Research Hypothesis In Psychology: Types, & Examples
Saul Mcleod, PhD
Editor-in-Chief for Simply Psychology
BSc (Hons) Psychology, MRes, PhD, University of Manchester
Saul Mcleod, PhD., is a qualified psychology teacher with over 18 years of experience in further and higher education. He has been published in peer-reviewed journals, including the Journal of Clinical Psychology.
Learn about our Editorial Process
Olivia Guy-Evans, MSc
Associate Editor for Simply Psychology
BSc (Hons) Psychology, MSc Psychology of Education
Olivia Guy-Evans is a writer and associate editor for Simply Psychology. She has previously worked in healthcare and educational sectors.
On This Page:
A research hypothesis, in its plural form “hypotheses,” is a specific, testable prediction about the anticipated results of a study, established at its outset. It is a key component of the scientific method .
Hypotheses connect theory to data and guide the research process towards expanding scientific understanding
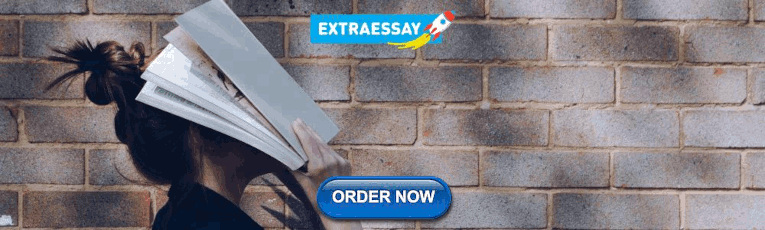
Some key points about hypotheses:
- A hypothesis expresses an expected pattern or relationship. It connects the variables under investigation.
- It is stated in clear, precise terms before any data collection or analysis occurs. This makes the hypothesis testable.
- A hypothesis must be falsifiable. It should be possible, even if unlikely in practice, to collect data that disconfirms rather than supports the hypothesis.
- Hypotheses guide research. Scientists design studies to explicitly evaluate hypotheses about how nature works.
- For a hypothesis to be valid, it must be testable against empirical evidence. The evidence can then confirm or disprove the testable predictions.
- Hypotheses are informed by background knowledge and observation, but go beyond what is already known to propose an explanation of how or why something occurs.
Predictions typically arise from a thorough knowledge of the research literature, curiosity about real-world problems or implications, and integrating this to advance theory. They build on existing literature while providing new insight.
Types of Research Hypotheses
Alternative hypothesis.
The research hypothesis is often called the alternative or experimental hypothesis in experimental research.
It typically suggests a potential relationship between two key variables: the independent variable, which the researcher manipulates, and the dependent variable, which is measured based on those changes.
The alternative hypothesis states a relationship exists between the two variables being studied (one variable affects the other).
A hypothesis is a testable statement or prediction about the relationship between two or more variables. It is a key component of the scientific method. Some key points about hypotheses:
- Important hypotheses lead to predictions that can be tested empirically. The evidence can then confirm or disprove the testable predictions.
In summary, a hypothesis is a precise, testable statement of what researchers expect to happen in a study and why. Hypotheses connect theory to data and guide the research process towards expanding scientific understanding.
An experimental hypothesis predicts what change(s) will occur in the dependent variable when the independent variable is manipulated.
It states that the results are not due to chance and are significant in supporting the theory being investigated.
The alternative hypothesis can be directional, indicating a specific direction of the effect, or non-directional, suggesting a difference without specifying its nature. It’s what researchers aim to support or demonstrate through their study.
Null Hypothesis
The null hypothesis states no relationship exists between the two variables being studied (one variable does not affect the other). There will be no changes in the dependent variable due to manipulating the independent variable.
It states results are due to chance and are not significant in supporting the idea being investigated.
The null hypothesis, positing no effect or relationship, is a foundational contrast to the research hypothesis in scientific inquiry. It establishes a baseline for statistical testing, promoting objectivity by initiating research from a neutral stance.
Many statistical methods are tailored to test the null hypothesis, determining the likelihood of observed results if no true effect exists.
This dual-hypothesis approach provides clarity, ensuring that research intentions are explicit, and fosters consistency across scientific studies, enhancing the standardization and interpretability of research outcomes.
Nondirectional Hypothesis
A non-directional hypothesis, also known as a two-tailed hypothesis, predicts that there is a difference or relationship between two variables but does not specify the direction of this relationship.
It merely indicates that a change or effect will occur without predicting which group will have higher or lower values.
For example, “There is a difference in performance between Group A and Group B” is a non-directional hypothesis.
Directional Hypothesis
A directional (one-tailed) hypothesis predicts the nature of the effect of the independent variable on the dependent variable. It predicts in which direction the change will take place. (i.e., greater, smaller, less, more)
It specifies whether one variable is greater, lesser, or different from another, rather than just indicating that there’s a difference without specifying its nature.
For example, “Exercise increases weight loss” is a directional hypothesis.
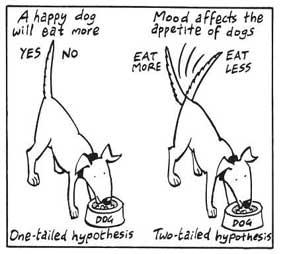
Falsifiability
The Falsification Principle, proposed by Karl Popper , is a way of demarcating science from non-science. It suggests that for a theory or hypothesis to be considered scientific, it must be testable and irrefutable.
Falsifiability emphasizes that scientific claims shouldn’t just be confirmable but should also have the potential to be proven wrong.
It means that there should exist some potential evidence or experiment that could prove the proposition false.
However many confirming instances exist for a theory, it only takes one counter observation to falsify it. For example, the hypothesis that “all swans are white,” can be falsified by observing a black swan.
For Popper, science should attempt to disprove a theory rather than attempt to continually provide evidence to support a research hypothesis.
Can a Hypothesis be Proven?
Hypotheses make probabilistic predictions. They state the expected outcome if a particular relationship exists. However, a study result supporting a hypothesis does not definitively prove it is true.
All studies have limitations. There may be unknown confounding factors or issues that limit the certainty of conclusions. Additional studies may yield different results.
In science, hypotheses can realistically only be supported with some degree of confidence, not proven. The process of science is to incrementally accumulate evidence for and against hypothesized relationships in an ongoing pursuit of better models and explanations that best fit the empirical data. But hypotheses remain open to revision and rejection if that is where the evidence leads.
- Disproving a hypothesis is definitive. Solid disconfirmatory evidence will falsify a hypothesis and require altering or discarding it based on the evidence.
- However, confirming evidence is always open to revision. Other explanations may account for the same results, and additional or contradictory evidence may emerge over time.
We can never 100% prove the alternative hypothesis. Instead, we see if we can disprove, or reject the null hypothesis.
If we reject the null hypothesis, this doesn’t mean that our alternative hypothesis is correct but does support the alternative/experimental hypothesis.
Upon analysis of the results, an alternative hypothesis can be rejected or supported, but it can never be proven to be correct. We must avoid any reference to results proving a theory as this implies 100% certainty, and there is always a chance that evidence may exist which could refute a theory.
How to Write a Hypothesis
- Identify variables . The researcher manipulates the independent variable and the dependent variable is the measured outcome.
- Operationalized the variables being investigated . Operationalization of a hypothesis refers to the process of making the variables physically measurable or testable, e.g. if you are about to study aggression, you might count the number of punches given by participants.
- Decide on a direction for your prediction . If there is evidence in the literature to support a specific effect of the independent variable on the dependent variable, write a directional (one-tailed) hypothesis. If there are limited or ambiguous findings in the literature regarding the effect of the independent variable on the dependent variable, write a non-directional (two-tailed) hypothesis.
- Make it Testable : Ensure your hypothesis can be tested through experimentation or observation. It should be possible to prove it false (principle of falsifiability).
- Clear & concise language . A strong hypothesis is concise (typically one to two sentences long), and formulated using clear and straightforward language, ensuring it’s easily understood and testable.
Consider a hypothesis many teachers might subscribe to: students work better on Monday morning than on Friday afternoon (IV=Day, DV= Standard of work).
Now, if we decide to study this by giving the same group of students a lesson on a Monday morning and a Friday afternoon and then measuring their immediate recall of the material covered in each session, we would end up with the following:
- The alternative hypothesis states that students will recall significantly more information on a Monday morning than on a Friday afternoon.
- The null hypothesis states that there will be no significant difference in the amount recalled on a Monday morning compared to a Friday afternoon. Any difference will be due to chance or confounding factors.
More Examples
- Memory : Participants exposed to classical music during study sessions will recall more items from a list than those who studied in silence.
- Social Psychology : Individuals who frequently engage in social media use will report higher levels of perceived social isolation compared to those who use it infrequently.
- Developmental Psychology : Children who engage in regular imaginative play have better problem-solving skills than those who don’t.
- Clinical Psychology : Cognitive-behavioral therapy will be more effective in reducing symptoms of anxiety over a 6-month period compared to traditional talk therapy.
- Cognitive Psychology : Individuals who multitask between various electronic devices will have shorter attention spans on focused tasks than those who single-task.
- Health Psychology : Patients who practice mindfulness meditation will experience lower levels of chronic pain compared to those who don’t meditate.
- Organizational Psychology : Employees in open-plan offices will report higher levels of stress than those in private offices.
- Behavioral Psychology : Rats rewarded with food after pressing a lever will press it more frequently than rats who receive no reward.


What Is A Research (Scientific) Hypothesis? A plain-language explainer + examples
By: Derek Jansen (MBA) | Reviewed By: Dr Eunice Rautenbach | June 2020
If you’re new to the world of research, or it’s your first time writing a dissertation or thesis, you’re probably noticing that the words “research hypothesis” and “scientific hypothesis” are used quite a bit, and you’re wondering what they mean in a research context .
“Hypothesis” is one of those words that people use loosely, thinking they understand what it means. However, it has a very specific meaning within academic research. So, it’s important to understand the exact meaning before you start hypothesizing.
Research Hypothesis 101
- What is a hypothesis ?
- What is a research hypothesis (scientific hypothesis)?
- Requirements for a research hypothesis
- Definition of a research hypothesis
- The null hypothesis
What is a hypothesis?
Let’s start with the general definition of a hypothesis (not a research hypothesis or scientific hypothesis), according to the Cambridge Dictionary:
Hypothesis: an idea or explanation for something that is based on known facts but has not yet been proved.
In other words, it’s a statement that provides an explanation for why or how something works, based on facts (or some reasonable assumptions), but that has not yet been specifically tested . For example, a hypothesis might look something like this:
Hypothesis: sleep impacts academic performance.
This statement predicts that academic performance will be influenced by the amount and/or quality of sleep a student engages in – sounds reasonable, right? It’s based on reasonable assumptions , underpinned by what we currently know about sleep and health (from the existing literature). So, loosely speaking, we could call it a hypothesis, at least by the dictionary definition.
But that’s not good enough…
Unfortunately, that’s not quite sophisticated enough to describe a research hypothesis (also sometimes called a scientific hypothesis), and it wouldn’t be acceptable in a dissertation, thesis or research paper . In the world of academic research, a statement needs a few more criteria to constitute a true research hypothesis .
What is a research hypothesis?
A research hypothesis (also called a scientific hypothesis) is a statement about the expected outcome of a study (for example, a dissertation or thesis). To constitute a quality hypothesis, the statement needs to have three attributes – specificity , clarity and testability .
Let’s take a look at these more closely.
Need a helping hand?
Hypothesis Essential #1: Specificity & Clarity
A good research hypothesis needs to be extremely clear and articulate about both what’ s being assessed (who or what variables are involved ) and the expected outcome (for example, a difference between groups, a relationship between variables, etc.).
Let’s stick with our sleepy students example and look at how this statement could be more specific and clear.
Hypothesis: Students who sleep at least 8 hours per night will, on average, achieve higher grades in standardised tests than students who sleep less than 8 hours a night.
As you can see, the statement is very specific as it identifies the variables involved (sleep hours and test grades), the parties involved (two groups of students), as well as the predicted relationship type (a positive relationship). There’s no ambiguity or uncertainty about who or what is involved in the statement, and the expected outcome is clear.
Contrast that to the original hypothesis we looked at – “Sleep impacts academic performance” – and you can see the difference. “Sleep” and “academic performance” are both comparatively vague , and there’s no indication of what the expected relationship direction is (more sleep or less sleep). As you can see, specificity and clarity are key.
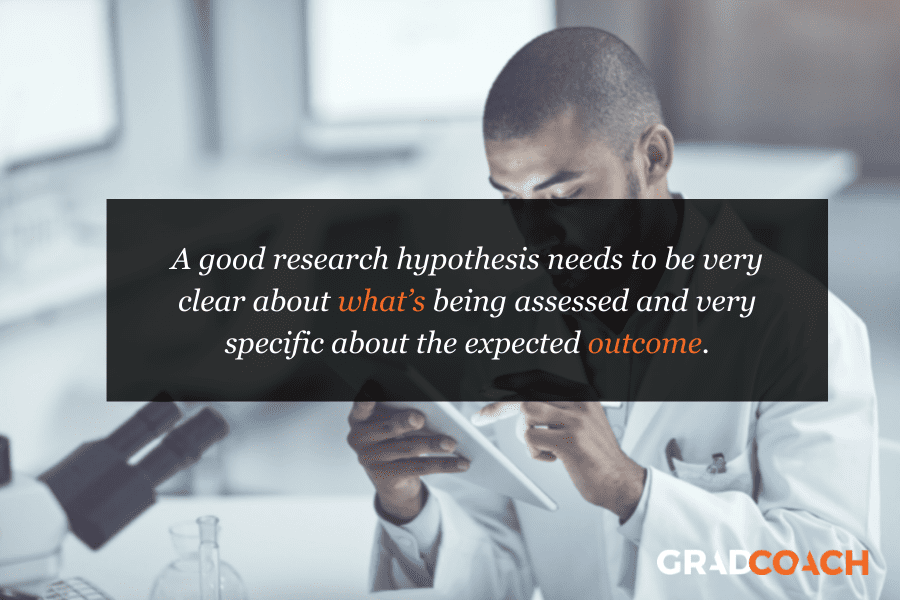
Hypothesis Essential #2: Testability (Provability)
A statement must be testable to qualify as a research hypothesis. In other words, there needs to be a way to prove (or disprove) the statement. If it’s not testable, it’s not a hypothesis – simple as that.
For example, consider the hypothesis we mentioned earlier:
Hypothesis: Students who sleep at least 8 hours per night will, on average, achieve higher grades in standardised tests than students who sleep less than 8 hours a night.
We could test this statement by undertaking a quantitative study involving two groups of students, one that gets 8 or more hours of sleep per night for a fixed period, and one that gets less. We could then compare the standardised test results for both groups to see if there’s a statistically significant difference.
Again, if you compare this to the original hypothesis we looked at – “Sleep impacts academic performance” – you can see that it would be quite difficult to test that statement, primarily because it isn’t specific enough. How much sleep? By who? What type of academic performance?
So, remember the mantra – if you can’t test it, it’s not a hypothesis 🙂
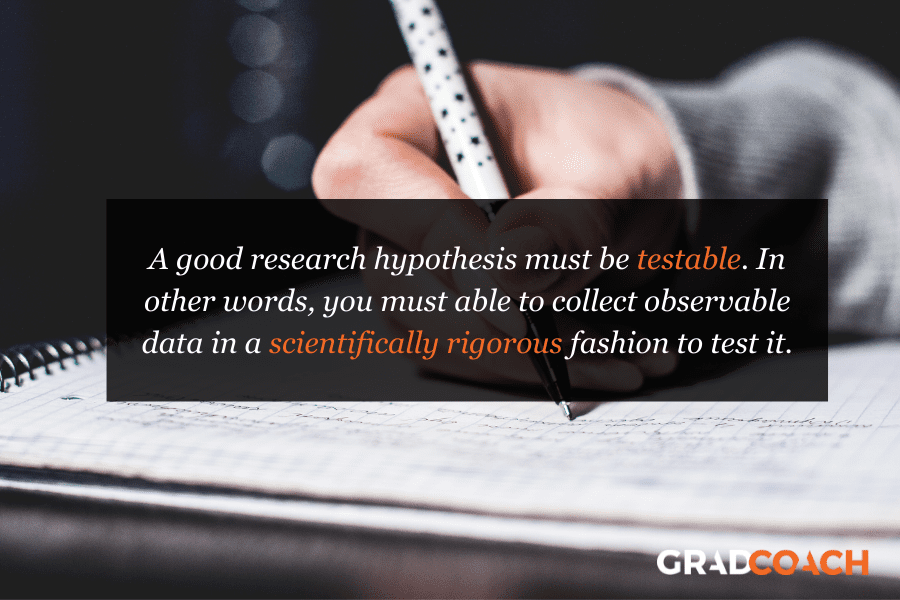
Defining A Research Hypothesis
You’re still with us? Great! Let’s recap and pin down a clear definition of a hypothesis.
A research hypothesis (or scientific hypothesis) is a statement about an expected relationship between variables, or explanation of an occurrence, that is clear, specific and testable.
So, when you write up hypotheses for your dissertation or thesis, make sure that they meet all these criteria. If you do, you’ll not only have rock-solid hypotheses but you’ll also ensure a clear focus for your entire research project.
What about the null hypothesis?
You may have also heard the terms null hypothesis , alternative hypothesis, or H-zero thrown around. At a simple level, the null hypothesis is the counter-proposal to the original hypothesis.
For example, if the hypothesis predicts that there is a relationship between two variables (for example, sleep and academic performance), the null hypothesis would predict that there is no relationship between those variables.
At a more technical level, the null hypothesis proposes that no statistical significance exists in a set of given observations and that any differences are due to chance alone.
And there you have it – hypotheses in a nutshell.
If you have any questions, be sure to leave a comment below and we’ll do our best to help you. If you need hands-on help developing and testing your hypotheses, consider our private coaching service , where we hold your hand through the research journey.
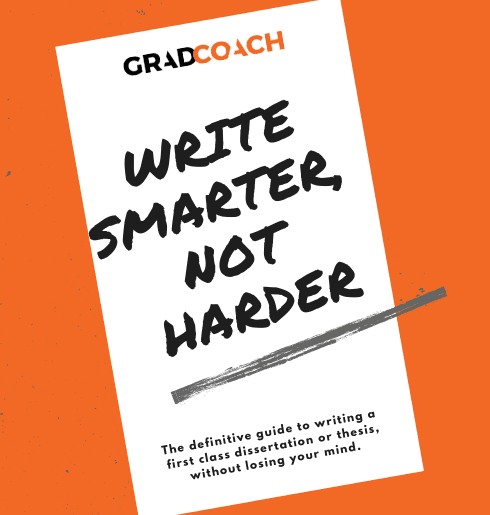
Psst… there’s more (for free)
This post is part of our dissertation mini-course, which covers everything you need to get started with your dissertation, thesis or research project.
You Might Also Like:
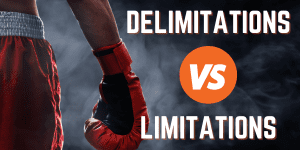
15 Comments
Very useful information. I benefit more from getting more information in this regard.
Very great insight,educative and informative. Please give meet deep critics on many research data of public international Law like human rights, environment, natural resources, law of the sea etc
In a book I read a distinction is made between null, research, and alternative hypothesis. As far as I understand, alternative and research hypotheses are the same. Can you please elaborate? Best Afshin
This is a self explanatory, easy going site. I will recommend this to my friends and colleagues.
Very good definition. How can I cite your definition in my thesis? Thank you. Is nul hypothesis compulsory in a research?
It’s a counter-proposal to be proven as a rejection
Please what is the difference between alternate hypothesis and research hypothesis?
It is a very good explanation. However, it limits hypotheses to statistically tasteable ideas. What about for qualitative researches or other researches that involve quantitative data that don’t need statistical tests?
In qualitative research, one typically uses propositions, not hypotheses.
could you please elaborate it more
I’ve benefited greatly from these notes, thank you.
This is very helpful
well articulated ideas are presented here, thank you for being reliable sources of information
Excellent. Thanks for being clear and sound about the research methodology and hypothesis (quantitative research)
I have only a simple question regarding the null hypothesis. – Is the null hypothesis (Ho) known as the reversible hypothesis of the alternative hypothesis (H1? – How to test it in academic research?
Trackbacks/Pingbacks
- What Is Research Methodology? Simple Definition (With Examples) - Grad Coach - […] Contrasted to this, a quantitative methodology is typically used when the research aims and objectives are confirmatory in nature. For example,…
Submit a Comment Cancel reply
Your email address will not be published. Required fields are marked *
Save my name, email, and website in this browser for the next time I comment.
- Print Friendly
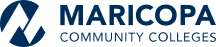
9 Chapter 9 Hypothesis testing
The first unit was designed to prepare you for hypothesis testing. In the first chapter we discussed the three major goals of statistics:
- Describe: connects to unit 1 with descriptive statistics and graphing
- Decide: connects to unit 1 knowing your data and hypothesis testing
- Predict: connects to hypothesis testing and unit 3
The remaining chapters will cover many different kinds of hypothesis tests connected to different inferential statistics. Needless to say, hypothesis testing is the central topic of this course. This lesson is important but that does not mean the same thing as difficult. There is a lot of new language we will learn about when conducting a hypothesis test. Some of the components of a hypothesis test are the topics we are already familiar with:
- Test statistics
- Probability
- Distribution of sample means
Hypothesis testing is an inferential procedure that uses data from a sample to draw a general conclusion about a population. It is a formal approach and a statistical method that uses sample data to evaluate hypotheses about a population. When interpreting a research question and statistical results, a natural question arises as to whether the finding could have occurred by chance. Hypothesis testing is a statistical procedure for testing whether chance (random events) is a reasonable explanation of an experimental finding. Once you have mastered the material in this lesson you will be used to solving hypothesis testing problems and the rest of the course will seem much easier. In this chapter, we will introduce the ideas behind the use of statistics to make decisions – in particular, decisions about whether a particular hypothesis is supported by the data.
Logic and Purpose of Hypothesis Testing
The statistician Ronald Fisher explained the concept of hypothesis testing with a story of a lady tasting tea. Fisher was a statistician from London and is noted as the first person to formalize the process of hypothesis testing. His elegantly simple “Lady Tasting Tea” experiment demonstrated the logic of the hypothesis test.
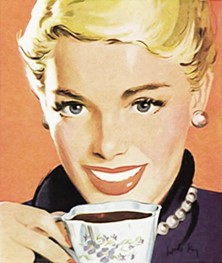
Figure 1. A depiction of the lady tasting tea Photo Credit
Fisher would often have afternoon tea during his studies. He usually took tea with a woman who claimed to be a tea expert. In particular, she told Fisher that she could tell which was poured first in the teacup, the milk or the tea, simply by tasting the cup. Fisher, being a scientist, decided to put this rather bizarre claim to the test. The lady accepted his challenge. Fisher brought her 8 cups of tea in succession; 4 cups would be prepared with the milk added first, and 4 with the tea added first. The cups would be presented in a random order unknown to the lady.
The lady would take a sip of each cup as it was presented and report which ingredient she believed was poured first. Using the laws of probability, Fisher determined the chances of her guessing all 8 cups correctly was 1/70, or about 1.4%. In other words, if the lady was indeed guessing there was a 1.4% chance of her getting all 8 cups correct. On the day of the experiment, Fisher had 8 cups prepared just as he had requested. The lady drank each cup and made her decisions for each one.
After the experiment, it was revealed that the lady got all 8 cups correct! Remember, had she been truly guessing, the chance of getting this result was 1.4%. Since this probability was so low , Fisher instead concluded that the lady could indeed differentiate between the milk or the tea being poured first. Fisher’s original hypothesis that she was just guessing was demonstrated to be false and was therefore rejected. The alternative hypothesis, that the lady could truly tell the cups apart, was then accepted as true.
This story demonstrates many components of hypothesis testing in a very simple way. For example, Fisher started with a hypothesis that the lady was guessing. He then determined that if she was indeed guessing, the probability of guessing all 8 right was very small, just 1.4%. Since that probability was so tiny, when she did get all 8 cups right, Fisher determined it was extremely unlikely she was guessing. A more reasonable conclusion was that the lady had the skill to tell the cups apart.
In hypothesis testing, we will always set up a particular hypothesis that we want to demonstrate to be true. We then use probability to determine the likelihood of our hypothesis is correct. If it appears our original hypothesis was wrong, we reject it and accept the alternative hypothesis. The alternative hypothesis is usually the opposite of our original hypothesis. In Fisher’s case, his original hypothesis was that the lady was guessing. His alternative hypothesis was the lady was not guessing.
This result does not prove that he does; it could be he was just lucky and guessed right 13 out of 16 times. But how plausible is the explanation that he was just lucky? To assess its plausibility, we determine the probability that someone who was just guessing would be correct 13/16 times or more. This probability can be computed to be 0.0106. This is a pretty low probability, and therefore someone would have to be very lucky to be correct 13 or more times out of 16 if they were just guessing. A low probability gives us more confidence there is evidence Bond can tell whether the drink was shaken or stirred. There is also still a chance that Mr. Bond was very lucky (more on this later!). The hypothesis that he was guessing is not proven false, but considerable doubt is cast on it. Therefore, there is strong evidence that Mr. Bond can tell whether a drink was shaken or stirred.
You may notice some patterns here:
- We have 2 hypotheses: the original (researcher prediction) and the alternative
- We collect data
- We determine how likley or unlikely the original hypothesis is to occur based on probability.
- We determine if we have enough evidence to support the original hypothesis and draw conclusions.
Now let’s being in some specific terminology:
Null hypothesis : In general, the null hypothesis, written H 0 (“H-naught”), is the idea that nothing is going on: there is no effect of our treatment, no relation between our variables, and no difference in our sample mean from what we expected about the population mean. The null hypothesis indicates that an apparent effect is due to chance. This is always our baseline starting assumption, and it is what we (typically) seek to reject . For mathematical notation, one uses =).
Alternative hypothesis : If the null hypothesis is rejected, then we will need some other explanation, which we call the alternative hypothesis, H A or H 1 . The alternative hypothesis is simply the reverse of the null hypothesis. Thus, our alternative hypothesis is the mathematical way of stating our research question. In general, the alternative hypothesis (also called the research hypothesis)is there is an effect of treatment, the relation between variables, or differences in a sample mean compared to a population mean. The alternative hypothesis essentially shows evidence the findings are not due to chance. It is also called the research hypothesis as this is the most common outcome a researcher is looking for: evidence of change, differences, or relationships. There are three options for setting up the alternative hypothesis, depending on where we expect the difference to lie. The alternative hypothesis always involves some kind of inequality (≠not equal, >, or <).
- If we expect a specific direction of change/differences/relationships, which we call a directional hypothesis , then our alternative hypothesis takes the form based on the research question itself. One would expect a decrease in depression from taking an anti-depressant as a specific directional hypothesis. Or the direction could be larger, where for example, one might expect an increase in exam scores after completing a student success exam preparation module. The directional hypothesis (2 directions) makes up 2 of the 3 alternative hypothesis options. The other alternative is to state there are differences/changes, or a relationship but not predict the direction. We use a non-directional alternative hypothesis (typically see ≠ for mathematical notation).
Probability value (p-value) : the probability of a certain outcome assuming a certain state of the world. In statistics, it is conventional to refer to possible states of the world as hypotheses since they are hypothesized states of the world. Using this terminology, the probability value is the probability of an outcome given the hypothesis. It is not the probability of the hypothesis given the outcome. It is very important to understand precisely what the probability values mean. In the James Bond example, the computed probability of 0.0106 is the probability he would be correct on 13 or more taste tests (out of 16) if he were just guessing. It is easy to mistake this probability of 0.0106 as the probability he cannot tell the difference. This is not at all what it means. The probability of 0.0106 is the probability of a certain outcome (13 or more out of 16) assuming a certain state of the world (James Bond was only guessing).
A low probability value casts doubt on the null hypothesis. How low must the probability value be in order to conclude that the null hypothesis is false? Although there is clearly no right or wrong answer to this question, it is conventional to conclude the null hypothesis is false if the probability value is less than 0.05 (p < .05). More conservative researchers conclude the null hypothesis is false only if the probability value is less than 0.01 (p<.01). When a researcher concludes that the null hypothesis is false, the researcher is said to have rejected the null hypothesis. The probability value below which the null hypothesis is rejected is called the α level or simply α (“alpha”). It is also called the significance level . If α is not explicitly specified, assume that α = 0.05.
Decision-making is part of the process and we have some language that goes along with that. Importantly, null hypothesis testing operates under the assumption that the null hypothesis is true unless the evidence shows otherwise. We (typically) seek to reject the null hypothesis, giving us evidence to support the alternative hypothesis . If the probability of the outcome given the hypothesis is sufficiently low, we have evidence that the null hypothesis is false. Note that all probability calculations for all hypothesis tests center on the null hypothesis. In the James Bond example, the null hypothesis is that he cannot tell the difference between shaken and stirred martinis. The probability value is low that one is able to identify 13 of 16 martinis as shaken or stirred (0.0106), thus providing evidence that he can tell the difference. Note that we have not computed the probability that he can tell the difference.
The specific type of hypothesis testing reviewed is specifically known as null hypothesis statistical testing (NHST). We can break the process of null hypothesis testing down into a number of steps a researcher would use.
- Formulate a hypothesis that embodies our prediction ( before seeing the data )
- Specify null and alternative hypotheses
- Collect some data relevant to the hypothesis
- Compute a test statistic
- Identify the criteria probability (or compute the probability of the observed value of that statistic) assuming that the null hypothesis is true
- Drawing conclusions. Assess the “statistical significance” of the result
Steps in hypothesis testing
Step 1: formulate a hypothesis of interest.
The researchers hypothesized that physicians spend less time with obese patients. The researchers hypothesis derived from an identified population. In creating a research hypothesis, we also have to decide whether we want to test a directional or non-directional hypotheses. Researchers typically will select a non-directional hypothesis for a more conservative approach, particularly when the outcome is unknown (more about why this is later).
Step 2: Specify the null and alternative hypotheses
Can you set up the null and alternative hypotheses for the Physician’s Reaction Experiment?
Step 3: Determine the alpha level.
For this course, alpha will be given to you as .05 or .01. Researchers will decide on alpha and then determine the associated test statistic based from the sample. Researchers in the Physician Reaction study might set the alpha at .05 and identify the test statistics associated with the .05 for the sample size. Researchers might take extra precautions to be more confident in their findings (more on this later).
Step 4: Collect some data
For this course, the data will be given to you. Researchers collect the data and then start to summarize it using descriptive statistics. The mean time physicians reported that they would spend with obese patients was 24.7 minutes as compared to a mean of 31.4 minutes for normal-weight patients.
Step 5: Compute a test statistic
We next want to use the data to compute a statistic that will ultimately let us decide whether the null hypothesis is rejected or not. We can think of the test statistic as providing a measure of the size of the effect compared to the variability in the data. In general, this test statistic will have a probability distribution associated with it, because that allows us to determine how likely our observed value of the statistic is under the null hypothesis.
To assess the plausibility of the hypothesis that the difference in mean times is due to chance, we compute the probability of getting a difference as large or larger than the observed difference (31.4 – 24.7 = 6.7 minutes) if the difference were, in fact, due solely to chance.
Step 6: Determine the probability of the observed result under the null hypothesis
Using methods presented in later chapters, this probability associated with the observed differences between the two groups for the Physician’s Reaction was computed to be 0.0057. Since this is such a low probability, we have confidence that the difference in times is due to the patient’s weight (obese or not) (and is not due to chance). We can then reject the null hypothesis (there are no differences or differences seen are due to chance).
Keep in mind that the null hypothesis is typically the opposite of the researcher’s hypothesis. In the Physicians’ Reactions study, the researchers hypothesized that physicians would expect to spend less time with obese patients. The null hypothesis that the two types of patients are treated identically as part of the researcher’s control of other variables. If the null hypothesis were true, a difference as large or larger than the sample difference of 6.7 minutes would be very unlikely to occur. Therefore, the researchers rejected the null hypothesis of no difference and concluded that in the population, physicians intend to spend less time with obese patients.
This is the step where NHST starts to violate our intuition. Rather than determining the likelihood that the null hypothesis is true given the data, we instead determine the likelihood under the null hypothesis of observing a statistic at least as extreme as one that we have observed — because we started out by assuming that the null hypothesis is true! To do this, we need to know the expected probability distribution for the statistic under the null hypothesis, so that we can ask how likely the result would be under that distribution. This will be determined from a table we use for reference or calculated in a statistical analysis program. Note that when I say “how likely the result would be”, what I really mean is “how likely the observed result or one more extreme would be”. We need to add this caveat as we are trying to determine how weird our result would be if the null hypothesis were true, and any result that is more extreme will be even more weird, so we want to count all of those weirder possibilities when we compute the probability of our result under the null hypothesis.
Let’s review some considerations for Null hypothesis statistical testing (NHST)!
Null hypothesis statistical testing (NHST) is commonly used in many fields. If you pick up almost any scientific or biomedical research publication, you will see NHST being used to test hypotheses, and in their introductory psychology textbook, Gerrig & Zimbardo (2002) referred to NHST as the “backbone of psychological research”. Thus, learning how to use and interpret the results from hypothesis testing is essential to understand the results from many fields of research.
It is also important for you to know, however, that NHST is flawed, and that many statisticians and researchers think that it has been the cause of serious problems in science, which we will discuss in further in this unit. NHST is also widely misunderstood, largely because it violates our intuitions about how statistical hypothesis testing should work. Let’s look at an example to see this.
There is great interest in the use of body-worn cameras by police officers, which are thought to reduce the use of force and improve officer behavior. However, in order to establish this we need experimental evidence, and it has become increasingly common for governments to use randomized controlled trials to test such ideas. A randomized controlled trial of the effectiveness of body-worn cameras was performed by the Washington, DC government and DC Metropolitan Police Department in 2015-2016. Officers were randomly assigned to wear a body-worn camera or not, and their behavior was then tracked over time to determine whether the cameras resulted in less use of force and fewer civilian complaints about officer behavior.
Before we get to the results, let’s ask how you would think the statistical analysis might work. Let’s say we want to specifically test the hypothesis of whether the use of force is decreased by the wearing of cameras. The randomized controlled trial provides us with the data to test the hypothesis – namely, the rates of use of force by officers assigned to either the camera or control groups. The next obvious step is to look at the data and determine whether they provide convincing evidence for or against this hypothesis. That is: What is the likelihood that body-worn cameras reduce the use of force, given the data and everything else we know?
It turns out that this is not how null hypothesis testing works. Instead, we first take our hypothesis of interest (i.e. that body-worn cameras reduce use of force), and flip it on its head, creating a null hypothesis – in this case, the null hypothesis would be that cameras do not reduce use of force. Importantly, we then assume that the null hypothesis is true. We then look at the data, and determine how likely the data would be if the null hypothesis were true. If the data are sufficiently unlikely under the null hypothesis that we can reject the null in favor of the alternative hypothesis which is our hypothesis of interest. If there is not sufficient evidence to reject the null, then we say that we retain (or “fail to reject”) the null, sticking with our initial assumption that the null is true.
Understanding some of the concepts of NHST, particularly the notorious “p-value”, is invariably challenging the first time one encounters them, because they are so counter-intuitive. As we will see later, there are other approaches that provide a much more intuitive way to address hypothesis testing (but have their own complexities).
Step 7: Assess the “statistical significance” of the result. Draw conclusions.
The next step is to determine whether the p-value that results from the previous step is small enough that we are willing to reject the null hypothesis and conclude instead that the alternative is true. In the Physicians Reactions study, the probability value is 0.0057. Therefore, the effect of obesity is statistically significant and the null hypothesis that obesity makes no difference is rejected. It is very important to keep in mind that statistical significance means only that the null hypothesis of exactly no effect is rejected; it does not mean that the effect is important, which is what “significant” usually means. When an effect is significant, you can have confidence the effect is not exactly zero. Finding that an effect is significant does not tell you about how large or important the effect is.
How much evidence do we require and what considerations are needed to better understand the significance of the findings? This is one of the most controversial questions in statistics, in part because it requires a subjective judgment – there is no “correct” answer.
What does a statistically significant result mean?
There is a great deal of confusion about what p-values actually mean (Gigerenzer, 2004). Let’s say that we do an experiment comparing the means between conditions, and we find a difference with a p-value of .01. There are a number of possible interpretations that one might entertain.
Does it mean that the probability of the null hypothesis being true is .01? No. Remember that in null hypothesis testing, the p-value is the probability of the data given the null hypothesis. It does not warrant conclusions about the probability of the null hypothesis given the data.
Does it mean that the probability that you are making the wrong decision is .01? No. Remember as above that p-values are probabilities of data under the null, not probabilities of hypotheses.
Does it mean that if you ran the study again, you would obtain the same result 99% of the time? No. The p-value is a statement about the likelihood of a particular dataset under the null; it does not allow us to make inferences about the likelihood of future events such as replication.
Does it mean that you have found a practially important effect? No. There is an essential distinction between statistical significance and practical significance . As an example, let’s say that we performed a randomized controlled trial to examine the effect of a particular diet on body weight, and we find a statistically significant effect at p<.05. What this doesn’t tell us is how much weight was actually lost, which we refer to as the effect size (to be discussed in more detail). If we think about a study of weight loss, then we probably don’t think that the loss of one ounce (i.e. the weight of a few potato chips) is practically significant. Let’s look at our ability to detect a significant difference of 1 ounce as the sample size increases.
A statistically significant result is not necessarily a strong one. Even a very weak result can be statistically significant if it is based on a large enough sample. This is why it is important to distinguish between the statistical significance of a result and the practical significance of that result. Practical significance refers to the importance or usefulness of the result in some real-world context and is often referred to as the effect size .
Many differences are statistically significant—and may even be interesting for purely scientific reasons—but they are not practically significant. In clinical practice, this same concept is often referred to as “clinical significance.” For example, a study on a new treatment for social phobia might show that it produces a statistically significant positive effect. Yet this effect still might not be strong enough to justify the time, effort, and other costs of putting it into practice—especially if easier and cheaper treatments that work almost as well already exist. Although statistically significant, this result would be said to lack practical or clinical significance.
Be aware that the term effect size can be misleading because it suggests a causal relationship—that the difference between the two means is an “effect” of being in one group or condition as opposed to another. In other words, simply calling the difference an “effect size” does not make the relationship a causal one.
Figure 1 shows how the proportion of significant results increases as the sample size increases, such that with a very large sample size (about 262,000 total subjects), we will find a significant result in more than 90% of studies when there is a 1 ounce difference in weight loss between the diets. While these are statistically significant, most physicians would not consider a weight loss of one ounce to be practically or clinically significant. We will explore this relationship in more detail when we return to the concept of statistical power in Chapter X, but it should already be clear from this example that statistical significance is not necessarily indicative of practical significance.
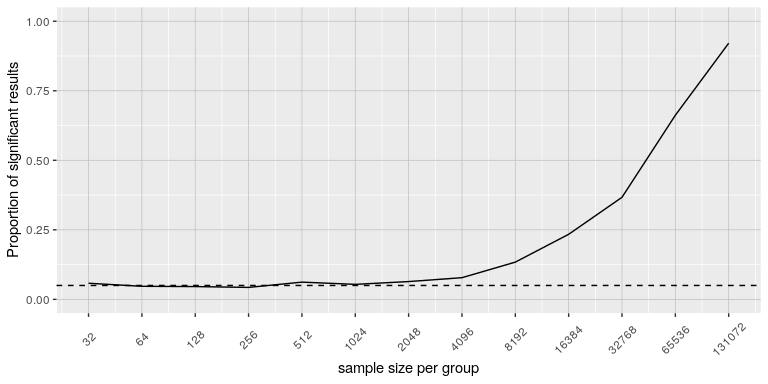
Figure 1: The proportion of significant results for a very small change (1 ounce, which is about .001 standard deviations) as a function of sample size.
Challenges with using p-values
Historically, the most common answer to this question has been that we should reject the null hypothesis if the p-value is less than 0.05. This comes from the writings of Ronald Fisher, who has been referred to as “the single most important figure in 20th century statistics” (Efron, 1998 ) :
“If P is between .1 and .9 there is certainly no reason to suspect the hypothesis tested. If it is below .02 it is strongly indicated that the hypothesis fails to account for the whole of the facts. We shall not often be astray if we draw a conventional line at .05 … it is convenient to draw the line at about the level at which we can say: Either there is something in the treatment, or a coincidence has occurred such as does not occur more than once in twenty trials” (Fisher, 1925 )
Fisher never intended p<0.05p < 0.05 to be a fixed rule:
“no scientific worker has a fixed level of significance at which from year to year, and in all circumstances, he rejects hypotheses; he rather gives his mind to each particular case in the light of his evidence and his ideas” (Fisher, 1956 )
Instead, it is likely that p < .05 became a ritual due to the reliance upon tables of p-values that were used before computing made it easy to compute p values for arbitrary values of a statistic. All of the tables had an entry for 0.05, making it easy to determine whether one’s statistic exceeded the value needed to reach that level of significance. Although we use tables in this class, statistical software examines the specific probability value for the calculated statistic.
Assessing Error Rate: Type I and Type II Error
Although there are challenges with p-values for decision making, we will examine a way we can think about hypothesis testing in terms of its error rate. This was proposed by Jerzy Neyman and Egon Pearson:
“no test based upon a theory of probability can by itself provide any valuable evidence of the truth or falsehood of a hypothesis. But we may look at the purpose of tests from another viewpoint. Without hoping to know whether each separate hypothesis is true or false, we may search for rules to govern our behaviour with regard to them, in following which we insure that, in the long run of experience, we shall not often be wrong” (Neyman & Pearson, 1933 )
That is: We can’t know which specific decisions are right or wrong, but if we follow the rules, we can at least know how often our decisions will be wrong in the long run.
To understand the decision-making framework that Neyman and Pearson developed, we first need to discuss statistical decision-making in terms of the kinds of outcomes that can occur. There are two possible states of reality (H0 is true, or H0 is false), and two possible decisions (reject H0, or retain H0). There are two ways in which we can make a correct decision:
- We can reject H0 when it is false (in the language of signal detection theory, we call this a hit )
- We can retain H0 when it is true (somewhat confusingly in this context, this is called a correct rejection )
There are also two kinds of errors we can make:
- We can reject H0 when it is actually true (we call this a false alarm , or Type I error ), Type I error means that we have concluded that there is a relationship in the population when in fact there is not. Type I errors occur because even when there is no relationship in the population, sampling error alone will occasionally produce an extreme result.
- We can retain H0 when it is actually false (we call this a miss , or Type II error ). Type II error means that we have concluded that there is no relationship in the population when in fact there is.
Summing up, when you perform a hypothesis test, there are four possible outcomes depending on the actual truth (or falseness) of the null hypothesis H0 and the decision to reject or not. The outcomes are summarized in the following table:
Table 1. The four possible outcomes in hypothesis testing.
- The decision is not to reject H0 when H0 is true (correct decision).
- The decision is to reject H0 when H0 is true (incorrect decision known as a Type I error ).
- The decision is not to reject H0 when, in fact, H0 is false (incorrect decision known as a Type II error ).
- The decision is to reject H0 when H0 is false ( correct decision ).
Neyman and Pearson coined two terms to describe the probability of these two types of errors in the long run:
- P(Type I error) = αalpha
- P(Type II error) = βbeta
That is, if we set αalpha to .05, then in the long run we should make a Type I error 5% of the time. The 𝞪 (alpha) , is associated with the p-value for the level of significance. Again it’s common to set αalpha as .05. In fact, when the null hypothesis is true and α is .05, we will mistakenly reject the null hypothesis 5% of the time. (This is why α is sometimes referred to as the “Type I error rate.”) In principle, it is possible to reduce the chance of a Type I error by setting α to something less than .05. Setting it to .01, for example, would mean that if the null hypothesis is true, then there is only a 1% chance of mistakenly rejecting it. But making it harder to reject true null hypotheses also makes it harder to reject false ones and therefore increases the chance of a Type II error.
In practice, Type II errors occur primarily because the research design lacks adequate statistical power to detect the relationship (e.g., the sample is too small). Statistical power is the complement of Type II error. We will have more to say about statistical power shortly. The standard value for an acceptable level of β (beta) is .2 – that is, we are willing to accept that 20% of the time we will fail to detect a true effect when it truly exists. It is possible to reduce the chance of a Type II error by setting α to something greater than .05 (e.g., .10). But making it easier to reject false null hypotheses also makes it easier to reject true ones and therefore increases the chance of a Type I error. This provides some insight into why the convention is to set α to .05. There is some agreement among researchers that level of α keeps the rates of both Type I and Type II errors at acceptable levels.
The possibility of committing Type I and Type II errors has several important implications for interpreting the results of our own and others’ research. One is that we should be cautious about interpreting the results of any individual study because there is a chance that it reflects a Type I or Type II error. This is why researchers consider it important to replicate their studies. Each time researchers replicate a study and find a similar result, they rightly become more confident that the result represents a real phenomenon and not just a Type I or Type II error.
Test Statistic Assumptions
Last consideration we will revisit with each test statistic (e.g., t-test, z-test and ANOVA) in the coming chapters. There are four main assumptions. These assumptions are often taken for granted in using prescribed data for the course. In the real world, these assumptions would need to be examined, often tested using statistical software.
- Assumption of random sampling. A sample is random when each person (or animal) point in your population has an equal chance of being included in the sample; therefore selection of any individual happens by chance, rather than by choice. This reduces the chance that differences in materials, characteristics or conditions may bias results. Remember that random samples are more likely to be representative of the population so researchers can be more confident interpreting the results. Note: there is no test that statistical software can perform which assures random sampling has occurred but following good sampling techniques helps to ensure your samples are random.
- Assumption of Independence. Statistical independence is a critical assumption for many statistical tests including the 2-sample t-test and ANOVA. It is assumed that observations are independent of each other often but often this assumption. Is not met. Independence means the value of one observation does not influence or affect the value of other observations. Independent data items are not connected with one another in any way (unless you account for it in your study). Even the smallest dependence in your data can turn into heavily biased results (which may be undetectable) if you violate this assumption. Note: there is no test statistical software can perform that assures independence of the data because this should be addressed during the research planning phase. Using a non-parametric test is often recommended if a researcher is concerned this assumption has been violated.
- Assumption of Normality. Normality assumes that the continuous variables (dependent variable) used in the analysis are normally distributed. Normal distributions are symmetric around the center (the mean) and form a bell-shaped distribution. Normality is violated when sample data are skewed. With large enough sample sizes (n > 30) the violation of the normality assumption should not cause major problems (remember the central limit theorem) but there is a feature in most statistical software that can alert researchers to an assumption violation.
- Assumption of Equal Variance. Variance refers to the spread or of scores from the mean. Many statistical tests assume that although different samples can come from populations with different means, they have the same variance. Equality of variance (i.e., homogeneity of variance) is violated when variances across different groups or samples are significantly different. Note: there is a feature in most statistical software to test for this.
We will use 4 main steps for hypothesis testing:
- Usually the hypotheses concern population parameters and predict the characteristics that a sample should have
- Null: Null hypothesis (H0) states that there is no difference, no effect or no change between population means and sample means. There is no difference.
- Alternative: Alternative hypothesis (H1 or HA) states that there is a difference or a change between the population and sample. It is the opposite of the null hypothesis.
- Set criteria for a decision. In this step we must determine the boundary of our distribution at which the null hypothesis will be rejected. Researchers usually use either a 5% (.05) cutoff or 1% (.01) critical boundary. Recall from our earlier story about Ronald Fisher that the lower the probability the more confident the was that the Tea Lady was not guessing. We will apply this to z in the next chapter.
- Compare sample and population to decide if the hypothesis has support
- When a researcher uses hypothesis testing, the individual is making a decision about whether the data collected is sufficient to state that the population parameters are significantly different.
Further considerations
The probability value is the probability of a result as extreme or more extreme given that the null hypothesis is true. It is the probability of the data given the null hypothesis. It is not the probability that the null hypothesis is false.
A low probability value indicates that the sample outcome (or one more extreme) would be very unlikely if the null hypothesis were true. We will learn more about assessing effect size later in this unit.
3. A non-significant outcome means that the data do not conclusively demonstrate that the null hypothesis is false. There is always a chance of error and 4 outcomes associated with hypothesis testing.
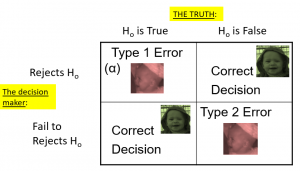
- It is important to take into account the assumptions for each test statistic.
Learning objectives
Having read the chapter, you should be able to:
- Identify the components of a hypothesis test, including the parameter of interest, the null and alternative hypotheses, and the test statistic.
- State the hypotheses and identify appropriate critical areas depending on how hypotheses are set up.
- Describe the proper interpretations of a p-value as well as common misinterpretations.
- Distinguish between the two types of error in hypothesis testing, and the factors that determine them.
- Describe the main criticisms of null hypothesis statistical testing
- Identify the purpose of effect size and power.
Exercises – Ch. 9
- In your own words, explain what the null hypothesis is.
- What are Type I and Type II Errors?
- Why do we phrase null and alternative hypotheses with population parameters and not sample means?
- If our null hypothesis is “H0: μ = 40”, what are the three possible alternative hypotheses?
- Why do we state our hypotheses and decision criteria before we collect our data?
- When and why do you calculate an effect size?
Answers to Odd- Numbered Exercises – Ch. 9
1. Your answer should include mention of the baseline assumption of no difference between the sample and the population.
3. Alpha is the significance level. It is the criteria we use when decided to reject or fail to reject the null hypothesis, corresponding to a given proportion of the area under the normal distribution and a probability of finding extreme scores assuming the null hypothesis is true.
5. μ > 40; μ < 40; μ ≠ 40
7. We calculate effect size to determine the strength of the finding. Effect size should always be calculated when the we have rejected the null hypothesis. Effect size can be calculated for non-significant findings as a possible indicator of Type II error.
Introduction to Statistics for Psychology Copyright © 2021 by Alisa Beyer is licensed under a Creative Commons Attribution-NonCommercial-ShareAlike 4.0 International License , except where otherwise noted.
Share This Book

Want to create or adapt books like this? Learn more about how Pressbooks supports open publishing practices.
6.1 Experiment Basics
Learning objectives.
- Explain what an experiment is and recognize examples of studies that are experiments and studies that are not experiments.
- Explain what internal validity is and why experiments are considered to be high in internal validity.
- Explain what external validity is and evaluate studies in terms of their external validity.
- Distinguish between the manipulation of the independent variable and control of extraneous variables and explain the importance of each.
- Recognize examples of confounding variables and explain how they affect the internal validity of a study.
What Is an Experiment?
As we saw earlier in the book, an experiment is a type of study designed specifically to answer the question of whether there is a causal relationship between two variables. Do changes in an independent variable cause changes in a dependent variable? Experiments have two fundamental features. The first is that the researchers manipulate, or systematically vary, the level of the independent variable. The different levels of the independent variable are called conditions. For example, in Darley and Latané’s experiment, the independent variable was the number of witnesses that participants believed to be present. The researchers manipulated this independent variable by telling participants that there were either one, two, or five other students involved in the discussion, thereby creating three conditions. The second fundamental feature of an experiment is that the researcher controls, or minimizes the variability in, variables other than the independent and dependent variable. These other variables are called extraneous variables. Darley and Latané tested all their participants in the same room, exposed them to the same emergency situation, and so on. They also randomly assigned their participants to conditions so that the three groups would be similar to each other to begin with. Notice that although the words manipulation and control have similar meanings in everyday language, researchers make a clear distinction between them. They manipulate the independent variable by systematically changing its levels and control other variables by holding them constant.
Internal and External Validity
Internal validity.
Recall that the fact that two variables are statistically related does not necessarily mean that one causes the other. “Correlation does not imply causation.” For example, if it were the case that people who exercise regularly are happier than people who do not exercise regularly, this would not necessarily mean that exercising increases people’s happiness. It could mean instead that greater happiness causes people to exercise (the directionality problem) or that something like better physical health causes people to exercise and be happier (the third-variable problem).
The purpose of an experiment, however, is to show that two variables are statistically related and to do so in a way that supports the conclusion that the independent variable caused any observed differences in the dependent variable. The basic logic is this: If the researcher creates two or more highly similar conditions and then manipulates the independent variable to produce just one difference between them, then any later difference between the conditions must have been caused by the independent variable. For example, because the only difference between Darley and Latané’s conditions was the number of students that participants believed to be involved in the discussion, this must have been responsible for differences in helping between the conditions.
An empirical study is said to be high in internal validity if the way it was conducted supports the conclusion that the independent variable caused any observed differences in the dependent variable. Thus experiments are high in internal validity because the way they are conducted—with the manipulation of the independent variable and the control of extraneous variables—provides strong support for causal conclusions.
External Validity
At the same time, the way that experiments are conducted sometimes leads to a different kind of criticism. Specifically, the need to manipulate the independent variable and control extraneous variables means that experiments are often conducted under conditions that seem artificial or unlike “real life” (Stanovich, 2010). In many psychology experiments, the participants are all college undergraduates and come to a classroom or laboratory to fill out a series of paper-and-pencil questionnaires or to perform a carefully designed computerized task. Consider, for example, an experiment in which researcher Barbara Fredrickson and her colleagues had college students come to a laboratory on campus and complete a math test while wearing a swimsuit (Fredrickson, Roberts, Noll, Quinn, & Twenge, 1998). At first, this might seem silly. When will college students ever have to complete math tests in their swimsuits outside of this experiment?
The issue we are confronting is that of external validity. An empirical study is high in external validity if the way it was conducted supports generalizing the results to people and situations beyond those actually studied. As a general rule, studies are higher in external validity when the participants and the situation studied are similar to those that the researchers want to generalize to. Imagine, for example, that a group of researchers is interested in how shoppers in large grocery stores are affected by whether breakfast cereal is packaged in yellow or purple boxes. Their study would be high in external validity if they studied the decisions of ordinary people doing their weekly shopping in a real grocery store. If the shoppers bought much more cereal in purple boxes, the researchers would be fairly confident that this would be true for other shoppers in other stores. Their study would be relatively low in external validity, however, if they studied a sample of college students in a laboratory at a selective college who merely judged the appeal of various colors presented on a computer screen. If the students judged purple to be more appealing than yellow, the researchers would not be very confident that this is relevant to grocery shoppers’ cereal-buying decisions.
We should be careful, however, not to draw the blanket conclusion that experiments are low in external validity. One reason is that experiments need not seem artificial. Consider that Darley and Latané’s experiment provided a reasonably good simulation of a real emergency situation. Or consider field experiments that are conducted entirely outside the laboratory. In one such experiment, Robert Cialdini and his colleagues studied whether hotel guests choose to reuse their towels for a second day as opposed to having them washed as a way of conserving water and energy (Cialdini, 2005). These researchers manipulated the message on a card left in a large sample of hotel rooms. One version of the message emphasized showing respect for the environment, another emphasized that the hotel would donate a portion of their savings to an environmental cause, and a third emphasized that most hotel guests choose to reuse their towels. The result was that guests who received the message that most hotel guests choose to reuse their towels reused their own towels substantially more often than guests receiving either of the other two messages. Given the way they conducted their study, it seems very likely that their result would hold true for other guests in other hotels.
A second reason not to draw the blanket conclusion that experiments are low in external validity is that they are often conducted to learn about psychological processes that are likely to operate in a variety of people and situations. Let us return to the experiment by Fredrickson and colleagues. They found that the women in their study, but not the men, performed worse on the math test when they were wearing swimsuits. They argued that this was due to women’s greater tendency to objectify themselves—to think about themselves from the perspective of an outside observer—which diverts their attention away from other tasks. They argued, furthermore, that this process of self-objectification and its effect on attention is likely to operate in a variety of women and situations—even if none of them ever finds herself taking a math test in her swimsuit.
Manipulation of the Independent Variable
Again, to manipulate an independent variable means to change its level systematically so that different groups of participants are exposed to different levels of that variable, or the same group of participants is exposed to different levels at different times. For example, to see whether expressive writing affects people’s health, a researcher might instruct some participants to write about traumatic experiences and others to write about neutral experiences. The different levels of the independent variable are referred to as conditions , and researchers often give the conditions short descriptive names to make it easy to talk and write about them. In this case, the conditions might be called the “traumatic condition” and the “neutral condition.”
Notice that the manipulation of an independent variable must involve the active intervention of the researcher. Comparing groups of people who differ on the independent variable before the study begins is not the same as manipulating that variable. For example, a researcher who compares the health of people who already keep a journal with the health of people who do not keep a journal has not manipulated this variable and therefore not conducted an experiment. This is important because groups that already differ in one way at the beginning of a study are likely to differ in other ways too. For example, people who choose to keep journals might also be more conscientious, more introverted, or less stressed than people who do not. Therefore, any observed difference between the two groups in terms of their health might have been caused by whether or not they keep a journal, or it might have been caused by any of the other differences between people who do and do not keep journals. Thus the active manipulation of the independent variable is crucial for eliminating the third-variable problem.
Of course, there are many situations in which the independent variable cannot be manipulated for practical or ethical reasons and therefore an experiment is not possible. For example, whether or not people have a significant early illness experience cannot be manipulated, making it impossible to do an experiment on the effect of early illness experiences on the development of hypochondriasis. This does not mean it is impossible to study the relationship between early illness experiences and hypochondriasis—only that it must be done using nonexperimental approaches. We will discuss this in detail later in the book.
In many experiments, the independent variable is a construct that can only be manipulated indirectly. For example, a researcher might try to manipulate participants’ stress levels indirectly by telling some of them that they have five minutes to prepare a short speech that they will then have to give to an audience of other participants. In such situations, researchers often include a manipulation check in their procedure. A manipulation check is a separate measure of the construct the researcher is trying to manipulate. For example, researchers trying to manipulate participants’ stress levels might give them a paper-and-pencil stress questionnaire or take their blood pressure—perhaps right after the manipulation or at the end of the procedure—to verify that they successfully manipulated this variable.
Control of Extraneous Variables
An extraneous variable is anything that varies in the context of a study other than the independent and dependent variables. In an experiment on the effect of expressive writing on health, for example, extraneous variables would include participant variables (individual differences) such as their writing ability, their diet, and their shoe size. They would also include situation or task variables such as the time of day when participants write, whether they write by hand or on a computer, and the weather. Extraneous variables pose a problem because many of them are likely to have some effect on the dependent variable. For example, participants’ health will be affected by many things other than whether or not they engage in expressive writing. This can make it difficult to separate the effect of the independent variable from the effects of the extraneous variables, which is why it is important to control extraneous variables by holding them constant.
Extraneous Variables as “Noise”
Extraneous variables make it difficult to detect the effect of the independent variable in two ways. One is by adding variability or “noise” to the data. Imagine a simple experiment on the effect of mood (happy vs. sad) on the number of happy childhood events people are able to recall. Participants are put into a negative or positive mood (by showing them a happy or sad video clip) and then asked to recall as many happy childhood events as they can. The two leftmost columns of Table 6.1 “Hypothetical Noiseless Data and Realistic Noisy Data” show what the data might look like if there were no extraneous variables and the number of happy childhood events participants recalled was affected only by their moods. Every participant in the happy mood condition recalled exactly four happy childhood events, and every participant in the sad mood condition recalled exactly three. The effect of mood here is quite obvious. In reality, however, the data would probably look more like those in the two rightmost columns of Table 6.1 “Hypothetical Noiseless Data and Realistic Noisy Data” . Even in the happy mood condition, some participants would recall fewer happy memories because they have fewer to draw on, use less effective strategies, or are less motivated. And even in the sad mood condition, some participants would recall more happy childhood memories because they have more happy memories to draw on, they use more effective recall strategies, or they are more motivated. Although the mean difference between the two groups is the same as in the idealized data, this difference is much less obvious in the context of the greater variability in the data. Thus one reason researchers try to control extraneous variables is so their data look more like the idealized data in Table 6.1 “Hypothetical Noiseless Data and Realistic Noisy Data” , which makes the effect of the independent variable is easier to detect (although real data never look quite that good).
Table 6.1 Hypothetical Noiseless Data and Realistic Noisy Data
One way to control extraneous variables is to hold them constant. This can mean holding situation or task variables constant by testing all participants in the same location, giving them identical instructions, treating them in the same way, and so on. It can also mean holding participant variables constant. For example, many studies of language limit participants to right-handed people, who generally have their language areas isolated in their left cerebral hemispheres. Left-handed people are more likely to have their language areas isolated in their right cerebral hemispheres or distributed across both hemispheres, which can change the way they process language and thereby add noise to the data.
In principle, researchers can control extraneous variables by limiting participants to one very specific category of person, such as 20-year-old, straight, female, right-handed, sophomore psychology majors. The obvious downside to this approach is that it would lower the external validity of the study—in particular, the extent to which the results can be generalized beyond the people actually studied. For example, it might be unclear whether results obtained with a sample of younger straight women would apply to older gay men. In many situations, the advantages of a diverse sample outweigh the reduction in noise achieved by a homogeneous one.
Extraneous Variables as Confounding Variables
The second way that extraneous variables can make it difficult to detect the effect of the independent variable is by becoming confounding variables. A confounding variable is an extraneous variable that differs on average across levels of the independent variable. For example, in almost all experiments, participants’ intelligence quotients (IQs) will be an extraneous variable. But as long as there are participants with lower and higher IQs at each level of the independent variable so that the average IQ is roughly equal, then this variation is probably acceptable (and may even be desirable). What would be bad, however, would be for participants at one level of the independent variable to have substantially lower IQs on average and participants at another level to have substantially higher IQs on average. In this case, IQ would be a confounding variable.
To confound means to confuse, and this is exactly what confounding variables do. Because they differ across conditions—just like the independent variable—they provide an alternative explanation for any observed difference in the dependent variable. Figure 6.1 “Hypothetical Results From a Study on the Effect of Mood on Memory” shows the results of a hypothetical study, in which participants in a positive mood condition scored higher on a memory task than participants in a negative mood condition. But if IQ is a confounding variable—with participants in the positive mood condition having higher IQs on average than participants in the negative mood condition—then it is unclear whether it was the positive moods or the higher IQs that caused participants in the first condition to score higher. One way to avoid confounding variables is by holding extraneous variables constant. For example, one could prevent IQ from becoming a confounding variable by limiting participants only to those with IQs of exactly 100. But this approach is not always desirable for reasons we have already discussed. A second and much more general approach—random assignment to conditions—will be discussed in detail shortly.
Figure 6.1 Hypothetical Results From a Study on the Effect of Mood on Memory

Because IQ also differs across conditions, it is a confounding variable.
Key Takeaways
- An experiment is a type of empirical study that features the manipulation of an independent variable, the measurement of a dependent variable, and control of extraneous variables.
- Studies are high in internal validity to the extent that the way they are conducted supports the conclusion that the independent variable caused any observed differences in the dependent variable. Experiments are generally high in internal validity because of the manipulation of the independent variable and control of extraneous variables.
- Studies are high in external validity to the extent that the result can be generalized to people and situations beyond those actually studied. Although experiments can seem “artificial”—and low in external validity—it is important to consider whether the psychological processes under study are likely to operate in other people and situations.
- Practice: List five variables that can be manipulated by the researcher in an experiment. List five variables that cannot be manipulated by the researcher in an experiment.
Practice: For each of the following topics, decide whether that topic could be studied using an experimental research design and explain why or why not.
- Effect of parietal lobe damage on people’s ability to do basic arithmetic.
- Effect of being clinically depressed on the number of close friendships people have.
- Effect of group training on the social skills of teenagers with Asperger’s syndrome.
- Effect of paying people to take an IQ test on their performance on that test.
Cialdini, R. (2005, April). Don’t throw in the towel: Use social influence research. APS Observer . Retrieved from http://www.psychologicalscience.org/observer/getArticle.cfm?id=1762 .
Fredrickson, B. L., Roberts, T.-A., Noll, S. M., Quinn, D. M., & Twenge, J. M. (1998). The swimsuit becomes you: Sex differences in self-objectification, restrained eating, and math performance. Journal of Personality and Social Psychology, 75 , 269–284.
Stanovich, K. E. (2010). How to think straight about psychology (9th ed.). Boston, MA: Allyn & Bacon.
Research Methods in Psychology Copyright © 2016 by University of Minnesota is licensed under a Creative Commons Attribution-NonCommercial-ShareAlike 4.0 International License , except where otherwise noted.
- Bipolar Disorder
- Therapy Center
- When To See a Therapist
- Types of Therapy
- Best Online Therapy
- Best Couples Therapy
- Best Family Therapy
- Managing Stress
- Sleep and Dreaming
- Understanding Emotions
- Self-Improvement
- Healthy Relationships
- Student Resources
- Personality Types
- Verywell Mind Insights
- 2023 Verywell Mind 25
- Mental Health in the Classroom
- Editorial Process
- Meet Our Review Board
- Crisis Support
How the Hawthorne Effect Works
Kendra Cherry, MS, is a psychosocial rehabilitation specialist, psychology educator, and author of the "Everything Psychology Book."
:max_bytes(150000):strip_icc():format(webp)/IMG_9791-89504ab694d54b66bbd72cb84ffb860e.jpg)
Nick David / Getty Images
- Does It Really Exist?
Other Explanations
- How to Avoid It
The Hawthorne effect is a term referring to the tendency of some people to work harder and perform better when they are participants in an experiment.
The term is often used to suggest that individuals may change their behavior due to the attention they are receiving from researchers rather than because of any manipulation of independent variables .
The Hawthorne effect has been widely discussed in psychology textbooks, particularly those devoted to industrial and organizational psychology . However, research suggests that many of the original claims made about the effect may be overstated.
History of the Hawthorne Effect
The Hawthorne effect was first described in the 1950s by researcher Henry A. Landsberger during his analysis of experiments conducted during the 1920s and 1930s.
Why Is It Called the Hawthorne Effect?
The phenomenon is named after the location where the experiments took place, Western Electric’s Hawthorne Works electric company just outside of Hawthorne, Illinois.
The electric company had commissioned research to determine if there was a relationship between productivity and work environments.
The original purpose of the Hawthorne studies was to examine how different aspects of the work environment, such as lighting, the timing of breaks, and the length of the workday, had on worker productivity.
Increased Productivity
In the most famous of the experiments, the focus of the study was to determine if increasing or decreasing the amount of light that workers received would have an effect on how productive workers were during their shifts. In the original study, employee productivity seemed to increase due to the changes but then decreased once the experiment was over.
What the researchers in the original studies found was that almost any change to the experimental conditions led to increases in productivity. For example, productivity increased when illumination was decreased to the levels of candlelight, when breaks were eliminated entirely, and when the workday was lengthened.
The researchers concluded that workers were responding to the increased attention from supervisors. This suggested that productivity increased due to attention and not because of changes in the experimental variables.
Findings May Not Be Accurate
Landsberger defined the Hawthorne effect as a short-term improvement in performance caused by observing workers. Researchers and managers quickly latched on to these findings. Later studies suggested, however, that these initial conclusions did not reflect what was really happening.
The term Hawthorne effect remains widely in use to describe increases in productivity due to participation in a study, yet additional studies have often offered little support or have even failed to find the effect at all.
Examples of the Hawthorne Effect
The following are real-life examples of the Hawthorne effect in various settings:
- Healthcare : One study found that patients with dementia who were being treated with Ginkgo biloba showed better cognitive functioning when they received more intensive follow-ups with healthcare professionals. Patients who received minimal follow-up had less favorable outcomes.
- School : Research found that hand washing rates at a primary school increased as much as 23 percent when another person was present with the person washing their hands—in this study, being watched led to improved performance.
- Workplace : When a supervisor is watching an employee work, that employee is likely to be on their "best behavior" and work harder than they would without being watched.
Does the Hawthorne Effect Exist?
Later research into the Hawthorne effect suggested that the original results may have been overstated. In 2009, researchers at the University of Chicago reanalyzed the original data and found that other factors also played a role in productivity and that the effect originally described was weak at best.
Researchers also uncovered the original data from the Hawthorne studies and found that many of the later reported claims about the findings are simply not supported by the data. They did find, however, more subtle displays of a possible Hawthorne effect.
While some additional studies failed to find strong evidence of the Hawthorne effect, a 2014 systematic review published in the Journal of Clinical Epidemiology found that research participation effects do exist.
After looking at the results of 19 different studies, the researchers concluded that these effects clearly happen, but more research needs to be done in order to determine how they work, the impact they have, and why they occur.
While the Hawthorne effect may have an influence on participant behavior in experiments, there may also be other factors that play a part in these changes. Some factors that may influence improvements in productivity include:
- Demand characteristics : In experiments, researchers sometimes display subtle clues that let participants know what they are hoping to find. As a result, subjects will alter their behavior to help confirm the experimenter’s hypothesis .
- Novelty effects : The novelty of having experimenters observing behavior might also play a role. This can lead to an initial increase in performance and productivity that may eventually level off as the experiment continues.
- Performance feedback : In situations involving worker productivity, increased attention from experimenters also resulted in increased performance feedback. This increased feedback might actually lead to an improvement in productivity.
While the Hawthorne effect has often been overstated, the term is still useful as a general explanation for psychological factors that can affect how people behave in an experiment.
How to Reduce the Hawthorne Effect
In order for researchers to trust the results of experiments, it is essential to minimize potential problems and sources of bias like the Hawthorne effect.
So what can researchers do to minimize these effects in their experimental studies?
- Conduct experiments in natural settings : One way to help eliminate or minimize demand characteristics and other potential sources of experimental bias is to utilize naturalistic observation techniques. However, this is simply not always possible.
- Make responses completely anonymous : Another way to combat this form of bias is to make the participants' responses in an experiment completely anonymous or confidential. This way, participants may be less likely to alter their behavior as a result of taking part in an experiment.
- Get familiar with the people in the study : People may not alter their behavior as significantly if they are being watched by someone they are familiar with. For instance, an employee is less likely to work harder if the supervisor watching them is always watching.
Many of the original findings of the Hawthorne studies have since been found to be either overstated or erroneous, but the term has become widely used in psychology, economics, business, and other areas.
More recent findings support the idea that these effects do happen, but how much of an impact they actually have on results remains in question. Today, the term is still often used to refer to changes in behavior that can result from taking part in an experiment.
Schwartz D, Fischhoff B, Krishnamurti T, Sowell F. The Hawthorne effect and energy awareness . Proc Natl Acad Sci U S A . 2013;110(38):15242-15246. doi:10.1073/pnas.1301687110
McCambridge J, Witton J, Elbourne DR. Systematic review of the Hawthorne effect: New concepts are needed to study research participation effects . J Clin Epidemiol . 2014;67(3):267-277. doi:10.1016/j.jclinepi.2013.08.015
Letrud K, Hernes S. Affirmative citation bias in scientific myth debunking: A three-in-one case study . Bornmann L, ed. PLoS ONE. 2019;14(9):e0222213. doi:10.1371/journal.pone.0222213
McCarney R, Warner J, Iliffe S, van Haselen R, Griffin M, Fisher P. The Hawthorne effect: a randomised, controlled trial . BMC Med Res Methodol . 2007;7:30. doi:10.1186/1471-2288-7-30
Pickering AJ, Blum AG, Breiman RF, Ram PK, Davis J. Video surveillance captures student hand hygiene behavior, reactivity to observation, and peer influence in Kenyan primary schools . Gupta V, ed. PLoS ONE. 2014;9(3):e92571. doi:10.1371/journal.pone.0092571
Understanding Your Users . Elsevier ; 2015. doi:10.1016/c2013-0-13611-2
Levitt S, List, JA. Was there really a Hawthorne effect at the Hawthorne plant? An analysis of the original illumination experiments . 2009. University of Chicago. NBER Working Paper No. w15016,
Levitt, SD & List, JA. Was there really a Hawthorne effect at the Hawthorne plant? An analysis of the original illumination experiments . American Economic Journal: Applied Economics. 2011;3:224-238. doi:10.2307/25760252
McCambridge J, de Bruin M, Witton J. The effects of demand characteristics on research participant behaviours in non-laboratory settings: A systematic review . PLoS ONE . 2012;7(6):e39116. doi:10.1371/journal.pone.0039116
Chwo GSM, Marek MW, Wu WCV. Meta-analysis of MALL research and design . System. 2018;74:62-72. doi:10.1016/j.system.2018.02.009
Gnepp J, Klayman J, Williamson IO, Barlas S. The future of feedback: Motivating performance improvement through future-focused feedback . PLoS One . 2020;15(6):e0234444. doi:10.1371/journal.pone.0234444
Hawthorne effect . The SAGE Encyclopedia of Educational Research, Measurement, and Evaluation. doi:10.4135/9781506326139.n300
Murdoch M, Simon AB, Polusny MA, et al. Impact of different privacy conditions and incentives on survey response rate, participant representativeness, and disclosure of sensitive information: a randomized controlled trial . BMC Med Res Methodol . 2014;14:90. doi:10.1186/1471-2288-14-90
Landy FJ , Conte JM. Work in the 21st Century: An Introduction to Industrial and Organizational Psychology . New York: John Wiley and Sons; 2010.
McBride DM. The Process of Research in Psychology . London: Sage Publications; 2013.
By Kendra Cherry, MSEd Kendra Cherry, MS, is a psychosocial rehabilitation specialist, psychology educator, and author of the "Everything Psychology Book."
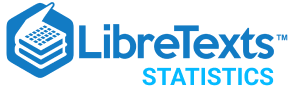
- school Campus Bookshelves
- menu_book Bookshelves
- perm_media Learning Objects
- login Login
- how_to_reg Request Instructor Account
- hub Instructor Commons
- Download Page (PDF)
- Download Full Book (PDF)
- Periodic Table
- Physics Constants
- Scientific Calculator
- Reference & Cite
- Tools expand_more
- Readability
selected template will load here
This action is not available.
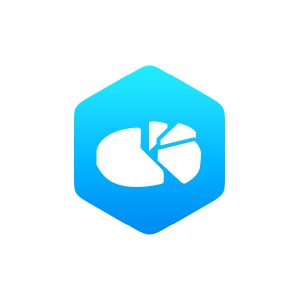
3.1: The Fundamentals of Hypothesis Testing
- Last updated
- Save as PDF
- Page ID 2883
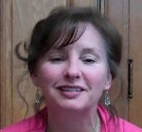
- Diane Kiernan
- SUNY College of Environmental Science and Forestry via OpenSUNY
The previous two chapters introduced methods for organizing and summarizing sample data, and using sample statistics to estimate population parameters. This chapter introduces the next major topic of inferential statistics: hypothesis testing.
A hypothesis is a statement or claim about a property of a population.
The Fundamentals of Hypothesis Testing
When conducting scientific research, typically there is some known information, perhaps from some past work or from a long accepted idea. We want to test whether this claim is believable. This is the basic idea behind a hypothesis test:
- State what we think is true.
- Quantify how confident we are about our claim.
- Use sample statistics to make inferences about population parameters.
For example, past research tells us that the average life span for a hummingbird is about four years. You have been studying the hummingbirds in the southeastern United States and find a sample mean lifespan of 4.8 years. Should you reject the known or accepted information in favor of your results? How confident are you in your estimate? At what point would you say that there is enough evidence to reject the known information and support your alternative claim? How far from the known mean of four years can the sample mean be before we reject the idea that the average lifespan of a hummingbird is four years?
Definition: hypothesis testing
Hypothesis testing is a procedure, based on sample evidence and probability, used to test claims regarding a characteristic of a population.
A hypothesis is a claim or statement about a characteristic of a population of interest to us. A hypothesis test is a way for us to use our sample statistics to test a specific claim.
Example \(\PageIndex{1}\):
The population mean weight is known to be 157 lb. We want to test the claim that the mean weight has increased.
Example \(\PageIndex{2}\):
Two years ago, the proportion of infected plants was 37%. We believe that a treatment has helped, and we want to test the claim that there has been a reduction in the proportion of infected plants.
Components of a Formal Hypothesis Test
The null hypothesis is a statement about the value of a population parameter, such as the population mean (µ) or the population proportion ( p ). It contains the condition of equality and is denoted as H 0 (H-naught).
H 0 : µ = 157 or H0 : p = 0.37
The alternative hypothesis is the claim to be tested, the opposite of the null hypothesis. It contains the value of the parameter that we consider plausible and is denoted as H 1 .
H 1 : µ > 157 or H1 : p ≠ 0.37
The test statistic is a value computed from the sample data that is used in making a decision about the rejection of the null hypothesis. The test statistic converts the sample mean ( x̄ ) or sample proportion ( p̂ ) to a Z- or t-score under the assumption that the null hypothesis is true. It is used to decide whether the difference between the sample statistic and the hypothesized claim is significant.
The p-value is the area under the curve to the left or right of the test statistic. It is compared to the level of significance (α).
The critical value is the value that defines the rejection zone (the test statistic values that would lead to rejection of the null hypothesis). It is defined by the level of significance.
The level of significance (α) is the probability that the test statistic will fall into the critical region when the null hypothesis is true. This level is set by the researcher.
The conclusion is the final decision of the hypothesis test. The conclusion must always be clearly stated, communicating the decision based on the components of the test. It is important to realize that we never prove or accept the null hypothesis. We are merely saying that the sample evidence is not strong enough to warrant the rejection of the null hypothesis. The conclusion is made up of two parts:
1) Reject or fail to reject the null hypothesis, and 2) there is or is not enough evidence to support the alternative claim.
Option 1) Reject the null hypothesis (H0). This means that you have enough statistical evidence to support the alternative claim (H 1 ).
Option 2) Fail to reject the null hypothesis (H0). This means that you do NOT have enough evidence to support the alternative claim (H 1 ).
Another way to think about hypothesis testing is to compare it to the US justice system. A defendant is innocent until proven guilty (Null hypothesis—innocent). The prosecuting attorney tries to prove that the defendant is guilty (Alternative hypothesis—guilty). There are two possible conclusions that the jury can reach. First, the defendant is guilty (Reject the null hypothesis). Second, the defendant is not guilty (Fail to reject the null hypothesis). This is NOT the same thing as saying the defendant is innocent! In the first case, the prosecutor had enough evidence to reject the null hypothesis (innocent) and support the alternative claim (guilty). In the second case, the prosecutor did NOT have enough evidence to reject the null hypothesis (innocent) and support the alternative claim of guilty.
The Null and Alternative Hypotheses
There are three different pairs of null and alternative hypotheses:
Table \(PageIndex{1}\): The rejection zone for a two-sided hypothesis test.
where c is some known value.
A Two-sided Test
This tests whether the population parameter is equal to, versus not equal to, some specific value.
Ho: μ = 12 vs. H 1 : μ ≠ 12
The critical region is divided equally into the two tails and the critical values are ± values that define the rejection zones.
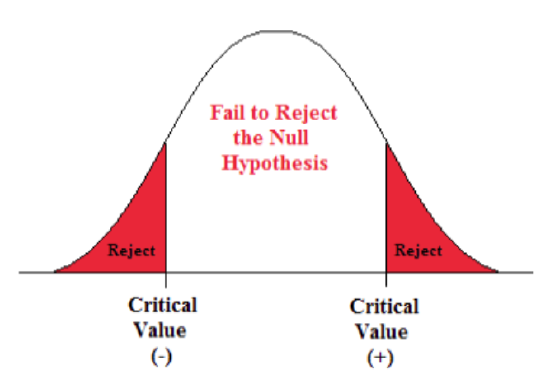
Example \(\PageIndex{3}\):
A forester studying diameter growth of red pine believes that the mean diameter growth will be different if a fertilization treatment is applied to the stand.
- Ho: μ = 1.2 in./ year
- H 1 : μ ≠ 1.2 in./ year
This is a two-sided question, as the forester doesn’t state whether population mean diameter growth will increase or decrease.
A Right-sided Test
This tests whether the population parameter is equal to, versus greater than, some specific value.
Ho: μ = 12 vs. H 1 : μ > 12
The critical region is in the right tail and the critical value is a positive value that defines the rejection zone.
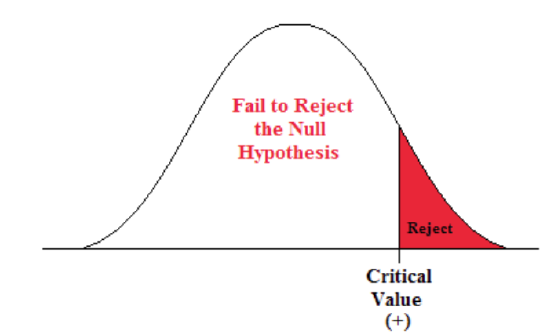
Example \(\PageIndex{4}\):
A biologist believes that there has been an increase in the mean number of lakes infected with milfoil, an invasive species, since the last study five years ago.
- Ho: μ = 15 lakes
- H1: μ >15 lakes
This is a right-sided question, as the biologist believes that there has been an increase in population mean number of infected lakes.
A Left-sided Test
This tests whether the population parameter is equal to, versus less than, some specific value.
Ho: μ = 12 vs. H 1 : μ < 12
The critical region is in the left tail and the critical value is a negative value that defines the rejection zone.
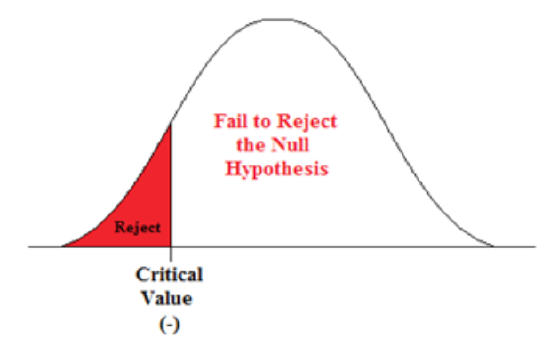
Example \(\PageIndex{5}\):
A scientist’s research indicates that there has been a change in the proportion of people who support certain environmental policies. He wants to test the claim that there has been a reduction in the proportion of people who support these policies.
- Ho: p = 0.57
- H 1 : p < 0.57
This is a left-sided question, as the scientist believes that there has been a reduction in the true population proportion.
Statistically Significant
When the observed results (the sample statistics) are unlikely (a low probability) under the assumption that the null hypothesis is true, we say that the result is statistically significant, and we reject the null hypothesis. This result depends on the level of significance, the sample statistic, sample size, and whether it is a one- or two-sided alternative hypothesis.
Types of Errors
When testing, we arrive at a conclusion of rejecting the null hypothesis or failing to reject the null hypothesis. Such conclusions are sometimes correct and sometimes incorrect (even when we have followed all the correct procedures). We use incomplete sample data to reach a conclusion and there is always the possibility of reaching the wrong conclusion. There are four possible conclusions to reach from hypothesis testing. Of the four possible outcomes, two are correct and two are NOT correct.
Table \(\PageIndex{2}\). Possible outcomes from a hypothesis test.
A Type I error is when we reject the null hypothesis when it is true. The symbol α (alpha) is used to represent Type I errors. This is the same alpha we use as the level of significance. By setting alpha as low as reasonably possible, we try to control the Type I error through the level of significance.
A Type II error is when we fail to reject the null hypothesis when it is false. The symbol β(beta) is used to represent Type II errors.
In general, Type I errors are considered more serious. One step in the hypothesis test procedure involves selecting the significance level ( α ), which is the probability of rejecting the null hypothesis when it is correct. So the researcher can select the level of significance that minimizes Type I errors. However, there is a mathematical relationship between α, β, and n (sample size).
- As α increases, β decreases
- As α decreases, β increases
- As sample size increases (n), both α and β decrease
The natural inclination is to select the smallest possible value for α, thinking to minimize the possibility of causing a Type I error. Unfortunately, this forces an increase in Type II errors. By making the rejection zone too small, you may fail to reject the null hypothesis, when, in fact, it is false. Typically, we select the best sample size and level of significance, automatically setting β.
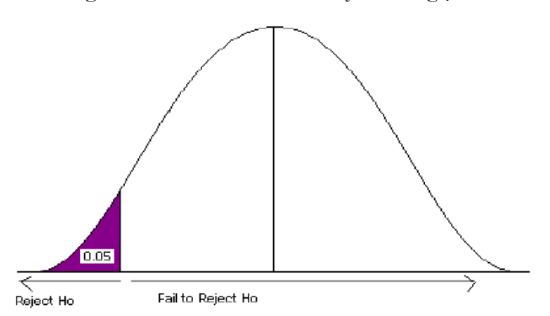
Power of the Test
A Type II error (β) is the probability of failing to reject a false null hypothesis. It follows that 1-β is the probability of rejecting a false null hypothesis. This probability is identified as the power of the test, and is often used to gauge the test’s effectiveness in recognizing that a null hypothesis is false.
Definition: power of the test
The probability that at a fixed level α significance test will reject H0, when a particular alternative value of the parameter is true is called the power of the test.
Power is also directly linked to sample size. For example, suppose the null hypothesis is that the mean fish weight is 8.7 lb. Given sample data, a level of significance of 5%, and an alternative weight of 9.2 lb., we can compute the power of the test to reject μ = 8.7 lb. If we have a small sample size, the power will be low. However, increasing the sample size will increase the power of the test. Increasing the level of significance will also increase power. A 5% test of significance will have a greater chance of rejecting the null hypothesis than a 1% test because the strength of evidence required for the rejection is less. Decreasing the standard deviation has the same effect as increasing the sample size: there is more information about μ.

- SAT Downloads
- SAT Study Guide
- Digital SAT Reading & Writing
- Digital SAT Math
- Digital SAT Mock Tests
- Digital SAT Flashcards
- SAT Reading
- SAT Writing & Language
- Multiple-Choice Tests
- Grid-Ins Tests
Digital SAT Reading and Writing Practice Question 113: Answer and Explanation
- Digital SAT Test
- Digital SAT Reading and Writing Tests
Question: 113
Researchers hypothesized that a decline in the population of dusky sharks near the mid-Atlantic coast of North America led to a decline in the population of eastern oysters in the region. Dusky sharks do not typically consume eastern oysters but do consume cownose rays, which are the main predators of the oysters.
Which finding, if true, would most directly support the researchers' hypothesis?
- A. Declines in the regional abundance of dusky sharks' prey other than cownose rays are associated with regional declines in dusky shark abundance.
- B. Eastern oyster abundance tends to be greater in areas with both dusky sharks and cownose rays than in areas with only dusky sharks.
- C. Consumption of eastern oysters by cownose rays in the region substantially increased before the regional decline in dusky shark abundance began.
- D. Cownose rays have increased in regional abundance as dusky sharks have decreased in regional abundance.
Correct Answer: D
Explanation:
Choice D is the best answer because it presents a finding that, if true, would most directly support the researchers' hypothesis about the connection between the dusky shark population decline and the eastern oyster population decline. The text indicates that although dusky sharks don't usually eat eastern oysters, they do consume cownose rays, which are the main predators of eastern oysters. An increase in the abundance of cownose rays in the region in response to a decline in the abundance of dusky sharks would directly support the researchers' hypothesis: a higher number of cownose rays would consume more eastern oysters, driving down the oyster population.
Choice A is incorrect because a finding that there's an association between a decline in the regional abundance of some of dusky sharks' prey and the regional abundance of dusky sharks wouldn't directly support the researchers' hypothesis that a decline in dusky sharks has led to a decline in eastern oysters in the region. Although such a finding might help explain why shark abundance has declined, it would reveal nothing about whether the shark decline is related to the oyster decline. Choice B is incorrect because a finding that eastern oyster abundance tends to be greater when dusky sharks and cownose rays are present than when only dusky sharks are present wouldn't support the researchers' hypothesis that a decline in dusky sharks has led to a decline in eastern oysters in the region. The text indicates that the sharks prey on the rays, which are the main predators of the oysters; if oyster abundance is found to be greater when rays are present than when rays are absent, that would suggest that rays aren't keeping oyster abundance down, and thus that a decline in rays' predators, which would be expected to lead to an increase in the abundance of rays, wouldn't bring about a decline in oyster abundance as the researchers hypothesize. Choice C is incorrect because a finding that consumption of eastern oysters by cownose rays increased substantially before dusky sharks declined in regional abundance wouldn't support the researchers' hypothesis that the decline in dusky sharks has led to a decline in eastern oysters in the region. Such a finding would suggest that some factor other than shark abundance led to an increase in rays' consumption of oysters and thus to a decrease in oyster abundance, thereby weakening the researchers' hypothesis.
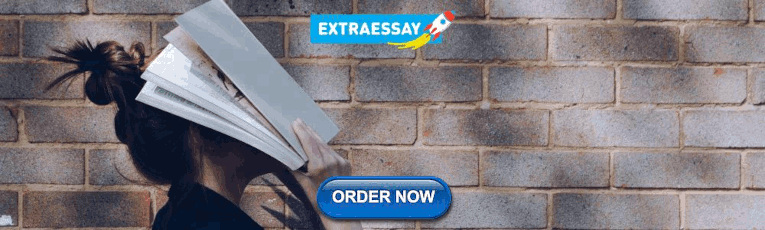
Test Information
- Use your browser's back button to return to your test results.
- Do more Digital SAT Reading and Writing Tests tests.
- Digital SAT Reading and Writing Test 1
- Digital SAT Reading and Writing Test 2
- Digital SAT Reading and Writing Test 3
- Digital SAT Reading and Writing Test 4
- Digital SAT Reading and Writing Test 5
- Digital SAT Reading and Writing Test 6
- Digital SAT Reading and Writing Test 7
- Digital SAT Reading and Writing Test 8
- Digital SAT Reading and Writing Test 9
- Digital SAT Reading and Writing Test 10
- Digital SAT Reading and Writing Test 11
- Digital SAT Reading and Writing Test 12
- Digital SAT Reading and Writing Test 13
- Digital SAT Reading and Writing Test 14
- Digital SAT Reading and Writing Test 15
- Digital SAT Reading and Writing Test 16
- Digital SAT Reading and Writing Test 17
- Digital SAT Reading and Writing Test 18
- Digital SAT Reading and Writing Test 19
- Digital SAT Reading and Writing Test 20
SAT Practice Tests
- Digital SAT Reading and Writing
- SAT Math Multiple-Choice
- SAT Math Grid-Ins
More Information
Switch to mobile version.
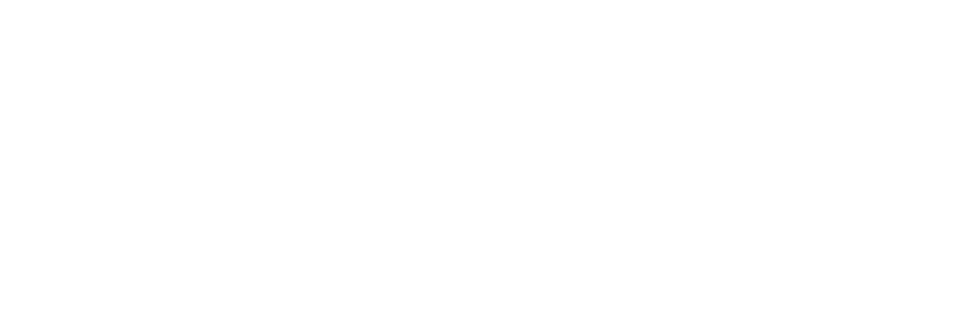
Want to create or adapt books like this? Learn more about how Pressbooks supports open publishing practices.
7 Chapter 7: Introduction to Hypothesis Testing
alternative hypothesis
critical value
effect size
null hypothesis
probability value
rejection region
significance level
statistical power
statistical significance
test statistic
Type I error
Type II error
This chapter lays out the basic logic and process of hypothesis testing. We will perform z tests, which use the z score formula from Chapter 6 and data from a sample mean to make an inference about a population.
Logic and Purpose of Hypothesis Testing
A hypothesis is a prediction that is tested in a research study. The statistician R. A. Fisher explained the concept of hypothesis testing with a story of a lady tasting tea. Here we will present an example based on James Bond who insisted that martinis should be shaken rather than stirred. Let’s consider a hypothetical experiment to determine whether Mr. Bond can tell the difference between a shaken martini and a stirred martini. Suppose we gave Mr. Bond a series of 16 taste tests. In each test, we flipped a fair coin to determine whether to stir or shake the martini. Then we presented the martini to Mr. Bond and asked him to decide whether it was shaken or stirred. Let’s say Mr. Bond was correct on 13 of the 16 taste tests. Does this prove that Mr. Bond has at least some ability to tell whether the martini was shaken or stirred?
This result does not prove that he does; it could be he was just lucky and guessed right 13 out of 16 times. But how plausible is the explanation that he was just lucky? To assess its plausibility, we determine the probability that someone who was just guessing would be correct 13/16 times or more. This probability can be computed to be .0106. This is a pretty low probability, and therefore someone would have to be very lucky to be correct 13 or more times out of 16 if they were just guessing. So either Mr. Bond was very lucky, or he can tell whether the drink was shaken or stirred. The hypothesis that he was guessing is not proven false, but considerable doubt is cast on it. Therefore, there is strong evidence that Mr. Bond can tell whether a drink was shaken or stirred.
Let’s consider another example. The case study Physicians’ Reactions sought to determine whether physicians spend less time with obese patients. Physicians were sampled randomly and each was shown a chart of a patient complaining of a migraine headache. They were then asked to estimate how long they would spend with the patient. The charts were identical except that for half the charts, the patient was obese and for the other half, the patient was of average weight. The chart a particular physician viewed was determined randomly. Thirty-three physicians viewed charts of average-weight patients and 38 physicians viewed charts of obese patients.
The mean time physicians reported that they would spend with obese patients was 24.7 minutes as compared to a mean of 31.4 minutes for normal-weight patients. How might this difference between means have occurred? One possibility is that physicians were influenced by the weight of the patients. On the other hand, perhaps by chance, the physicians who viewed charts of the obese patients tend to see patients for less time than the other physicians. Random assignment of charts does not ensure that the groups will be equal in all respects other than the chart they viewed. In fact, it is certain the groups differed in many ways by chance. The two groups could not have exactly the same mean age (if measured precisely enough such as in days). Perhaps a physician’s age affects how long the physician sees patients. There are innumerable differences between the groups that could affect how long they view patients. With this in mind, is it plausible that these chance differences are responsible for the difference in times?
To assess the plausibility of the hypothesis that the difference in mean times is due to chance, we compute the probability of getting a difference as large or larger than the observed difference (31.4 − 24.7 = 6.7 minutes) if the difference were, in fact, due solely to chance. Using methods presented in later chapters, this probability can be computed to be .0057. Since this is such a low probability, we have confidence that the difference in times is due to the patient’s weight and is not due to chance.
The Probability Value
It is very important to understand precisely what the probability values mean. In the James Bond example, the computed probability of .0106 is the probability he would be correct on 13 or more taste tests (out of 16) if he were just guessing. It is easy to mistake this probability of .0106 as the probability he cannot tell the difference. This is not at all what it means.
The probability of .0106 is the probability of a certain outcome (13 or more out of 16) assuming a certain state of the world (James Bond was only guessing). It is not the probability that a state of the world is true. Although this might seem like a distinction without a difference, consider the following example. An animal trainer claims that a trained bird can determine whether or not numbers are evenly divisible by 7. In an experiment assessing this claim, the bird is given a series of 16 test trials. On each trial, a number is displayed on a screen and the bird pecks at one of two keys to indicate its choice. The numbers are chosen in such a way that the probability of any number being evenly divisible by 7 is .50. The bird is correct on 9/16 choices. We can compute that the probability of being correct nine or more times out of 16 if one is only guessing is .40. Since a bird who is only guessing would do this well 40% of the time, these data do not provide convincing evidence that the bird can tell the difference between the two types of numbers. As a scientist, you would be very skeptical that the bird had this ability. Would you conclude that there is a .40 probability that the bird can tell the difference? Certainly not! You would think the probability is much lower than .0001.
To reiterate, the probability value is the probability of an outcome (9/16 or better) and not the probability of a particular state of the world (the bird was only guessing). In statistics, it is conventional to refer to possible states of the world as hypotheses since they are hypothesized states of the world. Using this terminology, the probability value is the probability of an outcome given the hypothesis. It is not the probability of the hypothesis given the outcome.
This is not to say that we ignore the probability of the hypothesis. If the probability of the outcome given the hypothesis is sufficiently low, we have evidence that the hypothesis is false. However, we do not compute the probability that the hypothesis is false. In the James Bond example, the hypothesis is that he cannot tell the difference between shaken and stirred martinis. The probability value is low (.0106), thus providing evidence that he can tell the difference. However, we have not computed the probability that he can tell the difference.
The Null Hypothesis
The hypothesis that an apparent effect is due to chance is called the null hypothesis , written H 0 (“ H -naught”). In the Physicians’ Reactions example, the null hypothesis is that in the population of physicians, the mean time expected to be spent with obese patients is equal to the mean time expected to be spent with average-weight patients. This null hypothesis can be written as:

The null hypothesis in a correlational study of the relationship between high school grades and college grades would typically be that the population correlation is 0. This can be written as
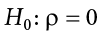
Although the null hypothesis is usually that the value of a parameter is 0, there are occasions in which the null hypothesis is a value other than 0. For example, if we are working with mothers in the U.S. whose children are at risk of low birth weight, we can use 7.47 pounds, the average birth weight in the U.S., as our null value and test for differences against that.
For now, we will focus on testing a value of a single mean against what we expect from the population. Using birth weight as an example, our null hypothesis takes the form:
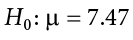
Keep in mind that the null hypothesis is typically the opposite of the researcher’s hypothesis. In the Physicians’ Reactions study, the researchers hypothesized that physicians would expect to spend less time with obese patients. The null hypothesis that the two types of patients are treated identically is put forward with the hope that it can be discredited and therefore rejected. If the null hypothesis were true, a difference as large as or larger than the sample difference of 6.7 minutes would be very unlikely to occur. Therefore, the researchers rejected the null hypothesis of no difference and concluded that in the population, physicians intend to spend less time with obese patients.
In general, the null hypothesis is the idea that nothing is going on: there is no effect of our treatment, no relationship between our variables, and no difference in our sample mean from what we expected about the population mean. This is always our baseline starting assumption, and it is what we seek to reject. If we are trying to treat depression, we want to find a difference in average symptoms between our treatment and control groups. If we are trying to predict job performance, we want to find a relationship between conscientiousness and evaluation scores. However, until we have evidence against it, we must use the null hypothesis as our starting point.
The Alternative Hypothesis
If the null hypothesis is rejected, then we will need some other explanation, which we call the alternative hypothesis, H A or H 1 . The alternative hypothesis is simply the reverse of the null hypothesis, and there are three options, depending on where we expect the difference to lie. Thus, our alternative hypothesis is the mathematical way of stating our research question. If we expect our obtained sample mean to be above or below the null hypothesis value, which we call a directional hypothesis, then our alternative hypothesis takes the form

based on the research question itself. We should only use a directional hypothesis if we have good reason, based on prior observations or research, to suspect a particular direction. When we do not know the direction, such as when we are entering a new area of research, we use a non-directional alternative:
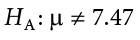
We will set different criteria for rejecting the null hypothesis based on the directionality (greater than, less than, or not equal to) of the alternative. To understand why, we need to see where our criteria come from and how they relate to z scores and distributions.
Critical Values, p Values, and Significance Level

The significance level is a threshold we set before collecting data in order to determine whether or not we should reject the null hypothesis. We set this value beforehand to avoid biasing ourselves by viewing our results and then determining what criteria we should use. If our data produce values that meet or exceed this threshold, then we have sufficient evidence to reject the null hypothesis; if not, we fail to reject the null (we never “accept” the null).
Figure 7.1. The rejection region for a one-tailed test. (“ Rejection Region for One-Tailed Test ” by Judy Schmitt is licensed under CC BY-NC-SA 4.0 .)
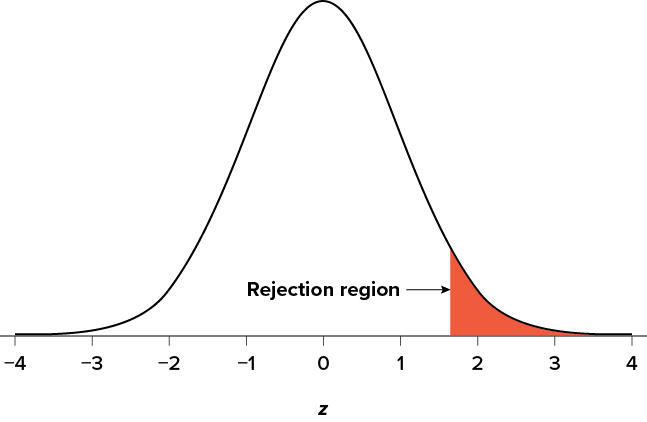
The rejection region is bounded by a specific z value, as is any area under the curve. In hypothesis testing, the value corresponding to a specific rejection region is called the critical value , z crit (“ z crit”), or z * (hence the other name “critical region”). Finding the critical value works exactly the same as finding the z score corresponding to any area under the curve as we did in Unit 1 . If we go to the normal table, we will find that the z score corresponding to 5% of the area under the curve is equal to 1.645 ( z = 1.64 corresponds to .0505 and z = 1.65 corresponds to .0495, so .05 is exactly in between them) if we go to the right and −1.645 if we go to the left. The direction must be determined by your alternative hypothesis, and drawing and shading the distribution is helpful for keeping directionality straight.
Suppose, however, that we want to do a non-directional test. We need to put the critical region in both tails, but we don’t want to increase the overall size of the rejection region (for reasons we will see later). To do this, we simply split it in half so that an equal proportion of the area under the curve falls in each tail’s rejection region. For a = .05, this means 2.5% of the area is in each tail, which, based on the z table, corresponds to critical values of z * = ±1.96. This is shown in Figure 7.2 .
Figure 7.2. Two-tailed rejection region. (“ Rejection Region for Two-Tailed Test ” by Judy Schmitt is licensed under CC BY-NC-SA 4.0 .)
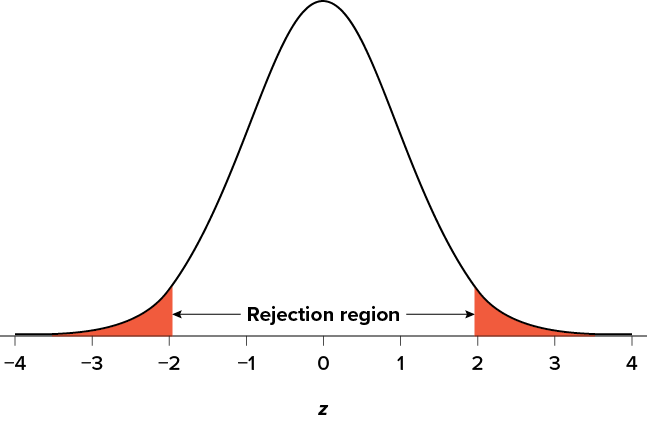
Thus, any z score falling outside ±1.96 (greater than 1.96 in absolute value) falls in the rejection region. When we use z scores in this way, the obtained value of z (sometimes called z obtained and abbreviated z obt ) is something known as a test statistic , which is simply an inferential statistic used to test a null hypothesis. The formula for our z statistic has not changed:
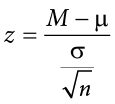
Figure 7.3. Relationship between a , z obt , and p . (“ Relationship between alpha, z-obt, and p ” by Judy Schmitt is licensed under CC BY-NC-SA 4.0 .)
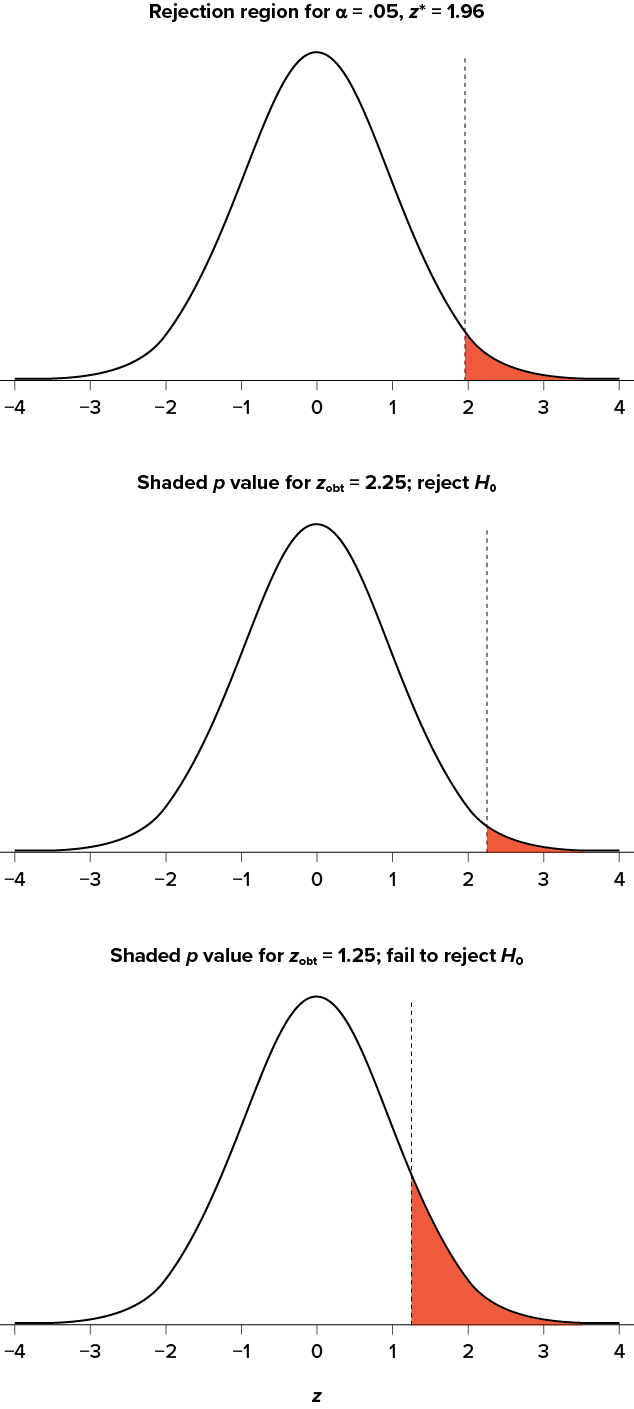
When the null hypothesis is rejected, the effect is said to have statistical significance , or be statistically significant. For example, in the Physicians’ Reactions case study, the probability value is .0057. Therefore, the effect of obesity is statistically significant and the null hypothesis that obesity makes no difference is rejected. It is important to keep in mind that statistical significance means only that the null hypothesis of exactly no effect is rejected; it does not mean that the effect is important, which is what “significant” usually means. When an effect is significant, you can have confidence the effect is not exactly zero. Finding that an effect is significant does not tell you about how large or important the effect is.
Do not confuse statistical significance with practical significance. A small effect can be highly significant if the sample size is large enough.
Why does the word “significant” in the phrase “statistically significant” mean something so different from other uses of the word? Interestingly, this is because the meaning of “significant” in everyday language has changed. It turns out that when the procedures for hypothesis testing were developed, something was “significant” if it signified something. Thus, finding that an effect is statistically significant signifies that the effect is real and not due to chance. Over the years, the meaning of “significant” changed, leading to the potential misinterpretation.
The Hypothesis Testing Process
A four-step procedure.
The process of testing hypotheses follows a simple four-step procedure. This process will be what we use for the remainder of the textbook and course, and although the hypothesis and statistics we use will change, this process will not.
Step 1: State the Hypotheses
Your hypotheses are the first thing you need to lay out. Otherwise, there is nothing to test! You have to state the null hypothesis (which is what we test) and the alternative hypothesis (which is what we expect). These should be stated mathematically as they were presented above and in words, explaining in normal English what each one means in terms of the research question.
Step 2: Find the Critical Values
Step 3: calculate the test statistic and effect size.
Once we have our hypotheses and the standards we use to test them, we can collect data and calculate our test statistic—in this case z . This step is where the vast majority of differences in future chapters will arise: different tests used for different data are calculated in different ways, but the way we use and interpret them remains the same. As part of this step, we will also calculate effect size to better quantify the magnitude of the difference between our groups. Although effect size is not considered part of hypothesis testing, reporting it as part of the results is approved convention.
Step 4: Make the Decision
Finally, once we have our obtained test statistic, we can compare it to our critical value and decide whether we should reject or fail to reject the null hypothesis. When we do this, we must interpret the decision in relation to our research question, stating what we concluded, what we based our conclusion on, and the specific statistics we obtained.
Example A Movie Popcorn
Our manager is looking for a difference in the mean weight of popcorn bags compared to the population mean of 8. We will need both a null and an alternative hypothesis written both mathematically and in words. We’ll always start with the null hypothesis:

In this case, we don’t know if the bags will be too full or not full enough, so we do a two-tailed alternative hypothesis that there is a difference.
Our critical values are based on two things: the directionality of the test and the level of significance. We decided in Step 1 that a two-tailed test is the appropriate directionality. We were given no information about the level of significance, so we assume that a = .05 is what we will use. As stated earlier in the chapter, the critical values for a two-tailed z test at a = .05 are z * = ±1.96. This will be the criteria we use to test our hypothesis. We can now draw out our distribution, as shown in Figure 7.4 , so we can visualize the rejection region and make sure it makes sense.
Figure 7.4. Rejection region for z * = ±1.96. (“ Rejection Region z+-1.96 ” by Judy Schmitt is licensed under CC BY-NC-SA 4.0 .)
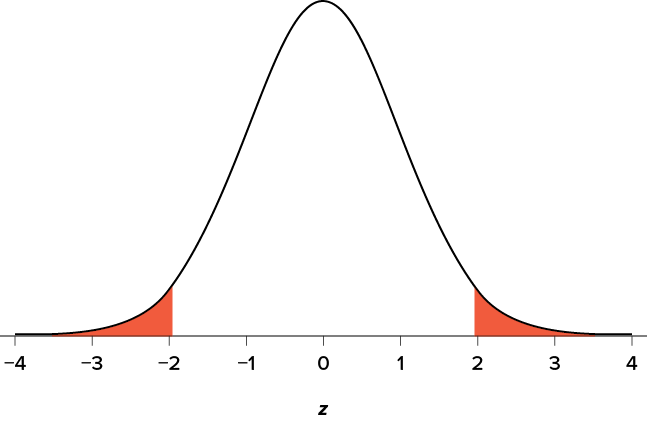
Now we come to our formal calculations. Let’s say that the manager collects data and finds that the average weight of this employee’s popcorn bags is M = 7.75 cups. We can now plug this value, along with the values presented in the original problem, into our equation for z :
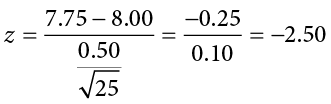
So our test statistic is z = −2.50, which we can draw onto our rejection region distribution as shown in Figure 7.5 .
Figure 7.5. Test statistic location. (“ Test Statistic Location z-2.50 ” by Judy Schmitt is licensed under CC BY-NC-SA 4.0 .)
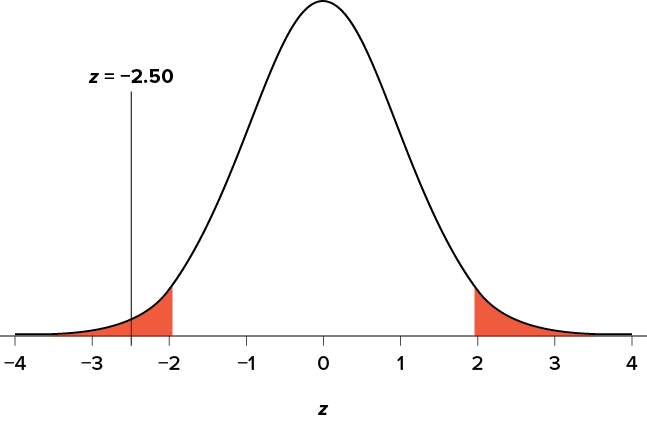
Effect Size
When we reject the null hypothesis, we are stating that the difference we found was statistically significant, but we have mentioned several times that this tells us nothing about practical significance. To get an idea of the actual size of what we found, we can compute a new statistic called an effect size. Effect size gives us an idea of how large, important, or meaningful a statistically significant effect is. For mean differences like we calculated here, our effect size is Cohen’s d :
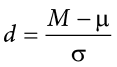
This is very similar to our formula for z , but we no longer take into account the sample size (since overly large samples can make it too easy to reject the null). Cohen’s d is interpreted in units of standard deviations, just like z . For our example:
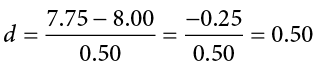
Cohen’s d is interpreted as small, moderate, or large. Specifically, d = 0.20 is small, d = 0.50 is moderate, and d = 0.80 is large. Obviously, values can fall in between these guidelines, so we should use our best judgment and the context of the problem to make our final interpretation of size. Our effect size happens to be exactly equal to one of these, so we say that there is a moderate effect.
Effect sizes are incredibly useful and provide important information and clarification that overcomes some of the weakness of hypothesis testing. Any time you perform a hypothesis test, whether statistically significant or not, you should always calculate and report effect size.
Looking at Figure 7.5 , we can see that our obtained z statistic falls in the rejection region. We can also directly compare it to our critical value: in terms of absolute value, −2.50 > −1.96, so we reject the null hypothesis. We can now write our conclusion:
Reject H 0 . Based on the sample of 25 bags, we can conclude that the average popcorn bag from this employee is smaller ( M = 7.75 cups) than the average weight of popcorn bags at this movie theater, and the effect size was moderate, z = −2.50, p < .05, d = 0.50.
Example B Office Temperature
Let’s do another example to solidify our understanding. Let’s say that the office building you work in is supposed to be kept at 74 degrees Fahrenheit during the summer months but is allowed to vary by 1 degree in either direction. You suspect that, as a cost saving measure, the temperature was secretly set higher. You set up a formal way to test your hypothesis.
You start by laying out the null hypothesis:

Next you state the alternative hypothesis. You have reason to suspect a specific direction of change, so you make a one-tailed test:

You know that the most common level of significance is a = .05, so you keep that the same and know that the critical value for a one-tailed z test is z * = 1.645. To keep track of the directionality of the test and rejection region, you draw out your distribution as shown in Figure 7.6 .
Figure 7.6. Rejection region. (“ Rejection Region z1.645 ” by Judy Schmitt is licensed under CC BY-NC-SA 4.0 .)
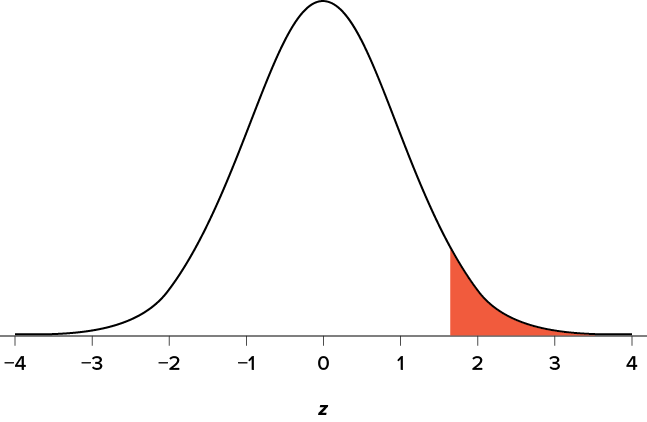
Now that you have everything set up, you spend one week collecting temperature data:
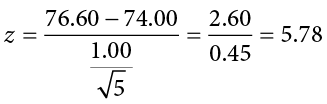
This value falls so far into the tail that it cannot even be plotted on the distribution ( Figure 7.7 )! Because the result is significant, you also calculate an effect size:
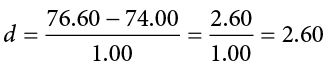
The effect size you calculate is definitely large, meaning someone has some explaining to do!
Figure 7.7. Obtained z statistic. (“ Obtained z5.77 ” by Judy Schmitt is licensed under CC BY-NC-SA 4.0 .)
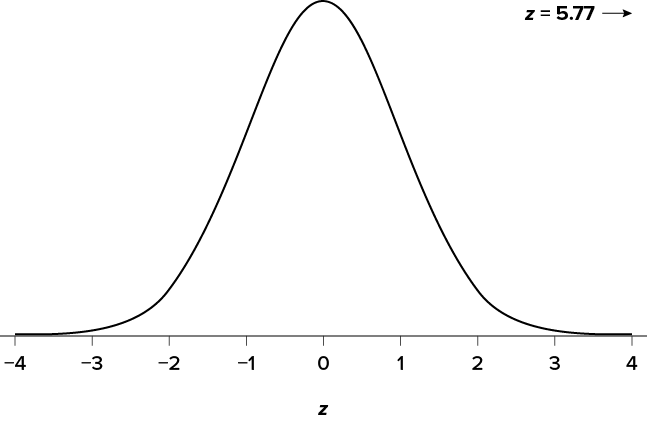
You compare your obtained z statistic, z = 5.77, to the critical value, z * = 1.645, and find that z > z *. Therefore you reject the null hypothesis, concluding:
Reject H 0 . Based on 5 observations, the average temperature ( M = 76.6 degrees) is statistically significantly higher than it is supposed to be, and the effect size was large, z = 5.77, p < .05, d = 2.60.
Example C Different Significance Level
Finally, let’s take a look at an example phrased in generic terms, rather than in the context of a specific research question, to see the individual pieces one more time. This time, however, we will use a stricter significance level, a = .01, to test the hypothesis.
We will use 60 as an arbitrary null hypothesis value:

We will assume a two-tailed test:
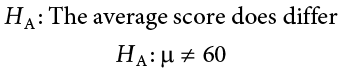
We have seen the critical values for z tests at a = .05 levels of significance several times. To find the values for a = .01, we will go to the Standard Normal Distribution Table and find the z score cutting off .005 (.01 divided by 2 for a two-tailed test) of the area in the tail, which is z * = ±2.575. Notice that this cutoff is much higher than it was for a = .05. This is because we need much less of the area in the tail, so we need to go very far out to find the cutoff. As a result, this will require a much larger effect or much larger sample size in order to reject the null hypothesis.
We can now calculate our test statistic. We will use s = 10 as our known population standard deviation and the following data to calculate our sample mean:
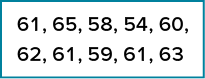
The average of these scores is M = 60.40. From this we calculate our z statistic as:
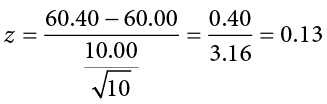
The Cohen’s d effect size calculation is:

Our obtained z statistic, z = 0.13, is very small. It is much less than our critical value of 2.575. Thus, this time, we fail to reject the null hypothesis. Our conclusion would look something like:
Fail to reject H 0 . Based on the sample of 10 scores, we cannot conclude that there is an effect causing the mean ( M = 60.40) to be statistically significantly different from 60.00, z = 0.13, p > .01, d = 0.04, and the effect size supports this interpretation.
Other Considerations in Hypothesis Testing
There are several other considerations we need to keep in mind when performing hypothesis testing.
Errors in Hypothesis Testing
In the Physicians’ Reactions case study, the probability value associated with the significance test is .0057. Therefore, the null hypothesis was rejected, and it was concluded that physicians intend to spend less time with obese patients. Despite the low probability value, it is possible that the null hypothesis of no true difference between obese and average-weight patients is true and that the large difference between sample means occurred by chance. If this is the case, then the conclusion that physicians intend to spend less time with obese patients is in error. This type of error is called a Type I error. More generally, a Type I error occurs when a significance test results in the rejection of a true null hypothesis.
The second type of error that can be made in significance testing is failing to reject a false null hypothesis. This kind of error is called a Type II error . Unlike a Type I error, a Type II error is not really an error. When a statistical test is not significant, it means that the data do not provide strong evidence that the null hypothesis is false. Lack of significance does not support the conclusion that the null hypothesis is true. Therefore, a researcher should not make the mistake of incorrectly concluding that the null hypothesis is true when a statistical test was not significant. Instead, the researcher should consider the test inconclusive. Contrast this with a Type I error in which the researcher erroneously concludes that the null hypothesis is false when, in fact, it is true.
A Type II error can only occur if the null hypothesis is false. If the null hypothesis is false, then the probability of a Type II error is called b (“beta”). The probability of correctly rejecting a false null hypothesis equals 1 − b and is called statistical power . Power is simply our ability to correctly detect an effect that exists. It is influenced by the size of the effect (larger effects are easier to detect), the significance level we set (making it easier to reject the null makes it easier to detect an effect, but increases the likelihood of a Type I error), and the sample size used (larger samples make it easier to reject the null).
Misconceptions in Hypothesis Testing
Misconceptions about significance testing are common. This section lists three important ones.
- Misconception: The probability value ( p value) is the probability that the null hypothesis is false. Proper interpretation: The probability value ( p value) is the probability of a result as extreme or more extreme given that the null hypothesis is true. It is the probability of the data given the null hypothesis. It is not the probability that the null hypothesis is false.
- Misconception: A low probability value indicates a large effect. Proper interpretation: A low probability value indicates that the sample outcome (or an outcome more extreme) would be very unlikely if the null hypothesis were true. A low probability value can occur with small effect sizes, particularly if the sample size is large.
- Misconception: A non-significant outcome means that the null hypothesis is probably true. Proper interpretation: A non-significant outcome means that the data do not conclusively demonstrate that the null hypothesis is false.
- In your own words, explain what the null hypothesis is.
- What are Type I and Type II errors?
- Why do we phrase null and alternative hypotheses with population parameters and not sample means?
- Why do we state our hypotheses and decision criteria before we collect our data?
- Why do you calculate an effect size?
- z = 1.99, two-tailed test at a = .05
- z = 0.34, z * = 1.645
- p = .03, a = .05
- p = .015, a = .01
Answers to Odd-Numbered Exercises
Your answer should include mention of the baseline assumption of no difference between the sample and the population.
Alpha is the significance level. It is the criterion we use when deciding to reject or fail to reject the null hypothesis, corresponding to a given proportion of the area under the normal distribution and a probability of finding extreme scores assuming the null hypothesis is true.
We always calculate an effect size to see if our research is practically meaningful or important. NHST (null hypothesis significance testing) is influenced by sample size but effect size is not; therefore, they provide complimentary information.

“ Null Hypothesis ” by Randall Munroe/xkcd.com is licensed under CC BY-NC 2.5 .)
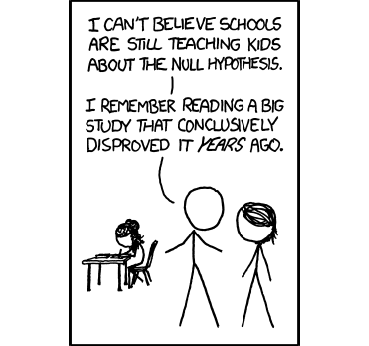
Introduction to Statistics in the Psychological Sciences Copyright © 2021 by Linda R. Cote Ph.D.; Rupa G. Gordon Ph.D.; Chrislyn E. Randell Ph.D.; Judy Schmitt; and Helena Marvin is licensed under a Creative Commons Attribution-NonCommercial-ShareAlike 4.0 International License , except where otherwise noted.
Share This Book
Module 6: Motivation in the Workplace
The hawthorne effect, learning outcome.
- Explain the role of the Hawthorne effect in management
During the 1920s, a series of studies that marked a change in the direction of motivational and managerial theory was conducted by Elton Mayo on workers at the Hawthorne plant of the Western Electric Company in Illinois. Previous studies, in particular Frederick Taylor’s work, took a “man as machine” view and focused on ways of improving individual performance. Hawthorne, however, set the individual in a social context, arguing that employees’ performance is influenced by work surroundings and coworkers as much as by employee ability and skill. The Hawthorne studies are credited with focusing managerial strategy on the socio-psychological aspects of human behavior in organizations.
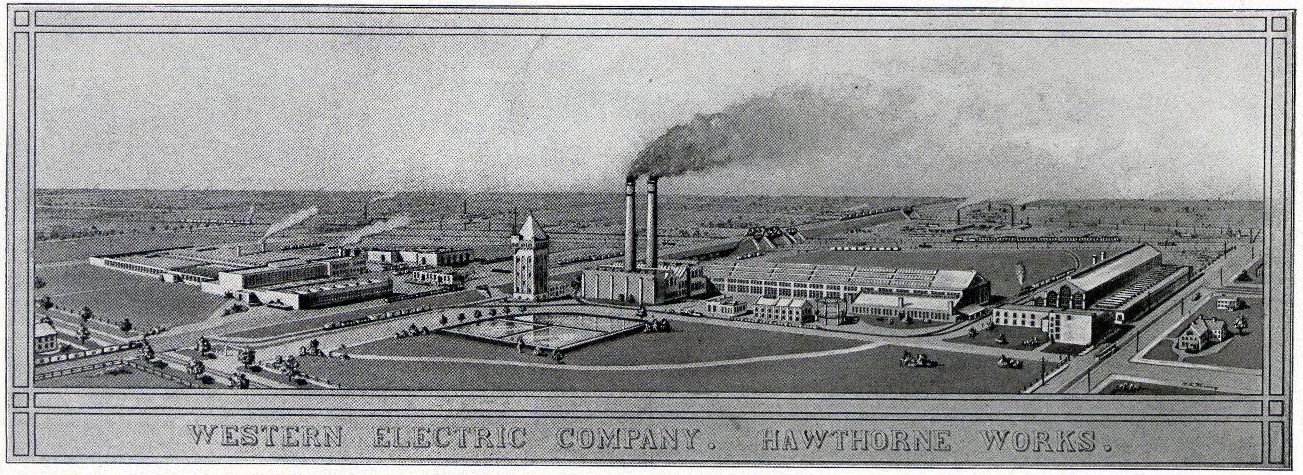
The following video from the AT&T archives contains interviews with individuals who participated in these studies. It provides insight into the way the studies were conducted and how they changed employers’ views on worker motivation.
The studies originally looked into the effects of physical conditions on productivity and whether workers were more responsive and worked more efficiently under certain environmental conditions, such as improved lighting. The results were surprising: Mayo found that workers were more responsive to social factors—such as their manager and coworkers—than the factors (lighting, etc.) the researchers set out to investigate. In fact, worker productivity improved when the lights were dimmed again and when everything had been returned to the way it was before the experiment began, productivity at the factory was at its highest level and absenteeism had plummeted.
What happened was Mayo discovered that workers were highly responsive to additional attention from their managers and the feeling that their managers actually cared about and were interested in their work. The studies also found that although financial incentives are important drivers of worker productivity, social factors are equally important.
Practice Question
There were a number of other experiments conducted in the Hawthorne studies, including one in which two women were chosen as test subjects and were then asked to choose four other workers to join the test group. Together, the women worked assembling telephone relays in a separate room over the course of five years (1927–1932). Their output was measured during this time—at first, in secret. It started two weeks before moving the women to an experiment room and continued throughout the study. In the experiment room, they were assigned to a supervisor who discussed changes with them and, at times, used the women’s suggestions. The researchers then spent five years measuring how different variables affected both the group’s and the individuals’ productivity. Some of the variables included giving two five-minute breaks (after a discussion with the group on the best length of time), and then changing to two ten-minute breaks (not the preference of the group).
Changing a variable usually increased productivity, even if the variable was just a change back to the original condition. Researchers concluded that the employees worked harder because they thought they were being monitored individually. Researchers hypothesized that choosing one’s own coworkers, working as a group, being treated as special (as evidenced by working in a separate room), and having a sympathetic supervisor were the real reasons for the productivity increase.
The Hawthorne studies showed that people’s work performance is dependent on social issues and job satisfaction. The studies concluded that tangible motivators such as monetary incentives and good working conditions are generally less important in improving employee productivity than intangible motivators such as meeting individuals’ desire to belong to a group and be included in decision making and work.
Contribute!
Improve this page Learn More
- Boundless Management. Provided by : Boundless. Located at : https://courses.lumenlearning.com/boundless-management/ . License : CC BY-SA: Attribution-ShareAlike
- Revision and adaptation. Authored by : Linda Williams and Lumen Learning. Provided by : Tidewater Community College. Located at : https://courses.lumenlearning.com/wmopen-introductiontobusiness/chapter/introduction-to-the-hawthorne-effect/ . License : CC BY-SA: Attribution-ShareAlike
- AT&T Archives: The Year They Discovered People. Provided by : AT&T Tech Channel. Located at : https://youtu.be/D3pDWt7GntI . License : All Rights Reserved . License Terms : Standard YouTube License
- Hawthorne Works. Provided by : Western Electric Company. Located at : https://commons.wikimedia.org/wiki/File:Hawthorne_Works_aerial_view_ca_1920_pg_2.jpg . License : Public Domain: No Known Copyright
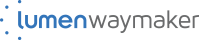
If you're seeing this message, it means we're having trouble loading external resources on our website.
If you're behind a web filter, please make sure that the domains *.kastatic.org and *.kasandbox.org are unblocked.
To log in and use all the features of Khan Academy, please enable JavaScript in your browser.
Official SAT Practice Test 1 Review
Course: official sat practice test 1 review > unit 1.
- Reviewing your SAT practice test
- Reading and Writing - Part 1
Reading and Writing - Part 2
- Math - Part 1
- Math - Part 2
Question 1 | Words in context
- (Choice A) overreacts A overreacts
- (Choice B) reciprocates B reciprocates
- (Choice C) retaliates C retaliates
- (Choice D) deviates D deviates
Question 2 | Words in context
- (Choice A) recognizable A recognizable
- (Choice B) intriguing B intriguing
- (Choice C) significant C significant
- (Choice D) useful D useful
Question 3 | Words in context
- (Choice A) responsiveness to A responsiveness to
- (Choice B) ambivalence toward B ambivalence toward
- (Choice C) renunciation of C renunciation of
- (Choice D) mastery over D mastery over
Question 4 | Words in context
- (Choice A) predatory A predatory
- (Choice B) obscure B obscure
- (Choice C) diverse C diverse
- (Choice D) localized D localized
Question 5 | Words in context
- (Choice A) prevail A prevail
- (Choice B) succumb B succumb
- (Choice C) diverge C diverge
- (Choice D) intersect D intersect
Question 6 | Words in context
- (Choice A) disorienting A disorienting
- (Choice B) tenuous B tenuous
- (Choice C) nuanced C nuanced
- (Choice D) unoriginal D unoriginal
Question 7 | Words in context
- (Choice A) unobtrusive A unobtrusive
- (Choice B) concealed B concealed
- (Choice C) approximate C approximate
- (Choice D) peripheral D peripheral
Question 8 | Words in context
- (Choice A) pragmatic A pragmatic
- (Choice B) controversial B controversial
- (Choice C) extensive C extensive
- (Choice D) universal D universal
Question 9 | Central ideas and details
- (Choice A) He asks about the meaning of the crowd’s shouting, even though he claims to know what the crowd wants. A He asks about the meaning of the crowd’s shouting, even though he claims to know what the crowd wants.
- (Choice B) He indicates a desire to speak to the crowd, even though the crowd has asked to speak to the Sub-Warden. B He indicates a desire to speak to the crowd, even though the crowd has asked to speak to the Sub-Warden.
- (Choice C) He expresses sympathy for the crowd’s demands, even though the crowd’s shouting annoys him. C He expresses sympathy for the crowd’s demands, even though the crowd’s shouting annoys him.
- (Choice D) He describes the crowd as being united, even though the crowd clearly appears otherwise. D He describes the crowd as being united, even though the crowd clearly appears otherwise.
Question 10 | Command of textual evidence
- (Choice A) Chambi took many commissioned portraits of wealthy Peruvians, but he also produced hundreds of images carefully documenting the peoples, sites, and customs of Indigenous communities of the Andes. A Chambi took many commissioned portraits of wealthy Peruvians, but he also produced hundreds of images carefully documenting the peoples, sites, and customs of Indigenous communities of the Andes.
- (Choice B) Chambi’s photographs demonstrate a high level of technical skill, as seen in his strategic use of illumination to create dramatic light and shadow contrasts. B Chambi’s photographs demonstrate a high level of technical skill, as seen in his strategic use of illumination to create dramatic light and shadow contrasts.
- (Choice C) During his lifetime, Chambi was known and celebrated both within and outside his native Peru, as his work was published in places like Argentina, Spain, and Mexico. C During his lifetime, Chambi was known and celebrated both within and outside his native Peru, as his work was published in places like Argentina, Spain, and Mexico.
- (Choice D) Some of the peoples and places Chambi photographed had long been popular subjects for Peruvian photographers. D Some of the peoples and places Chambi photographed had long been popular subjects for Peruvian photographers.
Question 11 | Command of textual evidence
- (Choice A) “The first collective I joined included many amazingly talented artists, and we enjoyed each other’s company, but because we had a hard time sharing credit and responsibility for our work, the collective didn’t last.” A “The first collective I joined included many amazingly talented artists, and we enjoyed each other’s company, but because we had a hard time sharing credit and responsibility for our work, the collective didn’t last.”
- (Choice B) “We work together, but that doesn’t mean that individual projects are equally the work of all of us. Many of our projects are primarily the responsibility of whoever originally proposed the work to the group.” B “We work together, but that doesn’t mean that individual projects are equally the work of all of us. Many of our projects are primarily the responsibility of whoever originally proposed the work to the group.”
- (Choice C) “Having worked as a member of a collective for several years, it’s sometimes hard to recall what it was like to work alone without the collective’s support. But that support encourages my individual expression rather than limits it.” C “Having worked as a member of a collective for several years, it’s sometimes hard to recall what it was like to work alone without the collective’s support. But that support encourages my individual expression rather than limits it.”
- (Choice D) “Sometimes an artist from outside the collective will choose to collaborate with us on a project, but all of those projects fit within the larger themes of the work the collective does on its own.” D “Sometimes an artist from outside the collective will choose to collaborate with us on a project, but all of those projects fit within the larger themes of the work the collective does on its own.”
Question 12 | Command of textual evidence
- (Choice A) Declines in the regional abundance of dusky sharks’ prey other than cownose rays are associated with regional declines in dusky shark abundance. A Declines in the regional abundance of dusky sharks’ prey other than cownose rays are associated with regional declines in dusky shark abundance.
- (Choice B) Eastern oyster abundance tends to be greater in areas with both dusky sharks and cownose rays than in areas with only dusky sharks. B Eastern oyster abundance tends to be greater in areas with both dusky sharks and cownose rays than in areas with only dusky sharks.
- (Choice C) Consumption of eastern oysters by cownose rays in the region substantially increased before the regional decline in dusky shark abundance began. C Consumption of eastern oysters by cownose rays in the region substantially increased before the regional decline in dusky shark abundance began.
- (Choice D) Cownose rays have increased in regional abundance as dusky sharks have decreased in regional abundance. D Cownose rays have increased in regional abundance as dusky sharks have decreased in regional abundance.
Question 13 | Command of textual evidence
- (Choice A) The folklore that the ethnographers collected included several songs written in the form of a décima , a type of poem originating in late sixteenth-century Spain. A The folklore that the ethnographers collected included several songs written in the form of a décima , a type of poem originating in late sixteenth-century Spain.
- (Choice B) Much of the folklore that the ethnographers collected had similar elements from region to region. B Much of the folklore that the ethnographers collected had similar elements from region to region.
- (Choice C) Most of the folklore that the ethnographers collected was previously unknown to scholars. C Most of the folklore that the ethnographers collected was previously unknown to scholars.
- (Choice D) Most of the folklore that the ethnographers collected consisted of corridos —ballads about history and social life—of a clearly recent origin. D Most of the folklore that the ethnographers collected consisted of corridos —ballads about history and social life—of a clearly recent origin.
Question 14 | Inferences
- (Choice A) individual male tanagers can engage in honest signaling without relying on carotenoid consumption. A individual male tanagers can engage in honest signaling without relying on carotenoid consumption.
- (Choice B) feather microstructures may be less effective than deeply saturated feathers for signaling overall fitness. B feather microstructures may be less effective than deeply saturated feathers for signaling overall fitness.
- (Choice C) scientists have yet to determine why tanagers have a preference for mates with colorful appearances. C scientists have yet to determine why tanagers have a preference for mates with colorful appearances.
- (Choice D) a male tanager’s appearance may function as a dishonest signal of the individual’s overall fitness. D a male tanager’s appearance may function as a dishonest signal of the individual’s overall fitness.
Question 15 | Inferences
- (Choice A) face-like stimuli are likely perceived as harmless by newborns of social species that practice parental care but as threatening by newborns of solitary species without parental care. A face-like stimuli are likely perceived as harmless by newborns of social species that practice parental care but as threatening by newborns of solitary species without parental care.
- (Choice B) researchers should not assume that an innate attraction to face-like stimuli is necessarily an adaptation related to social interaction or parental care. B researchers should not assume that an innate attraction to face-like stimuli is necessarily an adaptation related to social interaction or parental care.
- (Choice C) researchers can assume that the attraction to face-like stimuli that is seen in social species that practice parental care is learned rather than innate. C researchers can assume that the attraction to face-like stimuli that is seen in social species that practice parental care is learned rather than innate.
- (Choice D) newly hatched Testudo tortoises show a stronger preference for face-like stimuli than adult Testudo tortoises do. D newly hatched Testudo tortoises show a stronger preference for face-like stimuli than adult Testudo tortoises do.
Question 16 | Inferences
- (Choice A) digital technologies made it easier than it had been previously for authors to write very long works and get them published. A digital technologies made it easier than it had been previously for authors to write very long works and get them published.
- (Choice B) customers generally expected the cost of books to decline relative to the cost of other consumer goods. B customers generally expected the cost of books to decline relative to the cost of other consumer goods.
- (Choice C) publishers increased the variety of their offerings by printing more unique titles but also printed fewer copies of each title. C publishers increased the variety of their offerings by printing more unique titles but also printed fewer copies of each title.
- (Choice D) the costs of writing, editing, and designing a book were less affected by the technologies used than were the costs of manufacturing and distributing a book. D the costs of writing, editing, and designing a book were less affected by the technologies used than were the costs of manufacturing and distributing a book.
Question 17 | Boundaries
- (Choice A) compound, aluminum oxide A compound, aluminum oxide
- (Choice B) compound aluminum oxide, B compound aluminum oxide,
- (Choice C) compound, aluminum oxide, C compound, aluminum oxide,
- (Choice D) compound aluminum oxide D compound aluminum oxide
Question 18 | Form, structure, and sense
- (Choice A) are A are
- (Choice B) have been B have been
- (Choice C) were C were
- (Choice D) is D is
Question 19 | Boundaries
- (Choice A) biologist, Yuree Lee A biologist, Yuree Lee
- (Choice B) biologist Yuree Lee, B biologist Yuree Lee,
- (Choice C) biologist Yuree Lee C biologist Yuree Lee
- (Choice D) biologist, Yuree Lee, D biologist, Yuree Lee,
Question 20 | Boundaries
- (Choice A) food: A food:
- (Choice B) food, B food,
- (Choice C) food while C food while
- (Choice D) food D food
Question 21 | Form, structure, and sense
- (Choice B) is B is
- (Choice D) have been D have been
Question 22 | Boundaries
- (Choice A) equations, though: A equations, though:
- (Choice B) equations, though, B equations, though,
- (Choice C) equations. Though, C equations. Though,
- (Choice D) equations though D equations though
Question 23 | Transitions
- (Choice A) Specifically, A Specifically,
- (Choice B) Similarly, B Similarly,
- (Choice C) Nevertheless, C Nevertheless,
- (Choice D) Hence, D Hence,
Question 24 | Transitions
- (Choice A) However, A However,
- (Choice B) Additionally, B Additionally,
- (Choice C) In comparison, C In comparison,
- (Choice D) For example, D For example,
Question 25 | Transitions
- (Choice A) Subsequently, A Subsequently,
- (Choice B) Besides, B Besides,
- (Choice D) Thus, D Thus,
Question 26 | Rhetorical synthesis
- The Gullah are a group of African Americans who have lived in parts of the southeastern United States since the 18th century.
- Gullah culture is influenced by West African and Central African traditions.
- Louise Miller Cohen is a Gullah historian, storyteller, and preservationist.
- She founded the Gullah Museum of Hilton Head Island, South Carolina, in 2003.
- Vermelle Rodrigues is a Gullah historian, artist, and preservationist.
- She founded the Gullah Museum of Georgetown, South Carolina, in 2003.
- (Choice A) At the Gullah Museums in Hilton Head Island and Georgetown, South Carolina, visitors can learn more about the Gullah people who have lived in the region for centuries. A At the Gullah Museums in Hilton Head Island and Georgetown, South Carolina, visitors can learn more about the Gullah people who have lived in the region for centuries.
- (Choice B) Louise Miller Cohen and Vermelle Rodrigues have worked to preserve the culture of the Gullah people, who have lived in the United States since the 18th century. B Louise Miller Cohen and Vermelle Rodrigues have worked to preserve the culture of the Gullah people, who have lived in the United States since the 18th century.
- (Choice C) Since 2003, Louise Miller Cohen and Vermelle Rodrigues have worked to preserve Gullah culture through their museums. C Since 2003, Louise Miller Cohen and Vermelle Rodrigues have worked to preserve Gullah culture through their museums.
- (Choice D) Influenced by the traditions of West and Central Africa, Gullah culture developed in parts of the southeastern United States in the 18th century. D Influenced by the traditions of West and Central Africa, Gullah culture developed in parts of the southeastern United States in the 18th century.
Question 27 | Rhetorical synthesis
- The factors that affect clutch size (the number of eggs laid at one time) have been well studied in birds but not in lizards.
- A team led by Shai Meiri of Tel Aviv University investigated which factors influence lizard clutch size.
- Meiri’s team obtained clutch-size and habitat data for over 3,900 lizard species and analyzed the data with statistical models.
- Larger clutch size was associated with environments in higher latitudes that have more seasonal change.
- Lizards in higher-latitude environments may lay larger clutches to take advantage of shorter windows of favorable conditions.
- (Choice A) Researchers wanted to know which factors influence lizard egg clutch size because such factors have been well studied in birds but not in lizards. A Researchers wanted to know which factors influence lizard egg clutch size because such factors have been well studied in birds but not in lizards.
- (Choice B) After they obtained data for over 3,900 lizard species, researchers determined that larger clutch size was associated with environments in higher latitudes that have more seasonal change. B After they obtained data for over 3,900 lizard species, researchers determined that larger clutch size was associated with environments in higher latitudes that have more seasonal change.
- (Choice C) We now know that lizards in higher-latitude environments may lay larger clutches to take advantage of shorter windows of favorable conditions. C We now know that lizards in higher-latitude environments may lay larger clutches to take advantage of shorter windows of favorable conditions.
- (Choice D) Researchers obtained clutch-size and habitat data for over 3,900 lizard species and analyzed the data with statistical models. D Researchers obtained clutch-size and habitat data for over 3,900 lizard species and analyzed the data with statistical models.
Want to join the conversation?
- Upvote Button navigates to signup page
- Downvote Button navigates to signup page
- Flag Button navigates to signup page

2.4 Developing a Hypothesis
Learning objectives.
- Distinguish between a theory and a hypothesis.
- Discover how theories are used to generate hypotheses and how the results of studies can be used to further inform theories.
- Understand the characteristics of a good hypothesis.
Theories and Hypotheses
Before describing how to develop a hypothesis it is imporant to distinguish betwee a theory and a hypothesis. A theory is a coherent explanation or interpretation of one or more phenomena. Although theories can take a variety of forms, one thing they have in common is that they go beyond the phenomena they explain by including variables, structures, processes, functions, or organizing principles that have not been observed directly. Consider, for example, Zajonc’s theory of social facilitation and social inhibition. He proposed that being watched by others while performing a task creates a general state of physiological arousal, which increases the likelihood of the dominant (most likely) response. So for highly practiced tasks, being watched increases the tendency to make correct responses, but for relatively unpracticed tasks, being watched increases the tendency to make incorrect responses. Notice that this theory—which has come to be called drive theory—provides an explanation of both social facilitation and social inhibition that goes beyond the phenomena themselves by including concepts such as “arousal” and “dominant response,” along with processes such as the effect of arousal on the dominant response.
Outside of science, referring to an idea as a theory often implies that it is untested—perhaps no more than a wild guess. In science, however, the term theory has no such implication. A theory is simply an explanation or interpretation of a set of phenomena. It can be untested, but it can also be extensively tested, well supported, and accepted as an accurate description of the world by the scientific community. The theory of evolution by natural selection, for example, is a theory because it is an explanation of the diversity of life on earth—not because it is untested or unsupported by scientific research. On the contrary, the evidence for this theory is overwhelmingly positive and nearly all scientists accept its basic assumptions as accurate. Similarly, the “germ theory” of disease is a theory because it is an explanation of the origin of various diseases, not because there is any doubt that many diseases are caused by microorganisms that infect the body.
A hypothesis , on the other hand, is a specific prediction about a new phenomenon that should be observed if a particular theory is accurate. It is an explanation that relies on just a few key concepts. Hypotheses are often specific predictions about what will happen in a particular study. They are developed by considering existing evidence and using reasoning to infer what will happen in the specific context of interest. Hypotheses are often but not always derived from theories. So a hypothesis is often a prediction based on a theory but some hypotheses are a-theoretical and only after a set of observations have been made, is a theory developed. This is because theories are broad in nature and they explain larger bodies of data. So if our research question is really original then we may need to collect some data and make some observation before we can develop a broader theory.
Theories and hypotheses always have this if-then relationship. “ If drive theory is correct, then cockroaches should run through a straight runway faster, and a branching runway more slowly, when other cockroaches are present.” Although hypotheses are usually expressed as statements, they can always be rephrased as questions. “Do cockroaches run through a straight runway faster when other cockroaches are present?” Thus deriving hypotheses from theories is an excellent way of generating interesting research questions.
But how do researchers derive hypotheses from theories? One way is to generate a research question using the techniques discussed in this chapter and then ask whether any theory implies an answer to that question. For example, you might wonder whether expressive writing about positive experiences improves health as much as expressive writing about traumatic experiences. Although this question is an interesting one on its own, you might then ask whether the habituation theory—the idea that expressive writing causes people to habituate to negative thoughts and feelings—implies an answer. In this case, it seems clear that if the habituation theory is correct, then expressive writing about positive experiences should not be effective because it would not cause people to habituate to negative thoughts and feelings. A second way to derive hypotheses from theories is to focus on some component of the theory that has not yet been directly observed. For example, a researcher could focus on the process of habituation—perhaps hypothesizing that people should show fewer signs of emotional distress with each new writing session.
Among the very best hypotheses are those that distinguish between competing theories. For example, Norbert Schwarz and his colleagues considered two theories of how people make judgments about themselves, such as how assertive they are (Schwarz et al., 1991) [1] . Both theories held that such judgments are based on relevant examples that people bring to mind. However, one theory was that people base their judgments on the number of examples they bring to mind and the other was that people base their judgments on how easily they bring those examples to mind. To test these theories, the researchers asked people to recall either six times when they were assertive (which is easy for most people) or 12 times (which is difficult for most people). Then they asked them to judge their own assertiveness. Note that the number-of-examples theory implies that people who recalled 12 examples should judge themselves to be more assertive because they recalled more examples, but the ease-of-examples theory implies that participants who recalled six examples should judge themselves as more assertive because recalling the examples was easier. Thus the two theories made opposite predictions so that only one of the predictions could be confirmed. The surprising result was that participants who recalled fewer examples judged themselves to be more assertive—providing particularly convincing evidence in favor of the ease-of-retrieval theory over the number-of-examples theory.
Theory Testing
The primary way that scientific researchers use theories is sometimes called the hypothetico-deductive method (although this term is much more likely to be used by philosophers of science than by scientists themselves). A researcher begins with a set of phenomena and either constructs a theory to explain or interpret them or chooses an existing theory to work with. He or she then makes a prediction about some new phenomenon that should be observed if the theory is correct. Again, this prediction is called a hypothesis. The researcher then conducts an empirical study to test the hypothesis. Finally, he or she reevaluates the theory in light of the new results and revises it if necessary. This process is usually conceptualized as a cycle because the researcher can then derive a new hypothesis from the revised theory, conduct a new empirical study to test the hypothesis, and so on. As Figure 2.2 shows, this approach meshes nicely with the model of scientific research in psychology presented earlier in the textbook—creating a more detailed model of “theoretically motivated” or “theory-driven” research.

Figure 2.2 Hypothetico-Deductive Method Combined With the General Model of Scientific Research in Psychology Together they form a model of theoretically motivated research.
As an example, let us consider Zajonc’s research on social facilitation and inhibition. He started with a somewhat contradictory pattern of results from the research literature. He then constructed his drive theory, according to which being watched by others while performing a task causes physiological arousal, which increases an organism’s tendency to make the dominant response. This theory predicts social facilitation for well-learned tasks and social inhibition for poorly learned tasks. He now had a theory that organized previous results in a meaningful way—but he still needed to test it. He hypothesized that if his theory was correct, he should observe that the presence of others improves performance in a simple laboratory task but inhibits performance in a difficult version of the very same laboratory task. To test this hypothesis, one of the studies he conducted used cockroaches as subjects (Zajonc, Heingartner, & Herman, 1969) [2] . The cockroaches ran either down a straight runway (an easy task for a cockroach) or through a cross-shaped maze (a difficult task for a cockroach) to escape into a dark chamber when a light was shined on them. They did this either while alone or in the presence of other cockroaches in clear plastic “audience boxes.” Zajonc found that cockroaches in the straight runway reached their goal more quickly in the presence of other cockroaches, but cockroaches in the cross-shaped maze reached their goal more slowly when they were in the presence of other cockroaches. Thus he confirmed his hypothesis and provided support for his drive theory. (Zajonc also showed that drive theory existed in humans (Zajonc & Sales, 1966) [3] in many other studies afterward).
Incorporating Theory into Your Research
When you write your research report or plan your presentation, be aware that there are two basic ways that researchers usually include theory. The first is to raise a research question, answer that question by conducting a new study, and then offer one or more theories (usually more) to explain or interpret the results. This format works well for applied research questions and for research questions that existing theories do not address. The second way is to describe one or more existing theories, derive a hypothesis from one of those theories, test the hypothesis in a new study, and finally reevaluate the theory. This format works well when there is an existing theory that addresses the research question—especially if the resulting hypothesis is surprising or conflicts with a hypothesis derived from a different theory.
To use theories in your research will not only give you guidance in coming up with experiment ideas and possible projects, but it lends legitimacy to your work. Psychologists have been interested in a variety of human behaviors and have developed many theories along the way. Using established theories will help you break new ground as a researcher, not limit you from developing your own ideas.
Characteristics of a Good Hypothesis
There are three general characteristics of a good hypothesis. First, a good hypothesis must be testable and falsifiable . We must be able to test the hypothesis using the methods of science and if you’ll recall Popper’s falsifiability criterion, it must be possible to gather evidence that will disconfirm the hypothesis if it is indeed false. Second, a good hypothesis must be logical. As described above, hypotheses are more than just a random guess. Hypotheses should be informed by previous theories or observations and logical reasoning. Typically, we begin with a broad and general theory and use deductive reasoning to generate a more specific hypothesis to test based on that theory. Occasionally, however, when there is no theory to inform our hypothesis, we use inductive reasoning which involves using specific observations or research findings to form a more general hypothesis. Finally, the hypothesis should be positive. That is, the hypothesis should make a positive statement about the existence of a relationship or effect, rather than a statement that a relationship or effect does not exist. As scientists, we don’t set out to show that relationships do not exist or that effects do not occur so our hypotheses should not be worded in a way to suggest that an effect or relationship does not exist. The nature of science is to assume that something does not exist and then seek to find evidence to prove this wrong, to show that really it does exist. That may seem backward to you but that is the nature of the scientific method. The underlying reason for this is beyond the scope of this chapter but it has to do with statistical theory.
Key Takeaways
- A theory is broad in nature and explains larger bodies of data. A hypothesis is more specific and makes a prediction about the outcome of a particular study.
- Working with theories is not “icing on the cake.” It is a basic ingredient of psychological research.
- Like other scientists, psychologists use the hypothetico-deductive method. They construct theories to explain or interpret phenomena (or work with existing theories), derive hypotheses from their theories, test the hypotheses, and then reevaluate the theories in light of the new results.
- Practice: Find a recent empirical research report in a professional journal. Read the introduction and highlight in different colors descriptions of theories and hypotheses.
- Schwarz, N., Bless, H., Strack, F., Klumpp, G., Rittenauer-Schatka, H., & Simons, A. (1991). Ease of retrieval as information: Another look at the availability heuristic. Journal of Personality and Social Psychology, 61 , 195–202. ↵
- Zajonc, R. B., Heingartner, A., & Herman, E. M. (1969). Social enhancement and impairment of performance in the cockroach. Journal of Personality and Social Psychology, 13 , 83–92. ↵
- Zajonc, R.B. & Sales, S.M. (1966). Social facilitation of dominant and subordinate responses. Journal of Experimental Social Psychology, 2 , 160-168. ↵

Share This Book
- Increase Font Size

An official website of the United States government
The .gov means it’s official. Federal government websites often end in .gov or .mil. Before sharing sensitive information, make sure you’re on a federal government site.
The site is secure. The https:// ensures that you are connecting to the official website and that any information you provide is encrypted and transmitted securely.
- Publications
- Account settings
Preview improvements coming to the PMC website in October 2024. Learn More or Try it out now .
- Advanced Search
- Journal List
- HHS Author Manuscripts

More than Tuskegee: Understanding Mistrust about Research Participation
Darcell p. scharff.
a Department of Community Health, School of Public Health, Saint Louis University
Katherine J. Mathews
b Southern Illinois Healthcare Foundation
Pamela Jackson
c Washington University Alzheimer’s Disease Research Center
Jonathan Hoffsuemmer
d Center for Practice Excellence, Barnes-Jewish Hospital Research Center
Emeobong Martin
e Congressional Research Service
Dorothy Edwards
f Departments of Kinesiology, Neurology, Medicine and the Wisconsin Alzheimer’s Institute, University of Wisconsin-Madison
This paper describes results of a qualitative study that explored barriers to research participation among African American adults. A purposive sampling strategy was used to identify African American adults with and without previous research experience. A total of 11 focus groups were conducted. Groups ranged in size from 4–10 participants (N = 70). Mistrust of the health care system emerged as a primary barrier to participation in medical research among participants in our study. Mistrust stems from historical events including the Tuskegee syphilis study and is reinforced by health system issues and discriminatory events that continue to this day. Mistrust was an important barrier expressed across all groups regardless of prior research participation or socioeconomic status. This study illustrates the multifaceted nature of mistrust, and suggests that mistrust remains an important barrier to research participation. Researchers should incorporate strategies to reduce mistrust and thereby increase participation among African Americans.
Despite mandates by the federal government to ensure inclusion of women and minorities in all federally funded research, 1 African Americans continue to participate less frequently than Whites. Lower participation rates among African Americans have been reported across various study types ( e.g. , controlled clinical treatment trials, 2 , 3 intervention trials, 4 , 5 as well as studies on various disease conditions, including AIDS, 6 – 8 Alzheimer’s disease, 9 prostate cancer and other malignancies, 10 – 14 stroke, 15 and cardiovascular disease 16 ).
Several factors that affect the participation of African Americans in studies have been identified 17 , 18 including elements of study design, 19 – 21 logistical problems, low levels of health literacy, sociocultural factors, and specific attitudes that hinder research participation. 17 Mistrust of academic and research institutions and investigators is the most significant attitudinal barrier to research participation reported by African Americans. 8 , 17 , 22 – 29 Its etiology stems from historic events, but is also exacerbated by more current actions, 30 – 34 including socioeconomic and healthcare system inequities. 35 , 36
From a historical perspective, the Tuskegee syphilis study is widely recognized as a reason for mistrust because of the extent and duration of deception and mistreatment and the study’s impact on human subject review and approval. 37 – 39 However, the history of medical and research abuse of African Americans goes well beyond Tuskegee. Harriet Washington eloquently describes the history of medical experimentation and abuse, 40 demonstrating that mistrust of medical research and the health care infrastructure is extensive and persistent among African Americans and illustrating that more than four centuries of a biomedical enterprise designed to exploit African Americans is a principal contributor to current mistrust. As recently as the 1990s, unethical medical research involving African Americans has been conducted by highly esteemed academic institutions. For example, researchers at a prestigious U.S. university recruited African American boys into a study that hypothesized a genetic etiology of aggressive behavior. Through the use of monetary incentives, they were able to convince parents to enroll their sons in a study that included withdrawal from all medications (including asthma medications), ingesting a mono-amine (low protein) diet, an overnight stay (without parents), withholding of water, hourly blood draws, and the administration of fenfluramine, a drug known to increase serotonin levels and suspected to be associated with aggressive behaviors. In addition to these methods, several other significant human subject violations were cited, including restricting the recruitment to Black children. 40 It is fair to ask whether mistreatment of African Americans that has occurred more recently than the Tuskegee syphilis study is exacerbating mistrust today.
Attitudinal studies suggest that mistrust of clinical investigators is strongly influenced by sustained racial disparities in health, limited access to health care, and negative encounters with health care providers. 41 – 43 Beliefs about physician mistrust among African American patients are reinforced through differential treatment in comparison with Whites. Moreover, previous research indicates that a lack of cultural diversity and competence among physicians is a major contributor to African American mistrust of physicians. 36 , 44 , 45 Ethnic minority patients receive less information, empathy, and attention from their physicians regarding their medical care than their White counterparts. 46 Lack of information results in limited awareness, knowledge or understanding of the availability or value of medical research. 34 Further, studies have illustrated that African American patients are less likely to receive medical services than White patients with similar complaints and symptoms. 36
Attitudes of mistrust reflect perceptions about interpersonal and technical incompetence, physician focus on profit, and expectations of experimentation. 44 Several investigators have found that African Americans are more likely than age-, education-, and gender-matched Whites to believe that research findings will be used to reinforce negative stereotypes about their ethnic group 47 or will expose them to unnecessary risks. 25 , 48 Two separate studies examining barriers to African American participation in genetics research found that African American participants worried about the use of DNA data collected in biomedical research in later criminal investigations to implicate innocent people. 49 , 50 Moreover, the literature demonstrates that African Americans report concern that the findings associated with their participation will not benefit the African American community. 24 Finally, several studies suggest that investigators themselves often limit minority participation because they are less likely to ask minority patients to consider enrolling in clinical trials. 6 , 11 , 15 , 46 Despite these concerns, other research has shown that African Americans recognize the value and importance of clinical research and the possibility of new and better treatments for themselves and the African American community emerging from it. 17
This exploratory, qualitative study was undertaken to attempt to understand the barriers to research participation particular to African American adults who reside in a mid-size urban area. This study was designed to gain an in-depth understanding of the factors associated with participation/lack of participation in research studies, including more invasive biomarker and clinical treatment trials sponsored by Washington University, and Siteman Cancer Center. Both had been successful in recruitment of African Americans for surveys and screening studies, but had experienced less success in recruitment for invasive studies or clinical trials. The goal was to identify barriers to research participation, including more complex studies, and then use the findings to develop interventions to improve participation in both cancer and Alzheimer’s-related trials.
Participants and sampling
A purposive sample of African American adults was generated for participation in one of 11 focus groups (N=70). The focus groups were designed to be homogeneous on at least one of three characteristics: previous or current participation in research ( Yes / No ), age category (18–35, 36–55, older than 55 years) and gender and to represent a range of socioeconomic categories. We recruited individuals with and without previous research experience because previous studies have found differences by previous participation 51 , 52 and the researchers in the study groups had intentionally implemented strategies to enhance participation. Participants were recruited in four ways: 1) letters sent and phone calls made to participants enrolled in studies at the Alzheimer’s Disease Research Center at Washington University School of Medicine; 2) coordination with community members and leaders to identify potential participants; 3) advertisements placed in the local African American newspaper; and 4) recruitment flyers posted at a community health center.
The content of the focus group interview was generated by the project investigators, and questions were adapted by the moderator to be conversational. The flow of the questions followed a traditional focus group question format ( i.e. , introductory, key, ending, and summary) to ensure maximum participation by focus group attendees. 53 Probes or follow-up/clarification questions succeeded each of the three main-topic questions (barriers, facilitators, and suggestions to increase participation). Additionally, participants were probed about specific topics that were not always spontaneously generated by them ( e.g. , Does the recruiter need to be your doctor or African American? ).
Data collection
Each focus group lasted one and a half hours and was audio-taped. Recordings were transcribed by a professional transcriptionist. Moderators created an informal atmosphere so that participants felt comfortable sharing both positive and negative perceptions. The co-moderator took notes that reflected the tone and processes of each group, noting characteristics of the groups’ conversation (such as participation, signs of emotion, and non-verbal responses). Each participant received a $25 voucher to a local grocery store. A debriefing between moderators was conducted at the end of each group.
Data analysis
A grounded theory design guided data collection and analysis. Grounded theory is an inductive approach, meaning that there is no preconceived theory (about barriers to research participation, for example) that drives data collection, and the theory evolves from the data. 54 Theory emerges from systematic data collection and the observation of the interrelationships of categories of information. 55 , 56
As an initial step in the analysis, each of the analysis team members independently reviewed the focus group transcripts using a whole-text analysis, open-coding method 57 to identify themes around barriers and facilitators of research participation. Using the debriefing and the co-moderators’ notes, a summary of the group themes, dynamics, and demographic characteristics was then developed. The codes were developed from the major themes that emerged from the first phase of analysis, along with the original focus group guides. The list of theme-generated codes was compared with the original focus group interview guide and items that were not identified by the themes were added as codes. The co-moderator’s notes and debriefing notes were also used as validation of the codes. Additional codes were developed from the notes that were not reflected in the existing list.
In the next step of analysis, independent focused coding 58 occurred and inter-rater reliability was established. Where inconsistencies in the coding occurred, the raters came to consensus on discrepancies. Next, the coded transcripts were reviewed by the senior team member to ensure that the final list of codes adequately reflected the data. New codes were developed to capture new themes or ideas. QSR N6 qualitative software was used to code, retrieve, merge, and analyze chunks of data and annotate data about group dynamics (QSR International, Melbourne, Australia). Coded data were then reorganized into logical categories for presentation.
The final phase of analysis involved identifying significant themes from the data. Themes were considered significant if any of the following characteristics were observed: the theme was discussed frequently, extensive comments around the theme were made, intensity or passion around the theme became evident, and/or stories were used to specify the theme or indicate its relevance to the focus group participant.
Sample characteristics
Table 1 reports the demographic characteristics of focus group participants. All participants were African American adults residing in a mid-size urban area (population: 347,000 59 ). In groups one through four and seven, all participants had previous experience with research participation. Five of the nine groups were made up of women only. We were unable to create groups based on all of the predefined age categories. However, groups one to three mostly comprised older individuals and group 11 comprised individuals who were all under 30 years of age. Focus groups seven and eleven comprised individuals who had at least a bachelor’s degree and earned more than $30,000 per year. Groups five, nine, and ten comprised individuals who earned $30,000 or less each year. The Washington University Human Studies Committee approved all procedures. Informed consent was obtained from participants after the study was fully described.
Demographic characteristics of focus group participants
A number of barrier themes were identified in the study, including mistrust of researchers and the health care system, fear related to research participation, inadequate information about research and opportunities to participate, inconvenience, questionable reputation of the researcher or research institution, and logistical concerns. The themes related to mistrust surfaced as significant in frequency, intensity, extensiveness, and specificity. However, we focus here on mistrust, fear, and inadequate information, and their effects on research participation and their relationships to each other. (Other barrier themes and facilitators such as relevance to individuals and benefit to the African American community are fully discussed in a soon-to-be-published manuscript.) The findings are presented in the aggregate because there were no clear differences about mistrust that emerged by the types of groups, i.e. , there were no differences in mistrust, fear, and inadequate information by gender, education, income, or by prior participation in research.
In general, participants understood that medical research usually occurs within the context of the health care system, and it appeared that participants’ beliefs about one frequently informed their beliefs about the other. Focus group discussions about medical research regularly turned back to discussions about their experiences with the health care system. When this occurred, the moderator confirmed with participants the transferability of their beliefs about the health care system to beliefs about medical research. Additionally, overall, participants recognized that research is both important and necessary for scientists to learn better ways to treat and prevent disease. However, they gave strong voice to a number of barriers that prevent them from participation.
Participants associate the term “medical research” with terms that represent the negative connotations of research, such as experimentation, rats, and test tubes. Experimentation, it was said, is viewed in a particularly negative light, given the history of research in the African American community. More specifically, participants in every focus group suggested that medical research conjures up the term “guinea pig.” Many endorsed the view that this term applied specifically to African Americans (as opposed to other racial or ethnic groups) being used to test medications or procedures. A participant said,
One of the reasons most Black people are reluctant to get involved is suspicion. We’ve been kind of brainwashed, and we’re guinea pigs.
Mistrust of the health care system among African Americans in our sample is deeply ingrained and appears to cross socioeconomic lines, in that mistrust was identified as a barrier to participation in research in every group. In fact, most of the participants in Group 11 (all professionals of high socioeconomic status) discussed recent events that they directly or indirectly experienced in health care or research situations that exacerbated mistrust. For example, one participant described his experience with attempting to enter a study that was evaluating a treatment for razor burn. He asked several questions of the researchers as a way to determine whether to participate. The researchers, he explained, were surprised by the extent of the questions he posed. Additionally, when he informed the researchers that he used clippers to remove whiskers, the researchers were unfamiliar with this method and asked him to describe it. The gentleman decided not to participate: he saw that the researchers were unfamiliar with a technique for hair removal common in the African American community (and relevant for the study) and, consequently, concluded that they were unprepared for African American participants in the study.
Impact of Tuskegee
Participants explained that the lack of trust regarding the health care system among African Americans has historical roots: the Tuskegee syphilis study and others were either explicitly or implicitly referred to in every group. The impact of this event carries on throughout the generations, as this participant explained:
Just that awareness [about Tuskegee] is enough to stand up generation after generation.
Although most understood that men were not treated for syphilis in the Tuskegee study, many believe that men were both injected with the disease and not treated . One participant said,
Most people have gained information on the Tuskegee experiments where they injected these men with the syphilis virus.
This belief remains active within the Black community, regardless of age or socioeconomic status. For example, a young professional understood on the one hand that the government did not infect men with syphilis but believed otherwise given what was heard in the community.
And I think that over time the legend of Tuskegee is more palpable than what people know about what went down. I think I’ve always known. But I’ve always known that the government gave people syphilis, and this is not true.
Much of the mistrust expressed by participants is focused on the federal government as they recognize their role in the support of research studies such as the Tuskegee syphilis study. One participant said,
I think you have a lot of people who mistrust the government. You start looking at a lot of medical centers, there’s always going to be some link up the chain to some government entity.
Participants discussed how the government is supposed to have the best interest of its citizens in mind but has proven on a number of occasions that it does not:
You don’t know what they are giving you and what they’re experimenting on you. They are very secretive. They say one thing and might do another.
In fact, some participants believe that the government only stands to make money through research, especially research performed on underserved individuals.
Participants explained that the result of the Tuskegee syphilis study and other negative historical events have both a rational and emotional component. They argued that after the number of years during which African Americans have been deceived, it makes sense that they do not trust researchers and are not willing to participate in medical research:
It [Tuskegee] becomes a symbol of these two portions of my existence and it becomes a way for me to answer the question, why. So me participating in something else that might be like that, why would I do that to myself?
From an emotional perspective, participants described how the impact of historical and current events effect other decisions they make. One participant used a story to illustrate the depth of the emotion.
I sat in the driver’s license bureau for about 45 minutes and every Black person that was in there, they’d be like, “Would you like to be an organ donor?” And every Black person said no. And every person of another race they asked was like, “Yeah, no problem.” And I immediately said no. And this thing in my head was telling me they will misuse my organs. I don’t even know why I was so emotional.
The emotional toll that history plays on many African Americans was evident in many of the focus group discussions. Participants discussed the Tuskegee syphilis study with passion and provided examples illustrating how it (and other historical events) plays a role in who they are today.
It’s [Tuskegee] part of the sociological and theological question: who are we and why are we in this position?
One woman described being in Tuskegee when President Clinton officially apologized for the experiments on behalf of the U.S. governement. She talked about the fact that the university received a large sum of money in reparation, but that no amount of money could ever take away the hurt she saw first-hand from residents of the Tuskegee area.
I was actually there [Tuskegee] for the satellite telecast of the apology. I got to see some of the participants and it was pretty profound hearing what some of them had to go through. I know now the university has a whole new medical center and they got a lot of money, but that can’t make up for it. And you can’t go back and change what happened. I can’t speak for other people but that was a huge emotional experience for me.
No benefit to African American community
Participants indicated that their relationships with White America have historically been one-sided. They recounted stories in which they gave to White America and received nothing in return.
We were … a lot of Black people don’t ever encounter White people. Whenever I encounter White people, they’re coming to take. The only White person in my neighborhood is the insurance man, and he only takes my money.
In fact, participants indicated that research findings rarely benefit the African American community because they do not see the results of studies. They contend that research usually is conducted for the benefit of others, specifically, Whites.
I think the deception is when we read studies, they don’t relate to us. They don’t … I mean, they’re about another nationality. They’re not really for African Americans. And they don’t apply to us.
Additionally, many expressed that they have not seen any positive results from the research conducted in their community and, therefore, have no expectations:
And it goes back, if you find something, are we ever going to see it? So truly, why does my participation really matter for anything? If it’s not going to produce a product that I’m going to see, why should I be one of the study participants?
Some participants question the motives and practices of the researchers:
I guess it all ties in with the motives and the integrity of those doing the research. Are they doing it because of race, is it class, or is it a combination of both?
Many wondered how information is used by researchers:
How are you going to use this? It still comes back, to me, to that question, how much feeling, how much thought of life and value of life are they putting into this research?
Recent examples of racism or discrimination
Many participants described recent stories about the use of the health care system in which they or someone they know received poor quality health care or was treated disrespectfully. They emphasized that this type of mistreatment occurs today:
I’m not going to go into details. But he wasn’t treated properly, given the proper tests at this hospital. He’s in a coma to this day. And I guess because he’s a Black man. Like I said, I just haven’t seen it happen to White patients.
Such experiences create a lack of respect for health care providers that appears to be fairly prevalent in the community:
I think life experience. Working in the hospital, I have a great deal less respect for doctors and nurses.
Inadequate information
Participants explained that the deception experienced by the men in the Tuskegee syphilis study continues to be a factor for many African Americans today, especially men. Many expressed the belief that, just as the Tuskegee study participants were not informed, they too are not informed by health care providers today. Inadequate information exacerbates mistrust and creates the perception that there is something to hide. Many suggested that health care providers are dishonest, either by leaving out important information when obtaining consent or by misinforming them: I know as a Black American that we are not told all the time the correct truth.
Participants suggested that misinformation (or lack of information) has resulted in African Americans being enrolled in research studies without a full understanding of what their participation meant. They argued that researchers often target vulnerable individuals as study participants because they believe that these individuals are less likely to question them. For example, they talked about the fact that researchers often reach out to the homeless, prisoners, children, elderly, and impoverished when recruiting for studies. Using money as an incentive ensures that marginalized individuals will volunteer to participate in research studies.
I do know that when they offer money for research, they are trying to get probably a different clientele of people. If people are going to be paid a large amount of money, people who need money may be more likely to involve themselves in that
Overall, many participants believe that confusion about research and medical care stems from the lack of education and dissemination to the public. When information is presented, it is rarely done in way that is understandable, and therefore its use is limited.
Additionally, participants suggested that doctors, researchers, and others in health care do not always present information in a way that is respectful and understandable.
The words are important but it’s also the way you present those words, because they can say a lot of words but if it’s not presented correctly or with some kind of feeling that you have concern… just don’t talk to me.
Impact of mistrust
In view of widespread mistrust and suspicion in their community, African Americans in our study indicated that they tend to be reluctant to provide information about themselves. Many discussed the fear that personal information may be used against them at some later date. This belief has a historical etiology that has been sustained throughout the generations.
People are reluctant to go open up because of what’s happened to them. It’s just a fear that they don’t want anyone to know their business.
The deep beliefs described by participants have a major impact on willingness to participate in medical research, as illustrated by the following:
Normally, African Americans are, as you said before, suspicious. They have that first in their mind, well, what are these experimental drugs they’re using, because of the things that have happened in the past.
This study used qualitative methods to explore barriers to participation in research among African American adults who reside in a mid-size urban city. Mistrust was the primary concern voiced by study participants. Literature that describes and explores the role of mistrust among African Americans dates back to the early 1970s, just after the Tuskegee syphilis study became public and uses both quantitative 17 , 24 , 25 , 29 , 42 , 48 and qualitative 17 , 22 , 26 , 27 approaches to describe mistrust and understand its relationship to research participation. Our data support existing literature and deepen the understanding of how multifaceted this mistrust is, how it influences many parts of people’s lives, and how it creates a significant emotional burden. Indeed, our data remind us that ongoing experiences with the health care system perpetuate feelings of mistrust. This continues to reduce our ability to recruit African Americans into research studies and limiting the generalizability of current research findings.
Of particular interest is that the data indicate that barriers are common across multiple subgroups including those who had and had not previously participated in research studies, suggesting that merely participating in research is not enough to lessen mistrust. We intentionally created groups that consisted of individuals who had participated in research (from one of our centers), as there is some work that suggests that prior research participation increases the likelihood of participation. 51 , 52 Additionally, researchers in the study centers had developed multiple strategies to improve low participation among minorities The primary strategies in both cancer and Alzheimer’s disease were to develop long-term partnerships and improve access to quality care. The specific activities included (1) creating community advisory boards (2) delivering culturally targeted education programs (3) partnering with community-based organizations serving the African American community and (4) improving access to clinical care and support services. These findings suggest that previous participation in low risk research, such as survey or focus group studies, will not in and of itself increase participation in more invasive and higher risk studies.
Participants emphasized that historical events such as the Tuskegee syphilis study remain in the minds of many African Americans and often attributed mistrust to this history. History was discussed in every group and across socioeconomic statuses. Many described their beliefs that the federal government, responsible for the Tuskegee study, both injected syphilis into and withheld treatment from study participants beliefs learned from parents and grandparents. Even the more educated participants relate to this history and, although they recognize that Tuskegee participants were not injected, expressed continuing mistrust related to these past events. In fact, the emotional side of mistrust was particularly evident in groups constituted of higher-educated participants. This may reflect the fact that better-educated individuals have greater access to information, in general, and can therefore learn about specific events in more detail. This finding is illuminating because the research is mixed regarding the impact of education on mistrust 60 – 62 , and we could identify no other studies that clearly demonstrate that mistrust is similar across socioeconomic groups.
Although historical events such as Tuskegee foster mistrust, participants stressed that disrespect and discrimination towards African Americans continues to occur. Recent literature supports the view that current occasions of perceived or real racism or discrimination exacerbate mistrust. 63 The Institute of Medicine report on disparities of treatment by race suggests that much disparate care is due to discrimination, both conscious and unconscious. 36 VanRyn and colleagues describe the complex process of decision making that medical providers go through and suggest that perhaps false beliefs about individuals may result in disparate treatment. 64 Some studies, however, argue that participation in research increases discrimination by allowing investigators to highlight problems in the community ( i.e. , to emphasize negative traits of Black individuals and their communities). In fact, Nicholson and colleagues found that African Americans respond negatively to cancer disparity information and positively to messages of hope. 65
Perhaps the most disturbing instance of mistrust that participants described is the belief that information about research studies and their participation is withheld by researchers, which may be one factor that perpetuates feelings of mistrust. In particular, focus group participants indicated they are provided limited or inadequate information about their participation in research. Indeed, this may be one of the most enduring negative fallouts from the Tuskegee syphilis study and other unethical studies. However, these beliefs cannot just be attributed to historic events. For example, a recent study reported that over 50% of physicians prescribe placebos without thoroughly informing patients, suggesting that these beliefs may have merit. 66 As researchers continue to behave in a way that exacerbates mistrust, so will the fear about research among the African American community continue. In turn, there will continue to be low participation rates, resulting in studies that can only be generalized to the White majority. The resulting inability truly to understand the biological and social determinants of disease etiology and progression among minority populations will only deepen the existing disparities in health.
This study has limitations. Qualitative data are descriptive and are not meant to generalize to any broader population. Our goal in this study was to gain in-depth understanding about research participation from individuals who could speak from life experiences about the issue, therefore creating productive conversation. 53 Our data suggest the importance of working with and in the community as a way to understand perceptions specific to a particular community.
As is appropriate with focus group methodology, we developed and used a purposive sampling strategy. 53 We segmented groups by previous research participation, gender, and socioeconomic status. Our findings did not identify differences by segments, suggesting that previous research experience and/or higher socioeconomic status were not enough to change deep-seated beliefs. We were able to recruit a large number of groups, which allowed us to reach saturation (or, repetition) of themes. 55
Although researchers are adept at providing incentives and recruiting from community venues to enhance African Americans participation in studies, it is important to understand that these efforts are not enough to facilitate recruitment into many more involved clinical studies or trials. It is imperative that we understand and act specifically upon mistrust that this and other studies have reported. Several reports outline ways in which researchers and health care providers can gain the trust of community members. 4 , 18 , 24 , 67 – 69 For example, community-based participatory research (CBPR) models claim to improve community-research relationships 70 – 72 although they are still not widely used. 73 CBPR is a collaborative approach to research that equitably involves all partners in the research process, recognizing the unique strengths that each brings. 72 It stipulates that long term relationships develop and that knowledge is gained by both parties, which is used to improve health. 70 , 74 Cook recently conducted a review of CBPR projects that addressed health disparities. 75 In two-thirds of the studies, CBPR led to community actions to improve health. Studies that used qualitative methods were more acceptable to the community. In fact, community partners felt that the randomized controlled trials were too complex and were concerned that they withheld valuable interventions from the control group.
Principles of CBPR have been used by investigators to increase African American participation. One tool that has been used successfully is a community advisor board (CAB), which provides a window into the context in which many participants live, helps define the consent process, and creates relationships. 74 Several large research centers (Harvard, University of Pittsburg, Mayo Clinic) have created Community Research Advisory Boards (CRABs) to provide review and advice to investigators initiating more invasive studies and clinical trials. These boards review the project design and procedures to identify and address modifiable community-specific barriers to participation. Additionally, community boards and other groups promote regular, honest, and thorough dissemination of information about the research process. Studies also suggest that short and long-term outcomes must be communicated back to the community in order to gain and maintain trust. 25 , 76 Participants in our study confirmed this desire. Finally, it is strongly recommended that potential participants be given adequate time to make decisions about research participation. 25
Other ways to increase African American participants in research should also be considered. For example, in both this project and much of our other work, community members express the desire for researchers to have a presence in the community. Participants indicate that small group information sessions, co-led by researchers and community members, would be welcome. In some of our other work, we employ community members to help us carry out the research. Finally, as recommended by the Institute of Medicine and the Dept. of Health and Human Services, we must be diligent about encouraging African American (and other minority) students to continue their education to become scientists, thus increasing the proportion of underrepresented minorities in research positions. 36 , 77
In the early 1970s, the National Heart, Lung and Blood Institute established a program designed to increase minority participation. The most important strategy suggested by the report was soliciting contributions from community opinion leaders. 78 Why are researchers not implementing some of these strategies? Perhaps they have difficulty seeing the applicability of CBPR principles to clinical trials, as it has traditionally been used in public health and prevention studies. It is reasonable, however, to believe that researchers who conduct clinical trials can incorporate some of the CBPR assumptions and principles into their work. For example, researchers can attempt to understand the community and its “local theories,” or beliefs about determinants and solutions to problems. 79 Communication of study findings has also been shown to positively influence attitudes about participation and willingness to consider participation in future trials, even when the results are negative or inconclusive. 80 Participants in our study emphasized that when study findings are communicated back to the community, they should be presented in an understandable way, and also from a sense of interest and concern about the community. Teal and colleagues describe a framework for culturally competent communication, which includes communication repertoire, situational awareness, adaptability, and knowledge about core cultural issues. 81 Researchers should indicate how studies can potentially benefit the African American community. To close the loop, investigators can work to ensure that minority communities reap benefits from new research findings.
Investigators will continue to be limited in their ability to recruit study participants until they (the investigators) understand the depth of mistrust among many African Americans and its impact on access to health care, medical treatment, and research participation. Perhaps researchers are not as culturally competent as is necessary and continue to make decisions unconsciously based on race. 36 Our study suggests that the racism that was a community norm during the time Tuskegee syphilis study 82 persists, a position validated by the report issued by the Institute of Medicine . 36 This study reminds us that mistrust among African Americans, regardless of prior participation or socioeconomic status, continues and illuminates its multifaceted nature. Because of the recent and continued acknowledgement of health disparities that exist in African Americans, it is incumbent upon us to continue to explore and report the continuation of mistrust among African Americans related to research participation and to develop new and use existing strategies to enhance the trustworthiness of researchers and health care institutions. This study is a reminder about the significance of mistrust on research participation. It helps assure that investigators consider all of the issues related to mistrust as they embark upon studies, including informing community members about the potential impact of study findings on health disparities as part of outreach and recruitment. Unless researchers and practitioners acknowledge their roles in the development and continuation of disparities and create mechanisms to reduce mistrust, health disparities and limited research participation will continue.
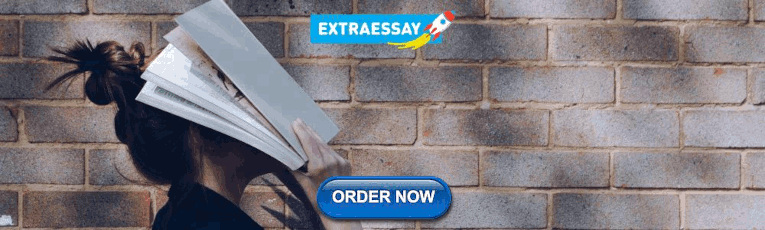
IMAGES
VIDEO
COMMENTS
1.) During studied of the effects of workplace conditions, the researchers manipulated several independent variables, such as levels of lighting, pay incentives, and break schedules. 2.) The researchers then measured the dependent variable, the speed at which workers did their jobs. Results: The workers' productivity increased when they were ...
The outcome factor; the variable that may change in response to manipulations of the independent variable. The measurable effect (variable), outcome, or response in which the research is interested. independent variable. The experimental factor that is manipulated; the variable whose effect is being studied. Stages of the Scientific Method. 1.
Study with Quizlet and memorize flashcards containing terms like 1. experiment 2. quasi-experiment 3. nonequivalent control groups, 1. nonequivalent control group posttest-only design 2. nonequivalent control group pretest/posttest design 3. nonequivalent control group interrupted time-series design 4. interrupted time-series design, 1. stable-baseline 2. multiple-baseline 3. reversal and more.
Examples. A research hypothesis, in its plural form "hypotheses," is a specific, testable prediction about the anticipated results of a study, established at its outset. It is a key component of the scientific method. Hypotheses connect theory to data and guide the research process towards expanding scientific understanding.
In many cases, researchers might draw a hypothesis from a specific theory or build on previous research. For example, prior research has shown that stress can impact the immune system. So a researcher might hypothesize: "People with high-stress levels will be more likely to contract a common cold after being exposed to the virus than people who ...
A research hypothesis (also called a scientific hypothesis) is a statement about the expected outcome of a study (for example, a dissertation or thesis). To constitute a quality hypothesis, the statement needs to have three attributes - specificity, clarity and testability. Let's take a look at these more closely.
The researchers hypothesized that physicians spend less time with obese patients. The researchers hypothesis derived from an identified population. In creating a research hypothesis, we also have to decide whether we want to test a directional or non-directional hypotheses. Researchers typically will select a non-directional hypothesis for a ...
Experiments have two fundamental features. The first is that the researchers manipulate, or systematically vary, the level of the independent variable. The different levels of the independent variable are called conditions. For example, in Darley and Latané's experiment, the independent variable was the number of witnesses that participants ...
Demand characteristics: In experiments, researchers sometimes display subtle clues that let participants know what they are hoping to find.As a result, subjects will alter their behavior to help confirm the experimenter's hypothesis.; Novelty effects: The novelty of having experimenters observing behavior might also play a role.This can lead to an initial increase in performance and ...
Components of a Formal Hypothesis Test. The null hypothesis is a statement about the value of a population parameter, such as the population mean (µ) or the population proportion (p).It contains the condition of equality and is denoted as H 0 (H-naught).. H 0: µ = 157 or H0 : p = 0.37. The alternative hypothesis is the claim to be tested, the opposite of the null hypothesis.
Question: 113. Researchers hypothesized that a decline in the population of dusky sharks near the mid-Atlantic coast of North America led to a decline in the population of eastern oysters in the region. Dusky sharks do not typically consume eastern oysters but do consume cownose rays, which are the main predators of the oysters.
This chapter lays out the basic logic and process of hypothesis testing. We will perform z tests, which use the z score formula from Chapter 6 and data from a sample mean to make an inference about a population.. Logic and Purpose of Hypothesis Testing. A hypothesis is a prediction that is tested in a research study. The statistician R. A. Fisher explained the concept of hypothesis testing ...
Researchers concluded that the employees worked harder because they thought they were being monitored individually. Researchers hypothesized that choosing one's own coworkers, working as a group, being treated as special (as evidenced by working in a separate room), and having a sympathetic supervisor were the real reasons for the ...
Researchers hypothesized that a decline in the population of dusky sharks near the mid-Atlantic coast of North America led to a decline in the population of eastern oysters in the region. Dusky sharks do not typically consume eastern oysters but do consume cownose rays, which are the main predators of the oysters.
Theories and Hypotheses. Before describing how to develop a hypothesis it is imporant to distinguish betwee a theory and a hypothesis. A theory is a coherent explanation or interpretation of one or more phenomena.Although theories can take a variety of forms, one thing they have in common is that they go beyond the phenomena they explain by including variables, structures, processes, functions ...
Determining statistical significance is important in being able to analyze data from experiments. An experiment was conducted to determine the impact of a medicine on illness recovery time. Researchers hypothesized that the medicinal treatment would decrease illness recovery time. Complete the paragraph to analyze the results of the experiment.
The variable hypothesized to cause or influence another is called: A) the dependent variable. B) the hypothetical variable. C) the correlation variable. ... Your text explains that the controversy surrounding research on human sexual behavior raises the issue of: A) the Hawthorne effect. B) value neutrality. C) casual logic. D) research design ...
The Hawthorne effect is, essentially, the positive effect on a person or group's behavior from knowingly being watched. It is a positive effect because when a person suspects they are being observed, they are more prone to act at a higher level of efficiency than they normally act. [2] Another way to interpret this is the failure on the part of ...
Study with Quizlet and memorize flashcards containing terms like The main tenet of Swiss physician Simon Tissot's degeneracy theory proposed that _____ was necessary for physical and sexual vigor and appearance characteristics such as beard growth in men. a. semen. b. testosterone. c. follicle-stimulating hormone. d. melatonin., Compared to heterosexual men and women, gay-identified men and ...
For example, researchers at a prestigious U.S. university recruited African American boys into a study that hypothesized a genetic etiology of aggressive behavior. Through the use of monetary incentives, they were able to convince parents to enroll their sons in a study that included withdrawal from all medications (including asthma medications ...
See Answer. Question: Determining statistical significance is important in being able to analyze data from experiments. An experiment was conducted to determine the impact of a medicine on illness recovery time. Researchers hypothesized that the medicinal treatment would decrease illness recovery time. Complete the paragraph to analyze the ...
An individual with an exceptional memory is identified. She is capable of recalling major events, the weather, and what she did on any given date. What research method is being used if a psychologist conducts an in-depth investigation of this individual including questionnaires, brain scans, and memory tests? a. Naturalistic observation, b.