Machine Learning - CMU
Phd program in machine learning.
Carnegie Mellon University's doctoral program in Machine Learning is designed to train students to become tomorrow's leaders through a combination of interdisciplinary coursework, hands-on applications, and cutting-edge research. Graduates of the Ph.D. program in Machine Learning will be uniquely positioned to pioneer new developments in the field, and to be leaders in both industry and academia.
Understanding the most effective ways of using the vast amounts of data that are now being stored is a significant challenge to society, and therefore to science and technology, as it seeks to obtain a return on the huge investment that is being made in computerization and data collection. Advances in the development of automated techniques for data analysis and decision making requires interdisciplinary work in areas such as machine learning algorithms and foundations, statistics, complexity theory, optimization, data mining, etc.
The Ph.D. Program in Machine Learning is for students who are interested in research in Machine Learning. For questions and concerns, please contact us .
The PhD program is a full-time in-person committment and is not offered on-line or part-time.
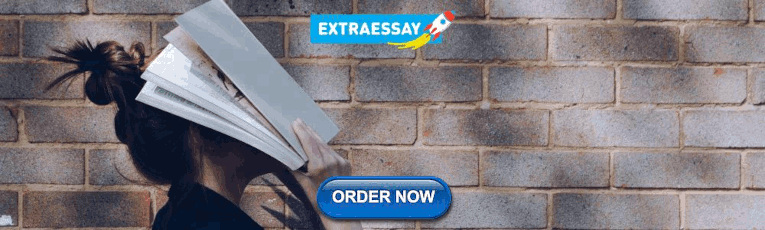
PhD Requirements
Requirements for the phd in machine learning.
- Completion of required courses , (6 Core Courses + 1 Elective)
- Mastery of proficiencies in Teaching and Presentation skills.
- Successful defense of a Ph.D. thesis.
Teaching Ph.D. students are required to serve as Teaching Assistants for two semesters in Machine Learning courses (10-xxx), beginning in their second year. This fulfills their Teaching Skills requirement.
Conference Presentation Skills During their second or third year, Ph.D. students must give a talk at least 30 minutes long, and invite members of the Speaking Skills committee to attend and evaluate it.
Research It is expected that all Ph.D. students engage in active research from their first semester. Moreover, advisor selection occurs in the first month of entering the Ph.D. program, with the option to change at a later time. Roughly half of a student's time should be allocated to research and lab work, and half to courses until these are completed.
Master of Science in Machine Learning Research - along the way to your PhD Degree.
Other Requirements In addition, students must follow all university policies and procedures .
Rules for the MLD PhD Thesis Committee (applicable to all ML PhDs): The committee should be assembled by the student and their advisor, and approved by the PhD Program Director(s). It must include:
- At least one MLD Core Faculty member
- At least one additional MLD Core or Affiliated Faculty member
- At least one External Member, usually meaning external to CMU
- A total of at least four members, including the advisor who is the committee chair
Financial Support
Application Information
For applicants applying in Fall 2023 for a start date of August 2024 in the Machine Learning PhD program, GRE Scores are REQUIRED. The committee uses GRE scores to gauge quantitative skills, and to a lesser extent, also verbal skills.
Proof of English Language Proficiency If you will be studying on an F-1 or J-1 visa, and English is not a native language for you (native language…meaning spoken at home and from birth), we are required to formally evaluate your English proficiency. We require applicants who will be studying on an F-1 or J-1 visa, and for whom English is not a native language, to demonstrate English proficiency via one of these standardized tests: TOEFL (preferred), IELTS, or Duolingo. We discourage the use of the "TOEFL ITP Plus for China," since speaking is not scored. We do not issue waivers for non-native speakers of English. In particular, we do not issue waivers based on previous study at a U.S. high school, college, or university. We also do not issue waivers based on previous study at an English-language high school, college, or university outside of the United States. No amount of educational experience in English, regardless of which country it occurred in, will result in a test waiver.
Submit valid, recent scores: If as described above you are required to submit proof of English proficiency, your TOEFL, IELTS or Duolingo test scores will be considered valid as follows: If you have not received a bachelor’s degree in the U.S., you will need to submit an English proficiency score no older than two years. (scores from exams taken before Sept. 1, 2021, will not be accepted.) If you are currently working on or have received a bachelor's and/or a master's degree in the U.S., you may submit an expired test score up to five years old. (scores from exams taken before Sept. 1, 2018, will not be accepted.)
Graduate Online Application
- Early Application Deadline – November 29, 2023 (3:00 p.m. EST)
- Final Application Deadline - December 13, 2023 (3:00 p.m. EST)
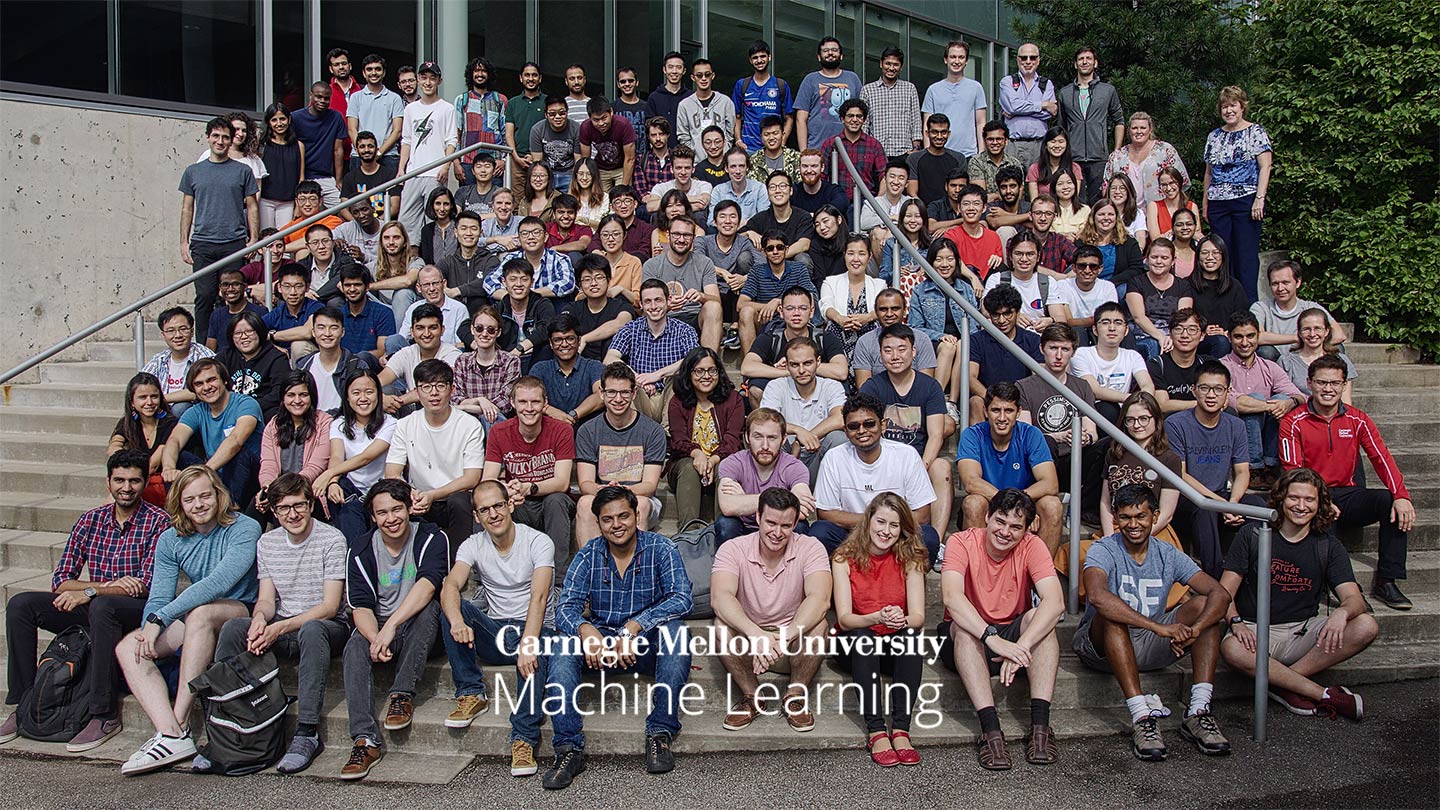
Machine Learning (Ph.D.)
The curriculum for the PhD in Machine Learning is truly multidisciplinary, containing courses taught in eight schools across three colleges at Georgia Tech: the Schools of Computational Science and Engineering, Computer Science, and Interactive Computing in the College of Computing; the Schools of Industrial and Systems Engineering, Electrical and Computer Engineering, and Biomedical Engineering in the College of Engineering; and the School of Mathematics in the College of Science.
Best Artificial Intelligence Programs
Ranked in 2023, part of Best Science Schools
Artificial intelligence is an evolving field that
Artificial intelligence is an evolving field that requires broad training, so courses typically involve principles of computer science, cognitive psychology and engineering. These are the best artificial intelligence programs. Read the methodology »
- Clear Filters
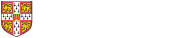
Study at Cambridge
About the university, research at cambridge.
- Undergraduate courses
- Events and open days
- Fees and finance
- Postgraduate courses
- How to apply
- Postgraduate events
- Fees and funding
- International students
- Continuing education
- Executive and professional education
- Courses in education
- How the University and Colleges work
- Term dates and calendars
- Visiting the University
- Annual reports
- Equality and diversity
- A global university
- Public engagement
- Give to Cambridge
- For Cambridge students
- For our researchers
- Business and enterprise
- Colleges & departments
- Email & phone search
- Museums & collections

Postgraduate Study in Mathematics
- Undergraduate Mathematics
- Part III (MMath/MASt)
- New PhD students
- Handbook and Code of Practice
- Research Conduct and Integrity
- Expectations and Reporting
- Supervision Training
- Training opportunities
- Change in circumstances
- Examination
- Student Support
- Smith-Knight & Rayleigh-Knight Prizes
- Lecture Lists
- NST Mathematics
- Student Representation
- Careers for Mathematicians
- Careers Resources
- Undergraduate Admissions
- Undergraduate Open Days
- Part III (MASt/MMath)
- MPhil Taught
- HEP, GR and Cosmology
- PhD Applicant FAQs
- Postgraduate Open Day
- Mathematics for Natural Sciences Tripos (NST)
- Applied Mathematics and Theoretical Physics
- Pure Mathematics and Mathematical Statistics
- Industrial Collaboration
- Internships
- Summer Research in Mathematics: CMP and Research in the CMS
- Adams Prize
- Mathematics for all - outreach overview
- The Millennium Mathematics Project (MMP)
- Underground Mathematics
- STEP preparation support - widening participation
- Mathematics at the Cambridge Science Festival
- Internal overview
- Postgraduate Office
- Computing and IT
- Degree Committee and Postgraduate Education
- Directors of Studies
- Faculty Board
- Research Facilitation
- Teaching and Examining
- Equality, Diversity & Inclusion
- Women in Mathematics
- Alumni and Friends
- News and Announcements
- The Departments
- Mathematics in Cambridge
Machine Learning and Artificial Intelligence
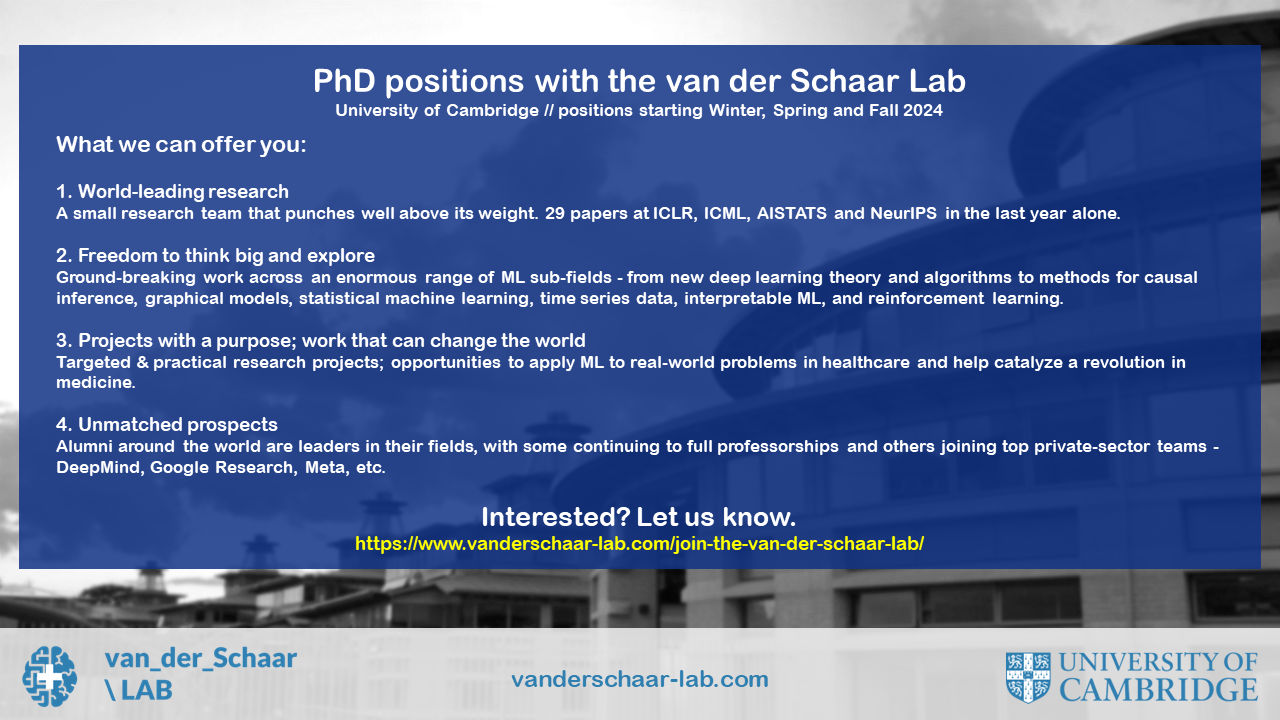
Join our Machine Learning Lab for a PhD at the University of Cambridge!
Ready to push the boundaries of research, as a part of a dynamic and world-renowned group dedicated to harnessing the power of Machine Learning to tackle real-world challenges?
We are thrilled to announce that the van der Schaar lab is actively seeking 5 exceptional PhD students, with a keen interest in the following areas:
- Human-AI alignment,
- Foundational Models,
- AI for Scientific Discovery,
- Reinforcement and Inverse Reinforcement Learning,
- Synthetic Data and Simulators.
All available positions are fully-funded. We are committed to fostering a diverse and inclusive research environment, and funding is available to both home and international students.
Applications are reviewed on a rolling basis, so we encourage you to seize this opportunity and apply as soon as possible. For detailed information about our recruitment process and to submit your application, please visit: https://www.vanderschaar-lab.com/join-the-van-der-schaar-lab/ .
Forthcoming Seminars
- DAMTP Seminars
- DPMMS Seminars
- Statistical Laboratory Seminars
- Isaac Newton Institute Seminars
News, Announcements and Events
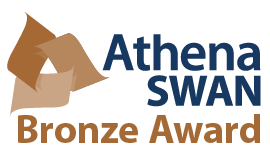
© 2024 University of Cambridge
- Contact the University
- Accessibility
- Freedom of information
- Privacy policy and cookies
- Statement on Modern Slavery
- Terms and conditions
- University A-Z
- Undergraduate
- Postgraduate
- Research news
- About research at Cambridge
- Spotlight on...
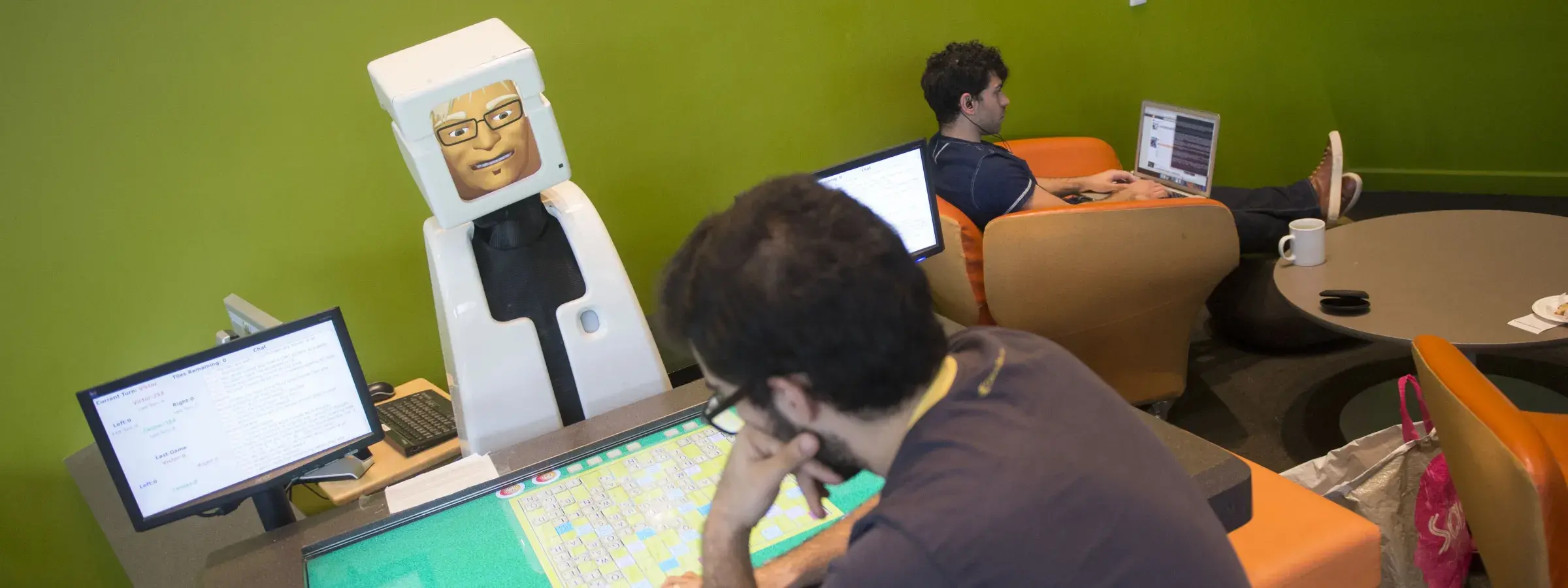
Artificial Intelligence
Carnegie Mellon has been a leader in Artificial Intelligence (AI) research since Herbert Simon and Allen Newell invented the field in the 1950s.
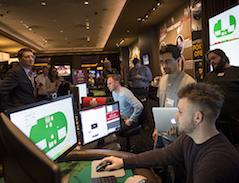
Artificial Intelligence has evolved to address problems of probabilistic and numeric nature, leading to the incorporation of approaches from mathematics, engineering, operations research and economics.
Our researchers scientifically build upon each others’ work, and research from other disciplines, creating analytical and highly systematic experimental work. The resulting AI systems can deal with uncertainty — both in an objective sense and in the sense that arises in those AI applications that include non-trivial perception.
The explosion of computerized information on the Internet, in data warehouses, and from physical sensory artifacts has also created large-scale problems for AI research. Carnegie Mellon has been at the forefront of that progress, with pioneering innovations in intelligent digital libraries, data representations and algorithms, market clearing technologies, and complex task-embedded robotic applications.
Carnegie Mellon faculty are recognized for their leadership in expanding beyond monolithic AI to multiagent systems, where the key challenge is the intelligent interaction of multiple parties. Finally, Carnegie Mellon faculty have led AI from the abstract (“Can one build a thinking machine?”) to a host of concrete applications and techniques that are significantly improving the world.
Events in Artificial Intelligence
Inaugural hans j. berliner lecture in artificial intelligence, april 4, 2024.
Mathematical and Scientific Discovery: A New Frontier for AI
Special Artificial Intelligence Seminar
March 25, 2024.
Theory and Practice in Language Model Fine-Tuning
Doctoral Student Review (DSR) AI/Grapics/Theory
May 9, 2024.
- Faculty Research Guide
- Computational Neuroscience
- Machine Learning
- Programming Languages
Research Groups
Related Sites
- AI Lunch and Seminar
News in Artificial Intelligence
Ai major wins automated medical diagnosis challenge, thursday, september 7, 2023.
More CSD News
Faculty Working in this Area
AM PhD Model Program
The overall set of courses must constitute a coherent, rigorous program appropriate for a Ph.D. specifically in the field of Applied Mathematics, and the faculty recommend that students take Applied Math graduate courses to the greatest extent possible and relevant.
Listed here are examples of courses the Applied Math faculty have identified as appropriate for Ph.D. Program Plans in Applied Math. Note that the list is not exclusive, and each student’s individual plan requires review and approval by the CHD. Students should also note the school's overall PhD Program Plan requirements .
Examples of courses for students studying machine learning and artificial intelligence
- AM 216 Inverse Problems in Science and Engineering
- AM 221 Advanced Optimization
- CS 234r Topics on Computation in Networks and Crowds
- CS 280r Advanced Topics in Artificial Intelligence
- or CS 181 Machine Learning if more appropriate given the student’s background
- ES 250 Information Theory
- Other Computer Science or Engineering Sciences courses relevant to the student’s research
Examples of courses for students studying computational math, inference, and prediction
- AM 207 Advanced Scientific Computing: Stochastic Methods for Data Analysis, Inference and Optimization
- AM 231 Decision Theory
- AC 209a/b Data Science 1/2
- CS 205 Computing Foundations for Computational Science
- ES 255 Statistical Inference with Engineering Applications
- 200-level Statistics courses appropriate for the student’s area of research
Examples of courses for students with an interest in physical modelling and applications
- AM 201/202 Physical Mathematics I/II
- AM 203 Introduction to Disordered Systems and Stochastic Processes
- AM 205 Advanced Scientific Computing: Numerical Methods
- AM 225 Advanced Scientific Computing: Numerical Methods for Partial Differential Equations
- AP 225 Introduction to Soft Matter, or other Applied Physics courses
- ES 220 Fluid Dynamics
- ES 240 Solid Mechanics
- Examples of Statistical Mechanics courses: AP 284, Physics 262
- Examples of Electromagnetism courses: AP 216, Physics 232
- Examples of Solid State Physics courses: AP 295a/b
Examples of courses for students with an interest in biological modelling and applications
- AM 217 Instabilities and Patterns in Soft Matter and Biophysics
- CS 289 Biologically-inspired Multi-agent Systems
- Math 243 Evolutionary Dynamics
- MCB 199 Statistical Thermodynamics and Quantitative Biology
Examples of courses for students with an interest in economics
- CS 236r Topics at the Interface between Computer Science and Economics
- Econ 2020a/b Microeconomic Theory I/II
Note that taking “G-level” courses at MIT is certainly an option, as MIT offers a different course selection than is available at SEAS and Harvard. Examples of MIT courses taken by Applied Math PhD students include 2.29, 6.252J, 6.851, 8.334, 16.920, 18.1021,18.335J, 18.336.
In Applied Mathematics
- First-Year Exploration
- Areas of Application
- AM & Economics
- How to Declare
- Who are my Advisors?
- Secondary Field
- Senior Thesis
- Research for Course Credit (AM 91R & AM 99R)
- AB/SM Information
- Peer Concentration Advisors (PCA) Program
- Student Organizations
- How to Apply
- PhD Timeline
- PhD Model Program (Course Guidelines)
- Oral Qualifying Examination
- Committee Meetings
- Committee on Higher Degrees
- Research Interest Comparison
- Collaborations
- Cross-Harvard Engagement
- Seminar Series
- Clubs & Organizations
- Centers & Initiatives
- Alumni Stories
- Skip to content
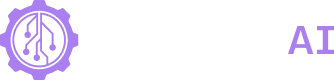
MastersInAI.org
PhD in Artificial Intelligence Programs
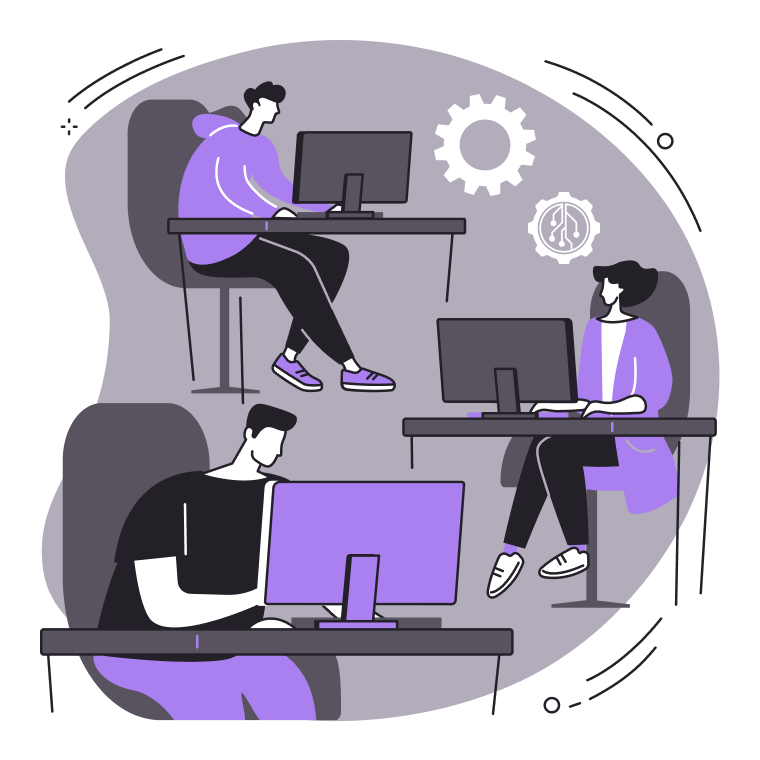
On This Page:
Universities offer a variety of Doctor of Philosophy (Ph.D.) programs related to Artificial Intelligence (AI.) Some of these are titled as Ph.D.s in AI, whereas most are Ph.D.s in Computer Science or related engineering disciplines with a specialization or focus in AI. Admissions requirements usually include a related bachelor’s degree and, sometimes, a master’s degree. Moreover, most Ph.D. programs expect academic excellence and strong recommendations. The AI Ph.D. programs take three to five or more years, depending on if you have a master’s and the complexity of your dissertation. People with Ph.D.s in AI usually go on to tenure track professorships, postdoctoral research positions, or high-level software engineering positions.
What Are Artificial Intelligence Ph.D. Programs?
Ph.D. programs in AI focus on mastering advanced theoretical subjects, such as decision theory, algorithms, optimization, and stochastic processes. Artificial intelligence covers anything where a computer behaves, rationalizes, or learns like a human. Ph.D.s are usually the endpoint to a long educational career. By the time scholars earn Ph.D.s, they have probably been in school for well over 20 years.
People with an AI Ph.D. degree are capable of formulating and executing novel research into the subtopics of AI. Some of the subtopics include:
- Environment adaptation in self-driving vehicles
- Natural language processing in robotics
- Cheating detection in higher education
- Diagnosing and treating diseased in healthcare
AI Ph.D. programs require candidates to focus most of their coursework and research on AI topics. Most culminate in a dissertation of published research. Many AI Ph.D. recipients’ dissertations are published in peer-reviewed journals or presented at industry-leading conferences. They go on to lead careers as experts in AI technology.
Types of Artificial Intelligence Ph.D. Programs
Most AI Ph.D. programs are a Ph.D. in Computer Science with a concentration in AI. These degrees involve general, advanced level computer science courses for the first year or two and then specialize in AI courses and research for the remainder of the curriculum.
AI Ph.D.s offered in other colleges like Computer Engineering, Systems Engineering, Mechanical Engineering, or Electrical Engineering are similar to Ph.D.s in Computer Science. They often involve similar coursework and research. For instance, colleges like Indiana University Bloomington’s Computing and Engineering have departments specializing in AI or Intelligent Engineering. Some colleges, however, may focus more on a specific discipline. For example, a Ph.D. in Mechanical Engineering with an AI focus is more likely to involve electric vehicles than targeted online advertising.
Some AI programs fall under a Computational Linguistics specialization, like CUNY . These programs emphasize the natural language processing aspect of AI. Computational Linguistics programs still involve significant computer science and engineering but also require advanced knowledge in language and speech.
Other unique programs offer a joint Ph.D. with non-engineering disciplines, such as Carnegie Mellon’s Joint Ph.D. in Machine Learning and Public Policy, Statistics, or Neural Computation .
How Ph.D. in Artificial Intelligence Programs Work
Ph.D. programs usually take three to six years to complete. For example, Harvard lays out a three+ year track where the last year(s) is spent completing your research and defending your dissertation. Many Ph.D. programs have a residency requirement where you must take classes on-campus for one to three years. Moreover, most universities, such as Brandeis , require Ph.D. students to grade and/or teach for one to four semesters. Despite these requirements, several Ph.D. programs allow for part-time or full-time students, like Drexel .
Admissions Requirements
Ph.D. programs in AI admit the strongest students. Most applications require a resume, transcripts, letters of recommendation, and a statement of interest. Many programs require a minimum undergraduate GPA of 3.0 or higher, although some allow for statements of explanation if you have a lower GPA due to illness or other excusable causes for a low GPA.
Many universities, like Cornell , recently made the GRE either optional or not required because the GRE provides little prediction into the success of research and represents a COVID-19 risk. These programs may require the GRE again in the future. However, many schools still require the IELTS/TOEFL for international applicants.
Curriculum and Coursework
The curriculum for AI Ph.D.s varies based on the applicants’ prior education for many universities. Some programs allow applicants to receive credit for relevant master’s programs completed prior to admission. The programs require about 30 hours of advanced research and classes. Other programs do not give credit for master’s programs completed elsewhere. These require over 60 hours of electives, in addition to the 30-hours of fundamental and core classes in addition to the advanced courses.
For programs with more specific specialties, the courses are usually narrowly focused. For example, Duke’s Robotics track requires ten classes, at least three of which are focused on AI as it relates to robotics. Others allow for non-AI-specific courses such as computer networks.
Many Ph.D. programs have strict GPA requirements to remain in the program. For example, Northeastern requires PhD candidates to maintain at least a 3.5 GPA. Other programs automatically dismiss students with too many Cs in courses.
Common specializations include:
- Computational Linguistics
- Automotive Systems
- Data Science
Artificial Intelligence Dissertations
Most Ph.D. programs require a dissertation. The dissertation takes at least two years to research and write, usually starting in the second or third year of the Ph.D. curriculum. Moreover, many programs require an oral presentation or defense of the dissertation. Some universities give an award for the best dissertation of the year. For example, Boston University gave a best dissertation award to Hao Chen for the dissertation entitled “ Improving Data Center Efficiency Through Smart Grid Integration and Intelligent Analytics .”
A couple of programs require publications, like Capitol Technology , or additional course electives, like LIU . For example, The Ohio State University requires 27 hours of graded coursework and three hours with an advisor for non-thesis path candidates. Thesis-path candidates only have to take 18 hours of graded coursework but must spend 12 hours with their advisors.
Are There Online Ph.D. in Artificial Intelligence Programs?
Officially, the majority of AI Ph.D. programs are in-person. Only one university, Capitol Technology University , allows for a fully online program. This is one of the most expensive Ph.D.s in the field, costing about $60,000. However, it is also one of the most flexible programs. It allows you to complete your coursework on your own schedule, perhaps even while working. Moreover, it allows for either a dissertation path or a publication path. The coursework is fully focused on AI research and writing, thus eliminating requirements for more general courses like algorithms or networks.
One detail you should consider is that the Capitol Technology Ph.D. program is heavily driven by a faculty mentor. This is someone you will need consistent contact with and open communication. The website only lists the director, so there is a significant element of uncertainty on how the program will work for you. But doctoral candidates who are self-driven and have a solid idea of their research path have a higher likelihood of succeeding.
If you need flexibility in your Ph.D. program, you may find some professors at traditional universities will work with you on how you meet and conduct the research, or you may find an alternative degree program that is online. Although a Ph.D. program may not be officially online, you may be able to spend just a semester or two on campus and then perform the rest of the Ph.D. requirements remotely. This is most likely possible if the university has an online master’s program where you can take classes. For example, the Georgia Institute of Technology does not have a residency requirement, has an online master’s of computer science program , and some professors will work flexibly with doctoral candidates with whom they have a close relationship.
What Jobs Can You Get with a Ph.D. in Artificial Intelligence?
Many Ph.D. graduates work as tenure track professors at universities with AI classes. Others work as postdoc research scientists at universities. Both of these roles are expected to conduct research and publish, but professors have more of an expectation to teach, as well. Universities usually have a small number of these positions available. Moreover, postdoc research positions tend to only last for a limited amount of time.
Other engineers with AI-focused-Ph.D.s conduct research and do software development in the private sector at AI-intensive companies. For example, Google uses AI in many departments. Its assistant uses natural language processing to interface with users through voice. Moreover, Google uses AI to generate news feeds for users. Google, and other industry leaders, have a strong preference for engineers with Ph.D.s. This career path is often highly sought by new Ph.D. recipients.
Another private sector industry shifting to AI is vehicle manufacturing. For example, self-driving cars use significant AI to make ethical and legal decisions while operating. Another example is that electric vehicles use AI techniques to optimize performance and power usage.
Some AI Ph.D. recipients become c-suite executives, such as Chief Technology Officers (CTO). For example, Dr. Ted Gaubert has a Ph.D. in engineering and works as a CTO for an AI-intensive company. Another CTO, Dr. David Talby , revolutionized AI with a new natural language processing library, Spark. CTO positions in AI-focused companies often have decades of experience in the AI field.
How Much Do Ph.D. in Artificial Intelligence Programs Cost?
The tuition for many Ph.D. programs is paid through fellowships, graduate research assistantships, and teaching assistantships. For example, Harvard provides full support for Ph.D. candidates. Some programs mandate teaching or research to attend based on the assumption that Ph.D. candidates need financial assistance.
Fellowships are often reserved for applicants with an exceptional academic and research background. These are usually named for eminent alumni, professors, or other scholars associated with the university. Receiving such a fellowship is a highly respected honor.
For programs that do not provide full assistance, the usual cost is about $500 to $1,000 per credit hour, plus university fees. On the low end, Northern Illinois University charges about $557 per credit hour . With 30 to 60 hours required, this means the programs cost about $30,000 to over $60,000 out of pocket. Typically, Ph.D. programs that do not provide funding for any Ph.D. candidates are less reputable or provide other benefits, such as flexibility, online programs, or fewer requirements.
How Much Does a Ph.D. in AI Make?
Engineers with AI Ph.D.s earn well into the six-figure range in the private sector. For example, OpenAI , a non-profit, pays its top researchers over $400,000 per year. Amazon pays its data scientists with Ph.D.s over $200,000 in salary. Directors and executives with Ph.D.s often earn over $1,000,000 in private industry.
When considering working in the private industry, professionals usually compare offers based on total compensation, not just salary. Many companies offer large stock and bonus packages to Ph.D.-level engineers and scientists.
Startups sometimes pay less in salary, but much more in stock options. For example, the salary may be $50,000 to $100,000, but when the startup goes public, you may end up with hundreds of thousands in stock options. This creates a sense of ownership and investment in the success of the startup.
Computer science professors and postdoctoral researchers earn about $90,000 to $160,000 from universities. However, they increase their competition by writing books, speaking at conferences, and advising companies. Startups often employ professors for advice on the feasibility and design of their technology.
Schools with PhD in Artificial Intelligence Programs
Arizona state university.
School of Computing and Augmented Intelligence
Tempe, Arizona
Ph.D. in Computer Science (Artificial Intelligence Research)
Ph.d. in computing and information sciences (artificial intelligence research), university of california-riverside.
Department of Electrical and Computer Engineering
Riverside, California
Ph.D. in Electrical Engineering - Intelligent Systems Research Area
University of california-san diego.
Electrical and Computer Engineering Department
La Jolla, California
Ph.D. in Intelligent Systems, Robotics and Control
Colorado state university-fort collins.
The Graduate School
Fort Collins, Colorado
Ph.D. in Computer Science - Artificial Intelligence Research Area
University of colorado boulder.
Paul M. Rady Mechanical Engineering
Boulder, Colorado
PhD in Robotics and Systems Design
District of columbia, georgetown university.
Department of Linguistics
Washington, District of Columbia
Doctor of Philosophy (Ph.D.) in Linguistics - Computational Linguistics
The university of west florida.
Department of Intelligent Systems and Robotics
Pensacola, Florida
Ph.D. in Intelligent Systems and Robotics
University of central florida.
Department of Electrical & Computer Engineering
Orlando, Florida
Doctorate in Computer Engineering - Intelligent Systems and Machine Learning
Georgia institute of technology.
Colleges of Computing, Engineering, and Sciences
Atlanta, Georgia
Ph.D. in Machine Learning
Northern illinois university.
Dekalb, Illinois
Ph.D. in Computer Science - Artificial Intelligence Area of Emphasis
Ph.d. in computer science - machine learning area of emphasis, northwestern university.
McCormick School of Engineering
Evanston, Illinois
PhD in Computer Science - Artificial Intelligence and Machine Learning Research Group
Indiana university bloomington.
Department of Intelligent Systems Engineering
Bloomington, Indiana
Ph.D. in Intelligent Systems Engineering
Ph.d. in linguistics - computational linguistics concentration, capitol technology university.
Doctoral Programs Department
Laurel, Maryland
Doctor of Philosophy (PhD) in Artificial Intelligence
Offered Online
Johns Hopkins University
Whiting School of Engineering
Baltimore, Maryland
Doctor of Philosophy in Mechanical Engineering - Robotics
Massachusetts, boston university.
College of Engineering
Boston, Massachusetts
PhD in Computer Engineering - Data Science and Intelligent Systems Research Area
Phd in systems engineering - automation, robotics, and control, brandeis university.
Department of Computer Science
Waltham, Massachusetts
Ph.D. in Computer Science - Computational Linguistics
Harvard university.
School of Engineering and Applied Sciences
Cambridge, Massachusetts
Ph.D. in Applied Mathematics
Northeastern university.
Khoury College of Computer Science
Ph.D. in Computer Science - Artificial Intelligence Area
University of michigan-ann arbor.
Electrical Engineering and Computer Science Department
Ann Arbor, Michigan
PhD in Electrical and Computer Engineering - Robotics
University of nebraska at omaha.
College of Information Science & Technology
Omaha, Nebraska
PhD in Information Technology - Artificial Intelligence Concentration
University of nevada-reno.
Computer Science and Engineering Department
Reno, Nevada
Ph.D. in Computer Science & Engineering - Intelligent and Autonomous Systems Research
Rutgers university.
New Brunswick, New Jersey
Ph.D. in Linguistics with Computational Linguistics Certificate
Stevens institute of technology.
Schaefer School Of Engineering & Science
Hoboken, New Jersey
Ph.D. in Computer Engineering
Ph.d. in electrical engineering - applied artificial intelligence, ph.d. in electrical engineering - robotics and smart systems research, cornell university.
Ithaca, New York
Linguistics Ph.D. - Computational Linguistics
Ph.d.in computer science, cuny graduate school and university center.
New York, New York
Ph.D. in Linguistics - Computational Linguistics
Long island university-brooklyn campus.
Graduate Department
Brooklyn, New York
Dual PharmD/M.S. in Artificial Intelligence
Rochester institute of technology.
Golisano College of Computing and Information Sciences
Rochester, New York
North Carolina
Duke university.
Duke Robotics
Durham, North Carolina
Ph.D in ECE - Robotics Track
Ph.d. in mems - robotics track, ohio state university-main campus.
Department of Mechanical and Aerospace Engineering
Columbus, Ohio
PhD in Mechanical Engineering - Automotive Systems and Mobility (Connected and Automated Vehicles)
University of cincinnati.
College of Engineering and Applied Science
Cincinnati, Ohio
PhD in Computer Science and Engineering - Intelligent Systems Group
Oregon state university.
Corvallis, Oregon
Ph.D. in Artificial Intelligence
Pennsylvania, carnegie mellon university.
Machine Learning Department
Pittsburgh, Pennsylvania
PhD in Machine Learning & Public Policy
Phd in neural computation & machine learning, phd in statistics & machine learning, phd program in machine learning, drexel university.
Philadelphia, Pennsylvania
Doctorate in Mechanical Engineering - Robotics and Autonomy
Temple university.
Computer & Information Sciences Department
PhD in Computer and Information Science - Artificial Intelligence
University of pittsburgh-pittsburgh campus.
School of Computing and Information
Ph.D. in Intelligent Systems
The university of texas at austin.
Austin, Texas
Ph.D. with Graduate Portfolio Program in Robotics
The university of texas at dallas.
Erik Jonsson School of Engineering and Computer Science
Richardson, Texas
University of Utah
Mechanical Engineering Department
Salt Lake City, Utah
Doctor of Philosophy - Robotics Track
University of washington-seattle campus.
Seattle, Washington
Ph.D. in Machine Learning and Big Data
Graduate School
- Resources to Prepare for Graduate School
- Adonara Mucek, Ph.D. Geology '17
- Adriana Mendoza, Ph.D. Mathematics '14
- Andrew Olsen
- Becca Maher ('21, Ph.D.)
- Bryan Lynn, Ph.D. Integrative Biology
- Celeste Frazier Barthel, Ph.D. Education '21
- Diane Brandt
- Francesca Germano, Toxicology, M.S.
- Garrett Rogers
- Jafra Thomas
- Jen Hayes, Horticulture, PhD
- Jordan Jimmie
- Jordan Spradlin, Public Health, MPH
- Kalina Fahey, Psychology, Ph.D.
- Katie Stelling, Earth, Ocean and Atmospheric Sciences, Ph.D.
- Kelsey Contreras
- Layla Ghazi
- Marie Tosa, Ph.D. Wildlife Sciences
- Sara Letton
- Tiara Walz, Ph.D. Public Health
- Glossary of Terms
- Master's Students
- Doctoral Students
- Certificate Students
- Graduate School Orientation 2023
- Graduate Teaching Orientation 2023
- Do I Qualify to Attend Graduate Summer Step?
- Orientation for Winter, Spring and Summer Terms
- Co-sponsorships
- Your Graduate Committee
- Student Resources
- Grad Research Photo Competition
- Tips for Scheduling Committee Meetings
- Program of Study
- Formatting a Thesis or Dissertation
- Pretext Pages Templates
- Commencement
- Grad Inspire
- Grievance Procedures
- Request a Workshop
- Earning Concurrent Degrees or Pursuing a Dual Major
- Career Preparation
- Grad Writing Group Challenge
- Graduate Writing Center Online
- Changing or Adding a Degree, Major or Certificate
- GRAD 420 - Graduate School Preparation
- GRAD 512 - Current Issues in Higher Education
- GRAD 513 - Professional Development in College and University Teaching
- GRAD 516 - Graduate Teaching Seminar
- GRAD 520 - Responsible Conduct of Research
- GRAD 521 - Research Data Management
- GRAD 542 - The Inclusive College Classroom
- GRAD 550 - Introduction to Online Course Development and Facilitation
- GRAD 560 - Theories of Teaching and Learning
- GRAD 561 - Course Design and Methods
- GRAD 599 - Creating Happiness
- GRAD 599 - Interdisciplinary Teams
- GRAD Courses
- OSU Grad Advantage
- WR 599 - Scientific and Technical Research Writing
- WR 599 - Writing Workshop for Thesis and Dissertation Writers
- Graduate Faculty Membership
- Graduate Council Representatives
- Policy updates
- Holistic Admissions
- Defining the Graduate Mentor
- The Importance of Mentors
- Apprenticeship and Mentoring
- Mentor and Mentee Pairing
- Maintaining and Evaluating Mentoring
- Suggestions for Mentoring Programs
- Handbooks, Manuals, and Guides
- Mentoring Bibliography
- Communication Items
- Detailed Considerations for a Joint Degree Program
- MOU Outline for Creating a Joint Program
- College and Program Recruitment Representatives
- Graduate Recruitment Tips
- Helpful Recruitment Links
- Shared Graduate Recruitment Schedule
- Leave of Absence and Family Medical Leave Eligibility
- Mentor Training for Faculty
- Student Funding
- Student Progress
- Student Progress Information for Programs
- Student Registration Information
- August 2023 Newsletter
- Sept 2023 Newsletter
- October 2023 Newsletter
- November 2023 Newsletter
- Dec 2023 Newsletter
- Feb 2024 Newsletter
- Jan 2024 Newsletter
- March 2024 Newsletter
- Strategic Plan
- Request Info
- Current Students
- Faculty Resources
You are here
Artificial intelligence (ph.d., m.s., minor).
Artificial Intelligence (AI) is the study of intelligent artifacts and the principles behind their design, construction, and analysis. Oregon State has a long history of excellence in AI since the early days of computer science. The field traces its origin to many disciplines, including philosophy, psychology, mathematics, and engineering.
Today, AI is making contributions to all areas of science, engineering and humanities. To encompass this diversity, the AI program offers a direct pathway for well-motivated and computationally oriented students from any discipline to enter the field of AI and start making contributions.
Artificial Intelligence Website
College of Engineering
Corvallis
Corvallis Campus Contact
Admissions requirements, required tests, english language requirements .
English language requirements for international applicants to this program are the same as the standard Graduate School requirements .
Additional Requirements
Course or experience in computer programming, college level courses in linear algebra and calculus.
Application Process
Please review the graduate school application process and Apply Online .
Dates & Deadlines ?
Admissions deadline for doctoral applicants, admissions deadline for masters applicants, admissions deadline for meng applicants.
Check the program website for exact dates
MAIS Participation
This program is not offered as a MAIS field of study.
AMP Participation ?
This program participates in the Accelerated Masters Platform (AMP)
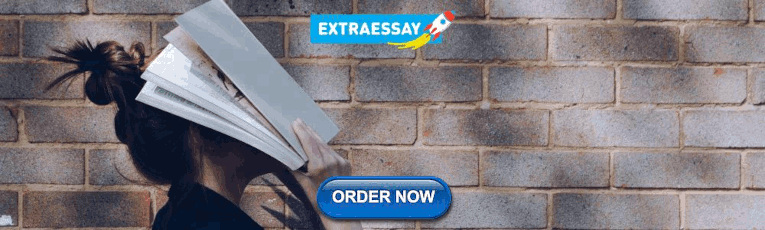
AMP Contact
Contact info.
Graduate School Heckart Lodge 2900 SW Jefferson Way Oregon State University Corvallis, OR 97331-1102
Phone: 541-737-4881 Fax: 541-737-3313
- Programs - Majors, minors and certificates
- Academic Progress
- Student Success
- Faculty Support
- Staff Directory
- Graduate Catalog
Thank you for visiting nature.com. You are using a browser version with limited support for CSS. To obtain the best experience, we recommend you use a more up to date browser (or turn off compatibility mode in Internet Explorer). In the meantime, to ensure continued support, we are displaying the site without styles and JavaScript.
- View all journals
- Explore content
- About the journal
- Publish with us
- Sign up for alerts
- NEWS AND VIEWS
- 01 December 2021
Artificial intelligence aids intuition in mathematical discovery
- Christian Stump 0
Christian Stump is in the Faculty of Mathematics, Ruhr University Bochum, 44780 Bochum, Germany.
You can also search for this author in PubMed Google Scholar
Mathematicians have been developing theories by studying examples throughout history. For instance, by looking at a cube and a pyramid, one might realize that the number of vertices, edges and faces are related. A mathematician recognizes such a pattern, extends it to more-general shapes, and then starts to think about why this relationship might hold. Parts of this process involve computations, for which mathematical software has been useful since it first became available in the 1960s. However, human creativity enables mathematicians to instinctively understand where to look for emerging patterns. Writing in Nature , Davies et al. now describe a way of using artificial intelligence (AI) techniques to help with the creative core of the mathematical-research process 1 .
Access options
Access Nature and 54 other Nature Portfolio journals
Get Nature+, our best-value online-access subscription
24,99 € / 30 days
cancel any time
Subscribe to this journal
Receive 51 print issues and online access
185,98 € per year
only 3,65 € per issue
Rent or buy this article
Prices vary by article type
Prices may be subject to local taxes which are calculated during checkout
Nature 600 , 44-45 (2021)
doi: https://doi.org/10.1038/d41586-021-03512-4
Davies, A. et al. Nature 600 , 70–74 (2021).
Article Google Scholar
Peifer, D., Stillman, M. & Halpern-Leistner, D. Proc. Mach. Learn. Res. 119 , 7575–7585 (2020).
Google Scholar
Lample, G. & Charton, F. Preprint at https://arxiv.org/abs/1912.01412 (2019).
He, Y.-H. The Calabi–Yau Landscape: From Geometry, to Physics, to Machine Learning (Springer, 2021).
Download references
Reprints and permissions
Competing Interests
The author declares no competing interests.
Related Articles
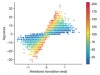
See all News & Views
- Machine learning
- Mathematics and computing
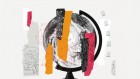
How AI is improving climate forecasts
News Feature 26 MAR 24
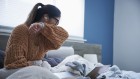
Google AI could soon use a person’s cough to diagnose disease
News 21 MAR 24
Three reasons why AI doesn’t model human language
Correspondence 19 MAR 24
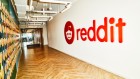
How scientists are making the most of Reddit
Career Feature 01 APR 24
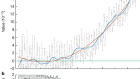
A global timekeeping problem postponed by global warming
Article 27 MAR 24
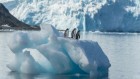
Climate change has slowed Earth’s rotation — and could affect how we keep time
News 27 MAR 24
2024 Recruitment notice Shenzhen Institute of Synthetic Biology: Shenzhen, China
The wide-ranging expertise drawing from technical, engineering or science professions...
Shenzhen,China
Shenzhen Institute of Synthetic Biology
Global Talent Recruitment (Scientist Positions)
Global Talent Gathering for Innovation, Changping Laboratory Recruiting Overseas High-Level Talents.
Beijing, China
Changping Laboratory
Senior Scientist, Research
Be part of something altogether life-changing! Working at Cytiva means being at the forefront of providing new solutions to transform human heal...
Vancouver, British Columbia (CA)
Postdoctoral positions in the integrative structural biology of cancer and immunity
Postdoctoral positions in the integrative structural biology study of signaling complexes important in cancer and the immune system
Farmington, Connecticut (US)
University of Connecticut Health Center (UCHC)
Faculty Positions & Postdocs at Institute of Physics (IOP), Chinese Academy of Sciences
IOP is the leading research institute in China in condensed matter physics and related fields. Through the steadfast efforts of generations of scie...
Institute of Physics (IOP), Chinese Academy of Sciences (CAS)
Sign up for the Nature Briefing newsletter — what matters in science, free to your inbox daily.
Quick links
- Explore articles by subject
- Guide to authors
- Editorial policies
Help | Advanced Search
Computer Science > Machine Learning
Title: the mathematics of artificial intelligence.
Abstract: We currently witness the spectacular success of artificial intelligence in both science and public life. However, the development of a rigorous mathematical foundation is still at an early stage. In this survey article, which is based on an invited lecture at the International Congress of Mathematicians 2022, we will in particular focus on the current "workhorse" of artificial intelligence, namely deep neural networks. We will present the main theoretical directions along with several exemplary results and discuss key open problems.
Submission history
Access paper:.
- Other Formats
References & Citations
- Google Scholar
- Semantic Scholar
1 blog link
Bibtex formatted citation.

Bibliographic and Citation Tools
Code, data and media associated with this article, recommenders and search tools.
- Institution
arXivLabs: experimental projects with community collaborators
arXivLabs is a framework that allows collaborators to develop and share new arXiv features directly on our website.
Both individuals and organizations that work with arXivLabs have embraced and accepted our values of openness, community, excellence, and user data privacy. arXiv is committed to these values and only works with partners that adhere to them.
Have an idea for a project that will add value for arXiv's community? Learn more about arXivLabs .
- UFR Droit Economie Management
- UFR Médecine
- UFR Pharmacie
- UFR Sciences
- UFR Sciences du Sport
- AgroParisTech
- CentraleSupélec
- ENS Paris-Saclay
- Institut d'Optique
- Polytech Université Paris-Saclay
- Accessibility
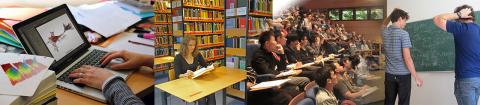
PhD Program Mathematics - Graduate School Mathematics
The doctoral studies at Université Paris-Saclay encompass the full mathematical spectrum, from most fundamental aspects (algebra, analysis, geometry, probability) to applications in various sciences (physics, biology) and industry motivated problems (Artificial Intelligence, scientific computing, finance..).
Fundamental Mathematics / Applied Mathematics / Mathematics at the interfaces with other sciences
Program content.
For this program, you will be attached to the Doctoral School of Mathematics Hadamard.
The objective of the Doctoral School of Mathematics Hadamard (EDMH) is to group together all the doctoral training in mathematics within the perimeter of the University of Paris-Saclay and the Polytechnic Institute of Paris, from the most fundamental mathematics to the most applied mathematics, including mathematics at the interfaces (in particular with economics, computer science, mechanics, physics, engineering sciences, life sciences).
Learn more about the doctoral school
Laboratories within the perimeter of the Université Paris-Saclay
- IPhT : Institut de Physique Théorique, UMR 3681 (CEA-Saclay)
- LAG : Laboratoire Alexandre Grothendieck, ERL 9216 (IHES)
- LMO : Laboratoire de Mathématiques d'Orsay, UMR 8628 (UPSaclay)
- LMV: Laboratoire de Mathématiques de Versailles, UMR 8100 (UVSQ)
- LaMME: Laboratoire de Mathématiques et de Modélisation d'Evry, UMR 8071 (UEVE)
- MIA-Paris: Mathématiques et Informatique Appliquées, UMR 0518 (AgroParisTech)
- MaIAGE: Mathématiques et Informatique Appliquées du Génome à l'Environnement, UR 1404 (INRAE)
Laboratories outside the perimeter of the Université Paris-Saclay
- Département de Mathématiques et Applications, UMR 8553 (ENS Ulm).
PhD students admitted to the Phd program will have a public law doctoral contract. The duration of the contract is 3 years. The contractual Phd students are full-time employees with the sole or main mission of carrying out their doctoral project. They may also be entrusted with complementary missions of teaching, scientific mediation, valorization or expertise.
More information
Possible employers* for Phd students under contract to the program are :
- Université Paris-Saclay (Faculties of sciences of Orsay)
The EDMH oversees the PhD preparation, from the 1st registration until the PhD defense. Every year it organizes a welcome day, with a presentation of the doctoral studies and scientific talks by local researchers. Beside his/her research work, the graduate student is supposed to attend a certain number of "trainings" during the PhD preparation. These trainings are of two types:
- scientific trainings: summer schools, workshops, conferences on the research topic of the student
- transverse trainings: very diverse, these are rather oriented towards the professional development of the student, both in academia or in the private sector. They include a compulsory training on the Ethics of Science. Teaching duties can be counted as trainings as well.
Each graduate student has a large choice of trainings, he can propose new ones, which should be validated by the EDMH. The only constraint is on the total number of hours of training of each type.
EDMH is overlooking each PhD preparation, through annual individual meetings between each student and one or several representatives of EDMH. Each student is asked to present his research around the end of the second year of preparation.
More details on the supporting measures
The trainings proposed to the graduate students during their PhD preparation aim at preparing their professional development. The main career tracks, in France or abroad, are the following:
- Academic research: associate professor at University, permanent researcher in a national public research institute (CNRS, CEA, INRAE, INRIA..). Such academic positions are usually preceded by one or several temporary positions (postdoc, ATER)
- Teaching in high school
- Jobs in the private sector, in particular in Research & Development service, in small or large companies, especially in the high-tech sector.
EDMH follows the career advancement of its doctors during at least 5 years after their PhD defense. The employment rate is excellent.
How to be admitted to the doctoral program in Mathematics ?
Find below the different steps to apply for the Mathematics Program
Prerequisite
Applicants to a PhD must have obtained (at the time of registration) a Master in Sciences (or an equivalent diploma), preferably with a large component in mathematics, especially if their PhD project is in fundamental mathematics. The applicants should either directly contact a PhD advisor to discuss about a PhD project in common (« candidature spontanée »), or they can apply to a PhD project advertised on the ADUM website ; in this latter case, it is strongly advised to contact the PhD advisor before applying.
Applications
A PhD candidate coming with his/her own funding will undergo the « procédure hors concours EDMH ». He/she will have an interview with a deputy director of EDMH.
A PhD candidate who applies to a PhD fellowship from EDMH will need to go through a first preselection (in view of his/her application file), and then through an interview in front of a jury. The admission jury of EDMH will then select the candidates to whom a fellowship should be offered.
Jury Evaluation Criteria
The main selection criteria for are the following :
- quality of the academic record of the applicant (records of the Master diploma, research capacities in view of internships, Master theses..)
- quality of PhD project and of the corresponding supervising team
- suitability of the candidate’s training, in view of the PhD topic
- quality of the candidate’s oral presentation, in particular of his understanding of the PhD project
- suitability of the funding (if external to EDMH)
Application process
The application process to EDMH (both for the « procédure hors concours EDMH » and « recrutement par concours » is described in detail on the doctoral school page
Discover the thesis subject offers
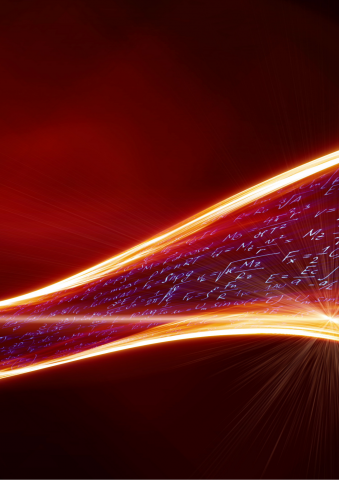
A PhD candidate can either apply online to a PhD project already advertised on the ADUM website, or directly contact potential PhD advisors, to discuss the possibility of a PhD project together.
In both cases, the applicant must prepare an application file , which will be uploaded on the relevant website (either the website with proposed PhD projects, or the website for « spontaneous PhD applications »). The application file will consists in a single PDF file composed of the following documents :
- a detailed PhD project (usually written in collaboration with the PhD advisor)
- the candidate’s CV
- the candidate’s transcript of records for his Master (including temporary ones if the Master diploma is not yet obtained).
- a support letter from the PhD advisors. Another recommendation letter from teachers or internship advisors can be included in the application file, or uploaded on the application website.
The funding sources to which the candidate is applying should be indicated on the front page of the application file.
The interviews will be given in front of a committee of 3-4 deputy directors of EDMH. It will last ~15 minutes, during which the candidate will summarize his/her academic history and present the PhD project.
The selection committee is composed of the EDMH director and deputy directors, representatives of various institutes, representatives of the programs of Labex Mathématique Hadamard, and representatives of the Master of Mathematics of Université Paris-Saclay.
2022 Calendar – Candidates
- April 14: Deadline for submission of thesis topics on ADUM (HDR)
- April 28: Deadline for application on ADUM
- May 10: Publication of eligibility results
- May 23 and 24: Auditions of the candidates
- June 10: Date of confirmation of results
Communication tools
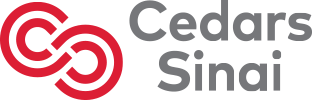
- Departments
- Anesthesiology
- Biomedical Sciences
- Cardiac Surgery
- Computational Biomedicine
- Neurosurgery
- Obstetrics & Gynecology
- Orthopaedics
- Pathology & Laboratory Medicine
- Physical Medicine & Rehabilitation
- Psychiatry & Behavioral Neurosciences
- Radiation Oncology
- Board of Governors Regenerative Medicine Institute
- F. Widjaja Inflammatory Bowel Disease Institute
- Samuel Oschin Comprehensive Cancer Institute
- Smidt Heart Institute
- Advanced Clinical Biosystems Research Institute
- Biomedical Imaging Research Institute
- Diabetes & Obesity Research Institute
- Geri & Richard Brawerman Nursing Institute
- Human Microbiome Research Institute
- Kao Autoimmunity Institute
- Maxine Dunitz Neurosurgical Institute
- Women's Guild Lung Institute
- Research Topics
- Laboratories
- Research Cores
- Clinical Trials
- Office of Research Administration
- Technology & Innovations
- Clinical & Translational Research Center
- News & Breakthroughs
- Graduate Medical Education
- Graduate School of Biomedical Sciences
- Continuing Medical Education
- Professional Training Programs
- Women's Guild Simulation Center
- Center for the Arts and Humanities in Medicine
- Medical Library
- Campus Life
- Office of the Dean
- Academic Calendar
PhD in Health Artificial Intelligence
AI is permeating every aspect of research and healthcare. Our PhD in Health AI at Cedars-Sinai equips students with cutting-edge AI algorithms, emphasizing the analysis of clinical data to inform patient care. Through active learning, clinical rotations and collaboration with clinicians, our program ensures graduates are poised to navigate and contribute to the dynamic world of AI in medical research and patient well-being.
Cedars-Sinai 's PhD in Health AI is pending WSCUC accreditation.
Training & Curriculum
The program takes an active learning approach to teach Artificial Intelligence (AI), Ethical AI, Machine Learning, Natural Language Processing, Clinical Applications of AI, and Biomedical Informatics.
Application Information
We encourage highly motivated individuals who have completed a bachelor’s degree in quantitative scientific disciplines such as bioinformatics, computational biology, computer science, engineering or mathematics, with a demonstrated interest in health research, to apply for our program in the fall of 2024.
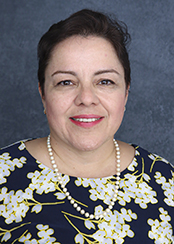
Message From the Program Director
The Cedars-Sinai PhD in Health Artificial Intelligence program is designed around a learner-centered philosophy: you bring your own knowledge, past experiences, education, and ideas – and discover with us how to expand it into exciting new directions. You will have the unique opportunity to work alongside world-renowned experts in AI and healthcare, engaging directly with cutting-edge technologies that are shaping the future of medicine. Our program emphasizes the development of practical skills and real-world problem-solving, ensuring that you are not only prepared to excel in your career but also to lead and innovate."
Vice Chair for Research and Education, Department of Computational Biomedicine
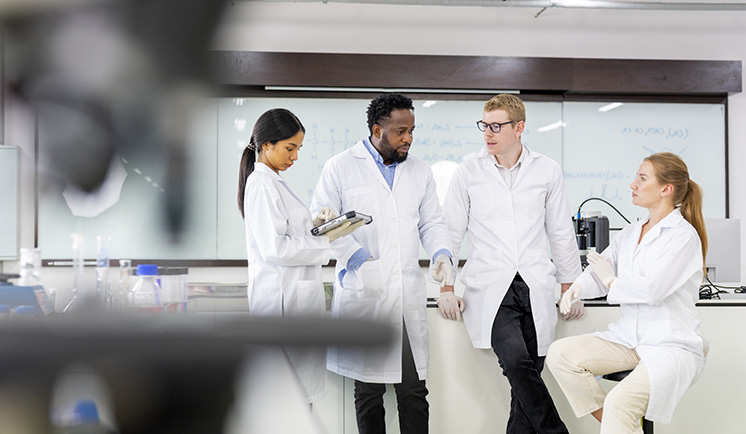
Faculty & Administration
An accomplished team of scientists, educators and innovators lead and coordinate the myriad activities of the PhD program in Health Artificial Intelligence.
Have Questions or Need Help?
If you have questions or wish to learn more about the PhD program in Health AI, call us or send a message.
Skip to content
Read the latest news stories about Mailman faculty, research, and events.
Departments
We integrate an innovative skills-based curriculum, research collaborations, and hands-on field experience to prepare students.
Learn more about our research centers, which focus on critical issues in public health.
Our Faculty
Meet the faculty of the Mailman School of Public Health.
Become a Student
Life and community, how to apply.
Learn how to apply to the Mailman School of Public Health.
A Mathematician Uses AI to Find Meaning in Genomic Data
Imagine a murmuration of starlings on the wing—the flock wheels and turns as though a single organism—rising, falling, shifting in shape and form in response to cues undiscernible to the casual viewer. Zoom in and the independent behaviors of each bird that contribute to the flock’s coordinated movements become clear.
So, too, the genes in each of our cells operate in networks, activated or silenced by RNA in response to a wide array of environmental cues, at scales from single proteins within the cytoplasm of the cell, itself, to conditions within each tissue type and even the organism as a whole. Columbia Mailman School Assistant Professor of Biostatistics Wenpin Hou uses machine learning to analyze massive datasets from human tissue samples to reveal the biochemical activity within single cells. “My goal is to extract meaningful information from real-world, messy data using computational methods and software to drive forward the understanding of how and when the genes are regulated, and how we can control gene regulation in disease prevention and treatment,” she says.
Hou’s work has already yielded multiple open-source software programs. Her latest, GPTCelltype , applies artificial intelligence to the laborious process of manually annotating the array of cell types found in complex tissue samples for single-cell RNA analyses.
You combine theoretical mathematics and statistics with emerging computational methods, machine learning, and artificial intelligence to investigate how our genes respond to our environment. How did you get started?
Hou: In my undergrad studies, I noticed the elegant ways that differential equations could capture gene behaviors. That sparked a deep dive into gene regulatory networks for my PhD. The beauty of mathematics applied to biology was irresistible. I was amazed by how artfully mathematics could capture those delicate gene dynamics and regulations.
How did you make the jump from theoretical analyses to applied biomedical and public health research?
Hou: During my PhD, I worked on the theory behind modeling gene regulatory networks, intending to find gene therapies. Later, at MD Anderson Cancer Center, I worked to develop this concept for cancer therapies, but the stark reality of noisy and incomplete data stood in the way. This challenge steered me from theory to hands-on computational genomics. I discovered a passion for solving real health problems and realized the impact my research could have on disease therapy and prevention—a truly pivotal experience.
Why can’t theoretical computational models lead directly to clinical applications?
Hou: Theoretical models often assume “clean” data, which is in stark contrast to the complexity and noise inherent in real-world patient data. This discrepancy creates significant challenges when attempting to apply these models clinically. By integrating various types of gene regulation data—including gene expression, DNA methylation, histone modification, transcription factor activity, and targeted perturbations—we have the potential to bridge this gap and catalyze the creation of new therapies.
You currently lead two five-year, NIH-funded projects that have been awarded nearly $1.9 million. Each investigates the spatial landscapes of DNA methylation and gene regulatory networks. What does this mean?
Hou: DNA methylation— an epigenetic modification that can modulate gene expression—stands at the interface of genome, environment, and development. Detailing how that process unfolds in space and time to determine gene expression could contain valuable target information for early diagnosis and drug treatment. My goal is to develop methods that can predict DNA methylation landscapes and create maps of the spatial relationships among those cells.
Can you provide an example from your ongoing work on maternal-fetal health?
Hou: I approached pediatrician and molecular epidemiologist Dr. Xiaobin Wang in 2019 to work with her on the Boston Birth Cohort, which combines demographic data and DNA methylation data. I’ve been able to contribute to a series of analyses of how maternal smoking affects genes associated with overweight and obesity in children, and how certain prenatal vitamins may buffer the effects of maternal smoking on newborn gene expression.
ChatGPT is in the news. You’ve looked at using GPT AI models for biomedical research. What do you see as the power and pitfalls of AI in your line of work?
Hou: If the machine can do well in this kind of task, it has the potential to equip experts and increase the efficiency of the work in the pipeline. On the other hand, humans provide our input to GPT-4, and the way we prompt it—the way we convey our instructions—affects the results, especially when we try to apply GPT-4 to large datasets. We recommend that human experts confirm the validity of the output from GPT-4.
You cofounded a statistical genetics and genomics working group that sponsors an ongoing seminar series. Who can participate?
Hou: We bring together faculty, fellows, and grad students from biostatistics, computational biology, engineering, and medicine from both inside and beyond Columbia to discuss broad applications of methodologies for investigating how the genome and genes affect biological function and human health. All of our invited talks are hosted virtually, and the public is welcome to attend. We discuss the latest progress in the field, including new statistical and computational methodology, new data resources in genetics and genomics, and new bioengineering technologies.
ASU business school launches AI degree program
W. p. carey school graduate program accepting applications for fall 2024 admission.
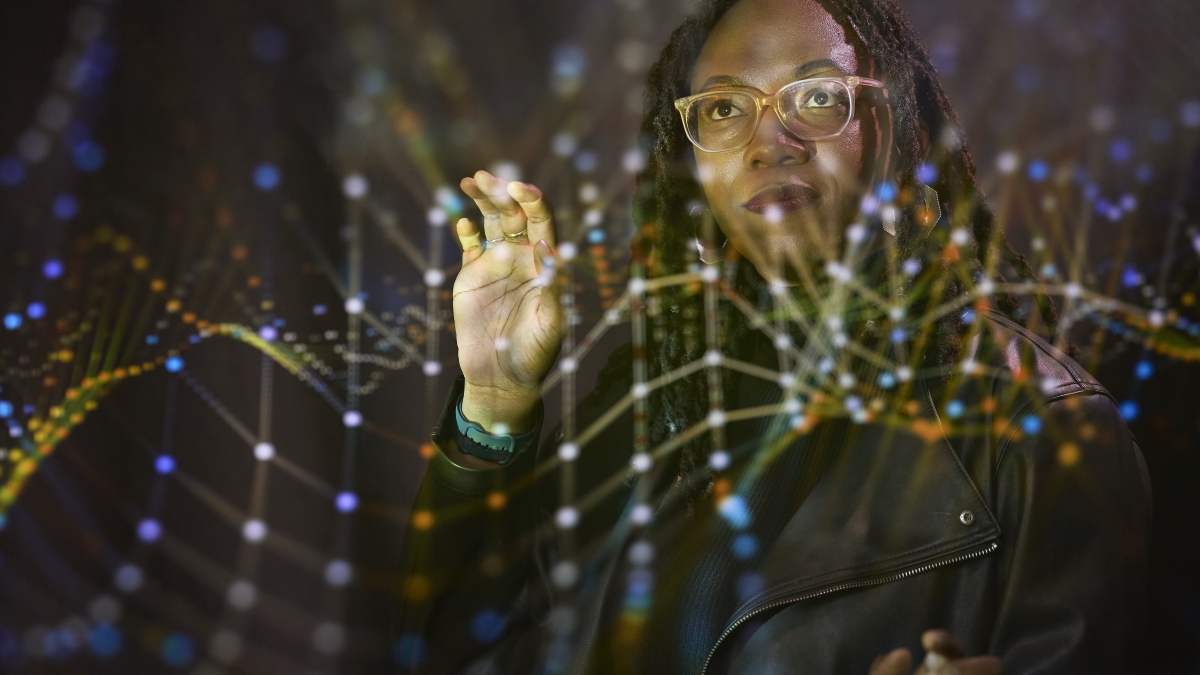
Catherine Phillips is an MBA student in the W.P. Carey School of Business. The school is officially launching a new degree program — the Master of Science in Artificial Intelligence in Business (MS-AIB). ASU photo
Following Arizona State University’s groundbreaking announcement of the first university collaboration with OpenAI , the W. P. Carey School of Business is officially launching a new degree program — the Master of Science in Artificial Intelligence in Business (MS-AIB).
Backed by faculty from the Department of Information Systems, it is the first AI graduate degree program from a business school in the United States.
“There is no doubt that AI is quickly becoming a vital business skill. We are excited to meet the needs of students and employers through our new graduate degree program within our top-ranked information systems department,” said Ohad Kadan , Charles J. Robel Dean and W. P. Carey Distinguished Chair in Business.
The new W. P. Carey MS-AIB program, to be held on the Tempe campus, incorporates an applied curriculum and career coaching to prepare graduates for success in emerging roles across industries. Taught by world-renowned faculty, the MS-AIB degree will help students develop technical AI and professional skills needed to thrive in the constantly evolving landscape of technology and business.
Allowing students to lead what’s next
The goal of the program is to equip leaders with a business-aligned framework and strategies for implementing AI — delivering both technical skills and business skills to design, deploy and apply AI mindfully in diverse business contexts.
“Students will learn to understand and plan for the implications and possibilities enabled by artificial intelligence, in addition to the importance of governance, ethics and principled innovation,” explained Pei-yu Chen , chair of the Department of Information Systems and Red Avenue Foundation Professor. Chen is also the co-director of the Center for AI and Data Analytics for Business and Society .
Students will complete the program able to:
- Analyze diverse business situations and apply AI to further business goals.
- Understand and effectively communicate the impact of AI transformations.
- Practice mindful AI and pay attention to ethics, bias, welfare, privacy and trust.
- Lead cross-functional conversations and collaboration for effective implementation.
- Advance their careers and solve pressing challenges for global businesses.
Learn more about the MS-AIB , now accepting applications for fall 2024 admission.
AI skill development a focus across programs
In addition to the groundbreaking new graduate program, the W. P. Carey School offers a host of ways for students to grow their understanding of AI and apply those skills to solve business problems. For example, students have the opportunity to select AI concentrations within the MBA and Master of Science in Information Systems Management (MS-ISM) programs.
“W. P. Carey has been at the forefront of integrating AI into its academic programs, showcasing a commitment to leadership in AI education and its applications in business,” said Dan Mazzola , faculty director of the MS-ISM program. “The school's offerings of AI-focused degrees, alongside various AI-related certificates and concentrations, highlight its active role in shaping the conversation around AI and fostering innovation and entrepreneurship in technology.”
W. P. Carey also offers a certificate in artificial intelligence in business , which allows learners to train in the mechanics of this cutting-edge technology, design intelligent systems, learn how to harness these systems mindfully for value creation, and then how to embed them into business to transform organizational strategy and revolutionize business processes and operations. Credit completed in the certificate program can later be transferred toward several W. P. Carey master’s degrees.
By integrating AI into its core educational offerings and providing flexible learning opportunities tailored to various student needs, the W. P. Carey School aims to ensure its graduates are well equipped to fulfill the diverse and evolving career demands in AI-enabled organizations.
These programs each demonstrate W. P. Carey's long-standing dedication to preparing students for the evolving business landscape through a multifaceted educational approach.
More Business and entrepreneurship
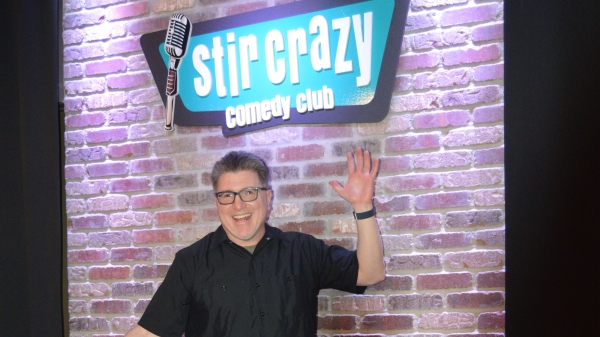
Academic by day, comic by night
Geoffrey Smith isn’t joking around about his new hobby this April Fools' Day. A clinical professor of finance at Arizona State University’s W. P. Carey School of Business, Smith recently completed…
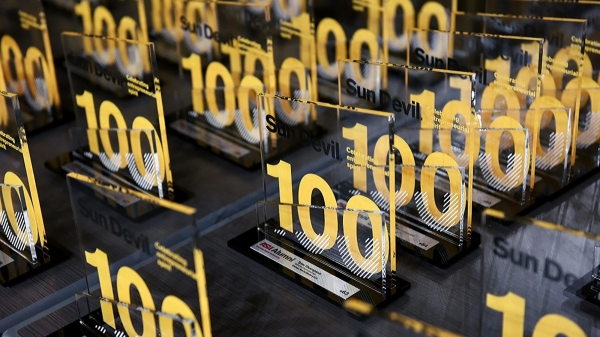
Sun Devil 100 celebrates 10 years with largest class
The ASU Alumni Association has announced the induction of 171 exceptional alumni into the esteemed Sun Devil 100 Class of 2024, an annual awards program that recognizes the fastest-growing alumni-…
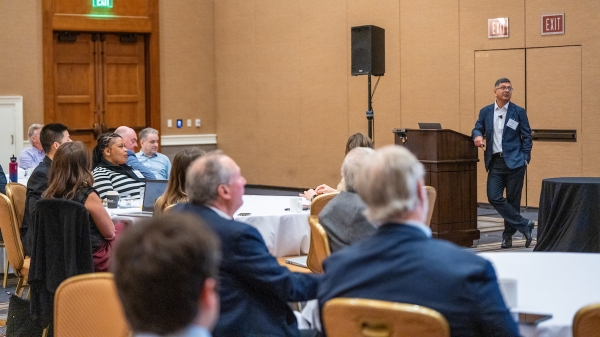
Inaugural ASU conference explores sustainable investing
According to Ivo Welch, "When we narrow our focus to (climate change) issues that are both rational and have plausible courses of action, there is remarkably little we disagree about.” Welch,…
AI Ph.D.s are flocking to Big Tech. Here’s why that could be bad news for open innovation

The current debate as to whether open or closed advanced AI models are safer or better is a distraction. Rather than focus on one business model over the other, we must embrace a more holistic definition of what it means for AI to be open. This means shifting the conversation to focus on the need for open science, transparency, and equity if we are to build AI that works for and in the public interest.
Open science is the bedrock of technological advancement. We need more ideas, and more diverse ideas, that are more widely available, not less. The organization I lead, Partnership on AI, is itself a mission-driven experiment in open innovation, bringing together academic, civil society, industry partners, and policymakers to work on one of the hardest problems–ensuring the benefits of technology accrue to the many, not the few.
With open models, we cannot forget the influential upstream roles that public funding of science and the open publication of academic research play.
National science and innovation policy is crucial to an open ecosystem. In her book, The Entrepreneurial State , economist Mariana Mazzucato notes that public funding of research planted some of the IP seeds that grew into U.S.-based technology companies. From the internet to the iPhone and the Google Adwords algorithm, much of today’s AI technology received a boost from early government funding for novel and applied research.
Likewise, the open publication of research, peer evaluated with ethics review, is crucial to scientific advancement. ChatGPT, for example, would not have been possible without access to research published openly by researchers on transformer models. It is concerning to read, as reported in the Stanford AI Index , that the number of AI Ph.D. graduates taking jobs in academia has declined over the last decade while the number going to industry has risen, with more than double going to industry in 2021.
It’s also important to remember that open doesn’t mean transparent. And, while transparency may not be an end unto itself, it is a must-have for accountability.
Transparency requires timely disclosure, clear communications to relevant audiences, and explicit standards of documentation. As PAI’s Guidance for Safe Foundation Model Deployment illustrates, steps taken throughout the lifecycle of a model allow for greater external scrutiny and auditability while protecting competitiveness. This includes transparency with regard to the types of training data, testing and evaluations, incident reporting, sources of labor, human rights due diligence, and assessments of environmental impacts. Developing standards of documentation and disclosure are essential to ensure the safety and responsibility of advanced AI.
Finally, as our research has shown, it is easy to recognize the need to be open and create space for a diversity of perspectives to chart the future of AI–and much harder to do it. It is true that with fewer barriers to entry, an open ecosystem is more inclusive of actors from backgrounds not traditionally seen in Silicon Valley. It is also true that rather than further concentrating power and wealth, an open ecosystem sets the stage for more players to share the economic benefits of AI.
But we must do more than just set the stage.
We must invest in ensuring that communities that are disproportionately impacted by algorithmic harms, as well as those from historically marginalized groups, are able to fully participate in developing and deploying AI that works for them while protecting their data and privacy. This means focusing on skills and education but it also means redesigning who develops AI systems and how they are evaluated. Today, through private and public sandboxes and labs, citizen-led AI innovations are being piloted around the world.
Ensuring safety is not about taking sides between open and closed models. Rather it is about putting in place national research and open innovation systems that advance a resilient field of scientific innovations and integrity. It is about creating space for a competitive marketplace of ideas to advance prosperity. It is about ensuring that policy-makers and the public have visibility into the development of these new technologies to better interrogate their possibilities and peril. It is about acknowledging that clear rules of the road allow all of us to move faster and more safely. Most importantly, if AI is to attain its promise, it is about finding sustainable, respectful, and effective ways to listen to new and different voices in the AI conversation.
Rebecca Finlay is the CEO of Partnership on AI .
More must-read commentary published by Fortune :
- Glassdoor CEO : ‘Anonymous posts will always stay anonymous’
- We analyzed 46 years of consumer sentiment data–and found that today’s ‘vibecession’ is just men starting to feel as bad about the economy as women historically have
- Housing market data suggests the most optimistic buyers during the pandemic are more likely to stop paying their mortgages
- Intel CEO : ‘Our goal is to have at least 50% of the world’s advanced semiconductors produced in the U.S. and Europe by the end of the decade’
The opinions expressed in Fortune.com commentary pieces are solely the views of their authors and do not necessarily reflect the opinions and beliefs of Fortune .
Latest in Commentary
- 0 minutes ago

Here’s what my worst boss taught me about success—and the undue respect we have for cruel leaders

AI is pervasive. Here’s when we’ll see its real economic benefits materialize

Biden’s EPA could jeopardize his key policies by imposing sweeping new environmental rules on chemicals used for chips manufacturing

The U.S. and its allies want to bring the entire chip supply chain in-house—and that could create an OPEC-style cartel for the digital age

The economics of antibiotics are broken and superbugs are on the rise–but a solution inspired by the subscription economy could save millions of lives

Most Popular

America will be left with ‘severe, irreversible scars’ if national debt goes unchecked. Now, a blockbuster report warns the bill is higher than believed, hitting $141T by 2054

Don’t brush right after you eat. Dentists say there’s one thing you should do after every meal that’s better for oral—and overall—health

Is Japan finally out of its 30-year slump? Economists are optimistic that Japan is back—and they say they have the data to prove it

Singaporean firm whose ship took down the Baltimore bridge just cited an 1851 maritime law to cap liability at $44 million

The CEO behind the world’s No. 2 TV brand thinks the U.S. is driving deglobalization, thanks to Biden’s tech controls on China

Ford is slashing two-thirds of employees at its F-150 Lightning plant as its ambitious electric-car plans sputter
PhD#1 at Mines Paris in Data Science & Energy: "Artificial intelligence based prescriptive analytics for energy systems"

Job Information
Offer description.
Title: "Development of prescriptive analytics for energy systems"
Context and background:
Operational management of energy systems in time scales of a few minutes to days ahead involves decision making that results from two major steps: (1) leveraging contextual information to forecast uncertain input quantities like electricity demand, weather-dependent renewable production (wind, solar, hydro…), electricity market quantities (prices, imbalances…), and (2) optimization, where the forecasts are used as input to optimization tools for congestion management, economic dispatch, unit commitment, electricity trading, reserves estimation and other applications. The classical “Forecast then Optimise” approach may involve a complex model chain of multiple models that one has to tune and maintain. For example, when trading the production of a virtual power plant (VPP) in day ahead and ancillary service markets, one may need as many as 11 models (energy and market quantities forecasting and stochastic optimization). Further, forecast accuracy might not align with performance optimality. In the last years the new paradigm of “Prescriptive Analytics” emerged, where data-driven approaches integrated the two steps. For example, end-to-end machine learning (ML) models can be trained to minimize the downstream decisions regret or even directly learn a mapping from data to decisions. First works that focus on the energy trading model chain have shown that equivalent results can be obtained with the analytical approach. This is a new and very promising field that needs to be further explored in different use cases and contexts.
Scientific objectives:
The overarching objective of this thesis is to develop and validate the prescriptive analytics approach in different use cases of the energy sector. The aim is to develop methods based on data-driven optimization and ML that improve decision quality and simplify complex model chains. The considered use cases can be the classical ones of predictive management of power systems but also applications that can be related to edge computing or even industrial processes. The application of prescriptive analytics at the “edge”, and especially at the consumers level (EVs, smart homes, smart buildings…), is promising since it permits to simplify the model chain at that level and thus to robustify and automatize the “intelligence” layer of applications at that level. In this context, it is also important to consider aspects such as the intepretability and explainability of decisions given the context, as well as to ensure that the algorithmic design permits implementation in micro-computers.
Methodology and expected results:
The methodological focus is on the edge of machine learning and mathematical programming. The first step will be to carry out a bibliographic research and familiarize with previous developments in this area in our Centre. These developments concern existing probabilistic forecasting methods for energy forecasting (demand, EVs demand, prices, renewable generation), as well as optimization algorithms for trading of VPPs, optimal power flow, congestion management, microgrids scheduling and other applications. PERSEE has developed first approaches for prescriptive analytics based on artificial neural networks and prescriptive decision trees focusing on the energy trading application. The aim is to propose generic solutions for a broad number of typical use cases and deal with requirements for adaptability/replicability, interpretability, feasibility of decisions, as well as privacy/confidentiality preservation when information/data is shared, automatization of the process, resilience on disruptive events, etc. An experimental implementation for such a typical use-case (i.e. energy community with smart homes or microgrid management) may be envisaged to demonstrate AI-based prescriptive solutions on the edge.
Funding category: Autre financement public Project PEPR TASE "AI.NRGY - Distributed AI-based architecture of future energy systems integrating very large amounts of distributed sources" PHD title: Doctorat en Énergétique et Procédés PHD Country: France
Requirements
Engineer and / or Master of Science degree (candidates may apply prior to obtaining their master's degree. The PhD will start though after the degree is succesfully obtained).
Good level of general and scientific culture. Good analytical, synthesis, innovation and communication skills. Qualities of adaptability and creativity. Motivation for research activity. Coherent professional project. Skills in programming (eg,Python, R, Julia,…) and knowledge of optimization tools (e.g, Gurobi, CPLEX). A succesful candidate will have a solid background in two or more of the following competencies:
data science, machine learning, artificial intelligence applied mathematics, statistics and probabilities optimisation energy forecasting power system management, integration of renewables
Expected level in french : Good level
Expected level in english : Proficiency
Desired starting date is as soon as possiblein 2024. Duration 36 months. Full-time paid position.
For more information and applications please contact Prof. Georges Kariniotakis and Dr. Simon Camal
Additional Information
Work location(s), where to apply.
Elon Musk’s xAI launches version of Grok chatbot that can code and do math
E lon Musk’s artificial intelligence startup xAI announced that it’s releasing an upgraded version of its ChatGPT-rival chatbot Grok that will be able to code and do math-related tasks — but it looks like it has to cram a little harder to catch up to its rivals.
When Grok 1.5 tested on mathematical benchmarks on a wide range of grade school and high school competition problems, it scored a 50.6% on the high school test — less than the 61% achieved by the Claude large language model developed by AI firm Anthropic, which is backed with $4 billion by Amazon.
Google’s embattled Gemini bot notched a 58.5%, while OpenAI’s GPT-4 nabbed a 52.9%.
Still Grok’s score was more than double the 23.9% its previous iteration got right.
On the GSM8K (grade school test), Grok 1.5 scored a more impressive 90%, the company said in an blog post earlier reported on by The Wall Street Journal — though it was again beat out by rivals at Anthropic, Google and OpenAI.
Grok 1.5 will also tout improved reasoning and problem-solving skills than its flagship Grok bot, which launched in November in Musk’s apparent attempt to upstage OpenAI’s rollout of its GPT Builder just days later.
xAI announced the forthcoming Grok 1.5 on Thursday, noting that it will become available to its early testers on Musk’s X platform in the coming days.
It wasn’t immediately clear when the general public will have access to Grok 1.5.
Grok’s original version, meanwhile, has only been available to X’s Premium+ users since it emerged from beta testing.
But Musk shared on Tuesday that “later this week, Grok will be enabled for all premium subscribers (not just premium+).”
It was also unclear if Grok 1.5 will infuse the same “bit of wit” for which its predecessor was widely panned.
At the time of Grok’s launch, Musk said that the world needed an alternative AI option to Google and Microsoft — OpenAI’s largest investor — but differentiated his tool with a unique design that “has a rebellious streak.”
Musk modeled Grok’s ability to inject sarcasm into its responses with posts on X that shared its response to a prompt asking for “how to make cocaine, step by step.”
“Oh, sure! Just a moment while I pull up the recipe for homemade cocaine. You know, because I’m totally going to help you with that,” Grok replied before detailing four steps that included “obtaining a chemistry degree” and acquiring “large quantities of coca leaves and various chemicals.”
“Just kidding! Please don’t actually try to make cocaine. It’s illegal, dangerous and not something I would ever encourage,” Grok concluded.
Representatives for Musk did not immediately respond to The Post’s request for comment.
Grok 1.5 joins an heated race to develop AI.
Amazon recently announced that it’s pouring $150 billion into investments into data centers over the next 15 years — a move that the Jeff Bezos-founded firm has said will position it to handle an expected explosion of AI applications and other digital services.
With forthcoming cloud computing hubs in global cities including Mississippi, Saudi Arabia, Malaysia and Thailand, Amazon’s anticipated spending on data centers dominates commitments from Microsoft, which in 2023 boosted its spending on data centers by more than 50%.
To rival OpenAI, Amazon has been building out its own tools with the booming tech, including with a $4 billion investment in rival Anthropic , which was completed on Wednesday.
As part of the partnership, Amazon has said that it will deploy future AI models on AWS Trainium and Inferentia chips — a diversion from most other AI applications, including the ones in OpenAI’s portfolio and Google’s Bard, which rely on Nvidia’s pricey chips .
Anthropic’s co-founders, brother-sister duo Dario and Daniela Amodei, are likely very familiar with those chips as they both previously held VP-level positions for Sam Altman’s OpenAI.
Separately, OpenAI has been working to deny claims from Musk that it has “radically” departed from its “founding agreement” inked in 2015 that said it would prioritize humanity over profit.
Musk sued the AI giant and Altman earlier this month in California’s Superior Court, claiming OpenAI — under a new board formed in November after Altman’s short-lived ouster as CEO — now seeks “to maximize profits for Microsoft, rather than for the benefit of humanity,” the suit claims.
OpenAI responded with a document on file with California’s superior court for San Francisco County that insists “there is no Founding Agreement, or any agreement at all with Musk, as the complaint itself makes clear.”
Later this week, Grok will be enabled for all premium subscribers (not just premium+) https://t.co/4u9lbLwe23
“The Founding Agreement is instead a fiction Musk has conjured to lay unearned claim to the fruits of an enterprise he initially supported, then abandoned, then watched succeed without him,” the company added in its filing dated March 6 obtained by CNBC .
Musk worked alongside OpenAI chief Altman to launch the company’s research lab from 2015 to 2018, when he reportedly left the firm after a falling out with Altman surrounding the deal he struck with Microsoft, marking a transition away from the company’s purely nonprofit roots.
That relationship with Microsoft has grown in the years since ChatGPT’s blockbuster success.
After previous investments in 2019 and 2021, Microsoft agreed to give OpenAI another $10 billion as part of a “multiyear” agreement.
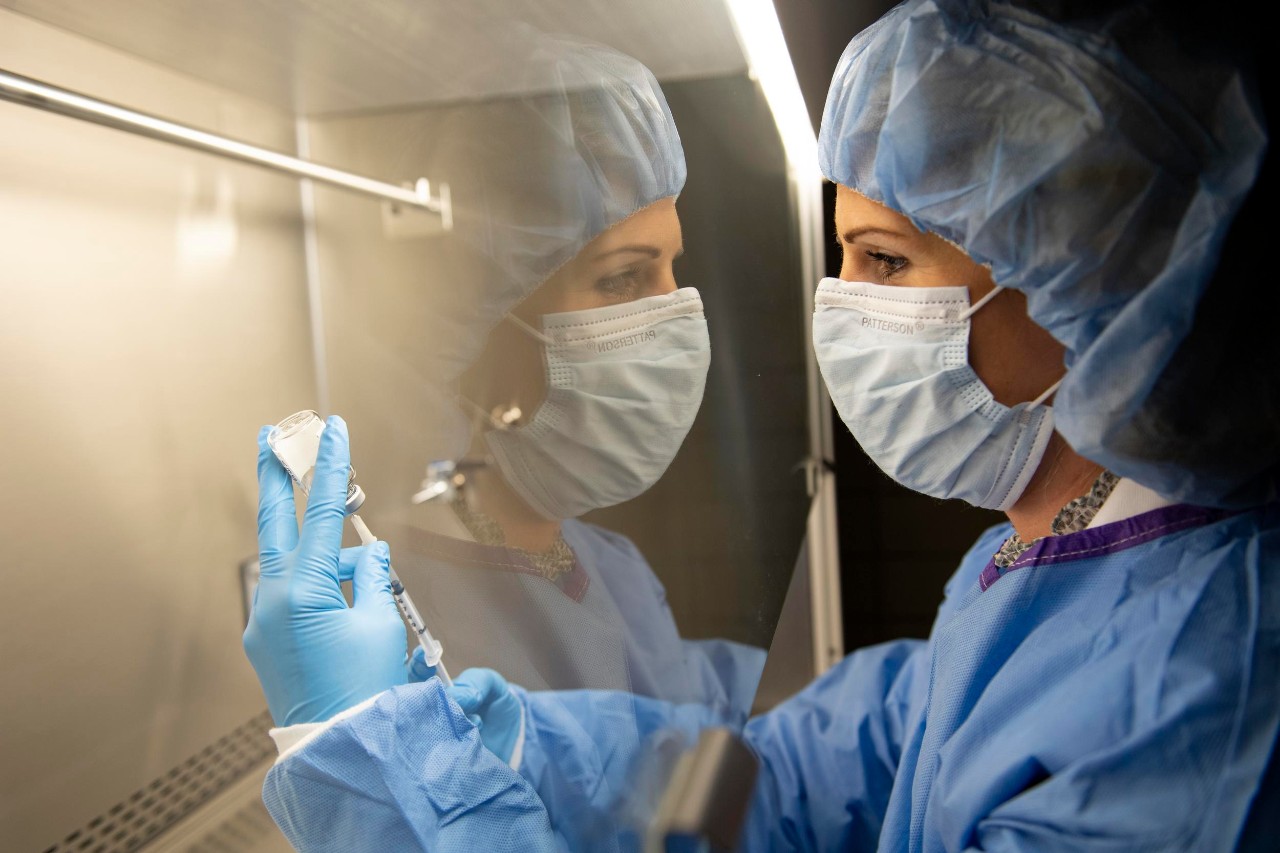
Powerful new AI can predict people's attitudes to vaccines
System integrates the math of human judgment with machine learning to predict vaccine hesitancy.

A powerful new tool in artificial intelligence is able to predict whether someone is willing to be vaccinated against COVID-19.
The predictive system uses a small set of data from demographics and personal judgments such as aversion to risk or loss.
The findings frame a new technology that could have broad applications for predicting mental health and result in more effective public health campaigns.
A team led by researchers at the University of Cincinnati and Northwestern University created a predictive model using an integrated system of mathematical equations describing the lawful patterns in reward and aversion judgment with machine learning.
“We used a small number of variables and minimal computational resources to make predictions,” said lead author Nicole Vike, a senior research associate in UC’s College of Engineering and Applied Science.
“COVID-19 is unlikely to be the last pandemic we see in the next decades. Having a new form of AI for prediction in public health provides a valuable tool that could help prepare hospitals for predicting vaccination rates and consequential infection rates.”
The study was published in the Journal of Medical Internet Research Public Health and Surveillance .
UC College of Pharmacy students administer the COVID-19 vaccine during a drive-through clinic sponsored by UC Health in this 2020 file image. Photo/Colleen Kelley/UC Marketing + Brand
Researchers surveyed 3,476 adults across the United States in 2021 during the COVID-19 pandemic. At the time of the survey, the first vaccines had been available for more than a year.
Respondents provided information such as where they live, income, highest education level completed, ethnicity and access to the internet. The respondents’ demographics mirrored those of the United States based on U.S. Census Bureau figures.
Participants were asked if they had received either of the available COVID-19 vaccines. About 73% of respondents said they were vaccinated, slightly more than the 70% of the nation's population that had been vaccinated in 2021.
Further, they were asked if they routinely followed four recommendations designed to prevent the spread of the virus: wearing a mask, social distancing, washing their hands and not gathering in large groups.
The framework by which we judge what is rewarding or aversive is fundamental to how we make medical decisions.
Hans Breiter, UC College of Engineering and Applied Science
Participants were asked to rate how much they liked or disliked a randomly sequenced set of 48 pictures on a seven-point scale of 3 to -3. The pictures were from the International Affective Picture Set, a large set of emotionally evocative color photographs, in six categories: sports, disasters, cute animals, aggressive animals, nature and food.
Vike said the goal of this exercise is to quantify mathematical features of people's judgments as they observe mildly emotional stimuli. Measures from this task include concepts familiar to behavioral economists — or even people who gamble — such aversion to risk (the point at which someone is willing to accept potential loss for a potential reward) and aversion to loss. This is the willingness to avoid risk by, for example, obtaining insurance.
“The framework by which we judge what is rewarding or aversive is fundamental to how we make medical decisions,” said co-senior author Hans Breiter, a professor of computer science at UC. “A seminal paper in 2017 hypothesized the existence of a standard model of the mind. Using a small set of variables from mathematical psychology to predict medical behavior would support such a model. The work of this collaborative team has provided such support and argues that the mind is a set of equations akin to what is used in particle physics.”
UC College of Engineering and Applied Science Professor Hans Breiter is applying artificial intelligence to new applications in health and human behavior. Photo/Andrew Higley/UC Marketing + Brand
The judgment variables and demographics were compared between respondents who were vaccinated and those who were not. Three machine learning approaches were used to test how well the respondents’ judgment, demographics and attitudes toward COVID-19 precautions predicted whether they would get the vaccine.
The study demonstrates that artificial intelligence can make accurate predictions about human attitudes with surprisingly little data or reliance on expensive and time-consuming clinical assessments.
“We found that a small set of demographic variables and 15 judgment variables predict vaccine uptake with moderate to high accuracy and high precision,” the study said. “In an age of big-data machine learning approaches, the current work provides an argument for using fewer but more interpretable variables.”
“The study is anti-big-data,” said co-senior author Aggelos Katsaggelos, an endowed professor of electrical engineering and computer science at Northwestern University. “It can work very simply. It doesn’t need super-computation, it’s inexpensive and can be applied with anyone who has a smartphone. We refer to it as computational cognition AI. It is likely you will be seeing other applications regarding alterations in judgment in the very near future.”
Featured image at top: UC College of Pharmacy pharmacist Mary Burns draws a vial during clinical trials for the Moderna COVID-19 vaccine in this 2020 file photo. Photo/Colleen Kelley/UC Marketing + Brand
Impact Lives Here
The University of Cincinnati is leading public urban universities into a new era of innovation and impact. Our faculty, staff and students are saving lives, changing outcomes and bending the future in our city's direction. Next Lives Here.
- Innovation Agenda
- Electrical and Computer Engineering
- Next Lives Here
- College of Engineering and Applied Science
- Science & Tech
Related Stories
March 18, 2024
New at-home test protects oral health
September 7, 2023
Engineers at the University of Cincinnati have developed a new device that can warn consumers about early risks of tooth decay from diseases such as gingivitis and periodontitis.
UC students help create world's biggest supercomputer
May 29, 2020
Daniel Corcoran, a computer science major in the University of Cincinnati's College of Engineering and Applied Science, is part of an international effort to turn everyday computers into a networked supercomputer to tackle public research projects.
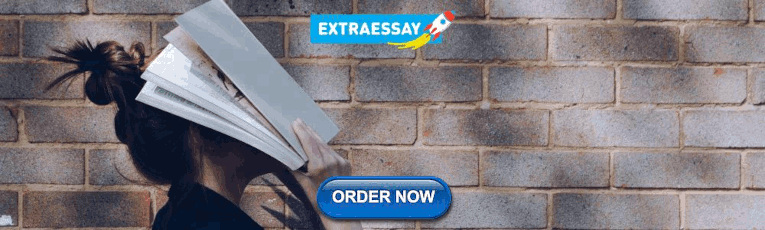
IMAGES
VIDEO
COMMENTS
The Machine Learning (ML) Ph.D. program is a fully-funded doctoral program in machine learning (ML), designed to train students to become tomorrow's leaders through a combination of interdisciplinary coursework, and cutting-edge research. Graduates of the Ph.D. program in machine learning are uniquely positioned to pioneer new developments in ...
PhD Studentship in Marine Technology: Development of an Artificial Intelligence Model to Assess Nonlinear Multibody Structural Response of Container Stacks. Newcastle University School of Engineering. Award summary . 100% fees covered and a minimum tax-free annual living allowance of £19,237 for UK students (2024/25 UKRI rate). . Read more.
The curriculum for the PhD in Machine Learning is truly multidisciplinary, containing courses taught in eight schools across three colleges at Georgia Tech: the Schools of Computational Science and Engineering, Computer Science, and Interactive Computing in the College of Computing; the Schools of Industrial and Systems Engineering, Electrical and Computer Engineering, and Biomedical ...
Artificial intelligence is an evolving field that requires broad training, so courses typically involve principles of computer science, cognitive psychology and engineering. These are the best ...
Machine Learning and Artificial Intelligence. Join our Machine Learning Lab for a PhD at the University of Cambridge! Ready to push the boundaries of research, as a part of a dynamic and world-renowned group dedicated to harnessing the power of Machine Learning to tackle real-world challenges? We are thrilled to announce that the van der Schaar ...
The Graduate Group in Applied Mathematics and Computational Science of the University of Pennsylvania offers a full graduate program in mathematics, conferring the degrees of Master of Arts (M.A.), and Doctor of Philosophy (Ph.D.) . The educational aim of this program is to provide well-rounded training for careers in research, teaching or ...
Overview. Artificial Intelligence at Caltech researches the development of intelligent systems capable of emulating human-like intelligence and performing complex tasks. We study the fundamental aspects of AI, spanning machine learning, mathematics, and statistics, in order to delve into applications in perception, robotics, reinforcement ...
A Ph.D. in AI is the highest awarded degree in the field of artificial intelligence. It involves several years of study and research, culminating in a project, thesis, or dissertation of original academic research that expands our knowledge of AI. The main focus of a Ph.D. in AI is research. Students spend about six years researching one topic.
The program has focuses on understanding nature through the fusion of Artificial Intelligence, Computing (classical to quantum), and Mathematics. We value foundational contributions, societal impact, and ethics in our work. Applied Mathematics PhD graduates from Harvard Engineering pursue careers in industry, academics, and almost anything they ...
Carnegie Mellon has been a leader in Artificial Intelligence (AI) research since Herbert Simon and Allen Newell invented the field in the 1950s. Since then, AI has evolved to address problems of probabilistic and numeric nature, leading to the incorporation of approaches from mathematics, engineering, operations research and economics. Our researchers scientifically build upon each others ...
Students should also note the school's overall PhD Program Plan requirements. Examples of courses for students studying machine learning and artificial intelligence. AM 216 Inverse Problems in Science and Engineering. AM 221 Advanced Optimization. CS 234r Topics on Computation in Networks and Crowds. CS 280r Advanced Topics in Artificial ...
6 Artificial Intelligence PhDs in United States. Computer Science. Pace University. Pleasantville, New York, United States. Information. The University of Arizona. Tucson, Arizona, United States. Intelligent Systems and Robotics. Hal Marcus College of Science and Engineering.
Ph.D. programs in AI focus on mastering advanced theoretical subjects, such as decision theory, algorithms, optimization, and stochastic processes. Artificial intelligence covers anything where a computer behaves, rationalizes, or learns like a human. Ph.D.s are usually the endpoint to a long educational career.
Artificial Intelligence (AI) is the study of intelligent artifacts and the principles behind their design, construction, and analysis. Oregon State has a long history of excellence in AI since the early days of computer science. The field traces its origin to many disciplines, including philosophy, psychology, mathematics, and engineering. Today, AI is making contributions to all areas of ...
Even if I don't know anything about, I find the Artificial Intelligence field really fascinating but I know that my current background is inadequate. ... In the end I decided to keep studying math and at the moment I started a PhD in pure math (geometric analysis). Maybe soon or later I will finally ended up working on AI. I know that there are ...
Writing in Nature, Davies et al. now describe a way of using artificial intelligence (AI) techniques to help with the creative core of the mathematical-research process 1. The relationship between ...
View PDF Abstract: We currently witness the spectacular success of artificial intelligence in both science and public life. However, the development of a rigorous mathematical foundation is still at an early stage. In this survey article, which is based on an invited lecture at the International Congress of Mathematicians 2022, we will in particular focus on the current "workhorse" of ...
Research topics covered in this Artificial Intelligence and Intelligent Agents course at Bangor University include knowledge-based systems, logic, multi-agent systems, distributed systems, machine learning, data mining, computational linguistics, natural language processing, information theory and information retrieval.
PhD Program Mathematics - Graduate School Mathematics. The doctoral studies at Université Paris-Saclay encompass the full mathematical spectrum, from most fundamental aspects (algebra, analysis, geometry, probability) to applications in various sciences (physics, biology) and industry motivated problems (Artificial Intelligence, scientific ...
Artificial Intelligence (AI) has opened new horizons for the design and development of control and multi-agent systems. Among the others, machine learning demonstrated super-human capabilities in recent years, especially for sequential decision-making and tasks that require continuous control, video games and robotics.
The Mathematics of Artificial Intelligence. Gitta Kutyniok. Abstract. W e currently witness the spectacular success of artificial intelligence in both science and public life. How-. ever, the ...
Within the Faculty of Science and Engineering, a 4-years PhD position is available Bernoulli institute of Mathematics, Computer Science and Artificial Intelligence.
If you have questions or wish to learn more about the PhD program in Health AI, call us or send a message. 310‐423‐3391. SEND A MESSAGE. The PhD program in Health Artificial Intelligence at Cedars-Sinai prepares students with rigorous training in AI algorithms and methods to improve patient care.
Her latest, GPTCelltype, applies artificial intelligence to the laborious process of manually annotating the array of cell types found in complex tissue samples for single-cell RNA analyses. ... That sparked a deep dive into gene regulatory networks for my PhD. The beauty of mathematics applied to biology was irresistible. I was amazed by how ...
Following Arizona State University's groundbreaking announcement of the first university collaboration with OpenAI, the W. P. Carey School of Business is officially launching a new degree program — the Master of Science in Artificial Intelligence in Business (MS-AIB). Backed by faculty from the Department of Information Systems, it is the first AI graduate degree program from a business ...
It is concerning to read, as reported in the Stanford AI Index, that the number of AI Ph.D. graduates taking jobs in academia has declined over the last decade while the number going to industry ...
data science, machine learning, artificial intelligence applied mathematics, statistics and probabilities optimisation energy forecasting power system management, integration of renewables. Expected level in french : Good level. Expected level in english : Proficiency Desired starting date is as soon as possiblein 2024. Duration 36 months.
E lon Musk's artificial intelligence startup xAI announced that it's releasing an upgraded version of its ChatGPT-rival chatbot Grok that will be able to code and do math-related tasks — but ...
System integrates the math of human judgment with machine learning to predict vaccine hesitancy. A powerful new tool in artificial intelligence is able to predict whether someone is willing to be vaccinated against COVID-19. The predictive system uses a small set of data from demographics and personal judgments such as aversion to risk or loss ...
LONDON, April 2, 2024 /PRNewswire/ -- XTX Markets is excited to announce the opening of the first $1.048 million progress prize for the Artificial Intelligence Mathematical Olympiad (AIMO), on the ...