This is where the search bar goes
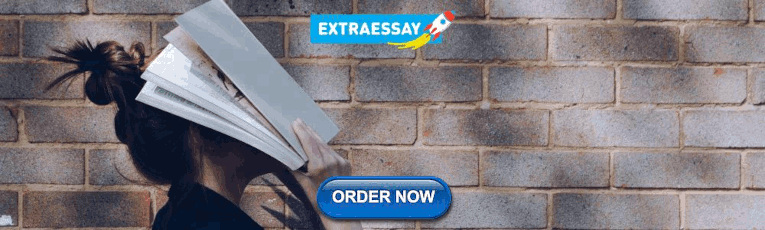
Case studies in data ethics
These studies provide a foundation for discussing ethical issues so we can better integrate data ethics in real life.

To help us think seriously about data ethics, we need case studies that we can discuss, argue about, and come to terms with as we engage with the real world. Good case studies give us the opportunity to think through problems before facing them in real life. And case studies show us that ethical problems aren’t simple. They are multi-faceted, and frequently there’s no single right answer. And they help us to recognize there are few situations that don’t raise ethical questions.
Princeton’s Center for Information Technology Policy and Center for Human Values have created four anonymized case studies to promote the discussion of ethics. The first of these studies, Automated Healthcare App , discusses a smartphone app designed to help adult onset diabetes patients. It raises issues like paternalism, consent, and even language choices. Is it OK to “nudge” patients toward more healthy behaviors? What about automatically moderating the users’ discussion groups to emphasize scientifically accurate information? And how do you deal with minorities who don’t respond to treatment as well? Could the problem be the language itself that is used to discuss treatment?
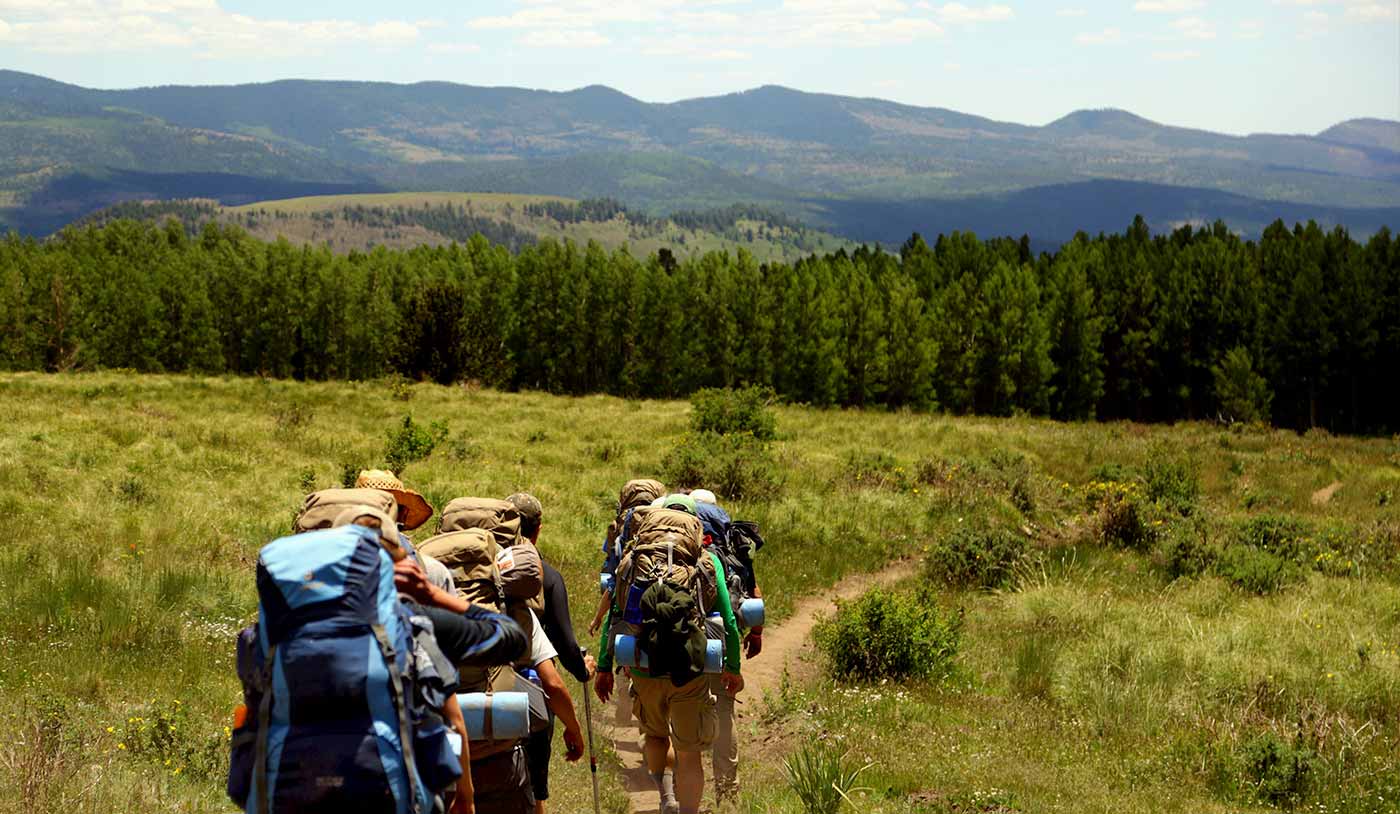
Learn faster. Dig deeper. See farther.
Join the O'Reilly online learning platform. Get a free trial today and find answers on the fly, or master something new and useful.
The next case study, Dynamic Sound Identification , covers an application that can identify voices, raising issues about privacy, language, and even gender. How far should developers go in identifying potential harm that can be caused by an application? What are acceptable error rates for an application that can potentially do harm? How can a voice application handle people with different accents or dialects? And what responsibility do developers have when a small experimental tool is bought by a large corporation that wants to commercialize it?
The Optimizing Schools case study deals with the problem of finding at-risk children in school systems. Privacy and language are again an issue; it also raises the issue of how decisions to use data are made. Who makes those decisions, and who needs to be informed about them? What are the consequences when people find out how their data has been used? And how do you interpret the results of an experiment? Under what conditions can you say that a data experiment has really yielded improved educational results?
The final case study, Law Enforcement Chatbots , raises issues about the tradeoff between liberty and security, entrapment, openness and accountability, and compliance with international law.
None of these issues are simple, and there are few (if any) “right answers.” For example, it’s easy to react against perceived paternalism in a medical application, but the purpose of such an application is to encourage patients to comply with their treatment program. It’s easy to object to monitoring students in a public school, but students are minors, and schools by nature handle a lot of private personal data. Where is the boundary between what is, and isn’t, acceptable? What’s important isn’t getting to the correct answer on any issue, but to make sure the issue is discussed and understood, and that we know what tradeoffs we are making. What is important is that we get practice in discussing ethical issues and put that practice to work in our jobs. That’s what these case studies give us.
Publications
- Analysis & Opinions
- News & Announcements
- Newsletters
- Policy Briefs & Testimonies
- Presentations & Speeches
- Reports & Papers
- Quarterly Journal: International Security
- Artificial Intelligence
- Conflict & Conflict Resolution
- Coronavirus
- Economics & Global Affairs
- Environment & Climate Change
- International Relations
- International Security & Defense
- Nuclear Issues
- Science & Technology
- Student Publications
- War in Ukraine
- Asia & the Pacific
- Middle East & North Africa
- North America
- South America
- Infographics & Charts

US-Russian Contention in Cyberspace
The overarching question imparting urgency to this exploration is: Can U.S.-Russian contention in cyberspace cause the two nuclear superpowers to stumble into war? In considering this question we were constantly reminded of recent comments by a prominent U.S. arms control expert: At least as dangerous as the risk of an actual cyberattack, he observed, is cyber operations’ “blurring of the line between peace and war.” Or, as Nye wrote, “in the cyber realm, the difference between a weapon and a non-weapon may come down to a single line of code, or simply the intent of a computer program’s user.”

The Geopolitics of Renewable Hydrogen
Renewables are widely perceived as an opportunity to shatter the hegemony of fossil fuel-rich states and democratize the energy landscape. Virtually all countries have access to some renewable energy resources (especially solar and wind power) and could thus substitute foreign supply with local resources. Our research shows, however, that the role countries are likely to assume in decarbonized energy systems will be based not only on their resource endowment but also on their policy choices.

What Comes After the Forever Wars
As the United States emerges from the era of so-called forever wars, it should abandon the regime change business for good. Then, Washington must understand why it failed, writes Stephen Walt.

Telling Black Stories: What We All Can Do
Full event video and after-event thoughts from the panelists.
- Defense, Emerging Technology, and Strategy
- Diplomacy and International Politics
- Environment and Natural Resources
- International Security
- Science, Technology, and Public Policy
- Africa Futures Project
- Applied History Project
- Arctic Initiative
- Asia-Pacific Initiative
- Cyber Project
- Defending Digital Democracy
- Defense Project
- Economic Diplomacy Initiative
- Future of Diplomacy Project
- Geopolitics of Energy Project
- Harvard Project on Climate Agreements
- Homeland Security Project
- Intelligence Project
- Korea Project
- Managing the Atom
- Middle East Initiative
- Project on Europe and the Transatlantic Relationship
- Security and Global Health
- Technology and Public Purpose
- US-Russia Initiative to Prevent Nuclear Terrorism
Special Initiatives
- American Secretaries of State
- An Economic View of the Environment
- Cuban Missile Crisis
- Russia Matters
- Thucydides's Trap
Analysis & Opinions - O'Reilly Media
- Mike Loukidos
- Hilary Mason
These studies provide a foundation for discussing ethical issues so we can better integrate data ethics in real life.
To help us think seriously about data ethics, we need case studies that we can discuss, argue about, and come to terms with as we engage with the real world. Good case studies give us the opportunity to think through problems before facing them in real life. And case studies show us that ethical problems aren't simple. They are multi-faceted, and frequently there's no single right answer. And they help us to recognize there are few situations that don't raise ethical questions.
Princeton's Center for Information Technology Policy and Center for Human Values have created four anonymized case studies to promote the discussion of ethics. The first of these studies, Automated Healthcare App , discusses a smartphone app designed to help adult onset diabetes patients. It raises issues like paternalism, consent, and even language choices. Is it OK to “nudge” patients toward more healthy behaviors? What about automatically moderating the users’ discussion groups to emphasize scientifically accurate information? And how do you deal with minorities who don’t respond to treatment as well? Could the problem be the language itself that is used to discuss treatment?
The next case study, Dynamic Sound Identification , covers an application that can identify voices, raising issues about privacy, language, and even gender. How far should developers go in identifying potential harm that can be caused by an application? What are acceptable error rates for an application that can potentially do harm? How can a voice application handle people with different accents or dialects? And what responsibility do developers have when a small experimental tool is bought by a large corporation that wants to commercialize it?
The Optimizing Schools case study deals with the problem of finding at-risk children in school systems. Privacy and language are again an issue; it also raises the issue of how decisions to use data are made. Who makes those decisions, and who needs to be informed about them? What are the consequences when people find out how their data has been used? And how do you interpret the results of an experiment? Under what conditions can you say that a data experiment has really yielded improved educational results?
The final case study, Law Enforcement Chatbots , raises issues about the tradeoff between liberty and security, entrapment, openness and accountability, and compliance with international law.
None of these issues are simple, and there are few (if any) "right answers." For example, it’s easy to react against perceived paternalism in a medical application, but the purpose of such an application is to encourage patients to comply with their treatment program. It’s easy to object to monitoring students in a public school, but students are minors, and schools by nature handle a lot of private personal data. Where is the boundary between what is, and isn’t, acceptable? What's important isn’t getting to the correct answer on any issue, but to make sure the issue is discussed and understood, and that we know what tradeoffs we are making. What is important is that we get practice in discussing ethical issues and put that practice to work in our jobs. That’s what these case studies give us.
Want to Read More?
The authors.

- Senior Fellow, Technology and Public Purpose Project
- Former Senior Fellow, Cyber Project
- Former U.S Chief Data Scientist
- Former CTO, Devoted Health
- Bio/Profile
- More by this author

Recommended
In the spotlight, most viewed.

Journal Article - Issues in Science and Technology
Nuclear Power Needs Leadership, but Not from the Military
- Michael J Ford
- Ahmed Abdulla
- M. Granger Morgan

Analysis & Opinions - The Washington Post
Don't Fear the TSA Cutting Airport Security. Be Glad That They're Talking about It.
- Bruce Schneier

Data's Day of Reckoning

Russia's Invasion of Ukraine and Its Impact on the Global Nuclear Order
- Mariana Budjeryn

Gaza Cease-fire Alone Won’t Repair Larger Enduring Rift
- Tarek Masoud

It’s the End of the Web as We Know It
- Judith Donath

Analysis & Opinions - New Straits Times
Gorbachev and the End of the Cold War
- Joseph S. Nye

Report - Belfer Center for Science and International Affairs
Challenging Biases and Assumptions in Analysis: Could Israel Have Averted Intelligence Failure?
- Beth Sanner
- Adam Siegel

Policy Brief - Quarterly Journal: International Security
Oil, Conflict, and U.S. National Interests
- Jeff D. Colgan
Belfer Center Email Updates
Belfer center of science and international affairs.
79 John F. Kennedy Street, Cambridge, MA 02138 (617) 495-1400
Data ethics: What it means and what it takes
Now more than ever, every company is a data company. By 2025, individuals and companies around the world will produce an estimated 463 exabytes of data each day, 1 Jeff Desjardins, “How much data is generated each day?” World Economic Forum, April 17, 2019. compared with less than three exabytes a decade ago. 2 IBM Research Blog , “Dimitri Kanevsky translating big data,” blog entry by IBM Research Editorial Staff, March 5, 2013.
With that in mind, most businesses have begun to address the operational aspects of data management—for instance, determining how to build and maintain a data lake or how to integrate data scientists and other technology experts into existing teams. Fewer companies have systematically considered and started to address the ethical aspects of data management, which could have broad ramifications and responsibilities. If algorithms are trained with biased data sets or data sets are breached, sold without consent, or otherwise mishandled, for instance, companies can incur significant reputational and financial costs. Board members could even be held personally liable. 3 Leah Rizkallah, “Potential board liability for cybersecurity failures under Caremark law,” CPO Magazine , February 22, 2022.
So how should companies begin to think about ethical data management? What measures can they put in place to ensure that they are using consumer, patient, HR, facilities, and other forms of data appropriately across the value chain—from collection to analytics to insights?
We began to explore these questions by speaking with about a dozen global business leaders and data ethics experts. Through these conversations, we learned about some common data management traps that leaders and organizations can fall into, despite their best intentions. These traps include thinking that data ethics does not apply to your organization, that legal and compliance have data ethics covered, and that data scientists have all the answers—to say nothing of chasing short-term ROI at all costs and looking only at the data rather than their sources.
In this article, we explore these traps and suggest some potential ways to avoid them, such as adopting new standards for data management, rethinking governance models, and collaborating across disciplines and organizations. This list of potential challenges and remedies is not exhaustive; our research base was relatively small, and leaders could face many other obstacles, beyond our discussion here, to the ethical use of data. But what’s clear from our research is that data ethics needs both more and sustained attention from all members of the C-suite, including the CEO.
Potential challenges for business leaders
What is data ethics.
We spoke with about a dozen business leaders and data ethics experts. In their eyes, these are some characteristics of ethical data use:
It preserves data security and protects customer information. The practitioners we spoke with tend to view cybersecurity and data privacy as part and parcel of data ethics. They believe companies have an ethical responsibility (as well as legal obligations) to protect customers’ data, defend against breaches, and ensure that personal data are not compromised.
It offers a clear benefit to both consumers and companies. “The consumer’s got to be getting something” from a data-based transaction, explained an executive at a large financial-services company. “If you’re not solving a problem for a consumer, you’ve got to ask yourself why you’re doing what you’re doing.” The benefit to customers should be straightforward and easy to summarize in a single sentence: customers might, for instance, get greater speed, convenience, value, or savings.
It offers customers some measure of agency. “We don’t want consumers to be surprised,” one executive told us. “If a customer receives an offer and says, ‘I think I got this because of how you’re using my data, and that makes me uncomfortable. I don’t think I ever agreed to this,’ another company might say, ‘On page 41, down in the footnote in the four-point font, you did actually agree to this.’ We never want to be that company.”
It is in line with your company’s promises. In data management, organizations must do what they say they will do—or risk losing the trust of customers and other key stakeholders. As one senior executive pointed out, keeping faith with stakeholders may mean turning down certain contracts if they contradict the organization’s stated data values and commitments.
There is a dynamic body of literature on data ethics. Just as the methods companies use to collect, analyze, and access data are evolving, so will definitions of the term itself. In this article, we define data ethics as data-related practices that seek to preserve the trust of users, patients, consumers, clients, employees, and partners. Most of the business leaders we spoke to agreed broadly with that definition, but some have tailored it to the needs of their own sectors or organizations (see sidebar, “What is data ethics?”). Our conversations with these business leaders also revealed the unintended lapses in data ethics that can happen in organizations. These include the following:
Thinking that data ethics doesn’t apply to your organization
While privacy and ethical considerations are essential whenever companies use data (including artificial-intelligence and machine-learning applications), they often aren’t top of mind for some executives. In our experience, business leaders are not intentionally pushing these thoughts away; it’s often just easier for them to focus on things they can “see”— the tools, technologies, and strategic objectives associated with data management—than on the seemingly invisible ways data management can go wrong.
In a 2021 McKinsey Global Survey on the state of AI , for instance, only 27 percent of some 1,000 respondents said that their data professionals actively check for skewed or biased data during data ingestion. Only 17 percent said that their companies have a dedicated data governance committee that includes risk and legal professionals. In that same survey, only 30 percent of respondents said their companies recognized equity and fairness as relevant AI risks. AI-related data risks are only a subset of broader data ethics concerns, of course, but these numbers are striking.
Thinking in silos: Legal, compliance, or data scientists have data ethics covered
Companies may believe that just by hiring a few data scientists, they’ve fulfilled their data management obligations. The truth is data ethics is everyone’s domain, not just the province of data scientists or of legal and compliance teams. At different times, employees across the organization—from the front line to the C-suite—will need to raise, respond to, and think through various ethical issues surrounding data. Business unit leaders will need to vet their data strategies with legal and marketing teams, for example, to ensure that their strategic and commercial objectives are in line with customers’ expectations and with regulatory and legal requirements for data usage.
As executives navigate usage questions, they must acknowledge that although regulatory requirements and ethical obligations are related, adherence to data ethics goes far beyond the question of what’s legal. Indeed, companies must often make decisions before the passage of relevant laws. The European Union’s General Data Protection Regulation (GDPR) went into effect only in May 2018, the California Consumer Privacy Act has been in effect only since January 2020, and federal privacy law is only now pending in the US Congress. Years before these and other statutes and regulations were put in place, leaders had to set the terms for their organizations’ use of data—just as they currently make decisions about matters that will be regulated in years to come.
Laws can show executives what they can do . But a comprehensive data ethics framework can guide executives on whether they should , say, pursue a certain commercial strategy and, if so, how they should go about it. One senior executive we spoke with put the data management task for executives plainly: “The bar here is not regulation. The bar here is setting an expectation with consumers and then meeting that expectation—and doing it in a way that’s additive to your brand.”
Chasing short-term ROI
Prompted by economic volatility, aggressive innovation in some industries, and other disruptive business trends, executives and other employees may be tempted to make unethical data choices—for instance, inappropriately sharing confidential information because it is useful—to chase short-term profits. Boards increasingly want more standards for the use of consumer and business data, but the short-term financial pressures remain. As one tech company president explained: “It’s tempting to collect as much data as possible and to use as much data as possible. Because at the end of the day, my board cares about whether I deliver growth and EBITDA.… If my chief marketing officer can’t target users to create an efficient customer acquisition channel, he will likely get fired at some point—or at least he won’t make his bonus.”
Looking only at the data, not at the sources
Ethical lapses can occur when executives look only at the fidelity and utility of discrete data sets and don’t consider the entire data pipeline. Where did the data come from? Can this vendor ensure that the subjects of the data gave their informed consent for use by third parties? Do any of the market data contain material nonpublic information? Such due diligence is key: one alternative data provider was charged with securities fraud for misrepresenting to trading firms how its data were derived. In that case, companies had provided confidential information about the performance of their apps to the data vendor, which did not aggregate and anonymize the data as promised. Ultimately, the vendor had to settle with the US Securities and Exchange Commission. 4 “SEC charges App Annie and its founder with securities fraud,” US Securities and Exchange Commission, September 14, 2021.
A few important building blocks
These data management challenges are common—and they are by no means the only ones. As organizations generate more data, adopt new tools and technologies to collect and analyze data, and find new ways to apply insights from data, new privacy and ethical challenges and complications will inevitably emerge. Organizations must experiment with ways to build fault-tolerant data management programs. These seven data-related principles, drawn from our research, may provide a helpful starting point.
Set company-specific rules for data usage
Leaders in the business units, functional areas, and legal and compliance teams must come together to create a data usage framework for employees—a framework that reflects a shared vision and mission for the company’s use of data . As a start, the CEO and other C-suite leaders must also be involved in defining data rules that give employees a clear sense of the company’s threshold for risk and which data-related ventures are OK to pursue and which are not.
Leaders must come together to create a data usage framework that reflects a shared vision and mission for the company’s use of data.
Such rules can improve and potentially speed up individual and organizational decision making. They should be tailored to your specific industry, even to the products and services your company offers. They should be accessible to all employees, partners, and other critical stakeholders. And they should be grounded in a core principle—for example, “We do not use data in any way that we cannot link to a better outcome for our customers.” Business leaders should plan to revisit and revise the rules periodically to account for shifts in the business and technology landscape.
Communicate your data values, both inside and outside your organization
Once you’ve established common data usage rules, it’s important to communicate them effectively inside and outside the organization. That might mean featuring the company’s data values on employees’ screen savers, as the company of one of our interview subjects has done. Or it may be as simple as tailoring discussions about data ethics to various business units and functions and speaking to their employees in language they understand. The messaging to the IT group and data scientists, for instance, may be about creating ethical data algorithms or safe and robust data storage protocols. The messaging to marketing and sales teams may focus on transparency and opt-in/opt-out protocols.
Organizations also need to earn the public’s trust. Posting a statement about data ethics on the corporate website worked for one financial-services organization. As an executive explained: “When you’re having a conversation with a government entity, it’s really helpful to be able to say, ‘Go to our website and click on Responsible Data Use, and you’ll see what we think.’ We’re on record in a way that you can’t really walk back.” Indeed, publicizing your company’s data ethics framework may help increase the momentum for powerful joint action, such as the creation of industry-wide data ethics standards.

Why digital trust truly matters
Build a diverse data-focused team.
A strong data ethics program won’t materialize out of the blue. Organizations large and small need people who focus on ethics issues; it cannot be a side activity. The work should be assigned to a specific team or attached to a particular role. Some larger technology and pharmaceutical companies have appointed chief ethics or chief trust officers in recent years. Others have set up interdisciplinary teams, sometimes referred to as data ethics boards, to define and uphold data ethics. Ideally, such boards would include representatives from, for example, the business units, marketing and sales, compliance and legal, audit, IT, and the C-suite. These boards should also have a range of genders, races, ethnicities, classes, and so on: an organization will be more likely to identify issues early on (in algorithm-training data, for example) when people with a range of different backgrounds and experiences sit around the table.
One multinational financial-services corporation has developed an effective structure for its data ethics deliberations and decision making. It has two main data ethics groups. The major decisions are made by a group of senior stakeholders, including the head of security and other senior technology executives, the chief privacy officer, the head of the consulting arm, the head of strategy, and the heads of brand, communications, and digital advertising. These are the people most likely to use the data.
Governance is the province of another group, which is chaired by the chief privacy officer and includes the global head of data, a senior risk executive, and the executive responsible for the company’s brand. Anything new concerning data use gets referred to this council, and teams must explain how proposed products comply with the company’s data use principles. As one senior company executive explains, “It’s important that both of these bodies be cross-functional because in both cases you’re trying to make sure that you have a fairly holistic perspective.”
As we’ve noted, compliance teams and legal counsel should not be the only people thinking about a company’s data ethics, but they do have an important role to play in ensuring that data ethics programs succeed. Legal experts are best positioned to advise on how your company should apply existing and emerging regulations. But teams may also want to bring in outside experts to navigate particularly difficult ethical challenges. For example, a large tech company brought in an academic expert on AI ethics to help it figure out how to navigate gray areas, such as the environmental impact of certain kinds of data use. That expert was a sitting but not voting member of the group because the team “did not want to outsource the decision making.” But the expert participated in every meeting and led the team in the work that preceded the meetings.
Engage champions in the C-suite
Some practitioners and experts we spoke with who had convened data ethics boards pointed to the importance of keeping the CEO and the corporate board apprised of decisions and activities. A senior executive who chaired his organization’s data ethics group explained that while it did not involve the CEO directly in the decision-making process, it brought all data ethics conclusions to him “and made sure he agreed with the stance that we were taking.” All these practitioners and experts agreed that having a champion or two in the C-suite can signal the importance of data ethics to the rest of the organization, put teeth into data rules, and support the case for investment in data-related initiatives.
Indeed, corporate boards and audit committees can provide the checks needed to ensure that data ethics are being upheld, regardless of conflicting incentives. The president of one tech company told us that its board had recently begun asking for a data ethics report as part of the audit committee’s agenda, which had previously focused more narrowly on privacy and security. “You have to provide enough of an incentive—a carrot or a stick to make sure people take this seriously,” the president said.
Consider the impact of your algorithms and overall data use
Organizations should continually assess the effects of the algorithms and data they use—and test for bias throughout the value chain. That means thinking about the problems organizations might create, even unwittingly, in building AI products. For instance, who might be disadvantaged by an algorithm or a particular use of data? One technologist we spoke with advises asking the hard questions: “Start your meetings about AI by asking, ‘Are the algorithms we are building sexist or racist?’”
Certain data applications require far greater scrutiny and consideration. Security is one such area. A tech company executive recalled the extra measures his organization took to prevent its image and video recognition products and services from being misused: “We would insist that if you were going to use our technology for security purposes, we had to get very involved in ensuring that you debiased the data set as much as possible so that particular groups would not be unfairly singled out.” It’s important to consider not only what types of data are being used but also what they are being used for—and what they could potentially be used for down the line.
Think globally
The ethical use of data requires organizations to consider the interests of people who are not in the room. Anthropologist Mary Gray, the senior principal researcher at Microsoft Research, raises questions about global reach in her 2019 book, Ghost Work . Among them: Who labeled the data? Who tagged these images? Who kept violent videos off this website? Who weighed in when the algorithm needed a steer?
Today’s leaders need to ask these sorts of questions, along with others about how such tech work happens. Broadly, leaders must take a 10,000-foot view of their companies as players in the digital economy, the data ecosystem, and societies everywhere. There may be ways they can support policy initiatives or otherwise help to bridge the digital divide, support the expansion of broadband infrastructure, and create pathways for diversity in the tech industry. Ultimately, data ethics requires leaders to reckon with the ongoing rise in global inequality—and the increasing concentration of wealth and value both in geographical tech hubs and among AI-enabled organizations. 5 For more on the concentration of value among AI-enabled firms, see Marco Iansiti and Karim R. Lakhani, Competing in the Age of AI: Strategy and Leadership When Algorithms and Networks Run the World , Boston: Harvard Business Review Press, 2020.
Embed your data principles in your operations
It’s one thing to define what constitutes the ethical use of data and to set data usage rules; it’s another to integrate those rules into operations across the organization. Data ethics boards, business unit leaders, and C-suite champions should build a common view (and a common language) about how data usage rules should link up to both the company’s data and corporate strategies and to real-world use cases for data ethics, such as decisions on design processes or M&A. In some cases, there will be obvious places to operationalize data ethics—for instance, data operations teams, secure-development operations teams, and machine-learning operations teams. Trust-building frameworks for machine-learning operations can ensure that data ethics will be considered at every step in the development of AI applications.
Regardless of which part of the organization the leaders target first, they should identify KPIs that can be used to monitor and measure its performance in realizing their data ethics objectives. To ensure that the ethical use of data becomes part of everyone’s daily work, the leadership team also should advocate, help to build, and facilitate formal training programs on data ethics.
Data ethics can‘t be put into practice overnight. As many business leaders know firsthand, building teams, establishing practices, and changing organizational culture are all easier said than done. What’s more, upholding your organization’s data ethics principles may mean walking away from potential partnerships and other opportunities to generate short-term revenues. But the stakes for companies could not be higher. Organizations that fail to walk the walk on data ethics risk losing their customers’ trust and destroying value.
Alex Edquist is an alumna of McKinsey’s Atlanta office; Liz Grennan is an associate partner in the Stamford, Connecticut, office; Sian Griffiths is a partner in the Washington, DC, office; and Kayvaun Rowshankish is a senior partner in the New York office.
The authors wish to thank Alyssa Bryan, Kasia Chmielinski, Ilona Logvinova, Keith Otis, Marc Singer, Naomi Sosner, and Eckart Windhagen for their contributions to this article.
This article was edited by Roberta Fusaro, an editorial director in the Waltham, Massachusetts, office.
Explore a career with us
Related articles.
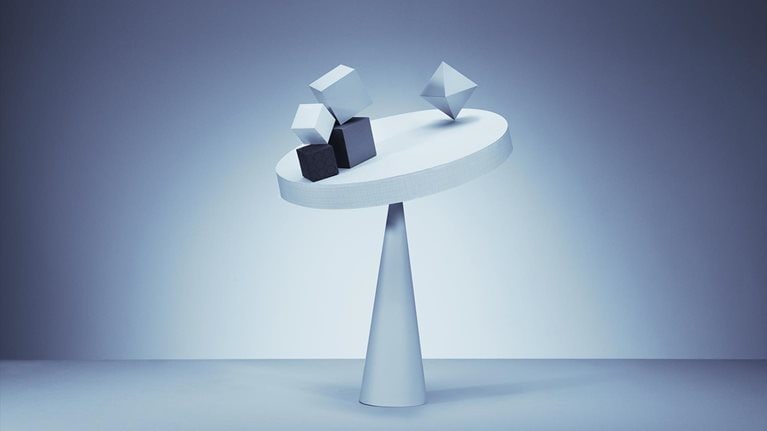
Getting to know—and manage—your biggest AI risks

Localization of data privacy regulations creates competitive opportunities

AI Ethics in today’s world
The Data Ethics Repository
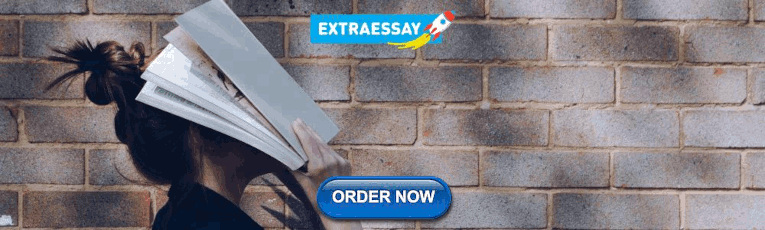
Crisis Data: An AI Ethics Case Study
Author: Irina Raicu
Publisher: Markkula Center for Applied Ethics
Publication Year: 2022
Summary: The following case study describes how there is an ethical concern with keeping data linked to mental health and using this data to receive monetary gain. The mental health hotline took the text messages from people who had reached out to them and made them anonymous. From there, they partnered with another company to use those messages to have a more comprehensive guide on how to provide care for those suffering from similar illnesses. This became a hot topic because there was pushback that the messages could be linked back to the original senders and therefore could impact their lives. It was also discussed how the organization was legally allowed to do this was through making users agree to their Terms, which linked to a 50-page document. This was considered to be somewhat ethically misleading due to the nature of the hotline, and critics questioned whether users following through in these instances are fully understood consent.
The Data Science Ethos
A structured approach to data science.
The Data Science Ethos operationalizes the responsibilities of data scientists - conducting outcomes-based research that is grounded in a thoughtful understanding of the human impacts and interactions of the work.
We offer practitioners structured ways of thinking about the social and ethical contexts relevant to each stage of the data science research process.
Getting Started
Learn about how to get started with the Data Science Ethos. What are the lenses and stages? How do the case studies help? How can I contribute or learn more?
Why the Data Science Ethos?
Learn about the origins of this project, dig into the concepts, and meet the interdisciplinary team involved in its creation.
Data Science Ethos - Announcement Webinar
The Data Science Ethos launched in May 2023 with an announcement webinar. Check out the recording of the webinar to learn more about the history of the Ethos, its implementation, and where we are taking it.

Jump to: Lenses
The lenses offer a structured approach to thinking about the ethical components at each stage of the data science lifecycle. Learn about how the lenses help contextualize the stages of data science research.

Jump To: Stages
Review the Research Lifecycle Stages - a familiar model for the data science research process. At each stage, we examine how the Lenses focus our attention on the social, human, and moral impacts and implications of research.

Jump To: Case Studies
Put the Lenses and Stages together with real-world research projects to see how social questions interact with the work of a data scientist.
Contribute a Case Study
The Data Science Ethos relies on individual contributions to provide case studies and teaching material.
To help contributors develop their case study, our six-step lifecycle captures the distinct, high-level stages of most data science projects and associates a robust conceptual framework that sheds light on the benefits and challenges of ethics-centered data science approaches.
Contact us to join the effort!
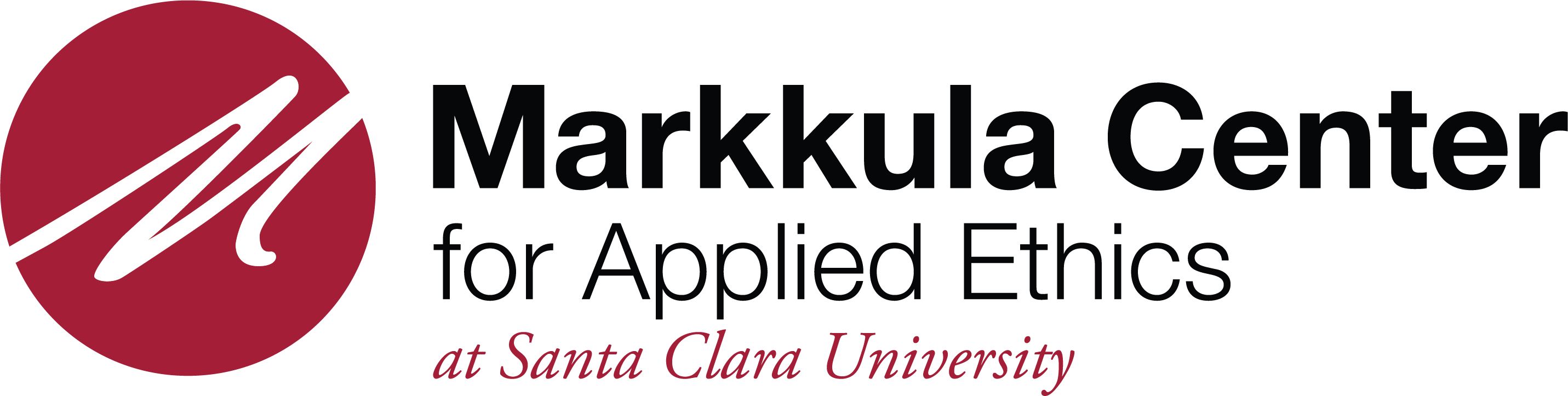
- Internet Ethics Cases
- Markkula Center for Applied Ethics
- Focus Areas
- Internet Ethics
Find ethics case studies on topics in Internet ethics including privacy, hacking, social media, the right to be forgotten, and hashtag activism. (For permission to reprint articles, submit requests to [email protected] .)
Ethical questions arise in interactions among students, instructors, administrators, and providers of AI tools.
What can we learn from the Tay experience, about AI and social media ethics more broadly?
Who should be consulted before using emotion-recognition AI to report on constituents’ sentiments?
When 'algorithm alchemy' wrongly accuses people of fraud, who is accountable?
Which stakeholders might benefit from a new age of VR “travel”? Which stakeholders might be harmed?
Ethical questions about data collection, data-sharing, access, use, and privacy.
As PunkSpider is pending re-release, ethical issues are considered about a tool that is able to spot and share vulnerabilities on the web, opening those results to the public.
With URVR recipients can capture and share 360 3D moments and live them out together.
VR rage rooms may provide therapeutic and inexpensive benefits while also raising ethical questions.
A VR dating app intended to help ease the stress and awkwardness of early dating in a safe and comfortable way.
- More pages:
Data Ethics Case Study Library
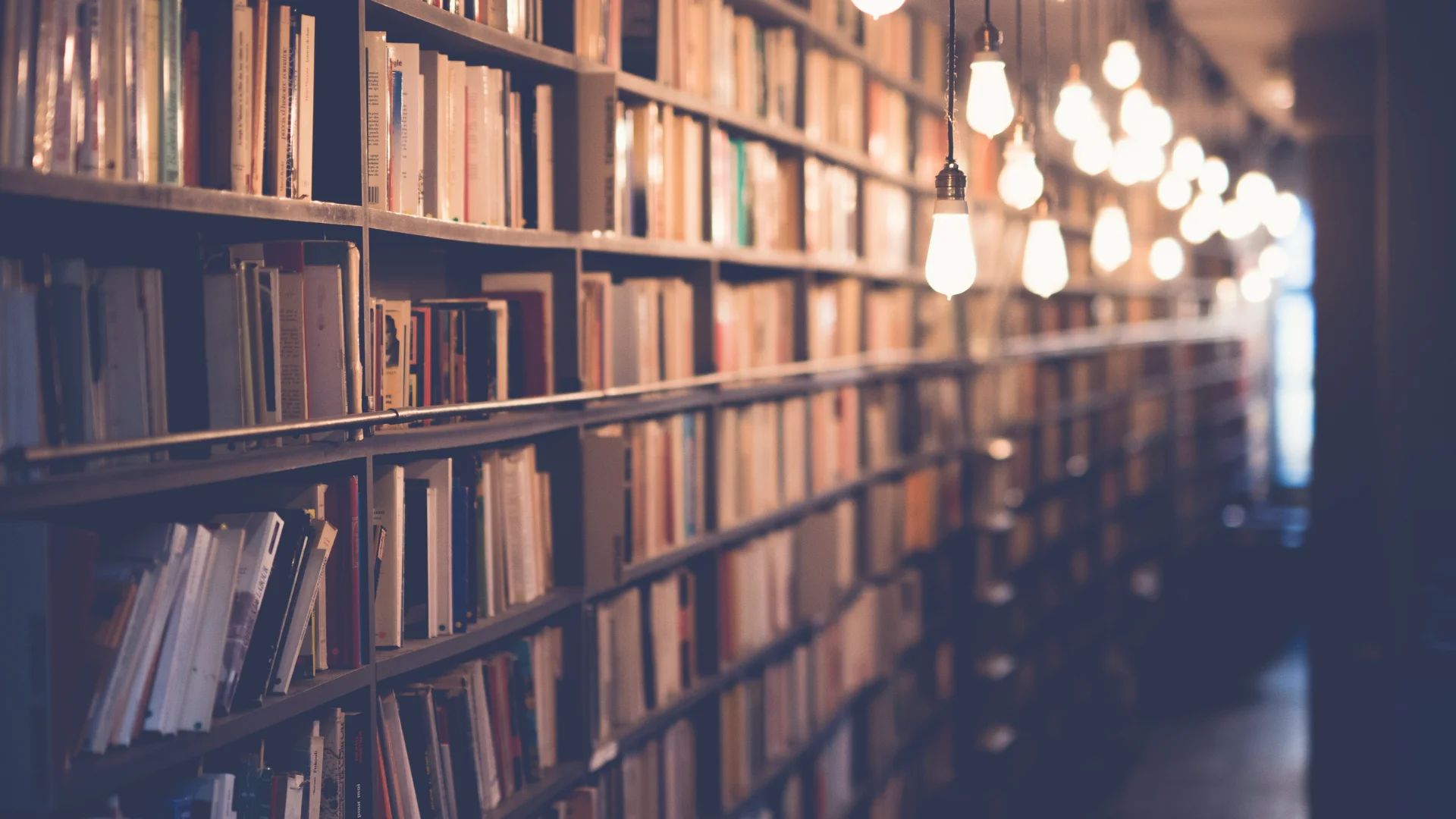
What is it?
LOTI have collected a range of case studies from different government organisations, both local, national and international, detailing different approaches to data ethics.
Why did we create it?
LOTI boroughs recognise that the data they collect and hold is one of their most valuable assets. Whilst this data can and will be used to improve residents’ lives, there are also risks of it not being used correctly. Beyond legal constraints, there are also ethical questions about how residents’ data should be used, and what processes and systems are needed to ensure organisations have a good data ethics practice.
These case studies map some of the practices that have emerged in the public sector in response to this challenge. As this is a new ethics domain for public sector across the world, we are still seeking to establish what exactly best practice is, and would look like in London. These case studies provide the foundation for our analysis and the subsequent support that we will deliver for LOTI boroughs as part of our ongoing data ethics investigation.
Who should use it?
Local authorities and other public sector organisations interested in learning about different approaches to data ethics, with an interest in setting up their own data ethics processes.
Case Studies:
Amsterdam’s Algorithm Register Amsterdam have created an Algorithm Register to provide transparency in a standard format about how data is being used in public services, along with a host of other complementary initiatives to be truly an ethical municipal authority.
Brent Council’s Data Ethics Governance Board Brent created a Data Ethics Board with the help of LOTI, which serves to provide the borough with advice from data ethics experts to guide their data projects in their ethical considerations.
Essex Council’s Essex Centre for Data Ethics (ECDA) Essex created a Centre for Data Ethics to serve as an independent and advisory ethics board.
Camden Council’s Data Charter Camden residents created a Data Charter for the borough, with a set of principles and recommendations for the Borough to adopt to ensure a transparent, accountable ethics practice on data.
The Metropolitan Police Service is working to build public trust through transparency The Metropolitan Police Service’s ambition is to develop a data ethics framework that is embedded in the organisational culture, and supports its work to build public trust and confidence through transparency in its decision making.
The National Health Service (NHS) is trialling Algorithmic Impact Assessments The NHS will be trialling an Algorithmic Impact Assessment (AIA) to ensure that those who seek to use its data to train their artificial intelligence applications are open about the design of their algorithms and what impact they will have on research outcomes.
Police Scotland is exploring how to institutionalise using data for public good Amongst various initiatives being explored by Police Scotland are publishing a Data Ethics Strategy and associated framework, integrating into the role of senior leaders, establishing a Scrutiny Group and embedding it into project management processes.
Transport for London (TfL) incorporate Ethics into their Data Privacy Impact Asessments (DPIAs) TfL have mirrored the Open Data Institute’s Data Ethics Canvas in its DPIA process. Each of the 15 elements of the Canvas are reflected in the DPIA so that privacy and ethics are as one.
Is this helpful?
- Our Approach
- Our Members
- Get in touch
- Get Online London
- Donate a device
- Become a hub
- Find your local service
Join the LOTI conversation
Sign up for our monthly newsletter to get the latest news and updates
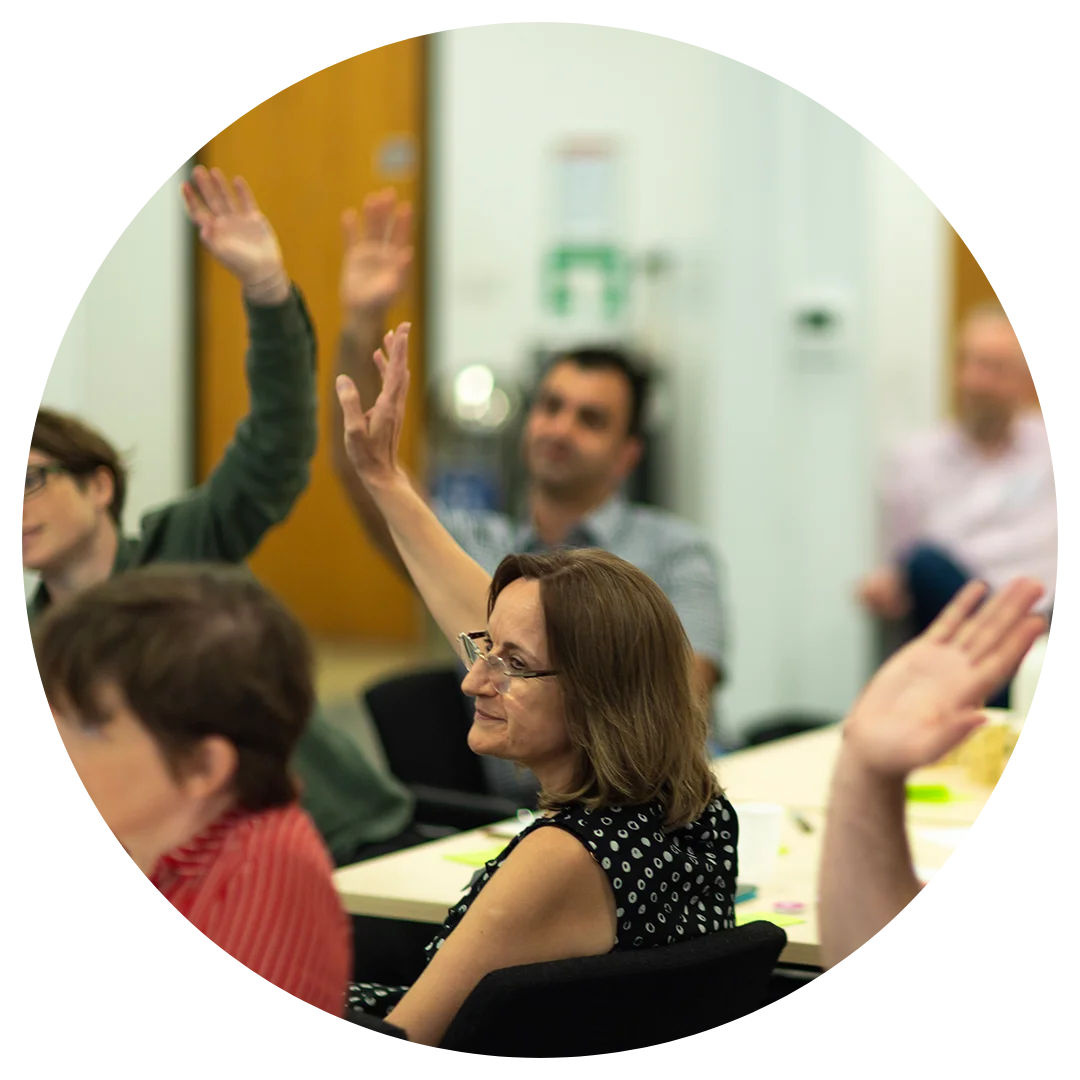
By submitting your email address you agree to the Terms
- Cookie Policy
Research Data Management Resources
- Why Manage Data?
- Analysis-ready Data Sets
- Data Documentation
- Data Management Plans
Data Ethics Case Studies
Responsible conduct of research, data integrity, what is de-identified data, how to de-identify data, federal resources, compliance with data security and breach notification laws, other resources at umass chan, do you need irb approval.
- File Management
- File Storage and Backup
- Sharing/ Publishing Data
- UMass Chan Campus Resources and Policies
- NEW! NIH Data Management and Sharing Policy
- Open and Publicly Available Data
- Training videos
Sally Gore, MS, MS LIS Manager, Research & Scholarly Communication Services [email protected]
Tess Grynoch, MLIS Research Data & Scholarly Communications Librarian [email protected]
Leah Honor, MLIS Research Data & Scholarly Communications Librarian [email protected]
Lisa Palmer, MSLS, AHIP Institutional Repository Librarian [email protected]
Retraction Watch

The ethical aspects of data are many. Examples include defining ownership of data, obtaining consent to collect and share data, protecting the identity of human subjects and their personal identifying information, and the licensing of data. Below are several ethics cases from Responsible Conduct of Research Casebook: Data Acquisition and Management a publication from the Office of Research Integrity at the U.S. Department of Health and Human Services.
There are generally four matters of data acquisition and management that need to be addressed at the outset of a study: (1) collection, (2) storage; (3) ownership, and 4) sharing. These cases and role play present common scenarios that occur at various stages of data acquisition and management. Issues discussed include acquiring sensitive data, sharing data with colleagues, and managing data collection processes.
Case One: A researcher wants to sequence the genomes of children with cancer, eventually making them publicly available online, but encounters issues with adequate data protection and parental consent.
Case Two: After working with her advisor to develop a sophisticated database, the postdoc wants access to the database in order to submit a grant proposal but runs into trouble when seeking the advisor’s permission.
Case Three: A post-doc has a novel idea after observing a procedure during residency, but he needs access to a large amount of clinical data, including medical record numbers, so that he can eventually recruit individuals to participate in his research.
Role Play: An assistant professor places her data on the NIH’s database of genotypes and phenotypes (dbGaP) only to find that a leading researcher has published a paper using the data shared in the NIH database before the one-year embargo period was up.
From the U.S. Office of Research Integrity's RCR Casebook Stories about Researchers Worth Sharing edited by James M. DuBois

All UMass Chan Morningside Graduate School of Biomedical Sciences Basic Science students and post docs must take CITI Training .
See also, RCR for Postdocs , a resource offered by the National Postdoctoral Association.
Actions that undermine data integrity include data fabrication , falsification , and misattribution . Some journals, such as the Journal of Cell Biology , have strict editorial policies regarding images and image manipulation that, if not followed, result in the rejection and/or retraction of papers.
Information from which all the following identifiers have been removed:
- Geographic subdivisions smaller than a state (except for 3-digit zip codes where the population is greater than 20,000)
- Dates other than year (except birth years that reveal an age of 90 or older, which must be aggregated so as to reveal only that the individual is age 90 or over)
- Names of relatives and employers
- Telephone and fax numbers
- E-mail addresses
- Social security numbers
- Medical record numbers
- Health plan beneficiary numbers
- Account numbers
- Certificate/license numbers
- Vehicle or other device serial numbers
- Internet protocol (IP) addresses
- Finger or voice prints
- Photographic images
- and any other unique identifying number, characteristic, or code
Resources from the UK Data Service:
- How to anonymize quantitative data
- How to anonymize qualitative data
- Health Information Privacy DHHS resources on HIPPA regulation.
- Office for Human Research Protections Policy and advocacy for subject involved in DHHS research.
- Office of Research Integrity Federal agency that oversees Public Health Services research integrity.
- NLM-Scrubber Freely available clinical text deidentification tool which uses an automated Safe Harbor process to deidentify the data. Review to ensure deidentification is complete is still required.
A Letter from UMass Chan Vice Chancellor on Data Security and Breach Notification Laws (June 6, 2013)

- Morningside Graduate School of Biomedical Sciences Research Ethics Certification requirements for Morningside Graduate School of Biomedical Sciences students.
- Research Compliance Within the Office of Research, this group oversees and maintains UMass Chan standards for research.
- IRB The IRB provides approval, guidance, and standards for human subjects research, and maintains a record of all human subject research conducted at UMass Chan.
- HIPAA Compliance Web Page Information about HIPAA regulations, including de-identification certification and authorization to disclose PHI for research forms.
- Thoughts on IRB vs Non-IRB Project Needs Judy Savageau, MPH (May, 2014) A decision to get IRB approval for research will depend on the complexities of your project. Judy Savageau, MPH, prepared a useful document to inform decisions and begin thiking about pursuing IRB approval for research projects.
- << Previous: Data Management Plans
- Next: File Management >>
- Last Updated: Apr 29, 2024 3:49 PM
- URL: https://libraryguides.umassmed.edu/research_data_management_resources

Ethics and Data Science
Main navigation.
New technologies often raise new moral questions. For example, the emergence of nuclear weapons placed great pressure on the distinction between combatants and non-combatants that had been central to the just war theory formulated in the middle ages. New theories were needed to reinterpret the meaning of this distinction in a nuclear age. With the emergence of new techniques of machine learning, and the possibility of using algorithms to perform tasks previously done by human beings, as well as to generate new knowledge, we again face a set of new ethical questions. These questions not only concern the possibility of harm by the misuse of data, but also questions of how to preserve privacy where data is sensitive, how to avoid bias in data selection, how to prevent disruption and “hacking” of data, and issues of transparency in data collection, research and dissemination. Underlying many of these questions is a larger question about who owns the data, who has the right of access to it, and under what conditions.
There are no currently agreed on responses to these questions. Nonetheless, it is extremely important to confront them and to attempt to work out shared ethical guidelines. Where agreement is not possible, it is important to attend to the competing values in place and to specifically articulate the underlying assumptions at work in different models. An interesting illustration involves the debate over fairness in models predicting the risk of recidivism among black and white defendants in Broward County Florida. Should a risk score be: equally accurate in predicting the likelihood of recidivism for members of different racial groups; assume that members of different groups have the same chance of being wrongly predicted to recidivate; or assume that failure to predict recidivism happens at the same rate across groups. Recent work has established that satisfying all three criteria at the same time would be impossible in most situations; meeting two will mean failing to comply with the third. So we need to decide which aspects of fairness are most important.
Developing a shared framework will take collaboration between programmers, statisticians, legal scholars and philosophers.
Contributors

- Business Essentials
- Leadership & Management
- Credential of Leadership, Impact, and Management in Business (CLIMB)
- Entrepreneurship & Innovation
- Digital Transformation
- Finance & Accounting
- Business in Society
- For Organizations
- Support Portal
- Media Coverage
- Founding Donors
- Leadership Team
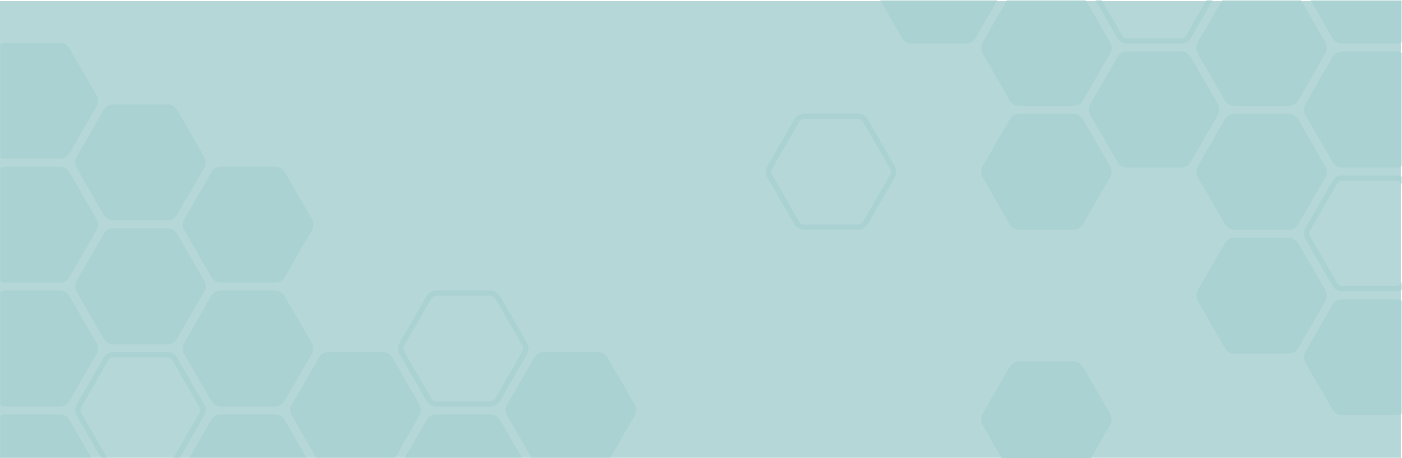
- Harvard Business School →
- HBS Online →
- Business Insights →
Business Insights
Harvard Business School Online's Business Insights Blog provides the career insights you need to achieve your goals and gain confidence in your business skills.
- Career Development
- Communication
- Decision-Making
- Earning Your MBA
- Negotiation
- News & Events
- Productivity
- Staff Spotlight
- Student Profiles
- Work-Life Balance
- AI Essentials for Business
- Alternative Investments
- Business Analytics
- Business Strategy
- Business and Climate Change
- Design Thinking and Innovation
- Digital Marketing Strategy
- Disruptive Strategy
- Economics for Managers
- Entrepreneurship Essentials
- Financial Accounting
- Global Business
- Launching Tech Ventures
- Leadership Principles
- Leadership, Ethics, and Corporate Accountability
- Leading with Finance
- Management Essentials
- Negotiation Mastery
- Organizational Leadership
- Power and Influence for Positive Impact
- Strategy Execution
- Sustainable Business Strategy
- Sustainable Investing
- Winning with Digital Platforms
5 Principles of Data Ethics for Business
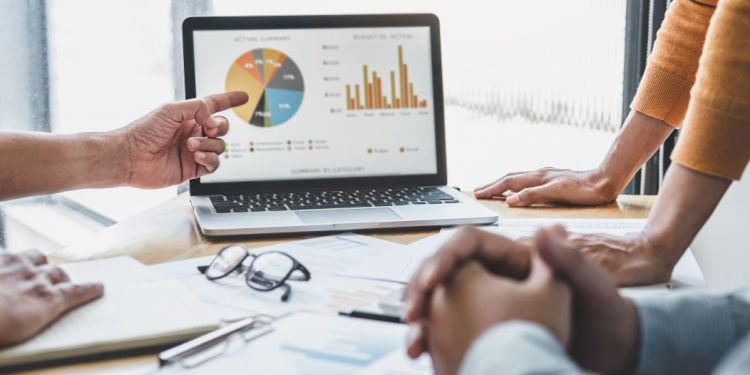
- 16 Mar 2021
Data can be used to drive decisions and make an impact at scale. Yet, this powerful resource comes with challenges. How can organizations ethically collect, store, and use data? What rights must be upheld? The field of data ethics explores these questions and offers five guiding principles for business professionals who handle data.
What Is Data Ethics?
Data ethics encompasses the moral obligations of gathering, protecting, and using personally identifiable information and how it affects individuals.
“Data ethics asks, ‘Is this the right thing to do?’ and ‘Can we do better?’” Harvard Professor Dustin Tingley explains in the Harvard Online course Data Science Principles .
Data ethics are of the utmost concern to analysts, data scientists, and information technology professionals. Anyone who handles data, however, must be well-versed in its basic principles.
For instance, your company may collect and store data about customers’ journeys from the first time they submit their email address on your website to the fifth time they purchase your product. If you’re a digital marketer, you likely interact with this data daily.
While you may not be the person responsible for implementing tracking code, managing a database, or writing and training a machine-learning algorithm, understanding data ethics can allow you to catch any instances of unethical data collection, storage, or use. By doing so, you can protect your customers' safety and save your organization from legal issues.
Here are five principles of data ethics to apply at your organization.
Access your free e-book today.
5 Principles of Data Ethics for Business Professionals
1. ownership.
The first principle of data ethics is that an individual has ownership over their personal information. Just as it’s considered stealing to take an item that doesn’t belong to you, it’s unlawful and unethical to collect someone’s personal data without their consent.
Some common ways you can obtain consent are through signed written agreements, digital privacy policies that ask users to agree to a company’s terms and conditions, and pop-ups with checkboxes that permit websites to track users’ online behavior with cookies. Never assume a customer is OK with you collecting their data; always ask for permission to avoid ethical and legal dilemmas.
2. Transparency
In addition to owning their personal information, data subjects have a right to know how you plan to collect, store, and use it. When gathering data, exercise transparency.
For instance, imagine your company has decided to implement an algorithm to personalize the website experience based on individuals’ buying habits and site behavior. You should write a policy explaining that cookies are used to track users’ behavior and that the data collected will be stored in a secure database and train an algorithm that provides a personalized website experience. It’s a user’s right to have access to this information so they can decide to accept your site’s cookies or decline them.
Withholding or lying about your company’s methods or intentions is deception and both unlawful and unfair to your data subjects.
Another ethical responsibility that comes with handling data is ensuring data subjects’ privacy. Even if a customer gives your company consent to collect, store, and analyze their personally identifiable information (PII) , that doesn’t mean they want it publicly available.
PII is any information linked to an individual’s identity. Some examples of PII include:
- Street address
- Phone number
- Social Security card
- Credit card information
- Bank account number
- Passport number
To protect individuals’ privacy, ensure you’re storing data in a secure database so it doesn’t end up in the wrong hands. Data security methods that help protect privacy include dual-authentication password protection and file encryption.
For professionals who regularly handle and analyze sensitive data, mistakes can still be made. One way to prevent slip-ups is by de-identifying a dataset. A dataset is de-identified when all pieces of PII are removed, leaving only anonymous data. This enables analysts to find relationships between variables of interest without attaching specific data points to individual identities.
Related: Data Privacy: 4 Things Every Business Professional Should Know
4. Intention
When discussing any branch of ethics, intentions matter. Before collecting data, ask yourself why you need it, what you’ll gain from it, and what changes you’ll be able to make after analysis. If your intention is to hurt others, profit from your subjects’ weaknesses, or any other malicious goal, it’s not ethical to collect their data.
When your intentions are good—for instance, collecting data to gain an understanding of women’s healthcare experiences so you can create an app to address a pressing need—you should still assess your intention behind the collection of each piece of data.
Are there certain data points that don’t apply to the problem at hand? For instance, is it necessary to ask if the participants struggle with their mental health? This data could be sensitive, so collecting it when it’s unnecessary isn’t ethical. Strive to collect the minimum viable amount of data, so you’re taking as little as possible from your subjects while making a difference.
Related: 5 Applications of Data Analytics in Health Care
5. Outcomes
Even when intentions are good, the outcome of data analysis can cause inadvertent harm to individuals or groups of people. This is called a disparate impact , which is outlined in the Civil Rights Act as unlawful.
In Data Science Principles, Harvard Professor Latanya Sweeney provides an example of disparate impact. When Sweeney searched for her name online, an advertisement came up that read, “Latanya Sweeney, Arrested?” She had not been arrested, so this was strange.
“What names, if you search them, come up with arrest ads?” Sweeney asks in the course. “What I found was that if your name was given more often to a Black baby than to a white baby, your name was 80 percent more likely get an ad saying you had been arrested.”
It’s not clear from this example whether the disparate impact was intentional or a result of unintentional bias in an algorithm. Either way, it has the potential to do real damage that disproportionately impacts a specific group of people.
Unfortunately, you can’t know for certain the impact your data analysis will have until it’s complete. By considering this question beforehand, you can catch any potential occurrences of disparate impact.
Ethical Use of Algorithms
If your role includes writing, training, or handling machine-learning algorithms, consider how they could potentially violate any of the five key data ethics principles.
Because algorithms are written by humans, bias may be intentionally or unintentionally present. Biased algorithms can cause serious harm to people. In Data Science Principles, Sweeny outlines the following ways bias can creep into your algorithms:
- Training: Because machine-learning algorithms learn based on the data they’re trained with, an unrepresentative dataset can cause your algorithm to favor some outcomes over others.
- Code: Although any bias present in your algorithm is hopefully unintentional, don’t rule out the possibility that it was written specifically to produce biased results.
- Feedback: Algorithms also learn from users’ feedback. As such, they can be influenced by biased feedback. For instance, a job search platform may use an algorithm to recommend roles to candidates. If hiring managers consistently select white male candidates for specific roles, the algorithm will learn and adjust and only provide job listings to white male candidates in the future. The algorithm learns that when it provides the listing to people with certain attributes, it’s “correct” more often, which leads to an increase in that behavior.
“No algorithm or team is perfect, but it’s important to strive for the best,” Tingley says in Data Science Principles. “Using human evaluators at every step of the data science process, making sure training data is truly representative of the populations who will be affected by the algorithm, and engaging stakeholders and other data scientists with diverse backgrounds can help make better algorithms for a brighter future.”

Using Data for Good
While the ethical use of data is an everyday effort, knowing that your data subjects’ safety and rights are intact is worth the work. When handled ethically, data can enable you to make decisions and drive meaningful change at your organization and in the world.
Are you interested in furthering your data literacy? Download our Beginner’s Guide to Data & Analytics to learn how you can leverage the power of data for professional and organizational success.

About the Author
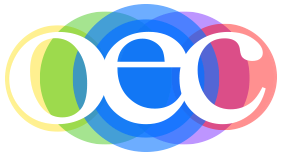
Site Search
- How to Search
- Advisory Group
- Editorial Board
- OEC Fellows
- History and Funding
- Using OEC Materials
- Collections
- Research Ethics Resources
- Ethics Projects
- Communities of Practice
- Get Involved
- Submit Content
- Open Access Membership
- Become a Partner
Overly Ambitious Researchers - Fabricating Data
A historical case study about the cases of Dr. John Darsee and Dr. Stephen Breuning who both were found to have fabricated data as part of their research.
This is one of six cases from Michael Pritchard and Theodore Golding's instructor guide, " Ethics in the Science Classroom ."
Categories Illustrated by This Case: Issues related to fraud in scientific research and its consequences.
1. Introduction
In recent years the National Science Foundation (NSF), the National Institutes of Health (NIH), the Public Health Services (PHS), the Office of Scientific Integrity (OSI), and various scientific organizations such as the National Academy of Sciences (NAS) have spent considerable time and effort in trying to agree on a definition of scientific misconduct . A good definition is needed in developing and implementing policies and regulations concerning appropriate conduct in research, particularly when federal funding is involved. This is an important area of concern because, although serious scientific misconduct itself may be infrequent, the consequences of even a few instances can be widespread.
Those cases that reach the public's attention can cause considerable distrust among both scientists and the public, however infrequent their occurrence. Like lying in general, we may wonder which scientific reports are tainted by misconduct, even though we may be convinced that relatively few are. Furthermore, scientists depend on each other's work in advancing their own. Building one's work on the incorrect or unsubstantiated data of others infects one's own research; and the chain of consequences can be quite lengthy, as well as very serious. This is as true of honest or careless mistakes as it is of the intentional distortion of data, which is what scientific misconduct is usually restricted to. Finally, of course, the public depends on the reliable expertise of scientists in virtually every area of health, safety, and welfare.
Although exactly what the definition of scientific misconduct should include is a matter of some controversy, all proposed definitions include the fabrication and falsification of data and plagiarism. As an instance of fraud, the fabrication of data is a particularly blatant form of misconduct. It lacks the subtlety of questions about interpreting data that pivot around whether the data have been fudged , or manipulated. Fabricating data is making it up, or faking it. Thus, it is a clear instance of a lie, a deliberate attempt to deceive others.
However, this does not mean that fabrications are easy to detect or handle effectively once they are detected; and this adds considerably to the mischief and harm they can cause. Two well-known cases illustrate this, both of which feature ambitious, and apparently successful, young researchers.
2. Background
Dr. John Darsee was regarded a brilliant student and medical researcher at the University of Notre Dame (1966-70), Indiana University (1970-74), Emory University (1974-9), and Harvard University (1979-1981). He was regarded by faculty at all four institutions as a potential "all-star" with a great research future ahead of him. At Harvard he reportedly often worked more than 90 hours a week as a Research Fellow in the Cardiac Research Laboratory headed by Dr. Eugene Braunwald. In less than two years at Harvard he was first author of seven publications in very good scientific journals. His special area of research concerned the testing of heart drugs on dogs.
3. The Darsee Case
All of this came to a sudden halt in May 1981, when three colleagues in the Cardiac Research Laboratory observed Darsee labeling data recordings 24 seconds, 72 hours, one week, and two weeks. In reality, only minutes had transpired. Confronted by his mentor Braunwald, Darsee admitted the fabrication; but he insisted that this was the only time he had done this, and that he had been under intense pressure to complete the study quickly. Shocked, Braunwald and Darsee's immediate supervisor, Dr. Robert Kroner, spent the next several months checking other research conducted by Darsee in their lab. Darsee's research fellowships were terminated, and an offer of a faculty position was withdrawn. However, he was allowed to continue his research projects at Harvard for the next several months (during which time Braunwald and Kroner observed his work very closely).
Hopeful that this was an isolated incident, Braunwald and Kroner were shocked again in October. A comparison of results from four different laboratories in a National Heart, Lung and Blood Institute (NHLBI) Models Study revealed an implausibly low degree of invariability in data provided by Darsee. In short, his data looked "too good." Since these data had been submitted in April, there was strong suspicion that Darsee had been fabricating or falsifying data for some time. Subsequent investigations seemed to indicate questionable research practices dating back as far as his undergraduate days.
What were the consequences of John Darsee's misconduct? Darsee, we have seen, lost his research position at Harvard, and his offer of a faculty position was withdrawn. The National Institutes of Health (NIH) barred him from NIH funding or serving on NIH committees for ten years. He left research and went into training as a critical care specialist. However, the cost to others was equally, if not more, severe. Harvard-affiliated Brigham and Women's Hospital became the first institution NIH ever required to return funds ($122,371) because of research involving fraudulent data. Braunwald and his colleagues had to spend several months investigating Darsee's research, rather than simply continuing the work of the Cardiac Research Laboratory. Furthermore, they were severely criticized for carrying on their own investigation without informing NIH of their concerns until several months later. The morale and productivity of the laboratory was damaged. A cloud of suspicion hung over all the work with which Darsee was associated. Not only was Darsee's own research discredited, but insofar as it formed an integral part of collaborative research, a cloud was thrown over published research bearing the names of authors whose work was linked with Darsee's.
The months of outside investigation also took others away from their main tasks and placed them under extreme pressure. Statistician David DeMets played a key role in the NIH investigation. Fifteen years later, he recalls the relief his team experienced when their work was completed. 50
For the author and the junior statistician, there was relief that the episode was finally over and we could get on with our careers, without the pressures of a highly visible misconduct investigation. It was clear early on that we had no room for error, that any mistakes would destroy the case for improbable data and severely damage our careers. Even without mistakes, being able to convince lay reviewers such as a jury using statistical arguments could still be defeating. Playing the role of the prosecuting statisticians was very demanding of our technical skills but also of our own integrity and ethical standards. Nothing could have adequately prepared us for what we experienced.
Braunwald notes some positive things that have come from the Darsee case. In addition to alerting scientists to the need for providing closer supervision of trainees and taking authorship responsibilities more seriously, the Darsee incident contributed to the development of guidelines and standards concerning research misconduct by PHS, NIH, NSF, medical associations and institutes, and universities and medical schools. However, he cautions that no protective system is able to prevent all research misconduct. In fact, he doubts that current provisions could have prevented Darsee's misconduct, although they might have resulted in earlier detection. Further, he warns that good science does not thrive in an atmosphere of heavy "policing" of one another's work. 51
The most creative minds will not thrive in such an environment and the most promising young people might actually be deterred from embarking on a scientific career in an atmosphere of suspicion. Second only to absolute truth, science requires an atmosphere of openness, trust, and collegiality.
Given this, it seems that William F. May is right in urging the need for a closer examination of character and virtue in professional life. 52 He says that an important test of character and virtue is what we do when no one is watching. The Darsee case and Brauwald's reflections seem to confirm this. If this is right, then it is important that attention be paid to these matters before college, by which time one's character is rather well set.
Many who are caught having engaged in scientific misconduct plead that they were under extreme pressure, needing to complete their research in order to meet the expectations of their lab supervisor, to meet a grant deadline, to get an article published, or to survive in the increasingly competitive world of scientific research. Although the immediate stakes are different, secondary school science students sometimes echo related concerns: "I knew how the experiment should have turned out, and I needed to support the right answer;" "I needed to get a good grade;" "I didn't have time to do it right; there's so much pressure." Often these thoughts are accompanied by another--namely, that this is only a classroom exercise and that, of course, one will not fabricate data when one becomes a scientist and these pressures are absent. What the Darsee case illustrates is that it is naive to assume such pressures will vanish. So, the time to begin dealing with the ethical challenges they pose is now, not later (when the stakes may be even higher).
4. The Bruening Case
In December 1983, Dr. Robert Sprague wrote an eight page letter, with 44 pages of appendices, to the National Institute of Mental Health (NIMH) documenting the fraudulent research of Dr. Stephen Breuning. 53 Breuning fabricated data concerning the effects psychotropic medication have on mentally retarded patients. Despite Breuning's admission of fabricating data only three months after Sprague sent his letter, the case was not finally resolved until July 1989. (Sprague credits media attention with speeding things along!) During that five and one-half year interval, Sprague himself was a target of investigation (in fact, he was the first target of investigation), he had his own research endeavors severely curtailed, he was subjected to threats of lawsuits, and he had to testify before a United States House of Representatives Committee. Most painful of all, Sprague's wife died in 1986 after a lengthy bout with diabetes. In fact, his wife's serious illness was one of the major factors prompting his whistleblowing to NIH. Realizing how dependent his diabetic wife was on reliable research and medication, Sprague was particularly sensitive to the dependency the mentally retarded, clearly a vulnerable population, have on the trustworthiness of not only their care givers, but also those who use them in experimental drug research.
Writing nine years after the closing of the Bruening case, Sprague obviously has vivid memories of the painful experiences he endured and of the potential harms to participants in Bruening's studies. However, he closes the account of his own experiences by reminding us of other victims of Bruening's misconduct--namely, psychologists and other researchers who collaborated with Bruening, but without being aware that he had fabricated data.
Dr. Alan Poling, one of those psychologists, writes about the consequences of Bruening's misconduct for his collaborators in research. Strikingly, Poling points out that between 1979 and 1983, Bruening was a contributor to 34% of all published research on the psychopharmacology of mentally retarded people. For those not involved in the research, initial doubts may, however unfairly, be cast on all these publications. For those involved in the research, efforts need to be made in each case to determine to what extent, if any, the validity of the research was affected by Bruening's role in the study. Even though Bruening was the only researcher to fabricate data, his role could contaminate an entire study. In fact, however, not all of Bruening's research did involve fabrication. Yet, convincing others of this is a time-consuming, demanding task. Finally, those who cited Bruening's publications in their own work may also suffer "guilt by association." As Poling points out, this is especially unfair in those instances where Bruening collaborations with others involved no fraud at all.
5. Readings
For readings on scientific integrity, including sections on the fabrication of data and a definition of scientific misconduct, see:
- Integrity and Misconduct in Research Washington, D.C.: U.S. Department of Health and Human Services, 1995.
- On Being a Scientist , 2 nd ed. (Washington, D.C.: National Academy Press, 1995)
- Honor in Science Research Triangle Park, NC: Sigma Xi, The Scientific Research Society, 1991.
Sources for information on the Darsee case include:
- Sharen Begley, with Phyllis Malamud and Mary Hager, "A Case of Fraud at Harvard," Newsweek , February 4, 1982, pp. 89-92.
- Richard Knox, The Harvard fraud case: where does the problem lie? , JAMA, Vol. 249, No. 14, April 3, 1983, pp. 1797-1807.
- Walter W. Stewart, The integrity of the scientific literature, Nature, Vol. 325, January 15, 1987, pp. 207-214.
- Eugene Brunwald, "Analysing scientific fraud", Nature , Vol. 325, January 15, 1987, pp. 215-216.
- Eugene Brunwald, "Cardiology: The John Darsee Experience", in David J. Miller and Michel Hersen, R esearch Fraud in the Behavioral and Biomedical Sciences (New York: John Wiley & Sons, Inc., 1992, pp. 55-79.
For readings on Bruening, see
- Sprague, Robert L., "The Voice of Experience," Science and Engineering Ethics , Vol. 4, Issue 1, 1998, p. 33.
- Poling, Alan, The Consequences of Fraud, in Miller and Hersen, pp. 140-157.
- The Miller and Hersen book includes other good essays on misconduct in science.
The Darsee and Bruening cases raise a host of ethical questions about the nature and consequences of scientific fraud:
- What kinds of reasons are offered for fabricating data?
- Which, if any, of those reasons are good reasons--i.e., reasons that might justify fabricating data?
- Who is likely to be harmed by fabricating data? Does actual harm have to occur in order for fabrication to be ethically wrong?
- What responsibilities does a scientist have for checking on the trustworthiness of the work of other scientists?
- What should a scientist do if he or she has reason to believe that another scientist has fabricated data?
- Why is honesty in scientific research important to the scientific community?
- Why is honesty in scientific research important for the public?
- What might be done to diminish the likelihood that research fraud occurs?
- What applications of the concerns raised in the above questions are there for teaching science classes in high school? Middle school? Elementary school?
- 50 Demets, David, "Statistics and Ethics in Medical Research," forthcoming in Science and Engineering Ethics. (P. 29 of draft.) At the 1994 Teaching Research Ethics for Faculty Workshop at Indiana University's Poynter Center, DeMets recounted in great detail the severe challenges he and his team of statisticians faced in carrying out their investigation.
- 51 Eugene Braunwald, "Cardiology: The John Darsee Experience," in David J. Miller and Michel Hersen, Research Fraud in the Behavioral and Biomedical Sciences (New York: John Wiley & Sons, Inc., 1992, pp. 55-79.
- 52 May, William F., "Professional Virtue and Self-regulation," in Joan Callahan, ed., Ethical Issues in Professional Life (New York: Oxford University Press, 1988), p. 408.
- 53 Sprague, Robert L., "The Voice of Experience," Science and Engineering Ethics, Vol. 4, Issue 1, 1998, p. 33.
Related Resources
Submit Content to the OEC Donate
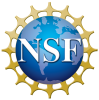
This material is based upon work supported by the National Science Foundation under Award No. 2055332. Any opinions, findings, and conclusions or recommendations expressed in this material are those of the author(s) and do not necessarily reflect the views of the National Science Foundation.
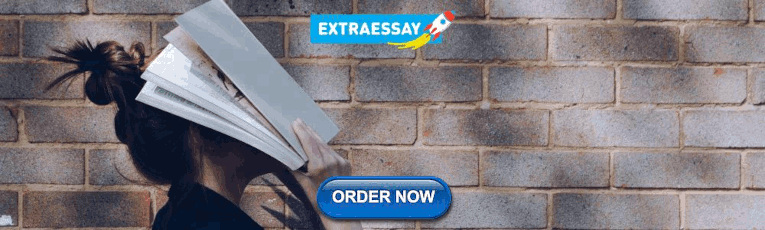
IMAGES
VIDEO
COMMENTS
Case studies in data ethics. These studies provide a foundation for discussing ethical issues so we can better integrate data ethics in real life. Check out the "Data Case Studies" lineup at the Strata Data Conference in New York, September 11-13, 2018. This post is part of a series on data ethics. To help us think seriously about data ethics ...
Apple's commitment to privacy. IBM's AI ethics. Microsoft's data governance. GDPR and data protection. Facebook and Cambridge Analytica scandal. Project Nightingale and Google. Toronto's Sidewalk Labs. Let's understand each one of them in detail. 1.
1. Target's pregnancy prediction. Let's first look at one of the most notorious examples of the potential of predictive analytics when studying big data ethics. It's well known that every time you go shopping, retailers are taking note of what you buy and when you're buying it.
These studies provide a foundation for discussing ethical issues so we can better integrate data ethics in real life. To help us think seriously about data ethics, we need case studies that we can discuss, argue about, and come to terms with as we engage with the real world. Good case studies give us the opportunity to think through problems ...
The Council for Big Data, Ethics, and Society has released three case studies (with more on the way) and has set a deadline of June 1, 2016, for any new submissions to its call for cases. 1) The Ethics of Using Hacked Data: Patreon's Data Hack and Academic Data Standards by Nathaniel Poor and Roei […]
In January 2022, Politico published an article about a nonprofit called Crisis Text Line, which offers support via text messages for people who are going through mental health crises. For years, the nonprofit had been collecting a database of messages exchanged, and used the data to triage the incoming calls for help and to train its volunteers ...
Data ethics boards, business unit leaders, and C-suite champions should build a common view (and a common language) about how data usage rules should link up to both the company's data and corporate strategies and to real-world use cases for data ethics, such as decisions on design processes or M&A.
Publisher: Markkula Center for Applied Ethics. Publication Year: 2022. Summary: The following case study describes how there is an ethical concern with keeping data linked to mental health and using this data to receive monetary gain. The mental health hotline took the text messages from people who had reached out to them and made them anonymous.
1 Introduction. Data ethics is a rapidly-developing yet inchoate subfield of research within the discipline of data science, which is itself rapidly-developing (Wender and Kloefkorn 2017 ). For example, the Data Science department at Stanford University lists "Ethics and Data Science" as one of its research areas: https://datascience ...
Data Science Needs Ethics • 3 minutes; Case Study: ... Who owns data about you? We'll explore that question in this module. A few examples of personal data include copyrights for biographies; ownership of photos posted online, Yelp, Trip Advisor, public data capture, and data sale. We'll also explore the limits on recording and use of data.
The Data Science Ethos relies on individual contributions to provide case studies and teaching material. To help contributors develop their case study, our six-step lifecycle captures the distinct, high-level stages of most data science projects and associates a robust conceptual framework that sheds light on the benefits and challenges of ethics-centered data science approaches.
Case Study 1. Fred and Tamara, a married couple in their 30's, are applying for a business loan to help them realize their long-held dream of owning and operating their own restaurant. Fred is a highly promising graduate of a prestigious culinary school, and Tamara is an accomplished accountant.
Markkula Center for Applied Ethics. Focus Areas. Internet Ethics. Internet Ethics Cases. Find ethics case studies on topics in Internet ethics including privacy, hacking, social media, the right to be forgotten, and hashtag activism. (For permission to reprint articles, submit requests to [email protected] .)
Case Studies: Amsterdam have created an Algorithm Register to provide transparency in a standard format about how data is being used in public services, along with a host of other complementary initiatives to be truly an ethical municipal authority. Brent created a Data Ethics Board with the help of LOTI, which serves to provide the borough ...
A data science framework has emerged and is presented in the remainder of this article along with a case study to illustrate the steps. This data science framework warrants refining scientific practices around data ethics and data acumen (literacy). A short discussion of these topics concludes the article. 2.
Summary. Most data and analytics leaders believe complying with rules ensures ethical use of D&A. It doesn't. Ethical use of D&A demands reflection on use cases, which enables decisions on their appropriateness. Highmark Health uses a decision-making system to encourage staff to reflect on their use of D&A.
Reidentification examples with deidentified data. In both examples, the adversary succeeded because key variables were available in both the deidentified data set (blue) and an identified auxiliary data set (red). ... This case study describes the privacy and ethics audit that we conducted as part of the Fragile Families Challenge.
The Ethics of Using Hacked Data: Patreon's Data Hack and Academic Data Standards1 Case Study 03.17.16 NATHANIEL POOR, PHD, ROEI DAVIDSON, PHD ... Another recent example is the hacking into citizens' cell phones by employees at Rupert Murdoch's News Corporation in the UK [6]. Both journalistic ethics and the law were violated, and both ...
CONCLUSION. Optimal ethical solutions should be sought on both a societal and inter-personal level. Governments should especially seek to ensure that persons vulnerable to becoming unwitting, or even witting research participants understand the risks they face. 49 This is especially important because Big Data studies might affect stigmatization, negatively target individuals, and even affect ...
Data Ethics Case Studies . The ethical aspects of data are many. Examples include defining ownership of data, obtaining consent to collect and share data, protecting the identity of human subjects and their personal identifying information, and the licensing of data.
Ethics and Data Science. New technologies often raise new moral questions. For example, the emergence of nuclear weapons placed great pressure on the distinction between combatants and non-combatants that had been central to the just war theory formulated in the middle ages. New theories were needed to reinterpret the meaning of this ...
Never assume a customer is OK with you collecting their data; always ask for permission to avoid ethical and legal dilemmas. 2. Transparency. In addition to owning their personal information, data subjects have a right to know how you plan to collect, store, and use it. When gathering data, exercise transparency.
Overly Ambitious Researchers - Fabricating Data. A historical case study about the cases of Dr. John Darsee and Dr. Stephen Breuning who both were found to have fabricated data as part of their research. This is one of six cases from Michael Pritchard and Theodore Golding's instructor guide, " Ethics in the Science Classroom ."