Thank you for visiting nature.com. You are using a browser version with limited support for CSS. To obtain the best experience, we recommend you use a more up to date browser (or turn off compatibility mode in Internet Explorer). In the meantime, to ensure continued support, we are displaying the site without styles and JavaScript.
- View all journals
- Explore content
- About the journal
- Publish with us
- Sign up for alerts
- Perspective
- Published: 23 February 2022
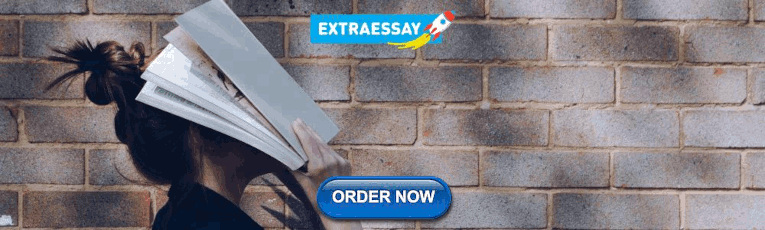
Responsible artificial intelligence in agriculture requires systemic understanding of risks and externalities
- Asaf Tzachor ORCID: orcid.org/0000-0002-4032-4996 1 , 2 ,
- Medha Devare ORCID: orcid.org/0000-0003-0041-4812 3 , 4 ,
- Brian King ORCID: orcid.org/0000-0002-7056-9214 3 ,
- Shahar Avin ORCID: orcid.org/0000-0001-7859-1507 1 &
- Seán Ó hÉigeartaigh ORCID: orcid.org/0000-0002-2846-1576 1 , 5
Nature Machine Intelligence volume 4 , pages 104–109 ( 2022 ) Cite this article
6678 Accesses
37 Citations
313 Altmetric
Metrics details
- Agriculture
- Science, technology and society
Global agriculture is poised to benefit from the rapid advance and diffusion of artificial intelligence (AI) technologies. AI in agriculture could improve crop management and agricultural productivity through plant phenotyping, rapid diagnosis of plant disease, efficient application of agrochemicals and assistance for growers with location-relevant agronomic advice. However, the ramifications of machine learning (ML) models, expert systems and autonomous machines for farms, farmers and food security are poorly understood and under-appreciated. Here, we consider systemic risk factors of AI in agriculture. Namely, we review risks relating to interoperability, reliability and relevance of agricultural data, unintended socio-ecological consequences resulting from ML models optimized for yields, and safety and security concerns associated with deployment of ML platforms at scale. As a response, we suggest risk-mitigation measures, including inviting rural anthropologists and applied ecologists into the technology design process, applying frameworks for responsible and human-centred innovation, setting data cooperatives for improved data transparency and ownership rights, and initial deployment of agricultural AI in digital sandboxes.
This is a preview of subscription content, access via your institution
Access options
Access Nature and 54 other Nature Portfolio journals
Get Nature+, our best-value online-access subscription
24,99 € / 30 days
cancel any time
Subscribe to this journal
Receive 12 digital issues and online access to articles
111,21 € per year
only 9,27 € per issue
Buy this article
- Purchase on Springer Link
- Instant access to full article PDF
Prices may be subject to local taxes which are calculated during checkout
Similar content being viewed by others
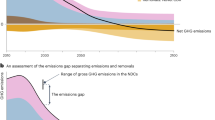
The carbon dioxide removal gap
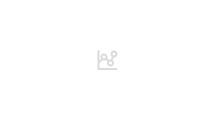
The impact of artificial intelligence on employment: the role of virtual agglomeration
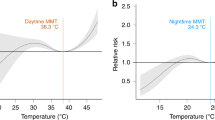
Heat health risk assessment in Philippine cities using remotely sensed data and social-ecological indicators
The State of Food Security and Nutrition in the World 2020: Transforming Food Systems for Affordable Healthy Diets (FAO, IFAD, UNICEF, WFP, WHO, 2020).
Cole, M. B., Augustin, M. A., Robertson, M. J. & Manners, J. M. The science of food security. NPJ Sci. Food 2 , 14 (2018).
Article Google Scholar
Matson, P. A., Parton, W. J., Power, A. G. & Swift, M. J. Agricultural intensification and ecosystem properties. Science 277 , 504–509 (1997).
Quinton, J. N., Govers, G., Van Oost, K. & Bardgett, R. D. The impact of agricultural soil erosion on biogeochemical cycling. Nat. Geosci. 3 , 311–314 (2010).
Singh, R. B. Environmental consequences of agricultural development: a case study from the Green Revolution state of Haryana, India. Agricult. Ecosyst. Environment 82 , 97–103 (2000).
The State of the World’s Plant Genetic Resources for Food and Agriculture (FAO, 2010).
Semchuk, K. M., Love, E. J. & Lee, R. G. Parkinson’s disease and exposure to agricultural work and pesticide chemicals. Neurology 42 , 1328–1328 (1992).
Campbell, J. In Topical Research Digest: Human Rights and Contemporary Slavery 131–141 (Univ. Denver, 2008).
Nguyen, H. Q. & Warr, P. Land consolidation as technical change: economic impacts in rural Vietnam. World Dev. 127 , 104750 (2020).
Nilsson, P. The role of land use consolidation in improving crop yields among farm households in Rwanda. J. Dev. Stud. 55 , 1726–1740 (2019).
Du, X., Zhang, X. & Jin, X. Assessing the effectiveness of land consolidation for improving agricultural productivity in China. Land Use Policy 70 , 360–367 (2018).
Schmitz, A., & Moss, C. B. Mechanized agriculture: Machine adoption, farm size, and labor displacement. AgBioForum 18 , 278–296 (2015).
Wilde, P. Food Policy in the United States: An Introduction (Routledge, 2013).
Tadele, Z. Orphan crops: their importance and the urgency of improvement. Planta 250 , 677–694 (2019).
Lugo-Morin, D. Indigenous communities and their food systems: a contribution to the current debate. J. Ethn. Food 7 , 6 (2020).
Akinola, R., Pereira, L. M., Mabhaudhi, T., de Bruin, F. M. & Rusch, L. A review of indigenous food crops in Africa and the implications for more sustainable and healthy food systems. Sustainability 12 , 3493 (2020).
Jose, S. Agroforestry for ecosystem services and environmental benefits: an overview. Agrofor. Syst. 76 , 1–10 (2009).
Talaviya, T., Shah, D., Patel, N., Yagnik, H. & Shah, M. Implementation of artificial intelligence in agriculture for optimisation of irrigation and application of pesticides and herbicides. Artif. Intell. Agricult. 4 , 58–73 (2020).
Google Scholar
Palacios-Lopez, A., Christiaensen, L., & Kilic, T. How much of the labor in African agriculture is provided by women? Food Policy 67, 52–63 (2017).
Alkon, A. H., & Agyeman, J. (eds) Cultivating Food Justice: Race, Class, and Sustainability (MIT Press, 2011).
Edmonds, E. V. & Pavcnik, N. The effect of trade liberalization on child labor. J. Int. Econ. 65 , 401–419 (2005).
Child Labour in Agriculture (International Labor Organization, 2021).
Lowder, S. K., Skoet, J. & Raney, T. The number, size, and distribution of farms, smallholder farms, and family farms worldwide. World Dev. 87 , 16–29 (2016).
Mehrabi, Z. et al. The global divide in data-driven farming. Nat. Sustain. 4 , 154–160 (2021).
Hennessy, T., Läpple, D. & Moran, B. The digital divide in farming: A problem of access or engagement? Appl. Econ. Persp. Policy 38 , 474–491 (2016).
Klerkx, L., Jakku, E., Labarthe, P. A review of social science on digital agriculture, smart farming and agriculture 4.0: New contributions and a future research agenda. NJAS Wageningen J. Life Sci. 90–91 , 100315 (2019).
Wolfert, S., Ge, L., Verdouw, C. & Bogaardt, M. J. Big data in smart farming–a review. Agric. Syst. 153 , 69–80 (2017).
Levins, R. & Cochrane, W. The treadmill revisited. Land Econ. 72 , 550–553 (1996).
Sontowski, S. et al. Cyber attacks on smart farming infrastructure. In 2020 IEEE 6th Int. Conf. on Collaboration and Internet Computing (CIC) 135–143 (IEEE, 2020).
Cyber-attack hits JBS meat works in Australia, North America. Reuters https://www.reuters.com/technology/cyber-attack-hits-jbs-meat-works-australia-north-america-2021-05-31/ (1 June 2021)
Sharma, A. $5.9 million ransomware attack on farming co-op may cause food shortage. Ars Technica https://arstechnica.com/information-technology/2021/09/5-9-million-ransomware-attack-on-farming-co-op-may-cause-food-shortage/ (21 September 2021)
Rahwan, I. et al. Machine behaviour. Nature 568 , 477–486 (2019).
Johnson, N. et al. Abrupt rise of new machine ecology beyond human response time. Sci. Rep. 3 , 2627 (2013).
Gold, E. R. The fall of the innovation empire and its possible rise through open science. Res. Policy 50 , 104226 (2021).
Majumdar, J., Naraseeyappa, S. & Ankalaki, S. Analysis of agriculture data using data mining techniques: application of big data. J. Big Data 4 , 20 (2017).
CGIAR GARDIAN Data Ecosystem https://gardian.bigdata.cgiar.org (CGIAR Platform for Big Data in Agriculture, 2021).
Yara and IBM. IBM https://www.ibm.com/services/client-stories/yara (accessed 18 August 2021).
Stilgoe, J., Owen, R. & Macnaghten, P. in The Ethics of Nanotechnology, Geoengineering and Clean Energy 347–359 (Routledge, 2020).
Theodorou, A. & Dignum, V. Towards ethical and socio-legal governance in AI. Nat. Mach. Intell. 2 , 10–12 (2020).
Kamle, S. & Ali, S. Genetically modified crops: detection strategies and biosafety issues. Gene 522 , 123–132 (2013).
Download references
Acknowledgements
This paper was made possible through the support of a grant from Templeton World Charity Foundation. The opinions expressed in this publication are those of the author(s) and do not necessarily reflect the views of Templeton World Charity Foundation.
Author information
Authors and affiliations.
Centre for the Study of Existential Risk, University of Cambridge, Cambridge, UK
Asaf Tzachor, Shahar Avin & Seán Ó hÉigeartaigh
School of Sustainability, Reichman University (IDC Herzliya), Herzliya, Israel
- Asaf Tzachor
Platform for Big Data in Agriculture, CGIAR, Cali, Colombia
Medha Devare & Brian King
International Institute for Tropical Agriculture, CGIAR, Ibadan, Nigeria
Medha Devare
Leverhulme Centre for the Future of Intelligence, University of Cambridge, Cambridge, UK
Seán Ó hÉigeartaigh
You can also search for this author in PubMed Google Scholar
Contributions
A.T., M.D., B.K., S.A. and S.Ó.H, developed the paper jointly and all contributed equally to the writing of the text.
Corresponding authors
Correspondence to Asaf Tzachor or Seán Ó hÉigeartaigh .
Ethics declarations
Competing interests.
The authors declare no competing interests.
Peer review
Peer review information.
Nature Machine Intelligence thanks Matthew McCabe and John Quinn for their contribution to the peer review of this work.
Additional information
Publisher’s note Springer Nature remains neutral with regard to jurisdictional claims in published maps and institutional affiliations.
Rights and permissions
Reprints and permissions
About this article
Cite this article.
Tzachor, A., Devare, M., King, B. et al. Responsible artificial intelligence in agriculture requires systemic understanding of risks and externalities. Nat Mach Intell 4 , 104–109 (2022). https://doi.org/10.1038/s42256-022-00440-4
Download citation
Received : 20 August 2021
Accepted : 02 January 2022
Published : 23 February 2022
Issue Date : February 2022
DOI : https://doi.org/10.1038/s42256-022-00440-4
Share this article
Anyone you share the following link with will be able to read this content:
Sorry, a shareable link is not currently available for this article.
Provided by the Springer Nature SharedIt content-sharing initiative
This article is cited by
Who is responsible for ‘responsible ai’: navigating challenges to build trust in ai agriculture and food system technology.
- Carrie S. Alexander
- Mark Yarborough
- Aaron Smith
Precision Agriculture (2024)
Large language models and agricultural extension services
Nature Food (2023)
Digital twins: a stepping stone to achieve ocean sustainability?
- Ofir Hendel
- Catherine E. Richards
npj Ocean Sustainability (2023)
Rewards, risks and responsible deployment of artificial intelligence in water systems
- Richard Fenner
Nature Water (2023)
Potential and limitations of digital twins to achieve the Sustainable Development Goals
- Soheil Sabri
- Michele Acuto
Nature Sustainability (2022)
Quick links
- Explore articles by subject
- Guide to authors
- Editorial policies
Sign up for the Nature Briefing: Anthropocene newsletter — what matters in anthropocene research, free to your inbox weekly.

The Role of Artificial Neuron Networks in Intelligent Agriculture (Case Study: Greenhouse)
- First Online: 01 September 2020
Cite this chapter
- Abdelkader Hadidi 5 ,
- Djamel Saba 5 &
- Youcef Sahli 5
Part of the book series: Studies in Computational Intelligence ((SCI,volume 912))
1245 Accesses
12 Citations
The cultivation under cover of fruits, vegetables, and floral species has developed from the traditional greenhouse to the agro-industrial greenhouse which is currently known for its modernity and its high level of automation (heating, misting system, air conditioning, control, regulation and command, supervision computer, etc.). New techniques have emerged, including the use of devices to control and regulate climatic variables in the greenhouse (temperature, humidity, CO 2 concentration, etc.). In addition, the use of artificial intelligence (AI) such as neural networks and/or fuzzy logic. Currently, the climate computer offers multiple services and makes it possible to solve problems relating to regulation, control, and commands. The main motivation in choosing an order by AI is to improve the performance of internal climate management, to move towards a control-command strategy to achieve a homogeneous calculation structure through a mathematical model of the process to be controlled, usable on the one hand for the synthesis of the controller and on the other hand by the simulation of the performances of the system. It is from this state, that begins this research work in this area include modelization an intelligent controller by the use of fuzzy logic.
This is a preview of subscription content, log in via an institution to check access.
Access this chapter
- Available as PDF
- Read on any device
- Instant download
- Own it forever
- Available as EPUB and PDF
- Compact, lightweight edition
- Dispatched in 3 to 5 business days
- Free shipping worldwide - see info
- Durable hardcover edition
Tax calculation will be finalised at checkout
Purchases are for personal use only
Institutional subscriptions
Abbreviations
Artificial Intelligence
Artificial Neural Networks
Carbon Dioxide
Evolution Algorithms
Food and Agriculture Organization of the United Nations
Fuzzy Logic
Genetic Algorithms
Information Technology
Linear Programming
Multi-Input Multi-Output
National Institute for Agronomic Research
Pseudo-Derivative Feedback
Polyethylene
Integral Controllers – Derivatives
Proportional-Integral-Plus
Polyvinyl Chloride
Single-Input, Single-Output
Temperature
Z. Li, J. Wang, R. Higgs et al., Design of an intelligent management system for agricultural greenhouses based on the internet of things, in Proceedings of the 2017 IEEE International Conference on Computational Science and Engineering and IEEE/IFIP International Conference on Embedded and Ubiquitous Computing, CSE and EUC (2017)
Google Scholar
D. Piscia, P. Muñoz, C. Panadès, J.I. Montero, A method of coupling CFD and energy balance simulations to study humidity control in unheated greenhouses. Comput. Electron. Agric. (2015). https://doi.org/10.1016/j.compag.2015.05.005
Article Google Scholar
E.J. van Henten, Greenhouse climate management : an optimal control approach. Agric. Eng. Phys. PE&RC (1994)
R. Ben Ali, S. Bouadila, A. Mami, Development of a fuzzy logic controller applied to an agricultural greenhouse experimentally validated. Appl. Therm. Eng. 141 , 798–810 (2018). https://doi.org/10.1016/J.APPLTHERMALENG.2018.06.014
T. Morimoto, Y. Hashimoto, An intelligent control technique based on fuzzy controls, neural networks and genetic algorithms for greenhouse automation. IFAC Proc. 31 , 61–66 (1998). https://doi.org/10.1016/S1474-6670(17)42098-2
Y. Lu, Artificial intelligence: a survey on evolution, models, applications and future trends. J. Manag. Anal (2019)
J.C. van Dijk, P. Williams, The history of artificial intelligence. Expert. Syst. Audit. (1990)
A. Benko, C. Sik Lányi, History of artificial intelligence, in: Encyclopedia of Information Science and Technology , 2nd edn (2011)
B. van Ginneken, Fifty years of computer analysis in chest imaging: rule-based, machine learning, deep learning. Radiol. Phys. Technol. (2017)
J. Ring, in We Were Yahoo! : From Internet Pioneer to the Trillion Dollar Loss of Google and Facebook
M. Haenlein, A. Kaplan, A brief history of artificial intelligence: On the past, present, and future of artificial intelligence. Calif. Manage. Rev. (2019). https://doi.org/10.1177/0008125619864925
S. Castellanos, Facebook AI Chief Yann LeCun Says Machines Are Decades Away From Matching the Human Brain—CIO Journal. Wall Str. J. (2017)
A. Wennberg, Food and Agriculture Organization of the United Nations, in Encyclopedia of Toxicology , 3rd edn (2014)
N. Radojević, D. Kostadinović, H. Vlajković, E. Veg, Microclimate control in greenhouses. FME Trans. (2014). https://doi.org/10.5937/fmet1402167R
C. Duarte-Galvan, I. Torres-Pacheco, R.G. Guevara-Gonzalez et al., Review advantages and disadvantages of control theories applied in greenhouse climate control systems. Spanish J. Agric. Res. (2012). https://doi.org/10.5424/sjar/2012104-487-11
N. Choab, A. Allouhi, A. El Maakoul et al., Review on greenhouse microclimate and application: design parameters, thermal modeling and simulation, climate controlling technologies. Sol. Energy 191 , 109–137 (2019). https://doi.org/10.1016/j.solener.2019.08.042
E. Iddio, L. Wang, Y. Thomas et al., Energy efficient operation and modeling for greenhouses: a literature review. Renew. Sustain. Energy Rev. 117 , 109480 (2020). https://doi.org/10.1016/J.RSER.2019.109480
G. Ted, in Greenhouse Management: A Guide to Greenhouse Technology and Operations . (Apex Publishers, USA, 2019)
Structure used as greenhouse roof frame, greenhouse roof frame, greenhouse framework, greenhouse, and greenhouse framework building method (2004)
G.-W. Bruns, Experiences on damages to roofing-materials and greenhouse construction. Acta Hortic 127–132. https://doi.org/10.17660/ActaHortic.1985.170.14
Greenhouse construction (1910)
C. von Zabeltitz, Greenhouse Structures, Integrated Greenhouse Systems for Mild Climates (Springer, Berlin, 2011), pp. 59–135
Chapter Google Scholar
S. Fang, C. Jie, I. Hideaki, in MIMO Systems . Lecture Notes in Control and Information Sciences (2017)
K.H. Ang, G. Chong, Y. Li, PID control system analysis, design, and technology. IEEE Trans. Control Syst. Technol. (2005). https://doi.org/10.1109/TCST.2005.847331
Industrial Process Automation Systems (2015)
O. Blial, M. Ben Mamoun, R. Benaini, An overview on SDN architectures with multiple controllers. J. Comput. Netw. Commun. (2016)
E. Camponogara, D. Jia, B.H. Krogh, S. Talukdar, Distributed model predictive control. IEEE Control Syst. (2002). https://doi.org/10.1109/37.980246
J.F. Cáceres, A.R. Kornblihtt, Alternative splicing: multiple control mechanisms and involvement in human disease. Trends Genet. 18 , 186–193 (2002). https://doi.org/10.1016/S0168-9525(01)02626-9
D. Saba, B. Berbaoui, H.E. Degha, F.Z. Laallam, A generic optimization solution for hybrid energy systems based on agent coordination. in eds. by A.E. Hassanien, K. Shaalan, T. Gaber, M.F. Tolba Advances in Intelligent Systems and Computing (Springer, Cham, Cairo, Egypte, 2018) pp. 527–536
D. Saba, H.E. Degha, B. Berbaoui et al., Contribution to the modeling and simulation of multiagent systems for energy saving in the habitat, in Proceedings of the 2017 International Conference on Mathematics and Information Technology , ICMIT (2017)
D. Saba, F.Z. Laallam, B. Berbaoui, F.H. Abanda, An energy management approach in hybrid energy system based on agent’s coordination, in Advances in Intelligent Systems and Computing , 533rd edn., ed. by A. Hassanien, K. Shaalan, T. Gaber, A.T.M. Azar (Springer, Cham, Cairo, Egypte, 2017), pp. 299–309
D. Saba, F.Z. Laallam, A.E. Hadidi, B. Berbaoui, Contribution to the management of energy in the systems multi renewable sources with energy by the application of the multi agents systems “MAS”. Energy Procedia 74 , 616–623 (2015). https://doi.org/10.1016/J.EGYPRO.2015.07.792
D. Saba, F.Z. Laallam, H.E. Degha et al., Design and development of an intelligent ontology-based solution for energy management in the home, in Studies in Computational Intelligence , 801st edn., ed. by A.E. Hassanien (Springer, Cham, Switzerland, 2019), pp. 135–167
D. Saba, R. Maouedj, B. Berbaoui, Contribution to the development of an energy management solution in a green smart home (EMSGSH), in Proceedings of the 7th International Conference on Software Engineering and New Technologies — ICSENT 2018 (ACM Press, New York, NY, USA, 2018), pp. 1–7
D. Saba, H.E. Degha, B. Berbaoui, R. Maouedj, Development of an Ontology Based Solution for Energy Saving Through a Smart Home in the City of Adrar in Algeria (Springer, Cham, 2018), pp. 531–541
M. Pöller, S. Achilles, Aggregated wind park models for analyzing power system dynamics, in 4th International Workshop on Large-scale Integration of Wind Power and Transmission Networks for Offshore Wind Farms (2003), pp. 1–10
D. Saba, F. Zohra Laallam, H. Belmili et al., Development of an ontology-based generic optimisation tool for the design of hybrid energy systems development of an ontology-based generic optimisation tool for the design of hybrid energy systems. Int. J. Comput. Appl. Technol. 55 , 232–243 (2017). https://doi.org/10.1504/IJCAT.2017.084773
D. Saba, F.Z. Laallam, A.E. Hadidi, B. Berbaoui, Optimization of a multi-source system with renewable energy based on ontology. Energy Procedia 74 , 608–615 (2015). https://doi.org/10.1016/J.EGYPRO.2015.07.787
V. Vanitha, P. Krishnan, R. Elakkiya, Collaborative optimization algorithm for learning path construction in E-learning. Comput. Electr. Eng. 77 , 325–338 (2019). https://doi.org/10.1016/J.COMPELECENG.2019.06.016
R.S. Epanchin-Niell, J.E. Wilen, Optimal spatial control of biological invasions. J. Environ. Econ. Manage. (2012). https://doi.org/10.1016/j.jeem.2011.10.003
M. Vassell, O. Apperson, P. Calyam et al., Intelligent dashboard for augmented reality based incident command response co-ordination, in 2016 13th IEEE Annual Consumer Communications and Networking Conference, CCNC 2016 (2016)
K. Lammari, F. Bounaama, B. Draoui, Interior climate control of Mimo green house model using PI and IP controllers. ARPN J. Eng. Appl. Sci. 12 (2017)
C.J. Taylor, P. Leigh, L. Price et al., Proportional-integral-plus (PIP) control of ventilation rate in agricultural buildings. Control Eng. Pract. (2004). https://doi.org/10.1016/S0967-0661(03)00060-1
M.-P. Raveneau, Effet des vitesses de dessiccation de la graine et des basses températures sur la germination du pois protéagineux
H.-J. Tantau, Greenhouse climate control using mathematical models. Acta Hortic 449–460 (1985). https://doi.org/10.17660/ActaHortic.1985.174.60
M. Trejo-Perea, G. Herrera-Ruiz, J. Rios-Moreno et al., Greenhouse energy consumption prediction using neural networks models. Int. J. Agric. Biol. (2009)
I. González Pérez, A. José, C. Godoy, Neural networks-based models for greenhouse climate control. J. Automática 1–5 (2018)
E.K. Burke, M. Hyde, G. Kendall et al., A classification of hyper-heuristic approaches (2010)
Genetic algorithms in search, optimization, and machine learning. Choice Rev. (1989). https://doi.org/10.5860/choice.27-0936
A. Konak, D.W. Coit, A.E. Smith, Multi-objective optimization using genetic algorithms: a tutorial. Reliab. Eng. Syst. Saf. (2006). https://doi.org/10.1016/j.ress.2005.11.018
Download references
Author information
Authors and affiliations.
Unité de Recherche en Energies Renouvelables en Milieu Saharien, URER-MS, Centre de Développement des Energies Renouvelables, CDER, 01000, Adrar, Algeria
Abdelkader Hadidi, Djamel Saba & Youcef Sahli
You can also search for this author in PubMed Google Scholar
Corresponding author
Correspondence to Djamel Saba .
Editor information
Editors and affiliations.
Information Technology Department, Faculty of Computers and Information, Cairo University, Giza, Egypt
Aboul Ella Hassanien
Department of Computer Science and Engineering, Faculty of Engineering, Manipal University, Jaipur, Rajasthan, India
Roheet Bhatnagar
Faculty of Science, Helwan University, Cairo, Egypt
Ashraf Darwish
Rights and permissions
Reprints and permissions
Copyright information
© 2021 The Editor(s) (if applicable) and The Author(s), under exclusive license to Springer Nature Switzerland AG
About this chapter
Hadidi, A., Saba, D., Sahli, Y. (2021). The Role of Artificial Neuron Networks in Intelligent Agriculture (Case Study: Greenhouse). In: Hassanien, A., Bhatnagar, R., Darwish, A. (eds) Artificial Intelligence for Sustainable Development: Theory, Practice and Future Applications. Studies in Computational Intelligence, vol 912. Springer, Cham. https://doi.org/10.1007/978-3-030-51920-9_4
Download citation
DOI : https://doi.org/10.1007/978-3-030-51920-9_4
Published : 01 September 2020
Publisher Name : Springer, Cham
Print ISBN : 978-3-030-51919-3
Online ISBN : 978-3-030-51920-9
eBook Packages : Intelligent Technologies and Robotics Intelligent Technologies and Robotics (R0)
Share this chapter
Anyone you share the following link with will be able to read this content:
Sorry, a shareable link is not currently available for this article.
Provided by the Springer Nature SharedIt content-sharing initiative
- Publish with us
Policies and ethics
- Find a journal
- Track your research
Harnessing Artificial Intelligence for Sustainable Agricultural Development in Africa: Opportunities, Challenges, and Impact
This paper explores the transformative potential of artificial intelligence (AI) in the context of sustainable agricultural development across diverse regions in Africa. Delving into opportunities, challenges, and impact, the study navigates through the dynamic landscape of AI applications in agriculture. Opportunities such as precision farming, crop monitoring, and climate-resilient practices are examined, alongside challenges related to technological infrastructure, data accessibility, and skill gaps. The article analyzes the impact of AI on smallholder farmers, supply chains, and inclusive growth. Ethical considerations and policy implications are also discussed, offering insights into responsible AI integration. By providing a nuanced understanding, this paper contributes to the ongoing discourse on leveraging AI for fostering sustainability in African agriculture.
Index Terms:
I introduction.
Africa’s rich agricultural heritage is at the forefront of a technological revolution, with artificial intelligence (AI) poised to address critical challenges in the sector [ 1 ] . The integration of AI technologies holds great promise for improving efficiency, increasing yields, and fostering sustainable agricultural practices. In Africa, agriculture is at a pivotal juncture, facing the dual challenges of ensuring food security for a growing population and mitigating the impact of climate change on traditional farming practices [ 2 ] . AI emerges as a transformative force capable of revolutionizing the sector by offering innovative solutions to age-old problems [ 3 ] . This article explores the multifaceted role of AI in shaping sustainable agricultural development across diverse regions of Africa, navigating through opportunities, challenges, and overall impact. The integration of AI technologies opens doors to precision farming, allowing farmers to optimize resource use through real-time insights into soil conditions, crop health, and weather patterns [ 4 ] . As demonstrated by Shaikh et al. precision farming not only increases yields but also contributes to resource conservation, making it a crucial element in the pursuit of sustainable agriculture.
However, the adoption of AI in African agriculture is not without its hurdles. Limited access to advanced technology and inadequate infrastructure in rural areas pose significant barriers to the widespread implementation of AI-driven solutions [ 5 ] . The digital divide exacerbates existing disparities, hindering the equitable distribution of technological benefits [ 6 ] . Addressing infrastructure challenges is essential to ensuring the transformative potential of AI reaches all corners of the agricultural sector in Africa. In addition, the success of AI applications in agriculture hinges on the availability and quality of data. Challenges related to data privacy, security, and the sheer volume of data required for accurate analyses must be systematically addressed. This article critically examines the data-related impediments to AI adoption in African agriculture, offering insights into strategies to enhance data quality, accessibility, and security for sustainable AI integration.
The proficiency of agricultural stakeholders in utilizing AI technologies is another significant challenge that merits attention. The dearth of skilled personnel capable of navigating the complexities of AI systems poses a considerable obstacle to the adoption of these technologies, particularly in rural communities [ 7 ] . The intersection of AI and agriculture in Africa represents a dynamic arena filled with both promise and obstacles. This introduction sets the stage for an in-depth exploration of how AI can be harnessed to propel sustainable agricultural development, navigating through the complex landscape of opportunities, challenges, and impact. Through a comprehensive analysis informed by the insights of various studies, this article aims to contribute to the evolving discourse on the integration of AI in African agriculture for the betterment of the continent’s food security and economic resilience.
The rest of the paper is structured as follows: section II we present opportunities presented by AI in African agriculture, section III discussion on challenges to AI adoption in agriculture , section IV impact on smallholder farmers and the agricultural value chain, section V ethical considerations and policy implications, section VI case studies: AI in action, section VII future trends and section VIII the conclusion.
II Opportunities Presented by AI in African Agriculture
The agricultural landscape in Africa is on the brink of a technological revolution, with AI emerging as a catalytic force offering a spectrum of transformative opportunities. As nations grapple with the dual challenges of feeding growing populations and ensuring the resilience of farming practices in the face of climate change, AI stands out as a beacon of innovation. This section delves into the vast array of opportunities that AI presents for agriculture in Africa, exploring key domains such as precision farming, crop monitoring, and climate-resilient practices.
II-A Precision Farming
AI-powered precision farming technologies offer the potential to optimize resource use by providing real-time insights into soil health [ 8 ] , crop conditions [ 9 ] , and weather patterns [ 10 ] . Precision farming, leveraging the capabilities of AI technologies, represents a groundbreaking approach to agricultural practices in Africa [ 11 ] . This innovative method integrates advanced data analytics, sensors, and machine learning algorithms to optimize farming processes, striking a balance between maximizing yields and minimizing resource use. At the core of precision farming is the ability to furnish farmers with unparalleled insights into their fields, facilitating informed real-time decision-making. AI algorithms play a pivotal role in processing data and providing precise recommendations for the application of fertilizers, pesticides, and irrigation [ 12 ] . This targeted approach enhances resource utilization efficiency and contributes to environmental sustainability by reducing the overall use of inputs. A significant aspect of precision farming involves the incorporation of autonomous machinery and robotic systems, capable of executing tasks such as planting, harvesting, and weeding with unparalleled precision [ 13 ] . These technologies not only alleviate farmers from labor-intensive tasks but also elevate the overall productivity of agricultural operations.
Precision farming holds promise in addressing the specific challenges faced by smallholder farmers in Africa [ 14 ] . By granting these farmers access to AI-driven tools for decision-making, market insights, and crop management, precision farming empowers them to compete in a progressively intricate agricultural landscape.Precision farming transcends being a mere technological upgrade; it serves as a transformative force aligning with the broader objectives of sustainable agriculture [ 15 ] . By promoting efficient resource use, minimizing environmental impact, and enhancing the economic viability of farming, precision farming, powered by AI, emerges as a cornerstone for the future of agriculture in Africa
II-B Crop Monitoring and Disease Prediction
AI algorithms can analyze satellite imagery and sensor data to monitor crop health, detect diseases early, and facilitate timely interventions [ 16 ] .The integration of AI in agriculture in Africa extends beyond productivity enhancement to encompass a pivotal opportunity in crop monitoring and disease prediction. This multifaceted application of AI technologies revolutionizes traditional farming practices, offering a proactive approach to safeguarding crops and ensuring food security in the face of evolving environmental challenges. One of the primary advantages of AI-powered crop monitoring lies in its ability to harness satellite imagery and sensor data for real-time analysis [ 17 ] . This capability allows farmers to obtain detailed insights into the health and condition of their crops, enabling timely and targeted interventions. The AI algorithms facilitates the detection of potential disease outbreaks and abnormalities in crop development, providing farmers with a valuable tool for preventing and mitigating crop losses.
The significance of AI-driven disease prediction becomes particularly pronounced in the context of changing climate patterns and the increasing prevalence of plant diseases [ 18 ] . By leveraging historical data, weather forecasts, and machine learning algorithms, AI can generate predictive models that enable farmers to anticipate disease outbreaks before they occur [ 19 ] . This predictive capability empowers farmers to implement preventive measures, ranging from targeted pesticide application to crop rotation strategies, thereby minimizing the impact of diseases on crop yields. AI’s role in disease prediction extends beyond individual farms to contribute to broader agricultural resilience. Integrating data from multiple sources allows for the creation of regional or even national disease prediction models, aiding in the development of effective strategies for disease control and management [ 20 ] . This collaborative and data-driven approach positions AI as a key ally in the collective efforts to safeguard agricultural productivity across diverse landscapes.
The practical implications of AI in crop monitoring and disease prediction are further underscored by its potential to enhance resource efficiency. By enabling targeted and precise interventions, farmers can optimize the use of inputs such as pesticides and fertilizers, resulting in cost savings and a reduction in environmental impact [ 21 ] . This resource-efficient approach aligns with broader sustainability goals in agriculture, highlighting the holistic impact of AI technologies. The opportunity presented by AI in crop monitoring and disease prediction is transformative for agriculture in Africa. Through real-time monitoring, timely interventions, and predictive modeling, AI equips farmers with the tools needed to navigate the complexities of modern farming, fostering resilience and ensuring sustainable food production.
II-C Climate-Resilient Agriculture
Climate change poses unprecedented challenges to global agriculture, and Africa is particularly vulnerable to the impacts of shifting weather patterns and extreme events. In this context, the adoption of climate-resilient agriculture emerges as a critical opportunity, leveraging AI to enhance the adaptive capacity of farming systems [ 22 ] . This paradigm shift goes beyond traditional agricultural practices, introducing innovative approaches that empower farmers to navigate the uncertainties associated with a changing climate. At the core of climate-resilient agriculture is the utilization of AI-driven predictive modeling and climate data analysis. These tools enable farmers to anticipate and respond to evolving climatic conditions, providing valuable insights for decision-making [ 23 ] . By integrating historical climate data, real-time observations, and advanced algorithms, AI contributes to the development of adaptive strategies that mitigate the impact of climate-related risks on crop yields and overall agricultural productivity.
In addition, AI facilitates the creation of precision climate-resilient farming practices tailored to local conditions [ 24 ] . Through the analysis of diverse data sources, including soil quality, precipitation patterns, and temperature variations, AI algorithms can recommend specific crops, planting times, and irrigation schedules that optimize resource use and enhance resilience [ 16 ] . This personalized approach is crucial in addressing the unique challenges faced by farmers in different regions, promoting sustainability and ensuring food security. The benefits of climate-resilient agriculture extend beyond immediate adaptation to climate change. By promoting sustainable land management practices and resource-efficient farming, AI contributes to the long-term viability of agricultural systems. This holistic approach aligns with global efforts to achieve environmental sustainability while meeting the increasing demands for food production.
In addition to on-farm applications, AI in climate-resilient agriculture plays a pivotal role in supporting broader policy initiatives and decision-making processes. By analyzing large-scale climate and agricultural data, AI contributes to the formulation of evidence-based policies that address the complex challenges of climate change in the agricultural sector [ 25 ] . This intersection of technology and policy enhances the overall resilience of agricultural systems on a systemic level. Moreover, climate-resilient agriculture empowered by AI fosters inclusivity by providing smallholder farmers with tools to adapt to changing climates. The accessibility of weather forecasts, crop management insights, and adaptive strategies through digital platforms ensures that farmers across diverse socio-economic backgrounds can benefit from the technological advancements. This inclusivity is vital for promoting equity and ensuring that the advantages of climate-resilient agriculture are shared by all stakeholders in the agricultural value chain.
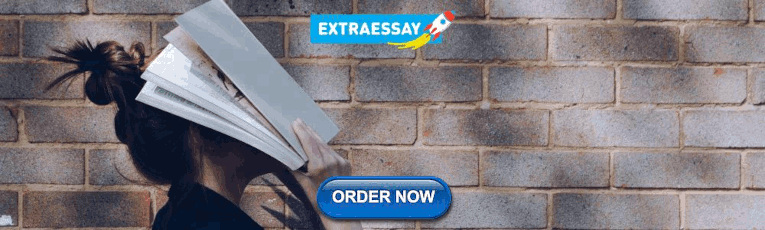
III Challenges to AI Adoption in Agriculture
The adoption of AI in agriculture across Africa faces a myriad of challenges that span technological, infrastructural, and socio-economic domains [ 26 ] . Limited access to advanced technology and insufficient digital infrastructure in rural areas hinders the widespread implementation of AI-driven solutions, exacerbating the existing digital divide [ 22 ] . Additionally, the scarcity of reliable and high-quality data, crucial for effective AI applications, poses a significant hurdle, with issues related to data privacy, security, and the sheer volume of data further complicating the adoption process [ 26 ] . The agricultural sector’s heavy reliance on smallholder farmers, many of whom lack the necessary resources and technical expertise, amplifies the challenge, requiring tailored strategies for capacity building and education [ 27 ] . Addressing these multifaceted challenges is essential for unlocking the full potential of AI in agriculture and ensuring its equitable and sustainable integration across the diverse landscapes of Africa.
III-A Technological Infrastructure
Technological infrastructure challenges pose a formidable barrier to the widespread adoption of AI in agriculture across Africa. In many rural areas, there is a notable lack of access to advanced technology, including the necessary hardware and connectivity, which impedes the deployment of AI-driven solutions. Limited internet connectivity and unreliable power supply further exacerbate these challenges, hindering the real-time data transmission and continuous operation essential for effective AI applications [ 28 ] . The absence of a robust technological foundation in rural settings not only impedes the implementation of AI technologies but also perpetuates a digital divide, preventing farmers from harnessing the transformative potential of AI for optimizing agricultural practices. Moreover, the absence of adequate technical support and training exacerbates technological infrastructure challenges. Many farmers in rural Africa lack the requisite knowledge and skills to operate and maintain AI-driven systems effectively. This knowledge gap extends beyond the farmers themselves to include local support networks, making it challenging to troubleshoot issues and ensure the sustainable integration of AI technologies. Bridging this gap requires targeted capacity-building initiatives and educational programs that empower farmers with the skills needed to leverage AI tools effectively, transforming technological challenges into opportunities for empowerment and innovation in African agriculture.
III-B Data Accessibility and Quality
The success of AI applications relies on quality data. Data accessibility and quality represent critical challenges to the successful adoption of AI in agriculture in Africa. Limited availability of reliable and high-quality data hampers the development and effectiveness of AI applications [ 28 ] . Many regions face data scarcity, with fragmented or outdated datasets, hindering the training and accuracy of AI algorithms. Additionally, issues related to data privacy and security further complicate the utilization of data for AI-driven solutions. Overcoming these challenges requires concerted efforts to establish comprehensive data collection systems, ensure data accuracy and relevance, and implement robust data governance frameworks. Addressing data-related hurdles is pivotal for unlocking the full potential of AI in agriculture, allowing for informed decision-making and sustainable resource management.
III-C Skill Gaps and Training
Skill gaps and the need for comprehensive training programs present significant challenges to the integration of AI in agriculture in Africa [ 28 ] . The agricultural sector, often dominated by smallholder farmers, lacks the technical expertise required to effectively implement and utilize AI-driven technologies. The dearth of skilled personnel capable of navigating the complexities of AI systems poses a considerable obstacle to the adoption of these transformative technologies. This skill gap extends not only to farmers but also to local support networks and extension services, emphasizing the need for targeted training initiatives that address the specific requirements of the agricultural context.
To address these skill gaps, strategic investments in capacity-building programs and educational resources are imperative. Tailored training initiatives must be designed to equip farmers with the necessary skills to operate and maintain AI technologies effectively. Additionally, comprehensive educational programs should be developed to empower agricultural stakeholders with a deeper understanding of AI applications, fostering a culture of innovation and adaptability in the face of evolving technological landscapes. Bridging the skill gap is crucial for ensuring that the benefits of AI in agriculture are accessible and maximized across diverse communities, contributing to increased productivity, sustainable practices, and economic resilience in the agricultural sector in Africa.
IV Impact on Smallholder Farmers and the Agricultural Value Chain
The adoption of AI in agriculture in Africa has a profound impact on smallholder farmers and the broader agricultural value chain [ 29 ] . Smallholder farmers, who often operate with limited resources, stand to benefit from AI technologies that offer solutions to long-standing challenges. AI applications in the agricultural value chain extend beyond on-farm activities to include areas such as logistics, market access, and decision support systems.
IV-A Empowering Smallholder Farmers
AI provides smallholder farmers with valuable insights, market information, and decision support tools, empowering them to make informed choices and enhance their livelihoods [ 30 ] . Empowering smallholder farmers through the strategic deployment of AI technologies is a transformative approach that holds the potential to revolutionize agriculture in Africa. Smallholder farmers, often operating with limited resources and facing numerous challenges, can benefit significantly from AI-driven solutions tailored to their unique needs. AI applications extend beyond the fields to address broader challenges faced by smallholder farmers, including market access and financial inclusion. AI-driven decision support systems provide valuable market insights, helping farmers make informed choices about crop selection, pricing strategies, and market timing [ 31 ] . Furthermore, innovative digital platforms, powered by AI, facilitate access to financial services, crop insurance, and market information, empowering smallholders to navigate market dynamics more effectively [ 32 ] . By bridging information gaps and enhancing market connectivity, AI contributes to the economic empowerment of smallholder farmers, positioning them as key players in the agricultural transformation in Africa.
IV-B Enhancing Supply Chain Efficiency
The integration of AI in agricultural supply chains represents a paradigm shift that significantly enhances efficiency across various stages of the value chain [ 33 ] . AI applications offer advanced analytics and decision support tools that streamline and optimize supply chain processes, contributing to improved overall efficiency and sustainability. One key area of impact is in logistics and distribution [ 34 ] . AI-driven predictive modeling helps optimize transportation routes, reducing delays and minimizing post-harvest losses. Smart logistics systems, empowered by AI, enable real-time monitoring of inventory levels and demand fluctuations, facilitating better inventory management and reducing waste.
Additionally, AI enhances the quality and accuracy of decision-making in supply chain management. Machine learning algorithms analyze historical data to predict market trends, allowing for more informed procurement, production, and distribution strategies. This not only minimizes the risk of overstocking or stockouts but also improves the overall responsiveness of the supply chain to changing market conditions [ 31 ] . AI can facilitate traceability and transparency throughout the supply chain. Through technologies like blockchain and data analytics, stakeholders can track the journey of agricultural products from farm to consumer. This not only ensures food safety but also builds trust among consumers by providing verifiable information about the origin, quality, and sustainability of the products [ 35 ] .
IV-C Inclusive Growth
The concept of inclusive growth in the context of agriculture in Africa involves fostering equitable development that benefits all stakeholders across the agricultural value chain [ 36 ] . AI plays a pivotal role in advancing inclusive growth by addressing disparities, promoting sustainability, and empowering marginalized groups. In smallholder farming communities, AI technologies, such as precision farming tools and data-driven decision support systems, empower farmers with valuable insights and resources, narrowing the information gap. This enables smallholders, often marginalized due to limited resources and access to information, to make informed decisions, optimize resource use, and improve yields [ 37 ] .
Inclusivity is further promoted through AI-driven financial services and market access platforms [ 38 ] . These technologies break down barriers for smallholder farmers by providing access to credit, insurance, and market information, thereby integrating them more effectively into the broader agricultural economy. This inclusivity is crucial for ensuring that the benefits of technological advancements are shared by all, contributing to poverty alleviation and economic empowerment. AI can supports gender inclusivity in agriculture by offering tools and technologies that cater to the specific needs of women in farming [ 39 ] . For instance, AI-driven mobile applications provide women farmers with tailored information on crop management, market prices, and financial services, enhancing their participation in decision-making processes [ 40 ] . Integration of AI in agriculture fosters inclusive growth by empowering smallholder farmers, breaking down informational and financial barriers, and ensuring that marginalized groups, including women, actively participate and benefit from the advancements in agricultural technologies. The inclusive adoption of AI holds the potential to create a more sustainable, resilient, and equitable agricultural landscape in Africa.
V Ethical Considerations and Policy Implications
The integration of AI in agriculture in Africa brings forth significant ethical considerations and necessitates thoughtful policy implications to ensure responsible and equitable deployment of these technologies. Ethical concerns include issues related to data privacy, as AI relies heavily on vast datasets, raising questions about how farmer data is collected, stored, and used. Transparent and ethical data governance frameworks are essential to protect farmers’ privacy rights and prevent misuse of sensitive information [ 41 ] . Another critical ethical consideration is the potential for technology-induced job displacement. As AI-driven technologies automate certain tasks, there is a risk of job losses in traditional agricultural roles. Policymakers must address these concerns through proactive measures such as reskilling programs and policies that promote the creation of new, technology-driven job opportunities within the agricultural sector [ 42 ] .
Moreover, fairness and inclusivity must be at the forefront of policy considerations. AI applications should be designed and deployed in ways that avoid reinforcing existing inequalities and ensure that benefits are distributed equitably among diverse communities. This involves crafting policies that prioritize access to AI technologies for smallholder farmers, women, and other marginalized groups, ensuring that they are active participants in and beneficiaries of the technological advancements [ 43 ] . Policy implications also extend to the need for regulatory frameworks that govern the development and use of AI in agriculture. These frameworks should establish standards for AI algorithms, ensuring transparency, accountability, and fairness. Policymakers should collaborate with stakeholders to create guidelines that foster innovation while safeguarding ethical considerations, ultimately promoting responsible and sustainable AI adoption in the agricultural sector. Balancing technological advancement with ethical considerations through well-crafted policies is essential for harnessing the full potential of AI in agriculture in a responsible and inclusive manner. By adhering to the below ethical AI practices, stakeholders in the agricultural sector can foster a responsible and sustainable integration of artificial intelligence, promoting positive outcomes for farmers, communities, and the environment.
V-A Ethical AI Practices
Ethical AI practices in agriculture involve a set of principles and guidelines aimed at ensuring responsible, transparent, and fair deployment of artificial intelligence technologies. These practices are essential for addressing potential ethical concerns and promoting positive outcomes in the agricultural sector.
Transparency and explainability necessitate transparency in the development and use of AI algorithms. Farmers and stakeholders should have clear insights into how AI-driven systems make decisions, fostering trust and accountability. Transparent AI practices enable users to understand the reasoning behind recommendations and predictions, ensuring that decision-making processes are explainable.
Data privacy and security is a fundamental ethical consideration. Ethical AI practices involve implementing robust data protection measures, ensuring that sensitive agricultural data is handled securely. Farmers should have control over their data, understanding how it is collected, stored, and utilized. Policies and technologies that prioritize data security contribute to responsible AI adoption.
Fairness and Bias Mitigation Ethical AI practices aim to eliminate biases in algorithms that may disproportionately impact certain groups or communities. Developers and policymakers should actively work to identify and rectify biases in AI models to ensure fair and equitable outcomes for all farmers, regardless of their background or location.
Inclusivity and Accessibility Ethical AI practices prioritize inclusivity, ensuring that AI technologies are accessible to all farmers, including smallholders and marginalized groups. Policies should focus on narrowing the digital divide and promoting equal access to AI-driven tools and resources. This inclusivity contributes to the democratization of technology benefits.
Human-Centric Design AI systems should be designed with a human-centric approach, considering the needs, values, and preferences of farmers. Ethical AI practices involve involving end-users in the development process, seeking feedback, and adapting technologies to align with the socio-cultural context of the agricultural communities.
Accountability and Responsibility Developers, providers, and users of AI technologies should be held accountable for the impact of their systems. Ethical AI practices involve establishing clear lines of responsibility, ensuring that errors or unintended consequences are addressed promptly. Policies and regulations should articulate accountability mechanisms to prevent misuse and promote responsible innovation.
Continuous Monitoring and Assessment Ethical AI practices require ongoing monitoring and assessment of AI systems throughout their lifecycle. Regular evaluations should be conducted to identify and rectify any ethical concerns that may arise as technology evolves. Continuous improvement ensures that AI technologies align with ethical standards and societal values.
V-B Policy Frameworks
Governments and international organizations need to collaborate in developing robust policy frameworks that support the responsible adoption of AI in agriculture [ 43 ] . Developing effective policy frameworks is crucial for guiding the responsible and equitable deployment of AI in agriculture. These frameworks aim to address ethical considerations, ensure transparency, and promote the positive impact of AI technologies in the agricultural sector. Here are key components of policy frameworks for AI in agriculture:
Ethical Guidelines Policy frameworks should establish clear ethical guidelines that govern the development, deployment, and use of AI technologies. These guidelines should encompass principles such as transparency, fairness, accountability, and inclusivity, ensuring that AI applications align with ethical standards.
Data Governance Policies should address the collection, storage, and use of agricultural data. This includes ensuring data privacy, securing sensitive information, and defining ownership rights. Clear regulations on data sharing and access can help foster collaboration while protecting the rights of farmers.
Transparency and Explainability The framework should require transparency in AI algorithms and decision-making processes. Developers and stakeholders should be obligated to provide clear explanations of how AI systems work, ensuring that users, including farmers, can understand and trust the technology.
Inclusivity and Accessibility Policies should promote inclusivity by addressing the digital divide and ensuring that AI technologies are accessible to all farmers, regardless of their size or location. Incentives and support programs can be included to facilitate the adoption of AI by smallholders and marginalized communities.
Accountability and Liability Policy frameworks must establish mechanisms for accountability and liability in case of unintended consequences or misuse of AI technologies. Clear guidelines on responsibilities for developers, users, and regulatory bodies can help prevent and address ethical breaches.
Stakeholder Engagement Involving all relevant stakeholders, including farmers, in the policy-making process is essential. This ensures that diverse perspectives are considered, and the policies align with the needs and values of the agricultural community.
Capacity Building and Training Policies should include provisions for capacity building and training programs to enhance the understanding and skills of farmers, extension workers, and other stakeholders in using AI technologies. This promotes responsible and effective adoption of AI in agricultural practices.
Monitoring and Evaluation Establishing mechanisms for continuous monitoring and evaluation of AI applications is critical. Regular assessments can identify potential ethical concerns, assess the impact of AI on farmers and the environment, and inform adjustments to the policy framework.
International Collaboration Given the global nature of AI technologies, policy frameworks should encourage international collaboration and information sharing. This ensures consistency in ethical standards, promotes interoperability, and prevents potential conflicts between different regulatory approaches.
Adaptive Approach The policy framework should be adaptive and flexible to accommodate the evolving nature of AI technologies. Regular reviews and updates are necessary to account for technological advancements, changing ethical standards, and the dynamic needs of the agricultural sector.
V-C Community Engagement and Participation
Community engagement and participation are integral aspects of the successful integration of AI in agriculture [ 44 ] . Involving local communities in the development, deployment, and decision-making processes ensures that AI technologies are tailored to meet the specific needs, challenges, and values of the agricultural stakeholders. We present some considerations for community engagement and participation in the context of AI in agriculture:
Needs Assessment : Conducting thorough needs assessments within the community helps identify specific challenges and opportunities. This ensures that AI applications address real and pressing issues faced by farmers, promoting relevance and effectiveness.
Educational Programs : Implementing educational programs is crucial for raising awareness and building the capacity of community members. Workshops, training sessions, and outreach initiatives can enhance the understanding of AI technologies, their benefits, and potential implications.
Consultative Decision-Making : Engaging communities in decision-making processes fosters a sense of ownership and empowerment. Inclusion in discussions about the adoption and use of AI technologies allows community members to express their concerns, preferences, and expectations.
Localization of Solutions : AI solutions should be adapted to local contexts, considering factors such as cultural practices, languages, and specific agricultural needs. Community engagement facilitates the localization of AI technologies, ensuring that they align with the unique characteristics of the farming community.
Partnerships with Local Organizations and community leaders helps build trust and ensures that AI initiatives are integrated into existing community structures. Local partnerships facilitate effective communication and implementation.
User-Friendly Interfaces will enable individuals with varying levels of technological literacy to access the services. This enhances the usability of AI tools among farmers and community members, promoting inclusivity.
Feedback Mechanisms : Establishing feedback mechanisms allows continuous communication between developers, policymakers, and the community. Regular feedback loops enable adjustments to AI applications based on user experiences, preferences, and emerging needs.
Cultural Sensitivity : Recognizing and respecting cultural nuances is critical in community engagement. AI solutions should be culturally sensitive, taking into account local traditions, beliefs, and social structures to ensure acceptance and avoid unintended consequences.
Empowerment Through Data Ownership : Community members should have a clear understanding of data ownership and usage policies. Empowering farmers with control over their data and ensuring transparency in data governance builds trust and mitigates potential ethical concerns.
Long-Term Collaboration : Community engagement is an ongoing process that extends beyond initial implementation. Long-term collaboration ensures that AI applications evolve with the changing needs of the community, fostering sustainability and resilience.
VI Case Studies: AI in Action
This section provides detailed case studies of successful AI implementations in African agriculture, highlighting diverse applications, challenges faced, and lessons learned.
VI-A Case Study 1: Kenya Agricultural Observatory Platform (KAOP)
Objective: To provide weather forecasts and advisories to farmers in Kenya that help them make better decisions with regard to farming.
The Kenya Agricultural Observatory Platform (KAOP) 1 1 1 https://www.kaop.co.ke/index.php is an active digital initiative developed by the Kenya Agricultural & Livestock Research Organization (KALRO) ICT, established on February 4, 2019. The platform focuses on providing actionable weather forecasts, agronomic advisories, and SMS alerts to farmers in Kenya, facilitating informed decision-making throughout the agricultural cycle. The system predicts precipitation and temperature for the next seven days and provides historical data for the last seven and 30 days using reverse geo-location. Agronomic advisories are generated for selected wards in Machakos and Kakamega counties, covering various stages of farming. The platform collaborates with stakeholders such as farmers, the private sector, policymakers, researchers, extension officers, and micro-financing institutions. The achievements include timely advisories on planting, addressing climate change challenges, and providing historical information. Challenges include the need for translation into local languages, and sustainability issues involve improving short-term and seasonal forecasts and mapping all counties in Kenya for more precise information based on ecological zones and value chains.
VI-B Case Study 2: Digital Agricultural Advisory Services (DAAS) in Ethiopia
Objective: Direct-to-farmer mobile-phone-based advisory approach to enhance AI adoption, reduce calf and cow mortality, and promote balanced feed diets..
The project addresses challenges in Ethiopia’s dairy sector, where indigenous breeds exhibit low milk productivity. The implementation includes leveraging Artificial Insemination (AI) to improve cross-breed animals’ share in the national herd. The initiative is led by PxD Ethiopia, collaborating with government stakeholders, research institutions, universities, and development partners. DAAS employs a direct-to-farmer mobile-phone-based advisory approach to enhance AI adoption, reduce calf and cow mortality, and promote balanced feed diets. The content is customized to individual cows’ needs, reproduction cycles, and regional factors. The project also extends its reach to AI technicians, ensuring the dissemination of crucial information on artificial insemination steps. To address gender-related disparities, the project encourages joint listening to advisory calls, recognizing the significant role of women farmers in dairy production.
VII Future Trends and Innovations
This section explores emerging trends and innovations in AI for agriculture in Africa, including advancements in technology, new applications, and potential breakthroughs on the horizon. Anticipating future trends and innovations in the realm of AI in African agriculture involves envisioning transformative developments that can contribute to sustainable, efficient, and inclusive agricultural practices. some of the potential future trends and innovations include:AI-Enabled Climate-Smart Agriculture, Blockchain for Supply Chain Transparency, AI-Driven Crop Breeding and Genetic Improvement, Robotics and Autonomous Farming, Digital Twins for Farm Management, AI in Aquaculture and Fisheries Management, Voice and Text-Based AI Interfaces for Farmers, AI for Natural Resource Management, Collaborative AI Platforms, Ethical AI Guidelines and Policies These potential future trends and innovations in AI for African agriculture reflect the ongoing evolution of technology to address the unique challenges and opportunities in the agricultural sector across the continent. It’s important to note that the successful implementation of these innovations will require collaboration among researchers, policymakers, technology developers, and local communities.
VIII Conclusion
As Africa charts its course towards sustainable agricultural development, the integration of AI emerges as a cornerstone. Addressing challenges, embracing opportunities, and establishing ethical and policy frameworks are imperative to unlock the full potential of AI in transforming agriculture and ensuring food security for future generations. The integration of AI in African agriculture promises transformative outcomes, as illustrated by case studies and future trends. These innovations, ranging from precision agriculture to blockchain-enabled supply chain transparency, have the potential to empower smallholder farmers, enhance productivity, and foster sustainable practices. However, successful implementation necessitates a focus on ethical considerations, transparent AI practices, and inclusive policies to ensure equitable benefits and mitigate potential risks. Looking forward, collaborative efforts, guided by responsible AI guidelines, can shape a more resilient and technology-driven agricultural landscape, contributing to increased productivity, climate resilience, and inclusive development across diverse farming communities in Africa.
- [1] L. Foster, K. Szilagyi, A. Wairegi, C. Oguamanam, and J. de Beer, “Smart farming and artificial intelligence in east africa: Addressing indigeneity, plants, and gender,” Smart Agricultural Technology , vol. 3, p. 100132, 2023.
- [2] M. A. Altieri and C. I. Nicholls, “The adaptation and mitigation potential of traditional agriculture in a changing climate,” Climatic change , vol. 140, pp. 33–45, 2017.
- [3] L. Ye, “From farm to future: Designing a roadmap for robotics in agriculture,” 2023.
- [4] T. A. Shaikh, T. Rasool, and F. R. Lone, “Towards leveraging the role of machine learning and artificial intelligence in precision agriculture and smart farming,” Computers and Electronics in Agriculture , vol. 198, p. 107119, 2022.
- [5] A. K. Sampene, F. O. Agyeman, B. Robert, and J. Wiredu, “Artificial intelligence as a path way to africa’s transformations,” Artificial Intelligence , vol. 9, no. 1, 2022.
- [6] E. O. Arakpogun, Z. Elsahn, R. B. Nyuur, and F. Olan, “Threading the needle of the digital divide in africa: The barriers and mitigations of infrastructure sharing,” Technological Forecasting and Social Change , vol. 161, p. 120263, 2020.
- [7] F. Pedro, M. Subosa, A. Rivas, and P. Valverde, “Artificial intelligence in education: Challenges and opportunities for sustainable development,” 2019.
- [8] Q. Chen, L. Li, C. Chong, and X. Wang, “Ai-enhanced soil management and smart farming,” Soil Use and Management , vol. 38, no. 1, pp. 7–13, 2022.
- [9] R. Singh, S. Srivastava, and R. Mishra, “Ai and iot based monitoring system for increasing the yield in crop production,” in 2020 International Conference on Electrical and Electronics Engineering (ICE3) . IEEE, 2020, pp. 301–305.
- [10] A. Chattopadhyay, E. Nabizadeh, and P. Hassanzadeh, “Analog forecasting of extreme-causing weather patterns using deep learning,” Journal of Advances in Modeling Earth Systems , vol. 12, no. 2, p. e2019MS001958, 2020.
- [11] E. McLennon, B. Dari, G. Jha, D. Sihi, and V. Kankarla, “Regenerative agriculture and integrative permaculture for sustainable and technology driven global food production and security,” Agronomy Journal , vol. 113, no. 6, pp. 4541–4559, 2021.
- [12] K. Jha, A. Doshi, P. Patel, and M. Shah, “A comprehensive review on automation in agriculture using artificial intelligence,” Artificial Intelligence in Agriculture , vol. 2, pp. 1–12, 2019.
- [13] T. Duckett, S. Pearson, S. Blackmore, B. Grieve, W.-H. Chen, G. Cielniak, J. Cleaversmith, J. Dai, S. Davis, C. Fox et al. , “Agricultural robotics: the future of robotic agriculture,” arXiv preprint arXiv:1806.06762 , 2018.
- [14] K. E. Giller, T. Delaune, J. V. Silva, K. Descheemaeker, G. van de Ven, A. G. Schut, M. van Wijk, J. Hammond, Z. Hochman, G. Taulya et al. , “The future of farming: Who will produce our food?” Food Security , vol. 13, no. 5, pp. 1073–1099, 2021.
- [15] H. Gosnell, N. Gill, and M. Voyer, “Transformational adaptation on the farm: Processes of change and persistence in transitions to ‘climate-smart’regenerative agriculture,” Global Environmental Change , vol. 59, p. 101965, 2019.
- [16] A. Kowalska and H. Ashraf, “Advances in deep learning algorithms for agricultural monitoring and management,” Applied Research in Artificial Intelligence and Cloud Computing , vol. 6, no. 1, pp. 68–88, 2023.
- [17] G. Obi Reddy, B. Dwivedi, and G. Ravindra Chary, “Applications of geospatial and big data technologies in smart farming,” in Smart Agriculture for Developing Nations: Status, Perspectives and Challenges . Springer, 2023, pp. 15–31.
- [18] A. Wongchai, D. rao Jenjeti, A. I. Priyadarsini, N. Deb, A. Bhardwaj, and P. Tomar, “Farm monitoring and disease prediction by classification based on deep learning architectures in sustainable agriculture,” Ecological Modelling , vol. 474, p. 110167, 2022.
- [19] H. Mishra and D. Mishra, “Artificial intelligence and machine learning in agriculture: Transforming farming systems,” Res. Trends Agric. Sci , vol. 1, pp. 1–16, 2023.
- [20] V. Balaska, Z. Adamidou, Z. Vryzas, and A. Gasteratos, “Sustainable crop protection via robotics and artificial intelligence solutions,” Machines , vol. 11, no. 8, p. 774, 2023.
- [21] R. Finger, S. M. Swinton, N. El Benni, and A. Walter, “Precision farming at the nexus of agricultural production and the environment,” Annual Review of Resource Economics , vol. 11, pp. 313–335, 2019.
- [22] M. J. Usigbe, S. Asem-Hiablie, D. D. Uyeh, O. Iyiola, T. Park, and R. Mallipeddi, “Enhancing resilience in agricultural production systems with ai-based technologies,” Environment, Development and Sustainability , pp. 1–29, 2023.
- [23] S. Vishnoi and R. K. Goel, “Climate smart agriculture for sustainable productivity and healthy landscapes,” Environmental Science & Policy , vol. 151, p. 103600, 2024.
- [24] A. Roy, S. Purkaystha, and S. Bhattacharyya, “Advancement in molecular and fast breeding programs for climate-resilient agriculture practices,” Harsh Environment and Plant Resilience: Molecular and Functional Aspects , pp. 73–98, 2021.
- [25] J. Cowls, A. Tsamados, M. Taddeo, and L. Floridi, “The ai gambit: leveraging artificial intelligence to combat climate change—opportunities, challenges, and recommendations,” Ai & Society , pp. 1–25, 2021.
- [26] H. F. Williamson, J. Brettschneider, M. Caccamo, R. P. Davey, C. Goble, P. J. Kersey, S. May, R. J. Morris, R. Ostler, T. Pridmore et al. , “Data management challenges for artificial intelligence in plant and agricultural research,” F1000Research , vol. 10, 2021.
- [27] C. Makate, “Effective scaling of climate smart agriculture innovations in african smallholder agriculture: A review of approaches, policy and institutional strategy needs,” Environmental science & policy , vol. 96, pp. 37–51, 2019.
- [28] E. O. Arakpogun, Z. Elsahn, F. Olan, and F. Elsahn, “Artificial intelligence in africa: Challenges and opportunities,” The fourth industrial revolution: Implementation of artificial intelligence for growing business success , pp. 375–388, 2021.
- [29] C. Ganeshkumar, S. K. Jena, A. Sivakumar, and T. Nambirajan, “Artificial intelligence in agricultural value chain: review and future directions,” Journal of Agribusiness in Developing and Emerging Economies , vol. 13, no. 3, pp. 379–398, 2023.
- [30] N. Gumbi, L. Gumbi, and H. Twinomurinzi, “Towards sustainable digital agriculture for smallholder farmers: A systematic literature review,” Sustainability , vol. 15, no. 16, p. 12530, 2023.
- [31] C. Vuppalapati, Machine Learning and Artificial Intelligence for Agricultural Economics: Prognostic Data Analytics to Serve Small Scale Farmers Worldwide . Springer Nature, 2021, vol. 314.
- [32] N. N. Thilakarathne, M. S. A. Bakar, P. E. Abas, and H. Yassin, “A cloud enabled crop recommendation platform for machine learning-driven precision farming,” Sensors , vol. 22, no. 16, p. 6299, 2022.
- [33] M. Lezoche, J. E. Hernandez, M. d. M. E. A. Díaz, H. Panetto, and J. Kacprzyk, “Agri-food 4.0: A survey of the supply chains and technologies for the future agriculture,” Computers in industry , vol. 117, p. 103187, 2020.
- [34] J.-W. Han, M. Zuo, W.-Y. Zhu, J.-H. Zuo, E.-L. Lü, and X.-T. Yang, “A comprehensive review of cold chain logistics for fresh agricultural products: Current status, challenges, and future trends,” Trends in Food Science & Technology , vol. 109, pp. 536–551, 2021.
- [35] K. Demestichas, N. Peppes, T. Alexakis, and E. Adamopoulou, “Blockchain in agriculture traceability systems: A review,” Applied Sciences , vol. 10, no. 12, p. 4113, 2020.
- [36] A. Devaux, M. Torero, J. Donovan, and D. Horton, “Agricultural innovation and inclusive value-chain development: a review,” Journal of Agribusiness in Developing and Emerging Economies , vol. 8, no. 1, pp. 99–123, 2018.
- [37] H. J. Smidt and O. Jokonya, “Factors affecting digital technology adoption by small-scale farmers in agriculture value chains (avcs) in south africa,” Information Technology for Development , vol. 28, no. 3, pp. 558–584, 2022.
- [38] M.-L. How, S.-M. Cheah, A. C. Khor, and Y. J. Chan, “Artificial intelligence-enhanced predictive insights for advancing financial inclusion: A human-centric ai-thinking approach,” Big Data and Cognitive Computing , vol. 4, no. 2, p. 8, 2020.
- [39] P. P. Grabowski, I. Djenontin, L. Zulu, J. Kamoto, J. Kampanje-Phiri, A. Darkwah, I. Egyir, and G. Fischer, “Gender-and youth-sensitive data collection tools to support decision making for inclusive sustainable agricultural intensification,” International Journal of Agricultural Sustainability , vol. 19, no. 5-6, pp. 359–375, 2021.
- [40] P. Varangis, J. Buchenau, T. Ono, R. Sberro-Kessler, and A. Okumura, “Women in agriculture using digital financial services: Lessons learned from technical assistance support to digifarm, fenix, and myagro,” 2021.
- [41] R. Dara, S. M. Hazrati Fard, and J. Kaur, “Recommendations for ethical and responsible use of artificial intelligence in digital agriculture,” Frontiers in Artificial Intelligence , vol. 5, p. 884192, 2022.
- [42] A. S. George and A. H. George, “Revolutionizing manufacturing: Exploring the promises and challenges of industry 5.0.” Partners Universal International Innovation Journal , vol. 1, no. 2, pp. 22–38, 2023.
- [43] A. Lauterbach, “Artificial intelligence and policy: quo vadis?” Digital Policy, Regulation and Governance , vol. 21, no. 3, pp. 238–263, 2019.
- [44] S. Camaréna, “Engaging with artificial intelligence (ai) with a bottom-up approach for the purpose of sustainability: Victorian farmers market association, melbourne australia,” Sustainability , vol. 13, no. 16, p. 9314, 2021.
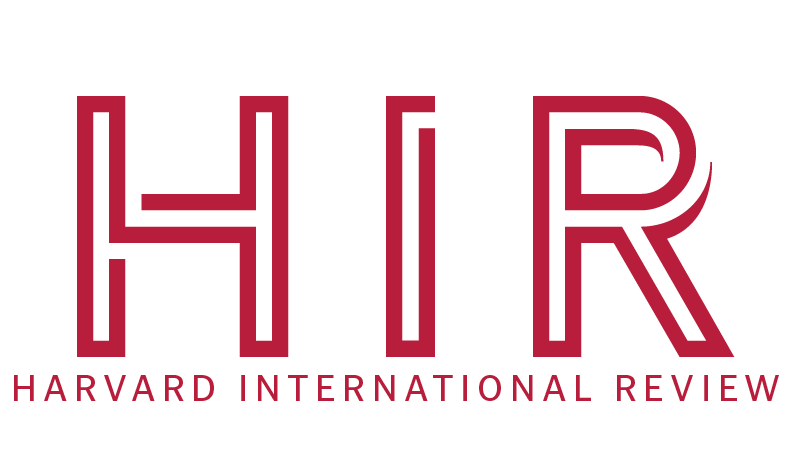
The Future of Farming: Artificial Intelligence and Agriculture
While artificial intelligence (AI) seemed until recently to be science fiction, countless corporations across the globe are now researching ways to implement this technology in everyday life. AI works by processing large quantities of data, interpreting patterns in that data, and then translating these interpretations into actions that resemble those of a human being. Scientists have used it to develop self-driving cars and chess-playing computers, but the technology has expanded into another domain: agriculture. AI has the potential to spur more efficient methods of farming in order to combat global warming, but only with expanded regulation of its development.
Global Warming and Agriculture: A Vicious Cycle
Global warming continues to threaten every aspect of our everyday lives, including crop production. It will reduce the soil moisture in areas close to the equator while leaving northern countries virtually unscathed, according to a study from Wageningen University. We are already seeing the impact of these modified growing conditions on our food production in the form of lower crop yields .
Reduced food production has an especially devastating impact on developing countries. Climate change causes the loss of 35 trillion consumable food calories per year and harms poorer countries who do not have the money to import food. The result is growing food insecurity. And rising sea levels only compound the problem. By the year 2100, sea levels are expected to rise by one meter, which will have a detrimental impact on growers on the coasts whose crops cannot survive in areas where the water is too salty.
However, agriculture is not just a victim of global warming, but also a cause. Agriculture is part of a vicious cycle in which farming leads to global warming, which in turn devastates agricultural production. The process of clearing land for agriculture results in widespread deforestation and contributes to 40 percent of global methane production. Therefore, to confront climate change, it is necessary to ensure reforestation—but how? What is the path to efficient, environmentally-conscious farming?
The Benefits of AI for Environmentally-Conscious Agriculture
This is where AI enters the scene. Farmers use AI for methods such as precision agriculture ; they can monitor crop moisture, soil composition, and temperature in growing areas, enabling farmers to increase their yields by learning how to take care of their crops and determine the ideal amount of water or fertilizer to use.
Furthermore, this technology may have the capacity to reduce deforestation by allowing humans to grow food in urban areas. One Israeli tech company used AI algorithms that create optimal light and water conditions to grow crops in a container small enough to be stored inside a home. The technology could be especially beneficial for countries in Latin America and the Caribbean, where much of the population lives in cities. Furthermore, the ability to grow food in pre-existing urban areas suggests that humans could become less dependent on deforestation for food production.
Additionally, AI can help locate and therefore protect carbon sinks , forest areas that absorb carbon dioxide from the atmosphere. Otherwise, continued efforts to clear these forests will release more carbon dioxide into the atmosphere. Furthermore, some AI is being developed that can find and target weeds in a field with the appropriate amount of herbicide, eliminating the need for farmers to spread chemicals across entire fields and pollute the surrounding ecosystem. Some countries are already implementing AI into their agricultural methods. Some farmers in Argentina are already using digital agriculture; there are already AI farms in China .
AI can also be used to curb global warming outside of agriculture. The technology can be used to monitor how efficiently buildings are using energy and monitor urban heat islands. Urban heat islands are first created when urban building materials like concrete and asphalt absorb heat, causing cities to grow hotter than the rest of their surroundings. People then rely more heavily on air conditioning throughout the day in order to stay cool, and the energy used for these services results in greater greenhouse gas emissions. Providing information about the location of these islands could help politicians determine what policies they should adopt to reduce emissions and encourage more efficient and environmentally-conscious city planning.
The Risks of AI
Nonetheless, AI is far from a silver bullet—it could actually contribute to global warming. Due to the large amount of data that AI needs to process, training a single AI releases five times the emissions that an average car would emit during its lifetime, thereby adding to the already substantial environmental impact of computing technology. Data storage and processing centers that deliver digital services like entertainment and cloud computing are already responsible for two percent of global greenhouse gas emissions, a number comparable to the overall percentage of pollution contributed by the aviation industry. Although this statistic may not seem overwhelming, it does suggest that the environmental costs of AI will need to be reduced before expanding the technology on a global scale. Some researchers are already working on developing a standard metric that researchers can use to compare how efficient their particular AI systems might be, ultimately encouraging innovators to create environmentally-friendly data-processing.
Further, securing access to AI on a global scale may pose some challenges. Countries will both need experts in the field who can successfully use the technology and internet connection, neither of which are always readily available. Therefore, in order for developing countries to take advantage of the benefits of AI and improve their food security, there will need to be a focus on developing the infrastructure necessary for internet access and teaching professionals how to use the technology. Additionally, AI can be expensive . Farmers might go into debt and will not be able to maintain the technology on their own as it suffers everyday wear-and-tear. Those unable to secure access to the technology will lose out to larger farms that can implement AI on a wide scale.
But farm owners themselves will not be the only ones faced with new pressures as a result of AI. New technologies will render many agricultural jobs obsolete as machines are able to accomplish the same tasks as humans. For example, China has created a seven-year pilot program that uses robots instead of humans to run farms. This program does not bode well for the future of jobs in agriculture: many of China’s 250 million farmers could lose their jobs due to increased automation.
Some may argue that the rise of automated jobs is not as threatening as it may seem, especially given the US agricultural labor shortage . However, the situation is not necessarily the same in other countries. Many countries in the Global South remain dependent on the agricultural sector because there are few job opportunities in urban areas. But if farmers can produce more food at a faster rate with machines, they will have an incentive to shift away from hiring humans, placing the livelihoods of many families at risk. Even if farmworkers do not lose their jobs, their wages could decline as they appear less efficient compared to their robot competitors. The result is chronic poverty and inequality.
Looking Forward: The Next Steps for AI in Agriculture
Given these concerns, AI cannot be the only response to climate change. These types of adaptive technologies can mitigate the consequences of climate change, but more sweeping measures are necessary to secure global access to food in the face of rising temperatures. If countries are to develop AI for use in agricultural sectors, global leaders must consider the potential costs, the role of legal institutions, and the environmental consequences of data processing before investing in the technology on a broader scale.
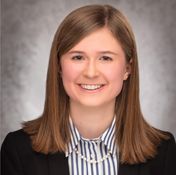
Sydney Young
Sydney is the former Director of Interviews and Perspectives at the HIR. She is interested in health, human rights, and social justice issues.
Recent Posts
In comparative perspective: interview with cofr president emeritus richard haass.
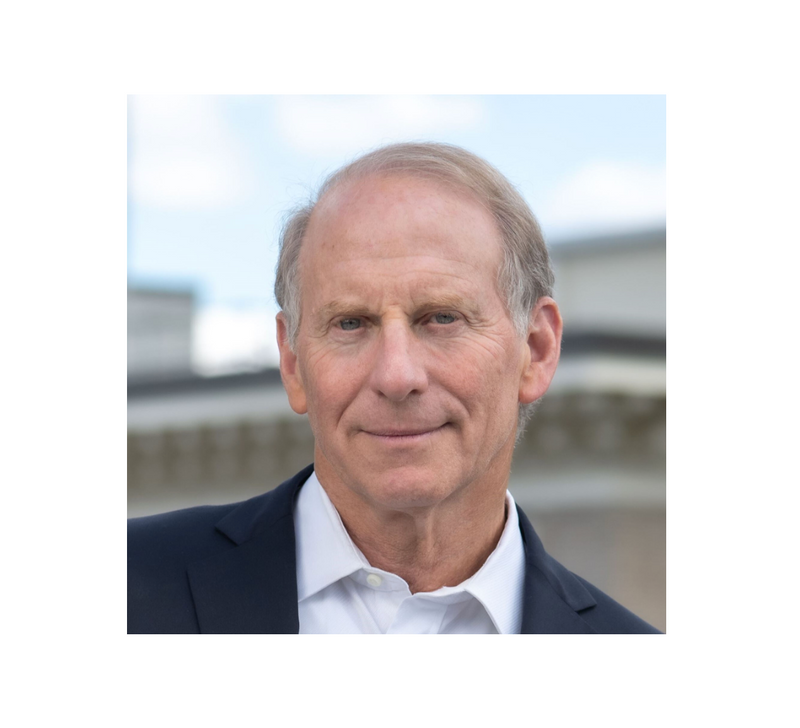
Bloodied Blossoms: Can Afghanistan Grow Past Its Opioid Industry?
The Consequences of a Burning Breadbasket
Botswana: The Pragmatic Path to Prosperity
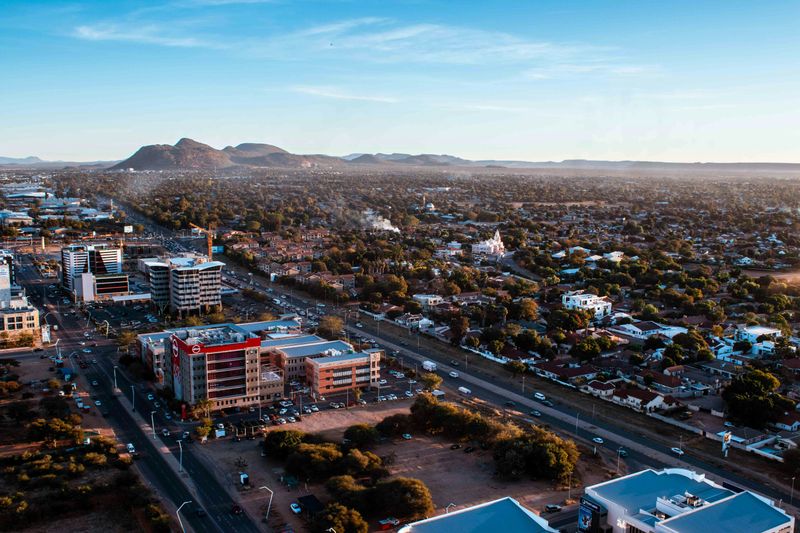
The United States of Europe and Liberalism in the 21st Century: Interview with Beate Meinl-Reisinger, chairwoman of Austria’s NEOS party
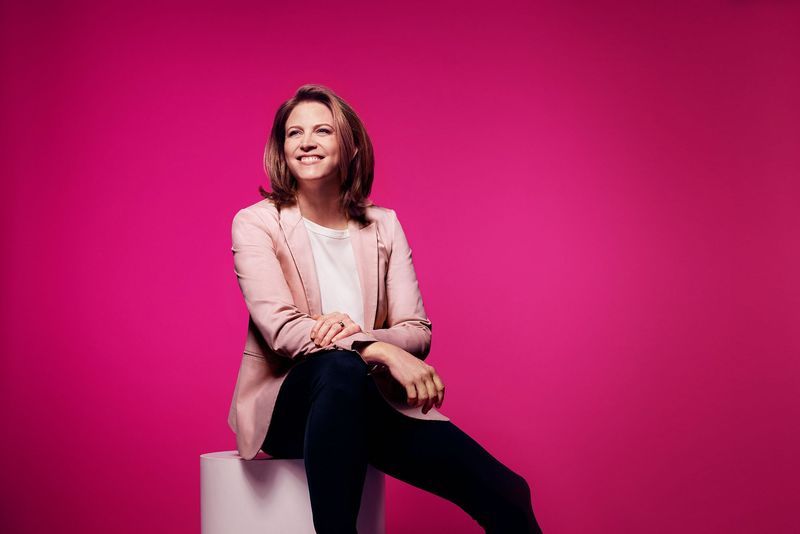
You Might Be Interested In
The artificial intelligence revolution in an unprepared world: china, the international stage, and the future of ai, cardano, crypto, and the developing world: interview with charles hoskinson, part 1, evolving technology, confidence-building measures, and autonomous weapons: interview with paul scharre.
To read this content please select one of the options below:
Please note you do not have access to teaching notes, artificial intelligence and new business models in agriculture: the “zero” case study.
Management Decision
ISSN : 0025-1747
Article publication date: 29 September 2023
This study aims to investigate the use of Artificial Intelligence (AI) applied to vertical farms to evaluate whether disrupting technology supports sustainability and increases strategic business model choices in the agricultural sector. The study responds through empirical analysis to the gap on the subject of AI-driven business models present in the growing sector literature.
Design/methodology/approach
The paper analyzes the case of “ZERO”, a company linked to the strategy innovation ecosystem of the Ca’ Foscari University of Venice, Italy. The empirical data were collected through a semi-structured questionnaire, interviews and the analysis of public news on the business model available in the analyzed case study. The research is empirical and uses exploratory, descriptive analysis to interpret the findings. The article focuses on the evaluation of AI impact on the agricultural sector and its potential to create new business models.
The study identified how AI can support the decision-making process leading to an increase in productivity, efficiency, product quality and cost reduction. AI helps increase these parameters through a continuous learning process and local production, and the possible decrease in prices directed toward the goal of zero km food with fresh products. AI is a winning technology to support the key elements of the vertical farm business model. However, it must be coupled with other devices, such as robots, sensors and drones, to collect enough data to enable continuous learning and improvement.
Research limitations/implications
The research supports new research trends in AI applied to agriculture. The major implication is the construction of ecosystems between farms, technology providers, policymakers, universities, research centers and local consumer communities.
Practical implications
The ZERO case study underlines the potential of AI as a destructive technology that, especially in vertical farms, eliminates external conditions by increasing productivity, reducing costs and responding to production needs with adequate consumption of raw materials, boosting both environmental and social sustainability.
Originality/value
The study is original, as the current literature presents few empirical case studies on AI-supporting business models in agriculture. The study also favors valuable strategic implications for the policies to be adopted in favor of new business models in agriculture.
- Business model
- Vertical farm
- Agriculture
- Decision-making
- Strategy innovation Srl
Cavazza, A. , Dal Mas, F. , Campra, M. and Brescia, V. (2023), "Artificial intelligence and new business models in agriculture: the “ZERO” case study", Management Decision , Vol. ahead-of-print No. ahead-of-print. https://doi.org/10.1108/MD-06-2023-0980
Emerald Publishing Limited
Copyright © 2023, Emerald Publishing Limited
Related articles
We’re listening — tell us what you think, something didn’t work….
Report bugs here
All feedback is valuable
Please share your general feedback
Join us on our journey
Platform update page.
Visit emeraldpublishing.com/platformupdate to discover the latest news and updates
Questions & More Information
Answers to the most commonly asked questions here

- Personal Finance
- Today's Paper
- Partner Content
- Entertainment
- Social Viral
- Pro Kabaddi League
Uttar Pradesh govt plans to promote agri startups, introduce AI in farming
The yogi adityanath government is also planning to host a global farmers' summit 'krishi bharat' in november 2024 in association with the confederation of indian industry.
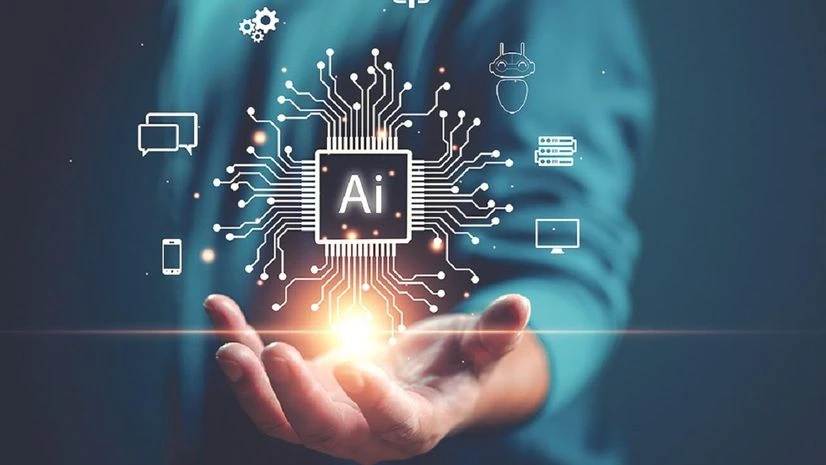)
Listen to This Article
Cii youth wing to hold national summit in chennai on december 21, 22, interim budget balances need for equitable growth, says cii president, budget 2024: a glance budget 2023 announcements for agriculture sector, msmes need to be future ready, foster development, says cii president, 67% of indian healthcare companies form esg boards: ey-cii report, every rupee invested in agri research yields rs 13.85, says study, madhya pradesh farmers struggle to make the most of soaring wheat prices, govt lifts ban on onion exports; imposes min export price of $550 per tonne, centre imposes 40% duty on export of onions with effect from may 4, sugarcane payments in uttar pradesh top rs 30,000 crore for 2023-24.
Don't miss the most important news and views of the day. Get them on our Telegram channel
First Published: May 10 2024 | 4:55 PM IST
Explore News
- Suzlon Energy Share Price Adani Enterprises Share Price Adani Power Share Price IRFC Share Price Tata Motors Share Price Tata Steel Share Price Yes Bank Share Price Infosys Share Price SBI Share Price Tata Power Share Price
- Latest News Company News Market News India News Politics News Cricket News Personal Finance Technology News World News Industry News Education News Opinion Shows Economy News Lifestyle News Health News
- Today's Paper About Us T&C Privacy Policy Cookie Policy Disclaimer Investor Communication GST registration number List Compliance Contact Us Advertise with Us Sitemap Subscribe Careers BS Apps
- Budget 2024 Lok Sabha Election 2024 IPL 2024 Pro Kabaddi League IPL Points Table 2024

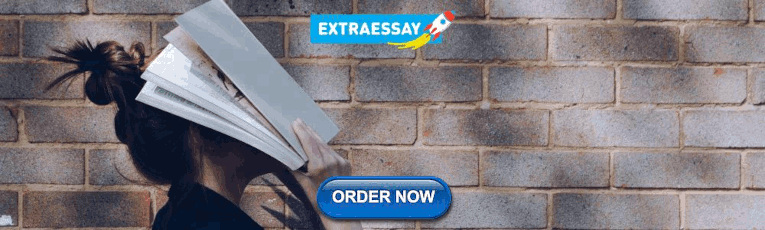
IMAGES
VIDEO
COMMENTS
The world's population has reached 8 billion and is projected to reach 9.7 billion by 2050, increasing the demand for food production. Artificial intelligence (AI) technologies that optimize resources and increase productivity are vital in an environment that has tensions in the supply chain and increasingly frequent weather events. This study performed a systemic review of the literature ...
The current article proposes the use of computer vision technologies and artificial intelligence in the agricultural sector based on the globally used AI technologies reported in the literature. Importantly, this review provides a detailed understanding of promising applications in agriculture. ... A case study of the Wadi Ouahrane basin in ...
agriculture sectors in the context of business model, SWO T, and PESTEL analysis. of blue river technology company. The study results found that main role of arti-. ficial intelligence in ...
2 The Artificial Intelligence for Agriculture Innovation (AI4AI) initiative 2.1 Context and approach 2.2 Vision and objectives 2.3 Structure and process ... linkages were the most favoured use case with the investor community. IoT- and AI-enabled warehousing and logistics systems, along
AI-driven crop monitoring in precision agriculture: A case study from the United States. Journal of Precision Agriculture, 15(2), 123-136. Harnessing the potential of AI in agriculture: A review ...
For this study research, we have observed different types of review of the literature all are related to artificial intelligence in agriculture [].Majorly the literature review collected from secondary sources like journals, research paper, etc., it has been covered use, challenges, and operation of AI in agriculture industry.
Global agriculture is poised to benefit from the rapid advance and diffusion of artificial intelligence (AI) technologies. AI in agriculture could improve crop management and agricultural ...
With the development of Artificial Intelligence (AI) models, there is a growing need to use them to achieve sustainable agriculture. The continuous enhancement of AI in agriculture, researchers have proposed many models in agriculture functions such as prediction,weed control, resource management, advance care of crops, and so on.
Integrating Artificial Intelligence (AI) in agriculture holds great promise for optimizing resource utilization, improving crop yields, and promoting sustainable practices. ... and Sustainable resource management by proposing a novel ETS framework for the responsible adoption of AI in agriculture and a case study of its application to achieve ...
The agricultural sector will face enormous challenges to feed a world population which, according to the FAO-UN, should reach 9.6 billion people by 2050, technological progress has worked considerably in the development of agricultural greenhouses [].They are becoming very sophisticated (accessories and accompanying technical equipment, control computer).
The world's population has reached 8 billion and is projected to reach 9.7 billion by 2050, increasing the demand for food production. Artificial intelligence (AI) technologies that optimize ...
Artificial Intelligence in agriculture has brought an agriculture revolution. This technology has protected the crop yield from various factors like the climate changes, population growth, employment issues and the food security problems. ... According to a study by researchers of the Indian Council for Agricultural Research, the country India ...
Africa's rich agricultural heritage is at the forefront of a technological revolution, with artificial intelligence (AI) poised to address critical challenges in the sector [].The integration of AI technologies holds great promise for improving efficiency, increasing yields, and fostering sustainable agricultural practices.
The Benefits of AI for Environmentally-Conscious Agriculture. This is where AI enters the scene. Farmers use AI for methods such as precision agriculture; they can monitor crop moisture, soil composition, and temperature in growing areas, enabling farmers to increase their yields by learning how to take care of their crops and determine the ...
The search key "Artificial intelligence AND Agriculture AND Business model" in the title, abstract or keywords, conducted on September, 13th 2022, led to 73 total contributions . ... Artificial Intelligence in Agriculture Sector: Case Study of Blue River Technology: 2021: Lecture Notes in Networks and Systems: Panpatte and Ganeshkumar (2021 ...
This study aims to investigate the use of Artificial Intelligence (AI) applied to vertical farms to evaluate whether disrupting technology supports sustainability and increases strategic business model choices in the agricultural sector. The study responds through empirical analysis to the gap on the subject of AI-driven business models present ...
DOI: 10.1007/978-981-15-9689-6_17 Corpus ID: 234339740; Artificial Intelligence in Agriculture Sector: Case Study of Blue River Technology @article{Panpatte2021ArtificialII, title={Artificial Intelligence in Agriculture Sector: Case Study of Blue River Technology}, author={Suraj Panpatte and C. Ganeshkumar}, journal={Lecture Notes in Networks and Systems}, year={2021}, url={https://api ...
1. Artificial Intelligence in Agriculture. Jiali Zha. 1 Moses Brown School, Providence, 02906, United States. Abstract. The application of AI in agriculture has been widely considered as one of ...
Artificial Intelligence and Internet of Things are contributing significantly in the area of modern agriculture by controlling and automating farming activities. Data generated by various sensors are of paramount importance and require to be managed and analysed using machine learning and deep learning based approaches to foresee upcoming ...
detected in these data sets. Artificial intelligence algorithms, and the models derived from them, are used as support systems for better decision making or, in some cases, are implemented in automatic control processes and robotics, to alleviate drudgery. In this study, sensing and data collection in different agri-food sectors are
Applications of artificial intelligence in agriculture. The AI in agriculture market is expected to grow from USD 1.7 billion in 2023 to USD 4.7 billion by 2028, according to MarketsandMarkets. ... In the case of big data, the data itself is not particularly useful. What matters is how it's processed and implemented. Big data for informed ...
Abstract — Artificial intelligence (AI) has advanced rapidly and is becoming a cornerstone. technology that drives innovation and efficiency in various industries. This paper examines. the real ...
The Uttar Pradesh government has partnered with the agriculture industry to bolster agri startups and introduce artificial intelligence (AI) in farming to boost rural economy and 'smart farming' practices. The Yogi Adityanath government is also planning to host a global farmers' summit ...
1. Introduction. Agriculture, as explained by (Da Silveira et al., 2021), involves growing crops, raising animals, and making products for people to eat.It ensures foods, helps rural areas grow, and reduces poverty. In today's world, advanced technologies like artificial intelligence and robotics are improving agriculture, making it more efficient and eco-friendlier.