Advertisement
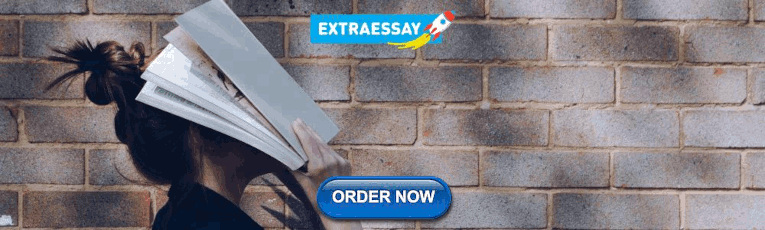
Exploring Student and Teacher Experiences in Hybrid Learning Environments: Does Presence Matter?
- Original Articles
- Published: 18 November 2021
- Volume 4 , pages 138–159, ( 2022 )
Cite this article
- Annelies Raes ORCID: orcid.org/0000-0001-9237-9385 1 , 2 , 3
31k Accesses
43 Citations
20 Altmetric
Explore all metrics
The global pandemic forced us to rethink education to fight Covid-19 and apply social distancing during lectures. Luckily, we could rely on earlier research into distance education in general, and more specifically, into synchronous hybrid learning. During synchronous hybrid learning both on-site and remote students are connected and taught synchronously in what we call at our university the ‘hybrid classroom’ or ‘hybrid lecture hall’. In order to further substantiate this potential new normal, research is needed to investigate the influencing factors of engagement and learning in these new environments from a student and teacher perspective. In this study, two different hybrid learning designs and practices are explored and analysed through the lens of the activity-centred analysis and design (ACAD) framework. Next to this more qualitative approach, this study also presents quantitative results on the effect of the level of presence (on-site versus remote, with or without interaction) on conceptual and affective outcomes. In terms of the student perspective, this study did not find any significant differences between physical and remote presence regarding conceptual understanding, yet significant differences were found in regard of affective engagement in favour of the on-site students and remote students having the opportunity to interact. In line with the ACAD framework, our research found that successful learning and teaching activities are interrelated with set, epistemic, and social design decisions.
Similar content being viewed by others
Here or There? Differences of On-Site and Remote Students’ Perceptions of Usability, Social Presence, Engagement, and Learning in Synchronous Hybrid Classrooms
Space and Time in Hybrid Teaching and Learning Environments: Two Cases and Design Principles
Designing Synchronous Hybrid Learning Spaces: Challenges and Opportunities
Avoid common mistakes on your manuscript.
Background of the Study
Over the course of the last 15 years, more attention has been devoted towards learning and teaching spaces, with many researchers claiming that flexible spaces better meet the needs of a diverse student population (Amoroso 2014 ; Joy et al. 2013 ; Wang et al. 2018 ). JISC, a not-for-profit company in the UK that was set up to provide computing support for education, suggested in their report ( 2006 ) that a learning space should be able to motivate learners and promote learning as an activity, support collaborative as well as formal practice, provide a personalized and inclusive environment, and be flexible in the face of changing needs.
In line with this, the European University Association (European University Association 2021 ) imagines future universities to be without walls, meaning that universities will be open and engaged in society whilst at the same time retaining their core values. They will provide an open, transformative space for common knowledge production through research, education, innovation and culture. It is stated that together with other societal stakeholders, they will shape the future of a knowledge-driven society (European University Association 2021 : 5).
These transformative spaces are also conceptualized as postdigital learning spaces, aiming to open up institutional spaces to form universities beyond traditional dichotomies (O’Bryrne and Pytash 2015 ). This means that in postdigital learning and teaching, physical environments merge with digital environments. As stated by Feenberg ( 2019 ), within postdigital learning spaces, the digital will be integrated and inherent in the everyday actions, interactions and experiences of teaching and learning.
In line with this societal and educational transition, KU Leuven, one of Europe’s oldest universities located in Belgium, started in 2016 with the research project TECOL (see: https://www.kuleuven-kulak.be/tecol ) to investigate how technology could be integrated to support future-proof teaching and learning. As KU Leuven is a multi-campus university spread across nine different cities, the project aimed to investigate how the various educational programs across the different campuses could become more flexible. In addition, the project aimed to investigate how the university would be able to better support expertise exchange and connectivity irrespective of place, in order to create a more open and multidisciplinary approach in which students get acquainted with different perspectives.
Creating hybridity starts with technical solutions and set-ups, and within the research and development project Lecture + (see https://www.imec-int.com/en/what-we-offer/research-portfolio/lecture ), a technological solution has been co-created and accordingly been called the hybrid classroom. The hybrid classroom connects both on-site students and individual remote students during synchronous teaching and learning (see Fig. 1 ). This classroom is operational since 2017 and aims to provide the pedagogical freedom to reach students and teachers from any place in the world, increasing societal access to education as well as improving the quality of education, as knowledge now transcends the boundaries of the classroom.
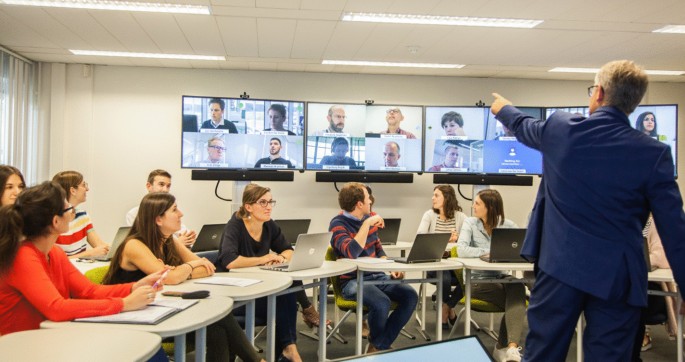
Hybrid classroom implemented at KU Leuven, Campus Kulak Kortrijk ©imec
Creating Conceptual Clarity
Although our university decided to conceptualize the concept of merging on-site and remote students at the same time as synchronous hybrid teaching and learning, many more concepts can be found in the literature that tries to capture the phenomenon of merging modes. Irvine ( 2020 ) emphasized the importance of focusing on the meaning of concepts in order to create a shared understanding for the future of our academic discourse. She provided an overview of the main terms, including hyflex, multi-access, (synchronous) blended and (synchronous) hybrid. The HyFlex (hybrid-flexible) model was developed by Beatty ( 2007 , 2019 ) and is described as a combination of hybrid, i.e. combining both online and face-to-face modalities, and flexible, as students may choose whether or not to attend face-to-face sessions. With support from the Canada Foundation for Innovation, Irvine introduced the multi-access learning model at the 2009 AACE EdMedia Conference and then expanded on the idea in a 2013 article (Irvine et al. 2013 ; Irvine 2020 ). The model identifies four levels of access: (1) face-to-face, (2) synchronous online, (3) asynchronous online and (4) open access. Hybrid and blended both refer to combining modes (Lakhal et al. 2017 ), but the term synchronously has later been added (see e.g. Shi et al. 2021 ; Zydney et al. 2020 ) as without this specification blended or hybrid are also used to describe learning scenarios operationalizing online and offline as dichotomies in which learning takes place either online or offline. Ladd ( 2020 ) (Dean of the San Francisco campus) for example mentioned in his article that he is reserving the term ‘hybrid’ for educational experiences where the students in a class group are either all online or all face-to-face in a classroom together. To describe a classroom which has both people online and in-person at the same time, Ladd ( 2020 ) put forth the term ‘concurrent classroom’. Other less common terms are synchromodal (Bell et al. 2014 ) and dual-mode teaching, meaning that both online and classroom-based instruction is provided in a course at the same time. The term dual-mode is used within CETL (Centre for the Enhancement of Teaching and Learning) at the University of Hong Kong. Recently, Nørgård ( 2021 ) also introduced the concept of hybridity, meaning that both physical and digital forms take place synchronously, for example through learning interactions that unfold as a coherent experience of being in multiple places at once.
Related Studies
Engagement within hybrid teaching and learning settings.
Although creating hybridity starts with technological solutions and set-ups, this is only one of the conditions creating the user experience within a certain setting, as no form of technology has the capacity to change practice (Stahl et al. 2014 ). In line with Goodyear et al. ( 2021 ), learning space is conceptualized as the ecosystem of learners, teachers, pedagogical practices, digital and material resources, buildings and furniture. This perspective aims to investigate the interrelations within this ecosystem. In that sense, it is crucial to investigate how people think about hybrid models of learning and teaching and to investigate how hybrid teaching and learning is experienced by both teachers and learners.
One of the main reasons why learning experience has been receiving much more attention over the last decade is that student engagement is regarded as one of the top factors affecting student performance (Furlong and Christenson 2008 ; Fredricks et al. 2004 ). Based on previous research and as shown in Fig. 2 , we can state that engagement involves three dimensions: (1) behavioural (e.g. attending lectures, asking question, participation in quizzes), (2) emotional (e.g. feelings of interest, sense of belonging, boredom, frustration or enjoyment) and (3) cognitive engagement (e.g. learning outcomes, metacognition and self-regulated learning). This three-component model has been used by several researchers in the past (e.g. Dobbins and Denton 2017 ; Gobert et al. 2015 ). In our research, we are particularly interested in unravelling emotional or affective engagement within the new learning spaces.
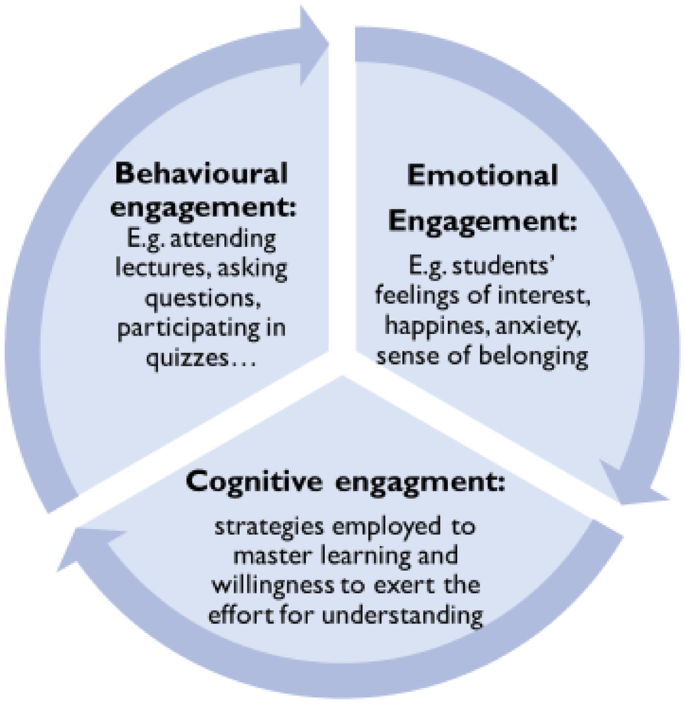
Three component model of engagement (Fredricks et al. 2004 )
As literature stresses that student engagement is influenced by contextual variations such as learning environments (Bond and Bedenlier 2019 ; Fredericks et al. 2004 ; Raes et al. 2020 ; Shi et al. 2021 ) or teacher strategies (Heilporn et al. 2021 ), it is important to investigate how student engagement as an affective learning outcome is influenced both by the design of the synchronous hybrid learning environment as well as by the type of participation, given that students can access the hybrid classrooms in different ways (see Figs. 3 , 4 and 5 ).
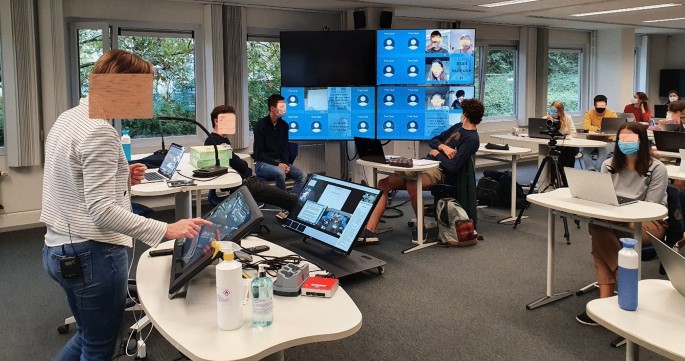
Hybrid classroom@KU Leuven, Campus Kulak Kortrijk
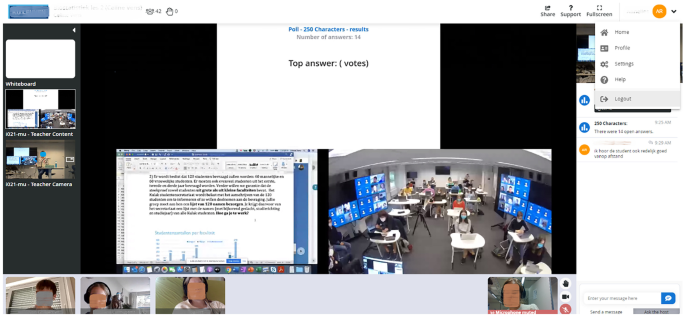
Hybrid classroom from the perspective of the remote participant
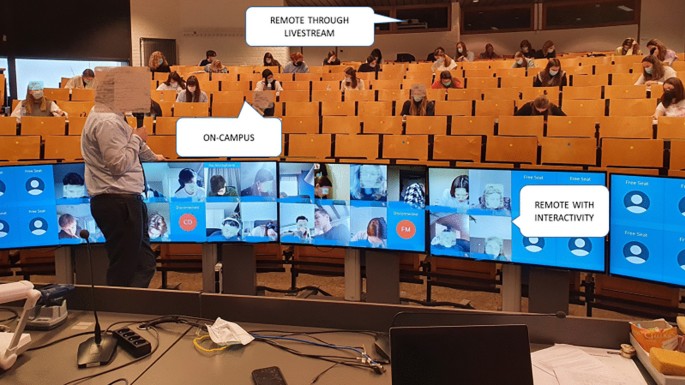
Hybrid lecture hall@KU Leuven, Campus Kulak Kortrijk
Previous research focusing on student engagement in hybrid classrooms is scarce (Raes et al. 2019 ; Lakhal et al. 2017 ; Miller et al. 2021 ), but the existing literature is consistent in that they all report that although hybrid education provides a lot of benefits, including flexibility, it brings many challenges, which are both pedagogical and technological in nature. The main pedagogical challenge reported in previous literature is that on-site students and remote students experience the lesson differently in the hybrid synchronous situation (Beatty 2007 , 2019 ; Szeto 2014 ; Zydney et al. 2019 ), although the Research & Development field strives for designing and implementing both pedagogical strategies and technological systems that enact comparable learning experiences (Butz et al. 2016 ; Butz and Stupnisky 2017 ; Cain et al. 2016 ). Previous research shows that remote learners feel a significant sense of distance towards their teacher and their face-to-face classmates (Ramsey et al. 2016 ). A qualitative study conducted by Olt ( 2018 ) concluded that the experience of the remote participant can be explained and understood by the concept of ‘ambiguity’ in regard to group membership, functionality of technology, and place. Also, Huang et al. ( 2017 ) showed that the remote students felt excluded from the chief class, because they were physically separated from the face-to-face class, especially when the remote class encounters technical difficulties without immediate support. In the study of Weitze et al. ( 2013 ), remote students indicated that it is difficult to alert the teacher that they want to answer a question, which makes them feel frustrated and uninvolved. Therefore, it is important to take this into consideration in the design of the classes and to be aware that remote students need to feel more invited into the class activity.
Designing for Effective Teaching and Learning
As stressed above, learning experience, and student engagement more particularly, is influenced by contextual variation. That same idea is shared by the founders of the activity-centred analysis and design (ACAD) framework (Carvalho and Goodyear 2014 ; Goodyear et al. 2021 ). Previous research has provided evidence on the fact that the way a learning environment is designed and which tools are provided matters for learning (Carvalho et al. 2020 ). The ACAD framework acknowledges the physical, epistemic and social situatedness of learning and conceptualizes this as set, epistemic and social design. Set design includes the specific tools, artefacts and learning space used to support learning and teaching. Epistemic design is related to the tasks or activities students are asked to do in service of learning, and social design is related to how students are grouped or how networks or communities are built. ACAD has previously been used to analyse emergent learning activity in both online and place-based spaces for networked learning. In this study, it will be used to describe the design of two synchronous hybrid learning practices and to analyse the results for student outcomes within these new settings.
Previous research within the context of hybrid education stresses that the loss of visual and audible cues, which normally are observable for the on-site students, impacts the learning and teaching experience (Weitze et al. 2013 ). In order to compensate for this loss and to mitigate for perceived distancing effects, it has been argued that teachers should frequently pause for questions throughout the lecture and be attentive to student responses (Heilporn et al. 2021 ; McGovern and Barnes 2009 ). In addition to this, students who attend the class remotely should perceive the same audio quality as students who are present face-to-face since the audio component has been found to be decisive for the success (Bower et al. 2015 ; Cunningham 2014 ). Irvine et al. ( 2013 ) found that synchronous hybrid tools that mimic face-to-face delivery can help students maintain closer connections with peers. This means that next to audio quality, good video quality and an optimal framing of the teacher are challenges for the set design.
Research Objective and Research Questions
In this paper, I present the results of an exploration of learning and teaching experiences within synchronous hybrid environments. Although the concept of the synchronous hybrid learning spaces had been developed before the pandemic happened, this study describes the exploration of two types of design which have been tested during the Covid-19 pandemic. As displayed in Figs. 3 and 4 , we differentiate between three levels of presence in the synchronous learning environment, i.e. (1) face-to-face or on campus, (2) remote, visible on the screen in the classroom and full interaction possible; (3) remote, following through livestream, not visible for the teacher and peers, not able to interact.
From a student perspective, I aimed to answer the following research questions:
How does the level of presence (i.e. physical or remote presence) affect students’ conceptual understanding?
How does the level of presence affect students’ emotional or affective engagement during learning?
According to students, what are the determining factors to be engaged during synchronous hybrid lectures?
From a teacher perspective, this study aimed to investigate to following research question:
What are teachers’ experiences during hybrid teaching and how does space affect their practice?
It has been claimed that it is important to investigate both the teacher perspective as well as the student perspective since what teachers do in their courses is linked with student engagement (Heilporn et al. 2021 ), but what teachers do or can do is also related to where they teach, i.e. the teaching space.
This study attempts to bridge this knowledge gap by presenting the student and teacher results of the exploration of two different postdigital learning spaces, where students engage with learning through different modes and with different levels of presence in the learning environment.
Methodology
Research design, participants and procedure.
This study is part of a larger university innovation, research and development project which is inspired on design-based research methodology in order to get a better theoretical understanding of effective teaching and learning in our new learning spaces and in order to be able to define evidence-based design-guidelines. The design-based research approach is frequently used in educational research conducted in authentic learning settings (McKenney and Reeves 2019 ). Design-based research (DBR) intends to produce new theories, artefacts and practices that account for and potentially impact learning and teaching in naturalistic settings (Barab and Squire 2004 ). It is characterized by its use of iterative cycles of design, enactment, analysis and redesign. As this paper presents teaching and learning in two different hybrid learning environments with differences in set-up and measured variables, this study needs to be viewed as a first exploration of these settings with the same research questions in mind.
In what follows, I focus firstly on the design in the two hybrid learning environments using the ACAD framework. This is followed by a description of the methodology.
Case Design Analysis 1: Problem-Based (Group) Learning in the Hybrid Classroom
The first case is visualized in Figs. 3 and 4 displaying the hybrid classroom from the perspective of the on-site student and the remote student. Data has been collected within the course on Biostatistics attended by third-year bachelor students within the Faculty of Medicine at KU Leuven, Campus Kulak Kortrijk. Within this course, 45 third-year bachelor students participated in the study. The teacher is a female professor who has been teaching this course for 5 years. She is open to innovation and has been involved in teacher-researcher collaboration since 2017, within the context of the larger research project. The data collection within the course on Biostatistics was organized in September 2020. In the hybrid classroom, students can follow the course on-site and at the same time, remote students can connect synchronously. In this case, students were randomly divided over the two conditions (on-site versus remote presence).
Epistemic Design
The learning activity organized in the hybrid classroom was built on the theory of delayed instruction or productive failure (Kapur 2016 ). The lecture started with a collaborative problem solving (CPS) task which was designed in line with the content of the lecture. Students were asked to set up a survey to evaluate the psychosocial wellbeing of students at campus. The focus of the task was not on how to formulate the questions of the survey, but rather on how to select the method of data collection, how to select a good sample, how to deal with randomness, how to deal with non-response, and how to solve the problem of over-and under-sampling through weights. In the second part of the lecture, the teacher taught the theory on sampling methods and bias in research including the input of the students.
Social Design
Group learning was mainly integrated in the first part of the lecture. The remote students formed groups based on the screen on which they were displayed. On-site students formed groups based on their seating. In the second part of the lecture, the teacher interacted with both on-site and remote students by launching several polls.
The hybrid classroom facilitates launching quizzes and polls by using the Wacom tablet. By means of the same Wacom the teacher can start and end break-sessions. After launching a quiz or poll, the answers of the remote students become visible on the screens. Also, the names of the remote students are visible on the screens which means that the teacher can easily address to a certain remote student. As a remote participant, it is possible to choose between different sources (e.g. whiteboard, teacher camera, teacher content), and it is possible to ask questions in the chat. As a remote student, it is possible to raise your hand, to share your content, to mute or unmute yourself and to turn off your camera. Because of a ceiling microphone remote students can easily hear on-site students talking.
Case Design Analysis 2: Hybrid Lecture Hall
The second case is visualized in Fig. 5 and consists of two lectures given within the same hybrid lecture hall, taught by two different professors within the Faculty of Law. Participants included 75 first-year bachelor students within the course on Contract law and 38 s-year bachelor students within the course on Family law. The Contract Law course is taught by a male professor and Family Law by a female professor. For both professors, it was the first time they participated in research within the context of the larger research project on learning spaces. Nevertheless both teachers were among the first users of the hybrid lecture hall. The data collection within the hybrid lecture hall was organized in March 2021.
Both lectures could be described as theoretical sessions focusing on knowledge transmission. Both teachers asked oral questions during the lecture, without using polling software.
Students had been asked to subscribe in advance and they could indicate how they would follow the course, choosing between three options: on-site, remote on-screen with interactivity or remote through livestream. Places for option 1 and option 2 were restricted because of Covid-19 restrictions. No group work or break-out sessions were integrated in the sessions.
Up to 60 students can follow the course remotely by being displayed on the screen in front. Students could also choose to follow the course synchronously through livestream. Students who followed the session through livestream could not connect to the on-site students or the remote students visible on the screens. Remote students visible on the screens could easily answer the teacher’s questions. Answers of remote students were audible through the boxes. As the hybrid lecture hall does not have a ceiling microphone, when on-site students answered to questions, this was not audible for remote students, unless the teacher passed on his/her microphone.
The three teachers who were responsible for the courses agreed to participate in the study to investigate student and teacher experiences during hybrid education. Their participation included data collection from their students and also required them to give an interview themselves. The research project ‘Measuring and optimizing students’ engagement in remote and (hybrid) virtual classrooms’ has been evaluated and approved by the Social and Societal Ethics Committee of the university (G- 2018 06 1264). In line with our research protocol, students could voluntarily participate in the study by signing an informed consent. A week before the start of the course, students were informed about the objectives of the study by means of a 5-min video, which had been shared on the learning management platform. In addition to this video, informed consents have been collected in advance of the first survey. Participants were informed that they were going to be videotaped, that data from self-reports and from the learning platform was going to be collected, and that all data was going to be processed anonymously. Students were asked permission to be photographed during the courses.
Data Collection and Measures
As explained earlier, the set-up and data collection for both cases were slightly different, but within both cases the main objective was to investigate differences in student experiences regarding the level of presence (on-site versus remote) and to unravel the determining factor for student engagement. Below, the measured variables are described per case analysis. In both cases, a mixed-method approach has been used which implies the combination of quantitative and qualitative research methods (Cohen et al. 2011 ). This approach strengthens the inferences in the analysis of student experiences in this new learning space.
Case Design Analysis 1
To answer research questions 1 to 3 focusing on the student perspective, quantitative data has been collected through a pre- and post-test. The pre-test aimed to measure prior knowledge on sampling methods and bias. The post-test aimed to measure the gain in conceptual knowledge and measured students’ intrinsic motivation, experienced relatedness and perceived value of past learning activities by means of validated scales based on the self-determination theory (Deci and Ryan 1985 ). At the end of the post-test, students could add open thoughts about the course and they were asked for suggestions for improvement.
Case Design Analysis 2
In terms of the courses given at the Faculty of Law, we were only able to conduct a post-intervention survey measuring student engagement, yet in this setting, we explored different scales to get a better sense of the emotional engagement in this new learning space. Next to the scales borrowed from the self-determination theory (Deci and Ryan 1985 ), case study 2 included the Eduflow scales Flow and Cognitive Absorption (Heutte et al. 2016 ) and a measurement of Sense of Presence (Barfield and Weghorst 1993 ; Dinh et al. 1999 ) and Sense of Belonging (Yorke 2016 ). The list of variables is presented in Table 1 , providing sample items for the different variables and the accompanying reliability scores. The Cronbach’s alphas regarding the different subscales indicate that the scales were reliable. At the end of the course, students could complete the digital survey supported by Qualtrics software in about 15 min by means of their smartphone or their personal computer. Qualitative data consisted of open answers given by the students at the end of the questionnaire, where they were asked about the determining factors for student engagement and about whether they had any open suggestions for improvement.
To answer research question 4 focusing on the teacher perspective, a semi-structured interview has been organized with each teacher separately in order to get insight into their experiences and thoughts about hybrid teaching and learning.
Data Analysis
Quantitative data has been analysed by means of the statistical software package SPSS. As we were particularly interested in possible differences between conditions, independent t -tests and ANOVAs have been conducted regarding the different dependent variables (see Table 1 ), including learning setting as a fixed factor. One sample t -test has been used to investigate if scores significantly differed from the neutral score. Regarding case study 1, where next to affective engagement also cognitive understanding has been measured, an analysis of covariance has been carried out to investigate the effect of the learning setting on learning gain.
Qualitative data including both written answers from students and teachers’ transcribed interviews have first been analysed inductively. Content analysis was used to generate common experiences and valuable feedback regarding their experience of learning and teaching in the hybrid classroom and to give a deeper insight into the quantitative data. Subsequently, students’ answers about the determinants for engagement have been clustered based on the ACAD framework, allowing us to better analyse the connections between design elements and students’ engagement.
How Does the Level of Presence Affect Students’ Conceptual Understanding?
The first research question could only be tested in the first experiment, which included the third-year bachelor students who had participated in the pre-test-post-test design. With regard to students’ conceptual understanding, an analysis of covariance revealed no differences between both conditions. This means that all students achieved significant learning gains and that remote and on-site students scored equally on the post-test (after controlling for pre-test scores) (see Table 2 ).
How Does the Level of Presence Affect Students’ Engagement during Learning?
Regarding students’ affective engagement, significant differences were found when comparing the different learning settings in both experiments. Table 3 presents the results revealed in case analysis 2 comparing three different levels of presence.
On-campus students showed to experience high levels of affective engagement operationalized as 1. enjoyment, 2. relatedness, 3. pressure, 4. value, 5. autotelic experience, 6. cognitive absorption, 7. sense of belonging and 8. sense of presence. Regarding on-site students ( N = 58), all means significantly differ from the neutral score. As for remote students, significant differences were found between visible and invisible remote students with significant higher engagement scores for students who were visible on the screens compared to students following the course through livestream.
Based on these findings and based on additional qualitative comments, we can state that the feature of the remote setting, i.e. being displayed in the classroom and being able to interact with the teacher, influences students’ engagement.
It’s easy to follow the lesson and it’s good to see other students as well. Because you turn on the camera and interact, you are obliged to be more attentive than you would be when following any other type of class remotely. (remote student, case analysis 1)
The results for sense of presence and sense of belonging show that it is easier to realize a sense of presence, conceived as a feeling of being in the classroom, than to create a sense of belonging, conceived as a feeling of being close and connected to your peers. It is important to note that during case analysis 2 no group work or breakout sessions were organized, whereas this was the case in case analysis 1. Qualitative data from this first analysis reveals that group work (as part of social design) might have influenced the sense of belonging.
I found it useful to work together in small groups online. You feel less alone and there is a good interaction with the professor. (remote student visible on screen with interaction, case analysis 1)
Moreover, qualitative feedback indicates that remote students were surprised that being at a physical distance did not preclude affective presence and connectivity:
Even though I was not physically present, I felt connected to the fellow students. (remote student visible on screen with interaction, case analysis 1)
Unfortunately, during case analysis 1, we did not collect scores for sense of presence and sense of belonging.
According to Students, What are the Determining Factors for Engagement during Synchronous Hybrid Lectures?
By means of an open question, all participants of case analysis 2 were asked to answer the following question: ‘What is, according to you, the most determining factor for engagement during the synchronous course?’. Ninety-six students answered this question and all answers were analysed and categorized based on the ACAD framework (Goodyear et al. 2021 ). Task or student activity-related answers were categorized under Epistemic Design. Answers related to the learning space, the set-up, and the features of the space, were categorized under Set Design. Finally, answers referring to peers and the community of learners were categorized under Social Design.
As displayed in Fig. 6 , 34 out of 96 (35%) students referred to the epistemic design as the most determining factor for engagement during a course, 31 (33%) referred to the set design, and 7 referred to social design. Some of the answers could be categorized under two categories. The following citation for example is both linked to set and social design:
Personally, what is determining for me, is feeling the ‘atmosphere’ in the auditorium, at home you cannot hear the conversation that is going on between your peers that are physically present at campus, that makes me feel excluded as a remote student. (remote student visible on screen with interaction, case analysis 2)
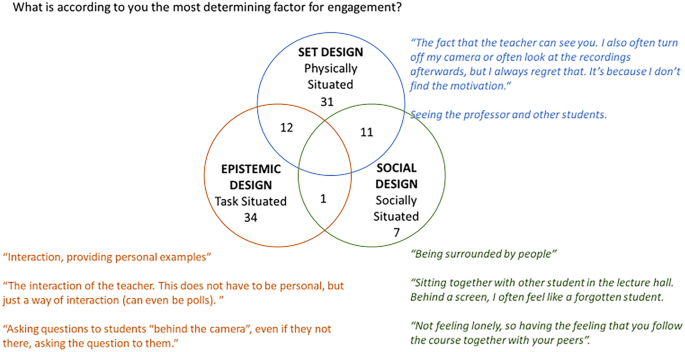
Students’ answers categorized based on the ACAD framework (Goodyear et al. 2021 )
A combination of set and epistemic design has been found as well, in the following statement:
What is determining for me, is being present in the room and experiencing a real-life lecture and being able to interact with the teacher who can ask you a question anytime. (remote student through livestream, case analysis 2)
How Does the Teaching Space Affect Teaching Practice?
Next to investigating students’ engagement in hybrid settings, additionally, this study sought to gain insight into the way in which teachers experience hybrid teaching and how space affects their practice. The results are given based on three 1-hour interviews with the professors who taught in the new learning context. These teachers have not only been teaching in the hybrid classroom and hybrid lecture hall shown respectively in Figs. 3 , 4 and 5 , but also used other solutions during the past year. At some times, strict lockdown requirements prevented students and teachers from coming to the campus altogether. During these times, teachers used systems like Blackboard (BB) Collaborate or Teams, both of which are provided at the institution and could be used from any place. Given the fact that these teachers have taught in many settings, they could easily come up with an answer to the question of what affects their teaching practice. Each interview started with an overview of the (anonymized) results collected in cooperation with their students. Next, it was stated that in the remaining time, we would focus on the teaching space instead of the learning space.
When comparing their teaching experience in the hybrid classroom/lecture hall with their experience using alternative systems (i.e. Teams and/or BB Collaborate), designed to teach from home, actually seeing the students was mentioned as the main advantage of the design of the hybrid classroom and hybrid lecture hall. Teachers mentioned that, in the alternative systems, students can see the presenting teacher in combination with the slides, but the teacher is not always able to see the students, or only a small number of them. Most of the time, they argue, students turn off their camera and their microphone. Teachers reveal that although it was indicated that students could ask questions using the chat, interaction was nearly non-existent.
One of the teachers described teaching from home and sitting behind the screen as cold and detached. That teacher also taught several classes just before the Christmas period in an empty auditorium, to which students were connected through livestream. That teacher mentioned that she had shared her feeling of loneliness with the students and once brought Christmas lights to decorate her desk to improve the astrosphere. According to that teacher, this small change to the teaching space really made a difference both for the students and the teacher. She also added that she even prefers livestream teaching over teaching behind a desk, as during livestream recording within an auditorium, students can at least see the posture of the teacher within the teaching environment and not only their face. In line with this, another teacher mentioned that (s)he likes to use a lot of gestures during teaching which do not come across as well when teaching behind a screen.
All the teachers revealed that teaching feels much more natural and intuitive in the hybrid classroom/hybrid lecture hall, because they have space to walk around, they are able to write something on a whiteboard, and, most importantly, they can interact with their students because they are visible on the screens. The teachers mentioned that they often tend to interact with their students based on their facial expressions: ‘You can see it on their faces if they do not understand something’. One of the teachers pointed out that when teaching a course without seeing your students, it is very difficult to evaluate the quality of your course. The teacher stated the following: ‘you are only able to evaluate your own performance, but it is so much nicer to get something in return and to feel and see to what extent your message has arrived and been understood by your students’.
When asking the teachers what would be their ideal scenario for a future without restrictions, the three teachers were all looking forward to teaching again in the auditorium with all students present. One of the teachers revealed that (s)he missed the crowded auditoria, sometimes so full that students even had to sit on the stairs. ‘This gives you the kick and the adrenaline you need to give a good performance; whether you are a speaker, athlete, teacher, or actor, having an audience is very important to perform’.
In all three of the interviews, the value and limitations of recording sessions was briefly discussed. A shared observation at our university, but also at universities worldwide, is that synchronous learning activities are attracting less and less students as students seem to prefer to watch lesson recordings. All teachers revealed that they agree that in some case recordings can be useful, for example for students who cannot attend the course if they combine work and study. Still, watching asynchronous recordings should not be seen as an equal alternative for attending the synchronous course.
Discussion and Conclusion
Looking into the literature on hybrid learning environments, it is generally viewed as both engaging and relevant due to its high level of flexibility (Nørgård 2021 ). More and more researchers claim that the nature and structure of future universities will be hybrid to better deal with changing contexts (Miller et al. 2021 ). This will entail that physical and digital learning and research environments must be designed in a holistic way in order to accommodate the different needs of a diverse university community. Hybrid learning environments allowing learners to attend synchronous online courses at home, at work or when traveling, are not new in the field. The last 15 years, new educational models have been proposed such as HyFlex learning and teaching conceptualized by Beatty ( 2007 , 2019 ) and multi-access education conceptualized by Irvine et al. ( 2013 ). Yet to be able to make thoughtful design decisions, more empirical research is needed about how these new learning spaces are experienced by both students and teachers, as the latter has often been neglected in previous research. Moreover, research is needed to inform pedagogy in these postdigital learning spaces. This paper aimed to fill this knowledge gap by both studying the learning and the teaching space through the lens of the ACAD framework. Since one of the key components for optimal experience is engagement, this study first investigated this multidimensional construct by quantitatively using different theoretical constructs. Second, by means of a qualitative approach, this study shed light on the determining factors to foster engagement during hybrid teaching and learning.
This research revolved around four research questions, three of them focusing on the student perspective, one of them focusing on the teacher perspective. Regarding the student perspective, this study did not find any significant differences between the levels of presence (i.e. physical and remote presence) in terms of conceptual understanding, yet significant differences were found regarding affective engagement, including intrinsic motivation, relatedness, experienced pressure, cognitive absorption, autotelic experience, sense of presence and sense of belonging. These findings are in line with previous research claiming that on-site students and remote students experience courses differently in the hybrid synchronous situation (Beatty 2007 , 2019 ; Szeto 2014 ; Zydney et al. 2019 ). Nevertheless, this study provided both quantitative and qualitative evidence that the design of the learning space really does matter to the remote experience. Students following the course through livestream without interaction or visibility to the teachers had the lowest engagement scores. Next, the qualitative data in relation to research question 3 showed that one out of three students referred to the set design as being determinant for being engaged during synchronous courses. This is in line with what previously has been claimed by Irvine et al. ( 2013 ), namely that synchronous hybrid tools that mimic face-to-face delivery can help students maintain closer connections. Yet, it is also known that having the innovative infrastructure will not guarantee anything. In line with the ACAD framework, successful learning is interrelated with set, epistemic and social design decisions, and this was also reflected in the results of this study. Two-thirds of the students indicated that the actions of the teacher during the course (epistemic design) and having the feeling that you are not alone (social design) are the most important for engendering engagement. Nonetheless, social design and epistemic design are closely interrelated with set design, as a certain teaching space can better support interaction and sense of belonging. Regarding our fourth research question, it has been explored how teachers experienced the teaching space, as those experiences have drastically changed over the course of the past year. The teachers expressed that they felt lucky to have experienced the new teaching spaces as they believe that the spaces supported them in qualitative teaching, and they believe that the facilities of the new spaces serve to engage both staff and students (Joy et al. 2013 ). Yet, we should be realistic in the sense that not every space will have all the facilities included. Next, when having huge student groups, the livestream option will still be used in future education. In these settings it will be important to incorporate live engagement, e.g. through polls and/or quizzes. Previous research has shown that using quizzes is effective for increasing engagement (Raes et al. 2020 ). This means that to design supportive hybrid learning and teaching it is crucial to take into consideration pedagogical, social and technical elements as being part of the epistemic, social and set design of a learning and teaching space.
Limitations and Suggestions for Future Research
Several limitations should be noted in this study. First of all, the case design analyses should be seen as explorations within the new settings and not as experiments which are comparable and generalizable. The differences between the cases do not allow the study to make generalizations to other postdigital learning spaces. Second, the present study only investigated academic performance in the first case analysis. It will be important to include academic performance in future research studies to investigate if our finding can be replicated. Although just a first step in investigating teaching and learning experiences in new synchronous hybrid learning settings, this paper highlights the importance of both the level of presence and the design of the space to support effective learning and teaching in hybrid learning spaces.
Amoroso, C. (2014). Hybrid by Choice. Increasing Engagement in a High Enrollment Course. Hybrid Pedagogy, 20 May. https://hybridpedagogy.org/hybrid-choice-increasing-engagement-high-enrollment-course/ . Accessed 14 November 2021.
Barab, S., & Squire, K. (2004). Design-based research: Putting a stake in the ground. Journal of the Learning Sciences , 13 (1), 1–14. https://doi.org/10.1207/s15327809jls1301_1 .
Article Google Scholar
Barfield, W., & Weghorst, S. (1993). The sense of presence within virtual environments: A conceptual framework. In G. Salvendy & M. Smith (Eds). Human-computer interaction: Applications and case studies (pp. 699-704). Amsterdam: Elsevier.
Google Scholar
Beatty, B. J. (2007). Hybrid classes with flexible participation options – If you build it , how will they come? Paper presented at the 2007 Association for Educational Communications and Technology Annual Convention (October). Anaheim, CA: Association for Educational Communications and Technology. http://citeseerx.ist.psu.edu/viewdoc/download?doi=10.1.1.457.495&rep=rep1&type=pdf . Accessed 14 November 2021.
Beatty, B. J. (2019). Hybrid-Flexible Course Design: Implementing student-directed hybrid classes . EdTech Books.
Bell, J., Sawaya, S., & Cain, W. (2014). Synchromodal classes: Designing for shared learning experiences between face-to- face and online students. International Journal of Designs for Learning, 5 (1). https://doi.org/10.14434/ijdl.v5i1.12657 .
Bond, M., & Bedenlier, S. (2019). Facilitating student engagement through educational technology: Towards a conceptual framework. Journal of Interactive Media in Education , 1 , 1–14. https://doi.org/10.5334/jime.528 .
Bower, M., Dalgarno, B., Kennedy, G. E., Lee, M. J. W., & Kenney, J. (2015). Design and implementation factors in blended synchronous learning environments: Outcomes from a cross-case analysis. Computers & Education, 86, 1-17. https://doi.org/10.1016/j.compedu.2015.03.006 .
Butz, N. T., & Stupnisky, R. H. (2017). Improving student relatedness through an online discussion intervention: The application of self-determination theory in synchronous hybrid programs. Computers and Education , 114 , 117–138. https://doi.org/10.1016/j.compedu.2017.06.006 .
Butz, N. T., Stupnisky, R. H., Pekrun, R., Jensen, J. L., & Harsell, D. M. (2016). The Impact of Emotions on Student Achievement in Synchronous Hybrid Business and Public Administration Programs: A Longitudinal Test of Control-Value Theory*. Decision Sciences Journal of Innovative Education , 14 (4), 441–474. https://doi.org/10.1111/dsji.12110 .
Cain, W., Bell, J., & Cheng, C. (2016). Implementing robotic telepresence in a synchronous hybrid course. In Proceedings of IEEE 16th International Conference on Advanced Learning Technologies, ICALT 2016. (pp. 171–175). New York: The Institute of Electrical and Electronics Engineers, Inc. https://doi.org/10.1109/ICALT.2016.79 .
Carvalho, L., & Goodyear, P. (2014). The architecture of productive learning networks. New York: Routledge.
Book Google Scholar
Carvalho, L., Nicholson, T., Yeoman, P., & Thibaut, P. (2020). Space matters: framing the New Zealand learning landscape. Learning Environments Research , 23 (3), 307–329. https://doi.org/10.1007/s10984-020-09311-4 .
Cohen, L., Manion, L., & Morrison, K. (2011). Research Methods in Education. 7th Ed. London: Routledge.
Cunningham, U. (2014). Teaching the Disembodied: Othering and Activity Systems in a Blended Synchronous Learning Situation. International Review of Research in Open and Distance Learning, 15 (6). https://doi.org/10.19173/irrodl.v15i6.1793 .
Deci, E. L., & Ryan, R. M. (1985). The general causality orientations scale - self-determination in personality. Journal of Research in Personality, 19 (2), 109–134. https://doi.org/10.1016/0092-6566(85)90023-6 .
Dinh, H. Q., Walker, N., Song, C., Kobayashi, A., & Hodges L.F. (1999). Evaluating the importance of multi-sensory input on memory and the sense of presence in virtual environments. In L. Rosenblum, P. Astheimer, & D. Teichmann (Eds.), Proceedings of the IEEE Virtual Reality 1999 (pp. 222-228). Los Alamitos, CA: The Institute of Electrical and Electronics Engineers, Inc. https://doi.org/10.1109/VR.1999.756955 .
Dobbins, C., & Denton, P. (2017). MyWallMate: An Investigation into the use of Mobile Technology in Enhancing Student Engagement. TechTrends , 61 (6), 541–549. https://doi.org/10.1007/s11528-017-0188-y .
European University Association. (2021). Universities without walls. A vision for 2030. Brussels: European University Association. https://eua.eu/downloads/publications/universities without walls a vision for 2030.pdf . Accessed 14 November 2021.
Feenberg, A. (2019). Postdigital or Predigital? Postdigital Science and Education , 1 (1), 8–9. https://doi.org/10.1007/s42438-018-0027-2 .
Fredricks, J. A., Blumenfeld, P. C., & Paris, A. H. (2004). School Engagement: Potential of the Concept, State of the Evidence. Review of Educational Research , 74 (1), 59–109. https://doi.org/10.3102/00346543074001059 .
Furlong, M. J., & Christenson, S. L. (2008). Engaging students at school and with learning: A relevant construct for all students. Psychology in the Schools , 45 (5), 365–368. https://doi.org/10.1002/pits.20302 .
Gobert, J. D., Baker, R. S., & Wixon, M. B. (2015). Operationalizing and Detecting Disengagement Within Online Science Microworlds. Educational Psychologist , 50 (1), 43–57. https://doi.org/10.1017/CBO9781107415324.004 .
Goodyear, P., Carvalho, L., & Yeoman, P. (2021). Activity-Centred Analysis and Design (ACAD): Core purposes, distinctive qualities and current developments. Educational Technology Research and Development , 0123456789. https://doi.org/10.1007/s11423-020-09926-7 .
Heilporn, G., Lakhal, S., & Bélisle, M. (2021). An examination of teachers’ strategies to foster student engagement in blended learning in higher education. International Journal of Educational Technology in Higher Education , 18 (1). https://doi.org/10.1186/s41239-021-00260-3 .
Heutte, J., Fenouillet, F., Martin-Krumm, C., Boniwell, I., & Csikszentmihalyi, M. (2016). Proposal for a conceptual evolution of the flow in education (EduFlow) model . Presented at 8th European Conference on Positive Psychology (ECPP2016), Angers, France. https://hal.archives-ouvertes.fr/hal-01470857 . Accessed 14 November 2021.
Huang, Y., Shu, F., Zhao, C., & Huang, J. (2017). Investigating and analyzing teaching effect of blended synchronous classroom. In Proceedings of 6th International Conference of Educational Innovation Through Technology (EITT) (pp. 134–135). New York: The Institute of Electrical and Electronics Engineers, Inc. https://doi.org/10.1109/EITT.2017.40 .
Irvine, V. (2020). The Landscape of Merging Modalities. Educause Review, 4, 40–58. https://er.educause.edu/articles/2020/10/the-landscape-of-merging-modalities . Accessed 9 May 2021.
Irvine, V., Code, J., & Richards, L. (2013). Realigning Higher Education for the 21st-Century Learner through Multi-Access Learning. Journal of Online Learning and Teaching , 9 (2).
JISC. (2006). Designing Spaces for Effective Learning A guide to 21st century learning space design. http://www.online-conference.net/jisc/content/designspaces.pdf . Accessed 14 November 2021.
Joy, M., Foss, J., King, E., Sinclair, J., Sitthiworachart, J., & Davis, R. (2013). Incorporating technologies into a flexible teaching space. British Journal of Educational Technology, 45 (2), 272-284. https://doi.org/10.1111/bjet.12040 .
Kapur, M. (2016). Examining Productive Failure, Productive Success, Unproductive Failure, and Unproductive Success in Learning. Educational Psychologist , 51 (2), 289–299. https://doi.org/10.1080/00461520.2016.1155457 .
Ladd, T. (2020). Optimizing concurrent classrooms: Teaching students in the room and online simultaneously. Forbes, 19 June. https://www.forbes.com/sites/tedladd/2020/06/19/optimizing-concurrent-classrooms-teaching-students-in-the-room-and-online-simultaneously/#50645ba93451 . Accessed 14 November 2021.
Lakhal, S., Bateman, D., & Bédard, J. (2017). Blended Synchronous Delivery Mode in Graduate Programs: A Literature Review and Its Implementation in the Master Teacher Program. Collected Essays on Learning and Teaching, 10 . http://dx.doi.org/10.22329/celt.v10i0.4747 .
Miller, A. N., Sellnow, D. D., & Strawser, M. G. (2021). Pandemic pedagogy challenges and opportunities: instruction communication in remote, HyFlex, and BlendFlex courses. Communication Education , 70 (2), 202–204. https://doi.org/10.1080/03634523.2020.1857418 .
McGovern, N., & Barnes, K. (2009). Lectures from My Living Room: A Pilot Study of Hybrid Learning from the Students’ Perspective. In F. L. Wang, J. Fong, L. Zhang, & V. S. K. Lee (Eds.), Hybrid Learning and Education (pp. 284–298). Berlin, Heidelberg: Springer. https://doi.org/10.1007/978-3-642-03697-2_27 .
McKenney, S., & Reeves, T. C. (2019). Conducting Educational Design Research . New York: Routledge.
Nørgård, R. T. (2021). Theorising hybrid lifelong learning. British Journal of Educational Technology . https://doi.org/10.1111/bjet.13121 .
O’Byrne, W. I., & Pytash, K. E. (2015). Hybrid and Blended Learning: Modifying Pedagogy Across Path, Pace, Time, and Place. Journal of Adolescent and Adult Literacy, 59 (2), 137-140. https://doi.org/10.1002/jaal.463 .
Olt, P. A. (2018). Virtually There: Distant Freshmen Blended in Classes through Synchronous Online Education. Innovative Higher Education , 43 (5), 381–395. https://doi.org/10.1007/s10755-018-9437-z .
Raes, A., Detienne, L., Windey, I., & Depaepe, F. (2019). A systematic literature review on synchronous hybrid learning: gaps identified. Learning Environments Research, 23 (3), 269–290. https://doi.org/10.1007/S10984-019-09303-Z .
Raes, A., Vanneste, P., Pieters, M., Windey, I., Van Den Noortgate, W., & Depaepe, F. (2020). Learning and instruction in the hybrid virtual classroom: An investigation of students’ engagement and the effect of quizzes. Computers and Education , 143 . https://doi.org/10.1016/j.compedu.2019.103682 .
Ramsey, D., Evans, J., & Levy, M. (2016). Preserving the seminar experience. Journal of Political Science Education, 12 (3), 256–267. https://doi.org/10.1080/15512169.2015.1077713 .
Shi, Y., Tong, M., & Long, T. (2021). Investigating relationships among blended synchronous learning environments, students’ motivation, and cognitive engagement: A mixed methods study. Computers and Education , 168 . https://doi.org/10.1016/j.compedu.2021.104193 .
Stahl, G., Koschmann, T., & Suthers, D. (2014). Computer-supported collaborative learning: An historical perspective. In R. K. Sawyer (Ed.), Cambridge handbook of the learning sciences, revised version (pp. 479–500). Cambridge, UK: Cambridge University Press.
Szeto, E. (2014). A Comparison of Online/Face-to-face Students’ and Instructor’s Experiences: Examining Blended Synchronous Learning Effects. Procedia - Social and Behavioral Sciences , 116 , 4250–4254. https://doi.org/10.1016/j.sbspro.2014.01.926 .
Wang, Q., Huang, C., & Quek, C. L. (2018). Students’ perspectives on the design and implementation of a blended synchronous learning environment. Australasian Journal of Educational Technology , 34 (1), 1–13. https://doi.org/10.14742/ajet.3404 .
Weitze, C. L., Ørngreen, R., & Levinsen, K. (2013). The Global Classroom Video Conferencing Model and First Evaluations. I M. Ciussi & M. Augier (Eds.), Proceedings of the 12th European Conference on E-Learning: SKEMA Business School, Sophia Antipolis France, 30-31 October 2013 (pp. 503-510). Reading, UK: Academic Conferences and Publishing International.
Yorke, M. (2016). The development and initial use of a survey of student ‘belongingness,’ engagement and self-confidence in UK higher education. Assessment & Evaluation in Higher Education, 41 (1), 154-166. https://doi.org/10.1080/02602938.2014.990415 .
Zydney, J. M., McKimm, P., Lindberg, R., & Schmidt, M. (2019). Here or There Instruction: Lessons Learned in Implementing Innovative Approaches to Blended Synchronous Learning. TECHTRENDS , 63 (2), 123–132. https://doi.org/10.1007/s11528-018-0344-z .
Zydney, J. M., Warner, Z., & Angelone, L. (2020). Learning through experience: Using design based research to redesign protocols for blended synchronous learning environments. Computers and Education , 143 . https://doi.org/10.1016/j.compedu.2019.103678 .
Download references
Acknowledgements
I would like to thank the professors and students from KU Leuven, campus Kulak Kortrijk for their participation and their thoughtful feedback regarding this research.
This study was carried out within imec’s Smart Education research programme, with support from the Flemish government and funding from KU Leuven University. This project was also supported by the French government through the Programme Investissement d’Avenir (I-SITE ULNE / ANR-16-IDEX-0004 ULNE) managed by the Agence Nationale de la Recherche.
Author information
Authors and affiliations.
Faculty of Psychology and Educational Sciences, KU Leuven Campus Kulak Kortrijk, Etienne Sabbelaan 51 - box 7800, 8500, Kortrijk, Belgium
Annelies Raes
Itec, Imec Research Group at KU Leuven, Kapeldreef 75, 3001, Leuven, Belgium
CIREL (Centre Interuniversitaire de Recherche en Education de Lille) (ULR 4354), Université de Lille, Villeneuve-d´Ascq, France
You can also search for this author in PubMed Google Scholar
Corresponding author
Correspondence to Annelies Raes .
Ethics declarations
Ethical approval and informed consent.
This research involves human participants, but this research project has been reviewed and approved by the Social and Societal Ethics Committee https://ppw.kuleuven.be/home/onderzoek/SMEC ). Informed consent was obtained from all individual participants included in the study.
Conflict of Interest
The author declares no competing interests.
Additional information
Publisher’s note.
Springer Nature remains neutral with regard to jurisdictional claims in published maps and institutional affiliations.
Rights and permissions
Reprints and permissions
About this article
Raes, A. Exploring Student and Teacher Experiences in Hybrid Learning Environments: Does Presence Matter?. Postdigit Sci Educ 4 , 138–159 (2022). https://doi.org/10.1007/s42438-021-00274-0
Download citation
Accepted : 03 November 2021
Published : 18 November 2021
Issue Date : January 2022
DOI : https://doi.org/10.1007/s42438-021-00274-0
Share this article
Anyone you share the following link with will be able to read this content:
Sorry, a shareable link is not currently available for this article.
Provided by the Springer Nature SharedIt content-sharing initiative
- Hybrid learning environments
- Learning space
- Teaching space
- User experience
- Find a journal
- Publish with us
- Track your research
MINI REVIEW article
The modality-specific learning style hypothesis: a mini-review.
- 1 Department of Psychology, Faculty of Social and Educational Sciences, Norwegian University of Science and Technology, Trondheim, Norway
- 2 Department of Neuromedicine and Movement Science, Faculty of Medicine and Health Sciences, Norwegian University of Science and Technology, Trondheim, Norway
The impact on learning outcome of tailoring instruction and teaching toward modality-specific learning style preferences has been researched and debated for decades. Several topical reviews have concluded that there is no evidence to support the meshing hypothesis and that it represents a persistent neuromyth in education. The concept, however, is still utilized in educational practice and favored by many academics. This mini-review presents literature, which has applied explicit and rigorous methodological criteria, in relation to the meshing hypothesis. In order to demonstrate evidence for the meshing hypothesis, studies had to screen participants for their preferred learning style, assign participants to matched or non-matched conditions, and then provide the same test to assess learning for all participants, as well as presenting statistical crossover-interaction effects. Across studies that have applied these methodological criteria, the overall effect sizes were very low and non-significant, indicating that there is still no replicable statistical evidence for enhanced learning outcome by aligning instruction to modality-specific learning styles.
Introduction
The concept of matching instructional strategies to an individual’s learning style in order to enhance learning outcome and achieve better academic success is a well-known concept among educators and the general population ( Pashler et al., 2008 ; Dekker et al., 2012 ; Howard-Jones, 2014 ). Learning styles are considered to have an impact in any learning situation regardless of content and this “refers to the concept that individuals differ in regard to what mode of instruction or study is most effective to them” ( Pashler et al., 2008 ). The term learning styles first appeared in the literature many decades ago (e.g., Thelen, 1954 ) and has been the focus of extensive research for the past three decades, especially in Western Europe and the United States ( Coffield et al., 2004 ).
Amongst a plethora of concepts and perspectives on learning styles (see Coffield et al., 2004 , for a tour de force on learning style concepts), one of the most cited and well-known learning style perspectives concerns modality-specific preferences ( Coffield et al., 2004 ; Howard-Jones, 2014 ; Cuevas, 2015 ). The overall prediction is that if individuals are given instruction in their preferred modality (visual, auditory, or kinesthetic), they will experience enhanced learning outcomes. This has been termed the meshing hypothesis ( Pashler et al., 2008 ). A related perspective that offers basically the same prediction states that people who are “verbalizers” will perform better if they are given verbal instructions and that “visualizers” will perform better if instructions are presented visually ( Massa and Mayer, 2006 ; Kollöffel, 2012 ). In either perspective, the instructional method should mesh with the preferred modality-specific learning style. The learning style concept, in general, and the meshing hypothesis, in particular, have been subjects of tremendous scrutiny in the recent years that continues to the present. Several independent authors have advanced the view that the latter represents a neuromyth , a term applied to educational applications argued to be based upon popular perspectives of brain functioning ( Geake, 2008 ; Riener and Willingham, 2010 ; Dekker et al., 2012 ; Howard-Jones, 2014 ; Newton, 2015 ; Newton and Miah, 2017 ). Typically, the evidence for neuromyths does not correspond to the findings of studies from cognitive psychology and the neurosciences, and sometimes the scientific evidence contradicts the brain-based claims ( Geake, 2008 ). In terms of the meshing hypothesis, the implicit assumption is that the learning material delivered via one sensory modality (i.e., visual, auditory, or kinesthetic) is processed in the brain independently from material delivered via other sensory modalities. However, substantial scientific evidence shows support for cross-modal processing and interconnectivity that contradicts the meshing perspective and demonstrates that input modalities in the brain are always interlinked ( Calvert et al., 2000 ).
The overall claim for improving learning by matching the mode of instruction to modality-specific learning preferences independent of both ability and content ( Riener and Willingham, 2010 ), as reflected by the meshing hypothesis, has also been scrutinized in several literature reviews. At first sight, modality-specific instruction appears to be supported by a large body of empirical literature ( Rohrer and Pashler, 2012 ). However, upon closer inspection, few of these studies have been found to have an appropriate research design ( Pashler et al., 2008 ). First, subjects need to be divided according to their preferred learning style, e.g., visual or auditory learners, based upon some sort of learning style assessment. Second, studies with an appropriate design must then randomize subjects (regardless of their assessed learning style) to receive either instruction tailored to their style or instruction tailored for other learning styles. This asserts that some subjects were presented with the “correct” kind of instruction (i.e., aligned with their preferred learning modality) and some with the “incorrect” instruction. Finally, all participants must be administered the same test to assess learning, and the results would support the efficacy of the practice of aligning instruction with modality-specific learning style if, and only if, the test scores reveal that, e.g., visual learners do better if instruction is presented visually rather than auditorily, and likewise, auditory learners do better if instruction is presented auditorily rather than visually (crossover-interaction effects; Pashler et al., 2008 ). In previous reviews, it has been stated repeatedly that there is a lack of studies that employ this rigorous design and that the few available at the time have, overall, generated no evidence to support the meshing hypothesis ( Coffield et al., 2004 ; Kozhevnikov, 2007 ; Pashler et al., 2008 ; Willingham et al., 2015 ).
The disappointing outcome of all these empirical and theoretical endeavors and efforts is that the modality-specific learning style concept is, as stated by Newton (2015) , thriving across all levels of education. This is reflected in the findings of 89% of research papers published from 2013 to 2015 and located in ERIC and PubMed databases support the application of learning styles to instructional methodology ( Newton, 2015 ). Furthermore, a survey by Dekker et al. (2012) showed that 93% of United Kingdom primary and secondary school teachers assumed that “individuals learn better when they receive information in their preferred learning style.” Later studies have revealed similar findings in other countries, K-12 teachers responding positively to statements favoring modality-specific learning styles ( Howard-Jones, 2014 ; Gleichgerrcht et al., 2015 ; Ferrero et al., 2016 ). In addition, when faculty working in higher education in the United States were given the following question: Does teaching to a student’s learning style enhance learning? , approximately two-thirds answered in the affirmative ( Dandy and Bendersky, 2014 ). At the institutional level, Meyer and Murrell (2014) found that, across 39 educational institutions in the United States, more than 70% taught “learning style theory” as a topic in teacher education.
A recent study showed a downward trend for the general belief in learning styles among academics working in higher education in the United Kingdom ( n = 114), although 58% still report believing in the concept and about a third report using learning styles actively in their work ( Newton and Miah, 2017 ). Thus, there appears to be widespread acceptance among educators, students, and academics globally and across all levels of education that the concept of learning styles is an established, textbook principle. Indeed, texts used in teacher education courses present learning style theory as a way to differentiate instruction for students ( Cuevas, 2015 ).
The presented considerations demonstrate that there exists a substantial continuum of perspectives on the application of modality-specific learning styles, ranging from viewing the concept as a neuromyth that should be abandoned in pedagogical practice to those who speak in favor of the concept and might use it as part of their routine practices. The principal aim of this mini-review is to provide a contribution toward narrowing this gap in perspectives by providing an updated overview of the available empirical studies that have applied rigorous methodological criteria as outlined by Pashler et al. (2008) . To the best of the authors’ knowledge, although previous reviews touching upon modality-specific learning styles have been both thorough and in-depth, they have been mostly narrative and have not been accompanied by a focus on specific effect sizes. This latter approach can be important for disentangling divergences in results, as there might be disagreements among studies. Pooling methodological and conceptually similar studies that all involve a certain degree of error allows for deriving an estimate of overall effect size that considers contrasting results from different studies. Such an update seems timely, given that several studies with methodological rigor have been published since the previous reviews.
Scope of the Mini-Review: Selection Criteria for Reporting of Evidence
The aim of this mini-review was to present literature in relation to the meshing hypothesis. Consequently, the authors independently performed database searches in EBSCO (including ERIC, Academic Search Complete, Psychology and Behavioral Sciences Collection) and Ovid (including Medline, EMBASE, and PsychINFO) using combinations of the terms learning styles ∗ , visual ∗ , and auditory ∗ . The searches were conducted up to January 2018. The reference lists from previous reviews were also examined, as well as citation-based searches in Google Scholar. A total of 1215 records were initially scanned, and 10 studies ( Constantinidou and Baker, 2002 ; Massa and Mayer, 2006 ; Kassaian, 2007 ; Korenman and Peynircioglu, 2007 ; Slack and Norwich, 2007 ; Tight, 2010 ; Kollöffel, 2012 ; Hansen and Cottrell, 2013 ; Rogowsky et al., 2015 ; Papanagnou et al., 2016 ) were found that had applied the appropriate methodology according to the criteria by Pashler et al. (2008) .
Tailoring Instruction for Modality-Specific Preferences: No Statistical Evidence for the Meshing Hypothesis
Statistical evidence for the meshing hypothesis could potentially be found in crossover-interaction effects, i.e., visual learners demonstrate improved learning if instruction is visual rather than auditory, and likewise, auditory learners show improvements if instruction is auditory rather than visual. The 10 publications amounted to 13 experiments, from which it was possible to extract means (SD) for computation of effect sizes (Hedges’ g ) for 11 of them. Altogether, 22 effect sizes from post-test data representing the differences in scores between the matched groups and the mismatched groups were analyzed by a random effects model. This resulted in a small and non-significant effect size for visual matching ( g = -0.09, 95% CI [-0.74–0.58], p = 0.80, n = 484) as well as for auditory matching ( g = -0.27, 95% CI [-0.87–0.32], p = 0.37, n = 356). In the paper by Constantinidou and Baker (2002) , the authors did not report data that allow for the computation of Hedges’ g . The authors did state, however, that no significant correlation between learning style and experimental task performance was found. Similarly, Papanagnou et al. (2016) reported only mean values for matched/non-matched learning outcomes and stated that both matched and non-matched groups achieved similar learning outcomes. Based on these data, it thus appears that there is no replicable evidence for a statistical crossover-interaction effect where participants systematically show higher learning outcomes when they are in a condition in which their preferred learning style modality matches the instructional mode and a lower learning outcome when there is a mismatch.
The overall (non-significant) effect sizes obtained across studies appears to be, by any standard, too small to be interpreted as signifying any modality-matching effect on learning outcomes. Although the interpretation of effect sizes is not a straightforward scientific endeavor ( Cohen, 1992 ), the effect size cut-offs indicating a practically relevant effect provided in the literature represent a much more substantial magnitude. For example, Ferguson (2009) recommended that a minimum effect size representing a “practically” significant effect amounts to g ≥ 0.41, and Hattie (2009) has advanced the view that effect sizes ≥0.40 represent a “hinge-point” at which deliberate interventions provide relevant outcomes for teaching and learning. Adding to the overall interpretation of the effect sizes obtained in the current meta-analysis, the 95% confidence intervals demonstrate crossings of zero both for the overall effect size and in data from some individual studies. This latter finding is a strong indicator that the null hypothesis (no effect of modality matching) should not be rejected ( Wilkinson et al., 1999 ).
An often-stated problem in the learning style literature is the plethora of inventories designed and applied for both research and commercial purposes ( Coffield et al., 2004 ; Peterson et al., 2009 ; Scott, 2010 ; Armstrong et al., 2012 ). At first sight, this might appear as a methodological challenge toward the pooling of results across studies. In particular, the VAKT classification vs. the verbalizer–visualizer dimension have previously been advocated as different and non-comparable approaches toward learning styles; e.g., it has been claimed that the verbalizer–visualizer dimension should be defined as a cognitive style and not included among the “family” of learning styles ( Massa and Mayer, 2006 ; Kollöffel, 2012 ). However, the latter perspective involves modality-specific content. Written material is considered proper instruction for verbalizers, as it is processed as spoken words, and therefore, a verbalizer can be considered synonymous to an auditory learner ( Felder and Silverman, 1988 ). Based on these contentions, there are strong theoretical arguments for a comparison of studies applying inventories based upon either perspective. Furthermore, rarely is any theoretical or methodological argument for the inclusion of a specific inventory in studies given, and in addition, some authors advance the view that one should apply the inventories that are most used (or most popular) in order to generate comparable results ( Hansen and Cottrell, 2013 ).
There still appear to be relatively few studies adhering strictly to the methodological criteria outlined by Pashler et al. (2008) . In particular, the participants’ learning styles are not necessarily established before they are separated into groups (e.g., Korenman and Peynircioglu, 2007 ), and participants can be randomly assigned to either one ( Massa and Mayer, 2006 ; Rogowsky et al., 2015 ) or all conditions (e.g., Kassaian, 2007 ). The only study located through the systematic literature search across six different databases and the screening of more than a 1000 records that was totally aligned with Pashler’s criteria was Rogowsky et al. (2015) . These authors report no statistically significant relationship or crossover-interaction effect between modality-specific learning styles and modes of instruction. Here, the authors assessed the participants’ learning styles and randomly assigned participants to either listening to a digital audiobook or reading an e-text, and all participants completed the same achievement test. Interestingly, the effect sizes from this latter study (visual-matching: g = -0.11, auditory-matching: g = -0.256) were similar to the overall effect size across studies.
The experimental tasks applied in studies varied considerably. The pooling of such various approaches can be justified by the modality-specific learning style theory. Here, the basic contention is that modality matching introduces more efficient learning irrespective of content and contexts. Indeed, the concept of a modality-specific learning style has been featured in the literature as a hardwired and more or less inherited preference in the cognitive system that should be taken into consideration in any learning situation ( Coffield et al., 2004 ). One methodological concern, however, arises when examining learning tasks more closely. It appears that some tasks have a “built-in” stronger visual or auditory component, which could potentially introduce an additive bias in favor of both a particular instructional mode and a learning style ( Fiorina et al., 2007 ; Hansen and Cottrell, 2013 ; Willingham et al., 2015 ). Although this could potentially lead to inflated effect sizes, the overall pattern of results across studies suggested no statistical effect of modality matching.
As stated in the introduction, the modality-specific learning style hypothesis is still a favored concept amongst the general public, educators, and in the research literature ( Pashler et al., 2008 ; Dekker et al., 2012 ; Howard-Jones, 2014 ). In previous reviews, it has been systematically addressed that there is, in general, no evidence to support the application of the learning style concept ( Coffield et al., 2004 ; Desmedt and Valcke, 2004 ; Kozhevnikov, 2007 ; Pashler et al., 2008 ; Peterson et al., 2009 ; Cuevas, 2015 ; Willingham et al., 2015 ). The present study responds to a call from the much-cited review (>1,500 citations in Google Scholar) of Pashler et al. (2008) , who stated that, in order for the learning styles hypothesis to be supported, several well-designed studies would have to test, amongst other elements, the modality-matching hypothesis and show significant interaction effects. Although the total number of studies ( n = 10) with appropriate methodology is not large at this time, the pattern of results clearly leans toward showing that tailoring instruction/teaching toward preferred modality-specific learning styles has no effect on learning outcome/rate.
Concluding Remarks
This mini-review has demonstrated that, across studies that have applied equivalent quantitative empirical research designs, no overall improvement in learning outcome when applying modality-specific matching of instruction was found. This conclusion of the presented meta-analysis of an element of the modality-specific learning style literature appears to add to further evidence-based refutations of the meshing hypothesis. Interestingly, some early meta-analysis on other elements of learning styles presented similar conclusions ( Tamir, 1985 ; Kavale and Forness, 1987 ). This appears in contrast to the recent literature review by Newton (2015) , in which it was demonstrated that a considerable percent (89%) of published studies in the period from 2013 to 2015 was positive toward learning styles. It thus appears important to continue to critically scrutinize different aspects of the learning style literature and to conduct pattern-type explanations ( Derry, 1999 ) involving conceptual syntheses of insights emerging from diverse disciplines. For example, connections have been found between visual-spatial strengths and superior abilities in other cognitive domains ( O’Boyle et al., 2005 ; Root-Bernstein et al., 2008 ). This latter work is not typically connected with modality-specific learning styles in the academic literature and highlights the need for further work on the credibility of the meshing hypothesis in order to prevent potential misuse of what might appear to be a persistent neuromyth.
Author Contributions
All authors listed have made a substantial, direct and intellectual contribution to the work, and approved it for publication.
Conflict of Interest Statement
The authors declare that the research was conducted in the absence of any commercial or financial relationships that could be construed as a potential conflict of interest.
Armstrong, S. J., Peterson, E. R., and Rayner, S. G. (2012). Understanding and defining cognitive style and learning style: a Delphi study in the context of educational psychology. Educ. Stud. 38, 449–455. doi: 10.1080/03055698.2011.643110
CrossRef Full Text | Google Scholar
Calvert, G. A., Campbell, R., and Brammer, M. J. (2000). Evidence from functional magnetic resonance imaging of crossmodal binding in human heteromodal cortex. Curr. Biol. 10, 649–657. doi: 10.1016/S0960-9822(00)00513-3
Coffield, F., Moseley, D., Hall, E., and Ecclestone, K. (2004). Learning Styles and Pedagogy in Post-16 Learning: A Systematic and Critical Review. Available at: http://www.hull.ac.uk/php/edskas/learning
Google Scholar
Cohen, J. (1992). A power primer. Psychol. Bull. 112, 155–159. doi: 10.1037/0033-2909.112.1.155
Constantinidou, F., and Baker, S. (2002). Stimulus modality and verbal learning performance in normal aging. Brain Lang. 82, 296–311. doi: 10.1016/S0093-934X(02)00018-4
Cuevas, J. (2015). Is learning styles-based instruction effective? A comprehensive analysis of recent research on learning styles. Theory Res. Educ. 13, 308–333. doi: 10.1177/1477878515606621
Dandy, K., and Bendersky, K. (2014). Student and faculty beliefs about learning in higher education: implications for teaching. Int. J. Teach. Learn. High. Educ. 26, 358–380.
Dekker, S., Lee, N. C., Howard-Jones, P., and Jolles, J. (2012). Neuromyths in education: prevalence and predictors of misconceptions among teachers. Front. Psychol. 3:429. doi: 10.3389/fpsyg.2012.00429
PubMed Abstract | CrossRef Full Text | Google Scholar
Derry, G. N. (1999). What Science is and How it Works. Princeton, NJ: Princeton University Press.
Desmedt, E., and Valcke, M. (2004). Mapping the learning styles “jungle”: an overview of the literature based on citation analysis. Educ. Psychol. 24, 445–464. doi: 10.1080/0144341042000228843
Felder, R. M., and Silverman, L. K. (1988). Learning and teaching styles in engineering education. Eng. Educ. 78, 674–681.
Ferguson, C. J. (2009). An effect size primer: a guide for clinicians and researchers. Prof. Psychol. Res. Pr. 40, 532–538. doi: 10.1037/a0015808
Ferrero, M., Garaizar, P., and Vadillo, M. A. (2016). Neuromyths in education: prevalence among Spanish teachers and an exploration of cross-cultural variation. Front. Hum. Neurosci. 10:496. doi: 10.3389/fnhum.2016.00496
Fiorina, L., Antonietti, A., Colombo, B., and Bartolomeo, A. (2007). Thinking style, browsing primes and hypermedia navigation. Comput. Educ. 49, 916–941. doi: 10.1016/j.compedu.2005.12.005
Geake, J. (2008). Neuromythologies in Education. Educ. Res. 50, 123–133. doi: 10.1080/00131880802082518
Gleichgerrcht, E., Luttges, B. L., Salvarezza, F., and Campos, A. L. (2015). Educational neuromyths among teachers in Latin America. Mind Brain Educ. 9, 170–178. doi: 10.1111/mbe.12086
Hansen, L., and Cottrell, D. (2013). An evaluation of modality preference using a “Morse code” recall task. J. Exp. Educ. 81, 123–137. doi: 10.1080/00220973.2012.678408
Hattie, J. (2009). Visible Learning: A Synthesis of Over 800 Meta-Analyses Relating to Achievement. Abingdon: Routledge.
Howard-Jones, P. A. (2014). Neuroscience and education: myths and messages. Nat. Rev. Neurosci. 15, 817–824. doi: 10.1038/nrn3817
Kassaian, Z. (2007). Learning styles and lexical presentation modes. Estud. Lingüíst. Inglesa Apl. 7, 53–78.
Kavale, K. A., and Forness, S. R. (1987). Substance over style: assessing the efficacy of modality testing and teaching. Except. Child. 54, 228–239. doi: 10.1177/001440298705400305
Kollöffel, B. (2012). Exploring the relation between visualizer–verbalizer cognitive styles and performance with visual or verbal learning material. Comput. Educ. 58, 697–706. doi: 10.1016/j.compedu.2011.09.016
Korenman, L. M., and Peynircioglu, Z. F. (2007). Individual differences in learning and remembering music: auditory versus visual presentation. J. Res. Music Educ. 55:48. doi: 10.1177/002242940705500105
Kozhevnikov, M. (2007). Cognitive styles in the context of modern psychology: toward an integrated framework of cognitive style. Psychol. Bull. 133, 464–481. doi: 10.1037/0033-2909.133.3.464
Massa, L. J., and Mayer, R. E. (2006). Testing the ATI hypothesis: should multimedia instruction accommodate verbalizer-visualizer cognitive style? Learn. Individ. Dif. 16, 321–335. doi: 10.1016/j.lindif.2006.10.001
Meyer, K. A., and Murrell, V. S. (2014). A national study of theories and their importance for faculty development for online teaching. J. Distance Learn. Adm. Contents 17, 1–15. doi: 10.1111/j.1365-2923.2012.04350.x
Newton, P. M. (2015). The learning styles myth is thriving in higher education. Front. Psychol. 6:1908. doi: 10.3389/fpsyg.2015.01908
Newton, P. M., and Miah, M. (2017). Evidence-based higher education-Is the learning styles ‘myth’ important? Front. Psychol. 8:444. doi: 10.3389/fpsyg.2017.00444
O’Boyle, M. W., Cunnington, R., Silk, T. J., Vaughan, D., Jackson, G., Syngeniotis, A., et al. (2005). Mathematically gifted male adolescents activate a unique brain network during mental rotation. Cogn. Brain Res. 25, 583–587. doi: 10.1016/j.cogbrainres.2005.08.004
Papanagnou, D., Serrano, A., Barkley, K., Chandra, S., Governatori, N., Piela, N., et al. (2016). Does tailoring instructional style to a medical student’s self-perceived learning style improve performance when teaching intravenous catheter placement? A randomized controlled study. BMC Med. Educ. 16:205. doi: 10.1186/s12909-016-0720-3
Pashler, H., McDaniel, M., Rohrer, D., and Bjork, R. (2008). Learning styles concepts and evidence. Psychol. Sci. Public Interest 9, 105–119. doi: 10.1111/j.1539-6053.2009.01038.x
Peterson, E. R., Rayner, S. G., and Armstrong, S. J. (2009). Researching the psychology of cognitive style and learning style: is there really a future? Learn. Individ. Dif. 19, 518–523. doi: 10.1016/j.lindif.2009.06.003
Riener, C., and Willingham, D. (2010). The myth of learning styles. Change 42, 32–35. doi: 10.1080/00091383.2010.503139
Rogowsky, B. A., Calhoun, B. M., and Tallal, P. (2015). Matching learning style to instructional method: effects on comprehension. J. Educ. Psychol. 107, 64–78. doi: 10.1037/a0037478
Rohrer, D., and Pashler, H. (2012). Learning styles: where’s the evidence? Med. Educ. 46, 634–635.
Root-Bernstein, R., Allen, L., Beach, L., Bhadula, R., Fast, J., Hosey, C., et al. (2008). Arts foster scientific success: avocations of nobel, national academy, royal society, and sigma xi members. J. Psychol. Sci. Technol. 1, 51–63. doi: 10.1891/1939-7054.1.2.51
Scott, C. (2010). The enduring appeal of ‘learning styles’. Aust. J. Educ. 54, 5–17. doi: 10.1177/000494411005400102
Slack, N., and Norwich, B. (2007). Evaluating the reliability and validity of a learning styles inventory: a classroom-based study. Educ. Res. 49, 51–63. doi: 10.1080/00131880701200765
Tamir, P. (1985). Meta-analysis of cognitive preferences and learning. J. Res. Sci. Teach. 22, 1–17. doi: 10.1002/tea.3660220101
Thelen, H. A. (1954). Dynamics of Groups at Work. Chicago, IL: Univeristy of Chicago Press.
Tight, D. G. (2010). Perceptual learning style matching and L2 vocabulary acquisition. Lang. Learn. 60, 792–833. doi: 10.1111/j.1467-9922.2010.00572.x
Wilkinson, L., Task Force on Statistical Inference, American Psychological Association, and Science Directorate (1999). Statistical methods in psychology journals: guidelines and explanations. Am. Psychol. 54, 594–604. doi: 10.1037/0003-066X.54.8.594
Willingham, D. T., Hughes, E. M., and Dobolyi, D. G. (2015). The scientific status of learning styles theories. Teach. Psychol. 42, 266–271. doi: 10.1007/s10459-009-9202-2
Keywords : modality-specific, instruction, teaching, learning styles, meshing hypothesis, neuromyth
Citation: Aslaksen K and Lorås H (2018) The Modality-Specific Learning Style Hypothesis: A Mini-Review. Front. Psychol. 9:1538. doi: 10.3389/fpsyg.2018.01538
Received: 17 April 2018; Accepted: 02 August 2018; Published: 21 August 2018.
Reviewed by:
Copyright © 2018 Aslaksen and Lorås. This is an open-access article distributed under the terms of the Creative Commons Attribution License (CC BY) . The use, distribution or reproduction in other forums is permitted, provided the original author(s) and the copyright owner(s) are credited and that the original publication in this journal is cited, in accordance with accepted academic practice. No use, distribution or reproduction is permitted which does not comply with these terms.
*Correspondence: Håvard Lorås, [email protected]
Disclaimer: All claims expressed in this article are solely those of the authors and do not necessarily represent those of their affiliated organizations, or those of the publisher, the editors and the reviewers. Any product that may be evaluated in this article or claim that may be made by its manufacturer is not guaranteed or endorsed by the publisher.
- Home
- University Colleges
- SUNY Brockport
- Brockport Education and Human Development Master's Theses
Campus Communities in SOAR
Show simple item record
A Research Review of Literature on Learning Modalities
Files in this item.

This item appears in the following Collection(s)
entitlement
Export search results
The export option will allow you to export the current search results of the entered query to a file. Different formats are available for download. To export the items, click on the button corresponding with the preferred download format.
By default, clicking on the export buttons will result in a download of the allowed maximum amount of items.
To select a subset of the search results, click "Selective Export" button and make a selection of the items you want to export. The amount of items that can be exported at once is similarly restricted as the full export.
After making a selection, click one of the export format buttons. The amount of items that will be exported is indicated in the bubble next to export format.
- Research article
- Open access
- Published: 06 February 2017
Blended learning effectiveness: the relationship between student characteristics, design features and outcomes
- Mugenyi Justice Kintu ORCID: orcid.org/0000-0002-4500-1168 1 , 2 ,
- Chang Zhu 2 &
- Edmond Kagambe 1
International Journal of Educational Technology in Higher Education volume 14 , Article number: 7 ( 2017 ) Cite this article
753k Accesses
220 Citations
37 Altmetric
Metrics details
This paper investigates the effectiveness of a blended learning environment through analyzing the relationship between student characteristics/background, design features and learning outcomes. It is aimed at determining the significant predictors of blended learning effectiveness taking student characteristics/background and design features as independent variables and learning outcomes as dependent variables. A survey was administered to 238 respondents to gather data on student characteristics/background, design features and learning outcomes. The final semester evaluation results were used as a measure for performance as an outcome. We applied the online self regulatory learning questionnaire for data on learner self regulation, the intrinsic motivation inventory for data on intrinsic motivation and other self-developed instruments for measuring the other constructs. Multiple regression analysis results showed that blended learning design features (technology quality, online tools and face-to-face support) and student characteristics (attitudes and self-regulation) predicted student satisfaction as an outcome. The results indicate that some of the student characteristics/backgrounds and design features are significant predictors for student learning outcomes in blended learning.
Introduction
The teaching and learning environment is embracing a number of innovations and some of these involve the use of technology through blended learning. This innovative pedagogical approach has been embraced rapidly though it goes through a process. The introduction of blended learning (combination of face-to-face and online teaching and learning) initiatives is part of these innovations but its uptake, especially in the developing world faces challenges for it to be an effective innovation in teaching and learning. Blended learning effectiveness has quite a number of underlying factors that pose challenges. One big challenge is about how users can successfully use the technology and ensuring participants’ commitment given the individual learner characteristics and encounters with technology (Hofmann, 2014 ). Hofmann adds that users getting into difficulties with technology may result into abandoning the learning and eventual failure of technological applications. In a report by Oxford Group ( 2013 ), some learners (16%) had negative attitudes to blended learning while 26% were concerned that learners would not complete study in blended learning. Learners are important partners in any learning process and therefore, their backgrounds and characteristics affect their ability to effectively carry on with learning and being in blended learning, the design tools to be used may impinge on the effectiveness in their learning.
This study tackles blended learning effectiveness which has been investigated in previous studies considering grades, course completion, retention and graduation rates but no studies regarding effectiveness in view of learner characteristics/background, design features and outcomes have been done in the Ugandan university context. No studies have also been done on how the characteristics of learners and design features are predictors of outcomes in the context of a planning evaluation research (Guskey, 2000 ) to establish the effectiveness of blended learning. Guskey ( 2000 ) noted that planning evaluation fits in well since it occurs before the implementation of any innovation as well as allowing planners to determine the needs, considering participant characteristics, analyzing contextual matters and gathering baseline information. This study is done in the context of a plan to undertake innovative pedagogy involving use of a learning management system (moodle) for the first time in teaching and learning in a Ugandan university. The learner characteristics/backgrounds being investigated for blended learning effectiveness include self-regulation, computer competence, workload management, social and family support, attitude to blended learning, gender and age. We investigate the blended learning design features of learner interactions, face-to-face support, learning management system tools and technology quality while the outcomes considered include satisfaction, performance, intrinsic motivation and knowledge construction. Establishing the significant predictors of outcomes in blended learning will help to inform planners of such learning environments in order to put in place necessary groundwork preparations for designing blended learning as an innovative pedagogical approach.
Kenney and Newcombe ( 2011 ) did their comparison to establish effectiveness in view of grades and found that blended learning had higher average score than the non-blended learning environment. Garrison and Kanuka ( 2004 ) examined the transformative potential of blended learning and reported an increase in course completion rates, improved retention and increased student satisfaction. Comparisons between blended learning environments have been done to establish the disparity between academic achievement, grade dispersions and gender performance differences and no significant differences were found between the groups (Demirkol & Kazu, 2014 ).
However, blended learning effectiveness may be dependent on many other factors and among them student characteristics, design features and learning outcomes. Research shows that the failure of learners to continue their online education in some cases has been due to family support or increased workload leading to learner dropout (Park & Choi, 2009 ) as well as little time for study. Additionally, it is dependent on learner interactions with instructors since failure to continue with online learning is attributed to this. In Greer, Hudson & Paugh’s study as cited in Park and Choi ( 2009 ), family and peer support for learners is important for success in online and face-to-face learning. Support is needed for learners from all areas in web-based courses and this may be from family, friends, co-workers as well as peers in class. Greer, Hudson and Paugh further noted that peer encouragement assisted new learners in computer use and applications. The authors also show that learners need time budgeting, appropriate technology tools and support from friends and family in web-based courses. Peer support is required by learners who have no or little knowledge of technology, especially computers, to help them overcome fears. Park and Choi, ( 2009 ) showed that organizational support significantly predicts learners’ stay and success in online courses because employers at times are willing to reduce learners’ workload during study as well as supervisors showing that they are interested in job-related learning for employees to advance and improve their skills.
The study by Kintu and Zhu ( 2016 ) investigated the possibility of blended learning in a Ugandan University and examined whether student characteristics (such as self-regulation, attitudes towards blended learning, computer competence) and student background (such as family support, social support and management of workload) were significant factors in learner outcomes (such as motivation, satisfaction, knowledge construction and performance). The characteristics and background factors were studied along with blended learning design features such as technology quality, learner interactions, and Moodle with its tools and resources. The findings from that study indicated that learner attitudes towards blended learning were significant factors to learner satisfaction and motivation while workload management was a significant factor to learner satisfaction and knowledge construction. Among the blended learning design features, only learner interaction was a significant factor to learner satisfaction and knowledge construction.
The focus of the present study is on examining the effectiveness of blended learning taking into consideration learner characteristics/background, blended learning design elements and learning outcomes and how the former are significant predictors of blended learning effectiveness.
Studies like that of Morris and Lim ( 2009 ) have investigated learner and instructional factors influencing learning outcomes in blended learning. They however do not deal with such variables in the contexts of blended learning design as an aspect of innovative pedagogy involving the use of technology in education. Apart from the learner variables such as gender, age, experience, study time as tackled before, this study considers social and background aspects of the learners such as family and social support, self-regulation, attitudes towards blended learning and management of workload to find out their relationship to blended learning effectiveness. Identifying the various types of learner variables with regard to their relationship to blended learning effectiveness is important in this study as we embark on innovative pedagogy with technology in teaching and learning.
Literature review
This review presents research about blended learning effectiveness from the perspective of learner characteristics/background, design features and learning outcomes. It also gives the factors that are considered to be significant for blended learning effectiveness. The selected elements are as a result of the researcher’s experiences at a Ugandan university where student learning faces challenges with regard to learner characteristics and blended learning features in adopting the use of technology in teaching and learning. We have made use of Loukis, Georgiou, and Pazalo ( 2007 ) value flow model for evaluating an e-learning and blended learning service specifically considering the effectiveness evaluation layer. This evaluates the extent of an e-learning system usage and the educational effectiveness. In addition, studies by Leidner, Jarvenpaa, Dillon and Gunawardena as cited in Selim ( 2007 ) have noted three main factors that affect e-learning and blended learning effectiveness as instructor characteristics, technology and student characteristics. Heinich, Molenda, Russell, and Smaldino ( 2001 ) showed the need for examining learner characteristics for effective instructional technology use and showed that user characteristics do impact on behavioral intention to use technology. Research has dealt with learner characteristics that contribute to learner performance outcomes. They have dealt with emotional intelligence, resilience, personality type and success in an online learning context (Berenson, Boyles, & Weaver, 2008 ). Dealing with the characteristics identified in this study will give another dimension, especially for blended learning in learning environment designs and add to specific debate on learning using technology. Lin and Vassar, ( 2009 ) indicated that learner success is dependent on ability to cope with technical difficulty as well as technical skills in computer operations and internet navigation. This justifies our approach in dealing with the design features of blended learning in this study.
Learner characteristics/background and blended learning effectiveness
Studies indicate that student characteristics such as gender play significant roles in academic achievement (Oxford Group, 2013 ), but no study examines performance of male and female as an important factor in blended learning effectiveness. It has again been noted that the success of e- and blended learning is highly dependent on experience in internet and computer applications (Picciano & Seaman, 2007 ). Rigorous discovery of such competences can finally lead to a confirmation of high possibilities of establishing blended learning. Research agrees that the success of e-learning and blended learning can largely depend on students as well as teachers gaining confidence and capability to participate in blended learning (Hadad, 2007 ). Shraim and Khlaif ( 2010 ) note in their research that 75% of students and 72% of teachers were lacking in skills to utilize ICT based learning components due to insufficient skills and experience in computer and internet applications and this may lead to failure in e-learning and blended learning. It is therefore pertinent that since the use of blended learning applies high usage of computers, computer competence is necessary (Abubakar & Adetimirin, 2015 ) to avoid failure in applying technology in education for learning effectiveness. Rovai, ( 2003 ) noted that learners’ computer literacy and time management are crucial in distance learning contexts and concluded that such factors are meaningful in online classes. This is supported by Selim ( 2007 ) that learners need to posses time management skills and computer skills necessary for effectiveness in e- learning and blended learning. Self-regulatory skills of time management lead to better performance and learners’ ability to structure the physical learning environment leads to efficiency in e-learning and blended learning environments. Learners need to seek helpful assistance from peers and teachers through chats, email and face-to-face meetings for effectiveness (Lynch & Dembo, 2004 ). Factors such as learners’ hours of employment and family responsibilities are known to impede learners’ process of learning, blended learning inclusive (Cohen, Stage, Hammack, & Marcus, 2012 ). It was also noted that a common factor in failure and learner drop-out is the time conflict which is compounded by issues of family , employment status as well as management support (Packham, Jones, Miller, & Thomas, 2004 ). A study by Thompson ( 2004 ) shows that work, family, insufficient time and study load made learners withdraw from online courses.
Learner attitudes to blended learning can result in its effectiveness and these shape behavioral intentions which usually lead to persistence in a learning environment, blended inclusive. Selim, ( 2007 ) noted that the learners’ attitude towards e-learning and blended learning are success factors for these learning environments. Learner performance by age and gender in e-learning and blended learning has been found to indicate no significant differences between male and female learners and different age groups (i.e. young, middle-aged and old above 45 years) (Coldwell, Craig, Paterson, & Mustard, 2008 ). This implies that the potential for blended learning to be effective exists and is unhampered by gender or age differences.
Blended learning design features
The design features under study here include interactions, technology with its quality, face-to-face support and learning management system tools and resources.
Research shows that absence of learner interaction causes failure and eventual drop-out in online courses (Willging & Johnson, 2009 ) and the lack of learner connectedness was noted as an internal factor leading to learner drop-out in online courses (Zielinski, 2000 ). It was also noted that learners may not continue in e- and blended learning if they are unable to make friends thereby being disconnected and developing feelings of isolation during their blended learning experiences (Willging & Johnson, 2009). Learners’ Interactions with teachers and peers can make blended learning effective as its absence makes learners withdraw (Astleitner, 2000 ). Loukis, Georgious and Pazalo (2007) noted that learners’ measuring of a system’s quality, reliability and ease of use leads to learning efficiency and can be so in blended learning. Learner success in blended learning may substantially be affected by system functionality (Pituch & Lee, 2006 ) and may lead to failure of such learning initiatives (Shrain, 2012 ). It is therefore important to examine technology quality for ensuring learning effectiveness in blended learning. Tselios, Daskalakis, and Papadopoulou ( 2011 ) investigated learner perceptions after a learning management system use and found out that the actual system use determines the usefulness among users. It is again noted that a system with poor response time cannot be taken to be useful for e-learning and blended learning especially in cases of limited bandwidth (Anderson, 2004 ). In this study, we investigate the use of Moodle and its tools as a function of potential effectiveness of blended learning.
The quality of learning management system content for learners can be a predictor of good performance in e-and blended learning environments and can lead to learner satisfaction. On the whole, poor quality technology yields no satisfaction by users and therefore the quality of technology significantly affects satisfaction (Piccoli, Ahmad, & Ives, 2001 ). Continued navigation through a learning management system increases use and is an indicator of success in blended learning (Delone & McLean, 2003 ). The efficient use of learning management system and its tools improves learning outcomes in e-learning and blended learning environments.
It is noted that learner satisfaction with a learning management system can be an antecedent factor for blended learning effectiveness. Goyal and Tambe ( 2015 ) noted that learners showed an appreciation to Moodle’s contribution in their learning. They showed positivity with it as it improved their understanding of course material (Ahmad & Al-Khanjari, 2011 ). The study by Goyal and Tambe ( 2015 ) used descriptive statistics to indicate improved learning by use of uploaded syllabus and session plans on Moodle. Improved learning is also noted through sharing study material, submitting assignments and using the calendar. Learners in the study found Moodle to be an effective educational tool.
In blended learning set ups, face-to-face experiences form part of the blend and learner positive attitudes to such sessions could mean blended learning effectiveness. A study by Marriot, Marriot, and Selwyn ( 2004 ) showed learners expressing their preference for face-to-face due to its facilitation of social interaction and communication skills acquired from classroom environment. Their preference for the online session was only in as far as it complemented the traditional face-to-face learning. Learners in a study by Osgerby ( 2013 ) had positive perceptions of blended learning but preferred face-to-face with its step-by-stem instruction. Beard, Harper and Riley ( 2004 ) shows that some learners are successful while in a personal interaction with teachers and peers thus prefer face-to-face in the blend. Beard however dealt with a comparison between online and on-campus learning while our study combines both, singling out the face-to-face part of the blend. The advantage found by Beard is all the same relevant here because learners in blended learning express attitude to both online and face-to-face for an effective blend. Researchers indicate that teacher presence in face-to-face sessions lessens psychological distance between them and the learners and leads to greater learning. This is because there are verbal aspects like giving praise, soliciting for viewpoints, humor, etc and non-verbal expressions like eye contact, facial expressions, gestures, etc which make teachers to be closer to learners psychologically (Kelley & Gorham, 2009 ).
Learner outcomes
The outcomes under scrutiny in this study include performance, motivation, satisfaction and knowledge construction. Motivation is seen here as an outcome because, much as cognitive factors such as course grades are used in measuring learning outcomes, affective factors like intrinsic motivation may also be used to indicate outcomes of learning (Kuo, Walker, Belland, & Schroder, 2013 ). Research shows that high motivation among online learners leads to persistence in their courses (Menager-Beeley, 2004 ). Sankaran and Bui ( 2001 ) indicated that less motivated learners performed poorly in knowledge tests while those with high learning motivation demonstrate high performance in academics (Green, Nelson, Martin, & Marsh, 2006 ). Lim and Kim, ( 2003 ) indicated that learner interest as a motivation factor promotes learner involvement in learning and this could lead to learning effectiveness in blended learning.
Learner satisfaction was noted as a strong factor for effectiveness of blended and online courses (Wilging & Johnson, 2009) and dissatisfaction may result from learners’ incompetence in the use of the learning management system as an effective learning tool since, as Islam ( 2014 ) puts it, users may be dissatisfied with an information system due to ease of use. A lack of prompt feedback for learners from course instructors was found to cause dissatisfaction in an online graduate course. In addition, dissatisfaction resulted from technical difficulties as well as ambiguous course instruction Hara and Kling ( 2001 ). These factors, once addressed, can lead to learner satisfaction in e-learning and blended learning and eventual effectiveness. A study by Blocker and Tucker ( 2001 ) also showed that learners had difficulties with technology and inadequate group participation by peers leading to dissatisfaction within these design features. Student-teacher interactions are known to bring satisfaction within online courses. Study results by Swan ( 2001 ) indicated that student-teacher interaction strongly related with student satisfaction and high learner-learner interaction resulted in higher levels of course satisfaction. Descriptive results by Naaj, Nachouki, and Ankit ( 2012 ) showed that learners were satisfied with technology which was a video-conferencing component of blended learning with a mean of 3.7. The same study indicated student satisfaction with instructors at a mean of 3.8. Askar and Altun, ( 2008 ) found that learners were satisfied with face-to-face sessions of the blend with t-tests and ANOVA results indicating female scores as higher than for males in the satisfaction with face-to-face environment of the blended learning.
Studies comparing blended learning with traditional face-to-face have indicated that learners perform equally well in blended learning and their performance is unaffected by the delivery method (Kwak, Menezes, & Sherwood, 2013 ). In another study, learning experience and performance are known to improve when traditional course delivery is integrated with online learning (Stacey & Gerbic, 2007 ). Such improvement as noted may be an indicator of blended learning effectiveness. Our study however, delves into improved performance but seeks to establish the potential of blended learning effectiveness by considering grades obtained in a blended learning experiment. Score 50 and above is considered a pass in this study’s setting and learners scoring this and above will be considered to have passed. This will make our conclusions about the potential of blended learning effectiveness.
Regarding knowledge construction, it has been noted that effective learning occurs where learners are actively involved (Nurmela, Palonen, Lehtinen & Hakkarainen, 2003 , cited in Zhu, 2012 ) and this may be an indicator of learning environment effectiveness. Effective blended learning would require that learners are able to initiate, discover and accomplish the processes of knowledge construction as antecedents of blended learning effectiveness. A study by Rahman, Yasin and Jusoff ( 2011 ) indicated that learners were able to use some steps to construct meaning through an online discussion process through assignments given. In the process of giving and receiving among themselves, the authors noted that learners learned by writing what they understood. From our perspective, this can be considered to be accomplishment in the knowledge construction process. Their study further shows that learners construct meaning individually from assignments and this stage is referred to as pre-construction which for our study, is an aspect of discovery in the knowledge construction process.
Predictors of blended learning effectiveness
Researchers have dealt with success factors for online learning or those for traditional face-to-face learning but little is known about factors that predict blended learning effectiveness in view of learner characteristics and blended learning design features. This part of our study seeks to establish the learner characteristics/backgrounds and design features that predict blended learning effectiveness with regard to satisfaction, outcomes, motivation and knowledge construction. Song, Singleton, Hill, and Koh ( 2004 ) examined online learning effectiveness factors and found out that time management (a self-regulatory factor) was crucial for successful online learning. Eom, Wen, and Ashill ( 2006 ) using a survey found out that interaction, among other factors, was significant for learner satisfaction. Technical problems with regard to instructional design were a challenge to online learners thus not indicating effectiveness (Song et al., 2004 ), though the authors also indicated that descriptive statistics to a tune of 75% and time management (62%) impact on success of online learning. Arbaugh ( 2000 ) and Swan ( 2001 ) indicated that high levels of learner-instructor interaction are associated with high levels of user satisfaction and learning outcomes. A study by Naaj et al. ( 2012 ) indicated that technology and learner interactions, among other factors, influenced learner satisfaction in blended learning.
Objective and research questions of the current study
The objective of the current study is to investigate the effectiveness of blended learning in view of student satisfaction, knowledge construction, performance and intrinsic motivation and how they are related to student characteristics and blended learning design features in a blended learning environment.
Research questions
What are the student characteristics and blended learning design features for an effective blended learning environment?
Which factors (among the learner characteristics and blended learning design features) predict student satisfaction, learning outcomes, intrinsic motivation and knowledge construction?
Conceptual model of the present study
The reviewed literature clearly shows learner characteristics/background and blended learning design features play a part in blended learning effectiveness and some of them are significant predictors of effectiveness. The conceptual model for our study is depicted as follows (Fig. 1 ):
Conceptual model of the current study
Research design
This research applies a quantitative design where descriptive statistics are used for the student characteristics and design features data, t-tests for the age and gender variables to determine if they are significant in blended learning effectiveness and regression for predictors of blended learning effectiveness.
This study is based on an experiment in which learners participated during their study using face-to-face sessions and an on-line session of a blended learning design. A learning management system (Moodle) was used and learner characteristics/background and blended learning design features were measured in relation to learning effectiveness. It is therefore a planning evaluation research design as noted by Guskey ( 2000 ) since the outcomes are aimed at blended learning implementation at MMU. The plan under which the various variables were tested involved face-to-face study at the beginning of a 17 week semester which was followed by online teaching and learning in the second half of the semester. The last part of the semester was for another face-to-face to review work done during the online sessions and final semester examinations. A questionnaire with items on student characteristics, design features and learning outcomes was distributed among students from three schools and one directorate of postgraduate studies.
Participants
Cluster sampling was used to select a total of 238 learners to participate in this study. Out of the whole university population of students, three schools and one directorate were used. From these, one course unit was selected from each school and all the learners following the course unit were surveyed. In the school of Education ( n = 70) and Business and Management Studies ( n = 133), sophomore students were involved due to the fact that they have been introduced to ICT basics during their first year of study. Students of the third year were used from the department of technology in the School of Applied Sciences and Technology ( n = 18) since most of the year two courses had a lot of practical aspects that could not be used for the online learning part. From the Postgraduate Directorate ( n = 17), first and second year students were selected because learners attend a face-to-face session before they are given paper modules to study away from campus.
The study population comprised of 139 male students representing 58.4% and 99 females representing 41.6% with an average age of 24 years.
Instruments
The end of semester results were used to measure learner performance. The online self-regulated learning questionnaire (Barnard, Lan, To, Paton, & Lai, 2009 ) and the intrinsic motivation inventory (Deci & Ryan, 1982 ) were applied to measure the constructs on self regulation in the student characteristics and motivation in the learning outcome constructs. Other self-developed instruments were used for the other remaining variables of attitudes, computer competence, workload management, social and family support, satisfaction, knowledge construction, technology quality, interactions, learning management system tools and resources and face-to-face support.
Instrument reliability
Cronbach’s alpha was used to test reliability and the table below gives the results. All the scales and sub-scales had acceptable internal consistency reliabilities as shown in Table 1 below:
Data analysis
First, descriptive statistics was conducted. Shapiro-Wilk test was done to test normality of the data for it to qualify for parametric tests. The test results for normality of our data before the t- test resulted into significant levels (Male = .003, female = .000) thereby violating the normality assumption. We therefore used the skewness and curtosis results which were between −1.0 and +1.0 and assumed distribution to be sufficiently normal to qualify the data for a parametric test, (Pallant, 2010 ). An independent samples t -test was done to find out the differences in male and female performance to explain the gender characteristics in blended learning effectiveness. A one-way ANOVA between subjects was conducted to establish the differences in performance between age groups. Finally, multiple regression analysis was done between student variables and design elements with learning outcomes to determine the significant predictors for blended learning effectiveness.
Student characteristics, blended learning design features and learning outcomes ( RQ1 )
A t- test was carried out to establish the performance of male and female learners in the blended learning set up. This was aimed at finding out if male and female learners do perform equally well in blended learning given their different roles and responsibilities in society. It was found that male learners performed slightly better ( M = 62.5) than their female counterparts ( M = 61.1). An independent t -test revealed that the difference between the performances was not statistically significant ( t = 1.569, df = 228, p = 0.05, one tailed). The magnitude of the differences in the means is small with effect size ( d = 0.18). A one way between subjects ANOVA was conducted on the performance of different age groups to establish the performance of learners of young and middle aged age groups (20–30, young & and 31–39, middle aged). This revealed a significant difference in performance (F(1,236 = 8.498, p < . 001).
Average percentages of the items making up the self regulated learning scale are used to report the findings about all the sub-scales in the learner characteristics/background scale. Results show that learner self-regulation was good enough at 72.3% in all the sub-scales of goal setting, environment structuring, task strategies, time management, help-seeking and self-evaluation among learners. The least in the scoring was task strategies at 67.7% and the highest was learner environment structuring at 76.3%. Learner attitude towards blended learning environment is at 76% in the sub-scales of learner autonomy, quality of instructional materials, course structure, course interface and interactions. The least scored here is attitude to course structure at 66% and their attitudes were high on learner autonomy and course interface both at 82%. Results on the learners’ computer competences are summarized in percentages in the table below (Table 2 ):
It can be seen that learners are skilled in word processing at 91%, email at 63.5%, spreadsheets at 68%, web browsers at 70.2% and html tools at 45.4%. They are therefore good enough in word processing and web browsing. Their computer confidence levels are reported at 75.3% and specifically feel very confident when it comes to working with a computer (85.7%). Levels of family and social support for learners during blended learning experiences are at 60.5 and 75% respectively. There is however a low score on learners being assisted by family members in situations of computer setbacks (33.2%) as 53.4% of the learners reported no assistance in this regard. A higher percentage (85.3%) is reported on learners getting support from family regarding provision of essentials for learning such as tuition. A big percentage of learners spend two hours on study while at home (35.3%) followed by one hour (28.2%) while only 9.7% spend more than three hours on study at home. Peers showed great care during the blended learning experience (81%) and their experiences were appreciated by the society (66%). Workload management by learners vis-à-vis studying is good at 60%. Learners reported that their workmates stand in for them at workplaces to enable them do their study in blended learning while 61% are encouraged by their bosses to go and improve their skills through further education and training. On the time spent on other activities not related to study, majority of the learners spend three hours (35%) while 19% spend 6 hours. Sixty percent of the learners have to answer to someone when they are not attending to other activities outside study compared to the 39.9% who do not and can therefore do study or those other activities.
The usability of the online system, tools and resources was below average as shown in the table below in percentages (Table 3 ):
However, learners became skilled at navigating around the learning management system (79%) and it was easy for them to locate course content, tools and resources needed such as course works, news, discussions and journal materials. They effectively used the communication tools (60%) and to work with peers by making posts (57%). They reported that online resources were well organized, user friendly and easy to access (71%) as well as well structured in a clear and understandable manner (72%). They therefore recommended the use of online resources for other course units in future (78%) because they were satisfied with them (64.3%). On the whole, the online resources were fine for the learners (67.2%) and useful as a learning resource (80%). The learners’ perceived usefulness/satisfaction with online system, tools, and resources was at 81% as the LMS tools helped them to communicate, work with peers and reflect on their learning (74%). They reported that using moodle helped them to learn new concepts, information and gaining skills (85.3%) as well as sharing what they knew or learned (76.4%). They enjoyed the course units (78%) and improved their skills with technology (89%).
Learner interactions were seen from three angles of cognitivism, collaborative learning and student-teacher interactions. Collaborative learning was average at 50% with low percentages in learners posting challenges to colleagues’ ideas online (34%) and posting ideas for colleagues to read online (37%). They however met oftentimes online (60%) and organized how they would work together in study during the face-to-face meetings (69%). The common form of communication medium frequently used by learners during the blended learning experience was by phone (34.5%) followed by whatsapp (21.8%), face book (21%), discussion board (11.8%) and email (10.9%). At the cognitive level, learners interacted with content at 72% by reading the posted content (81%), exchanging knowledge via the LMS (58.4%), participating in discussions on the forum (62%) and got course objectives and structure introduced during the face-to-face sessions (86%). Student-teacher interaction was reported at 71% through instructors individually working with them online (57.2%) and being well guided towards learning goals (81%). They did receive suggestions from instructors about resources to use in their learning (75.3%) and instructors provided learning input for them to come up with their own answers (71%).
The technology quality during the blended learning intervention was rated at 69% with availability of 72%, quality of the resources was at 68% with learners reporting that discussion boards gave right content necessary for study (71%) and the email exchanges containing relevant and much needed information (63.4%) as well as chats comprising of essential information to aid the learning (69%). Internet reliability was rated at 66% with a speed considered averagely good to facilitate online activities (63%). They however reported that there was intermittent breakdown during online study (67%) though they could complete their internet program during connection (63.4%). Learners eventually found it easy to download necessary materials for study in their blended learning experiences (71%).
Learner extent of use of the learning management system features was as shown in the table below in percentage (Table 4 ):
From the table, very rarely used features include the blog and wiki while very often used ones include the email, forum, chat and calendar.
The effectiveness of the LMS was rated at 79% by learners reporting that they found it useful (89%) and using it makes their learning activities much easier (75.2%). Moodle has helped learners to accomplish their learning tasks more quickly (74%) and that as a LMS, it is effective in teaching and learning (88%) with overall satisfaction levels at 68%. However, learners note challenges in the use of the LMS regarding its performance as having been problematic to them (57%) and only 8% of the learners reported navigation while 16% reported access as challenges.
Learner attitudes towards Face-to-face support were reported at 88% showing that the sessions were enjoyable experiences (89%) with high quality class discussions (86%) and therefore recommended that the sessions should continue in blended learning (89%). The frequency of the face-to-face sessions is shown in the table below as preferred by learners (Table 5 ).
Learners preferred face-to-face sessions after every month in the semester (33.6%) and at the beginning of the blended learning session only (27.7%).
Learners reported high intrinsic motivation levels with interest and enjoyment of tasks at 83.7%, perceived competence at 70.2%, effort/importance sub-scale at 80%, pressure/tension reported at 54%. The pressure percentage of 54% arises from learners feeling nervous (39.2%) and a lot of anxiety (53%) while 44% felt a lot of pressure during the blended learning experiences. Learners however reported the value/usefulness of blended learning at 91% with majority believing that studying online and face-to-face had value for them (93.3%) and were therefore willing to take part in blended learning (91.2%). They showed that it is beneficial for them (94%) and that it was an important way of studying (84.3%).
Learner satisfaction was reported at 81% especially with instructors (85%) high percentage reported on encouraging learner participation during the course of study 93%, course content (83%) with the highest being satisfaction with the good relationship between the objectives of the course units and the content (90%), technology (71%) with a high percentage on the fact that the platform was adequate for the online part of the learning (76%), interactions (75%) with participation in class at 79%, and face-to-face sessions (91%) with learner satisfaction high on face-to-face sessions being good enough for interaction and giving an overview of the courses when objectives were introduced at 92%.
Learners’ knowledge construction was reported at 78% with initiation and discovery scales scoring 84% with 88% specifically for discovering the learning points in the course units. The accomplishment scale in knowledge construction scored 71% and specifically the fact that learners were able to work together with group members to accomplish learning tasks throughout the study of the course units (79%). Learners developed reports from activities (67%), submitted solutions to discussion questions (68%) and did critique peer arguments (69%). Generally, learners performed well in blended learning in the final examination with an average pass of 62% and standard deviation of 7.5.
Significant predictors of blended learning effectiveness ( RQ 2)
A standard multiple regression analysis was done taking learner characteristics/background and design features as predictor variables and learning outcomes as criterion variables. The data was first tested to check if it met the linear regression test assumptions and results showed the correlations between the independent variables and each of the dependent variables (highest 0.62 and lowest 0.22) as not being too high, which indicated that multicollinearity was not a problem in our model. From the coefficients table, the VIF values ranged from 1.0 to 2.4, well below the cut off value of 10 and indicating no possibility of multicollinearity. The normal probability plot was seen to lie as a reasonably straight diagonal from bottom left to top right indicating normality of our data. Linearity was found suitable from the scatter plot of the standardized residuals and was rectangular in distribution. Outliers were no cause for concern in our data since we had only 1% of all cases falling outside 3.0 thus proving the data as a normally distributed sample. Our R -square values was at 0.525 meaning that the independent variables explained about 53% of the variance in overall satisfaction, motivation and knowledge construction of the learners. All the models explaining the three dependent variables of learner satisfaction, intrinsic motivation and knowledge construction were significant at the 0.000 probability level (Table 6 ).
From the table above, design features (technology quality and online tools and resources), and learner characteristics (attitudes to blended learning, self-regulation) were significant predictors of learner satisfaction in blended learning. This means that good technology with the features involved and the learner positive attitudes with capacity to do blended learning with self drive led to their satisfaction. The design features (technology quality, interactions) and learner characteristics (self regulation and social support), were found to be significant predictors of learner knowledge construction. This implies that learners’ capacity to go on their work by themselves supported by peers and high levels of interaction using the quality technology led them to construct their own ideas in blended learning. Design features (technology quality, online tools and resources as well as learner interactions) and learner characteristics (self regulation), significantly predicted the learners’ intrinsic motivation in blended learning suggesting that good technology, tools and high interaction levels with independence in learning led to learners being highly motivated. Finally, none of the independent variables considered under this study were predictors of learning outcomes (grade).
In this study we have investigated learning outcomes as dependent variables to establish if particular learner characteristics/backgrounds and design features are related to the outcomes for blended learning effectiveness and if they predict learning outcomes in blended learning. We took students from three schools out of five and one directorate of post-graduate studies at a Ugandan University. The study suggests that the characteristics and design features examined are good drivers towards an effective blended learning environment though a few of them predicted learning outcomes in blended learning.
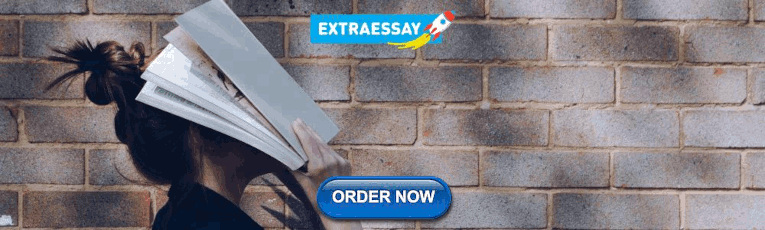
Student characteristics/background, blended learning design features and learning outcomes
The learner characteristics, design features investigated are potentially important for an effective blended learning environment. Performance by gender shows a balance with no statistical differences between male and female. There are statistically significant differences ( p < .005) in the performance between age groups with means of 62% for age group 20–30 and 67% for age group 31 –39. The indicators of self regulation exist as well as positive attitudes towards blended learning. Learners do well with word processing, e-mail, spreadsheets and web browsers but still lag below average in html tools. They show computer confidence at 75.3%; which gives prospects for an effective blended learning environment in regard to their computer competence and confidence. The levels of family and social support for learners stand at 61 and 75% respectively, indicating potential for blended learning to be effective. The learners’ balance between study and work is a drive factor towards blended learning effectiveness since their management of their workload vis a vis study time is at 60 and 61% of the learners are encouraged to go for study by their bosses. Learner satisfaction with the online system and its tools shows prospect for blended learning effectiveness but there are challenges in regard to locating course content and assignments, submitting their work and staying on a task during online study. Average collaborative, cognitive learning as well as learner-teacher interactions exist as important factors. Technology quality for effective blended learning is a potential for effectiveness though features like the blog and wiki are rarely used by learners. Face-to-face support is satisfactory and it should be conducted every month. There is high intrinsic motivation, satisfaction and knowledge construction as well as good performance in examinations ( M = 62%, SD = 7.5); which indicates potentiality for blended learning effectiveness.
Significant predictors of blended learning effectiveness
Among the design features, technology quality, online tools and face-to-face support are predictors of learner satisfaction while learner characteristics of self regulation and attitudes to blended learning are predictors of satisfaction. Technology quality and interactions are the only design features predicting learner knowledge construction, while social support, among the learner backgrounds, is a predictor of knowledge construction. Self regulation as a learner characteristic is a predictor of knowledge construction. Self regulation is the only learner characteristic predicting intrinsic motivation in blended learning while technology quality, online tools and interactions are the design features predicting intrinsic motivation. However, all the independent variables are not significant predictors of learning performance in blended learning.
The high computer competences and confidence is an antecedent factor for blended learning effectiveness as noted by Hadad ( 2007 ) and this study finds learners confident and competent enough for the effectiveness of blended learning. A lack in computer skills causes failure in e-learning and blended learning as noted by Shraim and Khlaif ( 2010 ). From our study findings, this is no threat for blended learning our case as noted by our results. Contrary to Cohen et al. ( 2012 ) findings that learners’ family responsibilities and hours of employment can impede their process of learning, it is not the case here since they are drivers to the blended learning process. Time conflict, as compounded by family, employment status and management support (Packham et al., 2004 ) were noted as causes of learner failure and drop out of online courses. Our results show, on the contrary, that these factors are drivers for blended learning effectiveness because learners have a good balance between work and study and are supported by bosses to study. In agreement with Selim ( 2007 ), learner positive attitudes towards e-and blended learning environments are success factors. In line with Coldwell et al. ( 2008 ), no statistically significant differences exist between age groups. We however note that Coldwel, et al dealt with young, middle-aged and old above 45 years whereas we dealt with young and middle aged only.
Learner interactions at all levels are good enough and contrary to Astleitner, ( 2000 ) that their absence makes learners withdraw, they are a drive factor here. In line with Loukis (2007) the LMS quality, reliability and ease of use lead to learning efficiency as technology quality, online tools are predictors of learner satisfaction and intrinsic motivation. Face-to-face sessions should continue on a monthly basis as noted here and is in agreement with Marriot et al. ( 2004 ) who noted learner preference for it for facilitating social interaction and communication skills. High learner intrinsic motivation leads to persistence in online courses as noted by Menager-Beeley, ( 2004 ) and is high enough in our study. This implies a possibility of an effectiveness blended learning environment. The causes of learner dissatisfaction noted by Islam ( 2014 ) such as incompetence in the use of the LMS are contrary to our results in our study, while the one noted by Hara and Kling, ( 2001 ) as resulting from technical difficulties and ambiguous course instruction are no threat from our findings. Student-teacher interaction showed a relation with satisfaction according to Swan ( 2001 ) but is not a predictor in our study. Initiating knowledge construction by learners for blended learning effectiveness is exhibited in our findings and agrees with Rahman, Yasin and Jusof ( 2011 ). Our study has not agreed with Eom et al. ( 2006 ) who found learner interactions as predictors of learner satisfaction but agrees with Naaj et al. ( 2012 ) regarding technology as a predictor of learner satisfaction.
Conclusion and recommendations
An effective blended learning environment is necessary in undertaking innovative pedagogical approaches through the use of technology in teaching and learning. An examination of learner characteristics/background, design features and learning outcomes as factors for effectiveness can help to inform the design of effective learning environments that involve face-to-face sessions and online aspects. Most of the student characteristics and blended learning design features dealt with in this study are important factors for blended learning effectiveness. None of the independent variables were identified as significant predictors of student performance. These gaps are open for further investigation in order to understand if they can be significant predictors of blended learning effectiveness in a similar or different learning setting.
In planning to design and implement blended learning, we are mindful of the implications raised by this study which is a planning evaluation research for the design and eventual implementation of blended learning. Universities should be mindful of the interplay between the learner characteristics, design features and learning outcomes which are indicators of blended learning effectiveness. From this research, learners manifest high potential to take on blended learning more especially in regard to learner self-regulation exhibited. Blended learning is meant to increase learners’ levels of knowledge construction in order to create analytical skills in them. Learner ability to assess and critically evaluate knowledge sources is hereby established in our findings. This can go a long way in producing skilled learners who can be innovative graduates enough to satisfy employment demands through creativity and innovativeness. Technology being less of a shock to students gives potential for blended learning design. Universities and other institutions of learning should continue to emphasize blended learning approaches through installation of learning management systems along with strong internet to enable effective learning through technology especially in the developing world.
Abubakar, D. & Adetimirin. (2015). Influence of computer literacy on post-graduates’ use of e-resources in Nigerian University Libraries. Library Philosophy and Practice. From http://digitalcommons.unl.edu/libphilprac/ . Retrieved 18 Aug 2015.
Ahmad, N., & Al-Khanjari, Z. (2011). Effect of Moodle on learning: An Oman perception. International Journal of Digital Information and Wireless Communications (IJDIWC), 1 (4), 746–752.
Google Scholar
Anderson, T. (2004). Theory and Practice of Online Learning . Canada: AU Press, Athabasca University.
Arbaugh, J. B. (2000). How classroom environment and student engagement affect learning in internet-basedMBAcourses. Business Communication Quarterly, 63 (4), 9–18.
Article Google Scholar
Askar, P. & Altun, A. (2008). Learner satisfaction on blended learning. E-Leader Krakow , 2008.
Astleitner, H. (2000) Dropout and distance education. A review of motivational and emotional strategies to reduce dropout in web-based distance education. In Neuwe Medien in Unterricht, Aus-und Weiterbildung Waxmann Munster, New York.
Barnard, L., Lan, W. Y., To, Y. M., Paton, V. O., & Lai, S. (2009). Measuring self regulation in online and blended learning environments’. Internet and Higher Education, 12 (1), 1–6.
Beard, L. A., Harper, C., & Riley, G. (2004). Online versus on-campus instruction: student attitudes & perceptions. TechTrends, 48 (6), 29–31.
Berenson, R., Boyles, G., & Weaver, A. (2008). Emotional intelligence as a predictor for success in online learning. International Review of Research in open & Distance Learning, 9 (2), 1–16.
Blocker, J. M., & Tucker, G. (2001). Using constructivist principles in designing and integrating online collaborative interactions. In F. Fuller & R. McBride (Eds.), Distance education. Proceedings of the Society for Information Technology & Teacher Education International Conference (pp. 32–36). ERIC Document Reproduction Service No. ED 457 822.
Cohen, K. E., Stage, F. K., Hammack, F. M., & Marcus, A. (2012). Persistence of master’s students in the United States: Developing and testing of a conceptual model . USA: PhD Dissertation, New York University.
Coldwell, J., Craig, A., Paterson, T., & Mustard, J. (2008). Online students: Relationships between participation, demographics and academic performance. The Electronic Journal of e-learning, 6 (1), 19–30.
Deci, E. L., & Ryan, R. M. (1982). Intrinsic Motivation Inventory. Available from selfdeterminationtheory.org/intrinsic-motivation-inventory/ . Accessed 2 Aug 2016.
Delone, W. H., & McLean, E. R. (2003). The Delone and McLean model of information systems success: A Ten-year update. Journal of Management Information Systems, 19 (4), 9–30.
Demirkol, M., & Kazu, I. Y. (2014). Effect of blended environment model on high school students’ academic achievement. The Turkish Online Journal of Educational Technology, 13 (1), 78–87.
Eom, S., Wen, H., & Ashill, N. (2006). The determinants of students’ perceived learning outcomes and satisfaction in university online education: an empirical investigation’. Decision Sciences Journal of Innovative Education, 4 (2), 215–235.
Garrison, D. R., & Kanuka, H. (2004). Blended learning: Uncovering its transformative potential in higher education. Internet and Higher Education, 7 (2), 95–105.
Goyal, E., & Tambe, S. (2015). Effectiveness of Moodle-enabled blended learning in private Indian Business School teaching NICHE programs. The Online Journal of New Horizons in Education, 5 (2), 14–22.
Green, J., Nelson, G., Martin, A. J., & Marsh, H. (2006). The causal ordering of self-concept and academic motivation and its effect on academic achievement. International Education Journal, 7 (4), 534–546.
Guskey, T. R. (2000). Evaluating Professional Development . Thousands Oaks: Corwin Press.
Hadad, W. (2007). ICT-in-education toolkit reference handbook . InfoDev. from http://www.infodev.org/en/Publication.301.html . Retrieved 04 Aug 2015.
Hara, N. & Kling, R. (2001). Student distress in web-based distance education. Educause Quarterly. 3 (2001).
Heinich, R., Molenda, M., Russell, J. D., & Smaldino, S. E. (2001). Instructional Media and Technologies for Learning (7th ed.). Englewood Cliffs: Prentice-Hall.
Hofmann, J. (2014). Solutions to the top 10 challenges of blended learning. Top 10 challenges of blended learning. Available on cedma-europe.org .
Islam, A. K. M. N. (2014). Sources of satisfaction and dissatisfaction with a learning management system in post-adoption stage: A critical incident technique approach. Computers in Human Behaviour, 30 , 249–261.
Kelley, D. H. & Gorham, J. (2009) Effects of immediacy on recall of information. Communication Education, 37 (3), 198–207.
Kenney, J., & Newcombe, E. (2011). Adopting a blended learning approach: Challenges, encountered and lessons learned in an action research study. Journal of Asynchronous Learning Networks, 15 (1), 45–57.
Kintu, M. J., & Zhu, C. (2016). Student characteristics and learning outcomes in a blended learning environment intervention in a Ugandan University. Electronic Journal of e-Learning, 14 (3), 181–195.
Kuo, Y., Walker, A. E., Belland, B. R., & Schroder, L. E. E. (2013). A predictive study of student satisfaction in online education programs. International Review of Research in Open and Distributed Learning, 14 (1), 16–39.
Kwak, D. W., Menezes, F. M., & Sherwood, C. (2013). Assessing the impact of blended learning on student performance. Educational Technology & Society, 15 (1), 127–136.
Lim, D. H., & Kim, H. J. (2003). Motivation and learner characteristics affecting online learning and learning application. Journal of Educational Technology Systems, 31 (4), 423–439.
Lim, D. H., & Morris, M. L. (2009). Learner and instructional factors influencing learner outcomes within a blended learning environment. Educational Technology & Society, 12 (4), 282–293.
Lin, B., & Vassar, J. A. (2009). Determinants for success in online learning communities. International Journal of Web-based Communities, 5 (3), 340–350.
Loukis, E., Georgiou, S. & Pazalo, K. (2007). A value flow model for the evaluation of an e-learning service. ECIS, 2007 Proceedings, paper 175.
Lynch, R., & Dembo, M. (2004). The relationship between self regulation and online learning in a blended learning context. The International Review of Research in Open and Distributed Learning, 5 (2), 1–16.
Marriot, N., Marriot, P., & Selwyn. (2004). Accounting undergraduates’ changing use of ICT and their views on using the internet in higher education-A Research note. Accounting Education, 13 (4), 117–130.
Menager-Beeley, R. (2004). Web-based distance learning in a community college: The influence of task values on task choice, retention and commitment. (Doctoral dissertation, University of Southern California). Dissertation Abstracts International, 64 (9-A), 3191.
Naaj, M. A., Nachouki, M., & Ankit, A. (2012). Evaluating student satisfaction with blended learning in a gender-segregated environment. Journal of Information Technology Education: Research, 11 , 185–200.
Nurmela, K., Palonen, T., Lehtinen, E. & Hakkarainen, K. (2003). Developing tools for analysing CSCL process. In Wasson, B. Ludvigsen, S. & Hoppe, V. (eds), Designing for change in networked learning environments (pp 333–342). Dordrecht, The Netherlands, Kluwer.
Osgerby, J. (2013). Students’ perceptions of the introduction of a blended learning environment: An exploratory case study. Accounting Education, 22 (1), 85–99.
Oxford Group, (2013). Blended learning-current use, challenges and best practices. From http://www.kineo.com/m/0/blended-learning-report-202013.pdf . Accessed on 17 Mar 2016.
Packham, G., Jones, P., Miller, C., & Thomas, B. (2004). E-learning and retention key factors influencing student withdrawal. Education and Training, 46 (6–7), 335–342.
Pallant, J. (2010). SPSS Survival Mannual (4th ed.). Maidenhead: OUP McGraw-Hill.
Park, J.-H., & Choi, H. J. (2009). Factors influencing adult learners’ decision to drop out or persist in online learning. Educational Technology & Society, 12 (4), 207–217.
Picciano, A., & Seaman, J. (2007). K-12 online learning: A survey of U.S. school district administrators . New York, USA: Sloan-C.
Piccoli, G., Ahmad, R., & Ives, B. (2001). Web-based virtual learning environments: a research framework and a preliminary assessment of effectiveness in basic IT skill training. MIS Quarterly, 25 (4), 401–426.
Pituch, K. A., & Lee, Y. K. (2006). The influence of system characteristics on e-learning use. Computers & Education, 47 (2), 222–244.
Rahman, S. et al, (2011). Knowledge construction process in online learning. Middle East Journal of Scientific Research, 8 (2), 488–492.
Rovai, A. P. (2003). In search of higher persistence rates in distance education online programs. Computers & Education, 6 (1), 1–16.
Sankaran, S., & Bui, T. (2001). Impact of learning strategies and motivation on performance: A study in Web-based instruction. Journal of Instructional Psychology, 28 (3), 191–198.
Selim, H. M. (2007). Critical success factors for e-learning acceptance: Confirmatory factor models. Computers & Education, 49 (2), 396–413.
Shraim, K., & Khlaif, Z. N. (2010). An e-learning approach to secondary education in Palestine: opportunities and challenges. Information Technology for Development, 16 (3), 159–173.
Shrain, K. (2012). Moving towards e-learning paradigm: Readiness of higher education instructors in Palestine. International Journal on E-Learning, 11 (4), 441–463.
Song, L., Singleton, E. S., Hill, J. R., & Koh, M. H. (2004). Improving online learning: student perceptions of useful and challenging characteristics’. Internet and Higher Education, 7 (1), 59–70.
Stacey, E., & Gerbic, P. (2007). Teaching for blended learning: research perspectives from on-campus and distance students. Education and Information Technologies, 12 , 165–174.
Swan, K. (2001). Virtual interactivity: design factors affecting student satisfaction and perceived learning in asynchronous online courses. Distance Education, 22 (2), 306–331.
Article MathSciNet Google Scholar
Thompson, E. (2004). Distance education drop-out: What can we do? In R. Pospisil & L. Willcoxson (Eds.), Learning Through Teaching (Proceedings of the 6th Annual Teaching Learning Forum, pp. 324–332). Perth, Australia: Murdoch University.
Tselios, N., Daskalakis, S., & Papadopoulou, M. (2011). Assessing the acceptance of a blended learning university course. Educational Technology & Society, 14 (2), 224–235.
Willging, P. A., & Johnson, S. D. (2009). Factors that influence students’ decision to drop-out of online courses. Journal of Asynchronous Learning Networks, 13 (3), 115–127.
Zhu, C. (2012). Student satisfaction, performance and knowledge construction in online collaborative learning. Educational Technology & Society, 15 (1), 127–137.
Zielinski, D. (2000). Can you keep learners online? Training, 37 (3), 64–75.
Download references
Authors’ contribution
MJK conceived the study idea, developed the conceptual framework, collected the data, analyzed it and wrote the article. CZ gave the technical advice concerning the write-up and advised on relevant corrections to be made before final submission. EK did the proof-reading of the article as well as language editing. All authors read and approved the final manuscript.
Competing interests
The authors declare that they have no competing interests.
Author information
Authors and affiliations.
Mountains of the Moon University, P.O. Box 837, Fort Portal, Uganda
Mugenyi Justice Kintu & Edmond Kagambe
Vrije Universiteit Brussel, Pleinlaan 2, Brussels, 1050, Ixelles, Belgium
Mugenyi Justice Kintu & Chang Zhu
You can also search for this author in PubMed Google Scholar
Corresponding author
Correspondence to Mugenyi Justice Kintu .
Rights and permissions
Open Access This article is distributed under the terms of the Creative Commons Attribution 4.0 International License ( http://creativecommons.org/licenses/by/4.0/ ), which permits unrestricted use, distribution, and reproduction in any medium, provided you give appropriate credit to the original author(s) and the source, provide a link to the Creative Commons license, and indicate if changes were made.
Reprints and permissions
About this article
Cite this article.
Kintu, M.J., Zhu, C. & Kagambe, E. Blended learning effectiveness: the relationship between student characteristics, design features and outcomes. Int J Educ Technol High Educ 14 , 7 (2017). https://doi.org/10.1186/s41239-017-0043-4
Download citation
Received : 13 July 2016
Accepted : 23 November 2016
Published : 06 February 2017
DOI : https://doi.org/10.1186/s41239-017-0043-4
Share this article
Anyone you share the following link with will be able to read this content:
Sorry, a shareable link is not currently available for this article.
Provided by the Springer Nature SharedIt content-sharing initiative
- Blended learning effectiveness
- Learner characteristics
- Design features
- Learning outcomes and significant predictors
Thank you for visiting nature.com. You are using a browser version with limited support for CSS. To obtain the best experience, we recommend you use a more up to date browser (or turn off compatibility mode in Internet Explorer). In the meantime, to ensure continued support, we are displaying the site without styles and JavaScript.
- View all journals
- Explore content
- About the journal
- Publish with us
- Sign up for alerts
- Published: 12 April 2024
Pretraining a foundation model for generalizable fluorescence microscopy-based image restoration
- Chenxi Ma 1 na1 ,
- Weimin Tan ORCID: orcid.org/0000-0001-7677-4772 1 na1 ,
- Ruian He 1 &
- Bo Yan ORCID: orcid.org/0000-0001-5692-3486 1
Nature Methods ( 2024 ) Cite this article
20 Altmetric
Metrics details
- Confocal microscopy
- Image processing
- Super-resolution microscopy
- Wide-field fluorescence microscopy
Fluorescence microscopy-based image restoration has received widespread attention in the life sciences and has led to significant progress, benefiting from deep learning technology. However, most current task-specific methods have limited generalizability to different fluorescence microscopy-based image restoration problems. Here, we seek to improve generalizability and explore the potential of applying a pretrained foundation model to fluorescence microscopy-based image restoration. We provide a universal fluorescence microscopy-based image restoration (UniFMIR) model to address different restoration problems, and show that UniFMIR offers higher image restoration precision, better generalization and increased versatility. Demonstrations on five tasks and 14 datasets covering a wide range of microscopy imaging modalities and biological samples demonstrate that the pretrained UniFMIR can effectively transfer knowledge to a specific situation via fine-tuning, uncover clear nanoscale biomolecular structures and facilitate high-quality imaging. This work has the potential to inspire and trigger new research highlights for fluorescence microscopy-based image restoration.
This is a preview of subscription content, access via your institution
Access options
Access Nature and 54 other Nature Portfolio journals
Get Nature+, our best-value online-access subscription
24,99 € / 30 days
cancel any time
Subscribe to this journal
Receive 12 print issues and online access
251,40 € per year
only 20,95 € per issue
Rent or buy this article
Prices vary by article type
Prices may be subject to local taxes which are calculated during checkout
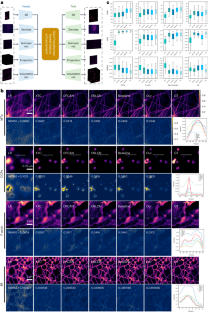
Similar content being viewed by others
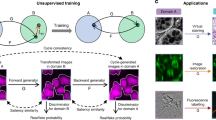
Unsupervised content-preserving transformation for optical microscopy
Xinyang Li, Guoxun Zhang, … Qionghai Dai
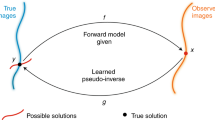
Applications, promises, and pitfalls of deep learning for fluorescence image reconstruction
Chinmay Belthangady & Loic A. Royer
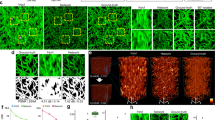
Deep learning enables reference-free isotropic super-resolution for volumetric fluorescence microscopy
Hyoungjun Park, Myeongsu Na, … Jong Chul Ye
Data availability
All training and testing data involved in the experiments come from existing literature and can be downloaded from the corresponding links provided in Supplementary Table 2 or via Zenodo at https://doi.org/10.5281/zenodo.8401470 (ref. 55 ).
Code availability
The PyTorch code of our UniFMIR, together with trained models, as well as some example images for inference are publicly available at https://github.com/cxm12/UNiFMIR ( https://doi.org/10.5281/zenodo.10117581 ) 56 . Furthermore, We also provide a live demo for UniFMIR at http://unifmir.fdudml.cn/ . Users can also access the colab at https://colab.research.google.com/github/cxm12/UNiFMIR/blob/main/UniFMIR.ipynb or use the steps in our GitHub documentation to run the demo locally. This newly built interactive software platform facilitates users to freely and easily use the pretrained foundation model. It also makes it easy for us to continuously train the foundation model with new data and share it with the community. Finally, we shared all models on BioImage.IO at https://bioimage.io/#/ . Data are available via Zenodo at https://doi.org/10.5281/zenodo.10577218 , https://doi.org/10.5281/zenodo.10579778 , https://doi.org/10.5281/zenodo.10579822 , https://doi.org/10.5281/zenodo.10595428 , https://doi.org/10.5281/zenodo.10595460 , https://doi.org/10.5281/zenodo.8420081 and https://doi.org/10.5281/zenodo.8420100 (refs. 57 , 58 , 59 , 60 , 61 , 62 , 63 ). We used the Pycharm software for code development.
Preibisch, S. et al. Efficient bayesian-based multiview deconvolution. Nat. Methods 11 , 645–648 (2014).
Article CAS PubMed PubMed Central Google Scholar
Gustafsson, N. et al. Fast live-cell conventional fluorophore nanoscopy with ImageJ through super-resolution radial fluctuations. Nat. Commun. 7 , 12471 (2016).
Arigovindan, M. et al. High-resolution restoration of 3D structures from widefield images with extreme low signal-to-noise-ratio. Proc. Natl Acad. Sci. USA 110 , 17344–17349 (2013).
Weigert, M. et al. Content-aware image restoration: pushing the limits of fluorescence microscopy. Nat. Methods 15 , 1090–1097 (2018).
Article CAS PubMed Google Scholar
Qiao, C. et al. Evaluation and development of deep neural networks for image super-resolution in optical microscopy. Nat. Methods 18 , 194–202 (2021).
Chen, J. et al. Three-dimensional residual channel attention networks denoise and sharpen fluorescence microscopy image volumes. Nat. Methods 18 , 678–687 (2021).
Wang, Z., Xie, Y. & Ji, S. Global voxel transformer networks for augmented microscopy. Nat. Mach. Intell. 3 , 161–171 (2021).
Article Google Scholar
Wang, Z. et al. Real-time volumetric reconstruction of biological dynamics with light-field microscopy and deep learning. Nat. Methods 18 , 551–556 (2021).
Li, X. et al. Reinforcing neuron extraction and spike inference in calcium imaging using deep self-supervised denoising. Nat. Methods 18 , 1395–1400 (2021).
Qiao, C. et al. Rationalized deep neural network for sustained super-resolution live imaging of rapid subcellular processes. Nat. Biotechol. 41 , 367–377 (2022).
Belthangady, C. & Royer, L. A. Applications, promises, and pitfalls of deep learning for fluorescence image reconstruction. Nat. Methods 16 , 1215–1225 (2019).
Wu, Y. & Shroff, H. Faster, sharper, and deeper: structured illumination microscopy for biological imaging. Nat. Methods 15 , 1011–1019 (2018).
Wu, Y. et al. Multiview confocal super-resolution microscopy. Nature 600 , 279–284 (2021).
Chen, R. et al. Single-frame deep-learning super-resolution microscopy for intracellular dynamics imaging. Nat. Commun. 14 , 2854 (2023).
Xu, Y. K. T. et al. Cross-modality supervised image restoration enables nanoscale tracking of synaptic plasticity in living mice. Nat. Methods 20 , 935–944 (2023).
Bommasani, R. et al. On the opportunities and risks of foundation models. Preprint at https://arxiv.org/abs/2108.07258 (2021).
Fei, N. et al. Towards artificial general intelligence via a multimodal foundation model. Nat. Commun. 13 , 3094 (2022).
Zhang, Y. et al. DialoGPT: large-scale generative pre-training for conversational response generation. In Proceedings of the 58th Annual Meeting of the Association for Computational Linguistics: System Demonstrations. 270–278 (2020).
Yang, Z. et al. Xlnet: generalized autoregressive pretraining for language understanding. In Conference on Neural Information Processing Systems (NeurIPS) (2019).
Dai, Z. et al. Coatnet: marrying convolution and attention for all data sizes. In IEEE/CVF Conference on Computer Vision and Pattern Recognition (CVPR) (2021).
Kirillov, A. et al. Segment anything. In Proceedings of the IEEE/CVF International Conference on Computer Vision , 4015–4026 (2023).
Achiam, J. et al. Gpt-4 technical report. Preprint at https://arxiv.org/abs/2303.08774 (2023).
Bao, F. et al. One transformer fits all distributions in multi-modal diffusion at scale. In International Conference on Machine Learning (ICML) (2023).
Bi, K. et al. Accurate medium-range global weather forecasting with 3D neural networks. Nature 619 , 533–538 (2023).
Singhal, K. et al. Large language models encode clinical knowledge. Nature 620 , 172–180 (2023).
Jiang, L. Y. et al. Health system-scale language models are all-purpose prediction engines. Nature 619 , 357–362 (2023).
Huang, Z. et al. A visual-language foundation model for pathology image analysis using medical twitter. Nat. Methods 29 , 2307–2316 (2023).
CAS Google Scholar
Zhou, Y. et al. A foundation model for generalizable disease detection from retinal images. Nature 622 , 156–163 (2023).
Moor, M. et al. Foundation models for generalist medical artificial intelligence. Nature 616 , 259–265 (2023).
Madani, A. et al. Large language models generate functional protein sequences across diverse families. Nature Biotechnol. 41 , 1099–1106 (2023).
Article CAS Google Scholar
Theodoris, C. V. et al. Transfer learning enables predictions in network biology. Nature 618 , 616–624 (2023).
Henighan, T. et al. Scaling laws for autoregressive generative modeling. Preprint at https://arxiv.org/abs/2010.14701 (2020).
Zamir, A. et al. Taskonomy: disentangling task transfer learning. In Twenty-Eighth International Joint Conference on Artificial Intelligence (IJCAI) , 3712–3722 (2019).
Liu, Z. et al. Swin transformer: hierarchical vision transformer using shifted windows. In IEEE/CVF Conference on Computer Vision and Pattern Recognition (CVPR) (2021).
Xia, B. et al. Efficient non-local contrastive attention for image super-resolution. In Association for the Advancement of Artificial Intelligence (AAAI) (2022).
Descloux, A., Grubmayer, K. S. & Radenovic, A. Parameter-free image resolution estimation based on decorrelation analysis. Nat. Methods 16 , 918–924 (2019).
Nieuwenhuizen, R. et al. Measuring image resolution in optical nanoscopy. Nat. Methods 10 , 557–562 (2013).
Culley, S. et al. Quantitative mapping and minimization of super-resolution optical imaging artifacts. Nat. Methods 15 , 263–266 (2018).
Li, X. et al. Three-dimensional structured illumination microscopy with enhanced axial resolution. Nat. Biotechnol. 41 , 1307–1319 (2023).
Spahn, C. et al. DeepBacs for multi-task bacterial image analysis using open-source deep learning approaches. Commun. Biol. 5 , 688 (2022).
Ouyang, W. et al. ShareLoc—an open platform for sharing localization microscopy data. Nat. Methods 19 , 1331–1333 (2022).
Zhang, X. C. et al. Zoom to learn, learn to zoom. In IEEE/CVF Conference on Computer Vision and Pattern Recognition (CVPR) (2019).
Nehme, E. et al. Deep-storm: super-resolution single-molecule microscopy by deep learning. Optica 5 , 458–464 (2018).
Guo, L. L. et al. EHR foundation models improve robustness in the presence of temporal distribution shift. Sci. Rep. 13 , 3767 (2023).
Liang, J. et al. Swinir: image restoration using swin transformer. In IEEE/CVF International Conference on Computer Vision Workshops (ICCVW) , 1833–1844 (2021).
Simonyan, K. & Zisserman, A. Very deep convolutional networks for large-scale image recognition. In International Conference on Machine Learning (ICLR) (2015).
Kingma, D. & Ba, J. Adam: a method for stochastic optimization. Preprint at https://arxiv.org/abs/1412.6980 (2014).
Wang, Z. et al. Image quality assessment: from error visibility to structural similarity. IEEE Trans. Image Process. 13 , 600–612 (2004).
Article PubMed Google Scholar
Abbe, E. Beiträge zur theorie des mikroskops und der mikroskopischen wahrnehmung. Archiv. f. Mikrosk. Anatomie 9 , 413–418 (1873).
Koho, S. et al. Fourier ring correlation simplifies image restoration in fluorescence microscopy. Nat. Commun. 10 , 3103 (2019).
Article PubMed PubMed Central Google Scholar
Baskin, C. et al. UNIQ: uniform noise injection for non-uniform quantization of neural networks. ACM Transactions on Computer Systems (TOCS) , 37 (1–4), 1–15 (2021).
Arganda, C. et al. Trainable weka segmentation: a machine learning tool for microscopy pixel classification. Bioinformatics 33 , 2424–2426 (2017).
Jacob, B. et al. Quantization and training of neural networks for efficient integer-arithmetic-only inference. In IEEE/CVF Conference on Computer Vision and Pattern Recognition (CVPR) , 2704–2713 (2018).
Ma, C., Tan, W., He, R. & Yan, B. UniFMIR: pre-training a foundation model for universal fluorescence microscopy image restoration (2023.10.03). Zenodo https://doi.org/10.5281/zenodo.8401470 (2023).
Ma, C., Tan, W., He, R., & Yan, B. UniFMIR: pre-training a foundation model for universal fluorescence microscopy image restoration (version 2023.11.13). Zenodo https://doi.org/10.5281/zenodo.10117581 (2023).
Ma, C., Tan, W., He, R. & Yan, B. UniFMIRProjectionOnFlyWing. Zenodo https://doi.org/10.5281/zenodo.10577218 (2024).
Ma, C., Tan, W., He, R. & Yan, B. UniFMIRDenoiseOnPlanaria. Zenodo https://doi.org/10.5281/zenodo.10579778 (2024).
Ma, C., Tan, W., He, R. & Yan, B. UniFMIRDenoiseOnTribolium. Zenodo https://doi.org/10.5281/zenodo.10579822 (2024).
Ma, C., Tan, W., He, R. & Yan, B. UniFMIRVolumetricReconstructionOnVCD. Zenodo https://doi.org/10.5281/zenodo.10595428 (2024).
Ma, C., Tan, W., He, R. & Yan, B. UniFMIRIsotropicReconstructionOnLiver. Zenodo https://doi.org/10.5281/zenodo.10595460 (2024) .
Ma, C., Tan, W., He, R. & Yan, B. UniFMIRSuperResolutionOnMicrotubules. Zenodo https://doi.org/10.5281/zenodo.8420081 (2023).
Ma, C., Tan, W., He, R. & Yan, B. UniFMIRSuperResolutionOnFactin. Zenodo https://doi.org/10.5281/zenodo.8420100 (2023).
Download references
Acknowledgements
We gratefully acknowledge support for this work provided by the National Natural Science Foundation of China (NSFC) (grant nos. U2001209 to B.Y. and 62372117 to W.T.) and the Natural Science Foundation of Shanghai (grant no. 21ZR1406600 to W.T.).
Author information
These authors contributed equally: Chenxi Ma, Weimin Tan.
Authors and Affiliations
School of Computer Science, Shanghai Key Laboratory of Intelligent Information Processing, Fudan University, Shanghai, China
Chenxi Ma, Weimin Tan, Ruian He & Bo Yan
You can also search for this author in PubMed Google Scholar
Contributions
B.Y. and W.T. supervised the research. C.M. and W.T. conceived of the technique. C.M. implemented the algorithm. C.M. and W.T. designed the validation experiments. C.M. trained the network and performed the validation experiments. R.H. implemented the interactive software platform and organized the codes and models. All authors had access to the study and wrote the paper.
Corresponding author
Correspondence to Bo Yan .
Ethics declarations
Competing interests.
The authors declare no competing interests.
Peer review
Peer review information.
Nature Methods thanks Ricardo Henriques and the other, anonymous, reviewer(s) for their contribution to the peer review of this work. Primary Handling Editor: Rita Strack, in collaboration with the Nature Methods team.
Additional information
Publisher’s note Springer Nature remains neutral with regard to jurisdictional claims in published maps and institutional affiliations.
Extended data
Extended data fig. 1 overall architecture of the unifmir..
The proposed UniFMIR approach is composed of three submodules: a multihead module, a Swin transformer-based feature enhancement module, and a multitail module. The numbers of parameters (M) and calculations (GFLOPs) required for the head, feature enhancement and tail modules for different tasks are marked below the structures of the respective modules. The input sizes and output sizes of training batches for different tasks are also marked below the images.
Extended Data Fig. 2 Network architecture of the Swin transformer-based feature enhancement module 46 .
The feature enhancement module consists of convolutional layers and a series of Swin transformer blocks (STB), each of which includes several Swin transformer layers (STL), a convolutional layer and a residual connection. The STL is composed of layer normalization operations, a multihead self-attention (MSA) mechanism and a multilayer perceptron (MLP). In the MSA mechanism, the input features are first divided into multiple small patches with a moving window operation, and then the self-attention in each patch is calculated to output features f out . The MLP is composed of two fully connected layers (FCs) and Gaussian-error linear unit (GELU) activation.
Extended Data Fig. 3 Generalization ability analysis of super-resolution on unseen modality of single-molecule localization microscopy data from the Shareloc platform 52 .
a, SR results obtained by the SOTA model (DeepSTORM 54 ), the pretrained UniFMIR model without fine-tuning, Baseline (same network structure as UniFMIR trained from scratch), and our fine-tuned UniFMIR model. The GT dSTORM images of microtubules stained with Alexa 647 in U2OS cells incubated with nocodazole and the input synthesized LR images are also shown. The PSNR/NRMSE results of the SR outputs obtained on n = 16 synthetic inputs are shown on the right. b, SR results obtained on the real-world wide-field images. The NRMSE values are depicted on the residual images under different SR results and the raw input images. The PSNR/NRMSE results on n = 9 real-world inputs are shown on the right. Box-plot elements are defined as follows: center line (median); box limits (upper and lower quartiles); whiskers (1.5x interquartile range). The line plots show the pixel intensities along the dashed lines in the corresponding images. Scale bar: 6.5 μ m.
Supplementary information
Supplementary information.
Supplementary Notes 1–5, Figs. 1–17 and Tables 1 and 2.
Reporting Summary
Rights and permissions.
Springer Nature or its licensor (e.g. a society or other partner) holds exclusive rights to this article under a publishing agreement with the author(s) or other rightsholder(s); author self-archiving of the accepted manuscript version of this article is solely governed by the terms of such publishing agreement and applicable law.
Reprints and permissions
About this article
Cite this article.
Ma, C., Tan, W., He, R. et al. Pretraining a foundation model for generalizable fluorescence microscopy-based image restoration. Nat Methods (2024). https://doi.org/10.1038/s41592-024-02244-3
Download citation
Received : 27 July 2023
Accepted : 13 March 2024
Published : 12 April 2024
DOI : https://doi.org/10.1038/s41592-024-02244-3
Share this article
Anyone you share the following link with will be able to read this content:
Sorry, a shareable link is not currently available for this article.
Provided by the Springer Nature SharedIt content-sharing initiative
Quick links
- Explore articles by subject
- Guide to authors
- Editorial policies
Sign up for the Nature Briefing newsletter — what matters in science, free to your inbox daily.


An official website of the United States government
The .gov means it’s official. Federal government websites often end in .gov or .mil. Before sharing sensitive information, make sure you’re on a federal government site.
The site is secure. The https:// ensures that you are connecting to the official website and that any information you provide is encrypted and transmitted securely.
- Publications
- Account settings
Preview improvements coming to the PMC website in October 2024. Learn More or Try it out now .
- Advanced Search
- Journal List
- v.7(8); 2021 Aug

Identifying learning styles and cognitive traits in a learning management system
Associated data.
The authors do not have permission to share data.
Investigating learner behavior is an increasingly important research topic in online learning. Learning styles and cognitive traits have been the subjects of research in this area. Although learning institutions use Learning Management Systems such as Moodle, Claroline, and Blackboard to facilitate teaching, the platforms do not have features for analyzing data and identifying behavior such as learning styles and cognitive traits. Instead, they only produce certain statistical reports from the daily access records. Even though complex models have been proposed in the literature, most studies are based on a single behavior such as learning styles or cognitive traits but not both. Only a few have investigated a combination of cognition-based theories such as working memory capacity and psychology-based ones such as learning styles. Thus, this study sought to answer the research question of whether it was possible to establish a methodology for the estimation of learning styles and cognitive traits from a learning management system. The study combined the Felder-Silverman Learning Style Model and Cognitive Trait Model as theoretical frameworks to identify behavior in a Learning Management System. This study designed a model for extracting records from Learning Management Systems access records to estimate learning style and cognitive traits. From this, a prototype was developed to estimate the learning style and cognitive traits for each student. The model was evaluated by administering manual tools to students in a classroom environment then comparing the results gathered against those estimated by the model. The results analyzed using Kappa statistics demonstrated the interrater reliability results were moderately in agreement. Taken together, these results suggest that it is possible to estimate the learning styles and cognitive traits of a learner in a Learning Management System. The information generated by the model can be used by tutors to provide a conducive online learning environment where learners with similar behavior ask each other for help. This can reduce the teaching load for online tutors because learners themselves act as a teaching resource. Information on learning styles and cognitive styles can also facilitate online group formation by isolating the individual factors that contribute to team success.
Learning style; Cognitive trait; Learner behavior; Learning management system; Learner modeling.
1. Introduction
Learner behavior modeling has received much attention over the last two decades ( Chrysafiadi and Virvou, 2013 ; Abyaa et al., 2019 ). Learner characteristics such as Learning styles (LS) and cognitive traits (CT) are the most prevalent topic in educational psychology texts ( Wininger et al., 2019 ). These characteristics measure learners’ psychological attitude towards learning ( Drachsler and Kirschner, 2012 ). A learner model is a system that collects and processes information on student behavior such as LS and CT. Knowledge of learner characteristics enables instruction designers to create relevant instructions for a target group. Although institutions use Learning Management Systems (LMS) such as Moodle, Claroline, and Blackboard ( Don, 2014 ) to facilitate teaching, the platforms do not have features for analyzing data and identifying behavior such as LS and CT. Most LMS have Sharable Content Object Reference Model (SCORM) specifications. SCORM format allows the creation of the learning contents that can be managed, re-used, and assembled in different learning platforms ( Varlamis and Apostolakis, 2006 ).
Recent developments in online learning renewed the interest in learner behavior modeling in an LMS. According to Blakemore et al. (1984) , learning style indicates the way a learner observes, interacts with, and answer back to learning content. Several examples of learning style models exist in the literature. Felder-Silverman Learning Style Model classifies learners as sensing or intuitive, verbal or visual, active or reflective, and sequential or global ( Felder, 1988 ); Myers Briggs Type Indicator classifies learners as extraversion or introversion, sensing or intuition, thinking or feeling, judging or perceiving ( Myers, 1995 ); Honey and Mumford model groups learners as an activist, reflector, theorists, and pragmatist ( Honey and Mumford, 1982 ); Kolb learning model groups learners as concrete experience or abstract conceptualization, active experimentation or reflective observation ( Kolb, 2015 ); VARK learning styles groups learners as visual, aural, read/write, and kinesthetic ( Fleming, 2014 ).
According to Zhong-Lin and Barbara (2007) cognitive style is a variation in an individual's manners of perceiving, remembering, and thinking. Kellogg (2012) identifies the main cognitive processes as sensation, attention, memory, learning, remembering, and forgetting. The studies in learner behavior modeling have used both manual and automatic methods to identify learner characteristics. In manual detection, a questionnaire corresponding to a learning style model, in which individuals fill their answers to identify their learning styles, is used. Cognitive assessment tests are also administered to identify different types of behavior such as memory, concentration, reasoning, and planning based. However, it is almost certain that manual measurements have psychometric flaws. They are prone to errors, require resources to administer and consume a lot of time to fill.
Concerning the automatic modeling method, the actual actions of students when accessing an online course are analyzed to infer students’ behavior. Several studies investigating learner behavior in LMS have been carried out. More recently, Şahin et al. (2020) utilized the ELECTRE TRI method to classify the learners based on the interaction data gathered from LMS and concluded that there was a correlation between the categories investigated and the real-life classification. In another related study, Ferreira et al. (2019) used machine learning algorithms to analyze data from the Moodle LMS and characterized the learning profiles of students according to the Felder-Silverman Learning Style Model (FSLSM). Troussas, Chrysafiadi, and Virvou (2020) combined the Visual, Auditory, Reading/Writing, and Kinesthetic (VARK) learning style model and the Herrmann Brain Dominance Instrument (HBDI) to model the sensory modalities of learning and the way of thinking. Even though complex models have been proposed in the literature, most studies are based on a single behavior such as learning style or cognitive traits but not both. Only a few such as Nakić et al. (2015) investigated a combination of cognition-based theories such as working memory capacity and psychology-based ones such as learning styles. Thus, the studies highlight the need for further research in learner behavior modeling.
It is worth noting that LS models have been criticized by some authors. For instance, Pashler et al. (2009) argued that many versions of learning styles models had not been tested at all. Kirschner (2017) further questioned the existence of students with diverse learning preferences. In contrast, Felder and Spurlin (2005) reported that the ILS was a reliable and valid tool for measuring learning styles. FSLSM has been used to date in several studies involving learner behavior modeling in LMS such as Aissaoui et al. (2018) , Abdelhadi et al. (2019) , and Ferreira et al. (2019) .
This study adopted a hybrid of the Felder-Silverman Learning Styles Model (FSLSM) and Cognitive Traits Model (CTM) as theoretical frameworks. Analysis from the literature shows the two are the most researched and validated with LMS to model CT ( Kinshuk, Lin and Mcnab, 2004 , 2006 ; Lin et al., 2007 ; Lin and Kao, 2018 ) and LS ( Graf et al., 2009 ; Graf et al., 2013 ; Aissaoui et al., 2018 ) thus acceptable.
The study sought to answer the research question: is it possible to establish a methodology for the estimation of learning styles and cognitive traits from LMS records? The study combined the Felder-Silverman Learning Style Model (FSLSM) and Cognitive Trait Model (CTM) to identify behavior in an LMS. FSLSM profiles a learner as active or reflective, sensing or intuitive, sequential or global, and visual or verbal ( Felder, 1988 ). The model uses a 44 - questions Index of Learning Styles Questionnaire (ILS) with 11 for each dimension as a manual measurement tool. A student selects choice a or b. The questionnaire score sheet classifies a respondent as 1–3 mild, 5–7 moderate, 9–11 strong preference for either dimension. The cognitive Trait Model (CTM) on the other hand profiles a learner based on associative learning ability, working memory capacity, inductive reasoning ability, and Information processing speed ( Graf et al., 2009 ).
The first objective of this study was to design a model based on LS and CT. The second objective was to develop a prototype implementing the model. The third objective was to evaluate the developed model using a case study and analyze the results. This paper presents a model for extracting records from LMS access records to estimate LS and CT based on FSLSM and CTM dimensions. A prototype was developed to estimate LS and CT for each student. The results evaluated using the Kappa statistic demonstrated the interrater reliability results were moderately in agreement.
The rest of the paper has been organized as follows. Section 2 discusses the proposed model. Section 3 discusses the implementation and evaluation. Section 4 discusses the results. Section 5 discusses the conclusions and suggests future work. Throughout this paper, the acronym LS will refer to learning styles. CT will refer to cognitive traits. CTM will refer to Cognitive Trait Model. FSLSM will refer to the Felder-Silverman Learning Style Model. SCORM will refer to the Sharable Content Object Reference Model. LMS will refer to learning management systems.
2. Proposed model
The initial objective of this study was to design a learner behavior model complementing LS with psychology-based ones such as CT. In this section, we present a model based on the FSLSM and CTM that analyses student access records generated by LMS to estimate LS and CT. As stated in the previous section, the two theories are well researched and validated with promising evaluation results in many similar studies such as Bernard et al. (2017) , Lin (2007) . Figure 1 illustrates the working mechanism of the model. First, the model extracts records of students’ access from learning content hosted in LMS. Second, it maps access patterns to relevant LS and CT dimensions described in FSLSM and CTM respectively. Third, it estimates and displays LS and CT for each student.

LS and CT detection process.
To understand the model implementation process, it is important to explain the log data used to implement the prototype. As indicated in Figure 1 above, the learning resources hosted in an LMS are the source of data for implementing and testing the model. These are electronic books prepared in SCORM format and hosted in an LMS. The first component of each book section contains introductions, objectives, and outlines of the topics covered. The second part of the book section covers the definition of concepts. The third section has topics and subtopics. These are pages with concepts and facts discussed in detail. Activity questions appear at the end of each topic. Finally, each section ends with a summary of the topics discussed. Some content pages consist of illustrations and pictures. Figure 2 illustrates the layout of an electronic learning module hosted on a Learning Management System. The navigation window displaying a list of book pages appears to the left. Learning content is displayed on the right when a user selects the page. The sample log data extracted from LMS records appear in table 12 in the appendices. The table shows the number of relevant content pages viewed and the times spent. These are introductions and overviews, definitions, topics, activities, summaries, and illustration pages viewed. The average grade a student scored in online tests and attempts was recorded. The table also indicates the average grade scored in online tests and the overall number of content pages in percentage viewed by a student. Table 13 in the appendices shows sample log data on content navigation. The table shows the ratio of pages that are viewed once or revisited by a student out of the total. These formed the dataset for identifying and estimating LS and CT as explained in section 3 .

Model architecture.
Figure 2 above shows the components of the model. LS and CT pattern extraction engines fetch relevant LS and CT from the LMS log database. Learning Style Generator (LSG) and Cognitive Trait Generator (CTG) engine receive data from pattern extraction component, calculate then map results to 3 item scale: 0.1–0.3 low, 0.4–0.6 moderate, and 0.7–1.0 high and 0.0 – no preference. The behavior for both LS and CT is combined and displayed for each student.
3. Implementation
The second objective was to develop a prototype implementing the above model. First, the study participants were identified. The participants accessed an online course hosted on an LMS for a 15-week semester. Records of the participant's interaction with the LMS were analyzed and used to implement the model. The following sections describe participants, course information, and the model development process.
3.1. Participants and course information
The study was conducted on the 600 first-year students taking Bachelor of medicine and bachelor of surgery degrees in the academic year 2017/2018 at the University of Nairobi. Usually, the students taking a degree in Bachelor of Medicine and Bachelor of Surgery at the University of Nairobi study a common medical psychiatry unit in behavioral science. The course unit is divided into 10 learning modules taken over one year. The learning modules are psychology, social processes, foundations of human behavior, social processes, sociology, social psychology, anthropology, physical illness causing the behavior, neurosciences, and healthcare systems. The learning modules are converted to SCORM format and hosted on a university LMS running on the Claroline open-source platform ( Claroline, 2017 ).
Figure 3 below shows an example of the course page interface in the LMS used in the study.

A course interface in SCORM format.
In Figure 3 above, the contents of the online learning modules are systematically arranged by instruction designers to enable easy navigation. Each learning module is divided into sections, topics, and subtopics. Activity questions are provided after each topic to test learners' understanding. The course contents have both textual and visual information. Figure 4 below shows content navigation statistics. The LMS tracking tool records the time taken on each page and the status as complete or incomplete. A single page viewed by a student is marked as complete or incomplete. The page that is viewed is assigned a numerical value of 100 %. Any page which is not visited is assigned status 0.

The content navigation statistics.
3.2. Data collection procedures
Once the online learning modules were hosted on the university LMS, the students accessed the psychology and sociology modules for a period of 15 – week semester. Two online tests in multiple-choice questions (MCQ) formats were administered to students on the LMS in the midsemester and at the end. Each test had 100 questions and students were instructed to answer all. The tests were scheduled to last for two hours. The LMS was programmed to automatically submit answers entered by each student when allocated time elapsed. The MCQs were preferred because the LMS could automatically mark and generate the mark sheet upon submission of the answers. Figure 5 a below shows sample questions.

a. sample MCQ.
As indicated in the sample mark sheet in Figure 5 b below, the LMS records the minimum, maximum, and average grades for each student. The time spent is also recorded for each student by the LMS.

b. The sample mark sheet.
Data was collected from the LMS and analyzed to identify learner behavior. Only students who viewed the online resources by visiting revision pages, outlines, summaries, and attempted quizzes were considered for the study. Approximately 200,000 log records of 311 students who satisfactorily accessed the learning modules for a 15-week semester were extracted from the system to create a dataset. Access records of instructional materials and tests were analyzed to identify patterns. The patterns were used as hints matching descriptions of FSLSM and CTM.
To evaluate the model, Index of Learning Styles (ILS) Questionnaires and Online Cognitive Multiple Choice Questions were also administered. The evaluation process is discussed in section 3.6 of this paper.
3.3. Extracting data patterns from log
The study analyzed navigation records on learning contents and online assessment tests to identify patterns from the LMS database. The annotations were done on the records to match the descriptions of FSLSM and CTM. Table 1 and Table 2 show how each of the patterns was mapped to FSLSM and CTM dimensions.
Table 1
Investigated patterns for LS. Visits indicate the number of times a relevant page is viewed. Time indicates how long the page was viewed.
Table 2
Investigated patterns for CT. Visits indicate the number of times a relevant page is viewed. Time indicates how long the page was viewed.
Table 1 above shows each dimension of FSLSM, description, learning object investigated and pattern extracted. According to FSLSM, an active learner likes trying out things; a reflective learner thinks about materials read. A student who spent time viewing content with activities was considered an active learner; a student who spent time on content with summaries, conclusions, and revisions was considered a reflective learner.
A sensing learner dislikes challenging tasks but is patient with details; an intuitive learner likes challenges but is impatient with details. A student who spent time viewing content with topics and subtopics was considered a sensing learner; a student who spends time on content with definitions and meanings was considered an intuitive learner.
A verbal learner likes text-based content; a visual learner likes illustrations and pictures. A student who spent time viewing content with text-based pages only was considered a verbal learner; a student who spends time on content with illustrations was considered a visual learner.
A global learner likes getting the full picture of the content; a sequential learner likes navigating content step by step. A student who spent time viewing content with introductions, overviews, and content outlines was considered a global learner; a student who followed the content pages systematically by accessing overviews, followed by topics then summary pages was considered a sequential learner.
Table 2 above shows each dimension of CTM, description, learning object investigated and pattern extracted.
According to CTM, an associative learner likes linking new to existing knowledge. The number of visits and time spent viewing already visited content pages was considered a sign of associative learning. A student who spent time viewing already read content was assumed to have a high associative learning ability.
Working memory capacity tests the ability to keep a limited amount of information for a brief period. The number and time spent navigating content page forward was considered signs of high working memory capacity. Such a student was able to concentrate on a reading task. A student who viewed the content without revisiting previously read pages was assumed to have a high working memory capacity.
Inductive reasoning is the ability to construct concepts from examples. The number of visits and time spent viewing content with examples was considered a sign of inductive reasoning. A student who spent time viewing content with examples was assumed to have inductive reasoning ability.
Information processing speed checks how fast the learner acquires and uses the information to make the correct decision. The number of attempts marks scored, and time spent on online tests was considered an indication of information processing speed. A student who took a short time on online tests with few attempts but scored high marks was assumed to have high information processing speed.
3.4. Behavior estimating from patterns
Using the automatic behavior estimation method proposed by Pham and Florea (2013) patterns above were used to estimate LS and CT by computing the average ratio of total learning objects accessed and time spent by each student. The following steps describe the estimation procedure.
3.4.1. Estimating LS
For matching pairs of learning preferences such as active-reflective, sensing-intuitive, sequential-global, and visual-verbal, the LS was computed based on the following steps:
- i. Time on learning objects relevant to an LS dimension (t) (e.g. active or reflective) out of total time spent in all objects (T) ∑ t ∑ T (e.g. active + reflective)
- ii. Number of visits on a learning object relevant to an LS (lo) (e.g. active or reflective) dimension out of total objects accessed (LO) ∑ l o ∑ L O (e.g. active + reflective)
- iii. Compute average ratio from step 2 a v e r a g e ( ∑ l o ∑ L O + ∑ t ∑ T )
- iv. Map to 3 item scale: 0.1–0.3 -low, 0.4–0.6 moderate and 0.7–1.0 high to get appropriate preference
Table 3 below shows the estimation procedure for each CT dimension.
Table 3
LS estimation functions.
3.4.2. Estimating CT
For matching pair of CT patterns such as pages revisited vs visited once for associative learning or forward vs backward navigation for working memory , the CT was estimated based on the following steps:
- i. Time on learning objects relevant to a CT dimension (t) (e.g. revisited vs visited once for associative learning or forward vs backward navigation) out of total time spent in all objects (T) ∑ t ∑ T (e.g. revisited + visited once for associative learning or forward + backward navigation)
- ii. Number of visits on a learning object relevant to CT (lo) (e.g. revisited vs visited once for associative learning or forward vs backward navigation)dimension out of total objects accessed (LO) ∑ l o ∑ L O e.g. revisited + visited once for associative learning or forward + backward navigation)
For inductive reasoning ability, summation a v e r a g e ( ti T + o O ) time spent on relevant objects (ti) and total objects accessed (o) out of the total time (T) and total objects (O) were considered. For information processing speed, summation a v e r a g e ( t T , a A , s S ) time spent (t), attempts (a), and score (s) out of the total time (T), total attempts (T), and the total score (T) for relevant objects were considered.
Table 4 shows the estimation procedure for each CT dimension.
CT estimation functions.
3.5. Prototype development
A web-based system was developed based on the above estimation functions. The prototype was developed using the MYSQL database and the PHP scripting language. The model initiates the connection to the MYSQ database, fetches data, and computes LS and CT then displays preference. The MYSQL database and the PHP scripting language are open-source tools thus available for use free of charge. Figure 6 below shows the model's interface functionality.

Model interface functionality.
From Figure 6 above, a user can initiate a search by entering the identification or registration number. As shown in Figure 6 above, a student with user id 224043, for instance, is strongly reflective 0.74 than active 0.26, sensing 0.74 than intuitive 0.31, visual 0.78 than verbal 0.15, sequential 0.85 than global 0.15, with strong associative learning 1.00, strong working memory capacity 0.99, moderate information processing speed 0.54 and weak inductive reasoning 0.33. A lecturer can use such information to provide appropriate learning materials, apply appropriate tutoring methods, and provide individualized attention matching learner behavior. A student on the other hand uses the above information for self-awareness to apply appropriate study methods and choose appropriate learning materials.
3.6. Evaluation
As mentioned in the introduction section, the third objective was to evaluate the developed model using a case study and analyze the results. The evaluation was done by administering manual tools to students then comparing the results gathered against those estimated by the model. The performance of the prototype was evaluated using experiments done with students in a classroom environment. A total of 200 students taking bachelor of medicine and bachelor of surgery participated. The group was part of the 311 students who had actively accessed the psychology and sociology learning modules and did online tests during course work. Only 200 students were available and readily accessible through the course lecturer. The first experiment was done to evaluate the learning style results generated by the prototype. Index of Learning Styles Questionnaires (ILS), a standard measurement tool for FSLSM were administered to students which they filled and returned. Learning styles for each student were calculated and analyzed. The second experiment was done to evaluate the cognitive traits generated by the system. Online Cognitive Multiple Choice Questions based on a method adapted from Cambridge Brain Sciences ( Cambridge Brain Sciences, 1998 ) were created and hosted in the university LMS. Four categories of tests were administered. Paired associate tests, spatial span tests, abstract reasoning tests, and mental speed tests were created on university learning management systems to evaluate associative learning ability, working memory capacity, inductive reasoning ability, and information processing speed respectively. Cognitive traits were determined in terms of marks scored in the test classified as low, moderate, and high. These were compared to CTM results predicted for the same students by the model.
The interrater reliability test was done to measure the agreement between the behavior gathered through the Index of Learning Styles Questionnaires (ILS), Online Cognitive Multiple Choice Questions, and those estimated by the model. The test was conducted to determine how well the implementation of the model was in agreement with the traditional methods. The reliability analysis was done using the Kappa statistic to determine consistency among raters. Kappa statistic as a method was selected because it is the metric frequently used to assess the agreement between two raters ( Cohen, 1960 ). The study opted for the kappa coefficient as the most suitable method for the assessment of the model because the extent of agreement between predictions made by the psychometric tools and the model could be established.
The analysis was performed using the Statistical Package for the Social Sciences (SPSS) software (version 1.1.463). According to Landis and Koch (1977) values of Kappa between less than 0 indicates there is no agreement. Values 0.0 and 0.20 indicates slight agreement. Values between 0.21 and 0.40 indicate fair agreement. Values between 0.41 and 0.60 indicate moderate agreement. Values between 0.61 and 0.80 indicate substantial agreement. Values between 0.81 and 1.00 indicate almost perfect agreement.
4. Results and discussions
As indicated in the previous section, the study was designed to compare the LS and CT estimated by the model against those gathered by the ILS and online cognitive tests.
Tables 5–11 in the appendices section show the interrater reliability analysis results for LS and CT. As shown in table 5, the results of the interrater reliability for the active-reflective raters were found to be Kappa = 0.260 (p < .0.018). This indicates a fair agreement between the students predicted by the model as active–reflective compared to the data collected through the ILS questionnaire. The results of the interrater reliability for the sensing-intuitive raters were found to be Kappa = 0.595 (p < .0.002) as shown in table 6. This indicates a moderate agreement between the students' LS predicted by the model as sensing–intuitive compared to the data gathered through the ILS questionnaire. The results of the interrater reliability for the sequential - global raters were found to be Kappa = 0.326 (p < .0.004) as shown in table 7. This indicates a fair agreement between the LS for students predicted by the model as sequential – global compared to the data gathered through the ILS questionnaire. The study did not do the interrater reliability test on the visual-verbal learning style since all students indicated a preference for visual content.
Concerning CT evaluation, the results of the interrater analysis of Kappa = 0.012 with p < 0.640 were recorded for associative learning ability as shown in table 8. This indicates a slight agreement between the behavior of students predicted by the model compared to the data gathered through the online cognitive multiple-choice questions. The results of the interrater analysis of Kappa = 0.455 with p < 0.067 were recorded for information processing speed as shown in table 9. This indicates a moderate agreement between the behavior of students predicted by the model compared to the data gathered through the online cognitive multiple-choice questions. The results of the interrater analysis of Kappa = 0.224 with p < 0.052 were recorded for inductive reasoning ability shown in table 10. This indicates a fair agreement between the behavior of students predicted by the model compared to the data gathered through the online cognitive multiple-choice questions. The results of the interrater analysis of Kappa = 0.455 with p < 0.067 were recorded for working memory capacity as shown in table 11. This indicates a moderate agreement between the behaviors of students predicted by the model compared to the data gathered through the online cognitive multiple-choice questions.
Interestingly, results based on LS are more statistically significant than CT. However, the results for associative learning ability and information processing speed were not statistically significant. A possible explanation for this could be the psychometric flaws associated with the measurement tools. It seems possible that some students experienced challenges when attempting the online cognitive tests used to access CT. Since the study was conducted on first-year students who were new to the university, it may be that some students were unable to use the computer well and this affected the response to the online tests.
The findings in this study support the previous research in this area which links LMS records to learner behavior such as LS and CT. The method validates the ideas of Şahin et al. (2020) who classified the learners based on the interaction data gathered from an LMS. The author analyzed the relationship between the classification based on the ELECTRE TRI method and the classification in real life. The ELECTRE TRI method is a multi-criteria decision-making technique designed to classify the learners based on the interaction data in different units. The classification results in the ELECTRE TRI method were compared to the real-life classification, which showed a medium-level correlation. This also supports our findings which showed that there was a moderate agreement between the behavior of students predicted by the proposed model compared to the data gathered through the online cognitive multiple-choice questions.
The results are also in agreement with Aissaoui et al. (2018) who extracted learning sequences from learners' log files and classified the extracted records according to FSLSM using clustering algorithms. The study reported 88.61 % 89.93 % 92.35 % 88.97 % accuracy, precision, recall, f-measure. One difference between this study and that of Aissaoui et al. (2018) is that the reliability analysis was done using the Kappa statistic while their study used precision, recall, and f-score. The prediction success rates in our study are, slightly lower than the previous studies cited above. This study proposed a mathematical method for estimating the learners’ behavior based on the time spent on the content and the number of objects visited while the previous studies used machine learning models. The results are expected to improve after further data cleaning to remove impurities from LMS records.
5. Conclusion
This study set out to determine a methodology for estimating learning styles and cognitive traits from LMS access records. In this paper, we presented a model for the automatic identification of learner behavior in LMS records. The model takes advantage of data collected from the LMS log, education theories, and literature-based methods to automatically estimate learning styles and cognitive traits. This brings forth a generic modeling architecture that developers can integrate with existing learning management system platforms to improve learner characterization. The interrater reliability test was done to compare the agreement between the behavior gathered through the Index of Learning Styles Questionnaires (ILS), Online Cognitive Multiple Choice Questions, and those estimated by the model. Taken together, these results suggest that it is possible to estimate the LS and CT an LMS. Whilst the study did not confirm a perfect match between behavior gathered through the Index of Learning Styles Questionnaires (ILS), Online Cognitive Multiple Choice Questions, and those estimated by the model, it did partially substantiate that the interrater reliability results were moderately in agreement. The results can be improved with more data cleaning and refinement.
The results of this study have implications for online instructional design and teaching. An online instructor can use the information generated to design and develop appropriate instructional materials matching appropriate LS and CT preferences. According to Churngchow et al. (2020) , learners from different disciplines have different learning styles. Such learners need the instructional materials matching their learning preferences. Malacapay (2019) reported that both visual and auditory learners learned best when subjected to audio-visual contents while kinesthetic learned best when exposed to actual objects. On the same note, an online instructor can use the information generated by the model to form online learning groups for students with similar LS and CT to improve learning outcomes. According to Mutrofin et al. (2017) , the group learning approach results in a better learning outcome than the lecture-discussion method. Thus an instructor can form online learning groups according to the respective preferences and prepare the learning materials relevant for each group. Research also reports that students find it easy to understand difficult concepts when they learn in groups ( Wikle and West, 2018 ). On the same note, an online instructor can use the information generated by the model to improve the social interaction among online learners. A recent study carried out by Mahmoud Hawa and Tılfarlıoğlu (2019) reported that the learners' preferred learning styles had a relationship with their self-efficacy and social interactions.
The study has some limitations. First, data used in the study was collected from log records of 311 students who satisfactorily accessed learning modules for a 15 – week semester. Second, the study evaluation was conducted only on 200 preclinical students. Further studies might be carried out with more students from other disciplines to further validate the findings. Another important issue to resolve for future studies is developing a real-time model integrated with an LMS to estimate LS and CT.
Future work involves automatically mapping e-Learning course content to respective learning styles and cognitive traits. Additional evaluations may also be carried out to properly validate the model.
Declarations
Author contribution statement.
C. Lwande, L. Muchemi, R. Oboko: Conceived and designed the experiments; Performed the experiments; Analyzed and interpreted the data; Wrote the paper.
Funding statement
This research did not receive any specific grant from funding agencies in the public, commercial, or not-for-profit sectors.
Data availability statement
Declaration of interests statement.
The authors declare no conflict of interest.
Additional information
No additional information is available for this paper.
Appendix A. Supplementary data
The following is the supplementary data related to this article:
- Abdelhadi A., Ibrahim Y., Nurunnabi M. Investigating engineering student learning style trends by using multivariate statistical analysis. Educ. Sci. 2019; 9 (1) [ Google Scholar ]
- Abyaa A., Khalidi Idrissi M., Bennani S. Learner modeling: a systematic review of the literature from the last 5 years. Educ. Technol. Res. Dev. 2019; 67 (5):1105–1143. [ Google Scholar ]
- Aissaoui O. El. A fuzzy classification approach for learning style prediction based on web mining technique in e-learning environments. Educ. Inform. Technol. 2018;(September) [ Google Scholar ]
- Bernard J. Learning style Identifier: improving the precision of learning style identification through computational intelligence algorithms. Expert Syst. Appl. 2017:75. [ Google Scholar ]
- Blakemore T., McCray P., Coker C. 1984. A Guide to Learning Style Assessment. Research and Training Center Research Report. [ Google Scholar ]
- Cambridge Brain Sciences. 1998. www.cambridgebrainsciences.com Available at: [ Google Scholar ]
- Chrysafiadi K., Virvou M. Student modeling approach: a literature review for the last decade. Expert Syst. Appl. 2013 [ Google Scholar ]
- Churngchow C. Appropriate learning management for students with different learning styles within a multicultural society at state-run universities in Thailand. Int. J. High. Educ. 2020; 9 (2):200–208. [ Google Scholar ]
- Claroline. 2017. https://www.claroline.net Available at: [ Google Scholar ]
- Cohen J. A coefficient of agreement for nominal scales. Educ. Psychol. Meas. 1960; 20 (1):37. [ Google Scholar ]
- Don M. List of corporate learning management systems. 2014. https://elearningindustry.com/list-corporate-learning-management-systems Available at:
- Drachsler H., Kirschner P.A. Springer; Boston MA: 2012. ‘Learner Characteristics’, Encyclopedia of the Sciences of Learning . [ Google Scholar ]
- Felder R.M. Learning and teaching styles in engineering education. Eng. Educ. 1988; 78 (7):674–681. http://www4.ncsu.edu/unity/lockers/users/f/felder/public/Papers/LS-1988.pdf Available at: [ Google Scholar ]
- Felder R.M., Spurlin J. Applications, reliability, and validity of the Index of learning styles. Int. J. Eng. 2005; 21 (1) 113–112. [ Google Scholar ]
- Ferreira L.D. Proceedings - Frontiers in Education Conference, FIE. IEEE; 2019. A comparative analysis of the automatic modeling of Learning Styles through Machine Learning techniques; pp. 1–8. [ Google Scholar ]
- Fleming N.D. The VARK modalities. 2014. https://web.archive.org/web/20150314235648/http://vark-learn.com/introduction-to-vark/the-vark-modalities/ Available at:
- Graf S. Automatic, global and dynamic student modeling in a ubiquitous learning environment. Knowl. Manag. E-Learn. 2009; 1 (1) [ Google Scholar ]
- Graf S. International journal of information systems and social change. Int. J. Inf. Syst. Soc. Change. 2013 https://econpapers.repec.org/article/iggjissc0/v_3a4_3ay_3a2013_3ai_3a1_3ap_3a85-100.htm IGI Pub. Available at: [ Google Scholar ]
- Honey P., Mumford A. Peter Honey; Berkshire: 1982. The Manual of Learning Styles. [ Google Scholar ]
- Kellogg R.T. second ed. Sage; London: 2012. Fundamentals of Cognitive Psychology. [ Google Scholar ]
- Kinshuk, Lin T., Mcnab P. Celda); 2004. Modelling Inductive Reasoning Ability for; pp. 343–349. [ Google Scholar ]
- Kinshuk, Lin T., Mcnab P. Adaptive support for inductive reasoning ability. In: Ma Z., editor. Web-Based Intelligent E-Learning Systems: Technologies and Applications. Idea Group Inc.; 2006. pp. 1–23. [ Google Scholar ]
- Kirschner P.A. Stop propagating the learning styles myth. Comput. Educ. 2017; 106 [ Google Scholar ]
- Kolb D. second ed. Pearson Education; 2015. Experiential Learning: Experience as the Source of Learning and Development. [ Google Scholar ]
- Landis J.R., Koch G.G. The measurement of observer agreement for categorical data. Biometrics. 1977; 33 (1):159. [ PubMed ] [ Google Scholar ]
- Lin F.-R., Kao C.-M. Mental effort detection using EEG data in E-learning contexts. Comput. Educ. 2018; 122 :63–79. [ Google Scholar ]
- Lin T.-Y. Massey University; Palmerston North, New Zealand: 2007. Cognitive trait model for adaptive learning environments: a thesis presented in partial fulfillment of the requirements for the degree of Doctor of Philosophy in Information System [i.e. Systems] https://mro.massey.ac.nz/handle/10179/1451 Massey University. Available at: [ Google Scholar ]
- Lin T., Kinshuk, Graf S. Proceedings - the 7th IEEE International Conference on Advanced Learning Technologies, ICALT 2007. IEEE Computer Society; 2007. Cognitive trait model and divergent associative learning; pp. 325–327. [ Google Scholar ]
- Mahmoud Hawa H., Tılfarlıoğlu F.Y. ‘Students’ learning styles, self-efficacy and its correlation with their social interaction’ Adv. Lang. Lit. Stud. 2019; 10 (4):42. [ Google Scholar ]
- Malacapay M.C. ‘Differentiated instruction in relation to pupils’ learning style’ Int. J. InStruct. 2019; 12 (4):625–638. [ Google Scholar ]
- Mutrofin The effect of instructional methods (Lecture-Discussion versus group discussion) and teaching talent on teacher trainees student learning outcomes. J. Educ. Pract. 2017; 8 (9):203–209. http://search.ebscohost.com/login.aspx?direct=true&db=eric&AN=EJ1138824&site=ehost-live Available at: [ Google Scholar ]
- Myers I.B. Davies-Black Publishing; 1995. Gifts Differing: Understanding Personality Type . Reprint. [ Google Scholar ]
- Nakić J., Granić A., Glavinić V. Anatomy of student models in adaptive learning systems: a systematic literature review of individual differences from 2001 to 2013. J. Educ. Comput. Res. 2015 [ Google Scholar ]
- Pashler H. Learning styles concepts and evidence. Psychol. Sci. Publ. Interest. 2009 Supplement. [ PubMed ] [ Google Scholar ]
- Pham Q.D., Florea A.M. A method for detection of learning styles in learning management systems. UPB Scient. Bull., Series C: Electr. Eng. 2013; 75 (4):3–12. [ Google Scholar ]
- Şahin M., Ulucan A., Yurdugül H. Learner Classification Based on Interaction Data in E-Learning Environments: the electre tri Method. Educ. Inform. Technol. 2020 [ Google Scholar ]
- Troussas C., Chrysafiadi K., Virvou M. Personalized tutoring through a stereotype student model incorporating a hybrid learning style instrument. Educ. Inf. Technol. 2020 https://link.springer.com/article/10.1007/s10639-020-10366-2#citeas Available at: [ Google Scholar ]
- Varlamis I., Apostolakis I. The present and future of standards for E-learning technologies. Interdiscipl. J. Knowl. Learn. Obj. 2006; 2 :59–76. http://citeseerx.ist.psu.edu/viewdoc/summary Available at: [ Google Scholar ]
- Wikle J.S., West R.E. An analysis of discussion forum participation and student learning outcomes. Int. J. e Learn. 2018; 18 (2):205–228. [ Google Scholar ]
- Wininger S.R. Prevalence of learning styles in educational psychology and introduction to education textbooks: a content analysis. Psychol. Learn. Teach. 2019; 18 (3):221–443. [ Google Scholar ]
- Zhong-Lin L., Barbara A.D. 2007. Cognitive Psychology, Scholarpedia. [ Google Scholar ]
Deep learning based synthesis of MRI, CT and PET: Review and analysis
Affiliations.
- 1 Department of Data Science and AI, Faculty of Information Technology, Monash University, Clayton VIC 3800, Australia. Electronic address: [email protected].
- 2 Monash Biomedical Imaging, Clayton VIC 3800, Australia.
- 3 Department of Medical Imaging and Radiation Sciences, Faculty of Medicine, Monash University, Clayton VIC 3800, Australia.
- 4 Bioengineering Department and Imperial-X, Imperial College London, W12 7SL, United Kingdom.
- 5 Department of Data Science and AI, Faculty of Information Technology, Monash University, Clayton VIC 3800, Australia.
- 6 Department of Data Science and AI, Faculty of Information Technology, Monash University, Clayton VIC 3800, Australia; Monash Biomedical Imaging, Clayton VIC 3800, Australia.
- PMID: 38052145
- DOI: 10.1016/j.media.2023.103046
Medical image synthesis represents a critical area of research in clinical decision-making, aiming to overcome the challenges associated with acquiring multiple image modalities for an accurate clinical workflow. This approach proves beneficial in estimating an image of a desired modality from a given source modality among the most common medical imaging contrasts, such as Computed Tomography (CT), Magnetic Resonance Imaging (MRI), and Positron Emission Tomography (PET). However, translating between two image modalities presents difficulties due to the complex and non-linear domain mappings. Deep learning-based generative modelling has exhibited superior performance in synthetic image contrast applications compared to conventional image synthesis methods. This survey comprehensively reviews deep learning-based medical imaging translation from 2018 to 2023 on pseudo-CT, synthetic MR, and synthetic PET. We provide an overview of synthetic contrasts in medical imaging and the most frequently employed deep learning networks for medical image synthesis. Additionally, we conduct a detailed analysis of each synthesis method, focusing on their diverse model designs based on input domains and network architectures. We also analyse novel network architectures, ranging from conventional CNNs to the recent Transformer and Diffusion models. This analysis includes comparing loss functions, available datasets and anatomical regions, and image quality assessments and performance in other downstream tasks. Finally, we discuss the challenges and identify solutions within the literature, suggesting possible future directions. We hope that the insights offered in this survey paper will serve as a valuable roadmap for researchers in the field of medical image synthesis.
Keywords: Generative deep-learning models; Medical image synthesis; Pseudo-CT; Synthetic MR; Synthetic PET.
Copyright © 2023 The Author(s). Published by Elsevier B.V. All rights reserved.
Publication types
- Deep Learning*
- Image Processing, Computer-Assisted / methods
- Magnetic Resonance Imaging
- Positron-Emission Tomography
- Tomography, X-Ray Computed
Help | Advanced Search
Computer Science > Computer Vision and Pattern Recognition
Title: vifnet: an end-to-end visible-infrared fusion network for image dehazing.
Abstract: Image dehazing poses significant challenges in environmental perception. Recent research mainly focus on deep learning-based methods with single modality, while they may result in severe information loss especially in dense-haze scenarios. The infrared image exhibits robustness to the haze, however, existing methods have primarily treated the infrared modality as auxiliary information, failing to fully explore its rich information in dehazing. To address this challenge, the key insight of this study is to design a visible-infrared fusion network for image dehazing. In particular, we propose a multi-scale Deep Structure Feature Extraction (DSFE) module, which incorporates the Channel-Pixel Attention Block (CPAB) to restore more spatial and marginal information within the deep structural features. Additionally, we introduce an inconsistency weighted fusion strategy to merge the two modalities by leveraging the more reliable information. To validate this, we construct a visible-infrared multimodal dataset called AirSim-VID based on the AirSim simulation platform. Extensive experiments performed on challenging real and simulated image datasets demonstrate that VIFNet can outperform many state-of-the-art competing methods. The code and dataset are available at this https URL .
Submission history
Access paper:.
- HTML (experimental)
- Other Formats
References & Citations
- Google Scholar
- Semantic Scholar
BibTeX formatted citation

Bibliographic and Citation Tools
Code, data and media associated with this article, recommenders and search tools.
- Institution
arXivLabs: experimental projects with community collaborators
arXivLabs is a framework that allows collaborators to develop and share new arXiv features directly on our website.
Both individuals and organizations that work with arXivLabs have embraced and accepted our values of openness, community, excellence, and user data privacy. arXiv is committed to these values and only works with partners that adhere to them.
Have an idea for a project that will add value for arXiv's community? Learn more about arXivLabs .
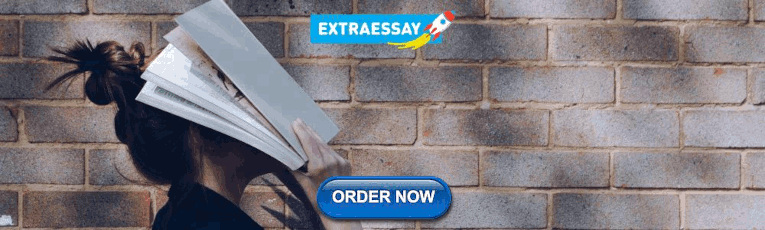
IMAGES
VIDEO
COMMENTS
The Learning Modality Change CoI Scale can be used to measure the impacts of learning modality changes on students' perceptions of cognitive, social, and teaching presence. ... Murphy R. Learning online: What research tells us about whether, when and how. Routledge; 2014. [Google Scholar] Muthén, L. K., & Muthén, B. O. (1998-2012). Mplus ...
Impact of Learning Modalities on Academic Success. Fabien Fenouillet & Jonathan Kaplan [[email protected]] Education and Training Research Centre [CREF - EA 1589] West Paris Nanterre - La Défense University [UPX] 200, avenue de la République F-92001 Nanterre, France. Phone: +33 970 448 282.
The rapid learning environment transition initiated by the COVID-19 pandemic impacted students' perception of, comfort with, and self-efficacy in the online learning environment. Garrison's Community of Inquiry framework provides a lens for examining students' online learning experiences through three interdependent elements: social presence, cognitive presence, and teaching presence ...
2. Research methodology. To systematically examine the teaching and learning modes adopted during and post-COVID-19 in higher education, we adopted a five-step procedure proposed by Fahimnia, Sarkis, and Eshragh (2015).The objective of our research is to examine the current body of literature and systematically identify research gaps objectively (García, Pino, Elkhwesky, & Salem, 2022; Salem ...
Blended learning and research issues. Blended learning (BL), or the integration of face-to-face and online instruction (Graham 2013), is widely adopted across higher education with some scholars referring to it as the "new traditional model" (Ross and Gage 2006, p. 167) or the "new normal" in course delivery (Norberg et al. 2011, p. 207).). However, tracking the accurate extent of its ...
While a diverse range of learning styles have been proposed, modality preference has received the most attention within educational research and practice. Supporters of this theory posit that each individual has a dominant sense and that when new material is presented in this preferred modality, learning is improved.
In the case of the online modality, we observed the highest scores in accessibility and knowledge in ICT but the worst score in participation. In this case, an average of 7.8 was reached. The blended learning modality reached an average of 8.1, obtaining scores around 7.5 and 9.3.
Fallace argues that the VAK learning style typology emerged from an unwarranted overextension of research on remedial reading; that is, the VAK typology was never meant to be applied to all students or to all subjects. Further, some of the earliest literature reviews of the effectiveness of VAK-specific teaching techniques consistently found ...
As blended learning combines traditional classroom with online instruction; there emerges a complexity in terms of variety and diversity of learning environments, learning design(s) and as well as [learning styles of] the students (Garrison & Kanuka, 2004; George, Walker & Keeffe, 2010; Vaughan, 2007) and narrow awareness. Similarly, cultural
The global pandemic forced us to rethink education to fight Covid-19 and apply social distancing during lectures. Luckily, we could rely on earlier research into distance education in general, and more specifically, into synchronous hybrid learning. During synchronous hybrid learning both on-site and remote students are connected and taught synchronously in what we call at our university the ...
The disappointing outcome of all these empirical and theoretical endeavors and efforts is that the modality-specific learning style concept is, as stated by Newton (2015), thriving across all levels of education. This is reflected in the findings of 89% of research papers published from 2013 to 2015 and located in ERIC and PubMed databases ...
1.1. Related literature. Online learning is a form of distance education which mainly involves internet‐based education where courses are offered synchronously (i.e. live sessions online) and/or asynchronously (i.e. students access course materials online in their own time, which is associated with the more traditional distance education).
A Research Review of Literature on Learning Modalities. Repository staff provided abstract to aid in discovery. In this paper, the author examines both the history of learning modalities in reading instruction and contemporary research in using modalities to strengthen learning. He finds that while learning modality is an interesting concept ...
1. Introduction. In the context of the rapidly developing scientific and technical revolution, the education and training sector has actively implemented tasks and solutions to enhance support management, teaching, learning, assessment, scientific research, and the application of information and communication technology (ICT) (Acosta et al., 2018; Baris, 2015; Bray and Tangney, 2017; Diabat ...
the online delivery modality. Some research has examined variability delivery modalities (i.e., online and F2F). between Elvers, Polzella, and Graetz [5] found no differences in procrastination in accessing course information, exam scores, or attitudes about the respective course (i.e., online or F2F). However, they did find a significant
Research design. This research applies a quantitative design where descriptive statistics are used for the student characteristics and design features data, t-tests for the age and gender variables to determine if they are significant in blended learning effectiveness and regression for predictors of blended learning effectiveness.
During the first semester of the Academic Year 2020-2021, this study sought to ascertain the various learning styles (visual, auditory, read/write, and kinesthetic) and preferred learning ...
The term "modular approach" refers to learning that takes the form of individualized instruction and allows students to use Self-Learning Modules (SLMs) in the print or advanced format/electronic ...
An exploratory sequential mixed methods research design was utilized. In the first stage of the study, the data was collected through an open- ended research interview protocol through google forms then, their responses were downloaded and extracted from the file for presentation, ... appropriate learning modality be used by the grade 6 ...
University of South Carolina
style', and for others it denotes preferred approaches to learning based on modality strengths" (Nel, 2008). Lawrence (1984) stated that the term learning style is used to "incorporate
Reference resolution is an important problem, one that is essential to understand and successfully handle context of different kinds. This context includes both previous turns and context that pertains to non-conversational entities, such as entities on the user's screen or those running in the background. While LLMs have been shown to be extremely powerful for a variety of tasks, their use in ...
The disappointing outcome of all these empirical and theoretical endeavors and efforts is that the modality-specific learning style concept is, as stated by Newton (2015), thriving across all levels of education. This is reflected in the findings of 89% of research papers published from 2013 to 2015 and located in ERIC and PubMed databases ...
To cover as many imaging modalities and fluorescence microscopy-based image restoration tasks as possible, we collected datasets from the literature (Supplementary Table 2) and grouped numerous ...
This study set out to determine a methodology for estimating learning styles and cognitive traits from LMS access records. In this paper, we presented a model for the automatic identification of learner behavior in LMS records. The model takes advantage of data collected from the LMS log, education theories, and literature-based methods to ...
Medical image synthesis represents a critical area of research in clinical decision-making, aiming to overcome the challenges associated with acquiring multiple image modalities for an accurate clinical workflow. This approach proves beneficial in estimating an image of a desired modality from a giv …
Image dehazing poses significant challenges in environmental perception. Recent research mainly focus on deep learning-based methods with single modality, while they may result in severe information loss especially in dense-haze scenarios. The infrared image exhibits robustness to the haze, however, existing methods have primarily treated the infrared modality as auxiliary information, failing ...