Thank you for visiting nature.com. You are using a browser version with limited support for CSS. To obtain the best experience, we recommend you use a more up to date browser (or turn off compatibility mode in Internet Explorer). In the meantime, to ensure continued support, we are displaying the site without styles and JavaScript.
- View all journals
- Explore content
- About the journal
- Publish with us
- Sign up for alerts
- Review Article
- Published: 17 May 2021
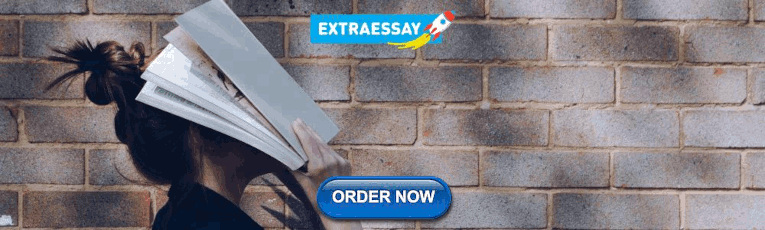
Health systems resilience in managing the COVID-19 pandemic: lessons from 28 countries
- Victoria Haldane ORCID: orcid.org/0000-0002-8674-4099 1 na1 ,
- Chuan De Foo ORCID: orcid.org/0000-0001-7254-4881 2 na1 ,
- Salma M. Abdalla 3 , 4 na1 ,
- Anne-Sophie Jung 5 na1 ,
- Melisa Tan 2 ,
- Shishi Wu 2 ,
- Alvin Chua 2 ,
- Monica Verma 2 ,
- Pami Shrestha 2 ,
- Sudhvir Singh 4 , 6 ,
- Tristana Perez 5 ,
- See Mieng Tan 2 ,
- Michael Bartos 4 , 7 ,
- Shunsuke Mabuchi 4 ,
- Mathias Bonk 4 , 8 ,
- Christine McNab 4 ,
- George K. Werner 4 ,
- Raj Panjabi 4 ,
- Anders Nordström 4 &
- Helena Legido-Quigley 2 , 4 , 5
Nature Medicine volume 27 , pages 964–980 ( 2021 ) Cite this article
93k Accesses
493 Citations
711 Altmetric
Metrics details
- Infectious diseases
- Public health
Health systems resilience is key to learning lessons from country responses to crises such as coronavirus disease 2019 (COVID-19). In this perspective, we review COVID-19 responses in 28 countries using a new health systems resilience framework. Through a combination of literature review, national government submissions and interviews with experts, we conducted a comparative analysis of national responses. We report on domains addressing governance and financing, health workforce, medical products and technologies, public health functions, health service delivery and community engagement to prevent and mitigate the spread of COVID-19. We then synthesize four salient elements that underlie highly effective national responses and offer recommendations toward strengthening health systems resilience globally.
Similar content being viewed by others
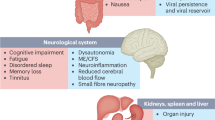
Long COVID: major findings, mechanisms and recommendations
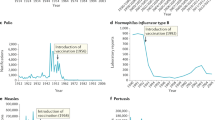
A guide to vaccinology: from basic principles to new developments
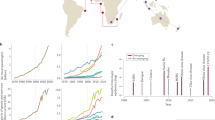
Infectious disease in an era of global change
COVID-19 has caused an unprecedented global crisis, including millions of lives lost, public health systems in shock and economic and social disruption, disproportionately affecting the most vulnerable. As of April 2021, there are over 140 million confirmed cases and over three million COVID-19 deaths globally 1 . While vaccination has commenced in numerous countries, new outbreaks and variants continue to emerge. At the same time, the global distribution of vaccines is marred by challenges of equity on top of logistical complications. Millions more are therefore still at risk of dying, facing significant morbidity or losing their livelihoods given the uncertain economic outlook.
The pandemic has challenged local, national, regional and global capacities to prepare and respond. The various national strategies taken to control viral transmission are widely debated 2 , 3 . However, the relative success of these strategies depends largely on how an existing health system is organized, governed and financed across all levels in a coordinated manner 4 . The pandemic has exposed the limitations of many health systems, including some that have been previously classified as high performing and resilient 5 . A comprehensive analysis of the resilience of health systems during the pandemic can therefore pinpoint important lessons and help strengthen countries’ preparedness, response and approach to future health challenges.
While resilience is a core concept in disaster risk reduction, its application to health systems is relatively new. It has been defined broadly as institutions’ and health actors’ capacities to prepare for, recover from and absorb shocks, while maintaining core functions and serving the ongoing and acute care needs of their communities 6 , 7 . During a crisis, a resilient health system is able to effectively adapt in response to dynamic situations and reduce vulnerability across and beyond the system. Experience from previous epidemics, such as Ebola, severe acute respiratory syndrome (SARS) and Middle East respiratory syndrome, emphasized the links between resilience and thwarting new outbreak transmission 8 .
Health systems resilience literature stresses that efforts should focus not only on absorbing unforeseen shocks precipitated by emerging health needs, but also on ensuring continuity in health improvement, sustaining gains in systems functioning and fostering people centeredness, while delivering high-quality care 9 , 10 . As COVID-19 has overwhelmed health systems worldwide, debates around resilience have become more urgent, and there is a need to better understand the elements of national responses through a resilience lens 11 , 12 . Thus, in this perspective, we use an expanded health systems resilience framework centered on community engagement to examine 28 national responses to COVID-19. This analysis provides insights into the policies countries implemented and how these were implemented to tackle the pandemic.
Conceptual framework
Our conceptual framework (Fig. 1 ) is grounded in the World Health Organization’s (WHO) health systems framework 13 . We develop the framework elements by adding public health functions, including testing, contact tracing, disease surveillance and non-pharmaceutical public health interventions, which often operate separately from health service delivery. Yet, they are critical both to pandemic responses and to ongoing population health. Similarly, health information systems are vital functions for both public health and health systems as, ideally, they should be integrated to capture data at individual, health system and population levels.
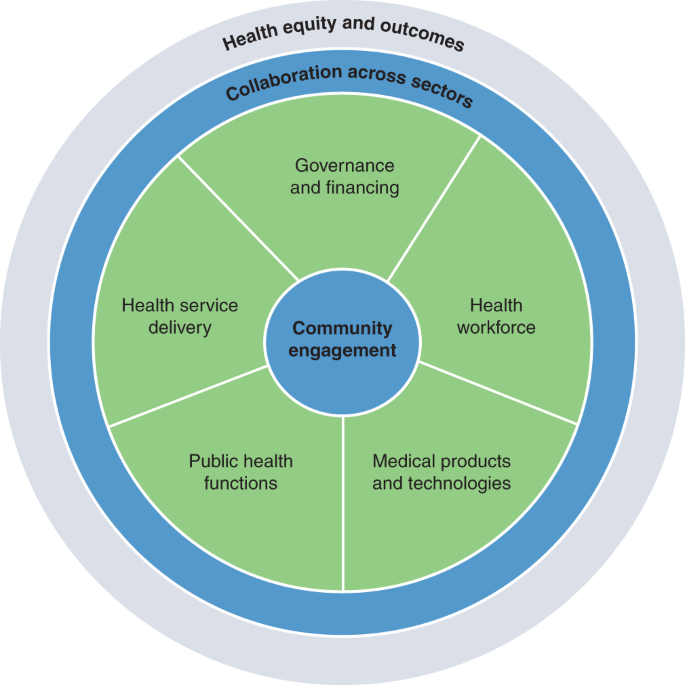
The scheme illustrates the components of the resilient health systems framework we developed based on the WHO’s health systems building blocks framework. The five elements of resilient health systems are centered around community engagement as core to all elements of health systems resilience.
We centered our analysis on community engagement as core to all elements of health systems resilience (see Box 1 for more information on the analytical approach). To serve communities in a more equitable manner and promote healthy societies, resilience must be developed with these communities and according to their needs. There can be no health systems resilience without community engagement across domains 14 . We also acknowledge the critical role of coordination with non-health sectors as essential to providing necessary supports to address the social determinants of health. Underpinning these elements are health equity and outcomes. Resilient health systems should aim to generate positive physical and mental health outcomes for all, including vulnerable and marginalized groups. In many countries, COVID-19 mortality rates have been disproportionately higher among older populations, minority ethnic groups, socioeconomically deprived populations and low-wage and migrant workers, emphasizing the interconnectedness between equity and health outcomes 15 .
Box 1 Analytical approach
The analysis presented in this review is based on a purposive selection of countries including positive and negative outliers in relation to reported COVID-19 deaths per capita among highly populous countries, as well as a selection of countries in the middle ground from different regions and with widely varying health systems and economic status. Figure 2 provides an overview of countries in our review. Countries were selected according to the recorded number of deaths attributed to COVID-19 per 100,000 inhabitants on 6 November 2020. Given the dynamic and continuously changing situation, the ranking of positive and negative outliers in terms of death per capita may have changed during the pandemic. The analysis is thus limited to this specific time period.
Five complementary methods were adopted to analyze national responses to COVID-19:
Literature review: using standardized methods, we identified peer-reviewed papers and public reports that examined national and subnational policy responses and extracted data for each country on five dimensions comprising 62 items. The dimensions and items were identified through a review of 14 existent frameworks.
Semi-structured interviews and national government submissions : to supplement the literature review, we asked the selected countries and country experts a set of questions related to the measures implemented to contain COVID-19 in their respective countries. A total of 45 interviews and written submissions were provided. Semi-structured interviews with COVID-19 national experts at the policy, operational and academic levels were recorded and transcribed in full. All interviews were coded through an inductive approach and thematic analysis, using QSR NVivo 12 software, drawing on techniques of the constant comparison method.
In-depth case studies of 6 countries to validate the data from the 28 countries.
Expert validation of country-specific data with country experts. Experts in countries were provided with the specific data for each of their respective countries to validate the data.
Expert validation through roundtable discussions with country experts. A total of 35 national and international experts in COVID-19 policies participated in two roundtable discussions.
COVID-19 responses in 28 countries
Using our framework, we organized our results beginning with domains often viewed as external to health, which are nevertheless central determinants of health systems resilience—governance, finance, collaboration across sectors and community engagement—before exploring domains more closely associated with traditional views of health and health systems—health service delivery, health workforce, medical products and technologies and public health functions. We offer illustrative examples of selected countries for each domain in Tables 1 – 6 . We analyzed 28 countries based on a purposive selection, including positive and negative outliers in relation to reported COVID-19 deaths per capita among highly populous countries, as well as a selection of countries in the middle ground (as of 6 November 2020). Figure 2 provides an overview of countries in our review.
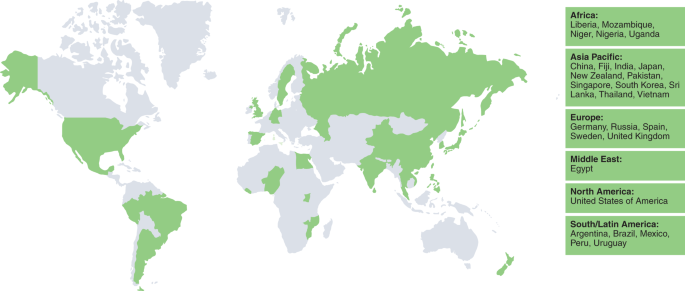
The map presents an overview of countries selected according to the recorded number of deaths attributed to COVID-19 per 100,000 inhabitants on 6 November 2020. Countries are listed according to region and in alphabetical order.
Governance, finance and collaboration across sectors
COVID-19 has made policymakers and the wider public acutely aware of the relationship between health systems, domestic economies and governance. Government decisions determine healthcare infrastructures, regulations and guidelines, defining access to medication and treatment, the provision of health coverage and the financing of these. Government responses to COVID-19, in the immediate term, have meant the difference between lockdown or business as usual, and have eroded or increased public trust. In the longer term, they have shaped national choices regarding private healthcare or universal health coverage (UHC) and strengthened or impoverished social safety nets that underpin health and well-being.
Overall, COVID-19 responses saw health policy moving beyond the remit of Ministries of Health and in doing so, draw on expertise from other ministries, particularly during the early response. Countries took whole-of-government approaches to strengthen health systems in response to COVID-19, particularly those with experience of other health-related disasters, such as Ebola. These decisions were, in most countries, made by translating evidence-based research into policies that preserve health system capacity, while protecting both public health and livelihoods. As such, most countries established temporary COVID-19 advisory groups to inform government decisions. However, in the majority of countries, the views represented on these committees were largely biomedical. More information on national responses from a governance and leadership perspective is available (V.H., A.-S.J., R. Neill, S.W. and M. Jamieson, unpublished data).
The COVID-19 response requires testing, treatment and vaccines to be financed with either a portion or all of these costs coming from public funds, and depends on healthcare infrastructures, workforce and supplies to provide much-needed surge capacity within health systems. Additionally, fiscal support measures, including relief packages aiming at helping businesses stay viable, protect jobs or provide financial aid to low-income households and the unemployed have been taken by many countries. These policies support people to adhere to public health guidance, with the aim of preventing infections, in turn mitigating the strain on the health system resulting from the need to deliver high-intensity COVID-19 care. To further enhance the reach of health services, many countries reviewed took specific actions to mitigate potential financial and physical barriers to care, such as covering part or all of the costs of COVID-19 care, and funding the establishment of testing and treatment centers in communities 16 . Importantly, the outcomes resulting from financial expenditures on health and well-being are only as good as the structures that support them. Thus, they require governance expertise across levels, sectors and domains and depend on a system’s ability to reach (vulnerable) populations.
Community engagement
Deep engagement with local communities is central to resilient health systems as a way to inform service delivery, decision-making and governance and to meet the needs of communities before, during and after crises. Community engagement strategies, such as building partnerships with local leaders and working alongside community members to tailor messages and campaigns are crucial during public health emergencies 17 . The range of non-pharmaceutical public health interventions employed in response to COVID-19, such as mask wearing and social distancing, rely on shared values and a sense of social responsibility within communities to break chains of viral transmission 18 .
Several countries reviewed engaged networks of community health workers (CHWs) to encourage active community participation in COVID-19 responses. Their roles range from creating awareness through door-to-door visits, supporting contact tracing efforts, maintaining essential health services, providing necessary medication to patients without COVID-19, surveillance or monitoring adherence to quarantine measures and assessing mental well-being 19 . They are also key to identifying and referring patients who face barriers to accessing healthcare services. For example, Thailand deployed over 1 million CHWs to disseminate and amplify messages widely in communities. Singapore deployed volunteers to educate seniors and help distribute daily necessities. Liberia further empowered community leaders by providing orientation on COVID-19 epidemiology to support containment efforts. However, many of these efforts depended on volunteers.
During early response efforts, a few countries conducted surveys to understand public sentiment regarding evolving measures. Japan conducted a survey in April 2020 to understand compliance with social distancing measures, using the results to inform response strategies. Governments also started multilingual hotlines to ensure comprehensive access to COVID-19 information. Other countries used social media platforms to engage communities. For example, the #TakeResponsibility campaign in Nigeria called on citizens to join forces and be proactive in taking greater individual and collective responsibility in controlling the spread of COVID-19 20 .
Health service delivery
Health systems globally have employed three common approaches to rapidly scale up health system infrastructure, namely by constructing new treatment facilities, converting public venues and reconfiguring existing medical facilities to provide care for patients with COVID-19. Thus, some of the health systems reviewed invested significant resources into rapidly creating dedicated field facilities. For example, in early 2020, China established two specialty field hospitals in under 2 weeks 21 . Where field hospitals were set up to house patients with COVID-19, countries often drew on their armed forces and military field hospital models, or adapted existing large public facilities. However, most health systems relied on a less resource-intensive approach that modified traditional healthcare facilities into dedicated COVID-19 care centers 22 . Other health systems relied on home care for patients with mild to moderate COVID-19, with facilities available if patients were unable to safely self-isolate within their homes. Additionally, the majority of reviewed countries canceled elective surgeries in an effort to ensure system capacity for COVID-19 care.
In many health systems, primary-care providers are the frontline of the health system providing continuous, coordinated and people-centered care. Primary care is an important point of COVID-19 triage, as well as the point at which most routine and acute care services are provided within communities 23 . In many of the countries reviewed, primary-care providers rapidly adopted and scaled up digital technology or telehealth services to provide ongoing and acute care while also triaging and referring persons with symptoms of COVID-19 to onward care 24 . Additionally, some countries complemented digital technology with proactive deployment of existing and new community health resources 25 . Community-based approaches developed with deep knowledge of local contexts are crucial to pandemic response and health systems resilience, particularly given the disproportionate impact of the pandemic on vulnerable groups 15 , 26 . While outside the reach of the health system in many countries, there have been extensive outbreaks in long-term care homes with devastating impacts on the health and well-being of high-risk older adults, long-term care patients and their families 27 . In response, most countries reviewed prioritized long-term care facilities and older adults for testing, surveillance and vaccine distribution, although often not until there had been high rates of mortality in these settings.
Health workforce
Resilient health systems manage crises by having an adequate, trained and willing workforce. Yet, in many countries, COVID-19 has spread quickly among health workers as they have been the most exposed to the virus, with data indicating that they have been disproportionately affected by the pandemic 28 . Health workforce challenges during COVID-19 include low staffing levels (particularly among nurses) and uneven geographical distribution, shortages of adequate personal protective equipment (PPE), limited testing capacity, insufficient training, social discrimination and attacks and poor mental health 29 .
As cases surged globally, most reviewed countries reallocated healthcare professionals, including primary-care workers, to emergency care wards, intensive care units (ICUs) and diagnosis and surveillance activities. Several recruitment strategies were implemented to increase the healthcare workforce. Retired, student or nonpracticing medical and paramedical professionals were asked to volunteer for healthcare tasks. For example, medical and nursing students were recruited and allowed to perform supervised work in different COVID-19 response capacities in countries such as Germany, Russia, Spain, the United Kingdom and Vietnam. Given these new roles or expanded job scopes, there was an immediate need for rapid and high-quality pandemic-related training of frontline healthcare workers, which was accomplished through virtual training courses in many countries.
Further measures were taken by countries to maintain, protect and support their healthcare workers in light of the physical and psychological strain of the pandemic. In some countries (for example, Japan, Mozambique, Singapore and South Korea), healthcare professionals were supported by measures such as organization of shifts to avoid extended hours without rest, leaves from duty for mental and physical recovery, accommodation near their workplaces to protect their families, and childcare. Additionally, most countries reported giving some form of financial support to their health workers, such as monetary incentives, bonuses, insurance, tax benefits, overtime pay, meal allowances, classification of their infections as an occupational disease or injury and declaring cause of death as work related. Several countries reported making psychological support available for health workers, such as counseling or trauma support, to maintain well-being and morale. Frontline staff and their families were especially vulnerable and were targeted for psychological interventions. Moreover, some countries launched social media campaigns that encourage people to show their pride, admiration and gratitude for healthcare workers to promote solidarity.
Medical products and technologies
High-quality prevention, diagnosis and management of COVID-19 require the ongoing development, production and sustained distribution of mass quantities of medical products and technologies. However, overreliance on a few countries for production, competition among countries and supply chain disruptions have caused global supply shortages. Some countries reviewed had national or regional stockpiles of PPE, including masks, gloves, face shields and gowns, which were used as a buffer while awaiting imported supplies or scale up of domestic production. Singapore, for example, drew from experience responding to SARS and preserved a national stockpile of medical products for up to 6 months 30 . To replenish stockpiles in Japan, medical product manufacturers were urged to boost production output, resulting in the tripling of production volume as factories operated 24 h a day 31 .
Governments also worked beyond the typical health sectors and developed guidelines and specifications for non-health sectors to supplement the existing medical product manufacturing lines. In India, automotive manufacturers were repurposed to produce low-cost ventilators and PPE 32 . Beyond industry, communities in some countries mobilized to bolster medical product supplies. Several countries had also relied on purchasing consortia, like the UN COVID-19 Supply Chain System and Africa Medical Supplies Platform, donations from development partners or grants and loans from other countries and international financial entities (that is, The World Bank and Asian Development Bank) to secure medical supplies 33 , 34 , 35 , 36 , 37 . Vaccine procurement is also an essential part of the medical supply chain, and countries have either made advance-purchase agreements or participated in the COVAX facility to ensure prompt access to the vaccine for their populations, although concerns remain about equitable access to vaccines in short supply.
In response to increased demand driven by widespread community transmission, countries enacted laws to prevent hoarding and exploitative pricing, as well as policies prohibiting export of medical supplies, while relaxing import licensing requirements and tariffs 38 . Within health facilities, measures including rational-use guidelines, per WHO recommendations, and postponement of nonemergency medical procedures helped stretch existing medical inventories 39 . Although supply chains began to stabilize during 2020, many points of care globally faced uncertain stocks and reports of counterfeit medical essentials highlighting the need for secure supply pipelines. Platforms that assist in monitoring logistics networks are integral in ensuring a steady and rapid flow of medical products and technologies, promoting transparency and ensuring better management of supply chains.
Public health functions
Public health interventions embedded within communities, such as testing, contact tracing, quarantine or self-isolation, and surveillance are crucial functions to break chains of transmission 40 . However, in many health systems, public health and health service delivery systems are siloed: their coordination is limited or ineffective, and they have separate referral processes and reporting systems, all of which serve to undermine health systems resilience 41 . Testing and contact tracing are a case in point that clearly illustrates why public health and health systems must act together in a coordinated manner.
Diagnostic tests to identify whether a person is, or has been, infected are foundational to infectious disease responses to pinpoint locations of spread, and provide care and treatment if it exists. Testing strategies are broadly classified as passive or proactive. Many of the countries reviewed relied on passive testing strategies, where symptomatic individuals self-present to a healthcare facility for testing after meeting certain criteria. However, some countries adopted proactive testing strategies characterized by programs tailored to the unique needs of specific populations as an important tool toward breaking chains of transmission and offering a clearer epidemiological picture 42 , 43 . Additionally, many countries rapidly decentralized testing capacity by strengthening or developing new laboratory networks.
Proactive testing must be accompanied by comprehensive contact tracing in partnership with communities. Contact tracing is the systematic process of following up with individuals who may have been exposed to COVID-19 44 . It can be characterized as either forward, aiming to find ‘downstream’ individuals who have been in contact with a person with COVID-19, or backwards, aiming to find an ‘upstream’ source of infection 45 . While most countries reviewed conducted forward contact tracing, Japan conducted backwards contact tracing measures aimed at identifying and ‘busting’ clusters by working with individuals to trace 14 d before symptom onset 46 . Contact tracing, particularly backwards tracing, is labor and time intensive and may be stigmatizing if not done with community engagement and consideration of at-risk and vulnerable groups 47 . The majority of countries reviewed introduced digital contact tracing tools. Even when fully operational, they may not be accessible, acceptable or feasible for use among those with limited access to, or concerns in using, adequate technology such as migrants, refugees or those experiencing homelessness, among others 48 .
Once cases and contacts are identified, self-isolation and quarantine measures are crucial to prevent onwards transmission and identify emergent cases. All countries reported on quarantine and isolation protocols. While necessary to outbreak management, unless done in coordination with communities, quarantine measures can have negative impacts on mental health and well-being, become a source of stigma and be deployed at the significant cost of human rights 49 . Some countries have implemented policies to provide social and economic assistance to those who must self-isolate or quarantine. Social supports range from services that ensure food and necessities during quarantine to dedicated quarantine or isolation facilities (for example, converted hotels, public facilities or purpose-built quarantine hospitals). Such self-isolation supports are recognized as integral to mitigating transmission, particularly among younger people and those working in high-exposure occupations, living in overcrowded housing or without a home 50 . However, to avoid negative unintended consequences, such facilities must be operationalized with a human rights focus 51 .
These efforts are enhanced by surveillance, including testing in areas or settings with outbreaks, to rapidly limit community circulation 52 . Given the high transmissibility of severe acute respiratory syndrome coronavirus 2, surveillance needs to be geographically comprehensive to provide accurate depictions of disease burden and epidemiology to prevent and mitigate community transmission 53 , 54 , 55 . As recommended by WHO guidelines, nearly all countries have adapted existing surveillance system infrastructure to collect information on COVID-19 cases 55 . However, surveillance based on case reporting may underestimate the epidemiological characteristics of COVID-19, given that stigma or other barriers may limit healthcare seeking, particularly in vulnerable populations 56 , 57 . Therefore, New Zealand, Sweden and the United States have additionally deployed syndromic surveillance, which monitors cases that meet the clinical definition of COVID-19 without confirmation by testing. By implementing active surveillance approaches, countries have expanded surveillance coverage from healthcare settings into communities, such as through primary care, thereby strengthening epidemiological surveillance among vulnerable populations.
Timely sharing of case-based data between public health and healthcare sectors is key to early detection of outbreaks, identification of changes in epidemiological trends and planning of health services 58 . This was facilitated by the use of innovative digital technology. For example, the China CDC launched a web-based infectious disease reporting system that allows real-time reporting of confirmed and suspected COVID-19 cases by healthcare providers 59 , 60 . Similar real-time surveillance and information systems are also used in Fiji, India, Japan and Vietnam.
Assessing health systems resilience is vital in helping policymakers plan for sustainable recovery and strengthen systems to better prepare and respond to current and future crises. Using an adapted and improved resilience framework, our review highlights many parallels in the measures implemented by countries in response to COVID-19. The similarities across countries with divergent health outcomes makes clear that there is no one silver bullet toward a resilient health system. Nevertheless, there are a number of characteristics of well-performing countries across the resilience determinants that stand out. These are summarized as four elements of resilience that are featured in highly effective country responses. These elements draw on the concept that resilient health systems are systems that: (1) activate comprehensive responses, which are responses that consider and address health and well-being as intertwined with social and economic considerations; (2) adapt capacity within and beyond the health system to meet the needs of communities; (3) preserve functions and resources within and beyond the health system to maintain pandemic-related and non-related routine and acute care; and (4) reduce vulnerability to catastrophic losses in communities, both in terms of health and well-being, as well as individual or household finances; all while continually learning, monitoring and adjusting in light of emerging evidence or the evolving epidemiological situation (Fig. 3 ).
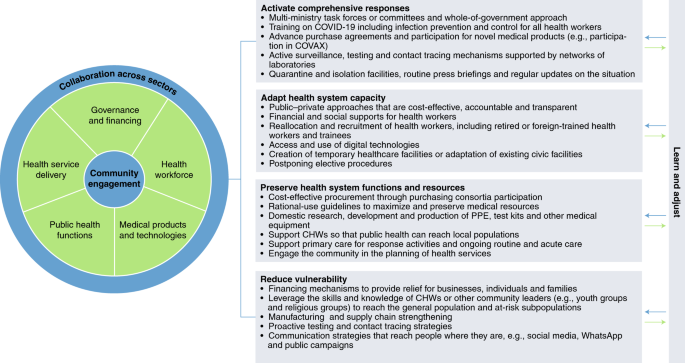
The framework presented expands upon and applies the determinants of health systems resilience framework to identify four resilience elements characteristic of highly effective country responses to COVID-19.
High-performing countries
High-performing countries activated comprehensive responses across the determinants’ domains, including through whole-of-government approaches and the creation of multi-ministry task forces, to ensure adequate translation of evidence into policy and practices that preserve health system capacity, while protecting public health and livelihoods. Specific measures taken include training health workers, bolstering public health functions (including offering designated isolation facilities, either for all or for those unable to safely self-isolate at home) and preparing for new technologies and medicines through purchase agreements, while also engaging communities through routine communications on the epidemiological situation and emergent policies.
These countries also learned from emerging evidence and adapted the capacity of their health system in response to the evolving epidemiological situation. This was achieved by increasing capacity in hospitals, through construction of makeshift hospitals or repurposing of existing health facilities or civic spaces. The health workforce in high-performing countries was expanded through reallocation and recruitment and supported through financial and social supports.
These countries took action to preserve health system functions and resources through purchasing consortia and rational-use guidelines to maximize available material resources such as PPE, as well as investing in domestic research, development and production of medical supplies, test kits and vaccines. Additionally, these countries protected health and well-being more broadly by ensuring health system functioning for non-COVID-19-related health services. High-performing countries supported primary care and CHWs to conduct COVID-19 screening, assessment and/or referral, while providing ongoing routine and acute care in communities.
High-performing countries also sought to reduce vulnerability across the resilience determinants by providing financial relief and social supports to complement proactive and robust testing and contact tracing in partnership with communities to ensure public health measures and safety net supports reached all groups.
Building resilient health systems
While some countries have demonstrated elements of resilience, as we highlight above, progress is limited in developing resilient health systems overall. Our review highlights six areas requiring urgent action to build resilient health systems globally.
First, COVID-19 responses provide a clear illustration of the importance of governance supported by scientific evidence and leadership willing to learn and adjust course for successful health systems that protect health and well-being. Enhancing resilience to future disease outbreaks requires longer-term work to create high-quality healthcare systems and build community trust. Our review emphasizes that governments are well advised to address COVID-19, and any future disease outbreak, through a whole-of-government approach that incorporates all sectors, engages relevant actors across all levels, including community and local authorities, and is based on strong and clear coordination that extends beyond early-stage emergency management 61 . Crucial to health systems resilience is that governance must consider the intersections of gender, racialization and human rights, and their impact on health and well-being before, during and after crises 62 , 63 , 64 . Urging governments to adopt such an approach, which COVID-19 has made clear is essential, is not a new proposal. Yet, our review highlights a lack of uniform appreciation or adoption of such an approach by countries.
Second, health systems need appropriate financing, not only to prepare for new pandemics, but also to ensure that at all times, all people have access to the health services they need, when and where they need them, without financial hardship, regardless of ability to pay 65 . This is the foundation of UHC. While many countries have provided subsidized COVID-19 testing and treatment, more must be done to ensure people are not pushed into poverty due to out-of-pocket spending on health. Investing in UHC not only protects people from health threats but also mitigates the social and economic burdens that have characterized COVID-19. Countries will have to revisit the thresholds of health expenditure that they are willing to invest to build resilient health systems, promote population health and protect communities against financial risk.
Third, while country capacities varied, the pandemic has demonstrated a need to invest in improving both the quantity and quality of health workers to better prepare for and respond to future pandemics. Our review highlights that resilient health systems are those that not only invest in pandemic-related planning and training of health workers, but also ensure their physical, mental and economic protection in the workplace and beyond. Emphasis should also be placed on community mobilization where adequately trained and supported CHWs are equipped to play substantial roles in outbreak response and community engagement, much as they have played a crucial role in tuberculosis and HIV/AIDS response efforts globally for decades 66 .
Fourth, in terms of access to medicines and products, the pandemic has made visible, yet again, the clearly identified and thoroughly debated challenges to global supply chains for medicines and products. These challenges range from limited manufacturing capacities to financing to equity in access. The early experience of COVAX, with some high-income countries bypassing the initiative, has demonstrated the glaring limitations in the current system.
Fifth, health service delivery, including non-COVID-19-related health services, has been directly threatened, and often compromised, at all levels by the demands of the pandemic, even in traditionally high-performing health systems. Our review emphasizes that bolstering system capacity requires strong and well-funded primary care, with a skilled and protected workforce, to ensure that high-quality care is delivered in communities, with strengthened linkages to public health systems. Similarly, the long-term care sector, and care for older adults, must be prioritized and better integrated into health service delivery and public health functions. This must be underpinned by a renewed commitment to UHC to ensure high-quality care for all.
Finally, public health functions, such as testing and contact tracing, that are delivered in coordination with the health service system, are cornerstones for successful COVID-19 responses. These approaches often depend on innovative digital technologies, which bear their own challenges, including the potential to exacerbate inequalities and be the vehicle for human rights violations 67 , 68 , 69 , 70 . As such, future investments in these technologies requires a more holistic approach—one that engages communities, particularly the most vulnerable—that takes into account the potential risks and considers how health systems can minimize harms from their use 71 .
Importantly, our analysis of country responses points toward foundational debates on how we understand and think about resilient health systems. Health system resilience as a concept must expand beyond technical and biomedical knowledge and actions, to engage with the broader social, economic and political factors in society. Such comprehensive understanding of resilience requires a systems approach and should be guided by equity concerns, which include concerns for gender, human rights and racialization in health and healthcare 12 , 72 . Further, resilience cannot be achieved solely through unidirectional and top-down approaches by governments and other entities. Resilience requires community engagement as much as regulations and hospital capacity. Community engagement and its interlinkage with community resilience is fundamental to managing not only health threats but also other threats, such as climate and environmental change 73 . Importantly, health systems resilience requires countries worldwide to be open to exchange of knowledge and expertise from regions such as Asia and Africa, which have effectively mobilized CHWs and communities to extend the reach, capacity and quality of their health systems.
Our findings and recommendations are not new, and there have been prior incremental moves to expand what constitutes, influences and governs health and healthcare. COVID-19 demands dynamic systemic transformation. The pandemic has fundamentally challenged health systems and the communities they serve globally. The effect of a major shock represented by the pandemic is to manifest the points where the system is weakest, and to demonstrate the interdependencies of a range of health, social and economic structures. While the evidence of system failures has come at a huge cost in human and monetary terms, it has also pointed to what needs to change. With over 3 million global deaths and pervasive social and economic costs, the pandemic must serve as a call for transformation and investment toward resilience and people centeredness, beginning with health systems. COVID-19 provides a renewed prospect for solidarity, both within and between countries. It also serves as a reminder that health is more than healthcare and that a whole-of-government approach to health and well-being is needed to create healthy populations able to collectively prevent and respond to crises, leaving no one behind.
World Health Organization. WHO Coronavirus Disease (COVID-19) Dashboard. https://covid19.who.int (2021).
Glover, R. E. et al. A framework for identifying and mitigating the equity harms of COVID-19 policy interventions. J. Clin. Epidemiol. 128 , 35–48 (2020).
Article Google Scholar
Baker, M. G., Wilson, N. & Blakely, T. Elimination could be the optimal response strategy for COVID-19 and other emerging pandemic diseases. BMJ 371 , m4907 (2020).
Etienne, C. F. et al. COVID-19: transformative actions for more equitable, resilient, sustainable societies and health systems in the Americas. BMJ Glob. Health 5 , e003509 (2020).
El Bcheraoui, C., Weishaar, H., Pozo-Martin, F. & Hanefeld, J. Assessing COVID-19 through the lens of health systems’ preparedness: time for a change. Global Health 16 , 112 (2020).
Kruk, M. E., Myers, M., Varpilah, S. T. & Dahn, B. T. What is a resilient health system? Lessons from Ebola. Lancet 385 , 1910–1912 (2015).
United Nations System Chief Executives Board for Coordination (CEB). United Nations plan of action on disaster risk reduction for resilience . https://www.preventionweb.net/files/49076_unplanofaction.pdf (2016).
Nuzzo, B. et al. What makes health systems resilient against infectious disease outbreaks and natural hazards? Results from a scoping review. BMC Public Health 19 , 1310 (2019).
Haldane, V., Ong, S. E., Chuah, F. L. & Legido-Quigley, H. Health systems resilience: meaningful construct or catchphrase? Lancet 389 , 1513 (2017).
Legido-Quigley, H. & Asgari, N. Resilient and people-centred health systems: progress, challenges and future directions in Asia (World Health Organization, 2018).
Legido-Quigley, H. et al. Are high-performing health systems resilient against the COVID-19 epidemic? Lancet 395 , 848–850 (2020).
Article CAS Google Scholar
Haldane, V. & Morgan, G. T. From resilient to transilient health systems: the deep transformation of health systems in response to the COVID-19 pandemic. Health Policy Plan. https://doi.org/10.1093/heapol/czaa169 (2020).
World Health Organization. Monitoring the building blocks of health systems: a handbook of indicators and their measurement strategies (2010).
Barker, K. M. et al. Community engagement for health system resilience: evidence from Liberia’s Ebola epidemic. Health Policy Plan. 35 , 416–423 (2020).
Shadmi, E. et al. Health equity and COVID-19: global perspectives. Int. J. Equity Health 19 , 1–16 (2020).
Monaghesh, E. & Hajizadeh, A. The role of telehealth during COVID-19 outbreak: a systematic review based on current evidence. BMC Public Health 20 , 1193 (2020).
Gilmore, B. et al. Community engagement for COVID-19 prevention and control: a rapid evidence synthesis. BMJ Glob. Health 5 , e003188 (2020).
Bonell, C. et al. Harnessing behavioural science in public health campaigns to maintain ‘social distancing’ in response to the COVID-19 pandemic: key principles. J. Epidemiol. Community Health 74 , 617–619 (2020).
PubMed PubMed Central Google Scholar
Ballard, M. et al. Prioritising the role of community health workers in the COVID-19 response. BMJ Glob. Health 5 , e002550 (2020).
Nigeria Centre for Disease Control. NCDC and UNICEF launch Chatbot to combat COVID-19 misinformation in Nigeria. https://ncdc.gov.ng/news/272/ncdc-and-unicef-launch-chatbot-to-combat-covid-19-misinformation-in-nigeria (2020).
Luo, H., Liu, J., Li, C., Chen, K. & Zhang, M. Ultra-rapid delivery of specialty field hospitals to combat COVID-19: lessons learned from the Leishenshan Hospital project in Wuhan. Autom. Constr. 119 , 103345 (2020).
Her, M. Repurposing and reshaping of hospitals during the COVID-19 outbreak in South Korea. One Health 10 , 100137 (2020).
World Health Organization. Coronavirus disease (COVID-19) technical guidance: patient management. http://www.who.int/emergencies/diseases/novel-coronavirus-2019/technical-guidance/patient-management (2020).
Ministry of Health New Zealand. COVID-19: advice for all health professionals. https://www.health.govt.nz/our-work/diseases-and-conditions/covid-19-novel-coronavirus/covid-19-information-health-professionals/covid-19-advice-all-health-professionals (2020).
Haldane, V. et al. National primary care responses to COVID-19: a rapid review of the literature. BMJ Open 10 , e041622 (2020).
World Health Organization Western Pacific. COVID-19: vulnerable and high risk groups. http://www.who.int/westernpacific/emergencies/covid-19/information/high-risk-groups (2020).
McMichael, T. M. et al. Epidemiology of COVID-19 in a long-term care facility in King County, Washington. N. Engl. J. Med. 382 , 2005–2011 (2020).
Bandyopadhyay, S. et al. Infection and mortality of healthcare workers worldwide from COVID-19: a systematic review. BMJ Glob. Health 5 , e003097(2020).
World Health Organization. Health workforce policy and management in the context of the COVID-19 pandemic response (2020).
Chua, A. Q. et al. Health system resilience in managing the COVID-19 pandemic: lessons from Singapore. BMJ Glob. Health 5 , e003317 (2020).
Ministry of Economy, T. and I. Current status of production and supply of face masks, antiseptics and toilet paper. https://www.meti.go.jp/english/covid-19/mask.html (2020).
Mukherjee, S. Maruti Suzuki to help produce ventilators, masks and protective equipment to fight against COVID-19 (2020).
World Bank. 100 Countries Get Support in Response to COVID-19 (2020).
World Bank. World Bank group’s operational response to COVID-19 (coronavirus)–projects list (2020).
World Health Organization. COVID-19 supply chain system: requesting and receiving supplies. http://www.who.int/publications/m/item/covid-19-supply-chain-system-requesting-and-receiving-supplies (2020).
ABOUT US. Africa Medical Supplies Platform. https://amsp.africa/about-us/
World Health Organization. UK Gives £1.3 million to fight COVID-19 in Uganda. https://www.afro.who.int/news/uk-gives-ps13-million-fight-covid-19-uganda (2020).
International Trade Center. COVID-19 temporary trade measures. https://www.macmap.org/covid19 (2020).
World Health Organization. Rational use of personal protective equipment for coronavirus disease (COVID-19) and considerations during severe shortages. https://www.who.int/publications/i/item/rational-use-of-personal-protective-equipment-for-coronavirus-disease-(covid-19)-and-considerations-during-severe-shortages (2020).
Koo, D., Felix, K., Dankwa-Mullan, I., Miller, T. & Waalen, J. A call for action on primary care and public health integration. Am. J. Public Health 102 , S307–S309 (2012).
Nishtar, S. The mixed health systems syndrome. Bull. World Health Organ. 88 , 74–75 (2010).
Veillard, J., Campbell, J., Mohpal, A. & Evans, T. Testing, testing, testing: an essential strategy for public health, vaccine deployment and economic reactivation during COVID-19. https://blogs.worldbank.org/latinamerica/testing-testing-testing-essential-strategy-public-health-vaccine-deployment-and/ (World Bank Blogs, 2020).
The World Bank. Population-level, national testing strategies for COVID-19: Latin America & the Caribbean. https://www.worldbank.org/en/region/lac/brief/population-level-national-testing-strategies-for-covid-19-latin-america-and-the-caribbean (2020).
World Health Organization. Contact tracing in the context of COVID-19. https://www.who.int/publications/i/item/contact-tracing-in-the-context-of-covid-19 (2020).
Endo, A. et al. Implication of backward contact tracing in the presence of overdispersed transmission in COVID-19 outbreaks. Wellcome Open Res . 5 , 239 (2020).
Lewis, D. Why many countries failed at COVID contact-tracing—but some got it right . Nature 588 , 384–387 (2020).
Megnin-Viggars, O., Carter, P., Melendez-Torres, G. J., Weston, D. & Rubin, G. J. Facilitators and barriers to engagement with contact tracing during infectious disease outbreaks: a rapid review of the evidence. PLoS ONE 15 , e0241473 (2020).
Klenk, M. & Duijf, H. Ethics of digital contact tracing and COVID-19: who is (not) free to go? Ethics Inf. Technol. https://doi.org/10.1007/s10676-020-09544-0 (2020).
Brooks, S. K. et al. The psychological impact of quarantine and how to reduce it: rapid review of the evidence. Lancet 395 , 912–920 (2020).
Cevik, M., Baral, S., Crozier, A. & Cassell, J. Support for self-isolation is critical in COVID-19 response. BMJ 372 , 224 (2021).
Social Science in Humanitarian Action. Key considerations: quarantine in the context of COVID-19. https://opendocs.ids.ac.uk/opendocs/bitstream/handle/20.500.12413/15133/SSHAP%20COVID-19%20Key%20Considerations%20Quarantine.pdf?sequence=24&isAllowed=y (2020).
Pung, R. et al. Investigation of three clusters of COVID-19 in Singapore: implications for surveillance and response measures. Lancet 395 , 1039–1046 (2020).
Hao, X. et al. Reconstruction of the full transmission dynamics of COVID-19 in Wuhan. Nature 584 , 420–424 (2020).
Hu, B., Guo, H., Zhou, P. & Shi, Z.-L. Characteristics of SARS-CoV-2 and COVID-19. Nat. Rev. Microbiol . 19 , 141–154 (2021).
World Health Organization. Public health surveillance for COVID-19: interim guidance (2020).
Chiolero, A., Santschi, V. & Paccaud, F. Public health surveillance with electronic medical records: at risk of surveillance bias and overdiagnosis. Eur. J. Public Health 23 , 350–351 (2013).
Alwan, N. A. Surveillance is underestimating the burden of the COVID-19 pandemic. Lancet 396 , e24 (2020).
Jajosky, R. A. & Groseclose, S. L. Evaluation of reporting timeliness of public health surveillance systems for infectious diseases. BMC Public Health 4 , 1–9 (2004).
Khan, M. S., Wu, S., Wang, X. & Coker, R. Optimising routine surveillance systems for informing tuberculosis control policies in China. Health Policy Plan. 32 , i12–i14 (2017).
China CDC. The launch of infectious disease reporting system for COVID-19 (2020).
WHO regional office for Europe. Strengthening the health system response to COVID-19: recommendations for the WHO European region (2020).
Davies, S. E. & Bennett, B. A gendered human rights analysis of Ebola and Zika: locating gender in global health emergencies; a gendered human rights analysis of Ebola and Zika: locating gender in global health emergencies. Int. Aff. 92 , 1041–1060 (2016).
Harman, S. Ebola, gender and conspicuously invisible women in global health governance. Third World Q 37 , 524–541 (2016).
Wenham, C., Smith, J. & Morgan, R., Gender and COVID-19 Working Group. COVID-19: the gendered impacts of the outbreak. Lancet 395 , 846–848 (2020).
World Health Organization. Universal health coverage (UHC). https://www.who.int/news-room/fact-sheets/detail/universal-health-coverage-(uhc) (2019).
Bhutta, Z. A., Lassi, Z., Pariyo, G. & Huicho, L. Global Experience of Community Health Workers for Delivery of Health Related Millennium Development Goals: a systematic review, country case studies, and recommendations for integration into national health systems. https://www.who.int/workforcealliance/knowledge/publications/alliance/Global_CHW_web.pdf (2010).
Keshet, Y. Fear of panoptic surveillance: using digital technology to control the COVID-19 epidemic. Isr. J. Health Policy Res. 9 , 67 (2020).
Enter the cyborgs: health and human rights in the digital age. Health Hum. Rights https://www.hhrjournal.org/2020/12/editorial-enter-the-cyborgs-health-and-human-rights-in-the-digital-age/ (2020).
Technology, health, and human rights: a cautionary tale for the post-pandemic world. Health Hum. Rights https://www.hhrjournal.org/2020/12/viewpoint-technology-health-and-human-rights-a-cautionary-tale-for-the-post-pandemic-world/ (2020).
Analyzing the human rights impact of increased digital public health surveillance during the COVID-19 crisis. Health Hum. Rights https://www.hhrjournal.org/2020/12/analyzing-the-human-rights-impact-of-increased-digital-public-health-surveillance-during-the-covid-19-crisis/ (2020).
Lal, A., Erondu, N. A., Heymann, D. L., Gitahi, G. & Yates, R. Fragmented health systems in COVID-19: rectifying the misalignment between global health security and universal health coverage. Lancet 397 , 61–67 (2021).
Baum, F. et al. Explaining COVID-19 performance: what factors might predict national responses? BMJ 372 , n91 (2021).
Ebi, K. L. & Semenza, J. C. Community-based adaptation to the health impacts of climate change. Am. J. Prev. Med. 35 , 501–507 (2008).
Download references
Acknowledgements
Data for this review were collected under the auspices of the Independent Panel for Pandemic Preparedness and Response. The analysis of this paper is separate from the Independent Panel’s Final Report and has been facilitated by the Independent Panel Secretariat. The Secretariat of the Independent Panel for Pandemic Preparedness and Response is independent and impartial. The views expressed in this work are solely that of the authors and do not represent the views of the Independent Panel for Pandemic Preparedness and Response.
Author information
These authors contributed equally: Victoria Haldane, Chuan De Foo, Salma M. Abdalla, Anne-Sophie Jung.
Authors and Affiliations
Institute of Health Policy, Management and Evaluation, University of Toronto, Toronto, Ontario, Canada
Victoria Haldane
Saw Swee Hock School of Public Health, Singapore, Singapore
Chuan De Foo, Melisa Tan, Shishi Wu, Alvin Chua, Monica Verma, Pami Shrestha, See Mieng Tan & Helena Legido-Quigley
School of Public Health, Boston University, Boston, MA, USA
Salma M. Abdalla
The Independent Panel for Pandemic Preparedness and Response Secretariat, Geneva, Switzerland
Salma M. Abdalla, Sudhvir Singh, Michael Bartos, Shunsuke Mabuchi, Mathias Bonk, Christine McNab, George K. Werner, Raj Panjabi, Anders Nordström & Helena Legido-Quigley
London School of Hygiene and Tropical Medicine, London, UK
Anne-Sophie Jung, Tristana Perez & Helena Legido-Quigley
Faculty of Medical and Health Sciences, University of Auckland, Auckland, New Zealand
Sudhvir Singh
School of Sociology, Australian National University, Canberra, New South Wales, Australia
Michael Bartos
Berlin Institute of Global Health, Berlin, Germany
Mathias Bonk
You can also search for this author in PubMed Google Scholar
Contributions
V.H., C.D.F., S.A., A.-S.J. and H.L.-Q. conceived and designed this Review. V.H., C.D.F., S.A., A.-S.J., M.M.J.T., S.W., A.C., M.V., P.S., S.M.T. and H.L.-Q. collected the data. V.H., C.D.F., S.A., A.-S.J., M.M.J.T., S.W., S.S. and H.L.-Q. analyzed the data and drafted the manuscript with input from all authors. All authors contributed to revision of the manuscript.
Corresponding author
Correspondence to Helena Legido-Quigley .
Ethics declarations
Competing interests.
All authors declare no competing interests.
Additional information
Peer review information Nature Medicine thanks Ole F. Norheim and Margaret Kruk for their contribution to the peer review of this work. Joao Monteiro was the primary editor on this article and managed its editorial process and peer review in collaboration with the rest of the editorial team.
Publisher’s note Springer Nature remains neutral with regard to jurisdictional claims in published maps and institutional affiliations.
Rights and permissions
Reprints and permissions
About this article
Cite this article.
Haldane, V., De Foo, C., Abdalla, S.M. et al. Health systems resilience in managing the COVID-19 pandemic: lessons from 28 countries. Nat Med 27 , 964–980 (2021). https://doi.org/10.1038/s41591-021-01381-y
Download citation
Received : 08 March 2021
Accepted : 29 April 2021
Published : 17 May 2021
Issue Date : June 2021
DOI : https://doi.org/10.1038/s41591-021-01381-y
Share this article
Anyone you share the following link with will be able to read this content:
Sorry, a shareable link is not currently available for this article.
Provided by the Springer Nature SharedIt content-sharing initiative
This article is cited by
Transforming health care systems towards high-performance organizations: qualitative study based on learning from covid-19 pandemic in the basque country (spain).
- Ane Fullaondo
- Irati Erreguerena
- Esteban de Manuel Keenoy
BMC Health Services Research (2024)
The response of the Military Health System (MHS) to the COVID-19 pandemic: a summary of findings from MHS reviews
- Alysa Pomer
- Satish Munigala
- Tracey Perez Koehlmoos
Health Research Policy and Systems (2024)
Involving community pharmacists in interprofessional collaboration in primary care: a systematic review
- Morgane Angibaud
- Maud Jourdain
- Jean-François Huon
BMC Primary Care (2024)
Perceived risk of COVID-19 hurts mental health: the mediating role of fear of COVID-19 and the moderating role of resilience
- Jialin Yang
BMC Psychiatry (2024)
Understanding health system resilience in responding to COVID-19 pandemic: experiences and lessons from an evolving context of federalization in Nepal
- Shophika Regmi
- Maria Paola Bertone
- Sushil Baral
Quick links
- Explore articles by subject
- Guide to authors
- Editorial policies
Sign up for the Nature Briefing newsletter — what matters in science, free to your inbox daily.

Digital Twins for Managing Health Care Systems: Rapid Literature Review
Affiliation.
- 1 School of Systems and Enterprises, Stevens Institute of Technology, Hoboken, NJ, United States.
- PMID: 35972776
- PMCID: PMC9428772
- DOI: 10.2196/37641
Background: Although most digital twin (DT) applications for health care have emerged in precision medicine, DTs can potentially support the overall health care process. DTs (twinned systems, processes, and products) can be used to optimize flows, improve performance, improve health outcomes, and improve the experiences of patients, doctors, and other stakeholders with minimal risk.
Objective: This paper aims to review applications of DT systems, products, and processes as well as analyze the potential of these applications for improving health care management and the challenges associated with this emerging technology.
Methods: We performed a rapid review of the literature and reported available studies on DTs and their applications in health care management. We searched 5 databases for studies published between January 2002 and January 2022 and included peer-reviewed studies written in English. We excluded studies reporting DT usage to support health care practice (organ transplant, precision medicine, etc). Studies were analyzed based on their contribution toward DT technology to improve user experience in health care from human factors and systems engineering perspectives, accounting for the type of impact (product, process, or performance/system level). Challenges related to the adoption of DTs were also summarized.
Results: The DT-related studies aimed at managing health care systems have been growing over time from 0 studies in 2002 to 17 in 2022, with 7 published in 2021 (N=17 studies). The findings reported on applications categorized by DT type (system: n=8; process: n=5; product: n=4) and their contributions or functions. We identified 4 main functions of DTs in health care management including safety management (n=3), information management (n=2), health management and well-being promotion (n=3), and operational control (n=9). DTs used in health care systems management have the potential to avoid unintended or unexpected harm to people during the provision of health care processes. They also can help identify crisis-related threats to a system and control the impacts. In addition, DTs ensure privacy, security, and real-time information access to all stakeholders. Furthermore, they are beneficial in empowering self-care abilities by enabling health management practices and providing high system efficiency levels by ensuring that health care facilities run smoothly and offer high-quality care to every patient.
Conclusions: The use of DTs for health care systems management is an emerging topic. This can be seen in the limited literature supporting this technology. However, DTs are increasingly being used to ensure patient safety and well-being in an organized system. Thus, further studies aiming to address the challenges of health care systems challenges and improve their performance should investigate the potential of DT technology. In addition, such technologies should embed human factors and ergonomics principles to ensure better design and more successful impact on patient and doctor experiences.
Keywords: digital health; digital twins; health care; health informatics; human factors; information management; literature synthesis; operational control; safety; scheduling and optimization; supply chain management; technology; well-being promotion.
©Safa Elkefi, Onur Asan. Originally published in the Journal of Medical Internet Research (https://www.jmir.org), 16.08.2022.
Publication types
- Delivery of Health Care*
- Open access
- Published: 12 October 2020
A systematic literature review of researchers’ and healthcare professionals’ attitudes towards the secondary use and sharing of health administrative and clinical trial data
- Elizabeth Hutchings ORCID: orcid.org/0000-0002-6030-954X 1 ,
- Max Loomes ORCID: orcid.org/0000-0003-1042-0968 2 ,
- Phyllis Butow ORCID: orcid.org/0000-0003-3562-6954 2 , 3 , 4 &
- Frances M. Boyle ORCID: orcid.org/0000-0003-3798-1570 1 , 5
Systematic Reviews volume 9 , Article number: 240 ( 2020 ) Cite this article
14k Accesses
9 Citations
5 Altmetric
Metrics details
A systematic literature review of researchers and healthcare professionals’ attitudes towards the secondary use and sharing of health administrative and clinical trial data was conducted using electronic data searching. Eligible articles included those reporting qualitative or quantitative original research and published in English. No restrictions were placed on publication dates, study design, or disease setting. Two authors were involved in all stages of the review process; conflicts were resolved by consensus. Data was extracted independently using a pre-piloted data extraction template. Quality and bias were assessed using the QualSyst criteria for qualitative studies. Eighteen eligible articles were identified, and articles were categorised into four key themes: barriers, facilitators, access, and ownership; 14 subthemes were identified. While respondents were generally supportive of data sharing, concerns were expressed about access to data, data storage infrastructure, and consent. Perceptions of data ownership and acknowledgement, trust, and policy frameworks influenced sharing practice, as did age, discipline, professional focus, and world region. Young researchers were less willing to share data; they were willing to share in circumstances where they were acknowledged. While there is a general consensus that increased data sharing in health is beneficial to the wider scientific community, substantial barriers remain.
Systematic review registration
PROSPERO CRD42018110559
Peer Review reports
Healthcare systems generate large amounts of data; approximately 80 mB of data are generated per patient per year [ 1 ]. It is projected that this figure will continue to grow with an increasing reliance on technologies and diagnostic capabilities. Healthcare data provides an opportunity for secondary data analysis with the capacity to greatly influence medical research, service planning, and health policy.
There are many forms of data collected in the healthcare setting including administrative and clinical trial data which are the focus of this review. Administrative data collected during patients’ care in the primary, secondary, and tertiary settings can be analysed to identify systemic issues and service gaps, and used to inform improved health resourcing. Clinical trials play an essential role in furthering our understanding of disease, advancing new therapeutics, and developing improved supportive care interventions. However, clinical trials are expensive and can take several years to complete; a frequently quoted figure is that it takes 17 years for 14% of clinical research to benefit the patient [ 2 , 3 ].
Those who argue for increased data sharing in healthcare suggest that it may lead to improved treatment decisions based on all available information [ 4 , 5 ], improved identification of causes and clinical manifestations of disease [ 6 ], and provide increased research transparency [ 7 ]. In rare diseases, secondary data analysis may greatly accelerate the medical community’s understanding of the disease’s pathology and influence treatment.
Internationally, there are signs of movement towards greater transparency, particularly with regard to clinical research data. This change has been driven by governments [ 8 ], peak bodies [ 9 ], and clinician led initiatives [ 5 ]. One initiative led by the International Council of Medical Journal Editors (ICMJE) now requires a data sharing plan for all clinical research submitted for publication in a member scientific journal [ 9 ]. Further, international examples of data sharing can be seen in projects such as The Cancer Genome Atlas (TCGA) [ 10 ] dataset and the Surveillance, Epidemiology, and End Results (SEER) [ 11 ] database which have been used extensively for cancer research.
However, consent, data ownership, privacy, intellectual property rights, and potential for misinterpretation of data [ 12 ] remain areas of concern to individuals who are more circumspect about changing the data sharing norm. To date, there has been no published synthesis of views on data sharing from the perspectives of diverse professional stakeholders. Thus, we conducted a systematic review of the literature on the views of researchers and healthcare professionals regarding the sharing of health data.
This systematic literature review was part of a larger review of articles addressing data sharing, undertaken in accordance with the PRISMA statement for systematic reviews and meta-analysis [ 13 ]. The protocol was prospectively registered on PROSPERO ( www.crd.york.ac.uk /PROSPERO, CRD42018110559).
The following databases were searched: EMBASE/MEDLINE, Cochrane Library, PubMed, CINAHL, Informit Health Collection, PROSPERO Database of Systematic Reviews, PsycINFO, and ProQuest. The final search was conducted on 21 October 2018. No date restrictions were placed on the search; key search terms are listed in Table 1 . Papers were considered eligible if they: were published in English; were published in a peer review journal; reported original research, either qualitative or quantitative with any study design, related to data sharing in any disease setting; and included subjects over 18 years of age. Systematic literature reviews were included in the wider search but were not included in the results. Reference list and hand searching were undertaken to identify additional papers. Papers were considered ineligible if they focused on electronic health records, biobanking, or personal health records or were review articles, opinion pieces/articles/letters, editorials, or theses from masters or doctoral research. Duplicates were removed and title and abstract and full-text screening were undertaken using the Cochrane systematic literature review program Covidence [ 14 ]. Two authors were involved in all stages of the review process; conflicts were resolved by consensus.
Quality and bias were assessed at a study level using the QualSyst system for quantitative and qualitative studies as described by Kmet et al. [ 15 ]. A maximum score of 20 is assigned to articles of high quality and low bias; the final QualSyst score is a proportion of the total, with a possible score ranging from 0.0 to 1.0 [ 15 ].
Data extraction was undertaken using a pre-piloted form in Microsoft Office Excel. Data points included author, country and year of study, study design and methodology, health setting, and key themes and results. Where available, detailed information on research participants was extracted including age, sex, clinical/academic employment setting, publication and grant history, career stage, and world region.
Quantitative data were summarised using descriptive statistics. Synthesis of qualitative findings used a meta-ethnographic approach, in accordance with guidelines from Lockwood et al. [ 16 ].The main themes of each qualitative study were first identified and then combined, if relevant, into categories of commonality. Using a constant comparative approach, higher order themes and subthemes were developed. Quantitative data relevant to each theme were then incorporated. Using a framework analysis approach as described by Gale et al. [ 17 ], the perspectives of different professional groups (researchers, healthcare professionals, data custodians, and ethics committees) towards data sharing were identified. Where differences occurred, they are highlighted in the results. Similarly, where systematic differences according to other characteristics (such as age or years of experience), these are highlighted.
This search identified 4019 articles, of which 241 underwent full-text screening; 73 articles met the inclusion criteria for the larger review. Five systematic literature reviews were excluded as was one article which presented duplicate results; this left a total of 67 articles eligible for review. See Fig. 1 for the PRISMA diagram describing study screening.
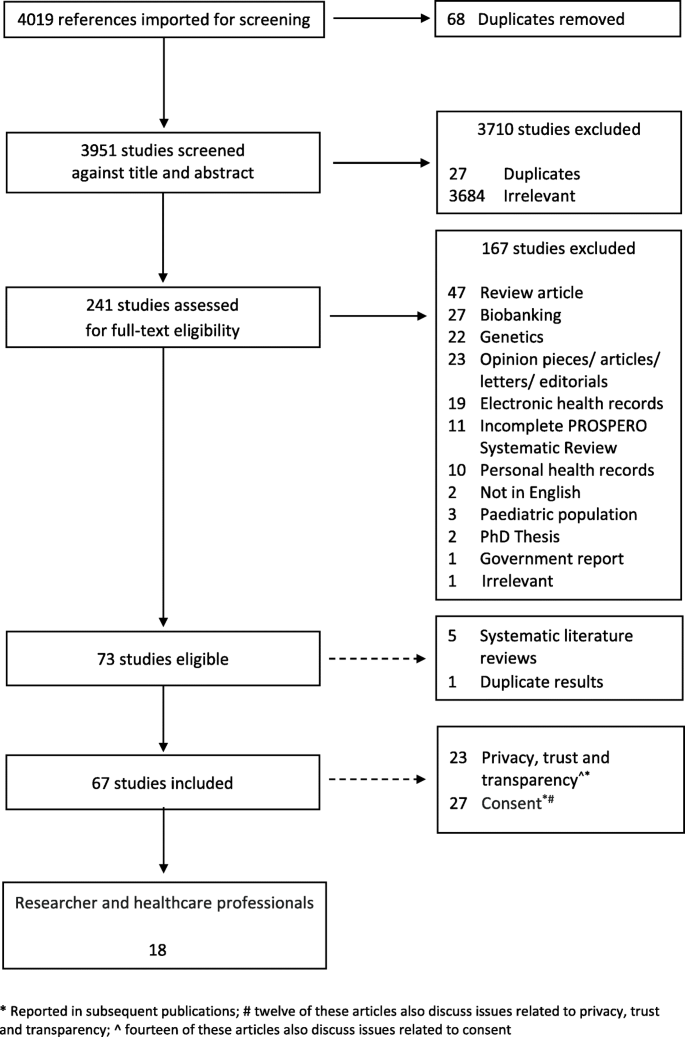
PRIMSA flow diagram (attached)
This systematic literature review was originally developed to identify attitudes towards secondary use and sharing of health administrative and clinical trial data in breast cancer. However, as there was a paucity of material identified specifically related to this group, we present the multidisciplinary results of this search, and where possible highlight results specific to breast cancer, and cancer more generally. We believe that the material identified in this search is relevant and reflective of the wider attitudes towards data sharing within the scientific and medical communities and can be used to inform data sharing strategies in breast cancer.
Eighteen [ 18 , 19 , 20 , 21 , 22 , 23 , 24 , 25 , 26 , 27 , 28 , 29 , 30 , 31 , 32 , 33 , 34 , 35 ] of the 67 articles addressed the perspectives of clinical and scientific researchers, data custodians, and ethics committees and were analysed for this paper (Table 2 ). The majority ( n = 16) of articles focused on the views of researchers and health professionals, [ 18 , 19 , 20 , 21 , 22 , 24 , 25 , 26 , 28 , 29 , 30 , 31 , 32 , 33 , 34 , 35 ], only one article focused on data custodians [ 27 ] and ethics committees [ 23 ] respectively. Four articles [ 18 , 19 , 21 , 35 ] included a discussion on the attitudes of both researchers and healthcare professionals and patients; only results relating to researchers/clinicians are included in this analysis (Fig. 1 ).
Study design, location, and disciplines
Several study methodologies were used, including surveys ( n = 11) [ 24 , 25 , 26 , 27 , 29 , 30 , 31 , 32 , 33 , 34 , 35 ], interviews and focus groups ( n = 6) [ 18 , 19 , 20 , 21 , 22 , 23 ], and mixed methods ( n = 1) [ 28 ]. Studies were conducted in a several countries and regions; a breakdown by country and study is available in Table 3 .
In addition to papers focusing on general health and sciences [ 18 , 21 , 22 , 24 , 25 , 26 , 29 , 30 , 31 , 32 , 33 , 34 ], two articles included views from both science and non-science disciplines [ 27 , 28 ]. Multiple sclerosis (MS) [ 19 ], mental health [ 35 ], and human immunodeficiency virus (HIV)/acquired immunodeficiency syndrome (AIDS)/tuberculosis (TB) [ 20 ] were each the subject of one article.
Study quality
Results of the quality assessment are provided in Table 2 . QualSyst [15] scores ranged from 0.7 to 1.0 (possible range 0.0 to 1.0). While none were blinded studies, most provided clear information on respondent selection, data analysis methods, and justifiable study design and methodology.
Four key themes, barriers, facilitators, access, and ownership were identified; 14 subthemes were identified. A graphical representation of article themes is presented in Fig. 2 . Two articles reflect the perspective of research ethics committees [ 23 ] and data custodians [ 27 ]; concerns noted by these groups are similar to those highlighted by researchers and healthcare professionals.
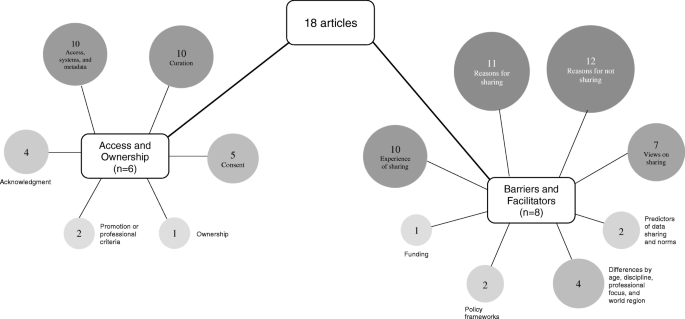
Graphic representation of key themes and subthemes identified (attached)
Barriers and facilitators
Reasons for not sharing.
Eleven articles identified barriers to data sharing [ 20 , 22 , 24 , 25 , 27 , 29 , 30 , 31 , 32 , 33 , 34 ]. Concerns cited by respondents included other researchers taking their results [ 24 , 25 ], having data misinterpreted or misattributed [ 24 , 27 , 31 , 32 ], loss of opportunities to maximise intellectual property [ 24 , 25 , 27 ], and loss of publication opportunities [ 24 , 25 ] or funding [ 25 ]. Results of a qualitative study showed respondents emphasised the competitive value of research data and its capacity to advance an individual’s career [ 20 ] and the potential for competitive disadvantage with data sharing [ 22 ]. Systematic issues related to increased data sharing were noted in several articles where it was suggested the barriers are ‘deeply rooted in the practices and culture of the research process as well as the researchers themselves’ [ 33 ] (p. 1), and that scientific competition and a lack of incentive in academia to share data remain barriers to increased sharing [ 30 ].
Insufficient time, lack of funding, limited storage infrastructure, and lack of procedural standards were also noted as barriers [ 33 ]. Quantitative results demonstrated that the researchers did not have the right to make the data public or that there was no requirement to share by the study sponsor [ 33 ]. Maintaining the balance between investigator and funder interests and the protection of research subjects [ 31 ] were also cited as barriers. Concerns about privacy were noted in four articles [ 25 , 27 , 29 , 30 ]; one study indicated that clinical researchers were significantly more concerned with issues of privacy compared to scientific researchers [ 25 ]. The results of one qualitative study indicated that clinicians were more cautious than patients regarding the inclusion of personal information in a disease specific registry; the authors suggest this may be a result of potential for legal challenges in the setting of a lack of explicit consent and consistent guidelines [ 19 ]. Researchers, particularly clinical staff, indicated that they did not see sharing data in a repository as relevant to their work [ 29 ]
Trust was also identified as a barrier to greater data sharing [ 32 ]. Rathi et al. identified that researchers were likely to withhold data if they mistrusted the intent of the researcher requesting the information [ 32 ]. Ethical, moral, and legal issues were other potential barriers cited [ 19 , 22 ]. In one quantitative study, 74% of respondents ( N = 317) indicated that ensuring appropriate data use was a concern; other concerns included data not being appropriate for the requested purpose [ 32 ]. Concerns about data quality were also cited as a barrier to data reuse; some respondents suggested that there was a perceived negative association of data reuse among health scientists [ 30 ].
Reasons for sharing
Eleven articles [ 19 , 20 , 21 , 22 , 24 , 25 , 29 , 30 , 31 , 32 , 33 ] discussed the reasons identified by researchers and healthcare professionals for sharing health data; broadly the principle of data sharing was seen as a desirable norm [ 25 , 31 ]. Cited benefits included improvements to the delivery of care, communication and receipt of information, impacts on care and quality of life [ 19 ], contributing to the advancement of science [ 20 , 24 , 29 ], validating scientific outputs, reducing duplication of scientific effort and minimising research costs [ 20 ], and promoting open science [ 31 , 32 ]. Professional reasons for sharing data included academic benefit and recognition, networking and collaborative opportunities [ 20 , 24 , 29 , 31 ], and contributing to the visibility of their research [ 24 ]. Several articles noted the potential of shared data for enabling faster access to a wider pool of patients [ 21 ] for research, improved access to population data for longitudinal studies [ 22 ], and increased responsiveness to public health needs [ 20 ]. In one study, a small percentage of respondents indicated that there were no benefits from sharing their data [ 24 ].
Analysis of quantitative survey data indicated that the perceived usefulness of data was most strongly associated with reuse intention [ 30 ]. The lack of access to data generated by other researchers or institutions was seen as a major impediment to progress in science [ 33 ]. In a second study, quantitative data showed no significant differences in reasons for sharing by clinical trialists’ academic productivity, geographic location, trial funding source or size, or the journal in which the results were published [ 32 ]. Attitudes towards sharing in order to receive academic benefits or recognition differed significantly based on the respondent’s geographic location; those from Western Europe were more willing to share compared to respondents in the USA or Canada, and the rest of the world [ 32 ].
Views on sharing
Seven articles [ 19 , 20 , 21 , 29 , 31 , 33 , 34 ] discussed researchers’ and healthcare professionals’ views relating to sharing data, with a broad range of views noted. Two articles, both qualitative, discussed the role of national registries [ 21 ], and data repositories [ 31 ]. Generally, there was clear support for national research registers and an acceptance for their rationale [ 21 ], and some respondents believed that sharing de-identified data through data repositories should be required and that when requested, investigators should share data [ 31 ]. Sharing de-identified data for reasons beyond academic and public health benefit were cited as a concern [ 20 ]. Two quantitative studies noted a proportion of researchers who believed that data should not be made available [ 33 , 34 ]. Researchers also expressed differences in how shared data should be managed; the requirement for data to be ‘gate-kept’ was preferred by some, while others were happy to relinquish control of their data once curated or on release [ 20 ]. Quantitative results indicated that scientists were significantly more likely to rank data reuse as highly relevant to their work than clinicians [ 29 ], but not all scientists shared data equally or had the same views about data sharing or reuse [ 33 ]. Some respondents argued that not all data were equal and therefore should only be shared in certain circumstances. This was in direct contrast to other respondents who suggested that all data should be shared, all of the time [ 20 ].
Differences by age, background, discipline, professional focus, and world region
Differences in attitudes towards shared data were noted by age, professional focus, and world region [ 25 , 27 , 33 , 34 ]. Younger researchers, aged between 20–39 and 40–49 years, were less likely to share their data with others (39% and 38% respectively) compared to other age groups; respondents aged over 50 years of age were more willing (46%) to share [ 33 ]. Interestingly, while less willing to share, younger researchers also believed that the lack of access to data was a major impediment to science and their research [ 33 ]. Where younger researchers were able to place conditions on access to their data, rates of willingness to share were increased [ 33 ].
Respondents from the disciplines of education, medicine/health science, and psychology were more inclined than others to agree that their data should not be available for others to use in the first place [ 34 ]. However, results from one study indicated that researchers from the medical field and social sciences were less likely to share compared to other disciplines [ 33 ]. For example, results of a quantitative study showed that compared to biologists, who reported sharing 85% of their data, medical and social sciences reported sharing their data 65% and 58% percent of the time, respectively [ 33 ].
One of the primary reasons for controlling access to data, identified in a study of data custodians, was due to a desire to avoid data misuse; this was cited as a factor for all surveyed data repositories except those of an interdisciplinary nature [ 27 ]. Limiting access to certain types of research and ensuring attribution were not listed as a concern for sociology, humanities or interdisciplinary data collections [ 27 ]. Issues pertaining to privacy and sensitive data were only cited as concerns for data collections related to humanities, social sciences, and biology, ecology, and chemistry; concerns regarding intellectual property were also noted [ 27 ]. The disciplines of biology, ecology, and chemistry and social sciences had the most policy restrictions on the use of data held in their repositories [ 27 ].
Differences in data sharing practices were also noted by world region. Respondents not from North American and European countries were more willing to place their data on a central repository; however, they were also more likely to place conditions on the reuse of their data [ 33 , 34 ].
Experience of data sharing
The experience of data sharing among researchers was discussed in nine articles [ 20 , 24 , 25 , 26 , 28 , 29 , 30 , 31 , 32 , 33 ]. Data sharing arrangements were highly individual and ranged from ad hoc and informal processes to formal procedures enforced by institutional policies in the form of contractual agreements, with respondents indicating data sharing behaviour ranging from sharing no data to sharing all data [ 20 , 26 , 31 ]. Quantitative data from one study showed that researchers were more inclined to share data prior to publication with people that they knew compared to those they did not; post publication, these figures were similar between groups [ 24 ]. While many researchers were prepared to share data, results of a survey identified a preference of researchers to collect data themselves, followed by their team, or by close colleagues [ 26 ].
Differences in the stated rate of data sharing compared to the actual rate of sharing [ 25 ] were noted. In a large quantitative study ( N = 1329), nearly one third of respondents chose not to answer whether they make their data available to others; of those who responded to the question, 46% reported they do not make their data electronically available to others [ 33 ]. By discipline, differences in the rate of refusal to share were higher in chemistry compared to non-science disciplines such as sociology [ 25 ]. Respondents who were more academically productive (> 25 articles over the past 3 years) reported that they have or would withhold data to protect research subjects less frequently than those who were less academically productive or received industry funding [ 32 ].
Attitudes to sharing de-identified data via data repositories was discussed in two articles [ 29 , 31 ]. A majority of respondents in one study indicated that de-identified data should be shared via a repository and that it should be shared when requested. A lack of experience in uploading data to repositories was noted as a barrier [ 29 ]. When data was shared, most researchers included additional materials to support their data including materials such as metadata or a protocol description [ 29 ].
Two articles [ 28 , 30 ] focused on processes and variables associated with sharing. Factors such as norms, data infrastructure/organisational support, and research communities were identified as important factors in a researcher’s attitude towards data sharing [ 28 , 30 ]. A moderate correlation between data reuse and data sharing suggest that these two variables are not linked. Furthermore, sharing data compared to self-reported data reuse were also only moderately associated (Pearson’s correlation of 0.25 ( p ≤ 0.001)) [ 26 ].
Predictors of data sharing and norms
Two articles [ 26 , 30 ] discussed the role of social norms and an individual’s willingness to share health data. Perceived efficacy and efficiency of data reuse were strong predictors of data sharing [ 26 ] and the development of a ‘positive social norm towards data sharing support(s)[ed] researcher data reuse intention’ [ 30 ] (p. 400).
Policy framework
The establishment of clear policies and procedures to support data sharing was highlighted in two articles [ 22 , 28 ]. The presence of ambiguous data sharing policies was noted as a major limitation, particularly in primary care and the increased adoption of health informatics systems [ 22 ]. Policies that support an efficient exchange system allowing for the maximum amount of data sharing are preferred and may include incentives such as formal recognition and financial reimbursement; a framework for this is proposed in Fecher et al. [ 28 ].
Research funding
The requirement to share data funded by public monies was discussed in one article [ 25 ]. Some cases were reported of researchers refusing to share data funded by tax-payer funds; reasons for refusal included a potential reduction in future funding or publishing opportunities [ 25 ].
Access and ownership
Articles relating to access and ownership were grouped together and seven subthemes were identified.
Access, information systems, and metadata
Ten articles [ 19 , 20 , 21 , 22 , 26 , 27 , 29 , 33 , 34 , 35 ] discussed the themes of access, information systems, and the use of metadata. Ensuring privacy protections in a prospective manner was seen as important for data held in registries [ 19 ]. In the setting of mental health, researchers indicated that patients should have more choices for controlling access to shared registry data [ 35 ]. The use of guardianship committees [ 19 ] or gate-keepers [ 20 ] was seen as important in ensuring the security and access to data held in registries by some respondents; however, many suggested that a researcher should relinquish control of the data collection once curated or released, unless embargoed [ 20 ]. Reasons for maintaining control over registry data included ensuring attribution, restricting commercial research, protecting sensitive (non-personal) information, and limiting certain types of research [ 27 ]. Concerns about security and confidentiality were noted as important and assurances about these needed to be provided; accountability and transparency mechanisms also need to be included [ 21 ]. Many respondents believed that access to the registry data by pharmaceutical companies and marketing agencies was not considered appropriate [ 19 ].
Respondents to a survey from medicine and social sciences were less likely to agree to have all data included on a central repository with no restrictions [ 33 ]; notably, this was also reflected in the results of qualitative research which indicated that health professionals were more cautious than patients about the inclusion of personal data within a disease specific register [ 19 ].
While many researchers stated that they commonly shared data directly with other researchers, most did not have experience with uploading data to repositories [ 29 ]. Results from a survey indicated that younger respondents have more data access restrictions and thought that their data is easier to access significantly more than older respondents [ 34 ]. In the primary care setting, concerns were noted about the potential for practitioners to block patient involvement in a registry by refusing access to a patient’s personal data or by not giving permission for the data to be extracted from their clinical system [ 21 ]. There was also resistance in primary care towards health data amalgamation undertaken for an unspecified purpose [ 22 ]; respondents were not in favour of systems which included unwanted functionality (do not want/need), inadequate attributes (capability and receptivity) of the practice, or undesirable impact on the role of the general practitioner (autonomy, status, control, and workflow) [ 22 ].
Access to ‘comprehensive metadata (is needed) to support the correct interpretation of the data’ [ 26 ] (p. 4) at a later stage. When additional materials were shared, most researchers shared contextualising information or a description of the experimental protocol [ 29 ]. The use of metadata standards was not universal with some respondents using their own [ 33 ].
Several articles highlighted the impact of data curation on researchers’ time [ 20 , 21 , 22 , 29 , 33 ] or finances [ 24 , 28 , 29 , 33 , 34 ]; these were seen as potential barriers to increased registry adoption [ 21 ]. Tasks required for curation included preparing data for dissemination in a usable format and uploading data to repositories. The importance of ensuring that the data is accurately preserved for future reuse was highlighted; it must be presented in a retriable and auditable manner [ 20 ]. The amount of time required to curate data ranged from ‘no additional time’ to ‘greater than ten hours’ [ 29 ]. In one study, no clinical respondent had their data in a sharable format [ 29 ]. In the primary care setting, health information systems which promote sharing were not seen as being beneficial if they required standardisation of processes and/or sharing of clinical notes [ 22 ]. Further, spending time on non-medical issues in a time poor environment [ 22 ] was identified as a barrier. Six articles described the provision of funding or technical support to ensure data storage, maintenance, and the ability to provide access to data when requested. All noted a lack of funding and time as a barrier to increased sharing data [ 20 , 24 , 28 , 29 , 33 , 34 ].
Results of qualitative research indicated a range of views regarding consent mechanisms for future data use [ 18 , 19 , 20 , 23 , 35 ]. Consenting for future research can be complex given that the exact nature of the study will be unknown, and therefore some respondents suggested that a broad statement on future data uses be included [ 19 , 20 ] during the consent process. In contrast, other participants indicated that the current consent processes were too broad and do not reflect patient preferences sufficiently [ 35 ]. The importance of respecting the original consent in all future research was noted [ 20 ]. It was suggested that seeking additional consent for future data use may discourage participation in the original study [ 20 ]. Differences in views regarding the provision of detailed information about sharing individual level data was noted suggesting that the researchers wanted to exert some control over data they had collected [ 20 ]. An opt-out consent process was considered appropriate in some situations [ 18 ] but not all; some respondents suggested that consent to use a patient’s medical records was not required [ 18 ]. There was support by some researchers to provide patients with the option to ‘opt-in’ to different levels of involvement in a registry setting [ 19 ]. Providing patients more granular choices when controlling access to their medical data [ 35 ] was seen as important.
The attitudes of ethics and review boards ( N = 30) towards the use of medical records for research was discussed in one article [ 23 ]. While 38% indicated that no further consent would be required, 47% required participant consent, and 10% said that the requirement for consent would depend on how the potentially identifying variables would be managed [ 23 ]. External researcher access to medical record data was associated with a requirement for consent [ 23 ].
Acknowledgement
The importance of establishing mechanisms which acknowledge the use of shared data were discussed in four articles [ 27 , 29 , 33 , 34 ]. A significant proportion of respondents to a survey believed it was fair to use other researchers’ data if they acknowledged the originator and the funding body in all disseminated work or as a formal citation in published works [ 33 ]. Other mechanisms for acknowledging the data originator included opportunities to collaborate on the project, reciprocal data sharing agreements, allowing the originator to review or comment on results, but not approve derivative works, or the provision of a list of products making use of the data and co-authorship [ 33 , 34 ]. In the setting of controlled data collections, survey results indicated that ensuring attribution was a motivator for controlled access [ 27 ]. Over half of respondents in one survey believed it was fair to disseminate results based either in whole or part without the data provider’s approval [ 33 ]. No significant differences in mechanisms for acknowledgement were noted between clinical and scientific participants; mechanisms included co-authorship, recognition in the acknowledgement section of publications, and citation in the bibliography [ 29 ]. No consentient method for acknowledging shared data reuse was identified [ 29 ].
Data ownership was identified as a potential barrier to increased data sharing in academic research [ 28 ]. In the setting of control of data collections, survey respondents indicated that they wanted to maintain some control over the dataset, which is suggestive of researchers having a perceived ownership of their research data [ 28 ]. Examples of researchers extending ownership over their data include the right to publish first and the control of access to datasets [ 28 ]. Fecher et al. noted that the idea of data ownership by the researcher is not a position always supported legally; ‘the ownership and rights of use, privacy, contractual consent and copyright’ are subsumed [ 28 ] (p. 15). Rather data sharing is restricted by privacy law, which is applied to datasets containing data from individuals. The legal uncertainty about data ownership and the complexity of law can deter data sharing [ 28 ].
Promotion/professional criteria
The role of data sharing and its relation to promotion and professional criteria were discussed in two articles [ 24 , 28 ]. The requirement to share data is rarely a promotion or professional criterion, rather the systems are based on grants and publication history [ 24 , 28 ]. One study noted that while the traditional link between publication history and promotion remains, it is ‘likely that funders will continue to get sub-optimal returns on their investments, and that data will continue to be inefficiently utilised and disseminated’ [ 24 ] (p. 49).
This systematic literature review highlights the ongoing complexity associated with increasing data sharing across the sciences. No additional literature meeting the inclusion criteria were identified in the period between the data search and the submission of this manuscript. Data gaps identified include a paucity of information specifically related to the attitudes of breast cancer researchers and health professionals towards the secondary use and sharing of health administrative and clinical trial data.
While the majority of respondents believed the principles of data sharing were sound, significant barriers remain: issues of consent, privacy, information security, and ownership were key themes throughout the literature. Data ownership and acknowledgement, trust, and policy frameworks influenced sharing practice, as did age, discipline, professional focus, and world region.
Addressing concerns of privacy, trust, and information security in a technologically changing and challenging landscape is complex. Ensuring the balance between privacy and sharing data for the greater good will require the formation of policy and procedures, which promote both these ideals.
Establishing clear consent mechanisms would provide greater clarity for all parties involved in the data sharing debate. Ensuring that appropriate consent for future research, including secondary data analysis and sharing and linking of datasets, is gained at the point of data collection, would continue to promote research transparency and provide healthcare professionals and researchers with knowledge that an individual is aware that their data may be used for other research purposes. The establishment of policy which supports and promotes the secondary use of data and data sharing will assist in the normalisation of this type of health research. With the increased promotion of data sharing and secondary data analysis as an established tool in health research, over time barriers to its use, including perceptions of ownership and concerns regarding privacy and consent, will decrease.
The importance of establishing clear and formal processes associated with acknowledging the use of shared data has been underscored in the results presented. Initiatives such as the Bioresource Research Impact Factor/Framework (BRIF) [ 36 ] and the Citation of BioResources in journal Articles (CoBRA) [ 37 ] have sought to formalise the process. However, increased academic recognition of sharing data for secondary analysis requires further development and the allocation of funding to ensure that collected data is in a usable, searchable, and retrievable format. Further, there needs to be a shift away from the traditional criteria of academic promotion, which includes research outputs, to one which is inclusive of a researcher’s data sharing history and the availability of their research dataset for secondary analysis.
The capacity to identify and use already collected data was identified as a barrier. Moves to make data findable, accessible, interoperable, and reusable (FAIR) have been promoted as a means to encourage greater accessibility to data in a systematic way [ 38 ]. The FAIR principles focus on data characteristics and should be interpreted alongside the collective benefit, authority to control, responsibility, and ethics (CARE) principles established by the Global Indigenous Data Alliance (GIDA) which a people and purpose orientated [ 39 ].
Limitations
The papers included in this study were limited to those indexed on major databases. Some literature on this topic may have been excluded if it was not identified during the grey literature and hand searching phases.
Implications
Results of this systematic literature review indicate that while there is broad agreement for the principles of data sharing in medical research, there remain disagreements about the infrastructure and procedures associated with the data sharing process. Additional work is therefore required on areas such as acknowledgement, curation, and data ownership.
While the literature confirms that there is overall support for data sharing in medical and scientific research, there remain significant barriers to its uptake. These include concerns about privacy, consent, information security, and data ownership.
Availability of data and materials
All data generated or analysed during this study are included in this published article.
Abbreviations
Bioresource Research Impact Factor/Framework
Collective benefit, authority to control, responsibility, and ethics
Citation of BioResources in journal Articles
Findable, accessible, interoperable, and reusable
Global Indigenous Data Alliance
Human immunodeficiency virus/acquired immunodeficiency
International Council of Medical Journal Editors
Multiple sclerosis
Surveillance, Epidemiology, and End Results
Tuberculosis
The Cancer Genome Atlas
Huesch MD, Mosher TJ. Using it or losing it? The case for data scientists inside health care. NEJM Catalyst. 2017.
Green LW. Closing the chasm between research and practice: evidence of and for change. Health Promot J Australia. 2014;25(1):25–9.
Article Google Scholar
Morris ZS, Wooding S, Grant J. The answer is 17 years, what is the question: understanding time lags in translational research. J R Soc Med. 2011;104(12):510–20.
Article PubMed PubMed Central Google Scholar
Goldacre B. Are clinical trial data shared sufficiently today? No. Br Med J. 2013;347:f1880.
Goldacre B, Gray J. OpenTrials: towards a collaborative open database of all available information on all clinical trials. Trials. 2016;17(1):164.
Kostkova P, Brewer H, de Lusignan S, Fottrell E, Goldacre B, Hart G, et al. Who owns the data? Open data for healthcare. Front Public Health. 2016;4.
Elliott M. Seeing through the lies: innovation and the need for transparency. Gresham College Lecture Series; 23 November 2016; Museum of London. 2016.
European Medicines Agency. Publication and access to clinical-trial data. London: European Medicines Agency; 2013.
Google Scholar
Taichman DB, Backus J, Baethge C, Bauchner H, de Leeuw PW, Drazen JM, et al. Sharing clinical trial data: a proposal from the International Committee of Medical Journal Editors. J Am Med Assoc. 2016;315(5):467–8.
Article CAS Google Scholar
National Institue of Health (NIH). The Cancer Genome Atlas (TCGA): program overview United States of America: National Institue of Health (NIH); 2019 [Available from: https://cancergenome.nih.gov/abouttcga/overview ].
National Institue of Health (NIH). Surveillance, Epidemiology, and End Results (SEER) Program Washington: The Government of United States of Ameica; 2019 [Available from: https://seer.cancer.gov ].
Castellani J. Are clinical trial data shared sufficiently today? Yes. Br Med J. 2013;347:f1881.
Moher D, Liberati A, Tetzlaff J, Altman DG, Group P. Preferred reporting items for systematic reviews and meta-analyses: the PRISMA statement. PLoS Med. 2009;6(7):e1000097–e.
Veritas Health Innovation. Covidence systematic review software. Melbourne: Cochrane Collaboration; 2018.
Kmet LM, Cook LS, Lee RC. Standard quality assessment criteria for evaluating primary research papers from a variety of fields; 2004.
Lockwood C, Munn Z, Porritt K. Qualitative research synthesis: methodological guidance for systematic reviewers utilizing meta-aggregation. Int J Evidence Based Healthcare. 2015;13(3):179–87.
Gale NK, Heath G, Cameron E, Rashid S, Redwood S. Using the framework method for the analysis of qualitative data in multi-disciplinary health research. BMC Med Res Methodol. 2013;13(1):117.
Asai A, Ohnishi M, Nishigaki E, Sekimoto M, Fukuhara S, Fukui T. Attitudes of the Japanese public and doctors towards use of archived information and samples without informed consent: preliminary findings based on focus group interviews. BMC Medical Ethics. 2002;3(1):1.
Article PubMed Central Google Scholar
Baird W, Jackson R, Ford H, Evangelou N, Busby M, Bull P, et al. Holding personal information in a disease-specific register: the perspectives of people with multiple sclerosis and professionals on consent and access. J Med Ethics. 2009;35(2):92–6.
Article CAS PubMed Google Scholar
Denny SG, Silaigwana B, Wassenaar D, Bull S, Parker M. Developing ethical practices for public health research data sharing in South Africa: the views and experiences from a diverse sample of research stakeholders. J Empiric Res Human Res Ethics. 2015;10(3):290–301.
Grant A, Ure J, Nicolson DJ, Hanley J, Sheikh A, McKinstry B, et al. Acceptability and perceived barriers and facilitators to creating a national research register to enable 'direct to patient' enrolment into research: the Scottish Health Research register (SHARE). BMC Health Serv Res. 2013;13(1):422.
Knight J, Patrickson M, Gurd B. Understanding GP attitudes towards a data amalgamating health informatics system. Electron J Health Inform. 2008;3(2):12.
Willison DJ, Emerson C, Szala-Meneok KV, Gibson E, Schwartz L, Weisbaum KM, et al. Access to medical records for research purposes: varying perceptions across research ethics boards. J Med Ethics. 2008;34(4):308–14.
Bezuidenhout L, Chakauya E. Hidden concerns of sharing research data by low/middle-income country scientists. Glob Bioethics. 2018;29(1):39–54.
Ceci SJ. Scientists' attitudes toward data sharing. Sci Technol Human Values. 1988;13(1-2):45–52.
Curty RG, Crowston K, Specht A, Grant BW, Dalton ED. Attitudes and norms affecting scientists’ data reuse. PLoS One. 2017;12(12):e0189288.
Article PubMed PubMed Central CAS Google Scholar
Eschenfelder K, Johnson A. The limits of sharing: controlled data collections. Proc Am Soc Inf Sci Technol. 2011;48(1):1–10.
Fecher B, Friesike S, Hebing M. What drives academic data sharing? PLoS One. 2015;10(2):e0118053.
Federer LM, Lu Y-L, Joubert DJ, Welsh J, Brandys B. Biomedical data sharing and reuse: attitudes and practices of clinical and scientific research staff. PLoS One. 2015;10(6):e0129506.
Joo S, Kim S, Kim Y. An exploratory study of health scientists’ data reuse behaviors: examining attitudinal, social, and resource factors. Aslib J Inf Manag. 2017;69(4):389–407.
Rathi V, Dzara K, Gross CP, Hrynaszkiewicz I, Joffe S, Krumholz HM, et al. Sharing of clinical trial data among trialists: a cross sectional survey. Br Med J. 2012;345:e7570.
Rathi VK, Strait KM, Gross CP, Hrynaszkiewicz I, Joffe S, Krumholz HM, et al. Predictors of clinical trial data sharing: exploratory analysis of a cross-sectional survey. Trials. 2014;15(1):384.
Tenopir C, Allard S, Douglass K, Aydinoglu AU, Wu L, Read E, et al. Data sharing by scientists: practices and perceptions. PLoS One. 2011;6(6):e21101.
Article CAS PubMed PubMed Central Google Scholar
Tenopir C, Dalton ED, Allard S, Frame M, Pjesivac I, Birch B, et al. Changes in data sharing and data reuse practices and perceptions among scientists worldwide. PLoS One. 2015;10(8):e0134826.
Grando MA, Murcko A, Mahankali S, Saks M, Zent M, Chern D, et al. A study to elicit behavioral health patients' and providers' opinions on health records consent. J Law Med Ethics. 2017;45(2):238–59.
Howard HC, Mascalzoni D, Mabile L, Houeland G, Rial-Sebbag E, Cambon-Thomsen A. How to responsibly acknowledge research work in the era of big data and biobanks: ethical aspects of the bioresource research impact factor (BRIF). J Commun Genetics. 2018;9(2):169–76.
Bravo E, Calzolari A, De Castro P, Mabile L, Napolitani F, Rossi AM, et al. Developing a guideline to standardize the citation of bioresources in journal articles (CoBRA). BMC Med. 2015;13:33.
Boeckhout M, Zielhuis GA, Bredenoord AL. The FAIR guiding principles for data stewardship: fair enough? Eur J Human Genetics. 2018;26(7):931–6.
Global Indigenous Data Alliance (GIDA). CARE principles for indigenous data governance GIDA; 2019 [Available from: https://www.gida-global.org/care ].
Download references
Acknowledgements
The authors would like to thank Ms. Ngaire Pettit-Young, Information First, Sydney, NSW, Australia, for her assistance in developing the search strategy.
This project was supported by the Sydney Vital, Translational Cancer Research, through a Cancer Institute NSW competitive grant. The views expressed herein are those of the authors and are not necessarily those of the Cancer Institute NSW. FB is supported in her academic role by the Friends of the Mater Foundation.
Author information
Authors and affiliations.
Northern Clinical School, Faculty of Medicine, University of Sydney, Sydney, Australia
Elizabeth Hutchings & Frances M. Boyle
Department of Psychology, The University of Sydney, Sydney, NSW, Australia
Max Loomes & Phyllis Butow
Centre for Medical Psychology & Evidence-Based Decision-Making (CeMPED), Sydney, Australia
Phyllis Butow
Psycho-Oncology Co-Operative Research Group (PoCoG), The University of Sydney, Sydney, NSW, Australia
Patricia Ritchie Centre for Cancer Care and Research, Mater Hospital, North Sydney, Sydney, Australia
Frances M. Boyle
You can also search for this author in PubMed Google Scholar
Contributions
EH, PB, and FB were responsible for developing the study concept and the development of the protocol. EH and ML were responsible for the data extraction and data analysis. FB and PB supervised this research. All authors participated in interpreting the findings and contributed the intellectual content of the manuscript. All authors have read and approved the manuscript.
Corresponding author
Correspondence to Elizabeth Hutchings .
Ethics declarations
Ethics approval and consent to participate.
Not applicable.
Consent for publication
Competing interests.
EH, ML, PB, and FB declare that they have no competing interests.
Additional information
Publisher’s note.
Springer Nature remains neutral with regard to jurisdictional claims in published maps and institutional affiliations.
Rights and permissions
Open Access This article is licensed under a Creative Commons Attribution 4.0 International License, which permits use, sharing, adaptation, distribution and reproduction in any medium or format, as long as you give appropriate credit to the original author(s) and the source, provide a link to the Creative Commons licence, and indicate if changes were made. The images or other third party material in this article are included in the article's Creative Commons licence, unless indicated otherwise in a credit line to the material. If material is not included in the article's Creative Commons licence and your intended use is not permitted by statutory regulation or exceeds the permitted use, you will need to obtain permission directly from the copyright holder. To view a copy of this licence, visit http://creativecommons.org/licenses/by/4.0/ . The Creative Commons Public Domain Dedication waiver ( http://creativecommons.org/publicdomain/zero/1.0/ ) applies to the data made available in this article, unless otherwise stated in a credit line to the data.
Reprints and permissions
About this article
Cite this article.
Hutchings, E., Loomes, M., Butow, P. et al. A systematic literature review of researchers’ and healthcare professionals’ attitudes towards the secondary use and sharing of health administrative and clinical trial data. Syst Rev 9 , 240 (2020). https://doi.org/10.1186/s13643-020-01485-5
Download citation
Received : 27 December 2019
Accepted : 17 September 2020
Published : 12 October 2020
DOI : https://doi.org/10.1186/s13643-020-01485-5
Share this article
Anyone you share the following link with will be able to read this content:
Sorry, a shareable link is not currently available for this article.
Provided by the Springer Nature SharedIt content-sharing initiative
- Secondary data analysis
Systematic Reviews
ISSN: 2046-4053
- Submission enquiries: Access here and click Contact Us
- General enquiries: [email protected]
A Systematic Literature Review of Digital Twin Research for Healthcare Systems: Research Trends, Gaps, and Realization Challenges
Ieee account.
- Change Username/Password
- Update Address
Purchase Details
- Payment Options
- Order History
- View Purchased Documents
Profile Information
- Communications Preferences
- Profession and Education
- Technical Interests
- US & Canada: +1 800 678 4333
- Worldwide: +1 732 981 0060
- Contact & Support
- About IEEE Xplore
- Accessibility
- Terms of Use
- Nondiscrimination Policy
- Privacy & Opting Out of Cookies
A not-for-profit organization, IEEE is the world's largest technical professional organization dedicated to advancing technology for the benefit of humanity. © Copyright 2024 IEEE - All rights reserved. Use of this web site signifies your agreement to the terms and conditions.
Log in using your username and password
- Search More Search for this keyword Advanced search
- Latest content
- For authors
- Browse by collection
- BMJ Journals More You are viewing from: Google Indexer
You are here
- Volume 14, Issue 4
- Carbon footprint of healthcare systems: a systematic review of evidence and methods
- Article Text
- Article info
- Citation Tools
- Rapid Responses
- Article metrics

- http://orcid.org/0000-0003-0180-2358 Mattis Keil 1 , 2 ,
- Leonie Frehse 3 ,
- Marco Hagemeister 3 ,
- Mona Knieß 3 ,
- Oliver Lange 1 , 4 ,
- Tobias Kronenberg 5 ,
- Wolf Rogowski 1
- 1 Department of Health Care Management, Institute of Public Health and Nursing Research, Health Sciences , University of Bremen , Bremen , Germany
- 2 Joint research cluster “Healthy City Bremen” of the University of Bremen, Bremen University of Applied Sciences and Apollon University of Applied Sciences Bremen , Bremen , Germany
- 3 Professional Public Decision Making, Faculty of Cultural Studies , University of Bremen , Bremen , Germany
- 4 Leibniz ScienceCampus Digital Public Health , Bremen , Germany
- 5 Department of Economics , Bochum University of Applied Sciences , Bochum , Germany
- Correspondence to Mattis Keil; m.keil{at}uni-bremen.de
Objective Given the demand for net-zero healthcare, the carbon footprint (CF) of healthcare systems has attracted increasing interest in research in recent years. This systematic review investigates the results and methodological transparency of CF calculations of healthcare systems. The methodological emphasis lies specifically on input–output based calculations.
Design Systematic review according to the Preferred Reporting Items for Systematic Reviews and Meta-Analyses guideline.
Data sources PubMed, Web of Science, EconBiz, Scopus and Google Scholar were initially searched on 25 November 2019. Search updates in PubMed and Web of Science were considered until December 2023. The search was complemented by reference tracking within all the included studies.
Eligibility criteria We included original studies that calculated and reported the CF of one or more healthcare systems. Studies were excluded if the specific systems were not named or no information on the calculation method was provided.
Data extraction and synthesis Within the initial search, two independent reviewers searched, screened and extracted information from the included studies. A checklist was developed to extract information on results and methodology and assess the included studies’ transparency.
Results 15 studies were included. The mean ratio of healthcare system emissions to total national emissions was 4.9% (minimum 1.5%; maximum 9.8%), and CFs were growing in most countries. Hospital care led to the largest relative share of the total CF. At least 71% of the methodological items were reported by each study.
Conclusions The results of this review show that healthcare systems contribute substantially to national carbon emissions, and hospitals are one of the main contributors in this regard. They also show that mitigation measures can help reduce emissions over time. The checklist developed here can serve as a reference point to help make methodological decisions in future research reports as well as report homogeneous results.
- decision making
- health services administration & management
- change management
- international health services
- organisation of health services
Data availability statement
All data relevant to the study are included in the article or uploaded as supplementary information. Data are available upon reasonable request. The data that support the findings of this study are available in online supplemental file 4 'System description and results' and online supplemental file 5 'Methods and transparency'. Further data are available from the corresponding author (MKeil), upon reasonable request.
This is an open access article distributed in accordance with the Creative Commons Attribution Non Commercial (CC BY-NC 4.0) license, which permits others to distribute, remix, adapt, build upon this work non-commercially, and license their derivative works on different terms, provided the original work is properly cited, appropriate credit is given, any changes made indicated, and the use is non-commercial. See: http://creativecommons.org/licenses/by-nc/4.0/ .
https://doi.org/10.1136/bmjopen-2023-078464
Statistics from Altmetric.com
Request permissions.
If you wish to reuse any or all of this article please use the link below which will take you to the Copyright Clearance Center’s RightsLink service. You will be able to get a quick price and instant permission to reuse the content in many different ways.
STRENGTHS AND LIMITATIONS OF THIS STUDY
The assessment of methodological choices and the transparency of methods when assessing the greenhouse gas (GHG) emissions of entire sectors in systematic reviews can help deepen our understanding of the results.
The systematic review of all available evidence on GHG emissions of and within healthcare can help to understand its impact and to identify reduction potentials.
This review was limited to articles in English and German, and excluded assessments, grey literature from public reports, and reports from statistical offices published in other languages.
Introduction
Climate change is one of the most pressing issues of our time. 1 Considering the correlation between the gross domestic product and carbon emissions, 2 the healthcare industry is likely an essential contributor to greenhouse gas (GHG) emissions. Demographic shifts and income effects have likely spurred greater demand for healthcare services, a trend projected to persist and further elevate the economic significance of the healthcare industry. 3 Evidence on healthcare’s GHG emissions is needed to understand its role better.
Methods for calculating a carbon footprint (CF) can be broadly categorised into bottom-up and top-down approaches. Bottom-up methods, such as process-based lifecycle assessments, require extensive data, which currently limits their application at a sectoral level. However, the CF of various sectors can be estimated using a more uncertain top-down methodology, providing a trade-off for broader coverage. In this case, emissions are divided according to the final demand or economic sectors of emission occurrence.
Input–output (I–O) analysis, which follows this approach, can be used to estimate sectoral CF. 4 Calculations of the CF use the static open-quantity I–O model in combination with an environmental extension. They rely on two fundamental building blocks: an I–O table and a demand vector. The I–O table describes the interactions between the sectors of production, often in monetary terms, and are usually constructed by national statistics offices. With additional information on their environmental impact, the emission intensity of a sector and its upstream production processes can be calculated. The demand vector represents the expenditures of the relevant sectors. For example, the demand vector of the healthcare sector includes expenditure on diesel fuel to power ambulances, electricity consumed by hospitals, and all other forms of energy. It may be necessary to synchronise the structures of the I–O table and the demand vector by balancing the definitions of different sectors and adjusting the level of sectoral aggregation.
I–O models can be grouped into single-region I–O (SRIO) and multi-region I–O (MRIO) models. SRIO models use I–O data from a single country, thus restricting their scope to domestic production and emissions only. MRIO models connect multiple I–O tables from multiple countries, and can thus account for different levels of production and ‘trade’ in emissions (ie, emissions occurring in one country related to the final demand of another country). The need for synchronised data from multiple countries complicates the development and update of the data of MRIO models.
The results of CF calculations for a specific sector can be influenced by methodological choices, including the selection between SRIO or MRIO models and the GHGs taken into account. Therefore, comprehensive reporting is needed to ensure the transparency of methodological choices, the data and the results. However, our search of the literature yielded neither a standardised procedure nor standardised reporting.
The aim of this study is to conduct a systematic review of research using I–O analysis to quantify the CF of systems, encompassing total CF, CF per capita, and its proportion relative to the national CF. Furthermore, data on emission trends over time, can deepen the understanding of the trajectory of the CF of healthcare systems. Finally, an assessment of the methodological choices and their transparency within the reviewed studies can help to discuss the state of the methodology and provides a foundation to discuss methodological differences between the studies.
Search strategy and selection criteria
This systematic review was performed by following the Preferred Reporting Items for Systematic Reviews and Meta-Analyses guidelines 5 (the checklist is provided in online supplemental file 1 ). The databases PubMed, Web of Science, EconBiz, Scopus and Google Scholar were searched for studies on 25 November 2019. The full search strategy is provided in online supplemental file 2 . The search was complemented by reference tracking within all the included studies. The updated search considered hits in PubMed and Web of Science up to December 2023.
Supplemental material
Following the screening of the titles and abstracts, studies were included for further investigation if they had (1) addressed the method of CF calculation, (2) addressed one or more healthcare systems or subsystems and (3) been written in English or German. A healthcare system was defined as the national healthcare system, federal system and/or state system. Single entities, such as individual hospitals, and specialised branches, such as dentistry, were excluded. In addition to the criteria used for screening the titles and abstracts of articles, full-text articles were excluded if they (1) did not name the specific healthcare (sub)system, (2) did not calculate the CF or (3) did not provide any information on the method of calculation used. In the initial search, two of the authors separately screened titles and abstracts, read the full text, extracted data and assessed the transparency. In the case of disagreement, decisions were made through discussion until a consensus was reached. During the search update these steps were conducted by one person.
Data extraction and analysis
The CF per capita, the contribution of healthcare to the country’s total CF emissions, and the origins of emissions were used as main results of the studies. The breakdown of the emission sources could be in scopes, demand categories or places of origin. The Greenhouse Gas Protocol Corporate Accounting and Reporting Standard 6 proposes three standardised scopes. Scope 1 represents direct emissions from owned or controlled sources, scope 2 represents indirect emissions generated by the purchased energy and scope 3 represents all indirect emissions that occur in the value chain. The categories of demand included the classes of expenditures of the demand vector, and the places of the origin of emissions were divided into hospitals, ambulatory services and so on.
In addition to evaluating their general characteristics and results, we developed and applied a checklist to assess the methodological transparency of the studies under consideration. We opted to use the term ‘transparency’ rather than ‘quality’ to address the issue that even a flawless study could receive a low score if the authors failed to adequately report their methodology. The checklist served as both a qualitative extraction tool and a quantitative transparency tool. The qualitative extraction tool facilitated the assessment of information from each included study, with responses to each criterion collected accordingly. As a quantitative transparency tool, it was evaluated whether the criteria were adequately addressed. When information was provided, the criterion was considered fulfilled, resulting in an increase in the transparency score. All criteria were weighted equally, therefore for each ‘fulfilled’ criterion one point was added to the transparency score, with a maximum of 17 points per study.
The utilisation of I–O data can introduce uncertainties into the assessment, given that the top-down approach relies on aggregated information from industrial sectors. When heterogeneous products with varying emission intensities are grouped into one industry, aggregation errors might occur: the average emission intensity of the aggregated industry would not appropriately reflect the emissions caused by the specific product within the industry. 7 Therefore, information on the extend of usage of I–O method (criterion 5), and the number of industry sectors (criterion 12) could help to understand the scope of this uncertainty.
The choice between MRIO and SRIO (criterion 11) can also help to understand the level of uncertainty. While MRIOs can account for differences between countries and trade between these countries, SRIO might provide a more detailed framework of the domestic economy. Finally, the specific source of the I–O tables (criterion 9) and emission data (criterion 13) can help the reader to assess the quality of the used data.
Similar to the I–O data, the level of aggregation within the demand data can impact the accuracy of the results. The number of demand or expenditure categories (criterion 8) can indicate on the level of aggregation and the source of demand data (criterion 6) could help to assess the quality of the data source. The quality of the outcomes is also influenced by the alignment between the temporal representativeness of the demand data (criterion 7) and the I–O data (criterion 10). Changes over time (eg, in technology, import and exports) can impact the results and in the best case both data sources refer to the same year. Finally, information on the matching process of demand categories and industry sectors, the publication of the concordance matrix (criterion 15), increases transparency for the reader.
The quantitative (criterion 16) and qualitative (criterion 17) assessment of uncertainty helps the readers to contextualise the results. A list of the included GHGs can indicate the scope of the study, in this case 0.5 were given, when the unit (typically CO 2 equivalents (CO 2 eq)) was mentioned and another 0.5 points if all included GHGs were listed. For the final transparency checklist, the criteria on outcomes ( table 1A ) and on methodology ( table 1B ) were combined. A more detailed description of the transparency criteria are provided in online supplemental file 3 .
- View inline
(A) Extracted outcomes. (B) Extracted methodological items
Emissions over time
To assess trends in GHG emissions of healthcare, data from all studies that reported total emissions for more than 1 year were taken. The data were normalised to the respective starting point of the report as a base year. Therefore, GHG emissions of time period t were divided by the GHG emissions of the base year t 0 and used in a descriptive analysis.
Patient and public involvement
A total of 4285 records were identified in the three searches ( figure 1 ). After removing duplicates and searching for eligible title, abstracts and full texts, 15 reports were included in this review ( figure 1 ). A summary of included studies is provided in table 2 . The detailed results of the data collection are listed in online supplemental files 4 and 5 .
- Download figure
- Open in new tab
- Download powerpoint
Preferred Reporting Items for Systematic Reviews and Meta-Analyses flow diagram, based on Page et al . 5
Characteristics and main results of the studies considered in this review
Characteristics of the studies considered
Eleven studies focused on a single national healthcare system, including England, 8 9 Japan, 10 USA, 11 12 Canada, 13 Scotland, 14 China, 15 Australia, 16 Austria 17 and the Netherlands. 18 The series of CFs from the Sustainable Development Unit of the English NHS was aggregated, and only the newest available report was cited. One study examined the healthcare system of the largest Australian state, New South Wales, 19 while three studies reported on healthcare systems in multiple countries. Pichler et al 20 reported results for 36 countries, Healthcare without Harm for 43 countries, 21 and the investigation by Lenzen et al 22 considered 189 countries.
Excluding the one that assessed the Scottish NHS, all studies were published after 2016. However, it is worth noting that the year of the analysis could be older. For instance, the study by Nansai et al 10 was published in 2020 but used demand data from 2011.
Differences in methodology and data
Eleven studies considered top-down data on emissions, while three studies employed bottom-up data on energy usage. 8 9 14 Steenmeijer et al 18 incorporated bottom-up data regarding the quantities of anaesthetic gases, inhalers and travel.
Most single-country studies used SRIO data from the respective governmental offices. In contrast, the studies on British and Dutch healthcare, and those that considered more than one country, used MRIO data. Additionally, Malik et al 23 used MRIO data, however, the database only included data from Australian regions. The EORA database emerged as the most frequently used MRIO database (three times), with one study each employing the WIOD database, the EXIOBASE database and the MRIO database provided by the British Department for the Environment, Food, and Rural Affairs.
The number of production sectors varied among the SRIO studies, ranging from 46 to 405 sectors. The MRIO studies typically used more extensive databases comprising approximately 15 000 sectors, although the MRIO study focusing on the UK considered 424 sectors.
All studies considered CO 2 emissions. However, only five studies considered the six GHGs covered in the Kyoto Protocol; three studies considered CO 2 , methane and nitrous oxide; two reported only that they had used CO 2 eq as unit; and two studies did not report any included GHG or the unit in which the outcomes were reported. The data on emissions were drawn mostly from national accounts in the case of SRIO databases and integrated accounts in the case of MRIO databases. One study did not report the source of its emission account data.
The demand data was taken either from official health expenditure accounts or from international organisations such as the WHO and the World Bank (which uses data provided by national offices and accounts). Lenzen et al 22 identified and directly used data on healthcare-related sectors from the MRIO database EORA. The number of reported expenditure accounts varied, mostly ranging from 13 to 19, although three studies reported fewer accounts. Weisz et al 17 used nine accounts, Wu 15 used eight accounts, and the study on the NHS in England employed five accounts. 9 Due to the distinct methodologies employed by Lenzen et al 22 and the structure of the EORA database, which reports country-specific sectors, they used 163 sectors from the EORA as demand data.
The time periods covered by the demand data were largely consistent with those covered by the respective I–O data. Some studies reporting outcomes for more than 1 year only used one reference year for the I–O database and adjusted the demand data for inflation. 11–13 The lag between the time at which the data were collected and the time of publication of the corresponding study ranged from 3 to 6 years, with deviations in the studies by Nansai et al , 10 Eckelman et al 12 (2 years) and in the report by the SDU. 9 The latter reported the CF periodically; the lag between the latest publication and the latest data was 1 year. 9 Further information on this is provided in online supplemental file 5 .
Five studies provided their concordance matrices, which link the categories of demand with the industrial sectors. The authors of one study had made their matrix available on request, and two articles had referred to a matrix previously used in another study. Five studies did not report their concordance matrices.
Reporting of the results
The origins of emissions were documented six times in the three scopes defined by the GHG protocol. Emission sources were reported eight times in the (sub)categories of final demand, such as hospitals or pharmaceuticals. Two studies reported the economic sector in which the emissions occurred, for example, the textile sector or the manufacture of fuels. Furthermore, three studies reported a breakdown of emissions by employing more than one reporting structure. Several differences were observed in the scopes of the reported results. Some studies directly referenced the GHG protocol while others reported emissions in divisions, such as travel, energy, procurement, etc. 47% of the articles did not normalise the results by reporting the CF per capita.
Overall transparency
Except for the three criteria ‘reporting of the concordance matrix’, ‘uncertainty analysis’ and ‘CF per capita’, all criteria were fulfilled by at least 75% of the studies ( figure 2 ). The studies fulfilled between 70.5% and 94% of all criteria with a mean of 85% ( figure 3 ). The full transparency assessment is provided in online supplemental file 6 .
Fulfilment rate of the transparency and reporting criteria.
Transparency score in percentage per article.
The results of the time series revealed successful efforts to mitigate the CF by the NHS in England and Scotland ( figure 4 ). In the nearly three decades from 1990 to 2019, the English NHS reduced its CF by roughly 25%. The four remaining countries (Japan, Canada, USA and Australia) examined in the studies considered here and the global trend showed increased CF due to healthcare ( figure 4 ). The annual increase in the CF ranged from 0.7% (USA, 2010–2018) to 3.8% (Japan, 2011–2015) over the observed period, with the CFs of Canada (1.9%, 2009–2015), USA (2.8%, 2011–2015) and Australia (2.9%, 2013–2015) in between these extremes. The global trend showed an increase in the CF of 2.7% per year from 2000 to 2015.
Emission trends over time. CF, carbon footprint.
The emission sources were mainly reported using the scope system from the GHG protocol or the categories of expenditure, that is, the categories of final demand. The largest dataset that used the categories of final demand was provided by Pichler et al , 20 who applied this to 36 countries and reported the average values. Medical retail (ie, provider of healthcare products without medical services, eg, pharmacies), hospitals and ambulatory healthcare services constituted 80% of the CF of healthcare, with medical retail contributing 33.1%, hospitals 28.6% and ambulatory healthcare services 18%. They also made a major contribution to the CF in Japan (hospitals, 25.1%; ambulatory services, 22.7%), USA in 2013 (hospital care, 36%; physician and clinical services, 12%) 11 and in 2018 (hospital care, 34.9%; physician and clinical services, 12.6%; ambulatory medical services, 4.8%), 13 Australia (public hospitals, 34.4%; private hospitals, 10.2%; ambulatory medical services, 15%), 16 China (public hospitals, 47%; private hospitals, 4%) 15 and Austria (hospitals, 32%; ambulatory services, 18%). 17 Other important categories of emissions were construction and pharmaceutical products, at around 10%, 11 16 20 with a higher share in China (pharmaceuticals, 18%; construction, 15%). 15
An alternative approach involved categorising emissions into direct emissions, indirect emissions through electricity production, and other indirect emissions. This division along these lines could also align with the three GHG protocol scopes.
By averaging data from 43 countries, HCWH reported a distribution of 17% for scope 1 emissions, 12% for scope 2 emissions and 71% for scope 3 emissions. 21 These findings, particularly the significance of scope 3 emissions, are corroborated by evidence from single-country studies. 8 11 12 14 24 The scope 3 emissions were further divided into those due to travel (patient and visitor travel, and staff commutes), production of pharmaceuticals, and medical instruments and equipment, which accounted for the largest share of scope 3 emissions.
Scotland’s scope 3 travel emissions in 2004 were 18% while those of England accounted for 13% in 2015 and 9.6% in 2018. 9 The share of emissions owing to pharmaceutical production ranged from 11% to 18%, and that owing to medical instruments and equipment accounted for 7%–10% of the total CF. 13 14 24
The ratio of emissions by the healthcare sector to the total CF in studies focused on a single country ranged from 2.7% in China in 2012 15 to 9.8% in the USA in 2013. 11 The three cross-national studies considered here estimated that healthcare had contributed 5.5% 20 on average to the national CF in 2014 and 4.4% in 2015. 22
Interpretation of results
The results indicate that healthcare significantly contributes to the CF, both in absolute numbers and in relation to a country’s overall emissions and its per capita emissions. However, the results varied among the studies, and their calculation methods were heterogeneous and frequently not fully transparent. The breakdown of the sources of emissions revealed the major contribution made by hospitals.
The time series results showed that the trend of emissions due to healthcare was positive in all the countries considered, that is, they were increasing, except in Scotland and England. These results align with the graphical results provided by Lenzen et al . 22 Furthermore, they indicated that the efforts of the British NHS systems to reduce their CF based on the Greener NHS programme was effective in reducing GHG emissions. The breakdown of the sources of emissions verified the important contribution of hospitals. However, hospitals provide the majority of medical care in many countries. Therefore, their large CF is not surprising but might motivate the relevant decision-makers to allocate scarce resources more efficiently. The breakdown further showed that a large portion of the CF of healthcare stemmed from scope 3 emissions. Decision-makers may conclude that the most considerable reduction in emissions can be obtained by considering staff and patient travel. Therefore, ‘greening’ the healthcare sector requires a sustainable transportation system and green healthcare goods.
Most data were from the Organisation for Economic Co-operation and Development (OECD) countries, China and India. The only exception was the work by Lenzen et al , 22 who considered 189 countries in their analysis. 22 However, even if the distribution of countries limits the representativeness of the results, the findings are consistent with the fact that OECD countries are the main emitters of GHGs.
While heterogeneity in methodology, in general, can lead to more robust results and a more informative perspective on the issue at hand, the differences in I–O methodologies to calculate the CF of healthcare may reduce the comparability of the results. However, the choice of method depends on the corresponding research question, for example, while SRIO may be more up-to date and include a more detailed description of the domestic production sectors, MRIO can account for international trade and differences in production emissions between countries.
Limitations
This review has several limitations. First, the review process used here was limited due to restrictions on the language used in the study and those related to access. Second, it is possible that further CF assessments exist which were published in the official languages of many countries in the grey literature, such as publications by national statistics offices or governmental agencies. Because this review included only publications in English and German, many such studies have likely been neglected. Third, the reporting scheme and transparency score used in this study may have limitations. Both were based only on a consensus among the authors. The instruments used to assess the quality of the published studies are typically chosen based on a broad consensus among experts, such as in the case of the Consolidated Health Economic Evaluation Reporting Standards. 25 However, we did not find similar guidance for I–O analyses. Finally, the review is limited as the studies only report averages instead of CIs or data ranges. Only Malik et al 16 report the 68% CI with a range of 20 748 kt CO 2 eq in the results (68% CI 25 398 kt CO 2 eq to 46 146 kt CO 2 eq). Therefore, the results presented in both the individual studies and in this review should not be regarded as precise measurements, but rather as indicative trends or directions.
Implications for further research
This review identified research gaps that should be investigated by future research. First, there is a need to assess the potential effects of efforts to reduce emissions on the system and pathways to a low-carbon healthcare system. Second, it should be examined errors of aggregation when using the I–O methodology in the healthcare context. Third, the differences in the outcomes when making different methodological choices (SRIO or MRIO, systemic boundaries, etc) should be analysed to guide future research.
The transparency checklist used in this study can serve as an initial reference point for future developments. For example, in the checklist’s current state, all criteria are weighted equally. However, some might be less crucial to delivering harmonised study findings. An extended consensus process with further experts is proposed to validate the checklist further and increase its value for research and practice.
Ethics statements
Patient consent for publication.
Not applicable.
Ethics approval
Acknowledgments.
We would like to thank Frauke Waßmuth for her help in the screening and extracting phase of the search update.
- Kronenberg T
- Wiedmann T ,
- Wood R , et al
- McKenzie JE ,
- Bossuyt PM , et al
- WBCSD and WRI
- Heijungs R ,
- Tennison I ,
- Roschnik S ,
- Ashby B , et al
- Malik A , et al
- Eckelman MJ ,
- Lagasse R , et al
- Sherman JD ,
- MacNeill AJ
- Health Facilities Scotland
- McAlister S , et al
- Pichler P-P ,
- Jaccard IS , et al
- Steenmeijer MA ,
- Rodrigues JFD ,
- Zijp MC , et al
- Carter S , et al
- Jaccard IS ,
- Weisz U , et al
- Karliner J ,
- Slotterback S ,
- Boyd R , et al
- Li M , et al
- du Plessis M , et al
- Husereau D ,
- Drummond M ,
- Augustovski F , et al
Supplementary materials
Supplementary data.
This web only file has been produced by the BMJ Publishing Group from an electronic file supplied by the author(s) and has not been edited for content.
- Data supplement 1
- Data supplement 2
- Data supplement 3
- Data supplement 4
- Data supplement 5
- Data supplement 6
X @MattisKeil
Contributors MKeil (guarantor): methodology, screening, formal analysis, writing – original draft, writing – review and editing, visualisation; LF, MH, MKnieß: methodology, screening, formal analysis, writing – original draft; OL: conceptualisation, methodology, writing – review and editing; TK: methodology, writing – review and editing. WR: conceptualising, methodology, writing – review and editing, supervision, project administration. All authors have read and approved the final manuscript for publication.
Funding This work was supported by the Leibniz ScienceCampus Bremen Digital Public Health (lsc-diph.de), which is jointly funded by the Leibniz Association (W4/2018), the Federal State of Bremen, and the Leibniz Institute for Prevention Research and Epidemiology (BIPS) for OL’s inputs to the research project. The funder had no role in the study design, the collection, analysis, interpretation or submission of the data. MKeil’s inputs were supported by the research cluster 'Health City Bremen' which is funded by the Federal State of Bremen. The funder had no role in the study design, the collection, analysis, interpretation or submission of the data.
Competing interests None declared.
Patient and public involvement Patients and/or the public were not involved in the design, or conduct, or reporting, or dissemination plans of this research.
Provenance and peer review Not commissioned; externally peer reviewed.
Supplemental material This content has been supplied by the author(s). It has not been vetted by BMJ Publishing Group Limited (BMJ) and may not have been peer-reviewed. Any opinions or recommendations discussed are solely those of the author(s) and are not endorsed by BMJ. BMJ disclaims all liability and responsibility arising from any reliance placed on the content. Where the content includes any translated material, BMJ does not warrant the accuracy and reliability of the translations (including but not limited to local regulations, clinical guidelines, terminology, drug names and drug dosages), and is not responsible for any error and/or omissions arising from translation and adaptation or otherwise.
Read the full text or download the PDF:
Advances, Systems and Applications
- Open access
- Published: 02 May 2024
Enhancing patient healthcare with mobile edge computing and 5G: challenges and solutions for secure online health tools
- Yazeed Yasin Ghadi 1 ,
- Syed Faisal Abbas Shah 2 ,
- Tehseen Mazhar 2 ,
- Tariq Shahzad 3 ,
- Khmaies Ouahada 3 &
- Habib Hamam 3 , 4 , 5 , 6
Journal of Cloud Computing volume 13 , Article number: 93 ( 2024 ) Cite this article
Metrics details
Patient-focused healthcare applications are important to patients because they offer a range of advantages that add value and improve the overall healthcare experience. The 5G networks, along with Mobile Edge Computing (MEC), can greatly transform healthcare applications, which in turn improves patient care. MEC plays an important role in the healthcare of patients by bringing computing resources to the edge of the network. It becomes part of an IoT system within healthcare that brings data closer to the core, speeds up decision-making, lowers latency, and improves the overall quality of care. While the usage of MEC and 5G networks is beneficial for healthcare purposes, there are some issues and difficulties that should be solved for the efficient introduction of this technological pair into healthcare. One of the critical issues that blockchain technology can help to overcome is the challenge faced by MEC in realizing the most potential applications involving IoT medical devices. This article presents a comprehensive literature review on IoT-based healthcare devices, which provide real-time solutions to patients, and discusses some major contributions made by MEC and 5G in the healthcare industry. The paper also discusses some of the limitations that 5G and MEC networks have in the IoT medical devices area, especially in the field of decentralized computing solutions. For this reason, the readership intended for this article is not only researchers but also graduate students.
Introduction
The IoT or the Internet of Things has introduced a fresh concept which is using wearable devices to upgrade healthcare services [ 1 ]. Involving data communication with the environment using interrelated physical objects and sensors, IoT constitutes a complex system [ 2 ]. Not only does IoT, but also 5G technology contributed significantly to the development of cooperation-based health technology solutions. The use of remote sensing in healthcare is vast and well-established from numerous case studies and practical implementations [ 3 ].
The innovation of healthcare IoT devices, such as the ability to monitor and record patients’ medical data that including electrocardiograms (ECG), blood pressure, and pulse measurements is an area at the forefront. Apart from these internal measurements, they also take into account external indicators of health which are humidity and temperature using sensors attached to patients; the collected data are then transmitted to health centres for further analysis, including telemedicine [ 4 ].
The Internet of Medical Things (IoMT) is made up of an assortment of devices such as mobiles, sensors, and wearables all developed to extract data from patients continuously across different environments [ 5 ]. Significantly, a slight delay in transmitting information can be fatal to a patient’s life. The combination of 5G technology and cloud computing is very effective in meeting latency criteria in healthcare applications [ 6 ].
An up-to-date innovation in the field of cloud computing is the introduction of Mobile Edge Computing (MEC), which redistributes computing resources from large-scale data centres to the edge nodes in a network [ 7 ]. MEC is important for mobile healthcare devices as it allows easy access to computation power by the users themselves. In this context, it consolidates all these mobile devices, applications, and data produced within healthcare environments, enabling nearly instantaneous processing at the source level [ 8 ].
In support of healthcare devices needing uninterrupted and reliable low-latency communications (URLLC), the cooperative utilization of 5G and MEC technologies is a way to go [ 9 ]. They ensure quick and flawless transmission of information between radio network services. Even though Medical IoT devices have limited computational power, MEC architecture can handle data processing and analysis at the edge of a network avoiding central server routing [ 10 ]. Such an approach offers several advantages in terms of time efficiency, cost minimization, and operational effectiveness [ 11 ]. However, data collection within healthcare in general and patient data, in particular, has been noted to contribute useful analysis by fusing details from different points thus obtaining a holistic picture of a patient’s health status [ 12 , 13 ]. Meanwhile, there are various challenges that MEC and 5G technologies must address so that healthcare systems can incorporate these technologies for the safety of patients [ 14 ]. Considering the sensitivity of health information and how it can put patients at risk if not carefully managed as well as infringe their privacy rights, security mechanisms must be embedded in MEC and IoT devices [ 15 ].
The contributions of this article are outlined as follows:
IoT devices enhance remote patient care, empowering physicians to monitor patient health and manage treatment plans remotely.
MEC and 5G networks augment patient privacy by localizing the processing of sensitive medical data, thereby reducing the exposure of raw data to public networks.
MEC optimizes the functioning of medical devices by offloading tasks to edge servers, which in turn improves resource utilization and treatment efficacy.
A significant challenge lies in securing sensitive medical information and maintaining its privacy at the network edge.
While blockchain has been noted for its potential in healthcare, particularly concerning security, the primary focus of this research is to elucidate the transformative impact of IoT-based healthcare devices integrated with MEC and the role of 5G technology in addressing security and privacy concerns.
The remaining article is structured as follows: Chap. 2 presents a concise literature review that acquaints readers with the historical context of IoT and MEC technology, including the emergence of 5G. Chapter 3 delineates the detailed methodology and research questions. Section 4 discusses the results and provides comprehensive responses to each question. Finally, Sect. 5 draws conclusions and suggests directions for future research.
Literature review
The Literature Review provides an in-depth examination of data offloading methods, MEC, and 5G networks, as they pertain to healthcare. Research has indicated that while mobile medical data can be transferred to the cloud for processing, analysis, and storage, the latency involved in transmitting data to remote clouds remains a concern, alongside the ongoing challenge of maintaining patient privacy [ 16 ]. These issues underscore the importance of developing more efficient and secure methods of data handling within the healthcare sector.
Advancements in mobile edge technology, as described in [ 17 ], have been shown to bring computing power closer to the user, thus improving the accessibility of information within the healthcare system. This paradigm shift towards edge computing is indicative of a broader trend of decentralization in data processing, emphasizing the proximity of computational resources to end-users.
The integration of MEC with healthcare devices has been shown to potentially revolutionize the field, as it allows for real-time data processing and analytics at the edge of the network [ 18 ]. By harnessing the power of MEC, healthcare applications can fully utilize the strengths of 5G by leveraging edge intelligence, network slicing, and distributed storage, which are pivotal for the next generation of healthcare systems [ 19 ].
Detailed examinations have been conducted on how edge and cloud computing can synergize to address operational challenges, with a special focus on optimization methods that address objectives and constraints of end-to-end application processing [ 20 ]. Security remains a paramount concern in the transmission of health data, and efforts have been made to enhance the security protocols and encryption methods to protect sensitive information [ 21 ].
Recent case studies and experiments have explored the implementation of MEC in various healthcare settings, with findings suggesting improvements in patient privacy and data latency reduction [ 22 ]. Additionally, the impact of 5G and MEC on telemedicine services has been examined, highlighting the benefits of improved remote patient monitoring and data security, though challenges related to network coverage and connectivity remain, particularly in rural and remote areas [ 23 ], as well as some technical issues [ 24 ].
It results that the current literature underscores the transformative potential of MEC and 5G in healthcare, pointing to improved efficiency, enhanced patient privacy, and increased reliability of healthcare services. However, there are still obstacles to overcome, including issues related to scalability, interoperability, and the consistent coverage of 5G networks. These challenges offer fertile ground for future research and innovation. Table 1 describes the existing literature review and key contributions and Figure 1 illustrates the IoT-based healthcare applications.
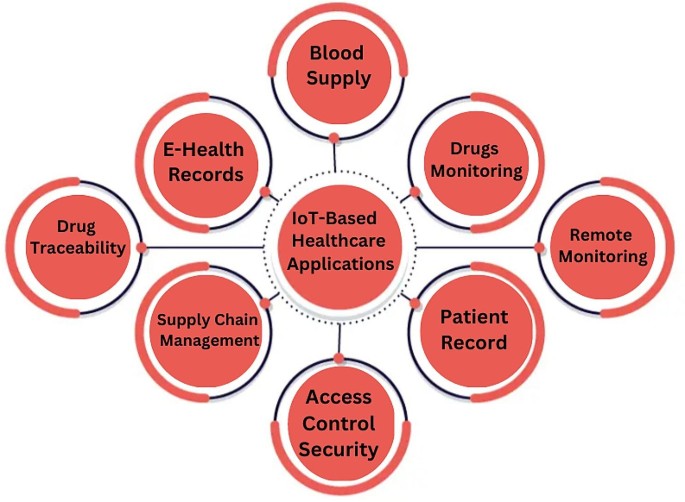
IoT-based healthcare applications
Figure 1 illustrates various IoT-based healthcare applications, demonstrating the diverse applications of these technologies in real-world healthcare settings.
Methods and techniques
The primary objective of this work is to construct a Systematic Literature Review (SLR) to identify, analyze, and gather real-world evidence on the usage of MEC in the healthcare sector and its integration with 5G networks to enhance patient care services. This study examines the healthcare sector’s advancements, particularly focusing on the contributions of MEC and 5G technologies, and how they facilitate IoT devices in healthcare systems. Additionally, it investigates the challenges faced by MEC systems and the potential solutions within the context of these technologies. The systematic review process is guided by targeted research questions, which are formulated to direct the investigation. Figure 2 illustrates the planning process of the methodology, and the specific research questions are delineated in Table 2 .
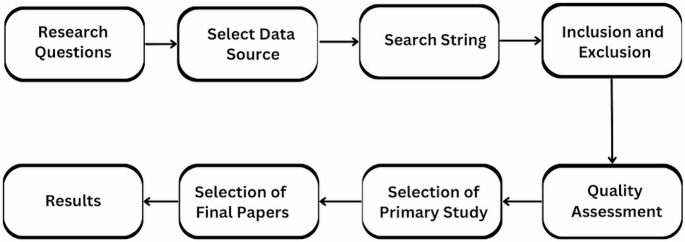
Proposed SLR
The research questions of Table 2 are designed to capture the breadth and depth of the MEC and 5G application in healthcare, ensuring that the review process is comprehensive and informative. The results of this SLR will contribute to a clearer understanding of the current state of MEC and 5G technologies in healthcare and will outline the avenues for future research and development.
Select data sources
The library or repository that serves as the source for search results is called a source. The following digital libraries were selected to extract key measurements, ACM Digital Library, Springer Digital Library, Science Direct, and IEEE Explore. To access previous studies, the full text of the literature should be searched. There are many research methods when searching for relevant information in any digital library. To find relevant information, it is necessary to change the search method to meet the requirements of the resources. Table 3 ; Fig. 3 show the illustration of the various data sources and the number of studies generated by the search queries.
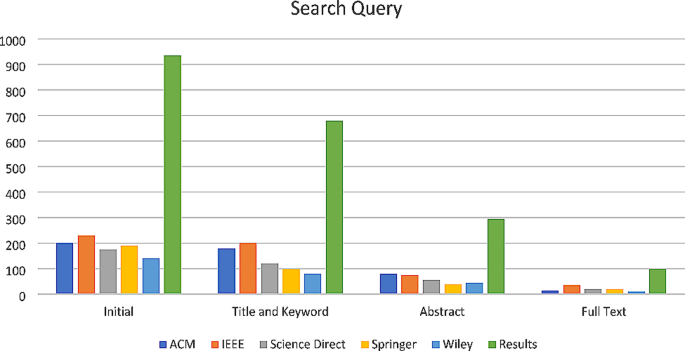
Search queries representation
Formulate search string
A search string is a carefully constructed set of keywords and search terms to find relevant information that answers the research question being examined. At this stage, search strings are created by focusing on specific terms and keywords and their definitions selected among the research questions, as shown in Table 4 . To complete the following search string, these keywords are combined using the “AND” and “OR” conditions in the order specified. Figure 4 respectively terms that affect the creation of search strings.
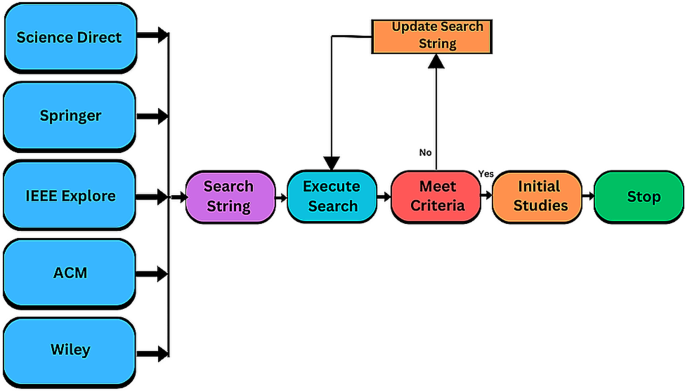
The process of formulating the search string
Define inclusion and exclusion criteria
Inclusion criteria are set to determine which studies are to be incorporated into the literature review. For this review, the following criteria have been established: All research must be published in English between 2017 and 2023. The primary focus is on the integration of MEC with 5G in the healthcare sector. To be included, a study must involve empirical research that addresses MEC, 5G, and their integration and application within healthcare. Research should also concentrate on the challenges related to MEC and 5G in the context of IoT in healthcare and their potential solutions. Only articles from reputable journals, books, or conferences are considered. Studies that do not meet these criteria are excluded. The exclusion criteria disqualify studies published before 2017 and those where the focus on MEC and 5G in the context of healthcare is of minor concern. Research lacking empirical analysis is also excluded.
Define quality assessment criteria
In systematic reviews and meta-analyses (SLRs), qualitative methods are used to assess the validity, reliability, and quality of the studies included. By applying these quality control methods, we ensure that the selected primary studies provide comprehensive information relevant to our research questions. Each study is evaluated against a set standard of quality control, which is detailed in Table 5 .
Primary study selection
The term “primary study” is used to describe specific topics covered in a book that examines a research question. The tollgate approach is a five-stage structured process that has been used to select previous studies for this review. Using this method, 100 original studies were carefully selected according to quality criteria. Figure 5 ; Table 6 represent the main works, and Fig. 6 shows the whole process in the diagram.
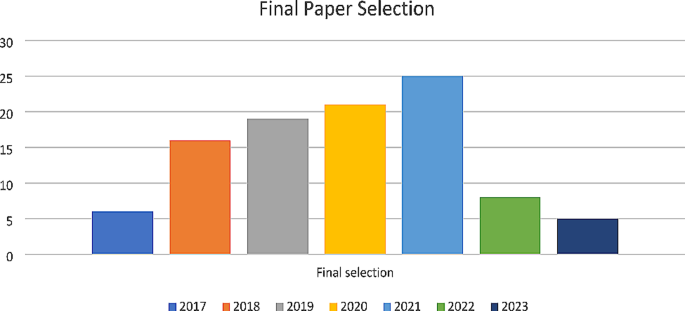
Final paper selection
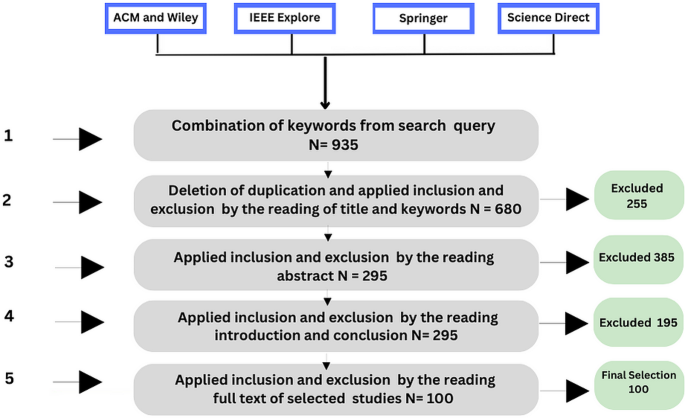
Prism diagram
Results and discussions
How do the mec and 5g network facilitate iot-based healthcare applications.
Healthcare applications using IoT can play an important role in monitoring and implementing various solutions. IoT devices can instantly collect patient data such as heart rate, blood pressure, and blood pressure [ 32 ]. Health apps can analyze this information and alert doctors or patients to any abnormalities and patterns that require attention. Patients with chronic diseases such as diabetes can benefit from IoT devices that constantly monitor their health. These applications can collect this information, analyze trends, and provide personalized insights and recommendations for better management [ 33 ]. IoT sensors can monitor the location and status of medical equipment to ensure efficient use and timely maintenance. IoT sensors can monitor temperature-sensitive drugs, vaccines, and blood products during storage and transportation [ 34 ]. Medical practices can instantly monitor temperature data and alert staff if temperatures fall outside acceptable ranges to keep things stable.
IoT-enabled chronic disease management helps patients with long-term health conditions such as diabetes, hypertension, and asthma track symptoms, keep their health metrics clean, and manage their business better [ 35 ]. These solutions include connected blood glucose monitors, blood pressure monitors, and spirometers, as well as mobile apps for self-management and remote monitoring. IoT-based chronic disease management can lead to better outcomes and lower healthcare costs by allowing patients to play an active role in health management [ 36 ].
Remote Patient Monitoring (RPM) solutions leverage IoT devices to remotely monitor patient vital signs, medication compliance, and other health metrics. These devices include wearable sensors, smart home monitoring systems, mobile health apps, and more [ 37 ]. Tracking patient health data in real-time allows healthcare providers to monitor patient conditions and intervene quickly if needed, leading to improved patient outcomes and reduced readmissions. The telemedicine platform uses IoT devices such as cameras, meters, and digital sensors to provide virtual consultations between patients and doctors [ 38 ]. These platforms facilitate remote diagnosis, treatment, and follow-up care, especially for rural or underserved patients. By integrating IoT sensors with telemedicine platforms, doctors can collect more information during virtual consultations, thereby improving the accuracy of diagnosis and quality of treatment [ 38 ].
IoT-based medication management helps patients adhere to medication regimens by providing alerts, dispensing medications at scheduled times, and monitoring compliance levels. These systems may include smart pill dispensers, prescription dispensers, and connected bottles. By tracking patients’ medication use in real time, doctors can detect adherence issues early and intervene to prevent medication errors or complications [ 39 ]. IoT-based asset tracking and management systems can help hospitals track the location and real-time monitoring of medical equipment, supplies, and personnel [ 40 ].
IoT sensors can also be used to monitor environmental factors such as air quality, humidity, and noise in healthcare facilities, and the environment where healthcare applications can reliably analyze this data is good for patient comfort and safety. IoT tags on surgical instruments can track their use, sterilization, and location in the hospital. Medical practices can reduce the risk of delays and errors in surgery by providing quick information on available equipment [ 41 ].
5G technology in wireless communication is the new generation of mobile phones. Among all existing mobile networks, 5G provides a high-speed network to everyone, anytime, anywhere. 5G is a bit different because of its new capabilities to connect people, and manage devices, equipment, and technology [ 42 ]. The category of 5G technology Enhance mobile broadband (eMBB) is a key application for 5G systems, which use massive multiple input, multiple output (MIMO) antennas, millimeter wave, and beamforming technology to deliver ultra-high-speed connectivity over large areas.
URLLC is another category of 5G networks that provides low latency, ultra-high reliability, and rich quality of service (QoS) that cannot be achieved with traditional mobile network architecture. URLLC is designed for on-demand real-time interaction such as remote surgery, vehicle-to-vehicle (V2V) communication, industry 4.0, smart grids, and intelligent transport systems. URLLC is designed for real-time needs such as remote surgery, vehicle-to-vehicle (V2V) connectivity, Industry 4.0, smart grids, and transportation systems. Another category of 5G network is Massive Machine Type Communications (MMTC) which enables long-range communications at very cost-effective and low power consumption.
MMTC provides high data services, low power consumption, and connectivity to IoT applications at lower costs for mobile users [ 43 ]. MMTC is used for high-speed connections between low-speed devices such as healthcare devices. MEC is an advanced version of cloud computing. It is designed to bring the power of cloud services closer to the user. When we think about IT, the first thing that comes to our mind is cloud computing, which is a well-known technology that offers many services to end users. MEC is the underlying technology that accommodates URLLC service latency. 5G can also rely on a variety of services that can be provided through network connections from different industries [ 44 ].
One of the key points of 5G is the network slicing that enables the work that needs to be done and resources are distributed using services to different services or tenants from the work available in the network. Network slicing creates multiple logical connections that meet specific needs. Network Slice Selection Function (NSSF) is a function that helps users select the appropriate network and deploy appropriate Access Management Functions (AMF). A MEC application located in the decentralized cloud of the MEC system can participate in one or more network connections established in the 5G core network [ 45 ].
To address the complexity of medical data transfer from patient wearable devices, 5G network slicing is becoming a catalyst for IoT innovation leading to new products and services in the medical field [ 46 ]. The combination of 5G and MEC can support applications and use cases that require reliable low-latency communications (URLLC). 5G wireless systems with MEC provide instant, low-latency, high-bandwidth access to radio network services. Medical IoT devices have limited computing power and cannot run demanding algorithms. In MEC architecture, the medical device offloads the epilepsy detection task to MEC servers [ 47 ].
Offloading to MEC servers is the process of moving some computing functions of mobile devices or endpoints to servers at the edge of the network [ 48 ]. Medical device outsourcing involves moving some computing functions or data processing from the medical device itself to other computing applications, such as MEC servers. This approach has many benefits regarding time efficiency, cost savings, and operational efficiency [ 49 ]. MEC servers use computing power at the edge of the network to collect and process data. Offloading can be used if the medical device has sufficient computing power to save device power and reduce task latency [ 50 ]. Energy saving is crucial for medical IoT devices that require long service life. Figure 7 shows the MEC architecture and its role in healthcare devices.
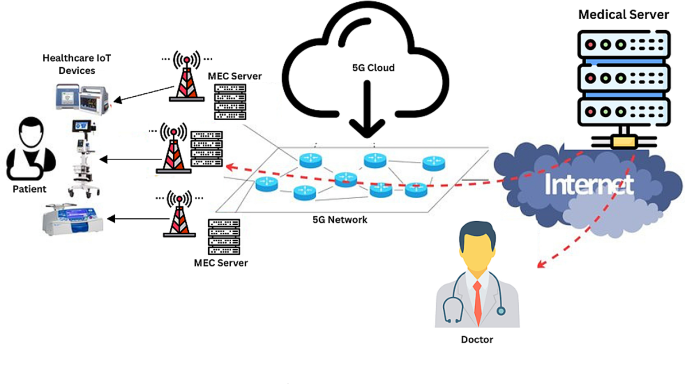
MEC architecture and healthcare devices
5G networks provide faster and more efficient data transfer. This means healthcare IoT devices can send and receive data quickly, allowing them to operate in a low-power state in less time [ 51 ]. MEC servers reduce data processing latencies by moving power consumption closer to the edge of the network. In healthcare applications, especially sensor-based applications, low latency is important for instant monitoring and decision-making [ 52 ]. MEC allows healthcare services to quickly process data without sending it remotely to cloud computing, resulting in faster response times. MEC servers enable local analysis and data collection. This reduces the risk of sending sensitive data long distances and provides greater control over the personal data of patients [ 19 , 53 ]. MEC servers usually have local storage capacity. MEC servers can store patient data directly on edge servers rather than relying on centralized cloud storage. This local storage provides rapid access to frequently requested patient information without the need to retrieve information from remote cloud servers [ 54 ].
What are the challenges in MEC and 5G networks in IoT Healthcare applications?
MEC and 5G networks present advanced potential to empower IoT healthcare applications [ 55 ]. Nonetheless, these technologies face several challenges that must be addressed to maximize their benefits in healthcare. Addressing these challenges will necessitate collaboration among technology developers, healthcare providers, regulators, and other stakeholders. Solutions may involve technological advancements, design improvements, and the establishment of best practices to ensure successful integration of MEC and 5G networks into IoT applications. Below are the key challenges identified, and Fig. 8 illustrates these challenges in the context of MEC and IoT-based healthcare applications.
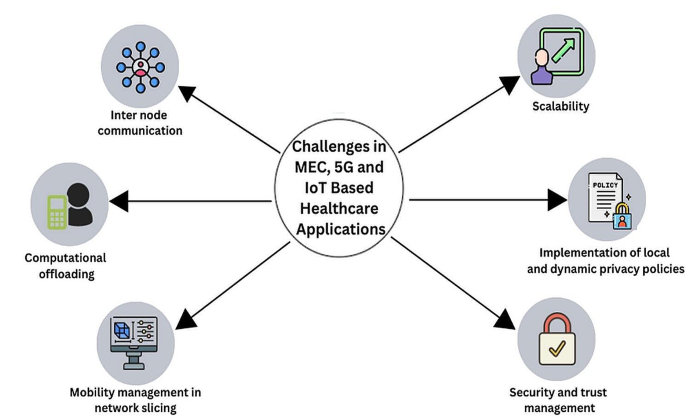
Challenges in MEC and 5G network in IoT healthcare applications
Inter nodes communication
The challenge of backhaul access in MEC and 5G networks involves ensuring robust connections among the MEC servers and the core network. As medical applications require high data transfer rates and increased numbers of connected devices, the backhaul networks need to be capable of handling significant bandwidth and capacity [ 56 ]. Addressing insufficient bandwidth is critical to prevent congestion, latency, and poor performance in healthcare IoT applications utilizing MEC and 5G networks. Moreover, healthcare applications demand real-time communication and data processing, making low-latency communication between nodes crucial for applications such as remote surgery and patient monitoring [ 57 , 58 , 59 , 60 ].
Computational offloading
In 5G networks and MEC environments, computational offloading decisions are pivotal. Deciding whether to process data at the edge or transfer it to a central cloud entails balancing latency, bandwidth, and cost. Improper offloading decisions can negatively affect performance and increase latency [ 61 ]. The challenge is to optimally allocate computing tasks to either the edge or local devices to ensure efficient resource utilization and minimize unnecessary data transfers [ 62 ].
Mobility management in network slicing
Network slicing, a pivotal feature of 5G, allows the creation of virtualized, dedicated network segments tailored to specific services [ 63 ]. Efficient mobility management between these slices is challenging, especially when ensuring uninterrupted service delivery for delay-sensitive medical applications such as remote monitoring [ 64 ]. Maintaining communication quality amidst changes in signal strength and minimizing interference are crucial to the success of 5G in healthcare applications [ 65 ].
Scalability
Scalability is a significant challenge for MEC and 5G networks, particularly concerning the capability of the system to accommodate an increasing number of devices, scale data volumes, and meet growing demand [80]. Scalability issues can lead to bottlenecks and reduced system efficiency, thereby impacting the real-time functionality of healthcare services [ 66 , 67 , 68 ].
Security and trust management
Solving security and trust issues comes through implementing a security framework that includes access, authentication, authorization, secure communication, and continuous monitoring [ 69 ]. Privacy breaches can lead to serious consequences, including identity theft, unauthorized access to medical information, and loss of patient trust [ 70 ]. Protecting medical information from unauthorized access and ensuring patient confidentiality is very difficult. Ensuring the integrity of medical records is crucial to prevent unauthorized falsification or alteration. Data integrity measures should be taken to detect and respond to adverse changes [ 71 ]. Attackers attack critical network or computing resources by sending requests at a faster rate than the MEC server can process, preventing other nodes from accessing the resources [ 72 ]. It is important to select and implement secure communication protocols between devices, edge servers, and the cloud in the middle. This includes protecting data during transmission and preventing man-in-the-middle attacks [ 73 ].
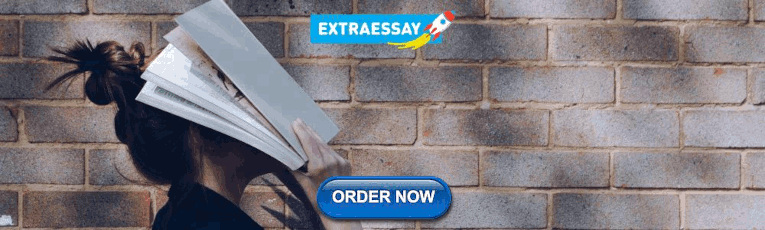
Implementation of local and dynamic privacy policies
Privacy policies for medical applications should be considered in the local context and consider factors such as region-specific regulations, cultural norms, and user preferences [ 74 ]. In the current system, privacy policies and guidelines are predefined at a global level and static over time. There is a need to find a mechanism to implement local and dynamic data protection policies. Failure to take local context into account can lead to non-compliance, ethical concerns, and potential user resistance due to cultural differences [ 75 ]. Failure to consider user-centric privacy can lead to a lack of user trust, reluctance to share data, and potential legal and ethical issues [ 76 ]. Existing privacy policies and guidelines in IoT systems need to be modified to support the adaptation of new technologies such as MEC. Inefficient use of edge computing to maintain privacy can lead to unnecessary data leakage and privacy risks [ 49 ].
Drawbacks of current technologies in healthcare
Current Technologies in Healthcare While discussing the potential of MEC and 5G in healthcare, it is crucial to acknowledge the challenges these technologies may face. For instance, the significant amount of data processed by these networks can cause delays, impacting the speed and efficiency of healthcare delivery [ 77 ]. Scalability solutions, such as distributed computing and network optimizations, are needed to ensure real-time data processing and timely access to patient information, which is critical for medical decisions.
The Implementation of MEC and 5G technologies must adhere to stringent data protection laws to safeguard patient privacy and prevent unauthorized access to medical information [ 78 ]. Developing strategies for integration with existing healthcare systems is essential for seamless interoperability and data exchange, requiring concerted efforts in terms of resources and development of middleware solutions and APIs [ 79 ].
Conclusion and future work
5G-MEC integration in the field of Internet of Things has opened up new ways for its deployment, notably in the health care industry. Both of these technologies are instrumental in delivering real-time, accurate medical data analysis and inter-device communication. Unfortunately, despite enormous potential, there are some operational difficulties limiting this promising collaboration.
The introduction of MEC and 5G technologies into the healthcare sector plays a crucial role in the development of patient care due to their advanced data management capabilities and improved connectivity for medical devices. Nevertheless, the implementation of these technologies is not devoid of challenges. Barriers such as scalability, data protection, and network stability remain an issue today.
An important area for future research is to find ways how these barriers can be overcome, given the comprehensive network of data flowing in a complicated systems environment; this includes, but is not limited to, developing effective security protocols and creating adaptive infrastructure that can handle the skyrocketing volume of data from contemporary healthcare applications.
In order to achieve optimal MEC and 5G, research needs to be a continuous process. One of the efforts should be simplified data transmission and processing while maintaining patient data privacy and system security at the highest level.
A pressing need exists for the evolution of novel technologies in support of health information systems that are responsive to the increasing sophistication of clinical networks. Not only will this serve as a means to safeguard patient records, but it will also keep health care delivery in line with advances in technology.
Dealing with these challenges will be an impetus for change, not only in the health system: as a result, it may transform the methods of care delivery, monitoring, and treatment management; this would contribute to enhancing global patients’ outcomes.
The process of developing these technologies continues. It portends the era where the health care system will be highly interconnected, efficacious, and trusted and make a tremendous contribution to those involved in caring as well as to those cared for.
Data availability
Not applicable.
Bhatt Y, Bhatt C (2017) Internet of things in healthcare Internet of things and big data technologies for next generation HealthCare, : pp. 13–33
Shafiq M et al (2022) The rise of internet of things: review and Open Research issues related to detection and Prevention of IoT-Based security attacks. Wirel Commun Mob Comput. https://doi.org/10.1155/2022/8669348
Article Google Scholar
Amani M et al (2020) Google earth engine cloud computing platform for remote sensing big data applications: a comprehensive review. IEEE J Sel Top Appl Earth Observations Remote Sens 13:5326–5350
Taiwo O, Ezugwu AE (2020) Smart healthcare support for remote patient monitoring during covid-19 quarantine. Inf Med Unlocked 20:100428
Shafiq M et al (2023) Advances in IoMT for Healthcare System. Sensors. https://doi.org/10.3390/s24010010
Balasundaram A et al (2023) Internet of things (IoT) based smart healthcare system for efficient diagnostics of health parameters of patients in emergency care. IEEE Internet Things J
Pham Q-V et al (2020) A survey of multi-access edge computing in 5G and beyond: fundamentals, technology integration, and state-of-the-art. IEEE Access 8:116974–117017
Mao Y et al (2017) Mobile edge computing: Survey and research outlook arXiv preprint arXiv:1701.01090, : pp. 1–37
Siddiqi MA, Yu H, Joung J (2019) 5G ultra-reliable low-latency communication implementation challenges and operational issues with IoT devices. Electronics 8(9):981
Liu Y et al (2020) Toward edge intelligence: Multiaccess edge computing for 5G and internet of things. IEEE Internet Things J 7(8):6722–6747
Kumar SM, Majumder D (2018) Healthcare solution based on machine learning applications in IOT and edge computing. Int J Pure Appl Math 119(16):1473–1484
Google Scholar
Premsankar G, Di Francesco M, Taleb T (2018) Edge computing for the internet of things: a case study. IEEE Internet Things J 5(2):1275–1284
Rajeswari D et al (2024) Structural health monitoring of aircraft through prediction of delamination using machine learning . PeerJ Computer Science, 10:e1955, 35 pages, doi.org/ https://doi.org/10.7717/peerj-cs.1955
Jagadeeswari V et al (2018) A study on medical internet of things and Big data in personalized healthcare system. Health Inform Sci Syst 6:1–20
Malik S et al (2022) Intelligent Load Balancing Framework for Fog enabled Communication in Healthcare . Electronics https://doi.org/10.3390/electronics11040566
Sajjad M et al (2022) Efficient Joint Key Authentication Model in E-Healthcare. CMC-Computers Mater Continua 71:2739–2753. https://doi.org/10.32604/cmc.2022.022706
He Q et al (2023) A blockchain-based scheme for secure data offloading in healthcare with deep reinforcement learning. IEEE/ACM Transactions on Networking
Tlemçani K et al (2023) An Advanced IoT-Based Architecture for Healthcare Systems: A Focus on Blockchain-based Edge Computing for Diabetes Management . in Proceedings of the 6th International Conference on Networking, Intelligent Systems & Security
Zhang Y et al (2020) Real-time remote health monitoring system driven by 5G MEC-IoT. Electronics 9(11):1753
Saeik F et al (2021) Task offloading in Edge and Cloud Computing: a survey on mathematical, artificial intelligence and control theory solutions. Comput Netw 195:108177
Saha R et al (2019) Privacy ensured ${e} $-healthcare for fog-enhanced IoT based applications. IEEE Access 7:44536–44543
Abdellatif AA et al (2019) Edge computing for smart health: context-aware approaches, opportunities, and challenges. IEEE Network 33(3):196–203
Roddy M et al (2019) 5G Network Slicing for Mission-critical use cases. 2019 IEEE 2nd 5G World Forum (5GWF). IEEE
Shafiei A et al (2021) A hybrid technique based on a genetic algorithm for fuzzy multiobjective problems in 5G, internet of things, and Mobile Edge Computing. Math Probl Eng. https://doi.org/10.1155/2021/9194578
Ranaweera P, Jurcut A, Liyanage M (2021) MEC-enabled 5G use cases: a survey on security vulnerabilities and countermeasures. ACM Comput Surv (CSUR) 54(9):1–37
Awad AI et al (2022) Utilization of mobile edge computing on the internet of Medical things: a survey. ICT Ex
Bishoyi PK, Misra S (2022) Towards energy-and cost-efficient sustainable MEC-assisted healthcare systems. IEEE Trans Sustainable Comput 7(4):958–969
Khan R et al (2019) A survey on security and privacy of 5G technologies: potential solutions, recent advancements, and future directions. IEEE Commun Surv Tutorials 22(1):196–248
Ning Z et al (2020) Mobile edge computing enabled 5G health monitoring for internet of medical things: a decentralized game theoretic approach. IEEE J Sel Areas Commun 39(2):463–478
Pustokhina IV et al (2020) An effective training scheme for deep neural network in edge computing enabled internet of medical things (IoMT) systems. IEEE Access 8:107112–107123
Al-Janabi S et al (2017) Survey of main challenges (security and privacy) in wireless body area networks for healthcare applications. Egypt Inf J 18(2):113–122
Ahmed A et al (2022) IoT-based real-time patients vital physiological parameters monitoring system using smart wearable sensors. Neural Comput Appl 34(22):19397–19673
Mohammed K et al (2019) Real-time remote-health monitoring systems: a review on patients prioritisation for multiple-chronic diseases, taxonomy analysis, concerns and solution procedure. J Med Syst 43:1–21
Bengiovanni T et al (2020) Risk management and healthcare: Iot technologies and smart monitoring system for a good cold chain management . in. 5th International Conference on Smart and Sustainable Technologies (SpliTech) . 2020. IEEE
Dadkhah M et al (2021) Use of internet of things for chronic disease management: an overview. J Med Signals Sens 11(2):138–157
Pradhan B, Bhattacharyya S, Pal K (2021) IoT-based applications in healthcare devices. J Healthc Eng 2021:1–18
Malviya R, Goyal P (2023) Remote patient monitoring: a computational perspective in Healthcare. CRC
Albahri AS et al (2021) IoT-based telemedicine for disease prevention and health promotion: state-of-the-art. J Netw Comput Appl 173:102873
Raisa FF et al (2023) IoT based medicine dispenser for improving medication adherence
Tsai M-H et al (2019) RFID medical equipment tracking system based on a location-based service technique. J Med Biol Eng 39:163–169
Ojuka DK, Okutoyi L, Otieno FC (2019) Communication in surgery for patient safety , in Vignettes in Patient Safety-Volume 4 . IntechOpen
Dangi R et al (2021) Study and investigation on 5G technology: a systematic review. Sensors 22(1):26
Sharma V et al (2018) Self-enforcing game theory-based resource allocation for LoRaWAN assisted public safety communications arXiv preprint arXiv:1804.07204
Kekki S et al (2018) MEC in 5G networks. ETSI White Paper 28(2018):p1–28
Vittal S, Singh MK, Franklin AA (2020) Adaptive network slicing with multi-site deployment in 5G core networks . in 6th IEEE Conference on Network Softwarization (NetSoft) . 2020. IEEE
Jain H, Chamola V, Jain Y (2021) 5G network slice for digital real-time healthcare system powered by network data analytics. Internet Things Cyber-Physical Syst 1:14–21
Hayyolalam V et al (2021) Edge-assisted solutions for IoT-based connected healthcare systems: a literature review. IEEE Internet Things J 9(12):9419–9443
Guo H et al (2018) Mobile-edge computation offloading for ultradense IoT networks. IEEE Internet Things J 5(6):4977–4988
Porambage P et al (2018) Survey on multi-access edge computing for internet of things realization. IEEE Commun Surv Tutorials 20(4):2961–2991
Islam A et al (2021) A survey on task offloading in multi-access edge computing. J Syst Architect 118:102225
Ahad A et al (2020) Technologies trend towards 5G network for smart health-care using IoT: a review. Sensors 20(14):4047
Alsiddiky A et al (2020) Priority-based data transmission using selective decision modes in wearable sensor based healthcare applications. Comput Commun 160:43–51
Mazhar T et al (2022) The role of ML, AI and 5G technology in smart energy and smart building management. Electronics 11(23):3960
Nguyen DC et al (2020) Blockchain and edge computing for decentralized EMRs sharing in federated healthcare . in GLOBECOM 2020–2020 IEEE Global Communications Conference . IEEE
Bishoyi PK, Misra S (2021) Enabling green mobile-edge computing for 5G-based healthcare applications. IEEE Trans Green Commun Netw 5(3):1623–1631
Deepa T, Chitra Kiran N (2019) A comprehensive research study on 5G backhauling: solutions, requirements and challenges. Commun Appl Electron 7(26):25–31
Nadeem L et al (2021) Integration of D2D, network slicing, and MEC in 5G cellular networks: Survey and challenges. IEEE Access 9:37590–37612
Peralta-Ochoa AM et al (2023) Smart Healthcare applications over 5G networks: a systematic review. Appl Sci 13(3):1469
Khujamatov K et al (2022) Device-to-device and millimeter waves communication for 5G healthcare informatics , in Blockchain Applications for Healthcare Informatics . Elsevier, pp 181–211
Ma Z et al (2019) High-reliability and low-latency wireless communication for internet of things: challenges, fundamentals, and enabling technologies. IEEE Internet Things J 6(5):7946–7970
Nencioni G, Garroppo RG, Olimid RF 5G multi-access edge computing: security, dependability, and performance arXiv preprint arXiv:2107.13374, 2021
Pereira RS et al (2020) RELIABLE: resource allocation mechanism for 5G network using mobile edge computing. Sensors 20(19):5449
Foukas X et al (2017) Network slicing in 5G: Survey and challenges. IEEE Commun Mag 55(5):94–100
Vecchiato R et al (2019) Technology foresight for growth and productivity: the design and implementation of a new foresight approach for UK SMEs
Spinelli F, Mancuso V (2020) Toward enabled industrial verticals in 5G: a survey on MEC-based approaches to provisioning and flexibility. IEEE Commun Surv Tutorials 23(1):596–630
Zyrianoff I et al (2018) Scalability of real-time iot-based applications for smart cities . in 2018 IEEE Symposium on Computers and Communications (ISCC) . IEEE
Bolettieri S, Bruno R, Mingozzi E (2021) Application-aware resource allocation and data management for MEC-assisted IoT service providers. J Netw Comput Appl 181:103020
Siriwardhana Y et al (2021) A survey on mobile augmented reality with 5G mobile edge computing: architectures, applications, and technical aspects. IEEE Commun Surv Tutorials 23(2):1160–1192
Tewari A, Gupta BB Security, privacy and trust of different layers in Internet-of-Things (IoTs) framework Future generation computer systems, 2020. 108: pp. 909–920
Keshta I, Odeh A (2021) Security and privacy of electronic health records: concerns and challenges. Egypt Inf J 22(2):177–183
Nowak TW et al (2021) Verticals in 5G MEC-use cases and security challenges. IEEE Access 9:87251–87298
Deng Y et al (2022) Resource provisioning for mitigating edge DDoS attacks in MEC-enabled SDVN. IEEE Internet Things J 9(23):24264–24280
Sabella D et al (2021) MEC security: Status of standards support and future evolutions. ETSI White Paper 46:1–26
Alahmari N et al (2023) Autonomous and Sustainable Service economies: Data-Driven optimization of Design and Operations through Discovery of Multi-perspective parameters. Sustainability 15(22):16003
Trakadas P et al (2019) Hybrid clouds for data-intensive, 5G-enabled IoT applications: an overview, key issues and relevant architecture. Sensors 19(16):3591
Bou Abdo J (2021) Prise de décision commerciale pour l’adoption du cloud computing: facteurs économiques et organisationnels. université Paris-Saclay
Khan D, Jung LT, Hashmani MA (2021) Systematic literature review of challenges in blockchain scalability. Appl Sci 11(20):9372
Yaqoob I et al (2022) Blockchain for healthcare data management: opportunities, challenges, and future recommendations. Neural Comput Appl, : p. 1–16
Baldimtsi F et al (2024) zkLogin: Privacy-Preserving Blockchain Authentication with Existing Credentials arXiv preprint arXiv:2401.11735
Download references
This work is supported by the research fund of the University of Johannesburg, South Africa.
Author information
Authors and affiliations.
Department of Computer Science and Software Engineering, Al Ain University, Abu Dhabi, 15322, UAE
Yazeed Yasin Ghadi
Department of Computer Science, Virtual University of Pakistan, Lahore, 54000, Pakistan
Syed Faisal Abbas Shah & Tehseen Mazhar
School of Electrical Engineering,Department of Electrical and Electronic Engineering Science, University of Johannesburg, Johannesburg, 2006, South Africa
Tariq Shahzad, Khmaies Ouahada & Habib Hamam
Faculty of Engineering, University of de Moncton, Moncton, NB, E1A3E9, Canada
Habib Hamam
Hodmas University College, Taleh Area, Mogadishu, Somalia
Bridges for Academic Excellence, Tunis, Centre-Ville, Tunisia
You can also search for this author in PubMed Google Scholar
Contributions
All authors have equal contribution.
Corresponding authors
Correspondence to Tehseen Mazhar or Tariq Shahzad .
Ethics declarations
Conflict of interest, additional information, publisher’s note.
Springer Nature remains neutral with regard to jurisdictional claims in published maps and institutional affiliations.
Rights and permissions
Open Access This article is licensed under a Creative Commons Attribution 4.0 International License, which permits use, sharing, adaptation, distribution and reproduction in any medium or format, as long as you give appropriate credit to the original author(s) and the source, provide a link to the Creative Commons licence, and indicate if changes were made. The images or other third party material in this article are included in the article’s Creative Commons licence, unless indicated otherwise in a credit line to the material. If material is not included in the article’s Creative Commons licence and your intended use is not permitted by statutory regulation or exceeds the permitted use, you will need to obtain permission directly from the copyright holder. To view a copy of this licence, visit http://creativecommons.org/licenses/by/4.0/ .
Reprints and permissions
About this article
Cite this article.
Ghadi, Y.Y., Shah, S.F.A., Mazhar, T. et al. Enhancing patient healthcare with mobile edge computing and 5G: challenges and solutions for secure online health tools. J Cloud Comp 13 , 93 (2024). https://doi.org/10.1186/s13677-024-00654-4
Download citation
Received : 11 January 2024
Accepted : 16 April 2024
Published : 02 May 2024
DOI : https://doi.org/10.1186/s13677-024-00654-4
Share this article
Anyone you share the following link with will be able to read this content:
Sorry, a shareable link is not currently available for this article.
Provided by the Springer Nature SharedIt content-sharing initiative
- Mobile edge computing (MEC)
- Healthcare device
- Integration
- Open access
- Published: 01 May 2024
Hospital performance evaluation indicators: a scoping review
- Shirin Alsadat Hadian ORCID: orcid.org/0000-0002-1443-1990 1 ,
- Reza Rezayatmand ORCID: orcid.org/0000-0002-9907-3597 2 ,
- Nasrin Shaarbafchizadeh ORCID: orcid.org/0000-0001-7104-2214 3 ,
- Saeedeh Ketabi ORCID: orcid.org/0000-0002-6778-5645 4 &
- Ahmad Reza Pourghaderi ORCID: orcid.org/0000-0003-2682-2160 5
BMC Health Services Research volume 24 , Article number: 561 ( 2024 ) Cite this article
Metrics details
Hospitals are the biggest consumers of health system budgets and hence measuring hospital performance by quantitative or qualitative accessible and reliable indicators is crucial. This review aimed to categorize and present a set of indicators for evaluating overall hospital performance.
We conducted a literature search across three databases, i.e., PubMed, Scopus, and Web of Science, using possible keyword combinations. We included studies that explored hospital performance evaluation indicators from different dimensions.
We included 91 English language studies published in the past 10 years. In total, 1161 indicators were extracted from the included studies. We classified the extracted indicators into 3 categories, 14 subcategories, 21 performance dimensions, and 110 main indicators. Finally, we presented a comprehensive set of indicators with regard to different performance dimensions and classified them based on what they indicate in the production process, i.e., input, process, output, outcome and impact.
The findings provide a comprehensive set of indicators at different levels that can be used for hospital performance evaluation. Future studies can be conducted to validate and apply these indicators in different contexts. It seems that, depending on the specific conditions of each country, an appropriate set of indicators can be selected from this comprehensive list of indicators for use in the performance evaluation of hospitals in different settings.
Peer Review reports
Healthcare is complex [ 1 ] and a key sector [ 2 ] that is now globally faced with problems of rising costs, lack of service efficiency, competition, and equity as well as responsiveness to users [ 3 ]. One estimate by the WHO has shown a yearly waste of approximately 20–40% of total healthcare resources because of inefficiency [ 4 ]. European countries have spent on average 9.6% of their gross domestic product (GDP) on healthcare in 2017 and 9.92% in 2019. Germany, France, and Sweden reported the highest healthcare expenditures in Europe in 2018 (between 10.9% and 11.5% of GDP) [ 5 ]. In the U.S., healthcare spending consumes 18% of the GDP, which is likely to eclipse $6 trillion by 2027 [ 6 ].
Hospitals, as the biggest consumers of health system budgets [ 7 ], are the major part of the health system [ 8 ]. In many countries 50–80% of the health sector budget is dedicated to hospitals [ 8 , 9 ]. As a result, hospital performance analysis is becoming a routine task for every hospital manager. On the one hand, hospital managers worldwide are faced with difficult decisions regarding cost reduction, increasing service efficiency, and equity [ 10 ]. On the other hand, measuring hospital efficiency is an issue of interest among researchers because patients demand high-quality care at lower expenses [ 11 ].
To address the above mentioned need to measure hospital performance, implementing an appropriate hospital performance evaluation system is crucial in any hospital. In doing so, hospital administrators use various tools to analyse and monitor hospital activities [ 1 ], which need well-defined objectives, standards and quantitative indicators [ 12 ]. The latter are used to evaluate care provided to patients both quantitatively and qualitatively and are often related to input, output, processes, and outcomes. These indicators can be used for continuous quality improvement by monitoring, benchmarking, and prioritizing activities [ 13 ]. These parameters are developed to improve health outcomes and to provide comparative information for monitoring and managing and formulating policy objectives within and across health services [ 12 ]. Studies thus far have used their own set of indicators while evaluating hospital performance, which could be context dependent. In addition, those studies have mostly used a limited set of indicators that focus on few dimensions (2–6 dimensions) of hospital performance [ 14 , 15 , 16 , 17 , 18 ].
Therefore, comprehensive knowledge of potential indicators that can be used for hospital performance evaluation is necessary. It would help choose appropriate indicators when evaluating hospital performance in different contexts. It would also help researchers extend the range of analysis to evaluate performance from a wider perspective by considering more dimensions of performance. Although performance is a very commonly used term, it has several definitions [ 19 , 20 ], yet, it is often misunderstood [ 21 ]. Therefore, some researchers have expressed confusion about the related terms and considered them interchangeable. These terms are effectiveness, efficiency, productivity, quality, flexibility, creativity, sustainability, evaluation, and piloting [ 21 , 22 , 23 ]. Thus, this scoping review aimed to categorize and present a comprehensive set of indicators that can be used as a suitable set for hospital performance evaluation at any needed level of analysis, i.e., clinical, para-clinical, logistical, or departmental, and relate those indicators to the appropriate performance dimensions. The uniqueness of this paper is that it provides its readers with a comprehensive collection of indicators that have been used in different performance analysis studies.
Materials and methods
We conducted a scoping review of a body of literature. The scoping review can be of particular use when the topic has not yet been extensively reviewed or has a complex or heterogeneous nature. This type of review is commonly undertaken to examine the extent, range, and nature of research activity in a topic area; determine the value and potential scope and cost of undertaking a full systematic review; summarize and disseminate research findings; and identify research gaps in the existing literature. As a scoping review provides a rigorous and transparent method for mapping areas of research, it can be used as a standalone project or as a preliminary step to a systematic review [ 24 ]. While a systematic review (qualitative or quantitative) usually addresses a narrow topic/scope and is a method for integrating or comparing findings from previous studies [ 25 ].
In our study, we used the Preferred Reporting Items for Systematic reviews and Meta-Analyses extension for Scoping Reviews (PRISMA-ScR) Checklist following the methods outlined by Arksey and O’Malley [ 26 ] and Tricco [ 27 ]. A systematic search for published and English-language literature on hospital performance evaluation models was conducted, using three databases, i.e., PubMed, Scopus, and Web of Science, from 2013 to January 2023. Initially, the identified keywords were refined and validated by a team of experts. Then, a combination of vocabularies was identified by the authors through a brainstorming process. The search strategy was formulated using Boolean operators. The title and abstract of the formulas were searched in the online databases. The search query for each database is presented in Table 1 .
In the screening process, relevant references related to hospital performance evaluation were screened and abstracted into researcher-developed Microsoft® Excel forms by dual independent reviewers and conflicting information was provided by other reviewers.
The inclusion criteria were as follows: focused only on the hospital setting, available full text and written in English. We excluded studies that focused on health organization indicators, not specifically on hospital indicators; articles without appropriate data (only focused on models and not indicators; or qualitative checklist questionnaires); and articles that focused only on clinical or disease-related indicators, not hospital performance dimensions, and provided very general items as indicators, not the domains of the indicators themselves. Then, a PRISMA-ScR Checklist was used to improve transparency in our review [ 28 ].
To extract the data, researcher-developed Microsoft® Excel forms (data tables) were designed. The following data were subsequently extracted into Microsoft®Excel for synthesis and evaluation: title, author, article year, country, indicator category, study environment (number of hospitals studied), study time frame, indicator name, number of indicators, indicator level (hospital level, department level), evaluation perspective (performance, productivity, efficiency, effectiveness, quality, cost, safety, satisfaction, etc. ) , study type (quantitative or qualitative), indicator subtype (input (structure), process, output (result), outcome and impact), and other explanations. To create a descriptive summary of the results that address the objectives of this scoping review, numerical summarization was also used.
The purpose of creating the main category and the evaluation perspective section was to develop them and create new categories, which focused on the type of indicators related to the performance term. For example, in the “Category” section, the names of the departments or wards of the hospital (such as hospital laboratories, pharmacies, clinical departments, and warehouses) and in the “Evaluation perspective” section, various terms related to the evaluation of hospital performance were extracted. These two types were used after extracting their information under the title “performance dimension”.
The indicators’ levels were collected to determine the level of performance evaluation with the relevant index. Some indicators were used to evaluate the performance of the entire hospital, some were used to evaluate the performance of hospital departments, and some were used to evaluate the performance at the level of a specific project. For example, several indicators (such as bed occupancy ratio, length of stay, and waiting time) were used to evaluate the performance of the entire hospital, and other indicators (such as laboratory department indicators, energy consumption indicators, and neonatal department indicators) were used only to measure the performance of specific departments. This sections were used under the title “category”. The “category” and “indicator’s name” sections were defined according to the results of the “subcategory” section.
The subtypes of indicators (input (structure), process, output(result), outcome and impact) were defined based on the chain model, and each of the selected indicators was linked to it (Appendix 1 ). As a result of the chain model, inputs were used to carry out activities, activities led to the delivery of services or products (outputs). The outputs started to bring about change (outcomes), and eventually, this (hopefully) contributed to the impact [ 29 ]. The classification of the set of input, process, output, outcome and impact indicators was such that readers could access these categories if necessary according to their chosen evaluation models. The term was used under the title “Indicators by types”.
The type of study was considered quantitative or qualitative for determining whether an indicator was able to perform calculations. In this way, readers can choose articles that use quantitative or qualitative indicators to evaluate hospital performance.
We included 91 full-text studies (out of 7475) in English published between 2013 and January 2023 (Fig. 1 ), approximately 40% of which were published between 2020 and 2023. More than 20% of the retrieved studies were conducted in Iran and USA.
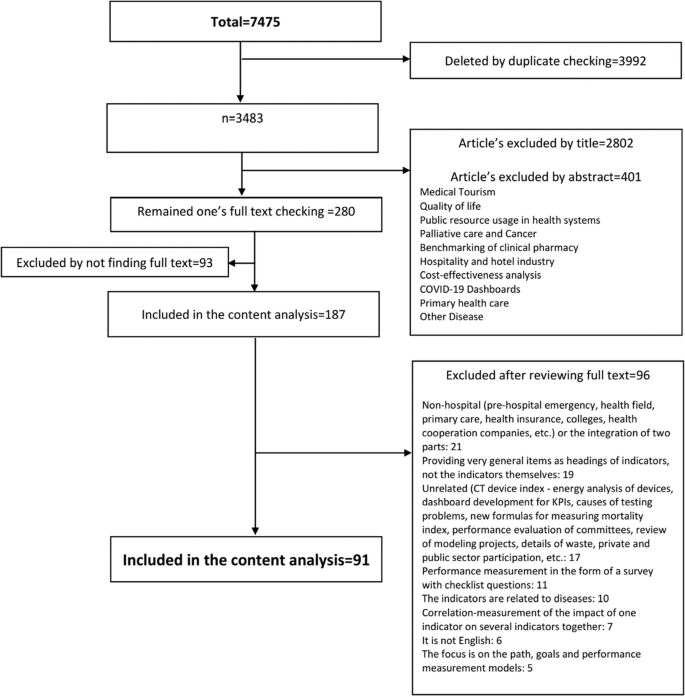
Study selection and data abstraction
Study characteristic
As shown in Table 2 , in 85% of the reviewed studies, a number of hospitals (1 to 3828 hospitals, 13,221 hospitals in total) were evaluated. More than 90% of the studies used a quantitative approach. In more than 70% of the studies, hospital evaluation occurred at the department level, which can also be divided into three levels: administrative, clinical ward, and paramedical department. In addition, the administrative departments consist of 13 departments, including financial management [ 48 , 55 , 61 , 67 , 68 , 80 , 83 , 109 , 113 ], supply chain management and warehouse [ 15 , 43 , 84 ], value-based purchasing [ 33 , 85 ], human resource management [ 97 , 101 ], medical equipment [ 32 , 87 ], health information management department [ 90 ], information systems [ 106 ], nutritional assessment [ 93 ], energy management [ 30 , 45 , 92 ], facility management [ 52 , 53 ], building sustainability and resilience [ 35 ], research activities [ 44 ], and education [ 107 ].
The clinical wards consisted of 8 wards, namely, emergency departments (EDs) [ 16 , 39 , 56 , 57 , 69 , 70 , 89 ], surgery departments [ 58 , 62 , 63 , 91 , 102 ], intensive care units (ICUs) [ 47 , 64 , 65 ], operating rooms (ORs) [ 38 , 88 , 108 ], surgical intensive care units (SICUs) [ 111 ], obstetrics and gynecology department [ 59 ], neonatal intensive care units (NICUs) [ 74 , 103 ] and quality of care [ 18 , 31 , 40 , 50 , 72 , 92 , 95 , 112 ] indicators. The paramedical departments consisted of 3 departments, pharmacy [ 60 , 76 , 98 ], laboratory and blood bank [ 37 , 42 , 43 , 49 ], and outpatient assessment [ 86 ] indicators.
With regard to data categorization, firstly, a total of 1204 indicators in 91 studies were extracted and after detailed examination, 43 indices (such as hospital ownership, level of care, admission process, and personal discipline) were removed due to their generality and impossibility of calculation in the hospital environment. Then, 1161 performance indicators were entered in this research and were categorized based on the performance criteria (more details about the indicators can be found in Appendix 1 ). Secondly, 145 functional dimensions, including divisions based on different departments and units of the hospital, were defined according to several focus group discussions with 5 health experts. Then, re-categorization and functional summarization were performed, after which 21 performance dimensions were finalized.
As shown in Table 4 , the 21 performance dimensions were divided into three parts: category, subcategory, and related indicators. Additionally, according to the hospital levels, there were three categories: ‘organizational management’, ‘clinical management’, and ‘administrative management’. Then, according to the type of indicators, fifteen subcategories were defined for the 110 selected main indicators.
Performance dimensions
The ‘productivity’ dimension focuses on indicators reflecting the macro-performance of the hospital, considering that this index is more effective and efficient. The ‘efficiency’ dimension focuses on general performance indicators for the optimal use of resources to create optimal output in the hospital. The ‘effectiveness’ dimension is a general performance indicator with an outcome view. The ‘speed’ dimension focuses on the indicators that show attention to the service delivery time and the speed of the procedures. The ‘development’ dimension focuses on matters related to employees’ and students’ training and related training courses. In terms of ‘safety’ dimension, there were issues related to patient safety, unwanted and harmful events, and hospital infections.
The “quality of work life” dimension emphasizes matters related to personnel volume and work conditions. The ‘quality’ dimension is related to the quality of service provided in different parts of the hospital and possible complications in improving the quality of services. The ‘satisfaction’ dimension focuses on the satisfaction of patients, employees, and their complaints. The ‘innovation’ dimension relates to the research process and its output. The ‘appropriateness’ dimension involves proper service from clinical departments, pharmaceutical services, and patient treatment. The ‘evaluation’ dimension focuses on the indicators related to the assessment scores of the para-clinical departments of the hospital.
The ‘profitability’ dimension focuses on the overall output indicators for income and profitability. The ‘cost’ dimension focuses on indicators related to general expenditures and the average cost per bed and patient and budgeting. The ‘economy’ dimension is related to financial rates and their indicators. The ‘coherence’ dimension emphasizes the indicators related to the continuity of the service delivery process. The ‘patient-centeredness’ dimension focuses on the indicators related to the patient’s experience of the facility, environment, treatment processes, communications, and relevant support for the patient. The ‘equity’ dimension studies indicators related to social and financial justice and life expectancy. The ‘relationship’ dimension evaluates the process of consultations and discussions required during the patients’ care provided by the treatment team. The ‘sustainability’ dimension focuses on indicators related to energy standards. The ‘flexibility’ dimension focuses on the hospital’s response to the crisis.
According to Table 4 , most studies focused on ‘efficiency’, ‘productivity’, ‘safety’ and ‘effectiveness’ as performance dimensions in 54, 53, 38 and 37 studies, respectively (40–70% of studies). In the ‘efficiency’ subcategory, resource management, supportive unit assessment, and human resource management indicators were the first to third most common indicators used in 26, 23 and 22 studies, respectively (approximately 25% of the studies).
In addition, for the ‘efficiency’ dimension, ‘medical staff numbers’, ‘emergency department bed numbers’, and ‘nonmedical staff numbers’ were reported in 16, 13, and 11 studies, respectively (between 20 and 30% of the studies). For the ‘productivity’ subcategory, ‘bed utilization rate’ and ‘service delivery and treatment’ were reported in 50% and 20% of the studies, respectively (46 and 19 out of 91).
Additionally, for the ‘productivity’ dimension, the ‘length of stay’ indicator was used more than others and reported in approximately 80% of the studies (43 out of 53), followed by the ‘bed occupancy rate’ in approximately 40% of the studies (21 out of 53). The ‘bed turnover ratio’ and ‘hospitalization rate’ were also reported in 12 studies. Furthermore, for ‘safety’ dimensions, all indicators were in the ‘patient safety’ subcategory, which has been reported in 38 studies, and ‘complications’, ‘accidents or adverse events’, and ‘incidents or errors rates’ were the most concentrated indicators by researchers in 13, 12, and 11 studies, respectively. The performance dimension of ‘effectiveness’ was presented in 37 studies (40%), with only two indicators, ‘mortality rate’ in 29 studies and ‘readmission rate’ in 23 studies.
Performance categories
Considering the three categories shown in Table 4 , ‘organizational management’ indicators were more commonly used among the other two categories (‘clinical’ and ‘administrative’) and were present in more than 85% of the studies (78 out of 91). Two categories, ‘clinical management’ and ‘administrative management’, were reported in 62 and 51 studies, respectively.
Performance subcategories
Considering the 14 subcategories shown in Table 4 , both the ‘bed utilization rate’ and ‘patient safety’ indicators were mentioned in 46 studies and were more common among the other subcategories. The second most common indicator of the ‘financial management’ subcategory was reported in 38 studies. At the third level, both the ‘human resource management’ and ‘time management’ indicators were presented in 31 studies. The ‘paramedical’ subcategory indicators were presented in less than 10% of the studies [ 60 , 96 , 97 , 98 , 106 , 113 ].
Performance indicators
According to the indicator columns in Table 3 , the most used indicators in reviewed studies were the length of stay, mortality rate, and readmission rate in 47%, 32%, and 25% of studies, respectively. Bed occupancy rate and non-personnel costs were reported in 23% of studies. Additionally, among the 110 indicators, 16 indicators, namely, the lab cancellation rate, exam-physician ratios, number of coded diagnoses, number of medical records, laboratory sample/report intervals, medical information request time, safety standards in the archives, nutritional risk screening, imaging quality control failures, errors in medical reports, average impact factor, nutritional measures, laboratory scoring, imaging inspection, discharge process and emergency response rate, were reported in less than 1% of the studies.
The classification of the indicators in Table 4 was performed based on the chain model, which included the input, process, output, outcome and impact. The assignment of the indicators to each category was performed according to the experts’ opinions. For instance, the number of publications by academic member of an academic hospital and the average impact factor of those publications were considered outcome indicators. As depicted in the Table 4 , most studies (80%) focused more on output indicators. Additionally, fifteen studies focused on introducing and extracting some of the input, process, output, outcome and impact indicators; among those, only one study [ 96 ] has examined the input, process, output and impact indicators simultaneously.
Additionally, in approximately 42% (36 out of 91) of the studies, the indicators’ definitions, formulas, or descriptions have been illustrated, while less than 10% of the studies have defined measuring units, standard or benchmark units for all studied indicators [ 15 , 43 , 45 , 51 , 52 , 57 , 67 ].
Overall, nine studies related to hospital performance evaluation were conducted using systematic review methodologies (five systematic reviews [ 16 , 29 , 30 , 56 , 113 ], two literature reviews [ 79 , 80 ], one narrative review [ 98 ] and one brief review [ 92 ]). Most of these studies focused on extracting performance indicators from one or more hospital departments (e.g., the emergency department) [ 16 , 56 ], hospital laboratory and radiology information systems [ 106 ], supply chain performance [ 29 ], resources and financial results and activity [ 113 ], hospital water consumption [ 30 ], and the pharmaceutical sector [ 98 ]. Other reviews included a three-step process to review, evaluate and rank these hospital indicators in a systematic approach [ 16 ], or to evaluate performance indicator models to create an interactive network and visualize the causal relationships between performance indicators [ 79 ]; moreover, some have focused on the importance of indicators to ensure adequate coverage of the relevant areas of health care services to be evaluated [ 92 ].
Only one scoping review aimed to identify current assessments of hospital performance and compared quality measures from each method in the context of the six qualitative domains of STEEEP (safety, timeliness, effectiveness, efficiency, equity, and patient-centeredness) of the Institute of Medicine (IOM) in accordance with Donabedian’s framework and formulating policy recommendations [ 115 ].
In addition, 21 studies divided performance indicators into 2 to 6 dimensions of performance. Also, the reviewed studies included 2–40 indicators in zero [ 29 , 30 , 98 ] to 6 domains [ 34 ]. Moreover, none of the studies have tried to comprehensively summarize and categorize the performance indicators in several categories, focusing on all the indicators reflecting the performance of the entire hospital organization, or the indicators of administrative units or clinical departments.
In this scoping review, a unique set of hospital performance evaluation indicators related to the various performance dimensions was categorized from 91 studies over the past ten years.
Similarly, in a study, 19 performance dimensions, 32 sub-dimensions, and 138 indicators were extracted from only six studies. Those dimensions were described by all studies included in the review, but only three studies specified the relevant indicators, and the list provided for all possible indicators was not comprehensive. Also, despite current review, there was no classification of indicators based on the hospital levels: managerial, clinical, or organizational levels [ 116 ]. Another study has similarly investigated the performance evaluation indicators of the hospital in such a way that among 42 studies, 111 indicators were presented in the four categories: input, output, outcome, and impact. But, there was no classification of indicators based on performance dimensions and hospital levels [ 117 ].
In this study, the importance of categorized indicators, for the first time to our knowledge, was determined based on their frequency of use in the published literature (Appendix 2 ). The ‘Organizational management’ indicators were the most common compared with the other two categories (‘clinical’ and ‘administrative’). It could be because of the fact that the indicators such as ‘bed occupancy rate’, ‘average length of stay’, ‘mortality rate’, ‘hospital infection rate’, and ‘patient safety’ are easier to be registered in hospital software compared to other indicators, and also they better reflect the overall performance of hospital. Thus, researchers are more interested in using these indicators.
Considering 14 subcategories, indicators related to three subcategories i.e. bed utilization, patient safety and financial management are the most frequent used indicators for hospital performance evaluation. It reflects the need of hospital managers to increase the profitability of hospital in one hand, and to control cost on the other hand. As a results, researchers have paid special attention to ‘cost income’, ‘profitability’, ‘economic’, etc., as indicators for evaluating hospital performance.
When considering indicators by type, more studies have focused on output indicators, while input indicators were the least common used. This might be because of the fact that at hospital level, it is difficult for managers to change those inputs such as ‘beds’, ‘human resources’, ‘equipment and facilities’. In addition, due to the complexity of interdepartmental relationships in hospitals, process indicators seemed to provide more variety for analysis than input indicators, so they were more often used. As mentioned above, output indicators were the most used indicators for hospital performance evaluation due to their ease of calculation and interpretation.
The main purpose of this paper was to identify a comprehensive set of indicators that can be used to evaluate hospital performance in various hospital settings by being distilled into a smaller and more related set of indicators for every hospital or department setting. future studies could be designed to validate each set of indicators in any specific context. In addition, they could investigate the relationship between the indicators and their outcomes of interest and the performance dimension each could address. This will enable hospital managers to build their own set of indicators for performance evaluation both at organization or at department level. Also it should be mentioned that.
Although some previous studies have provided definitions for each indicator and determined the standard criteria for them, this was not done in this study because the focus of this study was to provide a collection of all the indicators used in hospital performance evaluation, which resulted in the identification of more than a thousand indicators without limiting to specific country or context. So while preparing a smaller set of indicators, specific conditions of each country, such as the type of health system and its policy, the type of financing system, and the structure of services, should be taken into account to select appropriate indicators.
In addition, although it is important to examine the scope of each article to compare the list of indicators and the relationships between the dimensions of the hospital in terms of size and type and between the number and type of selected indicators, this was considered beyond the scope of this review due to the high number of indicators, which made the abovementioned investigations impossible. Future studies could do that while working with a smaller set of indicators.
This review aimed to categorize and present a comprehensive set of indicators for evaluating overall hospital performance in a systematic way. 1161 hospital performance indicators were drawn from 91 studies over the past ten years. They then were summarized into 110 main indicators, and categorized into three categories: 14 subcategories, and 21 performance dimensions This scoping review also highlighted the most frequent used indicators in performance evaluation studies which could reflect their importance for that purpose. The results of this review help hospital managers to build their own set of indicators for performance evaluation both at organization or at department level with regard to various performance dimensions.
As the results of this review was not limited to any specific country or context, specific conditions of each country, such as the type of health system and its policy, the type of financing system, and the structure of services, should be taken into account while selecting appropriate indicators as a smaller set of indicators for hospital performance evaluation in specific context.
Availability of data and materials
The datasets used and/or analyzed during the current study are available from the corresponding author on reasonable request.
Abbreviations
Gross domestic product
Preferred Reporting Items for Systematic reviews and Meta-Analyses extension for Scoping Reviews
Emergency departments
Intensive care unit
Operating room
Surgical intensive care unit
Neonatal intensive care unit
Readmission rate
Quality Control
Medication use evaluation
safety, timeliness, effectiveness, efficiency, equity, and patient-centeredness
Institute of Medicine
Abdullah A, Ahmad S, Athar MA, Rajpoot N, Talib F. Healthcare performance management using integrated FUCOM-MARCOS approach: the case of India. Int J Health Plann Manage. 2022;37(5):2635–68.
Article PubMed Google Scholar
Pestana M, Pereira R, Moro S. Improving health care management in hospitals through a productivity dashboard. J Med Syst. 2020;44(4):87.
Amos D. A practical framework for performance measurement of facilities management services in developing countries’ public hospitals. J Facil Manag. 2022;20(5):713–31.
Article Google Scholar
Ahmed S, Hasan MZ, MacLennan M, Dorin F, Ahmed MW, Hasan MM, et al. Measuring the efficiency of health systems in Asia: a data envelopment analysis. BMJ Open. 2019;9(3):e022155.
Article PubMed PubMed Central Google Scholar
Mitkova Z, Doneva M, Gerasimov N, Tachkov K, Dimitrova M, Kamusheva M, et al. Analysis of healthcare expenditures in Bulgaria. Healthc. 2022;10(2):274.
Patrinely JR, Walker SH, Glassman GE, Davis MJ, Abu-Ghname A, Khan U, et al. The importance of financial metrics in physician funding and performance evaluation. Plast Reconstr Surg. 2021;147:1213–8.
Article CAS PubMed Google Scholar
Buathong S, Bangchokdee S. The use of the performance measures in Thai public hospitals. ASIAN Rev Acc. 2017;25(4):472–85.
Google Scholar
Imani A, Alibabayee R, Golestani M, Dalal K. Key indicators affecting hospital efficiency: a systematic review. Front Public Heal. 2022;10:830102.
Mahdiyan S, Dehghani A, Tafti AD, Pakdaman M, Askari R. Hospitals’ efficiency in Iran: a systematic review and meta-analysis. J Educ Health Promot. 2019;8(1):126.
PubMed PubMed Central Google Scholar
Amos D, Musa ZN, Au-Yong CP. Performance measurement of facilities management services in Ghana’s public hospitals. Build Res Inf. 2020;48(2):218–38.
Feibert DC, Andersen B, Jacobsen P. Benchmarking healthcare logistics processes–a comparative case study of Danish and US hospitals. Total Qual Manag Bus Excell. 2019;30(1–2):108–34.
Gün I, Yilmaz F, Şenel IK. Efficiency analysis of health systems in world bank countries. Arch Heal Sci Res. 2021;8(2):147–52.
Breyer JZ, Giacomazzi J, Kuhmmer R, Lima KM, Hammes LS, Ribeiro RA, et al. Hospital quality indicators: a systematic review. Int J Health Care Qual Assur. 2019;32(2):474–87.
Regragui H, Sefiani N, Azzouzi H. Improving performance through measurement: the application of BSC and AHP in healthcare organization. In: Equipe De Recherche, Ingénierie, Innovation Et Management Des Systèmes Industriels, Université Abdelmalek Saadi. Tanger, Morocco: Institute of Electrical and Electronics Engineers Inc; 2018. p. 51–6.
Ghozali MT, Latifah DN, Darayani A. Analysis of Drug Supply Management of the Pharmacy Warehouse of Prof. Dr. Soerojo Mental Health Hospital, Magelang, Indonesia. Clin Schizophr Relat Psychoses. 2021;15:1–6.
Etu EE, Monplaisir L, Aguwa C, Arslanturk S, Masoud S, Markevych I, et al. Identifying indicators influencing emergency department performance during a medical surge: a consensus-based modified fuzzy Delphi approach. PLoS ONE. 2022;17(4 April):e0265101.
Article CAS PubMed PubMed Central Google Scholar
Lin C-Y, Shih F-C, Ho Y-H. Applying the balanced scorecard to build service performance measurements of medical institutions: An AHP-DEMATEL approach. Int J Environ Res Public Health. 2023;20(2):1022.
Backman C, Vanderloo S, Forster AJ. Measuring and improving quality in university hospitals in Canada: the collaborative for excellence in healthcare quality. Health Policy (New York). 2016;120(9):982–6.
Ghalem Â, Okar C, Chroqui R, Semma E. Performance: A concept to define. In: Performance: A concept to define. LOGISTIQUA 2016; 2016. p. 1–13.
Sonnentag S, Frese M. Performance Concepts and Performance Theory. In 2005. p. 1–25.
Tangen S. Demystifying productivity and performance. Int J Prod Perform Manag. 2005;54:34–46.
Elena-Iuliana I, Maria C. Organizational Performance – A Concept That Self-Seeks To Find Itself. Ann - Econ Ser Constantin Brancusi Univ Fac Econ. 2016;4(4):179–83.
Riratanaphong C, Van der Voordt T, Sarasoja A. Performance Measurement in the context of CREM and FM. In: Per Anker Jensen, Theo Van der Voordt CC, editor. The added value of facilities management: concepts, findings and perspectives. Lyngby Denmark: Polyteknisk Forlag; 2012. p. 1–21.
Pham M, Rajić A, Greig J, Sargeant J, Papadopoulos A, Mcewen S. A scoping review of scoping reviews: advancing the approach and enhancing the consistency. Res Synth Methods. 2014;5:371–85.
Chaney M. So you want to write a narrative review article? J Cardiothorac Vasc Anesth. 2021;35:3045–9.
Arksey H, O’Malley L. Scoping studies: towards a methodological framework. Int J Soc Res Methodol. 2005;8(1):19–32.
Tricco AC, Lillie E, Zarin W, O’Brien KK, Colquhoun H, Levac D, et al. PRISMA extension for scoping reviews (PRISMA-ScR): Checklist and explanation. Ann Intern Med. 2018;169(7):467–73.
Tricco A, Lillie E, Zarin W, O’Brien K, Colquhoun H, Levac D, et al. PRISMA extension for scoping reviews (PRISMA-ScR): Checklist and explanation. Ann Intern Med. 2018;169(7):467–73.
Dolatabad AH, Mahdiraji HA, Babgohari AZ, Garza-Reyes JA, Ai A. Analyzing the key performance indicators of circular supply chains by hybrid fuzzy cognitive mapping and Fuzzy DEMATEL: evidence from healthcare sector. Environ Dev Sustain. 2022;1–27.
Batista KJM, da Silva SR, Rabbani ERK, Zlatar T. Systematic review of indicators for the assessment of water consumption rates at hospitals. Water Supply. 2020;20(2):373–82.
Beta G, Role D, Berloviene D, Balkena Z. PATIENTS’ SATISFICATION AS THE QUALITY INDICATOR OF NURSING. In: Lubkina V, Kaupuzs A, Znotina D, editors. SOCIETY INTEGRATION EDUCATION, VOL VI: PUBLIC HEALTH AND SPORT, RESEARCHES IN ECONOMICS AND MANAGEMENT FOR SUSTAINABLE EDUCATION. 2020. p. 79–88.
Bhardwaj P, Joshi NK, Singh P, Suthar P, Joshi V, Jain YK, et al. Competence-based assessment of biomedical equipment management and maintenance system (e-Upkaran) using benefit evaluation framework. CUREUS J Med Sci. 2022;14(10):e30579.
Cheon O, Song M, Mccrea AM, Meier KJ. Health care in America: the relationship between subjective and objective assessments of hospitals. Int PUBLIC Manag J. 2021;24(5):596–622.
Craig KJT, McKillop MM, Huang HT, George J, Punwani ES, Rhee KB. US hospital performance methodologies: a scoping review to identify opportunities for crossing the quality chasm. BMC Health Serv Res. 2020;20(1):640.
Cristiano S, Ulgiati S, Gonella F. Systemic sustainability and resilience assessment of health systems, addressing global societal priorities: Learnings from a top nonprofit hospital in a bioclimatic building in Africa. Renew Sustain ENERGY Rev. 2021;141:110765.
Dadi D, Introna V, Santolamazza A, Salvio M, Martini C, Pastura T, et al. Private Hospital Energy Performance Benchmarking Using Energy Audit Data: An Italian Case Study. Energies. 2022;15(3):1–16.
Dawande PP, Wankhade RS, Akhtar FI, Noman O. Turnaround time: an efficacy measure for medical laboratories. CUREUS J Med Sci. 2022;14(9):e28824.
De Sousa LR, Mazzo A, De Almeida ACF, Tonello C, Lourençone LFM. Evaluation of quality indicators in the management of an operating room at a tertiary-level hospital. Med. 2022;55(1):1–8.
Drynda S, Schindler W, Slagman A, Pollmanns J, Horenkamp-Sonntag D, Schirrmeister W, et al. Evaluation of outcome relevance of quality indicators in the emergency department (ENQuIRE): study protocol for a prospective multicentre cohort study. BMJ Open. 2020;10(9):e038776.
Fekri O, Manukyan E, Klazinga N. Appropriateness, effectiveness and safety of care delivered in Canadian hospitals: a longitudinal assessment on the utility of publicly reported performance trend data between 2012–2013 and 2016–2017. BMJ Open. 2020;10(6):e035447.
Galloa AJO, Ramírez CA. Evaluating Colombian public hospitals productivity during 2004–2015. A luenberger-indicator approach. Rev Gerenc Y Polit Salud. 2020;19:1–23.
Gebreyes M, Sisay A, Tegen D, Asnake A, Wolde M. Evaluation of laboratory performance, associated factors and staff awareness towards achieving turnaround time in tertiary hospitals, Ethiopia. Ethiop J Health Sci. 2020;30(5):767–76.
Gnanaraj J, Kulkarni RG, Sahoo D, Abhishekh B. Assessment of the Key Performance Indicator Proposed by NABH in the Blood Centre of a Tertiary Health Care Hospital in Southern India. Indian J Hematol Blood Transfus. 2022;39:308–16.
Horenberg F, Lungu DA, Nuti S. Measuring research in the big data era: the evolution of performance measurement systems in the Italian teaching hospitals. Health Policy (New York). 2020;124(12):1387–94.
Hwang DK, Cho J, Moon J. Feasibility study on energy audit and data driven analysis procedure for building energy efficiency: bench-marking in Korean hospital buildings. Energies. 2019;14(15):3006.
Jaskova D. Efficiency of management, processes in a private hospital. Entrep Sustain Issues. 2021;9(1):436–46.
Jebraeily M, Valizadeh MA, Rahimi B, Saeidi S. The development of a clinical dashboard for monitoring of key performance indicators in ICU. J Iran Med Counc. 2022;5(2):308–17.
Kang Y, Kim M, Jung K. The equity of health care spending in South Korea: testing the impact of publicness. Int J Environ Res Public Health. 2020;17(5):1775.
Abou Tarieh RR, Zayyat R, Naoufal RN, Samaha HR. A case study exploring the impact of JCI standards implementation on staff productivity and motivation at the laboratory and blood bank. Heal Sci Rep. 2022;5(1):e497.
Kadoic N, Simic D, Mesaric J, Redep NB. Measuring quality of public hospitals in croatia using a multi-criteria Approach. Int J Environ Res Public Health. 2021;18:19.
Khalilabad T, Amir N, Asl P, Raeissi Shali M, Niknam N. Assessment of clinical and paraclinical departments of military hospitals based on the Pabon Lasso Model. J Educ Health Promot. 2020;9:1–6.
Lai JHK, Hou H, Edwards DJ, Yuen PL. An analytic network process model for hospital facilities management performance evaluation. Facilities. 2022;40(5–6):333–52.
Lai J, Yuen PL. Identification, classification and shortlisting of performance indicators for hospital facilities management. Facilities. 2021;39(1–2):4–18.
Lin CS, Chiu CM, Huang YC, Lang HC, Chen MS. Evaluating the operational efficiency and quality of Tertiary hospitals in Taiwan: the application of the EBITDA Indicator to the DEA Method and TOBIT Regression. Healthcare. 2022;10(1):58.
Matos R, Ferreira D, Pedro MI. Economic analysis of portuguese public hospitals through the construction of quality, efficiency, access, and financial related composite indicators. Soc Indic Res. 2021;157(1):361–92.
Morisod K, Luta X, Marti J, Spycher J, Malebranche M, Bodenmann P. Measuring health equity in emergency care using routinely collected data: a systematic review. Heal Equity. 2021;5(1):801–17.
Nik Hisamuddin R, Tuan Hairulnizam TK. Developing key performance indicators for emergency department of teaching hospitals: a mixed fuzzy Delphi and nominal group technique approach. Malays J Med Sci. 2022;29(2):114–25.
Ramírez Calazans A, Paredes Esteban RM, Grijalva Estrada OB, Ibarra Rodríguez MR. Assessment of quality indicators in pediatric major outpatient surgery. Influence of the COVID-19 pandemic. Cir Pediatr. 2023;36(1):17–21.
PubMed Google Scholar
Shaqura II, Gholami M, Akbari Sari A. Assessment of public hospitals performance in Gaza governorates using the Pabón Lasso Model. Int J Health Plann Manage. 2021;36(4):1223–35.
Al-Jazairi AS, Alnakhli AO. Quantifying clinical pharmacist activities in a tertiary care hospital using key performance indicators. Hosp Pharm. 2021;56(4):321–7.
Aloh HE, Onwujekwe OE, Aloh OG, Nweke CJ. Is bed turnover rate a good metric for hospital scale efficiency? A measure of resource utilization rate for hospitals in Southeast Nigeria. Cost Eff Resour Alloc. 2020;18(1):1–8.
Bari S, Incorvia J, Ahearn O, Dara L, Sharma S, Varallo J, et al. Building safe surgery knowledge and capacity in Cambodia: a mixed-methods evaluation of an innovative training and mentorship intervention. Glob Health Action. 2021;14(1):1998996.
Bari S, Incorvia J, Iverson KR, Bekele A, Garringer K, Ahearn O, et al. Surgical data strengthening in Ethiopia: results of a Kirkpatrick framework evaluation of a data quality intervention. Glob Health Action. 2021;14(1):1–11.
Bastos LSL, Hamacher S, Zampieri FG, Cavalcanti AB, Salluh JIF, Bozza FA. Structure and process associated with the efficiency of intensive care units in low-resource settings: an analysis of the CHECKLIST-ICU trial database. J Crit Care. 2020;59:118–23.
Bastos LSL, Wortel SA, de Keizer NF, Bakhshi-Raiez F, Salluh JIF, Dongelmans DA, et al. Comparing continuous versus categorical measures to assess and benchmark intensive care unit performance. J Crit Care. 2022;70:154063.
Kocisova K, Hass-Symotiuk M, Kludacz-Alessandri M. Use of the dea method to verify the performance model for hospitals. E M Ekon A Manag. 2018;21(4):125–40.
Lee D, Yu S, Yoon SN. Analysis of hospital management based on the characteristics of hospitals: focusing on financial indicators. Glob Bus Financ Rev. 2019;24(3):1–13.
Mirzaei A, Tabibi SJ, Nasiripour AA, Riahi L. Evaluating the feasibility of financial variables of health: A hospital administrator’s viewpoint. Galen Med J. 2016;5(1):25–30.
Middleton S, Gardner G, Gardner A, Considine J, FitzGerald G, Christofis L, et al. Are service and patient indicators different in the presence or absence of nurse practitioners? The EDPRAC cohort study of Australian emergency departments. BMJ Open. 2019;9(7):e024529.
Nobakht S, Jahangiri K, Hajinabi K. Correlation of performance indicators and productivity: A cross sectional study of emergency departments in Tehran, Iran during year 2016. Trauma Mon. 2018;23(5):1–6.
Nuti S, Grillo Ruggieri T, Podetti S. Do university hospitals perform better than general hospitals? A comparative analysis among Italian regions. BMJ Open. 2016;6(8):e011426.
Petrovic GM, Vukovic M, Vranes AJ. The impact of accreditation on health care quality in hospitals. Vojnosanit Pregl. 2018;75(8):803–8.
Pirani N, Zahiri M, Engali KA, Torabipour A. Hospital efficiency measurement before and after health sector evolution plan in Southwest of Iran: a DEA-panel data study. Acta Inf Med. 2018;26(2):106–10.
Profit J, Gould JB, Bennett M, Goldstein BA, Draper D, Phibbs CS, et al. The association of level of care with NICU quality. Pediatrics. 2016;137(3):44–51.
Rahimi H, Bahmaei J, Shojaei P, Kavosi Z, Khavasi M. Developing a strategy map to improve public hospitals performance with balanced scorecard and dematel approach. Shiraz E Med J. 2018;19(7):1–12.
Ahmed S, Hasan MZ, Laokri S, Jannat Z, Ahmed MW, Dorin F, et al. Technical efficiency of public district hospitals in Bangladesh: a data envelopment analysis. COST Eff Resour Alloc. 2019;17:17.
Rahman MH, Tumpa TJ, Ali SM, Paul SK. A grey approach to predicting healthcare performance. Meas J Int Meas Confed. 2019;134:307–25.
Sajadi HS, Sajadi ZS, Sajadi FA, Hadi M, Zahmatkesh M. The comparison of hospitals’ performance indicators before and after the Iran’s hospital care transformations plan. J Educ Health Promot. 2017;6:89.
Si S-L, You X-Y, Liu H-C, Huang J. Identifying key performance indicators for holistic hospital management with a modified DEMATEL approach. Int J Environ Res Public Health. 2017;14(8): 934.
Váchová L, Hajdíková T. Evaluation of Czech hospitals performance using MCDM methods. In: A SI, G WS, C D, editors. Department of exact methods, faculty of management, university of economics, Prague, Jarošovská 1117, Jindřichuv Hradec, vol. 37701. Czech Republic: Newswood Limited; 2017. p. 732–5.
Xenos P, Yfantopoulos J, Nektarios M, Polyzos N, Tinios P, Constantopoulos A. Efficiency and productivity assessment of public hospitals in Greece during the crisis period 2009–2012. Cost Eff Resour Alloc. 2017;15(1):6.
Zhang L, Liu R, Jiang S, Luo G, Liu H-C. Identification of key performance indicators for hospital management using an extended hesitant linguistic DEMATEL Approach. Healthc (Basel Switzerland). 2019;8(1):7.
Aksezer CS. A nonparametric approach for optimal reliability allocation in health services. Int J Qual Reliab Manag. 2016;33(2):284–94.
Cagliano AC, Grimaldi S, Rafele C. Assessing warehouse centralization and outsourcing in the healthcare sector: an Italian case study. In: Department of Management and Production Engineering, Politecnico Di Torino, Corso Duca Degli Abruzzi 24, Torino, 10129. Italy: AIDI - Italian Association of Industrial Operations Professors; 2017. p. 244–50.
Cefalu MS, Elliott MN, Setodji CM, Cleary PD, Hays RD. Hospital quality indicators are not unidimensional: a reanalysis of Lieberthal and Comer. Health Serv Res. 2019;54(2):502–8.
Gao H, Chen H, Feng J, Qin X, Wang X, Liang S, et al. Balanced scorecard-based performance evaluation of Chinese county hospitals in underdeveloped areas. J Int Med Res. 2018;46(5):1947–62.
Gonnelli V, Satta F, Frosini F, Iadanza E. Evidence-based approach to medical equipment maintenance monitoring. In: V HEO, V J, editors. University of Florence, Dept. of Information Engineering. Florence, Italy: Springer; 2017. p. 258–61.
Helkio P, Aantaa R, Virolainen P, Tuominen R. Productivity benchmarks for operative service units. ACTA Anaesthesiol Scand. 2016;60(4):450–6.
Khalifa M, Zabani I. Developing emergency room key performance indicators: What to measure and why should we measure it? J. M, A. H, P. G, A. K, M.S. H, editors. Vol. 226. King Faisal Specialist Hospital and Research Center, Jeddah, Saudi Arabia: IOS Press BV; 2016. p. 179–182.
Ajami S, Ebadsichani A, Tofighi S, Tavakoli N. Medical records department and balanced scorecard approach. J Educ Health Promot. 2013;2:7.
Bosse G, Mtatifikolo F, Abels W, Strosing C, Breuer J-P, Spies C. Immediate outcome indicators in perioperative care: a controlled intervention study on quality improvement in hospitals in Tanzania. PLoS One. 2013;8(6):e65428.
Hung K-Y, Jerng J-S. Time to have a paradigm shift in health care quality measurement. J Formos Med Assoc. 2014;113(10):673–9.
Jeejeebhoy KN, Keller H, Gramlich L, Allard JP, Laporte M, Duerksen DR, et al. Nutritional assessment: comparison of clinical assessment and objective variables for the prediction of length of hospital stay and readmission. Am J Clin Nutr. 2015;101(5):956–65.
Kittelsen SAC, Anthun KS, Goude F, Huitfeldt IMS, Häkkinen U, Kruse M, et al. Costs and quality at the hospital level in the nordic countries. Heal Econ (United Kingdom). 2015;24:140–63.
Koné Péfoyo AJ, Wodchis WP. Organizational performance impacting patient satisfaction in Ontario hospitals: a multilevel analysis. BMC Res Notes. 2013;6: 509.
Li CH, Yu CH. Performance evaluation of public non-profit hospitals using a BP Artificial neural network: the case of Hubei Province in China. Int J Environ Res Public Health. 2013;10(8):3619–33.
Liu K, Jain S, Shi J. Physician performance assessment using a composite quality index. Stat Med. 2013;32(15):2661–80.
Lloyd GF, Bajorek B, Barclay P, Goh S. Narrative review: Status of key performance indicators in contemporary hospital pharmacy practice. J Pharm Pract Res. 2015;45(4):396–403.
Mehrtak M, Yusefzadeh H, Jaafaripooyan E. Pabon Lasso and data envelopment analysis: a complementary approach to hospital performance measurement. Glob J Health Sci. 2014;6(4):107–16.
Mohammadi M, Ziapoor A, Mahboubi M, Faroukhi A, Amani N, Pour FH, et al. Performance evaluation of hospitals under supervision of Kermanshah medical sciences using pabonlasoty diagram of a five-year period (2008–2012). Life Sci J. 2014;11:77–81 ( 1 SPECL. ISSUE) ).
Niaksu O, Zaptorius J. Applying operational research and data mining to performance based medical personnel motivation system. In: Vilnius University, Institute of Mathematics and Informatics. Lithuania: IOS; 2014. p. 63–70.
Córdoba S, Caballero I, Navalón R, Martínez-Sánchez D, Martínez-Morán C, Borbujo J. Analysis of the surgical activity in the dermatology department of Fuenlabrada University Hospital, Madrid, Spain, between 2005 and 2010: determination of the standard operating times. Actas Dermosifiliogr. 2013;104(2):141–7.
Profit J, Kowalkowski MA, Zupancic JAF, Pietz K, Richardson P, Draper D, et al. Baby-MONITOR: a composite indicator of NICU Quality. Pediatrics. 2014;134(1):74–82.
Rabar D, Pap N. Evaluation of crotia’s regional hospital effiency: an application of data envelopment analysis . Bacher U, Barkovic D, Dernoscheg KH, LamzaMaronic M, Matic B, Runzheimer B, editors. Interdisciplinary Management Research IX. 2013;9:649–59.
Ramos MCA, da Cruz LP, Kishima VC, Pollara WM, de Lira ACO, Couttolenc BF. Performance evaluation of hospitals that provide care in the public health system, Brazil. Rev Saude Publica. 2015;49:1–9.
Schuers M, Joulakian MB, Griffon N, Pachéco J, Périgard C, Lepage E, et al. In: S IN, de PM AM, editors. Quality indicators from laboratory and radiology information systems. A. G. Volume 216. France: IOS; 2015. pp. 212–6. Department of Biomedical Informatics, Rouen University Hospital, Rouen Cedex, 76031,.
Tabrizi JS, Saadati M, Sadeghi-Bazargani H, Ebadi A, Golzari SEJ. Developing indicators to improve educational governance in hospitals. Clin Gov. 2014;19(2):117–25.
Costa A Jr, aS., Leão LE, Novais MA, Zucchi P. An assessment of the quality indicators of operative and non-operative times in a public university hospital. Einstein (Sao Paulo). 2015;13(4):594–9.
Coyne JS, Helton J. How prepared are US hospitals for the affordable care act? A financial condition analysis of US hospitals in 2011. J Health Care Finance. 2015;41(3).
Davis P, Milne B, Parker K, Hider P, Lay-Yee R, Cumming J, et al. Efficiency, effectiveness, equity (E-3). Evaluating hospital performance in three dimensions. Health Policy (New York). 2013;112(1–2):19–27.
Flatow VH, Ibragimova N, Divino CM, Eshak DSA, Twohig BC, Bassily-Marcus AM, et al. Quality outcomes in the surgical intensive care unit after electronic health record implementation. Appl Clin Inf. 2015;6(4):611–8.
Article CAS Google Scholar
Fonseca JRS, Ramos RMP, Santos AMP, Fonseca APSS. Policy effects on the quality of public health care: evaluating Portuguese public hospitals’ quality through customers’ views. Cent Eur J Public Policy. 2015;9(2):122–40.
Hadji B, Meyer R, Melikeche S, Escalon S, Degoulet P. Assessing the Relationships Between Hospital Resources and Activities: A Systematic Review. J Med Syst. 2014;38(10):1–21.
Hajduová Z, Herbrik G, Beslerová S. Application of DEA in the environment of Slovak hospitals. Invest Manag Financ Innov. 2015;12(4):148–53.
Thomas Craig KJ, McKillop MM, Huang HT, George J, Punwani ES, Rhee KB. U.S. hospital performance methodologies: a scoping review to identify opportunities for crossing the quality chasm. BMC Health Serv Res. 2020;20(1):640.
Carini E, Gabutti I, Frisicale EM, Di Pilla A, Pezzullo AM, de Waure C, et al. Assessing hospital performance indicators. What dimensions? Evidence from an umbrella review. BMC Health Serv Res. 2020;20(1):1038.
Rasi V, Delgoshaee B, Maleki M. Identification of common indicators of hospital performance evaluation models: a scoping review. J Educ Health Promot. 2020;9(1):63.
Xenos P, Yfantopoulos J, Nektarios M, Polyzos N, Tinios P, Constantopoulos A. Efficiency and productivity assessment of public hospitals in Greece during the crisis period 2009–2012. COST Eff Resour Alloc. 2017;15:15.
Shaqura II, Gholami M, Sari AA. Evaluation of performance at Palestinian public hospitals using Pabon Lasso model. Int J Health Plann Manage. 2021;36(3):896–910.
Li J, Seale H, Ray P, Wang Q, Yang P, Li S, et al. E-Health preparedness assessment in the context of an influenza pandemic: a qualitative study in China. BMJ Open. 2013;3(3):e002293.
Huang C-Y, Lai C-H. Effects of internal branding management in a hospital context. Serv Ind J. 2021;41(15–16):985–1006.
Download references
Acknowledgements
The authors are grateful for the support of the Vice Chancellor for Research of Isfahan University of Medical Sciences.
The present article is part of the result of a doctoral thesis approved by Isfahan University of Medical Sciences with code 55657 (IR.MUI.NUREMA.REC.1401.005), without financial source.
Author information
Authors and affiliations.
Student Research Committee, School of Management and Medical Information Sciences, Isfahan University of Medical Sciences, Isfahan, Iran
Shirin Alsadat Hadian
Health Management and Economics Research Center, Isfahan University of Medical Sciences, Isfahan, Iran
Reza Rezayatmand
Hospital Management Research Center, Health Management Research Institute, Iran University of Medical Sciences, Tehran, Iran
Nasrin Shaarbafchizadeh
Department of Management, Faculty of Administrative Sciences and Economics, University of Isfahan, Isfahan, Iran
Saeedeh Ketabi
School of Public Health and Preventive Medicine, Monash University, Victoria, Australia
Ahmad Reza Pourghaderi
You can also search for this author in PubMed Google Scholar
Contributions
Shirin Alsadat Hadian and Reza Rezayatmans and Saeedeh Ketabi: Study conceptualization and design. Acquisition of data: Shirin Alsadat Hadian, Reza Rezayatmand. Analysis and interpretation of data: Shirin Alsadat Hadian, Reza Rezayatmand, Nasrin Shaarbafchizadeh, Saeedeh Ketabi. Drafting of the manuscript: Shirin Alsadat Hadian, Reza Rezayatmand. Critical revision of the manuscript for important intellectual content: Reza Rezayatmand, Nasrin Shaarbafchizadeh, Saeedeh Ketabi, Ahmad Reza Pourghaderi.
Corresponding author
Correspondence to Reza Rezayatmand .
Ethics declarations
Ethics approval and consent to participate.
Not applicable.
Consent for publication
Competing interests.
The authors declare no competing interests.
Additional information
Publisher’s note.
Springer Nature remains neutral with regard to jurisdictional claims in published maps and institutional affiliations.
Supplementary Information
Supplementary material 1., supplementary material 2., supplementary material 3., rights and permissions.
Open Access This article is licensed under a Creative Commons Attribution 4.0 International License, which permits use, sharing, adaptation, distribution and reproduction in any medium or format, as long as you give appropriate credit to the original author(s) and the source, provide a link to the Creative Commons licence, and indicate if changes were made. The images or other third party material in this article are included in the article's Creative Commons licence, unless indicated otherwise in a credit line to the material. If material is not included in the article's Creative Commons licence and your intended use is not permitted by statutory regulation or exceeds the permitted use, you will need to obtain permission directly from the copyright holder. To view a copy of this licence, visit http://creativecommons.org/licenses/by/4.0/ . The Creative Commons Public Domain Dedication waiver ( http://creativecommons.org/publicdomain/zero/1.0/ ) applies to the data made available in this article, unless otherwise stated in a credit line to the data.
Reprints and permissions
About this article
Cite this article.
Hadian, S.A., Rezayatmand, R., Shaarbafchizadeh, N. et al. Hospital performance evaluation indicators: a scoping review. BMC Health Serv Res 24 , 561 (2024). https://doi.org/10.1186/s12913-024-10940-1
Download citation
Received : 03 January 2024
Accepted : 02 April 2024
Published : 01 May 2024
DOI : https://doi.org/10.1186/s12913-024-10940-1
Share this article
Anyone you share the following link with will be able to read this content:
Sorry, a shareable link is not currently available for this article.
Provided by the Springer Nature SharedIt content-sharing initiative
- Performance evaluation
BMC Health Services Research
ISSN: 1472-6963
- General enquiries: [email protected]
- Open access
- Published: 29 April 2024
What is context in knowledge translation? Results of a systematic scoping review
- Tugce Schmitt ORCID: orcid.org/0000-0001-6893-6428 1 ,
- Katarzyna Czabanowska 1 &
- Peter Schröder-Bäck 1
Health Research Policy and Systems volume 22 , Article number: 52 ( 2024 ) Cite this article
85 Accesses
4 Altmetric
Metrics details
Knowledge Translation (KT) aims to convey novel ideas to relevant stakeholders, motivating their response or action to improve people’s health. Initially, the KT literature focused on evidence-based medicine, applying findings from laboratory and clinical research to disease diagnosis and treatment. Since the early 2000s, the scope of KT has expanded to include decision-making with health policy implications.
This systematic scoping review aims to assess the evolving knowledge-to-policy concepts, that is, macro-level KT theories, models and frameworks (KT TMFs). While significant attention has been devoted to transferring knowledge to healthcare settings (i.e. implementing health policies, programmes or measures at the meso-level), the definition of 'context' in the realm of health policymaking at the macro-level remains underexplored in the KT literature. This study aims to close the gap.
A total of 32 macro-level KT TMFs were identified, with only a limited subset of them offering detailed insights into contextual factors that matter in health policymaking. Notably, the majority of these studies prompt policy changes in low- and middle-income countries and received support from international organisations, the European Union, development agencies or philanthropic entities.
Peer Review reports
Few concepts are used by health researchers as vaguely and yet as widely as Knowledge Translation (KT), a catch-all term that accommodates a broad spectrum of ambitions. Arguably, to truly understand the role of context in KT, we first need to clarify what KT means. The World Health Organization (WHO) defines KT as ‘the synthesis, exchange and application of knowledge by relevant stakeholders to accelerate the benefits of global and local innovation in strengthening health systems and improving people’s health’ [ 1 ]. Here, particular attention should be paid to ‘innovation’, given that without unpacking this term, the meaning of KT would still remain ambiguous. Rogers’ seminal work ‘Diffusion of Innovations’ [ 2 ] defines innovation as an idea, practice or object that is perceived as novel by individuals or groups adopting it. In this context, he argues that the objective novelty of an idea in terms of the amount of time passed after its discovery holds little significance [ 2 ]. Rather, it is the subjective perception of newness by the individual that shapes their response [ 2 ]. In other words, if an idea seems novel to individuals, and thereby relevant stakeholders according to the aforementioned WHO definition, it qualifies as an innovation. From this perspective, it can be stated that a fundamental activity of KT is to communicate ideas that could be perceived as original to the targeted stakeholders, with the aim of motivating their response to improve health outcomes. This leaves us with the question of who exactly these stakeholders might be and what kind of actions would be required from them.
The scope of stakeholders in KT has evolved over time, along with their prompted responses. Initially, during the early phases of KT, the focus primarily revolved around healthcare providers and their clinical decisions, emphasising evidence-based medicine. Nearly 50 years ago, the first scientific article on KT was published, introducing Tier 1 KT, which concentrated on applying laboratory discoveries to disease diagnosis or treatment, also known as bench-to-bedside KT [ 3 ]. The primary motivation behind this initial conceptualisation of KT was to engage healthcare providers as the end-users of specific forms of knowledge, primarily related to randomised controlled trials of pharmaceuticals and evidence-based medicine [ 4 ]. In the early 2000s, the second phase of KT (Tier 2) emerged under the term ‘campus-to-clinic KT’ [ 3 ]. This facet, also known as translational research, was concerned with using evidence from health services research in healthcare provision, both in practice and policy [ 4 ]. Consequently, by including decision-makers as relevant end-users, KT scholars expanded the realm of research-to-action from the clinical environment to policy-relevant decision-making [ 5 ]. Following this trajectory, additional KT schemes (Tier 3–Tier 5) have been introduced into academic discourse, encompassing the dissemination, implementation and broader integration of knowledge into public policies [ 6 , 7 ]. Notably, the latest scheme (Tier 5) is becoming increasingly popular and represents the broadest approach, which describes the translation of knowledge to global communities and aims to involve fundamental, universal change in attitudes, policies and social systems [ 7 ].
In other words, a noticeable shift in KT has occurred with time towards macro-level interventions, named initially as evidence- based policymaking and later corrected to evidence- informed policymaking. In parallel with these significant developments, various alternative terms to KT have emerged, including ‘implementation science’, ‘knowledge transfer’, and ‘dissemination and research use’, often with considerable overlap [ 8 ]. Arguably, among the plethora of alternative terms proposed, implementation science stands out prominently. While initially centred on evidence-based medicine at the meso-level (e.g. implementing medical guidelines), it has since broadened its focus to ‘encompass all aspects of research relevant to the scientific study of methods to promote the uptake of research findings into routine settings in clinical, community and policy contexts’ [ 9 ], closely mirroring the definition to KT. Thus, KT, along with activities under different names that share the same objective, has evolved into an umbrella term over the years, encompassing a wide range of strategies aimed at enhancing the impact of research not only on clinical practice but also on public policies [ 10 ]. Following the adoption of such a comprehensive definition of KT, some researchers have asserted that using evidence in public policies is not merely commendable but essential [ 11 ].
In alignment with the evolution of KT from (bio-)medical sciences to public policies, an increasing number of scholars have offered explanations on how health policies should be developed [ 12 ], indicating a growing focus on exploring the mechanisms of health policymaking in the KT literature. However, unlike in the earlier phases of KT, which aimed to transfer knowledge from the laboratory to healthcare provision, decisions made for public policies may be less technical and more complex than those in clinical settings [ 3 , 13 , 14 ]. Indeed, social scientists point out that scholarly works on evidence use in health policies exhibit theoretical shortcomings as they lack engagement with political science and public administration theories and concepts [ 15 , 16 , 17 , 18 ]; only a few of these works employ policy theories and political concepts to guide data collection and make sense of their findings [ 19 ]. Similarly, contemporary literature that conceptualises KT as an umbrella term for both clinical and public policy decision-making, with calls for a generic ‘research-to-action’ [ 20 ], may fail to recognise the different types of actions required to change clinical practices and influence health policies. In many respects, such calls can even lead to a misconception that evidence-informed policymaking is simply a scaled-up version of evidence-based medicine [ 21 ].
In this study, we systematically review knowledge translation theories, models and frameworks (also known as KT TMFs) that were developed for health policies. Essentially, KT TMFs can be depicted as bridges that connect findings across diverse studies, as they establish a common language and standardise the measurement and assessment of desired policy changes [ 22 ]. This makes them essential for generalising implementation efforts and research findings [ 23 ]. While distinctions between a theory, a model or a framework are not always crystal-clear [ 24 ], the following definitions shed light on how they are interpreted in the context of KT. To start with, theory can be described as a set of analytical principles or statements crafted to structure our observations, enhance our understanding and explain the world [ 24 ]. Within implementation science, theories are encapsulated as either generalised models or frameworks. In other words, they are integrated into broader concepts, allowing researchers to form assumptions that help clarify phenomena and create hypotheses for testing [ 25 ].
Whereas theories in the KT literature are explanatory as well as descriptive, KT models are only descriptive with a more narrowly defined scope of explanation [ 24 ]; hence they have a more specific focus than theories [ 25 ]. KT models are created to facilitate the formulation of specific assumptions regarding a set of parameters or variables, which can subsequently be tested against outcomes using predetermined methods [ 25 ]. By offering simplified representations of complex situations, KT models can describe programme elements expected to produce desired results, or theoretical constructs believed to influence or moderate observed outcomes. In this way, they encompass theories related to change or explanation [ 22 ].
Lastly, frameworks in the KT language define a set of variables and the relations among them in a broad sense [ 25 ]. Frameworks, without the aim of providing explanations, solely describe empirical phenomena, representing a structure, overview, outline, system or plan consisting of various descriptive categories and the relations between them that are presumed to account for a phenomenon [ 24 ]. They portray loosely-structured constellations of theoretical constructs, without necessarily specifying their relationships; they can also offer practical methods for achieving implementation objectives [ 22 ]. Some scholars suggest sub-classifications and categorise a framework as ‘actionable’ if it has the potential to facilitate macro-level policy changes [ 11 ].
Context, which encompasses the entire environment in which policy decisions are made, is not peripheral but central to policymaking, playing a crucial role in its conceptualisation [ 26 , 27 , 28 , 29 , 30 , 31 , 32 , 33 , 34 ]. In the KT literature, the term ‘context’ is frequently employed, albeit often with a lack of precision [ 35 ]. It tends to serve as a broad term including various elements within a situation that are relevant to KT in some way but have not been explicitly identified [36]. However, there is a growing interest in delving deeper into what context refers to, as evidenced by increasing research attention [ 31 , 32 , 37 , 38 , 39 , 40 , 41 ]. While the definition of context in the transfer of knowledge to healthcare settings (i.e. implementing health policies, programmes or measures at the meso-level) has been systematically studied [ 36 , 37 , 42 , 43 ], the question of how KT scholars detail context in health policymaking remains unanswered. With our systematic scoping review, we aim to close this gap.
While KT TMFs, emerged from evidence-based medicine, have historically depicted the use of evidence from laboratories or healthcare organisations as the gold standard, we aimed to assess in this study whether and to what extent the evolving face of KT, addressing health policies, succeeded in foregrounding ‘context’. Our objective was thus not to evaluate the quality of these KT TMFs but rather to explore how scholars have incorporated contextual influences into their reasoning. We conducted a systematic scoping review to explore KT TMFs that are relevant to agenda-setting, policy formulation or policy adoption, in line with the aim of this study. Therefore, publications related to policy implementation in healthcare organisations or at the provincial level, as well as those addressing policy evaluation, did not meet our inclusion criteria. Consequently, given our focus on macro-level interventions, we excluded all articles that concentrate on translating clinical research into practice (meso-level interventions) and health knowledge to patients or citizens (micro-level interventions).
Prior systematic scoping reviews in the area of KT TMFs serve as a valuable foundation upon which to build further studies [ 44 , 45 ]. Using established methodologies may ensure a validated approach, allowing for a more nuanced understanding of KT TMFs in the context of existing scholarly work. Our review methodology employed a similar approach to that followed by Strifler et al. in 2018, who conducted a systematic scoping review of KT TMFs in the field of cancer prevention and management, as well as other chronic diseases [ 44 ]. Their search strategy was preferred over others for two primary reasons. First, Strifler et al. investigated KT TMFs altogether, systematically and comprehensively. Second, unlike many other review studies on KT, they focused on macro-level KT and included all relevant keywords useful for the purpose of our study in their Ovid/MEDLINE search query [ 44 ]. For our scoping review, we adapted their search query with the assistance of a specialist librarian. This process involved eliminating terms associated with cancer and chronic diseases, removing time limitation on the published papers, and including an additional language other than English due to authors’ proficiency in German. We included articles published in peer-reviewed journals until November 2022, excluding opinion papers, conference abstracts and study protocols, without any restriction on publication date or place. Our search query is presented in Table 1 .
Following a screening methodology similar to that employed by Votruba et al. [ 11 ], the first author conducted an initial screening of the titles and abstracts of 2918 unique citations. Full texts were selected and scrutinised if they appeared relevant to the topics of agenda-setting, policy formulation or policy adoption. Among these papers, the first author also identified those that conceptualised a KT TMF. Simultaneously, the last author independently screened 2918 titles and abstracts, randomly selecting 20% of them to identify studies related to macro-level KT. Regarding papers that conceptualised a KT TMF, all those initially selected by the first author underwent a thorough examination by the last author as well. In the papers reviewed by these two authors of this study, KT TMFs were typically presented as either Tables or Figures. In cases where these visual representations did not contain sufficient information about ‘context’, the main body of the study was carefully scrutinised by both reviewers to ensure no relevant information was missed. Any unclear cases were discussed and resolved to achieve 100% inter-rater agreement between the first and second reviewers. This strategy resulted in the inclusion of 32 relevant studies. The flow chart outlining our review process is provided in Fig. 1 .
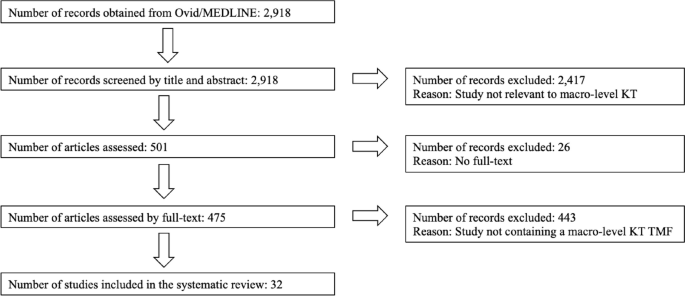
Flow chart of the review process
According to the results of our systematic scoping review (Table 2 ), the first KT TMF developed for health policies dates back to 2003, confirming the emergence of a trend that expanded the meaning of the term Knowledge Translation to include policymakers as end-users of evidence during approximately the same period. In their study, Jacobson et al. [ 46 ] present a framework derived from a literature review to enhance understanding of user groups by organising existing knowledge, identifying gaps and emphasising the importance of learning about new contexts. However, despite acknowledging the significance of the user group context, the paper lacks a thorough explanation of the authors’ understanding of this term. The second study in our scoping review provides some details. Recognising a shift from evidence-based medicine to evidence-based health policymaking in the KT literature, the article by Dobrow et al. from 2004 [ 30 ] emphasises the importance of considering contextual factors. They present a conceptual framework for evidence-based decision-making, highlighting the influence of context in KT. Illustrated through examples from colorectal cancer screening policy development, their conceptual framework emphasises the significance of context in the introduction, interpretation and application of evidence. Third, Lehoux et al. [ 47 ] examine the field of Health Technology Assessment (HTA) and its role in informing decision and policymaking in Canada. By developing a conceptual framework for HTA dissemination and use, they touch on the institutional environment and briefly describe contextual factors.
Notably, the first three publications in our scoping review are authored by scholars affiliated with Canada, which is less of a coincidence, given the role of Canadian Institutes of Health Research (CIHR), the federal funding agency for health research: The CIHR Act (Bill C-13) mandates CIHR to ensure that the translation of health knowledge permeates every aspect of its work [ 48 ]. Moreover, it was CIHR that coined the term Knowledge Translation, defining KT as ‘a dynamic and iterative process that includes the synthesis, dissemination, exchange and ethically sound application of knowledge to improve health, provide more effective health services and products, and strengthen the health care system’ [ 49 ] . This comprehensive definition has since been adapted by international organisations (IOs), including WHO. The first document published by WHO that utilised KT to influence health policies dates back to 2005, entitled ‘Bridging the “know-do” gap: Meeting on knowledge translation in global health’, an initiative that was supported by the Canadian Coalition for Global Health Research, the Canadian International Development Agency, the German Agency for Technical Cooperation and the WHO Special Programme on Research and Training in Tropical Diseases [ 1 ]. Following this official recognition by WHO, studies in our scoping review after 2005 indicate a noticeable expansion of KT, encompassing a wider geographical area than Canada.
The article of Ashford et al. from 2006 [ 50 ] discusses the challenge of policy decisions in Kenya in the health field being disconnected from scientific evidence and presents a model for translating knowledge into policy actions through agenda-setting, coalition building and policy learning. However, the framework lacks explicit incorporation of contextual factors influencing health policies. Bauman et al. [ 51 ] propose a six-step framework for successful dissemination of physical activity evidence, illustrated through four case studies from three countries (Canada, USA and Brazil) and a global perspective. They interpret contextual factors as barriers and facilitators to physical activity and public health innovations. Focusing on the USA, Gold [ 52 ] explains factors, processes and actors that shape pathways between research and its use in a summary diagram, including a reference to ‘other influences in process’ for context. Green et al. [ 4 ] examine the gap between health research and its application in public health without focusing on a specific geographical area. Their study comprehensively reviews various concepts of diffusion, dissemination and implementation in public health, proposing ways to blend diffusion theory with other theories. Their ‘utilization-focused surveillance framework’ interprets context as social determinants as structures, economics, politics and culture.
Further, the article by Dhonukshe-Rutten et al. from 2010 [ 53 ] presents a general framework that outlines the process of translating nutritional requirements into policy applications from a European perspective. The framework incorporates scientific evidence, stakeholder interests and the socio-political context. The description of this socio-political context is rather brief, encompassing political and social priorities, legal context, ethical issues and economic implications. Ir et al. [ 54 ] analyse the use of knowledge in shaping policy on health equity funds in Cambodia, with the objective of understanding how KT contributes to the development of health policies that promote equity. Yet no information on context is available in the framework that they suggest. A notable exception among these early KT TMFs until 2010 is the conceptual framework for analysing integration of targeted health interventions into health systems by Atun et al. [ 55 ], in which the authors provide details about the factors that have an influence on the process of bringing evidence to health policies. Focusing on the adoption, diffusion and assimilation of health interventions, their conceptual framework provides a systematic approach for evaluating and informing policies in this field. Compared to the previous studies discussed above, their definition of context for this framework is comprehensive (Table 2 ). Overall, most of the studies containing macro-level KT TMFs published until 2010 either do not fully acknowledge contextual factors or provide generic terms such as cultural, political and economic for brief description (9 out of 10; 90%).
Studies published after 2010 demonstrate a notable geographical shift, with a greater emphasis on low- and middle-income countries (LMICs). By taking the adoption of the directly observed treatment, short-course (DOTS) strategy for tuberculosis control in Mexico as a case study, Bissell et al. [ 56 ] examine policy transfer to Mexico and its relevance to operational research efforts and suggest a model for analysis of health policy transfer. The model interprets context as health system, including political, economic, social, cultural and technological features. Focusing on HIV/AIDS in India, Tran et al. [ 57 ] explore KT by considering various forms of evidence beyond scientific evidence, such as best practices derived from programme experience and disseminated through personal communication. Their proposed framework aims to offer an analytical tool for understanding how evidence-based influence is exerted. In their framework, no information is available on context. Next, Bertone et al. [ 58 ] report on the effectiveness of Communities of Practice (CoPs) in African countries and present a conceptual framework for analysing and assessing transnational CoPs in health policy. The framework organises the key elements of CoPs, linking available resources, knowledge management activities, policy and practice changes, and improvements in health outcomes. Context is only briefly included in this framework.
Some other studies include both European and global perspectives. The publication from Timotijevic et al. from 2013 [ 59 ] introduces an epistemological framework that examines the considerations influencing the policy-making process, with a specific focus on micronutrient requirements in Europe. They present case studies from several European countries, highlighting the relevance of the framework in understanding the policy context related to micronutrients. Context is interpreted in this framework as global trends, data, media, broader consumer beliefs, ethical considerations, and wider social, legal, political, and economic environment. Next, funded by the European Union, the study by Onwujekwe et al. [ 60 ] examines the role of different types of evidence in health policy development in Nigeria. Although they cover the factors related to policy actors in their framework for assessing the role of evidence in policy development, they provide no information on context. Moreover, Redman et al. [ 61 ] present the SPIRIT Action Framework, which aims to enhance the use of research in policymaking. Context is interpreted in this framework as policy influences, i.e. public opinion, media, economic climate, legislative/policy infrastructure, political ideology and priorities, stakeholder interests, expert advice, and resources. From a global perspective, Spicer et al. [ 62 ] explore the contextual factors that influenced the scale-up of donor-funded maternal and newborn health innovations in Ethiopia, India and Nigeria, highlighting the importance of context in assessing and adapting innovations. Their suggested contextual factors influencing government decisions to accept, adopt and finance innovations at scale are relatively comprehensive (Table 2 ).
In terms of publication frequency, the pinnacle of reviewed KT studies was in 2017. Among six studies published in 2017, four lack details about context in their KT conceptualisations and one study touches on context very briefly. Bragge et al. [ 5 ] brought for their study an international terminology working group together to develop a simplified framework of interventions to integrate evidence into health practices, systems, and policies, named as the Aims, Ingredients, Mechanism, Delivery framework, albeit without providing details on contextual factors. Second, Mulvale et al. [ 63 ] present a conceptual framework that explores the impact of policy dialogues on policy development, illustrating how these dialogues can influence different stages of the policy cycle. Similar to the previous one, this study too, lacks information on context. In a systematic review, Sarkies et al. [ 64 ] evaluate the effectiveness of research implementation strategies in promoting evidence-informed policy decisions in healthcare. The study explores the factors associated with effective strategies and their inter-relationship, yet without further information on context. Fourth, Houngbo et al. [ 65 ] focus on the development of a strategy to implement a good governance model for health technology management in the public health sector, drawing from their experience in Benin. They outline a six-phase model that includes preparatory analysis, stakeholder identification and problem analysis, shared analysis and visioning, development of policy instruments for pilot testing, policy development and validation, and policy implementation and evaluation. They provide no information about context in their model. Fifth, Mwendera et al. [ 66 ] present a framework for improving the use of malaria research in policy development in Malawi, which was developed based on case studies exploring the policymaking process, the use of local malaria research, and assessing facilitators and barriers to research utilisation. Contextual setting is considered as Ministry of Health (MoH) with political set up, leadership system within the MoH, government policies and cultural set up. In contrast to these five studies, Ellen et al. [ 67 ] present a relatively comprehensive framework to support evidence-informed policymaking in ageing and health. The framework includes thought-provoking questions to discover contextual factors (Table 2 ).
Continuing the trend, studies published after 2017 focus increasingly on LMICs. In their embedded case study, Ongolo-Zogo et al. [ 68 ] examine the influence of two Knowledge Translation Platforms (KTPs) on policy decisions to achieve the health millennium development goals in Cameroon and Uganda. It explores how these KTPs influenced policy through interactions within policy issue networks, engagement with interest groups, and the promotion of evidence-supported ideas, ultimately shaping the overall policy climate for evidence-informed health system policymaking. Contextual factors are thereby interpreted as institutions (structures, legacies, policy networks), interests, ideas (values, research evidence) and external factors (reports, commitments). Focusing on the ‘Global South’, Plamondon et al. [ 69 ] suggest blending integrated knowledge translation with global health governance as an approach for strengthening leadership for health equity action. In terms of contextual factors, they include some information such as adapting knowledge to local context, consideration of the composition of non-traditional actors, such as civil society and private sector, in governance bodies and guidance for meaningful engagement between actors, particularly in shared governance models. Further, Vincenten et al. [ 70 ] propose a conceptual model to enhance understanding of interlinking factors that influence the evidence implementation process. Their evidence implementation model for public health systems refers to ‘context setting’, albeit without providing further detail.
Similarly, the study by Motani et al. from 2019 [ 71 ] assesses the outcomes and lessons learned from the EVIDENT partnership that focused on knowledge management for evidence-informed decision-making in nutrition and health in Africa. Although they mention ‘contextualising evidence’ in their conceptual framework, information about context is lacking. Focusing on Latin America and the Caribbean, Varallyay et al. [ 72 ] introduce a conceptual framework for evaluating embedded implementation research in various contexts. The framework outlines key stages of evidence-informed decision-making and provides guidance on assessing embeddedness and critical contextual factors. Compared to others, their conceptual framework provides a relatively comprehensive elaboration on contextual factors. In addition, among all the studies reviewed, Leonard et al. [ 73 ] present an exceptionally comprehensive analysis, where they identify the facilitators and barriers to the sustainable implementation of evidence-based health innovations in LMICs. Through a systematic literature review, they scrutinise 79 studies and categorise the identified barriers and facilitators into seven groups: context, innovation, relations and networks, institutions, knowledge, actors, and resources. The first one, context, contains rich information that could be seen in Table 2 .
Continuing from LMICs, Votruba et al. [ 74 ] present in their study the EVITA (EVIdence To Agenda setting) conceptual framework for mental health research-policy interrelationships in LMICs with some information about context, detailed as external influences and political context. In a follow-up study, they offer an updated framework for understanding evidence-based mental health policy agenda-setting [ 75 ]. In their revised framework, context is interpreted as external context and policy sphere, encompassing policy agenda, window of opportunity, political will and key individuals. Lastly, to develop a comprehensive monitoring and evaluation framework for evidence-to-policy networks, Kuchenmüller et al. [ 76 ] present the EVIPNet Europe Theory of Change and interpret contextual factors for evidence-informed policymaking as political, economic, logistic and administrative. Overall, it can be concluded that studies presenting macro-level KT TMFs from 2011 until 2022 focus mainly on LMICs (15 out of 22; close to 70%) and the majority of them were funded by international (development) organisations, the European Commission and global health donor agencies. An overwhelming number of studies among them (19 out of 22; close to 90%) provide either no information on contextual details or these were included only partly with some generic terms in KT TMFs.
Our systematic scoping review suggests that the approach of KT, which has evolved from evidence-based medicine to evidence-informed policymaking, tends to remain closely tied to its clinical origins when developing TMFs. In other words, macro-level KT TMFs place greater emphasis on the (public) health issue at hand rather than considering the broader decision-making context, a viewpoint shared by other scholars as well [ 30 ]. One reason could be that in the early stages of KT TMFs, the emphasis primarily focused on implementing evidence-based practices within clinical settings. At that time, the spotlight was mostly on content, including aspects like clinical studies, checklists and guidelines serving as the evidence base. In those meso-level KT TMFs, a detailed description of context, i.e. the overall environment in which these practices should be implemented, might have been deemed less necessary, given that healthcare organisations, such as hospitals to implement medical guidelines or surgical safety checklists, show similar characteristics globally.
However, as the scope of KT TMFs continues to expand to include the influence on health policies, a deeper understanding of context-specific factors within different jurisdictions and the dynamics of the policy process is becoming increasingly crucial. This is even more important for KT scholars aiming to conceptualise large-scale changes, as described in KT Tier 5, which necessitate a thorough understanding of targeted behaviours within societies. As the complexity of interventions increases due to the growing number of stakeholders either affecting or being affected by them, the interventions are surrounded by a more intricate web of attitudes, incentives, relationships, rules of engagement and spheres of influence [ 7 ]. The persisting emphasis on content over context in the evolving field of KT may oversimplify the complex process of using evidence in policymaking and understanding the society [ 77 ]. Some scholars argue that this common observation in public health can be attributed to the dominance of experts primarily from medical sciences [ 78 , 79 , 80 ]. Our study confirms the potential limitation of not incorporating insights from political science and public policy studies, which can lead to what is often termed a ‘naïve’ conceptualisation of evidence-to-policy schemes [ 15 , 16 , 17 ]. It is therefore strongly encouraged that the emerging macro-level KT concepts draw on political science and public administration if KT scholars intend to effectively communicate new ideas to policymakers, with the aim of prompting their action or response. We summarised our findings into three points.
Firstly, KT scholars may want to identify and pinpoint exactly where a change should occur within the policy process. The main confusion that we observed in the KT literature arises from a lack of understanding of how public policies are made. Notably, the term ‘evidence-informed policymaking’ can refer to any stage of the policy cycle, spanning from agenda-setting to policy formulation, adoption, implementation and evaluation. Understanding these steps will allow researchers to refine their language when advocating for policy changes across various jurisdictions; for instance, the word ‘implementation’ is often inappropriately used in KT literature. As commonly known, at the macro-level, public policies take the form of legislation, law-making and regulation, thereby shaping the practices or policies to be implemented at the meso- and micro-levels [ 81 ]. In other words, the process of using specific knowledge to influence health policies, however evidence-based it might be, falls mostly under the responsibility and jurisdiction of sovereign states. For this reason, macro-level KT TMFs should reflect the importance of understanding the policy context and the complexities associated with policymaking, rather than suggesting flawed or unrealistic top-down ‘implementation’ strategies in countries by foregrounding the content, or the (public) health issue at hand.
Our second observation from this systematic scoping review points towards a selective perception among researchers when reporting on policy interventions. Research on KT does not solely exist due to the perceived gap between scientific evidence and policy but also because of the pressures the organisations or researchers face in being accountable to their funding sources, ensuring the continuity of financial support for their activities and claiming output legitimacy to change public policies [ 8 ]. This situation indirectly compels researchers working to influence health policies in the field to provide ‘evidence-based’ feedback on the success of their projects to donors [ 82 ]. In doing so, researchers may overly emphasise the content of the policy intervention in their reporting to secure further funding, while they underemphasis the contextual factors. These factors, often perceived as a given, might actually be the primary facilitators of their success. Such a lack of transparency regarding the definition of context is particularly visible in the field of global health, where LMICs often rely on external donors. It is important to note that this statement is not intended as a negative critique of their missions or an evaluation of health outcomes in countries following such missions. Rather, it seeks to explain the underlying reason why researchers, particularly those reliant on donors in LMICs, prioritise promoting the concept of KT from a technical standpoint, giving less attention to contextual factors in their reasoning.
Lastly, and connected to the previous point, it is our observation that the majority of macro-level KT TMFs fail to give adequate consideration to both power dynamics in countries (internal vs. external influences) and the actual role that government plays in public policies. Notably, although good policymaking entails an honest effort to use the best available evidence, the belief that this will completely negate the role of power and politics in decision-making is a technocratic illusion [ 83 ]. Among the studies reviewed, the framework put forth by Leonard et al. [ 73 ] offers the most comprehensive understanding of context and includes a broad range of factors (such as political, social, and economic) discovered also in other reviewed studies. Moreover, the framework, developed through an extensive systematic review, offers a more in-depth exploration of these contextual factors than merely listing them as a set of keywords. Indeed, within the domains of political science and public policy, such factors shaping health policies have received considerable scholarly attention for decades. To define what context entails, Walt refers in her book ‘Health Policy: An Introduction to Process and Power’ [ 84 ] to the work of Leichter from 1979 [ 85 ], who provides a scheme for analysing public policy. This includes i) situational factors, which are transient, impermanent, or idiosyncratic; ii) structural factors, which are relatively unchanging elements of the society and polity; iii) cultural factors, which are value commitments of groups; and iv) environmental factors, which are events, structures and values that exist outside the boundaries of a political system and influence decisions within it. His detailed sub-categories for context can be found in Table 3 . This flexible public policy framework may offer KT researchers a valuable approach to understanding contextual factors and provide some guidance to define the keywords to focus on. Scholars can adapt this framework to suit a wide range of KT topics, creating more context-sensitive and comprehensive KT TMFs.
Admittedly, our study has certain limitations. Despite choosing one of the most comprehensive bibliographic databases for our systematic scoping review, which includes materials from biomedicine, allied health fields, biological and physical sciences, humanities, and information science in relation to medicine and healthcare, we acknowledge that we may have missed relevant articles indexed in other databases. Hence, exclusively using Ovid/MEDLINE due to resource constraints may have narrowed the scope and diversity of scholarly literature examined in this study. Second, our review was limited to peer-reviewed publications in English and German. Future studies could extend our findings by examining the extent to which contextual factors are detailed in macro-level KT TMFs published in grey literature and in different languages. Given the abundance of KT reports, working papers or policy briefs published by IOs and development agencies, such an endeavour could enrich our findings and either support or challenge our conclusions. Nonetheless, to our knowledge, this study represents the first systematic review and critical appraisal of emerging knowledge-to-policy concepts, also known as macro-level KT TMFs. It successfully blends insights from both biomedical and public policy disciplines, and could serve as a roadmap for future research.
The translation of knowledge to policymakers involves more than technical skills commonly associated with (bio-)medical sciences, such as creating evidence-based guidelines or clinical checklists. Instead, evidence-informed policymaking reflects an ambition to engage in the political dimensions of states. Therefore, the evolving KT concepts addressing health policies should be seen as a political decision-making process, rather than a purely analytical one, as is the case with evidence-based medicine. To better understand the influence of power dynamics and governance structures in policymaking, we suggest that future macro-level KT TMFs draw on insights from political science and public administration. Collaborative, interdisciplinary research initiatives could be undertaken to bridge the gap between these fields. Technocratic KT TMFs that overlook contextual factors risk propagating misconceptions in academic circles about how health policies are made, as they become increasingly influential over time. Research, the systematic pursuit of knowledge, is neither inherently good nor bad; it can be sought after, used or misused, like any other tool in policymaking. What is needed in the KT discourse is not another generic call for ‘research-to-action’ but rather an understanding of the dividing line between research-to- clinical -action and research-to- political -action.
Availability of data and materials
Available upon reasonable request.
WHO. Bridging the ‘Know-Do’ Gap: Meeting on Knowledge Translation in Global Health : 10–12 October 2005 World Health Organization Geneva, Switzerland [Internet]. 2005. https://www.measureevaluation.org/resources/training/capacity-building-resources/high-impact-research-training-curricula/bridging-the-know-do-gap.pdf
Rogers EM. Diffusion of innovations. 3rd ed. New York: Free Press; 1983.
Google Scholar
Greenhalgh T, Wieringa S. Is it time to drop the ‘knowledge translation’ metaphor? A critical literature review. J R Soc Med. 2011;104(12):501–9.
Article PubMed PubMed Central Google Scholar
Green LW, Ottoson JM, García C, Hiatt RA. Diffusion theory and knowledge dissemination, utilization, and integration in public health. Annu Rev Public Health. 2009;30(1):151–74.
Article PubMed Google Scholar
Bragge P, Grimshaw JM, Lokker C, Colquhoun H, Albrecht L, Baron J, et al. AIMD—a validated, simplified framework of interventions to promote and integrate evidence into health practices, systems, and policies. BMC Med Res Methodol. 2017;17(1):38.
Zarbin M. What Constitutes Translational Research? Implications for the Scope of Translational Vision Science and Technology. Transl Vis Sci Technol 2020;9(8).
Hassmiller Lich K, Frerichs L, Fishbein D, Bobashev G, Pentz MA. Translating research into prevention of high-risk behaviors in the presence of complex systems: definitions and systems frameworks. Transl Behav Med. 2016;6(1):17–31.
Tetroe JM, Graham ID, Foy R, Robinson N, Eccles MP, Wensing M, et al. Health research funding agencies’ support and promotion of knowledge translation: an international study. Milbank Q. 2008;86(1):125–55.
Eccles MP, Mittman BS. Welcome to Implementation Science. Implement Sci. 2006;1(1):1.
Article PubMed Central Google Scholar
Rychetnik L, Bauman A, Laws R, King L, Rissel C, Nutbeam D, et al. Translating research for evidence-based public health: key concepts and future directions. J Epidemiol Community Health. 2012;66(12):1187–92.
Votruba N, Ziemann A, Grant J, Thornicroft G. A systematic review of frameworks for the interrelationships of mental health evidence and policy in low- and middle-income countries. Health Res Policy Syst. 2018;16(1):85.
Delnord M, Tille F, Abboud LA, Ivankovic D, Van Oyen H. How can we monitor the impact of national health information systems? Results from a scoping review. Eur J Public Health. 2020;30(4):648–59.
Malterud K, Bjelland AK, Elvbakken KT. Evidence-based medicine—an appropriate tool for evidence-based health policy? A case study from Norway. Health Res Policy Syst. 2016;14(1):15.
Borst RAJ, Kok MO, O’Shea AJ, Pokhrel S, Jones TH, Boaz A. Envisioning and shaping translation of knowledge into action: a comparative case-study of stakeholder engagement in the development of a European tobacco control tool. Health Policy. 2019;123(10):917–23.
Liverani M, Hawkins B, Parkhurst JO. Political and institutional influences on the use of evidence in public health policy: a systematic review. PLoS ONE. 2013;8(10): e77404.
Article CAS PubMed PubMed Central Google Scholar
Cairney P. The politics of evidence-based policy making, 1st ed. London: Palgrave Macmillan UK: Imprint: Palgrave Pivot, Palgrave Macmillan; 2016.
Parkhurst J. The Politics of Evidence: From evidence-based policy to the good governance of evidence [Internet]. Routledge; 2016. https://www.taylorfrancis.com/books/9781315675008
Cairney P, Oliver K. Evidence-based policymaking is not like evidence-based medicine, so how far should you go to bridge the divide between evidence and policy? Health Res Policy Syst. 2017;15(1):35.
Verboom B, Baumann A. Mapping the Qualitative Evidence Base on the Use of Research Evidence in Health Policy-Making: A Systematic Review. Int J Health Policy Manag. 2020;16.
Ward V, House A, Hamer S. Developing a framework for transferring knowledge into action: a thematic analysis of the literature. J Health Serv Res Policy. 2009;14(3):156–64.
Swinburn B, Gill T, Kumanyika S. Obesity prevention: a proposed framework for translating evidence into action. Obes Rev. 2005;6(1):23–33.
Article CAS PubMed Google Scholar
Damschroder LJ. Clarity out of chaos: Use of theory in implementation research. Psychiatry Res. 2020;283: 112461.
Birken SA, Rohweder CL, Powell BJ, Shea CM, Scott J, Leeman J, et al. T-CaST: an implementation theory comparison and selection tool. Implement Sci. 2018;13(1):143.
Nilsen P. Making sense of implementation theories, models and frameworks. Implement Sci. 2015;10(1):53.
Rapport F, Clay-Williams R, Churruca K, Shih P, Hogden A, Braithwaite J. The struggle of translating science into action: foundational concepts of implementation science. J Eval Clin Pract. 2018;24(1):117–26.
Hagenaars LL, Jeurissen PPT, Klazinga NS. The taxation of unhealthy energy-dense foods (EDFs) and sugar-sweetened beverages (SSBs): An overview of patterns observed in the policy content and policy context of 13 case studies. Health Policy. 2017;121(8):887–94.
Sheikh K, Gilson L, Agyepong IA, Hanson K, Ssengooba F, Bennett S. Building the field of health policy and systems research: framing the questions. PLOS Med. 2011;8(8): e1001073.
Tran NT, Hyder AA, Kulanthayan S, Singh S, Umar RSR. Engaging policy makers in road safety research in Malaysia: a theoretical and contextual analysis. Health Policy. 2009;90(1):58–65.
Walt G, Gilson L. Reforming the health sector in developing countries: the central role of policy analysis. Health Policy Plan. 1994;9(4):353–70.
Dobrow MJ, Goel V, Upshur REG. Evidence-based health policy: context and utilisation. Soc Sci Med. 2004;58(1):207–17.
Barnfield A, Savolainen N, Lounamaa A. Health Promotion Interventions: Lessons from the Transfer of Good Practices in CHRODIS-PLUS. Int J Environ Res Public Health. 2020;17(4).
van de Goor I, Hämäläinen RM, Syed A, Juel Lau C, Sandu P, Spitters H, et al. Determinants of evidence use in public health policy making: results from a study across six EU countries. Health Policy Amst Neth. 2017;121(3):273–81.
Article Google Scholar
Ornstein JT, Hammond RA, Padek M, Mazzucca S, Brownson RC. Rugged landscapes: complexity and implementation science. Implement Sci. 2020;15(1):85.
Seward N, Hanlon C, Hinrichs-Kraples S, Lund C, Murdoch J, Taylor Salisbury T, et al. A guide to systems-level, participatory, theory-informed implementation research in global health. BMJ Glob Health. 2021;6(12): e005365.
Pfadenhauer LM, Gerhardus A, Mozygemba K, Lysdahl KB, Booth A, Hofmann B, et al. Making sense of complexity in context and implementation: the Context and Implementation of Complex Interventions (CICI) framework. Implement Sci. 2017;12(1):21.
Rogers L, De Brún A, McAuliffe E. Defining and assessing context in healthcare implementation studies: a systematic review. BMC Health Serv Res. 2020;20(1):591.
Nilsen P, Bernhardsson S. Context matters in implementation science: a scoping review of determinant frameworks that describe contextual determinants for implementation outcomes. BMC Health Serv Res. 2019;19(1):189.
Arksey H, O’Malley L, Baldwin S, Harris J, Mason A, Golder S. Literature review report: services to support carers of people with mental health problems. 2002;182.
Tabak RG, Khoong EC, Chambers D, Brownson RC. Bridging research and practice. Am J Prev Med. 2012;43(3):337–50.
O’Donovan MA, McCallion P, McCarron M, Lynch L, Mannan H, Byrne E. A narrative synthesis scoping review of life course domains within health service utilisation frameworks. HRB Open Res. 2019.
Michie S, Johnston M, Abraham C, Lawton R, Parker D, Walker A, et al. Making psychological theory useful for implementing evidence based practice: a consensus approach. Qual Saf Health Care. 2005;14(1):26–33.
Bate P, Robert G, Fulop N, Øvretviet J, Dixon-Woods M. Perspectives on context: a collection of essays considering the role of context in successful quality improvement [Internet]. 2014. https://www.health.org.uk/sites/default/files/PerspectivesOnContext_fullversion.pdf
Ziemann A, Brown L, Sadler E, Ocloo J, Boaz A, Sandall J. Influence of external contextual factors on the implementation of health and social care interventions into practice within or across countries—a protocol for a ‘best fit’ framework synthesis. Syst Rev. 2019. https://doi.org/10.1186/s13643-019-1180-8 .
Strifler L, Cardoso R, McGowan J, Cogo E, Nincic V, Khan PA, et al. Scoping review identifies significant number of knowledge translation theories, models, and frameworks with limited use. J Clin Epidemiol. 2018;100:92–102.
Esmail R, Hanson HM, Holroyd-Leduc J, Brown S, Strifler L, Straus SE, et al. A scoping review of full-spectrum knowledge translation theories, models, and frameworks. Implement Sci. 2020;15(1):11.
Jacobson N, Butterill D, Goering P. Development of a framework for knowledge translation: understanding user context. J Health Serv Res Policy. 2003;8(2):94–9.
Lehoux P, Denis JL, Tailliez S, Hivon M. Dissemination of health technology assessments: identifying the visions guiding an evolving policy innovation in Canada. J Health Polit Policy Law. 2005;30(4):603–42.
Parliament of Canada. Government Bill (House of Commons) C-13 (36–2) - Royal Assent - Canadian Institutes of Health Research Act [Internet]. https://parl.ca/DocumentViewer/en/36-2/bill/C-13/royal-assent/page-31 . Accessed 1 Apr 2023.
Straus SE, Tetroe J, Graham I. Defining knowledge translation. CMAJ Can Med Assoc J. 2009;181(3–4):165–8.
Ashford L. Creating windows of opportunity for policy change: incorporating evidence into decentralized planning in Kenya. Bull World Health Organ. 2006;84(8):669–72.
Bauman AE, Nelson DE, Pratt M, Matsudo V, Schoeppe S. Dissemination of physical activity evidence, programs, policies, and surveillance in the international public health arena. Am J Prev Med. 2006;31(4):57–65.
Gold M. Pathways to the use of health services research in policy. Health Serv Res. 2009;44(4):1111–36.
Dhonukshe-Rutten RAM, Timotijevic L, Cavelaars AEJM, Raats MM, de Wit LS, Doets EL, et al. European micronutrient recommendations aligned: a general framework developed by EURRECA. Eur J Clin Nutr. 2010;64(2):S2-10.
Ir P, Bigdeli M, Meessen B, Van Damme W. Translating knowledge into policy and action to promote health equity: The Health Equity Fund policy process in Cambodia 2000–2008. Health Policy. 2010;96(3):200–9.
Atun R, de Jongh T, Secci F, Ohiri K, Adeyi O. Integration of targeted health interventions into health systems: a conceptual framework for analysis. Health Policy Plan. 2010;25(2):104–11.
Bissell K, Lee K, Freeman R. Analysing policy transfer: perspectives for operational research. Int J Tuberc Lung Dis Off J Int Union Tuberc Lung Dis. 2011;15(9).
Tran NT, Bennett SC, Bishnu R, Singh S. Analyzing the sources and nature of influence: how the Avahan program used evidence to influence HIV/AIDS prevention policy in India. Implement Sci. 2013;8(1):44.
Bertone MP, Meessen B, Clarysse G, Hercot D, Kelley A, Kafando Y, et al. Assessing communities of practice in health policy: a conceptual framework as a first step towards empirical research. Health Res Policy Syst. 2013;11(1):39.
Timotijevic L, Brown KA, Lähteenmäki L, de Wit L, Sonne AM, Ruprich J, et al. EURRECA—a framework for considering evidence in public health nutrition policy development. Crit Rev Food Sci Nutr. 2013;53(10):1124–34.
Onwujekwe O, Uguru N, Russo G, Etiaba E, Mbachu C, Mirzoev T, et al. Role and use of evidence in policymaking: an analysis of case studies from the health sector in Nigeria. Health Res Policy Syst. 2015;13(1):46.
Redman S, Turner T, Davies H, Williamson A, Haynes A, Brennan S, et al. The SPIRIT action framework: a structured approach to selecting and testing strategies to increase the use of research in policy. Soc Sci Med. 2015;136–137:147–55.
Spicer N, Berhanu D, Bhattacharya D, Tilley-Gyado RD, Gautham M, Schellenberg J, et al. ‘The stars seem aligned’: a qualitative study to understand the effects of context on scale-up of maternal and newborn health innovations in Ethiopia, India and Nigeria. Glob Health. 2016;12(1):75.
Mulvale G, McRae SA, Milicic S. Teasing apart “the tangled web” of influence of policy dialogues: lessons from a case study of dialogues about healthcare reform options for Canada. Implement Sci IS. 2017;12.
Sarkies MN, Bowles KA, Skinner EH, Haas R, Lane H, Haines TP. The effectiveness of research implementation strategies for promoting evidence-informed policy and management decisions in healthcare: a systematic review. Implement Sci. 2017;12(1):132.
Houngbo PTh, Coleman HLS, Zweekhorst M, De Cock Buning TJ, Medenou D, Bunders JFG. A Model for Good Governance of Healthcare Technology Management in the Public Sector: Learning from Evidence-Informed Policy Development and Implementation in Benin. PLoS ONE. 2017;12(1):e0168842.
Mwendera C, de Jager C, Longwe H, Hongoro C, Phiri K, Mutero CM. Development of a framework to improve the utilisation of malaria research for policy development in Malawi. Health Res Policy Syst. 2017;15(1):97.
Ellen ME, Panisset U, de AraujoCarvalho I, Goodwin J, Beard J. A knowledge translation framework on ageing and health. Health Policy. 2017;121(3):282–91.
Ongolo-Zogo P, Lavis JN, Tomson G, Sewankambo NK. Assessing the influence of knowledge translation platforms on health system policy processes to achieve the health millennium development goals in Cameroon and Uganda: a comparative case study. Health Policy Plan. 2018;33(4):539–54.
Plamondon KM, Pemberton J. Blending integrated knowledge translation with global health governance: an approach for advancing action on a wicked problem. Health Res Policy Syst. 2019;17(1):24.
Vincenten J, MacKay JM, Schröder-Bäck P, Schloemer T, Brand H. Factors influencing implementation of evidence-based interventions in public health systems—a model. Cent Eur J Public Health. 2019;27(3):198–203.
Motani P, Van de Walle A, Aryeetey R, Verstraeten R. Lessons learned from Evidence-Informed Decision-Making in Nutrition & Health (EVIDENT) in Africa: a project evaluation. Health Res Policy Syst. 2019;17(1):12.
Varallyay NI, Langlois EV, Tran N, Elias V, Reveiz L. Health system decision-makers at the helm of implementation research: development of a framework to evaluate the processes and effectiveness of embedded approaches. Health Res Policy Syst. 2020;18(1):64.
Leonard E, de Kock I, Bam W. Barriers and facilitators to implementing evidence-based health innovations in low- and middle-income countries: a systematic literature review. Eval Program Plann. 2020;82: 101832.
Votruba N, Grant J, Thornicroft G. The EVITA framework for evidence-based mental health policy agenda setting in low- and middle-income countries. Health Policy Plan. 2020;35(4):424–39.
Votruba N, Grant J, Thornicroft G. EVITA 2.0, an updated framework for understanding evidence-based mental health policy agenda-setting: tested and informed by key informant interviews in a multilevel comparative case study. Health Res Policy Syst. 2021;19(1):35.
Kuchenmüller T, Chapman E, Takahashi R, Lester L, Reinap M, Ellen M, et al. A comprehensive monitoring and evaluation framework for evidence to policy networks. Eval Program Plann. 2022;91: 102053.
Ettelt S. The politics of evidence use in health policy making in Germany—the case of regulating hospital minimum volumes. J Health Polit Policy Law. 2017;42(3):513–38.
Greer SL, Bekker M, de Leeuw E, Wismar M, Helderman JK, Ribeiro S, et al. Policy, politics and public health. Eur J Public Health. 2017;27(suppl 4):40–3.
Fafard P, Cassola A. Public health and political science: challenges and opportunities for a productive partnership. Public Health. 2020;186:107–9.
Löblová O. Epistemic communities and experts in health policy-making. Eur J Public Health. 2018;28(suppl 3):7–10.
Maddalena V. Evidence-Based Decision-Making 8: Health Policy, a Primer for Researchers. In: Parfrey PS, Barrett BJ, editors. Clinical Epidemiology: Practice and Methods. New York, NY: Springer; 2015. (Methods in Molecular Biology).
Louis M, Maertens L. Why international organizations hate politics - Depoliticizing the world [Internet]. London and New York: Routledge; 2021. (Global Institutions). https://library.oapen.org/bitstream/handle/20.500.12657/47578/1/9780429883279.pdf
Hassel A, Wegrich K. How to do public policy. 1st ed. Oxford: Oxford University Press; 2022.
Book Google Scholar
Walt G. Health policy: an introduction to process and power. 7th ed. Johannesburg: Witwatersrand University Press; 2004.
Leichter HM. A comparative approach to policy analysis: health care policy in four nations. Cambridge: Cambridge University Press; 1979.
Download references
Acknowledgements
Not applicable.
Author information
Authors and affiliations.
Department of International Health, Care and Public Health Research Institute – CAPHRI, Faculty of Health, Medicine and Life Sciences, Maastricht University, Maastricht, The Netherlands
Tugce Schmitt, Katarzyna Czabanowska & Peter Schröder-Bäck
You can also search for this author in PubMed Google Scholar
Contributions
TS: Conceptualization, Methodology, Formal analysis, Investigation, Writing—Original Draft; KC: Writing—Review & Editing; PSB: Validation, Formal analysis, Writing—Review & Editing, Supervision.
Corresponding author
Correspondence to Tugce Schmitt .
Ethics declarations
Ethics approval and consent to participate, consent for publication, competing interests, additional information, publisher's note.
Springer Nature remains neutral with regard to jurisdictional claims in published maps and institutional affiliations.
Rights and permissions
Open Access This article is licensed under a Creative Commons Attribution 4.0 International License, which permits use, sharing, adaptation, distribution and reproduction in any medium or format, as long as you give appropriate credit to the original author(s) and the source, provide a link to the Creative Commons licence, and indicate if changes were made. The images or other third party material in this article are included in the article's Creative Commons licence, unless indicated otherwise in a credit line to the material. If material is not included in the article's Creative Commons licence and your intended use is not permitted by statutory regulation or exceeds the permitted use, you will need to obtain permission directly from the copyright holder. To view a copy of this licence, visit http://creativecommons.org/licenses/by/4.0/ . The Creative Commons Public Domain Dedication waiver ( http://creativecommons.org/publicdomain/zero/1.0/ ) applies to the data made available in this article, unless otherwise stated in a credit line to the data.
Reprints and permissions
About this article
Cite this article.
Schmitt, T., Czabanowska, K. & Schröder-Bäck, P. What is context in knowledge translation? Results of a systematic scoping review. Health Res Policy Sys 22 , 52 (2024). https://doi.org/10.1186/s12961-024-01143-5
Download citation
Received : 26 June 2023
Accepted : 11 April 2024
Published : 29 April 2024
DOI : https://doi.org/10.1186/s12961-024-01143-5
Share this article
Anyone you share the following link with will be able to read this content:
Sorry, a shareable link is not currently available for this article.
Provided by the Springer Nature SharedIt content-sharing initiative
- Knowledge Translation
- Evidence-informed policymaking
- Health systems
Health Research Policy and Systems
ISSN: 1478-4505
- Submission enquiries: Access here and click Contact Us
- General enquiries: [email protected]
- Open access
- Published: 18 April 2024
Research ethics and artificial intelligence for global health: perspectives from the global forum on bioethics in research
- James Shaw 1 , 13 ,
- Joseph Ali 2 , 3 ,
- Caesar A. Atuire 4 , 5 ,
- Phaik Yeong Cheah 6 ,
- Armando Guio Español 7 ,
- Judy Wawira Gichoya 8 ,
- Adrienne Hunt 9 ,
- Daudi Jjingo 10 ,
- Katherine Littler 9 ,
- Daniela Paolotti 11 &
- Effy Vayena 12
BMC Medical Ethics volume 25 , Article number: 46 ( 2024 ) Cite this article
1145 Accesses
6 Altmetric
Metrics details
The ethical governance of Artificial Intelligence (AI) in health care and public health continues to be an urgent issue for attention in policy, research, and practice. In this paper we report on central themes related to challenges and strategies for promoting ethics in research involving AI in global health, arising from the Global Forum on Bioethics in Research (GFBR), held in Cape Town, South Africa in November 2022.
The GFBR is an annual meeting organized by the World Health Organization and supported by the Wellcome Trust, the US National Institutes of Health, the UK Medical Research Council (MRC) and the South African MRC. The forum aims to bring together ethicists, researchers, policymakers, research ethics committee members and other actors to engage with challenges and opportunities specifically related to research ethics. In 2022 the focus of the GFBR was “Ethics of AI in Global Health Research”. The forum consisted of 6 case study presentations, 16 governance presentations, and a series of small group and large group discussions. A total of 87 participants attended the forum from 31 countries around the world, representing disciplines of bioethics, AI, health policy, health professional practice, research funding, and bioinformatics. In this paper, we highlight central insights arising from GFBR 2022.
We describe the significance of four thematic insights arising from the forum: (1) Appropriateness of building AI, (2) Transferability of AI systems, (3) Accountability for AI decision-making and outcomes, and (4) Individual consent. We then describe eight recommendations for governance leaders to enhance the ethical governance of AI in global health research, addressing issues such as AI impact assessments, environmental values, and fair partnerships.
Conclusions
The 2022 Global Forum on Bioethics in Research illustrated several innovations in ethical governance of AI for global health research, as well as several areas in need of urgent attention internationally. This summary is intended to inform international and domestic efforts to strengthen research ethics and support the evolution of governance leadership to meet the demands of AI in global health research.
Peer Review reports
Introduction
The ethical governance of Artificial Intelligence (AI) in health care and public health continues to be an urgent issue for attention in policy, research, and practice [ 1 , 2 , 3 ]. Beyond the growing number of AI applications being implemented in health care, capabilities of AI models such as Large Language Models (LLMs) expand the potential reach and significance of AI technologies across health-related fields [ 4 , 5 ]. Discussion about effective, ethical governance of AI technologies has spanned a range of governance approaches, including government regulation, organizational decision-making, professional self-regulation, and research ethics review [ 6 , 7 , 8 ]. In this paper, we report on central themes related to challenges and strategies for promoting ethics in research involving AI in global health research, arising from the Global Forum on Bioethics in Research (GFBR), held in Cape Town, South Africa in November 2022. Although applications of AI for research, health care, and public health are diverse and advancing rapidly, the insights generated at the forum remain highly relevant from a global health perspective. After summarizing important context for work in this domain, we highlight categories of ethical issues emphasized at the forum for attention from a research ethics perspective internationally. We then outline strategies proposed for research, innovation, and governance to support more ethical AI for global health.
In this paper, we adopt the definition of AI systems provided by the Organization for Economic Cooperation and Development (OECD) as our starting point. Their definition states that an AI system is “a machine-based system that can, for a given set of human-defined objectives, make predictions, recommendations, or decisions influencing real or virtual environments. AI systems are designed to operate with varying levels of autonomy” [ 9 ]. The conceptualization of an algorithm as helping to constitute an AI system, along with hardware, other elements of software, and a particular context of use, illustrates the wide variety of ways in which AI can be applied. We have found it useful to differentiate applications of AI in research as those classified as “AI systems for discovery” and “AI systems for intervention”. An AI system for discovery is one that is intended to generate new knowledge, for example in drug discovery or public health research in which researchers are seeking potential targets for intervention, innovation, or further research. An AI system for intervention is one that directly contributes to enacting an intervention in a particular context, for example informing decision-making at the point of care or assisting with accuracy in a surgical procedure.
The mandate of the GFBR is to take a broad view of what constitutes research and its regulation in global health, with special attention to bioethics in Low- and Middle- Income Countries. AI as a group of technologies demands such a broad view. AI development for health occurs in a variety of environments, including universities and academic health sciences centers where research ethics review remains an important element of the governance of science and innovation internationally [ 10 , 11 ]. In these settings, research ethics committees (RECs; also known by different names such as Institutional Review Boards or IRBs) make decisions about the ethical appropriateness of projects proposed by researchers and other institutional members, ultimately determining whether a given project is allowed to proceed on ethical grounds [ 12 ].
However, research involving AI for health also takes place in large corporations and smaller scale start-ups, which in some jurisdictions fall outside the scope of research ethics regulation. In the domain of AI, the question of what constitutes research also becomes blurred. For example, is the development of an algorithm itself considered a part of the research process? Or only when that algorithm is tested under the formal constraints of a systematic research methodology? In this paper we take an inclusive view, in which AI development is included in the definition of research activity and within scope for our inquiry, regardless of the setting in which it takes place. This broad perspective characterizes the approach to “research ethics” we take in this paper, extending beyond the work of RECs to include the ethical analysis of the wide range of activities that constitute research as the generation of new knowledge and intervention in the world.
Ethical governance of AI in global health
The ethical governance of AI for global health has been widely discussed in recent years. The World Health Organization (WHO) released its guidelines on ethics and governance of AI for health in 2021, endorsing a set of six ethical principles and exploring the relevance of those principles through a variety of use cases. The WHO guidelines also provided an overview of AI governance, defining governance as covering “a range of steering and rule-making functions of governments and other decision-makers, including international health agencies, for the achievement of national health policy objectives conducive to universal health coverage.” (p. 81) The report usefully provided a series of recommendations related to governance of seven domains pertaining to AI for health: data, benefit sharing, the private sector, the public sector, regulation, policy observatories/model legislation, and global governance. The report acknowledges that much work is yet to be done to advance international cooperation on AI governance, especially related to prioritizing voices from Low- and Middle-Income Countries (LMICs) in global dialogue.
One important point emphasized in the WHO report that reinforces the broader literature on global governance of AI is the distribution of responsibility across a wide range of actors in the AI ecosystem. This is especially important to highlight when focused on research for global health, which is specifically about work that transcends national borders. Alami et al. (2020) discussed the unique risks raised by AI research in global health, ranging from the unavailability of data in many LMICs required to train locally relevant AI models to the capacity of health systems to absorb new AI technologies that demand the use of resources from elsewhere in the system. These observations illustrate the need to identify the unique issues posed by AI research for global health specifically, and the strategies that can be employed by all those implicated in AI governance to promote ethically responsible use of AI in global health research.
RECs and the regulation of research involving AI
RECs represent an important element of the governance of AI for global health research, and thus warrant further commentary as background to our paper. Despite the importance of RECs, foundational questions have been raised about their capabilities to accurately understand and address ethical issues raised by studies involving AI. Rahimzadeh et al. (2023) outlined how RECs in the United States are under-prepared to align with recent federal policy requiring that RECs review data sharing and management plans with attention to the unique ethical issues raised in AI research for health [ 13 ]. Similar research in South Africa identified variability in understanding of existing regulations and ethical issues associated with health-related big data sharing and management among research ethics committee members [ 14 , 15 ]. The effort to address harms accruing to groups or communities as opposed to individuals whose data are included in AI research has also been identified as a unique challenge for RECs [ 16 , 17 ]. Doerr and Meeder (2022) suggested that current regulatory frameworks for research ethics might actually prevent RECs from adequately addressing such issues, as they are deemed out of scope of REC review [ 16 ]. Furthermore, research in the United Kingdom and Canada has suggested that researchers using AI methods for health tend to distinguish between ethical issues and social impact of their research, adopting an overly narrow view of what constitutes ethical issues in their work [ 18 ].
The challenges for RECs in adequately addressing ethical issues in AI research for health care and public health exceed a straightforward survey of ethical considerations. As Ferretti et al. (2021) contend, some capabilities of RECs adequately cover certain issues in AI-based health research, such as the common occurrence of conflicts of interest where researchers who accept funds from commercial technology providers are implicitly incentivized to produce results that align with commercial interests [ 12 ]. However, some features of REC review require reform to adequately meet ethical needs. Ferretti et al. outlined weaknesses of RECs that are longstanding and those that are novel to AI-related projects, proposing a series of directions for development that are regulatory, procedural, and complementary to REC functionality. The work required on a global scale to update the REC function in response to the demands of research involving AI is substantial.
These issues take greater urgency in the context of global health [ 19 ]. Teixeira da Silva (2022) described the global practice of “ethics dumping”, where researchers from high income countries bring ethically contentious practices to RECs in low-income countries as a strategy to gain approval and move projects forward [ 20 ]. Although not yet systematically documented in AI research for health, risk of ethics dumping in AI research is high. Evidence is already emerging of practices of “health data colonialism”, in which AI researchers and developers from large organizations in high-income countries acquire data to build algorithms in LMICs to avoid stricter regulations [ 21 ]. This specific practice is part of a larger collection of practices that characterize health data colonialism, involving the broader exploitation of data and the populations they represent primarily for commercial gain [ 21 , 22 ]. As an additional complication, AI algorithms trained on data from high-income contexts are unlikely to apply in straightforward ways to LMIC settings [ 21 , 23 ]. In the context of global health, there is widespread acknowledgement about the need to not only enhance the knowledge base of REC members about AI-based methods internationally, but to acknowledge the broader shifts required to encourage their capabilities to more fully address these and other ethical issues associated with AI research for health [ 8 ].
Although RECs are an important part of the story of the ethical governance of AI for global health research, they are not the only part. The responsibilities of supra-national entities such as the World Health Organization, national governments, organizational leaders, commercial AI technology providers, health care professionals, and other groups continue to be worked out internationally. In this context of ongoing work, examining issues that demand attention and strategies to address them remains an urgent and valuable task.
The GFBR is an annual meeting organized by the World Health Organization and supported by the Wellcome Trust, the US National Institutes of Health, the UK Medical Research Council (MRC) and the South African MRC. The forum aims to bring together ethicists, researchers, policymakers, REC members and other actors to engage with challenges and opportunities specifically related to research ethics. Each year the GFBR meeting includes a series of case studies and keynotes presented in plenary format to an audience of approximately 100 people who have applied and been competitively selected to attend, along with small-group breakout discussions to advance thinking on related issues. The specific topic of the forum changes each year, with past topics including ethical issues in research with people living with mental health conditions (2021), genome editing (2019), and biobanking/data sharing (2018). The forum is intended to remain grounded in the practical challenges of engaging in research ethics, with special interest in low resource settings from a global health perspective. A post-meeting fellowship scheme is open to all LMIC participants, providing a unique opportunity to apply for funding to further explore and address the ethical challenges that are identified during the meeting.
In 2022, the focus of the GFBR was “Ethics of AI in Global Health Research”. The forum consisted of 6 case study presentations (both short and long form) reporting on specific initiatives related to research ethics and AI for health, and 16 governance presentations (both short and long form) reporting on actual approaches to governing AI in different country settings. A keynote presentation from Professor Effy Vayena addressed the topic of the broader context for AI ethics in a rapidly evolving field. A total of 87 participants attended the forum from 31 countries around the world, representing disciplines of bioethics, AI, health policy, health professional practice, research funding, and bioinformatics. The 2-day forum addressed a wide range of themes. The conference report provides a detailed overview of each of the specific topics addressed while a policy paper outlines the cross-cutting themes (both documents are available at the GFBR website: https://www.gfbr.global/past-meetings/16th-forum-cape-town-south-africa-29-30-november-2022/ ). As opposed to providing a detailed summary in this paper, we aim to briefly highlight central issues raised, solutions proposed, and the challenges facing the research ethics community in the years to come.
In this way, our primary aim in this paper is to present a synthesis of the challenges and opportunities raised at the GFBR meeting and in the planning process, followed by our reflections as a group of authors on their significance for governance leaders in the coming years. We acknowledge that the views represented at the meeting and in our results are a partial representation of the universe of views on this topic; however, the GFBR leadership invested a great deal of resources in convening a deeply diverse and thoughtful group of researchers and practitioners working on themes of bioethics related to AI for global health including those based in LMICs. We contend that it remains rare to convene such a strong group for an extended time and believe that many of the challenges and opportunities raised demand attention for more ethical futures of AI for health. Nonetheless, our results are primarily descriptive and are thus not explicitly grounded in a normative argument. We make effort in the Discussion section to contextualize our results by describing their significance and connecting them to broader efforts to reform global health research and practice.
Uniquely important ethical issues for AI in global health research
Presentations and group dialogue over the course of the forum raised several issues for consideration, and here we describe four overarching themes for the ethical governance of AI in global health research. Brief descriptions of each issue can be found in Table 1 . Reports referred to throughout the paper are available at the GFBR website provided above.
The first overarching thematic issue relates to the appropriateness of building AI technologies in response to health-related challenges in the first place. Case study presentations referred to initiatives where AI technologies were highly appropriate, such as in ear shape biometric identification to more accurately link electronic health care records to individual patients in Zambia (Alinani Simukanga). Although important ethical issues were raised with respect to privacy, trust, and community engagement in this initiative, the AI-based solution was appropriately matched to the challenge of accurately linking electronic records to specific patient identities. In contrast, forum participants raised questions about the appropriateness of an initiative using AI to improve the quality of handwashing practices in an acute care hospital in India (Niyoshi Shah), which led to gaming the algorithm. Overall, participants acknowledged the dangers of techno-solutionism, in which AI researchers and developers treat AI technologies as the most obvious solutions to problems that in actuality demand much more complex strategies to address [ 24 ]. However, forum participants agreed that RECs in different contexts have differing degrees of power to raise issues of the appropriateness of an AI-based intervention.
The second overarching thematic issue related to whether and how AI-based systems transfer from one national health context to another. One central issue raised by a number of case study presentations related to the challenges of validating an algorithm with data collected in a local environment. For example, one case study presentation described a project that would involve the collection of personally identifiable data for sensitive group identities, such as tribe, clan, or religion, in the jurisdictions involved (South Africa, Nigeria, Tanzania, Uganda and the US; Gakii Masunga). Doing so would enable the team to ensure that those groups were adequately represented in the dataset to ensure the resulting algorithm was not biased against specific community groups when deployed in that context. However, some members of these communities might desire to be represented in the dataset, whereas others might not, illustrating the need to balance autonomy and inclusivity. It was also widely recognized that collecting these data is an immense challenge, particularly when historically oppressive practices have led to a low-trust environment for international organizations and the technologies they produce. It is important to note that in some countries such as South Africa and Rwanda, it is illegal to collect information such as race and tribal identities, re-emphasizing the importance for cultural awareness and avoiding “one size fits all” solutions.
The third overarching thematic issue is related to understanding accountabilities for both the impacts of AI technologies and governance decision-making regarding their use. Where global health research involving AI leads to longer-term harms that might fall outside the usual scope of issues considered by a REC, who is to be held accountable, and how? This question was raised as one that requires much further attention, with law being mixed internationally regarding the mechanisms available to hold researchers, innovators, and their institutions accountable over the longer term. However, it was recognized in breakout group discussion that many jurisdictions are developing strong data protection regimes related specifically to international collaboration for research involving health data. For example, Kenya’s Data Protection Act requires that any internationally funded projects have a local principal investigator who will hold accountability for how data are shared and used [ 25 ]. The issue of research partnerships with commercial entities was raised by many participants in the context of accountability, pointing toward the urgent need for clear principles related to strategies for engagement with commercial technology companies in global health research.
The fourth and final overarching thematic issue raised here is that of consent. The issue of consent was framed by the widely shared recognition that models of individual, explicit consent might not produce a supportive environment for AI innovation that relies on the secondary uses of health-related datasets to build AI algorithms. Given this recognition, approaches such as community oversight of health data uses were suggested as a potential solution. However, the details of implementing such community oversight mechanisms require much further attention, particularly given the unique perspectives on health data in different country settings in global health research. Furthermore, some uses of health data do continue to require consent. One case study of South Africa, Nigeria, Kenya, Ethiopia and Uganda suggested that when health data are shared across borders, individual consent remains necessary when data is transferred from certain countries (Nezerith Cengiz). Broader clarity is necessary to support the ethical governance of health data uses for AI in global health research.
Recommendations for ethical governance of AI in global health research
Dialogue at the forum led to a range of suggestions for promoting ethical conduct of AI research for global health, related to the various roles of actors involved in the governance of AI research broadly defined. The strategies are written for actors we refer to as “governance leaders”, those people distributed throughout the AI for global health research ecosystem who are responsible for ensuring the ethical and socially responsible conduct of global health research involving AI (including researchers themselves). These include RECs, government regulators, health care leaders, health professionals, corporate social accountability officers, and others. Enacting these strategies would bolster the ethical governance of AI for global health more generally, enabling multiple actors to fulfill their roles related to governing research and development activities carried out across multiple organizations, including universities, academic health sciences centers, start-ups, and technology corporations. Specific suggestions are summarized in Table 2 .
First, forum participants suggested that governance leaders including RECs, should remain up to date on recent advances in the regulation of AI for health. Regulation of AI for health advances rapidly and takes on different forms in jurisdictions around the world. RECs play an important role in governance, but only a partial role; it was deemed important for RECs to acknowledge how they fit within a broader governance ecosystem in order to more effectively address the issues within their scope. Not only RECs but organizational leaders responsible for procurement, researchers, and commercial actors should all commit to efforts to remain up to date about the relevant approaches to regulating AI for health care and public health in jurisdictions internationally. In this way, governance can more adequately remain up to date with advances in regulation.
Second, forum participants suggested that governance leaders should focus on ethical governance of health data as a basis for ethical global health AI research. Health data are considered the foundation of AI development, being used to train AI algorithms for various uses [ 26 ]. By focusing on ethical governance of health data generation, sharing, and use, multiple actors will help to build an ethical foundation for AI development among global health researchers.
Third, forum participants believed that governance processes should incorporate AI impact assessments where appropriate. An AI impact assessment is the process of evaluating the potential effects, both positive and negative, of implementing an AI algorithm on individuals, society, and various stakeholders, generally over time frames specified in advance of implementation [ 27 ]. Although not all types of AI research in global health would warrant an AI impact assessment, this is especially relevant for those studies aiming to implement an AI system for intervention into health care or public health. Organizations such as RECs can use AI impact assessments to boost understanding of potential harms at the outset of a research project, encouraging researchers to more deeply consider potential harms in the development of their study.
Fourth, forum participants suggested that governance decisions should incorporate the use of environmental impact assessments, or at least the incorporation of environment values when assessing the potential impact of an AI system. An environmental impact assessment involves evaluating and anticipating the potential environmental effects of a proposed project to inform ethical decision-making that supports sustainability [ 28 ]. Although a relatively new consideration in research ethics conversations [ 29 ], the environmental impact of building technologies is a crucial consideration for the public health commitment to environmental sustainability. Governance leaders can use environmental impact assessments to boost understanding of potential environmental harms linked to AI research projects in global health over both the shorter and longer terms.
Fifth, forum participants suggested that governance leaders should require stronger transparency in the development of AI algorithms in global health research. Transparency was considered essential in the design and development of AI algorithms for global health to ensure ethical and accountable decision-making throughout the process. Furthermore, whether and how researchers have considered the unique contexts into which such algorithms may be deployed can be surfaced through stronger transparency, for example in describing what primary considerations were made at the outset of the project and which stakeholders were consulted along the way. Sharing information about data provenance and methods used in AI development will also enhance the trustworthiness of the AI-based research process.
Sixth, forum participants suggested that governance leaders can encourage or require community engagement at various points throughout an AI project. It was considered that engaging patients and communities is crucial in AI algorithm development to ensure that the technology aligns with community needs and values. However, participants acknowledged that this is not a straightforward process. Effective community engagement requires lengthy commitments to meeting with and hearing from diverse communities in a given setting, and demands a particular set of skills in communication and dialogue that are not possessed by all researchers. Encouraging AI researchers to begin this process early and build long-term partnerships with community members is a promising strategy to deepen community engagement in AI research for global health. One notable recommendation was that research funders have an opportunity to incentivize and enable community engagement with funds dedicated to these activities in AI research in global health.
Seventh, forum participants suggested that governance leaders can encourage researchers to build strong, fair partnerships between institutions and individuals across country settings. In a context of longstanding imbalances in geopolitical and economic power, fair partnerships in global health demand a priori commitments to share benefits related to advances in medical technologies, knowledge, and financial gains. Although enforcement of this point might be beyond the remit of RECs, commentary will encourage researchers to consider stronger, fairer partnerships in global health in the longer term.
Eighth, it became evident that it is necessary to explore new forms of regulatory experimentation given the complexity of regulating a technology of this nature. In addition, the health sector has a series of particularities that make it especially complicated to generate rules that have not been previously tested. Several participants highlighted the desire to promote spaces for experimentation such as regulatory sandboxes or innovation hubs in health. These spaces can have several benefits for addressing issues surrounding the regulation of AI in the health sector, such as: (i) increasing the capacities and knowledge of health authorities about this technology; (ii) identifying the major problems surrounding AI regulation in the health sector; (iii) establishing possibilities for exchange and learning with other authorities; (iv) promoting innovation and entrepreneurship in AI in health; and (vi) identifying the need to regulate AI in this sector and update other existing regulations.
Ninth and finally, forum participants believed that the capabilities of governance leaders need to evolve to better incorporate expertise related to AI in ways that make sense within a given jurisdiction. With respect to RECs, for example, it might not make sense for every REC to recruit a member with expertise in AI methods. Rather, it will make more sense in some jurisdictions to consult with members of the scientific community with expertise in AI when research protocols are submitted that demand such expertise. Furthermore, RECs and other approaches to research governance in jurisdictions around the world will need to evolve in order to adopt the suggestions outlined above, developing processes that apply specifically to the ethical governance of research using AI methods in global health.
Research involving the development and implementation of AI technologies continues to grow in global health, posing important challenges for ethical governance of AI in global health research around the world. In this paper we have summarized insights from the 2022 GFBR, focused specifically on issues in research ethics related to AI for global health research. We summarized four thematic challenges for governance related to AI in global health research and nine suggestions arising from presentations and dialogue at the forum. In this brief discussion section, we present an overarching observation about power imbalances that frames efforts to evolve the role of governance in global health research, and then outline two important opportunity areas as the field develops to meet the challenges of AI in global health research.
Dialogue about power is not unfamiliar in global health, especially given recent contributions exploring what it would mean to de-colonize global health research, funding, and practice [ 30 , 31 ]. Discussions of research ethics applied to AI research in global health contexts are deeply infused with power imbalances. The existing context of global health is one in which high-income countries primarily located in the “Global North” charitably invest in projects taking place primarily in the “Global South” while recouping knowledge, financial, and reputational benefits [ 32 ]. With respect to AI development in particular, recent examples of digital colonialism frame dialogue about global partnerships, raising attention to the role of large commercial entities and global financial capitalism in global health research [ 21 , 22 ]. Furthermore, the power of governance organizations such as RECs to intervene in the process of AI research in global health varies widely around the world, depending on the authorities assigned to them by domestic research governance policies. These observations frame the challenges outlined in our paper, highlighting the difficulties associated with making meaningful change in this field.
Despite these overarching challenges of the global health research context, there are clear strategies for progress in this domain. Firstly, AI innovation is rapidly evolving, which means approaches to the governance of AI for health are rapidly evolving too. Such rapid evolution presents an important opportunity for governance leaders to clarify their vision and influence over AI innovation in global health research, boosting the expertise, structure, and functionality required to meet the demands of research involving AI. Secondly, the research ethics community has strong international ties, linked to a global scholarly community that is committed to sharing insights and best practices around the world. This global community can be leveraged to coordinate efforts to produce advances in the capabilities and authorities of governance leaders to meaningfully govern AI research for global health given the challenges summarized in our paper.
Limitations
Our paper includes two specific limitations that we address explicitly here. First, it is still early in the lifetime of the development of applications of AI for use in global health, and as such, the global community has had limited opportunity to learn from experience. For example, there were many fewer case studies, which detail experiences with the actual implementation of an AI technology, submitted to GFBR 2022 for consideration than was expected. In contrast, there were many more governance reports submitted, which detail the processes and outputs of governance processes that anticipate the development and dissemination of AI technologies. This observation represents both a success and a challenge. It is a success that so many groups are engaging in anticipatory governance of AI technologies, exploring evidence of their likely impacts and governing technologies in novel and well-designed ways. It is a challenge that there is little experience to build upon of the successful implementation of AI technologies in ways that have limited harms while promoting innovation. Further experience with AI technologies in global health will contribute to revising and enhancing the challenges and recommendations we have outlined in our paper.
Second, global trends in the politics and economics of AI technologies are evolving rapidly. Although some nations are advancing detailed policy approaches to regulating AI more generally, including for uses in health care and public health, the impacts of corporate investments in AI and political responses related to governance remain to be seen. The excitement around large language models (LLMs) and large multimodal models (LMMs) has drawn deeper attention to the challenges of regulating AI in any general sense, opening dialogue about health sector-specific regulations. The direction of this global dialogue, strongly linked to high-profile corporate actors and multi-national governance institutions, will strongly influence the development of boundaries around what is possible for the ethical governance of AI for global health. We have written this paper at a point when these developments are proceeding rapidly, and as such, we acknowledge that our recommendations will need updating as the broader field evolves.
Ultimately, coordination and collaboration between many stakeholders in the research ethics ecosystem will be necessary to strengthen the ethical governance of AI in global health research. The 2022 GFBR illustrated several innovations in ethical governance of AI for global health research, as well as several areas in need of urgent attention internationally. This summary is intended to inform international and domestic efforts to strengthen research ethics and support the evolution of governance leadership to meet the demands of AI in global health research.
Data availability
All data and materials analyzed to produce this paper are available on the GFBR website: https://www.gfbr.global/past-meetings/16th-forum-cape-town-south-africa-29-30-november-2022/ .
Clark P, Kim J, Aphinyanaphongs Y, Marketing, Food US. Drug Administration Clearance of Artificial Intelligence and Machine Learning Enabled Software in and as Medical devices: a systematic review. JAMA Netw Open. 2023;6(7):e2321792–2321792.
Article Google Scholar
Potnis KC, Ross JS, Aneja S, Gross CP, Richman IB. Artificial intelligence in breast cancer screening: evaluation of FDA device regulation and future recommendations. JAMA Intern Med. 2022;182(12):1306–12.
Siala H, Wang Y. SHIFTing artificial intelligence to be responsible in healthcare: a systematic review. Soc Sci Med. 2022;296:114782.
Yang X, Chen A, PourNejatian N, Shin HC, Smith KE, Parisien C, et al. A large language model for electronic health records. NPJ Digit Med. 2022;5(1):194.
Meskó B, Topol EJ. The imperative for regulatory oversight of large language models (or generative AI) in healthcare. NPJ Digit Med. 2023;6(1):120.
Jobin A, Ienca M, Vayena E. The global landscape of AI ethics guidelines. Nat Mach Intell. 2019;1(9):389–99.
Minssen T, Vayena E, Cohen IG. The challenges for Regulating Medical Use of ChatGPT and other large Language models. JAMA. 2023.
Ho CWL, Malpani R. Scaling up the research ethics framework for healthcare machine learning as global health ethics and governance. Am J Bioeth. 2022;22(5):36–8.
Yeung K. Recommendation of the council on artificial intelligence (OECD). Int Leg Mater. 2020;59(1):27–34.
Maddox TM, Rumsfeld JS, Payne PR. Questions for artificial intelligence in health care. JAMA. 2019;321(1):31–2.
Dzau VJ, Balatbat CA, Ellaissi WF. Revisiting academic health sciences systems a decade later: discovery to health to population to society. Lancet. 2021;398(10318):2300–4.
Ferretti A, Ienca M, Sheehan M, Blasimme A, Dove ES, Farsides B, et al. Ethics review of big data research: what should stay and what should be reformed? BMC Med Ethics. 2021;22(1):1–13.
Rahimzadeh V, Serpico K, Gelinas L. Institutional review boards need new skills to review data sharing and management plans. Nat Med. 2023;1–3.
Kling S, Singh S, Burgess TL, Nair G. The role of an ethics advisory committee in data science research in sub-saharan Africa. South Afr J Sci. 2023;119(5–6):1–3.
Google Scholar
Cengiz N, Kabanda SM, Esterhuizen TM, Moodley K. Exploring perspectives of research ethics committee members on the governance of big data in sub-saharan Africa. South Afr J Sci. 2023;119(5–6):1–9.
Doerr M, Meeder S. Big health data research and group harm: the scope of IRB review. Ethics Hum Res. 2022;44(4):34–8.
Ballantyne A, Stewart C. Big data and public-private partnerships in healthcare and research: the application of an ethics framework for big data in health and research. Asian Bioeth Rev. 2019;11(3):315–26.
Samuel G, Chubb J, Derrick G. Boundaries between research ethics and ethical research use in artificial intelligence health research. J Empir Res Hum Res Ethics. 2021;16(3):325–37.
Murphy K, Di Ruggiero E, Upshur R, Willison DJ, Malhotra N, Cai JC, et al. Artificial intelligence for good health: a scoping review of the ethics literature. BMC Med Ethics. 2021;22(1):1–17.
Teixeira da Silva JA. Handling ethics dumping and neo-colonial research: from the laboratory to the academic literature. J Bioethical Inq. 2022;19(3):433–43.
Ferryman K. The dangers of data colonialism in precision public health. Glob Policy. 2021;12:90–2.
Couldry N, Mejias UA. Data colonialism: rethinking big data’s relation to the contemporary subject. Telev New Media. 2019;20(4):336–49.
Organization WH. Ethics and governance of artificial intelligence for health: WHO guidance. 2021.
Metcalf J, Moss E. Owning ethics: corporate logics, silicon valley, and the institutionalization of ethics. Soc Res Int Q. 2019;86(2):449–76.
Data Protection Act - OFFICE OF THE DATA PROTECTION COMMISSIONER KENYA [Internet]. 2021 [cited 2023 Sep 30]. https://www.odpc.go.ke/dpa-act/ .
Sharon T, Lucivero F. Introduction to the special theme: the expansion of the health data ecosystem–rethinking data ethics and governance. Big Data & Society. Volume 6. London, England: SAGE Publications Sage UK; 2019. p. 2053951719852969.
Reisman D, Schultz J, Crawford K, Whittaker M. Algorithmic impact assessments: a practical Framework for Public Agency. AI Now. 2018.
Morgan RK. Environmental impact assessment: the state of the art. Impact Assess Proj Apprais. 2012;30(1):5–14.
Samuel G, Richie C. Reimagining research ethics to include environmental sustainability: a principled approach, including a case study of data-driven health research. J Med Ethics. 2023;49(6):428–33.
Kwete X, Tang K, Chen L, Ren R, Chen Q, Wu Z, et al. Decolonizing global health: what should be the target of this movement and where does it lead us? Glob Health Res Policy. 2022;7(1):3.
Abimbola S, Asthana S, Montenegro C, Guinto RR, Jumbam DT, Louskieter L, et al. Addressing power asymmetries in global health: imperatives in the wake of the COVID-19 pandemic. PLoS Med. 2021;18(4):e1003604.
Benatar S. Politics, power, poverty and global health: systems and frames. Int J Health Policy Manag. 2016;5(10):599.
Download references
Acknowledgements
We would like to acknowledge the outstanding contributions of the attendees of GFBR 2022 in Cape Town, South Africa. This paper is authored by members of the GFBR 2022 Planning Committee. We would like to acknowledge additional members Tamra Lysaght, National University of Singapore, and Niresh Bhagwandin, South African Medical Research Council, for their input during the planning stages and as reviewers of the applications to attend the Forum.
This work was supported by Wellcome [222525/Z/21/Z], the US National Institutes of Health, the UK Medical Research Council (part of UK Research and Innovation), and the South African Medical Research Council through funding to the Global Forum on Bioethics in Research.
Author information
Authors and affiliations.
Department of Physical Therapy, Temerty Faculty of Medicine, University of Toronto, Toronto, Canada
Berman Institute of Bioethics, Johns Hopkins University, Baltimore, MD, USA
Bloomberg School of Public Health, Johns Hopkins University, Baltimore, MD, USA
Department of Philosophy and Classics, University of Ghana, Legon-Accra, Ghana
Caesar A. Atuire
Centre for Tropical Medicine and Global Health, Nuffield Department of Medicine, University of Oxford, Oxford, UK
Mahidol Oxford Tropical Medicine Research Unit, Faculty of Tropical Medicine, Mahidol University, Bangkok, Thailand
Phaik Yeong Cheah
Berkman Klein Center, Harvard University, Bogotá, Colombia
Armando Guio Español
Department of Radiology and Informatics, Emory University School of Medicine, Atlanta, GA, USA
Judy Wawira Gichoya
Health Ethics & Governance Unit, Research for Health Department, Science Division, World Health Organization, Geneva, Switzerland
Adrienne Hunt & Katherine Littler
African Center of Excellence in Bioinformatics and Data Intensive Science, Infectious Diseases Institute, Makerere University, Kampala, Uganda
Daudi Jjingo
ISI Foundation, Turin, Italy
Daniela Paolotti
Department of Health Sciences and Technology, ETH Zurich, Zürich, Switzerland
Effy Vayena
Joint Centre for Bioethics, Dalla Lana School of Public Health, University of Toronto, Toronto, Canada
You can also search for this author in PubMed Google Scholar
Contributions
JS led the writing, contributed to conceptualization and analysis, critically reviewed and provided feedback on drafts of this paper, and provided final approval of the paper. JA contributed to conceptualization and analysis, critically reviewed and provided feedback on drafts of this paper, and provided final approval of the paper. CA contributed to conceptualization and analysis, critically reviewed and provided feedback on drafts of this paper, and provided final approval of the paper. PYC contributed to conceptualization and analysis, critically reviewed and provided feedback on drafts of this paper, and provided final approval of the paper. AE contributed to conceptualization and analysis, critically reviewed and provided feedback on drafts of this paper, and provided final approval of the paper. JWG contributed to conceptualization and analysis, critically reviewed and provided feedback on drafts of this paper, and provided final approval of the paper. AH contributed to conceptualization and analysis, critically reviewed and provided feedback on drafts of this paper, and provided final approval of the paper. DJ contributed to conceptualization and analysis, critically reviewed and provided feedback on drafts of this paper, and provided final approval of the paper. KL contributed to conceptualization and analysis, critically reviewed and provided feedback on drafts of this paper, and provided final approval of the paper. DP contributed to conceptualization and analysis, critically reviewed and provided feedback on drafts of this paper, and provided final approval of the paper. EV contributed to conceptualization and analysis, critically reviewed and provided feedback on drafts of this paper, and provided final approval of the paper.
Corresponding author
Correspondence to James Shaw .
Ethics declarations
Ethics approval and consent to participate.
Not applicable.
Consent for publication
Competing interests.
The authors declare no competing interests.
Additional information
Publisher’s note.
Springer Nature remains neutral with regard to jurisdictional claims in published maps and institutional affiliations.
Rights and permissions
Open Access This article is licensed under a Creative Commons Attribution 4.0 International License, which permits use, sharing, adaptation, distribution and reproduction in any medium or format, as long as you give appropriate credit to the original author(s) and the source, provide a link to the Creative Commons licence, and indicate if changes were made. The images or other third party material in this article are included in the article’s Creative Commons licence, unless indicated otherwise in a credit line to the material. If material is not included in the article’s Creative Commons licence and your intended use is not permitted by statutory regulation or exceeds the permitted use, you will need to obtain permission directly from the copyright holder. To view a copy of this licence, visit http://creativecommons.org/licenses/by/4.0/ . The Creative Commons Public Domain Dedication waiver ( http://creativecommons.org/publicdomain/zero/1.0/ ) applies to the data made available in this article, unless otherwise stated in a credit line to the data.
Reprints and permissions
About this article
Cite this article.
Shaw, J., Ali, J., Atuire, C.A. et al. Research ethics and artificial intelligence for global health: perspectives from the global forum on bioethics in research. BMC Med Ethics 25 , 46 (2024). https://doi.org/10.1186/s12910-024-01044-w
Download citation
Received : 31 October 2023
Accepted : 01 April 2024
Published : 18 April 2024
DOI : https://doi.org/10.1186/s12910-024-01044-w
Share this article
Anyone you share the following link with will be able to read this content:
Sorry, a shareable link is not currently available for this article.
Provided by the Springer Nature SharedIt content-sharing initiative
- Artificial intelligence
- Machine learning
- Research ethics
- Global health
BMC Medical Ethics
ISSN: 1472-6939
- General enquiries: [email protected]
- - Google Chrome
Intended for healthcare professionals
- Access provided by Google Indexer
- My email alerts
- BMA member login
- Username * Password * Forgot your log in details? Need to activate BMA Member Log In Log in via OpenAthens Log in via your institution

Search form
- Advanced search
- Search responses
- Search blogs
- News & Views
- Health systems and...
Health systems and environmental sustainability: updating frameworks for a new era
- Related content
- Peer review
- Michael Padget , researcher 1 ,
- Michael A Peters , health specialist 2 ,
- Matthias Brunn , affiliated researcher 3 ,
- Dionne Kringos , associate professor 4 5 ,
- Margaret E Kruk , professor of health systems 6
- 1 Massachusetts General Hospital Center for the Environment and Health, Boston, MA, USA
- 2 Exemplars in Global Health, Gates Ventures, Seattle, USA
- 3 Laboratory for Interdisciplinary Evaluation of Public Policies (LIEPP), Sciences Po, Paris, France
- 4 Department of Public and Occupational Health, University of Amsterdam, Amsterdam, Netherlands
- 5 Amsterdam Public Health Research Institute, Amsterdam, Netherlands
- 6 Department of Global Health and Population, Harvard T H Chan School of Public Health, Boston, MA, USA
- Correspondence to: M Padget mpadget{at}mgh.harvard.edu
Michael Padget and colleagues argue that making environmental sustainability a measure of health system quality will support progress and help fulfil systems’ fundamental mission to protect and improve health
Healthcare systems around the world have a responsibility to “do no harm” but are responsible for substantial negative health effects through the production of greenhouse gases, particulate matter, pharmaceutical waste, and other environmental pollutants. 1 Recent studies estimate that the healthcare sector accounts for 4.6% of global greenhouse gases, and over 8% of these emissions in some countries. 2 3 Although high income countries produce most health system pollution, low and middle income countries also contribute through poor hazardous waste disposal practices and other carbon intensive healthcare activities. 4 5
Growing numbers of health systems have recognised the importance of their environmental impact and are acting to reduce it. To date, 82 countries, nearly half of which are classified as low or lower middle income by the World Bank, have signed an international pledge to develop climate resilient and low carbon health systems. 6 In early 2023, over 100 health systems in the US signed a health sector climate pledge endorsed by the White House to achieve net zero emissions by 2050. 7 Health systems in the UK and many other European countries have also developed extensive decarbonisation plans. 8 9
Despite these multiplying pledges and the urgency to act, real progress has been uneven. Simple and important actions, such as substituting highly polluting anaesthetic gases for equally effective, less polluting options, have not been universally adopted. 10 In clinical practice, heavy reliance on disposable devices persists even when moving to a reusable option is safe, less costly, and less polluting. 11 Education on the inter-relatedness of climate and health and the importance of health system sustainability remains rare in medical schools. 12
A major barrier to progress on environmental sustainability is the perception among some key stakeholders such as healthcare purchasers, managers, staff, and providers that environmental sustainability goals do not belong among fundamental health system priorities. In 2023, the Joint Commission, the largest and most important hospital accreditation organisation in the US, decided to make its environmental sustainability metrics optional after hospitals and health systems argued that the topic was not relevant when dealing with more urgent issues. 13 Health systems often consider environmental sustainability a “nice to have” that is combined with other social responsibility goals, or is encouraged only if it can be linked to cost savings. 14 Environmental topics may also be considered to be political or belonging to the realm of activism by healthcare workers. 15 This perception has hindered the advancement of environmental sustainability efforts along with the development of crucial tools, data, and research.
To fulfil the health system’s purpose of promoting health and to accomplish stated goals, environmental sustainability must be accepted as a central mission of the health system by all those involved, including governments, funders, healthcare providers, academia, regulatory bodies, and patient groups. To support this, we suggest that environmental sustainability be adopted as a core responsibility of the health system in all countries and included in a revised definition and framework for high quality health systems.
Embedding sustainability in health system quality
Health system quality frameworks help to conceptualise, prioritise, and operationalise the function and goals of the health system. Several widely used frameworks exist, including the World Health Organization’s (WHO) building blocks framework, which focuses on ensuring quality through adequate financing, workforce, information systems, medical products and technologies, leadership and governance, and service delivery. 16 This framework has been incorporated into common health system evaluation tools and influenced work worldwide on indicator development, reporting, research, and other system components. Another widely used framework was developed by Institute of Medicine (IOM), which focuses on delivering safe, effective, patient centred, timely, efficient, and equitable care. 17 Patient safety initiatives, which today represent a central focus of quality improvement efforts and measurement, were popularised in part by the inclusion of safety in the framework in 1999.
The framework for high quality health systems produced by the Lancet Global Health Commission on High Quality Health Systems in 2018 builds on the WHO, IOM, and other frameworks by focusing on system foundations, processes, and impacts. 18 All system components are guided by the principles of efficiency, resilience, equity, and people centredness. This holistic focus makes this framework useful for tackling the rising challenges of chronic and complex conditions. 19 20 21 The framework has also been incorporated into health services research in low and middle income countries and shaped discussions of care quality in WHO documents supporting improved primary care and maternal, newborn, and child health. 22 23 24
To achieve ambitious health systems goals and overcome current hurdles, environmental sustainability will need the support of quality frameworks and the corresponding quality infrastructure. Treating environmental sustainability as a health system quality issue would increase awareness and prioritisation of the topic; help to embed it into decision making across multiple levels; promote engagement and support from existing organisations involved in creating and maintaining quality standards such as healthcare inspectorates, funders, medical associations, and patient organisations; and stimulate progress on key topics such as the supply chain, care coordination, and prescribing. 25 Importantly, it would also provide a conceptual structure to facilitate discussions about how environmental sustainability relates to other system goals and priorities, particularly in cases where they are not aligned.
Adapting health system quality frameworks
The structure and multifaceted approach of the Lancet commission’s high quality health system framework make it well suited for the integration of environmental sustainability, which must be integrated into multiple components of the health system to be effective. In this framework, environmental sustainability would fit as a fifth overarching principle used to help guide the foundations, processes, and outcomes of care ( fig 1 ). Table 1 provides examples of how environmental sustainability can be linked conceptually or operationally to the components of this framework.

High quality health system framework including environmental sustainability as an overarching principle
- Download figure
- Open in new tab
- Download powerpoint
Examples of environmental sustainability links or work within each component of a high quality health system 18
- View inline
Applying an adapted high quality health system framework means that environmental sustainability should be considered across all health system components, from system planning to clinical care. Because sustainability is considered alongside other principles, actions may differ depending on the context. For example, in its guidance for climate resilient and environmentally sustainable healthcare facilities, WHO recommends assembling and training a multisectoral operative team, establishing a baseline, defining and prioritising short term and long term interventions, developing and implementing an improvement plan, and monitoring and evaluating improvements. 26 Each of these steps requires time and resources. In a health system with few resources that has not achieved sufficient equity or resilience but has a low environmental footprint, the relative benefit of investing in this strategy may be limited. In a higher resource setting where the system creates more pollution and has achieved greater overall performance, this approach may be more valuable. This does not mean that environmental sustainability is irrelevant in low resource settings since many examples exist of less polluting care or system options in these contexts that can also support patient care or other priorities. 5 27
Examples of environmental sustainability aligning with existing principles include reducing unnecessary care, which supports the principle of efficiency; use of telemedicine, which can support patient access and equity; and investment in primary care and public health, which may help avoid high resource care and improve health outcomes. In other cases, environmental sustainability may align with one principle but not another or be in direct conflict with other principles—for example, if a less polluting option increases costs. An adapted framework can help identify and highlight these examples.
Expanding the definition of health system quality to include environmental sustainability would also expand the role of existing organisations that measure quality, accredit and certify healthcare organisations, and provide education or conduct research on quality topics. Examples of this work may include the creation and reporting of new quality measures focused on environmental sustainability, education on environment and health topics as part of quality training and assessment, or inclusion of environmental effects into health technology assessments or other decision aids. Some of these efforts are already underway ( box 1 ), and frameworks can help organise, highlight, and expand these efforts. While many of the structures involved in quality would remain the same, participation and expertise from sectors not typically considered in quality such as building management, environmental services, purchasing, and others would be needed.
Current environmental sustainability efforts
Environmental sustainability in healthcare has been advancing quickly in the UK, where work and research are underway on purchasing criteria and supply chain, low carbon patient pathways and medicines, and specific specialty recommendations. 28 29 Notably, the Care Quality Commission in England, which ensures the quality of health provision through regular inspections and monitoring, has recently added environmental sustainability to its list of quality evaluation criteria 30
Internationally, WHO has published guidance on creating climate resilient and environmentally sustainable healthcare facilities, calling for comprehensive action across the health systems building blocks. 26 31 Although this guidance is structured on the WHO building blocks framework, environmental sustainability is not included in the actual framework
Aga Khan Health Services has been a pioneer in calculating carbon emissions, waste generation, and water use for its facilities, located in eight low and middle income countries. It has developed a carbon management tool with WHO, which it uses to implement activities to reduce carbon emissions in facility construction, operation, and service delivery 32
The SusQI approach developed by the Centre for Sustainable Healthcare in the UK provides a model for the integration of environmental sustainability into quality assessment, and its use has contributed to the expansion of sustainability work in the NHS 33
Action is needed now
Redefining models of quality and integrating them into health systems requires time and resources. Adding additional responsibilities to already overburdened healthcare staff and systems when they are recovering from the covid pandemic may not be ideal. Organisations involved in assessing and validating quality may also find this expansion of responsibilities difficult given the necessity for new expertise. However, health must remain a priority, even if this requires new concepts and practices at an inopportune time. Conversely, this period may present an opportunity for change as health systems are already considering fundamental questions of design and resilience. 34
Some may question the effectiveness of leveraging quality frameworks to advance environmental sustainability, particularly when other performance improvement efforts have stalled or led to unintended consequences, such as an excessive focus on measurement affecting patient care or increased costs. 35 36 Although the quality improvement movement may not have achieved all desired outcomes, it has been successful in increasing awareness, stimulating research, influencing policy, and encouraging investment and work on the topic. These same steps are desirable and necessary to achieve environmental sustainability in healthcare.
The actions and expertise needed to support environmental sustainability can also alleviate some of the unintended risks of quality work. Many effective actions to reduce the environmental impact of healthcare, such as capital investments or work with the supply chain, take place outside the realm of patient care; these can be cost saving and may be more straightforward to implement than many of the systematic or behaviour changes necessary for achieving traditional quality objectives such as reduced patient harm. Environmental goals that require behaviour change, such as reducing low value care, would probably face many of the same challenges as those of other quality improvement interventions and would require specific efforts to overcome them.
The speed and practicality of using quality frameworks might also be questioned. If decarbonisation is urgent, why should we spend time discussing concepts rather than acting? Including environmental sustainability in quality frameworks does not preclude action and has the potential to accelerate and expand ongoing efforts by providing a stronger conceptual foundation and basis for acting. Achieving environmental sustainability will take time and considerable effort. Creating a solid conceptual foundation capable of integrating multiple interests may ultimately lead to more consistent and streamlined decision making. Lastly, environmental sustainability has no expiration date and must remain a health system priority beyond specific target dates. Careful consideration of this topic today will ensure it is included in decision making now and into the future.
Our proposed framework adaptation focuses on environmental sustainability whereas WHO and others propose an approach combining both environmental sustainability and climate resilience. 31 However, the high quality health system framework’s existing principle of resilience includes the ability to adapt to changing contexts, including climate change. This same logic may not be applicable to other quality frameworks.
Forging consensus on sustainable health systems
Changing quality frameworks means first creating a consensus around these changes. The participation of organisations that define and diffuse healthcare quality such as the National Academy of Medicine, WHO, or the Organization for Economic Cooperation and Development is critical to the incorporation of environmental sustainability into these frameworks. Other groups such as the Lancet Commission on Sustainable Healthcare, the Centre for Sustainable Healthcare in the UK, or the Nordic Centre for Sustainable Healthcare can also support this approach.
This conceptual work is an important step in shaping opinions on environmental sustainability and providing a way forward, but it is far from the last step. Implementing the necessary changes requires action at all levels, including health providers, academics, administrators, patient groups, governmental bodies, and funding organisations. Importantly, progress will require collaboration with groups not typically considered as being within the medical sphere. Data will also be needed to support environmentally informed decision making and track progress. Resources such as the HealthcareLCA database or support from the Agency for Healthcare Research and Quality on measuring sustainability provide examples to be further developed. 37 38
Despite the considerable effort ahead, this work is urgent and necessary. Incorporating environmentally sustainability into health system frameworks is an important part of this critical journey.
Key messages
Environmental pollution from health systems contributes to large negative health effects
Progress on pledges to reduce pollution in the health sector has been slow because the topic is often seen as outside core health system responsibilities
Placing environmental sustainability as a core concept in health system quality frameworks can help support efforts to reduce health system pollution
The high quality health system framework provides an effective illustration of how environmental sustainability can be integrated into quality frameworks
Acknowledgments
This article has received support from the French National Research Agency (ANR) Investissement d’avenir programme as part of the IdEx Université Paris Cité (ANR-18-IDEX-0001).
Contributors and sources: MP is a health policy analyst and researcher with expertise in health system quality measurement and environmental sustainability in health systems. MAP is a health systems and policy researcher who works on multisectoral efforts to improve quality in health systems in low and middle income countries. MB is a researcher on comparative health policies and politics in Europe and works with MP on integrating environmental sustainability into health system decision making. DK and MEK are internationally recognised experts and researchers on health system quality. MP and MEK conceived the article. MP drafted the manuscript. All authors revised the manuscript critically for important intellectual content and approved the final version.
Competing interests: We have read and understood BMJ policy on declaration of interests and declare that MEK was chair of the Lancet commission that produced the high quality health system framework.
Provenance and peer review: Not commissioned; externally peer reviewed.
- Eckelman MJ ,
- Romanello M ,
- Napoli CD ,
- Pichler PP ,
- Ferronato N ,
- Rasheed FN ,
- Baddley J ,
- Prabhakaran P ,
- ↵ Alliance for Transformative Action on Climate and Health. Countries. 2023. https://www.atachcommunity.com/atach-community/countries/
- ↵ US Department of Health and Human Services. Health sector pledge. https://www.hhs.gov/climate-change-health-equity-environmental-justice/climate-change-health-equity/actions/health-sector-pledge/index.html
- ↵ NHS England. Delivering a “net zero” National Health Service. 2022. https://www.england.nhs.uk/greenernhs/publication/delivering-a-net-zero-national-health-service/
- ↵ Autorite de Sante H. Feuille de route santé-environnement. 2023. https://www.has-sante.fr/upload/docs/application/pdf/2023-11/feuille_de_route_sante_environnement_has.pdf
- Caviglia M ,
- Ucciero A ,
- Di Filippo A ,
- Barone-Adesi F
- MacNeill AJ ,
- Khanuja A ,
- Omrani OE ,
- Dafallah A ,
- Paniello Castillo B ,
- ↵ Kaplan S, Sadler B, Little K, Franz C, Orris P. Can sustainable hospitals help bend the health care cost curve? 2012 https://www.commonwealthfund.org/sites/default/files/documents/___media_files_publications_issue_brief_2012_nov_1641_kaplan_can_sustainable_hosps_bend_cost_curve_ib.pdf
- ↵ WHO. Monitoring the building blocks of health systems: a handbook of indicators and their measurement strategies. 2010. www.iniscommunication.com
- Institute of Medicine
- Arsenault C ,
- Mitgang E ,
- Nimako KT ,
- Qamruddin J
- Pokhrel S ,
- Chowdury M ,
- Roder-DeWan S ,
- Doubova SV ,
- Infante-Castañeda C ,
- Pérez-Cuevas R
- ↵ WHO. The Network for Improving Quality of Care for Maternal, Newborn, and Child Health: evolution, implementation and progress. 2017-2020 report. 2021. https://www.who.int/publications/i/item/9789240023741
- Sherman JD ,
- ↵ WHO. WHO guidance for climate-resilience and environmentally sustainable health care facilities. 2020. https://www.who.int/publications/i/item/9789240012226
- ↵ NHS England. Greener NHS: suppliers. 2023. https://www.england.nhs.uk/greenernhs/get-involved/suppliers/
- ↵ Greener Practice. High quality and low carbon asthma care. 2023. https://www.greenerpractice.co.uk/high-quality-and-low-carbon-asthma-care/
- ↵ Care Quality Commission. Environmental sustainability—sustainable development. 2023. https://www.cqc.org.uk/assessment/quality-statements/well-led/environmental-sustainability
- ↵ WHO. Operational framework for building climate resilient and low carbon health systems. 2015. https://www.who.int/publications/i/item/9789241565073
- Mortimer F ,
- Isherwood J ,
- Wilkinson A ,
- ↵ OECD. Ready for the next crisis? Investing in health system resilience. 2023.
- Braithwaite J ,
- Glasziou P ,
- Westbrook J
- Casalino LP
- ↵ Sampath B, Jensen M, Lenoci-Edwards J, Little K, Singh H, Sherman JD. Reducing healthcare carbon emissions: a primer on measures and actions for healthcare organizations to mitigate climate change. 2022. https://www.ahrq.gov/sites/default/files/wysiwyg/healthsystemsresearch/decarbonization/decarbonization.pdf
- ↵ Drew J, Rizan C. HealthcareLCA database. 2023. healthcarelca.com/database
Advertisement
Analysing the Efficiency of Health Systems: A Systematic Review of the Literature
- Systematic Review
- Open access
- Published: 28 December 2022
- Volume 21 , pages 205–224, ( 2023 )
Cite this article
You have full access to this open access article
- Rahab Mbau 1 ,
- Anita Musiega 1 , 2 ,
- Lizah Nyawira 1 ,
- Benjamin Tsofa 3 ,
- Andrew Mulwa 4 ,
- Sassy Molyneux 3 , 5 ,
- Isabel Maina 6 ,
- Julie Jemutai 1 ,
- Charles Normand 7 , 8 ,
- Kara Hanson 9 &
- Edwine Barasa ORCID: orcid.org/0000-0001-5793-7177 1 , 5
10k Accesses
16 Citations
28 Altmetric
Explore all metrics
Efficiency refers the use of resources in ways that optimise desired outcomes. Health system efficiency is a priority concern for policy makers globally as countries aim to achieve universal health coverage, and face the additional challenge of an aging population. Efficiency analysis in the health sector has typically focused on the efficiency of healthcare facilities (hospitals, primary healthcare facilities), with few studies focusing on system level (national or sub-national) efficiency. We carried out a thematic review of literature that assessed the efficiency of health systems at the national and sub-national level.
We conducted a systematic search of PubMed and Google scholar between 2000 and 2021 and a manual search of relevant papers selected from their reference lists. A total of 131 papers were included. We analysed and synthesised evidence from the selected papers using a thematic approach (selecting, sorting, coding and charting collected data according to identified key issues and themes).
There were more publications from high- and upper middle-income countries (53%) than from low-income and lower middle-income countries. There were also more publications focusing on national level (60%) compared to sub-national health systems’ efficiency. Only 6% of studies used either qualitative methods or mixed methods while 94% used quantitative approaches. Data envelopment analysis, a non-parametric method, was the most common methodological approach used, followed by stochastic frontier analysis, a parametric method. A range of regression methods were used to identify the determinants of health system efficiency. While studies used a range of inputs, these generally considered the building blocks of health systems, health risk factors, and social determinants of health. Outputs used in efficiency analysis could be classified as either intermediate health service outputs (e.g., number of health facility visits), single health outcomes (e.g., infant mortality rate) or composite indices of either intermediate outputs of health outcomes (e.g., Health Adjusted Life Expectancy). Factors that were found to affect health system efficiency include demographic and socio-economic characteristics of the population, macro-economic characteristics of the national and sub-national regions, population health and wellbeing, the governance and political characteristics of these regions, and health system characteristics.
This review highlights the limited evidence on health system efficiency, especially in low- and middle-income countries. It also reveals the dearth of efficiency studies that use mixed methods approaches by incorporating qualitative inquiry. The review offers insights on the drivers of the efficiency of national and sub-national health systems, and highlights potential targets for reforms to improve health system efficiency.
Similar content being viewed by others
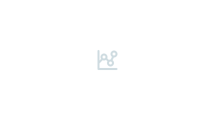
What is Qualitative in Qualitative Research
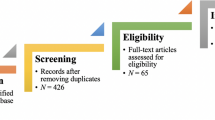
Criteria for Good Qualitative Research: A Comprehensive Review
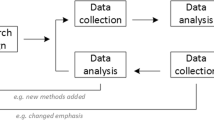
How to use and assess qualitative research methods
Avoid common mistakes on your manuscript.
1 Introduction
Health system efficiency is a priority concern for policy makers globally in the face of mounting health system expenditures [ 1 , 2 , 3 ]. Attainment of efficiency demonstrates good stewardship through good use of available resources and elimination of wastage [ 1 , 4 ]. It also inspires the willingness of governments and citizens to contribute resources towards Universal Health Coverage (UHC) [ 4 ]. Improved efficiency is recognised as a desirable goal of the health system [ 5 ] and an intermediate objective of health-financing policies that contributes towards the attainment of health system goals [ 6 ]. It is also a major criterion for priority setting by decision makers [ 7 ]. Given the scarcity of healthcare resources, it is imperative that health systems, in both high income (HIC) and low- and middle-income countries (LMICs), orient their operations towards using their resources efficiently to optimise the achievement of stated health system goals and promote financial sustainability in the long-term towards UHC [ 3 , 4 ].
Efficiency refers to the extent to which system objectives are met given the resources invested in the system [ 1 ]. Two types of efficiency, technical and allocative efficiency, have been distinguished [ 8 ]. Technical efficiency (TE) is achieved when resources are allocated such that outputs are maximised for a given level of inputs, or inputs are minimised for a given level of outputs [ 9 ]. Allocative efficiency (AE) is achieved when resources are allocated such that outputs are maximised for a given level of input cost, or input costs are minimised for a given level of outputs [ 9 ]. Allocative and technical efficiency together make the 'overall' efficiency of a system. Productivity, a concept related but different from efficiency, refers to the ratio of outputs to inputs in a production system [ 10 ]. This paper focuses on the efficiency of health systems rather than productivity.
It has been estimated that 20–40% of health system spending globally is wasted through inefficiency [ 11 ]. Such inefficiencies haemorrhage resources within the health sector and impede progress towards UHC [ 11 ]. While generating additional resources for health is crucial, improving the use of available resources in the health sector is argued by some as one of the a promising strategies towards expanding the fiscal space for health [ 11 , 12 , 13 ]. This is important both for LMICs that face constrained fiscal space as the support from donors reduces, and HICs as an aging population increases resource requirements in the health sector.
Efficiency measurement is therefore a key dimension of health system performance assessment. It requires the identification of the boundaries of the entity under scrutiny ranging from micro (provider-patient level) to meso (organisational) to macro (national or global) levels of the health system [ 4 , 14 ]. The chosen level of analysis should reflect an entity that will take accountability for the level of performance identified by the analysis [ 4 ]. Efficiency is increasingly assessed in healthcare, but most of these studies analyse efficiency at the meso-level of the health system involving healthcare organisations (such as hospitals and health centres) [ 4 , 10 , 15 ], with fewer examining the national or subnational level [ 10 ].
Understanding efficiency within the health system and the associated inputs, outputs/outcomes and determinants of efficiency can influence policy formulation and managerial decision-making [ 4 ]. This literature review aims to synthesise existing empirical evidence on efficiency at the health system level (national and sub-national rather than health facility level) to increase the understanding of the conceptualisation and determinants of health system efficiency.
2.1 Literature Search Strategy
We followed the Preferred Reporting Items for Systematic Review and Meta-Analysis (PRISMA) guidelines [ 16 ] in the reporting of this literature review. We searched two electronic databases: PubMed and Google scholar for published and grey literature using specific subject headings and free text terms. We ran the searches separately for these databases. Search terms included ‘efficiency’, ‘technical efficiency’, ‘data envelopment analysis’, ‘stochastic frontier analysis’, ‘health system’, ‘health sector’, ‘nation’, ‘sub nation’, ‘country’, ‘region’ and ‘state’. These search terms were used as key words in google scholar. We developed a Boolean algorithm to search PubMed was developed (Fig. 1 ). The last literature search was done in December 2021.
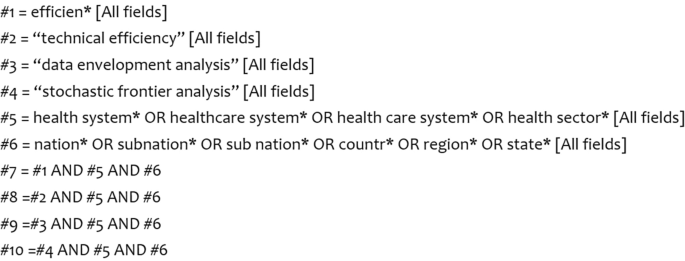
2.2 Article Selection
The electronic literature search identified 11,030 publications. Of these, 10,899 were rejected based on our eligibility criteria (Table 1 ). We retained 131 articles that met our inclusion criteria. We only included publications that met the inclusion criteria. We included publications that reported on empirical research on efficiency of health systems above the meso-level of the health system. We defined these levels to include jurisdictions such as sub national, national, regional and international health systems. We included publications that were published in English due to time and resource constraints that would otherwise be required for the translation of non-English publications. We did not use any restrictions on publication year, publication status, country income classification or study design. The publications that met the inclusion criteria were imported into EndNote X8. The article selection process is summarised in a search flow diagram in Fig. 2 . A total of 131 publications were retrieved and reviewed.
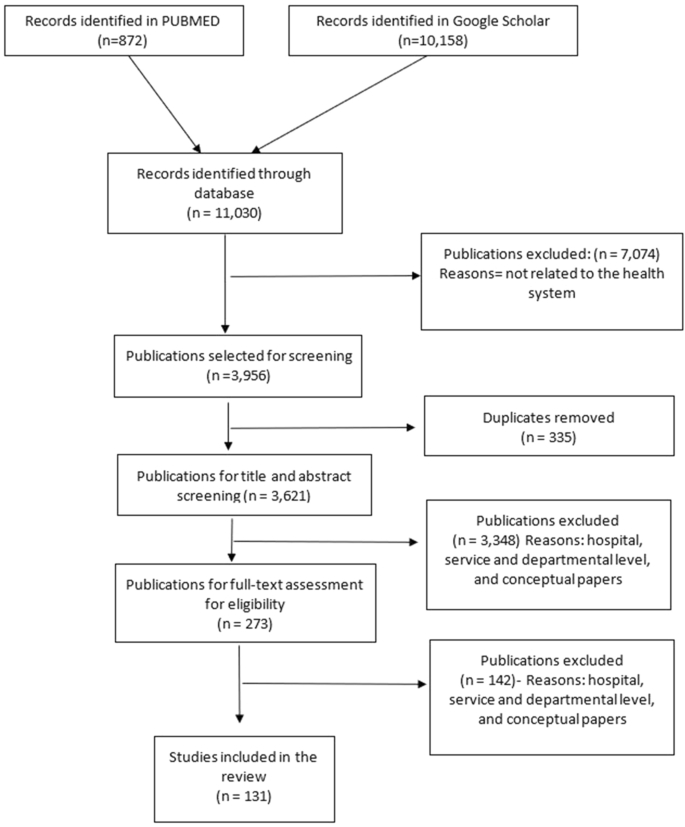
Search flow diagram
2.3 Quality Appraisal
We used the critical appraisal skills programme (CASP) tool to assess the quality of the identified publications [ 17 ]. CASP tool uses a checklist approach to assess the adequacy, trustworthiness and relevance of the evidence reported in the publications [ 18 , 19 ]. The CASP tool helps to reflect the character of the studies included in the review [ 17 ]. The results of the quality appraisal are indicated in Table 2 . All the publications were of sufficient quality to be included in the review.
2.4 Data Extraction
Two authors (RM and EB) performed data extraction of the retrieved publications using a thematic analysis approach. Specifically, RM and EB first extracted data from a subset of selected papers (25/131). The data extracted by the two authors was compared to establish concurrence on the extraction approach. RB thereafter concluded data extraction of the remaining papers. Thematic analysis is an analytic process that involves a systematic process of sifting, sorting, coding and charting collected data according to key issues and themes [ 20 ]. The first step in this analysis process involved familiarisation with the publications through reading and re-reading. This formed the beginning of the abstraction process. We used a data extraction form structured (Additional File 2) in line with the review question and efficiency concepts. This form was used as a data registry and a guide for the identification of inputs, outputs and determinants of efficiency within the health system. Second, after familiarisation, we applied codes, developed inductively and deductively, to data that we interpreted as important and relevant. We then grouped similar codes into categories or themes drawing upon: (a) a priori issues (those informed by the original research aims and researchers’ knowledge in the subject area), (b) emergent categories identified by the authors in the retrieved literature, and lastly, (3) analytical themes arising from similarities across the identified codes. We then charted the data into a framework matrix using Microsoft Excel. This allowed us to: (1) summarise the data by category, (2) identify patterns and linkages in our data, that is, findings that were similar and recurrent across selected papers, and (3) make comparisons across the papers, to identified both converging and diverging findings and seek explanations for this.
3.1 Characteristics of Selected Publications
The list of the selected publications is provided in Online Supplementary Material, File 1. Empirical literature on health system efficiency has expanded noticeably over the years with the highest number of the retrieved literature published in 2018 (Fig. 3 ). However, most of these studies (53%) presented findings of health system efficiency in upper middle-income and high-income countries, while 25% of the studies focused exclusively on lower middle-income countries and low-income countries, and another 19% focused on countries across all income groups (Table 3 ).
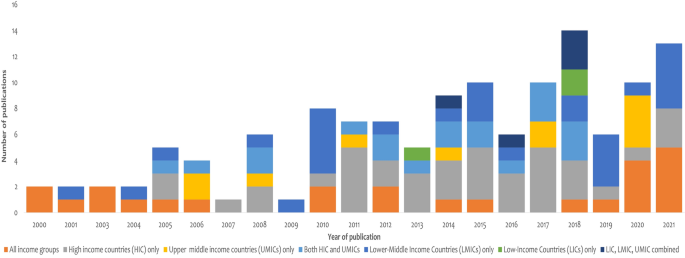
Number of publications published by income level classification and year
Sixty percent of all the retrieved publications examined efficiency at the national/country level. These included studies that examined a single country health system or several country health systems such as OECD countries [ 21 ], World Health Organization member states [ 22 ], Eastern European countries [ 23 ], Asian countries [ 24 , 25 ], Latin America and Caribbean countries [ 26 ] and Sub Saharan Africa [ 27 ]. Forty percent of the publications examined efficiency at sub national levels such as: (1) provinces in China [ 28 , 29 ] and South Africa [ 30 ]; regions in Saudi Arabia [ 31 ] and Switzerland [ 32 ]; municipalities in Brazil [ 33 ] and Finland [ 34 ]; and districts in India [ 35 ], Zambia [ 36 ] and Mozambique [ 37 ].
3.2 Conceptualisation of Efficiency at Health System Level in the Retrieved Literature
Following existing production literature described by Farrell [ 8 ], the majority of the authors of the retrieved literature explicitly defined efficiency as the extent to which desired health system goals were achieved given existing resources [ 38 , 39 , 40 , 41 ]. The literature conceptualised a health system as a production system that transformed inputs into desired outputs [ 42 ]. In most of the studies, this production system was considered as a single unit. In two studies, however, the health system was perceived to be composed of two subunits—a public health system (non-health facility-based health promotion and prevention services) and a medical care system (i.e., healthcare facilities) that offered population-based and individual-based care, respectively [ 43 , 44 ]. Both subunits contributed towards the efficiency of the overall health system [ 43 ]. In addition to inputs and outputs, the efficiency of the health system as a production “unit” was thought to be affected by contextual factors from within and outside of the health sector. These factors had different labels, including exogenous factors, explanatory factors, and determinants of efficiency.
3.3 Methods Used to Analyse Efficiency
Of the selected papers, 123 (94%) used purely quantitative approaches, five (4%) used purely qualitative approaches, and another three (2%) used mixed methods approaches. Quantitative approaches were used to measure the level and determinants of efficiency. Qualitative approaches were used to examine study participants’ perceptions about the objectives of the health system [ 41 , 45 ] and existence and nature of health system inefficiency and its determinants [ 41 , 45 , 46 , 47 , 48 ]. Beyond identification, qualitative approaches provided explanations of the relationship between identified determinants and health system efficiency [ 36 , 48 , 49 ]. Seventy-two (57%) of the publications that used pure quantitative approaches or mixed methods used cross-sectional quantitative data to estimate the level of efficiency in the health system. The remaining 54 (43%) of these papers used panel data with authors such as [ 27 , 50 , 51 ] indicating that panel data offer more accurate estimations of efficiency because of the richness of the data and consideration of the effect of time [ 52 ] precludes the need to impose assumptions on the error terms likely to be correlated with time. Of the papers that used panel data, 36 (67%) used the Malmquist productivity index (MPI) approach to measure efficiency changes over time, while 18 (33%) included time as a covariate in a regression analysis. Five publications (4%) employed qualitative approaches [ 36 , 46 , 53 , 54 , 55 ], while two studies (2%) used a mixed methods approach by combining both qualitative and quantitative methods [ 47 , 49 ].
Efficiency measurement in the retrieved literature was done using non-parametric (data envelopment analysis-DEA and Free disposal hull technique) and parametric methods (stochastic frontier analysis-SFA). DEA was the most used technique for measuring efficiency. DEA is a non-parametric linear programming method that assess the relative efficiency of production units by obtaining the ratio of a weighted sum of the outputs of a productive unit to a weighted sum of its inputs [ 56 ]. The DEA technique is relevant in the health sector given the complex nature of health systems where multiple inputs are utilised to produce multiple outputs. A key limitation of DEA is that its results may be influenced by measurement error or statistical noise given that DEA is non-stochastic. DEA ascribes deviations from the frontier entirely to inefficiency, even though these may be due to measurement errors. DEA was exclusively used in 95 (76%) of the selected papers and used in combination and compared with free disposal hull (FDH) or SFA in two (2%) papers respectively. SFA was the second most common approach, used exclusively in 23 (18%) papers and in combination and compared to FDH in one (1%) paper. SFA is a parametric method that uses regression analysis to estimate the production frontier, measuring the efficiency of a unit using the residuals from the estimated equation [ 57 ]. Its key advantage over DEA is that SFA explicitly accounts for measurement error. The DEA model decomposes the error term in a stochastic error component and an additional error term that represents systematic inefficiency. SFA is used because it accounts for random disturbances in the data [ 58 ]. Qualitative data were analysed using thematic analysis [ 36 ].
Determinants of health systems efficiency were identified in 72 (55%) of the selected papers. Methods used for the quantitative identification of the determinants of efficiency include: Bayesian linear regression [ 59 , 60 ], Tobit regression [ 60 , 61 ], truncated regression model [ 62 ], and multiple regression analysis [ 63 ]. These methods were second stage analysis in DEA or SFA.
3.3.1 Inputs, Their Definition and Reasons Why They Were Chosen
Inputs were defined as resources required to facilitate the production function of the health system [ 21 , 60 ]. These resources were considered to be within the control of the managers in the health system [ 33 ]. The list of inputs identified in the literature is provided in Table 4 . While different studies used different inputs, the inputs could be classified into three broad categories: health system building blocks, social determinants of health, and health risk factors. Among the health system building blocks, finances were the most common input, with 68% of the studies using this variable in the production function. This was followed by human resources for health (66%) and medical equipment (54%). In some of the studies, the number of beds was used as a proxy for capital investment in health production [ 62 , 64 , 65 ] because direct measurement of capital in healthcare was found to be problematic [ 62 ]. The number of health facilities was only used in 22% of the studies. Education, a social determinant of health, was used as an input in 15% of the studies. Health risk factor characteristics used as inputs included tobacco and alcohol consumption (5%).
The choice of inputs used in assessing efficiency was informed by various reasons. These included evidence of use of the input variables in previous efficiency studies, availability of the data, positive relationship with the outputs, frequency of data reporting on the variable, direct involvement of the input in the production of health, input that would allow cross-country comparisons of efficiency or whether the input could be standardised across the system to allow comparison. It also included whether the input variable could be consistently measured across the units being assessed, whether the influence of the variable on efficiency was within the control of the health system, and based on economic theory and wider literature, and opinions of experts and stakeholders in the system.
3.3.2 Outputs and Outcomes, Their Definition and Reasons Why They Were Chosen
Outputs used in the reviewed literature fall into three categories: intermediate health service outputs, single health outcomes, or composite indices of either intermediate outputs or health outcomes. While several authors indicate that a general consensus in existing literature puts health status of the population [ 60 ] as the single most important output of the system [ 66 ], its measurement has, however, remained difficult [ 60 ]. As indicated by [ 23 ], the distinction between output and outcome is often blurred leading authors to use the two terms interchangeably.
The list of outputs and outcomes identified in the literature is provided in Table 5 . Seventy percent of the publications included more than one output variable in their assessment of efficiency. Of the health outcome variable used, mortality rates and life expectancy were the most common (51%). Mortality rates were considered a good summary measure of overall population health [ 67 ] as well as the closest measurable indicator of the stated health system objectives [ 41 ]. Common intermediate health outputs used included outpatient and inpatient workload measures and maternal and child health services utilisation measures. Several studies used composite indices as output/outcome measures. For example Tandon et al. [ 68 ] used a weighted average of health system goals using disability adjusted life expectancy (DALE), health inequality, responsiveness level, responsiveness distribution and fair financing. Tandon et al. [ 69 ] created an outcome index by combining five indicators on immunisation coverage, skilled birth attendance, iodised salt content, catastrophic expenditure and life expectancy while Achoki et al. [ 70 ] use a composite metric for maternal and child health services made up of diphtheria, pertussis, tetanus vaccine-3 doses (DPT3) and measles immunisations, skilled birth attendance and malaria prevention.
The most common criterion that informed the choice of outputs used in a study was evidence of common use of the variable in previous studies [ 44 , 60 , 65 , 71 , 72 , 73 ]. This was indicated in 40% of the retrieved literature. Other criteria applied to select outputs included: (1) use of the variable by the ministry of health to monitor efficiency of the health system, for example, the hospital bed occupancy rate in Zambia [ 36 ]. (2) Relevance to millennium development goals related to reduction of maternal mortality and child mortality such as institutional delivery rate [ 74 ] or under-5-year-old mortality rates. (3) Relevance to the national government priorities such as primary healthcare agenda in India [ 74 ] or increased number of live births in Thailand [ 75 ] or access to quality and effective healthcare in Canada [ 41 ]. (4) Availability of data [ 52 , 76 ]. (5) Robustness of the indicators [ 77 , 78 , 79 ]. (6) Objectivity of the variables [ 80 ]. Relevance of the variable to the context [ 81 ]. (7) Routine collection of the data and its ability to allow for cross-unit comparison [ 66 , 82 ].
3.3.3 Exogenous or Environmental Variables, Their Definition and Reasons Why They Were Chosen
Exogenous variables refer to the factors that are not directly related to the resources in the sector in question but may have an effect on the relationship between the inputs and outputs of that sector [ 69 ]. These variables are recognised as the third variable for inclusion in efficiency measurement along with inputs and outputs [ 68 ]. Exogenous variables were thought to capture heterogeneity and explain some of the differences or dispersion in the efficiency levels of units under analysis [ 39 ]. Fifty-six percent of the retrieved publications considered the influence of exogenous variables on the efficiency of the units under consideration. However, only one author [ 53 ] provided a conceptual framework that shows the influence of environmental variables on a health system’s production function. The list of these variables is provided in Table 6 .
Exogenous variables were chosen based on: (1) evidence of use in previous studies [ 15 , 21 , 59 , 77 ]; this was the most common reason given by a third of all the authors who used exogenous variables in their analysis. (2) Completeness and consistency of reporting of the variable in question for the units under consideration [ 72 ]. And lastly, (3) evidence of their potential influence on efficiency [ 33 , 62 , 65 ]. Table 6 outlines the categories of exogenous variables used in the analysis. It will be evident that some factors are used either as inputs or as exogenous variables in different studies.
3.4 Efficiency of Health Systems
It is challenging to summarise and/or compare findings from the literature on the efficiency of health systems because of heterogeneity of methods. This includes differences in approach (qualitative and quantitative), selection of inputs, outputs, exogenous variables, and models. For instance, a sensitivity analysis of an efficiency analysis of 141 countries originally conducted by the World Health Organization (WHO) found that country rankings and efficiency scores were sensitive to the definition of efficiency and choice of model specification [ 63 ]. Qualitative papers focused on health system stakeholders’ views about the existence of inefficiency and sources of inefficiency in health systems. These are summarised in the next section. Quantitative approaches reported the level of health system efficiency as a proportion (with a range of 0–100) or an inefficiency score. For example, the most recent regional analysis of the efficiency the country health systems reported a mean efficiency of 80% (range 31–100%) for 45 African countries [ 27 ], 92% (range 81–91%) for 46 Asian countries [ 83 ], 93% (range 51–93%) for 27 Latin American countries, and 83% (range 54–94%) for 28 European countries [ 84 ]. The most recent global analysis of the efficiency of 140 country health systems reported a mean efficiency of 93% (range 71–100%), with the following regional means: African countries (86%), Asian countries (95%) South American countries (95%), and European countries (96%) [ 85 ]. An efficiency score of 100% denotes an efficient health system, while a score below 100% means that there is scope to improve efficiency by either producing more output or reducing inputs to achieve a score of 100%.
3.5 Factors Affecting the Efficiency of Health Systems
3.5.1 demographic characteristics of the population.
Several population/demographic characteristics were found to determine health system technical efficiency. One of these was population density. Some studies found that a high population density of a country or sub-national unit (region/district etc) was associated with increased technical efficiency. For instance, a study of the primary healthcare system in Chile found that a high population of primary healthcare catchment areas increased the technical efficiency regional health systems [ 61 ]. Ahmed et al. assessed the technical efficiency of the health systems of 46 Asian countries and found that countries having more than 200 people per square kilometre were more technically efficient compared with the countries with ≤ 100 population per square kilometre. Higher population densities increased the technical efficiency of regional health systems by reducing distances to populations and making it easier for health systems to organise and utilise their services infrastructure, and by reducing per capita cost of healthcare [ 33 ]. However, some studies reported a negative association between population density and health system technical efficiency. For instance, a study of Finnish municipalities found that large populations reduced the technical efficiency of municipalities and speculated that this could be because other factors related to population size such as quality differences, bureaucratic inefficiency, or unmeasured outputs [ 34 ]. A study in Kenya found that the technical efficiency of county health system was negatively associated with population density, and speculated that this was likely because higher population densities were not matched with healthcare resources and hence compromising health outcomes [ 86 ]. Another factor that was explored was the rural/urban distribution of the population. There is a general finding that regions with low urbanisation rates are likely to be less technically efficient [ 34 , 35 , 87 ]. This was because, among others, lower urbanisation was associated with lower unemployment rates and lower income levels that affect healthcare utilisation [ 88 ]. Population age structure was also explored; high proportions of the very young (children) or the very old reduced the technical efficiency of health systems because these vulnerable populations increased the cost of healthcare [ 33 , 61 ].
3.5.2 Socio-Economic Characteristics of the Population/Social Determinants of Health
Several socio-economic characteristics of the population were examined. Some studies reported that improved socio-economic status of the population is positively associated with health system technical efficiency. For instance, several studies found that increased per capita income of a country or regions population was associated with increased technical efficiency of the health system [ 89 ]. However, some studies reported a negative association between population income per capita and health system technical efficiency. This was thought to be because health systems whose catchment populations had higher income per capita were characterised by higher levels of overprovision and higher costs of care.
In addition to average income levels in a country, the distribution of incomes was also found to determine health system technical efficiency. High income inequality and poverty was associated with reduced technical efficiency. Bekarogu and Heffley found that increased poverty and income inequality affected the technical efficiency of health system by reducing the overall level of health system outcomes. A related socio-economic characteristic was employment status, where high unemployment rates were associated with reduced health system technical efficiency [ 34 ].
Several studies found that access to basic sanitation and clean water increased the technical efficiency of health systems. This was because improved sanitation improved health outcomes, which was linked to improved technical efficiency of the system. For example, Grigoli and Kapsoli [ 90 ] found that the percentage of the population with access to sanitation services was associated with an increase in technical efficiency, while Hassan et al. [ 91 ] found that the rate of access to drinking water decreased the incidence of water-related diseases such as cholera, fever and malaria, and was associated with increased technical efficiency.
Increased literacy was associated with increased technical efficiency of health systems [ 26 , 38 , 87 , 92 ]. For example, Ahmed et al. found that Asian countries with higher literacy levels have higher health system technical efficiency. This was thought to be because educated people more easily transform health information and knowledge into health outcomes [ 87 , 89 ].
3.5.3 Macro-Economic Characteristics
Findings on the effect of the size of a country’s economy on health system technical efficiency were mixed. Some studies found that higher country per capita gross domestic product (GDP) was associated with a more technically efficient delivery of healthcare [ 93 , 94 , 95 , 96 ]. This was thought to be because increased country wealth could translate to increased investments in the health sector as well as other sectors that impact on social determinants of health, with improved health and quality of life having a positive impact on overall health outcomes. For instance, some studies found that countries with good road infrastructure and good access to electricity were associated with increased technical efficiency of health systems [ 87 ]. However, other studies found that higher GDP per capita was associated with lower technical efficiency of health systems. This was thought to be because of the increased cost of healthcare because of overprovision [ 15 , 61 , 97 ] and higher relative prices of healthcare in richer countries [ 98 ].
3.5.4 Health and Wellbeing of the Population
Several aspects of the health and wellbeing of the population affected the technical efficiency of the health system. Generally, higher prevalence of chronic disease was associated with reduced health system technical efficiency. For instance, Novignon and Lawanson found that HIV/AIDS negatively affects technical efficiency of health systems in Africa, with a similar finding reported in Kenya [ 86 ]. Allin et al. found that an increase in the proportion of people with chronic conditions by 10% would decrease the technical efficiency score by between 10 and 18% in regional health systems in Canada. Further, health systems that serve populations with high levels of health risk factors such as smoking, alcohol consumption and obesity were likely to be less technically efficient [ 15 , 21 , 59 , 99 ]. For example, Bekaroglu and Heffley found that a high consumption of alcohol increases inefficiency by causing premature ill health and death. A high prevalence of chronic disease and health risk factors reduced health system outcomes and increased healthcare costs with negative impacts on health system efficiency.
3.5.5 Health System Characteristics
Several characteristics of health system functions were found to determine the efficiency of health systems. First, how health systems are financed affected health system efficiency in several ways. The fragmentation of financing arrangements, and specifically the presence of multiple health insurance firms, was negatively associated with health system efficiency [ 53 , 77 ]. The level of health expenditure also had an impact on health system efficiency. Total health expenditure as a share of GDP was positively associated with the technical efficiency of health systems [ 38 , 42 , 65 , 100 ]. The role of availability of funds was also highlighted in Kenya [ 47 , 48 ]. This was thought to be because greater healthcare spending was essential in improving health outcomes [ 65 ]. However, some studies found that higher levels of total health expenditure can be negatively associated with efficiency when the health system is characterised by unnecessary care and/or higher costs of care [ 14 , 15 , 40 , 48 ]. The source of funding for the health sector was also shown to affect technical efficiency. The share of public spending on healthcare was positively associated with health system technical efficiency [ 14 ]. Further, Increased population coverage with a prepayment health-financing mechanism (such as health insurance) was associated with increased technical efficiency of health systems [ 101 ]. An assessment in China found that provinces with a high proportion of out-of-pocket payments had lower technical efficiency [ 102 ]. However, some studies on the efficiency of OECD (Organisation for Economic Co-operation and Development) countries [ 98 , 101 ] have found that out of pocket payments in the form of co-payments were positively associated with health system efficiency in contexts that have adequate population coverage with prepayment mechanisms. This was because co-payments disincentivized unnecessary use of care. Public finance management arrangements also influenced health system efficiency. Enhanced capacity to execute budgets, flow of funds directly to providers, timeliness of funds disbursements to local authorities and health facilities, the flexibility of budgets, and the autonomy of local authorities and health facilities over resources enhanced efficiency [ 45 , 47 , 48 ].
With regard to the purchasing function of the health system, how healthcare providers were paid also affected health system efficiency. For instance, prospective payments such as capitation, rather than fee-for-service payments, were founds to be positively associated with health system efficiency in some studies because they disincentivised unnecessary care and provided purchasers with better control over costs [ 103 ]. In the Democratic republic of Congo, the introduction of a zero-margin policy for drug sales in the public sector reduced the incentive of healthcare providers to prescribe unnecessary medicines [ 53 ]. The design and implementation of benefit packages also affects health system efficiency. Chile, Mexico and Uruguay improved the efficiency of their health systems by prioritising health services that are cost-effective in their benefit packages [ 53 ].
The efficiency of health systems was also found to be affected by how users interacted with the health service providers. Health systems where patients exercised choice of health providers were associated with higher technical efficiency [ 59 ]. Gate keeping by primary-care providers, where a patient is required to have a referral from a general practitioner for non-emergency access to a specialist, enhanced health system efficiency by aligning the level of specialisation and cost of healthcare with healthcare needs, and reducing healthcare costs [ 103 ]. However, some studies found that gate keeping could reduce health system efficiency in settings where primary-care physicians had limited ability to coordinate the follow-up of patient care, or in settings where the health system’s capacity to provide secondary care was limited [ 77 ]. Inadequate health system capacity to provide specialised care resulted in long waiting times, and increased the utilisation of emergency departments and hospitalisations and hence resulting in inefficiency [ 77 ]. The effectiveness of gate keeping in enhancing health system efficiency was also dependent on whether it was accompanied by interventions to improve the availability and quality of secondary-care services [ 77 ]. Further, an interaction between price regulation and gate keeping has been reported. It has been observed that when healthcare prices are regulated, gate keeping may reduce efficiency by incentivising excessive specialisation of healthcare professionals to access higher fees [ 60 ]. It also incentivises general practitioners to make unnecessary referrals of patients to specialised care so as to minimise their (general practitioner) input costs [ 60 ].
On health governance, strong regulation of health system functions, and specifically price regulation, medicine use, and health workforce regulation were associated with increased technical efficiency [ 53 , 59 , 60 ]. In China and El Salvador, the introduction medicines regulations that strengthened price regulation, generic prescribing, and the enforcement of national essential drugs lists improved health system efficiency [ 53 ]. Improved coordination in the health sector, including the coordination of donor initiatives, was also associated with improved health system efficiency [ 45 , 53 ]. The Democratic Republic of Congo and Zambia realised improvement in health system efficiency by aligning and coordinating donor support with health sector priorities, and coordinating health sector planning, budgeting and resource allocation to reduce waste and duplication [ 36 , 53 ]. Beyond health sector coordination, multisectoral coordination and partnerships to tackle social determinants of health were thought to improve efficiency [ 46 ]. Some studies reported that decentralisation of health functions was associated with higher technical efficiency of national and sub-national health systems [ 59 ]. An assessment of the technical efficiency of healthcare systems of selected middle-income countries found that technical efficiency was enhanced by decentralisation, which enhanced the delivery of care in rural areas, and improved the responsiveness of health systems to community needs through improved community participation in healthcare decision making [ 104 ]. Effective performance monitoring and accountability was found to improve health system efficiency in Canada [ 46 ]. Leadership and management practices and capacity were also thought to be a determinant of health system efficiency [ 45 , 46 , 53 ]. An assessment of the technical efficiency of 27 Latin American and Caribbean countries found a positive association between governance quality and system technical efficiency [ 26 ]. Governance quality in the study was defined as a multidimensional index that included measures of government effectiveness, voice and accountability, rule of law, regulatory quality, political stability and absence of violence/terrorism, and control of corruption. Further, assessments of the technical efficiency of WHO member country health systems found that an increase in democratisation and freedom was associated with increased health system technical efficiency [ 39 , 94 ]. Corruption has also been found to be associated with reduced technical efficiency [ 69 , 93 ].
The availability and distribution of health system hardware such infrastructure, equipment and health commodities were associated with increased technical efficiency of health systems [ 83 ]. Inadequate availability of input led to an inefficient mix of inputs with negative impacts on health system efficiency. For example, an assessment of the technical efficiency of Asian country health systems found that the density of hospital beds had a positive association with technical efficiency [ 83 ]. An assessment in Canada found that increased inequitable distribution of health workers was associated with increased technical efficiency of national and sub-national health systems [ 15 ]. In Ethiopia, an increase in the number of primary healthcare facilities that was not matched with an increase in the number of health workers resulted in inefficiency [ 53 ]. The level and distribution of health workers affected health system efficiency. National and sub-national health systems that had inadequate numbers of health workers were less efficient [ 97 , 105 ]. Procurement inefficiencies were also identified. This included fragmented procurement of health commodities, high procurement prices, and supply chain challenges leading to delays in deliveries and stock-outs, and procurement corruption [ 45 , 47 , 53 ]. Verhoeven et al. [ 98 ] found that high spending on pharmaceuticals was associated with lower health system efficiency. This was thought to be because high pharmaceutical expenditure crowded out other healthcare inputs and hence reduced the efficient use of health resources.
Overprovision of health services (long lengths of stay, high referrals, high doctor consultations, high admission rates, inappropriate drug (such as antibiotic) use), an aspect of quality of care, was associated with reduced technical efficiency [ 15 , 61 , 97 ]. For example, Chai et al. found that negative association of admission rates with technical efficiency implied that a resource-intensive hospitalisation service use was harmful to health system technical efficiency. Ramirez-Valdivia et al. [ 61 ] found that increasing annual referrals to specialists increases the intechnical efficiency score. In China, the inappropriate use of drugs reduced health system efficiency [ 53 ].
The organisation of care to prioritise lower level basic care primary healthcare is associated with increased health system efficiency. Ding et al. [ 28 ] found that an increase in the proportion of medical services in primary facilities would increase the technical efficiency of provincial medical centres. The share of essential/basic services in benefit packages was positively associated with health system efficiency [ 53 , 98 ]. This was because essential/basic services were more cost-effective compared to advanced/expensive care. Further, health systems with a high share of basic care health workers (rather than specialists) were likely to be more efficient [ 15 , 59 ]. Policy reforms with a focus on expanding primary and community-based care, and engaging the community were shown to improve the technical efficiency of healthcare systems in OECD countries [ 43 ]. Further reforms geared on enhancing access to healthcare for the disadvantaged and vulnerable, and reducing inequality in access to healthcare services was associated with increased technical efficiency [ 43 ].
4 Discussion
Previous reviews of health system efficiency have either focused on the methods used in the analysis [ 106 , 107 , 108 ] or on synthesising the evidence on health facility level efficiency [ 107 , 109 ]. This study presents a systematic review of empirical studies that have examined the efficiency of national or sub-national health systems. Our findings show that there were more publications from high and upper middle-income countries than from low-income and lower middle-income countries. One of the factors that may have contributed to this is the availability of rich cross-country data on high income countries such as the ones held by OECD and EU, and health system observatory databases. It underlies the need for LMICs to set up mechanisms to collate and curate health system data that could be used for monitoring health system performance.
All the identified studies assessed technical efficiency and its determinants and used quantitative approaches except for five studies that used qualitative approaches and three that used mixed methods and considered efficiency more broadly (beyond technical). While quantitative approaches quantified the level and determinants of health system efficiency, they did not provide insights on the mechanisms by which determinants interact with the technical efficiency of health systems. Quantitative approaches were also limited to assessing quantifiable factors (typically health system hardware), and missed non-quantifiable, software aspects of health systems. On the other hand, qualitative approaches provided more information about how certain factors might affect health system efficiency. They also identified determinants of efficiency that are not easily quantifiable—software factors such as the role of leadership and management practices, and health sector coordination. This highlights the need for mixed methods approaches that incorporate the use of qualitative methods to undertake in-depth assessments of the interplay of factors that determine health system efficiency.
While studies used a range of inputs, these were generally the building blocks of health systems, health risk factors, and social determinants of health. The common justification for the use of specific inputs and outputs in efficiency analysis was the fact that it had been used by a similar analysis in other settings and data availability. This finding is similar to that of a review efficiency analysis of primary-care systems [ 107 ]. A limitation to this input/output selection approach is the likelihood that the selected inputs/outputs may not be relevant and suitable to characterising specific health systems. Exercises to engage health system decision makers and implementors in specific contexts to understand their health systems and identify context-appropriate inputs and outputs for efficiency analysis should be explored. Such efforts will require and inform the strengthening of data systems for health system performance monitoring and evaluations in specific contexts.
The findings on factors that determine the efficiency of health systems highlight several issues. First, that the efficiency of national and sub-national health system is partly determined by factors not easily influenced by health system policy makers. Broader contextual factors such as the demographic, individual and household socio-economic, macro-economic, and governance and political system characteristics of the national and sub-national unit are outside of the control of health system policy makers. This emphasises the fact that health systems are part of and are affected by the larger society that forms their contexts and underlies the need for countries to strengthen multisectoral coordination and approaches to health [ 110 , 111 ].
Second, the efficiency of national and sub-national health systems is also affected by the general health and wellbeing of populations. This underlies the need for health policy makers to prioritise investments in preventive and promotive interventions that reduce the risk of disease, and the management of chronic conditions to reduce their burden on health systems. Health system reforms aimed at promoting efficiency should not only focus on optimising health system functions to provide care for the sick, but also prioritise interventions to prevent and promote health and wellbeing of the population. Investing in preventive and promotive health interventions has been shown to be cost effective [ 112 ] and prioritising preventive and promotive health has been identified as an efficiency enhancing intervention by the WHO 2010 world health report [ 113 ].
Lastly, health system arrangements offer several policy levers for improving the efficiency of national and sub-national health systems. These factors are key because they are under the direct control of health system managers and policy makers and can be leveraged to enhance health system efficiency. On health governance, strengthening health sector leadership and management, enhancing health sector coordination as well as multisectoral coordination, decentralisation of health functions, and introducing/strengthening the regulation of health workers and healthcare costs/pricing should be considered. These findings resonate with those of other analyses. For instance, a review of 10 case studies of health system efficiency found that strengthening health sector regulation, pharmaceutical pricing, and enhancing donor coordination enhanced the efficiency of country health systems [ 114 ]. With regard to health financing, interventions include scaling-up prepayment health financing mechanisms and reducing the level of out-of-pocket payments. Purchasing and public finance management (PFM) reforms that include defining evidence-based, cost-effective benefit packages, reforming payment systems to transition to prospective payment mechanisms, strengthening budget execution and flexibility of budgets, the direct payment of healthcare providers (to improve provider autonomy, and flow of funds), health facility autonomy, and ensuring patient choice of health providers may promote the efficiency of health systems. Yip and Hafez [ 114 ] found that the introduction of evidence based benefit packages enhanced health system efficiency in Chile and Uruguay while scaling population coverage with prepayment financing systems was efficiency enhancing in Mexico. On health system hardware, investing in adequate levels of health workers, health commodities, and health infrastructure, as well as interventions to ensure equity (including geographical) in their distribution and access are key. Having an optimal mix of health system inputs has been identified by other studies as efficiency enhancing [ 113 ]. On service delivery, policy options include re-orienting health systems to prioritise primary health care and strengthening community health systems and strengthening gate keeping and referral systems. Investments are needed to support care at all levels, for example, increasing PHC financing, coverage of basic services, prioritising health workers providing primary healthcare. In Ethiopia, investment in health extension workers that provided community health services to catchment populations improved the efficiency of the health system by improving access to primary healthcare [ 53 ]. Further, interventions to strengthen quality of care and curb overprovision of unnecessary care should be considered. The WHO report on the ten leading causes of efficiency identified examples that include inappropriate hospital admissions, medical errors, and overuse of equipment, investigations, and procedures [ 113 ]. Further, the introduction of essential medicines list to guide medicine procurement was found to be efficiency enhancing in China [ 114 ].
Policy options will, however, need to appropriate for context and take a whole system view given that policy reforms are efficiency enhancing only if they are implemented in policy environments that they are aligned with or coherent. For instance, while increasing the level of financing to the health sector may enhance efficiency, this needs to be accompanied by reforms to contain overprovision/unnecessary care or price increases. While gate keeping by primary healthcare providers may enhance health system efficiency in contexts with good availability and quality of secondary care services, and controls that check against unnecessary referrals. Further, cost-sharing appears to be efficiency enhancing in settings in context with adequate prepayment health financing mechanisms. Policy interventions will therefore need to be contextualised for specific settings.
A potential limitation of this review is the likelihood of missing out key literature since we conducted searches on only two databases. Further, this review presents a qualitative evaluation of determinants of efficiency rather thana quantification of their impacts. Further research could therefore include meta-analysis of the impact of interventions to address health system efficiency.
5 Conclusion
This review highlights the asymmetry of evidence on health system efficiency between HICs and LMICs, with most studies focusing on HICs. It underscores the need to carry out studies to understand the levels and determinants of system level health system efficiency in LMICs. The review also reveals the dearth of efficiency studies that use mixed methods approaches by incorporating qualitative inquiry. While the standard quantitative approaches determine the level of efficiency and the factors that influence efficiency, they fall short in illuminating how and why certain factors influence health systems efficiency in certain contexts. There is therefore a need for mixed methods approaches to deepen the understanding of efficiency and its determinants in different settings. Lastly, the review offers insights on the drivers of the efficiency of national and sub-national health systems, highlights potential targets for reforms to improve health system efficiency.
World Health Organization. The World Health Report 2000. Health systems: improving performance. 2000.
Jacobs R, Smith PC, Street A. Measuring efficiency in health care: analytic techniques and health policy. Cambridge: Cambridge University Press; 2006.
Book Google Scholar
Hollingsworth B. Cost, production, efficiency, or effectiveness: where should we focus? Lancet Glob Health. 2013;1(5):e249–50.
Article PubMed Google Scholar
Cylus J, Papanicolas I, Smith PC. Health system efficiency. How to make measurement matter for policy and management. 2016.
World Health Organization. Everybody's business. Strengthening health systems to improve health outcomes. WHO's framework for action. 2007.
Kutzin J. Health financing policy: a guide for decision-makers. Health financing policy paper. Copenhagen, WHO Regional Office for Europe, 24, 2008.
Palmer S, Torgerson DJ. Definitions of efficiency. BMJ. 1999;318(7191):1136.
Article CAS PubMed PubMed Central Google Scholar
Farrell MJ. The measurement of productive efficiency. 1957.
Coelli TJ, et al. An introduction to efficiency and productivity analysis. 2nd ed. New York: Springer Science & Business Media; 2005.
Google Scholar
Hollingsworth B, Peacock S. Efficiency measurement in health and health care. Oxon: Routledge; 2008.
World Health Organization. Health systems financing: the path to universal coverage. 2010.
Tandon A, Cashin C. Assessing public expenditure on health from a fiscal space perspective. Washington, DC: World Bank; 2010. p. 1–84.
Powell-Jackson, T., K. Hanson, and D. McIntyre, Fiscal space for health: a review of the literature . 2012: London, UK.
Karpa W, Leśniowska J. Efficiency of Health Care Systems: Stochastic Frontier Analysis Including Innovation Component. Prace Naukowe Akademii im. Jana Długosza w Częstochowie. Pragmata Tes Oikonomias. 2014; (8): 159–67
Allin S, Grignon M, Wang L. The determinants of efficiency in the Canadian health care system. Health Econ Policy Law. 2016;11(1):39–65.
PRISMA. PRISMA-TRANSPARENT REPORTING of SYSTEMATIC REVIEWS and META-ANALYSES. 2015. http://www.prisma-statement.org/ . Accessed 31 Dec 2017.
Mijovic H, McKnight J, English M. What does the literature tell us about health workers’ experiences of task-shifting projects in sub-Saharan Africa? A systematic, qualitative review. J Clin Nurs. 2016;25(15–16):2083–100.
Article PubMed PubMed Central Google Scholar
CASP UK. Critical Appraisal Skills Programme (CASP) Check lists. 2017. http://www.casp-uk.net/casp-tools-checklists . Accessed 31 July 2017.
Hannes K. Chapter 4: Critical appraisal of qualitative research in Noyes J, Booth A, Hannes K, Harden A, Harris J, Lewin S, Lockwood C (editors), Supplementary Guidance for Inclusion of Qualitative Research in Cochrane Systematic Reviews of Interventions. 2011. http://cqrmg.cochrane.org/supplemental-handbook-guidance . Accessed 1 Sept 2015.
Ritchie J, Spencer L. Qualitative data analysis for applied policy research. In: Alan B, Robert GB, editors. Analyzing qualitative data. The qualitative researcher’s companion. Oxon: Routledge; 1994. p. 173–94.
Chapter Google Scholar
Bekaroglu C, Heffley D. A multi-stage efficiency analysis of OECD healthcare systems. J Manag Econ Res. 2018;16(2):264–85.
Evans DB, et al. The comparative efficiency of national health systems in producing health: an analysis of 191 countries. World Health Organization. 2000;29(29):1–36.
Aristovnik A. Measuring relative efficiency in health and education sector: the case of East European countries. Actual Probl Econ. 2012;10(136):305–14.
Kim Y, Park MJ, Atukeren E. Healthcare and Welfare Policy Efficiency in 34 Developing Countries in Asia. Int J Environ Res Public Health. 2020;17:4617.
Singh S, et al. Application of DEA-Based Malmquist Productivity Index on healthcare system efficiency of ASEAN Countries. Int J Health Plan Manag. 2020;36:1236–50.
Article Google Scholar
Moreno-Serraa R et al. An exploratory application of data envelopment analysis to the efficiency of health service coverage and access. Results for Development Institute. 2012.
Novignon J, Lawanson A. Efficiency of health systems in sub-Sahara Africa: a comparative analysis of time varying stochastic frontier models, MPRA paper 56897. Berlin: University Library of Munich; 2014.
Ding J, et al. Equity and efficiency of medical service systems at the provincial level of China’s mainland: a comparative study from 2009 to 2014. BMC Public Health. 2018;18(1):214.
Du T. Performance measurement of healthcare service and association discussion between quality and efficiency: evidence from 31 provinces of mainland china. Sustainability. 2017;10(1):74.
Ngobeni V, Breitenbach MC, Aye GC. Technical efficiency of provincial public healthcare in South Africa. Cost Eff Resour Alloc. 2020;18(3):1–19.
Mousa W, Aldehayyat JS. Regional efficiency of healthcare services in Saudi Arabia. Middle East Dev J. 2018;10(1):152–74.
Schleiniger R. Regional quantity, productivity and efficiency measures of the Swiss health care system. Swiss J Econ Stat. 2008;144(3):459–76.
Varela PS, de Andrade Martins G. Efficiency of primary health care spending by municipalities in the metropolitan region of Sao Paulo: a comparative analysis pf DEA models. Rev Bus. 2011;32(1):17–35.
Loikkanen HA, Susiluoto I. Cost efficiency of Finnish municipalities in basic service provision 1994–2002. 2005.
Purohit BC. Efficiency in health care sector in Bihar (India): An exploratory analysis using DEA. Afr J Health Econ. 2015;4(2):1–13.
Chansa C, et al. Exploring SWAp’s contribution to the efficient allocation and use of resources in the health sector in Zambia. Health Policy Plan. 2008;23(4):244–51.
Anselmi L, Lagarde M, Hanson K. The efficiency of the local health systems: investigating the roles of health administrations and health care providers. Health Econ Policy Law. 2018;13(1):10–32.
Evans DB, et al. Comparative efficiency of national health systems: cross national econometric analysis. BMJ. 2001;323(7308):307–10.
Kumbhakar SC. Efficiency and productivity of world health systems: where does your country stand? Appl Econ. 2010;42(13):1641–59.
Herrera S, Pang G. Efficiency of public spending in developing countries: an efficiency frontier approach. Washington, DC: The World Bank; 2005.
Allin S, et al. How can health system efficiency be improved in Canada? Healthc Policy. 2015;11(1):33.
PubMed PubMed Central Google Scholar
Popescu C, Asandului L, Fatulescu P. A data envelopment analysis for evaluating Romania’s health system. Procedia Soc Behav Sci. 2014;109:1185–9.
Ozcan YA, Khushalani J. Assessing efficiency of public health and medical care provision in OECD countries after a decade of reform. CEJOR. 2017;25(2):325–43.
Kujawska J. Efficiency of healthcare systems in european countries-the DEA network approach. Metody Ilościowe w Badaniach Ekonomicznych. 2018;19:60–70.
Nyawira L, et al. Examining health sector stakeholder perceptions on the efficiency of county health systems in Kenya. PLoS Glob Public Health. 2021;1(12):e0000077.
Allin S, et al. Improving health system efficiency: perspectives of decision makers. Healthc Q. 2017;20(1):10–3.
Moses MW, et al. Performance assessment of the county healthcare systems in Kenya: a mixedmethods analysis. BMJ Glob Health. 2021;6:e004707.
Zeng W, et al. Understanding the performance of county health service delivery in Kenya: a mixed-method analysis. Health Policy Plan. 2022;37(2):189–99.
Nannyonjo J, Okot N. Decentralization, local government capacity and efficiency of health service delivery in Uganda. J Afr Dev. 2013;15(1):125–58.
Hooda Y. Efficiency in expenditure on healthcare system at state level in India. Int J Adv Res Manag Soc Sci. 2018;7:34–41.
Purohit BC. Efficiency of social sector expenditure in India: a case of health and education in selected Indian states . Healthc Low Resource Settings. 2014;2(1):26–34
Felder S, Tauchmann H. Federal state differentials in the efficiency of health production in Germany: an artifact of spatial dependence? Eur J Health Econ. 2013;14(1):21–39.
Yip W, Hafez R, Organization WH. Improving health system efficiency: reforms for improving the efficiency of health systems: lessons from 10 country cases. World Health Organization. 2015.
Nyawira L, et al. Examining health sector stakeholder perceptions on the efficency of county ehalth systems in Kenya. PLoS Global Public Health. 2021;1(12):e0000077.
Zeng W, et al. Understanding the performance of county health service delivery in Kenya: a mixed-method analysis. Health Policy Plan. 2021;00:1–11.
Charnes A, Cooper W, Rhodes E. Measuring of efficiency of decision making units. Eur J Oper Res. 1978;2:429–39.
Aigner D, Lovell C, Schmidt P. Formulation and estimation of stochastic frontier production function models. J Econom. 1977;6:21–37.
Makiela K. State level efficiency measures for healthcare systems. 2010.
Şenel T, Cengiz MA. A bayesian approach for evaluation of determinants of health system efficiency using stochastic frontier analysis and beta regression . Comput Math Methods Med. 2016.
de Cos PH, Moral-Benito E. Determinants of health-system efficiency: evidence from OECD countries. Int J Health Care Finance Econ. 2014;14(1):69–93.
Ramírez-Valdivia MT, Maturana S, Salvo-Garrido S. A multiple stage approach for performance improvement of primary healthcare practice. J Med Syst. 2011;35(5):1015–28.
De Nicola A, Gitto S, Mancuso P. A two-stage DEA model to evaluate the efficiency of the Italian health system. 2011.
Gravelle H, et al. Comparing the efficiency of national health systems: a sensitivity analysis of the WHO approach. Appl Health Econ Health Policy. 2003;2(3):141–7.
PubMed Google Scholar
Purohit BC. Healthcare sector efficiency in Gujarat (India): an exploratory study using data envelopment analysis. Healthc Low Resour Settings. 2016;4(1). https://doi.org/10.4081/hls.2016.5525
See KF, Yen SH. Does happiness matter to health system efficiency? A performance analysis. Heal Econ Rev. 2018;8(1):33.
Sankar D, Kathuria V. Health system performance in rural India: efficiency estimates across states . Econ Political Wkly. 2004: 39(13):1427–33
Sinimole K. Evaluation of the efficiency of national health systems of the members of World Health Organization. Leadersh Health Serv. 2012;25(2):139–50.
Tandon A, et al. Measuring overall health system performance for 191 countries. Geneva: World Health Organization; 2000.
Tandon A. Measuring efficiency of macro systems: an application to Millennium Development Goal attainment. 2005.
Achoki T, et al. Technical and scale efficiency in the delivery of child health services in Zambia: results from data envelopment analysis. BMJ Open. 2017;7(1): e012321.
Stefko R, Gavurova B, Kocisova K. Healthcare efficiency assessment using DEA analysis in the Slovak Republic. Health Econ Rev. 2018;8(1):6.
Dash U, Vaishnavi SD, Muraleedharan VR. Technical efficiency in the use of healthcare resources: a case study of Tamil Nadu. Indian Econ Rev. 2008;43(1):69–82
Anton SG. Technical efficiency in the use of health care resources: a cross-country analysis. Ann Alexandru Ioan Cuza Univ Econ. 2013;60(1):1–12.
Kinfu Y, Sawhney M. Inefficiency, heterogeneity and spillover effects in maternal care in India: a spatial stochastic frontier analysis. BMC Health Serv Res. 2015;15(1):118.
Suraratdecha C, Okunade AA. Measuring operational efficiency in a health care system: a case study from Thailand. Health Policy. 2006;77(1):2–23.
Canagarajah S, Ye X. Public health and education spending in Ghana in 1992–98: issues of equity and efficiently, Vol. 2579. 2001; World Bank Publications.
Hadad S, Hadad Y, Simon-Tuval T. Determinants of healthcare system’s efficiency in OECD countries. Eur J Health Econ. 2013;14(2):253–65.
Ibrahim MD, Daneshvar S. Efficiency analysis of healthcare system in Lebanon using modified data envelopment analysis. J Healthc Eng. 2018;2018:2060138. https://doi.org/10.1155/2018/2060138 .
Porcelli F. Electoral accountability and local government efficiency: quasi-experimental evidence from the Italian health care sector reforms. Econ Gov. 2014;15(3):221–51.
Lee S, Kim C. Estimation of association between healthcare system efficiency and policy factors for public health. Appl Sci. 2018;8(12):2674.
González E, Cárcaba A, Ventura J. Value efficiency analysis of health systems: does public financing play a role? J Public Health. 2010;18(4):337–50.
Spinks J, Hollingsworth B. Cross-country comparisons of technical efficiency of health production: a demonstration of pitfalls. Appl Econ. 2009;41(4):417–27.
Ahmed S, et al. Measuring the efficiency of health systems in Asia: a data envelopment analysis. BMJ Open. 2019;9:e022155.
Moreno-Serra R, Anaya-Montes M, Smith PC. Potential determinants of health system efficiency: evidence from Latin America and the Caribbean. PLoS One. 2019;14(5): e0216620.
Zarulli V, et al. Health care system efficiency and life expectancy: a 140-country study. PLoS One. 2021;16(7): e0253450.
Barasa E, et al. Level and determinants of county health system technical efficiency in Kenya: two stage data envelopment analysis. Cost Eff Resour Alloc. 2021;19(78):1–11.
Purohit BC. Efficiency in healthcare sector in Assam: a sub-state level analysis. Online J Health Allied Sci. 2016;14(4):1.
Prachitha J, Shanmugam K. Efficiency of raising health outcomes in the Indian States. Chennai: Madras School of Economics; 2012.
Purohit BC. Efficiency of health care sector at sub-state level in India: a case of Punjab. Online J Health Allied Sci. 2009;8(3):212–25.
Grigoli F, Kapsoli J. Waste not, want not: the efficiency of health expenditure in emerging and developing economies. Rev Dev Econ. 2018;22(1):384–403.
Hassan CM, Djekonbe D, DRD T. The efficiency of the health system in Chad. 2018.
Hollingsworth B, Wildman J. The efficiency of health production: re-estimating the WHO panel data using parametric and non-parametric approaches to provide additional information. Health Econ. 2003;12(6):493–504.
Lagravinese R, Paradiso M, Mastromarco C. Social heterogeneity and efficiency in Italian health care system: a Simar–Wilson methodology analysis. Società Italiana degli Economisti Working Paper September, 2011. 15: p. 2011.
Greene W. Distinguishing between heterogeneity and inefficiency: stochastic frontier analysis of the World Health Organization’s panel data on national health care systems. Health Econ. 2004;13(10):959–80.
Ravangard R, et al. Factors affecting the technical efficiency of health systems: a case study of Economic Cooperation Organization (ECO) countries (2004–10). Int J Health Policy Manag. 2014;3(2):63.
Lagravinese R, Paradiso M, Mastromarco C. Social heterogeneity and efficiency in Italian healthcare system: a Simar–Wilson methodology analysis. 2011, Department of Economics, University of Bari
Chai P, Wan Q, Kinfu Y. Efficiency and productivity of health systems in prevention and control of non-communicable diseases in China, 2008–2015. Eur J Health Econ. 2021;22:267–79.
Verhoeven M, Gunnarsson V, Lugaresi S. The health sector in the Slovak Republic: efficiency and reform. IMF Working Papers, 2007. p. 1–25.
Jafarov E, Gunnarsson V. Efficiency of government social spending in Croatia. Financial Theory Pract. 2008;32(3):289–320.
Gong G, et al. Has the efficiency of China’s healthcare system improved after healthcare reform? A network data envelopment analysis and Tobit regression approach. Int J Environ Res Public Health. 2019;16:4847.
Wranik D. Healthcare policy tools as determinants of health-system efficiency: evidence from the OECD. Health Econ Policy Law. 2012;7(2):197–226.
Chai P, et al. Technical and scale efficiency of provincial health systems in China: a bootsrappin data envelopment analysis. BMJ Open. 2019;9:e027539.
Bhat VN. Institutional arrangements and efficiency of health care delivery systems. Eur J Health Econ. 2005;6(3):215–22.
Nassar H, et al. Technical efficiency of health-care systems in selected middle-income countries: an empirical investigation . Review of economics and political science. 2020;5(4):267–87.
Sousa A, et al. Measuring the efficiency of human resources for health for attaining health outcomes across subnational units in Brazil . World health report, 2006.
Varabyova Y, Müller J-M. The efficiency of health care production in OECD countries: a systematic review and meta-analysis of cross-country comparisons. Health Policy. 2016;120:252–63.
Pelone F, et al. Primary care efficiency measurement using data envelopment analysis: a systematic review. J Med Syst. 2015;39:156.
Hussey PS, et al. A systematic review of health care efficiency measures. Health Services Res. 2009;44(3):784–805.
Asbu EZ, Masri MD, Naboulsi MA. Determinants of hospital efficiency: a literature review . Int J Healthc. 2020;6(2).44–53.
Bennett S, Glandon D, Rasanathan K. Governing multisectoral action for health in low-income and middle-income countries: unpacking the problem and rising to the challenge. BMJ Glob Health. 2018;3(Suppl 4):e000880.
Tangcharoensathien V, et al. Multisectoral actions for health: challenges and opportunities in complex policy environments. Int J Health Policy Manag. 2017;6(7):359–63.
Merkur S, Sassi F, McDaid D. Promoting health, preventing disease: is there an economic case? WHO Regional Office for Europe: Denmark; 2013.
WHO. More health for the money, in World Health Report—Health Systems Financing; the path to universal coverage. 2010, World Health Organization: Geneva.
Yip W, Hafez R. Reforms for improving the efficiency of health systems: lessons from 10 country cases; 2015. World Health Organization.
Download references
Acknowledgements
This paper is published with the permission of the director KEMRI.
Author information
Authors and affiliations.
Health Economics Research Unit, KEMRI-Wellcome Trust Research Programme, Nairobi, Kenya
Rahab Mbau, Anita Musiega, Lizah Nyawira, Julie Jemutai & Edwine Barasa
Institute of Healthcare Management, Strathmore University, Nairobi, Kenya
Anita Musiega
Health Systems and Research Ethics Department, KEMRI-Wellcome Trust Research Programme, Kilifi, Kenya
Benjamin Tsofa & Sassy Molyneux
County Department of Health, Makueni County Government, Nairobi, Kenya
Andrew Mulwa
Centre for Tropical Medicine and Global Health, Nuffield department of Medicine, University of Oxford, Oxford, UK
Sassy Molyneux & Edwine Barasa
Health Financing Department, Ministry of Health, Nairobi, Kenya
Isabel Maina
Centre for Health Policy and Management, Trinity College, The University of Dublin, Dublin, Ireland
Charles Normand
Cicely Saunders Institute, Kings College London, London, UK
Faculty of Public Health and Policy, London School of Hygiene and Tropical Medicine, London, UK
Kara Hanson
You can also search for this author in PubMed Google Scholar
Corresponding author
Correspondence to Edwine Barasa .
Ethics declarations
Ethics approval and consent to participate.
Not applicable—this is a review of literature.
Consent for publication
Availability of data and materials.
All the data used for this study, as well as the coding framework used, are publicly available as Online Supplementary files.
Conflict of interests
All authors declare no competing interests.
This work was funded by a MRC/FCDO/ESRC/Wellcome Trust Health Systems Research Initiative (HSRI) Grant no MR/R01373X/1.
Authors’ contributions
EB, JJ, KH, AM, LN, IM, SM, BS, and CN conceived of the study. EB and RM collected data. EB and RM analysed the data and wrote the first version of the manuscript. All authors contributed to further analysis and writing of subsequent versions of the manuscript.
Supplementary Information
Below is the link to the electronic supplementary material.
Supplementary file1 (XLSX 26 kb)
Supplementary file2 (xlsx 9 kb), rights and permissions.
Open Access This article is licensed under a Creative Commons Attribution-NonCommercial 4.0 International License, which permits any non-commercial use, sharing, adaptation, distribution and reproduction in any medium or format, as long as you give appropriate credit to the original author(s) and the source, provide a link to the Creative Commons licence, and indicate if changes were made. The images or other third party material in this article are included in the article's Creative Commons licence, unless indicated otherwise in a credit line to the material. If material is not included in the article's Creative Commons licence and your intended use is not permitted by statutory regulation or exceeds the permitted use, you will need to obtain permission directly from the copyright holder. To view a copy of this licence, visit http://creativecommons.org/licenses/by-nc/4.0/ .
Reprints and permissions
About this article
Mbau, R., Musiega, A., Nyawira, L. et al. Analysing the Efficiency of Health Systems: A Systematic Review of the Literature. Appl Health Econ Health Policy 21 , 205–224 (2023). https://doi.org/10.1007/s40258-022-00785-2
Download citation
Accepted : 04 December 2022
Published : 28 December 2022
Issue Date : March 2023
DOI : https://doi.org/10.1007/s40258-022-00785-2
Share this article
Anyone you share the following link with will be able to read this content:
Sorry, a shareable link is not currently available for this article.
Provided by the Springer Nature SharedIt content-sharing initiative
- Find a journal
- Publish with us
- Track your research
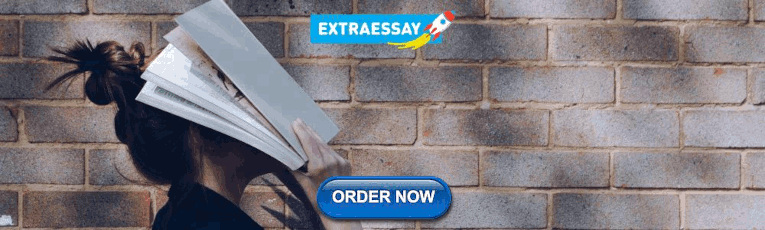
IMAGES
VIDEO
COMMENTS
Thus, through a comprehensive review of the extant literature, this study presents a critique of the health information system for healthcare to supplement the gap created as a result of the lack of an in-depth outlook of the current health information system from a holistic slant. From the studies, the health information system was ascertained ...
Health information systems (HIS) are critical systems deployed to help organizations and all stakeholders within the healthcare arena eradicate disjointed information and modernize health processes by integrating different health functions and departments across the healthcare arena for better healthcare delivery [1,2,3,4,5,6].Over time, the HIS has transformed significantly amidst several ...
Thus, through a comprehensive review of the extant literature, this study presents a critique of the health information system for healthcare to supplement the gap created as a result of the lack ...
Background Accelerated by the coronavirus disease 2019 (Covid-19) pandemic, major and lasting changes are occuring in healthcare structures, impacting people's experiences and value creation in all aspects of their lives. Information systems (IS) research can support analysing and anticipating resulting effects. Aim The purpose of this study is to examine in what areas health information ...
We present a systematic review of the literature on health information systems. ... Kumar et al. [24] identified research gaps in routine health information systems by means of a literature review for low- and middle-income countries. From 316 studies they identified obstacles related to data quality, data use, and system design. They present ...
As there is a lack of similar studies on the topic, this analysis uses a systematic literature review of articles published from 2014 to 2019 to identify the most common e-health practices used worldwide, as well as the main services provided, diseases treated, and the associated technologies that assist in e-health practices.
A systematic review of healthcare recommender systems: Open issues, challenges, and techniques. ... This study presents a systematic literature review (SLR) method for HRSs regarding 41 papers published between 2010 and 2021; the selected articles fall into five classes: collaborative-based, content-based, knowledge-based, context-based, and ...
5 Conclusions. The EHR is a complex system which manages the health information of the patients, the workflows on the health organizations and must be a tool for helping the clinical staff. The increase of the cost of the public healthcare made governments to think about strategies for doing it affordable.
2 Purpose of Study. The objective of this study is to conduct a systematic literature review of articles centering on the topic of healthcare automation to determine the role automated systems play in the healthcare industry and its relationship with human factors, patient safety, healthcare quality, and data management.
Health systems resilience is key to learning lessons from country responses to crises such as coronavirus disease 2019 (COVID-19). In this perspective, we review COVID-19 responses in 28 countries ...
The traditional healthcare system is facing various challenges. Chronic diseases are one of the big threats to human health, and are becoming a challenge to healthcare systems all over the world. 1 The rapidly growing aging population is becoming another challenge. The proportion of the world's population aged 60 years or above is expected to reach 21 percent in 2050. 2 Therefore, the ...
Adopting a comprehensive literature review approach that addresses well-known issues resulting from applying structured literature review approaches (e.g., Webster & Watson, ... HIS research is capable of theorizing on an ecosystem level (i.e., capturing the complexity of the socio-technical health system), but would benefit from increasing the ...
AI-based healthcare systems are rapidly evolving, particularly for early detection and diagnostic applications (Chen, 2018; ... methodology, and functionalities. Subsequently, the research classification framework was developed to review the literature related to the nature of how AI enables the healthcare sector in relation to the benefits ...
Efficiency analysis in the health sector has typically focused on the efficiency of healthcare facilities (hospitals, primary healthcare facilities), with few studies focusing on system level (national or sub-national) efficiency. We carried out a thematic review of literature that assessed the efficiency of health systems at the national and ...
The "Mobile Healthcare Management System (HMS)" is one of the main IoT apps that link the Internet to mobile sensors, people, clinicians, networks and other connected devices. The failed method ...
Methods: We performed a rapid review of the literature and reported available studies on DTs and their applications in health care management. We searched 5 databases for studies published between January 2002 and January 2022 and included peer-reviewed studies written in English. We excluded studies reporting DT usage to support health care ...
Given the long standing call for clinical care review, with limited literature to inform care review systems, we conducted a qualitative systematic review to identify characteristics discussed in existing models for care review. ... Spigelman AD, Swan J. Measuring clinical audit and peer review practice in a diverse health care setting. ANZ J ...
Abstract A systematic literature review of researchers and healthcare professionals' attitudes towards the secondary use and sharing of health administrative and clinical trial data was conducted using electronic data searching. Eligible articles included those reporting qualitative or quantitative original research and published in English. No restrictions were placed on publication dates ...
Areview of the literature will identify the range of approaches and offer a critique of their contribution to understanding. The systematic review of the literature in health and social care has a differ- ent focus. It aims to contribute to clinical practice through an assessment of the efficacy of a particular health care intervention and ...
Using the PRISMA approach, we present the first systematic literature review of digital twin (DT) research in healthcare systems (HSs). This endeavor stems from the pressing need for a thorough analysis of this emerging yet fragmented research area, with the goal of consolidating knowledge to catalyze its growth. Our findings are structured around three research questions aimed at identifying ...
Health Care Manage Rev 2019; 44: 30-40. Google Scholar. 3. ... a literature review. Health Informatics J 2010; ... et al. Progress on implementing and using electronic health record systems: Developments in OECD countries as of 2021 [Internet]. Paris: OECD iLibrary, 2023. [cited 2024 Mar 1]. Google Scholar. 22.
Objective Given the demand for net-zero healthcare, the carbon footprint (CF) of healthcare systems has attracted increasing interest in research in recent years. This systematic review investigates the results and methodological transparency of CF calculations of healthcare systems. The methodological emphasis lies specifically on input-output based calculations. Design Systematic review ...
can be explained as below, Personalized: The smart healthcare system is be able to provide a unique and suitable treatment. plan for each user based on the user's physical or spiritual condition ...
The Literature Review provides an in-depth examination of data offloading methods, MEC, and 5G networks, as they pertain to healthcare. Research has indicated that while mobile medical data can be transferred to the cloud for processing, analysis, and storage, the latency involved in transmitting data to remote clouds remains a concern, alongside the ongoing challenge of maintaining patient ...
Hospitals are the biggest consumers of health system budgets and hence measuring hospital performance by quantitative or qualitative accessible and reliable indicators is crucial. This review aimed to categorize and present a set of indicators for evaluating overall hospital performance. We conducted a literature search across three databases, i.e., PubMed, Scopus, and Web of Science, using ...
Knowledge Translation (KT) aims to convey novel ideas to relevant stakeholders, motivating their response or action to improve people's health. Initially, the KT literature focused on evidence-based medicine, applying findings from laboratory and clinical research to disease diagnosis and treatment. Since the early 2000s, the scope of KT has expanded to include decision-making with health ...
The ethical governance of Artificial Intelligence (AI) in health care and public health continues to be an urgent issue for attention in policy, research, and practice [1,2,3].Beyond the growing number of AI applications being implemented in health care, capabilities of AI models such as Large Language Models (LLMs) expand the potential reach and significance of AI technologies across health ...
Michael Padget and colleagues argue that making environmental sustainability a measure of health system quality will support progress and help fulfil systems' fundamental mission to protect and improve health Healthcare systems around the world have a responsibility to "do no harm" but are responsible for substantial negative health effects through the production of greenhouse gases ...
This review of the literature included empirical studies (qualitative and quantitative), literature reviews, and meta-analyses that assessed mental health in Black American adolescents. We focused on adolescents (ages 10-17) to inform prevention and intervention efforts.
We carried out a thematic review of literature that assessed the efficiency of health systems at the national and sub-national level. We conducted a systematic search of PubMed and Google scholar between 2000 and 2021 and a manual search of relevant papers selected from their reference lists. ... Zarulli V, et al. Health care system efficiency ...