Foundations of Clinical Research
This Harvard Medical School six-month, application-based certificate program provides the essential skill sets and fundamental knowledge required to begin or expand your clinical research career.

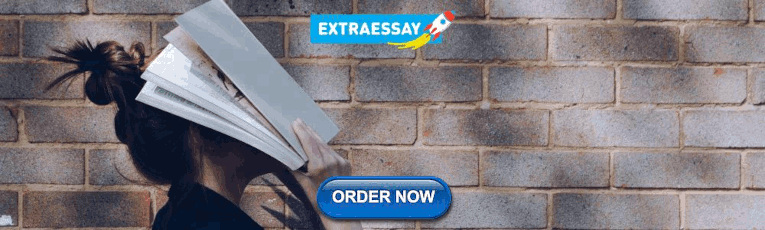
Associated Schools
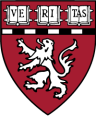
Harvard Medical School
What you'll learn.
Understand and apply the foundational concepts of biostatistics and epidemiology
Develop a research question and formulate a testable hypothesis
Design and begin to implement a clinical research study
Cultivate the skills required to present a clinical research study
Critically evaluate the research findings in medical literature
Synthesize crucial statistical analyses using Stata software
Course description
The Foundations of Clinical Research program is rooted in the belief that clinical research training is critical to professional development in health care. Clinical research training not only creates potential independent investigators, but also enables clinicians to advance their careers through a greater understanding of research evidence. Designed to provide learners with the foundational knowledge and skill sets required to produce high-quality clinical research, our program will lay the fundamental groundwork in epidemiology and biostatistics required for a multifaceted career in clinical research.
The overarching goal of the Foundations of Clinical Research program is to equip the next generation of researchers with the skill sets essential to evaluating evidence, understanding biostatistics, and beginning their clinical research careers. Our aim is to ensure that learners develop a strong foundation in the design, implementation, analysis and interpretation of clinical research studies.
During the program, our innovative active learning approach emphasizes the traditional tutorial system with weekly live video tutorials, seminars and symposia anchored by 3 live intense weekend online workshops. The Foundations of Clinical Research program’s six-month online curriculum emphasizes real-time skill-based learning.
Participants will be eligible for Associate Alumni status upon successful completion of the program. Early tuition and need-based tuition reductions may be available.
Course Outline
Live Workshops
The interactive workshop curriculum will focus on hands-on skill development through active learning. To that end, the intensive schedule is designed to accelerate the growth of high-yield clinical research skills via individual and team-based workshop exercises. Students will be immersed in a dynamic learning environment that encourages collaboration and collegial networking with faculty and peers.
Essential elements of the workshop include instruction and practical exercises in the core concepts of biostatistics, epidemiology and research question development, as well as critical assessment of the medical literature and practical training in statistical software using real-life datasets. In addition to providing training in mentorship, academic career development and leadership, we create a supportive and active learning environment where opportunities for knowledge retention and networking abound.
Live Symposia, Tutorials and Seminars
Symposia, tutorials and seminars are mandatory and will be delivered live online and organized according to eight specific clinical research topics.
Eight 3-Hour Symposia
- Instruction on a specific clinical research topic (e.g., cohort study design and interpretation)
- In-depth discussion on a related epidemiology concept (e.g., odds ratio)
- Hands-on guidance for implementing the related analysis with statistical programming in Stata
Eight 1-Hour Tutorials
- Interpret and report on papers related to the specific clinical research topic
Eight 1-Hour Special-Topic Seminars
- The biostatistical and epidemiological concepts to specific clinical research topics with concrete examples
Assignments
All students will be expected to complete all assignments by the due dates. Assignments will be graded as either “pass” or “fail.”
Individual Assignment 1
Individual Research Question and Study Design
- Generate a novel research question in the evidence-based PICO format
- Receive expert faculty review
Individual Assignment 2
Design, Implement and Present an Original Abstract
- Design and implement a clinical research study based on a publicly available dataset
- Analyze and create data visualizations via a user-friendly R Shiny web app
- Write a formal 350-word abstract suitable for submission to an international conference
- Present a digital poster to faculty at Workshop 3
Online Lectures
Research Study Introduction
- Designing a Clinical Research Study I–III
- Introduction to Evidence-Based Medicine, Systematic Review and Meta-Analysis
- Study Design 1 – Observational
- Study Design 2 – Randomized Controlled Trials
- Study Design 3 – Quasi-Experimental Studies
- Introduction to Biostatistics
- An Investigator’s Responsibility for Protection of Research Subjects
- How to Search PubMed
- Overview of Evidence-Based Medicine
Statistical Programming in Stata
- Loading Data
- Basic Programming Commands
- Data Cleansing
- Data Analytics I – Central Tendency
- Data Analytics II – Statistical Testing
- Data Analytics III – Regression Testing
Instructors
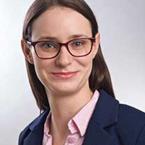
Jamie Robertson
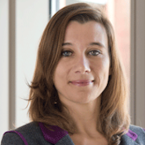
Djøra Soeteman
You may also like.
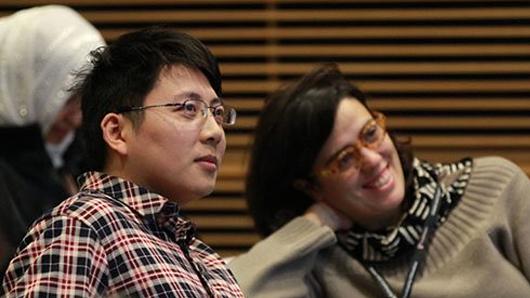
Global Clinical Scholars Research Training
This Harvard Medical School one-year, application-based certificate program provides advanced training in health care research and methods.
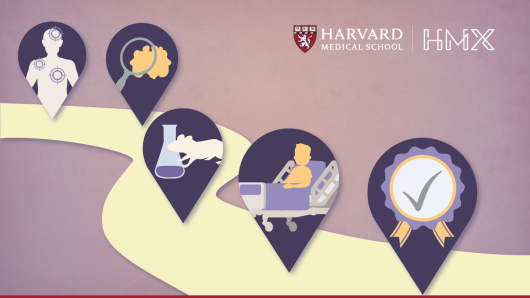
Clinical Drug Development
Learning about the process of clinical drug development has important implications for anyone working in health care and related sectors.
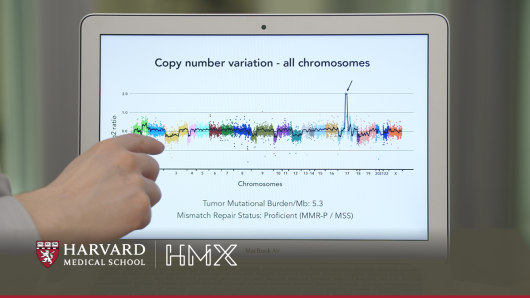
Cancer Genomics and Precision Oncology
Learn how cancer treatment is evolving due to advances in genetics..
A Nature Research Service
Clinical Research Methodology
Key features.
Supports clinicians with strategies to improve the impact and influence of their clinical studies
Includes a workbook with keypoints, activities to reinforce the content, and additional online resources
Interactive workshop where the trainer stimulates engagement and discussion with the attendees
1-day live workshop delivered by an expert trainer
Auditorium or classroom style
Available in-person or virtually
Up to 250 early-career clinical researchers
Masterclass in Clinical Research Methodology
Conducting clinical research has a tremendous impact on patient care and management as well as improving public health. Therefore, it is essential that the study is designed and conducted properly to maximize impact. This one-day workshop aims to give researchers the necessary skills to develop a robust clinical research study that will advance evidence-based medicine. Nature Masterclasses are available in-person and virtually.
Clinical Research Methodology workshop - led by experts
Planning a clinical study
- Discusses how to properly choose a research question that is necessary for the field
- Reviews efficient literature searching strategies and how to stay up-to-date
- Discusses focusing the research problem in a manageable and realistic manner
- Reviews different primary and secondary outcomes related to the research question that should be the focus of the study
Choosing the right study design
- Covers how to identify which study design is appropriate for the research problem and outcome
- Briefly introduces systematic reviews, randomised controlled trials (RCTs), cohort studies, case-controlled studies, cross-sectional studies, and case reports/series
- Highlights the advantages and limitations of the above that should be considered
- Discusses RCTs, given their important impact in clinical research
Quick Links
- UW Directory
- DOM Intranet
- DOM Web Services
- HMC MedConnection
Clinical Research Methods course
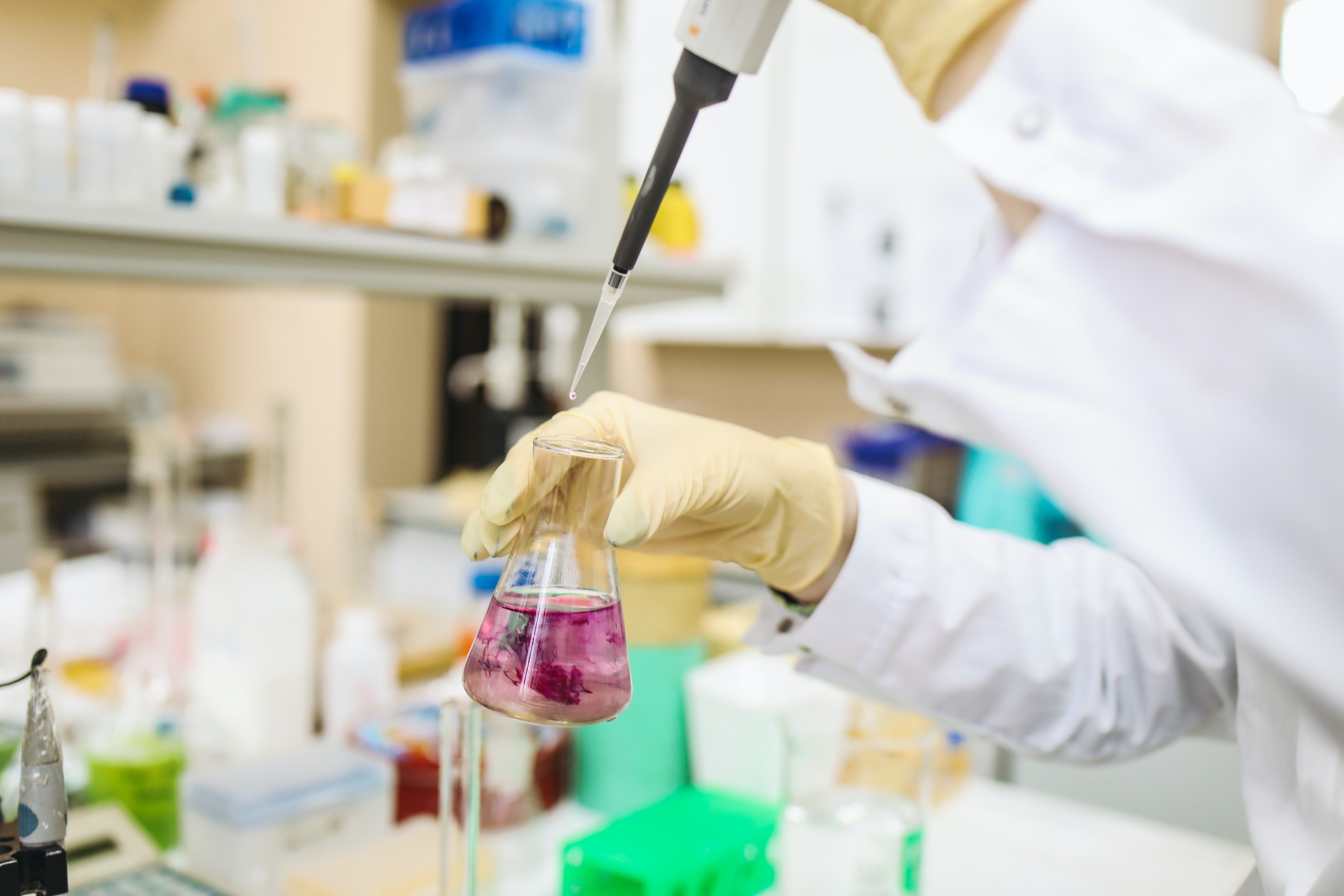
Fellows interested in clinical research are invited to join a fast-paced comprehensive course in clinical research methods.
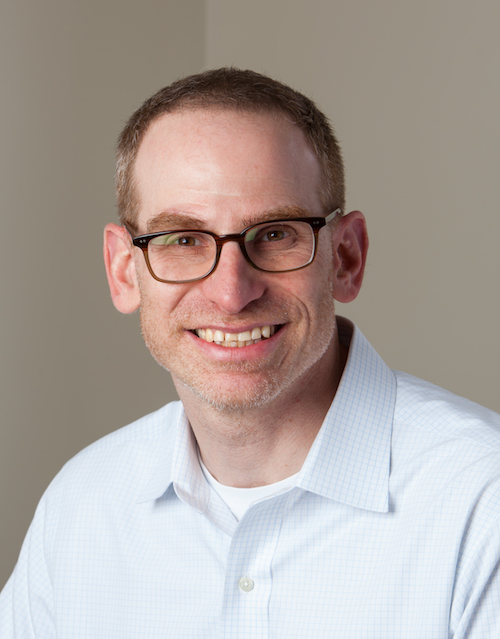
Registration now open
March 4-May 20, 2024, 12-1:30pm South Campus Center Room 348 Please register for this course.
The University of Washington is offering a fast-paced comprehensive course in clinical research methods geared toward fellows in medicine and pediatrics.
The 11-week course will teach fundamental concepts of Epidemiology and Biostatistics with direct application of these methods toward the interpretation of contemporary biomedical research.
The course will combine out of class reading and video content with in-class problem solving sessions and journal article appraisal.
Instructors
The course is taught by instructors in the Division of Nephrology and Kidney Research Institute. Dr. Bryan Kestenbaum , professor, is the course director and primary instructor. He will be assisted by Dr. Leila Zelnick , research associate professor, and Dr. David Prince , research scientist.
Topics to be covered by the course include:
Course logistics
Mondays, March 4 - May 20, 2024, 12-1:30pm (in-person)
South Campus Center Room 348
Precourse materials include written syllabi and short instructional videos.
Course Schedule 2024
Registration.

- NIH Employee Intranet
- Staff Directory
- En Español
OCRECO Home > Clinical Research Education > Introduction to the Principles and Practice of Clinical Research (IPPCR)
OFFICE OF CLINICAL RESEARCH EDUCATION AND COLLABORATION OUTREACH
- Clinical Research Education
- Funding Opportunities

Introduction to the Principles and Practice of Clinical Research (IPPCR)
- Course Information
- Description
- Registration
- Course Login
Description Important Dates General Information Course Objectives Individual (Non-Registered) Lecture Option Texbook Contact --> Welcome
The Introduction to the Principles and Practice of Clinical Research (IPPCR) course trains registrants on how to effectively and safely conduct clinical research. The course focuses on the spectrum of clinical research and the research process by highlighting biostatistical and epidemiologic methods, study design, protocol preparation, patient monitoring, quality assurance, ethical and legal issues, and much more.
Course Objectives
Provide an overview of basic biostatistical and epidemiologic methods involved in conducting clinical research.
Describe the principles involved in the ethical, legal, and regulatory issues in clinical human subjects research, including the role of Institutional Review Boards (IRBs).
Describe principles and issues involved in monitoring patient-oriented research.
Describe the infrastructure required in performing clinical research and the steps involved in developing and funding research studies.
Intended Audience
This course will be of interest to physicians, scientists, medical and dental students, nurses, public health professionals, and others conducting or planning a career in clinical research.
Course Directors
Social media links.
- Bookmark & Share
- E-mail Updates
Page Footer
- Visitor Information
- Privacy Notice
- Accessibility
- No Fear Act
- U.S. Department of Health and Human Services
- USA.gov – Government Made Easy
- HHS Vulnerability Disclosure
National Institutes of Health (NIH), 9000 Rockville Pike, Bethesda, Maryland 20892
NIH…Turning Discovery Into Health®

- Weill Cornell Medicine
Wayfinder menu
- National CTSA
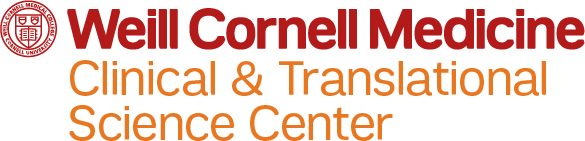
Clinical Research Methodology Curriculum
Application instructions.

The Clinical Research Methodology Curriculum (CRMC) is a one-year clinical research methodology for investigators with clinical research experience seeking to obtain up-to-date knowledge in the field of clinical research. It is conducted at Memorial Sloan Kettering Cancer Center to promote greater flexibility for trainees from across the CTSC partner institutes. The CRMC curriculum allow participants to either enroll in the entire program or audit specific components that address self-identified educational needs.
The Clinical Research Methodology Curriculum is currently accepting applications for the 2023-2024 academic year. The application deadline to submit is Friday, August 18, 2023 at 5:00PM .

Clinical & Translational Science Center 1300 York Ave., Box 149 New York, NY 10065
Home > Education & Training > Essentials of Clinical Research Course
Essentials of Clinical Research Course
The Essentials of Clinical Research course is designed for Stanford and CTSA-affiliated faculty and staff engaged in clinical research and consists of 10 sessions.
Email our Education & Training team
Register for:
2024 LIVE Essentials of Clinical Research (Jan. 11 – Mar. 14, 2024) Register Here
This course provides an overview of basic principles of clinical research design, including biostatistics; study design and interpretation of diagnostic and predictive test studies; and required and desired elements of clinical trial protocols. Participants will be introduced to the regulatory aspects of clinical research conduct and oversight, Good Clinical Practice (GCP) principles, and ethical dimensions of clinical research.
Course Details
The On-Demand Essentials of Clinical Research will be available starting May 1, 2024. These are the recorded seminars from Jan – Mar 2024. This information is for learning purposes only. An evaluation is requested at the end of the course. Presentations and resources are available. A certificate will not be issued.
Registration open for the 2024 Essentials of Clinical Research The course will be held in person with zoom option for our out-of-state participants. Note, the course takes place from 4:00 – 6:00p pacific time. While the course is recorded for course participant viewing, participants should plan to attend the LIVE sessions. Enrolled participants may be dropped from the course if there are more than 3 absences. Please complete the knowledge tests and evaluations within the allotted time frame to receive a course completion certificate, and CME credit, if offered. Continuing Medical Education (CME) credit is applicable towards clinician and nurse license renewals.
Find Syllabus here
Faculty Director
Steve Goodman, MD, MHS, PhD Professor (Epidemiology and Population Health) Associate Dean of Clinical and Translational Research
Certification of Completion (Live Course)
A Certificate of Completion is available to those who meet the following requirements:
- Attend a minimum of 8 sessions
- Complete a minimum of 8 session evaluations
- Take post-course knowledge assessment
Essentials of Clinical Research Course Syllabus
Sessions are taught by Stanford faculty and staff who are experts in the field of clinical research.
Upon course completion, attendees will have an understanding of how to:
- Design and analyze clinical research protocols.
- Comply with “Good Clinical Practice” guidelines for study conduct, data management, and relevant regulations.
- Apply the principles and practices underlying ethical and reproducible research.
If you have any questions, please contact Research Office Training
Additional Resources
ICCR Study Design and Performance
Clinical Research Operations Program
Other Education and Training Opporunities
Skip to content
Read the latest news stories about Mailman faculty, research, and events.
Departments
We integrate an innovative skills-based curriculum, research collaborations, and hands-on field experience to prepare students.
Learn more about our research centers, which focus on critical issues in public health.
Our Faculty
Meet the faculty of the Mailman School of Public Health.
Become a Student
Life and community, how to apply.
Learn how to apply to the Mailman School of Public Health.
Clinical Research Methods
Director: Todd Ogden, PhD
The Mailman School offers the degree of Master of Science in Biostatistics, with an emphasis on issues in the statistical analysis and design of clinical studies. The Clinical Research Methods track was conceived and designed for clinicians who are pursuing research careers in academic medicine. Candidacy in the CRM program is open to anyone who holds a medical/doctoral degree and/or has several years of clinical research experience.
Competencies
In addition to achieving the MS in Biostatistics core competencies, graduates of the 30 credit MS Clinical Research Methods Track develop specific competencies in data analysis and computing, public health and collaborative research, and data management. MS/CRM graduates will be able to:
Data Analysis and Computing
- Apply the basic tenets of research design and analysis for the purpose of critically reviewing research and programs in disciplines outside of biostatistics;
- Differentiate between quantitative problems that can be addressed with standard methods and those requiring input from a professional biostatistician.
Public Health and Collaborative Research
- Formulate and prepare a written statistical plan for analysis of public health research data that clearly reflects the research hypotheses of the proposal in a manner that resonates with both co-investigators and peer reviewers;
- Prepare written summaries of quantitative analyses for journal publication, presentations at scientific meetings, grant applications, and review by regulatory agencies;
Data Management
- Identify the uses to which data management can be put in practical statistical analysis, including the establishment of standards for documentation, archiving, auditing, and confidentiality; guidelines for accessibility; security; structural issues; and data cleaning;
- Differentiate between analytical and data management functions through knowledge of the role and functions of databases, different types of data storage, and the advantages and limitations of rigorous database systems in conjunction with statistical tools;
- Describe the different types of database management systems, the ways these systems can provide data for analysis and interact with statistical software, and methods for evaluating technologies pertinent to both; and
- Assess database tools and the database functions of statistical software, with a view to explaining the impact of data management processes and procedures on their own research.
Required Courses
The required courses enable degree candidates to gain proficiency in study design, application of commonly-used statistical procedures, use of statistical software packages, and successful interpretation and communication of analysis results. A required course may be waived for students with demonstrated expertise in that field of study. If a student places out of one or more required courses, that student must substitute other courses, perhaps a more advanced course in the same area or another elective course in biostatistics or another discipline, with the approval of the student’s faculty advisor.
The program, which consists of 30 credits of coursework and research, may be completed in one year, provided the candidate begins study during the summer semester of his or her first year. If preferred, candidates may pursue the MS/CRM on a part-time basis. The degree program must be completed within five years of the start date.
The curriculum, described below, is comprised of 24 credits of required courses, including a 3-credit research project (the “Master’s essay”) to be completed during the final year of study, and two electives of 6 credits. Note that even if a course is waived, students must still complete a minimum of 30 credits to be awarded the MS degree.
Commonly chosen elective courses include:
Master's Essay
As part of MS/CRM training, each student is required to register for the 3-credit Master's essay course (P9160). This course provides direct support and supervision for the completion of the required research project, or Master's essay, consisting of a research paper of publishable quality. CRM candidates should register for the Master's essay during the spring semester of their final year of study. Students are required to come to the Master's essay course with research data in hand for analysis and interpretation.
CRM graduates have written excellent Master's essays over the years, many of which were ultimately published in the scientific literature. Some titles include:
- A Comprehensive Analysis of the Natural History and the Effect of Treatment on Patients with Malignant Pleural Mesothelioma
- Prevalence and Modification of Cardiovascular Risk Factors in Early Chronic Kidney Disease: Data from the Third National Health and Nutrition Examination Survey
- Perspectives on Pediatric Outcomes: A Comparison of Parents' and Children's Ratings of Health-Related Quality of Life
- Clinical and Demographic Profiles of Cancer Discharges throughout New York State Compared to Corresponding Incidence Rates, 1990-1994
Sample Timeline
Candidates may choose to complete the CRM program track on a part-time basis, or complete all requirements within one year (July through May). To complete the degree in one year, coursework must commence during the summer term.
Note that course schedules change from year to year, so that class days/times in future years will differ from the sample schedule below; you must check the current course schedule for each year on the course directory page .
Paul McCullough Director of Academic Programs Department of Biostatistics Columbia University [email protected] 212-342-3417
More information on Admission Requirements and Deadlines.
Course Listings
INSTITUTE FOR CLINICAL RESEARCH EDUCATION (ICRE) COURSE DESCRIPTIONS
- Clinical Research (CLRES)
- Medical Education (MEDEDU)
- Descriptions of Courses Offered Through Other University Departments
- Online and hybrid courses
Externships, Practica, and Short Courses
If you wish to take a course as a nondegree student please click here for more information.
If you require a permission number to register please click here for more information.
ICRE Course Term Schedules
Disability Resources and Services
The ICRE supports and follows the diversity policies of the Office of Diversity , Health Sciences. Students needing support and/or accommodation may request it through the University's Office of Disability Resources and Services .
If you have a disability that requires special testing accommodations or other classroom modifications, you need to notify both the instructor and Disability Resources and Services no later than the second week of the term. You may be asked to provide documentation of your disability to determine the appropriateness of accommodations. To notify Disability Resources and Services, call 412-648-7890 (Voice or TTD) to schedule an appointment. The office is located in 140 William Pitt Union.
"The MS in Clinical Research gave me the chance to learn essential skills in data analysis, statistics, epidemiology, and clinical trial design from leading experts, all presented in a format that made it both enjoyable and relevant to my research interests."
- Anthony Lewis, MD, MS General Surgery Resident (PGY-5), University of Pittsburgh 2017 Master of Science in Clinical Research Graduate
Course List and Course Descriptions
Clinical Research Courses
back to top
Medical Education Courses
COURSES OFFERED ONLINE/HYBRID COURSES
- CLRES 2010: Clinical Research Methods
- CLRES 2040: Measurement in Clinical Research
- CLRES 2140: Best Practices in Clinical Research
- MEDEDU 2010: Clinical Research Methods
- MEDEDU 2040: Measurement in Clinical Research
ICRE students at all stages of their careers, in all programs, are encouraged to investigate these training opportunities offered through agencies, universities, and corporations.
- Columbia University’s SHARP (Skills for Health and Research Professionals) Training Program in the Mailman School of Public Health offers short, intensive boot camps and workshops that teach in-demand skills on relevant topics in research and education.
- The CTSA Center for Leading Innovation and Collaboration (CLIC) offers a wealth of training resources available to all investigators interested in translational research.
- CTSI of Southeast Wisconsin offers a wide variety of education and training opportunities including certificate programs, seminars, and lectures , and online Intellectual Property and Commercialization Training Modules .
- The Foundation for Advanced Education in the Sciences (FAES) at the NIH offers BioTech training programs and short training experiences on topics including CRISPR, TALENs, ZFNs, and super resolution microscopy.
- The Institute for Translational Epidemiology Short Course Program at the Mount Sinai School of Medicine offers ongoing short course offerings .
- The National Academies of Sciences, Engineering, and Medicine's Health and Medicine Division (HMD) offers many different types of activities, including workshops, forums, and consensus studies, all aimed at improving health.
- The National Cancer Institute's Division of Cancer Control & Population Sciences Implementation Science team coordinates and supports several training and educational activities.
- The National Cancer Institute's Graduate Student Recruiting Program (GSRP) is open to U.S. citizens and foreign nationals. The GSRP provides an opportunity to explore postdoctoral opportunities within the intramural research program.
- The NIH Clinical Center Office of Clinical Research Training and Medical Education (OCRTME) offers an extensive range of clinical research training to help prepare the next generation of clinician-scientists.
- The NIH Office of Behavioral and Social Sciences Research offers a wide variety of online training resources.
- The NIH Office of Intramural Training and Education offers workshops and training programs for trainees outside of NIH, as well as programs for prospective and current NIH trainees.
- Penn State College of Health and Human Development's Methodology Center offers workshops, talks, and trainings that are open to the public and led by Methodology Center researchers.
Other Courses at the University of Pittsburgh
ICRE students have the option to enroll in graduate-level courses offered in other departments at Pitt. ICRE students have taken courses in the following schools and departments:
- Graduate School of Public Health
- Interdisciplinary Biomedical Graduate Programs
- Center for Bioethics and Health Law
- School of Pharmacy
Institute for Clinical Research Education 200 Meyran Avenue, Suite 300 Pittsburgh, PA 15213
© 2021 University of Pittsburgh ICRE
- Open access
- Published: 22 April 2024
The effect of peer mentoring program on clinical academic progress and psychological characteristics of operating room students: a parallel randomized controlled trial
- Amin Sedigh 1 ,
- Sara Bagheri 2 ,
- Pariya Naeimi 3 ,
- Vahid Rahmanian 4 &
- Nader Sharifi 5
BMC Medical Education volume 24 , Article number: 438 ( 2024 ) Cite this article
Metrics details
One of the new educational systems is the mentorship method. This study aimed to investigate the effect of peer mentoring program on clinical academic progress and psychological characteristics of operating room students.
This research was a randomized controlled trial that was conducted on undergraduate students in the operating room department of Khomein Faculty of Medical Sciences, Markazi Province in Iran. The number of operating room students were 70 that were divided into intervention and control groups by random allocation using Permuted Block Randomization. Inclusion criteria included all operating room students who were in internship, and exclusion criteria included failure to complete the questionnaires. The data collection tools were the demographic questionnaire, Depression Anxiety Stress Scale, Rosenberg Self-Esteem Scale and Situational Motivational Scale. In the control group, clinical training was done in the traditional way. In the intervention group, training was done by peer mentoring method. The obtained data were analyzed using descriptive statistics, independent t-test, paired t-test, chi-square test, ANCOVA, univariable and multivariable linear regression.
The study revealed significant differences between the intervention and control groups. Post-intervention, the intervention group demonstrated substantial increases in self-confidence (mean difference = 5.97, p < 0.001) and significant reductions in stress levels (mean difference = -3.22, p < 0.001). Conversely, minimal changes were noted in the control group for both self-confidence (mean difference = 0.057, p = 0.934) and stress levels (mean difference = 0.142, p = 0.656). Although both groups experienced decreases in anxiety and depression levels, these changes were not statistically significant ( p > 0.05). Furthermore, the intervention significantly enhanced academic progress in the intervention group compared to the control group (mean difference = 20.31, p < 0.001).
The results showed that the implementation of the peer mentoring program was effective in improving academic progress, self-confidence, and reducing the stress of operating room students. Therefore, this educational method can be used in addition to the usual methods to improve the education of operating room students.
Peer Review reports
Introduction
Using effective training methods can increase people's motivation and commitment, increase productivity and reduce mistakes [ 1 ]. Clinical training is an important part of training in medical sciences, which plays an essential role in shaping the basic skills and professional abilities of students, including students of the operating room [ 2 , 3 ]. Learning and mastering work roles and tasks in the operating room environment is challenging; In addition, operating room students should be trained in many interventions in the surgical process before, during and after surgery [ 4 ].
Operating room students are affected by various stresses during the course of clinical training, and various contextual and environmental factors play a role in creating this stress [ 5 ]. The results of a study among nursing students showed the prevalence of depression, anxiety and stress symptoms to be 28.7%, 41.7% and 20.2%, respectively [ 6 ]. Also, studies have shown students' self-efficacy at an average level [ 7 ]. The experience of stress in the clinical environment can affect students' learning and acquisition of clinical skills and lead to a drop in their academic performance [ 8 , 9 ]. Considering the high level of stress and the fact that mistakes have no place in the operating room, it is important to pay attention to the quality of training of operating room students and to strengthen the knowledge and skills of future operating room personnel [ 10 ].
Learners and students prefer new educational methods to traditional and passive methods. Active approach is a form of teacher-learner interaction in which learners are no longer passive listeners, but active participants in the learning process [ 11 , 12 ]. The basis of active and comprehensive learning methods is that learning is based on experience and learners actively create knowledge based on their personal experience [ 13 , 14 , 15 ]. The importance of active learning has led professional associations and accreditation organizations, as well as organizations such as UNESCO, to recommend active learning methods in education [ 16 ].
One of the new educational systems is the mentorship method. In this educational method, the mentor and mentee establish a long-term relationship based on friendship with each other. Positive attitude, experience and volunteering are characteristics of mentorship [ 17 , 18 ]. For the first time, Whitman and Fife examined the peer teaching strategy in university education. In this method, higher year students teach practical and theoretical lessons to lower year students [ 19 , 20 ]. The implementation of the mentorship program increases self-confidence, emotional support, and increases students' interactions [ 21 , 22 ]. When students, despite having knowledge and ability in clinical practice, lack sufficient competence, the reason may be a lack of self-confidence, confidence in their own ability, or understanding of the necessary self-efficacy [ 23 , 24 ]. This study was conducted with the aim of investigating the effect of peer mentoring program on clinical academic progress and psychological characteristics of operating room students.
Study design
This research was a parallel randomized controlled trial that was conducted on undergraduate students in the operating room department of Khomein Faculty of Medical Sciences, Markazi Province in Iran from September 2022 to April 2023.
Participants
The number of operating room students were 70, who were included in the study by census method. Inclusion criteria included all operating room students who were in internship, and exclusion criteria included failure to complete the questionnaires.
Randomization and blindness
First, the students completed the written consent to participate in the study, and then they were divided into intervention and control groups by random allocation using Permuted Block Randomization [ 25 ]. Therefore, 35 participants were placed in each group. Then the participants of the intervention and control groups completed the questionnaires before the beginning of the internship. Due to the nature of the intervention in the present study, it was not possible to blind the subjects under the study. Therefore, blinding was performed on those who collected and recorded the data and those who performed the analysis. This research was designed and implemented according to the CONSORT guidelines (Fig. 1 ).
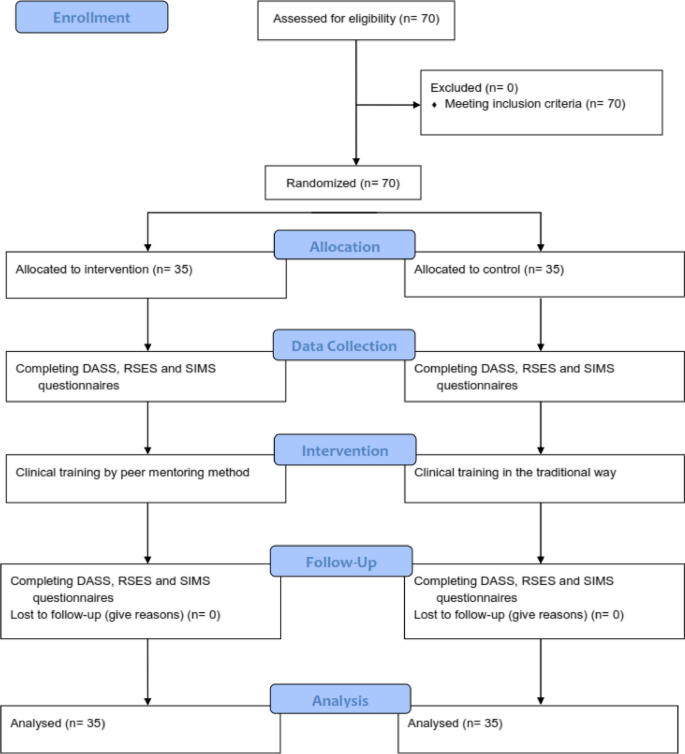
Consort -flow- diagram
Instrument and data collection
The demographic questionnaire included gender, age, marital status, economic status of the family, education level of parents and occupation of parents.
Depression Anxiety Stress Scale (DASS) consists of three subscales including 7 questions for each. Each question is scored from 0 (does not apply to me at all) to 3 (completely applies to me). Each of the areas of stress, anxiety and depression has 7 questions and the minimum score for each area is 0 and the maximum score is 21. The score of each area is obtained from the sum of the scores of the answers given to the questions of that area. Antony et al. analyzed the mentioned scale; The results of the correlation calculation indicated a correlation coefficient of 0.48 between the two factors of depression and stress, a correlation coefficient of 0.53 between anxiety and stress, and a correlation coefficient of 0.28 between anxiety and depression [ 26 ]. The reliability of this scale in Iran in a sample of 400 participants was reported as 0.7 for depression, 0.66 for anxiety and 0.76 for stress [ 27 ]. Also, in the validation study of this questionnaire in Iran by Sahebi et al. the reliability of this scale was investigated through internal consistency and its validity using factor analysis and criterion validity with the simultaneous implementation of Beck depression, Zang anxiety and perceived stress tests. In general, the obtained reliability and validity coefficients were very satisfactory and significant at the p < 0.001 level. The correlations between DASS depression subscale with Beck depression test were 0.70, DASS anxiety subscale with Zang anxiety test was 0.67, and DASS stress subscale with perceived stress test was 0.49. The internal consistency of DASS scales was also calculated using Cronbach's alpha and these results were obtained: depression 0.77, anxiety 0.79 and stress 0.78 [ 28 ].
Rosenberg Self-Esteem Scale (RSES) consists of 10 two-choice questions. Every statement that applies to the person receives the answer "I agree" and every statement that does not apply to the person receives the answer "I disagree". A positive answer to each of statements 1 to 5 will receive a positive score of one, a negative response to statements 1 to 5 will receive a negative score of one, a positive response to statements 6 to 10 will receive a negative score of one, and a negative response to statements 6 to 10 will receive a positive score of one. Then the total score is calculated. A positive score of 10 indicates the highest level of self-esteem, and a negative score of 10 indicates very low self-esteem. The retest correlation is in the range of 0.82–0.88 and the internal consistency coefficient or Cronbach's alpha is in the range of 0.77–0.88, this scale has satisfactory validity (0.77). It also has a high correlation with the New York and Guttman National Questionnaire in measuring self-esteem, so its content validity is also confirmed [ 29 ]. In Iran, Cronbach's alpha coefficients of 0.84 to 0.92 have been reported for this scale. Also, the reliability and validity of this tool has been checked by factor analysis, dichotomization and re-sampling methods, and the results show that this scale can be used in Iran as well [ 30 ].
The Situational Motivational Scale (SIMS): After confirming the content validity of the tool in Iran, its reliability has been confirmed by retest method (73.76) and Cronbach's alpha has been reported as 74–88%. The short form of this questionnaire was made by Bahrani in Shiraz. This questionnaire has 49 statements that are arranged on a Likert scale from completely disagree [ 1 ] to completely agree [ 5 ]. Reliability of the 49-question questionnaire used in this research was measured by Bahrani by retesting and calculating Cronbach's alpha. In the retest method, the reliability coefficient of the whole test was 0.95. Also, the internal consistency of the questionnaire was calculated as 0.77 [ 31 , 32 ].
Intervention program
In the control group, clinical training was done in the traditional way with the help of a trainer. In the intervention group, training was done by peer mentoring method with the help of fourth year operating room students and under the supervision of the instructor. Based on the overall GPA criteria, the first to sixth ranked students were selected as mentor students. Before using the students as mentors in the internship, 3 training sessions were held for them by the professors of the operating room.
In these meetings, the lesson plan of the internship course was fully explained based on the last chapter of the operating room field, and the necessary points regarding training and how to deal with students were explained.
Then, these students participated in three tests and the first to third students of each test were selected as mentors. Therefore, a total of nine students were selected as mentors. In the intervention group, internship training was carried out with the implementation of peer mentoring program during one academic semester. Students of the intervention group (35 participants) were placed in five groups of seven according to the internship program. The total training sessions of each group were 18 sessions, nine of which were conducted by the method of peer mentoring program. A total of 45 peer mentoring sessions were conducted for all groups. Each of the mentors mentored a seven-person group of mentees during nine sessions. At the beginning of each session, the mentor briefly explained the topics to the mentees according to the educational topics and guided them practically during the session. It should be noted that all the meetings were held under the supervision of the main teacher of the course and if necessary, this person provided the necessary guidance.
At the end of the academic semester, the Depression Anxiety Stress Scale, Rosenberg Self-Esteem Scale (RSES) and Situational Motivational Scale (SIMS) were completed again by the students of the intervention and control groups.
Statistical analysis
Stata software version 14 was used for the data analysis process. Initially, the data's normality was verified using the Kolmogorov–Smirnov test. The results were presented as mean, standard deviation, frequency, and percentage in the section on descriptive statistics.
The means of the study variable between the intervention and control groups were compared using an independent t-test, and the means before and after the intervention were compared using a paired t-test in the analytical statistics section. The Chi-square test was used to compare the associations between qualitative variables in the various groups.
The ANCOVA test was conducted after the intervention to control for any baseline differences in scores of self-confidence, stress, perceived anxiety, depression and academic progress between the two groups before the intervention (pre-test). This adjustment was made to account for any potential confounding factors that may have influenced the outcomes.
Univariable and multivariable linear regression by the backward method was applied to examine the association between self-confidence, stress, perceived anxiety, depression, gender, mother's education, father's education, family economic, and academic progress. A significance threshold of less than 0.05 was used.
The mean age of participants was 22.31 ± 2.59. Thirty-six individuals (51.4%) were female, and 50 individuals (71.4%) were single. Regarding education, 22 participants (31.4%) held diplomas from their fathers, and 21 participants (30%) held diplomas from their mothers. In terms of mothers' occupations, 35 individuals (52.9%) were housewives, and 31 individuals (44.3%) reported their family's economic status as medium (Table 1 ). On the other hand, there were no significant differences in age, gender, marital status, mothers' education, fathers' education, fathers' occupation, mothers' occupation, and family economic status between the intervention and control groups( p > 0.05) (Table 1 ). Also, in terms of variables of self-confidence, stress, anxiety, depression and academic progress between the intervention and control groups, no significant difference was observed before the intervention ( p > 0.05) (Table 2 ).
Before the intervention, high levels of stress (12.65; 12.25), anxiety (11.34; 11.02) and depression (10.08; 10.42) and low levels of self-confidence (1.31; 1.22) were observed in the intervention and control groups.
The results indicated a significant difference in the mean scores of self-confidence ( p < 0.001), stress ( p < 0.001), and academic progress ( p < 0.001), between the intervention and control groups after the educational intervention. Furthermore, this difference was also statistically significant in the intervention group before and after the educational intervention ( p < 0.05). However, there was no significant difference in the mean scores of anxiety and depression before and after the intervention, as well as in comparison with the control group ( p > 0.05) (Table 2 ).
The results showed significant differences between the intervention and control groups. Post-intervention, the intervention group showed substantial increases in self-confidence (mean difference = 5.97, p < 0.001) and significant reductions in stress levels (mean difference = -3.22, p < 0.001). In contrast, minimal changes were observed in the control group for both self-confidence (mean difference = 0.057, p = 0.934) and stress levels (mean difference = 0.142, p = 0.656). While both groups exhibited decreases in anxiety and depression levels, these changes were not statistically significant ( p > 0.05). Moreover, the intervention significantly improved academic progress in the intervention group compared to the control group (mean difference = 20.31, p < 0.001) (Table 2 ).
The ANCOVA test was used to compare the means of self-confidence, stress, anxiety, depression and academic progress in the two groups after adjusting the Pre-test as a covariate. Results showed there was a significant difference between the means in the self-confidence, stress and academic progress before and after intervention with adjusted pre- test score (before intervention) (Table 3 ).
The results of the univariate linear regression analysis showed that self-confidence and stress are associated with academic progress ( p < 0.05) (Table 4 ). Additionally, the results of the multiple regression analysis revealed that for a one-unit increase in the stress score, the mean academic progress score decreases by 0.520 (B = -0.520, P < 0.001). Furthermore, for a one-unit increase in age, the mean academic progress score increases by 0.220(B = 0.220, P = 0.029). Moreover, students whose fathers have university education have, on mean, a higher academic progress score compared to students whose fathers are illiterate, with an increase of 0.212 for each unit difference in paternal education level (B = 0.212, P = 0.036). According to the multiple regression model, 33.4% of the variations in academic progress can be predicted by stress, age, and father’s education (Table 4 ).
This research was conducted to determine the effect of peer mentoring program on clinical academic progress and psychological characteristics of operating room students.
The results showed that before the educational intervention, there was no significant difference between the control and intervention groups in demographic variables, academic progress, self-confidence, stress, anxiety and depression. It is noteworthy that according to the regression analysis, students whose fathers had a university education had a higher academic progress score compared to students whose fathers were illiterate.
The results of the study before the intervention show a high level of stress, anxiety and depression and a low level of self-confidence in students. Mohammadi's study showed the mean situational anxiety scores of the operating room students to be at a medium–high level [ 33 ]. Of course, according to Findik's study, the stress level of nursing students was low on the first day of operating room practice. It was found that students use the self-confidence approach in dealing with stress [ 34 ]. According to Norouzi's study, insufficient skills of students in communicating with staff, discrimination between paramedical students and assistants, lack of practical prerequisite skills, weak supportive performance of instructors and psychological needs are among the stressful factors of operating room students [ 3 ]. According to the students, practice with the support of staff and instructors in clinical training leads to better training. Improper interaction between staff and students negatively affects the clinical education process [ 35 , 36 ]. The results of Mohibi's research report the existence of discrimination as one of the main complaints of students in the clinical environment [ 37 ].
The results showed that training using the peer mentor method improved the mean scores of self-confidence, stress and academic progress variables in the intervention group after the educational intervention. Also, compared to the control group, the intervention group had achieved a significant improvement in the mentioned variables. In addition, the results showed that self-confidence and stress are related to academic progress, and as the stress score increases, the mean academic progress decreases. The results of Raymond's study showed that the implementation of the mentorship program was effective in reducing the stress and loneliness of first-year nursing students. In addition, an increase in their sense of self-efficacy and sense of psychological belonging was also reported [ 38 ]. According to Yoon's study, peer mentoring program increased students' self-confidence in basic nursing skills and critical thinking skills [ 39 ]. Considering that clinical educators play a fundamental role in controlling stress, creating a supportive environment and promoting students' self-confidence in the clinical learning environment [ 40 ], it seems that the use of students in the role of peer mentoring has been able to act as an important factor in increasing self-confidence, reducing stress and enjoying clinical experiences and thus improving their academic progress.
While in Walker's study, a significant reduction in the anxiety of a specific clinical situation was observed among nursing students who were guided by their peers [ 41 ], in the present study, no significant improvement was observed in the students' anxiety. It can be said that the special conditions of the operating room distinguish it from other clinical skills training departments, therefore peer training alone cannot be effective in reducing the anxiety of operating room students. Also, depression did not decrease significantly in any of the intervention and control groups. It should be said that anxiety and depression are more complex than stress and their reduction in operating room students requires the use of psychological interventions along with peer mentoring program.
Due to the limitation of the statistical population, sampling was not possible and the students were selected by census method. On the other hand, due to the special considerations of the operating room space, the implementation of the peer mentoring program faced limitations. Although the main teacher of the course was present in all the implementation sessions of the mentorship program, physicians and other clinical personnel did not trust the mentors to some extent.
Of course, the use of this training method could not be effective in reducing anxiety and depression, which can be aggravated as a result of working in the tense environment of the operating room, and it seems necessary to conduct more investigations in this field.
Availability of data and materials
The datasets generated and analyzed during the current study are not publicly available because they contain raw data from study participants, and sharing these data requires participants' permission. But are available from the corresponding author on reasonable request.
Erfani Khanghahi M, Ebadi Fard Azar F, Ebadi Fard Azar G. A Model of Effective Factors on Educational Transfer among Health Deputy Staff of Iran University of Medical Sciences. J Heal. 2020;11(2):203–12.
Article Google Scholar
Tazakori Z, Mehri S, Mobaraki N, Dadashi L, Ahmadi Y, Shokri F, et al. Factors affecting on quality of clinical education from perspectives of operating room students. J Heal Care. 2015;17(2):128–36.
Google Scholar
Norouzi N, Imani B. Clinical education stressors in operating room students: a qualitative study. Investig y Educ en Enfermería. 2021;39(1):e08.
Chevillotte J. Operating room nursing diploma soon to be accessible through competence validation. Rev Infirm. 2014;199:10.
Geraghty S, Speelman C, Bayes S. Fighting a losing battle: Midwives experiences of workplace stress. Women and Birth. 2019;32(3):e297-306.
Zeng Y, Wang G, Xie C, Hu X, Reinhardt JD. Prevalence and correlates of depression, anxiety and symptoms of stress in vocational college nursing students from Sichuan, China: a cross-sectional study. Psychol Health Med. 2019;24(7):798–811.
Abdal M, Alavi NM, Adib-Hajbaghery M. Clinical self-efficacy in senior nursing students: A mixed-methods study. Nurs midwifery Stud. 2015;4(3):e29143.
Mussi FC, da S Pires CG, da Silva RM, de Macedo TTS, de ST Santos CA. Stress level among undergraduate nursing students related to the training phase and sociodemographic factors. Rev Lat Am Enfermagem. 2020;28:e3209.
Hasson F, Slater PF, Guo XJ. Resilience, stress and well-being in undergraduate nursing students in China and the UK. Int J Res Nurs. 2021;12(1):11–20.
Mirbagher Ajorpaz N, Zagheri Tafreshi M, Mohtashami J, Zayeri F. Mentoring in training of operating room students: A systematic review. J Nurs Educ. 2016;5(3):47–54.
Nguyen T, Netto CLM, Wilkins JF, Bröker P, Vargas EE, Sealfon CD, et al. Insights into students’ experiences and perceptions of remote learning methods: From the COVID-19 pandemic to best practice for the future. In: Frontiers in Education. Frontiers; 2021. p. 91.
Kurganovna KD, Abdusalamovna AS, Sabirovna AN, Gafurovna AS. The Use Of Interactive Methods And Literary Lessons And High School Education. J Posit Sch Psychol. 2022;6(10):4328–32.
Hartikainen S, Rintala H, Pylväs L, Nokelainen P. The concept of active learning and the measurement of learning outcomes: A review of research in engineering higher education. Educ Sci. 2019;9(4):276.
Cho HJ, Zhao K, Lee CR, Runshe D, Krousgrill C. Active learning through flipped classroom in mechanical engineering: improving students’ perception of learning and performance. Int J Stem Educ. 2021;8:1–3.
Tudevdagva U, Heller A, Hardt W. An implementation and evaluation report of the active learning method eduscrum in flipped class. Int J Inf Educ Technol. 2020;10(9):649–54.
Lima RM, Andersson PH, Saalman E. Active Learning in Engineering Education: a (re) introduction. Eur J Eng Educ. 2017;2;42(1):1–4.
Fard ZR, Azadi A, Khorshidi A, Mozafari M, O’Connor T, Budri AMV, et al. A comparison of faculty led, mentorship program and peer mentoring on nursing students wound dressing clinical skills. Nurse Educ Today. 2020;89: 104378.
Mullen CA, Klimaitis CC. Defining mentoring: a literature review of issues, types, and applications. Ann N Y Acad Sci. 2021;1483(1):19–35.
Safari M, Yazdanpanah B, Islam-Nik PS. Comparison of midwifery students satisfaction with the teaching of gynecology and infertility by lecture and peer education. Armaghane Danesh. 2019;23(6):722–36.
Messerer DAC, Kraft SF, Horneffer A, Messerer LAS, Böckers TM, Böckers A. What factors motivate male and female Generation Z students to become engaged as peer teachers? A mixed-method study among medical and dental students in the gross anatomy course. Anat Sci Educ. 2022;15(4):650–62.
Ahmed M, Muldoon TJ, Elsaadany M. Employing faculty, peer mentoring, and coaching to increase the self-confidence and belongingness of first-generation college students in biomedical engineering. J Biomech Eng. 2021;143(12): 121001.
Davey Z, Jackson D, Henshall C. The value of nurse mentoring relationships: Lessons learnt from a work-based resilience enhancement programme for nurses working in the forensic setting. Int J Ment Health Nurs. 2020;29(5):992–1001.
Sadeghi A, Oshvandi K, Moradi Y. Explaining the inhibitory characteristics of clinical instructors in the process of developing clinical competence of nursing students: a qualitative study. J Fam Med Prim care. 2019;8(5):1664.
Gemuhay HM, Kalolo A, Mirisho R, Chipwaza B, Nyangena E. Factors affecting performance in clinical practice among preservice diploma nursing students in Northern Tanzania. Nurs Res Pract. 2019;2019:3453085.
Zarrabi M, Imanieh M, Zarrabi K, Masjedi M, Kojuri J, Amini M, et al. Designing and organizing mentoring at shiraz medical school and reinforcing deep knowledge–based education using mentoring. J Med Spirit Cultiv. 2017;26(3):228–36.
Antony MM, Bieling PJ, Cox BJ, Enns MW, Swinson RP. Psychometric properties of the 42-item and 21-item versions of the Depression Anxiety Stress Scales in clinical groups and a community sample. Psychol Assess. 1998;10(2):176.
Maleki A, Asghari M, Salari R. Credit terms of scale, depression, anxiety Vastrs DASS-21 in the Iranian populatio1. Maleki A, Asghari M, Salari R. Credit terms of scale, depression, anxiety Vastrs DASS-21 in the Iranian population. J Iran Psychol. 2005;1(4):9–12.
Sahebi A, Asghari MJ, Salari RS. Validation of depression anxiety and stress scale (DASS-21) for an Iranian population. J Iran Psychol. 2005;1(4):36–54.
Martín-Albo J, Núñez JL, Navarro JG, Grijalvo F. The Rosenberg Self-Esteem Scale: translation and validation in university students. Span J Psychol. 2007;10(2):458–67.
Amini Manesh S, Nazari AM, Moradi A, Farzad V. Youth online gaming addiction: the role of self esteem, anxiety and depression. Strateg Stud Youth Sport. 2014;13(25):97–112.
Bahrani M. The study of validity and reliability of Harter’s scale of educational motivation. J Psychol Stud. 2009;5(1):51–72.
Østerlie O, Løhre A, Haugan G. The Situational Motivational Scale (SIMS) in physical education: A validation study among Norwegian adolescents. Cogent Educ. 2019;6(1):1603613.
Mohammadi G, Tourdeh M, Ebrahimian A. Effect of simulation-based training method on the psychological health promotion in operating room students during the educational internship. J Educ Health Promot. 2019;8:172.
Findik UY, Ozbas A, Cavdar I, Topcu SY, Onler E. Assessment of nursing students’ stress levels and coping strategies in operating room practice. Nurse Educ Pract. 2015;15(3):192–5.
Al-Zayyat AS, Al-Gamal E. Perceived stress and coping strategies among J ordanian nursing students during clinical practice in psychiatric/mental health courses. Int J Ment Health Nurs. 2014;23(4):326–35.
Bazrafkan L, Najafi Kalyani M. Nursing students’ experiences of clinical education: A qualitative study. Investig y Educ en Enferm. 2018;36(3):e04.
Mohebbi Z, Rambod M, Hashemi F, Mohammadi HR, Setoudeh G, Najafi DS. View point of the nursing students on challenges in clinical training, Shiraz. Iran Hormozgan Med J. 2012;16(5):415–21.
Raymond JM, Sheppard K. Effects of peer mentoring on nursing students’ perceived stress, sense of belonging, self-efficacy and loneliness. J Nurs Educ Pr. 2017;8(1):16.
Yoon MO, Ju YS. The effects of peer mentoring learnings-based preclinical OSCE program on self-confidence on core basic nursing skills and critical thinking disposition for nursing student. J Digit Converg. 2017;15(7):285–95.
Arkan B, Ordin Y, Yılmaz D. Undergraduate nursing students’ experience related to their clinical learning environment and factors affecting to their clinical learning process. Nurse Educ Pract. 2018;29:127–32.
Walker D, Verklan T. Peer mentoring during practicum to reduce anxiety in first-semester nursing students. J Nurs Educ. 2016;55(11):651–4.
Download references
Acknowledgements
The authors of this study wish to express their gratitude to all the students, especially Miss Azadeh Nasiri and the officials of Khomein University of Medical Sciences.
Informed consent
All participants provided written informed consent.
This research was supported by Khomain University of Medical Sciences (No: 400000009).
Author information
Authors and affiliations.
Molecular and Medicine Research Center, Khomein University of Medical Sciences, Khomein, Iran
Amin Sedigh
Department of Medical Education, School of Medical Education and Learning Technologies, Shahid Beheshti University of Medical Sciences, Tehran, Iran
Sara Bagheri
Student Research Committee, Khomein University of Medical Sciences, Khomein, Iran
Pariya Naeimi
Department of Public Health, Torbat Jam Faculty of Medical Sciences, Torbat Jam, Iran
Vahid Rahmanian
Department of Public Health, Khomein University of Medical Sciences, Khomein, Iran
Nader Sharifi
You can also search for this author in PubMed Google Scholar
Contributions
Conceptualization: A S, S B; Data curation: A S, P N; Formal analysis: N SH, V R; Methodology: A S, S B, N SH; Project administration: A S, P N, N SH; Writing–original draft: N SH, V R; Writing–review & editing: all authors.
Corresponding author
Correspondence to Nader Sharifi .
Ethics declarations
Ethics approval and consent to participate.
Ethical approval was obtained from the Human Research Ethics Committee at the Khomain University of Medical Sciences (Code IR.KHOMEIN.REC.1400.010). All study participants provided written informed consent. Confidentiality and anonymity were ensured. All procedures performed in studies involving human participants were by the ethical standards of the institutional and national research committee and with the 1964 Helsinki Declaration.
Consent for publication
Not applicable.
Competing interests
The authors declare no competing interests.
Additional information
Publisher's note.
Springer Nature remains neutral with regard to jurisdictional claims in published maps and institutional affiliations.
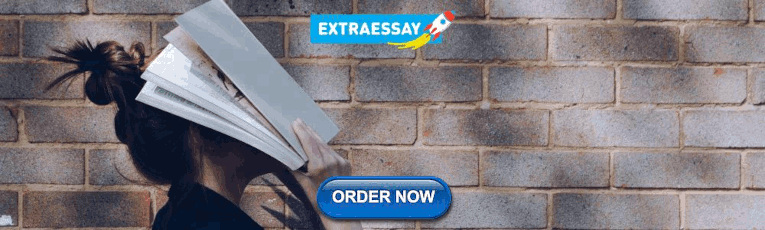
Rights and permissions
Open Access This article is licensed under a Creative Commons Attribution 4.0 International License, which permits use, sharing, adaptation, distribution and reproduction in any medium or format, as long as you give appropriate credit to the original author(s) and the source, provide a link to the Creative Commons licence, and indicate if changes were made. The images or other third party material in this article are included in the article's Creative Commons licence, unless indicated otherwise in a credit line to the material. If material is not included in the article's Creative Commons licence and your intended use is not permitted by statutory regulation or exceeds the permitted use, you will need to obtain permission directly from the copyright holder. To view a copy of this licence, visit http://creativecommons.org/licenses/by/4.0/ . The Creative Commons Public Domain Dedication waiver ( http://creativecommons.org/publicdomain/zero/1.0/ ) applies to the data made available in this article, unless otherwise stated in a credit line to the data.
Reprints and permissions
About this article
Cite this article.
Sedigh, A., Bagheri, S., Naeimi, P. et al. The effect of peer mentoring program on clinical academic progress and psychological characteristics of operating room students: a parallel randomized controlled trial. BMC Med Educ 24 , 438 (2024). https://doi.org/10.1186/s12909-024-05424-z
Download citation
Received : 29 December 2023
Accepted : 12 April 2024
Published : 22 April 2024
DOI : https://doi.org/10.1186/s12909-024-05424-z
Share this article
Anyone you share the following link with will be able to read this content:
Sorry, a shareable link is not currently available for this article.
Provided by the Springer Nature SharedIt content-sharing initiative
- Operating Room
BMC Medical Education
ISSN: 1472-6920
- Submission enquiries: [email protected]
- General enquiries: [email protected]
Loading metrics
Open Access
Peer-reviewed
Research Article
Large language models approach expert-level clinical knowledge and reasoning in ophthalmology: A head-to-head cross-sectional study
Roles Conceptualization, Data curation, Formal analysis, Funding acquisition, Investigation, Methodology, Project administration, Resources, Software, Supervision, Validation, Visualization, Writing – original draft, Writing – review & editing
* E-mail: [email protected] (AJT); [email protected] (DSJT)
Affiliations University of Cambridge School of Clinical Medicine, Cambridge, United Kingdom, Oxford University Clinical Academic Graduate School, University of Oxford, Oxford, United Kingdom

Roles Data curation, Investigation, Writing – review & editing
Affiliation University of Cambridge School of Clinical Medicine, Cambridge, United Kingdom
Affiliation Eye Institute, Cleveland Clinic Abu Dhabi, Abu Dhabi Emirate, United Arab Emirates
Roles Data curation, Investigation, Writing – original draft, Writing – review & editing
Affiliations University of Cambridge School of Clinical Medicine, Cambridge, United Kingdom, Department of Physiology, Development and Neuroscience, University of Cambridge, Cambridge, United Kingdom
Roles Data curation, Investigation
Affiliation West Suffolk NHS Foundation Trust, Bury St Edmunds, United Kingdom
Affiliation Manchester Royal Eye Hospital, Manchester University NHS Foundation Trust, Manchester, United Kingdom
Affiliation Birmingham and Midland Eye Centre, Sandwell and West Birmingham NHS Foundation Trust, Birmingham, United Kingdom
Affiliation Department of Ophthalmology, Chang Gung Memorial Hospital, Linkou Medical Center, Taoyuan, Taiwan
Affiliation Yong Loo Lin School of Medicine, National University of Singapore, Singapore
Roles Data curation, Investigation, Project administration, Writing – review & editing
Affiliation Bedfordshire Hospitals NHS Foundation Trust, Luton and Dunstable, United Kingdom
Affiliation Singapore Eye Research Institute, Singapore National Eye Centre, Singapore, Singapore
Roles Writing – review & editing
Affiliations Birmingham and Midland Eye Centre, Sandwell and West Birmingham NHS Foundation Trust, Birmingham, United Kingdom, Academic Unit of Ophthalmology, Institute of Inflammation and Ageing, University of Birmingham, Birmingham, United Kingdom
Roles Funding acquisition, Project administration
Affiliations Singapore Eye Research Institute, Singapore National Eye Centre, Singapore, Singapore, Duke-NUS Medical School, Singapore, Singapore, Byers Eye Institute, Stanford University, Palo Alto, California, United States of America
- [ ... ],
Roles Conceptualization, Formal analysis, Funding acquisition, Methodology, Project administration, Supervision, Writing – original draft, Writing – review & editing
Affiliations Birmingham and Midland Eye Centre, Sandwell and West Birmingham NHS Foundation Trust, Birmingham, United Kingdom, Academic Unit of Ophthalmology, Institute of Inflammation and Ageing, University of Birmingham, Birmingham, United Kingdom, Academic Ophthalmology, School of Medicine, University of Nottingham, Nottingham, United Kingdom
- [ view all ]
- [ view less ]
- Arun James Thirunavukarasu,
- Shathar Mahmood,
- Andrew Malem,
- William Paul Foster,
- Rohan Sanghera,
- Refaat Hassan,
- Sean Zhou,
- Shiao Wei Wong,
- Yee Ling Wong,
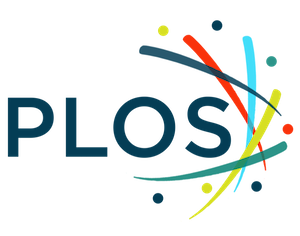
- Published: April 17, 2024
- https://doi.org/10.1371/journal.pdig.0000341
- Reader Comments
Large language models (LLMs) underlie remarkable recent advanced in natural language processing, and they are beginning to be applied in clinical contexts. We aimed to evaluate the clinical potential of state-of-the-art LLMs in ophthalmology using a more robust benchmark than raw examination scores. We trialled GPT-3.5 and GPT-4 on 347 ophthalmology questions before GPT-3.5, GPT-4, PaLM 2, LLaMA, expert ophthalmologists, and doctors in training were trialled on a mock examination of 87 questions. Performance was analysed with respect to question subject and type (first order recall and higher order reasoning). Masked ophthalmologists graded the accuracy, relevance, and overall preference of GPT-3.5 and GPT-4 responses to the same questions. The performance of GPT-4 (69%) was superior to GPT-3.5 (48%), LLaMA (32%), and PaLM 2 (56%). GPT-4 compared favourably with expert ophthalmologists (median 76%, range 64–90%), ophthalmology trainees (median 59%, range 57–63%), and unspecialised junior doctors (median 43%, range 41–44%). Low agreement between LLMs and doctors reflected idiosyncratic differences in knowledge and reasoning with overall consistency across subjects and types ( p >0.05). All ophthalmologists preferred GPT-4 responses over GPT-3.5 and rated the accuracy and relevance of GPT-4 as higher ( p <0.05). LLMs are approaching expert-level knowledge and reasoning skills in ophthalmology. In view of the comparable or superior performance to trainee-grade ophthalmologists and unspecialised junior doctors, state-of-the-art LLMs such as GPT-4 may provide useful medical advice and assistance where access to expert ophthalmologists is limited. Clinical benchmarks provide useful assays of LLM capabilities in healthcare before clinical trials can be designed and conducted.
Author summary
Large language models (LLMs) are the most sophisticated form of language-based artificial intelligence. LLMs have the potential to improve healthcare, and experiments and trials are ongoing to explore potential avenues for LLMs to improve patient care. Here, we test state-of-the-art LLMs on challenging questions used to assess the aptitude of eye doctors (ophthalmologists) in the United Kingdom before they can be deemed fully qualified. We compare the performance of these LLMs to fully trained ophthalmologists as well as doctors in training to gauge the aptitude of the LLMs for providing advice to patients about eye health. One of the LLMs, GPT-4, exhibits favourable performance when compared with fully qualified and training ophthalmologists; and comparisons with its predecessor model, GPT-3.5, indicate that this superior performance is due to improved accuracy and relevance of model responses. LLMs are approaching expert-level ophthalmological knowledge and reasoning, and may be useful for providing eye-related advice where access to healthcare professionals is limited. Further research is required to explore potential avenues of clinical deployment.
Citation: Thirunavukarasu AJ, Mahmood S, Malem A, Foster WP, Sanghera R, Hassan R, et al. (2024) Large language models approach expert-level clinical knowledge and reasoning in ophthalmology: A head-to-head cross-sectional study. PLOS Digit Health 3(4): e0000341. https://doi.org/10.1371/journal.pdig.0000341
Editor: Man Luo, Mayo Clinic Scottsdale, UNITED STATES
Received: July 31, 2023; Accepted: February 26, 2024; Published: April 17, 2024
Copyright: © 2024 Thirunavukarasu et al. This is an open access article distributed under the terms of the Creative Commons Attribution License , which permits unrestricted use, distribution, and reproduction in any medium, provided the original author and source are credited.
Data Availability: All data are available as supplementary information , excluding copyrighted material from the textbook used for experiments.
Funding: DSWT is supported by the National Medical Research Council, Singapore (NMCR/HSRG/0087/2018; MOH-000655-00; MOH-001014-00), Duke-NUS Medical School (Duke-NUS/RSF/2021/0018; 05/FY2020/EX/15-A58), and Agency for Science, Technology and Research (A20H4g2141; H20C6a0032). DSJT is supported by a Medical Research Council / Fight for Sight Clinical Research Fellowship (MR/T001674/1). These funders were not involved in the conception, execution, or reporting of this review.
Competing interests: AM is a member of the Panel of Examiners of the Royal College of Ophthalmologists and performs unpaid work as an FRCOphth examiner. DSWT holds a patent on a deep learning system to detect retinal disease. DSJT authored the book used in the study and receives royalty from its sales. The other authors have no competing interests to declare.
Introduction
Generative Pre-trained Transformer 3.5 (GPT-3.5) and 4 (GPT-4) are large language models (LLMs) trained on datasets containing hundreds of billions of words from articles, books, and other internet sources [ 1 , 2 ]. ChatGPT is an online chatbot which uses GPT-3.5 or GPT-4 to provide bespoke responses to human users’ queries [ 3 ]. LLMs have revolutionised the field of natural language processing, and ChatGPT has attracted significant attention in medicine for attaining passing level performance in medical school examinations and providing more accurate and empathetic messages than human doctors in response to patient queries on a social media platform [ 3 , 4 , 5 , 6 ]. While GPT-3.5 performance in more specialised examinations has been inadequate, GPT-4 is thought to represent a significant advancement in terms of medical knowledge and reasoning [ 3 , 7 , 8 ]. Other LLMs in wide use include Pathways Language Model 2 (PaLM 2) and Large Language Model Meta AI 2 (LLaMA 2) [ 3 ], [ 9 , p. 2], [ 10 ].
Applications and trials of LLMs in ophthalmological settings has been limited despite ChatGPT’s performance in questions relating to ‘eyes and vision’ being superior to other subjects in an examination for general practitioners [ 7 , 11 ]. ChatGPT has been trialled on the North American Ophthalmology Knowledge Assessment Program (OKAP), and Fellowship of the Royal College of Ophthalmologists (FRCOphth) Part 1 and Part 2 examinations. In both cases, relatively poor results have been reported for GPT-3.5, with significant improvement exhibited by GPT-4 [ 12 , 13 , 14 , 15 , 16 ]. However, previous studies are afflicted by two important issues which may affect their validity and interpretability. First, so-called ‘contamination’, where test material features in the pretraining data used to develop LLMs, may result in inflated performance as models recall previously seen text rather than using clinical reasoning to provide an answer. Second, examination performance in and of itself provides little information regarding the potential of models to contribute to clinical practice as a medical-assistance tool [ 3 ]. Clinical benchmarks are required to understanding the meaning and implications of scores in ophthalmological examinations attained by LLMs and are a necessary precursor to clinical trials of LLM-based interventions.
Here, we used FRCOphth Part 2 examination questions to gauge the ophthalmological knowledge base and reasoning capability of LLMs using fully qualified and currently training ophthalmologists as clinical benchmarks. These questions were not freely available online, minimising the risk of contamination. The FRCOphth Part 2 Written Examination tests the clinical knowledge and skills of ophthalmologists in training using multiple choice questions with no negative marking and must be passed to fully qualify as a specialist eye doctor in the United Kingdom.
Question extraction
FRCOphth Part 2 questions were sourced from a textbook for doctors preparing to take the examination [ 17 ]. This textbook is not freely available on the internet, making the possibility of its content being included in LLMs’ training datasets unlikely [ 1 ]. All 360 multiple-choice questions from the textbook’s six chapters were extracted, and a 90-question mock examination from the textbook was segregated for LLM and doctor comparisons. Two researchers matched the subject categories of the practice papers’ questions to those defined in the Royal College of Ophthalmologists’ documentation concerning the FRCOphth Part 2 written examination. Similarly, two researchers categorised each question as first order recall or higher order reasoning, corresponding to ‘remembering’ and ‘applying’ or ‘analysing’ in Bloom’s taxonomy, respectively [ 18 ]. Disagreement between classification decisions was resolved by a third researcher casting a deciding vote. Questions containing non-plain text elements such as images were excluded as these could not be inputted to the LLM applications.
Trialling large language models
Every eligible question was inputted into ChatGPT (GPT-3.5 and GPT-4 versions; OpenAI, San Francisco, California, United States of America) between April 29 and May 10, 2023. The answers provided by GPT-3.5 and GPT-4 were recorded and their whole reply to each question was recorded for further analysis. If ChatGPT failed to provide a definitive answer, the question was re-trialled up to three times, after which ChatGPT’s answer was recorded as ‘null’ if no answer was provided. Correct answers (‘ground truth’) were defined as the answers provided by the textbook and were recorded for every eligible question to facilitate calculation of performance. Upon their release, Bard (Google LLC, Mountain View, California, USA) and HuggingChat (Hugging Face, Inc., New York City, USA) were used to trial PaLM 2 (Google LLC) and LLaMA (Meta, Menlo Park, California, USA) respectively on the portion of the textbook corresponding to a 90-question examination, adhering to the same procedures between June 20 and July 2, 2023.
Clinical benchmarks
To gauge the performance, accuracy, and relevance of LLM outputs, five expert ophthalmologists who had all passed the FRCOphth Part 2 (E1-E5), three trainees (residents) currently in ophthalmology training programmes (T1-T3), and two unspecialised ( i . e . not in ophthalmology training) junior doctors (J1-J2) first answered the 90-question mock examination independently, without reference to textbooks, the internet, or LLMs’ recorded answers. As with the LLMs, doctors’ performance was calculated with reference to the correct answers provided by the textbook. After completing the examination, ophthalmologists graded the whole output of GPT-3.5 and GPT-4 on a Likert scale from 1–5 (very bad, bad, neutral, good, very good) to qualitatively appraise accuracy of information provided and relevance of outputs to the question used as an input prompt. For these appraisals, ophthalmologists were blind to the LLM source (which was presented in a randomised order) and to their previous answers to the same questions, but they could refer to the question text and correct answer and explanation provided by the textbook. Procedures are comprehensively described in the protocol issued to the ophthalmologists ( S1 Protocol ).
Our null hypothesis was that LLMs and doctors would exhibit similar performance, supported by results in a wide range of medical examinations [ 3 , 6 ]. Prospective power analysis was conducted which indicated that 63 questions were required to identify a 10% superior performance of an LLM to human performance at a 5% significance level (type 1 error rate) with 80% power (20% type 2 error rate). This indicated that the 90-question examination in our experiments was more than sufficient to detect ~10% differences in overall performance. The whole 90-question mock examination was used to avoid over- or under-sampling certain question types with respect to actual FRCOphth papers. To verify that the mock examination was representative of the FRCOphth Part 2 examination, expert ophthalmologists were asked to rate the difficulty of questions used here in comparison to official examinations on a 5-point Likert scale (“much easier”, “somewhat easier”, “similar”, “somewhat more difficult”, “much more difficult”).
Statistical analysis
Performance of doctors and LLMs were compared using chi-squared (χ 2 ) tests. Agreement between answers provided by doctors and LLMs was quantified through calculation of Kappa statistics, interpreted in accordance with McHugh’s recommendations [ 19 ]. To further explore the strengths and weaknesses of the answer providers, performance was stratified by question type (first order fact recall or higher order reasoning) and subject using a chi-squared or Fisher’s exact test where appropriate. Likert scale data corresponding to the accuracy and relevance of GPT-3.5 and GPT-4 responses to the same questions were analysed with paired t -tests with the Bonferroni correction applied to mitigate the risk of false positive results due to multiple-testing—parametric testing was justified by a sufficient sample size [ 20 ]. A chi-squared test was used to quantify the significance of any difference in overall preference of ophthalmologists choosing between GPT-3.5 and GPT-4 responses. Statistical significance was concluded where p < 0.05. For additional contextualisation, examination statistics corresponding to FRCOphth Part 2 written examinations taken between July 2017 and December 2022 were collected from Royal College of Ophthalmologists examiners’ reports [ 21 ]. These statistics facilitated comparisons between human and LLM performance in the mock examination with the performance of actual candidates in recent examinations. Failure cases where all LLMs provided an incorrect answer were appraised qualitatively to explore any specific weaknesses of the technology.
Statistical analysis was conducted in R (version 4.1.2; R Foundation for Statistical Computing, Vienna, Austria), and figures were produced in Affinity Designer (version 1.10.6; Serif Ltd, West Bridgford, Nottinghamshire, United Kingdom).
Questions sources
Of 360 questions in the textbook, 347 questions (including 87 of the 90 questions from the mock examination chapter) were included [ 17 ]. Exclusions were all due to non-text elements such as images and tables which could not be inputted into LLM chatbot interfaces. The distribution of question types and subjects within the whole set and mock examination set of questions is summarised in Table 1 and S1 Table alongside performance.
- PPT PowerPoint slide
- PNG larger image
- TIFF original image
Question subject and type distributions presented alongside scores attained by LLMs (GPT-3.5, GPT-4, LLaMA, and PaLM 2), expert ophthalmologists (E1-E5), ophthalmology trainees (T1-T3), and unspecialised junior doctors (J1-J2). Median scores do not necessarily sum to the overall median score, as fractional scores are impossible.
https://doi.org/10.1371/journal.pdig.0000341.t001
GPT-4 represents a significant advance on GPT-3.5 in ophthalmological knowledge and reasoning.
Overall performance over 347 questions was significantly higher for GPT-4 (61.7%) than GPT-3.5 (48.41%; χ 2 = 12.32, p <0.01), with results detailed in S1 Fig and S1 Table . ChatGPT performance was consistent across question types and subjects ( S1 Table ). For GPT-4, no significant variation was observed with respect to first order and higher order questions (χ 2 = 0.22, p = 0.64), or subjects defined by the Royal College of Ophthalmologists (Fisher’s exact test over 2000 iterations, p = 0.23). Similar results were observed for GPT-3.5 with respect to first and second order questions (χ 2 = 0.08, p = 0.77), and subjects (Fisher’s exact test over 2000 iterations, p = 0.28). Performance and variation within the 87-question mock examination was very similar to the overall performance over 347 questions, and subsequent experiments were therefore restricted to that representative set of questions.
GPT-4 compares well with other LLMs, junior and trainee doctors and ophthalmology experts.
Performance in the mock examination is summarised in Fig 1 —GPT-4 (69%) was the top-scoring model, performing to a significantly higher standard than GPT-3.5 (48%; χ 2 = 7.33, p < 0.01) and LLaMA (32%; χ 2 = 22.77, p < 0.01), but statistically similarly to PaLM 2 (56%) despite a superior score (χ 2 = 2.81, p = 0.09). LLaMA exhibited the lowest examination score, significantly weaker than GPT-3.5 (χ 2 = 4.58, p = 0.03) and PaLM-2 (χ 2 = 10.01, p < 0.01) as well as GPT-4.
Examination performance in the 87-question mock examination used to trial LLMs (GPT-3.5, GPT-4, LLaMA, and PaLM 2), expert ophthalmologists (E1-E5), ophthalmology trainees (T1-T3), and unspecialised junior doctors (J1-J2). Dotted lines depict the mean performance of expert ophthalmologists (66/87; 76%), ophthalmology trainees (60/87; 69%), and unspecialised junior doctors (37/87; 43%). The performance of GPT-4 lay within the range of expert ophthalmologists and ophthalmology trainees.
https://doi.org/10.1371/journal.pdig.0000341.g001
The performance of GPT-4 was statistically similar to the mean score attained by expert ophthalmologists ( Fig 1 ; χ 2 = 1.18, p = 0.28). Moreover, GPT-4’s performance exceeded the mean mark attained across FRCOphth Part 2 written examination candidates between 2017–2022 (66.06%), mean pass mark according to standard setting (61.31%), and the mean official mark required to pass the examination after adjustment (63.75%), as detailed in S2 Table . In individual comparisons with expert ophthalmologists, GPT-4 was equivalent in 3 cases (χ 2 tests, p > 0.05, S3 Table ), and inferior in 2 cases (χ 2 tests, p < 0.05; Table 2 ). In comparisons with ophthalmology trainees, GPT-4 was equivalent to all three ophthalmology trainees (χ 2 tests, p > 0.05; Table 2 ). GPT-4 was significantly superior to both unspecialised trainee doctors (χ 2 tests, p < 0.05; Table 2 ). Doctors were anonymised in analysis, but their ophthalmological experience is summarised in S3 Table . Unsurprisingly, junior doctors (J1-J2) attained lower scores than expert ophthalmologists (E1-E5; t = 7.18, p < 0.01), and ophthalmology trainees (T1-T3; t = 11.18, p < 0.01), illustrated in Fig 1 . Ophthalmology trainees approached expert-level scores with no significant difference between the groups ( t = 1.55, p = 0.18). None of the other LLMs matched any of the expert ophthalmologists, mean mark of real examination candidates, or FRCOphth Part 2 pass mark.
Expert ophthalmologists agreed that the mock examination was a faithful representation of actual FRCOphth Part 2 Written Examination papers with a mean and median score of 3/5 (range 2-4/5).
Results of pair-wise comparisons of examination performance between GPT-4 and the other answer providers. Significantly greater performance for GPT-4 is highlighted green, significantly inferior performance for GPT-4 is highlighted orange. GPT-4 was superior to all other LLMs and unspecialised junior doctors, and equivalent to most expert ophthalmologists and all ophthalmology trainees.
https://doi.org/10.1371/journal.pdig.0000341.t002
LLM strengths and weaknesses are similar to doctors.
Agreement between answers given by LLMs, expert ophthalmologists, and trainee doctors was generally absent (0 ≤ κ < 0.2), minimal (0.2 ≤ κ < 0.4), or weak (0.4 ≤ κ < 0.6), with moderate agreement only recorded for one pairing between the two highest performing ophthalmologists ( Fig 2 ; κ = 0.64) [ 19 ]. Disagreement was primarily the result of general differences in knowledge and reasoning ability, illustrated by strong negative correlation between Kappa statistic (quantifying agreement) and difference in examination performance (Pearson’s r = -0.63, p < 0.01). Answer providers with more similar scores exhibited greater agreement overall irrespective of their category (LLM, expert ophthalmologist, ophthalmology trainee, or junior doctor).
Agreement correlates strongly with overall performance and stratification analysis found no particular question type or subject was associated with better performance of LLMs or doctors, indicating that LLM knowledge and reasoning ability is general across ophthalmology rather than restricted to particular subspecialties or question types.
https://doi.org/10.1371/journal.pdig.0000341.g002
Stratification analysis was undertaken to identify any specific strengths and weaknesses of LLMs with respect to expert ophthalmologists and trainee doctors ( Table 1 and S4 Table ). No significant difference between performance in first order fact recall and higher order reasoning questions was observed among any of the LLMs, expert ophthalmologists, ophthalmology trainees, or unspecialised junior doctors ( S4 Table ; χ 2 tests, p > 0.05). Similarly, only J1 (junior doctor yet to commence ophthalmology training) exhibited statistically significant variation in performance between subjects ( S4 Table ; Fisher’s exact tests over 2000 iterations, p = 0.02); all other doctors and LLMs exhibited no significant variation (Fisher’s exact tests over 2000 iterations, p > 0.05). To explore whether consistency was due to an insufficient sample size, similar analyses were run for GPT-3.5 and GPT-4 performance over the larger set of 347 questions ( S1 Table ; S4 Table ). As with the mock examination, no significant differences in performance across question types ( S4 Table ; χ 2 tests, p > 0.05) or subjects ( S4 Table ; Fisher’s exact tests over 2000 iterations, p > 0.05) were observed.
LLM examination performance translates to subjective preference indicated by expert ophthalmologists.
Ophthalmologists’ appraisal of GPT-4 and GPT-3.5 outputs indicated a marked preference for the former over the latter, mirroring objective performance in the mock examination and over the whole textbook. GPT-4 exhibited significantly ( t -test with Bonferroni correction, p < 0.05) higher accuracy and relevance than GPT-3.5 according to all five ophthalmologists’ grading ( Table 3 ). Differences were visually obvious, with GPT-4 exhibiting much higher rates of attaining the highest scores for accuracy and relevance than GPT-3.5 ( Fig 3 ). This superiority was reflected in ophthalmologists’ qualitative preference indications: GPT-4 responses were preferred to GPT-3.5 responses by every ophthalmologist with statistically significant skew in favour of GPT-4 (χ 2 test, p < 0.05; Table 3 ).
Accuracy (A) and relevance (B) ratings were provided by five expert ophthalmologists for ChatGPT (powered by GPT-3.5 and GPT-4) responses to 87 FRCOphth Part 2 mock examination questions. In every case, the accuracy and relevance of GPT-4 is significantly superior to GPT-3.5 (t-test with Bonferroni correct applied, p < 0.05). Pooled scores for accuracy (C) and relevance (D) from all five raters are presented in the bottom two plots, with GPT-3.5 (left bars) compared directly with GPT-4 (right bars).
https://doi.org/10.1371/journal.pdig.0000341.g003
t-test results with Bonferroni correction applied showing the superior accuracy and relevance of GPT-4 responses relative to GPT-3.5 responses in the opinion of five fully trained ophthalmologists (positive mean differences favour GPT-4), and χ 2 test showing that GPT-4 responses were preferred to GPT-3.5 responses by every ophthalmologist in their blinded qualitative appraisals.
https://doi.org/10.1371/journal.pdig.0000341.t003
Failure cases exhibit no association with subject, complexity, or human answers.
The LLM failure cases—where every LLM provided an incorrect answer—are summarised in Table 4 . While errors made by LLMs were occasionally similar to those made by trainee ophthalmologists and junior doctors, this association was not consistent ( Table 4 ). There was no preponderance of ophthalmological subject or first or higher order questions in the failure cases, and questions did not share a common theme, sentence structure, or grammatical construct ( Table 4 ). Examination questions are redacted here to avoid breaching copyright and prevent future LLMs accessing the test data during pretraining but can be provided on request.
Summary of LLM failure cases, where all models provided an incorrect answer to the FRCOphth Part 2 mock examination question. No associations were found with human answers, complexity, subject, theme, sentence structure, or grammatic constructs.
https://doi.org/10.1371/journal.pdig.0000341.t004
Here, we present a clinical benchmark to gauge the ophthalmological performance of LLMs, using a source of questions with very low risk of contamination as the utilised textbook is not freely available online [ 17 ]. Previous studies have suggested that ChatGPT can provide useful responses to ophthalmological queries, but often use online question sources which may have featured in LLMs’ pretraining datasets [ 7 , 12 , 15 , 22 ]. In addition, our employment of multiple LLMs as well as fully qualified and training doctors provides novel insight into the potential and limitations of state-of-the-art LLMs through head-to-head comparisons which provide clinical context and quantitative benchmarks of competence in ophthalmology. Subsequent research may leverage our questions and results to gauge the performance of new LLMs and applications as they emerge.
We make three primary observations. First, performance of GPT-4 compares well to expert ophthalmologists and ophthalmology trainees, and exhibits pass-worthy performance in an FRCOphth Part 2 mock examination. PaLM 2 did not attain pass-worthy performance or match expert ophthalmologists’ scores but was within the spread of trainee doctors’ performance. LLMs are approaching human expert-level knowledge and reasoning in ophthalmology, and significantly exceed the ability of non-specialist clinicians (represented here by unspecialised junior doctors) to answer ophthalmology questions. Second, clinician grading of model outputs suggests that GPT-4 exhibits improved accuracy and relevance when compared with GPT-3.5. Development is producing models which generate better outputs to ophthalmological queries in the opinion of expert human clinicians, which suggests that models are becoming more capable of providing useful assistance in clinical settings. Third, LLM performance was consistent across question subjects and types, distributed similarly to human performance, and exhibited comparable agreement between other LLMs and doctors when corrected for differences in overall performance. Together, this indicates that the ophthalmological knowledge and reasoning capability of LLMs is general rather than limited to certain subspecialties or tasks. LLM-driven natural language processing seems to facilitate similar—although idiosyncratic—clinical knowledge and reasoning to human clinicians, with no obvious blind spots precluding clinical use.
Similarly dramatic improvements in the performance of GPT-4 relative to GPT-3.5 have been reported in the context of the North American Ophthalmology Knowledge Assessment Program (OKAP) [ 13 , 15 ]. State-of-the-art models exhibit far more clinical promise than their predecessors, and expectations and development should be tailored accordingly. Results from the OKAP also suggest that improvement in performance is due to GPT-4 being more well-rounded than GPT-3.5 [ 13 ]. This increases the scope for potential applications of LLMs in ophthalmology, as development is eliminating weaknesses rather than optimising in narrow domains. This study shows that well-rounded LLM performance compares well with expert ophthalmologists, providing clinically relevant evidence that LLMs may be used to provide medical advice and assistance. Further improvement is expected as multimodal foundation models, perhaps based on LLMs such as GPT-4, emerge and facilitate compatibility with image-rich ophthalmological data [ 3 , 23 , 24 ].
Limitations
This study was limited by three factors. First, examination performance is an unvalidated indicator of clinical aptitude. We sought to ameliorate this limitation by employing expert ophthalmologists, ophthalmology trainees, and unspecialised junior doctors answering the same questions as clinical benchmarks; and compared LLM performance to real cohorts of candidates in recent FRCOphth examinations. However, it remains an issue that comparable performance to clinical experts in an examination does not necessarily demonstrate that an LLM can communicate with patients and practitioners or contribute to clinical decision making accurately and safely. Early trials of LLM chatbots have suggested that LLM responses may be equivalent or even superior to human doctors in terms of accuracy and empathy, and experiments using complicated case studies suggest that LLMs operate well even outside typical presentations and more common medical conditions [ 4 , 25 , 26 ]. In ophthalmology, GPT-3.5 and GPT-4 have been shown to be capable of providing precise and suitable triage decisions when queried with eye-related symptoms [ 22 , 27 ]. Further work is now warranted in conventional clinical settings.
Second, while the study was sufficiently powered to detect a less than 10% difference in overall performance, the relatively small number of questions in certain categories used for stratification analysis may mask significant differences in performance. Testing LLMs and clinicians with more questions may help establish where LLMs exhibit greater or lesser ability in ophthalmology. Furthermore, researchers using different ways to categorise questions may be able to identify specific strengths and weaknesses of LLMs and doctors which could help guide design of clinical LLM interventions.
Finally, experimental tasks were ‘zero-shot’ in that LLMs were not provided with any examples of correctly answered questions before it was queried with FRCOphth questions from the textbook. This mode of interrogation entails the maximal level of difficulty for LLMs, so it is conceivable that the ophthalmological knowledge and reasoning encoded within these models is actually even greater than indicated by results here [ 1 ]. Future research may seek to fine-tune LLMs by using more domain-specific text during pretraining and fine-tuning, or by providing examples of successfully completed tasks to further improve performance in that clinical task [ 3 ].
Future directions
Autonomous deployment of LLMs is currently precluded by inaccuracy and fact fabrication. Our study found that despite meeting expert standards, state-of-the-art LLMs such as GPT-4 do not match top-performing ophthalmologists [ 28 ]. Moreover, there remain controversial ethical questions about what roles should and should not be assigned to inanimate AI models, and to what extent human clinicians must remain responsible for their patients [ 3 ]. However, the remarkable performance of GPT-4 in ophthalmology examination questions suggests that LLMs may be able to provide useful input in clinical contexts, either to assist clinicians in their day-to-day work or with their education or preparation for examinations [ 3 , 13 , 14 , 27 ]. Further improvement in performance may be obtained by specific fine-tuning of models with high quality ophthalmological text data, requiring curation and deidentification [ 29 ]. GPT-4 may prove especially useful where access to ophthalmologists is limited: provision of advice, diagnosis, and management suggestions by a model with FRCOphth Part 2-level knowledge and reasoning ability is likely to be superior to non-specialist doctors and allied healthcare professionals working without support, as their exposure to and knowledge of eye care is limited [ 27 , 30 , 31 ].
However, close monitoring is essential to avoid mistakes caused by inaccuracy or fact fabrication [ 32 ]. Clinical applications would also benefit from an uncertainty indicator reducing the risk of erroneous decisions [ 7 ]. As LLM performance often correlates with the frequency of query terms’ representation in the model’s training dataset, a simple indicator of ‘familiarity’ could be engineered by calculating the relative frequency of query term representation in the training data [ 7 , 33 ]. Users could appraise familiarity to temper their confidence in answers provided by the LLM, perhaps reducing error. Moreover, ophthalmological applications require extensive validation, preferably with high quality randomised controlled trials to conclusively demonstrate benefit (or lack thereof) conferred to patients by LLM interventions [ 34 ]. Trials should be pragmatic so as not to inflate effect sizes beyond what may generalise to patients once interventions are implemented at scale [ 34 , 35 ]. In addition to patient outcomes, practitioner-related variables should also be considered: interventions aiming to improve efficiency should be specifically tested to ensure that they reduce rather than increase clinicians’ workload [ 3 ].
According to comparisons with expert and trainee doctors, state-of-the-art LLMs are approaching expert-level performance in advanced ophthalmology questions. GPT-4 attains pass-worthy performance in FRCOphth Part 2 questions and exceeds the scores of some expert ophthalmologists. As top-performing doctors exhibit superior scores, LLMs do not appear capable of replacing ophthalmologists, but state-of-the-art models could provide useful advice and assistance to non-specialists or patients where access to eye care professionals is limited [ 27 , 28 ]. Further research is required to design LLM-based interventions which may improve eye health outcomes, validate interventions in clinical trials, and engineer governance structures to regulate LLM applications as they begin to be deployed in clinical settings [ 36 ].
Supporting information
S1 fig. chatgpt performance in questions taken from the whole textbook..
Mosaic plot depicting the overall performance of ChatGPT versions powered by GPT-3.5 and GPT-4 in 360 FRCOphth Part 2 written examination questions. Performance was significantly higher for GPT-4 than GPT-3.5, and was close to mean human examination candidate performance and pass mark set by standard setting and after adjustment.
https://doi.org/10.1371/journal.pdig.0000341.s001
S1 Table. Question characteristics and performance of GPT-3.5 and GPT-4 over the whole textbook.
Similar observations were noted here to the smaller mock examination used for subsequent experiments. GPT-4 performs to a significantly higher standard than GPT-3.5
https://doi.org/10.1371/journal.pdig.0000341.s002
S2 Table. Examination statistics corresponding to FRCOphth Part 2 written examinations sat between July 2017-December 2022.
https://doi.org/10.1371/journal.pdig.0000341.s003
S3 Table. Experience of expert ophthalmologists (E1-E5), ophthalmology trainees (T1-T3), and unspecialised junior doctors (J1-J2) involved in experiments.
https://doi.org/10.1371/journal.pdig.0000341.s004
S4 Table. Results of statistical tests of variation in performance between question subjects and types, for each trialled LLM, expert ophthalmologist, and trainee doctor.
Statistically significant results are highlighted in green.
https://doi.org/10.1371/journal.pdig.0000341.s005
S1 Protocol. Procedures followed by ophthalmologists to grade the output of GPT-3.5 and GPT-4 in terms of accuracy, relevance, and rater-preference of model outputs.
https://doi.org/10.1371/journal.pdig.0000341.s006
Acknowledgments
The authors extend their thanks to Mr Arunachalam Thirunavukarasu (Betsi Cadwaladr University Health Board) for his advice and assistance with recruitment.
- 1. Brown T, Mann B, Ryder N, Subbiah M, Kaplan JD, Dhariwal P, et al. Language Models are Few-Shot Learners. In: Advances in Neural Information Processing Systems [Internet]. Curran Associates, Inc.; 2020 [cited 2023 Jan 30]. p. 1877–901. Available from: https://papers.nips.cc/paper/2020/hash/1457c0d6bfcb4967418bfb8ac142f64a-Abstract.html
- 2. OpenAI. GPT-4 Technical Report [Internet]. arXiv; 2023 [cited 2023 Apr 11]. Available from: http://arxiv.org/abs/2303.08774
- View Article
- PubMed/NCBI
- Google Scholar
- 9. Google. PaLM 2 Technical Report [Internet]. 2023 [cited 2023 May 11]. Available from: https://ai.google/static/documents/palm2techreport.pdf
- 17. Ting DSJ, Steel D. MCQs for FRCOphth Part 2. Oxford University Press; 2020. 253 p.
- 21. Part 2 Written FRCOphth Exam [Internet]. The Royal College of Ophthalmologists. [cited 2023 Jan 30]. Available from: https://www.rcophth.ac.uk/examinations/rcophth-exams/part-2-written-frcophth-exam/
- Clinical Physics
- Translational Physics
- Proton Engineers
- Physics Residents
- Information Technology
- Past Members
Certification
Therapeutic Physics, American Board of Radiology
AAPM Jack Fowler Junior Investigators Award, 2004
Publications in Radiation Oncology and Medical Physics
Yan S, Lu HM, Flanz J, Adams J, Trofimov A, Bortfeld T. Reassessment of the necessity of the proton gantry: analysis of beam orientations from 4332 treatments at the F.H. Burr proton center over the past 10 years. International Journal of Radiation Oncology Biology Physics 2016
Moteabbed M, Trofimov A, Sharp GC, Wang Y, Zietman AL, Efstathiou JA, Lu HM. A prospective comparison of the effects of interfractional variations on proton therapy and IMRT for prostate cancer. International Journal of Radiation Oncology Biology Physics 2016
Patel AV, Lane AM, Morrison MA, Trofimov AV, Shih HA, Gragoudas ES, Kim IK. Visual Outcomes after Proton Beam Irradiation for Choroidal Melanomas Involving the Fovea. Ophthalmology 2015
M oteabbed M, Sharp GC, Wang Y, Trofimov A, Efstathiou JA, Lu HM. Validation of a deformable image registration technique for cone beam CT-based dose verification. Medical Physics 2015;42:196-205
Cheney MD, Chen YL, Lim R, Winrich BK, Grosu AL, Trofimov AV, Depauw N, Shih HA, Schwab JH, Hornicek FJ, DeLaney TF. 18F-FMISO PET/CT visualization of tumor hypoxia in patients with chordoma of the mobile and sacrococcygeal spine. International Journal of Radiation Oncology Biology Physics 2014
Safai S, Trofimov A, Adams JA, Engelsman M, Bortfeld T. The rationale for intensity-modulated proton therapy in geometrically challenging cases. Physics in Medicine and Biology 2013;58:6337-6353.
Giantsoudi D, Grassberger C, Craft D, Niemierko A, Trofimov A, Paganetti H. Linear energy transfer (LET)-Guided Optimization in intensity modulated proton therapy (IMPT): feasibility study and clinical potential. International Journal of Radiation Oncology Biology Physics 2013;87:216-222.
Wang. Y, Efstathiou JE, Lu H, Sharp GC, Trofimov A. Hypofractionated proton therapy for prostate cancer: dose delivery uncertainty due to inter-fractional motion. Medical Physics 2013;40:071714
Zeng C, Giantsoudi D, Grassberger C, Goldberg S, Niemierko A, Paganetti H, Efstathiou JA, Trofimov A. Maximizing the biological effect of proton dose delivered with scanned beams via inhomogeneous daily dose distributions. Medical Physics 2013;40:051708.
De Amorim Bernstein K, Sethi R, Trofimov A, Zeng C, Fullerton B, Yeap BY, Ebb D, Tarbell NJ, Yock TI, Macdonald SM. Early clinical outcomes using proton radiation for children with central nervous system atypical teratoid rhabdoid tumors. International Journal of Radiation Oncology Biology Physics 2013;86:114-20.
Trofimov A, Unkelbach J, DeLaney TF, Bortfeld T. Visualization of a variety of possible dosimetric outcomes in radiation therapy using dose-volume histogram bands. Practical Radiation Oncology 2012;2:164-171.
Chen W, Unkelbach J, Trofimov A, Madden T, Kooy H, Bortfeld T, Craft D. Including robustness in multi-criteria optimization for intensity-modulated proton therapy. Physics in Medicine and Biology 2012;57:591-608.
Wang Y, Efstathiou J, Sharp G, Lu HM, Ciernik IF, Trofimov A. Evaluation of the dosimetric impact of inter-fractional anatomical variations on prostate proton therapy using daily in-room CT images. Medical Physics 2011
Grassberger C, Trofimov A, Lomax A, Paganetti H. Variations in linear energy transfer within clinical proton therapy fields and the potential for biological treatment planning. International Journal of Radiation Oncology Biology Physics 2011
Trofimov A, NguyenPL, EfstathiouJA, Wang Y, LuHM, EngelsmanM, MerrickS, ChengCW, WongJR, ZietmanAL. Interfractional variations in the set-up of pelvic bony anatomy and soft tissue, and their implication on the delivery of proton therapy for localized prostate cancer. International Journal of Radiation Oncology Biology Physics 2011; 80:928-937.
Ding A, Gu J, Trofimov A, Xu XG. Monte Carlo calculation of imaging doses from diagnostic multi-detector CT and kilovoltage cone-beam CT as part of prostate cancer treatment plans. Medical Physics 2010; 37:6199-6204.
MacDonald SM, Trofimov A, Safai S, Adams J, Fullerton B, Ebb D, Tarbell NJ, Yock T. Proton Radiotherapy for Pediatric Central Nervous System Germ Cell Tumors: Early Clinical Outcomes. International Journal of Radiation Oncology Biology Physics 2011; 79:121-129
Nguyen PL, Chen RC, Hoffman KE, Trofimov A, Efstathiou JA, Coen JJ, Shipley WU, Zietman AL, Talcott JA. Rectal Dose-Volume Histogram Parameters Are Associated with Long-Term Patient-Reported Gastrointestinal Quality of Life After Conventional and High-Dose Radiation for Prostate Cancer: A Subgroup Analysis of a Randomized Trial. International Journal of Radiation Oncology Biology Physics 2010; 78:1081-5
Suit H, Delaney T, Goldberg S, Paganetti H, Clasie B, Gerweck L, Niemierko A, Hall E, Flanz J, Hallman J, Trofimov A. Proton vs carbon ion beams in the definitive radiation treatment of cancer patients. Radiotherapy and Oncology 2010; 95:3-22.
Kooy HM, Clasie BM, Lu HM, Madden TM, Bentefour H, Depauw N, Adams JA, Trofimov AV, Demaret D, Delaney TF, Flanz JB. A case study in proton pencil-beam scanning delivery. International Journal of Radiation Oncology Biology Physics. 2010; 76:624-30.
Efstathiou JA, Trofimov AV, Zietman AL. Life, liberty, and the pursuit of protons: an evidence-based review of the role of particle therapy in the treatment of prostate cancer. Cancer J. 2009; 15:312-8.
Seco J, Robinson D, Trofimov A, Paganetti H. Breathing interplay effects during proton beam scanning: simulation and statistical analysis. Physics in Medicine and Biology 2009; 54:N283-294.
Vrancic C, Trofimov A, Chan TCY, Sharp G, Bortfeld T. Experimental evaluation of a robust optimization method for IMRT of moving targets. Physics in Medicine and Biology 2009; 54: 2901-2914.
Bortfeld T, Chan TCY, Trofimov A, Tsitsiklis JN. Robust management of motion uncertainty in intensity-modulated radiation therapy. Operations Research 2008; 56:1461-1473
Nguyen PL, Trofimov A, Zietman AL. Proton beam or intensity-modulated therapy in the treatment of prostate cancer? Oncology 2008; 22:748-754.
Trofimov A, Vrancic C, Chan TCY, Sharp GC, Bortfeld T. Tumor trailing startegy for intensity-modulated radiation therapy of moving targets. Medical Physics 2008; 35:1718-1733
MacDonald SM, Safai S, Trofimov A, Wolfgang J, Fullerton B, Yeap BY, Bortfeld T, Tarbell NJ, Yock T. Proton radiotherapy for childhood ependymoma: initial clinical outcomes and dose comparisons. International Journal of Radiation Oncology Biology Physics 2008; 71:979-987
Suit H, Kooy H,Trofimov A, Farr J, Munzenrider J, DeLaney T, Loeffler J, Clasie B, Safai S, Paganetti H. Should positive phase III clinical trial data be required before proton beam therapy is more widely adopted? No. Radiotherapy and Oncology 2008; 86:148-153.
Trofimov A, Nguyen PL, Coen JJ, Doppke KP, Schneider RJ, Adams JA, Bortfeld TR, Zietman AL, DeLaney TF, Shipley WU. Radiotherapy treatment of early stage prostate cancer with IMRT and protons: a treatment planning comparsion. International Journal of Radiation Oncology Biology Physics 2007; 69:444-453 (follow-up: Letter to the Editor. In reply to Ms.Albertini et al. International Journal of Radiation Oncology Biology Physics 2007; 69:1334-1335)
Sharp GC, Lu HM, Trofimov A, Tang X, Jiang SB, Turcotte J, Gierga DP, Chen GTY, Hong TS. Assessing residual motion for gated proton-beam radiotherapy.Journal of Radiation Research 2007; 48:A55-59.
Censor Y, Bortfeld T, Martin B, Trofimov A. A unified approach for inversion problems in intensity-modulated radiation therapy. Physics in Medicine and Biology 2006; 51:2353-65.
Trofimov A, Rietzel E, Lu H, Martin B, Jiang S, Chen G, Bortfeld T. Temporo-spatial IMRT optimization: Concepts, implementation and initial results. Physics in Medicine and Biology 2005; 50:2779-98.
Paganetti H, Jiang H, Trofimov A. 4D Monte Carlo simulation of proton beam scanning: modeling of variations in time and space to study the interplay between scanning pattern and time-dependent patient geometry. Physics in Medicine and Biology 2005; 50:983-90.
DeLaney TF, Trofimov AV, Engelsman M, Suit HD. Advanced-technology radiation therapy in the management of bone and soft tissue sarcomas. Cancer Control 2005; 12:27-35
Weber DC, Trofimov AV, Delaney TF, Bortfeld T. A treatment planning comparison of intensity modulated photon and proton therapy for paraspinal sarcomas. International Journal of Radiation Oncology Biology Physics 2004; 58:1596-606.
Suit H, Goldberg S, Niemierko A, Trofimov A, Adams J, Paganetti H, Chen GTY, Bortfeld T, Rosenthal S, Loeffler J, DeLaney T. Protons to Replace Photon Beams in Radical Dose Treatments. Acta Oncologica 2003; 42:800-8.
Trofimov A, Bortfeld T. Optimization of beam parameters and treatment planning for intensity modulated proton therapy. Technology in Cancer Research and Treatment 2003; 2:437-44.
Trofimov A, Bortfeld T. Beam delivery sequencing for intensity modulated proton therapy. Physics in Medicine and Biology 2003; 48:1321-31.
Publications in High-Energy Physics (with g-2 Collaboration, Brookhaven National Laboratory)
Bennett GW, et al. Improved limit on the muon electric dipole moment. Physical Review D 2009; 80:052008.
Bennett GW et al. Search for Lorentz and CPT violation effects in muon spin precession. Physical Review Letters 2008; 100:091602.
Bennett GW et al Statistical equations and methods applied to the precision muon (g-2) experiment at BNL. Nuclear Instruments and Methods in Physics Research A 2007; 579:1096-1116.
Bennett GW et al. Final report of the E821 muon anomalous magnetic moment measurement at BNL. Physical Review D 2006; 73:072003.
Bennett GW et al. Measurement of the negative muon anomalous moment to 0.7 ppm. Physical Review Letters 2004; 92:161802.
Bennett GW et al. Measurement of the positive muon anomalous moment to 0.7 ppm. Physical Review Letters 2002; 89:101804.
Brown HN et al. Precise measurement of the positive muon anomalous magnetic moment. Physical Review Letters 2001; 86:2227-31.
Sedykh SA et al. Electromagnetic calorimeters for the BNL muon (g-2) experiment. Nuclear Instruments and Methods A 2000; 455:346-60.
Brown HN et al. Improved measurement of the positive muon anomalous magnetic moment. Physical Review D 2000; 62:091101.
Carey RM et al. New measurement of the anomalous magnetic moment of the positive muon. Physical Review Letters 1999; 82:1632-35.

REVIEW article
This article is part of the research topic.
Unveiling Inflammaging – Mechanistic Insights on Aging and Related Diseases
What improvements do general exercise training and traditional Chinese exercises have on Knee Osteoarthritis? A Narrative Review based on biological mechanisms and clinical efficacy Provisionally Accepted
- 1 Shandong Huayu University of Technology, China
- 2 Qufu Normal University, China
The final, formatted version of the article will be published soon.
Background: Knee osteoarthritis (KOA) is a disease that significantly affects the quality of life of patients, with a complex pathophysiology that includes degeneration of cartilage and subchondral bone, synovitis, and associations with mechanical load, inflammation, metabolic factors, hormonal changes, and aging. Objective: This article aims to comprehensively review the biological mechanisms and clinical effects of general exercise training and traditional Chinese exercises (such as Tai Chi and Qigong) on the treatment of KOA, providing references for the development of clinical exercise prescriptions. Methods: A systematic search of databases including PubMed, Web of Science, Google Scholar, and China National Knowledge Infrastructure (CNKI) was conducted, reviewing studies including randomized controlled trials (RCTs), observational studies, systematic reviews, and meta-analyses. Keywords included "knee osteoarthritis," "exercise therapy," "physical activity," and "traditional Chinese exercise.": General exercise training positively affects KOA by mechanisms such as promoting blood circulation, improving the metabolism of inflammatory factors, enhancing the expression of anti-inflammatory cytokines, and reducing cartilage cell aging. Traditional Chinese exercises, like Tai Chi and Qigong, benefit the improvement of KOA symptoms and tissue repair by regulating immune function and alleviating joint inflammation. Clinical studies have shown that both types of exercise can improve physical function, quality of life, and pain relief in patients with KOA. Both general exercise training and traditional Chinese exercises are non-pharmacological treatment options for KOA that can effectively improve patients' physiological function and quality of life. Future research should further explore the long-term effects and biological mechanisms of these exercise interventions and develop personalized exercise programs based on the specific needs of patients.
Keywords: General exercise training, traditional Chinese exercise, knee osteoarthritis, Biological mechanism, clinical efficacy
Received: 03 Mar 2024; Accepted: 22 Apr 2024.
Copyright: © 2024 Du, Fan and Kong. This is an open-access article distributed under the terms of the Creative Commons Attribution License (CC BY) . The use, distribution or reproduction in other forums is permitted, provided the original author(s) or licensor are credited and that the original publication in this journal is cited, in accordance with accepted academic practice. No use, distribution or reproduction is permitted which does not comply with these terms.
* Correspondence: Dr. Jianda Kong, Qufu Normal University, Qufu, 273165, Shandong Province, China
People also looked at
- Open access
- Published: 20 April 2024
Interpretable machine learning in predicting drug-induced liver injury among tuberculosis patients: model development and validation study
- Yue Xiao 1 ,
- Yanfei Chen 1 ,
- Ruijian Huang 1 ,
- Feng Jiang 1 ,
- Jifang Zhou 1 na1 &
- Tianchi Yang 2 na1
BMC Medical Research Methodology volume 24 , Article number: 92 ( 2024 ) Cite this article
71 Accesses
1 Altmetric
Metrics details
The objective of this research was to create and validate an interpretable prediction model for drug-induced liver injury (DILI) during tuberculosis (TB) treatment.
A dataset of TB patients from Ningbo City was used to develop models employing the eXtreme Gradient Boosting (XGBoost), random forest (RF), and the least absolute shrinkage and selection operator (LASSO) logistic algorithms. The model's performance was evaluated through various metrics, including the area under the receiver operating characteristic curve (AUROC) and the area under the precision recall curve (AUPR) alongside the decision curve. The Shapley Additive exPlanations (SHAP) method was used to interpret the variable contributions of the superior model.
A total of 7,071 TB patients were identified from the regional healthcare dataset. The study cohort consisted of individuals with a median age of 47 years, 68.0% of whom were male, and 16.3% developed DILI. We utilized part of the high dimensional propensity score (HDPS) method to identify relevant variables and obtained a total of 424 variables. From these, 37 variables were selected for inclusion in a logistic model using LASSO. The dataset was then split into training and validation sets according to a 7:3 ratio. In the validation dataset, the XGBoost model displayed improved overall performance, with an AUROC of 0.89, an AUPR of 0.75, an F1 score of 0.57, and a Brier score of 0.07. Both SHAP analysis and XGBoost model highlighted the contribution of baseline liver-related ailments such as DILI, drug-induced hepatitis (DIH), and fatty liver disease (FLD). Age, alanine transaminase (ALT), and total bilirubin (Tbil) were also linked to DILI status.
XGBoost demonstrates improved predictive performance compared to RF and LASSO logistic in this study. Moreover, the introduction of the SHAP method enhances the clinical understanding and potential application of the model. For further research, external validation and more detailed feature integration are necessary.
Peer Review reports
Drug-induced liver injury (DILI) presents significant challenges in the context of tuberculosis (TB) treatment. Anti-TB drugs exhibit noteworthy involvement in the occurrence of DILI [ 1 , 2 ], and the lack of certain early-detection biomarkers [ 3 ] further poses challenges to the timely diagnosis and management of DILI. This absence of early detection may result in treatment interruptions and failures amongst TB patients [ 4 , 5 ], impeding global TB eradication efforts [ 6 ]. In China, the elevated incidence rates of DILI in comparison to western nations highlight the potential involvement of traditional Chinese medicines (TCM) and herbal medicines in the development of DILI [ 7 ]. This requires addressing various challenges and complexities associated with DILI assessment in a comprehensive and objective manner. Therefore, the primary objective of this study is to develop an optimal predictive model for assessing DILI status, with a specific focus on TB patients within the Chinese context.
The emergence of machine learning (ML) algorithms presents an exciting opportunity to enhance DILI prediction models [ 8 ]. Among these, eXtreme Gradient Boosting (XGBoost) [ 9 ] and random forest (RF) [ 10 ] stand out as two widely-used ensemble learning techniques, each distinguished by its algorithmic approach and features. Selecting the most suitable option between them hinges on the particular characteristics of the data and the prediction objective. Therefore, it is often advisable to conduct experiments with both models to compare their performance.
Nevertheless, one of the primary challenges in implementing ML algorithms in clinical settings is interpreting the outcomes of the models [ 11 , 12 ]. The Shapley Additive exPlanations (SHAP) framework [ 13 ] provides insights into the influence of various features on model predictions and the effect of these features on the DILI status in individuals, thus bridging the interpretability gap.
This study focuses on the development and validation of a prediction model for DILI in the context of TB treatment by using advanced ML algorithms with SHAP interpretability. Through this endeavor, we aim to achieve a balance between accurate prediction and the interpretability of the model, which is crucial for its clinical application.
Data source
The study participants comprised individuals diagnosed with TB at specified hospitals in Ningbo from 1st January 2015 to 2nd January 2020, initially referred by the Chinese Center for Disease Control and Prevention (CDC) [ 14 ]. Thereafter, they were connected to administrative records obtained from the electronic health records (EHR) system employed by the local government [ 15 ]. The merged dataset comprised demographic information, hospitalization records (both inpatient and outpatient), laboratory tests, and medication profiles.
Exclusion criteria
To ensure consistency in the identification of covariates, individuals with only one health care encounter during the study period were excluded. Furthermore, individuals without ethnicity information and those under 18 years old at diagnosis were not included in the study. The exclusion criteria also filtered out misdiagnosed cases of DILI and liver injuries attributed to known factors like alcohol-related liver disease, non-alcoholic fatty liver disease (NAFLD), and viral hepatitis unrelated to drug-induced causes. The detailed flowchart is presented in Fig. 1 .
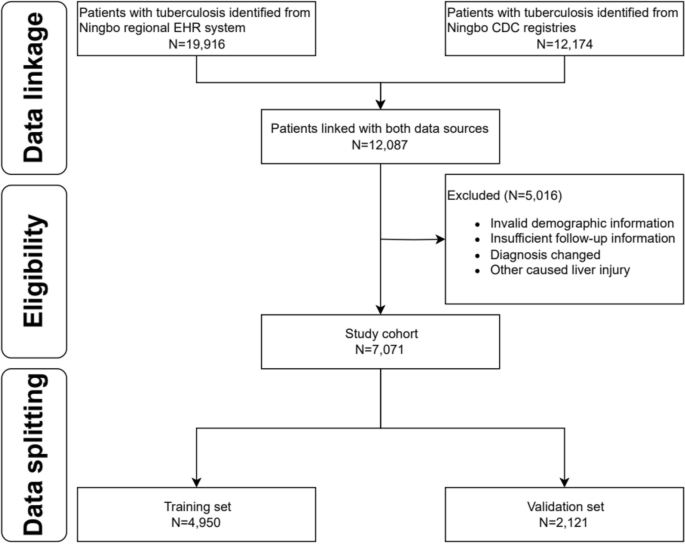
Study schema for subject selection. Abbreviations: EHR, Electronic healthcare record; CDC, Center for Disease Control and Prevention
Baseline laboratory result collection
For patients included in the study, we defined the baseline period for collecting laboratory test results as from January 1, 2015, to the day before the index diagnosis of pulmonary tuberculosis, as shown in Supplemental Fig. 1 . Additionally, liver function test indicators such as alanine transaminase (ALT) or alkaline phosphatase (ALP) were simultaneously examined.
To address the issue of varied baseline definitions in laboratory testing, we utilized two main strategies. Firstly, we employed a binary variable approach to categorize laboratory testing indicators as abnormal or normal, by comparing their values with predefined normal ranges. Secondly, we utilized ratio-based representation to quantify indicator abnormalities, such as calculating ALT multiples relative to the upper limit of the normal (ULN) range.
Factor identification
In our research, we followed the initial steps outlined in the high dimensional propensity score (HDPS) methodology by Schneeweiss et al. [ 16 ]. First, we identified 24 common factors, such as age and gender, to integrate into our models. We then categorized our data into four dimensions: outpatient records, inpatient records, laboratory test records, and medication records. Following the approach of Chen et al. [ 17 ], we identified the top 500 most prevalent codes within each dimension. Next, we evaluated code recurrence, classifying codes into three binary variables based on their frequency of occurrence over a 12-month baseline period. This yielded a total of 4*500*3 binary factors. Using a multiplicative model considering binary factor and DILI status, we prioritized covariates and selected the top 400 for inclusion in our final model based on an arbitrary cutoff recommendation [ 18 , 19 ]. Finally, considering the previously specified 24 variables, our model training ultimately involved incorporating a total of 424 factors.
DILI diagnostic process
The determination of DILI outcomes followed the revised criteria set forth by the Chinese Society of Hepatology (CSH) DILI consensus, as outlined in Supplemental Table 1 [ 20 ].
Extraction of features used in prediction model
The LASSO regression method, aimed at reducing the number of variables and preventing overfitting [ 21 ], was applied to extract significant features for constructing the logistic model. Additionally, both the XGBoost and RF algorithms come equipped with their own feature selection techniques tailored to enhance their respective models.
Statistical analysis
The study reported the features of both the non-DILI and DILI groups by mean and standard deviation (SD) or as numbers and percentages whenever necessary. Laboratory variables were represented in median and quartiles [ 22 ]. The Kruskal–Wallis rank sum test was used for continuous variables, while the chi-square test was used for categorical variables. These analyses were conducted using the statistical software packages SAS 9.4 and R 4.0.3. A statistically significant result was determined with a two-sided P -value below 0.05.
Data splitting
In order to create training and validation sets, a stratified random function in R randomly assigned records at a 7:3 ratio, following conventional practices.
Parameter optimization
To optimize the parameters of the XGBoost and RF models, a ten-fold cross-validation process combined with grid search [ 23 ] was employed. This approach entailed identifying the hyperparameter set that yielded the maximum receiver operating characteristic (ROC). A detailed breakdown of the grid search particulars and optimal results can be found in Supplemental Table 2 .
Model evaluation and interpretation
To assess the model's capacity to differentiate between positive and negative cases, we computed both the area under the receiver operating characteristic curve (AUROC) and the area under the precision recall curve (AUPR) [ 24 ]. Calibration was examined through reliability diagrams and Brier scores. Furthermore, the model's clinical utility was evaluated using decision curve analysis. The SHAP technique was utilized to delve deeper into variable contributions. A comprehensive overview of the workflow can be found in Supplemental Fig. 2 .
Participant and factor identification
The preliminary linkage of data yielded 12,087 instances. Following the application of exclusion criteria, a total of 7,071 subjects were identified as suitable for inclusion in the study.
During a one-year baseline period, we identified the 500 most prevalent codes across each data dimension (outpatient, inpatient, medication, and laboratory test) using the International Classification of Diseases-Tenth Revision (ICD-10), Current Procedural Terminology (CPT), and generic drug names. These items were then categorized into three binary variables: "ever occurring", "sporadically occurring", and "frequently occurring", indicating their recurrence. This process resulted in a total of 6,000 variables, from which the top 400 binary empirical variables were chosen based on their highest risk ratios associated with DILI status. Additionally, the final model incorporated 24 predefined baseline variables, such as gender, age, education level, medication, and maximum ratio of ULN for ALT, ALP, and Tbil, etc. Out of an initial pool of 424 features, 37 were selected for logistic model development using LASSO. The factors included in the LASSO logistic model are detailed in Supplemental Table 3 .
Epidemiology of DILI
The incidence of DILI was observed to be 16.3% overall, with a slightly higher observed in female patients (17.3% vs. 15.8%, p = 0.134). Detailed demographics and clinical information are outlined in Table 1 . Compared to non-DILI individuals, those with DILI demonstrated lower educational attainment and a higher incidence of abnormal baseline levels in ALT and ALP [ALT: 91 (7.9%) vs. 273 (4.6%), p < 0.001; ALP: 100 (8.7%) vs. 400 (6.8%), p = 0.023]. Individuals of middle age, females, and those with pre-existing chronic liver conditions were found to have a higher susceptibility to DILI. Significant associations with DILI were identified for certain drugs, including pyrazinamide (PZA), isoniazid (INH), traditional Chinese medicines (TCM), and hepatoprotective agents such as silymarin and glycyrrhetinic acid.
Model development and validation
The XGBoost and RF models were constructed using optimal parameters obtained through the previously mentioned GridSearchCV method. The LASSO logistic model was constructed with the aforementioned variables. Internal validation was conducted by partitioning validation sets, resulting in a comparison of model performance among the three models showcased in Table 2 . The XGBoost model exhibited slightly superior discriminatory ability when compared with the RF and LASSO logistic model, with AUROC values of 0.89 versus 0.88/0.85 and AUPR values of 0.75 versus 0.73/0.67, respectively, as shown in Figs. 2 and 3 . The RF model demonstrated increased recall with a score of 0.78, while the XGBoost model achieved the highest F1-score of 0.57. Calibration was evaluated through ten predictive probability-based bins and verified by the reliability diagram presented in Fig. 4 , supported by a Brier score of 0.08, indicating the impressive alignment in calibration between the XGBoost and LASSO logistic models. Extensive analysis of the decision curve revealed positive net benefits for all models. Notably, XGBoost models outperformed both the RF and LASSO logistic models within the threshold range of approximately 0.2 to 0.5, as demonstrated in Fig. 5 .
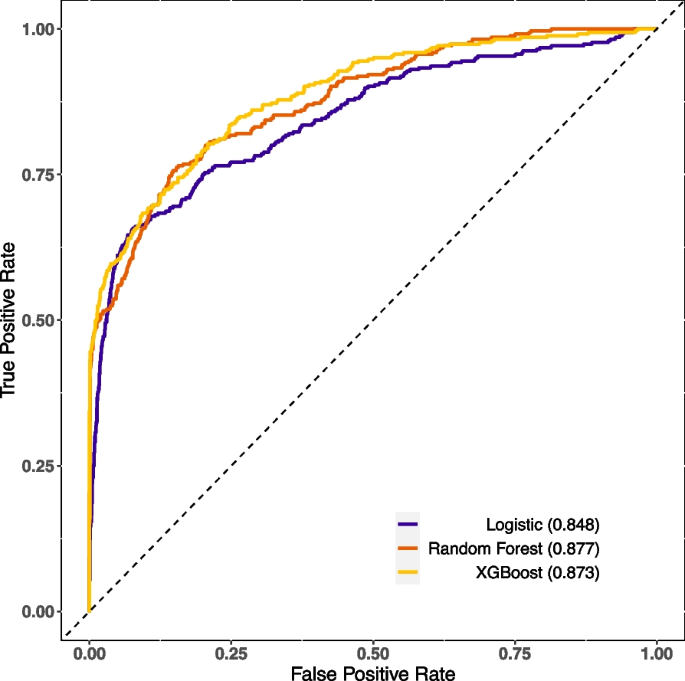
Comparison of the AUROC of the XGBoost, logistic and random forest in the validation set
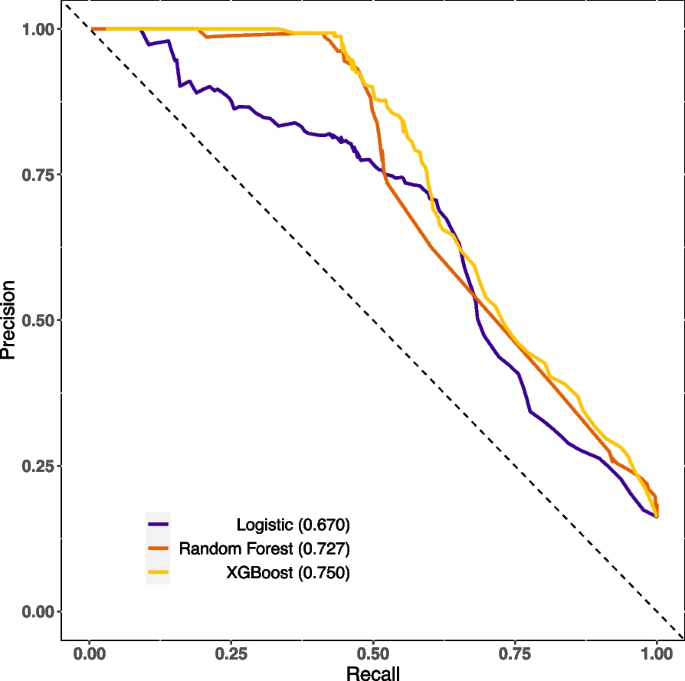
Comparison of the AUPR of the XGBoost, logistic and random forest in the validation set
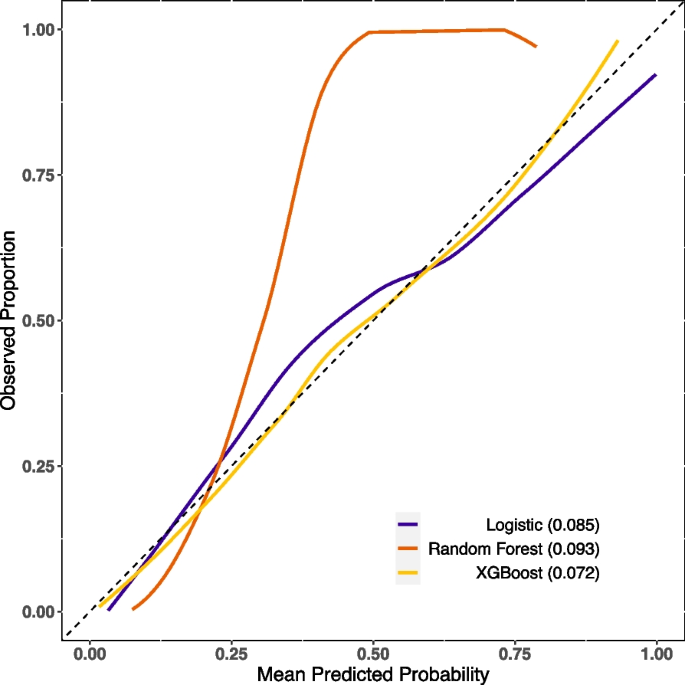
Comparison of the calibration curve of the XGBoost, logistic and random forest in the validation set
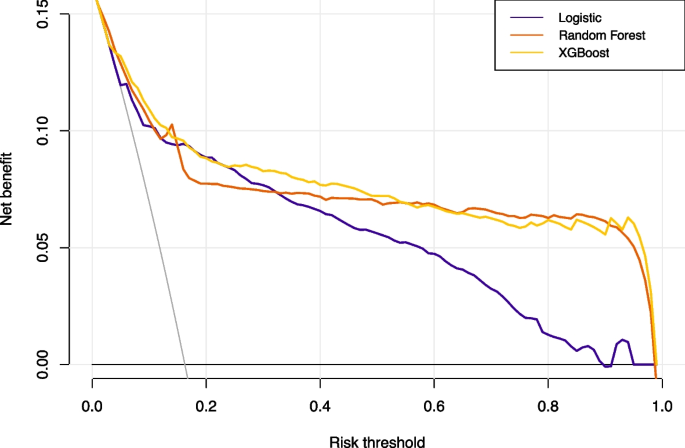
The decision curve of the XGBoost, logistic and random forest in the validation set
Model interpretation
Revealing the factors that influenced the outperformed model's predictions, Fig. 6 laid out the most paramount features of XGBoost (with feature importance > 0.01). Of note, historical occurrences of DILI, DIH, and fatty liver disease (FLD) during the baseline phase were consistently highlighted. Moreover, the ULN for ALT, ALP and Tbil were also identified as critical factors. The SHAP values calculated for the XGBoost model, as shown in Supplemental Fig. 3 , indicate that individuals who had chronic liver disease during baseline were more likely to be in DILI status. Interestingly, we found that those with a lower educational level were more susceptible to DILI status. To gain a deeper understanding of the underlying mechanism and the effects of features in the XGBoost model, we randomly selected two typical patients from the dataset. Furthermore, we created force plots to visualize their decision process, as illustrated in Supplemental Fig. 4 and Supplemental Fig. 5 . The average SHAP value was 0.168, where yellow indicates a positive impact and purple represents a negative impact. In Supplemental Fig. 4 , the identified patient with a SHAP value of 1.06, surpassing the average, is likely to develop DILI. The significant influencing factor is being diagnosed with DILI or DIH at least once during the baseline period. The same rationale applies to the identified patient as depicted in Supplemental Fig. 5 . Additionally, Supplemental Fig. 6 presents a force plot that captures the aggregate effect in the validation set.
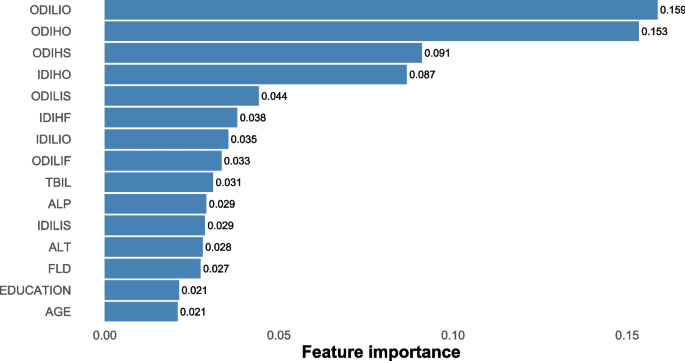
Top important features selected by XGBoost (> 0.01). Abbreviations: ODILIO, outpatient drug-induced liver injury, once occurring; ODIHO, outpatient drug induced hepatitis, once occurring; ODIHS, outpatient drug induced hepatitis, sporadically occurring; IDIHO, inpatient drug induced hepatitis, once occurring; ODILIS, outpatient drug induced liver injury, sporadically occurring; IDIHF, inpatient drug induced hepatitis, frequently occurring; IDILIO, inpatient drug induced liver injury, once occurring; ODILIF, outpatient drug induced liver injury, frequently occurring; TBIL, total bilirubin; ALP, alkaline phosphatase; IDILIS, inpatient drug induced liver injury, sporadically occurring; ALT, alanine aminotransferase; FLD, fatty liver disease
To our knowledge, this study represents the initial attempt to evaluate the prediction for DILI in an Asian population, predominantly of Han ethnicity, with TB using regional electronic health records. We observed slightly enhanced discrimination abilities in ML models compared to the logistic model. While logistic regression offers better clinical generalizability, it struggles with overfitting and handling missing variables, resulting in overall weaker performance than anticipated. In contrast, both XGBoost and RF employ more advanced techniques. XGBoost utilizes gradient boosting, progressively building weak learners and effectively capturing non-linear relationships with built-in regularization. On the other hand, RF, a bagging ensemble method, constructs independent decision trees on random subsets of data, resulting in robust averaging but with less explicit regularization. XGBoost excels in capturing intricate non-linear patterns, making it suitable for tasks involving complex and dynamic interactions like predicting DILI during TB treatment. Its training efficiency is also evident when handling large datasets. RF, with its robust averaging, is well-suited for further application in diverse datasets but may encounter challenges in effectively capturing subtle non-linear patterns among multiple explanatory variables.
Several prior studies have identified risk factors associated with DILI during TB treatment, involving chronic liver disease, specific drug combinations, age, and various demographic characteristics [ 25 , 26 , 27 ]. Lammert et al. [ 28 ] suggested an increased risk of DILI in patients with chronic liver disease indicative of NAFLD. Chang et al. [ 29 ] indicated a significant rise in hepatotoxicity risk associated with adding PZA to INH and RIF. Hosford et al. [ 30 ] established a notable elevation in hepatotoxicity risk among individuals over 60 years of age through a systematic literature review. Abbara et al. [ 2 ] found low patient weight, HIV-1 co-infection, higher baseline ALP levels, and alcohol intake were risk factors. Thus, in our model, we predefined enzyme levels, utilization of anti-TB drugs such as PZA, INH, and RIF, hepatoprotective agents such as silymarin and glycyrrhetinic acid, alcohol intake, and demographic variables such as age, gender, education level, ethnicity, profession as predictors. In the ultimate XGBoost model, the contribution weights for chronic liver disease, ULN of ALT, ALP, Tbil, and age surpass 0.01, consistent with earlier research discoveries.
Currently, a range of predictive models for DILI primarily operates at the molecular level in preclinical settings [ 31 ], utilizing diverse artificial intelligence assisted algorithms [ 32 ]. Minerali et al. [ 33 ] employed the Bayesian ML method, resulting in an AUROC of 0.81, 74% sensitivity, 76% specificity, and 75% accuracy. Xu et al. [ 34 ] proposed a deep learning model, achieving 87% accuracy, 83% sensitivity, 93% specificity, and an AUROC of 0.96. Dominic et al.'s Bayesian prediction model [ 35 ] demonstrated balanced performance with 86% accuracy, 87% sensitivity, 85% specificity, 92% positive predictive value, and 78% negative predictive value. In the clinical stage, only Zhong et al. introduced a single tree XGBoost model with 90% precision, 74% recall, and 76% classification accuracy for DILI prediction, using a clinical sample of 743 TB cases [ 36 ]. In our study, we leveraged regional healthcare data and employed the XGBoost algorithm. The model exhibited 76% recall, 82% specificity, and 81% accuracy in predicting DILI status. Our approach was proven robust, as evidenced by a mean AUROC of 0.89 and AUPR of 0.75 upon tenfold cross validation. During the clinical treatment stage, our model exhibited high levels of accuracy and interpretability.
The choice of a cutoff in a DILI prediction model is crucial and depends on specific study goals and requirements. Various studies have investigated optimal cutoff values in DILI prediction models to enhance understanding and prediction accuracy. For instance, in a study focused on drug-induced liver tumors, the maximum Youden index was utilized to determine the ideal cutoff point [ 37 ]. Another study, aimed at predicting DILI and cardiotoxicity, determined 0.4 as the optimal cutoff value using chemical structure and in vitro assay data [ 38 ]. Similarly, a system named DILIps, designed to predict DILI in drug safety, utilized the ROC curve to select the best cutoff value [ 39 ]. Given the imbalanced dataset in our study, we found the precision recall curve method seemed to be more appropriate. Additionally, considering the severe consequences of DILI, prioritizing the detection of DILI suggests choosing a lower cutoff to maximize sensitivity. Thus, in our study, we opted for the maximum Youden index as the best cutoff.
However, the acceptability of ML in the medical community faces a significant hurdle regarding interpretability, particularly in settings where clinical decisions are paramount. Our research employed SHAP strategies to illuminate the complex mechanisms of the XGBoost model.
Strengths and limitations
The study utilized a large dataset of over 7,000 TB patients to develop a robust model and comprehensively included clinical, demographic, and biochemical variables to improve predictive accuracy. Furthermore, the model incorporates SHAP analysis to improve interpretability. However, as we embark on the integration of ML into clinical settings, a vital concern persists regarding the generalizability of models [ 40 ]. While our model demonstrates enhanced predictive accuracy, it's important to recognize the inherent limitations stemming from the lack of external validation. Patient characteristics [ 41 ] and drug interactions [ 42 ] may differ widely across populations. This underscores the importance of validating models on diverse patient cohorts and geographical regions. Moreover, the study's reliance on a data-driven approach and the inherent complexity of integrating ML models into clinical practice present additional limitations [ 43 ]. Additionally, the dependence on clinical diagnosis for DILI and the potential influence of unmeasured variables on model accuracy are acknowledged. While the study's findings offer valuable insights, careful consideration is warranted when interpreting them.
Conclusions
XGBoost shows improved predictive performance compared to RF and LASSO logistics in this study. Moreover, introducing the SHAP method enhances the clinical understanding and potential application of the model. For further research, external validation and more detailed feature integration are necessary.
Code availability statement
To enhance reproducibility and facilitate peer review, we uploaded the code used for model fitting. The source code associated with this research is available on the GitHub repository ( https://github.com/cpu-pharmacoepi/TB-DILI ). For inquiries or assistance related to the code, please contact 1,020,202,[email protected].
Availability of data and materials
The datasets used and analyzed during the current study are available from the corresponding author on reasonable request. Data cannot be shared publicly because of privacy and confidentially of the TB patients in Ningbo, Zhejiang, China.
Abbreviations
Alkaline phosphatase
Alanine transaminase
Area under the precision recall curve
Area under the receiver operating characteristic curve
Center for Disease Control and Prevention
Current procedural terminology
Chinese Society of Hepatology
Drug-induced hepatitis
- Drug-induced liver injury
Electronic healthcare record
Fatty liver disease
High dimensional propensity score
International Classification of Diseases-Tenth Revision
International normalized ratio
Least Absolute Shrinkage and Selection Operator
- Machine learning
Nonalcoholic fatty liver disease
Pyrazinamide
Random Forest
Receiver operating characteristic
Standard deviation
Shapley Additive exPlanations
Standardized mean difference
- Tuberculosis
Total serum bilirubin
Traditional Chinese medicine
Upper limit of normal
EXtreme Gradient Boosting
Jiang F, Yan H, Liang L, et al. Incidence and risk factors of anti-tuberculosis drug induced liver injury (DILI): Large cohort study involving 4,652 Chinese adult tuberculosis patients. Liver Int. 2021;41(7):1565–75.
Article CAS PubMed Google Scholar
Abbara A, Chitty S, Roe JK, et al. Drug-induced liver injury from antituberculosis treatment: a retrospective study from a large TB center in the UK. BMC Infect Dis. 2017;17:231.
Article PubMed PubMed Central Google Scholar
Council for International Organizations Medical Sciences. Drug-induced liver injury. Geneva: CIMOS; 2020. Available from: https://cioms.ch/wp-content/uploads/2020/06/CIOMS_DILI_Web_16Jun2020.pdf . Accessed 01 Mar 2021
Nahid P, Dorman SE, Alipanah N, et al. Official American Thoracic Society/Centers for Disease Control and Prevention/Infectious Diseases Society of America Clinical Practice Guidelines: Treatment of Drug-Susceptible Tuberculosis. Clin Infect Dis. 2016;63(7):e147–95.
Stravitz RT. WM Lee. Acute liver failure The Lancet. 2019;394(10201):869–81.
CAS Google Scholar
World Health Organization. Global tuberculosis report. Geneva: WHO; 2020. Available from: https://www.who.int/tb/publications/global_report/en/ .
Shen T, Liu Y, Shang J, et al. Incidence and Etiology of Drug-Induced Liver Injury in Mainland China. Gastroenterology. 2019;156(8):2230-2241.e11.
Article PubMed Google Scholar
Sarker IH. Machine Learning: Algorithms, Real-World Applications and Research Directions. SN COMPUT. 2021;2:160.
Article Google Scholar
Chen T, Guestrin C. XGBoost: A Scalable Tree Boosting System. In: Proceedings of the 22nd ACM SIGKDD International Conference on Knowledge Discovery and Data Mining. New York: ACM; 2016;785–795.
Breiman L. Random Forests. Mach Learn. 2001;45:5–32.
Bjerregaard SS. Exploring predictors of welfare dependency 1, 3, and 5 years after mental health-related absence in Danish municipalities between 2010 and 2012 using flexible machine learning modelling. BMC Public Health. 2023;23(1):224.
Alan I, Andrew P, Catherine BH. Visualizing Variable Importance and Variable Interaction Effects in Machine Learning Models. J Comput Graph Stat. 2022;31(3):766–78.
Lu S, Chen R, Wei W, et al. Understanding Heart Failure Patients EHR Clinical Features via SHAP Interpretation of Tree-Based Machine Learning Model Predictions. AMIA Annu Symp Proc. 2022;2021:813–22.
PubMed PubMed Central Google Scholar
Jiang WX, Huang F, Tang SL, et al. Implementing a new tuberculosis surveillance system in Zhejiang, Jilin and Ningxia: improvements, challenges and implications for China’s National Health Information System. Infect Dis Poverty. 2021;10(1):22.
Liu Z, Zhang L, Yang Y, et al. Active Surveillance of Adverse Events Following Human Papillomavirus Vaccination: Feasibility Pilot Study Based on the Regional Health Care Information Platform in the City of Ningbo, China. J Med Internet Res. 2020;22(6): e17446.
Schneeweiss S. Automated data-adaptive analytics for electronic healthcare data to study causal treatment effects. Clin Epidemiol. 2018;10:771–88.
Chen Q, Hu A, Ma A, et al. Effectiveness of Prophylactic Use of Hepatoprotectants for Tuberculosis Drug-Induced Liver Injury: A Population-Based Cohort Analysis Involving 6,743 Chinese Patients. Front Pharmacol. 2022;20(13): 813682.
Polinski JM, Schneeweiss S, Glynn RJ, et al. Confronting “confounding by health system use” in Medicare Part D: comparative effectiveness of propensity score approaches to confounding adjustment. Pharmacoepidemiol Drug Saf. 2012;21(Suppl 2):90–8.
Schneeweiss S, Rassen JA, Glynn RJ, et al. High-dimensional propensity score adjustment in studies of treatment effects using health care claims data. Epidemiology. 2009;20(4):512–22.
Yu YC, Mao YM, Chen CW, et al. CSH guidelines for the diagnosis and treatment of drug-induced liver injury. Hepatol Int. 2017;11(3):221–41.
Sun L, Wang Q, Liu M, et al. Albumin binding function is a novel biomarker for early liver damage and disease progression in non-alcoholic fatty liver disease. Endocrine. 2020;69:294–302.
James G, Witten D, Hastie T, et al. An introduction to statistical learning: with applications in R. New York: Springer; 2013.
Book Google Scholar
Sattar N, Scherbakova O, Ford I, et al. Elevated alanine aminotransferase predicts new-onset type 2 diabetes independently of classical risk factors, metabolic syndrome, and C-reactive protein in the west of Scotland coronary prevention study. Diabetes. 2004;53(11):2855–60.
Coyner AS, Chen JS, Singh P, et al. Single-Examination Risk Prediction of Severe Retinopathy of Prematurity. Pediatrics. 2021;148(6): e2021051772.
Cao J, Mi Y, Shi C, et al. First-line anti-tuberculosis drugs induce hepatotoxicity: A novel mechanism based on a urinary metabolomics platform. Biochem Biophys Res Commun. 2018;497(2):485–91.
Tweed CD, Wills GH, Crook AM, et al. Liver toxicity associated with tuberculosis chemotherapy in the REMoxTB study. BMC Med. 2018;16(1):46.
Patterson B, Abbara A, Collin S, et al. Predicting drug-induced liver injury from anti-tuberculous medications by early monitoring of liver tests. J Infect. 2021;82(2):240–4.
Lammert C, Imler T, Teal E, et al. Patients With Chronic Liver Disease Suggestive of Nonalcoholic Fatty Liver Disease May Be at Higher Risk for Drug-Induced Liver Injury. Clin Gastroenterol Hepatol. 2019;17(13):2814–5.
Chang KC, Leung CC, Yew WW, et al. Hepatotoxicity of pyrazinamide: cohort and case-control analyses. Am J Respir Crit Care Med. 2008;177(12):1391–6.
Hosford JD, von Fricken ME, Lauzardo M, et al. Hepatotoxicity from antituberculous therapy in the elderly: a systematic review. Tuberculosis (Edinb). 2015;95(2):112–22.
Chen M, Bisgin H, Tong L, et al. Toward predictive models for drug-induced liver injury in humans: are we there yet? Biomark Med. 2014;8(2):201–13.
Vall A, Sabnis Y, Shi J, et al. The Promise of AI for DILI Prediction. Front Artif Intell. 2021;14(4): 638410.
Minerali E, Foil DH, Zorn KM, et al. Comparing Machine Learning Algorithms for Predicting Drug-Induced Liver Injury (DILI). Mol Pharm. 2020;17(7):2628–37.
Article CAS PubMed PubMed Central Google Scholar
Xu Y, Dai Z, Chen F, et al. Deep Learning for Drug-Induced Liver Injury. J Chem Inf Model. 2015;55(10):2085–93.
Williams DP, Lazic SE, Foster AJ, et al. Predicting Drug-Induced Liver Injury with Bayesian Machine Learning. Chem Res Toxicol. 2020;33(1):239–48.
Zhong T, Zhuang Z, Dong X, et al. Predicting Antituberculosis Drug-Induced Liver Injury Using an Interpretable Machine Learning Method: Model Development and Validation Study. JMIR Med Inform. 2021;9(7): e29226.
Linden A. Measuring diagnostic and predictive accuracy in disease management: an introduction to receiver operating characteristic (ROC) analysis. J Eval Clin Pract. 2006;12(2):132–9.
Ye L, Ngan DK, Xu T, et al. Prediction of drug-induced liver injury and cardiotoxicity using chemical structure and in vitro assay data. Toxicol Appl Pharmacol. 2022;1(454): 116250.
Liu Z, Shi Q, Ding D, et al. Translating clinical findings into knowledge in drug safety evaluation–drug induced liver injury prediction system (DILIps). PLoS Comput Biol. 2011;7(12): e1002310.
Fisher S, Rosella LC. Priorities for successful use of artificial intelligence by public health organizations: a literature review. BMC Public Health. 2022;22:2146.
Obermeyer Z, et al. Dissecting racial bias in an algorithm used to manage the health of populations. Science. 2019;366(6464):447–53.
Juurlink David N. Drug-drug interactions among elderly patients hospitalized for drug toxicity. JAMA. 2003;289(13):1652–8.
Luo W, Phung D, Tran T, et al. Guidelines for Developing and Reporting Machine Learning Predictive Models in Biomedical Research: A Multidisciplinary View. J Med Internet Res. 2016;18(12): e323.
Download references
Acknowledgements
The authors thank all staff of the tuberculosis control centers, designated hospitals, community health service centers, and township health centers in ten counties/districts from Ningbo for their hard work and help in collecting clinical data. We also thank our colleagues from Ningbo Health Information Center for providing clinically relevant data for this study.
Disclosure of AI tools
We hereby disclose that generative AI tools were not utilized in the preparation or analysis of data presented in this manuscript. All methodologies and analyses were conducted utilizing established statistical and machine learning techniques as outlined in the Method section.
This research was supported by Zhejiang Medical Research Project(2018KY733) and Natural Science Foundation of Ningbo (2019A610386, 2019A610385). The content is solely the responsibility of the authors and does not necessarily represent the official views of the funding agencies.
Author information
Jifang Zhou and Tianchi Yang are both authors contributed equally to this work and shared corresponding authorship.
Authors and Affiliations
School of International Pharmaceutical Business, China Pharmaceutical University, Nanjing, Jiangsu, China
Yue Xiao, Yanfei Chen, Ruijian Huang, Feng Jiang & Jifang Zhou
Institute of Tuberculosis Prevention and Control, Ningbo Municipal Center for Disease Control and Prevention, No.237, Yongfeng Road, Ningbo, Zhejiang, China
Tianchi Yang
You can also search for this author in PubMed Google Scholar
Contributions
All authors were involved in the design of the study, FJ and RH cleaned data and constructed the cohort; YC was involved in conceptualizing the study; YX and JZ were responsible for the analysis of the data and interpretation of the results.; YX, JZ and TY contributed to the drafting of the manuscript. All authors read and approved the final manuscript.
Corresponding authors
Correspondence to Jifang Zhou or Tianchi Yang .
Ethics declarations
Ethics approval and consent to participate.
All aspects of this study, including research methods were conducted in strict accordance with relevant guidelines and regulations. This study was conducted in compliance with the ethical principles outlined in the Declaration of Helsinki. All patient data in the database were de-identified, and this study was determined to be exempt by the Institutional Review Board of the Ningbo Municipal Center for Disease Control and Prevention. Written informed consent was waived for the present study. The institutional Review Board of the Ningbo Municipal Center for Disease Control and Prevention waived the need for informed consent.
Consent for publication
Not applicable.
Competing interests
The authors declare no competing interests.
Additional information
Publisher's note.
Springer Nature remains neutral with regard to jurisdictional claims in published maps and institutional affiliations.
Supplementary Information
Supplementary material 1., supplementary material 2., rights and permissions.
Open Access This article is licensed under a Creative Commons Attribution 4.0 International License, which permits use, sharing, adaptation, distribution and reproduction in any medium or format, as long as you give appropriate credit to the original author(s) and the source, provide a link to the Creative Commons licence, and indicate if changes were made. The images or other third party material in this article are included in the article's Creative Commons licence, unless indicated otherwise in a credit line to the material. If material is not included in the article's Creative Commons licence and your intended use is not permitted by statutory regulation or exceeds the permitted use, you will need to obtain permission directly from the copyright holder. To view a copy of this licence, visit http://creativecommons.org/licenses/by/4.0/ . The Creative Commons Public Domain Dedication waiver ( http://creativecommons.org/publicdomain/zero/1.0/ ) applies to the data made available in this article, unless otherwise stated in a credit line to the data.
Reprints and permissions
About this article
Cite this article.
Xiao, Y., Chen, Y., Huang, R. et al. Interpretable machine learning in predicting drug-induced liver injury among tuberculosis patients: model development and validation study. BMC Med Res Methodol 24 , 92 (2024). https://doi.org/10.1186/s12874-024-02214-5
Download citation
Received : 09 October 2023
Accepted : 10 April 2024
Published : 20 April 2024
DOI : https://doi.org/10.1186/s12874-024-02214-5
Share this article
Anyone you share the following link with will be able to read this content:
Sorry, a shareable link is not currently available for this article.
Provided by the Springer Nature SharedIt content-sharing initiative
- Logistic regression
- Retrospective study
BMC Medical Research Methodology
ISSN: 1471-2288
- General enquiries: [email protected]
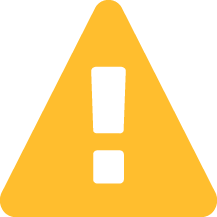
Study record managers: refer to the Data Element Definitions if submitting registration or results information.
Search for terms
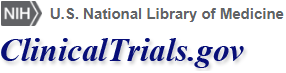
- Advanced Search
- See Studies by Topic
- See Studies on Map
- How to Search
- How to Use Search Results
- How to Find Results of Studies
- How to Read a Study Record

- Learn About Studies
- Other Sites About Studies
- Glossary of Common Site Terms

- Submit Studies to ClinicalTrials.gov PRS
- Why Should I Register and Submit Results?
- FDAAA 801 and the Final Rule
- How to Apply for a PRS Account
- How to Register Your Study
- How to Edit Your Study Record
- How to Submit Your Results
- Frequently Asked Questions
- Support Materials
- Training Materials

- Selected Publications
- Clinical Alerts and Advisories
- Trends, Charts, and Maps
- Downloading Content for Analysis

- ClinicalTrials.gov Background
- About the Results Database
- History, Policies, and Laws
- ClinicalTrials.gov Modernization
- Media/Press Resources
- Linking to This Site
- Terms and Conditions
- Search Results
- Study Record Detail
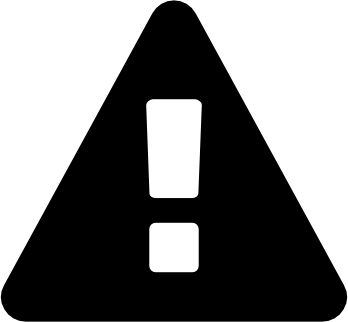
An Open Comparative Study of the Effectiveness and Incomparable Study of the Immunogenicity and Safety of the Vaccine (CoviVac) for Adults Aged 60 Years and Older
- Study Details
- Tabular View
- No Results Posted

Inclusion Criteria:
Volunteers must meet the following inclusion criteria:
Type of participants
• Healthy volunteers or volunteers with a history of stable diseases that do not meet any of the criteria for non-inclusion in the study.
Other inclusion criteria
- Written informed consent of volunteers to participate in a clinical trial
- Volunteers who are able to fulfill the Protocol requirements (i.e., fill out a self-observation Diary, come to control visits).
Exclusion Criteria:
SARS-CoV-2 infection • A case of established COVID-19 disease confirmed by PCR and/or ELISA in the last 6 months.
Diseases or medical conditions
- Serious post-vaccination reaction (temperature above 40 C, hyperemia or edema more than 8 cm in diameter) or complication (collapse or shock-like condition that developed within 48 hours after vaccination; convulsions, accompanied or not accompanied by a feverish state) to any previous vaccination.
- Burdened allergic history (anaphylactic shock, Quincke's edema, polymorphic exudative eczema, serum sickness in the anamnesis, hypersensitivity or allergic reactions to the introduction of any vaccines in the anamnesis, known allergic reactions to vaccine components, etc.).
- Guillain-Barre syndrome (acute polyradiculitis) in the anamnesis.
- The axillary temperature at the time of vaccination is more than 37.0 ° C.
- Acute infectious diseases (recovery earlier than 4 weeks before vaccination) according to anamnesis.
- Donation of blood or plasma (in the amount of 450 ml or more) less than 2 months before inclusion in the study.
- Severe and/or uncontrolled diseases of the cardiovascular, bronchopulmonary, neuroendocrine systems, gastrointestinal tract, liver, kidneys, hematopoietic, immune systems.
- Is registered at the dispensary for tuberculosis, leukemia, oncological diseases, autoimmune diseases.
- Any confirmed or suspected immunosuppressive or immunodeficiency condition in the anamnesis.
- Splenectomy in the anamnesis.
- Neutropenia (decrease in the absolute number of neutrophils less than 1000/mm3), agranulocytosis, significant blood loss, severe anemia (hemoglobin less than 80 g/l) according to anamnesis.
- Anorexia according to anamnesis.
Prior or concomitant therapy
- Vaccination with any vaccine carried out within 30 days before vaccination / the first dose of the studied vaccine or planned administration within 30 days after vaccination / the last dose of the studied vaccine.
- Prior vaccination with an experimental or registered vaccine that may affect the interpretation of the study data (any coronavirus or SARS vaccines).
- Long-term use (more than 14 days) of immunosuppressants or other immunomodulatory drugs (immunoregulatory peptides, cytokines, interferons, immune system effector proteins (immunoglobulins), interferon inducers (cycloferon) during the six months preceding the study, according to anamnesis.
- Treatment with systemic glucocorticosteroids (≥ 20 mg of prednisone, or an analog, for more than 15 days during the last month).
- Volunteers who received immunoglobulin preparations or blood transfusion during the last 3 months prior to the start of the study according to anamnesis.
Other non-inclusion criteria
• Participation in any other clinical trial within the last 3 months.
Exclusion criteria:
- Withdrawal of Informed consent by a volunteer;
- The volunteer was included in violation of the inclusion/non-inclusion criteria of the Protocol;
- Any condition of a volunteer that requires, in the reasoned opinion of a medical researcher, the withdrawal of a volunteer from the study;
- Taking unauthorized medications (see section 6.2);
- The volunteer refuses to cooperate or is undisciplined (for example, failure to attend a scheduled visit without warning the researcher and/or loss of communication with the volunteer), or dropped out of observation;
- For administrative reasons (termination of the study by the Sponsor or regulatory authorities), as well as in case of gross violations of the Protocol that may affect the results of the study.
- For Patients and Families
- For Researchers
- For Study Record Managers
- Customer Support
- Accessibility
- Viewers and Players
- Freedom of Information Act
- HHS Vulnerability Disclosure
- U.S. National Library of Medicine
- U.S. National Institutes of Health
- U.S. Department of Health and Human Services
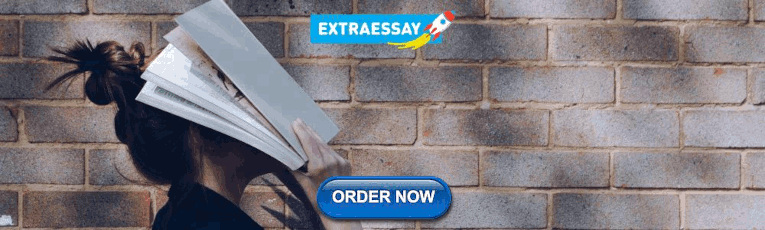
IMAGES
VIDEO
COMMENTS
Foundations of Clinical Research. This Harvard Medical School six-month, application-based certificate program provides the essential skill sets and fundamental knowledge required to begin or expand your clinical research career. Learn More. September 28, 2024 - April 6, 2025. $6,900 - $7,900.
The best free clinical research courses available are Clinical Data Management, Clinical Research, Research Methods, Researcher Management and Leadership Training, and Medical Research. All of these courses are free and offer a great introduction to the world of clinical research.
Understand and apply the principles of clinical trial design, such as using frameworks to create research questions and establish study objectives. Ensure diverse, representative study populations by studying participant selection criteria and recruitment and retention strategies. Minimize bias and ensure trial reliability and validity through ...
Masterclass in Clinical Research Methodology. Conducting clinical research has a tremendous impact on patient care and management as well as improving public health. Therefore, it is essential that the study is designed and conducted properly to maximize impact. This one-day workshop aims to give researchers the necessary skills to develop a ...
Best online courses in Clinical Research from Harvard, Stanford, University of Michigan, Johns Hopkins and other top universities around the world ... Learn practical methods for planning, collecting, storing, and disseminating data in clinical research. Increase productivity and improve your science. Vanderbilt University. 6 weeks.
Ph.D. in Clinical Investigation (PCI) The Ph.D. in Clinical Investigation provides rigorous advanced training that prepares you for an indepenedent research career in clinical and translational science.. The Einstein-Montefiore PCI track can prepare you to conduct research that will improve the health and welfare of society using clinical and translational research methodology.
Obtain the skills and knowledge necessary for a career in clinical research. Learn from world-renowned UCSF researchers online, from anywhere. For Institutions & Organizations. Offer online clinical research courses covering a wide array of topics, inlcuding clinical research methods, writing & publication, ethics and mentoring.
March 4-May 20, 2024, 12-1:30pm. South Campus Center Room 348. Please register. bit.ly. for this course. The University of Washington is offering a fast-paced comprehensive course in clinical research methods geared toward fellows in medicine and pediatrics. The 11-week course will teach fundamental concepts of Epidemiology and Biostatistics ...
Rigorous training delivered by world-class faculty. We offer a suite of online courses that provide rigorous training in clinical research, implementation sciences and mentoring to physicians, healthcare workers, community health partners and students. The modular design of our courses makes it easy to control the pace of the course while ...
This course presents an overview of the methodology that guides epidemiological and clinical research. Students explore common study designs, precision and accuracy in measurement, sample size estimation and tools, ways to identify and minimize study biases, and analysis and critique of studies.
Welcome. The Introduction to the Principles and Practice of Clinical Research (IPPCR) course trains registrants on how to effectively and safely conduct clinical research. The course focuses on the spectrum of clinical research and the research process by highlighting biostatistical and epidemiologic methods, study design, protocol preparation ...
The Clinical Research Methodology Curriculum is currently accepting applications for the 2023-2024 academic year. The application deadline to submit is Friday, August 18, 2023 at 5:00PM. View application instructions and eligibility criteria. The Clinical Research Methodology Curriculum (CRMC) is a one-year clinical research methodology for ...
There are 4 modules in this course. This course focuses on research methodologies. In this vein, the focus will be placed on qualitative and quantitative research methodologies, sampling approaches, and primary and secondary data collection. The course begins with a discussion on qualitative research approaches, looking at focus groups ...
Course Details. The On-Demand Essentials of Clinical Research will be available starting May 1, 2024. These are the recorded seminars from Jan - Mar 2024. This information is for learning purposes only. An evaluation is requested at the end of the course. Presentations and resources are available.
Core course discussing the elements of effective research design, including the basic concepts in clinical trials, the main aspects for different types of trials such as proof of principle stage, Phase I, II, III and IV, and understanding good clinical research methodology. Course will introduce and address issues, idea and outline of design ...
1. Design clinical research studies, 2. Conduct clinical trials, prospective and retrospective studies. 3. Understand the basics of Biostatistics including descriptive and analytic statistics. 4. You be well equipped to write any type of manuscript including Original Research, Case reports and Letter to editors. 5.
The Clinical Research Methods (CRM) track in Biostatistics responds to a pressing need for advanced training in clinical research design and analysis. As medical school curricula become increasingly full and apprenticeship prospects wane, pathways to becoming a clinical researcher have narrowed.
Clinical Research Methods. Director: Todd Ogden, PhD. The Mailman School offers the degree of Master of Science in Biostatistics, with an emphasis on issues in the statistical analysis and design of clinical studies. The Clinical Research Methods track was conceived and designed for clinicians who are pursuing research careers in academic medicine.
Clinical research methods provides an overview of the basic research strategies, methods, and goals of clinical research. Topics include study design, data analysis and interpretation, and determination of appropriate methodologies to answer different research questions. ... The goal of the course is to familiarize students with example ...
Alla Kholmogorova currently works at the Moscow State University of Psychology and Education (dean of the faculty of Counseling and Clinical Psychology). Alla does research in Health Psychology ...
One of the new educational systems is the mentorship method. This study aimed to investigate the effect of peer mentoring program on clinical academic progress and psychological characteristics of operating room students. This research was a randomized controlled trial that was conducted on undergraduate students in the operating room department of Khomein Faculty of Medical Sciences, Markazi ...
Introduction. Generative Pre-trained Transformer 3.5 (GPT-3.5) and 4 (GPT-4) are large language models (LLMs) trained on datasets containing hundreds of billions of words from articles, books, and other internet sources [1, 2].ChatGPT is an online chatbot which uses GPT-3.5 or GPT-4 to provide bespoke responses to human users' queries [].LLMs have revolutionised the field of natural language ...
Alexey V Trofimov. Chemiluminescence quantum yields for the reactions of permanganate with oxalic, tartaric, and citric acids; hydrazine; KBr; and FeSO4 in aqueous solutions of sulfuric acid have ...
Bennett GW et al Statistical equations and methods applied to the precision muon (g-2) experiment at BNL. Nuclear Instruments and Methods in Physics Research A 2007; 579:1096-1116. Bennett GW et al. Final report of the E821 muon anomalous magnetic moment measurement at BNL. Physical Review D 2006; 73:072003.
Objective: This article aims to comprehensively review the biological mechanisms and clinical effects of general exercise training and traditional Chinese exercises (such as Tai Chi and Qigong) on the treatment of KOA, providing references for the development of clinical exercise prescriptions. Methods: A systematic search of databases ...
Background The objective of this research was to create and validate an interpretable prediction model for drug-induced liver injury (DILI) during tuberculosis (TB) treatment. Methods A dataset of TB patients from Ningbo City was used to develop models employing the eXtreme Gradient Boosting (XGBoost), random forest (RF), and the least absolute shrinkage and selection operator (LASSO) logistic ...
Choosing to participate in a study is an important personal decision. Talk with your doctor and family members or friends about deciding to join a study. To learn more about this study, you or your doctor may contact the study research staff using the contacts provided below. For general information, Learn About Clinical Studies.