Click through the PLOS taxonomy to find articles in your field.
For more information about PLOS Subject Areas, click here .
Loading metrics
Open Access
Peer-reviewed
Research Article
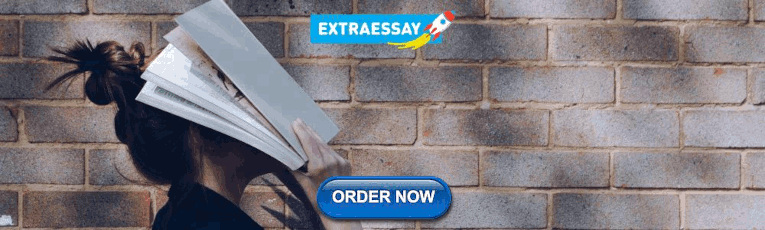
Mortality Burden and Socioeconomic Status in India
Affiliation Department of Global Health and Population, Harvard School of Public Health, Boston, Massachusetts, United States of America
* E-mail: [email protected]
Affiliation Department of Society, Human Development and Health, Harvard School of Public Health, Boston, Massachusetts, United States of America
- June Y. T. Po,
- S. V. Subramanian
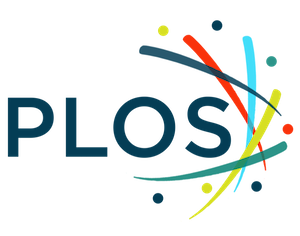
- Published: February 9, 2011
- https://doi.org/10.1371/journal.pone.0016844
- Reader Comments
The dimensions along which mortality is patterned in India remains unclear. We examined the specific contribution of social castes, household income, assets, and monthly per capita consumption to mortality differentials in India.
Methods and Findings
Cross-sectional data on 217 363 individuals from 41 554 households from the 2004–2005 India Human Development Survey was analyzed using multiple logistic regressions. Mortality differentials across social castes were attenuated after adjusting for household economic factors such as income and assets. Individuals living in the lowest income and assets quintiles had an increased risk of mortality with odds ratio (OR) of 1.66 (95% CI = 1.23–2.24) in the bottom income quintile and OR of 2.94 (95% CI = 1.66–5.22) in the bottom asset quintile. Counter-intuitively, individuals living in households with lowest monthly consumption per capita had significantly lower probability of death (OR = 0.27, 95% CI = 0.20–0.38).
Conclusions
Mortality burden in India is largely patterned on economic dimensions as opposed to caste dimensions, though caste may play an important role in predicting economic opportunities.
Citation: Po JYT, Subramanian SV (2011) Mortality Burden and Socioeconomic Status in India. PLoS ONE 6(2): e16844. https://doi.org/10.1371/journal.pone.0016844
Editor: Pieter van Baal, Erasmus University Rotterdam, Netherlands
Received: August 17, 2010; Accepted: January 16, 2011; Published: February 9, 2011
Copyright: © 2011 Po, Subramanian. This is an open-access article distributed under the terms of the Creative Commons Attribution License, which permits unrestricted use, distribution, and reproduction in any medium, provided the original author and source are credited.
Funding: No direct funding was available for this study. However, S. V. Subramanian is supported by the National Institutes of Health Career Development Award (NHLBI K25 HL081275). The funders had no role in study design, data collection and analysis, decision to publish, or preparation of the manuscript.
Competing interests: The authors have declared that no competing interests exist.
Introduction
Social class and economic well-being have been identified as important social determinants that shape health inequalities [1] , [2] , [3] , [4] , [5] . In India, social castes were previously considered as a proxy for socioeconomic status and poverty [6] . A nationally representative study on India based on the 1981 census indicated that under-five child mortality in the lower castes, Scheduled Tribes and Scheduled Castes, were significantly higher than upper social castes [7] . The 1998–1999 India National Family Health Survey (NFHS) demonstrated an increase in mortality rates of neonates, infants, and under five children in progressively disadvantaged social castes [8] , [9] . In more detail, a study on an urban population of North India provided evidence that cardiovascular-related mortality was more prevalent in higher social castes whereas infections-related mortality was more prevalent in lower social castes [10] . Similarly, based on the analysis of the 1998-99 NFHS data, mortality was found to differentially associate with household wealth and much less with caste [8] . In this study, we provide an update of the most recent association between social caste, economic factors and mortality in India using individual data from the India Human Development Survey 2004–2005.
Study Design and Data
The cross-sectional data was drawn from the India Human Development Survey (IHDS) [11] , a nationally representative, multi-topic survey collected from November 2004 to October 2005. It sampled 215 754 alive individuals from 41 554 rural and urban households in India. Villages and urban blocks formed the primary sampling unit consisted of 150–200 households, from which the sample of households was selected [11] . The survey response rates were calculated as 92% for the total sample [11] .
The lowest unit of observation was the individual member, including 1609 who died in the previous year. Data on age and gender for both living and deceased household members were collected. The deceased household members were assumed to have belonged in the similar caste and religion as other household members and benefited from similar quality of living based on shared household income, assets and consumption. One household was defined as a group of people living under one roof and sharing the same kitchen.
Outcome and Predictors
The study outcome measure was a dichotomous variable indicating whether an individual was dead (1) or alive (0).
Caste and religion of the household were self-identified by the head of household. The caste categories were separated into Brahmin, High Caste, Other Backward Classes, Scheduled Castes (Dalit), Scheduled Tribes (Adivasi), and No Caste. Other Backward Classes, Dalits and Adivasis are considered the lower, marginalized social groups in India [12] . The individuals in IHDS that self-identified as No Caste were further stratified according to their religion into Muslims, Christians and Sikhs and Jains combined.
Detailed household income data was collected from queries of over 50 different income sources. The queries were categorized into eight major household income types: family farm income, household agricultural wages, non-agricultural wages, salaries, net business income, sum household remittance, government benefits, and property and pensions. The current analysis used the aggregated total income data and divided it into quintile groups and a group that reported negative household income.
The variable for household assets was a score constructed from the summation of 22 equally weighted dichotomous items measuring household possessions of consumer goods and eight aspects of housing quality. The household asset score was divided into quintiles for the current analysis.
The consumption variable was constructed from a standard battery of 47 expenditure questions taken from the short form of India's National Sample Survey. These included 30 questions on monthly expenditure and 17 questions on annual expenditure reported for the previous year. The final consumption total was calculated as the sum of the expenditure on monthly items and one twelfth of the expenditure on annual items. The monthly consumption per capita was divided into quintiles for the current analysis.
Age was divided into six categories to capture the different stages of life course: infants (<1 year), young children (1–5 years), children or adolescents (6–18 years), young adults (19–44 years), middle-aged adults (45–64 years) and elderly ( = 65 years). Other predictors were gender and residency location. Residency location was divided into three categories: rural villages, urban neighborhoods that were not metropolitan cities (population 5000–100 000) and cities of Mumbai, Delhi, Kolkata, Chennai, Bangalore and Hyderabad (population >100 000). The data on residency location was based on the India Census 2001, where an urban neighborhood must have a minimum population of 5000, at least 75% of male working population engaged in non-agricultural pursuits and a population density of at least 400 persons per square kilometer.
Statistical Analysis
The probability of an individual being dead is π i . The parameter β 0 estimates the log odds of mortality for the reference group, and the parameter β estimates with maximum likelihood, the differential log odds of mortality associated with the predictor X, as compared to the reference group. Odds ratios (OR) and predicted probabilities (PP) with 95% CI were calculated. All analyses were performed using the statistical program SAS 9.2 ‘surveylogit’ procedure, adjusted for sample clustering at the level of primary sampling units.
We used multivariable regression models to explore the effects of social caste, household income, household assets, and monthly consumption per capita on mortality separately, while adjusting for gender, age and residency location. Secondly, we explored the associations of the above factors together as they mutually adjusted for each other. Furthermore, we explored these associations with regards to age-specific mortality by adding interaction terms between age and caste, income, assets and monthly consumption per capita. We also explored the effects of caste on mortality when modified by income, assets and monthly consumption per capita. Social castes were stratified into five groups to observe mortality differentials across asset quartiles in finer detail. Households were re-grouped into asset quartiles to ensure at least one death is present within each asset quartile stratified by caste.
Ethical Review
The India Human Development Survey was conducted under the scientific and administrative supervision of the National Council of Applied Economic Research, Delhi and the University of Maryland and was reviewed by the relevant ethics review board. Formal written consent was obtained for all the surveys. This study was reviewed by Harvard School of Public Health Institutional Review Board and was considered exempt from full review as it was based on an anonymous public use data set with no identifiable information on survey participants.
Descriptive Statistics
There were 217 363 individuals included in the analysis. There were 1609 individuals, 0.7% of the total sample, who died in the one year prior to household survey. The distributions of gender, religion, social castes, and quintiles of household income, household assets and monthly consumption per capita are listed in Table 1 .
- PPT PowerPoint slide
- PNG larger image
- TIFF original image
https://doi.org/10.1371/journal.pone.0016844.t001
Socioeconomic Differentials in Mortality
The conditional odds ratios (OR) and predicted probabilities (PP) of each subgroup is shown in Table 2 and Table S1 . The reference group represents a Hindu male between the ages of 19–44, living in a metropolitan city. He belongs to the High Caste and has household income, household assets and monthly consumption per capita of the highest quintile.
https://doi.org/10.1371/journal.pone.0016844.t002
Across social castes, we found significantly higher odds of mortality in Other Backward Classes, Scheduled Castes and Scheduled Tribes. However, after adjusting for all wealth factors: income, assets and consumption per capita, the associations was no longer statistically significant with the exception of Scheduled Castes (OR = 1.72, 95% CI = 1.23–2.41). Adjusting the effect of social caste on mortality with household income and asset ownership independently also resulted in the attenuation of caste effect on mortality except in Scheduled Castes ( Table S2 ). Within the Muslims, Christians, Sikhs and Jains populations outside the traditional social caste system, no significant patterning in risk of mortality was found.
We found a statistically significant association between household income and mortality. Compared to the top quintile, the third and fourth quintile displayed significant and progressively higher odds of mortality. Individuals living with household income at the bottom quintile had 76% higher odds of mortality (OR = 1.76, 95% CI = 1.35–2.29). Mortality differentials in lower income quintiles were attenuated after mutually adjusting for assets and expenditure, but remained statistically significant (OR = 1.66, 95% CI = 1.23–2.24).
Similar to associations with household income, individuals with household assets within the middle quintile and lower quintiles had higher odds of mortality. Individuals who had little or no ownership of household assets at the bottom quintile had odds of mortality substantially higher than the top quintile (OR = 2.38, 95% CI = 1.42–3.99), which increased to almost three times when adjusted for income and expenditure (OR = 2.94, 95% CI = 1.66–5.22).
Unlike household income and assets ownership, a decrease in monthly consumption per capita was associated with decreased odds of mortality. Individuals living in households with the lowest quintile of monthly consumption per capita were 73% less likely to die than individuals from the top consumption quintile (OR = 0.27, 95% CI = 0.20–0.38).
Interaction of Socioeconomic Factors with Age
The mortality odds of infants younger than one year were differentially associated with social castes. Furthermore, infants, young children and adolescents up to 18 years old had mortality odds that were differentially associated with quintiles of household assets. Mortality at age 65 and above was significantly associated with being in Scheduled Castes, the bottom income quintile and the bottom assets quintile ( Figure 1 , Figure 2 ). No apparent interaction between age and monthly consumption per capita was found ( Table 3 , Figure 3 ).
https://doi.org/10.1371/journal.pone.0016844.g001
https://doi.org/10.1371/journal.pone.0016844.g002
https://doi.org/10.1371/journal.pone.0016844.g003
https://doi.org/10.1371/journal.pone.0016844.t003
Interaction of Social Castes with Wealth Measures
There were significant interactions found between Scheduled Castes and asset ownership, but not with household income or monthly consumption per capita ( Table S3 ). Upon stratification of social castes, we found significant mortality differentials across asset quintiles in High Caste and in Scheduled Castes ( Table 4 ).
https://doi.org/10.1371/journal.pone.0016844.t004
Our analysis has the following findings related to patterns of mortality differential among socioeconomic groups in India. First, the mortality burden associated to lower castes was substantially attenuated after accounting for the individuals' household income and assets. Our analysis showed that infant mortality burden remained associated with social castes. This mirrored previous findings which suggested that there were differential attenuation by economic factors in mortality burdens across life stages [9] . In our case, the importance of economic factors was lesser in infants than older ages. Although lower castes such as Scheduled Castes and Scheduled Tribes are disadvantaged in terms of social standing and materialistic wealth [13] , recent studies supported views that economic well-being, such as standard of living, is a more favorable indicator of mortality and morbidity burden than social caste as an intrinsic risk factor [8] , [9] , [14] . Results from sensitivity analysis provided support that asset ownership, among the three wealth measures, was the most important underlying factor in the mortality differentials observed across social castes ( Table S2 ). Furthermore, individuals belonging to the High Caste and the bottom asset quartile suffered from a higher mortality gap than Other Backward Classes. This suggested that magnitude of inequality across asset ownership may be an additional risk factor ( Table 4 ).
In our study, we utilized three measure of economic well-being to unravel the associations of wealth and mortality patterns. Low household income and asset ownership continued to be strongly associated with increased risk of overall mortality, but asset ownership alone were strongly associated with age-specific mortality. Total household income reflected short term, self-reported wealth of a household. The benefit of higher income may not trickle down to all members of the household at different ages. The data did not reflect significant income effect on mortality risk across age groups ( Table 3 ).
Household asset ownership is a relatively accurate long-term reflection of a household's economic well-being than income. Asset ownership measure has lower recall bias; consumer goods and housing quality can easily be verified by survey administrators. Comparing the two different household wealth indicators, we observed differential mortality gaps across age groups, mainly concentrated in infants and elderly ( Figure 1 , Figure 2 ). Furthermore, the mortality gradient of the population over 64 years old was much greater when deprived of asset ownership than of high household income. This suggested that wealth in terms of monetary resources translated less readily to standard of living in elderly than ownership of household goods and housing quality.
Among different household goods and housing qualities, there exists a differential mortality risk reduction for different age groups as well. For instance, an electric fan may reduce risk of malarial infection in infants and young children who stay at home, but not for older children and adults. We observed significant interactions between asset quintiles and age groups 0–18 years but not with older adults, suggesting ownership of particular assets could affect age groups selectively ( Table 3 ).
Consumption captures monthly household expenditures such as staple food cost and seasonal or sporadic expenditures such as contributions to annual festivals, weddings and major medical expenses. Surprisingly, our findings showed significantly lower odds of mortality in individuals from lower quintiles of monthly consumption per capita. Higher quintiles of monthly consumption per capita captured poor households that might need to exert greater marginal effort and percentage of household wealth than rich households to obtain similar daily resources [15] . For example, a woman in an urban center could switch on a tap for potable water in the matter of seconds and a woman living in a rural village may require several hours each morning and afternoon to line up, pump and carry water for daily use, in turn reducing her available income-generating time. Correlations of consumption with income quintiles (r = 0.32) and asset quintiles (r = 0.48) were low, which suggested the subpopulations with low wealth measured by household total income and ownership of assets may not be the similar subpopulations that were consuming the least per capita monthly. On the other hand, high monthly consumption per capita was strongly associated with high mortality risk in elderly individuals ( Figure 3 ). This was likely due to the increased medical expenses at older ages.
The findings of our study need to be considered along with following limitations. Our 2004–2005 update of mortality differential across socioeconomic factors in India was based on cross-sectional survey data. Although it captured only a snap shot of India's mortality patterns, it reinforced previous findings from the NFHS 1998-1999 that economic well-being was a more robust determinant of mortality risk than social caste [8] , [16] . However, both findings related to mortality were influenced by recall bias of deaths within the household [17] . Age of the deceased and socioeconomically levels influence under-reporting of deaths differentially. The Sample Registration System (SRS), which is a large-scale demographic survey conducted in India, reports birth rate, death rate and other fertility and mortality indicators at the national and sub-national levels. The crude death rate from IHDS 2004-2005 was 7.4 deaths per 1000 population compared with 8.0 from the 2003 SRS ( http://censusindia.gov.in/vital_statistics/Vital_Rates/Vital_rates.aspx ; accessed on December 1, 2010). However, infant deaths from IHDS estimate was considerably lower than the SRS estimate. Furthermore, our measures do not capture all dimensions of socioeconomic status. Although multiple studies have illustrated strong link between education levels of the head of household and mother with childhood mortality [5] , [16] , [18] , [19] , education levels of individuals who died was not collected from IHDS, thus related aspects such as maternal education level and literacy rate were not included in our analysis. Given the above, conclusions drawn from the mortality analyses presented should qualitatively reflect the underlying patterns of mortality differences across social castes and household economic well-being [5] .
Our study suggests a gradual decrease in importance of social caste as an intrinsic mortality risk. Social caste influences individuals' opportunities to income-generating work and asset ownership. However when adjusted for these economic household measures, the importance of social caste attenuates while the economic measures remain strong indicators of the mortality gap. Overall, mortality differential in India remains salient. Social caste exerts strongest influence in mortality during the first year of life while economically disadvantaged households bear heavier burdens across several age groups.
Supporting Information
Predicted Probabilities of Mortality by Socioeconomic Factors, Adjusted for Gender, Age, Urban-Rural Status, Religion, Fixed Effects on States: Indian Human Development Survey, 2004-2005.
https://doi.org/10.1371/journal.pone.0016844.s001
Odds Ratio of Mortality by Social Castes, Adjusted for Income, Assets and Consumption per Capita, Fixed Effects on States: Indian Human Development Survey, 2004-2005.
https://doi.org/10.1371/journal.pone.0016844.s002
Statistical Significance ( p - value) of Mortality in Social Castes modified by Economic Factors, Adjusted for Gender, Urban-Rural Status, Fixed Effects on States: Indian Human Development Survey, 2004-2005.
https://doi.org/10.1371/journal.pone.0016844.s003
Acknowledgments
We are grateful for the India Human Development Survey data provided by Professor Sonalde Desai and the research teams from the University of Maryland and the National Council of Applied Economic Research, New Delhi.
Author Contributions
Conceived and designed the experiments: SVS. Performed the experiments: JP SVS. Analyzed the data: JP. Contributed reagents/materials/analysis tools: JP SVS. Wrote the paper: JP SVS.
- View Article
- Google Scholar
- 11. Desai S, Dubey A, Joshi BL, Sen M, Shariff A, et al. (2007) India Human Development Survey. New Delhi: University of Maryland and National Council of Applied Economic Research. 30 June 2009 ed.
- 13. Beteille A (1992) The Backward Classes in Contemporary India. New Delhi: Oxford University Press.
ORIGINAL RESEARCH article
A national-level analysis of life expectancy associated with the covid-19 pandemic in india.
- 1 Department of Health Economics, ICMR-National Institute for Research in Tuberculosis, Chennai, India
- 2 Department of Social-Behavioral Research, ICMR-National Institute for Research in Tuberculosis, Chennai, India
- 3 Department of Clinical Research, ICMR-National Institute for Research in Tuberculosis, Chennai, India
Background: From a demographic perspective, the impact of severe acute respiratory syndrome coronavirus 2 (SARS-CoV-2) on life expectancy is not clear. Hence, there is a need to study the number of years of life lost concerning the existing average life expectancy due to COVID-19 in India.
Objective: This study aimed to estimate the impact of life expectancy due to the COVID-19 pandemic in India.
Methodology: We considered day-wise age-specific mortality due to COVID-19 which was extracted from the COVID-19 data repository from March 11, 2020, to June 30, 2021, in India. All-cause mortality was collected from the United Nations population estimates. An abridged life table technique was utilized for calculating life expectancies based on all-cause mortality and mortality due to COVID-19. MortPak software was used to calculate the life expectancy with and without the COVID-19 pandemic. Life expectancy at birth in different age groups was estimated with respect to with and without COVID-19.
Results: A total of 399,459 deaths due to COVID-19 were distributed age wise, and their corresponding life expectancy was calculated. The general mortality was compared with COVID-19 mortality for the various age groups, and it was observed that mortality due to COVID-19 was significantly higher among the elderly age group [i.e., 45 to 60 years (36%) and > 60 years (51%)] when compared with < 25 years (1%) and 26–44 years (11%) (trend Chi-square 7.59; p = 0.001). The life expectancy without and with COVID-19 was 69.28 years and 69.16 years, respectively.
Conclusion: Overall, it was estimated that COVID-19 has an impact on life expectancy by 0.12 years during the study period. Even though mortality due to COVID-19 was high, factors such as lockdown, vaccination, and accidents also had an influence on mortality. Thus, there is a need to assess the impact of COVID-19 on life expectancy in future.
Introduction
Severe acute respiratory syndrome coronavirus 2 (SARS-CoV-2) emerged in Wuhan, Hubei, China, in late December 2019 ( 1 ) and was declared a pandemic by the World Health Organization ( 2 ). The SARS-CoV-2 belongs to the family of SARS and is the cause of respiratory disease known as coronavirus disease 2019 (COVID-19). The disease spreads from person to person through small droplets of an infected person's cough or sneeze, and these can settle down on the nearby object or area and can make it infectious. Symptoms including fever, cough, and pneumonia range from mild to severe ( 2 ). In addition to affecting public health, the COVID-19 pandemic globally sparked a severe demographic and socioeconomic crisis ( 2 ). The COVID-19 pandemic affected several countries, and it was the greatest threat to life expectancy, resulting in an unprecedented rise in mortality caused by COVID-19 and significant years of life lost.
Documented fatality due to COVID-19 from South Asia was 1% ( 3 , 4 ). In India, the first COVID-19 case was documented on January 27, 2020, in Kerala ( 5 ). India is the second most populous country in the world with a population of 1.35 billion, but India had a slow pace of COVID-19 spread over the first three months, from January 2020 to March 2020. A total of 399,459 deaths due to COVID-19 were reported from March 11, 2020, to June 30, 2021. Though India had the lowest mortality rate, it also has the highest recovery rate for COVID-19.
Life expectancy at birth is a commonly used indicator and a key summary measure of the health and well-being of the population. It is the widely used metric of population health and longevity. It refers to the average number of years a hypothetical cohort of people would live if they were to experience the death rates observed in a given period throughout their lifespan. The life expectancy is estimated based on the death rates for a given period. Over a period, life expectancy at birth increased significantly in most countries. It was reported that people in developed countries have higher life expectancy than others ( 6 ). Improvements in life expectancy among high-income countries were predominantly driven by gains made at older ages ( 7 ). Few countries experienced significant gains in life expectancy in the past decade ( 8 ), whereas other countries witnessed noticeable slowdowns in the pace of improvements and some countries stalls or temporary reversal ( 9 ). People with the COVID-19 infection are more prone to many life-threatening morbidities and mortalities. Almost 1.8 million estimated lives have been lost due to COVID-19 around the globe in 2020 ( 10 , 11 ). The COVID-19 pandemic has resulted in a change in the life expectancy of the population. COVID-19 mortality experience in the USA, Italy, North America, Europe, Colombia, Canada, and Liberia suggests that it has a major impact on 2020 life expectancy ( 12 ). The life expectancy losses are viewed as a cause of concern, and actual declines in life expectancy are alarming.
During the COVID-19 pandemic, life expectancy progress became more varied, and the pandemic triggered a global crisis posing additional challenges to population health. Mortality due to COVID-19 was higher in all age groups, and it was higher in females than in males and higher among the older population ( 13 ). In addition, the pandemic also indirectly increased other causes of death due to delay in diagnosis and treatment. Population-level studies on the impact of the COVID-19 pandemic through life expectancy loss were carried out in 29 countries ( 12 ). It was reported that the USA and Eastern European countries such as Lithuania, Bulgaria, and Poland experienced significant losses in life expectancy in 2020 ( 12 ).
From India, Suryakant Yadav et al. ( 14 ) reported the inequality of life expectancy between males and females and in different age groups using the Gini coefficient; Guru Vasishtha et al. ( 15 ) reported the impact of COVID-19 infection on life expectancy, premature mortality, and DALY from one state of India; and Jha et al. ( 16 ) reported the comparison of officially reported and estimated COVID-19 deaths. We do not have a population-level study on life expectancy for the entire country. Hence, this current study examines the impact of the COVID-19 pandemic on life expectancy in 2020 specific to India. We focused on the change in life expectancy in 2020 relevant to COVID-19.
Study setting
India is the second most populated country in the world accounting for one-sixth of the world's population. The present population of India in 2022 is 1,417,173,173, and the median age was 28.7 years. It is expected to become the first country to be home to more than 1.5 billion people by 2030. For people living in a resource-poor country with a high population density, planners recognized that population stabilization is an essential prerequisite for sustainable development. As per the National Population Policy, India has set the goal of achieving population stabilization by 2045. During the last five decades, there have been a sharp decline in mortality and a sustained decline in fertility. Recent trends show that India's population growth has already peaked and it is on the decline now. The total fertility rate declined significantly from 3.4 in 1993 to 2.2 in 2016 as per the National Family Health Survey 2015–2016. The ongoing global pandemic of COVID-19, which started at the end of 2019, and outbreaks have caused a significant number of deaths worldwide including India. Since then, India is one of the countries experiencing excess mortality caused by COVID-19 and has more than 10.3 million confirmed cases, and the case fatality rate was 1.4% ( 17 ). India ranks the third position globally in terms of deaths attributable to COVID-19 in 2020.
Source of data
Data for this study were collected from different secondary sources. These include the United Nations population estimates, the United Nations World Population Prospects, and the COVID-19 repository of the Center for Systems Science and Engineering (CSSE) at John Hopkins University. An age-specific all-cause mortality rate for the country for the year 2020–2021 was taken from the United Nations population estimates prepared by the Population Division of the Department of Economic and Social Affairs of the United Nations Secretariat ( 18 ). Information on the COVID-19-confirmed cases and deaths by age group and day wise was collected from the COVID-19 repository of the CSSE at John Hopkins University. The distribution of deaths due to COVID-19 provided by the COVID-19 repository of the CSSE was verified with COVID-19-India Application Programming Interface (API), Ministry of Health and Family Welfare, Government of India, portal available in the public domain ( 19 ). The estimated average years of life expectancy for India were collected from the macrotrends provided by the United Nations World Population Prospects from 1955 to 2020 ( 20 ).
Construction of life tables
A life table is a mathematical model that portrays mortality conditions at a particular time among a population and provides a basis for measuring longevity. It is based on age-specific mortality rates observed for a population for a particular year ( 21 ). In this current study, an abridged life table technique was used to calculate life expectancies based on all-cause mortality and mortality due to COVID-19. We formulated the following seven different columns to construct the life table: (1) an age-specific interval or period of life between two exact ages stated in years (x, x + n) . (2) the proportion of persons alive at the beginning of the age interval who die during the age interval ( n q x ). (3) the starting number of new born in the life table called the radix of the life table, usually set at 100,000, the number living at the beginning of the age interval, or the number surviving to the beginning of the age interval (l x ). (4) the number of persons in the cohort who die in the age interval x, x + n ( n d x ). (5) the number of years of life lived by the cohort within the indicated age interval x, x + n or person-years of life in the age interval ( n L x ). (6) the total person-years of life contributed by the cohort after attaining age x (T x ), and (7) the average number of years of life remaining for a person alive at the beginning of age interval x (e x 0 ).
Data analysis and statistical tools used
Data were entered in MS Excel. MortPak software (version 4.3) was used to estimate life expectancy at birth as well as for every 5-year age group at birth 0–1, 1–5 to 95—years. Trend Chi-square test was used to compare the life expectancy with and without COVID-19 at a 5% level of significance.
This study is merely based on a type of mathematical computation called the “differential method.” The differences in life expectancy can be explained based on observed changes in mortality ( 22 ).
Time period
We measured the variation of life expectancy in different age groups for the general population and the COVID-19-affected population from March 11, 2020, to June 30, 2021, for the first and the second waves.
Ethical considerations
This study considered secondary data which were collected from data repositories which are freely available in the public domain. Hence, ethical clearance is not required.
It was observed that overall life expectancy at birth was 69.28 years ( Supplementary Table S1 ). Life expectancy at birth will decrease as age increases (70.56 to 4.05 years). Life expectancy in different age groups was calculated ( Supplementary Table S2 ) based on 399,459 deaths due to COVID-19.
Comparison of general mortality with mortality due to COVID-19
The general mortality was compared with COVID-19 mortality for the various age groups ( Figure 1 ). It was observed that the mortality due to COVID-19 was significantly higher among the elderly age group [i.e., 45 to 60 years (36%) and > 60 years (51%)] when compared with < 25 years (1%) and 26–44 years (11%) (trend Chi-square 7.59; p = 0.001). The results highlighted that COVID-19 deaths significantly increase as age increases ( Supplementary Figure S1 ).
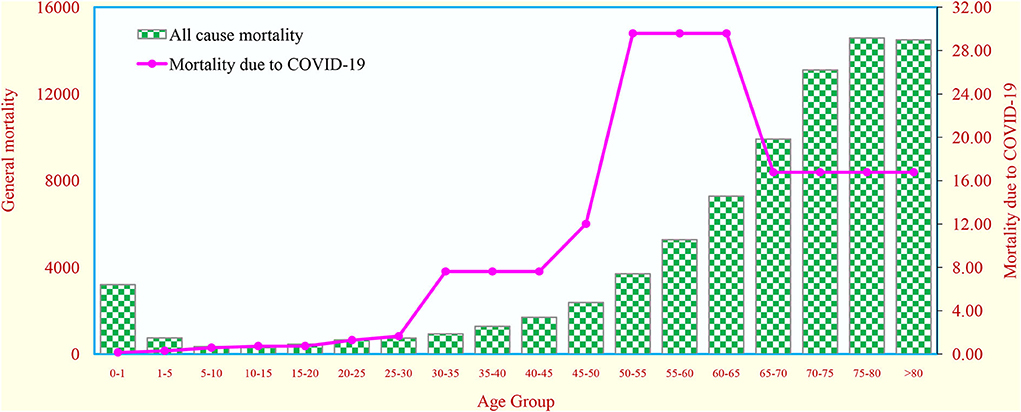
Figure 1 . Comparison of probability of death due to all–cause mortality with probability of death due to COVID−19 in different age groups in India.
Comparison of life expectancy at birth
We generated the abridged life table to compare life expectancy at birth for both all-cause mortality and COVID-19 mortality ( Supplementary Table S3 ). It was observed that there was a high mortality due to COVID-19 (0.39%). There was a decline of 43 days in life expectancy due to COVID-19 mortality ( Supplementary Table S4 ). The life expectancy without and with COVID-19 was 69.28 years and 69.16 years, respectively.
Comparison of age-specific life expectancy
We compared the life expectancy with and without COVID-19 in various age groups ( Supplementary Table S4 ). The life expectancy with COVID-19 in 0–1 year, 45–50 years, 60–65 years, 75–80 years, 85–90 years, and more than 90 years was 0.28, 0.98, 1.03, 0.99, 1.14, and 1.05 years, respectively, whereas the life expectancy without COVID−19 in 0–1 year, 45–50 years, 60–65 years, 75–80 years, 85–90 years, and more than 90 years was 0.16, 0.87, 0.93, 0.87, 0.92, and 0.64 years, respectively. The difference between the life expectancy with and without COVID−19 in 0–1 year, 45–50 years, 60–65 years, 75–80 years, 85–90 years, and more than 90 years was 0.12, 0.11, 0.10, 0.12, 0.22, and 0.41 years, respectively ( Figure 2 ).
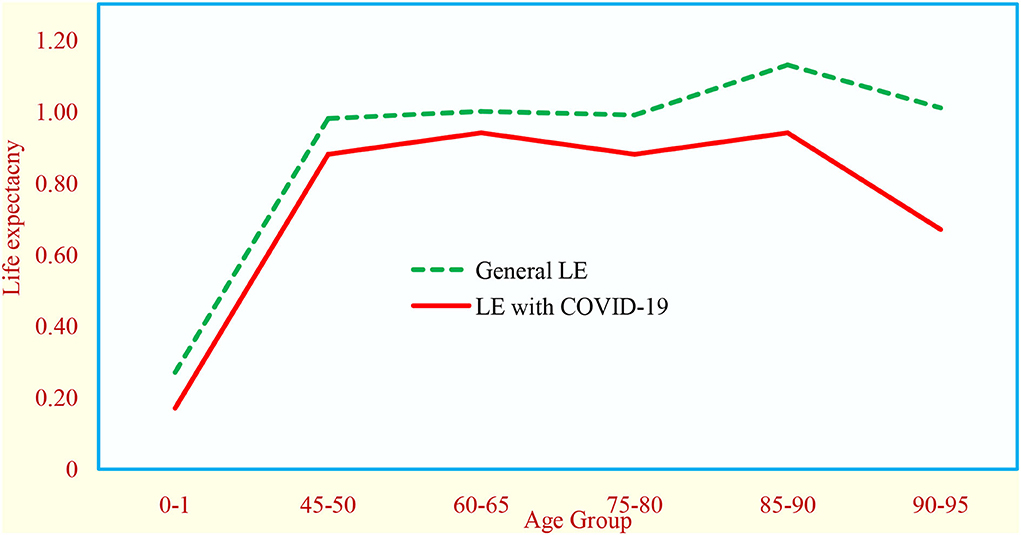
Figure 2 . Effect of COVID−19 on life expectancy at birth in India (June 2020 estimates).
Life expectancy in India over a period
From 1950 to 2021, life expectancy at birth increased from 36.98 to 69.28 years, while a gain of 32.3 years seems to be a significant increase, which represents a decrease in rate over a period ( Supplementary Figure S2 ) in India. From 1950 to 1970, there was an increasing trend (average 3.1 years), there was a decreasing trend during 1970–1985 (average 2.6 years), it was almost stable during 1985–2010 (average 2.1 years), and then, there was a decreasing trend (average 1.9 years) ( Figure 3 ) in the subsequent years.
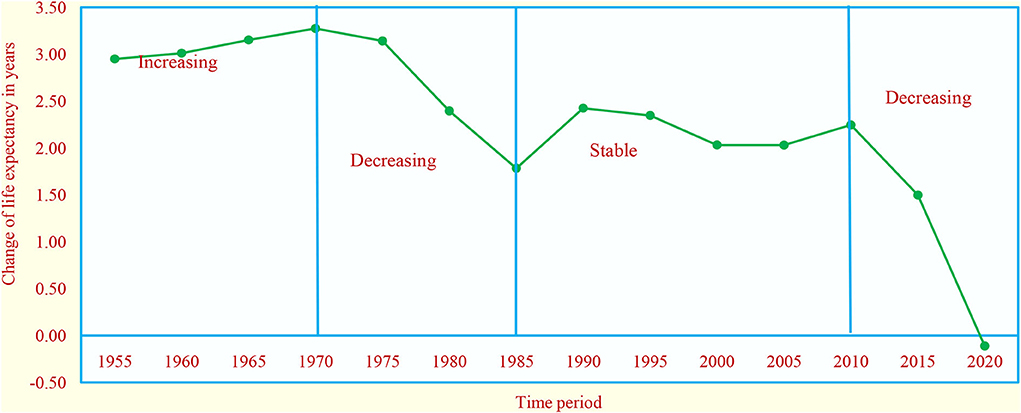
Figure 3 . Years of life expectancy change in five–year intervals in India.
The current study has documented the national–level analysis of life expectancy associated with the COVID−19 pandemic based on 399,459 reported deaths from 2020 to 2021. This study found that there is a decrease in life expectancy by 43 days (0.12 years) due to COVID−19. Similar studies from developed countries reported that those who were severely affected by COVID−19 have also lost significant years of life. It was estimated that there is a decline in life expectancy in Canada by 0.41 years ( 23 ), in Italy by 0.5 years ( 12 ), and in Columbia by 0.18 ( 12 ) years.
The other salient finding from our study was that there was a decrease in life expectancy in all the age groups. However, increased mortality among the elderly age group (i.e., more than 60 years) resulted in a decrease in life expectancy during the pandemic. A similar finding reported that more than one–third of COVID−19 deaths were in the age group of more than 65 years ( 24 ). As COVID−19 caused an excessive number of deaths in the elderly population, the number of years lost concerning the existing average life expectancy might be smaller than expected. As long as the prevalence does not exceed a particular threshold, the loss in life expectancy is minimal. With a prevalence rate of < 1%, the years of life lost are anticipated to be less than the yearly secular increase, which is approximately 0.2 in high–income countries. It is a known fact that the increase in mortality will result in a decline in period life expectancy. It was documented that in North America and Europe, the loss in period life expectancy would vary from 4 to 11 years at very high mortality due to COVID−19 ( 25 , 26 ). In India, Maharashtra was significantly affected by the COVID−19 pandemic and the impact on life expectancy at birth was estimated, and it is likely to reduce by 1.4 years ( 12 ). This was higher as compared to our current national–level estimate.
It is a well–known fact that as the age increases, there will be a considerable reduction in life expectancy. More than 60% of COVID−19 cases are in the age group of 30–64 years which is the economically productive segment of the population. From an economic perspective, health and longevity are very consequential. Health is believed to drive economic growth due to a healthier workforce that is more productive. The pandemic will have short– and long–term implications on socioeconomic determinants of health. In addition, a demographic perspective of the impact of COVID−19 on life expectancy also should be considered, as the mortality in a particular age group of the population may affect the structure of the population.
During the first wave due to the national lockdown and other preventive measures, there was not much death reported. Currently, India is facing the fourth wave, and the last two waves severely affected the country concerning the number of COVID−19 infections, where the disease remains a constant threat due to a large number of population, cities with compact settings, and inadequate healthcare facilities. The consequences of the COVID−19 pandemic, particularly in terms of mortality, will be reduced eventually by the existing interventions such as vaccination, XraySetu (AI–driven platform) for early identification of COVID−19 positivity, and rapid antigen test (RATs) COVID−19 home testing kit.
The life table technique is used by actuaries, demographers, and many others to study reproduction, migration, fertility, and population growth. It is also used to make a relative comparison of various measures of mortality such as death rate and expectations of life for two or more different groups of the population. The life table is accepted widely as an important tool in demographic and public health studies. In this current study, we used the life table technique to measure the impact of COVID−19 on life expectancy in India.
In this study, we are not able to calculate gender–specific life expectancy due to the non–availability of data. We employed data for a single cross section of the period from March 2020 to June 2021 to describe the current mortality pattern due to COVID−19. They are officially reported death data, but not actual death data. The main advantage is that it provides measures localized in time. Also, the data pertain to a limited period.
The life expectancy in India overall seemed to be radically increasing over a period, despite past experiences of the toll of deaths caused by many infectious diseases. The fluctuations in the life expectancy over a period were due to a number of infectious diseases such as HIV and influenza in India. In the USA and Liberia, previous epidemics such as the 1918 influenza pandemic and the 2014 Ebola virus outbreak resulted in the decline in life expectancy by 11.8 years and 1.6–5.6 years, respectively ( 27 ). The epidemiological transition can be controlled by reducing the deaths from endemic diseases through vaccination and immunization programs, better sanitation, better housing, social welfare programs, and improved health system practices through technological advancements and biomedical research.
Life expectancy is primarily a public health measure that can be compared across the nations and large subpopulation. It has been used as an indicator for both economic success and the effectiveness of medical care. The COVID−19 pandemic is the recent experience of high morbidity and mortality that will reverse the secular trend of increasing life expectancy, resulting in a drop in period life expectancy. This study found that there is a decrease in life expectancy by 43 days (0.12 years) due to COVID−19 during 2020. Even though mortality due to COVID−19 was high, factors such as lockdown, vaccination, and accidents also had an influence on mortality. Thus, there is a need to assess the impact of COVID−19 on life expectancy in future. Further, a comprehensive pandemic preparedness aimed at more resilient health systems, interventions on the reduction of premature mortality, and timely monitoring of excess mortality will help future policy interventions.
Data availability statement
The datasets presented in this study can be found in online repositories. The names of the repository/repositories and accession number(s) can be found in the article/ Supplementary material .
Author contributions
MM, PS, and NK were responsible for conceptualization of the study, data collection, supervision, project administration, and formal analysis. MM, PS, YA, and NK were responsible for the methodology. MM, PS, and YA were responsible for data collection. MM, NK, and CP were responsible for the original draft preparation. MM, PS, YA, NK, and CP were responsible for review and editing of the manuscript. All authors have read and agreed to publish the manuscript.
Conflict of interest
The authors declare that the research was conducted in the absence of any commercial or financial relationships that could be construed as a potential conflict of interest.
Publisher's note
All claims expressed in this article are solely those of the authors and do not necessarily represent those of their affiliated organizations, or those of the publisher, the editors and the reviewers. Any product that may be evaluated in this article, or claim that may be made by its manufacturer, is not guaranteed or endorsed by the publisher.
Supplementary material
The Supplementary Material for this article can be found online at: https://www.frontiersin.org/articles/10.3389/fpubh.2022.1000933/full#supplementary-material
1. Zhu N, Zhang D, Wang W, Li X, Yang B, Song J, et al. A novel coronavirus from patients with pneumonia in China, 2019. N Engl J Med. (2020) 382:727–33. doi: 10.1056/NEJMoa2001017
PubMed Abstract | CrossRef Full Text | Google Scholar
2. World Health Organisation. Coronavirus disease (COVID-19). World Health Organisation. (2020).
Google Scholar
3. Babu GR, Khetrapal S, John DA, Deepa R, Narayan KMV. Pandemic preparedness and response to COVID-19 in South Asian countries. Int J Infect Dis. (2021) 104:169–74. doi: 10.1016/j.ijid.2020.12.048
4. Ram VS, Babu GR, Prabhakaran D. COVID-19 pandemic in India. Eur Heart J . (2020) 41:3874–6. doi: 10.1093/eurheartj/ehaa493
5. Andrews MA, Areekal B, Rajesh KR, Krishnan J, Suryakala R, Krishnan B, et al. First confirmed case of COVID-19 infection in India: a case report. Indian J Med Res. (2020) 151:490–2. doi: 10.4103/ijmr.IJMR_2131_20
6. Yves Decady, Lawson Greenberg. Ninety years of change in life expectancy. Statistics Canada Catal . (2014) 82:1–8 Available online at: https://www150.statcan.gc.ca/n1/en/pub/82-624-x/2014001/article/14009-eng.pdf ?st=2oJBS-HR (accessed March 15, 2022).
7. Aburto JM, Villavicencio F, Basellini U, Kjærgaard S, Vaupel JW. Dynamics of life expectancy and life span equality. Proc Natl Acad Sci USA. (2020) 117:5250–59. doi: 10.1073/pnas.1915884117
8. Aburto JM, van Raalte A. Lifespan dispersion in times of life expectancy fluctuation: the case of central and Eastern Europe. Demography. (2018) 55:2071–96. doi: 10.1007/s13524-018-0729-9
9. Ho JY, Hendi AS. Recent trends in life expectancy across high income countries: retrospective observational study. BMJ. (2018) 362:k2562 doi: 10.1136/bmj.k2562
10. Dong E, Du H, Gardner L. An interactive web-based dashboard to track COVID-19 in real time. Lancet Infect Dis. (2020) 20:533–34. doi: 10.1016/S1473-3099(20)30120-1
11. World Health Organization. The True Death Toll of COVID-19: Estimating Global Excess Mortality. World Health Organization. (2020).
12. Manuel Aburto J, Schöley J, Kashnitsky I, Zhang L, Rahal C, Missov TI, et al. Quantifying impacts of the COVID-19 pandemic through life-expectancy losses: a population-level study of 29 countries. Int J Epidemiol. (2022) 51:63–74. doi: 10.1093/ije/dyab207
13. Jin JM, Bai P, He W, Wu F, Liu XF, Han DM, et al. Gender differences in patients with COVID-19: Focus on severity and mortality. Front Public Health. (2020) 8:152. doi: 10.3389/fpubh.2020.00152
14. Yadav S, Yadav PK, Yadav N. Impact of COVID-19 on life expectancy at birth in India: a decomposition analysis. BMC Public Health. (2021) 21:1906. doi: 10.1186/s12889-021-11690-z
15. Vasishtha G, Mohanty SK, Mishra US, et al. Impact of COVID-19 infection on life expectancy, premature mortality, and DALY in Maharashtra, India. BMC Infect Dis. (2021) 21:343 doi: 10.1186/s12879-021-06026-6
16. Jha P, Deshmukh Y, Tumbe C, Suraweera W, Bhowmick A, Sharma S, et al. mortality in India: National survey data and health facility deaths. Science. (2022) 375:667–71. doi: 10.1126/science.abm5154
17. Yadav S, Yadav PK, Kishore M, Unisa S, Shekhar C, Ram U, et al. Basic reproduction rate and case fatality rate of COVID-19: application of Meta-analysis. Demography India . (2020) 49:76–97 doi: 10.1101/2020.05.13.20100750
CrossRef Full Text | Google Scholar
18. United Nations. World Population Prospects 2019. Population Division of the Department of Economic and Social Affairs of United Nations Secretariat 2020 . Available online at: https://population.un.org/wpp (accessed January 17, 2022).
19. COVID19-India API. A Volunteer-Driven, Crowd-Sourced Database for COVID19 Stats & Patient Tracing In India (Online) . (2020). Available online at: https://api.covid19india.org/ (accessed January 20, 2022).
20. Macrotrends. India Life Expectancy 1950-2022 . Available online at: https://www.macrotrends.net/countries/IND/india/life-expectancy (accessed January 17, 2022).
21. Sociology Discussion. Life Table: Meaning, Types and Importance. Sociology Discussion . Available online at: www.sociologydiscussion.com/demography/population-growth/life-table-meaning-types-and-importance/3030 (accessed March 17, 2022).
22. Pollard JH. On the decomposition of changes in expectation of life and differentials in life expectancy. Demography. (1988) 25:265–76. doi: 10.2307/2061293
23. Patrice Dion. Demographic Documents: Reductions in Life Expectancy Directly Associated With COVID-19 in 2020. Statistics Canada 2021 . Available online at: https://www150.statcan.gc.ca/n1/pub/91f0015m/91f0015m2021002-eng.htm (accessed February 7, 2022).
24. Mohanty SK, Sahoo U, Mishra USDM. Age pattern of premature mortality under varying scenarios of COVID-19 Infection in India. MedRxiv. (2020). doi: 10.1101/2020.06.11.20128587
25. European mortality monitoring activity (EuroMOMO). Pooled Mortality Estimates From The EuroMOMO Network . EuroMOMO Bulletin 2020). Available online at: https://www.euromomo.eu/bulletins/2020-15 (accessed March 10, 2022).
26. Marois G, Muttarak R, Scherbov S. Assessing the potential impact of COVID-19 on life expectancy. PLoS ONE. (2020) 15:e0238678. doi: 10.1371/journal.pone.0238678
27. Helleringer S, Noymer A. Assessing the direct effects of the Ebola outbreak on life expectancy in Liberia, Sierra Leone and Guinea. PLoS Curr . (2015) 19:d1749574ea. doi: 10.1371/currents.outbreaks.01a99f8342b42a58d806d7d1749574ea
Keywords: COVID-19, life expectancy, longevity, mortality, India
Citation: Muniyandi M, Singh PK, Aanandh Y, Karikalan N and Padmapriyadarsini C (2022) A national-level analysis of life expectancy associated with the COVID-19 pandemic in India. Front. Public Health 10:1000933. doi: 10.3389/fpubh.2022.1000933
Received: 22 July 2022; Accepted: 26 September 2022; Published: 18 October 2022.
Reviewed by:
Copyright © 2022 Muniyandi, Singh, Aanandh, Karikalan and Padmapriyadarsini. This is an open-access article distributed under the terms of the Creative Commons Attribution License (CC BY) . The use, distribution or reproduction in other forums is permitted, provided the original author(s) and the copyright owner(s) are credited and that the original publication in this journal is cited, in accordance with accepted academic practice. No use, distribution or reproduction is permitted which does not comply with these terms.
*Correspondence: Malaisamy Muniyandi, muniyandi.m@icmr.gov.in
Disclaimer: All claims expressed in this article are solely those of the authors and do not necessarily represent those of their affiliated organizations, or those of the publisher, the editors and the reviewers. Any product that may be evaluated in this article or claim that may be made by its manufacturer is not guaranteed or endorsed by the publisher.
We use cookies. Read more about them in our Privacy Policy.
- Accept site cookies
- Reject site cookies
Search results:
- Afghanistan
- Africa (African Union)
- African Union
- American Samoa
- Antigua and Barbuda
- Bolivia (Plurinational State of)
- Bonaire, Sint Eustatius and Saba
- Bosnia and Herzegovina
- British Virgin Islands
- Brunei Darussalam
- Burkina Faso
- Cayman Islands
- Central Africa (African Union)
- Central African Republic
- Channel Islands
- China, Hong Kong Special Administrative Region
- China, Macao Special Administrative Region
- China, Taiwan Province of China
- Cook Islands
- Côte d'Ivoire
- Democratic People's Republic of Korea
- Democratic Republic of the Congo
- Dominican Republic
- Eastern Africa (African Union)
- El Salvador
- Equatorial Guinea
- Falkland Islands (Malvinas)
- Faroe Islands
- French Guiana
- French Polynesia
- Guinea-Bissau
- Humanitarian Action Countries
- Iran (Islamic Republic of)
- Isle of Man
- Kosovo (UNSCR 1244)
- Lao People's Democratic Republic
- Liechtenstein
- Marshall Islands
- Micronesia (Federated States of)
- Netherlands (Kingdom of the)
- New Caledonia
- New Zealand
- North Macedonia
- Northern Africa (African Union)
- Northern Mariana Islands
- OECD Fragile Contexts
- Papua New Guinea
- Philippines
- Puerto Rico
- Republic of Korea
- Republic of Moldova
- Russian Federation
- Saint Barthélemy
- Saint Helena, Ascension and Tristan da Cunha
- Saint Kitts and Nevis
- Saint Lucia
- Saint Martin (French part)
- Saint Pierre and Miquelon
- Saint Vincent and the Grenadines
- Sao Tome and Principe
- Saudi Arabia
- Sierra Leone
- Sint Maarten
- Solomon Islands
- South Africa
- South Sudan
- Southern Africa (African Union)
- State of Palestine
- Switzerland
- Syrian Arab Republic
- Timor-Leste
- Trinidad and Tobago
- Turkmenistan
- Turks and Caicos Islands
- United Arab Emirates
- United Kingdom
- United Republic of Tanzania
- United States
- Venezuela (Bolivarian Republic of)
- Virgin Islands U.S.
- Wallis and Futuna
- Western Africa (African Union)
Search countries
Search for data in 245 countries
- SDG Progress Data
- Child Marriage
- Immunization
- Benchmarking child-related SDGs
- Maternal and Newborn Health Disparities
- Continuity of essential health services
- Country profiles
- Interactive data visualizations
- Journal articles
- Publications
- Data Warehouse
- Côte d’Ivoire
- Democratic People’s Republic of Korea
- Lao People’s Democratic Republic
Key demographic indicators
1,428,627,663
Trends in under-five mortality rate in India
Child health, careseeking for ari – percentage of children (under age 5) with acute respiratory infection symptoms whom advice or treatment was sought from a health facility or provider, diarrhoea treatment – percentage of children (under age 5) with diarrhoea who received ors (packets or pre-packaged fluids), itn use by children – percentage of children (under age 5) who slept under an insecticide-treated mosquito net the night prior to the survey, percentage of households with at least one insecticide-treated mosquito net (itn), percentage of surviving infants who received the third dose of dtp-containing vaccine, percentage of children who received the 2nd dose of measles-containing vaccine, as per administered in the national schedule, child protection, percentage of children under age 5 whose births are registered, percentage of women (aged 20-24 years) married or in union before age 18, percentage of children (aged 5-17 years) engaged in child labour (economic activities and household chores), percentage of children (aged 1-14 years) who experienced any physical punishment and/or psychological aggression by caregivers, percentage of women (aged 15-49 years) who consider a husband to be justified in hitting or beating his wife for at least one of the specified reasons, percentage of men (aged 15-49 years) who consider a husband to be justified in hitting or beating his wife for at least one of the specified reasons, percentage of girls and women (aged 15-49 years) who have undergone female genital mutilation (fgm), child survival, under-five mortality rate, under-five deaths, infant mortality rate, neonatal mortality rate, under-five mortality rate (female), under-five mortality rate (male), early childhood, percentage of children aged 36-59 months attending an early childhood education programme, percentage of children (aged 24-59 months) with whom any adult household member has engaged in 4 or more activities to provide early stimulation and responsive care in the last 3 days, percentage of children (aged 24-59 months) whose father has engaged in 4 or more activities to provide early stimulation and responsive care in the last 3 days, percentage of children under age 5 who have 3 or more children’s books, percentage of children under age 5 who play with 2 or more types of playthings, percentage of children under age 5 left alone or under the supervision of another child younger than 10 years of age for more than 1 hour at least once in the last week, adjusted net attendance rate, one year before the official primary entry age, adjusted net attendance rate for children of primary school age, adjusted net attendance rate for adolescents of lower secondary school age, adjusted net attendance rate for youth of upper secondary school age, completion rate for children of primary school age, youth literacy rate for 15-24 years, per cent of pregnant women living with hiv receiving effective arvs for pmtct (excludes single-dose nevirapine), estimated number of new hiv infections (children aged 0-19 years), reported number of children (aged 0-14 years) receiving antiretroviral treatment (art), per cent of young people (aged 15-24 years) who were tested for hiv in the last 12 months and received the result of the last test (female), per cent of young people (aged 15-24 years) who were tested for hiv in the last 12 months and received the result of the last test (male), estimated number of new hiv infections (adolescents and young people aged 15-24 years), maternal and newborn health, postnatal care for mothers – percentage of women (aged 15-49 years) who received postnatal care within 2 days of giving birth (female), antenatal care 4+ visits – percentage of women (aged 15-49 years) attended at least four times during pregnancy by any provider (female), skilled birth attendant – percentage of deliveries attended by skilled health personnel (female), c-section rate – percentage of deliveries by cesarean section, early childbearing – percentage of women (aged 20-24 years) who gave birth before age 18 (female), maternal mortality ratio (number of maternal deaths per 100,000 live births) (female), postnatal care for newborns – percentage of newborns who have a postnatal contact with a health provider within 2 days of delivery, early initiation of breastfeeding, exclusive breastfeeding (0-5 months), continued breastfeeding (20-23 months), height-for-age <-2 sd (stunting), modeled estimates, vitamin a supplementation, two-dose coverage, iodized salt consumption (>0 ppm) among all tested households, proportion of population using safely managed sanitation services, proportion of population using basic sanitation services, proportion of population using at least basic sanitation services, proportion of population using limited sanitation services, drinking water, proportion of population using safely managed drinking water services, proportion of population using basic drinking water services, proportion of population using at least basic drinking water services, proportion of population using limited drinking water services, proportion of population using unimproved drinking water sources, proportion of population using surface water.
Since its inception in 1995, the Multiple Indicator Cluster Surveys, known as MICS, has become the largest source of statistically sound and internationally comparable data on women and children worldwide. In countries as diverse as Costa Rica, Mali and Qatar, trained fieldwork teams conduct face-to-face interviews with household members on a variety of topics – focusing mainly on those issues that directly affect the lives of children and women.
See MICS Surveys by Country | About MICS
Join our community
Receive the latest updates from the UNICEF Data team
- Don’t miss out on our latest data
- Get insights based on your interests
The dataset you are about to download is licensed under a Creative Commons Attribution 3.0 IGO license.
- Research article
- Open access
- Published: 21 August 2021
Preceding child survival status and its effect on infant and child mortality in India: An evidence from National Family Health Survey 2015–16
- Shobhit Srivastava 1 ,
- Shubhranshu Kumar Upadhyay 2 ,
- Shekhar Chauhan ORCID: orcid.org/0000-0002-6926-7649 3 &
- Manoj Alagarajan 4
BMC Public Health volume 21 , Article number: 1577 ( 2021 ) Cite this article
4378 Accesses
10 Citations
Metrics details
India has achieved impressive gains in child survival over the last two decades; however, it was not successful in attaining MDG 2015 goals. The study’s objective is to inquire how the survival status of the preceding child affects the survival of the next born child.
This is a retrospective analysis of data from the National Family Health Survey, 2015–16. Analysis was restricted to women with second or higher-order births because women with first-order births do not have a preceding child. Proportional hazards regression, also called the Cox regression model, has been used to carry out the analysis. Kaplan–Meier (K–M) survival curves were also generated, with a focus on preceding birth intervals.
Results found that female children were more likely to experience infant mortality than their male counterparts. Children born after birth intervals of 36+ months were least likely to experience infant mortality. Mother’s education and household wealth are two strong predictors of child survival, while the place of residence and caste did not show any effect in the Cox proportional model. Infant and child deaths are highly clustered among those mothers whose earlier child is dead.
Maternal childbearing age is still low in India, and it poses a high risk of infant and child death. Education is a way out, and there is a need to focus on girl’s education. The government shall also focus on raising awareness of the importance of spacing between two successive births. There is also a need to create a better health infrastructure catering to the needs of rich and poor people alike.
Peer Review reports
According to 2017 data, 4.1 million infants died, accounting for almost 75% of all fatalities among children under the age of five [ 1 ]. From 65 deaths per 1000 live births in 1990 to 29 deaths per 1000 live births in 2017, the global infant mortality rate has decreased [ 1 ]. Annual infant deaths have declined from 8.8 million in 1990 to 4.1 million in 2017 [ 1 ]. On the other hand, 5.4 million children under the age of five die in the same year, equating to 15,000 fatalities each day [ 2 ]. The risk of a child dying before completing five years of age is still highest in the WHO African Region (74 per 1000 live births), around eight times higher than that in the WHO European Region (9 per 1000 live births) [ 2 ]. Premature birth, pneumonia, congenital impairments, neonatal infections, malaria, sepsis, measles, delivery difficulties, and diarrhoea are all well-documented avoidable causes of mortality in children under five [ 3 , 4 ].
Over the previous two decades, India has made significant advances in child survival; nonetheless, it failed to meet the MDG 2015 targets. In India, the U5MR has decreased by 3% each year since 1990, from 114 per 1000 live births in 1990 to 39 in 2016 [ 5 ]. Similarly, IMR decreased from 81 to 34 per 1000 live births between 1990 and 2016 [ 5 ]. Children born to mothers who had completed eight years of schooling had a better probability of surviving, but children born to adolescent mothers had a greater risk of dying [ 6 ]. Some preventative strategies for child health included exclusive breastfeeding, high-quality complementary foods, correct micronutrient feeding, vitamin A and iron, sufficient handwashing, and complete vaccination [ 7 , 8 , 9 ].
The previous study found that the effect of short preceding intervals on children under age five years was mainly concentrated in the neonatal period [ 10 , 11 ]. Furthermore, previous research has shown that prenatal variables are more significant than postnatal ones in the causal pathway between early childhood mortality and early childbearing. If the gap between births was less than 24 months, the combined effects of pregnancy and breastfeeding on a mother’s physiology and nutritional condition might represent a mechanism through which short intervals impact child health. Low birth weight, preterm, and poor breastfeeding ability are all possible outcomes.
Even after adjusting for neonatal mortality, infants delivered after short birth intervals had a slightly greater probability of not being breastfed, which might imply that mothers who do not breastfeed have shorter birth intervals [ 12 ]. Longer birth intervals are associated with a decreased risk of child mortality. When the correlations were stratified by maternal completed fertility, the link between short birth intervals and child mortality vanished almost entirely for women with low completed fertility. Both shorter intervals and a higher risk of repeat infant mortality occur in families that have experienced one early infant death; the death of a second child after a short interval may not be due to the interval but to some other factor that also caused the first death and the shortened interval [ 13 ].
Short birth intervals may not deplete the mother’s nutritional resources to the degree that raises the child’s risk of death since low fertility women may have superior nutritional status and access to treatment. For high-fertility moms, nutritional status and access to treatment may be impacted from the outset [ 14 , 15 ]. Multiple births are associated with a greater risk of mortality, according to numerous research. Furthermore, household wealth is a major predictor of infant mortality, but the mother’s education and age at first birth were not shown to be significant predictors of death [ 16 ].
Previous literature confirms that first-born children of adolescent mothers are the most vulnerable to infant mortality and poor child health outcomes [ 17 ]. This issue can be confirmed by the fact that low maternal age is highly associated with the stunting of the child [ 17 ]. Offspring mortality had a U-shaped association with maternal age, as compared to the reference group of 20–24 years, younger (≤19 years) and older (≥35 years) maternal ages were associated with a higher risk of offspring mortality [ 18 ]. However, the higher age of the mother contributes to an increased risk of preterm delivery, which poses a high risk of infant mortality to the male gender than their female counterparts [ 19 ]. Various prior research has shown that birth order and infant and child mortality risk have a substantial association. The birth-order impact is said to be significantly greater in the post-neonatal period. The chance of dying increases as the birth order rises, and this relationship is further divided between male and female children.
Furthermore, the mother’s age and education and the child’s sex and birth weight all play a role in understanding the link between birth order and infant mortality [ 20 ]. Early neonatal, neonatal, post-neonatal, and infant mortality are all likely to have a U–shaped association with the mother’s age at birth [ 21 ]. Even after accounting for the previous birth–to–conception gap, the child’s birth order, and other possible confounders, the U-shaped connection remains [ 21 ]. Women’s education contributes to child survival through increasing health knowledge and giving them more influence over their children’s health decisions, as well as preventing and controlling childhood illnesses [ 6 , 8 , 22 , 23 , 24 , 25 ]. Education attainment has been noted to improve social and economic standards, further determining maternal health care utilization [ 24 ].
India can play a key role in global efforts to stop the preventable death of newborns and children under the age of five since it has the greatest number of deaths linked to newborns and children under the age of five. In the study of newborn and child mortality, the previous child survival status is a significant predictor [ 25 , 26 ], which further is linked to improved infant and child mortality [ 22 ]. Furthermore, previous studies have noted a higher prevalence of child death in families with a prior history of experiencing child loss [ 27 , 28 ]. While there is a plethora of studies on the factors that influence newborn and child mortality, there is little data on the relationship between previous child survival status and infant and child death. Only a small amount of study has been done on the link between the death of the previous child and the following impact on infant and child mortality.
Zenger (1993) describes the mechanism that stems from the death of a child may plausibly raise the risk of death of his or her subsequent sibling [ 29 ]. She only estimated either the previous sibling’s survival status or mother-related unobserved heterogeneity, and not both [ 29 ]. However, some other studies have included both [ 30 , 31 , 32 , 33 ]. Bolstad & Manda (2001), utilizing Malawi Demographic and Health Survey, learned that infant and child deaths tend to cluster in some families with a history of a previous child [ 30 ]. However, Sastry (1997) uncovered that childhood mortality clustering is mainly attributed to birth spacing and that death of the previous child followed by a shorter birth spacing period would be a great risk for the subsequent child when investigating the pathways of family-level clustering of childhood mortality in Northeast Brazil [ 33 ].. Guo (1993), using sibling data to estimate family mortality effects in Guatemala, estimated that each child loss in the family was associated with a 22% increase in the risk of death to the index child [ 32 ]. The study from Guo (1993) was different from this study as that study could link the risk of death of index child to several previous birth histories whereas, in this study, the risk is linked to only previous birth history [ 32 ]. Curtis et al. (1993) noted that birth interval between two successive births was an important predictor of childhood mortality in Brazil [ 31 ]. The risk of death of an index child attributed to the death of a previous child was higher when the birth spacing was low [ 31 ].
In light of the foregoing explanation, this study aimed to see how the survival status of the previous child influences the survival status of the next born child. The present study hypothesized that there was no significant link between the survival status of the previous child and the survival status of the following born child.
This is a retrospective data from the 2015–16 National Family Health Survey. The fourth in the NFHS series, the 2015–16 National Family Health Survey (NFHS-4), offers data on India’s population, health, and nutrition for each state and union territory [ 34 ]. All four NFHS surveys have been conducted under the stewardship of the Ministry of Health and Family Welfare (MoHFW), Government of India [ 34 ]. MoHFW designated the International Institute for Population Sciences (IIPS), Mumbai, as the nodal agency for all surveys [ 34 ]. The National Family Health Survey (NFHS) is a cross-sectional household survey performed on representative samples selected throughout India’s 36 states [ 34 ]. The birth history data set contains data on 1,315,617 infants born between 1970 and 2016 who were born to 476,619 women. On the entire birth history of mothers, we conducted two different analyses. The first analysis looked at newborn death, whereas the second looked at child death separately. In the previous five years, only singleton births were included in the study. Only children with birth orders of two or above are included in the sample. As a result, the study’s analytical sample size was 824,693 children aged 0 to 59 months in India.
Variable description
Outcome variables.
The current study had two outcome variables: infant (0–11 months) and child (12–59 months) mortality. Infant mortality refers to a child’s death before his first birthday, whereas child mortality refers to a child’s death from his first birthday to the day before his fifth birthday.
Explanatory variables
The key explanatory variables were any preceding child’s survival status (alive, dead), the child’s sex (male, female), maternal age at childbirth (18, 18–34, 35+), birth order (2, 3, and 4+), and the preceding birth interval (PBI), defined as the difference in months between the index child’s birth date and the preceding child’s birth date. It was categorized as a four-category variable (< 19 months, 18–23 months, 24–35 months, and 36 months), and the status of having multiple births was classified as (single and multiple). Other mothers socio-economic variables were taken into account, education (including illiteracy, primary, secondary, and higher education), religion (Hindu, Muslims, and Others), Caste (deprived: Scheduled Caste/Scheduled Tribe (SC/ST) and not-deprived: other than SC/ST), wealth index (poorest, poorer, middle, richer and richest), type of residence (urban and rural), regions of India (north, central, east, north-east, west and south).
Statistical analysis
The unadjusted estimates were derived using bivariate analysis. To achieve the objectives of the study, Cox regression (also known as proportional hazards regression) was employed.
In this study, infant and child fatalities were utilised to conduct the analysis. The Kaplan–Meier (K–M) survival curves for the PBI were also calculated [ 16 ]. The dataset was analysed, and the model’s fitness was assessed using non-parametric K–M survival curves [ 16 ]. The index child’s age (in months) was used as the time variable, and the child’s death was used as the censoring variable in the Cox models [ 16 ]. Fotso et al. (2016) also defined the hazard of mortality at any point in time t is given as [ 16 ]:
Where h (t) is the baseline hazard, representing the probability of the child dying before any exposure to X [ 16 ].
In this study, the proportionality assumptions of all Cox models were evaluated. The Schoenfeld proportionality test was used in this study [ 16 ], a technique for re-estimating the Cox proportional hazard model using residuals [ 16 ]. The multivariate analyses were carried out through four models [ 16 ]. The major explanatory variables (survival status of any previous child) was represented as a binary variable (alive or dead) in Model 1. Model 2 incorporates model 1 as well as five additional bio-demographic variables: the child’s gender, maternal age at childbirth, PBI, and single/multiple births. Model 3 incorporates the mother’s remaining background variables, such as education, religion, caste, wealth index, type of residence, and regions, into model 1. All of the additional variables from all three models are combined in Model 4. The data reported in the study were analysed using STATA 13.
Table 1 shows the percentage of infant and child deaths by their background characteristics in India. Births succeeded by a dead child had the highest infant (15.21) and child deaths (2.86). Infant deaths constitute more deaths of male children (4.98), while child mortality was higher among females (1.61). Higher infant (5.61) and child deaths (1.63) were noticed for maternal age below 18 years. Noticeably, 4+ birth order was associated with higher infant (6.43) and child mortality rates (1.93). Births with preceding birth intervals shorter than 19 months had the highest infant (10.22) and child mortality rate (2.36). A considerably higher number of infants (26.61) and child deaths (1.82) were recorded in the case of multiple births. Among maternal socioeconomic factors, the education of mother and household wealth index showed a negative association with infant and child mortality rates. A significantly higher number of infant and child deaths were found among children belonging to the Hindu religion (5.04 & 1.39 respectively) and deprived section of the society (5.31 & 1.67 respectively). Higher infant (5.29) and child deaths (1.51) were found in the rural areas compared to urban areas. Region-wise, the highest infant deaths were found in the central region (6.79), while a significant preponderance of child deaths was found in the central region of India (2.05).
Table 2 predicts the results of the hazard models on the determinants of infant mortality . In model 1, the partial effect of survival status of the preceding child was strong as the infants with the preceding child as dead were more likely to experience infant mortality (3.43*) than the alive ones. Model 2 includes bio-demographic factors along with the survival status of the preceding child. After the inclusion of other factors, the effect of survival status on infant death reduces to a certain extent (2.63*). Female children were more likely to experience infant mortality than their male counterparts (1.16*). Higher maternal age at childbirth (18–34) depicted a lower likelihood of infant deaths (0.85*) than the below 18 age group. Children born after a birth interval of 36+ months were less likely to experience infant mortality (0.61*) compared to a birth interval of less than 19 months. Lastly, multiple births showed more likelihood of infant mortality (4.56*) than single births. Model 3 includes the survival status of the preceding child along with the maternal socioeconomic factors. In model 3, the effect of the survival status of the preceding child on infant mortality remains substantial (3.08*), implying that infant mortality was more likely to occur among the births with a preceding dead child. Among these factors, place of residence came up as insignificant while the education of mother showed a negative relationship with infant mortality. It was seen that infants of educated mothers experienced lower mortality (0.46*) compared to infants whose mothers were illiterate. Infants belonging to other religions were more likely to experience mortality (1.13*) than their Hindu counterparts. Infant deaths were less likely to occur among the not-deprived caste category (0.96*) than the deprived ones. The household wealth index showed a negative association with infant mortality wherein the children from the wealth quintile were least likely to experience infant mortality than the poorest ones. Lastly, infants from the central region of India were more likely to experience mortality (1.34*), whereas the least likelihood was found in the western region (0.67*) compared to the northern region.
Model 4 includes all the factors into consideration, i.e., bio demographic and maternal socioeconomic factors. With the inclusion of all the factors, the effect of survival status of the preceding child decreases but remains significant (2.42*). Factors like caste and place of residence became insignificant in this full effect model. Like model 2, female infants were more likely to experience mortality (1.15*) as compared to male infants. Infants born to mothers of age 18–34 showed less likelihood of mortality (0.86*) as compared to mothers aged less than 18 years. Similar to model 2, infants with a birth interval of more than 36 months were less likely to die (0.29*) when compared to infants with a birth interval of less than 19 months. Education of mother and household wealth index showed a negative relationship with infant deaths.
Infants from the central region of India were more likely to experience mortality (1.33*) as compared to infants from the northern region. In contrast, the least likelihood of infant mortality was found in the western region (0.67*) compared to the northern region.
The results of the hazard models on the determinants of child mortality are shown in Table 3 . In model 1, the risk of dying in early childhood is about 2.79 times higher among children with a preceding dead child. This result changes marginally in model 2, where the risk of dying in early childhood is about 2.27 times higher among children with a preceding dead child. There is a significant difference between male and female child mortality; the latter is associated with statistically significant excess early childhood mortality (1.37*) compared to male children. Children born to mothers of age 18–34 years had a mortality rate that is nearly 20% lower (0.80*) than their counterparts. Children born at 4+ birth order experienced higher mortality than their respective counterparts (1.61*). Children born with a birth interval of more than 36+ months were 66% less likely to experience child mortality (0.34*). Multiple births continue to experience a mortality rate that is 1.72 times higher than single births. In model 3, the effect of the survival status of the preceding child was significantly associated with child mortality (2.33*). Mother’s education was significantly related to the risk of death among children as children whose mothers had higher education status were 63% less likely to experience child mortality than their counterparts. Children from other religions showed a 19% more likelihood of experiencing mortality than their Hindu counterparts. Lower risk of child mortality was observed among children belonging to the non-deprived section of the society (0.89*). Household wealth was negatively associated with child deaths as the richest children were 58% less likely to experience child mortality than the poorest lot. Region-wise association showed that children belonging to the central region were 56% more likely to experience child mortality than the children in the northern region. Model 4 is the full effect model, including all the factors, shows that the effect of survival status of preceding child on child mortality has decreased but is still significant (1.95*). The pattern of difference between male and female child mortality remains the same as model 2, where female gender was associated with statistically significant excess early childhood mortality (1.36*). Mother’s age at birth emerged to be significantly related to the risk of death in childhood, thereby depicting a lower risk of child mortality (0.68*) when the mother’s age was above 35 years. In the same model 2, children born at 4 + birth order experienced higher mortality than their respective counterparts (1.19*). Children born with a birth interval of more than 36+ months were 66% less likely to experience child mortality (0.34*).
Multiple births continue to experience a mortality rate that was 1.85 times higher than single births. Household wealth and education of mothers proved to be strong predictors of child mortality. Children from the richest households and born to educated mothers had a child mortality rate of about 57% and 77% lower than their counterparts from the poorest economic class and illiterate mothers, respectively. Region emerged as a strong predictor of infant mortality, with births from the central region recording child mortality about 55% higher than those from the north region.
Infant and child mortality trajectories by preceding child survival status were depicted in Fig. 1 and Fig. 2 , respectively. It was found that the probability of infant and child mortality was high among those children whose previous sibling was dead.
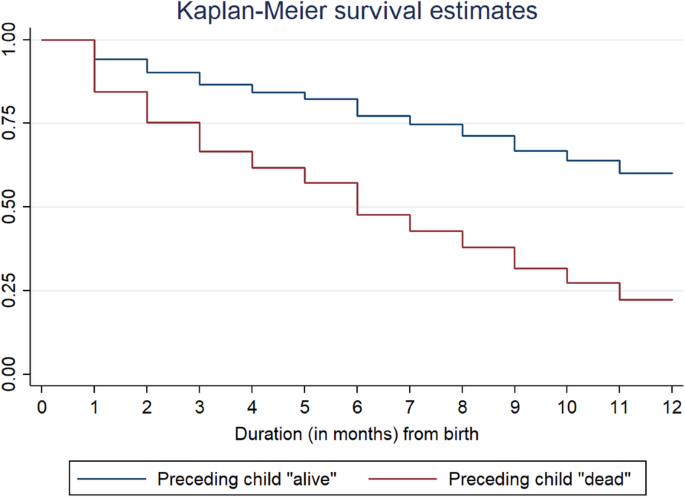
Kaplan-Meier survival estimates depicting survival curve for Infant mortality (0–11 months) by survival status of any preceding child, 2015–16 . Duration in months (from birth)
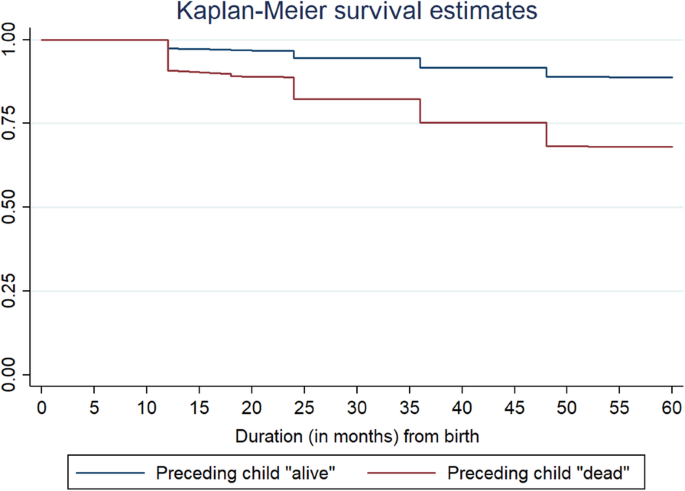
Kaplan-Meier survival estimates depicting survival curve for child mortality by survival status of any preceding child, 2015–16 . Duration in months (from birth)
This study shows how the preceding child’s survival status affects the survival status of the succeeding child. Other maternal bio-demographic and demographic factors are taken into consideration to carry out the analysis.
The socio-economic status of the mother plays a vital role in determining the survival status of the child. As found in the present study and evidence from other literature, infant and child survival is low among illiterate and poor mothers [ 35 ]. In the present study, it was found that the survival status of the preceding child plays a vital role in the survival status of the succeeding child. Similar results were depicted in other studies, too [ 36 , 37 ]. Mosley & Chen’s analytical framework for studying the determinants of child survival also found age, parity, and birth interval as determining factors for child survival [ 38 ]. It has been found that the effect of short preceding birth intervals on child mortality is strong when a preceding sibling dies [ 39 ]. This statement signifies that if the preceding birth interval is short, then chances of infant or child death are high, and if the child dies, then this forms a chain of death clustering among siblings having short PBI and dead siblings.
Over the last few years, hyped attention has been given to children born to mothers having a higher risk of repeated child loss. Researchers started taking an interest in related studies during the late 1930s when in 1936, Gardiner and Yerushalmy identified a repetitive pattern of fetal losses occurring to the same set of women [ 40 ]. Thereafter, another study following the suit examined that in mothers whose last pregnancy terminated in fetal or infant death, there was a tendency for the death to recur [ 41 , 42 , 43 , 44 ]. Studies carried out in developing countries also highlighted the association between previous child mortality and survival of subsequent children; and noted a lower risk of neonatal and postnatal mortality if the previous child survived to at least one year of age than if it was dead during infancy [ 45 , 46 , 47 ]. In the Indian context also, previous research highlighted a higher proportion of children dying to the mothers whose previous child was dead [ 48 ].
The results noted an increased risk of infant and child mortality among those whose previous sibling was dead. Several factors such as a lower level of mother’s education predispose the children to higher death risk during subsequent birth history [ 49 ]. Why would the death of a child leads to a higher risk of death for the next born child of the same mother is a very critical query to seek an answer. One plausible mechanism operates by the death of a child, shortening the time to the next birth. One possibility, also known as the fecundity hypothesis, is that the death of an infant results in the mother ceasing to breastfeed, and so they can conceive sooner than otherwise [ 50 , 51 ]. The alternate hypothesis, known as the replacement hypothesis, states that the death of a child leads parents to intentionally conceive sooner in a desire to replace their loss [ 52 ]. Following both the hypothesis mentioned above, it is clear that a shorter birth interval for the index child followed by the death of the previous child is a risk factor that could cause an elevated risk of death [ 53 ]. Several previous studies have outlined the risk of child death associated with shorter birth intervals [ 53 , 54 ]. However, a study noted that a child born after a short birth interval has higher chances to survive its infancy if the previous sibling died than if it survived [ 55 ]. A shorter birth interval history is more damaging to poor women, and the risk of child death is even higher [ 56 ]. This is so because poor women might be undernourished, and it takes time to recover physiologically from birth before the women could be ready for the subsequent pregnancy. Moreover, child death leaves a mother depressed, leading to compromise in the subsequent birth if the birth interval is shorter, leading to an increased risk of child death, known as the depression hypothesis [ 49 ].
First, women with short birth intervals have shorter recuperative intervals between the end of lactation and the start of a new pregnancy than women with longer intervals. The over-lap of gestation and lactation is particularly stressful to the mother and the child. Therefore, the joint effects of pregnancy and lactation on a mother’s physiology and nutritional status may be a mechanism through which short preceding intervals affect child-health status. This may result in low birth- weight children, prematurity, or impaired lactational abilities. Second, short birth intervals are associated with a higher risk of not attending prenatal care at all. This higher risk may be due to the lack of opportunities to attend antenatal clinics since there is a young child to care for, although controlling for the survival status of the previous child did not affect it. There may also be sociocultural or personal reasons for not attending prenatal care since a rapid return to pregnancy is often considered undesirable and perhaps embarrassing. Another explanation is that a higher proportion of premature deliveries among women having short birth intervals contributes to the lower proportion attending prenatal care among such women, particularly if women tend to make the first visit to antenatal clinics late in pregnancy. Women with long birth intervals (of three years or more) have moderately higher levels of attendance at prenatal care and are slightly more likely to deliver in institutions than women with shorter intervals. Both more opportunities and better motivation to have another child may contribute to these differences [ 12 ]. Children born with multiple births were more prone to die in infancy than those born as single children. Other studies also confirm that multiple births such as twins or triplets are at high risk of pregnancy and childbirth [ 57 ].
Similar to earlier studies, it was found that central India exhibits the highest IMR and child mortality, whereas southern and western regions perform better in the respective scenario [ 5 ]. It was further commented that IMR was higher in north India in comparison to south India. The bivariate and multivariate analysis show that illiteracy, working status of women, and low age at birth were the main mother-related covariates for a high IMR [ 58 ]. Girls die more commonly than boys due to existing underlying causes like inequality in health care; for example, fewer girls are vaccinated in health facilities [ 59 ]. RMNCH+A interventions were rolled out to achieve under-five mortality to 33/1000 and IMR to 25/1000 by 2017; however, the goals have not been achieved yet. The current rate of under-five and infant death is 39 and 34 per 1000, respectively, far behind the goals to be achieved [ 60 ]. Younger maternal age is associated with lower birth weight, preterm birth, and low nutritional status, leading to a higher risk of infant and child mortality. The children of teenage mothers aged ≤19 years had higher chances of preventable child death. Women belonging to low socio-economic tend to get pregnant early and find it challenging to take care of newborns due to poverty [ 61 ]. Finding from other studies also adds that the risk of under-five death is high among women aged 16 years or less [ 62 , 63 ].
Infant and child deaths are highly clustered among those mothers whose earlier child was dead. This signifies that some mothers are always at a disadvantageous stage in infant and child mortality. Moreover, as maternal childbearing age is still low in India, it poses a high risk of infant and child death. Mothers with higher birth order have a high risk of losing their children as it is challenging to take care of every child.
One of the study’s main limitations is the spacing between live births, i.e., the definition of interval ignores the abortion ((both induced and spontaneous) and stillbirths. It leads to difficulty as both reproductive failures and intentional pregnancy spacing are associated with increased intervals between live births. Another major limitation could be the unavailability of the sex history of the previous child who was dead. If sex were to be known, the study would have sought the attention of policymakers, given the high sex disparity in the country.
Education is a way out, and there is a need to focus on girl’s education. The government shall also focus on raising awareness on the importance of spacing between two successive births, as this will undoubtedly lower infant mortality and child mortality. There is also a need to create a better health infrastructure catering to the needs of rich and poor people alike. There is also a need to strengthen the current health system in India for safe perinatal care with community engagement. It has been noted in a previous study that the current numbers of sub-centres, PHCs, and CHCs are not sufficient to meet their population norms, and therefore there is a need to increase the number of such facilities across the country [ 24 ]. There is also a need for such interventions that would cater to maternal healthcare needs to improve child survival by upgrading infrastructure and improving human resource support during delivery care.
Availability of data and materials
The study utilizes secondary sources of data freely available in the public domain https://dhsprogram.com/methodology/survey/survey-display-355.cfm . The necessary ethical approval has been taken by the respective organisations involved in the data collection process.
Abbreviations
Millennium Development Goals
World Health Organization
Under-five Mortality Rate
Infant Mortality Rate
United Nations International Children’s Emergency Fund
National Family Health Survey
Ministry of Health and Family Welfare
International Institute for Population Sciences
Preceding Birth Interval
Scheduled Caste
Scheduled Tribe
Reproductive, Maternal, Newborn, Child and Adolescent Health
Primary Health Centre
Community Health Centre
WHO, “Newborns: improving survival and well-being,” 2020. Accessed: Jun. 13, 2021. [Online]. Available: https://www.who.int/news-room/fact-sheets/detail/newborns-reducing-mortality
WHO, “Children: improving survival and well-being,” 2020. Accessed: Jun. 13, 2021. [Online]. Available: https://www.who.int/news-room/fact-sheets/detail/children-reducing-mortality
Bryce J, Black RE, Walker N, Bhutta ZA, Lawn JE, Steketee RW. Can the world afford to save the lives of 6 million children each year? Lancet. 2005;365(9478):2193–200. https://doi.org/10.1016/S0140-6736(05)66777-3 .
Article PubMed Google Scholar
Mathers C. Ending preventable child deaths: addressing the high-risk days after birth. Lancet Glob Health. 2014;2(11):e617–8. https://doi.org/10.1016/S2214-109X(14)70333-X .
Bhatia M, Dwivedi LK, Ranjan M, Dixit P, Putcha V. Trends, patterns and predictive factors of infant and child mortality in well-performing and underperforming states of India: a secondary analysis using National Family Health Surveys. BMJ Open. 2019;9(3):e023875.
Article Google Scholar
Kumar P, Patel R, Chauhan S, Srivastava S, Khare A, Patel KK. Does socio-economic inequality in infant mortality still exists in India? An analysis based on National Family Health Survey 2005–06 and 2015–16. Clin Epidemiol Glob Health. 2021;9:116–22. https://doi.org/10.1016/j.cegh.2020.07.010 .
Chauhan S, Patel R, Bansod D. Are we ignoring the importance of sanitation while mourning the adverse pregnancy outcomes. Clin Mother Child Health. 2020;17(2):1–3.
Google Scholar
Patel R, Gupta A, Chauhan S, Bansod DW. Effects of sanitation practices on adverse pregnancy outcomes in India: a conducive finding from recent Indian demographic health survey. BMC Pregnancy Childbirth. 2019;19(1):1–12.
WHO, The world health report 2002: reducing risks, promoting healthy life . World Health Organization, 2002.
Gonçalves SD, Moultrie TA. Short preceding birth intervals and child mortality in Mozambique. Afr J Reprod Health. 2012;16(4):29–42.
PubMed Google Scholar
Hobcraft J, McDonald JW, Rutstein S. Child-spacing effects on infant and early child mortality. Popul Index. 1983;49(4):585–618. https://doi.org/10.2307/2737284 .
Boerma JT, Bicego GT. Preceding birth intervals and child survival: searching for pathways of influence. Stud Fam Plan. 1992;23(4):243–56. https://doi.org/10.2307/1966886 .
Article CAS Google Scholar
Winikoff B. The effects of birth spacing on child and maternal health. Stud Fam Plan. 1983;14(10):231–45. https://doi.org/10.2307/1965748 .
Kozuki N, Walker N. Exploring the association between short/long preceding birth intervals and child mortality: using reference birth interval children of the same mother as comparison. BMC Public Health. 2013;13(3):1–10.
Molitoris J. The effect of birth spacing on child mortality in Sweden, 1878-1926. Popul Dev Rev. 2017;43(1):61–82. https://doi.org/10.1111/padr.12050 .
Fotso JC, Cleland J, Mberu B, Mutua M, Elungata P. Birth spacing and child mortality: an analysis of prospective data from the Nairobi urban health and demographic surveillance system. J Biosoc Sci. 2013;45(6):779–98. https://doi.org/10.1017/S0021932012000570 .
J. E. Finlay, E. Özaltin, and D. Canning, “The association of maternal age with infant mortality, child anthropometric failure, diarrhoea and anaemia for first births: evidence from 55 low-and middle-income countries,” BMJ open , vol. 1, no. 2, 2011.
Sinha S, Aggarwal AR, Osmond C, Fall CH, Bhargava SK, Sachdev HS. Maternal age at childbirth and perinatal and under-five mortality in a prospective birth cohort from Delhi. Indian Pediatr. 2016;53(10):871–7. https://doi.org/10.1007/s13312-016-0950-9 .
Article PubMed PubMed Central Google Scholar
Astolfi P, Zonta LA. Risks of preterm delivery and association with maternal age, birth order, and fetal gender. Hum Reprod. 1999;14(11):2891–4. https://doi.org/10.1093/humrep/14.11.2891 .
Article CAS PubMed Google Scholar
Mishra SK, Ram B, Singh A, Yadav A. Birth order, stage of infancy and infant mortality in India. J Biosoc Sci. 2018;50(5):604–25. https://doi.org/10.1017/S0021932017000487 .
S. O. Rutstein and R. Winter, “The Effects of Fertility Behavior on Child Survival and Child Nutritional Status: Evidence from the Demographic and Health Surveys, 2006 to 2012,” USA, DHS Analytical Study, 2014. Accessed: Jun. 13, 2021. [Online]. Available: https://www.google.com/search?q=21.+Rutstein+SO%2C+Winter+R.+The+effects+of+fertility+behavior+on+child+survival+and+child+nutritional+status%3A+evidence+from+the+demographic+and+health+surveys%2C+2006+to+2012.+ICF+International%3B+2014.&rlz=1C1CHBF_enIN904IN904&oq=21.%09Rutstein+SO%2C+Winter+R.+The+effects+of+fertility+behavior+on+child+survival+and+child+nutritional+status%3A+evidence+from+the+demographic+and+health+surveys%2C+2006+to+2012.+ICF+International%3B+2014.&aqs=chrome..69i57j69i60.314j0j7&sourceid=chrome&ie=UTF-8
Ali B, Chauhan S. Inequalities in the utilisation of maternal health care in rural India: Evidences from National Family Health Survey III & IV. BMC Public Health. 2020;20(1):1–13.
Kumar S, Patel R, Chauhan S. Does land possession among working women empower them and improve their child health: a study based on National Family Health Survey-4. Child Youth Serv Rev. 2020;119:105697. https://doi.org/10.1016/j.childyouth.2020.105697 .
N. Singh, R. Patel, and S. Chauhan, “Geospatial analysis of utilization of maternal health care services in India,” GeoJournal, Apr. 2021, doi: https://doi.org/10.1007/s10708-021-10410-9 .
Upadhyay SK, Srivastava S, Patel R, Chauhan S, Alagarajan M. Is there any link between death of preceding child and child health care services utilization for subsequent birth? Indian J Pediatr. 2021;88(4):363–6. https://doi.org/10.1007/s12098-020-03452-3 .
Akinyemi JO, Bolajoko I, Gbadebo BM. Death of preceding child and maternal healthcare services utilisation in Nigeria: investigation using lagged logit models. J Health Popul Nutr. 2018;37(1):1–12.
L. K. Dwivedi and M. Ranjan, “Sibling death clustering among the tribes of central and eastern India: an application of random effects dynamic probit model,” in Demography and health issues , Springer, 2018, pp. 337–355.
Ranjan M, Dwivedi LK, Mishra R. Caste differentials in death clustering in central and eastern Indian states. J Biosoc Sci. 2018;50(2):254.
Zenger E. Siblings’ neonatal mortality risks and birth spacing in Bangladesh. Demography. 1993;30(3):477–88. https://doi.org/10.2307/2061652 .
Bolstad WM, Manda SO. Investigating child mortality in Malawi using family and community random effects. Null. 2001;96(453):12–9. https://doi.org/10.1198/016214501750332659 .
Curtis SL, Diamond I, McDonald JW. Birth interval and family effects on post-neonatal mortality in Brazil. Demography. 1993;30(1):33–43. https://doi.org/10.2307/2061861 .
Guo G. Use of sibling data to estimate family mortality effects in Guatemala. Demography. 1993;30(1):15–32. https://doi.org/10.2307/2061860 .
Sastry N. Family-level clustering of childhood mortality risk in Northeast Brazil. Popul Stud. Nov. 1997;51(3):245–61. https://doi.org/10.1080/0032472031000150036 .
B. Paswan, S. K. Singh, H. Lhungdim, and C. Shekhar, “National Family Health Survey (NFHS-4),” Mumbai, 2017.
Yaya S, Ekholuenetale M, Tudeme G, Vaibhav S, Bishwajit G, Kadio B. Prevalence and determinants of childhood mortality in Nigeria. BMC Public Health . 2017;17(1):485. https://doi.org/10.1186/s12889-017-4420-7 .
BROECK JVD, EECKELS R, MASSA G. Maternal determinants of child survival in a rural African community. Int J Epidemiol. Oct. 1996;25(5):998–1004. https://doi.org/10.1093/ije/25.5.998 .
Singhi S, Kumar R, Raina N, Kumar V. Determinants of infant and child mortality in rural Haryana. Indian J Pediatr. Nov. 1989;56(6):753–63. https://doi.org/10.1007/BF02724460 .
Hill K. Frameworks for studying the determinants of child survival. Bull World Health Organ. 2003;81(2):138–9.
PubMed PubMed Central Google Scholar
Conde-Agudelo A, Rosas-Bermudez A, Castaño F, Norton MH. Effects of birth spacing on maternal, perinatal, infant, and child health: a systematic review of causal mechanisms. Stud Fam Plan. 2012;43(2):93–114. https://doi.org/10.1111/j.1728-4465.2012.00308.x .
Gardiner EM, Yerushalmy J. Familial susceptibility to stillbirths and neonatal deaths. Am J Epidemiol. 1939;30(1):11–31.
Schlesinger ER, MAZUMDAR SM, Logrillo VM. Long-term trends in perinatal deaths among offspring of mothers with previous child losses. Am J Epidemiol. 1972;96(4):255–62. https://doi.org/10.1093/oxfordjournals.aje.a121456 .
Schlesinger ER, Allaway N. Trends in familial susceptibility to perinatal loss. American Journal of Public Health and the Nations Health. 1955;45(2):174–83. https://doi.org/10.2105/AJPH.45.2.174 .
Article CAS PubMed Central Google Scholar
Schlesinger ER, Allaway NC. Use of child loss data in evolving priorities in maternal health services. American Journal of Public Health and the Nations Health. 1957;47(5):570–7. https://doi.org/10.2105/AJPH.47.5.570 .
Shapiro S, Ross LJ, Levine HS. Relationship of selected prenatal factors to pregnancy outcome and congenital anomalies. American Journal of Public Health and the Nations Health. 1965;55(2):268–82. https://doi.org/10.2105/AJPH.55.2.268 .
Chowdhury AKM. Infants deaths, determinants and dilemmas (a cohort analysis for rural Bangladesh). Dacca: ICDDRB; 1981.
Stoeckel J, Chowdhury AA. Neo-natal and post-neo-natal mortality in a rural area of Bangladesh. Popul Stud. 1972;26(1):113–20. https://doi.org/10.1080/00324728.1972.10405206 .
Swenson I. Early childhood survivorship related to the subsequent interpregnancy interval and outcome of the subsequent pregnancy. J Trop Pediatr. 1978;24(3):103–6. https://doi.org/10.1093/tropej/24.3.103 .
Das N. An indirect approach to study inter-relationships between infant mortality and fertility. Demog India. 1975;4(2):449–56.
Arulampalam W, Bhalotra S. The linked survival prospects of siblings: evidence for the Indian states. Popul Stud. Jul. 2008;62(2):171–90. https://doi.org/10.1080/00324720802000176 .
Bongaarts J, Robert GP. Fertility, biology and behaviour: an analysis of the proximate determinants. NewYork: Elsevier; 1983. https://doi.org/10.1016/C2009-0-03021-9 .
Book Google Scholar
Kennedy KI, Visness CM. Contraceptive efficacy of lactational amenorrhoea. Lancet. 1992;339(8787):227–30. https://doi.org/10.1016/0140-6736(92)90018-X .
S. H. Preston, Reproductive Change in Developing Countries , vol. 18. Oxford University Press, 1985. Accessed: Jun. 14, 2021. [Online]. Available: https://www.cambridge.org/core/journals/journal-of-biosocial-science/article/abs/reproductive-change-in-developing-countries-insights-from-the-world-fertility-survey-edited-by-john-cleland-and-john-hobcraft-pp-301-oxford-university-press-oxford-1985-1500/F1DA5EB2CFFF85C3264E3E35FE302EC4 .
Arulampalam W, Bhalotra S. Sibling death clustering in India: state dependence versus unobserved heterogeneity. Journal of the Royal Statistical Society: Series A (Statistics in Society). 2006;169(4):829–48. https://doi.org/10.1111/j.1467-985X.2006.00425.x .
Alam N, David PH. INFANT AND CHILD MORTALITY IN BANGLADESH: AGE-SPECIFIC EFFECTS OF PREVIOUS CHILD’S DEATH. J Biosoc Sci. Jul. 1998;30(3):333–48. https://doi.org/10.1017/S0021932098003332 .
van Soest A, Saha UR. Relationships between infant mortality, birth spacing and fertility in Matlab, Bangladesh. PLOS ONE . 2018;13(4):e0195940. https://doi.org/10.1371/journal.pone.0195940 .
Article CAS PubMed PubMed Central Google Scholar
Rawlings JS, Rawlings VB, Read JA. Prevalence of low birth weight and preterm delivery in relation to the interval between pregnancies among white and black women. N Engl J Med. 1995;332(2):69–74. https://doi.org/10.1056/NEJM199501123320201 .
Uthman OA, Uthman MB, Yahaya I. A population-based study of effect of multiple birth on infant mortality in Nigeria. BMC Pregnancy Childbirth . 2008;8(1):41. https://doi.org/10.1186/1471-2393-8-41 .
Patel KK, Gouda J. “Infant Mortality in Northern and Southern Regions of India: Differentials and Determinants,” Soc Sci Spectrum. 2018;3(2):2.
Million Death Study Collaborators. Causes of neonatal and child mortality in India: a nationally representative mortality survey. Lancet. 2010;376(9755):1853–60. https://doi.org/10.1016/S0140-6736(10)61461-4 .
MoHFW, “A strategic approach to Reproductive, maternal, newborn, child and adolescent health,” MoHFW, India, 2013. Accessed: Jun. 13, 2021. [Online]. Available: https://www.proquest.com/openview/2316e318b96fcd035e985942b1268f51/1?pq-origsite=gscholar&cbl=2030549
Vidal e Silva SMC, et al. Factors associated with preventable infant death: a multiple logistic regression. Rev. Saúde Pública . 2018;52(3):1–11. https://doi.org/10.11606/s1518-8787.2018052000252 .
Fall CHD, et al. Association between maternal age at childbirth and child and adult outcomes in the offspring: a prospective study in five low-income and middle-income countries (COHORTS collaboration). Lancet Glob Health. 2015;3(7):e366–77. https://doi.org/10.1016/S2214-109X(15)00038-8 .
Neal S, Channon AA, Chintsanya J. The impact of young maternal age at birth on neonatal mortality: Evidence from 45 low and middle income countries. PLOS ONE . 2018;13(5):e0195731. https://doi.org/10.1371/journal.pone.0195731 .
Download references
Acknowledgements
The authors are thankful to David Jean Simon, Pantheon-Sorbonne University, Paris, France, for copyediting the manuscript.
Authors did not receive any funding to carry out this research.
Author information
Authors and affiliations.
Department of Mathematical Demography and Statistics, International Institute for Population Sciences, Mumbai, India
Shobhit Srivastava
Senior Resource Person, Knowledge Resource management, IED/ UNICEF, Lucknow, India
Shubhranshu Kumar Upadhyay
Department of Population Policies and Programmes, International Institute for Population Sciences, Mumbai, India
Shekhar Chauhan
Department of Development Studies, International Institute for Population Sciences, Mumbai, India
Manoj Alagarajan
You can also search for this author in PubMed Google Scholar
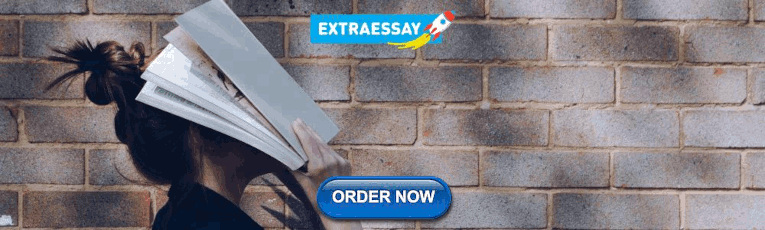
Contributions
The concept was drafted by SKU. SKU contributed to the analysis design. MA advised on the paper and assisted in paper conceptualization. SC and SS contributed in the comprehensive writing of the article. All authors read and approved the final manuscript.
Corresponding author
Correspondence to Shekhar Chauhan .
Ethics declarations
Ethics approval and consent to participate.
The data is freely available in the public domain, and survey agencies that conducted the field survey for the data collection have collected prior consent from the respondent. The local ethics committee of the International Institute for Population Sciences (IIPS), Mumbai, ruled that no formal ethics approval was required to carry out research from this data source.
Consent for publication
Not applicable.
Competing interests
The authors declare that they have no competing interests.
Additional information
Publisher’s note.
Springer Nature remains neutral with regard to jurisdictional claims in published maps and institutional affiliations.
Rights and permissions
Open Access This article is licensed under a Creative Commons Attribution 4.0 International License, which permits use, sharing, adaptation, distribution and reproduction in any medium or format, as long as you give appropriate credit to the original author(s) and the source, provide a link to the Creative Commons licence, and indicate if changes were made. The images or other third party material in this article are included in the article's Creative Commons licence, unless indicated otherwise in a credit line to the material. If material is not included in the article's Creative Commons licence and your intended use is not permitted by statutory regulation or exceeds the permitted use, you will need to obtain permission directly from the copyright holder. To view a copy of this licence, visit http://creativecommons.org/licenses/by/4.0/ . The Creative Commons Public Domain Dedication waiver ( http://creativecommons.org/publicdomain/zero/1.0/ ) applies to the data made available in this article, unless otherwise stated in a credit line to the data.
Reprints and permissions
About this article
Cite this article.
Srivastava, S., Upadhyay, S.K., Chauhan, S. et al. Preceding child survival status and its effect on infant and child mortality in India: An evidence from National Family Health Survey 2015–16. BMC Public Health 21 , 1577 (2021). https://doi.org/10.1186/s12889-021-11569-z
Download citation
Received : 03 November 2020
Accepted : 29 July 2021
Published : 21 August 2021
DOI : https://doi.org/10.1186/s12889-021-11569-z
Share this article
Anyone you share the following link with will be able to read this content:
Sorry, a shareable link is not currently available for this article.
Provided by the Springer Nature SharedIt content-sharing initiative
- Maternal and child health
BMC Public Health
ISSN: 1471-2458
- Submission enquiries: [email protected]
- General enquiries: [email protected]
Your Article Library
Mortality in india: trends and spatial patterns.
ADVERTISEMENTS:
Trends in Mortality Rates :
As anywhere else in the less developed parts of the world, mortality transition in India is a phenomenon of the twentieth century. However, India has the distinction of having experienced one of the earliest declines in mortality among the less developed countries. The less developed countries, in general, began experiencing decline in mortality levels only by the middle of the twentieth century. As against this, in India, the decline is found to have set in as early as in the 1920s. Prior to that, death rates were usually very high, often exceeding birth rates in the wake of famines and epidemics.
This excess of deaths over births occasionally resulted in the shrink in the population size of the country. The decade 1911-21, for instance, had witnessed a negative growth in population due to heavy loss of life in the wake of influenza epidemic that struck several areas in north India. It is, therefore, rightly remarked that the history of population growth in the country prior to 1921 is the history of a great fight against death.
It may be recalled that accurate estimates on trends in mortality in the country for much of the past, when death rates were very high and fluctuating, are not available. As noted earlier, although civil registration system has a longer history, its estimates are not reliable. Sample registration system, which is based on the principle of dual report system and which provides more reliable estimates, was introduced in the country only in the late 1960s.
Scholars have, however, derived estimated on trends in mortality in the country using some indirect measures. The present account of trends in mortality rates in the country is largely based on those estimates. Table 9.3 presents average annual death rate in India during the last one hundred years.

The table reveals that, prior to 1921 crude death rates in India were very high. Crude death rate, which remained around 45 per thousand persons during the early years of the twentieth century, is found to have come down to below 10 per thousand persons now. This decline in death rates was fraught with great amount of interruptions up to 1921. The decade 1911-21 is marked with a drastic increase in the death rate as compared to the previous decade due to the disastrous influenza epidemic of the year 1918, which claimed the lives of 15 million persons.
The death rate was indeed the highest ever known in the history of India’s population. Thereafter, the rates have declined continuously over the period. The first major decline occurred during the decade 1921-31 when the annual death rate came down to 36 per thousand persons. Decline in death rates, once begun, continued during each of the subsequent decades. From 1911-21 to 1971-81, i.e., over a period of nearly 60 years, death rate in the country is found to have declined by nearly 34 points. By the period 1996-98, it had reached a level that was less than 10 per thousand persons.
The latest estimate for the year 2000 indicates a death rate of 8.5. In other words, over a period of nearly 80 years, death rate in the country declined by a margin that had earlier taken more than 170 years in the developed nations. Obviously, the decline in the death rates in India, as also in other less developed countries, has been much faster than that in the developed countries. Since birth rates responded only with a time lag, and since the pace of decline in it was not as rapid as that in the death rates, India’s population began growing at increasing pace after 1921.
The rates of growth were conspicuously higher during the post-independence period. The pace in growth of population is, however, found to have slowed down since 1980s, thanks to a significant dent in fertility levels.

One of the main reasons of high mortality rates in the past has been a significantly higher incidence of deaths among infants and children in the country. Although, firm data on trends on mortality among infants and children are not available, it is understood that infant mortality rates in India during the early parts of the twentieth century were abnormally high. According to one estimate, infant mortality rates during the decade 1901-11 were as high as 290 for males and 284.6 for females.
Though, infant mortality has undergone impressive decline during the last century, percentage decline in it has been smaller than that in the general mortality rates. The current level of infant mortality, which stands at 68 per thousand live births, is still very high in the world. This means that while general mortality rate has responded to community health measures such as control of infectious and parasitic diseases, much is still desired for the improvement in mortality conditions among infants in the country.
As a result of very high mortality rates in the past, particularly among infants and children, average life expectancy at birth in India at the turn of the last century was one of the lowest in the recorded history of mankind. During much of the first half the century, average life expectancy in India remained below 30 years. In fact, during the first two decades of the century, it was only marginally above 20 years. Nevertheless, with improvements in general mortality conditions, life expectancy has recorded an increase in each successive decade.
Although, mortality conditions in the country have undergone impressive progress, death rate still remains higher than many of the developing countries of the world. Crude death rate of a little over 8 per thousand persons is still more than those in some other less developed countries in Asia like Sri Lanka, Maldives, Indonesia, Malaysia and Philippines.
The gap becomes wider if one compares the estimates on infant mortality rate. As noted already, infant mortality rate in the country still stands at 68 per thousand live births as against only 13 in Sri Lanka, 11 in Malaysia, 17 in Maldives and 26 in Philippines. Similar is the case of the differences in life expectancy. It can be argued that though mortality conditions in the country are far better now than that in the past, there is still a lot of scope for further improvement to ensure a speedy social and economic progress.
The rural areas, as will be seen later, report 48 per cent higher crude death rate than that in the urban areas. Similarly, population in the rural areas exhibits 70 per cent higher infant mortality rate than its counterpart in the urban areas. Remember that more than 70 per cent of the country’s population still resides in the villages. Thus, the health care measures have to be extended further to the rural areas, particularly among the weaker sections of the society, where mortality conditions are far worse despite significant strides made at the aggregate national level.
Mortality conditions in the country are marked with a great amount of differentials from one group to another. According to the findings of the NFHS-2, children born to women belonging to scheduled castes and scheduled tribes have significantly higher probability of dying during infancy and childhood (Table 9.5). Infant mortality and child mortality rates among households having low standard of living are almost twice as high as those of the high standard of living.
More striking difference is found to exist in the case of post-neonatal mortality rate. Households with low standard of living have three times as high post-neonatal mortality as those among households with high standard of living. Interestingly, similar pattern of the differentials exists in rural and urban areas separately. Obviously, child survival programmes need to be further intensified among specific social and economic groups in order to achieve further improvement in mortality conditions. This will go a long way in bringing down fertility rates in the country.

Spatial Patterns :
Table 9.6 presents the estimates on mortality rates in India for the year 2000. The table provides estimates for rural and urban separately. As is expected, there exists a sharp difference in the mortality rates between rural and urban areas. The difference is of a much greater magnitude in the case of infant mortality rate.
Crude death rate varies from a high of 10.5 in Orissa to a low of 3.9 in Chandigarh. If the range were viewed in absolute terms, it would appear that regional variation in crude death rates is of a smaller magnitude than that in crude birth rate. However, this conclusion turns out to be erroneous when we look at the coefficient of variation in birth and death rates among the states and union territories. Contrary to the suggestion of some scholars, the magnitude of regional variation in death rates is greater, albeit marginally, than that in the birth rates. The variation becomes even sharper in the case of infant mortality rate.
Among the major states, such as Orissa, Uttar Pradesh, Madhya Pradesh, Assam, Rajasthan and Bihar, crude death rates are higher than the nation’s average. It can be recalled that the states of Bihar, Madhya Pradesh, Rajasthan and Uttar Pradesh are also marked with very high birth rates. Thus, any improvement in mortality conditions in future in these states would mean further rise in the natural rate of growth in population. These states taken together account for a little less than one-fourth of the country’s population in 2001.
The future prospect of India’s demographic transition will continue to depend on the performance of vital transition in these states. It is interesting to note that Orissa, which appears at par with some of the most developed states in India in terms of fertility levels, reports the highest crude death rate in the country. Orissa is perhaps the only instance of such a mismatch between fertility and mortality transition. A high death rate in the state can largely be attributed to a very high incidence of mortality among infants and children.
The state reports the highest infant mortality rate also in the country. According to the findings of the NFHS-2 (1998-99), despite an overall improvement in infant and child mortality in the state, approximately one in every 12 children born during the preceding five years of survey died within five years of life, and more than one in every 10 children died before attaining the age of five.
Among the smaller states, Chhattisgarh, Jharkhand and Meghalaya report a higher crude death rate than the nation’s average. The first two of them were formerly parts of undivided Madhya Pradesh and Bihar respectively. A high crude birth rate in these states is, therefore, understandable. But, what is more striking is a relatively higher death rate in Meghalaya.
Among the northeastern states, Meghalaya appears conspicuous with a very high death rate. Strikingly, in terms of crude birth rate also, the state stands quite distinct from its neighbouring states. The southern states, in general, exhibit a lower death rate – the lowest being in the case of Kerala among the major states. Goa, a smaller state in the southwest, also shows a lower death rate than the nation’s average.
It is interesting to note that all the union territories reveal lower crude death rates than the nation’s average. Chandigarh, with a crude death rate of less than 4 per thousand persons, occupies the lowest position in the country. Among the union territories, the highest crude death rate is noticed in Dadar & Nagar Haveli, followed by Daman & Diu and Pondicherry.
One comes across more or less similar pattern with regard to the levels of infant mortality rates also. The states of Assam, Orissa, Madhya Pradesh, Rajasthan and Uttar Pradesh have a higher infant mortality rate than the nation’s average. Among the smaller states again, Jharkhand and Chhattisgarh report a higher infant mortality rates. On the other hand, Kerala, Goa, northeastern states like Manipur and Mizoram, and the union territories of Pondicherry, Chandigarh and A&N Islands report one of the lowest infant mortality rates in the country. Interestingly, all the union territories have a lower infant mortality rate than that at the aggregate level in the country.

It is generally argued that factors that cause high mortality rates in a population also contribute to a wide differential among different groups and between rural and urban areas. With rural-urban break up of mortality rates available for the states and union territories, it is worthwhile to examine the relationship between rural-urban differentials, on the one hand, and mortality levels, on the other.
In conformity with this generalization, there exists a positive and significant association between the two. The values of correlation coefficient work out to be 0.612, in the case of crude death rate, and 0.604 in the case of infant mortality rate. Thus, areas marked with high mortality levels are also marked with a wider differential between rural and urban mortality rates.
In the foregoing discussion we have examined the regional dimension of mortality conditions in the country using state level figures. India is a vast country with a great amount of variation in the determinants of mortality levels from one region to another. More often than not, this regional variation turns out to be as great within states as between them. State level patterns, thus, often mask regional variation at local levels. This necessitates a district-level analysis of mortality levels. However, district-level estimates of vital rates, as mentioned already, are not generally available.
The office of the Registrar General of India and also some independent authors, have attempted to derive district-level estimates on mortality rates. Rajan and Mohanachandran (1998) have recently worked out estimates on infant and child mortality rates in the districts of India using 1991 census data.
Although, mortality levels have undergone significant change since then, one can argue that its spatial patterns continue to be same. It is, therefore, worthwhile to examine spatial patterns in mortality levels in the country using this estimate. In the following paragraphs, a brief account of the spatial patterns in infant mortality rate is presented.
An examination of Figure 9.4 reveals that infant mortality rate is strikingly higher in the central parts of the country, lying mainly in the state of Madhya Pradesh. Of the total 24 districts reporting ‘very high’ infant mortality rates (i.e., 115 and above) as many as 15 districts are located in Madhya Pradesh (undivided) alone.
Moreover, 13 of them form a geographically contiguous pocket. To the north of this lies another pocket of ‘very high’ infant mortality rate in the central part of the upper Ganga plain in Uttar Pradesh (undivided). In the eastern part of the country, in Orissa, distinct pockets of ‘very high’ infant mortality can be seen both along the coast and in the upland plateaus. In addition, small patches of ‘very high’ infant mortality can also be observed in Arunachal Pradesh in the northeast.

These pockets of ‘very high’ infant mortality rates are surrounded by districts with ‘high’ infant mortality levels (i.e., between 90 and 114 infant deaths per thousand live births). On an average, the entire stretch from the southwestern Rajasthan in the west to Orissa in the east is marked with ‘high’ to ‘very high’ levels of infant mortality. The southern limit of this vast pocket is demarcated roughly by the northern boundaries of the states of Maharashtra and Andhra Pradesh.
Likewise, in the northwest, the spread is limited by a generally ‘low’ to ‘moderate’ levels of infant mortality rates (i.e., below 90 infant deaths per thousand live births) in the northern parts of Rajasthan and Punjab and Haryana plains. Remarkably, the eastern parts of Uttar Pradesh reveal somewhat better infant mortality conditions than their counterpart in the west. In Bihar (undivided), too, infant mortality rates are lower, and some districts, both in the plains and in Chotanagpur plateaus, report ‘moderate’ to ‘low’ level of infant mortality rates.
In the states of Maharashtra, Andhra Pradesh, Karnataka, Kerala and Tamil Nadu, infant mortality rates are found to vary from ‘low’ to ‘very low’ categories (less than 70 per thousand live births). However, in this peninsular region, the coastal districts, on an average, show a lower level of infant mortality than the interior districts. Thus, in the Karnataka plateaus and its adjacent western districts of Andhra Pradesh, infant mortality rates are somewhat higher. In Maharashtra, too, the Vidarbha region in the east is conspicuous with a higher infant mortality rate than its counterpart in the west.
Sex Differentials in Mortality :
So far we have examined various facets of mortality levels in India for the two sexes taken together. It is also important to look into the sex differentials in mortality rates in the country. Table 9.7 presents crude death and infant death rates by sex in India and its major states. The estimates pertain to the year 1998. Barring a few countries in the less developed parts, mainly in south and Southeast Asia, mortality rates among males exceed that of females throughout the world. Sex differentials in mortality in any population are the result of differential impact of a set of environmental and socio-economic factors.
A generally lower mortality rate among females in most of the populations may be attributed to their physiological superiority as well as a relatively lower extent of exposure to hazardous factors than males. Evidences indicate that women live longer than males throughout world. In India, the situation remained contrary to this general rule till recent past. In the country, more females died than males at each age until much of 1980s. The average life expectancy of females, thus, remained lower than that of males till late 1980s. During the early decades of the twentieth century, however, when death rates in the country were very high, female life expectancy was higher than that of males.
It appears that mortality transition in the initial stages were highly male selective. Life expectancy for males improved faster and remained higher than that of females for nearly six decades. With improvement in general mortality conditions of females, average life expectancy for females surpassed that of males for the first time after many decades sometime in the late 1980s. However, adverse sex differentials in mortality rates continue to exist in some age brackets mainly during the early stages of life and during childhood.

As revealed in Table 9.7, male death rate exceeds female death rate in all the major states of the country excepting Bihar and Uttar Pradesh. In other words, women continue to suffer from adverse mortality conditions in these two states. Further, average mortality conditions of females are only marginally better than that of males in the states like Gujarat, Haryana, Madhya Pradesh and Orissa. One could attribute this to a somewhat late start in mortality transition in these states.
The states of Kerala, Tamil Nadu, Andhra Pradesh and Maharashtra where mortality transition had an early start, on the other hand, report a markedly lower death rate among females than males. Among these states, Kerala has the largest gap between male and female death rates. The state has been known for a significantly higher status of women for quite some time. A greater prevalence of literacy among females and a better status of women are often cited as the main factors for demographic transition in the state.
Sex differentials in infant mortality, however, present a different picture. As noted above, more female babies continue to die before reaching age one, both in urban and rural areas. According to the SRS estimates, female infant mortality rate in the rural areas in 1998 was 79 as against 76 for males.
The corresponding figures for the urban areas were 42 and 49. It is, then, interesting to note that sex differential in infant mortality rate in the urban areas is of a significantly greater magnitude than that in the rural areas. While in the rural areas, female infant mortality rate was only 4 per cent as high as that of male infant mortality rate, the same is nearly 17 per cent higher in the urban areas.
The explanation for this can be sought in the differential impact of better social and economic conditions in the urban areas. It appears that better living conditions and availability of health care facilities in the urban areas have had sex selective impact leading to a greater reduction in death of male infants, who are otherwise more vulnerable to diseases and death in the early stages of life.
At the state level, one comes across a mixed picture relating to sex differentials in infant mortality rates. While some major states do report a higher incidence of death among female infants – in line with the pattern observed at the aggregate national level – others like Assam, Bihar, Karnataka, Kerala, Madhya Pradesh, Orissa and West Bengal show an opposite pattern.
Remarkably, the states reporting higher male infant death rates vary a great deal in terms of social and economic attributes as well as pace of transition in the levels of overall infant mortality rates. Further investigation based on primary level data at lower level will explain this unique sex differential in infant mortality rates in different parts of the country.
However, so far as the case of Kerala is concerned, it can be argued that with infant mortality rate at a very low level, and absence of any sex discrimination, the biological superiority of female infants gets fully reflected in the sex differential of mortality rate at this age.
Related Articles:
- 3 Different Ways to Measure the Mortality Rate
- Public Health: Infant Mortality Rate and Maternal Mortality Ratio
No comments yet.
Leave a reply click here to cancel reply..
You must be logged in to post a comment.
Essay on the History of Mortality Rate in India
The history of population growth in India before 1921 is the history of a great fight against death. Up to 1921, population growth in this subcontinent was very often marked by the heavy toll taken by famines and epidemics.
Millions of lives were lost during these massive calamities. Despite the fact that death rates are of central importance in the study of population growth in India, it is unfortunate that the actual death rates during those early periods, when these rates were very high and fluctuating, are not available, for the death registration system was highly inadequate.
Demographers have, however, attempted to estimate the death rates and the infant mortality rates and have constructed Life Tables for India on the basis of census statistics and other available material.
The trends in mortality in India may therefore be studied with the help of these three measures. The two most striking facts about this Table are: (1) the higher level of the Indian rate prior to 1921 (that is, between 40 and 50 per thousand populations) and (2) the decline in the death rates after 1921.
ADVERTISEMENTS:
It may be noted, however, that the average annual death rates tend to give a false impression as the decline in the death rates fluctuated a great deal.
During the decade 1911-1921, the disastrous influenza epidemic swept over India in 1918 and, according to the estimates of Kingsley Davis, this epidemic wiped out more than 15 million people.
That is the reason for the highest average annual death rate (48.6 per thousand populations) for that decade. It is also evident from Table 7.10 that India had made an impressive progress in her fight against death.
From 1911-1921 to 1971-1981, that is in 60 years, the average annual Indian death rate has declined from 48.6 per thousand populations to 14.9, a reduction of more than 69 per cent.
It may be observed from, which presents the estimated crude death rates from 1970 onwards, that there have been wide fluctuations in the crude death rates of India up to 1975, and that over a period of five years, a decline of only one point was achieved. In 1988, the death rate for India was 11, a decline of 3.2 points from 1978.
Two decades of the present century was very low because of poor mortality conditions. During 1911-1921, it was 19.4 years for males, 20.9 years for females and 20.1 years when both sexes were considered together.
These figures may be considered to be the lowest for the country, and one of the lowest anywhere in the world, (it may be recalled that in European countries even during the pre-Greek period in 3500 B.C., the average life span was 31 years.)
The mortality conditions in India have, however, improved over the years, and the average life expectancy has increased in each successive decade.
The main reason for the low life expectancy in India has been high infant mortality rates. During 1901-1911, these rates per thousand for males and females were 290.0 and 284.6 respectively.
In other words, one-fourth of the babies died before they completed their first year of life. Over the years, infant mortality rates in India have also declined, though they are still quite high. In 1982, this rate was 104.8 per thousand live births.
In the past, the fight against death in India was undertake in three important fields, namely, the elimination of wars and banditry, the control of famines and of epidemics.
The gains of these three points were “slow, hard and lengthy.” The fight however, is not yet over, though substantial reductions in mortality have been achieved.
Although the death rate in India has been considerably reduce it still remains very high as compared to that of other developing countries of Asia.
During 1980-85, the crude death rates per thousand populations for some Asian countries were as follows: Sri Lanka: 6.6; the Philippines: 8.4; West Malaysia: 6.5; and Singapore- 5.4. This rate for India in the same period was 12.3 per thousand populations.
The average expectation of life at birth for both and females is still very low in India, as compared to that in otherdeveloping Asian countries.
For instance, the average life span formales and females in Sri Lanka during 1980-85 was 67.0 years at 70.0 years respectively. Infant mortality rates in India, as has beenpointed out earlier, are much higher than those in many developing countries of the world.
It may be concluded from this discussion that though the present mortality conditions in India are much better than they were in the past, they are far from satisfactory, and there is much scope for improvements.
Related Articles:
- Essay on the Important of the Study of Infant Mortality
- 3 essential causes for the Decline in Death Rate in India
- Notes on the Difference between Mortality Rate of Urban-Rural Population
- Notes on the Recent Mortality Levels of the Population of the World
- Biology Difference Between
Difference Between Morbidity And Mortality
The terms morbidity and mortality are often related but not identical. Morbidity is the state of being unhealthy for a particular disease or situation, whereas mortality is the number of deaths that occur in a population. Read on to explore the difference between morbidity and mortality in detail.
Morbidity vs Mortality
What is morbidity.
Morbidity refers to the state of being unhealthy. It applies to all the people affected by a disease in a particular region. The morbidity rate refers to the number of people affected by a particular disease. This helps health officials to make risk management and adopt national health systems according to the needs of the population.
What is Mortality?
Mortality shows the number of deaths in a particular population. It is expressed as the number of deaths per 100,000 people per year. It is measured with the help of systems such as SAPS II and III, APACHE-II, Glasgow, Coma scale, etc.
To know more about the difference between morbidity and mortality, register at BYJU’S. Keep visiting BYJU’S for the latest updates on various Biology topics.
Some important links:
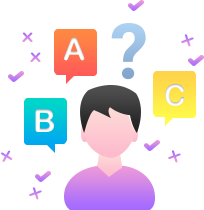
Put your understanding of this concept to test by answering a few MCQs. Click ‘Start Quiz’ to begin!
Select the correct answer and click on the “Finish” button Check your score and answers at the end of the quiz
Visit BYJU’S for all Biology related queries and study materials
Your result is as below
Request OTP on Voice Call
Leave a Comment Cancel reply
Your Mobile number and Email id will not be published. Required fields are marked *
Post My Comment

- Share Share
Register with BYJU'S & Download Free PDFs
Register with byju's & watch live videos.


An official website of the United States government
The .gov means it’s official. Federal government websites often end in .gov or .mil. Before sharing sensitive information, make sure you’re on a federal government site.
The site is secure. The https:// ensures that you are connecting to the official website and that any information you provide is encrypted and transmitted securely.
- Publications
- Account settings
Preview improvements coming to the PMC website in October 2024. Learn More or Try it out now .
- Advanced Search
- Journal List
- Wiley-Blackwell Online Open

Trends in maternal mortality in India over two decades in nationally representative surveys
1 Centre for Global Health Research, Unity Health Toronto and Dalla Lana School of Public Health, University of Toronto, Toronto Ontario, Canada
2 Department of Public Health and Mortality Studies, International Institute for Population Sciences, Mumbai India
3 Department of Internal Medicine, Western University, London Ontario, Canada
JW Snelgrove
4 Department of Obstetrics & Gynaecology, Mount Sinai Hospital, University of Toronto, Toronto Ontario, Canada
L Raveendran
5 UNICEF, New York New York, USA
Associated Data
Table S2 . Proportions of maternal death and health facility births, 1997–2018.
Data, partially derived from public domain resources, are available on request from the authors.
To assess national and regional trends and causes‐specific distribution of maternal mortality in India.
Nationally representative cross‐sectional surveys.
All of India from 1997 to 2020.
About 10 000 maternal deaths among 4.3 million live births over two decades.
We analysed trends in the maternal mortality ratio (MMR) from 1997 through 2020, estimated absolute maternal deaths and examined the causes of maternal death using nationally representative data sources. We partitioned female deaths (aged 15–49 years) and live birth totals, based on the 2001–2014 Million Death Study to United Nations (UN) demographic totals for the country.
Main outcome measures
Maternal mortality burden and distribution of causes.
The MMR declined in India by about 70% from 398/100 000 live births (95% CI 378–417) in 1997–98 to 99/100 000 (90–108) in 2020. About 1.30 million (95% CI 1.26–1.35 million) maternal deaths occurred between 1997 and 2020, with about 23 800 (95% CI 21 700–26 000) in 2020, with most occurring in poorer states (63%) and among women aged 20–29 years (58%). The MMRs for Assam (215), Uttar Pradesh/Uttarakhand (192) and Madhya Pradesh/Chhattisgarh (170) were highest, surpassing India’s 2016–2018 estimate of 113 (95% CI 103–123). After adjustment for education and other variables, the risks of maternal death were highest in rural and tribal areas of north‐eastern and northern states. The leading causes of maternal death were obstetric haemorrhage (47%; higher in poorer states), pregnancy‐related infection (12%) and hypertensive disorders of pregnancy (7%).
Conclusions
India could achieve the UN 2030 MMR goals if the average rate of reduction is maintained. However, without further intervention, the poorer states will not.
Tweetable abstract
We estimated that 1.3 million Indian women died from maternal causes over the last two decades. Although maternal mortality rates have fallen by 70% overall, the poorer states lag behind.
Introduction
The Government of India is a signatory to the United Nations (UN) Sustainable Development Goals (SDGs), which adopted a global maternal mortality ratio (MMR) target of fewer than 70 deaths per 100 000 live births by 2030. 1 This requires the reliable quantification of maternal deaths and trends and an understanding of the major causes of these deaths at the subnational level. India, similar to many countries with high maternal mortality, officially registers only a fraction of births, deaths and vital events. 2 , 3 Maternal deaths are concentrated in remote rural areas and are among the least likely to be recorded. 4 India, however, has had a functioning Sample Registration System (SRS) to monitor fertility and mortality covering over 1 million nationally representative homes for more than five decades.
The UN estimates that about 24 million children were born in 2017 in India, and about 35 000 mothers died during childbirth or shortly thereafter, giving an MMR of 145 per 100 000 live births. 5 This rate represented 12% of global maternal deaths. According to the World Health Organization (WHO), the worldwide MMR has fallen substantially from 342 in the year 2000 to 211 in 2017, reducing global maternal deaths from 451 000 to 295 000 during this period. 5 About 40% of this absolute decline was derived from fewer maternal deaths in India. 4
Here, we evaluate national and subnational levels and trends of maternal mortality over a 20‐year period in India by examining nearly 10 000 maternal deaths among 4.3 million live births identified in the SRS, and over 1500 maternal deaths assigned causes in the Million Death Study (MDS), a nationally representative, population‐based mortality survey. 6 , 7 We focus particularly on the rates of progress in the poorer states of India, which currently lag behind the national trends. A 2006 report, 4 based on maternal deaths captured in the SRS from 1997 to 2003, suggested that progress at that time was insufficient for India to meet the 2015 UN goals. We reassess that projection and point to how India can achieve the 2030 SDG 3.1.
Data source and design
We applied the WHO definitions of maternal death, namely the death of a woman while pregnant or within 42 days of terminating a pregnancy, irrespective of the site and duration of the pregnancy, from any cause related to or aggravated by the pregnancy or its management, but not from accidental or incidental causes. 8 We selected nationally representative data sources to assess MMRs, and estimate maternal deaths and cause distribution. We extracted ten periodic MMR estimates (representing 2‐ or 3‐year periods from 1997 to 2018) for all of India, for major regions and for individual states from the SRS Special Bulletins on Maternal Mortality, 9 which provide subnational MMR statistics.
The SRS is a large‐scale continuous demographic survey conducted by the Registrar General of India since 1971 that provides national‐ and state‐level birth and death estimates. The current SRS covers about 8.1 million people selected from 8847 sample units (rural villages or urban blocks) in all states and union territories. 10 , 11 We also assembled complimentary information from other nationally representative MMR estimates from the National Family Health Surveys (NFHS) for 1998–1999, 12 the Indian Institute of Health and Family Welfare survey, 13 and the model‐based WHO/UN MMR estimates from 2000 to 2017. 14 These provided relevant maternal mortality data, albeit with limitations in methodology and under‐reporting. We extracted MMR estimates for 2010–2013 in three rounds of the Annual Health Survey (AHS), representative for nine poorer states, 15 , 16 , 17 which permitted a regional comparison of AHS and SRS estimates for this group of states.
We created two main categories of states: ‘poorer’ states, comprising eight Empowered Action Group states plus Assam (EAGA); and ‘Other’ states, consisting of richer states and union territories in the south and other regions, as defined by the SRS. 9 Within EAGA, we regrouped six previously partitioned major states into their original (pre‐1991) states to enable the consistent monitoring of trends over the two decades in: Uttar Pradesh combined with Uttarakhand, Bihar combined with Jharkhand, Madhya Pradesh combined with Chhattisgarh, Rajasthan, Orissa and Assam.
Cause‐of‐death information (2007–2014) comes from MDS, a continuing, nationally representative, population‐based mortality survey conducted concurrently within the SRS. 18 , 19 The MDS collects data on the causes of death for all households in the SRS. We applied a modified WHO verbal autopsy tool that includes a local language narrative section describing the history prior to death, obtained from a family member of the deceased. We converted paper records to electronic records, and two trained general physicians independently and anonymously assigned an underlying cause of death using MDS guidelines. 20 We used International Classification of Diseases and Related Health Problems, 10th revision (ICD‐10) codes to determine the cause of death. 21 Any coding disagreements underwent a process of reconciliation and adjudication. The codes assigned by non‐specialist physicians were subsequently re‐reviewed by another group of specially trained obstetricians and physicians (RB, NC, MS, PS and JWS) to assign final maternal causes of death using the most recent WHO criteria. 22 A subteam (AS, TH, LR, NC and PS) reviewed the narratives to establish the planned and actual place of birth, and place of death, using a validated maternal data extraction tool. 23 Details of the MDS methodology, including the completeness of deaths captured and misclassification of causes, are published elsewhere. 3 , 24 , 25
We computed MMR as maternal deaths per 100 000 live births by pooling the respective SRS sample number of maternal deaths and live births for each state, and derived 95% CIs using the delta method with a variance of P f /[ N * (1 – P f ) 3 ] where Pf is the proportion of maternal deaths to live births. 5 , 26 , 27 For 2016–2018, we estimated the sample number of maternal deaths and live births by using the published SRS indicators for births and population, as the 2016–2018 SRS bulletins published only ratios and not the actual numbers of maternal deaths and live births. We projected MMRs for 2020 in India and major subnational regions (EAGA and other) assuming the average annual rate of reduction for the 20‐year period, with an upper and lower limit based on the 95% CI. 5 We excluded the 1997–1998 estimate for Gujarat because of an implausibly low MMR of 46. Finally, we used the trends over the study period to project MMRs in 2030 to determine the likelihood of meeting India’s goal for MMRs of <70 per 100 000 live births in the country as a whole and in each major state.
We estimated the absolute number of maternal deaths in India using UN Population Division 2019 estimates for deaths of women aged 15–49 years, and distributed the national total by state and region using the proportional distribution of MDS deaths, after applying survey weights. 28 We adjusted regional and state totals to match the UN national totals. This enabled the forward projections from 2016–2018 to 2019 and 2020. The use of UN demographic totals has the benefit of correcting for the slight undercounting in the ascertainment of adult female deaths in the SRS. 29
For a spatial analysis of the risk of maternal mortality, we geocoded maternal deaths and SRS live births for 2008–2011 to 7214 sampling units (after the exclusion of sampling units from smaller islands of India), as we had live birth data only at the SRS unit level for these years. We applied a geostatistical Bayesian Poisson model to estimate the spatially smoothed and risk‐adjusted MMR. 30 The geostatistical models adjusted for yearly time trends, urban/rural setting, subdistrict‐level female illiteracy, proportion of smokers from the sampling units and language regions (as a proxy for geographic regions). To account for potential spatial variation and sampling unit‐level variation, possibly as a result of factors that were not already included as covariates, we added smoothly varying spatial random effects (i.e. spatial autocorrelation or clustering) and independent unit‐level random effects. The geostatistical Bayesian model has been applied recently to snakebite mortality in India. 30 , 31 , 32
We analysed causes of maternal death from the MDS for 2007–2014 by extracting deaths that were assigned obstetric or pregnancy‐related ICD codes by trained physicians, according to WHO guidelines. We tabulated the main categories of maternal death causes and classified them further into two broad groups of direct and indirect causes. We assessed the distribution of causes of maternal deaths in India and compared this between poorer (Bihar and Uttar Pradesh) and richer (Maharashtra, Kerala and Tamil Nadu) states.
We included 9573 maternal deaths among 4.3 million live births reported in India through the SRS from 1997–1998 to 2016–2018. About 58% (5517) of maternal deaths occurred among women aged 20–29 years, peaking at the ages of 20–24 years (Table 1 ). Nearly two‐thirds of maternal deaths (6057) occurred in EAGA states, with Uttar Pradesh/Uttarakhand contributing about 22% (2059), followed by Bihar/Jharkhand, with 12% (1113). About 37% (3519) of all maternal deaths occurred in the other states, led by West Bengal and Karnataka (Table 2 ). The concentration of maternal deaths in EAGA states was substantially higher than the proportion of total live births in these states (44%).
Characteristics of maternal deaths and live births in the Sample Registration System, 1997–2018: sample age distribution of maternal deaths
Numbers may not add up because of rounding (%) in the SRS; 48 maternal deaths were missing age.
Characteristics of maternal deaths and live births in the Sample Registration System, 1997–2018: maternal deaths and live births distribution by major state and region, 1997–2018
Numbers may not add up because of rounding in the SRS results published for each state. *EAGA is the Empowered Action Group states plus Assam.
The MMR per 100 000 live births fell over the study period by more than 70%, from 398 (95% CI 378–417) in 1997–1998 to 113 (95% CI 103–123) in 2016–2018 (Figure 1 ), with an average annual rate of decline of 6.3% (95%CI 5.6–7.0%). Projecting the observed decline for a further 2 years, we estimate an MMR of 99 (95% CI 90–108) by 2020. Overall, we estimate that about 1.30 million (95% CI 1.26–1.35) maternal deaths occurred in India between 1997 and 2020. The EAGA states accounted for 952 000 maternal deaths, whereas about 317 400 maternal deaths occurred in the other states. The number of maternal deaths fell from 110 800 (95% CI 105 200–116 100) in 1997 to an estimated 23 800 (95% CI 21 700–26 000) in 2020 (Table S1 ). The proportion of maternal deaths among deaths in women aged 15–49 years fell from about 12% to below 4% during this time period. The MMR declines were broadly consistent with the WHO model‐based estimates (Figure 1 ) and correlated with the expansion of the percentage of births occurring in facilities as well as the decline in neonatal mortality (Pearson correlations of 93% and 98%, respectively, each P < 0.0001; Table S2 ).

Trends in maternal mortality ratio (MMR) from 1998 to 2018 and projection for 2020 for India. Each year on the horizontal axis represents the last of each three‐year group for each MMR, except for 1998, which represents the second of two years. The pink bands represent the United Nations (UN)/World Health Organization (WHO) estimates. NFHS‐2 is the National Family Health Survey, 2nd round, and IIHFW is the Indian Institute of Health and Family Welfare survey. Absolute maternal deaths are scaled to the UN demographic totals for females at 15–49 years of age.
The percentage declines in MMR were similar in the EAGA states and in the other states (Figure 2 ), averaging 5.9% (95% CI 5.0–6.8%) in EAGA and 6.3% (95%CI 5.2–7.5%) in the other states. Projected MMRs per 100 000 live births for 2020 were 143 (95% CI 127–159) for the EAGA states and 68 (95% CI 58–77) for the other states. This amounts to 17 900 (95% CI 16 300–19 600) maternal deaths in the EAGA states and 5800 (95% CI 5300–6400) maternal deaths in the other states. The MMR in the EAGA states remained about twice as high as in the other states throughout the two decades. The proportion of deaths for maternal causes among females aged 15–49 years in the EAGA states fell from 20% in 1997 to about 7% in 2018. From 1997 to 2001, maternal deaths in Rajasthan accounted for nearly a third of all deaths in females aged 15–49 years (Table S1 ). The two states with the largest declines in MMRs were Bihar/Jharkhand (76%) and Maharashtra (72%), and the two states with the smallest declines were Haryana (33%) and Punjab (54%; Table S1 ).

Trends in maternal mortality ratio from 1998 to 2018 and projection for 2020 for subnational regions of India. Each year on the horizontal axis represents the last of each three‐year group, except for 1998, which represents the last of two years. EAGA is the Empowered Action Group states plus Assam. Estimates for EAGA from the Annual Health Survey (AHS) data are for two‐year groups. Absolute maternal deaths are scaled to the United Nations demographic totals for females aged 15–49 years.
Between 2008 and 2011, three‐quarters of India’s maternal deaths identified in the MDS were clustered in rural areas of the EAGA states (Figure 3 ). After adjusting for female literacy, rural/urban status, smoking prevalence, language and spatial clustering effects, we noted particular ‘hotspots’ of higher risk‐adjusted maternal death rates in 2008–2011 (the period during which such analyses were possible) in central Uttar Pradesh, in Assam and in other north‐eastern states, and in the tribal areas that border Bihar, Madhya Pradesh and Chhattisgarh. During these years, over 22% of India’s estimated 26 million live births occurred in areas with a risk‐adjusted MMR of 70 or below, which is the SDG target for 2030. Over two‐thirds of births occurred in areas with a risk‐adjusted MMR ranging from 71 to 200, and 10% occurred in subareas with a risk‐adjusted MMR exceeding 201. The highest risk‐adjusted MMRs of ≥251 occurred in populations with only 0.7% of live births.

Spatial distribution of risk‐adjusted maternal mortality ratios (MMRs) in rural areas of India in 2008–2011. The risk of maternal death is adjusted for female literacy, rural/urban status, smoking prevalence, language and spatial clustering effects. States/union territories in India: AP, Andhra Pradesh; AR, Arunachal Pradesh; AS, Assam; BR, Bihar; CH, Chandigarh; CT, Chhattisgarh; DL, Delhi; GA, Goa; GJ, Gujarat; HR, Haryana; HP, Himachal Pradesh; JH, Jharkhand; JK, Jammu and Kashmir; KA, Karnataka; KL, Kerala; MP, Madhya Pradesh; MH, Maharashtra; MN, Manipur; ML, Meghalaya; MZ, Mizoram; NL, Nagaland; OR, Orissa; PB, Punjab; RJ, Rajasthan; SK, Sikkim; TN, Tamil Nadu; TR, Tripura; UL, Uttarakhand; UP, Uttar Pradesh; WB, West Bengal.
At the end of the study period, in 2016–2018, Assam had the highest MMR at 215, followed by Uttar Pradesh/Uttarakhand at 192. Maharashtra and Kerala had the lowest MMRs, at 46 and 43, respectively (Figure 4 ). Based on the trends in Figure 1 , the MMRs for Assam and Uttar Pradesh/Uttarakhand were about 9 years behind the national ratios of 2016–2018, and Madhya Pradesh/Chhattisgarh was 6 years behind. Of the national total of 23 800 maternal deaths projected for 2020, Uttar Pradesh/Uttarakhand had 7600 maternal deaths, followed by Bihar/Jharkhand with 3500 and Madhya Pradesh/Chhattisgarh with 3100 (Table S1 ).

Trends in maternal mortality ratio (MMR) in major states (1998–2018) and estimated maternal deaths (2020). Each line starts in 1998 and ends in 2018. *National MMR. The number of years lagging behind the 2018 national MMR is derived from the trends in Figure 1 . See Figure 3 for state abbreviations. The blue MMR trend lines represent trends in poorer states (the EAGA states).
At the current average rates of progress, we project that by 2030 India’s overall MMR will meet and exceed the UN SDG target, reaching an MMR of 52 (95% CI 47–56), based mostly on progress in the other states, which we project to reach an MMR of 35 (95% CI 30–40). By contrast, we project that the EAGA states will most likely not meet the SDG target, reaching an MMR of only 78 (95% CI 69–87). To examine the possible reported effects of the interruption of maternal services as a result of the COVID‐19 pandemic, 33 we posited that the MMR would remain unchanged for 2020 and 2021 and would then continue to decline at the previous rate of progress. In this scenario, by 2030 MMRs would reach 59 (95% CI 54–64) for India overall, 88 (95% CI 78–98) in the EAGA states and 40 (95% CI 34–46) in the other states.
A total of 1565 maternal deaths among 35 295 female deaths at ages 15–49 years recorded in the MDS from 2007 to 2014 were available for the analyses of cause of death. (The causes of maternal deaths from 2001 to 2003 have already been published. 34 ) These 2007–2014 deaths had similar age and regional distributions to the overall and periodic samples of maternal deaths in the SRS. The leading causes of death were obstetric haemorrhage (47%), pregnancy‐related infection (12%) and hypertensive disorders of pregnancy (7%) (Table 3 ). Nationally, obstetric haemorrhage caused about 11 200 (95% CI 10 600–11 900) maternal deaths in 2020, and pregnancy‐related infection caused 2800 (95% CI 2400–3300), hypertensive disorders caused 1600 (95% CI 1300–2000) and abortion (termination of pregnancy) caused 1200 (95% CI 900–1500) maternal deaths. The leading causes of maternal deaths were similarly distributed between the periods before (2007–2009) and after (2010–2013) the introduction of the National Health Mission, a national programme dedicated to improving maternal and child health outcomes. 35 , 36 Obstetric haemorrhage was the leading cause of maternal death in all states, but represented a notably higher proportion in Bihar, Uttar Pradesh and Uttarakhand than in Maharashtra, Tamil Nadu and Kerala (Figure 3 , ,5), 5 ), where the proportion of maternal deaths linked to hypertensive disorders was higher. Based on the review of the verbal autopsy narrative section, 457 (29%) of 1565 maternal deaths had planned to give birth at home, and of these 139 (30%) died in a health facility or en route. Of the 727 deaths of women who planned to give birth at a facility, 568 (78%) died in a facility, 68 (9%) died at home and 57 (8%) died en route to the facility (Table 4 ). Overall, about half of the maternal deaths occurred in health facilities (51.4%), about a third occurred at home (30.2%) or en route to a health facility (8.4%), and for the remainder, the place of death was unknown (10.0%).
Causes of maternal death in the Million Death Study from 2007 to 2014, and projected national totals for 2020
Sample proportions are weighted for sampling probability and national totals are rounded to the nearest hundred.

Causes of maternal death in India and in selected states, 2007–2014: sepsis (pregnancy‐related infections); others (other obstetric complications); HDP (hypertensive disorders of pregnancy).
Place of death by planned place of birth and location
Proportions are row percentages.
Main findings
This comprehensive documentation of nearly 10 000 maternal deaths using nationally representative data from India over a 20‐year period finds that maternal mortality has been substantial in India, totalling nearly 1.3 million deaths over this time period, but that MMRs have fallen by nearly 70% over the period. Although India fell short of the 2015 UN goal, it is likely to meet the 2030 goal of 70 or fewer maternal deaths per 100 000 live births. However, without further efforts in the poorest EAGA states – currently the location of over 60% of maternal deaths – India is unlikely to achieve the national goal of all states meeting the SDG. Indeed, these poorer states are particularly vulnerable to the disruption that the COVID‐19 pandemic might cause in the delivery of health services. Maternal deaths are concentrated in selected rural areas of Uttar Pradesh and Assam, and in tribal areas overlapping a few northern states, pointing to the need for focal intervention in these areas.
Strengths and limitations
Our analysis provides the largest study yet to document trends in maternal mortality in India. We use robust nationally representative survey data paired with geospatial analyses to show the marked variation in maternal death risk across the country. We demonstrate that the poorer states need to accelerate their rates of progress in reducing maternal mortality if the whole of India is to achieve the UN SDGs. Our analyses have some limitations, however. Maternal deaths remain a reasonably uncommon cause of death. Reliable measurement of trends requires large sample sizes. Indeed, NFHS surveys prior to 1997 showed increases in maternal mortality, but this was with fewer than 50 maternal deaths being available for analyses. 4 Hence, some of the state‐level estimates relying on small numbers of recorded deaths may be similarly unreliable. If the SRS had undercounted the overall number of female deaths at 15–49 years of age, we might have also undercounted the absolute maternal deaths (but less likely the MMR). However, independent demographic assessments find that the SRS has a high coverage of expected births, 37 but undercounts deaths in females older than 5 years by 8–11%. 38 , 39 , 40 We correct for this possible undercounting in absolute deaths by using the UN totals of deaths at 15–49 years of age, to which we apply the SRS proportions of deaths. The causes of death based on verbal autopsy are subject to misclassification. 25 However, we minimised such misclassification by having a central panel with at least two skilled obstetrical experts re‐review all maternal deaths. Overall, our analyses provide useful evidence to inform health policies and strategies for tackling maternal mortality in India.
Interpretation
The reductions in maternal deaths reflect successful initiatives by the government and stakeholders through the Child Survival and Safe Motherhood programme (1992), the Reproductive and Child Health Program (1997) and the National Health Mission (2005). 35 , 41 Our analyses reveal that MMR fell at a faster rate than neonatal mortality (in the first month of life), which is particularly sensitive to access to delivery in facilities. 24 , 42 However, although the reduced number of maternal deaths correlated with an expansion of the percentage of births occurring in facilities, driven mostly by the Janani Suraksha Yojana, a conditional cash transfer programme to increase deliveries in health facilities, maternal death declines did not accelerate after the introduction of this scheme. Our findings suggest that the quality of emergency obstetric care is a major driver of maternal mortality. Even planned facility births ended in a substantial proportion of deaths occurring at home or en route to facilities. Earlier analyses of maternal deaths by cause from 2001 to 2003 reached similar conclusions. 34 The high proportion of deaths occurring in facilities among women who planned to give birth in a health facility suggest unanticipated emergency obstetrical scenarios, particularly haemorrhage.
The leading cause of maternal death at the national and subnational level remains obstetric haemorrhage. 34 , 43 The proportion of deaths resulting from obstetric haemorrhage has increased in poorer states compared with 2001–2003 estimates, 34 suggesting that persistent underlying medical conditions, including severe anaemia, as well as delayed recognition and management, may exacerbate postpartum bleeding. 44 In rich states, hypertensive disorders of pregnancy caused a significant proportion of maternal deaths, highlighting a need to improve efforts to prevent, diagnose and treat this condition. Given the substantial interstate variations in maternal deaths, there may be a role for state‐specific maternal health interventions to reduce maternal mortality. 13 , 42 , 45
The COVID‐19 pandemic has the potential to reverse gains in maternal mortality in India by reducing access to antenatal care services and institutional births. However, representative national and regional level evidence on the impact on maternal mortality are lacking as data are still emerging. The effects of COVID‐19 on maternal health outcomes on health facilities in rural areas remain poorly understood. 46 , 47 Recent increases in maternal deaths reported to the national Health Management Information System were mostly linked to other/unknown causes, probably reflecting the effects of COVID‐19 infection among pregnant women. 47 It remains uncertain whether COVID‐19 effects have had an impact on maternal care prevention and treatment services, which would increase the MMR.
India has made great strides in implementing interventions that have improved maternal health in the country. However, attention is needed in three areas. First, we need policies that recognise and monitor the subnational disparities, particularly in the EAGA states and the rural and tribal areas, where the majority of maternal deaths occur (and which might be particularly affected by disruptions in delivery resulting from the COVID‐19 pandemic). 33 This argues for additional targeted efforts to improve access and address quality gaps in these hotspots. Second, educational programmes should inform women about the benefits of care and planned births at health facilities. Finally, for these areas and nationally a continuing reliable estimation of maternal deaths is needed, including ensuring that the SRS results on causes of death are published in a timely manner. Unfortunately, the last release of MDS mortality data was from 2014. Additional research in the EAGA states is required to identify the drivers of maternal mortality and effective interventions. By prioritising improvements in maternal health outcomes in the EAGA states, India can accelerate the overall decline in MMR and ensure that each state achieves the 2030 SDG goal and continues to improve beyond that date.
Disclosure of interests
None declared. Completed disclosure of interests form available to view online as supporting information.
Contribution to authorship
PJ conceived and developed the study idea and design. CM, AS, UR and SHF analysed the data. CM performed the literature review. NC, JWS, PS, RB, MS and PJ coded the causes. AS, PS, MS, TH and LR extracted data from the narratives. PJ and CM wrote the initial draft, and all authors revised and approved the final draft for publication.
Details of ethics approval
This study was approved by St. Michael’s Hospital Research Ethics Board (ref. no. REB 04‐268; 5 February 2004).
This study was supported by a Canadian Institutes of Health Research Foundation grant (FDN 154277). PJ is supported by a Canada Research Chair and Endowed Chair at the University of Toronto.
Acknowledgement
We thank Hellen Gelband for her helpful suggestions.
Data Availability Statement
Supporting information.
Table S1 . National and regional indicators for maternal mortality in India, 1997–2018.
Supplementary Material
Meh C, Sharma A, Ram U, Fadel S, Correa N, Snelgrove JW, Shah P, Begum R, Shah M, Hana T, Fu SH, Raveendran L, Mishra B, Jha P. Trends in maternal mortality in India over two decades in nationally representative surveys . BJOG 2022; 10.1111/1471-0528.16888. 129 :550–561. [ PMC free article ] [ PubMed ] [ CrossRef ] [ Google Scholar ]
- International edition
- Australia edition
- Europe edition
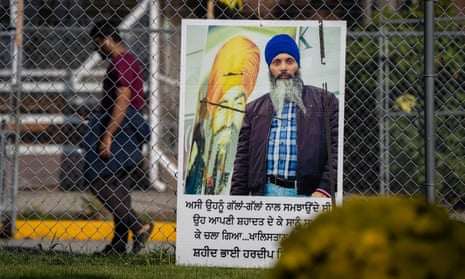
India blocks access to documentary about death of Sikh activist in Canada
CBC aired an investigation into the death of Hardeep Singh Nijjar, whose killing the Canadian government suspects India was behind
India has ordered prominent video-sharing platforms to block access to a new Canadian documentary probing the assassination of a prominent Sikh activist in Vancouver, in a move that highlights India’s mounting frustration with the allegations its government was behind the high-profile killing.
The request marks the second time in just over a year that India has sought to block a documentary critical of the Indian government or its leader, Narendra Modi. In 2023, India used emergency laws to block the distribution of the BBC documentary India: The Modi Question.
Last week, the CBC’s Fifth Estate, the national broadcaster’s investigative unit, aired Contract to Kill , a 43-minute documentary about the shooting death of Hardeep Singh Nijjar.
Nijjar, who held Canadian citizenship, was shot and killed in a parking lot in suburban Vancouver. The murder, and subsequent investigation by Canada’s national security apparatus, prompted the prime minister, Justin Trudeau, in September to tell the country’s parliament there were “credible allegations” that “agents of the Indian government” were behind the killing. India has rejected Canada’s allegations, and relations between the two countries remain strained.
Earlier this week, YouTube told the CBC that it had received an order from India’s ministry of electronics and information technology, requesting it to block access to the video of the story from its website.
The video-sharing platform said “the content has now been blocked from view” on the India YouTube country site, but it is still available outside the country.
In its request, the Indian government cited the country’s Information Technology Act of 2000 which gives it the power to “intercept, monitor or decrypt any information generated, transmitted, received or stored in any computer resource”.
India also made a request to the social media site X, formerly Twitter, requesting it block access to the documentary.
“Indian law obligates X to withhold access to this content in India; however, the content remains available elsewhere,” X said in an email to the CBC. “We disagree with this action and maintain that freedom of expression should extend to these posts. Following the Indian legal process, we are in current communication with the Indian authorities.”
The documentary includes security footage of Nijjar leaving the parking lot of the Guru Nanak Sikh Gurdwara temple on a June evening. As he approaches the exit, a white vehicle blocks his truck and two men, dressed in hooded sweatshirts, approach the truck and shoot Nijjar.
after newsletter promotion
“CBC News stands by its journalism on this story. To ensure fairness and balance, the documentary included a wide range of voices, witnesses and subject matter experts,” Chuck Thompson, CBC’s head of public affairs, said in a statement. “And, as is the case with all stories on the Fifth Estate, Contract to Kill was thoroughly researched, vetted by senior editorial leaders and meets our journalistic standards.”
Canada’s allegations that India was behind the assassination have yet to produce any arrests, despite an ongoing police investigation. The allegations also resurfaced this week when New Zealand’s deputy prime minister, Winston Peters, appeared to cast doubt on Canada’s claims.
According to the Indian Express newspaper , Peters made comments to the media outlet during a recent visit to India. “Where’s the evidence? Where’s the finding right here, right now? Well, there isn’t one,” Peters is reported to have said.
“New Zealand’s position on the allegations remains unchanged – if they are proven correct, then that would be of serious concern,” John Tulloch, the senior press secretary in Peters’ office, said in a statement. “The minister’s point is that this is an ongoing criminal investigation. It needs to run its course before clear conclusions can be drawn.”
- South and central Asia
Most viewed
- Share full article
Advertisement
Supported by
Guest Essay
The Spectacle of the Ambani Wedding Event Reveals India’s Inequality

By Sonia Faleiro
Ms. Faleiro is an author from India.
Rihanna, Mark Zuckerberg, bejeweled elephants and 5,500 drones. Those were some of the highlights of what is likely the most ostentatious pre-wedding ceremony the modern world has ever seen.
On a long weekend in early March, members of the global elite gathered to celebrate the impending nuptials of the billionaire business titan Mukesh Ambani’s youngest son, Anant, and Radhika Merchant. Monarchs, politicians and the ultrawealthy, including Bill Gates and Ivanka Trump, descended on an oil refinery city in the western Indian state of Gujarat for an event so extravagant, you’d be forgiven for thinking it was, well, a wedding. But that will take place in July. For the long windup to the big day, some of Bollywood’s biggest stars, though invited as guests, took to the stage to sing and dance in what amounted to a bending of the knee to India’s most powerful family.
Watching the event, I couldn’t help thinking of the 1911 durbar, or royal reception, when King George V was proclaimed emperor of India. Once India won its independence from Britain in 1947, it committed itself to becoming a democratic welfare state — an audacious experiment that resulted in what is now the world’s largest democracy. But in advance of this year’s general election, expected to begin in April, the Ambani-Merchant matrimonial extravaganza shows us where true power in India now lies: with a handful of people whose untrammeled wealth and influence have elevated them to the position of India’s shadow leaders.
It’s difficult to imagine the Ambani-Merchant wedding event in an India that isn’t ruled by Prime Minister Narendra Modi. It’s true that the Ambanis have been wealthy for years now and that accusations of favorable treatment from government authorities are not unique to this family or the Modi government. But no other prime minister in India’s history has been so openly aligned with big business, and never before has the concentration of wealth been more apparent. India’s richest 1 percent now own more than 40 percent of the country’s wealth, according to Oxfam. The country has the world’s largest number of poor, at 228.9 million . And according to a newly published study looking at 92 low- and middle-income countries, India had the third-highest percentage of “zero food” children — babies between 6 months and 23 months old who had gone a day or more without food other than breast milk at the time they were surveyed. Oxfam has described this new India as the “survival of the richest.”
For the uberwealthy, this presents a no-holds-barred opportunity to exert their power and influence. In 2017, Mr. Modi introduced a fund-raising mechanism called electoral bonds to allow unlimited anonymous donations to political parties. In the five years that followed, the prime minister’s Bharatiya Janata Party received $635 million in contributions through such bonds, 5.5 times as much as its closest rival, the Congress Party. The 2019 Indian general elections cost $8.6 billion, surpassing the estimated $6.5 billion spent on the 2016 U.S. presidential and congressional elections.
Analysis by three independent media organizations in India published on March 14 revealed that a company called Qwik Supply Chains purchased bonds in the scheme worth $50 million. One of the company’s three directors, reporters later uncovered, is also a director at several subsidiaries of Reliance, Mukesh Ambani’s megacompany. A spokesperson for Reliance said that Qwik is not a Reliance subsidiary and did not respond to further questioning from Reuters . The Indian Supreme Court has since struck down the electoral bond mechanism, calling it unconstitutional , but the delay in addressing the matter has most likely come too late to change the outcome of the forthcoming election, which is widely considered all but certain to go in Mr. Modi’s favor.
And judging by the omnipresence of Mr. Modi’s image — the state-funded publicity exercises focused on exalting him, the constant advertisements in newspapers and on TV, his image plastered on billboards and life-size cutouts everywhere from train stations to public parks — even those who wish to vote for other candidates might be forgiven for thinking there are none. With the help of a small group of business tycoons, led by Mr. Ambani, the prime minister has dominated the Indian media landscape. Since Mr. Modi came to power, Mr. Ambani has invested heavily in the media and now owns more than 70 outlets, including India’s biggest media conglomerate, which are followed by 800 million weekly viewers. Many of these outlets have been trumpeting Mr. Modi’s credentials and heaping praise on him.
Mr. Ambani has been relentless in expressing his gratitude to Mr. Modi for working in step with Reliance. In January, Mr. Ambani hailed Mr. Modi as the “most successful prime minister in India’s history.” Later that month, the tycoon’s family traveled to Ayodhya for the inauguration of the Ram Mandir temple complex, which is being built on the highly contentious grounds of a functioning mosque that was destroyed by a Hindu mob in 1992. The construction of the Ram Mandir is the epitome of Mr. Modi’s tenure, which has been defined by a violent and divisive Hindu majoritarianism. Mr. Ambani donated $300,000 toward the temple costs.
Mr. Modi was reportedly not present during the Ambani wedding event, leading to speculation on social media that he wanted to avoid further accusations of cronyism in an election year. But his hand was evident. Anant Ambani told the press that he in part chose his family’s hometown Jamnagar as his pre-wedding venue to honor the prime minister’s “Wed in India” call for young Indians to marry at home rather than abroad. Jamnagar is the location of Mukesh Ambani’s oil refinery, which is the largest in the world. And in a brazen misuse of public resources, the government temporarily turned the city’s small domestic airport into a designated international airport, clearing the way for guests to land their private aircraft. The government airport was expanded, staff numbers were increased , and the Indian Air Force deployed additional military personnel — all in the service of one event for one family.
Some Indians viewed the arrival of the world’s elite on their shores as a sign of their country’s growing prominence in the world. The occasion was treated as a national event, with “breaking news” and live feeds of the arrival and then departure of every celebrity, of the more than 1,000 guests who stayed in luxury tents and were provided with makeup artists and sari drapers and were reportedly served 2,500 dishes and of the lion-shaped diamond brooch on Anant Ambani’s suit and his Richard Mille wristwatch, worth an estimated $1 million, which even Mr. Zuckerberg appeared to covet .
But what viewers saw that long weekend in March wasn’t India at all but the playground of an oligarch. The festivities took place weeks before a national election, at a time when India’s democracy is teetering on an edge. Violence against Muslims, Christians and Dalits has been normalized. The harassment of journalists, the incarceration of human rights activists and police violence against protesters have all but wrenched the country from the path chosen by its founding leaders, who wished the people of India to enjoy a “sovereign, socialist, secular, democratic republic.” In 2022, Freedom House, the nonprofit organization that tracks democratic governance, downgraded India from “free” to “partly free.” This status remains unchanged.
The Ambanis are entitled to spend their money on whatever they want (except, perhaps, on electoral bonds). And this latest celebration, while lavish, wasn’t entirely atypical in India, where weddings are viewed as an opportunity to demonstrate status. The giddy young couple at the center of the spectacle are charming: Ms. Merchant is a trained classical dancer; her fiancé is fond of animals. It would be churlish not to celebrate their happiness.
But if the 1911 Delhi durbar was a symbol of British imperial power, then the Ambani pre-wedding event in Jamnagar symbolized the rise of Mr. Modi’s oligarchs. And if these few are thriving in their symbiotic relationship with the Indian prime minister, it comes at the cost of the nation’s experiment with building a democratic welfare state. That’s nothing to celebrate.
Sonia Faleiro is a writer and the founder of the literary mentorship program South Asia Speaks. Her most recent book is “The Good Girls: An Ordinary Killing.”
The Times is committed to publishing a diversity of letters to the editor. We’d like to hear what you think about this or any of our articles. Here are some tips . And here’s our email: [email protected] .
Follow The New York Times Opinion section on Facebook , Instagram , TikTok , X and Threads .
- Ground Reports
- 50-Word Edit
- National Interest
- Campus Voice
- Security Code
- Off The Cuff
- Democracy Wall
- Around Town
- PastForward
- In Pictures
- Last Laughs
- ThePrint Essential

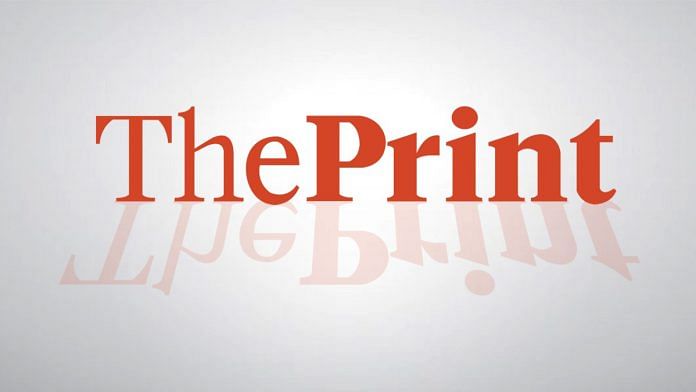
Jammu, March 20 (PTI) The Jammu and Kashmir Board of School Education (BOSE) was forced to postpone the Class 12th annual examination on Wednesday after the candidates were mistakenly given question papers of Class 11th across the Union Territory (UT).
The BOSE will investigate the circumstances that led to such a mistake, officials said.
The Class 12th students were mistakenly given question papers of Physical Education meant for Class 11th due to packaging error, the officials said.
The BOSE subsequently decided to postpone today’s Class 12th examination across the UT.
The Physical Education paper has been cancelled, and a new date will be announced soon, the official said. PTI AB AB KSS KSS
This report is auto-generated from PTI news service. ThePrint holds no responsibility for its content.
Subscribe to our channels on YouTube , Telegram & WhatsApp
Support Our Journalism
India needs fair, non-hyphenated and questioning journalism, packed with on-ground reporting. ThePrint – with exceptional reporters, columnists and editors – is doing just that.
Sustaining this needs support from wonderful readers like you.
Whether you live in India or overseas, you can take a paid subscription by clicking here .
LEAVE A REPLY Cancel
Save my name, email, and website in this browser for the next time I comment.
Most Popular
1st-time investor don’t take that influencer’s advice. small & mid-cap stocks a risky idea, here’s why, west bengal politics has to be de-brahminised. dalit aspirations get dismissed daily, ‘guruji ki bahu’ — who is sita soren & how her switch from jmm to bjp will electorally impact jharkhand.

Required fields are marked *
Copyright © 2024 Printline Media Pvt. Ltd. All rights reserved.
- Terms of Use
- Privacy Policy
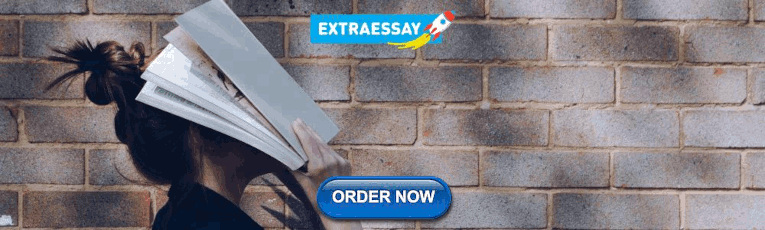
VIDEO
COMMENTS
When plague epidemics swept India in the second half of the 19 th century, there was an epidemiological need for mortality statistics; as a result, vital event registration systems were established. However, despite the existence of multiple sources of mortality statistics in many ministries/departments of the government, neither the number of deaths nor the causes of deaths reported annually ...
To fill the gaps in national-level estimates, we quantified COVID mortality in India using one independent and two government data sources. The first study is mortality reported in a nationally representative telephone survey conducted by CVoter, an established, independent, private polling agency, which launched the survey on a nonprofit basis to help track the pandemic [see materials and ...
Background The dimensions along which mortality is patterned in India remains unclear. We examined the specific contribution of social castes, household income, assets, and monthly per capita consumption to mortality differentials in India. Methods and Findings Cross-sectional data on 217 363 individuals from 41 554 households from the 2004-2005 India Human Development Survey was analyzed ...
1970-75 1976-80 1989-93 1993-97 1997-2001. Mortality Trends and Patterns in India a 285. early 1970s, about 40 per cent of men and 36 per cent of women. died during the ages 60-69 ...
Papers pertaining to India were filtered and studied. Search criteria were limited to titles, abstracts and keywords showing the words excess mortality, excess deaths, India, and COVID-19. Studies were filtered further with search within options in the search tools of the above-mentioned databases.
According to Unicef, India has the highest number of under-5 deaths, with a total of 1.08 million deaths in 2016. 5 It is one of the six countries that contribute to 50% of the world's under-5 mortality rate (U5MR). 5 On its own, India contributes to 19% of all under-5 deaths and 24% of all neonatal deaths. 5 However, infant mortality rate ...
Childhood mortality in India has declined substantially, especially after the 1990s (114 in 1990 to 39 children deaths per 1,000 live births in 2016). This remarkable improvement is the result of massive efforts to improve comprehensive maternal and child health programs and nationwide implementation of the national health mission. The latter ...
What new insights do these papers report on the mortality burden of India and its states? They offer a more fine-grained picture of long-term trends of cardiovascular diseases, respiratory diseases, and diabetes mortality in India. The India GBD Collaborators found that leading cardiovascular diseases—ischaemic heart disease and stroke—made ...
Though India had the lowest mortality rate, it also has the highest recovery rate for COVID-19. Life expectancy at birth is a commonly used indicator and a key summary measure of the health and well-being of the population. It is the widely used metric of population health and longevity. It refers to the average number of years a hypothetical ...
Changes in cause-specific neonatal and 1-59-month child mortality in India from 2000 to 2015: a nationally representative survey. Lancet. 2017 Sep 19. [Epub ahead of print].
MICS. Since its inception in 1995, the Multiple Indicator Cluster Surveys, known as MICS, has become the largest source of statistically sound and internationally comparable data on women and children worldwide. In countries as diverse as Costa Rica, Mali and Qatar, trained fieldwork teams conduct face-to-face interviews with household members ...
Cardiovascular diseases (CVDs) have now become the leading cause of mortality in India. A quarter of all mortality is attributable to CVD. Ischemic heart disease and stroke are the predominant causes and are responsible for >80% of CVD deaths. The Global Burden of Disease study estimate of age-standardized CVD death rate of 272 per 100 000 ...
Background India has achieved impressive gains in child survival over the last two decades; however, it was not successful in attaining MDG 2015 goals. The study's objective is to inquire how the survival status of the preceding child affects the survival of the next born child. Methods This is a retrospective analysis of data from the National Family Health Survey, 2015-16. Analysis was ...
Stroke is a significant global health problem and a major cause of mortality and morbidity in developed countries and increasingly in low-middle income countries (LMICs). 1 Seventy percent of strokes occur in LMICs, and the subsequent disease burden is greater than that of high-income countries. 2 Life expectancy in India has recently increased ...
where mortality decline took place over more than a century and a half. The improvement in mortality in India is also reflected in the rising expecta- tion of life at birth as presented in. Table 2. Over the last 75 years, the expecta- tion of life has more than doubled, and. the average expectation of life is now.
Although, firm data on trends on mortality among infants and children are not available, it is understood that infant mortality rates in India during the early parts of the twentieth century were abnormally high. According to one estimate, infant mortality rates during the decade 1901-11 were as high as 290 for males and 284.6 for females.
Suicide is a major public health problem, with far-reaching socioeconomic, political, and emotional consequences. Southeast Asia accounts for roughly 40% of the estimated 800 000 annual suicide deaths globally,1 and is the frontline for delivering on the aspirational Sustainable Development Goal (SDG) of a one-third reduction in the suicide death rate (SDR) by 2030.
Smoking. Until 2016, after China, India was the second largest consumer of tobacco. However, as per the report of Global Adult Tobacco Survey-2 in June 2017, there was a 6% decline in the prevalence of tobacco use among adults (>15 years) in India. 3 The smoking rates among men were constantly on decline since 1995-1996 till 2016-2017 and among women from 2.9% to 2% for the corresponding ...
Interpretation: The SBM period (2014-2020) in India witnessed accelerated reductions in infant mortality compared to the pre-SBM years (2003-2014), which corresponds with increased access to toilets. Based on our estimates, the provision of toilets at-scale may have contributed to saving an additional 32,000 to 35,000 infant lives annually.
In 1988, the death rate for India was 11, a decline of 3.2 points from 1978. Two decades of the present century was very low because of poor mortality conditions. During 1911-1921, it was 19.4 years for males, 20.9 years for females and 20.1 years when both sexes were considered together. These figures may be considered to be the lowest for the ...
Difference Between Morbidity And Mortality. The terms morbidity and mortality are often related but not identical. Morbidity is the state of being unhealthy for a particular disease or situation, whereas mortality is the number of deaths that occur in a population. Read on to explore the difference between morbidity and mortality in detail.
Apollo Radiology in India is leveraging these AI models for 3 million free screenings TB detection: A shortage of radiologists to analyze chest X-rays, crucial for TB screening, is a major hurdle.
New research found that fewer than 10 percent of countries and territories met World Health Organization guidelines for particulate matter pollution last year.
Introduction. The Government of India is a signatory to the United Nations (UN) Sustainable Development Goals (SDGs), which adopted a global maternal mortality ratio (MMR) target of fewer than 70 deaths per 100 000 live births by 2030. 1 This requires the reliable quantification of maternal deaths and trends and an understanding of the major causes of these deaths at the subnational level.
In an essay for the Los Angeles Times, Vankin shared her reluctance to read the obituaries and how the experience changed how she thinks about death. An AI-generated obituary for Deborah Vankin ...
Bulgarian-owned MV Ruen was hijacked in December 2023 in rare recent case of Somali piracy India's navy said it recaptured a ship from Somali pirates off the Indian coast on Saturday, rescuing ...
India has ordered prominent video-sharing platforms to block access to a new Canadian documentary probing the assassination of a prominent Sikh activist in Vancouver, in a move that highlights ...
India's richest 1 percent now own more than 40 percent of the country's wealth, according to Oxfam. The country has the world's largest number of poor, at 228.9 million .
Jammu, March 20 (PTI) The Jammu and Kashmir Board of School Education (BOSE) was forced to postpone the Class 12th annual examination on Wednesday after the candidates were mistakenly given question papers of Class 11th across the Union Territory (UT). The BOSE will investigate the circumstances that led to such a mistake, officials said. The […]