Research Design Review
A discussion of qualitative & quantitative research design, generalizability in case study research.
Portions of the following article are modified excerpts from Applied Qualitative Research Design: A Total Quality Framework Approach (Roller & Lavrakas, 2015, pp. 307-326)

One of the controversies associated with case study research designs centers on “generalization” and the extent to which the data can explain phenomena or situations outside and beyond the specific scope of a particular study. On the one hand, there are researchers such as Yin (2014) who espouse “analytical generalization” whereby the researcher compares (or “generalizes”) case study data to existing theory 1 . From Yin’s perspective, case study research is driven by the need to develop or test theory, giving single- as well as multiple-case study research explanatory powers — “Some of the best and most famous case studies have been explanatory case studies” (Yin, 2014, p. 7).
Diane Vaughan’s research is a case study referenced by Yin (2014) as an example of a single-case research design that resulted in outcomes that provided broader implications (i.e., “generalized”) to similar contexts outside the case. In both The Challenger Launch Decision: Risky Technology, Culture, and Deviance at NASA (1996) and “The Trickle-Down Effect: Policy Decisions, Risky Work, and the Challenger Tragedy” (1997), Vaughan describes the findings and conclusions from her study of the circumstances that led to the Challenger disaster in 1986. By way of scrutinizing archival documents and conducting interviews, Vaughan “reconstructed the history of decision making” and ultimately discovered “an incremental descent into poor judgment” (1996, p. xiii). More broadly, Vaughan used this study to illustrate “how deviance in organizations is transformed into acceptable behavior,” asserting, for example, that “administrators in offices removed from the hands-on risky work are easily beguiled by the myth of infallibility” (1997, p. 97).
In contrast to Yin (2014), there are researchers such as Stake (1995), who believes that the purpose of case study research is “particularization, not generalization” (p. 8), and Thomas (2010), who rejects the concept of theoretical generalizability in case study research, believing instead that “the goal of social scientific endeavor, particularly in the study of cases, should be exemplary knowledge . . . that can come from [the] case . . . rather than [from] its generalizability” (p. 576). Thomas goes further in asserting that simply attempting to generalize case study data will have the detrimental effect of dampening the researcher’s “curiosity and interpretation” of the outcomes.
So, the prospective case study researcher is left with somewhat of a dilemma:
- Is my goal to generalize my case study to some greater theory?
- Is my goal to envelop myself in this particular case in order to find in-depth meaning and derive valid interpretations of the data for this case, and not to apply my results to a preconceived theory?
- Or do I want to strike some kind of balance and focus my analysis on “both the emergent theory that is the research objective and the rich empirical evidence that supports the theory” (Eisenhardt & Graebner, 2007, p. 29)?
1 Smith (2018) provides a broader discussion of analytical generalization along with three other types of generalizability in qualitative research, i.e., naturalistic, transferable, and intersectional.
Eisenhardt, K. M., & Graebner, M. E. (2007). Theory building from cases: Opportunities and challenges. Academy of Management Journal , 50 (1), 25–32. https://doi.org/10.5465/AMJ.2007.24160888
Smith, B. (2018). Generalizability in qualitative research: misunderstandings, opportunities and recommendations for the sport and exercise sciences. Qualitative Research in Sport, Exercise and Health , 10 (1), 137–149. https://doi.org/10.1080/2159676X.2017.1393221
Stake, R. E. (1995). The art of case study research . Thousand Oaks, CA: Sage Publications.
Thomas, G. (2010). Doing case study: Abduction not induction, phronesis not theory. Qualitative Inquiry , 16 (7), 575–582. https://doi.org/10.1177/1077800410372601
Vaughan, D. (1996). The Challenger launch decision: Risky technology, culture, and deviance at NASA . Chicago, IL: The University of Chicago Press.
Vaughan, D. (1997). The trickle-down effect: Policy decisions, risky work, and the Challenger tragedy. California Management Review , 39 (2), 80–102.
Yin, R. K. (2014). Case study research: Design and methods (5th ed.). Thousand Oaks, CA: Sage Publications.
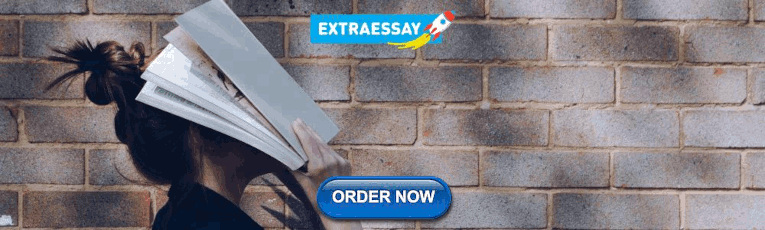
Share this:
- Click to share on Reddit (Opens in new window)
- Click to share on Twitter (Opens in new window)
- Click to share on LinkedIn (Opens in new window)
- Click to share on Facebook (Opens in new window)
- Click to share on Tumblr (Opens in new window)
- Click to email a link to a friend (Opens in new window)
- Click to print (Opens in new window)
One comment
Reblogged this on Managementpublic .
Like Liked by 1 person
Leave a comment Cancel reply
This site uses Akismet to reduce spam. Learn how your comment data is processed .

- Already have a WordPress.com account? Log in now.
- Subscribe Subscribed
- Copy shortlink
- Report this content
- View post in Reader
- Manage subscriptions
- Collapse this bar
Have a language expert improve your writing
Run a free plagiarism check in 10 minutes, generate accurate citations for free.
- Knowledge Base
- Research bias
- What Is Generalizability? | Definition & Examples
What Is Generalizability? | Definition & Examples
Published on October 8, 2022 by Kassiani Nikolopoulou . Revised on March 3, 2023.
Generalizability is the degree to which you can apply the results of your study to a broader context. Research results are considered generalizable when the findings can be applied to most contexts, most people, most of the time.
Generalizability is determined by how representative your sample is of the target population . This is known as external validity .
Table of contents
What is generalizability, why is generalizability important, examples of generalizability, types of generalizability, how do you ensure generalizability in research, other types of research bias, frequently asked questions about generalizability.
The goal of research is to produce knowledge that can be applied as widely as possible. However, since it usually isn’t possible to analyze every member of a population, researchers make do by analyzing a portion of it, making statements about that portion.
To be able to apply these statements to larger groups, researchers must ensure that the sample accurately resembles the broader population.
In other words, the sample and the population must share the characteristics relevant to the research being conducted. When this happens, the sample is considered representative, and by extension, the study’s results are considered generalizable.
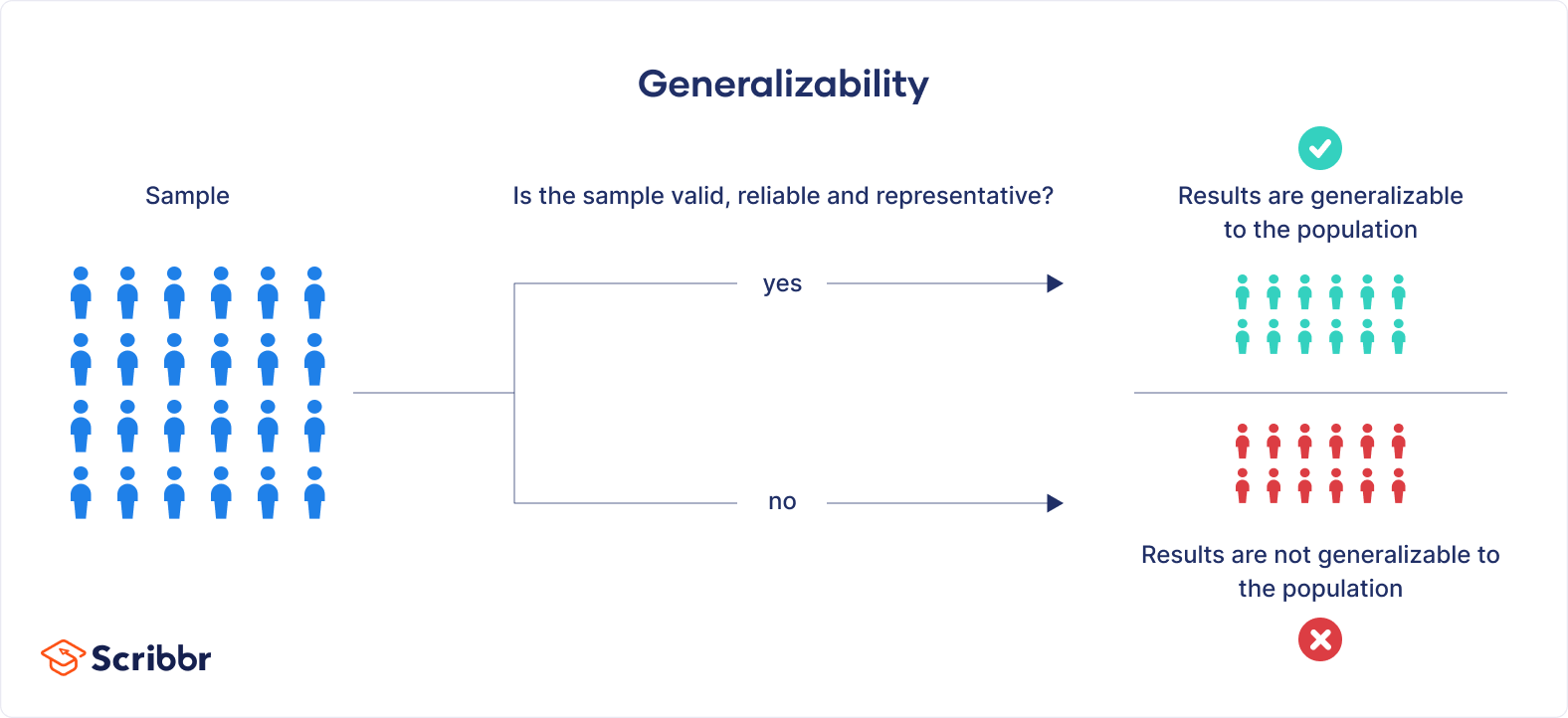
In general, a study has good generalizability when the results apply to many different types of people or different situations. In contrast, if the results can only be applied to a subgroup of the population or in a very specific situation, the study has poor generalizability.
Obtaining a representative sample is crucial for probability sampling . In contrast, studies using non-probability sampling designs are more concerned with investigating a few cases in depth, rather than generalizing their findings. As such, generalizability is the main difference between probability and non-probability samples.
There are three factors that determine the generalizability of your study in a probability sampling design:
- The randomness of the sample, with each research unit (e.g., person, business, or organization in your population) having an equal chance of being selected.
- How representative the sample is of your population.
- The size of your sample, with larger samples more likely to yield statistically significant results.
Generalizability is one of the three criteria (along with validity and reliability ) that researchers use to assess the quality of both quantitative and qualitative research. However, depending on the type of research, generalizability is interpreted and evaluated differently.
- In quantitative research , generalizability helps to make inferences about the population.
- In qualitative research , generalizability helps to compare the results to other results from similar situations.
Generalizability is crucial for establishing the validity and reliability of your study. In most cases, a lack of generalizability significantly narrows down the scope of your research—i.e., to whom the results can be applied.
Luckily, you have access to an anonymized list of all residents. This allows you to establish a sampling frame and proceed with simple random sampling . With the help of an online random number generator, you draw a simple random sample.
After obtaining your results (and prior to drawing any conclusions) you need to consider the generalizability of your results. Using an online sample calculator, you see that the ideal sample size is 341. With a sample of 341, you could be confident that your results are generalizable, but a sample of 100 is too small to be generalizable.
However, research results that cannot be generalized can still have value. It all depends on your research objectives .
You go to the museum for three consecutive Sundays to make observations.
Your observations yield valuable insights for the Getty Museum, and perhaps even for other museums with similar educational offerings.
There are two broad types of generalizability:
- Statistical generalizability, which applies to quantitative research
- Theoretical generalizability (also referred to as transferability ), which applies to qualitative research
Statistical generalizability is critical for quantitative research . The goal of quantitative research is to develop general knowledge that applies to all the units of a population while studying only a subset of these units (sample). Statistical generalization is achieved when you study a sample that accurately mirrors characteristics of the population. The sample needs to be sufficiently large and unbiased.
In qualitative research , statistical generalizability is not relevant. This is because qualitative research is primarily concerned with obtaining insights on some aspect of human experience, rather than data with solid statistical basis. By studying individual cases, researchers will try to get results that they can extend to similar cases. This is known as theoretical generalizability or transferability.
In order to apply your findings on a larger scale, you should take the following steps to ensure your research has sufficient generalizability.
- Define your population in detail. By doing so, you will establish what it is that you intend to make generalizations about. For example, are you going to discuss students in general, or students on your campus?
- Use random sampling . If the sample is truly random (i.e., everyone in the population is equally likely to be chosen for the sample), then you can avoid sampling bias and ensure that the sample will be representative of the population.
- Consider the size of your sample. The sample size must be large enough to support the generalization being made. If the sample represents a smaller group within that population, then the conclusions have to be downsized in scope.
- If you’re conducting qualitative research , try to reach a saturation point of important themes and categories. This way, you will have sufficient information to account for all aspects of the phenomenon under study.
After completing your research, take a moment to reflect on the generalizability of your findings. What didn’t go as planned and could impact your generalizability? For example, selection biases such as nonresponse bias can affect your results. Explain how generalizable your results are, as well as possible limitations, in the discussion section of your research paper .
Cognitive bias
- Confirmation bias
- Baader–Meinhof phenomenon
- Availability heuristic
- Halo effect
- Framing effect
Selection bias
- Sampling bias
- Ascertainment bias
- Attrition bias
- Self-selection bias
- Survivorship bias
- Nonresponse bias
- Undercoverage bias
- Hawthorne effect
- Observer bias
- Omitted variable bias
- Publication bias
- Pygmalion effect
- Recall bias
- Social desirability bias
- Placebo effect
Generalizability is important because it allows researchers to make inferences for a large group of people, i.e., the target population, by only studying a part of it (the sample ).
I nternal validity is the degree of confidence that the causal relationship you are testing is not influenced by other factors or variables .
External validity is the extent to which your results can be generalized to other contexts.
The validity of your experiment depends on your experimental design .
In the discussion , you explore the meaning and relevance of your research results , explaining how they fit with existing research and theory. Discuss:
- Your interpretations : what do the results tell us?
- The implications : why do the results matter?
- The limitation s : what can’t the results tell us?
Scope of research is determined at the beginning of your research process , prior to the data collection stage. Sometimes called “scope of study,” your scope delineates what will and will not be covered in your project. It helps you focus your work and your time, ensuring that you’ll be able to achieve your goals and outcomes.
Defining a scope can be very useful in any research project, from a research proposal to a thesis or dissertation . A scope is needed for all types of research: quantitative , qualitative , and mixed methods .
To define your scope of research, consider the following:
- Budget constraints or any specifics of grant funding
- Your proposed timeline and duration
- Specifics about your population of study, your proposed sample size , and the research methodology you’ll pursue
- Any inclusion and exclusion criteria
- Any anticipated control , extraneous , or confounding variables that could bias your research if not accounted for properly.
Cite this Scribbr article
If you want to cite this source, you can copy and paste the citation or click the “Cite this Scribbr article” button to automatically add the citation to our free Citation Generator.
Nikolopoulou, K. (2023, March 03). What Is Generalizability? | Definition & Examples. Scribbr. Retrieved April 15, 2024, from https://www.scribbr.com/research-bias/generalizability/
Is this article helpful?
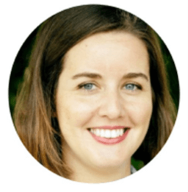
Kassiani Nikolopoulou
Other students also liked, external validity | definition, types, threats & examples, what is probability sampling | types & examples, what is quantitative research | definition, uses & methods.
This website may not work correctly because your browser is out of date. Please update your browser .
Analytical generalisation
Analytical generalisation involves making projections about the likely transferability of findings from an evaluation, based on a theoretical analysis of the factors producing outcomes and the effect of context.
Realist evaluation can be particularly important for this.
Analytic generalisation is distinct from statistical generalisation, in that it does not draw inferences from data to a population. Instead, analytic generalisation compares the results of a case study to a previously developed theory.
"A ... common concern about case studies is that they provide little basis for scientific generalization. "How can you generalize from a single case?" is a frequently heard question. ... In fact, scientific facts are rarely based on single experiments; they are usually based on a multiple set of experiments that have replicated-the same phenomenon under different conditions. The same approach can be used with multiple-case studies but requires a different concept of the appropriate research designs ... The short answer is that case studies, like experiments, are generalizable to theoretical propositions and not to populations or universes. In this sense, the case study, like the experiment, does not represent a "sample," and in doing a case study, your goal will be to expand and generalize theories (analytic generalization) and not to enumerate frequencies (statistical generalization)." (Yin, 2009: 15)
In the Encyclopedia of Case Study Research, Robert Yin describes the process of analytic generalisation as a two-step process: First, a conceptual claim is made by researchers which "show[s] how their case study findings bear upon a particular theory, theoretical construct, or theoretical ... sequence of events". Secondly, this theory is applied to implicate situations in which similar events might occur. (Yin, 2010)
Advice for using this method
- The argument or theory should be made clear at the beginning of the case study
- The argument should be grounded in a research literature rather than specific related to the case study
- Findings should show how the results of the case study either challenged or supported the theory or argument
- If the findings support the theory, a logical and sound argument needs to be made by researchers to show how these findings can be generalised to similar situations.
- Examining rival hypotheses will strengthen claims of analytical generalisation
- "Beyond making a claim, the generalizability of the findings from a single case study increases immeasurably if similar results have been found with other case studies—whether such studies already existed in the literature or were completed after the first case study." (Yin, 2010)
Written by Robert Yin, this entry gives a clear overview of analytic generalisation from case studies, where it is appropriate, and how to effectively apply it.
Providing a complete portal to the world of case study research, the Fifth Edition of Robert K. Yin’s bestselling text offers comprehensive coverage of the design and use of the case study method as a valid research tool.
Robert Yin (2010) references Allison and Zelikow's book as a strong example of analytic generalisation, as the authors conclude that the lessons learned in this crisis could be extrapolated to similar situations of confrontations between superpowers.
Yin, R. (1994) Case Study Research: Design and Methods. Sage Publications, Thousand Oaks, CA.
Yin, R. (2010). 'Analytic Generalization.' In Albert J. Mills, G. Durepos, & E. Wiebe (Eds.), Encyclopedia of Case Study Research . (pp. 21-23). Thousand Oaks, CA: SAGE Publications, Inc.
'Analytical generalisation' is referenced in:
Framework/guide.
- Rainbow Framework : Extrapolate findings
- Critical case sampling
Back to top
© 2022 BetterEvaluation. All right reserved.
Generalizing from Case Studies: A Commentary
- Regular Article
- Published: 01 September 2017
- Volume 52 , pages 94–103, ( 2018 )
Cite this article
- Ingrid de Saint-Georges ORCID: orcid.org/0000-0002-4357-0471 1
1130 Accesses
5 Citations
11 Altmetric
Explore all metrics
This commentary responds to the articles assembled for the thematic issue Self-identity on the move: methodological elaborations (IPBS, 51 (2), June 2017). The issue points in two directions. Firstly, the articles investigate the way individual self-identity develops in changing social and cultural environments, specifically in the contexts of family, youth and migration. Secondly, the special issue is also interested in methodological elaboration, more specifically the question of how one can generalize from individual case studies, especially when looking at complex, multiscale, semiotic processes. This commentary particularly addresses the second point and uses the various cases in this issue (i) to better understand something of the larger intellectual debate around the question of ‘generalizing from case studies’, and (ii) to reflect on writing as a tool for indexing generalization. The commentary highlights five textual moves the authors use to make their findings relevant beyond the specifics of the local study.
This is a preview of subscription content, log in via an institution to check access.
Access this article
Price includes VAT (Russian Federation)
Instant access to the full article PDF.
Rent this article via DeepDyve
Institutional subscriptions
This comprehensive work has already been carried out by Ramos ( 2017 ) who offers a thorough and critical overview of the same collection.
Abbott, A. D. (2004). Methods of Discovery: Heuristics for the Social Sciences . New York: W.W. Norton.
Google Scholar
Albert, I., & Barros Coimbra, S. (2017). Family cultures in the context of migration and ageing. Integrative Psychological and Behavioral Science, 51 , 205–222. https://doi.org/10.1007/s12124-017-9381-y .
Article PubMed Google Scholar
Baskerville, R., & Lee, A. S. (1999). Distinctions among different types of generalizing in information systems research. In O. Ngwenyama, L. D. Introna, M. Myers, & J. DeGross (Eds.), New Information Technologies in Organizational Processes (pp. 49–65). Dordrecht: Springer.
Chapter Google Scholar
Becker, H. S. (1998). Trick of the trade. How to think about your research while doing it . Chicago: University of Chicago Press.
Book Google Scholar
Becker, H. S. (2014). What About Mozart? What About Murder: Reasoning from Cases . London: The University of Chicago Press.
Berger, P., & Luckmann, T. (1966). The Social Construction of Reality: A Treatise in the Sociology of Knowledge . Garden City: Anchor Books.
Burawoy, M. (1998). The Extended Case Method. Sociological Theory, 16 (1), 4–33.
Article Google Scholar
Burgess, E. (1927). Statistics and Case Studies. Sociology and Social Research, 12 (2), 103–120.
Duff, P. (2008). Case Study Research in Applied Linguistics . New York & London: Taylor & Francis.
Eisenhart, M. (2009). Generalization from Qualitative Inquiry. In K. Ercikan & W.-M. Roth (Eds.), Generalizing from Educational Research. Beyond Qualitative and Quantitative Polarization (pp. 51–66). New York & London: Routledge.
Ferring, D. (2017). The family in us: Family story, family identity and self reproductive adaptive behavior. Integrative Psychological and Behavioral Science, 51 , 195–204. https://doi.org/10.1007/s12124-017-9383-9 .
Flyvbjerg, B. (2006). Five Misunderstandings About Case-Study Research. Qualitative Inquiry, 12 (2), 219–245.
Glaser, B. G. (2006). Generalizing: The descriptive struggle. Grounded Theory Review . An International Journal, 6 (1), 1–27.
Kennedy, M. M. (1979). Generalizing from Single Case Studies. Evaluation Quarterly, 3 (4), 661–678.
Kress, G. R., & Van Leeuwen, T. (1996). Reading images : the grammar of visual design . London: Routledge.
Lincoln, Y., & Guba, E. (1985). Naturalistic Inquiry . Beverly Hills: Sage Publications.
Maxwell, J. (2005). Qualitative research design: An interactive approach (2nd ed.). San Francisco: Jossey-Bass.
Maxwell, J. (2007). Types of Generalization in qualitative Research. Online document. https://www.tcrecord.org/Discussion.asp?i=3&vdpid=2761&aid=2&rid=12612&dtid=0 . Accessed 9 July 2017.
Mazur, B. C. (2002). Introduction to the talk 'The problem of Thinking Too Much' by Persi Diaconis. Paper presented at the 1865th Stated Meeting, House of the Academy. Online document. https://www.amacad.org/publications/bulletin/spring2003/diaconis.pdf Accessed 9 July 2017.
Merriam, S. (1998). Qualitative research and case study applications in education . San Fransisco: Jossey-Bass Publishers.
Moscovici, S. (1984). The phenomenon of social repressentation. In R. Farr & S. Moscovici (Eds.), Social representations (pp. 3–68). Cambridge: Cambridge University Press.
Murdock, E. (2017). Identity and its construal: Learning from Luxembourg. Integrative Psychological and Behavioral Sciences, 51 , 261–278. https://doi.org/10.1007/s12124-017-9385-7 .
Pearson Casanave, C., & Li, Y. (2015). Novices’ Struggles with Conceptual and Theoretical Framing in Writing Dissertations and Papers for Publication. Publica, 2015 (3), 104–119. https://doi.org/10.3390/publications3020104 .
Porter, T. M. (1995). Trust in Numbers: The Pursuit of Objectivity in Science and Public Life . Princeton: Princeton University Press.
Ramos, C. (2017). Piecing Together Ideas on Sociocultural Psychology and Methodological Approaches. Integrative Psychological and Behavioral Sciences, 51 , 279–284. https://doi.org/10.1007/s12124-017-9389-3 .
Steinberg, P. F. (2015). Can We Generalize from Case Studies? Global Environmental Politics, 15 (3), 152–175.
Swales, J. (1990). Genre Analysis. English in Academic and Research Settings . Cambridge: Cambridge University Press.
Thomas, G. (2011). A Typology for the Case Study in Social Science Following a Review of Definition, Discourse, and Structure. Qualitative Inquiry, 17 (6), 511–521.
Watzlawik, M., & Brescó de Luna, I. (2017). The self in movement: Being identified and identifying oneself in the process of migration and asylum seeking. Integrative Psychological and Behavioral Science, 51 , 244–260. https://doi.org/10.1007/s12124-017-9386-6 .
Weick, K. E. (1995). What Theory is Not, Theorizing Is. Administrative Science Quarterly, 40 (3), 385–390.
Weis, D., & Willems, H. (2017). Aggregation, validation, and generalization of qualitative data – Methodological and practical research strategies illustrated by the research process of an empirically based typology. Integrative Psychological and Behavioral Science, 51 , 223–243. https://doi.org/10.1007/s12124-016-9372-4 .
Wright Mills, C. (1959). On Intellectual Craftmanship. In C. Wright Mills (Ed.), The Sociological Imagination . Oxford: Oxford University Press.
Yin, R. K. (2014). Case Study Research . Los Angeles: Sage.
Zittoun, T. (2017). Modalities of generalization through single case studies. Integrative Psychological and Behavioral Science, 51 , 171–194.
Download references
Author information
Authors and affiliations.
Institute for Research on Multilingualism (MLing)/Research Unit on Education, Cognition, Culture & Society (ECCS), University of Luxembourg, Maison des Sciences Humaines, 11, Porte des Sciences, L-4366 Esch-sur-Alzette, Luxembourg City, Luxembourg
Ingrid de Saint-Georges
You can also search for this author in PubMed Google Scholar
Corresponding author
Correspondence to Ingrid de Saint-Georges .
Ethics declarations
I am the sole author of this article and I declare that I have no conflict of interest.
Ethical Approval
This article does not contain any studies with human participants or animals performed by the author.
Rights and permissions
Reprints and permissions
About this article
de Saint-Georges, I. Generalizing from Case Studies: A Commentary. Integr. psych. behav. 52 , 94–103 (2018). https://doi.org/10.1007/s12124-017-9402-x
Download citation
Published : 01 September 2017
Issue Date : March 2018
DOI : https://doi.org/10.1007/s12124-017-9402-x
Share this article
Anyone you share the following link with will be able to read this content:
Sorry, a shareable link is not currently available for this article.
Provided by the Springer Nature SharedIt content-sharing initiative
- Generalization
- Rhetorical moves
- Sociocultural psychology
- Find a journal
- Publish with us
- Track your research

An official website of the United States government
The .gov means it’s official. Federal government websites often end in .gov or .mil. Before sharing sensitive information, make sure you’re on a federal government site.
The site is secure. The https:// ensures that you are connecting to the official website and that any information you provide is encrypted and transmitted securely.
- Publications
- Account settings
Preview improvements coming to the PMC website in October 2024. Learn More or Try it out now .
- Advanced Search
- Journal List
- Front Psychol
Quantitative and Qualitative Approaches to Generalization and Replication–A Representationalist View
In this paper, we provide a re-interpretation of qualitative and quantitative modeling from a representationalist perspective. In this view, both approaches attempt to construct abstract representations of empirical relational structures. Whereas quantitative research uses variable-based models that abstract from individual cases, qualitative research favors case-based models that abstract from individual characteristics. Variable-based models are usually stated in the form of quantified sentences (scientific laws). This syntactic structure implies that sentences about individual cases are derived using deductive reasoning. In contrast, case-based models are usually stated using context-dependent existential sentences (qualitative statements). This syntactic structure implies that sentences about other cases are justifiable by inductive reasoning. We apply this representationalist perspective to the problems of generalization and replication. Using the analytical framework of modal logic, we argue that the modes of reasoning are often not only applied to the context that has been studied empirically, but also on a between-contexts level. Consequently, quantitative researchers mostly adhere to a top-down strategy of generalization, whereas qualitative researchers usually follow a bottom-up strategy of generalization. Depending on which strategy is employed, the role of replication attempts is very different. In deductive reasoning, replication attempts serve as empirical tests of the underlying theory. Therefore, failed replications imply a faulty theory. From an inductive perspective, however, replication attempts serve to explore the scope of the theory. Consequently, failed replications do not question the theory per se , but help to shape its boundary conditions. We conclude that quantitative research may benefit from a bottom-up generalization strategy as it is employed in most qualitative research programs. Inductive reasoning forces us to think about the boundary conditions of our theories and provides a framework for generalization beyond statistical testing. In this perspective, failed replications are just as informative as successful replications, because they help to explore the scope of our theories.
Introduction
Qualitative and quantitative research strategies have long been treated as opposing paradigms. In recent years, there have been attempts to integrate both strategies. These “mixed methods” approaches treat qualitative and quantitative methodologies as complementary, rather than opposing, strategies (Creswell, 2015 ). However, whilst acknowledging that both strategies have their benefits, this “integration” remains purely pragmatic. Hence, mixed methods methodology does not provide a conceptual unification of the two approaches.
Lacking a common methodological background, qualitative and quantitative research methodologies have developed rather distinct standards with regard to the aims and scope of empirical science (Freeman et al., 2007 ). These different standards affect the way researchers handle contradictory empirical findings. For example, many empirical findings in psychology have failed to replicate in recent years (Klein et al., 2014 ; Open Science, Collaboration, 2015 ). This “replication crisis” has been discussed on statistical, theoretical and social grounds and continues to have a wide impact on quantitative research practices like, for example, open science initiatives, pre-registered studies and a re-evaluation of statistical significance testing (Everett and Earp, 2015 ; Maxwell et al., 2015 ; Shrout and Rodgers, 2018 ; Trafimow, 2018 ; Wiggins and Chrisopherson, 2019 ).
However, qualitative research seems to be hardly affected by this discussion. In this paper, we argue that the latter is a direct consequence of how the concept of generalizability is conceived in the two approaches. Whereas most of quantitative psychology is committed to a top-down strategy of generalization based on the idea of random sampling from an abstract population, qualitative studies usually rely on a bottom-up strategy of generalization that is grounded in the successive exploration of the field by means of theoretically sampled cases.
Here, we show that a common methodological framework for qualitative and quantitative research methodologies is possible. We accomplish this by introducing a formal description of quantitative and qualitative models from a representationalist perspective: both approaches can be reconstructed as special kinds of representations for empirical relational structures. We then use this framework to analyze the generalization strategies used in the two approaches. These turn out to be logically independent of the type of model. This has wide implications for psychological research. First, a top-down generalization strategy is compatible with a qualitative modeling approach. This implies that mainstream psychology may benefit from qualitative methods when a numerical representation turns out to be difficult or impossible, without the need to commit to a “qualitative” philosophy of science. Second, quantitative research may exploit the bottom-up generalization strategy that is inherent to many qualitative approaches. This offers a new perspective on unsuccessful replications by treating them not as scientific failures, but as a valuable source of information about the scope of a theory.
The Quantitative Strategy–Numbers and Functions
Quantitative science is about finding valid mathematical representations for empirical phenomena. In most cases, these mathematical representations have the form of functional relations between a set of variables. One major challenge of quantitative modeling consists in constructing valid measures for these variables. Formally, to measure a variable means to construct a numerical representation of the underlying empirical relational structure (Krantz et al., 1971 ). For example, take the behaviors of a group of students in a classroom: “to listen,” “to take notes,” and “to ask critical questions.” One may now ask whether is possible to assign numbers to the students, such that the relations between the assigned numbers are of the same kind as the relations between the values of an underlying variable, like e.g., “engagement.” The observed behaviors in the classroom constitute an empirical relational structure, in the sense that for every student-behavior tuple, one can observe whether it is true or not. These observations can be represented in a person × behavior matrix 1 (compare Figure 1 ). Given this relational structure satisfies certain conditions (i.e., the axioms of a measurement model), one can assign numbers to the students and the behaviors, such that the relations between the numbers resemble the corresponding numerical relations. For example, if there is a unique ordering in the empirical observations with regard to which person shows which behavior, the assigned numbers have to constitute a corresponding unique ordering, as well. Such an ordering coincides with the person × behavior matrix forming a triangle shaped relation and is formally represented by a Guttman scale (Guttman, 1944 ). There are various measurement models available for different empirical structures (Suppes et al., 1971 ). In the case of probabilistic relations, Item-Response models may be considered as a special kind of measurement model (Borsboom, 2005 ).

Constructing a numerical representation from an empirical relational structure; Due to the unique ordering of persons with regard to behaviors (indicated by the triangular shape of the relation), it is possible to construct a Guttman scale by assigning a number to each of the individuals, representing the number of relevant behaviors shown by the individual. The resulting variable (“engagement”) can then be described by means of statistical analyses, like, e.g., plotting the frequency distribution.
Although essential, measurement is only the first step of quantitative modeling. Consider a slightly richer empirical structure, where we observe three additional behaviors: “to doodle,” “to chat,” and “to play.” Like above, one may ask, whether there is a unique ordering of the students with regard to these behaviors that can be represented by an underlying variable (i.e., whether the matrix forms a Guttman scale). If this is the case, we may assign corresponding numbers to the students and call this variable “distraction.” In our example, such a representation is possible. We can thus assign two numbers to each student, one representing his or her “engagement” and one representing his or her “distraction” (compare Figure 2 ). These measurements can now be used to construct a quantitative model by relating the two variables by a mathematical function. In the simplest case, this may be a linear function. This functional relation constitutes a quantitative model of the empirical relational structure under study (like, e.g., linear regression). Given the model equation and the rules for assigning the numbers (i.e., the instrumentations of the two variables), the set of admissible empirical structures is limited from all possible structures to a rather small subset. This constitutes the empirical content of the model 2 (Popper, 1935 ).

Constructing a numerical model from an empirical relational structure; Since there are two distinct classes of behaviors that each form a Guttman scale, it is possible to assign two numbers to each individual, correspondingly. The resulting variables (“engagement” and “distraction”) can then be related by a mathematical function, which is indicated by the scatterplot and red line on the right hand side.
The Qualitative Strategy–Categories and Typologies
The predominant type of analysis in qualitative research consists in category formation. By constructing descriptive systems for empirical phenomena, it is possible to analyze the underlying empirical structure at a higher level of abstraction. The resulting categories (or types) constitute a conceptual frame for the interpretation of the observations. Qualitative researchers differ considerably in the way they collect and analyze data (Miles et al., 2014 ). However, despite the diverse research strategies followed by different qualitative methodologies, from a formal perspective, most approaches build on some kind of categorization of cases that share some common features. The process of category formation is essential in many qualitative methodologies, like, for example, qualitative content analysis, thematic analysis, grounded theory (see Flick, 2014 for an overview). Sometimes these features are directly observable (like in our classroom example), sometimes they are themselves the result of an interpretative process (e.g., Scheunpflug et al., 2016 ).
In contrast to quantitative methodologies, there have been little attempts to formalize qualitative research strategies (compare, however, Rihoux and Ragin, 2009 ). However, there are several statistical approaches to non-numerical data that deal with constructing abstract categories and establishing relations between these categories (Agresti, 2013 ). Some of these methods are very similar to qualitative category formation on a conceptual level. For example, cluster analysis groups cases into homogenous categories (clusters) based on their similarity on a distance metric.
Although category formation can be formalized in a mathematically rigorous way (Ganter and Wille, 1999 ), qualitative research hardly acknowledges these approaches. 3 However, in order to find a common ground with quantitative science, it is certainly helpful to provide a formal interpretation of category systems.
Let us reconsider the above example of students in a classroom. The quantitative strategy was to assign numbers to the students with regard to variables and to relate these variables via a mathematical function. We can analyze the same empirical structure by grouping the behaviors to form abstract categories. If the aim is to construct an empirically valid category system, this grouping is subject to constraints, analogous to those used to specify a measurement model. The first and most important constraint is that the behaviors must form equivalence classes, i.e., within categories, behaviors need to be equivalent, and across categories, they need to be distinct (formally, the relational structure must obey the axioms of an equivalence relation). When objects are grouped into equivalence classes, it is essential to specify the criterion for empirical equivalence. In qualitative methodology, this is sometimes referred to as the tertium comparationis (Flick, 2014 ). One possible criterion is to group behaviors such that they constitute a set of specific common attributes of a group of people. In our example, we might group the behaviors “to listen,” “to take notes,” and “to doodle,” because these behaviors are common to the cases B, C, and D, and they are also specific for these cases, because no other person shows this particular combination of behaviors. The set of common behaviors then forms an abstract concept (e.g., “moderate distraction”), while the set of persons that show this configuration form a type (e.g., “the silent dreamer”). Formally, this means to identify the maximal rectangles in the underlying empirical relational structure (see Figure 3 ). This procedure is very similar to the way we constructed a Guttman scale, the only difference being that we now use different aspects of the empirical relational structure. 4 In fact, the set of maximal rectangles can be determined by an automated algorithm (Ganter, 2010 ), just like the dimensionality of an empirical structure can be explored by psychometric scaling methods. Consequently, we can identify the empirical content of a category system or a typology as the set of empirical structures that conforms to it. 5 Whereas the quantitative strategy was to search for scalable sub-matrices and then relate the constructed variables by a mathematical function, the qualitative strategy is to construct an empirical typology by grouping cases based on their specific similarities. These types can then be related to one another by a conceptual model that describes their semantic and empirical overlap (see Figure 3 , right hand side).

Constructing a conceptual model from an empirical relational structure; Individual behaviors are grouped to form abstract types based on them being shared among a specific subset of the cases. Each type constitutes a set of specific commonalities of a class of individuals (this is indicated by the rectangles on the left hand side). The resulting types (“active learner,” “silent dreamer,” “distracted listener,” and “troublemaker”) can then be related to one another to explicate their semantic and empirical overlap, as indicated by the Venn-diagram on the right hand side.
Variable-Based Models and Case-Based Models
In the previous section, we have argued that qualitative category formation and quantitative measurement can both be characterized as methods to construct abstract representations of empirical relational structures. Instead of focusing on different philosophical approaches to empirical science, we tried to stress the formal similarities between both approaches. However, it is worth also exploring the dissimilarities from a formal perspective.
Following the above analysis, the quantitative approach can be characterized by the use of variable-based models, whereas the qualitative approach is characterized by case-based models (Ragin, 1987 ). Formally, we can identify the rows of an empirical person × behavior matrix with a person-space, and the columns with a corresponding behavior-space. A variable-based model abstracts from the single individuals in a person-space to describe the structure of behaviors on a population level. A case-based model, on the contrary, abstracts from the single behaviors in a behavior-space to describe individual case configurations on the level of abstract categories (see Table 1 ).
Variable-based models and case-based models.
From a representational perspective, there is no a priori reason to favor one type of model over the other. Both approaches provide different analytical tools to construct an abstract representation of an empirical relational structure. However, since the two modeling approaches make use of different information (person-space vs. behavior-space), this comes with some important implications for the researcher employing one of the two strategies. These are concerned with the role of deductive and inductive reasoning.
In variable-based models, empirical structures are represented by functional relations between variables. These are usually stated as scientific laws (Carnap, 1928 ). Formally, these laws correspond to logical expressions of the form
In plain text, this means that y is a function of x for all objects i in the relational structure under consideration. For example, in the above example, one may formulate the following law: for all students in the classroom it holds that “distraction” is a monotone decreasing function of “engagement.” Such a law can be used to derive predictions for single individuals by means of logical deduction: if the above law applies to all students in the classroom, it is possible to calculate the expected distraction from a student's engagement. An empirical observation can now be evaluated against this prediction. If the prediction turns out to be false, the law can be refuted based on the principle of falsification (Popper, 1935 ). If a scientific law repeatedly withstands such empirical tests, it may be considered to be valid with regard to the relational structure under consideration.
In case-based models, there are no laws about a population, because the model does not abstract from the cases but from the observed behaviors. A case-based model describes the underlying structure in terms of existential sentences. Formally, this corresponds to a logical expression of the form
In plain text, this means that there is at least one case i for which the condition XYZ holds. For example, the above category system implies that there is at least one active learner. This is a statement about a singular observation. It is impossible to deduce a statement about another person from an existential sentence like this. Therefore, the strategy of falsification cannot be applied to test the model's validity in a specific context. If one wishes to generalize to other cases, this is accomplished by inductive reasoning, instead. If we observed one person that fulfills the criteria of calling him or her an active learner, we can hypothesize that there may be other persons that are identical to the observed case in this respect. However, we do not arrive at this conclusion by logical deduction, but by induction.
Despite this important distinction, it would be wrong to conclude that variable-based models are intrinsically deductive and case-based models are intrinsically inductive. 6 Both types of reasoning apply to both types of models, but on different levels. Based on a person-space, in a variable-based model one can use deduction to derive statements about individual persons from abstract population laws. There is an analogous way of reasoning for case-based models: because they are based on a behavior space, it is possible to deduce statements about singular behaviors. For example, if we know that Peter is an active learner, we can deduce that he takes notes in the classroom. This kind of deductive reasoning can also be applied on a higher level of abstraction to deduce thematic categories from theoretical assumptions (Braun and Clarke, 2006 ). Similarly, there is an analog for inductive generalization from the perspective of variable-based modeling: since the laws are only quantified over the person-space, generalizations to other behaviors rely on inductive reasoning. For example, it is plausible to assume that highly engaged students tend to do their homework properly–however, in our example this behavior has never been observed. Hence, in variable-based models we usually generalize to other behaviors by means of induction. This kind of inductive reasoning is very common when empirical results are generalized from the laboratory to other behavioral domains.
Although inductive and deductive reasoning are used in qualitative and quantitative research, it is important to stress the different roles of induction and deduction when models are applied to cases. A variable-based approach implies to draw conclusions about cases by means of logical deduction; a case-based approach implies to draw conclusions about cases by means of inductive reasoning. In the following, we build on this distinction to differentiate between qualitative (bottom-up) and quantitative (top-down) strategies of generalization.
Generalization and the Problem of Replication
We will now extend the formal analysis of quantitative and qualitative approaches to the question of generalization and replicability of empirical findings. For this sake, we have to introduce some concepts of formal logic. Formal logic is concerned with the validity of arguments. It provides conditions to evaluate whether certain sentences (conclusions) can be derived from other sentences (premises). In this context, a theory is nothing but a set of sentences (also called axioms). Formal logic provides tools to derive new sentences that must be true, given the axioms are true (Smith, 2020 ). These derived sentences are called theorems or, in the context of empirical science, predictions or hypotheses . On the syntactic level, the rules of logic only state how to evaluate the truth of a sentence relative to its premises. Whether or not sentences are actually true, is formally specified by logical semantics.
On the semantic level, formal logic is intrinsically linked to set-theory. For example, a logical statement like “all dogs are mammals,” is true if and only if the set of dogs is a subset of the set of mammals. Similarly, the sentence “all chatting students doodle” is true if and only if the set of chatting students is a subset of the set of doodling students (compare Figure 3 ). Whereas, the first sentence is analytically true due to the way we define the words “dog” and “mammal,” the latter can be either true or false, depending on the relational structure we actually observe. We can thus interpret an empirical relational structure as the truth criterion of a scientific theory. From a logical point of view, this corresponds to the semantics of a theory. As shown above, variable-based and case-based models both give a formal representation of the same kinds of empirical structures. Accordingly, both types of models can be stated as formal theories. In the variable-based approach, this corresponds to a set of scientific laws that are quantified over the members of an abstract population (these are the axioms of the theory). In the case-based approach, this corresponds to a set of abstract existential statements about a specific class of individuals.
In contrast to mathematical axiom systems, empirical theories are usually not considered to be necessarily true. This means that even if we find no evidence against a theory, it is still possible that it is actually wrong. We may know that a theory is valid in some contexts, yet it may fail when applied to a new set of behaviors (e.g., if we use a different instrumentation to measure a variable) or a new population (e.g., if we draw a new sample).
From a logical perspective, the possibility that a theory may turn out to be false stems from the problem of contingency . A statement is contingent, if it is both, possibly true and possibly false. Formally, we introduce two modal operators: □ to designate logical necessity, and ◇ to designate logical possibility. Semantically, these operators are very similar to the existential quantifier, ∃, and the universal quantifier, ∀. Whereas ∃ and ∀ refer to the individual objects within one relational structure, the modal operators □ and ◇ range over so-called possible worlds : a statement is possibly true, if and only if it is true in at least one accessible possible world, and a statement is necessarily true if and only if it is true in every accessible possible world (Hughes and Cresswell, 1996 ). Logically, possible worlds are mathematical abstractions, each consisting of a relational structure. Taken together, the relational structures of all accessible possible worlds constitute the formal semantics of necessity, possibility and contingency. 7
In the context of an empirical theory, each possible world may be identified with an empirical relational structure like the above classroom example. Given the set of intended applications of a theory (the scope of the theory, one may say), we can now construct possible world semantics for an empirical theory: each intended application of the theory corresponds to a possible world. For example, a quantified sentence like “all chatting students doodle” may be true in one classroom and false in another one. In terms of possible worlds, this would correspond to a statement of contingency: “it is possible that all chatting students doodle in one classroom, and it is possible that they don't in another classroom.” Note that in the above expression, “all students” refers to the students in only one possible world, whereas “it is possible” refers to the fact that there is at least one possible world for each of the specified cases.
To apply these possible world semantics to quantitative research, let us reconsider how generalization to other cases works in variable-based models. Due to the syntactic structure of quantitative laws, we can deduce predictions for singular observations from an expression of the form ∀ i : y i = f ( x i ). Formally, the logical quantifier ∀ ranges only over the objects of the corresponding empirical relational structure (in our example this would refer to the students in the observed classroom). But what if we want to generalize beyond the empirical structure we actually observed? The standard procedure is to assume an infinitely large, abstract population from which a random sample is drawn. Given the truth of the theory, we can deduce predictions about what we may observe in the sample. Since usually we deal with probabilistic models, we can evaluate our theory by means of the conditional probability of the observations, given the theory holds. This concept of conditional probability is the foundation of statistical significance tests (Hogg et al., 2013 ), as well as Bayesian estimation (Watanabe, 2018 ). In terms of possible world semantics, the random sampling model implies that all possible worlds (i.e., all intended applications) can be conceived as empirical sub-structures from a greater population structure. For example, the empirical relational structure constituted by the observed behaviors in a classroom would be conceived as a sub-matrix of the population person × behavior matrix. It follows that, if a scientific law is true in the population, it will be true in all possible worlds, i.e., it will be necessarily true. Formally, this corresponds to an expression of the form
The statistical generalization model thus constitutes a top-down strategy for dealing with individual contexts that is analogous to the way variable-based models are applied to individual cases (compare Table 1 ). Consequently, if we apply a variable-based model to a new context and find out that it does not fit the data (i.e., there is a statistically significant deviation from the model predictions), we have reason to doubt the validity of the theory. This is what makes the problem of low replicability so important: we observe that the predictions are wrong in a new study; and because we apply a top-down strategy of generalization to contexts beyond the ones we observed, we see our whole theory at stake.
Qualitative research, on the contrary, follows a different strategy of generalization. Since case-based models are formulated by a set of context-specific existential sentences, there is no need for universal truth or necessity. In contrast to statistical generalization to other cases by means of random sampling from an abstract population, the usual strategy in case-based modeling is to employ a bottom-up strategy of generalization that is analogous to the way case-based models are applied to individual cases. Formally, this may be expressed by stating that the observed qualia exist in at least one possible world, i.e., the theory is possibly true:
This statement is analogous to the way we apply case-based models to individual cases (compare Table 1 ). Consequently, the set of intended applications of the theory does not follow from a sampling model, but from theoretical assumptions about which cases may be similar to the observed cases with respect to certain relevant characteristics. For example, if we observe that certain behaviors occur together in one classroom, following a bottom-up strategy of generalization, we will hypothesize why this might be the case. If we do not replicate this finding in another context, this does not question the model itself, since it was a context-specific theory all along. Instead, we will revise our hypothetical assumptions about why the new context is apparently less similar to the first one than we originally thought. Therefore, if an empirical finding does not replicate, we are more concerned about our understanding of the cases than about the validity of our theory.
Whereas statistical generalization provides us with a formal (and thus somehow more objective) apparatus to evaluate the universal validity of our theories, the bottom-up strategy forces us to think about the class of intended applications on theoretical grounds. This means that we have to ask: what are the boundary conditions of our theory? In the above classroom example, following a bottom-up strategy, we would build on our preliminary understanding of the cases in one context (e.g., a public school) to search for similar and contrasting cases in other contexts (e.g., a private school). We would then re-evaluate our theoretical description of the data and explore what makes cases similar or dissimilar with regard to our theory. This enables us to expand the class of intended applications alongside with the theory.
Of course, none of these strategies is superior per se . Nevertheless, they rely on different assumptions and may thus be more or less adequate in different contexts. The statistical strategy relies on the assumption of a universal population and invariant measurements. This means, we assume that (a) all samples are drawn from the same population and (b) all variables refer to the same behavioral classes. If these assumptions are true, statistical generalization is valid and therefore provides a valuable tool for the testing of empirical theories. The bottom-up strategy of generalization relies on the idea that contexts may be classified as being more or less similar based on characteristics that are not part of the model being evaluated. If such a similarity relation across contexts is feasible, the bottom-up strategy is valid, as well. Depending on the strategy of generalization, replication of empirical research serves two very different purposes. Following the (top-down) principle of generalization by deduction from scientific laws, replications are empirical tests of the theory itself, and failed replications question the theory on a fundamental level. Following the (bottom-up) principle of generalization by induction to similar contexts, replications are a means to explore the boundary conditions of a theory. Consequently, failed replications question the scope of the theory and help to shape the set of intended applications.
We have argued that quantitative and qualitative research are best understood by means of the structure of the employed models. Quantitative science mainly relies on variable-based models and usually employs a top-down strategy of generalization from an abstract population to individual cases. Qualitative science prefers case-based models and usually employs a bottom-up strategy of generalization. We further showed that failed replications have very different implications depending on the underlying strategy of generalization. Whereas in the top-down strategy, replications are used to test the universal validity of a model, in the bottom-up strategy, replications are used to explore the scope of a model. We will now address the implications of this analysis for psychological research with regard to the problem of replicability.
Modern day psychology almost exclusively follows a top-down strategy of generalization. Given the quantitative background of most psychological theories, this is hardly surprising. Following the general structure of variable-based models, the individual case is not the focus of the analysis. Instead, scientific laws are stated on the level of an abstract population. Therefore, when applying the theory to a new context, a statistical sampling model seems to be the natural consequence. However, this is not the only possible strategy. From a logical point of view, there is no reason to assume that a quantitative law like ∀ i : y i = f ( x i ) implies that the law is necessarily true, i.e.,: □(∀ i : y i = f ( x i )). Instead, one might just as well define the scope of the theory following an inductive strategy. 8 Formally, this would correspond to the assumption that the observed law is possibly true, i.e.,: ◇(∀ i : y i = f ( x i )). For example, we may discover a functional relation between “engagement” and “distraction” without referring to an abstract universal population of students. Instead, we may hypothesize under which conditions this functional relation may be valid and use these assumptions to inductively generalize to other cases.
If we take this seriously, this would require us to specify the intended applications of the theory: in which contexts do we expect the theory to hold? Or, equivalently, what are the boundary conditions of the theory? These boundary conditions may be specified either intensionally, i.e., by giving external criteria for contexts being similar enough to the ones already studied to expect a successful application of the theory. Or they may be specified extensionally, by enumerating the contexts where the theory has already been shown to be valid. These boundary conditions need not be restricted to the population we refer to, but include all kinds of contextual factors. Therefore, adopting a bottom-up strategy, we are forced to think about these factors and make them an integral part of our theories.
In fact, there is good reason to believe that bottom-up generalization may be more adequate in many psychological studies. Apart from the pitfalls associated with statistical generalization that have been extensively discussed in recent years (e.g., p-hacking, underpowered studies, publication bias), it is worth reflecting on whether the underlying assumptions are met in a particular context. For example, many samples used in experimental psychology are not randomly drawn from a large population, but are convenience samples. If we use statistical models with non-random samples, we have to assume that the observations vary as if drawn from a random sample. This may indeed be the case for randomized experiments, because all variation between the experimental conditions apart from the independent variable will be random due to the randomization procedure. In this case, a classical significance test may be regarded as an approximation to a randomization test (Edgington and Onghena, 2007 ). However, if we interpret a significance test as an approximate randomization test, we test not for generalization but for internal validity. Hence, even if we use statistical significance tests when assumptions about random sampling are violated, we still have to use a different strategy of generalization. This issue has been discussed in the context of small-N studies, where variable-based models are applied to very small samples, sometimes consisting of only one individual (Dugard et al., 2012 ). The bottom-up strategy of generalization that is employed by qualitative researchers, provides such an alternative.
Another important issue in this context is the question of measurement invariance. If we construct a variable-based model in one context, the variables refer to those behaviors that constitute the underlying empirical relational structure. For example, we may construct an abstract measure of “distraction” using the observed behaviors in a certain context. We will then use the term “distraction” as a theoretical term referring to the variable we have just constructed to represent the underlying empirical relational structure. Let us now imagine we apply this theory to a new context. Even if the individuals in our new context are part of the same population, we may still get into trouble if the observed behaviors differ from those used in the original study. How do we know whether these behaviors constitute the same variable? We have to ensure that in any new context, our measures are valid for the variables in our theory. Without a proper measurement model, this will be hard to achieve (Buntins et al., 2017 ). Again, we are faced with the necessity to think of the boundary conditions of our theories. In which contexts (i.e., for which sets of individuals and behaviors) do we expect our theory to work?
If we follow the rationale of inductive generalization, we can explore the boundary conditions of a theory with every new empirical study. We thus widen the scope of our theory by comparing successful applications in different contexts and unsuccessful applications in similar contexts. This may ultimately lead to a more general theory, maybe even one of universal scope. However, unless we have such a general theory, we might be better off, if we treat unsuccessful replications not as a sign of failure, but as a chance to learn.
Author Contributions
MB conceived the original idea and wrote the first draft of the paper. MS helped to further elaborate and scrutinize the arguments. All authors contributed to the final version of the manuscript.
Conflict of Interest
The authors declare that the research was conducted in the absence of any commercial or financial relationships that could be construed as a potential conflict of interest.
Acknowledgments
We would like to thank Annette Scheunpflug for helpful comments on an earlier version of the manuscript.
1 A person × behavior matrix constitutes a very simple relational structure that is common in psychological research. This is why it is chosen here as a minimal example. However, more complex structures are possible, e.g., by relating individuals to behaviors over time, with individuals nested within groups etc. For a systematic overview, compare Coombs ( 1964 ).
2 This notion of empirical content applies only to deterministic models. The empirical content of a probabilistic model consists in the probability distribution over all possible empirical structures.
3 For example, neither the SAGE Handbook of qualitative data analysis edited by Flick ( 2014 ) nor the Oxford Handbook of Qualitative Research edited by Leavy ( 2014 ) mention formal approaches to category formation.
4 Note also that the described structure is empirically richer than a nominal scale. Therefore, a reduction of qualitative category formation to be a special (and somehow trivial) kind of measurement is not adequate.
5 It is possible to extend this notion of empirical content to the probabilistic case (this would correspond to applying a latent class analysis). But, since qualitative research usually does not rely on formal algorithms (neither deterministic nor probabilistic), there is currently little practical use of such a concept.
6 We do not elaborate on abductive reasoning here, since, given an empirical relational structure, the concept can be applied to both types of models in the same way (Schurz, 2008 ). One could argue that the underlying relational structure is not given a priori but has to be constructed by the researcher and will itself be influenced by theoretical expectations. Therefore, abductive reasoning may be necessary to establish an empirical relational structure in the first place.
7 We shall not elaborate on the metaphysical meaning of possible worlds here, since we are only concerned with empirical theories [but see Tooley ( 1999 ), for an overview].
8 Of course, this also means that it would be equally reasonable to employ a top-down strategy of generalization using a case-based model by postulating that □(∃ i : XYZ i ). The implications for case-based models are certainly worth exploring, but lie beyond the scope of this article.
- Agresti A. (2013). Categorical Data Analysis, 3rd Edn. Wiley Series In Probability And Statistics . Hoboken, NJ: Wiley. [ Google Scholar ]
- Borsboom D. (2005). Measuring the Mind: Conceptual Issues in Contemporary Psychometrics . Cambridge: Cambridge University Press; 10.1017/CBO9780511490026 [ CrossRef ] [ Google Scholar ]
- Braun V., Clarke V. (2006). Using thematic analysis in psychology . Qual. Res. Psychol . 3 , 77–101. 10.1191/1478088706qp063oa [ CrossRef ] [ Google Scholar ]
- Buntins M., Buntins K., Eggert F. (2017). Clarifying the concept of validity: from measurement to everyday language . Theory Psychol. 27 , 703–710. 10.1177/0959354317702256 [ CrossRef ] [ Google Scholar ]
- Carnap R. (1928). The Logical Structure of the World . Berkeley, CA: University of California Press. [ Google Scholar ]
- Coombs C. H. (1964). A Theory of Data . New York, NY: Wiley. [ Google Scholar ]
- Creswell J. W. (2015). A Concise Introduction to Mixed Methods Research . Los Angeles, CA: Sage. [ Google Scholar ]
- Dugard P., File P., Todman J. B. (2012). Single-Case and Small-N Experimental Designs: A Practical Guide to Randomization Tests 2nd Edn . New York, NY: Routledge; 10.4324/9780203180938 [ CrossRef ] [ Google Scholar ]
- Edgington E., Onghena P. (2007). Randomization Tests, 4th Edn. Statistics. Hoboken, NJ: CRC Press; 10.1201/9781420011814 [ CrossRef ] [ Google Scholar ]
- Everett J. A. C., Earp B. D. (2015). A tragedy of the (academic) commons: interpreting the replication crisis in psychology as a social dilemma for early-career researchers . Front. Psychol . 6 :1152. 10.3389/fpsyg.2015.01152 [ PMC free article ] [ PubMed ] [ CrossRef ] [ Google Scholar ]
- Flick U. (Ed.). (2014). The Sage Handbook of Qualitative Data Analysis . London: Sage; 10.4135/9781446282243 [ CrossRef ] [ Google Scholar ]
- Freeman M., Demarrais K., Preissle J., Roulston K., St. Pierre E. A. (2007). Standards of evidence in qualitative research: an incitement to discourse . Educ. Res. 36 , 25–32. 10.3102/0013189X06298009 [ CrossRef ] [ Google Scholar ]
- Ganter B. (2010). Two basic algorithms in concept analysis , in Lecture Notes In Computer Science. Formal Concept Analysis, Vol. 5986 , eds Hutchison D., Kanade T., Kittler J., Kleinberg J. M., Mattern F., Mitchell J. C., et al. (Berlin, Heidelberg: Springer Berlin Heidelberg; ), 312–340. 10.1007/978-3-642-11928-6_22 [ CrossRef ] [ Google Scholar ]
- Ganter B., Wille R. (1999). Formal Concept Analysis . Berlin, Heidelberg: Springer Berlin Heidelberg; 10.1007/978-3-642-59830-2 [ CrossRef ] [ Google Scholar ]
- Guttman L. (1944). A basis for scaling qualitative data . Am. Sociol. Rev . 9 :139 10.2307/2086306 [ CrossRef ] [ Google Scholar ]
- Hogg R. V., Mckean J. W., Craig A. T. (2013). Introduction to Mathematical Statistics, 7th Edn . Boston, MA: Pearson. [ Google Scholar ]
- Hughes G. E., Cresswell M. J. (1996). A New Introduction To Modal Logic . London; New York, NY: Routledge; 10.4324/9780203290644 [ CrossRef ] [ Google Scholar ]
- Klein R. A., Ratliff K. A., Vianello M., Adams R. B., Bahník Š., Bernstein M. J., et al. (2014). Investigating variation in replicability . Soc. Psychol. 45 , 142–152. 10.1027/1864-9335/a000178 [ CrossRef ] [ Google Scholar ]
- Krantz D. H., Luce D., Suppes P., Tversky A. (1971). Foundations of Measurement Volume I: Additive And Polynomial Representations . New York, NY; London: Academic Press; 10.1016/B978-0-12-425401-5.50011-8 [ CrossRef ] [ Google Scholar ]
- Leavy P. (2014). The Oxford Handbook of Qualitative Research . New York, NY: Oxford University Press; 10.1093/oxfordhb/9780199811755.001.0001 [ CrossRef ] [ Google Scholar ]
- Maxwell S. E., Lau M. Y., Howard G. S. (2015). Is psychology suffering from a replication crisis? what does “failure to replicate” really mean? Am. Psychol. 70 , 487–498. 10.1037/a0039400 [ PubMed ] [ CrossRef ] [ Google Scholar ]
- Miles M. B., Huberman A. M., Saldaña J. (2014). Qualitative Data Analysis: A Methods Sourcebook, 3rd Edn . Los Angeles, CA; London; New Delhi; Singapore; Washington, DC: Sage. [ Google Scholar ]
- Open Science, Collaboration (2015). Estimating the reproducibility of psychological science . Science 349 :Aac4716. 10.1126/science.aac4716 [ PubMed ] [ CrossRef ] [ Google Scholar ]
- Popper K. (1935). Logik Der Forschung . Wien: Springer; 10.1007/978-3-7091-4177-9 [ CrossRef ] [ Google Scholar ]
- Ragin C. (1987). The Comparative Method : Moving Beyond Qualitative and Quantitative Strategies . Berkeley, CA: University Of California Press. [ Google Scholar ]
- Rihoux B., Ragin C. (2009). Configurational Comparative Methods: Qualitative Comparative Analysis (Qca) And Related Techniques . Thousand Oaks, CA: Sage Publications, Inc; 10.4135/9781452226569 [ CrossRef ] [ Google Scholar ]
- Scheunpflug A., Krogull S., Franz J. (2016). Understanding learning in world society: qualitative reconstructive research in global learning and learning for sustainability . Int. Journal Dev. Educ. Glob. Learn. 7 , 6–23. 10.18546/IJDEGL.07.3.02 [ CrossRef ] [ Google Scholar ]
- Schurz G. (2008). Patterns of abduction . Synthese 164 , 201–234. 10.1007/s11229-007-9223-4 [ CrossRef ] [ Google Scholar ]
- Shrout P. E., Rodgers J. L. (2018). Psychology, science, and knowledge construction: broadening perspectives from the replication crisis . Annu. Rev. Psychol . 69 , 487–510. 10.1146/annurev-psych-122216-011845 [ PubMed ] [ CrossRef ] [ Google Scholar ]
- Smith P. (2020). An Introduction To Formal Logic . Cambridge: Cambridge University Press. 10.1017/9781108328999 [ CrossRef ] [ Google Scholar ]
- Suppes P., Krantz D. H., Luce D., Tversky A. (1971). Foundations of Measurement Volume II: Geometrical, Threshold, and Probabilistic Representations . New York, NY; London: Academic Press. [ Google Scholar ]
- Tooley M. (Ed.). (1999). Necessity and Possibility. The Metaphysics of Modality . New York, NY; London: Garland Publishing. [ Google Scholar ]
- Trafimow D. (2018). An a priori solution to the replication crisis . Philos. Psychol . 31 , 1188–1214. 10.1080/09515089.2018.1490707 [ CrossRef ] [ Google Scholar ]
- Watanabe S. (2018). Mathematical Foundations of Bayesian Statistics. CRC Monographs On Statistics And Applied Probability . Boca Raton, FL: Chapman And Hall. [ Google Scholar ]
- Wiggins B. J., Chrisopherson C. D. (2019). The replication crisis in psychology: an overview for theoretical and philosophical psychology . J. Theor. Philos. Psychol. 39 , 202–217. 10.1037/teo0000137 [ CrossRef ] [ Google Scholar ]
- Search Menu
- Browse content in Arts and Humanities
- Browse content in Archaeology
- Anglo-Saxon and Medieval Archaeology
- Archaeological Methodology and Techniques
- Archaeology by Region
- Archaeology of Religion
- Archaeology of Trade and Exchange
- Biblical Archaeology
- Contemporary and Public Archaeology
- Environmental Archaeology
- Historical Archaeology
- History and Theory of Archaeology
- Industrial Archaeology
- Landscape Archaeology
- Mortuary Archaeology
- Prehistoric Archaeology
- Underwater Archaeology
- Urban Archaeology
- Zooarchaeology
- Browse content in Architecture
- Architectural Structure and Design
- History of Architecture
- Residential and Domestic Buildings
- Theory of Architecture
- Browse content in Art
- Art Subjects and Themes
- History of Art
- Industrial and Commercial Art
- Theory of Art
- Biographical Studies
- Byzantine Studies
- Browse content in Classical Studies
- Classical History
- Classical Philosophy
- Classical Mythology
- Classical Literature
- Classical Reception
- Classical Art and Architecture
- Classical Oratory and Rhetoric
- Greek and Roman Papyrology
- Greek and Roman Epigraphy
- Greek and Roman Law
- Greek and Roman Archaeology
- Late Antiquity
- Religion in the Ancient World
- Digital Humanities
- Browse content in History
- Colonialism and Imperialism
- Diplomatic History
- Environmental History
- Genealogy, Heraldry, Names, and Honours
- Genocide and Ethnic Cleansing
- Historical Geography
- History by Period
- History of Emotions
- History of Agriculture
- History of Education
- History of Gender and Sexuality
- Industrial History
- Intellectual History
- International History
- Labour History
- Legal and Constitutional History
- Local and Family History
- Maritime History
- Military History
- National Liberation and Post-Colonialism
- Oral History
- Political History
- Public History
- Regional and National History
- Revolutions and Rebellions
- Slavery and Abolition of Slavery
- Social and Cultural History
- Theory, Methods, and Historiography
- Urban History
- World History
- Browse content in Language Teaching and Learning
- Language Learning (Specific Skills)
- Language Teaching Theory and Methods
- Browse content in Linguistics
- Applied Linguistics
- Cognitive Linguistics
- Computational Linguistics
- Forensic Linguistics
- Grammar, Syntax and Morphology
- Historical and Diachronic Linguistics
- History of English
- Language Evolution
- Language Reference
- Language Acquisition
- Language Variation
- Language Families
- Lexicography
- Linguistic Anthropology
- Linguistic Theories
- Linguistic Typology
- Phonetics and Phonology
- Psycholinguistics
- Sociolinguistics
- Translation and Interpretation
- Writing Systems
- Browse content in Literature
- Bibliography
- Children's Literature Studies
- Literary Studies (Romanticism)
- Literary Studies (American)
- Literary Studies (Asian)
- Literary Studies (European)
- Literary Studies (Eco-criticism)
- Literary Studies (Modernism)
- Literary Studies - World
- Literary Studies (1500 to 1800)
- Literary Studies (19th Century)
- Literary Studies (20th Century onwards)
- Literary Studies (African American Literature)
- Literary Studies (British and Irish)
- Literary Studies (Early and Medieval)
- Literary Studies (Fiction, Novelists, and Prose Writers)
- Literary Studies (Gender Studies)
- Literary Studies (Graphic Novels)
- Literary Studies (History of the Book)
- Literary Studies (Plays and Playwrights)
- Literary Studies (Poetry and Poets)
- Literary Studies (Postcolonial Literature)
- Literary Studies (Queer Studies)
- Literary Studies (Science Fiction)
- Literary Studies (Travel Literature)
- Literary Studies (War Literature)
- Literary Studies (Women's Writing)
- Literary Theory and Cultural Studies
- Mythology and Folklore
- Shakespeare Studies and Criticism
- Browse content in Media Studies
- Browse content in Music
- Applied Music
- Dance and Music
- Ethics in Music
- Ethnomusicology
- Gender and Sexuality in Music
- Medicine and Music
- Music Cultures
- Music and Media
- Music and Religion
- Music and Culture
- Music Education and Pedagogy
- Music Theory and Analysis
- Musical Scores, Lyrics, and Libretti
- Musical Structures, Styles, and Techniques
- Musicology and Music History
- Performance Practice and Studies
- Race and Ethnicity in Music
- Sound Studies
- Browse content in Performing Arts
- Browse content in Philosophy
- Aesthetics and Philosophy of Art
- Epistemology
- Feminist Philosophy
- History of Western Philosophy
- Metaphysics
- Moral Philosophy
- Non-Western Philosophy
- Philosophy of Language
- Philosophy of Mind
- Philosophy of Perception
- Philosophy of Science
- Philosophy of Action
- Philosophy of Law
- Philosophy of Religion
- Philosophy of Mathematics and Logic
- Practical Ethics
- Social and Political Philosophy
- Browse content in Religion
- Biblical Studies
- Christianity
- East Asian Religions
- History of Religion
- Judaism and Jewish Studies
- Qumran Studies
- Religion and Education
- Religion and Health
- Religion and Politics
- Religion and Science
- Religion and Law
- Religion and Art, Literature, and Music
- Religious Studies
- Browse content in Society and Culture
- Cookery, Food, and Drink
- Cultural Studies
- Customs and Traditions
- Ethical Issues and Debates
- Hobbies, Games, Arts and Crafts
- Lifestyle, Home, and Garden
- Natural world, Country Life, and Pets
- Popular Beliefs and Controversial Knowledge
- Sports and Outdoor Recreation
- Technology and Society
- Travel and Holiday
- Visual Culture
- Browse content in Law
- Arbitration
- Browse content in Company and Commercial Law
- Commercial Law
- Company Law
- Browse content in Comparative Law
- Systems of Law
- Competition Law
- Browse content in Constitutional and Administrative Law
- Government Powers
- Judicial Review
- Local Government Law
- Military and Defence Law
- Parliamentary and Legislative Practice
- Construction Law
- Contract Law
- Browse content in Criminal Law
- Criminal Procedure
- Criminal Evidence Law
- Sentencing and Punishment
- Employment and Labour Law
- Environment and Energy Law
- Browse content in Financial Law
- Banking Law
- Insolvency Law
- History of Law
- Human Rights and Immigration
- Intellectual Property Law
- Browse content in International Law
- Private International Law and Conflict of Laws
- Public International Law
- IT and Communications Law
- Jurisprudence and Philosophy of Law
- Law and Politics
- Law and Society
- Browse content in Legal System and Practice
- Courts and Procedure
- Legal Skills and Practice
- Primary Sources of Law
- Regulation of Legal Profession
- Medical and Healthcare Law
- Browse content in Policing
- Criminal Investigation and Detection
- Police and Security Services
- Police Procedure and Law
- Police Regional Planning
- Browse content in Property Law
- Personal Property Law
- Study and Revision
- Terrorism and National Security Law
- Browse content in Trusts Law
- Wills and Probate or Succession
- Browse content in Medicine and Health
- Browse content in Allied Health Professions
- Arts Therapies
- Clinical Science
- Dietetics and Nutrition
- Occupational Therapy
- Operating Department Practice
- Physiotherapy
- Radiography
- Speech and Language Therapy
- Browse content in Anaesthetics
- General Anaesthesia
- Neuroanaesthesia
- Clinical Neuroscience
- Browse content in Clinical Medicine
- Acute Medicine
- Cardiovascular Medicine
- Clinical Genetics
- Clinical Pharmacology and Therapeutics
- Dermatology
- Endocrinology and Diabetes
- Gastroenterology
- Genito-urinary Medicine
- Geriatric Medicine
- Infectious Diseases
- Medical Toxicology
- Medical Oncology
- Pain Medicine
- Palliative Medicine
- Rehabilitation Medicine
- Respiratory Medicine and Pulmonology
- Rheumatology
- Sleep Medicine
- Sports and Exercise Medicine
- Community Medical Services
- Critical Care
- Emergency Medicine
- Forensic Medicine
- Haematology
- History of Medicine
- Browse content in Medical Skills
- Clinical Skills
- Communication Skills
- Nursing Skills
- Surgical Skills
- Browse content in Medical Dentistry
- Oral and Maxillofacial Surgery
- Paediatric Dentistry
- Restorative Dentistry and Orthodontics
- Surgical Dentistry
- Medical Ethics
- Medical Statistics and Methodology
- Browse content in Neurology
- Clinical Neurophysiology
- Neuropathology
- Nursing Studies
- Browse content in Obstetrics and Gynaecology
- Gynaecology
- Occupational Medicine
- Ophthalmology
- Otolaryngology (ENT)
- Browse content in Paediatrics
- Neonatology
- Browse content in Pathology
- Chemical Pathology
- Clinical Cytogenetics and Molecular Genetics
- Histopathology
- Medical Microbiology and Virology
- Patient Education and Information
- Browse content in Pharmacology
- Psychopharmacology
- Browse content in Popular Health
- Caring for Others
- Complementary and Alternative Medicine
- Self-help and Personal Development
- Browse content in Preclinical Medicine
- Cell Biology
- Molecular Biology and Genetics
- Reproduction, Growth and Development
- Primary Care
- Professional Development in Medicine
- Browse content in Psychiatry
- Addiction Medicine
- Child and Adolescent Psychiatry
- Forensic Psychiatry
- Learning Disabilities
- Old Age Psychiatry
- Psychotherapy
- Browse content in Public Health and Epidemiology
- Epidemiology
- Public Health
- Browse content in Radiology
- Clinical Radiology
- Interventional Radiology
- Nuclear Medicine
- Radiation Oncology
- Reproductive Medicine
- Browse content in Surgery
- Cardiothoracic Surgery
- Gastro-intestinal and Colorectal Surgery
- General Surgery
- Neurosurgery
- Paediatric Surgery
- Peri-operative Care
- Plastic and Reconstructive Surgery
- Surgical Oncology
- Transplant Surgery
- Trauma and Orthopaedic Surgery
- Vascular Surgery
- Browse content in Science and Mathematics
- Browse content in Biological Sciences
- Aquatic Biology
- Biochemistry
- Bioinformatics and Computational Biology
- Developmental Biology
- Ecology and Conservation
- Evolutionary Biology
- Genetics and Genomics
- Microbiology
- Molecular and Cell Biology
- Natural History
- Plant Sciences and Forestry
- Research Methods in Life Sciences
- Structural Biology
- Systems Biology
- Zoology and Animal Sciences
- Browse content in Chemistry
- Analytical Chemistry
- Computational Chemistry
- Crystallography
- Environmental Chemistry
- Industrial Chemistry
- Inorganic Chemistry
- Materials Chemistry
- Medicinal Chemistry
- Mineralogy and Gems
- Organic Chemistry
- Physical Chemistry
- Polymer Chemistry
- Study and Communication Skills in Chemistry
- Theoretical Chemistry
- Browse content in Computer Science
- Artificial Intelligence
- Computer Architecture and Logic Design
- Game Studies
- Human-Computer Interaction
- Mathematical Theory of Computation
- Programming Languages
- Software Engineering
- Systems Analysis and Design
- Virtual Reality
- Browse content in Computing
- Business Applications
- Computer Security
- Computer Games
- Computer Networking and Communications
- Digital Lifestyle
- Graphical and Digital Media Applications
- Operating Systems
- Browse content in Earth Sciences and Geography
- Atmospheric Sciences
- Environmental Geography
- Geology and the Lithosphere
- Maps and Map-making
- Meteorology and Climatology
- Oceanography and Hydrology
- Palaeontology
- Physical Geography and Topography
- Regional Geography
- Soil Science
- Urban Geography
- Browse content in Engineering and Technology
- Agriculture and Farming
- Biological Engineering
- Civil Engineering, Surveying, and Building
- Electronics and Communications Engineering
- Energy Technology
- Engineering (General)
- Environmental Science, Engineering, and Technology
- History of Engineering and Technology
- Mechanical Engineering and Materials
- Technology of Industrial Chemistry
- Transport Technology and Trades
- Browse content in Environmental Science
- Applied Ecology (Environmental Science)
- Conservation of the Environment (Environmental Science)
- Environmental Sustainability
- Environmentalist Thought and Ideology (Environmental Science)
- Management of Land and Natural Resources (Environmental Science)
- Natural Disasters (Environmental Science)
- Nuclear Issues (Environmental Science)
- Pollution and Threats to the Environment (Environmental Science)
- Social Impact of Environmental Issues (Environmental Science)
- History of Science and Technology
- Browse content in Materials Science
- Ceramics and Glasses
- Composite Materials
- Metals, Alloying, and Corrosion
- Nanotechnology
- Browse content in Mathematics
- Applied Mathematics
- Biomathematics and Statistics
- History of Mathematics
- Mathematical Education
- Mathematical Finance
- Mathematical Analysis
- Numerical and Computational Mathematics
- Probability and Statistics
- Pure Mathematics
- Browse content in Neuroscience
- Cognition and Behavioural Neuroscience
- Development of the Nervous System
- Disorders of the Nervous System
- History of Neuroscience
- Invertebrate Neurobiology
- Molecular and Cellular Systems
- Neuroendocrinology and Autonomic Nervous System
- Neuroscientific Techniques
- Sensory and Motor Systems
- Browse content in Physics
- Astronomy and Astrophysics
- Atomic, Molecular, and Optical Physics
- Biological and Medical Physics
- Classical Mechanics
- Computational Physics
- Condensed Matter Physics
- Electromagnetism, Optics, and Acoustics
- History of Physics
- Mathematical and Statistical Physics
- Measurement Science
- Nuclear Physics
- Particles and Fields
- Plasma Physics
- Quantum Physics
- Relativity and Gravitation
- Semiconductor and Mesoscopic Physics
- Browse content in Psychology
- Affective Sciences
- Clinical Psychology
- Cognitive Psychology
- Cognitive Neuroscience
- Criminal and Forensic Psychology
- Developmental Psychology
- Educational Psychology
- Evolutionary Psychology
- Health Psychology
- History and Systems in Psychology
- Music Psychology
- Neuropsychology
- Organizational Psychology
- Psychological Assessment and Testing
- Psychology of Human-Technology Interaction
- Psychology Professional Development and Training
- Research Methods in Psychology
- Social Psychology
- Browse content in Social Sciences
- Browse content in Anthropology
- Anthropology of Religion
- Human Evolution
- Medical Anthropology
- Physical Anthropology
- Regional Anthropology
- Social and Cultural Anthropology
- Theory and Practice of Anthropology
- Browse content in Business and Management
- Business Ethics
- Business Strategy
- Business History
- Business and Technology
- Business and Government
- Business and the Environment
- Comparative Management
- Corporate Governance
- Corporate Social Responsibility
- Entrepreneurship
- Health Management
- Human Resource Management
- Industrial and Employment Relations
- Industry Studies
- Information and Communication Technologies
- International Business
- Knowledge Management
- Management and Management Techniques
- Operations Management
- Organizational Theory and Behaviour
- Pensions and Pension Management
- Public and Nonprofit Management
- Strategic Management
- Supply Chain Management
- Browse content in Criminology and Criminal Justice
- Criminal Justice
- Criminology
- Forms of Crime
- International and Comparative Criminology
- Youth Violence and Juvenile Justice
- Development Studies
- Browse content in Economics
- Agricultural, Environmental, and Natural Resource Economics
- Asian Economics
- Behavioural Finance
- Behavioural Economics and Neuroeconomics
- Econometrics and Mathematical Economics
- Economic History
- Economic Systems
- Economic Methodology
- Economic Development and Growth
- Financial Markets
- Financial Institutions and Services
- General Economics and Teaching
- Health, Education, and Welfare
- History of Economic Thought
- International Economics
- Labour and Demographic Economics
- Law and Economics
- Macroeconomics and Monetary Economics
- Microeconomics
- Public Economics
- Urban, Rural, and Regional Economics
- Welfare Economics
- Browse content in Education
- Adult Education and Continuous Learning
- Care and Counselling of Students
- Early Childhood and Elementary Education
- Educational Equipment and Technology
- Educational Strategies and Policy
- Higher and Further Education
- Organization and Management of Education
- Philosophy and Theory of Education
- Schools Studies
- Secondary Education
- Teaching of a Specific Subject
- Teaching of Specific Groups and Special Educational Needs
- Teaching Skills and Techniques
- Browse content in Environment
- Applied Ecology (Social Science)
- Climate Change
- Conservation of the Environment (Social Science)
- Environmentalist Thought and Ideology (Social Science)
- Natural Disasters (Environment)
- Social Impact of Environmental Issues (Social Science)
- Browse content in Human Geography
- Cultural Geography
- Economic Geography
- Political Geography
- Browse content in Interdisciplinary Studies
- Communication Studies
- Museums, Libraries, and Information Sciences
- Browse content in Politics
- African Politics
- Asian Politics
- Chinese Politics
- Comparative Politics
- Conflict Politics
- Elections and Electoral Studies
- Environmental Politics
- European Union
- Foreign Policy
- Gender and Politics
- Human Rights and Politics
- Indian Politics
- International Relations
- International Organization (Politics)
- International Political Economy
- Irish Politics
- Latin American Politics
- Middle Eastern Politics
- Political Behaviour
- Political Economy
- Political Institutions
- Political Methodology
- Political Communication
- Political Philosophy
- Political Sociology
- Political Theory
- Politics and Law
- Public Policy
- Public Administration
- Quantitative Political Methodology
- Regional Political Studies
- Russian Politics
- Security Studies
- State and Local Government
- UK Politics
- US Politics
- Browse content in Regional and Area Studies
- African Studies
- Asian Studies
- East Asian Studies
- Japanese Studies
- Latin American Studies
- Middle Eastern Studies
- Native American Studies
- Scottish Studies
- Browse content in Research and Information
- Research Methods
- Browse content in Social Work
- Addictions and Substance Misuse
- Adoption and Fostering
- Care of the Elderly
- Child and Adolescent Social Work
- Couple and Family Social Work
- Developmental and Physical Disabilities Social Work
- Direct Practice and Clinical Social Work
- Emergency Services
- Human Behaviour and the Social Environment
- International and Global Issues in Social Work
- Mental and Behavioural Health
- Social Justice and Human Rights
- Social Policy and Advocacy
- Social Work and Crime and Justice
- Social Work Macro Practice
- Social Work Practice Settings
- Social Work Research and Evidence-based Practice
- Welfare and Benefit Systems
- Browse content in Sociology
- Childhood Studies
- Community Development
- Comparative and Historical Sociology
- Economic Sociology
- Gender and Sexuality
- Gerontology and Ageing
- Health, Illness, and Medicine
- Marriage and the Family
- Migration Studies
- Occupations, Professions, and Work
- Organizations
- Population and Demography
- Race and Ethnicity
- Social Theory
- Social Movements and Social Change
- Social Research and Statistics
- Social Stratification, Inequality, and Mobility
- Sociology of Religion
- Sociology of Education
- Sport and Leisure
- Urban and Rural Studies
- Browse content in Warfare and Defence
- Defence Strategy, Planning, and Research
- Land Forces and Warfare
- Military Administration
- Military Life and Institutions
- Naval Forces and Warfare
- Other Warfare and Defence Issues
- Peace Studies and Conflict Resolution
- Weapons and Equipment

- < Previous chapter
- Next chapter >

14 Generalization, Case Studies, and Within-Case Causal Inference: Large-N Qualitative Analysis (LNQA)
Gary Goertz is Professor of Political Science at the Kroc Center for International Peace Studies at Notre Dame University. He is the author or editor of nine books and more than fifty articles and chapters on topics of international institutions, methodology, and conflict studies. His methodological research focuses on concepts and measurement along with set theoretic approaches, including “Explaining War and Peace: Case Studies and Necessary Condition Counterfactuals,” (2007), “Politics, Gender, and Concepts: Theory and Methodology” (2008), “A Tale of Two Cultures: Qualitative and Quantitative Research in the Social Sciences” (2012), and “Multimethod Research, Causal Mechanisms, and Case Studies: The Research Triad” (2017). The completely revised and rewritten edition of his (2005) concept book “Social science concepts and measurement” was published by Princeton in 2020.
Stephan Haggard is Krause Distinguished Professor at the School of Global Policy and Strategy at the University of California San Diego. His publications on international political economy include Pathways from the Periphery: The Newly Industrializing Countries in the International System (Cornell University Press, 1990); The Political Economy of the Asian Financial Crisis (Institute for International Economics, 2000); and Developmental States (Cambridge University Press, 2018). His work with Robert Kaufman on democratization, inequality, and social policy includes The Political Economy of Democratic Transitions (Princeton University Press, 1995); Democracy, Development and Welfare States: Latin America, East Asia, Eastern Europe (Princeton, 2008); Dictators and Democrats: Masses, Elites and Regime Change (Princeton, 2016) and Backsliding: Democratic Regress in the Contemporary World (Cambridge, 2020). His work on North Korea with Marcus Noland includes Famine in North Korea (Columbia University Press, 2007); Witness to Transformation: Refugee Insights into North Korea (Peterson Institute for International Economics, 2011); and Hard Target: Sanctions, Inducements and the Case of North Korea (Stanford University Press, 2017).
- Published: 23 February 2023
- Cite Icon Cite
- Permissions Icon Permissions
Abstract : Experiments no less than case studies always raise the question of generalization. This chapter discusses this problem and reviews a developing qualitative research practice that we call large-N qualitative analysis (LNQA). The core of the methodology lies in exploring postulated causal mechanisms within individual cases, but for a relatively large number of cases or even the entire population. The approach raises wider epistemological questions about how to generalize and the relationship between type and token causal inference.
Singular causal claims are primary. This is true in two senses. First, they are a necessary ingredient in the methods we use to establish generic causal claims. Even the methods that test causal laws by looking for regularities will not work unless some singular causal information is filled in first. Second, the regularities themselves play a secondary role in establishing a causal law. They are just evidence—and only one kind of evidence at that—that certain kinds of singular causal fact have happened. Nancy Cartwright
The particular and productive character of mechanisms in fact implies that we should think of causation as fundamentally a singular and intrinsic relation between events, rather than as something mediated by laws or universals. Stuart Glennan
Introduction
Philosophers of social science and causation have a long tradition of distinguishing between type versus token causal inference. Types are abstract and general; tokens are concrete particulars. As illustrated in the epigraphs to this chapter, we think that all causal regularities or generalizations ultimately rest on the effects that operate in individual cases. If an experiment shows that there is a significant average treatment effect, that must mean that there are individual cases in which the treatment influenced the outcome for that case. Although cast in probabilistic terms, the average treatment effect is ultimately a kind of summing up of individual level causal influences. If there was no causal effect at the case level, there could be no treatment effect at the population level.
In this chapter we pursue the notion that type causation is a generalization of token causal inference. In the social sciences, serious interest in the role of token causal inference received little methodological attention until qualitative and multimethod research took off in political science and sociology over the course of the 1990s. Process tracing and counterfactuals have been focal points in that literature. Process tracing explores the mechanism by which X produced or caused Y in an individual case. Similarly, counterfactual analysis focuses on the determinants of outcomes in individual cases by posing and then confirming or dismissing alternative explanations. This is typically counterfactual dependence with mechanisms . 1 We focus on what in the causal mechanism literature is often known at the “trigger” that is the initial factor in a causal mechanism. 2 The logic here is of sufficient conditions: the initial factor is sufficient to set the causal mechanism in motion. In this chapter we leave the causal mechanism in general as a black box and focus on the generalizability of the mechanism.
A central contention of this chapter is that both experiments and case studies face the problem of external validity or, what we prefer, the problem of generalization. How generalizable is the randomized experiment or case study? Experimentalists in political science have started to tackle this problem. The Metaketa project has repeated experiments across different countries to see how generalizable findings are ( Dunning et al. 2019 ). We are seeing a similar effort to think about generalization from case studies as well. A recent example is Kaplan ’s excellent book on civil society organizations in civil war (2017) . It starts with what we call a causal mechanism case study. The remainder of the book is preoccupied with how generalizable that mechanism is in Columbia as well as in other settings such as Syria and Afghanistan. Ziblatt’s (2017) analysis of the role of conservative parties in European democratization rests on an extensive causal mechanism case study of the UK as well as a comparison with Germany. In his last chapter, however, he provides additional case studies on other European countries and briefer discussions of transitions in Latin America, the Middle East, and Asia.
A new research practice has emerged in recent years both among multi-method and qualitative researchers: to multiply the number of qualitative case studies in order to strengthen causal inference that we call Large-N Qualitative Analysis (LNQA). Early examples of the work took a particular form: they sought to challenge prominent statistical or game-theoretical findings by showing that postulated causal relationships did not in fact hold when subjected to closer scrutiny via within-case causal inference; among the targets of this work were prominent accounts on inequality and democratization ( Haggard and Kaufman 2016 ), democratization and war ( Narang and Nelson 2009 ), the effect of audience costs on conflict ( Trachtenberg 2012 ), and the role that rebel victory plays in civil war settlements ( Wallensteen 2015 ; see Goertz 2017 , chapter 7 for a discussion). However, the approach has subsequently expanded to define a wider research methodology aimed not only at disconfirming existing analysis but supporting multimethod and in-depth case study work as well.
LNQA is clearly most conducive to the analysis of rare events, or those in which the N is small, such as famines, wars and civil wars, regime changes or the acquisition of nuclear weapons. The approach sometimes starts with statistical analysis, and thus takes a multimethod approach. Other examples, such as the work by Kaplan just cited, start with a single in-depth case study and then augments it with others. But the core of the approach is the use of a (relatively) large number of individual case studies, and even a whole population, in order to strengthen causal inference and generalizability.
To date, these practices have not been justified by reference to methodological works or even by methodological discussions (see however Haggard and Kaufman 2016 ; Goertz 2017 ). In the spirit of what Goertz calls “methodological ethnography,” this chapter outlines this approach and seeks to ground it theoretically. Based on practice both among experimentalists and those using case studies we argue that the logic of generalization at work is what we will call “absolute generalization” versus the statistical logic of comparison and relative generalization. Causal inference is strengthened via multiple within-case causal inferences rather than comparisons between control and treatment groups or other comparative approaches.
Toward the end of the chapter we explore some concrete examples of this methodology in action. We provide an extended discussion of two prominent books that have effectively employed the research methodology that we outline here. One is an international relations example, Sechser and Fuhrmann’s Nuclear weapons and coercive diplomacy , the other is from comparative politics, Ziblatt’s Conservative parties and the birth of democracy . They illustrate virtually all of the key features of LNQA.
Our analysis of case studies and generalization links very naturally to the philosophical literature on causal mechanisms. As we move through the methodological issues in a political science context we connect with familiar authors and works in the causal mechanism literature in philosophy. For example, our emphasis on within-case causal inference and generalization fits quite naturally with the requirement that causal explanation involve the analysis of mechanisms. As we shall see, “regularities”—be they observational or experimental—require more detailed analysis of mechanisms within cases.
Generalization and (External Validity, Extrapolation, Regularities, Transportability, Analytic Generalization, etc.)
The concepts of external validity, along with its partner internal validity, were introduced into the methodological literature in the classic Campbell and Stanley volume. Campbell and Stanley (1963) define external validity in terms of “generalizability: To what populations, settings, treatment variables, and measurement variables can [an] effect be generalized?”
As Shadish, Cook, and Campbell note, “Although internal validity may be the sine qua non of experiments, most researchers use experiments to make generalizable causal inferences” (2002, 18–20). However, experiments are generally seen as weak on external validity, in part because sample populations in lab experiments are not seen as representative ( Druckman and Kam 2011 ; Bardsley et al. 2010). However, the problem goes deeper and extends to field experiments as well. What is to assure that an experiment in one setting will necessarily yield the same result in a different one where context is fundamentally different?
In her discussion of external validity, McDermott provides the standard solution: “external validity results primarily from replication of particular experiments across diverse populations and different settings, using a variety of methods and measures” ( McDermott 2011 , 34). Literature reviews and meta-analysis attempt syntheses of these findings, and implicitly reach judgments of the extent to which diverse experimental findings should be considered robust. Recently, a major research project—the Metaketa project—has attempted to test some core propositions in political science through highly structured replications. While meta-analysis and replication have gotten more sophisticated, however, there is surprisingly little guidance on how such replications might produce higher or lower levels of generalization.
In their very nice review of the experimental literature on behavioral economics Bardsley et al. call these experiments aimed at increasing external validity “exhibits.” They define the exhibit as “a replicable experimental design that reliably produces some interesting result” ( Bardsley et al. 2010 , epub 409).
Among experimenters in political science the term “transportability” seems to have gained in popularity. We prefer the term generalization because external validity has other dimensions as well, such as how realistic the lab experiment might be. We think it is also the preferred language of those who do case studies: the question most often posed to such studies is exactly their generalizability.
For experimentalists, the definition of generalizability then becomes something like:
The extent to which the same treatment X = 1 produces a similarly significant average treatment effect Y = 1 un der some scope conditions S .
Our working definition of generalization in the case study context, by contrast, underlines the importance of token causal inference to the process of achieving external validity:
The same causal mechanism produces the same outcome based on valid within-case causal analysis in all or a high percentage of cases within some scope conditions S .
In the causal mechanism literature in philosophy this is the “regularity” condition that typically appears in conceptualizations of causal mechanisms.
In short, experiments and case studies have problems of generalizability. To be sure, these problems are subtly different. Experiments generate findings in the form of an average treatment effect, which may or may not extend to other settings. Within-case causal inference offers an explanation for a particular case but the mechanisms may or may not yield the same outcome in a different setting. When well done, however, both have high degrees of internal validity. But case studies are not alone in being vulnerable to the question of generalizability; experiments face this challenge too.
Absolute Generalizations
A core claim of this chapter is one about methodological ethnography. Scholars doing large-N qualitative analysis, working either with the entire population of relevant cases or a relatively large sample of them (roughly ten-plus case studies), often perform what we call in this section absolute tests. A claim is made about a causal relationship or the operation of a causal mechanism in law-like, sufficient-condition, or even necessary-and-sufficient condition, form: if X then Y . Conversely, a number of prominent disconfirmatory studies have tested law-like statements, showing that they in fact fail the sufficient or necessary-and-sufficient condition test when mechanisms are examined more carefully. Yet these practices are rarely if ever justified methodologically or with reference to a corresponding methodological literature. In this and the following section we take up the logic of absolute and relative generalizations, starting with a basic reduced-form example of the former, and then introducing relative as well as absolute tests and the crucial role of causal mechanisms in the method.
Table 14.1 presents our basic set up in a 2 × 2 table. A distinctive feature of the approach is both its consideration of the distribution of cases and the particular emphasis it places on the X = 1 column. X = 1 means the treatment has been given in an experimental context or that X has occurred in an observational setting. Two outcomes are then possible: the treatment has an effect (the ( 1 , 1 ) cell) or it doesn’t (the ( 1 , 0 ) cell). We call the ( 1 , 1 ) cell the causal mechanism cell, as consideration of cases from the cell are designed to test for the operation of the postulated causal mechanism. As we shall see below, the X = 0 column plays a role when we deal with equifinality, but not in the basic generalization of the causal mechanism.
Many multimethod and qualitative books in recent years include a core case study that illustrates the basic theory. In multimethod books these will sometimes follow the statistical analysis; in qualitative books they are more likely to lead and are often intensive, multi-chapter analyses. These cases inevitably come from the ( 1 , 1 ) cell; they are designed not just to illustrate but to test for the effect of the postulated causal mechanism in the nominally conforming cases.
In purely qualitative books, these central causal mechanism case studies generate the question about generalization which then occupies the latter part of the book. However, just looking at the ( 1 , 1 ) cell ignores the situation where the causal mechanism may not be working, which is critical to the generalizability question. This is the ( 1 , 0 ) cell of Table 14.1 , and we return to it in more detail below.
An example from Levy and Thompson illustrates the basics of the generalization logic and the utility of focusing on the X = 1 column with a classic hypothesis from realism in international politics. Levy and Thompson test one of the most influential theories in the history of international relations, balance of power theory. As they note in another article “The proposition that near-hegemonic concentrations of power in the system nearly always trigger a counter-balancing coalition of the other great powers has long been regarded as an ‘iron law’ by balance of power theorists” ( Levy and Thompson 2010 ). This “iron law” is a generalization in the terms of this chapter, a type-level causal claim, and one that is made in strong law-like or sufficient-condition form. A core version of the balance of power hypothesis involves balancing against hegemons: if there is a hegemon then other states will form an alliance to balance it. The short version of the hypothesis is “if hegemon, then balancing.”
The logic of “if treatment then outcome” suggests where we need to go to see how generalizable a causal mechanism case study might be. The “if” defines what we call an absolute generalization: if X = 1 then the outcome Y = 1 occurs.
Table 14.2 shows balancing 55 percent of the time if there is a hegemon. If the iron law with respect to balancing held, then the probability of balancing would be near 1.0, which is the common-sense meaning of an “iron law.” So this proposition is rejected because .55 is not near 1.0. 3
χ 2 = 28 , p = .000 , N = 445
If this were an experimental test, we would be asking whether the hegemon “treatment” were adequate to generate a statistically significant population-level effect or an average treatment effect. The comparative generalization test compares the percentages in the X = 1 versus the X = 0 column. This then generates well-known 2 × 2 statistics of association as well as average treatment effects.
In the relative test in Table 14.2 this becomes the bar of 30 percent in the nonhegemon column. This is of course why it is a relative test; it is the comparison of the percentages in the two columns as opposed to the absolute percentage in one column. Thus hegemonic balancing passes the relative test, i.e., significant χ 2 . But note that it does not pass the absolute test; the generalization is in fact quite weak. The χ 2 test, like most tests of two-way tables—is comparing percentages across columns, i.e., 30 percent is significantly different from 55 percent. But Levy and Thompson are not posing the question in relative terms; they are postulating a law-like regularity. Literature on scientific laws, e.g., Armstrong (1983) almost inevitably discusses them in terms of how many Y ’s are also an X an absolute as opposed to comparative framing. The famous democratic peace example is posed as follows: joint democracy triggers a mechanism (or mechanisms) for not-war 100 percent of the time. The hegemonic balancing hypothesis has the form of a sufficient condition: if hegemon then balancing.
Despite its failure to meet the conditions of an absolute test, does it nonetheless constitute a modestly important generalization? And can we draw a judgment to that effect without relying on statistical, comparative analyses? Those interested in necessary conditions or qualitative comparative analysis (QCA) have thought about this question, and about standards for absolute generalizations, in this case a sufficient condition generalization.
Within QCA there are some common standards, e.g., like p values, for saying there is significant support for a sufficient condition hypothesis. These tend to have a minimum bar of around 75–80 percent (often higher for necessary conditions; see Schneider and Wagemann 2012 ). Within QCA this constitutes the criterion for passing the sufficient condition test. Since the balancing hypothesis is a sufficient condition one, percentages above this or some other stipulated bar constitute passing the test. We call it an absolute test because it only uses information in the X = 1 column .
This example illustrates two key points. First, generalization from case studies is typically framed in terms of absolute not relative generalizations. Second, the absolute and relative criteria for judging generalization do not have to agree because they are different criteria. It is possible to have comparative effects that are significant and to also see strong absolute effects. However, two other outcomes are also possible. First, it is possible to have a clear a high absolute bar and still conclude that the relative evidence is weak. Conversely, the relative test might appear strong but there are too many falsifying cases (i.e., ( 1 , 0 ) cases) to satisfy an absolute criterion.
The hegemony example is a hypothesis that does not pass the absolute test; the generalization is weak. One might wonder whether this is “too hard” a test, and it could be in the social sciences if such tests were virtually impossible to pass. However the democratic peace examples suggests this is not the case; we see significant research programs in political science around generalizations of this sort. Below we consider a prominent book by Ziblatt in some detail and pursue this question. The basic hypotheses is “if strong conservative party before mass democratization then stable democracy.” Ziblatt does not do an explicit test such as those presented in our tables, and it is not clear exactly what population is fulfilling the “if” which defines X = 1 column, i.e., the scope conditions. Nonetheless, it appears from his discussion that the proposition would pass the absolute test for both pre-war European democratic experiences and a sample of post-war cases. Ziblatt discusses a number cases in varying degrees of detail but mentions no clear falsifying example.
Until this point, causal mechanisms and within-case causal inference have not made an appearance. Whether we are performing an absolute or relative test we are still looking at patterns across data and are not looking at causal inferences within any of the given cases. However, the set-up is critical because it tells us where to go to do the within-case causal inference. Again, this is the critical role of the X = 1 column in defining the population for generalization and thus for case selection.
Relative Tests and Within-Case Causal Inference
We shall treat the notion of a token cause to be roughly equivalent to within-case causal inference, which means making causal claims about individual cases. The modern philosophical literature on token causal inference, starting with Anscombe (1971) and Lewis (1973) , rests basically on the possibility of doing counterfactuals as a way of generating causal inference in individual cases. However, as Holland has famously discussed, this is “impossible”:
Fundamental Problem of Causal Inference. It is impossible to observe the value of Y t (i) and Yc ( i ) on the same unit and, therefore, it is impossible to observe the effect of t on i. ( Holland 1986 , 947) The important point is that the statistical solution replaces the impossible-to-observe causal effect of t on a specific unit with the possible-to-estimate average causal effect of t over a population of units. ( Holland 1986 , 947)
One natural consequence of this statement of the problem of inference is that it is “impossible” to do within-case causal inference because one cannot construct a real counterfactual case for the comparison. As Holland notes, the best we can do is to compare control groups with treatment groups, hopefully with randomization of treatments, and to derive average treatment effects in populations. Causal inference is based on cross-case comparisons. 4
The literature on process tracing and causal process observation, which adopts a mechanism approach to causation, rejects these assumptions. Rather, it assumes that token causal inference is possible. Overwhelming evidence from our everyday lives as well as natural science supports this claim. We assume that individual events can be explained “internally” without reference to population patterns. An example is the space shuttle Challenger explosion. Scientists devoted tremendous energy into why this single event happened, and in less than three years another Space Shuttle Discovery lifted off with a crew of five from Kennedy Space Center. Clearly, the teams investigating the initial failure believed it was possible to find the cause of the singular event that led to the explosion and confident they could prevent it from recurring.
Glennan makes this point in his discussion of the new mechanical philosophy, which is closely related to the move toward within-case causal inference. Based on the distribution of conforming and non-conforming cases in a statistical analysis such as Table 14.2 , we only have what he calls “a bare causal explanation”: “Bare causal explanations show what depends upon what without showing why or how this dependence obtains. The causal claims required are established, broadly speaking, by observational and experimental methods like Mill’s methods of agreement and difference or controlled experiments. Ontologically speaking, causal dependencies require the existence of mechanisms, but bare causal explanations are silent on what those mechanisms are” ( Glennan 2017 , 224).
He then discusses at some length an example from the history of medicine where Semmelweis linked the failure to wash hands and instruments to sepsis in Vienna hospitals: “Semmelweis sought to explain the epidemic of puerperal (or childbed) fever among mothers giving birth at the Vienna General Hospital during the 1840s. His first observation was that the division of the hospital to which the women were admitted appeared to be causally relevant, since the death rate from puerperal fever for women in the First Division was three to four times that of women admitted to the Second Division (6.8–11.4% versus 2.0–2.7%)” ( Glennan 2017 , 224). These statistics imply basically something like Table 14.2 .
In his specific example, Clara—a mother with puerperal fever contracted in the non-hygienic division of the hospital—clearly belongs in the ( 1 , 1 ) causal mechanism cell. She is definitely in the treatment group and perhaps even receives more specifically the treatment of unwashed hands and instruments. As Glennan notes this does not necessarily mean that she got the disease via those treatments:
Let us start with the single case. Suppose Clara contracted puerperal fever (call this event e). What caused her to contract it? A first explanation might simply be that Clara contracted puerperal fever because she delivered her baby in the First Division (call this c). If the claim that c caused e is true, that is, if there exists a mechanism by which c contributes to the production e, then that claim provides a bare causal explanation of e. Note that the mere fact that there is a higher incidence of puerperal fever in the First Division is not sufficient to guarantee there is such a mechanism, because it might be the case that that mechanism did not depend upon Clara’s being in the First Division. ( Glennan 2017 , 225)
The kicker comes in the final statement that he makes in discussing this example: “I would argue that until this generalization is attached to particular cases, there is no explanation” ( Glennan 2017 , 225).
The within-case causal inferences for cell ( 1 , 1 ) cases are important because, as in Table 14.2 , correlation is not causation, neither in observational nor experimental research: it is always an inference more or less well founded. In an experimental setting those individual cases in the ( 1 , 1 ) cell all count as evidence for the impact of the treatment. This is exactly the point Cartwright is making in her epigraph to this chapter. The average treatment effect must be built upon individual cases where the treatment caused the outcome at least in part.
The within-case causal analysis is thus an examination of cases in the ( 1 , 1 ) cell to ascertain whether the postulated causal mechanism is active; within-case causal inference as used here implies a focus on causal mechanisms.
With that framing, we can now turn to some examples from the increasing body of mixed-method and qualitative research that seeks to strengthen causal inference and generalization by conducting within-case causal inference on a large number of cases. We start with the famous theory of Acemoglu and Robinson that inequality affects the likelihood of democratization. We draw on the multi-method analysis of Haggard and Kaufman (2016) , which supplements statistical analysis with consideration of causal mechanisms in individual cases to see how this methodology plays out in one important substantive domain. We also use the case to again underline the difference between absolute and relative tests.
Acemoglu and Johnson’s theory is presented in formal terms, through a series of game theoretic models. They do not explicitly state their core arguments in absolute terms, but game theoretic models typically generate necessary and sufficient conditions claims and their models do show crisp equilibria that should rule out certain transition paths. However, their claims about inequality and how transitions occur can be put in probabilistic terms. First, they argue that transitions to democratic rule are more likely at moderate levels of inequality than in highly unequal or highly equal countries; at high levels of inequality, elites will resist the attendant distributional outcomes; at low levels of inequality, demands for redistribution via regime change are muted. As stated, however, levels of inequality constitute only a permissive condition for democratization. Acemoglu and Robinson also argue that inequality is ultimately related to democratization via the mechanism of mass mobilization; it is through mass mobilization or the exercise of what Acemoglu and Robinson call “de facto power,” that authoritarian rulers are ultimately dislodged. In the absence of such pressure, why would autocrats forego the advantages of incumbency?
We can now replicate the analysis on hegemony and balancing by taken data from Haggard and Kaufman (2016) on inequality and regime change, but now framing their findings both absolute and relative terms and adding in within-case causal analysis of the theory linking inequality and regime change via mass mobilization. This exercise is given in Table 14.3 . 5 Again, both claims derived from the Acemoglu and Robinson model are subject to scrutiny here: those having to do with the greater likelihood of transitions in medium-inequality countries; and that they should occur via mass mobilization.
χ 2 = 3.8 , p = .15 , N = 173
The absolute hypotheses would be that if there is medium-levels of inequality then there is democratization through the mechanism of mass mobilization. The relative hypotheses would be that they are more likely to occur in moderately unequal authoritarian regimes and via the mechanism of mass mobilization, ceteris paribus. Note that “relative” means relative to other paths to the outcome, and thus implicitly raises the issue of equifinality or other paths to the outcome.
Table 14.3 gives the same basic analysis as Table 14.2 above for the hegemony and balancing hypothesis. Now can begin to consider a stipulated causal mechanism. If a country is democratic for the entire inequality period, it is deleted; we are only considering the population of authoritarian regimes, seeing the conditions under which they might transition. We use the terciles of the inequality data for all authoritarian governments to constitute the inequality categories. Given that inequality changes quite slowly we have treated each country as one observation; we consider each of the three inequality categories as a “treatment.” If a country never changes inequality category and never has a transition, it counts as one observation of Y = 0 . If there is a transition, then that constitutes a positive value on Y for the whole inequality period. If a country’s level of inequality changes to another category (tercile), however, that constitutes a new treatment. It is thus possible that a given country constitutes one observation if its inequality category does not change or potentially three or four observations if it changes inequality categories. Thus the number of years per observation, i.e., country-inequality category, can vary significantly depending on how long the country stays within a given inequality category. However, the number of years in each inequality category overall is based on equal treatment: because we use the terciles of authoritarian regime years, the basic categories have basically the same number of years.
The X = 1 column focuses on the core Acemoglu and Robinson inequality hypothesis in its absolute form. The X = 0 as well as the X = 2 columns present the incidence of transitions which do not occur at intermediate levels of inequality. As with the hegemony example above the key thing is the percentage of democratizing cases in the X = 1 column. The medium inequality column does not pass the absolute test, with the proportion of cases being about one-third. For the relative test the χ 2 statistic for the table is not significant either indicating that the proportions for the other columns are not radically different. It is higher for the high inequality category, but there is no difference between the low inequality category and the medium at all.
The game theory model in the book might be read to make an absolute claim regarding the high and low inequality columns: there should be no transitions in these situations. This would be an absolute test with probability of 0.0. One can do a probabilistic test of these absolute hypotheses saying that if the probability is less than, say, .25 (symmetric to the .75 used for the QCA sufficiency bar), then it passes the absolute test for these columns. As is clear from the table these columns do not pass this absolute test.
However the mere incidence of cases across different levels of inequality does not test for the presence of the stipulated causal mechanism, namely, mass mobilization. The theory rests on the presumption—quite reasonable—that such transitions are not simply granted from above, but ultimately reflect the exercise of “de facto power” on the part of mass publics; in Przeworski’s (2009) terms, democracy is “conquered” not “granted.” The ( 1 , 1 ) cell for Acemoglu and Robinson (2006) is thus ultimately not only a cell in which there is an intermediate level of inequality and a transition, but one in which there is an intermediate level of inequality and in which the transition occurs through mass mobilization. Using the usual symbols for tracing a causal mechanism, this can be indicated as X → M → Y where X is moderate inequality and M is mobilization.
The within-case question is therefore whether the observed cases in the ( 1 , 1 ) cell were caused by inequality via mobilization; answering this question requires consideration of each observation individually, in short, token causal inference analysis. This could be done via process tracing, counterfactuals, or other within-case causal inference strategies; Haggard and Kaufman do it through construction of a qualitative data set that interrogates each case for the presence or absence of mass mobilization.
When using the within-case generalization strategy several things can happen. The first is that the cases in the ( 1 , 1 ) cell were generated via the mechanism of mobilization. These token analyses support the basic theory. But it could also be that an examination of the ( 1 , 1 ) cases reveals that moderate inequality leads to democratization but not via their proposed mechanism. We shall deal with this important issue in the next section, where we note that mobilization may appear as a mechanism in the X = 0 column as well as the X = 2 column. This could support the mobilization mechanism, but not the connection between democratization and a particular level of inequality and mass mobilization.
The causal mechanism test for generalization generally involves the simultaneous analysis of both X as well as the mechanism, M . In Table 14.4 we include those cases in the (1,1) cell that were generated by mobilization. We also include those in the other columns that were also generated by mass mobilization. In parentheses we have included the original Ns from Table 14.3 .
While Table 14.4 looks like a regular two-way table it is fundamentally different from Table 14.3 above. To emphasize this we have put the number of cases in which democratization occurs via mobilization in boldface. We do this to stress that these are counts of token, within-case causal inferences: the number of cases that exhibit the postulated mechanism. Unlike Table 14.3 , which might be used to make a causal inference, Table 14.4 is a summary of token causal inferences . The boldface numbers would be like summarizing the results of a number of experiments. The question then becomes does this summary of token causal inferences permit us to make a type causal inference about moderate inequality operating through the mechanism of mass mobilization?
χ 2 = 1.96 , p = .38 , N = 65
Note: Total transitions cases from Table 14.3 in parentheses.
Table 14.3 looks at the basic hypothesis with the mechanism still black boxed: ( X = 1 ) → ( Y = 1 ) . This happened 21 times. We now include the mobilization mechanism into the mix. This is asking if in these 21 cases we saw this: ( X = 1 ) → ( M = 1 ) → ( Y = 1 ) , where M = 1 means that the mobilization mechanism was part of the reason why Y occurred. Generating these counts requires process tracing and even counterfactual dependence Analysis, in short, token causal inference with respect to each case.
If we include mobilization token causal inferences into the mix, the data in Table 14.4 fails to show support either for the reduced-form inequality hypothesis nor for the mobilization mechanism. In other words, Table 14.4 shows that of the 21 ( X = 1 ) → ( Y = 1 ) cases only 8 had ( X = 1 ) → ( M = 1 ) → ( Y = 1 ) . Only 38 percent of the transitions in the medium-inequality category come via their postulated causal mechanism. Hence some other mechanism generated the outcome in the other 13 cases.
It is also worth noting that the percentage of mobilization mechanism cases is higher in the high inequality category than it is in the middle category. If one did a χ 2 statistic just comparing the middle category to extreme inequality separately the χ 2 statistic begins to look quite significant, but not in the direction that Acemoglu and Robinson suggest; more transitions are likely to take place via mass mobilization in the high-inequality cases, even though they were not expected to take place there at all! This suggests that mass mobilization may play a role in some democratic transitions, but again, that inequality does not appear to play a significant causal role.
We have framed our discussion of hegemonic balancing in terms of strong regularities with percentages of .75 greater. One can go in the other direction and ask the skeptics question about whether there is any evidence at all for the hypothesis in question. This is basically asking if the regularity is in fact near zero. So in the hegemonic balancing example one might claim that .55 is some evidence in favor of the theory even though it does not form an iron law.
In their statistical analysis of Acemoglu and Robinson Haggard and Kaufman basically rejected their hypothesis. So their conclusion based on the econometric model is that that there is no relationship between medium levels of inequality and democratization. But as we have seen once one has started to do within-case causal inference on individual cases that can change conclusions.
As we noted above, 8/21=38 percent of cases of medium inequality showed support for the hypothesis. So while it is not a strong regularity it is significantly greater than zero at the same time. So based on within-case causal inference analysis there is modest support for the hypothesis. This again is perhaps quite different than rejecting the hypothesis altogether based on the statistical analysis.
The analysis opens doors onto other lines of research. Following the logic of LNQA, we might investigate in much more detail the eight cases that support their mechanism. These are the confirming cases and it could be worth exploring how the details of these eight match the details and discussion of their mechanism. Haggard and Kaufman ultimately use the information they collect on so-called “distributive conflict transitions” to theorize about other causal factors that might influence this class of transitions; we return to this feature of the approach in more detail below.
Nonetheless, the findings are damning: Haggard and Kaufman—using a combination of statistical and within-case causal analysis—find at best modest support for the Acemoglu and Robinson theory, neither with respect to their claims about inequality nor their claims about the role of mass mobilization. How damning depends the extent to which Acemoglu and Robinson were claiming to providing the mechanism for democratization or a mechanism, i.e., 8/21 for the Acemoglu and Robinson mechanism and 13/21 for some other unspecified mechanism. There is certainly evidence for the latter but not the former, but note that their claim is now reduced to one of identifying a causal path that sometimes occurs, but not with overwhelming frequency.
To summarize, the LNQA methodology involves investigation of absolute tests to see if there is any prima facie evidence for a strong generalization. However, it can also be used to support relative generalizations, particularly in mixed method designs with complementary statistical analysis. The next move, however, is crucial: within-case causal inference to see if the hypothesized mechanisms are present or not in the cases. The absolute test focuses on X while the causal mechanism tests focus on the M . As can be seen, these are related but separate analyses; a reduced form finding may or may not be supported when we turn to evidence of the presence of the mechanism.
Equifinality and Multiple Pathways to the Outcome
Our analysis above only focused on the connection between a particular theoretical hunch linking moderate inequality, mass mobilization, and democratization. However, it is critical to understand how multiple causal paths can lead to the same outcome. How does the ever-present possibility of equifinality figure into the methodology? 6 Note that mass mobilization was seen to operate in less than half of the cases across all levels of inequality, implying that some other causal mechanism or mechanisms were at work in the democratization process. For example, a number of scholars have argued that international pressure is another cause of democratization.
The question of equifinality arises when there are cases in the X = 0 and Y = 1 cell. That means something other than X is causing Y . In general most scholars do not claim there is only one path to the outcome; in various ways they assume equifinality. For example, international relations scholars would probably find it objectionable to claim that hegemony is the only circumstance under which balancing could occur. Similarly, it would be odd to claim that democratization only occurs in moderately unequal countries—and through mass mobilization—when there are plenty of instances where this is manifestly is not the case. That is exactly what Table 14.3 shows.
In the discussion of balancing against hegemony, it is quite clear that the basic hypothesis was in fact posed as an absolute one. However, it might be the case that the argument is rather a relative one. In the democracy case, moderately unequal authoritarian countries are more likely to democratize than very equal or unequal countries. If posed in these terms, the hypothesis demands a relative test. Haggard and Kaufman provide an extensive set of relative tests using standard statistical techniques, such as standard panel designs and fixed and random effects models. These tests reject the relationship between inequality and democratization in this relative form as well.
The question here is how causal mechanism and token analysis might fit into a comparative, relative test? We have already suggested an answer: that while their particular theory linking moderate inequality to democratization through mass mobilization is rejected, it is the case that mass mobilization is the causal mechanism at work in over half of all transitions. Haggard and Kaufman go on to argue that mass mobilization may not be due not to inequality but to the robustness of social organization: mass mobilization is more likely where unions and other civil society organizations are present. In effect, Haggard and Kaufman also use the distribution of cases not simply to cast doubt on the Acemoglu and Robinson model, but also to identify alternative causal pathways to democracy: from social organization, through mass mobilization to democracy.
Let’s call this additional path Z , perhaps international pressure, social organization or some other mechanism. So now instead of a two-way table we would have a three-dimensional table with the third dimension being the alternative path to Y . This is in fact what Haggard and Kaufman do: they theorize that there are two causal pathways to democracy, one involving mass mobilization and the other not. They then go on to explore some of the underlying causal factors at work in cases not characterized by pressure from below, including international pressures and the calculations of incumbent elites.
Opening another theoretical front necessarily raises questions of overdetermination. Overdetermination means there are cases where X = 1 and Z = 1 are producing Y = 1 . The key point is that a particular case might lie on two or more pathways or have two mechanisms present at the same time. This can be thought of as ( X = 1 OR Z = 1) → Y . Equifinality can also occur at the mechanism level: ( X = 1) → ( M 1 OR M 2 ) → Y . One sees this frequently in quantitative articles where the author proposes multiple mechanisms that can explain the significant effect of X on Y.
As discussed in all the process tracing literature, a key role of this methodology is to evaluate and adjudicate between competing explanations at the level of the individual case. This can be at X–Z level or the M level. That is exactly the problem here. If possible it would be useful to determine the extent to which one pathway is really the dominant explanation or the other. Of course one might conclude that there is a mixed mechanism involving them both.
These overdetermined cases would be thrown out of statistical analyses because they are not informative. But within-case causal analysis allows the researcher to take a more nuanced approach. For example, within case causal analysis could conclude that the other factor was present (say international pressure), but had no causal influence over the outcome. For example, Schenoni and his colleagues (2019) looking at the resolution of territorial conflicts in Latin America argue that they result from the conjunction of militarization of the territorial dispute, regime change toward democracy, and international mediation. A possible critique is that they have omitted a core explanatory factor in the form of US hegemony. US hegemony is a Z variable in addition to their three X variables. In a series of within-case analyses they argue that this was not the case for the individual settlements that they study: US actions were not a cause of territorial conflict resolution. In the case of Haggard and Kaufman treatment of democratization, moderate inequality might be present, but within-case analyses argue that it had no causal impact on the outcome via the causal mechanisms stipulated by Acemoglu and Robinson; it was the other path—e.g., international pressure—which had causal effect. The key point is that moderate inequality can led to democratization via M 1 , the Acemoglu and Robinson mechanism, or via the M 2 via alternative mechanism.
As this short discussion stresses, the approach outlined here leads quite naturally to discussion of equifinality in a way that standard statistical tests do not. Balancing may be causally related to rising hegemons, but not only. Similarly, democracy maybe caused by mass mobilization, but not only. Considerations of equifinality lead the researcher to think about how to theorize alternative pathways and to establish the scope conditions under which one or another pathway emerges. To deal with issues of equifinality empirically also requires within-case causal analysis to disentangle the impact of confounders, which are in fact alternative pathways to Y.
The key point is that equifinality occurs both at the X--Z level as well as at the mechanism level. Within case analysis is essential to disentangling causal inference when there is potential overdetermination both at the X--Z level as well as at the M level.
Case Selection Strategies When There Are Too Many Cases for Intensive Within-Case Inference
Absolutely core to the within-case generalization strategy is establishing a list of cases where one should see the mechanism in action. This is the critical role of the if discussed above. Case selection establishes the universe of cases where one should see the mechanism in action based on the triggering conditions or whatever the scope of the mechanism might be. As practiced, a common feature of LNQA is the focus on rare events: the panel, typically a country-year panel—may include thousands of cells, but instances of the outcome are relatively rare. It might seem that the study of rare events would—virtually by definition—constitute an area of niche concern. In fact, nearly the opposite is the case. Many phenomena that are central to the disciplines of economics, political science and sociology are in fact rare events. In economics, examples include financial crises, episodes of unusually high growth, famines, or—rarer still—the emergence of international financial centers. In political science, transitions to and from democratic rule have been relatively infrequent, to which one could add coups, civil wars and—again, rarer still—social revolutions. International relations is similarly preoccupied with events that are fairly uncommon, most notably wars, but also phenomenon such as the acquisition of nuclear weapons or—again, rarer still—the rise or decline of hegemonic powers and possible reactions to those power shifts.
The precise definition of a rare event is of course relatively elastic, and practical considerations necessarily come into play. For example, the number of social revolutions in world history is relatively small, arguably less than ten. Considering such events permits much more complex causal arguments. Other events might be more common; for example, Haggard and Kaufman consider 78 discrete transitions, but focusing in on the presence or absence of a very particular causal mechanism. In the examples we discuss below, the number of cases considered falls in the 10–30 range. Ziblatt similarly looks at democratic transitions, making the claim initially around the European experience but then cautiously reaching beyond Europe in concluding chapters. Sechser and Fuhrmann consider an even smaller population of coercive nuclear threats. When the total number of cases in the X = 1 column is relatively small it becomes possible to examine them all in some detail via within-case causal inference. Sechser and Fuhrmann illustrate this very nicely by having a whole chapter devoted to the ( 1 , 0 ) cases and another chapter devoted to all the ( 1 , 1 ) cases. In a slightly different set up Ziblatt does more or less the same thing.
If one moves to phenomena in which the X = 1 cases are large, replicating within-case causal inference across all cases becomes impractical. There are two related approaches for addressing this problem, and they can be outlined by considering the democratic peace literature. The democratic peace illustrates a setting where the X = 1 column has many cases, all democratic dyads, and the outcome variable not-war (or peace), i.e., Y = 1 , is quite common. This means virtually by definition that democracy will pass the absolute test as described above. In this relatively common scenario both in the X = 0 and X = 1 columns you have very high percentages. That means both X = 1 and X = 0 pass the absolute test. In the QCA framework this would raise the question of potentially trivial sufficient conditions. After passing the absolute test one would move to the trivialness test which involves the other column (see any QCA textbook for procedures for dealing with this issue, e.g., Schneider and Wagemann 2012 ).
Another way to deal with these cases is to use the same basic logic with the Y = 1 row instead of the X = 1 column, where Y = 1 is no war. This makes it a necessary condition test, but by definition one with relatively few cases because Y , war, itself is rare. If Y = 1 is common then by definition Y = 0 (war in the democratic peace) is rare and the visibility of falsifying examples, i.e., (1,0) cell cases, goes up dramatically up. This can be seen in the democratic peace literature where there was a tremendous amount of attention given, by critics in particular, to potential falsifying cases of democracies fighting each other (e.g., Ray 1993 ).
When there are “a lot” of cases in the ( 1 , 1 ) as well as the falsifying ( 1 , 0 ) cell, making repeated within-case causal inference impractical, one could randomly select among the population of these relevant cases. Fearon and Laitin (2008) have argued for random case selection for case studies. There are few who have found their argument convincing. When they describe random selection it is among all of the cases in the 2 × 2 table. This has meant choosing cases that are not directly relevant to the causal mechanism because they are not in the X = 1 column or the causal mechanism ( 1 , 1 ) cell; (0, 0) cases are particularly unhelpful in this regard and indeed virtually useless. However, if one restricts the analysis to say, the causal mechanism cell, then random selection makes much more sense. One is randomly selecting among all the cases which are used to support the causal generalization in the experiment or statistical analysis. If they are found to comport with the hypothesis, it would increase confidence in it.
In short, although LNQA has emerged largely to address rare events, it is possible that the method could be extended to larger populations. If there are too many cases to examine individually in the X = 1 column or in the causal mechanism cell we think the first good response is to think about randomly selecting cases for intensive within-case analysis cases from the causal mechanism or (1,1) cell. Of course, this too is subject to practical constraints, but nevertheless is a very good starting point.
Large-N Qualitative Analysis: Some Examples
In this section we give two more extended examples of LNQA in practice. Our purposes are several. First, from the standpoint of our anthropological approach to the method, they show how wide-ranging the applications of this approach have been, showing up in fields as diverse as the study of nuclear weapons and historical analyses of democratization. In addition to reiterating our analysis of the method, these cases also show how it is used both in a multi-method context and where the central approach is rooted in case studies, in this case an historical analysis of democratization in the UK and Germany.
Sechser and Fuhrmann (2017) : Nuclear Weapons and Coercive Diplomacy
Sechser and Furhmann provide an example of a multimethod approach to LNQA. In the first half of the book, they undertake a large-N statistical analysis. They report on detailed statistical tests of the effect of possessing nuclear weapons on two outcomes: whether nuclear states make more effective compellent threats; and whether they achieve better outcomes in territorial disputes. Their statistical tests fail to find a nuclear advantage.
We ignore these chapters, however, and focus on the two main case study chapters which form at least half of the volume and which are structured along the lines we have outlined here. These chapters are self-consciously addressed to questions of the postulated causal mechanisms behind their “nuclear skepticism” argument, including particular questions about the credibility of nuclear threats and possible backlash effects of using them. They carefully delimit the scope of cases to those in which countries attempted nuclear coercion. They explicitly adopt the LNQA method we have outlined: “[W]e delve deeply into history’s most serious coercive nuclear crises. Coercive nuclear threats are rare: nuclear weapons have been invoked to achieve coercive goals less than two dozen times since 1945. We study each of these episodes, drawing on declassified documents when possible” ( Sechser and Fuhrmann 2017 , 20).
Thus the X = 1 cases are those of attempted nuclear coercion and brinksmanship. The outcome is whether the state was able to coerce the target into changing its behavior. It should be emphasized that their theory explains nuclear coercion threat failure. This makes failure the Y = 1 cases (we do this to remain consistent with what we have done throughout this chapter). It then makes complete sense that their first case study chapter involves the causal mechanism cases where a coercive threat was made but failed. The next chapter by contrast takes up potentially falsifying cases: cases where the threat was made and appeared to succeed, making it the ( 1 , 0 ) set of cases (threat, but success instead of failure). They use this chapter to show that—following detailed within-case causal inference—these nominally falsifying cases may not be falsifying after all.
Their justification for the approach fits the large-N qualitative analysis that we have outlined: “The purpose of quantitative analysis was to identify general trends—not to explain any single case. Even if the quantitative analysis suggests that our argument is generally correct, nuclear skepticism theory may fail to explain some cases. Why does this matter? The unexplained cases—often referred to as outliers—may be particularly salient” (p. 130). Put differently, Sechser and Fuhrmann underline that we do not simply want relative comparisons; we want convincing explanations of cases that are deemed important on substantive grounds.
They pay particular attention to case selection, and seek to consider the entire universe of cases in which states attempt nuclear coercion. They identify 13 cases that are clear examples and another six “borderline” cases; the two groups are pooled. These include the (1,1) cases which confirm their theory of nuclear coercion failure: “In this chapter, we discuss nine nuclear coercion failures. These are cases in which countries openly brandished nuclear weapons but still failed to achieve their coercive objectives” (p. 132). Case studies of these failure cases show the operation of the causal mechanisms postulated in their theory of coercion failure, such as the fact that the threats were not credible and were resisted by presumably weaker parties.
In the next chapter—appropriately called “Think Again: Reassessing Nuclear Victories,” they turn to the ( 1 , 0 ) cases: “cases in which nuclear blackmail seemingly worked” (p. 173). In these cases, causal mechanism analysis becomes central: the cases are designed to see if the mechanism proposed by nuclear coercion theorists really explains the outcome (i.e., that nuclear coercion “worked”). They could have probed for some conditional factors suggested by their theory that might have operated in these success cases, thus establishing scope conditions on their skepticism. But their within-case causal analysis concludes regarding the ( 1 , 0 ) cases that
in each instance, at least one of three factors mitigates the conclusion that a nuclear threat resulted in a coercive victory. First, factors other than nuclear weapons often played a significant role in states’ decisions to back down. Second, on close inspection, some crisis outcomes were not truly “victories” for the coercer. Third, when nuclear weapons have helped countries in crises, they have aided in deterrence rather than coercion. (p. 174)
By looking at the cases closely they in effect also identify measurement error. When they say that these were not cases of coercion success that means that that instead of being Y = 0 cases they are in fact Y = 1 cases in which nuclear coercion failed. Hence they are removed from the falsifying cases population. A related critique is that the outcome is not successful coercion but rather successful deterrence. This is more nuanced, but is arguing again that when Y is coercion success one should not count deterrence success as its equivalent. The treatment (attempted nuclear coercion) might produce other positive outcomes, but that is not what is being tested; the test is of the hypothesis that nuclear weapons can compel, not deter.
If one includes the clear 13 failure cases in the previous chapter, the absolute test will score at best 10 of 23 cases (44 percent). Once one takes mismeasurement into account, however, Sechser and Fuhrmann claim that not a single one of the apparently successful cases in fact constitutes a success. Their within-case analysis brings the total down from a potential 10 to zero. In other words, they find no clear-cut case of nuclear coercion success. This illustrates the potentially dramatic impact of doing causal mechanism, within-case causal analysis; purported causal mechanisms were found not to operate when interrogating the cases.
They end their book with this further reflection on the way generalizations can get drawn: “It is worth noting that the cases that provide the strongest support for the nuclear coercionist view—including the Cuban missile crisis—happened in the early days of the Cold War. … There is scant evidence that nuclear blackmail has worked since the collapse of the Soviet Union. The nuclear crises of the last quarter-century illustrate the coercive limits, rather than the virtues, of nuclear weapons.” They are clearly thinking about how generalizable their findings are over time. They think that they are generalizable, but not in the way that “coercionists” would think: it is hard to find any success case in the last 30 years of international politics, a strong law-like statement.
Ziblatt (2017) : Conservative Parties and the Birth of Democracy
Ziblatt’s influential, prize-winning book on the role of conservative parties and democratization is an example of a study that is built from the start around intensive examination of two cases, and then broadened out to consider other cases using the LNQA generalization strategy.
Ziblatt is interested in the role of conservative parties as countries move from authoritarian regimes to democracy. He argues that democracy was not the result of underlying structural factors, such as socioeconomic change, nor class pressures, whether from the middle or working classes. Rather he argues that democracy depended on how successfully conservative political parties were able to recast themselves to adapt to electoral pressure while holding off authoritarian tendencies in their own right wings. His two core case studies occupy most of the book and include the UK, where the conservative party recast itself, and Germany which saw failures in that regard. UK is discussed in chapters 3–5 , Germany appears in chapters 6–9 .
In the final chapter (appropriately titled “How Countries Democratize: Europe and Beyond”), he considers generalization case studies. The generalization goal of the final chapter is stated clearly in the introduction:
Strictly speaking this book’s argument has made sense of political developments within Britain and Germany between the middle of the nineteenth and the middle of the twentieth centuries. But a second purpose, as promised in the introduction, was that the interpretation of these specific historical experiences has general implications for how to think about the enduring impact of old-regime forces on democratization in other places and times. Is our understanding of the world in fact deepened when we widen our scope beyond the main cases we have studied? What more general implications can we draw? ( Ziblatt 2017 , 334–335)
In that final chapter he outlines the scope of these potential generalization case studies for Europe: “Table 10.1 provides a list of the major parties of the electoral right after 1918, noting which electoral right party had the greatest number of votes in the first postwar democratic elections, a score for the fragmentation of the right camp of parties in this period, and whether or not democracy survived the interwar years” (p. 336). He then proceeds to choose from these for case studies lasting a few pages. Then he considers the Latin American cases. Here the analysis is very short and arguably more superficial; however the purpose—as in Haggard and Kaufman—is focused: to test for the operation of the favored causal mechanism related to conservative parties.
For the European cases, he chooses causal mechanism cases for further generalization (aka “on-line” cases) from the (1,1) cell:
We begin by analyzing two “well-predicted” cases that appear to fit the framework: one where the right was relatively cohesive and democracy survived (Sweden), and one where it remained organizationally fractious and democracy ultimately collapsed (Spain).
That is, for his generalization case studies he starts by choosing a case that is close to UK—Sweden—and one that is seen as similar to Germany, Spain. The Sweden case study takes up four pages, while the Spanish one eight.
Ziblatt then moves to consider non-European cases. He summarizes the patterns briefly: “In the four countries where conservative political parties emerged before mass suffrage—Chile, Colombia, Costa Rica, and Uruguay—democratization even if predominately oligarchic at first, was on average more stable than in the rest of the region. By contrast, in the remaining twelve countries—Argentina, Brazil, Ecuador, Peru, and so on—where no conservative political party existed until after mass democratization, democracy was, on average, less durable” ( Ziblatt 2017 , 358–359). He then proceeds to spend a couple of pages on Argentina as the Latin American example. He then spends one paragraph on some Asian cases like South Korea and Taiwan and then two paragraphs on the Arab Spring, in each case testing for the presence of absence of conservative parties and the presence or absence of the outcome, democratization.
This example illustrates nicely that the case studies need not be treated equally. The amount of space devoted to each case study can be skewed as long as adequate information is provided to reach a reasonable conclusion with respect to the presence or absence of the postulated causal mechanism. The book is framed around two core causal mechanism case studies, followed by a series of generalization case studies that are unequal in length but nonetheless focused on the core causal relationship. In each generalization case study, the purpose is to focus on the postulated causal mechanism and see if it works in that case; generalization is enhanced by effectively “summing” these well-explained cases.
In this chapter we explore a research paradigm involving within-case causal inference and a strategy using case studies to support generalization claims about causal mechanisms. The most distinctive feature of the approach are two. The first is the effort to use a large number of cases, and ideally the entire population of the X = 1 cases (for sufficient conditions claims) or Y = 1 cases (for necessary conditions claims). The second is the systematic use of within-case causal inference as opposed to experimental designs—in which treatment is contrasted with control—or cross-case observational designs, such as those deployed in many studies in comparative politics and international relations.
This research design typically takes advantage of the fact that there are often relatively few cases in the causal mechanism cell. Going back to Ziblatt the universe for half of the book is mass democratization in Europe after 1918 and he is thus able to consider the causal effect of his chosen theory involving the timing of the emergence of conservative parties and their relative strength. Similarly with Sechser and Fuhrmann, the panels they use for their statistical analysis have as many as 6,500 observations in some models. But the number of cases in which nuclear states unambiguously made coercive nuclear threats is not more than a couple dozen. This makes the design described here a plausible alternative (for Ziblatt) or complement (for Sechser and Fuhrmann) to standard statistical analysis.
One might argue that absolute tests with just one variable are very unrealistic, particularly with a relatively high bar of 75 percent. A widely held intuition is that it is unlikely that many factors will pass this sort of law-like test; note that it is much stronger than claims that a given causal variable has at least a statistically significant effect on a population when potential confounds are either randomized away or controlled for. Not surprisingly, some of the early examples of this work were aimed at taking down expansive law-like claims with respect to a diverse array of outcomes, from the role of economic interests in the design of electoral systems to the role of audience costs in war.
A natural response is to say there must be other factors that are important in producing the outcome, and that one causal variable is not likely adequate to generate convincing explanation. QCA deals with this by focusing on interaction terms: it is only when there is an interaction of three or four causal factors that the outcome is very likely to occur and will pass the absolute test. Yet we think it can in fact be useful to identify necessary or sufficient conditions relationships between causal factors and outcomes. Moreover, the logic of absolute tests is the same whether the hypothesis rests on the operation of one variable or the interaction of four or five. And that logic is quite different from an average treatment effect logic.
As we have noted, this design has become quite standard practice in both case study–only books in recent years as well as those engaged in mixed-methods approaches. But the practice has yet to appear in the methodological literature (again, see Goertz 2017 , chapter 7). In almost all cases authors just “do it.” The fact that it seems not to have provoked any backlash on the part of reviewers, commissioning editors, or others seems to indicate that the logic has some resonance.
We have sought to extract some of the main features of this approach and link them to wider discussions about generalization in philosophy and the social sciences. First, and most obviously, it is best suited to analysis of rare events, which in fact figure quite prominently in the political science canon. Second, it rests on focused tests of postulated causal mechanisms; theory matters. And finally, it requires use of within-case causal inference techniques, including process-tracing predominantly but potentially within-case counterfactuals as well.
We can see several ways in which this work might be pushed forward. One interesting link is to discussions in the Bayesian tradition about what amount of case work might be adequate to reach closure. Dion (1998) nicely showed that in a Bayesian framework five or six consistent case studies can easily lead to 90 percent posterior confidence starting with a uniform prior. Clear theorizing in this area might reduce the demands of this approach with respect to the conduct of detailed cases studies and thus strengthen its appeal.
More work might also be done on a version of generalization which can be called extrapolation. Using the classic Shadish, Cook and Campbell (2002) units, treatment, outcomes, and settings (UTOS) framework we can ask to what extent when we move along some dimension of UTOS the same results apply. For example, the quest for external validity and generalization in experimental work has been about doing the experiment or treatment on new units. Extrapolation could be about changing the treatments gradually in some direction within populations of case studies as well.
Often a strong generalization that is empirically founded naturally leads to questions about extrapolation. The democratic peace is a well-founded empirical generalization. This should, but does not seem to have, lead to a question about how generalizable it is to less democratic countries. So as we extrapolate from democratic towards authoritarian how far does the democratic peace generalize and extrapolate? We think that this is a clear next step in the analysis of generalization and external validity.
This methodology also has potential applications for experiments and other “well specified” designs as well as large-N statistical analyses: matching, difference-in-difference, instrumental variables or regression discontinuity designs. Currently the solution for the generalization problem for experiments or quasi-experimental designs is just to do more of them. We know of almost nothing that systematically tries to analyze what constitutes successful generalization criteria; we can see room for parallel work looking at how experiments on a given issue aggregate across the X = 1 column. The critical cell is the causal mechanism ( 1 , 1 ) cell. One could easily take a random sample of the ( 1 , 1 ) cases in an experimental or quasi-experimental design to see if the causal mechanism is in fact present. This would represent an independent check on the statistical or experimental results.
We have barely begun to systematically analyze the crucial decisions and the crucial options in case study–generalization methodologies and in LNQA in particular. But our analysis suggests that there are a wide array of topics opened up by this research strategy that require more sustained analysis.
Acknowledgments
Thanks to Sharon Crasnow, Harold Kincaid, and Julian Reiss for comments on an earlier draft, and Terence Teo for help with the data analysis of democratization and inequality. Goertz thanks James Copestake and the University of Bath for providing a wonderful environment for writing this chapter. Thanks to the participants in the Handbook workshop at Washington State University as well for valuable feedback.
There is not agreement at all in the process tracing literature about counterfactuals. Some are opposed, some in favor.
We shall not deal with the more complex situation where there may be multiple factors, e.g., interactions, at the beginning of the chain.
In the philosophical literature on causal mechanisms one often reads about the initial conditions or a trigger which sets the causal mechanism in motion, for example: “Mechanisms are entities and activities organized such that they are productive of regular changes from start or set-up to finish or termination conditions.” ( Machamer et al. 2000 , 3; displayed definition). The key point is that there is some initial triggering condition which then generates the mechanism. This always has logical form if triggering condition then mechanism then outcome. The “regular changes” means a generally reliable causal mechanism.
An increasing popular approach to within-case causal inference is to create a “synthetic” counterfactual case based on the combination of real cases and then compare that case with the actual one. Abadie et al. (2015) construct a counterfactual Germany to explore the impact of German unification on economic growth. This counterfactual Germany is amalgam of “similar” countries such as France, Canada, etc.
Inequality data are the Gini market data from Solt 2020 . Transitions based on Haggard and Kaufman 2016 . Division into the three inequality categories is based on the terciles of the Gini inequality data for authoritarian regimes, 1980–2008, the terciles are divided at less than 42.6, and greater than 47.6. In the polity data we consider all –66, –77, and –88 observations as authoritarian.
As discussed in some detail in Goertz and Mahoney (2012) , equifinality is just assumed in many contexts. For example, it lies at the core of the INUS model. A non-INUS model is one like Y = X 1 AND X 2 , where X i are individually necessary and jointly sufficient for Y , there is only one path to Y . Why it is central in qualitative methods in general, and QCA in particular, is the idea that the number of mechanisms generating the outcome is limited to a few like in QCA and not a huge number like in general statistical models.
Abadie, A. , et al. 2015 . Comparative politics and the synthetic control method. American Journal of Political Science 59:495–510.
Google Scholar
Acemoglu, D. , and J. Robinson . 2006 . Economic origins of dictatorship and democracy . Cambridge: Cambridge University Press.
Google Preview
Anscombe, G. 1971 . Causality and determination . Cambridge: Cambridge University Press.
Armstrong, D. 1983 . What is a law of nature? Cambridge: Cambridge University Press.
Bardsley, N. , et al. 2010 . Experimental economics: Rethinking the rules . Princeton, NJ: Princeton University Press.
Campbell, D. , and J. Stanley . 1963 . Experimental and quasi-experimental designs for research . Chicago: Rand McNally.
Dion, D. 1998 . Evidence and inference in the comparative case study. Comparative Politics 30:127–145.
Druckman, J. , and C. Kam . 2011 . Students as experimental participants a defense of the “narrow data base”. In J. Druckman et al. (eds.) Cambridge handbook of experimental political science , pp. 41–57. Cambridge: Cambridge University Press.
Dunning, T. , et al. (eds.). 2019 . Information, accountability, and cumulative learning . Cambridge: Cambridge University Press.
Fearon, J. , and D. Laitin . 2008 . Integrating qualitative and quantitative methods. In J. Box-Steffensmier , H. Brady , and D. Collier (eds.) The Oxford handbook of political methodology , pp. 757–776. Oxford: Oxford University Press.
Glennan, S. 2017 . The new mechanical philosophy . Oxford: Oxford University Press.
Goertz, G. 2017 Multimethod research, causal mechanisms, and case studies: an integrated approach . Princeton, NJ: Princeton University Press.
Goertz, G. , and J. Mahoney . 2012 . A tale of two cultures: Qualitative and quantitative research in the social sciences . Princeton, NJ: Princeton University Press.
Haggard, S. , and R. Kaufman . 2016 . Dictators and democrats: Masses, elites, and regime change . Princeton, NJ: Princeton University Press.
Holland, P. 1986 . Statistics and causal inference (with discussion ). Journal of the American Statistical Association 81:945–960.
Kaplan, O. 2017 . Resisting war: How communities protect themselves . Cambridge: Cambridge University Press.
Levy, J. , and W. Thompson . 2005 . Hegemonic threats and great power balancing in Europe, 1495–1999. Security Studies 14:1–30.
Levy, J. , and W. Thompson . 2010 . Balancing at sea: Do states ally against the leading global power? International Security 35:7–43.
Lewis, D. 1973 . Counterfactuals . Cambridge, MA: Harvard University Press.
Machamer, P. , et al. 2000 . Thinking about mechanisms. Philosophy of Science 67:1–25.
McDermott, R. 2011 . Internal and external validity. In J. Druckman et al. (eds.) Cambridge handbook of experimental political science , pp. 27–40. Cambridge: Cambridge University Press.
Narang, V. , and R. Nelson . 2009 . Who are these belligerent democratizers? Reassessing the impact of democratization on war. International Organization 63:357–379.
Przeworski, A. 2009 . Conquered or granted? A history of suffrage extensions. British Journal of Political Science , 39:291–321.
Ray, J. 1993 . Wars between democracies: Rare or nonexistent? International Interactions 18:251–276.
Ripsman, N. 2016 . Peacemaking from above, peace from below: Ending conflict between regional rivals . Ithaca, NY: Cornell University Press.
Schenoni, L. et al. 2019 . Settling resistant disputes: The territorial boundary peace in Latin America. Manuscript. University of Notre Dame.
Schneider, C. , and C. Wagemann . 2012 . Set-theoretic methods for the social sciences: A guide to qualitative comparative analysis . Cambridge: Cambridge University Press.
Sechser, T. , and M. Fuhrmann . 2017 . Nuclear weapons and coercive diplomacy . Cambridge: Cambridge University Press.
Shadish, W. , T. Cook , and D. Campbell . 2002 . Experimental and quasi-experimental designs for general causal inference . Boston: Houghton Mifflin.
Solt, F. 2020 . Measuring income inequality across countries and over time: The standardized world income inequality database. Social Science Quarterly 101:1183–1199.
Trachtenberg, M. 2012 . Audience costs: An historical analysis. Security Studies 21:3–42.
Wallensteen, P. 2015 . Quality peace: Peacebuilding, victory and world order . Oxford: Oxford University Press.
Ziblatt, D. 2017 . Conservative parties and the birth of democracy . Cambridge: Cambridge University Press.
- About Oxford Academic
- Publish journals with us
- University press partners
- What we publish
- New features
- Open access
- Institutional account management
- Rights and permissions
- Get help with access
- Accessibility
- Advertising
- Media enquiries
- Oxford University Press
- Oxford Languages
- University of Oxford
Oxford University Press is a department of the University of Oxford. It furthers the University's objective of excellence in research, scholarship, and education by publishing worldwide
- Copyright © 2024 Oxford University Press
- Cookie settings
- Cookie policy
- Privacy policy
- Legal notice
This Feature Is Available To Subscribers Only
Sign In or Create an Account
This PDF is available to Subscribers Only
For full access to this pdf, sign in to an existing account, or purchase an annual subscription.
Generalization in quantitative and qualitative research: myths and strategies
Affiliation.
- 1 Humanalysis, Inc., Saratoga Springs, NY 12866, USA. [email protected]
- PMID: 20598692
- DOI: 10.1016/j.ijnurstu.2010.06.004
Generalization, which is an act of reasoning that involves drawing broad inferences from particular observations, is widely-acknowledged as a quality standard in quantitative research, but is more controversial in qualitative research. The goal of most qualitative studies is not to generalize but rather to provide a rich, contextualized understanding of some aspect of human experience through the intensive study of particular cases. Yet, in an environment where evidence for improving practice is held in high esteem, generalization in relation to knowledge claims merits careful attention by both qualitative and quantitative researchers. Issues relating to generalization are, however, often ignored or misrepresented by both groups of researchers. Three models of generalization, as proposed in a seminal article by Firestone, are discussed in this paper: classic sample-to-population (statistical) generalization, analytic generalization, and case-to-case transfer (transferability). Suggestions for enhancing the capacity for generalization in terms of all three models are offered. The suggestions cover such issues as planned replication, sampling strategies, systematic reviews, reflexivity and higher-order conceptualization, thick description, mixed methods research, and the RE-AIM framework within pragmatic trials.
Copyright 2010 Elsevier Ltd. All rights reserved.
- Evidence-Based Nursing
- Models, Nursing
- Nursing Research*
Advertisement
- Previous Article
- Next Article
Can We Generalize from Case Studies?
- Cite Icon Cite
- Permissions
- Article contents
- Figures & tables
- Supplementary Data
- Peer Review
- Search Site
Paul F. Steinberg; Can We Generalize from Case Studies?. Global Environmental Politics 2015; 15 (3): 152–175. doi: https://doi.org/10.1162/GLEP_a_00316
Download citation file:
- Ris (Zotero)
- Reference Manager
This article considers the role of generalization in comparative case studies, using as exemplars the contributions to this special issue on climate change politics. As a research practice, generalization is a logical argument for extending one’s claims beyond the data, positing a connection between events that were studied and those that were not. No methodological tradition is exempt from the requirement to demonstrate a compelling logic of generalization. The article presents a taxonomy of the logics of generalization underlying diverse research methodologies, which often go unstated and unexamined. I introduce the concept of resonance groups, which provide a causeway for cross-system generalization from single case studies. Overall the results suggest that in the comparative study of complex political systems, case study research is, ceteris paribus , on par with large-N research with respect to generalizability.
Client Account
Sign in via your institution, email alerts, related articles, related book chapters, affiliations.
- Online ISSN 1536-0091
- Print ISSN 1526-3800
A product of The MIT Press
Mit press direct.
- About MIT Press Direct
Information
- Accessibility
- For Authors
- For Customers
- For Librarians
- Direct to Open
- Open Access
- Media Inquiries
- Rights and Permissions
- For Advertisers
- About the MIT Press
- The MIT Press Reader
- MIT Press Blog
- Seasonal Catalogs
- MIT Press Home
- Give to the MIT Press
- Direct Service Desk
- Terms of Use
- Privacy Statement
- Crossref Member
- COUNTER Member
- The MIT Press colophon is registered in the U.S. Patent and Trademark Office
This Feature Is Available To Subscribers Only
Sign In or Create an Account
Thank you for visiting nature.com. You are using a browser version with limited support for CSS. To obtain the best experience, we recommend you use a more up to date browser (or turn off compatibility mode in Internet Explorer). In the meantime, to ensure continued support, we are displaying the site without styles and JavaScript.
- View all journals
- My Account Login
- Explore content
- About the journal
- Publish with us
- Sign up for alerts
- Open access
- Published: 19 October 2023
A taxonomy and review of generalization research in NLP
- Dieuwke Hupkes 1 ,
- Mario Giulianelli ORCID: orcid.org/0009-0004-1281-9686 2 ,
- Verna Dankers 3 ,
- Mikel Artetxe 4 ,
- Yanai Elazar 5 , 6 ,
- Tiago Pimentel ORCID: orcid.org/0000-0002-5159-4641 7 ,
- Christos Christodoulopoulos ORCID: orcid.org/0000-0001-7708-0051 8 ,
- Karim Lasri 9 ,
- Naomi Saphra 10 ,
- Arabella Sinclair 11 ,
- Dennis Ulmer 12 , 13 ,
- Florian Schottmann 14 , 15 ,
- Khuyagbaatar Batsuren ORCID: orcid.org/0000-0002-6819-5444 16 ,
- Kaiser Sun 17 ,
- Koustuv Sinha 17 ,
- Leila Khalatbari 18 ,
- Maria Ryskina ORCID: orcid.org/0009-0002-5936-1380 19 ,
- Rita Frieske ORCID: orcid.org/0000-0002-3921-3519 18 ,
- Ryan Cotterell 14 &
- Zhijing Jin ORCID: orcid.org/0000-0003-0238-9024 14 , 20
Nature Machine Intelligence volume 5 , pages 1161–1174 ( 2023 ) Cite this article
16k Accesses
1 Citations
202 Altmetric
Metrics details
- Computer science
- Language and linguistics
A preprint version of the article is available at arXiv.
The ability to generalize well is one of the primary desiderata for models of natural language processing (NLP), but what ‘good generalization’ entails and how it should be evaluated is not well understood. In this Analysis we present a taxonomy for characterizing and understanding generalization research in NLP. The proposed taxonomy is based on an extensive literature review and contains five axes along which generalization studies can differ: their main motivation, the type of generalization they aim to solve, the type of data shift they consider, the source by which this data shift originated, and the locus of the shift within the NLP modelling pipeline. We use our taxonomy to classify over 700 experiments, and we use the results to present an in-depth analysis that maps out the current state of generalization research in NLP and make recommendations for which areas deserve attention in the future.
Similar content being viewed by others
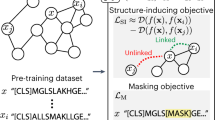
Structure-inducing pre-training
Matthew B. A. McDermott, Brendan Yap, … Marinka Zitnik
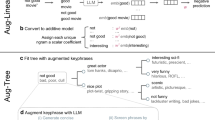
Augmenting interpretable models with large language models during training
Chandan Singh, Armin Askari, … Jianfeng Gao
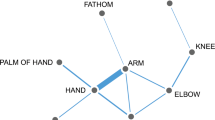
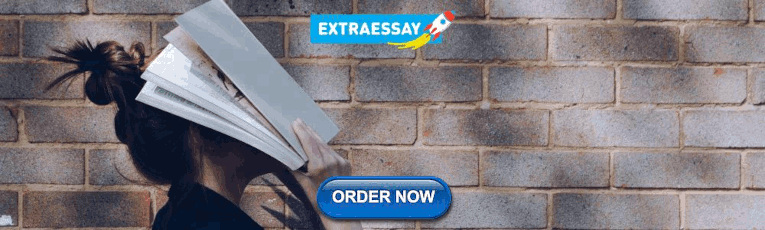
The Database of Cross-Linguistic Colexifications, reproducible analysis of cross-linguistic polysemies
Christoph Rzymski, Tiago Tresoldi, … Johann-Mattis List
Good generalization, roughly defined as the ability to successfully transfer representations, knowledge and strategies from past experience to new experiences, is one of the primary desiderata for models of natural language processing (NLP), as well as for models in the wider field of machine learning 1 , 2 . For some, generalization is crucial to ensure that models behave robustly, reliably and fairly when making predictions about data different from the data on which they were trained, which is of critical importance when models are employed in the real world. Others see good generalization as intrinsically equivalent to good performance and believe that, without it, a model is not truly able to conduct the task we intended it to. Yet others strive for good generalization because they believe models should behave in a human-like way, and humans are known to generalize well. Although the importance of generalization is almost undisputed, systematic generalization testing is not the status quo in the field of NLP.
At the root of this problem lies the fact that there is little understanding and agreement about what good generalization looks like, what types of generalization exist, how those should be evaluated, and which types should be prioritized in varying scenarios. Broadly speaking, generalization is evaluated by assessing how well a model performs on a test dataset, given the relationship of this dataset with the data on which the model was trained. For decades, it was common to exert only one simple constraint on this relationship: that the train and test data are different. Typically, this was achieved by randomly splitting the available data into training and test partitions. Generalization was thus evaluated by training and testing models on different but similarly sampled data, assumed to be independent and identically distributed (i.i.d.). In the past 20 years, we have seen great strides on such random train–test splits in a range of different applications (for example, refs. 3 , 4 ).
With this progress, however, came the realization that, for an NLP model, reaching very high or human-level scores on an i.i.d. test set does not imply that the model robustly generalizes to a wide range of different scenarios. We have witnessed a tide of different studies pointing out generalization failures in neural models that have state-of-the-art scores on random train–test splits (as in refs. 5 , 6 , 7 , 8 , 9 , 10 , to give just a few examples). Some show that when models perform well on i.i.d. test splits, they might rely on simple heuristics that do not robustly generalize in a wide range of non-i.i.d. scenarios 8 , 11 , over-rely on stereotypes 12 , 13 , or bank on memorization rather than generalization 14 , 15 . Others, instead, display cases in which performances drop when the evaluation data differ from the training data in terms of genre, domain or topic (for example, refs. 6 , 16 ), or when they represent different subpopulations (for example, refs. 5 , 17 ). Yet other studies focus on models’ inability to generalize compositionally 7 , 9 , 18 , structurally 19 , 20 , to longer sequences 21 , 22 or to slightly different formulations of the same problem 13 .
By showing that good performance on traditional train–test splits does not equal good generalization, these examples bring into question what kind of model capabilities recent breakthroughs actually reflect, and they suggest that research on the evaluation of NLP models is catching up with the fast recent advances in architectures and training regimes. This body of work also reveals that there is no real agreement on what kind of generalization is important for NLP models, and how that should be studied. Different studies encompass a wide range of generalization-related research questions and use a wide range of different methodologies and experimental set-ups. As of yet, it is unclear how the results of different studies relate to each other, raising the question of how should generalization be assessed, if not with i.i.d. splits? How do we determine what types of generalization are already well addressed and which are neglected, or which types of generalization should be prioritized? Ultimately, on a meta-level, how can we provide answers to these important questions without a systematic way to discuss generalization in NLP? These missing answers are standing in the way of better model evaluation and model development—what we cannot measure, we cannot improve.
Here, within an initiative called GenBench, we introduce a new framework to systematize and understand generalization research in an attempt to provide answers to the above questions. We present a generalization taxonomy, a meta-analysis of 543 papers presenting research on generalization in NLP, a set of online tools that can be used by researchers to explore and better understand generalization studies through our website— https://genbench.org —and we introduce GenBench evaluation cards that authors can use to comprehensively summarize the generalization experiments conducted in their papers. We believe that state-of-the-art generalization testing should be the new status quo in NLP, and we aim to lay the groundwork for facilitating that.
The GenBench generalization taxonomy
The generalization taxonomy we propose—visualized in Fig. 1 and compactly summarized in Extended Data Fig. 2 —is based on a detailed analysis of a large number of existing studies on generalization in NLP. It includes the main five axes that capture different aspects along which generalization studies differ. Together, they form a comprehensive picture of the motivation and goal of the study and provide information on important choices in the experimental set-up. The taxonomy can be used to understand generalization research in hindsight, but is also meant as an active device for characterizing ongoing studies. We facilitate this through GenBench evaluation cards, which researchers can include in their papers. They are described in more detail in Supplementary section B , and an example is shown in Fig. 2 . In the following, we give a brief description of the five axes of our taxonomy. More details are provided in the Methods .
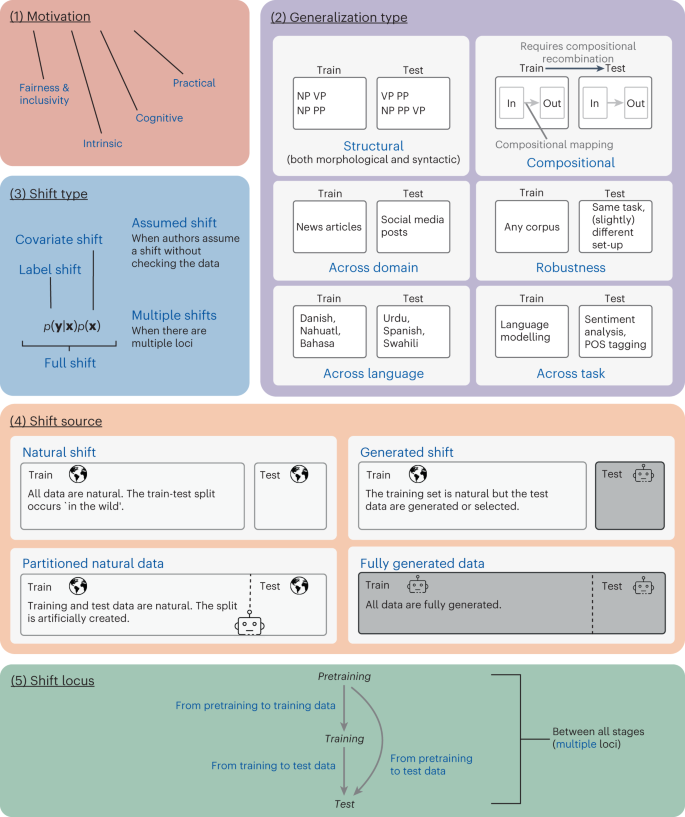
The generalization taxonomy we propose consists of five different (nominal) axes that describe (1) the high-level motivation of the work, (2) the type of generalization the test is addressing, (3) what kind of data shift occurs between training and testing and (4) what the source and (5) locus of this shift are. NP, noun phrase; VP, verb phrase; PP, prepositional phrase.
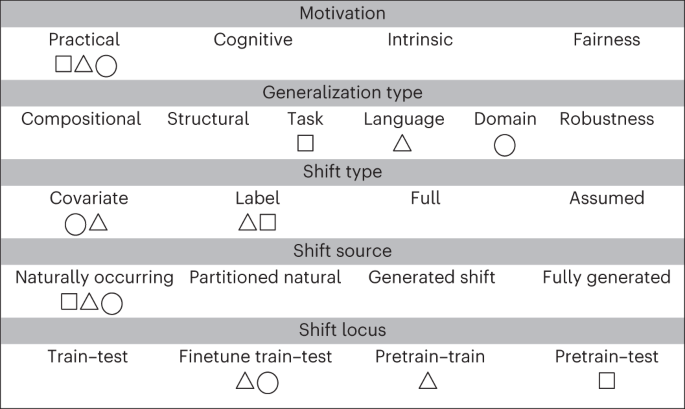
This example GenBench evaluation card describes a hypothetical paper with three different experiments. As can be seen in the first two rows, all experiments are practically motivated and test different types of generalization: cross-task generalization (square), cross-lingual generalization (triangle) and cross-domain generalization (circle). To do so, they use different data shifts and different loci. The task generalization experiment (square) involves a label shift from pretrain to test, the domain-generalization experiment (circle) a covariate shift in the finetuning stage, and the cross-lingual experiment (triangle) considers multiple shifts (covariate and label) across different stages of the modelling pipeline (pretrain–train and finetune train–test). All experiments use naturally occurring shifts. The LaTeX code for this card was generated with the generation tool at https://genbench.org/eval_cards .
The first axis of our taxonomy describes the high-level motivation for the study. The motivation of a study determines what type of generalization is desirable, as well as what kind of conclusions can be drawn from a model’s display or lack of generalization. Furthermore, the motivation of a study shapes its experimental design. It is therefore important for researchers to be explicitly aware of it, to ensure that the experimental set-up aligns with the questions they seek to answer. We consider four different types of motivation: practical, cognitive, intrinsic, and fairness and inclusivity.
Generalization type
The second axis in our taxonomy indicates the type of generalization the test is addressing. This axis describes on a high level what the generalization test is intended to capture, rather than considering why or how, making it one of the most important axes of our taxonomy. In the literature, we have found six main types of generalization: compositional generalization, structural generalization, cross-task generalization, cross-lingual generalization, cross-domain generalization and robustness generalization. Figure 1 (top right) further illustrates these different types of generalization.
The third axis in our taxonomy describes what kind of data shift is considered in the generalization test. This axis derives its importance from the fact that data shift plays an essential formal role in defining and understanding generalization from a statistical perspective, as well as from the fact that different types of shift are best addressed with different kinds of experimental set-up. On the data shift axis we consider three shifts, which are well-described in the literature: covariate, label and full shift. We further include assumed shift to denote studies that assume a data shift without properly justifying it. In our analysis, we mark papers that consider multiple shifts between different distributions involved in the training and evaluation process as having multiple shifts.
Shift source
The fourth axis of the taxonomy characterizes the source of the data shift used in the experiment. The source of the data shift determines how much control the experimenter has over the training and testing data and, consequently, what kind of conclusions can be drawn from an experiment. We distinguish four different sources of shift: naturally occurring shifts, artificially partitioned natural corpora, generated shifts and fully generated data. Figure 1 further illustrates these different types of shift source.
Shift locus
The last axis of our taxonomy considers the locus of the data shift, which describes between which of the data distributions involved in the modelling pipeline a shift occurs. The locus of the shift, together with the shift type, forms the last piece of the puzzle, as it determines what part of the modelling pipeline is investigated and thus the kind of generalization question that can be asked. On this axis, we consider shifts between all stages in the contemporary modelling pipeline—pretraining, training and testing—as well as studies that consider shifts between multiple stages simultaneously.
A review of generalization research in NLP
Using our generalization taxonomy, we analysed 752 generalization experiments in NLP, presented in a total of 543 papers from the anthology of the Association for Computational Linguistics (ACL) that have the (sub)words ‘generali(s ∣ z)ation’ or ‘generali(s ∣ z)e’ in their title or abstract. Aggregate statistics on how many such papers we found across different years is available in Fig. 3 . For details on how we selected and annotated the papers, see Supplementary section A . A full list of papers is provided in Supplementary section G , as well as on our website ( https://genbench.org ). On the same website, we also present interactive ways to visualize the results, a search tool to retrieve relevant citations, and a means to generate GenBench evaluation cards, which authors can add to their paper (or appendix) to comprehensively summarize the generalization experiments in their paper (for more information, see Supplementary section B ). In this section, we present the main findings of our analysis.
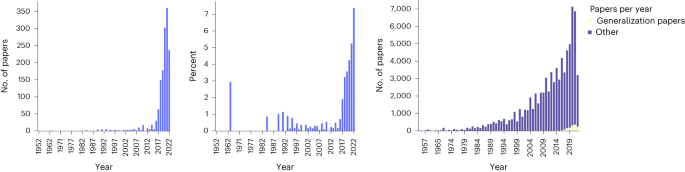
Visualization of the number of papers in the ACL anthology that contain the (sub)words ‘generalisation’, ‘generalization’, ‘generalise’ or ‘generalize’ in their title or abstract, over time, in absolute terms (left), percentually (middle) and compared to all papers (right). We see how both the absolute number of papers and the percentage of papers about generalization have starkly increased over time. On the right, we visualize the total number of papers and generalization papers published each year.
Source data
Overall trends on different axes.
We begin by discussing the overall frequency of occurrence of different categories on the five axes, without taking into account interactions between them. We plot the relative frequencies of all axis values in Fig. 4 and their development over time in Fig. 5 . Because the number of generalization papers before 2018 that are retrieved is very low (Fig. 3a ), we restricted the diachronic plots to the last five years. All other reported statistics are computed over our entire selection of papers.
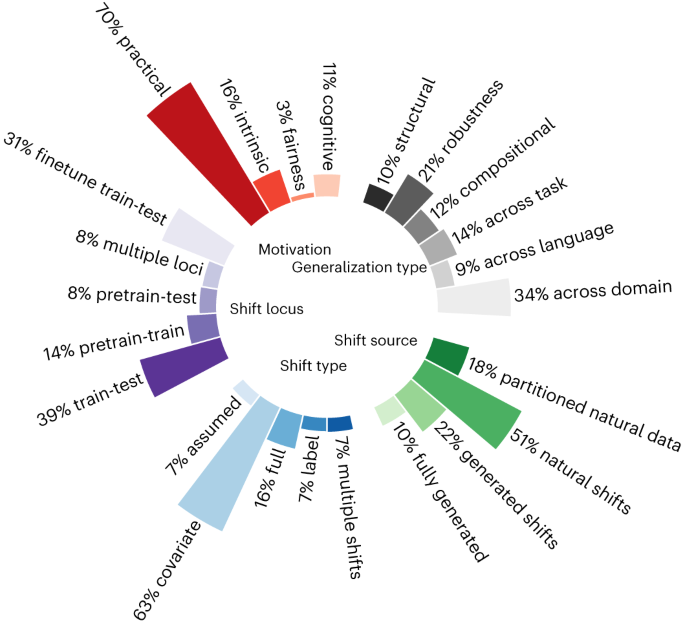
Visualization of the percentage of times each axis value occurs, across all papers that we analysed. Starting from the top left, shown clockwise, are the motivation, the generalization type, the shift source, the shift type and the shift locus.
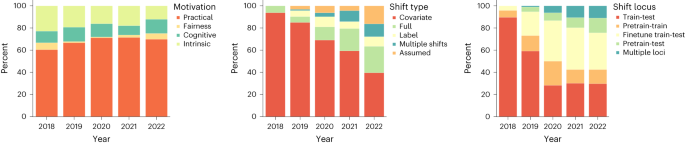
Trends from the past five years for three of the taxonomy’s axes (motivation, shift type and shift locus), normalized by the total number of papers annotated per year.
Motivations
As we can see in Fig. 4 (top left), by far the most common motivation to test generalization is the practical motivation. The intrinsic and cognitive motivations follow, and the studies in our Analysis that consider generalization from a fairness perspective make up only 3% of the total. In part, this final low number could stem from the fact that our keyword search in the anthology was not optimal for detecting fairness studies (further discussion is provided in Supplementary section C ). We welcome researchers to suggest other generalization studies with a fairness motivation via our website. However, we also speculate that only relatively recently has attention started to grow regarding the potential harmfulness of models trained on large, uncontrolled corpora and that generalization has simply not yet been studied extensively in the context of fairness. Overall, we see that trends on the motivation axis have experienced small fluctuations over time (Fig. 5 , left) but have been relatively stable over the past five years.
We find that cross-domain is the most frequent generalization type, making up more than 30% of all studies, followed by robustness, cross-task and compositional generalization (Fig. 4 ). Structural and cross-lingual generalization are the least commonly investigated. Similar to fairness studies, cross-lingual studies could be undersampled because they tend to use the word ‘generalization’ in their title or abstract less frequently. However, we suspect that the low number of cross-lingual studies is also reflective of the English-centric disposition of the field. We encourage researchers to suggest cross-lingual generalization papers that we may have missed via our website so that we can better estimate to what extent cross-lingual generalization is, in fact, understudied.
Data shift types (Fig. 4 ) are very unevenly distributed over their potential axis values: the vast majority of generalization research considers covariate shifts. This is, to some extent, expected, because covariate shifts are more easily addressed by most current modelling techniques and can occur between any two stages of the modelling pipeline, whereas label and full shifts typically only occur between pretraining and finetuning. More unexpected, perhaps, is the relatively high amount of assumed shifts, which indicate studies that claim to test generalization but do not explicitly consider how their test data relate to their training data. The percentage of such assumed shifts has increased over the past few years (Fig. 5 , middle). We hypothesize that this trend, which signals a movement of the field in the wrong direction, is predominantly caused by the use of increasingly large, general-purpose training corpora. Such large corpora, which are often not in the public domain, make it very challenging to analyse the relationship between the training and testing data and, consequently, make it hard to determine what kind of conclusions can be drawn from evaluation results. More promising, instead, is the fact that several studies consider multiple shifts, thus assessing generalization throughout the entire modelling pipeline.
On the shift source axis (Fig. 4 ) we see that almost half of the reviewed generalization studies consider naturally occurring shifts: natural corpora that are not deliberately split along a particular dimension. As discussed later in this section, this type of data source is most prevalent in cross-task and cross-domain generalization studies, for which such naturally different corpora are widely available. The next most frequent categories are generated shifts, where one of the datasets involved is generated with a specific generalization property in mind, and artificially partitioned natural data, describing settings in which all data are natural, but the way it is split between train and test is controlled. Fully generated datasets are less common, making up only 10% of the total number of studies.
Finally, for the locus axis (Fig. 4 ), we see that the majority of cases focus on finetune/train–test splits. Much fewer studies focus on shifts between pretraining and training or pretraining and testing. Similar to the previous axis, we observe that a comparatively small percentage of studies considers shifts in multiple stages of the modelling pipeline. At least in part, this might be driven by the larger amount of compute that is typically required for those scenarios. Over the past five years, however, the percentage of studies considering multiple loci and the pretrain–test locus—the two least frequent categories—have increased (Fig. 5 , right).
Interactions between axes
Next we consider interactions between different axes. Are there any combinations of axes that occur together very often or combinations that are instead rare? We discuss a few relevant trends and encourage the reader to explore these interactions dynamically on our website.
What data shift source is used for different generalization types?
In Fig. 6 (top left), we show the relative frequency of each shift source per generalization type. We can see that the shift source varies widely across different types of generalization. Compositional generalization, for example, is predominantly tested with fully generated data, a data type that hardly occurs in research considering robustness, cross-lingual or cross-task generalization. Those three types of generalization are most frequently tested with naturally occurring shifts or, in some cases, with artificially partitioned natural corpora. Structural generalization is the only generalization type that appears to be tested across all different data types. As far as we are aware, very few studies exist that directly compare results between different sources of shift—for example, to investigate to what extent results on generated shifts or fully generated data are indicative of performances on natural corpora (such as refs. 23 , 24 ). Such studies could provide insight into how choices in the experimental design impact the conclusions that are drawn from generalization experiments, and we believe that they are an important direction for future work.
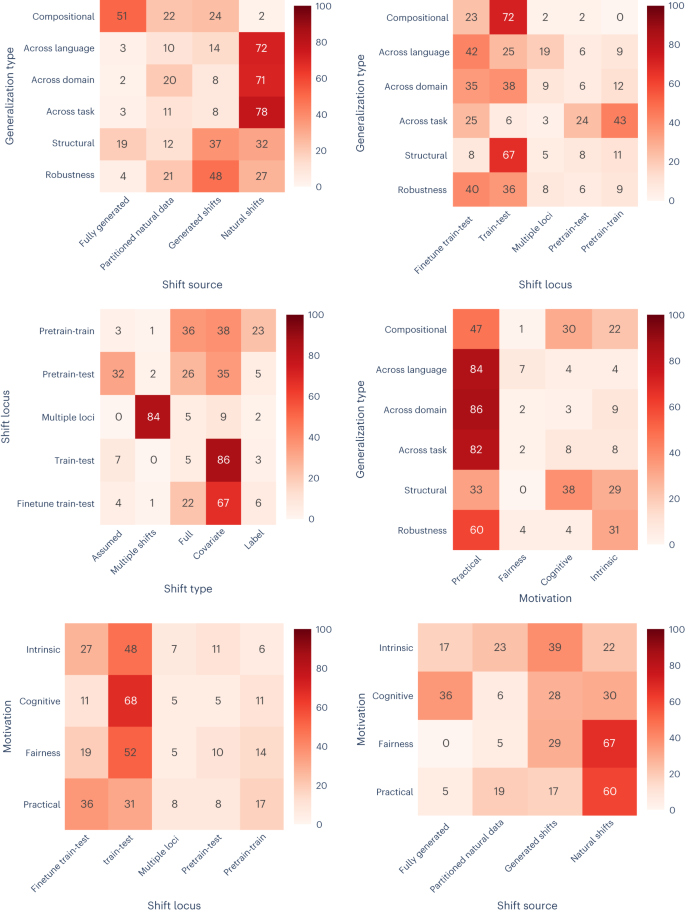
The interaction between occurrences of values on various axes of our taxonomy, shown as heatmaps. The heatmaps are normalized by the total row value to facilitate comparisons between rows. Different normalizations (for example, to compare columns) and interactions between other axes can be analysed on our website, where figures based on the same underlying data can be generated.
For which loci of shift are different generalization types studied?
Another interesting question to ask is for which locus different generalization types are considered (Fig. 6 , top right). We observe that only cross-task generalization is frequently investigated in the pretrain–train and pretrain–test stages. For all other types of generalization, the vast majority of tests are conducted in the train–test or finetune train–test stage. In some cases, these differences are to be expected: as general-purpose pretrained models are usually trained on very large, relatively uncontrolled corpora, investigating how they generalize to a different domain without further finetuning is hardly possible, and neither is evaluating their robustness, which typically also requires more detailed knowledge of the training data. The statistics also confirm the absence of studies that consider compositional generalization from pretraining to finetuning or from pretraining to training, which is philosophically and theoretically challenging in such set-ups because of their all-encompassing training corpora and the fact that in (large) language models, form and meaning are conflated in one space. A final observation is the relative underrepresentation of studies with multiple loci across all generalization types, especially given the large number of studies that consider generalization in the finetuning stage or with the pretrain–train locus. Those studies have included multiple training stages but considered generalization in only one of them. We hope to see this trend change in the future, with more studies considering generalization in the entire modelling pipeline.
Which types of data shift occur across different loci?
Another interesting interaction is the one between the shift locus and the data shift type. Figure 6 (centre left) shows that assumed shifts mostly occur in the pretrain–test locus, confirming our hypothesis that they are probably caused by the use of increasingly large, general-purpose training corpora. When such pretrained models are further finetuned, experiments often consider either a shift between pretraining and finetuning where new labels are introduced, or a covariate shift in the finetuning stage; as such, they do not require an in-depth understanding of the pretraining corpus. The studies that do investigate covariate or full shifts with a pretrain–train or pretrain–test are typically not studies considering large language models, but instead multi-stage processes for domain adaptation.
How does motivation drive generalization research?
To discuss the relationship between the motivation behind a study and the other axes, we focus on its interactions with generalization type, shift locus and shift source, as shown in the bottom right half of Fig. 6 . Considering first the relationship between motivation and generalization type (Fig. 6 , centre right), we see that cross-domain, robustness, cross-task and cross-lingual generalizations are predominantly motivated by practical considerations; robustness generalization studies are also frequently motivated by an interest in understanding how models work intrinsically. We find that compositional and structural generalization studies are both frequently driven by cognitive motivations, which is to be expected given the importance of these concepts in human cognition and intelligence (for example, ref. 25 ). The motivation given most frequently for compositional generalization, however, is a practical one. Although in human learning, compositionality is indeed often associated with important practical properties—speed of learning, powerful generalization—as far as we know, there is little empirical evidence that compositional models actually perform better on natural language tasks. A similar apparent mismatch can be observed in Fig. 6 (bottom right) when focusing on the practical motivation. Practical generalization tests are typically aimed at improving models or at being directly informative of a model’s applicability. Nonetheless, more than 20% of the practically motivated studies use either artificially partitioned natural data or even fully generated data. To what extent could their conclusions then actually be informative of models applied in practical scenarios? These apparent mismatches between the motivation and the experimental set-up illustrate the importance of the motivation axis in our taxonomy—being aware of and explicit about a study’s motivation ensures that its conclusions are indeed informative with respect to the underlying research question.
Another interesting observation that can be made from the interactions between motivation and shift locus is that the vast majority of cognitively motivated studies are conducted in a train–test set-up. Although there are many good reasons for this, conclusions about human generalization are drawn from a much more varied range of ‘experimental set-ups’. For example, any experiments done with adults can be thought of as more similar to tests with a finetune train–test or pretrain–test locus than to the train–test locus, as adults have a life-long experience over which the experimenter has little control beyond participant selection. On the one hand, this suggests that generalization with a cognitive motivation should perhaps be evaluated more often with those loci. On the other hand, it begs the question of whether the field could take inspiration from experiments on human generalization for the challenging effort of evaluating the generalization of large language models, trained on uncontrolled corpora, in a pretrain–test setting. Although there are, of course, substantial differences between the assumptions that can reasonably be made about the linguistic experiences of a human and the pretraining of a language model, we still believe that input from experts that have extensively considered human generalization would be beneficial to improve generalization testing in these more challenging set-ups.
In this Analysis we have presented a framework to systematize and understand generalization research. The core of this framework consists of a generalization taxonomy that can be used to characterize generalization studies along five dimensions. This taxonomy, which is designed based on an extensive review of generalization papers in NLP, can be used to critically analyse existing generalization research as well as to structure new studies. The five nominal axes of the taxonomy describe why a study is executed (the main motivation of the study), what the study intends to evaluate (the type of generalization it aims to solve) and how the evaluation is conducted (the type of data shift considered, the source of this data shift, and the locus in which the shift is investigated).
To illustrate the use and usefulness of our taxonomy, we analysed 543 papers from the ACL anthology about generalization. Through our extensive analysis, we demonstrated that the taxonomy is applicable to a wide range of generalization studies and were able to provide a comprehensive map of the field, observing overall patterns and making suggestions for areas that should be prioritized in the future. Our most important conclusions and recommendations are as follows:
The goal of a study is not always perfectly aligned with its experimental design. We recommend that future work should be more explicit about motivations and should incorporate deliberate assessments to ensure that the experimental set-up matches the goal of the study (for example, with the GenBench evaluation cards, as discussed in Supplementary section B ).
Cross-lingual studies and generalization studies motivated by fairness and inclusivity goals are underrepresented. We suggest that these areas should be given more attention in future work.
Papers that target similar generalization questions vary widely in the type of evaluation set-up they use. The field would benefit from more meta-studies that consider how the results of experiments with different experimental paradigms compare to one another.
The vast majority of generalization studies focus on only one stage of the modelling pipeline. More work is needed that considers generalization in all stages of training, to prioritize models whose generalizing behaviour persists throughout their training pipeline.
Recent popular NLP models that can be tested directly for their generalization from pretraining to testing are often evaluated without considering the relationship between the (pre)training and test data. We advise that this should be improved, and that inspiration might be taken from how generalization is evaluated in experiments with human participants, where control and access to the ‘pretraining’ data of a participant are unattainable.
Along with this Analysis we also launch a website , with (1) a set of visualization tools to further explore our results; (2) a search tool that allows researchers to find studies with specific features; (3) a contributions page, allowing researchers to register new generalization studies; and (4) a tool to generate GenBench evaluation cards, which authors can use in their articles to comprehensively summarize their generalization experiments. Although the review and conclusions presented in this Analysis are necessarily static, we commit to keeping the entries on the website up to date when new papers on generalization are published, and we encourage researchers to engage with our online dynamic review by submitting both new studies and existing studies we might have missed. By providing a systematic framework and a toolset that allow for a structured understanding of generalization, we have taken the necessary first steps towards making state-of-the-art generalization testing the new status quo in NLP. In Supplementary section E , we further outline our vision for this, and in Supplementary section D , we discuss the limitations of our work.
In this Analysis we propose a novel taxonomy to characterize research that aims to evaluate how (well) NLP models generalize, and we use this taxonomy to analyse over 500 papers in the ACL anthology. In this section, we describe the five axes that make up the taxonomy: motivation, generalization type, shift type, shift source and shift locus. A list of examples for every axis value is provided in Supplementary section C . More details about the procedure we used to annotate papers is available in Supplementary section A .
Motivation—what is the high-level motivation for a generalization test?
The first axis we consider is the high-level motivation or goal of a generalization study. We identified four closely intertwined goals of generalization research in NLP, which we refer to as the practical motivation, the cognitive motivation, the intrinsic motivation and the fairness motivation. The motivation of a study determines what type of generalization is desirable, shapes the experimental design, and affects which conclusions can be drawn from a model’s display or lack of generalization. It is therefore crucial for researchers to be explicit about the motivation underlying their studies, to ensure that the experimental set-up aligns with the questions they seek to answer. We now describe the four motivations we identified as the main drivers of generalization research in NLP.
One frequent motivation to study generalization is of a markedly practical nature. Studies that consider generalization from a practical perspective seek to assess in what kind of scenarios a model can be deployed, or which modelling changes can improve performance in various evaluation scenarios (for example, ref. 26 ). We provide further examples of research questions with a practical nature in Supplementary section C .
A second high-level motivation that drives generalization research is a cognitive one, which can be separated into two underlying categories. The first category is related to model behaviour and focuses on assessing whether models generalize in human-like ways. Human generalization is a useful reference point for the evaluation of models in NLP because it is considered to be a hallmark of human intelligence (for example, ref. 25 ) and, perhaps more importantly, because it is precisely the type of generalization that is required to successfully model natural language. The second, more deeply cognitively inspired category embraces work that evaluates generalization in models to learn more about language and cognition (for example, ref. 27 ). Studies in this category investigate what underlies generalization in computational models, not to improve the models’ generalization capabilities, but to derive new hypotheses about the workings of human generalization. In some cases, it might be difficult to distinguish cognitive from practical motivations: a model that generalizes like a human should also score well on practically motivated tests, which is why the same experiments can be motivated in multiple ways. In our axes-based taxonomy, rather than assuming certain experiments come with a fixed motivation, we rely on motivations provided by the authors.
A third motivation to evaluate generalization in NLP models, which cuts through the two previous motivations, pertains to the question of whether models learned the task we intended them to learn, in the way we intended the task to be learned. We call this motivation the intrinsic motivation. The shared presupposition underpinning this type of research is that if a model has truly learned the task it is trained to do, it should also be able to execute this task in settings that differ from the exact training scenarios. What changes, across studies, is the set of conditions under which a model is considered to have appropriately learned a task. Some examples are provided in Supplementary section C . In studies that consider generalization from this perspective, generalization failures are taken as proof that the model did not—in fact—learn the task as we intended it to learn it (for example, ref. 28 ).
Fairness and inclusivity
A last yet important motivation for generalization research is the desire to have models that are fair, responsible and unbiased, which we denote together as the fairness and inclusivity motivation. One category of studies driven by these concepts, often ethical in nature, asks questions about how well models generalize to diverse demographics, typically considering minority or marginalized groups (for example, ref. 5 ), or investigates to what extent models perpetuate (undesirable) biases learned from their training data (for example, ref. 17 ). Another line of research related to both fairness and inclusivity focuses on efficiency, both in terms of the amount of data that is required for a model to converge to a solution as well as the necessary amount of compute. In such studies, efficiency is seen as a correlate of generalization: models that generalize well should learn more quickly and require less data (for example, ref. 29 ). As such, they are more inclusively applicable—for instance to low-resource languages or demographic groups for which little data are available—they are more accessible for groups with smaller computational resources, and they have a lower environmental impact (for example ref. 30 ).
Generalization type—what type of generalization is a test addressing?
The second axis in our taxonomy describes, on a high level, what type of generalization a test is intended to capture, making it an important axis of our taxonomy. We identify and describe six types of generalization that are frequently considered in the literature.
Compositional generalization
The first prominent type of generalization addressed in the literature is compositional generalization, which is often argued to underpin humans’ ability to quickly generalize to new data, tasks and domains (for example, ref. 31 ). Although it has a strong intuitive appeal and clear mathematical definition 32 , compositional generalization is not easy to pin down empirically. Here, we follow Schmidhuber 33 in defining compositionality as the ability to systematically recombine previously learned elements to map new inputs made up from these elements to their correct output. For an elaborate account of the different arguments that come into play when defining and evaluating compositionality for a neural network, we refer to Hupkes and others 34 .
Structural generalization
A second category of generalization studies focuses on structural generalization—the extent to which models can process or generate structurally (grammatically) correct output—rather than on whether they can assign them correct interpretations. Some structural generalization studies focus specifically on syntactic generalization; they consider whether models can generalize to novel syntactic structures or novel elements in known syntactic structures (for example, ref. 35 ). A second category of structural generalization studies focuses on morphological inflection, a popular testing ground for questions about human structural generalization abilities. Most of this work considers i.i.d. train–test splits, but recent studies have focused on how morphological transducer models generalize across languages (for example, ref. 36 ) as well as within each language 37 .
Cross-task generalization
A third direction of generalization research considers the ability of individual models to adapt to multiple NLP problems—cross-task generalization. Cross-task generalization in NLP has traditionally been strongly connected to transfer and multitask learning 38 , in which the goal was to train a network from scratch on multiple tasks at the same time, or to transfer knowledge from one task to another. In that formulation, it was deemed an extremely challenging topic. This has changed with the relatively recent trend of models that are first pretrained with a general-purpose, self-supervised objective and then further finetuned, potentially with the addition of task-specific parameters that learn to execute different tasks using the representations that emerged in the pretraining phase. Rather than evaluating how learning one task can benefit another, this pretrain–finetune paradigm instead gives a central role to the question of how well a model that has acquired some general knowledge about language can successfully be adapted to different kinds of tasks (for example, refs. 4 , 39 ), with or without the addition of task-specific parameters.
Cross-lingual generalization
The fourth type of generalization we include is generalization across languages, or cross-lingual generalization. Research in NLP has been very biased towards models and technologies for English 40 , and most of the recent breakthroughs rely on amounts of data that are simply not available for the vast majority of the world’s languages. Work on cross-lingual generalization is thus important for the promotion of inclusivity and democratization of language technologies, as well as from a practical perspective. Most existing cross-lingual studies focus on scenarios where labelled data is available in a single language (typically English) and the model is evaluated in multiple languages (for example, ref. 41 ). Another way in which cross-lingual generalization can be evaluated is by testing whether multilingual models perform better than monolingual models on language-specific tasks as a result of being trained on multiple languages at the same time (for example, ref. 42 ).
Generalization across domains
The next category we include is generalization across domains, a type of generalization that is often required in naturally occurring scenarios—more so than the types discussed so far—and thus carries high practical relevance. Although there is no precise definition of what constitutes a domain, the term broadly refers to collections of texts exhibiting different topical and/or stylistic properties, such as different genres or texts with varying formality levels. We include also temporal generalization, where the training data are produced in a specific time period and the model is tested on data from a different time period, either in the future or in the past (for example, ref. 43 ), in the category of domain generalization. In the literature, cross-domain generalization has often been studied in connection with domain adaptation—the problem of adapting an existing general model to a new domain (for example, ref. 44 ).
Robustness generalization
The last category of generalization research we consider on the generalization type axis is robustness generalization, which concerns models’ ability to learn task solutions that abstract away from spurious correlations that may occur in the training data and that are aligned with the underlying generalizing solution that humans associate with the task (for example, ref. 28 ). Research on robustness generalization usually focuses on data shifts that stem from varying data collection processes, which are generally unintended and can be hard to spot. Current work therefore focuses on characterizing such scenarios and understanding their impact. Many of these studies show that models do not generalize in the way we would expect them to, because the training data was in some subtle manner not representative of the true task distribution. For example, they may focus on how models generalize in the face of annotation artefacts (for example, ref. 45 ), across static and non-static splits (for example, ref. 46 ) and when certain demographics are under- or over-represented in the training data (for example, ref. 17 ).
Shift type—what kind of data shift is considered?
We have seen that generalization tests differ in terms of their motivation and the type of generalization that they target. What they share, instead, is that they all focus on cases in which there is a form of shift between the data distributions involved in the modelling pipeline. In the third axis of our taxonomy, we describe the ways in which two datasets used in a generalization experiment can differ. This axis adds a statistical dimension to our taxonomy and derives its importance from the fact that data shift plays an essential role in formally defining and understanding generalization from a statistical perspective.
We formalize the differences between the test, training and potentially pretraining data involved in generalization tests as shifts between the respective data distributions:
These data distributions can be expressed as the product of the probability of the input data p ( x ) and the conditional probability of the output labels given the input data p ( y ∣ x ):
This allows us to define four main types of relation between two data distributions, depending on whether the distributions differ in terms of p ( x ), p ( y ∣ x ), both or none. Note that, for clarity, we focus on train–test shifts, as this is the most intuitive setting, but the shift types we describe in this section can be used to characterize the relationship between any two data distributions involved in a modelling pipeline. One of the four shift types constitutes the case in which there is no shift in data distributions—both p ( x tr ) = p ( x tst ) and p ( y tr | x tr ) = p ( y tst | x tst ). This matches the i.i.d. evaluation set-up traditionally used in machine learning. As discussed earlier, this type of evaluation, also referred to as within-distribution generalization, has often been reported not to be indicative of good performance for the more complex forms of generalization that we often desire from our models. We will not discuss this further here, but instead focus on the other three cases, commonly referred to as out-of-distribution (o.o.d.) shifts. In the following, we discuss the shift types we include in our taxonomy.
Covariate shift
The most commonly considered data distribution shift in o.o.d. generalization research is the one where p ( x tst ) ≠ p ( x tr ) but p ( y tst | x tst ) = p ( y tr | x tr ). In this scenario, often referred to as the covariate shift 47 , 48 , the distribution of the input data p ( x ) changes, but the conditional probability of the labels given the input—which describes the task—remains the same. Under this type of shift, one can evaluate if a model has learned the underlying task distribution while only being exposed to p ( x tr , y tr ).
Label shift
The second type of shift corresponds to the case in which the focus is on the conditional output distributions: p ( y tst | x tst ) ≠ p ( y tr | x tr ). We refer to this case as the label shift. Label shift can happen within the same task when there are inter-annotator disagreements, when there is a temporal shift in the data, or a change of domain (for example, the phrase ‘it doesn’t run’ can lead to different sentiment labels depending on whether it appears in a review for software or one for mascara). Label shift also occurs when there is a change in task, where it may even happen that not only the meaning of the labels, but the labels themselves change, for example, when shifting from language modelling (where the set of labels is the language vocabulary) to part-of-speech (POS) tagging.
The most extreme type of shift corresponds to the case in which p ( x ) and p ( y ∣ x ) change simultaneously: p ( x tst ) ≠ p ( x tr ) and p ( y tst | x tst ) ≠ p ( y tr | x tr ). We refer to this case as full shift. Full shifts may occur in language modelling tasks, where changes in the p ( x ) directly translate into changes in p ( y ∣ x ), when adapting to new language pairs in multilingual experiments (for example, ref. 49 ) or when entirely different types of data are used either for pretraining (for example, ref. 50 ) or for evaluation (for example, ref. 51 ).
Assumed shift
When classifying shifts in our Analysis, we mainly focus on cases where authors explicitly consider the relationship between the data distributions they use in their experiments, and the assumptions they make about this relationship are either well-grounded in the literature (for example, it is commonly assumed that switching between domains constitutes a covariate shift) or empirically verified. Nevertheless, we identify numerous studies that claim to be about generalization where such considerations are absent: it is assumed that there is a shift between train and test data, but this is not verified or grounded in previous research. We include this body of work in our Analysis and denote this type of shift with the label ‘assumed shift’.
Multiple shifts
Note that some studies consider shifts between multiple distributions at the same time, for instance to investigate how different types of pretraining architecture generalize to o.o.d. splits in a finetuning stage 52 or which pretraining method performs better cross-domain generalization in a second training stage 53 . In the GenBench evaluation cards, both these shifts can be marked (Supplementary section B ), but for our analysis in this section, we aggregate those cases and mark any study that considers shifts in multiple different distributions as multiple shift.
Shift source—how are the train and test data produced?
We have discussed what types of shift may occur in generalization tests. We now focus on how those shifts originated. Our fourth axis, graphically shown in Fig. 1 , concerns the source of the differences occurring between the pretraining, training and test data distributions. The source of the data shift determines how much control an experimenter has over the training and testing data and, consequently, what kind of conclusions can be drawn from a generalization experiment.
To formalize the description of these different sources of shift, we consider the unobserved base distribution, which describes all data considered in an experiment:
In this equation, the variable τ represents a data property of interest, with respect to which a specific generalization ability is tested. This can be an observable property of the data (for example, the length of an input sentence), an unobservable property (for example, the timestamp that defines when a data point was produced) or even a property relative to the model (architecture) under investigation (for example, τ could represent how quickly a data point was learned in relation to the overall model convergence). The base distribution over x , y and τ can be used to define different partition schemes to be adopted in generalization experiments. Formally, such a partitioning scheme is a rule \({f}:{{{\mathcal{T}}}}\to \{{\mathtt{true}},\; {\mathtt{false}}\}\) that discriminates data points according to a property \({{{\mathbf{\uptau }}}}\in {{{\mathcal{T}}}}\) . To investigate how a partitioning scheme impacts model behaviour, the pretraining, training and test distributions can be defined as
Using these data descriptions, we can now discuss four different sources of shifts.
Naturally occurring shifts
The first type of shift we include comprises the naturally occurring shifts, which naturally occur between two corpora. In this case, both data partitions of interest are naturally occurring corpora, to which no systematic operations are applied. For the purposes of a generalization test, experimenters have no direct control over the partitioning scheme f ( τ ). In other words, the variable τ refers to properties that naturally differ between collected datasets.
Artificially partitioned natural data
A slightly less natural set-up is one in which a naturally occurring corpus is considered, but it is artificially split along specific dimensions. In our taxonomy, we refer to these with the term ‘partitioned natural data’. The primary difference with the previous category is that the variable τ refers to data properties along which data would not naturally be split, such as the length or complexity of a sample. Experimenters thus have no control over the data itself, but they control the partitioning scheme f ( τ ).
Generated shifts
The third category concerns cases in which one data partition is a fully natural corpus and the other partition is designed with specific properties in mind, to address a generalization aspect of interest. We call these generated shifts. Data in the constructed partition may avoid or contain specific patterns (for example, ref. 18 ), violate certain heuristics (for example, ref. 8 ) or include unusually long or complex sequences (for example, ref. 54 ), or it may be constructed adversarially, generated either by humans 55 or automatically using a specific model (for example, ref. 56 ).
Fully generated
The last possibility is to use fully generated data. Generating data is often the most precise way of measuring specific aspects of generalization, as experimenters have direct control over both the base distribution and the partitioning scheme f ( τ ). Sometimes the data involved are entirely synthetic (for example, ref. 34 ); other times they are templated natural language or a very narrow selection of an actual natural language corpus (for example, ref. 9 ).
Locus of shift—between which data distributions does the shift occur?
The four axes that we have discussed so far demonstrate the depth and breadth of generalization evaluation research, and they also clearly illustrate that generalization is evaluated in a wide range of different experimental set-ups. They describe high-level motivations, types of generalization, data distribution shifts used for generalization tests, and the possible sources of those shifts. What we have not yet explicitly discussed is between which data distributions those shifts can occur—the locus of the shift. In our taxonomy, the shift locus forms the last piece of the puzzle, as it determines what part of the modelling pipeline is investigated and, with that, what kind of generalization questions can be answered. We consider shifts between all stages in the contemporary modelling pipeline—pretraining, training/finetuning and testing—as well as studies that consider shifts between multiple stages at the same time, as expressed by the data distributions that we have considered (for a graphical representation, see Extended Data Fig. 1 ).
We describe the loci of shift and how they interact with different components of the modelling pipeline with the aid of three modelling distributions. These modelling distributions correspond to the previously described stages—testing a model, training it, and potentially pretraining it:
In these equations, ϕ broadly denotes the training and pretraining hyperparameters, θ refers to the model parameters, and \({{{\mathcal{X}}}},\,{{{\mathcal{Y}}}}\) indicate sets of inputs ( x ) and their corresponding output ( y ). Equation ( 9 ) defines a model instance, which specifies the probability distribution over the target test labels \({{{{\mathcal{Y}}}}}_{{{{\rm{tst}}}}}\) , given the model’s parameters θ * and a set of test inputs \({{{{\mathcal{X}}}}}_{{{{\rm{tst}}}}}\) . Equation ( 10 ) defines a training procedure, by specifying a probability distribution over model parameters \({{{{\mathbf{\uptheta }}}}}^{* }\in {{\mathbb{R}}}^{d}\) given a training dataset \({{{{\mathcal{X}}}}}_{{{{\rm{tr}}}}},\,{{{{\mathcal{Y}}}}}_{{{{\rm{tr}}}}}\) , a set of training hyperparameters ϕ tr and a (potentially pretrained) model initialization \({\hat{{{{\mathbf{\uptheta }}}}}}\) . Finally, equation ( 11 ) defines a pretraining procedure, specifying a conditional probability over the set of parameters \({\hat{{{{\mathbf{\uptheta }}}}}}\) , given a pretraining dataset, a set of pretraining hyperparameters ϕ pr and a model initialization. Between which of these stages a shift occurs impacts which modelling distributions can be evaluated. We now discuss the different potential loci of shifts.
The train–test locus
Probably the most commonly occurring locus of shift in generalization experiments is the train–test locus, corresponding to the classic set-up where a model is trained on some data and then directly evaluated on a shifted (o.o.d.) test partition. In some cases, researchers investigate the generalization abilities of a single model instance (that is, a set of parameters θ *, as described in equation ( 9 )). Studies of this type therefore report the evaluation of a model instance—typically made available by others—without considering how exactly it was trained, or how that impacted the model’s generalization behaviour (for example, ref. 57 ). Alternatively, researchers might evaluate one or more training procedures, investigating if the training distribution results in model instances that generalize well (for example, ref. 58 ). Although these cases also require evaluating model instances, the focus of the evaluation is not on one particular model instance, but rather on the procedure that generated the evaluated model instances.
The finetune train–test locus
The second potential locus of shift—the finetune train–test locus—instead considers data shifts between the train and test data used during finetuning and thus concerns models that have gone through an earlier stage of training. This locus occurs when a model is evaluated on a finetuning test set that contains a shift with respect to the finetuning training data. Most frequently, research with this locus focuses on the finetuning procedure and on whether it results in finetuned model instances that generalize well on the test set. Experiments evaluating o.o.d. splits during finetuning often also include a comparison between different pretraining procedures; for instance, they compare how BERT models and RoBERTa models behave during finetuning, thus investigating both a pretrain–train shift and a finetune train–test shift at the same time.
The pretrain–train locus
A third possible locus of shift is the pretrain–train locus, between pretraining and training data. Experiments with this locus evaluate whether a particular pretraining procedure (equation ( 11 )) results in models (parameter sets \(\hat{{{{\mathbf{\uptheta }}}}}\) ) that are useful when further trained on different tasks or domains (for example, ref. 59 ).
The pretrain–test locus
Finally, experiments can have a pretrain–test locus, where the shift occurs between pretraining and test data. This locus occurs when a pretrained model is evaluated directly on o.o.d. data, without further training (that is, \({{{{\mathcal{X}}}}}_{{{{\rm{tr}}}}},\,{{{{\mathcal{Y}}}}}_{{{{\rm{tr}}}}}={{\emptyset}},\,{{\emptyset}}\) )—as frequently happens in in-context learning set-ups (for example, ref. 60 )—or when a pretrained model is finetuned on examples that are i.i.d. with respect to the pretraining data and then tested on out-of-distribution instances. The former case ( \({{{{\mathbf{\uptheta }}}}}^{* }={\hat{{{{\mathbf{\uptheta }}}}}}\) ) is similar to studies with only one training stage in the train–test locus, but distinguishes itself by the nature of the (pre)training procedure, which typically has a general-purpose objective, rather than being task-specific (for example, a language modelling objective).
Multiple loci
In some cases, one single study may investigate multiple shifts between different parts of the modelling pipeline. Multiple-loci experiments evaluate all stages of the modelling pipeline at once: they assess the generalizability of models produced by the pretraining procedure as well as whether generalization happens in the finetuning stage (for example, ref. 61 ). Although those can be separately annotated in GenBench evaluation cards, in the analysis section of this Analysis we take them all together in a single category and denote those studies to have multiple loci.
Data availability
The full annotated list of articles included in our survey is available through the GenBench website ( https://genbench.org/references ), where articles can be filtered through a dedicated search tool. This is an evolving survey: we encourage authors to submit new work and to request annotation corrections through our contributions page ( https://genbench.org/contribute ). The exact list used at the time of writing can be retrieved from https://github.com/GenBench/GenBench.github.io/blob/cea0bd6bd8af6f2d0f096c8f81185b1dfc9303b5/taxonomy_clean.tsv . We also release interactive tools to visualize the results of our survey at https://genbench.org/visualisation . Source data are provided with this paper.
Marcus, G. F. Rethinking eliminative connectionism. Cogn. Psychol. 37 , 243–282 (1998).
Article Google Scholar
Kirk, R., Zhang, A., Grefenstette, E. & Rocktäschel, T. A survey of generalisation in deep reinforcement learning. J. Artif. Intell. Res. https://doi.org/10.1613/jair.1.14174 (2023).
Chowdhery, A. et al. PaLM: scaling language modeling with pathways. J. of Mach. Learn. Res. 24 , 1–113 (2023).
Google Scholar
Devlin, J., Chang, M.-W., Lee, K. & Toutanova, K. BERT: pre-training of deep bidirectional transformers for language understanding. In Proc. 2019 Conference of the North American Chapter of the Association for Computational Linguistics: Human Language Technologies, Volume 1 (Long and Short Papers) (Burstein, J. et al eds) 4171–4186 (Association for Computational Linguistics, 2019); https://doi.org/10.18653/v1/N19-1423
Blodgett, S. L., Green, L. & O’Connor, B. Demographic dialectal variation in social media: a case study of African-American English. Jian Su, Kevin Duh, Xavier Carreras (eds). In Proc. 2016 Conference on Empirical Methods in Natural Language Processing (Su, J. et al eds) 1119–1130 (Association for Computational Linguistics, 2016); https://doi.org/10.18653/v1/D16-1120 . https://aclanthology.org/D16-1120
Plank, B. What to do about non-standard (or non-canonical) language in NLP. Preprint at arXiv https://doi.org/10.48550/arXiv.1608.07836 (2016).
Lake, B. & Baroni, M. Generalization without systematicity: on the compositional skills of sequence-to-sequence recurrent networks. In Proc. 35th International Conference on Machine Learning ( ICML ) 4487–4499 (International Machine Learning Society, 2018).
McCoy, T., Pavlick, E. & Linzen, T. Right for the wrong reasons: diagnosing syntactic heuristics in natural language inference. In Proc. 57th Annual Meeting of the Association for Computational Linguistics (Korhonen, A. et al eds.) 3428–3448 (Association for Computational Linguistics, 2019); https://doi.org/10.18653/v1/P19-1334 , https://aclanthology.org/P19-1334
Kim, N. & Linzen, T. COGS: a compositional generalization challenge based on semantic interpretation. In Proc. 2020 Conference on Empirical Methods in Natural Language Processing ( EMNLP ) (Webber, B. et al eds.) 9087–9105 (Association for Computational Linguistics, 2020); https://doi.org/10.18653/v1/2020.emnlp-main.731 , https://aclanthology.org/2020.emnlp-main.731
Khishigsuren, T. et al. Using linguistic typology to enrich multilingual lexicons: the case of lexical gaps in kinship. In Proceedings of the Thirteenth Language Resources and Evaluation Conference 2798-2807 (European Language Resources Association, 2022); https://aclanthology.org/2022.lrec-1.299
Kaushik, D., Hovy, E. & Lipton, Z. Learning the difference that makes a difference with counterfactually-augmented data. In International Conference on Learning Representations (2019).
Parrish, A. et al. BBQ: a hand-built bias benchmark for question answering. In Findings of the Association for Computational Linguistics (Muresan, S. et al eds.) 2086–2105 (Association for Computational Linguistics, 2022); https://doi.org/10.18653/v1/2022.findings-acl.165 , https://aclanthology.org/2022.findings-acl.165
Srivastava, A. et al. Beyond the imitation game: quantifying and extrapolating the capabilities of language models. Preprint at arXiv https://doi.org/10.48550/arXiv.2206.04615 (2022).
Razeghi, Y., Logan, R. L. IV, Gardner, M. & Singh, S. Impact of pretraining term frequencies on few-shot reasoning. In Findings of the Association for Computational Linguistics: EMNLP 2022 840-854 (Association for Computational Linguistics, 2022); https://aclanthology.org/2022.findings-emnlp.59.pdf
Lewis, P., Stenetorp, P. & Riedel, S. Question and answer test-train overlap in open-domain question answering datasets. In Proc. 16th Conference of the European Chapter of the Association for Computational Linguistics : Main Volume (Merlo, P. et al eds.) 1000–1008 (Association for Computational Linguistics, 2021); https://doi.org/10.18653/v1/2021.eacl-main.86 , https://aclanthology.org/2021.eacl-main.86
Michel, P. & Neubig, G. MTNT: a testbed for machine translation of noisy text. In Proc. 2018 Conference on Empirical Methods in Natural Language Processing (Riloff, E. et al eds.) 543–553 (Association for Computational Linguistics, 2018); https://doi.org/10.18653/v1/D18-1050 , https://aclanthology.org/D18-1050
Dixon, L., Li, J., Sorensen, J., Thain, N. & Vasserman, L. Measuring and mitigating unintended bias in text classification. In Proc. 2018 AAAI / ACM Conference on AI , Ethics and Society 67–73 (Association for Computing Machinery, 2018); https://doi.org/10.1145/3278721.3278729
Dankers, V., Bruni, E. & Hupkes, D. The paradox of the compositionality of natural language: a neural machine translation case study. In Proc. 60th Annual Meeting of the Association for Computational Linguistics ( Volume 1 : Long Papers ) (Muresan, S. et al eds.) 4154–4175 (Association for Computational Linguistics, 2022); https://doi.org/10.18653/v1/2022.acl-long.286 , https://aclanthology.org/2022.acl-long.286
Wei, J., Garrette, D., Linzen, T. & Pavlick, E. Frequency effects on syntactic rule learning in transformers. In Proc. 2021 Conference on Empirical Methods in Natural Language Processing (Moens, M.-F. et al eds.) 932–948 (Association for Computational Linguistics, 2021); https://doi.org/10.18653/v1/2021.emnlp-main.72 , https://aclanthology.org/2021.emnlp-main.72
Weber, L., Jumelet, J., Bruni, E. & Hupkes, D. Language modelling as a multi-task problem. In Proc. 16th Conference of the European Chapter of the Association for Computational Linguistics : Main Volume (Merlo, P. et al eds.) 2049–2060 (Association for Computational Linguistics, 2021); https://doi.org/10.18653/v1/2021.eacl-main.176 , https://aclanthology.org/2021.eacl-main.176
Raunak, V., Kumar, V., Metze, F. & Callan, J. On compositionality in neural machine translation. In NeurIPS 2019 Context and Compositionality in Biological and Artificial Neural Systems Workshop (2019); https://arxiv.org/abs/1911.01497
Dubois, Y., Dagan, G., Hupkes, D. & Bruni, E. Location attention for extrapolation to longer sequences. In Proc. 58th Annual Meeting of the Association for Computational Linguistics (Jurafsky, D. et al eds.) 403–413 (Association for Computational Linguistics, 2020); https://doi.org/10.18653/v1/2020.acl-main.39 , https://aclanthology.org/2020.acl-main.39
Chaabouni, R., Dessì, R. & Kharitonov, E. Can transformers jump around right in natural language? Assessing performance transfer from SCAN. In Proc. Fourth BlackboxNLP Workshop on Analyzing and Interpreting Neural Networks for NLP 136–148 (Association for Computational Linguistics, 2021); https://doi.org/10.18653/v1/2021.blackboxnlp-1.9 , https://aclanthology.org/2021.blackboxnlp-1.9
Sun, K., Williams, A. & Hupkes, D. A replication study of compositional generalization works on semantic parsing. In ML Reproducibility Challenge 2022. (2023); https://openreview.net/pdf?id=MF9uv95psps
Marcus, G. F. The Algebraic Mind : Integrating Connectionism and Cognitive Science (Linzen, T. et al eds.) (MIT Press, 2003).
Zhou, X., Elfardy, H., Christodoulopoulos, C., Butler, T. & Bansal, M. Hidden biases in unreliable news detection datasets. In Proc. 16th Conference of the European Chapter of the Association for Computational Linguistics : Main Volume (Merlo, P. et al eds.) 2482–2492 (Association for Computational Linguistics, 2021); https://doi.org/10.18653/v1/2021.eacl-main.211 , https://aclanthology.org/2021.eacl-main.211
Lakretz, Y. et al. Mechanisms for handling nested dependencies in neural-network language models and humans. Cognition 213 , 104699 (2021).
Talman, A. & Chatzikyriakidis, S. Testing the generalization power of neural network models across NLI benchmarks. In Proc. 2019 ACL Workshop BlackboxNLP : Analyzing and Interpreting Neural Networks for NLP (Linzen, T. et al eds.) 85–94 (Association for Computational Linguistics, 2019); https://doi.org/10.18653/v1/W19-4810 , https://aclanthology.org/W19-4810
Marcus, G. Deep learning: a critical appraisal. Preprint at arXiv https://doi.org/10.48550/arXiv.1801.00631 (2018).
Strubell, E., Ganesh, A. & McCallum, A. Energy and policy considerations for deep learning in NLP. In Proc. 57th Annual Meeting of the Association for Computational Linguistics (Korhonen, A. et al eds.) 3645–3650 (Association for Computational Linguistics, 2019); https://doi.org/10.18653/v1/P19-1355 , https://aclanthology.org/P19-1355
Fodor, J. A. & Pylyshyn, Z. W. Connectionism and cognitive architecture: a critical analysis. Cognition 28 , 3–71 (1988).
Montague, R. Universal grammar. Theoria 36 , 373–398 (1970).
Article MathSciNet MATH Google Scholar
Schmidhuber, J. Towards compositional learning in dynamic networks. Technical report (Istituto Dalle Molle di Studi sull’Intelligenza Artificiale (IDSIA), 1990).
Hupkes, D., Dankers, V., Mul, M. & Bruni, E. Compositionality decomposed: how do neural networks generalise? J. Artif. Intell. Res. 67 , 757–795 (2020).
Article MathSciNet Google Scholar
Jumelet, J., Denic, M., Szymanik, J., Hupkes, D. & Steinert-Threlkeld, S. Language models use monotonicity to assess NPI licensing. In Findings of the Association for Computational Linguistics (Zong, C. et al eds.) 4958–4969 (Association for Computational Linguistics, 2021); https://doi.org/10.18653/v1/2021.findings-acl.439 , https://aclanthology.org/2021.findings-acl.439
Pimentel, T. et al. SIGMORPHON 2021 shared task on morphological reinflection: generalization across languages. In Proc. 18th SIGMORPHON Workshop on Computational Research in Phonetics , Phonology and Morphology (Nicolai, G. et al eds.) 229–259 (Association for Computational Linguistics, 2021); https://doi.org/10.18653/v1/2021.sigmorphon-1.25 , https://aclanthology.org/2021.sigmorphon-1.25
Liu, L. & Hulden, M. Can a transformer pass the wug test? Tuning copying bias in neural morphological inflection models. In Proc. 60th Annual Meeting of the Association for Computational Linguistics ( Volume 2 : Short Papers ) (Muresan, S. et al eds.) 739–749 (Association for Computational Linguistics, 2022); https://doi.org/10.18653/v1/2022.acl-short.84 , https://aclanthology.org/2022.acl-short.84
Collobert, R. & Weston, J. A unified architecture for natural language processing: deep neural networks with multitask learning. In Proc. Twenty-Fifth International Conference on Machine Learning ( ICML 2008 ) Vol. 307 of ACM International Conference Proceeding Series (eds Cohen, W. W., McCallum, A. & Roweis, S. T.) 160–167 (ACM, 2008).
Radford, A. et al. Language models are unsupervised multitask learners. OpenAI blog 1 , 9 (2019).
Bender, E. M. On achieving and evaluating language-independence in NLP. Ling. Issues Lang. Technol. https://doi.org/10.33011/lilt.v6i.1239 (2011).
Wu, S. & Dredze, M. Beto, Bentz, Becas: the surprising cross-lingual effectiveness of BERT. In Proc. 2019 Conference on Empirical Methods in Natural Language Processing and the 9th International Joint Conference on Natural Language Processing ( EMNLP-IJCNLP ) (Inui, K. et al eds.) 833–844 (Association for Computational Linguistics, 2019); https://doi.org/10.18653/v1/D19-1077 , https://aclanthology.org/D19-1077
Zhang, B., Williams, P., Titov, I. & Sennrich, R. Improving massively multilingual neural machine translation and zero-shot translation. In Proc. 58th Annual Meeting of the Association for Computational Linguistics (Jurafsky, D. et al eds.) 1628–1639 (Association for Computational Linguistics, 2020); https://doi.org/10.18653/v1/2020.acl-main.148 , https://aclanthology.org/2020.acl-main.148
Lazaridou, A. et al. Mind the gap: assessing temporal generalization in neural language models. Adv. Neural Inf. Process. Syst. 34 , 29348–29363 (2021).
Daumé, H. III. Frustratingly easy domain adaptation. In Proc. 45th Annual Meeting of the Association of Computational Linguistics (Zaenen, A. et al eds.) 256–263 (Association for Computational Linguistics, 2007); https://aclanthology.org/P07-1033
Poliak, A., Naradowsky, J., Haldar, A., Rudinger, R. & Van Durme, B. Hypothesis only baselines in natural language inference. In Proc. Seventh Joint Conference on Lexical and Computational Semantics (Nissim, M. et al eds.) 180–191 (Association for Computational Linguistics, 2018); https://doi.org/10.18653/v1/S18-2023 , https://aclanthology.org/S18-2023
Gorman, K. & Bedrick, S. We need to talk about standard splits. In Proc. 57th Annual Meeting of the Association for Computational Linguistics (Korhonen, A. et al eds.) 2786–2791 (Association for Computational Linguistics, 2019); https://doi.org/10.18653/v1/P19-1267 , https://aclanthology.org/P19-1267
Storkey, A. When training and test sets are different: characterizing learning transfer. Dataset Shift Mach. Learn. 30 , 3–28 (2009).
Moreno-Torres, J. G., Raeder, T., Alaiz-Rodríguez, Rocío, Chawla, N. V. & Herrera, F. A unifying view on dataset shift in classification. Pattern Recogn. 45 , 521–530 (2012).
Kodner, J. et al. SIGMORPHON–UniMorph 2022 shared task 0: generalization and typologically diverse morphological inflection. In Proc. 19th SIGMORPHON Workshop on Computational Research in Phonetics , Phonology and Morphology (Nicolai, G. et al eds.) 176–203 (Association for Computational Linguistics, 2022); https://doi.org/10.18653/v1/2022.sigmorphon-1.19 , https://aclanthology.org/2022.sigmorphon-1.19
Papadimitriou, I. & Jurafsky, D. Learning music helps you read: using transfer to study linguistic structure in language models. In Proc. 2020 Conference on Empirical Methods in Natural Language Processing ( EMNLP ) (Webber, B. et al eds.) 6829–6839 (Association for Computational Linguistics, 2020); https://doi.org/10.18653/v1/2020.emnlp-main.554 , https://aclanthology.org/2020.emnlp-main.554
De Varda, A. & Zamparelli, R. Multilingualism encourages recursion: a transfer study with mBERT. In Proc. 4th Workshop on Research in Computational Linguistic Typology and Multilingual NLP (Vylomova, E. et al eds.) 1–10 (Association for Computational Linguistics, 2022); https://doi.org/10.18653/v1/2022.sigtyp-1.1 , https://aclanthology.org/2022.sigtyp-1.1
Li, B. et al. Quantifying adaptability in pre-trained language models with 500 tasks. In Proc. 2022 Conference of the North American Chapter of the Association for Computational Linguistics : Human Language Technologies (Carpuat, M. et al eds.) 4696–4715 (Association for Computational Linguistics, 2022); https://doi.org/10.18653/v1/2022.naacl-main.346 , https://aclanthology.org/2022.naacl-main.346
Wang, B., Lapata, M. & Titov, I. Meta-learning for domain generalization in semantic parsing. In Proc. 2021 Conference of the North American Chapter of the Association for Computational Linguistics : Human Language Technologies (Toutanova, K. et al eds.) 366–379 (Association for Computational Linguistics, 2021); https://doi.org/10.18653/v1/2021.naacl-main.33 , https://aclanthology.org/2021.naacl-main.33
Lakretz, Y., Desbordes, T., Hupkes, D. & Dehaene, S. Causal transformers perform below chance on recursive nested constructions, unlike humans. In Proceedings of the 29th International Conference on Computational Linguistics 3226–3232 (International Committee on Computational Linguistics, 2022); https://aclanthology.org/2022.coling-1.285
Kiela, D. et al. Dynabench: rethinking benchmarking in NLP. In Proc. 2021 Conference of the North American Chapter of the Association for Computational Linguistics : Human Language Technologies (Toutanova, K. et al eds.) 4110–4124 (Association for Computational Linguistics, 2021); https://doi.org/10.18653/v1/2021.naacl-main.324 , https://aclanthology.org/2021.naacl-main.324
Zellers, R., Bisk, Y., Schwartz, R. & Choi, Y. SWAG: a large-scale adversarial dataset for grounded commonsense inference. In Proc. 2018 Conference on Empirical Methods in Natural Language Processing (Riloff, E. et al eds.) 93–104 (Association for Computational Linguistics, 2018); https://doi.org/10.18653/v1/D18-1009 , https://aclanthology.org/D18-1009
Lakretz, Y. et al. The emergence of number and syntax units in LSTM language models. In Proc. 2019 Conference of the North American Chapter of the Association for Computational Linguistics : Human Language Technologies , Volume 1 ( Long and Short Papers ) (Burstein, J. et al eds.) 11–20 (Association for Computational Linguistics, 2019); https://doi.org/10.18653/v1/N19-1002 , https://aclanthology.org/N19-1002
Rae, J. W. et al. Scaling language models: methods, analysis and insights from training gopher. Preprint at arXiv https://doi.org/10.48550/arXiv.2112.11446 (2021).
Artetxe, M. et al. Efficient large scale language modeling with mixtures of experts. In Proceedings of the 2022 Conference on Empirical Methods in Natural Language Processing (Goldberg, Y. et al eds.) 11699-11732 (Association for Computational Linguistics, 2022); https://doi.org/10.18653/v1/2022.emnlp-main.804 , https://aclanthology.org/2022.emnlp-main.804/
Lin, Xi Victoria et al. Few-shot learning with multilingual generative language models. In Proceedings of the 2022 Conference on Empirical Methods in Natural Language Processing (Goldberg, Y. et al eds.) 9019–9052 (Association for Computational Linguistics, 2022); https://doi.org/10.18653/v1/2022.emnlp-main.616 , https://aclanthology.org/2022.emnlp-main.616/
Yanaka, H., Mineshima, K. & Inui, K. Exploring transitivity in neural NLI models through veridicality. In Proc. 16th Conference of the European Chapter of the Association for Computational Linguistics : Main Volume (Merlo, P. et al eds.) 920–934 (Association for Computational Linguistics, 2021); https://doi.org/10.18653/v1/2021.eacl-main.78 , https://aclanthology.org/2021.eacl-main.78
Download references
Acknowledgements
We thank A. Williams, A. Joulin, E. Bruni, L. Weber, R. Kirk and S. Riedel for providing feedback on the various stages of this paper, and G. Marcus for providing detailed feedback on the final draft. We also thank the reviewers of our work for providing useful comments. We thank E. Hupkes for making the app that allows searching through references, and we thank D. Haziza and E. Takmaz for other contributions to the website. M.G. was supported by the European Research Council (ERC) under the European Union’s Horizon 2020 research and innovation programme (grant agreement no. 819455). V.D. was supported by the UKRI Centre for Doctoral Training in Natural Language Processing, funded by the UKRI (grant no. EP/S022481/1) and the University of Edinburgh. N.S. was supported by the Hyundai Motor Company (under the project Uncertainty in Neural Sequence Modeling) and the Samsung Advanced Institute of Technology (under the project Next Generation Deep Learning: From Pattern Recognition to AI).
Author information
Authors and affiliations.
FAIR, Amsterdam, The Netherlands
Dieuwke Hupkes
University of Amsterdam, Amsterdam, The Netherlands
Mario Giulianelli
University of Edinburgh, Edinburgh, UK
Verna Dankers
Reka AI, Zarautz, Spain
Mikel Artetxe
Allen Institute for AI, Seattle, WA, USA
Yanai Elazar
University of Washington, Seattle, WA, USA
University of Cambridge, Cambridge, UK
Tiago Pimentel
Amazon, Edinburgh, UK
Christos Christodoulopoulos
École Normale Supérieure, Paris, France
Karim Lasri
Harvard University, Cambridge, MA, USA
Naomi Saphra
University of Aberdeen, Aberdeen, UK
Arabella Sinclair
IT University of Copenhagen, Copenhagen, Denmark
Dennis Ulmer
Pioneer Centre for Artificial Intelligence, Copenhagen, Denmark
ETH Zürich, Zurich, Switzerland
Florian Schottmann, Ryan Cotterell & Zhijing Jin
Textshuttle, Zurich, Switzerland
Florian Schottmann
National University of Mongolia, Ulaanbaatar, Mongolia
Khuyagbaatar Batsuren
FAIR, New York, NY, USA
Kaiser Sun & Koustuv Sinha
Hong Kong University of Science and Technology, Hong Kong, Hong Kong SAR, China
Leila Khalatbari & Rita Frieske
MIT, Cambridge, MA, USA
Maria Ryskina
Max Planck Institute for Intelligent Systems, Tübingen, Germany
Zhijing Jin
You can also search for this author in PubMed Google Scholar
Corresponding authors
Correspondence to Dieuwke Hupkes , Mario Giulianelli or Verna Dankers .
Ethics declarations
Competing interests.
The authors declare no competing interests.
Peer review
Peer review information.
Nature Machine Intelligence thanks Karin Verspoor and Raphaël Millière for their contribution to the peer review of this work. Primary Handling Editor: Jacob Huth, in collaboration with the Nature Machine Intelligence team.
Additional information
Publisher’s note Springer Nature remains neutral with regard to jurisdictional claims in published maps and institutional affiliations.
Extended data
Extended data fig. 1 different loci of splits, and the parts of the modelling pipeline for which they may investigate generalisation..
The shifts that characterise generalisation experiments in NLP can occur in different places in the modelling pipeline. In this figure, we visualise the three stages of the contemporary modelling pipeline: the pretraining stage, consisting of pretraining data as well as a pretraining procedure; the training stage, which involves training data, a pretrained model, and a training procedure; and finally, the test stage, in which an already trained model is tested on a test dataset. As visualised in this figure, shifts can occur between all or multiple of those stages, which allows to investigate different parts of the modelling pipeline.
Extended Data Fig. 2 A compact graphical representation of our proposed taxonomy of generalisation in NLP.
The generalisation taxonomy we propose consists of five different (nominal) axes, that describe the high-level motivation of the work (top, left), the type of generalisation the test is addressing (bottom, left); what kind of data shift occurs between training and testing (top, middle), and what the source (top, right) and locus of this shift (bottom, right) are.
Supplementary information
Supplementary information.
Supplementary sections A–F (Annotation set-up, Evaluation cards, Examples, Limitations, Future work) and references.
Supplementary Code 1
Code to generate year count visualizations (count_papers.py Fig. 3 ). Code to generate meta-analysis visualizations (generate_plots.ipynb Figs. 4 – 6 ).
Source Data Fig. 3, 4, 5, 6
Annotated list of all surveyed papers.
Rights and permissions
Open Access This article is licensed under a Creative Commons Attribution 4.0 International License, which permits use, sharing, adaptation, distribution and reproduction in any medium or format, as long as you give appropriate credit to the original author(s) and the source, provide a link to the Creative Commons license, and indicate if changes were made. The images or other third party material in this article are included in the article’s Creative Commons license, unless indicated otherwise in a credit line to the material. If material is not included in the article’s Creative Commons license and your intended use is not permitted by statutory regulation or exceeds the permitted use, you will need to obtain permission directly from the copyright holder. To view a copy of this license, visit http://creativecommons.org/licenses/by/4.0/ .
Reprints and permissions
About this article
Cite this article.
Hupkes, D., Giulianelli, M., Dankers, V. et al. A taxonomy and review of generalization research in NLP. Nat Mach Intell 5 , 1161–1174 (2023). https://doi.org/10.1038/s42256-023-00729-y
Download citation
Received : 22 December 2022
Accepted : 05 September 2023
Published : 19 October 2023
Issue Date : October 2023
DOI : https://doi.org/10.1038/s42256-023-00729-y
Share this article
Anyone you share the following link with will be able to read this content:
Sorry, a shareable link is not currently available for this article.
Provided by the Springer Nature SharedIt content-sharing initiative
Quick links
- Explore articles by subject
- Guide to authors
- Editorial policies
Sign up for the Nature Briefing: AI and Robotics newsletter — what matters in AI and robotics research, free to your inbox weekly.

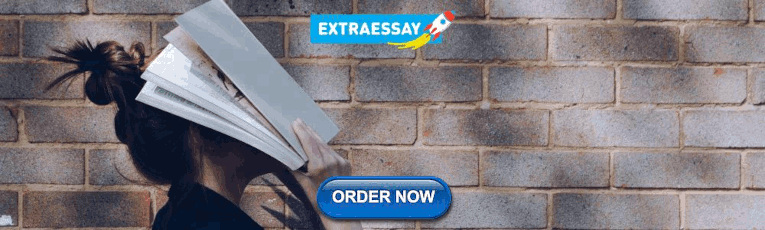
IMAGES
VIDEO
COMMENTS
One of the controversies associated with case study research designs centers on "generalization" and the extent to which the data can explain phenomena or situations outside and beyond the specific scope of a particular study. On the one hand, there are researchers such as Yin (2014) who espouse "analytical generalization" whereby the ...
Case study researchers often see their work praised for the richness of their data, but critiqued on the grounds that case study research is unable to illustrate anything beyond itself (Steinberg 2015, p. 152).The critique is raised frequently enough that case study researchers themselves regularly declare that 'generalization is their principle vulnerability' (Steinberg 2015, p. 155 ...
As we noted earlier, the cases studied by case study researchers are often. investigation has to be selective, and reliance is thereby placed on internal generalization.17 This can occur along ...
Revised on March 3, 2023. Generalizability is the degree to which you can apply the results of your study to a broader context. Research results are considered generalizable when the findings can be applied to most contexts, most people, most of the time. Example: Generalizability. Suppose you want to investigate the shopping habits of people ...
Validity and generalization continue to be challenging aspects in designing and conducting case study evaluations, especially when the number of cases being studied is highly limited (even limited to a single case). To address the challenge, this article highlights current knowledge regarding the use of: (1) rival explanations, triangulation ...
Analytical generalisation involves making projections about the likely transferability of findings from an evaluation, based on a theoretical analysis of the factors producing outcomes and the effect of context. Realist evaluation can be particularly important for this. Analytic generalisation is distinct from statistical generalisation, in ...
Abstract. The generalisability of case study findings is heavily criticised in the scientific community. This study attempts to answer to what extent generalisation is possible, through a ...
Case study research has been extensively used in numerous disciplines as a way to test and develop theory, add to humanistic understanding and existing experiences, and uncover the intricacies of complex social phenomena. ... This will allow for a more nuanced way to involve naturalistic generalizations in case study research that provides both ...
Discourses of Generalizing. Case study researchers often see their work praised for the richness of their data, but critiqued on the grounds that case study research is unable to illustrate anything beyond itself (Steinberg 2015, p. 152). The critique is raised frequently enough that case study researchers themselves regularly declare that ...
The case study as a key research method has often been criticized for generating results that are less generalizable than those of large-sample, quantitative methods. This paper clearly defines generalization and distinguishes it from other related concepts. Drawing on the literature, the author shows that case study results may be less ...
Generalization, which is an act of reasoning that involves drawing broad inferences from particular observations, is widely-acknowledged as a quality standard in quantitative research, but is more controversial in qualitative research. The goal of most qualitative studies is not to generalize but rather to provide a rich, contextualized ...
This "replication crisis" has been discussed on statistical, theoretical and social grounds and continues to have a wide impact on quantitative research practices like, for example, open science initiatives, pre-registered studies and a re-evaluation of statistical significance testing (Everett and Earp, 2015; Maxwell et al., 2015; Shrout ...
The potential for generalization of research findings is among the most divisive of concerns facing psychologists. An article by Roald, Køppe, Jensen, Hansen, and Levin argues that generalizability is not only a relevant concern but an inescapable dimension of qualitative research, directly challenging the view that generalization and generalizability apply only to quantitative research. Thus ...
Generalizability in qualitative research has been a controversial topic given that interpretivist scholars have resisted the dominant role and mandate of the positivist tradition within social sciences. Aiming to find universal laws, the positivist paradigm has made generalizability a crucial criterion for evaluating the rigor of quantitative ...
Other examples, such as the work by Kaplan just cited, start with a single in-depth case study and then augments it with others. But the core of the approach is the use of a (relatively) large number of individual case studies, and even a whole population, in order to strengthen causal inference and generalizability.
Case study research is variously referred to as a methodology, research design, method, research strategy, research approach, ... This tension is the source of the problem of generalization. A case study, no matter how insightful, is a study of a particular case (or a handful of particular cases). However, we are usually interested in it ...
Generalising from qualitative research (GQR) has been an abiding interest of the authors throughout their careers (Falk & Guenther, 2006; Guenther & Falk, 2019a, 2019b).Of all the projects, 54 have been evaluations in a variety of contexts across Australia and Indonesia (Arnott et al., 2012; Falk et al., 2006; Guenther et al., 2009).Commissioners invariably want answers to questions, but a sub ...
Although generalizability is not typically considered a feature or goal of qualitative research, it is an integral part of applying findings to advance knowledge in the counseling profession. First, we describe types of generalizability, the use of trustworthiness criteria, and strategies for maximizing generalizability within and across ...
Generalization, which is an act of reasoning that involves drawing broad inferences from particular observations, is widely-acknowledged as a quality standard in quantitative research, but is more controversial in qualitative research. The goal of most qualitative studies is not to generalize but rather to provide a rich, contextualized ...
Abstract. This article considers the role of generalization in comparative case studies, using as exemplars the contributions to this special issue on climate change politics. As a research practice, generalization is a logical argument for extending one's claims beyond the data, positing a connection between events that were studied and ...
text, sociological research should aim at constructing externally valid and unambiguous generalizations, even when these take a moderated form. Alternative Approaches to Qualitative Generalization One of the two main approaches to generalization in qualitative sociology has been to emphasize internal validity and proceed as though what really ...
In this article, we outline and seek to codify an emerging research practice that, following Fortna (Reference Fortna 2004), we call "Large-N Qualitative Analysis" or LNQA.Among its distinctive features are a focus on regularities rather than average treatment effects, an effort to conduct within-case causal inference with respect to all cases falling within stipulated scope conditions ...
The generalization taxonomy we propose—visualized in Fig. 1 and compactly summarized in Extended Data Fig. 2 —is based on a detailed analysis of a large number of existing studies on ...