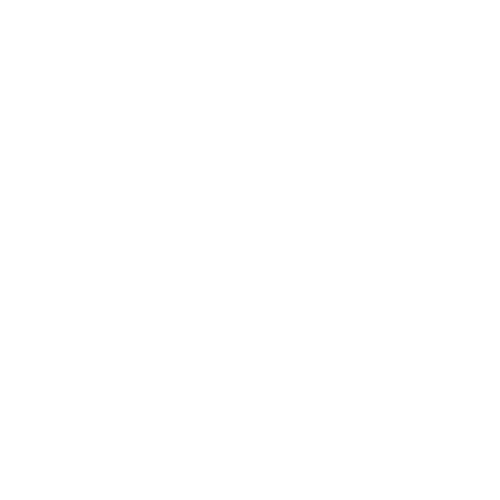
May 25, 2021
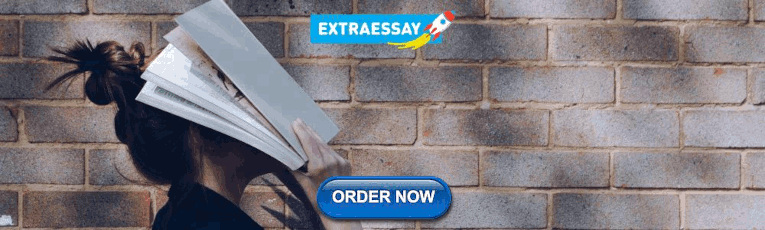
New study shows how to boost muscle regeneration and rebuild tissue
Salk research reveals clues about molecular changes underlying muscle loss tied to aging
Home - Salk News - New study shows how to boost muscle regeneration and rebuild tissue
LA JOLLA—One of the many effects of aging is loss of muscle mass, which contributes to disability in older people. To counter this loss, scientists at the Salk Institute are studying ways to accelerate the regeneration of muscle tissue, using a combination of molecular compounds that are commonly used in stem-cell research.
In a study published on May 25, 2021, in Nature Communications , the investigators showed that using these compounds increased the regeneration of muscle cells in mice by activating the precursors of muscle cells, called myogenic progenitors. Although more work is needed before this approach can be applied in humans, the research provides insight into the underlying mechanisms related to muscle regeneration and growth and could one day help athletes as well as aging adults regenerate tissue more effectively.
“Loss of these progenitors has been connected to age-related muscle degeneration,” says Salk Professor Juan Carlos Izpisua Belmonte , the paper’s senior author. “Our study uncovers specific factors that are able to accelerate muscle regeneration, as well as revealing the mechanism by which this occurred.”
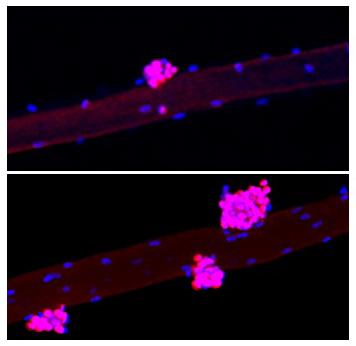
The compounds used in the study are often called Yamanaka factors after the Japanese scientist who discovered them. Yamanaka factors are a combination of proteins (called transcription factors) that control how DNA is copied for translation into other proteins. In lab research, they are used to convert specialized cells, like skin cells, into more stem-cell-like cells that are pluripotent, which means they have the ability to become many different types of cells.
“Our laboratory previously showed that these factors can rejuvenate cells and promote tissue regeneration in live animals,” says first author Chao Wang, a postdoctoral fellow in the Izpisua Belmonte lab. “But how this happens was not previously known.”
Muscle regeneration is mediated by muscle stem cells, also called satellite cells. Satellite cells are located in a niche between a layer of connective tissue (basal lamina) and muscle fibers (myofibers). In this study, the team used two different mouse models to pinpoint the muscle stem-cell-specific or niche-specific changes following addition of Yamanaka factors. They focused on younger mice to study the effects of the factors independent of age.
In the myofiber-specific model, they found that adding the Yamanaka factors accelerated muscle regeneration in mice by reducing the levels of a protein called Wnt4 in the niche, which in turn activated the satellite cells. By contrast, in the satellite-cell-specific model, Yamanaka factors did not activate satellite cells and did not improve muscle regeneration, suggesting that Wnt4 plays a vital role in muscle regeneration.
According to Izpisua Belmonte, who holds the Roger Guillemin Chair, the observations from this study could eventually lead to new treatments by targeting Wnt4.
“Our laboratory has recently developed novel gene-editing technologies that could be used to accelerate muscle recovery after injury and improve muscle function,” he says. “We could potentially use this technology to either directly reduce Wnt4 levels in skeletal muscle or to block the communication between Wnt4 and muscle stem cells.”
The investigators are also studying other ways to rejuvenate cells, including using mRNA and genetic engineering. These techniques could eventually lead to new approaches to boost tissue and organ regeneration.
Other authors included: Ruben Rabadan Ros, Paloma Martinez Redondo, Zaijun Ma, Lei Shi, Yuan Xue, Isabel Guillen-Guillen, Ling Huang, Tomoaki Hishida, Hsin-Kai Liao, Concepcion Rodriguez Esteban, and Pradeep Reddy of Salk; Estrella Nuñez Delicado of Universidad Católica San Antonio de Murcia in Spain; and Pedro Guillen Garcia of Clinica CEMTRO in Spain.
The work was funded by NIH-NCI CCSG: P30 014195, the Helmsley Trust, Fundacion Ramon Areces, Asociación de Futbolistas Españoles (AFE), Fundacion Pedro Guillen, Universidad Católica San Antonio de Murcia (UCAM), the Moxie Foundation and CIRM (GC1R-06673-B).
DOI: 10.1038/s41467-021-23353-z
PUBLICATION INFORMATION
Nature Communications
In vivo partial reprogramming of myofibers promotes muscle regeneration by remodeling the stem cell niche
Chao Wang, Ruben Rabadan Ros, Paloma Martinez Redondo, Zaijun Ma, Lei Shi, Yuan Xue, Isabel Guillen-Guillen, Ling Huang, Tomoaki Hishida, Hsin-Kai Liao, Estrella Nuñez Delicado, Concepcion Rodriguez Esteban, Pedro Guillen Garcia, Pradeep Reddy and Juan Carlos Izpisua Belmonte
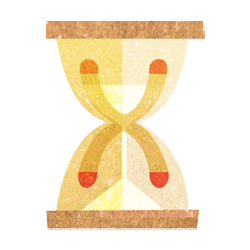
Healthy Aging
For more information.
Office of Communications Tel: (858) 453-4100 [email protected]
The Salk Institute For Biological Studies:
Unlocking the secrets of life itself is the driving force behind the Salk Institute. Our team of world-class, award-winning scientists pushes the boundaries of knowledge in areas such as neuroscience, cancer research, aging, immunobiology, plant biology, computational biology and more. Founded by Jonas Salk, developer of the first safe and effective polio vaccine, the Institute is an independent, nonprofit research organization and architectural landmark: small by choice, intimate by nature, and fearless in the face of any challenge.
Connect With Us
Keep in touch.
Inside Salk
Explore our magazine.
Every cure begins with you.
Latest discoveries, events & more.
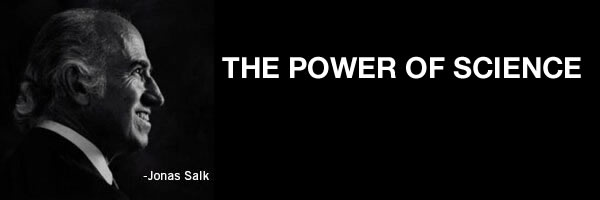
Your partnership allows our scientists to accelerate the pace of high-risk, high-reward discoveries that have the potential to benefit the health of all humanity-be it cancer, Alzheimer’s disease, climate change, infectious diseases or more.
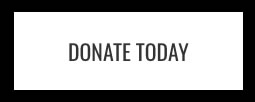
Systematic review and meta-analysis of protein intake to support muscle mass and function in healthy adults
Affiliations.
- 1 Exercise Metabolism Research Group, Department of Kinesiology, McMaster University, Hamilton, Ontario, Canada.
- 2 Laboratory of Investigation of Chronic Diseases, Department of Physiological Sciences, Federal University of Santa Catarina, Florianópolis, Brazil.
- 3 McMaster Evidence Review and Synthesis Centre, McMaster University, Hamilton, Ontario, Canada.
- 4 School of Nursing, Faculty of Health Sciences, McMaster University, Hamilton, Ontario, Canada.
- 5 Université Paris-Saclay, AgroParisTech, INRAE, UMR PNCA, Paris, France.
- 6 MRC Versus Arthritis Centre of Excellence for Musculoskeletal Ageing Research (CMAR), NIHR Biomedical Research Centre, School of Medicine, University of Nottingham, Nottingham, UK.
- 7 Abbott Nutrition, Research and Development, Granada, Spain.
- 8 Givaudan, Research and Development, Avignon, France.
- 9 International Flavors & Fragrances, Research and Development, St. Louis, MO, USA.
- 10 Fondazione Policlinico Universitario A. Gemelli IRCCS, Rome, Italy.
- PMID: 35187864
- PMCID: PMC8978023
- DOI: 10.1002/jcsm.12922
We performed a systematic review, meta-analysis, and meta-regression to determine if increasing daily protein ingestion contributes to gaining lean body mass (LBM), muscle strength, and physical/functional test performance in healthy subjects. A protocol for the present study was registered (PROSPERO, CRD42020159001), and a systematic search of Medline, Embase, CINAHL, and Web of Sciences databases was undertaken. Only randomized controlled trials (RCT) where participants increased their daily protein intake and were healthy and non-obese adults were included. Research questions focused on the main effects on the outcomes of interest and subgroup analysis, splitting the studies by participation in a resistance exercise (RE), age (<65 or ≥65 years old), and levels of daily protein ingestion. Three-level random-effects meta-analyses and meta-regressions were conducted on data from 74 RCT. Most of the selected studies tested the effects of additional protein ingestion during RE training. The evidence suggests that increasing daily protein ingestion may enhance gains in LBM in studies enrolling subjects in RE (SMD [standardized mean difference] = 0.22, 95% CI [95% confidence interval] 0.14:0.30, P < 0.01, 62 studies, moderate level of evidence). The effect on LBM was significant in subjects ≥65 years old ingesting 1.2-1.59 g of protein/kg/day and for younger subjects (<65 years old) ingesting ≥1.6 g of protein/kg/day submitted to RE. Lower-body strength gain was slightly higher by additional protein ingestion at ≥1.6 g of protein/kg/day during RE training (SMD = 0.40, 95% CI 0.09:0.35, P < 0.01, 19 studies, low level of evidence). Bench press strength is slightly increased by ingesting more protein in <65 years old subjects during RE training (SMD = 0.18, 95% CI 0.03:0.33, P = 0.01, 32 studies, low level of evidence). The effects of ingesting more protein are unclear when assessing handgrip strength and only marginal for performance in physical function tests. In conclusion, increasing daily protein ingestion results in small additional gains in LBM and lower body muscle strength gains in healthy adults enrolled in resistance exercise training. There is a slight effect on bench press strength and minimal effect performance in physical function tests. The effect on handgrip strength is unclear.
Keywords: Muscle mass; Muscle strength; Physical function; Protein quantity.
© 2022 The Authors. Journal of Cachexia, Sarcopenia and Muscle published by John Wiley & Sons Ltd on behalf of Society on Sarcopenia, Cachexia and Wasting Disorders.
Publication types
- Meta-Analysis
- Systematic Review
- Research Support, Non-U.S. Gov't
- Exercise Therapy
- Muscle Strength / physiology
- Randomized Controlled Trials as Topic
- Resistance Training*
Grants and funding
- 308584/2019-8/Brazilian National Council for Scientific and Technological Development (CNPq)

Team advances research on muscle health
An international team led by uOttawa Faculty of Medicine researchers have published findings that could contribute to future therapeutics for muscle degeneration due to old age, and diseases such as cancer and muscular dystrophy.
In a study appearing in the Journal of Cell Biology , which publishes peer-reviewed research on cellular structure and function, the authors said their work demonstrates the importance of the enzyme GCN5 in maintaining the expression of key structural proteins in skeletal muscle. Those are the muscles attached to bone that breathing, posture and locomotion all rely on.
"We found that if you delete GCN5 expression from muscle it will no longer be able to handle extreme physical stress," says Dr. Keir Menzies, a molecular biologist at the Faculty of Medicine's Biochemistry, Microbiology and Immunology department and cross-appointed as an associate professor at the Interdisciplinary School of Health Sciences.
Over the span of roughly five years, the uOttawa-led international collaboration painstakingly experimented with a muscle-specific mouse "knockout" of GCN5, a well-studied enzyme which regulates multiple cellular processes such as metabolism and inflammation. Through a series of manipulations, scientists produce lab mice in which specific genes are disrupted, or knocked out, to unveil animal models of human disease and better understand how genes work.
In this case, multiple experiments were done to examine the role the GCN5 enzyme plays in muscle fiber. What they found with this line of muscle-specific mouse knockouts was a notable decline in muscle health during physical stress, such as downhill treadmill running, a type of exercise known by athletes to cause micro-tears in muscle fibres to stimulate muscle growth. The lab animals' muscle fibers became dramatically weaker as they scurried downhill, like those of old mice, while wild-type mice were not similarly impacted.
Dr. Menzies, the senior author of the study, says the findings are akin to what is observed in advanced aging, or myopathies and muscular dystrophy, a group of genetic diseases that result in progressive weakness and loss of muscle mass. It was supported by human data, including an observed negative correlation between muscle fiber diameter and Yin Yang 1, a highly multifunctional protein that is pivotal to a slew of cellular processes and found by the Menzies lab to be a target of GCN5.
Ultimately, the team's research found that GCN5 boosts the expression of key structural muscle proteins, notably dystrophin, and a lack of it will reduce them.
This is significant because dystrophin is the body's most important protein for maintaining the membrane of muscle cells, serving as a kind of anchor and cushioning shock absorber in cells of muscles. Without it, muscles are very susceptible to physical stress, and the withering of muscles can lead to crippling and deadly consequences.
"Our publication shows that if you knock out GCN5 the one major thing we see is a lack of dystrophin, without seeing any real disruption of any other mechanisms," says Dr. Menzies. He noted that the paper also reaffirmed other research showing that GCN5 doesn't alter the content of muscle mitochondria, the powerhouses in cells, and another major influencer of muscle health.
The research builds on data showing that dystrophin is "important for maintaining general muscle integrity and muscle health," according to Dr. Menzies.
Dr. Menzies suggests the research could help to create a foundation for developing therapeutics down the line. "These findings may therefore be useful for the discovery of new therapeutics that regulate GCN5 activity, or its downstream targets, for maintaining healthy muscle during cancer, myopathies, muscular dystrophy or aging," he says.
- Muscular Dystrophy
- Fibromyalgia
- Chronic Fatigue Syndrome
- Medical Topics
- Stem cell treatments
- Encephalopathy
- Sore muscles after exercising
- Chemotherapy
- Cervical cancer
Story Source:
Materials provided by University of Ottawa . Original written by David McFadden. Note: Content may be edited for style and length.
Journal Reference :
- Gregory C. Addicks, Hongbo Zhang, Dongryeol Ryu, Goutham Vasam, Alexander E. Green, Philip L. Marshall, Sonia Patel, Baeki E. Kang, Doyoun Kim, Elena Katsyuba, Evan G. Williams, Jean-Marc Renaud, Johan Auwerx, Keir J. Menzies. GCN5 maintains muscle integrity by acetylating YY1 to promote dystrophin expression . Journal of Cell Biology , 2022; 221 (2) DOI: 10.1083/jcb.202104022
Cite This Page :
Explore More
- Fossil Frogs Share Their Skincare Secrets
- Fussy Eater? Most Parents Play Short Order Cook
- Precise Time Measurement: Superradiant Atoms
- Artificial Cells That Act Like Living Cells
- Affordable and Targeted Anticancer Agent
- This Alloy Is Kinky
- Giant Galactic Explosion: Galaxy Pollution
- Flare Erupting Around a Black Hole
- Two Species Interbreeding Created New Butterfly
- Warming Antarctic Deep-Sea and Sea Level Rise
Trending Topics
Strange & offbeat.
Articles on Muscle growth
Displaying all articles.
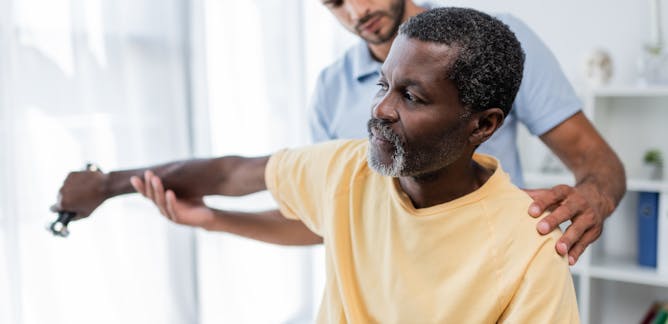
For cancer patients, maintaining muscle is vital to health and treatment, but staying strong is complicated
Meghan McCue , Laurentian University
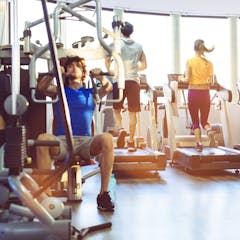
Cardio or weights first? A kinesiologist explains how to optimize the order of your exercise routine
Randal Claytor , Miami University
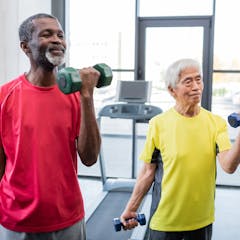
Am I too old to build muscle? What science says about sarcopenia and building strength later in life
David Scott , Deakin University and Robin M. Daly , Deakin University
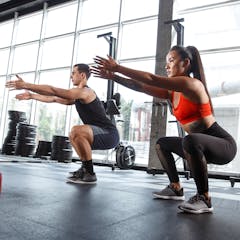
‘Training to failure’: debunking claims this popular weightlifting technique is the most effective for building muscle and strength
Rob Erskine , Liverpool John Moores University and Gerard McMahon , Ulster University
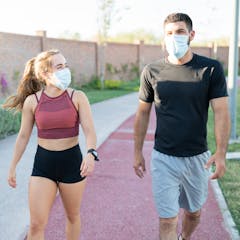
Walking workouts are great for heart, bone, and muscle health – and almost everyone can do it
Bradley Elliott , University of Westminster
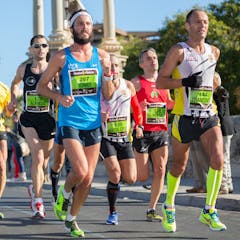
Exercise nutrition: whether you should eat before or after a workout depends on your fitness goals
Justin Roberts , Anglia Ruskin University
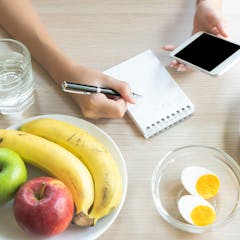
Calories or macros: nutritionist explains which works best for weight loss or building muscle
Emma Kinrade , Glasgow Caledonian University
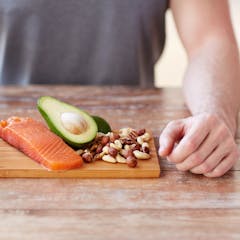
What’s the best source of protein for building muscle – meat or plants?
Oliver Witard , University of Stirling ; Kevin Tipton , University of Stirling , and Lee Hamilton , University of Stirling
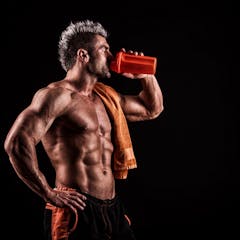
Gym workout advice: protein guidance looks wrong, our findings suggest
Lee Hamilton , University of Stirling ; Kevin Tipton , University of Stirling , and Oliver Witard , University of Stirling
Related Topics
- Sports training
- Weightlifting
- Weight loss
Top contributors
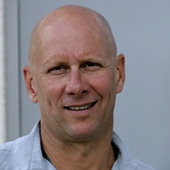
Professor of Sport, Health and Exercise Sciences, Durham University
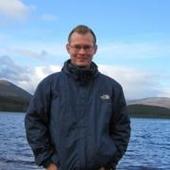
Lecturer in Exercise Physiology Across the Lifespan, Deakin University
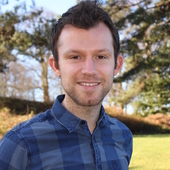
Senior Lecturer in Sport, Health & Exercise Science, University of Stirling
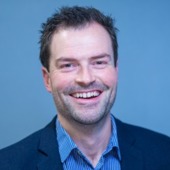
Associate Professor in Neuromuscular Physiology, Liverpool John Moores University
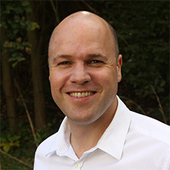
Professor of Nutritional Physiology, Anglia Ruskin University
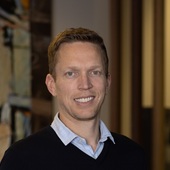
Associate Professor (Research) and NHMRC Emerging Leadership Fellow, Deakin University
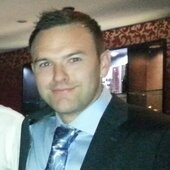
Lecturer Exercise Physiology, Ulster University
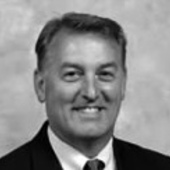
Associate Professor of Kinesiology, Nutrition and Health, Miami University
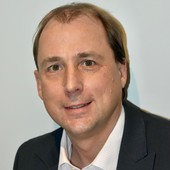
Professor of Exercise and Ageing, Institute for Physical Activity and Nutrition, Deakin University, Deakin University
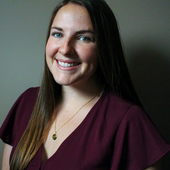
PhD Candidate, Biomolecular Sciences, Laurentian University
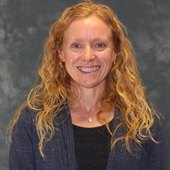
Lecturer in Nutrition and Dietetics, Glasgow Caledonian University
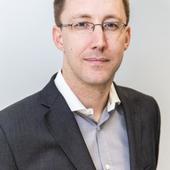
Senior Lecturer in Physiology, University of Westminster
- X (Twitter)
- Unfollow topic Follow topic
Thank you for visiting nature.com. You are using a browser version with limited support for CSS. To obtain the best experience, we recommend you use a more up to date browser (or turn off compatibility mode in Internet Explorer). In the meantime, to ensure continued support, we are displaying the site without styles and JavaScript.
- View all journals
- My Account Login
- Explore content
- About the journal
- Publish with us
- Sign up for alerts
- Open access
- Published: 22 April 2024
Multimodal cell atlas of the ageing human skeletal muscle
- Yiwei Lai 1 , 2 na1 ,
- Ignacio Ramírez-Pardo 3 , 4 na1 ,
- Joan Isern ORCID: orcid.org/0000-0002-1401-9779 4 na1 ,
- Juan An 1 , 2 , 5 , 6 na1 ,
- Eusebio Perdiguero 3 , 4 na1 ,
- Antonio L. Serrano 3 , 4 na1 ,
- Jinxiu Li 1 , 2 , 7 na1 ,
- Esther García-Domínguez ORCID: orcid.org/0000-0001-6276-4944 8 ,
- Jessica Segalés 3 ,
- Pengcheng Guo 1 , 2 , 9 ,
- Vera Lukesova 3 ,
- Eva Andrés 3 ,
- Jing Zuo 1 , 2 ,
- Yue Yuan 1 , 2 ,
- Chuanyu Liu ORCID: orcid.org/0000-0003-2258-0897 1 , 2 ,
- José Viña ORCID: orcid.org/0000-0001-9709-0089 8 ,
- Julio Doménech-Fernández ORCID: orcid.org/0000-0001-9488-8159 10 , 11 ,
- Mari Carmen Gómez-Cabrera ORCID: orcid.org/0000-0003-4000-1684 8 ,
- Yancheng Song 12 ,
- Longqi Liu ORCID: orcid.org/0000-0002-5828-5542 1 , 2 , 7 ,
- Xun Xu ORCID: orcid.org/0000-0002-5338-5173 1 , 2 , 7 ,
- Pura Muñoz-Cánoves ORCID: orcid.org/0000-0002-7533-9047 3 , 4 , 13 &
- Miguel A. Esteban ORCID: orcid.org/0000-0002-1426-6809 1 , 2 , 5 , 9 , 14
Nature ( 2024 ) Cite this article
1685 Accesses
124 Altmetric
Metrics details
- Cell biology
Muscle atrophy and functional decline (sarcopenia) are common manifestations of frailty and are critical contributors to morbidity and mortality in older people 1 . Deciphering the molecular mechanisms underlying sarcopenia has major implications for understanding human ageing 2 . Yet, progress has been slow, partly due to the difficulties of characterizing skeletal muscle niche heterogeneity (whereby myofibres are the most abundant) and obtaining well-characterized human samples 3 , 4 . Here we generate a single-cell/single-nucleus transcriptomic and chromatin accessibility map of human limb skeletal muscles encompassing over 387,000 cells/nuclei from individuals aged 15 to 99 years with distinct fitness and frailty levels. We describe how cell populations change during ageing, including the emergence of new populations in older people, and the cell-specific and multicellular network features (at the transcriptomic and epigenetic levels) associated with these changes. On the basis of cross-comparison with genetic data, we also identify key elements of chromatin architecture that mark susceptibility to sarcopenia. Our study provides a basis for identifying targets in the skeletal muscle that are amenable to medical, pharmacological and lifestyle interventions in late life.
Similar content being viewed by others
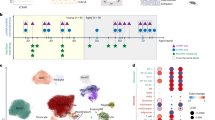
Human skeletal muscle aging atlas
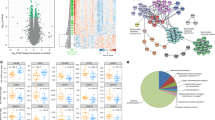
Mitochondrial oxidative capacity and NAD+ biosynthesis are reduced in human sarcopenia across ethnicities
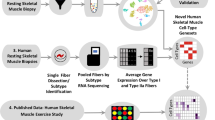
Single-cell transcriptional profiles in human skeletal muscle
Increased longevity demands new approaches to promote healthy ageing. Owing to its connections with other body tissues, the skeletal muscle is a major determinant of systemic health 1 , 2 . Accordingly, pronounced loss of skeletal muscle mass and function associated with ageing—termed sarcopenia—is not only a disabling event but also a critical catalysing step in the accelerated degenerating cascade of older people 1 . Sarcopenia often affects individuals aged over 80 years and is more pronounced in locomotor muscles due to their constant exposure to stress 1 .
Skeletal muscle comprises large multinucleated myofibres with distinct contractile and metabolic activities (slow twitch/oxidative, also known as type I myofibres; and fast twitch/glycolytic, also known as type II myofibres) controlled by the activity of motoneurons that contact the myofibres at the neuromuscular junction (NMJ) 5 , 6 . Muscles also contain a variety of less abundant mononucleated cells, including muscle stem cells (MuSCs, satellite cells), fibro-adipogenic progenitors (FAPs), adipocytes, fibroblast-like cells, immune cells, vascular cells and Schwann cells 3 , 4 . On average, lean muscle mass declines from 50% of the total body weight in young adults to 25% in individuals aged over 80 years 1 . Preservation of muscle mass and function during life requires appropriate interactions of myofibres with the nearby resident cell types 7 , 8 . Moreover, skeletal muscle has the ability to regenerate due to MuSCs, which are quiescent unless damage occurs 9 . Ageing negatively affects the overall multicellular cross-talk in the skeletal muscle niche as well as the relative cell numbers, and reduces the regenerative ability of MuSCs 9 . However, the underlying mechanisms remain poorly characterized at the molecular level, especially in humans, complicating the development of therapeutic approaches.
Here we aimed to generate a comprehensive transcriptomic and epigenomic cell atlas of the human locomotor skeletal muscle across different age groups and sexes, including individuals aged ≥84 years with signs of sarcopenia.
Multimodal atlas of human skeletal muscle
To investigate the molecular changes that occur in the human skeletal muscle with ageing, we obtained hindlimb muscle biopsies from 31 participants (17 male and 14 female) from Spain and China, who were divided into two age groups: adults (aged 15 to 46 years, n = 12) and older adults (aged 74 to 99 years, n = 19) of both sexes, with median ages of 36 and 84 years, respectively (Fig. 1a and Supplementary Table 1 ). We assessed muscle functionality using (1) the Barthel index, which measures the ability of an individual to carry out daily living activities and their degree of autonomy 10 ; and (2) the Charlson index, which predicts life expectancy on the basis of a person’s comorbidities 11 . Ageing was inversely and directly correlated with Barthel index and Charlson index scores, respectively, in both sexes (Extended Data Fig. 1a and Supplementary Table 1 ). Each biopsy was divided into various samples, which were (1) fixed with paraformaldehyde for histology; (2) snap-frozen in liquid nitrogen for single-nucleus RNA-sequencing (snRNA-seq) and single-nucleus assay for transposase-accessible chromatin using sequencing (snATAC-seq); and/or (3) freshly dissociated in single-cell suspensions for single-cell RNA sequencing (scRNA-seq). Morphological analysis confirmed the integrity of the tissue architecture in all cases, and of overt myofibre atrophy in older individuals (Extended Data Fig. 1b ). Senescent cells, as determined by senescence-associated β-galactosidase (SA-β-gal) staining, were not detected in the myofibre area in either adult or older adult muscle samples (Extended Data Fig. 1c ). Previous evidence has demonstrated the scarcity of senescent cells in both mouse and human unperturbed muscles 12 . We performed snRNA-seq and snATAC-seq analysis of whole samples, and scRNA-seq analysis of isolated mononucleated cells (Fig. 1a ). After quality control, the overall dataset contained 387,444 nuclei/cells corresponding to 22 individuals: 212,774 for snRNA-seq, 79,649 for scRNA-seq and 95,021 for snATAC-seq (Supplementary Table 2 ).
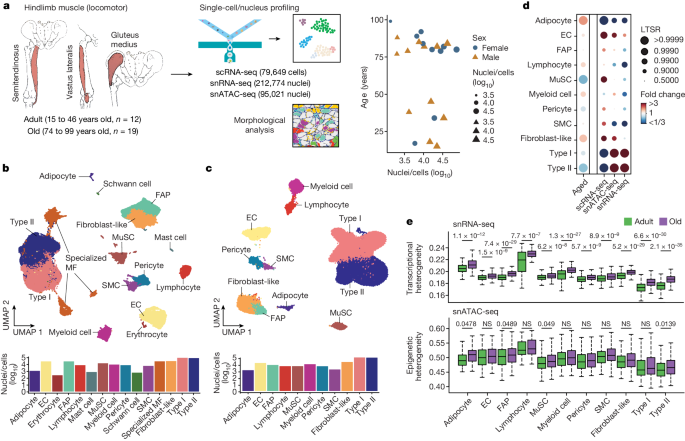
a , Schematic of the hindlimb skeletal muscle samples analysed in this study. The samples were obtained from 12 adult and 19 older adult (old) individuals (left). The samples were processed for single-nucleus or single-cell isolation for sc/snRNA-seq and/or snATAC-seq library construction (using the DNBelab C4 kit) and sequencing (top middle), or subjected to morphological analysis (bottom middle). Right, the sex, age and profiled nuclei/cells per individual. b , UMAP analysis of 292,423 sc/snRNA-seq profiles delineating 15 main skeletal muscle cell populations (top). Bottom, the number of nuclei/cells sequenced for each cell type. Dots and bars are coloured by cell type. MF, myofibre. c , UMAP analysis of 95,021 snATAC-seq profiles delineating 11 main skeletal muscle cell populations (top) at the chromatin level based on gene-activity scores of established marker genes. Bottom, the number of nuclei sequenced for each cell type. Dots and bars are coloured by cell type. d , The relative proportional changes of each cell type with ageing (column 1) and each single-cell modality (columns 2–4) considering co-variable factors as sex, ethnicity, omics technology and sequencing batch. The colour scale represents the fold change, and the dot size shows the probability of change (local true sign rate (LTSR)) calculated using a generalized linear mixed model with a Poisson outcome 14 . e , Quantification of the transcriptional (top) and epigenetic (bottom) heterogeneity by age group and cell type. n = 300 cells obtained by downsampling from the total captured cells in each cell type. For cell types with fewer than 300 cells, all cells were included for analysis. For the box plots, the centre line shows the median, the box limits show the upper and lower quartiles, and the whiskers show 1.5× the interquartile range. For e , P values were calculated using two-tailed Mann–Whitney U -tests.
Source Data
Uniform manifold approximation and projection (UMAP) visualization of the scRNA-seq and snRNA-seq (sc/snRNA-seq) datasets showed clusters representative of type I and II and specialized myonuclei in the multinucleated myofibre compartment 13 . Within the mononucleated cells, the major muscle-resident cell types were MuSCs, stromal cells (FAPs, fibroblast-like cells and adipocytes), vascular cells (pericytes, smooth muscle cells (SMCs) and endothelial cells (ECs)), immune cells (myeloid, lymphoid and mast cells) and glial cells (Schwann cells) 3 , 4 (Fig. 1b and Extended Data Fig. 1d ). Analysis of snATAC-seq data showed robust identification of the main cell types (Fig. 1c and Extended Data Fig. 1e,f ). Integration of the sc/snRNA-seq and snATAC-seq results showed a high correlation, indicating no obvious biases due to method, age, sex, ethnicity or muscle group (Extended Data Fig. 2a–c ). A generalized linear mixed model 14 that considered potentially clinically relevant factors (sex and ethnicity) and technical factors (omics dataset and sequencing batch) revealed age-related decreases in myonuclei, especially in type II myofibres, MuSCs and pericytes, and age-related increases in adipocytes, fibroblast-like cells and immune cells (Fig. 1d ). Cell proportion analyses for each individual in all omics datasets depicted similar results irrespective of sex (Extended Data Fig. 2d ). These analyses also highlighted that the snRNA-seq and snATAC-seq data are enriched for myonuclei, whereas scRNA-seq mostly captured mononucleated cells. Immunofluorescence validated the progressive changes of MuSCs, FAPs/fibroblast-like cells, adipocytes and immune cells with ageing (Extended Data Fig. 3 ). Notably, we noticed that most of the cell types showed increased transcriptional heterogeneity among individual cells/nuclei, which is an emerging feature of ageing 15 (Fig. 1e ). This was associated with variations in the levels of chromatin accessibility at these loci in the snATAC-seq data, together pointing to increased epigenetic instability that could facilitate cell identity drifts.
Changes in myonucleus composition
Our collection of human skeletal muscle samples constitutes a powerful resource tool to elucidate the molecular drivers and processes underlying muscle wasting in older people. We first dissected the heterogeneity of myonuclei in different ages by scoring the snRNA-seq data based on known myofibre-type-specific markers 6 (Supplementary Table 3 ). In addition to MYH7 + myonuclei (type I, TNNT1 + ), we identified the two known type II myonuclei ( TNNT3 + ) subtypes expressing either MYH2 (type IIA) or MYH1 (type IIX), as well as hybrid myonuclei simultaneously expressing two MYH genes, across individuals (Fig. 2a and Extended Data Fig. 4a–e ). Consistent with previous knowledge 6 , ageing induced a general decrease in type II myonuclei accompanied by a relative increase in type I myonuclei in both sexes, which translated into structural changes in the myofibres, as confirmed by immunofluorescence analysis (Fig. 2b and Extended Data Fig. 4f ). The decrease in type II myonuclei was more marked for the IIX subtype, followed by hybrid IIA/IIX myonuclei, and the extent of the changes was highly correlated with the age of the individual (Fig. 2b and Extended Data Fig. 4g ). We drew similar conclusions after analysing the snATAC-seq dataset.
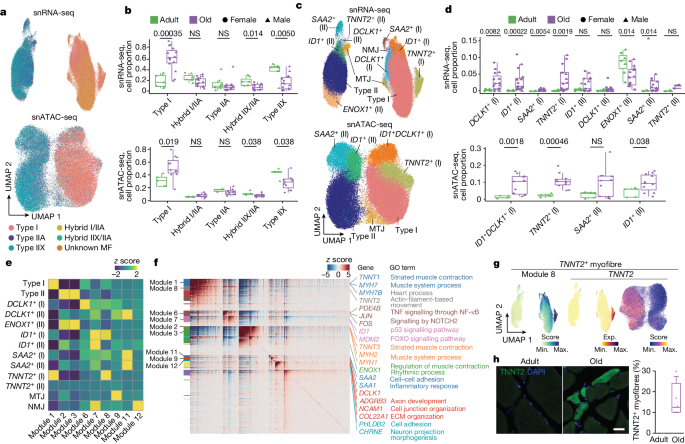
a , UMAP analysis of myonuclei in both snRNA-seq (top) and snATAC-seq (bottom) coloured according to their myofibre-type-specific classification. Each annotated population is correspondingly coloured in both datasets. b , Quantification of the myonucleus proportions in adult (green) and older adult (purple) individuals according to the classified myofibre type in snRNA-seq (top) and snATAC-seq (bottom). NS, not significant. For snRNA-seq, n = 7 adult individuals and n = 15 older adult individuals; for snATAC-seq, n = 5 adult individuals and n = 11 older adult individuals. c , UMAP analysis of myonucleus subpopulations of snRNA-seq (top) and snATAC-seq (bottom) data. Each annotated population is correspondingly coloured in both datasets. MTJ, myotendinous junction. d , As in b , quantification of the detected myonucleus subpopulation proportions in adult and older adult individuals. e , The scaled aggregated expression levels ( z score) in each myonucleus population for the co-expressing genes in each module. f , The scaled gene expression level ( z score) across co-expression modules. Selected enriched genes and their associated pathways (coloured according to module) are highlighted on the right. GO, Gene Ontology. g , UMAP analysis of the aggregated expression (exp.) level for module 8 (left), and TNNT2 gene expression (middle) and its gene score (right). h , Representative images (left) and corresponding quantification (right) of immunofluorescence analysis of TNNT2 + myofibres (TNNT2, green; nuclei, DAPI, blue) in adult (sample P9) and older adult (sample P29) individuals. Scale bar, 10 μm. n = 2 adult individuals and n = 4 older adult individuals. For the box plots, the centre line shows the median, the box limits show the upper and lower quartiles, and the whiskers show 1.5× the interquartile range. For b and d , P values were calculated using two-tailed Mann–Whitney U -tests.
Further snRNA-seq subclustering identified the presence of myonuclei specialized at the myotendinous junction and the NMJ in both main myofibre types (Fig. 2c and Extended Data Fig. 5a,b ). Myotendinous junction myonuclei exhibited enrichment in genes associated with cell–matrix interactions ( COL22A1 , ADGBR4 ), whereas NMJ myonuclei showed enrichment in genes linked to synaptic transmission responses ( PHLDB2 , CHRNE ). Importantly, subclustering identified other populations enriched in either adult or older adult muscle. For example, ENOX1 + myonuclei specific for type II myofibres were enriched in the adult group (median: adult, 9.13%; older adult, 4.21%). By contrast, TNNT2 + , ID1 + , DCLK1 + and SAA2 + myonuclei were enriched in the older group: (1) TNNT2 + and DCLK1 + myonuclei were primarily present in type I myofibres ( TNNT2 + : adult, 0.08%; older adult, 2.45%; DCLK1 + : adult, 0.27%; older adult, 2.27%); (2) ID1 + , in both types of myofibre (type I: adult, 0.17%; older adult, 1.47%; type II: adult, 0.47%; older adult, 0.76%); and (3) SAA2 + populations, mainly in type II (adult, 0.10%; older adult, 0.98%) (Fig. 2c,d ). Most of these myonuclear subpopulations were also detected by snATAC-seq subclustering and showed the same trend after ageing (Extended Data Fig. 5c ). All subpopulations were confirmed by Hotspot analysis 16 , which clusters gene expression profiles into modules (Fig. 2e–g , Extended Data Fig. 5d–f and Supplementary Table 4 ).
Consistent with the protective role of NADPH oxidases in skeletal muscle 17 , ENOX1 + myonuclei may represent a healthy type II myofibre population, as supported by the high expression levels of genes related to carbohydrate metabolism necessary for fast-twitch contraction (Fig. 2f and Extended Data Fig. 5a,b ). Cardiac troponin T (TNNT2) expression has been associated with denervation and ageing 18 . TNNT2 + myonuclei were enriched in genes associated with cardiac muscle contraction ( MYH6 , TNNT2 ), suggesting a loss of skeletal muscle sarcomere specification. TNNT2 expression in older myofibres was confirmed by immunofluorescence analysis (Fig. 2h ). DCLK1 encodes doublecortin-like kinase 1, which is involved in microtubule assembly and dynamics and is highly expressed in dystrophic regenerative (RegMyon) myonuclei 19 . ID1 is a transcription factor (TF) involved in BMP signalling that is associated with muscle atrophy in mice 20 . Serum amyloid A2 (encoded by SAA2 ) is a major acute-phase protein that is highly expressed in response to inflammation and chronic tissue injury 21 . ID1 + , DCLK1 + and SAA2 + older myonuclei expressed high levels of NMJ-related genes ( CHRNA1 , CHRNG , MUSK , COLQ ) and cell adhesion genes, such as members of the PCDHG gene family 22 (Fig. 2f and Extended Data Fig. 5a,b ), which may indicate a compensatory response for the loss of innervation. These subpopulations were also enriched for stress and pro-inflammatory genes ( FOS , JUN , EGR1 ) 23 and proteolysis genes ( FBXO32 , CTSD ) 24 . The increased presence of myofibres with signs of denervation in older muscle was validated by immunofluorescence analysis of NCAM1 5 (Extended Data Fig. 5g ).
General and myofibre-type-specific deterioration
To assess the stepwise transcriptional changes that skeletal myofibres undergo with ageing, we first determined the common differentially expressed genes (DEGs) between adult (aged ≤46 years), ‘old’ (aged 74–82 years) and ‘very old’ (aged ≥84 years) type I and type II myonucleus populations and performed functional enrichment analysis. The shared effects of ageing in older myonuclei comprised a downregulation of genes related to metabolism, including glucose metabolic processes ( SLC2A4 , PFKFB1 ) and TFs regulating lipid metabolism ( PPARGC1A , PPARA ), and sarcomeric genes, such as myosin and troponin genes (Extended Data Fig. 6a,b ). There was a strong correlation between an individual’s age and the downregulation of expression and chromatin accessibility of sarcomeric genes (Extended Data Fig. 6c and Supplementary Table 5a,b ). We also observed a general dysregulation of the circadian machinery in aged myonuclei: core clock genes such as PER1 , PER2 and RORA were downregulated, whereas CLOCK and BMAL1 (also known as ARNTL ) were upregulated, consistent with circadian misalignment with ageing 25 (Extended Data Fig. 6b ). Although transcriptional changes generally had a good match in the snATAC-seq data, circadian genes did not, indicating regulation at other levels. Other shared effects included upregulation of myofibre-atrophy-related processes, such as protein catabolism (lysosome, autophagy and the ubiquitin-proteasome system) and FOXO signalling 24 (Extended Data Fig. 6a ). Moreover, older myonuclei displayed an increased enrichment in TGFβ signalling and homophilic cell adhesion, suggesting an altered interaction with the myofibre environment. Importantly, comparative analysis by age groups revealed that the activation of pro-inflammatory signalling (TNF) 26 was persistently high in the myonuclei of individuals aged ≥84 years. Moreover, we observed a positive correlation with ageing of genes associated with muscle weakness such as increased PCDHGA1 and AMPD3 22 , 27 transcription and chromatin accessibility, albeit with higher variability at the level of chromatin accessibility (Extended Data Fig. 6c ).
To study the directionality of transcriptional variation in the myofibres, we analysed the pseudotime cell trajectories, observing a defined path with ageing in both type I and type II myonuclei (Fig. 3a ). The trajectory end points of these myonuclei corresponded to the transcriptional profiles of the new populations that emerged mostly in aged muscle. This trend was also evident when plotting specific skeletal muscle functions (grouped as scores) progressively affected by ageing, such as the sarcomeric apparatus or atrophy-related genes (Fig. 3b , Extended Data Fig. 6d and Supplementary Table 3 ). Notably, the trajectory of type I myonuclei with ageing was progressive, while that of type II myonuclei was abrupt (Fig. 3a ). This difference agrees with the greater sensitivity of type II myofibres to ageing, which results in their preferential loss. By contrast, type I myofibres persist in aged muscle and accumulate progressive damage that further boosts muscle dysfunction over time.
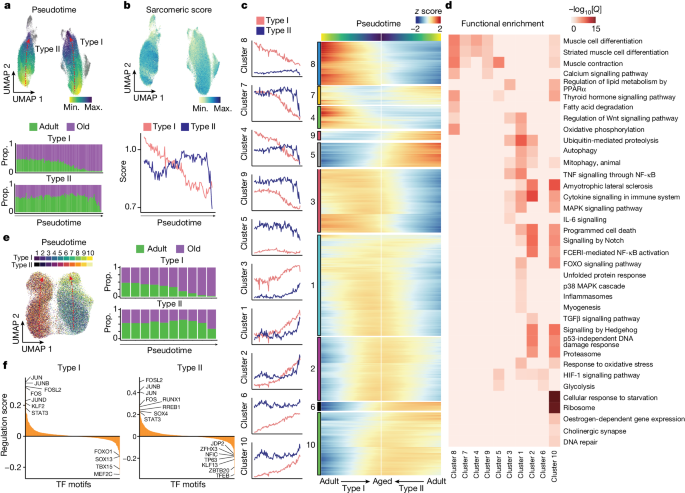
a , UMAP analysis of the ageing trajectory (pseudotime) (top) for type I and type II myonucleus populations in the snRNA-seq dataset. Dots are coloured by the projected pseudotime. The proportion (prop.) of adult (green) and older adult (purple) myonuclei in snRNA-seq data aligned along the type I (middle) and type II (bottom) myonucleus ageing trajectory (divided into 100 bins). b , UMAP analysis of the sarcomeric score for the myonuclei (top) and a line chart showing the average sarcomeric score for type I (red) and type II (blue) myonuclei along the ageing trajectory (bottom). The gene list for sarcomeric score is provided in Supplementary Table 3 . c , The module score for the gene clusters along the ageing trajectory for type I (red) and type II (blue) myonuclei (left). Right, the corresponding gene expression level ( z score). The gene list for each gene cluster is provided in Supplementary Table 6 . d , Functional enrichment analysis of each gene cluster obtained from c . Pathway significance (−log 10 [ Q ]) is depicted by the colour scale. A list of genes associated with each pathway is provided in Supplementary Table 6 . e , UMAP analysis of the ageing trajectory for type I and type II myonuclei in the snATAC-seq dataset, transferred from snRNA-seq data. The ageing trajectory was divided into ten bins (left). The proportion of adult (green) and older adult (purple) myonuclei in snATAC-seq aligned along the type I (top right) and type II (bottom right) pseudotime trajectory. f , The mean regulation score (log 10 -transformed) across all DORCs using the FigR 31 approach per TF for type I and type II myonuclei along the ageing trajectory. The regulation score ( y axis) discerns between TF activators (positive score) and repressors (negative score) for the mapped TF motifs.
Further analysis of the pseudotime showed ten major clusters of transcriptional variation, most of which reflected the progressive or abrupt course of degeneration in type I or type II myofibres, respectively (Fig. 3c and Supplementary Table 6 ). For example, the trajectories of the inflammasome ( NFKB1 , TXNIP ), autophagy ( NBR1 , ATG7 ) and oxidative stress response ( SOD2 , NFE2L2 ) genes increased steadily in type I myonuclei but more sharply in type II myonuclei (cluster 1) (Fig. 3d ). Pro-atrophic Notch signalling 28 ( HES1 , NOTCH2 ) increased with a similar trend in both type I and type II myofibres (clusters 1, 2 and 10) with ageing. IL-6 signalling ( IL6ST , SOCS3 ) was more clearly upregulated along the ageing trajectory in type I myonuclei (cluster 3). Moreover, both myofibre types showed an increased denervation signature (cholinergic synapse; ITPR1 , GNG12 ) (cluster 10). Consistent with the expression of DCLK1 in end-stage myonuclei, we also detected myonuclei with the RegMyon repair signature 19 (that is, MYF6 , DCLK1 , MYOG , RUNX1 ; Supplementary Table 3 ) at the end of the trajectory associated with ageing, which emerged progressively in type I myofibres and more abruptly in type II (Extended Data Fig. 6e ). This repair program probably arises in response to daily wear-and-tear microdamage in myofibres, which can be fixed by (1) MuSCs 9 or (2) intrinsic myonuclear self-repair mechanisms 29 . However, the chronic presence of this repair signature in aged myonuclei may indicate the persistence of myofibre damage and unsuccessful repair. Consistently, we detected a higher presence of FLNC + scars in aged muscle, which are indicative of ongoing myofibre self-repair 29 (Extended Data Fig. 6f ). These findings indicate that aged muscle is not able to cope with daily mild myofibre lesions.
As the largest human tissue, skeletal muscle is the main contributor to whole-body energy expenditure. Mitochondria are crucial for maintaining skeletal myofibre homeostasis and matching energy production through oxidative phosphorylation and fatty acid degradation 30 . The muscle ability to produce energy to sustain contraction substantially reduces with ageing, and defective mitochondria contribute to this phenomenon 30 , which we confirmed by succinate dehydrogenase (SDH) activity analysis of myofibres (Extended Data Fig. 6g ). Oxidative phosphorylation ( IDH2 , MDH1 ) and fatty acid degradation ( ACADM , ACAT1 ) were downregulated in the type I myonuclei ageing trajectory (cluster 8) (Fig. 3d and Extended Data Fig. 6h ). Unexpectedly, glycolysis ( PKM , HK1 ) was upregulated (cluster 6) in type I myonuclei at the late stage of the ageing trajectory (Extended Data Fig. 6i ). This may reflect a compensatory rearrangement in slow myofibres to prevent the loss of muscle capacity and produce energy during sustained contractions, which is substantially impaired in older individuals 30 .
GRNs in ageing myonuclei
To examine the cis -regulatory landscape of myonucleus degeneration, we defined the underlying gene regulatory networks (GRNs) using functional inference of gene regulation (FigR) 31 . We first integrated the snRNA-seq and snATAC-seq datasets using canonical correlation analysis (CCA), identifying the most likely paired nuclei using a constrained optimal cell mapping approach within the common CCA space (Extended Data Fig. 7a ). This yielded a consistent progressive or abrupt cell trajectory towards the ageing state for type I or type II myonuclei, respectively, in snATAC-seq (Fig. 3e ). Using these paired nuclei, we linked the snATAC-seq peaks to their target genes based on the peak-to-gene accessibility correlation. This identified a significant association of 28,193 unique chromatin accessibility peaks with 10,707 genes (permutation P < 0.05) in type I myofibres, and 27,901 peaks with 10,669 genes in type II myofibres. We defined the high density of the peak–gene interaction subset as domains of regulatory chromatin (DORCs) ( n > 6 significant peak–gene associations; type I, 8,370 peaks, 908 genes; type II, 7,879 peaks, 912 genes) (Extended Data Fig. 7b ). The list of DORC-associated genes included several stress TFs ( JUND , JUN , FOS , JUNB , EGR1 ) in both type I and type II myofibres. Notably, these gene loci opened their chromatin before the increase of gene expression, indicating a priming process and a stepwise transition to overt myonucleus degeneration (Extended Data Fig. 7c ). We next computed TF motif enrichments, considering both the expression patterns and the chromatin accessibility for all DORCs, to generate the regulation score representing the intersection of motif-enriched and RNA-correlated TFs. We distinguished dozens of putative transcriptional activators and repressors in type I or type II myonuclei along the ageing trajectory (Fig. 3f ). Among these, we observed that stress-related TFs (FOSL2, JUN, FOS, JUNB, STAT3) become upregulated and drive a coordinated gene expression program along the degeneration trajectory; this was confirmed by TF footprinting analysis (Extended Data Fig. 7d ). We also noticed that some of the enriched transcriptional repressors were myofibre-type-specific; for example, type I myofibres were enriched for TBX15, and type II myofibres were enriched for JDP2. These two TFs have been implicated in glycolytic myofibre metabolism 32 and cardiomyocyte protection by inhibiting AP-1 complex activity 33 , and their enrichment could account for myofibre-type-specific dysfunction in old age.
MuSC exhaustion with ageing by premature priming
The ability of MuSCs to transition on injury from their quiescent state to an activated state for tissue repair is substantially reduced with age 9 but the underlying mechanisms are poorly understood. A major confounding factor for assessing MuSCs using single-cell isolation protocols is that tissue dissociation induces stress 34 . To overcome this, we focused on snRNA-seq data (Extended Data Fig. 8a and Supplementary Table 3 ).
We identified three MuSC subpopulations expressing the TF PAX7 and one expressing MYOG . PAX7 + MuSCs were subclassified in different states according to previously defined markers 4 , 9 : calcitonin receptor ( CALCR ) for MuSCs in deep quiescence (qMuSCs); MYF5 for MuSCs early primed for activation (epMuSCs); and modest levels of MKI67 for MuSCs primed for late activation/proliferation (lpMuSCs) (Fig. 4a and Extended Data Fig. 8b,c ). epMuSCs were enriched for FOS , JUN and EGR , which have been described to allow a rapid MuSC exit from quiescence after muscle injury 34 . As MYOG + MuSCs were enriched for myogenic differentiation genes ( ACTC1 ), we termed them differentiating MuSCs (dMuSCs). All MuSC subtypes except for the scarce lpMuSCs could also be discerned in snATAC-seq.
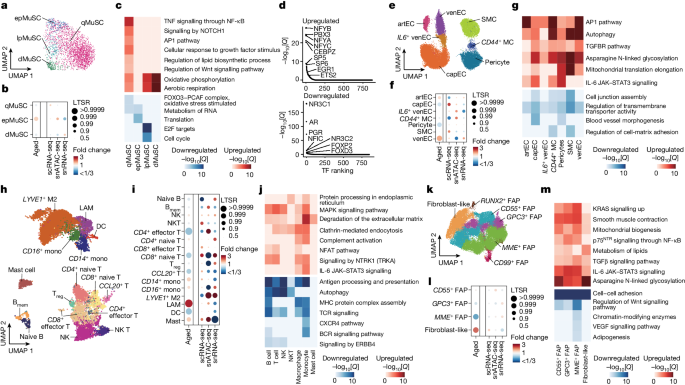
a , UMAP analysis of the detected MuSC subpopulations in snRNA-seq data. Dots are coloured according to cell type. b , The relative proportional changes of each MuSC subpopulation with ageing (column 1) and each single-cell modality (columns 2–4), considering co-variable factors (ethnicity, omics technology and sequencing batch). The colour scale represents the fold change, and the dot size shows the probability of change (LTSR) calculated using a generalized linear mixed model with a Poisson outcome 14 . c , Functional enrichment analysis of DEGs obtained between adult and older adult groups for each MuSC subpopulation. The colour scale represents the significance (−log 10 [ Q ]) of the enriched terms for upregulated (red) and downregulated (blue) genes with ageing. d , TF motif enrichment for upregulated (top) and downregulated (bottom) peaks in qMuSCs with ageing (older adult versus adult). TFs were plotted according to their rank ( x axis) and their associated −log 10 [ Q ] ( y axis). e , UMAP analysis as in a for vascular cell subpopulations. artEC, arteriole EC; venEC, venule EC; capEC, capillary EC; MC, mural cell subpopulations. f , The relative proportional changes as in b for vascular cell subpopulations. g , Functional enrichment analysis of DEGs (older adult versus adult) as in c for vascular cell subpopulations. h , UMAP analysis as in a for immune cell subpopulations. B mem , memory B cells; DC, dendritic cells; M2, M2-like macrophages; mono, monocytes; T reg , regulatory T cells. i , The relative proportional changes as in b for immune cell subpopulations. j , Functional enrichment analysis of DEGs (older adult versus adult) as in c for immune cell subpopulations. k , UMAP analysis as in a for stromal cell subpopulations. l , The relative proportional changes as in b for stromal cell subpopulations. m , Functional enrichment analysis of DEGs (older adult versus adult) as in c for stromal cell subpopulations.
Wider DEG and functional enrichment analysis showed that qMuSCs were enriched for extracellular matrix (ECM)-remodelling genes ( FBN1 , VIT , COL5A2 and CALCR ) and hormone nuclear receptors ( ESRRG , GHR ). This is consistent with the knowledge that the collagen V/CALCR axis and hormones help to maintain the pool of qMuSCs in mice 9 (Extended Data Fig. 8d ). Accordingly, snATAC-seq peaks in qMuSCs showed substantial enrichment in binding motifs for TFs related to growth hormone regulation 35 (PGR, NR3C1) in addition to myogenic functions (NFIC) 36 (Extended Data Fig. 8e ). epMuSCs were enriched for inflammation-related genes (cytokine and TNF signalling), cell growth (MYC targets) and autophagy. Moreover, they were enriched for binding motifs of the cofactor SMARCC1, FOS and JUNB, indicating higher readiness for activation, which is similar to in mice 34 . In addition to proliferation-related genes, lpMuSCs expressed genes involved in chromatin organization ( DNMT1 , HELLS , EZH2 ). In agreement with their gene expression pattern, dMuSCs had a higher enrichment of binding motifs for MYOG. Enhanced binding for JUNB and MYOG in epMuSCs and dMuSCs, respectively, was confirmed by footprinting analysis (Extended Data Fig. 8f ).
Although MuSC heterogeneity persisted with ageing, there was an increase in the proportion of epMuSCs in older muscle (Fig. 4b and Extended Data Fig. 8g ). In mice, a break of quiescence induced by changes in the niche accounts for the loss of MuSCs with age 9 . Thus, an increase in epMuSCs may be partly responsible for the loss of MuSCs in older human muscle. We confirmed the prevalence of FOS + MuSCs transiting to a primed state in older muscle using immunofluorescence (Extended Data Fig. 8h ). Pathways related to MuSC stemness, such as FOXO signalling for qMuSCs 9 , and proliferative capacity, such as translation for epMuSCs and cell cycle for lpMuSCs 9 , were diminished with ageing (Fig. 4c ). All aged MuSC subtypes except for epMuSCs displayed enhanced mitochondrial oxidative phosphorylation. A detailed analysis of qMuSCs from adult and older adult groups showed that downregulation of ECM-related processes ( ITGBL1 ) is progressively associated with ageing, whereas upregulation of myogenesis ( MEF2D ) peaked in qMuSCs from adults aged 74–82 years, and inflammatory and stress pathways (TNF/NF-κB and NFAT–JUN–FOS) peaked in qMuSCs from adults aged ≥84 years (Fig. 4c , Extended Data Fig. 8i,j and Supplementary Table 5c ). snATAC-seq analysis showed that older qMuSCs are enriched for binding motifs of TFs that regulate advanced myogenic stages, such as differentiation-related (NFYA, NFYB, NFYC) 37 and stress response (ETS2, EGR1) 34 TFs (Fig. 4d ). Conversely, motif enrichment of growth-hormone-related TFs (PGR, NR3C1, AR) in qMuSCs was lost with ageing. These findings suggest exhaustion and inability to respond to muscle injury or homeostatic body signals.
Pro-inflammatory and profibrotic responses
We next performed subclustering of mononucleated cells in the sc/snRNA-seq datasets. These resident cell types are not only crucial for overall skeletal muscle homeostasis but also support the regenerative activities of MuSCs after injury.
Within the vascular compartment, we identified four subtypes of ECs: (1) arterial ECs that express SEMA3G ; (2) capillary ECs that express CA4 ; (3) venous ECs (venECs) that express ACKR1 ; and (4) a subpopulation of venECs that express IL6 ( IL6 + venECs) (Fig. 4e and Extended Data Fig. 9a ). We also identified three subtypes of mural cells: (1) SMCs that express ACTA2 and MYH11 ; (2) pericytes that express HIGD1B and RGS5 ; and (3) mural cells that express CD44 . snATAC-seq analysis confirmed the same cell types (Extended Data Fig. 9b–d ). In older muscle, the proportion of capillary ECs and pericytes decreased, while that of arterial ECs and venECs increased (Fig. 4f and Extended Data Fig. 9e ). Vascular cell types downregulated genes related to cell junction assembly and transmembrane transporter activity with ageing, and upregulated inflammatory (IL-6 and AP-1 pathways), fibrotic (TGFβ pathway) and autophagy pathways (Fig. 4g ). We concluded that ageing alters the skeletal muscle vascular integrity by increasing pro-inflammatory and stress-related signals.
Among the immune cells, we identified different subpopulations of myeloid cells and lymphocytes from sc/snRNA-seq and snATAC-seq data, including CD14 + and FCGR3A + (CD16) monocytes that are endowed with distinct responses to different pathogens and stimuli 38 ; macrophages (lipid-associated macrophages (LAMs) and LYVE1 + macrophages) with yet-to-be characterized distinctive functions in skeletal muscle 3 ; mast cells; dendritic cells; B cells (naive and memory); natural killer (NK) cells; NK T cells; CD4 + T cells (effector CCR7 − , naive CCR7 + and regulatory IL2RA + ); CD8 + (effector CCR7 − and naive CCR7 + ); and a group of CCL20 + T cells 3 (Fig. 4h and Extended Data Fig. 9f–i ). Consistent with the increased inflammatory cell infiltration shown by histological analysis (Extended Data Fig. 3d–f ), mast cells, LAMs and monocytes increased in older muscle, while some of the T cell subtypes and dendritic cells decreased (Fig. 4i and Extended Data Fig. 9j ). Activated mast cells in skeletal muscle have been associated with cancer-induced muscle atrophy (cachexia) 39 . All of the immune cell subpopulations, except for mast cells, downregulated homeostatic immune functions with ageing, including antigen processing and presentation (MHC pathway, B cell receptor signalling and TCR signalling) (Fig. 4j ). Similarly, anti-inflammatory responses were downregulated in some immune cell types (signalling by ERBB4 in lymphoid cells), while pro-inflammatory ones were upregulated in others (IL-6 pathway in myeloid cells, complement activation and signalling by NTRK1 in myeloid cells and lymphocytes). Moreover, older immune cells were enriched for processes associated with phagocytosis (protein processing in the endoplasmic reticulum, clathrin-mediated endocytosis and degradation of the ECM), pointing to a predominantly activated status. Thus, in addition to a general increase in infiltrating immune cells with ageing, there is a switch towards a pro-inflammatory state, consistent with inflammation being a key driver of ageing 40 .
Within the stromal cell compartment, we identified various subtypes of fibro-adipogenic cells (FAPs), including CD55 + , CD99 + , GPC3 + , MME + and RUNX2 + , and fibroblast-like cells expressing THY1 (Fig. 4k and Extended Data Fig. 10a ). snATAC-seq analysis confirmed the predominance of fibroblast-like cells, CD55 + , MME + and GPC3 + FAPs in the muscle stroma (Extended Data Fig. 10b–d ). Fibroblast-like cells substantially increased with ageing, whereas MME + FAPs were diminished (Fig. 4l and Extended Data Fig. 10e ). MME + FAPs are a well-known dominant FAP subtype 41 and expressed genes related to adipogenic pathway, whereas CD99 + and GPC3 + FAPs were pro-inflammatory FAPs expressing CCL2 and CXCL14 (Extended Data Fig. 10a,f ). Fibroblast-like cells and CD55 + FAPs showed higher fibroblast activation traits (epithelial–mesenchymal transition, ECM organization) compared with other FAP subtypes 42 (Extended Data Fig. 10a ). RUNX2 + FAPs were enriched for SOX5 and are involved in migration and collagen production 43 . Older FAP subtypes largely shared an ageing signature characterized by the downregulation of growth factor pathways (VEGF and Wnt) and upregulation of profibrotic (TGFβ signalling) and pro-inflammatory (IL-6 signalling) pathways and asparagine N-linked glycosylation (Fig. 4m ). These results point to a shift in the stromal populations (especially CD55 + and fibroblast-like cells) towards an activation state characterized by active ECM remodelling.
Importantly, comparative analysis by age groups (individuals aged 15–46 years; 74–82 years; and ≥84 years) revealed that these muscle-resident cells (vascular, immune and stromal) displayed a peak enrichment of pro-inflammatory pathways (IL-6/AP-1 pathway) in the group aged 74–82 years, and of profibrotic pathways (TGFβ signalling) in the group aged ≥84 years (Extended Data Fig. 10g ). These non-myogenic populations, particularly the lymphocytes, also presented a moderate increase in the cell cycle inhibitor genes CDKN1A (p21) and CDKN1B (p27) (Extended Data Fig. 10h ).
Altered intercellular communication
Cells within a tissue communicate with each other through elaborated circuits 44 . How intercellular cross-talk in the human skeletal muscle niche is affected by ageing is largely unclear. To study this in an integrative manner, we used CellChat 45 .
Ligand–receptor interactions involved more dominantly mononucleated cells than myofibres, and the total number of interactions nearly doubled with ageing (Fig. 5a ). Interactions involving myeloid and lymphoid cells—and, to a lesser extent, FAPs, fibroblast-like cells and type I myofibres—increased more substantially with ageing compared with those of other cell types (Fig. 5b and Supplementary Table 7 ). On the basis of these results, we focused on three interaction categories as potential effectors of the muscle-wasting process caused by ageing: inflammation, ECM and growth factors (Fig. 5c ).
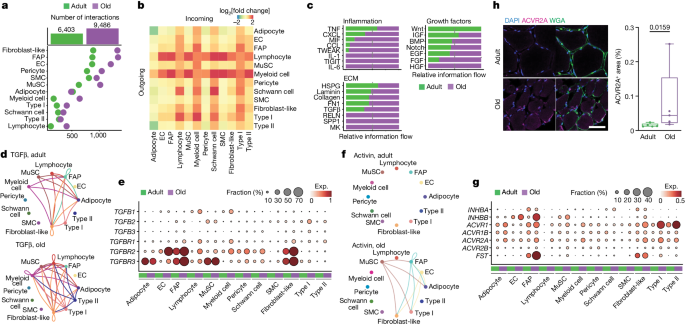
a , The number of predicted interactions (L–R pairs) for each cell type in the adult (green) and older adult (purple) age groups. b , The fold change (log 2 -transformed, colour scale) with ageing in the number of sent signals (outgoing, horizontal side) and received signals (incoming, vertical side) for each cell type. c , The sum of the interaction probability differences (relative information flow) among all pairs for each depicted group of interactions in the adult (green) and older adult (purple) age groups. Interactions are grouped according to the following categories: inflammation (top left), ECM (bottom left) and growth factors (right). d , The TGFβ signalling network in adult (top) and older adult (bottom); nodes represent cell types and edges represent the interactions among them. The edge width is proportional to the interaction probability. e , The expression levels of the genes associated with TGFβ signalling pathway in adult (green) and older adult (purple) muscles. The colour scale represents the average gene expression, and the dot size shows the percentage of cells expressing a given marker within the subpopulation. f , Signalling network, as in d , for the activin signalling pathway. g , The expression levels as in e for the activin signalling pathway and muscle-atrophy-related genes. h , Representative images (left) and corresponding quantification (right) of immunofluorescence analysis of ACVR2A + area (ACVR2A, magenta; cell membrane, WGA, green; nuclei, DAPI, blue) in adult (sample P5) and older adult (sample P28) individuals. Scale bar, 50 μm. n = 5 individuals for each age group. P values were calculated using two-tailed Mann–Whitney U- tests. For the box plots, the centre line shows the median, the box limits show the upper and lower quartiles, and the whiskers show 1.5× the interquartile range.
Although the transient concurrence of immune cells is required for efficient muscle repair, their persistence and the subsequent chronic inflammation is a major driver of dysfunction in aged muscle 40 . Among the inflammation-related communication networks, we observed enhanced secretion of chemokines and cytokines (TNF, CXCL and CCL family members, MIF, IL-1, IL-6) by immune and stromal cells, acting on a variety of cell types including the myofibres (Extended Data Fig. 11a–e ). For example, FAPs in older muscle expressed high levels of CXCL12 , which is a strong chemoattractant for immune cells 3 , suggesting the existence of inflammatory–fibrogenic feedback loops. Likewise, increased IL6R in myonuclei and IL6ST in other cell types with ageing may stimulate myofibre atrophy 8 . TNF was reduced with ageing in immune cells, but its receptors ( TNFRSF1A / B ) increased in different cell types.
Excessive ECM deposition, especially of collagen, perturbs skeletal muscle function and is a major hallmark of sarcopenia 8 . Sirius Red staining confirmed extensive fibrosis in older muscle as compared to in adult muscle (Extended Data Fig. 11f ). This phenomenon, and the subsequent expansion of the derived interactions with ageing, is consistent with the increase in FAPs and fibroblast-like cells, which are the major ECM producers in the skeletal muscle 42 . Indeed, we observed an increase in most collagens and fibronectin ( FN1 ) in FAPs or fibroblast-like cells, as well as in Schwann cells (Extended Data Fig. 11g ). Conversely, there was a downregulation of laminin components in FAPs, fibroblast-like cells and SMCs, concomitant with the downregulation of adhesion molecules ( ITGA7 ) in myofibres. This suggests a reduction in the basal lamina causing impaired vascular integrity. Immunofluorescence confirmed the exacerbated reduction of ITGA7 with ageing (Extended Data Fig. 11h ). Coinciding with the altered ECM composition, the major profibrotic factor TGFβ increased with ageing, produced mainly by immune cells ( TGFB1 ), MuSCs ( TGFB2 ) and type I myofibres ( TGFB2 , TGFB3 ), and acting through its receptors ( TGFBR1 , TGFBR2 , TGFBR3 ) on a variety of cell types, in particular FAPs, fibroblast-like cells, adipocytes and ECs (Fig. 5d,e ).
Among the growth factors implicated in muscle mass control, we observed a dysregulation of signalling mediated by activins 46 , 47 , IGF 46 , BMP 7 , Notch 28 and Wnt 48 factors (Extended Data Fig. 12a ). Proatrophic activin signalling 46 was upregulated with ageing, with activin receptors ( ACVR1 , ACVR1B , ACVR2A , ACVR2B ) upregulated in myonuclei, and the activin ligand INHBB in ECs, FAPs, MuSCs and fibroblast-like cells (Fig. 5f,g ). The increased expression of ACVR2A was validated by immunofluorescence analysis (Fig. 5h ). Notably, there was an increase in follistatin ( FST ) in FAPs, probably to counteract the proatrophic effects of activin signalling 46 . Higher levels of the Notch ligand DLL4 in ECs and the NOTCH2 receptor in older myofibres (Extended Data Fig. 12b ) may be related to the recently described EC–myofibre cross-talk in mice 28 . Moreover, IGF1 increased in FAPs, fibroblast-like and myeloid cells but decreased in MuSCs, whereas IGF2 decreased in ECs and FAPs, suggesting a differential downstream cascade of IGF signalling with ageing. Likewise, BMP4 decreased in stromal cells, while BMP5 increased in stromal, pericyte and Schwan cell populations. The shift of BMP ligands and the downregulation of hypertrophy-promoting BMP receptor ( BMPR1A ) 46 in type I and type II myofibres with ageing is probably involved in the loss of muscle mass with ageing (Extended Data Fig. 12c,d ). We also observed a reduction with ageing in WNT9A expressed mainly by type II myonuclei (Extended Data Fig. 12e ) and acting on a variety of cell types, in particular stromal cells. The Wnt pathway amplifiers LGR4 and LGR5 were differentially expressed in type II and type I myofibres, respectively, as reported in monkeys 49 , and decreased with ageing. Considering that WNT9A regulates NMJ development 48 , it is conceivable that alterations in this pathway result in abnormalities of NMJ myonuclei and muscle mass with ageing.
Linking inherited risk variants to cell types
Recent genome-wide association studies (GWASs) have revealed susceptibility loci associated with muscle weakness 50 . Correlations between candidate loci and susceptibility to sarcopenia in those reports reinforced the direct and indirect functional links of skeletal muscle with other body systems. Our integrated dataset provides a valuable opportunity for interpreting the functional impact of these risk variants at the cellular level. By aggregating fragments from all nuclei across cell types and age groups, we generated a union peak set containing 636,363 peaks, from which we identified 93,565 peaks enriched in individual cell types from all of the tested individuals (Extended Data Fig. 13a ). Adult and older adult individuals showed similarities and differences in the openness of these peaks, highlighting that epigenetic alterations are probably an important driver of muscle ageing and sarcopenia. To determine whether cell-type-specific accessible regions in the snATAC-seq data were enriched in GWAS variants for muscle strength and other phenotypes related to muscular diseases or metabolic function 50 , we performed linkage disequilibrium (LD) score regression (LDSC) analysis (Fig. 6a and Supplementary Table 8a ). For example, whereas lean body mass was enriched in type II myonuclei as expected, muscle-strength-related traits were unexpectedly enriched in aged fibroblast-like cells and FAPs but not in myofibres, supporting the idea that genetic variations can promote sarcopenia by altering intercellular communication networks. Impedance of leg was highly related to MuSCs in older people, and fracture resulting from a simple fall was associated with adult MuSCs and older type II myonuclei. Moreover, we noticed that sleep duration, creatinine and fasting glucose were related to myofibres, pointing to a potential role of these cell populations in body-level circadian rhythm regulation and metabolic regulation.
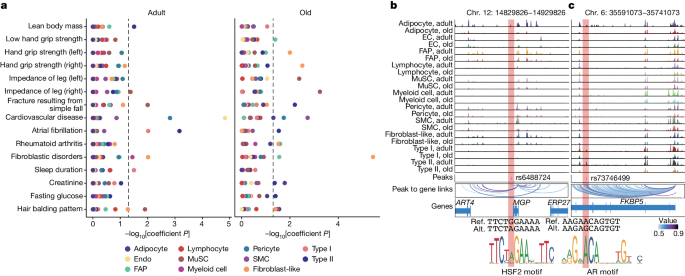
a , Differential enrichment (−log 10 [ P ]) for complex traits obtained by LDSC analysis of the snATAC-seq peaks mapped within each cell type between adult (left) and older adult (right) age groups. Dots are coloured by cell type. b , c , Genome browser tracks (top) showing the normalized aggregate signals associated to the genetic variant rs6488724 at the MGP locus ( b ) and rs73746499 at the FKBP5 locus ( c ) for each cell population (rows) in adult and older adult muscles. Obtained peaks at these loci were linked to corresponding genes. The association between peaks and genes is represented by the colour scale. The reference sequence (ref.) and the altered sequence (alt.) of the genetic variants and the motifs for HSF2 (gain of binding) and AR (loss of binding) are shown at the bottom.
As a proof of principle, we prioritized variants taken from low hand grip strength traits 50 and lean body mass traits 51 using a multitiered approach 52 (Extended Data Fig. 13b ). We overlapped lead variants and variants with high association (LD r 2 > 0.8) with cell-type-specific peaks, identifying 3,158 candidate variants (Extended Data Fig. 13c and Supplementary Table 8b–f ). Among others, we found rs1862574 in the GDNF locus in myofibres, which may affect muscle innervation. We also observed rs3008232 in TRIM63 (MuRF1), rs1281155 in ANGPL2 and rs571800667 in FOXO1 , which are critical drivers of muscle atrophy 24 , 53 . We next used the deltaSVM 54 framework to predict the impact of regulatory variants on the binding of TFs. We noticed that, in one of the potential causal variants (rs6488724), the overlapping peak is located in the promoter of MGP (Fig. 6b ), which is involved in myogenesis 55 . This single-nucleotide polymorphism (SNP) creates a G-to-A mutation that increases the binding affinity of HSF2, which participates in the transcriptional regulation of sarcomeric chaperones to maintain the contractile apparatus 56 . We also identified rs73746499, located in the intronic region of FKBP5 loci, in myofibre 57 (Fig. 6c ). This SNP creates an A-to-G mutation that disrupts the binding affinity of androgen receptor, one of the key TFs for maintaining muscle mass 58 . Notably, chromatin accessibility at the FKBP5 locus substantially decreased with ageing, consistent with the decrease in Fkbp5 expression in sarcopenic mice 57 .
Our reference atlas for human skeletal muscle ageing provides a compelling series of integrated cellular and molecular explanations for increased sarcopenia and frailty development in older individuals (Extended Data Fig. 13d ). Further exploration using our open and interactive online portal, the Human Muscle Ageing Cell Atlas (HMA) ( https://db.cngb.org/cdcp/hlma/ ), will generate additional insights.
Ageing leads to considerable alterations in the composition of myofibres and the characteristics of myonuclei. These changes include the loss and gain of specific myonucleus types, the emergence of new subtypes, and the alteration of gene programs and GRNs in a general or myofibre-type-specific manner. For example, we observed an overall activation of inflammatory and catabolic programs, impaired expression of contractile protein genes, altered myonuclear identity, upregulation of repair and innervation gene signatures in type I and II myonuclei, and the emergence of myonucleus subtypes associated with denervation. These phenomena may represent compensatory mechanisms, could be causal factors contributing to sarcopenia, or both. Notably, type I myonuclei undergo metabolic reprogramming towards a more glycolytic phenotype, probably counterbalancing the loss of oxidative capacity in resilient type I myofibres. By contrast, type II myonuclei exhibit increased glycogen depletion and protein catabolism processes, explaining their higher susceptibility to atrophy.
Quiescent MuSCs are substantially reduced in aged muscle, whereas resident non-myogenic cells are increased. Importantly, the remaining MuSCs undergo chronic activation of inflammatory and stress pathways, which could explain their failure to proliferate and differentiate 9 . These changes translate into MuSCs being more primed for activation, which may partly account for their exhaustion at an advanced age 9 . The alteration in the activity of TFs involved in the stress response and muscle maintenance probably contributes to the disruption of MuSC homeostasis. In stromal cells, ageing causes clear alterations in vascular integrity, with increased pro-inflammatory and chemoattractant signals, whereas immune cells increase in numbers and turn on inflammatory programs. Furthermore, during ageing, the heterogeneous population of FAPs switches from a proregenerative profile to a profibrotic one, accompanied by a higher presence of mature adipocytes. These changes may predispose the skeletal muscle to cellular senescence in the presence of overt damage, such as trauma 12 . In turn, this pro-inflammatory muscle state may also contribute to systemic inflammation (inflammageing) 40 and accelerate the overall body decline in older individuals. We conclude that the perturbed relationship of muscle cells with mononuclear cells in the niche and the imbalance between pro-fibrotic and pro-regenerative signals acts as a major cause of muscle dysfunction in old age. Comparison with GWAS datasets also enabled us to identify the potential relationship between genome architecture in different cell types and heritable susceptibility to sarcopenia.
Future expansions of HMA will include a larger cohort size and muscle samples from different origins, single-cell multiomics and high-definition spatially resolved technologies 59 . This may reveal differences in ethnic and sex groups unnoticed in the current study. Together, it may provide a window of opportunity for slowing down or even blocking sarcopenia, frailty and disability in older people, promoting healthier body ageing over a longer time and enhancing longevity. In addition to the ageing field, this atlas will be an important reference for future studies in patients with neuromuscular diseases.
Muscle biopsy and ethical clearance
Samples were taken during orthopaedic surgery with informed consent from the 18 patients in the European cohort and the 13 patients in the Asian cohort; for one individual below 18 years, the informed consent was obtained from the legally acceptable representative. The study was performed following the Declaration of Helsinki. Ethical approval was granted for the European cohort by the Research Ethics Committee of Hospital Arnau de Vilanova (CEIm 28/2019), and for the Asian cohort by the Institutional Ethics Committee of the First Affiliated Hospital/School of Clinical Medicine of Guangdong Pharmaceutical University, Guangzhou (China) (2020-ICE-90). Exclusion criteria were myopathy, haemiplegia or haemiparesis, rheumatoid arthritis or other autoimmune connective tissue disorders, inability to consent, prior hospital admission in the previous month or major surgery in the previous 3 months. For the European cohort, the individuals’ medical and functional states were assessed according to the Barthel index 10 and Charlson Index 11 . The Barthel index estimates the grade of dependency of the individual ranging from 0 (totally dependent) to 100 (independent). The Charlson Index indicates the grade of comorbidities associated with the individual and ranged from 0 (without comorbidity) to 6 (the individual with a higher number of comorbidities) in our samples. A list of detailed information for the individuals is provided in Supplementary Table 1 .
Animal experiment
C57Bl/6 (wild type) mice were bred and raised until 8–12 weeks of age at the animal facility of the Barcelona Biomedical Research Park (PRBB). They were housed in standard cages under a 12 h–12 h light–dark cycle and given unrestricted access to a standard chow diet. All experiments adhered to the ‘three Rs’ principle—replacement, reduction and refinement—outlined in Directive 63/2010 and its implementation in Member States. Procedures were approved by the PRBB Animal Research Ethics Committee (PRBB-CEEA) and the local government (Generalitat de Catalunya), following European Directive 2010/63/EU and Spanish regulations RD 53/2013. Both male and female mice were used for experiments and were maintained according to the Jackson Laboratory guidelines and protocols. Mice were randomly allocated to experimental or treatment groups. No blinding was used. No statistical methods were used to predetermine the sample size. Muscle injury was induced by intramuscular injection of CTX (Latoxan, L8102, 10 µM) and mice were euthanized at 7 days after injury as previously described 12 .
Muscle sample processing
Muscle samples were obtained in all cases by selecting a macroscopically healthy area of muscle, without signs of contusion or haematoma. A small portion of muscle was removed by blunt dissection following the course of the myofibres and avoiding the use of electrocautery. The samples were immediately processed into three groups and stored next to the operating room as follows: (1) fixed with paraformaldehyde before being mounted in OCT compound as described previously (for immunochemistry and immunofluorescence) 60 ; (2) immediately frozen in liquid nitrogen (for snRNA-seq and snATAC-seq); and (3) tissue-digested (for scRNA-seq).
Single-cell preparation from skeletal muscle
Before the experiment, the post-operative muscle was immediately transferred in prechilled Dulbecco’s modified Eagle’s medium (DMEM, Corning, 10-017-CVR). For single-cell isolation, adipose and tendon tissues were removed using forceps, the remained muscle chunks were mechanically shredded on ice in a 10 cm plate. Next, prechilled DMEM medium was added to the plate for collecting muscle tissues and transferred into a 50 ml tube. After standing for 3 min, the supernatant containing the remaining adipose tissues was discarded. The remained muscle tissues were transferred to a 15 ml tube for digestion in 5 ml tissue digestion buffer (0.2 mg ml −1 liberase (Roche, 5401119001), 0.4 μM CaCl 2 (Thermo Fisher Scientific, J63122AE), 5 μM MgCl 2 (Thermo Fisher Scientific, R0971), 0.2% BSA (Genview, FA016), 0.025% trypsin-EDTA (Thermo Fisher Scientific, 25300120). The muscles were digested in a shaking metal bath at 1000 rpm, 37 °C for 1 h, and mixed by inversion every 10 min. After all tissue pieces were digested, 3 ml of fetal bovine serum (FBS, Cellcook, CM1002L) was added to the mixture to terminate the digestion. The cell suspension was filtered through a 100 μm strainer, and centrifuged at 700 g for 10 min at 4 °C to pellet the cells. The cell pellet was then resuspended in 10 ml wash buffer (DMEM medium supplemented with 10% FBS) and filtered through a 40 μm strainer, then centrifuged at 700 g for 10 min at 4 °C to pellet the cells. The resultant single-cell suspensions were washed twice with prechilled PBS supplemented with 0.04% BSA and were used as input for scRNA-seq library construction.
Single-nucleus extraction from skeletal muscle
Single-nucleus isolation was performed as previously described 6 . In brief, tissues were thawed, minced and transferred to a 2 ml Dounce homogenizer (Sigma-Aldrich, D8938) with 1 ml of homogenization buffer A containing 250 mM sucrose (Sigma-Aldrich, S8501), 10 mg ml –1 BSA, 5 mM MgCl 2 , 0.12 U μl –1 RNasin (Promega, N2115) and 1× cOmplete Protease Inhibitor Cocktail (Roche, 11697498001). Frozen tissues were kept in an ice box and homogenized by 25–50 strokes of the loose pestle (pestle A), after which the mixture was filtered using a 100 µm cell strainer into a 1.5 ml tube. The mixture was then transferred to a clean 1 ml Dounce homogenizer with 750 μl of buffer A containing 1% Igepal (Sigma-Aldrich, CA630), and the tissue was further homogenized by 25 strokes of the tight pestle (pestle B). The mixture was then filtered through a 40 μm strainer into a 1.5 ml tube and centrifuged at 500 g for 5 min at 4 °C to pellet the nuclei. The pellet was resuspended in 1 ml of buffer B containing 320 mM sucrose, 10 mg ml −1 BSA, 3 mM CaCl 2 , 2 mM magnesium acetate, 0.1 mM EDTA (Thermo Fisher Scientific, 15575020), 10 mM Tris-HCl (Invitrogen, AM9856), 1 mM DTT (Invitrogen, 707265ML), 1× Complete Protease Inhibitor Cocktail and 0.12 U μl −1 RNasin. This was followed by centrifugation at 500 g for 5 min at 4 °C to pellet the nuclei. The nuclei were then washed twice with prechilled PBS supplemented with 0.04% BSA and finally resuspended in PBS at a concentration of 1,000 nuclei per μl for library preparation.
Library preparation and sequencing
Sc/snrna-seq library preparation.
scRNA-seq libraries were prepared using the DNBelab C Series Single-Cell Library Prep Set (MGI, 1000021082) 49 . In brief, the single-cell/nucleus suspensions were converted to barcoded scRNA-seq libraries through droplet encapsulation, emulsion breakage, mRNA-captured bead collection, reverse transcription, cDNA amplification and purification. Indexed sequencing libraries were constructed according to the manufacturer’s instructions. Library concentrations were quantified using the Qubit ssDNA Assay Kit (Thermo Fisher Scientific, Q10212). Libraries were sequenced using the DIPSEQ T1 sequencer.
snATAC-seq library preparation
snATAC-seq libraries were prepared using the DNBelab C Series Single-Cell ATAC Library Prep Set (MGI, 1000021878) 49 . In brief, nuclei were extracted from tissue using the same protocol described above. After Tn5 tagmentation, transposed single-nucleus suspensions were converted to barcoded snATAC-seq libraries through droplet encapsulation, pre-amplification, emulsion breakage, captured bead collection, DNA amplification and purification. Indexed libraries were prepared according to the manufacturer’s instructions. Concentrations were measured with a Qubit ssDNA Assay Kit. Libraries were sequenced by a BGISEQ-2000 sequencer.
sc/snRNA-seq raw data processing, clustering and cell type annotation
Raw data processing.
Raw sequencing reads were filtered, demultiplexed, and aligned to hg38 reference genome using a custom workflow ( https://github.com/MGI-tech-bioinformatics/DNBelab_C_Series_HT_scRNA-analysis-software ) 49 . For scRNA-seq, reads aligned to gene exons were counted. For snRNA-seq, reads aligned to gene loci, including both exons and introns, were counted. Doublets were identified and filtered by DoubletFinder (v.2.0.3) 61 . Ambient RNA for snRNA-seq was reduced using SoupX (v.1.4.8) 62 with the default settings.
Integration, clustering and cell type annotation
The resulting count matrix for cells/nuclei was filtered by the number of unique molecular identifiers (UMIs) > 1,000, gene > 500 and mitochondria content < 5%. Global clustering was performed using Scanpy (v.1.8.1) 63 in Python (v.3.7). Filtered data were normalized to total counts and log-transformed. The top 3,000 highly variable genes were selected, and the number of UMIs and the percentage of mitochondrial genes were regressed out. Each gene was scaled with the default options, followed by dimensionality reduction using principal component analysis. Batch effects between snRNA-seq and scRNA-seq were corrected using Harmony 64 . Next, the batch-effect-corrected top 30 principal components were used for generating the neighbourhood graph with the number of neighbours set at 10. The cell clustering was further performed with the Louvain algorithm and annotated by canonical markers, putative scRNA-seq- and snRNA-seq-derived myofibre fragments were removed from the analysis. For satellite cell, immune cell, vascular cell and stromal cell reclustering, cells/nuclei were subset from the global clustering object and processed according to the same procedure as described above. For the reclustering of myonuclei, data were processed in Seurat (v.4.0.2) 65 , and only snRNA-seq data were retained for further analysis. In brief, myonuclei data were subjected to SCTransform-based normalization, anchor identification between samples, integration, Louvain clustering and projection onto the UMAP space. Clustering results were further annotated by highly expressed genes.
Analysis of cell type composition variation in ageing
A generalized linear mixed model with a Poisson outcome 14 was used to model the effect of age on cell-type-specific counts as previously reported, accounting for the possible biological (sex, ethnicity) and technical (omics, sequencing batch) covariates. The effect of each biological/technical factor on cell type composition was estimated by the interaction term with the cell type. The fold change is relative to the grand mean and adjusted. The statistical significance of the fold change estimation was measured by the LTSR, which is the probability that the estimated direction of the effect is true. As an alternative method, the proportion for each population was estimated over the total number of nuclei/cells for a given dataset (Supplementary Table 9 ).
Transcriptional and epigenetic heterogeneity analysis
Transcriptional heterogeneity analysis was performed as previously described 66 . In brief, snRNA-seq data for each cell type in each age group were downsampled to 300 nuclei. For cell types with fewer than 300 nuclei, all nuclei were included for analysis. The resultant gene × cell matrix was further downsampled to make an equal number of UMI counts and cells between adult/older adult groups in each cell type. Next, all genes were ranked into ten blocks on the basis of the average expression value, and the 10% genes with the lowest coefficient of variation in each block were used to calculate the Euclidean distance between each cell. This Euclidean distance was used to measure transcriptional heterogeneity for each cell. For epigenetic heterogeneity, we adapted the same analysis method as transcriptional noise but using the rounded gene score matrix as input.
Myonucleus classification
Myonuclei were classified on the basis of previous markers associated with the described pure myofibre types (type I, type IIA and type IIX) and the hybrid myofibres (hybrid I/IIA, hybrid IIA/IIX). A module score was calculated for each myofibre type based on the expression of the following markers 67 : type I ( TNNT1 , MYH7 , MYH7B , TNNC1 , TNNI1 , and ATP2A2 ), type II ( TNNT3 , MYH1 , MYH2 , TNNC2 , TNNI2 , ATP2A1 ), type IIA ( MYH2 , ANKRD2 , NDUFA8 , MYOM3 , CASQ2 , HSPB6 , RDH11 , AIMP1 ) and type IIX ( MYH1 , MYLK2 , ACTN3 , MYBPC2 , PCYOX1 , CAPZA1 , CD38 , PDLIM7 , COBL , TMEM159 , HNRNPA1 , TFRC ). On the basis of these scores, myonuclei were first classified as type I, type II or hybrid I/IIa; thereafter, type II myonuclei were further classified as type IIA, type IIX or hybrid IIA/IIX. A residual amount of myonuclei remained unclassified due to the lower expression of these genes.
Differential gene expression and functional enrichment analysis
Seurat was used to compute the DEGs for each population and subpopulations between samples in the younger and older cohorts with the thresholds set at log 2 [fold change] > 0.25 and Q < 0.05 (Supplementary Table 10 ). For myofibre subpopulations, the thresholds were set at: log 2 [fold change] > 1 and Q < 0.05. The obtained DEGs for each comparison were used as input in Metascape online tool 68 to perform functional enrichment analysis, with a Q value threshold set at 0.05 (Supplementary Table 11 ). Heatmap results were plotted using pheatmap (v.1.0.12) in R.
Identification of coexpressing gene modules
Hotspot (v.1.1.1) 16 was used to compute coexpressing gene modules among myofibre populations. The normalized expression matrix for the top 5,000 variable genes, the RegMyon- 19 , sarcomeric- 67 and atrophy-related 24 genes (Supplementary Table 3 ) were used as input. In brief, the k -nearest neighbour graph was created using the create_knn_graph function with the parameters: n_neighbors = 30, and then genes with significant correlation ( Q < 0.05) were retained for further analysis. The modules were identified using the create_modules function with the parameters min_gene_threshold = 10 and fdr_threshold = 0.05.
Pseudotime analysis
For the myofibre degeneration trajectory, DCLK1 + (type I), ID1 + (type I), ID1 + (type II), ENOX1 + (type II) and other unperturbed myonuclei were selected for pseudotime analysis using Monocle3 69 . After trajectory construction, myonuclei were ordered by pseudotime, and the corresponding gene expression matrixes were aggregated into 100 bins. The top 4,000 variable genes in type I or type II myonucleus trajectory were selected and visualized by k -means clustering heat map ordered by the pseudotime.
Cell–cell interaction analysis
CellChat (v.1.1.0) 45 detected ligand–receptor interactions on integrated sc/snRNA-seq data according to the standard procedures. The expression matrix and the cell type information were imported to CellChat. Specialized myonuclei, mast cells and erythrocyte clusters were removed from the analyses due to the insufficient number of cells/nuclei or the disproportionate number of cells/nuclei between the younger and older cohorts. The overall communication probability among the cell clusters was calculated using the computeCommunProb function with a trim set at 0.1.
snATAC-seq data processing
Raw data processing, clustering and cell type annotation.
Raw sequencing reads were filtered, demultiplexed and aligned to the hg38 reference genome using PISA ( https://github.com/shiquan/PISA ) 70 . Fragment files for each library were generated for downstream analysis. The transcription start site enrichment score, number of fragments and doublet score for each nucleus were calculated using ArchR 71 . Nuclei with transcription start site enrichment scores < 8 and number of fragments < 1,000 were removed from the analysis. Doublets were filtered out using the filterDoublets function with the settings filterRatio = 2. We next performed latent-semantic-indexing-based dimensionality reduction on the 500 bp tiles across the genome using the addIterativeLSI function of ArchR. Anchors between the scATAC-seq and scRNA-seq/snRNA-seq datasets were identified and used to transfer cell type labels identified from the scRNA-seq/snRNA-seq data. For co-embedding of snRNA-seq/scRNA-seq and snATAC-seq data, an anchor-based integration approach was applied based on the sequencing techniques. Then, data were further subjected to batch correction by Harmony among samples. Pearson’s correlation between snRNA/scRNA-seq and snATAC-seq was performed based on the integrated assay.
Motif enrichment analysis
Before motif enrichment, a reproducible peak set was created in ArchR 71 using the addReproduciblePeakSet function based on cell types/subtypes. Differentially enriched peaks were identified using the getMarkerFeatures function with the thresholds set at log 2 [fold change] > 0.5 and Q < 0.1. The motif presence in the peak set was determined with the addMotifAnnotations function using CisBP motif database (v.2) 72 .
TF occupancy
TF occupancy was evaluated by footprinting analysis implemented in ArchR 71 . In brief, putative binding sites of selectively enriched motifs were first inferred using the addMotifAnnotations function. Next, footprintings for the putative TF-binding sites were calculated using the getFootprints function, in which the Tn5 insertion bias was taken into account. The results were further plotted using the plotFootprints function.
GRN analysis
Construction of the GRNs was performed using FigR 31 . In brief, we first sampled an equal number of nuclei (20,000) in snRNA-seq and snATAC-seq analysis of myofibre and performed data integration using scOptMatch implemented in FigR. For creating the co-embedding map in these two independent datasets, we first input the variable features taken from the snRNA-seq and snATAC-seq datasets to perform CCA using the RunCCA function in Seurat. After integration, pairs of ATAC–RNA cells were identified by geodesic distance-based pairing using the pairCells function, and unpaired cells were removed from the analysis. Significant ( P < 0.05) peak-to-gene associations were then identified among the cell pairs in type I or type II myonuclei. The DORCs were defined as peak-gene associations ≥ 6. For inference of the GRNs, the smoothed DORC score, RNA counts, snATAC-seq peak counts and the significant peak-to-gene associations were fed into runFigRGRN function, generating the GRNs. Next, the activators and repressors were identified by ranking the TFs by average regulation score.
GWAS analysis
Association of gwas traits with skeletal muscle cell types.
To identify trait/disease-relevant cell types, we performed LDSR analysis 73 , a method for partitioning heritability from GWAS summary statistics. In brief, differentially accessible peaks for each adult/older adult cell type were identified (log 2 [fold change] > 1 and Q < 0.01). The LDSC analysis was performed according to the standard workflow ( https://github.com/bulik/ldsc/wiki ). The summary statistics file for each trait was downloaded from the GWAS catalogue database 74 or published studies 50 , 51 (Supplementary Table 8a ).
Fine mapping of non-coding variants and predicting the effect of TF binding
Lead SNPs were taken from low-hand-grip strength and lean-body-mass traits 50 , 51 , 75 . FUMA, a web-based platform for GWAS analysis 76 , was used to identify high-correlation SNPs with an LD r 2 ≥ 0.8 with lead SNPs. High-correlation SNPs within ±50 bp of the differentially accessible peaks were identified for further analysis. The peak-to-gene associations were determined using addPeak2GeneLinks function in ArchR package in the integrative object. To identify SNPs that affect TF binding, we used two approaches, (1) gkm-SVM 54 and (2) SNP2TFBS 77 . For gkm-SVM, TF models were used from https://github.com/ren-lab/deltaSVM/tree/master/gkmsvm_models , and effective alleles were identified using the gkmExplain function 78 . For SNP2TFBS tools, the analysis was performed in the SNP2TFBS web interface ( https://ccg.epfl.ch/snp2tfbs/ ) following the tutorial.
Histology and immunofluorescence
Cryostat sections (10 μm thickness) were collected from muscles and stained with haematoxylin and eosin (Sigma-Aldrich, HHS80 and 45235) to assess tissue morphology or SA-β-gal (AppliChem, A1007,0001) for senescence cells with a modified staining protocol as described previously 12 , 79 . Histochemical SDH staining was assayed by placing the slides in a solution containing sodium succinate (Sigma-Aldrich, S2378) as a substrate and nitro-blue tetrazolium (Sigma-Aldrich, N6876) for visualization of the reaction for 1 h at 37 °C. The intensity and pattern of staining were evaluated using light microscopy 80 . Muscle collagen content was quantified after Sirius Red (Sigma-Aldrich, 365548) staining as previously described 81 . For immunofluorescence, the sections were air-dried, fixed, washed on PBS, permeabilized with Triton X-100 0.5% (Sigma-Aldrich, 11332481001) and incubated with primary antibodies (diluted as indicated below) after blocking with a high-protein-containing solution (BSA at 5%) (Sigma-Aldrich, A7906-100G) in PBS overnight at 4 °C. Subsequently, the slides were washed with PBS and incubated for 1 h at room temperature with the appropriate secondary antibodies diluted at 1:500; DAPI (Thermo Fisher Scientific, 62248) at 1:1,000 for nuclei; and WGA (Thermo Fisher Scientific, W11261) at 1:200 for cell/myofibre membrane. After washing, the tissue sections were mounted with Mowiol (Sigma-Aldrich, 81381) or Fluoromount-G (SoutherBiotech, 0100-01). Quantitative results for histology and immunofluorescence are listed in Supplementary Table 12 . Primary antibodies were as follows: PAX7 (DSHB, PAX7, 1:50), PDGFRa (eBioscience, 17-1401-81, 1:100), perilipin-1 (Cell Signalling, 9349, 1:100), filamin C (MyBiosource, MBS2026155, 1:100), TNNT2 (Bioss, BS-10648R, 1:100), CD11b (eBioscience, 14-0112-85, 1:100), CD3 (Invitrogen, 14-0038-82, 1:100), CD19 (eBioscience, 14-0199-82, 1:100), NCAM1 (Cell Sciences, MON9006-1, 1:100), MYH7 (MyHC type I) (DSHB, A4.840, 1:10), MyHC type IIA/IIX (DSHB, SC-71, 1:70), laminin-647 (Novus Biologicals, NB300-144AF647, 1:200), FOS (Cell Signalling, 2250S, 1:200), ACVR2A (R&D, AF340, 1:100), ITGA7 (BioCell Scientific, 10007, 1:100), dystrophin (Sigma-Aldrich, D8168, 1:100). Secondary antibodies were as follows: goat anti-mouse IgM (DyLight 550, Invitrogen, SA5-10151), goat anti-mouse IgG1 (Alexa Fluor 488, Invitrogen, A21121), goat anti-mouse IgG (Alexa Fluor 488, Invitrogen, A11001), goat anti-mouse IgG (Alexa Fluor 568, Invitrogen, A11004), goat anti-rabbit IgG (Alexa Fluor Plus 488, Invitrogen, A32731TR), goat anti-rabbit IgG (Alexa Fluor Plus 647, Invitrogen, A32733TR), donkey anti-goat IgG (Alexa Fluor Plus 647, Invitrogen, A32849TR), goat anti-rat IgG (Alexa Fluor 568, Invitrogen, A11077).
Digital image acquisition and processing
Immunohistochemistry images were acquired using an upright microscope (Leica DMR6000B) equipped with a DFC300FX camera, and, for immunofluorescence pictures, using a Hamamatsu ORCA-ER camera. Images were acquired using HCX PL Fluotar objectives (×10/0.30 NA, ×20/0.50 NA and ×40/0.75 NA) and LAS AF software (Leica, v.4.0). Immunofluorescence pictures were also obtained using the Nikon Ti2 fluorescence microscope with NIS Elements software (v.4.11.0), and a confocal microscope (Zeiss 980 Airyscan2) with ZenBlue software (v.3.5) and a ×20 air objective. The acquired images were composed, edited and analysed using Fiji (ImageJ, v.2.14.0/1,54f). To reduce background, brightness and contrast adjustments were applied to the entire image. Myofibre size was assessed using the MyoSight tool 34 , with a manual correction applied after automated outlining, and the cross-sectional area (CSA) was determined using Fiji. Signals of SA-β-gal, PAX7, PDGFRα, perilipin, CD11B, CD3, CD19, TNNT2, NCAM1, filamin C, SDH and FOS staining were manually counted in Fiji. The area of ACVR2A, Sirius Red and ITGA7 staining was calculated by normalizing the positive-signal area to the total imaged area in Fiji.
Statistical analysis
The sample size of each experimental group or number of independent experiments is described in the corresponding figure legend. The calculation method for P values is explained in the figure legends. The number of replicates for each experiment is presented in the figure legends. For Pearson’s correlation, statistical significance for positive or negative correlation (represented as the R value) was set at P < 0.05 and shading represents the 95% confidence interval along the correlation line (Supplementary Table 5 ). For the box plots, the central line shows the median, the box limits indicate the upper and lower quartiles, and the whiskers indicate 1.5× the interquartile range. Python, R or Prism (v.10) were used for statistical analyses.
Reporting summary
Further information on research design is available in the Nature Portfolio Reporting Summary linked to this article.
Data availability
All raw data have been deposited to CNGB Nucleotide Sequence Archive (CNP0004394, CNP0004395, CNP0004494 and CNP0004495). All processed data are available at the Human Muscle Ageing Cell Atlas database ( https://db.cngb.org/cdcp/hlma/ ). The data deposited and made public is compliant with the regulations of the Ministry of Science and Technology of the People’s Republic of China. Source data are provided with this paper.
Code availability
All data were analysed using standard programs and packages, as described in the Methods . Custom code supporting the current study was created for the processing of the sequencing data 49 ( https://github.com/MGI-tech-bioinformatics/DNBelab_C_Series_HT_scRNA-analysis-software ) and analysis of the generated data 82 ( https://github.com/123anjuan/HMA ).
Evans, W. J. et al. Sarcopenia: no consensus, no diagnostic criteria, and no approved indication—how did we get here? Geroscience 46 , 183–190 (2023).
Article PubMed PubMed Central Google Scholar
Wiedmer, P. et al. Sarcopenia—molecular mechanisms and open questions. Ageing Res. Rev. 65 , 101200 (2021).
Article CAS PubMed Google Scholar
Eraslan, G. et al. Single-nucleus cross-tissue molecular reference maps toward understanding disease gene function. Science 376 , eabl4290 (2022).
Article CAS PubMed PubMed Central Google Scholar
Perez, K. et al. Single nuclei profiling identifies cell specific markers of skeletal muscle aging, frailty, and senescence. Aging 14 , 9393–9422 (2022).
CAS PubMed PubMed Central Google Scholar
Soendenbroe, C., Andersen, J. L. & Mackey, A. L. Muscle-nerve communication and the molecular assessment of human skeletal muscle denervation with aging. Am. J. Physiol. Cell Physiol. 321 , C317–C329 (2021).
Murgia, M. et al. Single muscle fiber proteomics reveals fiber-type-specific features of human muscle aging. Cell Rep. 19 , 2396–2409 (2017).
Sartori, R. et al. Perturbed BMP signaling and denervation promote muscle wasting in cancer cachexia. Sci. Transl. Med. 13 , eaay9592 (2021).
Madaro, L. et al. Denervation-activated STAT3-IL-6 signalling in fibro-adipogenic progenitors promotes myofibres atrophy and fibrosis. Nat. Cell Biol. 20 , 917–927 (2018).
Sousa-Victor, P., García-Prat, L. & Muñoz-Cánoves, P. Control of satellite cell function in muscle regeneration and its disruption in ageing. Nat. Rev. Mol. Cell Biol. 23 , 204–226 (2022).
Stone, S. P., Ali, B., Auberleek, I., Thompsell, A. & Young, A. The Barthel index in clinical practice: use on a rehabilitation ward for elderly people. J. R. Coll. Physicians Lond. 28 , 419–423 (1994).
Charlson, M. E. et al. The Charlson comorbidity index is adapted to predict costs of chronic disease in primary care patients. J. Clin. Epidemiol. 61 , 1234–1240 (2008).
Moiseeva, V. et al. Senescence atlas reveals an aged-like inflamed niche that blunts muscle regeneration. Nature 613 , 169–178 (2023).
Article ADS CAS PubMed Google Scholar
Schiaffino, S. & Reggiani, C. Fiber types in mammalian skeletal muscles. Physiol. Rev. 91 , 1447–1531 (2011).
Yoshida, M. et al. Local and systemic responses to SARS-CoV-2 infection in children and adults. Nature 602 , 321–327 (2022).
Mendelsohn, A. R. & Larrick, J. W. Epigenetic drift is a determinant of mammalian lifespan. Rejuvenation Res. 20 , 430–436 (2017).
Article PubMed Google Scholar
DeTomaso, D. & Yosef, N. Hotspot identifies informative gene modules across modalities of single-cell genomics. Cell Syst. 12 , 446–456 (2021).
Xirouchaki, C. E. et al. Skeletal muscle NOX4 is required for adaptive responses that prevent insulin resistance. Sci. Adv. 7 , eabl4988 (2021).
Article ADS CAS PubMed PubMed Central Google Scholar
Xu, Z. et al. Cardiac troponin T and fast skeletal muscle denervation in ageing. J. Cachexia Sarcopenia Muscle 8 , 808–823 (2017).
Chemello, F. et al. Degenerative and regenerative pathways underlying Duchenne muscular dystrophy revealed by single-nucleus RNA sequencing. Proc. Natl Acad. Sci. USA 117 , 29691–29701 (2020).
Yilmaz, A. et al. MuSK is a BMP co-receptor that shapes BMP responses and calcium signaling in muscle cells. Sci. Signal. 9 , ra87 (2016).
Langhans, C. et al. Inflammation-induced acute phase response in skeletal muscle and critical illness myopathy. PLoS ONE 9 , e92048 (2014).
Article ADS PubMed PubMed Central Google Scholar
Hangelbroek, R. W. J. et al. Expression of protocadherin gamma in skeletal muscle tissue is associated with age and muscle weakness. J. Cachexia Sarcopenia Muscle 7 , 604–614 (2016).
Karakaslar, E. O. et al. Transcriptional activation of Jun and Fos members of the AP-1 complex is a conserved signature of immune aging that contributes to inflammaging. Aging Cell 22 , e13792 (2023).
Taillandier, D. & Polge, C. Skeletal muscle atrogenes: from rodent models to human pathologies. Biochimie 166 , 251–269 (2019).
Wolff, C. A. et al. Defining the age-dependent and tissue-specific circadian transcriptome in male mice. Cell Rep. 42 , 111982 (2023).
Stratos, I. et al. Inhibition of TNF-α restores muscle force, inhibits inflammation, and reduces apoptosis of traumatized skeletal muscles. Cells 11 , 2397 (2022).
Miller, S. G. et al. AMP deamination is sufficient to replicate an atrophy-like metabolic phenotype in skeletal muscle. Metabolism 123 , 154864 (2021).
Fujimaki, S. et al. The endothelial Dll4–muscular Notch2 axis regulates skeletal muscle mass. Nat. Metab. 4 , 180–189 (2022).
Roman, W. et al. Muscle repair after physiological damage relies on nuclear migration for cellular reconstruction. Science 374 , 355–359 (2021).
Gonzalez‐Freire, M. et al. Skeletal muscle ex vivo mitochondrial respiration parallels decline in vivo oxidative capacity, cardiorespiratory fitness, and muscle strength: The Baltimore Longitudinal Study of Aging. Aging Cell 17 , e12725 (2018).
Kartha, V. K. et al. Functional inference of gene regulation using single-cell multi-omics. Cell Genom. 2 , 100166 (2022).
Lee, K. Y. et al. Tbx15 controls skeletal muscle fibre-type determination and muscle metabolism. Nat. Commun. 6 , 8054 (2015).
Hill, C. et al. Inhibition of AP-1 signaling by JDP2 overexpression protects cardiomyocytes against hypertrophy and apoptosis induction. Cardiovasc Res. 99 , 121–128 (2013).
Machado, L. et al. Tissue damage induces a conserved stress response that initiates quiescent muscle stem cell activation. Cell Stem Cell 28 , 1125–1135 (2021).
Kim, J.-H. et al. Sex hormones establish a reserve pool of adult muscle stem cells. Nat. Cell Biol. 18 , 930–940 (2016).
MacQuarrie, K. L. et al. Comparison of genome-wide binding of MyoD in normal human myogenic cells and rhabdomyosarcomas identifies regional and local suppression of promyogenic transcription factors. Mol. Cell. Biol. 33 , 773–784 (2013).
Rigillo, G. et al. The transcription factor NF-Y participates to stem cell fate decision and regeneration in adult skeletal muscle. Nat. Commun. 12 , 6013 (2021).
Dick, S. A. et al. Three tissue resident macrophage subsets coexist across organs with conserved origins and life cycles. Sci. Immunol. 7 , eabf7777 (2022).
Widner, D. B. et al. Activated mast cells in skeletal muscle can be a potential mediator for cancer‐associated cachexia. J. Cachexia Sarcopenia Muscle 12 , 1079–1097 (2021).
Antuña, E. et al. Inflammaging: implications in sarcopenia. Int. J. Mol. Sci. 23 , 15039 (2022).
Fitzgerald, G. et al. MME+ fibro-adipogenic progenitors are the dominant adipogenic population during fatty infiltration in human skeletal muscle. Commun. Biol. 6 , 111 (2023).
Contreras, O., Rossi, F. M. V. & Theret, M. Origins, potency, and heterogeneity of skeletal muscle fibro-adipogenic progenitors—time for new definitions. Skelet. Muscle 11 , 16 (2021).
Shi, Y. et al. Transcription factor SOX5 promotes the migration and invasion of fibroblast-like synoviocytes in part by regulating MMP-9 expression in collagen-induced arthritis. Front. Immunol. 9 , 749 (2018).
Zhou, X. et al. Circuit design features of a stable two-cell system. Cell 172 , 744–757 (2018).
Jin, S. et al. Inference and analysis of cell-cell communication using CellChat. Nat. Commun. 12 , 1088 (2021).
Sartori, R., Romanello, V. & Sandri, M. Mechanisms of muscle atrophy and hypertrophy: implications in health and disease. Nat. Commun. 12 , 330 (2021).
Morvan, F. et al. Blockade of activin type II receptors with a dual anti-ActRIIA/IIB antibody is critical to promote maximal skeletal muscle hypertrophy. Proc. Natl Acad. Sci. USA 114 , 12448–12453 (2017).
Shen, C. et al. Motoneuron Wnts regulate neuromuscular junction development. eLife 7 , e34625 (2018).
Han, L. et al. Cell transcriptomic atlas of the non-human primate Macaca fascicularis. Nature 604 , 723–731 (2022).
Jones, G. et al. Genome-wide meta-analysis of muscle weakness identifies 15 susceptibility loci in older men and women. Nat. Commun. 12 , 654 (2021).
Zillikens, M. C. et al. Large meta-analysis of genome-wide association studies identifies five loci for lean body mass. Nat. Commun. 8 , 80 (2017).
Corces, M. R. et al. Single-cell epigenomic analyses implicate candidate causal variants at inherited risk loci for Alzheimer’s and Parkinson’s diseases. Nat. Genet. 52 , 1158–1168 (2020).
Zhao, J. et al. Age-dependent increase in angiopoietin-like protein 2 accelerates skeletal muscle loss in mice. J. Biol. Chem. 293 , 1596–1609 (2018).
Lee, D. et al. A method to predict the impact of regulatory variants from DNA sequence. Nat. Genet. 47 , 955–961 (2015).
Ahmad, S., Jan, A. T., Baig, M. H., Lee, E. J. & Choi, I. Matrix gla protein: an extracellular matrix protein regulates myostatin expression in the muscle developmental program. Life Sci. 172 , 55–63 (2017).
Karvinen, S. et al. Effects of intrinsic aerobic capacity, aging and voluntary running on skeletal muscle sirtuins and heat shock proteins. Exp. Gerontol. 79 , 46–54 (2016).
Lee, H. et al. MiR-141-3p promotes mitochondrial dysfunction in ovariectomy-induced sarcopenia via targeting Fkbp5 and Fibin. Aging 13 , 4881–4894 (2021).
Sakakibara, I. et al. Myofiber androgen receptor increases muscle strength mediated by a skeletal muscle splicing variant of Mylk4. iScience 24 , 102303 (2021).
Chen, A. et al. Spatiotemporal transcriptomic atlas of mouse organogenesis using DNA nanoball-patterned arrays. Cell 185 , 1777–1792 (2022).
García-Prat, L. et al. FoxO maintains a genuine muscle stem-cell quiescent state until geriatric age. Nat. Cell Biol. 22 , 1307–1318 (2020).
McGinnis, C. S., Murrow, L. M. & Gartner, Z. J. DoubletFinder: doublet detection in single-cell RNA sequencing data using artificial nearest neighbors. Cell Syst. 8 , 329–337 (2019).
Young, M. D. & Behjati, S. SoupX removes ambient RNA contamination from droplet-based single-cell RNA sequencing data. Gigascience 9 , giaa151 (2020).
Wolf, F. A., Angerer, P. & Theis, F. J. SCANPY: large-scale single-cell gene expression data analysis. Genome Biol. 19 , 15 (2018).
Korsunsky, I. et al. Fast, sensitive and accurate integration of single-cell data with Harmony. Nat. Methods 16 , 1289–1296 (2019).
Hao, Y. et al. Integrated analysis of multimodal single-cell data. Cell 184 , 3573–3587 (2021).
Angelidis, I. et al. An atlas of the aging lung mapped by single cell transcriptomics and deep tissue proteomics. Nat. Commun. 10 , 963 (2019).
Murgia, M. et al. Protein profile of fiber types in human skeletal muscle: a single-fiber proteomics study. Skelet. Muscle 11 , 24 (2021).
Zhou, Y. et al. Metascape provides a biologist-oriented resource for the analysis of systems-level datasets. Nat. Commun. 10 , 1523 (2019).
Cao, J. et al. The single cell transcriptional landscape of mammalian organogenesis. Nature 566 , 496–502 (2019).
Shi, Q., Liu, S., Kristiansen, K. & Liu, L. The FASTQ+ format and PISA. Bioinformatics 38 , 4639–4642 (2022).
Granja, J. M. et al. ArchR is a scalable software package for integrative single-cell chromatin accessibility analysis. Nat. Genet. 53 , 403–411 (2021).
Weirauch, M. T. et al. Determination and inference of eukaryotic transcription factor sequence specificity. Cell 158 , 1431–1443 (2014).
Consortium, R. et al. Partitioning heritability by functional annotation using genome-wide association summary statistics. Nat. Genet. 47 , 1228–1235 (2015).
Article PubMed Central Google Scholar
Sollis, E. et al. The NHGRI-EBI GWAS Catalog: knowledgebase and deposition resource. Nucleic Acids Res. 51 , D977–D985 (2022).
Trajanoska, K. et al. Assessment of the genetic and clinical determinants of fracture risk: genome wide association and Mendelian randomisation study. BMJ 362 , k3225 (2018).
Watanabe, K., Taskesen, E., van Bochoven, A. & Posthuma, D. Functional mapping and annotation of genetic associations with FUMA. Nat. Commun. 8 , 1826 (2017).
Kumar, S., Ambrosini, G. & Bucher, P. SNP2TFBS—a database of regulatory SNPs affecting predicted transcription factor binding site affinity. Nucleic Acids Res. 45 , D139–D144 (2017).
Shrikumar, A., Prakash, E. & Kundaje, A. GkmExplain: fast and accurate interpretation of nonlinear gapped k -mer SVMs. Bioinformatics 35 , i173–i182 (2019).
García-Prat, L. et al. Autophagy maintains stemness by preventing senescence. Nature 529 , 37–42 (2016).
Article ADS PubMed Google Scholar
Punsoni, M. et al. Succinate dehydrogenase B (SDHB) immunohistochemistry for the evaluation of muscle biopsies. Appl. Immunohistochem. Mol. Morphol. 25 , 645–650 (2017).
Pessina, P. et al. Novel and optimized strategies for inducing fibrosis in vivo: focus on Duchenne muscular dystrophy. Skelet. Muscle 4 , 7 (2014).
Lai, Y., Juan, A. & Ramírez-Pardo, I. Human Muscle Ageing Cell Atlas (HMA) code. Zenodo https://doi.org/10.5281/zenodo.10796317 (2024).
Download references
Acknowledgements
We thank all of the members of our teams for technical help and discussions; M. Raya for project coordination; A. Navarro for technical assistance; C. García-Domínguez for help in patient characterization, and V. A. Raker for editing. The members of Y.L. and M.A.E.’s team thank the staff at the China National GeneBank, H. Li and D. Zhu (Bioland Laboratory, Guangzhou, China) and M. Cao (Chinese University of Hong Kong, Hongkong, China) for their support. Work in the P.M.-C. laboratory was supported partly by Milky Way Research Foundation and UPGRADE-H2020-825825 at MELIS (recipient of a Maria de Maeztu Program for Units of Excellence to UPF (MDM-2014-0370)) and Altos Labs. Work in Y.L. and M.A.E.’s team was supported by the National Key Research and Development Program of China (2022YFC3400400), the National Natural Science Foundation of China (32200688, U20A2015, 32211530050), Guangdong Basic and Applied Basic Research Foundation (2021B1515120075, 2021A1515110180), Key Laboratory of Guangdong Higher Education Institutes (2021KSYS009), and Guangzhou Key Laboratory of Biological Targeting Diagnosis and Therapy (202201020379). Freshage Research Group was supported by the Instituto de Salud Carlos III CB16/10/00435 (CIBERFES); PID2022-142470OB-I00 (M.C.G-C) from the Spanish Ministry of Innovation and Science; PROMETEO: CIPROM/2022/56 (M.C.G.-C) from Consellería de Educación, Universidades, y Empleo de la Generalitat Valenciana; EU Funded H2020-DIABFRAIL-LATAM: 825546 (M.C.G.-C), Red EXERNET-RED DE EJERCICIO FISICO Y SALUD: RED2022-134800-T (M.C.G.-C) from the Spanish Ministry of Innovation and Science; Ramón Areces Foundation and Soria Melguizo Foundation (J.V.). I.R.-P. was supported by an FPI predoctoral fellowship (Ministry of Science, Spain). Y.S. was supported by the Guangdong Basic and Applied Basic Research Foundation (2020A1414040036). The schematic of Extended Data Fig. 13d was drawn using BioRender under the academic license terms (HI266XMRUN).
Author information
These authors contributed equally: Yiwei Lai, Ignacio Ramírez-Pardo, Joan Isern, Juan An, Eusebio Perdiguero, Antonio L. Serrano, Jinxiu Li
Authors and Affiliations
BGI Research, Hangzhou, China
Yiwei Lai, Juan An, Jinxiu Li, Pengcheng Guo, Jing Zuo, Yue Yuan, Chuanyu Liu, Longqi Liu, Xun Xu & Miguel A. Esteban
BGI Research, Shenzhen, China
Department of Medicine and Life Sciences, Universitat Pompeu Fabra (UPF), Barcelona, Spain
Ignacio Ramírez-Pardo, Eusebio Perdiguero, Antonio L. Serrano, Jessica Segalés, Vera Lukesova, Eva Andrés & Pura Muñoz-Cánoves
Altos Labs, San Diego Institute of Science, San Diego, CA, USA
Ignacio Ramírez-Pardo, Joan Isern, Eusebio Perdiguero, Antonio L. Serrano & Pura Muñoz-Cánoves
Laboratory of Integrative Biology, Guangzhou Institutes of Biomedicine and Health, Chinese Academy of Sciences, Guangzhou, China
Juan An & Miguel A. Esteban
School of Life Sciences, Division of Life Sciences and Medicine, University of Science and Technology of China, Hefei, China
College of Life Sciences, University of Chinese Academy of Sciences, Beijing, China
Jinxiu Li, Longqi Liu & Xun Xu
Freshage Research Group, Department of Physiology, Faculty of Medicine, University of Valencia and CIBERFES, Fundación Investigación Hospital Clínico Universitario/INCLIVA, Valencia, Spain
Esther García-Domínguez, José Viña & Mari Carmen Gómez-Cabrera
State Key Laboratory for Diagnosis and Treatment of Severe Zoonotic Infectious Diseases, Key Laboratory for Zoonosis Research of the Ministry of Education, Institute of Zoonosis, College of Veterinary Medicine, Jilin University, Jilin, China
Pengcheng Guo & Miguel A. Esteban
Servicio de Cirugía Ortopédica y Traumatología, Hospital Arnau de Vilanova y Hospital de Liria and Health Care Department Arnau-Lliria, Valencia, Spain
Julio Doménech-Fernández
Department of Orthopedic Surgery, Clinica Universidad de Navarra, Pamplona, Spain
Department of Orthopedics, The First Affiliated Hospital of Guangdong Pharmaceutical University, Guangzhou, China
Yancheng Song
ICREA, Barcelona, Spain
Pura Muñoz-Cánoves
The Fifth Affiliated Hospital of Guangzhou Medical University-BGI Research Center for Integrative Biology, The Fifth Affiliated Hospital of Guangzhou Medical University, Guangzhou, China
Miguel A. Esteban
You can also search for this author in PubMed Google Scholar
Contributions
P.M.-C. and M.A.E. supervised the study. Y.L., I.R.-P., J.I., E.P., A.L.S., P.M.-C. and M.A.E. conceptualized the study and wrote the manuscript. Y.L., I.R.-P., J.A., A.L.S., J.S., P.G., V.L. and E.A. performed the experiments. Y.L., I.R.-P., J.A. and J.L. analysed the data. E.G.-D., J.V., J.D.-F, M.C.G.-C. and Y.S. collected the muscle biopsies. J.Z., Y.Y. and C.L. provided technical support. L.L. and X.X. gave relevant advice. I.R.-P., J.I. and Y.L. drew the schematic. J.L. constructed the HMA website. J.V., J.D., M.C.G.-C. and Y.S. contributed equally.
Corresponding authors
Correspondence to Pura Muñoz-Cánoves or Miguel A. Esteban .
Ethics declarations
Competing interests.
Y.L., P.G., J.Z., Y.Y., C.L., L.L., X.X. and M.A.E. are employees of BGI Group. I.R.-P., J.I., E.P., A.L.S. and P.M.-C. are employees of Altos Labs. The other authors declare no competing interests.
Peer review
Peer review information.
Nature thanks Benjamin D. Cosgrove, Rong Fang, Vittorio Sartorelli and the other, anonymous, reviewer(s) for their contribution to the peer review of this work. Peer reviewer reports are available.
Additional information
Publisher’s note Springer Nature remains neutral with regard to jurisdictional claims in published maps and institutional affiliations.
Extended data figures and tables
Extended data fig. 1 functional characterization and major cell type identification in skeletal muscle across ageing..
a , Correlation plots of the Barthel index 10 (autonomy vs dependency assessment, top panel) and the Charlson index 11 (patient´s comorbidities, bottom panel) with the age of the individuals in both men (triangles) and women (circles). The shape and colour represent the sex group. R , Pearson correlation coefficient; P , two-sided P -value; centre represents the average value; shade indicates 95% confidence interval. b , Samples used in this study were subjected to H&E staining to assess their tissular integrity. One representative image from the tissue of each age group is depicted (top panel; adult, sample P13; old, sample P28). Scale bar, 50 μm. The myofibre size (Min Feret diameter) distribution was quantified between muscles from the two age groups (bottom panel). The colour represents the age group. n = 4 for each age group. c . Representative images of SA-β-gal staining of adult (sample P26), old (sample P28) uninjured human muscle (top panel) or of injured mouse muscle at 7 days post-cardiotoxin injury (7 DPI) as a positive control (bottom left panel). In each muscle section (overview and insets), muscle tissue is designated with the red dashed line, and the surrounding tissue is marked with an asterisk. Scale bars, 25 μm. Boxplot showing the SA-β-gal + cells quantification within the myofibre tissue where boxes represent the upper/lower quartiles, the line depicts the median and whiskers the 1.5 interquartile range. n = 4 individuals from each age group for human muscle, n = 7 for injured mouse muscle. d , Dot plot showing the expression level for representative marker genes in the indicated cell types. Colour scale represents the average gene expression, and dot size, the percentage of cells expressing a given marker within the subpopulation. e , Normalized genome browser track profiles in the proximal promoters of the indicated marker genes (columns) for the indicated cell types (rows) measured in snATAC-seq. f , Heatmap of marker genes (gene score, left panel) and marker peaks (differentially accessible peaks, right panel) for the indicated cell types measured in snATAC-seq. Colour scale represents the relative score ( Z -score) of each marker gene/peak.
Extended Data Fig. 2 Integration of multimodal single-cell data.
a , UMAP plots of the cells/nuclei from the integrated scRNA-seq, snRNA-seq and snATAC-seq datasets split by technologies. Dots are coloured by the cell types. b , Correlation heatmap for cell-type assignment between the sc/snRNA-seq and snATAC-seq datasets. Colour scale represents the computed Pearson correlation coefficient ( R ). c , UMAP plots of the cells/nuclei from the integrated scRNA-seq, snRNA-seq and snATAC-seq datasets grouped by sampling factors (age, ethnic, sex and muscle group). Dots are coloured by the sampling factors within each group comparison. d , Cell proportion analysis for the percentage of each cell population in the scRNA-seq (top), snRNA-seq (middle) and snATAC-seq (bottom) for each individual. The colour represents the age group and the shape represent the sex group. For scRNA-seq, n = 3 adult individuals and n = 4 old individuals; for snRNA-seq, n = 7 adult individuals and n = 15 old individuals; for snATAC-seq, n = 5 adult individuals and n = 11 old individuals. For boxplots, boxes represent the upper/lower quartiles, the line depicts the median and whiskers the 1.5 interquartile range.
Extended Data Fig. 3 Cell proportion changes of human skeletal muscle with ageing.
Representative images of immunofluorescence staining from adult (left) and old (right) muscle tissue sections for specific cell types (red), their respective quantifications by age groups, and correlation plots with the age of the individuals (right panels). a , MuSCs labelled with anti-PAX7 (adult, sample P5; old, sample P28); b , FAPs and fibroblast-like cells labelled with anti-PDGFRα (adult, sample P5; old, sample P28); c , Adipocytes labelled with anti-Perilipin (adult, sample P26; old, sample P28); d , macrophages labelled with anti-CD11B (adult, sample P9; old, sample P29) e , T cells labelled with anti-CD3 (adult, sample P9; old, sample P16); f , B cells labelled with anti-CD19 (adult, sample P5; old, sample P23). Scale bars, 50 μm in ( a , c ), and 25 μm in ( b, d-f ). Nuclei were counterstained with DAPI (blue). For PDGFRα and perilipin, n = 4 individuals for each group; for PAX7, CD11B, CD3 and CD19, n = 5 individuals for each group. For all boxplots, P values were calculated by a two-tailed Mann-Whitney U -test. For boxplots, boxes represent the upper/lower quartiles, the line depicts the median and whiskers the 1.5 interquartile range. For correlation plots, R , Pearson correlation coefficient; P , two-sided P -value; centre represents the average value; shade indicates 95% confidence interval.
Extended Data Fig. 4 Myofibre type classification and proportion assessment with ageing.
a , Schematic depicting the definition of myofibre types according to myosin (MYH) genes expression. b , UMAP plots of myonucleus populations coloured according to TNNT1 (type I myonuclei) and TNNT3 (type II myonuclei) gene expression (colour scale). c , UMAP plots of myonucleus populations coloured and grouped by sampling factors (ethnic, sex and muscle group) in the snRNA-seq dataset. d , UMAP plots of myonucleus populations belonging to adult (green) and old (purple) muscles in both snRNA-seq (left panel) and snATAC-seq (right panel) datasets. e , UMAP plots of myonucleus type score for each myofibre type from the snRNA-seq dataset (left panels) and the snATAC-seq dataset (right panels). Myonucleus type scores were calculated based on the gene expression (snRNA-seq) or gene score (snATAC-seq) of distinct MYH genes among other myofibre-type specific genes (Supplementary Table 3 ). Dots (myonuclei) are coloured according to their computed score. f , Representative images of immunofluorescence for myofibre typing from adult (left top, sample P13) and old (left bottom, sample P29) muscle tissue sections of type I (blue) and type II (red) myofibres delimited by laminin (white). Scale bars, 100 μm. Quantification graphs of average myofibre size (middle bottom), myofibre type proportion (middle top) and myofibre size distribution for type I (right top) and type II myofibres (right bottom). Myofibre size was measured as cross-sectional area (CSA). n = 4 individuals of each age group. For boxplots, boxes represent the upper/lower quartiles, the line depicts the median and whiskers the 1.5 interquartile range. P value was calculated by a two-tailed unpaired Student’s t-test. For barplots, data are presented as mean values +/− SEM. g , Correlation plots of the proportion of indicated myofibre types with ageing. The shape and colours represent the sex group. R , Pearson correlation coefficient; P , two-sided P value; centre represents the average value; shade indicates 95 th confidence interval.
Extended Data Fig. 5 Markers for defining specialized myonucleus subtypes.
a , Dot plot showing the expression levels for representative marker genes in all myonucleus subpopulations. Colour scale represents the average gene expression, and dot size, the percentage of cells expressing a given marker within the subpopulation. b , Heatmap depicting enriched pathways from DEGs obtained in each myonucleus population. Colour scale represents the significance (-log 10 Q ) of the enriched terms. c , Heatmap showing the gene score for DEGs (row) in each myonucleus population (columns) identified in snATAC-seq. Colour scale represents the scaled gene score ( Z -score) for each DEG. d-f , UMAP plots highlighting the aggregated gene module expression (left panel); gene expression (middle panel) and gene score (right panel) for the following myonucleus subpopulations: d , module 9 with ADGRB3 gene for MTJ population; and module 12 with PHLDB2 gene for NMJ population; e , module 3 with ENOX1 gene for ENOX1 + population; f , module 6 and 7 with FOS, ID1 and DCLK1 genes for ID1 + / DCLK1 + population; and module 11 with SAA2 genes for SAA2 + population. Dots (myonuclei) are coloured according to their computed score or gene expression value. g , Representative images (left panel) and the corresponding quantification (right panel) of immunofluorescence for denervated myofibres (NCAM1, red; myofibre membrane, laminin, green; nuclei, DAPI, blue) in adult (sample P9) and old (sample P28) individuals. n = 4 individuals of each age group. Scale bar, 10 μm. For boxplots, boxes represent the upper/lower quartiles, the line depicts the median and whiskers the 1.5 interquartile range. P value was calculated by a two-tailed Student’s t-test.
Extended Data Fig. 6 Functional processes driving type I and type II myofibre ageing.
a , Heatmap depicting enriched pathways of DEGs for both myonucleus types in adult (≤ 46 years), old (74-82 years) and very old (≥ 84 years) age groups. Pathway significance (-log 10 Q ) is represented by the colour scale. b , Dot plots showing the gene expression (snRNA-seq, left panel) or gene score (snATAC-seq, right panel) for selected genes (rows) in adult, old and very old type I (I) and type II (II) myonuclei. Colour scale represents the average gene expression, and dot size, the percentage of cells expressing a given marker within the subpopulation. c , Correlation plots of the expression levels for the given genes ( MYH1 , MYH7 , AMPD3, PCDHGA1 ) with the individual’s age, for type I (red) and type II (blue) myonuclei in snRNA-seq (left panels) and chromatin accessibility levels for the corresponding genes in snATAC-seq (right panels). R , Pearson correlation coefficient; P , two-sided P -value; centre represents the average value; shade indicates 95% confidence interval. d-e , UMAP plots (left) or line charts (right) showing the ( d ) atrophy score (atrophy-related genes 24 ; left panels) and ( e ) RegMyon score (regenerating myonucleus signature 19 ; right panels) for type I (red) and type II myonuclei (blue) along the ageing trajectory. Gene lists for atrophy score and RegMyon score can be found in Supplementary Table 3 . Dots (myonuclei) are coloured according to their computed score. f , Representative images (top panel), quantification comparing the age groups (bottom left panel) and correlation with the age of the individuals (bottom right panel) of immunofluorescence for filamin-C + myofibres (filamin-C, red; myofibre membrane, dystrophin, green; nuclei, DAPI, blue) (left panel) in adult (sample P13) and old (sample P28) individuals; boxplot showing the quantification for filamin-C + myofibres (middle panel); correlation plot of the proportion of filamin-C + myofibres. n = 4 individuals of each age group. Scale bar, 25 μm. For boxplots, boxes represent the upper/lower quartiles, the line depicts the median and whiskers the 1.5 interquartile range. P value was calculated by two-tailed Mann-Whitney U -test. For the correlation plot, R , Pearson correlation coefficient; P , two-sided P value; centre represents the average value; shade indicates a 95% confidence interval. g , Representative images (top panel), quantification comparing the age groups (bottom left panel) and correlation with the age of the individuals (bottom right panel) for succinate dehydrogenase (SDH) activity staining (blue) in adult (sample P13) and old (sample P28) individuals. n = 4 for the adult group and n = 5 for old group. Scale bar, 50 μm. For boxplots, boxes represent the upper/lower quartiles, the line depicts the median and whiskers the 1.5 interquartile range. P value was calculated by two-tailed Mann-Whitney U -test. For the correlation plot, R , Pearson correlation coefficient; P , two-sided P value; centre represents the average value; shade indicates 95% confidence interval. h-i , UMAP plots (left) or line charts (right) showing the oxidative phosphorylation scores ( h ) and the gene expression level of PKM ( i ) as a representative gene for glycolysis pathway for type I (red) and type II myonuclei (blue) along the ageing trajectory. Gene lists for oxidative phosphorylation scores can be found in Supplementary Table 3 . Dots (myonuclei) are coloured according to their computed score or gene expression value.
Extended Data Fig. 7 Gene regulatory network for ageing myofiber.
a , Pipeline scheme for constructing gene-regulatory network: (1) nuclei of snRNA-seq and snATAC-seq datasets were paired using scOptMatch 31 ; (2) identification of significant peak-to-gene associations for defining the domains of regulatory chromatin (DORC); (3) TF binding motif enrichment was evaluated in DORC regions; and (4) identification of potential TF regulators (repressors or activators) based on the correlation of TF expression and the motif enrichment. b , Correlation heatmap for the DORC expression (RNA) and chromatin accessibility (ATAC) for type I (top panel) and type II (bottom panel) myonuclei. Colour scale represents the computed Pearson correlation coefficient ( R ). c , Heatmap showing the smoothed normalized DORC accessibility (left panel), RNA expression (middle panel), and residual (DORC-RNA, right panel) levels for JUND, JUN, FOS, JUNB and EGR1 along the ageing trajectory for type I (top panel) and type II (bottom panel) myofibre. d , Tn5 bias-adjusted TF footprints for FOSL2 and STAT3 along the type I (left panel) and type II (right panel) ageing trajectory. The type I and type II myonuclei were classified into five proportions according to the pseudotime in the ageing trajectory. In each graph, the Tn5 bias-subtracted normalized insertions (y-axis) from the motif centre to 200 bp at each side (x-axis) were shown.
Extended Data Fig. 8 Multimodal human muscle stem cell atlas in ageing.
a , UMAP plots showing the level of stress index (top panels) and FOS (bottom panels) expression as representative genes contributing to this index for both scRNA-seq (left panels) and snRNA-seq (right panels) datasets. The gene list for the stress index can be found in Supplementary Table 3 . Dots (cells/nuclei) are coloured according to their computed score or gene expression value. b , UMAP plots of the detected MuSC subpopulations (top panels) and age groups (bottom panels) in sc/snRNA-seq (left panels) and snATAC-seq (right panels). Dots (cells/nuclei) are coloured by subpopulation (top panels) or age group (bottom panels). c , Dot plots showing the expression level for representative marker genes (columns) for MuSC subpopulations (rows) by snRNA-seq (top panel) and scRNA-seq (bottom panel). Colour scale represents the average gene expression, and dot size, the percentage of cells expressing a given marker within the subpopulation. d , Heatmaps showing the expression levels for DEGs (left panel, colour scale by Z -score) and the corresponding functional enrichment analysis (right panel, colour scale by -log 10 Q ) for each MuSC subpopulation in the snRNA-seq dataset. e , Heatmaps showing the marker peaks (left panel, colour scaled by Z -score) and the corresponding enriched TF motifs (right panel, colour scaled by -log 10 Q ) for each MuSC subpopulation in the snATAC-seq dataset. f , Tn5 bias-adjusted TF footprints for JUNB (top panel) and MYOG (bottom panel) in each MuSC subpopulation detected by snATAC-seq. In each graph, the Tn5 bias-subtracted normalized insertions (y-axis) from the motif centre to 200 bp at each side (x-axis) were shown. g , Cell proportion analysis for the percentage of each MuSC subpopulation in the snRNA-seq (top), scRNA-seq (middle) and snATAC-seq (bottom) for each individual. The colours represent the age group and the shapes represent the sex group. For scRNA-seq, n = 3 adult individuals and n = 4 old individuals; for snRNA-seq, n = 7 adult individuals and n = 15 old individuals; for snATAC-seq, n = 5 adult individuals and n = 11 old individuals. h , Representative images (left panel) and corresponding quantification (right panel) of immunofluorescence for primed MuSCs (PAX7, red; FOS, green; nuclei, DAPI, blue) in adult (sample P26, top left) and old (sample P28, bottom left) individuals. n = 4 individuals of each age group. Scale bar, 25 μm. For boxplots, boxes represent the upper/lower quartiles, the line depicts the median and whiskers the 1.5 interquartile range. P value was calculated by a two-tailed Student’s t-test. i , Heatmap depicting enriched pathways of the qMuSCs population in adult (≤ 46 years), old (74-82 years) and very old (≥ 84 years) age groups. Colour scale represents the significance (-log 10 Q ) of the enriched terms. j , Correlation plots of the expression levels for the given genes ( ITGBL1 , MEF2D and FOS ) with individuals’ age for qMuSCs. R , Pearson correlation coefficient; P , two-sided P -value; centre represents the average value; shade indicates 95% confidence interval.
Extended Data Fig. 9 Vascular and immune compartment atlas of the ageing human skeletal muscle.
a , Dot plot showing the expression levels for representative marker genes (columns) for each vascular cell subpopulation (rows). Colour scale represents the average gene expression, and dot size, the percentage of cells expressing a given marker within the subpopulation. b , UMAP plot of the detected vascular cell subpopulations by snATAC-seq. Dots were coloured by the cell types. c , Heatmaps of the marker genes (gene score, left panel) and marker peaks (differentially accessible peaks, right panel) for each vascular subpopulation measured in snATAC-seq. Colour scale represents the relative score ( Z -score) of each marker gene/peak. d , Heatmap showing the cell-type assignment correlation for vascular cell subpopulations between the sc/snRNA-seq and snATAC-seq datasets. Colour scale represents the computed Pearson correlation coefficient ( R ). e , Cell proportion analysis with the percentage of each vascular cell subpopulation for the scRNA-seq (top panel), snRNA-seq (middle panel) and snATAC-seq (bottom panel) for each individual. The colours represent the age group and the shapes represent the sex group. For scRNA-seq, n = 3 adult individuals and n = 4 old individuals; for snRNA-seq, n = 7 adult individuals and n = 15 old individuals; for snATAC-seq, n = 5 adult individuals and n = 11 old individuals. For boxplots, boxes represent the upper/lower quartiles, the line depicts the median and whiskers the 1.5 interquartile range. f , as in ( a ), for immune cell subpopulations. g , as in ( b ), for immune cell subpopulations. h , as in ( c ), for immune cell subpopulations. i , as in ( d ), for immune cell subpopulations. j , as in ( e ), for immune cell subpopulations. For scRNA-seq, n = 3 adult individuals and n = 4 old individuals; for snRNA-seq, n = 5 adult individuals and n = 13 old individuals; for snATAC-seq, n = 4 adult individuals and n = 10 old individuals.
Extended Data Fig. 10 Stromal compartment atlas of the ageing human skeletal muscle.
a , Dot plot showing the expression levels for representative marker genes for each stromal subpopulation (row). Colour scale represents the average gene expression, and dot size, the percentage of cells expressing a given marker within the subpopulation. b , UMAP plot of the detected stromal subpopulations in snATAC-seq. Dots were coloured by the cell types. c , Heatmaps of the marker genes (gene score, left panel) and marker peaks (differentially accessible peaks, right panel) for each identified population (columns). Colour scale represents the relative score ( Z -score) for each marker gene/peak. d , Heatmap showing the cell-type assignment correlation for stromal cell subpopulations. Colour scale represents the Pearson correlation coefficient ( R ). e , Cell proportion analysis with the percentage of each stromal cell subpopulation in the scRNA-seq (top), snRNA-seq (middle) and snATAC-seq (bottom) for each individual. The colours represent the age group and the shapes represent the sex group. For scRNA-seq, n = 3 adult individuals and n = 4 old individuals; for snRNA-seq, n = 7 adult individuals and n = 14 old individuals; for snATAC-seq, n = 5 adult individuals and n = 11 old individuals. For boxplots, boxes represent the upper/lower quartiles, the line depicts the median and whiskers the 1.5 interquartile range. f , Heatmap depicting significantly enriched pathways for DEGs in each stromal cell subpopulation. Colour scale represents the significance (-log 10 Q ) of the enriched terms. g , Heatmap depicting the enriched pro-inflammatory (IL6/AP1) and pro-fibrotic (TGFβ) pathways of the resident mononucleated cell subpopulations in adult (≤ 46 years), old (74-82 years) and very old (≥ 84 years) age groups. Colour scale represents the significance (-log 10 Q ) of the enriched terms. h , Dot plots showing the expression levels for the indicated cell-cycle inhibitor genes. Colour scale represents the average gene expression, and dot size, the percentage of cells expressing a given gene within the subpopulation.
Extended Data Fig. 11 Inflammatory and cell-ECM interaction networks of skeletal muscle with ageing.
a , Heatmaps of the incoming (white-blue scale, left panel) and outgoing (white-red scale, right panel) signals for adult (left side) and old (right side) for indicated signalling pathways. Colour scale represents the relative interaction intensity of each cell type for the represented pathways. b-c , ( b ) Chemokines (CCL, top panel; and CXCL, bottom panel) and ( c ) inflammatory factors (IL6, top panel; and TNF, bottom panel) signalling networks in adult (left panels) and old (right panels) groups. The nodes represent cell types and edges the interactions among them. Edge width is proportional to the interaction probability. Nodes and edges are coloured according to the cell type. d-e , Dot plot showing the expression level for the representative ( d ) chemokines and ( e ) inflammatory factors in adult (green) and old (purple) skeletal muscle cell populations. Colour scale represents the average gene expression, and dot size, the percentage of cells expressing a given gene within the subpopulation. f , Representative images (left panel) of adult (sample P26) and old (sample P28) muscles of Sirius red staining, quantification comparing the age groups (middle panel) and correlation with the age of the individuals (right panel). n = 4 individuals of each age group. Scale bar, 50 μm. For boxplots, boxes represent the upper/lower quartiles, the line depicts the median and whiskers the 1.5 interquartile range. P value was calculated by a two-tailed Mann-Whitney U -test. For the correlation plot, R , Pearson correlation coefficient; P , two-sided P value; centre represents the average value; shade indicates a 95% confidence interval. g , Heatmaps of the relative gene expression ( Z -score) of ECM-ligands (left panel) and ECM-receptors (right panel) in each major cell population in both adult (green) and old (purple) individuals. h , Representative images (left panel) and corresponding quantification (right panel) of immunofluorescence for ITGA7 + area (ITGA7, yellow; nuclei, DAPI, blue) in adult (sample P5) and old (sample P29) individuals. Scale bar, 50 μm. n = 4 individuals of each age group. For boxplots, boxes represent the upper/lower quartiles, the line depicts the median and whiskers the 1.5 interquartile range. P value was calculated by a two-tailed Student’s t-test.
Extended Data Fig. 12 Perturbed Notch, IGF, BMP and Wnt signalling networks in aged muscles.
a , Heatmaps of the incoming (white-blue scale, left panel) and outgoing (white-red scale, right panel) signals for adult (left side) and old (right side) for indicated signalling pathways. Colour scale represents the relative interaction intensity of each cell type for the represented pathways. b-e , Notch ( b ), IGF ( c ), BMP ( d ), and Wnt ( e ) signalling networks (top panels) and the expression levels for the corresponding representative genes in each signalling network (bottom panels). Signalling networks are depicted for adult (left top panels) and old (right top panels). The nodes represent cell types and edges the interactions among them. Edge width is proportional to the interaction probability. Nodes and edges are coloured according to the cell type. For dot plots, the colour scale represents the average gene expression and dot size the percentage of cells expressing a given gene within the cell type in adult (green) and old (purple) individuals.
Extended Data Fig. 13 GWAS analysis of ageing muscle and spatial distribution of the human skeletal muscle changes with ageing.
a , Heatmap of the ageing-related marker peaks in each cell type identified by snATAC-seq. Colour scale represents the relative score ( Z -score) of each marker peak. b , Pipeline scheme for identification of candidate sarcopenia-related genetic variants. Briefly, genetic variants with the high linkage disequilibrium (LD, r 2 ≥ 0.8) to reported lead variants were overlapped to cell-type defined marker peaks to identify candidate variants. Next, the potential effects of candidate variants were assessed by the following steps: 1) identification of the co-accessible peaks; 2) construction of peak to gene linkage 3) predicting the effect of genetic variants on TF binding. c , Genome browser tracks for each cell population in adult and old muscles showing the peaks associated with the identified SNPs rs1862574, rs3008232, rs1281155 and rs571800667 within the locus of GDNF , TRIM63 , ANGPTL2 and FOXO1 , respectively. Chromatin tracks show the linkages between the variant-containing peaks and the associated gene promoter. d , ( Adult ) Human skeletal muscles are mainly composed of syncytial multinucleated type II and type I myofibres, each of which is innervated by single motorneuron endplates whose axons are surrounded by Schwann cells. Muscle resident mononuclear cell populations, such as MuSCs, stromal cells (FAPs and fibroblast-like cells), immune cells (myeloid cells and lymphocytes) and vascular cells (ECs, SMCs and pericytes), contribute to muscle homeostasis. ( Old ) Human skeletal muscle ageing is characterized by a reduction in mass, increased inflammation and fibrosis. Myofibre numbers are reduced, particularly type II myofibres. In addition, myofibres tend to lose their innervation and change their characteristics, and new myonuclear populations arise with abnormal regenerative, degenerative and denervation traits. The MuSC population is reduced and enters a primed state, leading to exhaustion of the stem cell pool. Intramuscular adipocytes accumulate among muscle fibres, and stromal cells increase, with a shift towards a pro-fibrotic profile. In the vasculature, the proportion of pericytes and capillary ECs decrease, and vascular integrity is impaired. Immune cells (myeloid cells, lymphocytes and mast cells) infiltrate the muscle and acquire a pro-inflammatory profile. Collectively, these alterations and the associated abnormalities in signalling networks impact muscle characteristics and functions. The schematic was created with BioRender.com.
Supplementary information
Reporting summary, peer review file, supplementary table 1.
Donor information.
Supplementary Table 2
Library information.
Supplementary Table 3
Gene signatures.
Supplementary Table 4
Gene modules identified by Hotspot and the corresponding enriched pathways.
Supplementary Table 5
Correlation analysis identified age-related gene expression changes in type I and type II myofibre and MuSCs.
Supplementary Table 6
Gene clusters along the pseudotime trajectory for degenerating type I and type II myofibre and the corresponding enriched pathways.
Supplementary Table 7
Identified significant cell–cell interaction pairs in adult and old skeletal muscle.
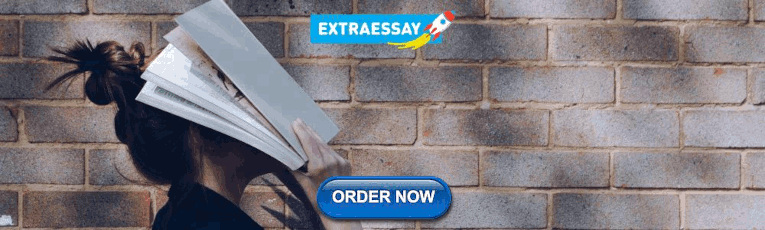
Supplementary Table 8
GWAS traits and identified sarcopenia-related candidate genetic variants.
Supplementary Table 9
Cell proportions in each individual across single-cell modalities.
Supplementary Table 10
List of DEGs.
Supplementary Table 11
Functional enrichment results.
Supplementary Table 12
Quantitative results for histology and immunofluorescence staining.
Source data
Source data fig. 1, source data fig. 2, source data fig. 3, source data fig. 4, source data fig. 5, source data fig. 6, source data extended data fig. 1, source data extended data fig. 2, source data extended data fig. 3, source data extended data fig. 4, source data extended data fig. 5, source data extended data fig. 6, source data extended data fig. 7, source data extended data fig. 8, source data extended data fig. 9, source data extended data fig. 10, source data extended data fig. 11, source data extended data fig. 12, rights and permissions.
Open Access This article is licensed under a Creative Commons Attribution 4.0 International License, which permits use, sharing, adaptation, distribution and reproduction in any medium or format, as long as you give appropriate credit to the original author(s) and the source, provide a link to the Creative Commons licence, and indicate if changes were made. The images or other third party material in this article are included in the article’s Creative Commons licence, unless indicated otherwise in a credit line to the material. If material is not included in the article’s Creative Commons licence and your intended use is not permitted by statutory regulation or exceeds the permitted use, you will need to obtain permission directly from the copyright holder. To view a copy of this licence, visit http://creativecommons.org/licenses/by/4.0/ .
Reprints and permissions
About this article
Cite this article.
Lai, Y., Ramírez-Pardo, I., Isern, J. et al. Multimodal cell atlas of the ageing human skeletal muscle. Nature (2024). https://doi.org/10.1038/s41586-024-07348-6
Download citation
Received : 24 August 2022
Accepted : 25 March 2024
Published : 22 April 2024
DOI : https://doi.org/10.1038/s41586-024-07348-6
Share this article
Anyone you share the following link with will be able to read this content:
Sorry, a shareable link is not currently available for this article.
Provided by the Springer Nature SharedIt content-sharing initiative
By submitting a comment you agree to abide by our Terms and Community Guidelines . If you find something abusive or that does not comply with our terms or guidelines please flag it as inappropriate.
Quick links
- Explore articles by subject
- Guide to authors
- Editorial policies
Sign up for the Nature Briefing newsletter — what matters in science, free to your inbox daily.

EVIDENCE BASED MUSCLE
BUILD MUSCLE THE SMART WAY
- GAIN MUSCLE
Debunking the Biggest Muscle Growth Myth: New Research
Debunking muscle growth myths: insights from new research summary.
- A recent review has debunked some of the biggest muscle growth myths. It challenges traditional beliefs about slow and controlled reps for optimal muscle growth; the review suggests that muscle growth can occur over a wide range of repetition durations (0.5 to 8 seconds) and that the tempo of repetitions, specifically the balance between slow eccentric and faster concentric phases, may not be as critical as previously thought for optimizing hypertrophy.
- Moreover, performing partial range of motion (ROM) exercises at longer muscle lengths could prove superior to full ROM exercises for promoting muscle hypertrophy. This indicates that we might need to reevaluate the traditional emphasis on full ROM for all exercises based on specific muscle groups and training goals.
- While important for biomechanical efficiency and injury prevention, exercise-specific kinematics, including detailed body positioning and movement patterns, may not directly correlate with muscle hypertrophy outcomes, indicating a need for more research on how strict versus non-strict repetition techniques affect muscle growth.
Muscle Growth Myths
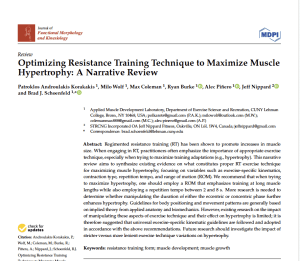
Muscle Growth Myth 1: Slow and Controlled Reps Are Key for Muscle Growth
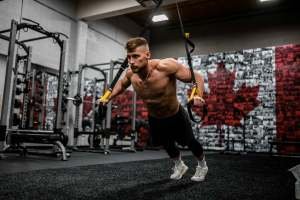
However, a review by Schoenfeld et al. discovered that muscle growth occurs across a broad range of repetition durations, from 0.5 to 8 seconds, suggesting that repetition tempo (i.e., lifting speed) may not be as critical as previously thought. (Schoenfeld et al., 2015) Wilk et al.’s findings propose that a combination of slower eccentric and faster concentric tempos could be optimal for muscle development. However, a definitive tempo recommendation remains elusive due to the diversity in study protocols.(Schoenfeld et al., 2015) These insights challenge the dogma that slow, controlled reps are superior, indicating that significant hypertrophy can occur within 2 to 8 seconds of repetition durations, regardless of whether the concentric or eccentric phase is extended.
Recommendations : To debunk this muscle growth myth, trainers should consider incorporating a variety of repetition tempos. The authors recommend a repetition tempo of 2-8 seconds to maximize hypertrophy, although it remains unclear whether extending a repetition’s concentric or eccentric phase will lead to greater hypertrophy.
Muscle Growth Myth 2: All Muscles Need to be trained with a Full Range of Motion for Optimal Muscle Growth
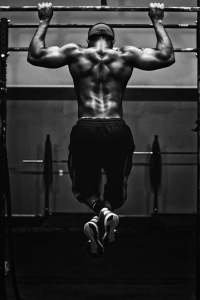
Recent reviews have sparked a reevaluation of the necessity for a full ROM in training. Wolf et al. found that a partial ROM performed at longer muscle lengths might be superior to a full ROM for hypertrophy. (Milo et al., 2023) Kassiano et al. corroborated this, showing that a partial ROM at long muscle lengths could promote optimal growth in muscles like the quadriceps, biceps, and triceps. (Kassiano et al., 2023) These findings suggest that employing a ROM that favors longer muscle lengths could be more beneficial than adhering strictly to a full ROM.
Recommendations : It’s time to move past this muscle growth myth by advocating for exercises that allow muscles to extend fully, particularly focusing on longer muscle lengths, to foster better hypertrophy results. The authors recommend employing a range of motion (ROM) that emphasizes training at long muscle lengths (deep stretch) to maximize muscle hypertrophy. They suggest using a full ROM, defined as the largest exercise-specific degree of ROM that can be achieved at each joint, to optimize hypertrophic outcomes.
Muscle Growth Myth 3: Exercise-Specific Kinematics Determine Muscle Growth
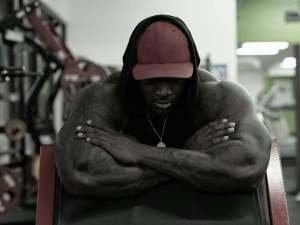
Strict adherence to these guidelines was also thought to maximize hypertrophy by ensuring that the target muscles are engaged correctly and fully. However, the evidence does not support the direct correlation between these strict kinematic guidelines and muscle hypertrophy.
Direct studies on the topic, such as one exploring the effect of different foot positions during calf training, indicate that while certain positions can influence hypertrophy in specific muscle areas, muscle activation alone may not be a reliable hypertrophy predictor.(Andersen et al., 2014; Martín-Fuentes et al., 2022; Nunes et al., 2020; Saeterbakken et al., 2021) This lack of direct evidence challenges the notion that strict adherence to specific kinematic guidelines is essential for hypertrophy, suggesting that there might be more flexibility in effective resistance training techniques than previously thought.
Muscle Growth Myths: Practical Applications
Given the current evidence, resistance training programs designed to maximize muscle hypertrophy should:
- Employ a ROM that allows a muscle to be fully stretched, accommodating for longer muscle lengths where possible.
- Utilize a repetition tempo that spans an overall duration of 2–8 seconds, allowing for various concentric and eccentric durations. This flexibility accommodates a wide spectrum of effective training methodologies.(Milo et al., 2023; Schoenfeld et al., 2015; Wilk et al., 2021)
Conclusions
In conclusion, debunking these muscle growth myths embraces a nuanced approach to resistance training that acknowledges the complexity of muscle hypertrophy. By adapting training techniques based on scientific evidence, practitioners can explore a broader range of effective strategies tailored to individual goals and needs.
Andersen, V., Fimland, M. S., Wiik, E., Skoglund, A., & Saeterbakken, A. H. (2014). Effects of Grip Width on Muscle Strength and Activation in the Lat Pull-Down. The Journal of Strength & Conditioning Research , 28 (4), 1135-1142. https://doi.org/10.1097/jsc.0000000000000232
Androulakis Korakakis, P., Wolf, M., Coleman, M., Burke, R., Piñero, A., Nippard, J., & Schoenfeld, B. J. (2024). Optimizing Resistance Training Technique to Maximize Muscle Hypertrophy: A Narrative Review. Journal of Functional Morphology and Kinesiology , 9 (1), 9. https://www.mdpi.com/2411-5142/9/1/9
Brad, S., James, F., Jozo, G., Cody, H., Eric, H., Stuart, P., James, S., & Andrew, V. (2021). Resistance Training Recommendations to Maximize Muscle Hypertrophy in an Athletic Population: Position Stand of the IUSCA. International Journal of Strength and Conditioning , 1 (1). https://doi.org/10.47206/ijsc.v1i1.81
Kassiano, W., Costa, B., Nunes, J. P., Ribeiro, A. S., Schoenfeld, B. J., & Cyrino, E. S. (2023). Which ROMs Lead to Rome? A Systematic Review of the Effects of Range of Motion on Muscle Hypertrophy. The Journal of Strength & Conditioning Research , 37 (5), 1135-1144. https://doi.org/10.1519/jsc.0000000000004415
Martín-Fuentes, I., Oliva-Lozano, J. M., & Muyor, J. M. (2022). Influence of Feet Position and Execution Velocity on Muscle Activation and Kinematic Parameters During the Inclined Leg Press Exercise. Sports Health , 14 (3), 317-327. https://doi.org/10.1177/19417381211016357
Milo, W., Patroklos, A.-K., James, F., Brad, S., & James, S. (2023). Partial Vs Full Range of Motion Resistance Training: A Systematic Review and Meta-Analysis. International Journal of Strength and Conditioning , 3 (1). https://doi.org/10.47206/ijsc.v3i1.182
Nunes, J. P., Costa, B. D. V., Kassiano, W., Kunevaliki, G., Castro-e-Souza, P., Rodacki, A. L. F., Fortes, L. S., & Cyrino, E. S. (2020). Different Foot Positioning During Calf Training to Induce Portion-Specific Gastrocnemius Muscle Hypertrophy. The Journal of Strength & Conditioning Research , 34 (8), 2347-2351. https://doi.org/10.1519/jsc.0000000000003674
Saeterbakken, A. H., Stien, N., Pedersen, H., Solstad, T. E., Cumming, K. T., & Andersen, V. (2021). The Effect of Grip Width on Muscle Strength and Electromyographic Activity in Bench Press among Novice- and Resistance-Trained Men. International Journal of Environmental Research and Public Health , 18 (12).
Schoenfeld, B. J. (2010). The Mechanisms of Muscle Hypertrophy and Their Application to Resistance Training. The Journal of Strength & Conditioning Research , 24 (10), 2857-2872. https://doi.org/10.1519/JSC.0b013e3181e840f3
Schoenfeld, B. J., Ogborn, D. I., & Krieger, J. W. (2015). Effect of Repetition Duration During Resistance Training on Muscle Hypertrophy: A Systematic Review and Meta-Analysis. Sports Medicine , 45 (4), 577-585. https://doi.org/10.1007/s40279-015-0304-0
Wilk, M., Zajac, A., & Tufano, J. J. (2021). The Influence of Movement Tempo During Resistance Training on Muscular Strength and Hypertrophy Responses: A Review. Sports Medicine , 51 (8), 1629-1650. https://doi.org/10.1007/s40279-021-01465-2
About The Author
Robbie Durand
I am an exercise physiologist dedicated to writing about health, fitness, nutrition, and resistance exercise. I have spent a large portion of my career studying muscle growth, fat loss, and nutrition.
See author's posts
Share this:
Related news.

Hardgainers Need More Sets for Muscle Growth (New Study)
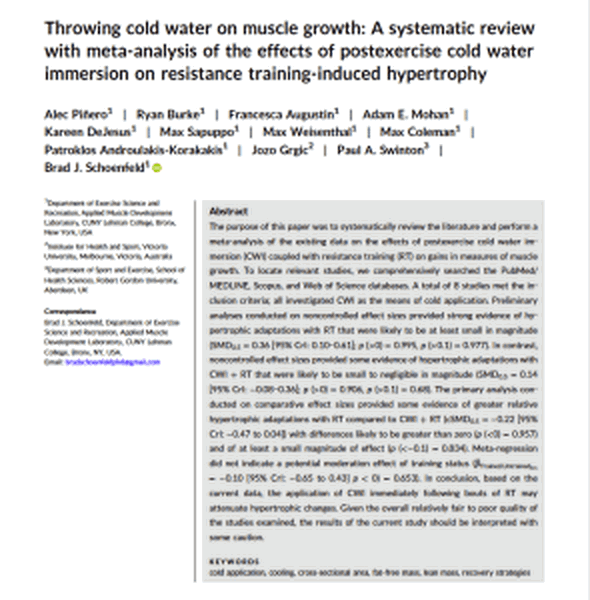
Ice Baths and Muscle Growth: 2024 Research Updates
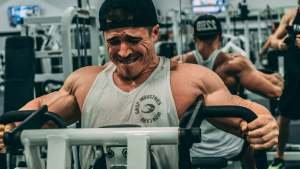
Rest Redistributed Sets: Shorter Rest, Same Gains!

Bodyweight Exercises vs. Gym Gains: Can You Ditch the Gym and Still Gain Muscle?
share this!
April 23, 2024
This article has been reviewed according to Science X's editorial process and policies . Editors have highlighted the following attributes while ensuring the content's credibility:
fact-checked
peer-reviewed publication
trusted source
New small molecule helps scientists study regeneration
by Friedrich Miescher Institute for Biomedical Research
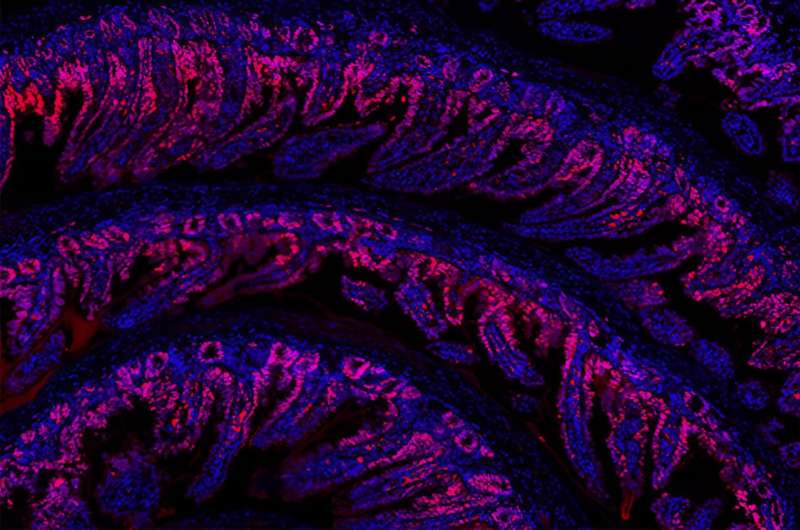
Regenerating damaged tissues or organs has been a dream of scientists for decades. Now, researchers at the FMI and Novartis Biomedical Research have discovered a new molecule that activates a protein involved in regeneration. The tool holds promise for advancing our understanding of how organisms repair damaged tissue.
During embryo development and throughout life, specific molecules act as signals that orchestrate cell growth and organ size. One of these molecules—a protein called YAP—not only tells cells to grow and multiply, it is also involved in healing wounds and repairing damaged tissues.
Given YAP's potential for promoting tissue regeneration, scientists at Novartis Biomedical Research sought to manipulate the protein's activity. To do so, they screened many synthetic small molecules and identified one, which they called NIBR-LTSi, that activated YAP.
To investigate how NIBR-LTSi affects tissue regeneration, the researchers teamed up with Clara Baader, a Ph.D. student in the Liberali group at the FMI. The Liberali group has significant expertise in establishing and using 3D models of the mouse gut, known as mouse intestinal organoids. These intestinal organoids accurately mimic the structure and function of the gut epithelium, a tissue that helps the body absorb nutrients from food and preserve the intestinal barrier.
Treating the intestinal organoids with NIBR-LTSi activated YAP and resulted in an increase in cell proliferation . The findings, published in Cell Stem Cell , hint that activating YAP may boost tissue regeneration.
However, as the organoids' regenerative potential increased, the number of mature, functional intestinal cells decreased, the researchers found. In the long run, this side effect may compromise the structure and function of the tissue, Baader cautions.
"The expertise of our lab has been instrumental in understanding the adverse effects, particularly on the intestine, observed with systemic YAP activation," she says.
Although more work is needed before small molecules like NIBR-LTSi can find applications in the clinic, the tool promises to advance our understanding of tissue regeneration by allowing scientists to target YAP in multiple experimental settings.
Journal information: Cell Stem Cell
Provided by Friedrich Miescher Institute for Biomedical Research
Explore further
Feedback to editors

Ancient giant tortoise fossils found in Colombian Andes
15 minutes ago

Emperor penguins perish as ice melts to new lows: Study
22 minutes ago

Artificial intelligence helps scientists engineer plants to fight climate change
11 hours ago

Ultrasensitive photonic crystal detects single particles down to 50 nanometers
12 hours ago

Scientists map soil RNA to fungal genomes to understand forest ecosystems

Researchers show it's possible to teach old magnetic cilia new tricks

Mantle heat may have boosted Earth's crust 3 billion years ago
13 hours ago

Study suggests that cells possess a hidden communication system

Researcher finds that wood frogs evolved rapidly in response to road salts

Imaging technique shows new details of peptide structures
Relevant physicsforums posts, the cass report (uk).
14 hours ago
Major Evolution in Action
Apr 22, 2024
If theres a 15% probability each month of getting a woman pregnant...
Apr 19, 2024
Can four legged animals drink from beneath their feet?
Apr 15, 2024
Mold in Plastic Water Bottles? What does it eat?
Apr 14, 2024
Dolphins don't breathe through their esophagus
More from Biology and Medical
Related Stories

Intestinal regeneration: Lessons from organoid research
Oct 8, 2020

Bile acids boost gut regeneration
Sep 18, 2020

Optimization of human small intestinal organoids
Aug 23, 2022

Early developmental spinal cord extracellular matrix can promote neural regeneration
Apr 3, 2024

Mini-guts reveal crucial forces that shape the intestinal lining
Jun 22, 2021

The future of replacement organs is (quite possibly) here: Robust human intestinal organoids created in a lab
Dec 5, 2022
Recommended for you

Vast DNA tree of life for plants revealed by global science team using 1.8 billion letters of genetic code
17 hours ago

Giant virus discovered in wastewater treatment plant infects deadly parasite
16 hours ago

Researchers uncover 'parallel universe' in tomato genetics
Let us know if there is a problem with our content.
Use this form if you have come across a typo, inaccuracy or would like to send an edit request for the content on this page. For general inquiries, please use our contact form . For general feedback, use the public comments section below (please adhere to guidelines ).
Please select the most appropriate category to facilitate processing of your request
Thank you for taking time to provide your feedback to the editors.
Your feedback is important to us. However, we do not guarantee individual replies due to the high volume of messages.
E-mail the story
Your email address is used only to let the recipient know who sent the email. Neither your address nor the recipient's address will be used for any other purpose. The information you enter will appear in your e-mail message and is not retained by Phys.org in any form.
Newsletter sign up
Get weekly and/or daily updates delivered to your inbox. You can unsubscribe at any time and we'll never share your details to third parties.
More information Privacy policy
Donate and enjoy an ad-free experience
We keep our content available to everyone. Consider supporting Science X's mission by getting a premium account.
E-mail newsletter
- Skip to primary navigation
- Skip to main content
- Skip to primary sidebar
The Online Home for Strength Sports
New Study on Protein Timing: Even 100 Grams Per Meal Isn’t Wasted
A new study out of the journal cell reports medicine might be the final nail in the coffin about protein timing..
As you’re reading these words, somewhere out there, an overly meticulous gym bro is shaving fine specks of protein powder off the top of his measuring scoop before taking it from the food scale to his blender. After all, the body can only absorb 25 to 30 grams of protein at a time, right? The big fella in the squat rack banging out sets of three-plate barbell rows may think so, but a recent study has another take on the issue.
The paper, conducted by Trommelen et al. and published on Dec. 19, 2023, in the journal Cell Reports Medicine , makes a strong argument in favor of putting as many scoops of your favorite whey protein powder into your shaker bottle as you want. The study appears to show that there’s no upper limit to the anabolic response generated by consuming as much as 100 grams of protein at a time .
[Related: Different Types of Protein Powder Explained: Which Is Right for You?]
Editor’s Note: The content on BarBend is meant to be informative in nature, but it should not be taken as medical advice . When starting a new training regimen and/or diet, it is always a good idea to consult with a trusted medical professional. We are not a medical resource. The opinions and articles on this site are not intended for use as diagnosis, prevention, and/or treatment of health problems. They are not substitutes for consulting a qualified medical professional.
What the Study Says
Trommelen & colleagues, the authors of the study titled “The anabolic response to protein ingestion during recovery from exercise has no upper limit in magnitude and duration in vivo in humans,” take a firm stance regarding the ongoing protein timing debate right from the get-go. They state in their work that “ The belief that anabolic response to feeding during post-exercise recovery has an upper limit … lacks scientific proof .” ( 1 ) To that end, they set about conducting an acute observational study of the effects of ingesting different amounts of dietary protein after a workout.
For context, the bodybuilding and fitness community seems to constantly be at war over the “optimal” amount of protein on a per-meal basis. Some data has thrown out numbers like 25 to 40 grams. ( 2 )
At the same time, other reputable sources argue that the body will eventually utilize however much protein it is provided, whether that is across one meal or six. ( 3 ) Here’s what the authors of this recent study did to find out the truth:
- Participants were randomly selected to receive 25 grams, 100 grams, or a placebo of milk-based protein after a strength training workout.
- It is argued that most studies supporting a so-called “upper limit” of usable protein ingestion don’t assess over a long enough time period to account for the digestion of larger protein-rich meals .
- The authors observed a “clear dose-dependent pattern in muscle protein synthesis rates over the entire 12-hour period…,” indicating that acute muscle protein synthesis rises to match how much protein is being consumed in a single sitting.
- The authors conclude by stating that, “the magnitude and duration of the anabolic response to protein ingestion is not restricted and has previously been underestimated in humans .”
Limitations
The authors make some strong claims in favor of the viability of high-protein meals. However, their data is not entirely infallible; there are some limitations to the utility of this study. For one, large anabolic responses don’t necessarily mean that all of that protein is being put to work to repair and grow muscle tissue . Certain studies have argued that elevated muscle protein synthesis and anabolic signaling may not be highly important for actual muscle hypertrophy . ( 4 )
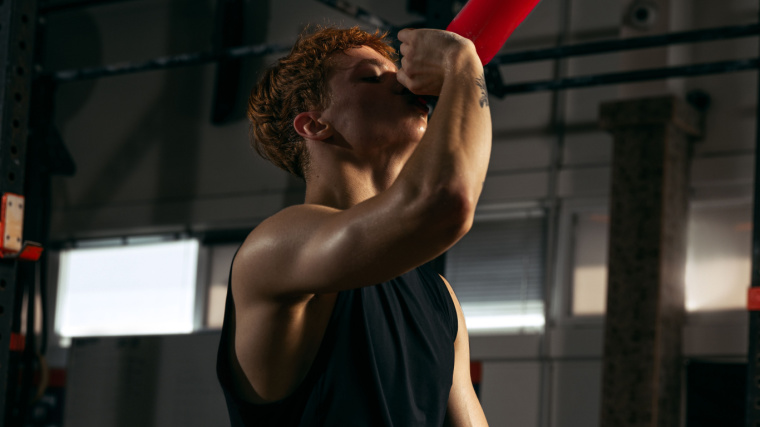
[Related: Best Meal Replacement Shakes for Bulking and Weight Loss ]
The authors may have opted out of making such a claim because this was not a longitudinal study . Longitudinal, or “over time,” data is more actionable. This study shows that there may not be an upper limit to single-meal protein ingestion. Notably, they also didn’t observe complete protein “saturation” with 100 grams, implying that even higher doses may also be fine.
Main Takeaways
This study by Trommelen & colleagues appears to be another notch in favor of protein frequency/timing not being as big of a deal. It’s still far from an open-and-shut case, especially if you’re the type of person who wants to optimize every aspect of your bodybuilding meal prep or dietary habits. Here are the study’s main takeaways:
- There appears to be no identifiable “cap” to the amount of protein your body can utilize if you extend the time frame long enough.
- Even 100 grams of protein ingested in one sitting won’t lead to amino acid oxidation or, in plain terms, wasting.
- If you don’t have the luxury of eating many small protein-rich meals daily, don’t worry about leaving gains on the table .
- Other studies still assert that more “moderate” per-meal levels of protein might be better for maximizing muscle protein synthesis, but the jury’s still out.
- Your time is probably better spent worrying about hitting a consistent protein-per-day benchmark . How much protein is needed to build muscle is a highly individualized question. If you need a hand to know what’s likely right fo you, try BarBend’s in-house protein calculator:
Protein Intake Calculator
Total calories: 1699 per day.
Exercise: 15-30 minutes of elevated heart rate activity. Intense exercise: 45-120 minutes of elevated heart rate activity. Very intense exercise: 2+ hours of elevated heart rate activity.
[Related: How To Bulk — the Ultimate Guide To Gaining Size ]
More Research Content
- A New Study Reveals 2 Training Techniques to Maximize Muscle Growth
- Stretching Can Directly Create Muscle Growth
- New Study Suggests Overhead Triceps Extensions Build More Muscle Than Pushdowns
- Trommelen, J., van Lieshout, G. A. A., Nyakayiru, J., Holwerda, A. M., Smeets, J. S. J., Hendriks, F. K., van Kranenburg, J. M. X., Zorenc, A. H., Senden, J. M., Goessens, J. P. B., Gijsen, A. P., & van Loon, L. J. C. (2023). The anabolic response to protein ingestion during recovery from exercise has no upper limit in magnitude and duration in vivo in humans. Cell reports. Medicine , 4 (12), 101324.
- Moore, D. R., Robinson, M. J., Fry, J. L., Tang, J. E., Glover, E. I., Wilkinson, S. B., Prior, T., Tarnopolsky, M. A., & Phillips, S. M. (2009). Ingested protein dose response of muscle and albumin protein synthesis after resistance exercise in young men. The American journal of clinical nutrition , 89 (1), 161–168.
- Macnaughton, L. S., Wardle, S. L., Witard, O. C., McGlory, C., Hamilton, D. L., Jeromson, S., Lawrence, C. E., Wallis, G. A., & Tipton, K. D. (2016). The response of muscle protein synthesis following whole-body resistance exercise is greater following 40 g than 20 g of ingested whey protein. Physiological reports , 4 (15), e12893.
- Damas, F., Phillips, S., Vechin, F. C., & Ugrinowitsch, C. (2015). A review of resistance training-induced changes in skeletal muscle protein synthesis and their contribution to hypertrophy. Sports medicine (Auckland, N.Z.) , 45 (6), 801–807.
Featured Image: Milan Ilic Photographer / Shutterstock
About Jake Dickson, NASM-CPT, USAW-L2
Jake is a graduate of the University of North Carolina at Wilmington with a B.S. in Exercise Science. He began his career as a weightlifting coach before transitioning into sports media to pursue his interest in journalism.
View All Articles
BarBend is an independent website. The views expressed on this site may come from individual contributors and do not necessarily reflect the view of BarBend or any other organization. BarBend is the Official Media Partner of USA Weightlifting.

- April 24, 2024 | Quantum Computing Meets Genomics: The Dawn of Hyper-Fast DNA Analysis
- April 24, 2024 | Scientists Turn to Venus in the Search for Alien Life
- April 24, 2024 | NASA Astronauts Enter Quarantine As Boeing Starliner Test Flight Approaches
- April 23, 2024 | Peeking Inside Protons: Supercomputers Reveal Quark Secrets
- April 23, 2024 | A Cheaper and More Sustainable Lithium Battery: How LiDFOB Could Change Everything
Muscle News
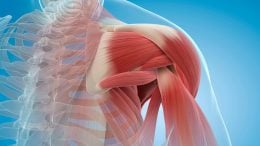
Health April 20, 2024
Revolutionary Study Reveals Why Our Muscles Weaken With Age
The first detailed cell atlas of aging human muscle uncovers the complex genetic and cellular activities involved in muscle degeneration and identifies ways to mitigate…
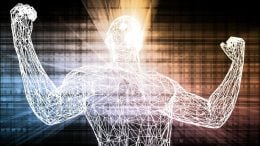
Health March 23, 2024
Natural Ingredient Found in Coffee Could Keep Your Muscles Young
A research consortium led by Nestlé Research in Switzerland and the Yong Loo Lin School of Medicine, National University of Singapore (NUS Medicine) made a…
Health March 1, 2024
Surprising Diet Change Boosts Muscle Strength and Slows Aging
NIH study suggests a small reduction in daily calories is beneficial for wellness. Reducing overall calorie intake may rejuvenate your muscles and activate biological pathways…
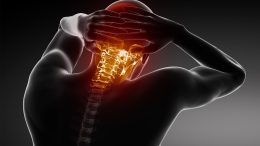
Health January 27, 2024
Neck Inflammation: The Hidden Culprit Behind Common Headaches
Researchers presenting at the annual meeting of the Radiological Society of North America (RSNA) have discovered objective evidence showing the involvement of neck muscles in…
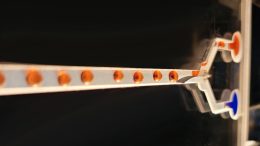
Technology January 26, 2024
Scientists Develop Artificial Muscle Device That Produces Force 34 Times Its Weight
Soft robots, medical devices, and wearable devices are now common in our daily routines. Researchers at KAIST have created a fluid switch that employs ionic…
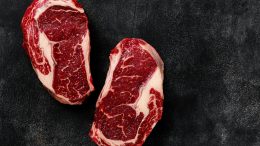
Health January 20, 2024
Meat vs. Plant: New Research Reveals a Clear Winner for Muscle Growth
Evidence contributes to the expanding body of research that indicates protein food sources are essential for building and maintaining muscle. Extensive studies have demonstrated that…
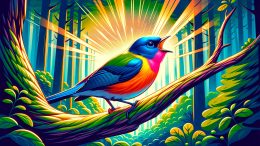
Science December 12, 2023
Melodic Muscles: The Impact of Daily Singing on Songbird Attractiveness
Research on songbirds demonstrates the critical need for daily singing to maintain vocal muscle strength and attractiveness, with implications for human vocal training and speech…
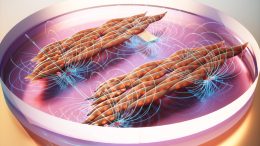
Biology November 14, 2023
Muscle Mat Magic: MIT Engineers a Hydrogel Gym for Cellular Fitness
The vibrating platform could be useful for growing artificial muscles to power soft robots and testing therapies for neuromuscular diseases. There’s no doubt that exercise…
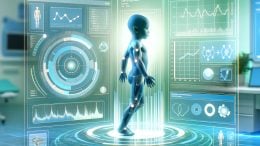
Health November 9, 2023
A New Frontier in Pediatric Care: AI-Driven Growth Charts for Muscle Mass
An analysis of MRI scans led by Brigham researchers using artificial intelligence resulted in the production of a reference growth standard and a fast, reproducible…
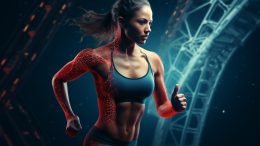
Biology October 30, 2023
Scientists Shed New Light on How Endurance Training Transforms Your Muscles
The more we exercise our endurance, the fitter we become – and so do our muscles. They adapt to the load and are able to…
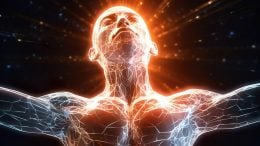
Health October 2, 2023
The Magic Number – New Research Sheds Light on How Often You Need To Exercise To Make It Worth It
A recent study provides insight into the frequency of exercise necessary to reap its benefits. Interested in the health advantages of exercise but not eager…
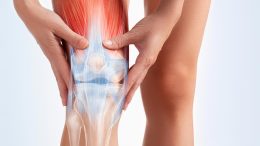
Health September 23, 2023
Muscling Through “Runner’s Knee” Myths: New Research Sheds Light on Chronic Knee Pain
The new study finds personalized rehabilitation may be needed to effectively address chronic knee pain. Neal Glaviano, assistant professor of kinesiology in the University of…
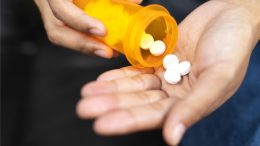
Health September 17, 2023
Researchers Discover Surprising Side Effect of Common Diabetes Drug
Diabetes might not be the first thing that comes to mind when considering muscle function. However, a widely used diabetes drug that controls blood sugar…
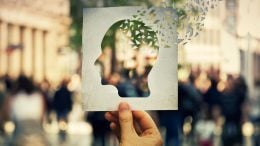
Health September 7, 2023
New Research: High Levels of Lean Muscle Might Protect Against Alzheimer’s Disease
Additional research is required to elucidate the potential biological pathways, according to the authors of the study. High levels of lean muscle could protect against…
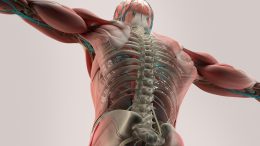
Health August 18, 2023
Unmasking the Long COVID Mystery: New Study Reveals Cause of Muscle Weakness
New research paves the way for new strategies to address long-term COVID-19 complications. Researchers from the University of Malta have identified a possible cause for…
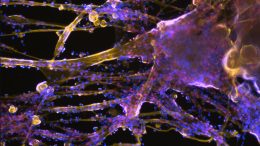
Biology August 17, 2023
Lab-Grown Muscles Breakthrough: The Future of Medicine and Meat
Professor Ori Bar-Nur and his colleagues at ETH Zurich are pioneering the cultivation of muscle cells in the lab, currently using mouse cells as their…
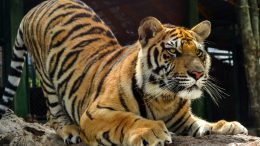
Science August 2, 2023
Big Paws, Bigger Problems: Why You Shouldn’t Declaw Tigers or Other Big Cats
A study shows that declawing significantly impacts the muscular capabilities of large cat species more than smaller cats. Despite it being illegal to surgically modify…
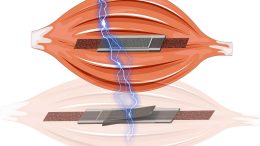
Technology July 17, 2023
Bionic Breakthrough: Revolutionary Self-Sensing Electric Artificial Muscles
Queen Mary University researchers have engineered a self-sensing, variable-stiffness artificial muscle that mimics natural muscle characteristics. The breakthrough has significant implications for soft robotics and…

An official website of the United States government
The .gov means it’s official. Federal government websites often end in .gov or .mil. Before sharing sensitive information, make sure you’re on a federal government site.
The site is secure. The https:// ensures that you are connecting to the official website and that any information you provide is encrypted and transmitted securely.
- Publications
- Account settings
Preview improvements coming to the PMC website in October 2024. Learn More or Try it out now .
- Advanced Search
- Journal List

Dietary Protein and Muscle Mass: Translating Science to Application and Health Benefit
John w. carbone.
1 School of Health Sciences, Eastern Michigan University, Ypsilanti, MI 48197, USA
Stefan M. Pasiakos
2 Military Nutrition Division, U.S. Army Research Institute of Environmental Medicine (USARIEM), Natick, MA 01760, USA; [email protected]
Adequate consumption of dietary protein is critical for the maintenance of optimal health during normal growth and aging. The current Recommended Dietary Allowance (RDA) for protein is defined as the minimum amount required to prevent lean body mass loss, but is often misrepresented and misinterpreted as a recommended optimal intake. Over the past two decades, the potential muscle-related benefits achieved by consuming higher-protein diets have become increasingly clear. Despite greater awareness of how higher-protein diets might be advantageous for muscle mass, actual dietary patterns, particularly as they pertain to protein, have remained relatively unchanged in American adults. This lack of change may, in part, result from confusion over the purported detrimental effects of higher-protein diets. This manuscript will highlight common perceptions and benefits of dietary protein on muscle mass, address misperceptions related to higher-protein diets, and comment on the translation of academic advances to real-life application and health benefit. Given the vast research evidence supporting the positive effects of dietary protein intake on optimal health, we encourage critical evaluation of current protein intake recommendations and responsible representation and application of the RDA as a minimum protein requirement rather than one determined to optimally meet the needs of the population.
1. Introduction
Consuming adequate dietary protein is critical for maintaining optimal health, growth, development, and function throughout life. Dietary protein requirements in healthy adults (≥19 years old) are dictated largely by body mass and lean body mass, as well as net energy balance and physical activity [ 1 ]. The Institute of Medicine (IOM) established the current Dietary Reference Intakes (DRIs) for protein in 2005, including the Estimated Average Requirement (EAR), Recommended Dietary Allowance (RDA), and the Acceptable Macronutrient Distribution Range (AMDR) [ 2 ]. The EAR for protein is 0.66 g per kg body mass per day (g/kg/d) and is defined as the minimum amount of protein expected to meet the individual indispensable amino acid requirements of 50% of the U.S. adult population. The RDA, however, is 0.8 g/kg/d, and reflects the minimum amount of dietary protein required to meet indispensable amino acid requirements, establish nitrogen balance, and prevent muscle mass loss for nearly the entire (i.e., 97.5%) U.S. adult population [ 2 , 3 ]. The RDA for American adults is similar to international adult protein recommendations established by the World Health Organization (0.83 g/kg/d) [ 4 ]. The current protein RDA, however, is often incorrectly applied when used as the definition of recommended intake, rather than its true designation as the required minimum intake. This misapplication is problematic for healthy populations and aging adults, and disadvantageous for those with pathophysiological conditions that would necessitate higher-protein needs.
Over the past decade, the potential muscle-related benefits achieved by consuming higher-protein diets (i.e., > RDA but within the AMDR) have become increasingly clear. Increased protein intake contributes to greater strength and muscle mass gains when coupled with resistance exercise [ 5 ], allows for greater muscle mass preservation when consumed during periods of negative energy balance [ 6 ], limits age-related muscle loss [ 7 ], and, to a lesser extent, provides a greater muscle protein synthetic response when evenly distributed across meals [ 5 , 8 ]. A prospective, cross-sectional analysis of the National Health and Nutrition Examination Survey (NHANES) database demonstrates inverse associations between animal and plant protein intake and waist circumference, body weight, and body mass index (BMI) [ 9 ]. Advances in this field of nutritional science have translated to a greater emphasis on higher-protein diets, protein quality, and supplemental protein in peer-reviewed literature, lay media, and the commercial food market. Despite greater awareness of how higher-protein diets might be advantageous for muscle mass, actual dietary patterns, particularly as they pertain to protein, have remained relatively unchanged in American adults as a whole [ 10 ]. The disparity between knowledge and action raises the question of whether this expanded understanding of dietary protein is truly meaningful if scientific data are not translated and ultimately reflected in nutrition guidance and, more importantly, in what people eat. As such, the purpose of this brief communication is to highlight common perceptions and benefits of dietary protein on muscle mass, to address misperceptions related to higher-protein diets, and to comment on the translation of academic advances to real-life application and health benefit.
2. Dietary Protein and Muscle Mass Perceptions
Skeletal muscle protein is dynamic and in constant flux, alternating between states of negative (i.e., muscle protein synthesis < muscle protein breakdown) and positive (i.e., muscle protein synthesis > muscle protein breakdown) protein balance, largely in response to fasting (i.e., postabsorptive) and feeding (i.e., postprandial), respectively. In the postabsorptive state, muscle protein serves as the primary repository of amino acids that is readily catabolized to release free amino acids that can be reincorporated into muscle protein or used to support other critical physiological needs, including serving as an energy substrate through carbon skeleton oxidation, as well as by providing gluconeogenic precursors to support euglycemia ( Figure 1 ). In addition, free amino acids derived from muscle protein breakdown are used in the synthesis of immune system components, plasma proteins, peptide hormones, and intra- and extracellular enzymes. Transient periods of negative protein balance in healthy adults are completely normal and reversed by feeding. The magnitude of the postprandial stimulation of muscle protein synthesis, suppression of muscle (and whole-body) protein breakdown, and shift to a positive protein balance is mediated by dietary protein content, protein quality (i.e., based on an individual protein’s digestibility and absorption kinetics, and abundance of indispensable amino acids), and the format in which protein is consumed (e.g., mixed-macronutrient meal, isolated supplemental intact protein, or free-form amino acids) [ 6 ]. The collective optimization of these protein intake-related factors can potentiate the beneficial effects of other protein kinetic stimuli, such as the mechanical and metabolic effects of resistance and aerobic exercise, respectively, resulting in the enhanced remodeling and repair of existing muscle proteins and synthesis of new muscle protein, providing the conditions for muscle maintenance and growth [ 11 ].

Energy and/or dietary protein restriction induce net muscle catabolism, releasing amino acids for energy production, gluconeogenesis, and synthesis of peptide hormones, plasma proteins, immune system components, and enzymes (representative examples, not an exhaustive list; not drawn to scale). AA, amino acids; ADH, antidiuretic hormone; hGH, human growth hormone; T3, triiodothyronine; T4, thyroxine.
2.1. Current Dietary Protein Recommendations
The current DRIs for protein have been in place since 2005 but are not without limitations. The EAR and RDA were derived from meta-analyses of nitrogen balance studies [ 12 ]. The nitrogen balance method has many limitations and tends to overestimate nitrogen intake (via diet) and underestimate nitrogen excretion (via urine, feces, sweat, and integumental loss), thus falsely illustrating nitrogen balance [ 13 ]. Nitrogen balance is also considered a crude measure that fails to provide any information as to what occurs within the system to modulate the body nitrogen pool and subsequent balance [ 14 , 15 ]. Likewise, the AMDR for protein (10–35% of total daily energy intake) was established by setting the lower end of the AMDR at the relative amount of protein believed to meet the set RDA of 0.8 g/kg/d, while the upper end is the mathematical difference achieved if carbohydrate (45–65% of energy) and fat (20–35% of energy) are consumed at the lower ends of their respective AMDR (i.e., 100% − 45% − 20% = 35% as the upper end of protein AMDR) [ 2 ]. Carbohydrate and fat are important energy substrates and energy balance is critical to optimal health, but this derivation raises uncertainty about the physiological relevance underlying a recommended upper limit for protein consumption at 35% of total energy intake.
Similarly, the RDA may be sufficient to meet the dietary protein needs of healthy, relatively sedentary young adults, though investigators have argued that this recommendation should be reconsidered based on data from studies demonstrating the inadequacy of the RDA within certain populations when compared to greater requirements derived from the indicator amino acid oxidation method [ 16 ]. Accordingly, internationally recognized professional organizations recommend protein intakes on the order of double the current RDA for physically active individuals, including the joint recommendation to consume protein between 1.2–2.0 g/kg/d established by the Academy of Nutrition and Dietetics, Dietitians of Canada, and the American College of Sports Medicine [ 17 ]. The International Society for Sports Nutrition also recommends protein intake at levels higher than the RDA for physically active individuals (1.4–2.0 g/kg/d) [ 1 ]. The definition of the protein RDA itself draws criticism given that it reflects the minimal amount of protein required to prevent deficiency, rather than an amount which may allow for optimal health. The AMDR does provide for more flexibility in dietary protein intake recommendations in the context of the complete diet, yet most American adults habitually consume protein on the lower end of this range (i.e., 14–16% of total energy intake) [ 10 ].
2.2. Dietary Protein and Physical Activity
The benefits of consuming protein following resistance exercise training have been well-documented, especially as they relate to muscle hypertrophy and function [ 18 ]. A recent meta-analysis showed significant positive associations between coupling resistance exercise with post-exercise protein ingestion and total fat-free mass, strength, as measured by one-repetition maximum, and muscle size, as measured by myofiber cross-sectional area [ 18 ]. The type and volume of exercise plays a determining role in muscle protein synthetic responses to post-exercise protein ingestion [ 19 , 20 ], as does age [ 21 ] and the training experience [ 18 ] of the individual. The type of protein consumed also factors into the net anabolic response, given that postprandial muscle protein and whole-body protein kinetic responses to free-form amino acids, isolated intact proteins, and mixed-macronutrient meals all differ [ 22 , 23 , 24 ]. As reflected in sports nutrition recommendations [ 1 , 17 ], holistic evaluation of varied experimental designs suggests that coupling post-resistance exercise protein ingestion (~20–30 g or 0.25–0.30 g/kg) with habitual protein intakes at ~1.6 g/kg/d promotes favorable muscle adaptations to exercise training [ 18 ].
2.3. Dietary Protein during Energy Deficit
Consuming higher amounts of protein during typical moderate energy-deficient weight loss diets (i.e., 500–750 kcal/d deficit [ 25 ]) preserves muscle mass in an otherwise catabolic physiological environment [ 6 ]. However, the protective effect of higher-protein diets on muscle and whole-body protein homeostasis is compromised as the severity of energy deficit increases beyond 40% of daily energy needs, as a greater proportion of dietary amino acids are oxidized for energy production, thereby minimizing amino acid availability to support protein balance [ 26 ] ( Figure 1 ). However, most adults rarely experience acute or sustained periods of severe energy deficit, except for perhaps acute fasting for religious reasons, poorly-constructed drastic weight loss plans, preparation and/or recovery from bariatric surgery, or scenarios where food availability is severely limited (e.g., victims of natural disasters, emergency responders, etc.). Regardless of the cause, these periods of severe energy deficit usually manifest only for short durations (e.g., 1–3 days) and, therefore, are likely physiologically tolerable. However, if energy expenditures are high and dietary energy and protein intake are limited for extended periods of time, for example during sustained, multi-stressor military operations [ 27 , 28 ], the consequences of severe energy deficit are much more problematic, especially if body mass and fat-free mass loss are so severe that immune system and muscle function and performance are compromised [ 29 , 30 , 31 ]. During those conditions, prioritizing energy intake, more so than focusing solely on protein per se, is vitally important to help prevent excessive muscle catabolism and conserve muscle function and performance. With moderate energy deficit, however, protein intakes on the order of double the current RDA (i.e., 1.6 g/kg/d) have proved efficacious in preserving muscle mass during weight loss [ 6 ].
2.4. Pathophysiological Conditions
Inadequate dietary protein intake challenges muscle and whole-body protein balance (i.e., protein synthesis = protein breakdown), negatively impacting muscle mass, function, adaptations to exercise, bone and calcium homeostasis, immune system response, fluid and electrolyte balance, enzyme production and activity, and hormone synthesis. In the absence of sufficient dietary protein intake, muscle is catabolized to provide amino acids to allow for continued endogenous protein synthesis in critical physiological tissues and organs [ 32 ] ( Figure 1 ). Certain pathophysiological conditions, such as burns [ 33 ], chronic obstructive pulmonary disease (COPD) [ 34 ], human immunodeficiency virus/acquired immunodeficiency syndrome (HIV/AIDS) [ 35 ], cancer [ 36 ], and sepsis [ 37 ], also challenge protein homeostasis, albeit the etiology and mechanisms for disrupted protein balance are generally much different from those in healthy adults [ 38 ]. Nevertheless, these conditions often induce muscle wasting, suggesting that greater dietary protein intakes may be warranted, with specific recommendations based on the individual patient and clinical scenario.
While much focus has been placed on adult protein needs in the context of these disease states, the potential benefits of higher-protein intakes extend across the lifespan. Muscle loss and failure-to-thrive are particularly worrisome in the pediatric population, a time typically characterized by rapid growth and development. Recent meta-analysis shows that higher protein intakes in critically ill pediatric patients are associated with positive protein balance, improved clinical outcomes, and lower mortality [ 39 ]. These effects manifest at intakes above 1.1 g/kg/d and become more prominent when protein intakes exceed 1.5 g/kg/d. Similarly, unintentional weight loss and decrements in muscle mass in the elderly are predictive of morbidity and mortality, particularly in institutionalized populations [ 40 , 41 , 42 ]. Provision of dietary protein at or above 1.2 g/kg/d is associated with reductions in unintentional weight loss [ 43 ]. Dietary protein supplementation, bringing daily protein intake to 1.5 g/kg, may also be beneficial in mitigating the detrimental body composition changes and muscle mass and function losses associated with sarcopenia [ 44 ]. Similarly, consideration should be made for the timing and method of delivery, with isolated, intact proteins providing for a greater anabolic response than mixed meals [ 45 ]. While advanced age does limit the postprandial anabolic response typically observed subsequent to protein feeding [ 46 , 47 ], regular intakes above the current protein RDA and consumption of at least 0.4 g/kg (i.e., 0.6 g/kg lean body mass) high quality protein at each meal appear to be critical contributors to preservation of muscle mass and strength that may limit frailty in older populations [ 42 , 46 , 48 ]. There is also some evidence to support the notion that even higher protein intakes (e.g., 70 g per meal) may be of benefit, in terms of suppressing whole-body proteolysis and enhancing net protein balance [ 49 , 50 ].
3. Protein Misconceptions and Reality
While the popularity of dietary protein has increased over the past decade or longer, largely because of its role in muscle health, there are still some in the media, clinical practice, and within academia [ 51 ] that perpetuate certain risks associated with the protein content of balanced mixed-diets for healthy adults. Common criticisms of greater protein intakes, or with the types of foods dietary protein is derived from, include the potential for detrimental effects of protein on bone, renal function, low-grade inflammation, cardiometabolic disease, and cancer risk. These concerns are generally unfounded with regard to the protein content of the diet and are antithetical to contemporary published data [ 52 , 53 , 54 , 55 , 56 , 57 , 58 , 59 ]. Their persistence, however, and the mislabeling of health detriments to protein itself, as opposed to the whole foods which contribute protein to the overall diet, may underlie lower dietary intakes and thereby contribute to suboptimal muscle integrity. While these associations have been corrected in the scientific literature, other concerns do warrant thoughtful consideration and should be debated in the context of whole foods rather than just ascribing protein foods to labels of “non-dairy animal,” “dairy,” and “plant,” without consideration of the other nutrients these foods provide and their possible links to health and disease [ 60 ].
Observations of hypercalciuria from nearly a century ago in those consuming greater amounts of meat raised concerns that higher protein intakes resulted in increased bone resorption and, therefore, diminished bone mineral density [ 61 ]. It was later hypothesized that greater intakes of sulfur-containing amino acids induce an acidemia that leads to increased bone resorption and calcium release from bone as a compensatory mechanism to buffer reductions in pH secondary to higher protein intake [ 2 ]. More recent data suggest that this conclusion is false, as well-controlled studies using stable isotope tracer techniques to assess calcium absorption have shown that the observed hypercalciuria secondary to higher protein intake results from increased calcium bioavailability and greater intestinal calcium absorption potentiated by protein [ 62 , 63 ]. Data from NHANES show that dietary acid load and bone mineral density are not related in adults who consume adequate calcium [ 64 ]. In fact, higher protein diets may actually protect against osteoporosis, in part, as a result of the increased hepatic release of insulin-like growth factor 1 (IGF-1) [ 65 ]. A recent meta-analysis demonstrated that those with the highest protein intakes had significantly lower hip fracture incidence relative to those with the lowest protein intakes, supporting the assertion that increased dietary protein intake may be protective and serve a critical role in accruing and maintaining bone mineral density [ 66 ]. The National Osteoporosis Foundation recognizes the potential benefit of dietary protein on bone, while advocating for continued research, particularly the execution of randomized controlled trials that account for dietary calcium intake [ 67 ].
Higher-protein diets have also been labeled as damaging to the kidneys. Increased amino acid intake can potentiate renal workload and should be reduced in the presence of established renal disease. However, otherwise healthy kidneys are well-capable of adapting to protein intakes above the RDA and within the AMDR. The kidneys are faced with increased nitrogenous waste as more amino acids are oxidized for energy and/or directed towards gluconeogenesis as the relative percentage of energy intake derived from protein increases. In a recent evaluation of NHANES data, protein intake was directly associated with blood urea nitrogen (BUN) concentrations, but those in the highest decile for protein intake (~1.4 g/kg/d) still exhibited normal BUN (14.8 ± 0.3; reference range, 7–20 mg/dL) [ 9 ]. This finding held true across non-dairy animal, animal, and plant protein sources and neither glomerular filtration rate (GFR) nor blood creatinine concentrations were related to dietary protein content. Similarly, a recent meta-analysis of randomized controlled trials with dietary protein interventions showed a small but positive relationship between higher protein intakes (i.e., ≥ 1.5 g/kg/d or ≥ 20% energy intake) and GFR [ 52 ]. Overall, current available data suggest higher-protein diets do increase renal workload, but they do not negatively impact kidney health nor increase the risk of developing chronic kidney disease in healthy adults.
Recent studies have raised concerns about the potential for higher-protein intakes to increase systemic inflammation. One large-scale investigation showed that those in the greatest high-sensitivity C-reactive protein (hs-CRP) serum concentration quartile also had higher relative protein intakes than the lowest hs-CRP quartile [ 68 ]. However, the mean differences in absolute and energy-adjusted protein intakes between the highest and lowest hs-CRP quartiles were only 1.0 and 1.2 g/d, respectively. Similarly, a large controlled dietary intervention study showed greater reductions in hs-CRP with lower-protein intakes (i.e., 10–15% vs. 23–28% total energy intake), although this protein-based difference was observed only in conjunction with a high-glycemic index diet and not with a low-glycemic index background [ 69 ]. In contrast, an analysis of the Framingham Heart Study Offspring Cohort shows an inverse association between dietary protein intake and inflammation and oxidative stress scores, derived from measures of nine inflammatory biomarkers [ 59 ]. This potential beneficial effect was observed for higher total and animal protein intakes but was even more pronounced with higher plant protein intakes.
Concern has also been raised regarding potential connections between dietary protein intake and risk of cardiometabolic disease and cancer. These associations are typically confounded by misrepresentation of foods labeled as “protein-rich” which may, by their nature, be overall less-healthful nutrient-sparse food options, providing high amounts of total and saturated fats and processing additives (e.g., nitrates, nitrites, sodium) [ 60 ]. To the best of our knowledge, there are no data demonstrating a well-defined association between dietary protein itself and cardiovascular disease [ 70 , 71 ] or type 2 diabetes mellitus [ 56 ]. Similarly, methionine restriction (e.g., vegan dietary pattern) may be a viable approach to limit carcinogenic processes and tumor growth [ 72 , 73 ], yet meta-analyses show no link between overall dietary protein intake and incidence of colorectal [ 57 ] or breast [ 58 ] cancers. Higher protein intakes may, however, exert a protective effect on post-diagnosis survival [ 74 ]. A greater emphasis must be placed on dietary protein consumption in the context of overall nutrient-dense, healthy food choices when considering relations to health and disease, as the aforementioned potential connections are influenced heavily by food item quality more so than macronutrient profile [ 55 , 75 ].
4. Translation and Application
An assessment of national dietary patterns shows that protein food intakes have remained relatively unchanged over the past decade (i.e., 5.79 ounce equivalents (2005–2006), 5.58 (2007–2008), 5.74 (2009–2010), 5.70 (2011–2012), 5.83 (2013–2014), 5.80 (2015–2016)), as intake data for the latest 2-year cycle are nearly identical to those from 10 years earlier [ 76 , 77 , 78 , 79 , 80 , 81 ]. This static intake pattern may relate to the regular presentation of recommended protein intakes in a g/d format [ 82 , 83 ], calculated from anthropometrics assumed when the RDA was crafted in 2005 (i.e., 70 kg male, 57 kg female [ 2 ]), despite the fact that contemporary measures are significantly different (i.e., 90 kg average male, 77 kg average female [ 84 ]). A present-day, moderately physically active, average adult male, consuming protein at the RDA, would have an intake below the low end of the AMDR while maintaining energy balance. In reality, most American adults consume ~14–16% of total energy as protein (1.0–1.5 g/kg/d, depending on age and sex) [ 10 ]; an amount greater than the current RDA, but supported as beneficial to muscle and overall health by contemporary research. In fact, the Healthy Vegetarian, Healthy Mediterranean-Style, and Healthy U.S.-Style eating patterns promoted in the 2015 Dietary Guidelines for Americans equate to protein intakes 1.55-, 1.94-, and 1.98-fold greater than the current RDA, respectively (theoretical 19–50 year old female consuming 2000 kcal/d) [ 85 ]. If the American adult population, as a whole, consumed protein at approximately 1.6 g/kg/d, as advocated in a recent review from one of the more prominent laboratory groups in this field [ 5 ], this would still represent an approximate 17–19% of total energy intake, certainly reasonable based on the current AMDR for protein. Indeed, even increasing to 2.5–3.0 g/kg/d would still fall within the 10–35% of total energy from protein suggested by the AMDR and would provide ample opportunity to optimize muscle health.
In addition to the dietary protein and skeletal muscle considerations, the protein leverage hypothesis suggests that protein under-consumption increases appetite drive in an effort to ensure sufficient amino acid intake [ 86 ]. The unfortunate effect of this response in the absence of increased protein intake is excess energy consumption and resultant positive energy balance. The fact that over 120 million Americans have some type of cardiovascular disease [ 87 ], over 29 million are believed to have type 2 diabetes mellitus [ 88 ], and approximately 5–7% of the young adult population meets the diagnostic criteria for metabolic syndrome [ 89 ] illustrates the need to change how we structure feeding recommendations and encourage compliance with dietary guidance. Improper application of the protein RDA in federal policy, which informs institutional feeding practices, can result in dietary protein intakes that may be sub-optimal for muscle growth and preservation and overall health. As an example, the National School Lunch Act (Section 17(o)(1)) requires that participating programs provide “approximately one-third of the daily recommended dietary allowance” [ 90 ]. In practice, this means that American school children are required to be provided with one-third of the minimum amount of dietary protein needed to prevent dysfunction, rather than one-third of the amount which may best support muscle mass, growth, and health. Given the relative cost of protein-rich foods and concerns for cost-efficiency amongst school breakfast and lunch program administrators [ 91 ], it is reasonable to expect that protein offerings are reflective of the minimum requirement, rather than robust provision for optimal health.
Certainly, food choices within macronutrient recommendations are critical, with a needed emphasis on nutrient-dense selections [ 92 ]. Similarly, we recognize the traditional association between higher-protein diets and higher meat consumption and the much-needed focus on sustainability and the potential environmental impact of our food sources. With these in mind, we strongly encourage the reevaluation of macronutrient recommendations to best reflect high quality science, basing them on experimental studies over observational data [ 93 ]. Implementing reliable macronutrient recommendations for both healthy and diseased populations at all stages of the lifecycle, which engender consumer confidence, can then be followed by greater emphasis on quality food choices within those guidelines. Such action would allow for dietary protein recommendations, and resultant public health policy, best designed to provide for muscle accretion, quality, and preservation throughout the lifespan. A realignment of macronutrient intake recommendations with contemporary research findings would create the foundation for advances in public health.
Acknowledgments
The authors like to thank Jillian Allen for her assistance with manuscript development.
Author Contributions
J.W.C. and S.M.P. conceptualized, wrote, reviewed, and approved this manuscript.
This research received no external funding.
Conflicts of Interest
The authors declare no conflict of interest. The opinions or assertions contained herein are the private views of the authors and are not to be construed as official or as reflecting the views of the Army or the Department of Defense. Any citations of commercial organizations and trade names in this report do not constitute an official Department of the Army endorsement of approval of the products or services of these organizations.
References and Notes
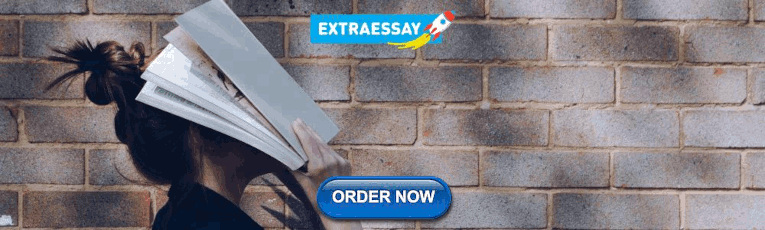
IMAGES
VIDEO
COMMENTS
1. Introduction. Resistance training (RT) is a primary exercise intervention used to develop strength and stimulate muscle hypertrophy. Increases in muscle mass constitute key components of conditioning in various sports due to the correlation between muscle cross-sectional area and muscle strength [1,2].Additionally, an increase in muscle mass is one of the goals of bodybuilding [], and many ...
1. Introduction. Muscle growth involves increasing muscular size, typically through strength training and protein supplementation [].Dietary protein intake supports skeletal muscle remodeling after exercise by stimulating muscle protein synthesis and can optimize resistance training-mediated increases in skeletal muscle size and strength [].Muscle growth has been an important issue in the ...
Using genetic approaches, researchers have demonstrated how a certain protein is involved in skeletal muscle growth. The findings open new avenues to develop drug targets for neuromuscular ...
LA JOLLA—One of the many effects of aging is loss of muscle mass, which contributes to disability in older people. To counter this loss, scientists at the Salk Institute are studying ways to accelerate the regeneration of muscle tissue, using a combination of molecular compounds that are commonly used in stem-cell research.
Oct. 15, 2019 — A research team found muscle cells treated with statins released the amino acid called glutamate at much higher levels than muscle cells that were untreated. As glutamate is a ...
Summary: MXene nanoparticle scaffolds have been shown to stimulate muscle growth, making them a promising option to treat muscle loss and damage. Now, researchers explain the molecular mechanisms ...
We performed a systematic review, meta-analysis, and meta-regression to determine if increasing daily protein ingestion contributes to gaining lean body mass (LBM), muscle strength, and physical/functional test performance in healthy subjects. A protocol for the present study was registered (PROSPER …
Team advances research on muscle health. Date: January 19, 2022. Source: University of Ottawa. Summary: Researchers have published findings that could contribute to future therapeutics for muscle ...
By Salk Institute May 29, 2021. Salk Institute scientists are studying ways to accelerate the regeneration of muscle tissue, using a combination of molecular compounds that are often used in stem-cell research. Salk Institute research reveals clues about molecular changes underlying muscle loss tied to aging. One of the many effects of aging is ...
Support for the repetition continuum is derived from the seminal work of DeLorme [], who proposed that high-load resistance exercise enhances muscle strength/power while low-resistance exercise improves muscular endurance, and that these loading zones are incapable of eliciting adaptations achieved by the other.Subsequent research by Anderson and Kearney from 1982 [] and Stone et al., 1994 ...
Therefore, the present special issue provides important contributions to research and clinical endeavors that aim to maintain muscle mass and function with age. The growth of new muscle and improvements in functionality (e.g., increased strength) are prime goals of many active individuals and especially athletes.
Skeletal muscle is the protein reservoir of our body and an important regulator of glucose and lipid homeostasis. Consequently, the growth or the loss of muscle mass can influence general ...
In fact, researchers observed a 47% higher muscle protein synthesis rate following consumption of the omnivorous meal with lean beef, compared with the whole food vegan meal that provided an equal amount of protein from plants. "While studies have previously assessed the impact of consuming isolated proteins, this research aims to mirror a ...
Muscle articles from across Nature Portfolio. Muscle is a soft tissue composed of elongated cells (muscle fibres) that produce force and motion through contraction. Involuntary cardiac and smooth ...
Creatine supplementation is the most popular ergonomic aid for athletes in recent years and is used for improving sport performance and muscle growth. However, creatine supplementation is not always effective in all populations. To address these discrepancies, numerous studies have examined the use of creatine supplementation for muscle growth. This scoping review aimed to investigate the ...
The establishment of the postnatal skeletal muscle stem cell pool is not well understood. Here the authors show a requirement for Nf1 to coordinate muscle fiber growth and stem cell quiescence ...
In this example, you'll take four seconds to lower the weight. 1: The second number is for the moment at the bottom of the rep. In this case, one second. 1: This is for the concentric (or ...
For cancer patients, maintaining muscle is vital to health and treatment, but staying strong is complicated. Meghan McCue, Laurentian University. Cachexia — significant loss of weight and muscle ...
Increased longevity demands new approaches to promote healthy ageing. Owing to its connections with other body tissues, the skeletal muscle is a major determinant of systemic health 1,2 ...
Here is what the latest research says. One 2020 meta-analysis published in the journal Nutrition Reviews found that protein intakes ranging from 0.5 to 3.5 g per kg of body weight can support ...
a) Volume: research has reported a graded dose-response relationship between resistance training weekly volume and muscle growth. Therefore, it would be recommended to prescribe 2-3 sets per exercise, covering at least 10 weekly sets for each muscle group, while greater weekly volume does not seem to offer additional hypertrophy benefits.
Debunking Muscle Growth Myths: Insights from New Research Summary. A recent review has debunked some of the biggest muscle growth myths. It challenges traditional beliefs about slow and controlled reps for optimal muscle growth; the review suggests that muscle growth can occur over a wide range of repetition durations (0.5 to 8 seconds) and that the tempo of repetitions, specifically the ...
Regenerating damaged tissues or organs has been a dream of scientists for decades. Now, researchers at the FMI and Novartis Biomedical Research have discovered a new molecule that activates a ...
Here are the study's main takeaways: There appears to be no identifiable "cap" to the amount of protein your body can utilize if you extend the time frame long enough. Even 100 grams of ...
Meat vs. Plant: New Research Reveals a Clear Winner for Muscle Growth Evidence contributes to the expanding body of research that indicates protein food sources are essential for building and maintaining muscle.
This is the long-held belief that: Training with low reps, for example a powerlifting type routine, is the best for increasing strength but not the best for adding size. Training with high reps on the other hand is best for muscular endurance and again not the best for size. As a result, there exists a hypertrophy rep range of roughly 6-12 reps ...
The EAR for protein is 0.66 g per kg body mass per day (g/kg/d) and is defined as the minimum amount of protein expected to meet the individual indispensable amino acid requirements of 50% of the U.S. adult population. The RDA, however, is 0.8 g/kg/d, and reflects the minimum amount of dietary protein required to meet indispensable amino acid ...