Cookies on NHS Jobs
We've put some small files called cookies on your device to help us make improvements to our site.
To improve our site we'd also like to use cookies which will send information to Google Analytics. You can read more about our cookies before you choose.
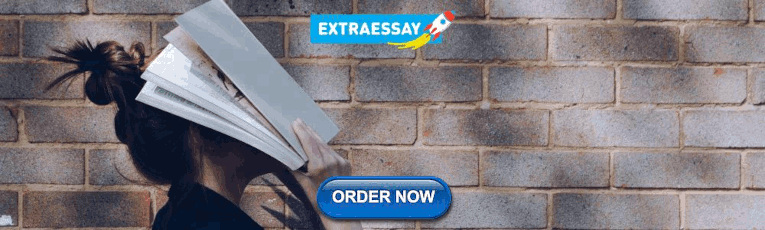
Research Support Officer
University Hospitals of Leicester NHS Trust
This job is now closed
Job summary
The Research Support Officer will work as part of a team of staff led by the Research Manager in the Hope Clinical Trials Centre.
The Hope Clinical Trials Centre is located at the Leicester Royal Infirmary and is a dedicated area for cancer patients participating in clinical research studies, particularly those at the early stages of development.
The department hosts approximately 40 staff members who hold either administrative or clinical positions but work closely together to provide the best possible care for our patients.
The Research Support Officer will be responsible for supporting researchers and clinical teams in the local set-up of clinical trials and will coordinate the process of Capacity and Capability assessment in order to receive Research & Innovation Authorisation to conduct the research at our centre.
Main duties of the job
The Research Support Officer will be responsible for ensuring that studies are reviewed, set up and submitted for R&I Authorisation in a timely manner.
Keeping abreast of legislation, the post-holder will also be responsible for implementing national best practice research management processes within the Trust in a streamlined manner.
The post-holder will be the day to day point of contact for investigators and external Sponsors and will be responsible for providing advice and support where necessary.
The post holder should be adaptable, flexible and show initiative. In addition they need to show good communication skills, be able to liaise with all levels of staff, demonstrate good organisational skills and attention to detail, have good time management skills and be flexible as the working hours may not be fixed.
Opportunities to develop expertise in other research specialties will be available. The post-holder may be required to work in other research areas across UHL if service needs arise.
We are one of the biggest and busiest NHS trusts in the country, incorporating the General, Glenfield and Royal Infirmary hospitals. We have our very own Children's Hospital and run one of the country's leading heart centres. Our team is made up of more than 17,000 staff providing a range of services primarily for the one million residents of Leicester, Leicestershire and Rutland. We work with partners at the University of Leicester and De Montfort University providing world-class teaching to nurture and develop the next generation of doctors, nurses and other healthcare professionals, many of whom go on to spend their working lives with us. Our purpose is to provide 'Caring at its best' and our staff have helped us create a set of values that embody who we are and what we're here to do. They are:
We focus on what matters most.
We treat others how we would like to be treated.
We are passionate and creative in our work.
We do what we say we are going to do.
We are one team and we are best when we work together
Our patients are at the heart of all we do and we believe that 'Caring at its best' is not just about the treatments and services we provide, but about giving our patients the best possible experience.
About the University Hospitals of Leicester NHS Trust (leicestershospitals.nhs.uk
Date posted
23 May 2023
Agenda for change
£28,407 to £34,581 a year per annum
Working pattern
Reference number.
358-5293120-CHU
Job locations
Leicester Royal Infirmary
Job description
Job responsibilities.
This is a unique and exciting opportunity for an individual experienced in research to join the Research & Innovation (R&I) research delivery workforce at University Hospitals of Leicester NHS Trust.
University Hospitals of Leicester hosts the NIHR Leicester Biomedical Research Centre and the NIHR Clinical Research Facilities including the Hope Clinical Trials Facility, a centre of excellence in oncology and haematology research and the Clinical Renal Research Unit, a leading centre in national recruitment of patients in clinical trials.
All research facilities are designed to deliver clinical effectiveness, safety and a positive patient/volunteer experience in a consistent way.
JOB SUMMARY
The Research Support Officer will be responsible for supporting researchers and clinical teams in the local set-up of clinical trials and will coordinate the process of Capacity and Capability assessment in order to receive R&I Authorisation.
Keeping abreast of legislation, the post-holder will also be responsible for implementing national best practice research management processes within the Trust. They will be responsible for ensuring that local integration of systems and processes are achieved so that individuals can benefit fully from this in a streamlined manner.
The Research Support Officer will be responsible for identifying and addressing Trust specific feasibility issues and ensuring that studies are reviewed, set up and submitted for R&I Authorisation in a timely manner.
INFORMATION MANAGEMENT
Ensure databases are completed accurately and maintained appropriately.
To work autonomously
Is organised and able to manage and prioritise own workload in order to meet specific deadlines.
Budget: Not applicable for role. Staff: Not applicable for role. Policy: Trust wide policies to be implemented. Communications: Good communication skills will be paramount to this position with the involvement of working across clinical business units to include physicians, MDT, research scientists, patients and carers.
KEY WORKING RELATIONSHIPS
Director of the HOPE Clinical Trials Centre Other senior Managers within the HOPE Clinical Trials Centre Finance and Contracts Manager Quality Assurance Manager All staff within the Clinical Trials Facility Investigators/Clinicians Other research associates/departments within the University of Leicester NHS Trust Other partners e.g NIHR Research Networks, ECMC Research active personnel & support services at UHL, University of Leicester, DeMontfort University UHL Research & Innovation Office Health Research Authority Research Ethics Committees External Organisations e.g. commercial companies Other Universities Other NHS Trusts
WORKING RELATIONSHIPS To develop and maintain a good working relationship with multi-professional teams within the speciality.
Be responsibility for ensuring effective communication between all service providers within the speciality.Actively participate in local and national networking groups to develop an expert level of knowledge in the speciality.
KEY RESULT AREAS
Research Management & Governance Work closely with colleagues in the Research Team to support implementation of research management processes within the Trust. Maintain a standard of conduct in accordance with trust policies Be accountable for actions and aware of limitations. Conduct financial reviews of clinical trials to ensure that they are adequately resourced. Contribute to the implementation of quality systems providing assurance to researchers that processes operate in accordance with the law, national and best practice. Support the Research Manager in providing assurances and evidence that research management processes are compliant with Governance Frameworks, relevant legislation including but not limited to the EU Directive, HRA, , REC, MHRA, ARSAC , Human Tissue Act, GTAC, CAG,MCA and the Department of Health. Provide specialist expert regulatory and governance advice and support by actively promoting the research management and governance process to deliver a smooth operation to researchers in all aspects of preparing study documentation for NHS authorisation, including but not limited to IRAS, the application process, ethics application process, finance and contracts, NHS indemnity, and highlighting where necessary requirements for Research Passports and Honorary Research Contracts. Assist researchers with applications that do not require NHS Ethics opinion or are exempt from HRA review, to ensure a smooth and streamlined approach to obtaining NHS Trust authorisation. Ensure that all projects have the necessary checks in place: e.g. HRA Approval, REC FIFO letter, MHRA, CAG, G-TAC, CMG approval, financial approval, relevant support services approvals etc. and that the required documentation is provided for review. Ensure all research applications/amendments are reviewed as appropriate in accordance with tight national deadlines, maintaining the Trusts reputation for expediency. Determine impact on Capacity and Capability and adopt a proactive approach in identifying and rectifying issues at an early stage, communicating with points of contact for individual research studies then facilitating best outcome. Signpost and highlight as appropriate when contracts are required to the Research Contracts Manager in order to ensure that all relevant contracts are in place prior to approval of a new research study or amendment at the Trust. Support the Research Manager to ensure that all research studies are and continue to be properly resourced and conducted according to applicable regulations Support the Research Manager, Quality Assurance Manager within the wider Hope Team in implementing Standard Operating Procedures, local guidance and other procedures based on national operating guidelines and current legislation by effectively communicating the purpose of the procedures and any changes to all staff involved with the Capacity and Capability Assessment processes for studies based in the Trust.
Information Management Ensure that spreadsheets and databases are completed accurately and maintained appropriately.Work autonomously to manage and prioritise own workload in order to meet specific internal and external deadlines.
Monitoring / Audit & Performance ManagementAssist in undertaking the administrative processes involved in preparing for, hosting and responding to inspections or audits by internal and external regulatory bodies, agencies and other organisations.
Ensure that researchers are familiar with and understand their responsibilities in respect of the delivery of recruitment targets with timelines.
Communication & Relationship Skills Develop and maintain constructive relationships with staff across the Trust as part of the review process, communicate directly with researchers, dealing with telephone or email queries and provide one to one advice or meetings, referring to agreed guidelines and using own initiative to action responses when appropriate.
Communicate directly with staff and agencies such as HRA assessors, research ethics committees, Universities, commercial companies, and Research Networks, as part of the capacity and capability review process and to raise overall awarenessAdapt appropriate methods of communication and persuasion skills to overcome strong resistance to bureaucratic processes.
Training & Education To assist the Research Manager & Quality Assurance Manager in identifying the learning needs of oneself and colleagues by seeking appropriate training that is relevant to the role.
R&I Directorate 1. Identify personal educational needs associated with participation in current clinical trials and ensure these are effectively communicated to the Research Nurse. Participates in the development of an agreed personal development plan to meet identified needs.
2. Ensure safe standards of practice through identification of areas of risk associated with participation in clinical trials. Ensure clinical trial protocols and appropriate professional guidelines are adhered to.
3. Participate in the implementation of research practice standards.
4. Responsible for remaining adequately informed of clinical trials, R&I activity and the Trust by attendance at team brief and using other appropriate forms of communication.
This job description indicates the main functions and responsibilities of the post. It is not intended to be a complete list. You may be required to undertake other duties from time to time as we may reasonably require.
You will be required to maintain compliance with all statutory and mandatory training requirements.
The link to the Trusts policies and procedures is:
https://secure.library.leicestershospitals.nhs.uk/PAGL/SitePages/Home.aspx
Person Specification
Commitment to trust values and behaviours.
- Must be able to demonstrate behaviours consistent with the Trust's Values and Behaviours
Training & Qualifications
- Educated to degree level or equivalent in related area (e.g. Science, Social Services, healthcare) or relevant experience ECDL or equivalent IT Skills
- Project Management Qualification.
- At least 3 years' experience of managing multiple projects / assignments in the NHS/Public Sector or Commercially relevant environment (e.g. CRO) Working with Databases and using reporting tools Experience of producing and delivering presentations Proven track record of working with the Research Governance Framework or with GAfREC. Knowledge of research with the NHS
- Previous experience of Research Ethics Committees Previous experience and participation in clinical audit e.g. MHRA inspection.*
Communication and relationship skills
- Excellent written and verbal skills Ability to work well independently and also as part of a team
- Knowledge of the Research Governance environment
Analytical and Judgement skills
- Attention to detail
- Understanding of Clinical Trials Regulations
- Ability to prioritise own workload. Ability to manage multiple deadlines
Planning and organisation skills
- Evidence of continued professional development Self-motivated and flexible Good influencing skills Ability to learn new skills and apply them appropriately in the workplace
Other requirements specific to the role
- Willingness to travel to training or research events external to UHL
Equality, Diversity and Inclusion
- Able to demonstrate a commitment and understanding of the importance of treating all individuals with dignity and respect appropriate to their individual needs. Willingness to travel between sites
Disclosure and Barring Service Check
This post is subject to the Rehabilitation of Offenders Act (Exceptions Order) 1975 and as such it will be necessary for a submission for Disclosure to be made to the Disclosure and Barring Service (formerly known as CRB) to check for any previous criminal convictions.
Certificate of Sponsorship
Applications from job seekers who require current Skilled worker sponsorship to work in the UK are welcome and will be considered alongside all other applications. For further information visit the UK Visas and Immigration website (Opens in a new tab) .
From 6 April 2017, skilled worker applicants, applying for entry clearance into the UK, have had to present a criminal record certificate from each country they have resided continuously or cumulatively for 12 months or more in the past 10 years. Adult dependants (over 18 years old) are also subject to this requirement. Guidance can be found here Criminal records checks for overseas applicants (Opens in a new tab) .
Employer details
Employer name, employer's website.
https://www.jobsatleicestershospitals.nhs.uk/ (Opens in a new tab)

For questions about the job, contact:
Operations Director - Cancer Research
01162585952
Supporting documents
Privacy notice.
University Hospitals of Leicester NHS Trust's privacy notice (opens in a new tab)

An official website of the United States government
The .gov means it’s official. Federal government websites often end in .gov or .mil. Before sharing sensitive information, make sure you’re on a federal government site.
The site is secure. The https:// ensures that you are connecting to the official website and that any information you provide is encrypted and transmitted securely.
- Publications
- Account settings
Preview improvements coming to the PMC website in October 2024. Learn More or Try it out now .
- Advanced Search
- Journal List
- Health Res Policy Syst

The organization and impacts of clinical research delivery workforce redeployment during the COVID-19 pandemic: a qualitative case study of one research-intensive acute hospital trust
Rachel faulkner-gurstein.
1 King’s College London, London, UK
2 National Institute for Health Research Biomedical Research Centre at Guy’s and St. Thomas’ NHS Foundation Trust and King’s College London, London, UK
David Wyatt
Hannah cowan.
3 Guy’s and St. Thomas’ NHS Foundation Trust, London, UK
Clair Harris
Charles wolfe, associated data.
The data generated and analysed during the current study are not publicly available, as they contain information that could compromise research participant privacy, but are available from the corresponding author on reasonable request.
COVID-19 has tested healthcare and research systems around the world, forcing the large-scale reorganization of hospitals, research infrastructure and resources. The United Kingdom has been singled out for the speed and scale of its research response. The efficiency of the United Kingdom’s research mobilization was in large part predicated on the pre-existing embeddedness of the clinical research system within the National Health Service (NHS), a public, free-at-point-of-delivery healthcare system. In this paper we discuss the redeployment of the clinical research workforce to support the pandemic clinical services, detailing the process of organizing this redeployment, as well as the impacts redeployment has had on both staff and research delivery at one research-intensive acute NHS trust in London.
A social science case study of one large research-active NHS trust drawing on data from an online questionnaire; participant observation of key research planning meetings; semi-structured interviews with staff involved in research; and document analysis of emails and official national and trust communications.
We found that at our case-study hospital trust, the research workforce was a resource that was effectively redeployed as part of the pandemic response. Research delivery workers were redeployed to clinical roles, to COVID-related research and to work maintaining the research system during the redeployment itself. Redeployed research workers faced some difficulties with technology and communication, but many had a positive experience and saw the redeployment as a significant and valuable moment in their career.
Conclusions
This study explicates the role of the research delivery workforce for the United Kingdom’s COVID response. Redeployed research workers facilitated the emergency response by delivering significant amounts of patient care. The public also benefited from having a well-developed research infrastructure in place that was able to flexibly respond to a novel virus. Many research workers feel that the NHS should provide more support for this distinctive workforce.
COVID-19 has tested healthcare and research systems around the world, forcing the large-scale reorganization of hospitals, research infrastructures and resources. The United Kingdom has been singled out by some commentators for the speed and scale of its research response, particularly regarding clinical trial setup, patient recruitment, and the delivery of globally important treatment and vaccine studies [ 1 – 8 ]. The efficiency of the United Kingdom’s research mobilization was in large part predicated on the pre-existing embeddedness of the clinical research system within the National Health Service (NHS), a public, free-at-point-of-delivery healthcare system. As we detail elsewhere, in addition to maintaining a routine level of service, hospitals have been tasked with delivering a high volume of emergency care alongside nationally prioritized COVID research, something which has required the large-scale reorganization of services, systems and working practices [ 9 ].
The research infrastructure in England and Wales comprises a network of institutions and organizations working to support clinical research within the NHS. While research is funded through a variety of governmental, commercial and charitable sources, the creation of the National Institute for Health and Care Research (NIHR) in 2006 was a key juncture in the development and coordination of a research system [ 10 ]. In particular, the NIHR has supported the establishment of 19 clinical research facilities (CRFs) and 20 biomedical research centres (BRCs) which provide support for experimental and translational research [ 3 ]. The NIHR also funds the Clinical Research Network (CRN), which funds and supports research staff embedded in NHS trusts across 30 different clinical specialties.
Roope et al. [ 11 ] noted that the COVID-19 pandemic has allowed us to see the “option value” of infrastructures like BRCs, CRFs and the CRN. By “option value”, Roope et al. refer to the potential value of having additional capacity that is dedicated to nonurgent work. They suggest that systems with option value are able to adapt faster and more flexibly to the needs of an unforeseen emergency, such as a global pandemic. In many ways, the United Kingdom’s research system’s response to the pandemic affirms Roope et al.’s analysis of the option value of additional capacity. But it is crucial not to lose sight of the complex reorganization of research staffing and resources that took place to facilitate the simultaneous delivery of frontline care and vital COVID research.
In this paper, we draw on original questionnaire and interview data, documentary analysis and our first-hand experience of working in a busy hospital during the first COVID surge to examine the work of research staff redeployment that allowed the United Kingdom’s research system to participate in the pandemic response. This dimension has not received the same level of public awareness as other aspects of the national and international response to COVID-19. Throughout the pandemic, the public have been informed through media reports of the stress and strain experienced by frontline care staff, with many working long hours and redeployed to different clinical areas. Clinical redeployments have also been described in several first-hand accounts (see for example [ 12 – 14 ]). But aside from a few exceptions in specialized professional literature (such as [ 14 ]), the patient-facing research delivery workforce has been largely absent from the discussion. This workforce includes research nurses and midwives and research allied health professionals (AHP), as well as clinical research practitioners (CRP), a newly emerging bespoke element of the research delivery workforce who do not deliver patient care outside the research pathway (for more details on the CRP role see [ 15 ]). Furthermore, accounts of research developments and breakthroughs tend to ignore the detail of how such work was delivered within these unprecedented times.
Our focus is on the redeployment of the patient-facing clinical research delivery workforce. We detail the process of organizing this redeployment, as well as the impacts redeployment has had on both staff and the clinical research delivery service at one research-intensive acute NHS foundation trust in London. We are focused specifically on three groups of research workers: those who were clinically redeployed as part of the emergency response to the pandemic, those who were redeployed to carry out urgent COVID-related research on COVID treatments and vaccines, and those who were not redeployed but were kept in place to maintain existing research.
Through our focus on the redeployment of the research delivery workforce we are able to shed light not only on the benefits and limitations of the local response to COVID-19, but also on wider questions around the existence and development of an agile, resilient and flexible research workforce, identified as a priority by the NIHR [ 16 – 18 ]. Our data also provide an opportunity to consider how we might change the way clinical research is organized and delivered in the United Kingdom going forward, in part due to the pace achieved in vaccine trials [ 19 ], and the change in practice this entailed. Yet, as our analysis demonstrates, while the clinical research delivery workforce are resilient and adaptable, some of the existing infrastructure and organizational practices required substantial effort to facilitate the COVID response and require further investment to underpin a system that supports agile working.
The research delivery workforce
The research delivery system within the NHS is organized to manage, mobilize and implement clinical research protocols effectively, safely and on time [ 15 ]. Research delivery teams including nurses, midwives, AHPs and CRPs are responsible for the patient-facing aspect of clinical research, from supporting participation, undertaking informed consent, administering trial procedures and observation through to supporting governance and accurate data collection. Participating in a research delivery team requires an in-depth understanding of research protocols, the establishment of ongoing relationships with participants and principal investigators (PI), and the completion of study-specific training before trial duties are delegated.
The clinical research delivery workforce has become a significant component of the NHS. This staffing category has undergone substantial expansion in recent years, although career paths within clinical research delivery are relatively underdeveloped. Before the COVID-19 pandemic, efforts were underway to grow and professionalize this workforce with the expansion of NIHR-funded career development opportunities for research nurses and midwives and the creation of a new professional register for CRPs. COVID-19 has underscored the value of the research delivery workforce to both the United Kingdom’s COVID response and its longer-term vision of a research-led NHS [ 17 ]. Taking stock of the United Kingdom’s research response to the pandemic, health ministers from England and the devolved nations issued a white paper on the future of clinical research delivery in the United Kingdom, outlining “a bold and ambitious vision for the future of clinical research delivery, which capitalises on innovation, is resilient in the face of future healthcare challenges and improves the lives of patients around the world” [ 20 ]. Realizing this ambitious vision requires a greater understanding of the research delivery workforce and the specific skills and contributions of its workers.
In this paper, we draw on data from a larger project which explored the impact of COVID-19 on the clinical research system and the contribution of the clinical research system to the pandemic response. Applying established social-scientific case study methods [ 21 , 22 ] to one NHS trust, this project employed an online questionnaire, participant observation of key research planning meetings, semi-structured interviews with staff involved in research, and document analysis of emails and official national and trust communications. In order to maintain the anonymity of research informants, we have chosen to call our case study site South London Acute Trust (SLAT). SLAT is a research-intensive trust, hosting a number of NIHR research infrastructure installations. By December 2020, SLAT had commenced research on 80 COVID studies, with more than 18 of these classed as Urgent Public Health (UPH). UPH studies are those reviewed by the office of the Chief Medical Officer and deemed of high importance to the pandemic response. UPH studies are to be resourced and prioritized by trusts across the country [ 23 ].
We collected data from four areas within the research infrastructure: central research oversight and governance, PIs, the research delivery workforce, and patient and public involvement (PPI) managers and participants. In this paper we focus specifically on findings from an analysis of the data derived from or about the patient-facing research delivery workforce. This workforce comprises 164 staff members across the trust, working in a variety of roles, including research nurses and midwives and CRPs.
Data were collected over a 6-month period between May 2020 and October 2020. On 18 May 2020 we distributed an online questionnaire to all research-involved staff at SLAT (approximately 700) using existing mailing lists. Using the SurveyMonkey online survey platform, the questionnaire was designed as a qualitative and descriptive data-gathering tool. Questions were not drawn from a validated question bank but were reviewed and tested with a small group of research staff for clarity and comprehensiveness before wider distribution. When the questionnaire closed on 10 June 2020, we had received 170 responses, an overall response rate of approximately 24%. On first appearance, this suggests a low response rate. However, among the patient-facing research delivery workforce ( n = 164) who are the focus of this paper, the response rate was 48% ( n = 79), representing 46% of our total respondents. Within this group, 49% were research nurses ( n = 39), 6% research midwives ( n = 5) and 15% CRPs ( n = 12). The remaining respondents were research AHPs ( n = 6, 8%), trial coordinators ( n = 9, 11%) or research support staff ( n = 8, 10%). This questionnaire provided a broad overview of key issues in the organization of the research workforce during the pandemic, and specifically asked about experiences of redeployment and working during the pandemic. This questionnaire also acted as a recruitment tool for semi-structured interviews that took place later.
Interview participants were also recruited through purposive and snowball sampling, aiming to gain a sample with maximum variation [ 24 ]. Our approach aimed to gather a diversity of redeployment experiences—for example, those redeployed to frontline care, redeployed to administrative roles, redeployed to COVID research, and those who for various reasons were not redeployed. Interviewees were asked about their experiences of working during the pandemic, what their work had entailed, whether and how their work had changed because of COVID, and their views on the longer-term impact of COVID on their future work and working practices.
In total we interviewed 24 participants, of whom nine were from the research delivery workforce (see Table Table1 1 for breakdown of research delivery workforce interviews). These nine interviews lasted between 33 and 104 minutes, averaging 45 minutes. Interviews took place over and were recorded through Microsoft Teams and were transcribed verbatim. The research delivery workers from whom we gathered either questionnaire or interview data represented the following staff groups:
Breakdown of research delivery workforce study participants
Questionnaire and interview data were supplemented by an analysis of documents and emails pertaining specifically to the organization of the COVID-19 response produced by SLAT for internal use. We also analysed documents produced by national bodies (for example, the NIHR and the Chief Medical Officer for England) related to national directives on COVID-19 and clinical research. Internal documents were obtained via email from SLAT’s Research and Development (R&D) department and publicly available material on the research shutdown and UPH studies was obtained via the NIHR website. We also observed key trust research governance and prioritization meetings. These meetings, occurring twice a week, determined which COVID studies to open and discussed how studies including UPH studies were to be resourced and staffed.
Data were managed and analysed through NVivo 12 software. Data were first analysed for a descriptive account of how processes changed and how the research system and workforce were impacted [ 25 ]. Data were then analysed thematically for an analytic account [ 26 ]. RFG, HC and DW independently analysed the data and then met to agree on codes and final themes. These themes and codes were then discussed and agreed with the wider project team.
Ethical approval for this research was granted by the North East – Newcastle & Tyneside 2 Research Ethics Committee (REC) (reference: 20/NE/0138). As part of our ethics approval, we have taken steps to ensure our informants’ anonymity in the presentation of qualitative data. Interview excerpts are identified by a letter to indicate their role category and a respondent number. “D” indicates research delivery staff, “P” indicates patient and public involvement managers, and “R” indicates research leaders/PIs. “Q” denotes comments from the questionnaire.
The process of redeployment
A reconstruction of the timeline of redeployment at SLAT suggests that while much of the process was organized quickly and reactively, with little time for detailed planning, it was successful because of the extensive, flexible, skilled workforce of research delivery staff that were in place at the trust. Redeployment drew on the specific skill set of research workers and was often crafted by them while in process. At the start of 2020, there were no redeployment guidelines, and research staff were not initially included in the trust’s emergency planning. But it immediately became clear that the defining feature of the research delivery workforce—that it operates at the nexus of care and research [ 15 , 27 ]—made it a valuable resource for responding to the pandemic.
COVID-19 became a notifiable disease in March 2020, and redeployment within the NHS began soon after. On 16 March, the Department of Health and Social Care and the NIHR issued guidance stating that all clinical research conducted within NHS sites was to be halted, with specific exemptions for trials considered urgent for patient safety and COVID-related research [ 28 ]. Responding to this decree, SLAT’s R&D department asked research teams to review their portfolios and identify those activities that needed to continue and those that could be paused, alongside guidance on maintaining protocol and regulatory adherence in exceptional circumstances. For the majority of the trust’s more than 500 trials, the research shutdown meant that patient recruitment was stopped, face-to-face assessments and follow-ups were discontinued or, where possible, done remotely, and the day-to-day operation of research ground to a halt.
At SLAT, services were rapidly reorganized to meet the anticipated clinical demand, and lists were compiled of staff available to be redeployed as hospital admissions and especially admissions to the critical care and intensive care units (ICU) began to rise. At the beginning of March 2020, as the United Kingdom was entering its first lockdown, a “command and control” structure was implemented by the trust in order to centralize the pandemic response. This involved central control by the trust’s executive of daily operations at the trust following established emergency protocols. The embedded clinical research system, with its facilities, equipment and many trained staff, came to be seen as a vital resource the trust could access to bolster already stretched clinical services [ 9 ]. Though coordination with the trust’s central command structure was initially patchy, communication quickly regularized, and engagement of the clinical research delivery workforce to support the clinical response was coordinated with the research workforce lead through the trust’s nursing workforce hub.
One early hurdle to the rapid redeployment of staff was identification. Heads of nursing were required to urgently collate and submit staff skill-set and information lists so redeployment could be centrally planned and coordinated. This information did not readily exist and so necessitated collection through the circulation of spreadsheets to all the directorate research teams, which were completed manually by team managers. The process generated a real-world representation of the staffing of research teams which differed from any one centrally held electronic record.
Although the trust were trying to manage the redeployment centrally, in practice local redeployment arrangements were common. Heads of nursing made a number of informal arrangements which facilitated rapidly moving research staff into areas of need. As one senior research matron describes:
Quite a few of my team are ICU nurses. I was literally rung and said, “They’re going tomorrow. This is their last day with you. They will be gone tomorrow.” And I lost four overnight. (D-4)
By 14 April 2020, according to administrative records, there had been extensive redeployment of both clinical and nonclinical research staff. Table Table2 2 provides a breakdown of how 152 members of the research delivery workforce were redeployed. In addition, the R&D team (covering those who work in research governance and research portfolio management) redeployed 31 staff members to act as ward clerks.
Total clinical and nonclinical research staff redeployment at SLAT on 14 April 2020
a NHS Nightingale London Hospital was a temporary hospital set up by NHS England for the COVID-19 pandemic
At the same time, in March 2020, some clinical research staff were redeployed to a new COVID research team. This group comprised a variety of staff, including managers, matrons, research nurses, CRPs and data managers. This team worked to extremely tight timelines to establish treatment trials and in-house COVID research. D-1 describes some of the challenges the COVID team faced from the outset:
We’ve got a team of 22 members of staff, which have been pulled together from numerous clinical areas. Originally there was one research nurse. And that team is now a team of 22. So that’s been the biggest challenge, I suppose, is that trying to bring staff members together and quickly induct them, and again you need to get them going straightaway. You need them in and delivering on this research because we needed to start the research while we’re right in the middle of the surge in numbers. That’s been different, in that you don’t slowly take on studies. You have studies that come, they need to be set up tomorrow, recruit the first patient by the end of the week. And you need staff to deliver, even though they might be feeling wobbly in their role … and needing actually to get to grip with quite complex studies very quickly. (D-1)
Despite the challenges they faced, the group quickly developed processes for consenting highly infectious patients, established a 7-day rota and other important new procedures that required staff with knowledge of guidelines and protocols to innovate and adapt within the constraints of the regulatory framework.
As the need became more urgent and all available registered nurses were asked to go to ICU, R&D advice was strengthened and teams were asked to stop all but essential activity. The redeployment process was concluded with a final engagement of research managers to provide justification for all remaining research staff, who made up what interviewees called the “skeleton staff” for management of essential activity. Although new research activity was stopped, safety monitoring of existing participants and dispensing of trial drugs was still required, which meant some research staff were left in place to fulfil these important tasks. In small teams this was often carried out by only one person, who might now be covering several studies.
By early May the pressure of new COVID admissions on the trust was declining, and focus began shifting to gradually repatriating staff and allowing rest. At the beginning of June the plan for the restart of research was issued and the decision was taken to formally second a dedicated team to manage the ongoing portfolio of COVID research. Staff were released gradually as secondees started, with staff working on the highest-priority research being released first. The last of the redeployed clinical research staff were released by 1 September 2020.
For research delivery staff at SLAT, the pandemic and the associated shutdown of non-COVID research meant a rapid and dramatic change to “business as usual”. Asked to identify the number of studies on which they were active on 1 January 2020, 64% of respondents said they were working on five or more studies, with 37% saying they worked on nine or more studies on that date. Compare that with the figure as of 10 June—before the restart of research at the trust—of just 12% of respondents working on five or more studies, 22% saying that they were working on one study, and a further 26% working on no studies at all. However, with 74% of research delivery staff saying that they were currently working on at least one study, it is clear that while the volume of research had decreased, research activities nevertheless continued throughout the shutdown, and the research workforce remained engaged.
Impacts of redeployment
Our data indicate that redeployment developed new skills and capacities, both for individual members of the research delivery workforce and for the research system as a whole. At the same time, it presented a number of challenges. A closer examination of some of the individual experiences of research delivery workers demonstrates both the new capacities acquired during redeployment and the challenges that this process entailed.
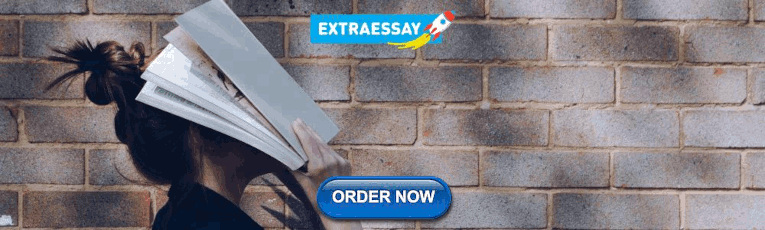
Working from home
Clinical research has hitherto been strongly tethered to clinical settings, but the response to the pandemic created capacities for new locational flexibility. As with other industries, in order to accommodate shielding and minimize the number of people in the workplace, certain forms of clinical research work moved to the home. Some research delivery staff remained in their team, but adapted to working from home, while other staff were both working from home and redeployed. What is clear is that all staff, even those who remained in post, experienced major disruptions to their work routines and to the substantive nature of the work carried out as a result of locational changes stemming from the pandemic. These disruptions reconfigured the way research was conducted at the trust, and may have far-reaching effects on the norms and processes that shape the research system into the future.
Government guidance on social distancing and avoiding unnecessary journeys preceded the official nationwide lockdown on 23 March 2020. By 17 March, research staff at SLAT were already being instructed to work from home where possible. By the time our questionnaire was conducted in June 2020, 43% of research delivery staff respondents reported that they were working from home for all or part of the week. This included staff who remained in their usual jobs but were now carrying out their work from home, as well as staff who had been redeployed to mainly administrative or clerical roles which they could perform from home. For many research delivery staff, working from home was not something routinely done before the pandemic, and it presented a set of unique challenges as well as some unexpected benefits.
A number of challenges emerged which are common to many industries that have suddenly shifted to working from home. These related to problems of technical connectivity, owing to variable internet connections or familiarity with remote working platforms. Also common to many was the problem of trying to maintain levels of productivity while not having any childcare, the feeling of working more hours, being isolated from colleagues, and not having any space away from work at home.
More specific to the work of research delivery, respondents identified issues with electronic or paper-based documentation systems that were not available from home. Accessing, storing and sending patient data is tightly controlled under the Data Protection Act 2018, and some staff found working within the guidelines complicated or even impossible to do remotely. One respondent noted:
Not all features of the clinical documentation system were accessible on my private laptop; therefore, I still had to come to SLAT sometimes to fulfil my role—even if I belong to the vulnerable group in regards to COVID. IT [information technology] services tried to help me, but I would have needed a trust laptop, which wasn't available. (Q-6)
Additionally, staff working from home had to keep up with the rapidly changing research context, which had increased the administrative burden linked to communication with colleagues—many of whom had been clinically redeployed—and patients, as well as keeping track of multiple protocol deviations and amendments.
However, for some staff the experience of working from home was not wholly negative. Some appreciated being able to remain active and contribute to the NHS’s pandemic response while shielding at home. Some appreciated the change of pace and being able to set their own schedule. One respondent suggested that “senior leadership should consider working from home as a truly viable option, rather than just a benefit to staff” (Q21), signalling the interest of some staff to continue home working after the pandemic. A shift to remote working could have wider implications for how research is organized and delivered at the trust, with the benefits of flexible hours and locations seen by some as an advantage to patients as well. As one respondent put it, “In the same vein, exploring remote options with research follow-ups would be more convenient for patients too” (Q112).
While it is too soon to say whether this shift to working from home is temporary, or what future regular home working for research delivery staff might look like, what has been made evident is that large volumes of work once performed exclusively on-site at the hospital have been transferred to remote working. This has opened up new possibilities and ways of thinking about how research delivery might be organized in the future.
Clinical redeployment
For many clinical research delivery staff, redeployment meant being sent to work on the clinical front line. Research nurses with ICU experience were sent to the ICU, and others were sent to work on COVID wards. Many clinical staff who were not registered nurses, including CRPs, were asked to support ICU by joining a “turning team”, a key resource-intensive intervention for the management of critically ill patients. While specific experiences varied across staff groups and locations, a common thread among our respondents was the need to quickly adapt to new ways of working in an intense clinical environment which was rapidly evolving.
Redeployment to the ICU occurred early during the first wave of the pandemic. Nurses, some of whom had not worked in ICU or on the wards in many years, found themselves thrust back to the front line. Respondents described feeling anxious about being redeployed, but also “duty-bound” (D-2) to answer the call of service. One research matron who manages a team of research nurses recounted what it was like to inform staff of their redeployment:
You had to go and talk to these people to say, “You are going back to ICU”. Now, they had all worked in ICU, but they’d left ICU because of a reason. Some of them didn’t like it. That was quite tough. You know, they were all great and they were all amazing and said, “Absolutely, we will go”. But there were lots of tears and people were scared, I think. At that point you had just heard the horror stories from Europe and everywhere and they didn’t really know what they were walking into. (D-4)
Even some nurses who had worked clinically in the more recent past felt daunted by the prospect of a return to clinical work under these conditions.
It was not just nurses who were sent to the clinical front line. CRPs who had never set foot in an ICU prior to the pandemic joined turning teams, where they were trained to turn COVID patients to the prone position, a manoeuvre which had been shown to improve respiratory failure and hypoxaemia [ 29 ]. A CRP describes her reaction to the news that she was to be redeployed to the turning team:
I’d never heard of proning before. So just went straight onto Google and Googled what proning was. And then, when we had the training session, we got to see what it actually was … I felt anxious. The weekend before the first day felt very anxious, just because it’s completely unknown to me. I’ve never set foot on an intensive care ward before, never worn PPE [personal protection equipment] before, you know. It was just all unknown. (D-5)
The result of redeployment for many staff was the opportunity to learn new skills or deepen expertise of existing ones. Of the 49 research delivery staff who answered the question on skill acquisition in the questionnaire, 59% (29) reported developing new skills through their redeployments. For some, this meant learning new computer programmes and refreshing “IT skills in use of clinical programmes that I don't need to use routinely in my research role” (Q-57). For others, redeployment meant developing new areas of clinical practice. D-4 was redeployed to a COVID research team; despite having worked in research for most of her career, she was pleased to acquire skills:
Some of the things were exciting. The first study we were doing was stem cell therapy. I’d never done that before. So, normally you probably have like 6 months to prepare for a study like that. I mean we had a week. And I hadn’t given an IV [intravenous] drug for about 10 years. The first drug I gave is stem cells. So, it was learning—it’s the steepest learning curve of my 30-year career, that first month. Every day, I had not got a clue what we were doing. (D-4)
Other interviewees also saw redeployment as potentially opening up new career trajectories:
I think there’s opportunities there for having some really good development pathways, just sort of understanding more what skills we want to develop in people. And knowing where those are amongst our teams. I think, workforce-wise, there’s loads and loads of opportunities. And really good links have been built up as well. (D-2)
For D-2, redeployment was an unprecedented opportunity for the development of new skills and career pathways.
Though redeployment was for many staff a difficult and stressful experience, many were generally positive about the opportunity to develop new skills it had provided. However, some staff were uncertain about their ability to build on these skills to advance their careers. This was particularly the case among CRPs whose uncertain career path was an issue which preceded the pandemic.
I’m a Band 6. So the next aim for me would obviously be to a Band 7. And within research, it is quite difficult once you’re at a 6 to get to a 7 because there’s minimal Band 7 roles in research. They do just get harder to progress at a certain point. So I think my trajectory is the same, because I’d probably still be in the same position without the pandemic. So you don’t think that the added clinical experience has made an impact on… No, because I’m not going to now decide to go into anything clinical. So, no, I don’t think so. The only thing it would add is, I think it does look really good on your CV [curriculum vitae] to have been involved with that. (D-5)
The experience of D-5, who had been redeployed to the turning team, illustrates a key issue for the research system. Redeployed staff acquired new skills and competencies, but since the health research system remains separate from clinical care, it is not always clear how workers will be able to apply and leverage these skills.
Holding the fort
While some of their colleagues were redeployed to COVID-specific roles, other redeployed staff were tasked with maintaining the research system that had been put on hold. As the pandemic continued, some research delivery staff had to remain “holding the fort” (D-2) in their research roles. Whilst there was a lighter workload as trial recruitment stopped for most studies and some questionnaire participants reported a decrease in workload, many others had to rapidly learn new skills to follow up and maintain the research process for patients already participating in trials. Some were the last member left on their team, and as such needed to both take on other colleagues’ studies and cover for those colleagues with more clinical skills who were sent to the front line. These staff who remained found an increase in stress and workload:
There has been a dramatic increase in stress, in part due to trying to keep up with ever-changing guidance. Also, as other members of the team have been redeployed and as patients feel scared, I have to work longer hours and pick up duties from where others have been redeployed, which involves learning about things I hadn't had to look at before very quickly, but am not compensated for this. (Q-7)
As one research nurse who was “holding the fort” suggested, “I’m just making sure that the patients are safe. Our main concern at the moment is, are they getting their medication, and are they safe.” (D-6).
These staff also had to quickly adapt to a number of significant procedural changes. Many patients on drug trials still had to gain access to these medications, so staff had to arrange couriers to deliver them to their doors. Scans were postponed or switched to a less time-consuming alternative where possible. Blood draws needed to be taken in health centres more local to participants to avoid travel out of the local area. Whilst some research visits still had to be conducted face-to-face, staff found switching most of their follow-ups online to be particularly challenging, especially when “dealing with anxious oncology patients, as well as my own anxieties” (Q121). Indeed, as R-5 suggested, sometimes it was “better to see patients face-to-face with particularly delicate news”, such as the discontinuation of cancer treatment.
Who gets to be an NHS hero?
Some redeployed research staff experienced a particular sort of stress tied to professional self-understanding and the strongly moralized narratives being promoted by the government, the media and the public at large. Despite contributing immense amounts of labour under difficult circumstances, some research delivery staff who had not been redeployed to the front line felt excluded from the “NHS heroes” public narrative which emerged early during the pandemic. Rather, as participant D-3 suggests, “There was shame and guilt if you weren’t going [to the front line]”. Other participants explained further:
I felt that, you know, really sort of useless being at home, and when there were so many brilliant colleagues that were out there really helping and getting involved right from the start. (P-1, shielded but continued work from home) I personally volunteered for two roles but was told I couldn't because I was a nurse [needed in her team]. Instead I did nothing and felt alienated from all those who were helping out. (Q-97)
For D-3, who manages research staff, “there was very much a feeling like being the major sitting three miles back from the front line”, even though they were “currently managing everything that’s left… covering all my staff who haven’t been redeployed”. For other staff involved in clinical research, the exclusion from the “NHS heroes” narrative was underlined by the fact that they did not entirely feel like a central part of the health system even before the pandemic.
For other staff, however, the experience of redeployment to the COVID research team or the clinical front line contributed to a strong feeling of pride at having been part of the NHS pandemic response, alongside clinical colleagues. Many respondents, especially CRPs and those whose work was typically confined to the spaces and routines of research, described a new sense of belonging within the trust.
I felt really privileged actually to be able to be there. You know, we were chatting and, in 20 years, when you look back and you think, what did you do in COVID, I would definitely say I was at the forefront doing research, which is lovely. (D-4)
The presence of research nurses, CRPs and other research staff on COVID wards and the high profile of the COVID research being undertaken at the hospital contributed to many clinical research staff feeling visible and appreciated in the eyes of colleagues, if not also by the wider public.
I think it’s been really good for research because it’s really raised the profile that research nurses and practitioners are clinical, patient-facing, and they have clinical skills and expertise to be able to meet the need of clinical areas during this pandemic. And that kind of flexibility and willingness of us to kind of have released staff and tried to release them quite quickly in response to that appropriately. (D-1)
Although the “NHS heroes” narrative emphasized the role of frontline care workers, others recognized that there were many different ways for research staff to contribute to supporting the health system during this exceptional time.
Discussion and conclusion
This study has examined redeployment of the research delivery workforce in one research-active NHS trust during the pandemic. Drawing on empirical data collected between May 2020 and October 2020 as well as wider documentary analysis, we have demonstrated that at SLAT, the research workforce was able to function as a resource that was effectively redeployed as part of the pandemic response. Redeployment was in many ways an improvised process, but it drew on the specific skill set and professional profile of the research delivery workforce. It presented some unique challenges for research workers but also created new capacities for the research system. Redeployed research workers faced some difficulties with technology, communication and stress, but many also had a positive experience and saw the redeployment as a significant and valuable moment in their career and a way to contribute to the national pandemic response.
Although one case study of one trust cannot yield data generalizable to all trusts, the validity of these findings is supported by the broad and varied qualitative data set upon which it is based. This study benefited from first-hand operational experience of the redeployment process, as well as multiple forms of qualitative data. Our broad sample of the research delivery workforce at SLAT allowed us to gain in-depth perspective on the pandemic response within the trust’s clinical research system.
At the same time, we recognize that this study has a number of limitations. Our sample was designed to be illustrative of a significant case, not representative of all cases. The location of the trust in central London, the size of the research workforce and the scale of research activity mean that our case is unlikely to be perfectly representative of trusts in other areas or with varying levels of research activity. Additionally, data collection was prospective rather than retrospective—data collection took place while the pandemic was ongoing. This meant that some day-to-day concerns may have been amplified. Seen retrospectively, our study participants might report a different set of experiences.
This study suggests a number of ways that, going forward, trusts might better draw upon and support their research workforces. One of the clearest lessons to be learned from this case is that the relationship between research and clinical care can be clarified and further developed. At SLAT, large amounts of research are carried out, but research is not as well integrated with clinical functions as it could be. During redeployment, this was reflected in some awkward initial stages where it was not clear what role research delivery workers would play. Our findings also suggest that trusts should start to think more holistically about research not as an exceptional activity but as part of the business as usual of the trust. Researchers constitute a trained, flexible workforce embedded in trust core function, supporting patients who choose research as part of their care pathway. The process of redeployment at SLAT provides support for Roope et al.’s “option value” argument [ 11 ]: the research delivery workforce did not represent unnecessary excess capacity but rather an agile, flexible and valuable resource that was able to mobilize effectively in an unprecedented emergency. Our study also speaks to the wider importance of an integrated and agile research infrastructure that sits outside of individual research projects. Zakaria et al. [ 30 ] discuss some of the key challenges of assessing the impact of research infrastructures. Acknowledging how impact assessment can often be focused on discrete research projects, Zakaria et al. call for debate in how we evaluate the impact of research infrastructures specifically. While our case study does not provide a framework to measure impact, it does demonstrate some of the key benefits and impacts of research infrastructures that may be overlooked in current impact assessment practices—in particular, the skills and adaptability of the research delivery workforce, the “option value” offered by both the research delivery workforce and the research infrastructure more broadly, which allows the system to support pressing and emerging research needs across the whole research portfolio, and support care delivery in emergencies.
In the exceptional circumstances of the COVID-19 pandemic, the research delivery workforce provided much-needed support to the NHS, but particularly in an NHS that is pushing to develop its research capacity and instil research into everyday NHS activity, using the skills and adaptability of the research infrastructure more broadly is not a solution to everyday staffing problems which span well beyond the confines of individual NHS trusts. Even in the case discussed here, the research delivery workforce and wider research infrastructure (including the R&D department) represent just 2% of the trust’s staff numbers.
Our case also suggests a number of specific ways that the research workforce could be supported. For example, during redeployment, CRPs ended up performing important clinical patient-facing roles. Recognizing this, trusts might seek to supplement their skills base, in order to make their skills more visible to supervisors and uniform across departments. Trusts might also engage in other forms of upskilling for research delivery workers. Many research staff appreciated the opportunity that redeployment gave them to learn new skills, interact with new colleagues and patients, and participate in new parts of the trust. After the pandemic, more avenues for skill acquisition and lateral working should be offered. This would build on the linkages between the often-siloed research system and the day-to-day clinical services at the trust and could be a benefit to both, and ultimately to patients.
At the same time, many research delivery workers, like their colleagues across the health service, experienced burnout, trauma and exhaustion. For some research delivery workers, this was exacerbated by the knowledge that the opportunities to build upon new skills acquired during redeployment are limited. Trusts could help support the research workforce by clarifying their career trajectories and ensuring that there are adequate forms of advancement and progression available to them. Work is being done here at a national level through the NIHR to develop the profession and professional identity of the CRP [ 31 ]. However, the pandemic has rendered issues around skills development and documentation and advancement within this workforce into sharper focus.
Above all, this study demonstrates the “option value” and potential of the research delivery workforce. Patients benefited from the care that redeployed research workers were able to offer. The public benefited from having a well-developed research infrastructure that could facilitate rapid respond to a novel virus. The NHS should recognize the value of this workforce and support it accordingly.
The pandemic represented a high-stakes stress test for the United Kingdom’s research infrastructure and the workforce that keeps it running. Our case suggests that this workforce successfully delivered crucial research as well as vital clinical support during a critical time. The health service and the British public only stand to benefit by investing in the development of and support for these unique medical workers.
Acknowledgements
The authors would like to acknowledge support from NIHR Biomedical Research Centre, Chris McKevitt, and would also like to extend our deepest thanks to the research delivery staff at SLAT, whose work has contributed so much to studies conducted on and during COVID, including ours.
Author contributions
Conceptualization: RF-G, DW, HC, CDAW. Data curation: RF-G, DW, HC. Formal analysis: RF-G, DW, HC. Funding acquisition: CDAW. Investigation: RF-G, DW, HC. Methodology: RF-G, DW, HC. Project administration: RF-G, DW, HC. Resources: RF-G, DW, HC. Validation: RF-G, DW, HC. Writing—original draft: RF-G, DW, HC, CH, NH. Writing—review and editing: RF-G, DW, HC, CW, CH, NH. All authors read and approved the final manuscript.
DW, RFG, HC and CW are all funded by the NIHR (http:// nihr.ac.uk/) Biomedical Research Centre at Guy’s and St Thomas’ NHS Foundation Trust and King’s College London (Grant number IS-BRC-1215-20006). The funder had no role in study design, data collection and analysis, decision to publish, or preparation of the manuscript. The views expressed are those of the authors and not necessarily those of the NHS, the NIHR or the Department of Health and Social Care. NH is a National Institute for Health Research (NIHR) 70@70 Senior Nurse and Midwife Research Leader. The views expressed in this article are those of the author and not necessarily those of the NIHR.
Availability of data and materials
Declarations.
Ethics approval for the study was granted by North East – Newcastle & North Tyneside 2 REC (reference: 20/NE/0138).
Not applicable.
The authors declare that they have no competing interests. The views expressed are those of the author(s) and not necessarily those of the NHS, the NIHR or the Department of Health.
Publisher's Note
Springer Nature remains neutral with regard to jurisdictional claims in published maps and institutional affiliations.
This paper is in the following e-collection/theme issue:
Published on 8.4.2024 in Vol 8 (2024)
Fast Healthcare Interoperability Resources–Based Support System for Predicting Delivery Type: Model Development and Evaluation Study
Authors of this article:

Original Paper
- João Coutinho-Almeida 1, 2, 3 , MSci, PharmD ;
- Alexandrina Cardoso 4 , PhD ;
- Ricardo Cruz-Correia 1, 2, 3 , PhD ;
- Pedro Pereira-Rodrigues 1, 2, 3 , PhD
1 Faculty of Medicine, University of Porto, Porto, Portugal
2 Centre for Health Technologies and Services Research, University of Porto, Porto, Portugal
3 Health Data Science, Faculty of Medicine, University of Porto, Porto, Portugal
4 Nursing School of Porto, Porto, Portugal
Corresponding Author:
João Coutinho-Almeida, MSci, PharmD
Faculty of Medicine, University of Porto
Alameda Professor Hernâni Monteiro
Porto, 4200-319
Phone: 351 225513600
Email: [email protected]
Background: The escalating prevalence of cesarean delivery globally poses significant health impacts on mothers and newborns. Despite this trend, the underlying reasons for increased cesarean delivery rates, which have risen to 36.3% in Portugal as of 2020, remain unclear. This study delves into these issues within the Portuguese health care context, where national efforts are underway to reduce cesarean delivery occurrences.
Objective: This paper aims to introduce a machine learning, algorithm-based support system designed to assist clinical teams in identifying potentially unnecessary cesarean deliveries. Key objectives include developing clinical decision support systems for cesarean deliveries using interoperability standards, identifying predictive factors influencing delivery type, assessing the economic impact of implementing this tool, and comparing system outputs with clinicians’ decisions.
Methods: This study used retrospective data collected from 9 public Portuguese hospitals, encompassing maternal and fetal data and delivery methods from 2019 to 2020. We used various machine learning algorithms for model development, with light gradient-boosting machine (LightGBM) selected for deployment due to its efficiency. The model’s performance was compared with clinician assessments through questionnaires. Additionally, an economic simulation was conducted to evaluate the financial impact on Portuguese public hospitals.
Results: The deployed model, based on LightGBM, achieved an area under the receiver operating characteristic curve of 88%. In the trial deployment phase at a single hospital, 3.8% (123/3231) of cases triggered alarms for potentially unnecessary cesarean deliveries. Financial simulation results indicated potential benefits for 30% (15/48) of Portuguese public hospitals with the implementation of our tool. However, this study acknowledges biases in the model, such as combining different vaginal delivery types and focusing on potentially unwarranted cesarean deliveries.
Conclusions: This study presents a promising system capable of identifying potentially incorrect cesarean delivery decisions, with potentially positive implications for medical practice and health care economics. However, it also highlights the challenges and considerations necessary for real-world application, including further evaluation of clinical decision-making impacts and understanding the diverse reasons behind delivery type choices. This study underscores the need for careful implementation and further robust analysis to realize the full potential and real-world applicability of such clinical support systems.
Introduction
The ability to provide care to both women and newborns during delivery is one of the most important aspects of health care and is often used as a metric to assess health care across different countries. Cesarean delivery is one of the most important aspects of delivering babies since it has a considerable impact on the mother’s health and well-being. Despite the increased prevalence of this procedure over the last few years, the reasons behind this trend still remain unclear. Reports suggest that this increment is a global phenomenon, with the rate of cesarean deliveries almost tripling from 6.7% to 19.1% between 1990 and 2014 [ 1 , 2 ]. Research on the impacts of cesarean deliveries has focused on the risk of infection, hemorrhage, organ injury, and complications related to anesthesia or blood transfusion [ 3 , 4 ]. There is also a higher risk of complications in subsequent pregnancies, such as uterine rupture, abnormal placental implantation, and the need for hysterectomy [ 5 , 6 ]. As for the infant, cesarean deliveries can lead to respiratory problems, asthma, and childhood obesity [ 5 ]. In light of this, in 2015, the World Health Organization stated that cesarean delivery rates higher than 10% were not associated with a reduction in maternal or newborn mortality, even though other complications could not be fully assessed [ 7 ]. In contrast, there is no evidence of the benefits of this procedure for women or babies when there is no clear medical need; therefore, it is paramount to focus on identifying and reducing such cases [ 2 ]. It was estimated that in 2018, there were 8.8 million unnecessary cesarean deliveries [ 8 ]. It was with this in mind that a committee was established in Portugal with the specific purpose of decreasing the percentage of cesarean deliveries nationwide. One of the policies resulting from this committee’s work was the reduction of government funding per inpatient cesarean delivery episode for hospitals with rates of cesarean deliveries above 25%; as of 2020, the number of cesarean deliveries in Portugal stands at approximately 36.3%, nearing the all-time high of 36.9% in 2009 [ 9 ]. Furthermore, studies have shown that several countries could benefit from similar policies [ 8 ]. A quantitative analysis estimated that a reduction in cesarean deliveries could save millions of dollars [ 10 ] worldwide. Therefore, lowering the proportion of cesarean deliveries can yield health and financial benefits for both institutions and patients alike. With these considerations in mind, we developed a machine learning, algorithm-based support system to assist clinical teams in identifying cases of potentially unnecessary cesarean deliveries. As such, in this paper, we propose to (1) elaborate on how clinical decision support systems for cesarean deliveries can be developed using interoperability standards; (2) understand, based on the data collected, which features have the most significant impact on predicting delivery type; (3) conduct a concise economic analysis to assess the potential financial impact of implementing the proposed clinical decision support tool; and (4) compare the system’s output with clinicians’ responses.
Rationale and Related Work
Regarding the related work, several teams already tackled the potential of predicting the delivery type before birth. We found studies related to predicting a successful vaginal birth after a previous cesarean delivery, such as the work of Lipschuetz et al [ 11 ], where a gradient boosting method was used to predict such an event using prenatal data to do so. Grobman et al [ 12 ] performed a similar study with a multivariable logistic regression model. Different modalities of data were also used to predict delivery type. Fergus et al [ 13 ] introduced a method of predicting delivery type using fetal heart rate signals. Similarly, the work from Saleem et al [ 14 ] proposed a method for predicting delivery type using interactions between the fetal heart rate and maternal uterine contraction. Finally, some studies focus on predicting the delivery mode, such as the work of Ullah et al [ 15 ], where a boosting algorithm was used to predict a delivery mode with enriched data sets. In addition, Gimovsky et al [ 16 ] introduced decision trees to predict cesarean deliveries by physician group with an area under the receiver operating characteristic curve (AUROC) of 0.73. The works of Rossi et al [ 17 ] resulted in a 7-variable model with an AUROC of 0.78, and the works of Guedalia et al [ 18 ] resulted in a model with an AUROC of 0.82, reaching 0.93 with a first cervical examination. Finally, the works of Meyer et al [ 19 ] focused on selecting something suitable for a trial of labor after cesarean delivery with an area under the precision recall curve graph around 0.351. However, to the best of our knowledge, there was no model tested in clinical practice with an interoperable format of communication such as Fast Healthcare Interoperability Resources (FHIR), which tried to not only predict delivery type but also provide support about possibly wrong deliveries, and none with simulation about financial implication, making our paper a potential novelty on different dimensions.
Data were retrospectively collected from 9 different public Portuguese hospitals across the country, focusing on obstetric information and encompassing maternal data, various fetal data points, and the method of delivery retrospectively. The inclusion criteria are all mothers with a registered outcome of the pregnancy from 2019 to 2020. There were no exclusion criteria. Each institution used identical electronic health record software, ensuring that the data columns remained consistent.
Clinical Comparison
The clinical comparison was performed by sending questionnaires to clinicians with a relationship with obstetrics to assess 10 patients, with only access to the variables used by the model, and to answer 3 questions for each. The first question was to give a score from 1 to 10 of how likely it was that a patient would give birth through cesarean delivery, the second question was to select the feature or variable that most influenced the decision, and the third question was to select which feature they would require to make a better assessment. We sent the questionnaire to 20 people and obtained 6 answers, totaling 60 patient assessments. For these 10 patients, we also predicted the delivery type using our model to compare it with the clinicians’ answers. These patients were new and were not seen by the model during the training phase.
All null representations were standardized. Data were prepossessed by removing features with high missing rates (>90% overall). The imputation process was performed using the k-nearest neighbor imputation method (for continuous variables) or a new category (NULLIMP) for categorical variables. Weight was categorized into percentiles defined specifically for Portuguese babies [ 20 ]. For this study, the birth type was reduced to binary. All assisted birth were merged into vaginal birth, and cesarean delivery remained as the other class. Procedures and diagnoses were also used and were encoded as binary features, and we took the time to analyze each one of them to avoid leakage because there were procedures obviously related to cesarean deliveries and vaginal deliveries. Feature creation was performed through the free-text variable related to the prescribed medication. Medicine names were collected from it and converted into Anatomical Therapeutic Chemical classification group level 4, which represents chemical subgroups. We also created some new features from data in the data set, namely new categories related to the labor and condition of the baby. In addition, data quality issues were addressed, such as impossible values that were transformed into null values. The main variables affected by data quality were BMI or weight and gestational age. The data were split into training and test sets in a 0.75:0.25 manner. From the overall data sets that comprised over 200 columns, only a few columns were selected (see Table 1 ). We used a mixture of features selected by surveying the literature [ 21 - 23 ] and features with a high correlation with the outcome. The tested models were logistic regression, decision tree, random forest, 3 different boosting methods (as implemented by extreme gradient boosting [XGBoost], light gradient-boosting machine [LightGBM], and scikit learn), and a linear model based on stochastic gradient descent.
a Mode, number, and percentage for categorical variables.
b Mean and SD for continuous variables.
c N/A: not applicable.
The evaluation was performed with repeated stratified cross-validation with 10 splits and 2 repetitions, with 2 full cycles of dividing the training set into 10 equal parts and using 9 as the training set and 1 as the validation set. The results are shown in Table 2 . The application programming interface (API) for serving the prediction model was developed using FastAPI. We wrote all the code in Python (version 3.9.7; Python Software Foundation).
a AUROC: area under the receiver operating characteristic curve.
b XGBoost: extreme gradient boosting.
c Not available.
d AdaBoost: Adaptive Boosting.
e LightGBM: light gradient-boosting machine.
Ethical Considerations
This study received institutional review board approval from all hospitals included in this study with the following references: Centro Hospitalar São João (08/2021), Centro Hospitalar Baixo Vouga (12-03-2021), Unidade Local de Saúde de Matosinho (39/CES/JAS), Hospital da Senhora da Oliveira (85/2020), Centro Hosptilar Tamega Sousa (43/2020), Centro Hospitalar Vila Nova de Gaia/Espinho (192/2020), Centro Hospitalar entre Douro e Vouga (CA-371/2020-0t_MP/CC), and Unidade Local de Saúde do Alto Minho (11/2021). All methods were carried out per relevant guidelines and regulations. The need for informed consent was waived by the ethics committee.
Descriptive Statistics
The number of samples varied across the hospitals, ranging from 2364 to 18,177. Distributions of the selected variables are presented in Table 1 . The sum of all samples was 73,351. The outcome variable distribution is stated in Table 3 .
The AUROC is presented in Table 2 for the best hyper-parameters found for each algorithm in the training data. All models used the variables indicated in Table 1 .
While XGBoost was the best-performing algorithm, we selected LightGBM [ 24 ] because of its speed and lower memory requirements, which we believe are better suited for deployment in a low-hardware environment. The threshold selected for deploying the model was 0.7457, which rendered the metrics in the test set, as shown in Table 4 .
The purpose of this model is to serve as an API for usage within a health care institution and to act as a supplementary clinical decision support tool for obstetrics teams. For this to happen, a health information system must make the requests to the API. Even though a concrete, vendor-specific information model and input health information system were used, we hope to create a more interoperable clinical decision support system that can be used by every system that acts on birth and obstetrics departments. Therefore, we built it around the HL7 FHIR standard (R5 version) to simplify the method of interacting with the API. This decision, opposed as to using a proprietary model for the data, sits upon the usage of FHIR resources: bundle and observation for request and returning the result as a message through a custom operation called “$predict.” It is intended to publish the profiles of these objects to facilitate access to the API using standardized mechanisms and data models. The current build of the profiles can be seen in the published FHIR implementation guide where the current specifications are described in detail [ 25 ]. The process is illustrated in Figure 1 . We deployed this model in production in a single hospital without a user interface, collecting only the data and predictions for later discussion and analysis. We collected 3231 requests. During this period, 123 (3.8%) alarms were triggered. From this, we tried to understand the level of certainty for the decision and check the difference from the threshold of these alarms. The distance to the threshold for 73 was lower than 0.1 and was bigger than 0.1 for 50 (1.55%) cases.

The median scores given by each clinician are presented in Figure 2 . We also predicted the result using our model as stated in Figure 2 . The model misclassified only 1 record (ID 4). As for the analysis of missing features for the responders, they were divided into 3 categories: (1) existent in the data set but not included in the model, (2) nonexistent in the data set, and (3) existent in the data set and included but that particular information was not filled for the patient assessed. Out of 60 responders, this rendered a total of 37 (62%) with nonexistent features and 23 (38%) with existent features but no information was provided at that moment. No feature mentioned existed in the data set but had not been included in the model. From the 37 nonexistent features, 14 (38%) were new clinical assessments, 14 (38%) were linked to information from previous births, 5 (15%) were connected to more in-depth information about provided information (ie, a motive for induction), and 4 (11%) were related to the mother’s choice (if she wanted a cesarean delivery). As for feature importance, from the 60 answers, 33 (55%) stated that labor was the most important factor. Further, 9 (15%) stated the number of previous vaginal births, 5 (8%) stated the evolution of weight, and another 5 (8%) stated the number of previous cesarean deliveries as being the most important. The remaining 8 (14%) were various features, such as BMI, neuroaxis techniques, gestational age, and weight of the mother. Of all of these, 54 (90%) were in the top 10 features of the model.

Potential Financial Impact
The financial support provided to public hospitals in Portugal is partially tied to the rate of cesarean deliveries. To assess the potential impact of this mechanism on Portuguese public hospitals, we conducted a simulation. We got data for every public hospital for the last 12 months and applied a 3.8% reduction (the rate of warnings triggered in the new data set) and recalculated the rate of cesarean deliveries. The increase in support was calculated by the state-mandated rate as shown in Table 5 . With this new rate, we observed that implementing our tool would result in financial benefits for 30% (15/48) of the public hospitals. Specifically, 5 hospitals would begin receiving support instead of no support at all. Further, 3 hospitals would experience a doubling of their financial benefit, while 2 hospitals would see a 50% increase. Furthermore, 1 hospital would receive an additional one-third of financial support. If we assumed that only half of the warnings found in the new data were actually true (1.9%), we found that only 6 hospitals would be benefited: 3 from 0 to 0.25, 2 from 0.25 to 0.50, and 1 from 0.50 to 0.75.
Principal Findings
The first thing to address about this model is the number of biases that we introduced in the model by choice. We joined all vaginal delivery types into a single category (assisted and nonassisted), which introduces a bias since these delivery modes are indeed different. Second, the fact that we want to predict if the delivery type was wrongly chosen, mainly for the case of a cesarean delivery that did not need to be so, is also a bias. We used this approach because the initially collected data did not have the representation of such events. Thus, the biases of possibly wrong delivery types were present in the training data. We attempted to minimize this issue by selecting a threshold that gave the model higher sensitivity than specificity so that only large probabilities would trigger an alarm for human consideration. Parallel to this, we are starting to gather labeled cases, with the help of clinicians to create a better training data set. Furthermore, since the data were collected from different hospitals, differences in the data input can also occur. Even though the health information system is the same, the processes that originate the data and are being used for secondary purposes could introduce several biases in the data. This is an issue that was accepted from the start regarding the mechanism of data collection and model training. Despite this, we reached a model with a very high AUROC (88%, 95% CI 0.8795-0.8815), which is encouraging when compared to the state-of-the-art, which is between 0.73 and 0.82 [ 16 - 18 ]. Moreover, assuming that more data are provided, and proper labeling is done regarding the outcome variable (such as a clinical evaluation of needless cesarean deliveries) is added as well, a better model could be developed.
Regarding the preliminary clinical evaluation, it was only possible to obtain an overview of the possible comparison due to the number of responders. Despite that, the results are encouraging, since the model seems to behave better than humans with the data provided. However, this is a biased vision since clinicians in the real world have access to more data and information than the model has. It is encouraging, but caution is advised before more tests and evaluations are done. As for the deployment, future work could be the improvement of the API to map all variables to an ontology such as SNOMED CT or similar, making it easier for every system and person to access it and obtain a suggestion of the delivery type. Finally, we believe the assessment can be improved. A more robust clinical assessment is necessary, as well as a thorough analysis of the impact of the tool in the real world, since we need to create the bridge between the results of the model and how clinical decisions are affected by it. A full cost-effectiveness analysis is also necessary to understand the real-world impact of the model. Further, 1 interesting result is the fact that 38% of the answers regarding the most important data element missing from the patient record refer to data that are being collected but was missing for that specific patient, raising an important question about data input methodology, interoperability, and quality. If we cannot have access to data when these matter the most, these can become meaningless. Missing data are a problem of biomedical data as a whole. However, when specifically targeted at machine learning usage of this data for predicting something, we did not find any work comparing them with clinicians. However, we did find reporting of similar missing values in obstetrics data [ 27 ] and we also found works of a similar nature using machine learning models with robust handling of missing data such as XGBoost [ 28 ] to counter this problem. This indicates that our model has the potential to counter the missing data problem as well since LightGBM can also handle missing data natively.
Conclusions
We believe we have developed a robust system capable of detecting potentially incorrect cesarean delivery decisions, which could positively impact real-world medical practice. However, before implementation, several challenges must be addressed, particularly the need for further evaluation of the system’s impact on clinical decision-making and the reasons underlying suboptimal delivery-type decisions. Cesarean deliveries may be performed for various reasons, from a mother’s preference to a decision made by the obstetrics team. This system is not designed to impede medical practice or to highlight flawed decisions, potentially scrutinizing specific professionals. Such caution is necessary when implementing systems like these. While having a high AUROC is beneficial, the real-world impact is another consideration. The assumptions and biases associated with autonomous systems supporting clinical practice must be carefully considered. Nonetheless, the metrics and results we have achieved so far are promising for positively influencing health and economic outcomes.
Acknowledgments
The authors would like to acknowledge the support of Component 5—Capitalization and Business Innovation, integrated in the Resilience Dimension of the Recovery and Resilience Plan within the scope of the Recovery and Resilience Mechanism (MRR) of the European Union, framed in the NextGenerationEU, for the period 2021-2026, within project HfPT (41).
Data Availability
The data cannot be publicly available due to privacy and ethical restrictions. The code is available [ 29 ].
Conflicts of Interest
None declared.
- Betrán AP, Ye J, Moller AB, Zhang J, Gülmezoglu AM, Torloni MR. The increasing trend in caesarean section rates: global, regional and national estimates: 1990-2014. PLoS One. 2016;11(2):e0148343. [ FREE Full text ] [ CrossRef ] [ Medline ]
- Chen I, Opiyo N, Tavender E, Mortazhejri S, Rader T, Petkovic J, et al. Non-clinical interventions for reducing unnecessary caesarean section. Cochrane Database Syst Rev. 2018;9(9):CD005528. [ FREE Full text ] [ CrossRef ] [ Medline ]
- Cook JR, Jarvis S, Knight M, Dhanjal MK. Multiple repeat caesarean section in the UK: incidence and consequences to mother and child. A national, prospective, cohort study. BJOG. 2013;120(1):85-91. [ CrossRef ] [ Medline ]
- Marshall NE, Fu R, Guise JM. Impact of multiple cesarean deliveries on maternal morbidity: a systematic review. Am J Obstet Gynecol. 2011;205(3):262.e1-262.e8. [ CrossRef ] [ Medline ]
- Keag OE, Norman JE, Stock SJ. Long-term risks and benefits associated with cesarean delivery for mother, baby, and subsequent pregnancies: systematic review and meta-analysis. PLoS Med. 2018;15(1):e1002494. [ FREE Full text ] [ CrossRef ] [ Medline ]
- Timor-Tritsch IE, Monteagudo A. Unforeseen consequences of the increasing rate of cesarean deliveries: early placenta accreta and cesarean scar pregnancy. A review. Am J Obstet Gynecol. 2012;207(1):14-29. [ CrossRef ] [ Medline ]
- World Health Organization Human Reproduction Programme‚ 10 April 2015. WHO statement on caesarean section rates. Reprod Health Matters. 2015;23(45):149-150. [ FREE Full text ] [ CrossRef ] [ Medline ]
- Hoxha I, Fink G. Caesarean sections and health financing: a global analysis. BMJ Open. 2021;11(5):e044383. [ FREE Full text ] [ CrossRef ] [ Medline ]
- Cesarianas nos hospitais (%). PORDATA. 2022. URL: https://tinyurl.com/54dru8fm [accessed 2024-02-28]
- Callander E, Shand A, Ellwood D, Fox H, Nassar N. Financing maternity and early childhood healthcare in the Australian healthcare system: costs to funders in private and public hospitals over the first 1000 days. Int J Health Policy Manag. 2021;10(9):554-563. [ FREE Full text ] [ CrossRef ] [ Medline ]
- Lipschuetz M, Guedalia J, Rottenstreich A, Persky MN, Cohen SM, Kabiri D, et al. Prediction of vaginal birth after cesarean deliveries using machine learning. Am J Obstet Gynecol. 2020;222(6):613.e1-613.e12. [ CrossRef ] [ Medline ]
- Grobman WA, Lai Y, Landon MB, Spong CY, Leveno KJ, Rouse DJ, et al. Development of a nomogram for prediction of vaginal birth after cesarean delivery. Obstet Gynecol. 2007;109(4):806-812. [ CrossRef ] [ Medline ]
- Fergus P, Hussain A, Al-Jumeily D, Huang DS, Bouguila N. Classification of caesarean section and normal vaginal deliveries using foetal heart rate signals and advanced machine learning algorithms. Biomed Eng Online. 2017;16(1):89. [ FREE Full text ] [ CrossRef ] [ Medline ]
- Saleem S, Naqvi SS, Manzoor T, Saeed A, Rehman NU, Mirza J. A strategy for classification of "vaginal vs. cesarean section" delivery: bivariate empirical mode decomposition of cardiotocographic recordings. Front Physiol. 2019;10:246. [ FREE Full text ] [ CrossRef ] [ Medline ]
- Ullah Z, Saleem F, Jamjoom M, Fakieh B. Reliable prediction models based on enriched data for identifying the mode of childbirth by using machine learning methods: development study. J Med Internet Res. 2021;23(6):e28856. [ FREE Full text ] [ CrossRef ] [ Medline ]
- Gimovsky AC, Zhuo D, Levine JT, Dunn J, Amarm M, Peaceman AM. Benchmarking cesarean delivery rates using machine learning-derived optimal classification trees. Health Serv Res. 2022;57(4):796-805. [ FREE Full text ]
- Rossi RM, Requarth E, Warshak CR, Dufendach KR, Hall ES, DeFranco EA. Risk calculator to predict cesarean delivery among women undergoing induction of labor. Obstet Gynecol. 2020;135(3):559-568. [ CrossRef ] [ Medline ]
- Guedalia J, Lipschuetz M, Novoselsky-Persky M, Cohen SM, Rottenstreich A, Levin G, et al. Real-time data analysis using a machine learning model significantly improves prediction of successful vaginal deliveries. Am J Obstet Gynecol. Sep 2020;223(3):437.e1-437.e15. [ CrossRef ] [ Medline ]
- Meyer R, Hendin N, Zamir M, Mor N, Levin G, Sivan E, et al. Implementation of machine learning models for the prediction of vaginal birth after cesarean delivery. J Matern Fetal Neonatal Med. 2022;35(19):3677-3683. [ CrossRef ] [ Medline ]
- Sousa-Santos RF, Miguelote RF, Cruz-Correia RJ, Santos CC, Bernardes JFMAL. Development of a birthweight standard and comparison with currently used standards. what is a 10th centile? Eur J Obstet Gynecol Reprod Biol. 2016;206:184-193. [ FREE Full text ] [ CrossRef ] [ Medline ]
- Irwinda R, Hiksas R, Lokeswara AW, Wibowo N. Maternal and fetal characteristics to predict c-section delivery: a scoring system for pregnant women. Womens Health (Lond). 2021;17:17455065211061969. [ FREE Full text ] [ CrossRef ] [ Medline ]
- De Ramón Fernández A, Fernández DR, Sánchez MTR. Prediction of the mode of delivery using artificial intelligence algorithms. Comput Methods Programs Biomed. 2022;219:106740. [ FREE Full text ] [ CrossRef ] [ Medline ]
- Parveen R, Khakwani M, Naz A, Bhatti R. Analysis of cesarean sections using Robson's Ten Group classification system. Pak J Med Sci. 2021;37(2):567-571. [ FREE Full text ] [ CrossRef ] [ Medline ]
- Ke G, Meng Q, Finley T, Wang T, Chen W, Ma W, et al. LightGBM: a highly efficient gradient boosting decision tree. NeurIPS Proc. 2017;30:3146-3154.
- Obstetrics Machine Learning IG. URL: https://build.fhir.org/ig/joofio/obs-cdss-fhir/ [accessed 2024-02-28]
- Termos referência para contratualização de cuidados de saúde no SNS para 2023. ACSS. 2023. URL: https://tinyurl.com/nn3xj7hf [accessed 2024-02-28]
- Venkatesh KK, Strauss RA, Grotegut CA, Heine RP, Chescheir NC, Stringer JSA, et al. Machine learning and statistical models to predict postpartum hemorrhage. Obstet Gynecol. 2020;135(4):935-944. [ FREE Full text ] [ CrossRef ] [ Medline ]
- Bitar G, Liu W, Tunguhan J, Kumar KV, Hoffman MK. A machine learning algorithm using clinical and demographic data for all-cause preterm birth prediction. Am J Perinatol. Dec 4, 2023. [ CrossRef ] [ Medline ]
- obs-ml-analysis. GitHub. URL: https://github.com/joofio/obs-ml-analysis [accessed 2024-02-29]
Abbreviations
Edited by A Mavragani; submitted 30.10.23; peer-reviewed by S Matathil, M Roqueta Vall-llosera; comments to author 02.01.24; revised version received 04.01.24; accepted 06.02.24; published 08.04.24.
©João Coutinho-Almeida, Alexandrina Cardoso, Ricardo Cruz-Correia, Pedro Pereira-Rodrigues. Originally published in JMIR Formative Research (https://formative.jmir.org), 08.04.2024.
This is an open-access article distributed under the terms of the Creative Commons Attribution License (https://creativecommons.org/licenses/by/4.0/), which permits unrestricted use, distribution, and reproduction in any medium, provided the original work, first published in JMIR Formative Research, is properly cited. The complete bibliographic information, a link to the original publication on https://formative.jmir.org, as well as this copyright and license information must be included.
We've detected unusual activity from your computer network
To continue, please click the box below to let us know you're not a robot.
Why did this happen?
Please make sure your browser supports JavaScript and cookies and that you are not blocking them from loading. For more information you can review our Terms of Service and Cookie Policy .
For inquiries related to this message please contact our support team and provide the reference ID below.
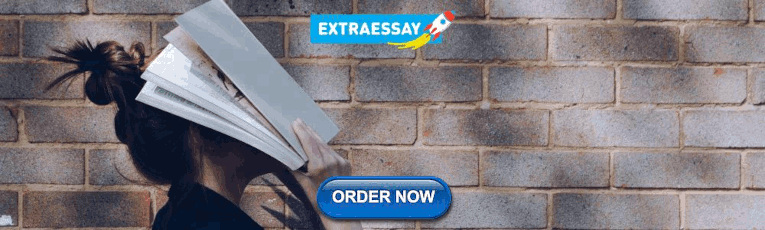
IMAGES
VIDEO
COMMENTS
4 Dec 2023. Current Coordinator in Coventry, England, England. Limited, nothing particularly special on offer. 10 Sep 2023. Current Graduate Student in Coventry, England, England. Its a flexible and positive workplace. Coventry University is now hiring a Research Delivery Support Officer in Coventry, England. View job listing details and apply now.
2,342 Research Support Officer jobs available on Indeed.com. Apply to Philanthropy Manager, Program Officer, Chief Information Officer and more!
Posted 12:45:38 PM. Fixed-term contract ending December 2023 The Research Delivery Support Officer plays a vital…See this and similar jobs on LinkedIn.
The role of Research Projects Support Officer provides administrative and coordination support to two key ... Program Support and Delivery • Provide project support, communication and coordination requirements under the guidance of the Senior Manager Research Implementation, Distributed Leadership Program Managers and ...
Apply to Research Delivery Support Officer jobs now hiring in Coventry on Indeed.com, the worlds largest job site.
111 Research and support officer jobs in United States. Most relevant. Military Service - U.S. Air Force. 4.1. Military Service - U.S. Air Force, Biomedical Laboratory Officer. United States. Certification as a Medical Technologist or Medical Laboratory Scientist or certification as a Specialist in Blood Banking (SBB) from the American Society ...
The Research Delivery Support Officer provides support to Principal Investigators (PIs) on projects that have been identified through a risk assessment, to ensure research excellence outputs are achieved; income generation to the University Group is maximised and to ensure
Research Delivery Support Officers provide support to Principal Investigators (PIs) on projects to ensure research excellence outputs are achieved, income generation to the University Group is maximised and to ensure the delivery meets University objectives and the requirements of the funder. The position sits within Research Delivery Support ...
Apply to Research Delivery Support Officer jobs now hiring on Indeed.com, the worlds largest job site.
Accomplished professional with a diverse background in research support, operations administration, and marketing co-ordination. Also has a proven track record of delivering excellence in each role.<br><br>Skills include:<br>- providing essential support to research initiatives,<br>- collaborating with researchers, academics and external partners,<br>- facilitating smooth project execution,<br ...
Research is a strategic priority area for Coventry University; significant investment has been made into facilities, research staff and research support services. To support planned future growth the university is expanding its portfolio of research and facilities and has a range of vacancies across our research centres. It is an exciting time ...
Research Delivery Officer (Horizon Scanning) TLT. Hybrid remote in Bristol. Part-time +1. Hybrid remote. The KM team, with the firms Knowledge Lawyers deliver both legal and business research to support client activity, bids & tenders as well as sector and ...
Research Delivery Support Officer. Coventry, England. Employer Est.:£29K - £39K . Unfortunately, this job posting is expired. Don't worry, we can still help! Below, please find related information to help you with your job search. Suggested Searches. uber driver. non cdl driver.
Research Delivery Support Officer Coventry University Jan 2022 - Present 2 years 2 months. Coventry, England, United Kingdom Project Coordinator Coventry University Nov 2014 - Present 9 years 4 months. View Lindsay's full profile See who you know in common Get introduced ...
I am passionate about carrying out social research that indeed is likely to improve the well-being of society. I have a great deal of interest in studying South Asian women and ethnic minorities with issues regarding family, employment, education, gender equality, gender-based violence and immigration. | Learn more about Arifa Choudhoury's work experience, education, connections & more by ...
The Research Support Officer will work as part of a team of staff led by the Research Manager in the Hope Clinical Trials Centre. ... This is a unique and exciting opportunity for an individual experienced in research to join the Research & Innovation (R&I) research delivery workforce at University Hospitals of Leicester NHS Trust.
Shared by Thilini Fernando. I am an experienced project coordinator with a proven track record of successfully delivering and supporting<br>research and development projects across the world. My 15 years of experience in planning and coordinating projects within the UN, NGO, and academic sectors have given me a good understanding of the UK ...
We also analysed documents produced by national bodies (for example, the NIHR and the Chief Medical Officer for England) related to national directives on COVID-19 and clinical research. ... and engagement of the clinical research delivery workforce to support the clinical response was coordinated with the research workforce lead through the ...
The Department offers the opportunity for a wide range of clinical interests and support for research. The Emergency Department sees presentations approaching 46,000 per annum. We currently have a team of 16 SMOs, 15 registrars and 15 House Officers. We have ACEM accreditation for 24 months of training in Emergency Medicine.
Background: The escalating prevalence of cesarean delivery globally poses significant health impacts on mothers and newborns. Despite this trend, the underlying reasons for increased cesarean delivery rates, which have risen to 36.3% in Portugal as of 2020, remain unclear. This study delves into these issues within the Portuguese health care context, where national efforts are underway to ...
Research Delivery Support Officer. University of Hertfordshire. Sep 2022 - Present 1 year 3 months. Hatfield, England, United Kingdom.
Inspiring and developing junior officers through innovative, research-based approaches The Center for Junior Officers leads the Army in creating and modeling the delivery of high-quality content that maximizes human potential in Army junior officers. ... I was an Executive Officer in the Forward Support Company (FSC) and deployed advanced ...
The US Navy is pushing back the estimated first delivery of its next-generation nuclear-armed submarine by 12-16 months, the most significant schedule slip to date for the service's top ...
Research Delivery Support Officer. Sep 2022 - Present11 months. Providing strategic and operational post-award support for the portfolio of research studies within the School of Health and Social Work. Monitoring expenditure, invoicing and contract matters. Co-ordinating with colleagues across the Research Grants, Research Finance, Legal and ...