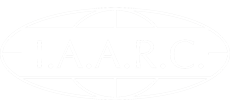
The International Association for Automation and Robotics in Construction
Publications / 2014 Proceedings of the 31st ISARC, Sydney, Australia
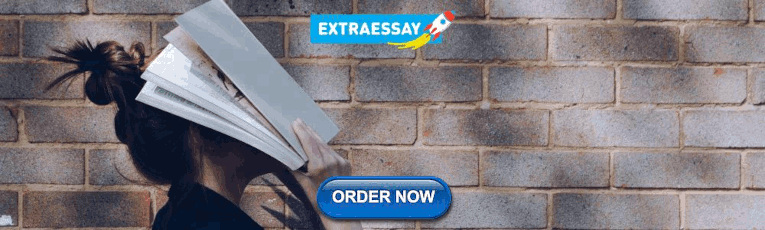
5D BIM: A Case Study of an Implementation Strategy in the Construction Industry
Several factors have contributed to the growth of BIM usage in the global construction industry, including availability of appropriate software and hardware tools, the opportunity to minimize errors, waste & cost and increasingly competitive markets [1][2]. An emerging approach in the construction industry is the use of 5D-BIM, by combining the traditional three dimensions of a BIM with the schedule as the fourth dimension and cost estimate as the fifth dimension [3]. This approach allows the contractors to better predict the cost of the project, the time-line of the project when these expenses are anticipated to occur, while simultaneously allowing the schedule to be optimized by considering the quantities of materials derived from the model and the productivity rate of construction crews. On the one hand 5D-BIM methods provide an excellent opportunity to connect processes in design, cost and construction methods; on the other hand they call for a significant shift in the way construction companies operate. It is unrealistic to commit extensive resources for a large construction company, towards a re-alignment of their internal processes, while continuing to be competitive and profitable. This paper considers how one construction company in the United States adopted the 5D-BIM methods, the challenges faced in implementing it within the company and the lessons learned in the process. A case-study method was used in an effort to understand the paradigm shift within the company in adopting 5D-BIM. Interviews with key personnel within the company were conducted and content analysis of the data was performed to describe the results. The results from this study show the intricacies of implementing a technology driven paradigm shift to a population of construction experts with a not-so erstwhile philosophy of success within the construction industry. The captains within the company communicated a great appreciation for the 5D-BIM concepts but were unwilling to delve into it whole-heartedly. Several reasons contributed to this attitude, including a reluctance to change by some, motivation to demonstrate immediate profitability and the lack of demand for such endeavours from the owner/designer community. However there were also personnel within the company that quickly adapted to the 5D way of thinking about construction and were keen to proceed with using the methodology on future projects. The advantages of using 5D-BIM and the problems encountered in implementing it are analysed in the results section of the paper.
- Best Paper Awards
- Tucker-Hasegawa Award
- Chapters & Innovation Hubs
- ISARC 2020 Online – Keynotes
- Past ISARC 2020 Online
- Privacy Policy
- Organization
- Past ISARC Locations
- Author Instructions
- Conference Venue
- IAARC Ethics and Malpractice Statement
- Important Dates
- Paper and Presentation Types
- Prepare Your Stay
- Publications Organizing Team
- Review and Publication Process
- Topics Framework
- Travel Visa
- Visit the Region
- ICRA Workshop Papers
- Publications Abstract Page
- Publications Search Result
- Related Journals
© 1984-2024 The International Association for Automation and Robotics in Construction (IAARC)
To read this content please select one of the options below:
Please note you do not have access to teaching notes, implementing 5d bim on construction projects: contractor perspectives from the uk construction sector.
Journal of Engineering, Design and Technology
ISSN : 1726-0531
Article publication date: 11 May 2020
Issue publication date: 28 October 2020
The purpose of this paper is to report on primary research findings that sought to investigate and analyse salient issues on the implementation of 5D building information modelling (BIM) from the UK contractors’ perspective. Previous research and efforts have predominantly focussed on the use of technologies for cost estimation and quantity takeoff within a more traditional-led procurement, with a paucity of research focussing on how 5D BIM could facilitate costing within contractor-led procurement. This study fills this current knowledge gap and enhances the understanding of the specific costing challenges faced by contractors in contractor-led projects, leading to the development of 5D framework for use in future projects.
Design/methodology/approach
To develop a fully detailed understanding of the challenges and issues being faced in this regard, a phenomenological, qualitative-based study was undertaken through interviews involving 21 participants from UK-wide construction organisations. A thematic data analytical process was applied to the data to derive key issues, and this was then used to inform the development of a 5D-BIM costing framework.
Multi-disciplinary findings reveal a range of issues faced by contractors when implementing 5D BIM. These exist at strategic, operational and technological levels which require addressing successful implementation of 5D BIM on contractor-led projects adhering to Level 2 BIM standards. These findings cut across the range of stakeholders on contractor-led projects. Ultimately, the findings suggest strong commitment and leadership from organisational management are required to facilitate cost savings and generate accurate cost information.
Practical implications
This study highlights key issues for any party seeking to effectively deploy 5D BIM on a contractor-led construction project. A considerable cultural shift towards automating and digitising cost functions virtually, stronger collaborative working relationship relative to costing in design development, construction practice, maintenance and operation is required.
Originality/value
By analysing findings from primary research data, the work concludes with the development of a 5D BIM costing framework to support contractor-led projects which can be implemented to ensure that 5D BIM is successfully implemented.
- Building information modelling (BIM)
- BIM Level 2
- Contractor-led project
- Costing framework
Moses, T. , Heesom, D. and Oloke, D. (2020), "Implementing 5D BIM on construction projects: contractor perspectives from the UK construction sector", Journal of Engineering, Design and Technology , Vol. 18 No. 6, pp. 1867-1888. https://doi.org/10.1108/JEDT-01-2020-0007
Emerald Publishing Limited
Copyright © 2020, Emerald Publishing Limited
Related articles
We’re listening — tell us what you think, something didn’t work….
Report bugs here
All feedback is valuable
Please share your general feedback
Join us on our journey
Platform update page.
Visit emeraldpublishing.com/platformupdate to discover the latest news and updates
Questions & More Information
Answers to the most commonly asked questions here
Open Access is an initiative that aims to make scientific research freely available to all. To date our community has made over 100 million downloads. It’s based on principles of collaboration, unobstructed discovery, and, most importantly, scientific progression. As PhD students, we found it difficult to access the research we needed, so we decided to create a new Open Access publisher that levels the playing field for scientists across the world. How? By making research easy to access, and puts the academic needs of the researchers before the business interests of publishers.
We are a community of more than 103,000 authors and editors from 3,291 institutions spanning 160 countries, including Nobel Prize winners and some of the world’s most-cited researchers. Publishing on IntechOpen allows authors to earn citations and find new collaborators, meaning more people see your work not only from your own field of study, but from other related fields too.
Brief introduction to this section that descibes Open Access especially from an IntechOpen perspective
Want to get in touch? Contact our London head office or media team here
Our team is growing all the time, so we’re always on the lookout for smart people who want to help us reshape the world of scientific publishing.
Home > Books > Smart Cities and Construction Technologies
5D BIM Applications in Quantity Surveying: Dynamo and 3D Printing Technologies
Submitted: 30 April 2019 Reviewed: 16 January 2020 Published: 13 May 2020
DOI: 10.5772/intechopen.91221
Cite this chapter
There are two ways to cite this chapter:
From the Edited Volume
Smart Cities and Construction Technologies
Edited by Sara Shirowzhan and Kefeng Zhang
To purchase hard copies of this book, please contact the representative in India: CBS Publishers & Distributors Pvt. Ltd. www.cbspd.com | [email protected]
Chapter metrics overview
1,511 Chapter Downloads
Impact of this chapter
Total Chapter Downloads on intechopen.com

Total Chapter Views on intechopen.com
Overall attention for this chapters
Digital construction is increasingly introduced to the architecture, engineering, and construction (AEC) industry. The fifth dimension of Building Information Modeling (5D BIM) has a significant contribution to the AEC industry, such as managing time and costs and resources management. However, 5D BIM has not fulfilled its promise completely. The major challenge of implementing the 5D BIM technology and applications is the interoperability between technologies and the low accuracy of measurement estimations. Dynamo is adopted in this chapter for detailed quantity measurement. Three-dimensional printing (3DP) is one of the additive manufacturing technologies which is recommended to be used in construction. 3DP is aiming to create complex and customized geometries. This chapter demonstrates how to apply the Dynamo scripts of detailed quantity take-off for estimating the volume of elements created by 3DP. Dynamo as a quantity take-off Add-in in Revit is always used for detailed quantity take-off or precise model created. After the detailed quantity take-off from Revit, the data can be exported into Excel extension, which allows the quantity surveyors to insert the price per unit to the file and to generate a Bill of Quantity (BOQ). This chapter will offer a procedure for applying Dynamo through two selected case studies.
- quantity surveyor
- decision-making
- quantity extraction
- data-driven procedure
Author Information
- Faculty of Built Environment, University of New South Wales, Australia
Sara Shirowzhan
Samad m.e. sepasgozar, alireza kaboli.
- Australian Cladding Specialists, Australia
*Address all correspondence to: [email protected]
1. Introduction
Building Information Modeling (BIM) has been adopted in the AEC industry for a long time, especially in the offsite (prefabrication) construction [ 1 , 2 ]. 5D BIM is Building Information Modeling that includes a 3D model plus scheduling (4D) and cost management (5D) [ 3 ]. 5D BIM is an advanced technology in the AEC industry, which can be used for managing time, cost, and resources; it can even handle the logistical site plan. 5D BIM can help quantity surveyors review alternative designs during the early stage of the project as a decision-making tool [ 4 ], since the 5D BIM can quickly extract approximated quantity from different 3D BIM models and then add the 4D schedule to finalise the 5D cost budget. However, the development of 5D BIM is hampered [ 3 ], by many reasons, such as the high initial implementation costs [ 5 ] but low accuracy [ 3 ]. Moreover, the current 5D BIM software and applications cannot fulfil the subcontractors’ requirements which are focused on detailed works, such as external cladding. They are appropriate for the rough cost estimation of a large scope, which is satisfied with the requirements from project planners and head contractors. The quantity take-off of current major 5D BIM applications or software is approximate quantity instead of exact quantity due to the Level of Development (LOD) limitation. Dynamo is more appropriate for the detailed quantity take-off, such as three-dimensional printing (3DP) elements. 3DP is used to print precise and complex elements [ 6 ].
Hall and Tewdwr-Jones [ 7 ] demonstrated that the lack of cooperation and difficulty of information sharing are the major reasons for the communication issue during the entire project life cycle. However, the BIM cannot always guarantee the communication to be effective [ 5 ]. The chapter will investigate how Dynamo Revit can be used for detailed quantity take-off and how to analyse the combination of 5D BIM quantity take-off appliances (Dynamo) with 3DP. A face-to-face semi-structured interview with regard to the case study will be conducted. Scopus database was the main source used for literature review in this chapter, since it has a relatively wide range of data [ 8 ], especially for BIM and the 3DP.
Figure 1 visualizes the geographical locations of the 5D BIM papers published in different countries or territories. The nodes present the number of papers that contributed to each country or territory. These nodes are grouped into two groups with different colors by running the Modularity program. The Modularity program implements a community detection algorithm, called the Louvain method [ 9 ].

Geographical locations of articles contribution.
2. Aims and objectives
Objective 1: To identify the advantages and challenges of the 5D BIM implementation in the AEC industry.
Objective 2: To evaluate the implementation of 3DP in the AEC industry.
Objective 3: To develop a 5D BIM-3DP integrated workflow for the project’s cost analysis.
3. Literature review
‘A BIM is a shared knowledge resource for information about a facility forming a reliable basis for decisions during its life cycle; defined as existing from earliest conception to demolition’ [ 10 ]. Regarding BIM, 4D BIM is about scheduling, 5D BIM is used for the cost analysis, 6D BIM is for sustainability analysis, and 7D is about the facility management [ 11 ]. BIM generates a comprehensive environment for a project and also assigns personnel responsibilities [ 12 ]. The AEC industry is always low in productivity and lacks cooperation and innovation. BIM has the potential to solve these challenges [ 13 , 14 ].
3.2 5D BIM implementation advantages
As a useful information platform, 5D BIM is not only utilized during the project construction process but also during the entire life cycle. For example, BIM can share and update the drawings and specifications easily in the cloud database, and then 5D BIM can generate more consistent and accurate cost estimation. BIM platform provides a smooth flow of information sharing among stakeholders to transfer the information quicker and easier among multidisciplines [ 15 ] to reduce errors or unnecessary works. Additionally, 5D BIM can show a clear budget and construction progresses to participants [ 3 ]. Moreover, 5D BIM is time efficient for alternative design analysis and decisions at the early stage. 5D BIM often conducted the cost management and cost analysis in other software or application. 5D BIM can better monitor the project costs not only in the short term but also in the long term by including related information and resources during [ 16 ].
3.3 5D BIM implementation challenges
The challenges of 5D BIM adoption are, for instance, software securing, training investment, and low time efficiency, lacking standards of software compatibility [ 16 ]. Sattineni and Macdonald [ 3 ] reported that 5D BIM always has high initial installation costs and requires new expertise, such as computer experts, compared to traditional construction. Thus, the company is unwilling to change [ 3 ]. The other limitation of 5D BIM is the low LOD, which cannot extract the detailed data from the 3D model for cost estimation. Aibinu and Venkatesh [ 5 ] stated there are several difficulties of 5D BIM used by the quantity surveying organization, such as the low LOD of a model. This statement is based on 40 responses received from their 180 QS web survey, and two in-depth interviews are conducted. Therefore, due to the lack of detailed information, the team always spent longer time to make sure the quantity take-off is accurate [ 3 , 5 ].
BIM-based clash detection is a quantity take-off method, which was introduced by Khosakitchalert et al. [ 4 ] to enhance the level of the quantity take-off accuracy of the layered structure. BIM-based clash detection approach extracts quantity information by geometry data of the model, such as the area and the name of the target objects [ 4 ]. Dynamo-extension is one Add-in of the Revit, and it calculates the quantity for the components by script [ 4 ]. Khosakitchalert et al. [ 4 ] stated that the LOD of the layered structures is low, such as walls, which causes low accuracy of the quantity take-off [ 4 ]. Different layers of the components have different dimensions due to the overlapped connection requirements [ 4 ]. Revit supports two different quantity take-offs, one is the material take-off and the other one is the quantity take-off [ 4 ]. The quantity take-off is based on elements, and the material take-off is based on material information [ 4 ]. The data or 3D model can be processed by creating an algorithm in Dynamo [ 4 ]. Dynamo cannot conduct quantity take-off for one element when various materials are used in this element [ 4 ]. Besides, there is rare software available for higher-level BIM functions at the same time [ 4 ]. The common 5D BIM or BIM software is not suitable for the detailed quantity take-off, for instance, cladding tasks. Since architectures and designers always add many details into the drawings instead of models, most of the subcontractor companies used BIM only for the 3D model visualization. Some subcontractors do not share the information with the crew members timely, and the designers might not be willing to share the models with the construction team. It is easy to case unneeded work due to inefficient communication and inconsistent drawings. In addition, the design drawings and specifications may conflict, which is caused by the errors or data missing and ultimately leads to inaccurate cost estimation [ 17 , 18 ]. Furthermore, the model’s errors may be not revised timely, which will affect the accuracy of the quantity take-off [ 3 ]. Mayouf et al. [ 17 ] also stated that the 5D BIM implementation requires a comprehensive workflow and information. One of the difficulties of quantity surveyors’ work is the poor quality of design documentation. Multidisciplinary and collaborative approaches play an essential role in improving the efficiency of communication and the quality of the documentations [ 17 ].
The BIM models are not designed for the quantity take-off or the cost estimation, so some details will not be shown in the models. It is a significant issue for the quantity surveying companies [ 5 ]. Thus, it is tough to extract the detailed quantity from elements for quantity estimation. 5D BIM provides timely communication but cannot guarantee the quality of the communication. At the end of 2016, Software Advice (UK) analysed and reported that 50% of the investigated small and medium enterprises still conduct the cost estimation manually [ 16 ]. There are many limitations of CUBIT adoption in New Zealand and Australia, such as low accuracy of quantity take-off or detailed quantity missing [ 16 , 19 ]. CUBIT Buildsoft is developed by MiTek (an Australian company) and used in Australia, New Zealand, the United Kingdom, and Ireland [ 16 ].
There are other challenges of 5D BIM applied in a project, such as the standardization [ 20 ] and high training costs that some organizations may not able to afford [ 20 ].
Different from academia, the industry believes BIM needs a process change approach than information—drive approach [ 17 ]. Table 1 shows the academic paper reviewing the possibility of integrating Dynamo with other tools. These papers were selected from the Scopus database since they focus on the Dynamo Revit. The Dynamo is the essential step for the 5D BIM-3DP integrated workflow. Table 2 presents those papers which focused on sustainability applications. In both tables, green color means the article is related to Dynamo and Revit for the quantity take-off; blue color means partially related.
Summary of papers examines in dynamo and Revit applications.
Summary of papers focusing on sustainability issues by implementing dynamo and Revit applications.
3.4 3D printing
Contour crafting (CC) was developed by researchers at Loughborough University, UK [ 38 , 39 ]. The conventional construction methods damage the environment [ 40 ] with lower efficiency, and it is hard to achieve a project on time and within budget without compromising the quality [ 41 , 42 ]. 3DP can reduce the cost, shorten construction duration, and minimize waste. At the same time, 3DP is efficient and sustainable and can achieve customization [ 43 ]. Additionally, Tay et al. [ 44 ] reviewed 115 relevant articles in the Web of Science and Science Direct and stated 3DP could achieve higher productivity with lower investment cost and waste. Tay et al. [ 44 ] also indicated that the 3DP is appropriate for flexible component customization. Sakin and Kiroglu [ 45 ] stated the 3DP might be developed along with the traditional construction method.
However, the 3DP is also not fulfilled its promise completely [ 46 ]. Although the 3DP can reduce the labour to save the costs, the more equipment is required in the construction site, and the depreciation costs also need to be considered. Therefore, it is hard to state if the 3DP is saving money or not. The material technology is a significant challenge for 3DP development. Gosselin et al. [ 47 ] are against to the idea that the 3DP can achieve the feasibility ‘free-form’ structures. For instance, if 3DP material is clay, then the suspended parts cannot stand immediately after the printing without any support (see Figure 2 ). Figure 2 shows the sagging section (red square) of a clay 3DP model. The 3DP challenges are summarized into (1) material development, (2) reinforcement implementation, and (3) process parameter optimization, such as the flow rate of material and print speed [ 44 ]. Since the materials are the major challenge of 3DP development, the 5D BIM-3DP integrated workflow in this chapter focuses on the material quantity take-off.

Column models in laboratory scale with 30 degrees.
3.5 BIM-3DP integration
There are many projects adopted by BIM technologies to combinate with offsite construction. Offsite construction is also named prefabrication construction, which is a method to produce the standardized components under the controlled environment to ensure the project’s quality and efficiency [ 48 ]. 3DP is very similar to offsite construction. Although 3DP lacks the corresponding regulations and standards, and the data exchange efficiency might be improved after combinating with the BIM, 3DP is time efficient and sustainable and can achieve customization [ 43 ] than the offsite construction. Thus, BIM and also 5D BIM can integrate with 3DP instead of offsite construction. BIM can automate the 3DP since it can store the equipment and manufacturing information, such as printer control data [ 44 ]. The integration of the multi-nozzle with a hybrid 3DP system is useful for concrete structures with various materials and elements, such as rebar. The combination of 3DP with BIM can monitor the variables in the construction site [ 49 , 50 ]. Also, this 5D BIM-3DP integrated workflow can achieve a simple customized building structure [ 44 ] in a laboratory scale and be brought to the construction site or meeting for communication quality improvement.
4. Methodology
The methods in this section are two case studies. Case study 1 is aiming to apply the 5D BIM quantity take-off to the multilayer wall to get all the layers’ quantity information at the same time. The second case study is to apply 5D BIM to the 3DP elements. The builder and stakeholders cannot get all the layers’ quantity information at the same time by using the conventional 5D BIM applications or software. This chapter adopts the Dynamo script principle that is mentioned in Khosakitchalert et al. [ 4 ], to design a script for all the layers’ quantity take-off from one multilayer wall at the same time, to detect the meticulous differences among the layers. Furthermore, this script can be used to extract the detailed quantities from the 3DP elements.
Figure 3 is only a simple script to show the area quantity extracted from the existing models. Also, Dynamo can be used to create a precise 3D model by node (units in Dynamo script) (see Figure 4 ). Later, the 3D model’s dimensions can be revised or changed precisely through the nodes in the core category of the Dynamo library. Each node is a function or order of the executed commands [ 4 ].

Dynamo-extension in the Revit for detailed quantity take-off.

Dynamo and fusion quantity take-off.
The floor is just an example to show the basic concept for the entire programming.
Select the ‘Select Model Elements’ node from the Dynamo library, and select the target element in the 3D model.
Select the ‘Watch’ node to list the detail code of each item.
Connect the ‘Watch’ node to the ‘Element. Get Parameter Value By Name’ node. The results listed below are the area (parameter name) of each visible element.
In this scenario, the value or the outcome of the parameter name is area.
Select the ‘Math Sum’ node to calculate the total area of the 3D model.
This programming is also suitable for the users to gain other material information, such as the type of material.
5. Case studies
Two case studies are contained in this section. One is applied this detailed quantity take-off to one target multilayer wall in the facade case study; the other one is regarding the combination of 5D BIM-3DP integrated workflow.
5.1 Case study 1: multilayer wall quantity take-off
In the facade installation project, there is an external wall consisting of different layers such as cladding, sub-framing, waterproofing membrane, stud-framing, insulation, and plasterboard. However, the area quantity (m2) of these layers cannot be the same, especially when it connects with other walls. The accuracy of the element’s quantity take-off in this project may decrease by the LOD issue [ 4 ]. For instance, the quantity take-off could be accurate for doors, windows, or structural framing, but not the external cladding, or internal plasterboard, where there are many details. Figure 5 shows different wall types at two different levels.

Detail plan and section drawings. (a) Planning drawing levels 02, 04, and 06. (b) Planning drawing levels 03 and 05.
The highlighted area is wall type 8.25, and according to the wall type schedule document, it consists of external cladding, sub-framing, waterproofing membrane, stud wall, insulation, and plasterboard. The Dynamo script can help to extract the element quantity. For example, if quantity surveyors allow that the area of wall type 8.25 is 500, all the other layers of this wall should be 500 m2 as well ( Figure 5a ). However, wall type 9.03a ( Figure 5b ) on the right side of wall type 8.25 is a concrete wall. It has the plasterboard inside. However, wall type 8.16 ( Figure 5b ) is behind wall type 9.03a (and perpendicular to it), which reduced the quantity of plasterboard on wall type 9.03a compared to the other layers. The conventional 5D BIM estimating software, such as Cubit, cannot detect these detail differences. Thus, the quantity information of some walls’ layers might be slightly inaccurate if we use conventional 5D BIM software to get the quantities. Although it is helpful to use BIM for estimating as it reduces time and human mistakes, it is essential to consider its limitation for some specific works. The Dynamo in this case study can detect a small difference between the wall layers. The quantity take-off for each layer would be more accurate than the conventional 5D BIM estimating software.
Figure 6 is shown how to use the Dynamo script to extract the detailed quantity of a multilayer wall. Users can add extra nodes from the Dynamo library for other purposes. This Dynamo script was developed based on the script in Figure 3 . The updated Dynamo script can list all the target layers’ information of multiple walls at the same time, including the name, width, and area of each layer. The rest of the script is the same with Figure 3 . Figure 6 shows that the area of each layer’s quantity is slightly different: the gypsum wallboard is 14.89 m 2 , but the other two are 15.74 m 2 . It states the Dynamo script can be accurate on two decimal places for the quantity estimation. For instance, in this case study, the differences between 14.89 m 2 and 15.74 m 2 are 0.85 m 2 . Figure 6 is only shown one multilayer wall’s Dynamo script. The users can create as many as groups they want to check the entire project walls’ layer information at the same time. The quantity data can be exported into an Excel extension, which allows the quantity surveyors to insert the price per unit to the file and to generate a Bill of Quantity (BOQ).

The quantity take-off for multiple layers of the wall through dynamo.
5.2 Case study 2: 5D BIM-3DP workflow
Research designed a workflow that combines the 5D BIM with 3DP by Dynamo. As mentioned above, the 3DP might be applied to tradition construction [ 45 ]. The 3DP technologies are advance on small-size components, which requires an accurate quantity take-off. This 5D BIM-3DP integrated workflow can be used to estimate the 3DP elements’ quantities, to improve the accuracy of the cost estimation. In addition, the 3DP can print scaled-down models of some special parts to improve communication efficiency. The Dynamo script for the quantity take-off is based on the script in Figures 3 and 6 . Sometimes, the LOD of the Revit architecture model is not enough to create the 3D model. The users also can use the Dynamo script to create a model (see Figure 4 ) and then measure it.
Figure 4 demonstrated the integration of the 5D BIM (Dynamo for quantity take-off) with the 3DP.
Create a 3DP model using Dynamo (see Figure 4 ) or Fusion 360 (Step 4). Fusion 360 is more suitable in the manufacturing industry, which is appropriate for accurate quantity take-off [ 51 ]. The Dynamo script in Figure 4 is a process for model creation since the quantity take-off script is the same as in Figures 3 and 6 . The model should be imported into Navisworks by ‘.rvt’ or ‘.rfa’ field extension.
Conduct the clash detection in Navisworks; 4D schedule information in the Navisworks is added into BOQ [ 52 ]. Then, the users can revise the model in Revit based on the clash detection results. The 3D model should be saved as ‘.stl’ extension in Revit (by installing a plugin—STL Exporter [ 53 ]) and Fusion 360.
Input the 3D model into Simply3D .STL extension, and the model would be sliced into the 2D printable layers; the G-coding will be generated automatically.
The models also can be created by Fusion 360 as mentioned above, and then repeat step 3 and make sure the models are ready to be printed. The quantity information of the models also can be found in Fusion 360. However, this software is more suitable for manufacturing industry’s models.
Import the G-coding into the 3D printer by USB, and the final models can be printed.
The schedule, and other cost information, such as the density of the ink materials, is collected.
Generate and finalize a BOQ of 3DP samples based on the quantity take-off results from the Dynamo (the same steps as in Figures 3 and 6 ) and other cost information.
Final physical models in the desired scale are printed.
6. Findings
Conventional 5D BIM software and applications cannot extract the small element’s quantities accurately at the same time. 5D BIM-3DP integrated workflow has been introduced based on the nature of 5D BIM and 3DP. The users can use the Dynamo to extract accurate quantities from small and precise elements at the same time. For instance, in case study 1—Multilayer wall quantity take-off—the Dynamo script can list the precise quantity of each layer of the multilayer wall at the same time. It is easier for the quantity surveyors, builders, and contractors to understand and check the construction details and then improve the accuracy of the cost estimation and project quality. Also, case study 2—5D BIM-3DP workflow—demonstrated this workflow also can be applied to the 3DP components.
Also, the Dynamo can help the users grab the other information quickly, such as the type of material. It helps the BOQ preparation and decision-making at the early stage of the project.
In the AEC industry, communication misunderstanding is a significant issue. It is because everyone is thinking in different ways. This 5D BIM-3DP integrated workflow can also be used to print and estimate the scaled-down or laboratory scale models for the complicated or specific components as the communication tool in the construction site or meeting to enhance the communication efficiency.
7. Conclusion
This chapter summarized the advantages and challenges of 5D BIM in the AEC industry. Also, the current conventional 5D BIM applications and software are not suitable for the detailed quantity take-off. The Dynamo, which is one of the Revit Add-ins, can help the users to extract the accurate quantity take-off. For instance, the Dynamo script in case study 1 lists the precise quantities of each layer of the multilayer wall at the same time. In addition, the status of the 3DP in the AEC industry had been evaluated. Since the 3DP has the potential to develop with the traditional construction industry, and 3DP in advance on the small and precise elements printing, the accuracy quantity take-off is very essential. The Dynamo also can be used to create and estimate the 3DP elements and components. The 5D BIM-3DP integrated workflow introduced in this chapter can help the quantity surveyors to get more accuracy quantity take-off for the 3DP. Moreover, the 5D BIM-3DP integrated workflow has the potential to reduce errors and misunderstanding during the communication. Besides, the highly accurate estimation of a 3D model at the early stage of the project would help the users to choose the best alternative design. The 3DP scaled-down samples can be used to deliver and exchange the participant’s idea during the meeting or in the construction site, which can improve communication efficiency. This 5D BIM-3DP integrated workflow is mainly focused on material quantity. Although the 5D BIM-3DP integration can reduce the labour to save the costs, more equipment is required in the construction site and the depreciation costs also need to be considered. Thus, the cost pattern may be changed. Thus, the other costs, such as equipment and labour salary, should be considered and investigated in the future as well.
- 1. Yin X, Liu H, Chen Y, Al-Hussein M. Building information modelling for off-site construction: Review and future directions. Automation in Construction. 2019; 101 :72-91
- 2. Li CZ, Hong J, Xue F, Shen GQ, Xu X, Mok MK. Schedule risks in prefabrication housing production in Hong Kong: A social network analysis. Journal of Cleaner Production. 2016; 134 :482-494
- 3. Sattineni A, Macdonald JA. 5D-BIM: A case study of an implementation strategy in the construction industy. In: ISARC. Proceedings of the International Symposium on Automation and Robotics in Construction, Vol. 31. IAARC Publications; 2014. p. 1
- 4. Khosakitchalert C, Yabuki N, Fukuda T. The accuracy enhancement of architectural walls quantity takeoff for schematic BIM models. In: ISARC Proceedings of the International Symposium on Automation and Robotics in Construction, Vol. 35. IAARC Publications; 2018. pp. 1-8
- 5. Aibinu A, Venkatesh S. Status of BIM adoption and the BIM experience of cost consultants in Australia. Journal of Professional Issues in Engineering Education and Practice. 2013; 140 (3):04013021
- 6. Ma GW, Wang L, Ju Y. State-of-the-art of 3D printing technology of cementitious material—An emerging technique for construction (in English). Science China-Technological Sciences. 2018; 61 (4):475-495. DOI: 10.1007/s11431-016-9077-7
- 7. Hall P, Tewdwr-Jones M. Urban and Regional Planning. Routledge; 2010
- 8. Mongeon P, Paul-Hus A. The journal coverage of web of science and scopus: A comparative analysis. Scientometrics. 2016; 106 (1):213-228
- 9. Start TQ. Gephi Tutorial Quick Start [Online]. 2010. Available from: https://gephi.org/tutorials/gephi-tutorial-quick_start.pdf
- 10. Moynihan GP, Harsh C. Current state of construction project delivery methods. In: IIE Annual Conference. Proceedings. Institute of Industrial and Systems Engineers (IISE); 2015. p. 174
- 11. A. A. Digital. "05. All the BIM Dimensions. Available from: http://www.axdstudio.com/bim-dimensions/ [Accessed: 10 September 2019]
- 12. Ding Z, Liu S, Liao L, Zhang L. A digital construction framework integrating building information modeling and reverse engineering technologies for renovation projects. Automation in Construction. 2019; 102 :45-58
- 13. Arayici Y, Egbu C, Coates S. Building information modelling (BIM) implementation and remote construction projects: Issues, challenges, and critiques. Journal of Information Technology in Construction. 2012; 17 :75-92
- 14. Elmualim A, Gilder J. BIM: Innovation in design management, influence and challenges of implementation. Architecture, Engineering & Design. 2014; 10 (3–4):183-199
- 15. Grilo A, Jardim-Goncalves R. Value proposition on interoperability of BIM and collaborative working environments. Automation in Construction. 2010; 19 (5):522-530
- 16. Mesároš P, Smetanková J, Mandičák T. The fifth dimension of BIM–implementation survey. IOP Conference Series: Earth and Environmental Science. 2019; 222 (1):012003
- 17. Mayouf M, Gerges M, Cox S. 5D BIM: An investigation into the integration of quantity surveyors within the BIM process. Journal of Engineering Design and Technology. 2019; 17 (3):537-553
- 18. Wu S, Ginige K, Wood G, Jong SW. How can building information modelling (BIM) support the new rules of measurement (NRM1). Royal Institution of Chartered Surveyors Research Paper; 2014
- 19. Harrison C, Thurnell D. BIM implementation in a New Zealand consulting quantity surveying practice. International Journal of Construction Supply Chain Management. 2015; 5 (1):1-15
- 20. Smith P. Project cost management with 5D BIM. Procedia-Social and Behavioral Sciences. 2016; 226 :193-200
- 21. Likhitruangsilp V, Handayani TN, Ioannou PG, Yabuki N. A BIM-enabled system for evaluating impacts of construction change orders. In: Construction Research Congress 2017; 2018. pp. 622-631
- 22. Song FC, Xie LB, Li MC, Liu JT, Zhang GQ. Collaborative design of complex overpass based on BIM technology (in Chinese). Shenyang Gongye Daxue Xuebao. 2018; 40 (6):692-696. DOI: 10.7688/j.issn.1000-1646.2018.06.17
- 23. Pocobelli DP, Boehm J, Bryan P, Still J, Grau-Bové J. Building information models for monitoring and simulation data in heritage buildings. International Archives of the Photogrammetry, Remote Sensing and Spatial Information Sciences-ISPRS Archives. 2018:909-916
- 24. Peters B. Defining environments: Understanding architectural performance through modelling, simulation and visualisation (in English). Architectural Design. 2018; 88 (1):82-91. DOI: 10.1002/ad.2262
- 25. Sadeghi M, Elliott JW, Porro N, Strong K. Developing building information models (BIM) for building handover, operation and maintenance (in English). Journal of Facilities Management. 2019; 17 (3):301-316. DOI: 10.1108/JFM-04-2018-0029
- 26. May R. Engineering urban complexity: Bespoke integrated design (in English). Architectural Design. 2015; 85 (6):104-109. DOI: 10.1002/ad.1986
- 27. Kensek K, Ding Y, Longcore T. Green building and biodiversity: Facilitating bird friendly design with building information models (in English). Journal of Green Building. 2016; 11 (2):116-130. DOI: 10.3992/jgb.11.2.116.1
- 28. Yang X, Lu YC, Murtiyoso A, Koehl M, Grussenmeyer P. HBIM modeling from the surface mesh and its extended capability of knowledge representation (in English). ISPRS International Journal of Geo-Information. 2019; 8 (7):301. DOI: 10.3390/ijgi8070301
- 29. Collins J. Incorporating BIM into architectural precast concrete fabrication. ISARC. Proceedings of the International Symposium on Automation and Robotics in Construction. IAARC Publications; 2016. p. 1
- 30. Yang X, Koehl M, Grussenmeyer P. Mesh-to-BIM: From segmented mesh elements to BIM model with limited parameters; 2018
- 31. Shen YT, Lu PW. Development of Kinetic Facade Units with BIM-Based Active Control System for the Adaptive Building Energy Performance Service; 2016
- 32. Ignatova E, Zotkin S, Zotkina I. The extraction and processing of BIM data. IOP Conference Series: Materials Science and Engineering. IOP Publishing; 2018. p. 062033
- 33. Bueno C, Pereira LM, Fabricio MM. Life cycle assessment and environmental-based choices at the early design stages: An application using building information modelling (in English). Architecture, Engineering & Design. 2018; 14 (5):332-346. DOI: 10.1080/17452007.2018.1458593
- 34. Shahsavari F, Koosha R, Yan W. In: Schnabel MA, Fukuda T, Haeusler MH, editors. Uncertainty and Sensitivity Analysis Using Building Information Modeling. Vol. 1. The Association for Computer-Aided Architectural Design Research in Asia (CAADRIA); 2019. pp. 615-624. Available from: https://www2.scopus.com/inward/record.uri?eid=2-s2.0-85068415632&partnerID=40&md5=8896bc32678a7ca87796eaef94e99021 ; https://www2.scopus.com/inward/record.uri?eid=2-s2.0-85068415632&partnerID=40&md5=8896bc32678a7ca87796eaef94e99021
- 35. Kensek KM. Integration of environmental sensors with BIM: Case studies using Arduino, Dynamo, and the Revit API (in English). Informes de la Construcción. 2014; 66 (536):e044. DOI: 10.3989/ic.13.151
- 36. Kensek KM. Teaching visual scripting in BIM: A case study using a panel controlled by solar angles (in English). Journal of Green Building. 2018; 13 (1):115-137. DOI: 10.3992/1943-4618.13.1.113
- 37. Kensek K. Visual programming for building information modeling: Energy and shading analysis case studies (in English). Journal of Green Building. 2015; 10 (4):28-43. DOI: 10.3992/jgb.10.4.28
- 38. Lim S, Buswell RA, Le TT, Austin SA, Gibb AG, Thorpe T. Developments in construction-scale additive manufacturing processes. Automation in Construction. 2012; 21 :262-268
- 39. Le TT, Austin SA, Lim S, Buswell RA, Gibb AG, Thorpe T. Mix design and fresh properties for high-performance printing concrete. Materials and Structures. 2012; 45 (8):1221-1232
- 40. Hwang B-G, Zhao X, See YL, Zhong Y. Addressing risks in green retrofit projects: The case of Singapore. Project Management Journal. 2015; 46 (4):76-89
- 41. Bock T. The future of construction automation: Technological disruption and the upcoming ubiquity of robotics. Automation in Construction. 2015; 59 :113-121
- 42. Wu P, Xia B, Pienaar J, Zhao X. The past, present and future of carbon labelling for construction materials—A review. Building and Environment. 2014; 77 :160-168
- 43. Hager I, Golonka A, Putanowicz R. 3D printing of buildings and building components as the future of sustainable construction? Procedia Engineering. 2016; 151 :292-299
- 44. Tay YWD, Panda B, Paul SC, Noor Mohamed NA, Tan MJ, Leong KF. 3D printing trends in building and construction industry: A review. Virtual and Physical Prototyping. 2017; 12 (3):261-276
- 45. Sakin M, Kiroglu YC. 3D printing of buildings: Construction of the sustainable houses of the future by BIM. Energy Procedia. 2017; 134 :702-711
- 46. Jung Y, Joo M. Building information modelling (BIM) framework for practical implementation. Automation in Construction. 2011; 20 (2):126-133
- 47. Gosselin C, Duballet R, Roux P, Gaudillière N, Dirrenberger J, Morel P. Large-scale 3D printing of ultra-high performance concrete–a new processing route for architects and builders. Materials & Design. 2016; 100 :102-109
- 48. Liu H, Holmwood B, Sydora C, Singh G, Al-Hussein M. Optimizing multi-wall panel configuration for panelized construction using BIM. In: Proceedings of the 2017 International Structural Engineering & Construction Conference (ISEC), Valencia, Spain; July 2017. pp. 24-29
- 49. Azhar S. Building information modeling (BIM): Trends, benefits, risks, and challenges for the AEC industry. Leadership and Management in Engineering. 2011; 11 (3):241-252
- 50. Bryde D, Broquetas M, Volm JM. The project benefits of building information modelling (BIM). International Journal of Project Management. 2013; 31 (7):971-980
- 51. A. F. 360. Product Development has Changed. So Should the Tools. Available from: https://www.autodesk.com.au/products/fusion-360/overview [Accessed: 11 September 2019]
- 52. Moledina MMG, Pin GW, Enegbuma WI, Ali KN, Adenuga K. Building information modelling technological innovations in industrialised building systems cost estimation. In: 2017 International Conference on Research and Innovation in Information Systems (ICRIIS). IEEE; 2017. pp. 1-6
- 53. Autodesk. Export your Revit Model to a STL File. Available from: https://knowledge.autodesk.com/support/revit-products/learn-explore/caas/CloudHelp/cloudhelp/2020/ENU/Revit-AddIns/files/GUID-93AE8701-3958-43E9-8D95-0C1650B88061-htm.html [Accessed: 10 September 2019]
© 2020 The Author(s). Licensee IntechOpen. This chapter is distributed under the terms of the Creative Commons Attribution-NonCommercial 4.0 License , which permits use, distribution and reproduction for non-commercial purposes, provided the original is properly cited.
Continue reading from the same book
Edited by Sara Shirowzhan
Published: 13 May 2020
By Mohsen Ghobadi and Samad M.E. Sepasgozar
1448 downloads
By Aman Kumar and Jasvir Singh Rattan
1250 downloads
By Faham Tahmasebinia, Kevin Yu, Jiachen Bao, George ...
826 downloads

- Tips & Tricks
- Inside Bluebeam
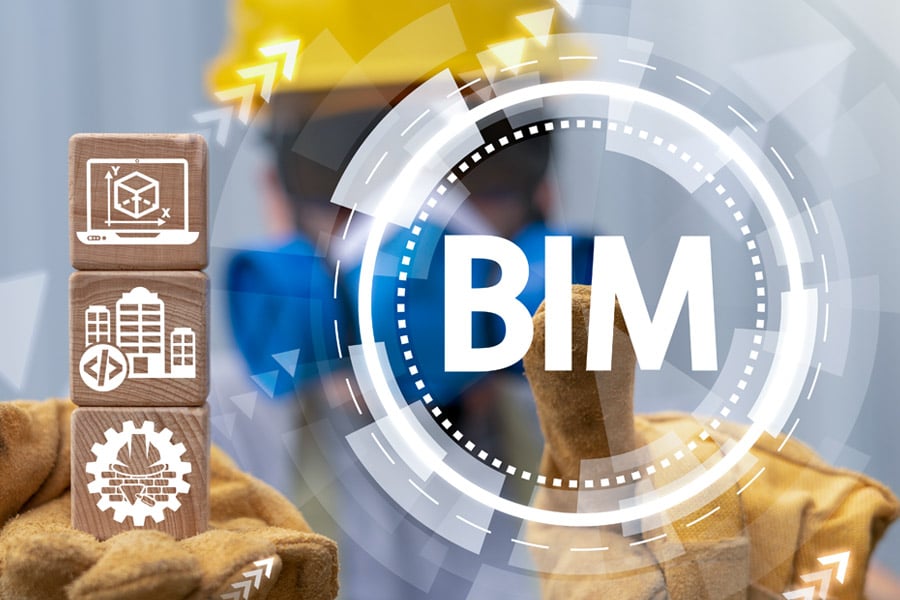
Using 5D BIM – a contractor’s view
- BY Hamish Champ
- March 24, 2021
Sessions vs. Projects: When to Use One, the Other—or Both
Land development quantities in bluebeam, why this woman chose a career in construction, why smaller contractors are uniquely positioned to benefit from construction technology.
The construction industry recognises it needs to grasp the potential offered by new technologies, including modern methods of construction, if it is to meet the demand for new homes, offices, commercial space and infrastructure projects.
Construction is still a hugely labour-intensive undertaking, yet the need for efficiencies, timely and secure delivery, and building integrity mean an increasingly digital future for much of the sector is inevitable; this has been evident with an increase in the use of BIM – building information modelling.
Using 3D renditions of a design via BIM means architects and contractors can understand how the design and proposed end product works; teams can play around with it, identifying strengths and weaknesses before getting on site.
What Does BIM Offer?
Using BIM helps to highlight costs and cost savings, lead to shorter project lifecycles, better communications between project participants and higher-quality end results.
But as project owners and contractors often use different platforms that do not sync with one another, there is consequently no single source that provides an integrated, real-time view of project design, cost and its schedule.
Yet there are solutions, not least with 5D BIM. A five-dimensional representation of a project’s physical and functional characteristics, 5D BIM allows stakeholders to identify, research and record the impact any changes will have on a project’s cost and construction timetable.
Getting Onboard with 5D BIM
For its benefits to be fully realised , 5D BIM needs to be incorporated from the design stage, along with the adoption of standardised design and data-reporting formats compatible with BIM.
But more and more firms are recognising its benefits.
Given 5D BIM’s increasingly popularity, Bluebeam asked Kyle Mattingly, virtual design and construction manager at US contractor Barton Malow, to explain why his company uses the technology.
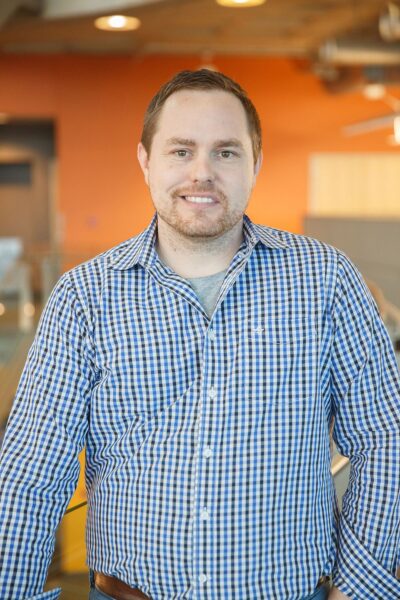
Why do you use 5D BIM alongside tools like Bluebeam Revu?
5D allows us to verify quantities alongside our precon and estimating teams. If a model is fully enough developed it is an easy double check of an estimator’s takeoff.
How does 5D BIM work compared with the earlier versions?
The concept of early 5D BIM remains the same today; the largest difference is adoption. Large companies like Barton Malow cannot quickly incorporate a change that completely swaps the method our estimators use to take off building projects. However, over time the technology is tested and implemented on a percentage of projects and those estimators become comfortable with the quantities extracted from the model.
Would you be able to give examples of where it helps you with workflows and helps to complete a project on time and within budget?
The best use case for a 5D BIM cost model is when we are provided a fully developed design model during our pricing exercises. Elements in the model that are most often correct are items like square footage of roofs, walls, floors, etc. We can easily take off counts for windows and doors, and quickly report out quantities for cubic yards of concrete for footings, foundations and slabs.
Where does the data you use within 5D BIM come from, and how does the system use that data?
The 3D model is generated from software that has built-in properties. Not all 3D modelling software is created equal; a Revit model is created with families with the properties of each element backed into the 3D components. Conversely a ‘sketchup’ model would give us little value from a 5D BIM perspective, since it is created with simple solids and no inherent properties.
Does 5D BIM help you with things like bids for work, or a job changing midway through delivery?
The bid phase is where 5D is most valuable. It assists us in rapidly doing takeoffs of quantities, so our estimators use Bluebeam Revu for quantity takeoffs, which is a quick and efficient process. They can simply put costs next to those elements, or it gives us a quick measurement to compare to the quantities that our estimators have provided.
How do you share data using 5D BIM? Is it done any differently from previous versions?
Collaboration is extremely important in sharing the data that we extract from these models. We use web-based software called Assemble which allows us to share links with our team members and allows them to see the full 5D BIM model and all the quantities and lists that we generate.
5D BIM can provide a continuous – and updated – assessment of costs throughout the life of a project. Presumably it is very helpful for your cost managers with budgets, etc.?
Pulling quantities early in design provides little useful data. However, some elements can be used early and then monitored at each design iteration for major changes. All design drawings should be generated from these models, so it is very likely that a simple change in the drawing of a window size might go unnoticed on a paper drawing, unless it is specifically called out. However, if we use the ‘model compare’ features of a 5D BIM software system like Assemble, we are able to identify each and every change no matter how minor or large.
Lastly, there is much talk about the future of BIM, of a 6D BIM. What do you think will happen with the technology? How else can it improve working practices and make delivery of ‘on-time, on-budget’ schemes more commonplace?
5D and 6D BIM are an extension of the properties and data that we are able to extract from the models. While 5D is mostly associated with costs, 6D is mostly used for its additional properties. Elements in the model have baked-in information on specific pieces of equipment. Properties like make, model, purchase date, install date, install contractor, warranty periods, specs and much more can all be baked into that 3D model and then pushed into an owner’s facility management program.
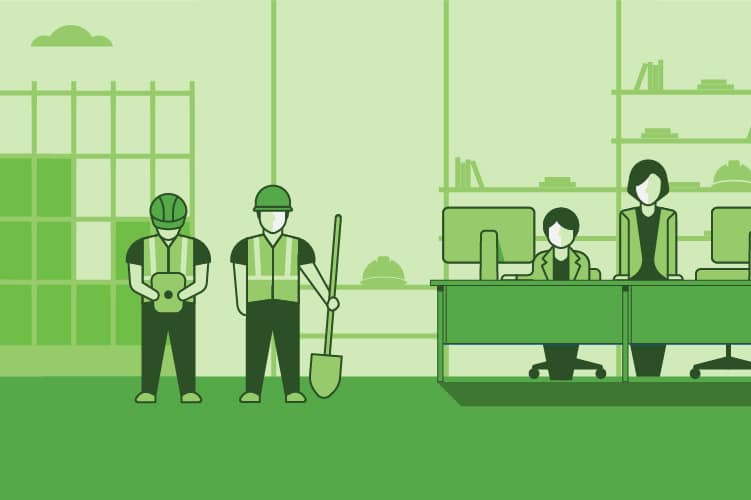
Find Out How Barton Malow Is Becoming More Environmentally Sustainable
- Tagged under: 5D , BIM , Customer Success , Stories from the UK & Ireland
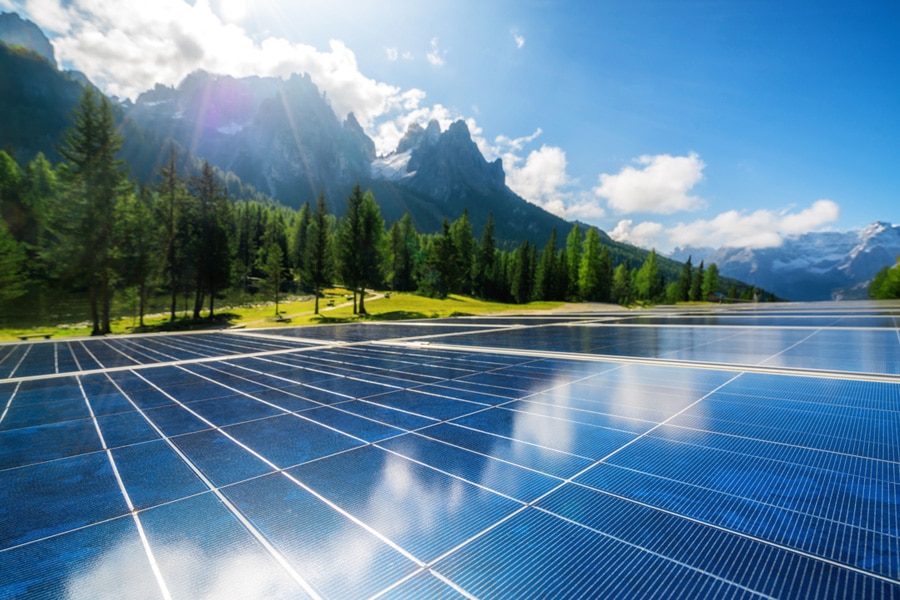
Technology Advancements in Green Building
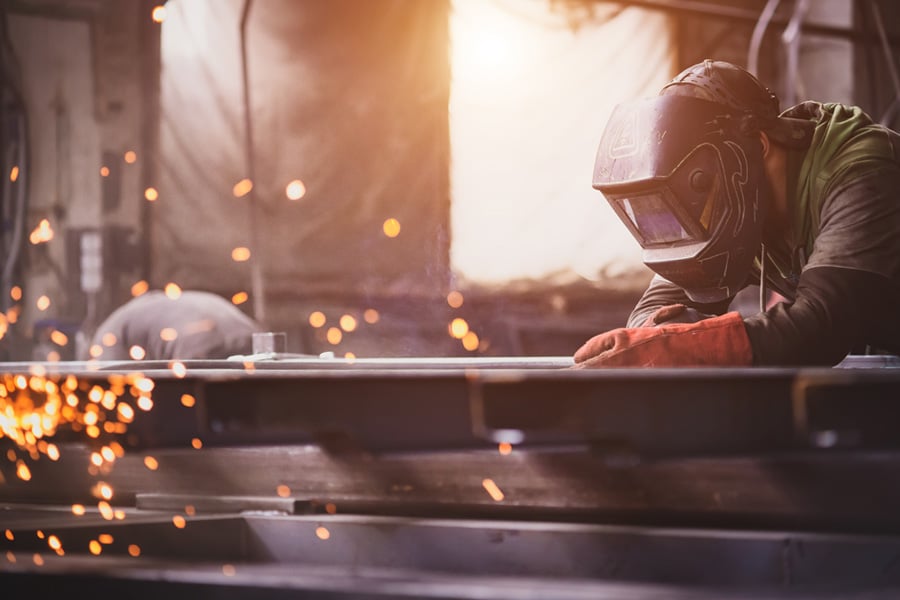
6 Bluebeam Features Perfect for Steel Professionals
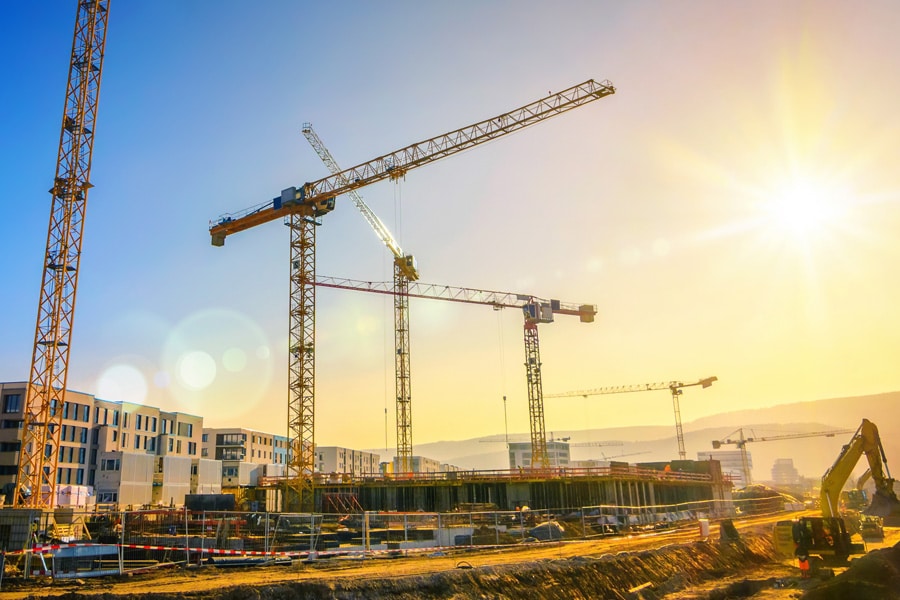
Transforming Waste Management: The TRUE Certification Initiative in Construction
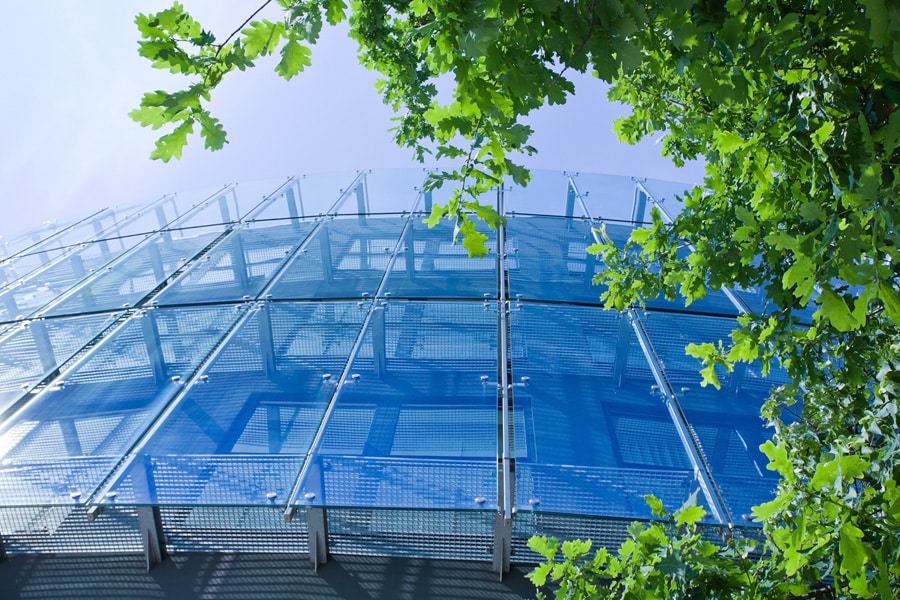
5 Key Trends in the Future of Green Building
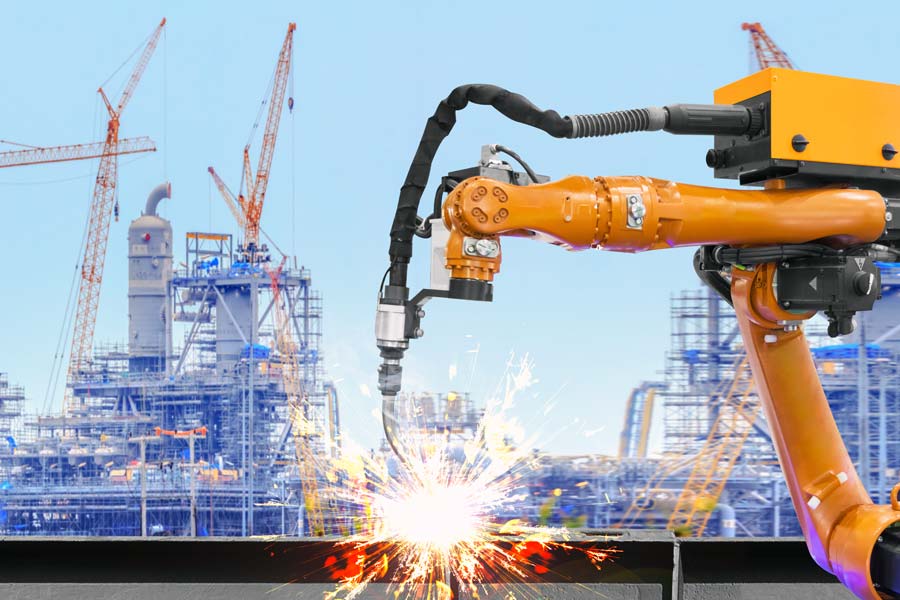
Can Automation Improve Construction Productivity?
Can the increased use of automation bolster the construction industry’s efforts to improve efficiency?
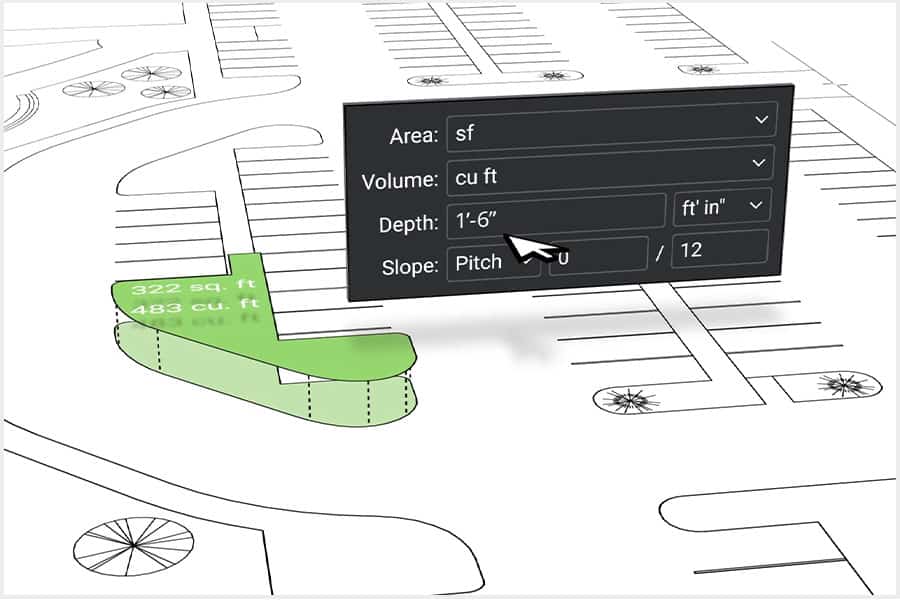
Improved Measurement Tools Help Estimators, Enhance Quantity Takeoffs
Construction professionals now can set scale across multiple drawings in Revu 20.2 and apply length units independently of page scale
Select Region

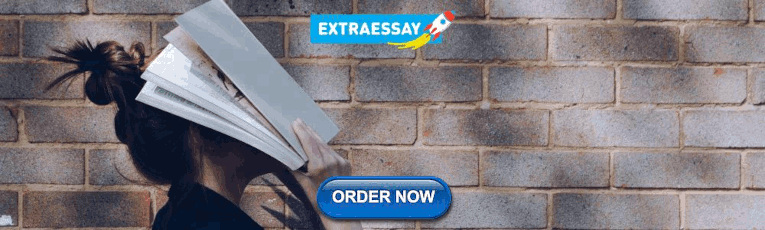
Machine learning-integrated 5D BIM informatics: building materials costs data classification and prototype development
- Technical paper
- Open access
- Published: 16 April 2022
- Volume 7 , article number 215 , ( 2022 )
Cite this article
You have full access to this open access article
- Saeed Banihashemi ORCID: orcid.org/0000-0002-7438-1235 1 ,
- Saeed Khalili 2 ,
- Moslem Sheikhkhoshkar 3 &
- Abdulwahed Fazeli 3
4361 Accesses
8 Citations
Explore all metrics
Non-informatics cost estimation is a tedious process and requires substantial amount of time and manual operations. However, BIM adoption approaches have attracted significant attention with this respect. Since BIM models are object-based with built-in parametric information, it is easier to capture the quantities of building elements and deliver more accurate estimates with less errors and omissions. As most of the current cost estimation standards are designed and developed based on old-fashioned construction project delivery systems, a lack of compatibility between their classification and BIM-based informatics is observed. This study, therefore, aims to develop an informatics framework to integrate a cost estimation standard with BIM in order to expedite the 5D BIM process and enhance the digital transformation practices in construction projects. The developed framework is considered to be a new approach which can automatically estimate the cost of building elements using machine learning-integrated algorithms and MATLAB engine for its effective implementation.
Similar content being viewed by others
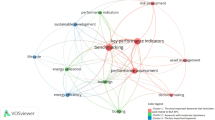
Building Condition Indicators Analysis for BIM-FM Integration
Raquel Matos, Hugo Rodrigues, … Fernanda Rodrigues
Quantity Take-Off Process Supported by Building Information Modeling (BIM) Methodology
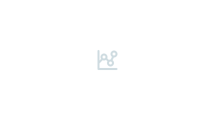
A Case Study on BIM Object-Based Earned Value and Process Management in Highway Construction
Yun-ok Kim, Dong-hyuk Lee & Du-hee Park
Avoid common mistakes on your manuscript.
Introduction
One of the critical and vital processes in construction projects is a precise cost estimate. As the project becomes larger and more complex, the more accurate estimate of the cost of a project, over time, becomes a major factor in the project success [ 1 ]. Cost estimation refers to the process of calculating and projecting the costs of three main parts including all building materials, labors and equipment within the scope of the project [ 2 , 3 ]. It involves performing quantity takeoff (QTO), which is a list of quantities of the items and materials needed for a project. Cost items contain the quantity and unit price of particular resources, connected with one or several tasks [ 4 ]. The cost of each task is estimated by multiplying the required quantities with their unit cost items and the total cost is, then, the sum of corresponding cost items [ 5 , 6 ]. Since the cost estimation is considered as one of the main parts of the projects and decision-making at the conceptual stage, its accuracy plays a key role in the success and quality of the project [ 7 , 8 ], while cost overrun is the major problem, particularly in projects with tight budgets. Indeed, the cost overrun can trigger the failure of projects [ 9 ] in developing countries like Iran where its economy suffers from huge inflation and uncertainty [ 10 , 11 , 12 ]. On the other hand, the cost estimation process is still labor-intensive and error-prone [ 13 ]. In fact, estimators should manually take off the information from printed CAD drawings and 2D-based documents. This process is not only error prone and time consuming but also it is heavily relied on the estimators’ knowledge and experience. So, it makes it difficult to process in large and complex projects [ 14 , 15 , 16 ]. Another challenging task is when estimators may need to identify different design conditions in their projects [ 17 ]. Especially, if the design changes, estimators should manually determine affected costs and adjust tasks and resources based on the updated project design. This is mainly error prone and leads to inconsistencies and inefficiencies of cost estimation process [ 18 ]. Therefore, there is still a need for practical frameworks and prototypes to accurately and precisely classify building cost informatics, estimate the total cost of the project and automate its estimation process [ 2 ].
The advent of the BIM can resolve many of the problems caused by the use of traditional estimation methods [ 19 , 20 ]. BIM offers a powerful solution to consistently produce takeoffs, counts and measurements through the updated model [ 6 , 21 ]. As opposed to traditional methods, all of the cost estimation data can be exchanged through different software with the help of collaborative platforms and open data exchanges standard for BIM such as Industry Foundation Class (IFC) [ 22 , 23 ]. This information exchange can significantly reduce the amount of workloads and human-oriented errors [ 24 , 25 ]. Furthermore, BIM is a digital representation of physical and functional traits of facilities. The product centric and object-oriented information of the BIM model is enriched with parametric building objects as properties [ 26 , 27 ]. All of these information can be extracted from BIM models for future analyses and so, the BIM model can have the ability to assist decision making in different areas of construction [ 14 , 28 , 29 ]. Ultimately, Davidson [ 30 ] indicates that BIM has the ability to takeoff the cost analysis at any stage of a project lifecycle.
Since the traditional BIM-based cost estimation requires human interference and there is no standardized cost specification for being connected with each building element, the BIM-based cost estimation is still a time-consuming, error-prone process and suffers from a low return on investment [ 31 , 32 ]. Therefore, this study aims to eliminate the human intervention by developing a machine learning and 5D BIM-integrated cost estimation prototype which classifies building cost elements, links with the 5D BIM process and enhances the adaptation of the cost classification systems (Iranian Cost Estimation Standard, in this case) with BIM-based informatics. This research also extends a new approach to the automated cost estimation process through applying a machine learning-integrated method. In which, first, an informatics prototype is built, so that all construction cost items can be classified, collected, developed and transferred to the Excel software. Second, the database of BIM is connected and updated through the Iranian Cost Estimation Standard (ICES) [ 33 ], and finally, the developed application extracts QTO from each item-cost and estimates the cost of the whole project. Furthermore, in order to automate the process, a suite of machine learning; Artificial Neural Network (ANN) algorithms including Multilayer Perceptron (MLP), Radial Basis Function (RBF) and Adaptive Neuro Fuzzy Inference System (ANFIS) is developed and applied via setting the parameters of the project as the inputs and the result of costs as the outputs.
Literature review
There are many studies which emphasize on and present the BIM-based cost estimation process. Balali, Zalavadia [ 34 ] proposed a virtual reality (VR)-based cost estimation approach for improving the ability of design changes and cost updates during preliminary phase of the project. However, the main approach only represents elemental costs of the project and it fails to estimate the cost of labors or equipment in the project. Bademosi, Tayeh [ 35 ] also developed a framework that utilizes VR as a method of visualization in order to improve the efficiency of cost estimation process and concluded that using VR can significantly reduce the time of estimation process as well as streamline the process of interactive estimates for the project. proposed a knowledge-based approach for automating the process of code-compliant QTO using the combinations of standard method of measurement rules and semantic information. Despite the effectiveness of the proposed method, the main approach still needs human intervention for extracting the QTO-related information which is prone to errors and faults. Valentini, Mirarchi [ 36 ] compared two different methods of cost estimation including traditional and BIM-based methods and found that using BIM can both increase the precision and reduce the cost of the process. developed a BIM-based system for analyzing incremental costs of prefabricated projects in China. This two-dimensional incremental cost index system was validated in a real project in the Shanghai, China. developed a BIM based method for estimating the cost of steel frames in the early phase of building projects. The proposed integrated steel design approach can estimate the whole costs of steel frames including materials, fabrications and erection. However, the main framework can be only limited to steel projects while there are many other types of projects like wood and concrete projects.
Akanbi and Zhang [ 37 ] developed a semantic natural language processing-based approach which can automate the process of information extraction and matching in the main process of cost estimation. Khosakitchalert, Yabuki [ 38 ] proposed an automatic approach for improving the accuracy of QTO in the BIM model for compound elements. The proposed method lays the groundwork for more optimized cost estimation as many overlapping regions are eliminated in this process. Wang, Wang [ 39 ] developed a new framework for cost estimation which combines project schedules from work breakdown structure (WBS) and cost information from cost breakdown structure (CBS) in the BIM area. Firstly, all quantities for one cost item in the BIM are taken off; then, the pop-up menus are represented for estimating the cost of that item. This utilizes keynotes, assembly codes and family types in the BIM and the user can manually explore the names items and gain the cost of the desired items. However, despite the practicality of the proposed method, the model has less user-friendly interface which complicates the application of the model. In addition, the user must have sufficient knowledge of BIM for efficient performance of the model. Wu, Wang [ 40 ] developed a new cost estimation framework which enables the designers to estimate the cost of structural elements in the preliminary stage of the project by linking ETABS and BIM model. The proposed method is, then, examined through a reinforced concrete building and its compliance with Taiwanese architectural laws and guidelines. Ma, Liu [ 13 ] developed an ontology-based framework that streamlines the adoption of the formulized representation of cost specifications as to improving the efficiency of cost specifications implementation in computer programs. The main advantage of this study is its capability to adapt to the other cost specifications based on the bill of quantity method. Ma and Liu [ 41 ] proposed a new approach to identify and derive the construction information in order to increase the automation of cost estimation for tendering of building projects based on the BIM-based design. Joosung and Jaejun [ 42 ] developed a 4D simulation framework which can seek out more efficient management in modular construction projects. According to this methodology, projects can benefit from the reduction in time and cost required for module yields. Zanni, Sharpe [ 43 ] examined the process of integration between the whole lifecycle cost and BIM. The method only concentrates on the emerging private rental sector since the built-to-rent market is repeatable task and similar workflow pattern. The proposed model has the potential of improving BIM lifecycle management via an augmented decision-making approach which is integral to the natural design development process. Fazeli, Dashti [ 44 ] developed a BIM-based cost estimation method which can link Iranian cost specifications with MasterFormat and UniFormat.
There are also other studies which focus on the application of machine learning in the process of cost estimation. Jiang [ 45 ] proposed the deployment of ANN in cost estimation and compared the results with RBF neural networks. The results indicate the higher performance of ANN in comparison with RBF neural networks. To optimize the accuracy of the model, the paper also examined other types of projects. Huang and Hsieh [ 46 ] proposed a BIM-based method for prediction of labor costs using the combination of random forest and simple linear regression in construction projects. The proposed method was then tested through nineteen real projects in Taiwan. Chandanshive and Kambekar [ 47 ] examined the potential of multilayer feed forward ANN along with a backpropagation learning algorithm for cost estimation in 78 building projects in India. To prevent overfitting of the model and improvement in the generalization competency of neural networks, the paper also used early stopping and Bayesian regularization approaches. Elghaish, Abrishami [ 48 ] developed an approach for the process of cost estimation using the combinations of 5D BIM and integrated project delivery. This study also used Monte Carlo simulation, target value design and activity-based costing in the main process of cost estimation which can make the process unique. Bala, Ahmad Bustani [ 49 ] used backpropagation ANN for predicting the cost of building projects in Nigeria. Despite the effectiveness of the model, the study only takes the institutional type of projects into account and there is a void for estimating other type of projects. Cheng, Tsai [ 50 ] applied the hybrid of ANN with fuzzy logic as a tool for reducing uncertainties in the process of cost estimation. The results confirmed the effectiveness of the model combination in comparison with only ANN approach for predicting the cost of projects in early phase of the project.
With regards to the literature review, the following limitations still exist in the previous studies. Firstly, although the previous approaches aim to propose a new method for cost estimation, few of them apply standardized resource specifications such as ICES items. ICES not only considers the cost of materials, but it also takes into account the cost of labors and equipment which can make the process more accurate and precise along with the full rich of standardized items which can be updated annually. Furthermore, the holistic view on the literature indicates that there is still a room of improvement for BIM-based cost informatics classification and estimation processes, particularly through the application of machine learning tools and their proper integration with the national cost guidelines to increase the digital transformation uptake in the construction industry. Finally, few studies try to classify different building elements in the project and integrate the classified system with machine learning algorithms to make the process more automated and deliver superior results.
Methodology
This study aims to develop a machine learning-integrated 5D-BIM informatics prototype for estimating the cost of buildings and validating the process through using ICES. Figure 1 demonstrates the research design of this work in which the process starts with a quantitative analysis and the workflow design, so that the BIM-based cost estimation is produced. Then, machine learning algorithms are developed through the simulation method involving several calculations and formulas in the MATLAB engine. Through the simulation, researchers are enabled to test the influences of causes and effects on various variables and likelihood reactions of different scenarios [ 51 ]. In this phase, a workflow should be developed to integrate machine learning with BIM. The algorithms are then implemented through training datasets by machine learning, and the automated cost estimation process is generated. According to Hancock and Algozzine [ 52 ], a viable case study demonstrates the values and performance of the workflow, models or algorithms implemented within a real-life setting. The algorithms are, hence, performed on a practical case study for training machine learning and developing an automated cost estimation through BIM. Finally, the control dataset is utilized to test the process and find the optimum performance for machine learning algorithms.
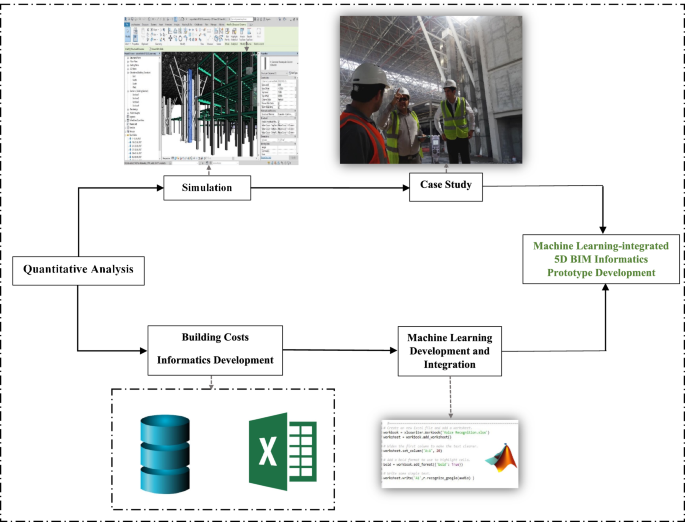
Research design
The practical steps for this research design include 6 main technical phases. Firstly, a database of ICES items is created in Excel and automatically imported into BIM authoring tool; Revit to align the materials information along with their extra data. After that, the user should create elements based on the generated Iranian materials and the system will then estimate the cost of the building through ANN and show the results in both BIM and Excel in the form of reports. However, one of the main challenging tasks for the proposed method is to adjust the elements based on ICES items. Therefore, this study also implements machine learning for facilitating the process of cost estimation of every element by using MATLAB functions. By doing so, the user can estimate cost of the project in the shorter time. So, a BIM-based extension with six major functions is required to be developed in Revit to facilitate and automate the cost estimation process. The development of the proposed approach, as represented in Fig. 2 , is explained through the following six steps.
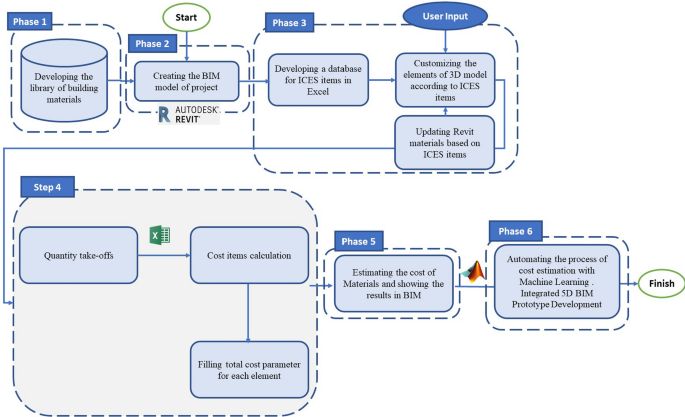
5D BIM prototype development
Phase 1: developing the library of building materials
Since Revit software has relatively modest number of building components, a comprehensive library should be created by designers to develop a BIM model with high details of information and components. This library of components enables designers to develop a BIM model with full details. It is also imperative for a quick and accurate cost estimation according to their price list. The designer can define different scenarios for the project by changing the different components (see “Appendix 1 ”) and it will help stakeholders for efficient future decision in terms of cost saving. The components of a project are provided in the formats of.rvt and.rfa. So, the designer can download them from formal webpages such as SmartBIM or ArCat libraries and import them in the Revit file projects.
Phase 2: creating the BIM model of project
3D model is considered as one of the main benefits of BIM. This evident merit can greatly assist all practitioners of projects to detect clashes of building and include data for each component. This information is needed for an accurate cost estimation of the project, and therefore, a 3D model should be created for capturing all quantities of objects.
Phase 3: developing a database for ICES items in Excel
For estimating the cost of a project in Iran, it is needed to apply Iranian cost items in the BIM model. For doing so, a database of ICES items is created in the Excel environment in this phase. This database includes all information of ICES items including Name, ID, ICES Description and unit costs.
The ICES database includes 909 items which is mainly used for the cost estimation of structural and architectural elements of projects. For better understanding about the process, the process is described in the form of a sample wall. Figure 3 shows items of ICES for this sample Wall. Table 1 also indicates all Excel information of ICES items of this sample wall which is extracted from the main database. The first column is the name of ICES items and the second, third, fourth and fifth columns are ICES items, unit cost, formulas and description, respectively.
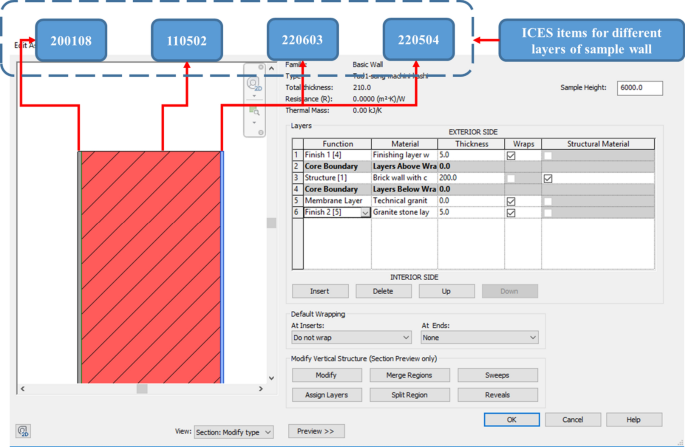
Different layers for a sample wall
Updating Revit materials based on ICES items
In this phase, a plugin is created to import all created ICES items in the BIM environment. By activating this plugin, Revit calls the Excel file, the information of ICES are automatically imported into Revit and the respected table is updated. So, 909 items of ICES were added to the Revit material list. Figure 4 shows the updated table of Revit after implementing the created plugin. As shown in Fig. 4 , the name of materials, cost parameters and unit costs are corresponded to their ICES names and Keynotes of materials in Revit.
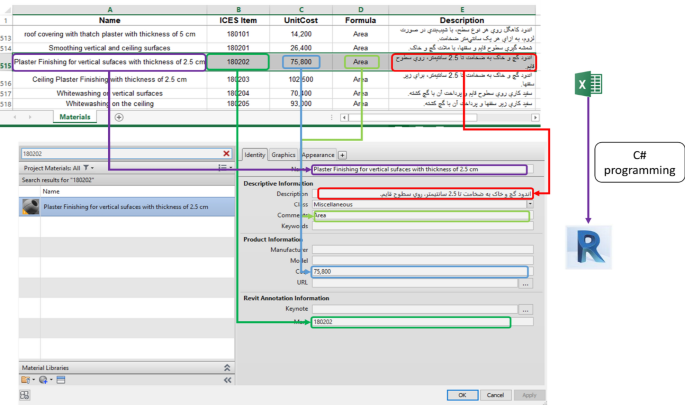
Updated database of ICES items in Revit materials
Customizing the elements of 3D model according to ICES items
By compiling the database of ICES items in Revit, the user updates the elements based on ICES items. For doing so, the user must update the layers of components according to ICES items. Figure 5 shows how the user can add ICES items in the sample wall. As it can be shown in this Fig. 5 , the wall has four layers and each layer is chosen from the ICES item database.
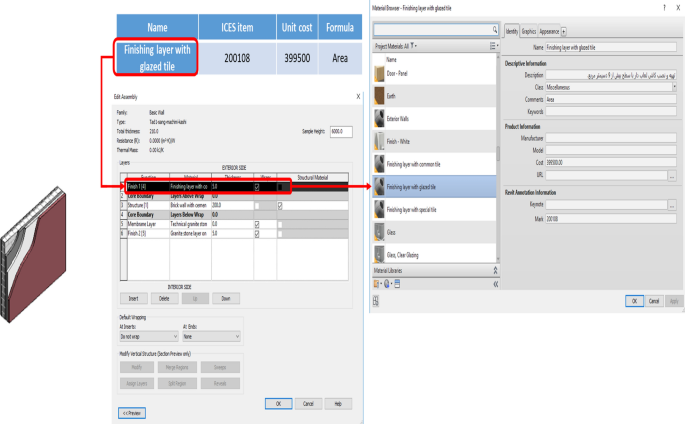
Phase 4: quantity takeoffs
In this phase, all quantities of materials are taken-off from Revit files and sent into the Excel. So, all required data for cost estimation such as cost items and unit costs in the ICES along with the geometric information such as area, volume and length of building elements are exported and categorized based on BIM parameters (Fig. 6 ).
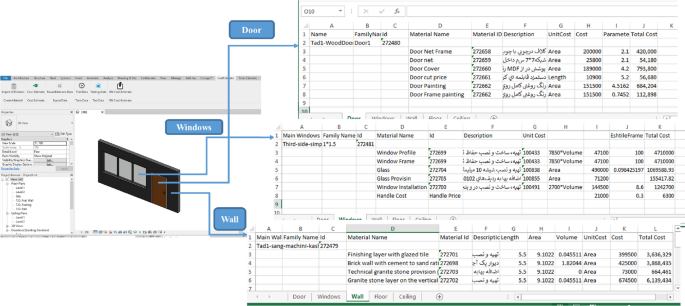
Cost estimation process and reporting to Excel file
Cost items calculation
In this step, all required calculations are performed by multiplying all related ICES cost items, corresponding ICES unit costs and their appropriate quantities of components. There are different units in the ICES items including square meter, meter, cubic meter and kilogram. It should be considered that all of these processes were done before by using formula instead of units of ICES items (Table 1 ). Figure 6 demonstrates a cost estimation for the sample wall along with their doors and windows. As can be seen in Figs. 4 , 6 , categories are estimated including floor, wall, door and windows and for each of them, a separated sheet is created and the results of each category and costs are shown in the Excel.
Filling total cost parameter for each element
In this step, the generated total cost parameter is filled by the function of “Cost-Estimate” in the developed extension. For doing so, first, all cost information of elements is summed up in the Excel. The resulted cost data are then set in the total cost parameter for every component. This parameter is needed for any future cost prediction using ANN algorithms. These data would be considered as the output data for machine learning algorithms and would be exported to MATLAB for getting run (Fig. 7 ).
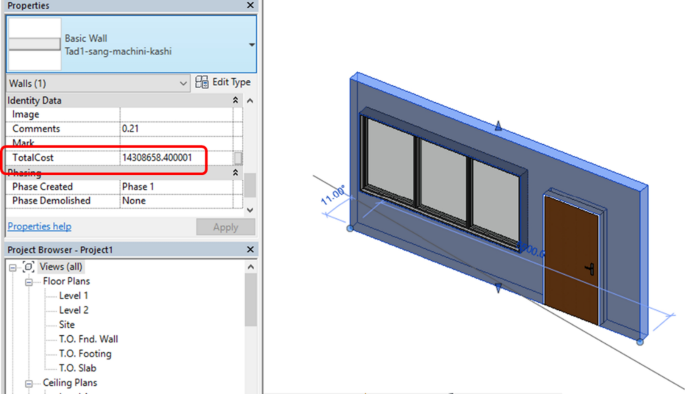
The resulted total cost for each component in Revit
Phase 5: estimating the cost of materials and showing the results in BIM
In the phase 5, the total cost of different categories of building components is shown in the Revit environment using the “Cost-Estimate” function. As being explained in the former steps, all data of elements are sorted according to the Revit parametric elements and so, the cost figures are summed up to deliver the total cost estimate (Fig. 8 ). The framework also exports the whole reports of the BIM-based cost estimation to the excel software. This report can help cost estimators to show all stakeholders of the project the details of cost estimation. With using this report, cost estimators can easily change their desired ICES items for each element using the associated ID and if there is any error including wrong ICES items or wrong type of elements, they can easily fix that problem. In fact, the main objective of this step is to reduce errors resulting from staff interventions during selecting ICES items in the phase 3 of the study.
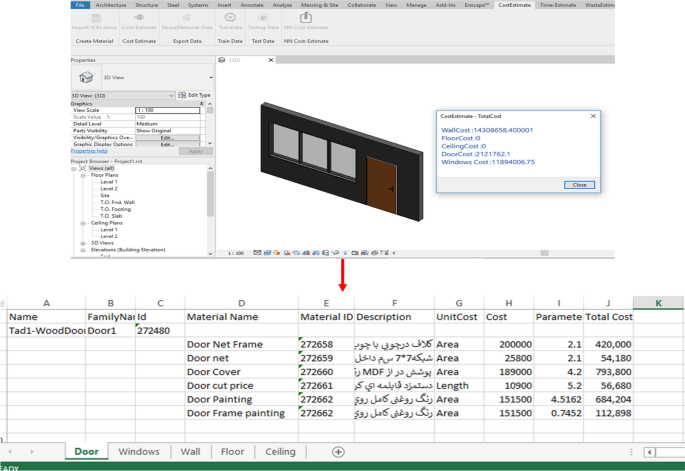
Total cost representation
Phase 6: automating the process of cost estimation with machine learning-integrated 5D BIM prototype development
The main objective of this phase is to develop an algorithm for facilitating the process of cost estimation of each element in the project using the neural network for training data. Since the process of selecting ICES items for different elements in the project was time-consuming and error-prone, this step can help all stakeholders of construction projects to automatically estimate the cost of elements. In addition, for understanding the performance of different algorithms of neural network, three versions of neural networks are examined including MLP, RBF and ANFIS. Figure 9 shows the overview of implementation of the neural network algorithms in MATLAB.
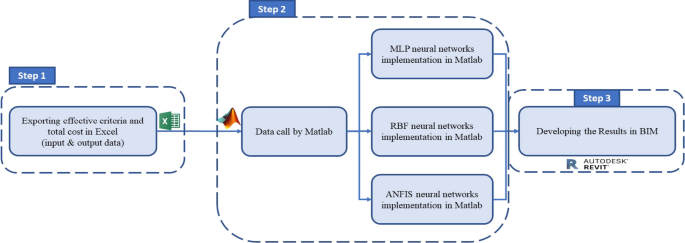
Machine learning implementation process in MATLAB
Step 1: exporting effective criteria and total cost in Excel (input and output data)
The main challenge for this step is to identify main effective criteria which considerably impact the cost of compound elements. For doing this, the digitization technique was applied and the components were corresponded to detect significant criteria of the total cost of elements. Figure 10 demonstrates a sample wall number 163. As it can be shown, the first number (1) is gypsum wall which is located as the below finishing layer of wall, the second number (6) represents engineering brick which is the structural layer of wall and the third number (3) demonstrates the trowel finish concrete wall which is laid in the upper layer of wall finishing. The similar method is applied for all other building components, parameters and cost elements.
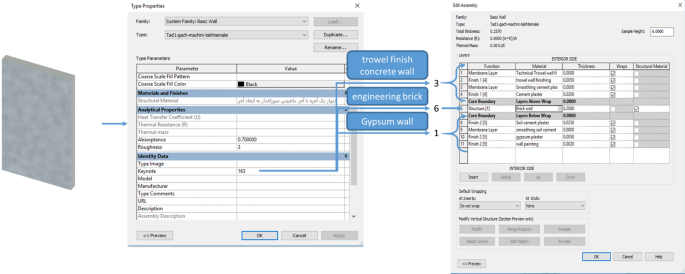
Classification of compound elements in Revit
The total cost parameter is also exported from Revit into Excel. This parameter is the output for neural network algorithms. All outputs are exported into the Excel using “NeuralNetwork-Data” function in the extension.
Step 2: data call by MATLAB
MATLAB is selected in this study because it benefits from the powerful and flexible engine and is linked well with BIM models [ 53 ]. By having the data in Excel, another plugin is created which can transfer them to MATLAB, implement neural network algorithms and finally show the results in the BIM. Hence, the implementation of the ANN algorithms encompassing MLP, RBF and ANFIS are explained in the following three steps.
MLP neural networks implementation in MATLAB
The MLP network is known to be a prevalent and one of the most prominent networks applied in neural networks. By MLP, the bias terms and weighted sum of the inputs pass to an activation level by a transfer function in order to generate an output. The units are arranged in a feed-forward layered topology coined as the feed forward neural network. MLP has some features including any number of inputs, hidden layers with any number of units, linear combination functions in the input layers, activation functions in the hidden layers and any number of outputs with any activation function [ 54 ]. Each layer involves a set of neurons and nodes. The input and output layers are linked by a hidden layer. All connections between the input layers and hidden layers contain the input weight matrix and all connections between hidden layers and output layers encompass the output weight matrix [ 55 ]. Each neuron, by computing the weighted sum of its input ( \(x_{j}\) , for j = 1,2 ,…, n ), utilizes a nonlinear activation function in order to achieve the output signal of y as Eq. 1 :
where f is the sigmoid activation function which is used in most feed-forward neural network applications. The function is demonstrated as Eq. 2 :
After clicking on the “TrainData” function in the developed extension, Revit asks from users to choose which algorithm should be implemented. By choosing MLP algorithm function, the data are transferred and processed in MATLAB and MATLAB sends back the processed data to Revit. Figure 11 shows how this back-and-forth application works.
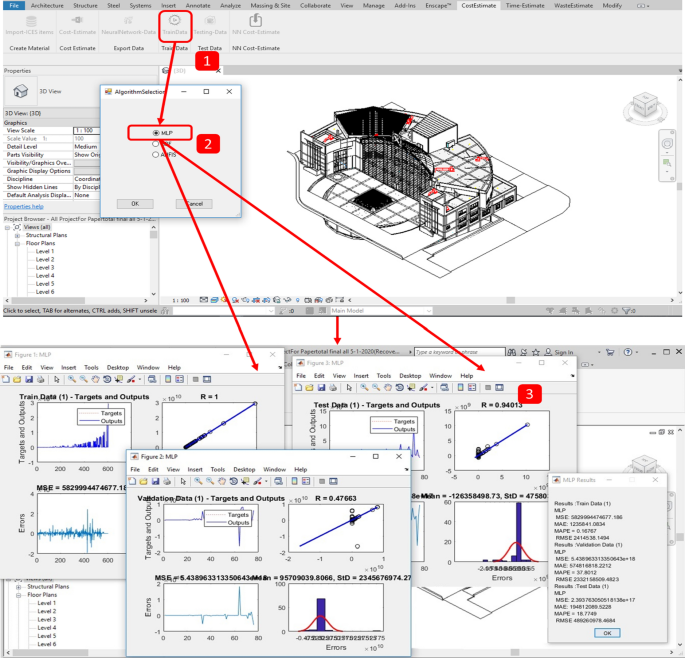
Back and forth MLP implementation
RBF neural networks implementation in MATLAB
RBF neural networks as the pattern recognition functions were proposed by several researchers to develop training methods and increase the precision of the algorithm in comparison with other neural networks [ 55 ]. The RBF networks is well working at modelling nonlinear function approximations. One-stage-training can achieve a good result rather than applying a repetitive process in MLP, as well as early learning of the application [ 56 ]. It is also used in the data classification, system modelling and control [ 55 ]. It includes one input layer, one hidden layer with a nonlinear RBF activation function along with one linear output layer. Each RBF has two key parameters, representing the location of the functions center and its deviation or width. The hidden unit calculates the gap between an input data and the center of its RBF. There also exists a single hidden layer and two sets of weights, connecting hidden layers with input and output layers. The weights which connect hidden layer with input layer include the parameters of basis functions. The weights are applied to constitute linear combinations of the activations of the basis functions (hidden units) to produce the network output [ 54 ]. The output of the network is as Eq. 3 :
where M is the number of basic functions, x is the input data vector, \(w_{kj}\) represents a weighted connection between the basic function and output layer, and \(\phi_{j}\) is the nonlinear function of the j th unit, which is typically a Gaussian function (Eq. 4 ):
where x and µ are the input and the center of RBF unit, respectively, and \(\sigma_{j}\) is the spread of the Gaussian basis function.
By clicking on the “TrainData” function in the developed extension, the same process as MLP gets activated.
ANFIS neural networks implementation in MATLAB
ANFIS is a combination of neural networks and fuzzy systems that contain the benefits of both developed by [ 57 , 58 ]. Its structure is based on the feed forward neural network system where each layer is formed from the neuro-fuzzy system components [ 55 ]. For facilitating the learning and adaptation process, the ANFIS performs according to the fuzzy surgeon model in an application of adaptive system [ 59 ]. It makes ANFIS modeling more systematic and without necessity for expert knowledge. Figure 12 represents a surgeon fuzzy system that includes two input parameters, one output parameter and two rules [ 55 , 60 ]. The corresponding ANFIS Architecture is also indicated in Fig. 12 [ 54 , 55 ]. Its rules are as follows:
If x is \(A_{1}\) and y is \(B_{1}\) Then f = \(p_{1}\) x + \(q_{1}\) y + \(r_{1}\)
If x is \(A_{2}\) and y is \(B_{2}\) Then f = \(p_{2}\) x + \(q_{2}\) y + \(r_{2}\)
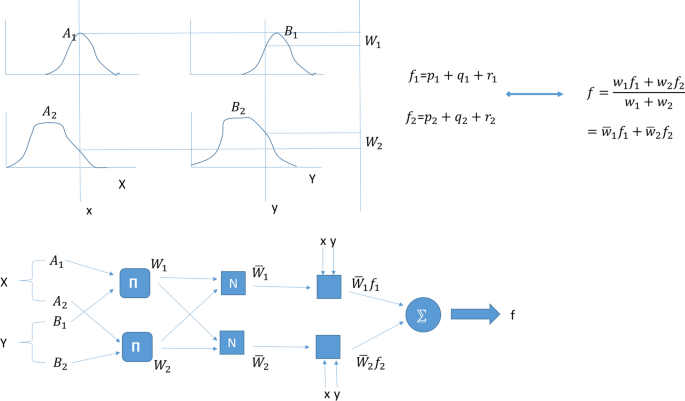
ANFIS process in MATLAB [ 54 , 55 ]
where x and y are the inputs, \(A_{i}\) and \(B_{i}\) are the fuzzy sets, \(f_{i}\) is the output, and \(p_{i}\) , \(q_{i}\) , and \(r_{i}\) are the design parameters determined during training [ 57 ]. It has five layers in which each output signal is entered into the nodes of the next layer.
The layers from the input to output are as follows [ 55 ]:
Layer 1, Fuzzy layer, includes the membership functions. Triangular and bell-shaped functions are the most popular membership functions in this layer. Every node in this layer is an adaptive node.
Layer 2, product layer, represents the strength of each rule. In fact, it evaluates the grade of activation of incorporated rules. Every node in this layer is a fixed node.
Layer 3, normalized layer, represents the normalized strength of each rule. The i th node computes the ratio of the i th rules firing strength. Every node in this layer is a fixed node.
Layer 4, defuzzied layer, contains a linear combination of multiplied inputs by the normalized strength w. It produces the output of layers.
Layer 5, total output layer, calculates the sum of the outputs in layer 4 (Fig. 12 ).
The same back-and-forth method is run for the ANFIS as well.
Step 3: developing the results in BIM
In this step, the results of the desired algorithm trained by MATLAB are shown for the user in Revit. Therefore, 4 values are shown including train and test charts, all data results from the algorithms and the measure of errors in the algorithms by using different methods including mean square error (MSE), mean absolute error (MAE), mean absolute percentage error (MAPE) and root-mean-square error (RMSE) for all charts. Figure 11 shows the sample charts of MLP algorithm after implementing the framework. As it can be shown, for each chart, there are number of tables. The first table is the data of training or test in MATLAB, the second one is to show the linear regression for results, the third one is to demonstrate errors and the final one shows the variants of errors. All of these processes are automatically implemented on the “TrainData” function in the developed extension. It should be considered that MATLAB can save the trained data. So, for testing the trained data for different algorithms, another plugin has been created. This plugin enables BIM engineers to figure out the best performing neural network, the one which performs as close as that of BIM-based cost estimation (Fig. 13 ).
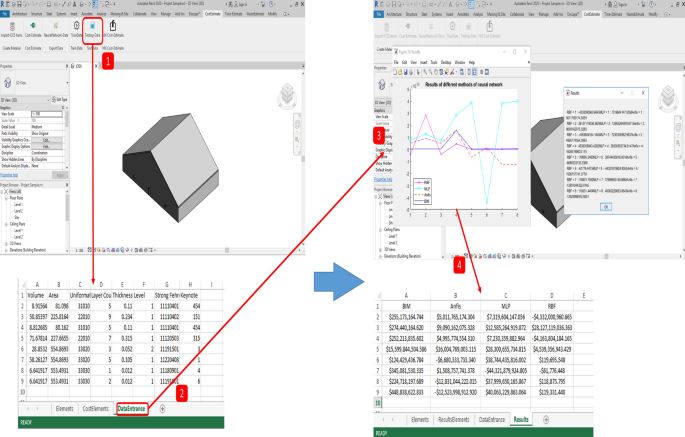
Testing data framework process
An application called “Testing-Data” has been produced in order to estimate and compare the cost of elements using the trained data with BIM-based cost estimation. The plugin extracts data from the new 3D model and the user can finally estimate the cost of elements using trained data. This application can give an estimation cost by different methods in the form of charts along with the reports in MATLAB (Fig. 13 ).
By finding out the best ANN algorithm, there is no need to repeat the whole previous process of examining different types of neural networks and BIM engineers can simply estimate the cost of building elements in the future projects using “NN Cost Estimate” plugin. In fact, this tool estimates the cost of elements using the trained algorithm and associates the results to each building element. Figure 14 shows how this plugin works. As it can be seen, firstly, BIM engineers need to choose the best neural network algorithm for the current project and then a new parameter called “NN-estimation” is generated and the estimated cost is drawn for each element. The generated plugin also delivers the total cost of each element.
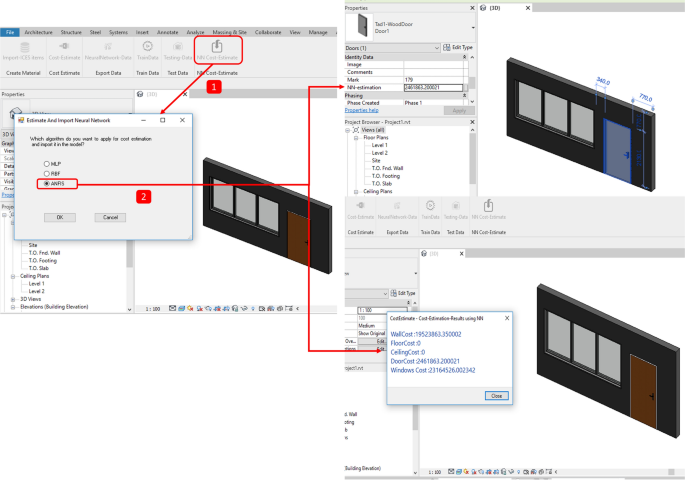
Importing the algorithms estimation results
The generated prototype
In order to implement the proposed method, an extension and compilation of 6 plugins is developed. Figure 14 demonstrates an overview of the generated prototype. As it can be shown, the first plugin called “ImportICES” sends ICES items into Revit using the ICES database created in Excel. The second plugin CostEstimate” is to estimate the cost of each element and produce a report to the user. This plugin also creates a total cost parameter for each element in the project and fills in the blank parameters with estimated costs. The next part of the plugin, “TrainData” application, is for sending created data and implementing ANN algorithms in MATLAB. The next plugin is to test new data and enable BIM engineers to test different algorithms in different projects and select the most suitable one for that project. The final plugin is for the cost estimation using the algorithm and importing results to the Revit (Fig. 15 ).
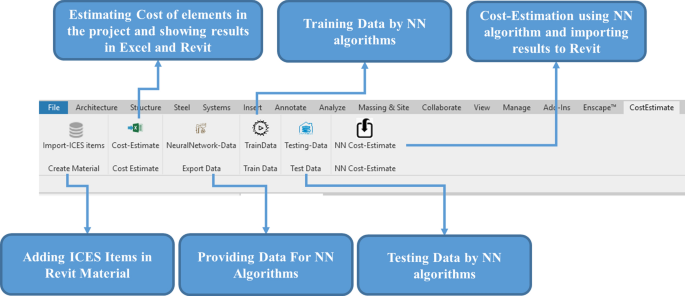
The overview of generated prototype in Revit
Prototype validation
To validate the developed prototype, a real Construction Engineering Organization Office located in Qom, a city of Iran, was used. This building can accommodate around 900 people. The project includes four main floors with one basement for parking and a facility room. The construction site of the project has a total of 6800 square meters. Figure 16 depicts an overview of the project.
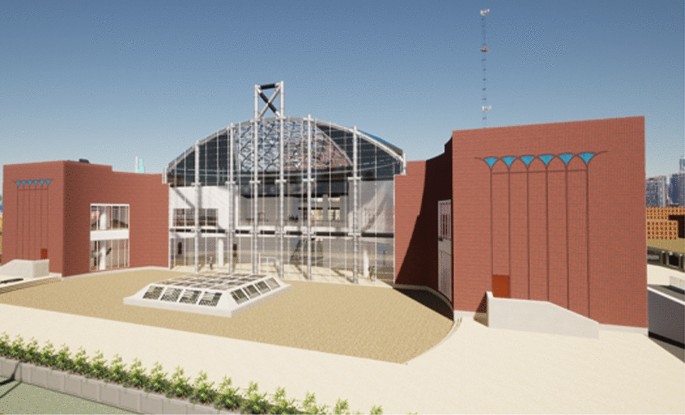
The overview of the case study
For estimating the cost of the project, all ICES items in the project are imported using “ImportICES item” plugin. Then, the user must determine appropriate ICES items for each element of the project. After doing this, the user can apply the “cost estimate” plugin for the project and the plugin, then, estimates the cost and shows a popup window for summarized cost estimation report. The plugin also reports the full ICES items along with the details in the Excel file. It also produces a parameter which is named the total cost for each element and the user can see the cost by clicking on each. Figure 17 shows how the user should choose ICES items for the elements, and Fig. 18 demonstrates the summarized cost estimation reports. It should be noticed that the most recent ICES version of 2020 was used for this case study [ 33 ].
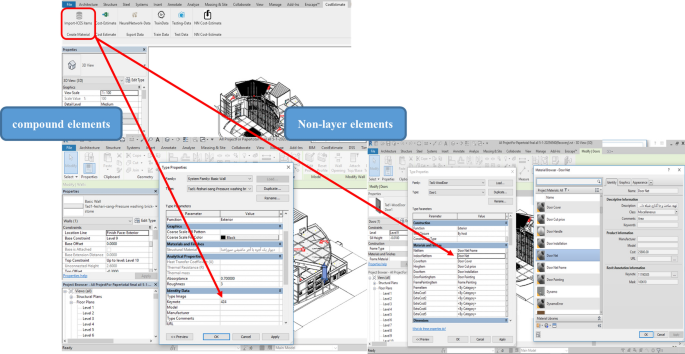
Selecting ICES items by user
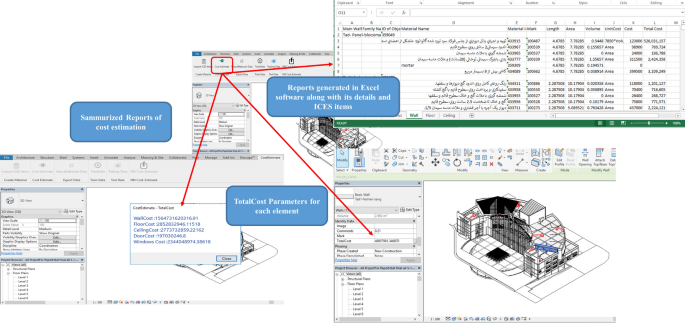
Results application by the prototype
Table 2 shows the cost of each category of the project elements. As it can be seen, the cost of the wall category is 1.564 E+12 Rials which constitutes the largest cost of the project. Floor and windows are in the second to the third ranks by representing 2.852 E+9 Rials, and 2.344 E+9 Rials, respectively. Door components make up the least amount of the cost by 1.970 E+8 Rials.
The main challenge for the developed prototype is to manually select the ICES items which is a very time consuming and error-prone task. The main solution for this issue is to estimate the cost of elements using machine learning approach. Therefore, all data of the elements in the project are applied as the training data for ANN. So, all data were extracted from Revit and imported into Excel using “NeuralNetwork Data” plugin. By its activation, all required inputs and outputs of the project were imported in the “Elements” and “CostElements” of the Excel and as a result, a record of 756 items of elements along with their costs were sent to Excel. Figure 19 demonstrates the overview of the created Excel.
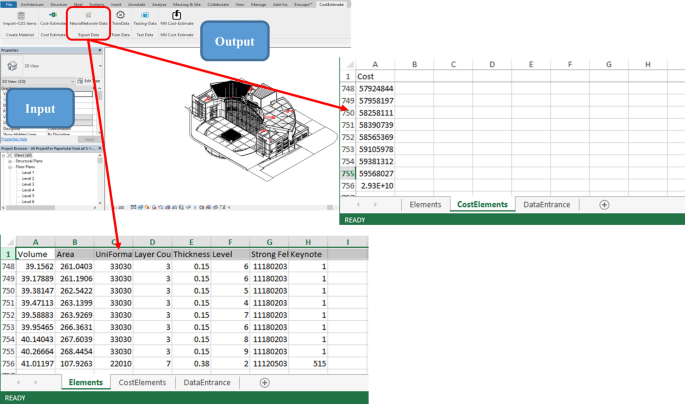
Extracting data for machine learning algorithms
After compiling the dataset for neural network, the machine learning algorithms using MATLAB engine are utilized. In fact, by “TrainingData” plugin, the user must choose the desired algorithm and MATLAB finds the Excel generated data, implements the algorithm and trains the data. In this study, all algorithms were conducted for the case study. Figure 20 shows the results of MLP algorithm. As it can be shown on the Figure, 80% of the data were used for training and 10% was dedicated for the validation and test, respectively. To assess the performance of the algorithm, four major indicators of MSE, MAE, MAPE and RMSE were applied. The MSE, MAE, MAPE and RMSE for the test data were found as 1.36 E+16, 40.13 E+6, 1.9983 and 11.664 E+7, respectively.
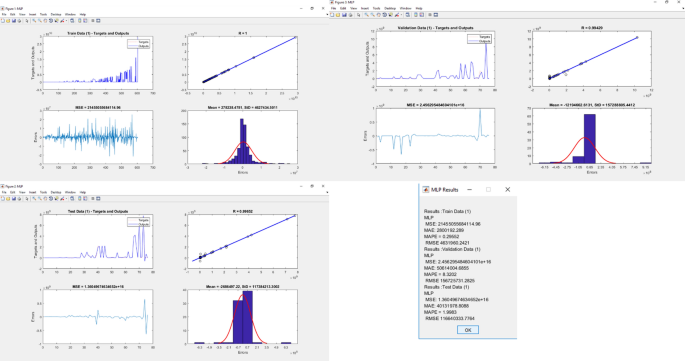
MLP algorithm results
Figure 21 demonstrates the application of RBF algorithm on the data. The data were performed by the maximum neurons of 525 neurons. 80% of data were utilized for training and the rest was used for testing. As it is shown in Fig. 20 , the MSE, MAE, MAPE and RMSE performance indexes of the algorithm for testing data are 1.58 E+17, 99.07 E+6, 1.57 and 39.79 E+7, respectively.
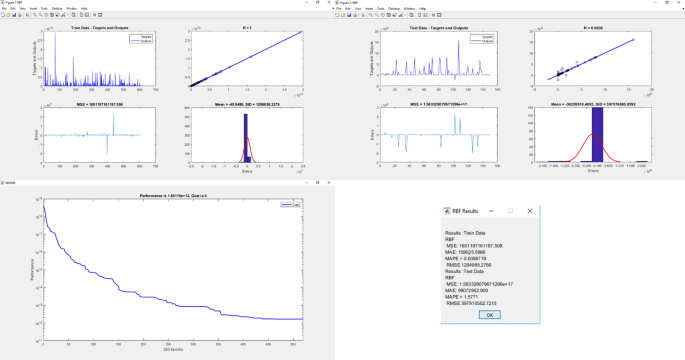
RBF algorithm results
Figure 22 indicates the results of the ANFIS. The initial fuzzy inference system for data was performed by the maximum iteration of 450 through the surgeon function. 70% of data were utilized for training and the rest was used for testing. As it is seen in this Figure, the MSE, MAE, MAPE and RMSE performance indexes of algorithm for testing data are 5.91 E+17, 414.51 E+6, 37.59 and 76.90 E+7, respectively.
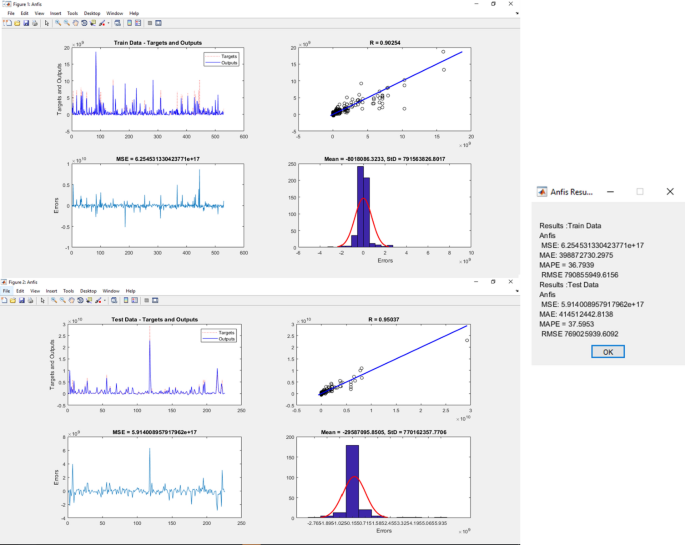
ANFIS algorithm results
Once the data were trained, the algorithms are prepared by adding new inputs and comparing the outputs for the validation stage. In fact, the main objective of this step is to compare different types of neural networks and choose the optimized algorithm for cost estimation. For doing so, new inputs were added in the” Entrance Data” sheet of the Excel.
By generating new inputs, the “TestingData” plugin was applied for estimating the cost of elements using trained algorithms. The plugin, first, estimated the cost of elements using BIM and, then, predicted the costs using trained Data. Figure 23 shows the generated results produced by different algorithms. As it is inferred from this Figure, the best algorithm which generated the most optimum results is ANFIS. It has perfectly generated the cost estimates matched with the 5D BIM system estimation. Then, RBF is in the next place and MLP shows the worst results. It can be shown from this figure that despite the good results of training, the solo application of neural networks cannot, accurately, estimate the cost of building elements in this prototype. This can be due to the insufficient number of data which should be used for training of this type of algorithm. However, ANFIS has performed very superior against RBF and MLP and also delivered the results well-aligned with 5D BIM. It is, therefore, a recommended machine learning tool for 5D BIM integration.
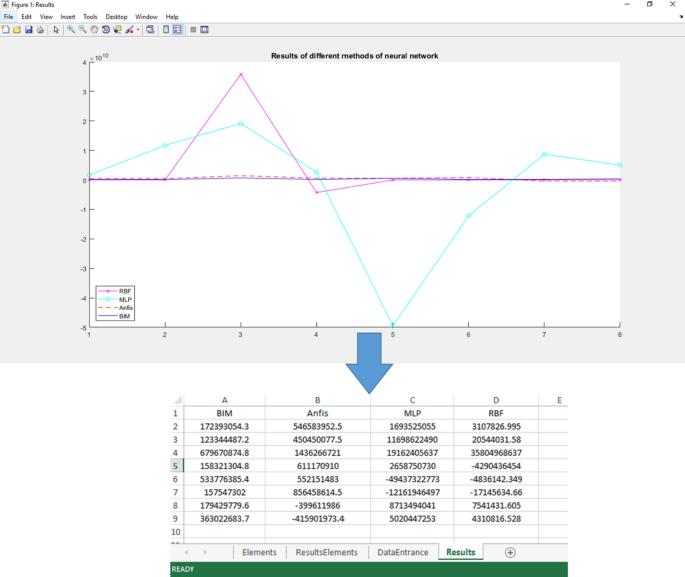
The performance results of algorithms
By choosing ANFIS as the best algorithm, there is no need to repeat the process of testing different algorithms and BIM engineers can choose ANFIS, hereafter, as the most optimized algorithm for cost estimation of the future projects. Therefore, a one-floor residential house, located in Tehran, is chosen for cost estimation applying this algorithm. Figure 24 shows the result of cost estimation using ANFIS on this case study.
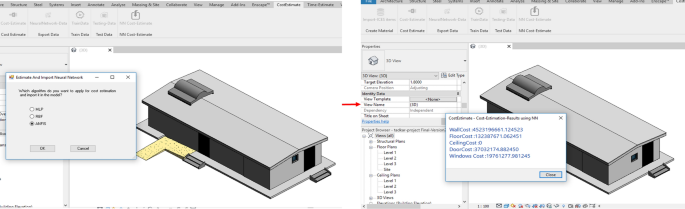
Cost Estimation using ANFIS
Table 3 compares the results of the ANFIS case study (Fig. 24 ) with the BIM-based cost estimation which is the same as the planned cost estimation of the building elements. The comparison indicates the acceptable performance for the Wall category where there is around 13% of variation between the ANFIS and BIM whereas the variations for the other elements including floor, doors and windows are higher. This finding can be in view of benefiting from the larger number of data for the wall category and having smaller dataset of floor, windows and doors in the applied case study. All in all, ANFIS has indicated a good performance in estimating the cost of elements, while there is still a room for better performance by expanding the dataset for more enhanced training.
Discussion and contribution
The lack of connection between BIM and cost specifications is one of the main barriers for adoption of BIM in the construction industry of developing countries such as Iran. Therefore, this paper aimed to bridge the gap of connecting building cost specifications and BIM-based system. ICES items are used as the representative of specification where BIM engineers can apply the similar system for other BIM-integrated applications. Previous studies made attempt to separately estimate the cost of materials, equipment and labors. However, this study develops an integrated system in which all of the building cost items are considered. Furthermore, non-informatics project cost estimation process suffers from a great deal of time and errors. Thus, there is a research priority to develop a classified building cost component and integrate with 5D BIM in order to facilitate the procedure of cost estimation. This necessity is particularly significant where BIM-driven parametric capabilities can alleviate this error-prone process. Therefore, this study developed a new informatics prototype which can connect ICES items with BIM authoring tools. However, it should be noted that this process was still semi-automatic and involved handiworks as users, cost estimators and BIM managers should designate and choose appropriate ICES items for each element in projects. So, cost estimation could be still time-consuming and error-prone. In order to solve this problem, this study employed machine learning and ANN algorithms in order to fully automate the process. Thus, three main types of ANN including MLP, RBF and ANFIS were implemented and their performance was tested and compared via the real-life construction case study. The results indicated the most optimum performance for the ANFIS algorithm in comparison with the other ANN algorithms. The reason is in light of the integration of fuzzy and neural networks in the ANFIS which declines the negative effects of the black box mechanism of neural networks. However, the validation comparison between ANFIS and BIM-based cost estimation implies that the algorithm should be trained with larger and more expanded datasets in order to deliver more accurate and closer results with that of BIM system.
In addition to the above advantages, cost estimators can be benefited from the following items:
Cost estimators can automatically find out the quantities of all construction elements along with the cost estimation details in the form of reports and schedules.
A prototype application is developed which can use a formulated process map and algorithms in the process of cost estimation. The process can significantly decrease estimators’ workloads and errors.
The total project cost can be identified in the preliminary steps of the project development.
Traditional cost estimation process involves selecting individual elements, using a tool to determine the dimensions for the takeoff, and inputting the quantities into the QTO list. These shortcomings require estimators, engineers and construction managers to spend a substantial amount of time, errors and omissions. However, using BIM systems can facilitate the process of accurate QTO, as they are enriched by object-based and built-in parametric information. Although many studies have been done in the area of implementing BIM approach in the cost estimation, very little is known regarding an integrated and standardized BIM-based system for cost estimation using neural networks algorithms in the design phase of construction projects. This is particularly prominent for those developing countries such as Iran, where the BIM implementation level and construction digital transformation are in the embryonic steps.
This research aimed to develop a new cost estimation informatics prototype which integrates building cost components of ICES items with BIM. The automation process was also sought through the ANN algorithms including MLP, RBF and ANFIS. The proposed prototype not only introduces the standardized and integrated BIM-based system in the preliminary phase of a construction project, but it also makes a greater stride for encouraging cost specifications to be integrated with BIM. Progressively, a BIM-based application in Revit environment was also developed to facilitate the process of cost estimation and in continue, the MATLAB was used to automate the process by implementing neural network algorithms on the data.
The developed prototype was further examined through a real-life construction case study. For automating the cost estimation process, the results of the case study were utilized for training ANN algorithms. The research also compared and examined different neural networks. The results show that ANFIS delivers the best results and is the most aligned algorithm with the BIM-based cost estimation system, as compared to the other algorithms. Despite the relatively good performance of the other algorithms, the sole neural network system failed to accurately estimate the cost of components vis-à-vis that of BIM and ANFIS-integrated system. For boosting the performance of those algorithms, it is recommended to add the number of training data, separate different components and train each separately for getting better results.
In addition, only four categories of building elements including wall, floor, door and windows were included in this study, while there are other building elements which can be considered too. The current study only takes into account the architectural and structural parts of ICES items whereas there are other parts in the ICES such as electrical and mechanical elements which can be included in the future works. Moreover, there are some coefficients in the ICES to apply the rate of inflation in building elements during the construction phase of a project. It is recommended to incorporate these coefficients in the future works of cost estimation.
Lee G, Park HK, Won JS (2012) 3D City project-Economic impact of BIM-assisted design validation. Autom Constr 22:577–586
Article Google Scholar
Elfaki AO, Alatawi S, Abushandi E (2014) Using intelligent techniques in construction project cost estimation: 10-year survey. Adv Civ Eng
Ma Z, Wei Z, Zhang X (2013) Semi-automatic and specification-compliant cost estimation for tendering of building projects based on IFC data of design model. Autom Constr 30:126–135
Simpson A et al. (2018) Asset information requirements guide: information required for the operation and maintenance of an asset
Xu S et al (2016) Computers in Industry A framework for integrating syntax, semantics and pragmatics for computer-aided professional practice: with application of costing in construction industry. Comput Ind 83:28–45
Liu X, Li Z, Jiang S (2016) Ontology-based representation and reasoning in building construction cost estimation in China. Fut Internet 8(3):39
Yarnold J et al. (2021) Building and construction quality: systematic literature review, thematic and gap analysis. Int J Build Pathol Adapt
Akbari S et al. (2020) Dynamic sustainable success prediction model for infrastructure projects: a rough set based fuzzy inference system. Constr Innov
Elmousalami HH (2020) Artificial intelligence and parametric construction cost estimate modeling: state-of-the-art review. J Constr Eng Manag 146(1):03119008
Khanzadi M, Sheikhkhoshkar M, Banihashemi S (2020) BIM applications toward key performance indicators of construction projects in Iran. Int J Constr Manag 20(4):305–320
Google Scholar
Hosseini MR et al. (2016) Building information modeling (BIM) in Iran: an exploratory study. J Eng Project Prod Manag
Banihashemi S et al (2017) Critical success factors (CSFs) for integration of sustainability into construction project management practices in developing countries. Int J Project Manage 35(6):1103–1119
Ma Z, Liu Z, Wei Z (2016) Formalized representation of specifications for construction cost estimation by using ontology. Comput-Aided Civ Infrastruct Eng 31(1):4–17
Liu H, Lu M, Al-Hussein M (2016) Ontology-based semantic approach for construction-oriented quantity take-off from BIM models in the light-frame building industry. Adv Eng Inform 30(2):190–207
Monteiro A, Martins JP (2013) A survey on modeling guidelines for quantity takeoff-oriented BIM-based design. Autom Constr 35:238–253
Banihashemi S, Zarepour Sohi S (2022) Data-centric regenerative built environment: big data for sustainable regeneration
Shourangiz E et al. (2011) Flexibility of BIM towards design change. In: 2nd international conference on construction and project management. IPEDR ACSIT Press, Singapore
Staub-French S et al (2003) A generic feature-driven activity-based cost estimation process. Adv Eng Inform 17(1):23–39
National Institute of Building Sciences C. (2007) National building information modeling standards: overview principles, and methodologies. NBIMS, United States, vol 1
Banihashemi S et al. (2019) Multi-sided platforms: a business model for BIM adoption in built environment SMEs. In: HCI in business, government and organizations. Information systems and analytics. HCII 2019. Lecture Notes in Computer Science. Springer, pp 22–32
Ferdosi H et al. (2022) BIM applications in sustainable construction: scientometric and state-of-the-art review. International Journal of Construction Management
Building Smart International, L.T.D. (2016) IFC overview
Oraee M et al (2017) Where the gaps lie: ten years of research into collaboration on BIM-enabled construction projects. Constr Econ Build 17(1):121–139
Estimator (2016) Graphisoft .
ToCoMan (2016) Tocosoft .
Banihashemi S, Tabadkani A, Hosseini MR (2018) Integration of parametric design into modular coordination: a construction waste reduction workflow. Autom Constr 88:1–12
Hosseini MR et al (2016) BIM adoption within Australian small and medium-sized enterprises (SMEs): an innovation diffusion model. Constr Econ Build 16(3):71–86
Banihashemi S (2017) Active BIM with artifical intelligence for energy optimisation in buildings. University of Technology Sydney
Banihashemi S, Ding G, Wang J (2015) Developing a framework of artificial intelligence application for delivering energy efficient buildings through active BIM. In: RICS COBRA 2015. RICS
Davidson AR (2009) A study of the deployment and impact of Building Information Modelling software in the construction industry. e-Engineering--Journal of Undergraduate Research (University of Leeds). London: Available at: http://www.engineering.leeds.ac.uk/eengineering . e-Engineering–Journal of Undergraduate Research (University of Leeds). London
Sompolgrunk A, Banihashemi S, Mohandes SR (2021) Building information modelling (BIM) and the return on investment: a systematic analysis. Construction Innovation
Sompolgrunk A et al. (2022) An integrated model of BIM return on investment for Australian small- and medium-sized enterprises (SMEs). Engineering, Construction and Architectural Management
ICES. Iranian Cost Estimation Standard (2022) http://sama.mporg.ir/sites/publish/SitePages/ZabetehView.aspx?mdid=5577
Balali V, Zalavadia A, Heydarian A (2020) Real-time interaction and cost estimating within immersive virtual environments. J Constr Eng Manag 146(2):10
Bademosi FM, Tayeh R, Issa RRA (2019) An immersive approach to construction cost estimating, in computing in civil engineering 2019, pp 48–54
Valentini V, Mirarchi C, Pavan A (2017) Comparison between traditional and digital preliminary cost-estimating approaches. Innov Infrast Solut 2(1):1–8
Akanbi T, Zhang JS (2021) Design information extraction from construction specifications to support cost estimation. Autom Constr 131:14
Khosakitchalert C, Yabuki N, Fukuda T (2020) Automated modification of compound elements for accurate BIM-based quantity takeoff. Autom Constr 113:23
Wang K-c et al. (2016) Applying building information modeling to integrate schedule and cost for establishing construction progress curves. Autom Constr
Wu C-L, Wang KC, Wang WC (2018) A BIM-based conceptual cost estimation model considering structural analysis and design. In: ISARC. proceedings of the international symposium on automation and robotics in construction, vol 35, pp 1–8
Ma Z, Liu Z (2014) BIM-based intelligent acquisition of construction information for cost estimation of building projects. Procedia Eng 85:358–367
Joosung L, Jaejun K (2017) BIM-based 4D simulation to improve module manufacturing productivity for sustainable building projects
Zanni M et al (2019) Developing a methodology for integration of whole life costs into bim processes to assist design decision making. Buildings 9(5):114–114
Fazeli A et al. (2020) An integrated BIM-based approach for cost estimation in construction projects. Engineering, Construction and Architectural Management
Jiang Q (2019) Estimation of construction project building cost by back-propagation neural network. J Eng Des Technol 18(3):601–609
Huang CH, Hsieh SH (2020) Predicting BIM labor cost with random forest and simple linear regression. Autom Constr 118:16
Chandanshive V, Kambekar AR (2019) Estimation of building construction cost using artificial neural networks. J Soft Comput Civ Eng 3(1):91–107
Elghaish F et al (2021) Revolutionising cost structure for integrated project delivery: a BIM-based solution. Eng Constr Archit Manag 28(4):1214–1240
Bala K, Ahmad Bustani S, Shehu Waziri B (2014) A computer-based cost prediction model for institutional building projects in Nigeria: an artificial neural network approach. J Eng Des Technol 12(4):519–530
Cheng M-Y, Tsai H-C, Sudjono E (2010) Conceptual cost estimates using evolutionary fuzzy hybrid neural network for projects in construction industry. Expert Syst Appl 37(6):4224–4231
Prive NC, Errico RM (2016) Observing system simulation experiments: an overview. Maryland University, College Park
Hancock D, Algozzine B (2016) Doing case study research: a practical guide for beginning researchers. Teachers College Press
Fazeli A et al. (2019) BIM-integrated TOPSIS-Fuzzy framework to optimize selection of sustainable building components. Int J Constr Manag:1–20
Kashi H, Emamgholizadeh S, Ghorbani H (2014) Estimation of soil infiltration and cation exchange capacity based on multiple regression, ANN (RBF, MLP), and ANFIS models. Commun Soil Sci Plant Anal 45(9):1195–1213
Amid S, Mesri Gundoshmian T (2017) Prediction of output energies for broiler production using linear regression, ANN (MLP, RBF), and ANFIS models. Environ Prog Sustain Energy 36(2):577–585
Venkatesan P, Anitha S (2006) Application of a radial basis function neural network for diagnosis of diabetes mellitus. Curr Sci 91(9):1195–1199
Jang JR (1993) ANFIS: adaptive-network-based fuzzy inference system. IEEE Trans Syst Man Cybern 23(3):665–685
Wali WA et al (2012) Online genetic-ANFIS temperature control for advanced microwave biodiesel reactor. J Process Control 22(7):1256–1272
Mousavi SM et al (2021) An intelligent hybrid classification algorithm integrating fuzzy rule-based extraction and harmony search optimization: medical diagnosis applications. Knowl-Based Syst 220:106943
Riahi-Madvar H et al (2019) Comparative analysis of soft computing techniques RBF, MLP, and ANFIS with MLR and MNLR for predicting grade-control scour hole geometry. Eng Appl Comput Fluid Mech 13(1):529–550
Download references
Open Access funding enabled and organized by CAUL and its Member Institutions.
Author information
Authors and affiliations.
University of Canberra, Canberra, Australia
Saeed Banihashemi
Bana Yar Mehraz Iranian Company, Tehran, Iran
Saeed Khalili
Iran University of Science and Technology, Tehran, Iran
Moslem Sheikhkhoshkar & Abdulwahed Fazeli
You can also search for this author in PubMed Google Scholar
Corresponding author
Correspondence to Saeed Banihashemi .
Ethics declarations
Conflict of interest.
All authors acknowledge that there is no conflict of interest for this publication.
Appendix 1: Applying different scenarios in the cost estimation using the current methodology
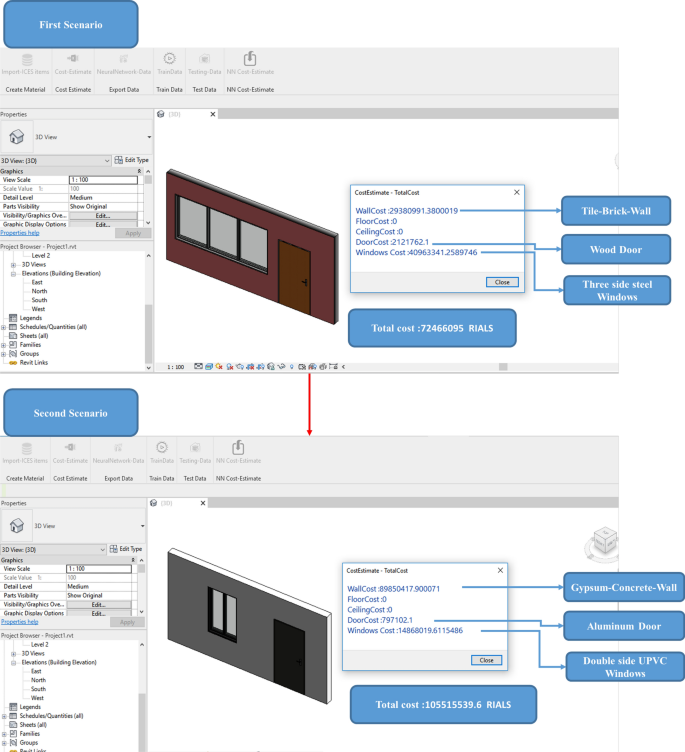
Rights and permissions
Open Access This article is licensed under a Creative Commons Attribution 4.0 International License, which permits use, sharing, adaptation, distribution and reproduction in any medium or format, as long as you give appropriate credit to the original author(s) and the source, provide a link to the Creative Commons licence, and indicate if changes were made. The images or other third party material in this article are included in the article's Creative Commons licence, unless indicated otherwise in a credit line to the material. If material is not included in the article's Creative Commons licence and your intended use is not permitted by statutory regulation or exceeds the permitted use, you will need to obtain permission directly from the copyright holder. To view a copy of this licence, visit http://creativecommons.org/licenses/by/4.0/ .
Reprints and permissions
About this article
Banihashemi, S., Khalili, S., Sheikhkhoshkar, M. et al. Machine learning-integrated 5D BIM informatics: building materials costs data classification and prototype development. Innov. Infrastruct. Solut. 7 , 215 (2022). https://doi.org/10.1007/s41062-022-00822-y
Download citation
Received : 01 December 2021
Accepted : 28 March 2022
Published : 16 April 2022
DOI : https://doi.org/10.1007/s41062-022-00822-y
Share this article
Anyone you share the following link with will be able to read this content:
Sorry, a shareable link is not currently available for this article.
Provided by the Springer Nature SharedIt content-sharing initiative
- Building information modelling
- Machine learning
- Cost estimation standard
- Find a journal
- Publish with us
- Track your research
- AU Overview

Estimating and Scheduling Integration: Automated 5D BIM Case Study
Description, key learnings.
- See a VICO 5D demo for a health care project: top down method (importing models from Revit)
- Come and see a VICO 5D demo for a residential project: Bottom-up method (import schedules from P6)
- Look at logistics tracking: Equipment sets linking various platforms assisted by Dynamo
- Learn about automated takeoffs and customized reporting templates
Academia.edu no longer supports Internet Explorer.
To browse Academia.edu and the wider internet faster and more securely, please take a few seconds to upgrade your browser .
Enter the email address you signed up with and we'll email you a reset link.
- We're Hiring!
- Help Center
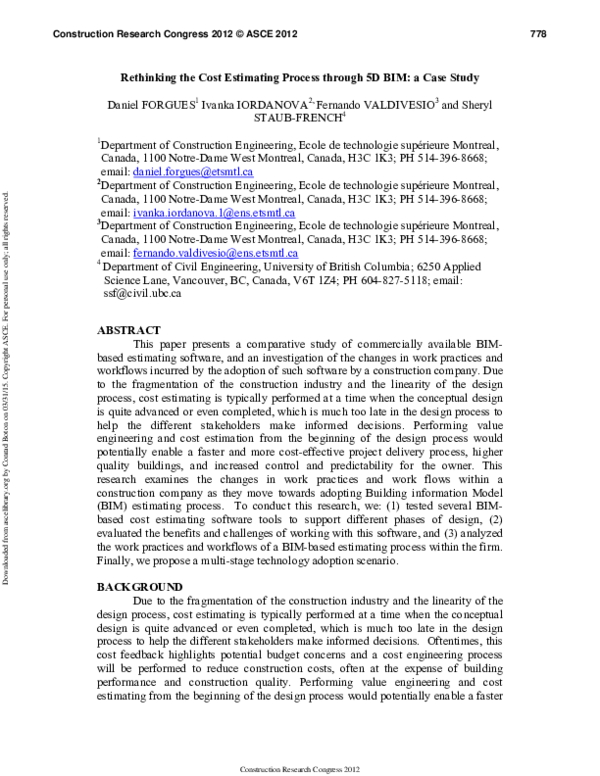
Rethinking the Cost Estimating Process through 5D BIM: A Case Study

Related Papers
johnson adafin
Accuracy of early cost estimates is a major concern for clients and cost engineers. Several researchers have long expressed their concern about cost estimating inaccuracies by recognising that the accuracy achieved in cost estimating has been less than desirable. The essence of having accurate design stage cost estimate as a reliable budgetary tool is defeated if the factors that affect design stage cost estimating accuracy and the critical methods for improving the subject are not given adequate consideration in the estimation process. Hence, project objectives regarding cost, time and quality targets are threatened. This paper first identifies various factors affecting design stage cost estimating accuracy in construction practice. Also, it provides a review of the influencing methods for improving design stage cost estimating accuracy of building projects by demonstrating the theoretical context. The study is a literature-based theoretical exploration, and a preliminary part of a...
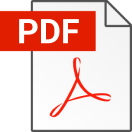
David Blackwood
This thesis describes a programme of research work which investigated the potential for the application of rational approaches, using historic cost data, to the determination of realistic fee estimates for design work in the construction industry. The development of the following research methodology for the programme of work, which was initiated at the request of industrial collaborators, is described and justified. A survey of design practices is described that enabled a model of the cost estimation process to be developed. This was tested using a large sample of design organisations and proved to be valid. There was clear evidence in the survey of a polarisation in the use of two forms of cost estimation, with intuitive approaches being used frequently and rational data driven approach being seldom, if ever, used. It was also shown that there was a widely held view amongst designers that design work was not suited to accurate cost estimation and rigorous cost control and, consequ...
Raja Bukhary
The accuracy of the cost estimates is very important in the initial stage because it will determine the viability of the project and provide the project budget. Therefore, Inaccuracy of the cost estimates beyond the acceptable range will cause the cost overrun in the later stage. This research aims to recommend BIM to be applied as a part of the cost management process and to improve the cost estimating at the initial/design stage. In addition, this research will investigate the factors influencing the accuracy, why BIM not been applied to reduce the inaccuracy and how BIM can be integrated into cost estimates at the initial / design stage. This research was carried out via literature review, questionnaire survey and semi-structured interview. It was indicated that insufficient information related to the project, poor communication between project team and complexity of design and construction are the three most factors influenced the accuracy of cost estimates. While collaborating ...
Journal of Civil Engineering and Architecture
Journal of Civil Engineering and Architecture DPC
Since the construction industry has been adopting Building Information Modeling (BIM) as the standard practice for design, engineering, and fabrication in the recent decade, many Construction Management (CM) programs at U.S. universities have started to introduce BIM for cost estimating in their curriculum. Although considered as the fifth dimension beyond 3D and schedule, BIM for cost estimating in many cases is still used merely as an alternative model-based quantity takeoff method to the traditional plan-based approach. The disconnection between automated quantity takeoff and cost estimating, however, still exists, and the benefits of the BIM process in a project life cycle can not be fully understood by CM students without realizing its impact in the preconstruction phase. To bridge these gaps in a CM curriculum, an Advanced Cost Estimating course for CM programs has been developed that focuses on integrating BIM in both the takeoff and estimating process. The new course streamlines the connection between model-based quantity takeoff and cost estimating with the help of a combination of multiple construction software programs. Through the integration between the software, quantity data from a BIM model can be seamlessly transferred to a construction cost database for bid pricing and reporting. This paper presents the development of the new Advanced Cost Estimating course as a case study, including its objectives, layout, and assessment methods, and provides empirical and valuable insights on how to integrate BIM in a cost estimating course for a CM curriculum.
Advances in Informatics and Computing in Civil and Construction Engineering
Mariangela Zanni
Impact of Sustainability on Project Evaluation
chika Udeaja
Saifulnizam Mohamad
Value Management (VM) has been proven to provide a structured framework, together with other supporting tools and techniques, that facilitate effective decision-making in many types of projects, thus achieving ‘best value’ for clients. One of the major success factors of VM in achieving better project objectives for clients is through the provision of beneficial input by multi-disciplinary team members being involved in critical decision-making discussions during the early stage of construction projects. This paper describes a doctoral research proposal based on the application of VM in design and build construction projects, especially focussing on the design stage. The research aims to study the effects of implementing VM in design and build construction projects, in particular how well the methodology addresses issues related to cost overruns resulting from poor coordination and overlooking of critical constructability issues amongst team members in construction projects in Malaysia. It is proposed that through contractors’ early involvement during the design stage, combined with the use of the VM methodology, particularly as a decision-making tool, better optimization of construction cost can be achieved, thus promoting more efficient and effective constructability. The main methods used in this research involve a thorough literature study, semi-structured interviews, and a survey of major stakeholders, a detailed case study and a VM workshop and focus group discussions involving construction professionals in order to explore and possibly develop a framework and a specific methodology for the facilitating successful application of VM within design and build construction projects.
Milad Torabi
This paper examines the global issues related to the role of project cost management professionals in the implementation and evolution of Building Information Modeling (BIM) in the construction industry. The paper is based on a review of current industry trends and issues with BIM implementation and detailed interviews with quantity surveying firms in Australia. BIM involves more than just 3D modelling and is also commonly defined in further dimensions such as 4D (time), 5D (cost) and even 6D (as-built operation). 4D links information and data in the 3D object model with project programming and scheduling data and facilitates the simulation analysis of construction activities. 5D integrates all of this information with cost data such as quantities, schedules and prices. 6D represents the as-built model that can then be used during the operational stages of the facility. This paper explores the importance for project cost management professionals to be integrally involved across all project phases and to embrace the 5th dimension to become key players in the BIM environment-the '5D Project Cost Manager'. The literature review and industry interviews will examine the issues associated with this and will identify leading edge best practices in the field by professional project cost management firms. The paper concludes with the findings that the greatest value with the modern day project cost manager lies in their ability to be 5D literate and to be able to utilise electronic models to provide detailed 5D estimates and living cost plans in real time.
Tyler Weisberg
We propose that a systems engineering methodology may be applied in an effective interactive design environment for lifecycle cost estimation and value optimization in the context of a manufacturing enterprise. In order to optimize a product design for value, engineering and manufacturing businesses need to be able to estimate accurately product lifecycle costs during the early design phases of its development, because this is when the majority of these costs are determined. Systems engineering defines realizing value as meeting stakeholder requirements and emphasizes formalizing these in order to link coherently the individual estimated costs of a design to the needs it fulfils. Furthermore, formalized requirement and design parameters are suitable for modelling and simulation, and we envision a systems model implemented within existing knowledge-based engineering tools embedded in a design environment. The results of this model may support design decisions, as well as reinforce sy...
International Journal of Construction Engineering and Management
Saeed Bozorgmehr Nia
Accurate cost estimation is crucial during the pre-construction stage of a building project as it provides valuable insights for overall cost control. This study focuses on the reliability assessment of conceptual cost estimates to enable effective budget management. Early-stage cost estimates help identify potential cost overruns, allowing stakeholders to explore alternative design options. However, due to incomplete design details, a large contingency percentage is typically allocated (around 10%) during this stage. As the project progresses, the contingency decreases, and estimate accuracy improves. Project owners, managers, and stakeholders must ensure that the design aligns with the estimated project budget to avoid overruns, cancellations, or re-bidding. This research proposes a Conceptual Cost Estimate Reliability Index (CCERI) that incorporates the weighted influence of 20 factors affecting cost estimate quality. The weights are derived from expert opinions and experience. Analysis using cost data from 100 building projects validates the CCERI. Results indicate that conceptual cost estimates with a CCERI score below 2800 are highly likely to exceed a 10% error margin, suggesting their unreliability. The CCERI score provides decision-makers with an understanding of the estimate's reliability and supports decision-making processes. Furthermore, the CCERI, along with the relative importance weights of factors, helps estimators modify and re-estimate conceptual cost estimates, reducing risks and facilitating successful project management. The study incorporates the Analytic Hierarchy Process (AHP) to assign weights to the factors influencing cost estimate reliability. The research also utilizes the Welch ANOVA test to assess statistical differences between different classes of cost estimates. Microsoft Azure Cloud is employed for data storage and analysis, ensuring scalability and efficiency in handling the large dataset.
RELATED PAPERS
Internal Medicine today Journal (IMTJ)
Delia Dumitras
L'année psychologique
Pierre Perruchet
Fabio Cypriano
Majallah-i ḥifāẓat-i giyāhān
Mehrdad Daryaei
septi eka wardani
European Journal of Clinical Pharmacology
Indian Phytopathology
Subhash Bhardwaj
Revista De Investigacion Linguistica
Alicia López
Sri Raharso
MORUFF MUDASIRU
Prof Dr Awaluddin Mohamed Shaharoun
Amazonia: Landscape and Species Evolution
Francisca Felix Leite
Foremost Journal
Neni Nurkhamidah
JUDIKA (JURNAL PENDIDIKAN UNSIKA)
oswar mungkasa
Journal of Magnetism and Magnetic Materials
Mohamad Ali Bijarchi
Revista Brasileira de Direito Urbanístico | RBDU
Debora Ungaretti
International Journal of Digital Earth
Dilene do Nascimento
Deborah Santesso Bonnas
FORHUM International Journal of Social Sciences and Humanities
Tania Briseño
tyghfg hjgfdfd
Robert JM Brummer
Arquivo Brasileiro de Medicina Veterinária e Zootecnia
Julieta Rodini Engracia de Moraes
See More Documents Like This
- We're Hiring!
- Help Center
- Find new research papers in:
- Health Sciences
- Earth Sciences
- Cognitive Science
- Mathematics
- Computer Science
- Academia ©2024
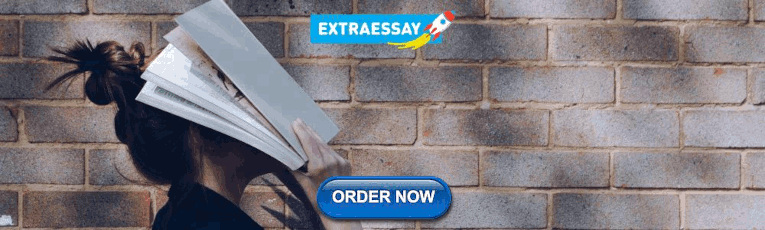
IMAGES
VIDEO
COMMENTS
Despite this, several studies focused around 5D BIM do not consider the 4D BIM as the point of departure, but often limit the methodologies for cost estimation at 3D model and cost information [15 ...
This paper considers how one construction company in the United States adopted the 5D-BIM methods, the challenges faced in implementing it within the company and the lessons learned in the process. A case-study method was used in an effort to understand the paradigm shift within the company in adopting 5D-BIM.
Ultimately, the findings suggest strong commitment and leadership from organisational management are required to facilitate cost savings and generate accurate cost information.,This study highlights key issues for any party seeking to effectively deploy 5D BIM on a contractor-led construction project.
The level of 5D BIM implementation in different countries around the world is still low, whether in the ... an increase in the satisfaction of the user of BIM digital tools. In each case study, the database and 5D tool was different, and various degrees of 5D BIM implementation can be observed: from the lowest, which can be seen in the micro ...
This research examines the changes in work practices and work flows within a construction company as they move towards adopting Building information Model (BIM) estimating process. To conduct this research, we: (1) tested several BIM-based cost estimating software tools to support different phases of design, (2) evaluated the benefits and ...
Furthermore, recent studies on 5D BIM are also at the exploration stage from three aspects, such as (a) specific case studies and applications of 5D BIM on construction costs and its key performance indicators ; (b) technical development on the software or plug-in for decommission process , lifecycle assessment and multimodels for data exchange ...
TLDR. This case study presents the use of BIM / VDC tools and processes for the coordination of MEP systems on a $96.9M healthcare project in Northern California, USA and discusses the challenges project team members faced, the specific quantitative and qualitative benefits, and the lessons that the project team learned. 304.
Case study research 5D-BIM implementation at HOAR construction may involve the investigation of a single case or company were explored. multiple cases and can be categorised as 'descriptive', 'explanatory' or 'exploratory' in nature. A descriptive The data from the interviews was transcribed case study is used to describe a ...
The purpose of the study was to understand various stakeholders' role on 5D BIM platform, the process involved in 5D BIM, challenges observed in those process and benefits achieved as compared to the traditional approach. ... T. & Stein, C. (2010, July). Case study of using an integrated 5D system in a large hospital construction project. In ...
5D BIM Implementation The development of 5D (Cost) capabilities is gaining momentum and leading edge project cost management firms are starting to realize the competitive advantages by embracing this ‘new-age’ approach to cost management. A major catalyst for the profession using this technology occurred in 2008 in the United States.
Interoperability and workflow between BIM 3D, 5D and cost estimating Figure 2 presents the BIM-based estimating workflows tested during the case study:-During the schematic design stage: starting ...
The second case study is to apply 5D BIM to the 3DP elements. The builder and stakeholders cannot get all the layers' quantity information at the same time by using the conventional 5D BIM applications or software. This chapter adopts the Dynamo script principle that is mentioned in Khosakitchalert et al. , to design a script for all the ...
5D-BIM: a case study of an implementation strategy in the construction industry. Int. Symp. Autom. Robot. Constr. Min. (ISARC 2014) (2014) View more references. ... An innovative method for project control in LNG project through 5D CAD: A case study. Automation in Construction, Volume 45, 2014, pp. 126-135.
The best use case for a 5D BIM cost model is when we are provided a fully developed design model during our pricing exercises. Elements in the model that are most often correct are items like square footage of roofs, walls, floors, etc. We can easily take off counts for windows and doors, and quickly report out quantities for cubic yards of ...
5D BIM is more than a construction and project management tool. It encompasses scheduling of material delivery and implementation, labor requirements and tasks, and project phasing. It also plays a vital communication and collaboration role, spotting and troubleshooting bottlenecks, clashes, or other problems across the entire project team ...
A case-study method was used in an effort to understand the paradigm shift within the company in adopting 5D-BIM. Interviews with key personnel within the company were conducted and content ...
The 5D BIM Workflow in VICO starts at the creation of a new project and ends with reporting and data mining.When start gearing up for a new project, 2D drawings and 3D models are added to the Document Registry for version control and change management.
Therefore, this study aims to eliminate the human intervention by developing a machine learning and 5D BIM-integrated cost estimation prototype which classifies building cost elements, links with the 5D BIM process and enhances the adaptation of the cost classification systems (Iranian Cost Estimation Standard, in this case) with BIM-based ...
This workshop will explore how estimating and scheduling can be further integrated within your project team to ensure costs are accurate, while capitalizing on the latest 5D BIM, project controls, risk analysis, and CPM methods. Additionally, we will present a case study for logistics tracking using equipment sets on various platforms assisted ...
Interoperability and workflow between BIM 3D, 5D and cost estimating Figure 2 presents the BIM-based estimating workflows tested during the case study: - During the schematic design stage: starting from a D-Profiler parametric schematic model and importing it into Revit.
5D BIM applications use 3D models (Frei et al., 2013) developed by the designers to generate outputs (e.g. QTOs, bills, etc.), but the role of quantity surveyors do not seem to be well-defined during the pre-5D BIM application process in terms of outlining the data/information required.
Consequently, the successful implementation of BIM with automated 4D/5D simulation and analysis stands as a critical milestone in advancing the capabilities of the AEC industry, ensuring greater ...
5D BIM - Cost Modelling 6D BIM - Environmental Sustainability Assessment ... Bradley A, Hyatt P, Leed A. 2011. A case study in integrating lean, green and BIM into an undergraduate construction management scheduling course. In: Proceedings of the 47th Annual International Associated Schools of Construction Conference, Lincoln, Omaha, Ascpro