Thank you for visiting nature.com. You are using a browser version with limited support for CSS. To obtain the best experience, we recommend you use a more up to date browser (or turn off compatibility mode in Internet Explorer). In the meantime, to ensure continued support, we are displaying the site without styles and JavaScript.
- View all journals
- My Account Login
- Explore content
- About the journal
- Publish with us
- Sign up for alerts
- Data Descriptor
- Open access
- Published: 29 March 2024
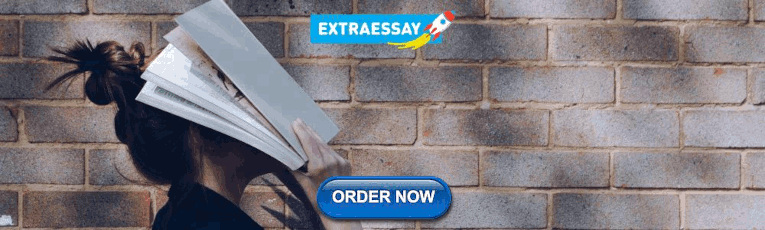
A unified dataset for the city-scale traffic assignment model in 20 U.S. cities
- Xiaotong Xu ORCID: orcid.org/0000-0001-7577-6194 1 ,
- Zhenjie Zheng 1 ,
- Zijian Hu 1 ,
- Kairui Feng ORCID: orcid.org/0000-0001-8978-2480 2 &
- Wei Ma 1 , 3
Scientific Data volume 11 , Article number: 325 ( 2024 ) Cite this article
648 Accesses
Metrics details
- Engineering
City-scale traffic data, such as traffic flow, speed, and density on every road segment, are the foundation of modern urban research. However, accessing such data on a city scale is challenging due to the limited number of sensors and privacy concerns. Consequently, most of the existing traffic datasets are typically limited to small, specific urban areas with incomplete data types, hindering the research in urban studies, such as transportation, environment, and energy fields. It still lacks a city-scale traffic dataset with comprehensive data types and satisfactory quality that can be publicly available across cities. To address this issue, we propose a unified approach for producing city-scale traffic data using the classic traffic assignment model in transportation studies. Specifically, the inputs of our approach are sourced from open public databases, including road networks, traffic demand, and travel time. Then the approach outputs comprehensive and validated citywide traffic data on the entire road network. In this study, we apply the proposed approach to 20 cities in the United States, achieving an average correlation coefficient of 0.79 in average travel time and an average relative error of 5.16% and 10.47% in average travel speed when compared with the real-world data.
Similar content being viewed by others
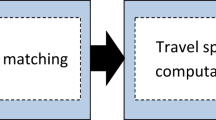
Urban link travel speed dataset from a megacity road network
Feng Guo, Dongqing Zhang, … Zhaoxia Guo
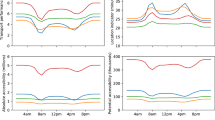
A fine resolution dataset of accessibility under different traffic conditions in European cities
Aris Christodoulou, Lewis Dijkstra, … Hugo Poelman
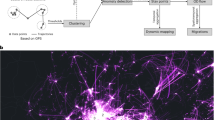
Defining a city — delineating urban areas using cell-phone data
Lei Dong, Fabio Duarte, … Carlo Ratti
Background & Summary
City-scale traffic data, including traffic flow, speed, and density on every road segment of the entire road network, are foundational inputs and building blocks for modern urban research. These traffic datasets offer an overview of urban mobility, facilitating a better understanding of traffic conditions and travelers’ behaviors in a city. Utilizing the city-scale traffic data, policymakers could develop appropriate transport policies and strategies to mitigate traffic congestion 1 , 2 . Additionally, the traffic data can also be used to evaluate the noise and air pollution caused by vehicles in urban areas 3 , 4 , 5 , which are important in enhancing public health and environmental conditions 6 , 7 , 8 . Furthermore, it assists in formulating energy-efficient traffic management and control strategies that can substantially reduce energy consumption 9 , 10 , 11 . In view of this, it is of great importance to produce and publish open-access traffic datasets on a city scale to support related studies in interdisciplinary research.
However, it is challenging to directly collect the traffic data on every road segment on the entire road network. This is because the traffic data are typically collected from various traffic sensors (e.g., loop detectors, CCTV cameras), which are usually insufficient to cover the entire network due to the associated high installation and maintenance costs. For instance, there are over 30,000 links on the road network of Hong Kong, but less than 10% of the links (i.e., 2,800) are equipped with volume detectors 12 . Moreover, data missing or data measurement errors are inevitable problems due to various factors such as sensor failures, software malfunctions, and weak communication signal transmission 13 , 14 . For example, existing studies indicate that approximately 30% of the freeway sensors in California Performance Measurement System (PeMS: https://pems.dot.ca.gov/ ) are not working properly, resulting in data missing 15 , 16 . More importantly, directly observing the traffic conditions may not be sufficient since the underlying mechanism of the traffic dynamics is not reflected. For example, a reduction in traffic speed indicates congestion, while it is still not clear how the congestion is formed 17 .
To address the above challenges, many urban planning or transport departments utilize traffic modeling techniques to estimate the city-scale traffic data in a generative manner. Specifically, the traffic assignment model 18 , which is a mature model that has been studied extensively in the transportation field, is adopted to estimate the city-scale traffic states. The input of the traffic assignment model only includes the Origin-Destination (OD) demand information and network structure, both of which are public and openly available. Then, the model outputs the city-scale traffic dataset. Traffic assignment models utilize OD data to predict traffic flow and route choices for individual travelers, relying on either predefined or data-driven behavioral models. By modeling the interactions between travelers’ behaviors and traffic congestion, the traffic assignment model searches for the equilibrium condition that mimics real-world traffic conditions. Traffic assignment models can often serve as the primary tool for local governments to assess the potential impact of changes in land use or road network expansions on both local and global traffic conditions. These models are indispensable because they inherently focus on optimizing travel decisions for local residents, aligning with their individual preferences. This capability enables the model to predict changes in agent-level behavior in situations that may not be fully reflected in the available data. Moreover, traffic assignment models demonstrate robust predictive capabilities for estimating future traffic conditions. For example, Metropolitan Planning Organizations (MPOs) in urban areas of the United States would utilize travel survey data, such as the National Household Travel Survey (NHTS: https://nhts.ornl.gov/ ), to produce traffic data for each local urban area that represent residents’ travel patterns 19 . However, these traffic assignment models and data are usually maintained by public agencies and generally not available to most researchers or the public due to difficulties in information sharing or privacy concerns 20 , 21 . Furthermore, the data used in traffic assignment models are under the ownership of various institutions and lack standardization in terms of their structures, granularity, and output formats. As a result, the data are restricted to a few researchers and it is challenging to access the necessary data for traffic assignment models across cities from official sources. Given the above, there is still a notable absence of city-scale traffic datasets that include multiple major cities within one geographic and cultural region, adhere to consistent standards, collect and validate information on a uniform scale, provide comprehensive data types, and meet high-quality standards for public availability.
Although there are a few publicly available datasets 22 , 23 concerning urban areas (see Table 1 ), the reliability and completeness of these datasets limit their applications across broader urban studies, especially in fields like energy, environment, and public health 24 , 25 . The limitations come from the following aspects: First, the existing traffic datasets typically cover some important traffic segments for a single city rather than a city-scale traffic dataset for multiple cities. Second, these current datasets often lack the necessary input, including road network data and corresponding OD data, directly usable for traffic assignment models. Third, these datasets often suffer from incomplete data types and lack of timely updating, resulting in limited convenience when utilizing them. In other words, these datasets are often collected by different researchers or volunteers several years ago, leading to a lack of uniformity in the data types and formats, as well as infrequent updates and maintenance. Fourth, these datasets frequently lack comprehensive validation across multiple variables or fail to offer adequate tools for predicting traffic features from behavioral data. For example, a dataset that includes OD numbers may result in unrealistic traffic flow predictions when attempting to utilize a traffic assignment model. In light of these mentioned facts, currently, there is no unified and well-validated traffic dataset available for multiple cities that covers the entire urban road network at a citywide scale, which hinders the feasibility of conducting comprehensive urban studies across cities to unearth novel discoveries.
To facilitate convenient access to citywide traffic assignment models and data for researchers from different domains besides transportation fields, this study provides a unified traffic dataset for traffic assignment models in 20 representative U.S. cities, with populations ranging from 0.3 million to over 8.8 million. Specifically, we first obtain the input of the model by fusing multiple open public data sources, including OpenStreetMap, The Longitudinal Employer-Household Dynamics Origin-Destination Employment Statistics (LODES), Waze, and TomTom. Then, we employ a grid-search method to fine-tune the parameters and generate the final traffic dataset for each city. The real world’s average travel time and traffic speed serve as validation criteria to ensure a reliable and effective traffic dataset for multiple cities. The validation results demonstrate that our approach can successfully produce the dataset with an average correlation coefficient of 0.79 for average travel time and an average error of 5.16% and 10.47% for average travel speed between real-world data and our data. Finally, we upload the validated traffic dataset and the code used in this study to a public repository.
To sum up, we utilize the static traffic assignment model, leveraging annually aggregated statistical data and open public data sources, to offer a city-scale traffic dataset for macroscopic urban research. It is worth noting that the approach provided in this study can also be applied to other cities. A comprehensive workflow of processing multi-source open public datasets to acquire this dataset is provided in Fig. 1 .
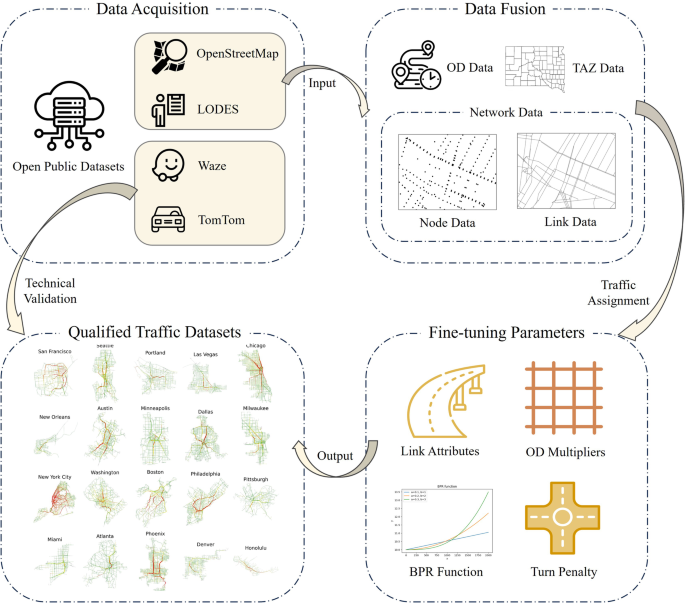
The workflow of obtaining unified and validated traffic datasets from multi-source open public datasets.
Creating a unified traffic dataset in multiple cities involves four main procedures: (1) the identification of representative cities; (2) the acquisition of corresponding input data from multi-source open public datasets; (3) the fusion of the obtained data; and (4) the implementation of traffic assignment, along with parameters calibration. The main procedures are illustrated accordingly below.
Identification of representative cities
In this study, we select a total of 20 representative cities in the United States and generate corresponding traffic datasets using the proposed approach. To ensure diversity and exemplarity among the selected cities, we primarily consider factors such as geographic location, urban scale, topography, and traffic conditions during the commute. Our selection includes a range of cities, including megacities like New York City, as well as several large cities such as Chicago and Philadelphia. We also included smaller but equally representative cities such as Honolulu. The topography of these cities also varies widely. For example, New York and San Francisco are separated by several rivers and rely on critical bridges and tunnels for commuting, while Las Vegas and Phoenix have relatively flat and continuous terrain, with surface transportation playing a predominant role.
Basic information of the 20 representative cities in the United States is given in Table 2 . The population and land area data in the year 2020 are sourced from the U.S. Census Bureau ( https://www.census.gov/ ) while the congestion ranking information in the year 2022 is from TomTom ( https://www.tomtom.com/traffic-index/ranking/ ). Their geospatial distribution is shown in Fig. 2 .
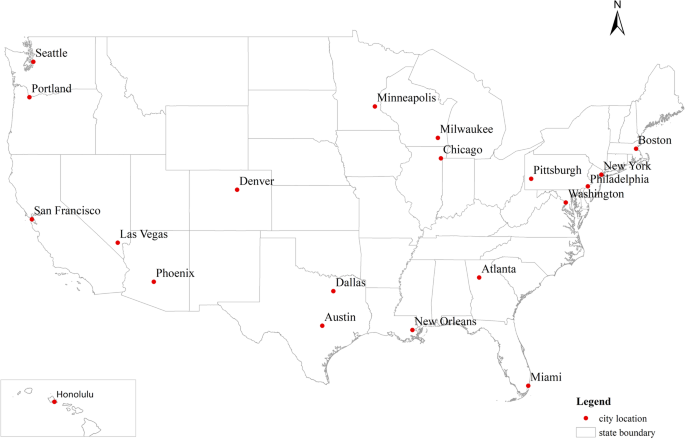
The geospatial distribution of 20 representative U.S. cities.
Data acquisition
The road network structure and travel demand are two crucial inputs for traffic assignment. In this study, we derive these data from public open-source datasets. This section provides a brief overview of the data acquisition procedures.
Road networks
First, the road network structures of the 20 cities are generated from the OpenStreetMap (OSM: https://www.openstreetmap.org/ ) database, which is an open-source mapping platform that provides crowd-sourced road network geographic information, including network topology, road attributes, and connectivity information. By leveraging OSM data, researchers gain convenient access to a comprehensive and up-to-date depiction of the network structure, which facilitates the research in urban studies 26 , 27 , 28 , 29 . The road attributes are also sourced from OSM. After the implementation of cleaning and integration procedures, these processed data can serve as the input for the traffic assignment. A summary of the road network data is given in Table 3 .
Specifically, we employ a Python package named osmnx 30 ( https://github.com/gboeing/osmnx ) to download the OSM data. We then use another Python package called osm2gmns 31 ( https://github.com/jiawlu/OSM2GMNS ) to extract the nodes and links on the road network from the OSM data and save them into separate CSV files in GMNS format 32 , 33 . We use five main link types including ‘motorway’, ‘trunk’, ‘primary’, ‘secondary’, and ‘tertiary’ to implement the traffic assignment. For each link type, we initiate the corresponding road attributes, including parameters such as road capacity, speed limits, the number of lanes, and so on. For the nodes, each node represents the intersection between two links and contains a unique identifier along with latitude and longitude information. By establishing the connectivity between nodes and links through their corresponding relationships, the network topology and road attributes can be constructed. We use the graphing functions of osmnx to visualize the constructed road networks of 20 representative U.S. cities in Fig. 3 .
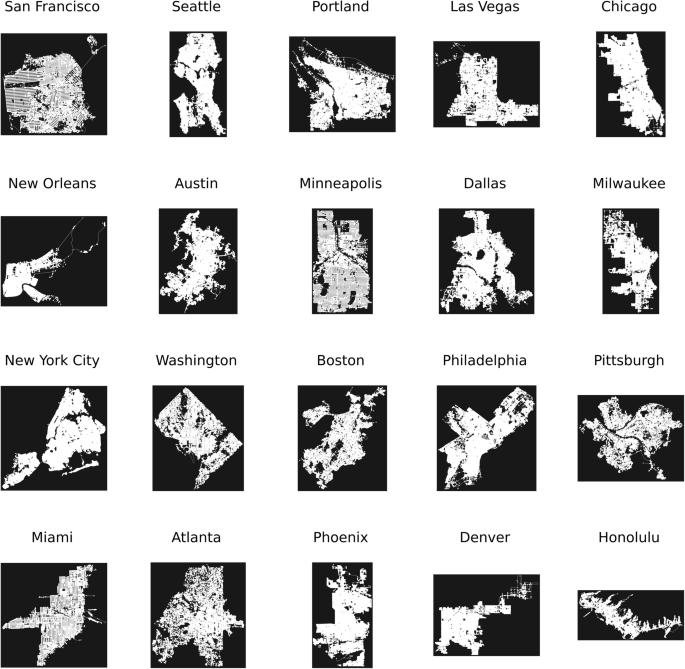
Road networks of 20 representative U.S. cities extracted from OpenStreetMap.
Travel demand
We then estimate the travel demand, another essential input data for traffic assignment, using the data from the LODES dataset ( https://lehd.ces.census.gov/data/lodes/ ) provided by the U.S. Census Bureau. The LODES dataset includes commuting data for the workforce in all states across the United States over multiple years, which have been widely used in existing studies 34 . LODES data collection involves employers reporting employee details to state workforce agencies, including work and home locations. The U.S. Census Bureau collaborates with state agencies to process and anonymize this data. It’s then used to create Origin-Destination (OD) pairs. This dataset, at the finest granularity of block level, documents the block code for both workplace census and residence census, along with the corresponding total number of jobs. Essentially, the LODES dataset provides an excellent representation of the trip distributions of the U.S. working population that can be used to construct the OD matrix. In this study, we mainly focus on producing the traffic dataset for the year 2019 and the commuting OD data in that year are collected. Moreover, the data collection process is performed at the block level, resulting in the OD data between blocks.
Travel time and speed
We collect data from two open-source dataset platforms, namely TomTom ( https://www.tomtom.com/traffic-index/ranking/ ) and Waze ( https://www.waze.com/live-map/ ), as two indicators of travel time and average speed respectively for our dataset validation. The detailed procedures of data collection can be found in the subsequent sec:Technical ValidationTechnical Validation section.
Data fusion
In this section, we integrate the road network data and OD data to unify the data format. Since the origins and destinations in the OD matrix are not associated with network nodes, it is infeasible to directly take these data as input for the traffic assignment. Therefore, we need to establish a connection between network nodes and blocks. After establishing the connection, we can employ the traffic assignment model to identify appropriate travel paths and allocate traffic flow to the respective links.
To be specific, we begin by aggregating the OD data from its minimum granularity at the block level to a higher level, namely, the tract level. According to the United State Bureau 35 , 36 , 37 , blocks are statistical units with small areas, generally defined to contain between 600 and 3,000 people, whereas tracts composed of multiple blocks are relatively larger and typically have a population size ranging from 1,200 to 8,000 people. In order to achieve a balance between computational complexity and accuracy, we consider tracts as an ideal basic unit for the traffic assignment, which is similar to the existing studies 38 , 39 . This implies that we use the tract as a Traffic Analysis Zone (TAZ) in the traffic assignment model.
Then, the geographical location of each TAZ is determined as the average coordinates of all the blocks within a tract. These TAZs (also called centroids) are generated and stored in the existing node file labeled with a unique identifier. Finally, we generate connectors to bridge the TAZs and network nodes. These connectors can be regarded as a special type of links that are generated from each TAZ center to their neighbor links. Moreover, these connectors are incorporated into the existing links labeled with a unique identifier. As a result, the commuting trips could start from the origin TAZ, traverse a connector to access the nearby road network, choose a suitable path, and then use another connector to reach the destination TAZ.
Traffic assignment
In this section, we use the traffic assignment model to produce the dataset based on the User Equilibrium (UE) 40 . To be specific, we formulate the UE using an optimization model and calibrate four categories of parameters used in the model. Using the network structure and OD demand as input, the model would output the traffic flow, speed, and density on each link. Moreover, we mainly focus on the static traffic assignment and do not consider the influence of temporal variations on traffic conditions.
User equilibrium
All travelers naturally make decisions to minimize their own travel costs (either travel time or equivalent monetary value). Wardrop’s First Principle 41 posits that when every traveler seeks to minimize their individual travel costs, traffic flow eventually stabilizes. In this equilibrium state, the travel costs on all utilized paths become equal and minimized. Meanwhile, the travel costs on unused paths for any given OD pair are greater than or equal to those on the used paths. In other words, a steady-state traffic condition is reached only when no traveler can improve his or her travel time by unilaterally changing routes. The satisfaction of Wardrop’s first principle is commonly referred to as User Equilibrium (UE).
The physical transport network including road segments and intersections in an urban area can be represented as a graph structure G ( N , A ) containing a link set A and a node set N . For each link α ∈ A , it has the link flow x a and the link travel cost t a respectively. For each node r , s ∈ N , it is defined as the TAZ that generates or attracts traffic demand. Therefore, the mathematical formulation of the traffic assignment model under the UE condition 42 can be expressed as follows:
where t a ( x a ) denotes the link performance function that indicates the travel cost on link a when the traffic flow is x a . \({f}_{k}^{rs}\) represents the traffic flow on path k connecting origin r and destination s . q rs indicates the number of trips from origin r to destination s . \({\delta }_{ka}^{rs}\) is a binary variable indicates whether link a is part of path k between origin r and destination s . Equation ( 2 ) imposes the flow conservation constraints. Equation ( 3 ) expresses the relationship between link flow and path flow. Please refer to the book Urban Transportation Networks 40 for details.
Once the traffic flow on each link is determined, the total travel time, denoted as \({c}_{k}^{rs}\) , for a specific path k can be calculated by summing the travel time of each link along this path, which can be formulated as follows:
Although the above optimization model has been proven to be a strict convex problem with a unique solution for traffic flow on links 40 , the computational cost of finding the optimal solution would significantly increase when dealing with large-scale city road networks. To alleviate the computational burden, a bi-conjugate Frank-Wolfe algorithm 43 , 44 is employed to find the optimal solution. In order to enable convenient usage of the provided dataset by users from various disciplines and allow them to easily modify the core parameter settings of the traffic assignment process according to their research needs, we employ two traffic modeling platforms to generate the final dataset. Subsequent users can either directly view the dataset in a no-code format or quickly adjust parameters through a low-code approach to conduct scenario testing under different scenarios. Specifically, a commercial software (named TransCAD ) and an open-source Python package for transportation modeling (named AequilibraE ) are utilized simultaneously in this study. For both platforms, the maximum assignment iteration time and the convergence criteria are set to 500 and 0.001, respectively. The results of the traffic assignment model in 20 U.S. cities are shown in Fig. 4 .
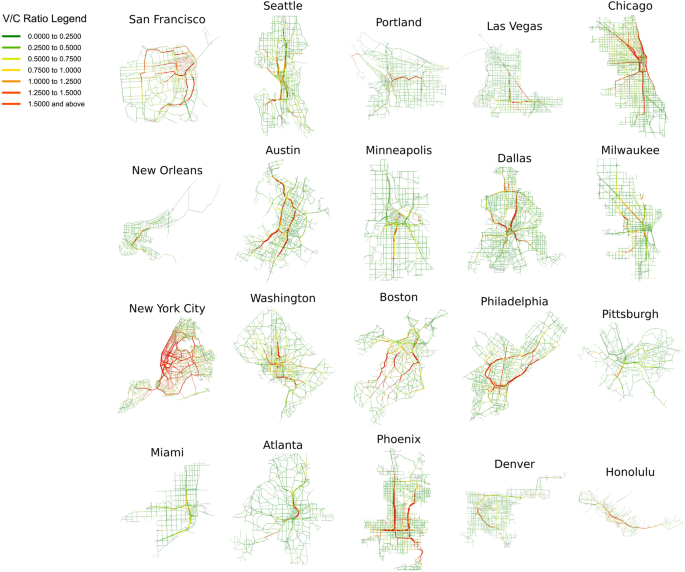
Results of the traffic assignment model in 20 representative U.S. cities.
Parameters calibration
The traffic conditions on the network are influenced by many factors related to traffic supply and demand. Consequently, the traffic assignment model would be impacted and output different results. Since the disturbances in the transport system are nonlinear and challenging to quantify, it is difficult to establish a deterministic mapping relationship between various influencing factors and the results of the traffic assignment model. Therefore, we adopt a grid-search approach to calibrate four common categories of factors that are closely related to the traffic assignment model. We determine the final model by continuously fine-tuning various parameters associated with the traffic assignment model until the transport system reaches the UE condition. In this study, we introduce four categories of factors including road attributes, travel demand, impedance function, and turn penalty, as outlined below.
Road attributes
We categorize the entire road network into three major types, namely expressways, arterial highways, and local roads. Capacity and free flow speed of each road type are two parameters identified to be calibrated. Based on the experimental results, the appropriate range of road capacity for expressways is between 1800 veh/h/lane and 2200 veh/h/lane, while the range for free flow speed is from 65 km/h to 90 km/h. In the case of highways, the corresponding capacity value falls within the range of 1500 veh/h/lane to 2000 veh/h/lane, and the free flow speed value ranges from 40 km/h to 65 km/h. As for local roads, their capacity varies from 600 veh/h/lane to 1500 veh/h/lane, while the suitable speed ranges between 25 km/h and 45 km/h. The detailed information for each type of road can be found in Table 4 .
The OD travel demand is another significant factor influencing the outcome of the traffic assignment. In this study, we aim to simulate the traffic conditions during the peak hours. As mentioned above, the OD demand matrix is derived from the total number of jobs in the United States in 2019, generated from LODES datasets. Although it is reasonable to assume that commuting travel accounts for the majority during peak hours, such demand cannot reflect the actual traffic conditions. Therefore, it is necessary to adjust the initial OD demand, considering variations in transport modes, travel departure time, and carpooling availability during commuting to work. To address this issue, we introduce an OD multiplier to estimate the actual traffic demand during the commuting time. We find that stable results can be obtained when the parameter ranges from 0.55 to 0.65. We show the travel demand and the percentage of internal travel within each TAZ in Fig. 5 .
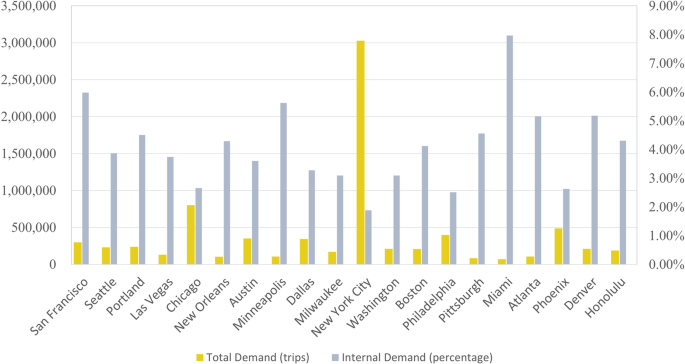
Total travel demand and the percentage of internal travel demand for 20 U.S. cities.
Link performance function
The link performance function, also known as the impedance function or volume delay function, refers to the relationship between travel time and traffic flow on a road. Typically, travel time increases non-linearly with the increase in traffic flow, which also significantly affects the traffic assignment. One of the most commonly adopted functions in the literature is called the Bureau of Public Roads (BPR) function 45 , which is expressed as follows:
In the function above, t indicates the actual travel time on the road while t 0 represents the free flow travel time on the corresponding road. v and c are the traffic flow and capacity of the road, respectively. α and β are parameters needed to be fine-tuned. We find that the results are satisfactory when parameter α ranges from 0.15 to 0.6 while parameter β changes from 1.2 to 3. The specific values of parameters for each city are provided in Table 5 .
Turn penalty
The turning delay at intersections is also a significant factor that should not be dismissed. When vehicles pass through road intersections, their speed typically decreases, either due to signal control or the necessity to make turns. However, this behaviour cannot be adequately represented in solving traffic assignment problems. To ensure that the results of the traffic assignment model are in accordance with real-world scenarios, we uniformly set corresponding parameters for all junctions to simulate the turning delay effects. In other words, the turn penalty parameters are an average value for the turning delay at all intersections in the road network and these intersection types include signal-controlled intersections, roundabouts, yield or stop intersections, and others. Specifically, the time delay for right turns varies between 0 and 0.25 minutes, while the penalty for making a left turn ranges from 0 to 0.35 minutes. The delay for through traffic is between 0 and 0.15 minutes. U-turn is prohibited in the traffic assignment simulation. The specific parameter setting is demonstrated in Table 5 .
Data Records
We share the traffic dataset on a public repository (Figshare 46 ). In this dataset, each folder, named after the city, contains the input and output of the traffic assignment model specific to that city. We elaborate on the details as follows:
This folder contains all the input data required for the traffic assignment model, namely the OD demand data and network data. The network data contains both node and link files in a CSV format. The data in this file folder specifically includes the following contents:
the initial network data obtained from OSM
the visualization of the OSM data
processed node/link/od data
The detailed meanings of the fields contained in different input data are given in Table 6 .
TransCAD results
This folder contains all the input data required for the traffic assignment model in TransCAD, as well as the corresponding output data. The data in this file folder specifically includes the following contents:
cityname.dbd: geographical network database of the city supported by TransCAD
cityname_link.shp/cityname_node.shp: network data supported by the GIS software, which can be imported into TransCAD manually
od.mtx: OD matrix supported by TransCAD
LinkFlows.bin/LinkFlows.csv: results of the traffic assignment model by TransCAD
ShortestPath.mtx/ue_travel_time.csv: the travel time (in minutes) between OD pairs by TransCAD
The detailed meanings of the fields contained in output data generated from TransCAD are given in Table 7 .
AequilibraE results
This folder contains all the input data required for the traffic assignment model in AequilibraE, as well as the corresponding output data. The data in this file folder specifically includes the following contents:
cityname.shp: shapefile network data of the city support by QGIS or other GIS software
od_demand.aem: OD matrix supported by AequilibraE
network.csv: the network file used for traffic assignment in AequilibraE
assignment_result.csv: results of the traffic assignment model by AequilibraE
The detailed meanings of the fields contained in output data generated from AequilibraE are given in Table 8 .
Technical Validation
To ensure the consistency between the traffic assignment model’s output and real-world traffic conditions, we conduct validation using two different public open sources of traffic data. Specifically, the travel time between different OD pairs and the overall average travel speed are employed as two validation indicators to ensure the reliability and accuracy of the provided dataset. The validation results are shown in Tables 9 , 10 and we can see that the provided dataset for each city is accurate and valid.
Travel time
In examining the travel time metric, we obtain the travel time between different OD pairs both from traffic assignment models and map service providers. As for the model side, the travel time under both UE and free flow conditions are calculated respectively using traffic assignment models. First, under UE conditions, the travel time between different OD pairs could be generated by summing the link travel time determined by the corresponding assigned traffic flow along the shortest path as shown in Eq. ( 5 ). Then, under free flow conditions, the travel time between OD pairs is the travel time associated with the shortest path, disregarding congestion on road segments. Furthermore, the average value of Travel Time (in minutes) under UE conditions (UETT) as well as free flow conditions (FFTT) for all OD pairs can be expressed as follows:
where \({c}_{ue}^{rs}\) and \({c}_{ff}^{rs}\) denote the travel time between origin r and destination s under the UE and free flow conditions respectively. Additionally, the difference as well as the ratio between these two types of travel time give the average travel delay (in minutes) and delay factor for each city.
In terms of the real-world data for validation, since nowadays many map service providers have the capability to offer travel time estimates between two location points at different departure times based on users’ historical navigation records, in this study, we choose Waze as the data source to obtain the actual travel time between each OD pair by using its WazeRouteCalculator API ( https://github.com/kovacsbalu/WazeRouteCalculator ) with Python code.
The results of travel time are shown in Table 9 . It can be seen that Honolulu experiences the least travel time under free flow conditions, at about 8.70 minutes, while Minneapolis has the shortest average travel time during commuting hours, at about 10.25 minutes. Minneapolis also has the lowest delay travel time among all cities, merely 0.47 minutes, indicating that the commuting travel time in this city is almost the same as the travel time under free flow conditions. In contrast, New York City experiences significant delays, with a delay time of 24.47 minutes, revealing that the travel time during peak periods in New York is more than double that of the free flow condition. In terms of the delay factor, New York City has the highest value, reaching 2.24, followed by Chicago with a value of 1.65. Minneapolis and Pittsburgh have the lowest delay factor values, both at 1.05.
To evaluate the results, we use the Pearson Correlation Coefficient (PCC) 47 to measure the correlation between the actual travel time and the travel time produced by our model. The PCC r xy is defined as follows:
where r xy denotes the Pearson’s Correlation Coefficient. x i and y i are the individual sample points indexed with i . n represents the sample size.
Since the turning penalties are not incorporated in the traffic assignment algorithm of AequilibraE, the parameter settings in TransCAD and AequilibraE are not identical. Consequently, results of the two platforms are not entirely consistent. Considering the more comprehensive parameter settings in TransCAD, we adopt the results of TransCAD as the primary benchmark. We perform PCC analysis between Waze and TransCAD, as well as between TransCAD and AequilibraE, with the evaluation results presented in Table 9 .
From the correlation analysis, we can find that all correlation coefficients R 2 are greater than 0.7, which confirms the accuracy and reliability of the results to some extent. We also visualize the correlation coefficient for each city in Fig. 6 . It can be seen that the simulated travel time is consistent with the travel time in the real world.
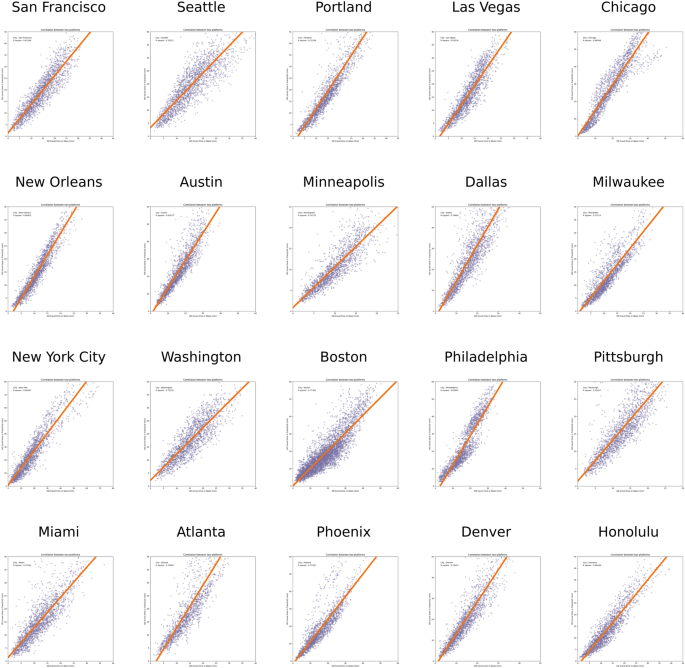
Correlation analysis results between Waze and TransCAD.
Average speed
The overall average speed of the entire road network is another important indicator for validation. In this study, we use the speed data collected from TomTom Traffic Index as the actual speed to validate our model. We first calculate the average link-based speed of our model through dividing Vehicle Hours Travelled (VHT) by Vehicle Kilometers Travelled (VKMT). Then, the average OD-based speed values are derived from the ratio of distance to travel time between each OD pair. The Mean Absolute Percent Errors (MAPE) and Mean Absolute Errors (MAE) for both the link-based speed and the OD-based speed are used to measure the reliability of our model:
where y i is the actual observed value, \({\widehat{y}}_{i}\) is the predicted value, and n is the number of samples.
The results are summarized in Table 10 . We find that the average MAPE and MAE values for the link-based speed metric are 5.16% and 1.77 km/h, respectively. Moreover, the average MAPE and MAE values for the OD-based speed indicator are 10.47% and 3.82 km/h, respectively. This implies that our approach can produce satisfactory and reliable results.
Network traffic impact on model performance
To validate the effectiveness and robustness of our model across cities, we further investigate how traffic conditions of a city affect the model performance. The MAE and MAPE values for link-based average speed metrics obtained in Table 10 are used to evaluate the model performance. The traffic conditions are characterized by two different indicators. One is the ratio of the total OD travel demand to the number of links for the entire road network, which can characterize the average OD demand and represent the traffic conditions of a city. The other is the average speed (km/h) in rush hour obtained from TomTom (refer to Table 10 ). If the values of average traffic demand are large, it reveals a congested city network experiencing substantial traffic demand, exemplified by cities like New York and San Francisco. Conversely, a small value suggests a city road network with low traffic demand, as observed in cities like Atlanta and Dallas. We can draw similar conclusions with respect to the average traffic speed.
The results are shown in Fig. 7 . The red dashed line represents the linear regression trendline that has been fitted to the data points. The R 2 values of Fig. 7a and Fig. 7b are 0.0049 and 0.0218, respectively. This implies that there is no evident relationship between the model performance and the varying traffic demand of the network. Similarly, the R 2 values of Fig. 7c and Fig. 7d are 0.0212 and 0.0177, respectively. This suggests that the model performance is not affected by the varying traffic speeds in different cities. In summary, the proposed model exhibits low sensitivity to variations in city traffic conditions and achieves satisfactory performance across cities.
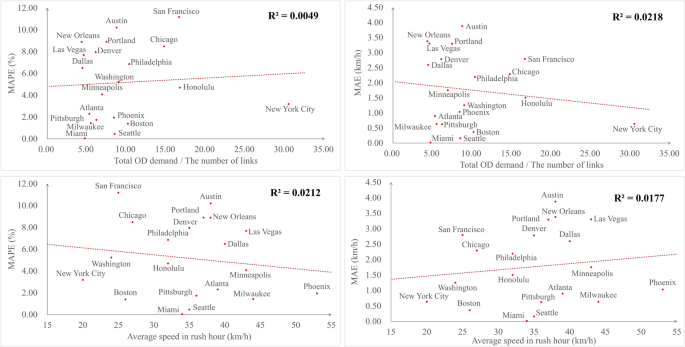
The model performance in relation to different traffic conditions for 20 U.S. cities. ( a ) The MAPE values (%) regarding the average OD demand for different cities. ( b ) The MAE values (km/h) regarding to the average OD demand for different cities. ( c ) The MAPE values (%) regarding the average speed for different cities. ( d ) The MAE values (km/h) regarding the average speed for different cities.
Usage Notes
The acquisition of OD data is crucial in performing the traffic assignment and producing the citywide traffic dataset. In this study, we utilize the commuting OD data (LODES) provided by the U.S. Census Bureau to generate the OD matrix. For cities in other countries, OD data can be substituted with alternative open data sources, such as OD data provided by TomTom ( https://developer.tomtom.com/od-analysis/documentation/product-information/introduction ).
Moreover, we use the average traffic time and average travel speed between different OD pairs in the real world to validate the results of our approach, ensuring its reliability and accuracy. If additional data sources are available, such as traffic flow data obtained from traffic detectors, we can also use the corresponding data to further evaluate the effectiveness of the provided dataset.
It is worth noting that the provided dataset is mainly used for macroscopic urban research and policy development across interdisciplinary studies. In view of this, the given dataset provides full spatial coverage of the entire road network, unlike existing traffic datasets that focus on specific areas. Hence, the provided traffic dataset and existing traffic datasets complement each other, which can better facilitate research in urban studies. Specifically, the full spatial coverage of the provided dataset makes it valuable for comprehensive macroscopic urban research and policy development, making a notable contribution to the literature, such as public transport planning, road expansions, the determination of bus routes, the estimation of the transport-related environmental impact and so on. In contrast, existing traffic datasets (e.g., PeMS) may exhibit incomplete spatial coverage, making them less suitable for the aforementioned macroscopic urban studies. Actually, the datasets containing fine-grained temporal information are more suitable for investigating regional traffic dynamics by leveraging the spatiotemporal relationship between the traffic data, such as traffic prediction, spatiotemporal propagation of shockwaves, calibration of fundamental diagrams, traffic data imputation, and so on.
In this study, the provided dataset lacks fine-grained temporal information due to the limited availability of input data. To fully understand dynamic traffic patterns, it is essential to consider both spatial and temporal dimensions within the traffic data. Consequently, developing a dynamic traffic assignment model that effectively captures the spatiotemporal interdependencies of traffic data is important. Moreover, employing daily traffic data for more fine-grained validation would enhance further urban research.
Code availability
The guidelines for data retrieval and utilization have been uploaded to GitHub 48 . The specific contents comprise:
1. Input data introduction.ipynb : A brief introduction and data demonstration about the input data for the traffic assignment process in the dataset.
2. A guide for TransCAD users.md : It is a guide for users who want to view and modify the dataset in the Graphical User Interface (GUI) of TransCAD.
3. AequilibraE_assignmnet.py : A Python code file for users who want to get access to the traffic assignment results by using the AqeuilibraE.
Huang, Y., Weng, Y., Wu, W. & Chen, B. Control strategies for solving the problem of traffic congestion. IET Intelligent Transport Systems 10 , 642–648 (2016).
Article Google Scholar
Tajdari, F. & Roncoli, C. Adaptive traffic control at motorway bottlenecks with time-varying fundamental diagram. IFAC-PapersOnLine 54 , 271–277 (2021).
Guo, B. et al . Uncovering China’s transport CO 2 emission patterns at the regional level. Energy Policy 74 , 134–146 (2014).
Article CAS Google Scholar
Donchenko, V. et al . Estimated atmospheric emission from motor transport in Moscow based on transport model of the city. Transportation Research Procedia 14 , 2649–2658 (2016).
Aminzadegan, S., Shahriari, M., Mehranfar, F. & Abramović, B. Factors affecting the emission of pollutants in different types of transportation: A literature review. Energy Reports 8 , 2508–2529 (2022).
Wang, X., Rodrguez, D. A., Sarmiento, O. L. & Guaje, O. Commute patterns and depression: Evidence from eleven Latin American cities. Journal of Transport & Health 14 , 100607 (2019).
Dam, P. et al . COVID-19: Impact on transport and mental health. Journal of Transport & Health 19 , 100969 (2020).
Conceição, M. A. et al . The effect of transport infrastructure, congestion and reliability on mental wellbeing: A systematic review of empirical studies. Transport Reviews 43 , 264–302 (2023).
Achour, H. & Belloumi, M. Investigating the causal relationship between transport infrastructure, transport energy consumption and economic growth in Tunisia. Renewable and Sustainable Energy Reviews 56 , 988–998 (2016).
Wang, Y., Li, K., Xu, X. & Zhang, Y. Transport energy consumption and saving in China. Renewable and Sustainable Energy Reviews 29 , 641–655 (2014).
Adams, S., Boateng, E. & Acheampong, A. O. Transport energy consumption and environmental quality: Does urbanization matter? Science of the Total Environment 744 , 140617 (2020).
Article ADS CAS PubMed Google Scholar
Transport Department, The Government of the Hong Kong Special Administrative Region. Intelligent Road Network Package (IRNP). https://www.td.gov.hk/en/public_services/intelligent_road_network_package/index.html (2023).
Chen, X., He, Z. & Sun, L. A Bayesian tensor decomposition approach for spatiotemporal traffic data imputation. Transportation Research Part C: Emerging Technologies 98 , 73–84 (2019).
Liang, Y., Zhao, Z. & Sun, L. Memory-augmented dynamic graph convolution networks for traffic data imputation with diverse missing patterns. Transportation Research Part C: Emerging Technologies 143 , 103826 (2022).
Rajagopal, R. & Varaiya, P. P. Health of California’s loop detector system. Tech. Rep. (2007).
Yang, Y., Yang, H. & Fan, Y. Networked sensor data error estimation. Transportation Research Part B: Methodological 122 , 20–39 (2019).
Johari, M., Keyvan-Ekbatani, M., Leclercq, L., Ngoduy, D. & Mahmassani, H. S. Macroscopic network-level traffic models: Bridging fifty years of development toward the next era. Transportation Research Part C: Emerging Technologies 131 , 103334 (2021).
Patriksson, M. The Traffic Assignment Problem: Models and Methods (Courier Dover Publications, 2015).
Horowitz, A. Statewide Travel Forecasting Models, NCHRP Synthesis of Highway Practices N 358. Transportation Research Board, Washington DC (2006).
Xie, H., Kulik, L. & Tanin, E. Privacy-aware traffic monitoring. IEEE Transactions on Intelligent Transportation Systems 11 , 61–70 (2009).
Google Scholar
Xiao, W., Zhang, H., Wen, Q. & Li, W. Passive RFID-supported source location privacy preservation against global eavesdroppers in WSN. In 2013 5th IEEE International Conference on Broadband Network & Multimedia Technology , 289–293 (IEEE, 2013).
Nourmohammadi, F., Mansourianfar, M., Shafiei, S., Gu, Z. & Saberi, M. An open GMNS dataset of a dynamic multi-modal transportation network model of Melbourne, Australia. Data 6 , 21 (2021).
Yap, W. & Biljecki, F. A global feature-rich network dataset of cities and dashboard for comprehensive urban analyses. Scientific Data 10 , 667 (2023).
Article PubMed PubMed Central Google Scholar
Rey, D., Bar-Gera, H., Dixit, V. V. & Waller, S. T. A branch-and-price algorithm for the bilevel network maintenance scheduling problem. Transportation Science 53 , 1455–1478 (2019).
Loder, A., Ambühl, L., Menendez, M. & Axhausen, K. W. Understanding traffic capacity of urban networks. Scientific Reports 9 , 16283 (2019).
Article ADS PubMed PubMed Central Google Scholar
Fonte, C. C. et al . Using openstreetmap to create land use and land cover maps: Development of an application. In Geospatial Intelligence: Concepts, Methodologies, Tools, and Applications , 1100–1123 (IGI Global, 2019).
Vargas-Munoz, J. E., Srivastava, S., Tuia, D. & Falcao, A. X. OpenStreetMap: Challenges and opportunities in machine learning and remote sensing. IEEE Geoscience and Remote Sensing Magazine 9 , 184–199 (2020).
Grinberger, A. Y., Schott, M., Raifer, M. & Zipf, A. An analysis of the spatial and temporal distribution of large-scale data production events in OpenStreetMap. Transactions in GIS 25 , 622–641 (2021).
Atwal, K. S., Anderson, T., Pfoser, D. & Züfle, A. Predicting building types using OpenStreetMap. Scientific Reports 12 , 19976 (2022).
Article ADS CAS PubMed PubMed Central Google Scholar
Boeing, G. OSMnx: New methods for acquiring, constructing, analyzing, and visualizing complex street networks. Computers, Environment and Urban Systems 65 , 126–139 (2017).
Lu, J. & Zhou, X. S. Virtual track networks: A hierarchical modeling framework and open-source tools for simplified and efficient connected and automated mobility (CAM) system design based on general modeling network specification (GMNS). Transportation Research Part C: Emerging Technologies 153 , 104223 (2023).
Li, P. & Zhou, X. Path4GMNS. https://github.com/jdlph/Path4GMNS (2023).
Zhou, X. & Taylor, J. DTALite: A queue-based mesoscopic traffic simulator for fast model evaluation and calibration. Cogent Engineering 1 , 961345 (2014).
McKinney, K. L., Green, A. S., Vilhuber, L. & Abowd, J. M. Total error and variability measures for the quarterly workforce indicators and LEHD origin-destination employment statistics in OnTheMap. Journal of Survey Statistics and Methodology 9 , 1146–1182 (2021).
Morrill, R., Cromartie, J. & Hart, G. Metropolitan, urban, and rural commuting areas: Toward a better depiction of the United States settlement system. Urban Geography 20 , 727–748 (1999).
Logan, J. R., Xu, Z. & Stults, B. J. Interpolating US decennial census tract data from as early as 1970 to 2010: A longitudinal tract database. The Professional Geographer 66 , 412–420 (2014).
Smith, R. M. & Blizard, Z. D. A census tract level analysis of urban sprawl’s effects on economic mobility in the United States. Cities 115 , 103232 (2021).
Abdel-Aty, M., Lee, J., Siddiqui, C. & Choi, K. Geographical unit based analysis in the context of transportation safety planning. Transportation Research Part A: Policy and Practice 49 , 62–75 (2013).
Gunier, R. B., Hertz, A., Von Behren, J. & Reynolds, P. Traffic density in California: socioeconomic and ethnic differences among potentially exposed children. Journal of Exposure Science & Environmental Epidemiology 13 , 240–246 (2003).
Sheffi, Y. Urban transportation networks , vol. 6 (Prentice-Hall, Englewood Cliffs, NJ, 1985).
Wardrop, J. G. Road paper. some theoretical aspects of road traffic research. Proceedings of the Institution of Civil Engineers 1 , 325–362 (1952).
Boyce, D. Beckmann’s transportation network equilibrium model: Its history and relationship to the Kuhn–Tucker conditions. Economics of Transportation 2 , 47–52 (2013).
Mitradjieva, M. & Lindberg, P. O. The stiff is moving–Conjugate direction Frank-Wolfe methods with applications to traffic assignment. Transportation Science 47 , 280–293 (2013).
Zill, J. C., Camargo, P., Daisy, N. S. & Veitch, T. Toll choice and stochastic user equilibrium: ticking all the boxes. Transportation Research Record 2673 , 930–940 (2019).
Manual, T. A. Bureau of Public Roads, US Dept. Commerce, Urban Planning Division, Washington, DC, USA (1964).
Xu, X., Zheng, Z., Hu, Z., Feng, K. & Ma, W. A unified and validated traffic dataset for 20 U.S. cities. Figshare https://doi.org/10.6084/m9.figshare.24235696 (2023).
Edwards, A. L. An introduction to linear regression and correlation. The Correlation Coefficient 33 , 46 (1976).
Xu, X., Zheng, Z., Hu, Z., Feng, K. & Ma, W. A unified and validated traffic dataset for 20 U.S. cities. https://github.com/xuxiaotong/A_unified_and_validated_traffic_dataset_for_20_U.S._cities (2023).
Transportation Networks for Research Core Team. Transportation Networks for Research. https://github.com/bstabler/TransportationNetworks (2023).
The Institute for Transport Planning and Systems ETH Zurich. UTD19. https://utd19.ethz.ch/index.html (2023).
California Department of Transportation (Caltrans). Caltrans Performance Measurement System (PeMS). https://pems.dot.ca.gov/ (2023).
Department for Transport (UK). Road Traffic Statistics. https://roadtraffic.dft.gov.uk/downloads/ (2023).
California Department of Transportation (Caltrans). Traffic Volumes (Annual Average Daily Traffic (AADT)). https://gisdata-caltrans.opendata.arcgis.com/datasets/d8833219913c44358f2a9a71bda57f76_0/about (2023).
New York City Department of Transportation (NYC DOT). Traffic Volume Counts. https://data.cityofnewyork.us/Transportation/Traffic-Volume-Counts/btm5-ppia/about_data (2023).
Transport Department (HK). Traffic Data of Strategic/Major Roads. https://data.gov.hk/en-data/dataset/hk-td-sm_4-traffic-data-strategic-major-roads (2023).
Yan, H. City-scale vehicle trajectory data from traffic camera videos. Figshare https://doi.org/10.6084/m9.figshare.c.6676199.v1 (2023).
Yu, F. et al . City-scale vehicle trajectory data from traffic camera videos. Scientific Data 10 , 711 (2023).
Wang, Y. et al . City-scale holographic traffic flow data based on vehicular trajectory resampling. Figshare https://doi.org/10.6084/m9.figshare.c.5796776.v1 (2022).
Wang, Y. et al . City-scale holographic traffic flow data based on vehicular trajectory resampling. Scientific Data 10 , 57 (2023).
Download references
Acknowledgements
The work described in this paper was supported by the National Natural Science Foundation of China (No. 52102385), grants from the Research Grants Council of the Hong Kong Special Administrative Region, China (Project No. PolyU/25209221 & PolyU/15206322), and a grant from Dean’s Reserve at the Hong Kong Polytechnic University (Project No. P0034271). The authors would like to thank Prof. Xuesong Zhou for providing constructive suggestions and active discussions regarding the data.
Author information
Authors and affiliations.
Department of Civil and Environmental Engineering, The Hong Kong Polytechnic University, Hong Kong, 999077, China
Xiaotong Xu, Zhenjie Zheng, Zijian Hu & Wei Ma
The Department of Civil and Environmental Engineering, Princeton University, Princeton, 08544, USA
Kairui Feng
The Hong Kong Polytechnic University Shenzhen Research Institute, Shenzhen, Guangdong, 518000, China
You can also search for this author in PubMed Google Scholar
Contributions
X.X. conceived the study, curated data, developed methodology, conducted experiment and wrote the original draft. Z.Z. conceived the study, developed methodology, coded for the data acquisition, reviewed and edited writing. Z.H. coded for the data acquisition. K.F. conceived the study, contributed to the original data, reviewed and edited writing. W.M. conceived the study, acquired funding, developed methodology and supervised the study. All authors reviewed and agreed on the final manuscript.
Corresponding authors
Correspondence to Zhenjie Zheng or Wei Ma .
Ethics declarations
Competing interests.
The authors declare no competing interests.
Additional information
Publisher’s note Springer Nature remains neutral with regard to jurisdictional claims in published maps and institutional affiliations.
Rights and permissions
Open Access This article is licensed under a Creative Commons Attribution 4.0 International License, which permits use, sharing, adaptation, distribution and reproduction in any medium or format, as long as you give appropriate credit to the original author(s) and the source, provide a link to the Creative Commons licence, and indicate if changes were made. The images or other third party material in this article are included in the article’s Creative Commons licence, unless indicated otherwise in a credit line to the material. If material is not included in the article’s Creative Commons licence and your intended use is not permitted by statutory regulation or exceeds the permitted use, you will need to obtain permission directly from the copyright holder. To view a copy of this licence, visit http://creativecommons.org/licenses/by/4.0/ .
Reprints and permissions
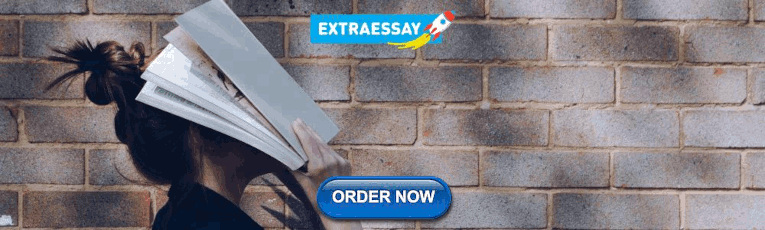
About this article
Cite this article.
Xu, X., Zheng, Z., Hu, Z. et al. A unified dataset for the city-scale traffic assignment model in 20 U.S. cities. Sci Data 11 , 325 (2024). https://doi.org/10.1038/s41597-024-03149-8
Download citation
Received : 27 November 2023
Accepted : 18 March 2024
Published : 29 March 2024
DOI : https://doi.org/10.1038/s41597-024-03149-8
Share this article
Anyone you share the following link with will be able to read this content:
Sorry, a shareable link is not currently available for this article.
Provided by the Springer Nature SharedIt content-sharing initiative
Quick links
- Explore articles by subject
- Guide to authors
- Editorial policies
Sign up for the Nature Briefing newsletter — what matters in science, free to your inbox daily.

- Help & FAQ
Traffic assignment: A survey of mathematical models and techniques
- Civil and Urban Engineering
- Center for Interacting Urban Networks
Research output : Chapter in Book/Report/Conference proceeding › Chapter
This chapter presents the fundamentals of the theory and techniques of traffic assignment problem. It first presents the steady-state traffic assignment problem formulation which is also called static assignment, followed by Dynamic Traffic Assignment (DTA), where the traffic demand on the network is time varying. The static assignment problem is shown in a mathematical programming setting for two different objectives to be satisfied. The first one where all users experience same travel times in alternate used routes is called user-equilibrium and another setting called system optimum in which the assignment attempts to minimize the total travel time. The alternate formulation uses variational inequality method which is also presented. Dynamic travel routing problem is also reviewed in the variational inequality setting. DTA problem is shown in discrete and continuous time in terms of lumped parameters as well as in a macroscopic setting, where partial differential equations are used for the link traffic dynamics. A Hamilton–Jacobi- based travel time dynamics model is also presented for the links and routes, which is integrated with the macroscopic traffic dynamics. Simulation-based DTA method is also very briefly reviewed. This chapter is taken from the following Springer publication and is reproduced here, with permission and with minor changes: Pushkin Kachroo, and Neveen Shlayan, “Dynamic traffic assignment: A survey of mathematical models and technique,” Advances in Dynamic Network Modeling in Complex Transportation Systems (Editor: Satish V. Ukkusuri and Kaan Özbay) Springer New York, 2013. 1-25.
Publication series
Asjc scopus subject areas.
- Control and Systems Engineering
- Automotive Engineering
- Aerospace Engineering
- Industrial and Manufacturing Engineering
Access to Document
- 10.1007/978-3-319-69231-9_2
Other files and links
- Link to publication in Scopus
- Link to the citations in Scopus
Fingerprint
- Mathematical models Engineering & Materials Science 100%
- Travel time Engineering & Materials Science 56%
- Time varying networks Engineering & Materials Science 25%
- Mathematical programming Engineering & Materials Science 21%
- User experience Engineering & Materials Science 18%
- Partial differential equations Engineering & Materials Science 18%
- Dynamic models Engineering & Materials Science 14%
T1 - Traffic assignment
T2 - A survey of mathematical models and techniques
AU - Kachroo, Pushkin
AU - Özbay, Kaan M.A.
N1 - Publisher Copyright: © Springer International Publishing AG, part of Springer Nature 2018.
N2 - This chapter presents the fundamentals of the theory and techniques of traffic assignment problem. It first presents the steady-state traffic assignment problem formulation which is also called static assignment, followed by Dynamic Traffic Assignment (DTA), where the traffic demand on the network is time varying. The static assignment problem is shown in a mathematical programming setting for two different objectives to be satisfied. The first one where all users experience same travel times in alternate used routes is called user-equilibrium and another setting called system optimum in which the assignment attempts to minimize the total travel time. The alternate formulation uses variational inequality method which is also presented. Dynamic travel routing problem is also reviewed in the variational inequality setting. DTA problem is shown in discrete and continuous time in terms of lumped parameters as well as in a macroscopic setting, where partial differential equations are used for the link traffic dynamics. A Hamilton–Jacobi- based travel time dynamics model is also presented for the links and routes, which is integrated with the macroscopic traffic dynamics. Simulation-based DTA method is also very briefly reviewed. This chapter is taken from the following Springer publication and is reproduced here, with permission and with minor changes: Pushkin Kachroo, and Neveen Shlayan, “Dynamic traffic assignment: A survey of mathematical models and technique,” Advances in Dynamic Network Modeling in Complex Transportation Systems (Editor: Satish V. Ukkusuri and Kaan Özbay) Springer New York, 2013. 1-25.
AB - This chapter presents the fundamentals of the theory and techniques of traffic assignment problem. It first presents the steady-state traffic assignment problem formulation which is also called static assignment, followed by Dynamic Traffic Assignment (DTA), where the traffic demand on the network is time varying. The static assignment problem is shown in a mathematical programming setting for two different objectives to be satisfied. The first one where all users experience same travel times in alternate used routes is called user-equilibrium and another setting called system optimum in which the assignment attempts to minimize the total travel time. The alternate formulation uses variational inequality method which is also presented. Dynamic travel routing problem is also reviewed in the variational inequality setting. DTA problem is shown in discrete and continuous time in terms of lumped parameters as well as in a macroscopic setting, where partial differential equations are used for the link traffic dynamics. A Hamilton–Jacobi- based travel time dynamics model is also presented for the links and routes, which is integrated with the macroscopic traffic dynamics. Simulation-based DTA method is also very briefly reviewed. This chapter is taken from the following Springer publication and is reproduced here, with permission and with minor changes: Pushkin Kachroo, and Neveen Shlayan, “Dynamic traffic assignment: A survey of mathematical models and technique,” Advances in Dynamic Network Modeling in Complex Transportation Systems (Editor: Satish V. Ukkusuri and Kaan Özbay) Springer New York, 2013. 1-25.
UR - http://www.scopus.com/inward/record.url?scp=85047219067&partnerID=8YFLogxK
UR - http://www.scopus.com/inward/citedby.url?scp=85047219067&partnerID=8YFLogxK
U2 - 10.1007/978-3-319-69231-9_2
DO - 10.1007/978-3-319-69231-9_2
M3 - Chapter
AN - SCOPUS:85047219067
T3 - Advances in Industrial Control
BT - Advances in Industrial Control
PB - Springer International Publishing

Dynamic Traffic Assignment
Early Experiences
Current Practices
Research Needs
Page categories
Activity Based Models
Network Assignment
Topic Circles
More pages in this category:
(opens new window) is a hot topic in travel forecasting.
# Background
Traditional user equilibrium highway assignment models predict the effects of congestion and the routing changes of traffic as a result of that congestion. They neglect, however, many of the details of real-world traffic operations, such as queuing, shock waves, and signalization. Currently, it is common practice to feed the results of user equilibrium traffic assignments into dynamic network models as a mechanism for evaluating these policies. The simulation models themselves, however, do not predict the routing of traffic, and therefore are unable to account for re-routing owing to changes in congestion levels or policy, and can be inconsistent with the routes determined by the assignment. Dynamic network models overcome this dichotomy by combining a time-dependent shortest path algorithm with some type of simulation (often meso or macroscopic) of link travel times and delay. In doing so it allows added reality and consistency in the assignment step, as well as the ability to evaluate policies designed to improve traffic operations. These are some of the main benefits of dynamic network models .
DTA models can generally be classified by how they model link or intersection delay. Analytical DTA models treat it in the same manner as static equilibrium assignment models, with no explicit representation of signals. Link capacity functions, often similar or identical to those used in static assignment, are used to calculate link travel times. Analytical models have been widely used in research and for real-time control system applications. Simulation-based DTA models include explicit representation of traffic control devices. Such models require detailed signal parameters to include phasing, cycle length, and offsets for each signal in the network. Delay is calculated for each approach, with vehicles moving from one link to the next only if available downstream capacity is available. The underlying traffic model is often different, but at the network level such models behave in a similar fashion.
Demand is specified in the form of origin–destination matrices for short time intervals, typically 15 minutes each. Trips are typically randomly loaded onto the network during each time interval. As with traffic microsimulation models, adequate downstream capacity must be present to load the trips onto the network. The shortest paths through time and space are found for each origin–destination pair, and flows loaded to these paths. A generalized flowchart of the process is shown below.

As with static assignment models, the process shown above is iteratively solved until a stable solution is reached. The memory and computing requirements of DTA, however, are orders of magnitude larger than for static assignment, reducing the number of iterations and paths that can be kept in memory. Instead of a single time period, as with static assignment, DTA models must store data for each time interval as well. A three-hour static assignment would involve only one time interval. A DTA model of the same period, however, might require 12 intervals, each 15 minutes in duration. These are all in addition to the memory requirements imposed by the number of user classes and zones.
# Early Experiences
Research into DTA dates back several decades, but was largely limited to academics working on its formulation and theoretical aspects. DTA overcomes the limitations of static assignment models, although at the cost of increased data requirements and computational burden. Moreover, software platforms capable of solving the DTA problem for large urban systems and experience in their use are recent developments.
(opens new window) has been successfully applied to a large subarea of Calgary and to analyses of the Rue Notre-Dame in Montreal. Although user group presentations of both applications have been made, and reported very encouraging results, the work is currently unpublished and inaccessible except through contact with the developers.
(opens new window) . The network from the Atlanta Regional Commission (ARC) regional travel model formed the starting point for the DTA network. Intersections were coded, centroid connectors were re-defined, and network coding errors were corrected. A signal synthesizer derived locally optimal timing parameters for more than 2,200 signalized intersections in the network. Trip matrices from the ARC model were divided into 15-minute intervals for the specification of demand. Approximately 40 runs of the model were required to diagnose coding and software errors. Unfortunately, the execution time for the model was approximately one week per run. The resulting model eventually validated well to observed conditions; however, the length of time required to render it operational and the run time required prevented it from being used in studies as originally intended. Subsequent work by the developer has resulted in substantial reductions in run time, but this remains a significant issue that must be overcome before such models can be more widely used.
# Current Practices
# research needs.
A number of cities are currently testing DTA models, but are not far enough along in their work to share even preliminary results. At least a dozen such cases are known to be in varying stages of planning or execution, suggesting that the use of DTA models in planning applications is about to expand dramatically. However, in addition to the issue of long run times, a number of other issues must be addressed before such models are likely to be widely adopted:
- Criteria for the validation of such models have not been widely accepted. The paucity of traffic counts in most urban areas, and especially at 15, 30, or 60 minute intervals, is a significant barrier to definitive assessment of these models.
← Network assignment Land Use-Transport Modeling →
This site uses cookies to learn which topics interest our readers.
Search form
- Publications
- Get Involved
- Planned Giving
Russian Offensive Campaign Assessment, July 4, 2023
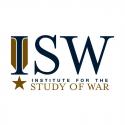
Karolina Hird, Grace Mappes, Nicole Wolkov, Angelica Evans, and Frederick W. Kagan
July 4, 2023, 8:35pm ET
Click here to see ISW’s interactive map of the Russian invasion of Ukraine. This map is updated daily alongside the static maps present in this report.
Click here to access ISW’s archive of interactive time-lapse maps of the Russian invasion of Ukraine. These maps complement the static control-of-terrain map that ISW produces daily by showing a dynamic frontline. ISW will update this time-lapse map archive monthly.
Note: The data cutoff for this product was 2pm ET on July 4. ISW will cover subsequent reports in the July 5 Russian Offensive Campaign Assessment.
Ukrainian forces appear to be focusing on creating an asymmetrical attrition gradient that conserves Ukrainian manpower at the cost of a slower rate of territorial gains, while gradually wearing down Russian manpower and equipment. Ukrainian National Security and Defense Council Secretary Oleksiy Danilov reported on July 4 that Ukrainian forces are performing their main task of destroying Russian manpower, equipment, fuel depots, artillery, and air defenses and that a “war of destruction is equal to a war of kilometers.”[1] Danilov’s assessment underlines the prioritization of Ukraine’s ongoing campaign to attrit Russian manpower and assets over attempting to conduct massive sweeping mechanized maneuvers to regain large swaths of territory rapidly. NATO Military Committee Chair Admiral Bob Bauer reported on July 3 that Ukrainian forces are correct to proceed cautiously and avoid high casualties in the counteroffensive and acknowledged that the counteroffensive is difficult due to landmines and other obstacles up to 30km deep into Russian-occupied territory.[2] Bauer stated that Ukrainian forces should not face criticism or pressure for moving slowly.
Ukrainian forces have liberated territory in multiple areas of the front since the start of the counteroffensive in early June. Ukrainian Deputy Defense Minister Hanna Malyar reported on July 3 that Ukrainian forces have liberated a total of 37.4 square kilometers in eastern and southern Ukraine in the past week.[3] Ukrainian forces are continuing to make steady, gradual advances.
The current pace of Ukrainian operations is not indicative of a stalemate or evidence that Ukraine cannot retake large areas. Ukrainian forces conducted slow and gradual interdiction campaigns against Russian concentration areas in east (left) bank Kherson Oblast and limited ground attacks on the west (right) bank between August and November of 2022, before finally forcing the Russian withdrawal from the right bank in mid-November.[4] The situation in southern Ukraine is different, of course, because there is no natural bottleneck of the sort created by Russian reliance on the two bridges over the Dnipro. The Ukrainian counter-offensive in Kherson nevertheless alternated phases of relatively rapid advance with long periods of preparation, combat focused on attritting Russian forces, and limited gains that ultimately made Russian positions on the west bank of the river untenable. By contrast, the Russian winter-spring offensive culminated in just over one month without making significant gains along the Luhansk-Kharkiv Oblast border.[5] The current Ukrainian counter-offensive is less dramatic and rapid than the one that liberated much of Kharkiv Oblast, more successful than the failed Russian winter offensive, and generally most like the slower but ultimately successful Kherson counteroffensive in its pace and initial progress.
Ukrainian forces continued counteroffensive operations on at least four sectors of the front and advanced on July 4. The Russian Ministry of Defense (MoD) claimed that Russian forces repelled Ukrainian ground attacks in the Lyman direction.[6] The Ukrainian General Staff reported that Ukrainian forces continue counteroffensive operations in the Bakhmut area, in the western Donetsk-Zaporizhia Oblast border area, and in western Zaporizhia Oblast.[7] Ukrainian military officials stated that Ukrainian forces have made some unspecified advances on Bakhmut’s northern and southern flanks, and a prominent Russian milblogger also claimed that Ukrainian forces advanced north of Bakhmut.[8] Ukrainian Tavrisk Group of Forces Spokesperson Valery Shershen stated that Ukrainian forces advanced up to two kilometers in the western Donetsk-Zaporizhia Oblast border area, and a Russian milblogger claimed that Ukrainian forces reached Pryyutne, 15 kilometers southwest of Velyka Novosilka in western Donetsk Oblast.[9] Geolocated footage confirms that Ukrainian forces made additional advances south of Orikhiv in western Zaporizhia Oblast.[10]
Russian and Ukrainian officials escalated their rhetoric surrounding the situation at the Zaporizhzhia Nuclear Power Plant (ZNPP) on July 4, but Russia is likely focused on accusing Ukraine of irresponsible actions around the ZNPP including setting conditions for a possible false flag attack . Russia remains unlikely to generate a radiological incident at the ZNPP at this time. The Ukrainian General Staff reported on July 4 that Ukrainian officials have begun preparations for a potential Russian provocation at the ZNPP “in the near future” and warned that Russian forces placed objects “resembling explosive devices” on the outer roofs of the ZNPP’s third and fourth reactors in order to blame damage to these areas on Ukrainian shelling.[11] Ukrainian President Volodymyr Zelensky echoed this statement in his nightly address on July 4, and other Ukrainian military sources warned of possible Russian provocations at the plant.[12] As ISW has previously reported, it is unlikely that limited Russian sabotage at the ZNPP that Russia could hope to blame on Ukraine would be able to generate a massive radiological incident, as the ZNPP’s reactors were constructed to withstand considerable damage.[13] Ukrainian military sources reiterated this assessment and noted that even if the purported explosive devices detonate, the damage would not harm the reactor but would rather create the false impression that Ukrainian forces had shelled the reactors.[14] Advisor to the head of Russian nuclear energy operator Rosenergoatom, Renat Karchaa, also claimed on July 4 that Ukraine is planning to strike the ZNPP overnight on July 4-5.[15] ISW has previously assessed that such provocative Russian statements, and even the possibility of a tangible provocation at the plant, are likely part of a Russian wider information operation meant to accuse Ukraine of irresponsibility at the ZNPP ahead of the upcoming NATO summit and dissuade Ukrainian forces from conducting counteroffensive operations against occupied Zaporizhia Oblast.[16]
The reported reorganization of Russian internal security organs suggests that the Kremlin has not yet concluded that it has effectively neutralized the threats of future armed rebellions following the Wagner Group’s June 23-24 rebellion. Russian outlet Vedomosti reported on July 3, citing internal law enforcement sources, that Russian law enforcement authorities are considering reassigning the “Grom” special units of the Russian Federal Drug Control Service (part of the Ministry of Internal Affairs) to Rosgvardia (Russian National Guard).[17] Vedomosti noted that this reported change follows Russian President Vladimir Putin’s meeting with heads of various Russian law enforcement agencies on June 26 in the wake of the Wagner armed rebellion.[18] Several Russian sources spoke out against the reported transfer of ”Grom” to Rosgvardia, citing overall poorer equipment, training, and leadership quality.[19] Vedomosti claimed that Alexander Khinstein, former advisor to Rosgvardia Head Viktor Zolotov, warned that the assignment of ”Grom” units to Rosgvardia would be a ”dangerous experiment.”[20] The alleged restructuring of Russia’s internal security forces suggests that the Kremlin is working to build an effective anti-rebellion force following Wagner’s armed rebellion. The fact that these purported changes are happening following the rebellion indicates that the Kremlin was correctly dissatisfied with the performance of security forces, which failed to stop or even contest Wagner’s march on Moscow, and suggests that the Kremlin has not ruled out the risk of future such rebellions.
Russian authorities are absolving Wagner Group financier Yevgeny Prigozhin of financial responsibility for damages caused by the Wagner Group rebellion and reportedly returned significant liquid assets to Prigozhin, possibly as part of the deal negotiated between Putin, Prigozhin, and Belarusian dictator Alexander Lukashenko. The Rostov-on-Don administration claimed that the total damages from Prigozhin’s rebellion amounted to 92.5 million rubles (roughly $1 million), and that the administration will not recover damages from Prigozhin or the Wagner Group.[21] St. Petersburg news outlet Fontanka claimed, citing internal sources, that Russian authorities returned over 10 billion rubles (roughly $111 million) in cash, five gold bars, and hundreds of thousands of US dollars in cash to Prigozhin on July 2 that authorities had seized from Prigozhin-affiliated facilities in St. Petersburg on June 24.[22] Fontanka claimed that authorities only reversed their decision to hold onto Prigozhin‘s liquid assets on July 2 but did not specify a reason for the reversal. The legal basis that Russian authorities would have had for seizing Prigozhin’s assets remains unclear in any case, as Russian authorities dropped criminal charges against Prigozhin for the rebellion.[23] A prominent Russian milblogger claimed that part of Prigozhin’s liquid assets were supposed to be compensation to the families of Russian pilots whom Wagner forces killed during the rebellion, but it is now uncertain whether Wagner will make those payments.[24] The milblogger assessed that Wagner will likely use at least part of the returned assets to support transferring Wagner Group personnel to Belarus.
The official Chechen response to an attack against a Russian opposition journalist in Chechnya may impact Chechen Republic Head Ramzan Kadyrov’s standing in the Russian ultranationalist information space. Russian opposition outlet Novaya Gazeta reported on July 4 that unspecified, masked actors in Grozny, Chechnya intercepted a car containing one of its journalists, Yelena Milashina, severely assaulted Milashina, destroyed her equipment and documents, and warned Milashina against writing “anything.”[25] Milashina traveled to Chechnya in order to cover the trial of Zarema Musayeva, the mother of an exiled Chechen opposition activist, and the attackers also assaulted Musayeva’s lawyer, Alexander Nemov, who was in the car with Milashina. Chechen courts sentenced Musayeva to five and a half years in prison on July 5 for alleged fraud and attacking Chechen authorities, but some Russian opposition voices claimed that Chechen authorities prosecuted Musayeva due to her son‘s activism.[26] Prominent Russian ultranationalist voices seized on Milashina’s attack despite its lack of relevance to the war in Ukraine likely out of concern for broader press censorship.[27] The voices condemned attacks against journalists – including Milashina – as unacceptable even though they disagree with Milashina.[28] The Russian Union of Journalists and the Russian Human Rights Council both issued statements of condemnation and opened investigations into the attack.[29]
Kadyrov’s prominence in the broader Russian information space will likely force Kadyrov to choose between preserving his regime and his support in the ultranationalist information space, however. Kadyrov’s response was a brief acknowledgment that the relevant Chechen authorities are investigating the ”incident” - a response inconsistent in tone and content with Kadyrov’s usual flamboyant, long-winded messaging.[30] Kadyrov previously condemned Milashina as a ”terrorist” and demanded her detention, which is largely consistent with his overall effort to retain his authoritarian rule in Chechnya.[31] If Kadyrov supports the investigation into Milashina’s attack, he risks undermining his domestic regime and crackdowns against Chechen opposition voices. But if Kadyrov refuses to support the investigation, then he risks undermining his standing within an information space that is hypersensitive to the prospect of increased censorship. Kadyrov already struggles to balance these dual aims in his force arrayment in Ukraine; Kadyrov portrays Akhmat forces as capable fighters against Ukraine but has simultaneously largely avoided committing them intensive and attritional combat, and some Russian milbloggers have complained that Chechen forces are distracted posing online while other Russian forces actually fight.[32] Chechen forces notably failed to engage Prigozhin’s rebels despite ostentatiously mobilizing and moving ostensibly to fight them, although Putin might have directed Kadyrov to avoid combat with Wagner forces.[33]
Russia is reportedly forming a new combined arms army as part of the Northern Fleet, likely in order to posture its preparedness against NATO. Russian news outlet Izvestia reported that Russian Ministry of Defense (MoD) sources claimed that the existing 14th Army Corps of the Northern Fleet will be reformed into the new combined arms army with motorized rifle brigades, divisions, and regiments subordinate to it.[34] Izvestia suggested that the 14th Army Corps‘ 200th and 80th Brigades will be reorganized into a division under the new combined arms army.[35] Russian army corps before the 2022 invasion of Ukraine existed only within fleets and largely performed the same functions as combined arms armies. The reported decision to form a new combined arms army is thus likely posturing ahead of the NATO summit on July 11-12 intended to show Russia’s military response to the accession of Finland and possibly Sweden to the alliance. The promotion of the 14th Army Corps to a combined arms army level will not by itself increase Russian combat capacity, and it is unclear where the Russian military leadership could find the personnel and equipment that would be needed for the new organization to generate a material difference.
The Russian Ministry of Defense (MoD) claimed that Ukrainian forces conducted a drone attack on Moscow Oblast and Novaya Moskva on July 4. The Russian MoD claimed that Russian air defenses shot down and electronic warfare suppressed five of five Ukrainian drones.[36] Russian milbloggers claimed that Russian air defenses destroyed two drones near Valuevo, electronic warfare suppressed one in the Odinstovo Raion, one drone fell near Krivosheino, and one flew toward a military unit in Kubinka - likely the Russian airbase there.[37] One Russian milblogger claimed that Ukrainian forces may have intended to strike Vnukovo Airport, and Moscow Mayor Sergei Sobyanin announced that Russian authorities temporarily redirected some flights from Vnukovo Airport in response to the drones.[38] Another milblogger claimed that Ukrainian forces may have conducted the drone attack in retaliation for an alleged Russian strike on a Ukrainian Security Services (SBU) building in Sumy Oblast.[39]
Key Takeaways:
- Ukrainian forces appear to be focusing on creating an asymmetrical attrition gradient that conserves Ukrainian manpower at the cost of a slower rate of territorial gains, while gradually wearing down Russian manpower and equipment. The current pace of Ukrainian operations is not indicative of a stalemate or evidence that Ukraine cannot retake large areas.
- Ukrainian forces continued counteroffensive operations on at least four sectors of the front and advanced on July 4.
- Russian and Ukrainian officials escalated their rhetoric surrounding the situation at the Zaporizhzhia Nuclear Power Plant (ZNPP) on July 5, but Russia is likely focused on accusing Ukraine of irresponsible actions around the ZNPP including setting conditions for a possible false flag attack. Russia remains unlikely to generate a radiological incident at the ZNPP at this time.
- The reported reorganization of Russian internal security organs suggests that the Kremlin has not yet concluded that it has effectively neutralized the threats of future armed rebellions following the Wagner Group’s June 23-24 rebellion.
- Russian authorities are absolving Wagner Group financier Yevgeny Prigozhin of financial responsibility for damages caused by the Wagner Group rebellion and reportedly returned significant liquid assets to Prigozhin, possibly as part of the deal negotiated between Putin, Prigozhin, and Belarusian dictator Alexander Lukashenko.
- The official Chechen response to an attack against a Russian opposition journalist in Chechnya may impact Chechen Republic Head Ramzan Kadyrov’s standing in the Russian ultranationalist information space.
- Kadyrov’s prominence in the broader Russian information space will likely force Kadyrov to choose between preserving his regime and his support in the ultranationalist information space, however.
- Russia is reportedly forming a new combined arms army as part of the Northern Fleet, likely in order to posture its preparedness against NATO.
- The Russian Ministry of Defense (MoD) claimed that Ukrainian forces conducted a drone attack on Moscow Oblast and Novaya Moskva on July 4.
- Russian conducted limited ground attacks along the Kupyansk-Svatove-Kreminna line and south of Kreminna.
- Russian sources claimed that Ukrainian forces conducted limited ground attacks along the Kupyansk-Svatove-Kreminna line.
- Russian and Ukrainian forces escalated ground attacks in the Bakhmut area.
- Russian forces continued ground attacks along the Avdiivka-Donetsk City line on July 4.
- Russian and Ukrainian forces conducted ground attacks in western Donetsk Oblast.
- Russian sources claimed that Ukrainian forces continued counteroffensive operations near Orikhiv in western Zaporizhia Oblast.
- Russia continues efforts to mobilize its defense industrial base (DIB).
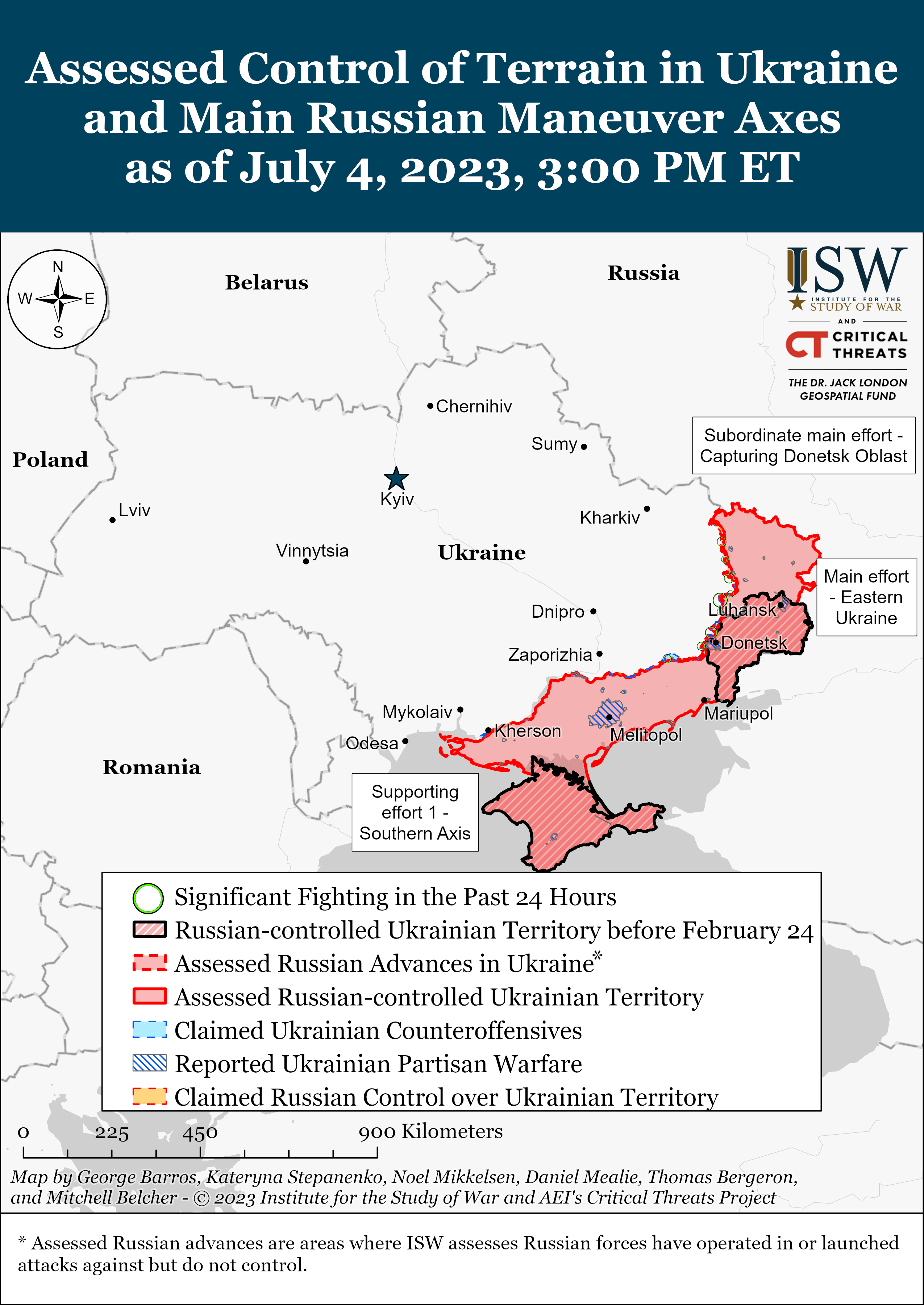
We do not report in detail on Russian war crimes because these activities are well-covered in Western media and do not directly affect the military operations we are assessing and forecasting. We will continue to evaluate and report on the effects of these criminal activities on the Ukrainian military and the Ukrainian population and specifically on combat in Ukrainian urban areas. We utterly condemn these Russian violations of the laws of armed conflict, Geneva Conventions, and humanity even though we do not describe them in these reports.
- Russian Main Effort – Eastern Ukraine (comprised of two subordinate main efforts)
- Russian Subordinate Main Effort #1 – Capture the remainder of Luhansk Oblast and push westward into eastern Kharkiv Oblast and encircle northern Donetsk Oblast
- Russian Subordinate Main Effort #2 – Capture the entirety of Donetsk Oblast
- Russian Supporting Effort – Southern Axis
- Russian Mobilization and Force Generation Efforts
- Activities in Russian-occupied areas
Russian Main Effort – Eastern Ukraine
Russian Subordinate Main Effort #1 – Luhansk Oblast (Russian objective: Capture the remainder of Luhansk Oblast and push westward into eastern Kharkiv Oblast and northern Donetsk Oblast)
The Ukrainian General Staff reported on July 4 that Russian sabotage and reconnaissance groups made unsuccessful attempts to cross the northern international border between Ukraine and Russia in unspecified areas in the Siversk and Slobozhansk directions.[40]
Russian forces conducted limited ground attacks along the Kupyansk-Svatove-Kreminna line and south of Kreminna on July 4. Geolocated footage published on July 4 shows that Russian forces made limited advances east of Nevske (18km northwest of Kreminna).[41] The Ukrainian General Staff reported that Russian forces conducted unsuccessful offensive operations near Novoselivske (15km northwest of Svatove), Novovodyane (16km southwest of Svatove), Dibrova (7km southwest of Kreminna), the Serebrianske forest area (10km south of Kreminna), and Vesele (30km south of Kreminna).[42] A Russian milblogger claimed that Russian and Ukrainian forces attempted to advance in the Svatove direction and that Russian forces conducted attacks near Kuzemivka (14km northwest of Svatove).[43] Ukrainian Eastern Group of Forces Spokesperson Colonel Serhiy Cherevaty reported on June 3 that Russian forces have about 180,000 troops in the area of responsibility of the Ukrainian Eastern Group of Forces, 120,000 of which are operating in the Kupyansk-Lyman direction, including Airborne (VDV) forces, mechanized units, BARS (Russian Combat Reserve) units, Territorial Defense units, and Storm-Z assault units.[44] Footage published on July 4 purportedly shows the 123rd Motorized Rifle Brigade (2nd Luhansk People’s Republic Army Corps) operating near Spirne (25km south of Kreminna).[45]
Russian sources claimed that Ukrainian forces conducted limited ground attacks along the Kupyansk-Svatove-Kreminna line on July 4. The Russian Ministry of Defense (MoD) claimed that Russian forces repelled a Ukrainian attack near Novoselivske, Novovodyane, and Yampolivka (16km west of Kreminna).[46] Russian milbloggers claimed that Ukrainian forces conducted unsuccessful ground attacks from Torske (16km west of Kreminna) and that artillery and UAV units of the Russian 120th Guards Artillery Brigade (41st Combined Arms Army, Central Military District) repelled Ukrainian attacks near Dibrova.[47]
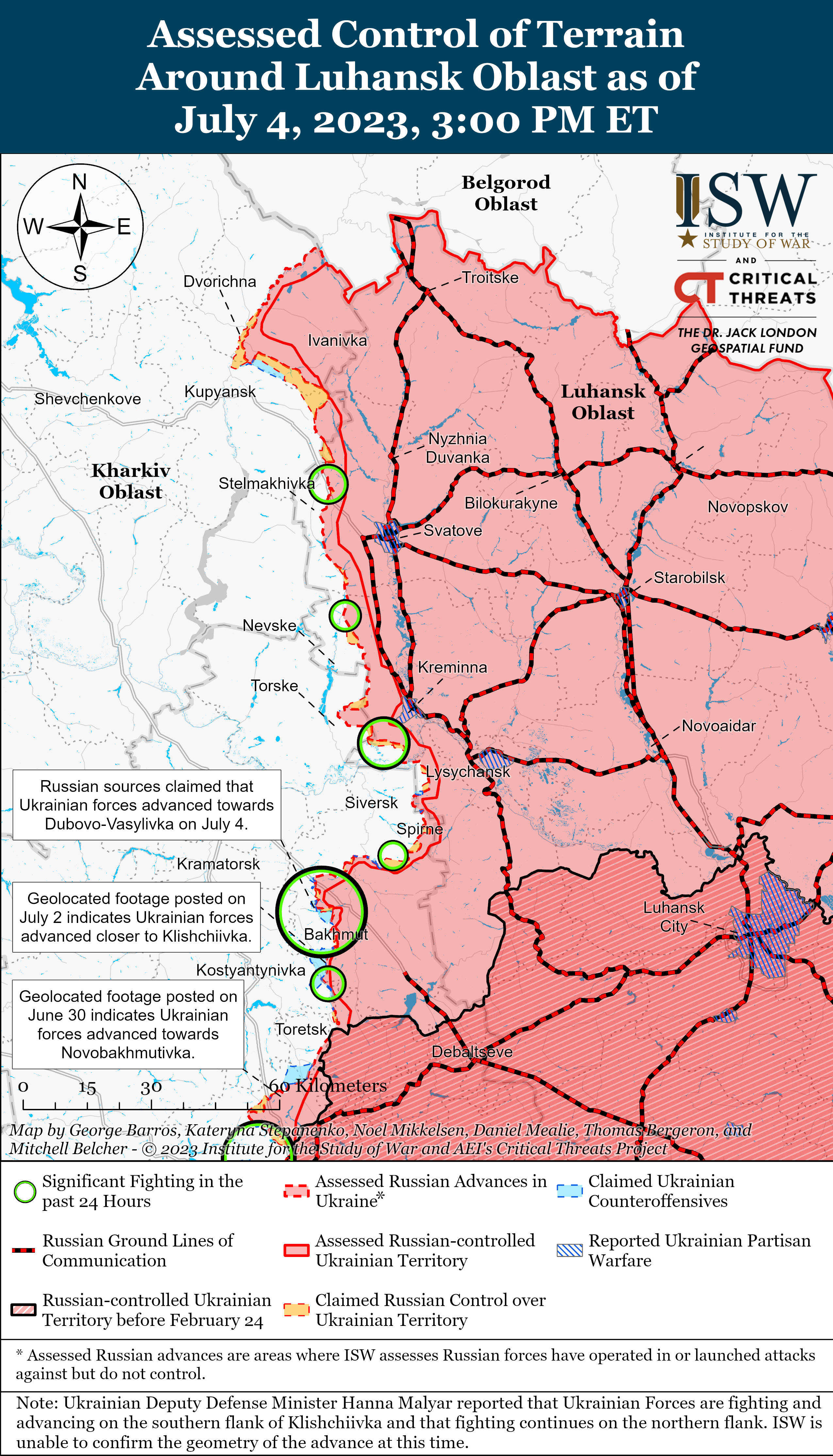
Russian Subordinate Main Effort #2 – Donetsk Oblast (Russian Objective: Capture the entirety of Donetsk Oblast, the claimed territory of Russia’s proxies in Donbas)
Russian and Ukrainian forces escalated ground attacks in the Bakhmut area on July 4. Ukranian Deputy Defense Minister Hanna Malyar reported that the situation in Bakhmut has escalated, and that Russian and Ukrainian forces are dueling for the initiative and control of terrain.[48] Malyar also stated that Ukrainian forces are advancing on the southern flank of Klishchiivka (7km southwest of Bakhmut) and that fighting continues on Klishchiivka’s northern flank.[49] The Ukrainian General Staff reported that Ukrainian forces repelled nine Russian attacks near Hryhorivka (8km northwest of Bakhmut), Bohdanivka (5km northwest of Bakhmut), Ivanivske (6km west of Bakhmut), west of Yahidne (2km north of Bakhmut), and southeast of Bila Hora (15km southwest of Bakhmut).[50] The Ukrainian General Staff also reported that Ukrainian forces conducted offensive operations north and south of Bakhmut, entrenching themselves in new positions.[51] Russian milbloggers claimed that Ukrainian forces advanced near Dubovo-Vasylivka (6km northwest of Bakhmut).[52] A Russian milblogger also claimed that Ukrainian forces conducted offensive operations near Klishchiivka, Ozarianivka (16km southwest of Bakhmut), and Kurdiumivka (12km southwest of Bakhmut).[53] The milblogger claimed that Russian forces held their positions and counterattacked from Berkhivka (6km north of Bakhmut) and along the M-03 highway in the direction of Minkivka (13km northwest of Bakhmut).[54] The Russian Ministry of Defense (MoD) claimed that units of the Russian Southern Group of Forces repelled 10 Ukrainian attacks near Orikhovo-Vasylivka (11km northwest of Bakhmut), Yahidne, and Klishchivka.[55]
Pervasive issues with Russian combat capabilities likely continue to affect the ability of Russian forces to defend against Ukrainian counterattacks in the Bakhmut area. Former Russian officer and prominent critical milblogger Igor Girkin claimed that Ukrainian forces are advancing north of Bakhmut where understaffed units of the Russian 3rd Army Corps (Western Military District) have been deployed.[56] ISW previously reported the formation and failure of the 3rd Army Corps, a new formation created in 2022 that was decimated during its first deployment to Kharkiv Oblast in September 2022 and again in its subsequent deployments to the Bakhmut area.[57] ISW previously assessed that issues with the ad hoc commitment of various depleted force groupings to the Bakhmut axis, alongside apparent command and control failures, were likely preventing Russian forces in the area from conducting sound defensive operations and would likely offer Ukrainian forces opportunities to exploit with limited counterattacks.[58]
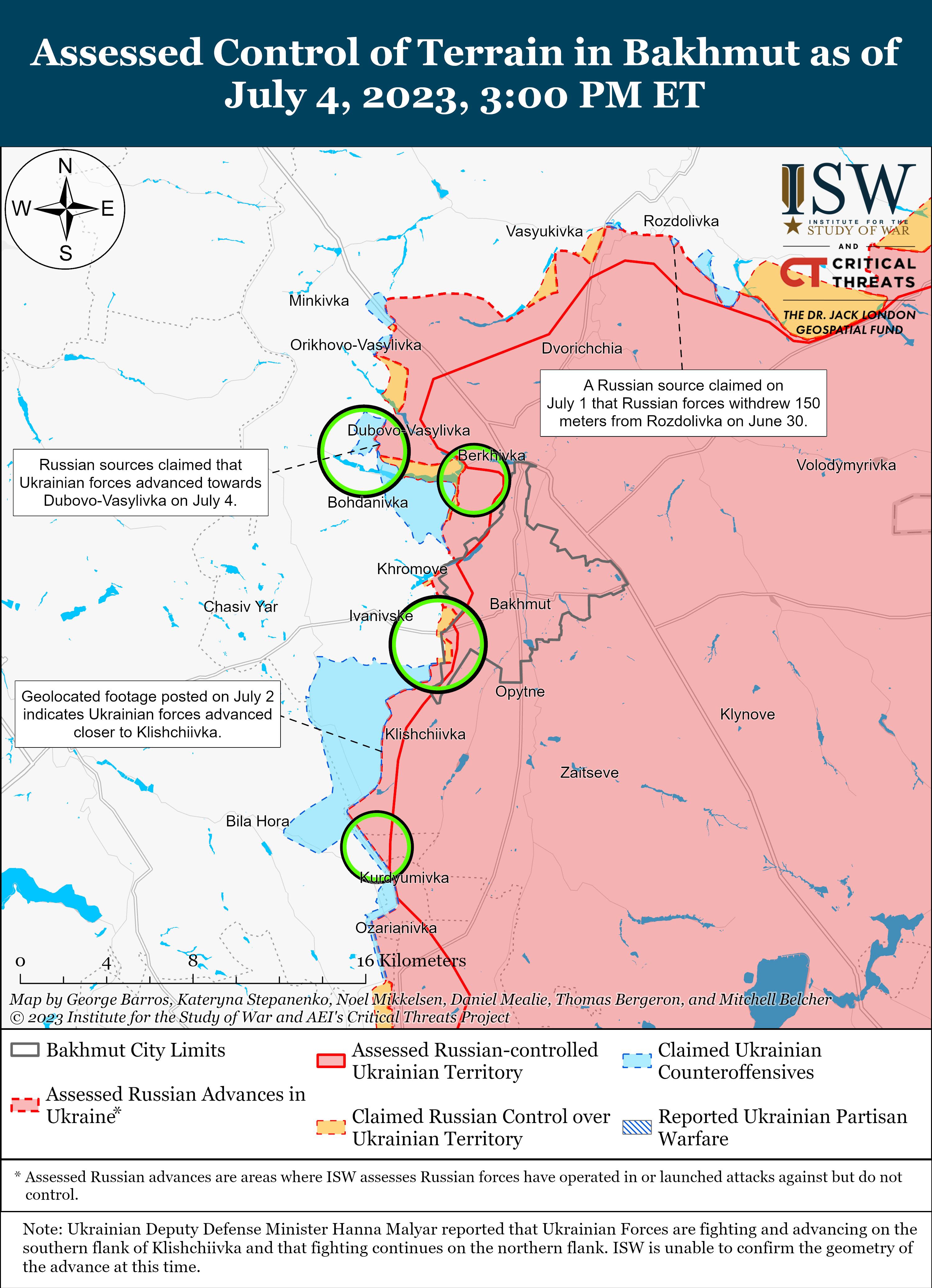
Russian forces continued ground attacks along the Avdiivka-Donetsk City line on July 4. The Ukrainian General Staff reported that Ukrainian forces defended against Russian offensive operations near Avdiivka and repelled 15 Russian ground attacks near Marinka (on the southwestern outskirts of Donetsk City).[59] A Russian milblogger claimed that Ukrainian forces conducted unsuccessful offensive operations in the Avdiivka direction.[60] Another milblogger claimed that Russian forces carried out unsuccessful offensive operations in Marinka and on the southwestern approach to Avdiivka.[61]
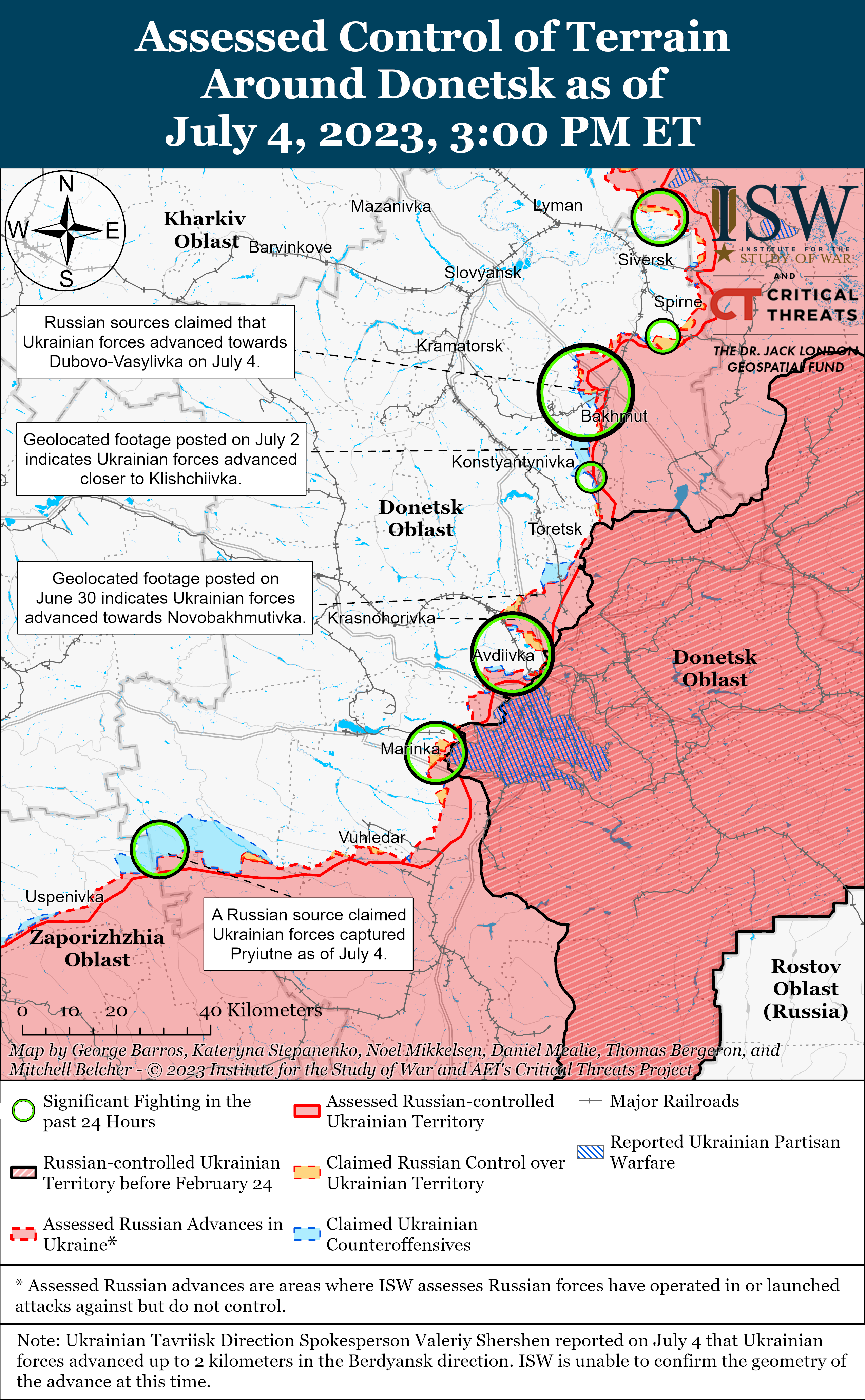
Russian Supporting Effort – Southern Axis (Russian objective: Maintain frontline positions and secure rear areas against Ukrainian strikes)
Russian and Ukrainian forces conducted ground attacks in western Donetsk Oblast on July 4. The Ukrainian General Staff reported that Russian forces conducted unsuccessful offensive operations near Rivnopil, 10km southwest of Velyka Novosilka along the western Donetsk-eastern Zaporizhia Oblast border.[62] The Russian Ministry of Defense (MoD) claimed that Russian forces repelled Ukrainian attacks in the Vuhledar area east of Velyka Novosilka and south of Velyka Novosilka near Urozhaine.[63] A Russian milblogger claimed that Ukrainian forces reached the borders of Pryyutne, 15km southwest of Velyka Novosilka in eastern Zaporizhia Oblast.[64] Ukrainian Tavrisk Direction Spokesperson Valeriy Shershen noted on July 4 that Ukrainian forces have advanced up to 2km into Russian defenses in an unspecified area of the Berdyansk (western Donetsk-eastern Zaporizhia oblasts) direction.[65]
Russian sources claimed that Ukrainian forces continued counteroffensive operations near Orikhiv in western Zaporizhia Oblast on July 4. Geolocated footage posted on July 4 shows elements of the 810 th Naval Infantry Brigade (Black Sea Fleet) and 58th Combined Arms Army (Southern Military District) shelling Ukrainian positions south of Orikhiv, indicating that Ukrainian forces have advanced to within 2km north of Robotyne.[66] Russian milbloggers claimed that Ukrainian forces continued attacks towards Robotyne but that elements of the 70th Motorized Rifle Regiment (42nd Motorized Rifle Division, 58th Combined Arms Army, Southern Military District) repelled Ukrainian attacks west of Robotyne.[67] Russian milbloggers additionally claimed that several small Ukrainian assault groups launched an attack southwest of Orikhiv towards the Pyatykhatyky-Zherebryanky line (about 25km southwest of Orikhiv) and reported that elements of the Crimea and Sudoplatov volunteer battalions and the 429th Motorized Rifle Regiment (19th Motorized Rifle Division, 58th Combined Arms Army, Southern Military District) are defending in this area.[68]
Ukrainian forces conducted a strike in the Russian rear of occupied Zaporizhia Oblast on July 4. Geolocated footage posted on July 4 shows the aftermath of a reported Ukrainian Storm Shadow missile strike on an unspecified Russian warehouse facility in Yakymivka, about 23km southwest of Melitopol along the T2209 Melitopol-Chonhar highway.[69] Russian sources additionally claimed that Ukrainian forces struck Vasylivka (35km north of Melitopol along the E105 highway).[70]
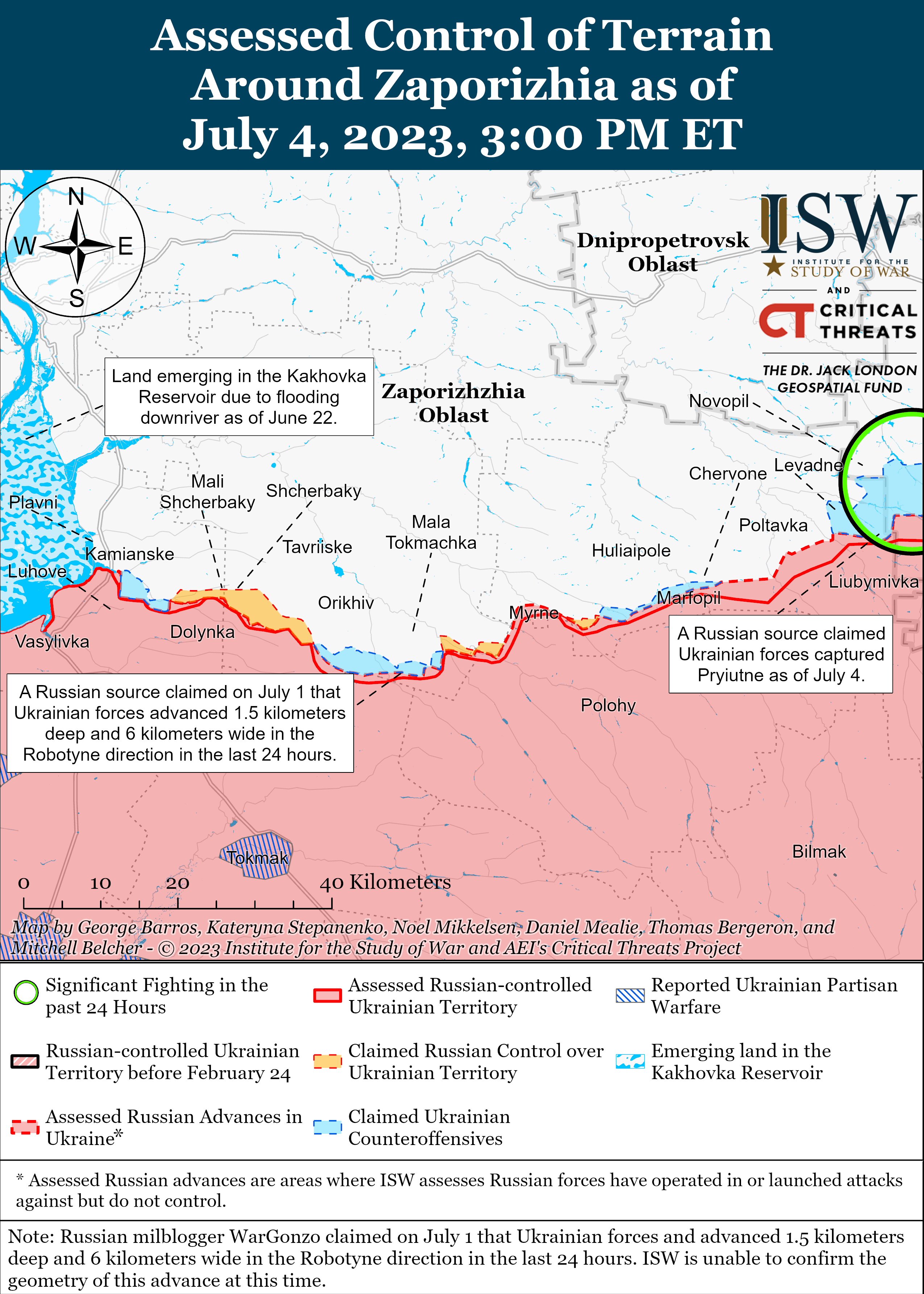
Russian sources continued to claim that Ukrainian forces are active near the Antonivsky Bridge on the east (left) bank of Kherson Oblast despite the Russian MoD’s efforts to claim that Russian forces have full control of this area.[71] One Russian milblogger claimed that there are heavy battles ongoing near the Antonivsky Bridge, and another warned that Ukrainian troops are regrouping and replenishing units to prepare for further attacks across the Dnipro River.[72] The Russian MoD claimed on July 1 that Russian troops fully restored their positions along the eastern shoreline of the Dnipro River, but milbloggers have continued to warn that Ukrainian forces maintain a presence on the east bank and are preparing for additional attacks.[73] Ukrainian Southern Operational Command Spokesperson Nataliya Humenyuk also noted that Russian forces near the Dnipro River are trying to retake positions previously flooded by the explosion of the Kakhovka Hydroelectric Power Plant (KHPP) dam.[74]
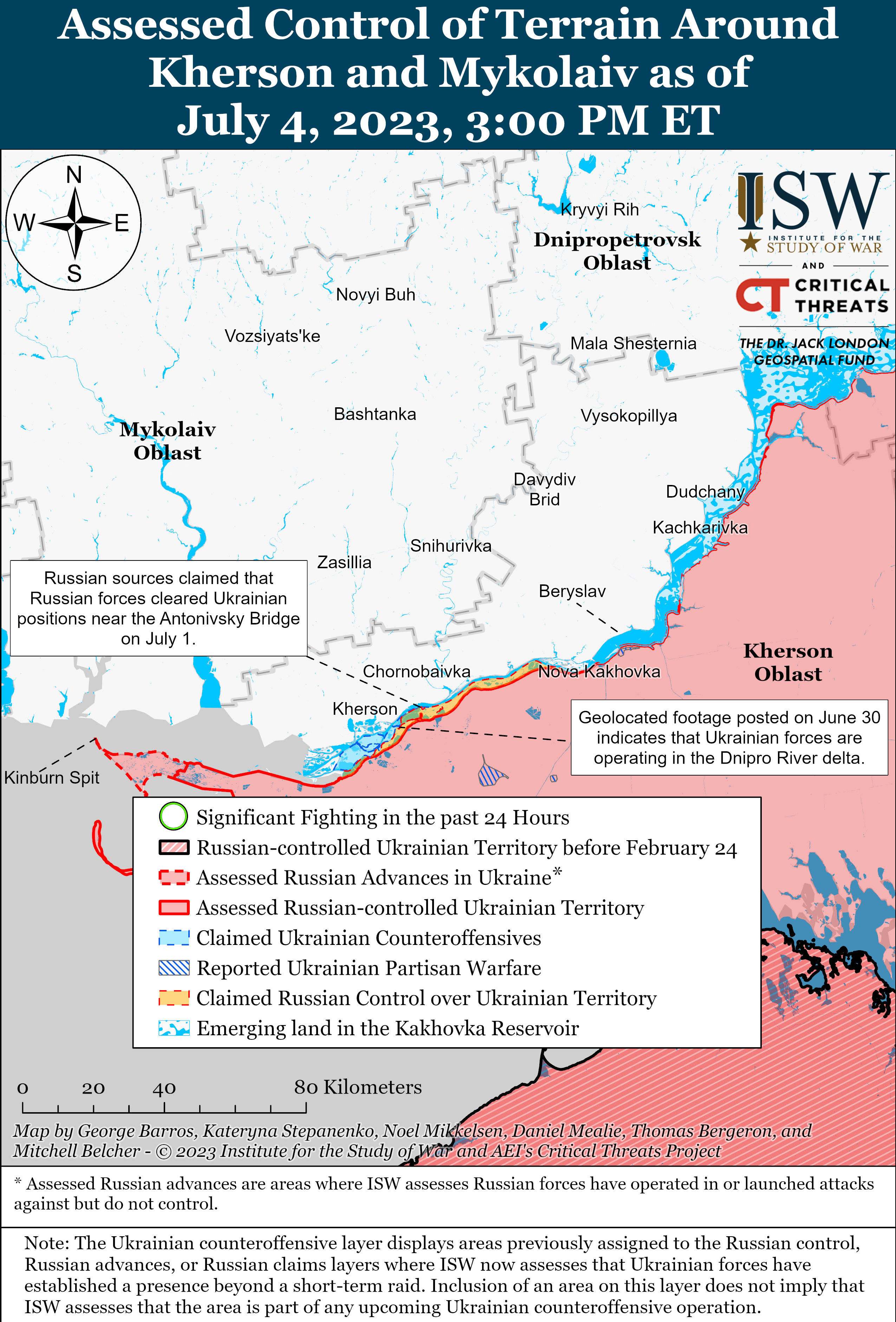
An influx of Russian tourists to Crimea is generating serious traffic jams along one of Russia’s most important ground lines of communication, prompting Putin and other senior Russian officials to direct state resources to help tourists move closer to a zone of active hostilities. Russian Transport Minister Vitaly Saveliev met with Russian President Vladimir Putin on July 4 to report on the situation at the entrance to the Kerch Strait Bridge and to ask for increased ferry crossings to reduce traffic jams.[75] Putin called for maximizing the use of ferries to ”normalize” the transport situation across the Kerch Strait and indicated that the Russian MoD should also lend transport assets to the area.[76] A Russian milblogger claimed that the traffic at the entrance of the Kerch Strait Bridge in Krasnodar Krai has increased by 40% since July 1 and is expected to increase further in the coming days as the summer tourist season is in full swing.[77] Another Russian milblogger called on the Black Sea Fleet to provide two large landing ships for the crossing of civilian vehicles to solve traffic issues and emphasized that Russian authorities have seriously underestimated the desire of Russians to continue vacationing in occupied Crimea despite ongoing hostilities.[78] Russian authorities are dealing with pervasive civilian and transport issues to Crimea partially because of their continued refusal to fully mobilize Russian society onto a wartime footing, resulting in the continued promotion of tourism to occupied Crimea despite the fact it is a legitimate rear-area target for continued Ukrainian strikes.
Russian Mobilization and Force Generation Efforts (Russian objective: Expand combat power without conducting general mobilization)
Russia continues efforts to mobilize its defense industrial base (DIB). Russian Defense Minister Sergey Shoigu, Industry and Trade Minister Denis Manturov, and unspecified other military leaders and DIB representatives discussed the implementation of the Russian state defense order to increase DIB production, but the Russian Ministry of Defense (MoD) did not provide details on the topics discussed or agreed plans.[79] Yelabuga, Tatarstan regional entity “Alabuga Start” advertised a program for women aged 16 to 22 to develop careers building drones.[80] The program claims to offer benefits including a 52,000 ruble ($577) monthly salary, training, housing, relocation aid, and opportunities for further education. ISW has previously reported that a Russo-Iranian contract is providing for the manufacture of Shahed drones in the Alabuga Special Economic Zone (SEZ).[81]
Russian officials continue to posture Russia as able to generate enough manpower to maintain the war effort in Ukraine. Russian Security Council Deputy Chairperson Dmitry Medvedev claimed that Russian forces have recruited over 185,000 contract and conscripted personnel since January 1, 2023, 109,000 of whom are in reserve.[82] Medvedev claimed that Russian forces recruited 1,400 people per day for contract service in June 2023.
Russia continues efforts to expand international military cooperation. Russian Navy Commander-in-Chief Nikolai Yevmenov and Chinese Defense Minister Li Shangfu met in Beijing on July 3 and discussed ongoing mutual cooperation and organizing joint military exercises.[83] Russian Deputy Defense Minister Colonel General Alexander Fomin met with Kuwaiti Army Assistant Chief of Staff Brigadier General Fawaz Al-Harbi in Moscow on July 4 and confirmed Russian and Kuwaiti intent to further defense cooperation.[84]
Activities in Russian-occupied areas (Russian objective: Consolidate administrative control of annexed areas; forcibly integrate Ukrainian civilians into Russian sociocultural, economic, military, and governance systems)
Russian officials continue to deport Ukrainian children to Russia under the guise of providing pediatric healthcare. Russian Commissioner for Children's Rights Maria Lvova-Belova claimed on July 4 that Russian authorities sent 23 disabled children from occupied Donetsk Oblast to a rehabilitation center in Krasnogorsk, Moscow Oblast and 12 children to a rehabilitation center in Elektrostal, Moscow Oblast.[85] Lvova-Belova claimed that Russian authorities plan to send about 370 more children in at least four more trips to rehabilitation centers by the end of 2023.[86] Lvova-Belova did not specify if the children have returned or will return to occupied Donetsk Oblast. ISW has previously reported on Russian authorities using access to pediatric healthcare as a guise to deport children in occupied Ukraine to Russia.[87]
Significant activity in Belarus (Russian efforts to increase its military presence in Belarus and further integrate Belarus into Russian-favorable frameworks).
ISW will continue to report daily observed Russian and Belarusian military activity in Belarus, as part of ongoing Kremlin efforts to increase their control over Belarus and other Russian actions in Belarus.
Nothing significant to report.
Note: ISW does not receive any classified material from any source, uses only publicly available information, and draws extensively on Russian, Ukrainian, and Western reporting and social media as well as commercially available satellite imagery and other geospatial data as the basis for these reports. References to all sources used are provided in the endnotes of each update.
[1] https://twitter.com/OleksiyDanilov/status/1676116133819170817
[2] https://www.reuters.com/world/europe/ukraine-right-be-cautious-with-counter-offensive-top-nato-official-says-2023-07-03/
[3] https://t.me/annamaliar/899
[4] https://www.understandingwar.org/backgrounder/russian-offensive-campaign-assessment-august-30 ; https://www.understandingwar.org/backgrounder/russian-offensive-campaign-assessment-november-13
[5] https://www.understandingwar.org/backgrounder/russian-offensive-campaign-assessment-march-15-2023 ; https://understandingwar.org/backgrounder/russian-offensive-campaign-assessment-february-8-2023
[6] https://t.me/mod_russia/28072
[7] https://www.facebook.com/GeneralStaff.ua/posts/pfbid02A52T2ugTVhiavau9ev...
[8] https://armyinform.com dot ua/2023/07/04/syly-oborony-prodovzhuyut-vesty-nastupalni-operacziyi-na-bahmutskomu-melitopolskomu-i-berdyanskomu-napryamkah/; https://t.me/strelkovii/5874 ; https://t.me/annamaliar/903
[9] https://suspilne dot media/521387-na-berdanskomu-napramku-zaporizkoi-oblasti-vijskovi-zsu-prosunulisa-do-dvoh-kilometriv-vpered/; https://www.facebook.com/PresscentrTavria/videos/932914671129838/
[10] https://twitter.com/GeoConfirmed/status/1676197514641387521; https://t.me/wargonzo/13655
[11] https://www.facebook.com/GeneralStaff.ua/posts/pfbid02ABo9LEqPDXC5mk6WF1Y8DnZ7p1DBLvQt2gWt1TwufuY7FfCaM7yYvYP6eBhn7uYHl
[12] https://www.president.gov dot ua/en/news/svit-bachit-sho-yedinim-dzherelom-nebezpeki-dlya-zaporizkoyi-84065; https://t.me/AFUStratCom/18805 ; https://t.me/spravdi/31142
[13] https://isw.pub/UkrWar112122 ; https://isw.pub/UkrWar070123 ; https://isw.pub/UkrWar062223
[14] https://www.reuters.com/world/europe/russia-ukraine-accuse-each-other-pl...
[15] https://www.reuters.com/world/europe/russia-ukraine-accuse-each-other-pl...
[16] https://isw.pub/RusCampaignSept22 ;
[17] https://www.vedomosti dot ru/politics/articles/2023/07/04/983567-vedomstva-prorabativayut-vopros-o-perepodchinenii-politseiskogo-spetsnaza-rosgvardii
[18] https://www.vedomosti dot ru/politics/articles/2023/07/04/983567-vedomstva-prorabativayut-vopros-o-perepodchinenii-politseiskogo-spetsnaza-rosgvardii; http://kremlin dot ru/events/president/news/71530; https://t.me/mod_russia/27870
[19] https://t.me/dva_majors/20216; https://t.me/dva_majors/20220 ; https://t.me/notes_veterans/10678 ; https://t.me/rosich_ru/44861
[20] https://www.vedomosti dot ru/politics/articles/2023/07/04/983567-vedomstva-prorabativayut-vopros-o-perepodchinenii-politseiskogo-spetsnaza-rosgvardii
[21] https://www.kavkazr dot com/a/administratsiya-rostova-ne-budet-trebovatj-vozmescheniya-uscherba-ot-prigozhina-posle-myatezha-v-gorode/32488862.html; https://meduza dot io/news/2023/07/03/vlasti-rostova-na-donu-otsenili-pochti-v-sto-millionov-rubley-uscherb-gorodu-ot-myatezha-chvk-vagnera; https://rtvi dot com/news/glava-rostova-na-donu-rasskazal-o-summe-ushherba-ot-myatezha-chvk-vagner/
[22] https://www.fontanka dot ru/2023/07/04/72460373/
[23] https://www.understandingwar.org/backgrounder/russian-offensive-campaign...
[24] https://t.me/boris_rozhin/91216
[25] https://t.me/novaya_pishet/41030 ; https://meduza dot io/news/2023/07/04/v-chechne-napali-na-zhurnalistku-novoy-gazety-elenu-milashinu-i-advokata-aleksandra-nemova-ih-zhestko-izbili-zhurnalistke-slomali-paltsy
[26] https://www.themoscowtimes dot com/2023/07/04/chechen-court-sentences-mother-of-prominent-activist-to-55-years-in-prison-a81728
[27] https://tass dot ru/obschestvo/18182187; https://t.me/sashakots/40758; https://t.me/MedvedevVesti/14692
[28] https://tass dot ru/obschestvo/18182187; https://t.me/sashakots/40758 ; https://t.me/MedvedevVesti/14692
[29] https://tass dot ru/obschestvo/18182187
[30] https://t.me/RKadyrov_95/3743;
[31] https://meduza dot io/news/2022/02/03/obozrevatel-novoy-gazety-elena-milashina-uedet-iz-rossii-posle-ugroz-kadyrova; https://meduza dot io/news/2023/07/04/razberemsya-ramzan-kadyrov-o-napadenii-na-elenu-milashinu-i-aleksandra-nemova; https://www.understandingwar.org/backgrounder/russian-offensive-campaign-assessment-december-10 ; https://www.understandingwar.org/sites/default/files/Russian%20Offensive%20Campaign%20Assessment%20April%2016.pdf
[32] https://www.understandingwar.org/backgrounder/russian-offensive-campaign-assessment-june-5-2023 ; https://www.understandingwar.org/backgrounder/russian-offensive-campaign-assessment-may-31-2023 ; https://www.understandingwar.org/backgrounder/russian-offensive-campaign-assessment-june-2-2023 ; https://www.understandingwar.org/backgrounder/russian-offensive-campaign-assessment-june-24-2023
[33] https://www.understandingwar.org/sites/default/files/Russian%20Offensive%20Campaign%20Assessment%2C%20May%2031%2C%202023%20PDF.pdf ; https://www.understandingwar.org/backgrounder/russian-offensive-campaign...
[34] https://iz dot ru/1538203/roman-kretcul-aleksei-ramm/poliarnyi-okrug-v-sostave-severnogo-flota-sozdadut-obshchevoiskovuiu-armiiu
[35] https://iz dot ru/1538203/roman-kretcul-aleksei-ramm/poliarnyi-okrug-v-sostave-severnogo-flota-sozdadut-obshchevoiskovuiu-armiiu
[36] https://t.me/mod_russia/28057 ; https://t.me/mod_russia/28057; https://t.me/severrealii/18203 ; https://t.me/shot_shot/53667 ; https://t.me/shot_shot/53668 ; https://t.me/svobodnieslova/2376 ; https://t.me/bazabazon/19166 ; https://t.me/sotaproject/62267; https://t.me/vrogov/10743; https://t.me/istories_media/2957; https://t.me/astrapress/31717; https://t.me/astrapress/31721; https://t.me/astrapress/31723; https://t.me/astrapress/31724 ; https://t.me/voenkorKotenok/48938 ; https://t.me/milinfolive/103099 ; https://t.me/milinfolive/103100 ; https://t.me/milinfolive/103102 ; https://t.me/milinfolive/103107
[37] https://t.me/rybar/49303; https://t.me/RVvoenkor/48650; https://t.me/russkiy_opolchenec/37131 ; https://gfsis.org.ge/maps/russian-military-forces
[38] https://t.me/rybar/49303; https://t.me/mos_sobyanin/5854
[39] https://t.me/milinfolive/103104
[40] https://www.facebook.com/GeneralStaff.ua/posts/pfbid02A52T2ugTVhiavau9ev...
[41] https://twitter.com/GeoConfirmed/status/1676238337202331648?s=20
[42] https://www.facebook.com/GeneralStaff.ua/posts/pfbid02A52T2ugTVhiavau9ev...
[43] https://t.me/boris_rozhin/91146
[44] https://armyinform.com dot ua/2023/07/03/vorog-namagayetsya-nastupaty-na-lymano-kupyanskomu-napryamku-sergij-cherevatyj/
[45] https://t.me/sons_fatherland/10707
[46] https://t.me/mod_russia/28072
[47] https://t.me/wargonzo/13644 ; https://t.me/rybar/49287; https://t.me/kremlinprachka/24720
[48] https://t.me/annamaliar/903
[49] https://t.me/annamaliar/903
[50] https://www.facebook.com/GeneralStaff.ua/posts/pfbid02pkdwwHKJ5eZcJc9FQ6u2pJAfj4vkxsmmVQTVdqqNbJFGN4KTPEKy7wywg3ATtH9ol
[51] https://www.facebook.com/GeneralStaff.ua/posts/pfbid02A52T2ugTVhiavau9evCzYCUKw7ZJdEccNPotT5BoeWZaKcopvwQGEWDWtirJjwTfl
[52] https://t.me/grey_zone/19409 ; https://t.me/strelkovii/5874
[53] https://t.me/wargonzo/13644
[54] https://t.me/wargonzo/13644
[55] https://t.me/mod_russia/28072
[56] https://t.me/strelkovii/5874
[57] https://www.understandingwar.org/backgrounder/russian-offensive-campaign... ; https://understandingwar.org/backgrounder/russian-offensive-campaign-ass...
[58] https://understandingwar.org/backgrounder/russian-offensive-campaign-assessment-may-10-2023
[59] https://www.facebook.com/GeneralStaff.ua/posts/pfbid02A52T2ugTVhiavau9evCzYCUKw7ZJdEccNPotT5BoeWZaKcopvwQGEWDWtirJjwTfl ; https://www.facebook.com/GeneralStaff.ua/posts/pfbid02pkdwwHKJ5eZcJc9FQ6u2pJAfj4vkxsmmVQTVdqqNbJFGN4KTPEKy7wywg3ATtH9ol ; https://www.facebook.com/GeneralStaff.ua/posts/pfbid02A52T2ugTVhiavau9evCzYCUKw7ZJdEccNPotT5BoeWZaKcopvwQGEWDWtirJjwTfl
[60] https://t.me/boris_rozhin/91146
[61] https://t.me/wargonzo/13644
[62] https://www.facebook.com/GeneralStaff.ua/posts/pfbid02pkdwwHKJ5eZcJc9FQ6u2pJAfj4vkxsmmVQTVdqqNbJFGN4KTPEKy7wywg3ATtH9ol
[63] https://t.me/mod_russia/28072; https://t.me/mod_russia/28066
[64] https://t.me/wargonzo/13644
[65] https://suspilne dot media/521387-na-berdanskomu-napramku-zaporizkoi-oblasti-vijskovi-zsu-prosunulisa-do-dvoh-kilometriv-vpered/ ; https://www.facebook.com/PresscentrTavria/videos/932914671129838/
[66] https://twitter.com/GeoConfirmed/status/1676197514641387521 ; https://t.me/wargonzo/13655
[67] https://t.me/RVvoenkor/48680 ; https://t.me/berloga_life/13918; https://t.me/RVvoenkor/48664
[68] https://t.me/rybar/49306; https://t.me/batalyon15/2205; https://t.me/batalyon15/2202; https://t.me/batalyon15/2200; https://t.me/negumanitarnaya_pomosch_Z/8536; https://t.me/voin_dv/3520; https://t.me/rusich_army/9742; https://t.me/rusich_army/9741; https://t.me/rusich_army/9739; https://t.me/RVvoenkor/48667
[69] https://t.me/vrogov/10736 ; https://twitter.com/GeoConfirmed/status/1676220226902294528?s=20; https://t.me/rybar/49304
[70] https://t.me/vrogov/10733 ; https://t.me/vrogov/10734; https://t.me/rybar/49304
[71] https://understandingwar.org/backgrounder/russian-offensive-campaign-ass...
[72] https://t.me/boris_rozhin/91146; https://t.me/readovkanews/61932; https://t.me/dva_majors/20233 ; https://t.me/dva_majors/20234 ; https://t.me/dva_majors/20196 ; https://t.me/russkiy_opolchenec/37139
[73] https://understandingwar.org/backgrounder/russian-offensive-campaign-assessment-july-1-2023 ; https://understandingwar.org/backgrounder/russian-offensive-campaign-assessment-july-2-2023 ; https://understandingwar.org/backgrounder/russian-offensive-campaign-ass...
[74] https://suspilne dot media/521107-v-lavah-armii-rf-pevna-panika-tomu-voni-tak-golosno-kricat-pro-antonivskij-mist-gumenuk-pro-situaciu-na-hersonsini/
[75] https://tass dot ru/obschestvo/18188277
[76] https://tass dot ru/obschestvo/18188277
[77] https://t.me/southtower/8821
[78] https://t.me/boris_rozhin/91215
[79] https://t.me/mod_russia/28067
[80] https://t.me/rusich_army/9749; https://t.me/AlabugaService/57
[81] https://isw.pub/UkrWar070323
[82] https://tass dot ru/armiya-i-opk/18189843 ; https://ria dot ru/20230704/kontraktniki-1882224486.html
[83] http://www.mod.gov dot cn/gfbw/qwfb/16234721.html; https://www.bloomberg.com/news/articles/2023-07-03/china-says-it-wants-m...
[84] https://t.me/mod_russia/28080
[85] https://t.me/malvovabelova/1675
[86] https://t.me/malvovabelova/1675
[87] https://www.understandingwar.org/backgrounder/russian-offensive-campaign-assessment-may-30-2023 ; https://www.understandingwar.org/backgrounder/russian-offensive-campaign-assessment-march-11-2023

Data-Driven Traffic Assignment: A Novel Approach for Learning Traffic Flow Patterns Using Graph Convolutional Neural Network
- Published: 24 July 2023
- Volume 5 , article number 11 , ( 2023 )
Cite this article
- Rezaur Rahman 1 &
- Samiul Hasan 1
434 Accesses
3 Citations
Explore all metrics
We present a novel data-driven approach of learning traffic flow patterns of a transportation network given that many instances of origin to destination (OD) travel demand and link flows of the network are available. Instead of estimating traffic flow patterns assuming certain user behavior (e.g., user equilibrium or system optimal), here we explore the idea of learning those flow patterns directly from the data. To implement this idea, we have formulated the traditional traffic assignment problem (from the field of transportation science) as a data-driven learning problem and developed a neural network-based framework known as Graph Convolutional Neural Network (GCNN) to solve it. The proposed framework represents the transportation network and OD demand in an efficient way and utilizes the diffusion process of multiple OD demands from nodes to links. We validate the solutions of the model against analytical solutions generated from running static user equilibrium-based traffic assignments over Sioux Falls and East Massachusetts networks. The validation results show that the implemented GCNN model can learn the flow patterns very well with less than 2% mean absolute difference between the actual and estimated link flows for both networks under varying congested conditions. When the training of the model is complete, it can instantly determine the traffic flows of a large-scale network. Hence, this approach can overcome the challenges of deploying traffic assignment models over large-scale networks and open new directions of research in data-driven network modeling.
This is a preview of subscription content, log in via an institution to check access.
Access this article
Price includes VAT (Russian Federation)
Instant access to the full article PDF.
Rent this article via DeepDyve
Institutional subscriptions
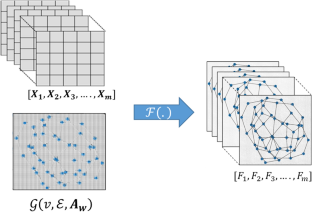
Similar content being viewed by others
Modeling Urban Traffic Data Through Graph-Based Neural Networks
Predicting Taxi Hotspots in Dynamic Conditions Using Graph Neural Network
MTGCN: A Multitask Deep Learning Model for Traffic Flow Prediction
Data availability.
The data that support the findings of this study are available from the corresponding author, [[email protected]], upon reasonable request.
Abdelfatah AS, Mahmassani HS (2002) A simulation-based signal optimization algorithm within a dynamic traffic assignment framework 428–433. https://doi.org/10.1109/itsc.2001.948695
Alexander L, Jiang S, Murga M, González MC (2015) Origin-destination trips by purpose and time of day inferred from mobile phone data. Transp Res Part C Emerg Technol 58:240–250. https://doi.org/10.1016/j.trc.2015.02.018
Article Google Scholar
Atwood J, Towsley D (2015) Diffusion-convolutional neural networks. Adv Neural Inf Process Syst. https://doi.org/10.5555/3157096.3157320
Ban XJ, Liu HX, Ferris MC, Ran B (2008) A link-node complementarity model and solution algorithm for dynamic user equilibria with exact flow propagations. Transp Res Part B Methodol 42:823–842. https://doi.org/10.1016/j.trb.2008.01.006
Bar-Gera H (2002) Origin-based algorithm for the traffic assignment problem. Transp Sci 36:398–417. https://doi.org/10.1287/trsc.36.4.398.549
Article MATH Google Scholar
Barthélemy J, Carletti T (2017) A dynamic behavioural traffic assignment model with strategic agents. Transp Res Part C Emerg Technol 85:23–46. https://doi.org/10.1016/j.trc.2017.09.004
Ben-Akiva ME, Gao S, Wei Z, Wen Y (2012) A dynamic traffic assignment model for highly congested urban networks. Transp Res Part C Emerg Technol 24:62–82. https://doi.org/10.1016/j.trc.2012.02.006
Billings D, Jiann-Shiou Y (2006) Application of the ARIMA Models to Urban Roadway Travel Time Prediction-A Case Study. Systems, Man and Cybernetics, 2006. SMC’06. IEEE International Conference on 2529–2534
Boyles S, Ukkusuri SV, Waller ST, Kockelman KM (2006) A comparison of static and dynamic traffic assignment under tolls: a study of the dallas-fort worth network. 85th Annual Meeting of 14
Brandes U (2001) A faster algorithm for betweenness centrality. J Math Sociol 25:163–177. https://doi.org/10.1080/0022250X.2001.9990249
Cai P, Wang Y, Lu G, Chen P, Ding C, Sun J (2016) A spatiotemporal correlative k-nearest neighbor model for short-term traffic multistep forecasting. Transp Res Part C Emerg Technol 62:21–34. https://doi.org/10.1016/j.trc.2015.11.002
Chien SI-J, Kuchipudi CM (2003) Dynamic travel time prediction with real-time and historic data. J Transp Eng 129:608–616. https://doi.org/10.1061/(ASCE)0733-947X(2003)129:6(608)
Cui Z, Henrickson K, Ke R, Wang Y (2018a) Traffic graph convolutional recurrent neural network: a deep learning framework for network-scale traffic learning and forecasting. IEEE Trans Intell Transp Syst. https://doi.org/10.1109/TITS.2019.2950416
Cui Z, Ke R, Pu Z, Wang Y (2018b) Deep bidirectional and unidirectional LSTM recurrent neural network for network-wide traffic speed prediction. International Workshop on Urban Computing (UrbComp) 2017
Cui Z, Lin L, Pu Z, Wang Y (2020) Graph Markov network for traffic forecasting with missing data. Transp Res Part C Emerg Technol 117:102671. https://doi.org/10.1016/j.trc.2020.102671
Defferrard M, Bresson X, Vandergheynst P (2016) Convolutional neural networks on graphs with fast localized spectral filtering. Adv Neural Inf Process Syst 3844–3852
Deshpande M, Bajaj PR (2016) Performance analysis of support vector machine for traffic flow prediction. 2016 International Conference on Global Trends in Signal Processing, Information Computing and Communication (ICGTSPICC) 126–129. https://doi.org/10.1109/ICGTSPICC.2016.7955283
Elhenawy M, Chen H, Rakha HA (2014) Dynamic travel time prediction using data clustering and genetic programming. Transp Res Part C Emerg Technol 42:82–98. https://doi.org/10.1016/j.trc.2014.02.016
Foytik P, Jordan C, Robinson RM (2017) Exploring simulation based dynamic traffic assignment with large scale microscopic traffic simulation model
Friesz TL, Luque J, Tobin RL, Wie BW (1989) Dynamic network traffic assignment considered as a continuous time optimal control problem. Oper Res 37:893–901. https://doi.org/10.2307/171471
Article MathSciNet MATH Google Scholar
Gundlegård D, Rydergren C, Breyer N, Rajna B (2016) Travel demand estimation and network assignment based on cellular network data. Comput Commun 95:29–42. https://doi.org/10.1016/j.comcom.2016.04.015
Guo S, Lin Y, Feng N, Song C, Wan H (2019) Attention based spatial-temporal graph convolutional networks for traffic flow forecasting. Proceed AAAI Conf Artif Intell 33:922–929. https://doi.org/10.1609/aaai.v33i01.3301922
Guo K, Hu Y, Qian Z, Sun Y, Gao J, Yin B (2020) Dynamic graph convolution network for traffic forecasting based on latent network of Laplace matrix estimation. IEEE Trans Intell Transp Syst 23:1009–1018. https://doi.org/10.1109/tits.2020.3019497
Guo K, Hu Y, Qian Z, Liu H, Zhang K, Sun Y, Gao J, Yin B (2021) Optimized graph convolution recurrent neural network for traffic prediction. IEEE Trans Intell Transp Syst 22:1138–1149. https://doi.org/10.1109/TITS.2019.2963722
Hammond DK, Vandergheynst P, Gribonval R (2011) Wavelets on graphs via spectral graph theory. Appl Comput Harmon Anal 30:129–150. https://doi.org/10.1016/j.acha.2010.04.005
He X, Guo X, Liu HX (2010) A link-based day-to-day traffic assignment model. Transp Res Part B 44:597–608. https://doi.org/10.1016/j.trb.2009.10.001
Huang Y, Kockelman KM (2019) Electric vehicle charging station locations: elastic demand, station congestion, and network equilibrium. Transp Res D Transp Environ. https://doi.org/10.1016/j.trd.2019.11.008
Innamaa S (2005) Short-term prediction of travel time using neural networks on an interurban highway. Transportation (amst) 32:649–669. https://doi.org/10.1007/s11116-005-0219-y
Jafari E, Pandey V, Boyles SD (2017) A decomposition approach to the static traffic assignment problem. Transp Res Part b Methodol 105:270–296. https://doi.org/10.1016/j.trb.2017.09.011
Janson BN (1989) Dynamic traffic assignment for urban road networks. Transp Res Part B Methodol 25:143–161
Ji X, Shao C, Wang B (2016) Stochastic dynamic traffic assignment model under emergent incidents. Procedia Eng 137:620–629. https://doi.org/10.1016/j.proeng.2016.01.299
Jiang Y, Wong SC, Ho HW, Zhang P, Liu R, Sumalee A (2011) A dynamic traffic assignment model for a continuum transportation system. Transp Res Part b Methodol 45:343–363. https://doi.org/10.1016/j.trb.2010.07.003
Kim H, Oh JS, Jayakrishnan R (2009) Effects of user equilibrium assumptions on network traffic pattern. KSCE J Civ Eng 13:117–127. https://doi.org/10.1007/s12205-009-0117-5
Kim TS, Lee WK, Sohn SY (2019) Graph convolutional network approach applied to predict hourly bike-sharing demands considering spatial, temporal, and global effects. PLoS ONE 14:e0220782. https://doi.org/10.1371/journal.pone.0220782
Kipf TN, Welling M (2016) Semi-supervised classification with graph convolutional networks. 5th International Conference on Learning Representations, ICLR 2017- Conference Track Proceedings
LeBlanc LJ, Morlok EK, Pierskalla WP (1975) An efficient approach to solving the road network equilibrium traffic assignment problem. Transp Res 9:309–318. https://doi.org/10.1016/0041-1647(75)90030-1
Lee YLY (2009) Freeway travel time forecast using artifical neural networks with cluster method. 2009 12th International Conference on Information Fusion 1331–1338
Leon-Garcia A, Tizghadam A (2009) A graph theoretical approach to traffic engineering and network control problem. 21st International Teletraffic Congress, ITC 21: Traffic and Performance Issues in Networks of the Future-Final Programme
Leurent F, Chandakas E, Poulhès A (2011) User and service equilibrium in a structural model of traffic assignment to a transit network. Procedia Soc Behav Sci 20:495–505. https://doi.org/10.1016/j.sbspro.2011.08.056
Li Y, Yu R, Shahabi C, Liu Y (2018) Diffusion convolutional recurrent neural network: Data-driven traffic forecasting. 6th International Conference on Learning Representations, ICLR 2018-Conference Track Proceedings 1–16
Li G, Knoop VL, van Lint H (2021) Multistep traffic forecasting by dynamic graph convolution: interpretations of real-time spatial correlations. Transp Res Part C Emerg Technol 128:103185. https://doi.org/10.1016/j.trc.2021.103185
Lin L, He Z, Peeta S (2018) Predicting station-level hourly demand in a large-scale bike-sharing network: a graph convolutional neural network approach. Transp Res Part C 97:258–276. https://doi.org/10.1016/j.trc.2018.10.011
Liu HX, Ban X, Ran B, Mirchandani P (2007) Analytical dynamic traffic assignment model with probabilistic travel times and perceptions. Transp Res Record 1783:125–133. https://doi.org/10.3141/1783-16
Liu Y, Zheng H, Feng X, Chen Z (2017) Short-term traffic flow prediction with Conv-LSTM. 2017 9th International Conference on Wireless Communications and Signal Processing, WCSP 2017-Proceedings. https://doi.org/10.1109/WCSP.2017.8171119
Lo HK, Szeto WY (2002) A cell-based variational inequality formulation of the dynamic user optimal assignment problem. Transp Res Part b Methodol 36:421–443. https://doi.org/10.1016/S0191-2615(01)00011-X
Luo X, Li D, Yang Y, Zhang S (2019) Spatiotemporal traffic flow prediction with KNN and LSTM. J Adv Transp. https://doi.org/10.1155/2019/4145353
Ma X, Tao Z, Wang Y, Yu H, Wang Y (2015) Long short-term memory neural network for traffic speed prediction using remote microwave sensor data. Transp Res Part C Emerg Technol 54:187–197. https://doi.org/10.1016/j.trc.2015.03.014
Ma X, Dai Z, He Z, Ma J, Wang Y, Wang Y (2017) Learning traffic as images: a deep convolutional neural network for large-scale transportation network speed prediction. Sensors 17:818. https://doi.org/10.3390/s17040818
Mahmassani HaniS (2001) Dynamic network traffic assignment and simulation methodology for advanced system management applications. Netw Spat Econ 1:267–292. https://doi.org/10.1023/A:1012831808926
Merchant DK, Nemhauser GL (1978) A model and an algorithm for the dynamic traffic assignment problems. Transp Sci 12:183–199. https://doi.org/10.1287/trsc.12.3.183
Mitradjieva M, Lindberg PO (2013) The stiff is moving—conjugate direction Frank-Wolfe methods with applications to traffic assignment * . Transp Sci 47:280–293. https://doi.org/10.1287/trsc.1120.0409
Myung J, Kim D-K, Kho S-Y, Park C-H (2011) Travel time prediction using k nearest neighbor method with combined data from vehicle detector system and automatic toll collection system. Transp Res Record 2256:51–59. https://doi.org/10.3141/2256-07
Nie YM, Zhang HM (2010) Solving the dynamic user optimal assignment problem considering queue spillback. Netw Spat Econ 10:49–71. https://doi.org/10.1007/s11067-007-9022-y
Peeta S, Mahmassani HS (1995) System optimal and user equilibrium time-dependent traffic assignment in congested networks. Ann Oper Res. https://doi.org/10.1007/BF02031941
Peeta S, Ziliaskopoulos AK (2001) Foundations of dynamic traffic assignment: the past, the present and the future. Netw Spat Econ 1:233–265
Peng H, Wang H, Du B, Bhuiyan MZA, Ma H, Liu J, Wang L, Yang Z, Du L, Wang S, Yu PS (2020) Spatial temporal incidence dynamic graph neural networks for traffic flow forecasting. Inf Sci (n y) 521:277–290. https://doi.org/10.1016/j.ins.2020.01.043
Peng H, Du B, Liu M, Liu M, Ji S, Wang S, Zhang X, He L (2021) Dynamic graph convolutional network for long-term traffic flow prediction with reinforcement learning. Inf Sci (n y) 578:401–416. https://doi.org/10.1016/j.ins.2021.07.007
Article MathSciNet Google Scholar
Polson NG, Sokolov VO (2017) Deep learning for short-term traffic flow prediction. Transp Res Part C Emerg Technol 79:1–17. https://doi.org/10.1016/j.trc.2017.02.024
Primer A (2011) Dynamic traffic assignment. Transportation Network Modeling Committee 1–39. https://doi.org/10.1016/j.trd.2016.06.003
PyTorch [WWW document], 2016. URL https://pytorch.org/ . Accessed 10 Jun 2020
Rahman R, Hasan S (2018). Short-term traffic speed prediction for freeways during hurricane evacuation: a deep learning approach 1291–1296. https://doi.org/10.1109/ITSC.2018.8569443
Ran BIN, Boyce DE, Leblanc LJ (1993) A new class of instantaneous dynamic user-optimal traffic assignment models. Oper Res 41:192–202
Sanchez-Gonzalez A, Godwin J, Pfaff T, Ying R, Leskovec J, Battaglia PW (2020) Learning to simulate complex physics with graph networks. 37th International Conference on Machine Learning, ICML 2020 PartF16814, 8428–8437
Shafiei S, Gu Z, Saberi M (2018) Calibration and validation of a simulation-based dynamic traffic assignment model for a large-scale congested network. Simul Model Pract Theory 86:169–186. https://doi.org/10.1016/j.simpat.2018.04.006
Sheffi Y (1985) Urban transportation networks. Prentice-Hall Inc., Englewood Cliffs. https://doi.org/10.1016/0191-2607(86)90023-3
Book Google Scholar
Tang C, Sun J, Sun Y, Peng M, Gan N (2020) A General traffic flow prediction approach based on spatial-temporal graph attention. IEEE Access 8:153731–153741. https://doi.org/10.1109/ACCESS.2020.3018452
Teng SH (2016) Scalable algorithms for data and network analysis. Found Trends Theor Comput Sci 12:1–274. https://doi.org/10.1561/0400000051
Tizghadam A, Leon-garcia A (2007) A robust routing plan to optimize throughput in core networks 117–128
Transportation Networks for Research Core Team (2016) Transportation Networks for Research [WWW Document]. 2016. URL https://github.com/bstabler/TransportationNetworks (Accessed 8 Jul 2018)
Ukkusuri SV, Han L, Doan K (2012) Dynamic user equilibrium with a path based cell transmission model for general traffic networks. Transp Res Part b Methodol 46:1657–1684. https://doi.org/10.1016/j.trb.2012.07.010
Waller ST, Fajardo D, Duell M, Dixit V (2013) Linear programming formulation for strategic dynamic traffic assignment. Netw Spat Econ 13:427–443. https://doi.org/10.1007/s11067-013-9187-5
Wang HW, Peng ZR, Wang D, Meng Y, Wu T, Sun W, Lu QC (2020) Evaluation and prediction of transportation resilience under extreme weather events: a diffusion graph convolutional approach. Transp Res Part C Emerg Technol 115:102619. https://doi.org/10.1016/j.trc.2020.102619
Wardrop J (1952) Some theoretical aspects of road traffic research. Proc Inst Civil Eng Part II 1:325–378. https://doi.org/10.1680/ipeds.1952.11362
Watling D, Hazelton ML (2003) The dynamics and equilibria of day-to-day assignment models. Netw Spat Econ. https://doi.org/10.1023/A:1025398302560
Wu CH, Ho JM, Lee DT (2004) Travel-time prediction with support vector regression. IEEE Trans Intell Transp Syst 5:276–281. https://doi.org/10.1109/TITS.2004.837813
Yasdi R (1999) Prediction of road traffic using a neural network approach. Neural Comput Appl 8:135–142. https://doi.org/10.1007/s005210050015
Yu B, Song X, Guan F, Yang Z, Yao B (2016) k-nearest neighbor model for multiple-time-step prediction of short-term traffic condition. J Transp Eng 142:4016018. https://doi.org/10.1061/(ASCE)TE.1943-5436.0000816
Yu B, Yin H, Zhu Z (2018) Spatio-temporal graph convolutional networks: a deep learning framework for traffic forecasting, in: Proceedings of the Twenty-Seventh International Joint Conference on Artificial Intelligence. International Joint Conferences on Artificial Intelligence Organization, California pp. 3634–3640. https://doi.org/10.24963/ijcai.2018/505
Zhang Y, Haghani A (2015) A gradient boosting method to improve travel time prediction. Transp Res Part C Emerg Technol 58:308–324. https://doi.org/10.1016/j.trc.2015.02.019
Zhang S, Tong H, Xu J, Maciejewski R (2019) Graph convolutional networks: a comprehensive review. Comput Soc Netw. https://doi.org/10.1186/s40649-019-0069-y
Zhao L, Song Y, Zhang C, Liu Y, Wang P, Lin T, Deng M, Li H (2020) T-GCN: a temporal graph convolutional network for traffic prediction. IEEE Trans Intell Transp Syst 21:3848–3858. https://doi.org/10.1109/TITS.2019.2935152
Zhou J, Cui G, Zhang Z, Yang C, Liu Z, Wang L, Li C, Sun M (2020) Graph neural networks: a review of methods and applications. AI Open. https://doi.org/10.1016/j.aiopen.2021.01.001
Download references
This study was supported by the U.S. National Science Foundation through the grant CMMI #1917019. However, the authors are solely responsible for the facts and accuracy of the information presented in the paper.
Author information
Authors and affiliations.
Department of Civil, Environmental, and Construction Engineering, University of Central Florida, Orlando, FL, USA
Rezaur Rahman & Samiul Hasan
You can also search for this author in PubMed Google Scholar
Contributions
The authors confirm their contribution to the paper as follows: study conception and design: RR, SH; analysis and interpretation of results: RR, SH; draft manuscript preparation: RR, SH. All authors reviewed the results and approved the final version of the manuscript.
Corresponding author
Correspondence to Rezaur Rahman .
Ethics declarations
Conflict of interest.
The authors declare that they have no conflict of interest.
Additional information
Publisher's note.
Springer Nature remains neutral with regard to jurisdictional claims in published maps and institutional affiliations.
Appendix A: modeling traffic flows using spectral graph convolution
In spectral graph convolution, a spectral convolutional filter is used to learn traffic flow patterns inside a transportation network in response to travel demand variations. The spectral filter is derived from spectrum of the Laplacian matrix, which consists of eigenvalues of the Laplacian matrix. So to construct the spectrum, we must calculate the eigenvalues of` the Laplacian matrix. For a symmetric graph, we can compute the eigenvalues using Eigen decomposition of the Laplacian matrix. In this problem, we consider the transportation network as a symmetric-directed graph, same number of links getting out and getting inside a node, which means the in-degree and out-degree matrices of the graph are similar. Thus, the Laplacian matrix of this graph is diagonalizable as follows using Eigen decomposition
where \(\boldsymbol{\Lambda }\) is a diagonal matrix with eigenvalues, \({\lambda }_{0},{\lambda }_{1},{\lambda }_{2}, . . . ,{\lambda }_{N}\) and \({\varvec{U}}\) indicates the eigen vectors, \({u}_{0},{u}_{1},{u}_{2}, . . . ,{u}_{N}\) . Eigen values represent characteristics of transportation network in terms of strength of a particular node based on its position, distance between adjacent nodes, and dimension of the network. The spectral graph convolution filter can be defined as follows:
where \(\theta\) is the parameter for the convolution filter shared by all the nodes of the network and \(K\) is the size of the convolution filter. Now the spectral graph convolution over the graph signal ( \({\varvec{X}})\) is defined as follows:
According to spectral graph theory, the shortest path distance i.e., minimum number of links connecting nodes \(i\) and \(j\) is longer than \(K\) , such that \({L}^{K}\left(i, j\right) = 0\) (Hammond et al. 2011 ). Consequently, for a given pair of origin ( \(i\) ) and destination ( \(j)\) nodes, a spectral graph filter of size K has access to all the nodes on the shortest path of the graph. It means that the spectral graph convolution filter of size \(K\) captures flow propagation through each node on the shortest path. So the spectral graph convolution operation can model the interdependency between a link and its \(i\) th order adjacent nodes on the shortest paths, given that 0 ≤ i ≤ K .
The computational complexity of calculating \({{\varvec{L}}}_{{\varvec{w}}}^{{\varvec{k}}}\) is high due to K times multiplication of \({L}_{w}\) . A way to overcome this challenge is to approximate the spectral filter \({g}_{\theta }\) with Chebyshev polynomials up to ( \(K-1\) )th order (Hammond et al. 2011 ). Defferrard et al. (Defferrard et al. 2016 ) applied this approach to build a K -localized ChebNet, where the convolution is defined as
in which \(\overline{{\varvec{L}} }=2{{\varvec{L}}}_{{\varvec{s}}{\varvec{y}}{\varvec{m}}}/{{\varvec{\uplambda}}}_{{\varvec{m}}{\varvec{a}}{\varvec{x}}}-{\varvec{I}}\) . \(\overline{{\varvec{L}} }\) represents a scaling of graph Laplacian that maps the eigenvalues from [0, \({\uplambda }_{max}\) ] to [-1,1]. \({{\varvec{L}}}_{{\varvec{s}}{\varvec{y}}{\varvec{m}}}\) is defined as symmetric normalization of the Laplacian matrix \({{{\varvec{D}}}_{{\varvec{w}}}}^{-1/2}{{\varvec{L}}}_{{\varvec{w}}}{{\boldsymbol{ }{\varvec{D}}}_{{\varvec{w}}}}^{-1/2}.\) \({T}_{k}\) and θ denote the Chebyshev polynomials and Chebyshev coefficients. The Chebyshev polynomials are defined recursively by \({T}_{k}\left(\overline{{\varvec{L}} }\right)=2x{T}_{k-1}\left(\overline{{\varvec{L}} }\right)-{T}_{k-2}\left(\overline{{\varvec{L}} }\right)\) with \({T}_{0}\left(\overline{{\varvec{L}} }\right)=1\) and \({T}_{1}\left(\overline{{\varvec{L}} }\right)=\overline{{\varvec{L}} }\) . These are the basis of Chebyshev polynomials. Kipf and Welling (Kipf and Welling 2016 ) simplified this model by approximating the largest eigenvalue \({\lambda }_{max}\) of \(\overline{L }\) as 2. In this way, the convolution becomes
where Chebyshev coefficient, \(\theta ={\theta }_{0}=-{\theta }_{1}\) , All the details about the assumptions and their implications of Chebyshev polynomial can be found in (Hammond et al. 2011 ). Now the simplified graph convolution can be written as follows:
Since \({\varvec{I}}+{{{\varvec{D}}}_{{\varvec{w}}}}^{-1/2}{{\varvec{A}}}_{{\varvec{w}}}{{\boldsymbol{ }{\varvec{D}}}_{{\varvec{w}}}}^{-1/2}\) has eigenvalues in the range [0, 2], it may lead to exploding or vanishing gradients when used in a deep neural network model. To alleviate this problem, Kipf et al. (Kipf and Welling 2016 ) use a renormalization trick by replacing the term \({\varvec{I}}+{{{\varvec{D}}}_{{\varvec{w}}}}^{-1/2}{{\varvec{A}}}_{{\varvec{w}}}{{\boldsymbol{ }{\varvec{D}}}_{{\varvec{w}}}}^{-1/2}\) with \({{\overline{{\varvec{D}}} }_{{\varvec{w}}}\boldsymbol{ }\boldsymbol{ }}^{-1/2}{\overline{{\varvec{A}}} }_{{\varvec{w}}}{{\boldsymbol{ }\overline{{\varvec{D}}} }_{{\varvec{w}}}}^{-1/2}\) , with \({\overline{{\varvec{A}}} }_{{\varvec{w}}}={{\varvec{A}}}_{{\varvec{w}}}+{\varvec{I}}\) , similar to adding a self-loop. Now, we can simplify the spectral graph convolution as follows:
where \({\varvec{\Theta}}\in {{\varvec{R}}}^{{\varvec{N}}\times {\varvec{N}}}\) indicates the parameters of the convolution filter to be learnt during training process. From Eq. 21 , we can observe that spectral graph convolution is a special case of diffusion convolution (Li et al. 2018 ), but the only difference is that in spectral convolution, we symmetrically normalized the adjacency matrix.
Rights and permissions
Springer Nature or its licensor (e.g. a society or other partner) holds exclusive rights to this article under a publishing agreement with the author(s) or other rightsholder(s); author self-archiving of the accepted manuscript version of this article is solely governed by the terms of such publishing agreement and applicable law.
Reprints and permissions
About this article
Rahman, R., Hasan, S. Data-Driven Traffic Assignment: A Novel Approach for Learning Traffic Flow Patterns Using Graph Convolutional Neural Network. Data Sci. Transp. 5 , 11 (2023). https://doi.org/10.1007/s42421-023-00073-y
Download citation
Received : 21 May 2023
Revised : 09 June 2023
Accepted : 13 June 2023
Published : 24 July 2023
DOI : https://doi.org/10.1007/s42421-023-00073-y
Share this article
Anyone you share the following link with will be able to read this content:
Sorry, a shareable link is not currently available for this article.
Provided by the Springer Nature SharedIt content-sharing initiative
- Traffic assignment problem
- Data-driven method
- Deep learning
- Graph convolutional neural network
- Find a journal
- Publish with us
- Track your research
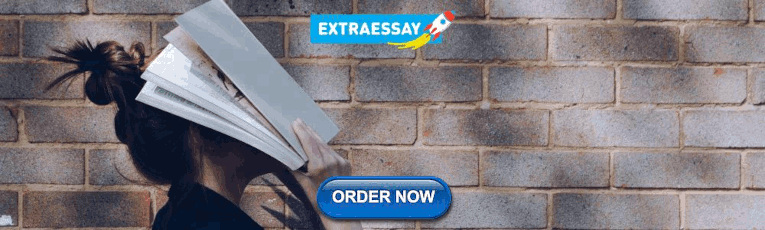
IMAGES
VIDEO
COMMENTS
To overcome these shortcomings, Static Traffic Assignment with Queuing (STAQ): an assignment model for road traffic within strategic transport models was developed as an alternative to the traditional STA model, providing more accuracy on congested networks without reducing robustness, applicability and accountability and without increasing ...
Static traffic assignment models with queues Traditional static TA models, governed by link performance functions, impose no capacity nor storage constraints. This results in link flows possibly exceeding capacities and no explicit queue formation. Such unconstrained models are widely applied in practice, but the desire for static capacity ...
To sum up, we utilize the static traffic assignment model, leveraging annually aggregated statistical data and open public data sources, to offer a city-scale traffic dataset for macroscopic urban ...
This section describes twoformulations for static traffic assignment, namely the User Equilibrium (UE) and System Optimum (SO) assignment. Traffic assignment is defined as the basic problem of finding the link flows given anorigin‐destination trip matrix and a set of link or marginal link travel times, as illustrated inFig. 3. The solution of ...
traffic assignment approach, and with an approximation to DTA using an add-in for TransCAD software. A novel demand profiling algorithm based on piecewise linear curves is developed, and a method to enable reasonable comparisons of static traffic assignment and the TransCAD add-in is provided.
The remainder of this chapter is organized as follows. The basic introduction to Dynamic Traffic Assignment (DTA) is provided in Sect. 2.1.Section 2.2 deals with the use of mathematical programming methodology for static traffic assignment. The user-equilibrium and system optimal formulations of the traffic assignment problem are discussed in the section.
Here, effort has been made to study the Static Traffic Assignment (STA) and Dynamic Traffic Assignment (DTA) with special focus on limitations of STA. Discover the world's research 25+ million members
The static traffic assignment problem (STAP) describes how traffic demand distributes itself across the routes and edges (i.e. streets) of a network in a time-independent setting [ 6 ]. The key inputs are: Cost functions c i for each edge i that describe how the travel time on that edge increases with the flow x i.
This research focus has been diverted to dynamic traffic assignment (DTA), which is to manage traffic in a network through real-time measurement, detection, communication, information provision, and control. Rapid urban growth is resulting into increase in travel demand and private vehicle ownership in urban areas. In the present scenario the existing infrastructure has failed to match the ...
Abstract. This chapter presents the fundamentals of the theory and techniques of traffic assignment problem. It first presents the steady-state traffic assignment problem formulation which is also called static assignment, followed by Dynamic Traffic Assignment (DTA), where the traffic demand on the network is time varying.
Based on the consideration of the temporal dimension, traffic assignment models are classified into three categories: dynamic traffic assignment (DTA), semi-dynamic traffic assignment, and static traffic assignment (STA) models (Bliemer et al., 2017).
Accurate static traffic assignment models are important tools for the assessment of strategic transportation policies. In this article we present a novel approach to partition road networks through network modularity to produce data-driven static traffic assignment models from loop detector data on large road systems. The use of partitioning allows the estimation of the key model input of ...
In this paper we propose a decentralized approach for traffic assignment on a regional network, defined as a large-scale network containing smaller urban networks. The "centralized " approach for ...
Static traffic assignment models with queues. Traditional static TA models, governed by link performance functions, impose no capacity nor storage constraints. This results in link flows possibly exceeding capacities and no explicit queue formation. Such unconstrained models are widely applied in practice, but the desire for static capacity ...
Now, after decades of research and intensive market readiness developments, dynamic traffic assignment (DTA) models have become a viable modeling option. DTA models supplemental existing travel forecasting models and microscopic traffic simulation models. Travel forecasting models represent the static regional travel analysis capability ...
Dynamic Traffic Assignment. Dynamic network assignment models (also referred to as dynamic traffic assignment models or DTA) capture the changes in network performance by detailed time-of-day, and can be used to generate time varying measures of this performance. They occupy the middle ground between static macroscopic traffic assignment and ...
ABSTRACT. This paper describes the road traffic assignment model Static Traffic Assignment with Queuing (STAQ) that was developed for situations where both static (STA) and dynamic (DTA) traffic assignment models are insufficient: strategic applications on large-scale congested networks. The paper demonstrates how the model overcomes ...
detectors, and 6,000 traffic lights, allows us to provide rea-ltime response to traffic . situatoni s throughout the city rather than waitni g for Muscovites to ca ell mergency responders, law enforcement, or others to resolve issues. The mayor of Moscow was personalyl involved in deveopil ng and implementni g the traffci -mpi rovement measures
The traffic assignment model is a static user equilibrium that interacts with the discrete choice model through level of service variables. An iterative algorithm was implemented and applied in a simple network and a more realistic network. The results indicate that the quantity of ride sharing drivers is a key parameter to the service success ...
These maps complement the static control-of-terrain map that ISW produces daily by showing a dynamic frontline. ISW will update this time-lapse map archive monthly. ... warned that the assignment of "Grom" units to Rosgvardia would be a "dangerous experiment."[20] The alleged restructuring of Russia's internal security forces suggests ...
Dance Studio Traffic-Jam, Moscow, Russia. 32 likes. School
The traffic pattern, which is resulted from the UE assignment, was compared with the real location-based data gathered by image processing of real-time traffic maps, which are calibrated by ANPR data. Fig. 1 illustrates a static assignment comparison analysis of automatic number-plate recognition data and location-based data model.
Here is the 4K time lapse video I made at August 12, 2020.You can get the RAW-files and craft your own version. Also you can use it any purposes. Just visit ...
One of the major issues with the static traffic assignment solution is that it assumes constant OD demand, whereas traffic demand may significantly change over time, which makes it unsuitable to deploy for real-world traffic scenario. Static traffic assignment problem fails to capture traffic dynamics of a network, which motivated researchers ...