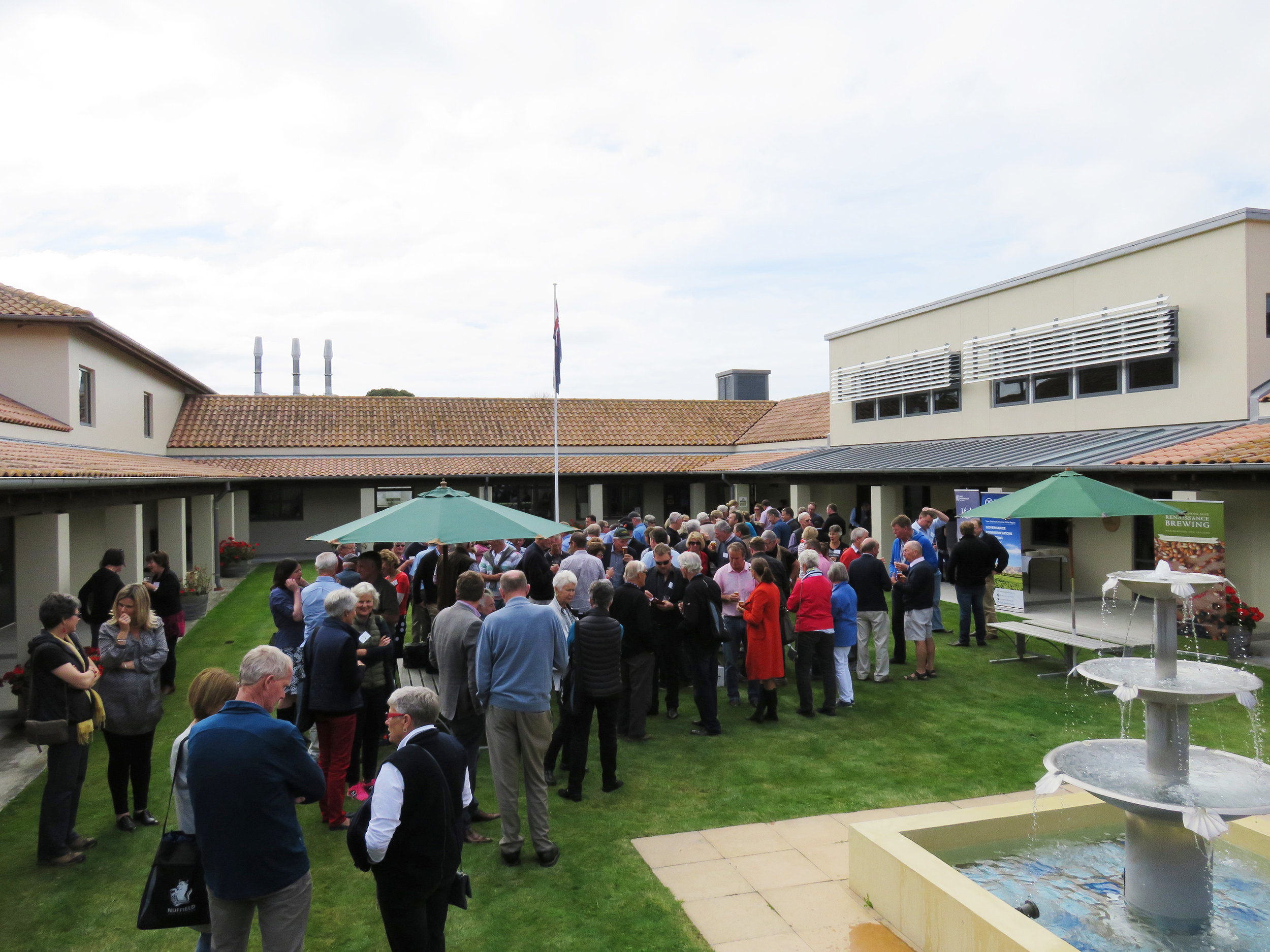
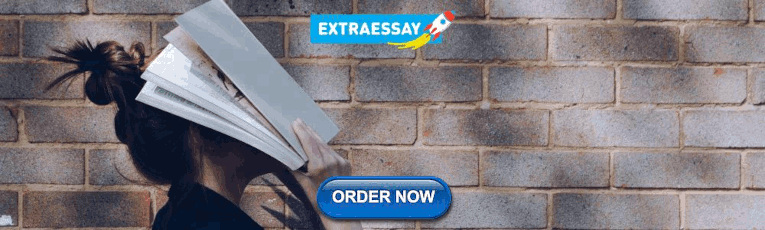
MRC was established in 1984 to support the need for locally based scientific research to grow the regional economy.
The Marlborough Research Centre (MRC) is the legal entity. The Trust owns and manages MRC properties in Blenheim and Grovetown and MRC works closely with the Marlborough District Council, Crown Research Institutes and other key sector governmental groups and organisations. The main office for MRC is located at 85 Budge Street .
MRC’s main campus is at 85 Budge Street, Blenheim. The Budge Street campus has offices, laboratories, teaching winery and a lecture theatre. This campus is shared with Plant & Food Research, NZ Wine, Wine Marlborough and NZ Dryland Forests Initiative.
MRC’s Grovetown Park campus is 2km away, on State Highway 1. This is a commercial campus with tenants ranging from the Ministry for Primary Industries, Hill Laboratories and commercial businesses. The adjacent 10-hectare Rowley Vineyard at Grovetown is the site for research trials and a sauvignon blanc vineyard.
Marlborough Research Centre
Marlborough District Council is a funding partner of the Marlborough Research Centre (MRC), a non-profit research organisation operating from two campuses located in Blenheim.
MRC's principal objective is to facilitate industry research, particularly in primary and secondary sectors that are of strategic importance to the region. The Centre has links with Nelson Marlborough Institute of technology (NMIT), Massey University, FoodHQ and key primary sector organisations such as NZ Winegrowers and Plant & Food Research.
MRC operates a Food & Beverage Innovation Cluster, which provides funding, specialist support and market insights to members.
Gerald Hope - Chief Executive Marlborough Research Centre Email Gerald Hope
Mandy Mitchell - Executive Administrator Marlborough Research Centre Email Mandy Mitchell
Postal Address
Marlborough Research Centre 85 Budge Street Blenheim 7201 New Zealand Phone: +64 3 577 2370 View the Marlborough Research Centre website
Other useful websites
- Food HQ New Zealand
- Massey University School of Food & Advanced Technology
- Nelson Marlborough Institute of Technology
- New Zealand Winegrowers / Wine Research Centre
- Plant & Food Research
- Wine Marlborough
Share this page
- Search Menu
- Volume 6, Issue 1, 2024 (In Progress)
- Volume 5, Issue 2, 2023
- Advance articles
- Special Issues
- Author Guidelines
- Open Access
- Submission Site
- Self-Archiving Policy
- Submit to our special issues
- Why Publish?
- About in silico Plants
- About Annals of Botany Company
- Editorial Board
- Advertising and Corporate Services
- Journals on Oxford Academic
- Books on Oxford Academic
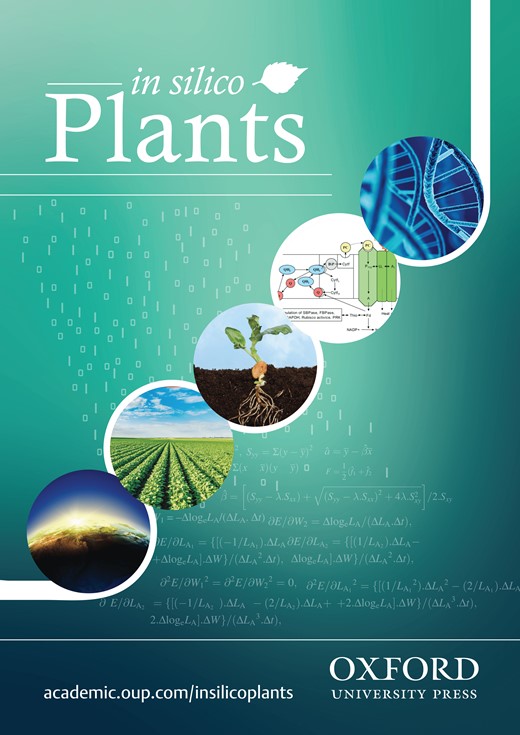
Article Contents
1. introduction, 2. materials and methods, 4. discussion, 5. conclusions and future prospects, supporting information, acknowledgements, sources of funding, conflict of interest, contributions by the authors, data availability, literature cited.
- < Previous
Developing perennial fruit crop models in APSIM Next Generation using grapevine as an example

- Article contents
- Figures & tables
- Supplementary Data
Junqi Zhu, Amber Parker, Fang Gou, Rob Agnew, Linlin Yang, Marc Greven, Victoria Raw, Sue Neal, Damian Martin, Michael C T Trought, Neil Huth, Hamish Edward Brown, Developing perennial fruit crop models in APSIM Next Generation using grapevine as an example, in silico Plants , Volume 3, Issue 2, 2021, diab021, https://doi.org/10.1093/insilicoplants/diab021
- Permissions Icon Permissions
A new model for grapevines ( Vitis vinifera ) is the first perennial fruit crop model using the Agricultural Production System sIMulator (APSIM) Next Generation framework. Modules for phenology, light interception, carbohydrate allocation, yield formation and berry composition were adapted or added into APSIM Next Generation to represent the nature of fruit-bearing vines. The simulated grapevine phenological cycle starts with the dormancy phase triggered by a critical photoperiod in autumn, and then goes through the subsequent phenophases sequentially and finally returns to dormancy for a new cycle. The canopy microclimate module within APSIM Next Generation was extended to allow for row crop light interception. The carbohydrate arbitrator was enhanced to consider both sink strength and sink priority to reflect carbohydrate reserve as a concurrent competing sink. Weather conditions and source–sink ratio at critical developmental stages were used to determine potential grapevine yield components, e.g. bunch number, berry number and berry fresh weight. The model was calibrated and tested extensively using four detailed data sets. The model captured the variations in the timing of measured budburst, flowering and véraison over 15 seasons across New Zealand for five different varieties. The calculated seasonal dynamics of light interception by the row and alley were consistent with field observations. The model also reproduced the dynamics of dry matter and carbohydrate reserve of different organs, and the wide variation in yield components caused by seasonal weather conditions and pruning regimes. The modelling framework developed in this work can also be used for other perennial fruit crops.
Perennial crops represent a long-term investment by any landowner. There are fewer opportunities to change location, genotype and plant configuration to adapt to climate compared with annual crops. As such, reliable models for evaluating options at establishment and for ongoing management would be valuable aids for decision-making. However, in contrast with annual crops where a large amount of modelling work has been undertaken on assessing the yield and adaptation options under climate change ( Schlenker and Roberts 2009 ; Challinor et al. 2014 ), less work has been undertaken on the perennial crops ( Santos et al. 2011 ; Bock et al. 2013 ). The research on perennial crop models lags behind the annual crop models largely owing to the physiological complexity that regulate yield formation and the difficulties and high cost of data collection.
Grapevine ( Vitis vinifera ) is one of the most economically important perennial fruit crops worldwide, used both as table grapes and for wine production ( OIV 2020 ). It has played an important cultural role in many parts of the world. Because of its global economic importance and being found in diverse growing climates, the grapevine has emerged as a model perennial fruit crop species. There have been a large number of scientific studies from genomics to production practices carried out on grapevines over the years. The grapevine reaches reproductive maturity in 4–5 years after planting, and may remain economically productive for 20–60 years depending on management.
The key attributes required of a grapevine model include: (i) A reproductive cycle from inflorescence initiation to harvest, lasting about 15–18 months. Grapevines initiate inflorescence primordia in the axial buds of each shoot in the summer of the year prior to which they flower. (ii) The buds undergo a dormancy phase in winter and this breaks in spring. A period of intense vegetative growth follows after budburst. Initial vegetative growth is supported by the internal carbohydrate reserves until the vine achieves a net carbohydrate gain around flowering. (iii) Vegetative and reproductive growth occur concurrently. The reproductive stage occurs during the flowering process of the inflorescences. Some growers perform a top pruning around this time to reduce the carbohydrate competition between vegetative and reproductive growth. (iv) The number of berries that set and continue to grow is determined shortly after flowering. Fruit clusters gradually become the primary sink for photosynthates during development.
Several functional–structural plant (FSP) models with explicit descriptions of grapevine canopy architecture have been developed. These often focus on certain functional processes such as vegetative growth ( Louarn et al. 2008b ; Schmidt et al. 2019 ), light interception ( Louarn et al. 2008a ), canopy photosynthesis and transpiration ( Prieto et al. 2012 ; Zhu et al. 2018 ), plant water status ( Zhu et al. 2018 ; Albasha et al. 2019 ) and the effects of whole-plant carbohydrate and water status on berry water and sugar uptake ( Zhu et al. 2019 ). Those models allow us to better understand the functioning of a grapevine plant, and often the important processes highlighted by FSP models can be summarized by functional relationships for including in other models, e.g. farming system models. A farming system can be defined as an arrangement of land, crops, livestock, other capital goods and labour put together for the primary purpose of producing plant and animal products for consumption ( Woodward et al. 2008 ). Farming systems models contain interconnected biophysical and management modules which are able to simulate agricultural systems comprising soil, crop, tree, pasture and livestock, and have the flexibility to integrate non-biological farm resources such as availability of water or farm machinery ( Holzworth et al. 2014 ). Farming systems models are essential tools for investigating the effects of climate and management on crop and livestock growth, productivity and the system’s water and nitrogen balances ( Holzworth et al. 2018 ; Keating 2020 ). Thus, compared to FSP models and crop models which only focus on crop growth and development, farming system models have advantages in assessing the behaviour at farm level, such as large-scale water and nutrient balances with the consideration of spatial variability of farming types, soil and climate conditions.
A number of farming or cropping system grapevine models have been developed ( Moriondo et al. 2015 ), namely VineLogic ( Godwin et al. 2002 ), STICS grapevine model ( Brisson et al. 2003 , 2009 ), Cropsyst ( Stöckle et al. 2003 ), Gutierrez et al. (1985) , Williams et al. (1985) , VIMO ( Wermelinger et al. 1991 ), Bindi et al. (1997) , NVINE ( Nendel and Kersebaum 2004 ), SWAP ( Ben-Asher et al. 2006 ), Vitisim ( Lakso et al. 2008 ), WALIS ( Celette et al. 2010 ). The STICS grapevine model has the most functionalities among all the listed models. It can simulate the light interception by the grapevine rows based on geometry description, water balance of the whole-vineyard and the grapevine yield ( Valdés-Gómez et al. 2009 ; Fraga et al. 2016 ). However, it has some limitations as well. For instance, it does not capture seasonal variations of yield, or consider the availability of carbohydrate on leaf area and root expansion (see details in the Discussion section). Furthermore, a crop model is generally built to address certain objectives of a study ( Van Ittersum et al. 2003 ) and therefore would need to be adapted for other applications. Such an approach may be difficult in existing grapevine farming system models.
The Agricultural Production System sIMulator Next Generation (APSIM Next Generation) ( Holzworth et al. 2018 ) platform has advantages over other modelling platforms, allowing scientists to construct and configure a crop model visually, by dragging and dropping components in the user interface (UI) ( Brown et al. 2014 ). It combines a range of tools in the UI to assist model developers to build, run, test, evaluate and auto-document the model ( Fig. 1 ; see Supporting Information—Fig. S1 ). The APSIM Next Generation plant modelling framework provides a collection of generic software classes, e.g. plant organs and functions that can be assembled and parameterized in different ways to create different plant models ( Brown et al. 2018 ). It means that dynamic composition of lower-level processes and organ classes (e.g. photosynthesis, leaf) into larger constructions, e.g. wheat model, can be achieved by the model developer without additional coding. Graphs and statistics for assessing model performance can also be constructed in the UI. However, no formal perennial fruit crop model has been developed in APSIM Next Generation using the plant modelling framework.

Illustration of the APSIM Next Generation UI. This illustrates the basic structure of the grapevine model (left panel), the configuration of the leaf and berry module (middle panel) and the configuration of structure and trunk module (right panel). Configurations can be easily adapted based on the needs of the users. More screenshots of the UI relating to the basic simulation set-up for a vineyard, list of model simulations and validations, sensibility to water stress and a simulation example of bringing sheep into the vineyard are in Supporting Information—Fig. S3 .
The aim of this study was to: (i) develop a perennial fruit crop model in APSIM Next Generation using grapevine as a model plant; (ii) improve the carbohydrate allocation scheme for perennial crops; (iii) improve the light interception calculation for row-planted trees/vines; (iv) improve the representation of phenology, leaf cohort and perennial organ set-ups in APSIM Next Generation in general. The APSIM grapevine model developed in this study can be used to explore the long-term dynamics of phenology, canopy development, and yield and carbohydrate reserves under different pruning systems at different locations, and later be used to assess the environmental footprint of the viticultural practices, e.g. water and nutrient footprint, and the suitability of integrated grapevine–livestock farming systems.
2.1 Model description
A series of developments were undertaken to adapt the APSIM Next Generation source code to enable simulation of perennial fruit crops. The purpose of the model presented here was to simulate the phenology, canopy development, light interception, carbohydrate assimilate and allocation, carbohydrate storage and remobilization, water uptake, organ growth and yield formation in a row-planted vineyard ( Fig. 2 ; see Supporting Information—Method S1 APSIM Next Generation grapevine model documentation). The basic principle of the model is that phenology is largely driven by temperature and growth is dynamically driven by both environmental factors and differential allocation of carbohydrate among organs. It includes the following novel features:
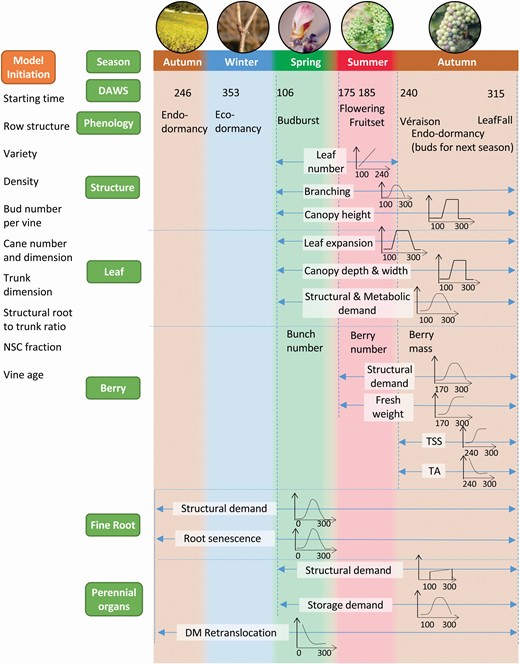
Illustration of the main processes happening in different modules according to the phenology timeline. The model follows the traditional calculation loop: phenology, potential organ growth, light interception, carbohydrate assimilation and allocation, and actual organ growth in a row-planted vineyard. The basic principle of the model is that phenology is largely driven by temperature and growth is dynamically driven by both environmental factors and differential allocation of carbohydrates among organs. Users first need to initialize the vineyard and vine condition in the tree initiation method (organ box) before running any simulation. Green boxes on the left list the main modules in the model. The main processes in each module are listed, with arrows indicating the start and end time of the process and a drawing indicating the dynamics of the process. DAWS represents days after winter solstice and the values indicated are the mean DAWS for each phenological event for Sauvignon blanc in Marlborough, New Zealand. DM represents dry matter and NSC represents non-structural carbohydrate. Perennial organs include cane, trunk and structural root.
A new carbohydrate allocation method to distribute carbohydrate to structural and non-structural organ components simultaneously based on sink strength and priority
A yield module that uses the weather conditions in both the previous and current season as well as the carbohydrate status to determine potential bunch number per shoot, berry number per bunch and berry weight
A row and alley strip configuration to represent a typical vineyard set-up with rows of grape vines growing in a strip of bare ground, interspersed with alleys of short grass [see Supporting Information—Fig. S2 ] . Roots from each strip are present beneath their neighbouring strip and compete for water and nutrients
An adapted row light interception method based on that of Goudriaan (1977) to partition light interception between the row canopy and the alley
Flexibility in simulating different cane- or spur-pruned training systems by controlling the cane or cordon number, bud number per vine, dynamics of canopy height, canopy width and depth in the UI ( Fig. 2 ). New training systems can be added in the UI through management scripts.
An adapted phenology method that defines the start of endo-dormancy by critical photoperiod and then goes through each phenology phase progressively. Budburst was used for triggering canopy growth instead of crop emergence as in annual crops ( Fig. 1 )
A flexible parameter optimization procedure that calls APSIM Next Generation through R bash command and optimizes model parameters through using an R package.
2.1.1 Phenology module.
The phenology module starts with dormancy phase of the buds. This is followed by budding, flowering, fruit setting, berry development and leaf death phases ( Fig. 1 ). The dormancy phase include two stages: endo-dormancy and eco-dormancy. Endo-dormancy is defined as the period when buds are dormant because of physiological conditions, and eco-dormancy is defined when buds remain dormant because of unfavourable environmental conditions ( Sarvaš 1974 ). The budburst stage in the budding phase occurs when eco-dormancy is broken. For consistency in calculating the phenology stage in both the Southern and Northern Hemispheres, we use days after winter solstice (DAWS) as the standard time in the model.
We assume the endo-dormancy stage of the bud starts in autumn when photoperiod drops below a critical value ( Camargo-Alvarez et al. 2020 ). The critical value depends on grape variety and was set to 13.1 h in the current model based on the findings of Camargo-Alvarez et al. (2020) . Three budburst approaches were tested using a phenology data set: (i) forcing-only approach, which only describes the release of eco-dormancy; (ii) chilling–forcing, which describes the release of endo- and eco-dormancy in sequence; (iii) chilling–forcing–overlap, which describes the release of endo- and eco-dormancy in sequence plus the effect of chilling units on forcing accumulation through implementation of an exponential decrease function. The chilling–forcing approach was selected in the current grapevine model because it enabled the best predictions of the timing of budburst. In this approach, endo-dormancy is completed when the chilling requirement is satisfied, and eco-dormancy is completed when sufficient heat units are accumulated to force budburst ( Chuine 2000 ). This approach has two major assumptions: (i) Chilling must be fulfilled before budburst. However, it has been shown that buds with insufficient chilling can still burst, although this often results in a delayed, desynchronized and low percentage of budburst ( Dokoozlian 1999 ). (ii) Temperature is the only driver for budburst while all other factors are not limiting budburst, e.g. carbohydrate reserves and water supply. Bonada et al. (2020) showed that severe water deficit in winter and spring in Australia can delay the budburst. Thus, the budburst functions may need to be adjusted if other factors were limiting budburst based on their local production conditions. A negative sigmoid function was selected for simulating the responses of chilling units ( R c , Eq. (1)) and a growing degree approach was used to calculate forcing heat unit ( R f , Eq. (2)).
where T a is the hourly air temperature and T b is the base temperature above which forcing heat unit will be accumulated. R c ( T a ) and R f ( T a ) are calculated at hourly intervals, and then summed and averaged daily to give the daily chill and heat unit accumulation. In APSIM Next Generation, hourly temperature is interpolated based on the method described in Goudriaan and Van Laar (1994) . Values for c , d and T b were fitted for each variety.
The flowering, fruit setting and berry development phases were simulated using a physiological development day (D s ) target which is the number of days the phase would take to complete at optimal temperatures. The daily increment of development days ( d D) is calculated using the Wang–Engle temperature response function (Eqs (3) and (4)), which returns a value of 1 when temperatures are optimal and a value between 0 and 1 at sub- and supra-optimal temperatures ( Wang and Engel 1998 ). Development days were used for all other temperature-dependent processes, e.g. leaf appearance and expansion.
where T a is air temperature, T min is the minimum temperature, T opt is the optimum temperature and T max is the maximum temperature. d D is calculated at hourly intervals, using interpolated hourly T a data, and these values are averaged to give d D. The Wang-Engel function in APSIM Next Generation included one additional variable T ref (reference temperature) and returned the ratio of d D( T a ) and d D( T ref ) as the final results. When T ref equals to T opt , it has the same result as the original function in Wang and Engel (1998) . Parameters were optimized for achieving the best prediction for flowering and véraison simultaneously.
After the summer solstice, the photoperiod decreases. When photoperiod decreases to a critical value, the bud for the following season’s growth enters into endo-dormancy. However, the whole-plant phenology cannot be set to endo-dormancy at this time because the current year’s shoots and berries are still growing. Although it would be tidy to develop two different phenological clocks, one for the bud and one for the plant in growth, we decided to work around it by resetting the plant phenology phase into endo-dormancy at the winter pruning event, which was defined in the management script with user input time. The chilling accumulation between the critical photoperiod and the pruning event was captured by a function and then added to the total chill accumulation in the endo-dormancy phase.
2.1.2 Structure module.
The structure module keeps track of the structure of the canopy, e.g. bud number per vine, main-stem leaf appearance, branching and canopy height ( Fig. 1 ). Main-stem leaf appearance is modelled with the appearance of a new leaf every time d D accumulation exceeds the current phyllochron (the D s interval between two successive leaves). Leaf appearance occurs between budburst and véraison with a phyllochron of 1.64 for the first five leaves to appear, increasing to 1.82 for the 10th leaf onwards ( Greer and Weston 2010 ).
Branching rate was calculated as the product of three components: potential branching rate, the effect of leaf area per vine and the effect of retained bud number per metre row. Parameters were optimized based on the retained node number experiment (see detailed description of the experiment in Data sets used for model calibration and validation).
2.1.3 Row-planted crop light interception method.
The row-planted crop light interception method developed by Goudriaan (1977) and Pronk et al. (2003) , and later widely used for intercrop studies ( Zhang et al. 2008 ; Gou et al. 2017 ) was implemented in APSIM Next Generation. The light interception method assumes that on a daily basis, the light intensity from all incoming directions is the same ( Goudriaan 1977 ). It can be considered as a uniform overcast sky where only diffuse radiation is presented. This assumption enables easy spatial integration over all directions without the need to consider time of day. Furthermore, it represents the row canopy as a cube and assumes leaves are randomly distributed within the cube ( Pronk et al. 2003 ). Based on those assumptions, the light interception by the row canopy can be expressed as a function of canopy width, canopy depth, the distance between two rows, leaf area of the row crops and light extinction coefficient [see Supporting Information—Fig. S2 ] . This light interception method is used when the field is configured by two rectangular zones. Row orientation is not accounted in the light interception calculation. If the canopy of a training system cannot be assumed to be a cube (e.g. tatura training system), new light interception methods can be added in the APSIM Next Generation source code ( https://github.com/APSIMInitiative/-ApsimX/ ), see details in 4.1 Canopy development and radiation interception.
Several modifications were made in the calculation compared with the method described in Gou et al. (2017) in order to capture the vineyard/orchard canopy features. For instance, for a vertical-shoot-positioned (VSP) grapevine, all the leaves were accumulated in the top part of the canopy with a bare trunk at the bottom. The original model assumed that the base of all canopies was at ground level. We removed this constraint by extending equations to allow it to start from any height.
Canopy depth and canopy width were used to calculate the light interception by the grapevine row instead of using canopy height and row width [see Supporting Information—Fig. S2 ] . Agricultural Production System sIMulator Next Generation distinguished canopy height from canopy depth. Canopy height was defined as the distance from the top of the canopy to the ground, while canopy depth was defined as the distance from the top of the canopy to the bottom of the canopy excluding the trunk part where there is no leaf. Canopy width was estimated by the width used in the summer trimming practice and can be viewed as the width where 95 % of leaves were covered.
2.1.4 Carbohydrate allocation method.
All organ classes in APSIM Next Generation use the same biomass subclass to keep track of their live and dead biomass status. The biomass subclass currently deals with dry matter (DM) and nitrogen (N) content of organs. Each of these components of biomass were separated into three types of pools represented by the properties ( see Supporting Information—Method S1 3.12 Arbitrator and Fig. 2 ):
StructuralDM and N are essential for the growth of the organ. They remain within the organ once they have been allocated, and pass from Live to Dead pools as the organ senesces.
MetabolicDM and N are essential for crop growth and their concentrations can influence the function of organs, e.g. MetabolicN content is a main factor to determine leaf photosynthetic efficiency. MetabolicDM and MetabolicN may be reallocated (moved to another organ upon senescence of this organ) or translocated (moved to other organs at any time when supplies do not meet their structural and metabolic DM demands).
Non-structural DM and N are non-essential to the function of an organ and they may be reallocated or translocated.
The DM allocation arbitrator steps through a series of calculations to determine the supply of biomass available for allocation each day. Biomass supply includes reallocations from senescing organs, photosynthesis fixation and remobilization of non-structural reserves, and they are used in sequence (see detailed description in Brown et al. (2019) ).
The existing allocation methods in APSIM Next Generation allocate DM to non-structural reserves only when all other carbohydrate demands are satisfied. This is not true for perennial crops, where trunk and root reserves are important competing sinks all the time. Therefore, a new allocation method, Q-Priority-then-Relative-Allocation, was developed based upon the existing Priority-then-Relative-Allocation method, where priority used only maintenance demand in APSIM Next Generation ( Brown et al. 2019 ). The Q-Priority-then-Relative-Allocation method is based on the common carbohydrate pool assumption that the ability of developing plant organs to attract carbohydrates is independent of the topological position of the organs. However, the priority for different DM demand varies. The use of the priority parameter was adapted from the mathematical kiwifruit model ( Buwalda 1991 ) and the functional–structural kiwifruit model ( Cieslak et al. 2011 ). In the Q-Priority-then-Relative-Allocation method, DM was allocated in two steps. In the first step, an allocation was calculated based on the relative DM demand and priority parameter ( q ) of each biomass type ( c ) for each organ ( o , Eq. (5)):
where P r o p [ o ] c is the proportion of DM allocated to organ o and biomass type c , and q is the corresponding priority parameter.
If there is still biomass unallocated and demand unsatisfied, a second step is carried out where the remaining biomass is allocated based on the relative demand without the priority to ensure every organ has a chance to fulfil their full demand. Thus, the priority factor limits growth only when there is a carbohydrate deficit.
Growth respiration coefficient was set as 0.2 gDM gDM −1 for all organs except berry, which had a much smaller growth respiration (0.02 gDM gDM −1 ) ( Dai et al. 2009 ). Maintenance respiration for different components was set as in Zhu et al. (2019) . The exponential response of maintenance respiration to temperature was set according to the Arrhenius equation ( Poni et al. 2006 ). The temperature sensitivity coefficients Q 10 [-] were 1.9 for stem, 1.77 for berry and 1.66 for leaf ( Poni et al. 2006 ). Q 10 was defined as the increase in the respiration rate resulting from a temperature increase of 10 °C.
2.1.5 Leaf module.
The grapevine model uses the phytomer-based Leaf organ class that simulates the expansion and senescence of cohorts of leaves at each position on the primary shoot and estimates the number of leaves in each cohort based on branching rates. Cohort leaves are treated the same as main-stem leaves appearing at the same time.
The maximum area of each cohort is currently set as a function of leaf rank. The growth duration, lag duration, senescence duration and minimum and maximum specific leaf area (SLA [m 2 g −1 ]) were parameterized based on our field experiment (see Section 2.2.4). For correctly modelling leaf senescence and the dynamics of leaf area, a photoperiod acceleration effect was added. The photoperiod acceleration effect also reflected the effects of the time of harvest on leaf senescence, as we observed that leaves would stay green much longer if the fruit were not harvested ( see Supporting Information—Method S1 3.5 Leaf module). Other leaf properties were parameterized to ensure no nitrogen on leaf expansion in the current grapevine model, as the nitrogen dynamic parts require further calibration.
The potential total DM demand of each leaf was calculated as the product of the daily leaf area increase (constrained by stress) and mean specific leaf area. The total DM demand was then divided into structural and metabolic demands based on the fraction of structural DM in total leaf weight. The priority factor q for leaf structural and metabolic demand was set to a high value (1.6) as it is the immediate source of photosynthates and has the highest priority early in the season ( Buwalda 1991 ; Lakso et al. 2008 ). Non-structural reserves were not considered in the current leaf model. At leaf senescence, metabolic leaf DM was reallocated to other organs (DMReallocationFactor = 1).
The dynamics of seasonal canopy width and canopy depth were defined in the leaf class based on our field observation. A canopy pruning event was simulated each summer, which removed 20 % of leaf and shoot biomass. The pruning time and intensity can be configured easily in the management script for each simulation. For current simulations in the test set, the same pruning dates and intensities were used, as we do not have the exact pruning information for each site and year.
2.1.6 Cane, trunk and structural root module.
Cane, trunk and structural root models were all represented by the Generic Organ class, which has properties of biomass status and daily biomass demand and supply ( Fig. 2 ; see Supporting Information—Method S1 3.7 to 3.9). The structural DM demand of Cane and Trunk (Eq. (6)) were calculated by their radius ( r [m]) derived from their DM and length, daily growth rate of the radius ( dr/dt [m day −1 ]), length ( l [m]) and wood density ( ρ [g m −3 ]) ( Cieslak et al. 2011 ):
where i is organ type, q str, i is the priority factor for structural DM demand for a certain organ, determined as 0.7 for all three organs (see Materials and Methods section). Daily growth rate of the radius was calculated based on the trunk circumference increment in 14 years measured on 800 field-grown irrigated Sauvignon blanc vines.
The structural carbohydrate demand of the structural root was calculated by the structural demand of the trunk times the structural root/trunk ratio (set to one in this model). The structural root/trunk ratio may vary between vineyards and training systems and further work is required to capture this in the model. The non-structural carbohydrate (NSC) DM demand was modelled as an active competing sink ( Cieslak et al. 2011 ), and the parameters were kept the same for those three organs. NSC included total soluble carbohydrates and starch. The rate of non-structural DM synthesis depended on organ size and limited by overloading (Eq. (7)):
The equation limited carbohydrate storage owing to non-structural DM overloading and the organ storage capacity, which was proportional to the current organ structural biomass, C max,NSC is the maximum non-structural DM per unit of structural DM, set to 0.26 based on field trunk and root samples of Sauvignon blanc ( Greven et al. 2016 ). q NSC was set to 0.61 throughout the growing season. k syn is the daily non-structural DM synthesis rate per unit of structural biomass, which is represented by a beta growth function in Eq. (8) (Yin et al. 2003) for capturing the fast recovery of carbohydrate reserves after flowering ( Greven et al. 2016 ; Seleznyova et al. 2018 ). Parameters were optimized based on the measured dynamics of NSC in trunk and root by Greven et al. (2016) (see Materials and Methods section):
where k m a x is the maximum non-structural DM synthesis rate, 0.03 [g g −1 ]. t e is the number of development days from budburst to the day when k max is reached, 54 D s (around véraison). t m is the time when maximum increase rate occurs, 34.4 D s . The daily accrual of t is calculated as for phenological development after budburst.
For potential carbohydrate retranslocation, 3.7 % of the total carbohydrate storage in each organ per day (DM retranslocation factor) was set available to be retranslocated. Actual retranslocation depends on the daily carbohydrate supply and demand differences. Carbohydrate supplies from organ senescence and photosynthesis will be used first before using carbohydrate storage.
The biomass of retained cane for the following season was reset by the winter pruning events based on input cane diameter in the UI for the cane-pruned vines. The biomass of trunk and structural root keeps growing each year. In addition, retained cane can be parameterized as cordon and keep growing each year as well.
2.1.7 Annual shoot module.
Shoot was represented by a Generic Organ class as well. The structural biomass demand of the shoot was calculated as the daily delta of a beta growth function, which was fitted to individual shoot weight as a function of development days, and then multiplied by the shoot population per square metre and priority factor for shoot demand (Eq. (9); see Supporting Information—Method S1 3.5). Shoot population per square metre was calculated internally based on shoot number per vine and vine density.
where StructuralDM s( t ) [g m −2 ] is the structural DM of all shoots at development day t from budburst. Here shoot refers to the first year shoot stem and does not include leaves. SP is the shoot population per square metre. StructuralDM max [g] is the fitted maximum structural DM per shoot under four-cane pruned vines. t e is the time when StructuralDM max is reached (79.2 D s ), t m is the time when maximum growth rate occurs (43 D s ). Note the shoot DM includes both the primary and secondary shoots. The priority factor for shoot structural DM demand was set to a very low value (4e-3) to capture the reduction of shoot biomass under high shoot density per metre caused by both smaller primary shoots and fewer lateral shoots ( Greven et al. 2014 ). Parameters for shoot non-structural DM demand, e.g. k syn and priority factors were set as half the values for Cane.
2.1.8 Root module.
The root module was parameterized to represent the fine roots of the plant, which are responsible for the uptake of water and nitrogen ( see Supporting Information—Method S1 3.11). The root module calculates root growth in terms of rooting depth, biomass accumulation and subsequent root length density in each soil layer. A proportion of the fine roots can be allocated to different zones as well, e.g. inter-row.
Only structural DM demand was considered for the fine root, which was estimated by current root DM and relative root growth rate (gDM gDM −1 day −1 ). The daily loss of roots was calculated using a SenescenceRate function. All senesced material was automatically detached and added to the soil fresh organic matter. Fine roots often represents less than 20 % of the total root biomass ( Buwalda 1993 ). Based on the data of Lakso et al. (2008) , we set the initial fine root DM to be 60 g per vine (converted into g m −2 in the model based on population density). The annual fluctuation of the fine root DM was captured by dynamics of the relative growth rate and senescence rate. The dynamics of relative growth rate and senescence rate throughout the growing season were added based on the data presented in Comas et al. (2005) . The priority factor for root DM demand was set to the same as for leaf DM demand. The perennial structural roots were separated from the root module because they have different functions and the separation makes the parameterization easier, e.g. root length growth.
Root length growth was calculated using the daily DM partitioned to fine roots and specific root length (SRL [m g −1 ]). Root proliferation in layers was calculated using an approach similar to the generalized equimarginal criterion used in economics. The uptake of water and N per unit root length was used to partition new root material into layers of higher ‘return on investment’.
2.1.9 Berry module.
The berry module was represented by a reproductive organ class. Final yield per vine was calculated as the product of berry number per vine and berry fresh weight. Both berry fresh weight and dry weight were calculated dynamically ( see Supporting Information—Method S1 3.10).
The berry number per vine was calculated by shoots per vine (as a function of retained node number), bunches per shoot and berries per bunch. Strong seasonal variations could be found in bunch number, berry number and berry fresh weight especially in cool-climate viticulture regions. The seasonal variation was captured by including the effects of weather conditions at critical periods around flowering of the previous and current season (see details in Zhu et al. (2020) ). Parameters were readjusted in the current model as the calculation of development days was updated.
The methods for determining bunch number per vine in Zhu et al. (2020) were further generalized into the product of bunch number per shoot and shoot number per vine to account for different numbers of retained nodes per vine. Mean inflorescence number per node counted on 80 nodes per plot during the flowering assessment in the regional phenology and yield monitoring data set (see Section 2.1) were used for model calibration. Mean daily maximum temperature (TmaxIni) and daily radiation during the inflorescence initiation were used for predicting mean bunch number per shoot for the following season. TmaxIni and mean daily maximum (TmaxFlow) and minimum temperature around the flowering period of the current season were used to predict berry number per bunch. TmaxFlow, mean daily radiation intensity and cumulated rainfall around flowering, and cumulated rainfall after flowering and before véraison were chosen to predict the potential berry fresh weight. Furthermore, in the current model, carbohydrate effects represented by total carbohydrate supply and demand were added in the calculation of bunch number, berry number and potential berry fresh weight. The yield estimation method assumes that the potential yield components can be estimated based on the weather and carbohydrate conditions at certain critical periods, e.g. inflorescence initiation, while other periods have no effect. It has been shown that weather and carbohydrate conditions pre-budburst have strong effects on flower number ( Eltom et al. 2017 ), and potentially on bunch number as well. However, our data set is insufficient to quantify such responses.
The dynamic of berry fresh weight was calculated based on the development days from flowering using the beta growth function (Eq. (8)). Potential berry fresh weight ( k max in Eq. (8)) was adjusted at véraison based on weather conditions and source/sink ratios in each season.
Total berry potential DM demand was determined based on berry number and the mean DM demand of individual berries described using the beta growth function (Eq. (8)). Parameters were determined based on Section 2.1. The priority factor for berry DM demand was set to one.
Total soluble solids (TSS in °Brix) after véraison was calculated based on the ratio of berry dry weight to fresh weight through an empirical relationship between water content and TSS derived by Garcia de Cortazar-Atauri et al. (2009) . Total titratable acid concentration (TA in g L −1 tartaric acid equivalents) was simulated through a negative exponential equation (Eq. (10)):
where TA max is the TA observed at véraison, 30.3 g L −1 in our data set, TA min is the fitted minimum TA, 7.8 g L −1 . t is the cumulated development days after véraison and r is the TA degradation rate with t , 0.0923 D s −1 .
2.2 Data sets used for model calibration and validation
2.2.1 data set 1: regional phenology and yield monitoring..
This data set was used for calibrating parameters related to phenology, bunch number, berry weight, berry TSS and berry TA. Starting in 2004, phenology data (budburst, flowering and fruit development), yield per vine, bunch number, berry weight and berry TSS and TA were collected from Sauvignon blanc vines in four commercial vineyards in Marlborough ( Table 1 , detailed descriptions of the experiment and yield calibration procedure in Zhu et al. (2020) ). The trial was expanded to four other regions and varieties in 2014: Gisborne (two Chardonnay vineyards), Hawke’s Bay (two Chardonnay, two Merlot, one Sauvignon blanc, one Pinot gris), Marlborough (two Chardonnay, two Pinot gris, two Pinot noir, eight Sauvignon blanc), Waipara Valley North Canterbury (one Pinot noir, one Sauvignon blanc) and Central Otago (four Pinot noir). In 2014 the regions represented by the data set accounted for 93 % of the New Zealand national vineyard area, and the five varieties in the data set represented also accounted for 93 % of the national varietal mix. The phenology of Sauvignon blanc was calibrated with the four Marlborough long-term sites, namely Upper Brancott, Western Wairau, Seaview Awatere and Central Rapaura, and validated with the remaining sites. For other varieties, all data were used for calibration.
List of data set used in model calibration and validation.
a The regional phenology and yield data set were used for calibration and validation of the phenology and yield module.
b The retained node number and post-harvest defoliation data sets were used for calibration and validation of the yield estimation, leaf area, carbon allocation between different components, and the dynamics of organ biomass and NSC.
c The MRL_Rapaura_Irrigation data set was used for calibrating the fraction of radiation interception, the water use and water uptake competition between vine row and alley. It was also used for validation of leaf area, carbon allocation between different components and the dynamics of organ biomass.
Four 32-berry samples were collected weekly immediately prior to véraison and this continued until harvest, to determine the berry weight and TSS for each site and cultivar combination. TA was measured only for Marlborough Sauvignon blanc. A threshold soluble solids concentration of 8 °Brix was used as an alternate measure of the midpoint (50 %) of véraison ( Parker et al. 2014 ). Yield, TSS, and TA prediction were calibrated only for the four long-term Sauvignon blanc sites.
2.2.2 Data set 2: retained node number trial.
The effects of retained node number per vine on leaf appearance, leaf area and biomass allocation were calibrated with the data from the retained node number trial ( Greven et al. 2014 ; Greven et al. 2015 ). Three-year-old Sauvignon blanc grapevines (clone UCD1MS on Schwarzmann rootstock, Vitis riparia × V. rupestris ) growing on a well-drained silt-loam soil (Wairau series) at the Rowley Crescent vineyard [41.49S; 173.95E] were selected for the trial in July 2006. Vines were planted with 2.8 ×1.8 m row-by-vine spacing. The vines, which had been previously pruned to four canes of 12 nodes (48 nodes), were cane-pruned to five different numbers of nodes per vine: 24, 36, 48, 60 or 72 by retaining either two, three, four, five or six 12-node canes per vine, respectively. The node treatment was repeated on the same vines each winter during pruning time for four consecutive years.
Early-season primary shoot vigour was assessed by taking detailed pre-flowering measurements of shoot length, shoot diameter (at third internode) and leaf number. Furthermore, shoot lengths were continuously monitored by a timelapse outdoor PlantCam (Wingscapes WSCA04) and manually measured from the photograph based on a reference ruler in the images. Leaf area per vine was measured by point quadrat after fruit set and by whole-vine destructive sampling at harvest. A random 30-berry sample was collected weekly from 50 % véraison and this sampling continued until harvest to determine the berry weight and TSS from a four-vine bay (plot). At harvest, all bays were hand harvested, with yield and total bunch number per plot (bay) recorded. Final berry weight was determined from a randomly collected 100-berry sample. Shoot number, shoot and cane fresh weight were assessed after harvest in winter.
Each season, during late winter, samples were taken from the trunk of one grapevine in each research plot for the assessment of overwinter carbohydrates reserves. A small 5-mm trunk corer was used to extract a piece of trunk wood that was quickly returned to the laboratory and freeze dried. Once freeze dried, samples were ground into powders and kept at −20 °C until analyses were performed.
2.2.3 Data set 3: post-harvest defoliation trial.
Starting in October 2008, a trial for studying the effects of post-harvest defoliation on carbohydrate reserves was conducted in the neighbouring rows of the retained node number trial. Two pruning treatments were compared: 48 nodes and 72 nodes ( Greven et al. 2016 ). Eight carbohydrates measurements were taken throughout the season, starting at five-leaf stage, then at bloom, lag phase, véraison, mid-ripening, harvest, leaf fall and dormancy. These measurements were conducted for four years. Samples from both trunk and root were collected. Root samples were taken from a mixture of old and young roots varying from 1 to 5 mm in diameter at a depth of ~150 mm. The results of this trial were grouped with the data from the retained node number trial for simplifying the simulation set-up.
2.2.4 Data set 4: MRL_Rapaura_Irrigation.
The canopy development, dynamics of leaf and shoot biomass were validated by data from a regulated deficit irrigation trial at Central Rapaura Marlborough (noted as MRL_Rapaura_Irrigation) [41.47 S; 173.89 E] from 2001 to 2005 ( Greven et al. 2005 ). The experimental results showed little differences in canopy development, dynamics of leaf and shoot biomass among different irrigation treatments; thus, we grouped the results and only used the mean treatment values. The comparison of the dynamics of soil water content in vine row and in alley between observation and simulation is shown in Supporting Information—Method S1 6.1.5 and 6.1.6 soil water.
The trial was conducted on four-cane pruned Sauvignon blanc vines (Telek 5C rootstock) grown on Wairau silt loam. In total, 72 vines were monitored for leaf, shoot and berry development. Canopy density was measured using the point quadrat with biweekly or monthly intervals. One or two destructive harvests of all leaves and shoots to establish the total leaf area and shoot biomass on the vines were done in mid-January or February. Fruit composition changes (TSS, TA and pH) were monitored weekly from five weeks before harvest. At harvest, bunch number per vine, bunch weight and berry fresh weight were determined for each plot.
2.3 Model calibration methods
Model parameters were determined based on data sets one to three where possible, e.g. parameters linked with phenology, leaf appearance rate and the dynamics of biomass demand of shoot and berry ( Table 2 ). Data set 4 was mainly used for validation. When parameters were not measured directly or linked with whole-plant function, e.g. q str, i (priority coefficient) they were optimized through the DEoptim function in R ( Ardia et al. 2011 ).
A partial list of model parameters and variables. A complete description of model parameters and variables was provided in Supporting Information—Method S1 APSIM Next Generation grapevine model documentation. A reference was provided for some parameter or variable names, while the remainder was calibrated based on the corresponding data set listed in Table 1 .
A link between APSIM Next Generation and R programming language was created through the APSIM Next Generation edit feature. The APSIM Next Generation edit feature enables users to edit the parameters through bash commands. An R script was developed where we can call APSIM Next Generation, pass the parameters and assess the performances of the model under new parameter values. The script was then coupled in the optimization function (DEoptim) for improving the model performances through parameter optimization. The criteria for optimization were set to minimize the sum of squares between observed values and predicted values when one observed variable was of concern. When there was more than one variables of concern, the criteria were set to minimize the sum of squares of normalized differences between observed and simulated values (Eq. (11)):
where i is the sample number (or time point), n the total number of measurements, j is the variable number, m the total number of variables in concern. X sim, j , i is the simulated value for variable j and sample number (time point) i , and X obs, j , i is the observed value for variable j and sample number (time point) i .
Variables that were less influenced by other processes were calibrated first. In general, the model calibration and validation were conducted in sequence of phenology (budburst, flowering and véraison), canopy height and width, leaf appearance rate, leaf area via changing branching rate and leaf extension duration, the biomass of different organs via radiation use efficiency (RUE) and carbohydrate allocation priority (with yield components as input in management script), NSC, bunch number, berry number and berry weight. Several iterations were undertaken, as there are correlations between different parameters.
Goodness-of-fit was assessed by root mean square error (RMSE) of the concerned variables and coefficients (intercept, slope and R 2 ) of linear regressions between observed and simulated values:
where i is the sample number, n the total number of measurements. X sim, i is the simulated value and X obs, i is the observed value. The unit of RMSE is the same as that of the investigated variable.
2.4 Simulation set-up
An initiation method (treeInitiation) was developed in each simulation in a management script for initializing the vineyard and vine conditions. Users can specify the variety, the starting date of the simulation, vine age, vine distance, bud number per vine, cane (or cordon for spur-pruned vine) number per vine, cane diameter, cane length, trunk diameter, trunk length, ratio of structural root biomass to trunk biomass, fraction of NSC in trunk, structural root and cane. The width of vine row and alley was defined in the soil zone.
Bunch number per node and berry number per bunch were determined/influenced by vine carbohydrate status and weather conditions in the season prior to the season of harvest. They were input for the first year of a simulation. As such, the yield result from the first year should be treated with caution as it does not reflect the model’s predictive mechanisms. We suggest starting the simulation one season earlier than the first observation season.
For the moment, the yield module has only been calibrated for irrigated and fertilized Sauvignon blanc grapes. Cane and trunk biomass were estimated based on the input dimension and default wood density in the management script. Non-structural carbohydrate content was calculated accordingly based on the fraction of NSC input for each organ. The age of the vine can be used in the calculation of some age-related attributes, e.g. canopy height and width. After initiation, the simulation can run continuously for the whole experimental period, e.g. 15 seasons for the phenology monitoring trial.
Soil physical parameters, water, nitrogen and organic matter properties can be specified for each simulation. For the current data sets, the root module was conducting calculations of water and nitrogen uptake, and the nitrogen arbitrator was automatically conducting nitrogen allocation and nitrogen-limited growth for each organ in the background. However, water and nitrogen stress on canopy and fruit growth were minimized by irrigation and fertilizer input in the currently simulation. A detail sensitivity analysis of the performance of the model outputs to days of interval between two irrigation events without the influence of rain (rainfall was removed in the script) were shown in Supporting Information—Method S1 8.1 Irrigation Response.
3.1 Phenology
Budburst, flowering and véraison dates for Sauvignon blanc in eight sites across New Zealand were simulated well ( Fig. 3 ), although the temperature profile varied greatly among sites owing to local terrain and distance to the ocean. For the calibration data set, the RMSEs were 4.1 days (based on date) for budburst, 3.1 days for flowering and 6.8 days for véraison for the calibration data set. For the validation data set, the RMSEs were 4.9 for budburst, 4.2 for flowering and 5.3 for véraison.

Verification and validation of the simulated budburst (A), flowering (B) and véraison (C) date expressed as DAWS for eight Sauvignon blanc sites across New Zealand. The solid black line is the one-to-one line between predictions and observations, and the red line is the linear regression between the two. HB represents Hawke’s Bay, MRL represents Marlborough, WV represents Waipara Valley. The phenology module was calibrated with the four Marlborough long-term sites, namely Upper Brancott, Western Wairau, Seaview Awatere and Central Rapaura, and validated with the remaining sites.
The model was parameterized for four other varieties: Chardonnay, Merlot, Pinot gris and Pinot noir [see Supporting Information—Fig. S3 ] . The overall RMSEs for those four varieties were 6.5 for budburst, 4.85 for flowering and 6.5 for véraison.
3.2 Leaf number and leaf area dynamics
The rates of leaf appearance, leaf expansion and branching had considerable effects on the leaf area dynamics. The performance of the model in those three aspects was evaluated by the dynamics of leaf number per main shoot ( Fig. 4A ), leaf number per whole shoot [see Supporting Information—Fig. S4 ] , individual leaf size [see Supporting Information—Fig. S5 ] , leaf area of the main shoot and leaf area of the lateral shoot ( Fig. 4C and D ). The rate of leaf appearance was similar across different retained node number treatments except for the 72-node treatment, which had fewer leaves on the main shoot in the 2009/10 season ( Fig. 4A ). This was not captured by the model, as only a constant leaf appearance rate was used in the model. The leaf area per vine did not differ greatly between different treatments in either observed or simulated values. However, a big drop in leaf area of the lateral shoot under high retained node number per vine was revealed by the model ( Fig. 4D ). This was captured through branching rate in the Structure Module. The sudden drop in leaf area around 200 DAWS was caused by the summer pruning events.

Model verification of vineyard leaf number per main shoot (A), leaf area per vine (B), leaf area on main shoots (C) and lateral shoots (D) in the retained node number trial in the season 2009/10. The sudden drop in leaf area around 200 DAWS was caused by the summer pruning events.
3.3 Radiation interception
Radiation interception is an important aspect of the model performance, and together with RUE they drive the DM production and carbohydrate accumulation. The newly implemented row-planted crop light interception method captured the seasonal dynamics of light interception in different years in the MRL_Rapaura_Irrigation trial ( Fig. 5 ). The corresponding dynamics of leaf area are shown in Supporting Information—Fig. S4 . Measured and simulated fractions of light interception increased rapidly from budburst (~100 DAWS) and reached a maximum fraction of light interception of 53 % around 200 DAWS. The measured fraction of light interception by light sensor in the alley was slightly higher than the simulated values. The deviation between the simulated values and observed values could be caused by the light interception by trunks and canes, which would cause a reduction in the radiation arriving at inter-row but would not increase DM production. The optimized RUE based on the observed leaf, shoot and berry biomass before flowering was 0.8 gDM MJ −1 , and after flowering was 0.9 gDM MJ −1 .

Model validation of the fraction of light interception by grapevine canopy in the Central Rapaura Vineyard in 2003-2004 (A) and in 2004-2005 (B). Points were observed values and lines were simulated ones. The decrease in the fraction of light interception around 200 DAWS in both observed data sets was due to summer pruning events.
3.4 Dynamics of the DM of different organs
Simulated leaf DM per shoot ( Fig. 6A , R 2 = 0.87), shoot DM per shoot ( Fig. 6B , R 2 = 0.84), and berry DM per vine ( Fig. 6C , R 2 = 0.82) were well aligned with the observed values for both retained node number trial (model calibration) and the MRL_Rapaura_Irrigation trial (model validation). The DM per shoot decreased rapidly with increasing number of nodes per vine. The mean shoots DM reduced from 35 g per shoot under 24 nodes treatment to 12 g per shoot under 72 nodes treatment at leaf fall.

Verification and validation of leaf DM per grapevine shoot (A), shoot DM per shoot (B), berry DM per vine (C). Shoot DM is the stem part and does not include leaf DM. The dynamics of DM were calibrated based on the data from the retained node number trial (MRL_Rowley), and validated with the data from the MRL_Rapaura_Irrigation trial. The solid black line is the one-to-one line between predictions and observations, and the red line is the linear regression between the two.
The seasonal patterns of DM dynamics of different organs were reproduced by the model ( Fig. 7 ). Initially, about 90 % of the DM were stored in trunk and structural root. After budburst, the stored non-structural DM was translocated into the newly growing leaf, shoot and berry ( Fig. 7A ). With photosynthesis and the support of stored DM, leaf and shoot DM increased rapidly. Leaf DM reached its peak at the end of January. Total berry DM increased rapidly after flowering and became the largest biomass pool at harvest ( Fig. 7B ).
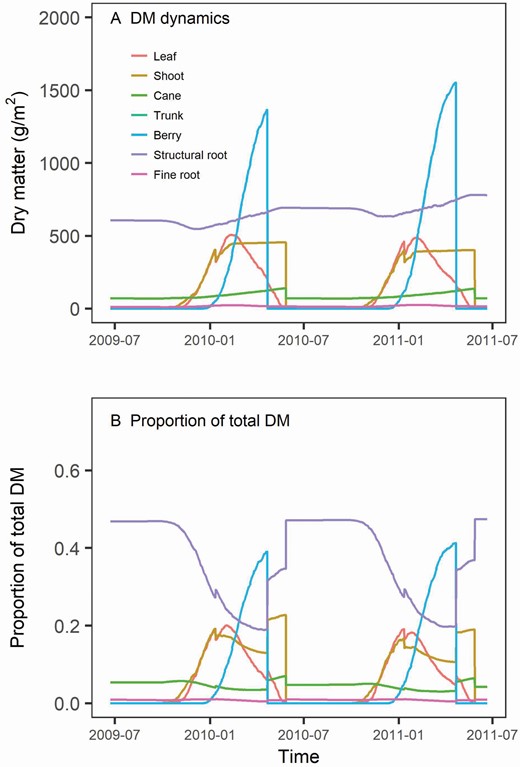
The dynamics of the dry weight of each grapevine organ (A) and proportion of each organ in the total plant DM in the 48-retained node number treatment (four-cane) (B). The DM was expressed in g m −2 , which was the default unit in APSIM. One vine occupies 1.62 m 2 in this simulation set-up (1.8 m vine spacing × 0.9 m width of the vine row). The lines of trunk were overwritten by the lines for structural root, as we use a biomass ratio of 1 between structural root and trunk, and the same DM demand parameters for them.
The DM retranslocation before flowering decreased NSC concentration in the trunk and structural root rapidly ( Fig. 8 ). The NSC pool started to replenish after flowering, mainly in competition with berry growth, as leaf and shoot DM demands had greatly decreased ( Figs 7 and 8 ). The rate of NSC replenishment slowed down during véraison but increased quickly when the berry DM demand went down, and leaf DM retranslocation accelerated during leaf senescence.
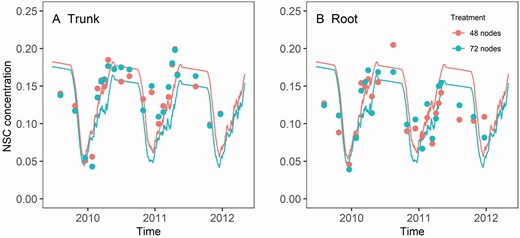
The dynamics of the NSC concentration in grapevine trunk (A) and root (B) with different retained node number per vine. Lines are predictions and points are observed data. The trial started in 2006 when the vines were 3 years old. Detail NSC measurement started in 2009 when the vines were 6 years old. Root samples were taken from a mixture of old and younger roots varying from 1 to 5 mm in diameter at a depth of ~150 mm.
3.5 Yield components
Wide variations in bunch number per vine (35–110), berry number per bunch (40–90) and yield per vine (3.5–13 kg) caused by seasonal fluctuations and retained node number per vine were captured by the model ( Fig. 9 ). For the calibration data set, the RMSEs were 5.6 for bunch number per vine, 7.7 for berry number per bunch and 1.3 kg for yield per vine. For the validation data set, the RMSEs were 6.3 for bunch number per vine, 9.8 for berry number per bunch and 2.0 kg per vine for yield per vine. The model underestimated these attributes at high values, e.g. 10–13 kg yield per vine, compared with the observations.
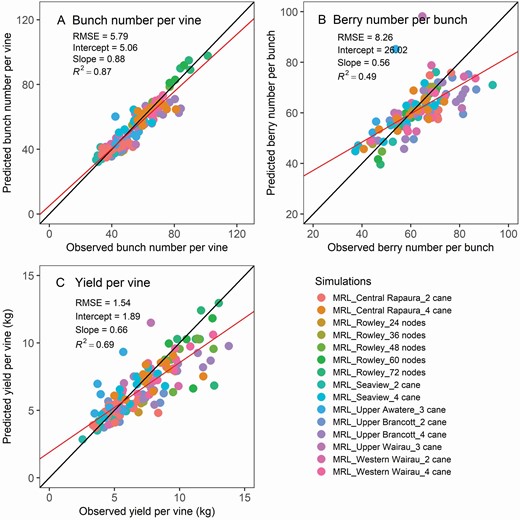
Model verification and validation of the bunch number per vine (A), berry number per bunch (B) and yield per vine (C) for seven Sauvignon blanc sites and five different retained cane number treatments in Marlborough, New Zealand. The solid black line is the one-to-one line between predictions and observations, and the red line is the linear regression between the two. The yield module was calibrated with the four Marlborough long-term sites, namely Upper Brancott, Western Wairau, Seaview Awatere and Central Rapaura, and validated with the remaining sites ( Table 1 ). Hawke’s Bay Bridge pa and Waipara Valley Glasneven were not included in the yield comparison because retained number of canes per vine changes from year to year and even within the same year owing to management decisions on yield control.
3.6 Berry composition
The model satisfactorily captured the dynamics in berry fresh weight ( Fig. 10A , R 2 = 0.75), berry TSS ( Fig. 10B , R 2 = 0.56) and berry TA ( Fig. 10C , R 2 = 0.7).
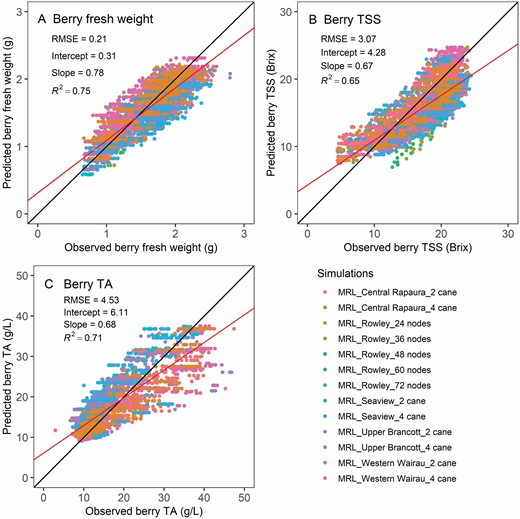
Model verification and validation of the single-berry fresh weight (A), total soluble solids (TSS, B) and total titratable acid concentration (TA in g L −1 tartaric acid equivalents, C). Data are for five Sauvignon blanc sites and five different retained cane number treatments in Marlborough, New Zealand. The solid black line is the one-by-one line between predictions and observations, and the red line is the linear regression between the two. The berry properties simulations were calibrated with the four Marlborough long-term sites, namely Upper Brancott, Western Wairau, Seaview Awatere and Central Rapaura, and validated with the remaining sites.
Two seasons (2016/17, and 2017/18) were selected to have a close check of the simulated dynamics of berry fresh weight, TSS and TA [see Supporting Information—Figs S6 – S8 ] . The season of 2016/17 had slightly higher cumulated growing degree days (°Cd) (>10 °C) from October to April than the long-term average (1299 °Cd versus 1228 °Cd). However, this season had more rainfall between véraison to harvest than the long-term average (240 mm versus 100 mm). The season of 2017/18 was warm (1455 °Cd) and berries were harvested in the first week of April.
The fresh weight of berries increased rapidly after fruit set. At the population level this presented a sigmoid shape [see Supporting Information—Fig. S6 ] , while at an individual level this has been shown to be a double sigmoid curve ( Garcia de Cortazar-Atauri et al. 2009 ). The model effectively captured the dynamics of berry fresh weight and final weight [see Supporting Information—Fig. S6 ] .
The model captured the high TSS at the same DAWS in the season of 2017/18 compared with 2016/17 [see Supporting Information—Fig. S7 ] ; however, the accuracy was largely affected by the predicted véraison time, which triggers the start of TSS calculation in the model. The model satisfactorily reproduced the degradation of TA after véraison [see Supporting Information—Fig. S8 ] . However, large errors can be expected when there is a big difference between predicted and observed véraison time [see Supporting Information—Fig. S8 ] . Strong effects of site and year were also found on the dynamics of berry fresh weight [see Supporting Information—Fig. S9 ] .
A new grapevine model was developed in APSIM Next Generation, which uses a version control system to ensure model reliability and a modern distribution system to ensure users can easily access models and receive updates. It has auto-documentation and a user-friendly interface whereby developers can drag and drop different modules and functions to represent the physiological processes. The UI is at the level of zero programming-skill requirement, which will enable more scientists to contribute to model development. All source code is open and is available on the APSIM initiative repository.
The grapevine model covered all phenological phases of development and captured the variations in phenological time under wide climatic conditions. The newly added row-planted crop radiation model captured the seasonal radiation interception pattern of a vertical shoot position training system and provided a framework for modelling the effects of vineyard configuration and alley management. The model captured the DM dynamics of different organs and the wide variations in yield components caused by different seasonal weather conditions and pruning strategies (e.g. different retained node number). The grapevine model represents an important advance as it is the first perennial fruit crop to be implemented into APSIM and it provides a useful template for the development of models for other perennial fruit crops.
4.1 Canopy development and radiation interception
Apart from two structural grapevine models that simulated leaf area at the individual leaf level ( Lecoeur et al. 2011 ; Schmidt et al. 2019 ), all other process-based grapevine models simulated leaf area at the canopy scale through different methods. For example, Bindi et al. (1997) calculated total leaf area based on the number of leaves that had appeared. In the STICS grapevine model, leaf area growth was driven by temperature, phenological stages and stresses, with an empirical plant density-dependent function representing inter-plant competitions. A few other grapevine models have calculated the increase in the leaf surface based on the biomass allocated to leaves and SLA ( Wermelinger et al. 1991 ; Nendel and Kersebaum 2004 ).
The current APSIM grapevine model took the ‘leaf-to-leaf’ approach, using phyllochrons to control the leaf initiation, leaf appearance and duration of leaf expansion to simulate the dynamics of potential individual leaf area. Actual leaf area depended on biomass allocation, SLA and other abiotic stresses, e.g. water stress. The variation in SLA caused by the ratio of structural and non-structural weight was captured by maximum and minimum SLA. Furthermore, for correctly simulating leaf area on lateral shoots, we controlled the branching rate through an empirical relationship between bud number per metre row and percentage of reduction in branching rate ( Fig. 4 ). Further development could replace this empirical relationship with sounder physiological controls, e.g. source/sink ratio, and add the branching pattern caused by succession of phytomer types (P0–P1–P2) ( Louarn et al. 2007 ).
The dynamics of leaf area was tightly linked with radiation interception, and the Beer–Lambert law was often used for the interception calculation ( Bindi et al. 1997 ). However, grapevines are usually planted in rows with substantial alley space in between. This violates the homogenous assumption of the Beer–Lambert law. Several approaches have been developed based on canopy height and width, and canopy porosity, to account for such heterogeneity ( Brisson et al. 2003 ; Oyarzun et al. 2007 ), but are not yet available in APSIM. Here, we added the row-planted crop radiation interception methods developed by Goudriaan (1977) and Pronk et al. (2003) into APSIM. We also adapted the method to account for three conditions: (i) canopy width is the same as row width, and leaves are distributed evenly across the plant height vertically ( Zhang et al. 2008 ; Gou et al. 2017 ). (ii) Canopy width is smaller than the row width and leaves are concentrated in the top part of canopy, e.g. hedge-pruned vines. (iii) Canopy width is larger than the row width and leaves are concentrated in the top part of the canopy, e.g. apple orchards. The current method is also applicable to conditions where gaps within the row are not negligible, by considering the canopy as an array of cuboids as shown in Pronk et al. (2003) on the conifer eastern white cedar ( Thuja occidentalis ‘Brabant’). For conditions where the canopy shape largely violates the cube assumption, other light interception methods for heterogeneous canopy need be implemented in the APSIM source code. de Castro and Fetcher (1998) developed a method to deal with three-dimensional (3D) canopy analytically. They divided the canopy into many cubic cells, and then calculated the probability that a beam would penetrate to any given cell without being intercepted by the foliage in the path, using an exponential extinction function. Another promising approach was to transform the 3D canopy light interception problem into a two-dimensional (2D) problem by using the shadow area and intensity on the ground at any given time ( Rojo et al. 2020 ).
4.2 Carbohydrate allocation and carbohydrate reserves
Three approaches have mainly been used for simulating carbohydrate allocation in existing grapevine models: (i) Harvest index or fruit biomass index. For instance, Bindi et al. (1997) assumed the ratio of fruit dry weight and total aboveground biomass increased linearly during the reproductive phase. (ii) Partitioning coefficient. For example, Nendel and Kersebaum (2004) used a partitioning coefficient for biomass allocation and the partitioning coefficient changes according to phenological stages. (iii) Relative sink strength ( Gutierrez et al. 1985 ; Wermelinger et al. 1991 ; Lecoeur et al. 2011 ). Carbohydrate reserves in perennial structures were rarely considered and only a few models considered the perennial reserves in different organs as a whole ( Brisson et al. 2009 ; Pallas et al. 2011 ).
We expanded the existing carbohydrate allocation approaches as follows by: (i) simulating both NSC synthesis and hydrolysis explicitly in separated organs, e.g. cane, trunk and structural root, based on the approach presented in L-kiwi ( Cieslak et al. 2011 ). (ii) Carbohydrate reserve (starch synthesis) was simulated as an active competing sink instead of allocating carbohydrate to reserve only when there was surplus carbohydrate. To achieve this, a new allocation method (Q-Priority-then-Relative-Allocation) was added to the PMF arbitrator to account for sink priority and sink strength simultaneously ( Buwalda 1991 ; Cieslak et al. 2011 ). The new approach effectively captured the seasonal dynamics of NSC concentrations in both trunk and structural root compared with our field observations ( Fig. 8 ). The pattern of the dynamics of NSC concentration depends on the rate of NSC synthesis and hydrolysis, but is strongly influenced by the rate of NSC consumption in spring and the rate of carbohydrate assimilation by leaf as well. The transition point of NSC from decrease to increase indicates when the vine achieves a net carbohydrate gain.
4.3 Variations in yield
Until now variations in yield and yield components, namely bunch number per vine, berry number per bunch and berry weight, were not well represented in process-based grapevine models ( Moriondo et al. 2015 ). As discussed above, the DM accumulation of the fruit compartment was often simplified and the variation in berry number per vine was not considered. The STICS grapevine model did calculate the berry number per square metre during the fruit setting period (a thermal time duration). During this period, on each day, the number of set fruit was calculated using the potential number of set fruit per inflorescence and per degree day, the daily development rate (degree day), the number of inflorescences per plant (input or calculated based on plant carbohydrate status at the early stage), the plant density, the trophic stress index and the frost stress index acting on fruit from flowering ( Brisson et al. 2009 ). As thermal time would linearize the temperature effect, the final total berry number will be similar each year if the carbohydrate status is similar. Thus, the STICS grapevine model did not account for the effects of weather conditions around flowering on bunch number per vine, berry number per bunch and potential berry fresh weight. This may not be a problem when applying the model in hot climate conditions where temperature does not limit the inflorescence initiation ( Sadras and Soar 2009 ). However, for cool-climate viticulture, remarkable seasonal variations in yield, up to 100 %, can be found ( Zhu et al. 2020 ). Much of this can be attributed to temperatures at inflorescence initiation and flowering, although inadequate carbohydrate reserves can result in inflorescence abortion and reductions in bunch weight ( Eltom et al. 2013 ; Greven et al. 2014 ). We simulated the seasonal variations in bunch number per vine, berry number per bunch and berry weight by integrating the statistical yield model developed by Zhu et al. (2020) with the process-based model which added additional carbohydrate effects to the statistical yield model. The approach accounted for 74 % of the variations in bunch number per vine, 46 % in berry number per bunch and 54 % in yield per vine across seven sites with different weather conditions and retained nodes per vine, even with the same parameter set.
When validating the model, we found that some high-yield sites and years were underestimated. This was probably caused by underestimating the shoot number per vine. High-yield vineyards often have high vine capacity with large trunks, and a high proportion of vigorous shoots growing from adventitious buds (uncounted at winter pruning) or growing from secondary and tertiary buds of the counted buds. Including more detailed site information like the soil physical properties, soil nutrients and water information into the model for accounting for variations in vine capacity, shoot number per vine, and leaf area development, are likely to further improve the prediction. For correctly simulating those aspects, further work needs to be done in calibrating the water and nutrient uptake and competition with the inter-row cover crops in the current grapevine model. Furthermore, updating the model with real-time information, e.g. shoot and inflorescence number in spring, flowering and véraison date, would largely improve the model accuracy for the predictions of leaf area, yield, berry TSS and berry TA and could assist in-season management decisions.
A grapevine model has been developed and is the first perennial fruit crop model in APSIM Next Generation. It has all the structures and functions to simulate the phenology, radiation interception, leaf growth, DM production, carbohydrate and nitrogen allocation and storage, and yield formation of a perennial plant. The model can be easily adapted in the UI to reflect different process formulations (e.g. yield formation), and to simulate different cultivars or VSP training systems without changing the source code. This extends the usability of the model under different conditions. The model performed well accounting for the effects of weather conditions on phenology across eight sites in New Zealand and the yield components in the Marlborough region. It can be used to explore the long-term suitability of different crop loads, based on the stability of the yield between years and the carbohydrate reserves in winter. Further studies can be done on extending the abilities of the model to simulate water and nutrient competition between the grapevine and the understory plants and their effects on grapevine development. The modelling framework developed in this study can also be able to be applied to other perennial fruit crops as shown by our preliminary modelling work on apple ( Malus domestica ), peach ( Prunus persica ) and kiwifruit ( Actinidia deliciosa ). Once the grapevine model is validated across a broader range of training systems, climatic conditions and varieties, it will become a promising tool for illustrating the impacts of climate change on current viticulture practices and for bridging the gap between real-time field data and decisions that growers often face.
The following additional information is available in the online version of this article—
Method S1. Agricultural Production System sIMulator (APSIM) Next Generation grapevine model documentation.
Figure S1. Illustration of the set-up of a vertical-shoot-positioned vineyard where our data were collected and definition of different distances.
Figure S2. Screenshots of Agricultural Production System sIMulator (APSIM) Next Generation user interface showing the basic simulation set-up for a vineyard, list of model simulations and validations and a simulation example of bringing sheep into the vineyard, and sensitivity to water stress.
Figure S3. Model verification of the simulated budburst, flowering and véraison dates for Chardonnay, Merlot, Pinot gris and Pinot noir across New Zealand.
Figure S4. Model validation of the leaf per shoot, leaf area per vine, leaf area on main shoots and lateral shoots in the MRL_Rapaura_Irrigation trial.
Figure S5. Illustration of the dynamics of leaf size in different cohort groups in the Central Rapaura phenology and yield monitoring vineyard in the 2004/05 season.
Figure S6. Model verification of the dynamics of berry fresh weight for the four long-term monitoring Marlborough (New Zealand) Sauvignon blanc sites in the 2016/17 and 2017/18 seasons.
Figure S7. Model verification of the dynamics of berry total soluble solids (°Brix) for the four long-term Marlborough (New Zealand) Sauvignon blanc sites in the 2016/17 and 2017/18 seasons.
Figure S8. Model verification of the dynamics of berry titratable acid concentration for the four long-term Marlborough (New Zealand) Sauvignon blanc sites in the 2016/17 and 2017/18 seasons.
Figure S9. The effects of sites and year on the simulated dynamics of berry fresh weight and final berry weight.
The authors would like to thank all the staff at The New Zealand Institute for Plant and Food Research in Hawke’s Bay, Marlborough and Central Otago, New Zealand, and wine company staff in Gisborne and the Waipara Valley who conducted the phenology monitoring, berry sampling and hand harvesting. Special thanks go to Rafidah Horner, Franziska Grab, Trevor Skilton, Rachel Bishell, Isabelle Sorensen, Ross Marshall, Chelle Gandell, Sarah Philips and Julian Smith. The authors also thank Dean Holzworth, Drew Holzworth and the APSIM initiative team for their support during model development; Jian Liu and Eric Burgueño for their help in developing the model optimization script; Stewart Field and Harris Lin for constructive comments on the manuscript; Pernod Ricard Winemakers, Delegat Limited, Indevin Group Limited, Craggy Range, Constellation Brands, Sileni Estates, Villa Maria Estate, Treasury Wine Estates, Terra Sancta, Mount Dunstan Estate, Peregrine Wines and Mt Difficulty Wines for allowing the monitoring to take place on their vineyards; the PFR internal Science Publication Office for their help in revising the manuscript; and Tony Corbett for improving the illustration of the model structure.
Over the period 2004–2020, the experimental work was funded by the New Zealand Ministry of Business, Innovation and Employment (MBIE, UOAX0404, C06X0004), New Zealand Winegrowers/Sustainable Farming Fund (NZW08-337, NZW14-100) and Sustainable Winegrowing New Zealand, Marlborough Research Centre Trust and Marlborough District Council. The modelling work was funded by the PFR Grape and Wine Research programme through the MBIE Strategic Science Investment Fund.
The authors declare that there is no conflict of interest.
Conceptualization, J.Z., A.P., R.A., D.M., M.C.T.T. and H.E.B.; software and methodology, J.Z., A.P., N.H. and H.E.B.; data curation, R.A., L.Y., M.G., V.R., S.N., M.C.T.T., J.Z.; data analyses, J.Z., F.G., A.P. and R.A.; writing—original draft preparation, J.Z.; writing—review and editing, J.Z., F.G., M.G., A.P., R.A., D.M., M.C.T.T., N.H., L.Y., V.R., S.N. and H.E.B.; visualization, J.Z. and F.G.; project administration, D.M. and J.Z.
The APSIM grapevine model, data used for model development and source code are publicly available in the APSIM Next Generation git repository: https://github.com/APSIMInitiative/-ApsimX/tree/master/Tests/Validation/Grapevine . The R code for plotting and analysis are available upon request.
Albasha R , Fournier C , Pradal C , Chelle M , Prieto JA , Louarn G , Simonneau T , Lebon E . 2019 . HydroShoot: a functional-structural plant model for simulating hydraulic structure, gas and energy exchange dynamics of complex plant canopies under water deficit—application to grapevine ( Vitis vinifera ) . In Silico Plants 1 : diz007 ; doi: 10.1093/insilicoplants/diz007 .
Google Scholar
Ardia D , Boudt K , Carl P , Mullen KM , Peterson BG . 2011 . Differential evolution with DEoptim: an application to non-convex portfolio optimization . R Journal 3 : 27 – 34 .
Ben-Asher J , Van Dam J , Feddes RA , Jhorar R . 2006 . Irrigation of grapevines with saline water: II. Mathematical simulation of vine growth and yield . Agricultural Water Management 83 : 22 – 29 .
Bindi M , Miglietta F , Gozzini B , Orlandini S , Seghi L . 1997 . A simple model for simulation of growth and development in grapevine ( Vitis vinifera L.). 1. Model description . VITIS-Journal of Grapevine Research 36 : 67 .
Bock A , Sparks TH , Estrella N , Menzel A . 2013 . Climate-induced changes in grapevine yield and must sugar content in Franconia (Germany) between 1805 and 2010 . PLoS One 8 : e69015 .
Bonada M , Edwards EJ , McCarthy MG , Sepúlveda GC , Petrie PR . 2020 . Impact of low rainfall during dormancy on vine productivity and development . Australian Journal of Grape and Wine Research 26 : 325 – 342 .
Brisson N , Gary C , Justes E , Roche R , Mary B , Ripoche D , Zimmer D , Sierra J , Bertuzzi P , Burger P , Bussiere F , Cabidoche YM , Cellier P , Debaeke P , Gaudillere JP , Henault C , Maraux F , Seguin B , Sinoquet H . 2003 . An overview of the crop model STICS . European Journal of Agronomy 18 : 309 – 332 .
Brisson N , Launay M , Mary B , Beaudoin N . 2009 . Conceptual basis, formalisations and parameterization of the STICS crop model. Editions QUAE, INRA, Versailles .
Google Preview
Brown H , Huth N , Holzworth D . 2018 . Crop model improvement in APSIM: using wheat as a case study . European Journal of Agronomy 100 : 141 – 150 .
Brown HE , Huth NI , Holzworth DP , Teixeira EI , Zyskowski RF , Hargreaves JNG , Moot DJ . 2014 . Plant modelling framework: software for building and running crop models on the APSIM platform . Environmental Modelling & Software 62 : 385 – 398 .
Brown HE , Huth NI , Holzworth DP , Teixeira EI , Wang E , Zyskowski RF , Zheng B . 2019 . A generic approach to modelling, allocation and redistribution of biomass to and from plant organs . In Silico Plants 1 : diy004 ; doi: 10.1093/insilicoplants/diy004 .
Buwalda J . 1991 . A mathematical model of carbon acquisition and utilisation by kiwifruit vines . Ecological Modelling 57 : 43 – 64 .
Buwalda JG . 1993 . The carbon costs of root systems of perennial fruit crops . Environmental and Experimental Botany 33 : 131 – 140 .
Camargo-Alvarez H , Salazar-Gutiérrez M , Keller M , Hoogenboom G . 2020 . Modeling the effect of temperature on bud dormancy of grapevines . Agricultural and Forest Meteorology 280 : 107782 .
Celette F , Ripoche A , Gary C . 2010 . WaLIS—a simple model to simulate water partitioning in a crop association: the example of an intercropped vineyard . Agricultural Water Management 97 : 1749 – 1759 .
Challinor AJ , Watson J , Lobell DB , Howden S , Smith D , Chhetri N . 2014 . A meta-analysis of crop yield under climate change and adaptation . Nature Climate Change 4 : 287 .
Chuine I . 2000 . A united model for budburst of trees . Journal of Theoretical Biology 2007 : 337 – 347 .
Cieslak M , Seleznyova AN , Hanan J . 2011 . A functional-structural kiwifruit vine model integrating architecture, carbon dynamics and effects of the environment . Annals of Botany 107 : 747 – 764 .
Comas LH , Anderson LJ , Dunst RM , Lakso AN , Eissenstat DM . 2005 . Canopy and environmental control of root dynamics in a long-term study of Concord grape . New Phytologist 167 : 829 – 840 .
Dai ZW , Vivin P , Robert T , Milin S , Li SH , Génard M . 2009 . Model-based analysis of sugar accumulation in response to source–sink ratio and water supply in grape ( Vitis vinifera ) berries . Functional Plant Biology 36 : 527 .
de Castro F , Fetcher N . 1998 . Three dimensional model of the interception of light by a canopy . Agricultural and Forest Meteorology 90 : 215 – 233 .
Dokoozlian N . 1999 . Chilling temperature and duration interact on the budbreak of Perlette’grapevine cuttings . HortScience 34 : 1 – 3 .
Eltom M , Trought M , Winefield C . 2013 . The effects of cane girdling before budbreak on shoot growth, leaf area and carbohydrate content of Vitis vinifera L. Sauvignon Blanc grapevines . Functional Plant Biology 40 : 749 – 757 .
Eltom M , Trought MC , Agnew R , Parker A , Winefield CS . 2017 . Pre‐budburst temperature influences the inner and outer arm morphology, phenology, flower number, fruitset, TSS accumulation and variability of Vitis vinifera L. Sauvignon Blanc bunches . Australian Journal of Grape and Wine Research 23 : 280 – 286 .
Fraga H , Garcia de Cortazar Atauri I , Malheiro AC , Santos JA . 2016 . Modelling climate change impacts on viticultural yield, phenology and stress conditions in Europe . Global Change Biology 22 : 3774 – 3788
Garcia de Cortazar-Atauri I , Brisson N , Ollat N , Jacquet O , Payan JC . 2009 . Asynchronous dynamics of grapevine ( Vitis vinifera ) maturation: experimental study for a modelling approach . Journal International des Sciences de la Vigne et du Vin 43 : 83 – 97 .
Godwin D , White R , Sommer KJ , Walker R , Goodwin I , Clingeleffer P . 2002 . VineLOGIC-a model of grapevine growth development and water use . Dundon C , Hamilton R , Johnstone R , Partridge S (Eds.), Managing Water. Australian Society of Viticulture and Oenology Inc: 46-50. Adelaide Australia.
Gou F , van Ittersum MK , Simon E , Leffelaar PA , van der Putten PEL , Zhang L , van der Werf W . 2017 . Intercropping wheat and maize increases total radiation interception and wheat RUE but lowers maize RUE . European Journal of Agronomy 84 : 125 – 139 .
Goudriaan J . 1977 . Crop micrometeorology: a simulation study: Pudoc, Center for Agricultural Publishing and Documentation, Wageningen, The Netherlands. .
Goudriaan J , Van Laar H . 1994 . Modelling potential crop growth processes: textbook with exercises . Wageningen, The Netherlands : Springer .
Greer DH , Weston C . 2010 . Effects of fruiting on vegetative growth and development dynamics of grapevines ( Vitis vinifera cv. Semillon) can be traced back to events at or before budbreak . Functional Plant Biology 37 : 756 – 766 .
Greven MM , Bennett JS , Neal SM . 2014 . Influence of retained node number on Sauvignon Blanc grapevine vegetative growth and yield . Australian Journal of Grape and Wine Research 20 : 263 – 271 .
Greven M , Green S , Neal S , Clothier B , Neal M , Dryden G , Davidson P . 2005 . Regulated deficit irrigation (RDI) to save water and improve Sauvignon Blanc quality? Water Science and Technology 51 : 9 – 17 .
Greven MM , Neal SM , Hall AJ , Bennett JS . 2015 . Influence of retained node number on Sauvignon Blanc grapevine phenology in a cool climate . Australian Journal of Grape and Wine Research 21 : 290 – 301 .
Greven MM , Neal SM , Tustin DS , Boldingh H , Bennett J , Vasconcelos MC . 2016 . Effect of postharvest defoliation on carbon and nitrogen resources of high-yielding Sauvignon blanc grapevines . American Journal of Enology and Viticulture 67 : 315 – 326 .
Gutierrez A , Williams D , Kido H . 1985 . A model of grape growth and development: the mathematical structure and biological considerations 1 . Crop Science 25 : 721 – 728 .
Holzworth DP , Huth NI , Devoil PG , Zurcher EJ , Herrmann NI , McLean G , Chenu K , van Oosterom EJ , Snow V , Murphy C , Moore AD , Brown H , Whish JPM , Verrall S , Fainges J , Bell LW , Peake AS , Poulton PL , Hochman Z , Thorburn PJ , Gaydon DS , Dalgliesh NP , Rodriguez D , Cox H , Chapman S , Doherty A , Teixeira E , Sharp J , Cichota R , Vogeler I , Li FY , Wang EL , Hammer GL , Robertson MJ , Dimes JP , Whitbread AM , Hunt J , van Rees H , McClelland T , Carberry PS , Hargreaves JNG , MacLeod N , McDonald C , Harsdorf J , Wedgwood S , Keating BA . 2014 . APSIM - evolution towards a new generation of agricultural systems simulation . Environmental Modelling & Software 62 : 327 – 350 .
Holzworth D , Huth NI , Fainges J , Brown H , Zurcher E , Cichota R , Verrall S , Herrmann NI , Zheng B , Snow V . 2018 . APSIM Next Generation: overcoming challenges in modernising a farming systems model . Environmental Modelling & Software 103 : 43 – 51 .
Keating BA . 2020 . Crop, soil and farm systems models – science, engineering or snake oil revisited . Agricultural Systems 184 : 102903 .
Lakso AN , Intrigliolo D , Eissenstat DM . 2008 . Modeling concord grapes with “VitiSim”, a simplified carbon balance model: understanding pruning effects . Acta Horticulturae 803 : 243 – 250 .
Lecoeur JRM , Poir-Lassus R , Christophe AL , Pallas BT , Casadebaig P , Debaeke P , Vear F , Guilioni L . 2011 . Quantifying physiological determinants of genetic variation for yield potential in sunflower. SUNFLO: a model-based analysis . Functional Plant Biology 38 : 246 – 259 .
Louarn G , Dauzat J , Lecoeur J , Lebon E . 2008a . Influence of trellis system and shoot positioning on light interception and distribution in two grapevine cultivars with different architectures: an original approach based on 3D canopy modelling . Australian Journal of Grape and Wine Research 14 : 143 – 152 .
Louarn G , Guedon Y , Lecoeur J , Lebon E . 2007 . Quantitative analysis of the phenotypic variability of shoot architecture in two grapevine ( Vitis vinifera ) cultivars . Annals of Botany 99 : 425 – 437 .
Louarn G , Lecoeur J , Lebon E . 2008b . A three-dimensional statistical reconstruction model of grapevine ( Vitis vinifera ) simulating canopy structure variability within and between cultivar/training system pairs . Annals of Botany 101 : 1167 – 1184 .
Moriondo M , Ferrise R , Trombi G , Brilli L , Dibari C , Bindi M . 2015 . Modelling olive trees and grapevines in a changing climate . Environmental Modelling & Software . doi: 10.1016/j.envsoft.2014.12.016 .
Nendel C , Kersebaum K . 2004 . A simple model approach to simulate nitrogen dynamics in vineyard soils . Ecological Modelling 177 : 1 – 15 .
OIV. 2020 . Current situation of the vitivinicultural sector at a global level. Paris : OIV .
Oyarzun RA , Stöckle CO , Whiting MD . 2007 . A simple approach to modeling radiation interception by fruit-tree orchards . Agricultural and Forest Meteorology 142 : 12 – 24 .
Pallas B , Loi C , Christophe A , Cournede PH , Lecoeur J . 2011 . Comparison of three approaches to model grapevine organogenesis in conditions of fluctuating temperature, solar radiation and soil water content . Annals of Botany 107 : 729 – 745 .
Parker AK , Hofmann RW , van Leeuwen C , McLachlan ARG , Trought MCT . 2014 . Leaf area to fruit mass ratio determines the time of veraison in Sauvignon Blanc and Pinot Noir grapevines . Australian Journal of Grape and Wine Research 20 : 422 – 431 .
Poni S , Palliotti A , Bernizzoni F . 2006 . Calibration and evaluation of a STELLA software-based daily CO 2 balance model in Vitis vinifera L . Journal of the American Society for Horticultural Science 131 : 273 – 283 .
Prieto JA , Louarn G , Perez PeÑA J , Ojeda H , Simonneau T , Lebon E . 2012 . A leaf gas exchange model that accounts for intra-canopy variability by considering leaf nitrogen content and local acclimation to radiation in grapevine ( Vitis vinifera L.) . Plant, Cell & Environment 35 : 1313 – 1328 .
Pronk AA , Goudriaan J , Stilma E , Challa H . 2003 . A simple method to estimate radiation interception by nursery stock conifers: a case study of eastern white cedar . NJAS - Wageningen Journal of Life Sciences 51 : 279 – 295 .
Rojo F , Dhillon R , Upadhyaya S , Jenkins B . 2020 . Development of a dynamic model to estimate canopy par interception . Biosystems Engineering 198 : 120 – 136 .
Sadras VO , Soar CJ . 2009 . Shiraz vines maintain yield in response to a 2–4 °C increase in maximum temperature using an open-top heating system at key phenostages . European Journal of Agronomy 31 : 250 – 258 .
Santos JA , Malheiro AC , Karremann MK , Pinto JG . 2011 . Statistical modelling of grapevine yield in the Port Wine region under present and future climate conditions . International Journal of Biometeorology 55 : 119 – 131 .
Sarvaš R . 1974 . Investigations on the annual cycle of development of forest trees II. Autumn dormancy and winter dormancy . Communicationes Instituti Forestalis Fenniae 84 : 1 – 101 .
Schlenker W , Roberts MJ . 2009 . Nonlinear temperature effects indicate severe damages to US crop yields under climate change . Proceedings of the National Academy of Sciences of the United States of America 106 : 15594 – 15598 .
Schmidt D , Bahr C , Friedel M , Kahlen K . 2019 . Modelling approach for predicting the impact of changing temperature conditions on grapevine canopy architectures . Agronomy-Basel 9 : 426 .
Seleznyova AN , Saei A , Han L , van Hooijdonk BM . 2018 . From field data to modelling concepts: building a mechanistic FSPM for apple . In: 6th International Symposium on Plant Growth Modeling, Simulation, Visualization and Applications, PMA 2018 . Hefei, China : Institute of Electrical and Electronics Engineers .
Stöckle CO , Donatelli M , Nelson R . 2003 . CropSyst, a cropping systems simulation model . European Journal of Agronomy 18 : 289 – 307 .
Valdés-Gómez H , Celette F , de Cortázar-Atauri IG , Jara-Rojas F , Ortega-Farías S , Gary C . 2009 . Modelling soil water content and grapevine growth and development with the STICS crop-soil model under two different water management strategies . Oeno One 43 : 13 – 28 .
Van Ittersum MK , Leffelaar PA , Van Keulen H , Kropff MJ , Bastiaans L , Goudriaan J . 2003 . On approaches and applications of the Wageningen crop models . European Journal of Agronomy 18 : 201 – 234 .
Wang E , Engel T . 1998 . Simulation of phenological development of wheat crops . Agricultural Systems 58 : 1 – 24 .
Wermelinger B , Baumgärtner J , Gutierrez AP . 1991 . A demographic model of assimilation and allocation of carbon and nitrogen in grapevines . Ecological Modelling 53 : 1 – 26 .
Williams DLEW , Barnett W , Kelley K , McKenry M . 1985 . Validation of a model for the growth and development of the Thompson seedless grapevine. I. Vegetative growth and fruit yield . American Journal of Enology and Viticulture 36 : 275 – 282 .
Woodward SJR , Romera AJ , Beskow WB , Lovatt SJ . 2008 . Better simulation modelling to support farming systems innovation: review and synthesis . New Zealand Journal of Agricultural Research 51 : 235 – 252 .
Yin X , Goudriaan J , Lantinga EA , Vos J , Spiertz HJ . 2003 . A flexible sigmoid function of determinate growth . Annals of Botany 91 : 361 – 371 .
Zhang L , van der Werf W , Bastiaans L , Zhang S , Li B , Spiertz JHJ . 2008 . Light interception and utilization in relay intercrops of wheat and cotton . Field Crops Research 107 : 29 – 42 .
Zhu J , Dai Z , Vivin P , Gambetta GA , Henke M , Peccoux A , Ollat N , Delrot S . 2018 . A 3-D functional-structural grapevine model that couples the dynamics of water transport with leaf gas exchange . Annals of Botany 121 : 833 – 848 .
Zhu J , Fraysse R , Trought MCT , Raw V , Yang L , Greven M , Martin D , Agnew R . 2020 . Quantifying the seasonal variations in grapevine yield components based on pre- and post-flowering weather conditions . Oeno One 54 :213–230.
Zhu J , Genard M , Poni S , Gambetta GA , Vivin P , Vercambre G , Trought MCT , Ollat N , Delrot S , Dai Z . 2019 . Modelling grape growth in relation to whole-plant carbon and water fluxes . Journal of Experimental Botany 70 : 2505 – 2521 .
Supplementary data
Email alerts, citing articles via.
- Recommend to your Librarian
- Journals Career Network
Affiliations
- Online ISSN 2517-5025
- Copyright © 2024 AoB Company
- About Oxford Academic
- Publish journals with us
- University press partners
- What we publish
- New features
- Open access
- Institutional account management
- Rights and permissions
- Get help with access
- Accessibility
- Advertising
- Media enquiries
- Oxford University Press
- Oxford Languages
- University of Oxford
Oxford University Press is a department of the University of Oxford. It furthers the University's objective of excellence in research, scholarship, and education by publishing worldwide
- Copyright © 2024 Oxford University Press
- Cookie settings
- Cookie policy
- Privacy policy
- Legal notice
This Feature Is Available To Subscribers Only
Sign In or Create an Account
This PDF is available to Subscribers Only
For full access to this pdf, sign in to an existing account, or purchase an annual subscription.
Primary Navigation
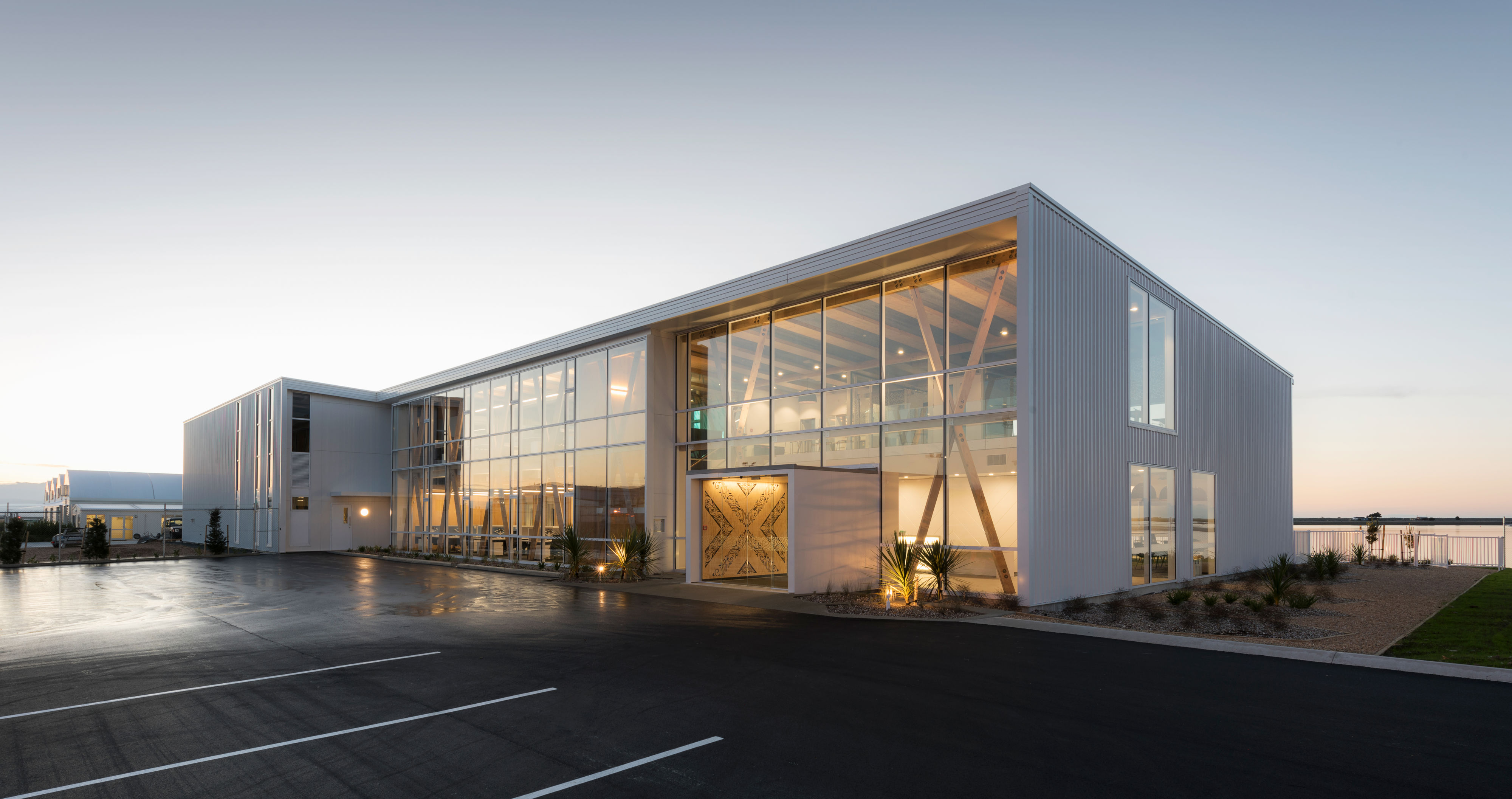
Interested in working with us?
Please provide a brief description of your business enquiry and we’ll be back in touch as soon as possible.
Other ways to contact us
For all other enquiries please email: [email protected] .
See our current vacancies on our Job portal . You can also create a profile and sign up for alerts so you'll be kept up to date on roles that might fit your skill set.
Māori engagement
To get in touch with our Māori Strategy, Partnerships & Enterprise Team please email: [email protected] .
Plant varieties
Find more information on Plant & Food Research bred plant varieties please see our Plant varieties page.
Visiting our sites
See the locations of all our sites on the Locations page.
OIA requests
As a Crown Research Institute, Plant & Food Research is subject to the New Zealand Official Information Act (OIA). To contact us with a request under the OIA, please email: [email protected] .
For more information about the OIA, who can submit a request and the types of information that can be requested, please visit the Ombudsman's website.
In-mouth attributes driving perceived quality of Pinot noir wines: Sensory and chemical characterisation
Affiliations.
- 1 AGLS Faculty, Lincoln University, Christchurch, New Zealand.
- 2 AGLS Faculty, Lincoln University, Christchurch, New Zealand. Electronic address: [email protected].
- 3 Institute of Plant and Food Research, Marlborough, New Zealand.
- 4 Institute of Plant and Food Research, Palmerston North, New Zealand.
- 5 Tripwire Wine Consulting Ltd. & Misha's Vineyard, Central Otago, New Zealand.
- 6 School of Chemical Sciences, The University of Auckland, New Zealand.
- 7 Centre des Sciences du Goût et de l'Alimentation, AgroSup Dijon, CNRS, INRAE, Université Bourgogne Franche-Comté, F-21000 Dijon, France.
- PMID: 34600667
- DOI: 10.1016/j.foodres.2021.110665
We investigated perception of mouthfeel in Pinot noir wines and its physicochemical determinants. Seventeen wine professionals judged 18 Pinot noir wines via two tasting tasks, Descriptive Rating and Directed Sorting, the latter based on perception of in-mouth attributes. Selected chemical measures of the same 18 wines were determined. Sensory results demonstrated that mouthfeel attributes driving high quality were smoothness/silky/velvety, volume/fullness/roundness, overall body, and viscosity/mouth-coating, while the taste of bitterness and perceived tannin harshness drove low quality judgments. Perceived astringency and expressions of tannin (e.g., soft; harsh; fine) drove sorting behaviour of participants. Partial least squares regression (PLSR) was used to associate perceived in-mouth phenomena and chemical composition. The phenolic profile, in particular tannin concentration and structure, was the most important predictor of astringency and its sub-components. These results have important implications for wine producers aiming to enhance perceived quality in their Pinot noir wines.
Keywords: Chemistry; In-mouth characteristics; Pinot noir; Sensory; Wine.
Copyright © 2021 Elsevier Ltd. All rights reserved.
Publication types
- Research Support, Non-U.S. Gov't
- Tannins / analysis
- Wine* / analysis
- Domestic Students
- International Students
Viticulture & Winemaking
NMIT offers three different Certificate programmes and a Bachelor of Viticulture and Winemaking. The Certificate courses prepare you for work in cellar or vineyard operations, while the three-year Degree programme covers everything you need for a budding career as a winemaker or vineyard manager. In the vintage season alone Marlborough has over 8,000 jobs available, see the New Zealand Wine (external link) site for more information.
At NMIT students have the chance to grow their own grapes at the on-campus research vineyard, make their own wine in the research winery with a micro-vinification unit, practice wine analysis, and develop wine sensory analysis skills in the high spec sensory laboratory.
With an unbeatable location and flexible study options to suit all learners, NMIT is an innovative place to start on the path to an exciting career in viticulture and winemaking.
The Marlborough region is the country’s largest winegrowing area and is at the forefront of New Zealand’s wine industry growth, producing over 75% of the country's wine and having more than 568 independent growers.
While studying you will be enabled to build close links with the local wineries and vineyards with field trips to experience all stages of wine production. Winemakers, viticulturists and industry specialists also come in as guest speakers.
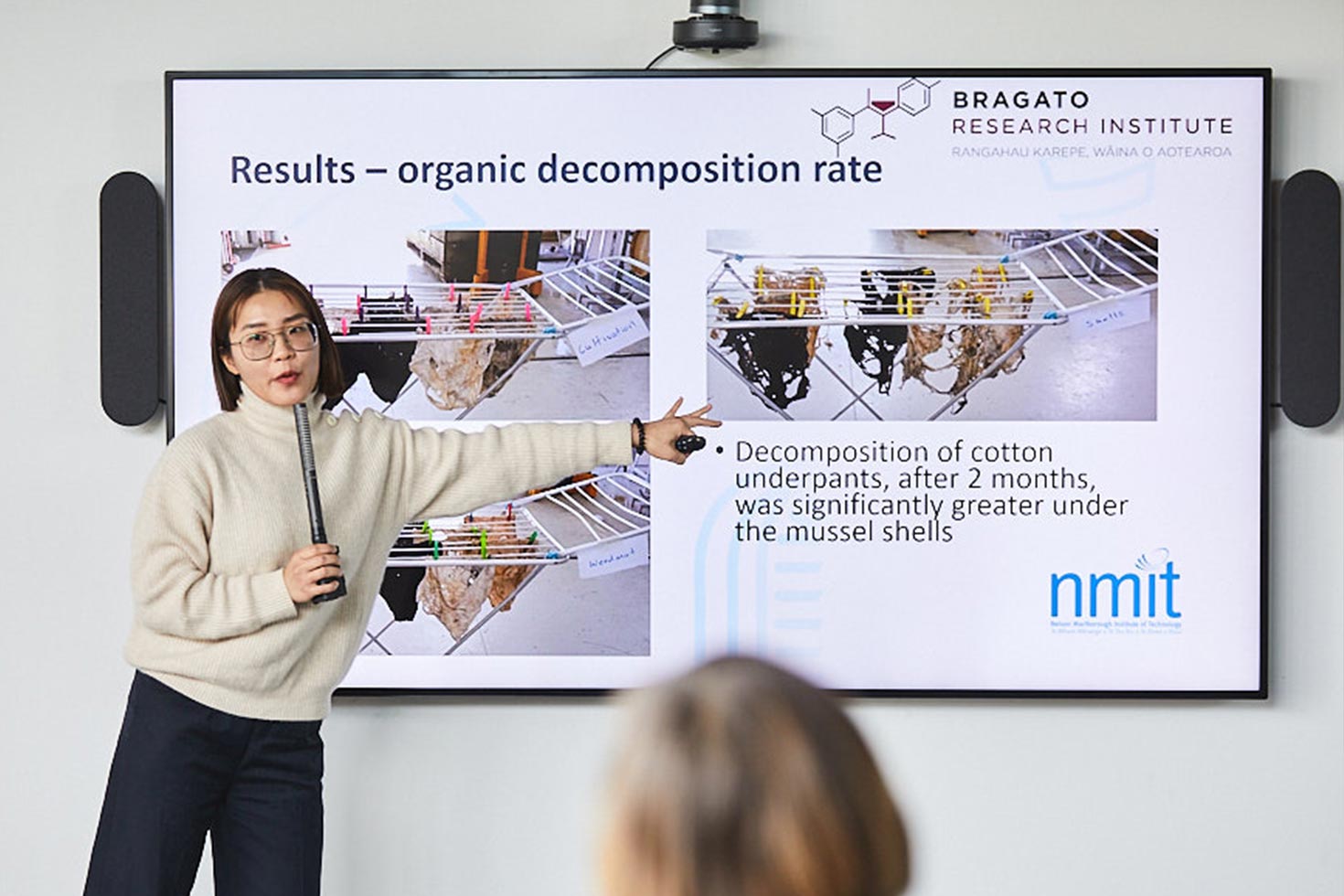
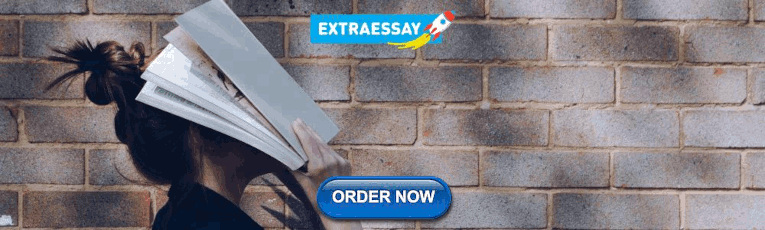
Upcoming programme intakes
Related programmes
Bachelor of viticulture and winemaking, new zealand diploma in primary industry business management, new zealand diploma in horticulture production (winegrowing), new zealand certificate in cellar operations, new zealand certificate in horticulture production (wine growing), new zealand certificate in primary industry skills - viticulture, new zealand certificate in primary industry skills - logistics and distribution, what's happening, recent graduates thank local industry, nmit at winepro, nmit at the marlborough wine and food festival, experience: cellar operations.

An official website of the United States government
Here’s how you know
Official websites use .gov A .gov website belongs to an official government organization in the United States.
Secure .gov websites use HTTPS A lock ( Lock A locked padlock ) or https:// means you’ve safely connected to the .gov website. Share sensitive information only on official, secure websites.
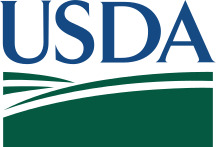
Animal and Plant Health Inspection Service
Updates on H5N1 Beef Safety Studies
May 1, 2024: USDA’s Food Safety and Inspection Service (FSIS) is announcing results from its testing of retail ground beef. FSIS collected 30 samples of ground beef from retail outlets in the states with dairy cattle herds that had tested positive for the H5N1 influenza virus at the time of sample collection. The samples were sent to APHIS’ National Veterinary Services Laboratories (NVSL) for PCR testing. On May 1, NVSL reported that all samples tested negative for H5N1. These results reaffirm that the meat supply is safe.
USDA is confident that the meat supply is safe. USDA has a rigorous meat inspection process, where USDA’s Food Safety and Inspection Service (FSIS) veterinarians are present at all federal livestock slaughter facilities. FSIS inspects each animal before slaughter, and all cattle carcasses must pass inspection after slaughter and be determined to be fit to enter the human food supply.
While we have multiple safeguards in place to protect consumers, we recommend consumers properly handle raw meats and cook to a safe internal temperature. Cooking to a safe internal temperature kills bacteria and viruses in meat. Specific recommendations are available online at: Safe Minimum Internal Temperature Chart | Food Safety and Inspection Service (usda.gov) .
Ongoing Research
To verify the safety of the meat supply in the context of H5N1, USDA’s FSIS, APHIS, and Agricultural Research Service (ARS) are working on three separate beef safety studies related to avian influenza in meat from dairy cattle. These studies are taking place in the interest of scientific inquiry and reaffirm consumer confidence.
- Samples of ground beef obtained at retail in the affected States: Final results were posted on May 1, 2024. Samples were collected at retail outlets in the States in which dairy cattle herds have tested positive for H5N1 influenza virus. The samples were analyzed by APHIS using polymerase chain reaction (PCR), to indicate whether any viral particles were present. No virus particles were found to be present .
- Beef muscle sampling of cull dairy cows condemned at select FSIS-inspected slaughter facilities: FSIS is currently collecting muscle samples at FSIS-inspected slaughter facilities of cull dairy cattle that have been condemned for systemic pathologies. The samples will be analyzed by APHIS using PCR to determine presence of viral particles. The results are forthcoming and will be posted as soon as they become available.
- Ground beef cooking study: ARS will be conducting a beef cooking study and will be using a virus surrogate in ground beef and cooking it at different temperatures to determine log-reduction of the virus. The results will be posted as soon as they become available.
share this!
April 26, 2024
This article has been reviewed according to Science X's editorial process and policies . Editors have highlighted the following attributes while ensuring the content's credibility:
fact-checked
peer-reviewed publication
trusted source
How bad are invasive plants for birds? Research suggests large-scale removal may not have intended benefits
by Great Hollow Nature Preserve & Ecological Research Center
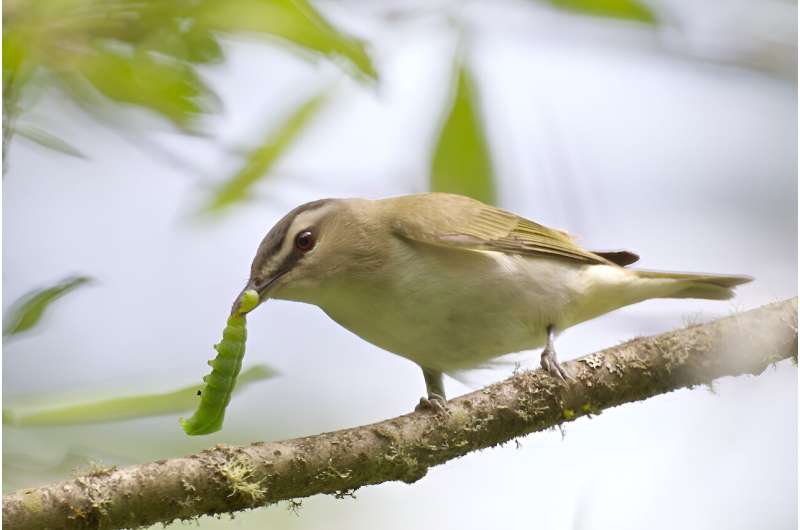
A prevailing opinion in land management is that non-native invasive plants are of no ecological value and they significantly diminish habitat quality for wildlife. Conservation practitioners allocate significant resources to invasive plant removal, often relying on surrounding native plants to passively fill the void. However, evidence that this practice improves food abundance or quality for wildlife is surprisingly limited.
In a new study published in the journal Biological Invasions , researchers in Connecticut, U.S. show that some of the most vilified invasive plants in northeastern U.S. forests may actually be of comparable value to native plants as foraging resources for insectivorous birds, and large-scale invasive plant removal on behalf of these birds may not have the intended benefits.
The research team from Great Hollow Nature Preserve and Ecological Research Center (New Fairfield, CT, U.S.) and Wesleyan University (Middletown, CT, U.S.) conducted a large-scale bird-exclusion experiment in which they compared arthropod biomass and bird foraging intensity among four species of non-native, invasive woody plants and six of the most dominant native species in an 800-acre forest preserve in western Connecticut.
They also measured the protein content (percent elemental nitrogen) of the arthropods on these plants as an indicator of food quality since protein strongly mediates food selection by breeding birds and is critical to offspring development. The study involved the collection, identification, and isotopic analysis of more than 17,000 arthropods from the branches of 240 trees and shrubs, half of which were covered with bird-exclusion netting.
The non-native invasive plants in the study included Japanese barberry, Morrow's honeysuckle, burning bush, and autumn olive while the natives included striped maple, shadbush, musclewood, witch-hazel, sweet birch, and American beech. In the common stewardship practice of removing invasives without actively planting and fostering natives afterwards, these six native species are among those most likely to fill the void left by invasive plant removal in secondary growth forests in southern New England.
They are therefore among the most realistic alternatives to invasive plants facing managers of such forests, as opposed to oaks, which are generally considered high-quality sources of insect prey for wildlife, but have been regenerating poorly and steeply declining in the eastern U.S. for nearly a century.
Unexpectedly, all lines of evidence in the study suggested the invasive plants were comparable to the natives in their value as foraging resources for birds. Arthropod biomass and protein content were broadly similar between the native and non-native species, and in turn, the birds foraged just as intensively on the non-native plants as they did on the natives.
Non-native honeysuckle stood out as having particularly high prey biomass and quality and frequent visitation by foraging birds, while Japanese barberry generally ranked the lowest.
"Our results indicate that it should first be demonstrated, not assumed, that invasive plants are inferior resources for birds compared to the dominant native plants in the community before land managers undertake costly removal efforts," said Dr. Chad Seewagen, one of the study's authors.
"Clearly some invasives are worse than others and it's not as simple as all invasive plants must go. While we certainly do not suggest that invasive plants have no negative ecological impacts, our study shows that coexisting native plants are not always superior resources for wildlife and that context is important.
"Managers need to know whether the native plants that are most likely to replace removed invasives are really of greater value to the wildlife for which they are managing the habitat, and if that effort is worth the cost and disturbance."
The study recommends a more nuanced approach to invasive plant management in eastern North American forests, where the services provided by non- native plants are considered against the backdrop of the native plant community in which they have become established.
Journal information: Biological Invasions
Provided by Great Hollow Nature Preserve & Ecological Research Center
Explore further
Feedback to editors

New Nevada experiments aim to improve monitoring of nuclear explosions
4 minutes ago

Lake tsunamis pose significant threat under warming climate

'Degree of Kevin Bacon' gene provides possible basis for central players in group connectedness

Physicists pioneer new quantum sensing platform
5 minutes ago

Cold sintering may rescue plastic, ceramics, battery components from landfills
7 minutes ago

New mirror that can be flexibly shaped improves X-ray microscopes
15 minutes ago

Astronomers inspect population of young stellar objects in open cluster NGC 346

The BREAD Collaboration is searching for dark photons using a coaxial dish antenna
2 hours ago

Research quantifies 'gap' in carbon removal for first time—shows countries need more awareness, ambition and action
4 hours ago

China sends a probe to get samples from the less-explored far side of the moon
5 hours ago
Relevant PhysicsForums posts
The cass report (uk).
May 1, 2024
Is 5 milliamps at 240 volts dangerous?
Apr 29, 2024
Major Evolution in Action
Apr 22, 2024
If theres a 15% probability each month of getting a woman pregnant...
Apr 19, 2024
Can four legged animals drink from beneath their feet?
Apr 15, 2024
Mold in Plastic Water Bottles? What does it eat?
Apr 14, 2024
More from Biology and Medical
Related Stories
Invasive japanese barberry drives down invertebrate richness and abundance.
Sep 4, 2019

Native forest plants rebound when invasive shrubs are removed
May 10, 2019

Invasive shrub has no effect on prey abundance or physiological condition of migratory songbird
May 5, 2020

Deer prefer native plants leaving lasting damage on forests
Oct 6, 2017

Gardeners can help identify potentially invasive plants
Feb 27, 2024

Invasive rodent research may help protect Hawaiian forests
Feb 5, 2024
Recommended for you

Research shows bumblebee nests are overheating due to climate change, threatening future populations
9 hours ago

Study finds human noise negatively impacts cricket survival and reproduction
16 hours ago

Bigger brains allow cliff-nesting seagull species to survive and thrive in urban environments
17 hours ago

For microscopic organisms, ocean currents act as 'expressway' to deeper depths, study finds

Pet parrots prefer live video-calls over watching pre-recorded videos of other birds
May 2, 2024
Let us know if there is a problem with our content
Use this form if you have come across a typo, inaccuracy or would like to send an edit request for the content on this page. For general inquiries, please use our contact form . For general feedback, use the public comments section below (please adhere to guidelines ).
Please select the most appropriate category to facilitate processing of your request
Thank you for taking time to provide your feedback to the editors.
Your feedback is important to us. However, we do not guarantee individual replies due to the high volume of messages.
E-mail the story
Your email address is used only to let the recipient know who sent the email. Neither your address nor the recipient's address will be used for any other purpose. The information you enter will appear in your e-mail message and is not retained by Phys.org in any form.
Newsletter sign up
Get weekly and/or daily updates delivered to your inbox. You can unsubscribe at any time and we'll never share your details to third parties.
More information Privacy policy
Donate and enjoy an ad-free experience
We keep our content available to everyone. Consider supporting Science X's mission by getting a premium account.
E-mail newsletter
April 15, 2024
New MSU dairy, greenhouse facilities critical to ag research, food production
MSU broke ground on two new infrastructure projects that are critical to the future of Michigan food production and agriculture. Construction began on an updated, state-of-the-art Dairy Cattle Teaching and Research Center, as well as substantial renovations and additions to the Plant Science Greenhouses.
The new and renovated spaces will expand research capacity for MSU scientists in the colleges of Agriculture and Natural Resources, Natural Science and Veterinary Medicine, including research funded by MSU AgBioResearch.
The State of Michigan provided $53 million for partial funding of each project. Both projects received strong bipartisan support from Michigan’s agricultural community.
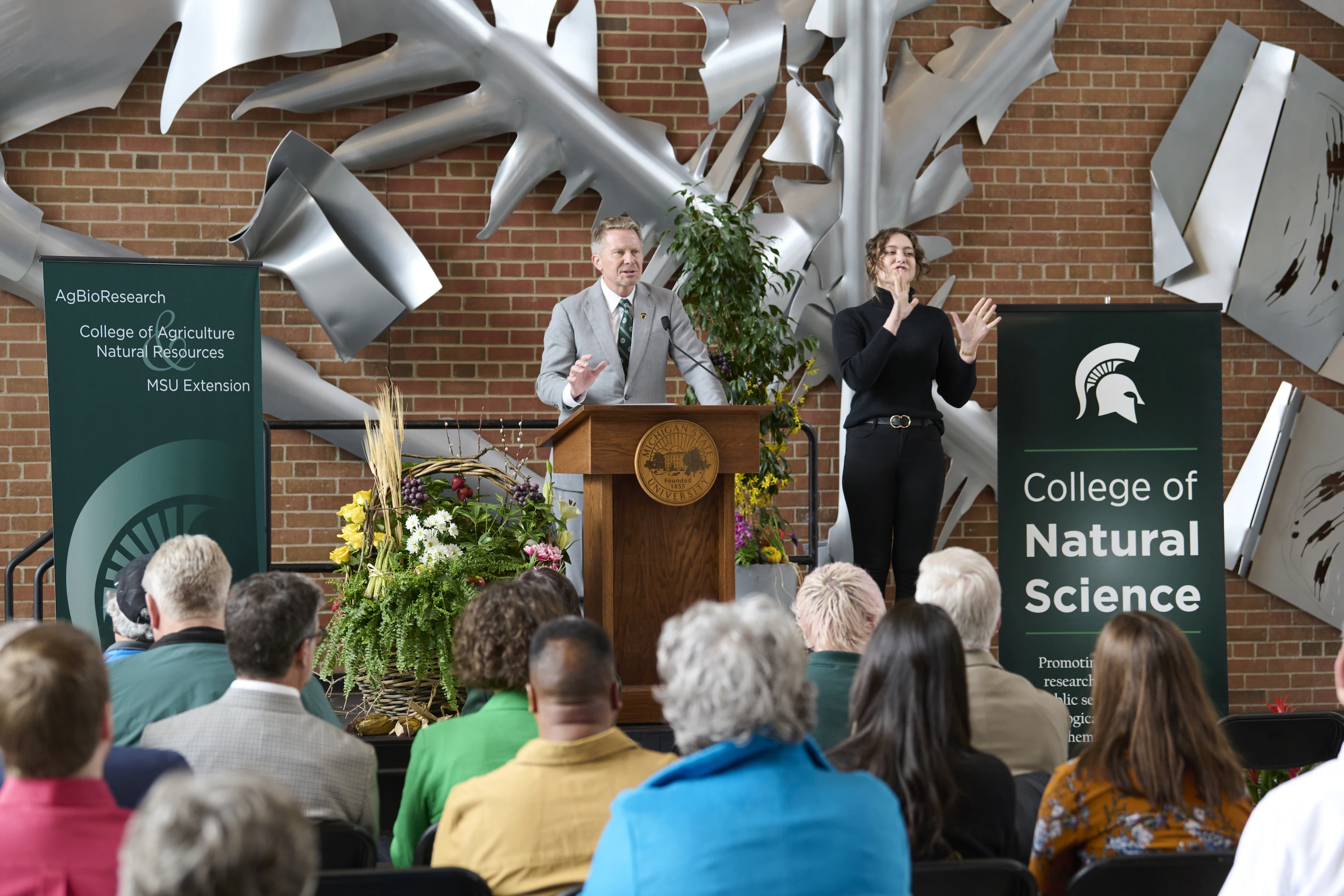
MSU and community leaders attended the Dairy Cattle Teaching and Research Center groundbreaking on Thursday, April 11, and the greenhouse groundbreaking on Friday, April 12.
“Not only do these two facilities play a vital role in supporting our agricultural partners with cutting-edge knowledge, but they are crucial assets to develop the next generation of dairy industry and plant science leaders,” said MSU President Kevin M. Guskiewicz. “These much-needed facility enhancements will serve MSU students, scientists and stakeholders well into the future, and we are grateful to the Legislature and governor for their support.”
“These two projects are a testament to the power of collaboration, uniting stakeholders across multiple sectors to advance sustainability in plant science best practices and high-quality dairy production,” said MSU Chair Dan Kelly. “These projects support our world-class scientists and students and empower them to work alongside the agricultural community, driving innovations that promote productivity and sustainability.”
New Dairy Cattle Teaching and Research Center
The existing MSU Dairy Cattle Teaching and Research Center currently houses 250 dairy cattle and supports the research of faculty in the colleges of Agriculture and Natural Resources and Veterinary Medicine. The new dairy facility will expand research capacity by increasing herd size to 680 in addition to creating modernized barns, feed centers, milking parlors and laboratories. The new facility also will provide spaces for student instruction. The project is budgeted at $75 million.
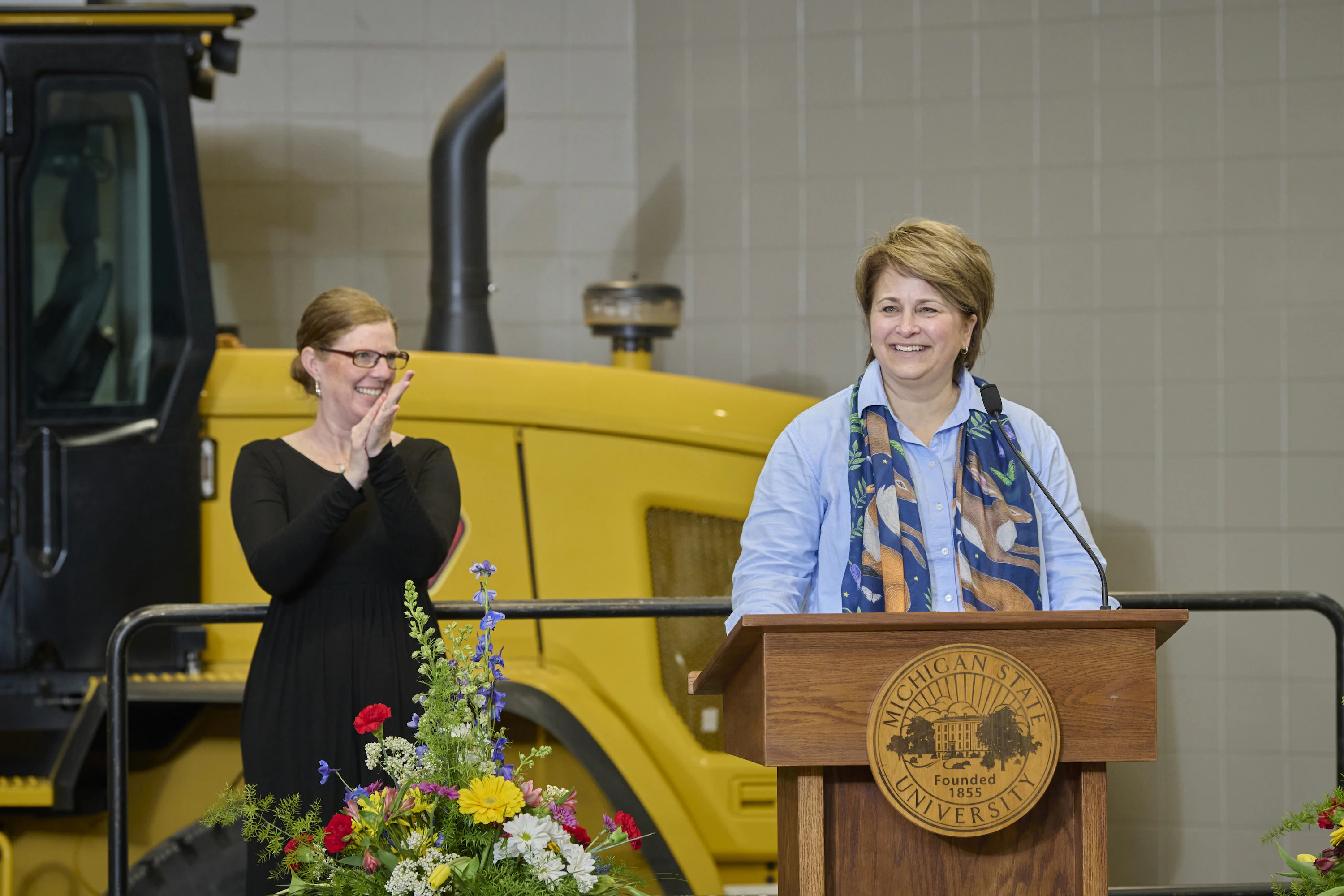
“This new dairy facility will stand as a testament to the power of partnerships,” said Kelly Millenbah, dean of the College of Agriculture and Natural Resources. “These spaces for research, education and outreach will serve Michigan’s farmers and develop tomorrow’s workforce, and we’re so grateful for this investment in the future of agriculture at Michigan State.”
The College of Veterinary Medicine also will benefit from the new research center.
“The dairy industry has advanced well beyond the center’s current capacity, particularly in regard to research potential and teaching modern production practices,” said Doug Freeman, interim dean of the College of Veterinary Medicine. “As we train the next generation of veterinarians, the center will allow them to develop significantly enhanced skills in dairy production medicine, which will enable them to serve clients and better protect local and global food systems.”
Plant Science Greenhouses
MSU’s Plant Science Greenhouses are a research and teaching hub for 70 faculty and 600 students in the colleges of Agriculture and Natural Resources and Natural Science. The renovations and additions have a project budget of $35 million and will include state-of-the-art environmental controls and energy-efficient LED lighting configured to external climate conditions. The project also includes a new headhouse, which will provide administrative and research space.
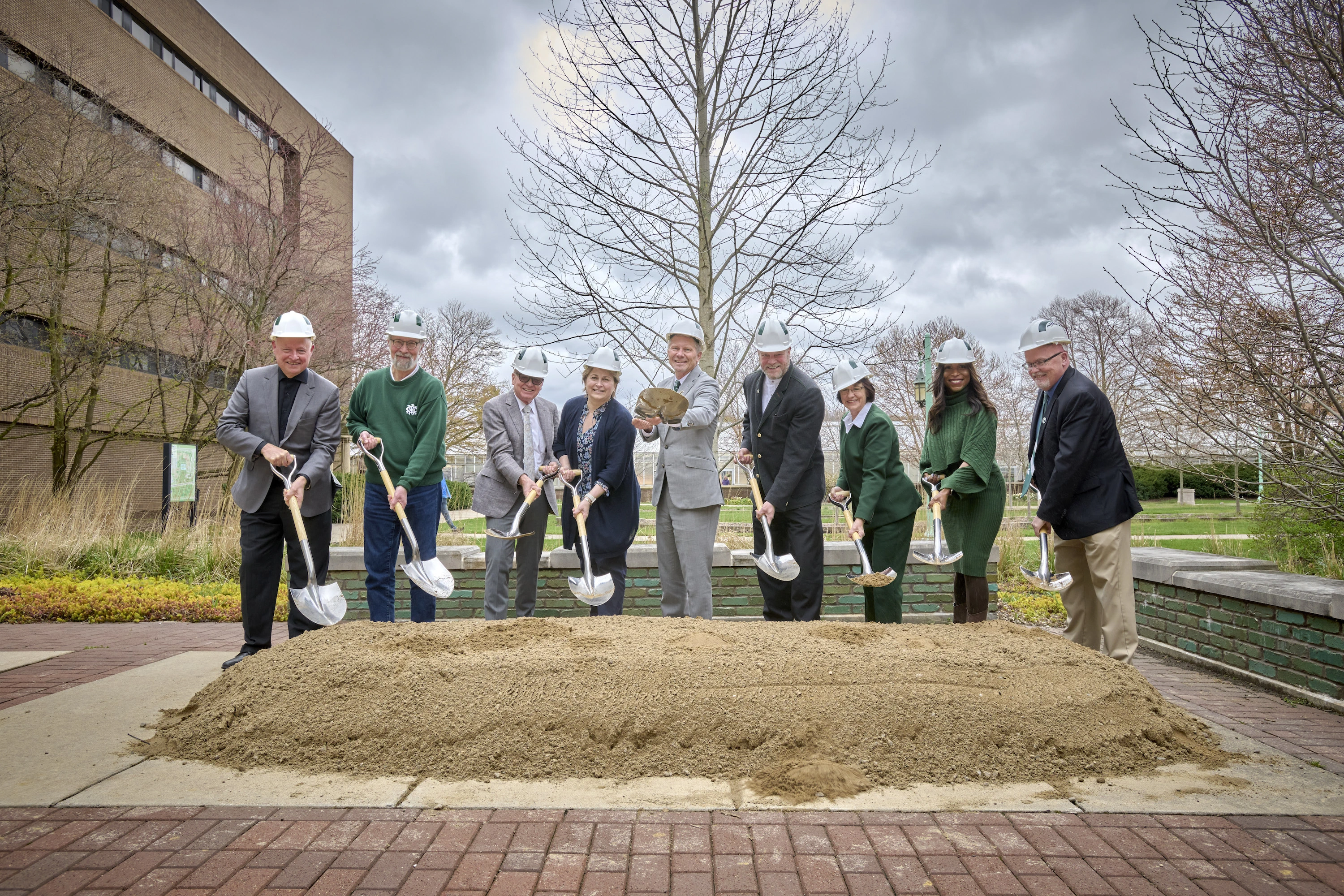
“MSU is a world-leading center for plant sciences research that drives innovation in the agricultural economy and addresses the impacts of a changing climate and emerging diseases, pests and contaminants,” said Phillip Duxbury, dean of the College of Natural Science. “Well-controlled greenhouse environments are essential to maintaining MSU’s leadership in these areas and we are very grateful to Michigan’s political leaders, the board and MSU leadership for supporting these high-impact upgrades.”
By: Kim Ward
Media Contacts
Related campus stories, university activities board celebrates 60 years of spring arts and crafts show in may, msu law is number one in national 2023-24 moot court competitions.
- Graduate voice: The history and future of the Campus Arboretum at 150 years
more content from this collection
Spartan innovation feeds Michigan’s food and agriculture industry
Growing success: spartans support first-generation farmers, the hidden complexity of the montmorency tart cherry genome, michigan fruit growers seek solutions for spotted wing drosophila, new msu research helps better quantify soil carbon stock changes, the future of agriculture: msu researchers are developing sustainable solutions to feed a fast-changing world, podcast: agricultural innovation working to feed a growing population in the face of climate challenges, gov. whitmer appoints msu’s wu to agriculture commission, helping michigan growers pick the best hard cider apples, good food that is better for the environment, spotting tar spot disease sooner, see full collection.
May 2, 2024
May 1, 2024
Renaissance scientists in the Mendoza lab tackle climate change
Msutoday weekly update.
The MSUToday Weekly Update email showcases how Spartans are making a difference through academic excellence, research impact and community outreach. Get inspired by these stories of innovation, collaboration and determination. Plus, enjoy photos and videos of campus and more MSU content to help keep you connected to the Spartan community.
Connect With Us
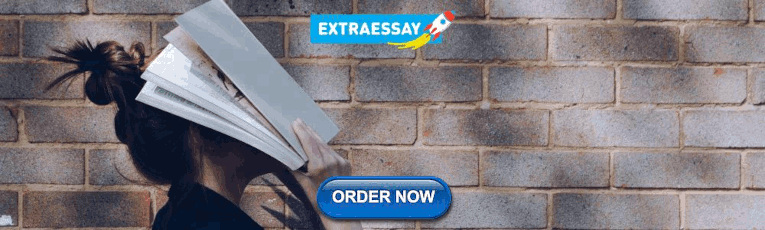
IMAGES
VIDEO
COMMENTS
With our partners, we use world-leading science to improve the way they grow, fish, harvest, prepare and share food. Every day, we have 1000 people working across Aotearoa New Zealand and the world to help deliver healthy foods from the world's most sustainable systems. Our smart green future starts here.
At Plant & Food Research, we believe science can create a better future. By finding smarter, greener options today, we're helping secure the world we want to live in tomorrow. With our partners, we use world-leading science to improve the way they grow, fish, harvest, prepare and share food. Every day, we have 1000 people working across ...
The New Zealand Institute for Plant and Food Research Limited | 19,686 followers on LinkedIn. A smart green future. Together. | At Plant & Food Research, we believe science can create a better future. ... Blenheim, Marlborough 7201, NZ Get directions 55 Old Mill Road, RD3 Motueka , 7198, NZ Get directions 297 Akersten Street, Port Nelson ...
Development of the Experimental Future Vineyard is projected to cost $3.3 million including $770,000 reallocated from the Government's earlier Kanoa Provincial Growth Fund loan to the Marlborough Research Centre. Plant & Food Research will operate the facility and develop research programmes in collaboration with the Marlborough Research ...
The New Zealand Institute for Plant and Food Research Limited will operate the facility and develop research programmes in collaboration with the Marlborough Research Centre, national and local ...
Plant & Food Research - The Marlborough Research Centre Trust ... Latest News
This campus is shared with Plant & Food Research, NZ Wine, Wine Marlborough and NZ Dryland Forests Initiative. MRC's Grovetown Park campus is 2km away, on State Highway 1. This is a commercial campus with tenants ranging from the Ministry for Primary Industries, Hill Laboratories and commercial businesses. ... Marlborough Research Centre, 85 ...
Marlborough District Council is a funding partner of the Marlborough Research Centre (MRC), a non-profit research organisation operating from two campuses located in Blenheim. ... FoodHQ and key primary sector organisations such as NZ Winegrowers and Plant & Food Research. MRC operates a Food & Beverage Innovation Cluster, which provides ...
In 1999 Dion joined the Plant & Food Research team in Marlborough and has been working with horticultural crops in Marlborough ever since. Skills and Expertise. Plant Pathology.
In 1999 Dion joined the Plant & Food Research team in Marlborough and has been working with horticultural crops in Marlborough ever since. View profile. Members (3) Andrew McLachlan.
New Zealand Institute for Plant and Food Research Ltd, Marlborough Wine Research Centre, PO Box 845, Blenheim, New Zealand. Dr Rob Bramley, fax +61 8 8303 8936, email [email protected]. Search for more papers by this author. M.C.T. TROUGHT. New Zealand Institute for Plant and Food Research Ltd, Marlborough Wine Research Centre, PO Box 845 ...
The New Zealand Institute for Plant and Food Research Limited (PFR), Marlborough Research Centre, PO Box 845, Blenheim 7240, New Zealand. ... The New Zealand Institute for Plant and Food Research Limited, Canterbury Agriculture & Science Centre, Private Bag 4704, Christchurch 8140,
OIA requests. As a Crown Research Institute, Plant & Food Research is subject to the New Zealand Official Information Act (OIA). To contact us with a request under the OIA, please email: [email protected]. For more information about the OIA, who can submit a request and the types of information that can be requested, please visit the Ombudsman's website.
For over 25 years The New Zealand Institute for Plant & Food Research Ltd (PFR) and its antecedents have operated a network of weather stations in the main New Zealand horticultural regions.
3 Institute of Plant and Food Research, Marlborough, New Zealand. 4 Institute of Plant and Food Research, Palmerston North, New Zealand. 5 Tripwire Wine Consulting Ltd. & Misha's Vineyard, Central Otago, New Zealand. 6 School of Chemical Sciences, The University of Auckland, New Zealand.
A single Marlborough isolate from the Plant & Food Research collection was found to be Cytospora chrysosperma by ITS sequencing (Table 2). Pestalotioid fungi Single isolates of a Pestalotiopsis sp. and Truncatella angustata (morphologically identified) from New Zealand grapevines are present in ICMP ( Table 2 ).
for The New Zealand Institute for Plant and . Food Research Limited (Plant & Food Research) for the financial year ending 30 June 2023. ... Co-located at Marlborough Research . Centre. Includes 10ha of research farm. RESEARCH ; PLANTINGS STAFF. Research; 11. General. 2. Total. 13. 15/ 16/ Australia. 15. South Australia. 16. Queensland.
The centre is a collaboration between NMIT, universities, Bragato Research Institute, Plant and Food Research, the Marlborough District Council and the owner, the Marlborough Research Centre Trust. With a supportive network of scientists conducting innovative research in viticulture and winemaking on campus, NMIT students are fortunate to rub ...
Experience: Plant and Food Research · Location: Blenheim, Marlborough, New Zealand · 83 connections on LinkedIn. View Lily Stuart's profile on LinkedIn, a professional community of 1 billion members.
Plant & Food Research, formerly DSIR is a New Zealand-based science company providing research and development that adds value to fruit, vegetable, crop and food products. In Auckland there are research teams covering horticulture, cropping, wasp and other insect control, plant disease, landcare and air and water pollution by chemicals. ...
What's New. May 1, 2024: USDA's Food Safety and Inspection Service (FSIS) is announcing results from its testing of retail ground beef. FSIS collected 30 samples of ground beef from retail outlets in the states with dairy cattle herds that had tested positive for the H5N1 influenza virus at the time of sample collection.
CSIRO Food Futures Flagship and CSIRO Ecosystem Sciences, PMB 2, Glen Osmond, SA 5064, Australia. New Zealand Institute for Plant and Food Research Ltd, Marlborough Wine Research Centre, PO Box 845, Blenheim, New Zealand. Dr Rob Bramley, fax +61 8 8303 8936, email [email protected] Search for more papers by this author
The research team from Great Hollow Nature Preserve and Ecological Research Center (New Fairfield, CT, U.S.) and Wesleyan University (Middletown, CT, U.S.) conducted a large-scale bird-exclusion ...
MSU's Plant Science Greenhouses are a research and teaching hub for 70 faculty and 600 students in the colleges of Agriculture and Natural Resources and Natural Science. The renovations and additions have a project budget of $35 million and will include state-of-the-art environmental controls and energy-efficient LED lighting configured to ...