.
Article PubMed CAS Google Scholar
Download references
Author information
Authors and affiliations.
L. L. Thurstone Psychometric Laboratory, Department of Psychology, University of North Carolina, Chapel Hill, NC, USA
A. T. Panter
You can also search for this author in PubMed Google Scholar
Corresponding author
Correspondence to A. T. Panter .
Editor information
Editors and affiliations.
National Institute of Mental Health, Executive Blvd. 6001, Bethesda, 20892-9641, Maryland, USA
Willo Pequegnat
Ellen Stover
Delafield Place, N.W. 1413, Washington, 20011, District of Columbia, USA
Cheryl Anne Boyce
Rights and permissions
Reprints and permissions
Copyright information
© 2010 Springer Science+Business Media, LLC
About this chapter
Cite this chapter.
Panter, A.T. (2010). Writing the Data Analysis Plan. In: Pequegnat, W., Stover, E., Boyce, C. (eds) How to Write a Successful Research Grant Application. Springer, Boston, MA. https://doi.org/10.1007/978-1-4419-1454-5_22
Download citation
DOI : https://doi.org/10.1007/978-1-4419-1454-5_22
Published : 20 August 2010
Publisher Name : Springer, Boston, MA
Print ISBN : 978-1-4419-1453-8
Online ISBN : 978-1-4419-1454-5
eBook Packages : Medicine Medicine (R0)
Share this chapter
Anyone you share the following link with will be able to read this content:
Sorry, a shareable link is not currently available for this article.
Provided by the Springer Nature SharedIt content-sharing initiative
- Publish with us
Policies and ethics
- Find a journal
- Track your research
Have a language expert improve your writing
Run a free plagiarism check in 10 minutes, generate accurate citations for free.
- Knowledge Base
The Beginner's Guide to Statistical Analysis | 5 Steps & Examples
Statistical analysis means investigating trends, patterns, and relationships using quantitative data . It is an important research tool used by scientists, governments, businesses, and other organizations.
To draw valid conclusions, statistical analysis requires careful planning from the very start of the research process . You need to specify your hypotheses and make decisions about your research design, sample size, and sampling procedure.
After collecting data from your sample, you can organize and summarize the data using descriptive statistics . Then, you can use inferential statistics to formally test hypotheses and make estimates about the population. Finally, you can interpret and generalize your findings.
This article is a practical introduction to statistical analysis for students and researchers. We’ll walk you through the steps using two research examples. The first investigates a potential cause-and-effect relationship, while the second investigates a potential correlation between variables.
Table of contents
Step 1: write your hypotheses and plan your research design, step 2: collect data from a sample, step 3: summarize your data with descriptive statistics, step 4: test hypotheses or make estimates with inferential statistics, step 5: interpret your results, other interesting articles.
To collect valid data for statistical analysis, you first need to specify your hypotheses and plan out your research design.
Writing statistical hypotheses
The goal of research is often to investigate a relationship between variables within a population . You start with a prediction, and use statistical analysis to test that prediction.
A statistical hypothesis is a formal way of writing a prediction about a population. Every research prediction is rephrased into null and alternative hypotheses that can be tested using sample data.
While the null hypothesis always predicts no effect or no relationship between variables, the alternative hypothesis states your research prediction of an effect or relationship.
- Null hypothesis: A 5-minute meditation exercise will have no effect on math test scores in teenagers.
- Alternative hypothesis: A 5-minute meditation exercise will improve math test scores in teenagers.
- Null hypothesis: Parental income and GPA have no relationship with each other in college students.
- Alternative hypothesis: Parental income and GPA are positively correlated in college students.
Planning your research design
A research design is your overall strategy for data collection and analysis. It determines the statistical tests you can use to test your hypothesis later on.
First, decide whether your research will use a descriptive, correlational, or experimental design. Experiments directly influence variables, whereas descriptive and correlational studies only measure variables.
- In an experimental design , you can assess a cause-and-effect relationship (e.g., the effect of meditation on test scores) using statistical tests of comparison or regression.
- In a correlational design , you can explore relationships between variables (e.g., parental income and GPA) without any assumption of causality using correlation coefficients and significance tests.
- In a descriptive design , you can study the characteristics of a population or phenomenon (e.g., the prevalence of anxiety in U.S. college students) using statistical tests to draw inferences from sample data.
Your research design also concerns whether you’ll compare participants at the group level or individual level, or both.
- In a between-subjects design , you compare the group-level outcomes of participants who have been exposed to different treatments (e.g., those who performed a meditation exercise vs those who didn’t).
- In a within-subjects design , you compare repeated measures from participants who have participated in all treatments of a study (e.g., scores from before and after performing a meditation exercise).
- In a mixed (factorial) design , one variable is altered between subjects and another is altered within subjects (e.g., pretest and posttest scores from participants who either did or didn’t do a meditation exercise).
- Experimental
- Correlational
First, you’ll take baseline test scores from participants. Then, your participants will undergo a 5-minute meditation exercise. Finally, you’ll record participants’ scores from a second math test.
In this experiment, the independent variable is the 5-minute meditation exercise, and the dependent variable is the math test score from before and after the intervention. Example: Correlational research design In a correlational study, you test whether there is a relationship between parental income and GPA in graduating college students. To collect your data, you will ask participants to fill in a survey and self-report their parents’ incomes and their own GPA.
Measuring variables
When planning a research design, you should operationalize your variables and decide exactly how you will measure them.
For statistical analysis, it’s important to consider the level of measurement of your variables, which tells you what kind of data they contain:
- Categorical data represents groupings. These may be nominal (e.g., gender) or ordinal (e.g. level of language ability).
- Quantitative data represents amounts. These may be on an interval scale (e.g. test score) or a ratio scale (e.g. age).
Many variables can be measured at different levels of precision. For example, age data can be quantitative (8 years old) or categorical (young). If a variable is coded numerically (e.g., level of agreement from 1–5), it doesn’t automatically mean that it’s quantitative instead of categorical.
Identifying the measurement level is important for choosing appropriate statistics and hypothesis tests. For example, you can calculate a mean score with quantitative data, but not with categorical data.
In a research study, along with measures of your variables of interest, you’ll often collect data on relevant participant characteristics.
Prevent plagiarism. Run a free check.
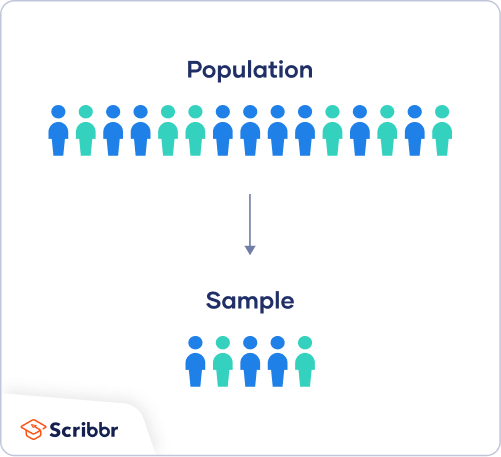
In most cases, it’s too difficult or expensive to collect data from every member of the population you’re interested in studying. Instead, you’ll collect data from a sample.
Statistical analysis allows you to apply your findings beyond your own sample as long as you use appropriate sampling procedures . You should aim for a sample that is representative of the population.
Sampling for statistical analysis
There are two main approaches to selecting a sample.
- Probability sampling: every member of the population has a chance of being selected for the study through random selection.
- Non-probability sampling: some members of the population are more likely than others to be selected for the study because of criteria such as convenience or voluntary self-selection.
In theory, for highly generalizable findings, you should use a probability sampling method. Random selection reduces several types of research bias , like sampling bias , and ensures that data from your sample is actually typical of the population. Parametric tests can be used to make strong statistical inferences when data are collected using probability sampling.
But in practice, it’s rarely possible to gather the ideal sample. While non-probability samples are more likely to at risk for biases like self-selection bias , they are much easier to recruit and collect data from. Non-parametric tests are more appropriate for non-probability samples, but they result in weaker inferences about the population.
If you want to use parametric tests for non-probability samples, you have to make the case that:
- your sample is representative of the population you’re generalizing your findings to.
- your sample lacks systematic bias.
Keep in mind that external validity means that you can only generalize your conclusions to others who share the characteristics of your sample. For instance, results from Western, Educated, Industrialized, Rich and Democratic samples (e.g., college students in the US) aren’t automatically applicable to all non-WEIRD populations.
If you apply parametric tests to data from non-probability samples, be sure to elaborate on the limitations of how far your results can be generalized in your discussion section .
Create an appropriate sampling procedure
Based on the resources available for your research, decide on how you’ll recruit participants.
- Will you have resources to advertise your study widely, including outside of your university setting?
- Will you have the means to recruit a diverse sample that represents a broad population?
- Do you have time to contact and follow up with members of hard-to-reach groups?
Your participants are self-selected by their schools. Although you’re using a non-probability sample, you aim for a diverse and representative sample. Example: Sampling (correlational study) Your main population of interest is male college students in the US. Using social media advertising, you recruit senior-year male college students from a smaller subpopulation: seven universities in the Boston area.
Calculate sufficient sample size
Before recruiting participants, decide on your sample size either by looking at other studies in your field or using statistics. A sample that’s too small may be unrepresentative of the sample, while a sample that’s too large will be more costly than necessary.
There are many sample size calculators online. Different formulas are used depending on whether you have subgroups or how rigorous your study should be (e.g., in clinical research). As a rule of thumb, a minimum of 30 units or more per subgroup is necessary.
To use these calculators, you have to understand and input these key components:
- Significance level (alpha): the risk of rejecting a true null hypothesis that you are willing to take, usually set at 5%.
- Statistical power : the probability of your study detecting an effect of a certain size if there is one, usually 80% or higher.
- Expected effect size : a standardized indication of how large the expected result of your study will be, usually based on other similar studies.
- Population standard deviation: an estimate of the population parameter based on a previous study or a pilot study of your own.
Once you’ve collected all of your data, you can inspect them and calculate descriptive statistics that summarize them.
Inspect your data
There are various ways to inspect your data, including the following:
- Organizing data from each variable in frequency distribution tables .
- Displaying data from a key variable in a bar chart to view the distribution of responses.
- Visualizing the relationship between two variables using a scatter plot .
By visualizing your data in tables and graphs, you can assess whether your data follow a skewed or normal distribution and whether there are any outliers or missing data.
A normal distribution means that your data are symmetrically distributed around a center where most values lie, with the values tapering off at the tail ends.
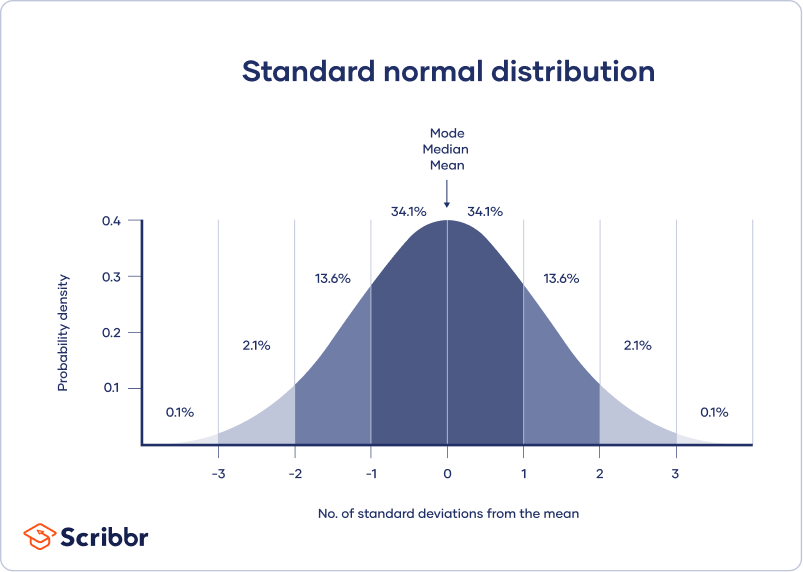
In contrast, a skewed distribution is asymmetric and has more values on one end than the other. The shape of the distribution is important to keep in mind because only some descriptive statistics should be used with skewed distributions.
Extreme outliers can also produce misleading statistics, so you may need a systematic approach to dealing with these values.
Calculate measures of central tendency
Measures of central tendency describe where most of the values in a data set lie. Three main measures of central tendency are often reported:
- Mode : the most popular response or value in the data set.
- Median : the value in the exact middle of the data set when ordered from low to high.
- Mean : the sum of all values divided by the number of values.
However, depending on the shape of the distribution and level of measurement, only one or two of these measures may be appropriate. For example, many demographic characteristics can only be described using the mode or proportions, while a variable like reaction time may not have a mode at all.
Calculate measures of variability
Measures of variability tell you how spread out the values in a data set are. Four main measures of variability are often reported:
- Range : the highest value minus the lowest value of the data set.
- Interquartile range : the range of the middle half of the data set.
- Standard deviation : the average distance between each value in your data set and the mean.
- Variance : the square of the standard deviation.
Once again, the shape of the distribution and level of measurement should guide your choice of variability statistics. The interquartile range is the best measure for skewed distributions, while standard deviation and variance provide the best information for normal distributions.
Using your table, you should check whether the units of the descriptive statistics are comparable for pretest and posttest scores. For example, are the variance levels similar across the groups? Are there any extreme values? If there are, you may need to identify and remove extreme outliers in your data set or transform your data before performing a statistical test.
From this table, we can see that the mean score increased after the meditation exercise, and the variances of the two scores are comparable. Next, we can perform a statistical test to find out if this improvement in test scores is statistically significant in the population. Example: Descriptive statistics (correlational study) After collecting data from 653 students, you tabulate descriptive statistics for annual parental income and GPA.
It’s important to check whether you have a broad range of data points. If you don’t, your data may be skewed towards some groups more than others (e.g., high academic achievers), and only limited inferences can be made about a relationship.
A number that describes a sample is called a statistic , while a number describing a population is called a parameter . Using inferential statistics , you can make conclusions about population parameters based on sample statistics.
Researchers often use two main methods (simultaneously) to make inferences in statistics.
- Estimation: calculating population parameters based on sample statistics.
- Hypothesis testing: a formal process for testing research predictions about the population using samples.
You can make two types of estimates of population parameters from sample statistics:
- A point estimate : a value that represents your best guess of the exact parameter.
- An interval estimate : a range of values that represent your best guess of where the parameter lies.
If your aim is to infer and report population characteristics from sample data, it’s best to use both point and interval estimates in your paper.
You can consider a sample statistic a point estimate for the population parameter when you have a representative sample (e.g., in a wide public opinion poll, the proportion of a sample that supports the current government is taken as the population proportion of government supporters).
There’s always error involved in estimation, so you should also provide a confidence interval as an interval estimate to show the variability around a point estimate.
A confidence interval uses the standard error and the z score from the standard normal distribution to convey where you’d generally expect to find the population parameter most of the time.
Hypothesis testing
Using data from a sample, you can test hypotheses about relationships between variables in the population. Hypothesis testing starts with the assumption that the null hypothesis is true in the population, and you use statistical tests to assess whether the null hypothesis can be rejected or not.
Statistical tests determine where your sample data would lie on an expected distribution of sample data if the null hypothesis were true. These tests give two main outputs:
- A test statistic tells you how much your data differs from the null hypothesis of the test.
- A p value tells you the likelihood of obtaining your results if the null hypothesis is actually true in the population.
Statistical tests come in three main varieties:
- Comparison tests assess group differences in outcomes.
- Regression tests assess cause-and-effect relationships between variables.
- Correlation tests assess relationships between variables without assuming causation.
Your choice of statistical test depends on your research questions, research design, sampling method, and data characteristics.
Parametric tests
Parametric tests make powerful inferences about the population based on sample data. But to use them, some assumptions must be met, and only some types of variables can be used. If your data violate these assumptions, you can perform appropriate data transformations or use alternative non-parametric tests instead.
A regression models the extent to which changes in a predictor variable results in changes in outcome variable(s).
- A simple linear regression includes one predictor variable and one outcome variable.
- A multiple linear regression includes two or more predictor variables and one outcome variable.
Comparison tests usually compare the means of groups. These may be the means of different groups within a sample (e.g., a treatment and control group), the means of one sample group taken at different times (e.g., pretest and posttest scores), or a sample mean and a population mean.
- A t test is for exactly 1 or 2 groups when the sample is small (30 or less).
- A z test is for exactly 1 or 2 groups when the sample is large.
- An ANOVA is for 3 or more groups.
The z and t tests have subtypes based on the number and types of samples and the hypotheses:
- If you have only one sample that you want to compare to a population mean, use a one-sample test .
- If you have paired measurements (within-subjects design), use a dependent (paired) samples test .
- If you have completely separate measurements from two unmatched groups (between-subjects design), use an independent (unpaired) samples test .
- If you expect a difference between groups in a specific direction, use a one-tailed test .
- If you don’t have any expectations for the direction of a difference between groups, use a two-tailed test .
The only parametric correlation test is Pearson’s r . The correlation coefficient ( r ) tells you the strength of a linear relationship between two quantitative variables.
However, to test whether the correlation in the sample is strong enough to be important in the population, you also need to perform a significance test of the correlation coefficient, usually a t test, to obtain a p value. This test uses your sample size to calculate how much the correlation coefficient differs from zero in the population.
You use a dependent-samples, one-tailed t test to assess whether the meditation exercise significantly improved math test scores. The test gives you:
- a t value (test statistic) of 3.00
- a p value of 0.0028
Although Pearson’s r is a test statistic, it doesn’t tell you anything about how significant the correlation is in the population. You also need to test whether this sample correlation coefficient is large enough to demonstrate a correlation in the population.
A t test can also determine how significantly a correlation coefficient differs from zero based on sample size. Since you expect a positive correlation between parental income and GPA, you use a one-sample, one-tailed t test. The t test gives you:
- a t value of 3.08
- a p value of 0.001
The final step of statistical analysis is interpreting your results.
Statistical significance
In hypothesis testing, statistical significance is the main criterion for forming conclusions. You compare your p value to a set significance level (usually 0.05) to decide whether your results are statistically significant or non-significant.
Statistically significant results are considered unlikely to have arisen solely due to chance. There is only a very low chance of such a result occurring if the null hypothesis is true in the population.
This means that you believe the meditation intervention, rather than random factors, directly caused the increase in test scores. Example: Interpret your results (correlational study) You compare your p value of 0.001 to your significance threshold of 0.05. With a p value under this threshold, you can reject the null hypothesis. This indicates a statistically significant correlation between parental income and GPA in male college students.
Note that correlation doesn’t always mean causation, because there are often many underlying factors contributing to a complex variable like GPA. Even if one variable is related to another, this may be because of a third variable influencing both of them, or indirect links between the two variables.
Effect size
A statistically significant result doesn’t necessarily mean that there are important real life applications or clinical outcomes for a finding.
In contrast, the effect size indicates the practical significance of your results. It’s important to report effect sizes along with your inferential statistics for a complete picture of your results. You should also report interval estimates of effect sizes if you’re writing an APA style paper .
With a Cohen’s d of 0.72, there’s medium to high practical significance to your finding that the meditation exercise improved test scores. Example: Effect size (correlational study) To determine the effect size of the correlation coefficient, you compare your Pearson’s r value to Cohen’s effect size criteria.
Decision errors
Type I and Type II errors are mistakes made in research conclusions. A Type I error means rejecting the null hypothesis when it’s actually true, while a Type II error means failing to reject the null hypothesis when it’s false.
You can aim to minimize the risk of these errors by selecting an optimal significance level and ensuring high power . However, there’s a trade-off between the two errors, so a fine balance is necessary.
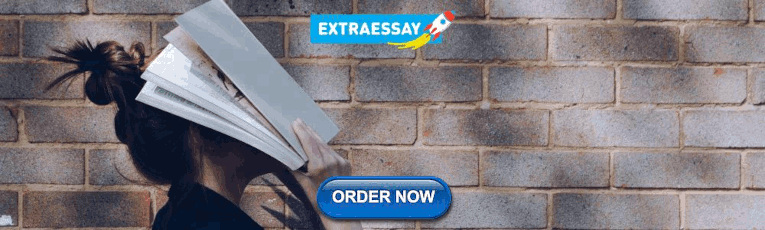
Frequentist versus Bayesian statistics
Traditionally, frequentist statistics emphasizes null hypothesis significance testing and always starts with the assumption of a true null hypothesis.
However, Bayesian statistics has grown in popularity as an alternative approach in the last few decades. In this approach, you use previous research to continually update your hypotheses based on your expectations and observations.
Bayes factor compares the relative strength of evidence for the null versus the alternative hypothesis rather than making a conclusion about rejecting the null hypothesis or not.
If you want to know more about statistics , methodology , or research bias , make sure to check out some of our other articles with explanations and examples.
- Student’s t -distribution
- Normal distribution
- Null and Alternative Hypotheses
- Chi square tests
- Confidence interval
Methodology
- Cluster sampling
- Stratified sampling
- Data cleansing
- Reproducibility vs Replicability
- Peer review
- Likert scale
Research bias
- Implicit bias
- Framing effect
- Cognitive bias
- Placebo effect
- Hawthorne effect
- Hostile attribution bias
- Affect heuristic
Is this article helpful?
Other students also liked.
- Descriptive Statistics | Definitions, Types, Examples
- Inferential Statistics | An Easy Introduction & Examples
- Choosing the Right Statistical Test | Types & Examples
More interesting articles
- Akaike Information Criterion | When & How to Use It (Example)
- An Easy Introduction to Statistical Significance (With Examples)
- An Introduction to t Tests | Definitions, Formula and Examples
- ANOVA in R | A Complete Step-by-Step Guide with Examples
- Central Limit Theorem | Formula, Definition & Examples
- Central Tendency | Understanding the Mean, Median & Mode
- Chi-Square (Χ²) Distributions | Definition & Examples
- Chi-Square (Χ²) Table | Examples & Downloadable Table
- Chi-Square (Χ²) Tests | Types, Formula & Examples
- Chi-Square Goodness of Fit Test | Formula, Guide & Examples
- Chi-Square Test of Independence | Formula, Guide & Examples
- Coefficient of Determination (R²) | Calculation & Interpretation
- Correlation Coefficient | Types, Formulas & Examples
- Frequency Distribution | Tables, Types & Examples
- How to Calculate Standard Deviation (Guide) | Calculator & Examples
- How to Calculate Variance | Calculator, Analysis & Examples
- How to Find Degrees of Freedom | Definition & Formula
- How to Find Interquartile Range (IQR) | Calculator & Examples
- How to Find Outliers | 4 Ways with Examples & Explanation
- How to Find the Geometric Mean | Calculator & Formula
- How to Find the Mean | Definition, Examples & Calculator
- How to Find the Median | Definition, Examples & Calculator
- How to Find the Mode | Definition, Examples & Calculator
- How to Find the Range of a Data Set | Calculator & Formula
- Hypothesis Testing | A Step-by-Step Guide with Easy Examples
- Interval Data and How to Analyze It | Definitions & Examples
- Levels of Measurement | Nominal, Ordinal, Interval and Ratio
- Linear Regression in R | A Step-by-Step Guide & Examples
- Missing Data | Types, Explanation, & Imputation
- Multiple Linear Regression | A Quick Guide (Examples)
- Nominal Data | Definition, Examples, Data Collection & Analysis
- Normal Distribution | Examples, Formulas, & Uses
- Null and Alternative Hypotheses | Definitions & Examples
- One-way ANOVA | When and How to Use It (With Examples)
- Ordinal Data | Definition, Examples, Data Collection & Analysis
- Parameter vs Statistic | Definitions, Differences & Examples
- Pearson Correlation Coefficient (r) | Guide & Examples
- Poisson Distributions | Definition, Formula & Examples
- Probability Distribution | Formula, Types, & Examples
- Quartiles & Quantiles | Calculation, Definition & Interpretation
- Ratio Scales | Definition, Examples, & Data Analysis
- Simple Linear Regression | An Easy Introduction & Examples
- Skewness | Definition, Examples & Formula
- Statistical Power and Why It Matters | A Simple Introduction
- Student's t Table (Free Download) | Guide & Examples
- T-distribution: What it is and how to use it
- Test statistics | Definition, Interpretation, and Examples
- The Standard Normal Distribution | Calculator, Examples & Uses
- Two-Way ANOVA | Examples & When To Use It
- Type I & Type II Errors | Differences, Examples, Visualizations
- Understanding Confidence Intervals | Easy Examples & Formulas
- Understanding P values | Definition and Examples
- Variability | Calculating Range, IQR, Variance, Standard Deviation
- What is Effect Size and Why Does It Matter? (Examples)
- What Is Kurtosis? | Definition, Examples & Formula
- What Is Standard Error? | How to Calculate (Guide with Examples)

Data Analysis Plan Template
Define research objectives, identify data sources, plan data collection method, define sample size and sampling procedure, approval: research design.
- Define Research Objectives Will be submitted
- Identify Data Sources Will be submitted
- Plan Data Collection Method Will be submitted
- Define Sample Size and Sampling Procedure Will be submitted
Collect Data
Prepare and clean data.
- 1 Remove irrelevant data
- 2 Remove redundant data
- 3 Filter outliers
Conduct Preliminary Analysis
Identify and address data quality issues.
- 1 Inaccurate data
- 2 Incomplete data
- 3 Inconsistent data
- 4 Missing data
- 5 Erroneous data
Approval: Initial Findings
- Collect Data Will be submitted
- Prepare and Clean Data Will be submitted
- Conduct Preliminary Analysis Will be submitted
- Identify and Address Data Quality Issues Will be submitted
Conduct Advanced Analysis
- 1 Regression analysis
- 2 Predictive modeling
- 3 Machine learning algorithms
Interpret Data Analysis Results
Formulate conclusions, prepare analysis report, approval: final report.
- Conduct Advanced Analysis Will be submitted
- Interpret Data Analysis Results Will be submitted
- Formulate Conclusions Will be submitted
- Prepare Analysis Report Will be submitted
Present Results to Stakeholders
Approval: presentation.
- Present Results to Stakeholders Will be submitted
Take control of your workflows today.
More templates like this.
Join thousands of product people at Insight Out Conf on April 11. Register free.
Insights hub solutions
Analyze data
Uncover deep customer insights with fast, powerful features, store insights, curate and manage insights in one searchable platform, scale research, unlock the potential of customer insights at enterprise scale.
Featured reads
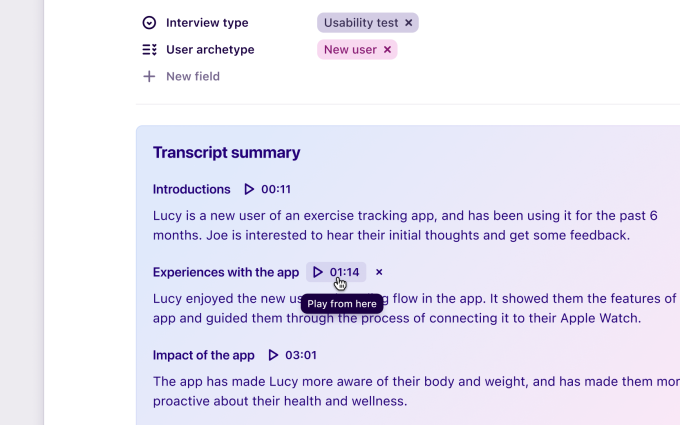
Tips and tricks
Make magic with your customer data in Dovetail
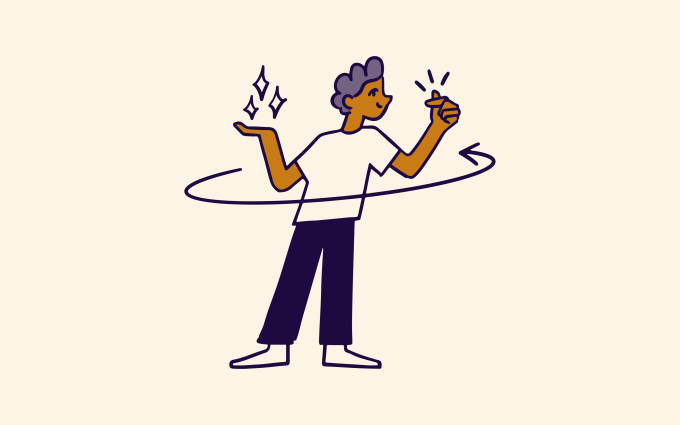
Four ways Dovetail helps Product Managers master continuous product discovery
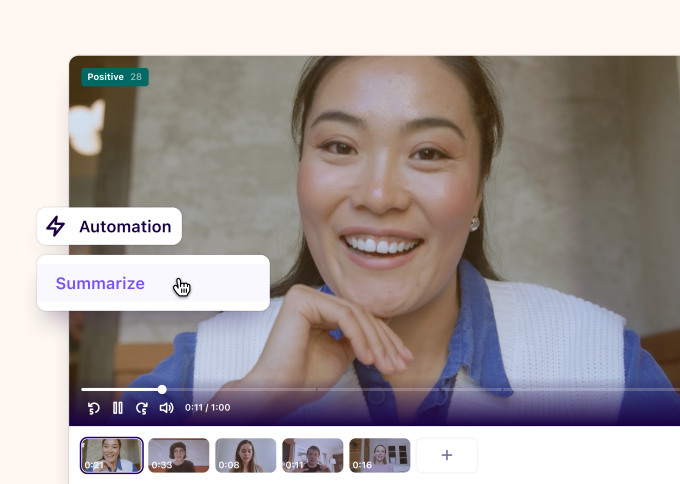
Product updates
Dovetail retro: our biggest releases from the past year
Events and videos
© Dovetail Research Pty. Ltd.
How to write a research plan: Step-by-step guide
Last updated
30 January 2024
Reviewed by
Today’s businesses and institutions rely on data and analytics to inform their product and service decisions. These metrics influence how organizations stay competitive and inspire innovation. However, gathering data and insights requires carefully constructed research, and every research project needs a roadmap. This is where a research plan comes into play.
There’s general research planning; then there’s an official, well-executed research plan. Whatever data-driven research project you’re gearing up for, the research plan will be your framework for execution. The plan should also be detailed and thorough, with a diligent set of criteria to formulate your research efforts. Not including these key elements in your plan can be just as harmful as having no plan at all.
Read this step-by-step guide for writing a detailed research plan that can apply to any project, whether it’s scientific, educational, or business-related.
- What is a research plan?
A research plan is a documented overview of a project in its entirety, from end to end. It details the research efforts, participants, and methods needed, along with any anticipated results. It also outlines the project’s goals and mission, creating layers of steps to achieve those goals within a specified timeline.
Without a research plan, you and your team are flying blind, potentially wasting time and resources to pursue research without structured guidance.
The principal investigator, or PI, is responsible for facilitating the research oversight. They will create the research plan and inform team members and stakeholders of every detail relating to the project. The PI will also use the research plan to inform decision-making throughout the project.
- Why do you need a research plan?
Create a research plan before starting any official research to maximize every effort in pursuing and collecting the research data. Crucially, the plan will model the activities needed at each phase of the research project.
Like any roadmap, a research plan serves as a valuable tool providing direction for those involved in the project—both internally and externally. It will keep you and your immediate team organized and task-focused while also providing necessary definitions and timelines so you can execute your project initiatives with full understanding and transparency.
External stakeholders appreciate a working research plan because it’s a great communication tool, documenting progress and changing dynamics as they arise. Any participants of your planned research sessions will be informed about the purpose of your study, while the exercises will be based on the key messaging outlined in the official plan.
Here are some of the benefits of creating a research plan document for every project:
Project organization and structure
Well-informed participants
All stakeholders and teams align in support of the project
Clearly defined project definitions and purposes
Distractions are eliminated, prioritizing task focus
Timely management of individual task schedules and roles
Costly reworks are avoided
- What should a research plan include?
The different aspects of your research plan will depend on the nature of the project. However, most official research plan documents will include the core elements below. Each aims to define the problem statement, devising an official plan for seeking a solution.
Specific project goals and individual objectives
Ideal strategies or methods for reaching those goals
Required resources
Descriptions of the target audience, sample sizes, demographics, and scopes
Key performance indicators (KPIs)
Project background
Research and testing support
Preliminary studies and progress reporting mechanisms
Cost estimates and change order processes
Depending on the research project’s size and scope, your research plan could be brief—perhaps only a few pages of documented plans. Alternatively, it could be a fully comprehensive report. Either way, it’s an essential first step in dictating your project’s facilitation in the most efficient and effective way.
- How to write a research plan for your project
When you start writing your research plan, aim to be detailed about each step, requirement, and idea. The more time you spend curating your research plan, the more precise your research execution efforts will be.
Account for every potential scenario, and be sure to address each and every aspect of the research.
Consider following this flow to develop a great research plan for your project:
Define your project’s purpose
Start by defining your project’s purpose. Identify what your project aims to accomplish and what you are researching. Remember to use clear language.
Thinking about the project’s purpose will help you set realistic goals and inform how you divide tasks and assign responsibilities. These individual tasks will be your stepping stones to reach your overarching goal.
Additionally, you’ll want to identify the specific problem, the usability metrics needed, and the intended solutions.
Know the following three things about your project’s purpose before you outline anything else:
What you’re doing
Why you’re doing it
What you expect from it
Identify individual objectives
With your overarching project objectives in place, you can identify any individual goals or steps needed to reach those objectives. Break them down into phases or steps. You can work backward from the project goal and identify every process required to facilitate it.
Be mindful to identify each unique task so that you can assign responsibilities to various team members. At this point in your research plan development, you’ll also want to assign priority to those smaller, more manageable steps and phases that require more immediate or dedicated attention.
Select research methods
Research methods might include any of the following:
User interviews: this is a qualitative research method where researchers engage with participants in one-on-one or group conversations. The aim is to gather insights into their experiences, preferences, and opinions to uncover patterns, trends, and data.
Field studies: this approach allows for a contextual understanding of behaviors, interactions, and processes in real-world settings. It involves the researcher immersing themselves in the field, conducting observations, interviews, or experiments to gather in-depth insights.
Card sorting: participants categorize information by sorting content cards into groups based on their perceived similarities. You might use this process to gain insights into participants’ mental models and preferences when navigating or organizing information on websites, apps, or other systems.
Focus groups: use organized discussions among select groups of participants to provide relevant views and experiences about a particular topic.
Diary studies: ask participants to record their experiences, thoughts, and activities in a diary over a specified period. This method provides a deeper understanding of user experiences, uncovers patterns, and identifies areas for improvement.
Five-second testing: participants are shown a design, such as a web page or interface, for just five seconds. They then answer questions about their initial impressions and recall, allowing you to evaluate the design’s effectiveness.
Surveys: get feedback from participant groups with structured surveys. You can use online forms, telephone interviews, or paper questionnaires to reveal trends, patterns, and correlations.
Tree testing: tree testing involves researching web assets through the lens of findability and navigability. Participants are given a textual representation of the site’s hierarchy (the “tree”) and asked to locate specific information or complete tasks by selecting paths.
Usability testing: ask participants to interact with a product, website, or application to evaluate its ease of use. This method enables you to uncover areas for improvement in digital key feature functionality by observing participants using the product.
Live website testing: research and collect analytics that outlines the design, usability, and performance efficiencies of a website in real time.
There are no limits to the number of research methods you could use within your project. Just make sure your research methods help you determine the following:
What do you plan to do with the research findings?
What decisions will this research inform? How can your stakeholders leverage the research data and results?
Recruit participants and allocate tasks
Next, identify the participants needed to complete the research and the resources required to complete the tasks. Different people will be proficient at different tasks, and having a task allocation plan will allow everything to run smoothly.
Prepare a thorough project summary
Every well-designed research plan will feature a project summary. This official summary will guide your research alongside its communications or messaging. You’ll use the summary while recruiting participants and during stakeholder meetings. It can also be useful when conducting field studies.
Ensure this summary includes all the elements of your research project. Separate the steps into an easily explainable piece of text that includes the following:
An introduction: the message you’ll deliver to participants about the interview, pre-planned questioning, and testing tasks.
Interview questions: prepare questions you intend to ask participants as part of your research study, guiding the sessions from start to finish.
An exit message: draft messaging your teams will use to conclude testing or survey sessions. These should include the next steps and express gratitude for the participant’s time.
Create a realistic timeline
While your project might already have a deadline or a results timeline in place, you’ll need to consider the time needed to execute it effectively.
Realistically outline the time needed to properly execute each supporting phase of research and implementation. And, as you evaluate the necessary schedules, be sure to include additional time for achieving each milestone in case any changes or unexpected delays arise.
For this part of your research plan, you might find it helpful to create visuals to ensure your research team and stakeholders fully understand the information.
Determine how to present your results
A research plan must also describe how you intend to present your results. Depending on the nature of your project and its goals, you might dedicate one team member (the PI) or assume responsibility for communicating the findings yourself.
In this part of the research plan, you’ll articulate how you’ll share the results. Detail any materials you’ll use, such as:
Presentations and slides
A project report booklet
A project findings pamphlet
Documents with key takeaways and statistics
Graphic visuals to support your findings
- Format your research plan
As you create your research plan, you can enjoy a little creative freedom. A plan can assume many forms, so format it how you see fit. Determine the best layout based on your specific project, intended communications, and the preferences of your teams and stakeholders.
Find format inspiration among the following layouts:
Written outlines
Narrative storytelling
Visual mapping
Graphic timelines
Remember, the research plan format you choose will be subject to change and adaptation as your research and findings unfold. However, your final format should ideally outline questions, problems, opportunities, and expectations.
- Research plan example
Imagine you’ve been tasked with finding out how to get more customers to order takeout from an online food delivery platform. The goal is to improve satisfaction and retain existing customers. You set out to discover why more people aren’t ordering and what it is they do want to order or experience.
You identify the need for a research project that helps you understand what drives customer loyalty. But before you jump in and start calling past customers, you need to develop a research plan—the roadmap that provides focus, clarity, and realistic details to the project.
Here’s an example outline of a research plan you might put together:
Project title
Project members involved in the research plan
Purpose of the project (provide a summary of the research plan’s intent)
Objective 1 (provide a short description for each objective)
Objective 2
Objective 3
Proposed timeline
Audience (detail the group you want to research, such as customers or non-customers)
Budget (how much you think it might cost to do the research)
Risk factors/contingencies (any potential risk factors that may impact the project’s success)
Remember, your research plan doesn’t have to reinvent the wheel—it just needs to fit your project’s unique needs and aims.
Customizing a research plan template
Some companies offer research plan templates to help get you started. However, it may make more sense to develop your own customized plan template. Be sure to include the core elements of a great research plan with your template layout, including the following:
Introductions to participants and stakeholders
Background problems and needs statement
Significance, ethics, and purpose
Research methods, questions, and designs
Preliminary beliefs and expectations
Implications and intended outcomes
Realistic timelines for each phase
Conclusion and presentations
How many pages should a research plan be?
Generally, a research plan can vary in length between 500 to 1,500 words. This is roughly three pages of content. More substantial projects will be 2,000 to 3,500 words, taking up four to seven pages of planning documents.
What is the difference between a research plan and a research proposal?
A research plan is a roadmap to success for research teams. A research proposal, on the other hand, is a dissertation aimed at convincing or earning the support of others. Both are relevant in creating a guide to follow to complete a project goal.
What are the seven steps to developing a research plan?
While each research project is different, it’s best to follow these seven general steps to create your research plan:
Defining the problem
Identifying goals
Choosing research methods
Recruiting participants
Preparing the brief or summary
Establishing task timelines
Defining how you will present the findings
Get started today
Go from raw data to valuable insights with a flexible research platform
Editor’s picks
Last updated: 21 December 2023
Last updated: 16 December 2023
Last updated: 17 February 2024
Last updated: 19 November 2023
Last updated: 5 March 2024
Last updated: 15 February 2024
Last updated: 11 March 2024
Last updated: 12 December 2023
Last updated: 6 March 2024
Last updated: 10 April 2023
Last updated: 20 December 2023
Latest articles
Related topics, log in or sign up.
Get started for free
Ask Yale Library
My Library Accounts
Find, Request, and Use
Help and Research Support
Visit and Study
Explore Collections
Research Data Management: Plan for Data
- Plan for Data
- Organize & Document Data
- Store & Secure Data
- Validate Data
- Share & Re-use Data
- Data Use Agreements
- Research Data Policies
What is a Data Management Plan?
Data management plans (DMPs) are documents that outline how data will be collected , stored , secured , analyzed , disseminated , and preserved over the lifecycle of a research project. They are typically created in the early stages of a project, and they are typically short documents that may evolve over time. Increasingly, they are required by funders and institutions alike, and they are a recommended best practice in research data management.
Tab through this guide to consider each stage of the research data management process, and each correlated section of a data management plan.
Tools for Data Management Planning
DMPTool is a collaborative effort between several universities to streamline the data management planning process.
The DMPTool supports the majority of federal and many non-profit and private funding agencies that require data management plans as part of a grant proposal application. ( View the list of supported organizations and corresponding templates.) If the funder you're applying to isn't listed or you just want to create one as good practice, there is an option for a generic plan.
Key features:
Data management plan templates from most major funders
Guided creation of a data management plan with click-throughs and helpful questions and examples
Access to public plans , to review ahead of creating your own
Ability to share plans with collaborators as well as copy and reuse existing plans
How to get started:
Log in with your yale.edu email to be directed to a NetID sign-in, and review the quick start guide .
Research Data Lifecycle
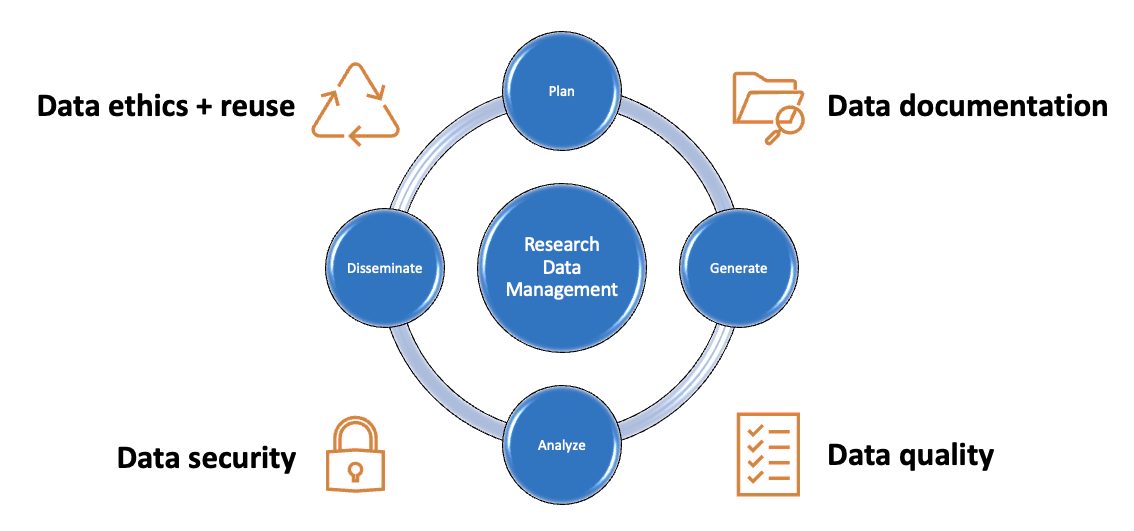
Additional Resources for Data Management Planning
- << Previous: Overview
- Next: Organize & Document Data >>
- Last Updated: Sep 27, 2023 1:15 PM
- URL: https://guides.library.yale.edu/datamanagement

Site Navigation
P.O. BOX 208240 New Haven, CT 06250-8240 (203) 432-1775
Yale's Libraries
Bass Library
Beinecke Rare Book and Manuscript Library
Classics Library
Cushing/Whitney Medical Library
Divinity Library
East Asia Library
Gilmore Music Library
Haas Family Arts Library
Lewis Walpole Library
Lillian Goldman Law Library
Marx Science and Social Science Library
Sterling Memorial Library
Yale Center for British Art
SUBSCRIBE TO OUR NEWSLETTER
@YALELIBRARY

Yale Library Instagram
Accessibility Diversity, Equity, and Inclusion Giving Privacy and Data Use Contact Our Web Team
© 2022 Yale University Library • All Rights Reserved
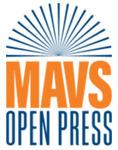
Want to create or adapt books like this? Learn more about how Pressbooks supports open publishing practices.
18.3 Preparations: Creating a plan for qualitative data analysis Learning Objectives
Learning objectives.
Learners will be able to…
- Identify how your research question, research aim, sample selection, and type of data may influence your choice of analytic methods
- Outline the steps you will take in preparation for conducting qualitative data analysis in your proposal
Now we can turn our attention to planning your analysis. The analysis should be anchored in the purpose of your study. Qualitative research can serve a range of purposes. Below is a brief list of general purposes we might consider when using a qualitative approach.
- Are you trying to understand how a particular group is affected by an issue?
- Are you trying to uncover how people arrive at a decision in a given situation?
- Are you trying to examine different points of view on the impact of a recent event?
- Are you trying to summarize how people understand or make sense of a condition?
- Are you trying to describe the needs of your target population?
If you don’t see the general aim of your research question reflected in one of these areas, don’t fret! This is only a small sampling of what you might be trying to accomplish with your qualitative study. Whatever your aim, you need to have a plan for what you will do once you have collected your data.
Decision Point: What are you trying to accomplish with your data?
- Consider your research question. What do you need to do with the qualitative data you are gathering to help answer that question?
To help answer this question, consider:
- What action verb(s) can be associated with your project and the qualitative data you are collecting? Does your research aim to summarize, compare, describe, examine, outline, identify, review, compose, develop, illustrate, etc.?
- Then, consider noun(s) you need to pair with your verb(s)—perceptions, experiences, thoughts, reactions, descriptions, understanding, processes, feelings, actions responses, etc.
Iterative or linear
We touched on this briefly in Chapter 17 about qualitative sampling, but this is an important distinction to consider. Some qualitative research is linear , meaning it follows more of a traditionally quantitative process: create a plan, gather data, and analyze data; each step is completed before we proceed to the next. You can think of this like how information is presented in this book. We discuss each topic, one after another.
However, many times qualitative research is iterative , or evolving in cycles. An iterative approach means that once we begin collecting data, we also begin analyzing data as it is coming in. This early and ongoing analysis of our (incomplete) data then impacts our continued planning, data gathering and future analysis. Again, coming back to this book, while it may be written linear, we hope that you engage with it iteratively as you are building your proposal. By this we mean that you will revisit previous sections so you can understand how they fit together and you are in continuous process of building and revising how you think about the concepts you are learning about.
As you may have guessed, there are benefits and challenges to both linear and iterative approaches. A linear approach is much more straightforward, each step being fairly defined. However, linear research being more defined and rigid also presents certain challenges. A linear approach assumes that we know what we need to ask or look for at the very beginning of data collection, which often is not the case.
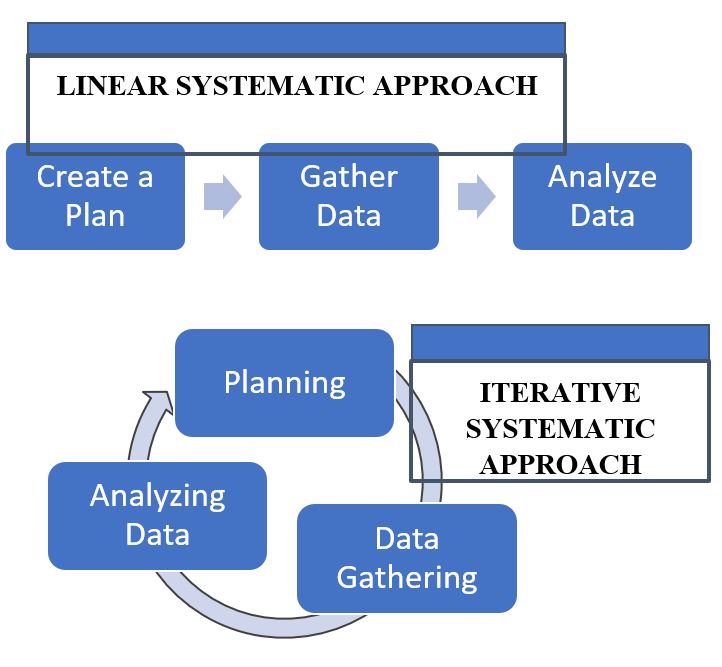
With iterative research, we have more flexibility to adapt our approach as we learn new things. We still need to keep our approach systematic and organized, however, so that our work doesn’t become a free-for-all. As we adapt, we do not want to stray too far from the original premise of our study. It’s also important to remember with an iterative approach that we may risk ethical concerns if our work extends beyond the original boundaries of our informed consent and IRB agreement. If you feel that you do need to modify your original research plan in a significant way as you learn more about the topic, you can submit an addendum to modify your original application that was submitted. Make sure to keep detailed notes of the decisions that you are making and what is informing these choices. This helps to support transparency and your credibility throughout the research process.
Decision Point: Will your analysis reflect more of a linear or an iterative approach?
- What justifies or supports this decision?
Think about:
- Fit with your research question
- Available time and resources
- Your knowledge and understanding of the research process
Reflexive Journal Entry Prompt
- What evidence are you basing this on?
- How might this help or hinder your qualitative research process?
- How might this help or hinder you in a practice setting as you work with clients?
Acquainting yourself with your data
As you begin your analysis, you need to get to know your data. This usually means reading through your data prior to any attempt at breaking it apart and labeling it. You might read through a couple of times, in fact. This helps give you a more comprehensive feel for each piece of data and the data as a whole, again, before you start to break it down into smaller units or deconstruct it. This is especially important if others assisted us in the data collection process. We often gather data as part of team and everyone involved in the analysis needs to be very familiar with all of the data.
Capturing your reaction to the data
During the review process, our understanding of the data often evolves as we observe patterns and trends. It is a good practice to document your reaction and evolving understanding. Your reaction can include noting phrases or ideas that surprise you, similarities or distinct differences in responses, additional questions that the data brings to mind, among other things. We often record these reactions directly in the text or artifact if we have the ability to do so, such as making a comment in a word document associated with a highlighted phrase. If this isn’t possible, you will want to have a way to track what specific spot(s) in your data your reactions are referring to. In qualitative research we refer to this process as memoing . Memoing is a strategy that helps us to link our findings to our raw data, demonstrating transparency. If you are using a Computre-Assisted Qualitative Data Analysis Software ( CAQDAS) software package, memoing functions are generally built into the technology.
Capturing your emerging understanding of the data
During your reviewing and memoing you will start to develop and evolve your understanding of what the data means. This understanding should be dynamic and flexible, but you want to have a way to capture this understanding as it evolves. You may include this as part of your memoing or as part of your codebook where you are tracking the main ideas that are emerging and what they mean. Figure 18.3 is an example of how your thinking might change about a code and how you can go about capturing it. Coding is a part of the qualitative data analysis process where we begin to interpret and assign meaning to the data. It represents one of the first steps as we begin to filter the data through our own subjective lens as the researcher. We will discuss coding in much more detail in the sections below covering various different approaches to analysis.
Decision Point: How to capture your thoughts?
- What will this look like?
- How often will you do it?
- How will you keep it organized and consistent over time?
In addition, you will want to be actively using your reflexive journal during this time. Document your thoughts and feelings throughout the research process. This will promote transparency and help account for your role in the analysis.
For entries during your analysis, respond to questions such as these in your journal:
- What surprises you about what participants are sharing?
- How has this information challenged you to look at this topic differently?
- Where might these have come from?
- How might these be influencing your study?
- How will you proceed differently based on what you are learning?
By including community members as active co-researchers, they can be invaluable in reviewing, reacting to and leading the interpretation of data during your analysis. While it can certainly be challenging to converge on an agreed-upon version of the results; their insider knowledge and lived experience can provide very important insights into the data analysis process.
Determining when you are finished
When conducting quantitative research, it is perhaps easier to decide when we are finished with our analysis. We determine the tests we need to run, we perform them, we interpret them, and for the most part, we call it a day. It’s a bit more nebulous for qualitative research. There is no hard and fast rule for when we have completed our qualitative analysis. Rather, our decision to end the analysis should be guided by reflection and consideration of a number of important questions. These questions are presented below to help ensure that your analysis results in a finished product that is comprehensive, systematic, and coherent.
Have I answered my research question?
Your analysis should be clearly connected to and in service of answering your research question. Your examination of the data should help you arrive at findings that sufficiently address the question that you set out to answer. You might find that it is surprisingly easy to get distracted while reviewing all your data. Make sure as you conducted the analysis you keep coming back to your research question.
Have I utilized all my data?
Unless you have intentionally made the decision that certain portions of your data are not relevant for your study, make sure that you don’t have sources or segments of data that aren’t incorporated into your analysis. Just because some data doesn’t “fit” the general trends you are uncovering, find a way to acknowledge this in your findings as well so that these voices don’t get lost in your data.
Have I fulfilled my obligation to my participants?
As a qualitative researcher, you are a craftsperson. You are taking raw materials (e.g. people’s words, observations, photos) and bringing them together to form a new creation, your findings. These findings need to both honor the original integrity of the data that is shared with you, but also help tell a broader story that answers your research question(s).
Have I fulfilled my obligation to my audience?
Not only do your findings need to help answer your research question, but they need to do so in a way that is consumable for your audience. From an analysis standpoint, this means that we need to make sufficient efforts to condense our data. For example, if you are conducting a thematic analysis, you don’t want to wind up with 20 themes. Having this many themes suggests that you aren’t finished looking at how these ideas relate to each other and might be combined into broader themes. Having these sufficiently reduced to a handful of themes will help tell a more complete story, one that is also much more approachable and meaningful for your reader.
In the following subsections, there is information regarding a variety of different approaches to qualitative analysis. In designing your qualitative study, you would identify an analytical approach as you plan out your project. The one you select would depend on the type of data you have and what you want to accomplish with it.
Key Takeaways
- Qualitative research analysis requires preparation and careful planning. You will need to take time to familiarize yourself with the data in general sense before you begin analyzing.
- Once you begin your analysis, make sure that you have strategies for capture and recording both your reaction to the data and your corresponding developing understanding of what the collective meaning of the data is (your results). Qualitative research is not only invested in the end results but also the process at which you arrive at them.
Decision Point: When will you stop?
- How will you know when you are finished? What will determine your endpoint?
- How will you monitor your work so you know when it’s over?
A research process where you create a plan, you gather your data, you analyze your data and each step is completed before you proceed to the next.
An iterative approach means that after planning and once we begin collecting data, we begin analyzing as data as it is coming in. This early analysis of our (incomplete) data, then impacts our planning, ongoing data gathering and future analysis as it progresses.
The point where gathering more data doesn't offer any new ideas or perspectives on the issue you are studying. Reaching saturation is an indication that we can stop qualitative data collection.
Memoing is the act of recording your thoughts, reactions, quandaries as you are reviewing the data you are gathering.
These are software tools that can aid qualitative researchers in managing, organizing and manipulating/analyzing their data.
A document that we use to keep track of and define the codes that we have identified (or are using) in our qualitative data analysis.
Part of the qualitative data analysis process where we begin to interpret and assign meaning to the data.
A research journal that helps the researcher to reflect on and consider their thoughts and reactions to the research process and how it may be shaping the study
Doctoral Research Methods in Social Work Copyright © by Mavs Open Press. All Rights Reserved.
Share This Book
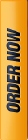
- IND +91 8754467066
- UK +44 - 753 714 4372
- [email protected]
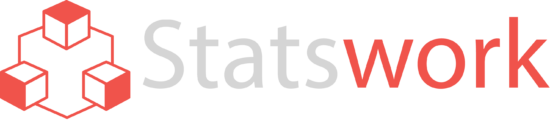
Data Analysis Plan for Quantitative Analysis:
Five steps used for analysis the quantitative data.

Our Statistical Services
We offer end-to-end quantitative data analysis.
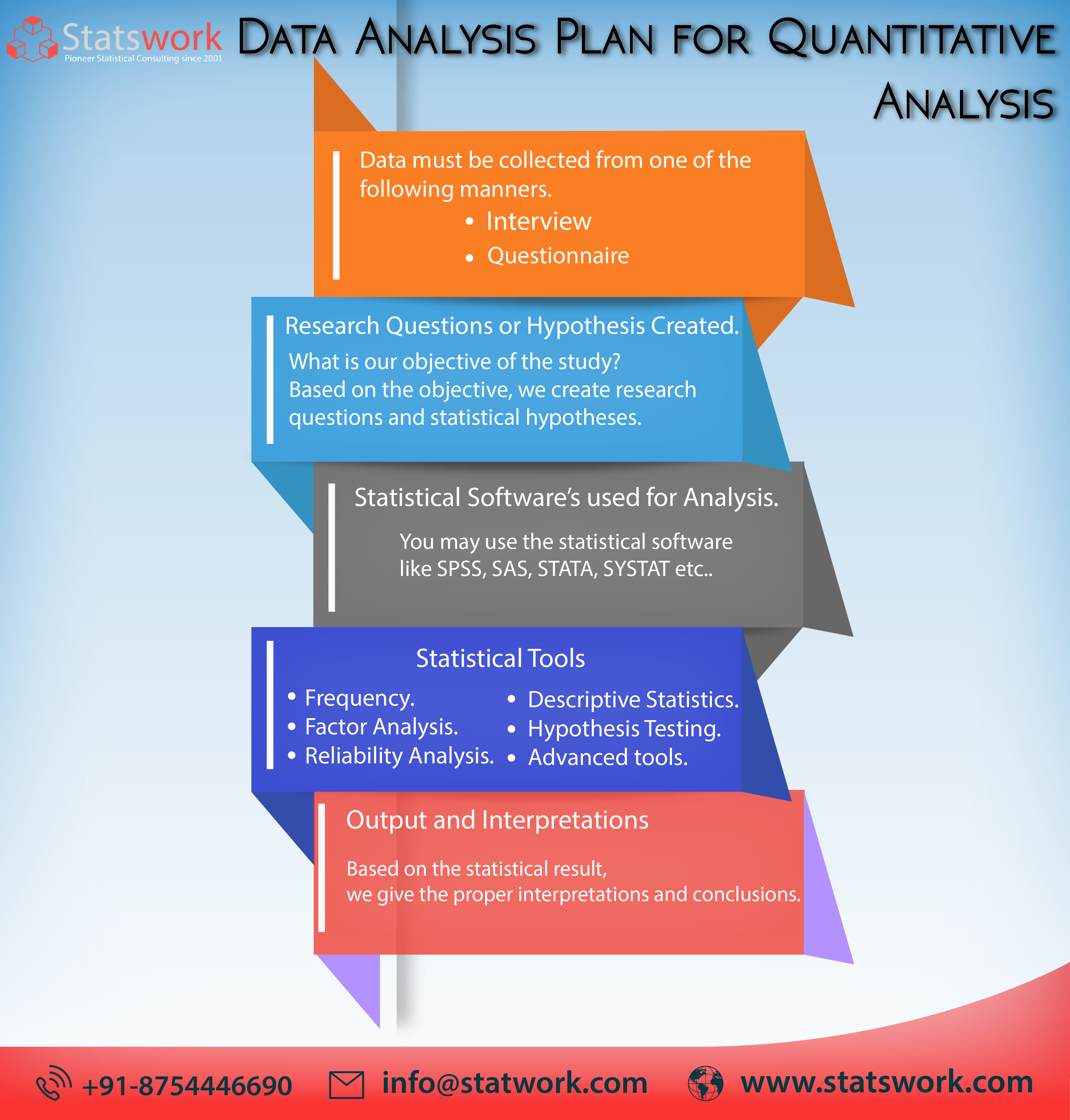
There are five steps used for analysis the quantitative data . Those are described in the below steps
Data must be collected from one of the following manners:
- Face to face interview
- Telephone interview
- Computer Assisted Personal Interview
Questionnaire
- Paper-pencil questionnaire
- Web based questionnaire
Research questions or hypothesis created
What is our objective of the study? Based on the objective, we create research questions and statistical hypotheses.
Statistical Software’s used for analysis
You may use the statistical software like SPSS , SAS, STATA, SYSTAT etc..
Statistical Tools
- Factor Analysis
- Reliability Analysis
- Descriptive Statistics
- Hypothesis Testing
- Parametric Tests
- Independent sample t test
- Paired sample t test
- Pearson Correlation Coefficient
- Regression Analysis
- Non-parametric Tests
- Mann-whitney U test
- Wilcoxon-signed ranked test
- Kruskal wallis test
- Spearman correlation
- Advanced tools
- SEM Analysis
Output and Interpretations
Based on the statistical result, we give the proper interpretations and conclusions
MAIN SERVICES
Directories & resources.
DataAnalysis Plan for qualitative research
Data Analysis Plan for quantitative Research
Data Analysis Planfor ANOVA
Data AnalysisPlan for Man-Whitney
Statistics SampleWork Database
Sample Size calculator
Power Calculation
Harvard Reference Generator
Vancouver Reference Generator
APA reference Generator
Financial RatioCalculator
Get the Help from Professional Statisticians & Biostatisticians.
Statswork popup.

- Privacy Overview
- Strictly Necessary Cookies
- 3rd Party Cookies
This website uses cookies so that we can provide you with the best user experience possible. Cookie information is stored in your browser and performs functions such as recognising you when you return to our website and helping our team to understand which sections of the website you find most interesting and useful.
Strictly Necessary Cookie should be enabled at all times so that we can save your preferences for cookie settings.
If you disable this cookie, we will not be able to save your preferences. This means that every time you visit this website you will need to enable or disable cookies again.
This website uses Google Analytics to collect anonymous information such as the number of visitors to the site, and the most popular pages.
Keeping this cookie enabled helps us to improve our website.
Please enable Strictly Necessary Cookies first so that we can save your preferences!
Log in using your username and password
- Search More Search for this keyword Advanced search
- Latest content
- For authors
- Browse by collection
- BMJ Journals More You are viewing from: Google Indexer
You are here
- Volume 14, Issue 3
- What impact has the Centre of Research Excellence in Digestive Health made in the field of gastrointestinal health in Australia and internationally? Study protocol for impact evaluation using the FAIT framework
- Article Text
- Article info
- Citation Tools
- Rapid Responses
- Article metrics

- http://orcid.org/0000-0002-8647-5933 Natasha Koloski 1 , 2 , 3 ,
- Kerith Duncanson 1 , 4 ,
- http://orcid.org/0000-0003-1374-5565 Shanthi Ann Ramanathan 1 , 4 ,
- Melanie Rao 4 ,
- Gerald Holtmann 3 , 5 ,
- Nicholas J Talley 1 , 4
- 1 School of Medicine and Public Health , University of Newcastle , Callaghan , New South Wales , Australia
- 2 School of Health & Behavioural Sciences , University of Queensland , St Lucia , Queensland , Australia
- 3 Department of Gastroenterology & Hepatology , Princess Alexandra Hospital , Woolloongabba , Queensland , Australia
- 4 Hunter Medical Research Institute , Newcastle , New South Wales , Australia
- 5 School of Medicine , University of Queensland , St Lucia , Queensland , Australia
- Correspondence to Nicholas J Talley; nicholas.talley{at}newcastle.edu.au
Introduction The need for public research funding to be more accountable and demonstrate impact beyond typical academic outputs is increasing. This is particularly challenging and the science behind this form of research is in its infancy when applied to collaborative research funding such as that provided by the Australian National Health and Medical Research Council to the Centre for Research Excellence in Digestive Health (CRE-DH).
Methods and analysis In this paper, we describe the protocol for applying the Framework to Assess the Impact from Translational health research to the CRE-DH. The study design involves a five-stage sequential mixed-method approach. In phase I, we developed an impact programme logic model to map the pathway to impact and establish key domains of benefit such as knowledge advancement, capacity building, clinical implementation, policy and legislation, community and economic impacts. In phase 2, we have identified and selected appropriate, measurable and timely impact indicators for each of these domains and established a data plan to capture the necessary data. Phase 3 will develop a model for cost–consequence analysis and identification of relevant data for microcosting and valuation of consequences. In phase 4, we will determine selected case studies to include in the narrative whereas phase 5 involves collation, data analysis and completion of the reporting of impact.
We expect this impact evaluation to comprehensively describe the contribution of the CRE-DH for intentional activity over the CRE-DH lifespan and beyond to improve outcomes for people suffering with chronic and debilitating digestive disorders.
Ethics and dissemination This impact evaluation study has been registered with the Hunter New England Human Research Ethics Committee as project 2024/PID00336 and ethics application 2024/ETH00290. Results of this study will be disseminated via medical conferences, peer-reviewed publications, policy submissions, direct communication with relevant stakeholders, media and social media channels such as X (formely Twitter).
- Protocols & guidelines
- Irritable Bowel Syndrome
- Inflammatory bowel disease
This is an open access article distributed in accordance with the Creative Commons Attribution Non Commercial (CC BY-NC 4.0) license, which permits others to distribute, remix, adapt, build upon this work non-commercially, and license their derivative works on different terms, provided the original work is properly cited, appropriate credit is given, any changes made indicated, and the use is non-commercial. See: http://creativecommons.org/licenses/by-nc/4.0/ .
https://doi.org/10.1136/bmjopen-2023-076839
Statistics from Altmetric.com
Request permissions.
If you wish to reuse any or all of this article please use the link below which will take you to the Copyright Clearance Center’s RightsLink service. You will be able to get a quick price and instant permission to reuse the content in many different ways.
STRENGTHS AND LIMITATIONS OF THIS STUDY
This protocol provides a prospective view of the application of the Framework to Assess the Impact of Translational health research to the Centre for Research Excellence in Digestive Health (CRE-DH with the explicit aim of optimising research impact and providing direction for future digestive health planning and prioritisation.
This protocol describes three validated methods of impact assessment including the Payback Framework that describes impact using quantified metrics in different domains, economic analyses to quantify the return on research investment and narratives to describe the pathway to impact and provide qualitative evidence of impact.
There is always a lag in the health research translation process resulting in delays in reporting the full extent of research impact. This lag will limit the reporting of the longer-term benefits of the CRE-DH, for which evidence will not be available.
Introduction
Chronic gastrointestinal (GI) diseases are a major health burden in Australia and worldwide. 1 2 More than one-third of Australians experience chronic or relapsing unexplained GI symptoms. 3 4 In half of these cases, symptoms are serious enough to require a medical consultation usually at a general practitioner clinic or an emergency department. These cases also currently make up half of all referrals to GI specialists. 5 For the majority of cases, however, no structural or biochemical abnormality is found after comprehensive and costly diagnostic workup resulting in a diagnosis of a disorder of gut-brain interaction (DGBI) most notably irritable bowel syndrome (IBS) or functional dyspepsia. 6 7 Currently, there is no cure and for DGBIs treatment approaches are suboptimal, leading to frequent healthcare consultations by these patients. 8 IBS alone has been estimated to cost more than US$41 billion annually in the USA. 2 For other chronic GI conditions, including gastro-oesophageal reflux disease and inflammatory bowel disease (IBD), the prevalence is increasing, placing pressure on the healthcare system. 9 10 Chronic GI diseases are also associated with significantly impaired quality of life, reduced work productivity, work absenteeism, relationship problems, higher levels of psychological distress and extraintestinal symptoms. 11–16
While there have been impressive advancements into the underlying pathology of chronic GI diseases in recent years, 17 18 there have been delays in the development of novel, pathology-based, subtyping of DGBI to facilitate improved integrated care and rationalised therapeutic strategies in clinical practice. This critical need was recognised by the Australian National Health and Medical Research Council (NHMRC) which funded the Centre for Research Excellence in Digestive Health (CRE-DH) from 2019 to 2024. The CRE’s vision is to advance the understanding, identification and treatment of chronic digestive diseases by implementing a risk-based and pathophysiology-based categorisation of patients and targeted treatments that are suitable for all sectors of the healthcare system (including primary care).
The specific objectives of the CRE scheme are to improve health-related outcomes and enhance translation of research outcomes into policy and/or practice while also building capacity in the health and medical research workforce. 19 This is aligned with the NHMRC definition of the impact of research as ‘the verifiable outcomes that research makes to knowledge, health, the economy and/or society, and not the prospective or anticipated effects of the research’. 20 However, the NHMRC also recognises that ‘the relationship between research and impact is often indirect, non-linear and not well understood and depends on complex interactions and collaboration across the health innovation system. 20 ’ This emphasis on research impact arises from the growing pressure on grant funding bodies to be accountable for taxpayer-funded research and provide evidence of the wider benefits of research above and beyond traditional academic outputs (eg, publications). Examples include evidence of translation to new drugs and devices, changes to policy and practice and ultimately the social and economic impacts on society including the return on research investment, in order to support continued research funding.
In light of the complexities involved in assessing the impact from research, a myriad of Research Impact Assessment Frameworks (RIAFs) have been developed that provide a conceptual framework and methods against which the translation and impact of research can be assessed. 21 22 However, most RIAFs tend to focus on specific research studies rather than research programmes such as CREs and are typically used retrospectively to justify past research investments. In contrast, the Framework to Assess the Impact from Translational health research (FAIT), developed by a team of health economists and health and medical researchers from the Hunter Medical Research Institute, is prospective in design and incorporates monitoring and feedback with the specific aim of increasing translation and impact. 23 Ramanathan et al applied FAIT to the CRE in Stroke Rehabilitation and Brain Recovery and assessed its validity and feasibility. 24 Overall, they found FAIT allowed a wide range of impacts to be reliably reported beyond the standard academic achievements. Thus, to take advantage of FAIT’s comprehensive design and prospective application, and allow for better benchmarking with other CREs, we have selected FAIT to assess the impact of the CRE-DH. This paper describes the protocol of a mixed methods study to:
Demonstrate the research impact and monetise the return on investment in the CRE-DH.
Provide a prospective view of optimising research impact.
Assess the suitability of FAIT.
The anticipated outcomes will be greater transparency and translation of research within CRE-DH, and the data will set the direction for future digestive health planning and prioritisation. In addition, this paper will contribute to this growing area of research impact assessment.
We prospectively applied FAIT to measure the impact of the CRE-DH. FAIT incorporates three validated methods of impact assessment. The Payback Framework describes impact within domains of benefit. Within FAIT, it has been modified to capture impact using quantitative indicators rather than qualitative data. Economic analyses are applied to quantify the return on research investment and narratives are used to describe the pathway to impact and provide qualitative evidence of impact. The assessment of the suitability of FAIT will take the form of a facilitated discussion among authors, at the conclusion of the impact evaluation, to identify the strengths and limitations of FAIT in the context of its application to the CRE and to make suggestions, if appropriate, for its future application
Details of FAIT have been previously published. 23
The setting is the CRE-DH, which is composed of senior, mid-career, early career and student researchers, clinicians, consumers and other key stakeholders in the fields of gastroenterology, immunology, microbiology, epidemiology, dietetics, psychology and biostatistics primarily from four major research centres across Australia. These include the University of Newcastle and Macquarie University in New South Wales, Princess Alexandra Hospital and University of Queensland in Queensland, and Monash University in Victoria, along with substantial international contributions from the University of Leuven in Belgium, McMaster University in Canada, Mayo Clinic in USA and Kings College in the UK. The CRE-DH researchers pool their highly complementary expertise and capabilities for projects within the CRE-DH, which facilitates recruitment of large representative patient cohorts, the availability of cutting-edge methodologies and translation of findings into practice and policy. The CRE-DH was funded ($A2.5 million) from 2019 to 2024.
Participants
These include a mix of experienced, early career and student researchers associated with the CRE-DH and end users of the findings and outputs of the CRE-DH including other DGBI researchers, patients, consumers more broadly, clinicians, health services, policy-makers and industry partners.
Patient and public involvement
Development of the FAIT model involved extensive and broad end user engagement including interviews with the following key stakeholder groups—researchers from across the research spectrum, multiple Australian medical research institutes, health and medical research funders including the NHMRC, Australian Research Council, The Medical Research Futures Fund, NSW Office for Health and Medical Research, Brunel University, UK and Karolinska Institute, Sweden who were leaders in the field at the time and policy-makers. All interviews were conducted by staff from the Health Economics and Impact team at HMRI and covered attitudes to impact measurements, barriers and enablers, what was being done at the time and opinions about what should be done. There was a diversity of views and differences which were reconciled by designing a comprehensive framework (FAIT) that addressed all their needs. There is an absolute bias to selecting and reporting metrics for which there are data and this is addressed by impact planning that ensures as much data as possible is collected from the start. Other ways this bias is mitigated is by expressing the limitations and bias inherent in an impact assessment framework like FAIT.
This was supplemented by broad consumer representation on the CRE-DH advisory board that provided feedback at all stages of CRE-DH impact framework development. The use of the existing Payback domains and input from consumers with a range of conditions and experiences will ensure that the metrics selected reflect a broad range of potential impacts beyond academic impacts.
The study involves a five-stage sequential mixed method design, summarised as follows:
Phase 1: Development of a programme logic model (PLM) to map the pathway to impact and establish domains of benefit and aspirational impacts.
Phase 2: Identifying and selecting appropriate, measurable and timely impact indicators for each of these domains and establishing a data plan to capture the necessary data.
Phase 3: Developing a model for the cost–consequence analysis and identification of relevant data for micro costing and valuation of consequences (where appropriate).
Phase 4: Determining selected case studies to include in the narrative including the data collection for these.
Phase 5: Collation, data analysis and completion of the reporting of impact using the three methods.
Phase 1: development of a logic model to map the pathway to impact and establish domains of benefit
A PLM is a critical component of any FAIT impact assessment. The PLM used in FAIT is a map that follows the pathway from the need for the CRE through its aims, activities, outputs and aspirational impacts. The CRE-DH logic model ( figure 1 ) shows how the needs and aims drive CRE activities. These activities should produce outputs that, when used by an end user, creates an opportunity for the generation of impact. These impacts are articulated as both short-term and medium-long-term impacts under broad domains of benefit such as impacts on knowledge advancement, capacity building, clinical implementation, policy legislation, community and economic impacts. While the PLM appears linear, its application over the lifetime of the CRE-DH will most likely be non-linear and subject to change.
- Download figure
- Open in new tab
- Download powerpoint
Logic model for the CRE-DH. CRE-DH, Centre for Research Excellence in Digestive Health; DGBI, disorder of gut brain interaction; GI, gastrointestinal; QOL, quality of life; TGA, Therapeutic Goods Administratio; EMCR, Early/Mid career researchers
Phase 2: identifying and selecting appropriate, measurable and timely impact indicators for each of these domains and establishing a data plan to capture the necessary data
The PLM ( figure 1 ) identifies the Payback domains of benefits under which the CRE’s impact will be assessed. Impact metrics have been developed and customised for the CRE-DH taking into account their appropriateness for the CRE-DH and its aims and their ability to be measured in a timely manner. Table 1 shows the list of Payback metrics under each domain for which evidence is captured.
- View inline
Payback metrics table for the CRE-DH
Routine monitoring of implementation embedded into each project stream
The purpose of this data collection method is to collect quantitative data to monitor and measure the impact of specific studies within the CRE-DH and its capacity building and translational activities. Initial data collection involves annual distribution of a CRE-DH impact data survey via REDCap to chief investigators and associate investigators to be populated for all their CRE-DH affiliated researchers. Results of the survey are being collated into an Excel file that includes individual spreadsheets that are aligned with impact indicators. Additional data are being retrieved from available sources including publicly available online data from researchers’ university profiles, data collected for triannual CRE-DH advisory board meetings, through ethics systems, publication tracking and evaluation of CRE-DH organised capacity building and translational activities. The Excel spreadsheets for each project stream are being emailed annually to each CI to add any data that has not been captured using the above methods.
Reports during the regular team meetings
This data collection method aims to collect quantitative and qualitative data to monitor and measure the translation, implementation and impact of CRE-DH that are not obtained from routine monitoring. The data are collected online by accessing the recorded monthly CRE-DH meeting minutes and added to project stream spreadsheets or flagged for further discussion in semistructured interviews for vignettes or case study examples of CRE-DH impact, described as part of phase 4.
Phase 3: developing a model for the cost–consequence analysis and identification of relevant data for microcosting and valuation of consequences (where appropriate)
To determine whether the cost associated with the delivery and participation in activities associated with the CRE-DH and the consequences achieved represent a good return on investment, a cost–consequence analysis will be undertaken. 25
First, we will detail out the activities funded by the NHMRC investment. Second, we will microcost any activity and other costs not covered by the US$2.5 million NHMRC research investment and add these to the NHMRC investment as implementation costs. This will include costing all in-kind investigator time and capacity building participation time not directly funded by the CRE monies.
Microcosting data will involve a log of all intervention activities including the individual’s involved, their roles and wages and the time taken for implementation. Other resources such as travel and consumables will also be costed. The proportion of cost attributable to CRE-DH activity will be estimated where feasible.
In collaboration with the lead investigators of the CRE-DH, the consequences of the CRE-DH will be established including the consequences that cannot be monetised and appear in their natural units in the Payback metrics table. For those consequences that can be monetised, economic methods will be employed to adequately monetise their value and determine the appropriate level of attribution to the CRE-DH. This will include a search of the literature for established values for these consequences (where they occur), clearly defined assumptions about these values and sensitivity analyses to account for any variance in these values. Given that CRE-DH activity will be occurring concurrently with other research activities supported by the research institutions from which CRE-DH researchers are affiliated, attribution of consequences (eg, leveraged funding) will take this into account. Where practical, researchers will be asked for their own assessment of CRE-DH attribution to a particular consequence or a conservative attribution percentage will be applied to avoid overclaiming the consequences and impacts of CRE-DH. All values will be converted into Australian dollars and valued in the year that the final analysis is conducted.
Phase 4: determining selected case studies to include in the narrative including the data collection for these
During the course of the CRE-DH, the pathways to adoption of the outputs will be documented by the team and team meetings will be used to highlight potential case studies that can be developed to demonstrate outstanding impacts of the CRE-DH or case studies that describe key learnings. Semistructured interviews will be conducted to collect relevant data that will inform these case studies. It is anticipated that these interviews will be with CRE-DH researchers and key end users, where appropriate.
Semistructured interviews involving CRE-DH staff, collaborative investigators, advisory group members and other key stakeholders
Qualitative data will be collected, to provide context and a richer, more comprehensive overall understanding of the impact of the CRE-DH. Topics of interest will be flagged through the quantitative data collection and in meeting discussions, based on the underlying question of ‘How did this publication, conference presentation, collaboration, capacity building activity or project lead to an impactful outcome that would not have been achieved without the CRE-DH?’ Interviews will be facilitated by the HMRI FAIT team, who have expertise in qualitative data collection for impact evaluation. These data will be narratively synthesised and triangulated with quantitative data and incorporated into impact evaluation reporting within the narrative method and include specific quotes from the researchers and end-users.
Impact assessment data will be collected for the 5-year period from November 2019 to October 2024.
Phase 5: collation, data analysis and completion of the reporting of impact using the three FAIT methods
The data collected over the course of the CRE-DH using the various methods described above will be reported using the FAIT scorecard format. 23
Results for the metrics table will be collated and where bibliometric results are required, a cut-off date will be established after which time, the results will not be updated. The cost–consequence will be reported by way of a cost–consequence table that will only include the consequences that can be monetised. Other consequences will be reported in their natural units in the Payback metrics tables. The narratives will be reported as vignettes highlighting some of the outstanding achievements of the CRE-DH including the pathway to translation and impact.
Ethics and dissemination
This impact evaluation study has been registered with Hunter New England Human Research Ethics Committee as project 2024/PID00336 and ethics application 2024/ETH00290. Results of this study will be disseminated via medical conferences, peer-reviewed publications, policy submissions, direct communication with relevant stakeholders, media and social media channels such as X (formerly Twitter).
This protocol aims to define and describe processes to collect, collate and synthesise data for the CRE-DH to evaluate the impact of the CRE-DH from inception in November 2019 to final data collection in mid-2024 for reporting of outcomes in October 2024. We plan to operationalise this protocol as a mixed-methods study by applying a PLM to the original aims and needs identified in our CRE-DH application, to use that modelling to review CRE-DH progress towards our aims, and to inform prospective direction for the CRE-DH based on ongoing progress and at specified annual data collection review time points. Therefore, our impact evaluation will be an organic, prospective, informative and responsive process, as well as providing an overall final and retrospective account of CRE-DH impact by the end of 2024. Impact will be reported and used to inform future funding applications and direction for digestive health research in Australia, and position the CI, AI and affiliate team as leaders in the field internationally. This impact evaluation will also inform future directions for DGBI and other digestive diseases research, which we expect to overlap and integrate more with related fields such as immune and microbiome research in coming years. The prospective design of our impact evaluation will facilitate expansion into new fields throughout the life of the CRE-DH, which will enhance translation potential, impact and transformative research and clinical practice change.
Although, there are other frameworks from various medical fields 26 to assess evaluation of research outcomes, this evaluation applied the FAIT to the CRE-DH with the explicit aim of optimising research impact and providing direction for future digestive health planning and prioritisation.
Despite the benefits of comprehensively assessing the impact of the CRE-DH using three distinct methods namely quantified impact metrics, a cost–consequence analysis and a narrative of the impact there are some potential risks and limitations. These include (1) Lag in translation could impact on the ability to capture and demonstrate longer-term impacts. (2) Data collection for impact reporting while feasible, does require additional commitment by CRE partners to ensure it is comprehensive and complete. Therefore, this could be seen as an added administrative burden and may not be completed as required. However, the desire to continue the collaboration and the fact that CRE affiliates have been engaged with the impact assessment from the start should provide a counterbalance to the burden. The inclusion of the HMRI Research Impact Team as expert advisors will also ensure that multiple strategies previously used in other CRE impact assessments are employed to enhance data collection. (3) Attribution of impacts is challenging and will have to rely on researchers to attribute the contribution of CRE-DH to a particular consequence. (4) Selection of case studies means other potential impact stories may be foregone.
The novelty of this work is that the application of FAIT is still very much in its infancy with only two protocol papers (both using very different framings for the application) 24 27 and only one results paper published. 28 There is still much to learn and reflect on in the application of such a comprehensive framework, and this protocol paper will provide a useful roadmap for other GI research collaborations planning formal impact evaluations. A deepened understanding about what enhances the impact of a CRE will only be possible when we have benchmarked protocols and outcomes. We will then have the ability to undertake meta-analyses to ascertain what works under what circumstances in order to further enhance the impact in a large and complex research collaborative such as a CRE. Contribution to a larger bank of metrics will give visibility to the potential capacity and capability impacts from CREs.
This study will capture outputs and impacts that have been initiated or enhanced as a result of the CRE-DH’s collaborative efforts of basic scientists, allied health and medical clinician researchers, translational scientists, consumers and advisors across the spectrum from animal, preclinical laboratory research to health service delivery from acute to integrated and primary care settings. All costs for CRE-DH activity will be valued and where possible, the economic analysis will monetise reportable CRE-DH outcomes and impacts. If this is not possible, these impacts will be reported in their natural units. We expect this impact evaluation to comprehensively describe the contribution of the CRE-DH to a range of impacts including any improved outcomes for people suffering with chronic and debilitating digestive disorders. The impact evaluation will inform future directions for digestive health research and assessment of its impact.
Ethics statements
Patient consent for publication.
Not applicable.
- Camilleri M ,
- Williams DE
- Talley NJ ,
- Burke C , et al
- Koloski NA ,
- Thompson WG ,
- Heaton KW ,
- Smyth GT , et al
- Chang L , et al
- Stanghellini V ,
- Hasler WL , et al
- Tornkvist NT ,
- Whitehead WE , et al
- Sepanlou SG ,
- Ikuta K , et al
- Li Z , et al
- Knowles SR ,
- Wilding H , et al
- Loundou A ,
- Hamdani N , et al
- Barberio B ,
- Black CJ , et al
- Canavan C ,
- Pimentel M ,
- Lazaridis N ,
- Germanidis G
- ↵ Available : https://www.nhmrc.gov.au/funding/find-funding/centres-research-excellence
- ↵ Available : https://www.nhmrc.gov.au/research-policy/research-translation-and-impact/research-impact
- Ramanathan S ,
- Angell B , et al
- Bauman AE ,
- Searles A ,
- Attia J , et al
- Deeming S , et al
- Brazier J ,
- Ratcliffe J ,
- Saloman J , et al
- Moldovan F ,
- Moldovan L ,
- Bernhardt J , et al
Twitter @Ramanathan
Contributors NK was involved in conceptualisation, methodology, project administration, writing of the original draft, revisions and editing. KD contributed to conceptualisation, writing of the original draft,revisions and editing. SAR was involved in the conceptualisation, methodology and writing of the original draft. MR, GH and NT were involved in the writing of the original draft, revisions and editing. In addition, GH and NT were involved in funding acquisition and resources.
Funding This work was supported by National Health and Medical Research Council of Australia, APP1170893.
Competing interests NK, KD, SAR and MR disclose no conflicts. NT is Emeritus Editor-in-Chief of Medical Journal of Australia, Section Editor of Up to Date and has research collaborations with Intrinsic Medicine (human milk oligosaccharide), Alimentry (gastric mapping) and is a consultant for Agency for Health Care Research and Quality (fiber and laxation), outside the submitted work. In addition, he has licenced Nepean Dyspepsia Index (NDI) to MAPI, and Talley Bowel Disease Questionnaire licensed to Mayo/Talley, 'Diagnostic marker for functional gastrointestinal disorders' Australian Provisional Patent Application 2021901692, 'Methods and compositions for treating age-related neurodegenerative disease associated with dysbiosis' US Patent Application No. 63/537,725. GH received unrestricted educational support from the Falk Foundation. Research support was provided via the Princess Alexandra Hospital, Brisbane by GI Therapies, Takeda Development Center Asia, Eli Lilly Australia, F. Hoffmann-La Roche, MedImmune, Celgene, Celgene International II Sarl, Gilead Sciences, Quintiles, Vital Food Processors, Datapharm Australia Commonwealth Laboratories, Prometheus Laboratories, FALK GmbH & Co KG, Nestle, Mylan and Allergan (prior to acquisition by AbbVie). GH is also a patent holder for a biopsy device to take aseptic biopsies (US 20150320407 A1).
Patient and public involvement Patients and/or the public were involved in the design, or conduct, or reporting, or dissemination plans of this research. Refer to the Methods section for further details.
Provenance and peer review Not commissioned; externally peer reviewed.
Read the full text or download the PDF:
ACR Responds to NIH Strategic Plan for Data Science
The page you recommended will be added to the "what others are reading" feed on "My ACR".
The page you bookmarked will be added to the "my reading list" feed on "My ACR".
The American College of Radiology® (ACR®) recently submitted comments in response to a National Institutes of Health (NIH) request for information (RFI) that sought input regarding the NIH Strategic Plan for Data Science 2023–2028. NIH sought input on its five goals of the strategic plan:
- Goal 1: Improve Capabilities to Sustain the NIH Policy for Data Management and Sharing.
- Goal 2: Develop Programs to Enhance Human Derived Data for Research.
- Goal 3: Provide New Opportunities in Software, Computational Methods and Artificial Intelligence.
- Goal 4: Support for a Federated Biomedical Research Data Infrastructure.
- Goal 5: Strengthen a Broad Community in Data Science.
In the submitted comments, ACR agreed with the concepts to promote trustworthy artificial intelligence (AI) in the strategic plan but highlighted the need for NIH to clarify how data collected from medical images used in research projects and AI training will be handled. Additionally, ACR noted the public distrust of data sharing, specifically in the context of AI and machine learning, and encouraged clear communication to explain patient confidentiality safeguards, as well as the significance of the development of new systems to advance and monitor novel treatments and diagnostics.
ACR looks forward to continued collaborations with the NIH and serving as a resource for the agency.
For more information, contact Katie Grady , ACR Government Affairs Director.
ORIGINAL RESEARCH article
Culturally responsive leadership: a critical analysis of one school district's five-year plan provisionally accepted.
- 1 University of North Texas, United States
- 2 Loyola University Maryland, United States
The final, formatted version of the article will be published soon.
Centering the need for culturally responsive leadership (CRL), this study engages in a critical analysis of one large urban school district's 5-year plan that aims to be culturally responsive and equity focused. We first define the various facets of CRL, connect its major components to culturally responsive teaching/pedagogy (CRTP) and student voice (SV), and offer an original, integrative framework as a tool for analysis. We argue that CRL is not enough on its own and needs more than the commitment of principals to reach its maximum potential. We also provide recommendations on what needs to happen to make culturally responsive schooling a reality for students and their communities.
Keywords: culturally responsive leadership 1, Culturally Responsive Teaching 2, culturally responsive teaching 3, student voice 4, equity 5, capacity building 6 KM: Conceptualization, Data curation, analysis
Received: 13 Feb 2024; Accepted: 21 Mar 2024.
Copyright: © 2024 Mansfield and Lambrinou. This is an open-access article distributed under the terms of the Creative Commons Attribution License (CC BY) . The use, distribution or reproduction in other forums is permitted, provided the original author(s) or licensor are credited and that the original publication in this journal is cited, in accordance with accepted academic practice. No use, distribution or reproduction is permitted which does not comply with these terms.
* Correspondence: Prof. Katherine C. Mansfield, University of North Texas, Denton, United States
People also looked at
Next-gen B2B sales: How three game changers grabbed the opportunity
Driven by digitalized operating models, B2B sales have seen sweeping changes over the recent period amid rising customer demand for more seamless and transparent services. 1 “ The multiplier effect: How B2B winners grow ,” McKinsey, April 13, 2023. However, many industrial companies are failing to keep pace with their more commercially focused peers and, as a result, are becoming less competitive in terms of performance and customer services.
The most successful B2B players employ five key tactics to sharpen their sales capabilities: omnichannel sales teams; advanced sales technology and automation; data analytics and hyperpersonalization; tailored strategies on third-party marketplaces; and e-commerce excellence across the full marketing and sales funnel. 2 “ The multiplier effect: How B2B winners grow ,” McKinsey, April 13, 2023.
Companies using all of these tactics are twice as likely to see more than 10 percent market share growth than companies focusing on just one. 3 “ The multiplier effect: How B2B winners grow ,” McKinsey, April 13, 2023. However, implementation is not as simple, requiring a strategic vision, a full commitment, and the right capabilities to drive change throughout the organization. Various leading European industrial companies—part of McKinsey’s Industrial Gamechangers on Go-to-Market disruption in Europe—have achieved success by implementing the first three of these five sales tactics.
Omnichannel sales teams
The clearest rationale for accelerating the transition to omnichannel go-to-market is that industry players demand it. In 2017, only about 20 percent of industrial companies said they preferred digital interactions and purchases. 4 Global B2B Pulse Survey, McKinsey, April 30, 2023. Currently, that proportion is around 67 percent. In 2016, B2B companies had an average of five distinct channels; by 2021, that figure had risen to ten (Exhibit 1).
Excelling in omnichannel means enabling customers to move easily between channels without losing context or needing to repeat information. Companies that achieve these service levels report increased customer satisfaction and loyalty, faster growth rates, lower costs, and easier tracking and analysis of customer data. Across most of these metrics, the contrast with analogue approaches is striking. For example, B2B companies that successfully embed omnichannel show EBIT growth of 13.5 percent, compared to the 1.8 percent achieved by less digitally enabled peers. Next to purely digital channels, inside sales and hybrid sales are the most important channels to deliver an omnichannel experience.
Differentiating inside versus hybrid sales
Best-in-class B2B sellers have achieved up to 20 percent revenue gains by redefining go-to-market through inside and hybrid sales. The inside sales model cannot be defined as customer service, nor is it a call center or a sales support role—rather, it is a customer facing, quota bearing, remote sales function. It relies on qualified account managers and leverages data analytics and digital solutions to optimize sales strategy and outreach through a range of channels (Exhibit 2).
The adoption of inside sales is often an advantageous move, especially in terms of productivity. In fact, inside sales reps can typically cover four times the prospects at 50 percent of the cost of a traditional field rep, allowing the team to serve many customers without sacrificing quality of service. 5 McKinsey analysis. Top performing B2B companies are 50 percent more likely to leverage inside sales.
Up to 80 percent of a company’s accounts—often smaller and medium-sized customers, accounting for about half of revenues—can be covered by inside sales teams. 6 Industry expert interviews; McKinsey analysis. The remaining 20 percent often require in-person interactions, triggering the need for hybrid sales. This pertains to highly attractive leads as well.
Hybrid sales is an innovative model combining inside sales with traditional in-person interactions. Some 85 percent of companies expect hybrid sales will be the most common job role within three years. 7 Global B2B Pulse Survey, McKinsey, December 2022. Hybrid is often optimal for bigger accounts, as it is flexible in utilizing a combination of channels, serving customers where they prefer to buy. It is scalable, thanks to the use of remote and online sales, and it is effective because of the multiplier effect of numerous potential interactions. Of companies that grew more than 10 percent in 2022, 57 percent had adopted a hybrid sales model. 8 Global B2B Pulse, April 2023.
How an industrial automation solution player implemented game-changing inside sales
In 2019, amid soaring digital demand, a global leader in industrial digital and automation solutions saw an opportunity to deliver a cutting-edge approach to sales engagement.
As a starting point, the company took time to clearly define the focus and role of the inside sales team, based on product range, customer needs, and touchpoints. For simple products, where limited customer interaction was required, inside sales was the preferred go-to-market model. For more complex products that still did not require many physical touchpoints, the company paired inside sales teams with technical sales people, and the inside sales group supported fields reps. Where product complexity was high and customers preferred many touch points, the inside sales team adopted an orchestration role, bringing technical functions and field sales together (Exhibit 3).
The company laid the foundations in four key areas. First, it took time to sketch out the model, as well as to set targets and ensure the team was on board. As in any change program, there was some early resistance. The antidote was to hire external talent to help shape the program and highlight the benefits. To foster buy-in, the company also spent time creating visualizations. Once the team was up and running, early signs of success created a snowball effect, fostering enthusiasm among both inside sales teams and field reps.
Second, the company adopted a mantra: inside sales should not—and could not—be cost saving from day one. Instead, a significant part of the budget was allocated to build a tech stack and implement the tools to manage client relationships. One of the company’s leaders said, “As inside sales is all about using tech to obtain better outcomes, this was a vital step.”
The third foundational element was talent. The company realized that inside sales is not easy and is not for everyone—so finding the right people was imperative. As a result, it put in place a career development plan and recognized that many inside sales reps would see the job as a stepping stone in their careers. Demonstrating this understanding provided a great source of motivation for employees.
Finally, finding the right mix of incentives was key. The company chose a system based on compensation and KPI leading and lagging indicators. Individual incentives were a function of whether individuals were more involved with closing deals or supporting others, so a mix of KPIs was employed. The result was a more motivated salesforce and productive cooperation across the organization.
Advanced sales technology and automation
Automation is a key area of advanced sales technology, as it is critical to optimizing non-value adding activities that currently account for about two-thirds of sales teams’ time. More than 30 percent of sales tasks and processes are estimated to be partially automatable, from sales planning through lead management, quotation, order management, and post-sales activities. Indeed, automation leaders not only boost revenues and reduce cost to serve—both by as much as 20 percent—but also foster customer and employee satisfaction. (Exhibit 4). Not surprisingly, nine out of ten industrial companies have embarked on go-to-market automation journeys. Still, only a third say the effort has achieved the anticipated impact. 9 McKinsey analysis.
Leading companies have shown that effective automation focuses on four areas:
- Lead management: Advanced analytics helps teams prioritize leads, while AI-powered chatbots contact prospective customers via text or email and schedule follow-up calls at promising times—for example, at the beginning or end of the working day.
- Contract drafting: AI tools automate responses to request for proposal (RFP) inquiries, based on a predefined content set.
- Invoice generation: Companies use robotic process automation to process and generate invoices, as well as update databases.
- Sales commission planning: Machine learning algorithms provide structural support, for example, to optimize sales commission forecasting, leading up to a 50 percent decline in time spent on compensation planning.
How GEA seized the automation opportunity
GEA is one of the world’s most advanced suppliers of processing machinery for food, beverages, and pharmaceuticals. To provide customers with tailored quotes and services, the company launched a dedicated configure, price, quote (CPQ) system. The aim of the system was to enable automated quote creation that would free up frontline sales teams to operate independently from their back office colleagues. This, in turn, would boost customer interaction and take customer care to the next level.
The work began with a bottom-up review of the company’s configuration protocols, ensuring there was sufficient standardization for the new system to operate effectively. GEA also needed to ensure price consistency—especially important during the recent supply chain volatility. For quotations, the right template with the correct conditions and legal terms needed to be created, a change that eventually allowed the company to cut its quotation times by about 50 percent, as well as boost cross-selling activities.
The company combined the tools with a guided selling approach, in which sales teams focused on the customers’ goals. The teams then leveraged the tools to find the most appropriate product and pricing, leading to a quote that could be enhanced with add-ons, such as service agreements or digital offerings. Once the quote was sent and agreed upon, the data automatically would be transferred from customer relationship management to enterprise resource planning to create the order. In this way, duplication was completely eliminated. The company found that the sales teams welcomed the new approach, as it reduced the time to quote (Exhibit 5).
Data analytics and hyperpersonalization
Data are vital enablers of any go-to-market transformation, informing KPIs and decision making across operations and the customer journey. Key application areas include:
- lead acquisition, including identification and prioritization
- share of wallet development, including upselling and cross-selling, assortment optimization, and microsegmentation
- pricing optimization, including market driven and tailored pricing, deal scoring, and contract optimization
- churn prediction and prevention
- sales effectiveness, so that sales rep time allocations (both in-person and virtual) are optimized, while training time is reduced
How Hilti uses machine data to drive sales
Hilti is a globally leading provider of power tools, services, and software to the construction industry. The company wanted to understand its customers better and forge closer relationships with them. Its Nuron battery platform, which harvests usage data from tools to transform the customer experience and create customer-specific insights, provided the solution.
One in three of Hilti’s frontline staff is in daily contact with the company’s customers, offering advice and support to ensure the best and most efficient use of equipment. The company broke new ground with its intelligent battery charging platform. As tool batteries are recharged, they transfer data to the platform and then to the Hilti cloud, where the data are analyzed to produce actionable insights on usage, pricing, add-ons, consumables, and maintenance. The system will be able to analyze at least 58 million data points every day.
Armed with this type of data, Hilti provides customers with advanced services, offering unique insights so that companies can optimize their tool parks, ensuring that the best tools are available and redundant tools are returned. In the meantime, sales teams use the same information to create deep insights—for example, suggesting that companies rent rather than buy tools, change the composition of tool parks, or upgrade.
To achieve its analytics-based approach, Hilti went on a multiyear journey, moving from unstructured analysis to a fully digitized approach. Still, one of the biggest learnings from its experience was that analytics tools are most effective when backed by human interactions on job sites. The last mile, comprising customer behavior, cannot be second guessed (Exhibit 6).
In the background, the company worked hard to put the right foundations in place. That meant cleaning its data (for example, at the start there were 370 different ways of measuring “run time”) and ensuring that measures were standardized. It developed the ability to understand which use cases were most important to customers, realizing that it was better to focus on a few impactful ones and thus create a convincing offering that was simple to use and effective.
A key element of the rollout was to ensure that employees received sufficient training— which often meant weeks of engagement, rather than just a few hours. The work paid off, with account managers now routinely supported by insights that enrich their interactions with customers. Again, optimization was key, ensuring the information they had at their fingertips was truly useful.
Levers for a successful transformation
The three company examples highlighted here illustrate how embracing omnichannel, sales technology, and data analytics create market leading B2B sales operations. However, the success of any initiative will be contingent on managing change. Our experience in working with leading industrial companies shows that the most successful digital sales and analytics transformations are built on three elements:
- Strategy: As a first step, companies develop strategies starting from deep customer insights. With these, they can better understand their customers’ problems and identify what customers truly value. Advanced analytics can support the process, informing insights around factors such as propensity to buy and churn. These can enrich the company’s understanding of how it wants its go-to-market model to evolve.
- Tailored solutions: Customers appreciate offerings tailored to their needs. 10 “ The multiplier effect: How B2B winners grow ,” McKinsey, April 13, 2023. This starts with offerings and services, extends to pricing structures and schemes, and ways of serving and servicing. For example, dynamic pricing engines that model willingness to pay (by segment, type of deal, and route to market) may better meet the exact customer demand, while serving a customer completely remotely might better suit their interaction needs, and not contacting them too frequently might prevent churn more than frequent outreaches. Analytics on data gained across all channels serves to uncover these needs and become hyperpersonalized.
- Single source of truth: Best-in-class data and analytics capabilities leverage a variety of internal and external data types and sources (transaction data, customer data, product data, and external data) and technical approaches. To ensure a consistent output, companies can establish a central data repository as a “single source of truth.” This can facilitate easy access to multiple users and systems, thereby boosting efficiency and collaboration. A central repository also supports easier backup, as well as data management and maintenance. The chances of data errors are reduced and security is tightened.
Many companies think they need perfect data to get started. However, to make productive progress, a use case based approach is needed. That means selecting the most promising use cases and then scaling data across those cases through speedy testing.
And with talent, leading companies start with small but highly skilled analytics teams, rather than amassing talent too early—this can allow them to create an agile culture of continual improvement and cost efficiency.
As shown by the three companies discussed in this article, most successful B2B players employ various strategies to sharpen their sales capabilities, including omnichannel sales teams; advanced sales technology and automation; and data analytics and hyperpersonalization. A strategic vision, a full commitment, and the right capabilities can help B2B companies deploy these strategies successfully.
Paolo Cencioni is a consultant in McKinsey’s Brussels office, where Jacopo Gibertini is also a consultant; David Sprengel is a partner in the Munich office; and Martina Yanni is an associate partner in the Frankfurt office.
The authors wish to thank Christopher Beisecker, Kate Piwonski, Alexander Schult, Lucas Willcke, and the B2B Pulse team for their contributions to this article.
Explore a career with us
Related articles.

The multiplier effect: How B2B winners grow
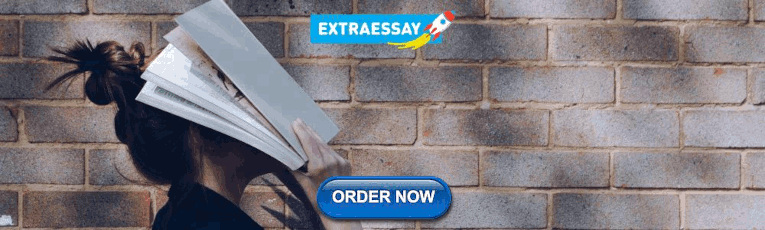
COMMENTS
A good data analysis plan should summarize the variables as demonstrated in Figure 1 below. Figure 1. Presentation of variables in a data analysis plan. 5. Statistical software. There are tons of software packages for data analysis, some common examples are SPSS, Epi Info, SAS, STATA, Microsoft Excel.
A Data Analysis Plan (DAP) is about putting thoughts into a plan of action. Research questions are often framed broadly and need to be clarified and funnelled down into testable hypotheses and action steps. The DAP provides an opportunity for input from collaborators and provides a platform for training. Having a clear plan of action is also ...
A data analysis plan is a roadmap for how you're going to organize and analyze your survey data—and it should help you achieve three objectives that relate to the goal you set before you started your survey: Answer your top research questions. Use more specific survey questions to understand those answers. Segment survey respondents to ...
The first step in a data analysis plan is to describe the data collected in the study. This can be done using figures to give a visual presentation of the data and statistics to generate numeric descriptions of the data. ... Sutton J, Austin Z. Qualitative research: data collection, analysis, and management. Can J Hosp Pharm. 2014;68(3):226 ...
analysis plan: example. • The primary endpoint is free testosterone level, measured at baseline and after the diet intervention (6 mo). • We expect the distribution of free T levels to be skewed and will log-transform the data for analysis. Values below the detectable limit for the assay will be imputed with one-half the limit.
Definition of research in data analysis: According to LeCompte and Schensul, research data analysis is a process used by researchers to reduce data to a story and interpret it to derive insights. The data analysis process helps reduce a large chunk of data into smaller fragments, which makes sense. Three essential things occur during the data ...
Analysis Plan and Manage Data. The main tasks are as follows: 1. Create an analysis plan • Identify research questions and/or hypotheses. • Select and access a dataset. • List inclusion/exclusion criteria. • Review the data to determine the variables to be used in the main analysis. • Select the appropriate statistical methods and ...
A data analysis plan is a roadmap for how you can organise and analyse your survey data. Learn how to write an effective survey data analysis plan today. ... It allows you to make sense of the information gathered and answer your key research questions. But when the data comes rolling in, you may feel a little overwhelmed. It's often hard to ...
A statistical analysis plan (SAP) is a document that specifies the statistical analysis that will be performed on a given dataset. It serves as a comprehensive guide for the analysis, presenting a clear and organized approach to data analysis that ensures the reliability and validity of the results. SAPs are most widely used in research, data ...
Data Analysis Plan: Ultimate Guide and Examples. Learn the post survey questions you need to ask attendees for valuable feedback. Once you get survey feedback, you might think that the job is done. The next step, however, is to analyze those results. Creating a data analysis plan will help guide you through how to analyze the data and come to ...
The data analysis plan flows from the research question, is integral to the study design, and should be well conceptualized prior to beginning data collection. In this section, we will walk through the basics of quantitative and qualitative data analysis to help you understand the fundamentals of creating a data analysis plan.
The last step of designing your research is planning your data analysis strategies. In this video, we'll take a look at some common approaches for both quant...
22.1 Writing the Data Analysis Plan. Congratulations! You have now arrived at one of the most creative and straightforward, sections of your grant proposal. You and your project statistician have one major goal for your data analysis plan: You need to convince all the reviewers reading your proposal that you would know what to do with your data ...
analytic plan for your grant application. Your data analytic plan has a story line with a beginning, middle, and end. The reviewers who will be evaluating your work will want to hear your complete story of what you plan to do given the many different assessments you will collect. 22.2 Before You Begin Writing Your challenge is to demonstrate to ...
Step 1: Write your hypotheses and plan your research design. To collect valid data for statistical analysis, you first need to specify your hypotheses and plan out your research design. Writing statistical hypotheses. The goal of research is often to investigate a relationship between variables within a population. You start with a prediction ...
Define Research Objectives Clearly outline the specific goals and objectives of the data analysis plan. Identify what you want to achieve and how the results will contribute to the overall research. Consider the impact of these objectives on decision-making processes and future actions. Specify the metrics or indicators you will utilize to measure success and
What you'll learn. In today's data-driven world, businesses are looking to make the most effective decisions backed by data. In this course, Designing and Executing an Exploratory Data Analysis Research Plan, you'll gain the ability to create research reports to present to business executives to start the foundation of answering a data science problem.
Start by defining your project's purpose. Identify what your project aims to accomplish and what you are researching. Remember to use clear language. Thinking about the project's purpose will help you set realistic goals and inform how you divide tasks and assign responsibilities.
This article is a practical guide to conducting data analysis in general literature reviews. The general literature review is a synthesis and analysis of published research on a relevant clinical issue, and is a common format for academic theses at the bachelor's and master's levels in nursing, physiotherapy, occupational therapy, public health and other related fields.
The data analysis plan refers to articulating how your data will be cleaned, transformed, and analyzed. All scientific research is replicable, and to be replicable you need to give the reader the roadmap of how you managed your data and conducted the analyses. Each of the following areas could be added into a data analysis plan.
Tab through this guide to consider each stage of the research data management process, and each correlated section of a data management plan. Tools for Data Management Planning The DMPTool allows you to create data management plans from templates based on funder requirements using a quick-and-easy click-through wizard.
Some qualitative research is linear, meaning it follows more of a traditionally quantitative process: create a plan, gather data, and analyze data; each step is completed before we proceed to the next. You can think of this like how information is presented in this book.
Quantitative Data Collection Tools >> Analytical Data Analysis Plan Quantitative Research >> Data Analysis Plan for Quantitative Analysis: There are five steps used for analysis the quantitative data. Those are described in the below steps . Step (i) Data must be collected from one of the following manners:
Methods and analysis In this paper, we describe the protocol for applying the Framework to Assess the Impact from Translational health research to the CRE-DH. The study design involves a five-stage sequential mixed-method approach. In phase I, we developed an impact programme logic model to map the pathway to impact and establish key domains of benefit such as knowledge advancement, capacity ...
The American College of Radiology® (ACR®) recently submitted comments in response to a National Institutes of Health (NIH) request for information (RFI) that sought input regarding the NIH Strategic Plan for Data Science 2023-2028. NIH sought input on its five goals of the strategic plan: Goal 1: Improve Capabilities to Sustain the NIH Policy for Data Management and Sharing.
Each year, somewhere between 700,000 and 1,000,000 people in the United States fall in the hospital. A fall may result in fractures, lacerations, or internal bleeding, leading to increased health care utilization. Research shows that close to one-third of falls can be prevented. Fall prevention involves managing a patient's underlying fall risk factors and optimizing the hospital's physical ...
CHICAGO, March 18, 2024 — An analysis of over 20,000 U.S. adults found that people who limited their eating across less than 8 hours per day, a time-restricted eating plan, were more likely to die from cardiovascular disease compared to people who ate across 12-16 hours per day, according to preliminary research presented at the American ...
Centering the need for culturally responsive leadership (CRL), this study engages in a critical analysis of one large urban school district's 5-year plan that aims to be culturally responsive and equity focused. We first define the various facets of CRL, connect its major components to culturally responsive teaching/pedagogy (CRTP) and student voice (SV), and offer an original, integrative ...
The adoption of inside sales is often an advantageous move, especially in terms of productivity. In fact, inside sales reps can typically cover four times the prospects at 50 percent of the cost of a traditional field rep, allowing the team to serve many customers without sacrificing quality of service. 5 McKinsey analysis. Top performing B2B companies are 50 percent more likely to leverage ...