Data Security and Privacy: Concepts, Approaches, and Research Directions
Ieee account.
- Change Username/Password
- Update Address
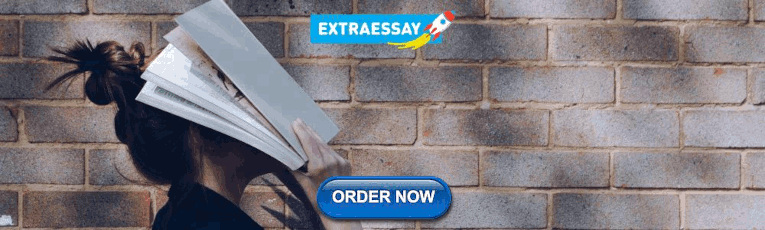
Purchase Details
- Payment Options
- Order History
- View Purchased Documents
Profile Information
- Communications Preferences
- Profession and Education
- Technical Interests
- US & Canada: +1 800 678 4333
- Worldwide: +1 732 981 0060
- Contact & Support
- About IEEE Xplore
- Accessibility
- Terms of Use
- Nondiscrimination Policy
- Privacy & Opting Out of Cookies
A not-for-profit organization, IEEE is the world's largest technical professional organization dedicated to advancing technology for the benefit of humanity. © Copyright 2024 IEEE - All rights reserved. Use of this web site signifies your agreement to the terms and conditions.
Thank you for visiting nature.com. You are using a browser version with limited support for CSS. To obtain the best experience, we recommend you use a more up to date browser (or turn off compatibility mode in Internet Explorer). In the meantime, to ensure continued support, we are displaying the site without styles and JavaScript.
- View all journals
- My Account Login
- Explore content
- About the journal
- Publish with us
- Sign up for alerts
- Open access
- Published: 17 April 2019
The GDPR and the research exemption: considerations on the necessary safeguards for research biobanks
- Ciara Staunton ORCID: orcid.org/0000-0002-3185-440X 1 ,
- Santa Slokenberga ORCID: orcid.org/0000-0002-5621-8485 2 &
- Deborah Mascalzoni 3
European Journal of Human Genetics volume 27 , pages 1159–1167 ( 2019 ) Cite this article
21k Accesses
63 Citations
23 Altmetric
Metrics details
- Health policy
The General Data Protection Regulation (GDPR) came into force in May 2018. The aspiration of providing for a high level of protection to individuals’ personal data risked placing considerable constraints on scientific research, which was contrary to various research traditions across the EU. Therefore, along with the set of carefully outlined data subjects’ rights, the GDPR provides for a two-level framework to enable derogations from these rights when scientific research is concerned. First, by directly invoking provisions of the GDPR on a condition that safeguards that must include ‘technical and organisational measures’ are in place and second, through the Member State law. Although these derogations are allowed in the name of scientific research, they can simultaneously be challenging in light of the ethical requirements and well-established standards in biobanking that have been set forth in various research-related soft legal tools, international treaties and other legal instruments. In this article, we review such soft legal tools, international treaties and other legal instruments that regulate the use of health research data. We report on the results of this review, and analyse the rights contained within the GDPR and Article 89 of the GDPR vis-à-vis these instruments. These instruments were also reviewed to provide guidance on possible safeguards that should be followed when implementing any derogations. To conclude, we will offer some commentary on limits of the derogations under the GDPR and appropriate safeguards to ensure compliance with standard ethical requirements.
Similar content being viewed by others
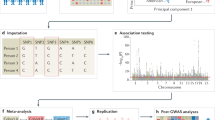
Genome-wide association studies
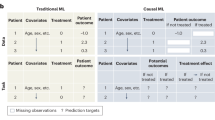
Causal machine learning for predicting treatment outcomes
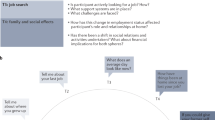
Interviews in the social sciences
Introduction.
The General Data Protection Regulation (GDPR) seeks to ensure the free movement of data throughout the European Union (EU) and give expression to the right to personal data protection within and beyond the EU, as long as an EU data subject’s data or data collected in the EU are being processed. It details, the lawful basis of the processing of data (Article 6) and delineates prohibitions for processing special categories of data, such as health and genetic data (Article 9), sets out the conditions for consent (Article 7), outlines the individual rights of data subjects (Articles 13–22), and provides data subjects with a mechanism to enforce their rights (Articles 77–84).
The EU aspiration to provide a high level of protection to individuals’ personal data risked placing considerable constraints on scientific research, which was contrary to various research traditions across the EU. Therefore, along with the set of carefully outlined data subjects’ rights, the GDPR also provides for a two-level framework to enable derogations from these rights when scientific research is concerned. First, by directly invoking provisions of the GDPR on a condition that derogations be subject to safeguards that must include ‘technical and organisational measures’, and second, through the Member State law and subject to safeguards.
Although these derogations are allowed in the name of scientific research, they can simultaneously be seen as challenging in light of the ethical requirements and long-standing protection standards for participants in biobanking that have been established in various research-related soft legal tools, international treaties and other legal instruments. In relation to the use of broad consent, the GDPR itself explicitly references such standards and Recital 33 states that it is permitted to give ‘consent to certain areas of scientific research when in keeping with recognised ethical standards for scientific research’. The recognition of ethical standards is here related to broad consent and there is no explicit requirement to consider derogations in line with these ‘recognised ethical standards for scientific research’. Hence, research that could be deemed legal under the GDPR or Member State laws that permits further derogations, might not necessarily be in line with ethical standards that are currently required by research ethics committees (RECs). In other words, a gap between the ethical standard and legal requirements could emerge.
In this article, we review such soft legal tools, international treaties and other legal instruments (collectively referred to here as ‘instruments’) that regulate the use of health research data. We report on the results of this review, and analyse the rights contained within the GDPR and Article 89 of the GDPR vis-à-vis these instruments. These instruments were also reviewed to provide guidance on possible safeguards that should be followed when implementing any derogations. To conclude, we will offer some commentary on limits of the derogations under the GDPR and appropriate safeguards to ensure compliance with standard ethical requirements.
The ethical concerns related to biobanking
Ethical consideration for biobank-based biomedical research strike a balance between the societal need for scientific development and individual’s dignity and autonomy. The Taipei declaration states that ‘[r]esearch should pursue science advancement and public health development while respecting the dignity, autonomy, privacy and confidentiality of individuals’. Those rights do not include only the direct risks for individuals of being re-identified as ‘the rights to autonomy, privacy and confidentiality also entitle individuals to exercise control over the use of their personal data and biological material’.
In fact concerns about research aims may be unrelated to ‘re-identifiability’ but rather related to possible uses in research, potentially against one person’s ethical beliefs (use of health and genetic data to profile families and new generations, gender profiling, race profiling, communities discrimination, biological weapons based on genetic specificities etc.). Concerns about possible actors involved in the use of data (insurances, private companies etc.) are also major issues as an individual’s willingness to participate in research is often based on trust in specific institutions.
Biobank research is based on long-term organised collections of data and samples that can potentially be used for very diverse research aims. At the time of the collection it is unlikely that the researcher can carefully and truly inform participants on all possible future research uses, but it is possible to provide good information on the governance of data. Recent instruments show that consent (previously the main legal basis in which to lawfully conduct research) has been in fact paired with strong governance measures and third party oversight mechanisms to be adopted by institutions to regulate sharing, accessing and use of the data. Making public governance procedures on decision making is part of the trustworthiness of an institution and has ‘replaced’ in some cases specific consent as a hardly implementable option [ 1 , 2 ]. Strong governance and ethical oversight has been proposed to support the oversight of waivers of consent in the biobank practice [ 3 ] along with forms of ongoing dynamic options [ 4 ]. Detailed governance information comprising oversight policies are usually available to participants at the time of consent.
Processing of data including secondary uses is a very sensitive area in research, not only from a legal point of view but also from an ethical one. Those concerns lead to a great emphasis on principles of transparency, trust and partnership and to the development of models for ongoing information and dynamic consent accounting for changing landscapes of uses including secondary findings [ 5 ].
The GDPR provisions on research are built on exceptions and national derogations to a law that otherwise is committed to paying great attention to human rights. However, it is unclear whether those provisions are balanced by appropriate safeguards or if they are challenging recent advancement in ethics, leading one to question whether a project that is legally compliant under the GDPR is in fact ethically compliant.
The ethical rip-off: GDPR perspectives on data subject and biobanking
Lawful processing of data under the gdpr.
Article 6 GDPR sets forth legality requirements, but these requirements must be viewed jointly with the special protection afforded to special categories of data, including health and genetic data under Article 9 GDPR. Generally for biobanking purposes, the following three lawfulness basis are of particular relevance. First, the data subject’s consent under Article 6 (1)(a). Second, the performance of a task carried out in the public interest under Article 6(1)(e). Third, processing is necessary for the purposes of the legitimate interests pursued by the controller or by a third party under Article 6(1)(f), except where such interests are overridden by the interests or fundamental rights and freedoms of the data subject, which require protection of personal data, in particular where the data subject is a child.
A distinction can be drawn between primary research and secondary biomedical research based on data and samples. While lawful basis of primary research could be consent based, which might not necessarily be so for secondary use of personal data or research using residual biological material. In the latter cases, the claim of legitimate interest is of particular importance. When data are not processed based on the individual’s consent, the requirements set in Article 6(4) shall be met, which includes the existence of appropriate safeguards.
Article 9(1) prohibits the processing of special information that includes genetic information, but Article 9(2)(j) allows for processing the genetic data as part of a special category of data if ‘processing is necessary for archiving purposes in the public interest, scientific or historical research purposes or statistical purposes.’ Furthermore, additional measures could be taken under Article 9(4) GDPR, through which Article 9(2) GDPR scope may be implicitly expanded allowing further conditions for processing health and genetic data, or constraining it. When applying Article 9(2)(j), this processing must be ‘in accordance with Article 89(1) based on Union or Member State law, which shall be proportionate to the aim pursued, respect the essence of the right to data protection and provide for suitable and specific measures to safeguard the fundamental rights and the interests of the data subject.’ In other words, for example, a Member State may attribute particular value to biobank research; they may limit a data subjects right to control the use of their data in research by removing the consent requirement for the processing of genetic data in biobanking, provided the national law respects the principle of proportionality, the essence of the right of data protection, and provides for suitable and specific measures to safeguard the rights and interests of data subjects. Yet, as further below is discussed, the GDPR is not very informative on these measures.
Individual rights and research-related derogations under GDPR
The GDPR provides data subjects with a number of rights. In biobanking, the following are of key importance: right to information (in particular, Art. 12–14), access rights (Art. 15), right to rectification (Art. 16), right to erasure (Art. 17), right to restriction of processing (Art. 18), right to data portability (Art. 20), right to object (Art. 21). Additional protective measures include, for example, a notification entitlement, providing the data subject has triggered it (Art. 19). Further to these rights and protection measures, at the data subject’s disposal is access to justice, including remedies, liability and penalties (Art. 77–84). However, this set of rights does not seem to be at the data subject’s disposal in all cases in biobank research. The exact set of rights at the data subject’s disposal depends on several circumstances, key being whether the Member State has derogated from the GDPR provisions under Article 89.2 GDPR, and/or the biobank relies on derogations through directly invoking the GDPR provisions under Article 89(1) GDPR and subsequent provisions throughout the GDPR.
Article 89(1) GDPR enables processing of genetic data for scientific research purposes, if there are appropriate safeguards for the rights and freedoms of the data subject. While the GDPR does not exhaustively specify what those safeguards are, it indicates their purpose is to ‘ensure that technical and organisational measures are in place in particular in order to ensure respect for the principle of data minimisation.’ These measures may include pseudonymisation provided it enables meeting the intended research purposes. In situations where data are not collected directly from the subject (e.g., residual material use), data subject’s right to information under Article 14 could be derogated from. Other rights that can be derogated from include the right to erasure under Article 17, as well as the right to object under Article 21.
In addition to these rights, EU or Member State law may provide for derogations from a set of other rights, provided that appropriate safeguards for the rights and freedoms of the data subject are in place. These derogations relate to the data subject’s access rights (Art. 15), right to rectification (Art. 16), right to restriction of processing (Art. 18), as well as right to object (Art. 21), and as specified in Article 89(2) GDPR, they can be applied if the derogations are necessary for research purposes.
While the data subject could remain with the data portability rights and additional protective measures, namely, the notification entitlement, these measures have a rather limited scope. First, the notification entitlement is closely related to information. If the data subject’s right to information is waived, the notification entitlement under Article 19 could be affected. Data portability, however, has a rather limited scope and it is inapplicable if biobank research relates to the performance of a task carried out in the public interest, as stated in Article 20 [ 6 ].
The potential scope of the research exemptions by directly invoking the GDPR and through the Member State laws that enables further derogations is so wide that, if applied to its full extent, not only is a data subject’s consent not necessary for the processing of personal data for research, but also the data subject can be stripped from a number of rights and others can be rendered ineffective, leaving the data subject with an enforcement mechanism only. This means, if, for example, data subjects do become aware of processing their data for biobank research, they might have no right to access information on this research or object to the research. The data subject could have no right to restrict the use of their data for research, correct any errors or request to erase the data. They would, however, be able to lodge a complaint with the data protection authority, and thus could retain some oversight [ 7 ]. However, one can then question the effectiveness of such a mechanism from a data subject’s perspective if there are no rights on the part of data subject what to oversee. The research exemption could thus undo many of the stated aims of the GDPR for research, and put at stake its own objective—the protection of privacy.
Soft legal tools, international treaties and other legal instruments (hereinafter collectively called ‘instruments’) that are influential on the regulation of health research were identified. Our review was limited to instruments that may be relevant to some or all Member States of the EU, which are either directly applicable to the Member States or to certain professions within the Member States. Our review did not include international consortia guidelines. A codebook was developed by CS and DM. All instruments were imported into Nvivo 11 and coded using thematic content analysis. A summary of the reviewed legally binding instruments can be found in Table 1 , instruments binding on particular bodies can be found in Table 2 and legally persuasive instruments can be found in Table 3 .
While the GDPR grants a number of rights to the data subjects (and simultaneously takes them away for research purposes), the focus of the other instruments is on the safeguards that must be in place for research participant data. Two exceptions are the right to information and the right to access.
Right to information
The requirements around information in the reviewed instruments is generally bound up with the consent requirements. All instruments, with the exception of the 2007 OECD Guidelines, have requirements regarding consent. It is clear from the instruments reviewed that the preference is in favour of specific, informed and written consent, where possible. Information is requested in all guidelines and should be given in plain language.
All legally binding instruments refer to the importance of informed consent, but do not necessarily set it as an obligatory pre-condition for secondary research. In addition, the GDPR introduces the possibility for electronic online consent as a viable option, provided the consent is clear and concise (Recital 32). Under the GDPR, a data subject must be informed about (among others) the identity and contact details of the data controller, the data protection officer, the purposes for which the data will be processed, the recipients of the data, the duration of storage and the right to withdraw consent if consent is the lawful basis of processing (Article 13). The 2016 Council of Europe Recommendation requires that participants be informed of the conditions applicable to the storage of the materials, including access and possible transfer policies and any relevant conditions governing the use of the materials, including re-contact and feedback (Article 10). The Council of Europe 2018 Convention mandates data subjects to be told the legal basis and the purposes of the intended processing, the categories of personal data processed, the recipients or categories of recipients of the personal data, and their rights as a data subject (Article 8).
Eminent guidance comes from the World Medical Association (WMA), historically among the first institutions to convey ethical rules for research. Its instruments sets professional standards for doctors, and are of importance in the regulation of health research globally. The Declaration of Helsinki requires that participants be informed of the aims of the research, methods, sources of funding, any possible conflict of interests, benefits and risks, institutional affiliations of the researchers and any other relevant information (Principle 26). The WMA Taipei Declaration seeks to regulate health databases and biobanks and provides more details on the requirements for consent: participants have to be informed about the purpose, the risks and burdens, storage and use of data and material, the nature of the data or material to be collected, the procedures for return of results including incidental findings, the rules of access to the health database or biobank, the protection of privacy, the governance arrangements, procedures to inform participants about the impact of anonymisation of data, their fundamental rights and safeguards as established in the Declaration, and when applicable, commercial use and benefit sharing, intellectual property issues and the transfer of data or material to other institutions or third countries (Article 12).
Allied with this extensive right to information, are the provisions on the right to withdraw consent and the obligation to inform data subjects about this right. Article 7(3) of the GDPR states that data subjects can withdraw their consent at any time and that it ‘shall be as easy to withdraw as to give consent’. The UNESCO Declaration on Bioethics (Article 6(1)), the Taipei Declaration (Article 15) and the 2017 OECD Recommendation (Article 5(2)) states that there must be procedures in place to accommodate withdrawal of consent. CIOMS states that participants need to be informed of their right to withdraw their consent, procedures need to be put in place, any withdrawal should be formalised and written, and future use of data are not permitted after this withdrawal.
Most instruments do recognise the limits on the withdrawal of consent. The 2009 OECD Guideline states that at the time of consent participants must be informed about the limits of a withdrawal of consent (Principle 4.G) as does the 2016 Council of Europe Recommendation (Article 13). Specifically, this can only be done for identified genetic data (UNESCO Declaration on Genetic Data, 2016 CoE Recommendation). It is not clear whether these limitations go beyond the practical limitation of the non-removal of anonymous data. Withdrawal of consent is thus seen of importance in both legally binding and other instruments where the legal processing of data are based on consent, but the limits on this withdrawal is recognised. Instruments that are of persuasive value state that the limits on the withdrawal of consent should be communicated to the participants.
Right to access
The GDPR, the Council of Europe 2018 Recommendation, the Taipei Declaration, the Oviedo Convention, the UNESCO Declaration on Genetic Data and the Council of Europe Recommendation all discuss the right of an individual to access their data, but there subtle differences. Under the GDPR, the data subject has the right to access information about their personal data including confirmation as to whether a data controller is processing their personal data and the purpose, other recipients of their personal data including to third countries (and the safeguards in place), where the data controller obtained the data when the data were not collected from the data subject, and expected storage period or the criteria to determine the storage period (Article 15). Article 15(3) also gives the data subject the right to access a copy of their personal data that is being processed. The Oviedo Convention states that individuals are ‘entitled to know any information collected about his or her health’ (Article 10(2)).
The WMA Taipei Declaration provides that individuals have the right to request and be provided with information about their data and requires health databases to adopt measures so that they can inform individuals about their activities (Article 14).
The 2017 OECD Guideline states that individuals must be provided with ‘information about the processing of their personal health data, including possible lawful access by third parties, the underlying objectives behind the processing, the benefits of the processing’. The Council of Europe also specifies that the management and use of the data should be made available to ‘persons’ concerned’, but this refers to the data collection in general and not the individual data (Article 6(7)). The 2006 UNESCO Declaration states that no one should be denied access to their data ‘unless domestic law limits such access in the interest of public health, public order or national security’ (Article 13).
There are limitations on this right to access. The GDPR states that the right to access does not apply when the data are anonymous and the right to access does fall within one of the possible national derogations under Article 89(2). The Oviedo Convention simply states that the right to access may be restricted in ‘exceptional’ cases with no further elaboration. The UNESCO Declaration on Genetic Data also states that the right to access does not apply when the data are anonymous and that the right to access can be limited by law in ‘the interest of public health, public order or national security’ (Article 13).
Some instruments also touch on feedback to participants as distinct from access to data. Generally, where it is discussed, a policy on feedback is required, but not necessarily that feedback must take place. Discussion on feedback of findings is confined to the non-binding instruments. The 2009 OCED Guidelines discusses ‘feedback’ to participants. It does not mandate that there is feedback to participants, only that there is a policy in place (Principle 4.9) and the results that can be feedback (Principle 4.14). The annotations to the Guideline do state that participants should be provided with information about the type of research that may be carried out on the data, whether it will be used for commercial research and if it will be transferred abroad. The 2016 CoE Recommendation similarly states that there must be a policy in place on feedback of findings and the importance of counselling (Article 17).
Right to rectification and right to erasure
The GDPR is alone in discussing the right to rectification and the right to erasure. None of the other instruments reviewed discussed these rights. The right to erasure may be linked to a right to withdraw from research as the withdrawal from any study may include the erasure of a participants’ data, but any recognised right to erasure would go further than a right to withdrawal. The right to withdrawal is arguably limited to ongoing and future research, whereas a right to erasure would include the removal from future, ongoing and past research, including potentially published research.
The right to data portability and the right to object
Article 20 of the GDPR gives data subjects the right to move their data from one data controller to another. The 2017 OECD Recommendation is the only other regulation reviewed that provides that data subjects should be permitted to request the sharing of their data for health-related purposes, and if this is rejected, they must be provided with a legal basis for that decision (Section 5(ii)(a) and (b)).
Article 21 of the GDPR provides data subjects with the right to object to the processing of their personal data, including for research purposes. Once again, the 2017 OECD Recommendation is the only regulation reviewed that states that where consent is not the lawful basis of processing, individuals should be able to object to the processing of their personal information. If this cannot be honoured, they should be provided with a relevant legal basis for the decision (Section 5(ii) (a) and (b)).
Safeguards to protect data subjects
Article 89(1) of the GDPR states that safeguards ‘shall ensure that technical and organisational measures are in place in particular in order to ensure respect for the principle of data minimisation’. These measures ‘may include pseudonymisation’, but offer no further insight into what they may also be.
Looking at the instruments reviewed, the importance of clear governance procedures (and by implication, transparency) is essential in the oversight of the use and re-use of data. This is of particular importance when the data subject has not provided specific consent to the use of the data. This may be in a manner prescribed by law, a requirement of institutional oversight that may include approval by an ethics committee or some other body, requirement of safeguards, or a combination. As outlined in Table 4 , there are three levels of oversight or protections discussed in the instruments: a legislative framework, institutional oversight that includes independent ethical review and provides for other safeguards.
The instruments reviewed have a different aim than the GDPR [ 8 ], are specifically developed for research and they do provide additional guidance that should be followed to ensure that biobanks meet standard ethical practice. First, although the derogations under the GDPR are potentially quite wide ranging, the instruments reviewed put some limits on these derogations, specifically in relation to the right to information and the right to access. Regarding the right to information, data subjects should be informed about re-contact, feedback, storage, and withdrawal of consent and any possible limits. Similarly, the instruments reviewed do strongly suggest that data subjects should be able to access their personal data, but a distinction is drawn between access of data and feedback of findings/results. The instruments do not mandate feedback of findings, but rather state that a policy should be in place. Whether there is in practice any real distinction between access of genetic data and feedback of findings, it is clear that at a minimum, biobanks should have a policy on feedback of findings in place stating whether or not this is foreseen.
Second, the instruments do provide guidance on possible safeguards. Article 89(1) and Article 89(2) speaks of the importance of the rights and freedoms of the data subject’ and this should be considered when invoking the research exemption. However, there is little guidance within the GDPR itself on striking the balance between research and individual rights. The words ‘in particular’ and ‘may include’ in Article 89(1) of the GDPR indicates that the safeguards include but are not confined to technical and organisational measures and pseudonymisation. The Article 29 WP (now the European Data Protection Board) states that safeguards could include ‘Information Security Management Systems (e.g., ISO/IEC standards) based on the analysis of information resources and underlying threats, measures for cryptographic protection during storage and transfer of sensitive data, requirements for authentication and authorisation, physical and logical access to data, access logging and others’ [ 9 ]. However, data protection is much more than a technical issue requiring technical solutions and the Article 29 WP has also spoken of the need for ‘additional legal, organisational and technical safeguards’ [ 9 ]. The safeguards must respond to the multitude of legal, ethical and social risks that are associated with the sharing of data. These risks are not static and change over time. Thus, any safeguards must be dynamic and responsive to an evolving science. In determining the safeguards that should be adopted, this review makes it clear that any derogations are subject to two pertinent factors.
First the importance of clear and transparent policies on a multitude of issues is evident. These policies may include policies on data transfer, feedback of findings, storage of data, withdrawal of consent, re-contact of data subjects, access requests from third parties, access requests of data subjects, governance, and (where applicable) intellectual property and commercial use. It is essential that biobanks have these policies in place and that they are publicly available. Having these policies available at the outset is also in line with the GDPR’s policy of privacy by design and default.
Second, it is evident that clear and transparent governance procedures that oversee the use of data are essential in protecting the rights of the data subject. Once again this is in line with the GDPR and the importance of transparency. A coherent and robust governance structure is key in fostering trust and trustworthiness that is so important in biobanking [ 10 ]. What emerges from the instruments reviewed is that there are broadly three levels to a governance structure that Member States should follow: a national legal and ethical framework; independent and interdisciplinary review and oversight of the research; and local policies on data sharing and protection.
At a minimum, national legislation should provide for the legal basis of processing of personal data for research purposes. The legislation should also mandate for local, independent approval and oversight prior to the use of data in research. Generally this will be in the form of institutional research ethics review. However, while each research project that seeks to collect and use personal data are currently subject to independent ethics review, the subsequent use and access of this data may not, with no body ensuring that the rights of data subjects are protected. Controlling access to the subsequent use of data are essential to ensure that there are no undue risks to the participant, and this review points to the importance of independent review of access requests for data. An independent body is best placed and likely to have the necessary expertise to consider the potential heterogeneous risks of an access request and these risks should not outweigh the benefits [ 11 ].
Third, each biobank must have transparent policies in place regarding the use of personal data. They should include but are not limited to policies on access, information, use and re-use of data, transfer to third parties and feedback of findings. These policies must be made publically available and submitted to a local ethics committee as part of the research protocol.
Finally, members of an ethics committee may be faced with a situation whereby a study under review meets the requirements and derogations under the GDPR, but have ethical concerns about the research. A resolution to this gap between the law and ethics may in part depended on the national legal order that is in place, but the purpose of such committees is to ensure the ethical conduct of research. The law is a minimal standard to which researchers must comply with and the Article 29 Working Party Guidelines on consent state that (where consent is the legal basis for processing) ‘consent for the use of personal data should be distinguished from other consent requirements that serve as an ethical standard or procedural obligation’ [ 12 ]. Ethics committees are not necessarily under the obligation to approve research that meets this legal threshold, but fails to meet ethical criteria.
The GDPR provides for derogations on certain individual rights for research on two separate grounds, but they must be subject to safeguards and provided for by Member State law. There is little insight or guidance contained within the GDPR as to the appropriate safeguards that must be in place, which is alarming considering the potential scope of the derogations. This review makes it clear that a full implementation of the derogations as provided for under the GDPR may render the research unethical and not in line with individuals interests. These instruments also suggest that clear governance procedures and policies on the use and re-use of personal data can go some way towards ensuring that there are necessary safeguards in place to ensure the protection of personal data. This would also ensure that any derogations continue to be in line with the GDPR’s transparency requirements and privacy by design and default. By following these necessary safeguards, biobanks can ensure that they may continue to conduct research, which ensuring the protection of personal data. In this way, research will not trump data protection, but there will be a balance of the (at times) two competing interests [ 13 ].
Mascalzoni D, Dove ES, Rubinstein Y, Dawkins H, Kole A, McCormack P, et al. International Charter of principles for sharing bio-specimens and data. Eur J Hum Genet. 2015;23:721–8.
Article Google Scholar
Boers S, van Delden J, Bredenoord A. Broad consent is consent for governance. Am J Bioethics. 2015;15:53–5.
Gainotti S, Turner C, Woods S, Kole A, McCormack P, Lochmuller H, et al. Improving the informed consent process in international collaborative rare disease research: effective consent for effective research. Eur J Hum Genet. 2016;24:1248–54.
Article CAS Google Scholar
Kaye J, Whitley EA, Lund D, Morrison M, Teare H, Melham K. Dynamic consent: a patient interface for twenty-first century research networks. Eur J Hum Genet. 2015;23:141–6.
Budin-Ljøsne I, Teare H, Kaye J, Beck S, Beate Bentzen H, Caenazzo, et al. Dynamic consent: a potential solution to some of the challenges of modern biomedical research. BMC Medical Ethics. 2017;18:4.
Chassang G, Southerington T, Tzortzatou O, Boeckhout M, Slokenberga S. Data portability in health research and biobanking : legal benchmarks for appropriate implementation. Eur Data Protection Law Rev. 2018;3:296–307.
Slokenberga S, Reichel J, Niringiye R, Croxton T, Swanepoel C, Okal J. EU data transfer rules and African legal realities: is data exchange for biobank research realistic? Int Data Privacy Law. 2018. https://doi.org/10.1093/idpl/ipy010.
Slokenberga S. Biobanking between the EU and third countries — can data sharing be facilitated via soft regulatory tools? Eur J Health Law. 2018;25:517–36. https://doi.org/10.1163/15718093-12550397
Article 29 Data Protection Working Party. Advice paper on special categories of data (“sensitive data”). Ares (2011) 444105.
Whitley EA, Kanellopoulou N, Kaye J. Consent and research governance in Biobanks: evidence from focus-groups with medical researchers. Public Health Genomics. 2012;15:232–42.
Shabani M, Borry P. Rules for processing genetic data for research purposes in view of the new EU General Data Protection Regulation. Eur J Hum Genet. 2018;26:149–56.
Article 29 Data Protection Working Party. Guidelines on consent underRegulation 2016/679. WP259 rev.01.
Mascalzoni D, Beate Bentzen H, Budin-Ljøsne I, Bygrave LA, Bell J, Dove ES, et al. Are requirements to deposit data in research repositories compatible with the European Union’s general data protection regulation? Ann Intern Med. 2019;170:332–4.
Download references
Acknowledgements
The authors thank the Department of Innovation, Research and University of the Autonomous Province of Bozen/Bolzano for covering the Open Access publication costs.
CS declares no financial support for this work. SS declares financial support by Stakeholder-informed ethics for new technologies with high socio-economic and human rights impact (SIENNA) H2020 project, grant agreement no. 741716. DM declares financial support by RD-Connect.
Author information
Authors and affiliations.
School of Law, Middlesex University, London and Centre for Biomedicine, EURAC, Bolzano, Italy
- Ciara Staunton
Faculty of Law, Lund University and Center for Research Ethics and Bioethics Uppsala University Sweden, Uppsala, Sweden
- Santa Slokenberga
Centre for Biomedicine, EURAC, Bolzano and CRB Uppsala University Sweden, Uppsala, Sweden
Deborah Mascalzoni
You can also search for this author in PubMed Google Scholar
Corresponding author
Correspondence to Ciara Staunton .
Ethics declarations
Conflict of interest.
The authors declare that they have no conflict of interest.
Additional information
Publisher’s note: Springer Nature remains neutral with regard to jurisdictional claims in published maps and institutional affiliations.
Rights and permissions
Open Access This article is licensed under a Creative Commons Attribution 4.0 International License, which permits use, sharing, adaptation, distribution and reproduction in any medium or format, as long as you give appropriate credit to the original author(s) and the source, provide a link to the Creative Commons license, and indicate if changes were made. The images or other third party material in this article are included in the article’s Creative Commons license, unless indicated otherwise in a credit line to the material. If material is not included in the article’s Creative Commons license and your intended use is not permitted by statutory regulation or exceeds the permitted use, you will need to obtain permission directly from the copyright holder. To view a copy of this license, visit http://creativecommons.org/licenses/by/4.0/ .
Reprints and permissions
About this article
Cite this article.
Staunton, C., Slokenberga, S. & Mascalzoni, D. The GDPR and the research exemption: considerations on the necessary safeguards for research biobanks. Eur J Hum Genet 27 , 1159–1167 (2019). https://doi.org/10.1038/s41431-019-0386-5
Download citation
Received : 18 July 2018
Revised : 05 March 2019
Accepted : 07 March 2019
Published : 17 April 2019
Issue Date : August 2019
DOI : https://doi.org/10.1038/s41431-019-0386-5
Share this article
Anyone you share the following link with will be able to read this content:
Sorry, a shareable link is not currently available for this article.
Provided by the Springer Nature SharedIt content-sharing initiative
This article is cited by
Specific measures for data-intensive health research without consent: a systematic review of soft law instruments and academic literature.
- Julie-Anne R. Smit
- Menno Mostert
- Johannes J. M. van Delden
European Journal of Human Genetics (2024)
Ethical and social reflections on the proposed European Health Data Space
- Mahsa Shabani
GBA/GBN-position on the feedback of incidental findings in biobank-based research: consensus-based workflow for hospital-based biobanks
- Joerg Geiger
- Joerg Fuchs
- Roland Jahns
European Journal of Human Genetics (2023)
Embedding artificial intelligence in society: looking beyond the EU AI master plan using the culture cycle
- Simone Borsci
- Ville V. Lehtola
- Raul Zurita-Milla
AI & SOCIETY (2023)
Quick links
- Explore articles by subject
- Guide to authors
- Editorial policies

- Open access
- Published: 01 March 2021
Research under the GDPR – a level playing field for public and private sector research?
- Paul Quinn ORCID: orcid.org/0000-0002-6243-765X 1
Life Sciences, Society and Policy volume 17 , Article number: 4 ( 2021 ) Cite this article
8597 Accesses
8 Citations
1 Altmetric
Metrics details
Scientific research is indispensable inter alia in order to treat harmful diseases, address societal challenges and foster economic innovation. Such research is not the domain of a single type of organization but can be conducted by a range of different entities in both the public and private sectors. Given that the use of personal data may be indispensable for many forms of research, the data protection framework will play an important role in determining not only what types of research may occur but also which types of actors may carry it out. This article looks at the role the EU’s General Data Regulation plays in determining which types of actors can conduct research with personal data. In doing so it focuses on the various legal bases that are available and attempts to discern whether the GDPR can be said to favour research in either the public or private domains. As this article explains, the picture is nuanced, with either type of research actor enjoying advantages and disadvantages in specific contexts.
1. Introduction
Research is without doubt of elemental importance to the wellbeing and advancement of any society (Mirowski and Sent 2002 ). It contributes to scientific knowledge, economic growth and can be used to address serious societal problems. Whilst the traditional image of research is that of a university research group or university hospital, the reality is that research has always and will always be conducted by a variety of actors. A large range of private entities, varying from small organisations to large and powerful tech and social media giants are continuously engaged in research. Whilst the motives of such research may often be more commercial in nature, it is nonetheless indispensable for innovation and economic growth.
The use of data is central to all forms of research. This often includes personal data. The ability of researchers to access personal data, is often a key factor in determining whether various forms of research are able to proceed (Heffetz and Ligett 2014 ). Regulatory frameworks, including data protection frameworks therefore play an important role in many instances in determining not only what forms of research may be conducted, but also what types of researchers are able to carry them out (Dalle Molle Araujo Dias 2017 ). This article looks at the importance of the EU’s General Data Protection Regulation (GDPR) in determining what types of research can be performed with personal data. In particular it focuses on the legal bases that exist within the GDPR that can be used for such purposes. As this paper discusses, the GDPR is, in general, friendly to research and presents a number of different options in terms of legal bases (informed consent being only one) that can be used in various contexts. This article aims to demonstrate which legal bases may be of most use to various types of research actor and aims in particular to contrast the position of researchers based in public sector institutions like universities with others working in the private sector e.g. for large commercial entities.
Section 2 of this article outlines the importance of personal data and consequently data protection frameworks for research. As section 3 discusses, this importance is only likely to increase given the increasingly data hungry nature of modern research, depending on inter alia access to forms of big data (Akoka et al. 2017 ). Sections 4 and 5 outline the research friendly nature of the GDPR and the various options it presents for those wishing to conduct research with personal data. These legal bases may be more appropriate for use in certain contexts and by certain types of actors than others. Whilst as sections 6 and 7 discuss, certain legal bases (e.g. allowing for research that is in the ‘public interest’ or for ‘scientific research’) may be in theory open to both public and private actors alike, the de facto reality surrounding their use means that private sector entities may find it more difficult to use them. This can be compared to the possibility to process personal data for ‘legitimate interests’. This can allow research in a number of important contexts but is in general only available to private entities and can not be used by public institutions. A further important possibility is the seemingly very broad option to further process any personal data a data controller may be in possession of for purposes of scientific research so long as it had a valid legal base for the possession of the data in the first place.
As this paper discusses, it is also important to consider the de facto context of various types of research entities. Large commercial entities (e.g. tech giants, social media platforms, or eCommerce vendors) may possess enormous pools of big data they have generated in relation to their clients. This may provide a significant practical advantage in certain conexts over researchers in public institutions. University researchers may by contrast often be dependent upon agreements with external parties to obtain research data. As sections 6 &7 discuss, these variations mean that the opportunities the GDPR provides for research are nuanced and may in reality not be equally available to all types of research actors. The answer to the question of whether there is a level playing field for research actors based in public and private research contexts is therefore complex, with neither enjoying a definitive advantage.
2. The use of personal data is becoming more common and complex in the age of big data
The importance of data protection frameworks to research has increased greatly in recent times. This is because more and more research is conducted using personal data and also because data protection frameworks have become more complex and far reaching. These changes are linked to the increasing ability to digitize more and more personal data and to share it in a world of ever increasing interconnectivity (Meszatos and Ho 2018 ). In the medical field for example patient dossiers are now routinely stored as Electronic Health Records (EHRs). Such data now forms a rich source of research data for a variety of researchers interested in investigating medical issues (Jensen et al. 2012 ). EHRs are but one example however. A wealth of diverse sources of data have emerged that can be of use for research (Connelly et al. 2016 ). These can vary from social media activity, phone tracking and efforts at citizen science (where individuals collect their own data for research purposes) (Corrales et al. 2017 ; Quinn 2018 ). Footnote 1 The ability to collect and combine any of these forms of ‘big data’ mean that researchers have a wealth of data to analyze for various research purposes. At the same time, computing power has been increasing enormously, meaning that the various analytical processes that can be applied to such data has increased greatly. This has led to an explosion of research that is solely data (or computational) based (Quinn and Quinn 2018 ). Footnote 2 Such research often does not depend on physically measuring or making impositions on individuals or objects, but rather makes use of pre-existing data. The use of such large forms of secondary data may have considerable advantages, including the analytic power that such large datasets often bring and also potentially the ability to avoid problems surrounding the primary collection of data, e.g. administrational, practical, ethical etc. Whilst this world of readily available big data might facilitate research in many ways it also brings more research within the purview of data protection. This is primarily for two reasons:
More personal data that ever before is now available.
In a digitized world individuals are able to upload and store data (on a permanent basis) to an extent that was not possible in the past. This ranges from official and important sources such as EHRs to apparently innocuous efforts at self-quantification (Swan 2013 ). It may include data taken from mobile phones (that can be linked to them) or relate to the information they post to social media. In other instances, it could come from a long history of online purchases. The continued creation, storage and ability to share such data means provides a rich source of material for researchers. The ability of researchers to access such data may vary depending on the type of entity they are and the context of the research in question (further discussed in section 6).
Many forms of big data may contain personal data (even where not immediately obvious).
In the big data world it may not always be intuitively obvious whether large data sets contain personal data within them. It may only become obvious after careful and considered inspection of the data in question. Such data may often be large, heterogeneous and unstructured. This means that it may contain personal data in ways that are not immediately obvious (Mai 2016 ). Discerning whether data is of a personal nature or not is of immense importance. The GDPR confirms that where data is anonymous it is not of application. Footnote 3 This means that researchers processing truly anonymous data do not have to concern themselves with the requirements of the GDPR (Quinn 2017 ). Footnote 4 By contrast however, where data in question is personal, the GDPR is of full application (no matter how pseudonymous or encrypted the data in question maybe). Footnote 5 Given that the bar for anonymization has been seemingly set very high, it is important not to rule out that any dataset may contain personal data even where this is not intuitively obvious. Footnote 6 This is especially true with large data sets. This is because it may be possible to combine various elements within a large data set or with data that is readily available elsewhere in order to arrive at conclusions about potentially identifiable individuals. Advances in computing power and in analytic software only increases such a possibility. Given that data is only going to become ‘bigger’, that computing power is only going to increase and that the availability of complimentary data is only going to grow, the likelihood of any large data set containing personal data is likely to increase. This factor means that it is becoming increasingly difficult to render datasets anonymous whilst allowing them to retain any useful value (e.g. for AI analysis).
3. The implications of data protection for research
A. personal data and harms.
Researchers and societies in general have long been aware of the potential for physical harms to be produced from experimentation in humans (Freidenfelds and Brandt 1996 ; Rothstein 2010 ; Drabiak 2017 ). In recent decades, and with scientific research becoming both more complex in its use of data (including data relating to human beings) an increasing awareness has been developing of harms that can be produced from the use of information in research. This has led to the creation of various strategies and approaches that have been formulated to regulate the use of personal data inter alia in research. Some of these may apply specifically to researchers whilst others may apply to other domains from which researchers may draw their data. Confidentiality laws may for instance limit the ability of researchers to obtain personal data (e.g. from medical professionals) (Berman 2002 ). Other approaches such as ethical or deontological codes may attempt to restrict what researchers can do with data in terms of research (discussed further in section 6) (Tene and Polonetsky 2016 ). Whilst such codes may not always be considered as law, they have had and continue to have an important role in regulating the use of data in research (see section 6).
In recent decades however and particularly within Europe, data protection frameworks have come to be seen as the most important form of regulation relating to the use of personal data. They are both general and of far reaching application, applying in most contexts where personal data is used, including for the purposes of research. Starting with Directive 95/46/EC Footnote 7 and more recently the General Data Protection Regulation (GDPR), Footnote 8 the European Union has effectively crafted a (mostly) harmonized regime across Europe regulating the use of personal data. Footnote 9 Data protection has arguably risen from a position of relative obscurity, to become the central regulatory framework concerning the use of personal data in research. In doing so it has arguably come to overshadow older legal regimes (e.g. related to confidentiality) that may apply. The GDPR, being the legislative initiative that is both the most harmonizing and of the most general application, is of particular importance to researchers active across numerous domains (Quinn and Quinn 2018 ; Peloquin et al. 2020 ). Footnote 10 In terms of its general application, it does not apply to specific contexts, but rather to most instances where personal data is being processed (including but thus not limited to research). If the GDPR is of application personal data can only be processed if certain conditionality is met. Footnote 11 Some of the most prominent forms of conditionality relate to:
Data protection principles
The GDPR foresees a number of important principles which controllers must adhere to when processing personal data. They are of general application and usually apply whatever the legal base is for the processing of question. The principles themselves are somewhat abstract and the application to a particular context will require reflection on the part of the data controller (Mondshein and Cosimo 2019 ). Data minimization for example obliges data controllers to collect no more personal data than they need for the processing operation that is envisaged. The storage limitation principle requires controllers to delete data once they are no longer needed for the purposes that were originally envisaged. Other principles require data controllers to ensure that their processing operations are secure, transparent and that privacy and data protection are taken into account at all stages of processing and planning (Forgo 2017 ). One important principle which the GDPR allows to be applied differently in instances of scientific research is that of purpose limitation. In particular, the GDPR allows researchers more room in terms of the description of the purpose of processing they must present to the data subject. This can be used to allow a broader form of consent for the use of personal data in scientific research than may be possible for other forms of processing. Footnote 12
Data subject rights
Data controllers must facilitate a number of important data subject rights, including in research contexts. Footnote 13 These rights may, depending on the context in question, be onerous to facilitate and may entail the creation of various administrative structures. This can be costly and time consuming for researchers. Notable data subject rights include a right of ‘erasure’ (commonly known as ‘the right to be forgotten’), a right ‘to object to processing’, a right of ‘data portability’. In forms of research that require complicated forms of big data facilitation of such rights may be complex and are once again likely to require careful consideration of the context in question. One important consideration for researchers is that the legal base selected for the processing of personal data in research may determine the extent to which data subject rights apply given the ability the GDPR allows Member States to limit them in instances of scientific research (discussed further in section 4.b).
Administrative requirements
The GDPR foresees a number of important administrative requirements that are likely to apply to researchers, particularly when they use sensitive data. This includes the need to appoint a Data Protection Officer and potentially the need to perform a data protection impact assessment (Quinn and Quinn 2018 ). In addition to the requirements outlined in the GDPR, Member states may add further requirements, in particular for the processing of sensitive forms of data. Footnote 14
The need for a legal base
The above requirements will apply to most forms of processing. Before processing of personal data can be contemplated however, it is necessary to ensure that there is a legal base for processing. Footnote 15 A legal base represents a set of conditions or a context in which the processing of certain form of personal data are permitted. Without an applicable legal base researchers can not process personal data for research or any other reason. One of the most well known is that of ‘informed consent’. Footnote 16 As sections 4 and 5 describes this forms the legal base for many forms of research. The GDPR, like its predecessor, also foresees a range legal bases that do not require consent. Some of these will permit research in circumstances where obtaining consent from data subjects is not desirable or even possible. Each of these bases will be outlined and presented further in sections 4 and 5.
Researchers conducting research with personal data must take into account all of the elements outlined in (i)-(iv) above. Whilst each represents an important factor that should not be understated, a full analysis of them is beyond the scope of this paper. The remaining focus will be on (iv), i.e. the need for a valid legal base. A considered examination of this requirement is interesting from the perspective of this paper because the various legal bases that exist within the GDPR may not be equally available to all types of research entities. Subsequent sections of this paper will analysis this in the context of modern research in order to highlight the existence and consequent importance of such differences.
4. The GDPR provides a wealth of legal bases for researchers
A. several bases are available to varying types of actor.
The increasing engagement of research by data protection frameworks such as the GDPR discussed in section 3 means that the GDPR will consequently become more determinative of what forms of research are permitted and how they should be conducted. Whilst the GDPR was not created solely for the purposes of research, it was clearly a significant form of processing that was envisaged by the those responsible for creating it (Nyren et al. 2014 ). Footnote 17 Importantly, it does not attempt to shoehorn all forms of scientific research into one single legal context but rather appears to recognize that scientific research, and the various motivations behind it, are extremely heterogenous. A ‘one size fits all’ notion of scientific research does not exist. Research varies in terms of its goal (‘blue skies’, ‘not for profit’, ‘commercial’ etc.), the identity of the those carrying it out (universities, hospitals, large commercial actors) and the various data subjects involved (varying from vulnerable individuals to well informed consumers) (Shmueli and Greene 2018 ). The regulation accordingly foresees several legal bases that can be utilized by individuals or entities wising to conduct research. This seeming assortment of options is important given the diverse nature of scientific research. As recital 159 of the GDPR states:
“For the purposes of this Regulation, the processing of personal data for scientific research purposes should be interpreted in a broad manner including for example technological development and demonstration, fundamental research, applied research and privately funded research”
This points to a very open mind in terms of what the drafters of the GDPR regarded as ‘research’, with a concept that does not discriminate between research of varying types (e.g. public, private or commercially motivated). Various research activities may also be permitted under grounds that are not specifically related to research. The GDPR is accordingly not lacking in terms of potential legal bases that may be available to researchers in various contexts. On the contrary, it can almost be considered to contain an ‘embarrassment of riches’ in terms of potential legal bases that can be used for research involving personal data. The most prominent are described below (legal bases or sensitive data are discussed in section 5).
The use of consent
Consent is perhaps the most well known legal base for justifying data processing in general. It can be used to justify the processing of personal data in an almost undefinable range of circumstances where data subjects have provided their consent. This includes a wide spectrum of research contexts in both the public and private sector. The GDPR demands that such consent must represent a clear and unambiguous indication of a data subject’s wishes and that it must be informed. It is defined in the GDPR as. Footnote 18
“any freely given, specific, informed and unambiguous indication of the data subject's wishes by which he or she, by a statement or by a clear affirmative action, signifies agreement to the processing of personal data relating to him or her”
Consent therefore can not be passive and individuals must receive a minimum of information in order to be able to provide their consent. In this regard, article 13 of the GDPR specifies a number of elements that must be explained to data subjects including, the purposes of the processing in question, the identity of the data controller and how long data will be kept for (Staunton et al. 2019 ). Footnote 19
Importantly the GDPR arguably foresees a slightly looser from of consent for scientific research than is available elsewhere. This is outlined by recital 33 which states that the purpose of processing can be described in less concrete terms for instances of scientific research. This may allow researchers some room where the nature of their research makes this difficult e.g. using AI or other computational processes with various forms of big data. This extra room should not be interpreted as a carte blanche for researchers. The purposes of research must nonetheless be described to a sufficient level. Footnote 20 Where this is not possible consent should not be used as a legal base for processing.
Although these conditions may not seem onerous, complying with them can produce considerable difficulties for researchers. Contacting (and even identifying) all potential data subjects may for example difficult, especially where research involved very large data sets. This may entail the use of complex administrative processes and consequentially further costs (Jamrozik 2004 ). Even where it is possible it may introduce an element of bias, where individuals who refuse consent may represent a statistically important group (Rothstein and Shoben n.d. ). For these reasons, the use of consent may not be practical in all instances where research is to be carried out.
Reasons of public interest
In many instances it may not be possible (or even desirable) to gain the consent of data subjects. The data subjects may be too many, too difficult to reach or the type of processing involved may be too difficult to explain. In certain contexts consent may not be ethically appropriate because of power imbalances (e.g. where the relationship between the data controller and subject makes it difficult for the latter to withhold consent) (Solove 2013 ; Corrigan 2003 ). Footnote 21 Numerous forms of research in the public interest fall into this latter category (Donnelly and McDonagh 2019 ). Footnote 22 This includes research needed to better organize public services or other forms of governance. In order to facilitate such forms processing (which are in the public interest) the GDPR foresees a particular legal base (described in article 6(e)) for processing in this context. Another important feature of this legal base that may often make it attractive to researchers is that Member States can, through legislation, limit a number of data subject rights normally incumbent upon instances of processing of personal data (discussed further below in (b)). This includes in a number of important areas relevant to research covering both a number of data protection principles (e.g. storage limitation) and data subject rights (e.g. the right to be forgotten). Footnote 23 In addition to obviating the need to obtain consent, the use of such a legal base can be highly advantageous to researchers in a number of contexts given that it may remove serious burdens upon researchers (i.e. the need to comply with strict understandings of various data processing principles or data subject rights) (Quinn and Quinn 2018 ).
Whilst being seemingly wide in terms of its scope, this legal base should not be viewed as being available for all forms of research. There are important limitations. Most importantly, it is only available where there is specific (European) Union or Member State law available (Donnelly and McDonagh 2019 ). This usually means that legislation must exist that identifies the controller in question as being able to carry out the type of processing in question. The use of this base represents one of the areas of data protection law where a considerable margin is left to the Member States to determine the specifics. As a result, there may be considerable variation in the exact nature of this base and its availability to researchers across Europe. As section 6 discusses, the legislation available in the various Member States may vary in terms of the extent it can be used by commercial forms of research. In some Member States legislation may be phrased in a manner that seemingly limits the applicability of this legal base to commercially motivated forms of research. Where such legislation does not exist, or is not applicable to a certain research context (i.e. commercially motivated research), this legal base for processing personal data will not be available. In addition, the use of this option must be necessary (and thus also proportional) in a specific context. Footnote 24 In other words it can not be simply opted for because of the innate advantages it may offer, but only when consent is clearly not appropriate.
Processing is necessary for the performance of a contract/ of a legal obligation
The GDPR recognizes forms of personal data processing are implicit to the execution of many forms of contract. Having to continually ask for consent from data subjects would be inefficient and make the goal (which both parties had contracted for difficult to achieve). Whilst one can imagine certain contexts where consumers contract with certain commercial entities to conduct research on their data, this type of relationship is not common in the public sector. Footnote 25 Similarly the GDPR recognizes that data controllers may have to comply with legal requirements in ways that involve the processing of data. This could be for instance when they are ordered by the courts to hand over certain data or when they are forced to defend themselves by legal proceedings (including where started by the data subject). Whilst such a potential ground may have societal importance, it is hard to see its potential relevance in permitting scientific research.
Legitimate interests
One legal base that is likely to be of more relevance to research is that of ‘legitimate interests’. Footnote 26 This represents an extremely broad option for non-governmental or public entities to process personal data when it is needed to do so in their interests (Donnelly and McDonagh 2019 ). Footnote 27 Where available it means that consent will not be required for processing of sensitive data (Olly 2018 ). This could for example include any number of instances where large commercial entities may wish to conduct further research on their customers’ data so that they can improve services to them, reduce the risk of criminality or other harms or improve their general commercial strategy. The concept of legitimate interests does however have some important limitations, some of which are outlined in recital 47 of the regulation. It states:
“The legitimate interests of a controller, including those of a controller to which the personal data may be disclosed, or of a third party, may provide a legal basis for processing, provided that the interests or the fundamental rights and freedoms of the data subject are not overriding, taking into consideration the reasonable expectations of data subjects based on their relationship with the controller. Such legitimate interest could exist for example where there is a relevant and appropriate relationship between the data subject and the controller in situations such as where the data subject is a client or in the service of the controller. At any rate, the existence of a legitimate interest would need careful assessment including whether a data subject can reasonably expect at the time and in the context of the collection of the personal data that processing for that purpose may take place.”
The GDPR thus demonstrates that the relationship between the data controller and the data subject is central in determining how far the ground of legitimate interest can be used to justify processing of personal data inter alia for research purposes. Where the relationship is of greater proximity and transparency it will be easier for proposed research use of personal data to be foreseeable. Foreseeability (or ‘reasonable expectations’ as the GDPR describes) is crucial because it allows data subjects to exercise their data rights (e.g. the right to object or the right of erasure) in instances where they may want to stop forms of processing that are likely to be conducted under the guise of legitimate interests. Footnote 28
Conversely, when the relationship between data subject and controller is more convoluted and less transparent, it will be more difficult to argue that the research use of personal data is foreseeable in terms of the legitimate interests of the data controller. The use of legitimate interests might be more acceptable for example when a social media giant conducts research with its clients’ data in order to find ways to prevent future account hacking. Research of this type is arguably foreseeable and even to be expected by the data subject. Research by an another organization which only had a transient link with certain data subjects and for purposes that had a limited connection the original relationship might however be difficult to justify. In addition, as the EDPS pointed out in its opinion on scientific research (A Preliminary Opinion on data protection and scientific research”, European Data Protection Supervisor 2020 ), data controllers must perform a balancing exercise when discerning what is permissible in terms of their legitimate interests. This test was previously outlined by the Article 29 working party in 2014. Footnote 29 It is complex and involves balancing the legitimate interest sought by the data controller against the fundamental rights and freedoms of data subjects. This will involve taking into account the importance of the processing in question (to both the data controller and society at large) and balancing this against the interests of the data subject, including taking into account and potential privacy harms. Research that has a high value to the controller or to society in general will carry a higher weight in such a balancing exercise.
B. Informational obligations/data subject rights may vary according to the legal base used
In discerning what requirements may be attached to a particular legal base it is important to take into account the informational obligations and data subject rights that may be applicable in each instance.
Informational obligations connected to the use of differing legal bases
In terms of informational obligations, two GDPR articles are of particular importance. Article 13 outlines what information must be provided when data subjectd provide their consent for the processing in question. This includes information such as the name and contact details of the data controller, the aims of the processing and further information concerning the potential exercise of their data subjects rights. In general compliance with these requirements is not particularly difficult. This is because such information can be provided at the same time a data subject is providing consent (e.g. with a physical or electronic consent form). This is obviously not the case for the other legal bases described above where the data subject does not provide consent. In such instances article 14 comes into play, outlining certain forms of information that should be provided when consent has not been obtained from data subjects for the processing in question. Complying with article 14 in many instances of scientific research is however likely to be more problematic than may be the case for article 13. In many instances this may for the same reasons outlined above that make obtaining consent itself difficult. This may be because it may be difficult to contact all data subjects or systematically gain consent from them. Having to contact data subjects even in instances where consent is not used as the legal basis for processing would often present serious problems for researchers that opt for other bases such as public interest or legitimate interests (where such bases are indeed available). The GDPR appears to recognize that this may be a problem in inter alia research stating that the requirements of article 14 do not need to be met where Footnote 30 :
“the provision of such information proves impossible or would involve a disproportionate effort, in particular for processing for archiving purposes in the public interest, scientific or historical research purposes or statistical purposes, subject to the conditions and safeguards referred to in Article 89 (1) or in so far as the obligation referred to in paragraph 1 of this Article is likely to render impossible or seriously impair the achievement of the objectives of that processing.”
This exception seemingly applies different tests to different research contexts, including the legal base that is opted for. For public interest or scientific research, the bar appears to be the creation of ‘disproportionate effort’. For other forms of processing it appears to be set higher i.e. ‘to render impossible or seriously impair’. This is important given that all of the legal bases discussed above may be used for research. Accordingly research that uses legitimate interest as a base will face a higher bar to demonstrate that they can use the exception outlined in Article 14 to not provide information to research subjects (i.e. they will need to demonstrate serious impairment or impossibility). Difficulty or high cost would not seem to be valid reasons. As Ducato states (Ducato 2020 ): Footnote 31
“This is not in any case a blanket exception and requires a balancing assessment. First of all, the “impossibility” and “disproportionate effort” must be tailored to “the number of data subjects, the age of the data and any appropriate safeguards”. Second, the EDPB has further stressed the need for the controller to evaluate “the effort involved for the data controller to provide the information to the data subject against the impact and effects on the data subject if he or she was not provided with the information”. This will consist at least of making the information publicly available (e.g. publication on website, newspapers, etc.)”
The room accorded to data controllers (including to researchers) by article 14(5) should thus not be overestimated. The author would argue that this will be particularly the case for research by private entities under the guise of legitimate interests. In situations where the ‘legitimate interests’ base are most likely to be applicable, i.e. where the data subject has reasonable expectations that research with their data may occur there will more often than not be a link between data controller and subject (e.g. customer/client) that will arguably make it difficult to claim that providing the information outlined in article 14 of the GDPR will render the research in question possible. Where this is the case the advantages of using legitimate interests as a legal base over consent will, to a large extent, be negated (given the need to provide sufficient information to the data subject).
Data Subject Rights may vary with certain legal bases
A somewhat similar situation also applies in terms of data subject rights. This is because article 89 of the GDPR permits member states to limit the application of data subject rights where research is for public interest or scientific research purposes (Article 89(2) states “Where personal data are processed for scientific or historical research purposes or statistical purposes, Union or Member State law may provide for derogations from the rights referred to in Articles 15, 16, 18 and 21 subject to the conditions and safeguards referred to in paragraph 1 of this Article in so far as such rights are likely to render impossible or seriously impair the achievement of the specific purposes, and such derogations are necessary for the fulfilment of those purposes. Footnote 32 In particular this relates to the rights to access, rectify, restrict and object to the data processing in question. Footnote 33 Facilitating each of these rights may create difficulties and burdens for researchers, depending on the particular research context in question. This may entail structuring some research differently or even making some research more expensive. Compliance will likely entail the need to for important administrative infrastructures and measures that will allow researchers to comply with data subject requests (Quinn and Quinn 2018 ). Footnote 34
Given this, the ability to use a legal base where such rights have been restricted may be an important advantage. The GDPR allows Member States to restrict the availability of such rights where processing is for “scientific or historical research purposes or statistical purposes”. Footnote 35 The choice of words here is interesting because it seemingly corresponds with the description of the legal bases outlined in article 9(2) (discussed further below in section 5) or potentially the option for further processing for scientific research (discussed in (C.) directly below). This appears to indicate that that this ability only applies where one of these options are chosen and not others such as ‘consent’ or ‘legitimate interests’. In terms of the former this is logical given the importance of data subject rights - it would surely be inappropriate if they could be restricted in too wide a manner. Given this, the need for Member State legislation, that would only apply in specific contexts is important (for more see the discussion in section 4.A) because such legislation will only apply in certain circumstances where the limitation of such key rights could be considered appropriate. Footnote 36 In many Member States such legislation may for instance only be applicable where research is perceived as being in the public interest (Dove and Chen 2019 ). Another important factor is that article 89 only appears to offer such limitations to be possible when “appropriate safeguards” are in place. In the research context this arguably relates to adequate forms of ethical governance, something that public sector research institutions are more likely to have in place than those in the private sector. Given these factors, it can be considered that public sector research organizations are more likely to be able to benefit from the ability to relax data subject rights than commercial entities. As discussed below however the second potential option of a valid legal base existing simply for ‘further processing for scientific research’ is more puzzling and raises serious questions about the potential limitation of data subject rights in a wide range of contexts.
C. The possibility of ‘further processing’ for scientific research
In addition to the legal bases outlined the above the GDPR contains one extremely broad an important provision that should be considered alongside the availability of legal bases that could be used for scientific research. This relates to article 5(1)(b) which states that personal data must be:
“collected for specified, explicit and legitimate purposes and not further processed in a manner that is incompatible with those purposes; further processing for archiving purposes in the public interest, scientific or historical research purposes or statistical purposes shall, in accordance with Article 89(1), not be considered to be incompatible with the initial purposes (‘purpose limitation’)”
This provision is remarkable in its potential breadth. Unlike many of the elements of article 6 discussed in part (a) above, it seemingly does not create a separate legal base for the processing of personal data. Rather, it appears to indicate that if a data controller has a valid legal basis for possessing personal data in the first place, it can subsequently process the data in question for purposes of scientific research. It can arguably be interpreted in one of two ways. The first is that no legal basis is required for the processing of personal data for the purposes of scientific research as long as there was a prior legal base for the collection of the data in the first place. The second is that the original legal base is still valid for such forms of processing, even where the legal base itself and the original context of data collection seemingly had little to do with scientific research. The first would be perhaps the most conceptually remarkable because it departs from the general approach within the GDPR that a specific legal basis is always required for any form of processing. Doubt may be cast over this interpretation given that the drafters of the GDPR did not opt make a legal basis in article 6 of ‘further processing for scientific research’ (i.e. one that does not require member state legislation).
The practical ramifications of this provision are however likely to be even more important that the conceptual confusion it creates. An expansive reading would seemingly indicate that a data controller that is legally in possession of personal data can carry out scientific research with it. This seemingly includes an almost indescribably wide range of contexts throughout both the public and private sectors. In terms of the latter for example the frequency and variety of contacts that individuals have with various commercial entities mean that their personal data could be used for a potentially enormous range of processing activity that could be termed as scientific research. In discerning just how broad this possibility might be it is important to consider the broad understanding of the term scientific research which the GDPR calls for (discussed in sections 3.A). This view of what can constitute scientific research means that many forms of processing that might not have been traditionally thought of as scientific research may be categorised as such by the GDPR. This includes for example efforts at product and service innovation by private enterprises, something that in the modern world could take an almost indescribable variety of forms.
It must be acknowledged however that article 5(1)(b) does contain some limiting factors that will serve to restrict its use to a certain extent. This includes references to processing “in accordance with article 89(1)”. As discussed this entails the need to have the necessary organisational measures (including potential systems of ethical review). Such structures may entail financial and administrative costs and may also impose additional requirements on the desired research in question. Whilst these may pose major difficulties for smaller entities, it is likely that larger financial entities for example will be able to put into place such measures (though doubt may be raised about their integrity and transparency) in many instances that will be seen as capable of demonstrating compliance with article article 89(1). This may for instance include ethical policies and procedures for review of processing activities.
Another important limiting factor is the need to comply with the informational requirements of Article 14 of the GDPR (as discussed in section 3.B above). This relates to a requirement to impart information about the processing activities to the data subjects concerned. This inter alia allows data subjects to invoke their data subject rights should they wish to do so (including potentially the right to be forgotten). Footnote 37 Whist this may form an important impediment in a number of contexts (where efforts must be made to contact various data subjects) this burden is significantly eased by the extra room permitted to scientific researchers by the GDPR in interpreting this requirement. As section 4.B discusses this requirement can be dispensed with when it requires a ‘disproportionate effort’.
Despite this limitations however the possibilities provided by article 5(1)(b) are enormous and are poorly clarified by the regulation itself.. A simple understanding of the wording used would seem to indicate that any instance of legal processing of personal data will give an entire range of entities the problems to conduct a variety of further processing under the umbrella of scientific research. Given the wide vision the GDPR seems to have of what can constitute scientific research it could be argued that this potential breath of this provision raises some serious concerns. This could for example include a wide spectrum of processing, much of which may only be loosely associated with conventional notions of scientific research (i.e. to include product innovation and better customer targeting/service delivery). Given that many commercial entities already legally possess an enormous range of their clients’ data, article 5(1)(b) seemingly provides them with an enormous potential to further use it under the guise of scientific research. Given such concerns, it is unfortunate that the EDPS chose not to deliver further commentary in its recent opinion on scientific processing. Footnote 38 It did however state that such commentary would be forthcoming in the future.
5. Potential legal bases for research with sensitive data
A. many forms of research may use sensitive data.
The GDPR foresees a special regime for sensitive data (termed ‘special categories’ of data). This includes a different set of legal bases for controllers that wish to process such forms of data. For researchers it is important to be aware of the existence of sensitive data and that different conditions may apply to their processing than other forms of personal data. Such data is defined by the GDPR as:
“personal data revealing racial or ethnic origin, political opinions, religious or philosophical beliefs, or trade union membership, and the processing of genetic data, biometric data for the purpose of uniquely identifying a natural person, data concerning health or data concerning a natural person's sex life or sexual orientation shall”
The breath of the definition of sensitive data is important. Not only are each of the elements described in article 9 of enormous potential breadth, many are likely to include forms of data that are of immense, social, societal and economic importance. They are therefore likely to be of interest to researchers (Quinn and Malgeri forthcoming-a ). Footnote 39 Health data is an important example and provides a good illustration of the potential breadth of sensitive data. The concept of health data itself goes far beyond medical data or medical dossiers and has been argued (by the article 29 working party) to include all personal data that is capable of providing an indication of the health status of specific individuals. This includes not only data relating to illness or health problems that an individual might have, but also data that provides an indication that an individual will develop a health problem in the future and even information that indicates a specific individual is simply ‘healthy’. Footnote 40 Given that various pieces of information that individually say nothing about an individual’s health status can be combined to provide such an indication, many forms of big data may be health data (often without being that being readily apparent). As a consequence, many forms of data that are sought after by researchers are likely to contain health data, whether researchers are aware of this or not. This goes far beyond traditional (and more obvious sources of health data) such as official medical records and can include (less obvious sources of health data) questionnaires, to information about movement (including from GPS mobile data) and social media. Data from such sources can be combined with increasing ease (due to increased computer power, the increased availability of complimentary forms of (often big) data and ever more powerful analytical software (often using artificial intelligence) to reveal information about the health status of specific individuals (Article 29 Working Party Opinion on Anonymisation Techniques 2014 ). An analogous form of reasoning can also be applied to the other forms of sensitive data described in article 9 of the GDPR (Quinn and Malgeri forthcoming-b ). In most cases (excluding genetic and biometric data), various elements that might not be themselves sensitive in nature, can be combined to provide inferences that may be of a sensitive nature. As a result of these factors, the proportion of data that researchers use in the future that is of a sensitive nature is only likely to increase.
The use of sensitive data brings with it a number of extra burdens. This includes the likely need to appoint a Data Protection Officer (DPO) and perform a Data Protection Impact Assessment (DPIA) (Kloza et al. 2017 ). Footnote 41 Each of these will impose added administrative burdens on those who wish to process sensitive data, including for research purposes (Quinn and Quinn 2018 ). Footnote 42 Whilst these are important requirements that should not be underestimated, they will not be explored further in this article given they apply to most forms of research with sensitive data irrespective of the identity of the data controller or the legal base used. Again, as with non-sensitive data, the remaining focus will be on the legal bases available to researchers given that they may in reality be differently available to different types of actors engaged in research.
B. A number of important legal bases are relevant for research with sensitive data
There are a number of important bases that are likely to be relevant for research with sensitive data. As with bases for non sensitive data, the reality is that some of these grounds are more likely to be available to some actors than others. The most important grounds for research are:
Explicit consent
As for non-sensitive personal data, consent is usually considered as one of the most important bases for the use of sensitive data (Corrigan 2003 ). Not only is the legal underpinning of this base relatively easy to understand, it is often considered the most ethically favorable option where it is possible (Mostert et al. 2016 ). For this reason, the use of consent as a legal base is often considered as the default options by researchers. Ethics committees will often expect it to be used where it is possible. The existence of two forms of consent (one for sensitive data one for non-sensitive data) has been a feature of the European data protection framework since Directive 95/46/EC (Quinn et al. 2013 ). Footnote 43 The directive foresaw a looser form of implicit consent for non-sensitive personal data and a more demanding form of ‘explicit consent’ for the use of sensitive data. In areas such as health care, such a division was important and was often understood in national law as requiring signed, written consent. The GDPR has however ‘muddied the water’ in terms of the difference between explicit and non-explicit consent. In particular, it has strengthened the requirements around consent for non-sensitive data, essentially making implied consent impossible. In place of this an ‘unambiguous indication’ is required. Footnote 44 At the same time, the GDPR makes clear that explicit consent need not be written, though a record that such consent has been given must be kept. Footnote 45 The difference that remains is seemingly the requirement that data subjects acknowledge they are giving explicit consent, i.e. that a clear recognition that a formal act of consent is being given. This will usually entail explicit acknowledgement by the data subject that consent is being given. As with the use of consent as a legal base for the processing of non-sensitive data, one of the most important benefits of using consent as a legal base for the processing of sensitive data is its versatility. As a legal base it is not intended to be applicable only to a specific context or setting but is extremely versatile, capable of being applicable to numerous contexts, including scientific research. Similarly, it is available to all the various types of entities that might want to conduct research throughout both the public and private spheres (and everything in between). This is not the case for many of the other legal bases that can be used to process sensitive data for research, which may be more or less suited to certain types of actors (discussed further below).
The scientific research exception
As with consent for the processing of non sensitive data (see section 4), the use of ‘explicit consent’ for research problems may raise serious issues in some contexts (Corrigan 2003 ). Unlike non-sensitive data which sees a general base for processing in the public interest, the GDPR foresees a series of more precise (at least in a thematic sense) legal bases that relate to specific contexts for the processing of sensitive data. One of these is for the “purposes of archiving or scientific research” (Donnelly and McDonagh 2019 ). In the literature this if often termed ‘the scientific research exception’ as it is often viewed as an alternative to the basic presumption that explicit consent is the default legal base to be used for research purposes involving sensitive data (Carter et al. 2015 ). Footnote 46 As with the use of the public interest legal base for non sensitive data (see section 4), there is however important conditionality attached (Quinn 2017 ; Hallinan and Friedewald 2015 ). Footnote 47 Footnote 48 Again, the use of such a base should be outlined in Member State or Union law and be both necessary and proportional. This means there should be a valid reason for not using other bases such as explicit consent. In most cases relevant legislation should outline what type of actors can make use of this legal base and in which circumstances. Sometimes such legislation can grant a broad discretion to certain entities (e.g. universities), whilst in other instances it may focus only on specific actors (e.g. certain governmental bodies) (Taylor et al. 2018 ). Given the need for legislation and all of the complexities surrounding it, it is more likely that such an option be available for large public entities (e.g. universities, health care actors) than for private entities. As section 6 discusses, the availability of the scientific research exception can vary between countries, with certain Member States seemingly more willing to let commercial entities avail themselves of this possibility (though it may still be harder for them to do so than it is for public sector entities).
Public health /substantial public interest
In addition to the scientific research exception there exist other bases that, although are not explicitly directed towards research, could in appropriate circumstances be used to conduct research in certain contexts. This includes for reasons of ‘public health’ and ‘substantial public interest’. Footnote 49 Again, as, with the scientific research base, these bases allow the processing of sensitive data without consent where there is specific legislation in national or EU law allowing so. This may, as section 4 discussed be necessary in contexts where consent is clearly inappropriate because of imbalanced power relations. Although not specifically intended to deal with research, it is possible that these legal bases can be used to cover research when it is intended to meet an aim falling within this area. Footnote 50 This could for example include research into numerous public health issues, ranging from issues associated with obesity, to the spread of pandemics (including currently with COVID-19). The ability to conduct such forms of research is important in inter alia allowing governments and public authorities to respond to serious challenges that can arise. Once again, the requirement for existing legislation specifying when such processing can occur will significantly restrict the types of entities that can avail themselves of this option. It should also be noted that the terms ‘substantial public interest’ outlined for sensitive data denotes a significantly higher threshold than ‘in the public interest’ (which is available for the processing of non-sensitive data). This will restrict the availability of this legal base further. This may for example mean that it may not be considered a suitable base for forms of blue skies research where the direct benefit in terms of public interest may not be immediately clear (Hallinan 2020 ). Footnote 51 The same is likely to be true for research that has a primarily commercial motivation.
Data that is manifestly made public
One controversial ground for processing sensitive data relates to sensitive data that has been “manifestly made public”. This base is particularly striking given that it is not available for the processing of non-sensitive data which is arguably of a far higher risk. Unfortunately the GDPR does not clearly elaborate the limits of this legal ground. The European Data protection Supervisor has recently provided guidance on when this base can be used stating:
“Special categories of data may be processed if the data subject has manifestly made them public. EU data protection authorities have argued that this provision has to be ‘interpreted to imply that the data subject was aware that the respective data will be publicly available which means to everyone’ including, in this case, researchers, and that, ‘In case of doubt, a narrow interpretation should be applied, as the assumption is that the data subject has voluntarily given up the special protection for sensitive data by making them available to the public including authorities’. Publishing personal data in a biography or an article in the press is not the same as posting a message on a social media page.” Footnote 52
Whilst this clarification is not extensive, it confirms two important things. The first is that sensitive data can indeed be processed when it has been made manifestly public by the data subject. This clarification is important given the ambiguity discussed above (i.e. that a similar base is not available for non-sensitive data). The second is that the provision should be interpreted with care in an extremely context dependent manner. The most important issue is one of awareness and whether a data subject can have expected that a particular use was made of his or her data. This question of awareness is complex in the context of research. One might ask in which situations individuals make their details available in the genuine understanding both that they are made public and that they may be used for research purposes? Is it realistic for example to expect individuals to realise that when they place their data on a web site it may be harvested by complex web crawling operations, packaged into large big data sets and subjected to research (with a diverse range of motives) with various forms of novel AI based techniques? (Massimo 2016 ) Whilst the answer may be yes for some individuals who have specific awareness of these areas, for most people the answer will likely be no. It is important to remember that given modern computing power, and the interconnectedness of the online world, the risks of harms occurring with research on sensitive data may be difficult to envisage, especially in the long term. Imagine for instance genetic data that is published online. The variety of likely unknown developments in the science of genetics make it extremely difficult to predict the nature and scope of future research (Quinn and Quinn 2018 ). Footnote 53 Given this, the author would argue that, unless made public in areas where awareness of research was indeed evident (e.g. particular online fora, websites communities etc.) it would not be prudent to assume ‘awareness’ of potential research (and especially complex research) in a way that the EDPS appears to be demanding. Footnote 54 A better approach would be to only extract data in instances where it had been accompanied by some sort of explicit definition of ‘public availability’, including importantly in the context of this paper, for research purposes. This will however have the obvious effect of limiting the potential use of this legal base.
6. An unlevel playing field?
The forging analysis demonstrates the wealth of options that the GDPR provides for research. The regulation can not be considered hostile to research, nor does it attempt to promote a ‘one size fits all’ approach to all forms of research. Its drafters clearly intended to provide opportunities for various actors, in various contexts to conduct research when certain forms of conditionality are met. This raises the questions as to whether various types of actors enjoy a level playing field in terms of their ability to conduct research with personal (including potentially sensitive) data. This question is important because the world of research is not populated by uniform actors operating in similar contexts but rather, a diverse array of actors operating in vastly different contexts. Innovative and socially useful research is not the domain of one particular entity or class of actor but can be conducted by a variety of entities (Hartley et al. 2017 ). This can vary from the traditional university research department, to medical institutions, to small business to large and enormously powerful commercial entities (Maroto et al. 2016 ). Each of these operates in different environments, with access to varying levels of resources and possessing different abilities to access personal data. Some of these are described below.
A. Universities
Consent as a default base that is not always appropriate
Universities represent the classic image of a research institution. Often publicly funded (or at least heavily subsidized) they are usually perceived (erroneously in some cases) as carrying out research not primarily because of a commercial interest but to advance scientific understanding or to address an important public interest need. Given the nature of their work they may be expected to undertake research that would not otherwise occur in the private sector. Such research may be unlikely to deliver an immediate financial return (Watts 2016 ).
Consent is the most important legal base for research with personal data in universities (Mostert et al. 2016 ). Its versatility allows it to be deployed in vastly different contexts with different types of research subjects and research of vastly differing aims. Universities as mature research institutions often have well developed and complex systems of ethical review (Vadeboncoeur et al. 2016 ; Kohn and Shore 2017 ). Such processes may be required by a university’s articles of establishment, demanded by funding bodies or obliged by national legislation. Most research carried out involving human participants or the use of personal data will be expected to gain approval by a university ethics body before commencement. In most instances, researchers at such institutions will depart from the expectation that where possible consent should be used as the legal basis for research using personal data. As the author has discussed in a previous paper, ethics bodies may push this attitude (sometimes too aggressively) where consent may not be appropriate. This may on occasion force a ‘consent or anonymize dilemma’ on researchers (Quinn 2017 ).
Although consent is useful, and in many cases clearly the preferable choice of legal base for ethical or practical reasons it is in many occasions not suitable (Donnelly and McDonagh 2019 ). As with other forms of research, universities are increasingly using large and complex forms of data. This may involve collecting or further processing personal data that relates to numerous data subjects. Assembling the types of large and often heterogeneous data needed for many forms of modern research may present a range of practical, ethical and legal problems, particularly where consent is used as a legal base. As section 4 discusses, in research with many data subjects it may be practically difficult to physically contact all research participants or gather them together in a way that is possible to obtain informed consent. Even where it is, problems with power relations or vulnerable data subjects (e.g. children) may render consent ethically undesirable. In addition, university researchers may want to reuse data for subsequent analysis, in a way that is materially different than the initial research that they conducted (Mcguire et al. 2008 ). In such cases, re-obtaining consent for all data subjects may be a disruptive exercise. The availability of the a legal base that does not require consent is thus important for many forms of research.
The importance of the scientific research exception
Legislation exits in all EU Member States that permits universities to process both non sensitive and sensitive personal data for aims of scientific research (Molnár-Gábor 2018 ). Footnote 55 This allows universities to process under the public interest or scientific research grounds described in sections 4 and 5 respectively. Such legislation can be broad, not referring to specific forms of personal data or it may refer to specific types of personal data “e.g. genetic data” (Taylor et al. 2018 ). Such legislation can thus in theory present an extremely broad ground for the universities to base their research upon. Whilst the availability of such legislation is a welcome and broad ground for researchers, using it is often not so simple. This is because university researchers will usually have to obtain ethical approval and explain why they are not using consent as a legal basis. As section 5 discussed, some ethics bodies may be more hostile than others concerning the use of the scientific research exception (or more specifically the processing of personal data in the absence of consent) (Mostert et al. 2016 ). Ethics bodies are for a number of reasons (many of which are correct) likely to greatly privilege research that is able to gain the consent of data subjects. This may lead to situations where researchers are not able to use the scientific research exception found within the GDPR, even though consent may not be suitable. In other instances they may be pressured into gaining some form of consent even where the scientific research exception is used (in such cases the use of consent represents an exercise in ethical compliance and does not need to meet the requirements of consent as a legal base as laid out in the GDPR). Footnote 56 Such factors can arguably lead to certain forms of research being disincentivized (i.e. where gaining any form of consent is truly problematic) and mean that in reality the availability of the scientific research exception may be less than that which would be apparent from a simple reading the GDPR itself.
B. Other public bodies
As with universities, a large variety of other public organizations may have to conduct research on personal data for a variety of reasons. This could range from public health bodies that attempt to model and predict the scale of potential or impending epidemics or other public health threats to bodies intended to regulate health and safety in the work place (Srncova et al. 2019 ). Research may be important in developing new strategies to safeguard the public from various existing or potential future harms. An obvious example is readily available in the ongoing COVID-19 pandemic (Malgeri 2020 ). In other cases it may be difficult to draw the line between research and important managerial activities, including the organisation of public services (Klievink et al. 2017 ). Conducting such activities inevitably requires forms of research that will, on certain occasions require the processing of personal data. In many cases consent may not be feasible for many of the reasons discussed above in section 4. Footnote 57 As section 4 & 5 describe, the GDPR accordingly foresees a number of legal bases that are more likely to be applicable. These include (as with universities) the public interest base (for non-sensitive data), the public health, substantial public interest and scientific research bases (for sensitive data). Footnote 58 In such instances where legislation outlines that a particular public body can conduct certain forms of research using personal data it can do so without consent. Depending on the body in question, it may or may not have ethical review procedures in place. In some cases ethical or deontological codes make exist that provide guidance or complaint mechanisms may exist to hear from individuals that feel that an incorrect course of action has been taken. Whilst providing an important form of redress, such procedures may not be as systematic or exert as high a level of scrutiny as usually occurs with university ethical review.
C. Commercial entities
Private entities (with some prominent philanthropic exceptions), will usually conduct research because of commercial or financial motivations. They may do this to be able to better deliver products to their customers, to better understand their tastes and desires and to be able target them with advertising more correctly (Moore and Tambini 2018 ). The role of large commercial entities in research is going to become ever more important in the future given the enormous amounts of data they are able to gather. Tech giants and social media companies (e.g. Google, Amazon and Facebook) are able to gather and store enormous quantities of customer data on an ongoing basis (Sharon 2016 ). This provides an incredible pool of data upon which such entities can perform research for a variety of reasons. Indeed, it is their ability to access and utilize such enormous amounts of data for innovative purposes that has largely been responsible for the atmospheric increase in their value as commercial entities (Klievink et al. 2017 ). This ability has stemmed from the range of complex services these companies are able to offer a large range of clients on a global basis. Such organizations are thus able to ‘harvest data in a manner and on a scale that university research groups can in general only dream of. This ability is only likely to increase further in the future as a consequence of increasing technological progression and the consequent generation of data (inter alia through developments such as IoT).
In terms of legal bases, the picture for commercial entities may be somewhat different. Whilst they will also have the default option of using consent as a base for processing, the availability of some of the other options described above may be limited. This is not because the GDPR makes a distinction about such bases being available for only public sector bodies, but more to do with the practical realties of facilitating the use of these legal bases. This is in particular related to the need for law outlining who can do what type of processing (where for instance the scientific research exception is used). Whilst large and important public sector entities such as universities, university hospitals and large research institutions may have been considered important enough to merit legislation facilitating the use of a personal data for research, this may not be the case for commercial entities (Taylor et al. 2018 ). Footnote 59 The chances that specific laws will be made facilitating the use of public interest bases by commercial organizations is small. In most cases, the interests of large commercial entities will not be seen as being in the public interest, and attempting to make the case that it is would likely come at a political price (For an alternative view see the report composed by (EPRS) - European Parliamentary Research Service Scientific Foresight Unit (STOA) 2019 ). Footnote 60 This is especially true in an age where the suspicion of the power and reach of such entities is growing stronger. As a result, and even in cases where a large commercial entity may claim to want to act in the public interest in a philanthropic way, it is unlikely that legislation will be created to facilitate processing of personal data without consent. For smaller commercial entities the creation of such legislation is even less likely given that the necessary legislative time is not likely to be accorded in order to facilitate the activities of smaller organization. Where such specific legislation is not available it may often be difficult for commercial entities to rely on exceptions that have been crafted primarily with the public sector in mind. This is particularly true for the ‘public interest’ based exceptions including the ‘scientific research’ exception available for sensitive data. Some states (e.g. the UK) (ICO report n.d. ) Footnote 61 only allow its use where it is demonstrably in the public interest. Whilst it is of course possible that some forms of commercially motivated forms of scientific research will be in the public interest (e.g. pharmaceutical research), it is likely that most forms will not be able to meet such a test. Others may even require approval of specialist committees (e.g. Ireland) (Dove and Chen 2019 ). Footnote 62 Whilst there are certain exceptions, the general rule of thumb is that it will be more difficulty for private or commercial entities to avail themselves of ‘the research exception’ outlined in the GDPR.
As section 4 discussed however, commercial entities have a number of other legal bases available to them that may not be so readily available to the public sector. These include research performed in order to ‘fulfill contract obligations’ which may be relevant where individuals have contracted with a commercial entity directly to perform research or for other services where research may be clearly implicit. Footnote 63 More important however is the potential use of the ‘legitimate interest’ grounds and the possibility for ‘further processing for scientific research (discussed in section 4.C).
Unlike the public interest based options, legitimate interests is not permitted as a legal basis for public entities and is available only for private sector data controllers. Footnote 64 As discussed in section 4 this basis permits an enormous range of processing that will likely encompass many forms of research. Its use is limited by the need to conduct a balancing exercise, taking into account the interests of data subjects and weighing it against the legitimate interest sought by the data controller. Central to deciding whether such a balance supports a particular instance of processing is how foreseeable the type of processing is on the part of the data subject. Where it is clear that such a form of processing can be expected it will be easier to argue that the balancing exercise supports processing in the legitimate interests of the data controller. The logic to this is that where such a form of processing is foreseeable (or within the ‘legitimate expectations’ of data subject as the Article 29 Working Party described it), Footnote 65 individuals can choose not to provide data to the data controller in question or, where the data controller is already in possession of it, exercise their rights as a data subject to prevent the type of processing in question (this may include exercising one’s right to object or have their data deleted). Accordingly, the GDPR makes it clear that where there is a clear relationship between the two parties (e.g. customer and client) the chances will be greater that a particular form of processing will be foreseeable. By extension, where data controllers make such activities known to their clients (e.g. through privacy polices) it will be easier to argue that processing under the guise of ‘legitimate interests’ is permissible. Where such a relationship exists, it will also be easier for data controllers to comply with their requirements vis-à-vis article 14 GDPR. As section 4 discussed, these relate to the details of the processing operation that should be provided to data subjects when their data has not be obtained through consent.
In terms of the second option described above, i.e. ‘further processing for scientific research’ the scope of use may even be wider. This potential possibility is less well defined, either in the GDPR or in any form of expert guidance that has been given under its authority. Even if its use is to be subject to further (as of yet not clear) restrictions, its potential for use may be extremely significant for similar reasons to that of ‘legitimate interests’ (i.e.; given the potential amount of data that many entities have) and also the potential flexibility of the concept of ‘scientific research’ under the GDPR. There are some subtle differences however. On the one hand, some of the restrictions applicable to the use of ‘legitimate interests’ base are seemingly not applicable to the ‘further processing’ option. This includes requirements to demonstrate legitimate expectations on the part of the data controller (including for example a relationship of proximity). On the other hand, the further processing option, unlike legitimate interests’ is subject to the requirement of compliance with article 89 (i.e. the need to create organizational structures to safeguard the rights of data subjects – discussed in section 4.C.). Notwithstanding the urgent need for further clarification of the extent of this processing option, it is clear that its availability alongside the ‘legitimate interests’ provides enormous possibilities for many types of actor, particularly in the research sector.
Given that large tech and social media giants have hundreds of millions of users with which they often have a customer-client relationship (and often with carefully crafted privacy policies), they can be argued to possess unparalleled pools of data upon which they may be able to perform research under the guise of ‘legitimate interests’ or ‘further processing for scientific research’. Firms such as Amazon have evolved and expanded continuously based upon innovate commercial research carried out on customer data. This has allowed the company inter alia to better target particular products based on its customers online behavior. This provides such entities with a source of data upon which they can perform research that is often simply not available for most universities. With the exception of university hospitals and their relationship with patients, most universities do not have vast repositories of client data available. By contrast, they often have to request data from external entities and may thus be greatly beholden to their willingness to co-operate. This is an important de facto limitation that acts to lessen the seemingly wide latitude granted by the GDPR’s research exemption.
7. Are private sector interests favoured by the GDPR?
The discussion above raises questions as to whether private sector researchers enjoy an advantage over their public sector equivalents. In particular one might ask whether the ability of private entities to use legitimate interests trumps public sector research’s likelier easier access to the GDPR’s ‘research exception’? Similarly, whist not only available to private sector interests, one might ask whether the possibility of ‘further processing for scientific research’ is more likely to be available to private sector interests in many instances? In asking these question it is necessary to take into account the de facto context researchers are likely to find themselves in. The situation of considerable data availability for large private entities can be contrasted with public sector research institutions, which may often not have direct access to the data they need for research (this is particularly true for universities). There is nothing in the GDPR to compel external entities to hand over data that may be useful for research, even where its processing may be legitimate for purposes of scientific research. External entities may refuse because of perceived commercial interests, or because they must submit such decisions to their own form of ethical oversight (e.g. hospitals) (Markoff 2012 ). Footnote 66 An increasing dependency on big research data arguably makes research institutions such as universities more dependent on external big datasets. Researchers may find it difficult or impossible to assemble these alone and are likely to become increasingly dependent on agreements with external entities to provide data that is necessary for research (Hao 2020 ). Footnote 67 In this modern big data context, the possibility to process personal data under the GDPR is only one part of the overall picture. Having the theoretical legal possibility to process such data for research ends arguably means very little if researchers have no access to the data in the first place.
This situation described above can be contrasted with the situation that commercial entities find themselves in. Whilst they may be limited by the GDPR or Member State legislation in terms of their ability to take personal data from disparate sources, for which they have a limited connection or relationship with the data subject, this may not be a serious problem when the controller in question is in possession of large amounts of data concerning individuals with which it does have such relationship. This may be the case where particular data controllers have large bases of customers to whom they provide goods and/or services to. As section 6 discusses, large online entities that sell goods or provide online services (e.g. social media) are likely to be in such a position. Such actors may as a result legitimately have access to heterogenous forms of data from numerous data subjects spread over many locations. The research value of such data may be immense and is only likely to increase further given never ending augmentation of both the volume and variety of the data in question.
The factors above could arguably seem to indicate a real de facto advantage in the ability of private sector entities to conduct research the era of online connectivity and big data. The sheer amount of data that many large commercial actors may possess, taken together with the existence of a legal grounds such as ‘legitimate interests’ or ‘further processing for scientific research’ and the relative weakness of their forms of ethical review may often provide an advantage in terms of the ability to conduct research, particularly with big data. It is important however to remember however that these grounds are not available for the processing of sensitive data (for research or any other purposes). Footnote 68 The reason for this is that they are not listed in Article 9. This is unlike the major grounds commonly used by public sector actors which are outlined both in Article 6 (for non sensitive data) and Article 9. The effect of this means legitimate interests can only be used as a legal basis for processing sensitive data if it can be combined with a legal basis outlined in article 9 and which is clearly applicable. Footnote 69 The result is that without being able to depend on another basis for processing sensitive data, legitimate interests/further processing for scientific research alone will not be sufficient. This factor renders the available advantage to private sector researchers of these options less significant than it might seem, especially given the importance of sensitive data to many forms of research.
This creates an extremely important limitation to the advantages private sector entities may possess in terms of their research potential when compared to public sector entities such as universities. This is because whilst they may have a certain de facto advantage in their ability to conduct research with non-sensitive data, this advantage disappears with regards to sensitive data. Given the wide ranging description of sensitive data (outlined in section 4), this is a considerable disadvantage. Forms of sensitive data are often those that are the most interesting to researchers, especially in the type of research for which there is the most societal/economic demand (Quinn and Quinn 2018 ). Health data for instance is of immeasurable importance to many forms of research. In addition, the increasing importance of big data to modern research, which is becoming increasingly computational in nature means that more and more research will in general be using sensitive data. This is because as section 5 identifies, it is becoming increasingly likely that many forms of big research data will contain sensitive data due the possibility to draw sensitive inferences by combining various elements within it (even where this might not be intuitively obvious) (Quinn and Malgeri forthcoming-a ). A reduced ability to process sensitive research data (without resorting to consent as a legal base) is therefore an important de facto impediment to research in the private sector that should not be underestimated. This places a severe limitation on the ability of private sector researchers to be able to rely on ‘legitimate interests’ as a base in the future, especially in areas of highly valued research (that often require sensitive data).
The aim of this paper has been to discern to what extent there is a level playing field for both private and public based researche entities in terms of their abilities to use the legal bases made available in the GDPR for research. The reality is a nuanced picture. As this paper shows, it is necessary not only to look at a particular base as it exists on paper, but to envisage how it is likely to be used in reality. This is important because even if a legal base is capable of being used for research purposes in theory the reality may, depending upon the context in question, be very different. This may be particularly true if one compares public research institutions (e.g. universities) to private commercial entities where different types of actor may be able to make more use of the legal bases within the GDPR that can be used for research.
Whilst (assuming there is no serious imbalance in power relations) consent as a legal base is available to all types of entities wishing to conduct research (i.e. both public and private), the same may not be true for other legal bases that are important for research. This incudes bases for processing in the public interest (non sensitive data) and for scientific research (sensitive data). Both of these can be used to justify processing personal data for research purposes in various contexts. Both are in theory open to public and private research institutions alike. This appearance of equal opportunity however does not hold up to scrutiny in the real world context. This largely because the GDPR mandates that the use of such bases must be further clarified in Union or national law. In reality, this often translates into a need for national legislation outlining what types of entities can use these legal bases and in which contexts. Such legislation is important in allowing universities and other public research bodies to conduct research where for instance obtaining consent may not be viable (or desirable). The extent to which such a possibility is available to private sector actors depending on the wording of a particular Member State’s law. In many instances it might not be suitable for the type of ‘research activities’ that private entities often conduct. Such legislation may for instance specify certain types of entities (e.g. public sector bodies) that can avail themselves of a research exemption. Others may require that research be considered in the ‘public interest’, something not necessarily true of much private sector research.
Whilst private sector research may be disadvantaged in terms of its ability to utilise these bases, it has others which are not available to researchers acting in the public sector. This most notably includes the ground of ‘legitimate interest’. This allows data controllers to process personal data (including for research purposes) when it is in their interest to do so. This can only be done however where such processing is foreseeable for the data subject and it is necessary and proportional taking into account the interests of the data subjects. Whilst this does not provide private entities with a carte blanche to take personal data from anywhere and use it for research; it does mean that in many cases where such entities have a large base of customers or clients that they will be able to use their data for research. This includes instances where the motivation behind the research is primarily commercial in nature. Entities such as tech and social media companies that dispose of enormous and growing quantities of customer data will therefore possess enormous pools of big data that are very high in research potential. This can be contrasted many public research actors that are dependent on the cooperation of external parties to obtain research data.
Another important factor that should not be underestimated is that private entities are unlikely to have as rigorous procedures of ethical review as public research entities. Ethical review committees are unlikely to have as strong an institutional underpinning in the former type of organisation. Where they do exist such procedures are unlikely to be as transparent as they are in their public sector counterparts. In entities such as universities, ethics committees have an extremely powerful role in deciding whether research can go ahead. They will often heavily scrutinize research proposals, in particular those that do not seek to base themselves upon informed consent (i.e. utilising a public interest or a scientific research ground as described above). Ethics bodies may often be reticent to allow such research to proceed, in particular where there is not an extremely good reason for not using informed consent as a basis. This factor, taken together with the availability of the ‘legitimate interest’ ground for private entities (which is not available for public research institutions) and the comparative superior access that some commercial organisations may have to large pools of customer data mean that the de facto advantage public institutions possess over private sector competitors to conduct research is less than it would appear on a simple reading of the GDPR.
In other important contexts however this advantage may be more important given that the legitimate interests base it not sufficient alone to allow the processing of sensitive forms of data. This limitation also applies to the broad possibility for ‘further processing for scientific research’. This is an important limitation that should be taken into account when looking at the overall ability of private entities to conduct research with personal data. The use of sensitive data is indispensable to research in a number of areas that may have a high economic value and/or societal importance. Not being able to use the legal bases it can for research with non-sensitive data to conduct research with sensitive data means that private entities will often be forced to rely on explicit consent as a base for processing where universities may be able to use one of the research exceptions outlined in the GDPR. This may especially be the case in certain Member States where legislation has been formulated in a way so that the GDPR’s research exception is only available for research that is perceived to be in the ‘public interest’.
Given the continuing evolution of both big data and the research that it can facilitate this limitation of the legitimate interests base may become increasingly important, even in contexts where there is no express intention to use big data. This is because the larger a data set is, the greater the chances are that that the data in question will contain sensitive data. This is especially true with modern forms of big data that are at the heart many forms of innovative research. The never ending advances in computer power and associated refinement of analytical software will make it increasingly likely that sensitive data can be found within many forms of big data. Data that is seemingly innocuous and not personal in isolation may, when processed with other forms of data, reveal information that is personal and sensitive in nature. This problem will arguably become increasingly important for research in the future and mean that researchers (in both the public and private realms) will have to seek a legal base for processing sensitive data. This situation will arguably increase the importance of public/private sector collaboration in research in order to combine the data gathering abilities of the private sector with the research options foreseen within the GDPR for sensitive that are often more available for publicly funded research entities (where indeed such research in the public interest).
The author of this paper suggests that an urgent priority is to clarify the scope of make use of the seemingly extremely broad possibility the GDPR allows for ‘further processing for purposes of scientific research’. Further clarifications on this last ground are needed quickly given the concerningly apparent wide remit it presents data controllers to further process personal data for purposes of scientific research if they are already legally in possession of the data in question. It is unfortunate that such guidance was not provided in the EDPS opinion on scientific research. One can only hope that such guidance is forthcoming quickly.
Availability of data and materials
For a rich an interesting analysis of the changing landscape in this area see: Corrales. M, Fenwick. M, Forgo. F, “New Technology, Big Data and the Law”, Springer, (2017). See also: Quinn. P, “Is the GDPR and Its Right to Data Portability a Major Enabler of Citizen Science?”, Global Jurist, (2018), DOI: https://doi.org/10.1515/gj-2018-0021
This is noticeable in fields such as genetics where study that is primarily computational in nature is becoming of increasing importance. See: Quinn. P & Quinn. L, “Big Genetic data and its big data protection challenges”, Computer Law and Security Review, (2018), 5, 34, p1000–1018
GDPR recital 26
As the author pointed out in a previous paper however, discerning whether data is truly anonymous may be a difficult task. In addition, where true anonymity is achieved it may often render data useless in research terms. See: Quinn. P, “The Anonymisation of Research Data — A Pyric Victory for Privacy that Should Not Be Pushed Too Hard by the EU Data Protection Framework?”, European Journal of Health Law, (2017), 24, 4, p1–21
The GDPR (in recital 26) unlike the previous directive 95/46/EC confirms explicitly that pseudonymized data (unlike anonymized data) is indeed personal data.
Article 29 Working Party Opinion 05/2014 on Anonymisation Techniques, Adopted on 10 April 2014, 0829/14/EN WP216
Directive 95/46/EC on the protection of individuals with regard to the processing of personal data and on the free movement of such data, is a European Union directive adopted in 1995 which regulates the processing of personal data within the European Union (EU). It was superseded by the GDPR, see Fn 23
Regulation (EU) 2016/679 of the European Parliament and of the Council of 27 April 2016 on the protection of natural persons with regard to the processing of personal data and on the free movement of such data, and repealing Directive 95/46/EC
Important exceptions remain, as will be discussed in section 5. These notably include exceptions for some forms of sensitive data such as health for which the GDPR (article 9(4)) allows an Member States to create further potentially divergent regulation.
Quinn. P & Quinn. L, “Big Genetic data and its big data protection challenges”, Computer Law and Security Review, (2018), 5, 34, p1000–1018 Despite the harmonizing effect of the GDPR, concerns still remain concerning the manner in which individual Member States are able to employ their own interpretation of key concepts. For more see: Peloquin. D, DiMaio. M, Bierer. B, Barnes, M, “Disruptive and avoidable: GDPR challenges to secondary research uses of data”, European Journal of Human Gentics, (2020), 28, 697–705
There are some important exceptions to the application of the GDPR however. This includes most criminal justice related activities. Article 2(2) for instances states that the regulation does not apply to activities “by competent authorities for the purposes of the prevention, investigation, detection or prosecution of criminal offences or the execution of criminal penalties, including the safeguarding against and the prevention of threats to public security.”
GDPR Recital 33
Data Subject rights are outlined in chapter 3 (articles 12–20) of the GDPR
Article 9(4) of the GDPR for example allows Member States to create additional requirements for the processing of various forms of sensitive data.
This is confirmed by Article 5(a) which calls for all processing of personal data to be “lawful”
Article 6 outlines consent as a legal base for the processing of data. Article 7 outlines some of the requirements attached to consent. As section 5 will discuss, article 9 outlines “explicit consent” as as legal basis for the processing of special categories of data.
Numerous concerns were raised on the part of research scientists during the lengthy negotiation process between various EU institutions for the GDPR. See for example: Nyren. O, Stenbeck. M, Groberg. H, “The European Parliament proposal for the new EU General Data Protection Regulation may severely restrict European epidemiological research”, European Journal of Epidemiology (2014), 29, p-227-230
GDPR Article 4(11)
“Under the GDPR, a data subject must inter alia be informed about the identity and contact details of the data controller, the data protection officer, the purposes for which the data will be processed, the recipients of the data, the duration of storage and the right to withdraw consent if consent is the lawful basis of processing”. See Staunton. C, Slokenberga. S, Mascalzoni. D, “The GDPR and the research exemption: considerations on the necessary safeguards for research biobanks”, European Journal of Genetics (2019), 27, p1159–1167
Article 29 Working Party Guidelines on consent under Regulation 2016/679 (2017) 17/EN WP259 p28: When regarded as a whole, the GDPR cannot be interpreted to allow for a controller to navigate around the key principle of specifying purposes for which consent of the data subject is asked
Corrigan. O, “Empty ethics: the problem with informed consent” Sociology of Health & Illness, 25, 3, p768–792; Solove. D, “Introduction: Privacy Self-Management and the Consent Dilemma” Harvard Law Review, (2013), 126, p1880–1903. GDPR recital 43 states “… In order to ensure that consent is freely given, consent should not provide a valid legal ground for the processing of personal data in a specific case where there is a clear imbalance between the data subject and the controller, in particular where the controller is a public authority and it is therefore unlikely that consent was freely given in all the circumstances of that specific situation”
The use of this legal base for research has been confirmed by the Article 29 working party (albeit discussing the applicability of Directive 95/46/EC). See: Article 29 Data Protection Working Party, Opinion 06/2014 on the notion of legitimate interests of the data controller under Article 7 of Directive 95/46/EC, European Commission, 9 April 2014 22. This matter is also discussed in Donnelly. M & McDonagh. M, “Health Research, Consent and the GDPR Exemption”, European Journal of Health Law, (2019), 26,2, p97–119 in section 3.1
GDPR Article 6(3)
The need for necessity and proportionality in the processing of personal data in the public interest has long been established by the European Court of Human Rights. A foundational case was S and Marper v United Kingdom [2008] ECHR 1581 where the requirements of necessity and proportionality were placed alongside the need for legality i.e. the existence of legislation outlining the processing in question.
One important area where this legal base can not be used is in research with sensitive (or special data). As section § discusses further, article 9, which outlines the bases for the processing of sensitive data does not include processing for contractual reasons. This means another base must be sought e.g. explicit consent. This could for example be the case with online commercial genealogical research databases. In such instances companies operating such a business model will need to also secure explicit consent to process sensitive data. (in addition to any contractual agreement they have to conduct research.)
For a broad (though pre GDPR) discussion of the concept of legitimate interests see: Article 29 Data Protection Working Party, Opinion 06/2014 on the notion of legitimate interests of the data controller under Article 7 of Directive 95/46/EC, European Commission, 9 April 2014
Donnelly. M & McDonagh. M, “Health Research, Consent and the GDPR Exemption”, European Journal of Health Law, (2019), 26,2, 97–119. The Article 29 working party did outline some potential room for public entities to use legitimate interests as a legal base where they are not carrying out a public function. (See WP29 Opinion on Legitimate Interests, p26). The author would argue that the applicability of this to research matters is likely to be limited.
See Article 29 Opinion on legitimate intersts, p.40
See Article 29 Opinion on legitimate interests, p.30
GDPR Article 14(5)b
Ducato. R, “Data protection, scientific research, and the role of information” CRIDES Working Paper Series, (2020) no. 1/2020, Computer Law and Security Review, forthcoming. Available at: file:///C:/Users/Pc/AppData/Local/Packages/Microsoft.MicrosoftEdge_8wekyb3d8bbwe/TempState/Downloads/2020%20-%20CRIDES%20WPS_1_2020_Ducato%20(1).pdf In this quote the author is referring to EDPS, Preliminary opinion on data protection and scientific research, p. 20.
Article 89(2) states “Where personal data are processed for scientific or historical research purposes or statistical purposes, Union or Member State law may provide for derogations from the rights referred to in Articles 15, 16, 18 and 21 subject to the conditions and safeguards referred to in paragraph 1 of this Article in so far as such rights are likely to render impossible or seriously impair the achievement of the specific purposes, and such derogations are necessary for the fulfilment of those purposes.
These rights are outlined in GDPR article 15, 16, 18 and 21 respectively.
The author discusses these difficulties in the context of genetic research in: Quinn. P & Quinn. L, “Big Genetic data and its big data protection challenges”, Computer Law and Security Review, (2018), 5, 34, p1000–1018
GDPR Article 89(2)
One important effect however of the requirement of national law, is that the extent to which rights are restricted may vary from one Member State to another, negating to a certain extent the general harmonizing mission of the GDPR. This means that the ability to limit data subject rights may vary considerably from one Member State to another.
See section 4.B where data subject rights are discussed
EDPS opinion on Scientific Research, page 16
The changing nature of sensitive data and its importance to researchers is reviewed by the author in and a colleague in Quinn. P & Malgeri. G, “Sensitive Data – Fast becoming a paper tiger (forthcoming)
This notion is extremely wide. See Article 29 Data Protection Working Party, Advice Paper on Special Categories Of Data (‘Sensitive Data’). See also annex to letter written by the Article 29 Working Party to the European Commissionon Feburary 5th 2014 concerning the interpretation of health data, available at: http://ec. europa.eu/justice/data-protection/article-29/documentation/ otherdocument/files/2015/20150205 _ letter _ art29wp _ ec _ health _ data _ after _ plenary _ annex _ en.pdf
For more on the complexities of performing data protection impact assessments in various circumstances see: Kloza, D, Van Dijk,N, Gellert, R, Böröcz, I, Tanas,A, Mantovani, E, Quinn, P. (Brussels Laboratory for Data Protection & Privacy Impact Assessments (d.pia.lab)),Data protection impact assessments in the European Union: complementing the new legal framework towards a more robust protection of individuals d.pia.lab PolicyBrief No.1/2017,2017,ISSN2565–9936.
For a discussion on the impact of such research in the are of genomics see Quinn. P & Quinn. L, “Big Genetic data and its big data protection challenges”, Computer Law and Security Review, (2018), 5, 34, p1000–1018
In Directive 95/46/EC the legal bases for the processing of sensitive data were outlined in article 8. For more see also: Quinn. P, Habbig. A, Mantovani. E, De Hert. P “The data protection and medical device frameworks—obstacles to the deployment of mHealth across Europe?“, European journal of health law, (2013) 20 (2), 185–204
GDPR Article 4(11) defines consent as Consent of the data subject means any freely given, specific, informed and unambiguous indication of the data subject’s wishes by which he or she, by a statement or by a clear affirmative action, signifies agreement to the processing of personal data relating to him or her.
GDPR Article 7(1)
For more on the consent/ anonymize dichotomy and the problems it can cause for research see: Carter., P Laurie. G, Dixon-Woods. M “The social licence for research: why care.data ran into trouble”, Journal of Medical Ethics, (2015), 41, p404–409
Hallinan. D & Friedewald. M,. “Open consent, biobanking and data protection law: can open consent be ‘informed’ under the forthcoming data protection regulation?”, Life Sciences, Society and Policy, (2015) 11:1. doi: https://doi.org/10.1186/s40504-014-0020-9 ; Quinn. P, “The Anonymisation of Research Data — A Pyric Victory for Privacy that Should Not Be Pushed Too Hard by the EU Data Protection Framework?”, European Journal of Health Law, (2017), 24,4, pp1–21
The GDPR foresees legal bases for the processing of sensitive data for reasons of ‘substantial public interest’ (article 9(g) and ‘public health (article 9(i)).
For more see ‘EPDPS Opinion on Scientific Research’, p23
For more discussion of the concept of substantial public interest see: Hallinan. D, “Broad consent under the GDPR: an optimistic perspective on a bright future”, Life Sciences, Society and Policy, (2020), 16, 1, https://doi.org/10.1186/s40504-019-0096-3
EDPS opinion on scientific research, P19
For more on the potentials problems of publicly available research information in the context of genetic research see: Quinn. P & Quinn. L, “Big Genetic data and its big data protection challenges”, Computer Law and Security Review, (2018), 5, 34, p1000–1018
The EDPS for example notes cautiously “Publishing personal data in a biography or an article in the press is not the same as posting a message on a social media page.” See EDPS opinion on scientific research, p19
For a discussion on the German perspective for example see Molnár-Gábor. F, “Germany: a fair balance between scientific freedom and data subjects’ rights?” Human Genetics (2018) 137:619–626
The European Data Protection Supervisor recognised that some form of consent might even be desirable even where it is not the legal base relied upon for the processing of personal data. In such instances the use of consent is supplemental and mainly for ethical purposes. See EDPS opinion on scientific research, p20
For more see the discussion in Fn39 concerning recital 43 of the GDPR.
See sections 4 & 5.
In the UK for example, national legislation is seen as providing a broad discretion for public sector organizations to process genetic data without consent.
For an alternative view see the report composed by (EPRS) - European Parliamentary Research Service Scientific Foresight Unit (STOA) “How the General Data Protection Regulation changes the rules for scientific research” PE 634.447 – July 2019 p66
In the UK the Relevant Legislation is the Data Protection Act (2018). The UK’s information commissioner has also stated that researchers who want to use personal data without consent must establish that the research in question is in the public interest. See ICO report “Guide to the General Data Protection Regulation (GDPR)” (n 14), p 284. Available at: https://ico.org.uk/media/for-organisations/guide-to-the-general-data-protection-regulation-gdpr-1-0.pdf
In Ireland the primary legislation connected to the GDPR is the Data Protection Act 2018. It is accompanied by the Health Research Regulations 2018 which regulate research using health data. For further analysis see: Dove. E & Chen. J “Should consent for data processing be privileged in health research? A comparative legal analysis” International Data Privacy Law, (2019), https://doi.org/10.1093/idpl/ipz023 see p8-10 Under Irish law data controllers must seek permission from the minister of Health who will issue a decision vis-à-vis an appointed committee. An application must be accompanied by “written information demonstrating that the public interest in carrying out the health research significantly outweighs the public interest in requiring the explicit consent of the data subject under Regulation 3(1)(e) together with a statement setting out the reasons why it is not proposed to seek the consent of the data subject for the purposes of the health research.”
GDPR Articles 6(b) and 6(c)
GDPR Article 6(1)(f)
See: Article 29 Data Protection Working Party, Opinion 06/2014 on the notion of legitimate interests of the data controller under Article 7 of Directive 95/46/EC, European Commission, 9 April 2014, p40
This is issue was highlighted by the New York Times as far Back as 2012. See Markoff. J “Troves of Personal Data, Forbidden to Researchers”, Published 21 May 2012 Available at: https://www.nytimes.com/2012/05/22/science/big-data-troves-stay-forbidden-to-social-scientists.html
This has been demonstrated during the COVID-19 pandemic. See Hao. K, “How Facebook and Google are helping the CDC forecast coronavirus” Published Online on MIT Technology Review, 09 April 2020. Available at: https://www.technologyreview.com/2020/04/09/998924/facebook-and-google-share-data-to-forecast-coronavirus/
The same is true for other bases that, as section 4 discusses, could be potentially applicable to research also (e.g. processing to meet a contractual obligation).
See WP29 Opinion on Legitimate Interests, p15. The Working party stated (in the context of Directive 95/46/EC) “a controller processing special categories of data may never invoke solely a legal ground under Article 7 to legitimize a data processing activity. Where applicable, Article 7 will not prevail but always apply in a cumulative way with Article 8 to ensure that all relevant safeguards and measures are complied with”
Abbreviations
Data protection impact assessment
Data Protection Officer
European Data Protection Supervisor
Electronic Health Record
European Union
General Data Protection Regulation
“A Preliminary Opinion on data protection and scientific research”, European Data Protection Supervisor, 6 th Janurary 2020. Available at: https://edps.europa.eu/sites/edp/files/publication/20-01-06_opinion_research_en.pdf
Akoka, J., I. Comyn-Wattiau, and N. Laoufi. 2017. Research on big data – A systematic mapping study. Computer Standards & Interfaces 54 (2): 105–115.
Article Google Scholar
2014. Article 29 Working Party Opinion on Anonymisation Techniques. 0829/14/ENWP216, p. 3.
Berman, J. 2002. Confidentiality issues for medical data miners. Artificial Intelligence in Medicine 26 (1–2): 25–36.
Carter, P., G. Laurie, and M. Dixon-Woods. 2015. The social licence for research: why care.data ran into trouble. Journal of Medical Ethics 41: 404–409.
Connelly, R., C. Playford, V. Gayle, and C. Dibden. 2016. The role of administrative data in the big data revolution in social science research. Social Science Research 59: 1–12.
Corrales, M., M. Fenwick, and F. Forgo. 2017. New technology, big data and the law . Springer.
Corrigan, O. 2003. Empty ethics: The problem with informed consent. Sociology of Health & Illness 25: p768–p792.
Dalle Molle Araujo Dias, R. 2017. The potential impact of the EU general data protection regulation on pharmacogenomics research. Medicine and Law 36 (2): 43–58.
Google Scholar
Donnelly, M., and M. McDonagh. 2019. Health Research, consent and the GDPR exemption. European Journal of Health Law 26 (2): 97–119 in section 3.1.
Dove, E., and J. Chen. 2019. Should consent for data processing be privileged in health research? A comparative legal analysis. International Data Privacy Law https://doi.org/10.1093/idpl/ipz023 .
Drabiak, K. 2017. Caveat emptor: How the intersection of big data and consumer genomics exponentially increases information privacy risks. Health Matrx 27: 143–228.
Ducato R. 2020. Data protection, scientific research, and the role of information . CRIDES working paper series. no. 1/2020, Computer Law and Security Review, forthcoming. Available at: file:///C:/Users/Pc/AppData/Local/Packages/Microsoft.MicrosoftEdge_8wekyb3d8bbwe/TempState/Downloads/2020%20-%20CRIDES%20WPS_1_2020_Ducato%20(1).pdf
Dummy, 2021
European Parliamentary Research Service Scientific Foresight Unit (STOA). How the general data protection regulation changes the rules for scientific research . PE 634.447 – July 2019. 66.
Forgo, N. 2017. The principle of purpose limitation and big data. In New technology, big data and the law , ed. M. Corrales, M. Fenwick, and F. Forgo. Springer.
Freidenfelds, L., and A. Brandt. 1996. Commentary: Research ethics after world war II: The insular culture of biomedicine. Kennedy Institute of Ethics Journal 6 (3): 239–243.
Hallinan, D. 2020. Broad consent under the GDPR: An optimistic perspective on a bright future. Life Sciences, Society and Policy 16: 1 https://doi.org/10.1186/s40504-019-0096-3 .
Hallinan, D., and M. Friedewald. 2015. Open consent, biobanking and data protection law: Can open consent be ‘informed’ under the forthcoming data protection regulation? Life Sciences, Society and Policy 11 (1). https://doi.org/10.1186/s40504-014-0020-9 .
Hao. K. How Facebook and Google are helping the CDC forecast coronavirus . Published Online on MIT Technology Review, 09 April 2020. Available at: https://www.technologyreview.com/2020/04/09/998924/facebook-and-google-share-data-to-forecast-coronavirus/
Hartley, J., J. Alford, E. Knies, and S. Douglas. 2017. Towards an empirical research agenda for public value theory. Public Management Review 19 (5): 670–685.
Heffetz, O., and K. Ligett. 2014. Privacy and data-based research. Journal of Economic Perspectives 28: s75–s98.
ICO report. Guide to the general data protection regulation (GDPR) . (n 14), p 284. Available at: https://ico.org.uk/media/for-organisations/guide-to-the-general-data-protection-regulation-gdpr-1-0.pdf
Jamrozik, K. 2004. Research ethics paperwork: What is the plot we seem to have lost? BMJ 329 (7460): 286–287.
Jensen, J., L. Jensen, and S. Brunak. 2012. Mining electronic health records: Towards better research applications and clinical care. Nature Reviews Genetics 13: 395–405.
Klievink, B., B. Romijn, S. Cunningham, and H. De Bruijn. 2017. Big data in the public sector: Uncertainties and readiness. Information Systems Frontiers 19: 267–283.
Kloza, D, Van Dijk, N, Gellert, R , Böröcz, I, Tanas A, Mantovani E, Quinn P. (Brussels Laboratory for Data Protection & Privacy Impact Assessments (d.pia.lab)). Data protection impact assessments in the European Union: complementing the new legal framework towards a more robust protection of individuals d.pia.lab PolicyBrief No.1/2017, 2017, ISSN2565–9936.
Kohn, T., and C. Shore. 2017. The ethics of university ethics committees. In Death of the Public University , ed. S. Wright and C. Shore, 229–249. Berghahn Books.
Mai, J. 2016. Big data privacy: The datafication of personal information. The Information Society 32 (3): 192–199.
Malgeri, G. 2020. Data protection and research: A vital challenge in the era of Covid-19 pandemic. Computer Law and Security Review . https://doi.org/10.1016/j.clsr.2020.105431 .
Markoff. J. Troves of personal data, forbidden to researchers . Published 21 May 2012. Available at: https://www.nytimes.com/2012/05/22/science/big-data-troves-stay-forbidden-to-social-scientists.html
Maroto, A., J. Gallego, and L. Rubalcaba. 2016. Publicly funded R&D for public sector performance and efficiency: Evidence from Europe. R and D Management 46 (S2): 564–578.
Massimo, B. 2016. Accessing online data: Web-crawling and information-scraping techniques to Automate the assembly of research data. Journal of Business Logistics 37 (1): 36–42.
Mcguire, A., J. Hamilton, R. Lunstroth, L. Mccullough, and A. Goldman. 2008. DNA data sharing: Research participants’ perspectives. Genetics in Medicine 10: 46–53.
Meszatos, J., and C. Ho. 2018. Big data and scientific research: The secondary use of personal data under the research exemption in the GDP. Hungarian Journal of Legal Studies 59 (4): 403–419.
Mirowski, P., and E. Sent. 2002. Science bought and sold : Essays in the economics of science . University of Chicago Press.
Molnár-Gábor, F. 2018. Germany: A fair balance between scientific freedom and data subjects’ rights? Human Genetics 137: 619–626.
Mondshein, C., and C. Cosimo. 2019. The EU’s general data protection regulation (GDPR) in a research context. In Fundamentals of clinical data science , ed. P. Kubben, M. Dumontier, and A. Dekker. Springer.
Moore, M., and D. Tambini. 2018. Digital dominance: The power of Google, Amazon, Facebook, and Apple . Oxford University Press.
Mostert, M., A. Bredenoord, M. Biesaart, and J. Van Delden. 2016. Big data in medical research and EU data protection law: Challenges to the consent or anonymise approach. European Journal of Human Genetics 24: 956–960.
Nyren, O., M. Stenbeck, and H. Groberg. 2014. The European Parliament proposal for the new EU general data protection regulation may severely restrict European epidemiological research. European Journal of Epidemiology 29: 227–230.
Olly, J. 2018. Businesses retreating from consent under GDPR . London: International Financial Law Review Available at https://search.proquest.com/openview/1243289302ad38c65af22160c5008a1f/1?pq-origsite=gscholar&cbl=36341 .
Peloquin, D., M. DiMaio, B. Bierer, and M. Barnes. 2020. Disruptive and avoidable: GDPR challenges to secondary research uses of data. European Journal of Human Gentics 28: 697–705.
Quinn & Malgeri, (forthcoming-b).
Quinn, P. 2017. The Anonymisation of research data — A pyric victory for privacy that should not be pushed too hard by the EU data protection framework? European Journal of Health Law 24 (4): 1–21.
Quinn, P. 2018. Is the GDPR and its right to data portability a major enabler of citizen science? Global Jurist . https://doi.org/10.1515/gj-2018-0021 .
Quinn, P., A. Habbig, E. Mantovani, and P. De Hert. 2013. The data protection and medical device frameworks—Obstacles to the deployment of mHealth across Europe? European Journal of Health Law 20 (2): 185–204.
Quinn, P., and G. Malgeri. forthcoming-a. Sensitive data – Fast becoming a paper tiger .
Quinn, P., and L. Quinn. 2018. Big genetic data and its big data protection challenges. Computer Law and Security Review 5 (34): 1000–1018.
Rothstein, A., and A. Shoben. Does consent Bias research? The American Journal of Bioethics 13 (4): 27–37.
Rothstein, M. 2010. Is Deidentification sufficient to protect health privacy in research? American Journal of Bioethics 10 (9): 3–11.
Sharon, T. 2016. The Googlization of health research: From disruptive innovation to disruptive ethics. Personalized Medicine 13: 6. https://doi.org/10.2217/pme-2016-0057 .
Shmueli. G, & Greene. T . 2018. Analyzing the impact of GDPR on data scientists using the InfoQ framework . Available at SSRN: https://ssrn.com/abstract=3183625 or https://doi.org/10.2139/ssrn.3183625 .
Solove, D. 2013. Introduction: Privacy self-management and the consent dilemma. Harvard Law Review 126: 1880–1903.
Srncova, Z., R. Babela, R. Mamrilla, and Z. Balazova. 2019. GDPR implementation in public health. International Health Journal 1: 15–17.
Staunton, C., S. Slokenberga, and D. Mascalzoni. 2019. The GDPR and the research exemption: Considerations on the necessary safeguards for research biobanks. European Journal of Genetics 27: 1159–1167.
Swan, M. 2013. The quantified self: Fundamental disruption in big data science and biological discovery. Big Data 1 (2) Available at: https://doi.org/10.1089/big.2012.0002 .
Taylor M,·Wallace S, Prictor P “United Kingdom: Transfers of genomic data to third countries”, Human Genetics, (2018), 137, 637–645 In the UK for example, national legislation is seen as providing a broad discretion for public sector organizations to process genetic data without consent.
Tene, O., and J. Polonetsky. 2016. Beyond IRBs: Ethical guidelines for data research. Washington and Lee Law Review 72 (3): 458–471.
Vadeboncoeur, C., N. Townsend, C. Foster, and M. Sheehan. 2016. Variation in university research ethics review: Reflections following an inter-university study in England. Research Ethics 12 (4): 217–233.
Watts, R. 2016. Thinking about the Public University. In Public universities, Managerialism and the value of higher education , 26–67. Springer.
Download references
Acknowledgments
Author information, authors and affiliations.
Vrije Universiteit Brussel (VUB), Brussels, Belgium
You can also search for this author in PubMed Google Scholar
Contributions
The author has written the entire paper alone. The author(s) read and approved the final manuscript.
Corresponding author
Correspondence to Paul Quinn .
Ethics declarations
Competing interests, additional information, publisher’s note.
Springer Nature remains neutral with regard to jurisdictional claims in published maps and institutional affiliations.
Rights and permissions
Open Access This article is licensed under a Creative Commons Attribution 4.0 International License, which permits use, sharing, adaptation, distribution and reproduction in any medium or format, as long as you give appropriate credit to the original author(s) and the source, provide a link to the Creative Commons licence, and indicate if changes were made. The images or other third party material in this article are included in the article's Creative Commons licence, unless indicated otherwise in a credit line to the material. If material is not included in the article's Creative Commons licence and your intended use is not permitted by statutory regulation or exceeds the permitted use, you will need to obtain permission directly from the copyright holder. To view a copy of this licence, visit http://creativecommons.org/licenses/by/4.0/ . The Creative Commons Public Domain Dedication waiver ( http://creativecommons.org/publicdomain/zero/1.0/ ) applies to the data made available in this article, unless otherwise stated in a credit line to the data.
Reprints and permissions
About this article
Cite this article.
Quinn, P. Research under the GDPR – a level playing field for public and private sector research?. Life Sci Soc Policy 17 , 4 (2021). https://doi.org/10.1186/s40504-021-00111-z
Download citation
Received : 03 August 2020
Accepted : 04 January 2021
Published : 01 March 2021
DOI : https://doi.org/10.1186/s40504-021-00111-z
Share this article
Anyone you share the following link with will be able to read this content:
Sorry, a shareable link is not currently available for this article.
Provided by the Springer Nature SharedIt content-sharing initiative
Life Sciences, Society and Policy
ISSN: 2195-7819
- Submission enquiries: Access here and click Contact Us
- General enquiries: [email protected]
Cybersecurity, Data Privacy and Blockchain: A Review
- Review Article
- Open access
- Published: 12 January 2022
- Volume 3 , article number 127 , ( 2022 )
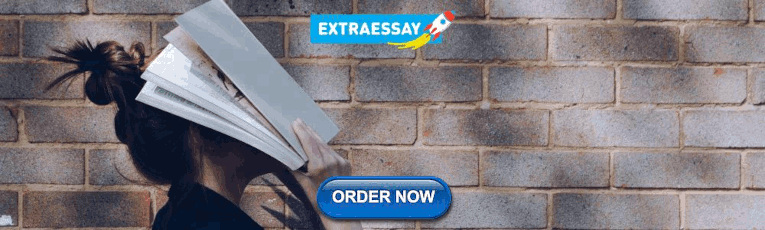
Cite this article
You have full access to this open access article
- Vinden Wylde 1 ,
- Nisha Rawindaran 1 ,
- John Lawrence 1 ,
- Rushil Balasubramanian 1 ,
- Edmond Prakash ORCID: orcid.org/0000-0001-9129-0186 1 ,
- Ambikesh Jayal 2 ,
- Imtiaz Khan 1 ,
- Chaminda Hewage 1 &
- Jon Platts 1
21k Accesses
48 Citations
Explore all metrics
In this paper, we identify and review key challenges to bridge the knowledge-gap between SME’s, companies, organisations, businesses, government institutions and the general public in adopting, promoting and utilising Blockchain technology. The challenges indicated are Cybersecurity and Data privacy in this instance. Additional challenges are set out supported by literature, in researching data security management systems and legal frameworks to ascertaining the types and varieties of valid encryption, data acquisition, policy and outcomes under ISO 27001 and the General Data Protection Regulations. Blockchain, a revolutionary method of storage and immutability, provides a robust storage strategy, and when coupled with a Smart Contract, gives users the ability to form partnerships, share information and consent via a legally-based system of carrying out business transactions in a secure digital domain. Globally, ethical and legal challenges significantly differ; consent and trust in the public and private sectors in deploying such defensive data management strategies, is directly related to the accountability and transparency systems in place to deliver certainty and justice. Therefore, investment and research in these areas is crucial to establishing a dialogue between nations to include health, finance and market strategies that should encompass all levels of society. A framework is proposed with elements to include Big Data, Machine Learning and Visualisation methods and techniques. Through the literature we identify a system necessary in carrying out experiments to detect, capture, process and store data. This includes isolating packet data to inform levels of Cybersecurity and privacy-related activities, and ensuring transparency demonstrated in a secure, smart and effective manner.
Similar content being viewed by others
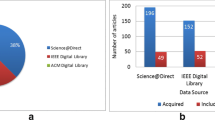
Blockchain smart contracts: Applications, challenges, and future trends
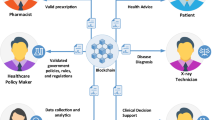
Blockchain for healthcare data management: opportunities, challenges, and future recommendations
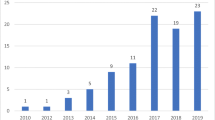
Security, Privacy and Risks Within Smart Cities: Literature Review and Development of a Smart City Interaction Framework
Avoid common mistakes on your manuscript.
Introduction
With the recent emphasis on societies in increasing their dependency on cloud technologies, coupled with the human need to communicate and share data via digital networks, Internet of Things (IoT) devices to include smart phones, industrial and domestic appliances, continue to be a necessary function in conducting business. Social exchanges and transactional types of data for example, drive the financial markets thus facilitating in the swift development of emerging technologies at an ever faster rate to keep up with supply and demand trends. In a domestic setting, the sharing of digital media (videos, music, pictures, documents (data)) through messaging services to enhance subject areas such as information technology, sport, social sciences, education and health for example, IoT devices enable the efficient and effective transfer of data world-wide instantly via the Internet of Everything (IoE) via the cloud. In an industrial context, Smart Sensors, Application Programming Interfaces (API) and IoT networks facilitate remote working across digital boundaries globally.
These potentially devastating instances of data sharing and/or criminality, influence the confidentiality and protections set out by governments, businesses and organisations, culminating in legal and ethical disputes with significant financial ramifications due to Denial of Service (DDoS) attacks for example, that would damage and disrupt entire business data architectures, infrastructures networks and services on a large scale. Consequently, with society relying more and more on the exchange and processing of Personal Identifiable Information (PII) via IoT, trust in renowned institutions and government organisations to include broadcast and digital media outlets becomes a main issue. As a user chooses to share social network, personal and confidential information whilst shopping on-line for example, they should be aware of the nature and intent of cyber-criminality and have faith in the criminal justice system of a given territory.
On the other hand, for businesses, organisations, government bodies and academic institutions to be able to freely validate and authenticate their data in the service of societies globally, Artificial Intelligence (AI), Big Data (BD), Blockchain (BC) Combined Technologies and methodologies, contribute significantly in mitigating cyber-crime, whilst providing legal bodies the power to hold companies, organisation and institutions to account. One such method is the Smart Contract (SC) for example, and when utilised in the drafting and consenting of a legal document or digital certificate, provides an evidence-based transparent method in enhancing the legal credibility and value of a financial transaction. As a function of BC, the SC is validated, implemented then shared across a Pier-to-Pier (P2P) network as a Distributed Ledger Technology (DLT) for all parties to see which provides transparency and accountability.
- Cybersecurity
When utilising elements of cybersecurity, these technical requirements facilitate in the effective management of IoT hardware and software operations, physical interfaces and internal policy development. Additionally, the management system ISO 27001 supports network communication protocols, data access control and cryptography (i.e., password encryption), that contribute in ensuring a robust and secure communication method inclusive of cybersecurity staff training; all whilst minimising network communication attacks in the presence of malicious third-parties [ 1 ].
However, to harness and derive value from the volume, variety and veracity of data available, concepts such as BD, AI and Machine Learning (ML) utilise prescribed algorithms and analysis techniques across vast quantities of public, private and sensitive data through digital networks, that exponentially increases the risk of data breaches, viruses and malicious attacks. In other words, in successfully utilising these technologies in the legal acquisition and processing of data from the public and private sectors, also to include practical user measures, potentially reveals challenges and vulnerabilities that can further expose a user or group to cyber-criminality.
Data Privacy
Additionally, the ISO 27001 framework functions in conjunction with the General Data Protection Regulation (GDPR) Regulation (EU) 2016/679, and Data Protection Act 2018 c. 12 (DPA), in facilitating personal data controls and measures in the UK and European Unions (EU) digital boundaries. In processing medical data for example, a mandatory Data Protection Impact Assessment (DPIA) is undertaken in identifying and establishing the risks alongside eight core principles to include; lawful and ethical methods of data acquisition, data storage security and duration, fair use, and for data to be kept within specified locations and regions [ 2 ].
In utilising these legal frameworks and management systems, tracking tools such as ‘cookies’ for example may utilise the aforementioned AI, ML and algorithmic analysis unlawfully, and as a result, a user may not be aware of the tracking nature and capabilities contained within the software for analysis and marketing purposes. Additionally, without user consent, the awareness and continual levels of maintenance required of said cookies, that are a necessary function in surfing the web, could expose business networks to anti-forensic methods, legal jurisdiction matters, system hardware and Service Level Agreement (SLA) breaches, which compound over time and further aggravate technical, legal and ethical challenges in operating IoT devices in a compliant, safe and secure business environment.
Furthermore, when utilising in a healthcare service context, a SC policy with cryptography as a cybersecurity control method, gives transparency, protected agency and responsibility to the public, financial markets, business professionals and legal representatives, in conducting valid and transparent actions or investigations on behalf of the directorate or client. When this method is applied retrospectively, it also gives accountability in upholding vigilance and resilience when managing cyberspace, an operators duty of care and consideration of confidential data breaches, its sharing, and ramifications of exposing vast amounts of confidential National Health Service (NHS) patient data for example [ 3 ].
Blockchain Security
BC based functions, methods and systems utilise concepts like Cryptocurrency (i.e., Bitcoin and Etherium) as an alternative to fiat currencies, representative consensus protocols, anonymous signatures, off-chain storage and non-interactive zero-knowledge proofs. These concepts provide validity, anonymity, and transparency when coupled with inner corporate or organisational audit, policy deployment, healthcare provider and security service function of carrying out legal and domestic activities. This system is trustless by design and offers promise for equitable and transparent transactions.
As per all the above, this review and study proposes an intelligent framework to aide in the identification and detection of compromised network packet data. The use of BC and SC are to be utilised as an information carrier (data) and for evaluation, validation and testing with pre-prescribed control protocols. Then, to conduct a literature review in ascertaining current methodologies, techniques and protocols in aiding the development of said framework. To minimise human intervention, an intelligent automated approach is utilised in the capturing of network data at pre-determined intervals. Ultimately, the data events are tested against a framework with analysis of findings to demonstrate comprehensive framework feasibility (see Fig. 1 ).
Cybersecurity refers to: “a measure for protecting computer systems, networks, and information from disruption or unauthorized access, use, disclosure, modification or destruction” [ 4 ]. Therefore, in trying to understand cybersecurity and its applications towards IoT and smart devices, brings additional questions that need analysis through various notions of cyberspace. One solution is unifying all the terminologies above to bring together the importance of understanding where network intrusion comes from, how it is detected, and how prevention of cyber threats occur. When looking at prevention, AI and ML uses could also potentially contribute to the rise in using this technology to secure and protect data [ 5 ].
Cybersecurity IoT and ML
As Information Technology (IT) facilities expanded, overall digital technology saw growth in more devices being introduced and connected to the internet, so that access to data is freely available to allow for more activities to be undertaken. These activities allow for outcomes to be predicted [ 6 ]. Therefore, in response, various ML mathematical algorithms allow for classification usage such as Support Vector Machines (SVM), Decision Trees and Neural Networks. These algorithms all compound and highlight how data is treated and managed to produce an outcome, and predictability that is required to contribute to economic growth as societies move forward. ML capabilities go far beyond the expectations of conquering human hobbies, but lends further into everyday chores and events in daily lives.
Other real-life examples of ML usage rest in many industries focusing on identifying fake news, implementation of spam filters, identifying fraudulent or criminal activities online, and improving marketing campaigns. These large quantities of data are often private and sensitive, whilst travelling through Cyberspace transferring data along the way. Disadvantageously, this existence of cyberspace creates a wider security attack surface for potential malicious activities to occur. This demonstrates that human factors and the large influence it has on the security of IoT [ 7 ] is highly impactful.
Humans’ perceptions of security and privacy concerning these devices are also a subject to be discussed, for example, the concept of ‘Cookies’ as a tracking tool for online web surfing, and its safety measures, which are often shoehorned as a debate in itself, and the awareness of how it should be used has been seen through glazed eyes [ 8 ]. However, recent reports suggest that many contributory questions arise from understanding IoT and the safety net around it, and how humans cope and live alongside IoT. Anti-forensic methods, jurisdiction and Service Level Agreements (SLA) for example, all further aggravate technical, privacy, security, and legal challenges. In addition, the presence of GDPR and IoT, coupled with the human factors involved, present immense challenges in keeping these devices safe and secure.
Cybersecurity and SMEs
UK Small to Medium Enterprises (SME’s) have always seen challenges in understanding cybersecurity due to the increase in threats that have risen in recent years. The European Commission’s employment criterion for an SME minimum cyber-criterion is that for any business that employs less than 250 people [ 9 ]. The challenges faced are both operational and commercial in SMEs using Intrusion Detection mechanisms coupled together with AI and ML techniques in the protection of their data.
SMEs intrusion, detection, and prevention methods has become a priority in the realisation of keeping their data secure and safe with the integration of real-world objects and IoT, with understanding how ML techniques and AI can help secure zero-day attacks. Rawindaran et al. [ 1 ] took particular interest in the SME market and showcased an experimental scenario in which the intrusion, detection and prevention models were compared, and the views of the SME examined. The study looked at the various approaches in identifying ways to detect and protect any intrusions coming into the network and what operating devices would help in this process. The paper also explored the understanding in trying to protect the data and how government policies and procedures such as GDPR in the UK/EU, could assist towards this process [ 10 ].
Cybersecurity and SME Attacks
Rawindaran et al. [ 11 ] further examined the impact of how threat levels of attacks such as Ransomware, Phishing, Malware, and Social-engineering amongst others, were compared between an Open-Source device, such as SNORT and pfSense, and Commercial Network Intrusion Detection (NIDs) such as Cisco. There were three different NIDs and their features were compared. It was concluded that whilst SNORT and pfSense were free to use from the Open-Source market, it required a certain level of expertise to implement and embed the rules into a business solution. It was also noted that Cisco, due to their engineering expertise and their position as market leaders in the industry, were able to embed these free rules and use it to their advantage.
What emerged from this study was how businesses and organisations with the help of government policies and processes, needed to work together to combat these hackers, malicious actors, and their bots, and manage and stay ahead of the game [ 4 ]. The paper also discussed various ML approaches such as signature based models and anomaly based rules used by these devices to combat these attacks [ 12 ].
Additionally, signature based models could only detect attacks that were known, whereas anomaly-based systems were able to detect unknown attacks [ 13 ]. Anomaly-based NIDs made it possible to detect attacks whose signatures were not included in rule files. Unfortunately, due to the maturity of Anomaly NIDs, the costs were still very high to run and required computing power that were unrealistic in the SME environment. Anomaly based NIDs whilst still in its infancy, require a deeper analysis and future study.
Rawindaran’s study provided perspectives on better comparisons and relative conclusions and how it was important to explore further both the empirical as well as in scenario analysis for different dimensions, the nature and context of cyber security in the current world of internet and cyber connections. Rawindaran also explored how ML techniques have become vital in the growth and dependencies of these SMEs in the UK in their operations and commercial environment. This study took on an initial look at success stories from big technology companies such as Amazon, Google, and Facebook, in their use of ML techniques for their cybersecurity [ 14 ]. The methodology adopted in this study focused on structured survey questions on a selected sample number of respondents and directed its questions to the SMEs management, technical and non-technical professionals.
Cybersecurity and ML to Mitigate Attacks
Rawindaran et al., found that awareness of ML and its uses is still on a learning curve and has yet to be defined. The study brought to surface the three main categories of ML that being Supervised Learning, Unsupervised Learning and Reinforcement Learning and the algorithms that sit behind them [ 15 ]. Examples of Supervised Learning included real life predictive text in tweets in Twitter and product reviews in Amazon and eBay, calculating temperature, insurance premiums, pricing, and number of workers to the revenue of a business.
Examples of Unsupervised Learning include examples include identifying fake news, implementation of spam filter, identifying fraudulent or criminal activity online, and marketing campaigns. Reinforcement Learning shows example of playing a video game that provides a reward system when the algorithm takes an action. Each learning method used algorithms that helped with calculations and predictions and a dataset that helped in the development and structures of its uses. It also deducted and quantified examples and showed strength in the SMEs perception and awareness towards ML and its uses.
The methods of ML and its algorithms lead into the focus of this study in which SMEs were given the opportunity to make themselves aware of these algorithms that exist within their own cybersecurity software package. Further the analysis of this study showed the existence of these algorithms such as Neural Networks, Support Vector Machines, Deep Networks and Bayesian, however most of these were cleverly embedded within the software used [ 16 ].
The initial idea of using an Intrusion, Detection and Prevention System (IDPS) method, from either a commercial or Open-Source device to protect the data of the SME, comes with the knowledge of ML and AI. As hackers become increasingly clever and the uses of bots take over, their ‘attacking’ methods, as protectors of the systems, society has had to lean on ML and AI technology to help. An IDPS system is able to help through the use of ML, to learn about malicious patterns compared to valid patterns on the internet. These various approaches are needed to protect and shield data. ML through anomaly detection, proved to be more effective in its zero-day detection than that of signature based in its effectiveness towards cybersecurity and adoption within the UK SMEs. There is a significant gap that needs to be fulfilled by perhaps more variations in the devices used for SMEs such as opensource and voluntary participants from knowledge of the community to keep future proofing these devices.
Cybersecurity and Adversarial ML
With the increased use of ML in Intrusion Detection Systems (IDS) and IDPS systems within cyber security packages of SME communities, there suddenly lies the introduction of a new type of attack called Adversarial Machine Learning (AML) [ 1 ]. In a paper by Anthi et al. [ 17 ] states that with the introduction of ML IDSs, comes the creation of additional attack vectors specifically trying to break the ML algorithms and causing a bypass to these IDS and IDPS systems. This causes the learning models of ML algorithms subject to cyber-attacks, often referred to as AML.
These AMLs are thought to be detrimental as they can cause further delayed attack detection which could result in infrastructure damages, financial loss, and even loss of life. As [ 17 ] suggests, the emergence of Industrial Control Systems (ICS) plays a critical part on national infrastructure such as manufacturing, power/smart grids, water treatment plants, gas and oil refineries, and health-care. With ICS becoming more integrated and connected to the internet, the degree of remote access and monitoring functionalities increases thus becoming a vulnerable point target for cyber war. Additionally, with ICS more prone to targeted attacks, new IDS systems have been used to cater for the niche market of ICS, thus introducing vulnerabilities in particular to the training model of ML.
With the introduction of these new IDSs, has also introduced new attack vectors into the mix. The definition of AML provided by Anthi states that: “The act of deploying attacks towards machine learning-based systems is known as Adversarial Machine Learning (AML) and its aim is to exploit the weaknesses of the pre-trained model which has ’blind spots’ between data points it has seen during training”.
This is challenging as ML usage in IDS is becoming a tool used in daily attack detection. The study showed how AML is used to target supervised models by generating adversarial samples and exploring and penetrating classification behaviours. This was utilised by the use of authentic power system datasets to train and test supervised machine learning classifiers through its vulnerabilities. The two popular methods that were used in AML testing were automatically generated perturbed samples that were the Fast Gradient Sign Method (FGSM) and the Jacobian based Saliency Map Attack (JSMA).
Both methods showed how AML was used in penetration of systems through ML training models leading onto cyber-attacks. In another study by Catak et al. [ 18 ], further explored the security problems associated with AML, this time through the networks of 6G applications in communicative technology, that focused on deep learning methods and training. With the rapid development and growth of deep learning and its algorithms in the future technology pipeline of 6G was to further understand the security concerns around it.
Cataks’ paper [ 18 ] produced faulty results through manipulation of deep learning models for 6G applications to understand AML attacks using Millimetre Wave (mmWave) beam prediction in this case. AML mitigation and preventative methods were also used to try and stop these attacks from occurring for 6G security in mmWave beam prediction application with fast gradient sign method attack. In conclusion to Cataks’ paper found that several iterations of introducing faulty results gave a more secure outcome of the performance and security of the device. ML deep learning methods and algorithms were able to use these faulty results in altering the adversarial training approach. This increased the RF beam-forming prediction performance and created a more accurate predictor in identifying these attacks against the ML applications use.
Cybersecurity: Summary
As with any new technology that stems to improve the cyber highways in lessening the effects of cyber-attacks, it is always coupled by the counterattack measure within this space. Being aware of these adversaries and future research will help reduce, or at least control the level of attacks being present in any cyberspace and landscape moving forward. The recognition of funding gaps that could be fulfilled by the government to support SMEs in the form of grants, subsidies, and similar financial assistance, through various public sector policies is also an important route to consider. Awareness and training for all SME management and their staff is important to understand the basic and perhaps advanced appreciation of cybersecurity through the eyes of ML and AI.
Whilst technology giants might lead the path in its implementation of ML and cybersecurity through its many variations of intrusion, detection, and prevention methods, it is these firms that will set precedence and bring awareness down to a SME level and the importance of ML in keeping our cyber world safe. Understanding whilst ML is increasing in usage through IDS and IDPS systems to reduce the cyber attack footprint, means that the rise in AML also is something to be concerned about.
An example in GDPR Recital 4 and in the proceeding Directive 1995/46/EC Recital 2, a main objective “the processing of personal data should be designed to serve mankind”. For this purpose, the Data Controller ensures legal compliance and legal justification of data processing out of necessity (not only processing convenience) and proportionality. For the acquisition of high-risk health data for example, GDPR mandates that a DPIA is carried out to mitigate risk and assess risk level to include if the data should be processed or not [ 19 ]. With data protection law, the UK and EU demonstrate cooperation, ethics, transparency with robust control methods in mitigating data privacy breaches. However, this also brings attention to the range of legal frameworks and the general movement of people globally. This should inform governments and business in data protection strategies.
Data Privacy: Legal Frameworks [UK-EU]
Between the UK and EU, the Data Protection Act 2018 (DPA) and General Data Protection Regulations 2016 (GDPR) function together in overseeing how businesses, organisations and governments, utilise personal data. Eight key objectives guide anyone responsible for the handing and processing of personal data, and strictly imposes that data has to be lawful [acquisition], fair, accurate and up-to-date, not kept longer than needed, kept safe and secure, and not to be transferred outside the European Economic Area (EEA). By design, GDPR encompasses human rights with additional data collecting and processing principles (e.g. purpose, data-types and processing duration) [ 20 ].
Data Privacy: SARS-Cov-2: Covid-19
In supporting the effort in mitigating disease transmission from the coronavirus pandemic (Covid-19), the cloud, cell-networks and IoT devices such as smart-phones, sensors and domestic appliances, continue to play a vital role in a wide range of global Tracing-Testing-Tracking programs. Many different approaches are adopted by global communities in minimising person-to-person transmission [ 21 , 22 ]. This demonstrates that in response to the pandemic, coupled with the urgency in developing and deploying digital solutions, data privacy implications become ever more challenging with increasing data privacy risks. As a result, the handling of personal data [acquisition] research has developed and expanded [ 23 ].
However, in mitigating data privacy risks under adverse social and environmental conditions, it is not simply a matter of deploying digital solutions. The challenges presented in terms of service delivery (consistency, proportionality and transparency), also potentially increases the risk of data privacy breaches. Therefore, in terms of scalability via the cloud, partnerships between populations, businesses and governments could harmonise policy development and implementation with digital solutions.
Data Privacy: Consent—Contact Tracing Apps
In a Republic of Ireland survey conducted with over 8000 participants, it was found that 54% would accept using a contact tracing app. Similarly, in the UK from a survey of 2000 participants found that 55% would accept using a government-controlled app, with higher uptake specifically for the NHS contact tracing app [ 21 ]. This information demonstrates a lack of app uptake in the remaining 45% of the British population that could undermine a governments ability in effectively handling data collection and the processing of critical medical information.
In contrast, other countries infer citizen consent when data collection is initiated for the public good. Meaning that private parties’ access to data is also endorsed by governments. Amnesty International (2020) also brings attention to many instances of questionable data privacy practices throughout numerous countries [ 21 ]. The examples potentially show the scale of data protection perceptions and attitudes and how they are interpreted, thus justifying a more focused and intensive approach to data privacy collaborative research. By analysing a variety of legal and regulatory frameworks, solutions and practices in a pandemic or crisis situation, we can learn how to effectively apply powerful and scalable outcomes. For example, robust and transparent data is necessary for the urgently needed Covid-19 vaccine distribution efforts for each nation [ 24 ].
Transparency: NHS Test-Trace App
In response to the pandemic, the UK Government and NHS X (Digital) contact tracing app, aided by the private sector, brought into question their overall GDPR utility and compliance. Sub-contractors and companies that represent NHS X are also considered as processors of data, which bring additional GDPR compliance pressures. In this instance, the NHS X app code and DPIA was voluntarily submitted to the Information Commissioners Office (ICO) without the data store. This potentially highlights a lack of transparency with GDPR compliance, health surveillance capabilities and data storage capacities. The Joint Committee on Human Rights (JCHR) for example, were concerned at the rapid development and deployment of the contact tracing app in March 2020 [ 19 ].
Data Storage and Identification
Clear definitions and solutions are needed for data and storage methods. Currently, obtaining an integrated and comprehensive view of (1) internal organisational personal data storage, (2) full organisational content comprehension of regulation, and (3) an auditable trail of necessary data processing activities [ 20 ]. Although GDPR compliance has significantly enhanced personal data protection (e.g. PII, PII sharing via add and marketing, collecting and sharing location data, child PII sharing, law enforcement, and data aggregation), more research is needed in facilitating a users right to erasure, to update and delete data and to completely satisfy the GDPR promise [ 25 ].
Accountability and Traceability: BC & SC
To aide government transparency and societal trust, part of a solution is robust data privacy and accountability policies. Antal et al., discusses how BC can be effective in traceability, transparency, vaccine ID, assurances of it’s delivery, storage to include self-reporting of side effects. The authors implement a BC strategy using the inherent integrity and immutability of BC with ’in case of beneficiary registration for vaccination’ provision, thus eliminating identity impersonations and identity theft [ 26 ].
An example from Honduras demonstrates how a Toronto-based technology launched ’Civitas’, with user and government linked ID on a BC-based network. The BC contains the necessary data for determining when an individual can buy medicine, go food shopping, and also data to inform government agencies in resource and deployment strategies [ 27 ]. The GDPR for example, would conflict with this contact tracing methodology. More specifically, the right for a user to be forgotten (Article 17: Right to Erasure) due to BC immutability, and processing speed that would also inhibit BC network uptake and scalability.
However, BC in this case could operate within the confines of management and governance of BD repositories and warehouses whilst leveraging SC to enhance accountability, transparency and consistency in the appropriate forum.
Trust: Vaccine Hesitancy in UK Households
Whilst a global effort was underway in mass vaccination programs, the UK strategy highlighted disparities from a lack of public engagement between public health bodies and ethnic minorities from historic mistrust and a lack of understanding in technology [ 24 , 28 ]. Additional hesitancy included acute and chronic health effects from the vaccine.
A UK survey from 2020 for example, illustrated how Black, Asian, Minorities and Ethnic (BAME) communities had high vaccine hesitancy rates, when compared to white ethnic populations [ 28 ]. In Robertson 2021, the authors state that “Herd immunity may be achievable through vaccination in the UK but a focus on specific ethnic minority and socioeconomic groups is needed to ensure an equitable vaccination program” [ 29 ]. Including a more targeted approach to mental illness and disability [ 30 ].
Data Privacy—Summary
In a global setting, is it possible to ethically and accurately collect data [also without consent] whilst also providing legibility for effective data collection, resource allocation and deployment strategies? A small part of the solution is in gaining a populations’ trust in technologies such as NHS app uptake, and for future research in global deployment strategies. This means a wide-ranging and continual assessment of legal frameworks and outcomes between companies, organisations and institutions for long-term data privacy planning. Strategies also include ensuring groups and individuals have faith in their data integrity in the cloud.
As necessary components of GDPR, the collecting, processing and deleting data remain a challenge. The enable user to fully engage with confidence, education and engagement with minorities, and with mental illnesses is an effective way to provide group assurances. As with different countries, data protection concepts and public engagement practices vary significantly. For anticipating any future disaster or pandemic scenario, it is clear that accountability through public engagement should help restore national and international trust. Also research needs to be undertaken to design and promote a flexible and global strategy to encompass technical solutions, operational resource strategy, and policy development. This would enhance data protection objectives, build population trust in government monitored apps and ultimately provide a successful and robust global protection strategy.
Blockchain for Security
Blockchain—integrity of data.
BC is one of the most commonly discussed DLT for ensuring the integrity of data storage and exchange in trust-less and distributed environments. It is a P2P decentralized distributed ledger [ 31 ] that can enable trusted data exchanges among untrusted participants in a network. BC systems such as Ethereum and Hyperledger fabric, have become popular BC frameworks for many BC-based software applications. Core features of BC such as immutability and decentralization are recognized by many sectors such as healthcare and finance to improve their operations. Although BC is a relatively new technology—just over a decade old—it seems to be revolutionary and there is a substantial number of research articles and white papers to justify this remark.
Blockchain—Cybersecurity
It is important to answer how emerging technologies such as BC can offer solutions to mitigate emerging cybersecurity threats and there is great research interest to study how BC can provide foundations for robust internet security infrastructures [ 32 ]. Many of the articles propose frameworks, prototypes and experimental beta BC-based solutions to problems in complex computing systems. Most of these experimental solutions are developed on Ethereum and Hyperledger fabric. In the case of Hyperledger fabric for example, this is due to its ease of software development, extensive customisability and interactivity.
Although Bitcoin is a most popular BC network, it has many cons such as its latency and great resource requirement. Some of practical solutions among them use innovative techniques to resolve critical cybersecurity issues. However, they imply infeasible changes to the existing system infrastructures that are difficult to readily test for efficiency and effectiveness when compared with conventional cybersecurity frameworks [ 33 ].
Blockchain—IoT
In our increasingly interconnected IoT world, there is a great need to improve cybersecurity. As explained in [ 34 , 35 ], cyber-attacks that exploit vulnerabilities in IoT devices raise serious concern and demand for appropriate mitigation strategies to tackle these threats. Ensuring integrity of data management and malware detection/prevention is an exciting topic of research [ 36 ].
It should be noted here that BC cannot eliminate cyber risks, but it can significantly minimize cyber threats with its core features. While most IT systems are built with cybersecurity frameworks that use advanced cryptographic techniques, they rely on centralized third-party intermediaries such as certificate authorities to ensure the integrity of their data management. Malicious parties can exploit weaknesses in such relationships to disrupt/penetrate these systems with cyber threats such as DDoS attack, malware, ransomware, etc.
Blockchain—Protocols
BC can resolve these issues due to its decentralization; it eliminates single points of failures and the need for third-party intermediaries in IT systems and ensures the integrity of data storage and exchange with encryption and hash functions [ 37 ] so that data owners can completely audit their data in the systems.
A BC network with many mutually trustless nodes is more secure than a network with few nodes that rely on trusted/semi-trusted centralized third-party intermediaries because, in a BC network, every node has a complete copy of the unique record of all transactions in the network that is maintained with the network consensus protocol. The robustness of a BC network i.e. its safety and security, depends on its decentralization, and this depends on its governance and consensus protocols. A good comparative study of DLT consensus protocols is provided by Shahaab et al. [ 38 ].
Blockchain—Summary
What are some future research directions and challenges for BC and Cybersecurity?
Consensus Protocols: Generally, public BC networks have high latency due to their consensus protocols. This makes them a non-starter for applications in real-time environment. Research on consensus protocols should be holistic and consider both, hardware and software, for such environments [ 39 ].
Cryptocurrencies: more research on cryptoassets is needed to tackle challenges to legal enforcement and forensics - both domestic and international—that enable cybercriminal activity such as terrorism financing.
IoT: As explained in [ 40 ], consortium BC networks can be used to improve the overall internet connectivity and access. Future research on IoT-BC integration should demonstrate feasible implementations that can be evaluated and compared with existing IoT solutions. They should also quantitatively study fault tolerance, latency, efficiency, etc. of BC-based IoT networks.
Data Analytics: BC can ensure the integrity of data and with AI/BD analytics it can be used to reduce risks and fraudulent activities in B2B networks. Hyperledger fabric is a DLT project that can be used for this relatively unexplored research areas.
Cybersecurity, Data Privacy and Blockchain
As stated in [ 41 ], BC-based digital services offer transparency, accountability and trust, however not one size fits all, as there are paradoxes between cybersecurity, GDPR compliance and the operation of BC. Haque et al., demonstrate in a systematic literature review regarding GDPR-BC compliance and highlights six major categories that are:
Data modification and deletion (Articles 16–18)
Default protection by design (Article 25)
Controllers/processors responsibilities (Articles 24, 26 and 28)
Consent management (Article 7)
Lawfulness and principles (Articles 5, 6 and 12)
Territorial scope (Article 3)
Haque et al. [ 41 ] states that use-cases of BC should be retrospectively applied in a way that can be made compliant to GDPR. The literature review also highlighted additional GDPR-BC research domains that include areas such as smart cities, information governance, healthcare, financial data and personal identity.
GDPR vs Blockchain
Vast amounts of PII are being collected, screened, and utilsed illegally due to cyber-espionage, phishing, spamming identity theft, and malpractice. BC on the other hand, due to the immutability in design and utility in tracking, storing and distributing DLT data, can clash with GDPR, especially with the “Right to be forgotten: Article 17”, including various rights to erasure [ 42 ]. Al-Zaben et al., proposes a framework that is on a separate off-chain mechanism that stores PII and non-PII in a different location. It is best to design and regulate network participation in fulfilling GDPR requirements, although not a perfect fit, this example shows how by design, a compliant use-case can be augmented in fulfilling parts of GDPR.
Ransomware Defense vs Blockchain
In [ 43 ], their paper describes that for malicious software to use configuration commands or information, malware has to be able to connect to the original owner. Therefore, a fairly new principle of domain generation is proposed, in that actively deployed ransomware is utilised to track user coordinates based on transactional data in a bitcoin BC. The gives a malware author the ability to dynamically change and update locations of servers in realtime.
Supply Chain Attack vs Blockchain
Recent and alarming increases in supply chain cyber attacks, has given various implementation strategies of BC in security of IoT data, that generally produces positive outcomes due to the transparency and traceability elements inherent in the technology by design. This paper highlights and discusses challenges to include many BC based systems in various industries, and focuses on the pharmaceutical supply chain. In conclusion, [ 44 ] states that the application of BCT can enhance supply chain security via authenticity and confidentiality principles.
Data Storage vs Blockchain
Due to the full-replication data storage mechanism in existing BC technologies, this produces scalability problems due to copying at each node, thus increases overall storage per-block [ 45 ]. Additionally, this mechanism can limit throughput in a permissioned BC. A novel storage system is proposed to enhance scalability by integrating erasure coding that can reduce data acquisition per block and enlarge overall storage capacity.
Of the many challenges that face legal, operational and performance criteria with utilising BC, it is clear to see that as we gather more and more personal data, endure more cyber attacks, and encounter storage disadvantages, many proposed frameworks seek to provide solutions that are only a part of compounding and escalating situation. The transactional speed and scalability of technologies such as BC, can hinder data protection rights, focused cyber-attacks, and the ability to update and track users, however there are advantages in creating separate mechanisms that when produced as a whole, that can indeed support data verification, transparency and accountability in many industries.
Results: Brief Overview of Intelligent Framework
Key Data Management Architecture Components: Fig. 1 shows the block diagram of the proposed framework. Key components of the framework are explained and synthesised in the following paragraphs.
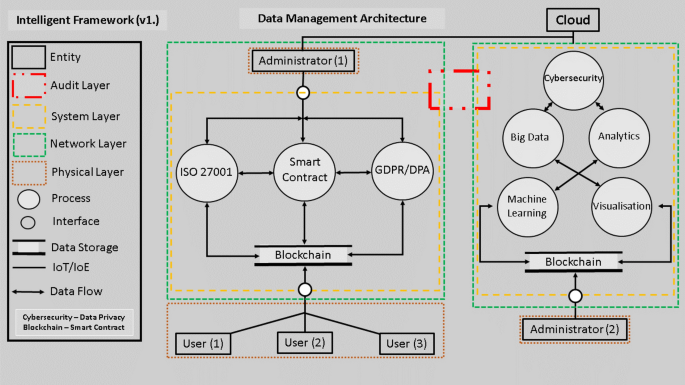
Data flow audit mechanism
Blockchain: Data Storage and Immutability
To provide system accountability, transparency and traceability from network system traffic point of view, an article by Kumar et al., 2020 demonstrates how DLT systems are applied in e-commerce to include health medicines, security devices, food products to ensure BC technological and e-commerce sustainability. Also, [ 46 ] presents a study that explores the potential of DLT in the publication industry and present a technological review. The studies demonstrate how research is being explored and influencing DLTs globally alongside their synergies of application across academic, private and public sectors.
Standardisation of IoT Interface Portal
For purposes of legal acquisition and processing of data with consent, users can connect from IoT smart devices and appliances, such as; smart phones, sensors, tablets and user desktops. User applications and interfaces also provide a level of protection by design in most cases, however the applications can also compound and conflict with each other to produce security vulnerabilities (e.g. Cookies). Networks include; Cellular, Local and Personal Area Networks (PAN/LAN), Low Power Wide Area Networks (LPWAN) and Campus Area Network (CAN) carrier methods operate and maintain IoT system stability. Some IoT devices are capable of ensuring seamless connectivity in data access. However, at the point of access, a user interfaces with a given IoT device could be one of multiple architectures that present challenges in correctly identifying and processing data in a legal, reliable and consistent fashion. Therefore an overarching framework to ensure a standardised system whilst mitigating risk (security Vulnerabilities) is catered for in utilising network protocols with a prescribed profile limited to key information such as, Personal Identification Number (PIN), Account Number and password encryption.
Administrator 1: Public LAN/WLAN/CAN
A main purpose here is the execution of network communication protocols for the processing and or keeping (storage) of PII and data access control to include cryptography. At the level of an SME, the types of regulatory compliance’s necessary to operate as a business include a retrospective and current auditable trail to demonstrate good practices. A selection of operational scenarios are to be emulated (e.g. from case law) in the preparation of codifying, selecting and the setting of chosen principles, standards and legal frameworks. Other objectives to explore include, Confidentiality, Integrity, Availability and Data Minimisation. As shown in [ 47 ], stakeholders are required to initialise and validate a product block, this activates the wallet, to include pseudo-identity generation with a public and private key pair. The keys are utilised for signature and verification processes. Here, administrator 1 oversees and combines the execution of network communication policies to govern a user or a given set of protocols.
Administrator 2: Private LAN Network
The function of the administrator here is to utilise criteria to facilitate accountability, transparency and traceability from network system traffic. Data entry points provide group integrity as each user, or entry, is available for all to see. More fundamentally, this data will help inform, develop, calibrate and test the setting of audit and assessment parameters. The information is then combined, contrasted and compared to the Administrator 1 data collection. Resulting information then updates the Valid Data Acquisition IDPS System and Cyber-Detection Methods (e.g. Packet Sniffing) of Network Packet Data communication protocols with data effective access control. In this case, Administrator 2 provides an array of users insights into the performance of ISO 27001 and DPA/GDPR policies to identify optimum operational cost in various prescribed operating scenarios. Through analysis with tools such as BD Analytics and ML for example, nuanced data, pattern identification and aggregation provides a basis for speculation as to an ideal operating system from within a business.
Smart Contract: Agreement or Terms of Contract
Unfortunately, maintaining these systems incur at significant cost, on the other hand, these systems also cut out the “middle-man” and save resources to empower individuals and business owners. For example, individual and group scenarios are negotiated and interpreted between users in partnerships. In emulating this function, key objectives are identified and embedded from legal frameworks to produce an automatic transaction protocol with consensus in the implementation of a codex (e.g. OPCODES). Therefore, a codex of legal precedent and statutory instrumental data protection, data operation and dissemination laws will be emulated to start. The codex is the library and framework that enables partners to equitably participate in a sustainable and trust-less operational environment. In utilising ISO 27001 for example, a collection of policies are negotiated and agreed upon prior to formally undertaking a contract between parties. Therefore, GDPR and ISO 27001 are transcribed, layered and mapped with verification mechanisms derived from case-law and by design into a SC agreement. This dynamic process forms the centre of any given exchange or process of data acquisition and data dissemination.
To enable an effective cybersecurity strategy for SME’s and alike, government and private sector finance initiatives are key. This includes awareness and training for management, with oversight and additional support for staff to incorporate ML and AI into the workplace more effectively. Intrusion, detection and prevention policy from SME to government level can then flourish in promoting and sustaining the full benefits and protections of cybersecurity from cyber-criminality. However, for global data security coverage, the concept in itself is interpreted differently as the legal, ethical and consensual implementation challenges remain formidable as a result. Acquiring personal data from regional divisions to aide authorities in resource strategy at this scale, requires trust in institutions and technologies to be fully beneficial to all.
Accountability and transparency efforts also require the continual assessment of legal frameworks, systems and outcomes, with generous investment from public and private sectors. Public awareness, perception and confidence levels in the justice system through transparency and education, with focus to include mental illness and minority group engagement policies, can benefit societies substantially. The earlier proposed framework from research, demonstrates a robust and complex strategy, however looking to the future, BC network latency present real-time challenges to assist SME technology adoption. Increasing digitalisation and decentralisation leads to diverse communications, hence creating a wider array of participants to collaborate and share. However, these digital systems are not mature in terms of security and inevitably create attack space for attackers.
In this review paper, we highlighted several security problems that arise in digital systems, computation data and associated trust mechanisms. These challenges have resulted in evolution of technical solutions. Current solutions are so diverse that range from preliminary in small organisations to the state-of-the-art in mega-organisations. The cyber landscape is likely to change even further that necessitates robust solutions. This paper also brings in research from different collaborators with the potential to identify the challenges and move in the direction of designing novel solutions. This we believe as a result, will enhance and lead to secure cyber systems which achieve data security comprehensiveness.
Rawindaran N, Jayal A, Prakash E. Artificial intelligence and machine learning within the context of cyber security used in the UK SME Sector. In: AMI 2021— the 5th advances in management and innovation conference 2021. Cardiff Metropolitan University. 2021.
Wylde V, Prakash E, Hewage C, Jon. Platts. Covid-19 Crisis: Is our Personal Data Likely to be Breached? In AMI 2021 - The 5th Advances in Management and Innovation Conference 2021. Cardiff Metropolitan University, 2021.
Balasubramanian R, Prakash E, Khan I, Platts J. Blockchain technology for healthcare. In: AMI 2021—the 5th advances in management and innovation conference 2021. Cardiff Metropolitan University; 2021.
Gallaher MP, Link AN, Rowe B. Cyber security: economic strategies and public policy alternatives. Chentanham: Edward Elgar Publishing; 2008.
Google Scholar
Zarpelão BB, Miani RS, Kawakani CT, de Alvarenga SC. A survey of intrusion detection in Internet of Things. J Netw Comp Appl. 2017;84:25–37.
Article Google Scholar
Are Your Operational Decisions Data-Driven? 2021. https://www.potentiaco.com/what-is-machine-learning-definition-typesapplications-and-examples/ . Accessed 11 Jul 2021.
Biju SM, Mathew A. Internet of Things (IoT): securing the next frontier in connectivity. ISSN. 2020.
Cahn A, Alfeld S, Barford P, Muthukrishnan S. An empirical study of web cookies. In: Proceedings of the 25th international conference on world wide web; 2016. pp. 891–901.
Cressy R, Olofsson C. European SME Financing: An Overview. Small Business Economics, 1997. pp 87–96.
General Data Protection Regulations (GDPR). https://ico.org.uk/for-organisations/guide-to-dataprotection/guide-to-the-general-data-protectionregulation-gdpr/ . Accessed 16-10-2020.
Roesch M, et al. SNORT: lightweight intrusion detection for networks. Lisa. 1999;99:229–38.
Dunham K, Melnick J. Malicious bots: an inside look into the cyber-criminal underground of the internet. Boca Raton: Auerbach Publications; 2008.
Book Google Scholar
Kabiri P, Ghorbani AA. Research on intrusion detection and response: a survey. Int J Netw Secur. 2005;1(2):84–102.
Fraley JB, Cannady J. The promise of machine learning in cybersecurity. In: SoutheastCon 2017, IEEE; 2017. pp. 1–6.
Buczak AL, Guven E. A survey of data mining and machine learning methods for cyber security intrusion detection. IEEE Commun Surv Tutor. 2015;18(2):1153–76.
Machine learning algorithm cheat sheet for azure machine learning designer. 2021. https://docs.microsoft.com/en-us/azure/machine-learning/algorithm-cheat-sheet . Accessed 3- Mar 2021.
Anthi E, Williams L, Rhode M, Burnap P, Wedgbury A. Adversarial attacks on machine learning cybersecurity defences in industrial control systems. J Inf Secur Appl. 2021;58:102717.
Catak E, Catak FO, Moldsvor A. Adversarial machine learning security problems for 6G: mmWave beam prediction use-case. arXiv:2103.07268 .2021.
Guinchard A. Our digital footprint under Covid-19: should we fear the UK digital contact tracing app? Int Rev Law Comput Technol. 2021;35(1):84–97.
Tran J, Ngoc C. GDPR handbook for record of processing activities. Case: the color club A/S. 2020.
Raman R, Achuthan K, Vinuesa R, Nedungadi P. COVIDTAS COVID-19 tracing app scale-an evaluation framework. Sustainability. 2021;13(5):2912.
Juneidi JS. Covid-19 tracing contacts apps: technical and privacy issues. Int J Adv Soft Comput Appl. 2020;12:3.
Majeed A. Towards privacy paradigm shift due to the pandemic: a brief perspective. Inventions. 2021;6(2):24.
Black M, Lee A, Ford J. Vaccination against COVID-19 and inequalities-avoiding making a bad situation worse. Public health in practice. England: Elsevier; 2021.
Zaeem RN, Barber SK. The effect of the GDPR on privacy policies: recent progress and future promise. ACM Trans Mgmt Inf Syst. 2020;12(1):1–20.
Antal CD, Cioara T, Antal M, Anghel I. Blockchain platform for COVID-19 vaccine supply management. 2021. arXiv:2101.00983 .
How Blockchain is helping in the fight against Covid-19. 2021. https://www.lexology.com/library/detail.aspx?g=8b5ef0f0-05b3-4909-b5d5-da7bd57f0381 . Accessed 24 Apr 2021.
Razai MS, Osama T, McKechnie D, Majeed A. Covid-19 vaccine hesitancy among ethnic minority groups. 2021.
Robertson E, Reeve KS, Niedzwiedz CL, Moore J, Blake M, Green M, Katikireddi SV, Benzeval MJ. Predictors of COVID-19 vaccine hesitancy in the UK Household Longitudinal Study. Brain Behavior Immunity. 2021.
MacKenna B, Curtis HJ, Morton CE, Inglesby P, Walker AJ, Morley J, Mehrkar A, Bacon S, Hickman G, Bates C, et al. Trends, regional variation, and clinical characteristics of COVID-19 vaccine recipients: a retrospective cohort study in 23.4 million patients using OpenSAFELY. 2021.
Zheng Z, Xie S, Dai H, Chen X, Wang H. An overview of blockchain technology: architecture, consensus, and future trends. In: 2017 IEEE international congress on big data (BigData Congress); 2017. pp. 557–64.
Salman T, Zolanvari M, Erbad A, Jain R, Samaka M. Security services using blockchains: a state of the art survey. IEEE Commun Surv Tutor. 2019;21(1):858–80.
Zhang R, Xue R, Liu L. Security and privacy on blockchain. ACM Comput Surv. 2019;52:3.
Pinno OJA, Gregio ARA, De Bona LCE. ControlChain: blockchain as a central enabler for access control authorizations in the IoT. In: GLOBECOM 2017—2017 IEEE global communications conference; 2017. pp. 1–6.
Mandrita B, Junghee L, Choo KKR. A blockchain future for internet of things security: a position paper. Dig Commun Netw. 2018;4(3):149–60.
Kshetri N. Blockchain’s roles in strengthening cybersecurity and protecting privacy. Celebrating 40 years of telecommunications policy—a retrospective and prospective view. Telecommun Policy. 2017;41(10):1027–38.
Ali M, Nelson J, Shea R, Freedman Freedman MJ. Blockstack: a global naming and storage system secured by blockchains. In: 2016 USENIX annual technical conference (USENIX ATC 16), pp 181–194. Denver, CO, 2016. USENIX:Association. 2016.
Shahaab A, Lidgey B, Hewage C, Khan I. Applicability and appropriateness of distributed ledgers consensus protocols in public and private sectors: a systematic review. IEEE Access. 2019;7:43622–36.
Taylor PJ, Dargahi T, Dehghantanha A, Prizi RM, Choo KKR. A systematic literature review of blockchain cybersecurity. Dig Commun Netw. 2020;6(2):147–56.
Alphand O, Amoretti M, Claeys T, Dall’Asta S, Duda A, Ferrari G, Rousseau F, Tourancheau B, Veltri L, Zanichelli F. IoT Chain: a blockchain security architecture for the internet of things. In: 2018 IEEE wireless communications and networking conference (WCNC); 2018. pp. 1–6.
Haque AB, Najmul Islam S, Hyrynsalmi AKM, Naqvi B, Smolander K. GDPR compliant blockchains-a systematic literature review. IEEE Access. 2021;9:50593–606.
Al-Zaben N, Hassan O, Mehedi M, Yang J, Lee NY, Kim CS. General data protection regulation complied blockchain architecture for personally identifiable information management. In: 2018 international conference on computing, electronics communications engineering (iCCECE); 2018. pp. 77–82.
Pletinckx S, Trap C, Doerr C. Malware coordination using the blockchain: an analysis of the cerber ransomware. In: 2018 IEEE conference on communications and network security (CNS); 2018. pp. 1–9.
Johny S, Priyadharsini C. Investigations on the implementation of blockchain technology in supplychain network. In: 2021 7th international conference on advanced computing and communication systems (ICACCS); 2021. pp. 1–6.
Qi X, Zhang Z, Jin C, Zhou A. A reliable storage partition for permissioned blockchain. IEEE Trans Knowl Data Eng. 2021;33(1):14–27.
Paruln K, Gulshan K, Geetha G. Exploring the potential of distributed ledger technology in publication industry—a technological review. In: CEUR Workshop Proceedings. 2021.
Kumar G, Saha R, Buchanan WJ, Geetha G, Thomas R, Rai MK, Kim T, Alazab M. Decentralized accessibility of e-commerce products through blockchain technology. Sustain Cities Soc. 2020;62:102361.
Download references
Author information
Authors and affiliations.
Cardiff School of Technologies, Cardiff Metropolitan University, CF5 2YB, Cardiff, UK
Vinden Wylde, Nisha Rawindaran, John Lawrence, Rushil Balasubramanian, Edmond Prakash, Imtiaz Khan, Chaminda Hewage & Jon Platts
School of Information Systems and Technology, University of Canberra, Bruce, ACT 2617, Australia
Ambikesh Jayal
You can also search for this author in PubMed Google Scholar
Corresponding author
Correspondence to Edmond Prakash .
Ethics declarations
Conflict of interest.
Authors declare that they have no conflicts of interest.
Additional information
Publisher's note.
Springer Nature remains neutral with regard to jurisdictional claims in published maps and institutional affiliations.
This article is part of the topical collection “Cyber Security and Privacy in Communication Networks” guest edited by Rajiv Misra, R. K. Shyamsunder, Alexiei Dingli, Natalie Denk, Omer Rana, Alexander Pfeiffer, Ashok Patel and Nishtha Kesswani.
Rights and permissions
Open Access This article is licensed under a Creative Commons Attribution 4.0 International License, which permits use, sharing, adaptation, distribution and reproduction in any medium or format, as long as you give appropriate credit to the original author(s) and the source, provide a link to the Creative Commons licence, and indicate if changes were made. The images or other third party material in this article are included in the article's Creative Commons licence, unless indicated otherwise in a credit line to the material. If material is not included in the article's Creative Commons licence and your intended use is not permitted by statutory regulation or exceeds the permitted use, you will need to obtain permission directly from the copyright holder. To view a copy of this licence, visit http://creativecommons.org/licenses/by/4.0/ .
Reprints and permissions
About this article
Wylde, V., Rawindaran, N., Lawrence, J. et al. Cybersecurity, Data Privacy and Blockchain: A Review. SN COMPUT. SCI. 3 , 127 (2022). https://doi.org/10.1007/s42979-022-01020-4
Download citation
Received : 04 August 2021
Accepted : 03 January 2022
Published : 12 January 2022
DOI : https://doi.org/10.1007/s42979-022-01020-4
Share this article
Anyone you share the following link with will be able to read this content:
Sorry, a shareable link is not currently available for this article.
Provided by the Springer Nature SharedIt content-sharing initiative
- Data privacy
- Smart Contracts
Advertisement
- Find a journal
- Publish with us
- Track your research
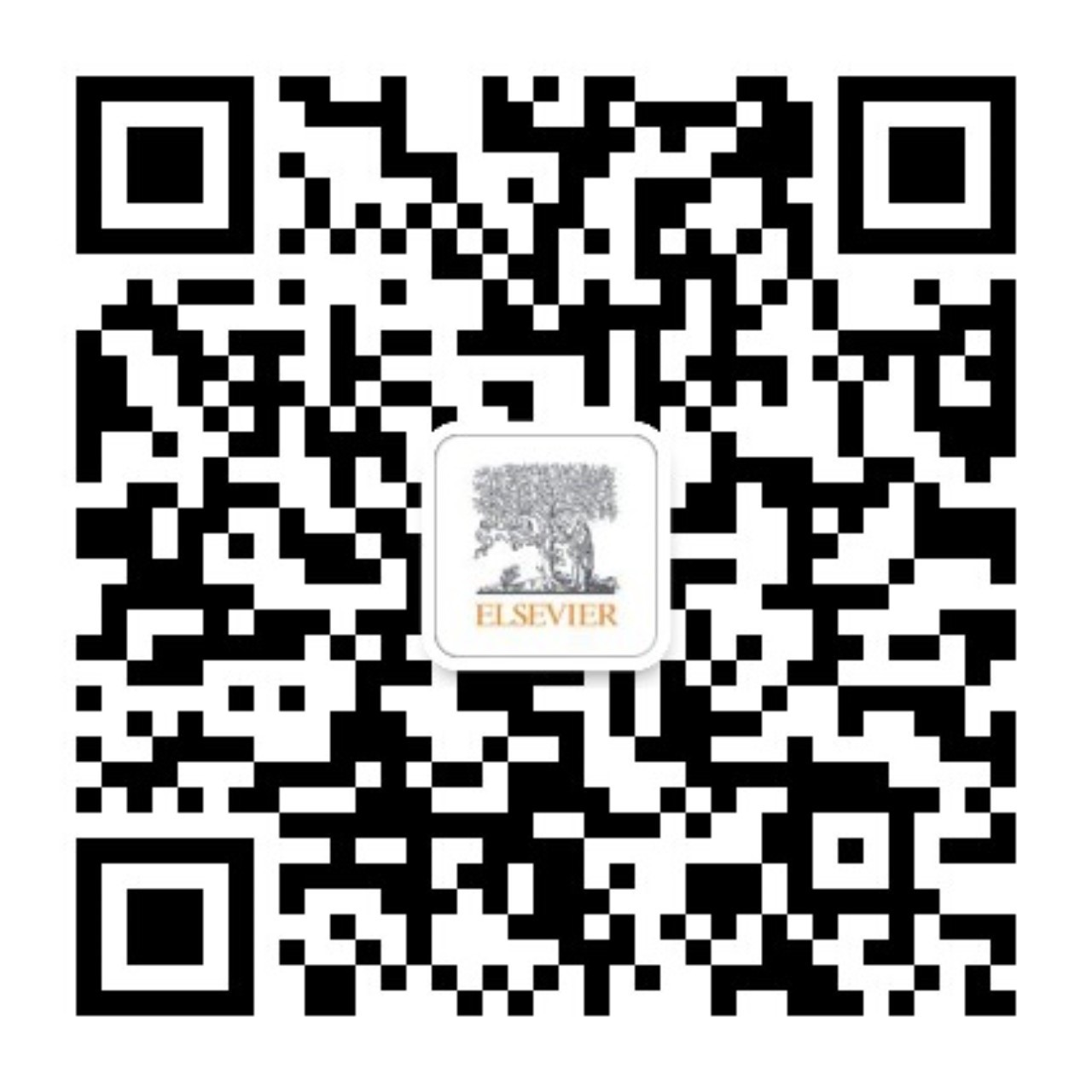
- Research Process
Confidentiality and Data Protection in Research
- 4 minute read
- 47.6K views
Table of Contents
Data protection issues in research remain at the top of researchers’ and institutional awareness, especially in this day and age where confidential information can be hacked and disseminated. When you are conducting research on human beings, whether its clinical trials or psychological inquiries, the importance of privacy and confidentiality cannot be understated. In the past, it was as easy as a lockable file cabinet. But now, it’s more and more challenging to maintain confidentiality and data protection in research.
In this article, we’ll talk about the implications of confidentiality in research, and how to protect privacy and confidentiality in research. We’ll also touch on ways to secure electronically stored data, as well as third-party data protection services.
Data Protection and Confidentiality in Research
How can you protect privacy and confidentiality in research? The answer, in some ways, is quite simple. However, the means of protecting sensitive data can often, by design, be complex.
In the research time, the Principal Investigator is ultimately responsible for the integrity of the stored data. The data protections and confidentiality protocols should be in place before the project starts, and includes aspects like theft, loss or tampering of the data. The easy way to do this is to limit access to the research data. The Principal Investigator should limit access to this information to the fewest individuals possible, including which research team members are authorized to manage and access any data.
For example, any hard-copies of notebooks, questionnaires, surveys and other paper documentation should be kept in a secure location, where there is no public access. A locked file cabinet, away from general access areas of the institution, for instance. Names and other personal information can be coded, with the encoding key kept in a separate and secure location.
It is the Principal Investigator’s responsibility to make sure that every member of the research team is fully trained and educated on the importance of data protection and confidentiality, as well as the procedures and protocols related to private information.
Check more about the Team Structure and Responsibilities .
Implications of Confidentiality in Research
Even if paper copies of questionnaires, notes, etc., are stored in a safe, locked location, typically all of that information is also stored in some type of electronic database. This fulfills the need to have data available for statistical analysis, as well as information accessible for developing conclusions and implications of the research project.
You’ve certainly heard about the multitude of data breaches and hacks that occur, even in highly sophisticated data protection systems. Since research projects can often involve data around human subjects, they can also be a target to hackers. Restoring, reproducing and/or replacing data that’s been stolen, including the time and resources needed to do so, can be prohibitively expensive. That doesn’t even take into consideration the cost to the human subjects themselves.
Therefore, it’s up to the entire research team to ensure that data, especially around the private information of human beings, is strongly protected.
How Can Electronic Data Be Protected?
Frankly, it’s easier said than done to ensure confidentiality and the protection of research data. There are several well-established protocols, however, that can guide you and your team:
- Just like for any hard-copy records, limit who has access to any electronic records to the bare minimum
- Continually evaluate and limit access rights as the project proceeds
- Protect access to data with strong passwords that can’t be easily hacked, and have those passwords change often
- Access to data files should be done through a centralized, protected process
- Most importantly, make sure that wireless devices can’t access your data and your network system
- Protect your data system by updating antivirus software for every computer that has access to the data and confidential information
- If your data system is connected via the cloud, use a very strong firewall, and test it regularly
- Use intrusion detection software to find any unauthorized access to your system
- Utilize encryption software, electronic signatures and/or watermarking to keep track of any changes made to data files and authorship
- Back up any and all electronic databases (on and offsite), and have hard and soft copies of every aspect of your data, analysis, etc.
- When applicable, make sure any data is properly and completely destroyed
Check more about: Why Manage Research Data?
Using Third-Party Data Protection Services
If your institution does not have built-in systems to assure confidentiality and data protection in research, you may want to consider a third party. An outside information technology organization, or a team member specifically tasked to ensure data protection, might be a good idea. Also look into different protections that are often featured within database programs themselves.
Elsevier Author Services
Helping you publish your research is our job. If you need assistance with translating services, proofreading, editing, graphics and illustrations services, look no further than Elsevier Author Services .
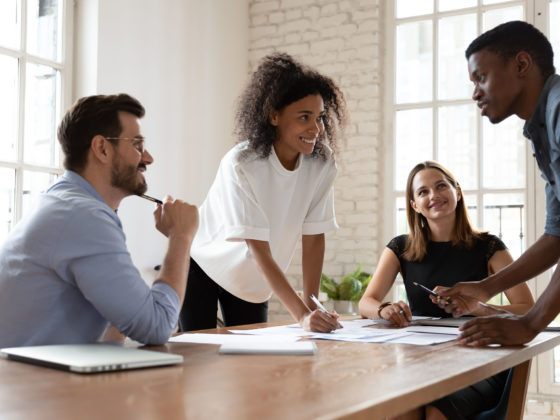
Research Team Structure
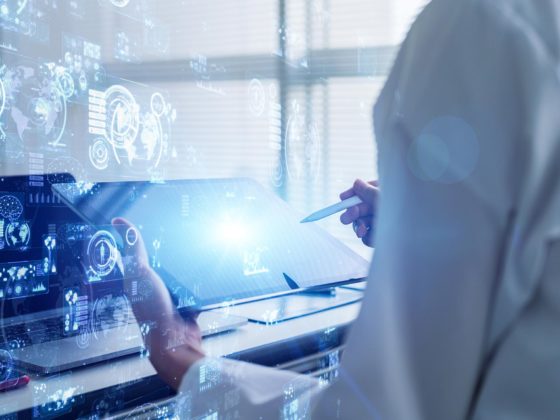
- Publication Process
Research Data Storage and Retention
You may also like.
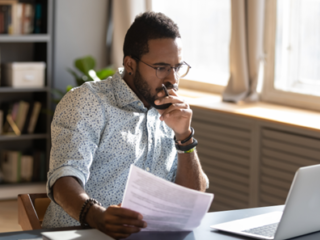
Descriptive Research Design and Its Myriad Uses
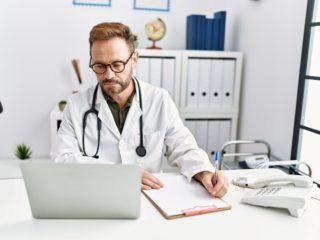
Five Common Mistakes to Avoid When Writing a Biomedical Research Paper
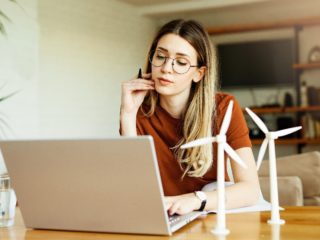
Making Technical Writing in Environmental Engineering Accessible
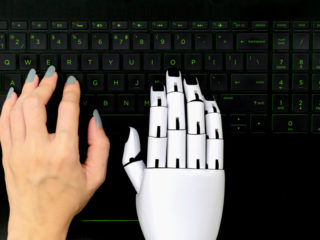
To Err is Not Human: The Dangers of AI-assisted Academic Writing
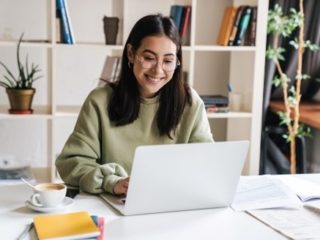
When Data Speak, Listen: Importance of Data Collection and Analysis Methods
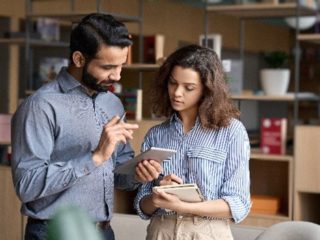
Choosing the Right Research Methodology: A Guide for Researchers
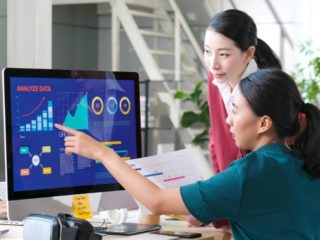
Why is data validation important in research?
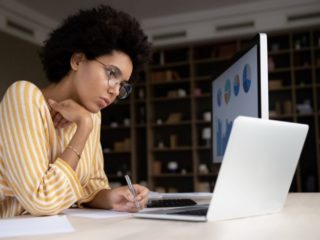
Writing a good review article
Input your search keywords and press Enter.
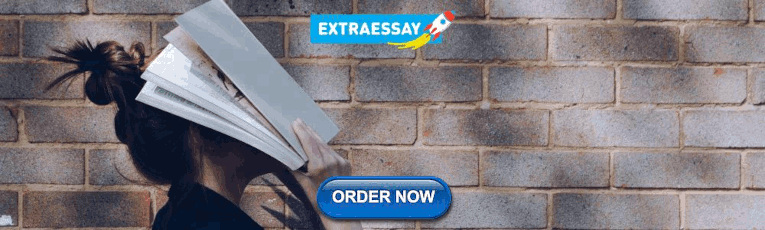
IMAGES
VIDEO
COMMENTS
Introduction. This paper aims to critically assess the information duties set out in the General Data Protection Regulation (GDPR) and national adaptations when the purpose of processing is scientific research. Due to the peculiarities of the legal regime applicable to the research context, information about the processing plays a crucial role ...
Three strategies are summarized in this section. The first is for clinical data and provides a practical user access rating system, and the second is majority for genomic data and designs a network architecture to address both security access and potential risk of privacy disclosure and reidentification.
Data are today an asset more critical than ever for all organizations we may think of. Recent advances and trends, such as sensor systems, IoT, cloud computing, and data analytics, are making possible to pervasively, efficiently, and effectively collect data. However for data to be used to their full power, data security and privacy are critical. Even though data security and privacy have been ...
Five major streams of research inform our work in this paper: (1) technology adoption model (TAM), (2) consumer privacy paradox, (3) service failure, (4) protection motivation theory (PMT), and (5) trust. ... Third, most research on data breaches has focused mainly on post-breach analysis, that is, the impact of data breach. ... Both CCPA and ...
This paper focuses on privacy and security concerns in Big Data. This paper also covers the encryption techniques by taking existing methods such as differential privacy, k-anonymity, T-closeness, and L-diversity.Several privacy-preserving techniques have been created to safeguard privacy at various phases of a large data life cycle.
The issue of data protection in research is becoming of pivotal importance, in particular in the last months with the pandemic emergency of COVID-19. 1 Studying the development of the outbreak on affected populations under a scientific and statistic perspective is necessary to understand the trend of contagion, the effectiveness of social distancing measures, the most vulnerable people who are ...
Research ethics moved beyond discussion of ethics codes when the prospect of data protection legislation arose. Much of the discussion in the 1980s sought to distance data collection in market research from other commercial practices, and in doing so make the case for exclusion of "legitimate" market research from legislation.
In this way, research will not trump data protection, but there will be a balance of the ... Article 29 Data Protection Working Party. Advice paper on special categories of data ("sensitive data
33 LIBE Compromise, proposal for a Data Protection Regulation (this paper refers to the unofficial Consolidated Version after LIBE Committee Vote, provided by the Rapporteur, General Data Protection Regulation, 22 October 2013. The European Parliament is an EU body with legislative, supervisory, and budgetary responsibilities. ... Amsterdam Law ...
The increased dependency on technology brings national security to the forefront of concerns of the 21st century. It creates many challenges to developing and developed nations in their effort to counter cyber threats and adds to the inherent risk factors associated with technology. The failure to securely protect data would potentially give rise to far-reaching catastrophic consequences ...
Scientific research is indispensable inter alia in order to treat harmful diseases, address societal challenges and foster economic innovation. Such research is not the domain of a single type of organization but can be conducted by a range of different entities in both the public and private sectors. Given that the use of personal data may be indispensable for many forms of research, the data ...
The issue of data protection in research is becoming of piv-. otal importance, in particular in the last months with the pan-. demic emergency of COVID-19.1. Studying the development of. the ...
Abstract. Against the background of the centrality of data for contemporary economies, the chapter contributes to a better understanding and contextualization of data protection and its interfaces ...
Purpose of the Study. This paper aims to examine the two case studies of the United States and India to show. that they do not have adequate data protection regulations to provide the right to privacy and. suggest ways that these two countries may move further towards the path of adopting adequate.
February 2019 regarding data protection at the EUI. President's Decision 10/2019 has adapted the EUI's Data Protection Policy to the new General Data Protection Regulation (GDPR) and Regulation 1725/2018. The goal of this guide is also to provide researchers with a handy tool to guide them through the daily work on their research project.
With data protection law, the UK and EU demonstrate cooperation, ethics, transparency with robust control methods in mitigating data privacy breaches. ... relatively new technology—just over a decade old—it seems to be revolutionary and there is a substantial number of research articles and white papers to justify this remark. Blockchain ...
provides an introduction to data protection laws and an overview of considerations for Congress. (For a more detailed analysis, see CRS Report R45631, Data Protection Law: An Overview, by Stephen P. Mulligan, Wilson C. Freeman, and Chris D. Linebaugh.) Defining Data Protection As a legislative concept, data protection melds the fields of
This chapter explores the alignment of the EU data protection and consumer protection policy agendas through a discussion of the reference to the Unfair Contract Terms Directive in Recital 42 of the General Data Protection Regulation. ... Australian National University College of Law Legal Studies Research Paper Series. Subscribe to this free ...
This paper is divided into three parts with the first discussing the rights of users and responsibilities of companies as well as the established regulations in the protection of data. The second part of this work considers the issues surrounding data privacy and data protection and the challenges faced in ensuring the safety of users ...
This paper describes privacy issues in big data analysis and. elaborates on two case studies (government-funded projects 2,3 ) in order to elucidate how legal priv acy requirements can be met. in ...
The rest of this paper is organized as follows: Section 3 of the paper describes the research methodology that consists of inclusion, exclusion criteria, quality assessment criteria, study selection process, research questions, and data extraction process. Also, we discuss assumptions and requirements for data protection in the cloud.
In the research time, the Principal Investigator is ultimately responsible for the integrity of the stored data. The data protections and confidentiality protocols should be in place before the project starts, and includes aspects like theft, loss or tampering of the data. The easy way to do this is to limit access to the research data.
The research provisions. This guidance discusses the research provisions in the UK GDPR and the DPA 2018 in detail. It is aimed at DPOs and those with specific data protection responsibilities in organisations undertaking research, archiving or processing for statistical purposes. It provides guidance on how these provisions work and sets out ...