Research Methods for Business
Definition…
Sampling is the process
of selecting a small number of elements
from a larger defined target group (Population)
of elements such that
the information gathered
from the small group will allow judgments
to be made about the larger groups .
Sampling is the act, process, or technique of selecting a suitable sample , or a representative part of a population for the purpose of determining parameters or characteristics of the whole population .
Purpose Of Sampling …
To draw conclusions about populations from samples, which enables us to determine a population`s characteristics by directly observing only a portion (or sample) of the population. We obtain a sample rather than a complete enumeration (a census ) of the population for many reasons.
6 MAIN REASONS FOR SAMPLING…
- . Timeliness
- . The large size of many populations
- . Inaccessibility of some of the population
- . Destructiveness of the observation
REASONS FOR SAMPLING…
- Economy - taking a sample requires fewer resources than a census.
- Time factor - a sample may provide you with needed information quickly.
- The very large populations - many populations about which inferences must be made are quite large
- The partly accessible populations- There are some populations that are so difficult to get access to that only a sample can be used.
- The destructive nature of the observation- sometimes the very act of observing the desired characteristic of a unit of the population destroys it for the intended use.
- Accuracy and sampling- A sample may be more accurate than a census. A sloppily conducted census can provide less reliable information than a carefully obtained sample.
Important terminologies...
- . Population
- . Sampling Unit
The population refers to the entire group of people, events or things of interest that the researcher wishes to investigate.
- If an organizational consultant is interested in studying the effects of a four-day work week on the white-coller workers in a telephone company in Ireland. Then all white-coller workers in that company will make up the population .
- If regulators wants to know how patients in nursing homes run by a company in France, then all the patients in all the nursing homes run by them will form the population. If however, the regulators are interested only in one particular nursing home run by that company, then only the patients in that particular nursing home will make the population.
An element is the
single member of the population.
- If 1000 blue-coller workers in a particular organization are working and an researcher is interested to know the satisfaction level of these workers then each member (blue-coller) of the particular organization will be considered as element.
- Census is a count of all elements in the human population .
A sample is a subset of the population. it comprises some members from it.
- . If 200 members are drawn/selected from a population of 1000 blue-coller workers to
study the desire outcome, then 200 members form the sample for the study .
- . If there are 145 patients in a hospital and 40 of them are to be surveyed by the hospital administrator to assess there level of satisfaction with the treatment received, then these 40 members will be called the sample .
A sample is thus a subgroup or subset of the population. By studying the sample, the researcher should be able to draw conclusions that are generalizable to the population of interest.
Sampling Unit
The sample unit is the element or the set of elements that is available for selection in some stage of the sampling process.
Example of sampling units in a multi stage sample are city blocks, house hold, and individuals with in the households.
A subject is a single member of the sample just as an element is a single member of the population.
- . If 200 members from the total population of 1000 blue-coller workers form the sample for the study. Then each blue-coller worker in the sample is a subject.
- . If there are 145 patients in a hospital and 40 of them are to be surveyed by the hospital administrator to assess there level of satisfaction with the treatment received, then each member from sample of 40 will be called the subject.
Representative of Sampling...�
- Choosing the right sample cannot be overemphasized.
- If we choose the sample in a scientific way, we can be reasonably sure that sample statistics (Mean, Standard Deviation, (S) Variation in the sample ) and population parameters (Mean (u), Standard Deviation, Variation in the sample ) are close to each others. �
Acknowledgments to Uma Sekaran
What is a Good Sample?
- . Accurate: absence of bias
- . Precise estimate: sampling error
Sampling error is any type of bias
that is attributable to mistakes
in either drawing a sample or
determining the sample size.
Sampling Process…
Define the Population
Determine the Sampling Frame
Select Sampling Technique(s)
Determine the Sample Size
Execute the Sampling Process
Defining Population of Interest…
Population of interest is entirely dependent on Management Problem, Research Problems, and Research Design.
Some Bases for Defining Population:
- . Geographic Area (Pakistan, Punjab, Banking sector, Our Institute etc.)
- . Demographics (Gender, Age, Color, Height etc.)
- . Usage/Lifestyle
- . Awareness
Sampling Frame …
A list of population elements (people, companies, houses, cities, etc.) from which units to be sampled can be selected.
- Difficult to get an accurate list.
- Sample frame error occurs when certain elements of the population are accidentally omitted or not included on the list.
Sampling Methods/Techniques
Probability
Nonprobability
Sampling Methods/Techniques/Types
Sampling Techniques
Convenience
Other Sampling
Simple Random
Probability Sampling Designs
A probability sample is one that gives every member of the population a known chance of being selected .
All are selected randomly.
- Simple random sampling - anyone
- Systematic sampling
- Stratified sampling - different groups (ages)
- Proportionate
- Cluster sampling - different areas (cities)
Simple Random Sampling
Simple random sampling is a method of probability sampling in which every unit has an equal nonzero
chance of being selected
- Each element in the population has a known and equal probability of selection.
- This implies that every element is selected independently of every other element.
Systematic Sampling
Systematic Random Sampling is a method of probability sampling in which the defined target population is ordered and the sample is selected according to position using a skip interval.
- The sample is chosen by selecting a random starting point and then picking every i th element in succession from the sampling frame.
- The sampling interval, i, is determined by dividing the population size N by the sample size n and rounding to the nearest integer.
- For example, there are 100,000 elements in the population and a sample of 1,000 is desired. In this case the sampling interval, i, is 100. A random number between 1 and 100 is selected. If, for example, this number is 23, the sample consists of elements 23, 123, 223, 323, 423, 523, and so on.
Stratified Sampling
Stratified Random Sampling is a method of probability sampling in which the population is divided into different subgroups and samples are selected from each
- A two-step process in which the population is partitioned into subpopulations.
- Divide the target population into homogeneous subgroups or strata
- Draw random samples fro each stratum
- Combine the samples from each stratum into a single sample of the target population
- A major objective of stratified sampling is to increase precision without increasing cost.
Cluster Sampling
- The target population is first divided into mutually exclusive and collectively exhaustive subpopulations, or clusters.
- Then a random sample of clusters is selected, based on a probability sampling technique.
- For each selected cluster, either all the elements are included in the sample (one-stage) or a sample of elements is drawn probabilistically (two-stage).
- Elements within a cluster should be as heterogeneous as possible, but clusters themselves should be as homogeneous as possible. Ideally, each cluster should be a small-scale representation of the population.
- In probability proportionate to size sampling, the clusters are sampled with probability proportional to size. In the second stage, the probability of selecting a sampling unit in a selected cluster varies inversely with the size of the cluster.
Nonprobability Sampling
… Nonprobability sample is an arbitrary grouping that limits the use of some statistical tests. It is not selected randomly.
Classifications of Nonprobability Sampling
Convenience Sampling
- Judgment Sampling
Quota Sampling
Snowball Sampling
Convenience sampling attempts to obtain a sample of convenient elements. Often, respondents are selected because they happen to be in the right place at the right time.
- Use of students, and members of social organizations
- Mail intercept interviews without qualifying the
respondents.
- “people on the street” interviews
Judgmental Sampling
Judgmental sampling is a form of convenience sampling in which the population elements are selected based on the judgment of the researcher.
- Test markets
- Engineers selected in industrial marketing research
- Expert witnesses used in court
Quota sampling may be viewed as two-stage restricted judgmental sampling.
- The first stage consists of developing control categories, or quotas, of population elements.
- In the second stage, sample elements are selected based on convenience or judgment.
Population Sample� composition composition� Control �Characteristic Percentage Percentage Number� Sex� Male 48 48 480� Female 52 52 520� ____ ____ ____� 100 100 1000
In Snowball Sampling , an initial group of respondents is selected, usually at random.
- After being interviewed, these respondents are asked to identify others who belong to the target population of interest.
- Subsequent respondents are selected based on the referrals.
Factors to Consider in Sample Design
Research objectives
Degree of accuracy
Knowledge of
target population
Research scope
Determining Sample Size
- How many completed questionnaires do we need to have a representative sample?
- Generally the larger the better, but that takes more time and money.
- Answer depends on :
- How different or dispersed the population is.
- Desired level of confidence.
- Desired degree of accuracy.
In conclusion, it can be said that using a sample in research saves mainly on money and time, if a suitable sampling strategy is used, appropriate sample size selected and necessary precautions taken to reduce on sampling and measurement errors, then a sample should yield valid and reliable information .
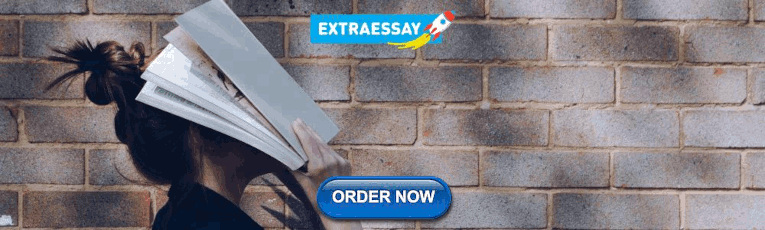
Have a language expert improve your writing
Run a free plagiarism check in 10 minutes, generate accurate citations for free.
- Knowledge Base
Methodology
- Sampling Methods | Types, Techniques & Examples
Sampling Methods | Types, Techniques & Examples
Published on September 19, 2019 by Shona McCombes . Revised on June 22, 2023.
When you conduct research about a group of people, it’s rarely possible to collect data from every person in that group. Instead, you select a sample . The sample is the group of individuals who will actually participate in the research.
To draw valid conclusions from your results, you have to carefully decide how you will select a sample that is representative of the group as a whole. This is called a sampling method . There are two primary types of sampling methods that you can use in your research:
- Probability sampling involves random selection, allowing you to make strong statistical inferences about the whole group.
- Non-probability sampling involves non-random selection based on convenience or other criteria, allowing you to easily collect data.
You should clearly explain how you selected your sample in the methodology section of your paper or thesis, as well as how you approached minimizing research bias in your work.
Table of contents
Population vs. sample, probability sampling methods, non-probability sampling methods, other interesting articles, frequently asked questions about sampling.
First, you need to understand the difference between a population and a sample , and identify the target population of your research.
- The population is the entire group that you want to draw conclusions about.
- The sample is the specific group of individuals that you will collect data from.
The population can be defined in terms of geographical location, age, income, or many other characteristics.
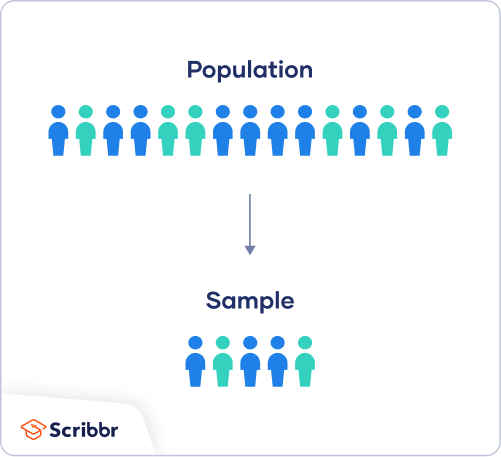
It is important to carefully define your target population according to the purpose and practicalities of your project.
If the population is very large, demographically mixed, and geographically dispersed, it might be difficult to gain access to a representative sample. A lack of a representative sample affects the validity of your results, and can lead to several research biases , particularly sampling bias .
Sampling frame
The sampling frame is the actual list of individuals that the sample will be drawn from. Ideally, it should include the entire target population (and nobody who is not part of that population).
Sample size
The number of individuals you should include in your sample depends on various factors, including the size and variability of the population and your research design. There are different sample size calculators and formulas depending on what you want to achieve with statistical analysis .
Receive feedback on language, structure, and formatting
Professional editors proofread and edit your paper by focusing on:
- Academic style
- Vague sentences
- Style consistency
See an example
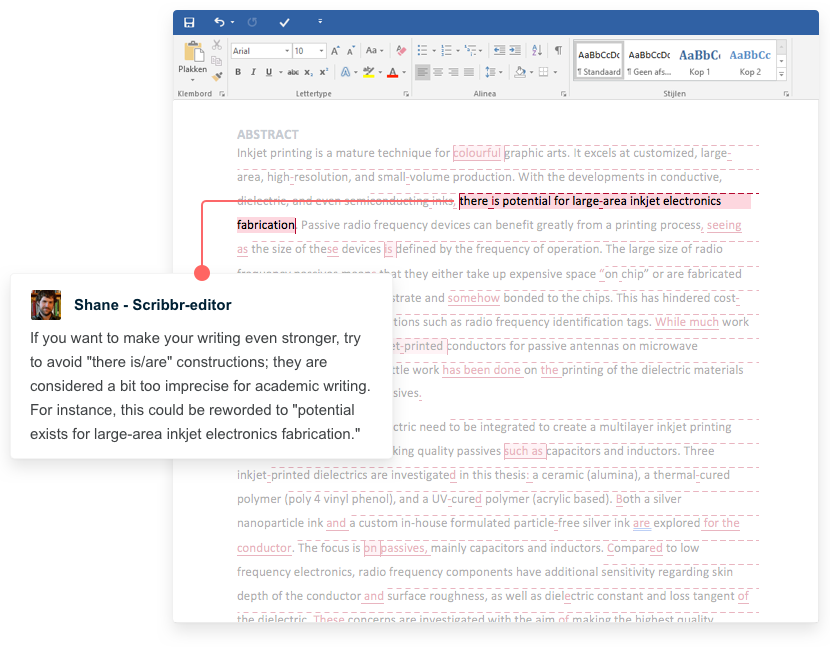
Probability sampling means that every member of the population has a chance of being selected. It is mainly used in quantitative research . If you want to produce results that are representative of the whole population, probability sampling techniques are the most valid choice.
There are four main types of probability sample.
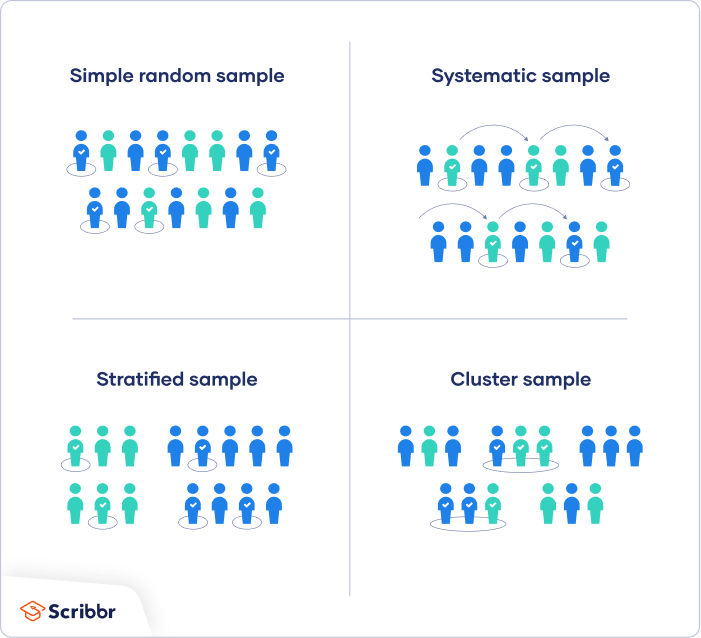
1. Simple random sampling
In a simple random sample, every member of the population has an equal chance of being selected. Your sampling frame should include the whole population.
To conduct this type of sampling, you can use tools like random number generators or other techniques that are based entirely on chance.
2. Systematic sampling
Systematic sampling is similar to simple random sampling, but it is usually slightly easier to conduct. Every member of the population is listed with a number, but instead of randomly generating numbers, individuals are chosen at regular intervals.
If you use this technique, it is important to make sure that there is no hidden pattern in the list that might skew the sample. For example, if the HR database groups employees by team, and team members are listed in order of seniority, there is a risk that your interval might skip over people in junior roles, resulting in a sample that is skewed towards senior employees.
3. Stratified sampling
Stratified sampling involves dividing the population into subpopulations that may differ in important ways. It allows you draw more precise conclusions by ensuring that every subgroup is properly represented in the sample.
To use this sampling method, you divide the population into subgroups (called strata) based on the relevant characteristic (e.g., gender identity, age range, income bracket, job role).
Based on the overall proportions of the population, you calculate how many people should be sampled from each subgroup. Then you use random or systematic sampling to select a sample from each subgroup.
4. Cluster sampling
Cluster sampling also involves dividing the population into subgroups, but each subgroup should have similar characteristics to the whole sample. Instead of sampling individuals from each subgroup, you randomly select entire subgroups.
If it is practically possible, you might include every individual from each sampled cluster. If the clusters themselves are large, you can also sample individuals from within each cluster using one of the techniques above. This is called multistage sampling .
This method is good for dealing with large and dispersed populations, but there is more risk of error in the sample, as there could be substantial differences between clusters. It’s difficult to guarantee that the sampled clusters are really representative of the whole population.
In a non-probability sample, individuals are selected based on non-random criteria, and not every individual has a chance of being included.
This type of sample is easier and cheaper to access, but it has a higher risk of sampling bias . That means the inferences you can make about the population are weaker than with probability samples, and your conclusions may be more limited. If you use a non-probability sample, you should still aim to make it as representative of the population as possible.
Non-probability sampling techniques are often used in exploratory and qualitative research . In these types of research, the aim is not to test a hypothesis about a broad population, but to develop an initial understanding of a small or under-researched population.
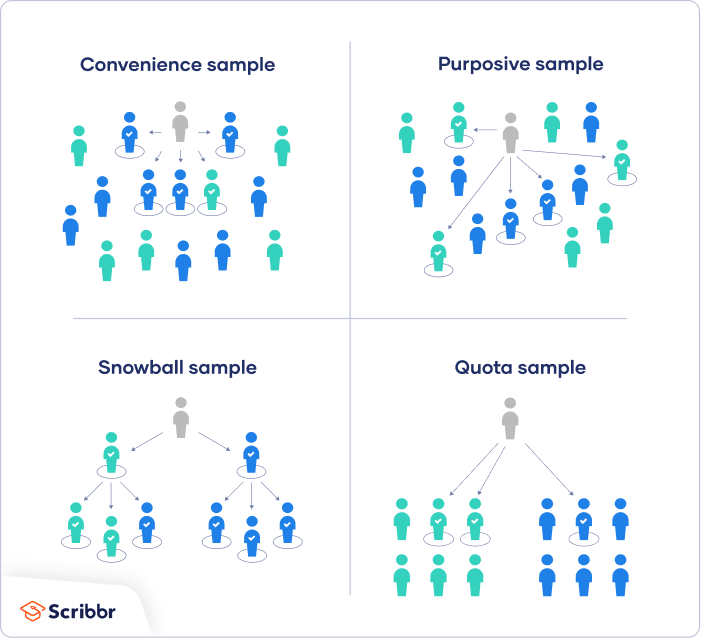
1. Convenience sampling
A convenience sample simply includes the individuals who happen to be most accessible to the researcher.
This is an easy and inexpensive way to gather initial data, but there is no way to tell if the sample is representative of the population, so it can’t produce generalizable results. Convenience samples are at risk for both sampling bias and selection bias .
2. Voluntary response sampling
Similar to a convenience sample, a voluntary response sample is mainly based on ease of access. Instead of the researcher choosing participants and directly contacting them, people volunteer themselves (e.g. by responding to a public online survey).
Voluntary response samples are always at least somewhat biased , as some people will inherently be more likely to volunteer than others, leading to self-selection bias .
3. Purposive sampling
This type of sampling, also known as judgement sampling, involves the researcher using their expertise to select a sample that is most useful to the purposes of the research.
It is often used in qualitative research , where the researcher wants to gain detailed knowledge about a specific phenomenon rather than make statistical inferences, or where the population is very small and specific. An effective purposive sample must have clear criteria and rationale for inclusion. Always make sure to describe your inclusion and exclusion criteria and beware of observer bias affecting your arguments.
4. Snowball sampling
If the population is hard to access, snowball sampling can be used to recruit participants via other participants. The number of people you have access to “snowballs” as you get in contact with more people. The downside here is also representativeness, as you have no way of knowing how representative your sample is due to the reliance on participants recruiting others. This can lead to sampling bias .
5. Quota sampling
Quota sampling relies on the non-random selection of a predetermined number or proportion of units. This is called a quota.
You first divide the population into mutually exclusive subgroups (called strata) and then recruit sample units until you reach your quota. These units share specific characteristics, determined by you prior to forming your strata. The aim of quota sampling is to control what or who makes up your sample.
If you want to know more about statistics , methodology , or research bias , make sure to check out some of our other articles with explanations and examples.
- Student’s t -distribution
- Normal distribution
- Null and Alternative Hypotheses
- Chi square tests
- Confidence interval
- Quartiles & Quantiles
- Cluster sampling
- Stratified sampling
- Data cleansing
- Reproducibility vs Replicability
- Peer review
- Prospective cohort study
Research bias
- Implicit bias
- Cognitive bias
- Placebo effect
- Hawthorne effect
- Hindsight bias
- Affect heuristic
- Social desirability bias
Prevent plagiarism. Run a free check.
A sample is a subset of individuals from a larger population . Sampling means selecting the group that you will actually collect data from in your research. For example, if you are researching the opinions of students in your university, you could survey a sample of 100 students.
In statistics, sampling allows you to test a hypothesis about the characteristics of a population.
Samples are used to make inferences about populations . Samples are easier to collect data from because they are practical, cost-effective, convenient, and manageable.
Probability sampling means that every member of the target population has a known chance of being included in the sample.
Probability sampling methods include simple random sampling , systematic sampling , stratified sampling , and cluster sampling .
In non-probability sampling , the sample is selected based on non-random criteria, and not every member of the population has a chance of being included.
Common non-probability sampling methods include convenience sampling , voluntary response sampling, purposive sampling , snowball sampling, and quota sampling .
In multistage sampling , or multistage cluster sampling, you draw a sample from a population using smaller and smaller groups at each stage.
This method is often used to collect data from a large, geographically spread group of people in national surveys, for example. You take advantage of hierarchical groupings (e.g., from state to city to neighborhood) to create a sample that’s less expensive and time-consuming to collect data from.
Sampling bias occurs when some members of a population are systematically more likely to be selected in a sample than others.
Cite this Scribbr article
If you want to cite this source, you can copy and paste the citation or click the “Cite this Scribbr article” button to automatically add the citation to our free Citation Generator.
McCombes, S. (2023, June 22). Sampling Methods | Types, Techniques & Examples. Scribbr. Retrieved April 14, 2024, from https://www.scribbr.com/methodology/sampling-methods/
Is this article helpful?
Shona McCombes
Other students also liked, population vs. sample | definitions, differences & examples, simple random sampling | definition, steps & examples, sampling bias and how to avoid it | types & examples, what is your plagiarism score.
Academia.edu no longer supports Internet Explorer.
To browse Academia.edu and the wider internet faster and more securely, please take a few seconds to upgrade your browser .
Enter the email address you signed up with and we'll email you a reset link.
- We're Hiring!
- Help Center
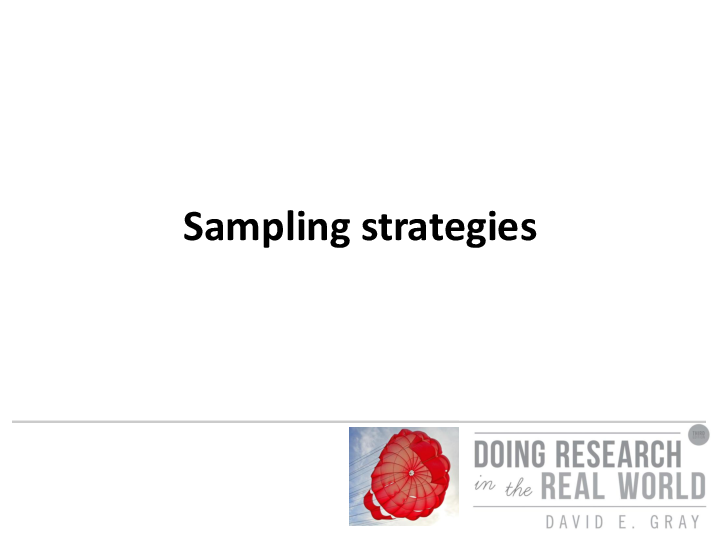
Chapter 9: Sampling strategies

2014, Doing Research in the Real World, 3rd edn
Related Papers
Dr. VISHAL VARIA
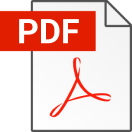
christopher masebo
Magdalena Saldaña
Random sampling is the procedure in which all members of a population have a known, nonzero chance of being selected. When samples are randomly chosen, researchers can use sample data to make educated guesses about the population. Likewise, random samples allow the calculation of sampling errors with a certain level of confidence. Simple random sampling, systematic random sampling, stratified random sampling and cluster sampling are the most common random sampling designs.
PIJUSH KHAN
Omid Tajik , Jawad Golzar
Simple random sampling is a widely utilized sampling method in quantitative studies with survey instruments. It is asserted that simple random sampling is favorable in homogeneous and uniformly selected populations. In this selection method, all the individuals have an equal opportunity to participate in the study where the selection process is entirely based on luck. The simple random sampling has benefits and drawbacks associated with it. It ensures unbiased, representative, and equal probability of the population; on the other hand, it can be cumbersome, rarely supported with readily available list of population, and challenging when population is heterogeneous and widely dispersed.
Adv Bukhari
Bruk Anteneh
william winkler
Shevoni Wisidagama
stanley igwe
The paper aims expose the similarities and differences between the two sampling techniques mentioned above and would further prove via the many defects of the cluster sampling technique that stratified sampling is more advantageous. However, other than jump straight to the distinction as the paper aims to showcase it is proper that a brief explication of the need for sampling as well as other kinds of sampling not mentioned as these insights would aid to shed better light on the differences between the cluster and stratified sampling types.
Loading Preview
Sorry, preview is currently unavailable. You can download the paper by clicking the button above.
RELATED PAPERS
SSRN Electronic Journal
Sebastian Hoenen
Rocznik Ziem Zachodnich
Marek Mazurkiewicz
IRJET Journal
luciano galeotti
Luciano Galeotti
Vira Liubchenko
Aggiornamenti Sociali
Paolo Foglizzo
Journal of Cardiovascular Magnetic Resonance
Michael Ith
Pollarise GROUPS
Rosa Arce-Ruiz
Interface - Comunicação, Saúde, Educação
Leticia Nolasco
Antigua Matanza
AUDITING AKUNTANSI
Lilis Wahyu Ningsih
Manhaj: Jurnal Penelitian dan Pengabdian Masyarakat
rini fitria
Chemical Communications
peter timmerman
awen Syahputra
The Journal of Organic Chemistry
Claudio F Lima
Salima Taibi
JURNAL AGRIMANSION
Hearing Research
Sharif Razzaque
Bali Medika Jurnal
Ni Kadek Wulandari
Subterranean Biology
Pier Mauro Giachino
Kevin Knuth
Pablo Román Rodriguez
Jasmeena Qadir
GIRUM TEFERA
RELATED TOPICS
- We're Hiring!
- Help Center
- Find new research papers in:
- Health Sciences
- Earth Sciences
- Cognitive Science
- Mathematics
- Computer Science
- Academia ©2024
- Privacy Policy
Buy Me a Coffee

Home » Sampling Methods – Types, Techniques and Examples
Sampling Methods – Types, Techniques and Examples
Table of Contents

Sampling refers to the process of selecting a subset of data from a larger population or dataset in order to analyze or make inferences about the whole population.
In other words, sampling involves taking a representative sample of data from a larger group or dataset in order to gain insights or draw conclusions about the entire group.
Sampling Methods
Sampling methods refer to the techniques used to select a subset of individuals or units from a larger population for the purpose of conducting statistical analysis or research.
Sampling is an essential part of the Research because it allows researchers to draw conclusions about a population without having to collect data from every member of that population, which can be time-consuming, expensive, or even impossible.
Types of Sampling Methods
Sampling can be broadly categorized into two main categories:
Probability Sampling
This type of sampling is based on the principles of random selection, and it involves selecting samples in a way that every member of the population has an equal chance of being included in the sample.. Probability sampling is commonly used in scientific research and statistical analysis, as it provides a representative sample that can be generalized to the larger population.
Type of Probability Sampling :
- Simple Random Sampling: In this method, every member of the population has an equal chance of being selected for the sample. This can be done using a random number generator or by drawing names out of a hat, for example.
- Systematic Sampling: In this method, the population is first divided into a list or sequence, and then every nth member is selected for the sample. For example, if every 10th person is selected from a list of 100 people, the sample would include 10 people.
- Stratified Sampling: In this method, the population is divided into subgroups or strata based on certain characteristics, and then a random sample is taken from each stratum. This is often used to ensure that the sample is representative of the population as a whole.
- Cluster Sampling: In this method, the population is divided into clusters or groups, and then a random sample of clusters is selected. Then, all members of the selected clusters are included in the sample.
- Multi-Stage Sampling : This method combines two or more sampling techniques. For example, a researcher may use stratified sampling to select clusters, and then use simple random sampling to select members within each cluster.
Non-probability Sampling
This type of sampling does not rely on random selection, and it involves selecting samples in a way that does not give every member of the population an equal chance of being included in the sample. Non-probability sampling is often used in qualitative research, where the aim is not to generalize findings to a larger population, but to gain an in-depth understanding of a particular phenomenon or group. Non-probability sampling methods can be quicker and more cost-effective than probability sampling methods, but they may also be subject to bias and may not be representative of the larger population.
Types of Non-probability Sampling :
- Convenience Sampling: In this method, participants are chosen based on their availability or willingness to participate. This method is easy and convenient but may not be representative of the population.
- Purposive Sampling: In this method, participants are selected based on specific criteria, such as their expertise or knowledge on a particular topic. This method is often used in qualitative research, but may not be representative of the population.
- Snowball Sampling: In this method, participants are recruited through referrals from other participants. This method is often used when the population is hard to reach, but may not be representative of the population.
- Quota Sampling: In this method, a predetermined number of participants are selected based on specific criteria, such as age or gender. This method is often used in market research, but may not be representative of the population.
- Volunteer Sampling: In this method, participants volunteer to participate in the study. This method is often used in research where participants are motivated by personal interest or altruism, but may not be representative of the population.
Applications of Sampling Methods
Applications of Sampling Methods from different fields:
- Psychology : Sampling methods are used in psychology research to study various aspects of human behavior and mental processes. For example, researchers may use stratified sampling to select a sample of participants that is representative of the population based on factors such as age, gender, and ethnicity. Random sampling may also be used to select participants for experimental studies.
- Sociology : Sampling methods are commonly used in sociological research to study social phenomena and relationships between individuals and groups. For example, researchers may use cluster sampling to select a sample of neighborhoods to study the effects of economic inequality on health outcomes. Stratified sampling may also be used to select a sample of participants that is representative of the population based on factors such as income, education, and occupation.
- Social sciences: Sampling methods are commonly used in social sciences to study human behavior and attitudes. For example, researchers may use stratified sampling to select a sample of participants that is representative of the population based on factors such as age, gender, and income.
- Marketing : Sampling methods are used in marketing research to collect data on consumer preferences, behavior, and attitudes. For example, researchers may use random sampling to select a sample of consumers to participate in a survey about a new product.
- Healthcare : Sampling methods are used in healthcare research to study the prevalence of diseases and risk factors, and to evaluate interventions. For example, researchers may use cluster sampling to select a sample of health clinics to participate in a study of the effectiveness of a new treatment.
- Environmental science: Sampling methods are used in environmental science to collect data on environmental variables such as water quality, air pollution, and soil composition. For example, researchers may use systematic sampling to collect soil samples at regular intervals across a field.
- Education : Sampling methods are used in education research to study student learning and achievement. For example, researchers may use stratified sampling to select a sample of schools that is representative of the population based on factors such as demographics and academic performance.
Examples of Sampling Methods
Probability Sampling Methods Examples:
- Simple random sampling Example : A researcher randomly selects participants from the population using a random number generator or drawing names from a hat.
- Stratified random sampling Example : A researcher divides the population into subgroups (strata) based on a characteristic of interest (e.g. age or income) and then randomly selects participants from each subgroup.
- Systematic sampling Example : A researcher selects participants at regular intervals from a list of the population.
Non-probability Sampling Methods Examples:
- Convenience sampling Example: A researcher selects participants who are conveniently available, such as students in a particular class or visitors to a shopping mall.
- Purposive sampling Example : A researcher selects participants who meet specific criteria, such as individuals who have been diagnosed with a particular medical condition.
- Snowball sampling Example : A researcher selects participants who are referred to them by other participants, such as friends or acquaintances.
How to Conduct Sampling Methods
some general steps to conduct sampling methods:
- Define the population: Identify the population of interest and clearly define its boundaries.
- Choose the sampling method: Select an appropriate sampling method based on the research question, characteristics of the population, and available resources.
- Determine the sample size: Determine the desired sample size based on statistical considerations such as margin of error, confidence level, or power analysis.
- Create a sampling frame: Develop a list of all individuals or elements in the population from which the sample will be drawn. The sampling frame should be comprehensive, accurate, and up-to-date.
- Select the sample: Use the chosen sampling method to select the sample from the sampling frame. The sample should be selected randomly, or if using a non-random method, every effort should be made to minimize bias and ensure that the sample is representative of the population.
- Collect data: Once the sample has been selected, collect data from each member of the sample using appropriate research methods (e.g., surveys, interviews, observations).
- Analyze the data: Analyze the data collected from the sample to draw conclusions about the population of interest.
When to use Sampling Methods
Sampling methods are used in research when it is not feasible or practical to study the entire population of interest. Sampling allows researchers to study a smaller group of individuals, known as a sample, and use the findings from the sample to make inferences about the larger population.
Sampling methods are particularly useful when:
- The population of interest is too large to study in its entirety.
- The cost and time required to study the entire population are prohibitive.
- The population is geographically dispersed or difficult to access.
- The research question requires specialized or hard-to-find individuals.
- The data collected is quantitative and statistical analyses are used to draw conclusions.
Purpose of Sampling Methods
The main purpose of sampling methods in research is to obtain a representative sample of individuals or elements from a larger population of interest, in order to make inferences about the population as a whole. By studying a smaller group of individuals, known as a sample, researchers can gather information about the population that would be difficult or impossible to obtain from studying the entire population.
Sampling methods allow researchers to:
- Study a smaller, more manageable group of individuals, which is typically less time-consuming and less expensive than studying the entire population.
- Reduce the potential for data collection errors and improve the accuracy of the results by minimizing sampling bias.
- Make inferences about the larger population with a certain degree of confidence, using statistical analyses of the data collected from the sample.
- Improve the generalizability and external validity of the findings by ensuring that the sample is representative of the population of interest.
Characteristics of Sampling Methods
Here are some characteristics of sampling methods:
- Randomness : Probability sampling methods are based on random selection, meaning that every member of the population has an equal chance of being selected. This helps to minimize bias and ensure that the sample is representative of the population.
- Representativeness : The goal of sampling is to obtain a sample that is representative of the larger population of interest. This means that the sample should reflect the characteristics of the population in terms of key demographic, behavioral, or other relevant variables.
- Size : The size of the sample should be large enough to provide sufficient statistical power for the research question at hand. The sample size should also be appropriate for the chosen sampling method and the level of precision desired.
- Efficiency : Sampling methods should be efficient in terms of time, cost, and resources required. The method chosen should be feasible given the available resources and time constraints.
- Bias : Sampling methods should aim to minimize bias and ensure that the sample is representative of the population of interest. Bias can be introduced through non-random selection or non-response, and can affect the validity and generalizability of the findings.
- Precision : Sampling methods should be precise in terms of providing estimates of the population parameters of interest. Precision is influenced by sample size, sampling method, and level of variability in the population.
- Validity : The validity of the sampling method is important for ensuring that the results obtained from the sample are accurate and can be generalized to the population of interest. Validity can be affected by sampling method, sample size, and the representativeness of the sample.
Advantages of Sampling Methods
Sampling methods have several advantages, including:
- Cost-Effective : Sampling methods are often much cheaper and less time-consuming than studying an entire population. By studying only a small subset of the population, researchers can gather valuable data without incurring the costs associated with studying the entire population.
- Convenience : Sampling methods are often more convenient than studying an entire population. For example, if a researcher wants to study the eating habits of people in a city, it would be very difficult and time-consuming to study every single person in the city. By using sampling methods, the researcher can obtain data from a smaller subset of people, making the study more feasible.
- Accuracy: When done correctly, sampling methods can be very accurate. By using appropriate sampling techniques, researchers can obtain a sample that is representative of the entire population. This allows them to make accurate generalizations about the population as a whole based on the data collected from the sample.
- Time-Saving: Sampling methods can save a lot of time compared to studying the entire population. By studying a smaller sample, researchers can collect data much more quickly than they could if they studied every single person in the population.
- Less Bias : Sampling methods can reduce bias in a study. If a researcher were to study the entire population, it would be very difficult to eliminate all sources of bias. However, by using appropriate sampling techniques, researchers can reduce bias and obtain a sample that is more representative of the entire population.
Limitations of Sampling Methods
- Sampling Error : Sampling error is the difference between the sample statistic and the population parameter. It is the result of selecting a sample rather than the entire population. The larger the sample, the lower the sampling error. However, no matter how large the sample size, there will always be some degree of sampling error.
- Selection Bias: Selection bias occurs when the sample is not representative of the population. This can happen if the sample is not selected randomly or if some groups are underrepresented in the sample. Selection bias can lead to inaccurate conclusions about the population.
- Non-response Bias : Non-response bias occurs when some members of the sample do not respond to the survey or study. This can result in a biased sample if the non-respondents differ from the respondents in important ways.
- Time and Cost : While sampling can be cost-effective, it can still be expensive and time-consuming to select a sample that is representative of the population. Depending on the sampling method used, it may take a long time to obtain a sample that is large enough and representative enough to be useful.
- Limited Information : Sampling can only provide information about the variables that are measured. It may not provide information about other variables that are relevant to the research question but were not measured.
- Generalization : The extent to which the findings from a sample can be generalized to the population depends on the representativeness of the sample. If the sample is not representative of the population, it may not be possible to generalize the findings to the population as a whole.
About the author
Muhammad Hassan
Researcher, Academic Writer, Web developer
You may also like

Probability Sampling – Methods, Types and...

Quota Sampling – Types, Methods and Examples

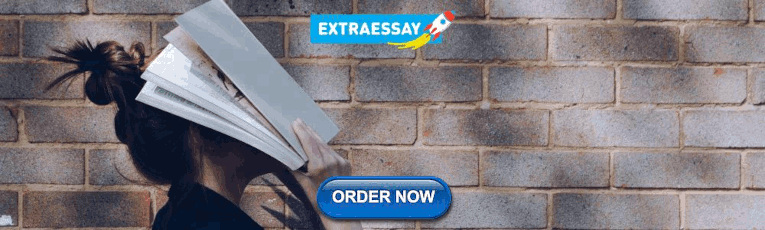
Simple Random Sampling – Types, Method and...

Convenience Sampling – Method, Types and Examples

Purposive Sampling – Methods, Types and Examples

Systematic Sampling – Types, Method and Examples
Please log in to save materials. Log in
- Resource Library
- Research Methods
- VIVA Grant Recipients
- Vgr-social-work-research
Education Standards
Radford university.
Learning Domain: Social Work
Standard: Basic Research Methodology
Lesson 10: Sampling in Qualitative Research
Lesson 11: qualitative measurement & rigor, lesson 12: qualitative design & data gathering, lesson 1: introduction to research, lesson 2: getting started with your research project, lesson 3: critical information literacy, lesson 4: paradigm, theory, and causality, lesson 5: research questions, lesson 6: ethics, lesson 7: measurement in quantitative research, lesson 8: sampling in quantitative research, lesson 9: quantitative research designs, powerpoint slides: sowk 621.01: research i: basic research methodology.
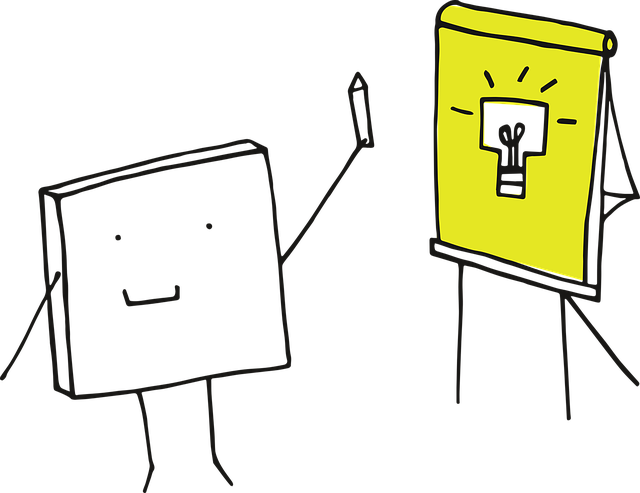
The twelve lessons for SOWK 621.01: Research I: Basic Research Methodology as previously taught by Dr. Matthew DeCarlo at Radford University. Dr. DeCarlo and his team developed a complete package of materials that includes a textbook, ancillary materials, and a student workbook as part of a VIVA Open Course Grant.
The PowerPoint slides associated with the twelve lessons of the course, SOWK 621.01: Research I: Basic Research Methodology, as previously taught by Dr. Matthew DeCarlo at Radford University.
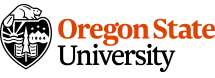
Chapter 20. Presentations
Introduction.
If a tree falls in a forest, and no one is around to hear it, does it make a sound? If a qualitative study is conducted, but it is not presented (in words or text), did it really happen? Perhaps not. Findings from qualitative research are inextricably tied up with the way those findings are presented. These presentations do not always need to be in writing, but they need to happen. Think of ethnographies, for example, and their thick descriptions of a particular culture. Witnessing a culture, taking fieldnotes, talking to people—none of those things in and of themselves convey the culture. Or think about an interview-based phenomenological study. Boxes of interview transcripts might be interesting to read through, but they are not a completed study without the intervention of hours of analysis and careful selection of exemplary quotes to illustrate key themes and final arguments and theories. And unlike much quantitative research in the social sciences, where the final write-up neatly reports the results of analyses, the way the “write-up” happens is an integral part of the analysis in qualitative research. Once again, we come back to the messiness and stubborn unlinearity of qualitative research. From the very beginning, when designing the study, imagining the form of its ultimate presentation is helpful.
Because qualitative researchers are motivated by understanding and conveying meaning, effective communication is not only an essential skill but a fundamental facet of the entire research project. Ethnographers must be able to convey a certain sense of verisimilitude, the appearance of true reality. Those employing interviews must faithfully depict the key meanings of the people they interviewed in a way that rings true to those people, even if the end result surprises them. And all researchers must strive for clarity in their publications so that various audiences can understand what was found and why it is important. This chapter will address how to organize various kinds of presentations for different audiences so that your results can be appreciated and understood.
In the world of academic science, social or otherwise, the primary audience for a study’s results is usually the academic community, and the primary venue for communicating to this audience is the academic journal. Journal articles are typically fifteen to thirty pages in length (8,000 to 12,000 words). Although qualitative researchers often write and publish journal articles—indeed, there are several journals dedicated entirely to qualitative research [1] —the best writing by qualitative researchers often shows up in books. This is because books, running from 80,000 to 150,000 words in length, allow the researcher to develop the material fully. You have probably read some of these in various courses you have taken, not realizing what they are. I have used examples of such books throughout this text, beginning with the three profiles in the introductory chapter. In some instances, the chapters in these books began as articles in academic journals (another indication that the journal article format somewhat limits what can be said about the study overall).
While the article and the book are “final” products of qualitative research, there are actually a few other presentation formats that are used along the way. At the very beginning of a research study, it is often important to have a written research proposal not just to clarify to yourself what you will be doing and when but also to justify your research to an outside agency, such as an institutional review board (IRB; see chapter 12), or to a potential funder, which might be your home institution, a government funder (such as the National Science Foundation, or NSF), or a private foundation (such as the Gates Foundation). As you get your research underway, opportunities will arise to present preliminary findings to audiences, usually through presentations at academic conferences. These presentations can provide important feedback as you complete your analyses. Finally, if you are completing a degree and looking to find an academic job, you will be asked to provide a “job talk,” usually about your research. These job talks are similar to conference presentations but can run significantly longer.
All the presentations mentioned so far are (mostly) for academic audiences. But qualitative research is also unique in that many of its practitioners don’t want to confine their presentation only to other academics. Qualitative researchers who study particular contexts or cultures might want to report back to the people and places they observed. Those working in the critical tradition might want to raise awareness of a particular issue to as large an audience as possible. Many others simply want everyday, nonacademic people to read their work, because they think it is interesting and important. To reach a wide audience, the final product can look like almost anything—it can be a poem, a blog, a podcast, even a science fiction short story. And if you are very lucky, it can even be a national or international bestseller.
In this chapter, we are going to stick with the more basic quotidian presentations—the academic paper / research proposal, the conference slideshow presentation / job talk, and the conference poster. We’ll also spend a bit of time on incorporating universal design into your presentations and how to create some especially attractive and impactful visual displays.
Researcher Note
What is the best piece of advice you’ve ever been given about conducting qualitative research?
The best advice I’ve received came from my adviser, Alford Young Jr. He told me to find the “Jessi Streib” answer to my research question, not the “Pierre Bourdieu” answer to my research question. In other words, don’t just say how a famous theorist would answer your question; say something original, something coming from you.
—Jessi Streib, author of The Power of the Past and Privilege Lost
Writing about Your Research
The journal article and the research proposal.
Although the research proposal is written before you have actually done your research and the article is written after all data collection and analysis is complete, there are actually many similarities between the two in terms of organization and purpose. The final article will (probably—depends on how much the research question and focus have shifted during the research itself) incorporate a great deal of what was included in a preliminary research proposal. The average lengths of both a proposal and an article are quite similar, with the “front sections” of the article abbreviated to make space for the findings, discussion of findings, and conclusion.
Figure 20.1 shows one model for what to include in an article or research proposal, comparing the elements of each with a default word count for each section. Please note that you will want to follow whatever specific guidelines you have been provided by the venue you are submitting the article/proposal to: the IRB, the NSF, the Journal of Qualitative Research . In fact, I encourage you to adapt the default model as needed by swapping out expected word counts for each section and adding or varying the sections to match expectations for your particular publication venue. [2]
You will notice a few things about the default model guidelines. First, while half of the proposal is spent discussing the research design, this section is shortened (but still included) for the article. There are a few elements that only show up in the proposal (e.g., the limitations section is in the introductory section here—it will be more fully developed in the conclusory section in the article). Obviously, you don’t have findings in the proposal, so this is an entirely new section for the article. Note that the article does not include a data management plan or a timeline—two aspects that most proposals require.
It might be helpful to find and maintain examples of successfully written sections that you can use as models for your own writing. I have included a few of these throughout the textbook and have included a few more at the end of this chapter.
Make an Argument
Some qualitative researchers, particularly those engaged in deep ethnographic research, focus their attention primarily if not exclusively on describing the data. They might even eschew the notion that they should make an “argument” about the data, preferring instead to use thick descriptions to convey interpretations. Bracketing the contrast between interpretation and argument for the moment, most readers will expect you to provide an argument about your data, and this argument will be in answer to whatever research question you eventually articulate (remember, research questions are allowed to shift as you get further into data collection and analysis). It can be frustrating to read a well-developed study with clear and elegant descriptions and no argument. The argument is the point of the research, and if you do not have one, 99 percent of the time, you are not finished with your analysis. Calarco ( 2020 ) suggests you imagine a pyramid, with all of your data forming the basis and all of your findings forming the middle section; the top/point of the pyramid is your argument, “what the patterns in your data tell us about how the world works or ought to work” ( 181 ).
The academic community to which you belong will be looking for an argument that relates to or develops theory. This is the theoretical generalizability promise of qualitative research. An academic audience will want to know how your findings relate to previous findings, theories, and concepts (the literature review; see chapter 9). It is thus vitally important that you go back to your literature review (or develop a new one) and draw those connections in your discussion and/or conclusion. When writing to other audiences, you will still want an argument, although it may not be written as a theoretical one. What do I mean by that? Even if you are not referring to previous literature or developing new theories or adapting older ones, a simple description of your findings is like dumping a lot of leaves in the lap of your audience. They still deserve to know about the shape of the forest. Maybe provide them a road map through it. Do this by telling a clear and cogent story about the data. What is the primary theme, and why is it important? What is the point of your research? [3]
A beautifully written piece of research based on participant observation [and/or] interviews brings people to life, and helps the reader understand the challenges people face. You are trying to use vivid, detailed and compelling words to help the reader really understand the lives of the people you studied. And you are trying to connect the lived experiences of these people to a broader conceptual point—so that the reader can understand why it matters. ( Lareau 2021:259 )
Do not hide your argument. Make it the focal point of your introductory section, and repeat it as often as needed to ensure the reader remembers it. I am always impressed when I see researchers do this well (see, e.g., Zelizer 1996 ).
Here are a few other suggestions for writing your article: Be brief. Do not overwhelm the reader with too many words; make every word count. Academics are particularly prone to “overwriting” as a way of demonstrating proficiency. Don’t. When writing your methods section, think about it as a “recipe for your work” that allows other researchers to replicate if they so wish ( Calarco 2020:186 ). Convey all the necessary information clearly, succinctly, and accurately. No more, no less. [4] Do not try to write from “beginning to end” in that order. Certain sections, like the introductory section, may be the last ones you write. I find the methods section the easiest, so I often begin there. Calarco ( 2020 ) begins with an outline of the analysis and results section and then works backward from there to outline the contribution she is making, then the full introduction that serves as a road map for the writing of all sections. She leaves the abstract for the very end. Find what order best works for you.
Presenting at Conferences and Job Talks
Students and faculty are primarily called upon to publicly present their research in two distinct contexts—the academic conference and the “job talk.” By convention, conference presentations usually run about fifteen minutes and, at least in sociology and other social sciences, rely primarily on the use of a slideshow (PowerPoint Presentation or PPT) presentation. You are usually one of three or four presenters scheduled on the same “panel,” so it is an important point of etiquette to ensure that your presentation falls within the allotted time and does not crowd into that of the other presenters. Job talks, on the other hand, conventionally require a forty- to forty-five-minute presentation with a fifteen- to twenty-minute question and answer (Q&A) session following it. You are the only person presenting, so if you run over your allotted time, it means less time for the Q&A, which can disturb some audience members who have been waiting for a chance to ask you something. It is sometimes possible to incorporate questions during your presentation, which allows you to take the entire hour, but you might end up shorting your presentation this way if the questions are numerous. It’s best for beginners to stick to the “ask me at the end” format (unless there is a simple clarifying question that can easily be addressed and makes the presentation run more smoothly, as in the case where you simply forgot to include information on the number of interviews you conducted).
For slideshows, you should allot two or even three minutes for each slide, never less than one minute. And those slides should be clear, concise, and limited. Most of what you say should not be on those slides at all. The slides are simply the main points or a clear image of what you are speaking about. Include bulleted points (words, short phrases), not full sentences. The exception is illustrative quotations from transcripts or fieldnotes. In those cases, keep to one illustrative quote per slide, and if it is long, bold or otherwise, highlight the words or passages that are most important for the audience to notice. [5]
Figure 20.2 provides a possible model for sections to include in either a conference presentation or a job talk, with approximate times and approximate numbers of slides. Note the importance (in amount of time spent) of both the research design and the findings/results sections, both of which have been helpfully starred for you. Although you don’t want to short any of the sections, these two sections are the heart of your presentation.
Fig 20.2. Suggested Slideshow Times and Number of Slides
Should you write out your script to read along with your presentation? I have seen this work well, as it prevents presenters from straying off topic and keeps them to the time allotted. On the other hand, these presentations can seem stiff and wooden. Personally, although I have a general script in advance, I like to speak a little more informally and engagingly with each slide, sometimes making connections with previous panelists if I am at a conference. This means I have to pay attention to the time, and I sometimes end up breezing through one section more quickly than I would like. Whatever approach you take, practice in advance. Many times. With an audience. Ask for feedback, and pay attention to any presentation issues that arise (e.g., Do you speak too fast? Are you hard to hear? Do you stumble over a particular word or name?).
Even though there are rules and guidelines for what to include, you will still want to make your presentation as engaging as possible in the little amount of time you have. Calarco ( 2020:274 ) recommends trying one of three story structures to frame your presentation: (1) the uncertain explanation , where you introduce a phenomenon that has not yet been fully explained and then describe how your research is tackling this; (2) the uncertain outcome , where you introduce a phenomenon where the consequences have been unclear and then you reveal those consequences with your research; and (3) the evocative example , where you start with some interesting example from your research (a quote from the interview transcripts, for example) or the real world and then explain how that example illustrates the larger patterns you found in your research. Notice that each of these is a framing story. Framing stories are essential regardless of format!
A Word on Universal Design
Please consider accessibility issues during your presentation, and incorporate elements of universal design into your slideshow. The basic idea behind universal design in presentations is that to the greatest extent possible, all people should be able to view, hear, or otherwise take in your presentation without needing special individual adaptations. If you can make your presentation accessible to people with visual impairment or hearing loss, why not do so? For example, one in twelve men is color-blind, unable to differentiate between certain colors, red/green being the most common problem. So if you design a graphic that relies on red and green bars, some of your audience members may not be able to properly identify which bar means what. Simple contrasts of black and white are much more likely to be visible to all members of your audience. There are many other elements of good universal design, but the basic foundation of all of them is that you consider how to make your presentation as accessible as possible at the outset. For example, include captions whenever possible, both as descriptions on slides and as images on slides and for any audio or video clips you are including; keep font sizes large enough to read from the back of the room; and face the audience when you are.
Poster Design
Undergraduate students who present at conferences are often encouraged to present at “poster sessions.” This usually means setting up a poster version of your research in a large hall or convention space at a set period of time—ninety minutes is common. Your poster will be one of dozens, and conference-goers will wander through the space, stopping intermittently at posters that attract them. Those who stop by might ask you questions about your research, and you are expected to be able to talk intelligently for two or three minutes. It’s a fairly easy way to practice presenting at conferences, which is why so many organizations hold these special poster sessions.
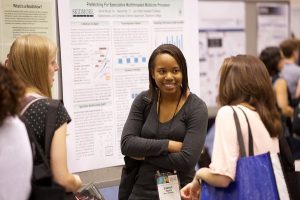
A good poster design will be immediately attractive to passersby and clearly and succinctly describe your research methods, findings, and conclusions. Some students have simply shrunk down their research papers to manageable sizes and then pasted them on a poster, all twelve to fifteen pages of them. Don’t do that! Here are some better suggestions: State the main conclusion of your research in large bold print at the top of your poster, on brightly colored (contrasting) paper, and paste in a QR code that links to your full paper online ( Calarco 2020:280 ). Use the rest of the poster board to provide a couple of highlights and details of the study. For an interview-based study, for example, you will want to put in some details about your sample (including number of interviews) and setting and then perhaps one or two key quotes, also distinguished by contrasting color background.
Incorporating Visual Design in Your Presentations
In addition to ensuring that your presentation is accessible to as large an audience as possible, you also want to think about how to display your data in general, particularly how to use charts and graphs and figures. [6] The first piece of advice is, use them! As the saying goes, a picture is worth a thousand words. If you can cut to the chase with a visually stunning display, do so. But there are visual displays that are stunning, and then there are the tired, hard-to-see visual displays that predominate at conferences. You can do better than most presenters by simply paying attention here and committing yourself to a good design. As with model section passages, keep a file of visual displays that work as models for your own presentations. Find a good guidebook to presenting data effectively (Evergreen 2018 , 2019 ; Schwabisch 2021) , and refer to it often.
Let me make a few suggestions here to get you started. First, test every visual display on a friend or colleague to find out how quickly they can understand the point you are trying to convey. As with reading passages aloud to ensure that your writing works, showing someone your display is the quickest way to find out if it works. Second, put the point in the title of the display! When writing for an academic journal, there will be specific conventions of what to include in the title (full description including methods of analysis, sample, dates), but in a public presentation, there are no limiting rules. So you are free to write as your title “Working-Class College Students Are Three Times as Likely as Their Peers to Drop Out of College,” if that is the point of the graphic display. It certainly helps the communicative aspect. Third, use the themes available to you in Excel for creating graphic displays, but alter them to better fit your needs . Consider adding dark borders to bars and columns, for example, so that they appear crisper for your audience. Include data callouts and labels, and enlarge them so they are clearly visible. When duplicative or otherwise unnecessary, drop distracting gridlines and labels on the y-axis (the vertical one). Don’t go crazy adding different fonts, however—keep things simple and clear. Sans serif fonts (those without the little hooks on the ends of letters) read better from a distance. Try to use the same color scheme throughout, even if this means manually changing the colors of bars and columns. For example, when reporting on working-class college students, I use blue bars, while I reserve green bars for wealthy students and yellow bars for students in the middle. I repeat these colors throughout my presentations and incorporate different colors when talking about other items or factors. You can also try using simple grayscale throughout, with pops of color to indicate a bar or column or line that is of the most interest. These are just some suggestions. The point is to take presentation seriously and to pay attention to visual displays you are using to ensure they effectively communicate what you want them to communicate. I’ve included a data visualization checklist from Evergreen ( 2018 ) here.
Ethics of Presentation and Reliability
Until now, all the data you have collected have been yours alone. Once you present the data, however, you are sharing sometimes very intimate information about people with a broader public. You will find yourself balancing between protecting the privacy of those you’ve interviewed and observed and needing to demonstrate the reliability of the study. The more information you provide to your audience, the more they can understand and appreciate what you have found, but this also may pose risks to your participants. There is no one correct way to go about finding the right balance. As always, you have a duty to consider what you are doing and must make some hard decisions.
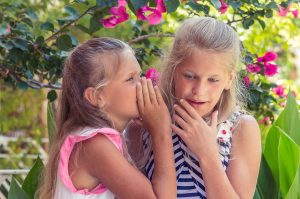
The most obvious place we see this paradox emerge is when you mask your data to protect the privacy of your participants. It is standard practice to provide pseudonyms, for example. It is such standard practice that you should always assume you are being given a pseudonym when reading a book or article based on qualitative research. When I was a graduate student, I tried to find information on how best to construct pseudonyms but found little guidance. There are some ethical issues here, I think. [7] Do you create a name that has the same kind of resonance as the original name? If the person goes by a nickname, should you use a nickname as a pseudonym? What about names that are ethnically marked (as in, almost all of them)? Is there something unethical about reracializing a person? (Yes!) In her study of adolescent subcultures, Wilkins ( 2008 ) noted, “Because many of the goths used creative, alternative names rather than their given names, I did my best to reproduce the spirit of their chosen names” ( 24 ).
Your reader or audience will want to know all the details about your participants so that they can gauge both your credibility and the reliability of your findings. But how many details are too many? What if you change the name but otherwise retain all the personal pieces of information about where they grew up, and how old they were when they got married, and how many children they have, and whether they made a splash in the news cycle that time they were stalked by their ex-boyfriend? At some point, those details are going to tip over into the zone of potential unmasking. When you are doing research at one particular field site that may be easily ascertained (as when you interview college students, probably at the institution at which you are a student yourself), it is even more important to be wary of providing too many details. You also need to think that your participants might read what you have written, know things about the site or the population from which you drew your interviews, and figure out whom you are talking about. This can all get very messy if you don’t do more than simply pseudonymize the people you interviewed or observed.
There are some ways to do this. One, you can design a study with all of these risks in mind. That might mean choosing to conduct interviews or observations at multiple sites so that no one person can be easily identified. Another is to alter some basic details about your participants to protect their identity or to refuse to provide all the information when selecting quotes . Let’s say you have an interviewee named “Anna” (a pseudonym), and she is a twenty-four-year-old Latina studying to be an engineer. You want to use a quote from Anna about racial discrimination in her graduate program. Instead of attributing the quote to Anna (whom your reader knows, because you’ve already told them, is a twenty-four-year-old Latina studying engineering), you might simply attribute the quote to “Latina student in STEM.” Taking this a step further, you might leave the quote unattributed, providing a list of quotes about racial discrimination by “various students.”
The problem with masking all the identifiers, of course, is that you lose some of the analytical heft of those attributes. If it mattered that Anna was twenty-four (not thirty-four) and that she was a Latina and that she was studying engineering, taking out any of those aspects of her identity might weaken your analysis. This is one of those “hard choices” you will be called on to make! A rather radical and controversial solution to this dilemma is to create composite characters , characters based on the reality of the interviews but fully masked because they are not identifiable with any one person. My students are often very queasy about this when I explain it to them. The more positivistic your approach and the more you see individuals rather than social relationships/structure as the “object” of your study, the more employing composites will seem like a really bad idea. But composites “allow researchers to present complex, situated accounts from individuals” without disclosing personal identities ( Willis 2019 ), and they can be effective ways of presenting theory narratively ( Hurst 2019 ). Ironically, composites permit you more latitude when including “dirty laundry” or stories that could harm individuals if their identities became known. Rather than squeezing out details that could identify a participant, the identities are permanently removed from the details. Great difficulty remains, however, in clearly explaining the theoretical use of composites to your audience and providing sufficient information on the reliability of the underlying data.
There are a host of other ethical issues that emerge as you write and present your data. This is where being reflective throughout the process will help. How and what you share of what you have learned will depend on the social relationships you have built, the audiences you are writing or speaking to, and the underlying animating goals of your study. Be conscious about all of your decisions, and then be able to explain them fully, both to yourself and to those who ask.
Our research is often close to us. As a Black woman who is a first-generation college student and a professional with a poverty/working-class origin, each of these pieces of my identity creates nuances in how I engage in my research, including how I share it out. Because of this, it’s important for us to have people in our lives who we trust who can help us, particularly, when we are trying to share our findings. As researchers, we have been steeped in our work, so we know all the details and nuances. Sometimes we take this for granted, and we might not have shared those nuances in conversation or writing or taken some of this information for granted. As I share my research with trusted friends and colleagues, I pay attention to the questions they ask me or the feedback they give when we talk or when they read drafts.
—Kim McAloney, PhD, College Student Services Administration Ecampus coordinator and instructor
Final Comments: Preparing for Being Challenged
Once you put your work out there, you must be ready to be challenged. Science is a collective enterprise and depends on a healthy give and take among researchers. This can be both novel and difficult as you get started, but the more you understand the importance of these challenges, the easier it will be to develop the kind of thick skin necessary for success in academia. Scientists’ authority rests on both the inherent strength of their findings and their ability to convince other scientists of the reliability and validity and value of those findings. So be prepared to be challenged, and recognize this as simply another important aspect of conducting research!
Considering what challenges might be made as you design and conduct your study will help you when you get to the writing and presentation stage. Address probable challenges in your final article, and have a planned response to probable questions in a conference presentation or job talk. The following is a list of common challenges of qualitative research and how you might best address them:
- Questions about generalizability . Although qualitative research is not statistically generalizable (and be prepared to explain why), qualitative research is theoretically generalizable. Discuss why your findings here might tell us something about related phenomena or contexts.
- Questions about reliability . You probably took steps to ensure the reliability of your findings. Discuss them! This includes explaining the use and value of multiple data sources and defending your sampling and case selections. It also means being transparent about your own position as researcher and explaining steps you took to ensure that what you were seeing was really there.
- Questions about replicability. Although qualitative research cannot strictly be replicated because the circumstances and contexts will necessarily be different (if only because the point in time is different), you should be able to provide as much detail as possible about how the study was conducted so that another researcher could attempt to confirm or disconfirm your findings. Also, be very clear about the limitations of your study, as this allows other researchers insight into what future research might be warranted.
None of this is easy, of course. Writing beautifully and presenting clearly and cogently require skill and practice. If you take anything from this chapter, it is to remember that presentation is an important and essential part of the research process and to allocate time for this as you plan your research.
Data Visualization Checklist for Slideshow (PPT) Presentations
Adapted from Evergreen ( 2018 )
Text checklist
- Short catchy, descriptive titles (e.g., “Working-class students are three times as likely to drop out of college”) summarize the point of the visual display
- Subtitled and annotations provide additional information (e.g., “note: male students also more likely to drop out”)
- Text size is hierarchical and readable (titles are largest; axes labels smallest, which should be at least 20points)
- Text is horizontal. Audience members cannot read vertical text!
- All data labeled directly and clearly: get rid of those “legends” and embed the data in your graphic display
- Labels are used sparingly; avoid redundancy (e.g., do not include both a number axis and a number label)
Arrangement checklist
- Proportions are accurate; bar charts should always start at zero; don’t mislead the audience!
- Data are intentionally ordered (e.g., by frequency counts). Do not leave ragged alphabetized bar graphs!
- Axis intervals are equidistant: spaces between axis intervals should be the same unit
- Graph is two-dimensional. Three-dimensional and “bevelled” displays are confusing
- There is no unwanted decoration (especially the kind that comes automatically through the PPT “theme”). This wastes your space and confuses.
Color checklist
- There is an intentional color scheme (do not use default theme)
- Color is used to identify key patterns (e.g., highlight one bar in red against six others in greyscale if this is the bar you want the audience to notice)
- Color is still legible when printed in black and white
- Color is legible for people with color blindness (do not use red/green or yellow/blue combinations)
- There is sufficient contrast between text and background (black text on white background works best; be careful of white on dark!)
Lines checklist
- Be wary of using gridlines; if you do, mute them (grey, not black)
- Allow graph to bleed into surroundings (don’t use border lines)
- Remove axis lines unless absolutely necessary (better to label directly)
Overall design checklist
- The display highlights a significant finding or conclusion that your audience can ‘”see” relatively quickly
- The type of graph (e.g., bar chart, pie chart, line graph) is appropriate for the data. Avoid pie charts with more than three slices!
- Graph has appropriate level of precision; if you don’t need decimal places
- All the chart elements work together to reinforce the main message
Universal Design Checklist for Slideshow (PPT) Presentations
- Include both verbal and written descriptions (e.g., captions on slides); consider providing a hand-out to accompany the presentation
- Microphone available (ask audience in back if they can clearly hear)
- Face audience; allow people to read your lips
- Turn on captions when presenting audio or video clips
- Adjust light settings for visibility
- Speak slowly and clearly; practice articulation; don’t mutter or speak under your breath (even if you have something humorous to say – say it loud!)
- Use Black/White contrasts for easy visibility; or use color contrasts that are real contrasts (do not rely on people being able to differentiate red from green, for example)
- Use easy to read font styles and avoid too small font sizes: think about what an audience member in the back row will be able to see and read.
- Keep your slides simple: do not overclutter them; if you are including quotes from your interviews, take short evocative snippets only, and bold key words and passages. You should also read aloud each passage, preferably with feeling!
Supplement: Models of Written Sections for Future Reference
Data collection section example.
Interviews were semi structured, lasted between one and three hours, and took place at a location chosen by the interviewee. Discussions centered on four general topics: (1) knowledge of their parent’s immigration experiences; (2) relationship with their parents; (3) understanding of family labor, including language-brokering experiences; and (4) experiences with school and peers, including any future life plans. While conducting interviews, I paid close attention to respondents’ nonverbal cues, as well as their use of metaphors and jokes. I conducted interviews until I reached a point of saturation, as indicated by encountering repeated themes in new interviews (Glaser and Strauss 1967). Interviews were audio recorded, transcribed with each interviewee’s permission, and conducted in accordance with IRB protocols. Minors received permission from their parents before participation in the interview. ( Kwon 2022:1832 )
Justification of Case Selection / Sample Description Section Example
Looking at one profession within one organization and in one geographic area does impose limitations on the generalizability of our findings. However, it also has advantages. We eliminate the problem of interorganizational heterogeneity. If multiple organizations are studied simultaneously, it can make it difficult to discern the mechanisms that contribute to racial inequalities. Even with a single occupation there is considerable heterogeneity, which may make understanding how organizational structure impacts worker outcomes difficult. By using the case of one group of professionals in one religious denomination in one geographic region of the United States, we clarify how individuals’ perceptions and experiences of occupational inequality unfold in relation to a variety of observed and unobserved occupational and contextual factors that might be obscured in a larger-scale study. Focusing on a specific group of professionals allows us to explore and identify ways that formal organizational rules combine with informal processes to contribute to the persistence of racial inequality. ( Eagle and Mueller 2022:1510–1511 )
Ethics Section Example
I asked everyone who was willing to sit for a formal interview to speak only for themselves and offered each of them a prepaid Visa Card worth $25–40. I also offered everyone the opportunity to keep the card and erase the tape completely at any time they were dissatisfied with the interview in any way. No one asked for the tape to be erased; rather, people remarked on the interview being a really good experience because they felt heard. Each interview was professionally transcribed and for the most part the excerpts are literal transcriptions. In a few places, the excerpts have been edited to reduce colloquial features of speech (e.g., you know, like, um) and some recursive elements common to spoken language. A few excerpts were placed into standard English for clarity. I made this choice for the benefit of readers who might otherwise find the insights and ideas harder to parse in the original. However, I have to acknowledge this as an act of class-based violence. I tried to keep the original phrasing whenever possible. ( Pascale 2021:235 )
Further Readings
Calarco, Jessica McCrory. 2020. A Field Guide to Grad School: Uncovering the Hidden Curriculum . Princeton, NJ: Princeton University Press. Don’t let the unassuming title mislead you—there is a wealth of helpful information on writing and presenting data included here in a highly accessible manner. Every graduate student should have a copy of this book.
Edwards, Mark. 2012. Writing in Sociology . Thousand Oaks, CA: SAGE. An excellent guide to writing and presenting sociological research by an Oregon State University professor. Geared toward undergraduates and useful for writing about either quantitative or qualitative research or both.
Evergreen, Stephanie D. H. 2018. Presenting Data Effectively: Communicating Your Findings for Maximum Impact . Thousand Oaks, CA: SAGE. This is one of my very favorite books, and I recommend it highly for everyone who wants their presentations and publications to communicate more effectively than the boring black-and-white, ragged-edge tables and figures academics are used to seeing.
Evergreen, Stephanie D. H. 2019. Effective Data Visualization 2 . Thousand Oaks, CA: SAGE. This is an advanced primer for presenting clean and clear data using graphs, tables, color, font, and so on. Start with Evergreen (2018), and if you graduate from that text, move on to this one.
Schwabisch, Jonathan. 2021. Better Data Visualizations: A Guide for Scholars, Researchers, and Wonks . New York: Columbia University Press. Where Evergreen’s (2018, 2019) focus is on how to make the best visual displays possible for effective communication, this book is specifically geared toward visual displays of academic data, both quantitative and qualitative. If you want to know when it is appropriate to use a pie chart instead of a stacked bar chart, this is the reference to use.
- Some examples: Qualitative Inquiry , Qualitative Research , American Journal of Qualitative Research , Ethnography , Journal of Ethnographic and Qualitative Research , Qualitative Report , Qualitative Sociology , and Qualitative Studies . ↵
- This is something I do with every article I write: using Excel, I write each element of the expected article in a separate row, with one column for “expected word count” and another column for “actual word count.” I fill in the actual word count as I write. I add a third column for “comments to myself”—how things are progressing, what I still need to do, and so on. I then use the “sum” function below each of the first two columns to keep a running count of my progress relative to the final word count. ↵
- And this is true, I would argue, even when your primary goal is to leave space for the voices of those who don’t usually get a chance to be part of the conversation. You will still want to put those voices in some kind of choir, with a clear direction (song) to be sung. The worst thing you can do is overwhelm your audience with random quotes or long passages with no key to understanding them. Yes, a lot of metaphors—qualitative researchers love metaphors! ↵
- To take Calarco’s recipe analogy further, do not write like those food bloggers who spend more time discussing the color of their kitchen or the experiences they had at the market than they do the actual cooking; similarly, do not write recipes that omit crucial details like the amount of flour or the size of the baking pan used or the temperature of the oven. ↵
- The exception is the “compare and contrast” of two or more quotes, but use caution here. None of the quotes should be very long at all (a sentence or two each). ↵
- Although this section is geared toward presentations, many of the suggestions could also be useful when writing about your data. Don’t be afraid to use charts and graphs and figures when writing your proposal, article, thesis, or dissertation. At the very least, you should incorporate a tabular display of the participants, sites, or documents used. ↵
- I was so puzzled by these kinds of questions that I wrote one of my very first articles on it ( Hurst 2008 ). ↵
The visual presentation of data or information through graphics such as charts, graphs, plots, infographics, maps, and animation. Recall the best documentary you ever viewed, and there were probably excellent examples of good data visualization there (for me, this was An Inconvenient Truth , Al Gore’s film about climate change). Good data visualization allows more effective communication of findings of research, particularly in public presentations (e.g., slideshows).
Introduction to Qualitative Research Methods Copyright © 2023 by Allison Hurst is licensed under a Creative Commons Attribution-ShareAlike 4.0 International License , except where otherwise noted.
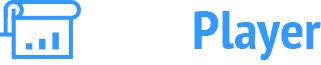
- My presentations
Auth with social network:
Download presentation
We think you have liked this presentation. If you wish to download it, please recommend it to your friends in any social system. Share buttons are a little bit lower. Thank you!
Presentation is loading. Please wait.
Lecture Notes on Research Methodology
Published by Eileen Garrison Modified over 6 years ago
Similar presentations
Presentation on theme: "Lecture Notes on Research Methodology"— Presentation transcript:
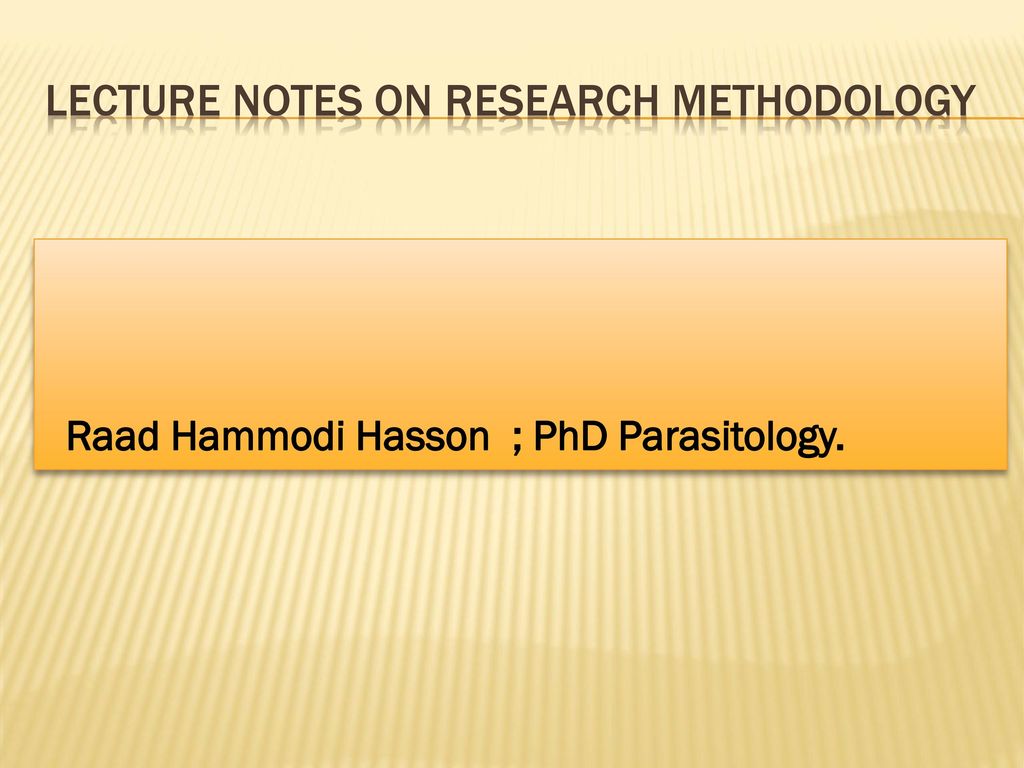
Introduction to Research Methodology
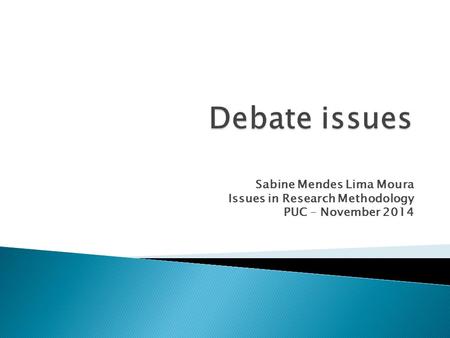
Sabine Mendes Lima Moura Issues in Research Methodology PUC – November 2014.
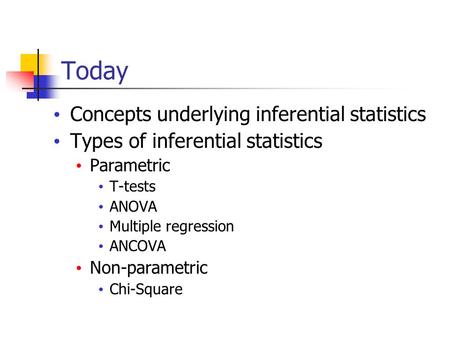
Today Concepts underlying inferential statistics
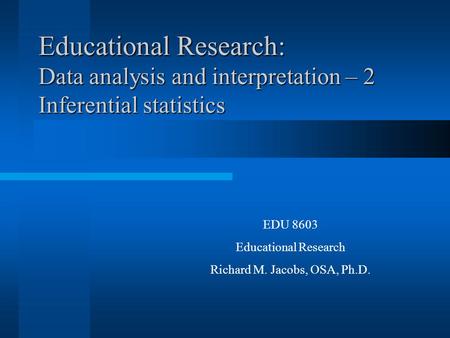
Richard M. Jacobs, OSA, Ph.D.
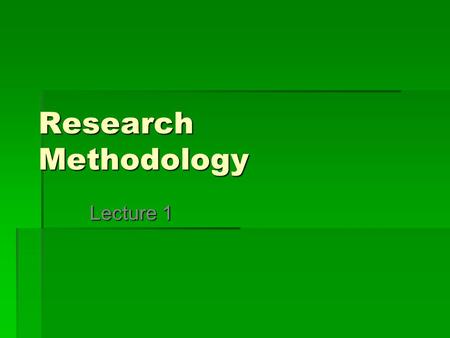
Research Methodology Lecture 1.
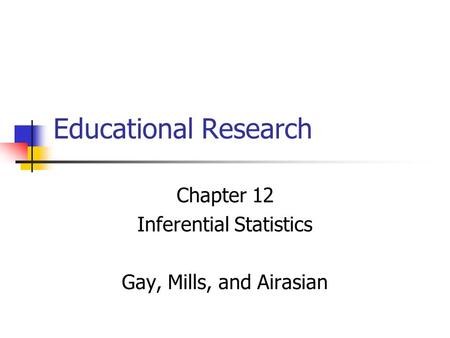
Chapter 12 Inferential Statistics Gay, Mills, and Airasian
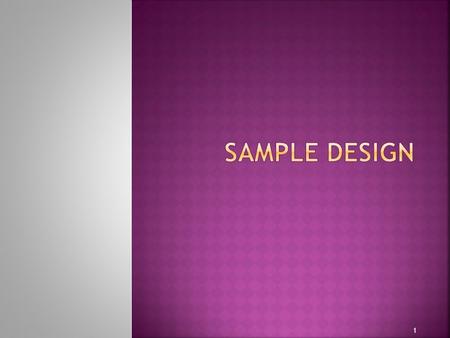
Sample Design.
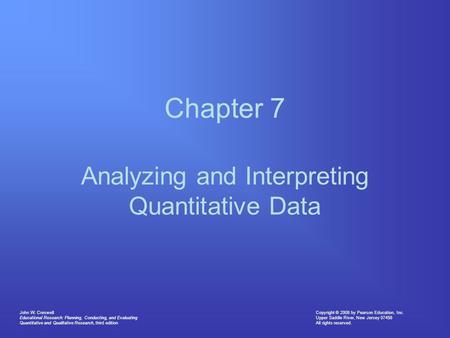
Copyright © 2008 by Pearson Education, Inc. Upper Saddle River, New Jersey All rights reserved. John W. Creswell Educational Research: Planning,
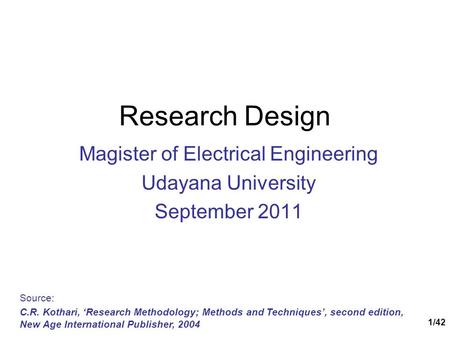
Magister of Electrical Engineering Udayana University September 2011
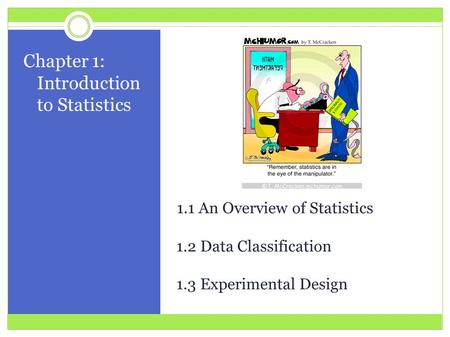
Chapter 1: Introduction to Statistics
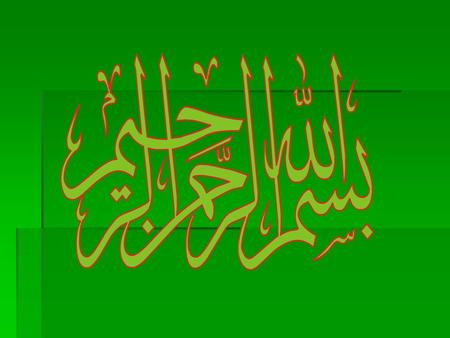
RESEARCH A systematic quest for undiscovered truth A way of thinking
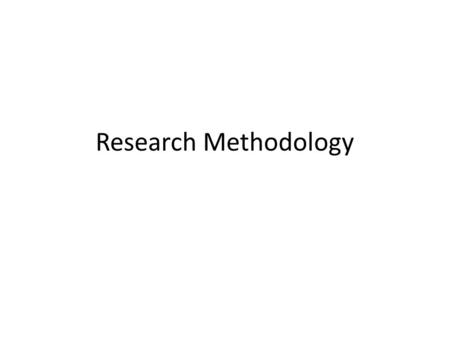
Research Methodology.
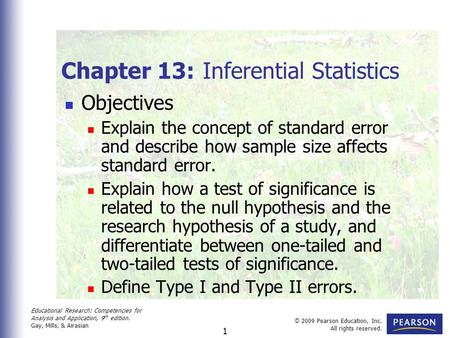
Educational Research: Competencies for Analysis and Application, 9 th edition. Gay, Mills, & Airasian © 2009 Pearson Education, Inc. All rights reserved.
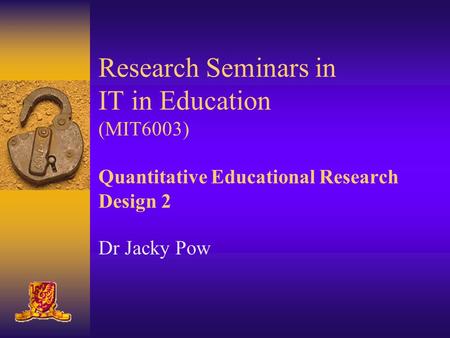
Research Seminars in IT in Education (MIT6003) Quantitative Educational Research Design 2 Dr Jacky Pow.
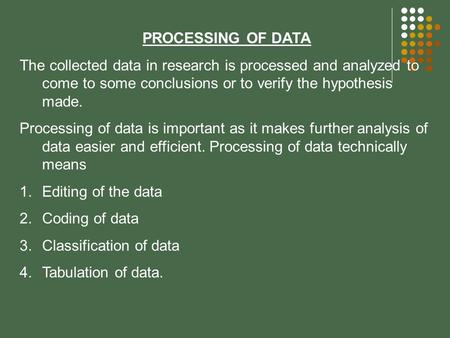
PROCESSING OF DATA The collected data in research is processed and analyzed to come to some conclusions or to verify the hypothesis made. Processing of.
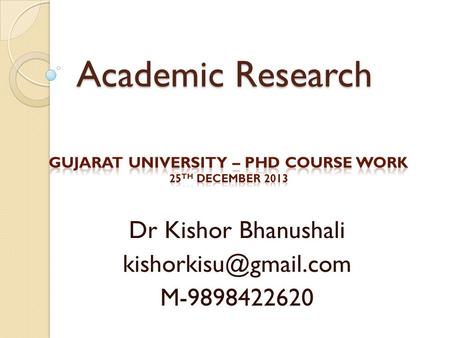
Academic Research Academic Research Dr Kishor Bhanushali M
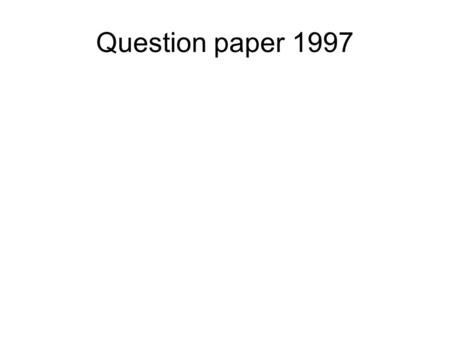
Question paper 1997.
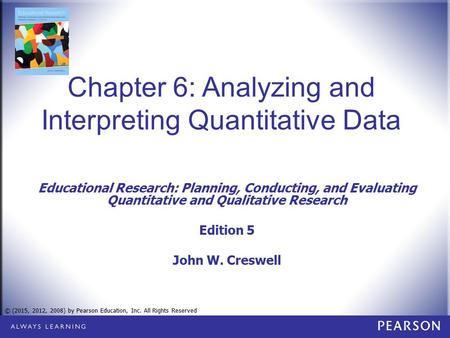
Chapter 6: Analyzing and Interpreting Quantitative Data
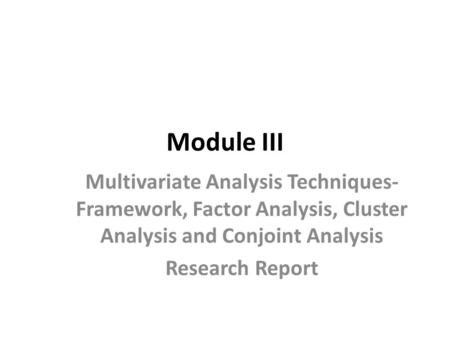
Module III Multivariate Analysis Techniques- Framework, Factor Analysis, Cluster Analysis and Conjoint Analysis Research Report.
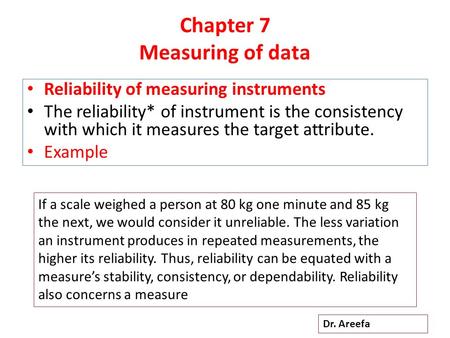
Chapter 7 Measuring of data Reliability of measuring instruments The reliability* of instrument is the consistency with which it measures the target attribute.
About project
© 2024 SlidePlayer.com Inc. All rights reserved.
Got any suggestions?
We want to hear from you! Send us a message and help improve Slidesgo
Top searches
Trending searches
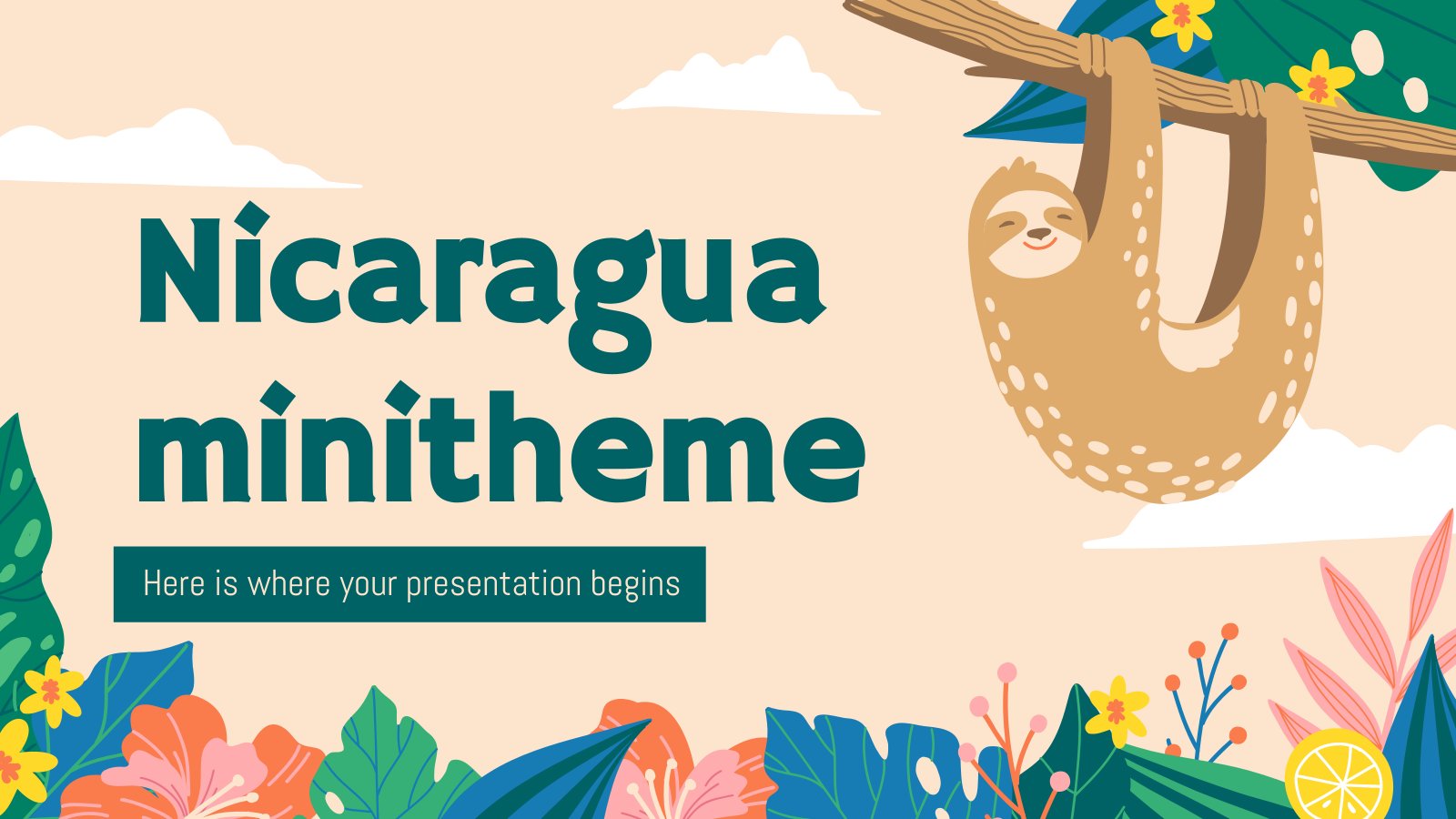
24 templates
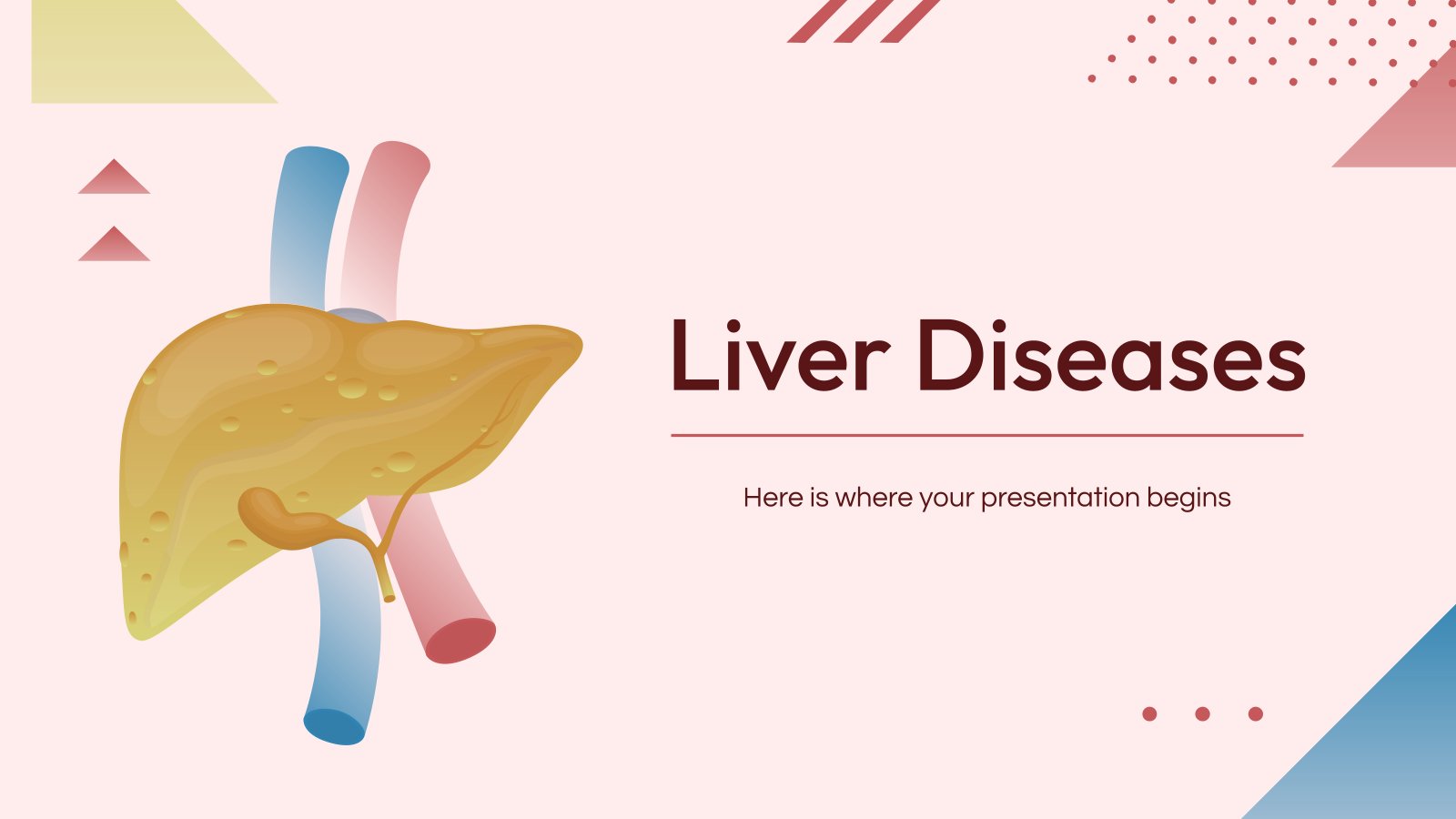
10 templates
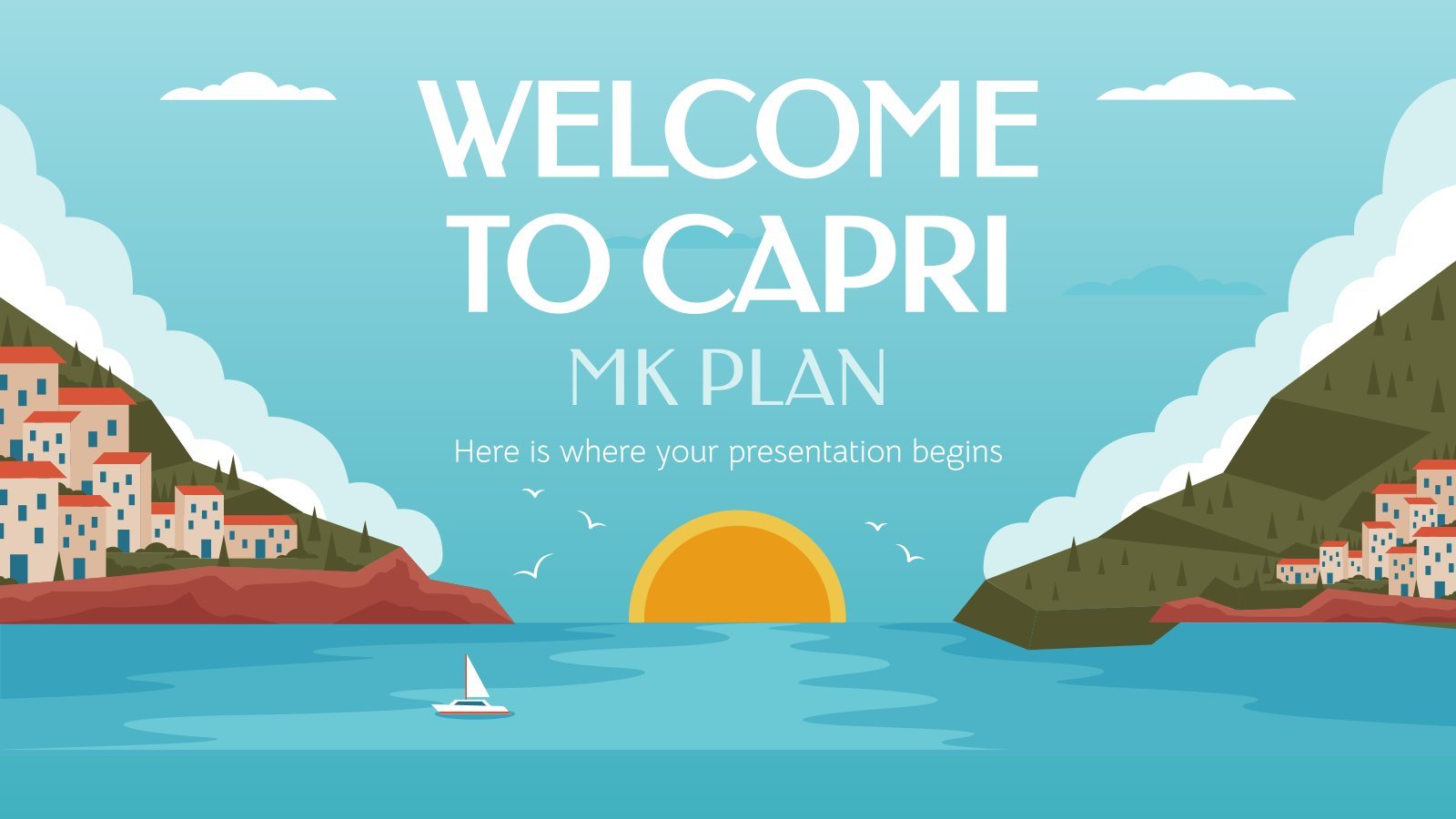
20 templates

education technology
181 templates
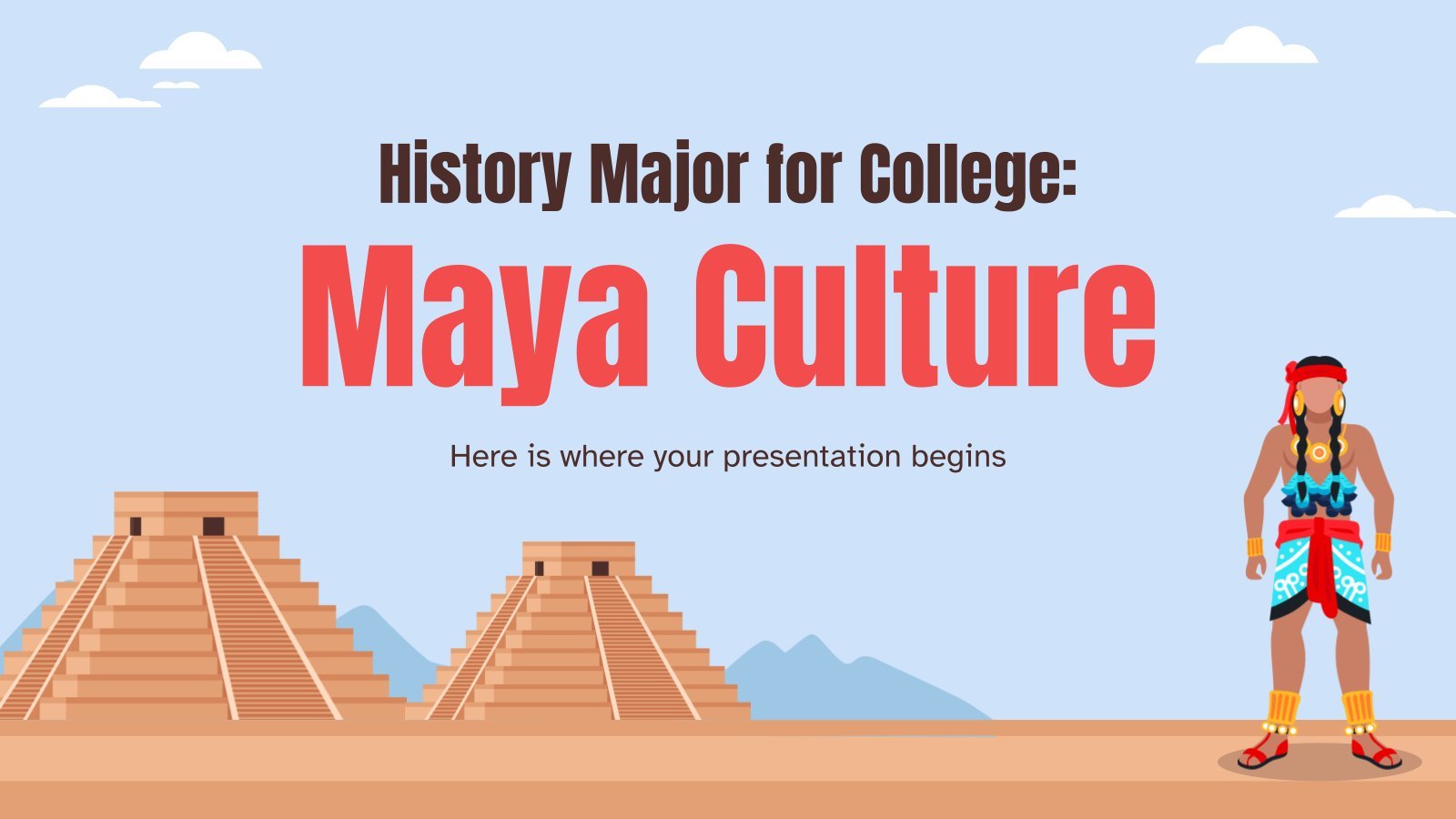
23 templates
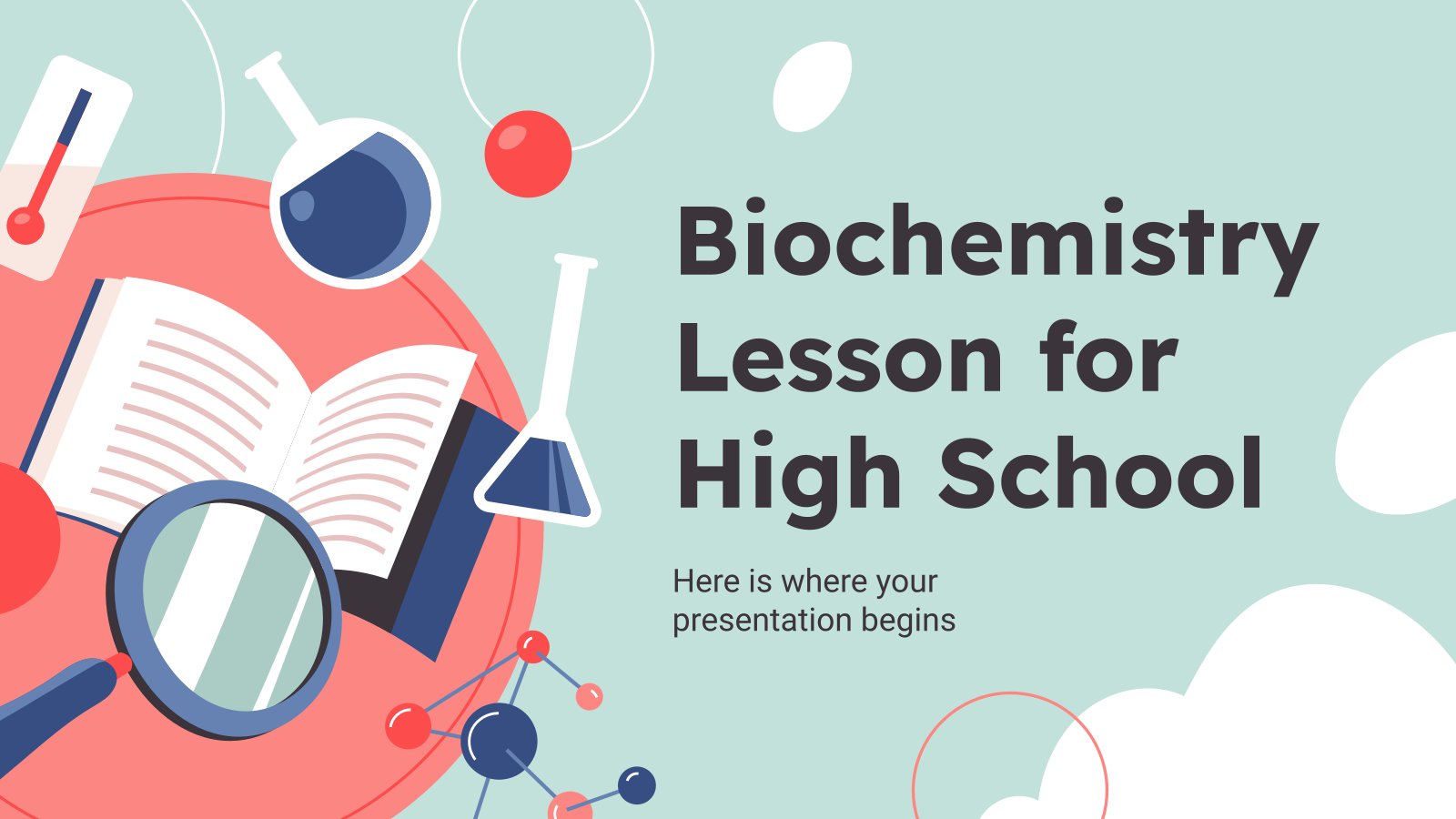
biochemistry
37 templates
Research Methodology Workshop
Research methodology workshop presentation, premium google slides theme and powerpoint template.
The backbone of any scientific inquiry: the methodology. A systematic process of collecting, analyzing, and interpreting data to draw conclusions about a particular subject matter. However, many researchers struggle with selecting the appropriate research design, sampling methods, data collection, and analysis techniques. But your workshop is ready to tackle that issue so as to help researchers to get started! With this template and its captivating design, you'll get everyone paying close attention to your explanations. Remember that you can customize everything!
Features of this template
- 100% editable and easy to modify
- 31 different slides to impress your audience
- Contains easy-to-edit graphics such as graphs, maps, tables, timelines and mockups
- Includes 500+ icons and Flaticon’s extension for customizing your slides
- Designed to be used in Google Slides and Microsoft PowerPoint
- 16:9 widescreen format suitable for all types of screens
- Includes information about fonts, colors, and credits of the resources used
What are the benefits of having a Premium account?
What Premium plans do you have?
What can I do to have unlimited downloads?
Don’t want to attribute Slidesgo?
Gain access to over 22500 templates & presentations with premium from 1.67€/month.
Are you already Premium? Log in
Related posts on our blog
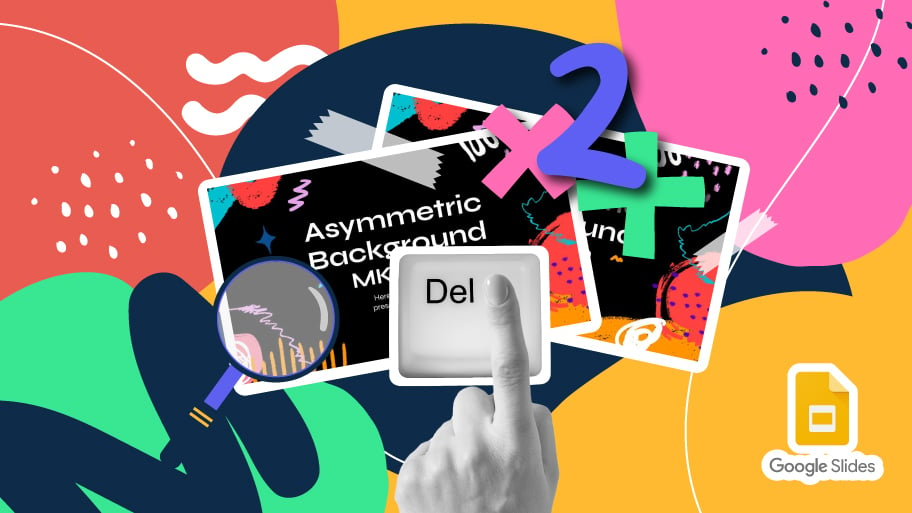
How to Add, Duplicate, Move, Delete or Hide Slides in Google Slides
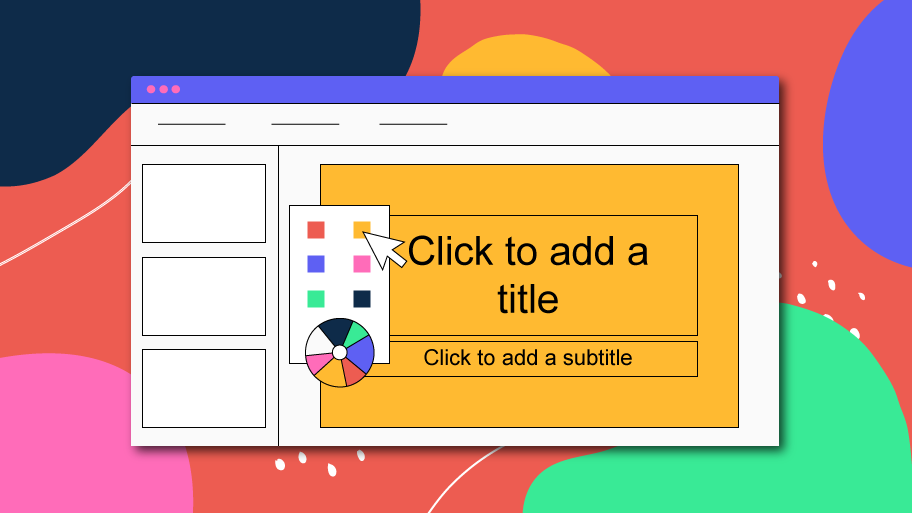
How to Change Layouts in PowerPoint
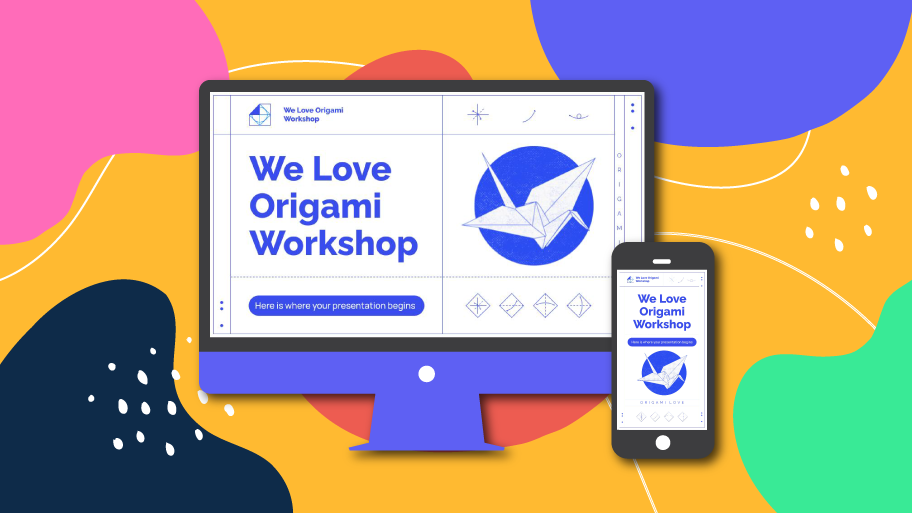
How to Change the Slide Size in Google Slides
Related presentations.
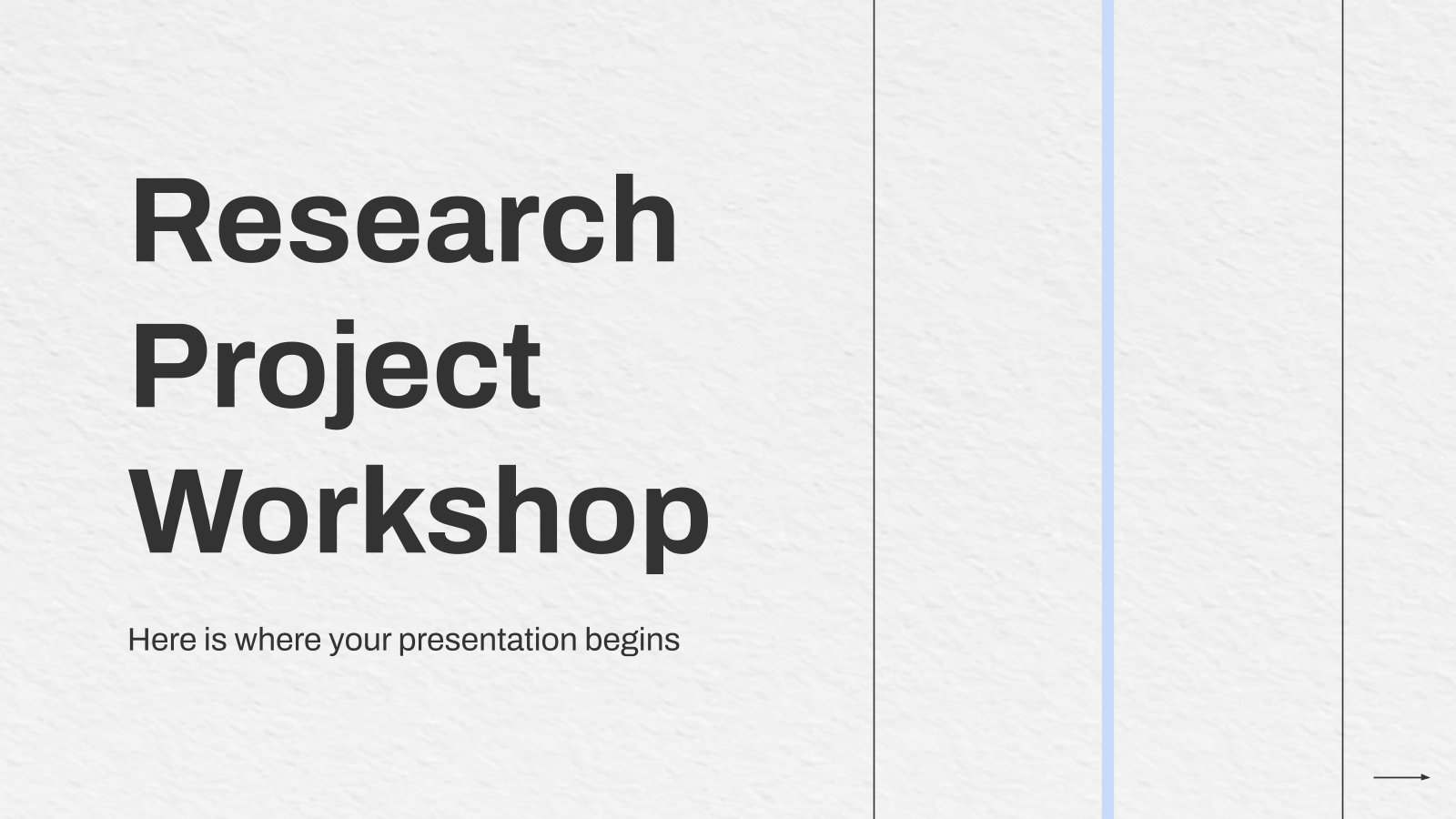
Premium template
Unlock this template and gain unlimited access
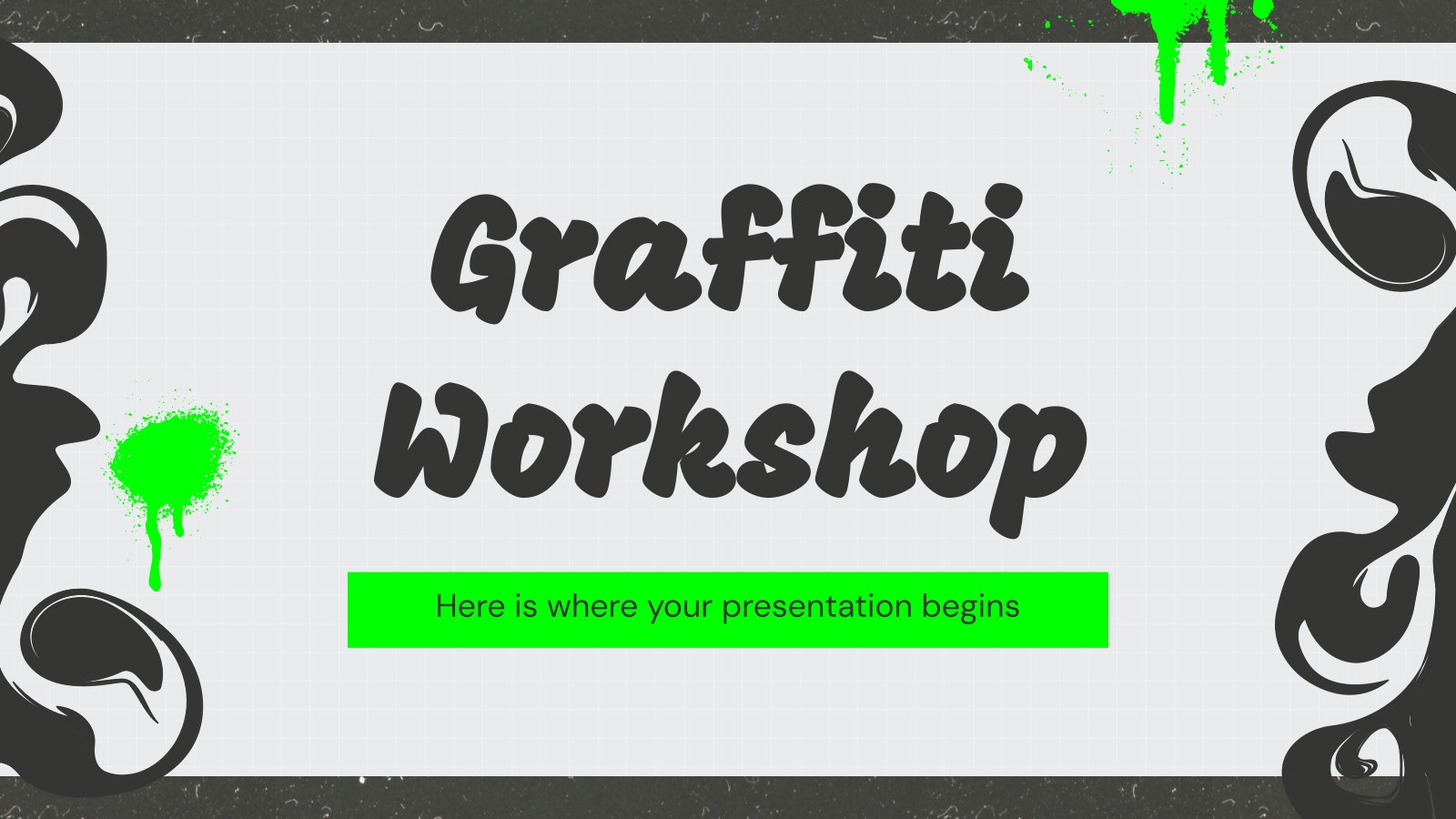
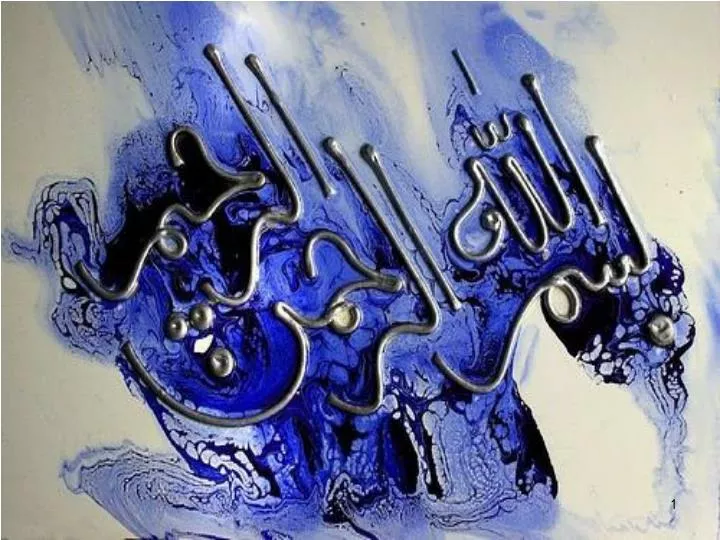
Sampling Business Research Methods
Aug 01, 2014
410 likes | 996 Views
Sampling Business Research Methods . 2. Sampling. Sampling : The process of selecting a sufficient number of elements from the population, so that results from analyzing the sample are generalizable to the population. OR
Share Presentation
- measuring students
- random choice
- much easier
- random sampling strategy
- various subgroups
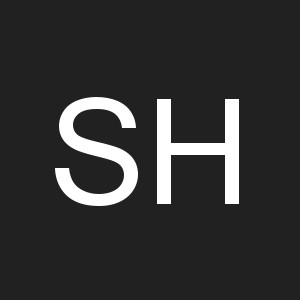
Presentation Transcript
Sampling Business Research Methods 2
Sampling • Sampling: The process of selecting a sufficient number of elements from the population, so that results from analyzing the sample are generalizable to the population. OR • The basic idea of sampling is that by selecting some of the elements in population, we draw conclusions about the entire population.
Populationrefers to the totality of people, events, things of interest or objects (which may be individuals, households, organizations, countries etc.) that the researcher wishes to investigate. E.g. All office workers in the firm compose a population of interest; all 4,000 files define a population of interest. • An elementis a single member of the population. • Element is the unit of study; it may be a person or may be something else. • E.g.: Each staff member questioned about an optimal promotional strategy is a population element. • Each advertising account analyzed is an element of an account population • Each ad is an element of a population of advertisements
Census • A census is a count of all the elements in a population; • If 4,000 files define the population, a census would obtain information from every one of them. • Sample: A subset of the population selected to investigate the properties of the population. Because populations are often extremely large, or even infinite, it is usually impossible – for cost and practical reasons – to take measurements on every element of the population. For this reason, more often, we draw a sample and generalize from the properties of the sample to the broader population. • Sampling unit:The element or set of elements that is available for selection in some stage of the sampling process. • A subject is a single member of the sample, just as an element is a single member of the population.
What is a Good Sample? • Sampling is acceptable only when it adequately reflects the population from which it is drawn; • No sample is a perfect representation of its population • The ultimate test of a sample design is how well it represents the characteristics of the population it purports to represents. • In measurement terms, the sample must be valid. • Validity of a sample depends on two considerations: • Accuracy and • Precision
Questions • You wish to study the care arrangements of at government hospitals in Islamabad and Rwp. • Find out the opinions of workers in a factory on changed working arrangements • Measuring students’ satisfaction level about teaching in the MBA/BBA/BS/MS programs • Find out the changing attitude of Pakistanis towards immigration to Australia, NZ, USA, UK.
Advantages of Sampling • Sometimes there is a need for sampling. Suppose we want to inspect the eggs, the bullets, the missiles and the tires of some firm. The study may be such that the objects are destroyed during the process of inspection. Sampling plays a key role in this process. • Sampling saves money as it is much cheaper to collect the desired information from a small sample than from the whole population. • Sampling saves a lot of time and energy as the needed data are collected and processed much faster than census information. • Sampling makes it possible to obtain more detailed information from each unit of the sample as collecting data from a few units of the population. • Sampling has much smaller “non-response”, following up of which is much easier. • The most important advantage of sampling is that it provides a valid measure of reliability for the sample estimates. • Sample data is also used to check the accuracy of the census data.
The Sampling Process • Major steps in sampling: • Define the population (elements, geographic boundaries, and time) • Determine the sample frame • Determine the sampling design • Determine the appropriate sample size • Execute the sampling process
Steps in sampling process • The population is defined in terms of element, units, time, etc. • Sampling unit: Maybe on a geographical basis such as state, province, district, village, etc. • Sampling frame: To prepare the source list to cover all the samples in the sampling frame that is as true a representative of the population as possible. • Size of the sample: Number of items to be selected from the universe to constitute a sample. Sampling Techniques • Probability versus nonprobability sampling • Probability sampling: elements in the population have a known and non-zero chance of being chosen
Sampling Techniques • Probability Sampling • Simple Random Sampling • Systematic Sampling • Stratified Random Sampling • Cluster Sampling • Nonprobability Sampling • Convenience Sampling • Judgment Sampling • Quota Sampling
Simple Random Sampling • Procedure • Each element has a known and equal chance of being selected • Characteristics • Highly generalizable • Easily understood • Reliable population frame necessary
Simple random sampling (all members have equal chance of being selected)
Systematic Sampling • Procedure • Each nth element, starting with random choice of an element between 1 and n • Characteristics • Easier than simple random sampling • Systematic biases when elements are not randomly listed
Systematic sampling (systematic sampling involves selecting every nth case within a defined population)
Cluster Sampling • Procedure • Divide of population in clusters • Random selection of clusters • Include all elements from selected clusters • Characteristics • Intercluster homogeneity • Intracluster heterogeneity • Easy and cost efficient • Low correspondence with reality
Cluster sampling (cluster sampling involves surveying whole clusters of the population selected through a defined random sampling strategy.)
Stratified Sampling • Procedure • Divide of population in strata • Include all strata • Random selection of elements from strata • Proportionate • Disproportionate • Characteristics • Interstrata heterogeneity • Intrastratum homogeneity • Includes all relevant subpopulations
Stratified random sampling(Dividing your population into various subgroups and then taking a simple random sample within each.)
Thanks to Allah
- More by User
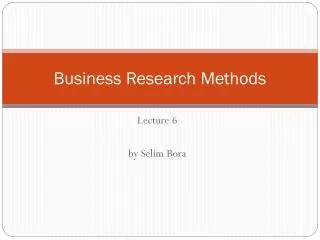
Business Research Methods
Business Research Methods Lecture 6 by Selim Bora Graphs The distribution of a variable tells us what values it takes and how often it takes these values. To see what data say, start with graphs. Pie chart and bar graphs are two illustrative types.
1.1k views • 9 slides
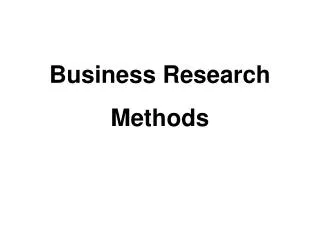
Business Research Methods. Course Title: Business Research Methods Text Book: Research Methodology: A step-By-Step Guide For Beginners by Ranjit Kumar Must Read Books: Business Research Methods by William G. Zikmund 7. ed. Reference Books:
2.5k views • 21 slides
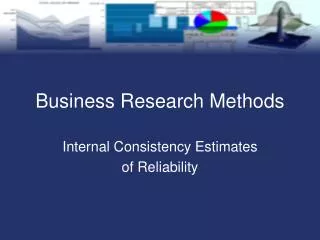
Business Research Methods. Internal Consistency Estimates of Reliability. Introduction Multiple-item or multiple-observation scales are often developed to assess characteristics about individuals. One important element in deciding the value of such a scale is its reliability and validity.
516 views • 27 slides
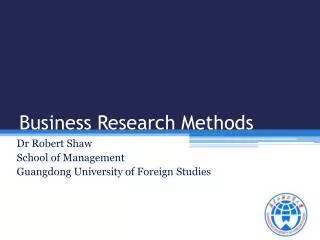
Business Research Methods. Dr Robert Shaw School of Management Guangdong University of Foreign Studies. Today. The hegemony of science Three kinds of research New directions in business research How to write How to publish. 1 . The hegemony of science. The word “hegemony”
678 views • 34 slides
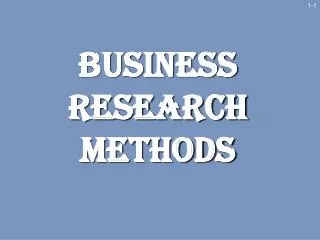
Business research methods
Business research methods. Chapter 1. Research in Business. Why Study Business Research?. Why Study Business Research?. Business research provides information to guide business decisions. Why Study Business Research?. Information and competitive advantage .
1.13k views • 18 slides
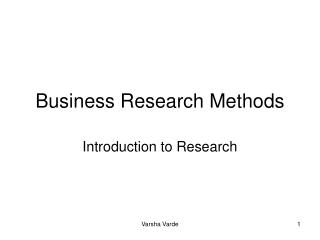
Business Research Methods. Introduction to Research. A Corporate Problem. Newly appointed VP (Finance) strongly believes that staffing her accounts dept only with CAs (instead of commerce or management graduates) will grossly improve its performance.
877 views • 40 slides
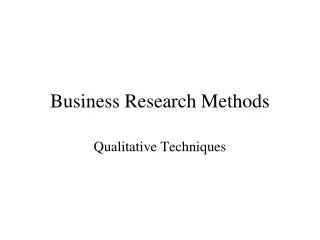
Business Research Methods. Qualitative Techniques. Qualitative versus Quantitative Research. Purpose Exploratory versus descriptive and conclusive Small versus large samples Broad range of questioning versus structured questions Subjective interpretation versus statistical analysis.
865 views • 38 slides
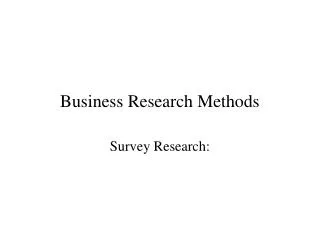
Business Research Methods. Survey Research:. Survey Research. Survey research methods are used for primary data collection Surveys ask a respondent for information using verbal or written questioning
935 views • 71 slides
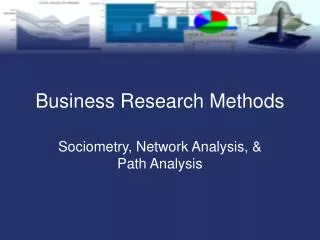
Business Research Methods. Sociometry, Network Analysis, & Path Analysis.
418 views • 16 slides
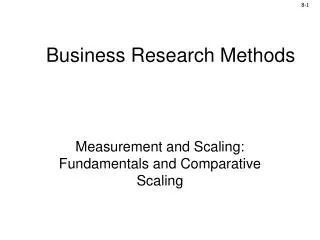
Business Research Methods. Measurement and Scaling: Fundamentals and Comparative Scaling. Measurement and Scaling. Measurement means assigning numbers or other symbols to characteristics of objects according to certain prespecified rules.
741 views • 45 slides
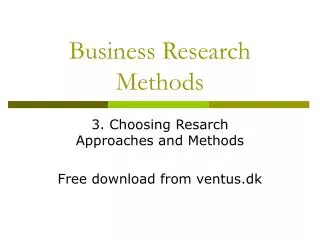
Business Research Methods. 3. Choosing Resarch Approaches and Methods Free download from ventus.dk. Filters. What is ”Reality” People who are directly experiencing an event look at it with filters adjusted to the situation Researcher look at the event with their searching filters
2.56k views • 8 slides
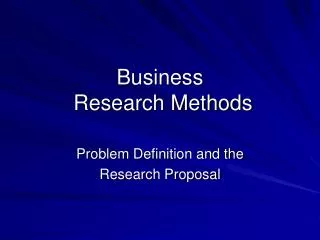
Business Research Methods. Problem Definition and the Research Proposal. A Sea Horse’s Tale. Selection of exploratory research technique. Problem discovery. Problem Discovery and Definition. Sampling. Selection of exploratory research technique. Probability. Nonprobability.
656 views • 37 slides
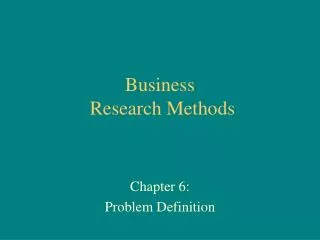
Business Research Methods. Chapter 6: Problem Definition. A Sea Horse’s Tale. Selection of exploratory research technique. Problem discovery. Problem Discovery and Definition. Sampling. Selection of exploratory research technique. Probability. Nonprobability. Secondary
509 views • 34 slides
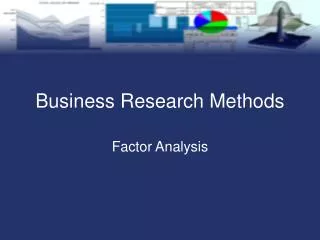
Business Research Methods. Factor Analysis.
492 views • 38 slides
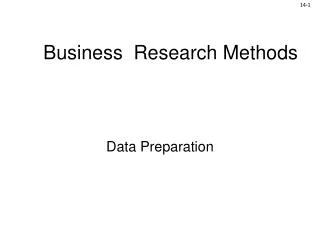
Business Research Methods. Data Preparation. Data Preparation. Data preparation refers to the process of ensuring the accuracy of the data and their conversion from raw form into classified forms appropriate for analysis. Validation. Editing. Coding. Data Entry.
461 views • 17 slides
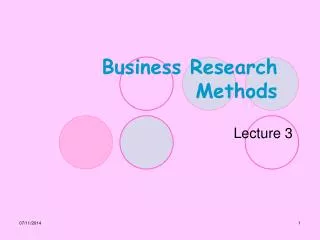
Business Research Methods. Lecture 3. Accessing and reviewing literature as part of research. Lecture Outline: Why is it so important in research? What is a literature review? Identifying research questions/ objectives How to put a lit. review together Writing critically, building argument.
393 views • 16 slides
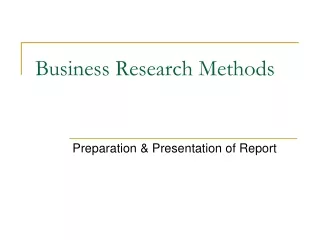
Business Research Methods. Preparation & Presentation of Report. Preparing & Presenting Report. This is the last stage of research process It Involves effective communication of research findings
486 views • 40 slides
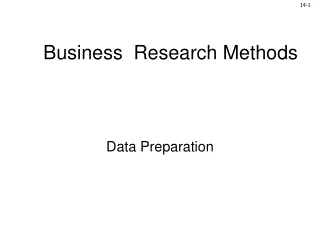
451 views • 17 slides
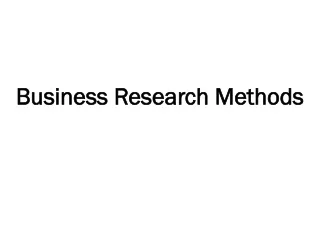
2.34k views • 21 slides
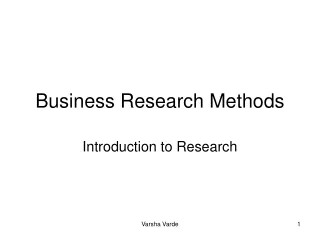
532 views • 40 slides
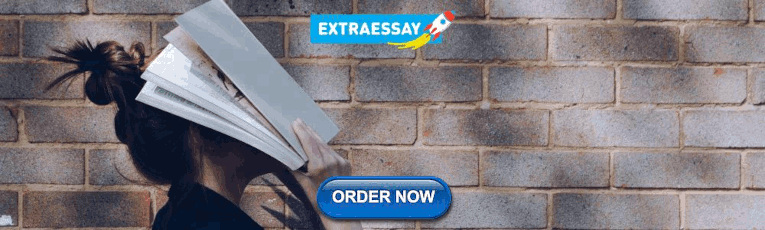
IMAGES
VIDEO
COMMENTS
63 likes • 38,403 views. Mumbai University. Follow. Sampling is an important process of research work. Education. 1 of 18. Download Now. Download to read offline. Sampling in research - Download as a PDF or view online for free.
Systematic Random Sampling is a method of probability sampling in which the defined target population is ordered and the sample is selected according to position using a skip interval.. The sample is chosen by selecting a random starting point and then picking every i th element in succession from the sampling frame.; The sampling interval, i, is determined by dividing the population size N by ...
The sample is selected because they are convenient. It is a nonprobability method. Often used during preliminary research efforts to get an estimate without incurring the cost or time required to select a random sample Judgment Sampling Judgment sampling is a common nonprobability method. The sample is selected based upon judgment.
Sampling methods are crucial for conducting reliable research. In this article, you will learn about the types, techniques and examples of sampling methods, and how to choose the best one for your study. Scribbr also offers free tools and guides for other aspects of academic writing, such as citation, bibliography, and fallacy.
Simple random sampling is a widely utilized sampling method in quantitative studies with survey instruments. It is asserted that simple random sampling is favorable in homogeneous and uniformly selected populations. In this selection method, all the individuals have an equal opportunity to participate in the study where the selection process is ...
13 The Sampling Process Sampling is the process of selecting a sufficient number of right elements from the population so, the major steps in the sampling include. 1.Defining the population 2.Determine the sample process 3.Determine the sampling design 4.Determine the appropriate sample size 5.Execute the sampling process. 14 The Sampling Process.
research methods - sampling techniques.ppt - Free download as Powerpoint Presentation (.ppt), PDF File (.pdf), Text File (.txt) or view presentation slides online. Research Methods
This is often used to ensure that the sample is representative of the population as a whole. Cluster Sampling: In this method, the population is divided into clusters or groups, and then a random sample of clusters is selected. Then, all members of the selected clusters are included in the sample. Multi-Stage Sampling: This method combines two ...
DeCarlo and his team developed a complete package of materials that includes a textbook, ancillary materials, and a student workbook as part of a VIVA Open Course Grant. The PowerPoint slides associated with the twelve lessons of the course, SOWK 621.01: Research I: Basic Research Methodology, as previously taught by Dr. Matthew DeCarlo at ...
Systematic random sampling is a method of probability sampling in which the defined target population is ordered and the 1st unit of sample is selected at random and rest of the sample is selected according to position using a skip interval (every Kth item) K = N n Where, K = Sampling/ Skip interval N = Universe/ Population Size n = Sample Size.
However, most often the researcher studies a 'sample' of the population because it is impractical, too large, difficult, or time and resource consuming to study the entire population. From the ...
Findings from qualitative research are inextricably tied up with the way those findings are presented. These presentations do not always need to be in writing, but they need to happen. Think of ethnographies, for example, and their thick descriptions of a particular culture. Witnessing a culture, taking fieldnotes, talking to people—none of ...
New York: Prentice-Hall, 1960. Download ppt "Lecture Notes on Research Methodology". 1 Research Methodology: An Introduction: MEANING OF RESEARCH: Research in common parlance refers to a search for knowledge. Once can also define research as a scientific & systematic search for pertinent information on a specific topic.
Sampling in Quantitative Research • Accessible population • The population of people available for a study • Target population • The entire population in which the researcher is interested and to which he/she wants to generalize the results. Sampling Plans • A sample is a subset of the population • A sample should be representative ...
Presentation Transcript. SAMPLINGMETHODS Dr. KANUPRIYA CHATURVEDI. LEARNING OBJECTIVES • Learn the reasons for sampling • Develop an understanding about different sampling methods • Distinguish between probability & non probability sampling • Discuss the relative advantages & disadvantages of each sampling methods.
The backbone of any scientific inquiry: the methodology. A systematic process of collecting, analyzing, and interpreting data to draw conclusions about a particular subject matter. However, many researchers struggle with selecting the appropriate research design, sampling methods, data collection, and analysis techniques. But your workshop is ...
Sampling Business Research Methods 2. Sampling • Sampling: The process of selecting a sufficient number of elements from the population, so that results from analyzing the sample are generalizable to the population. OR • The basic idea of sampling is that by selecting some of the elements in population, we draw conclusions about the entire population.