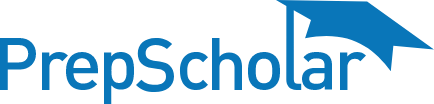
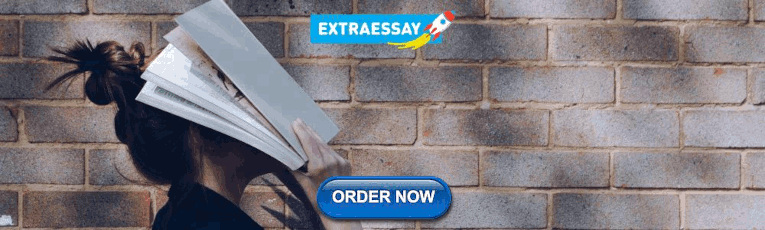
Choose Your Test
Sat / act prep online guides and tips, 113 great research paper topics.
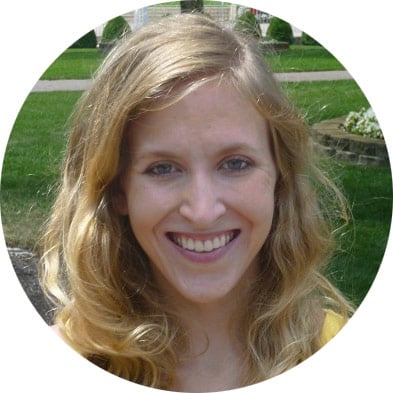
General Education
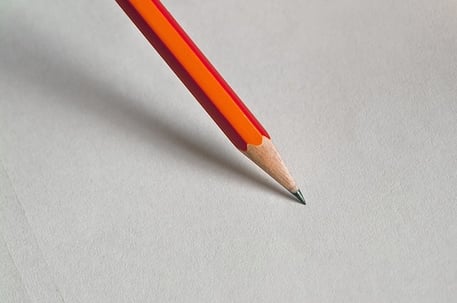
One of the hardest parts of writing a research paper can be just finding a good topic to write about. Fortunately we've done the hard work for you and have compiled a list of 113 interesting research paper topics. They've been organized into ten categories and cover a wide range of subjects so you can easily find the best topic for you.
In addition to the list of good research topics, we've included advice on what makes a good research paper topic and how you can use your topic to start writing a great paper.
What Makes a Good Research Paper Topic?
Not all research paper topics are created equal, and you want to make sure you choose a great topic before you start writing. Below are the three most important factors to consider to make sure you choose the best research paper topics.
#1: It's Something You're Interested In
A paper is always easier to write if you're interested in the topic, and you'll be more motivated to do in-depth research and write a paper that really covers the entire subject. Even if a certain research paper topic is getting a lot of buzz right now or other people seem interested in writing about it, don't feel tempted to make it your topic unless you genuinely have some sort of interest in it as well.
#2: There's Enough Information to Write a Paper
Even if you come up with the absolute best research paper topic and you're so excited to write about it, you won't be able to produce a good paper if there isn't enough research about the topic. This can happen for very specific or specialized topics, as well as topics that are too new to have enough research done on them at the moment. Easy research paper topics will always be topics with enough information to write a full-length paper.
Trying to write a research paper on a topic that doesn't have much research on it is incredibly hard, so before you decide on a topic, do a bit of preliminary searching and make sure you'll have all the information you need to write your paper.
#3: It Fits Your Teacher's Guidelines
Don't get so carried away looking at lists of research paper topics that you forget any requirements or restrictions your teacher may have put on research topic ideas. If you're writing a research paper on a health-related topic, deciding to write about the impact of rap on the music scene probably won't be allowed, but there may be some sort of leeway. For example, if you're really interested in current events but your teacher wants you to write a research paper on a history topic, you may be able to choose a topic that fits both categories, like exploring the relationship between the US and North Korea. No matter what, always get your research paper topic approved by your teacher first before you begin writing.
113 Good Research Paper Topics
Below are 113 good research topics to help you get you started on your paper. We've organized them into ten categories to make it easier to find the type of research paper topics you're looking for.
Arts/Culture
- Discuss the main differences in art from the Italian Renaissance and the Northern Renaissance .
- Analyze the impact a famous artist had on the world.
- How is sexism portrayed in different types of media (music, film, video games, etc.)? Has the amount/type of sexism changed over the years?
- How has the music of slaves brought over from Africa shaped modern American music?
- How has rap music evolved in the past decade?
- How has the portrayal of minorities in the media changed?
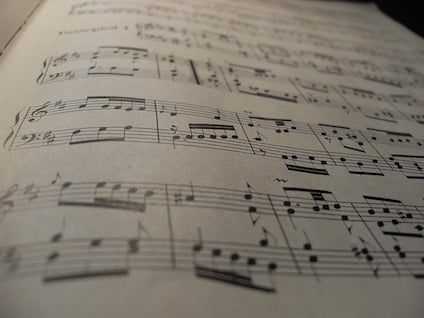
Current Events
- What have been the impacts of China's one child policy?
- How have the goals of feminists changed over the decades?
- How has the Trump presidency changed international relations?
- Analyze the history of the relationship between the United States and North Korea.
- What factors contributed to the current decline in the rate of unemployment?
- What have been the impacts of states which have increased their minimum wage?
- How do US immigration laws compare to immigration laws of other countries?
- How have the US's immigration laws changed in the past few years/decades?
- How has the Black Lives Matter movement affected discussions and view about racism in the US?
- What impact has the Affordable Care Act had on healthcare in the US?
- What factors contributed to the UK deciding to leave the EU (Brexit)?
- What factors contributed to China becoming an economic power?
- Discuss the history of Bitcoin or other cryptocurrencies (some of which tokenize the S&P 500 Index on the blockchain) .
- Do students in schools that eliminate grades do better in college and their careers?
- Do students from wealthier backgrounds score higher on standardized tests?
- Do students who receive free meals at school get higher grades compared to when they weren't receiving a free meal?
- Do students who attend charter schools score higher on standardized tests than students in public schools?
- Do students learn better in same-sex classrooms?
- How does giving each student access to an iPad or laptop affect their studies?
- What are the benefits and drawbacks of the Montessori Method ?
- Do children who attend preschool do better in school later on?
- What was the impact of the No Child Left Behind act?
- How does the US education system compare to education systems in other countries?
- What impact does mandatory physical education classes have on students' health?
- Which methods are most effective at reducing bullying in schools?
- Do homeschoolers who attend college do as well as students who attended traditional schools?
- Does offering tenure increase or decrease quality of teaching?
- How does college debt affect future life choices of students?
- Should graduate students be able to form unions?
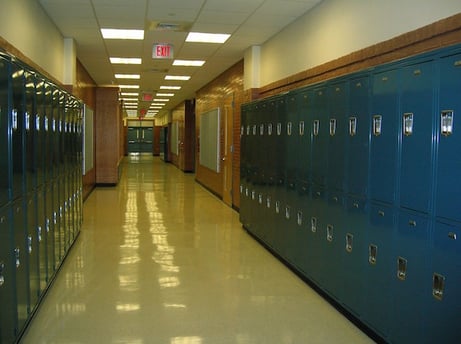
- What are different ways to lower gun-related deaths in the US?
- How and why have divorce rates changed over time?
- Is affirmative action still necessary in education and/or the workplace?
- Should physician-assisted suicide be legal?
- How has stem cell research impacted the medical field?
- How can human trafficking be reduced in the United States/world?
- Should people be able to donate organs in exchange for money?
- Which types of juvenile punishment have proven most effective at preventing future crimes?
- Has the increase in US airport security made passengers safer?
- Analyze the immigration policies of certain countries and how they are similar and different from one another.
- Several states have legalized recreational marijuana. What positive and negative impacts have they experienced as a result?
- Do tariffs increase the number of domestic jobs?
- Which prison reforms have proven most effective?
- Should governments be able to censor certain information on the internet?
- Which methods/programs have been most effective at reducing teen pregnancy?
- What are the benefits and drawbacks of the Keto diet?
- How effective are different exercise regimes for losing weight and maintaining weight loss?
- How do the healthcare plans of various countries differ from each other?
- What are the most effective ways to treat depression ?
- What are the pros and cons of genetically modified foods?
- Which methods are most effective for improving memory?
- What can be done to lower healthcare costs in the US?
- What factors contributed to the current opioid crisis?
- Analyze the history and impact of the HIV/AIDS epidemic .
- Are low-carbohydrate or low-fat diets more effective for weight loss?
- How much exercise should the average adult be getting each week?
- Which methods are most effective to get parents to vaccinate their children?
- What are the pros and cons of clean needle programs?
- How does stress affect the body?
- Discuss the history of the conflict between Israel and the Palestinians.
- What were the causes and effects of the Salem Witch Trials?
- Who was responsible for the Iran-Contra situation?
- How has New Orleans and the government's response to natural disasters changed since Hurricane Katrina?
- What events led to the fall of the Roman Empire?
- What were the impacts of British rule in India ?
- Was the atomic bombing of Hiroshima and Nagasaki necessary?
- What were the successes and failures of the women's suffrage movement in the United States?
- What were the causes of the Civil War?
- How did Abraham Lincoln's assassination impact the country and reconstruction after the Civil War?
- Which factors contributed to the colonies winning the American Revolution?
- What caused Hitler's rise to power?
- Discuss how a specific invention impacted history.
- What led to Cleopatra's fall as ruler of Egypt?
- How has Japan changed and evolved over the centuries?
- What were the causes of the Rwandan genocide ?
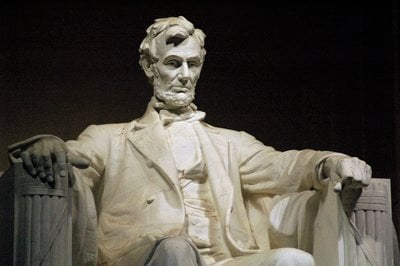
- Why did Martin Luther decide to split with the Catholic Church?
- Analyze the history and impact of a well-known cult (Jonestown, Manson family, etc.)
- How did the sexual abuse scandal impact how people view the Catholic Church?
- How has the Catholic church's power changed over the past decades/centuries?
- What are the causes behind the rise in atheism/ agnosticism in the United States?
- What were the influences in Siddhartha's life resulted in him becoming the Buddha?
- How has media portrayal of Islam/Muslims changed since September 11th?
Science/Environment
- How has the earth's climate changed in the past few decades?
- How has the use and elimination of DDT affected bird populations in the US?
- Analyze how the number and severity of natural disasters have increased in the past few decades.
- Analyze deforestation rates in a certain area or globally over a period of time.
- How have past oil spills changed regulations and cleanup methods?
- How has the Flint water crisis changed water regulation safety?
- What are the pros and cons of fracking?
- What impact has the Paris Climate Agreement had so far?
- What have NASA's biggest successes and failures been?
- How can we improve access to clean water around the world?
- Does ecotourism actually have a positive impact on the environment?
- Should the US rely on nuclear energy more?
- What can be done to save amphibian species currently at risk of extinction?
- What impact has climate change had on coral reefs?
- How are black holes created?
- Are teens who spend more time on social media more likely to suffer anxiety and/or depression?
- How will the loss of net neutrality affect internet users?
- Analyze the history and progress of self-driving vehicles.
- How has the use of drones changed surveillance and warfare methods?
- Has social media made people more or less connected?
- What progress has currently been made with artificial intelligence ?
- Do smartphones increase or decrease workplace productivity?
- What are the most effective ways to use technology in the classroom?
- How is Google search affecting our intelligence?
- When is the best age for a child to begin owning a smartphone?
- Has frequent texting reduced teen literacy rates?
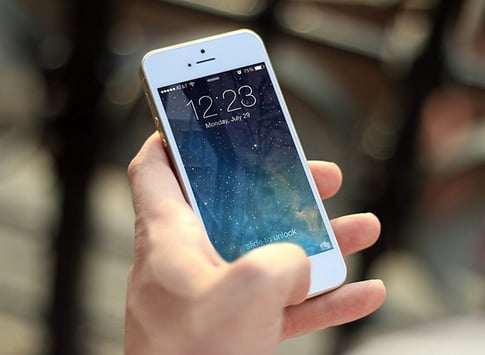
How to Write a Great Research Paper
Even great research paper topics won't give you a great research paper if you don't hone your topic before and during the writing process. Follow these three tips to turn good research paper topics into great papers.
#1: Figure Out Your Thesis Early
Before you start writing a single word of your paper, you first need to know what your thesis will be. Your thesis is a statement that explains what you intend to prove/show in your paper. Every sentence in your research paper will relate back to your thesis, so you don't want to start writing without it!
As some examples, if you're writing a research paper on if students learn better in same-sex classrooms, your thesis might be "Research has shown that elementary-age students in same-sex classrooms score higher on standardized tests and report feeling more comfortable in the classroom."
If you're writing a paper on the causes of the Civil War, your thesis might be "While the dispute between the North and South over slavery is the most well-known cause of the Civil War, other key causes include differences in the economies of the North and South, states' rights, and territorial expansion."
#2: Back Every Statement Up With Research
Remember, this is a research paper you're writing, so you'll need to use lots of research to make your points. Every statement you give must be backed up with research, properly cited the way your teacher requested. You're allowed to include opinions of your own, but they must also be supported by the research you give.
#3: Do Your Research Before You Begin Writing
You don't want to start writing your research paper and then learn that there isn't enough research to back up the points you're making, or, even worse, that the research contradicts the points you're trying to make!
Get most of your research on your good research topics done before you begin writing. Then use the research you've collected to create a rough outline of what your paper will cover and the key points you're going to make. This will help keep your paper clear and organized, and it'll ensure you have enough research to produce a strong paper.
What's Next?
Are you also learning about dynamic equilibrium in your science class? We break this sometimes tricky concept down so it's easy to understand in our complete guide to dynamic equilibrium .
Thinking about becoming a nurse practitioner? Nurse practitioners have one of the fastest growing careers in the country, and we have all the information you need to know about what to expect from nurse practitioner school .
Want to know the fastest and easiest ways to convert between Fahrenheit and Celsius? We've got you covered! Check out our guide to the best ways to convert Celsius to Fahrenheit (or vice versa).
These recommendations are based solely on our knowledge and experience. If you purchase an item through one of our links, PrepScholar may receive a commission.
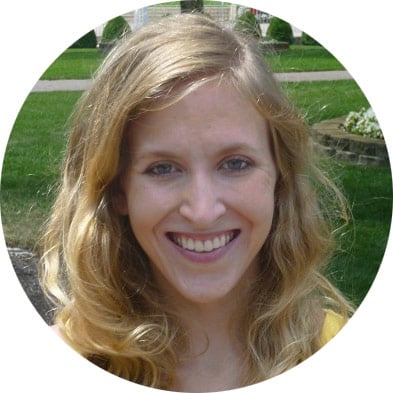
Christine graduated from Michigan State University with degrees in Environmental Biology and Geography and received her Master's from Duke University. In high school she scored in the 99th percentile on the SAT and was named a National Merit Finalist. She has taught English and biology in several countries.
Student and Parent Forum
Our new student and parent forum, at ExpertHub.PrepScholar.com , allow you to interact with your peers and the PrepScholar staff. See how other students and parents are navigating high school, college, and the college admissions process. Ask questions; get answers.

Ask a Question Below
Have any questions about this article or other topics? Ask below and we'll reply!
Improve With Our Famous Guides
- For All Students
The 5 Strategies You Must Be Using to Improve 160+ SAT Points
How to Get a Perfect 1600, by a Perfect Scorer
Series: How to Get 800 on Each SAT Section:
Score 800 on SAT Math
Score 800 on SAT Reading
Score 800 on SAT Writing
Series: How to Get to 600 on Each SAT Section:
Score 600 on SAT Math
Score 600 on SAT Reading
Score 600 on SAT Writing
Free Complete Official SAT Practice Tests
What SAT Target Score Should You Be Aiming For?
15 Strategies to Improve Your SAT Essay
The 5 Strategies You Must Be Using to Improve 4+ ACT Points
How to Get a Perfect 36 ACT, by a Perfect Scorer
Series: How to Get 36 on Each ACT Section:
36 on ACT English
36 on ACT Math
36 on ACT Reading
36 on ACT Science
Series: How to Get to 24 on Each ACT Section:
24 on ACT English
24 on ACT Math
24 on ACT Reading
24 on ACT Science
What ACT target score should you be aiming for?
ACT Vocabulary You Must Know
ACT Writing: 15 Tips to Raise Your Essay Score
How to Get Into Harvard and the Ivy League
How to Get a Perfect 4.0 GPA
How to Write an Amazing College Essay
What Exactly Are Colleges Looking For?
Is the ACT easier than the SAT? A Comprehensive Guide
Should you retake your SAT or ACT?
When should you take the SAT or ACT?
Stay Informed

Get the latest articles and test prep tips!
Looking for Graduate School Test Prep?
Check out our top-rated graduate blogs here:
GRE Online Prep Blog
GMAT Online Prep Blog
TOEFL Online Prep Blog
Holly R. "I am absolutely overjoyed and cannot thank you enough for helping me!”
Navigating the Research Process: Research Topic - Examples
- Do You Have a Topic?
- Topic Background Reading
- Research Topic - Examples
- Gathering Background Information
- Different Resource Types
- Levels of Resources
- Developing Key Terms
- Using Key Terms
- Where to Search
- Filters, Sorting, and Saving
- Revising Your Topic
- Search Results Analysis
- Revising Your Search
- Evaluating Resources
- Answering the Research Question
- Citing Sources
- Determining Needed Information
- Writing Your Paper
Developing a Research Topic: Examples
When you have the topic(s) or variables operationalized or specific enough, you can use the connections you decided on to link them together. For example, if you decided to focus on pornography viewing habits among veterans with PTSD, this would contain three research topics or concepts to study–that is, PTSD, veterans, and pornography (viewing). If you want to just look at what the research says in general (such as in a literature review), you can turn that into a question such as “What does the research say about pornography viewing habits among veterans with PTSD?”
Note that this would be a fairly narrow topic. You could broaden it by looking at just pornography viewing among people with PTSD or pornography viewing in any veterans.
If you want to look at other variables such as spiritual well-being, you would need to think about how they might link together. For example, your research statement would be “What is the relationship between the pornography viewing habits in veterans with PTSD and their spiritual well-being?” But it could also be, “What is the difference between veterans with PTSD, veterans without PTSD, and non-veterans on pornography viewing habits on spiritual well-being?” Deciding how they are linked together and their relationships makes the research sentence.
In the first case, you are looking at the relationship between pornography (viewing), veterans, and PTSD, and you are looking at the impact that the pornography (viewing) has on veterans with PTSD especially in relation to their spiritual well-being. You are also looking at the relationships between spiritual well-being, veterans, and PTSD as well. These become the areas of focus for the literature review.
Click here to go to the next part.
Click here to go back to the Home page and flowchart.
- << Previous: Developing a Research Topic
- Next: Gathering Background Information >>
- Last Updated: Apr 29, 2024 3:24 PM
- URL: https://library.divinemercy.edu/research_process
- USC Libraries
- Research Guides
Organizing Your Social Sciences Research Paper
- Independent and Dependent Variables
- Purpose of Guide
- Design Flaws to Avoid
- Glossary of Research Terms
- Reading Research Effectively
- Narrowing a Topic Idea
- Broadening a Topic Idea
- Extending the Timeliness of a Topic Idea
- Academic Writing Style
- Applying Critical Thinking
- Choosing a Title
- Making an Outline
- Paragraph Development
- Research Process Video Series
- Executive Summary
- The C.A.R.S. Model
- Background Information
- The Research Problem/Question
- Theoretical Framework
- Citation Tracking
- Content Alert Services
- Evaluating Sources
- Primary Sources
- Secondary Sources
- Tiertiary Sources
- Scholarly vs. Popular Publications
- Qualitative Methods
- Quantitative Methods
- Insiderness
- Using Non-Textual Elements
- Limitations of the Study
- Common Grammar Mistakes
- Writing Concisely
- Avoiding Plagiarism
- Footnotes or Endnotes?
- Further Readings
- Generative AI and Writing
- USC Libraries Tutorials and Other Guides
- Bibliography
Definitions
Dependent Variable The variable that depends on other factors that are measured. These variables are expected to change as a result of an experimental manipulation of the independent variable or variables. It is the presumed effect.
Independent Variable The variable that is stable and unaffected by the other variables you are trying to measure. It refers to the condition of an experiment that is systematically manipulated by the investigator. It is the presumed cause.
Cramer, Duncan and Dennis Howitt. The SAGE Dictionary of Statistics . London: SAGE, 2004; Penslar, Robin Levin and Joan P. Porter. Institutional Review Board Guidebook: Introduction . Washington, DC: United States Department of Health and Human Services, 2010; "What are Dependent and Independent Variables?" Graphic Tutorial.
Identifying Dependent and Independent Variables
Don't feel bad if you are confused about what is the dependent variable and what is the independent variable in social and behavioral sciences research . However, it's important that you learn the difference because framing a study using these variables is a common approach to organizing the elements of a social sciences research study in order to discover relevant and meaningful results. Specifically, it is important for these two reasons:
- You need to understand and be able to evaluate their application in other people's research.
- You need to apply them correctly in your own research.
A variable in research simply refers to a person, place, thing, or phenomenon that you are trying to measure in some way. The best way to understand the difference between a dependent and independent variable is that the meaning of each is implied by what the words tell us about the variable you are using. You can do this with a simple exercise from the website, Graphic Tutorial. Take the sentence, "The [independent variable] causes a change in [dependent variable] and it is not possible that [dependent variable] could cause a change in [independent variable]." Insert the names of variables you are using in the sentence in the way that makes the most sense. This will help you identify each type of variable. If you're still not sure, consult with your professor before you begin to write.
Fan, Shihe. "Independent Variable." In Encyclopedia of Research Design. Neil J. Salkind, editor. (Thousand Oaks, CA: SAGE, 2010), pp. 592-594; "What are Dependent and Independent Variables?" Graphic Tutorial; Salkind, Neil J. "Dependent Variable." In Encyclopedia of Research Design , Neil J. Salkind, editor. (Thousand Oaks, CA: SAGE, 2010), pp. 348-349;
Structure and Writing Style
The process of examining a research problem in the social and behavioral sciences is often framed around methods of analysis that compare, contrast, correlate, average, or integrate relationships between or among variables . Techniques include associations, sampling, random selection, and blind selection. Designation of the dependent and independent variable involves unpacking the research problem in a way that identifies a general cause and effect and classifying these variables as either independent or dependent.
The variables should be outlined in the introduction of your paper and explained in more detail in the methods section . There are no rules about the structure and style for writing about independent or dependent variables but, as with any academic writing, clarity and being succinct is most important.
After you have described the research problem and its significance in relation to prior research, explain why you have chosen to examine the problem using a method of analysis that investigates the relationships between or among independent and dependent variables . State what it is about the research problem that lends itself to this type of analysis. For example, if you are investigating the relationship between corporate environmental sustainability efforts [the independent variable] and dependent variables associated with measuring employee satisfaction at work using a survey instrument, you would first identify each variable and then provide background information about the variables. What is meant by "environmental sustainability"? Are you looking at a particular company [e.g., General Motors] or are you investigating an industry [e.g., the meat packing industry]? Why is employee satisfaction in the workplace important? How does a company make their employees aware of sustainability efforts and why would a company even care that its employees know about these efforts?
Identify each variable for the reader and define each . In the introduction, this information can be presented in a paragraph or two when you describe how you are going to study the research problem. In the methods section, you build on the literature review of prior studies about the research problem to describe in detail background about each variable, breaking each down for measurement and analysis. For example, what activities do you examine that reflect a company's commitment to environmental sustainability? Levels of employee satisfaction can be measured by a survey that asks about things like volunteerism or a desire to stay at the company for a long time.
The structure and writing style of describing the variables and their application to analyzing the research problem should be stated and unpacked in such a way that the reader obtains a clear understanding of the relationships between the variables and why they are important. This is also important so that the study can be replicated in the future using the same variables but applied in a different way.
Fan, Shihe. "Independent Variable." In Encyclopedia of Research Design. Neil J. Salkind, editor. (Thousand Oaks, CA: SAGE, 2010), pp. 592-594; "What are Dependent and Independent Variables?" Graphic Tutorial; “Case Example for Independent and Dependent Variables.” ORI Curriculum Examples. U.S. Department of Health and Human Services, Office of Research Integrity; Salkind, Neil J. "Dependent Variable." In Encyclopedia of Research Design , Neil J. Salkind, editor. (Thousand Oaks, CA: SAGE, 2010), pp. 348-349; “Independent Variables and Dependent Variables.” Karl L. Wuensch, Department of Psychology, East Carolina University [posted email exchange]; “Variables.” Elements of Research. Dr. Camille Nebeker, San Diego State University.
- << Previous: Design Flaws to Avoid
- Next: Glossary of Research Terms >>
- Last Updated: May 1, 2024 9:25 AM
- URL: https://libguides.usc.edu/writingguide
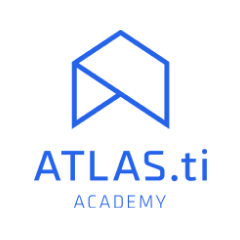
Variables in Research | Types, Definiton & Examples
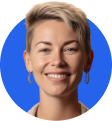
Introduction
What is a variable, what are the 5 types of variables in research, other variables in research.
Variables are fundamental components of research that allow for the measurement and analysis of data. They can be defined as characteristics or properties that can take on different values. In research design , understanding the types of variables and their roles is crucial for developing hypotheses , designing methods , and interpreting results .
This article outlines the the types of variables in research, including their definitions and examples, to provide a clear understanding of their use and significance in research studies. By categorizing variables into distinct groups based on their roles in research, their types of data, and their relationships with other variables, researchers can more effectively structure their studies and achieve more accurate conclusions.
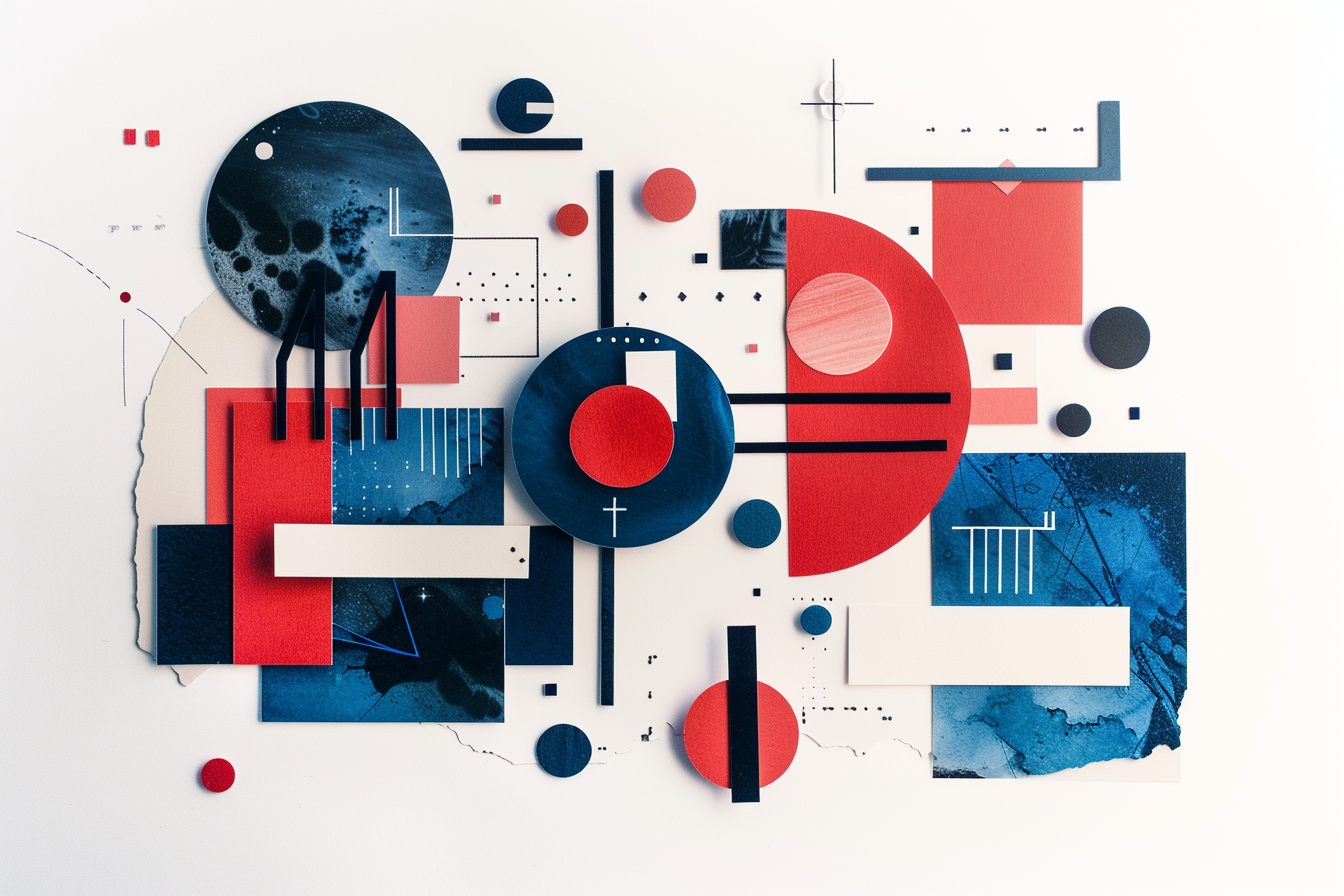
A variable represents any characteristic, number, or quantity that can be measured or quantified. The term encompasses anything that can vary or change, ranging from simple concepts like age and height to more complex ones like satisfaction levels or economic status. Variables are essential in research as they are the foundational elements that researchers manipulate, measure, or control to gain insights into relationships, causes, and effects within their studies. They enable the framing of research questions, the formulation of hypotheses, and the interpretation of results.
Variables can be categorized based on their role in the study (such as independent and dependent variables ), the type of data they represent (quantitative or categorical), and their relationship to other variables (like confounding or control variables). Understanding what constitutes a variable and the various variable types available is a critical step in designing robust and meaningful research.
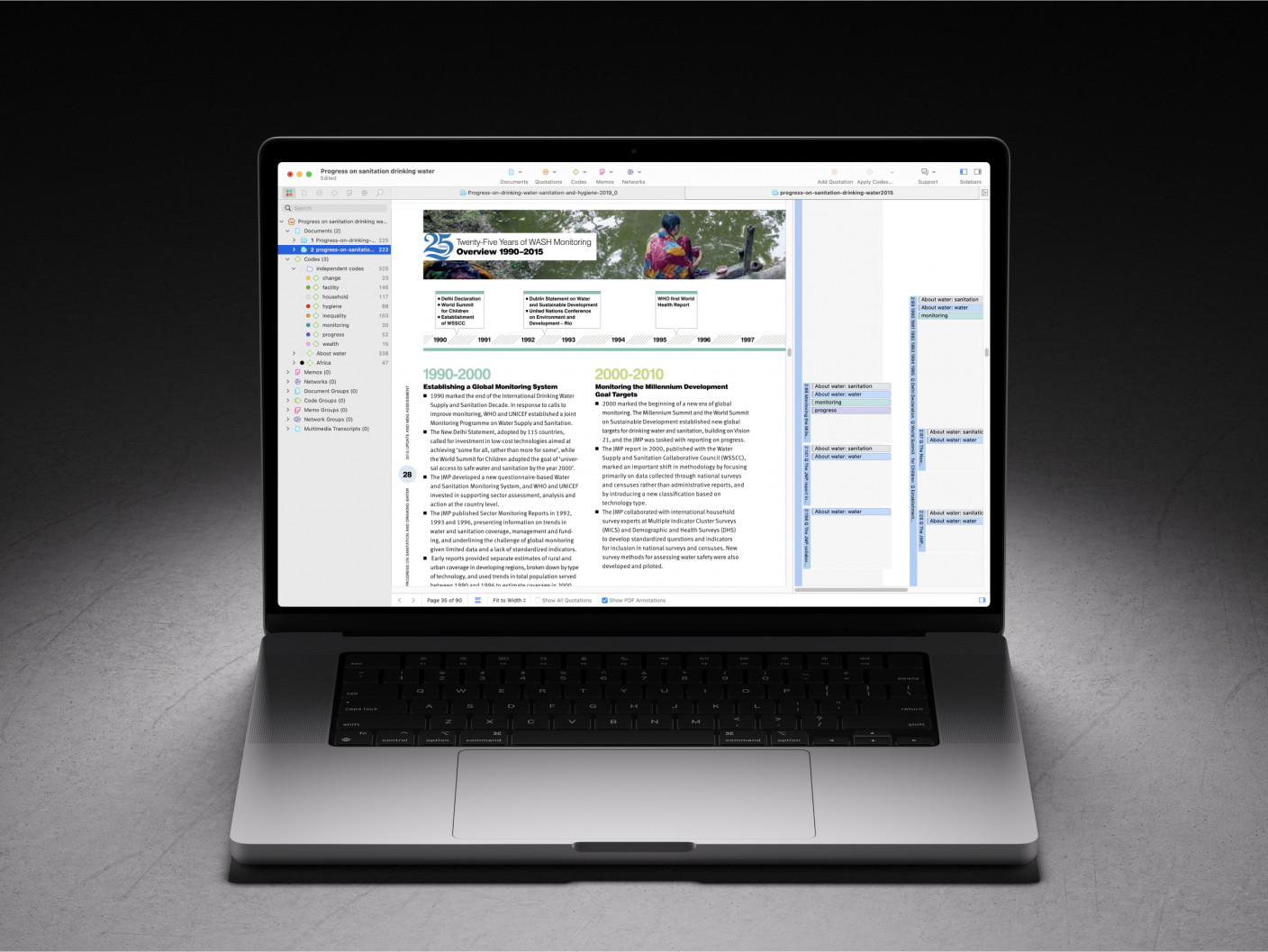
ATLAS.ti makes complex data easy to understand
Turn to our powerful data analysis tools to make the most of your research. Get started with a free trial.
Variables are crucial components in research, serving as the foundation for data collection , analysis , and interpretation . They are attributes or characteristics that can vary among subjects or over time, and understanding their types is essential for any study. Variables can be broadly classified into five main types, each with its distinct characteristics and roles within research.
This classification helps researchers in designing their studies, choosing appropriate measurement techniques, and analyzing their results accurately. The five types of variables include independent variables, dependent variables, categorical variables, continuous variables, and confounding variables. These categories not only facilitate a clearer understanding of the data but also guide the formulation of hypotheses and research methodologies.
Independent variables
Independent variables are foundational to the structure of research, serving as the factors or conditions that researchers manipulate or vary to observe their effects on dependent variables. These variables are considered "independent" because their variation does not depend on other variables within the study. Instead, they are the cause or stimulus that directly influences the outcomes being measured. For example, in an experiment to assess the effectiveness of a new teaching method on student performance, the teaching method applied (traditional vs. innovative) would be the independent variable.
The selection of an independent variable is a critical step in research design, as it directly correlates with the study's objective to determine causality or association. Researchers must clearly define and control these variables to ensure that observed changes in the dependent variable can be attributed to variations in the independent variable, thereby affirming the reliability of the results. In experimental research, the independent variable is what differentiates the control group from the experimental group, thereby setting the stage for meaningful comparison and analysis.
Dependent variables
Dependent variables are the outcomes or effects that researchers aim to explore and understand in their studies. These variables are called "dependent" because their values depend on the changes or variations of the independent variables.
Essentially, they are the responses or results that are measured to assess the impact of the independent variable's manipulation. For instance, in a study investigating the effect of exercise on weight loss, the amount of weight lost would be considered the dependent variable, as it depends on the exercise regimen (the independent variable).
The identification and measurement of the dependent variable are crucial for testing the hypothesis and drawing conclusions from the research. It allows researchers to quantify the effect of the independent variable , providing evidence for causal relationships or associations. In experimental settings, the dependent variable is what is being tested and measured across different groups or conditions, enabling researchers to assess the efficacy or impact of the independent variable's variation.
To ensure accuracy and reliability, the dependent variable must be defined clearly and measured consistently across all participants or observations. This consistency helps in reducing measurement errors and increases the validity of the research findings. By carefully analyzing the dependent variables, researchers can derive meaningful insights from their studies, contributing to the broader knowledge in their field.
Categorical variables
Categorical variables, also known as qualitative variables, represent types or categories that are used to group observations. These variables divide data into distinct groups or categories that lack a numerical value but hold significant meaning in research. Examples of categorical variables include gender (male, female, other), type of vehicle (car, truck, motorcycle), or marital status (single, married, divorced). These categories help researchers organize data into groups for comparison and analysis.
Categorical variables can be further classified into two subtypes: nominal and ordinal. Nominal variables are categories without any inherent order or ranking among them, such as blood type or ethnicity. Ordinal variables, on the other hand, imply a sort of ranking or order among the categories, like levels of satisfaction (high, medium, low) or education level (high school, bachelor's, master's, doctorate).
Understanding and identifying categorical variables is crucial in research as it influences the choice of statistical analysis methods. Since these variables represent categories without numerical significance, researchers employ specific statistical tests designed for a nominal or ordinal variable to draw meaningful conclusions. Properly classifying and analyzing categorical variables allow for the exploration of relationships between different groups within the study, shedding light on patterns and trends that might not be evident with numerical data alone.
Continuous variables
Continuous variables are quantitative variables that can take an infinite number of values within a given range. These variables are measured along a continuum and can represent very precise measurements. Examples of continuous variables include height, weight, temperature, and time. Because they can assume any value within a range, continuous variables allow for detailed analysis and a high degree of accuracy in research findings.
The ability to measure continuous variables at very fine scales makes them invaluable for many types of research, particularly in the natural and social sciences. For instance, in a study examining the effect of temperature on plant growth, temperature would be considered a continuous variable since it can vary across a wide spectrum and be measured to several decimal places.
When dealing with continuous variables, researchers often use methods incorporating a particular statistical test to accommodate a wide range of data points and the potential for infinite divisibility. This includes various forms of regression analysis, correlation, and other techniques suited for modeling and analyzing nuanced relationships between variables. The precision of continuous variables enhances the researcher's ability to detect patterns, trends, and causal relationships within the data, contributing to more robust and detailed conclusions.
Confounding variables
Confounding variables are those that can cause a false association between the independent and dependent variables, potentially leading to incorrect conclusions about the relationship being studied. These are extraneous variables that were not considered in the study design but can influence both the supposed cause and effect, creating a misleading correlation.
Identifying and controlling for a confounding variable is crucial in research to ensure the validity of the findings. This can be achieved through various methods, including randomization, stratification, and statistical control. Randomization helps to evenly distribute confounding variables across study groups, reducing their potential impact. Stratification involves analyzing the data within strata or layers that share common characteristics of the confounder. Statistical control allows researchers to adjust for the effects of confounders in the analysis phase.
Properly addressing confounding variables strengthens the credibility of research outcomes by clarifying the direct relationship between the dependent and independent variables, thus providing more accurate and reliable results.
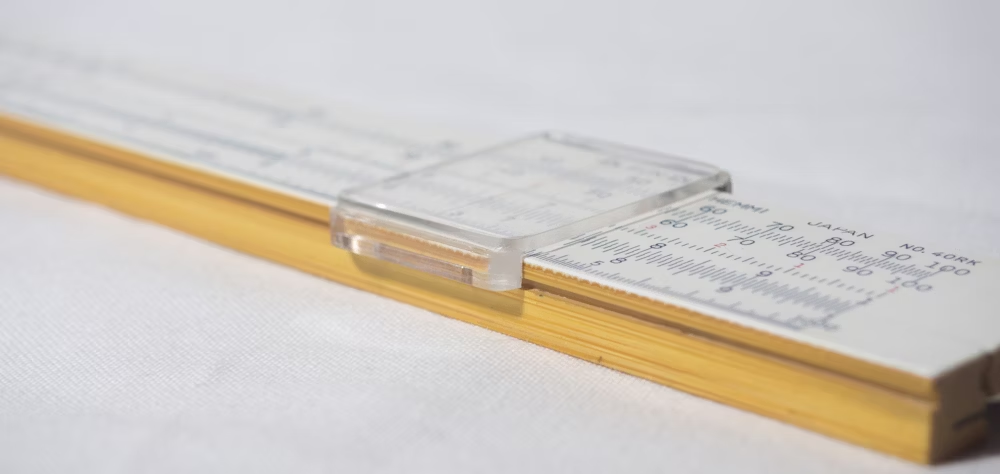
Beyond the primary categories of variables commonly discussed in research methodology , there exists a diverse range of other variables that play significant roles in the design and analysis of studies. Below is an overview of some of these variables, highlighting their definitions and roles within research studies:
- Discrete variables : A discrete variable is a quantitative variable that represents quantitative data , such as the number of children in a family or the number of cars in a parking lot. Discrete variables can only take on specific values.
- Categorical variables : A categorical variable categorizes subjects or items into groups that do not have a natural numerical order. Categorical data includes nominal variables, like country of origin, and ordinal variables, such as education level.
- Predictor variables : Often used in statistical models, a predictor variable is used to forecast or predict the outcomes of other variables, not necessarily with a causal implication.
- Outcome variables : These variables represent the results or outcomes that researchers aim to explain or predict through their studies. An outcome variable is central to understanding the effects of predictor variables.
- Latent variables : Not directly observable, latent variables are inferred from other, directly measured variables. Examples include psychological constructs like intelligence or socioeconomic status.
- Composite variables : Created by combining multiple variables, composite variables can measure a concept more reliably or simplify the analysis. An example would be a composite happiness index derived from several survey questions .
- Preceding variables : These variables come before other variables in time or sequence, potentially influencing subsequent outcomes. A preceding variable is crucial in longitudinal studies to determine causality or sequences of events.
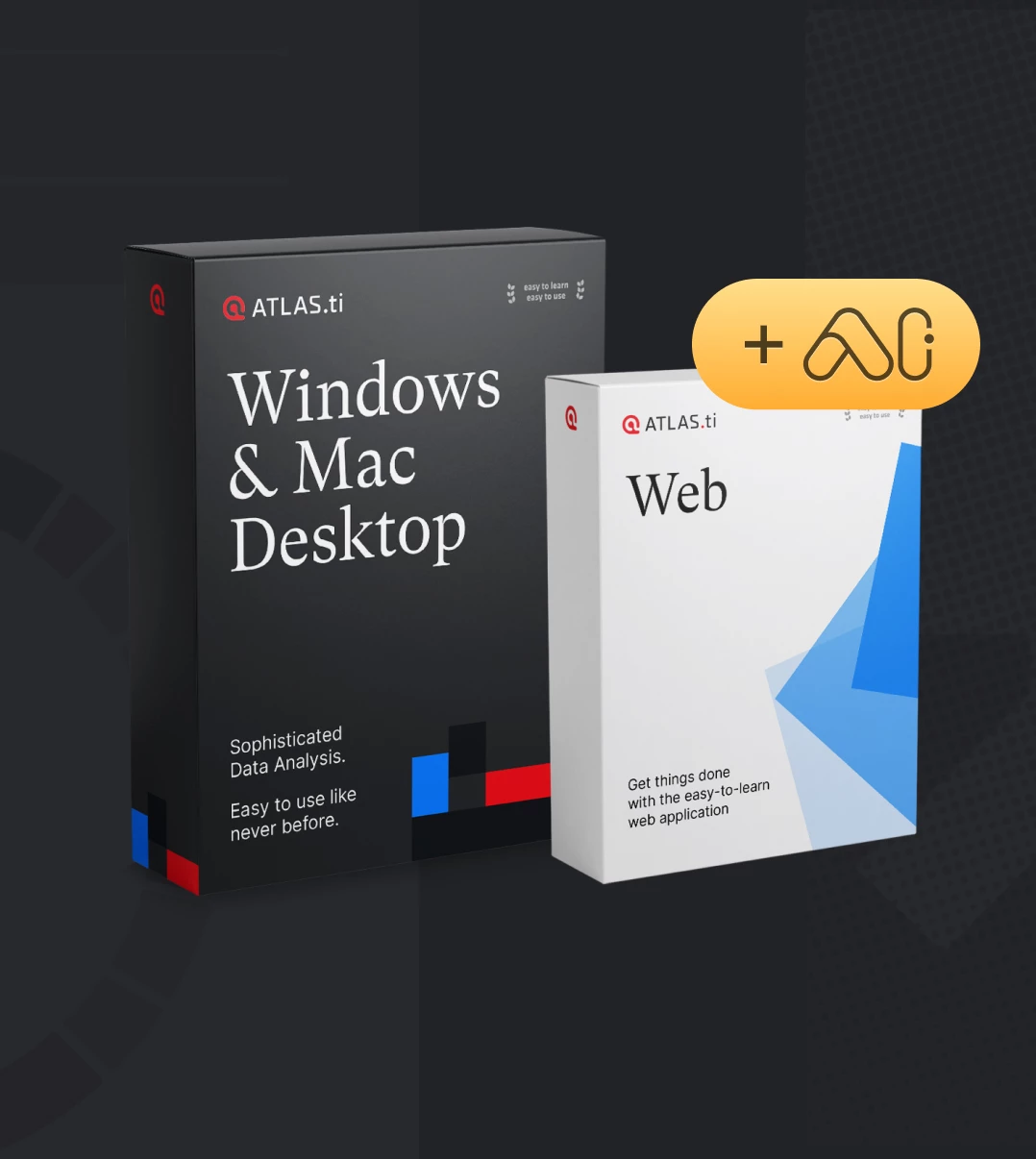
Master qualitative research with ATLAS.ti
Turn data into critical insights with our data analysis platform. Try out a free trial today.
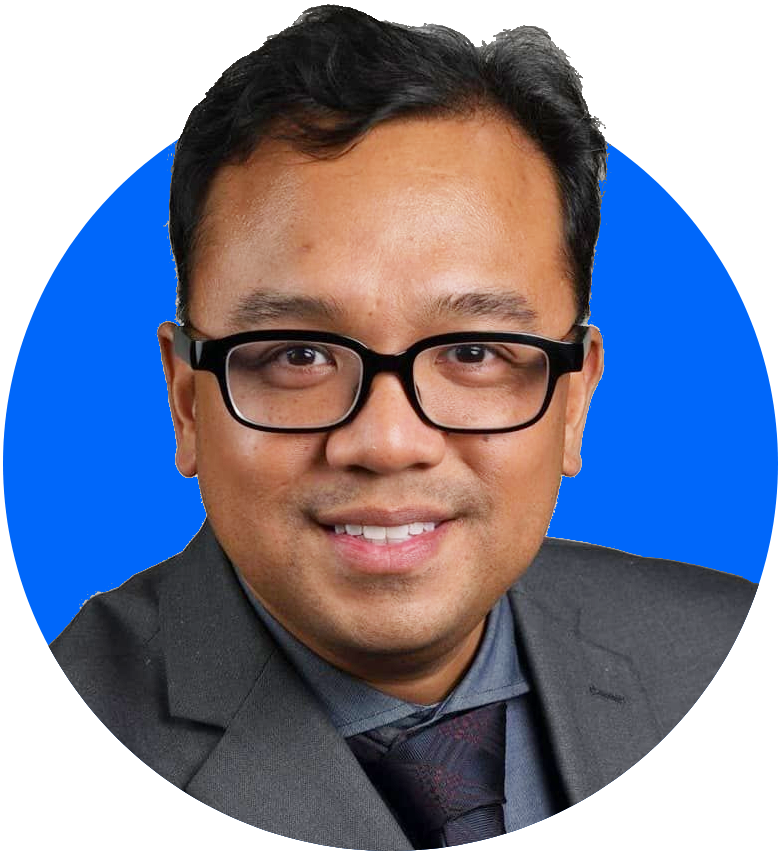
What are Examples of Variables in Research?
Table of contents, introduction.
In writing your thesis, one of the first terms that you encounter is the word variable. Failure to understand the meaning and the usefulness of variables in your study will prevent you from doing excellent research. What are variables, and how do you use variables in your research?
I explain this key research concept below with lots of examples of variables commonly used in a study.
You may find it challenging to understand just what variables are in research, especially those that deal with quantitative data analysis. This initial difficulty about variables becomes much more confusing when you encounter the phrases “dependent variable” and “independent variable” as you go deeper in studying this vital concept of research, as well as statistics.
Understanding what variables mean is crucial in writing your thesis proposal because you will need these in constructing your conceptual framework and in analyzing the data that you have gathered.
Therefore, it is a must that you should be able to grasp thoroughly the meaning of variables and ways on how to measure them. Yes, the variables should be measurable so that you will use your data for statistical analysis.
I will strengthen your understanding by providing examples of phenomena and their corresponding variables below.
Definition of Variable
Variables are those simplified portions of the complex phenomena that you intend to study. The word variable is derived from the root word “vary,” meaning, changing in amount, volume, number, form, nature, or type. These variables should be measurable, i.e., they can be counted or subjected to a scale.
The next section provides examples of variables related to climate change , academic performance, crime, fish kill, crop growth, and how content goes viral. Note that the variables in these phenomena can be measured, except the last one, where a bit more work is required.
Examples of Variables in Research: 6 Phenomena
The following are examples of phenomena from a global to a local perspective. The corresponding list of variables is given to illustrate how complex phenomena can be broken down into manageable pieces for better understanding and to subject the phenomena to research.
Phenomenon 1: Climate change
Examples of variables related to climate change :
- temperature
- the amount of carbon emission
- the amount of rainfall
Phenomenon 2: Crime and violence in the streets
Examples of variables related to crime and violence :
- number of robberies
- number of attempted murders
- number of prisoners
- number of crime victims
- number of laws enforcers
- number of convictions
- number of carnapping incidents
Phenomenon 3: Poor performance of students in college entrance exams
Examples of variables related to poor academic performance :
- entrance exam score
- number of hours devoted to studying
- student-teacher ratio
- number of students in the class
- educational attainment of teachers
- teaching style
- the distance of school from home
- number of hours devoted by parents in providing tutorial support
Phenomenon 4: Fish kill
Examples of variables related to fish kill :
- dissolved oxygen
- water salinity
- age of fish
- presence or absence of parasites
- presence or absence of heavy metal
- stocking density
Phenomenon 5: Poor crop growth
Examples of variables related to poor crop growth :
- the amount of nitrogen in the soil
- the amount of phosphorous in the soil
- the amount of potassium in the ground
- frequency of weeding
- type of soil
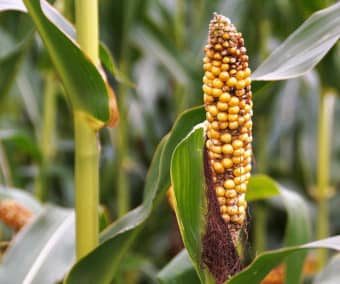
Phenomenon 6: How Content Goes Viral
- interesting,
- surprising, and
- causing physiological arousal.
Notice in the above variable examples that all the factors listed under the phenomena can be counted or measured using an ordinal, ratio, or interval scale, except for the last one. The factors that influence how content goes viral are essentially subjective.
But researchers devised ways to measure those variables by grouping the respondents’ answers on whether content is positive, interesting, prominent, among others (see the full description here ).
Thus, the variables in the last phenomenon represent the nominal scale of measuring variables .
The expected values derived from these variables will be in terms of numbers, amount, category, or type. Quantified variables allow statistical analysis . Variable descriptions, correlations, or differences are then determined.
Difference Between Independent and Dependent Variables
Which of the above examples of variables are the independent and the dependent variables?
Independent Variables
The independent variables are those variables that may influence or affect the other variable, i.e., the dependent variable.
For example, in the second phenomenon, i.e., crime and violence in the streets, the independent variables are the number of law enforcers. If there are more law enforcers, it is expected that it will reduce the following:
- number of robberies,
- number of attempted murders,
- number of prisoners,
- number of crime victims, and
- the number of carnapping incidents.
The five variables listed under crime and violence in the streets as the theme of a study are all dependent variables.
Dependent Variables
The dependent variable, as previously mentioned, is the variable affected or influenced by the independent variable.
For example, in the first phenomenon on climate change, temperature as the independent variable influences sea level rise, the dependent variable. Increased temperature will cause the expansion of water in the sea. Thus, sea-level rise on a global scale will occur.
I will leave the classification of the other variables to you. Find out whether those are independent or dependent variables. Note, however, that some variables can be both independent or dependent variables, as the context of the study dictates.
Finding the relationship between variables
How will you know that one variable may cause the other to behave in a certain way?
Finding the relationship between variables requires a thorough review of the literature . Through a review of the relevant and reliable literature, you will find out which variables influence the other variable. You do not just guess relationships between variables. The entire process is the essence of research.
At this point, I believe that the concept of the variable is now clear to you. Share this information with your peers, who may have difficulty in understanding what the variables are in research.
Related Posts
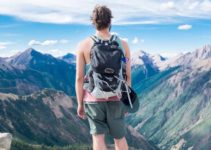
Thinking “Beyond” vs “Outside” the Box
Researcher’s euphoria: discovering something new, defining a research topic for your thesis, about the author, patrick regoniel.
Dr. Regoniel, a faculty member of the graduate school, served as consultant to various environmental research and development projects covering issues and concerns on climate change, coral reef resources and management, economic valuation of environmental and natural resources, mining, and waste management and pollution. He has extensive experience on applied statistics, systems modelling and analysis, an avid practitioner of LaTeX, and a multidisciplinary web developer. He leverages pioneering AI-powered content creation tools to produce unique and comprehensive articles in this website.
128 Comments
Your question is unclear to me Biyaminu. What do you mean? If you want to cite this, see the citation box after the article.
- Pingback: Quantitative Research Design: 4 Common Ways to Gather Your Data Efficiently October 15, 2020
Dear Calvin, when you state your research objectives that’s where you will know if you need to use variables or not.
Great work. I’d just like to know in which situations are variables not used in scientific research please. thank you.
- Pingback: Nonparametric Tests: 8 Important Considerations Before Using Them October 11, 2020
I salute your work, before I was have no enough knowledge about variable I think I was claimed from my lecturers, but the real meaning I was in the mid night. thanks
Thank you very much for your nice NOTE! I have a question: Can you please give me any examples of variables in students’ indiscipline?
A well articulated exposition! Pls, I need a simple guide on the variables of the following topic : IMPACT OF TAX REFORMS ON REVENUE GENERATION IN NIGERIA: A CASE STUDY OF KOGI STATE. THANKS A LOT.
thanks for the explanation a bout variables. keep on posting information a bout reseach on my email.
This was extremely helpful and easy to digest
Dear Hamse, That depends on what variables you are studying. Are you doing a study on cause and effect?
Dear Sophia and Hamse,
As I mentioned earlier, please read the last part of the above article on how to determine the dependent and independent variables.
CHALLENGES FACING DEVELOPMENT OF COOPERATIVE MOVEMENT IN TANA RIVER COUNTY
What is the IV and DV of this Research topic?
You can see in the last part of the above article an explanation about dependent and independent variables.
Dear Maur, what you just want to do is to describe the challenges. No need for a conceptual framework.
Hey, I really appreciate your explanation however I’m having a hard time figuring out the IV and DV on the topic about fish kill, can you help me?
I am requested to write 50 variables in my research as per my topic which is about street vending. I am really clueless.
Hi Regoniel…your articles are much more guiding….pls am writing my thesis on impact of insurgency on Baga Road fish market Maiduguri.
How will my conceptual framework looks like What do I need to talk on
Dear Alhaji, just be clear about what you want to do. Your research question must be clearly stated before you build your conceptual framework.
- Pingback: How to Analyze Frequency Data | SimplyEducate.Me December 4, 2018
Thanks so much ! This article is so much simple to my understanding. A friend of my referred me to this site and I am so greatful. Please Sir, when writing the dependent and independent variables should it be in a table form ?
Dear Grace, Good day. I don’t understand what you mean. But if your school requires that the independent and dependent variables be written in table form, I see no problem with that. It’s just a way for you to clearly show what variables you are analyzing. And you need to justify that.
Can you please give me what are the possible variables in terms of installation of street lights along barangay roads of calauan, laguna: an assessment?
Hello sir, sorry to bother you but what are the guidelines for writing a good report
Guidelines for writing a good research report?
- Privacy Policy

Home » Variables in Research – Definition, Types and Examples
Variables in Research – Definition, Types and Examples
Table of Contents

Variables in Research
Definition:
In Research, Variables refer to characteristics or attributes that can be measured, manipulated, or controlled. They are the factors that researchers observe or manipulate to understand the relationship between them and the outcomes of interest.
Types of Variables in Research
Types of Variables in Research are as follows:
Independent Variable
This is the variable that is manipulated by the researcher. It is also known as the predictor variable, as it is used to predict changes in the dependent variable. Examples of independent variables include age, gender, dosage, and treatment type.
Dependent Variable
This is the variable that is measured or observed to determine the effects of the independent variable. It is also known as the outcome variable, as it is the variable that is affected by the independent variable. Examples of dependent variables include blood pressure, test scores, and reaction time.
Confounding Variable
This is a variable that can affect the relationship between the independent variable and the dependent variable. It is a variable that is not being studied but could impact the results of the study. For example, in a study on the effects of a new drug on a disease, a confounding variable could be the patient’s age, as older patients may have more severe symptoms.
Mediating Variable
This is a variable that explains the relationship between the independent variable and the dependent variable. It is a variable that comes in between the independent and dependent variables and is affected by the independent variable, which then affects the dependent variable. For example, in a study on the relationship between exercise and weight loss, the mediating variable could be metabolism, as exercise can increase metabolism, which can then lead to weight loss.
Moderator Variable
This is a variable that affects the strength or direction of the relationship between the independent variable and the dependent variable. It is a variable that influences the effect of the independent variable on the dependent variable. For example, in a study on the effects of caffeine on cognitive performance, the moderator variable could be age, as older adults may be more sensitive to the effects of caffeine than younger adults.
Control Variable
This is a variable that is held constant or controlled by the researcher to ensure that it does not affect the relationship between the independent variable and the dependent variable. Control variables are important to ensure that any observed effects are due to the independent variable and not to other factors. For example, in a study on the effects of a new teaching method on student performance, the control variables could include class size, teacher experience, and student demographics.
Continuous Variable
This is a variable that can take on any value within a certain range. Continuous variables can be measured on a scale and are often used in statistical analyses. Examples of continuous variables include height, weight, and temperature.
Categorical Variable
This is a variable that can take on a limited number of values or categories. Categorical variables can be nominal or ordinal. Nominal variables have no inherent order, while ordinal variables have a natural order. Examples of categorical variables include gender, race, and educational level.
Discrete Variable
This is a variable that can only take on specific values. Discrete variables are often used in counting or frequency analyses. Examples of discrete variables include the number of siblings a person has, the number of times a person exercises in a week, and the number of students in a classroom.
Dummy Variable
This is a variable that takes on only two values, typically 0 and 1, and is used to represent categorical variables in statistical analyses. Dummy variables are often used when a categorical variable cannot be used directly in an analysis. For example, in a study on the effects of gender on income, a dummy variable could be created, with 0 representing female and 1 representing male.
Extraneous Variable
This is a variable that has no relationship with the independent or dependent variable but can affect the outcome of the study. Extraneous variables can lead to erroneous conclusions and can be controlled through random assignment or statistical techniques.
Latent Variable
This is a variable that cannot be directly observed or measured, but is inferred from other variables. Latent variables are often used in psychological or social research to represent constructs such as personality traits, attitudes, or beliefs.
Moderator-mediator Variable
This is a variable that acts both as a moderator and a mediator. It can moderate the relationship between the independent and dependent variables and also mediate the relationship between the independent and dependent variables. Moderator-mediator variables are often used in complex statistical analyses.
Variables Analysis Methods
There are different methods to analyze variables in research, including:
- Descriptive statistics: This involves analyzing and summarizing data using measures such as mean, median, mode, range, standard deviation, and frequency distribution. Descriptive statistics are useful for understanding the basic characteristics of a data set.
- Inferential statistics : This involves making inferences about a population based on sample data. Inferential statistics use techniques such as hypothesis testing, confidence intervals, and regression analysis to draw conclusions from data.
- Correlation analysis: This involves examining the relationship between two or more variables. Correlation analysis can determine the strength and direction of the relationship between variables, and can be used to make predictions about future outcomes.
- Regression analysis: This involves examining the relationship between an independent variable and a dependent variable. Regression analysis can be used to predict the value of the dependent variable based on the value of the independent variable, and can also determine the significance of the relationship between the two variables.
- Factor analysis: This involves identifying patterns and relationships among a large number of variables. Factor analysis can be used to reduce the complexity of a data set and identify underlying factors or dimensions.
- Cluster analysis: This involves grouping data into clusters based on similarities between variables. Cluster analysis can be used to identify patterns or segments within a data set, and can be useful for market segmentation or customer profiling.
- Multivariate analysis : This involves analyzing multiple variables simultaneously. Multivariate analysis can be used to understand complex relationships between variables, and can be useful in fields such as social science, finance, and marketing.
Examples of Variables
- Age : This is a continuous variable that represents the age of an individual in years.
- Gender : This is a categorical variable that represents the biological sex of an individual and can take on values such as male and female.
- Education level: This is a categorical variable that represents the level of education completed by an individual and can take on values such as high school, college, and graduate school.
- Income : This is a continuous variable that represents the amount of money earned by an individual in a year.
- Weight : This is a continuous variable that represents the weight of an individual in kilograms or pounds.
- Ethnicity : This is a categorical variable that represents the ethnic background of an individual and can take on values such as Hispanic, African American, and Asian.
- Time spent on social media : This is a continuous variable that represents the amount of time an individual spends on social media in minutes or hours per day.
- Marital status: This is a categorical variable that represents the marital status of an individual and can take on values such as married, divorced, and single.
- Blood pressure : This is a continuous variable that represents the force of blood against the walls of arteries in millimeters of mercury.
- Job satisfaction : This is a continuous variable that represents an individual’s level of satisfaction with their job and can be measured using a Likert scale.
Applications of Variables
Variables are used in many different applications across various fields. Here are some examples:
- Scientific research: Variables are used in scientific research to understand the relationships between different factors and to make predictions about future outcomes. For example, scientists may study the effects of different variables on plant growth or the impact of environmental factors on animal behavior.
- Business and marketing: Variables are used in business and marketing to understand customer behavior and to make decisions about product development and marketing strategies. For example, businesses may study variables such as consumer preferences, spending habits, and market trends to identify opportunities for growth.
- Healthcare : Variables are used in healthcare to monitor patient health and to make treatment decisions. For example, doctors may use variables such as blood pressure, heart rate, and cholesterol levels to diagnose and treat cardiovascular disease.
- Education : Variables are used in education to measure student performance and to evaluate the effectiveness of teaching strategies. For example, teachers may use variables such as test scores, attendance, and class participation to assess student learning.
- Social sciences : Variables are used in social sciences to study human behavior and to understand the factors that influence social interactions. For example, sociologists may study variables such as income, education level, and family structure to examine patterns of social inequality.
Purpose of Variables
Variables serve several purposes in research, including:
- To provide a way of measuring and quantifying concepts: Variables help researchers measure and quantify abstract concepts such as attitudes, behaviors, and perceptions. By assigning numerical values to these concepts, researchers can analyze and compare data to draw meaningful conclusions.
- To help explain relationships between different factors: Variables help researchers identify and explain relationships between different factors. By analyzing how changes in one variable affect another variable, researchers can gain insight into the complex interplay between different factors.
- To make predictions about future outcomes : Variables help researchers make predictions about future outcomes based on past observations. By analyzing patterns and relationships between different variables, researchers can make informed predictions about how different factors may affect future outcomes.
- To test hypotheses: Variables help researchers test hypotheses and theories. By collecting and analyzing data on different variables, researchers can test whether their predictions are accurate and whether their hypotheses are supported by the evidence.
Characteristics of Variables
Characteristics of Variables are as follows:
- Measurement : Variables can be measured using different scales, such as nominal, ordinal, interval, or ratio scales. The scale used to measure a variable can affect the type of statistical analysis that can be applied.
- Range : Variables have a range of values that they can take on. The range can be finite, such as the number of students in a class, or infinite, such as the range of possible values for a continuous variable like temperature.
- Variability : Variables can have different levels of variability, which refers to the degree to which the values of the variable differ from each other. Highly variable variables have a wide range of values, while low variability variables have values that are more similar to each other.
- Validity and reliability : Variables should be both valid and reliable to ensure accurate and consistent measurement. Validity refers to the extent to which a variable measures what it is intended to measure, while reliability refers to the consistency of the measurement over time.
- Directionality: Some variables have directionality, meaning that the relationship between the variables is not symmetrical. For example, in a study of the relationship between smoking and lung cancer, smoking is the independent variable and lung cancer is the dependent variable.
Advantages of Variables
Here are some of the advantages of using variables in research:
- Control : Variables allow researchers to control the effects of external factors that could influence the outcome of the study. By manipulating and controlling variables, researchers can isolate the effects of specific factors and measure their impact on the outcome.
- Replicability : Variables make it possible for other researchers to replicate the study and test its findings. By defining and measuring variables consistently, other researchers can conduct similar studies to validate the original findings.
- Accuracy : Variables make it possible to measure phenomena accurately and objectively. By defining and measuring variables precisely, researchers can reduce bias and increase the accuracy of their findings.
- Generalizability : Variables allow researchers to generalize their findings to larger populations. By selecting variables that are representative of the population, researchers can draw conclusions that are applicable to a broader range of individuals.
- Clarity : Variables help researchers to communicate their findings more clearly and effectively. By defining and categorizing variables, researchers can organize and present their findings in a way that is easily understandable to others.
Disadvantages of Variables
Here are some of the main disadvantages of using variables in research:
- Simplification : Variables may oversimplify the complexity of real-world phenomena. By breaking down a phenomenon into variables, researchers may lose important information and context, which can affect the accuracy and generalizability of their findings.
- Measurement error : Variables rely on accurate and precise measurement, and measurement error can affect the reliability and validity of research findings. The use of subjective or poorly defined variables can also introduce measurement error into the study.
- Confounding variables : Confounding variables are factors that are not measured but that affect the relationship between the variables of interest. If confounding variables are not accounted for, they can distort or obscure the relationship between the variables of interest.
- Limited scope: Variables are defined by the researcher, and the scope of the study is therefore limited by the researcher’s choice of variables. This can lead to a narrow focus that overlooks important aspects of the phenomenon being studied.
- Ethical concerns: The selection and measurement of variables may raise ethical concerns, especially in studies involving human subjects. For example, using variables that are related to sensitive topics, such as race or sexuality, may raise concerns about privacy and discrimination.
About the author
Muhammad Hassan
Researcher, Academic Writer, Web developer
You may also like

Control Variable – Definition, Types and Examples

Moderating Variable – Definition, Analysis...

Categorical Variable – Definition, Types and...

Independent Variable – Definition, Types and...

Ratio Variable – Definition, Purpose and Examples

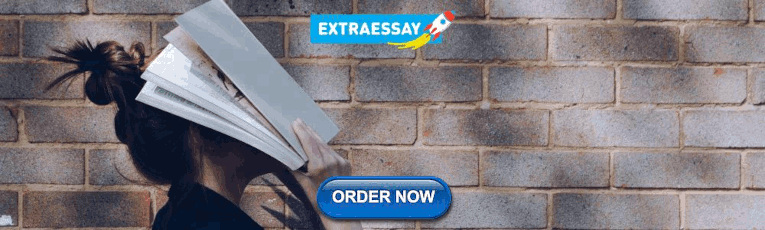
Ordinal Variable – Definition, Purpose and...
- Bipolar Disorder
- Therapy Center
- When To See a Therapist
- Types of Therapy
- Best Online Therapy
- Best Couples Therapy
- Best Family Therapy
- Managing Stress
- Sleep and Dreaming
- Understanding Emotions
- Self-Improvement
- Healthy Relationships
- Student Resources
- Personality Types
- Guided Meditations
- Verywell Mind Insights
- 2024 Verywell Mind 25
- Mental Health in the Classroom
- Editorial Process
- Meet Our Review Board
- Crisis Support
Clinical Psychology Research Topics
Stumped for ideas? Start here
Kendra Cherry, MS, is a psychosocial rehabilitation specialist, psychology educator, and author of the "Everything Psychology Book."
:max_bytes(150000):strip_icc():format(webp)/IMG_9791-89504ab694d54b66bbd72cb84ffb860e.jpg)
Emily is a board-certified science editor who has worked with top digital publishing brands like Voices for Biodiversity, Study.com, GoodTherapy, Vox, and Verywell.
:max_bytes(150000):strip_icc():format(webp)/Emily-Swaim-1000-0f3197de18f74329aeffb690a177160c.jpg)
Clinical psychology research is one of the most popular subfields in psychology. With such a wide range of topics to cover, figuring out clinical psychology research topics for papers, presentations, and experiments can be tricky.
Clinical Psychology Research Topic Ideas
Topic choices are only as limited as your imagination and assignment, so try narrowing the possibilities down from general questions to the specifics that apply to your area of specialization.
Here are just a few ideas to start the process:
- How does social media influence how people interact and behave?
- Compare and contrast two different types of therapy . When is each type best used? What disorders are best treated with these forms of therapy? What are the possible limitations of each type?
- Compare two psychological disorders . What are the signs and symptoms of each? How are they diagnosed and treated?
- How does "pro ana," "pro mia," " thinspo ," and similar content contribute to eating disorders? What can people do to overcome the influence of these sites?
- Explore how aging influences mental illness. What particular challenges elderly people diagnosed with mental illness face?
- Explore factors that influence adolescent mental health. Self-esteem and peer pressure are just a couple of the topics you might explore in greater depth.
- Explore the use and effectiveness of online therapy . What are some of its advantages and disadvantages ? How do those without technical literacy navigate it?
- Investigate current research on the impact of media violence on children's behavior.
- Explore anxiety disorders and their impact on daily functioning. What new therapies are available?
- What are the risk factors for depression ? Explore the potential risks as well as any preventative strategies that can be used.
- How do political and social climates affect mental health?
- What are the long-term effects of childhood trauma? Do children continue to experience the effects later in adulthood? What treatments are available for PTSD (post-traumatic stress disorder) in childhood ?
- What impact does substance use disorder have on the family? How can family members help with treatment?
- What types of therapy are most effective for childhood behavioral issues ?
Think of books you have read, research you have studied, and even experiences and interests from your own life. If you've ever wanted to dig further into something that interested you, this is a great opportunity. The more engaged you are with the topic, the more excited you will be to put the work in for a great research paper or presentation.
Consider Scope, Difficulty, and Suitability
Picking a good research topic is one of the most important steps of the research process. A too-general topic can feel overwhelming; likewise, one that's very specific might have limited supporting information. Spend time reading online or exploring your library to make sure that plenty of sources to support your paper, presentation, or experiment are available.
If you are doing an experiment , checking with your instructor is a must. In many cases, you might have to submit a proposal to your school's human subjects committee for approval. This committee will ensure that any potential research involving human subjects is done in a safe and ethical way.
Once you have chosen a topic that interests you, run the idea past your course instructor. (In some cases, this is required.) Even if you don't need permission from the instructor, getting feedback before you delve into the research process is helpful.
Your instructor can draw from a wealth of experience to offer good suggestions and ideas for your research, including the best available resources pertaining to the topic. Your school librarian may also be able to provide assistance regarding the resources available for use at the library, including online journal databases.
Kim WO. Institutional review board (IRB) and ethical issues in clinical research . Korean Journal of Anesthesiology . 2012;62(1):3-12. doi:10.4097/kjae.2012.62.1.3
By Kendra Cherry, MSEd Kendra Cherry, MS, is a psychosocial rehabilitation specialist, psychology educator, and author of the "Everything Psychology Book."
61 intriguing psychology research topics to explore
Last updated
11 January 2024
Reviewed by
Brittany Ferri, PhD, OTR/L
Psychology is an incredibly diverse, critical, and ever-changing area of study in the medical and health industries. Because of this, it’s a common area of study for students and healthcare professionals.
We’re walking you through picking the perfect topic for your upcoming paper or study. Keep reading for plenty of example topics to pique your interest and curiosity.
- How to choose a psychology research topic
Exploring a psychology-based topic for your research project? You need to pick a specific area of interest to collect compelling data.
Use these tips to help you narrow down which psychology topics to research:
Focus on a particular area of psychology
The most effective psychological research focuses on a smaller, niche concept or disorder within the scope of a study.
Psychology is a broad and fascinating area of science, including everything from diagnosed mental health disorders to sports performance mindset assessments.
This gives you plenty of different avenues to explore. Having a hard time choosing? Check out our list of 61 ideas further down in this article to get started.
Read the latest clinical studies
Once you’ve picked a more niche topic to explore, you need to do your due diligence and explore other research projects on the same topic.
This practice will help you learn more about your chosen topic, ask more specific questions, and avoid covering existing projects.
For the best results, we recommend creating a research folder of associated published papers to reference throughout your project. This makes it much easier to cite direct references and find inspiration down the line.
Find a topic you enjoy and ask questions
Once you’ve spent time researching and collecting references for your study, you finally get to explore.
Whether this research project is for work, school, or just for fun, having a passion for your research will make the project much more enjoyable. (Trust us, there will be times when that is the only thing that keeps you going.)
Now you’ve decided on the topic, ask more nuanced questions you might want to explore.
If you can, pick the direction that interests you the most to make the research process much more enjoyable.
- 61 psychology topics to research in 2024
Need some extra help starting your psychology research project on the right foot? Explore our list of 61 cutting-edge, in-demand psychology research topics to use as a starting point for your research journey.
- Psychology research topics for university students
As a university student, it can be hard to pick a research topic that fits the scope of your classes and is still compelling and unique.
Here are a few exciting topics we recommend exploring for your next assigned research project:
Mental health in post-secondary students
Seeking post-secondary education is a stressful and overwhelming experience for most students, making this topic a great choice to explore for your in-class research paper.
Examples of post-secondary mental health research topics include:
Student mental health status during exam season
Mental health disorder prevalence based on study major
The impact of chronic school stress on overall quality of life
The impacts of cyberbullying
Cyberbullying can occur at all ages, starting as early as elementary school and carrying through into professional workplaces.
Examples of cyberbullying-based research topics you can study include:
The impact of cyberbullying on self-esteem
Common reasons people engage in cyberbullying
Cyberbullying themes and commonly used terms
Cyberbullying habits in children vs. adults
The long-term effects of cyberbullying
- Clinical psychology research topics
If you’re looking to take a more clinical approach to your next project, here are a few topics that involve direct patient assessment for you to consider:
Chronic pain and mental health
Living with chronic pain dramatically impacts every aspect of a person’s life, including their mental and emotional health.
Here are a few examples of in-demand pain-related psychology research topics:
The connection between diabetic neuropathy and depression
Neurological pain and its connection to mental health disorders
Efficacy of meditation and mindfulness for pain management
The long-term effects of insomnia
Insomnia is where you have difficulty falling or staying asleep. It’s a common health concern that impacts millions of people worldwide.
This is an excellent topic because insomnia can have a variety of causes, offering many research possibilities.
Here are a few compelling psychology research topics about insomnia you could investigate:
The prevalence of insomnia based on age, gender, and ethnicity
Insomnia and its impact on workplace productivity
The connection between insomnia and mental health disorders
Efficacy and use of melatonin supplements for insomnia
The risks and benefits of prescription insomnia medications
Lifestyle options for managing insomnia symptoms
The efficacy of mental health treatment options
Management and treatment of mental health conditions is an ever-changing area of study. If you can witness or participate in mental health therapies, this can make a great research project.
Examples of mental health treatment-related psychology research topics include:
The efficacy of cognitive behavioral therapy (CBT) for patients with severe anxiety
The benefits and drawbacks of group vs. individual therapy sessions
Music therapy for mental health disorders
Electroconvulsive therapy (ECT) for patients with depression
- Controversial psychology research paper topics
If you are looking to explore a more cutting-edge or modern psychology topic, you can delve into a variety of controversial and topical options:
The impact of social media and digital platforms
Ever since access to internet forums and video games became more commonplace, there’s been growing concern about the impact these digital platforms have on mental health.
Examples of social media and video game-related psychology research topics include:
The effect of edited images on self-confidence
How social media platforms impact social behavior
Video games and their impact on teenage anger and violence
Digital communication and the rapid spread of misinformation
The development of digital friendships
Psychotropic medications for mental health
In recent years, the interest in using psychoactive medications to treat and manage health conditions has increased despite their inherently controversial nature.
Examples of psychotropic medication-related research topics include:
The risks and benefits of using psilocybin mushrooms for managing anxiety
The impact of marijuana on early-onset psychosis
Childhood marijuana use and related prevalence of mental health conditions
Ketamine and its use for complex PTSD (C-PTSD) symptom management
The effect of long-term psychedelic use and mental health conditions
- Mental health disorder research topics
As one of the most popular subsections of psychology, studying mental health disorders and how they impact quality of life is an essential and impactful area of research.
While studies in these areas are common, there’s always room for additional exploration, including the following hot-button topics:
Anxiety and depression disorders
Anxiety and depression are well-known and heavily researched mental health disorders.
Despite this, we still don’t know many things about these conditions, making them great candidates for psychology research projects:
Social anxiety and its connection to chronic loneliness
C-PTSD symptoms and causes
The development of phobias
Obsessive-compulsive disorder (OCD) behaviors and symptoms
Depression triggers and causes
Self-care tools and resources for depression
The prevalence of anxiety and depression in particular age groups or geographic areas
Bipolar disorder
Bipolar disorder is a complex and multi-faceted area of psychology research.
Use your research skills to learn more about this condition and its impact by choosing any of the following topics:
Early signs of bipolar disorder
The incidence of bipolar disorder in young adults
The efficacy of existing bipolar treatment options
Bipolar medication side effects
Cognitive behavioral therapy for people with bipolar
Schizoaffective disorder
Schizoaffective disorder is often stigmatized, and less common mental health disorders are a hotbed for new and exciting research.
Here are a few examples of interesting research topics related to this mental health disorder:
The prevalence of schizoaffective disorder by certain age groups or geographic locations
Risk factors for developing schizoaffective disorder
The prevalence and content of auditory and visual hallucinations
Alternative therapies for schizoaffective disorder
- Societal and systematic psychology research topics
Modern society’s impact is deeply enmeshed in our mental and emotional health on a personal and community level.
Here are a few examples of societal and systemic psychology research topics to explore in more detail:
Access to mental health services
While mental health awareness has risen over the past few decades, access to quality mental health treatment and resources is still not equitable.
This can significantly impact the severity of a person’s mental health symptoms, which can result in worse health outcomes if left untreated.
Explore this crucial issue and provide information about the need for improved mental health resource access by studying any of the following topics:
Rural vs. urban access to mental health resources
Access to crisis lines by location
Wait times for emergency mental health services
Inequities in mental health access based on income and location
Insurance coverage for mental health services
Systemic racism and mental health
Societal systems and the prevalence of systemic racism heavily impact every aspect of a person’s overall health.
Researching these topics draws attention to existing problems and contributes valuable insights into ways to improve access to care moving forward.
Examples of systemic racism-related psychology research topics include:
Access to mental health resources based on race
The prevalence of BIPOC mental health therapists in a chosen area
The impact of systemic racism on mental health and self-worth
Racism training for mental health workers
The prevalence of mental health disorders in discriminated groups
LGBTQIA+ mental health concerns
Research about LGBTQIA+ people and their mental health needs is a unique area of study to explore for your next research project. It’s a commonly overlooked and underserved community.
Examples of LGBTQIA+ psychology research topics to consider include:
Mental health supports for queer teens and children
The impact of queer safe spaces on mental health
The prevalence of mental health disorders in the LGBTQIA+ community
The benefits of queer mentorship and found family
Substance misuse in LQBTQIA+ youth and adults
- Collect data and identify trends with Dovetail
Psychology research is an exciting and competitive study area, making it the perfect choice for projects or papers.
Take the headache out of analyzing your data and instantly access the insights you need to complete your next psychology research project by teaming up with Dovetail today.
Get started today
Go from raw data to valuable insights with a flexible research platform
Editor’s picks
Last updated: 21 December 2023
Last updated: 16 December 2023
Last updated: 6 October 2023
Last updated: 25 November 2023
Last updated: 12 May 2023
Last updated: 15 February 2024
Last updated: 11 March 2024
Last updated: 12 December 2023
Last updated: 18 May 2023
Last updated: 6 March 2024
Last updated: 10 April 2023
Last updated: 20 December 2023
Latest articles
Related topics, log in or sign up.
Get started for free
- Open access
- Published: 01 May 2024
Quantifying reciprocal relationships between poverty and health: combining a causal loop diagram with longitudinal structural equation modelling
- Laurens Reumers 1 ,
- Niels Hameleers 1 ,
- Henk Hilderink 2 ,
- Marleen Bekker 3 ,
- Maria Jansen 1 , 4 &
- Dirk Ruwaard 1
International Journal for Equity in Health volume 23 , Article number: 87 ( 2024 ) Cite this article
Metrics details
This study takes on the challenge of quantifying a complex causal loop diagram describing how poverty and health affect each other, and does so using longitudinal data from The Netherlands. Furthermore, this paper elaborates on its methodological approach in order to facilitate replication and methodological advancement.
After adapting a causal loop diagram that was built by stakeholders, a longitudinal structural equation modelling approach was used. A cross-lagged panel model with nine endogenous variables, of which two latent variables, and three time-invariant exogenous variables was constructed. With this model, directional effects are estimated in a Granger-causal manner, using data from 2015 to 2019. Both the direct effects (with a one-year lag) and total effects over multiple (up to eight) years were calculated. Five sensitivity analyses were conducted. Two of these focus on lower-income and lower-wealth individuals. The other three each added one exogenous variable: work status, level of education, and home ownership.
The effects of income and financial wealth on health are present, but are relatively weak for the overall population. Sensitivity analyses show that these effects are stronger for those with lower incomes or wealth. Physical capability does seem to have strong positive effects on both income and financial wealth. There are a number of other results as well, as the estimated models are extensive. Many of the estimated effects only become substantial after several years.
Conclusions
Income and financial wealth appear to have limited effects on the health of the overall population of The Netherlands. However, there are indications that these effects may be stronger for individuals who are closer to the poverty threshold. Since the estimated effects of physical capability on income and financial wealth are more substantial, a broad recommendation would be that including physical capability in efforts that are aimed at improving income and financial wealth could be useful and effective. The methodological approach described in this paper could also be applied to other research settings or topics.
Introduction
The relationship between poverty and health has long been recognised. In 1924, a study on health differences between different groups of economic status states that “[t]hese data afford statistical evidence of what has in general been accepted, viz, that there is more sickness and a higher death rate among the poor than among the well-to-do” [ 1 ], p. 13. This indicates that as early as hundred years ago now, the existence of a relationship between poverty and health had been well-established.
In a broader context, poverty is regarded as one of the most prominent social determinants of health (SDoH) [ 2 , 3 ]. SDoH are described by the World Health Organization as “the conditions in which people are born, grow, work, live, and age, and the wider set of forces and systems shaping the conditions of daily life” [ 4 ]. They are influential factors in shaping health outcomes [ 2 , 5 , 6 ], ubiquitous, and understanding them is therefore of great value for advancing public health. In order to study how SDoH affect health outcomes and how these outcomes may potentially be influenced, models containing causal mechanisms are warranted. Merely testing for correlations between a SDoH and health outcomes brings little understanding of what is actually happening and the correlations may largely be explained by confounders. Conversely, simply adding control variables to an analysis is likely to ‘close off’ (i.e. completely eliminate and disregard) mechanisms through which the SDoH affects health outcomes and therefore result in grossly underestimating effects [ 7 ]. Modelling the causal mechanisms of SDoH is difficult and subjected to two main challenges.
The first challenge is how to formulate a theoretical model that reflects expectations about real-world dynamics before moving towards quantification [ 8 ]. We addressed this first challenge in an earlier phase of this research project as we constructed a causal loop diagram (CLD) (an extensive, qualitative model of a system) using the participatory method of group model building with system dynamics [ 9 ]. The model from this study is focused on the relationships between poverty and health and provides the conceptual starting point for the present study.
The second challenge lies in quantitatively estimating the interrelationships between the variables from a CLD that depicts a complex system. In the present study, we wanted to know how SDoH can have an impact on health – and vice versa – through pathways involving multiple variables. As indicated, this was done using a CLD that was constructed in a previous study [ 9 ], but adaptations were needed for this model to be quantifiable. One difficulty in this is that the pathways between SDoH and health outcomes usually involve a number of interrelated variables and (sometimes ambiguous) relationships between those variables [ 10 ]. The complexity that results from having more than just a few (both independent and dependent) variables in a model – often with time lags [ 6 ] – makes quantifying them difficult. Such a complex set of interrelationships is one of the reasons why the ties between a SDoH and health outcomes can be considered to constitute a wicked problem [ 11 ]. The process of quantifying the interrelationships between poverty and health as modelled in the CLD in the previous study [ 9 ] is described in this paper.
The variables in the model will often not only affect other variables, but also indirectly influence themselves, which means that there is simultaneity. Simultaneity is understood as X influencing Y, while Y in return influences X [ 12 ], forming a feedback loop. Considering feedback loops is important for understanding how a system as a whole behaves over time and central to system dynamics [ 13 ]. For a qualitative conceptual model, this poses no fundamental methodological difficulties. However, for a quantitative model, such reciprocity violates the assumption of independence between the errors of the predictor and of the outcome variable [ 14 ] that statistical methods such as (multiple) regression depend on. Furthermore, all arrows in a CLD imply causal effects, so it is therefore crucial to use methods that can be used to make inferences about causal direction and not just show correlations.
The issues mentioned above can be addressed or circumvented using longitudinal structural equation modelling (SEM) [ 15 ]. SEM includes a range of methods suited for estimating complex model structures. While cross-sectional SEMs are, as noted by Uleman et al. [ 16 ], not suited to properly deal with feedback loops and with causal direction, longitudinal SEMs do incorporate temporal precedence and can circumvent those problems. This is done based on the logic that if B happens after A, it is not possible for B to cause A, which is further elaborated on in step 3 in the methods section of this paper.
The body of literature on the relationships between poverty and health is quite extensive.. The relationships between the financial side of socioeconomic position (SEP) and health have been the topic of a number of studies that use longitudinal SEM (e.g., [ 17 , 18 , 19 ]), and especially in the (health) economic literature there have been many studies estimating causal relationships between SEP and health using longitudinal designs (see e.g., [ 20 ] for an overview). Existing studies tend to focus on either one direction – the effect of SEP on health (behaviour) or vice versa (e.g., [ 21 ]), sometimes using instrumental variables (e.g., [ 22 , 23 ]) – and usually focus on direct relationships between SEP and health without specifying mechanisms [ 24 ]. This is a suitable approach for studying to what extent the two affect each other. However, the goal of the present paper is also to elaborate on how poverty and health affect each other. In order to do this, mediating variables specifying mechanisms and a feedback loop structure can be used. This study demonstrates an approach that addresses both difficulties mentioned in this introduction section (obtaining a complex conceptual model and quantifying it) by combining system dynamics, longitudinal SEM, a number of variables in feedback loops, and mediation analysis.
Research question
The research question for this study is: ‘how and to what extent do poverty and health affect each other and are these findings in line with a CLD constructed by stakeholders?’ An additional aim of the study is providing an account on how this study’s methodological approach can be employed, which is intended as a starting point for others who might be interested in using it as well. While this study explicitly considers poverty and health, the approach itself is highly generally applicable and could be used for other SDoH as well and even outside of health-related research.
While many of these methodological elaborations are aimed at other researchers who are interested in quantification of SDoH, there are two other intended audiences for this paper. One audience consists of researchers who are not necessarily involved with quantitative research, but are interested in the topic of poverty and health, or SDoH in general. Most of this article will likely hold value for this group as well, though especially steps 3 and 4 of the Methods section may contain more information than is required. A final intended audience is that of policymakers. We think that especially Table 2 (including the extended one in Additional file 3 ) and the Discussion section contain insights that may be useful for this audience.
In this section, we describe the basic steps that were taken and the challenges encountered during adapting and quantifying the CLD, and how various issues were resolved. Some of the more technical considerations that are part of the steps of this methodological approach can be found in Additional file 1 .
Population and setting
The setting in which this study is conducted is the country of The Netherlands and the population is defined as all inhabitants of The Netherlands. The CLD that is taken as conceptual model for quantification (Fig. 1 ) was built in the Dutch city of Utrecht, where there is particular regard for both poverty and health and involvement of stakeholders from societal practice in this process. The national level of analysis for quantification was chosen because of data availability and potential generalisability of results.
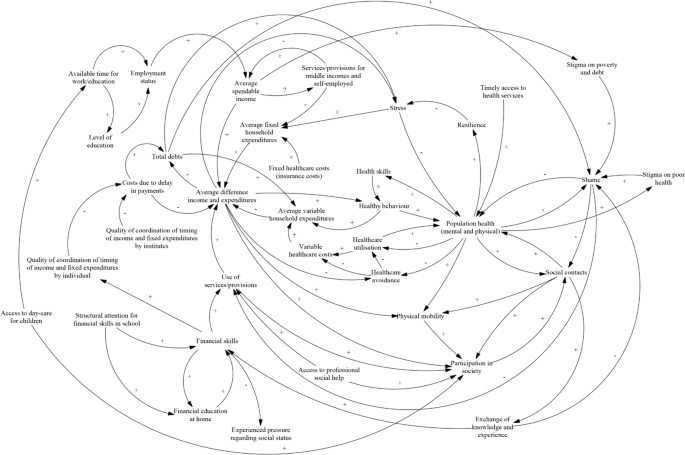
The causal loop diagram constructed using group model building in the previous study [ 9 ]
Data and sample
Individual-level data were used for quantification of the model, because the effects also occur on this level [ 25 ]. These data were derived from two datasets. The first of the two datasets is a large Dutch national survey, the Longitudinal Internet studies for the Social Sciences (LISS) panel, which contains data on thousands of individuals (exact sample size depends on the topics and wave selected – the health dataset from 2015 includes 6,009 respondents, for example) from all over The Netherlands [ 26 ]. A number of topics in this panel are relevant for this study, such as health, social integration and leisure, and other variables regarding personality and behaviour. Longitudinal datasets containing variables on all of these topics, which is essential for this study, are very scarce. For the original sample and first two replacement recruitments (2007–2012), random sampling was used. The third and fourth replacement recruitments (2013–2017) were drawn using stratified sampling, in an effort to maximise the sample’s representativeness. A comparison of population and sample characteristics is available [ 27 ]. The second dataset that is used is the official Dutch national registry data, managed by Statistics Netherlands (CBS) [ 28 ], which is used to complement the LISS survey data. These are data on all persons who officially live in the country, are longitudinal and cover the entire population with only little missing data. This data source was mainly used because it holds accurate and objective information on financial data, both on the individual and household levels.
The analyses in this study have been conducted using annual data from 2015 to 2019 from both datasets. For 2014 and several previous years, there were various crucial variables missing from the data, which is why 2015 was chosen as the first year for the analysis. The years 2020 and onwards were excluded from analysis because the outbreak of the coronavirus was assumed to influence the variables in the study in an idiosyncratic way that could not be controlled for. For an individual to be included in this study’s sample, just one of the questions on mental health or physical capability in any of the five included survey waves has to be answered and there has to be data on their financial position for at least one of those five years. No further exclusion criteria were applied. This resulted in a sample size of 6,581 individuals.
Steps to a quantified model
Quantification of the model can be roughly divided into five steps: adapting the CLD, measurement models and operationalisation, selecting the type of model, building the structural model, and interpretation of results. These steps were not as chronological and separated from each other as may seem from the descriptions below, but more of an iterative process with decisions made in later steps sometimes making it necessary to return to earlier ones to make adjustments. Constructing the measurement models and operationalising the variables were taken together as one step, as they are so interwoven that they were largely done at the same time. Figure 2 visualises the steps in a flowchart.
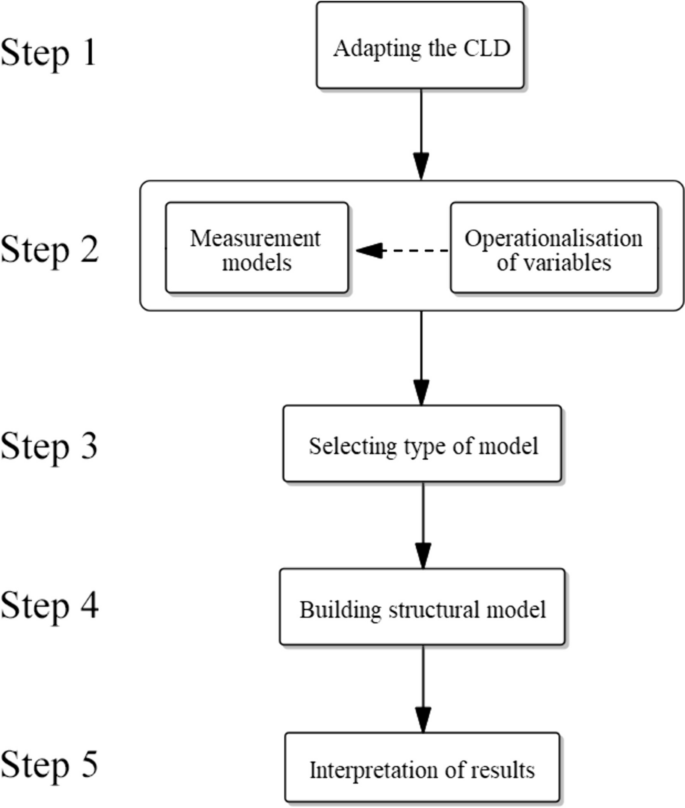
Flowchart showing the five steps of the quantification process
Adapting the CLD for quantification (step 1)
The stakeholders’ CLD had to be simplified before quantification was possible. As there will be a limit to the number of variables that can be taken into account in such a quantified model, some decisions had to be made: which variables should be included and which are to be omitted, or merged, in favour of simplification? In the validation phase in the previous study, after the CLD was constructed by stakeholders who were heavily involved in practice and policy regarding poverty and health, a meeting with scientific experts was organised (see that study for more details [ 9 ]). In this meeting, experts were asked to highlight core elements of the model and to name any alterations they would expect to be necessary for the model to better reflect reality. Their input was helpful while adapting the model to make it quantifiable in the present study and led to making the distinction between physical and mental health. Furthermore, variables that were part of many and major feedback loops were given priority over variables that were not part of any or only ‘peripheral’ feedback loops. This led to a first version of a simplified CLD (Fig. 3 ). This version contains the variables of mental health, physical health, poverty, healthy behaviour, social contacts, participation in society, shame, and work status. The variable of stress was taken as being part of mental health – as stress is one of the items that will make up the latent variable of mental health, as will be further explained in the next two sections. The variables of home ownership and level of education were also mentioned by the experts as being potentially influential and are included in separate sensitivity analyses.
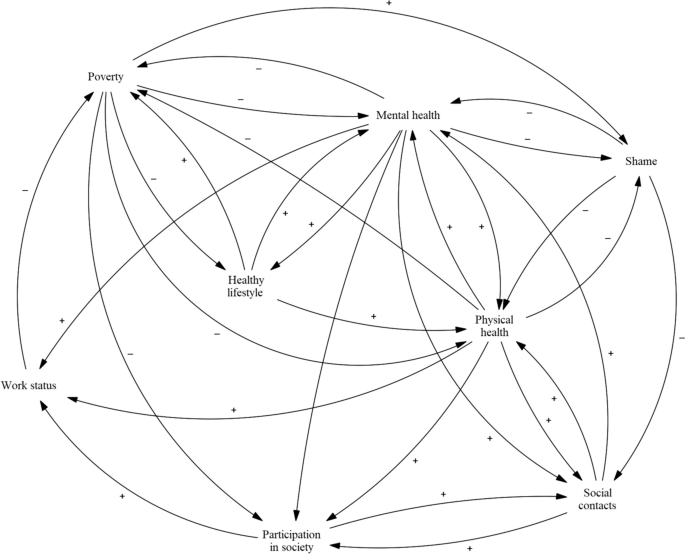
An adaptation of the causal loop diagram, based on comments from scientific experts and literature
Measurement models and operationalisation of variables (step 2)
Measurement models
This study makes use of observed variables as well as latent constructs (in this paper, the terms latent variable, latent construct, and underlying construct are used interchangeably). Some variables are considered to be directly observable: the measurement is the actual value of the variable and not just an approximation of it. In this study, the variables age and sex could be regarded as being measured directly.
Latent constructs are variables that cannot be directly observed and measured, because they are comprised of more than is directly measurable. Mental health is an example of such a variable that cannot be captured in just one observed indicator; it contains multiple facets and is a complex construct. A one-item operationalisation might simply consist of a respondent’s self-rated mental health, but this is not a direct measurement of mental health itself. Neither are more specific survey items pertaining to the respondent’s mental health. Such operationalisations would be accompanied with fair amounts of measurement error [ 29 ]. This measurement error would attenuate the variable’s relationships in quantitative models, meaning that all estimates of effects tend to be biased – all effects would be weakened. By using multiple indicators together – indicators that are expected to be influenced by the latent variable – a more complete picture of the respondents overall mental health was given and measurement error removed. In this study, mental health and physical capability were included as latent variables, using confirmatory factor analysis (CFA) [ 30 ]. See Fig. 4 for a schematic depiction of the latent variable of mental health and Additional file 2 for a more detailed description of the operationalisation of these two latent variables.
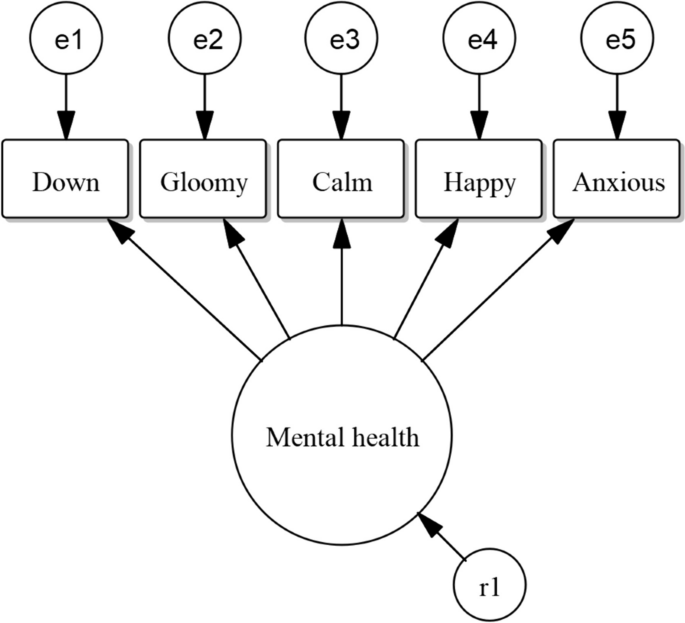
Measurement model (CFA) for latent variable mental health at one time point, using the five MHI-5 items [ 31 ]. The error terms of the indicators ‘e1’ to ‘e5’ are also shown, as well as the residual ‘r1’ for the latent variable
In some other cases, more practical reasons led to the choice to not construct a latent variable for a particular variable. Acceptable multiple indicators measuring the variables of satisfaction with social contacts and satisfaction with leisure time were not found in the available data. Additional elaboration on what is required for multiple indicators to be deemed acceptable and reliable can be found in Additional file 1 . For the construct of poverty, which theoretically and logically consists of multiple facets that do not indicate one common underlying construct, neither option (single item or latent variable) seemed acceptable. In response to this, both income and assets were included as single items measuring separate (observed) variables covering two different aspects of the construct. A person who has either a high income and few assets, or much financial wealth but little or no income, will normally not be seen as living in poverty. For this reason, the decision was made to include both variables in the model. Separating a construct into multiple single-item variables was also done for unhealthy behaviour. CFA showed that the available items were not suited for constructing one latent variable, and using a single observed variable would not be adequate either. Therefore, three separate observed variables were included as proxy variables for unhealthy behaviour: Body Mass Index (BMI, as a proxy for diet and physical activity), alcohol use, and smoking.
Operationalisation of variables
Below, brief descriptions of the items that were used for operationalising each variable are given. A more detailed overview can be found in Additional file 2 . The items taken from LISS data are mental and physical health indicators, social contacts, health behaviour, and leisure time. The CBS data used were income, assets and debts, employment status, and migration status. The variables age and sex were operationalised using both LISS and CBS data.
Physical health was eventually included in the form of the more limited construct of physical capability (Additional file 1 details why this was done), meaning whether and how easily someone is capable of executing several specific physical tasks. For physical capability, four indicators relating to activities of daily living were used, with an ordinal scale from 0 (“not” [capable]) to 4 (“without any trouble”). These items measure the difficulty with which respondents can walk up a flight of stairs, wash or bathe themselves, walk 100 m, and carry a bag of 5 kg. Those four items were chosen because they are similar to items from the SF-36 questionnaire [ 32 ] and all have high factor loadings when put in a CFA, indicating that they measure the same underlying construct. The latent variable of mental health was measured with five observed variables, each with an ordinal scale from 0 to 5 (from “never” to “continuously", with the category reflecting the best mental health coded as 5). The five items that were used considered feeling down, gloominess, calmness, happiness, and anxiety – all five of the indicators used in the MHI-5 questionnaire [ 31 ]. As physical capability and mental health are both latent variables, they have no native scales. However, the observed indicators from which the latent variables are inferred, of course do have scales and the latent variables’ scales are derived from theirs [ 33 ] (see Additional file 1 ).
The variables of income and financial wealth are taken to be observable and measured using single indicators. For income, household income was adjusted for household size and composition – by using equivalence factors as provided by Statistics Netherlands [ 34 ] – and then expressed in percentages of the low-income threshold, which is corrected for inflation annually [ 35 ]. To illustrate, in 2015, the threshold was €1,030 for a one-person household and €1,930 for a household consisting of a couple with two children. Finally, these percentages were divided by 100 so that a score of 1 means an income of exactly one time the low-income threshold. Financial wealth was operationalised as total household assets minus household debts ( not corrected for household size or composition) – excluding assets and debts relating to the respondent’s own home, as these are not directly spendable. It was then corrected for inflation, so that it is expressed in ‘2015 euros’. Then, it was divided by the median value for 2015, which is €21,414, so a value of 1 indicates one time the median household wealth of 2015. This was done to improve comparability and obtain similar standard error sizes across variables. Finally, in order to limit the impact of extreme outliers, cut-off points were placed at both plus and minus 50 times the median value, which limited the scores (and with that the impact) of the wealthiest individuals, fluctuating between 1.1 to 1.3 percent of the sample for each year.
For satisfaction with social contacts and satisfaction with leisure time, single-indicator measurements were used. Respondents were asked to indicate their satisfaction with both constructs on a scale of 0 (“not at all satisfied”) to 10 (“completely satisfied”). BMI was calculated using self-reported height and weight. Alcohol use was operationalised as the number of days per week a respondent drinks. Smoking was recoded into an ordinal variable with three categories: no smoking, smoking up to 5 units (cigarette, cigar, or pipe) per week, and smoking over 5 units per week.
All exogenous variables included in the models are time-invariant, which mean they are fixed and stay the same over the years. Operationalisations for these variables – age, sex, and migration background, and work status (also see point 4 of this section), level of education, and home ownership for the sensitivity analyses – can be found in Additional file 2 .
At the end of the operationalisation phase, the model constructed in this study contains the variables mental health, physical capability, income, financial wealth, satisfaction with social contacts, satisfaction with leisure time, BMI, alcohol use, and smoking (see Fig. 5 ). The variables shame and participation in society were excluded, due to various issues. Participation in society has a conceptual overlap with the variables of satisfaction with social contacts and work status, which would have become problematic. Additionally, data availability was an issue for both shame and participation in society. Age, sex, and migration background were included as control variables.
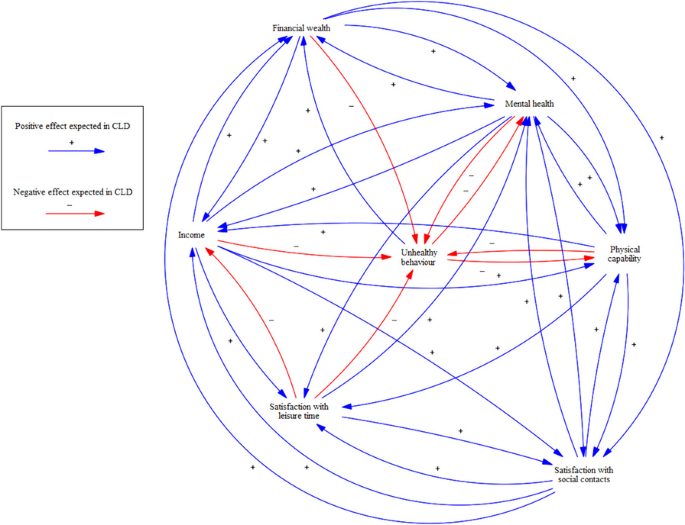
Final CLD structure with all variables and hypothesised effects that were eventually quantified. The three variables that are used to indicate unhealthy behaviour (BMI, alcohol use, and smoking) are not shown separately in this diagram for simplicity’s sake
Selecting the type of longitudinal SEM model and the estimator (step 3)
The type of SEM that was used in this study is a cross-lagged panel model (CLPM), following Orth et al.’s discussion on different types of longitudinal SEM and their different purposes and interpretations [ 36 ]. A CLPM considers both between-subject (i.e. between-person) and within-subject (i.e. within-person) variance together, which can be an advantage if one is interested in long-term effects. In addition, a CLPM can relatively easily capture the strength of each separate effect in a single coefficient, and can be used to cautiously model Granger-causal relationships. Granger-causality is based on the logic that because a variable logically cannot influence any variable’s past values, the directionality of effects can be ascertained, under the assumption that there are no omitted confounding variables [ 37 ]. Of course, there may still be omitted confounding variables, so an actual causal relationship between the variables cannot be ascertained. Lastly, the estimator selected for this study’s models is weighted least squares, which is suitable because of the presence of ordinal endogenous observed variables. More on choosing an estimators can be read in Additional file 1 .
Building the structural model and invariance testing (step 4)
A SEM can consist of two parts: a measurement model that constitutes any latent variables (and is optional) and a structural model that reflects how the variables in the model affect each other. The structural model in this study uses annual data, which means that every time interval has the length of one year. For instance, mental health in 2016 (t1) can be influenced by mental health in 2015 (t0) and income in 2015. Figure 6 shows a simplified diagram with just these two variables at three time points; the full model with all nine endogenous variables over five time points would be too large and cluttered to meaningfully depict.. The influence a variable has on its own subsequent value is the autoregression ( a and b in Fig. 6 ); the influences it has on other variables are cross-lags ( c and d in Fig. 6 ). All effects in the models in this study are entered as linear effects, in order to keep their complexity manageable, but it is possible to enter nonlinear effects into similar models. In Additional file 1 , more elaboration on the CLPM structure and its components can be found. Lastly, there can still be confounders present in the model, which is the reason why the results can be interpreted as Granger-causal and not causal effects. If there are no confounders, it would be an actual causal effect, but this is not testable and probably not realistic in any real-world applications. As is the case with ordinary regression models, including variables in the model that are expected to influence a relationship of interest decreases the likelihood of important missing confounders. Since an aim of the stakeholders’ CLD was to attempt to identify and incorporate the most relevant variables on this topic, the SEM based on that CLD is likely to ‘control’ for at least some influential confounders.
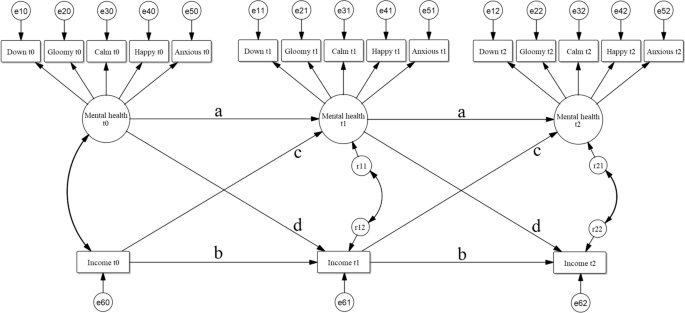
Two-variable CLPM structure with one latent variable and one observed continuous variable at three time points. The error terms (e) of the observed indicators are allowed to covary over time (not shown for simplicity’s sake). The residuals (r) are allowed to covary with the residuals of other variables at the same time point
Putting equality constraints over time on the regression coefficients (the relationships labelled with the same letter in Fig. 6 .) did not decrease the fit of our model and were therefore retained. For the structural model, two further considerations are important: whether a variable is endogenous (dependent, or ‘Y’) or exogenous (independent, or ‘X’) and what the level of measurement of the variables is. All three of these topics are discussed in Additional file 1 .
In addition, multiple sensitivity analyses were run. Their purpose is two-fold: they show whether effects are similar across models with different configurations of structural model or different subpopulations, and in doing so can also help shed some light on what may have caused results that were not the same as in the CLD. The primary model includes data on a sample drawn from the entire population of The Netherlands. It is however plausible that effects could be different for individuals in households with lower incomes or wealth. For this reason, separate analyses were run with two subsets of the sample. The first is the group whose household income is 2.5 times the low-income threshold (€30,900 for a one-person household in 2015) or less in at least two of the measurement years. The second group consists of those with a household financial net wealth (excluding worth of one’s home and mortgage) of two times the full sample’s median wealth (€41,828 in 2015) or less, in at least one measurement year. These thresholds are as low as they could be while retaining samples that are large enough to allow the model to be estimated.
In the CLD that was constructed by stakeholders, employment status was included as an exogenous variable (here meaning: not being part of the model’s feedback loops); whereas in the subsequent scientific expert meeting, the expectation was formulated that work status (having paid work) would have an important role in the system [ 9 ]. However, including it as an endogenous variable was problematic: due to its dichotomous nature, latent-response scales could not be linked over time. In order to do so, at least two thresholds would be needed [ 38 ], while a dichotomous variable has only one. Following the stakeholders’ CLD, a sensitivity analysis that includes work status as an exogenous and time-invariant variable was done. This was not done in the primary model, as the variable may well be influenced by the other variables in the model, as suggested in the scientific expert meeting [ 9 ]. If it is indeed part of any mediation mechanisms, it would not be truly exogenous, which could be problematic because it could largely ‘filter out’ effects where work status is a mediator [ 7 ]. However, the sensitivity analysis can be used to indicate possible confounding influences from work status. Two similar separate sensitivity analyses were then also conducted with the variables of level of education and home ownership added as exogenous variables, as a check to see how including them might affect the model. Information on the operationalisations of these variables can be found in Additional file 2 . Finally, satisfaction with leisure time was included in the model partly because it was mentioned by the stakeholders building the CLD, but also because it may account for some of the (adverse) effects from work status.
Interpretation of the model (step 5)
After estimating the model, it is used for two purposes: interpreting the direct and indirect effects from one variable to other variables and testing the expected effects from the adapted CLD that was primarily based on expectations held by stakeholders (Fig. 5 ). Direct effects, the effects that were estimated in the SEM, exclusively consider a one-year time lag and no mediation via other variables in the model and therefore provide only a limited part of the overall picture. Including indirect effects enables the calculation of total effects (the sum of the direct effect and all indirect effects) from one variable on another, which are dependent on the length of the time frame that is selected. In this study, effects were calculated for until eight years after the model start point. Although it is possible to calculate effects beyond that, the assumption that extrapolation over time is indeed valid would get increasingly difficult to defend as the time frame gets longer. This is why the longest time frame used in this study is eight years, which is twice the interval between the first and last observed time point. Total effects are calculated for each permutation of variables. Additionally, this is done for the effect of a variable on itself at later time points. Effect sizes are shown using unstandardised and standardised coefficients.
Unstandardised (‘raw’) coefficients are expressed in their own units and are therefore not directly comparable to each other. These coefficients do, however, usually have real-world meaning: for instance, an increase of once the low-income threshold (€12,360 for a one-person household in 2015) could lead to an increase of β times the median wealth (€21,414 in 2015) in a subsequent year, where β is the unstandardised coefficient. In order to get a feeling for the magnitude of the effects, one has to keep in mind the units in which the variables are expressed. These can be found earlier in this methods section, under the sub-heading ‘Operationalisation of variables’, and in Additional file 2 . Standardised coefficients are also reported, as is usual.
Using the results as a test of the a priori expected effects from the CLD is relatively more clear-cut than interpreting the coefficients by themselves, but still warrants attention. A CLD is constructed having direct effects in mind, so a comparison between the CLD and direct effects is in order. However, as described earlier, total effects show more of the full picture than direct effects do, so it seems useful to also involve these in the interpretation. Additionally, there are unstandardised and standardised coefficients that do not necessarily produce the same qualitative conclusions, and five sensitivity analyses were also run in this study. We opted to compare the CLD with expected relationships (Fig. 5 ) to unstandardised and standardised total effects from all six models and to the standardised direct effects from the primary model. This is done in the next section.
Total effects between the main variables of interest
The primary model and all sensitivity models were estimated for a one-year lag (the direct effects) up to an eight-year lag. The primary model indicates good fit (robust model fit indices: CFI 0.966, TLI 0.966, RMSEA 0.037, SRMR 0.046), as do the sensitivity analyses (fit indices available in Additional file 3 ). In the text below, unstandardised and standardised regression coefficients from this model are presented. For brevity’s sake, this is done only for the lag that shows the strongest effect, but for the main variables of interest, the full results from the primary model with comparison to expectations are shown in Table 1 . A summary of effects between these variables from the primary model and the five sensitivity analyses can be found in Table 2 . The results from all models for all estimated effects and model fit indices are provided in Additional file 3 . The raw lavaan (the modelling software package used in this study [ 39 , 40 ]) output for the primary model can be found in Additional file 4 , and the polychoric correlation matrix for all observed variables in this model in Additional file 5 .
Mental health in this model is clearly affected by physical capability. The (unstandardised and standardised) effect of physical capability on mental health increases steadily over time and is strongest after eight years (.131 and .145). This indicates that a one-unit increase in physical capability leads to an increase of .131 points on the latent mental health variable. Income has a smaller effect on mental health, with .039 and .045 after eight years. The unstandardised effect is over twice as strong for the lower-income group (.082), but the standardised effect is similar (0.054) to that in the primary model. The effect of financial wealth on mental health is very small (.002 and .013 after two years).
Physical capability in turn is influenced by mental health, which has a modest but clear effect on it, with .089 and .081 after seven years. The effect of income on physical capability is small in the primary model (.020 and .020 after three years). It is much stronger in three sensitivity analyses, especially the analysis with only the lower financial wealth group (.091 and .079 after eight years). The unstandardised effect of financial wealth on physical capability is small, but seems much larger when standardised (.015 and .112 after eight years). The latter is likely a result of the much larger standard deviation of financial wealth, which illustrates why it is generally advisable to not only interpret standardised effects.
Income is most strongly affected by physical capability, with an effect of .199 and .191 after eight years. Mental health displays a small effect on income (.016 and .014 after eight years). In the analysis with only the lower financial wealth group, this effect is a little over twice as strong (.038 and .040 after eight years). For financial wealth on income, the unstandardised coefficient again is small, but the standardised coefficient more substantial (.122) – once more a coefficient that is likely inflated by the larger standard deviation of financial wealth.
Financial wealth displays a prominent influence from physical capability, even though the direct effect was not modelled, with coefficients of 1.888 and .246 after eight years. This effect is decidedly less prominent in some of the sensitivity analyses, but is still strong in all of them. Mental health has a negative effect on financial wealth of -.587 and -.069 after eight years. This is the only one of the effects between the four main variables that shows the opposite of what was expected beforehand. The sensitivity analyses all show a moderately to almost entirely diminished effect (though it does remain negative in all of them), which suggests that this unexpected effect may largely be explained by the elements added in these analyses, such as work or education. Lastly, for the effect of income on financial wealth, the coefficients are .239 and .032 after two years, after which it shows a clear decrease to almost zero after eight years. This suggests short-term returns which are cancelled out in the long run. This decrease is much less strong in nearly all of the sensitivity analyses.
Notable total effects of and on other variables
Some of the effects involving other variables in the model also warrant being mentioned, because they are either strongly in line or in contradiction with the formulated expectations. In the primary model, effects that are clearly in line with the expectations are those of physical capability on smoking (negative), smoking on physical capability and on financial wealth (both negative). The results from the primary model seem to contradict expectations in the effects of income and mental health on smoking (both positive) and of BMI on smoking and alcohol use (both negative). Not all of these effects are the same in all of the sensitivity analyses. More detailed model results can be found in Additional file 3 .
Comparing standardised direct effects to expectations
In the adapted CLD, 50 direct effects were predicted (treating BMI, alcohol use, and smoking as separate variables). The standardised estimates for these effects are shown in Fig. 7 , along with the effects between the variables making up ‘unhealthy behaviour’. For clarity’s sake, only the endogenous variables are shown in this figure. Of the 50 predicted effects, 27 effects are significant and in line with prior expectations, 17 are non-significant, and six are significant and opposite to expectations.
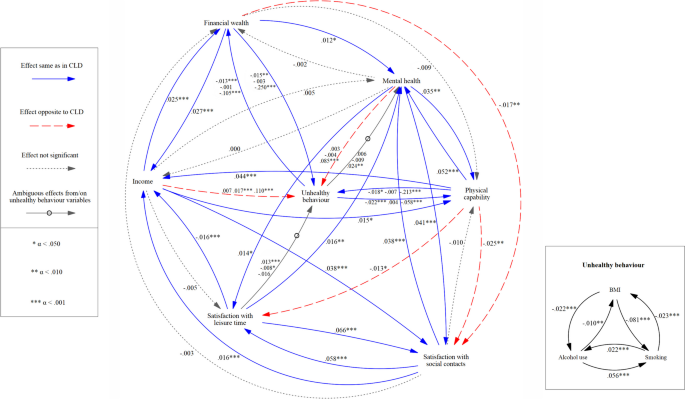
Model with standardised direct effects from the primary model. The three coefficients accompanying every arrow from and to unhealthy behaviour indicate individual relationships with BMI, alcohol use, and smoking, in that order. There were no predictions in the CLD regarding the relationships between these variables making up unhealthy behaviour (the box in the bottom right corner), so these were not compared to any prior expectations
Four variables and their effects on each other were found to be crucial in the interrelationships between poverty and health: mental health, physical capability, income, and financial wealth. Of the 11 expected effects between these variables, seven are significant and in line with expectations and four are non-significant. Between mental health, physical capability, income, and financial wealth, only five of a possible 11 direct effects are significant (and all in line with expectations). These are the effects of mental health on physical capability and vice versa, physical capability on income and vice versa, and financial wealth on mental health. A possible direct effect of physical capability on financial wealth was not included in the original CLD and therefore not estimated.
Summary of results
Regarding the research question – ‘how and to what extent do poverty and health affect each other and are these findings in line with a CLD constructed by stakeholders?’ – there are two clear insights that can be highlighted in summary. First, improving physical capability seems to be very beneficial to both financial wealth and income, as well as to mental health and healthy behaviour. Second, income and especially financial wealth seem to have limited effects on the health of the overall population. However, there are indications that income and financial wealth are more important for the health of people in lower-income and lower-wealth groups and their effects may be even stronger for people living in poverty.
Interpretation of model results
In the primary model, most effects between mental health, physical capability, income, and financial wealth are modest to small. The clearest exceptions to this are the effects from physical capability on the other three variables, though one should keep in mind that a change of 1 unit on the scale of physical capability is a relatively large change due to its small standard deviation. Nevertheless, a one-unit change of physical capability leads to a predicted .12 unit increase in mental health, a .20 unit increase in income (approximately €2,500 annually for a one-person household) and a 1.9 unit increase in financial wealth after eight years (approximately €43,000 for a household), which seems substantial. All of these effects remain substantial in all sensitivity analyses. This suggests that improving physical capability may be an effective way to improve both mental health as well as income and financial wealth. The effects of income on financial wealth and that of mental health on physical capability are also relatively substantial and stable, and in line with expectations.
Conversely, among the main variables of interest, there are two results from the primary model that were unexpected based on the CLD. First, the estimated effects of income and financial wealth on the health variables in the population as a whole are smaller than one might expect. The effect of income on mental health is much more strongly positive in the lower-income group; the effect of income on physical capability is also much stronger in the model with only the lower-income group but especially in that with only the lower-wealth group. These findings indicate that income may not be too consequential in determining the mental health and physical capability of the well-to-do part of the population, but is important for those who have a lower income or less financial wealth. Money may simply have more value for health per euro for these groups. Unlike the effects of income, the effect of financial wealth on mental health and physical capability is small in all models, and only the effect on physical capability in the standardised model seems substantial. It could be that this is different for even more specific groups, for example those with an income below the low-income threshold, but this was not tested in this study. Second, the negative effect of mental health on financial wealth is the opposite of what was expected based on the CLD. However, this effect has mostly dissipated in the sensitivity analysis that includes work status as exogenous variable, suggesting that factors having to do with having work or experiencing work pressure may play some confounding role here.
Strengths and limitations
This study addresses the question of how poverty and health are interrelated. In doing so, it introduces a methodological approach that combines four elements that are not usually used in conjunction: a CLD built by stakeholders, a longitudinal SEM design, a large number of endogenous variables with reciprocity, and mediation analysis. The approach entails a chain of decisions addressing methodological challenges in data and analysis. Estimating a complex system of endogenous variables has two main benefits: it generates more detailed insights about relationships that include mediation mechanisms and it removes confounding effects of a number of variables without eliminating mechanisms in which these variables are mediators. Doing so does require a conceptual model of relevant variables and how these are connected with each other. Using a causal loop diagram as qualitative input for the structure of the model and a set of expectations provides a non-arbitrary starting point from which to test these expectations [ 9 ]. Additionally, the longitudinal SEM design has multiple advantages. SEM allows the use of latent variables and for endogenous variables to also serve as mediators. A longitudinal SEM makes it possible to cautiously infer directionality, in a Granger-causal [ 37 ] way. It permits one to draw conclusions such as that, assuming no unmeasured confounders, the effect of physical capability on financial wealth is clear and strong, while the effect of financial wealth on physical capability is quite small. Moreover, the models resulting from this study can be used to make multi-year projections – with and without external interventions – thereby informing decision-making processes. Such projections can be tailored to fit specific (sub)populations simply by using aggregated-level population characteristics, where available.
There are of course also multiple considerations, assumptions, and limitations present in the study and the chosen design. Many choices are necessarily made during the entire modelling process, from the start of the construction of the CLD to the final quantification steps. A first limitation relates to the construction of the CLD and is addressed at length in the article that describes that previous part of the process [ 9 ]. In short, it entails that the CLD was built by a limited group of participating stakeholders (although with experience in practice and policy) within a specific setting (the city of Utrecht). While this is a non-arbitrary way to obtain a conceptual model, different participants or a different setting could yield a different model. Rather than introducing any model as the model of the issue, it should be presented as being a model. An important advantage of using SEM is that the conceptual model as a whole can be tested empirically, using model fit indices. However, this does not mean that it can be tested whether a model is ‘true’ or not – only how well the model structure fits the real-world data [ 41 ].
The second limitation concerns issues related to the operationalisations of physical capability and of poverty. After consideration, physical capability was included as a variable that represents a part of physical health. It has a more limited scope than physical health in general and for this reason may produce different results than broader operationalisations would. The concept of poverty was captured by considering both income and financial wealth and does not include household expenditures. The latter are more difficult to operationalise. Additionally, higher-income households might have higher expenditures as well. A household with much financial wealth and/or a very high income that chooses to spend more than their income can hardly be said to be living in poverty. Having higher expenditures or even a negative income-expenditures balance would therefore not necessarily be a good indicator for poverty, although these factors can contribute to perceived income inadequacy, which is reported to have health effects as well [ 28 ]. To retain as much information as possible, income and financial wealth were included as continuous variables.
That brings us to a third limitation: besides effects having been constrained in several other ways, they are also assumed to be linear. This means that the effect of X on Y is the same for each value of X, so a euro of income is modelled to matter as much to someone who earns €20,000 euros a year as it does to someone who earns €200,000. This assumption is necessary to avoid unmanageable model complexity, but it is not always equally plausible. By also running sensitivity analyses for only lower-income and lower-wealth groups, the moderating effect of having a low income (as a dichotomous property) on the effects can be shown. This provides insight into how effects are different for these groups, such as that income seems to have effects on both mental health and physical capability that are approximately twice as strong for the lower-income group. Such sensitivity analyses however do not show the direct effects of the dichotomous low-income variable on the other variables. Alternatively, differences between these two sensitivity models and the primary model can be interpreted as showing nonlinearity in the effects of income and wealth on other variables. Something that should also be noted is that in order to make the selection for the lower-income and lower-wealth groups for the sensitivity analyses in this study, cut-off points were set at 2.5 times the low-income threshold and two times the sample’s median wealth, respectively. These cut-off points could not be set lower in order to maintain a sufficient sample to estimate the model, but a model in which it is possible to narrow down the groups further might potentially produce stronger effects still. Finally, it seems likely that at a high enough income level, any further increases in income no longer lead to significant improvements in health. The results from this study reflect a diminishing incremental effect of income on mental health, but do not show how the effect’s gradient is shaped. It could be that the effect gradually diminishes, but it is similarly possible that there are threshold effects present. This applies to any of the relationships in the model, as the model was built with the assumption of linear effects. Studying incrementally diminishing effects in depth was not a main focus of this study, but could be done by constructing models that use an extensive multiple group or nonlinear design.
A fourth limitation is the possibility of unmeasured confounding effects. To counter these, this study includes a good number of relevant endogenous variables. By including them as endogenous, they are not merely ‘controlled for’ but can actively contribute in mediation mechanisms. The variable of work status was a particularly challenging variable. The stakeholders’ CLD and the participants from the scientific expert meeting included the variable as exogenous and endogenous, respectively. The dichotomous nature of the variable made it impossible to test the assumption of threshold invariance over time [ 38 ] and to include it in the model as varying over time (as would be necessary for a endogenous variable). At the same time, including it as exogenous in the primary model would be problematic if it was in reality endogenous. Therefore, it was included as a time-invariant exogenous variable in a sensitivity analysis, in order to examine possible effects that this variable has on the rest of the model, as was also done for level of education and home ownership. This is a limitation to the results of the study, however, and some mediation effects may be ‘filtered out’ of the results . Furthermore, the primary model and the sensitivity analyses show multiple alternatives with good and similar model fit (see Additional file 3 ) and this may create uncertainty regarding which one is the ‘best one’. The short answer is that this depends on the intended purpose. The primary model is most suited for application to the population as a whole, while the models focused on the lower-income and lower-wealth groups are intended to provide information on a more specific target group. It should be noted that the results from the model with only the lower-wealth group strikingly deviate from all the other models. This could be due to actual and clear differences between the groups, but it may be wise to approach this model more cautiously until there is more evidence to support its claims. Lastly, the models that control for work status, level of education, and home ownership remove these variables as possible unmeasured confounders. Conversely, relationships in which those variables are part of the mechanism itself (as mediator) are ‘closed off’, so are likely to be underestimated. This should be kept in mind when using one of these models.
A fifth and final limitation is that the specific model design may influence findings, in that a CLPM requires the specification of the length of the time lag to be estimated. In this study, this time lag had the length of one year. However, it is possible for this lag to be longer or shorter in reality. Any relationships that have an actual time lag that is in reality (much) longer than this, may have been underestimated. Conversely, if the actual lag is shorter, data points closer to each other would be needed to detect effects. Effects with a shorter-term lag than is modelled are also potentially underestimated, which would make the results presented in this study more likely to be conservative estimates than inflated ones.
In short, several key findings can be summarised from this study. There is an indication that physical capability (but not mental health) may have a substantial influence on income and financial wealth, and also on mental health. Financial wealth has some effect on physical capability in the models, but not on mental health. Income does not show a clear effect on either mental health or physical capability for the general population, but there are indications that income and financial wealth are more important for the health of lower-income and lower-wealth groups and their effects may be even stronger for people living in poverty. A broad recommendation from this study would be that physical capability is also considered in activities that are intended to improve income, financial wealth, or mental health.
Regarding the methodological approach, there are lessons to be drawn from this study as well. A positive one is that the quantification of a CLD can be done using longitudinal SEM, even while preserving a large part of the complexity and the feedback loops. Naturally, there are a number of assumptions and limitations inherent to achieving this. In the end, a model is always a simplified approximation of reality and is therefore ‘wrong’ by default [ 42 ]. However, the models from this study have been able to show quantitative estimations and generate insights and evidence that would otherwise not have been found, providing an indication of how mechanisms between poverty and health may operate.
Availability of data and materials
Any requests for additional information regarding this study can be addressed to the corresponding author. LISS data is free and can be accessed for non-commercial research. The CBS data that was used is non-public microdata. Under certain conditions, these microdata are accessible for statistical and scientific research. For further information: [email protected].
The software used for the estimation of structural equation models in this paper was the R package ‘lavaan’ (version 0.6–5; R version 4.2.3). Data transformations were done using SPSS version 25. The figures shown in this paper (Figs. 1 , 2 , 3 , 4 , 5 , 6 and 7 ) were made in Vensim PLE version 9.3.5 (developed by Ventana Systems), which is available for Windows and Macintosh OS (X) from https://vensim.com . This version of Vensim is free for academic use, but requires a paid license for non-academic use.
Abbreviations
Social determinant(s) of health
- Causal loop diagram
Structural equation model(ling)
Longitudinal Internet studies for the Social Sciences
Central Bureau voor de Statistiek (Statistics Netherlands)
Confirmatory factor analysis
Mental Health Inventory 5
Body Mass Index
Cross-lagged panel model
Comparative fit index
Tucker-Lewis index
Root mean square error of approximation
Standardised root mean square residual
Degrees of freedom
Collins SD. Economic status and health: a review and study of the relevant morbidity and mortality data. 1924.
Google Scholar
Ratcliff KS. The social determinants of health: looking upstream. Cambridge: Polity; 2017.
Singh GK, Daus GP, Allender M, Ramey CT, Martin EK, Perry C, Andrew A, Vedamuthu IP. Social determinants of health in the United States: addressing major health inequality trends for the nation, 1935–2016. Int J MCH AIDS. 2017;6(2):139.
Article PubMed PubMed Central Google Scholar
World Health Organization. Social determinants of health. https://www.who.int/health-topics/social-determinants-of-health . Accessed 19 Feb 2024.
Hood CM, Gennuso KP, Swain GR, Catlin BB. County health rankings: relationships between determinant factors and health outcomes. Am J Prev Med. 2016;50(2):129–35.
Article PubMed Google Scholar
Marmot M, Atkinson A, Bell J, Black C, Broadfoot P, Cumberlege J, et al. Fair Society, Healthy Lives (The Marmot Review): Strategic Review of Health Inequalities in England post-2010. 2010.
Pearl J, Mackenzie D. The book of why: the new science of cause and effect. New York: Basic Books; 2018.
Popper K. Conjectures and refutations: the growth of scientific knowledge. London: Routledge; 1963.
Reumers L, Bekker M, Hilderink H, Jansen M, Helderman J-K, Ruwaard D. Qualitative modelling of social determinants of health using group model building: the case of debt, poverty, and health. Int J Equity Health. 2022;21(1):1–12.
Article Google Scholar
Dahlgren G, Whitehead M. Policies and strategies to promote social equity in health. 1991.
Rittel HW, Webber MM. Dilemmas in a general theory of planning. Policy Sci. 1973;4(2):155–69.
Antonakis J, Bendahan S, Jacquart P, Lalive R. Causality and endogeneity: problems and solutions. Oxf Handb Leadersh Organ. 2014;1:93–117.
Sterman JD. System dynamics modeling: tools for learning in a complex world. Calif Manag Rev. 2001;43(4):8–25.
Williams MN, Grajales CAG, Kurkiewicz D. Assumptions of multiple regression: correcting two misconceptions. Pract Assess Res Eval. 2013;18(1):11.
Martens MP, Haase RF. Advanced applications of structural equation modeling in counseling psychology research. Couns Psychol. 2006;34(6):878–911.
Uleman JF, Melis RJ, Quax R, van der Zee EA, Thijssen D, Dresler M, van de Rest O, van der Velpen IF, Adams HH, Schmand B. Mapping the multicausality of Alzheimer’s disease through group model building. GeroScience. 2021;43(2):829–43.
Mudd AL, van Lenthe FJ, Verra SE, Bal M, Kamphuis CB. Socioeconomic inequalities in health behaviors: exploring mediation pathways through material conditions and time orientation. Int J Equity Health. 2021;20:1–13.
Halleröd B, Gustafsson J-E. A longitudinal analysis of the relationship between changes in socio-economic status and changes in health. Soc Sci Med. 2011;72(1):116–23.
Ahrenfeldt LJ, Möller S. The reciprocal relationship between socioeconomic status and health and the influence of sex: a European SHARE-analysis based on structural equation modeling. Int J Environ Res Public Health. 2021;18(9): 5045.
O’Donnell O, Van Doorslaer E, Van Ourti T. Health and inequality. In: Atkinson AB, Bourguignon F, editors. Handbook of income distribution, vol 2. Amsterdam: Elsevier; 2015. p. 1419–533.
Allanson P, Petrie D. Longitudinal methods to investigate the role of health determinants in the dynamics of income-related health inequality. J Health Econ. 2013;32(5):922–37.
Van Kippersluis H, Galama TJ. Why the rich drink more but smoke less: the impact of wealth on health behaviors. 2013.
Downward P, Rasciute S, Kumar H. Health, subjective financial situation and well-being: a longitudinal observational study. Health Qual Life Outcomes. 2020;18:1–9.
Michaud P-C, Van Soest A. Health and wealth of elderly couples: causality tests using dynamic panel data models. J Health Econ. 2008;27(5):1312–25.
Reumers LM, Bekker MP, Jansen MW, Hilderink HB, Helderman J-K, Ruwaard D. Quantitative health impact assessment methodology for societal initiatives: a scoping review. Environ Impact Assess Rev. 2021;86:106509.
Centerdata. LISS panel. https://www.centerdata.nl/en/liss-panel . Accessed 19 Feb 2024.
Centerdata. About the panel. https://www.lissdata.nl/about-panel . Accessed 19 Feb 2024.
CBS. Bevolkingsstatistiek. https://www.cbs.nl/nl-nl/onze-diensten/methoden/onderzoeksomschrijvingen/korte-onderzoeksbeschrijvingen/bevolkingsstatistiek . Accessed 19 Feb 2024.
Kline RB. Beyond significance testing: statistics reform in the behavioral sciences. Washington, D.C: American Psychological Association; 2013.
Book Google Scholar
Little TD. Longitudinal structural equation modeling. New York: Guilford Press; 2013.
Berwick DM, Murphy JM, Goldman PA, Ware JE, Barsky AJ, Weinstein MC. Performance of a five-item mental health screening test. Med Care. 1991;29(2):169–76.
Article CAS PubMed Google Scholar
Rand Corporation. 36-Item short form survey instrument (SF-36). https://www.rand.org/health-care/surveys_tools/mos/36-item-short-form/survey-instrument.html . Accessed 19 Feb 2024.
Klopp E, Klößner S. The impact of scaling methods on the properties and interpretation of parameter estimates in structural equation models with latent variables. Struct Equ Modeling Multidiscip J. 2021;28(2):182–206.
CBS. Welvaart in Nederland 2019: Bijlagen. https://longreads.cbs.nl/welvaartinnederland-2019/bijlagen . Accessed 7 Feb 2024.
CBS. Inhatab: Inkomen van huishoudens. https://www.cbs.nl/nl-nl/onze-diensten/maatwerk-en-microdata/microdata-zelf-onderzoek-doen/microdatabestanden/inhatab-inkomen-van-huishoudens . Accessed 19 Feb 2024.
Orth U, Clark DA, Donnellan MB, Robins RW. Testing prospective effects in longitudinal research: comparing seven competing cross-lagged models. J Personal Soc Psychol. 2021;120(4):1013.
Seth A. Granger causality. Scholarpedia. 2007;2(7):1667.
Mehta PD, Neale MC, Flay BR. Squeezing interval change from ordinal panel data: latent growth curves with ordinal outcomes. Psychol Methods. 2004;9(3): 301.
Rosseel Y. lavaan tutorial. https://lavaan.ugent.be/tutorial/cat.html . Accessed 19 Feb 2024.
Rosseel Y. Lavaan: an R package for structural equation modeling. J Stat Softw. 2012;48:1–36.
West SG, Taylor AB, Wu W. Model fit and model selection in structural equation modeling. Handb Struct Equation Model. 2012;1:209–31.
Box GE. Robustness in the strategy of scientific model building. In: Launer RL, Wilkinson GN, editors. Robustness in statistics. New York: Academic Press; 1979. p. 201–36.
Chapter Google Scholar
Download references
Acknowledgements
We would like to thank Jan-Kees Helderman of Radboud University for helping in multiple stages of the study, especially by giving feedback and for his support with the data. We also thank Coen Hacking for his assistance in drafting an early version of our total effects calculations, which helped point us in the right direction.
In this paper we make use of data of the LISS (Longitudinal Internet studies for the Social Sciences) panel administered by Centerdata (Tilburg University, The Netherlands). The LISS panel data were collected by Centerdata through its MESS project funded by the Netherlands Organization for Scientific Research.
Results based on calculations by the authors of this paper, using non-public microdata from Statistics Netherlands (CBS).
This study was funded by The Netherlands Organisation for Health Research and Development (ZonMw: 531005012).
Author information
Authors and affiliations.
Department of Health Services Research, Care and Public Health Research Institute (CAPHRI), Maastricht University, Maastricht, The Netherlands
Laurens Reumers, Niels Hameleers, Maria Jansen & Dirk Ruwaard
National Institute for Public Health and the Environment, Bilthoven, The Netherlands
Henk Hilderink
Chair Group Health and Society, Center for Space, Place and Society, Wageningen University and Research, Wageningen, The Netherlands
Marleen Bekker
Academic Collaborative Center for Public Health, Public Health Service South Limburg, Heerlen, The Netherlands
Maria Jansen
You can also search for this author in PubMed Google Scholar
Contributions
LR co-conceptualised the study, co-designed the research approach, examined methodological considerations, executed the SEM-modelling, and wrote the original draft of this paper. NH co-conceptualised the study, co-designed the research approach, examined methodological considerations (including about SEM), programmed total effects calculations, and co-wrote the paper. HH and DR co-conceptualised the study, co-wrote the paper, and were involved in detailed supervision. MB and MJ co-conceptualised the study, co-edited the paper, and were involved in supervision.
Corresponding author
Correspondence to Laurens Reumers .
Ethics declarations
Ethics approval and consent to participate.
Formal ethics approval was requested from the Faculty of Health, Medicine and Life Sciences Research Ethics Committee of Maastricht University. This committee reviewed the application and decided that no ethics approval was required for this study.
Consent for publication
The respondents whose data was used in this study have all explicitly consented to their data being used in this way. First, LISS panel respondents have all given their explicit permission for their responses to be used for scientific, policy, and social research. Second, only respondents who have also explicitly given explicit consent for their CBS registry data to be used in conjunction with their LISS panel responses were included in this study.
Competing interests
The authors declare no competing interests.
Additional information
Publisher’s note.
Springer Nature remains neutral with regard to jurisdictional claims in published maps and institutional affiliations.
Supplementary Information
Supplementary material 1., supplementary material 2., supplementary material 3., supplementary material 4., supplementary material 5., rights and permissions.
Open Access This article is licensed under a Creative Commons Attribution 4.0 International License, which permits use, sharing, adaptation, distribution and reproduction in any medium or format, as long as you give appropriate credit to the original author(s) and the source, provide a link to the Creative Commons licence, and indicate if changes were made. The images or other third party material in this article are included in the article's Creative Commons licence, unless indicated otherwise in a credit line to the material. If material is not included in the article's Creative Commons licence and your intended use is not permitted by statutory regulation or exceeds the permitted use, you will need to obtain permission directly from the copyright holder. To view a copy of this licence, visit http://creativecommons.org/licenses/by/4.0/ . The Creative Commons Public Domain Dedication waiver ( http://creativecommons.org/publicdomain/zero/1.0/ ) applies to the data made available in this article, unless otherwise stated in a credit line to the data.
Reprints and permissions
About this article
Cite this article.
Reumers, L., Hameleers, N., Hilderink, H. et al. Quantifying reciprocal relationships between poverty and health: combining a causal loop diagram with longitudinal structural equation modelling. Int J Equity Health 23 , 87 (2024). https://doi.org/10.1186/s12939-024-02172-w
Download citation
Received : 18 August 2023
Accepted : 04 April 2024
Published : 01 May 2024
DOI : https://doi.org/10.1186/s12939-024-02172-w
Share this article
Anyone you share the following link with will be able to read this content:
Sorry, a shareable link is not currently available for this article.
Provided by the Springer Nature SharedIt content-sharing initiative
- Financial wealth
- Social determinants of health
- Quantification
- Longitudinal
- Structural equation modelling
- Latent variables
- Indirect effects
International Journal for Equity in Health
ISSN: 1475-9276
- General enquiries: [email protected]

Research Topics & Ideas: Environment
100+ Environmental Science Research Topics & Ideas
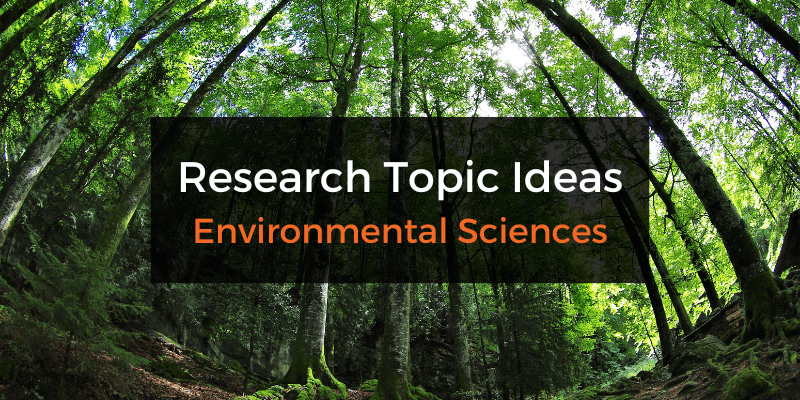
Finding and choosing a strong research topic is the critical first step when it comes to crafting a high-quality dissertation, thesis or research project. Here, we’ll explore a variety research ideas and topic thought-starters related to various environmental science disciplines, including ecology, oceanography, hydrology, geology, soil science, environmental chemistry, environmental economics, and environmental ethics.
NB – This is just the start…
The topic ideation and evaluation process has multiple steps . In this post, we’ll kickstart the process by sharing some research topic ideas within the environmental sciences. This is the starting point though. To develop a well-defined research topic, you’ll need to identify a clear and convincing research gap , along with a well-justified plan of action to fill that gap.
If you’re new to the oftentimes perplexing world of research, or if this is your first time undertaking a formal academic research project, be sure to check out our free dissertation mini-course. Also be sure to also sign up for our free webinar that explores how to develop a high-quality research topic from scratch.
Overview: Environmental Topics
- Ecology /ecological science
- Atmospheric science
- Oceanography
- Soil science
- Environmental chemistry
- Environmental economics
- Environmental ethics
- Examples of dissertations and theses
Topics & Ideas: Ecological Science
- The impact of land-use change on species diversity and ecosystem functioning in agricultural landscapes
- The role of disturbances such as fire and drought in shaping arid ecosystems
- The impact of climate change on the distribution of migratory marine species
- Investigating the role of mutualistic plant-insect relationships in maintaining ecosystem stability
- The effects of invasive plant species on ecosystem structure and function
- The impact of habitat fragmentation caused by road construction on species diversity and population dynamics in the tropics
- The role of ecosystem services in urban areas and their economic value to a developing nation
- The effectiveness of different grassland restoration techniques in degraded ecosystems
- The impact of land-use change through agriculture and urbanisation on soil microbial communities in a temperate environment
- The role of microbial diversity in ecosystem health and nutrient cycling in an African savannah
Topics & Ideas: Atmospheric Science
- The impact of climate change on atmospheric circulation patterns above tropical rainforests
- The role of atmospheric aerosols in cloud formation and precipitation above cities with high pollution levels
- The impact of agricultural land-use change on global atmospheric composition
- Investigating the role of atmospheric convection in severe weather events in the tropics
- The impact of urbanisation on regional and global atmospheric ozone levels
- The impact of sea surface temperature on atmospheric circulation and tropical cyclones
- The impact of solar flares on the Earth’s atmospheric composition
- The impact of climate change on atmospheric turbulence and air transportation safety
- The impact of stratospheric ozone depletion on atmospheric circulation and climate change
- The role of atmospheric rivers in global water supply and sea-ice formation
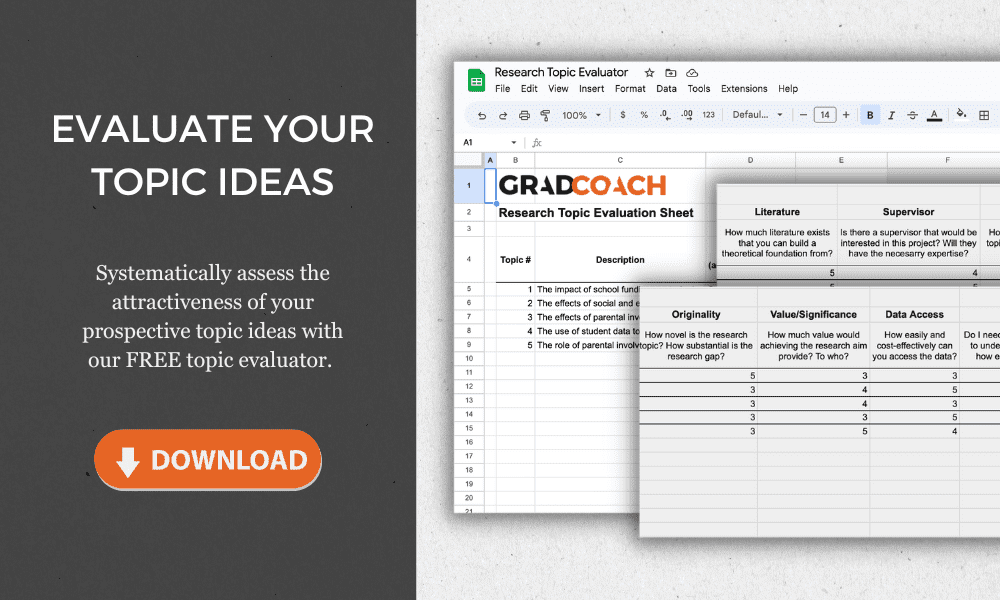
Topics & Ideas: Oceanography
- The impact of ocean acidification on kelp forests and biogeochemical cycles
- The role of ocean currents in distributing heat and regulating desert rain
- The impact of carbon monoxide pollution on ocean chemistry and biogeochemical cycles
- Investigating the role of ocean mixing in regulating coastal climates
- The impact of sea level rise on the resource availability of low-income coastal communities
- The impact of ocean warming on the distribution and migration patterns of marine mammals
- The impact of ocean deoxygenation on biogeochemical cycles in the arctic
- The role of ocean-atmosphere interactions in regulating rainfall in arid regions
- The impact of ocean eddies on global ocean circulation and plankton distribution
- The role of ocean-ice interactions in regulating the Earth’s climate and sea level
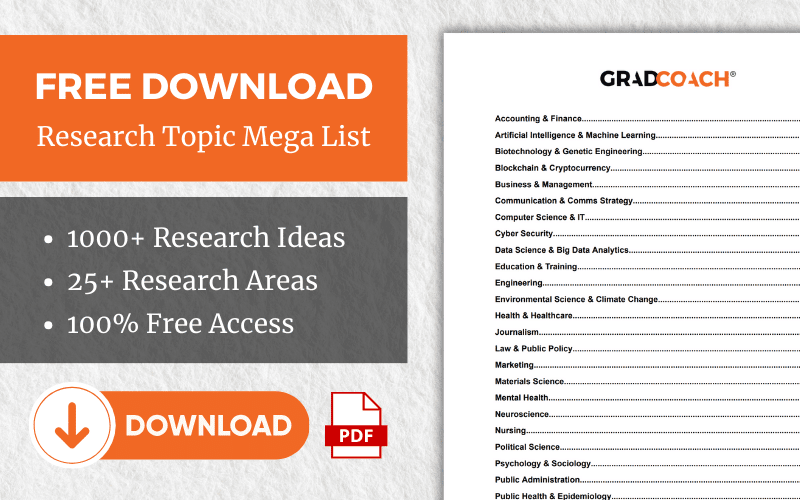
Tops & Ideas: Hydrology
- The impact of agricultural land-use change on water resources and hydrologic cycles in temperate regions
- The impact of agricultural groundwater availability on irrigation practices in the global south
- The impact of rising sea-surface temperatures on global precipitation patterns and water availability
- Investigating the role of wetlands in regulating water resources for riparian forests
- The impact of tropical ranches on river and stream ecosystems and water quality
- The impact of urbanisation on regional and local hydrologic cycles and water resources for agriculture
- The role of snow cover and mountain hydrology in regulating regional agricultural water resources
- The impact of drought on food security in arid and semi-arid regions
- The role of groundwater recharge in sustaining water resources in arid and semi-arid environments
- The impact of sea level rise on coastal hydrology and the quality of water resources
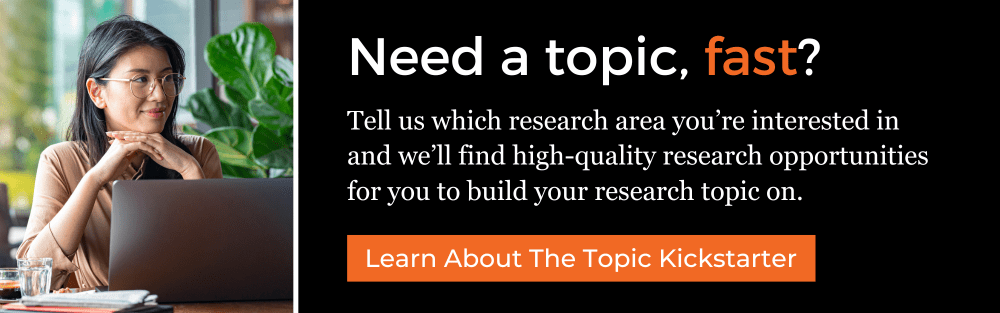
Topics & Ideas: Geology
- The impact of tectonic activity on the East African rift valley
- The role of mineral deposits in shaping ancient human societies
- The impact of sea-level rise on coastal geomorphology and shoreline evolution
- Investigating the role of erosion in shaping the landscape and impacting desertification
- The impact of mining on soil stability and landslide potential
- The impact of volcanic activity on incoming solar radiation and climate
- The role of geothermal energy in decarbonising the energy mix of megacities
- The impact of Earth’s magnetic field on geological processes and solar wind
- The impact of plate tectonics on the evolution of mammals
- The role of the distribution of mineral resources in shaping human societies and economies, with emphasis on sustainability
Topics & Ideas: Soil Science
- The impact of dam building on soil quality and fertility
- The role of soil organic matter in regulating nutrient cycles in agricultural land
- The impact of climate change on soil erosion and soil organic carbon storage in peatlands
- Investigating the role of above-below-ground interactions in nutrient cycling and soil health
- The impact of deforestation on soil degradation and soil fertility
- The role of soil texture and structure in regulating water and nutrient availability in boreal forests
- The impact of sustainable land management practices on soil health and soil organic matter
- The impact of wetland modification on soil structure and function
- The role of soil-atmosphere exchange and carbon sequestration in regulating regional and global climate
- The impact of salinization on soil health and crop productivity in coastal communities
Topics & Ideas: Environmental Chemistry
- The impact of cobalt mining on water quality and the fate of contaminants in the environment
- The role of atmospheric chemistry in shaping air quality and climate change
- The impact of soil chemistry on nutrient availability and plant growth in wheat monoculture
- Investigating the fate and transport of heavy metal contaminants in the environment
- The impact of climate change on biochemical cycling in tropical rainforests
- The impact of various types of land-use change on biochemical cycling
- The role of soil microbes in mediating contaminant degradation in the environment
- The impact of chemical and oil spills on freshwater and soil chemistry
- The role of atmospheric nitrogen deposition in shaping water and soil chemistry
- The impact of over-irrigation on the cycling and fate of persistent organic pollutants in the environment
Topics & Ideas: Environmental Economics
- The impact of climate change on the economies of developing nations
- The role of market-based mechanisms in promoting sustainable use of forest resources
- The impact of environmental regulations on economic growth and competitiveness
- Investigating the economic benefits and costs of ecosystem services for African countries
- The impact of renewable energy policies on regional and global energy markets
- The role of water markets in promoting sustainable water use in southern Africa
- The impact of land-use change in rural areas on regional and global economies
- The impact of environmental disasters on local and national economies
- The role of green technologies and innovation in shaping the zero-carbon transition and the knock-on effects for local economies
- The impact of environmental and natural resource policies on income distribution and poverty of rural communities
Topics & Ideas: Environmental Ethics
- The ethical foundations of environmentalism and the environmental movement regarding renewable energy
- The role of values and ethics in shaping environmental policy and decision-making in the mining industry
- The impact of cultural and religious beliefs on environmental attitudes and behaviours in first world countries
- Investigating the ethics of biodiversity conservation and the protection of endangered species in palm oil plantations
- The ethical implications of sea-level rise for future generations and vulnerable coastal populations
- The role of ethical considerations in shaping sustainable use of natural forest resources
- The impact of environmental justice on marginalized communities and environmental policies in Asia
- The ethical implications of environmental risks and decision-making under uncertainty
- The role of ethics in shaping the transition to a low-carbon, sustainable future for the construction industry
- The impact of environmental values on consumer behaviour and the marketplace: a case study of the ‘bring your own shopping bag’ policy
Examples: Real Dissertation & Thesis Topics
While the ideas we’ve presented above are a decent starting point for finding a research topic, they are fairly generic and non-specific. So, it helps to look at actual dissertations and theses to see how this all comes together.
Below, we’ve included a selection of research projects from various environmental science-related degree programs to help refine your thinking. These are actual dissertations and theses, written as part of Master’s and PhD-level programs, so they can provide some useful insight as to what a research topic looks like in practice.
- The physiology of microorganisms in enhanced biological phosphorous removal (Saunders, 2014)
- The influence of the coastal front on heavy rainfall events along the east coast (Henson, 2019)
- Forage production and diversification for climate-smart tropical and temperate silvopastures (Dibala, 2019)
- Advancing spectral induced polarization for near surface geophysical characterization (Wang, 2021)
- Assessment of Chromophoric Dissolved Organic Matter and Thamnocephalus platyurus as Tools to Monitor Cyanobacterial Bloom Development and Toxicity (Hipsher, 2019)
- Evaluating the Removal of Microcystin Variants with Powdered Activated Carbon (Juang, 2020)
- The effect of hydrological restoration on nutrient concentrations, macroinvertebrate communities, and amphibian populations in Lake Erie coastal wetlands (Berg, 2019)
- Utilizing hydrologic soil grouping to estimate corn nitrogen rate recommendations (Bean, 2019)
- Fungal Function in House Dust and Dust from the International Space Station (Bope, 2021)
- Assessing Vulnerability and the Potential for Ecosystem-based Adaptation (EbA) in Sudan’s Blue Nile Basin (Mohamed, 2022)
- A Microbial Water Quality Analysis of the Recreational Zones in the Los Angeles River of Elysian Valley, CA (Nguyen, 2019)
- Dry Season Water Quality Study on Three Recreational Sites in the San Gabriel Mountains (Vallejo, 2019)
- Wastewater Treatment Plan for Unix Packaging Adjustment of the Potential Hydrogen (PH) Evaluation of Enzymatic Activity After the Addition of Cycle Disgestase Enzyme (Miessi, 2020)
- Laying the Genetic Foundation for the Conservation of Longhorn Fairy Shrimp (Kyle, 2021).
Looking at these titles, you can probably pick up that the research topics here are quite specific and narrowly-focused , compared to the generic ones presented earlier. To create a top-notch research topic, you will need to be precise and target a specific context with specific variables of interest . In other words, you’ll need to identify a clear, well-justified research gap.
Need more help?
If you’re still feeling a bit unsure about how to find a research topic for your environmental science dissertation or research project, be sure to check out our private coaching services below, as well as our Research Topic Kickstarter .
Need a helping hand?
You Might Also Like:
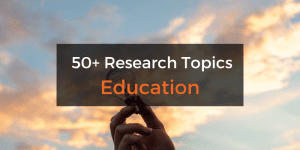
research topics on climate change and environment
I wish to learn things in a more advanced but simple way and with the hopes that I am in the right place.
Thank so much for the research topics. It really helped
the guides were really helpful
Research topics on environmental geology
Thanks for the research topics….I need a research topic on Geography
I want the research on environmental planning and management
I want a topic on environmental sustainability
It good coaching
Submit a Comment Cancel reply
Your email address will not be published. Required fields are marked *
Save my name, email, and website in this browser for the next time I comment.
- Print Friendly
Numbers, Facts and Trends Shaping Your World
Read our research on:
Full Topic List
Regions & Countries
- Publications
- Our Methods
- Short Reads
- Tools & Resources
Read Our Research On:
What the data says about crime in the U.S.
A growing share of Americans say reducing crime should be a top priority for the president and Congress to address this year. Around six-in-ten U.S. adults (58%) hold that view today, up from 47% at the beginning of Joe Biden’s presidency in 2021.
We conducted this analysis to learn more about U.S. crime patterns and how those patterns have changed over time.
The analysis relies on statistics published by the FBI, which we accessed through the Crime Data Explorer , and the Bureau of Justice Statistics (BJS), which we accessed through the National Crime Victimization Survey data analysis tool .
To measure public attitudes about crime in the U.S., we relied on survey data from Pew Research Center and Gallup.
Additional details about each data source, including survey methodologies, are available by following the links in the text of this analysis.
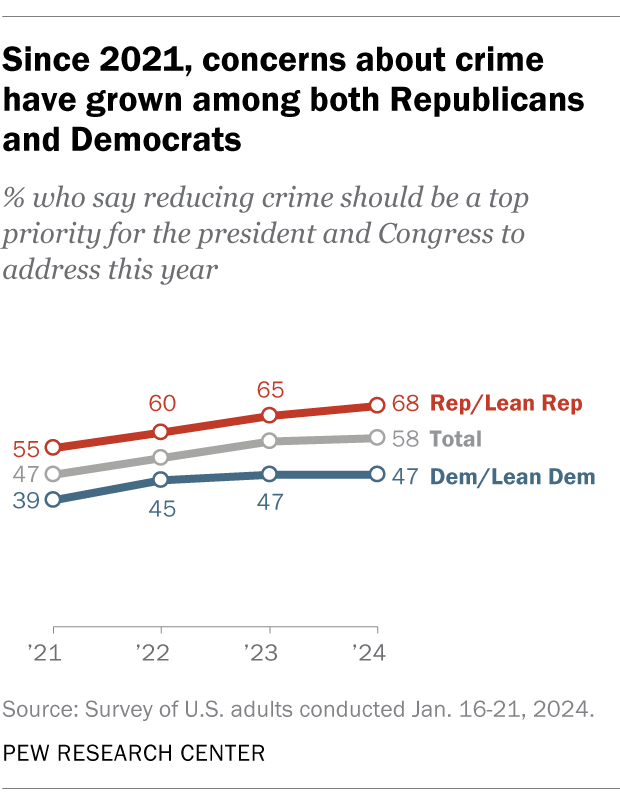
With the issue likely to come up in this year’s presidential election, here’s what we know about crime in the United States, based on the latest available data from the federal government and other sources.
How much crime is there in the U.S.?
It’s difficult to say for certain. The two primary sources of government crime statistics – the Federal Bureau of Investigation (FBI) and the Bureau of Justice Statistics (BJS) – paint an incomplete picture.
The FBI publishes annual data on crimes that have been reported to law enforcement, but not crimes that haven’t been reported. Historically, the FBI has also only published statistics about a handful of specific violent and property crimes, but not many other types of crime, such as drug crime. And while the FBI’s data is based on information from thousands of federal, state, county, city and other police departments, not all law enforcement agencies participate every year. In 2022, the most recent full year with available statistics, the FBI received data from 83% of participating agencies .
BJS, for its part, tracks crime by fielding a large annual survey of Americans ages 12 and older and asking them whether they were the victim of certain types of crime in the past six months. One advantage of this approach is that it captures both reported and unreported crimes. But the BJS survey has limitations of its own. Like the FBI, it focuses mainly on a handful of violent and property crimes. And since the BJS data is based on after-the-fact interviews with crime victims, it cannot provide information about one especially high-profile type of offense: murder.
All those caveats aside, looking at the FBI and BJS statistics side-by-side does give researchers a good picture of U.S. violent and property crime rates and how they have changed over time. In addition, the FBI is transitioning to a new data collection system – known as the National Incident-Based Reporting System – that eventually will provide national information on a much larger set of crimes , as well as details such as the time and place they occur and the types of weapons involved, if applicable.
Which kinds of crime are most and least common?
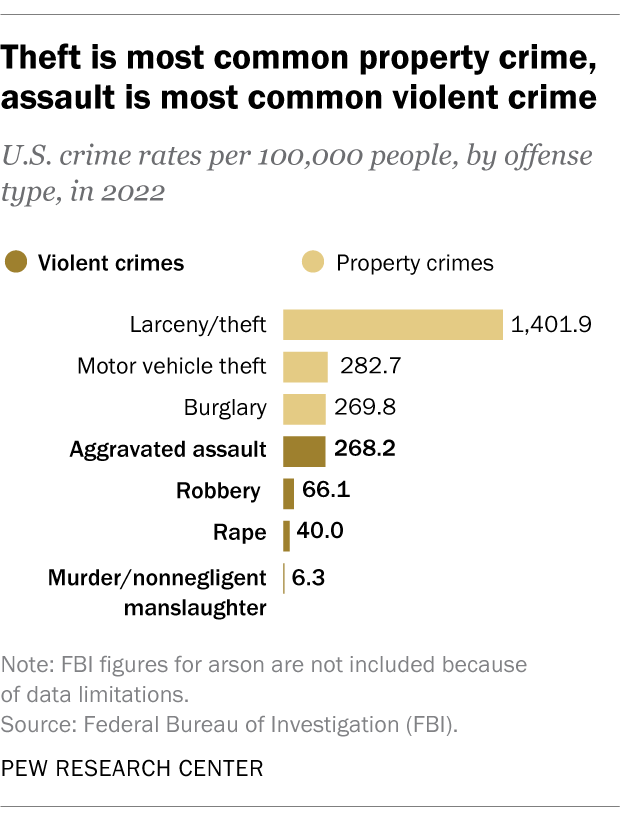
Property crime in the U.S. is much more common than violent crime. In 2022, the FBI reported a total of 1,954.4 property crimes per 100,000 people, compared with 380.7 violent crimes per 100,000 people.
By far the most common form of property crime in 2022 was larceny/theft, followed by motor vehicle theft and burglary. Among violent crimes, aggravated assault was the most common offense, followed by robbery, rape, and murder/nonnegligent manslaughter.
BJS tracks a slightly different set of offenses from the FBI, but it finds the same overall patterns, with theft the most common form of property crime in 2022 and assault the most common form of violent crime.
How have crime rates in the U.S. changed over time?
Both the FBI and BJS data show dramatic declines in U.S. violent and property crime rates since the early 1990s, when crime spiked across much of the nation.
Using the FBI data, the violent crime rate fell 49% between 1993 and 2022, with large decreases in the rates of robbery (-74%), aggravated assault (-39%) and murder/nonnegligent manslaughter (-34%). It’s not possible to calculate the change in the rape rate during this period because the FBI revised its definition of the offense in 2013 .
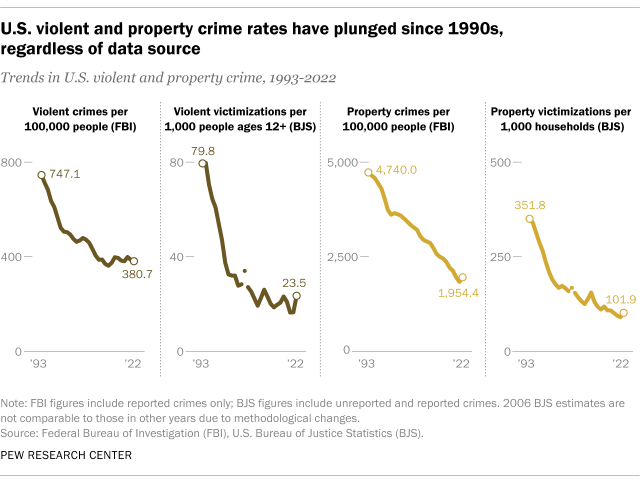
The FBI data also shows a 59% reduction in the U.S. property crime rate between 1993 and 2022, with big declines in the rates of burglary (-75%), larceny/theft (-54%) and motor vehicle theft (-53%).
Using the BJS statistics, the declines in the violent and property crime rates are even steeper than those captured in the FBI data. Per BJS, the U.S. violent and property crime rates each fell 71% between 1993 and 2022.
While crime rates have fallen sharply over the long term, the decline hasn’t always been steady. There have been notable increases in certain kinds of crime in some years, including recently.
In 2020, for example, the U.S. murder rate saw its largest single-year increase on record – and by 2022, it remained considerably higher than before the coronavirus pandemic. Preliminary data for 2023, however, suggests that the murder rate fell substantially last year .
How do Americans perceive crime in their country?
Americans tend to believe crime is up, even when official data shows it is down.
In 23 of 27 Gallup surveys conducted since 1993 , at least 60% of U.S. adults have said there is more crime nationally than there was the year before, despite the downward trend in crime rates during most of that period.
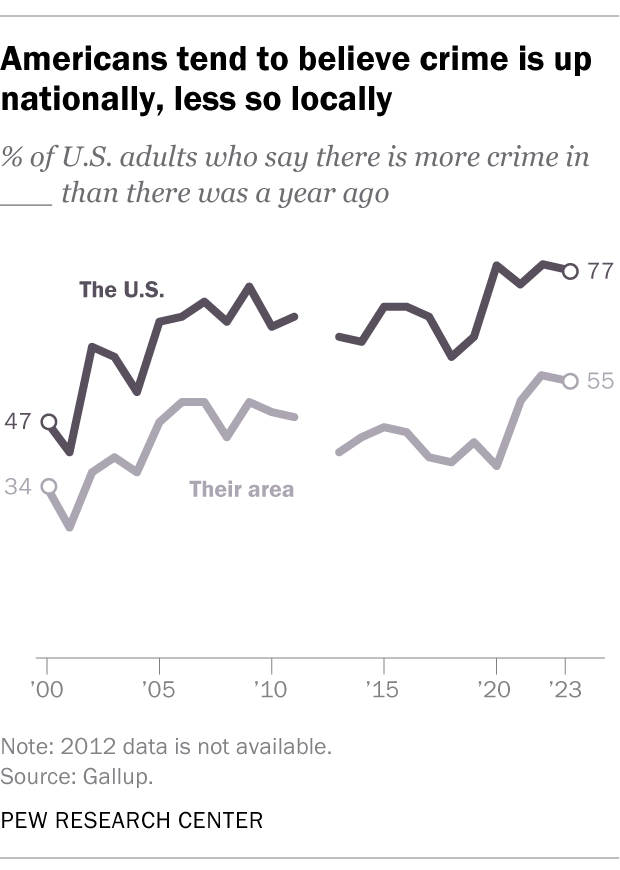
While perceptions of rising crime at the national level are common, fewer Americans believe crime is up in their own communities. In every Gallup crime survey since the 1990s, Americans have been much less likely to say crime is up in their area than to say the same about crime nationally.
Public attitudes about crime differ widely by Americans’ party affiliation, race and ethnicity, and other factors . For example, Republicans and Republican-leaning independents are much more likely than Democrats and Democratic leaners to say reducing crime should be a top priority for the president and Congress this year (68% vs. 47%), according to a recent Pew Research Center survey.
How does crime in the U.S. differ by demographic characteristics?
Some groups of Americans are more likely than others to be victims of crime. In the 2022 BJS survey , for example, younger people and those with lower incomes were far more likely to report being the victim of a violent crime than older and higher-income people.
There were no major differences in violent crime victimization rates between male and female respondents or between those who identified as White, Black or Hispanic. But the victimization rate among Asian Americans (a category that includes Native Hawaiians and other Pacific Islanders) was substantially lower than among other racial and ethnic groups.
The same BJS survey asks victims about the demographic characteristics of the offenders in the incidents they experienced.
In 2022, those who are male, younger people and those who are Black accounted for considerably larger shares of perceived offenders in violent incidents than their respective shares of the U.S. population. Men, for instance, accounted for 79% of perceived offenders in violent incidents, compared with 49% of the nation’s 12-and-older population that year. Black Americans accounted for 25% of perceived offenders in violent incidents, about twice their share of the 12-and-older population (12%).
As with all surveys, however, there are several potential sources of error, including the possibility that crime victims’ perceptions about offenders are incorrect.
How does crime in the U.S. differ geographically?
There are big geographic differences in violent and property crime rates.
For example, in 2022, there were more than 700 violent crimes per 100,000 residents in New Mexico and Alaska. That compares with fewer than 200 per 100,000 people in Rhode Island, Connecticut, New Hampshire and Maine, according to the FBI.
The FBI notes that various factors might influence an area’s crime rate, including its population density and economic conditions.
What percentage of crimes are reported to police? What percentage are solved?
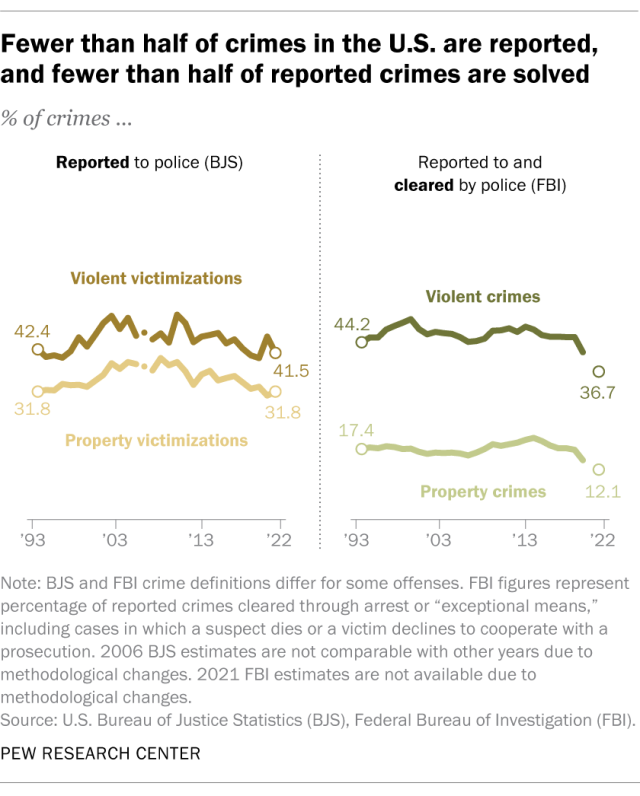
Most violent and property crimes in the U.S. are not reported to police, and most of the crimes that are reported are not solved.
In its annual survey, BJS asks crime victims whether they reported their crime to police. It found that in 2022, only 41.5% of violent crimes and 31.8% of household property crimes were reported to authorities. BJS notes that there are many reasons why crime might not be reported, including fear of reprisal or of “getting the offender in trouble,” a feeling that police “would not or could not do anything to help,” or a belief that the crime is “a personal issue or too trivial to report.”
Most of the crimes that are reported to police, meanwhile, are not solved , at least based on an FBI measure known as the clearance rate . That’s the share of cases each year that are closed, or “cleared,” through the arrest, charging and referral of a suspect for prosecution, or due to “exceptional” circumstances such as the death of a suspect or a victim’s refusal to cooperate with a prosecution. In 2022, police nationwide cleared 36.7% of violent crimes that were reported to them and 12.1% of the property crimes that came to their attention.
Which crimes are most likely to be reported to police? Which are most likely to be solved?
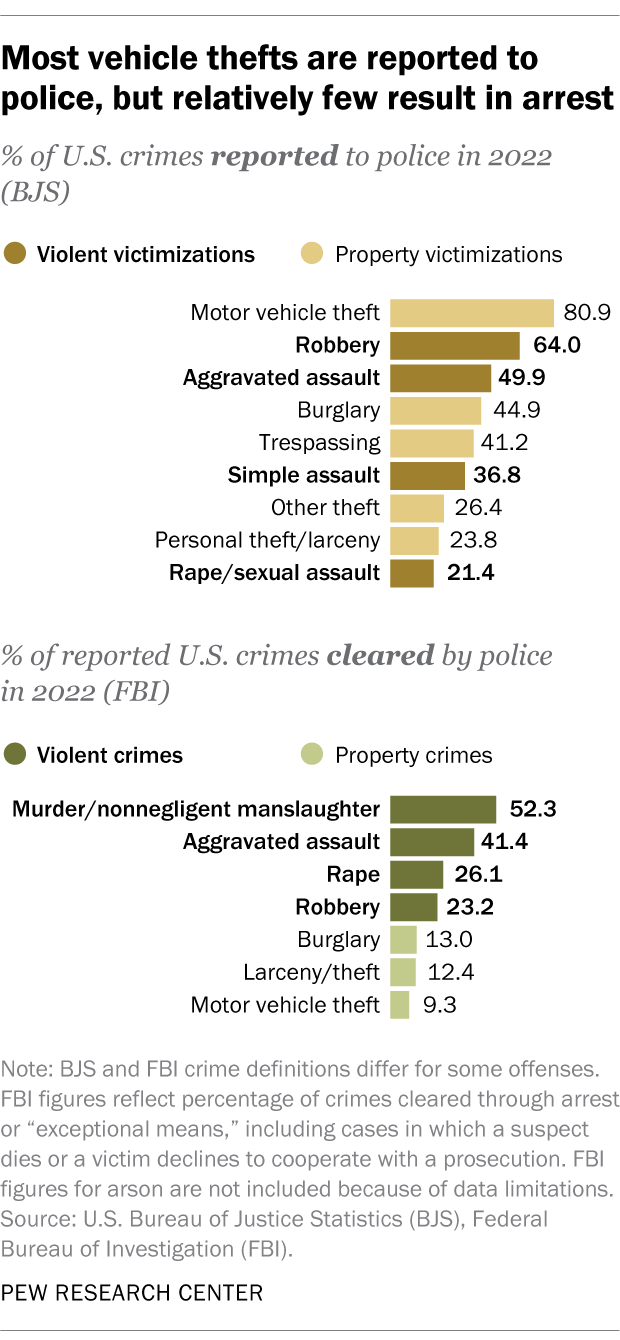
Around eight-in-ten motor vehicle thefts (80.9%) were reported to police in 2022, making them by far the most commonly reported property crime tracked by BJS. Household burglaries and trespassing offenses were reported to police at much lower rates (44.9% and 41.2%, respectively), while personal theft/larceny and other types of theft were only reported around a quarter of the time.
Among violent crimes – excluding homicide, which BJS doesn’t track – robbery was the most likely to be reported to law enforcement in 2022 (64.0%). It was followed by aggravated assault (49.9%), simple assault (36.8%) and rape/sexual assault (21.4%).
The list of crimes cleared by police in 2022 looks different from the list of crimes reported. Law enforcement officers were generally much more likely to solve violent crimes than property crimes, according to the FBI.
The most frequently solved violent crime tends to be homicide. Police cleared around half of murders and nonnegligent manslaughters (52.3%) in 2022. The clearance rates were lower for aggravated assault (41.4%), rape (26.1%) and robbery (23.2%).
When it comes to property crime, law enforcement agencies cleared 13.0% of burglaries, 12.4% of larcenies/thefts and 9.3% of motor vehicle thefts in 2022.
Are police solving more or fewer crimes than they used to?
Nationwide clearance rates for both violent and property crime are at their lowest levels since at least 1993, the FBI data shows.
Police cleared a little over a third (36.7%) of the violent crimes that came to their attention in 2022, down from nearly half (48.1%) as recently as 2013. During the same period, there were decreases for each of the four types of violent crime the FBI tracks:
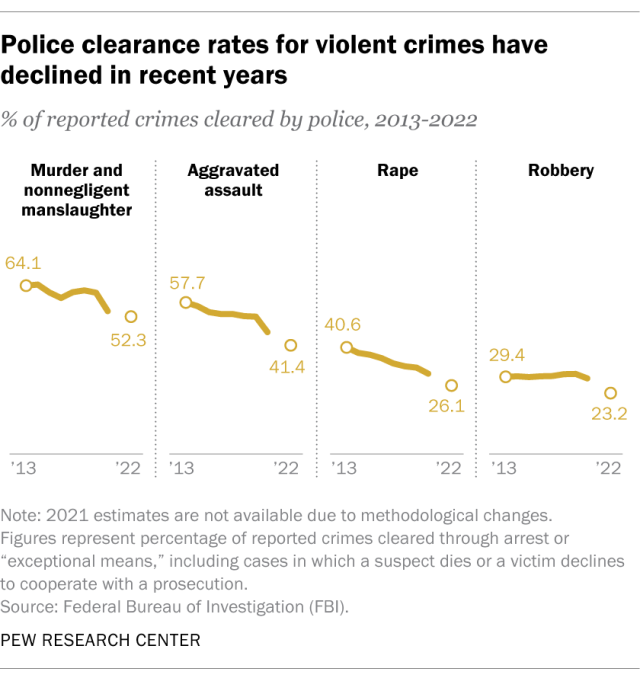
- Police cleared 52.3% of reported murders and nonnegligent homicides in 2022, down from 64.1% in 2013.
- They cleared 41.4% of aggravated assaults, down from 57.7%.
- They cleared 26.1% of rapes, down from 40.6%.
- They cleared 23.2% of robberies, down from 29.4%.
The pattern is less pronounced for property crime. Overall, law enforcement agencies cleared 12.1% of reported property crimes in 2022, down from 19.7% in 2013. The clearance rate for burglary didn’t change much, but it fell for larceny/theft (to 12.4% in 2022 from 22.4% in 2013) and motor vehicle theft (to 9.3% from 14.2%).
Note: This is an update of a post originally published on Nov. 20, 2020.
- Criminal Justice
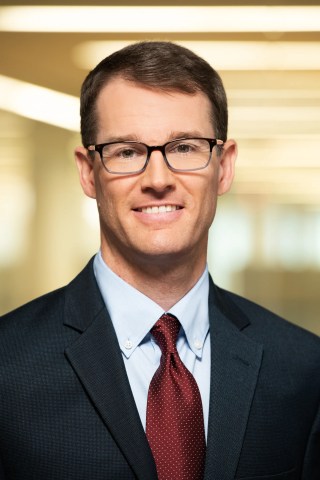
John Gramlich is an associate director at Pew Research Center .
8 facts about Black Lives Matter
#blacklivesmatter turns 10, support for the black lives matter movement has dropped considerably from its peak in 2020, fewer than 1% of federal criminal defendants were acquitted in 2022, before release of video showing tyre nichols’ beating, public views of police conduct had improved modestly, most popular.
1615 L St. NW, Suite 800 Washington, DC 20036 USA (+1) 202-419-4300 | Main (+1) 202-857-8562 | Fax (+1) 202-419-4372 | Media Inquiries
Research Topics
- Age & Generations
- Coronavirus (COVID-19)
- Economy & Work
- Family & Relationships
- Gender & LGBTQ
- Immigration & Migration
- International Affairs
- Internet & Technology
- Methodological Research
- News Habits & Media
- Non-U.S. Governments
- Other Topics
- Politics & Policy
- Race & Ethnicity
- Email Newsletters
ABOUT PEW RESEARCH CENTER Pew Research Center is a nonpartisan fact tank that informs the public about the issues, attitudes and trends shaping the world. It conducts public opinion polling, demographic research, media content analysis and other empirical social science research. Pew Research Center does not take policy positions. It is a subsidiary of The Pew Charitable Trusts .
Copyright 2024 Pew Research Center
Terms & Conditions
Privacy Policy
Cookie Settings
Reprints, Permissions & Use Policy
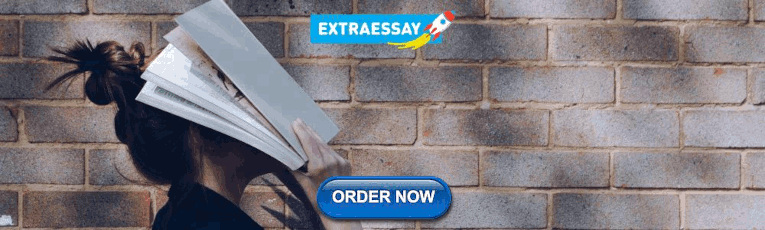
COMMENTS
Quantitative research involves collecting and analyzing numerical data to identify patterns, trends, and relationships among variables. This method is widely used in social sciences, psychology, economics, and other fields where researchers aim to understand human behavior and phenomena through statistical analysis. If you are looking for a quantitative research topic, there are numerous areas ...
113 Great Research Paper Topics. Posted by Christine Sarikas. General Education. One of the hardest parts of writing a research paper can be just finding a good topic to write about. Fortunately we've done the hard work for you and have compiled a list of 113 interesting research paper topics. They've been organized into ten categories and ...
A strong research topic comprises three important qualities: originality, value and feasibility.. Originality - a good topic explores an original area or takes a novel angle on an existing area of study.; Value - a strong research topic provides value and makes a contribution, either academically or practically.; Feasibility - a good research topic needs to be practical and manageable ...
Developing a Research Topic: Examples. When you have the topic (s) or variables operationalized or specific enough, you can use the connections you decided on to link them together. For example, if you decided to focus on pornography viewing habits among veterans with PTSD, this would contain three research topics or concepts to study-that is ...
Examples. Discrete variables (aka integer variables) Counts of individual items or values. Number of students in a class. Number of different tree species in a forest. Continuous variables (aka ratio variables) Measurements of continuous or non-finite values. Distance.
Sometimes coming up with a research topic or question is really straightforward. Someone may tell you explicitly what they want you to study such as: Did the program reduce delin- ... two variables (X and Y ) but does not purport to make any causal claims between them. In other words, correlational research questions might ask things like: What ...
INTRODUCTION. Scientific research is usually initiated by posing evidenced-based research questions which are then explicitly restated as hypotheses.1,2 The hypotheses provide directions to guide the study, solutions, explanations, and expected results.3,4 Both research questions and hypotheses are essentially formulated based on conventional theories and real-world processes, which allow the ...
Dependent Variable The variable that depends on other factors that are measured. These variables are expected to change as a result of an experimental manipulation of the independent variable or variables. It is the presumed effect. Independent Variable The variable that is stable and unaffected by the other variables you are trying to measure.
Revised on June 22, 2023. A correlational research design investigates relationships between variables without the researcher controlling or manipulating any of them. A correlation reflects the strength and/or direction of the relationship between two (or more) variables. The direction of a correlation can be either positive or negative.
Examples of categorical variables include gender (male, female, other), type of vehicle (car, truck, motorcycle), or marital status (single, married, divorced). These categories help researchers organize data into groups for comparison and analysis. Categorical variables can be further classified into two subtypes: nominal and ordinal.
Qualitative research is a methodological approach that involves gathering and analyzing non-numerical data to understand and interpret social phenomena. Unlike quantitative research, which emphasizes the collection of numerical data through surveys and experiments, qualitative research is concerned with exploring the subjective experiences ...
As you can see, these research topics are a lot more focused than the generic topic ideas we presented earlier. So, in order for you to develop a high-quality research topic, you'll need to get specific and laser-focused on a specific context with specific variables of interest. In the video below, we explore some other important things you ...
Research methods are specific procedures for collecting and analyzing data. Developing your research methods is an integral part of your research design. When planning your methods, there are two key decisions you will make. First, decide how you will collect data. Your methods depend on what type of data you need to answer your research question:
Research Topic Independent Variable Dependent Variable; All Research Topics: Manipulated by the researcher. Measured by the researcher. All Research Topics: What is being changed.
Definition of Variable. Examples of Variables in Research: 6 Phenomena. Phenomenon 1: Climate change. Phenomenon 2: Crime and violence in the streets. Phenomenon 3: Poor performance of students in college entrance exams. Phenomenon 4: Fish kill. Phenomenon 5: Poor crop growth. Phenomenon 6: How Content Goes Viral.
Examples: Education. Next, let's look at some potential research questions within the education, training and development domain. How does class size affect students' academic performance in primary schools? This example research question targets two clearly defined variables, which can be measured and analysed relatively easily.
Categorical Variable. This is a variable that can take on a limited number of values or categories. Categorical variables can be nominal or ordinal. Nominal variables have no inherent order, while ordinal variables have a natural order. Examples of categorical variables include gender, race, and educational level.
Clinical Psychology Research Topic Ideas. Topic choices are only as limited as your imagination and assignment, so try narrowing the possibilities down from general questions to the specifics that apply to your area of specialization. Here are just a few ideas to start the process:
Examples of systemic racism-related psychology research topics include: Access to mental health resources based on race. The prevalence of BIPOC mental health therapists in a chosen area. The impact of systemic racism on mental health and self-worth. Racism training for mental health workers.
Published on February 3, 2022 by Pritha Bhandari. Revised on June 22, 2023. In research, variables are any characteristics that can take on different values, such as height, age, temperature, or test scores. Researchers often manipulate or measure independent and dependent variables in studies to test cause-and-effect relationships.
The "Big 3" Variables. Variables can be a little intimidating for new researchers because there are a wide variety of variables, and oftentimes, there are multiple labels for the same thing. To lay a firm foundation, we'll first look at the three main types of variables, namely: Independent variables (IV) Dependant variables (DV) Control ...
For the structural model, two further considerations are important: whether a variable is endogenous (dependent, or 'Y') or exogenous (independent, or 'X') and what the level of measurement of the variables is. All three of these topics are discussed in Additional file 1. In addition, multiple sensitivity analyses were run.
The emergence of e-commerce reshaped the traditional trade models, also playing a significant role in meeting the UN sustainable development goals. According to the UN, sustained growth and social development must include resilient infrastructure, foster innovation, allow for better access to information and communications technology, and universal and affordable internet infrastructure. This ...
Topics & Ideas: Ecological Science. The impact of land-use change on species diversity and ecosystem functioning in agricultural landscapes. The role of disturbances such as fire and drought in shaping arid ecosystems. The impact of climate change on the distribution of migratory marine species.
Both the FBI and BJS data show dramatic declines in U.S. violent and property crime rates since the early 1990s, when crime spiked across much of the nation. Using the FBI data, the violent crime rate fell 49% between 1993 and 2022, with large decreases in the rates of robbery (-74%), aggravated assault (-39%) and murder/nonnegligent ...
The first question asks for a ready-made solution, and is not focused or researchable. The second question is a clearer comparative question, but note that it may not be practically feasible. For a smaller research project or thesis, it could be narrowed down further to focus on the effectiveness of drunk driving laws in just one or two countries.