Wind Energy Systems
Ieee account.
- Change Username/Password
- Update Address
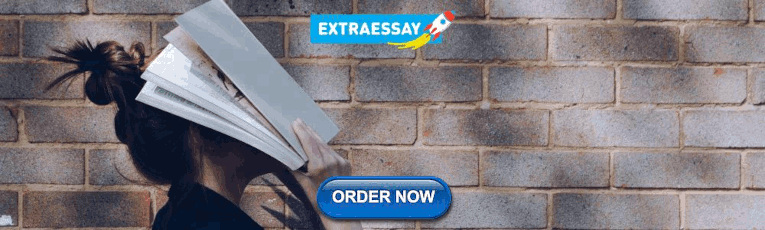
Purchase Details
- Payment Options
- Order History
- View Purchased Documents
Profile Information
- Communications Preferences
- Profession and Education
- Technical Interests
- US & Canada: +1 800 678 4333
- Worldwide: +1 732 981 0060
- Contact & Support
- About IEEE Xplore
- Accessibility
- Terms of Use
- Nondiscrimination Policy
- Privacy & Opting Out of Cookies
A not-for-profit organization, IEEE is the world's largest technical professional organization dedicated to advancing technology for the benefit of humanity. © Copyright 2024 IEEE - All rights reserved. Use of this web site signifies your agreement to the terms and conditions.
- Original Research Article
- Open access
- Published: 17 August 2020
Wind turbine performance analysis for energy cost minimization
- Yassine Charabi ORCID: orcid.org/0000-0003-2054-688X 1 &
- Sabah Abdul-Wahab 2
Renewables: Wind, Water, and Solar volume 7 , Article number: 5 ( 2020 ) Cite this article
38k Accesses
24 Citations
3 Altmetric
Metrics details
A Correction to this article was published on 17 January 2021
This article has been updated
The use of wind energy worldwide has overgrown in recent years to reduce greenhouse gas emissions. Wind power is free, but the installation and maintenance of wind turbines remain very costly. The size of the installation of the wind turbine is not only determined by wind statistics at a given location, but also by turbine infrastructure and maintenance costs. The payback time of the turbine is dependent on turbine energy costs. This study estimates the wind power generation capacity of Northern and Southern Oman and discusses the selection of the most economical, efficient and reliable wind turbines in Oman. HOMER Pro Software was used in this paper to evaluate the wind energy data in the north and south of Oman and to provide well-informed guidance on the most suitable turbines for the power needs of each area. Six different standard wind turbines were measured and compared in terms of the cost of energy and performance. The simulation analysis reveals that the DW54 turbine is the best possible turbine to generate electricity in northern Oman at $0.119/kW. Due to the difference in the wind regime between the north and the south of Oman, the simulation showed that the Hummer H25.0–200 kW turbine is the best option for south Oman with power generation at $0.070/kW. The northern wind turbine plant can efficiently contribute to decarbonization of the energy sector in Oman, with a potential reduction of CO 2 emission approximately 19,000 tons/year in comparison to natural gas and 28,000 tons/year in comparison to diesel. In the Southern Power Plant, carbon emissions are reduced by 18,000 and 12,000 tons/year compared to diesel and natural gas.
Introduction
The rise in global temperature and severe climate change worldwide has increased environmental concerns. Nowadays, more than 90% of the world’s electricity comes from fossil fuels (World-Bank 2015 ), and that energy production plays a vital role in global warming. Any changes in this field can have a significant impact on the environment. Numerous researchers, therefore, have attempted to change or alleviate the negative impacts of global warming, with much of this effort coming from the energy sector (Ghodsi et al. 2019 ; Khare et al. 2016 ; Sahu et al. 2018 ). In comparison to fossil fuels, the impact of renewable energy sources on the environment is negligible. These sources, for example, have no direct CO 2 or NOx emissions. From solar panels to wind turbine generators, a wide range of devices can convert ambient energy into a more useful form, like electricity (Charabi et al. 2019 ). Among these devices, wind turbines are some of the most popular and accessible methods of converting ambient energy to electricity (Yang et al. 2018 ). However, wind energy, like most other sources of renewable energy, has high capital costs, but during the past decade, this trend has changed tremendously. Statics show that the cost of wind production has dropped enormously in recent years, from two million dollars per M.W. to one million in the last decade (Moné 2017 ). This achievement has made it possible to see wind power plants with increasing frequency in both developed and developing countries (Sahu 2018 ).
As a Middle Eastern, oil-dependent country, Oman has started in a new direction on its path of development. The country is trying to change its electricity production industry from one that is entirely oil-based to one that is more reliant on sustainable “greener” energy sources (Abdul-Wahab et al. 2019a ; Al-Suleiman et al. 2019 ). The main two options for this plan are solar and wind energy. Although Oman’s sunny weather provides a unique opportunity for solar energy generation, the country’s wind power potential must not be neglected. As of this article’s writing, Oman has no industrial wind power stations, and the country’s wind turbines are mainly used for research purposes. However, this situation is changing, beginning with developing an understanding of the country’s wind power potential. An incorrect estimation of wind energy needs or the use of low-performance equipment not only reduces the benefits of the project, but also might lead to economic disaster (Dolatabadi et al. 2017 ).
Over the last decade, considerable information on wind resource mapping across Oman has been accumulated to stimulate the deployment of wind power (Al-Yayai and Charabi 2015 ; Al-Yahyai et al. 2012 , 2013 ; Charabi et al. 2011 ; Al Yahyai el al. 2010). Despite the availability of wind mapping information, the deployment of wind energy across Oman is still lagging due to the lack of accurate information on turbine energy cost. Without access to sound information on the cost of wind power technology, it is difficult for decision-makers, if not impossible; to evaluate which wind turbine technologies will most fit their national circumstances. The fast growth and cost reductions in the installed wind energy technologies mean that even data aged one or 2 years will substantially overestimate the cost of power from wind energy technologies. There is also a significant amount of perceived knowledge about the cost and performance of wind power generation technologies that are not accurate or is misleading. Significant knowledge of the cost and performance of wind generation technologies is also viewed that is not right or misleading. This paper fills a significant information gap because there is a lack of precise, comparable, and the latest data on the costs and performance of wind turbines in Oman.
Studies on the viability and economic potential of wind energy have recently spread worldwide.
Kumar and Gaddada ( 2015 ) have explored the outputs of four statistical methods to evaluate Weibull parameters for wind energy applications in four selected sites, located in northern Ethiopia. Gaddada and Kodicherla ( 2016 ) have evaluated wind power capacity and wind energy cost estimates for electricity generation systems in eight selected locations in Tigray (Ethiopia). Kodicherla et al. ( 2017 ) explored the potential of wind energy and developed an economic assessment of the water pumping system in various wind power conversion systems. In three selected Fiji Island stations, Kodicherla et al. ( 2018 ) have investigated the potential of wind power-assisted wind hydrogen production using different types of turbines. The literature also reflects different foci around wind turbines. Many researchers have worked on defining the shape and structure of wind turbines and their effects on aerodynamics (Cai 2019 ; Nema et al. 2009 ; Akpinar and Akpinar 2006 ). Others have tried to improve the performance of current turbines by optimizing placement and hub height (Abdul-Wahab et al. 2019b ; Elkinton et al. 2008 ).
Despite these efforts, the stochastic nature of wind speed makes wind energy generation difficult for some places (Padrón et al. 2019 ). A deep understanding of the specifications of each wind turbine and complete statistical data on wind velocity in any given location can begin to address this problem. These data must be processed and matched to a potential turbine to give a realistic and feasible answer to the suitability of any given piece of wind power equipment. In this paper, HOMER Pro software (HOMER Energy L.L.C., Boulder, Colorado, U.S.A.) was used to analyze wind data for the north and south of Oman and make a well-informed recommendation on the most suitable turbines for each region’s power needs. HOMER Pro software can combine data associated with wind regime, the specifications of wind turbines, and the power demands of consumers to estimate the cost of producing energy using different generators.
In this study, the researchers tried to estimate the potential for wind energy production in Oman’s north and south and suggest the feasibility of using wind turbines in the country. To this end, the performance of six different popular wind turbines was calculated and compared. By considering the performance and cost of energy (C.O.E.), suggestions on the best possible turbines for the north and south of Oman are provided.
Study areas
As has been mentioned previously, two locations were selected for the wind power plants. The northern site was located in Al Batinah North Governorate (24° 42′ 23″ N 56° 28′ 48″ E). The southern site was Mirbat, Dhofar Governorate (16° 58′ 22″ N 54° 42′ 56″ E) (Fig. 1 ). Both plants are located in rural areas with low populations and, therefore, low power demands. Population, power consumption per capita and power consumption patterns change power demands in an area. Demand also changes daily, hourly, and even in the summer and winter. The last reported data from Oman show that each Omani annually consumes around 6550 kWh on average (S.A.O.C 2017 ). Based on this information and the population of the area, the size of the wind power plant is considered at 10 MW. This size can cover current electricity consumption and any possible future growth. Even with a highly accurate prediction, real conditions can have unexpected variations. In order to consider this variation, the monthly 2% day-to-day random variability and 2% time-to-time step of random variability was considered. Figure 2 shows the power consumption patterns in Oman’s households. As can be seen in the figure, April to October is Oman’s summer season and has high electricity demand, while in wintertime, November to March, the power demand decreases significantly. The high demand for energy by cooling systems in the long summer of Oman is the main reason for this trend.
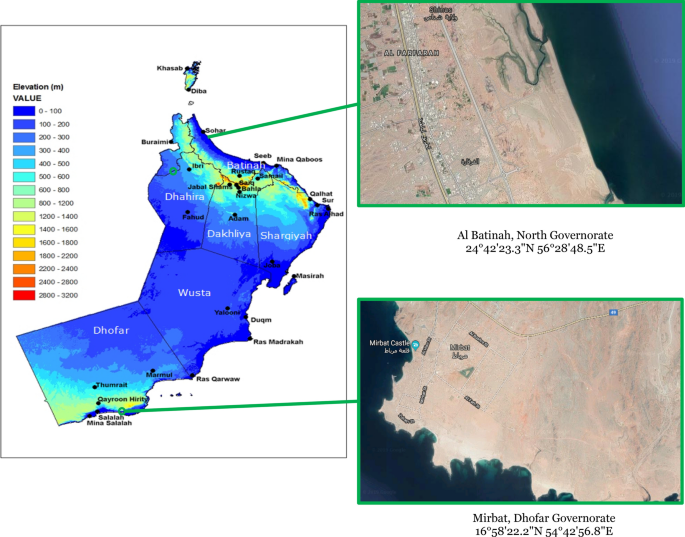
The location of the wind farms in north and south of Oman
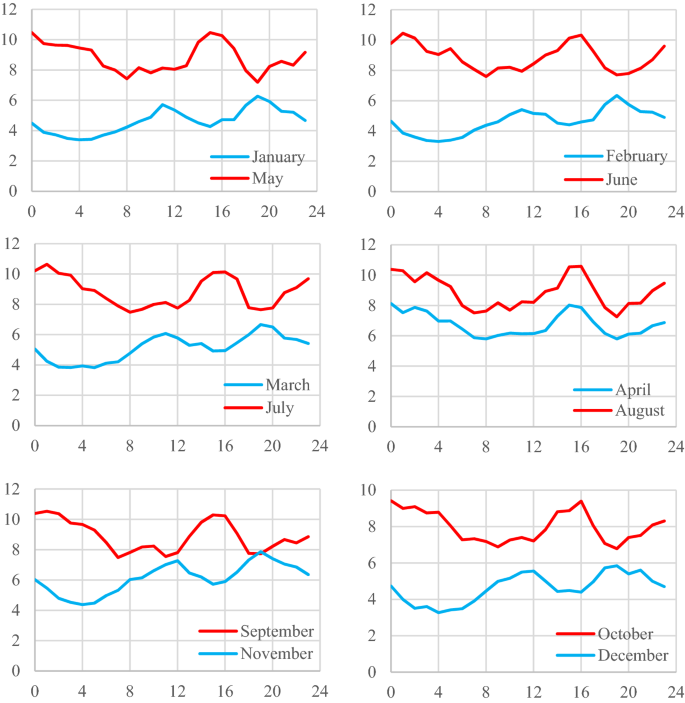
Monthly average of power demand (MW)
HOMER software
Wind turbine performance analysis.
A realistic estimation of power production requires accurate statistical data on wind velocity for an extended period, like a year or more, if possible. The accuracy of the output results entirely depends on the accuracy of this information. Wind velocity is usually measured on an hourly basis. Due to the high number of measurements in a calendar year, however, further processing for such an extended period would be time-consuming and difficult. Therefore, when making calculations based on such large data sets, the average wind velocity is usually used to reduce the processing load. Although using the monthly average seems practical, such a simple average can be misleading. For instance, by using a wind velocity of 0 m/s for 50% of the time and using a velocity of 6 m/s for the rest of the time, the simple average of the wind velocity would be 3 m/s.
Considering a wind turbine with a maximum output power of 3 m/s, the output performance would be wrongly calculated at 100% all day long. Such a system would have 100% output at 50% of the time at best. In order to address such miscalculations, in this research, the two-parameter Weibull distribution was used (Wang et al. 2018 ). In this method, both wind velocity and its probability over time are considered, and the distribution of the wind velocity is used for the following calculations (Moein et al. 2018 ). The probability density (f) and cumulative distribution (F) of the wind based on Weibull distribution are:
where c is the Weibull scale (m/s), and k is the Weibull shape factor.
The different wind turbines on the market have very different specifications. Considering and analyzing all of these turbines in this paper is not possible. Six of the most popular turbines on the market were selected and analyzed in order to make the article descriptive, rational, and practical. In some countries, other brands and models of turbines might be more popular, but the present approach can be used in those countries, too. In making this comparison, the C.O.E. production for each turbine must be calculated and compared carefully. Moreover, the whole system of a wind power plant consisting of one or more turbines must be able to handle the load demand of consumers with no or limited access to the main power line, for such a scenario where there is no access to the power grid, the power generation system has to be equipped with a sufficiently sized battery bank or a fossil fuel generator to cover non-windy hours or days. In order to simplify the problem and eliminate the calculation of fossil fuel generators, the system under consideration was conceptualized as having up to a 10% deficiency in a limited number of days. In real conditions, this amount of energy can be obtained from the main power lines (if accessible) or local generators. However, in this article, further calculations based on these generators were not considered.
Wind speed calculations represent the first phase of the HOMER Pro simulation. The wind velocity was measured and recorded every hour for 1 year. The system measured wind speed at a 10-m height above the sea level, which is the standard height for the measurement. Table 1 shows a sample of the measurements from the northern site for 1 week. For the calculation of the velocity at a different height (based on the height of each wind turbine), the measured values must be modified as in Eq. ( 3 ):
where \(V_{\text{Turbine}}\) and \(V\) show the wind velocity at the turbine and standard anemometer height, \(Z_{\text{Turbine}}\) and \(Z_{\text{anm}}\) are the height of the turbine and the anemometer (m) and \(Z_{0}\) is the surface roughness (m). Surface roughness characterizes the roughness of the field around the turbine. In this project, based on the local properties of the site location, \(Z_{0}\) was considered 0.03 m, which indicates a smooth field with some crops and no trees or buildings in the surrounding area (Homer-Energy 2016 ).
By combining the Weibull equation and Eq. ( 3 ), the average wind velocity can be written as:
And the output power in a wind turbine can be written in the form:
where \(\tau\) is the time, \(C_{p}\) is the turbine’s nominal capacity, and \(f_{v}\) is the wind velocity distribution.
The producers also provide the power curve of each turbine by testing different wind velocities. The power curve shows the real output power of the system in different ranges of wind velocity. Figure 3 shows the power curves of the six selected turbines with data extracted from the producers’ datasheet for the following turbine models:
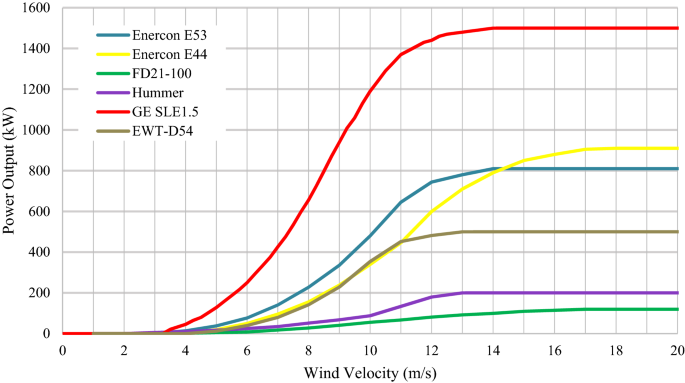
The power curves of the selected turbines
GE 1.5 SLE (GE Power, Schenectady, New York, USA).
Enercon E44 (Enercon, Aurich, Germany).
Enercon E53 (Enercon, Aurich, Germany).
FD21-100 (Enercon, Aurich, Germany).
EWT DW54 (Emergya Wind Turbines Pvt. Ltd., Amersfoort, The Netherlands);
Hummer H25.0–200 kW (Anhui Hummer Dynamo Co., Ltd., Hefei, Anhui, People’s Republic of China).
Economic analysis
In project planning, economic analysis is the most critical factor in decision-making. In this study, an economic analysis was the only indicator considered to show the feasibility of wind projects. Economic feasibility incorporates long-term performance, pointing to the best possible option among the wind turbines. In order to make an accurate estimation of economic feasibility, the total cost of the project must be calculated, including the capital cost (initial cost of the construction and devices), replacement cost as necessary, and maintenance costs. Operation costs should also be considered for the whole project. However, due to the low cost of operation in wind turbines, the operation cost can be considered part of maintenance costs. By accurately estimating these costs, the price of power generation per kW can be estimated. This price is a suitable indicator for choosing the best possible turbine for a wind power plant. In this research, the cost of energy (C.O.E.) per kW was the distinguishing feature considered among the turbines studied. HOMER sensitivity and optimization algorithms were used to select the best wind turbine (Pahlavan et al. 2018 ; Vahdatpour et al. 2017 ). The equations of the method of optimal system measuring, which has a minimum amount of total net present cost (N.P.C.), are as follows:
where C ann,total , C.R.F. i and R proj are the total annual cost, cost recovery factor, real interest rate and lifetime of the project, respectively.
All costs and incomes are evaluated at a constant interest rate over the year. The actual interest rate resulting from inflation is calculated and the effect of the change in interest rate on final N.P.C. is applied to purpose of influencing inflation in calculations. The cost recovery factor (C.R.F.), which indicates the cost recovery over the N years, is calculated as follows:
Software is able to calculate the annual interest rate through the following equation:
Also, the cost of per kW of energy during the lifetime of the project is obtained by software from the following equation:
In the above equation, E Load served is the real electric load in the hybrid system by unit kW/year.
Table 2 shows all costs associated with the selected turbines and which include:
The Capital cost is the initial purchase price,
The Replacement cost is the cost of replacing the generator at the end of its lifetime, the O&M cost is the annual cost of operating and maintaining the generator.
No energy battery storage system storage was taken into consideration for the current simulation focusing on the selection of the best wind turbine, and an annual interest rate of 6% was taken into account.
Results and discussion
Comparison between the proposed wind turbines.
Implementing big data associated with turbine measurements and specifications is difficult. HOMER Pro helps analyze this data and simulate plans for 20 years. The results of the simulation for each turbine are presented in Table 3 .
The main findings from the turbines simulation were as follows:
G.E. Energy 1.5 SLE This turbine is designed and manufactured by G.E. Power, a subsidiary of the General Electric Energy Company, and is a 1500-kW-rated power producer. This model has the highest power output among the selected turbines. It has a three-blade rotor with a 77-m diameter and 85-m hub height. The cut-in wind velocity for this model is 3 m/s, and the cut-off speed is 25 m/s. Cut-in and cut-off velocities can have a significant impact on the performance of the turbine. A turbine with a lower cut-off speed has the advantage of generating power in lower wind speed locations, like the north of Oman. The results of the simulation show that the C.O.E. for this turbine is USD$0.171 for each kW of energy in the north and USD$0.089 in the south. This cost contains the USD$1.75 million dollar maintenance cost for 20 years of operation and a capital cost of USD$3.38 million.
Enercon E44 This turbine, produced in Germany, has the second-highest power output of those considered, with a 900-kW-rated generator, 55-m hub height, and 44-m blade size. This Enercon production has a minimum cut-off wind velocity of 3 m/s, and a 28 m/s maximum cut-off. The HOMER Pro results showed that, by considering the capital cost of USD$2.34m and a maintenance cost of around USD$1 million, the C.O.E. would be USD$0.303 for each kW of energy in the north and USD$0.135/kW in the south.
Enercon E53 This turbine has a 53-m rotor diameter and 800 kW power production potential. Due to the lower power output, this model has lower capital and maintenance costs. Considering all of the costs of the turbine, the system would be able to generate power at USD$0.163/kW and USD$0.088/kW in the north and south, respectively.
FD21 - 100 This Enercon model uses GHREPOWER production with 100-kW output power. The lower output power makes it suitable for smaller wind power plants. FD21-100 has a 3–25 m/s range of working speed, and its highest possible hub height is 42 m. The HOMER Pro software simulation for this turbine showed that the C.O.E. would reach up to USD$0.290 per kW in the north and USD$0.144 kW in the south. In comparison to other turbines, this model has the highest cost of power generation for both locations.
DW54 This turbine is a 500-kW generator designed and produced by Energy Wind Technology (E.W.T.) in Amersfoort, The Netherlands. It has a 54-m rotor diameter and a working velocity between 3 and 10 m/s. With a USD$1.2 million capital cost and USD$750,000 maintenance cost over 20 years, the power generation cost would be USD$0.119/kW. This cost is the lowest possible for generating power in the north of Oman. However, the simulation showed that, due to differences in the wind regime in the north and south, this model is not the best possible option for the south. Each kW of energy produced in the south would cost USD$0.071. However, with its C.O.E., this model is the second best possible turbine for Oman’s north.
Hummer H25.0 – 200 K.W. This model is a 200-kW-rated wind turbine produced by the Anhui Hummer Dynamo Company of Hefei, China. In comparison to other analyzed turbines, this model has a lower cut-in wind velocity by 2.5 m/s and a smaller blade size (12 m). The simulation showed that while the capital cost of the turbine could be as low as USD$300,000, this model’s C.O.E. is not the best for all situations. In the north, power production would cost USD$0.132/kW. While this price is not the best possible option for the north, the results for the south are different. The simulation showed that the turbine would have the best possible results in the south among the selected models, generating power at USD$0.070/kW.
Considering the above-mentioned findings, the DW54 turbine is the best possible turbine for the north of Oman. On the other hand, the Hummer H25.0–200 KW turbine is the best option for Oman’s south. These models can generate electricity at the lowest possible cost. Figure 4 shows the graph of energy production cost for each turbine in the northern and southern sites.
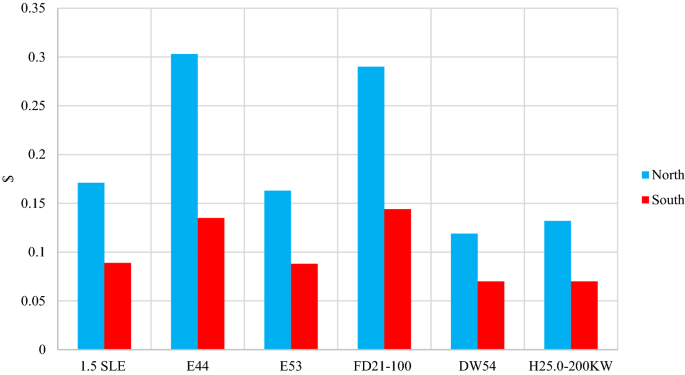
Cost of electricity for different turbines
Advantages of provided wind turbines over natural gas and diesel generators
The current power plants in Oman mostly use natural gas for electricity production. On the other hand, for off-grid consumers (some rural regions), the diesel generators are the primary source of electricity. It is clear that fossil fuel generators emit pollutant gases into the atmosphere and have negative impacts on the environment. In short, the diesel generator’s gas emission is calculated using the same energy production as the best wind turbines. For comparison, the unmet electrical load of wind turbines is considered (Fig. 5 ). Table 4 shows the emitted pollutant gases over one year of use. As it can be seen in Table 3 , the wind turbine power plant in the north can stop the CO 2 emission approximately 19,000 ton/year in comparison to natural gas and 28,000 ton/year in comparison to diesel. In the southern power plant, the reduced gas emission in comparison to diesel and natural gas are 18,000 and 12,000 ton/year, respectively.
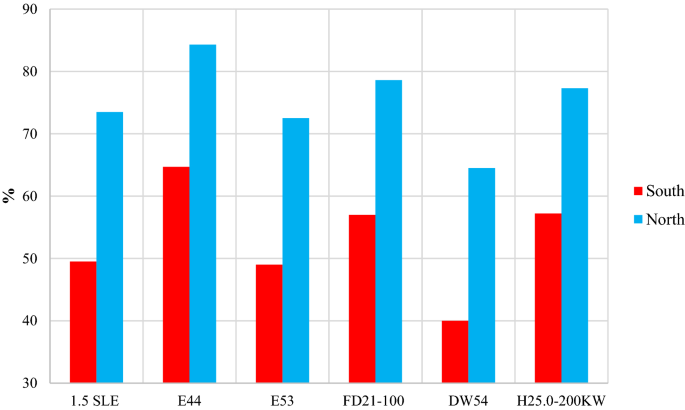
Unmet electrical loads for different turbines
In this study, the feasibility of using wind energy as a source of power production was calculated by collecting and analyzing hourly data on wind regimes over a 1-year period. HOMER Pro software was used to calculate the C.O.E. production of six different wind turbines, in order to select the most suitable wind turbine for two distinct locations in the north and south of Oman. The study’s main findings can be summarized as follows:
DW54 turbine produced by Energy Wind Technology in Amersfoort, The Netherlands, would have the best performance for Oman’s northern regions and can generate the cheapest possible energy from wind at $0.119/kW.
H25.0–200 kW turbine manufactured by the Anhui Hummer Dynamo Company of Hefeit, China, gives the best C.O.E. production for the southern regions of Oman and the lowest possible wind energy can be produced at $0.70/KW.
The difference of the wind regime between the northern and southern parts of Oman and the power curves of the turbines are the main reasons for the selection of two different wind turbines form different manufacturers.
The northern wind turbine plant is estimated to decrease CO 2 emissions by around 19,000 tons per year, compared to natural gas, while diesel emissions by around by 28,000 tons per year.
The southern wind turbines have a potential carbon emission reduction of about 18,000 and 12,000 tons per year compared to diesel and natural gas.
The application of the turbine selection using the HOMER Model described in this paper determined that the H25.0–200 kW turbine selected for the southern parts of Oman has a C.O.E. that is 58.8% lower than the DW54 turbine that was selected for the northern parts of the country. The application of the method followed in this research by developers during the planning stage could significantly improve the financial performance of their investment. Similarly, such techniques could be added to tools such as WAsP to improve decision-making during the initial planning stage.
Availability of data and materials
Data are openly available with HOMER software. HOMER uses the monthly average wind speeds, plus four parameters (Weibull k, 1-h autocorrelation factor, Diurnal pattern strength and Hour of peak wind speed) to synthesize wind data for simulation.
Change history
17 january 2021.
An amendment to this paper has been published and can be accessed via the original article.
Abdul-Wahab, S., Charabi, Y., Al-Mahruqi, A. M., et al. (2019a). Selection of the best solar photovoltaic (P.V.) for Oman. Solar Energy, 188, 1156–1168.
Article Google Scholar
Abdul-Wahab, S., Fessehaye, M., Yetilmezsoy, K., et al. (2019b). Optimization of an inverted multi-stage double slope solar still: An environmentally friendly system for seawater purification. Desalination and water treatment, 141, 42–50.
Akpinar, E. K., & Akpinar, S. (2006). An assessment of wind turbine characteristics and wind energy characteristics for electricity production. Energy Sources, Part A433A: Recovery, Utilization, and Environmental Effects + A465, 28, 941–953.
Al-Suleiman, S., Fessehaye, M., Yetilmezsoy, K., Al-Ghafir, A., Al-Brashdi, A., Al-Hajri, N., & Al-Bulushi, S. (2019). Optimization of an inverted multi-stage double slope solar still: an environmentally friendly system for seawater purification. Desalination and Water Treatment , 141 , 42–50. https://doi.org/10.5004/dwt.2019.23474 .
Al-Yahyai, S., Charabi, Y., Al Badi, A., & Gastli, A. (2013). Wind resource assessment using numerical weather prediction models and multi-criteria decision making technique: Case Study Masirah Island, Oman. International Journal of Renewable Energy Technology. . https://doi.org/10.1504/IJRET.2013.051070 .
Al-Yahyai, S., Charabi, Y., Gastli, A., & Al-Badi, A. (2012). Wind farm land suitability indexing using multi-criteria analysis. Renewable Energy, 44, 80–87. https://doi.org/10.1016/j.renene.2012.01.004 .
Al-Yayai, S., & Charabi, Y. (2015). Assessment of large-scale wind energy potential in the emerging city of Duqm (Oman). Renewable and Sustainable Energy Reviews, 47, 438–447. https://doi.org/10.1016/j.rser.2015.03.024 .
Cai X, Zhang Y, Ding W, et al. (2019) The aerodynamic performance of H-type darrieus V.A.W.T. rotor with and without winglets: C.F.D. simulations. Energy Sources, Part A: Recovery, Utilization, and Environmental Effects: 1–12.
Charabi, Y., Al Hinai, A., Al-Yahyai, S., et al. (2019). Offshore wind potential and wind atlas over the Oman Maritime Zone. Energy, Ecology and Environment, 4, 1–14.
Charabi, Y., Al-Yhyai, S., & Gastli, A. (2011). Evaluation of NWP performance for wind energy resources assessment in Oman. Renewable and Sustainable Energy Reviews, 15 (3), 1545–1555. https://doi.org/10.1016/j.rser.2010.11.055 .
Dolatabadi, A., Mohammadi-Ivatloo, B., Abapour, M., et al. (2017). Optimal stochastic design of wind integrated energy hub. IEEE Transactions on Industrial Informatics, 13, 2379–2388.
Elkinton, C. N., Manwell, J. F., & McGowan, J. G. (2008). Algorithms for offshore wind farm layout optimization. Wind Engineering, 32, 67–84.
Gaddada, S., & Kodicherla, S. P. K. (2016). Wind energy potential and cost estimation of wind energy conversion systems (W.E.C.S.s) for electricity generation in the eight selected locations of Tigray region (Ethiopia). Renewables, 2016 (3), 10. https://doi.org/10.1186/s40807-016-0030-8 .
Ghodsi, M., Ziaiefar, H., Mohammadzaheri, M., et al. (2019). Modeling and characterization of permendur cantilever beam for energy harvesting. Energy, 176, 561–569.
Homer-Energy (2016) HOMER pro version 3.7 user manual. HOMER Energy: Boulder, CO, U.S.A.
Khare, V., Nema, S., & Baredar, P. (2016). Solar–wind hybrid renewable energy system: A review. Renewable and Sustainable Energy Reviews, 58, 23–33.
Kodicherla, S. P. K., Gaddada, S., & Shaik, N. (2017). Wind energy potential and economic evaluation of WPS using WECSs in three selected locations of Northern Ethiopia. African Journal of Science Technology Innovation and Development., 9 (2), 179–187. https://doi.org/10.1080/20421338.2017.1303990 .
Kodicherla, S. P. K., Kan, C., & Nanduri, P. M. B. R. K. (2018). Likelihood of wind energy assisted hydrogen production in three selected stations of Fiji Islands. International Journal of Ambient Energy, 41 (7), 823–832. https://doi.org/10.1080/01430750.2018.1492444 .
Kumar, K. S. P., & Gaddada, S. (2015). Statistical scrutiny of Weibull parameters for wind energy potential appraisal in the area of northern Ethiopia. Renewables, 2, 14. https://doi.org/10.1186/s40807-015-0014-0 .
Moein, M., Pahlavan, S., Jahangiri, M., & Alidadi Shamsabadi, A. (2018). Finding the minimum distance from the national electricity grid for the cost-effective use of diesel generator-based hybrid renewable systems in Iran. Journal of Renewable Energy and Environment, 5 (1), 8–22. https://doi.org/10.30501/jree.2018.88377 .
Moné C, Hand M, Bolinger M, et al. (2017) 2015 cost of wind energy review. National Renewable Energy Lab.(NREL), Golden, CO (United States).
Nema, P., Nema, R., & Rangnekar, S. (2009). A current and future state of art development of hybrid energy system using wind and PV-solar: A review. Renewable and Sustainable Energy Reviews, 13, 2096–2103.
Padrón, I., Avila, D., Marichal, G. N., et al. (2019). Assessment of hybrid renewable energy systems to supplied energy to autonomous desalination systems in two islands of the Canary Archipelago. Renewable and Sustainable Energy Reviews, 101, 221–230.
Pahlavan, S., Jahangiri, M., Sahamsabadi, A. A., & Ariae, A. R. (2018). Assessment of PV-based CHP system: The effect of heat recovery factor and fuel type. Journal of Energy Mangement and Technology., 3 (1), 40–47.
Google Scholar
Sahu, B. K. (2018). Wind energy developments and policies in China: A short review. Renewable and Sustainable Energy Reviews, 81, 1393–1405.
S.A.O.C. (2017) 7 Years Statement of Oman Power & Water Production. Oman Power & Water Procurement Co.
Sahu A, Gupta S, Singh VK, et al. (2018) Design of permanent magnet synchronous generator for wind energy conversion system. Advances in Smart Grid and Renewable Energy. Springer, 23–32.
Vahdatpour, S., Adfar, S. B., Siampour, L., Veisi, E., & Jahangiri, M. (2017). Evaluation of off-grid hybrid renewable systems in the four climate regions of Iran. Journal of Renewable Energy and Environment., 4 (1), 61–70.
Wang, L., Yuan, J., Cholette, M. E., et al. (2018). Comparative study of discretization method and Monte Carlo method for wind farm layout optimization under Weibull distribution. Journal of Wind Engineering and Industrial Aerodynamics, 180, 148–155.
World-Bank. (2015). Renewable energy consumption (% of total final energy consumption) . World Bank: Sustainable Energy for All database.
Yang, B., Yu, T., Shu, H., et al. (2018). Robust sliding-mode control of wind energy conversion systems for optimal power extraction via nonlinear perturbation observers. Applied Energy, 210, 711–723.
Download references
Acknowledgements
The authors would like to thank the anonymous reviewers for their insightful suggestions and careful reading of the manuscript.
The authors received no specific funding for this work.
Author information
Authors and affiliations.
Center for Environmental Studies and Research, Sultan Qaboos University, P.O. Box 32, 123, Muscat, Oman
Yassine Charabi
Department of Mechanical and Industrial Engineering, Sultan Qaboos University, Muscat, Oman
Sabah Abdul-Wahab
You can also search for this author in PubMed Google Scholar
Contributions
The first and the second author contributed to the design and implementation of the research, to the analysis of the results and to the writing of the manuscript. All authors read and approved the final manuscript.
Corresponding author
Correspondence to Yassine Charabi .
Ethics declarations
Competing interests.
The authors declare no competing interests.
Additional information
Publisher's note.
Springer Nature remains neutral with regard to jurisdictional claims in published maps and institutional affiliations.
Rights and permissions
Open Access This article is licensed under a Creative Commons Attribution 4.0 International License, which permits use, sharing, adaptation, distribution and reproduction in any medium or format, as long as you give appropriate credit to the original author(s) and the source, provide a link to the Creative Commons licence, and indicate if changes were made. The images or other third party material in this article are included in the article's Creative Commons licence, unless indicated otherwise in a credit line to the material. If material is not included in the article's Creative Commons licence and your intended use is not permitted by statutory regulation or exceeds the permitted use, you will need to obtain permission directly from the copyright holder. To view a copy of this licence, visit http://creativecommons.org/licenses/by/4.0/ .
Reprints and permissions
About this article
Cite this article.
Charabi, Y., Abdul-Wahab, S. Wind turbine performance analysis for energy cost minimization. Renewables 7 , 5 (2020). https://doi.org/10.1186/s40807-020-00062-7
Download citation
Received : 28 May 2020
Accepted : 10 August 2020
Published : 17 August 2020
DOI : https://doi.org/10.1186/s40807-020-00062-7
Share this article
Anyone you share the following link with will be able to read this content:
Sorry, a shareable link is not currently available for this article.
Provided by the Springer Nature SharedIt content-sharing initiative
- Wind turbine Homer Pro
- Wind energy
- Load demand
- Cost of energy
- Sultanate of Oman
Thank you for visiting nature.com. You are using a browser version with limited support for CSS. To obtain the best experience, we recommend you use a more up to date browser (or turn off compatibility mode in Internet Explorer). In the meantime, to ensure continued support, we are displaying the site without styles and JavaScript.
- View all journals
- My Account Login
- Explore content
- About the journal
- Publish with us
- Sign up for alerts
- Open access
- Published: 22 October 2021
Geophysical constraints on the reliability of solar and wind power worldwide
- Dan Tong ORCID: orcid.org/0000-0003-3787-0707 1 , 2 , 3 ,
- David J. Farnham ORCID: orcid.org/0000-0002-6690-4251 3 ,
- Lei Duan ORCID: orcid.org/0000-0002-6540-1847 3 ,
- Qiang Zhang ORCID: orcid.org/0000-0002-8376-131X 1 ,
- Nathan S. Lewis 3 , 4 ,
- Ken Caldeira ORCID: orcid.org/0000-0002-4591-643X 3 , 5 &
- Steven J. Davis ORCID: orcid.org/0000-0002-9338-0844 2 , 3 , 6
Nature Communications volume 12 , Article number: 6146 ( 2021 ) Cite this article
51k Accesses
86 Citations
556 Altmetric
Metrics details
- Climate change
- Climate-change mitigation
- Renewable energy
If future net-zero emissions energy systems rely heavily on solar and wind resources, spatial and temporal mismatches between resource availability and electricity demand may challenge system reliability. Using 39 years of hourly reanalysis data (1980–2018), we analyze the ability of solar and wind resources to meet electricity demand in 42 countries, varying the hypothetical scale and mix of renewable generation as well as energy storage capacity. Assuming perfect transmission and annual generation equal to annual demand, but no energy storage, we find the most reliable renewable electricity systems are wind-heavy and satisfy countries’ electricity demand in 72–91% of hours (83–94% by adding 12 h of storage). Yet even in systems which meet >90% of demand, hundreds of hours of unmet demand may occur annually. Our analysis helps quantify the power, energy, and utilization rates of additional energy storage, demand management, or curtailment, as well as the benefits of regional aggregation.
Similar content being viewed by others
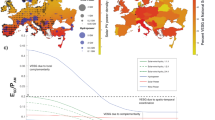
Spatiotemporal management of solar, wind and hydropower across continental Europe
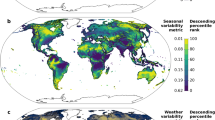
Identification of reliable locations for wind power generation through a global analysis of wind droughts
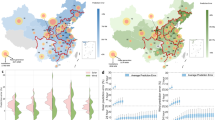
Inherent spatiotemporal uncertainty of renewable power in China
Introduction.
Stabilizing mean global temperatures requires a global transition to energy systems with near-zero (or net-negative) carbon dioxide equivalent emissions 1 , 2 , 3 . In cost-optimized scenarios that accomplish this transition, solar and wind resources often supply a large share (e.g., >60%) of electricity 4 , 5 , 6 , 7 , 8 , 9 , 10 . Designing and operating a highly reliable electricity system that is dependent on such large shares of wind and solar generation can be a challenge, however, due to the variable and uncertain nature of solar and wind resources 11 , 12 . The efficacy of meeting electricity demands with generation from solar and wind resources depends on factors such as location and weather; the area over which generating assets are distributed; the mix and magnitude of solar and wind generation capacities; the availability of energy storage; and firm generation capacity 11 , 12 , 13 , 14 , 15 , 16 . Meanwhile, reliability standards in industrialized countries are typically very high (e.g., targeting <2–3 h of unplanned outages per year, or ~99.97% 17 ). Resource adequacy planning standards for “1-in-10” are also high: in North America (BAL-502-RF-03) 18 , generating resources must be adequate to provide no more than 1 day of unmet electricity demand—or in some cases 1 loss of load event—in 10 years (i.e., 99.97% or 99.99%, respectively) 19 .
Here, we present a systematic analysis of the ability of specified amounts of solar and wind generation to meet electricity demands in 42 major countries across a range of assumptions associated with transmission, energy storage, and generation amounts. In particular, we assess spatial and temporal gaps between electricity demand and the availability of solar and wind resources, which represent gaps that must be filled by other non-emitting generation technologies or operating strategies in reliable electricity systems based on zero-carbon sources. The complementarity of renewable energy sources for this study is defined as a hybridization of solar-wind resources over a given area (here, countries), which we estimate by the Kendall correlation coefficient of these resources across 39-years of resource data 20 . Our goal is to identify the opportunities, complementarity, and challenges of variable renewable resources in greater detail than can be done by integrated assessment models that have multi-year time steps. Our results do not account for realistic power system specifications. Rather, we examine fundamental geophysical constraints on wind- and solar-dominated power systems independent of cost estimates. Note that we do not mean to suggest that the temporal variability of such resources would ever make it physically impossible to meet a given electricity demand (with enough capacity the solar and wind resources would be able to meet demand), but rather the extent to which such variability may determine the economic or socio-political feasibility of reliable systems. Our results will thus continue to be informative even as technological and socio-political feasibility evolves.
Details of our analytical approach are in the “Methods” section. In summary, we use 39 years (1980–2018) of gridded (0.5° × 0.625°) and hourly reanalysis data 21 , 22 and actual/projected hourly electricity demand from a single recent year to evaluate the adequacy of solar and wind resources to meet electricity demand in each of 42 major countries (data sources and countries are listed in Supplementary Data 1 ). First, hourly, area-weighted capacity factors for both solar and wind resources are calculated over each country (or region), assuming perfect transmission within the country or region. Then we exogenously specify (1) the mix of solar and wind generation, (2) the overall level of annual generation from these sources, and (3) the capacity of energy storage, and analyze the ability of the specified technologies to meet hourly demand. We analyze systems ranging from 100% solar (no wind) to 100% wind (no solar), in which total annual generation ranges from equal to annual demand (“1x generation”) to up to three times annual demand (“3x generation”), and in which available energy storage ranges from none (“0 h”) to 12 h of mean demand (“12 h”). In addition, we simulate the impacts of different demands (i.e., demand load profiles) and technologies (i.e., single-axis and dual-axis solar tracking systems) on electricity system reliabilities as sensitivity tests. The number of countries, years of reanalysis data, and different system configurations we analyze require computation and analysis of ~300,000 year-long simulations.
Resources and demand variability
Figure 1 shows the seasonal and daily variability of solar and wind resources and electricity demand in the six countries with the greatest electricity demand on every continents except Antarctica (results from six other major countries and continent-level aggregated regions are shown in Supplementary Figs. 1 and 2 , respectively). Solar and wind consistently peak in summer and winter, respectively, in countries of the Northern Hemisphere (seasons are reversed in countries of the Southern Hemisphere; Fig. 1a–f ). The seasonal cycles of solar and wind thus suggest potential complementarity in many countries (e.g., China, Fig. 1a ; and Germany, Fig. 1b ). However, during the 39-year period, interannual variability of wind is consistently much greater than that of solar in most countries (Fig. 1a–f ), though the magnitude of these resources’ variability differs substantially between two particular countries. For example, Germany’s small area (0.36 million km 2 ) and high latitude (centroid 51.2 °N) result in large interannual variations in both solar (measured by the robust coefficient of variation 23 ; RCoV = 58.8%) and wind resources (RCoV = 47.2%, Fig. 1b ), whereas solar resource variability is very low (RCoV = 6.6%) in the larger and tropical country of Brazil (8.52 million km 2 and centroid 14.2 °S; Fig. 1e ). Wind resources are also more variable than solar resources on the time scale of days to weeks in each country, which acts to limit and undermine the resources’ seasonal complementarity. Electricity demand profiles for each country are determined by factors such as economic conditions, prevailing weather conditions and consumer usage patterns 24 . Therefore, electricity demand for two countries can have unique seasonal shapes and a range of variabilities even if they have similar wind and solar resources. For example, seasonal variability of demand in France (RCoV = 14.4%; Supplementary Fig. 1e ) is greater than that in Germany (RCoV = 7.4%; Fig. 1b ), despite the countries’ similar wind and solar resource profiles.
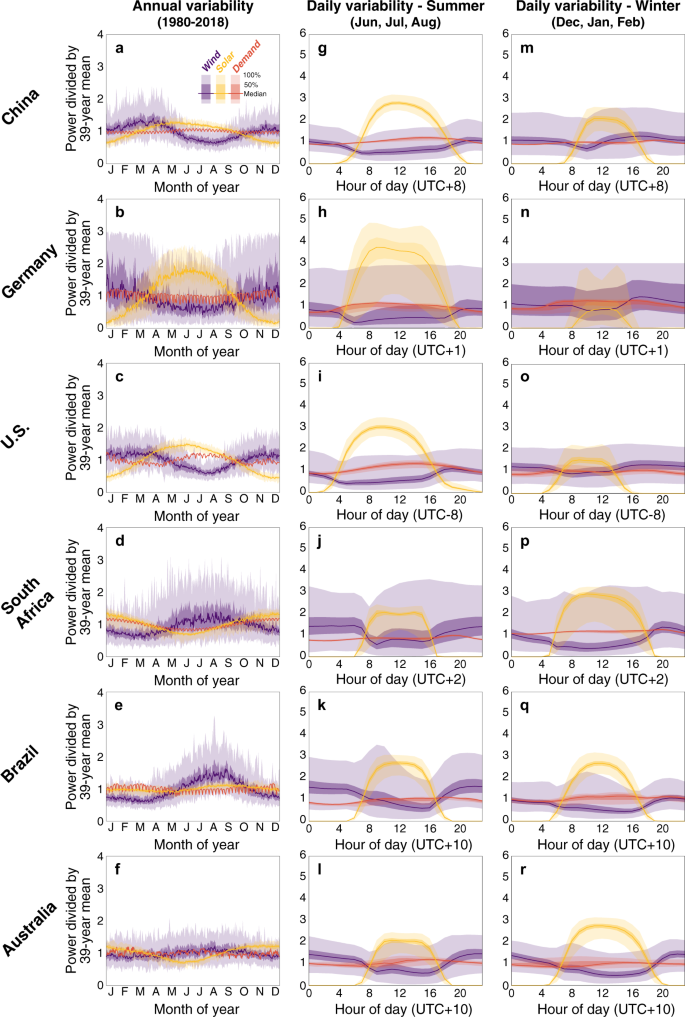
Climatological variability of the area-weighted median power from solar (orange) and wind (blue) resources for the selected country from six continents during the 39-year period 1980–2018. The countries (from the top row to the bottom row) are China ( a , g , m ), Germany ( b , h , n ), contiguous U.S. ( c , i , o ), South Africa ( d , j , p ), Brazil ( e , k , q ), and Australia ( f , l , r ). The left column ( a – f ) depicts the daily and seasonal variability, the middle column ( g – l ) depicts hourly summer (June, July, and August) variability, and the right column ( m – r ) depicts hourly winter (December, January, and February) variability. The lines represent the median, the dark shading represents the inner 50% of observations (25th to 75th percentile) and the light shading represents the outer 50% of observations (0th to 100th percentile) of the daily averaged value for that same day in each of the 39 years of record. Red curves in each panel represent electricity demand for a single, most recent, available year for each country. The time of day shown is the local time of each country and its relation to Coordinated Universal Time (UTC) is shown. Note that the middle of local time zones has been selected for the countries with multiple time zones. The solar, wind, and demand data are each normalized by dividing by their respective 39-year mean value.
Daily cycles of solar and wind resources in each country are also somewhat complementary. Wind power usually peaks at night and rarely falls to zero when resources are aggregated over an entire country. This daily cycle is not substantially different during the summer and winter months (comparing Fig. 1g–l with Fig. 1m–r ). Thirty-four (of the 42) countries have higher average wind power availability during the nighttime than during the daytime. Solar power peaks in the middle of the day and drops off sharply to zero at dusk. The amplitude and duration of the daily cycles for solar power availability is consistently different during the summer and winter months across countries (Fig. 1g–l versus Fig. 1m–r ). The daily cycle of solar resources is a barrier to realizing reliable solar-dominated electricity systems without energy storage and/or complementary wind generation to meet demand during the hours when the solar resource is not available. In addition, given our assumption of single-axis solar tracking, available solar power tends to be flat for several hours around its daytime peak during the daily cycles (Fig. 1g–r ), though in some countries (e.g., Germany, South Africa, Australia) there is a consistent dip near noon, perhaps related to our adjustments of the direct radiation (details in Supplementary Note 1 ). Kendall’s correlation coefficients of solar and wind resources in the 42 main countries range from −0.91 to −0.83 (see Supplementary Data 2 ), another indication of good complementarity (where −1 is the best possible complementarity) 20 .
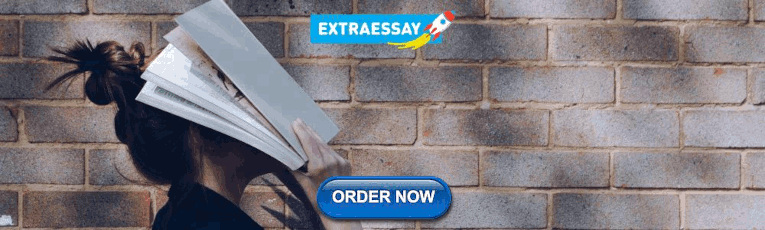
The most reliable generation systems
The colors in Fig. 2 show the reliability of electricity systems (i.e., the average percentage of electricity demand that is met each year from 1980 to 2018) based only on solar and wind resources for 18 major countries (4 from each of Asia, Europe, Africa, and the Americas, and 2 from Oceania; horizontal axes of each panel), according to: the mix of solar and wind generation (vertical axes), the level of annual generation relative to annual demand (1x in left panels and 1.5x in right panels), and the capacity of energy storage relative to mean electricity demand (0, 3, and 12 h in the first, second, and third rows of panels, respectively). Results for 24 other countries are shown in Supplementary Fig. 3 and Supplementary Data 3 . Figure 2a shows that without any excess annual generation or energy storage (assuming perfect national transmission), the most reliable mixes (white circles) of solar and wind generation could potentially meet 72–91% (average 83%) of electricity demand in these countries. Under these generation and storage assumptions, the most reliable solar-wind generation mixes range from 65 to 85% wind power (73% on average), with countries with substantial desert (like Algeria, Egypt, South Africa) favoring slightly more solar and less wind (65–70% wind) and with higher-latitude countries like Russia and Canada favoring more wind (85% wind; Fig. 2a ).
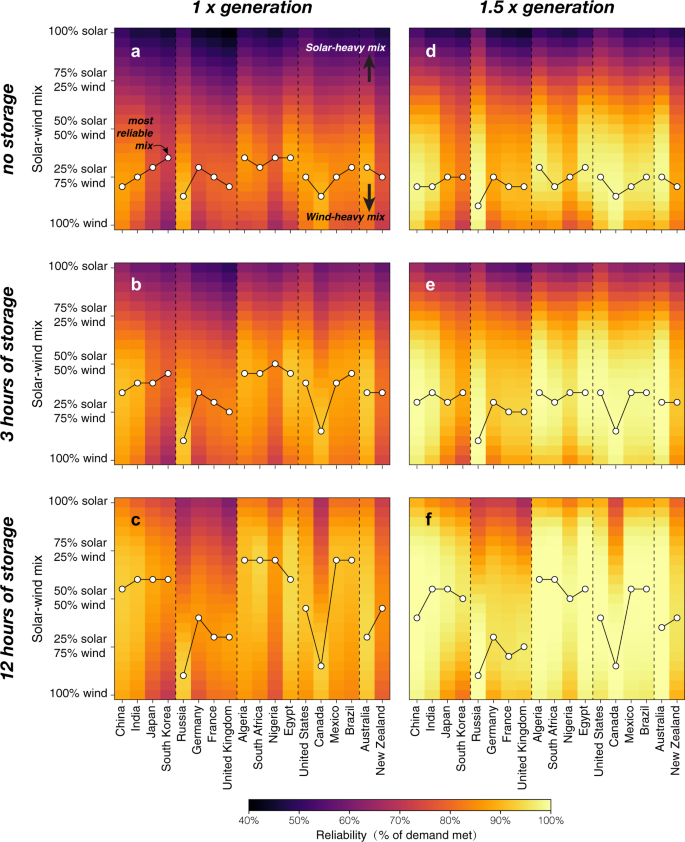
Shading in each panel represents the 39-year average estimated reliability (% of total annual electricity demand met) by a mix of solar and wind resources ranging from 100% solar to 100% wind (every 5% change for solar-wind generation mix). 18 main countries are chosen to show their ability to meet total annual electricity demand, including 16 main countries from four continents (Asia, Europe, Africa, and the Americas) and 2 main countries from Oceania. The white circles represent the highest reliability within each country under 21 sets of solar and wind generation mix (called the most reliable mix). Storage and generation quantities are varied in each panel: a 1x generation without storage; b 1x generation with 3 h of storage; c 1x generation with 12 h of storage; d 1.5x generation without storage; e 1.5x generation with 3 h of storage; and f 1.5x generation with 12 h of storage.
Adding 3 h of energy storage, but still without excess annual generation, increases the reliability so that the most reliable mixes (white circles) meet 78–93% (average 87%) of electricity demand. The share of solar generation in these most reliable mixes increases to 15–50% (36% on average; Fig. 2b ). However, the share of solar generation increases less, or even decreases, in higher-latitude countries like Russia, Canada, and Germany (Fig. 2b ). These trends continue as more storage is added, so that with 12 h of energy storage and no excess annual generation, 83–94% (average 90%) of electricity demand is met with mixes of 10–70% solar power (49% on average; Fig. 2c ).
If generating capacities are instead increased so that annual generation exceeds annual demand in each country by 50% (i.e., 1.5x generation), but without energy storage, the most reliable mixes meet 83–99% (average 94%) of electricity demand. The 1.5x generation most reliable mixes are substantially more reliable than in the 1x generation systems but include more wind power: 70–90% wind power (78% on average; Fig. 2d ). These “overbuilt” systems are more reliable in all of these 18 countries than the systems with 12 h of energy storage but no excess generation (Fig. 2c ). Adding energy storage to systems whose generation is 1.5x annual demand again increases both the system reliability (89–100%, average 98%) and the share of solar generation (most reliable mixes have 10–60% solar power, 36% on average; Fig. 2e, f ).
The unmet demand
The scatter plots in Fig. 3 show the relationships among reliability, energy storage, excess annual generation, and countries’ land area for the most reliable solar-wind mixes of all 42 countries analyzed (see relationships with a log y-axis in Supplementary Fig. 4 ). The linear fits in each panel show that solar-wind systems are generally less reliable in countries with smaller land areas (e.g., Fig. 3a ). Specifically, our results across countries indicate that the reliability of solar-wind systems that lack energy storage increases by 7.2% for every factor of 10 increase in land area; this relationship further suggests the improvement in system reliability that might be expected by expanding transmission systems within large countries. However, excess annual generation tends to alleviate the disadvantage of small country area more than energy storage (this can be seen by comparing the slopes of the linear fits in panels of Fig. 3c and d ). In addition, within each country, to compare the gains in reliability from excess annual generation and energy storage, a nonlinear function was fit to the reliability given the land area, the level of annual generation, and the capacity of energy storage (see Supplementary Information ). Our results indicate that a 10% increase in excess annual generation is equivalent to 3.9 h of storage (Supplementary Note 2 ).
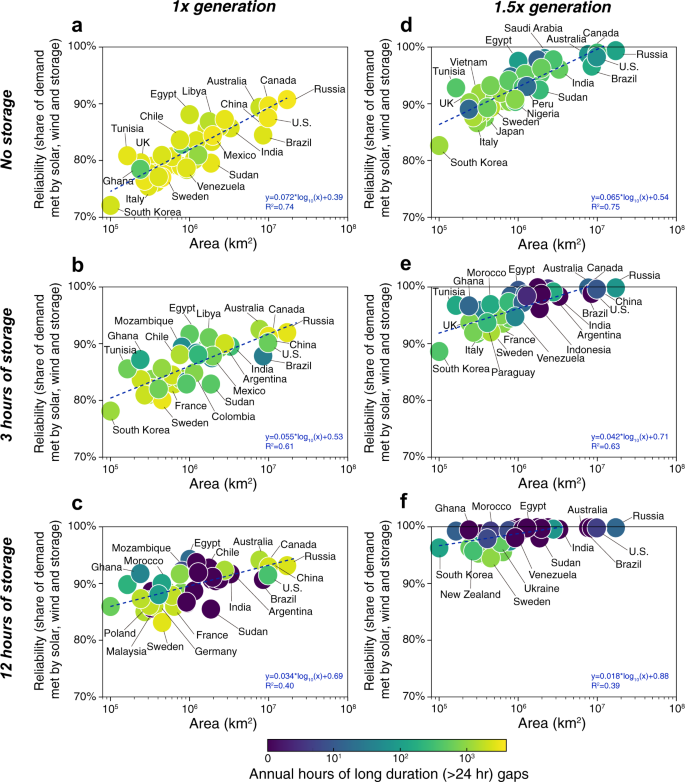
Shading of bubbles represents the annual average hours of long-duration (>24 h) power supply gaps. Storage and generation quantities are varied in each panel: a 1x generation without storage; b 1x generation with 3 h of storage; c 1x generation with 12 h of storage; d 1.5x generation without storage; e 1.5x generation with 3 h of storage; and f 1.5x generation with 12 h of storage.
Figure 3 also points to the nature of systems’ unreliability: the color of bubbles indicates the average number of events in which there would be unmet demand in each of at least 24 contiguous hours (i.e., “long-duration gaps”). In systems that meet >95% of a countrie’s demand, dozens of such long-duration gaps often remain each year (yellow and green circles). In some countries, excess annual generation reduces the number of such long-duration gaps more than adding 12 h of energy storage (e.g., compare Sweden, Australia, Canada, and Russia in Fig. 3c and d ).
Figure 4 further characterizes the magnitude and duration of unmet demand in 16 major countries (removing two African countries from the 18 countries shown in Fig. 1 for figure symmetry; in descending order of their land area), with curves showing gaps of different system configurations sorted by their magnitude and according to the number of hours each year that such a gap occurred (power supply gap represents the fraction of unmet demand to the total demand in that hour averaging over 1980–2018; see relationships with a log y-axis in Supplementary Fig. 5 ). For example, the pale purple curves show that systems with no excess annual generation and 12 h of energy storage consistently have gaps in which >50% of demand is unmet for >1000 h per year (Fig. 4 ). Pale green curves show that systems with 50% excess annual generation and 12 h of energy storage may have much smaller and shorter gaps in some countries (e.g., <10% of demand unmet in fewer than 100 h per year in Russia, China, and Australia), but the gaps may still be >20% of demand for tens of hours or more in countries with relatively large land areas (e.g., Canada, Brazil, India, and Mexico) and >60% of demand for several hundred hours per year in countries with smaller areas (e.g., France, Japan, Germany, New Zealand, the U.K., and South Korea; Fig. 4 ). Indeed, in smaller countries, substantial gaps (>30% of demand for >20 h per year; pale orange curves in Fig. 4 ) remain in systems even with 12 h of energy storage and annual generation that is 3x annual demand.
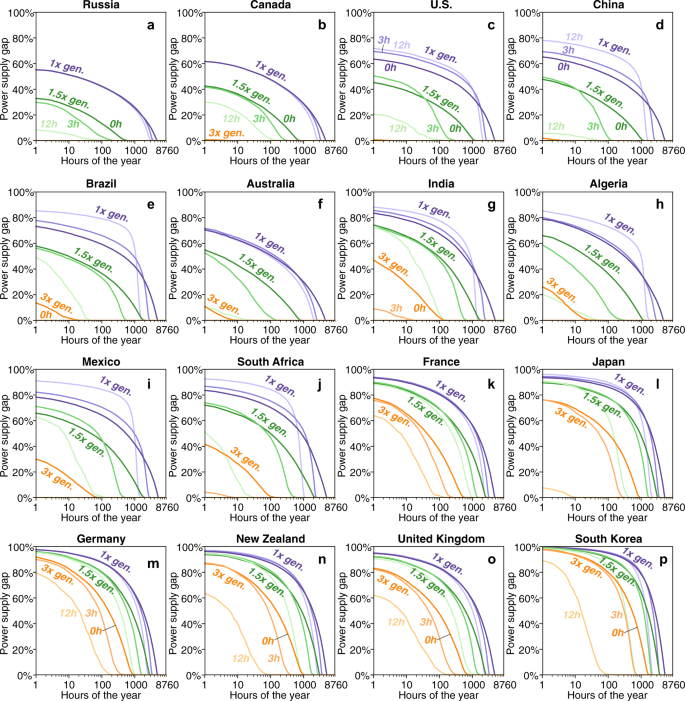
Areas under each curve show the share and hours of unmet electricity demand of the most reliable solar-wind systems in selected countries assuming specified storage and generation quantities: a Russia; b Canada; c contiguous U.S.; d China; e Brazil; f Australia; g India; h Algeria; i Mexico; j South Africa; k France; l Japan; m Germany; n New Zealand; o United Kingdom; p South Korea (see data in Supplementary Data 6 ). Color of lines represents different generation quantities: 1x generation in purple, 1.5x generation in green, and 3x generation in orange. Shading of lines represents different storage quantities: darkest shading represents without storage, medium shading represents 3 h of storage, and lightest shading represents 12 h of storage. Note that the y-axis of power supply gap represents the fraction of unmet demand to the total demand in that hour.
Benefits from sharing resources of multiple nations
We also evaluate the reliability benefits of regional electricity interconnections whereby the solar and wind resources of multiple nations are pooled and shared, again assuming perfect transmission within these regions. The maps in Fig. 5 present the effects of such spatial aggregation, showing the highest reliability of solar-wind generation with no excess annual generation or energy storage at the national level (Supplementary Data 7 ; Fig. 5a ), as well as when a system is aggregated into 19 separate, contiguous multinational regions (Fig. 5b ; categorization in Supplementary Data 4 ) and 6 continents (Supplementary Data 7 ; Fig. 5c ). Each step produces substantial improvements in reliability, with >89.8% of hourly demand met everywhere when resources are aggregated at the continental level (Fig. 5c ). Figure 5c also indicates the additional reliability gains in these systems that would be achieved as a consequence of specific intercontinental connections. Supplementary Fig. 6 shows that the supply gaps in continental-scale solar-wind systems might be entirely eliminated in Africa, Asia, and South America, and limited to <2% of demand and 49, 26, and 13 h in Europe, Oceania, and North America, respectively, given excess annual generation of 50% and 12 h of storage. Substantial supply gaps remain for continental-scale systems when excess annual generation and energy storage are not available (Supplementary Fig. 7 ).
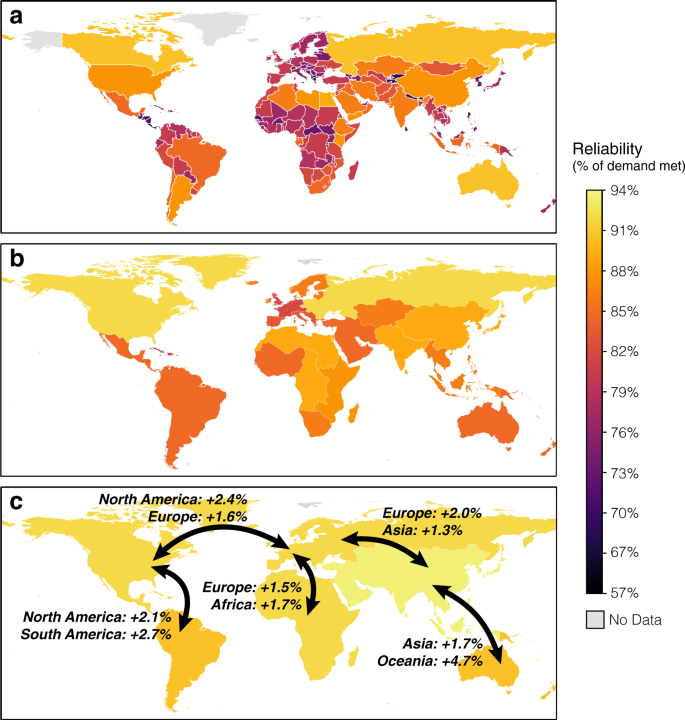
Maps show the reliability (i.e., hourly averaged resource adequacy) at country/region scale ( a ; Supplementary Data 4 ), the subcontinent scale ( b ; 19 multinational regions, and listed in the SI), and at continental scale ( c ; 6 continents: Asia, Europe, Africa, North America, South America, and Oceania). We also evaluated the reliability of the power supply system assuming several intercontinental connections (shown as the arrows: Asia–Oceania, Europe–Asia, Europe–Africa, North America–Europe, and North America–South America). The added reliabilities for each continental power system under various connections are labeled.
Our results suggest that, neglecting transmission constraints, with systems sized to meet time-integrated annual electricity demand, major countries’ solar and wind resources could meet at least 72% of instantaneous electricity demand without excess annual generation or energy storage. For instance, in the contiguous U.S., a solar and wind power system could provide ~85% of total electricity demand, which is consistent with the prior studies and reports 12 , 25 . Solar and wind resources can achieve greater levels of reliability by adding energy storage, increasing deployed capacities (i.e., generating electricity in excess of annual demand), or pooling resources of contiguous, multinational regions 26 . However, the marginal improvements in reliability related to these options differ considerably across countries and regions, according to their land area, location, and geophysical resources (Supplementary Figs. 8 and 9 ).
In small, high-latitude countries, the highest reliability systems are usually wind-heavy (e.g., as high as 95% wind power), with particularly large reliability gains achievable by regional aggregation. In contrast, the most reliable systems in temperate/tropical countries tend to include more solar. Meanwhile, the most reliable systems are not always the same systems that would minimize the frequency of long-duration (≥24 h) power supply gaps (Supplementary Fig. 9 ). In general, more solar in the wind-solar mix reduces the frequency of long-duration gaps. Although reasonably high levels of reliability can be reached by solar-wind resources alone, the defining challenge of such systems are the longer-duration gaps, often associated with extreme weather episodes. For instance, historical solar and wind resources data in Germany reveal that there were nearly 2 weeks in which dispatchable generation had to cover practically all of the demand because of a period with very low solar and wind power availability (called “dark doldrums”) 27 . Although with vast enough wind and solar capacity it might still be possible to meet demand in all hours, the required capacity increases exponentially after a point that depends on the renewable resources of that country, and it is this geophysically-dependent point that will largely determine the cost-effectiveness of highly-reliable, renewables-based electricity systems. Although dispatchable fossil fuel generators with 100% effective carbon capture storage (CCS) could provide system reliability without emissions 2 , such underutilized and capital-intensive backup electricity would require higher investments and variable costs. In contrast, combustion turbines or combined cycle plants burning carbon-neutral biogas, syngas, or hydrogen might have comparatively low capital costs, but would require additional and large capital investments to produce such fuels (e.g., biodigestion, direct air capture, Fischer-Tropsch, and/or electrolysis). Sector-coupling or right-sizing of these net-zero emissions fuel-production facilities could nonetheless make infrequent operation of generators feasible 28 . More firm generation would mean less solar and wind capacity in a given system, which might or might not be cost-effective depending on technology costs. But many jurisdictions and advocates are interested in “maxing out” solar and wind. Our results are especially relevant in that context, highlighting the implications of country-level differences in the variability of solar and wind resources, including how much storage and firm generation might be required to ensure resource adequacy. Although our methods are simple and transparent, our goals and findings are remarkably consistent with much more complex approaches. For example, the recently published Net-Zero America report includes a cost-optimized “all-renewables” scenario which decarbonizes U.S. electricity without nuclear or CCS: by 2050, ~81.6% of primary energy in the E + RE + scenario is from solar and wind 29 .
Our analysis has important limitations and uncertainties. To improve the generality of our results, our analysis focuses exclusively on geophysical constraints and does not consider economic feasibility. As noted throughout, our reliability estimates are a best case given the assumption that electricity can be transmitted losslessly throughout a region of interest. Also, we use area-weighted averages for solar and wind generation potential without regard to existing protections or uses. This use of area-weighted averages affects our estimates in two important ways. First, our estimates may include areas where currently generation cannot be sited. Second, our derivation of solar and wind capacity factors implies uniform distribution of wind and solar generation technology (i.e., a horizontal single-axis tracking system applied in this work), which does not allow us to select locations with particularly high capacity factors or to strategically select a set of locations whose generating potential is mutually negatively correlated. This second point has the effect of making our estimates for the efficacy of solar and wind resources to meet electricity demand more conservative by using the horizontal single-axis tracking system compared to the dual-axis solar tracking systems. For this case, dual-axis solar tracking systems are added to test the impacts on the system reliabilities (see Supplementary Note 3 ), we find that different solar tracking systems have very small impacts on the electricity system reliabilities and the reliability change ratios are within ±5% under the 1x generation system and less sensitive under 3x generation system (Supplementary Fig. 10 ). However, either method to calculate capacity factors of national and regional area-weighted averages may also reduce the resource variability and thereby increase estimates of reliability. Third, hourly variations of solar and wind capacity factors in the reanalysis data MERRA-2 we used may be biased. A new analysis based on a different and independent reanalysis product, ERA5 30 , 31 , has been added and compared to the original results (see Supplementary Note 3 and Supplementary Figs. 11 - 12 ). Our estimates of the system reliabilities by using ERA5 data in the 42 major countries are in good agreement with results of MERRA-2: under 1x generation and the most reliable mixes without storage, reliability under the different loads varies on average from −9.4 to 1.3% (see Supplementary Fig. 9a ). The differences are similar in systems with excess generation (Figs. S11b-c). We also compared the magnitude and duration of unmet demand in 16 major countries like Fig. 4 (see Supplementary Fig. 12 ). The data products of MERRA-2 and ERA5 both can essentially capture the number of hours each year that such a gap occurred. By contrast, the MERRA-2 data has a better performance of meeting hourly demand in larger countries (i.e., Russia and Canada) but a similar performance in small countries (i.e., United Kingdom). The somewhat different patterns of resource variability in the two datasets do not alter our main conclusions.
Our estimates show that the marginal reliability benefit of increased capacity of storage or increased overbuild of wind/solar declines steadily. Under a given capacity of energy storage (e.g., 3 h), our results of 1x, 1.5x, and 3x generation show that the first 10% excess generation increase is larger than the next 10% excess generation increase (i.e., the marginal benefit for system reliability decreases as excess generation increases). As might be expected, the diminishing marginal benefits between excess generation and increased storage apply in both directions. Our fitting model performs well across different nations, overbuild levels, and storage levels. The differences in reliability between the estimates and the model predicted values are between −5.5 and 5.8% and ~80% of the differences are within ±2%, with no systematic bias related to region or the magnitude of overbuild or storage. Nonetheless, our model and conclusions are limited by our experimental design and the discrete levels of excess generation (1x, 1.5x, and 3x) and storage (0, 3, and 12 h) we evaluated.
We compare the reliability improvements obtainable by energy storage, excess capacity, and regional aggregation but not the relative costs of the different options. For example, the energy storage capacities we consider are in some cases quite large: energy storage equal to 12 h of mean electricity demand in the contiguous U.S., Germany, and Japan represents 5.6, 0.7, and 1.4 TWh, respectively (Supplementary Data 5 ). These combined storage capacities represent ~35 times the capacity of Li-ion batteries produced globally to date 32 , and more than 200 times the pumped hydro storage capacities that now exist in those countries 33 . The feasibility of 12 or more hours of energy storage may depend on continued innovation and learning related to the associated materials and technologies 34 , 35 , 36 , 37 . Similarly, the feasibility of pooling solar and wind resources over national or multinational regions may depend on both technological advances that reduce the costs, losses, and risks of power transmission 38 , 39 , 40 as well as shifts in the socio-political support for such infrastructure 41 , 42 . In addition, setting up purely solar and wind supplied electricity systems requires a large number of solar panels and wind turbines to be installed, and we do not incorporate the impacts or interactions (e.g., wakes) from these hypothetical build-outs. Last, in this work, only 1-year of demand data is employed to assess the geophysical constraints of 39-year solar and wind resources. On one hand, we understand that the hourly patterns of countries electricity demand will of course change over time with changes in population, economic activities, power generation structure, and technology. For example, stronger positive correlation between solar/wind availability and demand may be observed as renewable energy gradually dominates the power system. However, our analysis compares resources and demand in different years and at the country-level, which should preclude any bias related to specific subnational weather events. On the other hand, electricity demand profile may also dramatically change with future high electrification. We therefore perform additional analysis using the demand pattern from the future high electrification scenario (i.e., combining the high electrification scenario and rapid technology advancement) 43 and use the results to discuss the sensitivity of our results to such different load profiles (see Supplementary Fig. 13 ), and the results of this test for the U.S. show that reliability is not especially sensitive to the high electrification demand profile: under 1x generation without storage, reliability under the different renewable mixes varies on average from −1 to 2.5%. The differences are even smaller in solar-heavy systems with excess generation. In addition, we test the sensitivity of our results to such changes in demand by simulating the reliability of U.S. resources in meeting current loads from each other region (see Supplementary Note 4 ). These tests show that reliability is not especially sensitive to demand profiles: under 1x generation and the most reliable mixes without storage, reliability under the different loads varies on average from −9 to 2% (see Supplementary Fig. 14a ). The differences become even smaller in systems with excess generation (Supplementary Fig. 14 ).
Despite these simplifying assumptions, our results offer insights from those provided by multi-year time step integrated assessment modes (IAMs) or hourly, cost-optimized energy system models. Specifically, hourly resolution over several decades allows us to evaluate the adequacy of regional solar and wind resources independent of costs. For example, cost-optimizing models which either require renewables sources to meet a very high share of demand or else assume extremely cheap renewable costs generally find substantial increases in system costs related to, e.g., energy storage. Our geophysically-focused results help to explain such results irrespective of cost assumptions. Indeed, we compare the estimates of reliability and capacities in this study with several techno-economic studies that have used independent approaches to model regional solar- and wind-dominated electricity systems in detail 29 , 44 , 45 . In each case, focusing on the U.S., these studies find that the share of non-emitting (or carbon neutral) electricity contributed by solar and wind in cost-optimized systems is typically ~80%, with the residual demand for non-emitting generation met by firmer renewables such as biomass, hydroelectricity, and geothermal 29 , 44 , 45 .
Variable solar and wind energy are projected by many to be the dominant sources of electricity in net-zero emissions energy systems of the future. With solar and wind capacities sized such that total annual generation meets total annual demand, seasonal and daily complementarities of these resources make them capable of meeting three-quarters of hourly electricity demand in larger countries. Increasing the share of demand that can be met by solar and wind generation will require either “overbuilding” (i.e., excess annual generation), the introduction of large-scale energy storage, and/or aggregating resources across multinational regions (Supplementary Data 6 ). We highlight the geophysical considerations related to these options, but economics and geopolitics will also strongly influence which strategies are ultimately adopted and are therefore important topics for further research. Our analysis for the 39-year record of solar and wind resources is in part to obtain a statistically significant analysis of interannual variability and rare events (such as prolonged storms). Establishing estimates for interannual variability and the frequency of rare events that impact solar and wind generation potential is important when considering the lifetime of the capital asset stock in an electricity grid and requires the use of many years of data. Our normalized analysis of the reliability for purely solar and wind supplied electricity system would apply as well to a system with other slowly time-varying generation (e.g., coal, hydro, geothermal, or nuclear) because the variability of solar and wind generation and related long-duration gaps in electricity supply will have to be managed either by ramping backup technologies up and down or by curtailing excess solar and wind generation. Our results reveal national and regional differences in solar and wind resources that may be useful to decision makers and researchers prioritizing their investments in pursuit of reliable and cost-effective electricity systems based predominantly on solar and wind energy.
Hourly solar and wind capacity factors
The capacity factor describes the actual energy output as compared to the systems’ rated energy output (power capacity multiplied by 1 h) 12 . To calculate the wind and solar capacity factors for this study, we first obtained the hourly climatology data from the Modern-Era Retrospective analysis for Research and Application, Version-2 (MERRA-2) reanalysis product, which spans 39 years (1980–2018) and has a horizontal resolution of 0.5° by latitude [−90–90°] and 0.625° by longitude [−180–179.375°] with 361 × 576 grid cells worldwide 21 , 22 . Here we used the surface incoming shortwave flux [W m −2 ] (variable name: SWGDN), top-of-atmosphere incoming shortwave flux [W m −2 ] (variable name: SWTDN), and surface air temperature [K] (variable name: T) for deriving solar capacity factors; and wind speed at 100 m [m s −1 ], estimated based on wind speed at 10 m and 50 m (variable names: U10M, V10M, U50M, and V50M) and a power-law relationship, to derive wind capacity factors. Wind and solar capacity factors were calculated for each grid cell and each hour. Each raw data point (an hourly energy density (solar) or wind speed (wind) value at a specific location and time) was then converted into the corresponding capacity factor based on the following procedures.
For solar capacity factor, we first calculated the solar zenith angle and the solar incidence angle for each grid based on the latitude/longitude location and local time 46 , 47 , and then estimated the in-panel solar radiation 48 . Here we separated the direct and diffuse solar radiation components based on an empirical piecewise model 49 that takes into account both ratios of surface to top-of-atmosphere solar radiation (i.e., the clearness index) and the local zenith angle. We assumed a horizontal single-axis tracking system (north-south direction) with a tilt of the solar panel to be 0° and a maximum tuning angle of 45°. Solar power output from a given panel is calculated using the performance model described by Huld et al. 50 and Pfenninger and Staffell 51 , which considers both the surrounding temperature and the effect of irradiance. It is noted that we assumed the single-axis trackers for calculating solar capacity factors, which may be unsuitable enough for the small countries such as Japan, South Korea, and United Kingdom. These small countries do not have enough uncommitted land area for that and are going very likely to have to favor no tracking with rooftop photovoltaic system. Therefore, we further assessed the impacts of different solar tracking systems (i.e., single-axis and dual-axis for both a horizontal and a vertical axis) on the electricity system reliability. The detailed comparisons are shown in the SI (Supplementary Note 3 and Supplementary Fig. 10 ).
For wind capacity factor, by assuming a wind turbine hub height of 100 m, the raw wind speed data is first interpolated to 100 m by employing a power law, based on wind speed at 10 and 50 m. The 100-m-height wind speed is estimated by employing the following Eqs. ( 1 ) and ( 2 ):
where \(i,\alpha\) represent grid and alpha exponent for wind profile, and \({U}_{10},{U}_{50},\) and \({U}_{100}\) represent wind speed at 10, 50, and 100 m.
The wind capacity factor calculation employed a piecewise function consisting of four parts: (i) below a cut-in speed ( \({u}_{{ci}}\) ) of 3 m s −1 the capacity factor is zero, (ii) between the cut-in speed of 3 m s −1 and rated speed ( \({u}_{r}\) ) of 12 m s −1 the capacity factor is \({{u}_{{ci}}}^{3}/{{u}_{r}}^{3}\) , (iii) between the rated speed of 12 m s −1 and the cut-out speed ( \({u}_{{co}}\) ) of 25 m s −1 the capacity factor is 1.0, and (iv) above the cut-out speed of 25 m s −1 the capacity factor is zero 12 , 52 , 53 . The process yielded the solar and wind capacity factors for each grid cell and each hour.
An area-weighed mean hourly energy generation profile was created for the solar and wind resources individually for each region of interest. In this work, hourly solar and wind capacity factors for 168 countries/regions were produced. Capacity factors derived from reanalysis data were known to differ from real-world systems 12 , 54 , and thus these calculated capacity factors from the reanalysis dataset were rescaled. That is, the reanalysis data were used herein only for reflecting the temporal and spatial characteristics of the resource. For consistency, we normalized capacity factor values using the 25th percentile calculated capacity factor data for a region of interest due to data availability of real-world wind and solar capacity factors from public datasets or reports for all the countries and regions of interest. Our estimates represent real-world wind and solar capacity factors that are in good agreement with available observational data 55 . We then obtained the time-series hourly normalized wind and solar capacity factor dataset at the country/region level.
Country-level hourly electricity demand data
In this work, country-level hourly electricity demand data were estimated in various ways, such as from government and electricity market websites, public power systems datasets, and previous studies (Supplementary Data 1 ). As shown in Supplementary Data 1 , we compiled 168 countries and regions’ demand data, including real-word hourly demand data of 62 countries and regions, and projected hourly demand data of the rest due to data availability 56 . Toktarova et al. developed a multiple linear regression model to project electricity demand in hourly resolution for all countries globally by incorporating 57 real load data profiles of diverse countries to analyze the cyclical pattern of the data. In addition, given the different self-consistent continuous gapless time series of hourly electricity demand among different countries and regions, a single latest year of hourly electricity demand data was used in our following simulations to investigate the impact of diversity of solar and wind resources across years on power system reliability for countries and regions with available real-world electricity data. For the rest of the countries and regions, we chose the hourly electricity demand data of the most recent year of future (i.e., the year of 2020) from the projection model 56 . We herein obtained the country-level demand dataset by joining the 1-year hourly demand data together 39 times to form a 39-year record consistent with the resource data. In addition, for regional demand data, we combined all the demand data of available countries within the according region at hourly scale to represent the temporal characteristics.
Simulation design
A set of forward simulations were performed to track the ability of wind, solar installed capacity, and energy storage, if present, to meet demand in every hour. In this study, we used a Macro Energy Model (MEM), which is developed for optimizing electricity system (or electricity and fuels) without considering any spatial variation, policy, capacity markets 57 . Without considering any power system cost, generation technology, and transmission loss, we modeled the idealized hourly power supply process through dispatching wind and solar energy, as well as charging or discharging of storage, if present. Here we specified the wind and solar installed capacity, and storage capacity under the various capacity mixes of solar and wind fractions (i.e., every 5% change of solar fraction from 0% solar and 100% wind to 100% solar and 0% wind) and different levels of excess annual generation (i.e., 1x, 1.5x, and 3x generation) and energy storage (i.e., maximun 3 and 12 h of charging time) assumptions. The installed capacities for solar, wind, and storage for individual countries/regions are estimated using the Eqs. ( 3 )–( 5 ).
where \(y\) and \(s\) represent the year and size, respectively. \({{{{Capacity}}}}_{{{{solar}}}}\) , \({{{{Capacity}}}}_{{{{wind}}}}\) , and \({{{{Capacity}}}}_{{{{{{{\mathrm{storage}}}}}}}}\) represent the solar, wind, and storage capacities, respectively. \({{{{{\rm{SF}}}}}}\) represents the fraction of energy generated from solar (from 0 to 100% at intervals of 5%); \({{{{{\rm{OB}}}}}}\) represents the overbuilding of capacity, equaling 1, 1.5, or 3; \({{{{{\rm{Pwr}}}}}}\_{{{{{\rm{avg}}}}}}\) represents the mean power demand; \({{{{{\rm{Hrs}}}}}}\) represents the total hours in the year; \({{{{{\rm{CF}}}}}}_{{solar}}\) and \({{{{{{\rm{CF}}}}}}}_{{{{wind}}}}\) represent normalized capacity factors of solar and wind, respectively. And \({{{{{\rm{Bat}}}}}}\) represents battery storage, equaling 0 (i.e., no storage), 3, or 12.
When storage was assumed to be available, we assumed the initial status of storage was the same as the final status for each year, which means the charging and discharging process is balanced. We also assumed a storage charging round-trip efficiency and storage decay rate of, respectively, 90% and 1.14 × 10 −6 per hour (i.e., 1% of stored electricity lost per month) 2 , reflecting the high-end performance of current batteries 58 , 59 . Dispatchable energy used to charge a battery (called the maximum hourly storage charging) was no more than the storage power rating, equaling storage capacity divided by storage charging time.
Given the restriction of computing resources, we chose ten major countries by comprehensively considering the electricity demand and growth domestic product (GDP) from each continent except Oceania (i.e., Asia, Africa, Europe, and the America), within which only two main countries were selected (i.e., Australia and New Zealand). For each main country, 21 sets of the solar and wind mix from 0% solar and 100% wind to 100% solar and 0% wind with 5% change under 3 groups of overbuilt (1x, 1.5x, and 3x generation) and 3 groups of storage (no storage, 3 h, and 12 h of storage) were simulated, totaling 7938 simulations for all the main countries. To investigate the ability to supply power at multinational regions, continental, and intercontinental scales, we further applied the same simulation design for the main countries to multinational regions, continents, and multi-continental regions (Supplementary Data 4 ). In addition, except the abovementioned main countries, 103,194 one-year simulations consisting of 21 sets of the solar and wind mix with no excess generation or energy storage, were added for each of the remaining 126 countries worldwide.
Hourly electricity supply process
For only solar-wind electricity systems without storage, in a given hour, the MEM model estimates the ability of power to be produced by assessing whether dispatchable solar and wind energy is no less than electricity demand. Excess solar and wind energy can be curtailed due to no available storage. 100% reliability results if the solar and wind power supply system can meet all the electricity demand in every hour of the simulation.
When storage is assumed to be available in a given hour, if the solar and wind energy could meet the electricity demand, storage would be charged with excess solar and wind generation, if available, until the storage is full under the constraint of the maximum hourly storage charging, after which solar and wind energy can be curtailed. In contrast, if wind and solar energy cannot meet electricity demand, storage would be discharged to fill the power supply gap until storage is emptied or the power supply gap is filled.
Here, we define reliability assuming electricity systems use only wind/solar/storage resources to meet current demand for electricity services. If one allows for other backup electricity (e.g., using natural gas with or without CCS), then issues of reliability with excess annual generation and/or storage are largely moot.
Data availability
The electricity demand, solar, and wind capacity factors data generated for this study have been deposited in Dantong2021/Geophysical_constraints: Data of electricity demand, solar and wind capacity factors (v1.0). Zenodo. https://doi.org/10.5281/zenodo.5463202 .
Code availability
The Macro Electricity Model (MEM) code is available on GitHub via https://github.com/ClabEnergyProject/MEM .
Rogelj, J. et al. Energy system transformations for limiting end-of-century warming to below 1.5 °C. Nat. Clim. Change 5 , 519–527 (2015).
Article ADS Google Scholar
Davis, S. J. et al. Net-zero emissions energy systems. Science 360 , eaas9793 (2018).
Article PubMed CAS Google Scholar
Tong, D. et al. Committed emissions from existing energy infrastructure jeopardize 1.5 °C climate target. Nature 572 , 373–377 (2019).
Article CAS PubMed PubMed Central Google Scholar
Luderer, G. et al. The role of renewable energy in climate stabilization: results from the EMF27 scenarios. Clim. Change 123 , 427–441 (2014).
Millstein, D., Wiser, R., Bolinger, M. & Barbose, G. The climate and air-quality benefits of wind and solar power in the United States. Nat. Energy 2 , 17134 (2017).
Pehl, M. et al. Understanding future emissions from low-carbon power systems by integration of life-cycle assessment and integrated energy modelling. Nat. Energy 2 , 939–945 (2017).
Article ADS CAS Google Scholar
Grubler, A. et al. A low energy demand scenario for meeting the 1.5 °C target and sustainable development goals without negative emission technologies. Nat. Energy 3 , 515–527 (2018).
Rogelj, J. et al. In Global warming of 1.5 °C. An IPCC Special Report on the Impacts of Global warming of 1.5 °C above Pre-Industrial Levels and Related Global Greenhouse Gas Emission Pathways, in the Context of Strengthening the Global Response to the Threat of Climate Change, Sustainable Development, and Efforts to Eradicate Poverty (eds Masson-Delmotte, V. et al.) https://www.ipcc.ch/site/assets/uploads/sites/2/2019/05/SR15_Chapter2_Low_Res.pdf (2018).
Rogelj, J. et al. Scenarios towards limiting global mean temperature increase below 1.5 °C. Nat. Clim. Change 8 , 325–332 (2018).
Luderer, G. et al. Environmental co-benefits and adverse side-effects of alternative power sector decarbonization strategies. Nat. Commun. 10 , 1–13 (2019).
Article CAS Google Scholar
Drechsler, M. et al. Efficient and equitable spatial allocation of renewable power plants at the country scale. Nat. Energy 2 , 17124 (2017).
Shaner, M. R., Davis, S. J., Lewis, N. S. & Caldeira, K. Geophysical constraints on the reliability of solar and wind power in the United States. Energy Environ. Sci. 11 , 914–925 (2018).
Article Google Scholar
Jacobson, M. Z. et al. 100% clean and renewable wind, water, and sunlight all-sector energy roadmaps for 139 countries of the world. Joule 1 , 108–121 (2017).
Heard, B. P., Brook, B. W., Wigley, T. M. L. & Bradshaw, C. J. A. Burden of proof: a comprehensive review of the feasibility of 100% renewable-electricity systems. Renew. Sust. Energ. Rev. 76 , 1122–1133 (2017).
Arbabzadeh, M., Sioshansi, R., Johnson, J. X. & Keoleian, G. A. The role of energy storage in deep decarbonization of electricity production. Nat. Commun. 10 , 1–11 (2019).
CAS Google Scholar
Sepulveda, N. A., Jenkins, J. D., de Sisternes, F. J. & Lester, R. K. The role of firm low-carbon electricity resources in deep decarbonization of power generation. Joule 2 , 2403–2420 (2018).
Budischak, C. et al. Cost-minimized combinations of wind power, solar power and electrochemical storage, powering the grid up to 99.9% of the time. J. Power Sources 225 , 60–74 (2013).
Planning Resource Adequacy Analysis, Assessment and Documentation (Standard BAL-502-RF-03). https://www.nerc.com/pa/Stand/Reliability%20Standards/BAL-502-RF-03.pdf (2017).
Carden. K., Wintermantel, N. & Pfeifenberger J. The Economics of Resource Adequacy Planning: Why Reserve Margins Are Not Just About Keeping the Lights On (National Regulatory Research Institute, 2011).
Jurasz, J., Canales, F. A., Kies, A., Guezgouz, M. & Beluco, A. A review on the complementarity of renewable energy sources: concept, metrics, application and future research directions. Sol. Energy 195 , 703–724 (2020).
Modern-Era Retrospective analysis for Research and Applications, Version 2 (MERRA-2). https://gmao.gsfc.nasa.gov/reanalysis/MERRA-2/ (2021).
Gelaro, R. et al. The modern-era retrospective analysis for research and applications, version 2 (MERRA-2). J. Clim. 30 , 5419–5454 (2017).
Article ADS PubMed Google Scholar
Lee, J. C. Y., Fields, M. J. & Lundquist, J. K. Assessing variability of wind speed: comparison and validation of 27 methodologies. Wind Energy Sci . 3 , 845–868 (2018).
Hyndman, R. J. & Fan, S. Density forecasting for long-term peak electricity demand. IEEE T. Power Syst. 25 , 1142–1153 (2010).
Mai, T., Sandor, D., Wiser, R. & Schneider, T. Renewable Electricity Futures Study: Executive Summary Vol. 55, https://www.nrel.gov/docs/fy13osti/52409-ES.pdf (2012).
Brinkerink, M., Gallachóir, B. Ó. & Deane, P. A comprehensive review on the benefits and challenges of global power grids and intercontinental interconnectors. Renew. Sust. Energ. Rev. 107 , 274–287 (2019).
Craig, M. Germany’s worse-case scenario in the power sector. https://energytransition.org/2017/07/germanys-worse-case-scenario-in-the-power-sector/#more-15335 (2017).
Dowling, J. A. et al. Role of long-duration energy storage in variable renewable electricity systems. Joule 4 , 1907–1928 (2020).
Larson, E. et al. Net-Zero America: Potential Pathways, Infrastructure, and Impacts . Interim report, Princeton University, Princeton, NJ (2020).
Jourdier, B. Evaluation of ERA5, MERRA-2, COSMO-REA6, NEWA and AROME to simulate wind power production over France. Adv. Sci. Res. 17 , 63 (2020).
Sianturi, Y., Marjuki & Sartika, K. Evaluation of ERA5 and MERRA2 reanalyses to estimate solar irradiance using ground observations over Indonesia region. AIP Conf. Proc. 2223 , 020002 (2020).
Battery Megafactory Forecast: 400% Increase in Capacity to 1 TWh by 2028. https://www.visualcapitalist.com/battery-megafactory-forecast-1-twh-capacity-2028/ (2018).
The International Renewable Energy Agency (IRENA): Renewable Capacity Statistics 2018. https://www.hydroreview.com/2018/04/10/data-source-irena-releases-renewable-capacity-statistics-2018-report/#gref (2018).
Braff, W. A., Mueller, J. M. & Trancik, J. E. Value of storage technologies for wind and solar energy. Nat. Clim. Change 6 , 964–969 (2016).
Kittner, N., Lill, F. & Kammen, D. M. Energy storage deployment and innovation for the clean energy transition. Nat. Energy 2 , 17125 (2017).
Sepulveda, N. A., Jenkins, J. D., Edington, A., Mallapragada, D. S. & Lester, R. K. The design space for long-duration energy storage in decarbonized power systems. Nat. Energy 6 , 506–516 (2021).
Ziegler, M. S. et al. Storage requirements and costs of shaping renewable energy toward grid decarbonization. Joule 3 , 2134–2153 (2019).
Wu, G. C. et al. Strategic siting and regional grid interconnections key to low-carbon futures in African countries. Proc. Natl Acad. Sci. USA 114 , E3004–E3012 (2017).
CAS PubMed PubMed Central Google Scholar
Clack, C. T. M. et al. Evaluation of a proposal for reliable low-cost grid power with 100% wind, water, and solar. Proc. Natl Acad. Sci. USA 114 , 6722–6727 (2017).
Article ADS CAS PubMed PubMed Central Google Scholar
Barasa, M., Bogdanov, D., Oyewo, A. S. & Breyer, C. A cost optimal resolution for Sub-Saharan Africa powered by 100% renewables in 2030. Renew. Sust. Energ. Rev. 92 , 440–457 (2018).
Geels, F. W., Sovacool, B. K., Schwanen, T. & Sorrell, S. The socio-technical dynamics of low-carbon transitions. Joule 1 , 463–479 (2017).
Lauber, V. & Jacobsson, S. The politics and economics of constructing, contesting and restricting socio-political space for renewables—The German Renewable Energy Act. Environ. Innov. Soc. Tr. 18 , 147–163 (2016).
Mai, T. et al. Electrification Futures Study: Scenarios of Electric Technology Adoption and Power Consumption for the United States . National Renewable Energy Laboratory. NREL/TP-6A20-71500. https://doi.org/10.2172/1459351 (2018).
Williams, J. H. et al. Carbon-neutral pathways for the United States. AGU Adv. 2 , e2020AV000284 (2021).
MacDonald, A. E. et al. Future cost-competitive electricity systems and their impact on US CO 2 emissions. Nat. Clim. Change 6 , 526–531 (2016).
Braun, J. E. & Mitchell, J. C. Solar geometry for fixed and tracking surfaces. Sol. Energy 31 , 439–444 (1983).
Meeus, J. H. Astronomical Algorithms (Willmann-Bell, Incorporated, 1991).
Perez, R., Ineichen, P., Seals, R., Michalsky, J. & Stewart, R. Modeling daylight availability and irradiance components from direct and global irradiance. Sol. Energy 44 , 271–289 (1990).
Reindl, D. T., Beckman, W. A. & Duffie, J. A. Diffuse fraction correlations. Sol. Energy 45 , 1–7 (1990).
Huld, T., Gottschalg, R., Beyer, H. G. & Topič, M. Mapping the performance of PV modules, effects of module type and data averaging. Sol. Energy 84 , 324–338 (2010).
Pfenninger, S. & Staffell, I. Long-term patterns of European PV output using 30 years of validated hourly reanalysis and satellite data. Energy 114 , 1251–1265 (2016).
Bett, P. E. & Thornton, H. E. The climatological relationships between wind and solar energy supply in Britain. Renew. Energy 87 , 96–110 (2016).
Rose, S. & Apt, J. Quantifying sources of uncertainty in reanalysis derived wind speed. Renew. Energy 94 , 157–165 (2016).
Staffell, I. & Pfenninger, S. Using bias-corrected reanalysis to simulate current and future wind power output. Energy 114 , 1224–1239 (2016).
Form EIA-860 detailed data with previous form data (EIA-860A/860B). https://www.eia.gov/electricity/data/eia860/ (2019).
Toktarova, A., Gruber, L., Hlusiak, M., Bogdanov, D. & Breyer, C. Long term load projection in high resolution for all countries globally. Int. J. Elec. Power 111 , 160–181 (2019).
Macro Energy Model (MEM). https://github.com/ClabEnergyProject/MEM (2019).
Pellow, M. A., Emmott, C. J., Barnhart, C. J. & Benson, S. M. Hydrogen or batteries for grid storage? A net energy analysis. Energy Environ. Sci. 8 , 1938–1952 (2015).
Wang, H., Jiang, Y. & Manthiram, A. Long cycle life, low self-discharge sodium–selenium batteries with high selenium loading and suppressed polyselenide shuttling. Adv. Energy Mater. 8 , 1701953 (2018).
Download references
Acknowledgements
This work was supported by the National Natural Science Foundation of China (41921005). D.T., D.J.F., L.D., and K.C. were supported by the Carnegie Institution for Science endowment and a gift from Gates Ventures, Inc. S.J.D. was supported by the US National Science Foundation (Innovations at the Nexus of Food, Energy and Water Systems (INFEWS) grant EAR 1639318).
Author information
Authors and affiliations.
Ministry of Education Key Laboratory for Earth System Modeling, Department of Earth System Science, Tsinghua University, Beijing, 100084, China
Dan Tong & Qiang Zhang
Department of Earth System Science, University of California, Irvine, CA, 92697, USA
Dan Tong & Steven J. Davis
Department of Global Ecology, Carnegie Institution for Science, Stanford, CA, 94035, USA
Dan Tong, David J. Farnham, Lei Duan, Nathan S. Lewis, Ken Caldeira & Steven J. Davis
Division of Chemistry and Chemical Engineering, California Institute of Technology, Pasadena, CA, 91125, USA
Nathan S. Lewis
Breakthrough Energy, 4110 Carillon Pt., Kirkland, WA, 98033, USA
Ken Caldeira
Department of Civil and Environmental Engineering, University of California, Irvine, CA, 92697, USA
Steven J. Davis
You can also search for this author in PubMed Google Scholar
Contributions
K.C., N.S.L., S.J.D., and D.T. designed the study. D.T. performed the analyses, with support from D.J.F. on simulations and L.D. on resources estimates, and from S.J.D., Q.Z., N.S.L., and K.C. on analytical approaches. D.T. and S.J.D. led the writing with input from all coauthors.
Corresponding author
Correspondence to Dan Tong .
Ethics declarations
Competing interests.
The authors declare no competing interests.
Additional information
Peer review information Nature Communications thanks Jesse Jenkin and the other, anonymous, reviewer(s) for their contribution to the peer review of this work. Peer reviewer reports are available.
Publisher’s note Springer Nature remains neutral with regard to jurisdictional claims in published maps and institutional affiliations.
Supplementary information
Supplementary information, peer review file, description of additional supplementary files, supplementary data 1, supplementary data 2, supplementary data 3, supplementary data 4, supplementary data 5, supplementary data 6, rights and permissions.
Open Access This article is licensed under a Creative Commons Attribution 4.0 International License, which permits use, sharing, adaptation, distribution and reproduction in any medium or format, as long as you give appropriate credit to the original author(s) and the source, provide a link to the Creative Commons license, and indicate if changes were made. The images or other third party material in this article are included in the article’s Creative Commons license, unless indicated otherwise in a credit line to the material. If material is not included in the article’s Creative Commons license and your intended use is not permitted by statutory regulation or exceeds the permitted use, you will need to obtain permission directly from the copyright holder. To view a copy of this license, visit http://creativecommons.org/licenses/by/4.0/ .
Reprints and permissions
About this article
Cite this article.
Tong, D., Farnham, D.J., Duan, L. et al. Geophysical constraints on the reliability of solar and wind power worldwide. Nat Commun 12 , 6146 (2021). https://doi.org/10.1038/s41467-021-26355-z
Download citation
Received : 24 February 2021
Accepted : 24 September 2021
Published : 22 October 2021
DOI : https://doi.org/10.1038/s41467-021-26355-z
Share this article
Anyone you share the following link with will be able to read this content:
Sorry, a shareable link is not currently available for this article.
Provided by the Springer Nature SharedIt content-sharing initiative
This article is cited by
Amplified positive effects on air quality, health, and renewable energy under china’s carbon neutral target.
Nature Geoscience (2024)
- Anders Wörman
- Ilias Pechlivanidis
- Cintia Bertacchi Uvo
Communications Engineering (2024)
An assessment of different transition pathways to a green global economy
- Soheil Shayegh
- Severin Reissl
- Matteo Calcaterra
Communications Earth & Environment (2023)
Reliance on fossil fuels increases during extreme temperature events in the continental United States
- Pierre Gentine
Global transcontinental power pools for low-carbon electricity
- Haozhe Yang
- Ranjit Deshmukh
- Sangwon Suh
Nature Communications (2023)
By submitting a comment you agree to abide by our Terms and Community Guidelines . If you find something abusive or that does not comply with our terms or guidelines please flag it as inappropriate.
Quick links
- Explore articles by subject
- Guide to authors
- Editorial policies
Sign up for the Nature Briefing newsletter — what matters in science, free to your inbox daily.

- Election 2024
- Entertainment
- Newsletters
- Photography
- Personal Finance
- AP Investigations
- AP Buyline Personal Finance
- AP Buyline Shopping
- Press Releases
- Israel-Hamas War
- Russia-Ukraine War
- Global elections
- Asia Pacific
- Latin America
- Middle East
- Election Results
- Delegate Tracker
- AP & Elections
- Auto Racing
- 2024 Paris Olympic Games
- Movie reviews
- Book reviews
- Personal finance
- Financial Markets
- Business Highlights
- Financial wellness
- Artificial Intelligence
- Social Media
New industry readies for launch as researchers hone offshore wind turbines that float
Researchers are racing to create large wind turbines and floating platforms, bringing energy from offshore wind closer to reality. (AP Video: Robert Bukaty)
FILE - The University of Maine’s first prototype of an offshore wind turbine is seen in this Sept. 20, 2013 file photo, near Castine Maine. (AP Photo/Robert F. Bukaty, files)
- Copy Link copied
Habib Dagher, director of the advanced composites center, center, works with research assistants Ryan Thornton, left, and Mitchell Kelley, at the University of Maine, Wednesday, March 27, 2024, at the University of Maine, in Orono, Maine. (AP Photo/Robert F. Bukaty)
A 13-foot-tall wind turbine is tested for stability in a wave pool, Wednesday, March 27, 2024, at the University of Maine, in Orono, Maine. The waves simulate worst possible conditions for an offshore wind turbine on a 1:70 scale. (AP Photo/Robert F. Bukaty)
A computer graphic shows stability tests on a 13-foot-tall wind turbine in a wave pool, Wednesday, March 27, 2024, at the University of Maine, in Orono, Maine. (AP Photo/Robert F. Bukaty)
A 13-foot-tall wind turbine floats in a wave pool, Wednesday, March 27, 2024, at the University of Maine, in Orono, Maine. At UMaine more than 50 engineers comprise the largest floating offshore wind team in the U.S.(AP Photo/Robert F. Bukaty)
Anthony Viselli, manager of offshore model testing and structure design, left, and Habib Dagher, director of the advanced composites center, examine used anchor cables used for securing floating offshore wind turbines to the ocean floor, Wednesday, March 27, 2024, at the University of Maine, in Orono, Maine. (AP Photo/Robert F. Bukaty)
A bank of powerful fans and 16 mechanical paddles are used to simulate in a pool the worst conditions at sea, Wednesday, March 27, 2024, at the University of Maine, in Orono, Maine. (AP Photo/Robert F. Bukaty)
A figure represents a human standing on a vertical column used to stabilize a floating wind turbine in the wave pool, Wednesday, March 27, 2024, at the University of Maine, in Orono, Maine. (AP Photo/Robert F. Bukaty)
ORONO, Maine (AP) — As waves grew and gusts increased, a wind turbine bobbed gently, its blades spinning with a gentle woosh. The tempest reached a crescendo with little drama other than splashing water.
The uneventful outcome is exactly what engineers aimed for.
The demonstration featuring a 13-foot-tall (4-meter-tall) floating wind turbine in an indoor pool aimed to ensure it can withstand the strain of powerful water and wind when much larger versions are deployed in the ocean.
It’s the University of Maine’s contribution to a worldwide race to improve floating machines to tap wind that blows across deeper waters offshore, too deep to attach turbines to the seabed with permanent pilings.
In the next decade, UMaine researchers said, they envision turbine platforms floating in the ocean beyond the horizon, stretching more than 700 feet (210 meters) skyward and anchored with mooring lines.
“These structures are massive,” said Anthony Viselli, chief engineer for offshore wind technology at the university’s Advanced Composites Center, after the demonstration wrapped up. “These would be some of the largest moving structures that humankind has endeavored to create. And there would be many of them.”
As the technology advances, dozens of designs are being promoted by experts who see floating wind turbines as a way to address climate change by shifting away from burning fossil fuels.
Floating turbines are the only way some countries and U.S. states can capture offshore wind energy on a large scale. In the U.S. alone, 2.8 terawatts of wind energy potential blows over ocean waters too deep for traditional turbines that affix to the ocean floor, according to the National Renewable Energy Laboratory . That’s enough to power 350 million homes — more than double the number of existing homes in the U.S.
The first floating wind farm started operating off Scotland’s coast in 2017. In the United States, the Department of Interior two weeks ago proposed the first floating wind energy auctions for the Gulf of Maine, following lease auctions for the West Coast that began in 2022 . The nearly 1 million acres up for auction off the New England coast could generate enough clean wind energy to power more than 5 million local homes, the department said.
UMaine is home to the nation’s largest team of engineers dedicated to floating offshore wind. Other big players include Equinor, which has installed a demonstration floating project of the coast of Norway; global company Principle Power, which has installed small-scale projects off Scotland and Portugal; and SBM Offshore, which has a demonstration project off France.
Floating offshore wind is still a nascent industry, however, making it expensive.
The Norwegian company Equinor postponed its Trollvind floating initiative , citing technology availability, rising costs and a strained timetable to deliver on the original concept.
Danish wind energy developer Ørsted decided to focus its efforts on fixed-bottom turbines, foregoing deeper offshore regions including Japan, Norway, Spain, Portugal and the U.S. West Coast. “We care a lot about affordability of renewable power, and floating wind is a lot more expensive than bottom-fixed,” said CEO Mads Nipper.
But others are moving forward.
Gazelle Wind Power is developing a modular platform system to make manufacture and assembly cost-effective and efficient.
“This is a global problem and this is an ideal solution in order to deliver power to shore,” said Gazelle Wind Power CEO Jon Salazar.
UMaine launched its first floating prototype a decade ago and became a surprise global leader after a study showed that the Gulf of Maine had the wind energy potential of 156 nuclear power plants, due to fast, consistent wind.
The state could meet all its home heating needs and power every car — if they were all electric vehicles — by tapping just 3% of that water. That improves the odds of successfully sharing the resource with fishermen, recreational boaters, the military and, of course, marine life . Indeed, the federal government’s lease proposal spares Maine’s key lobstering grounds from development, removing a potential obstacle.
Trailblazers in offshore wind are benefiting from work done by the oil industry, which engineered floating oil and gas rigs, said Habib Dagher, director of the Advanced Composites Center.
The university’s wind wave basin, which looks something like a swimming pool with wave and wind generators that can mimic ocean conditions up to a 500-year storm, takes that work to the next level.
On a recent day, the semi-submersible floating turbine was tethered to the bottom of the basin. Its 1:70 scale represented a real turbine standing about 800 feet (240 meters) tall atop a platform in the ocean. The goal is to have industrial scale turbines of 15 to 20 megawatts each, Dagher said.
Size and efficiency are keys to profitability. Larger wind turbines mean fewer are needed, reducing construction, installation and maintenance costs, Viselli said. With greater size and efficiency, developers envision only about 50 turbines needed to produce about the same amount of electricity as a nuclear power plant.
Full-size turbines generate peak power starting at about 20 mph (32 kph). In powerful storms, they shut down automatically to avoid stressing the equipment or breaking. The mooring lines tethered to the ocean floor are made of rope nearly thick as a telephone pole and under heavy tension. That makes them safer for marine mammals.
For all the turbine technology, the platforms developed by UMaine can be built locally with concrete, a simple material that’s readily available. The university already has partners around the world interested in licensing its technology. The state of Maine plans to develop a port facility in the Searsport area to build the floating bases and attach turbines before sending them into the Gulf of Maine.
A brand new industry means some experiments in design will succeed and some will fail. And there is work to ensure that wind farms are good neighbors, overcoming objections from others using the ocean.
“We’re going to have some problems and we have to figure out how to roll up our sleeves and solve these problems,” Dagher said. “And I think we have no choice as a society but to do that.”
McDermott reported from Providence, Rhode Island.
The Associated Press’ climate and environmental coverage receives financial support from multiple private foundations. AP is solely responsible for all content. Find AP’s standards for working with philanthropies, a list of supporters and funded coverage areas at AP.org .

EDITORIAL article
This article is part of the research topic.
Ionospheric signatures of magnetic reconnection in Earth’s magnetosphere
Editorial: Ionospheric Signatures of Magnetic Reconnection in Earth's Magnetosphere Provisionally Accepted
- 1 Goddard Space Flight Center, National Aeronautics and Space Administration, United States
- 2 Lockheed Martin Solar and Astrophysics Laboratory (LMSAL), United States
- 3 Independent Contractor, Canada
The final, formatted version of the article will be published soon.
emergence of a "standard model" of magnetic reconnection describing the conversion of magnetic energy 5 into plasma energy at spatially localized diffusion regions (e.g., see the review by (Hesse and Cassak, 2019)). The first paper in our collection, by (Fasel et al., 2024), presents evidence that Poleward Moving Auroral elevation angle of the PMAF, as observed by the MSP), with respect to time: the PMAF-SLOPE. The 33 PMAF-SLOPE is then plotted as a function of both the solar wind speed and the X (GSE) component of 34 the solar wind velocity vector, showing a very strong correlation in both cases. This strongly suggests 35 that the PMAF is a set of open field lines that maintains a connection to the reconnection source for some 36 period of time as it is convected Earthward over the polar cap by the solar wind. More observations such as 37 these, in which multiple ionospheric signatures are correlated with solar wind parameters, will be needed 38 to constrain global MHD models of three-dimensional magnetopause reconnection. Further, predicting 39 the auroral emission associated with PMAF will require extending MHD models to incorporate particle 40 precipitation from the reconnection sites to the ionosphere. One might expect reconnection generated particle precipitation to have an impact on the ionospheric 42 conductance, which in turn will impact ionospheric currents and electric fields in an observable way. Currently, global MHD models of Earth's magnetosphere do not incorporate all the kinetic processes in the 44 chain of events leading from magnetospheric particle precipitation and FAC to ionospheric currents and
Keywords: magnetic reconnection, aurora, ionospheric precipitation, Global Magnetosphere Simulations, field aligned currents
Received: 10 May 2024; Accepted: 14 May 2024.
Copyright: © 2024 Dorelli, Connor, Petrinec and Murphy. This is an open-access article distributed under the terms of the Creative Commons Attribution License (CC BY) . The use, distribution or reproduction in other forums is permitted, provided the original author(s) or licensor are credited and that the original publication in this journal is cited, in accordance with accepted academic practice. No use, distribution or reproduction is permitted which does not comply with these terms.
* Correspondence: Mx. John C. Dorelli, Goddard Space Flight Center, National Aeronautics and Space Administration, Greenbelt, United States
People also looked at
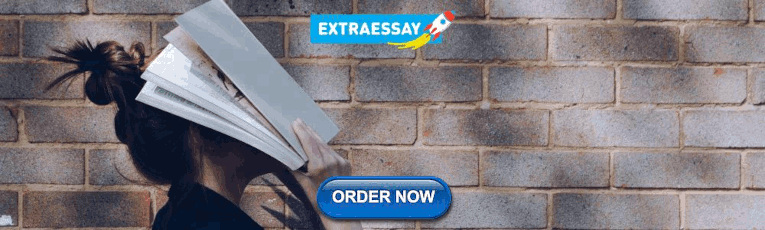
COMMENTS
Wind Energy: A Review Paper. Atul Kumar Muhammad Zafar Ullah Khan Bishwajeet Pandey. M S College, University of Management & Gyancity Rese arch Lab, 1 Motihari, India Technology, Sialkot Campus ...
This study applied a rigorous and reproducible SLNA methodology related to wind power production that aims to answer six research questions formulated at the introduction. Table 13 summarizes the outputs obtained for each one of the Research Questions (RQs) drawn in the Introduction. Table 13.
This paper reviews the wind energy technologies used, mainly focusing on the types of turbines used and their future scope. Further, the paper briefly discusses certain future wind generation technologies, namely airborne, offshore, smart rotors, multi-rotors, and other small wind turbine technologies.
Drawing from a recent international workshop, we identify three grand challenges in wind energy research that require further progress from the scientific community: (i) improved understanding of the physics of atmospheric flow in the critical zone of wind power plant operation, (ii) materials and system dynamics of individual wind turbines, and (iii) optimization and control of fleets of wind ...
Wind power now represents a major and growing source of renewable energy. Large wind turbines (with capacities of up to 6-8 MW) are widely installed in power distribution networks. Increasing numbers of onshore and offshore wind farms, acting as power plants, are connected directly to power transmission networks at the scale of hundreds of megawatts. As its level of grid penetration has ...
Wind energy's contribution to primary energy supply has increased in the past decade, while technology costs have decreased faster than predicted. New work finds that recent expert estimates of ...
This paper details improving a wind turbine blade's aerodynamic, aero-acoustic, and structural properties under different operating conditions, focusing especially on active and passive flow control devices and biomimetic adaptations. ... The current state of wind energy research reveals that the focus of a comprehensive review is frequently ...
Wind energy is a virtually carbon-free and pollution-free electricity source, with global wind resources greatly exceeding electricity demand. Accordingly, the installed capacity of wind turbines ...
Wind Energy offers a major forum for the reporting of advances in this rapidly developing technology with the goal of realising the world-wide potential to harness clean energy from land-based and offshore wind. The journal aims to reach all those with an interest in this field from academic research, industrial development through to applications, including individual wind turbines and ...
In this editorial paper, the progress and future outlook of wind energy research in two main aspects are discussed. The first aspect is in the area of wind turbine design and computations which covers engineering modeling and high-fidelity approaches. The second part of the paper discusses the usage of data-driven approaches in wind energy ...
To analyze which type of wind turbine best fits the wind resources of the investigated location in terms of power, this paper has reviewed and compared six wind turbines with three different ...
This work is adapted from two chapters in "Wind Energy for the Rest of Us" by the first author and summarizes the key characteristics of wind turbine development in tabular form, showing that the technology has converged to a common configuration: Horizontal-axis wind turbines with a three-blade rotor upwind of the tower.We introduce the metric of specific area (m 2;/kW) as a defining ...
In this research paper, wind turbines and solar modules are combined with pumped hydro storage, batteries, and green hydrogen. Energy management strategies are described for five different scenarios of hybrid renewable energy systems, based on single or hybrid storage technologies. ... Wind energy is an important renewable energy source. Rotor ...
2.1 Doubly fed induction generator-based turbine technologies. The DFIG (its configuration is shown in Figure 3) is the most recognized technology yet, and it has been installed widely since 2000.This wind energy harvesting technology uses both multiple- and single-gearbox systems; but the system with multiple gearboxes had widely gained acceptance until recent years, while single-gearbox ...
Wind publishes full research papers, communication letters and review articles. There is no restriction on the length of papers and no additional charges for color figures. ... Professor Chen is the leader of the Wind Power System Research Program at the Department of Energy Technology, Aalborg University. His main current research interests ...
A typical wind turbine is a complex piece of equipment that integrates thousands of devices and components to generate energy from the wind. From the late 1990s to the present, average turbine generation capacity has expanded considerably to supply the global demand for clean energy, with offshore-commissioned turbines expected to reach around 15 MW of nominal power by the year 2025.
This work is adapted from two chapters in "Wind Energy for the Rest of Us" by the first author and summarizes the key characteristics of wind turbine development in tabular form, showing that the technology has converged to a common configuration: Horizontal-axis wind turbines with a three-blade rotor upwind of the tower.We introduce the metric of specific area (m 2 /kW) as a defining ...
HOMER Pro Software was used in this paper to evaluate the wind energy data in the north and south of Oman and to provide well-informed guidance on the most suitable turbines for the power needs of each area. ... This price is a suitable indicator for choosing the best possible turbine for a wind power plant. In this research, the cost of energy ...
Here we specified the wind and solar installed capacity, and storage capacity under the various capacity mixes of solar and wind fractions (i.e., every 5% change of solar fraction from 0% solar ...
The aim of this review paper is to study the behaviour of the Vertical Axis Wind Turbine, present. technological state, new finding through modelling work and future direction of VAWTs. 1.6 ...
It reviews around 160 papers (2016 onwards). The papers were searched in Google Scholar, Web of Science (WoS) and Scopus websites using search keywords like 'condition monitoring wind turbine', 'artificial intelligence-based classification', 'artificial intelligence-based regression', and so on. The results were filtered by year ...
Lidar Buoy Deployment. In September 2020, WETO leveraged funding from the Bureau of Ocean Energy Management (BOEM) to deploy two offshore wind research buoys off the coast of California in water that is 2,000- 3,000 feet deep. This marks the first time the buoys are gathering meteorological and oceanographic measurements off the West Coast.
The utilization of wind power all-DC systems with DC collection and transmission is an effective solution for the extensive development of wind power in deep-sea areas. However, in the event of faults occurring in wind power all-DC systems, the fault propagation speed is extremely rapid, with a wide-ranging impact, and to date, there are no complete DC engineering references available.
Abstract. Vertical Axis Wind Turbine (VAWT) is relatively simple to implement in urban areas on ground or/and building-roofs, the development of appropriate design of VAWT will open new opportunities for the large-scale acceptance of these machines. The primary objective of this research was to design and modeling of a small-scale VAWT, which ...
Considering the physical characteristics of wind turbine wing icing, ... SUBMIT PAPER. Wind Engineering. Impact Factor: 1.5 / 5-Year Impact Factor: 1.4 . JOURNAL HOMEPAGE. SUBMIT PAPER. ... The focus of our research was on the equivalent particle roughness height correction model, and we numerically analyzed the two icing processes (frost ice ...
Floating turbines are the only way some countries and U.S. states can capture offshore wind energy on a large scale. In the U.S. alone, 2.8 terawatts of wind energy potential blows over ocean waters too deep for traditional turbines that affix to the ocean floor, according to the National Renewable Energy Laboratory.That's enough to power 350 million homes — more than double the number of ...
Considering the physical characteristics of wind turbine wing icing, icing synthesis rate, and icing type, we selected the icing type and surface roughness of ice-coated blades as sensitive parameters. The focus of our research was on the equivalent particle roughness height correction model, and we numerically analyzed the two icing processes (frost ice and clear ice) on wind turbine blade ...
The results highlighted a decrease in wind power density that ranged from −1.14 % to −0.36 % per decade, but this decrease would not significantly affect China's wind energy future. Another research was done on offshore wind power density globally by comparing the projection data of 20 years between 2080 and 2099 and 1980-1999 at ...
emergence of a "standard model" of magnetic reconnection describing the conversion of magnetic energy 5 into plasma energy at spatially localized diffusion regions (e.g., see the review by (Hesse and Cassak, 2019)). The first paper in our collection, by (Fasel et al., 2024), presents evidence that Poleward Moving Auroral elevation angle of the PMAF, as observed by the MSP), with respect to ...
From the starting point of the wind energy which it has with the wind flow, the kinetic energy of the mass in movement is presented with the equation: (4.2) E k = 1/2 m v 2 The power of wind is the speed of transport or the transformation of energy presented in the following equation: (4.3) P = dE dt = 1 2 v 2 dm dt Since the velocity of mass flow is: (4.4) dm dt = ρ A dx dt