- Search Menu
- Advance articles
- Editor's Choice
- Author Guidelines
- Submission Site
- Open Access
- Why Publish?
- About Migration Studies
- Editorial Board
- Call for Papers
- Advertising and Corporate Services
- Journals Career Network
- Self-Archiving Policy
- Dispatch Dates
- Journals on Oxford Academic
- Books on Oxford Academic

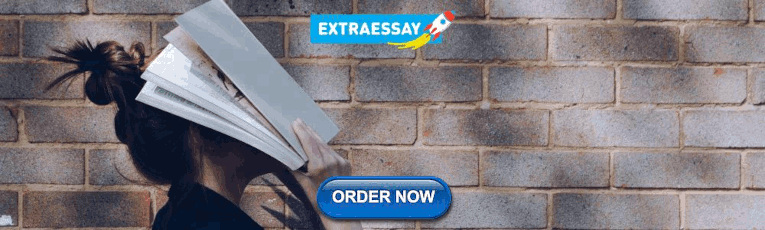
Editor-in-Chief
Gerasimos Tsourapas
About the journal
Migration Studies is an international refereed, online only journal dedicated to advancing scholarly understanding of the determinants, processes and outcomes of human migration in all its manifestations.
Learn more.
Latest articles
Editor's choice.
Why submit to Migration Studies ?
Discover our author benefits and why you should publish with the journal.
High Impact Research
Keep up to date with the latest research your peers are reading and citing, with a collection of the most cited articles published in Migration Studies in recent years.

Special Issues
Migration Studies regularly publishes impactful, topical special collections – browse our special collections here .
Forced Migration and Refugee Studies
Explore a collection of leading refugee studies research from Oxford University Press books, journals, and online resources. This collection covers a diverse breadth of regions and topics, including climate migrants, asylum policy in rich democracies, and the impact of COVID-19 on refugees.
Browse the collection
Published on the OUPblog

To dream or not to dream
Elzbieta M. Gozdziak asks, what are the effects of immigration status and parental influence on Latino children’s access to education.
Read blog post and article

Schrödinger’s immigrant
Getting rid of Schrödinger’s immigrant. Read the blog post from Migration Studies' Editor-in-Chief, Alan Gamlen.

Immigrant immigration researchers
Magdalena Nowicka asks, do immigrant researchers know more?
Read her blog post and article .

Economic migration and happiness
David Bartram discusses why economic migration may not lead to happiness.
Read his blog post and article .

Recommend to your library
Fill out our simple online form to recommend Migration Studies to your library.
Recommend now

Email alerts
Register to receive table of contents email alerts as soon as new issues of Migration Studies are published online.

Migration Studies Prize for Best Article
Congratulations to Marie Laurence Flahaux and Simona Vezzoli on winning the 2018 Migration Studies Prize for Best Article for their paper, 'Examining the role of border closure and post-colonial ties in Caribbean migration'. Read the 2018 Prize Winning Paper | Read past winners of the Prize for Best Article
Related Titles
- Recommend to your Library
Affiliations
- Online ISSN 2049-5846
- Copyright © 2024 Oxford University Press
- About Oxford Academic
- Publish journals with us
- University press partners
- What we publish
- New features
- Open access
- Institutional account management
- Rights and permissions
- Get help with access
- Accessibility
- Advertising
- Media enquiries
- Oxford University Press
- Oxford Languages
- University of Oxford
Oxford University Press is a department of the University of Oxford. It furthers the University's objective of excellence in research, scholarship, and education by publishing worldwide
- Copyright © 2024 Oxford University Press
- Cookie settings
- Cookie policy
- Privacy policy
- Legal notice
This Feature Is Available To Subscribers Only
Sign In or Create an Account
This PDF is available to Subscribers Only
For full access to this pdf, sign in to an existing account, or purchase an annual subscription.
Thank you for visiting nature.com. You are using a browser version with limited support for CSS. To obtain the best experience, we recommend you use a more up to date browser (or turn off compatibility mode in Internet Explorer). In the meantime, to ensure continued support, we are displaying the site without styles and JavaScript.
- View all journals
- My Account Login
- Explore content
- About the journal
- Publish with us
- Sign up for alerts
- Open access
- Published: 07 September 2023
World’s human migration patterns in 2000–2019 unveiled by high-resolution data
- Venla Niva ORCID: orcid.org/0000-0002-1456-4881 1 na1 ,
- Alexander Horton ORCID: orcid.org/0000-0003-3067-7138 1 ,
- Vili Virkki ORCID: orcid.org/0000-0002-2603-3420 1 ,
- Matias Heino 1 ,
- Maria Kosonen 1 ,
- Marko Kallio ORCID: orcid.org/0000-0002-6917-7790 1 , 2 ,
- Pekka Kinnunen ORCID: orcid.org/0000-0003-0373-2795 1 ,
- Guy J. Abel ORCID: orcid.org/0000-0002-4893-5687 3 , 4 , 5 ,
- Raya Muttarak 6 ,
- Maija Taka ORCID: orcid.org/0000-0002-6147-9137 1 ,
- Olli Varis 1 &
- Matti Kummu ORCID: orcid.org/0000-0001-5096-0163 1 na1
Nature Human Behaviour volume 7 , pages 2023–2037 ( 2023 ) Cite this article
15k Accesses
4 Citations
273 Altmetric
Metrics details
- Climate-change adaptation
- Climate-change impacts
- Socioeconomic scenarios
Despite being a topical issue in public debate and on the political agenda for many countries, a global-scale, high-resolution quantification of migration and its major drivers for the recent decades remained missing. We created a global dataset of annual net migration between 2000 and 2019 (~10 km grid, covering the areas of 216 countries or sovereign states), based on reported and downscaled subnational birth (2,555 administrative units) and death (2,067 administrative units) rates. We show that, globally, around 50% of the world’s urban population lived in areas where migration accelerated urban population growth, while a third of the global population lived in provinces where rural areas experienced positive net migration. Finally, we show that, globally, socioeconomic factors are more strongly associated with migration patterns than climatic factors. While our method is dependent on census data, incurring notable uncertainties in regions where census data coverage or quality is low, we were able to capture migration patterns not only between but also within countries, as well as by socioeconomic and geophysical zonings. Our results highlight the importance of subnational analysis of migration—a necessity for policy design, international cooperation and shared responsibility for managing internal and international migration.
Similar content being viewed by others
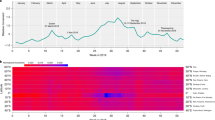
Mapping global variation in human mobility
Moritz U. G. Kraemer, Adam Sadilek, … John S. Brownstein
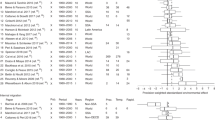
A meta-analysis of country-level studies on environmental change and migration
Roman Hoffmann, Anna Dimitrova, … Jonas Peisker
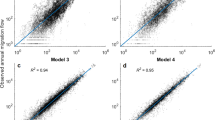
Gravity models do not explain, and cannot predict, international migration dynamics
Robert M. Beyer, Jacob Schewe & Hermann Lotze-Campen
Since the 1990s, human migration has been one of the top public concerns and political agenda items in Europe and North America 1 . Millions of people have been forced to flee due to conflicts while also millions have voluntarily moved to urban areas seeking better economic prospects. Around the world, diverse environmental factors, such as droughts, floods and other natural hazards also push people to move. Most of this mobility takes place within a short distance, making internal migration the most prevalent form of migration across the globe 2 . Indeed, climate-induced migration is shown to be more common within national borders 3 . Yet, public attention tends to focus on international migration including both voluntary and forced migration. Subnational information about the estimates of the number of migrants (and immobile persons), their origin and destination and the conditions of migration are much needed for planning of urban services and infrastructure as well as rural development 4 . Understanding migration patterns across spatial scales—including its conditions, magnitude and impact—is thus fundamental for policy design.
Whilst subnational (5 arcmin, ~10 km resolution) decadal estimates of net migration for three decades between 1970 and 2000 are available 5 , global-scale data on subnational migration for more recent years are sparse. One study 6 provides a more recent estimate of migration at grid cell level for 1975–2015 (5 year interval) but the baseline data are derived from national-level birth and death data. Other estimates describe global international migration by using national-level data 7 , 8 or internal migration at the national level, based on national census data. These studies suffer from a long time interval between census years (typically 10 years) 9 . The coarse spatial and temporal resolutions of these data hinder the ability to conduct gridded migration trend analyses over time.
Our study aims to address these research gaps by developing a detailed annual net migration dataset, by collecting, gap-filling and harmonizing (1) comprehensive national-level birth and death rate datasets for 216 countries or sovereign states; and (2) subnational data for births (covering 163 countries, divided into 2,555 administrative units) and deaths (123 countries, 2,067 administrative units) (Extended Data Fig. 1 ; Methods ). In doing so, we provide a detailed analysis of the spatiotemporal development of (1) the magnitude of net migration and (2) its impact on population growth over the past two decades. Firstly, for magnitude, we collected reported data from various sources to create an annual net migration dataset for 2000–2019, using national and subnational birth and death data, downscaled to 5 arcmin resolution (~10 km at the equator) with selected socioeconomic variables (Fig. 1 ; Methods ). These data enable us to perform analyses on net migration trends and patterns from local to global scales. Our gridded net migration data allow, for instance, comparing the intensity of net migration and its trends at several administrative scales. It is also possible to analyse the types of sending and receiving areas (rural or urban) at multiple scales (regional, national (administrative 0), provincial (administrative 1) and communal (administrative 2)) over the past two decades. Indeed, there is no such systematic global-scale classification on, for instance, which urban areas are net senders and which rural areas are net receivers. Here, we present rigorous analyses of the distribution of the types of origin and destination areas.
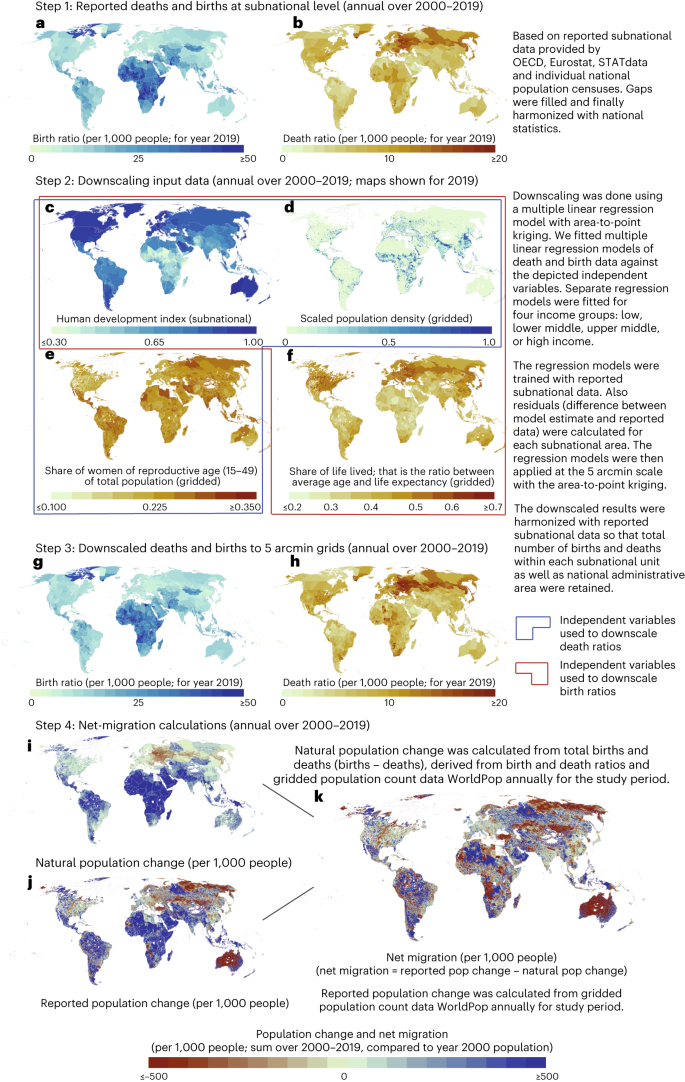
a , b , Reported annual subnational birth ( a ) and death rates ( b ) for 2000–2019 are based on various global and national datasets with some of the intermediate results ( Methods ). c – f , For downscaling to 5 arcmin grid level, we used four annual gridded or subnational datasets of ( c ) human development index, ( d ) scaled population density, ( e ) share of reproductive women and ( f ) share of life lived in each grid cell, as detailed in the panels. g – i , The annual downscaled birth ( g ) and death ( h ) rates allowed us to estimate the natural population change for each year ( i ). j , k , When combining this with reported population change based on WorldPop 55 ( j ), we were able to calculate annual net migration for 2000–2019 ( k ). See Methods for more details.
Our paper also contributes to analysing the impact of human migration on population change. Using our annual gridded dataset to map migration in parallel with demographic and geophysical data (Extended Data Figs. 2 and 3 ), we were able to assess the impact of net migration on rural and urban population change at national, subnational and communal levels and across different societal and climatic conditions. Understanding the contribution of migration to population change is crucial because migration affects sending and receiving societies in various ways. In terms of economic consequences, migration influences socioeconomic development of both sending and receiving areas—for example, by increasing productivity in receiving areas and reducing income inequalities across countries through remittances 10 , 11 . Nevertheless, migration can also cause considerable pressure on the infrastructure and services of the receiving areas 4 and consequently exacerbate the vulnerability of migrants 12 , 13 . However, few empirical studies have analysed the impact of migration on population change at the global scale over the past decades. Our analysis thus provides a solid quantitative foundation towards understanding the extended societal impacts caused by migration.
We first developed a gridded global net migration dataset at annual timesteps for 2000–2019 (Fig. 1 ). This here-developed dataset (openly available at https://doi.org/10.5281/zenodo.7997134 ) was constructed from subnational (administrative 1) birth and death rate data collected across 2,555 and 2,067 administrative units, respectively (Fig. 1a–b ; Methods ), downscaled to 5 arcmin resolution with rasterized socioeconomic data developed in this study and finally adjusted to match the subnational data collected (Fig. 1c–f ; Methods ).
Our birth and death rate data revealed considerable intranational heterogeneities, particularly in large countries, such as Russia, the United States, China, Brazil and India (Fig. 1g–h ). This highlights the importance of using subnational (particularly downscaled) data instead of national data (as used in ref. 6 ) for understanding global population dynamics. These downscaled birth and death data then allowed us to estimate natural change in population (deaths subtracted from births) for each year and grid cell (Fig. 1i ). When combined with reported annual population change over the same time period (Fig. 1j ), we were able to estimate annual net migration in each grid cell (Fig. 1k ) using a similar method to that of ref. 5 ( Methods ). Here, net migration can be either negative (more people out-migrating than in-migrating) or positive (more people in-migrating than out-migrating).
It should be noted that our data are prone to uncertainties that originate from collected subnational data but propagate to all derived data products—including birth and death rates, natural change in population, as well as net migration estimates. Subject to data availability, we performed a partial validation for our data products by comparing gridded data with subnational (mostly administrative 2 level) data ( Methods; Supplementary Table 2 and Supplementary Figs. 1 – 6 ). However, this validation cannot capture areas where uncertainties may be the highest—that is, areas in which the collected census data are not available or of poor quality, for example, those suffering from sporadic census years or changing subnational administrative units. To ensure global spatial and temporal coverage, we applied a series of adjustments and corrections to the data ( Methods ). Nevertheless, higher uncertainties remain in some countries (such as those in Africa, the Middle East and parts of Asia) than in others (such as those in Europe and much the Americas). As proxies of original data quality, we provide the description, resolution, timespan and sources of each collected dataset in the Supplementary Data .
Magnitude of global net migration
Temporal dynamics of net migration depend on scale.
To assess global migration dynamics, we aggregated net migration at three spatial levels: communal (administrative level 2), provincial (administrative level 1) and national (administrative level 0) (Fig. 2 ). This approach allowed us to compare the magnitude of migration that occurs at different spatial levels. Our results show that migration patterns vary remarkably across nations. On a national scale over the entire 20 year study period, net migration was positive (that is, in-migration was greater than out-migration) in Australia, North America, as well as parts of Europe and the Middle East—all being areas that have attracted either asylum or job seekers or both (Fig. 2 and Extended Data Fig. 4 ). Net migration was negative in countries like Syria, Lithuania, Zimbabwe, Venezuela and Guyana (Fig. 2e )—in line with previous assessments from Venezuela and Syria, where millions of people have fled a humanitarian crisis and conflict 14 , 15 , 16 and also from Lithuania and Zimbabwe where numerous people have out-migrated in search of better economic prospects 17 , 18 .
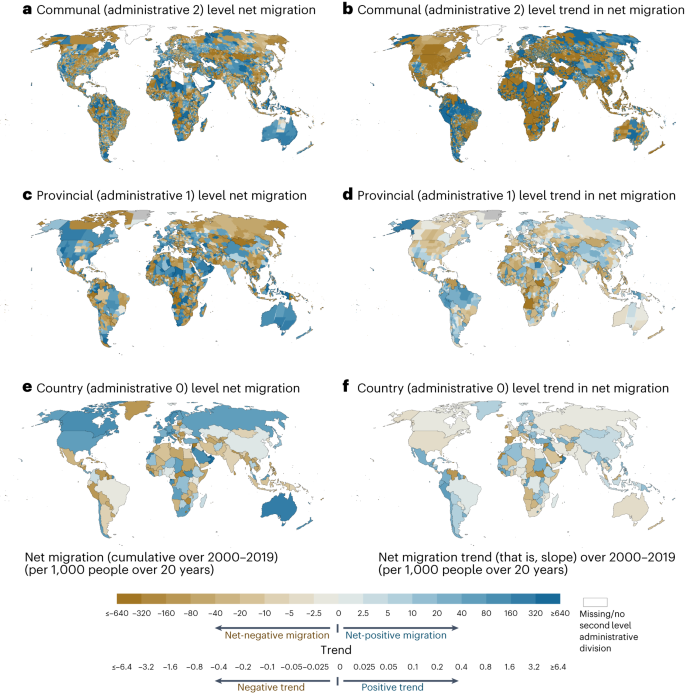
a , c , e , Sum of annual net migration over 2000–2019 is shown for communal (administrative 2) ( a ), provincial (administrative 1) ( c ) and national (administrative 0) ( e ) levels. b , d , f , Net migration trend (slope) over 2000–2019 is shown for communes ( b ), provinces ( d ) and countries ( f ). The trend was determined by calculating the slope of linear regression line. Negative net migration refers to a situation in which more people out-migrate than in-migrate and positive net migration refers to a situation in which more people in-migrate than out-migrate. With our online net migration explorer tool, it is possible to explore these patterns for each year and subnational unit: https://wdrg.aalto.fi/global-net-migration-explorer/ .
At a provincial level, the migration patterns reflect the prevalence of internal migration in many countries, as can be observed from both net-positive and net-negative provinces. For example, in China, the coastal areas show positive net migration while negative net migration (out-migration is greater than in-migration) was observed in many inland provinces (Fig. 2c ). This is consistent with the well-founded internal migration patterns in China where labour migration is concentrated towards urban, coastal areas 19 . The same applies to many other countries, such as the United States, where urban centres are attracting people from other states and abroad 20 , 21 . When assessing net migration at even finer spatial detail (communal level), interesting patterns start to arise. For example, in the United States, many states with positive net migration (Fig. 2c ) are characterized by mainly negative county-level net migration (Fig. 2a ). See our online net migration explorer tool for more detail: https://wdrg.aalto.fi/global-net-migration-explorer/
Our dataset also allowed us to explore the temporal dynamics of net migration over the study period (2000–2019) across three administrative levels. We assessed the trend of net migration at each level over the study period (2000–2019) by using linear regression. The results follow a similar pattern as cumulative net migration where the trend changes according to the administrative level. Further, the results show interesting patterns of where net migration has a negative trend and where it has a positive trend over the past two decades. In North America, for instance, net migration shows a declining trend in almost all regions, excluding small pockets in the southwest (Fig. 2b,d ). The same applies to South America, especially Brazil and Chile, where net migration has been on a growing trend in the northern parts of these countries, while in the south the trend has been declining; and Australia, where the trend of net migration has been positive in the middle parts, while being negative in the coastal regions of the continent (Fig. 2b,d ).
Rural and urban migration show high global variation
We further assessed the development of net migration by studying how net migration differs in rural and urban areas (Extended Data Fig. 5 ). Urban areas often receive migrants from rural areas— the so-called ‘urban pull–rural push’ situation 22 . We assessed if this holds true across 12 world regions (Extended Data Fig. 4 ) and for each country at three administrative levels by using the GADM delineation (national, administrative level 0; provincial, administrative level 1; and communal, administrative level 2). Here, we combined our net migration dataset with an urban extent dataset for 2000–2019 that was created for this study. The urban extent dataset maps urban areas on the basis of scaled population density and share of urban population at national level, annually for 2000–2019 ( Methods ; Extended Data Fig. 3 ).
Our data show that when aggregated globally, urban net migration was positive (more in-migrants than out-migrants) throughout the study period (2000–2019), while rural net migration remained negative (except for years 2010 and 2012) (Fig. 3m ). The magnitude of global net migration ranged annually from near-zero to around three net migrants per 1,000 people (Fig. 3m ). Notable spatiotemporal variation between rural and urban net migration was evident at the regional level (Fig. 3a–l ) as well as at national, provincial and communal levels (Extended Data Fig. 6 ). However, no considerable change in net migration rates towards urban or rural areas was observed in any region between 2000 and 2019. Both rural and urban net migration were negative (down to approximately −10 net migrants per 1,000 people) nearly throughout the study period in Central America (Fig. 3a ), whereas in North America (Fig. 3g ) and Oceania (Fig. 3h ), total net migration was steadily positive (with a constant magnitude of at least +5 net migrants per 1,000 people). In East Asia (Fig. 3b ), net migration was negative in rural areas and positive in urban areas (with magnitudes <5 net migrants per 1,000 people) while in other regions, the pattern was more complex (Fig. 3 ).
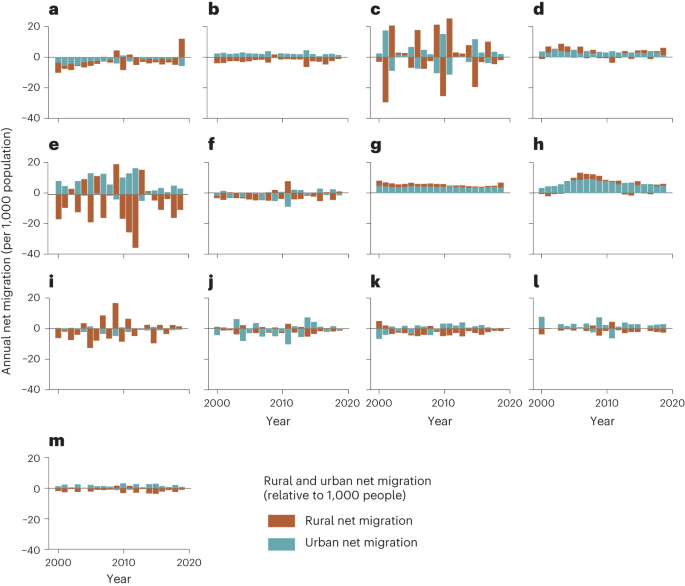
a – m , The regional sums for each year (Central America ( a ), East Asia ( b ), Eastern Europe ( c ), Europe ( d ), Middle East ( e ), North Africa ( f ), North America ( g ), Oceania ( h ), South American ( i ), South Asia ( j ), Southeast Asia ( k ) and Sub-Saharan Africa ( l )) and the annual global sum ( m ) of urban and rural net migration. Urban and rural net migration are reported per 1,000 urban or rural inhabitants in each region. The regional division follows the UN country grouping (Extended Data Fig. 4 ). See gridded net migration in rural and urban areas in Extended Data Fig. 5 .
In Eastern Europe and the Middle East, rural and urban net migration rates fluctuated annually, especially during the years preceding the Arab Spring, followed by massive rural out-migration and urban in-migration between 2011 and 2013—with magnitudes up to almost +40 net migrants per 1,000 people and down to −20 net migrants per 1,000 people (Fig. 3e ). Out-migration from Syria was among the largest in the world between 2010 and 2015, during when more than 2 million people left to neighbouring countries Turkey and Lebanon 2 , 14 , 23 . This explains a sharp influx of migrants to rural and urban areas in Eastern Europe group that includes Turkey (Fig. 3c ). Although our results align with previous estimates of migration in the Middle East, these regions are prone to high uncertainties in the data.
Net-receiving provinces contain a third of global population
When focusing on national and subnational scales, the global urban pull–rural push pattern (Fig. 3m ) becomes patchier (Fig. 4 ). At the national scale, 36% of global population (in 2019) lived in countries where this pattern was evident. These countries include the Nordics and several countries in Africa, Southeast and East Asia (Fig. 4c ). However, at the subnational scale, many more people lived in provinces and communes which were either net receivers or net senders, as presented by negative or positive net migration in both urban and rural areas. Such provinces were located in the United States, Canada and Australia, while Russia, the northeast of the United States, Mexico and the Balkans, for example, accommodated multiple net-sending provinces (Fig. 4b ).
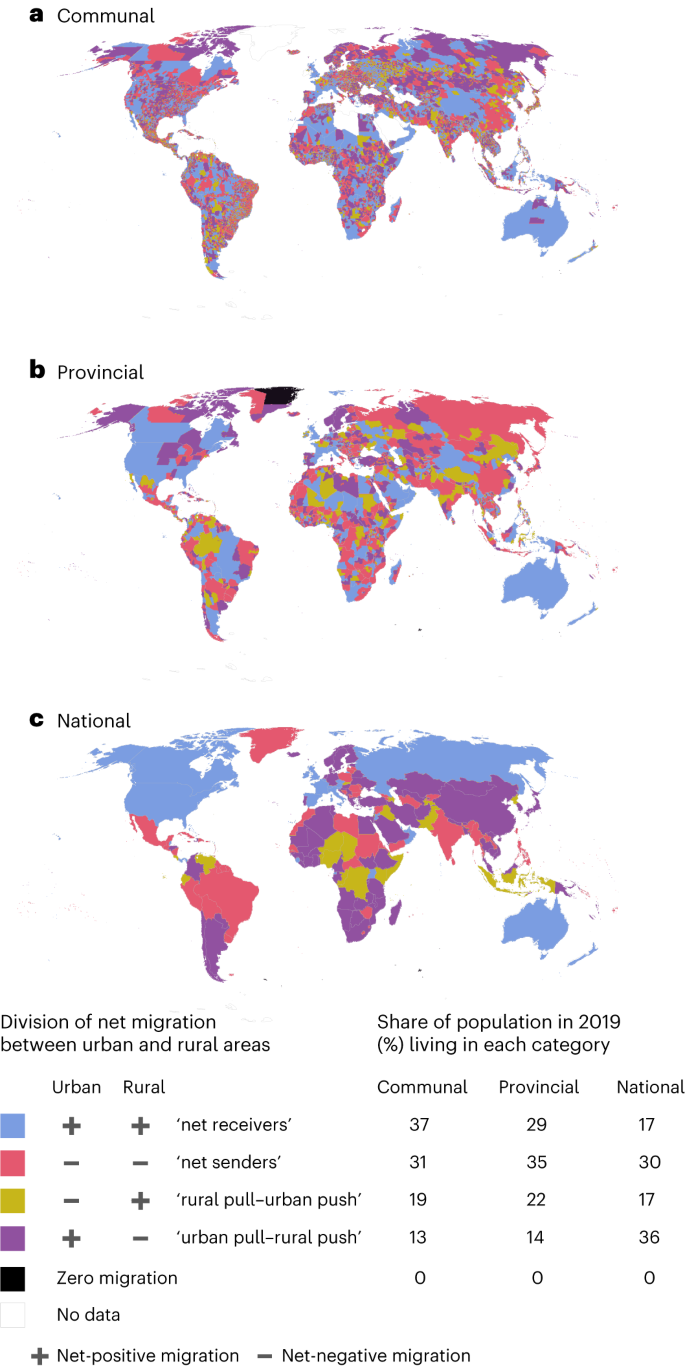
a – c , The communal ( a ), provincial ( b ) and national ( c ) levels. Each administrative unit was categorized into one of the four classes on the basis of the ‘direction’ of migration in rural and urban areas. For example, if net migration in an administrative unit was positive in both urban and rural areas, then that unit would be categorized as a net receiver, whereas a unit in which urban net migration was positive and rural net migration negative would be categorized as urban pull–rural push. The share of population living in each category was calculated for each administrative level. For instance, 36% of global population lived in communes where both urban and rural net migration were positive (net-receiving communes). See net migration in rural and urban areas by different administrative levels in Extended Data Fig. 6 .
A situation where urban net migration was negative and rural net migration positive (rural pull–urban push) was observed in few locations, such as certain provinces of Indonesia, Congo, Venezuela and Pakistan (Fig. 4b ), covering in total 22% of the world’s population. Notably, 37% of the global population lived in the urban and rural areas of net-receiving communes. Extensive rural in-migration is probably explained by interprovincial and intercommunal migration between rural areas and immigration from other countries. Studies show that a trend of rural–urban migration is shifting towards more complex mobility patterns, of which rural–rural mobility is one of the most prevalent types of internal migration. Especially in Sub-Saharan Africa, people tend to move between rural areas as seasonal circular migration and for economic diversification, given better access to land or job prospects than in cities 24 , 25 . In Europe, a similar pattern appears in rural areas attracting workers in the agricultural sector within the same country or from abroad 26 . Large urban agglomerates may also push people to move to rural areas in search of more affordable housing (counter-urbanization; see ref. 27 for counter-urbanization in Australia and ref. 28 for the United States). It should be noted that the results are strongly influenced by the delineation of urban areas ( Methods ).
Impact of migration on population change
Migration often accelerates urban population growth.
Net migration taking place in densely populated areas can be relatively large compared to natural population change (Figs. 1i,k and 2a ). In such cases, large-scale migration can strain natural and human resources, as well as infrastructure, which are insufficient to serve a steep surge in population, particularly in urban areas 4 , 29 . On the other hand, migration can potentially help ageing societies like those in Europe to maintain their work force 30 , 31 . To empirically examine the role of migration in population change globally, we compared natural population change (deaths subtracted from births) with reported population change in rural and urban areas at the three administrative levels.
We found that about half of the global urban population lived in areas that were affected by positive net migration in a way that positive net migration added to a naturally growing urban population. Notably, in some urban areas—especially in the Nordics, Germany, Austria and Spain—positive net migration even shifted a naturally decreasing population towards growth (Fig. 5c,e ). In very few countries, positive net migration slowed down natural decline in urban population but this affected urban areas accounting only for ~1% of global urban population. About 39–44% of the urban population lived in areas affected by negative net migration that slowed down urban population growth (Fig. 5 ). There were fewer cases (0–6% of urban population) where naturally growing urban population declined due to intensive out-migration. Such areas could be detected mainly at communal level in countries such as France, Italy, the United States and India.
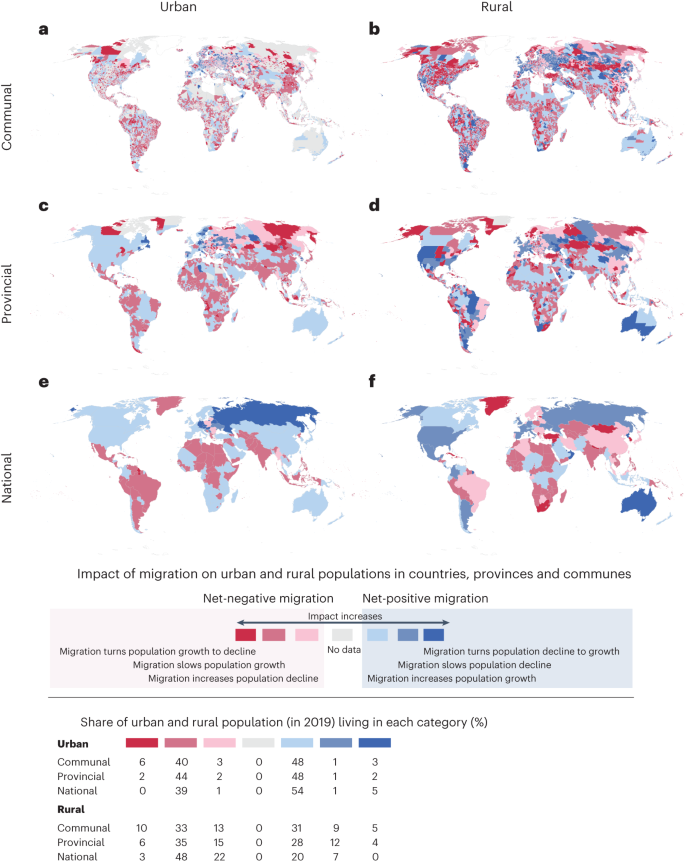
a – f , The communal ( a , b ), provincial ( c , d ) and national ( e , f ) levels for urban ( a , c , e ) and rural ( b , d , f ) areas. Impact is divided into seven categories by comparing total population change, net migration and natural population change (growth or decline). Total population change includes both net migration and natural change. Natural change is measured with births and deaths, that is population change without migration. For example, net migration slows down urban population decline when urban net migration is positive but total urban population change is negative ( Methods ). Share of global urban and rural population living in each category shows the share of global urban or rural inhabitants living in areas under each type of impact.
Negative net migration impacted rural areas more often than urban areas: 10% of global rural population lived in communes where out-migration turned rural population growth to a total population decline (Fig. 5b ). Approximately half of the global rural population lived in countries where rural population growth was slowed down by negative net migration, whereas one-fifth of rural population lived in countries where negative net migration accelerated the prevailing decline in population (Fig. 5f ). Yet, positive net migration propelled rural population growth in multiple provinces and communes accounting for around a third of global rural population. In Russian provinces and communes bordering Kazakhstan, for instance, in-migration of skilled workers from Kazakhstan potentially explains the accelerated rural population growth or slowed rural population decline 32 .
Human development is associated with migration more than aridity
Global migration is known to be driven by both socioeconomic and environmental factors 3 , 13 . Here, we analyse how total, urban and rural net migration co-occur with different socioclimatic conditions, dividing the globe into 100 socioclimatic bins (Extended Data Fig. 7 ) on the basis of the level of aridity, human development (measured by human development index (HDI); Extended Data Fig. 8 ) and population. Each bin accommodates ~1% of the global population ( Methods ).
Our results show that, over the past 20 years, high net migration often co-occurs with high HDI and high aridity, which is evident especially in urban areas (Fig. 6a ). For instance, urban areas in the Arabian Peninsula, arid parts of North America, Australia, Argentina and the Mediterranean region had high (mostly above +60 net migrants per 1,000 people over the study period) positive net migration rates (Fig. 6a ). High positive net-migration rates in many Middle Eastern countries, such as Qatar, Oman and Saudi Arabia (Fig. 2e ), are explained by large labour migration particularly from Asian and African countries 33 . On the other hand, in Australia and North America, the ‘preference of low-density living’ 34 can explain positive net migration in rural areas. Notably, high HDI is common for these regions whereas the range of aridity is wider.
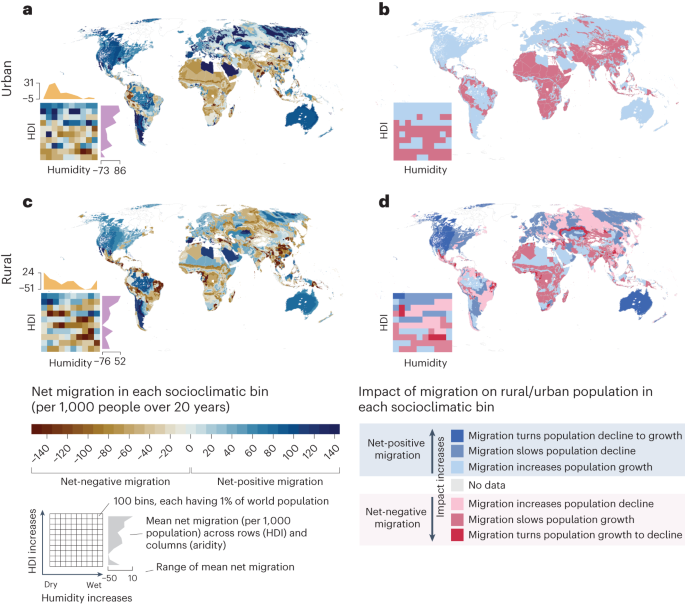
a – d , For each socioclimatic bin, urban net migration per 1,000 urban population summed over the study period ( a ), the impact of urban net migration on urban population change in each socioclimatic bin ( b ), rural net migration per 1,000 rural population summed over the study period ( c ) and the impact of rural net migration on rural population change ( d ). Here, net migration per urban or rural population was calculated as a zonal sum of net migration in urban ( a ) and rural ( c ) areas in each bin and then divided with the respective urban or rural population count in the respective bin. The maps are spatial representations of the heatmaps. Socioclimatic bins are based on socioeconomic and climatic conditions represented by human development and aridity indices ( Methods ; Extended Data Figs. 7 and 8 ).
In terms of negative net migration, global out-migration hotspots (areas with substantial negative net migration) are located in socioclimatic conditions with middle-level aridity and human development. This was especially the case in rural areas (Fig. 6c ). High negative net migration rates could be observed in regions like Central America, northeastern Brazil, Central Africa and Southeast Asia (mostly below −60 net migrants per 1,000 people over the study period). This aligns with recent studies showing that most migration is originating from areas where people have sufficient capacity to move and use migration as a form of adaptation to unfavourable environmental conditions 3 .
Positive net migration often co-occurs with high HDI
When comparing natural population growth with net migration across different socioclimatic conditions, we found that the contribution of migration to population change ( Methods ) was more strongly associated with the level of human development than with aridity (Fig. 6b,d ). Positive net migration often contributed to increasing population growth, slowing population decline or shifting declining population to growth in areas with relatively high human development. Our results show that the climatic factor, as measured by the level of aridity, has a weaker association with the impact of migration on population growth. Therefore, migration can accelerate or slow down population growth in a wide range of climatic conditions.
For instance, most of Europe, North America and Australia, which are regions with high human development, experienced increasing urban population due to positive net migration (Fig. 6b ). Notably, the capacity to cope with the pressure on physical and social infrastructure from population growth is high in these regions. On the other hand, many urban regions in West, East and South Africa, Arabia, as well as India, Bangladesh, China and Southeast Asia had positive net migration accelerating their natural population growth, while they also have more limited capacity (HDI) to cope with a growing population (Fig. 6b ). Urban regions with the lowest human development level experienced negative net migration and consequently a slowing down of natural population growth. While this may indicate that out-migration from those areas is alleviating the pressure caused by natural population growth, it can also reflect urban out-migration pushed by urban poverty.
Finally, the role of migration in rural population change follows a general pattern in which regions with higher human development level are impacted by positive net migration. Declining rural populations turned to growth due to positive net migration in North America and Australia (Fig. 6d ), whereas rural population decline was slowed down by positive net migration in most of Europe, parts of Russia and South America.
Discussion and concluding remarks
Despite recent progress in estimating global international 7 , 8 , 35 , 36 and internal migration 9 , 37 , global migration datasets often suffer from poor spatial and/or temporal resolutions. Our annual gridded net migration data cover the entire globe, allowing the analysis of both local and regional net migration patterns at various geospatial scales. Using these data, we have quantified the magnitude and impact of net migration over the past 20 years in three administrative levels and in socioclimatic zones. Our analysis highlights the importance of considering the spatial scale when analysing migration patterns. We showed that global net migration patterns depend strongly on the scale of analysis, both for the magnitude of migration and also for the trend (Fig. 2 ). Within the study period of 2000–2019, net global urban migration has been predominantly positive while net rural migration has been negative (Fig. 3m ), aligning with previous urbanization literature 38 . Further, we showed that the volume and impact of migration are positively associated with the level of human development (positive net migration often co-occurs with high HDI) and this association is stronger than that of climate and migration (Fig. 6 ).
Previous studies argue that migration often originates from areas where people have sufficient capacity to move and to use migration as one form of adaptation to unfavourable environmental conditions, often by migrating to urban areas 29 , 39 , 40 , 41 . Our analysis provides a global quantification of this argument and shows that rural out-migration hotspots were located in socioclimatic conditions with a middle level of aridity and human development (Fig. 6c,d ). This corresponds to about 50% of the global urban population who experienced accelerated population growth in the past two decades (Fig. 5 ). Notably, a relatively large volume of positive urban net migration took place in locations with medium-to-high levels of human development, indicating higher capacity to cope with additional pressures (Fig. 6a,b ).
Despite accelerating urbanization over the past 20 years, positive net migration has increased population growth and helped to slow down population decline in many rural areas (Figs. 5 and 6c,d ). Rural–rural migration, especially over short distances, is common particularly in smaller countries with few provincial and communal urban centres that attract migrants from other parts of the country 7 . Our results show that areas where migration slowed rural population decline were located in high-income countries (Fig. 6d ), and some countries may have reached a point where urbanization has turned to counter-urbanization 34 . On the other hand, the share of people living in urban areas is expected to grow, especially in lower income regions, such as Africa 42 . With the global average temperatures continuing to rise, impoverished urban areas are particularly vulnerable to the impact of extreme climate events, given lower capacity to manage population growth and to provide basic services and infrastructure 43 . Increased climatic hazards such as heatwaves, water stress, floods and droughts will increase the vulnerability of people living in the outskirts of poor urban agglomerates, which are already the predominant destinations for migrants, particularly in Africa 44 . Notably, such developments are visible in our study. For instance, migration-induced urban population growth over the past two decades was visible around the fast-growing cities of Nigeria, Angola, Kenya and Tanzania, where the capacity to cope with the accelerating population growth was remarkably low (Figs. 5 and 6 ).
While our study sheds light on global migration trends and patterns at various spatial scales, there are several limitations. First, given that our data derive net migration from the difference between total and natural population change, it is impossible to distinguish between different types of migrants such as refugees, internally displaced persons or economic migrants. Our data mask out important individual migration events and external shocks, such as conflict, altogether by only describing whether an area experienced more in-migration than out-migration, or vice versa, without being able to differentiate the dynamics of migration flows in and/or out of an area 45 . This can be particularly relevant, for instance, in areas under prolonged conflicts in our study period, such as Afghanistan, Syria and Iraq. Further, our analysis used long-term averages of climatic and socioeconomic conditions, thus masking any interannual or intra-annual variation of net migration related to sudden shocks, such as extreme weather events.
It is also important to note that the data collected from national censuses and other databases ( Methods ; Extended Data Fig. 1 ) were available at different temporal resolutions. Data for low-income countries were scarcer, while the information from high-income countries was generally more detailed. In some cases, the sources for births and deaths information were also different. To minimize uncertainties, the produced birth and death grids were adjusted so that the subnational sum of births and deaths matched with the collected subnational (administrative level 1) birth and death counts ( Supplementary Data ). Further, subnational data at administrative level 1 were harmonized with national data; that is, we ensured that the sum of births and deaths at the grid level agree at the national level with United Nations (UN) statistics. We validated the WorldPop data, downscaled births and deaths data as well as the produced net migration data against reported values, mostly at administrative level 2 (finer level than used for creating the gridded datasets). Validations show that the data are in line with reported data and thus indicate good performance of our downscaling method (Supplementary Figs. 1 – 6 and Supplementary Table 2 ). To smooth over inaccuracies, we recommend aggregating the data over some spatial or temporal units (for example, administrative/environmental zoning, 5 year period instead of an annual timestep).
Here, we have quantified the magnitude and impacts of net migration over the past two decades. In the light of our results, we emphasize the importance of subnational analysis of migration and, with our data, we provide various future research openings in studying complex human mobilities inside and between administrative boundaries and in other zones—combined with the co-occurrence of extreme weather, disasters, conflicts and migration events, for example. Capturing migration patterns not only between countries but also within countries and socioeconomic and geophysical zonings is essential for policy design, international cooperation and shared responsibility—all essential for managing internal and international migration and other mobilities 29 . Finally, our results underline the necessity of coupling environmental drivers with proxies of human development. Internal and international migration are expected to increase in unprecedentedly complex and rapidly changing socioclimatic conditions 46 and thus, increasing the empirical understanding of the global patterns of migration and its multifaceted drivers is very important 47 .
The overall workflow is illustrated in Fig. 1 , while a more detailed methodological explanation is given below.
Data processing for harmonized subnational data
We first collected national and subnational data for 1990–2019. For births, we used two global databases, namely STATcompiler 48 and Eurostat 49 , as well as national census data. Altogether we found subnational (administrative level 1) data for 163 countries which were divided altogether for 2,555 administrative units (Extended Data Fig. 1 ). For deaths, we used two databases, namely OECD regional stats 50 and Eurostat 51 , as well as national census data. For deaths, we found subnational data for 124 countries and 2,067 administrative units. For both data (births and deaths) and for each country, we evaluated the best-available data source (by the number of available years and missing data entries, if multiple data sources were available). The datasets used are described in the Supplementary Data and origin of data for each country illustrated in Extended Data Fig. 1 , which also shows the administrative units used in STATcompiler 52 , Eurostat 53 and OECD 54 .
As data were available either as a crude birth or death rate or as a number of births or deaths, we needed to harmonize the data so that all observations would be as a rate (births or deaths per 1,000 people). For this, we aggregated population data for each administrative unit and for each year, using WorldPop 55 gridded 1 km data for 2000–2019 and HYDE 3.2 (ref. 56 ) for 1990–2000. HYDE data were bias-corrected with delta change method 57 to be consistent with WorldPop data. Although WorldPop data were available also for the year 2020 at the time of data processing, our analysis required computing population change that could not be done for the 2020 WorldPop data and thus, final data are limited to 2019. While our final dataset covers years 2000–2019, collecting data for the previous decade made interpolations between years possible.
Once all data were transformed to rates, we filled missing data entries. Some data entries were missing due to changes in administrative units and temporal gaps in used datasets. For changes in administrative boundaries, we used Administrative Divisions of Countries (Statoids) database 58 . We processed the missing data according to following types (see list of subnational areas in each category in the Supplementary Data ):
Combine (number of cases in birth dataset ( n births = 11; n deaths = 11). In subnational units with low population (typically <50,000)—with available data for the number of births or deaths—the birth or death rates ended up differing substantially from the surrounding administrative units. In these cases, we ‘combined’ the unit with a neighbouring larger subnational unit so that the rate was calculated for both, using the combined number of births or deaths and population—that is, they ended having the same rate. For example, we combined Ulaanbaatar (Mongolia) with the surrounding Töv province, as rates in Ulaanbaatar were not realistic (probably because of how the population in the capital is defined).
Split ( n births = 15; n deaths = 14). In cases when a larger administrative unit was split to two or more subnational units during the extended study period and parts of the data were given only for the larger unit, we estimated birth or death rates before the split using the birth or death rate of the first timestep when these were split to separate units. We did this by calculating the combined birth or death rate, using subnational population and then used this to calculate the ratio between combined birth or death rate and each individual administrative unit birth or death rate. These were then multiplied with the ‘combined’ birth or death rate for years before the split. This way, we were able to estimate the birth or death rate for all individual administrative units for the time in which they were still one unit. For example, Panamá subnational unit was split in 2014 into two units: Panamá and Panamá Oeste. Birth rate data exist for combined Panamá over 2001–2013 and then for both split units from the year 2014 onwards. We thus first calculated the combined birth rate (in this case) for year 2014 using subnational population:
This \({\rm{birthRate}}_{\rm{comb}_{2014}}\) was then used to estimate the rates for each subnational unit separately:
and these were finally used to estimate the birth rate for each subnational unit for pre-2014 period (example for year 2013 given below):
where birthRate_Panama(combined)_2013 is the reported birth rate for the combined administrative unit for year 2013 (before the split).
Part of the data missing ( n births = 31; n deaths = 19). In cases of gaps in the reported data, we used the neighbouring area with the least difference in births or deaths as the scaling neighbour to scale the missing data. For this, we used the data from the closest year to the missing data year, for which data existed for both administrative units. We then calculated the ratio between these administrative units and used this ratio to estimate the missing data:
No data at all ( n births = 24; n deaths = 29). For some subnational units (often remote islands or other remote areas with very low population), no data existed. In this case, we used national average values.
Once we had filled the missing data entries, we interpolated and extrapolated the years for which there were no data available for any subnational unit, using interpolation and harmonization methods adapted from ref. 59 . Shortly, we first interpolated missing national values between the years with available data using linear interpolation. Then, we extrapolated missing data points either before the first reported year or after the last one. For extrapolation, we used macroregional ( n = 12; see ref. 59 ) trends that were calculated using population-weighted national birth and death rates of those countries with full data coverage over the extended study period (1990–2019).
We then used the interpolated and extrapolated subnational birth and death rates to calculate population-weighted national average birth and death rates for each country and year. These rates were then compared with reported national values (compiled from World Bank and UN databases), resulting in an annual ratio between the reported death and birth rates and the ones calculated from subnational units. When we then used this national ratio to correct the bias of the subnational-level birth and death rates, we ensured that our data matched with reported data at the national level.
As the final result, we attained gap-filled harmonized full time series for all subnational areas for which data were available as well as for those countries for which no subnational data were found (Extended Data Fig. 1 ). We produced a combined dataset (both gridded and polygon formats) for both birth and death rates, which consisted of subnational values for those areas where it was available and national values for others.
Downscaling to gridded level
We used multiple linear regression together with area-to-point kriging (ATPK) 60 , 61 to downscale the subnational birth and death rates to 5 arcmin (~10 km at the equator) resolution. The downscaling was done so that the average birth and death rates (total births and deaths of an area) were maintained at the administrative unit (subnational or national, depending on the source data) scale 62 . For the regression model, we used ‘relative population density’ (for urbanization proxy) and ‘subnational human development index’ data as independent variables in downscaling both births and deaths. Income and mean years of schooling, both being indicators of HDI, have been shown to correlate well with babies per woman at national scale 63 and ref. 5 used population density as a proxy for estimating the difference in natural population increase between urban and rural areas. For births, these were used together with gridded data of ‘share of women of reproductive age (15–49 years) of total population’ and for deaths with gridded data of ‘ratio of average age and life expectancy’. The ‘share of women of reproductive age (15–49 years) of total population’ is also used by ref. 64 to downscale births to gridded scale—although they did it only for one year and selected countries. For the independent variables used for downscaling, we used annual datasets for 2000–2019. These data were produced for this analysis by using openly available global datasets. Data preparations for the independent variables are described below, followed by the downscaling method in details.
Data preparation of independent variables for downscaling
Human development index.
We used tabulated subnational HDI data from ref. 65 to create a gridded and gap-filled gridded subnational HDI dataset for each year over 2000–2019, at 5 arcmin resolution. We used a similar method to fill missing years and rasterize the data as ref. 59 use in their gridded HDI dataset.
Relative population density
Rather than using global population density or night light data as the proxy of urbanization rate, we used population density scaled between 0 and 1 for each country. Using the WorldPop dataset 55 , we first extracted the 5th and 95th percentiles of population density by country across all years from 2000 to 2020, after the lowest population density cells (population density < 1 person per km 2 ) were omitted. We then scaled population densities within each country and year between 0 and 1, such that:
where popdScaled is the scaled population density for a year, popd is the unscaled population density for that year, popd95th is the 95th percentile population density for the country in question (using all years) and popd5th is the 5th percentile population density (using all years).
The scaled population density then indicates the degree of urbanization an area has undergone relative to the maximum state of urbanization experienced by that country across 2000–2020.
Share of women of reproductive age of total population
We used WorldPop ‘Age and sex structures’ dataset 66 to count the number of women of reproductive age (15–49 years). The data provide annual estimates globally for each 30 arcsec grid cell for the “[…] total number of people per grid square broken down by sex and age groupings (including 0–1 and by 5-year up to 80+)” (ref. 67 ). We aggregated these data to 5 arcmin resolution and divided this with the aggregated (from 30 arcsec to 5 arcmin) total population count of each grid cell using WorldPop ‘Population counts’ data 55 . This resulted in the share of women of reproductive age for each grid cell at 5 arcmin resolution. We repeated this for years 2000, 2005, 2010, 2015 and 2020 and interpolated the missing years to get annual gridded data for 2000–2020 (see map of the last timestep in Fig. 1e ).
Ratio of average age and life expectancy
To first calculate the average age of population in each grid cell, we used the same WorldPop ‘Age and sex structures’ dataset 66 that was used to compute the ‘share of women of reproductive age (15–49 years) of total population’ (see above). The data include the number of males and females by age group and thus, it allowed us to estimate the average age (for example, for the age group 5–9 years, we used an average age of 7 years). However, the dataset does not specify the average age for the age group 80+ and, thus, we needed to use UN population prospects national-level data to estimate it for each country and year and separately for females and males. From the national-level data, we first estimated the average age of that age group (separately for females and males) and then combined that with the grid cell level data—that is, assuming that in each grid cell of a country in question, the share of population over 80 years old had an average age equal to national average. As a result, we reached an estimate of the average age for each 5 arcmin grid cell. We then used the life expectancy component of subnational HDI data from ref. 65 . To fill missing years and rasterize the data, we used a method similar to the one ref. 59 use in their gridded HDI dataset. Finally, we took the ratio of these two datasets, resulting in a ratio of average age and life expectancy—the percentage of how much of their expected length of life has an average person lived—for each year over our study period 2000–2019.
Downscaling methods
To downscale the collated census data from the subnational administrative boundary scale to 5 arcmin gridded scale, we fitted multiple linear regression models of death and birth rates against global independent variables introduced above. Separate regression models were fitted for four income groups, where grouping was based on their relative income band 68 : low, lower middle, upper middle or high income. We first applied these regression models at the subnational administrative boundary scale to assess model performance and to calculate residuals (differences between model estimates and reported data) for each subnational administrative area. We then applied the regression models at the 5 arcmin scale and adjusted the estimates using an ATPK for the residuals calculated at the subnational administrative boundary scale. Finally, the downscaled estimates were adjusted to ensure that the total number of births and deaths matched the original data when aggregated to the subnational administration boundary scale 62 . Below, each step is presented in more detail.
Linear regression models
On the basis of the dataset from the World Bank 68 , we split countries into four income bands, marking them as low, lower middle, upper middle or high income. For each income bracket, we fitted a different regression model to capture the differing relationships between birth or death rates and independent variables.
Both birth and death rate models used the scaled population density and HDI data as predictor variables, with birth rate models additionally including the ‘share of women of reproductive age (15–49 years) of total population’ variable, whilst death rate models additionally included the ‘ratio of average age and life expectancy’ variable. The predictor variables were aggregated at the subnational administrative boundary scale by taking average values, which were then used for regression against either birth rates or death rates across all years (2000–2019).
We applied these regression models to predict birth and death rates for each grid cell, aggregated the cell-wise values at the subnational scale by weighting them with population and then assessed model performance and calculated residuals against the collated census data. We found that the models predicted birth and death rates well, with the coefficient of determinations being 0.74 and 0.60, respectively (Supplementary Table 1 ).
ATPK of the residuals and final population-weighted adjustment
Having calculated the residuals between modelled estimates of birth and death rates at the subnational scale and the collated census data, we performed an ATPK procedure to distribute these residuals across a 5 arcmin point mesh following the method described in ref. 69 . These grid-scale distributions of the residuals were then added to a grid-scale application of the regression models to give a first approximation for the downscaled datasets of both birth and death rates.
Finally, we adjusted the downscaled approximations to ensure that the total number of births or deaths represented in the downscaled dataset matched the collated census data at the subnational scale by applying equation ( 8 ) to each grid cell:
where R i adjusted is the adjusted rate (birth or death) in the grid cell, R i is the approximated rate in the grid cell, j = 1 …n are the grid cells that fall within each subnational administrative boundary, p j is the population within grid cell j taken from WorldPop and C n is the census data birth or death rate for the subnational boundary containing cells j = 1 …n .
Net migration data
The downscaled gridded births and deaths data (Fig. 1g,h ) were then used to calculate natural population change (deaths subtracted from births) (Fig. 1i ). Together with annual global gridded population count for 2000–2020 55 (Fig. 1j ), the natural population change data allowed us to calculate net migration for each year and grid cell (Fig. 1k ) as ’natural population change’ subtracted from ‘reported population change’.
Validation of data
We validated all downscaled datasets (WorldPop data and gridded births, deaths and net migration) with subnational and/or national observations. Subnational observations at administrative level 2 were collected from OECD 50 , 70 , Eurostat 49 , 51 , 71 , national statistical service of South Korea 72 and from ref. 73 . National observations were collected from the UN 74 . Here, it should be noted that the subnational validation data for population, births and deaths were collected at administrative level 2 (communal) whereas level 1 (provincial) data were used for producing and harmonizing the gridded datasets. Level 1 (provincial) data were used in validating the net migration estimates.
WorldPop data were validated against OECD data 70 which reports population counts for 1,818 subnational units globally. WorldPop data were first aggregated to the subnational units and then compared with OECD data through correlation analysis. Validation results are presented in detail in Supplementary Fig. 1 and Supplementary Table 2 .
Downscaled births and deaths
Downscaled births and deaths were validated against Eurostat subnational data ( n = 1,504) and OECD subnational data ( n = 1,883), respectively. Gridded birth and death rates were first aggregated to the respective subnational units and then compared with the observed values. Validation results are provided in the Supplementary Fig. 2 and Supplementary Table 2 .
Net migration
For validating net migration data, we used national-level data provided by the UN 74 and subnational observations collected from ref. 73 for the United States (counties; n = 3,056), national statistical service of South Korea (provinces; n = 16) (ref. 72 ) and Eurostat for Europe (districts; n = 1,522) (ref. 71 ). Gridded net migration data were first aggregated to the national/subnational units and then compared with the observations in the respective resolution. Subnational validation was conducted for both the total net migration count as well as the rate (net migration per population). Total net migration for a unit was first obtained by aggregating (zonal sum) the gridded net migration estimates over a subnational unit. Annual rate of net migration per population for a subnational unit could then be calculated by dividing both the aggregate sum of net migration and the observed net migration by the aggregate sum of population count (here, WorldPop data were used) of the respective subnational unit. Additionally, cumulative sums of net migration were calculated for both the estimated (here-produced) and observed net migration data. Cumulative sums were calculated for years 2000–2005, 2006–2010, 2011–2015, 2015–2020 (5 year sums) and for years 2000–2010 and 2011–2020 (10 year sums). Rates for the accumulated net migration counts were calculated by dividing the accumulated sum by the respective 5 or 10 year population average in the given subnational unit. Validation results and country-specific details are provided in Supplementary Figs. 3 – 6 and Supplementary Table 2 .
Urban extent data
Existing mappings of global urban areas include exercises like the one mapping global human settlements and urban centres by using a ‘degree of urbanization’ approach that divides human settlements into rural, semi-urban and urban areas on the basis of population size, population and the density of built-up areas. However, these data cover only a few years 75 . A recent urban extent dataset developed by using night light data also covers only one timestep 76 , while the most up-to-date time-series data of global urban extent cover nearly 100 years (2010–2100) (ref. 77 ), hence missing a decade from our study period.
Thus, we created urban extent data for each country for each year by using gridded relative population density (see above) and the share of urban population of total population for each country and year. The tabulated annual share of urban population in each country was acquired from the UN World Urbanization Prospects 42 . By going through each country cell by cell, the share of urban population was used to find a population density threshold where urban population turns to rural. Here, it was assumed that all urban area cells were more densely populated than all rural area cells. In other words, the calculation propagated in descending order of population density so that the sparsest-populated cell within urban extent was assumed to be more densely populated than the densest-populated cell not within the urban extent.
This was done by first calculating the total and urban population for each country:
Then, a threshold where urban population turns to rural was defined by calculating the cumulative population count. Starting from the cells with highest relative population density, population count in each grid cell in each country was summed until the cumulative count met the urban population defined above (urbanPop). All cells in the cumulative count were assigned value 1 (urban) and the rest were assigned value 0 (rural).
Zonal statistics: administrative areas and socioclimatic bins
For the three administrative levels, we used the Global Administrative Areas (GADM) levels 0, 1 and 2 for national, provincial and communal areas, respectively.
All zonal statistics in the study were done with the zonal tool in terra package (v.1.5-12) in R 78 . Socioclimatic bins were created by using global gridded data of aridity (global aridity index 79 ), human development (human development index; see above) and population counts for 2000–2019 (ref. 55 ). For the climatic factor, aridity index—a ratio of potential evaporation to precipitation—was chosen to capture the different atmospheric and land surface processes shaping terrestrial dryness. Aridity index has been used to assess desertification under climate change 80 . Here, the zonal analyses were conducted by using a long-term estimate of net migration (accumulated sum over the study period 2000–2019), which is why the impacts of short-term events, such as natural disasters, are not visible in the analysis.
Our binning divides global inhabited areas into 100 socioclimatologically analogous zones, which have similar human development and climatic conditions. The binning was conducted in two steps. First, we divided all considered grid cells into ten population-weighted quantiles on the basis of HDI. After that, each HDI quantile was again divided into ten population-weighted quantiles on the basis of aridity. All values in the first aridity quantile of the first HDI quantile would fall under the first bin (bin no. 0), while values in the second aridity quantile of the first HDI quantile would go in the second bin (bin no. 1). The division of bins is illustrated in Extended Data Fig. 7 . This division ensured that each bin incorporates ~1% of the global population. The binning represented as a heatmap could then be transformed into a map representation (Extended Data Fig. 8c ). Urban and rural areas in each bin could be extracted by using the urban extent data (see above). Global maps and description of the preprocessing of both human development, aridity index and socioclimatic bins are available in Extended Data Fig. 8 .
Impact calculations
Impact of total, rural and urban migration on respective populations was calculated for each administrative unit, as well as for each socioclimatic bin. First, migration data and population counts were aggregated for each spatial unit for each year (2000–2019) by using zonal sum. Then, total population change between 2019 and 2000 and accumulated net migration were calculated for each unit:
Population change without migration (natural population change) could then be calculated by subtracting cumulative net migration from total population change:
The impact of migration on population was deducted by comparing population change to cumulative net migration by using the following criteria:
Direction of migration
The direction of migration was studied in rural and urban areas at three administrative levels. Here, accumulated rural and urban net migration over 2000–2019 were calculated and compared to define the direction of migration according to the following criteria:
Reporting summary
Further information on research design is available in the Nature Portfolio Reporting Summary linked to this article.
Data availability
All the data used in this study are publicly available. The resultant datasets are available at the following open-access repository https://doi.org/10.5281/zenodo.7997134 , including the following datasets: annual global net migration rates at grid scale as a multiband GeoTIFF with 5 arcmin resolution for 2000–2019; annual global net migration rates for adm0, adm1 and adm2 levels as polygon layers (gpkg-files); and annual global birth and death rates as multiband GeoTIFFs with 5 arcmin resolution for 2000–2019. Data are visualized in online net migration explorer at https://wdrg.aalto.fi/global-net-migration-explorer/ . Data underlying the web application are available in the repository with all other data.
Code availability
The analysis was performed using RStudio (R v.4.1.2). The code is available at https://github.com/mattikummu/global_net_migration.git . The online net migration explorer was conducted using RStudio (R v.4.2.2). The code is available at https://github.com/vvirkki/net-migration-explorer.git .
World Migration Report 2020 (International Organization for Migration, 2019).
Human migration. Nature 543 , 21 (2017).
Hoffmann, R., Dimitrova, A., Muttarak, R., Crespo Cuaresma, J. & Peisker, J. A meta-analysis of country-level studies on environmental change and migration. Nat. Clim. Change 10 , 904–912 (2020).
Hoffmann, R. & Muttarak, R. Policy Brief: Environment, Migration and Urbanisation: Challenges and Solutions for Low-and Middle-Income Countries (Task Force 10: Migration) (Think20, 2021).
de Sherbinin, A. et al. Global Estimated Net Migration Grids by Decade: 1970–2000 (NASA Socioeconomic Data and Applications Center, 2015).
Alessandrini, A., Ghio, D. & Migali, S. Estimating Net Migration at High Spatial Resolution, EUR 30261 EN (European Union, 2020).
Abel, G. J. & Sander, N. Quantifying global international migration flows. Science 343 , 1520–1522 (2014).
Article CAS PubMed Google Scholar
Abel, G. J. & Cohen, J. E. Bilateral international migration flow estimates for 200 countries. Sci. Data 6 , 82 (2019).
Article PubMed PubMed Central Google Scholar
Bell, M. et al. Internal migration data around the world: assessing contemporary practice. Popul. Space Place 21 , 1–17 (2015).
Article Google Scholar
Benveniste, H., Cuaresma, J. C., Gidden, M. & Muttarak, R. Tracing international migration in projections of income and inequality across the Shared Socioeconomic Pathways. Clim. Change 166 , 39 (2021).
Clemens, M. A. & Pritchett, L. Income per natural: measuring development for people rather than places. Popul. Dev. Rev. 34 , 395–434 (2008).
de Sherbinin, A. et al. Migration and risk: net migration in marginal ecosystems and hazardous areas. Environ. Res. Lett. 7 , 045602 (2012).
Niva, V. et al. Global migration is driven by the complex interplay between environmental and social factors. Environ. Res. Lett. 16 , 114019 (2021).
Abel, G. J., Brottrager, M., Crespo Cuaresma, J. & Muttarak, R. Climate, conflict and forced migration. Glob. Environ. Change 54 , 239–249 (2019).
Muttarak, R., Abel, G. J., Crespo Cuaresma, J. & Brottrager, M. Commentary: Recent progress and future directions for research related to migration and conflict. Glob. Environ. Change 71 , 102401 (2021).
Selby, J., Dahi, O. S., Fröhlich, C. & Hulme, M. Climate change and the Syrian civil war revisited. Polit. Geogr. 60 , 232–244 (2017).
Sipavičienė, A. & Stankūnienė, V. in Coping with Emigration in Baltic and East European Countries Ch. 3 (OECD Publishing, 2013).
Zanamwe, L. & Devillard, A. Migration in Zimbabwe: A Country Profile 2009 (International Organization for Migration, 2010).
Qi, W., Abel, G. J. & Liu, S. Geographic transformation of China’s internal population migration from 1995 to 2015: insights from the migration centerline. Appl. Geogr. 135 , 102564 (2021).
Plane, D. A., Henrie, C. J. & Perry, M. J. Migration up and down the urban hierarchy and across the life course. Proc. Natl Acad. Sci. USA 102 , 15313–15318 (2005).
Article CAS PubMed PubMed Central Google Scholar
Molloy, R., Smith, C. L. & Wozniak, A. Internal migration in the United States. J. Econ. Perspect. 25 , 173–196 (2011).
Jedwab, R., Christiaensen, L. & Gindelsky, M. Demography, urbanization and development: rural push, urban pull and … urban push? J. Urban Econ. 98 , 6–16 (2017).
Valenta, M., Jakobsen, J., Župarić-Iljić, D. & Halilovich, H. Syrian refugee migration, transitions in migrant statuses and future scenarios of Syrian mobility. Refug. Surv. Q. 39 , 153–176 (2020).
Mercandalli, S. et al. Rural Migration in sub−Saharan Africa: Patterns, Drivers and Relation to Structural Transformation (FAO and CIRAD, 2019).
Steinbrink, M. & Niedenführ, H. Africa on the Move: Migration, Translocal Livelihoods and Rural Development in Sub-Saharan Africa (Springer International Publishing, 2020).
Natale, F., Kalantaryan, S., Scipioni, M., Alessandrini, A. & Pasa, A. Migration in EU Rural Areas (Publications Office of the European Union, 2019).
Buckle, C. & Osbaldiston, N. Editorial introduction: counter-urbanisation in contemporary Australia: a review of current issues and events. Aust. Geogr. 53 , 347–362 (2022).
Clark, S. L. In search of housing: urban families in rural xontexts. Rural Sociol. 77 , 110–134 (2012).
Adger, W. N. et al. Urbanization, migration and adaptation to climate change. One Earth 3 , 396–399 (2020).
Marois, G., Bélanger, A. & Lutz, W. Population aging, migration and productivity in Europe. Proc. Natl Acad. Sci. USA 117 , 7690–7695 (2020).
Coleman, D. The demographic effects of international migration in Europe. Oxf. Rev. Econ. Policy 24 , 452–476 (2008).
Nikiforova, E. & Brednikova, O. On labor migration to Russia: Central Asian migrants and migrant families in the matrix of Russia’s bordering policies. Polit. Geogr. 66 , 142–150 (2018).
Malit, F. & Al Youha, A. Kenyan Migration to the Gulf Countries: Balancing Economic Interests and Worker Protection (MPI, 2016).
Rees, P. et al. The impact of internal migration on population redistribution: an international comparison. Popul. Space Place 23 , e2036 (2017).
Abel, G. J. Non-zero trajectories for long-run net migration assumptions in global population projection models. DemRes 38 , 1635–1662 (2018).
Abel, G. J. Estimates of global bilateral migration flows by gender between 1960 and 20151. Int. Migr. Rev. 52 , 809–852 (2018).
Bell, M. et al. Cross-national comparison of internal migration: issues and measures. J. R. Stat. Soc. A 165 , 435–464 (2002).
Birch, E. L. & Wachter, S. M. Global Urbanization (Univ. Pennsylvania Press, 2011).
Black, R., Bennett, S. R. G., Thomas, S. M. & Beddington, J. R. Migration as adaptation. Nature 478 , 447–449 (2011).
Cattaneo, C. Migrant networks and adaptation. Nat. Clim. Change 9 , 907–908 (2019).
Gemenne, F. & Blocher, J. How can migration serve adaptation to climate change? Challenges to fleshing out a policy ideal. Geogr. J. 183 , 336–347 (2017).
World Urbanization Prospects: The 2018 Revision (ST/ESA/SER.A/420) (United Nations, 2019).
Niasse, M. & Varis, O. Quenching the thirst of rapidly growing and water-insecure cities in sub-Saharan Africa. Int. J. Water Resour. Dev. 36 , 505–527 (2020).
Henderson, J. V., Storeygard, A. & Deichmann, U. Has climate change driven urbanization in Africa? J. Dev. Econ. 124 , 60–82 (2017).
Article PubMed Google Scholar
Rogers, A. Requiem for the net migrant. Geogr. Anal. 22 , 283–300 (1990).
Xu, C., Kohler, T. A., Lenton, T. M., Svenning, J.-C. & Scheffer, M. Future of the human climate niche. Proc. Natl Acad. Sci. USA 117 , 11350–11355 (2020).
Boas, I. et al. Climate migration myths. Nat. Clim. Change 9 , 901–903 (2019).
The DHS Program (STATcompiler, accessed February 2021); http://www.statcompiler.com
Live Births and Crude Birth Rate (Eurostat, accessed February 2021); https://ec.europa.eu/Eurostat/databrowser/
Regional Statistics, Mortality Crude Rates by Cause of Death, Large TL2 Regions, Small TL3 Region (OECD, accessed February 2021); https://doi.org/10.1787/region-data-en
Deaths and Crude Death Rate (Eurostat, accessed February 2021); https://ec.europa.eu/Eurostat/databrowser/
The Demographic and Health Surveys Program (Spatial Data Repository, accessed May 2021); https://spatialdata.dhsprogram.com/home/
Geodata for NUTS (Nomenclature of Territorial Units for Statistics) (Eurostat, accessed May 2021); https://ec.europa.eu/Eurostat/web/gisco/geodata/reference-data
Geospatial Data of the Large TL2 Regions (OECD, accessed May 2021); https://www.oecd.org/regional/regional-statistics/
WorldPop Hub (WorldPop, accessed 2021); https://doi.org/10.5258/SOTON/WP00647
Klein Goldewijk, K., Beusen, A., Doelman, J. & Stehfest, E. New anthropogenic land use estimates for the Holocene; HYDE 3.2. Earth Syst. Sci. Data 9 , 927–953 (2017).
Gleick, P. H. Methods for evaluating the regional hydrologic impacts of global climatic changes. J. Hydrol. 88 , 97–116 (1986).
Administrative Divisions of Countries (Statoids, accessed May 2021); http://statoids.com
Kummu, M., Taka, M. & Guillaume, J. H. A. Gridded global datasets for gross domestic product and human development index over 1990–2015. Sci. Data 5 , 180004 (2018).
Kyriakidis, P. C. & Geostatistical, A. Framework for area-to-point spatial interpolation. Geogr. Anal. 36 , 259–289 (2004).
Google Scholar
Murakami, D. & Tsutsumi, M. Area-to-point parameter estimation with geographically weighted regression. J. Geogr. Syst. 17 , 207–225 (2015).
Kallio, M. et al. Unpacking dasymetric modelling to correct spatial bias in environmental model outputs. Environ. Model. Softw. 157 , 105511 (2022).
Babies per Woman Compared to Mean Years in School and Income (Gapminder, accessed May 2022); www.gapminder.org
James, W. H. M. et al. Gridded birth and pregnancy datasets for Africa, Latin America and the Caribbean. Sci. Data 5 , 180090 (2018).
Smits, J. & Permanyer, I. The subnational human development database. Sci. Data 6 , 190038 (2019).
Pezzulo, C. et al. Subnational mapping of population pyramids and dependency ratios in Africa and Asia. Sci. Data 4 , 170089 (2017).
WorldPop Hub (WorldPop, accessed February 2021); https://doi.org/10.5258/SOTON/WP00654
Fantom, N. & Serajuddin, U. The World Bank’s Classification of Countries by Income (World Bank, 2016); https://doi.org/10.1596/1813-9450-7528
Chen, Y., Zhang, R., Ge, Y., Jin, Y. & Xia, Z. Downscaling census data for gridded population mapping with geographically weighted area-to-point regression kriging. IEEE Access 7 , 149132–149141 (2019).
Population by 5-year Age Groups, Small Regions TL3 (OECD, accessed February 2021); https://doi.org/10.1787/region-data-en
Population Change—Demographic Balance and Crude Rates at Regional Level (NUTS 3) (Eurostat, accessed February 2023); https://ec.europa.eu/Eurostat/databrowser/
Number of Internal Migrants for City, County and District (KOSIS, accessed February 2023); https://kosis.kr/statHtml/statHtml.do?orgId=101&tblId=DT_1B26001_A01&conn_path=I2&language=en
Winkler, R., Johnson, K., Cheng, C., Voss, P. & Curtis, K. County-Specific Net Migration by Five-Year Age Groups, Hispanic Origin, Race and Sex: 2000-2010: Version 1 (ICPSR, 2013); https://doi.org/10.3886/ICPSR34638.V1
World Population Prospects 2022: Data for Net Migration Rate (Per 1,000 Population) (UN, accessed February 2023); https://data.un.org/
Pesaresi, M., Florczyk, A., Schiavina, M., Melchiorri, M. & Maffenini, L. GHS Settlement Layers, Updated and Refined REGIO Model 2014 in Application to GHS-BUILT R2018A and GHS-POP R2019A, Multitemporal (1975-1990-2000-2015) (European Commission, Joint Research Centre, accessed April 2022); https://doi.org/10.2905/42E8BE89-54FF-464E-BE7B-BF9E64DA5218
Zhou, Y. et al. A global map of urban extent from nightlights. Environ. Res. Lett. 10 , 054011 (2015).
Gao, J. & O’Neill, B. C. Mapping global urban land for the 21st century with data-driven simulations and Shared Socioeconomic Pathways. Nat. Commun. 11 , 2302 (2020).
Hijmans, R. J. terra: Spatial data analysis. R package version 1.4-11 (2021).
Trabucco, A. & Zomer, R. Global aridity index and potential evapotranspiration (ET0) climate database v2. figshare https://doi.org/10.6084/m9.figshare.7504448.v3 (2019).
Cherlet, M. et al. World Atlas of Desertification: Rethinking Land Degradation and Sustainable Land Management 3rd edn. (Publications Office of the European Union, 2018); https://www.preventionweb.net/publication/world-atlas-desertification-rethinking-land-degradation-and-sustainable-land-management
Download references
Acknowledgements
This study was funded by Maa- ja vesitekniikan tuki ry, the European Research Council under the European Union’s Horizon 2020 research and innovation programme (SOS.aquaterra project; grant no. 819202), the European Research Council under the European Union’s Horizon 2020 research and innovation programme (POPCLIMA project, grant no. 101002973), the Aalto University School of Engineering, the Academy of Finland (TREFORM project; grant no. 339834), the Academy of Finland (WATVUL project; grant no. 317320) and the National Science Foundation of China funding research fund for International Young Scientists (grant no. 41950410572). The funders had no role in study design, data collection and analysis, decision to publish or preparation of the manuscript. We would like to thank M. Jalava from Aalto University for help with issues related to computations and setting up a server for the web application.
Open Access funding provided by Aalto University.
Author information
These authors contributed equally: Venla Niva, Matti Kummu.
Authors and Affiliations
Water and Environmental Engineering Research Group, School of Engineering, Aalto University, Espoo, Finland
Venla Niva, Alexander Horton, Vili Virkki, Matias Heino, Maria Kosonen, Marko Kallio, Pekka Kinnunen, Maija Taka, Olli Varis & Matti Kummu
Geoinformatics Research Group, School of Engineering, Aalto University, Espoo, Finland
Marko Kallio
International Institute for Applied Systems Analysis, Laxenburg, Austria
Guy J. Abel
Asian Demographic Research Institute, Shanghai University, Shanghai, China
Faculty of Social Sciences, The University of Hong Kong, Pokfulam, Hong Kong
Department of Statistical Sciences, University of Bologna, Bologna, Italy
Raya Muttarak
You can also search for this author in PubMed Google Scholar
Contributions
V.N. and M. Kummu designed the research. Data collection and processing was led by M. Kummu and M. Kosonen with help from A.H., V.V., M.H., M. Kallio, P.K. and V.N. V.N. and M. Kummu performed the analysis with help from A.H. V.V. created the online net migration explorer. V.N. and M. Kummu created the illustrations. All authors discussed the methods and results and helped shape the research and analysis. V.N. and M. Kummu took the lead in writing the manuscript with important contributions from all authors.
Corresponding authors
Correspondence to Venla Niva or Matti Kummu .
Ethics declarations
Competing interests.
The authors declare no competing interests.
Peer review
Peer review information.
Nature Human Behaviour thanks Elin Charles-Edwards, Yuehong Chen and the other, anonymous, reviewer(s) for their contribution to the peer review of this work. Peer reviewer reports are available.
Additional information
Publisher’s note Springer Nature remains neutral with regard to jurisdictional claims in published maps and institutional affiliations.
Extended data
Extended data fig. 1 origin of datasets used..
Origin of the datasets used for a) birth rates and b) death rates. See Methods for more details. Sources for the data are provided in Supplementary Data Table. The geospatial files used in the maps are from The DHS Program 52 , Eurostat 53 , OECD 54 and GADM ( www.gadm.org ).
Extended Data Fig. 2 Input variables used for downscaling birth and death rates.
(a) Human Development Index, (b) population density, scaled between 0–1, (c) share of women of reproductive age (15–49) of total population and (d) share of life lived for an average person. Each dataset includes annual values for each year over 2000–2019 – shown here are the data for the last timestep, that is year 2019.
Extended Data Fig. 3 Urban and rural areas.
Example of (a) global urban areas in 2019 and in three timesteps (b: 2000, c: 2010, d: 2019) in the South China Sea around the Malaysian peninsula.
Extended Data Fig. 4 Regional division.
Regional division based on the UN country grouping.
Extended Data Fig. 5 Net migration in rural and urban areas.
Net migration in urban and rural grid cells in three timesteps: 2000 (a,b), 2010 (c,d) and 2019 (e,f).
Extended Data Fig. 6 Cumulative net migration in urban and rural areas.
Cumulative net migration (per 1000 urban and rural inhabitants in each administrative unit) in urban and rural areas over 2000–2019. Three administrative levels are shown: a-b: communal (admin 2 level), c-d: provincial (admin 1 level) and e-f: national (admin 0 level).
Extended Data Fig. 7 Schematic illustration of creating the socioeconomic bins.
Illustration of how the Human Development Index (HDI) and Aridity (AI) were combined to create socioeconomic bins. Each bin includes ca 1% of global population.
Extended Data Fig. 8 Socioclimatic bins with the input data for them.
Global maps of (a) human development index, (b) global aridity index and (c) socioclimatic bins. Each bin includes ca 1% of global population. See Methods for more details.
Supplementary information
Supplementary information.
Supplementary Text, Figs. 1–6 and Tables 1 and 2.
Reporting Summary
Peer review file, supplementary data.
Description, resolution, timespan and sources of each collected dataset.
Rights and permissions
Open Access This article is licensed under a Creative Commons Attribution 4.0 International License, which permits use, sharing, adaptation, distribution and reproduction in any medium or format, as long as you give appropriate credit to the original author(s) and the source, provide a link to the Creative Commons license, and indicate if changes were made. The images or other third party material in this article are included in the article’s Creative Commons license, unless indicated otherwise in a credit line to the material. If material is not included in the article’s Creative Commons license and your intended use is not permitted by statutory regulation or exceeds the permitted use, you will need to obtain permission directly from the copyright holder. To view a copy of this license, visit http://creativecommons.org/licenses/by/4.0/ .
Reprints and permissions
About this article
Cite this article.
Niva, V., Horton, A., Virkki, V. et al. World’s human migration patterns in 2000–2019 unveiled by high-resolution data. Nat Hum Behav 7 , 2023–2037 (2023). https://doi.org/10.1038/s41562-023-01689-4
Download citation
Received : 05 July 2022
Accepted : 01 August 2023
Published : 07 September 2023
Issue Date : November 2023
DOI : https://doi.org/10.1038/s41562-023-01689-4
Share this article
Anyone you share the following link with will be able to read this content:
Sorry, a shareable link is not currently available for this article.
Provided by the Springer Nature SharedIt content-sharing initiative
Quick links
- Explore articles by subject
- Guide to authors
- Editorial policies
Sign up for the Nature Briefing newsletter — what matters in science, free to your inbox daily.

- Original Article
- Open access
- Published: 21 December 2021
Measuring migration 2.0: a review of digital data sources
- Jasper Tjaden ORCID: orcid.org/0000-0002-5054-3535 1 , 2
Comparative Migration Studies volume 9 , Article number: 59 ( 2021 ) Cite this article
8166 Accesses
10 Citations
40 Altmetric
Metrics details
This article has been updated
The interest in human migration is at its all-time high, yet data to measure migration is notoriously limited. “Big data” or “digital trace data” have emerged as new sources of migration measurement complementing ‘traditional’ census, administrative and survey data. This paper reviews the strengths and weaknesses of eight novel, digital data sources along five domains: reliability, validity, scope, access and ethics. The review highlights the opportunities for migration scholars but also stresses the ethical and empirical challenges. This review intends to be of service to researchers and policy analysts alike and help them navigate this new and increasingly complex field.
Introduction
International interest in measuring human migration is at an all-time high. The number of people living in a country other than their country of birth reached an estimated 272 million in 2019, an increase of 51 million from 2010 (UN DESA, 2019 ). The number of forcibly displaced people due to conflicts and disasters is at its historic high (UNHCR 2020 ). International migration is expected to continue increasing given higher levels of interconnectedness in the world due to improved communication and transport systems, protracted crises producing displacement, and structural changes such as climate change and population growth in certain world regions.
Governments worldwide have a keen interest in anticipating future migration flows and understanding the drivers of migration to plan ahead, allocate funds, attract workers and students, use remittances, facilitate migrant integration, and manage public opinion, among other issues. The increased demand for systematic measurement from policymakers has also manifested itself in two landmark policy frameworks adopted in the last decade: The Global Compact on Migration (GCM) and the Sustainable Development Goals. Footnote 1
Second, in step with the increased salience of migration in policy circles, migration research output has grown dramatically (Pisarevskaya et al., 2019 ). Between 1960 and 1980, the number of academic journals on related subjects quadrupled. In 2020, the International Organization for Migration (IOM) identified over 130 migration-related journals publishing more than 2000 journal articles in English, French or Spanish (IOM, 2020 ).
Policy and scholarly interest both rely on fundamental measurements of migration, i.e. how many people migrate (flows) or have migrated (stocks) within a specific time frame. Yet, the popularity and relevance of migration has outpaced substantial improvements in the systematic measurement of migration, especially at the global level. Indeed, the demand for ‘evidence’ has revived long-standing calls for better data on international migration which experts have been lamenting for decades (Bilsborrow et al., 1997 ; Clemens et al., 2009 ; Laczko, 2016 ; Lemaitre, 2005 ; Willekens et al., 2017 ). Footnote 2
This is the context in which a set of new data sources emerged providing migration researchers of all disciplines with new opportunities to measure migration. The arrival of “innovative data sources”—often referred to as “Big Data” or “digital trace data”—have been described as a “migration data revolution” (Laczko & Rango, 2014 ) and bears much potential to complement traditional migration data (Cesare et al., 2018 ; Sîrbu et al., 2021). At the same time, digital data present a host of new ethical challenges for researchers that are of great concern (Beduschi, 2020 ; Brayne, 2018 ; Hayes, 2017 ; Latonero & Kift, 2018 ; Leese et al., 2021 ; Molnar, 2019 ; Zwitter, 2014 ).
As new researchers and policymakers flock to the field of migration and the empirical study of migration diversifies, there is a need to explain and review new migration data sources to provide a better understanding of their respective limitations and strengths. This paper reviews eight data sources in terms of their reliability, validity, scope for research, access and ethics. The aim is to familiarize experienced and incoming migration scholars with new approaches. The review should be considered an attempt to contribute towards a broader process of interdisciplinary dialogue and expanding the empirical toolbox in migration studies.
Analytical framework
Defining migration.
Before mapping novel data sources, it is important to first define migration for the purpose of this study and clearly delineate the scope of the review.
Important aspects of definitions of migration are space (internal or domestic vs. international/ cross-border migration), time (short term vs. long term), type (e.g. labour, irregular, forced, family, education) and form (flows vs. stocks) (Bilsborrow, 2016 ; UN DESA, 2017 ). This review takes a broad and inclusive view in line with its aim to describe a menu of novel data sources for diverse groups of migration scholars and research interests. Here, migration will be defined as the changing of residence of an individual within or outside the boundaries of a country for longer than three months. While this definition is broad, it excludes certain types of mobility such as travel for the purpose of recreation, holiday, visits to friends or relatives, business, medical treatment or religious pilgrimage (which usually do not imply a change of residence). Footnote 3 The review considers data sources providing information on both migration flows (i.e. the number of migrants entering and leaving (inflow and outflow) a country over the course of a specific period, for example, one year) and migration stocks (i.e. the total number of migrants present in a given location at a particular point in time) (see Global Migration Group, 2017 ). The review considers any form and channel of migration including, among others, labour, family, forced and irregular migration and is not restricted geographically. In addition, to actual observed migration, the review also considers proxies for migration; such as, migration intentions, plans, desires, or aspirations that are commonly used to predict a future change of residence (Tjaden et al., 2019 ). Footnote 4 The review does not include how novel data can be used to research other fields of interest to migration scholars such as integration, the causes of migration, communication, or the impact of migration on society. These fields are not primarily concerned with or rely on (directly or indirectly) inferring migration flows or stocks from data.
Defining “novel” data sources
What are these “new” data sources and what makes them “newer” than the “old” sources? Two popular concepts are helpful to delineate traditional from “innovative” data sources for migration: “big data” and “digital trace data”.
Big data is commonly defined by the “three V’s”: volume, velocity, variety. Volume refers to the magnitude of data. However, there is “little consensus around the fundamental question of how big the data has to be to qualify as “big data” (Gandomi & Haider, 2015 : 137). Velocity refers to the rate at which data are generated which has dramatically increased with the proliferation of digital devices such as smartphones and sensors. Variety refers to the type of data that is being generated. Big data often includes numerical data, text data, images, audio and geo-location data. These Vs are useful to describe many of the sources that are commonly associated with big data such as social media platforms (Facebook, Twitter, Twitter, Instagram etc.) and online search platforms (google). These data compile millions of records about their users ranging from location, online activity to demographic user profile information. At the same time, this information is accessible in real-time, sometimes even publicly through application programming interfaces (APIs). “Big data” includes social media data but is not limited to it. Google, for example, offers a search service which does not operate as a social platform.
Digital trace data are the “results of social interaction via digital tools and spaces as well as digital records of other culturally relevant materials, such as archived newspapers and Google searches including data from popular social networking sites (such as Facebook or Twitter), personal blogs, collaborative online spaces (such as Wikipedia), and data derived from mobile phone or credit card usage” (Cesare et al., 2018 : 1980).
The terms “big data” and “digital trace data” refer largely to the same type of sources. However, while term “big data” highlights the type of data that is produced, the term “digital trace” data focuses on how the data is produced, i.e. through using digital devices (Cesare et al., 2018 ; Hughes et al., 2016 ; Sîrbu et al., 2020 ).
New data sources are often collected by private companies for the purpose of offering services to customers. In contrast, “traditional” sources of migration data such as censuses, administrative data and surveys are traditionally collected or made available by government agencies or (publicly funded) research institutes. Footnote 5 This has far-reaching ethical and empirical implications which will be discussed in later sections.
Review criteria
The review will discuss new data sources along five domains: (1) Reliability—the consistency and reproducibility of migration measurements, (2) validity—the accuracy of migration measures and the extent to which data allows to capture the intended concepts used by migration researchers, (3) scope—the breadth and depth of migration-related research that could be explored based on the respective data source, (4) accessibility—the degree to which data is accessible to researchers, and lastly, (5) ethics –the potential risk of violations of data privacy, consent and data protection principles in the data generation process and potential risk of (unintended) harm for research subjects as a result of analysis produced based on new data sources (e.g. Beduschi, 2020 ; Brayne, 2018 ; Cesare et al., 2018 ; Hayes, 2017 ; Latonero & Kift, 2018 ; Leese et al., 2021 ; Molnar, 2019 ; Zwitter, 2014 ).
Review of innovative data sources to measure migration
Mobile phones: call detail records and gps data from smartphone operating systems.
Mobile phone Call Detail Records (CDR) can track the approximate location of individuals and, as a result, display movements across space by capturing the call signal sent to cell towers for each outgoing and incoming call (Williams et al., 2015 ). All caller details are anonymized. Some telecommunications providers are amenable to social research as well, and often provide documented and anonymized digital trace data from their customers to researchers interested in analysing these data (e.g. Cesare et al., 2018 ; Chi et al., 2020 ).
Reliability CDR provides reliable measures of migration in terms of consistency over time. Movements are recorded automatically as required by operating the telephone network. An advantage in terms of reliability is that the information on location does not rely on self-reports by individuals, which may be subject to response biases (a common issue in surveys). However, reliability issues may apply when using CDR data from different operators. This is a common issue because most countries have several telecommunication companies. As consumers switch services, measures of movement over time become less reliable. As a result, CDR is often used on narrowly defined locations and limited time frames.
Validity The key disadvantage is that such data refers to mobile devices, not individuals as such. It is possible that individuals will share the same device, or gift it to others. Furthermore, many migrants may change devices and/or SIM cards after migrating to other countries, given that service providers offer deals limited to particular countries. Therefore, most contributions using phone data have analysed mobility within narrow geographic units (cities, regions) rather than movements across borders. Furthermore, CDRs can be biased because locations are only recorded when calls are made leaving blank spots in the migration process. Footnote 6
Scope While CDR data are usually more helpful for identifying internal (sub-national) migration patterns, Footnote 7 in some cases they can also be used to measure international migration at the sub-regional level, particularly when combined with other sources. For example, CDR have been used to track internal displacement following natural disasters such as the Haiti and Nepal earthquakes (Bengtsson et al., 2011 ), and the combination of CDR with satellite data can help to map movements between cross-border communities (Hughes et al., 2016 ). Recent work has leveraged Google Location History data for analysis on migration flows. Google Location History is collected through smartphones that operate the Google Android system and through Google services used through smartphones (e.g. Google Maps or Gmail). Pilot research suggests that this novel source of information could provide information about international migration through ‘fine scale mobility with rare, long distance and international trips’ documented through changes in location by users (Ruktanonchai et al., 2018 ). Using the same data, Kraemer et al. ( 2020 ) described ‘global human mobility patterns, aggregated from over 300 million smartphone users’. According to the authors, the data cover nearly all countries and 65% of earth’s populated surface, including cross-border movements and international migration. The advantage of CDR and location data through smartphone use for measuring migration is the timeliness and detail regarding the location. As such, phone records are particularly useful for studying sudden movements in defined geographic locations. Fast evolving migration situations are difficult to capture with “traditional” data sources such as sample surveys and administrative data, and impossible using censuses.
Without linking mobile phone records to other data sources, CDR provides a limited scope for migration scholars. The only information available is time and location. The type, channel and motivation for a change in location remains unobserved. It thus remains unclear who moved, why people moved, where they wanted to go, who they travelled with, through which channel they travelled, and whether they are likely to stay in their current location. This lack of context information is a key shortcoming compared to “traditional” sample survey research.
Accessibility CDR data is not commonly available to researchers and access depends on willingness of telecommunication companies to collaborate. Different operators in different countries may need to comply with different data protection legislation limiting the extent and level of detail of data that can be shared. In addition, access is often tied to large fees.
Ethics CDR data poses serious ethical concerns. When entering a mobile phone contract or installing a smartphone operating system, many users may not be aware that their location data is collected and analysed for various purposes (Beduschi, 2020 ; Brayne, 2018 ; Molnar, 2019 ). Such data uses are often hidden in the fine print. Since telecommunication and smartphone operating system providers are often private companies, there is a lack of transparency of what companies do with the data. In many countries, governments can mandate companies to provide access to data, for example, for the purpose of criminal investigations (Brayne, 2018 ). In the field of migration, the granular CDR data can be used to target humanitarian assistance to specific populations in specific locations, however, it could also be used by authorities for enforcing immigration policies, border protection and identifying individuals entering or residing in a country with an irregular status.
Social media
Geo-located social media activity, such as activity on Facebook (Zagheni et al., 2017 ), Twitter (Chi et al., 2020 ; Fiori et al., 2017 ; Martin et al., 2020 ; UN Global Pulse, 2017 ; Zagheni et al., 2014 ), Skype (Kikas et al., 2015 ), or LinkedIn (State et al., 2014 ), have been used to infer migration flows and stocks based on the location where users log in or information on location provided by the users themselves through geo-tagged posts or profile information (e.g. nationality or birthplace).
Movement is usually inferred based on changes users make to their self-reported location on the respective platform, or changes in location of log-ins. For example, data from the Facebook advertising platform can yield information on ‘home country’ and country of current residence. This means that Facebook could be used as a ‘real-time census’ to estimate, among other things, the number of users classified by the social media platform as ‘expats’ (users living in a country other than their ‘home country’) at the national or global level at a certain point in time (Zagheni et al., 2017 ). Using changes in Facebook users’ locations over time, others have identified the increase in the number of Venezuelan migrants in Spain in early 2018, confirmed by official statistics from the Spanish National Statistical Office (Spyratos et al., 2019 ).
Reliability There are a host of reliability concerns involved in measuring migration using social media data. First, certain segments of the population may be over- or under-represented (for instance, on average, young people are more likely to use Facebook than older people). Footnote 8 Second, even frequent users may choose not to provide information on their past and current location. Certain types of migrants may deliberately avoid providing information on their location on social networks. Third, it is difficult to verify whether changes in location are accurate, given that this information is sometimes self-reported on a voluntary basis. Fourth, the user base of social media providers constantly changes, which complicates analysis of trends over time (see e.g. Cesare et al., 2018 ).
Validity With many kinds of social media data, there is a lack of transparency on key measures relevant for migration are generated. For example, there is limited information on how Facebook identifies who is an “expat” or how it labels users as speakers of a different language. This complicates meaningful interpretation of migration patterns observable in the data.
Scope The advantage of geo-located social media data is that, in many countries, certain social media platforms are wildly popular, so that real-time data on large volumes of movements can potentially be accessed. Such data may be particularly useful to study broader migration trends. The level of detail provided by geo-coded social media data is limited in many cases but more extensive compared to CDR data. For example, Facebook provides aggregate-level information on the number of users with specific characteristics such as age, gender, or even education and income proxies as well as a vast range of preferences (measured via users’ “likes” of particular pages). Changes in the characteristics of the number of people living in a specific place are used by researchers to infer ‘migration flows’, assuming that changes in the ‘stock’ of people that report that they live somewhere necessitates that people moves from countries with lower stock numbers to countries with higher stock numbers. Information on friendship networks across countries—recently made available by Facebook—may be used in the future to forecast cross-country migration trends (Tjaden et al., 2021 ). Despite availability of additional characteristics, the data provide no information about the causes, means, or consequences of migration. There are attempts by governments, law enforcement agencies, international organization and research institutes to monitor the social media activity of migrants before, during and after migration to understand changes in migration patterns (Brenner & Frouws, 2019 ; Dekker et al., 2018 ; Sanchez et al., 2018 ). Footnote 9
Access Many social media companies offer public APIs to allow access to certain parts of their data to researchers. In many cases (e.g. Facebook, Twitter), access can be obtained at no cost which is a substantial advantage over traditional sources such as censuses, administrative data and survey. However, access modalities can change at any given time because data is provided by private companies, rather than taxpayer-funded government or research bodies that are mandated to provide systematic data over time.
Ethics Users of social media are often unaware of the data that is being collected about them and there is a general lack of understanding how such data is and can be used by companies themselves or third parties (Cesare et al., 2018 ; Zwitter, 2014 ). Migration enforcement agencies may use such data for surveillance purposes, which are particularly serious in contexts of irregular migration and forced displacement. Agencies could monitor communication of specific groups or individuals on Twitter and Facebook to identify irregular migrants and track them during their journeys. Companies such as Facebook, however, only allow access to anonymized, aggregate level data to researchers which limits the possibility of using data to harm individuals. Any information on narrowly defined locations and groups becomes inaccessible if the underlying target population decreases beyond a threshold that risks identifying any specific individuals. However, this does not apply to attempts to monitor public communication in social media groups indicating changes in migration patterns. The European Asylum Support Office (EASO) has suspended its efforts to monitor communication of migrants on social media following concerns by the EU own data protection body. Footnote 10
Email IP addresses
Repeated logins to the same website and IP addresses from e-mail activity have also been used to estimate international mobility patterns and users’ likelihood to move to another country (Zagheni & Weber, 2012 ). Rather than self-reported location by the user, certain online services such as email providers collect data on where users log into their accounts.
Reliability and validity The same limitation in terms of reliability and validity apply compared to social media data. Similar to log-ins to social media, log-ins to emails are usually recorded via devices (IP addresses) not necessarily people. For example, it is possible—yet presumably rare—that various people use the same email account which will distort any aggregate measure of migration.
Scope The scope of potential migration analysis is further reduced in the case of email log-ins given that additional socio-demographic and socio-economic information about the users (which are available for Facebook) is lacking or not publicly accessible.
Access Most email providers also do not provide public APIs that make data available to researchers. Email communication is considered personal and private communication whereas some communication on social media platforms is (intentionally or unintentionally) made public by users.
Ethics Similar to social media data, there are issues concerning consent and data privacy. Users may not be aware that email providers track their location. In other cases, governmental enforcement agencies may mandate companies to share content of emails of specific individuals for the purpose of criminal investigations or intelligence which bears the potential for misuse also in case of migrants in irregular settings (Brayne, 2018 ).
Online search data
Online search data has also been used more recently to study migration. Records on Google searchers, for example, have been explored to forecast the number of arrivals of asylum-seekers in Europe (Connor 2017) or internal migration within the U.S. (Lin et al., 2019 ). Search data generated through Google’s online search platform for migration can be exploited to measure migration intentions and predict subsequent emigration flows (Böhme et al., 2018 ; UN Global Pulse, 2014 ). For example, researchers retrieve data on how many times individuals in country A have ‘googled’ a term that the researcher believes to indicate an intention to migrate (to country B)—for example, ‘jobs’, ‘visa’, or the name of the destination country.
Reliability Google Searches are recorded consistently and provide high reliability in terms of the measure as such. The main advantage to Search Data is that Google’s search engine is widely used across the globe and has been successfully used to study other social behaviours (e.g. flu outbreaks). Despite broad coverage, important countries (i.e. China) are missing entirely. Issues of reliability emerge regarding applicability across various country contexts, languages and specific populations. Preliminary research in this area suggests that online searches (e.g. via Goole searchers) are related to actual movement at the aggregate level, yet the selection of specific search terms in various country contexts appears to be highly important. Syrians looking for ways to flee to Europe ‘google’ different terms than Canadians looking for a job in the US. The meaning of the same search terms may also vary in different languages. Overall, this means that Google searches may be indicative of migration from a certain country to another country, but difficult to scale up to multiple migration contexts (see Tjaden et al., 2021 ).
Validity Online search data has one obvious shortcoming: ‘searching’ is not ‘doing’. Just because someone looks up information on another country or, more explicitly, gathers information on how to move to another country, does not mean that they will actually move. Search data (similarly to survey data on emigration intentions) are a ‘pre-behavioural’ proxy for actual migration. Some studies suggest that intentions are a good predictor for eventual migration (Van Dalen & Henkens, 2013 ; Tjaden et al., 2019 ), but research also suggests that the strength of the predictor varies considerably based on where migrants are from and where they want to go (Tjaden et al., 2019 ).
Scope A major disadvantage of Google search data is the high level of aggregation at which data is made available. Search data is made available at the population level for countries or, in certain countries like the US, for subregions. Search data does not include any additional information about those who show interest in migrating, and thus renders any individual-level analysis impossible.
Access Google search data is freely and publicly accessible via the Google Trends platform and API.
Ethics The potential risk of misuse of data is limited given the high level of aggregation and anonymity of data which the company makes available. Serious concerns would arise when data for specific locations and IP addresses is used to infer individual level migration behaviour. Google itself is analysing individual-level location data to provide targeted advertisements to users who use their search engine. However, there is a lack of transparency in terms of the conditions under which such data may be shared with governments or other third parties. In addition, usual concerns around unawareness among users about the usage of their data apply.
Bibliometric data
Bibliometrics is a field of research that uses statistical methods to systematically analyse publications records (books, articles etc.). One sub-field of bibliometrics—scientometrics—is the analysis of scientific publications. Detailed information about academic output is recorded and made accessible through scientific databases (e.g. Scopus, Web of Science, Google scholar and others). This information has been used to model the international mobility of academics (Czaika & Orazbayev, 2018 ; Laudel, 2003 ; Moed & Halevi, 2014 ; Sudakova & Tarasyev, 2019 ; Wang et al., 2019 ). Changes in the researchers’ affiliation to institutions located in different countries indicates migration.
Reliability Measuring migration through changes in affiliations is consistent and reliable. Scientists have an interest to publish their work in recognized journals and books, institutions have an interest that researchers indicate their home institution, and most research outlets make it mandatory for authors to provide this information. Nevertheless, the data is sensitive to the accuracy of self-reported data which can be outdated.
Validity Migration analysis based on bibliometric data has the potential to collect additional context information including socio-demographic characteristics of the professionals (age, gender, ethnic origin, for example, may be inferred based on name recognition algorithms and web scraping individual professionals’ web pages). Additional information about the universities, faculty and chair may be matched with additional effort.
Scope The drawback of this data source is its restriction to a narrowly defined group of professionals (i.e. academics) where public access to their affiliation is the norm. However, it may be possible to extend this approach to other fields of professionals where public information on affiliations is common (i.e. athletes, musicians etc.).
Access Bibliographic data has become available through the digitalization of entire libraries, records of publishers, academic journals, and ambitious projects such as Google Books and Google Scholar that aim to record any academic publications that is published. Most academics provide their affiliations publicly to gain visibility and broaden their reach.
Ethics Compared to previously described sources, ethical concerns are limited because the personal information used for analysis is provided voluntarily and knowingly. The population is restricted to regular labour migrants which limits the potential for misuse by authorities.
Remote sensing technologies
Remote sensing is an umbrella term for collecting information about something without making physical contact. In current usage, remote sensing refers to the use of satellite or aircraft-based sensor technologies (i.e. drones). Remote sensing is commonly used in geography, earth sciences, climate research, agricultural studies, wildlife studies, military, and intelligence gathering, but also increasingly for urban planning, tourism, commerce, and various humanitarian applications (Miller et al., 2019 ). Changes in human activity visible in the images (i.e. settlements, refugee camps, light emissions at night) can be used to infer mobility.
Reliability If applied consistently, the approach to measuring migration using remote sensing technology by averaging physical quantities over pixels can yield reliable migration measures. Algorithms automatically detect changes in visual patterns on satellite or drone images over time. For example, the population size of settlements can be estimated by counting rooftops visible on satellite/drone images. Depending on the proximity and resolution of the image, individuals within certain localities can be identified. Comparing images over time can be used to estimate immigration and emigration into a certain, narrowly defined, location.
Validity The obvious downside of satellite and drone images for measuring migration is that no additional individual-level information about migrants is available: Who is moving, from where, to where, how etc. By itself, remote sensing provides information on how many tents, rooftops or individuals are present in a certain locality, but no information about what happened when there are less dots and shadows the next time new images become available.
Scope There is a rapidly growing number of examples with relevance for migration studies. First, drones and satellite images inform policies and direct aid to refugees. For instance, the United Nations Institute for Training and Research (UNITAR) mapped refugee camps in Jordan and elsewhere with its Operational Satellite Applications Programme. Footnote 11 Civil society organizations such as Human Rights Watch or Amnesty International use satellite imagery to document humanitarian needs of displaced populations at borders or in refugee camps by measuring the growth of settlements. Footnote 12 In this case, satellite images are providing an indication of where aid and assistance are most needed (Bitelli et al., 2017 ; Quinn et al., 2018 ; Shatnawi et al., 2020 ; Tiede et al., 2017 ).
Satellite imagery also forms a key part of the ‘smart border’ agenda, which attempt to use modern technology to improve border management around the world and track ‘illegal’ crossings. Systems relying on remote sensing were developed “to assist border authorities with more effective surveillance and reliable decision-making support” (Al Fayez et al., 2019 ). In contrast, civil society organizations use the same technology to monitor deaths and violations of migrants' rights at the maritime borders of the EU. Footnote 13
For the moment, remote sensing appears to be most useful for informing operations on the ground (managing refugee camps, targeting humanitarian assistance, managing borders etc.) and less for research on migration per se. The technology can also be used to monitor slow onset emigration rates due to changes in climate which can also be inferred from images.
Access With improvements in the quality and accessibility of satellite imagery (Popkin, 2018 ) provided by the European Space Agency, NASA, and others, researchers are also exploring ways to use remote sensing data to measure human migration globally. Public and private bodies offer access to satellite imagery for research purposes and tech companies offer cloud computing power to conduct complex and demanding analyses within minutes. Footnote 14 Depending on the specific data provider, access can be free of charge to research institutes or come with a fee.
Ethics Ethical issues are a key concern for remote censoring technologies because information is collected without the knowledge or consent of individuals. New high-resolution satellite imagery and drone images can identify individuals using face recognition technology. Law enforcement, policing and intelligence agencies use such approaches (Brayne, 2018 ; Hayes, 2017 ; Leese et al., 2021 ; Molnar, 2019 ) which raises serious concerns regarding the situation in undemocratic countries with low data protection standards and policies aiming to suppress and control groups in society. Drones may also be increasingly operated by companies in addition to governments which raises concerns over unknown privacy violations by non-governmental actors.
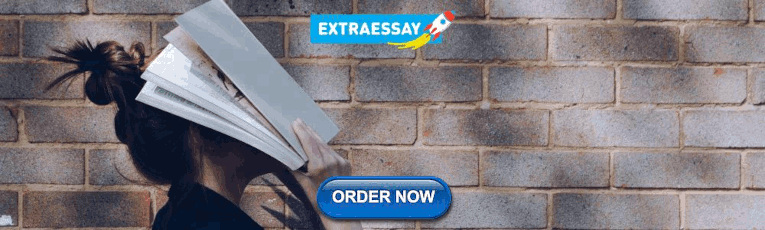
International air travel
Upon first view, international air passenger traffic belongs to the realm of tourism and transport studies, not migration (see Sect. 2.1.). However, there have been attempts to use this information to infer migration flows. For example. Gabrielli et al. ( 2019 ) used dyadic monthly air passenger traffic between 239 countries and territories worldwide from January 2010 to March 2018 to estimate the number of passengers on commercial flights operated globally. The study explored whether a surplus in travel (increase in travel from A to B but no increase in return travel from B to A within a year) can be linked to migration flows.
Reliability Air passenger data is highly standardized and consistent as it is subject to international industry standards.
Validity Passenger data does not measure migration directly and can only be used to infer different types of migration by inference. Since air passengers data does not allow to track individual passengers or specific cohorts on the basis of their date of entry, researchers need to make assumptions about the length of stay of the passengers. This is problematic because the publicly available data does not indicate who the passengers are, how long they will stay in the country, on which visa they are travelling etc. In addition, flight passenger data is a selective picture of global mobility. 44 percent of registered cross-border travels occur through commercial flights, and that this proportion increases at rising distances between countries (Recchi et al., 2019 ).
Scope Overall, the data may be used to estimate international migration flows if combined with additional data sources. At the moment, the research is still in its exploratory stage and the methods appear underdeveloped. In the future, this approach may bear the potential to measure the level of visa overstayers between countries, one indicator of irregular migration.
Access Air passenger data is collected by flight companies which some make available for purchase. The EU recently made a public and free dataset available. Footnote 15
Ethics Ethical concerns regarding flight data are limited in its current state of the available data. Flight data is aggregated at the country and month level and anonymized. Currently, any misuse for the disadvantage for individuals is unlikely.
Online news data
New advances in technology have made available online news aggregators such as Google News or the Global Database of Events, Language, and Tone (GDELT). Footnote 16 Such platforms monitor the world's news media from nearly every corner of every country in print, broadcast, and web formats. This data has the potential to capture acts of past or prospective migration that were not covered in traditional sources such as administrative data or surveys.
Reliability Migration measures based on online news aggregator data can be considered reliable to the extent that algorithms deriving information on migration apply consistently across all countries and news sources. The issue is that the success of the algorithm in detecting migration may vary by country, by quality of the news outlets, by language and type of migration to be covered. In addition, algorithms may capture the same migration events several times as the same event may have been covered by several news outlets.
Validity The large volume of news articles required to collect information on migration encourages researchers to use language processing algorithms. The emerging evidence is still unclear on how accurately such algorithms may detect events that actually capture migration.
Scope Approaches are still very recent but several uses of this data are available. Carammia et al., ( 2020 ) have used the GDELT database to measure political, social, economic “push factor” events that could motivate people to leave their country. In combination with other data sources, they attempt to forecast displacement and migration with a view to set up early warning systems currently under development in the EU. Footnote 17 In a similar vain, the Internal Displacement Monitoring Centre (IDMC) uses the GDELT database to track internal displacement. Footnote 18 The IOM is also experimenting with such data to improve analysis of the number of migrants that went missing along their journeys, such as the IOM’s missing migrants project (Borja & Black, 2021 ). Apart from eye-witness reports, news articles are the main way to systematically collect data on migrant fatalities and bring light to this tragic topic for policymakers.
Access GDELT and Google News can be accessed online free of charge for researchers.
Ethics Ethical concerns arise in countries with low standards of journalism and data privacy. It is possible, for example, that the identity of individual migrants is revealed in a news article and picked up by automated text analysis. In theory, this information could be used by enforcement agencies to press charges in case of irregular migration or used by smugglers for debt collection. Even when interviewees provide consent for their personal information to be used, they may not be aware that their information may enter migration databases. Such abuses are possible with traditional media sources, however, digital applications may exacerbate the problem by providing cheaper, faster and broader access to data.
Discussion and conclusion
This review highlighted both the enormous opportunity of “big data” and “digital trace data” to complement traditional sources of migration data (see Cesare et al., 2018 ; Hilbert, 2016 ; Hughes et al., 2016 ; Laczko & Rango, 2014 ; Rango & Vespe, 2017 ; Sîrbu et al., 2021 ) and the main challenges and risks associated with such data. Several broader conclusions can be drawn from the above discussion on the eight discussed sources (see Table 1 for a summary):
The main advantages of digital data sources for migration scholars are captured by the first two V’s of the “3 V’s definition” of big data introduced in Sect. 3.2 : Volume and velocity. In some cases, digital data sources provide information of millions of individuals in almost real-time. This provides migration researchers with the possibility explore migration trends where administrative data sources, surveys and censuses (sources traditionally used for inferring migration) are not available (such as in many low-income contexts), not accessible or too slow (for example in contexts of displacement and forced migration that are unfolding rapidly). Another key advantage is its granularity by providing “high-resolution” information. Digital data sources, especially approaches leveraging remote sensing technologies or mobile cell phones, often allow researchers to zoom into migration events at the sub-national, sub-regional or even local level. Nationally representative survey data, for example, often lacks sufficient sample size to disaggregate to the level of regions, districts or cities.
Unlike “traditional” sources, new data sources often make no distinction in terms of the “legal residence status” of individuals. Anyone using a digital service in included in the data. As a result, the volume, granularity and status-agnostics of many digital data sources offer new opportunities to collect information on “hard-to-reach” populations such as recent migrants, displaced or forced migrants and irregular migrants which are often excluded from ‘official’ data sources such as population registers or surveys (Cesare et al., 2018 ; Massey & Capoferro 2004 ; Reichel & Morales, 2017 ). Lastly, a key advantage to migration scholars is the fact that many data sources are accessible online and free of charge.
The review has also highlighted major challenges associated with using digital data sources for inferring migration. ‘Digital trace’ data is largely collected by private companies who offer services to their users and use user data to target advertisements or sell data to third parties. These data are not designed for research purposes. This has important implications both on ethical (Beduschi, 2020 , Brayne, 2018 ; Cesare et al., 2018 ; Hayes, 2017 , Latonero & Kift, 2018 ; Leese et al., 2021 ; Molnar, 2019 ; Sîrbu et al., 2020 ; Zwitter, 2014 ) and empirical grounds in terms of reliability and validity of migration measurement (Cesare et al., 2018 , Ruel et al., 2016 ; Sîrbu et al., 2020 ).
First, there are severe ethical concerns regarding the use of digital data sources including the limited awareness of users regarding the extent of and purposes for which their data is being used and the risk of harm for individual migrants in cases where information is used by law enforcement, border management, intelligence agencies or smugglers (Brayne, 2018 ; Hayes, 2017 ; Molnar, 2019 ). The first rule that a researcher must follow is to acknowledge that data are people and can do harm (Sîrbu et al., 2021 ). Data may include information on particular vulnerable groups such as refugees, irregular migrants, persons displaced by disasters. Some may be persecuted by authorities in origin and destination countries. Researchers must ensure ethical standards for data use that protect vulnerable groups from identification and possible discrimination (Cesare et al., 2018 ).
Violations of data privacy and protection standards are especially concerning in undemocratic countries with low data protection standards, limited rule-of-law, and a lack of democratic norms. In extreme cases, new technologies can enable “digital authoritarianism” and “Orwellian state surveillance” (Dragu & Lupu, 2020 ). Three examples illustrate the extent of real risks: China’s social credit system, for example, leverages smartphone location data, social media communication, travel records, purchase records, camera data and facial recognition, among others, in combination with various administrative records to assign a social credit to its citizen. Low scores could be used to prevent access to a passport or visa needed to leave the country. Footnote 19 After departure of US troops, the Taliban have reportedly considered using US-made digital identity technology to persecute Afghans who have worked with the international coalition. Funded by millions of donor funding, Afghanistan’s National Statistics and Information Authority launched a digital biometric identity card including fingerprints, iris scans and a photograph, as well as voter registration databases. Footnote 20 In 2018, Bangladesh shared hundreds of thousands of data of Rohingya refugees collected by UNHCR with Myanmar then used to facilitate potential repatriation. Footnote 21
Unlike states, researchers often do not have access to individual-level information from digital sources, however, they must be aware of the potential harms for individuals and groups when using digital data sources and must review the data providers’ data protection and privacy standards. Researchers are advised to seek ethical approval by a scientific committee when dealing with digital data and migration.
Apart from ethics, the review has highlighted many empirical challenges. Reliability and validity of digital data sources for inferring migration must be considered when engaging in research. It is often not transparent how exactly key measures of migration are generated (i.e. Facebook, Google). There are also concerns regarding “generalizability” of digital data as the user base of various digital services is often selective and does not represent the general population at large (Cesare et al., 2018 ; Sîrbu et al., 2020 ). Digital data is often made available at highly aggregated levels. This severely limits the analytical potential for migration scholars who are often interested in measuring migration at the individual (micro) level. Moreover, digital data often remains “thin” offering very few additional information beyond time and location such as socio-demographic or socio-economic characteristics of migration, the reasons and channels of migration or the expected duration of stay. This further limits its analytical use especially if the data is not combined with other data sources. Traditional surveys usually do not face these issues (but many others) as they are tailored for answering specific research questions.
Lastly, many populations are excluded from a variety of digital data sources despite technological advances worldwide. For those data relying in digital traces such as social media or online searches, it is still a long way to obtain a comprehensive picture of global mobility as smartphone user penetration reached 38.5 percent in 2020 and half the world population is still offline today. Footnote 22
Looking ahead, several trends are already unfolding. First, new breakthroughs in measuring migration research will stem from a combination of different sources (e.g. Alexander et al., 2020 ; Sîrbu et al., 2020 ; Snijders et al., 2012 ). Given the ‘representativeness’ issue of digital trace data, traditional data is needed for “ground truthing” (i.e. cross-validating data by comparing it with other ‘official’ data sources). To address, the “thinness” of digital data, combining data provides large opportunities to add richness to the analysis. One example is using social media, online search, or mobile phone data to locate migration events or patterns and then target surveys in certain geographies or adjust administrative data collection accordingly (Alexander et al., 2020 ).
The second trend is further convergence and integration of academic disciplines around the issue of measuring migration (e.g. Miller et al., 2019 ). Different disciplines such as earth sciences, climate research, security studies, tourism and transport studies, computer science, sociology, economics, demography, ethnography, library sciences and political science bring different tools, methodologies and technologies to the table which will likely see the field become even more interdisciplinary. As a result, more interdisciplinary dialogue is needed to advance the field.
As the field is changing at a dizzying speed, this paper attempted to provide a brief overview and reflection on the main new digital data sources. The aim of this review was to provide incoming migration researchers with a menu of options and seasoned researchers with an update on new approaches. The information provided assists researchers in making difficult trade-offs when approaching their research question and policy analysts with a broad understanding of the limitations of the data they use.
The review has two obvious limitations. First, given the complex and rapidly growing field it seems near impossible to cover every existing approach and to cover the literature in all its breath. The review is focused on the main approaches without any claim to be exhaustive. Second, the field is rapidly evolving. This means that new research will have become available already by the time of publication.
The toolbox for migration researchers will become bigger, more diverse, but also more powerful due to new opportunities of digital data. Despite the ‘gold rush’ on big and digital data, the review also cautioned migration scholars in view of the many ethical and empirical obstacles for inferring migration based on digital data sources. The review aimed to contribute to a balanced understanding of these new data sources to facilitate knowledge accumulation and interdisciplinary dialogue in the field of migration studies.
Availability of data and materials
Not applicable.
Change history
13 may 2022.
In the online version of this article, there was a typo in the funding note. The article has been updated.
The GCM is the first comprehensive, inter-governmentally negotiated (non-binding) treaty on migration adopted in 2018 by 152 countries, highlights the importance of data and evidence throughout its 23 objectives. The first goal of the GCM itself is improving migration data across the board. The SDGs are the follow-up to the UN Millennium Goals and feature several migration-relevant goals and include indicators to measure progress towards them. New approaches to measuring migration based on “innovative” data sources have mainly been pioneered by academic scholars, yet some of this work has long caught the attention of key national and international governmental stakeholders. In 2009, the United Nations Global Pulse initiative was launched to use new data sources for analysis of development projects and processes, including migration issues. In 2018, the European Commission, in partnership with the IOM Global Migration Data Analysis Centre, launched the ‘Big Data for Migration Alliance’ with the aim of sharing knowledge on data innovation in the field of migration, providing technical support to local and national administrations interested in using new data sources, and testing new data applications for specific policy needs.
There are exceptions. The collection, harmonisation and estimation of migration data within the EU (Poulain et al., 2006 ; Raymer et al., 2013 ) and compilation of data on migration flows in Latin America (Lemaitre, 2005 ) are key achievements. Data on migration in many non-OECD countries remains limited.
Mobility (migration regardless of distance and borders) more broadly has traditionally been the focus of transport studies and urban planning (e.g. Pappalardo et al., 2015 ; Song et al., 2010 ).
There has been some indication that intentions (also expressed in Google searches) are in fact a relevant predictor of future migration both at the national and international levels (e.g. Van Dalen & Henkens, 2013 ; Tjaden et al., 2019 ; Böhme et al., 2018 ).
Censuses are population counts and are used to calculate ‘stocks’ of migrants (Bilsborrow et al., 1997 ; Global Migration Group, 2017 ; White, 2016 ). Administrative data are collected by governments for the purpose of providing certain services or enforcing certain laws and, thus, may include records of migrants subject to a particular law or service. They may include population registers; visas; immigration, expatriation and asylum records; border entries; work permit registers; tax records, and health insurance or social insurance records (Bilsborrow, 2016 ; Global Migration Group, 2017 ; Lemaitre, 2005 ; Poulain et al., 2006 ; Willekens et al., 2017 ). Sample surveys are a common approach to measuring migration, especially in research, given its’ flexible choice of location, target group and thematic scope (Bilsborrow, 2016 ; Bilsborrow et al., 1997 ; Fawcett & Arnold, 1987 ; Goldstein & Goldstein, 1981 ).
Extended Detail Records (XDRs) and Control Plane Records (CPRs) also record locations when something is downloaded or phones are switching antennas providing more complete coverage.
Measuring human mobility (within countries, regions or cities) is an exploding field of research spanning physics and network science, to data mining, and has fueled advances from public health to transportation engineering, urban planning, official statistics and the design of smart cities (see e.g. Song et al., 2010 ; Pappalardo et al., 2015 ).
Researchers are working to address the methodological challenges associated with such (‘self-selection’) bias, and results look promising (Spyratos et al., 2019 ; Zagheni et al., 2017 ; Hughes et al., 2016 ).
Europol, Fontex and EASO as well as EU member states have been actively been monitoring communication in Facebook groups relevant for organizing migration, for example, from the Middle East and Africa to the EU. Information on prices of forged documents or sea transform offered by smugglers provide indication on changes in the volume and direction of migration flows. EASO was stopped collecting the data by the EU’s own data protection watchdog, see https://euobserver.com/investigations/146856 . In theory, it is possible to collect information on users’ profiles and monitor where those users report their location online a year later. This controversial approach has been tried successfully, yet no public evidence has been released.
https://euobserver.com/investigations/146856
https://unitar.org/sustainable-development-goals/satellite-analysis-and-applied-research
https://earthi.space/blog/satellite-solution-refugee-crisis/
https://www.hrw.org/news/2016/09/07/jordan-new-satellite-images-syrians-stranded-border
http://www.satsentinel.org/
https://www.unglobalpulse.org/2020/02/pulsesatellite-a-collaboration-tool-using-human-ai-interaction-to-analyse-satellite-imagery/
For example, governments use drone imagery to monitor immigration attempts in the Mediterranean Sea and at Europe’s land borders (Bhadwal et al., 2019 ; Dijstelbloem, 2017 ) or to monitor border crossing attempts at the Mexican-US border (see https://www.gao.gov/assets/690/682842.pdf ). Greek and EU authorities use satellite imagery to monitor crossing attempts (ibid.). NGOs and universities also use the same information to document human rights abuses by, for example, using satellite imagery and other evidence to reconstruct the journey of a boat with migrants that lost dozens passengers upon its return to Tripoli (Dijstelbloem, 2017 ). Although the boat had been spotted by several aircraft and vessels, no rescue operation had been mounted.
See for example https://earth.esa.int/eogateway/ ; https://search.earthdata.nasa.gov/search ; https://earthexplorer.usgs.gov/
See the EU Knowledge Centre for Migration and Demography’s Dynamic Data Hub, at: https://bluehub.jrc.ec.europa.eu/migration/app/?state=5d6005b30045242cabd750a2 .
GDELT is a repository of 316 types of geolocated event reported in the world’s broadcast, print and web media, in 100 languages.
Frontex, EASO and JRC are all developing early warning, forecasting and foresight approaches.
https://www.internal-displacement.org/expert-opinion/idetect-how-technology-and-collaboration-between-innovators-can-help-ensure-no-one
The system has reportedly been used to suppress and control the Uighur minority in China’s Xinjiang region. Analysis to linked data from various digital data sources alerts enforcement agencies at checkpoints when Uighurs approach the limits of their neighbourhood. (see Ross Andersen in the Atlantic: “The panopticon is already here”, available at https://www.theatlantic.com/magazine/archive/2020/09/china-ai-surveillance/614197/
See Emrys Schoemaker, 7 September 2021 in the Guardian: “The Taliban are showing us the dangers of personal data falling into the wrong hands “, available at https://www.theguardian.com/global-development/2021/sep/07/the-taliban-are-showing-us-the-dangers-of-personal-data-falling-into-the-wrong-hands
See Human Rights Watch on 21 June 2021: “UN Shared Rohingya Data Without Informed Consent”, available from https://www.hrw.org/news/2021/06/15/un-shared-rohingya-data-without-informed-consent
https://data.worldbank.org/indicator/IT.NET.USER.ZS
Al Fayez, F., Hammoudeh, M., Adebisi, B., & Abdul Sattar, K. N. (2019). Assessing the effectiveness of flying ad hoc networks for international border surveillance, International Journal of Distributed Sensor Networks , 15 (7), 1–12.
Alexander, M., Polimis, K., & Zagheni, E. (2020). Combining social media and survey data to Nowcast migrant stocks in the United States. arXiv preprint https://arxiv.org/abs/2003.02895 .
Beduschi, A. (2020). International migration management in the age of artificial intelligence. Migration Studies . https://doi.org/10.1093/migration/mnaa003 .
Bengtsson, L., Lu, X., Thorson, A., Garfield, R., & Von Schreeb, J. (2011). Improved response to disasters and outbreaks by tracking population movements with mobile phone network data: a post-earthquake geospatial study in Haiti. PLoS Medicine, 8 (8), e1001083.
Bhadwal, N., Madaan, V., Agrawal, P., Shukla, A., & Kakran, A. (2019). Smart border surveillance system using wireless sensor network and computer vision. In 2019 international conference on Automation, Computational and Technology Management (ICACTM) (pp. 183–190). London. https://doi.org/10.1109/ICACTM.2019.8776749 .
Bilsborrow, R. E. (2016). Concepts, definitions and data collection approaches. In M. J. White (Ed.), International handbook of migration and population distribution (pp. 109–156). Springer.
Google Scholar
Bilsborrow, R. E., Hugo, G., & Oberai, A. S. (1997). International migration statistics: Guidelines for improving data collection systems . International Labour Organization.
Bitelli, G., Eleias, M., Franci, F., & Mandanici, E. (2017). VHR satellite imagery for humanitarian crisis management: A case study. In: Fifth international conference on remote sensing and geoinformation of the environment (RSCy2017) , 10444, p. 104440T. International Society for Optics and Photonics.
Böhme, J., Gröger, A., & Stöhr, T. (2018). Searching for a better life: Predicting international migration with online search keywords. Journal of Development Economics, 142, 1–14.
Borja, A. G., & Black, J. (2021). Measuring migrant deaths and disappearances. Forced Migration Review, 66 , 58–60.
Brayne, S. (2018). The criminal law and law enforcement implications of big data. Annual Review of Law and Social Science, 14 , 293–308.
Article Google Scholar
Brenner, Y., & Frouws, B. (2019). Hype or hope? Evidence on use of smartphones & social media in mixed migration. Mixed Migration Centre, Geneva.
Carammia, M., Iacus, S. M., & Wilkin, T. (2020). Forecasting asylum-related migration flows with machine learning and data at scale. arXiv preprint arXiv:2011.04348 .
Cesare, N., Lee, H., McCormick, T., Spiro, E., & Zagheni, E. (2018). Promises and pitfalls of using digital traces for demographic research. Demography, 55 (5), 1979–1999.
Chi, G., Lin, F., Chi, G., & Blumenstock, J. (2020). A general approach to detecting migration events in digital trace data`. PLoS ONE, 15 (10), e0239408.
Clemens, M., Summers, L. H., & Santo Tomas, P. A. (2009). Migrants count: Five steps towards better migration data. In Report of the commission on international migration data for development research and policy. Center for Global Development.
Czaika, M., & Orazbayev, S. (2018). The globalisation of scientific mobility, 1970–2014. Applied Geography, 96 , 1–10.
Dekker, R., Engbersen, G., Klaver, J., & Vonk, H. (2018). Smart refugees: How Syrian asylum migrants use social media information in migration decision-making. Social Media+ Society , 4 (1), 2056305118764439.
Dijstelbloem, H. (2017). Migration tracking is a mess. Nature, 543 (7643), 32–34.
Dragu, T., & Lupu, Y. (2021). Digital authoritarianism and the future of human rights. International Organization , 75 (4), 991–1017.
Fawcett, J. T., & Arnold, F. (1987). The role of surveys in the study of international migration: An appraisal. International Migration Review, 21 (4), 1523–1540.
Fiori, L., Abel, G., Cai, J., Zagheni, E., Weber, I., & Vinué, G. (2017). Using Twitter data to estimate the relationship between short-term mobility and long-term migration. In Proceedings of the 2017 ACM on web science conference (pp. 103–110).
Gabrielli, L., Deutschmann, E., Natale, F., Recchi, E., & Vespe, M. (2019). Dissecting global air traffic data to discern different types and trends of transnational human mobility. EPJ Data Science, 8 (1), 26.
Gandomi, A., & Haider, M. (2015). Beyond the hype: Big data concepts, methods, and analytics. International Journal of Information Management, 35 (2), 137–144.
Global Migration Group. (2017). Handbook for improving the production and use of migration data for development. In Global Knowledge Partnership for Migration and Development (KNOMAD) . World Bank.
Goldstein, S., & Goldstein, A. (1981). Surveys of migration in developing countries: A methodological review. Papers of the East-West Population Institute, 71 , 120.
Hayes, B. (2017). Migration and data protection: Doing no harm in an age of mass displacement, mass surveillance and “big data”. International Review of the Red Cross , 99 (904), 1Ý79–209.
Hilbert, M. (2016). Big data for development: A review of promises and challenges. Development Policy Review, 34 (1), 135–174.
Hughes, C., Zagheni, E., Abel, G. J., Sorichetta, A., Wi’sniowski, A., Weber, I., & Tatem, A. J. (2016). Inferring migrations: Traditional methods and new approaches based on mobile phone, social media, and other big data: Feasibility study on inferring (labour) mobility and migration in the European Union from Big Data and Social Media Data . European Commission.
IOM (2020). ‘Chapter 3: Migration Research and Analysis: Growth, Reach and Recent Contributions’. In: World Migration Report 2020 . Geneva.
Kikas, R., Dumas, M., & Saabas, A. (2015). Explaining international migration in the skype network: The role of social network features. In: Proceedings of the 1st ACM Workshop on Social Media World Sensors (pp. 17–22).
Kraemer, M. U., Sadilek, A., Zhang, Q., Marchal, N. A., Tuli, G., Cohn, E. L., Hswen, Y., Perkins, T. A., Smith, D. L., Reiner Jr. R. C., & Brownstein, J. S. (2020). Mapping global variation in human mobility. Nature Human Behaviour, 4 (8), 800–810.
Laczko, F. (2016). Improving Data on International Migration and Development: Towards a Global Action Plan?’ Conference Paper, Improving Data on International Migration – Towards Agenda 2030 and the Global Compact on Migration , 1–3 December, 2016, German Federal Foreign Office.
Laczko, F., & Rango, M. (2014). Can big data help us achieve a “Migration Data Revolution”? Migration Policy Practice (IOM), 4 (2), 20–29.
Latonero, M., & Kift, P. (2018). On digital passages and borders: Refugees and the new infrastructure for movement and control. Social Media+ Society , 4 (1), 1–11.
Laudel, G. (2003). Studying the brain drain: Can bibliometric methods help? Scientometrics, 57 (2), 215–237.
Leese, M., Noori, S., & Stephan S. (2021). Data matters: The politics and practices of digital border and migration management. Geopolitics . https://doi.org/10.1080/14650045.2021.1940538 .
Lemaitre, G. (2005). The comparability of international migration statistics: Problems and prospects. Statistics Brief, 9 , 1–8.
Lin, A. Y., Cranshaw, J., & Counts, S. (2019). Forecasting US domestic migration using internet search queries. In: The world wide web conference (pp. 1061–1072).
Martín, Y., Cutter, S. L., Li, Z., Emrich, C. T., & Mitchell, J. T. (2020). Using geotagged tweets to track population movements to and from Puerto Rico after hurricane Maria. Population and Environment, 1 (1), 1–24.
Massey, D., & Capoferro, C. (2004). Measuring undocumented migration. The International Migration Review, 38 (3), 1075–1102.
Miller, H. J., Dodge, S., Miller, J., & Bohrer, G. (2019). Towards an integrated science of movement: Converging research on animal movement ecology and human mobility science. International Journal of Geographical Information Science, 33 (5), 855–876.
Moed, H. F., & Halevi, G. (2014). A bibliometric approach to tracking international scientific migration. Scientometrics, 101 (3), 1987–2001.
Molnar, P. (2019). Technology on the margins: AI and global migration management from a human rights perspective. Cambridge International Law Journal, 8 (2), 305–330.
Pappalardo, L., Simini, F., Rinzivillo, S., Pedreschi, D., Giannotti, F., & Barabási, A. L. (2015). Returners and explorers dichotomy in human mobility. Nature Communications, 6 (1), 1–8.
Pisarevskaya, A., Levy, N., Scholten, P., & Jansen, J. (2019). Mapping migration studies: An empirical analysis of the coming of age of a research field. Migration Studies, 8 (3), 455–481.
Popkin, G. (2018). Technology and satellite companies open up a world of data. Nature, 557 (7706), 745–748.
Poulain, M., Perrin, N., & Singleton, A. (2006). Towards harmonised european statistics on international migration . Presses Universitaires de Louvain.
Quinn, J. A., Nyhan, M. M., Navarro, C., Coluccia, D., Bromley, L., & Luengo-Oroz, M. (2018). Humanitarian applications of machine learning with remote-sensing data: Review and case study in refugee settlement mapping. Philosophical Transactions of the Royal Society A: Mathematical, Physical and Engineering Sciences , 376 (2128). 1–16.
Rango, M., & Vespe, M. (2017). Big data and alternative data sources on migration: from case-studies to policy support. Summary Report. Ispra: European Commission Joint Research Centre.
Raymer, J., Wiśniowski, A., Forster, J. J., Smith, P. W., & Bijak, J. (2013). Integrated modeling of European migration. Journal of the American Statistical Association, 108 (503), 801–819.
Recchi, E., Deutschmann, E., & Vespe, M. (2019). Estimating transnational human mobility on a global scale. Robert Schuman Centre for Advanced Studies Research Paper No. RSCAS, 30.
Reichel, D., & Morales, L. (2017). Surveying immigrants without sampling frames—Evaluating the success of alternative field methods. ComparatIve Migration Studies, 5 (1), 1.
Ruel, E., Wagner III, W., & Gillespie, B. (2016). The quality of measurement: reliability and validity. The practice of survey research: Theory and applications.
Ruktanonchai, N. W., Ruktanonchai, C. W., Floyd, J. R., & Tatem, A. J. (2018). Using Google Location History data to quantify fine-scale human mobility. International Journal of Health Geographics, 17 (1), 1–13.
Sanchez, G., Hoxhaj, R., Nardin, S., Geddes, A., Achilli, L., & Kalantaryan, S. (2018). A study of the communication channels used by migrants and asylum seekers in Italy, with a particular focus on online and social media.
Shatnawi, N., Weidner, U., & Hinz, S. (2020). Monitoring urban expansion as a result of refugee fluxes in North Jordan using remote sensing techniques. Journal of Urban Planning and Development, 146 (3), 1–32.
Sîrbu, A., Andrienko, G., Andrienko, N., Boldrini, C., Conti, M., Giannotti, F., & Pappalardo, L. (2020). Human migration: the big data perspective. International Journal of Data Science and Analytics, 11 (0), 1–20. https://doi.org/10.1007/s41060-020-00213-5 .
Snijders, C., Matzat, U., & Reips, U. D. (2012). Big data: Big gaps of knowledge in the field of internet science. International Journal of Internet Science, 7 (1), 1–5.
Song, C., Qu, Z., Blumm, N., & Barabási, A. L. (2010). Limits of predictability in human mobility. Science, 327 (5968), 1018–1021.
Spyratos, S., Vespe, M., Natale, F., Weber, I., Zagheni, E., & Rango, M. (2019) ‘Quantifying International Human Mobility Patterns Using Facebook Network Data. PLoS ONE , 14 (10), 1–22.
State, B., Rodriguez, M., Helbing, D., & Zagheni, E. (2014). Migration of professionals to the U.S.: Evidence from linkedin data. In L. M. Aiello, & D. McFarland (Eds.), 6th international conference on social informatics , SocInfo (pp. 531–543). Springer.
Sudakova, A. E., & Tarasyev, A. A. (2019). Digitalization and scientometrics in assessing the migration of scientists. In International scientific and practical conference on digital economy (ISCDE 2019). Atlantis Press.
Tiede, D., Krafft, P., Füreder, P., & Lang, S. (2017). Stratified template matching to support refugee camp analysis in OBIA workflows. Remote Sensing, 9 (4), 326.
Tjaden, J., Arau, A., Nuermaimaiti, M., Cetin, I., Acostamadiedo, E., & M. Rango (2021). Using “Big Data” to forecast migration—A tale of high expectations, promising results and a long road ahead’. MEDIUM. Available at https://medium.com/@UNmigration/using-big-data-to-forecast-migration-8c8e64703559 .
Tjaden, J., Auer, D., & Laczko, F. (2019). Linking migration intentions with flows: Evidence and potential use. International Migration, 57 , 36–57.
UN DESA. (2017). ‘UN Handbook on Measuring International Migration through Population Censuses’. In: Economic and Social Affairs . New York.
UN DESA. (2019). International Migration 2019. Department of Economic and Social Affairs, Population Division, New York City.
UN Global Pulse. (2014). Estimating Migration Flows Using Online Search Data. Global Pulse Project Series , 4 , 1–2.
UN Global Pulse. (2017) Social media and forced displacement: Big data analytics & machine-learning , Geneva.
UNHCR. (2020). UNHCR Global Trends - Forced displacement in 2020. United Nations High Commissioner for Refugees, Geneva.
Van Dalen, H. P., & Henkens, K. (2013). Explaining emigration intentions and behaviour in the Netherlands, 2005–2010. Population Studies, 67 (2), 225–241.
Wang, Y., Luo, H., & Shi, Y. (2019). Complex network analysis for international talent mobility based on bibliometrics. International Journal of Innovation Science, 11 (3), 419–435.
White, M. J. (Ed.). (2016). International Handbook of Migration and Population Distribution (vol. 6) . Springer.
Willekens, F., Massey, D., & Raymer, J. (2017). International migration under the microscope. Science, 352 (6288), 897–899.
Williams, N. E., Thomas, T. A., Dunbar, M., Eagle, N., & Dobra, A. (2015). Measures of human mobility using mobile phone records enhanced with GIS Data. PLoS ONE, 10 (7), 1–16.
Zagheni, E., Garimella, V. R. K., Ingmar, W., & State, B. (2014). Inferring international and internal migration patterns from twitter data. In Proceedings of the 23rd International Conference on World Wide Web (pp. 439–44). ACM Press.
Zagheni, E., & Weber, I. (2012). You are where you e-mail: Using e-mail data to estimate international migration rates. In Proceedings of the 4th annual ACM web science conference (pp. 348–351). ACM Press.
Zagheni, E., Weber, I., & Gummadi, K. (2017). Leveraging Facebook’s advertising platform to monitor stocks of migrants. Population and Development Review, 43 , 721–734.
Zwitter, A. (2014). Big data ethics. Big Data and Society, 1 (2), 2053951714559253.
Download references
Acknowledgements
The author would like to thank Marzia Rango (IOM), Emilio Zagheni (Max Planck Institute for Demographic Research), Ingmar Weber (Qatar Computing Research Institute) and Teddy Wilkin (EASO) for numerous discussions and presentations on the potential of digital trace data for measuring migration which motivated the idea for this paper.
Open Access funding enabled and organized by Projekt DEAL.
Author information
Authors and affiliations.
Department of Economics and Social Sciences, University of Potsdam, August-Bebel-Str.89, 14482, Potsdam, Germany
Jasper Tjaden
International Organization for Migration’s (IOM) Global Migration Data Analysis Centre (GMDAC), Berlin, Germany
You can also search for this author in PubMed Google Scholar
Contributions
Single authorship/ not applicable. Author read and approved the final manuscript.
Corresponding author
Correspondence to Jasper Tjaden .
Ethics declarations
Competing interests.
The author confirms no competing interests.
Additional information
Publisher's note.
Springer Nature remains neutral with regard to jurisdictional claims in published maps and institutional affiliations.
Rights and permissions
Open Access This article is licensed under a Creative Commons Attribution 4.0 International License, which permits use, sharing, adaptation, distribution and reproduction in any medium or format, as long as you give appropriate credit to the original author(s) and the source, provide a link to the Creative Commons licence, and indicate if changes were made. The images or other third party material in this article are included in the article's Creative Commons licence, unless indicated otherwise in a credit line to the material. If material is not included in the article's Creative Commons licence and your intended use is not permitted by statutory regulation or exceeds the permitted use, you will need to obtain permission directly from the copyright holder. To view a copy of this licence, visit http://creativecommons.org/licenses/by/4.0/ .
Reprints and permissions
About this article
Cite this article.
Tjaden, J. Measuring migration 2.0: a review of digital data sources. CMS 9 , 59 (2021). https://doi.org/10.1186/s40878-021-00273-x
Download citation
Received : 21 October 2020
Accepted : 02 November 2021
Published : 21 December 2021
DOI : https://doi.org/10.1186/s40878-021-00273-x
Share this article
Anyone you share the following link with will be able to read this content:
Sorry, a shareable link is not currently available for this article.
Provided by the Springer Nature SharedIt content-sharing initiative
- Digital trace
- Measurement
Advertisement
A systematic review of climate migration research: gaps in existing literature
- Review Paper
- Open access
- Published: 16 April 2022
- Volume 2 , article number 47 , ( 2022 )
Cite this article
You have full access to this open access article
- Rajan Chandra Ghosh ORCID: orcid.org/0000-0001-9027-6649 1 , 2 &
- Caroline Orchiston ORCID: orcid.org/0000-0002-3171-2006 1
10k Accesses
9 Citations
12 Altmetric
Explore all metrics
Climatic disasters are displacing millions of people every year across the world. Growing academic attention in recent decades has addressed different dimensions of the nexus between climatic events and human migration. Based on a systematic review approach, this study investigates how climate-induced migration studies are framed in the published literature and identifies key gaps in existing studies. 161 journal articles were systematically selected and reviewed (published between 1990 and 2019). Result shows diverse academic discourses on policies, climate vulnerabilities, adaptation, resilience, conflict, security, and environmental issues across a range of disciplines. It identifies Asia as the most studied area followed by Oceania, illustrating that the greatest focus of research to date has been tropical and subtropical climatic regions. Moreover, this study identifies the impact of climate-induced migration on livelihoods, socio-economic conditions, culture, security, and health of climate-induced migrants. Specifically, this review demonstrates that very little is known about the livelihood outcomes of climate migrants in their international destination and their impacts on host communities. The study offers a research agenda to guide academic endeavors toward addressing current gaps in knowledge, including a pressing need for global and national policies to address climate migration as a significant global challenge.
Similar content being viewed by others
Climate-Conflict-Migration Nexus: An Assessment of Research Trends Based on a Bibliometric Analysis
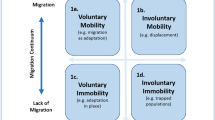
Scales and sensitivities in climate vulnerability, displacement, and health
Lori M. Hunter, Stephanie Koning, … Jamon Van Den Hoek
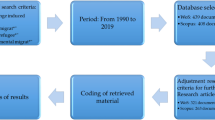
Climate change-induced migration: a bibliometric review
Juan Milán-García, José Luis Caparrós-Martínez, … Jaime de Pablo Valenciano
Avoid common mistakes on your manuscript.
Introduction
Population displacement can be driven by climatic hazards such as floods, droughts (hydrologic), and storms (atmospheric), and geophysical hazards such as earthquakes, volcanic eruptions, and tsunami (Smith and Smith 2013 ). The interactions between natural hazard events, and social, political, and human factors, frequently act to intensify the negative effects of climatic and geophysical hazards, leading to political and social unrest, increased social vulnerability, and human suffering. As a consequence of these adverse effects, people migrate from their native land, causing stress, uncertainty, and loss of lives and properties. However, such migration can also have positive impacts on migrants’ lives. For example, migrants may be able to diversify their livelihood and have greater access to education or healthcare.
In 2020, 30.7 million people from 149 countries and territories were displaced due to different natural disasters. Among them, climatic disasters were solely responsible for displacing 30 million people within their own country, with the highest recorded displacement occurring in 2010 when 38.3 million people were displaced (IDMC 2021a ; IOM 2021 ). It is difficult to estimate the actual number of people that moved due to the impacts of climate change (Mcleman 2019 ), because peoples’ migration decisions are triggered by a range of contextual factors (de Haas 2021 ). Nevertheless, the Internal Displacement Monitoring Centre (IDMC) states that approximately 283.4 million people were displaced internally between the years 2008 and 2020 because of climatic disasters across the globe (Table 1 ). This number represents almost 89% of the total disaster-induced displacement that occurred during this timeframe (IDMC 2021a ).
People who move from their homes due to climate-driven hazards are described in a range of ways, including climate migrants, environmental migrants, climate refugees, environmental refugees, and so on (Perkiss and Moerman 2018 ). The process of migration related to climate-driven hazards is variously described as environmental migration, environmental displacement, climate-induced migration or climigration (Bronen 2008 ).
In this research, we focus on climate-induced migration more specifically induced by slow-onset climatic disasters (sea-level rise, drought, salinity etc.), rapid onset extreme climatic events (storms, floods etc.), or both (precipitation, erosion etc.). This study investigates how climate change-induced migration studies are framed in the existing literature and identifies key gaps in the published literature.
There is a significant ongoing debate about the links between climate change and human migration in the academic literature. Some researchers strongly believe that climate change directly causes people to move, whereas the others argue that climate change is just one of the contextual factors in peoples’ migration decisions (Laczko and Aghazarm 2009 ). Although there are scholarly opinions that call into question climate change as a primary cause of migration (Black 2001 ; Black et al. 2011 ; McLeman 2014 ), there is also evidence that climate change causes severe environmental effects and exacerbates the vulnerabilities of people that force them to leave their place of living (Bronen and Chapin 2013 ; Laczko and Aghazarm 2009 ; McLeman 2014 ).
Moreover, the relationship between the adverse effects of climate change and different types of human mobility (migration, displacement, or planned relocation) has become increasingly recognized in recent years (Kälin and Cantor 2017 ). It is assumed in general that the number of climate displaced people is likely to increase in future (Mcleman 2019 ; Wilkinson et al. 2016 ), and climate change could permanently displace an estimated 150 million to nearly 1 billion people as a critical driver by 2050 (Held 2016 ; Perkiss and Moerman 2018 ). As the number of climate migrants increases rapidly in some areas of the world (IDMC 2017 ), it is now confirmed as a significant global challenge (Apap 2019 ) and recognized as a considerable threat to human populations (Ionesco et al. 2017 ).
Climate migration has multifaceted impacts on peoples’ livelihoods. Being displaced from their home, people migrate within their own country, described as internal migration, or across borders to other countries known as international migration. Internal movements of climate migrants occur mostly to nearby major cities or large urban centers (Poncelet et al. 2010 ). Climate migrants who try to move internationally are significantly challenged by two different security problems. Firstly, they cannot live in their own homeland because of worsening climatic impacts and are forced to leave their ancestral land. Secondly, they cannot move to other countries quickly to find a safer place because, according to international law, climate migrants are not refugees and they are not supported by the UN Refugee Convention or any international formal protection policies (Apap 2019 ; Mcleman 2019 ). In this situation, they live with significant livelihood uncertainty. The United Nation’s Sustainable Development Goals (SDGs) and the Sendai Framework for Disaster Risk Reduction (SFDRR) recognize them as a key group that is highly exposed and vulnerable because of their circumstances (Ionesco et al. 2017 ). Hence, policy development to address complex climate migration issues has become an emerging priority around the globe (Apap 2019 ).
In order to address this global challenge, there has been growing academic and policy attention focused on regional (Kampala Convention-2009 by African Union), national (Nansen Initiative—2012 by Norway and Switzerland), and international (Global Compact for Safe, Orderly and Regular Migration- 2018 by United Nations) levels of climate-induced migration in recent years. Myers’s ( 2002 ) seminal article signposted environmentally driven migration as one of the most significant challenges of the twenty-first century, and later, similar assumptions were made by Christian Aid (Baird et al. 2007 ), IOM (Brown 2008 ), and Care International (Warner et al. 2009 ). Such predictions led to a proliferation of the academic discourse on migration, focused on national and international security, policy frameworks, and human rights (Boncour and Burson 2009 ). Other studies have focused on vulnerability assessment, risk reduction, adaptation, resettlement, relocation, sustainability, and resilience, considering pre-, during and post-disaster circumstances of climate migration (Bronen 2011 ; Bronen and Chapin 2013 ; IDMC 2019 ; IOM 2021 ; King et al. 2014 ).
This research contributes to the discourse by identifying the gaps in the published literature regarding climate migration. A systematic literature review was undertaken to shed light on the current extent of academic literature, including gaps in knowledge to develop a climate migration research agenda. Two notable review papers provided a solid foundation for this endeavor. First, Piguet et al. ( 2018 ) developed a comprehensive review of publications on environment-induced migration from a global perspective based on a bibliographic database—CliMig. Their detailed mapping of environmentally induced migration research focused on five categories of climatic hazards (droughts, floods, hurricanes, sea-level rise, and rainfall); however, it did not include salinity and erosion which are also climate-driven and has direct effects on internal and international migration (Chen and Mueller 2018 ; Mallick and Sultana 2017 ; Rahman and Gain 2020 ).
The second key review paper was by Obokata et al. ( 2014 ), which provided an evidence-based explanation of the environmental factors leading to migration, and the non-environmental factors that influence the migration behaviors of people. Their scope of analysis was limited to international migration and excluded other types of migration, such as internal climate-induced migration.
Although migration, or more specifically environmental migration, was occurring over many decades of the twentieth century, the IPCC First Assessment report was released in 1990, which presented the first indications of the risks of climate change-induced human movement (IPCC 1990 ). This milestone report then stimulated the academic discourse, and consequently, a rapid increase in climate migration publication resulted. For this reason, the current study undertook a systematic review of literature across three decades beginning in 1990 and ending in 2019. This study aims to understand how the published literature has framed the climate-induced migration discourse. This paper identifies the key gaps in existing scholarship in this field and proposes a research agenda for future consideration on current and emerging climate migration issues.
In the following section, we outline the systematic review method and identify how journal articles were searched, selected, reviewed, and analyzed. In the next section, we present the results of this study. Results are organized into four subsections that illustrate the reviewed literature in the following ways—spatial and temporal trends, disciplinary foci, triggering forces of migration, and other key issues. Finally, we conclude by identifying research gaps, addressing the limitations of this study, and presenting a research agenda.
Methodology
We have adopted a systematic review methodology for this study because it provides an …overall picture of the evidence in a topic area which is needed to direct future research efforts (Petticrew and Robert 2006 ). Systematic reviews reduce the bias of a traditional narrative review, although it is challenging to eliminate researcher bias while interpreting and synthesizing results (Doyle et al. 2019 ). It also limits systematic bias by identifying, evaluating, and synthesizing all relevant studies to answer specific questions or sets of questions, and produces a scientific summary of the evidence in any research area (Petticrew and Robert 2006 ). Moreover, systematic reviews effectively address the research question and identify knowledge gaps and future research priorities (Mallett et al. 2012 ). We have adopted this approach following the methodology developed by Berrang-Ford et al. ( 2011 ) which was tested in the field of environmental and climate change studies, with measurable outcomes. We have conducted the review following these four steps—article search, selection, review, and analysis (Fig. 1 ).
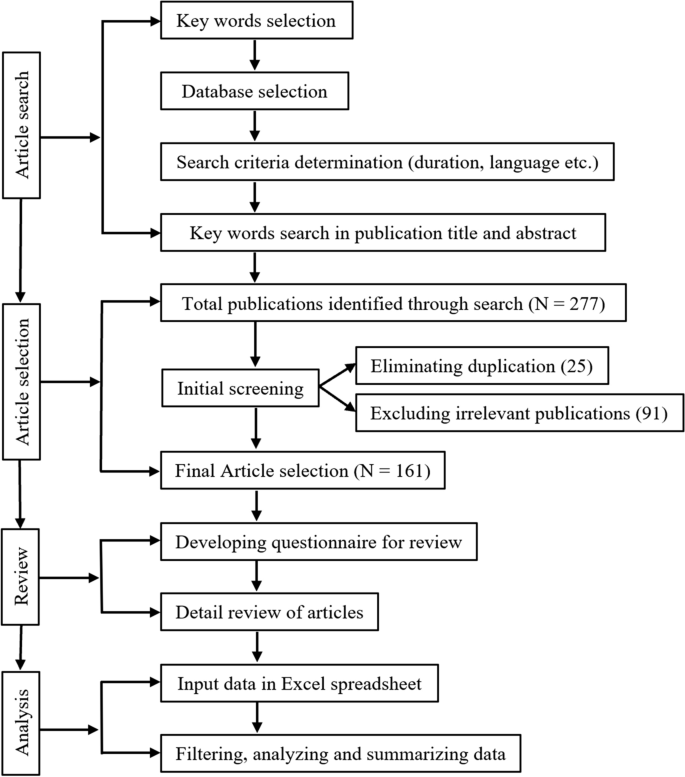
Systematic review flowchart
Article search
We conducted a comprehensive literature search to identify the published academic literature on climate-induced migration to develop a clear understanding of this field of study. We identified sixteen commonly used keywords to search for articles that are predominantly used in the literature. ProQuest central database was selected and used in consultation with a skilled subject librarian to search for the relevant articles for this study. We conducted this literature search in July 2019 using the key thesaurus terms, presented in Table 2 . All keywords were then searched individually in the publication’s title and abstract. We only considered English language peer-reviewed articles for this study, published between the years 1990 and 2019 (up to June).
Article selection
The main purpose of this process was to ensure the selection of appropriate literatures for further analysis. We approached the Preferred Reporting Items for Systematic Reviews and Meta Analyses (PRISMA), a systematic evaluation tool, which was also used by Huq et al. ( 2021 ). In stage one of the selection process, 277 articles were counted based on our search criteria. In stage two, we excluded 25 duplicates, and 252 articles remained for further assessment. In the third and final stage of the detailed assessment of each paper, we identified a further 91 publications that were not relevant to our study but appeared in our searched list because search terms were briefly mentioned in their title and/or abstract without being described in further detail. As these articles did not fit with the aim and content of this research, we excluded those 91 and selected a final 161 articles for this study.
Article review
All the selected articles were then considered for detailed review in order to achieve the purpose of the study. A questionnaire (Online Attachment—A) was developed partially following Berrang-Ford et al. ( 2011 ); Obokata et al. ( 2014 ) and Piguet et al. ( 2018 ) to investigate how climate migration studies are framed in the published literature. Then each article was reviewed in detail in response to the individual parameters of the questionnaire such as general information ( article title, authors name, publication year, journal, discipline, content ), methodological approach ( qualitative, quantitative, mixed ), focused study areas ( country, climatic zones ), source of migrants ( rural, urban ), migration types ( internal, international ), impacts of climate migration ( social, economic, political, health, cultural, environmental, security ), causes of migration ( climatic: flood, sea-level rise, drought etc ., other: socio-economic, political, cultural ), target communities ( displaced community, receiving community ), and livelihoods ( housing, income, employment, etc . ) of climate migrants described in the publications.
Article analysis
All the data were recorded in Microsoft Office Excel spreadsheets. Relevant data for each parameter were filtered, analyzed, and summarized using the necessary Excel tools. Referencing was compiled through Mendeley Desktop.
Spatial and temporal trend
General information.
In this section, the publication date of the reviewed articles was used in order to identify the development of the academic discourse in climate migration studies over the last three decades (1990–2019). Results show the increasing focus of academic attention on this area of research over that timeframe. The study found only four publications between the years 1990 and 1999. During 2000–2009, an additional 16 articles were published, which was followed by an almost 90 percent (141 publications) increase in reviewed articles over the period of 2010–2019 (Table 3 ).
Reviewed study areas
In 84 reviewed articles, the study reported research focused on a particular location, and in some cases, they considered two or more areas for their research. Therefore, multiple counting for each study has been considered, which represents all the continents except Antarctica. The analysis shows that Asia (38%) is the continent with the greatest number of climate migration studies, followed by Oceania (20%), North America (17%), and Africa (14%). In contrast, Europe and South America have received less attention, with 7% and 5%, respectively. Table 4 presents the distribution of study areas by continent focused on the reviewed papers.
Climatic zones of the reviewed studies
This study identified the climatic zones of the study areas in order to find out which zones are most commonly studied among the reviewed studies. We adopted the climatic zones of the world from Peel et al. ( 2007 ), which is the updated version of Koppen’s climate classification, and categorizes the world climate into five major zones, i.e., (i) tropical, (ii) arid, (iii) temperate, (iv) cold, and (v) polar. This review shows that 86 publications mentioned their study areas, equating to 54% of the total reviewed papers. Among them, 81% referred to a specific region as their study area. The study areas were then classified into the above-mentioned climatic zones with one reference offered randomly for each country as an example of the range of research that has been conducted.
This study reveals that 49% of this group (among 81%) focused on tropical climatic areas such as Bangladesh (Islam et al. 2014 ), Cambodia (Jacobson et al. 2019 ), Kiribati (Bedford et al. 2016 ), Papua New Guinea (Connell and Lutkehaus 2017 ), Philippines (Tanyag 2018 ), Tuvalu (Locke 2009 ), and Vanuatu (Perumal 2018 ) among others, and 16% focused on arid climatic zones such as African Sahel (McLeman and Hunter 2010 ), Israel (Weinthal et al. 2015 ), Peru (Scheffran 2008 ), and Senegal (Nawrotzki et al. 2016a , b ). In addition to these, 13% of authors focused on temperate regions, i.e., Mexico (Nawrotzki et al. 2016a , b ), Nepal (Chapagain and Gentle 2015 ), Taiwan (Kang 2013 ), UK (Abel et al. 2013 ), and the USA (Rice et al. 2015 ) for their study and 3% focused on cold climatic areas, i.e., Alaska: USA (Marino and Lazrus 2015 ), Canada (Omeziri and Gore 2014 ), and northern parts of China (Ye et al. 2012 ). No studies were found based on polar regions (Fig. 2 ). Some studies did not specify a region or country of study but instead focused on broader regions such as Africa (White 2012 ), Asia–Pacific (Mayer 2013 ), Europe (Werz and Hoffman 2016 ), Latin America (Wiegel 2017 ), and Pacific (Hingley 2017 ).
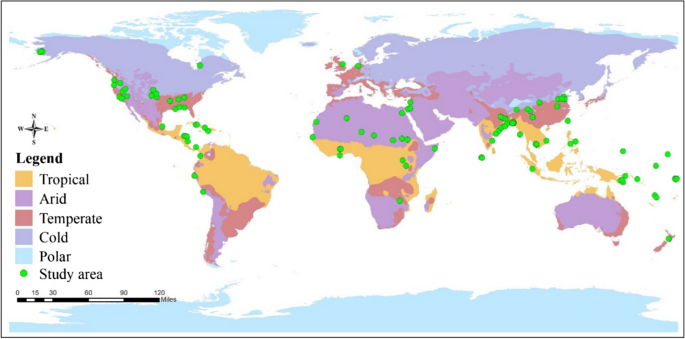
Climatic zones of the reviewed study areas-adopted from Peel (2007)
Migration types and sources of climate migrants
Migration types here refer to whether migration was internal (within a country or region) or international (across borders), and sources of climate migrants refer to people from rural or urban source regions. Most authors (73%) mentioned nothing regarding migration types, but a quarter (27%) explicitly discussed internal or international migration. Among them, 11% described climate migration within countries and 10% investigated cross-border migration. Some authors (6%) were concerned with both internal and international climate migration. Source regions for climate migrants were not often considered, with only 19 publications mentioning the origin of migrants. Among these, 11 articles stated that migration occurred from rural areas, and two publications discussed migration from urban areas. Also, six articles described climate migration from both rural and urban areas.
Disciplinary foci
Research discipline.
This study reveals that climate migration studies are becoming more focal issues in different research disciplines that include more than 40 subject areas. Hence, we developed a typology for the reviewed articles based on the relevant research themes. The typology consists of six research disciplines, each of which includes different subjects, as follows.
Social sciences: Social sciences, Sociology, Political Science, International Relations, Comprehensive Works, Population Studies, Anthropology, Social Services and Welfare, History, Philosophy, Ethnic Interests, Civil Rights, Women's Studies
Geography and environment: Meteorology, Environmental Studies, Energy, Conservation, Earth Sciences, Geography, Agriculture, Geology, Biology, Archaeology, Pollution
Business studies and development: Management, Business and Economics, International Commerce, International Development and Assistance, Economics, Insurance, Investments, Accounting
Law, policy, and planning: Law, Military, Civil Defense, Criminology and Security, Environmental policy
Health and medical science: Public Health, Psychology, Medical Sciences, Physical Fitness, and Hygiene
Other: Literature, Library and Information Sciences, Physics, Technology
Among the reviewed publications, some articles were discussed from the perspective of one particular discipline, while others came from two or more disciplines. Therefore, multiple counting for each discipline was considered during the analysis. The study reveals that Social Science covers the highest percentage of publications (41%), followed by Geography and Environment (30%), Business Studies and development (10%), Law, policy and planning (9%), and Health and medical science (7%). Only 2% of publications are not covered by any of these disciplines.
Primary research themes
The authors discussed a diverse range of themes in the reviewed articles. Key themes have been classified into eight categories based on their topics and focusing subjects. Some of the publications focused on multiple themes, which were counted separately under each theme. Most of the authors (27%) focused on Politics and policy issues, and almost a fifth (18%) of total articles focused on the themes of population, health, and development issues. Human rights, conflicts, and security issues were discussed in 16% of papers, and climate, vulnerability, adaptation, and resilience topics were the focus of 12% of publications. In 11% of publications, the authors focused on identity and cultural issues, and socio-economic topics comprised a further 9% of the total. Environmental issues were discussed by 4% of reviewed articles and 3% of publications did not fit into any of the above categories and are described as Other.
Methodological approaches
This review identified that researchers applied both qualitative and quantitative methods in climate migration research. A total of 82% of the reviewed articles used qualitative methodologies, and 9% quantitative. In addition to these, 9% of articles used mixed methods in climate migration research. Of those who used qualitative studies, most were review-based (86%), comprising systematic review, empirical evidence-based review, critical synthesis review, critical discourse review, and policy review. Only 14% of qualitative studies used interview methods (7%), case studies (6%), and focus group discussion (1%). Data sources reported in the reviewed literature for the quantitative research included secondary data (73%), historical data (13%), remote sensing data (7%), and survey data (7%).
Triggering forces of migration
Climatic causes of migration.
The reviewed publications outlined a range of different causes of climate migration. This study reveals nineteen climate-related causes of migration. We merged these causes into eight categories, defined as (i) climate change (climate change, global warming, temperature, environmental change, climate-induced natural disaster, meteorological events, extreme weather, heatwave), (ii) flood, (iii) sea-level rise (sea-level rise, melting glacier), (iv) drought (drought, desertification), (v) storm (storm, cyclone, hurricane, typhoon), (vi) salinity (salinity, tidal surge), (vii) precipitation-induced landslide, and (viii) erosion (coastal erosion, river erosion). “Climate change” is defined as a separate category because some publications named climate change as an overarching driver of migration, rather than specifying any particular hazard. In 70 publications, authors mentioned particular climatic events that were solely responsible for human migration, and 53 of these articles predominantly identified climate change as the main driver of migration, followed by sea-level rise (6), drought (4), flood (3), storm (2), and precipitation-induced landslide (2). In the remaining articles, scholars identified two or more climatic events that were collectively responsible for human displacement. Based on these articles, multiple counting for each climatic event was considered and the results show that climate change was the most commonly cited cause in 126 articles, along with other climatic causes. The authors also identified sea-level rise, drought, flood, and storms as the significant drivers of peoples’ migration along with other climatic drivers, which were mentioned in 51, 46, 44, and 43 articles, respectively. Precipitation-induced landslide and erosion were recognized in 17 and 12 articles, respectively, as the causes of human displacement, whereas eight articles identified salinity as the main reason.
Influencing causes of migration
Although this review was focused on identifying the climatic causes of human displacement, some other causes emerged during the analysis that also influence migration. In 68 publications, economic, social, environmental, political, cultural, and psychological causes were stated as drivers of migration, in addition to the climatic causes. Among these, economic causes (32%) have been identified as the most common driver, followed by social (25%) and environmental (22%) causes. Some articles described political causes (16%), and the remainder mentioned cultural (3%) and psychological (1%) drivers of migration.
Other key issues
- Impacts of climate migration
One of the key findings of this review concerns the impacts of climate migration. In 48 publications, authors described a range of different impacts caused by climate migration, such as social, economic, political, health, cultural, environmental, and security. All the impacts were identified based on the location of climate migrants which are classified into the following three categories: (i) impacts on the place of origin, (ii) impacts on the place of destination, and (iii) impacts on both origin and destination. The review demonstrates that the impacts of climate migration were more frequently identified for the place of origin rather than for the destination. In the place of origin, authors discussed the economic, social, and cultural impacts, compared to political, security, health, and environmental impacts. In contrast, in the destination, scholars were more focused on security and cultural impacts. Overall, security, cultural and economic impacts were the most frequently discussed themes by the authors of reviewed literature in comparison with other impacts (Table 5 ).
Discussed communities
More than half of the reviewed articles ( N = 81) described climate migrants and/or their receiving communities. In most of the discussions, authors talked about both displaced and host communities together (57%). In more than two-fifths of articles, they considered only displaced communities (42%). In contrast, none of the authors of the reviewed literature discussed host communities in detail in their publications, except Dorent ( 2011 ). Only a few authors briefly mentioned host communities during the discussion of climate migration impacts.
Livelihoods of climate migrants
This review demonstrates that the overall livelihood of climate migrants has not been a key focus in any of the reviewed literature. However, a few separate parameters of livelihoods, including housing, income and employment, health, access to resources, and education were mentioned in 23 articles. The analysis shows that the livelihoods of migrants in their place of origin (71%) were more likely to be considered compared to their destination (11%). In some articles (18%), authors addressed the livelihoods of climate migrants considering both their place of origin and destination. In total, all the articles which considered livelihoods had a specific focus on internal migration, and none mentioned the livelihoods of climate migrants in terms of international migration.
Discussion and research gaps
Climate change-induced migration is neither new (Nagra 2017 ), nor a future hypothetical phenomenon—it is a current reality (Coughlin 2018 ). This review provides a comprehensive analysis of how this field of study is framed in the existing literature. The academic discourse on human migration due to climate change is suggestive of a long-standing causal connection, which is hard to dissociate (Milán-García et al. 2021 ; Parrish et al. 2020 ; Piguet et al. 2011 ).
The review of spatial and temporal trends of climate-induced migration studies illustrates the growth in the field since the release of 1st IPCC report in 1990. In addition, this review has explored some basic questions that are useful to guide future research in this field of study, for instance, which study areas have received greater or lesser focus? Where are these study areas located in relation to global climatic zones? How are people migrating, i.e., internally, or internationally? What are the spatial sources of climate-induced migrants, i.e., rural, or urban environments?
This review also demonstrates that the expansion of climate migration research increased rapidly after 2000, although the studies in this field began before 2000 (Table 3 ). It denotes that the global academia and policymakers have emphasized their focus on this topic in recent decades (Milán-García et al. 2021 ; Piguet et al. 2011 ). Moreover, this review identifies the Asia–Pacific region as the global ‘hotspot’ of climate migration research (Table 4 ). This reflects the IDMC ( 2019 ) report that states more than 80% of the total displacement between 2008 and 2018 occurred within this region. Moreover, a significant proportion of global environmental displacement will continue to occur in the Asia–Pacific region (Mayer, 2013 ). Therefore, this region could be considered as a critical ‘living laboratory’ for future climate migration research.
Climate migration is mostly occurring internally (IDMC 2021a ; Laczko and Aghazarm 2009 ), and in recent years, it has been widely acknowledged in the policy areas (Fussell et al. 2014 ; The World Bank 2018 ). Nevertheless, this study reveals that only a quarter of the reviewed studies for example, Chapagain and Gentle ( 2015 ), Islam et al. ( 2014 ), and Prasain ( 2018 ) have considered the migration types (internal or international) and sources (rural or urban) of climate migrants in their research. Thus, this review identifies the gap and need for contributions to the academic discourse that investigate migration types, the origin of migrants, and their patterns of migration.
The review of the disciplinary foci of climate-induced migration literature reveals that a broader range of disciplines are now focusing on this research topic, which suggests that greater interdisciplinarity is developing in the discourse. IDMC ( 2021b ) data presented in Table 1 show that climate-induced disasters are displacing millions of people every year, but surprisingly none of the reviewed publications appeared under the subject category of disaster management in the database. This reflects the emergent nature of the academic discourse on climate migration and disaster management, which includes recent studies by Ye et al. ( 2012 ), Tanyag ( 2018 ), and Hamza et al. ( 2017 ). In addition, politics and policy issues regarding climate migration were discussed by scholars; however, no country-specific policies were found during the review that considered both the origin and host communities of climate migrants.
Campbell ( 2014 ) argues that there is insufficient empirical evidence within climate migration research. However, this review reveals that research in this area has been undertaken using a range of methodologies, from qualitative (review, case study, interview, focus group discussion etc.) to quantitative (based on survey data, secondary data, historical data, and remote sensing data), which has produced a strong foundation of work to guide future pathways for interdisciplinary climate migration research. A significant proportion of the research to date has been review-based. Also, there is a lack of empirical studies in this research field that consider the application of geographic information system and remote sensing.
It is clear from reviewing the triggering forces of climate-induced migration literature that climatic events are dominantly responsible for climate migration, which is supported by Rahman and Gain ( 2020 ), Connell and Lutkehaus ( 2017 ), Gemenne ( 2015 ), and Kniveton et al. ( 2012 ). Despite this, there are some other influencing push and/or pull factors such as socio-economic, political, cultural, etc., which are likely to compound (or be compounded by) climate impacts, to trigger the migration process (Black et al. 2011 ; de Haas 2011 , 2021 ; Fussell et al. 2014 ). While there remains ample anecdotal evidence of the relationship between climate change impacts and migration, the specific reasons for people to decide to migrate are interwoven with indirect pressures, such as livelihood disruption, poverty, war, or disaster (Werz and Hoffman 2016 ). Moreover, why people choose to stay at their places is also essential in the context of creeping environmental and climate-induced migration (Mallick and Schanze 2020 ).
One of the other key issues reviewed in this study is that the literature to date fails to build an understanding of the impacts of climate migration on both the origin (source regions) and destination of the climate migrants. There are very few studies such as Comstock and Cook ( 2018 ), Maurel and Tuccio ( 2016 ), Pryce and Chen ( 2011 ), Rahaman et al. ( 2018 ), Rice et al. ( 2015 ), and Schwan and Yu ( 2017 ) that investigate different aspects of socio-economic impacts (housing, health, social, economic, etc.) of climate migration in the destination region, and this presents a clear gap in knowledge that requires further study. Also, no current research has been identified during the review that focused on the environmental impacts of climate migration.
In addition, this review identifies that there was less attention paid to the impacts of climate migration on host communities compared to displaced populations in their new locations. Given that migration will continue to increase globally, there is likely to be a growing need to understand the range of potential impacts on host communities. Although some countries and regions are developing policies to manage internal migration, there are no formal protection policies for cross-border climate migration (Nishimura 2015 ; OHCHR 2018 ; Olsson 2015 ; Zaman 2021 ). Therefore, policy arrangements for managing the needs of climate displaced people in their new communities need to be developed to account for issues related to impacts, livelihoods, community cohesion, and cultural diversity and values. Future research should address the significant gap in understanding the livelihoods of climate migrants in their cross border or international destination. More specifically, in developed countries where the employment sector is more formalized, there is less room for informal economic practices that are common in developing contexts. More formal employment arrangements make it challenging for migrants to establish new livelihoods, alongside other challenges such as language barriers, and other financial, social, cultural and well-being issues.
Limitations and future research scope
Limitations of this study.
There are some limitations to this systematic review; firstly, this review used ProQuest as the sole database for the analysis, and future work could extend the scope to include other major databases. Secondly, this study only considered English language literature, and there are likely to be significant publications in other languages relating to climate migration that were not included in this analysis. Thirdly, looking at pre-1990 or post-2019 literature could add more exciting findings to the search list, which would provide more informative literature. Finally, the outputs of this review are limited to the nature of the search terms, and thus, if other words or texts such as climate-induced relocation or mobility were used, it might extend the range of the review.
Toward a research agenda for climate migration
This review has highlighted several exciting future research opportunities that will build on the strong foundation of work over the past decades in the field of climate migration studies. These include the following research themes; (i) a richer understanding of the full range of impacts (such as social, economic, environmental, and cultural) of climate migration on host communities; (ii) in-depth analysis of the livelihoods of climate-induced migrants in their new destination; (iii) evidence-based research on internal and international climate migration with their sources; (iv) long-term migration policy development at national, regional, or international levels considering both climate migrants and host communities; (v) scope and application of geographic information systems and remote sensing in this area of research, and (vi) developing sustainable livelihood frameworks for climate migrants. The authors believe that academic contributions to these research themes will drive climate migration challenges toward long-term solutions, particularly in those countries that are going to be hosting increasing numbers of climate migrants in future.
This study aimed to understand the past three decades of academic endeavor on climate migration and to identify the gaps in the existing literature in order to inform a research agenda for future research. Climate change, climate-induced migration, and climate migrants are now considered significant global challenges. Climate migrants are identified as a vulnerable group, and a consideration of issues for this group is essential in addressing the goals of the SDGs and SFDRRR. There is a growing body of knowledge that reflects the global relevance of climate migration as a major current and future challenge (Boncour and Burson 2009 ). Addressing the issues and challenges of this form of migration will improve the survival and certain resettlement rights of climate migrants (Miller 2017 ). Therefore, this review contributes a research agenda for future climate migration studies. This study has revealed a critical need to establish a universally agreed definition of ‘climate-induced migrants’ and ‘climate-induced migration,’ which remains unclear to date. Lack of clarity only acts to reduce the visibility of issues related to climate-induced migration. In addition, there is a crucial need to improve the evidence base for climate-induced migration by improving current global datasets, to inform local, regional, and global policy development. Policies need to be future-looking in preparation for a rapid and significant increase in climate-related migration across the globe, within and across national borders. For instance, it is important for receiving countries to anticipate an upsurge in migration by developing appropriate policies to support new migrants, particularly regarding visa and immigration arrangements. Addressing current gaps in knowledge will lead to improved pathways to manage this global migration challenge, which is now a critical need if we are to achieve a sustainable future in a climate-challenged world.
Data availability
Data are available from the corresponding author upon reasonable request.
Abel G, Bijak J, Findlay A, Mccollum D, Winiowski A (2013) Forecasting environmental migration to the United Kingdom: an exploration using Bayesian models. Popul Environ 35(2):183–203. https://doi.org/10.1007/s11111-013-0186-8
Article Google Scholar
Apap J (2019) The concept of ‘climate refugee’: towards a possible definition. https://www.europarl.europa.eu/RegData/etudes/BRIE/2018/621893/EPRS_BRI(2018)621893_EN.pdf . Accessed 26 Aug 2021
Baird R, Migiro K, Nutt D, Kwatra A, Wilson S, Melby J, Pendleton A, Rodgers M, Davison J (2007) Human tide: the real migration crisis. https://www.christianaid.org.uk/sites/default/files/2017-08/human-tide-the-real-migration-crisis-may-2007.pdf . Accessed 11 Sep 2021
Bedford R, Bedford C, Corcoran J, Didham R (2016) Population change and migration in Kiribati and Tuvalu, 2015–2050: Hypothetical scenarios in a context of climate change. N Z Popul Rev 42:103–134
Google Scholar
Berrang-Ford L, Ford J, Paterson J (2011) Are we adapting to climate change? Glob Environ Change 21(1):25–33. https://doi.org/10.1016/j.gloenvcha.2010.09.012
Black R (2001) Environmental refugees: myth or reality? In: UNHCR: New Issues in Refugee Research (No. 34). https://www.unhcr.org/research/working/3ae6a0d00/environmental-refugees-myth-reality-richard-black.html . Accessed 04 Sep 2021
Black R, Adger WN, Arnell NW, Dercon S, Geddes A, Thomas D (2011) The effect of environmental change on human migration. Glob Environ Change 21(SUPPL. 1):S3–S11. https://doi.org/10.1016/j.gloenvcha.2011.10.001
Boncour P, Burson B (2009) Climate change and migration in the South Pacific region: policy perspectives. Policy Q 5(4):13–20. https://doi.org/10.26686/pq.v5i4.4312
Bronen R (2008) Alaskan communities’ rights and resilience. Forced Migr Rev 31:30–32
Bronen R (2011) Climate-induced community relocations: creating an adaptive governance framework based in human rights doctrine. N Y Univ Rev Law Soc Change 35(2):357–407
Bronen R, Chapin FS (2013) Adaptive governance and institutional strategies for climate-induced community relocations in Alaska. Proc Natl Acad Sci USA 110(23):9320–9325. https://doi.org/10.1073/pnas.1210508110
Brown O (2008) Migration and climate change. In: IOM Migration Research Series (No. 31). https://publications.iom.int/books/mrs-no-31-migration-and-climate-change . Accessed 11 Sep 2021
Campbell JR (2014) Climate-change migration in the Pacific. Contemp Pac 26(1):1–28. https://doi.org/10.1353/cp.2014.0023
Chapagain B, Gentle P (2015) Withdrawing from agrarian livelihoods: environmental migration in Nepal. J Mt Sci 12(1):1–13. https://doi.org/10.1007/s11629-014-3017-1
Chen J, Mueller V (2018) Coastal climate change, soil salinity and human migration in Bangladesh. Nat Clim Change 8(11):981–985. https://doi.org/10.1038/s41558-018-0313-8
Comstock AR, Cook RA (2018) Climate change and migration along a Mississippian periphery: a fort ancient example. Am Antiq 83(1):91–108. https://doi.org/10.1017/aaq.2017.50
Connell J, Lutkehaus N (2017) Environmental refugees? A tale of two resettlement projects in coastal Papua New Guinea. Aust Geogr 48(1):79–95. https://doi.org/10.1080/00049182.2016.1267603
Coughlin J (2018) The Figure of the “Climate Refugee” in Inger Elisabeth Hansen’s Å resirkulere lengselen: avrenning foregår (2015). Scand Can Stud 25:68–90
de Haas H (2011) The determinants of international migration: conceptualising policy, origin and destination effects. IMI Working Pap Ser 32(2011):35
de Haas H (2021) A theory of migration: the aspirations-capabilities framework. Comp Migr Stud 9(1):1–35. https://doi.org/10.1186/S40878-020-00210-4
Dorent N (2011) Transitory cities: emergency architecture and the challenge of climate change. Development 54(3):345–351. https://doi.org/10.1057/dev.2011.60
Doyle EEH, Johnston DM, Smith R, Paton D (2019) Communicating model uncertainty for natural hazards: a qualitative systematic thematic review. Int J Disaster Risk Reduct 33:449–476. https://doi.org/10.1016/j.ijdrr.2018.10.023
Fussell E, Hunter LM, Gray CL (2014) Measuring the environmental dimensions of human migration: the demographer’s toolkit. Glob Environ Change 28(1):182–191. https://doi.org/10.1016/J.GLOENVCHA.2014.07.001
Gemenne F (2015) One good reason to speak of “climate refugees.” Forced Migr Rev 49:70–71
Hamza M, Koch I, Plewa M (2017) Disaster-induced displacement in the Caribbean and the Pacific. Forced Migr Rev 56:62–64
Held D (2016) Climate change, migration and the cosmopolitan dilemma. Global Pol 7(2):237–246. https://doi.org/10.1111/1758-5899.12309
Hingley R (2017) “Climate refugees”: an oceanic perspective. Asia Pac Policy Stud 4(1):158–165. https://doi.org/10.1002/app5.163
Huq ME, Sarker MNI, Prasad R, Hossain MA, Rahman MM, Al-Dughairi AA (2021) Resilience for disaster management: opportunities and challenges. In: Alam GMM, Erdiaw-Kwasie MO, Nagy GJ, Filho WL (eds) Climate vulnerability and resilience in the global south : human adaptations for sustainable futures, 1st edn. Springer, Cham, pp 425–442. https://doi.org/10.1007/978-3-030-77259-8_22
Chapter Google Scholar
IDMC (2017) Global Disaster Displacement Risk: A Baseline for Future Work. https://www.internal-displacement.org/publications/global-disaster-displacement-risk-a-baseline-for-future-work . Accessed 09 Sep 2021
IDMC (2019) Disaster displacement: a global review 2008–2018. https://environmentalmigration.iom.int/disaster-displacement-global-review-2008-2018 . Accessed 08 Sep 2021
IDMC (2021a) GRID 2021a: Internal displacement in a changing climate. Global Report on Internal Displacement, Internal Displacement Monitoring Centre. https://www.internal-displacement.org/global-report/grid2021/ . Accessed 14 June 2021
IDMC (2021b) IDMC Query Tool—Disaster. Global Internal Displacement Database. https://www.internal-displacement.org/database/displacement-data . Accessed 09 Dec 2021
IOM (2021) Environmental Migration: Recent Trends. Migration Data Portal, International Organization for Migration. https://migrationdataportal.org/themes/environmental_migration_and_statistics . Accessed 31 Aug 2021
Ionesco D, Mokhnacheva D, Gemenne F (2017) The atlas of environmental migration, 1st edn. Routledge, London. https://doi.org/10.4324/9781315777313
Book Google Scholar
IPCC (1990) IPCC First Assessment Report Overview. https://www.ipcc.ch/assessment-report/ar1/ . Accessed 10 Sep 2021
Islam MM, Sallu S, Hubacek K, Paavola J (2014) Migrating to tackle climate variability and change? Insights from coastal fishing communities in Bangladesh. Clim Change 124:733–746. https://doi.org/10.1007/s10584-014-1135-y
Jacobson C, Crevello S, Chea C, Jarihani B (2019) When is migration a maladaptive response to climate change? Reg Environ Change 19(1):101–112. https://doi.org/10.1007/s10113-018-1387-6
Kälin W, Cantor D (2017) The RCM Guide: a novel protection tool for cross-border disaster-induced displacement in the Americas. Forced Migr Rev 56:58–61
Kang MJ (2013) From original homeland to “permanent housing” and back: the post-disaster exodus and reconstruction of south Taiwan’s indigenous communities. Soc Sci Dir 2(4):85–105. https://doi.org/10.7563/SSD_02_04_08
King D, Bird D, Haynes K, Boon H, Cottrell A, Millar J, Okada T, Box P, Keogh D, Thomas M (2014) Voluntary relocation as an adaptation strategy to extreme weather events. Int J Disaster Risk Reduct 8:83–90. https://doi.org/10.1016/j.ijdrr.2014.02.006
Kniveton DR, Smith CD, Black R (2012) Emerging migration flows in a changing climate in dryland Africa. Nat Clim Change. https://doi.org/10.1038/nclimate1447
Laczko F, Aghazarm C (2009) Introduction and overview: enhancing the knowledge base. In: Laczko F, Aghazarm C (eds) Migration, environment and climate change: assessing the evidence. International Organization for Migration, Le Grand-Saconnex, pp 7–40
Locke JT (2009) Climate change-induced migration in the Pacific Region: sudden crisis and long-term developments. Geogr J 175(3):171–180. https://doi.org/10.1111/j.1475-4959.2009.00317.x
Mallett R, Hagen-Zanker J, Slater R, Duvendack M (2012) The benefits and challenges of using systematic reviews in international development research. J Dev Eff 4(3):445–455. https://doi.org/10.1080/19439342.2012.711342
Mallick B, Schanze J (2020) Trapped or voluntary? Non-migration despite climate risks. Sustainability 12(11):1–6. https://doi.org/10.3390/su12114718
Mallick B, Sultana Z (2017) Livelihood after relocation-evidences of Guchchagram project in Bangladesh. Soc Sci 6(3):1–19. https://doi.org/10.3390/socsci6030076
Marino E, Lazrus H (2015) Migration or forced displacement? The complex choices of climate change and disaster migrants in Shishmaref, Alaska and Nanumea, Tuvalu. Hum Organ 74(4):341–350. https://doi.org/10.17730/0018-7259-74.4.341
Maurel M, Tuccio M (2016) Climate instability, urbanisation and international migration. J Dev Stud 52(5):735–752. https://doi.org/10.1080/00220388.2015.1121240
Mayer B (2013) Environmental migration in the Asia-Pacific region: could we hang out sometime? Asian J Int Law 3(1):101–135. https://doi.org/10.1017/S204425131200029X
Mcleman R (2019) International migration and climate adaptation in an era of hardening borders. Nat Clim Change 9(12):911–918. https://doi.org/10.1038/s41558-019-0634-2
McLeman RA (2014) Climate and human migration: past experiences, future challenges. Cambridge University Press, Cambridge. https://doi.org/10.1017/CBO9781139136938
McLeman RA, Hunter LM (2010) Migration in the context of vulnerability and adaptation to climate change: insights from analogues. Wiley Interdiscip Rev Clim Change 1(3):450–461. https://doi.org/10.1002/wcc.51
Milán-García J, Caparrós-Martínez JL, Rueda-López N, de Pablo Valenciano J (2021) Climate change-induced migration: a bibliometric review. Glob Health 17(1):1–10. https://doi.org/10.1186/S12992-021-00722-3
Miller DS (2017) Climate refugees and the human cost of global climate change. Environ Justice 10(4):89–92. https://doi.org/10.1089/env.2017.29027.dm
Myers N (2002) Environmental refugees: a growing phenomenon of the 21st century. Philos Trans R Soc B Biol Sci 357(1420):609–613. https://doi.org/10.1098/rstb.2001.0953
Nagra S (2017) The Oslo principles and climate change displacement: missed opportunity or misplaced expectations? Carbon Clim Law Rev CCLR 11(2):120–135. https://doi.org/10.21552/cclr/2017/2/8
Nawrotzki RJ, Runfola DM, Hunter LM, Riosmena F (2016a) Domestic and International Climate Migration from Rural Mexico. Hum Ecol 44(6):687–699. https://doi.org/10.1007/s10745-016-9859-0
Nawrotzki RJ, Schlak AM, Kugler TA (2016b) Climate, migration, and the local food security context: introducing Terra Populus. Popul Environ 38(2):164–184. https://doi.org/10.1007/s11111-016-0260-0
Nishimura L (2015) ‘Climate change migrants’: impediments to a protection framework and the need to incorporate migration into climate change adaptation strategies. Int J Refug Law 27(1):107–134. https://doi.org/10.1093/ijrl/eev002
Obokata R, Veronis L, McLeman R (2014) Empirical research on international environmental migration: a systematic review. Popul Environ 36(1):111–135. https://doi.org/10.1007/s11111-014-0210-7
OHCHR (2018) The slow onset effects of climate change and human rights protection for cross-border migrants. https://www.ohchr.org/Documents/Issues/Migration/OHCHR_slow_onset_of_Climate_Change_EN.pdf . Accessed 10 Dec 2021
Olsson L (2015) Environmental migrants in international law: An assessment of protection gaps and solutions. Environmental Migration Portal: Climate Change, Displacement. https://environmentalmigration.iom.int/environmental-migrants-international-law-assessment-protection-gaps-and-solutions . Accessed 10 Dec 2021
Omeziri E, Gore C (2014) Temporary measures: Canadian refugee policy and environmental migration. Refuge 29(2):43–53. https://doi.org/10.25071/1920-7336.38166
Parrish R, Colbourn T, Lauriola P, Leonardi G, Hajat S, Zeka A (2020) A critical analysis of the drivers of human migration patterns in the presence of climate change: a new conceptual model. Int J Environ Res Public Health 17(17):1–20. https://doi.org/10.3390/ijerph17176036
Peel MC, Finlayson BL, Mcmahon TA (2007) Updated world map of the Köppen-Geiger climate classification. Hydrol Earth Syst Sci 11(5):1633–1644. https://doi.org/10.5194/hess-11-1633-2007
Perkiss S, Moerman L (2018) A dispute in the making. Account Audit Account J 31(1):166–192. https://doi.org/10.1108/AAAJ-06-2016-2582
Perumal N (2018) The place where I live is where I belong: community perspectives on climate change and climate-related migration in the Pacific island nation of Vanuatu. Island Stud J 13(1):45–64. https://doi.org/10.24043/isj.50
Petticrew M, Robert H (2006) Systematic reviews in the social sciences: a practical guide. Blackwell Publishing, Hoboken. https://doi.org/10.1002/9780470754887
Piguet E, Pécoud A, de Guchteneire P (2011) Migration and climate change: an overview. Refug Surv Q 30(3):1–23. https://doi.org/10.1093/rsq/hdr006
Piguet E, Kaenzig R, Guélat J (2018) The uneven geography of research on “environmental migration.” Popul Environ 39(4):357–383. https://doi.org/10.1007/s11111-018-0296-4
Poncelet A, Gemenne F, Martiniello M, Bousetta H (2010) A country made for disasters: environmental vulnerability and forced migration in Bangladesh. In: Afifi T, Jäger J (eds) Environment, forced migration and social vulnerability. Springer, Berlin, pp 211–222. https://doi.org/10.1007/978-3-642-12416-7
Prasain S (2018) Climate change adaptation measure on agricultural communities of Dhye in Upper Mustang, Nepal. Clim Change 148:279–291. https://doi.org/10.1007/s10584-018-2187-1
Pryce G, Chen Y (2011) Flood risk and the consequences for housing of a changing climate: an international perspective. Risk Manage 13(4):228–246. https://doi.org/10.1057/rm.2011.13
Rahaman MA, Rahman MM, Bahauddin KM, Khan S, Hassan S (2018) Health disorder of climate migrants in Khulna City: an urban slum perspective. Int Migr. https://doi.org/10.1111/imig.12460
Rahman MS, Gain A (2020) Adaptation to river bank erosion induced displacement in Koyra Upazila of Bangladesh. Prog Disaster Sci 5:1000552. https://doi.org/10.1016/j.pdisas.2019.100055
Rice JL, Burke BJ, Heynen N (2015) Knowing climate change, embodying climate praxis: experiential knowledge in Southern Appalachi. Ann Assoc Am Geogr 105(2):253–262. https://doi.org/10.1080/00045608.2014.985628
Scheffran J (2008) Climate change and security. Bull Atom Sci 64(2):19–25. https://doi.org/10.2968/064002007
Schwan S, Yu X (2017) Social protection as a strategy to address climate-induced migration. Int J Clim Change Strateg Manage 10(1):43–64. https://doi.org/10.1108/IJCCSM-01-2017-0019
Smith K, Smith K (2013) Environmental hazards: assessing risk and reducing disaster, 6th edn. Routledge, London. https://doi.org/10.4324/9780203805305
Tanyag M (2018) Resilience, female altruism, and bodily autonomy: disaster-induced displacement in Post-Haiyan Philippines. Signs J Women Cult Soc 43(3):563–585. https://doi.org/10.1086/695318
The World Bank (2018) Groundswell: preparing for internal climate migration. https://www.worldbank.org/en/news/infographic/2018/03/19/groundswell---preparing-for-internal-climate-migration . Accessed 29 June 2021
Warner K, Ehrhart C, de Sherbinin A, Adamo S, Chai-Onn T (2009) In search of shelter: mapping the effects of climate change on human migration and displacement. https://www.care.org/wp-content/uploads/2020/05/CC-2009-CARE_In_Search_of_Shelter.pdf . Accessed 11 Sept 2021
Weinthal E, Zawahri N, Sowers J (2015) Securitizing water, climate, and migration in Israel, Jordan, and Syria. Int Environ Agreem Polit Law Econ 15(3):293–307. https://doi.org/10.1007/s10784-015-9279-4
Werz M, Hoffman M (2016) Europe’s twenty-first century challenge: climate change, migration and security. Eur View 15(1):145–154. https://doi.org/10.1007/s12290-016-0385-7
White G (2012) Climate change and migration: security and borders in a warming world. Oxford University Press, Oxford. https://doi.org/10.1093/acprof:oso/9780199794829.001.0001
Wiegel H (2017) Refugees of rising seas. Science 357(6346):41. https://doi.org/10.1126/science.aan5667
Wilkinson E, Kirbyshire A, Mayhew L, Batra P, Milan A (2016) Climate-induced migration and displacement: closing the policy gap. https://www.odi.org/sites/odi.org.uk/files/resource-documents/10996.pdf . Accessed 12 March 2021
Ye Y, Fang X, Khan MAU (2012) Migration and reclamation in Northeast China in response to climatic disasters in North China over the past 300 years. Reg Environ Change 12(1):193–206. https://doi.org/10.1007/s10113-011-0245-6
Zaman ST (2021) Legal protection for the cross-border climate-induced population movement in south asia: exploring a durable solution. J Environ Law Litig 36:187–235
Download references
Acknowledgements
The authors would like to thank Dr Douglas Hill, Dr Ashraful Alam and Dr Bishawjit Mallick for their feedback on the initial draft of this article.
This research has been supported by a University of Otago Doctoral Scholarship. Open Access funding is enabled and organized by CAUL and its Member Institutions.
Author information
Authors and affiliations.
Centre for Sustainability, School of Geography, University of Otago, Dunedin, 9016, New Zealand
Rajan Chandra Ghosh & Caroline Orchiston
Department of Emergency Management, Faculty of Environmental Science and Disaster Management, Patuakhali Science and Technology University, Patuakhali, 8602, Bangladesh
Rajan Chandra Ghosh
You can also search for this author in PubMed Google Scholar
Contributions
Both authors have contributed to the study conception and design. RCG performed the literature search, collected and analyzed the data, and prepared the first draft of the manuscript. CO critically reviewed the manuscript. Both authors have read and approved the final manuscript.
Corresponding author
Correspondence to Rajan Chandra Ghosh .
Ethics declarations
Conflict of interest.
The authors have no financial or non-financial interests to disclose.
Supplementary Information
Below is the link to the electronic supplementary material.
Supplementary file1 (DOCX 14 kb)
Rights and permissions.
Open Access This article is licensed under a Creative Commons Attribution 4.0 International License, which permits use, sharing, adaptation, distribution and reproduction in any medium or format, as long as you give appropriate credit to the original author(s) and the source, provide a link to the Creative Commons licence, and indicate if changes were made. The images or other third party material in this article are included in the article's Creative Commons licence, unless indicated otherwise in a credit line to the material. If material is not included in the article's Creative Commons licence and your intended use is not permitted by statutory regulation or exceeds the permitted use, you will need to obtain permission directly from the copyright holder. To view a copy of this licence, visit http://creativecommons.org/licenses/by/4.0/ .
Reprints and permissions
About this article
Ghosh, R.C., Orchiston, C. A systematic review of climate migration research: gaps in existing literature. SN Soc Sci 2 , 47 (2022). https://doi.org/10.1007/s43545-022-00341-8
Download citation
Received : 23 September 2021
Accepted : 28 March 2022
Published : 16 April 2022
DOI : https://doi.org/10.1007/s43545-022-00341-8
Share this article
Anyone you share the following link with will be able to read this content:
Sorry, a shareable link is not currently available for this article.
Provided by the Springer Nature SharedIt content-sharing initiative
- Climate-induced migration
- Climate-induced migrants
- Livelihoods
- Systematic review
- Find a journal
- Publish with us
- Track your research

An official website of the United States government
The .gov means it’s official. Federal government websites often end in .gov or .mil. Before sharing sensitive information, make sure you’re on a federal government site.
The site is secure. The https:// ensures that you are connecting to the official website and that any information you provide is encrypted and transmitted securely.
- Publications
- Account settings
Preview improvements coming to the PMC website in October 2024. Learn More or Try it out now .
- Advanced Search
- Journal List
- Wiley-Blackwell Online Open

Anticipating sea‐level rise and human migration: A review of empirical evidence and avenues for future research
Sem j. duijndam.
1 Institute for Environmental Studies (IVM), Vrije Universiteit Amsterdam, Amsterdam The Netherlands
Wouter J. W. Botzen
2 Utrecht University School of Economics (U.S.E.), Utrecht University, Utrecht The Netherlands
3 Risk Management and Decision Processes Center, The Wharton School, University of Pennsylvania, Philadelphia Pennsylvania, USA
Liselotte C. Hagedoorn
Jeroen c. j. h. aerts.
4 Deltares, Delft The Netherlands
Associated Data
Data sharing is not applicable to this article as no new data were created or analyzed in this study.
Sea‐level rise (SLR) threatens millions of people living in coastal areas through permanent inundation and other SLR‐related hazards. Migration is one way for people to adapt to these coastal changes, but presents an enormous policy challenge given the number of people affected. Knowledge about the relationship between SLR‐related hazards and migration is therefore important to allow for anticipatory policymaking. In recent years, an increasing number of empirical studies have investigated, using survey or census data, how SLR‐related hazards including flooding, salinization, and erosion together with non‐environmental factors influence migration behavior. In this article, we provide a systematic literature review of this empirical work. Our review findings indicate that flooding is not necessarily associated with increased migration. Severe flood events even tend to decrease long‐term migration in developing countries, although more research is needed to better understand the underpinnings of this finding. Salinization and erosion do generally lead to migration, but the number of studies is sparse. Several non‐environmental factors including wealth and place attachment influence migration alongside SLR‐related hazards. Based on the review, we propose a research agenda by outlining knowledge gaps and promising avenues for future research on this topic. Promising research avenues include using behavioral experiments to investigate migration behavior under future SLR scenarios, studying migration among other adaptation strategies, and complementing empirical research with dynamic migration modeling. We conclude that more empirical research on the SLR‐migration nexus is needed to properly understand and anticipate the complex dynamics of migration under SLR, and to design adequate policy responses.
This article is categorized under:
- Climate Economics < Aggregation Techniques for Impacts and Mitigation Costs
- Vulnerability and Adaptation to Climate Change < Learning from Cases and Analogies
- Assessing Impacts of Climate Change < Evaluating Future Impacts of Climate Change
Sea‐level rise (SLR) threatens millions of people living in coastal areas. Migration is one way for people to adapt to SLR‐related hazards, with major implications for people and policy. A more comprehensive understanding of migration dynamics under SLR is therefore vital.

Photo: Flood event in Jakarta, Indonesia, 2017. Photo source: World Meteorological Organization on Flickr https://tinyurl.com/8azc3vnr
1. INTRODUCTION
According to the Intergovernmental Panel on Climate Change (IPCC) sixth assessment report, global mean sea levels have risen by around 0.2 m since 1900 (IPCC, 2021 ). Because of accelerating climatic change, the yearly rate of sea‐level rise has increased from 1.3 mm over the period 1901–1971 to 3.7 mm over the period 2006–2018, and is expected to increase further in the decades to come. Global mean sea‐level rise by 2100 relative to 1995–2014 is expected to be between 0.3 and 1 m depending on greenhouse gas emission scenarios. Higher levels approaching 2 m by 2100 and 5 m by 2150 cannot be ruled out because of deep uncertainty about ice sheet processes (IPCC, 2021 ). Sea‐level rise (SLR) posits an enormous threat to coastal populations, as it will lead to more frequent and intense flooding from storm surges, tidal extremes, waves, and backwater effects, and to coastal and riverbank erosion, water and soil salinization, and in some cases permanent inundation (Alam et al., 2016 ; Ketabchi et al., 2016 ; Nicholls & Cazenave, 2010 ; Vitousek et al., 2017 ). Next to climate‐induced SLR, other (non‐climatic) processes can further exacerbate these hazards, such as subsidence, dam construction, and industrial (dredging) activities (Dunn et al., 2018 , 2019 ; Nicholls, Lincke, et al., 2021 ; Vasilopoulos et al., 2021 ). Economic development and population growth are expected to exacerbate vulnerability to SLR, although projections of these processes are uncertain as well (Jongman et al., 2012 ; Nicholls, Hanson, et al., 2021 ). These impacts affect coastal societies in numerous ways, such as by reducing agricultural production, threatening freshwater supplies, and damaging and destroying properties and critical infrastructure (Wrathall et al., 2019 ).
SLR‐related hazards could potentially displace tens of millions of people living in coastal areas by the end of this century (Hino et al., 2017 ; Lincke & Hinkel, 2021 ; Nicholls et al., 2011 ). Depending on population growth, more than one billion people could be populating the low‐elevation coastal zone and be vulnerable to SLR‐related hazards (Hauer, 2017 ; Neumann et al., 2015 ). However, other common and more restricted measures to assess “at‐risk” populations, including the 100‐year floodplain and areas inundated under SLR, suggest a much lower number (Hauer et al., 2020 ). Although of the SLR‐related hazards flooding threatens the greatest number of people, livelihoods of millions of people are also threatened by salinization and erosion impacts (Chen & Mueller, 2018 ; Hinkel et al., 2013 ; Nicholls et al., 2018 ). Policy measures focused on protection (i.e., armoring coasts to prevent SLR hazards) or accommodation (i.e., adaptation measures that facilitate living with SLR hazards) can mitigate the impacts of SLR. However, the high costs of these measures make it unlikely that they will be implemented along all threatened coasts (Hauer et al., 2020 ). Especially in developing countries and along low‐populated coastlines, protection is in many areas projected to be economically unfavorable under 21st century SLR (Lincke & Hinkel, 2018 ). When protection and accommodation are not feasible, retreat might be the only option left for adapting to SLR. Retreat can be centrally planned with the goal to relocate affected populations to safer areas, but as such government‐led resettlements are often expensive, complicated, and contested, its applicability seems limited to smaller populations highly vulnerable to SLR (Hauer et al., 2020 ; Wilmsen & Webber, 2015 ). The largest share of future SLR‐related migration will therefore likely be the result of people that autonomously decide to migrate in anticipation of, or in reaction to, SLR‐related hazards (McLeman, 2014 ). Such migration can be facilitated by government policy, for instance by prohibiting development in flood‐prone areas, not investing in flood protection, and property buyouts (Siders, 2013 ).
The locations from which, and how many, people will eventually migrate as a result of SLR and where they would migrate to is difficult to predict. Nevertheless, gaining a better understanding of future migration flows is of critical academic and policy relevance. It enables anticipatory policy planning to reduce the societal and economic costs of migration, can provide better insights into the welfare impacts of climate change, and allows for improved understanding and modeling of future coastal risk. Besides uncertainty about future SLR and policy responses, an important reason why forecasting migration under SLR is so difficult is the complexity of the migration decision‐making process, where next to SLR‐related hazards also demographic, cultural, economic, social, political, and individual/household‐level factors play a role (Black et al., 2011 ; Hauer et al., 2020 ; Wrathall et al., 2019 ). At present, economic and social motives instead of environmental concerns are often the main reason for people to migrate, also in vulnerable locations (Adger et al., 2021 ; Nicholls et al., 2020 ; Safra de Campos et al., 2020 ). Despite this complexity, we can already learn how SLR might influence migration from the growing empirical literature that investigates, using survey or census data, how SLR‐related hazards including flooding (Bohra‐Mishra et al., 2014 ; Call et al., 2017 ; Codjoe et al., 2017 ), erosion (Bernzen et al., 2019 ; Goldbach, 2017 ), and salinization (Bernzen et al., 2019 ; Chen & Mueller, 2018 , 2019 ) impact migration or migration intentions. Although these hazards may not always be directly caused by SLR in the specific study settings, they reflect hazards that will be exacerbated by SLR, and are therefore relevant analogies.
This article presents the first systematic literature review that brings together these empirical studies. Our review focuses on those studies employing quantitative multivariate regression approaches because, despite the great value of qualitative research, this restriction facilitates the comparison of research findings and the isolation of SLR‐related hazards from other factors influencing the migration decision‐making process (Kaczan & Orgill‐Meyer, 2020 ). While previous review studies have been done on the impact of climate‐related events on human migration (see, e.g., Berlemann & Steinhardt, 2017 ; Black et al., 2013 ; Kaczan & Orgill‐Meyer, 2020 ; Klaiber, 2014 ), none of them centered around the impacts of SLR‐related hazards and as a result many relevant studies were excluded from these reviews. Moreover, the majority of the 15 studies included in this review were published since 2017, which indicates that reviews published before are outdated and miss many new studies. Kaczan and Orgill‐Meyer ( 2020 ) explicitly recognize the need for a systematic review of the impacts of SLR on migration, but yet do not consider these impacts themselves in their review of quantitative empirical studies on climate‐driven migration. Our study aims to fill this gap in the literature. Notwithstanding SLR is primarily a future affair, a “wait and see” approach is not an attractive option; instead the information available now can be utilized for making informed choices about how to anticipate and manage SLR‐induced migration.
To capitalize on the available information and to guide future research, this article provides two main outputs. First, embedding the findings from our literature review in a conceptual framework of human migration, adapted from Black et al. ( 2011 ) and Hauer et al. ( 2020 ), emerging patterns on the impact of SLR‐related hazards on human migration are discussed, as well as the role of non‐environmental factors. Second, this article proposes a research agenda for future empirical research on SLR and migration. Although this article takes stock of current knowledge, the evidence available is still sparse and confined to limited geographical contexts, which highlights the need for more empirical work on this topic (McLeman, 2014 ). We also argue that future empirical research should be more closely aligned to promising migration modeling approaches, such as agent‐based models (ABMs). ABMs can simulate the expected number of migrants under different SLR scenarios, where they come from and go to, and when they leave, while considering complex individual‐level and/or household‐level migration decision making processes (Kniveton et al., 2011 ; Speelman, 2015 ; Thober et al., 2018 ; Wrathall et al., 2019 ). This is relevant for policy making and goes beyond what can be achieved by empirical survey research alone (Williams et al., 2017 ; Wrathall et al., 2019 ). Key decisions that have to be made by developers of ABMs are the variables to include in the model and the decision‐making rules that agents (simulated individuals or households) follow. In the proposed research agenda, we discuss how empirical research can aid in making these decisions more well‐informed and improving modeling outcomes.
The remainder of this article is structured as follows. Section 2 discusses the conceptual framework. Section 3 explains the methodology used for the systematic literature review. Section 4 presents and discusses the review results. Section 5 proposes a research agenda for future empirical research on SLR and human migration. Section 6 concludes.
2. CONCEPTUAL FRAMEWORK
Migration can be defined as the permanent or semi‐permanent change of residence (Lee, 1966 ). 1 Studying the impacts of environmental change on migration has become of increasing relevance due to climate change and widespread environmental degradation (McLeman & Gemenne, 2018 ). While initially studied predominantly by scholars from the broader natural sciences, the environmental migration literature is increasingly integrated with concepts, theories, and methodologies from the social sciences, and it is acknowledged that environmental factors are just one of many factors that influence the migration decision (Adger et al., 2018 , 2021 ; Hunter et al., 2015 ; Nicholls et al., 2020 ; Safra de Campos et al., 2020 ).
Figure 1 presents a conceptual framework of migration under SLR‐related hazards. This framework is modified from the original work of Black et al. ( 2011 ), and the later adaptation of Hauer et al. ( 2020 ) who applied the framework to SLR‐induced migration. The main benefit of this framework is that it recognizes the complexity of the migration decision‐making process and the associated drivers of migration (Hunter et al., 2015 ). In our adapted version, we take advantage of the richness of non‐environmental factors proposed by Black et al. ( 2011 ) and the application to SLR by Hauer et al. ( 2020 ). The framework depicts five macro drivers of migration in the context of SLR: economic, political, social, demographic, and SLR‐related hazards as a subset of environmental drivers. SLR‐related hazards in the conceptual framework and in the remainder of this article refer to hazards that are expected to be exacerbated by climate‐induced SLR, being flooding, salinization, and erosion. However, these hazards may also have other (non‐climatic) causes such as subsidence, dam construction, and industrial (dredging) activities (Dunn et al., 2018 , 2019 ; Nicholls, Lincke, et al., 2021 ; Vasilopoulos et al., 2021 ). Environmental drivers of migration refer to the hazard people face (Black et al., 2011 ). Hence, studying people's migration response to (perceptions of) SLR‐related hazards, whether or not caused by climate‐induced SLR, provides us with important lessons on how people may respond to similar hazards under increased future SLR. SLR‐related hazards in the framework can influence migration directly or indirectly through the other four drivers. Within the pentagon, spatiotemporal variabilities in the migration response are shown. SLR‐related hazards can drive migration gradually (e.g., salinization) or suddenly (e.g., flooding from storm surges), and migration can be in anticipation or in reaction to SLR‐related hazards. Furthermore, both actual and perceived levels of risk can play a role. Whether drivers indeed translate to migration behavior is mediated by personal/household characteristics such as education, wealth, and preferences, and by intervening obstacles and facilitators such as the costs of migration, protection or retreat policies, and social networks. The framework reflects that both structural forces and human agency shape migration decisions, and it can be applied to different spatiotemporal types of migration. This conceptualization helps to classify and interpret the findings of our systematic literature review, and to identify knowledge gaps and fruitful avenues for future research.

Migration in the context of SLR‐related hazards, modified from Black et al. ( 2011 ) and Hauer et al. ( 2020 )
To synthesize current knowledge on the impact of SLR‐related hazards on migration, this study conducts a systematic review of the empirical literature. Appendix S1 provides a detailed description of the article inclusion criteria and the search and screening procedure of the review. To be included in the review, the following article inclusion criteria had to be met. First, the research should analyze migration intentions or actual migration as the dependent variable. Second, the research should study the effects of SLR‐related hazards, as an independent variable, on actual migration or migration intentions. Both studies looking at coastal and riverine flooding are included, as SLR through backwater effects can also increase the incidence and extent of upstream river flooding (Ikeuchi et al., 2015 ). Third, the study should utilize individual‐level or household‐level data. Fourth, data should be analyzed using multivariate regression analysis.
We conducted the systematic literature search over the period November and December 2020 to identify peer‐reviewed scientific articles meeting all the inclusion criteria. A search term including keywords on SLR, migration, and empirical research methods was used and yielded 5353 articles. These articles were subsequently screened and filtered for eligibility following a three‐step procedure: title analysis, abstract and keyword analysis, and finally a thorough full‐text analysis. This resulted in 13 studies meeting all the inclusion criteria. We then screened the references of these 13 studies and the references of a selection of review articles dealing with migration and the environment, which yielded an additional two studies. The article selection process is summarized in Figure 2 .

Selection process of articles included in the systematic literature review
4. LESSONS FROM EMPIRICAL RESEARCH ON SLR ‐RELATED HAZARDS AND HUMAN MIGRATION
4.1. characteristics of articles included in the review.
Table 1 provides an overview of the 15 articles included in the review, incorporating the following characteristics: (a) author(s) and year, (b) study area, (c) SLR‐related hazards studied, (d) how migration is measured, (e) type of migration studied in terms of migration destination and whether migration is temporary or permanent, and (f) survey or census data characteristics. The publication years of the articles included in this review lie between 2008 and 2020, with the majority of studies being published in the period 2017–2020. Geographically, the articles cover seven countries, being Bangladesh (five studies), the United States (four studies), Indonesia (two studies), Ghana (two studies), Australia (one study), Canada (one study), and Pakistan (one study). Most case studies comprise (at least partly) the coastal zone, except two. Regarding socio‐economic context, six studies are situated in developed countries, and nine studies in developing countries. In terms of SLR‐related hazards, the effect of flooding on migration is investigated in all included studies, and to a lesser extent salinization (three studies) and erosion (two studies) are also researched. For flood type, six studies focus on coastal flooding, three studies on riverine flooding, while six studies do not distinguish between flood types.
Overview of studies included in the review
Abbreviations: HH, households; HY, household‐years; IN, individuals.
The studies either use actual migration data (nine studies) or gauge migration intentions in survey questionnaires (six studies). Six out of the fifteen studies specify the migration destination (i.e., whether migration is characterized as short‐distance or long‐distance, internal, or international). Nine studies do not make this specification in their regression analyses, among which are all studies that investigate migration intentions. Six studies also specify the temporal dimension of migration (i.e., whether migration is temporary or permanent). 2 Lastly, studies differ in terms of whether they use cross‐sectional data (nine) or panel data (six) for their regression analyses.
4.2. SLR ‐related hazards and human migration
Table 2 presents the results of the 15 studies included in the review. The results are separately presented for the impacts of flooding, salinization, and erosion. Because of the heterogeneity in research approaches, the specific measurements of the independent variables are also described in the table. For each finding, the sign of the effect is shown, where a “+” indicates a positive impact on migration, and a “–” a negative impact. In addition, the significance level of the result is shown ( p < 0.10, p < 0.05, or p < 0.01). 3 Sometimes a range of significance levels is provided in case a study presents results of multiple model estimations. Non‐significant results are denoted by NS. Results are separated by migration destination, if applicable.
Empirical evidence of relationship between SLR‐related hazards and human migration
Note : −/+ is the sign of the effect, significance level in parentheses, NS means effect is not significant. Results displayed in merged cells indicate that the measure encompasses multiple migration destinations.
Abbreviations: CM, migration destination combined or unspecified; INTM, international migration; LIM, long‐distance internal migration; SIM, short‐distance internal migration.
4.2.1. Flooding
All studies included in this review studied the effect of flooding on migration. Different types of flood indicators are studied: flood experience, flood severity or frequency, economic damages from flooding, geographical indicators of flood risk, and flood risk perceptions. Of the eight flood experience indicators five are reported as insignificant, two as having a positive impact on migration, and one as having a negative impact on migration. Positive impacts of flood experience on migration are found in US studies by Paxson and Rouse ( 2008 ) and Schwaller et al. ( 2020 ), for experience with home flooding specifically. Home flooding can cause serious economic damage and experiencing it first‐hand can strongly increase risk perceptions of flooding, as explained by the availability heuristic (Botzen et al., 2021 ), which can promote migration behavior. However, for the other studies we find insignificant or even negative impacts of flood experience on migration. This discrepancy might be due to the fact that these studies were conducted in developing countries (except for Haney, 2019 , who finds a positive, albeit insignificant, effect on migration intentions in a Canadian study). People in developing countries are often more dependent on coastal and riverine resources for their livelihoods, and also face higher financial constraints, which may limit their ability to migrate. These financial constraints can be further exacerbated by flood events. This is exemplified by Call et al. ( 2017 ) who find that a river flood event in the month of occurrence significantly reduces temporary migration in Bangladesh, with the odds of migration being 17% lower in a month of flooding compared with a month without flooding. Besides financial constraints, the flood type studied could also explain the divergence in results. River flooding, as opposed to coastal flooding, provides freshwater that can boost agricultural productivity and may reduce migration in agricultural households (Banerjee, 2010 ; Chen & Mueller, 2019 ). As will be explained in Section 5 in greater detail, more research evidence is needed to validate and clarify this possible multidirectional relationship between flooding and migration.
A similar discrepancy between developed and developing country case studies can be observed when we look at indicators of flood severity or frequency. Studies focusing on Bangladesh, Indonesia, and Pakistan find that more severe floods in terms of deaths, destroyed houses, or exposed households significantly decrease long‐term migration (Bohra‐Mishra et al., 2014 ; Chen & Mueller, 2019 ; Mueller et al., 2014 ). Contrastingly, studies in Australia and New York find that more severe or frequent flooding increases intentions to migrate (Boon, 2014 ; Buchanan et al., 2019 ). However, the measurement of migration could also play a role here. Namely, the studies that focused on developing countries looked at actual migration flows, while those focusing on developed countries studied migration intentions. Flood type (coastal or riverine), which in some of these studies is unspecified, could also moderate the effects found. Nonetheless, this does not undermine the finding that severe flooding tends to restrict long‐term migration in developing country contexts. Although severe flooding can generate large temporary population displacements in developing countries (IDMC, 2017 ), displacement may often not result in permanent migration because people prefer to return to their place of residence for social or economic reasons, or because severe floods deplete household resources to finance migration (McLeman, 2014 ). Looking at less severe “moderate” flood events, Gray and Mueller ( 2012 ) find that this increases short‐distance migration and decreases long‐distance migration in Bangladesh. In contrast to severe flood events, moderate flood events might leave enough household resources to allow for short‐distance migration (Kaczan & Orgill‐Meyer, 2020 ). In light of the discussion on the possibly important role of financial constraints in developing countries, it is surprising that Bohra‐Mishra et al. ( 2014 ) and Gray and Mueller ( 2012 ) find that indicators of economic losses from flooding are insignificantly or weakly positively associated with migration. However, both studies use measures of absolute economic losses. Absolute economic losses from flooding may be higher for richer households, for whom migration may still be affordable.
Risk measured in terms of the distance people live from rivers and coasts is significantly associated with migration in three out of four studies looking at this geographical indicator. Goldbach ( 2017 ) and Bernzen et al. ( 2019 ) find in, respectively, Bangladesh and Indonesian case studies that people living closer to a coast or river are more likely to migrate. In contrast, Call et al. ( 2017 ) in a Bangladesh case study find that people living closer to a river are less likely to migrate. The choice of living close to coastal or riverine waterbodies reflects a trade‐off between greater economic and livelihood opportunities and increased flooding and other SLR‐related hazards. However, riverine flooding also comes with benefits by irrigating the land with freshwater (Banerjee, 2010 ). These benefits of riverine flooding may explain the positive effect found by Call et al. ( 2017 ), who focus exclusively on the distance to rivers.
Two studies in our review investigate the relationship between migration and self‐reported risk perception. Haney ( 2019 ) finds that people in the Canadian inland city of Calgary who believe that flooding will become more frequent in the future do not report higher migration intentions, while people who worry about future flooding affecting their neighborhood are significantly more likely to intend to migrate. In fact, worried individuals report a 67% lower likelihood of staying in their neighborhood in the coming year, and a 50% lower likelihood of staying for the coming 5 years, compared with non‐worried individuals. Song and Peng ( 2017 ) in a US study find that both a belief that SLR is occurring and a belief that SLR will intensify extreme weather events is significantly positively associated with migration intentions. Both findings illustrate the important role that risk perceptions can play alongside actual risk, as is also pointed out in Figure 1 . The influence of risk perceptions is particularly relevant for SLR, which main impacts lie in the future and can be gauged by self‐reported risk perceptions.
Chen and Mueller ( 2019 ), Gray and Mueller ( 2012 ), and Mueller et al. ( 2014 ) compare the migration impacts of flooding by gender. Chen and Mueller ( 2019 ) find that in Bangladesh more severe flooding decreases migration among men, but not among women. Gray and Mueller ( 2012 ) in another Bangladesh study find that indicators of flood severity are negatively associated with migration among men, albeit insignificantly, while they tend to be positively associated with migration among women, and significantly so for moderate flood severity. Mueller et al. ( 2014 ) find that flooding in Pakistan significantly deters short‐distance migration for both males and females but find no significant effects for long‐distance migration. The finding of the two Bangladesh studies that flooding has more negative effects on migration for men than for women is somewhat unexpected, given that men generally have more migration decision‐making power than women in developing countries, and men are also more likely than women to move for economic purposes (Kaczan & Orgill‐Meyer, 2020 ). It could be that migration decreases more among men than among women when communities have to be rebuild after a severe flood event. In addition, migration for marriage, one of the most important reasons for migration among Bangladeshi women, might be little affected or even increase after flooding as it can be seen as a risk diversification strategy for resource‐constrained households (Rosenzweig & Stark, 1989 ).
Whether studies focus on migration intention or on actual migration also seems to matter for the study outcomes. In studies that investigate migration intention, the effects of the flooding indicators are either insignificant or positive. Studies that investigate actual migration find that flooding can both decrease and increase migration, depending on the type of flood indicator and study context. Besides variations in study context, the intention–behavior gap could be a reason for the observed differences, as intentions do not always lead to action (Echegaray & Hansstein, 2017 ). Although people might intend to leave because of flooding, they may change their minds over time or find themselves physically, financially, or in any other way constrained in their migration decision. These diverging findings could also disclose that flooding impacts migration over longer time horizons than shortly after flooding events; flooding can influence migration intentions or migration decisions, but this might not yet be revealed through actual migration behavior. Reduced (financial) capability to migrate shortly after flood events can be one reason for this (Kaczan & Orgill‐Meyer, 2020 ).
4.2.2. Salinization and erosion
In contrast to flooding, much less research has been done on the impact of salinization on migration, and the studies included in this review are confined to Bangladesh. However, the results that are available provide a more consistent picture of the relationship between migration and salinization than is the case for flooding. In most cases, experience with or being geographically at risk of salinization increases migration (Bernzen et al., 2019 ; Chen & Mueller, 2018 , 2019 ). Although Chen and Mueller ( 2018 ) find that salinization deters international migration overall, salinization still has a significant positive influence on international migration to nearby countries in South Asia. Perhaps negative economic effects as a result of salinization can obstruct long‐distance international moves. Chen and Mueller ( 2019 ) find that a one standard deviation increase in soil salinity increases migration from coastal Bangladesh to India and other countries in the region by a factor of 18, leading to an expected yearly increase in outmigration of 17,874 people to India alone (based on 2011 data). Chen and Mueller ( 2019 ) also examine whether the impact of salinization differs by gender, but find that salinization increases migration among both men and women. Salinization in Bangladesh greatly reduces yields of rice and other important crops, and as a result threatens agricultural production and people's livelihoods (Clarke et al., 2015; Hussain et al., 2018). There is now a widespread conversion from paddy cultivation to aquaculture in saline‐affected areas in the country, increasing rural unemployment and poverty, and stimulating out‐migration (Lázár et al., 2020 ; Nicholls et al., 2018 ). This can all explain the strong positive effect of salinization on migration found in our review. In addition, while sudden financial losses from extreme flooding events can deter people's capability to migrate, salinization is more of a gradual process where people have the time to plan migration and collect the necessary resources (Chen & Mueller, 2019 ; Kaczan & Orgill‐Meyer, 2020 ). These findings on the impact of salinization in coastal Bangladesh correspond to Li et al. (2014) who in a case study in inland China find that individuals who experienced problematic salinization as a result of excessive groundwater extraction were much more inclined to migrate than individuals who did not experience these difficulties.
Only two studies in this review looked at how erosion in coastal areas influences migration. Goldbach ( 2017 ) finds that coastal erosion does not affect migration in both Ghana and Indonesia. A reason for this insignificant effect could be that coastal erosion only impacts migration for people living directly adjacent to the coast, while in the study of Goldbach ( 2017 ) most respondents lived a few kilometers away from the shoreline. In another study, Bernzen et al. ( 2019 ) focused on loss of arable land in Bangladesh and find that this does affect migration. Over 93% of respondents reported that riverbank erosion was the reason for the arable land loss. Future SLR, by intensifying the hydrological cycle and river flooding, is expected to enhance the loss of arable land as a result of riverbank erosion (Alam et al., 2016 ). Loss of arable land impedes agricultural and housing opportunities and, consequently, may force people to migrate (Islam & Rashid, 2011). In fact, in Bernzen et al. ( 2019 ) those who experienced arable land loss were 2.5 times more likely to migrate than those who did not. In the scientific and in the public discourse flooding as a result of SLR captures most attention (Nicholls, 2011 ), but the previous findings illustrate that salinization and erosion and their relationship with migration should be seriously considered as well.
4.3. Other factors that influence migration
Table A1 in the Appendix presents an overview of non‐environmental factors and their effects on migration, as obtained from the results of the 15 studies included in our review. These factors cover many, but certainly not all, elements shown in the conceptual framework in Figure 1 . Especially information on the impact of macrolevel factors, such as government policies, is still lacking. We only discuss factors that have been investigated in at least two study settings, so that the patterns found are more reliable.
We identify several factors that play a role in explaining migration behavior next to SLR‐related hazards. First, individuals with higher income/wealth are in most cases more likely to migrate than poorer individuals. Although the poor and marginalized are often hit hardest by natural disasters, a lack of economic resources can inhibit their capability to migrate, which can be further exacerbated by natural hazard events (Black et al., 2011 ; Kaczan & Orgill‐Meyer, 2020 ). However, Gray and Mueller ( 2012 ) find that coming from a richer household significantly increases migration among men but not among women in Bangladesh, reflecting potential gender inequalities in the capability to capitalize on higher household resources. Second, homeowners are less likely to migrate. Homeownership indicates a substantial economic investment that ties people to their place of residence, and may also indicate place attachment (Haney, 2019 ). Third, and related to the previous point, place attachment is associated with a lower intention to migrate, which is a commonly held view in the literature (Adams & Kay, 2019 ; Swapan & Sadeque, 2021 ). Fourth, two US studies find that black people are more likely to migrate than people of other ethnicities (Paxson & Rouse, 2008 ; Song & Peng, 2017 ). This could reflect that black communities in the United States are disproportionally located in areas most vulnerable to climate change (Shepard & Corbin‐Mark, 2009 ), as well as that black people are more likely to be affected by the phenomenon of climate gentrification (Shokry et al., 2020 ). This raises an important issue, and policy should prevent that already existing inequalities will be aggravated by SLR. Fifth, people with social networks outside of their place of residence are more inclined to migrate. External social networks reduce information costs and make it easier for migrants to integrate into their new destination, and are central in network theories of migration (Haug, 2008 ; McLeman, 2014 ; Ryan, 2011 ). Finally, individual preferences including risk aversion and impatience have been found to lower the likelihood of migration. This can be explained by the fact that migration involves a high level of uncertainty, and it might take time before one can reap the benefits of a migration investment (Goldbach, 2017 ). We observe no clear influence of age, education, or gender. Although younger, male, and higher educated individuals are often depicted as being more likely to take part in environmental migration, especially internationally (Kaczan & Orgill‐Meyer, 2020 ; Obokata et al., 2014 ), this general pattern is not observed for the studies included in this review.
5. A RESEARCH AGENDA FOR FUTURE EMPIRICAL RESEARCH ON SLR AND MIGRATION
The previous section presented the results of a systematic literature review concerning empirical research on the impact of SLR‐related hazards on human migration. Based on these results, the following section proposes a research agenda by discussing the main gaps that still exist in the literature and fruitful avenues for future research. With this research agenda, we aim to help bring the science forward and promote research that provides policymakers and other stakeholders with the information they need to anticipate and manage SLR‐related migration. The research agenda discusses seven main themes, identifying research avenues for: (a) empirical research for varying geographical contexts and SLR risks, (b) studying a broader set of explanatory variables, (c) utilizing behavioral experiments and risk perception data, (d) differentiating impacts of SLR for subpopulations, (r) defining migration specifics, (f) researching migration together with other adaptation options, and (g) making empirical research complementary to dynamic migration modeling. Consistent with our systematic literature review, this research agenda focuses on quantitative research. Although not discussed in detail here, we acknowledge the importance of qualitative research. Qualitative research allows for a more in‐depth and nuanced understanding of migration motives, and is of high value in identifying the role of non‐environmental factors like gender and culture, and how these factors interact with environmental change such as SLR (Gioli & Milan, 2018 ; McLeman & Gemenne, 2018 ; Zickgraf, 2019 ). In addition, qualitative work offers important insights and experiences from marginalized and indigenous people (Albert et al., 2018 ; Farbotko, 2018 ), whose specific views are not reflected in our review study.
- Empirical research for varying geographical contexts and SLR risks . Despite the wealth of knowledge gained from the 15 articles included in the systematic literature review, our understanding of the issue is still limited. One major limitation is the geographical scope of the current literature. Only seven country contexts are included in our review. Quantitative empirical research is lacking in some of the countries expected to be among the hardest hit by SLR, including China, India, Vietnam, and the African countries of Egypt and Nigeria (Hauer et al., 2020 ). Studies are also lacking in several developed countries with considerable population numbers in the low‐elevation coastal zone, such as the Netherlands and the United Kingdom (Neumann et al., 2015 ). Migration is generally a less pressing issue in these contexts because coastal protection is put in place and planned for, and is often economically feasible under future SLR scenarios (Lincke & Hinkel, 2018 ; McEvoy et al., 2021 ). For instance, in the Netherlands coastal protection currently insulates the population from SLR changes and flood risk perceptions are generally very low (Kellens et al., 2013 ; Mol et al., 2020 ). Such case study locations would only become of substantial relevance for SLR migration research would protection fail or risk perceptions increase for other reasons like public awareness programs. Another limitation of present research is the uneven distribution of SLR‐related hazards that are studied, with erosion and salinization being understudied compared with flooding. This balance should be improved as worldwide many countries already experience the impacts of salinization as well as coastal and riverbank erosion, which will increase under future SLR (Das et al., 2014 ; Mentaschi et al., 2018 ; Smajgl et al., 2015 ). Future studies focusing on flooding should differentiate between the type of flood event, as the impacts of coastal compared with riverine flooding may be different, sometimes interacting in coastal zones, but are often not accounted for in current studies. These studies could also help to validate and clarify the multidirectional relationship between flooding and migration suggested from the findings of our review, disentangling the role of possibly decisive factors such as flood type and financial constraints. Finally, when studying the impacts of SLR‐related hazards on migration, studies should consider not only the role of climate‐induced SLR but also that of other (non‐climatic) factors driving these hazards, including subsidence (Nicholls, Lincke, et al., 2021 ).
- Broadening of the set of explanatory variables . The conceptual framework portrayed in Figure 1 theorizes the factors that can be important in explaining migration in the context of SLR. However, many of these factors are not or barely analyzed in the empirical literature. Macrolevel drivers, including economic, social, demographic, and political drivers, are especially understudied. Of particular importance is the impact of government policies on migration decisions, such as adaptation or disaster insurance policies (Wrathall et al., 2019 ). As these are important tools that governments could use to steer migration, research is needed to provide insights into their effectiveness. Besides using data from available datasets, the effects of macrolevel variables can also be tested in behavioral field experiments or by collecting survey data on people's perceptions, which could be necessary if the geographical scope of the study does not include variation in macrolevel context. Future research could also focus more on the influence of intervening obstacles or facilitators of migration. This includes the costs of migration, which is considered to be of high importance in the migration decision. However, in the literature its impact is often only gauged indirectly by looking at household wealth measures (Kaczan & Orgill‐Meyer, 2020 ). The effect of pull, compared with push, factors also deserves more scholarly attention.
- Utilization of behavioral experiments and risk perception data . Many of the studies included in the literature review presented in this article have analyzed how SLR‐related hazards (i.e., flooding, salinization, and erosion) in the past have influenced migration. Although learning from the past gives us important insights, the dynamics of these hazards under future SLR will be markedly different and so will be their impact on migration. When flooding becomes more frequent and severe, and erosion and salinization increases, migration thresholds may be reached that have not been reached in the past. Currently, knowledge about migration thresholds for people under climate change and SLR is increasing but still limited, and more research is needed on what levels and manifestations of SLR trigger (inevitable) migration (Hauer et al., 2020 ; McLeman, 2018 ). To circumvent the drawbacks of using data on past migration, behavioral experiments and risk perception studies can be utilized. However, these studies are currently underrepresented in the literature. Research on risk perceptions can help us better understand how SLR already influences migration also beyond hazard exposure or impacts, for instance by studying if people who understand or are worried about SLR are more likely to migrate from vulnerable areas. This also allows for the incorporation of local knowledge in addition to scientific measures of SLR risk (McMichael et al., 2021 ). This can also improve our understanding of anticipatory migration patterns, instead of reactive migration where most studies so far have focused on. Behavioral experiments, on the other hand, can be utilized to identify when thresholds are reached and people start to migrate. This can be done by presenting respondents with migration and adaptation choices under realistic future SLR scenarios for permanent inundation, flooding, salinization, and/or erosion and analyzing the choices they make. Choice experiments are an example of behavioral experiments that could be used for such purposes (Dachary‐Bernard et al., 2019 ; Train, 2009 ). Results of behavioral experiments could provide pivotal information on when thresholds are reached for people to migrate, and consequently on when to anticipate migration under different SLR scenarios. Longitudinal, in addition to cross‐sectional, studies could help identify if results differ before and after experience with SLR‐related events such as flooding, or whether people actually migrate when they experience the SLR‐related hazards they were presented with in hypothetical experiments (Bubeck et al., 2012 ).
- Differentiation of the impacts of SLR for subpopulations . The impacts of SLR on migration are not expected to be uniform across populations. For instance, vulnerable population groups like women, the elderly, the poor, and minorities, tend to be less capable of acting on climate risks, especially in developing countries (Gioli & Milan, 2018 ; Otto et al., 2017 ; Rahman, 2013 ). However, this heterogeneity is not properly accounted for in the analytical strategies of most of the quantitative empirical literature, which is characterized by the use of linear regressions on the full sample. Better accounting for population heterogeneity is imperative, and prevents oversimplification of the environment‐migration relationship. To do this, future studies could estimate separate regressions for different population groups, such as is done by Mueller et al. ( 2014 ) for gender, or include interaction effects between SLR and socio‐demographic indicators (e.g., interacting soil salinity with income). It should be taken into account that for reliable results these analyses might require large sample sizes.
- Defining migration specifics . Migration specifics, including when, where and for how long people migrate, are often not well defined, whereas this is pivotal in properly understanding migration dynamics and their causal factors. For instance, only 6 out of the 15 studies in our review specify the migration destination or whether migration is temporary or permanent. Especially migration intention research tends to ignore migration specifics, where often broad dichotomous questions are analyzed on whether respondents plan to migrate in the future, but without considering its particularities. Migration intention research can help identify migration thresholds under future SLR, but loses much of its value when we do not know what this migration will look like. Another important aspect in this domain is that of migrant agency, which is about the agency or freedom an individual/household has in the migration decision‐making process (Bakewell, 2010 ; McLeman, 2014 ). Migrant agency represents a continuum with fully voluntary and involuntary (forced) migration as the two extremes. The degree of migrant agency in the context of SLR risk may vary (McLeman, 2014 ), and is important to examine in future research.
- Researching migration as part of a broader array of adaptation options . Most studies research migration as the only outcome, whereas people can adapt to SLR in many other ways than migration, such as by flood proofing their houses or by cultivating saline‐tolerant crops (Jamero et al., 2017 ; McLeman, 2014 ). Therefore, one of the greatest challenges for future research is to break the silos of the migration and mainstream adaptation literature, and to bundle strengths to explain how people respond to SLR risk. Compared with the migration literature, the literature on in‐situ adaptation to SLR‐related hazards is much larger and further developed, with most attention being focused on flood adaptation (Bubeck et al., 2012 ; Koerth et al., 2017 ; Paik et al., 2020 ). Combining both strands of literature could provide important insights into the circumstances under which people will implement private adaptation measures to protect themselves against SLR, when people will decide to migrate, how these relate to each other, and which response will be more likely under future SLR scenarios. A choice experiment analysis in the United States by Buchanan et al. ( 2019 ) where respondents under different flood scenarios choose whether to take up insurance, elevate their homes, or migrate, provides a good example of such an integrative approach.
- Making empirical research complementary to migration modeling research . It is of high policy relevance to obtain reliable forecasts on the key dimensions of human migration under future SLR: how many people will migrate, where they will migrate to, the timing of their migration, and their socio‐economic situation before and after migration (McLeman & Gemenne, 2018 ; Wrathall et al., 2019 ). Dynamic migration modeling approaches can be employed to obtain such forecasts, and examples include gravity models (Afifi & Warner, 2008 ), radiation models (Davis et al., 2018 ), machine learning models (Robinson et al., 2020 ), and agent‐based models (Bell et al., 2021 ). Although all these model types have their merits, in the remainder our focus will be on agent‐based models (ABMs) because of their unique capability to model complex individual decision‐making processes while incorporating socio‐ecological interactions at the individual/household level (Klabunde & Willekens, 2016 ). ABMs can take into account heterogeneity in the characteristics of individuals/households, and aggregate micro‐level results to population‐level migration outcomes (Klabunde & Willekens, 2016 ; Kniveton et al., 2011 ; Thober et al., 2018 ; Wrathall et al., 2019 ). The use of ABMs has become increasingly popular among migration scholars, and a few ABM studies have simulated migration under future SLR (e.g., Bell et al., 2021 ; Hassani‐Mahmooei & Parris, 2012 ). Empirical studies, like the studies included in our review, have an important role to play in developing realistic ABMs, and as a result in making their outcomes useful for policymakers (Gray et al., 2017 ; Schlüter et al., 2017 ; Williams et al., 2017 ). We highlight two ways in which empirical research can contribute, both reflecting key decisions that have to be made by ABM modelers. First, ABM modelers select and parametrize a set of variables that influence migration behavior in the model. Quantitative survey‐based research can facilitate this by providing insights into the main factors that influence migration and their effect sizes. Second, ABM modelers decide about the behavioral decision‐rules that agents follow (i.e., how individuals/households make decisions in the model), which are preferably guided by behavioral decision theory (Groeneveld et al., 2017 ). The choice and formalization of a theory can determine to a large extent the outcomes of the model, and it is therefore important that a theory is chosen that is most appropriate for the specific research context (Klabunde & Willekens, 2016 ; Schlüter et al., 2017 ; Wens et al., 2020 ). Some of the most commonly used behavioral theories in social‐ecological ABMs include economic theories such as Subjective Expected Utility Theory and Prospect Theory and psycho‐sociological theories such as the Theory of Planned Behavior and Protection Motivation Theory (Aerts, 2020 ; Schlüter et al., 2017 ). Empirical survey‐based research can aid in the choice and the subsequent calibration of a behavioral theory. For instance, our review of the empirical literature illustrates that economic wealth can play an important role in whether someone migrates after SLR disasters. This seems to indicate that people's (economic) capability to migrate is an important factor in the migration decision, which is one of the key elements in the Theory of Planned Behavior and Protection Motivation Theory, and can be incorporated in economic decision models as budget constraints. Applying behavioral theories that approach the true complexity of human behavior would be an important step in designing realistic ABMs, as at present still much of the ABM literature is not firmly grounded in behavioral theory or assumes rational agent behavior (Gray et al., 2017 ; Groeneveld et al., 2017 ; Schlüter et al., 2017 ). There is also potential in integrating the migration and adaptation literature in ABM modeling, so that future pathways of both SLR‐induced adaptation and migration can be simulated. Indeed, much ABM development is already going on in the flood adaptation literature (Haer et al., 2019 ; Zhuo & Han, 2020 ), and we encourage exploring cross‐fertilizations between both strands of research.
6. CONCLUSION
SLR presents a substantial threat to hundreds of millions of people living in coastal areas. Migration is one way for people to adapt, and may increasingly become the preferred option when SLR impacts intensify in the future. Knowledge about migration dynamics under SLR is important to allow for anticipatory policymaking and to better understand the welfare impacts of climate change. In this article, we present the results of a systematic review of the empirical literature and bring together existing knowledge on the relationship between migration behavior and SLR‐related hazards including flooding, salinization, and erosion. This review adds to existing reviews on climate change and human migration by focusing specifically on SLR‐related hazards, which is valuable given the growth in empirical studies on this topic in recent years. Findings from our review indicate no clear association between flooding and higher levels of migration. Severe flooding is often even associated with decreased levels of long‐term migration in developing countries, although the underpinnings of this finding remain unclear from present research. Salinization and erosion have been investigated in fewer studies, in which they tend to be associated with increased levels of migration. Important factors that influence migration alongside SLR‐related hazards include income, homeownership, place attachment, race, social networks, and risk attitudes.
Despite an increase in scholarly attention in recent years, the number of studies on SLR‐related hazards and migration is still limited and there are promising ways to improve upon existing findings. Therefore, we also presented a research agenda outlining knowledge gaps in the literature and fruitful avenues for future research. We highlight that dynamics of SLR‐related hazards such as flooding will be markedly different under future SLR than they were in the past. As a result, we encourage research to investigate how people will likely respond to future SLR scenarios, for instance by utilizing behavioral experiments. We also encourage scholars to clearly define migration specifics, expand the geographic contexts and explanatory variables that are investigated, differentiate impacts of SLR for subpopulation groups, and study migration as part of a broader array of adaptation strategies that people can choose. Finally, we discuss how empirical research can aid agent‐based modeling, which is a promising approach to forecast migration flows under future SLR scenarios. What is clear is that more empirical research is needed if we want to properly understand the complex dynamics of migration under SLR and anticipate it in a timely manner. We hope that our work can give directions for such endeavors.
CONFLICT OF INTEREST
The authors have declared no conflicts of interest for this article.
AUTHOR CONTRIBUTIONS
Sem Duijndam: Conceptualization (lead); data curation (lead); formal analysis (lead); investigation (lead); methodology (lead); validation (equal); visualization (lead); writing – original draft (lead); writing – review and editing (lead). Wouter Botzen: Conceptualization (supporting); data curation (supporting); formal analysis (supporting); investigation (supporting); methodology (supporting); validation (equal); visualization (supporting); writing – original draft (supporting); writing – review and editing (supporting). Liselotte Hagedoorn: Conceptualization (supporting); data curation (supporting); formal analysis (supporting); investigation (supporting); methodology (supporting); validation (equal); visualization (supporting); writing – original draft (supporting); writing – review and editing (supporting). Jeroen Aerts: Conceptualization (supporting); data curation (supporting); formal analysis (supporting); funding acquisition (lead); investigation (supporting); methodology (supporting); project administration (lead); validation (equal); visualization (supporting); writing – original draft (supporting); writing – review and editing (supporting).
RELATED WIRE s ARTICLES
A mobilities perspective on migration in the context of environmental change
Spatial and temporal ways of knowing sea level rise: Bringing together multiple perspectives
Integrating new sea‐level scenarios into coastal risk and adaptation assessments: An ongoing process
Supporting information
Appendix S1 Supporting Information
ACKNOWLEDGMENT
This research received funding from the European Research Council through the ERC Advanced Grant project COASTMOVE (grant number 884442).
Other variables and their influence on human migration in the context of SLR
Influence of other variables on human migration in the context of SLR
Duijndam, S. J. , Botzen, W. J. W. , Hagedoorn, L. C. , & Aerts, J. C. J. H. (2022). Anticipating sea‐level rise and human migration: A review of empirical evidence and avenues for future research . Wiley Interdisciplinary Reviews: Climate Change , 13 ( 1 ), e747. 10.1002/wcc.747 [ PMC free article ] [ PubMed ] [ CrossRef ] [ Google Scholar ]
Edited by: Julie Rozenberg, Domain Editor and Mike Hulme, Editor‐in‐Chief
Funding information European Research Council, ERC Advanced Grant COASTMOVE, Grant/Award Number: 884442
1 Migration as a result of SLR‐related hazards is characterized as environmental migration. We define environmental migrants in this article following the definition of Kaczan and Orgill‐Meyer ( 2020 ) and the International Organization for Migration ( 2009 ): “Environmentally induced migrants are persons or groups of persons who, for compelling reasons of sudden or progressive changes in the environment that adversely affect their lives or living conditions, are obliged to leave their habitual homes, or choose to do so, either temporarily or permanently, and who move either within their country or abroad” (Kaczan & Orgill‐Meyer, 2020 , p. 282).
2 Most migration intention studies do not specify whether migration is temporary or permanent, and only investigate whether respondents intend to “migrate” from their place of residence. Some studies focusing on actual migration flows do also not make this specification.
3 We do not report the size of the regression coefficients. Because studies did not report standardized regression coefficients, the size of the regression coefficients depends on the scales and units of the independent variables used in the specific studies, which impedes a meaningful comparison across studies. We therefore recommend future research to report standardized regression coefficients.
DATA AVAILABILITY STATEMENT
Further reading.
- Foresight . (2011). Migration and global environmental change: Future challenges and opportunities . Government Office for Science. [ Google Scholar ]
- McLeman, R. , & Gemenne, F. (Eds.). (2018). Routledge handbook of environmental displacement and migration . Routledge. [ Google Scholar ]
- Adams, H. , & Kay, S. (2019). Migration as a human affair: Integrating individual stress thresholds into quantitative models of climate migration . Environmental Science & Policy , 93 , 129–138. 10.1016/j.envsci.2018.10.015 [ CrossRef ] [ Google Scholar ]
- Adger, N. , de Campos, R. S. , Codjoe, S. N. A. , Siddiqui, T. , Hazra, S. , Das, S. , Adams, H. , Gavonel, M. F. , Mortreux, C. , & Abu, M. (2021). Perceived environmental risks and insecurity reduce future migration intentions in hazardous migration source areas . One Earth , 4 ( 1 ), 146–157. 10.1016/j.oneear.2020.12.009 [ CrossRef ] [ Google Scholar ]
- Adger, N. , de Campos, R. S. , & Mortreux, C. (2018). Mobility, displacement and migration, and their interactions with vulnerability and adaptation to environmental risks. In McLeman R. & Gemenne F. (Eds.), Routledge handbook of environmental displacement and migration (pp. 29–41). Routledge. [ Google Scholar ]
- Aerts, J. C. J. H. (2020). Integrating agent‐based approaches with flood risk models: A review and perspective . Water Security , 11 , 100076. 10.1016/j.wasec.2020.100076 [ CrossRef ] [ Google Scholar ]
- Afifi, T. , & Warner, K. (2008). The impact of environmental degradation on migration flows across countries . UNU‐EHS Working Papers. [ Google Scholar ]
- Alam, G. M. , Alam, K. , & Mushtaq, S. (2016). Influence of institutional access and social capital on adaptation decision: Empirical evidence from hazard‐prone rural households in Bangladesh . Ecological Economics , 130 , 243–251. 10.1016/j.ecolecon.2016.07.012 [ CrossRef ] [ Google Scholar ]
- Albert, S. , Bronen, R. , Tooler, N. , Leon, J. , Yee, D. , Ash, J. , Boseto, D. , & Grinham, A. (2018). Heading for the hills: Climate‐driven community relocations in the Solomon Islands and Alaska provide insight for a 1.5°C future . Regional Environmental Change , 18 ( 8 ), 2261–2272. 10.1007/s10113-017-1256-8 [ CrossRef ] [ Google Scholar ]
- Bakewell, O. (2010). Some reflections on structure and agency in migration theory . Journal of Ethnic and Migration Studies , 36 ( 10 ), 1689–1708. 10.1080/1369183X.2010.489382 [ CrossRef ] [ Google Scholar ]
- Banerjee, L. (2010). Effects of flood on agricultural productivity in Bangladesh . Oxford Development Studies , 38 ( 3 ), 339–356. 10.1080/13600818.2010.505681 [ CrossRef ] [ Google Scholar ]
- Bell, A. , Wrathall, D. J. , Mueller, V. , Chen, J. , Oppenheimer, M. , Hauer, M. , Adams, H. , Kulp, S. , Clark, P. U. , Fussell, E. , Magliocca, N. , Xiao, T. , Gilmore, E. A. , Abel, K. , Call, M. , & Slangen, A. B. A. (2021). Migration towards Bangladesh coastlines projected to increase with sea‐level rise through 2100 . Environmental Research Letters , 16 ( 2 ), 024045. 10.1088/1748-9326/abdc5b [ CrossRef ] [ Google Scholar ]
- Berlemann, M. , & Steinhardt, M. F. (2017). Climate change, natural disasters, and migration—A survey of the empirical evidence . CESifo Economic Studies , 63 ( 4 ), 353–385. 10.1093/cesifo/ifx019 [ CrossRef ] [ Google Scholar ]
- Bernzen, A. , Jenkins, J. , & Braun, B. (2019). Climate change‐induced migration in coastal Bangladesh? A critical assessment of migration drivers in rural households under economic and environmental stress . Geosciences , 9 ( 1 ), 51. 10.3390/geosciences9010051 [ CrossRef ] [ Google Scholar ]
- Black, R. , Adger, W. N. , Arnell, N. W. , Dercon, S. , Geddes, A. , & Thomas, D. (2011). The effect of environmental change on human migration . Global Environmental Change , 21 , S3–S11. 10.1016/j.gloenvcha.2011.10.001 [ CrossRef ] [ Google Scholar ]
- Black, R. , Arnell, N. W. , Adger, W. N. , Thomas, D. , & Geddes, A. (2013). Migration, immobility and displacement outcomes following extreme events . Environmental Science & Policy , 27 , S32–S43. 10.1016/j.envsci.2012.09.001 [ CrossRef ] [ Google Scholar ]
- Bohra‐Mishra, P. , Oppenheimer, M. , & Hsiang, S. M. (2014). Nonlinear permanent migration response to climatic variations but minimal response to disasters . Proceedings of the National Academy of Sciences , 111 ( 27 ), 9780–9785. 10.1073/pnas.1317166111 [ PMC free article ] [ PubMed ] [ CrossRef ] [ Google Scholar ]
- Boon, H. J. (2014). Disaster resilience in a flood‐impacted rural Australian town . Natural Hazards , 71 ( 1 ), 683–701. 10.1007/s11069-013-0935-0 [ CrossRef ] [ Google Scholar ]
- Botzen, W. , Duijndam, S. , & van Beukering, P. (2021). Lessons for climate policy from behavioral biases towards COVID‐19 and climate change risks . World Development , 137 , 105214. 10.1016/j.worlddev.2020.105214 [ PMC free article ] [ PubMed ] [ CrossRef ] [ Google Scholar ]
- Bubeck, P. , Botzen, W. J. W. , & Aerts, J. C. J. H. (2012). A review of risk perceptions and other factors that influence flood mitigation behavior: Review of flood risk perceptions . Risk Analysis , 32 ( 9 ), 1481–1495. 10.1111/j.1539-6924.2011.01783.x [ PubMed ] [ CrossRef ] [ Google Scholar ]
- Buchanan, M. K. , Oppenheimer, M. , & Parris, A. (2019). Values, bias, and stressors affect intentions to adapt to coastal flood risk: A case study from New York city . Weather, Climate, and Society , 11 ( 4 ), 809–821. 10.1175/WCAS-D-18-0082.s1 [ CrossRef ] [ Google Scholar ]
- Call, M. A. , Gray, C. , Yunus, M. , & Emch, M. (2017). Disruption, not displacement: Environmental variability and temporary migration in Bangladesh . Global Environmental Change , 46 , 157–165. 10.1016/j.gloenvcha.2017.08.008 [ PMC free article ] [ PubMed ] [ CrossRef ] [ Google Scholar ]
- Chen, J. , & Mueller, V. (2018). Coastal climate change, soil salinity and human migration in Bangladesh . Nature Climate Change , 8 ( 11 ), 981–985. 10.1038/s41558-018-0313-8 [ CrossRef ] [ Google Scholar ]
- Chen, J. , & Mueller, V. (2019). Climate‐induced cross‐border migration and change in demographic structure . Population and Environment , 41 ( 2 ), 98–125. 10.1007/s11111-019-00328-3 [ CrossRef ] [ Google Scholar ]
- Clarke, D., Williams, S., Jahiruddin, M., Parks, K., & Salehin, M. (2015). Projections of on‐farm salinity in coastal Bangladesh. Environmental Science: Processes & Impacts , 17(6), 1127‐1136. 10.1039/C4EM00682H [ PubMed ] [ CrossRef ] [ Google Scholar ]
- Codjoe, S. N. A. , Nyamedor, F. H. , Sward, J. , & Dovie, D. B. (2017). Environmental hazard and migration intentions in a coastal area in Ghana: A case of sea flooding . Population and Environment , 39 ( 2 ), 128–146. 10.1007/s11111-017-0284-0 [ CrossRef ] [ Google Scholar ]
- Dachary‐Bernard, J. , Rey‐Valette, H. , & Rulleau, B. (2019). Preferences among coastal and inland residents relating to managed retreat: Influence of risk perception in acceptability of relocation strategies . Journal of Environmental Management , 232 , 772–780. 10.1016/j.jenvman.2018.11.104 [ PubMed ] [ CrossRef ] [ Google Scholar ]
- Das, T. K. , Haldar, S. K. , Das Gupta, I. , & Sen, S. (2014). River Bank erosion induced human displacement and its consequences . Living Reviews in Landscape Research , 8 , 5–35. 10.12942/lrlr-2014-3 [ CrossRef ] [ Google Scholar ]
- Davis, K. F. , Bhattachan, A. , D'Odorico, P. , & Suweis, S. (2018). A universal model for predicting human migration under climate change: Examining future sea level rise in Bangladesh . Environmental Research Letters , 13 ( 6 ), 064030. 10.1088/1748-9326/aac4d4 [ CrossRef ] [ Google Scholar ]
- Dunn, F. E. , Darby, S. E. , Nicholls, R. J. , Cohen, S. , Zarfl, C. , & Fekete, B. M. (2019). Projections of declining fluvial sediment delivery to major deltas worldwide in response to climate change and anthropogenic stress . Environmental Research Letters , 14 ( 8 ), 084034. 10.1088/1748-9326/ab304e [ CrossRef ] [ Google Scholar ]
- Dunn, F. E. , Nicholls, R. J. , Darby, S. E. , Cohen, S. , Zarfl, C. , & Fekete, B. M. (2018). Projections of historical and 21st century fluvial sediment delivery to the Ganges‐Brahmaputra‐Meghna, Mahanadi, and Volta deltas . Science of the Total Environment , 642 , 105–116. 10.1016/j.scitotenv.2018.06.006 [ PubMed ] [ CrossRef ] [ Google Scholar ]
- Echegaray, F. , & Hansstein, F. V. (2017). Assessing the intention‐behavior gap in electronic waste recycling: The case of Brazil . Journal of Cleaner Production , 142 , 180–190. 10.1016/j.jclepro.2016.05.064 [ CrossRef ] [ Google Scholar ]
- Farbotko, C. (2018). Voluntary immobility: Indigenous voices in the Pacific . Forced Migration Review , 57 , 81–83. [ Google Scholar ]
- Gioli, G. , & Milan, A. (2018). Gender, migration and (global) environmental change. In McLeman R. & Gemenne F. (Eds.), Routledge handbook of environmental displacement and migration (pp. 135–150). Routledge. [ Google Scholar ]
- Goldbach, C. (2017). Out‐migration from coastal areas in Ghana and Indonesia—The role of environmental factors . CESifo Economic Studies , 63 ( 4 ), 529–559. 10.1093/cesifo/ifx007 [ CrossRef ] [ Google Scholar ]
- Gray, C. , & Mueller, V. (2012). Natural disasters and population mobility in Bangladesh . Proceedings of the National Academy of Sciences , 109 ( 16 ), 6000–6005. 10.1073/pnas.1115944109 [ PMC free article ] [ PubMed ] [ CrossRef ] [ Google Scholar ]
- Gray, J. , Hilton, J. , & Bijak, J. (2017). Choosing the choice: Reflections on modelling decisions and behaviour in demographic agent‐based models . Population Studies , 71 , 85–97. 10.1080/00324728.2017.1350280 [ PubMed ] [ CrossRef ] [ Google Scholar ]
- Groeneveld, J. , Müller, B. , Buchmann, C. M. , Dressler, G. , Guo, C. , Hase, N. , Hoffmann, F. , John, F. , Klassert, C. , Lauf, T. , Liebelt, V. , Nolzen, H. , Pannicke, N. , Schulze, J. , Weise, H. , & Schwarz, N. (2017). Theoretical foundations of human decision‐making in agent‐based land use models: A review . Environmental Modelling & Software , 87 , 39–48. 10.1016/j.envsoft.2016.10.008 [ CrossRef ] [ Google Scholar ]
- Haer, T. , Botzen, W. J. W. , & Aerts, J. C. J. H. (2019). Advancing disaster policies by integrating dynamic adaptive behaviour in risk assessments using an agent‐based modelling approach . Environmental Research Letters , 14 ( 4 ), 044022. 10.1088/1748-9326/ab0770 [ CrossRef ] [ Google Scholar ]
- Haney, T. J. (2019). Move out or dig in? Risk awareness and mobility plans in disaster‐affected communities . Journal of Contingencies and Crisis Management , 27 ( 3 ), 224–236. 10.1111/1468-5973.12253 [ CrossRef ] [ Google Scholar ]
- Hassani‐Mahmooei, B. , & Parris, B. W. (2012). Climate change and internal migration patterns in Bangladesh: An agent‐based model . Environment and Development Economics , 17 ( 6 ), 763–780. 10.1017/S1355770X12000290 [ CrossRef ] [ Google Scholar ]
- Hauer, M. E. (2017). Migration induced by sea‐level rise could reshape the US population landscape . Nature Climate Change , 7 ( 5 ), 321–325. 10.1038/nclimate3271 [ CrossRef ] [ Google Scholar ]
- Hauer, M. E. , Fussell, E. , Mueller, V. , Burkett, M. , Call, M. , Abel, K. , McLeman, R. , & Wrathall, D. (2020). Sea‐level rise and human migration . Nature Reviews Earth & Environment , 1 ( 1 ), 28–39. 10.1038/s43017-019-0002-9 [ CrossRef ] [ Google Scholar ]
- Haug, S. (2008). Migration networks and migration decision‐making . Journal of Ethnic and Migration Studies , 34 ( 4 ), 585–605. 10.1080/13691830801961605 [ CrossRef ] [ Google Scholar ]
- Hinkel, J. , Nicholls, R. J. , Tol, R. S. J. , Wang, Z. B. , Hamilton, J. M. , Boot, G. , Vafeidis, A. T. , McFadden, L. , Ganopolski, A. , & Klein, R. J. T. (2013). A global analysis of erosion of sandy beaches and sea‐level rise: An application of DIVA . Global and Planetary Change , 111 , 150–158. 10.1016/j.gloplacha.2013.09.002 [ CrossRef ] [ Google Scholar ]
- Hino, M. , Field, C. B. , & Mach, K. J. (2017). Managed retreat as a response to natural hazard risk . Nature Climate Change , 7 ( 5 ), 364–370. 10.1038/nclimate3252 [ CrossRef ] [ Google Scholar ]
- Hunter, L. M. , Luna, J. K. , & Norton, R. M. (2015). Environmental dimensions of migration . Annual Review of Sociology , 41 ( 1 ), 377–397. 10.1146/annurev-soc-073014-112223 [ PMC free article ] [ PubMed ] [ CrossRef ] [ Google Scholar ]
- Hussain, M., Ahmad, S., Hussain, S., Lal, R., Ul‐Allah, S., & Nawaz, A. (2018). Rice in Saline Soils: Physiology, Biochemistry, Genetics, and Management. Advances in Agronomy , 148, 231‐287. 10.1016/bs.agron.2017.11.002 [ CrossRef ] [ Google Scholar ]
- IDMC . (2017). Global report of internal displacement . https://reliefweb.int/sites/reliefweb.int/files/resources/2017-GRID.pdf
- Islam, M. F. , & Rashid, A. B. (2011). Riverbank erosion displacees in Bangladesh: Need for institutional response and policy intervention . Bangladesh Journal of Bioethics , 2 ( 2 ), 4–19. 10.3329/bioethics.v2i2.9540 [ CrossRef ] [ Google Scholar ]
- Ikeuchi, H. , Hirabayashi, Y. , Yamazaki, D. , Kiguchi, M. , Koirala, S. , Nagano, T. , Kotera, A. , & Kanae, S. (2015). Modeling complex flow dynamics of fluvial floods exacerbated by sea level rise in the Ganges–Brahmaputra–Meghna Delta . Environmental Research Letters , 10 ( 12 ), 124011. 10.1088/1748-9326/10/12/124011 [ CrossRef ] [ Google Scholar ]
- IOM . (2009). Migration, environment and climate change: Assessing the evidence . International Organization for Migration. [ Google Scholar ]
- IPCC . (2021). Climate change 2021: The physical science basis. Contribution of working group I to the sixth assessment report of the intergovernmental panel on climate change . Cambridge University Press. [ Google Scholar ]
- Jongman, B. , Ward, P. J. , & Aerts, J. C. J. H. (2012). Global exposure to river and coastal flooding: Long term trends and changes . Global Environmental Change , 22 ( 4 ), 823–835. 10.1016/j.gloenvcha.2012.07.004 [ CrossRef ] [ Google Scholar ]
- Kaczan, D. J. , & Orgill‐Meyer, J. (2020). The impact of climate change on migration: A synthesis of recent empirical insights . Climatic Change , 158 , 281–300. 10.1007/s10584-019-02560-0 [ CrossRef ] [ Google Scholar ]
- Kellens, W. , Terpstra, T. , & De Maeyer, P. (2013). Perception and communication of flood risks: A systematic review of empirical research . Risk Analysis: An International Journal , 33 ( 1 ), 24–49. 10.1111/j.1539-6924.2012.01844.x [ PubMed ] [ CrossRef ] [ Google Scholar ]
- Ketabchi, H. , Mahmoodzadeh, D. , Ataie‐Ashtiani, B. , & Simmons, C. T. (2016). Sea‐level rise impacts on seawater intrusion in coastal aquifers: Review and integration . Journal of Hydrology , 535 , 235–255. 10.1016/j.jhydrol.2016.01.083 [ CrossRef ] [ Google Scholar ]
- Klabunde, A. , & Willekens, F. (2016). Decision‐making in agent‐based models of migration: State of the art and challenges . European Journal of Population , 32 ( 1 ), 73–97. 10.1007/s10680-015-9362-0 [ PMC free article ] [ PubMed ] [ CrossRef ] [ Google Scholar ]
- Klaiber, H. A. (2014). Migration and household adaptation to climate: A review of empirical research . Energy Economics , 46 , 539–547. 10.1016/j.eneco.2014.04.001 [ CrossRef ] [ Google Scholar ]
- Kniveton, D. , Smith, C. , & Wood, S. (2011). Agent‐based model simulations of future changes in migration flows for Burkina Faso . Global Environmental Change , 21 , S34–S40. 10.1016/j.gloenvcha.2011.09.006 [ CrossRef ] [ Google Scholar ]
- Koerth, J. , Vafeidis, A. T. , & Hinkel, J. (2017). Household‐level coastal adaptation and its drivers: A systematic case study review . Risk Analysis , 37 ( 4 ), 629–646. 10.1111/risa.12663 [ PubMed ] [ CrossRef ] [ Google Scholar ]
- Jamero, L. , Onuki, M. , Esteban, M. , Billones‐Sensano, X. K. , Tan, N. , Nellas, A. , Takagi, H. , Thao, N. D. , & Valenzuela, V. P. (2017). Small‐Island communities in The Philippines prefer local measures to relocation in response to sea‐level rise . Nature Climate Change , 7 ( 8 ), 581–586. 10.1038/nclimate3344 [ CrossRef ] [ Google Scholar ]
- Lázár, A. N. , Nicholls, R. J. , Hall, J. W. , Barbour, E. J. , & Haque, A. (2020). Contrasting development trajectories for coastal Bangladesh to the end of century . Regional Environmental Change , 20 ( 3 ), 93. 10.1007/s10113-020-01681-y [ CrossRef ] [ Google Scholar ]
- Lee, E. (1966). A theory of migration . Demography , 3 ( 1 ), 47–57. [ Google Scholar ]
- Li, Y., López‐Carr, D., & Chen, W. (2014). Factors Affecting Migration Intentions in Ecological Restoration Areas and Their Implications for the Sustainability of Ecological Migration Policy in Arid Northwest China. Sustainability , 6(12), 8639‐8660. 10.3390/su6128639 [ CrossRef ] [ Google Scholar ]
- Lincke, D. , & Hinkel, J. (2018). Economically robust protection against 21st century sea‐level rise . Global Environmental Change , 51 , 67–73. 10.1016/j.gloenvcha.2018.05.003 [ CrossRef ] [ Google Scholar ]
- Lincke, D. , & Hinkel, J. (2021). Coastal migration due to 21st century sea‐level rise . Earth's Futures , 9 ( 5 ), 1–14. 10.1029/2020EF001965 [ CrossRef ] [ Google Scholar ]
- McEvoy, S. , Haasnoot, M. , & Biesbroek, R. (2021). How are European countries planning for sea level rise? Ocean & Coastal Management , 203 , 105512. 10.1016/j.ocecoaman.2020.105512 [ CrossRef ] [ Google Scholar ]
- McLeman, R. (2014). Climate and human migration: Past experiences, future challenges . Cambridge University Press. [ Google Scholar ]
- McLeman, R. (2018). Thresholds in climate migration . Population and Environment , 39 ( 4 ), 319–338. 10.1007/s11111-017-0290-2 [ CrossRef ] [ Google Scholar ]
- McLeman, R. , & Gemenne, F. (2018). Environmental migration research: Evolution and current state of the science. In McLeman R. & Gemenne F. (Eds.), Routledge handbook of environmental displacement and migration (pp. 3–16). Routledge. [ Google Scholar ]
- McMichael, C. , Kothari, U. , McNamara, K. E. , & Arnall, A. (2021). Spatial and temporal ways of knowing sea level rise: Bringing together multiple perspectives . WIREs Climate Change , 12 ( 3 ), 1–15. 10.1002/wcc.703 [ CrossRef ] [ Google Scholar ]
- Mentaschi, L. , Vousdoukas, M. I. , Pekel, J.‐F. , Voukouvalas, E. , & Feyen, L. (2018). Global long‐term observations of coastal erosion and accretion . Scientific Reports , 8 ( 1 ), 12876. 10.1038/s41598-018-30904-w [ PMC free article ] [ PubMed ] [ CrossRef ] [ Google Scholar ]
- Mol, J. M. , Botzen, W. J. W. , Blasch, J. E. , & Moel, H. (2020). Insights into flood risk misperceptions of homeowners in the Dutch River Delta . Risk Analysis , 40 ( 7 ), 1450–1468. 10.1111/risa.13479 [ PMC free article ] [ PubMed ] [ CrossRef ] [ Google Scholar ]
- Mueller, V. , Gray, C. , & Kosec, K. (2014). Heat stress increases long‐term human migration in rural Pakistan . Nature Climate Change , 4 ( 3 ), 182–185. 10.1038/nclimate2103 [ PMC free article ] [ PubMed ] [ CrossRef ] [ Google Scholar ]
- Neumann, B. , Vafeidis, A. T. , Zimmermann, J. , & Nicholls, R. J. (2015). Future coastal population growth and exposure to sea‐level rise and coastal flooding—A global assessment . PLoS One , 10 ( 3 ), e0118571. 10.1371/journal.pone.0118571 [ PMC free article ] [ PubMed ] [ CrossRef ] [ Google Scholar ]
- Nicholls, R. (2011). Planning for the impacts of sea level rise . Oceanography , 24 ( 2 ), 144–157. 10.5670/oceanog.2011.34 [ CrossRef ] [ Google Scholar ]
- Nicholls, R. , & Cazenave, A. (2010). Sea‐level rise and its impact on coastal zones . Science , 328 ( 5985 ), 1517–1520. 10.1126/science.1185782 [ PubMed ] [ CrossRef ] [ Google Scholar ]
- Nicholls, R. J. , Adger, W. N. , Hutton, C. W. , & Hanson, S. E. (2020). Deltas in the Anthropocene . Springer Nature. 10.1007/978-3-030-23517-8 [ CrossRef ] [ Google Scholar ]
- Nicholls, R. J. , Hanson, S. E. , Lowe, J. A. , Slangen, A. B. A. , Wahl, T. , Hinkel, J. , & Long, A. J. (2021). Integrating new sea‐level scenarios into coastal risk and adaptation assessments: An ongoing process . WIREs Climate Change , 12 ( 3 ), 1–27. 10.1002/wcc.706 [ CrossRef ] [ Google Scholar ]
- Nicholls, R. J. , Hutton, C. W. , Adger, W. N. , Hanson, S. E. , Rahman, M. M. , & Salehin, M. (2018). Ecosystem services for well‐being in deltas: Integrated assessment for policy analysis . Springer International Publishing , 1–593. 10.1007/978-3-319-71093-8 [ CrossRef ] [ Google Scholar ]
- Nicholls, R. J. , Lincke, D. , Hinkel, J. , Brown, S. , Vafeidis, A. T. , Meyssignac, B. , Hanson, S. E. , Merkens, J.‐L. , & Fang, J. (2021). A global analysis of subsidence, relative sea‐level change and coastal flood exposure . Nature Climate Change , 11 , 338–342. 10.1038/s41558-021-00993-z [ CrossRef ] [ Google Scholar ]
- Nicholls, R. J. , Marinova, N. , Lowe, J. A. , Brown, S. , Vellinga, P. , de Gusmão, D. , Hinkel, J. , & Tol, R. S. J. (2011). Sea‐level rise and its possible impacts given a “beyond 4°C world” in the twenty‐first century . Philosophical Transactions of the Royal Society A: Mathematical, Physical and Engineering Sciences , 369 ( 1934 ), 161–181. 10.1098/rsta.2010.0291 [ PubMed ] [ CrossRef ] [ Google Scholar ]
- Obokata, R. , Veronis, L. , & McLeman, R. (2014). Empirical research on international environmental migration: A systematic review . Population and Environment , 36 ( 1 ), 111–135. 10.1007/s11111-014-0210-7 [ PMC free article ] [ PubMed ] [ CrossRef ] [ Google Scholar ]
- Otto, I. M. , Reckien, D. , Reyer, C. P. O. , Marcus, R. , Le Masson, V. , Jones, L. , Norton, A. , & Serdeczny, O. (2017). Social vulnerability to climate change: A review of concepts and evidence . Regional Environmental Change , 17 ( 6 ), 1651–1662. 10.1007/s10113-017-1105-9 [ CrossRef ] [ Google Scholar ]
- Paik, S. , Le, D. T. P. , Nhu, L. T. , & Mills, B. F. (2020). Salt‐tolerant rice variety adoption in the Mekong River Delta: Farmer adaptation to sea‐level rise . PLoS One , 15 ( 3 ), e0229464. 10.1371/journal.pone.0229464 [ PMC free article ] [ PubMed ] [ CrossRef ] [ Google Scholar ]
- Paxson, C. , & Rouse, C. E. (2008). Returning to New Orleans after hurricane Katrina . American Economic Review , 98 ( 2 ), 38–42. 10.1257/aer.98.2.38 [ PMC free article ] [ PubMed ] [ CrossRef ] [ Google Scholar ]
- Rahman, M. (2013). Climate change, disaster and gender vulnerability: A study on two divisions of Bangladesh . American Journal of Human Ecology , 2 ( 2 ), 72–82. 10.11634/216796221302315 [ CrossRef ] [ Google Scholar ]
- Robinson, C. , Dilkina, B. , & Moreno‐Cruz, J. (2020). Modeling migration patterns in the US under sea level rise . PLoS One , 15 ( 1 ), e0227436. 10.1371/journal.pone.0227436 [ PMC free article ] [ PubMed ] [ CrossRef ] [ Google Scholar ]
- Rosenzweig, M. R. , & Stark, O. (1989). Consumption smoothing, migration, and marriage: Evidence from rural India . Journal of Political Economy , 97 ( 4 ), 905–926. 10.1086/261633 [ CrossRef ] [ Google Scholar ]
- Ryan, L. (2011). Migrants' social networks and weak ties: Accessing resources and constructing relationships post‐migration . The Sociological Review , 59 ( 4 ), 707–724. 10.1111/j.1467-954X.2011.02030.x [ CrossRef ] [ Google Scholar ]
- Safra de Campos, R. , Codjoe, S. N. A. , Adger, W. N. , Mortreux, C. , Hazra, S. , Siddiqui, T. , Das, S. , Atiglo, D. Y. , Bhuiyan, M. R. A. , Rocky, M. H. , & Abu, M. (2020). Where people live and move in deltas. In Nicholls R. J., Adger W. N., Hutton C. W., & Hanson S. E. (Eds.), Deltas in the Anthropocene (pp. 153–177). Springer Nature. 10.1007/978-3-030-23517-8_7 [ CrossRef ] [ Google Scholar ]
- Schlüter, M. , Baeza, A. , Dressler, G. , Frank, K. , Groeneveld, J. , Jager, W. , Janssen, M. A. , McAllister, R. R. J. , Müller, B. , Orach, K. , Schwarz, N. , & Wijermans, N. (2017). A framework for mapping and comparing behavioural theories in models of social‐ecological systems . Ecological Economics , 131 , 21–35. 10.1016/j.ecolecon.2016.08.008 [ CrossRef ] [ Google Scholar ]
- Schwaller, N. L. , Kelmenson, S. , BenDor, T. K. , & Spurlock, D. (2020). From abstract futures to concrete experiences: How does political ideology interact with threat perception to affect climate adaptation decisions? Environmental Science & Policy , 112 , 440–452. 10.1016/j.envsci.2020.07.001 [ CrossRef ] [ Google Scholar ]
- Shepard, P. M. , & Corbin‐Mark, C. (2009). Climate Justice . Environmental Justice , 2 ( 4 ), 163–166. 10.1089/env.2009.2402 [ CrossRef ] [ Google Scholar ]
- Shokry, G. , Connolly, J. J. , & Anguelovski, I. (2020). Understanding climate gentrification and shifting landscapes of protection and vulnerability in green resilient Philadelphia . Urban Climate , 31 , 100539. 10.1016/j.uclim.2019.100539 [ CrossRef ] [ Google Scholar ]
- Siders, A. (2013). Managed coastal retreat: A legal handbook on shifting development away from vulnerable areas . SSRN Electronic Journal , 1–140. 10.2139/ssrn.2349461 [ CrossRef ] [ Google Scholar ]
- Smajgl, A. , Toan, T. Q. , Nhan, D. K. , Ward, J. , Trung, N. H. , Tri, L. Q. , Tri, V. P. D. , & Vu, P. T. (2015). Responding to rising sea levels in the Mekong delta . Nature Climate Change , 5 ( 2 ), 167–174. 10.1038/nclimate2469 [ CrossRef ] [ Google Scholar ]
- Song, J. , & Peng, B. (2017). Should we leave? Attitudes towards relocation in response to sea level rise . Water , 9 ( 12 ), 941. 10.3390/w9120941 [ CrossRef ] [ Google Scholar ]
- Speelman, L. H. (2015). Empirical analyses of migration in small islands: The role of environmental and social factors (PhD Thesis). University of Southampton .
- Swapan, M. S. H. , & Sadeque, S. (2021). Place attachment in natural hazard‐prone areas and decision to relocate: Research review and agenda for developing countries . International Journal of Disaster Risk Reduction , 52 , 101937. 10.1016/j.ijdrr.2020.101937 [ CrossRef ] [ Google Scholar ]
- Thober, J. , Schwarz, N. , & Hermans, K. (2018). Agent‐based modeling of environment‐migration linkages: A review . Ecology and Society , 23 ( 2 ), art41. 10.5751/ES-10200-230241 [ CrossRef ] [ Google Scholar ]
- Train, K. (2009). Discrete choice methods with simulation . Cambridge University Press. [ Google Scholar ]
- Vasilopoulos, G. , Quan, Q. L. , Parsons, D. R. , Darby, S. E. , Tri, V. P. D. , Hung, N. N. , Haigh, I. D. , Voepel, H. E. , Nicholas, A. P. , & Aalto, R. (2021). Establishing sustainable sediment budgets is critical for climate‐resilient mega‐deltas . Environmental Research Letters , 16 ( 6 ), 064089. 10.1088/1748-9326/ac06fc [ CrossRef ] [ Google Scholar ]
- Vitousek, S. , Barnard, P. L. , Fletcher, C. H. , Frazer, N. , Erikson, L. , & Storlazzi, C. D. (2017). Doubling of coastal flooding frequency within decades due to sea‐level rise . Scientific Reports , 7 ( 1 ), 1399. 10.1038/s41598-017-01362-7 [ PMC free article ] [ PubMed ] [ CrossRef ] [ Google Scholar ]
- Wens, M. , Veldkamp, T. I. E. , Mwangi, M. , Johnson, J. M. , Lasage, R. , Haer, T. , & Aerts, J. C. J. H. (2020). Simulating small‐scale agricultural adaptation decisions in response to drought risk: An empirical agent‐based model for semi‐arid Kenya . Frontiers in Water , 2 , 15. 10.3389/frwa.2020.00015 [ CrossRef ] [ Google Scholar ]
- Williams, N. E. , O'Brien, M. L. , & Yao, X. (2017). Using survey data for agent‐based modeling: Design and challenges in a model of armed conflict and population change. In Grow A. & van Bavel J. (Eds.), Agent‐based modelling in population studies (pp. 159–184). Springer. [ Google Scholar ]
- Wilmsen, B. , & Webber, M. (2015). What can we learn from the practice of development‐forced displacement and resettlement for organised resettlements in response to climate change? Geoforum , 58 , 76–85. 10.1016/j.geoforum.2014.10.016 [ CrossRef ] [ Google Scholar ]
- Wrathall, D. J. , Mueller, V. , Clark, P. U. , Bell, A. , Oppenheimer, M. , Hauer, M. , Kulp, S. , Gilmore, E. , Adams, H. , Kopp, R. , Abel, K. , Call, M. , Chen, J. , DeSherbinin, A. , Fussell, E. , Hay, C. , Jones, B. , Magliocca, N. , Marino, E. , … Warner, K. (2019). Meeting the looming policy challenge of sea‐level change and human migration . Nature Climate Change , 9 ( 12 ), 898–901. 10.1038/s41558-019-0640-4 [ CrossRef ] [ Google Scholar ]
- Zhuo, L. , & Han, D. (2020). Agent‐based modelling and flood risk management: A compendious literature review . Journal of Hydrology , 591 , 125600. 10.1016/j.jhydrol.2020.125600 [ CrossRef ] [ Google Scholar ]
- Zickgraf, C. (2019). Keeping people in place: Political factors of (Im)mobility and climate change . Social Sciences , 8 ( 8 ), 228. 10.3390/socsci8080228 [ CrossRef ] [ Google Scholar ]
Read our research on: Gun Policy | International Conflict | Election 2024
Regions & Countries
Immigration & migration, what we know about unauthorized immigrants living in the u.s..
The unauthorized immigrant population in the United States reached 10.5 million in 2021. That was a modest increase over 2019 but nearly identical to 2017.
8 facts about recent Latino immigrants to the U.S.
Among asian americans, u.s.-born children of immigrants are most likely to have hidden part of their heritage, 11 facts about hispanic origin groups in the u.s., sign up for our weekly newsletter.
Fresh data delivery Saturday mornings
All Immigration & Migration Publications
How temporary protected status has expanded under the biden administration.
Since January 2021, the Biden administration has greatly expanded the number of immigrants who are eligible for Temporary Protected Status.
Key facts about Asian Americans living in poverty
Burmese (19%) and Hmong Americans (17%) were among the Asian origin groups with the highest poverty rates in 2022.
How Hispanic Americans Get Their News
U.S.-born Latinos mostly get their news in English and prefer it in English, while immigrant Latinos have much more varied habits.
State of the Union 2024: Where Americans stand on the economy, immigration and other key issues
Ahead of President Joe Biden's third State of the Union address Americans are focused on the health of the economy and immigration.
Latinos’ Views on the Migrant Situation at the U.S.-Mexico Border
U.S. Hispanics are less likely than other Americans to say increasing deportations or a larger wall along the border will help the situation.
U.S. Christians more likely than ‘nones’ to say situation at the border is a crisis
Majorities of White Christian groups say the large number of migrants seeking to enter at the border with Mexico is a “crisis” for the United States.
Americans’ Top Policy Priority for 2024: Strengthening the Economy
Growing shares of Republicans rate immigration and terrorism as top priorities for the president and Congress this year.
How Americans View the Situation at the U.S.-Mexico Border, Its Causes and Consequences
Just 18% of U.S. adults say the government is doing a good job dealing with the large number of migrants at the border. Eight-in-ten say it is doing a bad job, including 45% who say it's doing a very bad job.
Migrant encounters at the U.S.-Mexico border hit a record high at the end of 2023
The U.S. Border Patrol had nearly 250,000 encounters with migrants crossing into the United States from Mexico in December 2023.
Language and Traditions Are Considered Central to National Identity
Across more than 20 countries surveyed, a median of 91% say being able to speak their country’s most common language is important for being considered a true national. And 81% say sharing their country’s customs and traditions is important for true belonging.
Refine Your Results
About Pew Research Center Pew Research Center is a nonpartisan fact tank that informs the public about the issues, attitudes and trends shaping the world. It conducts public opinion polling, demographic research, media content analysis and other empirical social science research. Pew Research Center does not take policy positions. It is a subsidiary of The Pew Charitable Trusts .
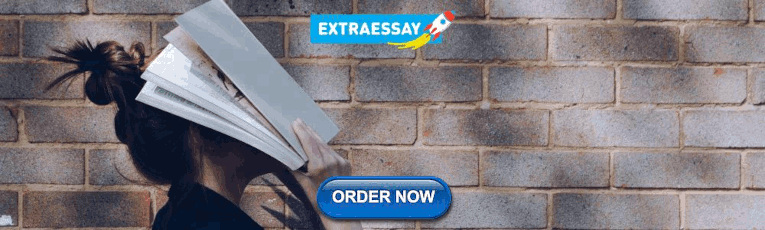
IMAGES
VIDEO
COMMENTS
This paper (1) develops a theoretical model of refugee migration that builds on existing research in early warning and preventive diplomacy, and (2) empirically tests this model in order to assess ...
The paper has been finalized thanks to the MADE (Migration as Development) project funded by the European Research Council under the European Community's Horizon 2020 Programme (H2020/2015-2020) / ERC Grant Agreement 648496, conducted at the International Migration Institute (IMI) now located at the University of Amsterdam.
Policy Research Working Paper No. 8376, World Bank, Washington, DC. Crossref. Google Scholar. ... Motivations for migration: An assessment and a value-expectancy research model. In Migration decision making: Multidisciplinary approaches to microlevel studies in developed and developing countries, eds. De Jong G. F., Gardner R. W., 13-58. New ...
1. Introduction. Migration studies have developed rapidly as a research field in recent decades. It encompasses studies on all types of international and internal migration, migrants, and migration-related diversity (King, 2002; Scholten, 2018).Many scholars have observed the increase in the volume of research on migration (Massey et al., 1998; Bommes and Morawska, 2005; Scholten et al., 2015).
For this paper, the population at risk is approximated as the start of period population based on estimates produced by the United Nations Population Division ... The availability of interval-based origin-destination flow data for migration opens new research avenues, including the application of a suite of tools and metrics developed in the ...
Forced Migration and Refugee Studies. Explore a collection of leading refugee studies research from Oxford University Press books, journals, and online resources. This collection covers a diverse breadth of regions and topics, including climate migrants, asylum policy in rich democracies, and the impact of COVID-19 on refugees.
Our paper also contributes to analysing the impact of human migration on population change. Using our annual gridded dataset to map migration in parallel with demographic and geophysical data ...
Migration research began to formalise and expand in the 1950s and 1960s (Greenwood & Hunt, 2003; Pedraza-Bailey, 1990). A noteworthy turning point for the field was the debate around assimilation which gathered pace throughout the 1950s and is perhaps most notably exemplified by Gordon's typology of this concept.
This paper elaborates an aspirations-capabilities framework to advance our understanding of human mobility as an intrinsic part of broader processes of social change. In order to achieve a more meaningful understanding of agency and structure in migration processes, this framework conceptualises migration as a function of aspirations and capabilities to migrate within given sets of perceived ...
The Impact of International Migration on Inclusive Growth: A Review. by Zsoka Koczan, Giovanni Peri, Magali Pinat, and Dmitriy Rozhkov. IMF Working Papers describe research in progress by the author(s) and are published to elicit comments and to encourage debate.
Abstract. Growing social and economic inequalities, and consequently, unfulfilled life aspirations trigger the migration intentions of millions, if not billions of people around the world. Surveys by Gallup World Poll suggest that more than 750 million adults would like to migrate if they had the chance to do so (Esipova et al., 2018).
The interviews also stressed the importance of deconstructing migration research. After the interviews, four participatory workshops were conducted in Spain aiming to explore the needs emerged in the interviews in detail, and to create together: (a) a possible structure for the digital tools; (b) and a co-created guidelines for conducting new ...
By and large, migration has positive economic impacts on the migrant household, the sending country as well as the receiving country. The paper is organized as follows. Section 2 provides a discussion of the development implications, first economic and then social impacts, of migration for origin countries.
Migration and Development is a peer-reviewed journal that publishes high-quality empirical and theoretical original research and review articles pertaining to all domains of Migration. The journal publishes scholarly articles that address the migration discourse as well as advance the discussions on migration-related policies both on a global and national scale.
The Journal of International Migration and Integration (JIMI) is a multidisciplinary peer-reviewed scholarly journal that publishes original research papers and policy discussions that enhance the understanding of immigration, settlement and integration and that contribute to policy development. The Journal consistently covers an array of ...
The interest in human migration is at its all-time high, yet data to measure migration is notoriously limited. "Big data" or "digital trace data" have emerged as new sources of migration measurement complementing 'traditional' census, administrative and survey data. This paper reviews the strengths and weaknesses of eight novel, digital data sources along five domains: reliability ...
Recent research, however, suggests that there is a positive relationship between migration and trade, as well as other forms of internationalization, such as foreign direct investment and offshoring. This paper aims to provide a comprehensive yet accessible overview of the literature on the role of migration in international trade and investment.
This paper identifies the key gaps in existing scholarship in this field and proposes a research agenda for future consideration on current and emerging climate migration issues. In the following section, we outline the systematic review method and identify how journal articles were searched, selected, reviewed, and analyzed.
In this paper, we analyze the relationship between development and outgoing international student mobility (ISM) for the years 2003-2018 using data from UNESCO. Starting from migration transition theory, we expect that development and outgoing migration follows an inverted U-shape due to changes in capabilities and aspirations of populations.
Migration intention research can help identify migration thresholds under future SLR, but loses much of its value when we do not know what this migration will look like. Another important aspect in this domain is that of migrant agency, which is about the agency or freedom an individual/household has in the migration decision‐making process ...
The migration rate and the level of resentments towards migrants are an important issue in modern civilisation. The infamous EU refugee crisis caught many countries unprepared, leading to sporadic ...
frontier and circulation), but recent migration research focuses almost exclusively on one kind: ... Th e paper proceeds in two parts. First, we briefly review how patterns of migration within .
According to the International Organisation for Migration (IOM), global forced migration has reached levels not seen in more than five decades ().The Global Trends Report from the United Nations High Commissioner for Refugees (UNHCR, 2018) noted that the global population of forcibly displaced people in 2017 exceeded the population of the United Kingdom.
The research topic of the paper is focused on the strategies and approaches that researcher can used in investigation of various aspects regarding migration. The central research question is: what ...
Language and Traditions Are Considered Central to National Identity. Across more than 20 countries surveyed, a median of 91% say being able to speak their country's most common language is important for being considered a true national. And 81% say sharing their country's customs and traditions is important for true belonging.
marked both by an increase in involuntary return migration as well as the steep rise of children and young adults caught in its wake.², ³ Although this is a global issue affecting the flow of migration in many countries, much of the attention and research on return migration focuses on the relationship between the U.S. and Mexico.