Cancer Biology
Focus areas.
The mission of the Department of Cancer Biology is to identify and understand the causes of cancer, to develop innovative approaches to reduce cancer incidence, to create and test novel and more effective therapies, and to translate these findings into clinical care for the benefit of patients.
Research in our department is highly collaborative and is potentiated through close interactions with other basic science departments and translated through clinical collaborations.
Our faculty members are aligned into eight research focus areas that address cancer development, progression and treatment.
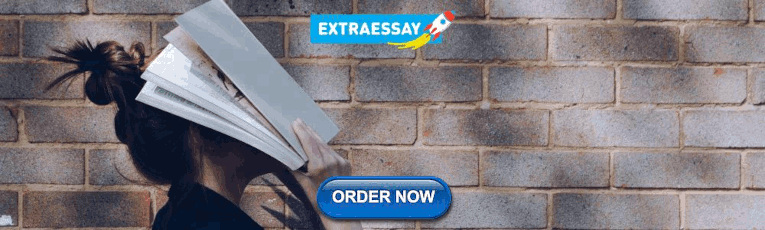
Cancer disparities
While cancer affects everyone, certain groups have higher rates of cancer cases, deaths and health complications. These differences can be associated with genetics, sex, racial and ethnic populations, socioeconomic status, or specific geographic areas. Studying the factors that lead to cancer disparities leads to more-effective prevention and treatment approaches for the affected populations.
- Read more about the cancer disparities focus area .
Cancer stem cells
The stem cell theory of cancer proposes that among the many different types of cells within a cancer, there exists a subpopulation of cells called cancer stem cells that multiply indefinitely, are resistant to chemotherapy, and are thought to be responsible for relapse after therapy. Cancer stem cells also give rise to highly metastatic cells that spread to other organs and tissues within the body. A deeper understanding of cancer stem cells is leading to better implementation of existing anti-cancer therapies and identification of new approaches that target the cancer stem cells specifically.
- Read more about the cancer stem cells focus area .
Cancer systems biology
Cancer is a complex disease with many molecular, genetic and cellular causes. Considering these causes as a system of interactions can lead to a better understanding of the processes involved in the development of cancer and response to therapies. Cancer systems biology integrates advanced experimental models, insights from genome sequencing and other large-scale data projects, and computational models to create unified models of cancer behavior.
- Read more about the cancer systems biology focus area .
Oncogenic gene dysregulation and carcinogenesis
Cancer initiates from genetic alterations, including mutations, deletions and copy number gains, that function to activate cancer-promoting pathways or to block processes that normally inhibit cancer development. Through better understanding of pro- and anti-cancer signaling processes and how they become dysregulated in carcinogenesis and tumor progression, our team can improve biological tools for clinicians and devise molecularly targeted therapies to intervene.
- Read more about the oncogenic gene dysregulation and carcinogenesis focus area .
Precision cancer medicine and translational therapeutics
Cancers develop and respond to therapies differently from one patient to the next. A better understanding of the specific processes driving cancer growth and spread in an individual patient allows for tailored therapeutic strategies that are more effective with minimal side effects. Profiling the mutations and abnormalities that drive a tumor, in combination with development of experimental models that assess the specific responses of cancer cells to therapeutics that target those abnormalities, has dramatically improved outcomes in many cancer types.
- Read more about the precision cancer medicine and translational therapeutics focus area .
Tumor immunology and immunotherapy
Therapeutic strategies that stimulate the immune system to target cancer cells can lead to long-lasting tumor regression and minimize relapse. Integrated efforts of laboratory researchers and clinicians are leading to improved knowledge of how the immune system interacts with cancer cells and how immune processes can be intentionally manipulated for therapeutic effect.
- Read more about the tumor immunology and immunotherapy focus area .
Tumor invasion and metastasis
A fundamental property of malignant tumor cells is the ability to invade surrounding tissues and to metastasize to other organs. These abilities underlie the majority of cancer-associated deaths. While invasion and metastasis are often thought of as the final stages of tumor development, recent studies have shown that tumor spread can occur even at early stages of tumor development, sometimes even before the primary tumor has been identified. Development of therapies targeting invasion and metastasis has the promise to significantly reduce cancer mortality.
- Read more about the tumor invasion and metastasis focus area .
Tumor microenvironment
Tumors require complex interactions with surrounding blood vessels, immune cells, supportive tissue structures and cell types that are distinct to the tumor site in order to grow, become invasive and metastasize. Tumors influence their microenvironment by releasing soluble signals that lead to degradation and remodeling of the tissue structures that constrain their growth. Targeting the interactions of tumors with the microenvironment is an important and developing area of study.
- Read more about the tumor microenvironment focus area .
More about research at Mayo Clinic
- Research Faculty
- Laboratories
- Core Facilities
- Centers & Programs
- Departments & Divisions
- Clinical Trials
- Institutional Review Board
- Postdoctoral Fellowships
- Training Grant Programs
- Publications
Mayo Clinic Footer
- Request Appointment
- About Mayo Clinic
- About This Site
Legal Conditions and Terms
- Terms and Conditions
- Privacy Policy
- Notice of Privacy Practices
- Notice of Nondiscrimination
- Manage Cookies
Advertising
Mayo Clinic is a nonprofit organization and proceeds from Web advertising help support our mission. Mayo Clinic does not endorse any of the third party products and services advertised.
- Advertising and sponsorship policy
- Advertising and sponsorship opportunities
Reprint Permissions
A single copy of these materials may be reprinted for noncommercial personal use only. "Mayo," "Mayo Clinic," "MayoClinic.org," "Mayo Clinic Healthy Living," and the triple-shield Mayo Clinic logo are trademarks of Mayo Foundation for Medical Education and Research.
Support Biology
Dei council and dei faculty committee, biology diversity community, mit biology catalyst symposium, honors and awards, employment opportunities, faculty and research, current faculty, in memoriam, areas of research, biochemistry, biophysics, and structural biology, cancer biology, cell biology, computational biology, human disease, microbiology, neurobiology, stem cell and developmental biology, core facilities, video gallery, faculty resources, undergraduate, why biology, undergraduate testimonials, major/minor requirements, general institute requirement, advanced standing exam, transfer credit, current students, subject offerings, research opportunities, biology undergraduate student association, career development, why mit biology, diversity in the graduate program, nih training grant, career outcomes, graduate testimonials, prospective students, application process, interdisciplinary and joint degree programs, living in cambridge, graduate manual: key program info, graduate teaching, career development resources, biology graduate student council, biopals program, postdoctoral, life as a postdoc, postdoc associations, postdoc testimonials, workshops for mit biology postdocs entering the academic job market, responsible conduct of research, postdoc resources, non-mit undergraduates, bernard s. and sophie g. gould mit summer research program in biology (bsg-msrp-bio), bsg-msrp-bio gould fellows, quantitative methods workshop, high school students and teachers, summer workshop for teachers, mit field trips, leah knox scholars program, additional resources, mitx biology, biogenesis podcast, biology newsletter, department calendar, ehs and facilities, graduate manual, resources for md/phd students, preliminary exam guidelines, thesis committee meetings, guidelines for graduating, mentoring students and early-career scientists, remembering stephen goldman (1962 – 2022).
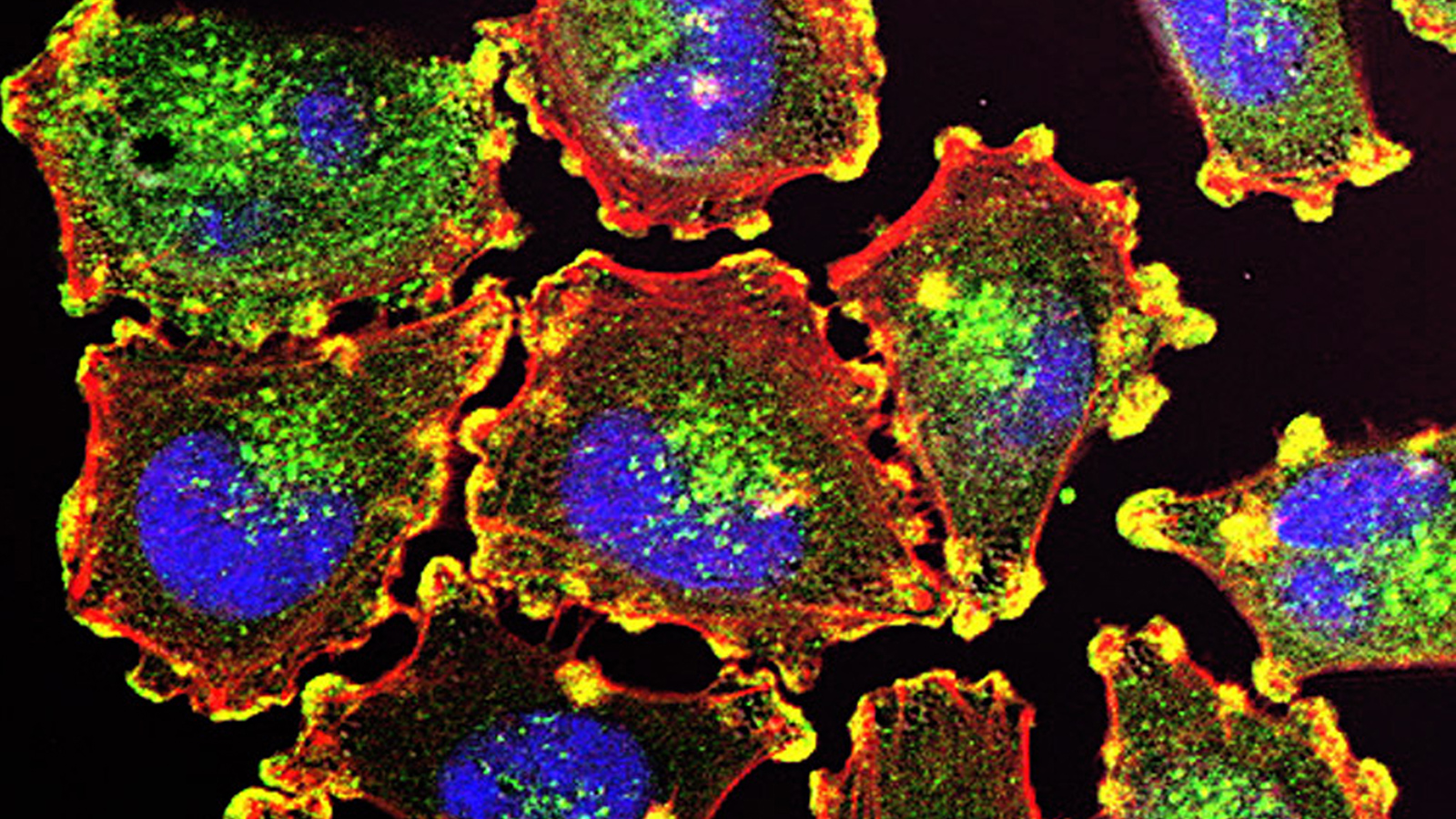
cancer gene discovery • tumorigenesis • cancer therapy and resistance • oncogenes • tumor suppressor genes • cancer models • growth control and cell proliferation • metastasis • cell proliferation • cell death • cell-cell and cell-matrix interactions • microenvironment •DNA repair and replication • transcription • chromosome stability • metabolism • immunology and cancer • immunotherapy • cancer stem cells
Eliezer Calo
Lindsay case, jianzhu chen, michael t. hemann, whitney henry, david housman, richard o. hynes, tyler jacks, sally kornbluth, douglas lauffenburger, jacqueline lees, alison e. ringel, francisco j. sánchez-rivera, phillip a. sharp, yadira soto-feliciano, stefani spranger, matthew vander heiden, robert a. weinberg, michael b. yaffe, omer h. yilmaz, richard a. young.
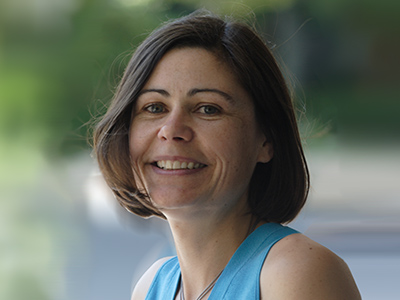
Jacqueline Lees develops mouse and zebrafish models, identifying the molecular pathways leading to tumor formation.
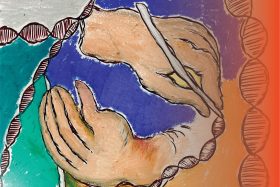
Scientists develop a rapid gene-editing screen to find effects of cancer mutations
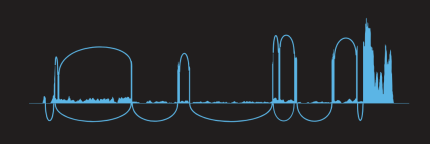
News brief: Calo Lab
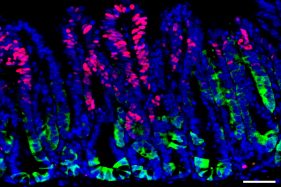
How early-stage cancer cells hide from the immune system
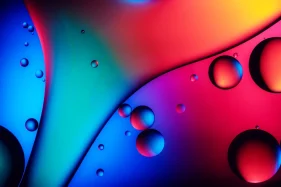
How phase separation is revolutionizing biology
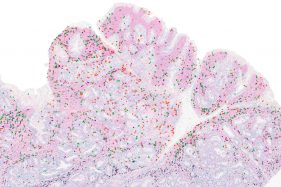
Study explains why certain immunotherapies don’t always work as predicted
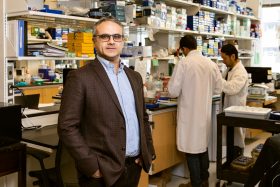
Exploring the links between diet and cancer
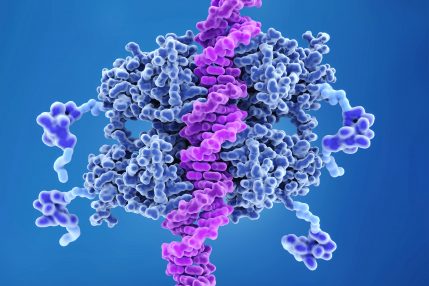
Gene-editing technique could speed up study of cancer mutations
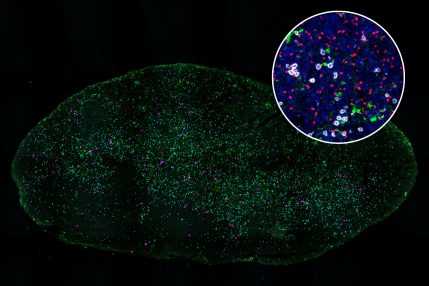
Why lung cancer doesn’t respond well to immunotherapy
Cancer Cell Biology Research
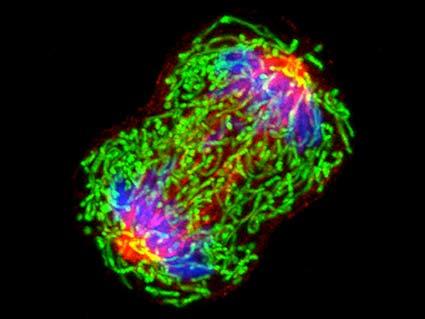
A dividing breast cancer cell.
Research in cancer cell biology seeks to define the biological basis underlying the differences between normal cells and cancerous cells. This includes studies of the fundamental mechanisms that drive pre-cancer states, oncogenic transformation, and that support tumor growth and behavior. Mechanistic understanding of this biology and the fundamental processes governing transformation, including the role of aging, gender, and ethnic disparities, are critical for identifying molecular targets for therapeutic or preventive interventions.
Research in this area is supported and directed by the Cancer Cell Biology Branch (CCBB) .
Cancer Cell Metabolism
Research in cancer cell metabolism focuses on altered cellular metabolic pathways that support the cancer phenotype, which is characterized by unchecked cell proliferation, resistance to metabolic and oxidative stress, evasion of programmed cell death, reduced dependence on growth factor signals, insensitivity to growth inhibitory signals, and resistance to therapeutic interventions.
Key research areas include:
- Oncogenic reprogramming of cellular metabolism (e.g., the Warburg Effect, glutamine addiction, upregulated/deregulated fatty acid metabolism)
- The links between protein translation, ribosome biogenesis, and metabolism
- Tumor metabolite profiling and characterization
- Regulation and mechanisms of nutrient, metabolic intermediate, and ion transport in cancer cells
Emerging areas in cancer metabolism include biological functions of metabolic intermediates, the molecular link between body homeostasis and cancer cell biology, mechanisms underlying the intersection between obesity and cancer, the metabolic plasticity of cancer cells, the mechanisms through which diet and fasting affect cancer initiation and maintenance, and the molecular mechanisms that lead to cancer cachexia.
Cancer Cell Stress Responses
Research in cancer cell stress responses focuses on the cell’s reaction to intrinsic and environmental stressors that determine whether a cell will die or adapt to survive. Examples of the types of stress included in this research area are oxidative stress, oncogenic stress, accumulation of unfolded or misfolded proteins, hypoxia, metal ions, chemotherapy, and inflammation.
- Mechanisms of cell death (e.g., apoptosis, necrosis/necroptosis, autophagy, anoikis, ferroptosis, and other forms of programmed/non-programmed cell death)
- Recycling of cellular components in response to stress (e.g., autophagy, mitophagy, lipophagy)
- ER stress and the unfolded protein response
- Exosome release as a mediator of cellular stress response and intercellular communications
- Altered processing of growth factors and their associated receptors
- Mechanisms of cellular control of toxic byproducts from biological processes (e.g., redox control)
Emerging areas relevant to this research include mechanisms of metal ions homeostasis, such as iron and copper, and their associated cellular targets and functions, and understanding the global effects of metal ions accumulation.
Organelle Biology
Research in the area of organelle biology investigates the mechanisms and role of dysregulated organelle biology in driving or supporting the cancer phenotype.
- Dysregulation of organelle biogenesis and function (e.g., mitochondria, endoplasmic reticulum, Golgi, lysosomes, lipid droplets, peroxisomes, endosomes, and cilia)
- Processing and trafficking of intracellular membranes and proteins
- Endocytosis and endosome sorting and recycling
- Interactions between nuclear-encoded oncogenic proteins and mitochondrial function
- Role of cell organelles in cancer-associated phenotypes
Emerging areas relevant to this research include regulation of mitochondrial growth and division, energy-independent functions of mitochondria, and the intersection between organelle structure/morphology and the phenotypic state or function of cancer cells.
Cancer Cell Cycle Control
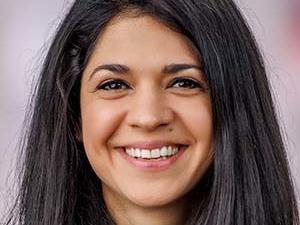
Dr. Sita Kugel Investigates the Biology of Pancreatic Cancer and Cholangiocarcinoma
Cell cycle dysregulation is a hallmark of cancer, and cell cycle components have been aggressively
targeted in chemotherapeutic strategies. Research in this area focuses on altered cell cycle regulation and its contribution to oncogenic transformation and tumor maintenance.
- Characterization of factors that regulate cell cycle, mitosis, cytokinesis, centrosome duplication, and DNA replication in cancer cells
- Alternative, kinase-independent functions of cell cycle regulators
- Mechanisms that alter protein stability and function of cell cycle components in cancer cells
- Understanding the biological effects of cell cycle inhibitors in tumors, either alone or in combination with other therapies
Emerging areas relevant to this research include the elucidation of nutrient-sensing cell cycle checkpoints, understanding mechanisms that allow for the bypass of cell cycle checkpoints, and exploration of combination therapies with CDK inhibitors for certain cancers.
Post-transcriptional Regulations Influencing Cancer
Research in this area investigates the wide-ranging mechanisms and functional effects of post-transcriptional regulations that affect the cancer phenotype.
- Altered mechanisms and regulations of RNA stability, splicing, modifications, transport, and mRNA translation
- Regulation and mechanisms of alternative splicing in cancer
- The role of non-coding RNAs and RNA binding proteins in the regulation of splicing, modifications, transport, translation, and mRNA stability
- Translation factors that act as oncogenes or tumor suppressors
- Changes in protein maturation and stability, including diverse post-translation modifications (e.g., phosphorylation, acetylation, methylation, hydroxylation, ubiquitylation, sumoylation, neddylation, and glycosylation), as well as modifications of signaling effectors (e.g., promotors and drivers of tumorigenesis or cancer progression)
Emerging areas relevant to this research include the study of chemical modifications to RNAs and protein molecules, including writers, erasers, and readers of such modifications, that affect their stability, trafficking, RNA splicing and translation, and protein function, the development of novel technologies for efficient profiling of these modifications, and the interplay of different modifications and their alterations in cancer.
Basic Mechanisms of Cell Transformation
Research in this area includes mechanisms and effectors that govern the transition from normal cell to pre-cancer, early lesion, and cancer cell, as well as the identification of early biological events in transformation. Studies cover the role of tumor-initiating cells, field cancerization, and diverse signaling pathways governing cell fate determination and tumor formation. Research also examines the functions and regulations of oncogenes and tumor suppressor genes/proteins.
- Functional and molecular characterization of oncogenes and tumor suppressors and their affected pathways
- Oncogenic signal transduction and their rewiring
- The biology of tumor-initiating cells and cancer stem cells
- Role of developmental and cell differentiation programs in preneoplasia and cancer
- Senescence as an oncogenic or tumor suppressive mechanism, the relationship between quiescence and senescence states, and the relationship between senescence, aging, and cancer
Emerging areas relevant to this research include understanding lineage affiliation of stem and progenitor cells and its role in oncogenesis, characterizing the actual cell targets for oncogenic transformation, and deciphering the functional effects of multiple mutations in normal cells and their role in transformation.
Biospecimen Resources to Support Cancer Biology Research
Research in this area includes the development of projects that encompass the collection, storage, processing, and dissemination of human biological specimens—including nucleic acids and tissue arrays—and associated data for studies of human cancer biology, particularly early events in cancer formation and pre-neoplasia.
- Open access
- Published: 15 April 2024
Machine-learning analysis reveals an important role for negative selection in shaping cancer aneuploidy landscapes
- Juman Jubran 1 na1 ,
- Rachel Slutsky 2 na1 ,
- Nir Rozenblum 2 ,
- Lior Rokach 3 ,
- Uri Ben-David 2 na2 &
- Esti Yeger-Lotem ORCID: orcid.org/0000-0002-8279-7898 1 , 4 na2
Genome Biology volume 25 , Article number: 95 ( 2024 ) Cite this article
949 Accesses
10 Altmetric
Metrics details
Aneuploidy, an abnormal number of chromosomes within a cell, is a hallmark of cancer. Patterns of aneuploidy differ across cancers, yet are similar in cancers affecting closely related tissues. The selection pressures underlying aneuploidy patterns are not fully understood, hindering our understanding of cancer development and progression.
Here, we apply interpretable machine learning methods to study tissue-selective aneuploidy patterns. We define 20 types of features corresponding to genomic attributes of chromosome-arms, normal tissues, primary tumors, and cancer cell lines (CCLs), and use them to model gains and losses of chromosome arms in 24 cancer types. To reveal the factors that shape the tissue-specific cancer aneuploidy landscapes, we interpret the machine learning models by estimating the relative contribution of each feature to the models. While confirming known drivers of positive selection, our quantitative analysis highlights the importance of negative selection for shaping aneuploidy landscapes. This is exemplified by tumor suppressor gene density being a better predictor of gain patterns than oncogene density, and vice versa for loss patterns. We also identify the importance of tissue-selective features and demonstrate them experimentally, revealing KLF5 as an important driver for chr13q gain in colon cancer. Further supporting an important role for negative selection in shaping the aneuploidy landscapes, we find compensation by paralogs to be among the top predictors of chromosome arm loss prevalence and demonstrate this relationship for one paralog interaction. Similar factors shape aneuploidy patterns in human CCLs, demonstrating their relevance for aneuploidy research.
Conclusions
Our quantitative, interpretable machine learning models improve the understanding of the genomic properties that shape cancer aneuploidy landscapes.
Introduction
Aneuploidy, defined as an abnormal number of chromosomes or chromosome-arms within a cell, is a characteristic trait of human cancer [ 1 ]. Aneuploidy is associated with patient prognosis and with response to anticancer therapies [ 2 , 3 ], indicating that it can play a driving role in tumorigenesis. It is well established that the fitness advantage conferred by specific aneuploidies depends on the genomic, environmental, and developmental contexts [ 1 ]. One important cellular context is the cancer tissue of origin; aneuploidy patterns are cancer type-specific, and cancers that originate from related tissues tend to exhibit similar aneuploidy patterns [ 2 , 4 , 5 ]. Nonetheless, the selection pressures that shape the aneuploidy landscapes of human tumors are not fully understood, and it is not clear why some chromosome-arm gains and losses would recur in some tumor types but not in others.
Several non-mutually exclusive explanations have been previously provided in an attempt to explain the tissue selectivity of aneuploidy patterns. First, the densities of oncogenes (OGs) and tumor suppressor genes (TSGs) are enriched in chromosome-arms that tend to be gained or lost, respectively, potentially due to the cumulative effect of altering multiple such genes at the same time [ 6 ]. As cell proliferation is controlled in a tissue-dependent manner, the relative importance of OGs and TSGs varies across tissues, so that the density of tissue-specific driver genes can help predict aneuploidy patterns [ 7 ]. Second, some recurrent aneuploidies reflect the chromosome arm-wide gene expression patterns that characterize their normal tissue of origin, suggesting that chromosome-arm gains and losses may ‘hardwire’ pre-existing gene expression patterns [ 8 ]. Third, several strong cancer driver genes have been shown to underlie the recurrent aneuploidy of the chromosome-arms on which these genes reside; prominent examples are the tumor suppressors TP53 and PTEN , which have been shown to drive the recurrent loss of chromosome-arm 17p in leukemia and that of 10q in glioma, respectively [ 9 , 10 , 11 ]. Fourth, it has been recently proposed that somatic amplifications, including chromosome-arm gains, are positively selected in cancer evolution in order to buffer gene inactivation of haploinsufficient genes in mutation-prone regions [ 12 ].
Notably, each previous study focused on a separate aspect of tissue specificity; therefore, the relative contribution of each factor to shaping the overall aneuploidy landscape of human tumors is currently unknown. Furthermore, whether any additional tissue-specific traits could also play a major role in driving aneuploidy patterns remains an open question. Importantly, previous studies focused on the role of positive selection in driving the gain or the loss of specific chromosome-arms in specific tumor types. However, unlike point mutations in specific genes, aneuploidies come with a strong fitness cost [ 1 , 13 ]. Therefore, whereas positive selection greatly outweighs negative selection in shaping the landscape of point mutations in cancer, as evaluated by a refined version of the normalized ratio of non-synonymous to synonymous mutations [ 14 ], both positive selection and negative selection may be important for shaping the landscape of aneuploidy. Indeed, a recent study showed that negative selection could determine the boundaries of recurrent cancer copy number alterations [ 15 ]. It is therefore necessary to consider the balance between positive and negative selection in shaping the aneuploidy landscapes of human cancer.
Machine learning (ML) methods have been applied to study a variety of biological and medical questions where heterogeneous large-scale data are available [ 16 ]. In the context of cancer, supervised ML methods were applied to predict cancer driver genes [ 17 , 18 ], to distinguish between cancer types [ 19 , 20 ], and to predict gene dependency in tumors [ 21 ]. However, ML has not been applied to investigate the observed patterns of aneuploidy in human cancer. Whereas ML has been frequently used for prediction and often regarded as a black box, recent advancements have allowed more insight into the factors that underlie prediction. For example, Shapley Additive exPlanations algorithm (SHAP) [ 22 , 23 ] estimates the importance and relative contribution of each of the features utilized by the model to the model’s decisions.
Here, we present a novel ML approach to elucidate the factors that underlie the cancer type-specific patterns of aneuploidy. For this, we constructed separate ML models for chromosome-arm gain and loss, whereby each of 39 chromosome-arms within 24 cancer types was associated with 20 types of features corresponding to various genomic attributes of chromosome-arms, normal tissues, primary tumors, and cancer cell lines (CCLs). Our approach is focused on interpretation rather than prediction of aneuploidy recurrence patterns. Interpretation of the gain and loss models for aneuploidy in primary tumors captured known genomic features that had been previously reported to shape aneuploidy landscapes, supporting the models’ validity. Furthermore, these analyses suggested that negative selection played a greater role than positive selection in this process and revealed paralog compensation as an important contributor to cancer type-specific aneuploidy patterns, in both primary tumors and CCLs. Lastly, we experimentally validated a specific aneuploidy driver using genetically engineered isogenic human cells.
Constructing machine learning models to classify cancer aneuploidy patterns
To create a supervised classification ML model that predicts the recurrence pattern of aneuploidy across cancer types, we built a large‐scale dataset consisting of labels and features per instance of chromosome-arm and cancer type. For each instance, the label indicated whether the chromosome-arm was recurrently gained, lost, or remained neutral in that cancer. Labels were determined according to Genomic Identification of Significant Targets in Cancer (GISTIC2.0) [ 24 ]. We focused on 24 cancer types for which transcriptomic data of normal tissues of origin was available from the Genotype-Tissue Expression Consortium (GTEx) ([ 25 ] ( Methods ). In total, 199 instances of chromosome-arm and cancer type were labeled as gained, 307 were labeled as lost, and 430 were labeled as neutral (Fig. 1 A).
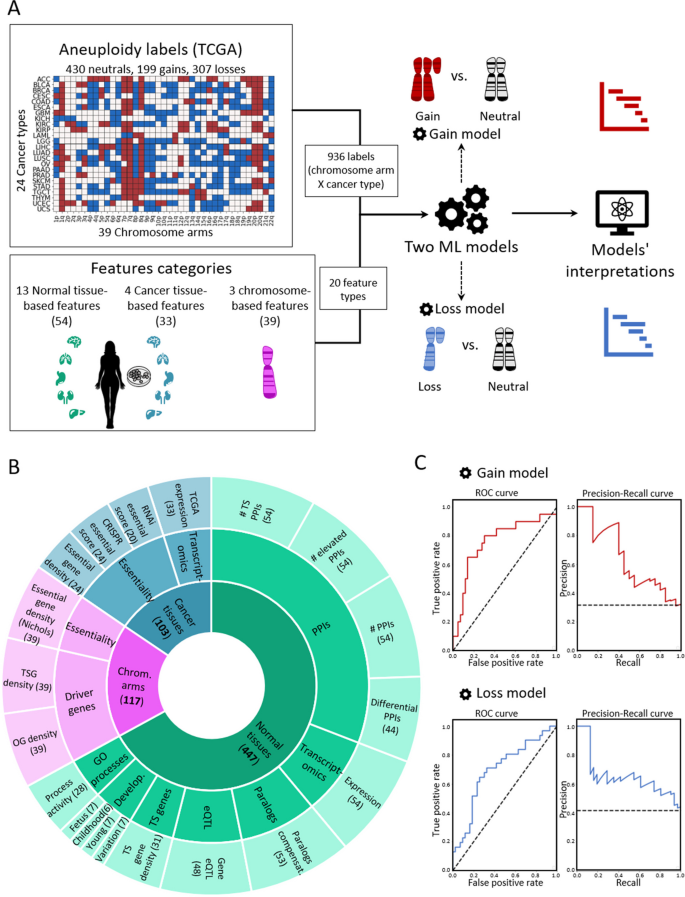
A machine learning (ML) approach for predicting aneuploidy in cancer. A Schematic view of the ML model construction. Labels represent aneuploidy status of each chromosome arm in 24 cancer types (abbreviation of cancer types detailed in Additional file 2 : Table S1), classified as gained (red, n = 199), lost (blue, n = 307), or neutral (white, n = 430). Features consist of 20 types of features pertaining to chromosome-arms, normal tissues and cancer tissues (see B ). Two separate ML models were constructed to predict gained and lost chromosome-arms (gain model and loss model). Each model was analyzed to estimate the contribution of the features to the predicted outcome. B The features analyzed by the ML model. The inner layer shows feature categories: chromosome arms (purple), cancer tissues (primary tumors and CCLs, blue), and normal tissues (green). The middle layer shows the sub-categories of the features. Chromosome-arm features include essentiality and driver genes features. Cancer-tissue features include transcriptomics and essentiality features. Normal-tissue features include protein–protein interactions (PPIs), transcriptomics, paralogs, eQTL, tissue-specific (TS) genes, development, and GO processes features. The outer layer represents all 20 feature types that were analyzed by the model. Numbers in parentheses indicate the number of tissues, organs, or cell lines from which cancer and normal tissue features were derived, or the number of chromosome-arms from which chromosome-arm features were derived. C The performance of the ML models as evaluated by the area under the receiver-operating characteristic curve (auROC, left) and the precision recall curve (auPRC, right) using tenfold cross-validation. Gain model (gradient boosting): auROC = 74% and auPRC = 63% (expected 32%). Loss model (XGBoost): auROC = 70% and auPRC = 63% (expected 42%)
Next, we defined three categories of features (Fig. 1 B; Methods ). The first category, denoted ‘chromosome-arms’, contained features of chromosome-arms that are independent of cancer type. Chromosome-arm features included the density of OGs, the density of TSGs [ 6 ], and the density of essential genes [ 26 ] per chromosome-arm. The second category, denoted ‘cancer tissues’, contained features pertaining to chromosome-arms in primary tumors and CCLs. It included features pertaining to expression of genes in primary tumors and essentiality of genes in CCLs. Expression levels of genes in each chromosome-arm per cancer type were obtained from The Cancer Genome Atlas (TCGA, https://www.cancer.gov/tcga ). Gene essentiality scores were obtained from the Cancer Dependency Map (DepMap) [ 27 ]. In total, this category included 103 omics-based readouts ( Methods ). The third category, denoted ‘normal tissues’, contained features pertaining to chromosome-arms in normal tissues from which cancer types originated (e.g., colon tissue was matched with colon adenocarcinoma, Additional file 2 : Table S1). Features of normal tissues included expression levels of genes located on each chromosome-arm in the respective normal tissue, their tissue protein–protein interactions (PPIs) [ 28 , 29 ], and their tissue-specific biological process activities [ 30 ]. It also included tissue-specific dosage relationships between paralogous genes, denoted ‘paralog compensation’ [ 31 , 32 ]. In total, this category included 447 tissue-based properties ( Methods ). To enhance our understanding of cancer and tissue selectivity, feature values of cancer and normal tissues were transformed from absolute to relative; for example, instead of indicating the absolute expression level of a gene in a given normal tissue, the expression feature was set to the expression level of the gene in the given tissue relative to its expression levels in all tissues (Additional file 1 : Fig. S1). Each chromosome-arm was then assigned with a feature value that was inferred from the values of its genes ( Methods , Additional file 1 : Fig. S2).
To fit the features dataset and the labels dataset, we further transformed the features dataset, such that each instance of chromosome-arm and cancer type was associated with features corresponding to the chromosome-arm, cancer type, and matching normal tissue ( Methods ). In total, the dataset included 20 types of features per chromosome-arm and cancer type: 3 in the chromosome-arm category, 4 in the cancer tissues category, and 13 in the normal tissues category (Fig. 1 B). We assessed the similarity between every pair of features using Spearman correlation (Additional file 1 : Fig. S3A). Most features did not correlate with each other (Additional file 1 : Fig. S3B). Among the correlated feature pairs were PPI-related features and expression in normal adult and developing tissues features (Additional file 1 : Fig. S3A). Lastly, we assessed the similarity between instances of chromosome-arm and cancer type by their feature values using principal component analysis (PCA) (Additional file 1 : Fig. S3C). Instances did not cluster by their aneuploidy pattern (gain/loss/neutral), suggesting that a more complex model is needed to classify the different patterns.
With these labels and features of each chromosome-arm and cancer type, we set out to construct two separate ML models to predict chromosome-arm gain and loss patterns across cancer types (denoted as the ‘gain model’ and the ‘loss model’, respectively; Fig. 1 A). Each model was trained and tested on data of gained (or lost) chromosome-arms versus neutral chromosome-arms. We employed five different ML methods ( Methods ) and assessed the performance of each method by using tenfold cross-validation and calculating average area under the receiver operating characteristic (auROC) and average area under the precision-recall curve (auPRC) (Additional file 1 : Fig. S4A,B). Logistic regression showed similar results to a random prediction, with auROC of 54% for each model (Additional file 1 : Fig. S4), indicating that the relationships between features and labels are non-linear. Decision tree methods that can capture such relationships [ 33 , 34 ], including gradient boosting, XGBoost, and random forest, performed better than logistic regression and similarly to each other (Additional file 1 : Fig. S4). Best performance in the gain model was achieved by gradient boosting method, with auROC of 74% and auPRC of 63% (expected: 32%) (Fig. 1 C). Best performance in the loss model was achieved by XGBoost, with auROC of 70% and auPRC of 63% (expected: 42%) (Fig. 1 C).
Revealing the top contributors to cancer aneuploidy patterns
The main purpose of our models was to identify the features that contribute the most to the recurrence patterns of aneuploidy observed in human cancer, which could illuminate the factors at play. To this aim, we used the SHAP (Shapley Additive exPlanations) algorithm [ 22 , 23 ], which estimates the importance and relative contribution of each feature to the model’s decision and ranks them accordingly. We applied SHAP separately to the gain model and to the loss model ( Methods ).
In the gain model, the topmost features were TSG density and OG density (Fig. 2 A,B). As expected, these features showed opposite directions: TSG density was low in gained chromosome-arms, whereas OG density was high, in line with previous observations [ 6 , 7 ] (Fig. 2 B). Importantly, this analysis revealed that the impact of TSGs on the gain model’s decision was twice larger than that of OGs (Fig. 2 A), highlighting the importance of negative selection for shaping cancer aneuploidy patterns. The third most important feature was TCGA expression, which quantified the expression of arm-residing genes in the given cancer type relative to their expression in other cancers. Notably, expression levels were obtained only from samples where the chromosome-arm was not gained or lost ( Methods ). This analysis revealed that, across cancer types, chromosome-arms that tend to be gained exhibit higher expression of genes even in neutral cases, consistent with a previous recent study [ 8 ]. This confirms that the genes on gained chromosome-arms are preferentially important for the specific cancer types in which these gains are recurrent. Congruently, PPIs and normal tissue expression—features of normal tissues—were also among the ten top-contributing features (Fig. 2 A). The estimated importance of all features in the gain model is shown in Additional file 1 : Fig. S5A.
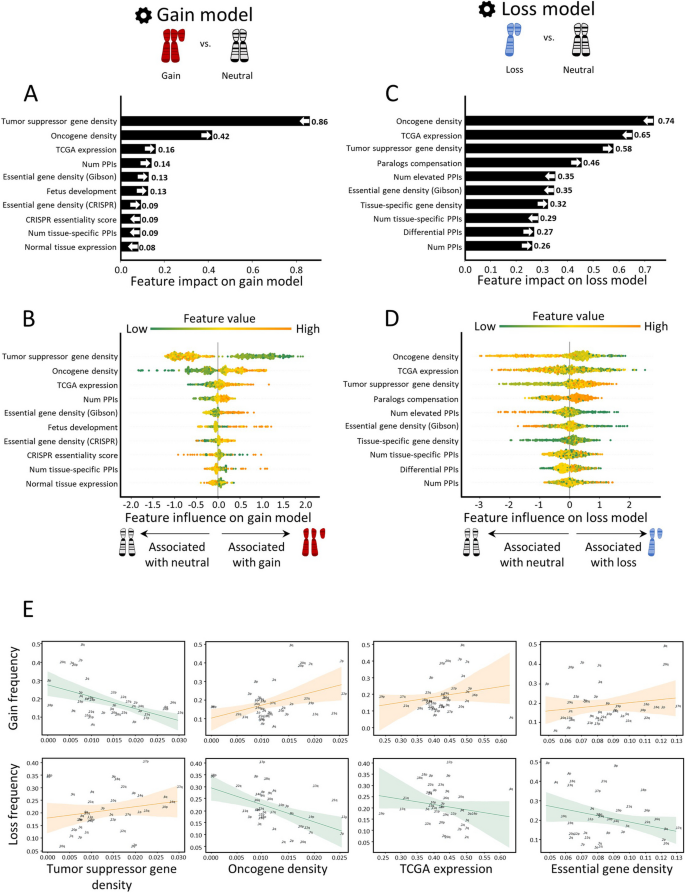
Quantitative views into the ten topmost contributing features of the gain and loss models. Features are ordered from bottom to top by their increased average absolute contribution to the model, as calculated by SHAP. A The average absolute contribution of each feature to the gain model. The directionality of the feature (i.e., whether high feature values correspond to gain or neutral) is represented by an arrow. B A detailed view of the contribution of each feature to the gain model. Per feature, each dot represents the contribution per instance of a chromosome-arm and cancer type pair. The dots are spread based on whether they were classified as neutral (left) or gain (right) by the model. Instances are colored by the feature value (green-to-orange scale denotes low-to-high value). The order (height) of each feature is the same as in A . C Same as panel A for the loss model. D Same as panel B for the loss model. E The correlations between top contributing features and the frequencies of chromosome-arm gains and losses, as measured by Spearman correlation. P -values were adjusted for multiple hypothesis testing using Benjamini–Hochberg procedure. Negative correlation between TSG density and gain frequency ( ρ = − 0.52, adjusted p = 0.006). Positive correlation between TSG density and loss frequency ( ρ = 0.3, adjusted p = 0.17). Positive correlation between OG density and gain frequency ( ρ = 0.25, adjusted p = 0.18). Negative correlation between OG density and loss frequency ( ρ = − 0.47, adjusted p = 0.01). Positive correlation between TCGA expression and gain frequency ( ρ = 0.29, adjusted p = 0.14). Negative correlation between TCGA expression and loss frequency ( ρ = − 0.33, adjusted p = 0.12). Positive correlation between essential gene density and gain frequency ( ρ = 0.16, adjusted p = 0.37). Negative correlation between essential gene density and loss frequency ( ρ = − 0.1, adjusted p = 0.5)
The loss model shared the same top three features, yet with opposite directions and different ranks (Fig. 2 C,D). OG density ranked first, was low in lost chromosome-arms, whereas TSG density ranked third, was high (Fig. 2 D), in line with previous observations [ 6 , 7 ]. In contrast to the gain model, in the loss model, the impact of OG density on the model’s decision was larger than that of TSG density, again in line with negative selection as an important force in cancer aneuploidy evolution. TCGA expression (computed from samples where the chromosome-arm was not lost or gained, see Methods ) ranked second: chromosome-arms with highly-expressed genes tended not to be recurrently lost, in line with negative selection. Another top feature that showed opposite directions between the gain and loss model was essential gene density [ 26 ]. As expected, essential gene density was low in lost chromosome-arms, in line with negative selection against losing copies of essential genes [ 26 , 27 , 35 ]. The estimated importance of all features in the loss model is shown in Additional file 1 : Fig. S5B.
To examine the direct relationships between high-ranking features and aneuploidy recurrence patterns, we assessed the correlations between these features and aneuploidy prevalence ( Methods ). In accordance with the SHAP analysis, the negative correlation between TSG density and chromosome-arm gain ( ρ = − 0.52, adjusted p = 0.0006, Spearman correlation; Fig. 2 E) was much stronger and more significant than the positive correlation between OG density and chromosome-arm gain ( ρ = 0.25, adjusted p = 0.12, Spearman correlation; Fig. 2 E). Similarly, the negative correlation between OG density and chromosome-arm loss ( ρ = − 0.47, adjusted p = 0.003, Spearman correlation; Fig. 2 E) was much stronger and more significant than the positive correlation between TSG density and chromosome-arm loss ( ρ = 0.3, adjusted p = 0.067, Spearman correlation; Fig. 2 E). TCGA expression and essential gene density were correlated with chromosome-arm gain, and anticorrelated with chromosome-arm loss, albeit to a lesser extent (Fig. 2 E, Additional file 1 : Fig. S6). Also showing positive correlations with gains and negative correlations with losses were features derived from expression levels in normal adult and developing tissues, certain PPI-related features, and additional essentiality features (Additional file 1 : Fig. S6). However, these correlations were weaker than the correlations described above. Altogether, correlation analyses supported the relationships between top features of each model and aneuploidy patterns.
The robust impact of top contributors to cancer aneuploidy patterns
Next, we asked if the above results were sensitive to our model construction schemes. We first tested the robustness of the models to internal parameters used to generate the features ( Methods ). We therefore recreated features upon modifying internal parameters and repeated model construction and interpretation ( Methods ). We found that feature importance was robust to these changes (Additional file 1 : Fig. S7, Additional file 3 : Table S2). Second, we tested the robustness of the results upon tuning the hyperparameters of each model ( Methods , Additional file 1 : Fig. S8). The top contributing features of each model were retained following hyperparameter tuning, supporting their reliability (Additional file 1 : Fig. S8C). We also checked whether the same top features would be recognized upon modeling one type of chromosome-arm event versus all other events. Applying the same approaches, we constructed two additional ML models. One model classified chromosome-arm gain versus no-gain (i.e., chromosome-arm loss or neutrality). Another model classified chromosome-arm loss versus no-loss (i.e., chromosome-arm gain or neutral). These additional models performed similarly to their respective models (Additional file 1 : Fig. S9). SHAP analysis of the two additional models revealed that feature importance was very similar between these models and the original models, which compared gained and lost chromosome-arms only to neutral chromosome-arms (Additional file 1 : Fig. S9).
We next tested whether the results were driven by a small subset of chromosome-arm and cancer type instances. For that, per model, we identified chromosome-arm and cancer type instances with the top contributions to the five topmost important features ( Methods , Additional file 4 : Table S3A,B, Additional file 5 : Table S4A,B). Most instances contributed to at least one of these features, and none of the instances contributed to all five (Additional file 5 : Table S4C). Next, we focused on chromosome-arm and cancer type instances that were top contributors to at least three of the five features (4.3% and 1.9% of the pairs in the gain and loss models, respectively). We tested their impact on the model by excluding them from the dataset and repeating the construction and interpretation of each model without them. The revised gain model retained its five topmost important features, though their ranking slightly changed (the third and fifth features switched). The revised loss model retained its four topmost important features (the fifth and seventh features switched) (Additional file 1 : Fig. S10). This suggests that the general effect of the features was not driven by a small subset of instances.
Lastly, we expanded our analyses to address whole-chromosome gains and losses. For this, we updated the features dataset to refer to whole-chromosome and cancer type instances ( Methods ). For example, the feature TSG density was updated to refer to the entire chromosome. Likewise, we updated the aneuploidy status of whole-chromosome and cancer type instances using data from GISTIC ( Methods ). This resulted in a dataset of 78 whole-chromosome gains, 151 whole-chromosome loss, and 299 neutral cases. Next, we used these data to train a whole-chromosome gain (trisomy) model and a whole-chromosome loss (monosomy) model. Model training and assessment were similar to the chromosome-arm gain and loss models. Specifically, we employed five different ML methods and assessed their performance using fivefold cross-validation. Best performance for the trisomy model was achieved by random forest, with auROC of 69% and auPRC of 47% (expected 21%; Additional file 1 : Fig. S11A). Best performance for the monosomy model was achieved by XGBoost, with auROC of 71% and auPRC of 59% (expected 34%; Additional file 1 : Fig. S11D). Performances were somewhat weaker than the chromosome-arm models, in accordance with the training data being almost twofold smaller. Lastly, we interpreted each model using SHAP. In the trisomy model, the topmost feature was TSG density and its impact was over twofold larger than the impact of other features, similarly to the chromosome-arm gain model (Additional file 1 : Fig. S11B,C). Other strong features of the chromosome-arm gain model, TCGA expression and OG density, ranked fifth and sixth, yet preserved their directionality. In the monosomy model, top features included OG density, TCGA expression, and paralogs compensation, fitting with the chromosome-arm loss model (Additional file 1 : Fig. S11E,F). The feature TSG density was ranked eight, yet preserved its directionality, similarly to the remaining features. Altogether, these results suggest that negative selection is an important factor in shaping both chromosome-arm and whole-chromosome aneuploidy patterns.
Similar features shape aneuploidy patterns in human cancer cell lines and in human tumors
Next, we aimed to test whether similar features also shape aneuploidy patterns in CCLs. We collected data of aneuploidy patterns of all chromosome-arms in CCLs [ 36 ] and analyzed 10 cancer types with matched normal tissue data from GTEx [ 25 ] ( Methods ). Similar to the analysis of cancer tissues, we labeled each instance of chromosome-arm and CCL as recurrently gained (59 instances), recurrently lost (45 instances), or neutral (286 instances) and updated the features associated with cancer types according to the CCL data ( Methods ). We then applied the gain and loss ML models, which were trained on primary tumor data, to identify determinants of aneuploidy patterns of CCLs ( Methods ). The performance of the models was at least as good as for primary tumors (gain model: auROC = 83% and auPRC = 49% (expected 15%); loss model: auROC = 76% and auPRC = 45% (expected 11%), Fig. 3 A). These results indicate that similar factors affect aneuploidy in cancers and in CCLs, consistent with the highly similar aneuploidy patterns observed in tumors and in CCLs [ 36 , 37 ].
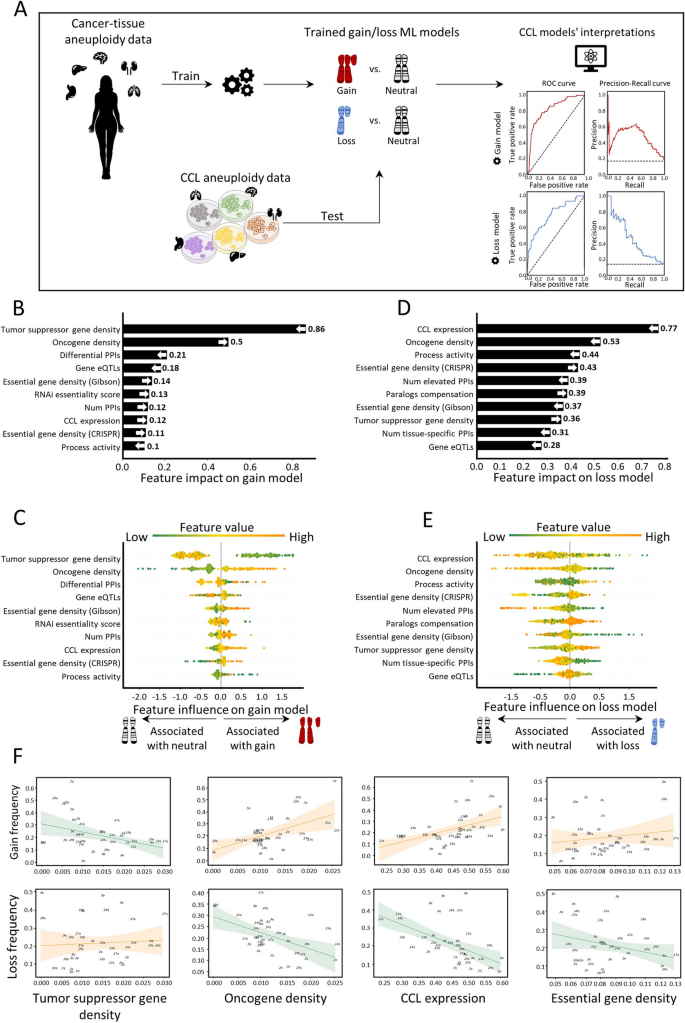
Aneuploidy patterns in CCLs and primary tumors are shaped by similar features. A The ML scheme for analysis of aneuploidy patterns in CCLs. The gain and loss models that were trained on aneuploidy patterns in primary tumors were applied to aneuploidy patterns in CCLs. Performance was measured using tenfold cross-validation. Gain model (gradient boosting): auROC = 83%, auPRC = 49% (expected 15%). Loss model (XGBoost): auROC = 76%, auPRC = 45% (expected 11%). B The average absolute contribution of the ten topmost features to the gain model (see legend of Fig. 2 A). The order and directionality of the features generally agree with the gain model in primary tumors. C A detailed view of the contribution of the ten topmost features to the gain model (see legend of Fig. 2 B). D Same as B for the loss model. The order and directionality of the features generally agree with the loss model in primary tumors. E Same as panel C for the loss model. F The correlations between top contributing features and the frequencies of chromosome-arm gains and losses, as measured by Spearman correlation. p -values were adjusted for multiple hypothesis testing using Benjamini–Hochberg procedure. Negative correlation between TSG density and gain frequency ( ρ = − 0.37, adjusted p = 0.04). Positive correlation between TSG density and loss frequency ( ρ = 0.17, adjusted p = 0.32). Positive correlation between OG density and gain frequency ( ρ = 0.44, adjusted p = 0.012). Negative correlation between OG density and loss frequency ( ρ = − 0.28, adjusted p = 0.13). Positive correlation between CCL expression and gain frequency ( ρ = 0.53, adjusted p = 0.002). Negative correlation between CCL expression and loss frequency ( ρ = − 0.6, adjusted p = 0.0006). Positive correlation between essential gene density and gain frequency ( ρ = 0.18, adjusted p = 0.33). Negative correlation between essential gene density and loss frequency ( ρ = − 0.17, adjusted p = 0.32)
We next used SHAP to assess the contribution of each feature to each of the models. TSG density and OG density remained the top contributing features for the gain model. Consistent with our results in primary tumors, the contribution of TSG density was much stronger than that of OG density, confirming the role of negative selection (Fig. 3 B,C). In the loss model, the ranking of top features was slightly different than in primary tumors (Fig. 3 D). Expression in CCL was the top feature, such that recurrently lost chromosome-arms were associated with lower gene expression in neutral cases. OG density was one of the strongest contributing features for the loss model whereas TSG density had weaker contribution, again in line with negative selection playing an important role in shaping cancer aneuploidy landscapes (Fig. 3 D,E). Certain features of normal tissues were also highly ranked. The contribution of essential gene density was also consistent with its impact in primary tumors (Fig. 3 B,C).
As with the primary tumors, correlation analyses supported the contributions of the different features. CCL expression was highly correlated with chromosome-arm gain and anticorrelated with chromosome-arm loss ( ρ = 0.54, adjusted p = 0.02, and ρ = − 0.6, adjusted p = 0.0006, respectively; Fig. 3 F). Negative correlations were also observed between TSG density and gain frequency ( ρ = − 0.37, adjusted p = 0.04, Spearman correlation; Fig. 3 F) and between OG density and loss frequency ( ρ = − 0.28, adjusted p = 0.1, Spearman correlation; Fig. 3 F). Altogether, these results indicate that despite the continuous evolution of aneuploidy throughout CCL culture propagation [ 38 ], similar features drive aneuploidy recurrence patterns in primary tumors and in CCLs.
Chromosome 13q aneuploidy patterns are tissue-specific, and KLF5 is a driver of 13q gain in colorectal cancer
In human cancer, a chromosome-arm is either recurrently gained across cancer types or it is recurrently lost across cancer types, but rarely is a chromosome-arm both gained in some cancer types and lost in others [ 4 , 5 ]. An intriguing exception is chr13q. Of all chromosome-arms, chr13q is the chromosome-arm with the highest density of tumor suppressor genes (Fig. 2 E). It is therefore not surprising that chr13q is recurrently lost across multiple cancer types (with a median of 30% of the tumors losing one copy of 13q across cancer types) [ 4 , 5 ]. Interestingly, however, chr13q is recurrently gained in human colorectal cancer (in 58% of the samples), suggesting that it can confer a selection advantage to colorectal cells in a tissue-specific manner. Indeed, when comparing colorectal tumors and colorectal cancer cell lines against all other cancer types, chr13q was the top differentially affected chromosome-arm (Fig. 4 A,B). We therefore set out to study the basis for this unique tissue-specific aneuploidy pattern.
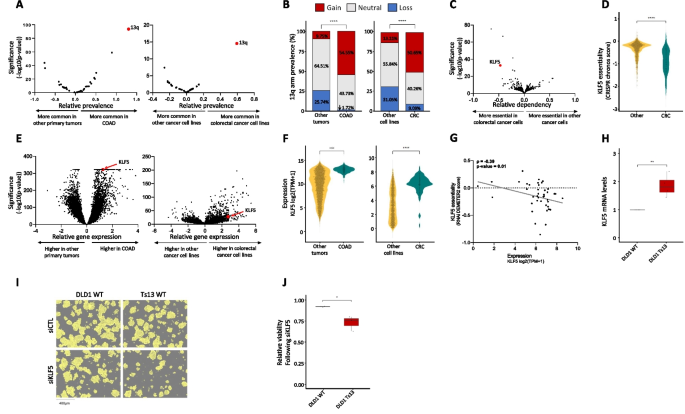
KLF5 is a potential driver of chromosome 13q gain in human colorectal cancer. A Comparison of the prevalence of chromosome-arm aneuploidies in colorectal tumors against all other tumors (left) and colorectal cancer cell lines against all other cancer cell lines (right). On the right side are the aneuploidies that are more common in colorectal cancer, and on the left side are the ones that are less common in colorectal cancer. Chromosome-arm 13q (in red) is the top differential aneuploidy in colorectal cancer. B Comparison of the prevalence of 13q aneuploidy between colorectal tumors and all other tumors (left) and between colorectal cancer cell lines and all other cancer cell lines (right). ****, p < 0.0001 and ****, p < 0.0001; Chi-square test. C Genome-wide comparison of differentially essential genes between colorectal cancer cell lines ( n = 85) and all other cancer cell lines ( n = 1407). On the right side are the genes that are more essential in other cancer cell lines, and on the left side are those that are more essential in colorectal cancer, based on a genome-wide CRISPR/Cas9 knockout screens [ 39 ]. The x -axis presents the effect size (i.e., the differential response between colorectal cell lines and other cell lines), and the y -axis presents the significance of the difference (-log10( p -value)). KLF5 (in red) is the second most differentially essential gene in colorectal cancer cell lines. D Comparison of the sensitivity to CRISPR knockout of KLF5 between colorectal cancer cell lines ( n = 59) and all other cancer cell lines ( n = 1041). ****, p < 0.0001; two-tailed Mann–Whitney test. E Genome-wide comparison of differentially expressed genes between colorectal tumors ( n = 434) and all other tumors (on the left, n = 11,060) and between colorectal cancer cell lines ( n = 85) and all other cancer cell lines (on the right, n = 1407). On the right side are the genes that are over-expressed in colorectal cancer and on the left side are those that are over-expressed in other cell lines. KLF5 (in red) significantly over-expressed in colorectal cancer. F Comparison of KLF5 mRNA levels between colorectal tumors ( n = 434) and all other tumors on the left ( n = 11,060) and between colorectal cancer cell lines ( n = 85) and all other cancer cell lines (on the right, n = 1407). ****, p < 0.0001; two-tailed Mann–Whitney test. G Correlation between KLF5 mRNA expression and the sensitivity to KLF5 knockdown, showing that higher KLF5 expression is associated with increased sensitivity to its RNAi-mediated knockdown. ρ = − 0.39, p = 0.01; Spearman correlation. H Comparison of KLF5 mRNA levels between DLD1-WT (without trisomy of chromosome 13) and DLD1-Ts13 (with trisomy of chromosome 13) colorectal cancer cells. **, p = 0.0025; one-sample t -test. I Representative images of DLD1-WT and DLD1-Ts13 cells treated with siRNA against KLF5 . DLD1-Ts13 cells proliferated more slowly, as previously reported, but were more sensitive to the knockdown after accounting for their basal proliferation rate. Cell masking (shown in yellow) was performed using live cell imaging (IncuCyte) following 72 h of treatment. Scale bar 400µm. J Quantification of the relative response to KLF5 knockdown between DLD1-WT and DLD1-Ts13, as evaluated by quantifying cell viability in cells treated with siRNA against KLF5 versus a control siRNA for 72 h. n = 3 independent experiments. *, p = 0.0346; one-sided paired t -test
We performed a genome-wide comparison of differentially essential genes between colorectal cell lines and all other cell lines. The two top genes, which are much more essential in colorectal cancer cells than in other cancer types, were CTNNB1 and KLF5 (Fig. 4 C). Of particular interest is KLF5 , which is located on chr13q and colorectal cancer cell lines are significantly more sensitive to its knockout (Fig. 4 D). KLF5 was reported to be tumor-suppressive in the context of several cancer types, such as breast and prostate [ 40 , 41 ]. In colon cancer, however, not only is KLF5 important for tissue identity [ 42 ], but it was also reported to be haploinsufficient [ 43 ], potentially explaining why loss of chr13q is so rare in colorectal cancer. In line with a potential driving role in the recurrence of chr13q gain in colorectal cancer, KLF5 was among the most significantly overexpressed genes in colorectal tumors and in colorectal cell lines versus all other cancer types (Fig. 4 E,F). Furthermore, KLF5 expression levels correlated with the cells’ sensitivity to its knockdown (Fig. 4 G). To confirm the association between chr13q gain and KLF5 expression and dependency, we next turned to an isogenic system of human colon cancer cells (DLD1) into which trisomy 13 had been introduced (DLD1-Ts13) [ 44 ]. Using this unique experimental system, we confirmed that trisomy 13 results in overexpression of KLF5 (Fig. 4 H) and increased sensitivity to its siRNA-mediated genetic depletion (Fig. 4 I,J and Additional file 1 : Fig. S12, Additional file 1 : Fig. S13). This differential response was specific to KLF5 , as the trisomy did not affect the sensitivity of the cells to a control siRNA (Additional file 1 : Fig. S14), to knockdown of an unrelated gene residing on chr13q ( NEK3 ; Additional file 1 : Fig. S15), or to knockdown of another transcription factor that plays a role in colon development and is located on another chromosome ( TTC7A , located on chr2p; Additional file 1 : Fig. S16). We, therefore, propose that KLF5 contributes to the uniquely variable pattern of chr13q aneuploidy across cancer types.
Paralog compensation is an important feature shaping tissue-specific aneuploidy patterns
One of the topmost contributing features to the chromosome-arm loss model in primary tumors and in CCLs, as well as to the whole-chromosome loss model, was paralog compensation. It was previously shown that while loss of genes with paralogs was less detrimental than loss of singleton genes [ 45 ], the impact of gene loss in a specific condition depends on the expression level of its paralog [ 46 ]. The paralog compensation feature was therefore designed to quantify the expression ratio between two paralogs. Specifically, higher values of this feature for a given gene correspond to a higher expression of the paralog relative to the gene ( Methods ). Previous studies of hereditary disease genes showed that lower paralog compensation in a tissue was associated with disease manifestation in that tissue [ 31 , 32 ]. Paralog compensation was also shown in cancer tissues: In CCLs, essentiality of a gene was decreased with an increased expression of its paralog [ 27 , 46 , 47 ]. In primary tumors, paralog compensation was shown to be associated with increased prevalence of non-synonymous mutations [ 48 ] and to correlate with the prevalence of homozygous gene deletion [ 49 ]. However, the contribution of paralog compensation to aneuploidy has not been studied to date.
Paralog compensation ranked fourth and sixth in the loss models of primary tumors and CCLs, respectively (Fig. 2 C, Fig. 3 D). In both, chromosome-arm loss was associated with higher paralog compensation, suggesting that loss is facilitated by higher relative expression of paralogs (Fig. 2 D, Fig. 3 E). We also analyzed the correlations between the frequency of chromosome-arm loss and paralog compensation ( Methods , Fig. 5 A). Indeed, the frequency of chromosome-arm loss was positively correlated with paralog compensation in both primary tumors and in CCLs ( ρ = 0.26 and ρ = 0.46, respectively, Spearman correlation; Fig. 5 A).
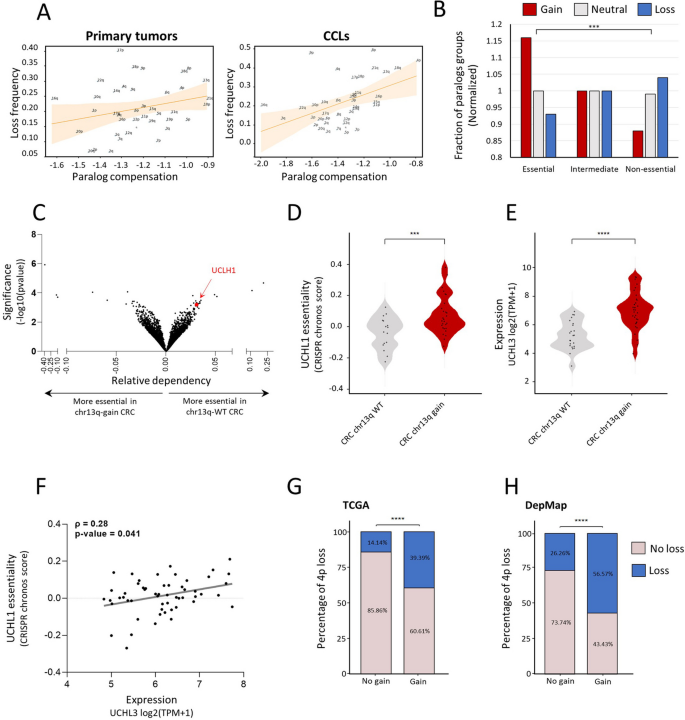
Paralog compensation is an important feature shaping tissue-specific aneuploidy patterns. A The correlation between paralog compensation values and loss frequency of chromosome arms in primary tumors (left, ρ = 0.26, adjusted p = 0.18, Spearman correlation) and in CCLs (right, ρ = 0.46, adjusted p = 0.01, Spearman correlation). B A view into the aneuploidy patterns of paralogs of recurrently lost genes. Recurrently lost genes were divided into essential, intermediate, and non-essential groups. Paralogs of essential genes were more frequently gained, whereas paralogs of non-essential genes were more frequently lost. C Genome-wide comparison of differentially essential genes in colorectal cell lines with chr13q gain ( n = 39) versus chr13q-WT colorectal cell lines ( n = 25). On the right side are the genes that are more essential in chr13q-WT cells, and on the left side those that are more essential in chr13q-gain cells, based on a genome-wide CRISPR/Cas9 knockout screens [ 39 ]. The x -axis presents the effect size (i.e., the differential response between chr1q-WT and chr13q-gain colorectal cell lines) and the y -axis presents the significance of the difference (-log10(p-value)). UCHL1 (in red) is one of the top genes identified to be more essential in chr13q-WT cells. D Comparison of the sensitivity to CRISPR knockout of UCHL1 between colorectal cell lines with ( n = 28) and without chr13q gain ( n = 16). ***, p = 0.0003; two-tailed Mann–Whitney test. E Comparison of UCHL3 mRNA expression between colorectal cell lines with ( n = 34) and without chr13q gain ( n = 23). ****, p < 0.0001; two-tailed Mann–Whitney test. F Correlation between UCHL3 mRNA expression and the sensitivity to UCHL1 knockout, showing that higher UCHL3 mRNA levels are associated with reduced sensitivity to UCHL1 knockout. ρ = 0.28, p = 0.041; Spearman correlation. G Comparison of the prevalence of chr4p loss between human primary colorectal tumors with and without chr13q gain. ****, p < 0.0001, Chi-square test. H Comparison of the prevalence of chr4p loss between human colorectal cancer cell lines with and without chr13q gain. ****, p < 0.0001, Chi-square test
Next, we tested whether paralog compensation, namely gain or overexpression of paralogs, could indeed facilitate chromosome-arm loss. We started by grouping genes in recurrently lost chromosome-arms into essential, intermediate, or non-essential, according to their essentiality in CCLs [ 27 ] ( Methods ). We then associated each gene with the aneuploidy status of the chromosome-arm of its paralog, namely whether the chromosome-arm of the paralog was gained, lost, or remained neutral in the corresponding CCL ( Methods , Additional file 1 : Fig. S17A). The fraction of genes with paralogs on neutral chromosome-arms was similar in all essentiality groups (Fig. 5 B). In contrast, the fraction of gained paralogs was highest in the group of essential genes and lowest in the group of non-essential genes. This suggests that the loss of essential genes is more likely accompanied by the gain of their paralogs. Likewise, the fraction of lost paralogs was lowest in the group of essential genes and highest in the group of non-essential genes ( p = 2.38e − 24, Chi-square test; Fig. 5 B). This suggests that the loss of essential genes is less likely to be accompanied by the loss of their paralog. The same trend was shown upon comparing the distribution of essentiality scores between genes with gained paralogs versus genes with lost paralogs ( p = 9.2e − 16, KS test; Additional file 1 : Fig. S17B). Hence, paralog compensation can facilitate chromosome-arm loss.
Next, we decided to identify a specific example. In human colon cancer, the long arm of chromosome 13 (chr13q) is commonly gained, as described above, whereas the short arm of chromosome 4 (chr4p) is commonly lost [ 5 , 37 ]. We analyzed the association between chr13q-residing genes and the essentiality of their paralogs, revealing UCHL3 (chr13q)- UCHL1 (chr 4p) as the most significant correlation (Additional file 6 : Table S5 and Fig. 5 C). Human colon cancer cell lines with chr13q gain were less sensitive to CRISPR/Cas9-mediated knockout of UCHL1 (Fig. 5 D). Consistently, chr13q-gained cell lines had significantly higher mRNA levels of UCHL3 (Fig. 5 E), and the expression of UCHL3 was significantly correlated with the essentiality of UCHL1 (Fig. 5 F). We hypothesized that the relationship between these paralogs may affect the co-occurrence patterns of the chromosome-arms on which they reside. Indeed, both in primary human colon cancer and in colon cancer cell lines, loss of chr4p was significantly more prevalent when chr13q was gained (Fig. 5 G,H). Together, these results demonstrate that paralog compensation can be affected by—and contribute to the shaping of—aneuploidy patterns.
Recurrent aneuploidy patterns are an intriguing phenomenon that is only partly understood. Several previous studies characterized the unique patterns of aneuploidy in cancer [ 4 , 5 , 50 ] or attempted to identify the driving role of a specific aberration in a specific cancer context [ 9 , 51 , 52 , 53 , 54 ]. Attempts to explain copy number patterns in cancer focused on specific pre-defined aspects, such as the specific boundaries of the alterations [ 15 ], the densities of OGs and TSGs on the aberrant chromosomes [ 6 , 7 ] or the gene expression changes that they induce [ 8 ], and these aspects were interrogated using statistical methods and correlation analyses. Here, in contrast, we studied this phenomenon using an unbiased ML-based approach. As with other ML applications, it allowed us to study multiple aspects simultaneously. Yet, unlike classical ML-based studies that mainly aim to improve prediction, for example by using deep learning to predict gene dependency in tumors [ 21 ], our focus was on interpretability. In fact, we built chromosome-arm gains and loss models only to then identify factors that shape aneuploidy patterns. Interpretable ML was recently applied to reveal genetic attributes that contribute to the manifestation of Mendelian diseases [ 55 ]. In this study, we applied interpretable ML for the first time in the context of aneuploidy and at chromosome-arm resolution.
The capability of ML to concurrently assess multiple features opened the door for assessing the relevance of features that have not been rigorously studied to date, such as paralog compensation. Yet, ML has its limitations. Mainly, the number of features that could be analyzed depends on the size of the labeled dataset [ 56 ], which, in aneuploidy, was restricted by the number of chromosome-arms and cancer types. We therefore analyzed 20 types of features and tested linear regression and tree-based ML methods, which, unlike deep learning, are suitable for this size of data. Following prediction, our main goal was to assess the relative contribution of each feature to the model’s decision and its directionality using SHAP. Nevertheless, SHAP results should be interpreted with caution. First, SHAP assumes feature independence, although features could be correlated with each other or confounded. Importantly, we found that only a small subset of features correlated with each other, and they did not include the topmost contributing features (Additional file 1 : Fig. S3A). Second, the top contributing factors could be correlated with prediction strength, rather than being causal. Lastly, due to the hierarchical nature of decision trees, features that are located low in the decision tree explain only a small fraction of the cases. To estimate feature contribution and directionality more broadly, we explicitly correlated feature values with chromosome-arm gain and loss frequency, finding support for their broad relevance (Fig. 2 E, Additional file 1 : Fig. S6). We also conducted multiple analyses that tested the robustness of the results to the models’ construction schemes (Additional file 1 : Fig. S7, S8), the modeled events (one event versus rest, Additional file 1 : Fig. S9; whole-chromosome, Additional file 1 : Fig. S11), or to a subset of the chromosome-arm and cancer type instances (Additional file 1 : Fig. S10). The different analyses repeatedly revealed the same factors at play, supporting the reliability of our results.
The features that we studied included known and previously underexplored attributes of chromosome-arms, healthy tissues and cancer cells (Fig. 1 A,B). OG and TSG densities, which have previously been observed to be enriched on gained and lost chromosome-arms, respectively [ 6 , 7 ], were top contributing features in both models, thereby supporting the validity of our approach (Fig. 2 A,C). In the gain model in particular, their contribution was over 2.6 and 5 times stronger, respectively, than any other feature (Fig. 2 A). As our TSG and OG features were cancer-independent, their importance may explain the observation that certain chromosome-arms tend to be either gained or lost across multiple cancer types [ 4 , 5 ]. Their relative contribution, however, was surprising. In both models, negative associations were much stronger than positive associations: OG density contributed to chromosome-arm loss more than TSG density, implying that it was more important to maintain OGs than to lose TSG (Fig. 2 B,D). The reciprocal relationship was true for chromosome-arm gain, as it was more important to maintain TSGs than to gain OGs (Fig. 2 A,C). These results were validated using correlation analyses (Fig. 2 E) and were recapitulated in CCLs (Fig. 3 ) and in the analysis of whole-chromosome gains and losses (Additional file 1 : Fig. S11). Together, they highlight the importance of negative selection for shaping cancer aneuploidy landscapes [ 1 , 15 ].
A known factor that contributed to both models was gene expression in primary tumors (TCGA expression, Fig. 2 ) and in CCLs (CCL expression, Fig. 3 ). This result suggests that cancers tend to gain chromosome-arms that are enriched for highly-expressed genes and tend to lose chromosome-arms that are enriched for lowly expressed genes. A Similar trend was shown recently for gene expression in normal tissues [ 8 ]. Our approach was capable of comparing the relative contributions of both features. We found that the contribution of gene expression in normal tissue was lower than that in cancer tissues, as also evident by its lower correlation with the frequencies of chromosome-arm gains and losses (Additional file 1 : Fig. S6). Nevertheless, other features that were derived from gene expression in normal tissues ranked highly, such as the number of PPIs in the gain model and paralog compensation in the loss model, and hence expression in normal tissues is also important (Fig. 2 ).
A previously under-explored feature that we considered was paralog compensation. Paralog compensation was shown to play a role in the manifestation of Mendelian and complex diseases [ 31 , 32 ] and in the dispensability of genes in tumors [ 48 , 49 ] and CCLs [ 27 , 46 , 47 ], but was not studied in the context of aneuploidy. Here, paralog compensation was among the top contributors to the loss model (Fig. 2 C, Fig. 3 D). The directionality of this feature and correlation analyses showed that, relative to genes located on neutral chromosome-arms, genes located on lost chromosome-arms tend to have higher compensation by paralogs (Fig. 5 A). This suggests that chromosome-arm loss is facilitated, or better tolerated, through paralogs’ expression. We also showed that the more essential recurrently lost genes are, the more likely they are to be associated with gains of paralog-bearing chromosome-arms (Fig. 5 B). We further demonstrated this for a specific example (the UCHL3 - UCHL1 paralog pair; Fig. 5 ). Overall, our analysis reveals that compensation between paralogs through expression or chromosome-arm gain plays an important role in shaping the landscape of chromosome-arm loss.
Combining the different results, our models reveal a previously under-appreciated role for negative selection in driving human cancer aneuploidy. This was evident by the tendency not to lose chromosome arms with high OG density, high frequency of essential genes, or low compensation by paralogs, and not to gain chromosome arms with high TSG density (Fig. 6 ). Previous studies have shown that positive selection outweighs negative selection in shaping the point mutation landscape of human tumors [ 14 ]. However, the strong fitness cost associated with aneuploidy suggests that the aneuploidy landscape of tumors might be strongly affected by negative selection as well (reviewed in [ 1 ]). Interestingly, evidence for the involvement of negative selection in shaping the copy number alteration (CNA) landscapes of tumors has been proposed in a recent study that analyzed CNA length distributions across human tumors [ 15 ]. Our study thus lends further independent support to the importance of negative selection in shaping the landscape of aneuploidy across human cancers (Fig. 6 ).
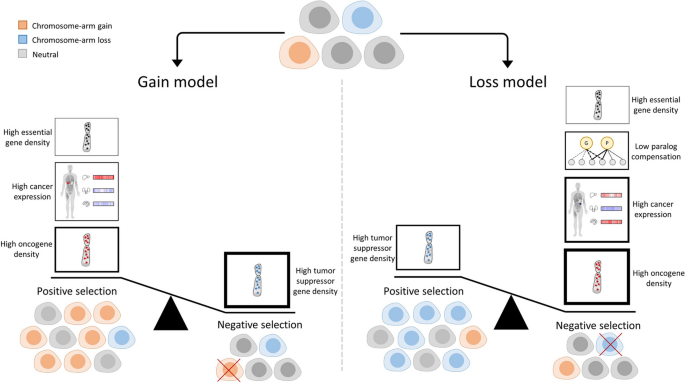
A schematic presentation of the results of the study. Cancer evolution is shaped by negative and positive selection leading to enrichment or depletion of cells with distinct aneuploidy patterns. In the gain model (left), main contributors to positive selection of gained chromosome arms are: (1) high oncogene density, (2) high expression of genes in the cancer tissue, and (3) high essential gene density. A major contributor to negative selection is high tumor suppressor gene density. Importantly, the density of TSGs is more important than the density of OGs for predicting chromosome-arm gains. In the loss model (right), a main contributor to positive selection of lost chromosome arms is high tumor suppressor gene density. Major contributors to negative selection are high oncogene density, high expression of genes in the cancer tissue, low compensation by paralogs, and high density of essential genes. In both models, the features associated with negative selection have higher overall contribution than features associated with positive selection. The thickness of the borders of the boxes reflects the relative contribution of the features to the model
Our genome-wide analysis could be expanded in future studies in several ways: (1) While we focused on the top-contributing features, other features, such as PPIs that contributed to both gain and loss models, are also relevant and remain to be studied in depth. (2) It will be interesting to consider additional types of aneuploidy, such as tetrasomies, and explore how whole-genome doubling affects the importance of the features in shaping the aneuploidy landscapes of tumors. (3) Tumors often exhibit heterogeneous (mosaic) aneuploidy patterns [ 57 , 58 , 59 , 60 ]. Our analyses were entirely based on bulk-population data, and our results therefore describe the selection pressures that shape the landscape of clonal aneuploidies. As more single-cell omics data becomes available, it will be interesting to also study the selection pressures that shape subclonal aneuploidy patterns. (4) Aneuploidies do not always arise independently, so that chromosome-arm events can co-occur or be mutually exclusive [ 37 ]. We show that only a small fraction of chromosome-arm events co-occur (Additional file 7 : Table S6), suggesting that their effect on our models would likely be small. Nonetheless, considering co-occurrence patterns could further refine the models.
Lastly, we explored one example of a unique aneuploidy pattern (chr13q) that is recurrently altered in opposite directions in different cancer types. In line with tumor suppressors and oncogenes being a major feature explaining aneuploidy patterns, we identified KLF5 as a colorectal-specific dependency gene. Using an isogenic system of colorectal cancer cells with/without gain of chr13, we experimentally demonstrated that this aneuploidy is associated with increased expression and increased essentiality of KLF5 . The finding that colorectal cells with trisomy 13 are more sensitive to KLF5 depletion suggests positive selection for its gain, on top of a potential negative selection against a deleterious loss. We therefore propose that KLF5 might explain why chr13q is commonly gained and rarely lost in colorectal cancer, unlike its recurrent loss across multiple other cancer types.
Overall, our study provides novel insights into the forces that shape the tissue-specific patterns of aneuploidy observed in human cancer and demonstrates the value of applying ML approaches to dissect this complicated question. Our results suggest that aneuploidy patterns are shaped by a combination of tissue-specific and non-tissue-specific factors. Negative selection in general and paralog compensation in particular play a major role in shaping the aneuploidy landscapes of human cancer and should therefore be computationally modeled and experimentally studied in the research of cancer aneuploidy.
Chromosome-arm aneuploidy patterns per cancer
Chromosome-arm events per cancer were defined according to GISTIC2.0 [ 24 ] for all (39) chromosome-arms in 24 cancer types for which data of the normal tissue of origin was available from GTEx [ 25 ]. GISTIC2.0 computed the probability of chromosome-arm events by comparing the observed frequency to the expected rate, while considering chromosome-arm length and other parameters [ 61 ]. A chromosome-arm was considered as gained or lost in a specific cancer if the q -value of its amplification or deletion, respectively, was lower than 0.05. Otherwise, the chromosome-arm was considered as neutral. In case the q -value of both amplification and deletion was lower than 0.05, decision was made based on the lower q -value. In case of a tie, the more frequent event was selected. GISTIC2.0 data, including q -values and frequencies, were downloaded from ref. [ 62 ]. Lastly, we analyzed co-incidence probabilities of chromosome-arm events per cancer. Co-incidence probabilities for chromosome-arms and cancers in our dataset were obtained from [ 37 ].The median fraction of chromosome-arm pairs with significant co-incidence per cancer was 2.05% (Additional file 7 : Table S6). Hence, the impact of co-incidence on the models is expected to be small.
We also carried separate analyses of gain and loss of whole-chromosomes. A whole-chromosome was considered as gained if the q -value of the amplification of its two arms was lower than 0.05. Likewise, a whole-chromosome was considered as lost if the q -value of the deletion of its two arms was lower than 0.05.
Construction of a features dataset of instances of chromosome-arm and cancer type pairs
For each chromosome-arm and cancer, we created features that were inferred from data of chromosome-arms, genes, cancer tissues and CCLs, and normal tissues (Fig. 1 B, Additional file 2 : Table S1). A schematic pipeline of the dataset construction appears in Additional file 1 : Fig. S1. The different types of features are described below.
Features of chromosome-arms
Each chromosome-arm was associated with three types of features, including oncogene density, tumor suppressor gene density, and essential gene density. Oncogene density and tumor suppressor gene density per chromosome-arm were obtained from Davoli et al. [ 6 ]. Data of essential genes was obtained from Nichols et al. [ 26 ], where a gene was considered essential if its essentiality probability was > 0.8. The density of essential genes per chromosome-arm was calculated as the fraction of essential genes out of the protein-coding genes on that chromosome-arm. Next, we associated each instance of chromosome-arm and cancer type with features of that chromosome-arm.
Features of cancer tissues
Each instance of chromosome-arm and cancer type was associated with four types of cancer-related features, including transcriptomics, essentiality by CRISPR or RNAi in CCLs, and cancer-specific density of essential genes. Transcriptomics was based on transcriptomic profiles of 33 cancer types from TCGA [ 63 ] that were obtained from GDC Xena Hub v18.0 (updated 2019–08-28). Per cancer, we associated each gene with its median expression level in samples of that cancer. To avoid expression bias due to chromosome-arm gain or loss, the median expression of each gene was computed from samples where the chromosome-arm harboring the gene was neutral according to Taylor et al. [ 5 ]. Essentiality by CRISPR was based on CRISPR screens of 24 CCLs from the DepMap portal version 21Q1. Essentiality by RNAi was based on RNAi data of 20 CCLs from DepMap [ 27 ]. In each of these datasets, the score of each gene indicated the change, relative to control, in the growth rate of the cell line upon gene inactivation via CRISPR or RNAi. Accordingly, genes with negative scores were essential for the growth of the respective cell line. We associated each gene with its median essentiality score based on either CRISPR or RNAi per cell line. To reflect gene essentiality more intuitively, we reversed the direction of the scores (multiplied them by − 1), so that more essential genes had higher scores. To avoid bias due to chromosome-arm gain or loss, the median essentially of each gene was computed from samples where the chromosome-arm harboring the gene was neutral [ 5 ]. Cancer-specific density of essential genes was calculated as the fraction of essential genes (CRISPR-based essentiality score > 0.5) in a given CCL out of the protein-coding genes residing on that chromosome-arm.
Features of normal tissues
Each instance of chromosome-arm and cancer type was associated with 13 types of features that were derived from [ 55 ]. We associated each cancer type with the normal tissue in which it originates (Additional file 2 : Table S1).
Transcriptomics
Data of normal tissues included transcriptomic profiles of 54 adult human tissues measured via RNA-sequencing from GTEx v8 [ 25 ]. Each gene was associated with its median expression in each adult human tissue. Genes with median TPM > 1 in a tissue were considered as expressed in that tissue.
Tissue-specific genes
Per gene, we measured its expression in a given tissue relative to other tissues using z -score calculation. Genes with z -score > 2 were considered tissue-specific. Lastly, we associated each chromosome-arm and tissue with the density of tissue-specific genes.
PPI features
Each gene was associated with the set of its PPI partners. We included only partners with experimentally detected interactions that were obtained from MyProteinNet web-tool [ 64 ]. Per each tissue, we associated each gene with four PPI-related features:
“Number PPIs” was set to the number of PPI partners that were expressed in that tissue.
“Number elevated PPIs” relied on preferential expression scores computed according to [ 28 ] and was set to the number of PPI partners that were preferentially expressed in that tissue (preferential expression > 2, [ 65 ].
“Number tissue-specific PPIs” was set to the number of PPI partners that were expressed in that tissue and in at most 20% of the tissues.
“Differential PPIs” relied on differential PPI scores per tissue from The DifferentialNet Database [ 28 ] and was set to gene’s median differential PPI score per tissue. If the gene was not expressed in a given tissue, its feature values in that tissue were set to 0.
Differential process activity features
Differential process activity scores per gene and tissue were obtained from [ 30 ]. The score of a gene in a given tissue was set to the median differential activity of the Gene Ontology (GO) processes involving that gene. The differential activity was relative to the activity of the same processes in other tissues.
eQTL features
eQTLs per gene and tissue were obtained from GTEx [ 25 ]. Each gene was associated with the p -value its eGene in that tissue.
Paralog compensation features
Each gene was associated with its best matching paralog according to Ensembl-BioMart. Per tissue, the gene score was set to the median expression ratio of the gene and its paralog, as described in [ 31 , 32 ]. Accordingly, high values mark genes with low paralog compensation.
Development features
Transcriptomic data of seven human organs measured at several time points during development were obtained from [ 66 ]. We united time points into time periods including fetal (4–20 weeks post-conception), childhood (newborn, infant, and toddler), and young (school, teenager and young adult). Per organ, we associated each gene with its median expression level per period. Next, we created an additional feature that reflected the expression variability of each gene across periods.
Transforming gene features into chromosome-arm features
Some of the features described above referred to genes. To create chromosome-arm-based features, we grouped together genes that were located on the same chromosome-arm [ 67 ]. Next, to highlight differences between tissues, for each feature, we associated a gene with its value in that tissue relative to other tissues. Features that were already tissue-relative, including “Differential PPIs” and “Differential process activity,” were maintained. Other features were converted into tissue-relative values via a z -score calculation (see Eq. 1 ). Lastly, per feature, we ranked genes by their tissue-relative score and associated each chromosome-arm with the median score of the genes ranking at the top 10% (Additional file 1 : Fig. S2). Transcriptomic features in the testis and whole blood were highly distinct from other tissues; we normalized all transcriptomic features per tissue. To reflect paralog compensation more intuitively, we reversed the direction of the resulting features (multiplied them by − 1), so that genes with higher compensation had higher scores.
T denotes the set of tissues, G denotes the set of genes, v denotes the value of the feature, and σ denotes the standard deviation.
Construction of the final dataset
The features described above referred to chromosome-arms in cancers, CCLs, and normal tissues. To create chromosome-arm features per cancer, we associated each cancer with the chromosome-arm features of its tissue of origin and CCL (Additional file 2 : Table S1). For features of normal tissues where multiple sub-regions were sampled (e.g., skin sun-exposed and not sun-exposed, or brain sub-regions), we set the chromosome-arm values to their median across sub-regions. The final dataset contained features for all 936 instances of 39 chromosome-arms and 24 cancers for which the cancer’s normal tissue of origin was available in GTEx [ 25 ] (Additional file 2 : Table S1). We assessed the similarity between every pair of features using Spearman correlation (Additional file 1 : Fig. S3A). We assessed whether chromosome-arm and cancer type instances had similar feature values using PCA (Additional file 1 : Fig. S3C).
ML application to model chromosome-arm and cancer aneuploidy
Below we describe the ML method used for aneuploidy classification and the SHAP (SHapley Additive exPlanations) analysis of feature importance that was used to interpret the resulting models.
Aneuploidy ML classification models
We constructed two ML models: a gain model that compared between gained and unchanged (neutral) chromosome-arms and a loss model that compared between lost and unchanged (neutral) chromosome-arm.
ML comparison and implementation
Per model, we tested several ML methods, including logistic regression, XGBoost, gradient boosting, random forest, and bagging. All ML methods were implemented using the Scikit-learn python package [ 68 ], except for XGB, which was implemented using the Scikit-learn API of the XGBoost package [ 69 ]. To assess the performance of each model, we used tenfold cross-validation. Then, we calculated the au-ROC and the au-PRC. Each point on the curve corresponded to a particular cutoff that represented a trade-off between sensitivity and specificity and between precision and recall, respectively.
SHAP analysis of feature importance
To measure the contribution and importance of the different features, we used SHAP algorithm [ 70 ]. SHAP is a game-theoretic approach to explain the output of ML models: for each feature, SHAP assigns a contribution value to each instance of chromosome-arm and cancer type. It then estimates the contribution of that feature to the model by the average absolute SHAP values of all instances. Per model, we created the SHAP plots corresponding to feature contribution and directionality. In both, features were ordered by their importance to the model (top meaning most contributing). We also visualized the directionality of each feature using arrows in the SHAP bar plot. The direction of the arrow showed whether the highest values of that feature (top 50%) corresponded to a chromosome-arm event (gain or loss, right) or to neutrality (left).
Robustness analyses
We analyzed the robustness of the models and their interpretation with respect to internal parameters used to generate the features and the hyperparameters of the ML models. For feature generation, we used top 10% of genes with highest values to calculate each gene-based chromosome-arm feature. We therefore reconstructed features by also using the top 1%, 5%, 15%, and 20% of the genes. We then assessed the performance of each method using tenfold cross-validation. In all cases, method performance was similar (Additional file 3 : Table S2). SHAP analysis of the best performing method per case showed similar results with respect to the topmost contributing features and their directionality (Additional file 1 : Fig. S7). For robustness to parameter choices, we tuned the hyperparameters per ML method separately for the gain model and for the loss model, and repeated model construction and interpretation. Tuning was optimized for precision and performed using the “RandomizedSearch” function of sklearn python package, with number of sampled parameters (iterations, n_iter) set to 200 and tenfold cross-validation. Best parameters per method and model and their performance appear in Additional file 1 : Fig. S8A,B. Performance was only slightly improved, and interpretation of the best performing models revealed similar results (Additional file 1 : Fig. S8C).
Lastly, we tested if the most important features per model were driven by a small subset of chromosome-arm and cancer type instances. For that, per model, we focused on the five most important features and identified instances with the top contributions to these features. An instance was considered a top contributor if its SHAP value for that feature that was among the 10% positive SHAP values (i.e., was a potential driver of the gain or loss) or the 10% negative SHAP values (i.e., was a potential driver of neutrality). The SHAP value for each instance and feature appears in Additional file 4 : Table S3. The list of instances and the features that they contributed to appears in Additional file 5 : Table S4. We then associated each instance with the number of features in which it was a top contributor. Next, we tested the impact of the strongest potential driver instances on the five most important features of the model. This was done by excluding from the dataset chromosome-arm and cancer type instances that were top contributors to at least three of the five features and repeating the construction and interpretation of each model using the revised dataset.
Correlation analysis
We correlated between feature values and the frequency of chromosome-arm gain or loss. The frequency of chromosome-arm gain/loss in cancers was obtained from GISTIC2.0 [ 24 ]. The frequency of chromosome-arm gain/loss in CCLs were obtained from [ 37 ]. Per chromosome-arm, its gain (loss) frequency was set to the median gain (loss) across cancers or CCLs. The feature value was set to median across cancers or CCLs. We used Spearman correlation, and p -values were adjusted using Benjamini–Hochberg procedure [ 71 ].
Paralog compensation analysis
For each cancer type and chromosome-arm, we considered all paralog pairs in which one of the genes resides on that chromosome-arm. We focused on recurrently lost genes per cancer type as defined by GISTIC2.0 [ 24 ]. We divided those genes by their minimal CRISPR essentiality score in CCLs that match the same cancer type (Additional file 2 : Table S1). Genes with a score ≤ − 0.5 were considered essential, and genes with a score ≥ − 0.3 were considered non-essential. Other genes were considered intermediate. Per gene, we checked whether its paralog was recurrently gained, lost, or neutral, in the same cancer, as detailed in Additional file 1 : Fig. S17A.
Chromosome-arm aneuploidy patterns in CCLs
Aneuploidy patterns were available for all (39) chromosome-arms in 14 CCLs from [ 37 ]. A chromosome-arm was considered as gained or lost in a CCL if the q -value of its amplification or deletion, respectively, was smaller than 0.15 (in case of ties, decision was made based on the lower q -value). In case of equal significant q -values, a chromosome-arm was considered as gained or lost based on their frequencies. Otherwise, the chromosome-arm was considered as neutral.
Construction of a feature dataset of instances of chromosome-arm and CCL pairs
The features dataset was similar to the dataset created for cancers, with the following exceptions. In features of cancer tissues, we replaced the transcriptomic features of cancers with transcriptomic features of CCLs. We obtained transcriptomic data of 25 CCLs from DepMap [ 27 ] and constructed the feature values per chromosome-arm and CCL as described above per chromosome-arm and cancer. Development features were removed since only a small number of CCLs had a matching organ. The final dataset contained features for all instances of 39 chromosome-arms and 10 CCLs for which the cancer’s normal tissue of origin was available in GTEx.
Cell culture
DLD1-WT cells and DLD1-Ts13 cells were cultured in RPMI-1640 (Life Technologies) with 10% fetal bovine serum (Sigma-Aldrich) and 1% penicillin–streptomycin-glutamine (Life Technologies). Cells were incubated at 37 °C with 5% CO2 and passaged twice a week using Trypsin–EDTA (0.25%) (Life Technologies). Cells were tested for mycoplasma contamination using the MycoAlert Mycoplasma Detection Kit (Lonza), according to the manufacturer’s instructions.
Cells were harvested using Bio-TRI® (Bio-Lab) and RNA was extracted following manufacturer’s protocol. cDNA was amplified using GoScript™ Reverse Transcription System (Promega) following manufacturer’s protocol. qRT-PCR was performed using Sybr® green, and quantification was performed using the ΔCT method. The following primer sequences were used: human KLF5 , forward, 5' ACACCAGACCGCAGCTCCA 3' and reverse 5' TCCATTGCTGCTGTCTGATTTGTAG 3', human NEK3 , forward, 5’ TACCCAAATGTGCCTTGGAG 3’, reverse 5’ ATCGGATTGGAGAGAAGACG 3’, human TTC7A , forward 5’ CTCGTGACCTGCAGACAAG 3’, reverse 5’ GGCTCCTAAAGTCTCCCAGC 3’.
siRNA transfection
For siRNA experiments, cells were plated in 96-well plates at 6000 cells per well and treated with compounds 24 h later. The cells were transfected with 15 nM siRNA against KLF5 (ONTARGETplus SMART-POOL®, Dharmacon) or with a control siRNA at the respective concentration (ONTARGETplus SMART-POOL®, Dharmacon) using Lipofectamine® RNAiMAX (Invitrogen) following the manufacturer’s protocol. Alternatively, for siRNA experiments against NEK3 and TTC7A , and for additional KLF5 experiments, cells were plated in 6-well plates at 400,000 cells per well and treated with compounds 24 h later. The cells were transfected with 30 nM against NEK3 and TTC7A or with 5 nM and 10 nM against KLF5 ; 48 h post seeding, the cells were split and plated in 96-wells at 10,000 cells per well. The effect of the knockdown against KLF5 , NEK3 , or TTC7A on cell viability/proliferation was measured by live cell imaging using Incucyte® (Satorius) or by the MTT assay (Sigma M2128) at 72 h (or at the indicated time point) post-transfection; 500 µg/mL MTT salt was diluted in complete medium and incubated at 37°C for 2 h. Formazan crystals were extracted using 10% Triton X-100 and 0.1 N HCl in isopropanol, and color absorption was quantified at 570 nm and 630 nm (Alliance Q9, Uvitec).
Cancer cell line and tumor data analysis
mRNA gene expression values, arm-level CNAs, CRISPR, and RNAi dependency scores (Chronos and DEMETER2 scores, respectively) were obtained from DepMap 22Q4 release ( www.depmap.org ). Effect size, p -values, and q -values (Fig. 4 A,C,E, Fig. 5 C) were taken directly from DepMap and were calculated as described in Tsherniak et al. TCGA mRNA gene expression values were obtained using the Xena browser [ 63 ]. Tumor arm-level alterations were retrieved from Taylor et al. 2018, Cancer Cell. Effect size, Spearman’s R and p -values in Fig. 4 G and Fig. 5 F were calculated using R functions. All colorectal cancer cell lines ( n = 85) and colorectal tumors ( n = 434) were included in the analyses.
The analyses that led to our choice of the paralog pair UCHL3 - UCHL1 are summarized in Additional file 6 : Table S5. In the left column are the paralogs that reside on chr-13q, which is frequently gained; in the adjacent column are the respective paralogs that reside on commonly lost chromosomes. The following columns describe the Spearman correlation between each paralog pair and the respective p -value. The right-hand columns describe the effect size of chr-13q paralogs’ gene expression between CRC cell lines with and without chr13q gain. Our criteria for finding appropriate paralog pairs for further analysis were as follows: firstly, to have a high expression of the chr-13q paralogs in CRC cell lines. Secondly, we aimed to reach a significant correlation between chr13q-residing genes and the essentiality of their paralogs.
Statistical analyses
Statistical analysis was performed using GraphPad PRISM® 9.1 software. Details of the statistical tests were reported in figure legends. Error bars represent SD. All experiments were performed in at least three biological replicates.
Availability of data and materials
The code for all the analyses is available on GitHub [ 72 ]. The datasets that were processed to build the dataset for the ML methods are available on Zenodo [ 73 ]. This includes features of normal tissues that were extracted from TRACE [ 74 ], TCGA expression data of the different cancer types that were obtained from Xena [ 75 ], and CRISPR and RNAi datasets that were obtained from DepMap [ 76 ].
Ben-David U, Amon A. Context is everything: aneuploidy in cancer. Nat Rev Genet. 2020;21(1):44–62.
Article CAS PubMed Google Scholar
Shukla A, Nguyen THM, Moka SB, Ellis JJ, Grady JP, Oey H, et al. Chromosome arm aneuploidies shape tumour evolution and drug response. Nat Commun. 2020;11(1):449.
Article CAS PubMed PubMed Central Google Scholar
Vasudevan A, Baruah PS, Smith JC, Wang Z, Sayles NM, Andrews P, et al. Single-Chromosomal gains can function as metastasis suppressors and promoters in colon cancer. Dev Cell. 2020;52(4):413–28 e6.
Ben-David U, Ha G, Tseng YY, Greenwald NF, Oh C, Shih J, et al. Patient-derived xenografts undergo mouse-specific tumor evolution. Nat Genet. 2017;49(11):1567–75.
Taylor AM, Shih J, Ha G, Gao GF, Zhang X, Berger AC, et al. Genomic and functional approaches to understanding cancer aneuploidy. Cancer Cell. 2018;33(4):676–89 e3.
Davoli T, Xu AW, Mengwasser KE, Sack LM, Yoon JC, Park PJ, et al. Cumulative haploinsufficiency and triplosensitivity drive aneuploidy patterns and shape the cancer genome. Cell. 2013;155(4):948–62.
Sack LM, Davoli T, Li MZ, Li Y, Xu Q, Naxerova K, et al. Profound tissue specificity in proliferation control underlies cancer drivers and aneuploidy patterns. Cell. 2018;173(2):499–514 e23.
Patkar S, Heselmeyer-Haddad K, Auslander N, Hirsch D, Camps J, Bronder D, et al. Hard wiring of normal tissue-specific chromosome-wide gene expression levels is an additional factor driving cancer type-specific aneuploidies. Genome Med. 2021;13(1):93.
Liu Y, Chen C, Xu Z, Scuoppo C, Rillahan CD, Gao J, et al. Deletions linked to TP53 loss drive cancer through p53-independent mechanisms. Nature. 2016;531(7595):471–5.
Zhou XP, Li YJ, Hoang-Xuan K, Laurent-Puig P, Mokhtari K, Longy M, et al. Mutational analysis of the PTEN gene in gliomas: molecular and pathological correlations. Int J Cancer. 1999;84(2):150–4.
Verhaak RG, Hoadley KA, Purdom E, Wang V, Qi Y, Wilkerson MD, et al. Integrated genomic analysis identifies clinically relevant subtypes of glioblastoma characterized by abnormalities in PDGFRA, IDH1, EGFR, and NF1. Cancer Cell. 2010;17(1):98–110.
Alfieri F, Caravagna G, Schaefer MH. Cancer genomes tolerate deleterious coding mutations through somatic copy number amplifications of wild-type regions. Nat Commun. 2023;14(1):3594.
Sheltzer JM, Amon A. The aneuploidy paradox: costs and benefits of an incorrect karyotype. Trends Genet. 2011;27(11):446–53.
Martincorena I, Raine KM, Gerstung M, Dawson KJ, Haase K, Van Loo P, et al. Universal patterns of selection in cancer and somatic tissues. Cell. 2017;171(5):1029–41 e21.
Shih J, Sarmashghi S, Zhakula-Kostadinova N, Zhang S, Georgis Y, Hoyt SH, et al. Cancer aneuploidies are shaped primarily by effects on tumour fitness. Nature. 2023;619(7971):793–800.
Zitnik M, Nguyen F, Wang B, Leskovec J, Goldenberg A, Hoffman MM. Machine learning for integrating data in biology and medicine: principles, practice, and opportunities. Inf Fusion. 2019;50:71–91.
Article PubMed Google Scholar
Han Y, Yang J, Qian X, Cheng WC, Liu SH, Hua X, et al. DriverML: a machine learning algorithm for identifying driver genes in cancer sequencing studies. Nucleic Acids Res. 2019;47(8): e45.
Luo P, Ding Y, Lei X, Wu FX. deepDriver: predicting cancer driver genes based on somatic mutations using deep convolutional neural networks. Front Genet. 2019;10:13.
Mostavi M, Chiu YC, Chen Y, Huang Y. CancerSiamese: one-shot learning for predicting primary and metastatic tumor types unseen during model training. BMC Bioinformatics. 2021;22(1):244.
Article PubMed PubMed Central Google Scholar
Ramirez R, Chiu YC, Hererra A, Mostavi M, Ramirez J, Chen Y, et al. Classification of cancer types using graph convolutional neural networks. Front Phys. 2020;8:203.
Chiu Y-C, Zheng S, Wang L-J, Iskra BS, Rao MK, Houghton PJ, et al. Predicting and characterizing a cancer dependency map of tumors with deep learning. Science Advances. 2021;7(34):eabh1275.
Lundberg SM, Lee S-I. A unified approach to interpreting model predictions. Adv Neural Inf Process Syst. 2017;30(9):4768–77.
Google Scholar
Rodriguez-Perez R, Bajorath J. Interpretation of compound activity predictions from complex machine learning models using local approximations and Shapley values. J Med Chem. 2020;63(16):8761–77.
Mermel CH, Schumacher SE, Hill B, Meyerson ML, Beroukhim R, Getz G. GISTIC2.0 facilitates sensitive and confident localization of the targets of focal somatic copy-number alteration in human cancers. Genome Biol. 2011;12(4):R41.
GTEx Consortium. The GTEx Consortium atlas of genetic regulatory effects across human tissues. Science. 2020;369(6509):1318–30.
Article Google Scholar
Nichols CA, Gibson WJ, Brown MS, Kosmicki JA, Busanovich JP, Wei H, et al. Loss of heterozygosity of essential genes represents a widespread class of potential cancer vulnerabilities. Nat Commun. 2020;11(1):2517.
Tsherniak A, Vazquez F, Montgomery PG, Weir BA, Kryukov G, Cowley GS, et al. Defining a cancer dependency map. Cell. 2017;170(3):564–76 e16.
Basha O, Argov CM, Artzy R, Zoabi Y, Hekselman I, Alfandari L, et al. Differential network analysis of multiple human tissue interactomes highlights tissue-selective processes and genetic disorder genes. Bioinformatics. 2020;36(9):2821–8.
Greene CS, Krishnan A, Wong AK, Ricciotti E, Zelaya RA, Himmelstein DS, et al. Understanding multicellular function and disease with human tissue-specific networks. Nat Genet. 2015;47(6):569–76.
Sharon M, Vinogradov E, Argov CM, Lazarescu O, Zoabi Y, Hekselman I, et al. The differential activity of biological processes in tissues and cell subsets can illuminate disease-related processes and cell-type identities. Bioinformatics. 2022;38(6):1584–92.
Barshir R, Hekselman I, Shemesh N, Sharon M, Novack L, Yeger-Lotem E. Role of duplicate genes in determining the tissue-selectivity of hereditary diseases. PLoS Genet. 2018;14(5): e1007327.
Jubran J, Hekselman I, Novack L, Yeger-Lotem E. Dosage-sensitive molecular mechanisms are associated with the tissue-specificity of traits and diseases. Comput Struct Biotechnol J. 2020;18:4024–32.
Kingsford C, Salzberg SL. What are decision trees? Nat Biotechnol. 2008;26(9):1011–3.
Kotsiantis SB. Decision trees: a recent overview. Artif Intell Rev. 2013;39:261–83.
McFarland JM, Ho ZV, Kugener G, Dempster JM, Montgomery PG, Bryan JG, et al. Improved estimation of cancer dependencies from large-scale RNAi screens using model-based normalization and data integration. Nat Commun. 2018;9(1):4610.
Cohen-Sharir Y, McFarland JM, Abdusamad M, Marquis C, Bernhard SV, Kazachkova M, et al. Aneuploidy renders cancer cells vulnerable to mitotic checkpoint inhibition. Nature. 2021;590(7846):486–91.
Prasad K, Bloomfield M, Levi H, Keuper K, Bernhard SV, Baudoin NC, et al. Whole-genome duplication shapes the aneuploidy landscape of human cancers. Cancer Res. 2022;82(9):1736–52.
Ben-David U, Siranosian B, Ha G, Tang H, Oren Y, Hinohara K, et al. Genetic and transcriptional evolution alters cancer cell line drug response. Nature. 2018;560(7718):325–30.
Dempster JM, Boyle I, Vazquez F, Root DE, Boehm JS, Hahn WC, et al. Chronos: a cell population dynamics model of CRISPR experiments that improves inference of gene fitness effects. Genome Biol. 2021;22(1):343.
Chen C, Bhalala HV, Qiao H, Dong JT. A possible tumor suppressor role of the KLF5 transcription factor in human breast cancer. Oncogene. 2002;21(43):6567–72.
Ma J-B, Bai J-Y, Zhang H-B, Jia J, Shi Q, Yang C, et al. KLF5 inhibits STAT3 activity and tumor metastasis in prostate cancer by suppressing IGF1 transcription cooperatively with HDAC1. Cell Death Dis. 2020;11(6):466.
Luo Y, Chen C. The roles and regulation of the KLF5 transcription factor in cancers. Cancer Sci. 2021;112(6):2097–117.
McConnell BB, Bialkowska AB, Nandan MO, Ghaleb AM, Gordon FJ, Yang VW. Haploinsufficiency of Kruppel-like factor 5 rescues the tumor-initiating effect of the Apc(Min) mutation in the intestine. Cancer Res. 2009;69(10):4125–33.
Rutledge SD, Douglas TA, Nicholson JM, Vila-Casadesus M, Kantzler CL, Wangsa D, et al. Selective advantage of trisomic human cells cultured in non-standard conditions. Sci Rep. 2016;6:22828.
Chen WH, Zhao XM, van Noort V, Bork P. Human monogenic disease genes have frequently functionally redundant paralogs. PLoS Comput Biol. 2013;9(5): e1003073.
Wang T, Birsoy K, Hughes NW, Krupczak KM, Post Y, Wei JJ, et al. Identification and characterization of essential genes in the human genome. Science. 2015;350(6264):1096–101.
Ito T, Young MJ, Li R, Jain S, Wernitznig A, Krill-Burger JM, et al. Paralog knockout profiling identifies DUSP4 and DUSP6 as a digenic dependence in MAPK pathway-driven cancers. Nat Genet. 2021;53(12):1664–72.
Zapata L, Pich O, Serrano L, Kondrashov FA, Ossowski S, Schaefer MH. Negative selection in tumor genome evolution acts on essential cellular functions and the immunopeptidome. Genome Biol. 2018;19(1):1–17.
de Kegel B, Ryan CJ. Paralog dispensability shapes homozygous deletion patterns in tumor genomes. Mol Syst Biol. 2023;19(12):e11987. https://doi.org/10.15252/msb.202311987 .
Zack TI, Schumacher SE, Carter SL, Cherniack AD, Saksena G, Tabak B, et al. Pan-cancer patterns of somatic copy number alteration. Nat Genet. 2013;45(10):1134–40.
Cai Y, Crowther J, Pastor T, Abbasi Asbagh L, Baietti MF, De Troyer M, et al. Loss of chromosome 8p governs tumor progression and drug response by altering lipid metabolism. Cancer Cell. 2016;29(5):751–66.
Girish V, Lakhani AA, Thompson SL, Scaduto CM, Brown LM, Hagenson RA, et al. Oncogene-like addiction to aneuploidy in human cancers. Science. 2023;381(6660):eadg4521.
Zhao X, Cohen EEW, William WN Jr, Bianchi JJ, Abraham JP, Magee D, et al. Somatic 9p24.1 alterations in HPV(-) head and neck squamous cancer dictate immune microenvironment and anti-PD-1 checkpoint inhibitor activity. Proc Natl Acad Sci U S A. 2022;119(47):e2213835119.
Ben-David U, Ha G, Khadka P, Jin X, Wong B, Franke L, et al. The landscape of chromosomal aberrations in breast cancer mouse models reveals driver-specific routes to tumorigenesis. Nat Commun. 2016;7:12160.
Simonovsky E, Sharon M, Ziv M, Mauer O, Hekselman I, Jubran J, et al. Predicting molecular mechanisms of hereditary diseases by using their tissue-selective manifestation. Mol Syst Biol. 2023;19(8):e11407. https://doi.org/10.15252/msb.202211407 .
Hua J, Xiong Z, Lowey J, Suh E, Dougherty ER. Optimal number of features as a function of sample size for various classification rules. Bioinformatics. 2005;21(8):1509–15.
Bakker B, Taudt A, Belderbos ME, Porubsky D, Spierings DC, de Jong TV, et al. Single-cell sequencing reveals karyotype heterogeneity in murine and human malignancies. Genome Biol. 2016;17(1):115.
Gao R, Bai S, Henderson YC, Lin Y, Schalck A, Yan Y, et al. Delineating copy number and clonal substructure in human tumors from single-cell transcriptomes. Nat Biotechnol. 2021;39(5):599–608.
Gao R, Davis A, McDonald TO, Sei E, Shi X, Wang Y, et al. Punctuated copy number evolution and clonal stasis in triple-negative breast cancer. Nat Genet. 2016;48(10):1119–30.
Gavish A, Tyler M, Greenwald AC, Hoefflin R, Simkin D, Tschernichovsky R, et al. Hallmarks of transcriptional intratumour heterogeneity across a thousand tumours. Nature. 2023;618(7965):598–606.
Beroukhim R, Getz G, Nghiemphu L, Barretina J, Hsueh T, Linhart D, et al. Assessing the significance of chromosomal aberrations in cancer: methodology and application to glioma. Proc Natl Acad Sci U S A. 2007;104(50):20007–12.
Center BITGDA. SNP6 copy number analysis (GISTIC2). Broad Institute of MIT and Harvard. 2016. https://gdac.broadinstitute.org/runs/analyses__latest/reports/cancer/STAD-TP/CopyNumber_Gistic2/nozzle.html .
Goldman MJ, Craft B, Hastie M, Repecka K, McDade F, Kamath A, et al. Visualizing and interpreting cancer genomics data via the Xena platform. Nat Biotechnol. 2020;38(6):675–8.
Basha O, Flom D, Barshir R, Smoly I, Tirman S, Yeger-Lotem E. MyProteinNet: build up-to-date protein interaction networks for organisms, tissues and user-defined contexts. Nucleic Acids Res. 2015;43(W1):W258–63.
Sonawane AR, Platig J, Fagny M, Chen C-Y, Paulson JN, Lopes-Ramos CM, et al. Understanding tissue-specific gene regulation. Cell Rep. 2017;21(4):1077–88.
Cardoso-Moreira M, Halbert J, Valloton D, Velten B, Chen C, Shao Y, et al. Gene expression across mammalian organ development. Nature. 2019;571(7766):505–9.
Cunningham F, Allen JE, Allen J, Alvarez-Jarreta J, Amode MR, Armean IM, et al. Ensembl 2022. Nucleic Acids Res. 2022;50(D1):D988–95.
Pedregosa F, Varoquaux G, Gramfort A, Michel V, Thirion B, Grisel O, et al. Scikit-learn: machine learning in Python. The Journal of machine Learning research. 2011;12:2825–30.
Chen T, Guestrin C, editors. Xgboost: a scalable tree boosting system. Proceedings of the 22nd acm sigkdd international conference on knowledge discovery and data mining; 2016. p. 785–94. https://doi.org/10.1145/2939672.2939785 .
Lundberg SM, Erion G, Chen H, DeGrave A, Prutkin JM, Nair B, et al. From local explanations to global understanding with explainable AI for trees. Nat Mach Intell. 2020;2(1):56–67.
Benjamini Y, Hochberg Y. Controlling the false discovery rate: a practical and powerful approach to multiple testing. J Roy Stat Soc: Ser B (Methodol). 1995;57(1):289–300.
Jubran J, Yeger-Lotem E. Machine-learning analysis of factors that shape cancer aneuploidy landscapes reveals an important role for negative selection. GitHub https://github.com/JumanJubran/AneuploidyML .
Jubran J, Yeger-Lotem E. Machine-learning analysis of factors that shape cancer aneuploidy landscapes reveals an important role for negative selection. Zenodo. https://zenodo.org/records/8199048 .
Simonovsky E, Yeger-Lotem E. Predicting molecular mechanisms of hereditary diseases by using their tissue-selective manifestation. Datasets. Zenodo. https://zenodo.org/records/10115922 .
Goldman MJ, Craft B, Hastie M, Repecka K, McDade F, Kamath A, et al. Visualizing and interpreting cancer genomics data via the Xena platform. Datasets. Xena. https://xenabrowser.net/datapages/?hub=https://gdc.xenahubs.net:443 .
Tsherniak A, Vazquez F, Montgomery P, Weir B, Kryukov G, Cowley G. Defining a cancer dependency map. Datasets. DepMap. https://depmap.org/portal/download/all/ .
Download references
Acknowledgements
The authors would like to thank Jason Sheltzer for providing DLD1-WT and DLD1 Ts13 cell lines.
J.J. wishes to thank the Baroness Ariane de Rothschild Women Doctoral Program.
Peer review information
Andrew Cosgrove was the primary editor of this article and managed its editorial process and peer review in collaboration with the rest of the editorial team.
Review history
The review history is available as Additional File 8 .
This study was funded by the Israel Science Foundation [401/22 to E.Y.-L.] and by a Ben-Gurion University grant [to E.Y.-L.]. Work in the Ben-David lab is supported by the European Research Council Starting Grant (grant #945674 to U.B.-D.), the Israel Science Foundation (grant #1805/21 to U.B.-D.), the Israel Cancer Research Fund (Project Grant to U.B.-D.), and the BSF Project Grant (grant #2019228 to U.B.-D.), and by the EMBO Young Investigator Program (to U.B.-D.).
Author information
Juman Jubran and Rachel Slutsky are equally contributing first authors.
Uri Ben-David and Esti Yeger-Lotem are equally contributing last authors.
Authors and Affiliations
Department of Clinical Biochemistry and Pharmacology, Ben-Gurion University of the Negev, 84105, Beer Sheva, Israel
Juman Jubran & Esti Yeger-Lotem
Department of Human Molecular Genetics and Biochemistry, Faculty of Medicine, Tel Aviv University, Tel Aviv, Israel
Rachel Slutsky, Nir Rozenblum & Uri Ben-David
Department of Software & Information Systems Engineering, Ben-Gurion University of the Negev, 84105, Beer Sheva, Israel
Lior Rokach
The National Institute for Biotechnology in the Negev, Ben-Gurion University of the Negev, 84105, Beer Sheva, Israel
Esti Yeger-Lotem
You can also search for this author in PubMed Google Scholar
Contributions
U.B.-D. and E.Y.-L. conceived and oversaw the study. J.J. designed and performed the computational analyses and developed and interpreted the ML models. R.S. designed and performed the UCHL1 and KLF5 DepMap data analyses and the in vitro experiments. N.R. assisted with the in vitro experiments. L.R. advised on the ML analyses. J.J., R.S., U.B.-D., and E.Y.-L. analyzed and interpreted the data and wrote the manuscript. All authors reviewed and approved the manuscript.
Authors’ Twitter handles
Twitter handles: @yegerlotemlab (Esti Yeger-Lotem), @BenDavidLab (Uri Ben-David).
Corresponding authors
Correspondence to Uri Ben-David or Esti Yeger-Lotem .
Ethics declarations
Ethics approval and consent to participate.
Ethics approval is not applicable.
Competing interests
The authors declare that they have no competing interests.
Additional information
Publisher’s note.
Springer Nature remains neutral with regard to jurisdictional claims in published maps and institutional affiliations.
Supplementary Information
Additional file 1: supplementary figures..
This file contains Supplementary Figures S1-S17.
Additional file 2: Table S1.
Association of TCGA cancer types with normal tissues-of-origin and matching cell lines.
Additional file 3: Table S2.
The auROC and auPRC performance of ML models whose features were calculated using distinct percentages of genes.
Additional file 4: Table S3.
SHAP value per feature of each instance of chromosome-arm and tumor type in the gain and loss models.
Additional file 5: Table S4.
Potential driver instances of each feature in the gain and loss models, and their frequencies.
Additional file 6: Table S5.
Correlations between chr-13q residing genes and the essentiality of their paralogs.
Additional file 7: Table S6.
Co-incidence of arm-level events in the different cancer types, and their frequencies.
Additional file 8.
Rights and permissions.
Open Access This article is licensed under a Creative Commons Attribution 4.0 International License, which permits use, sharing, adaptation, distribution and reproduction in any medium or format, as long as you give appropriate credit to the original author(s) and the source, provide a link to the Creative Commons licence, and indicate if changes were made. The images or other third party material in this article are included in the article's Creative Commons licence, unless indicated otherwise in a credit line to the material. If material is not included in the article's Creative Commons licence and your intended use is not permitted by statutory regulation or exceeds the permitted use, you will need to obtain permission directly from the copyright holder. To view a copy of this licence, visit http://creativecommons.org/licenses/by/4.0/ . The Creative Commons Public Domain Dedication waiver ( http://creativecommons.org/publicdomain/zero/1.0/ ) applies to the data made available in this article, unless otherwise stated in a credit line to the data.
Reprints and permissions
About this article
Cite this article.
Jubran, J., Slutsky, R., Rozenblum, N. et al. Machine-learning analysis reveals an important role for negative selection in shaping cancer aneuploidy landscapes. Genome Biol 25 , 95 (2024). https://doi.org/10.1186/s13059-024-03225-7
Download citation
Received : 05 July 2023
Accepted : 26 March 2024
Published : 15 April 2024
DOI : https://doi.org/10.1186/s13059-024-03225-7
Share this article
Anyone you share the following link with will be able to read this content:
Sorry, a shareable link is not currently available for this article.
Provided by the Springer Nature SharedIt content-sharing initiative
Genome Biology
ISSN: 1474-760X
- Submission enquiries: [email protected]
- General enquiries: [email protected]
Thank you for visiting nature.com. You are using a browser version with limited support for CSS. To obtain the best experience, we recommend you use a more up to date browser (or turn off compatibility mode in Internet Explorer). In the meantime, to ensure continued support, we are displaying the site without styles and JavaScript.
- View all journals
- Explore content
- About the journal
- Publish with us
- Sign up for alerts
Collection 11 March 2020
Top 100 in Cancer
This collection highlights our most downloaded* cancer papers published in 2019. Featuring authors from around the world, these papers feature valuable research from an international community.
*Data obtained from SN Insights which is based on Digital Science’s Dimensions.
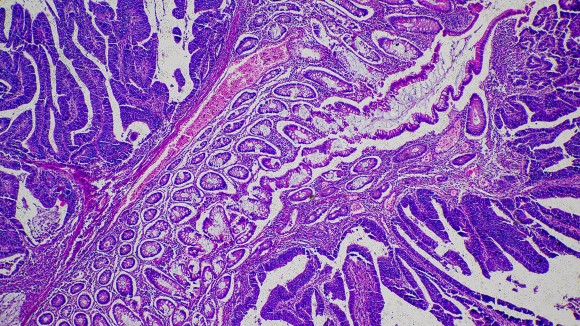
A landmark in drug discovery based on complex natural product synthesis
- Satoshi Kawano
- Yoshito Kishi
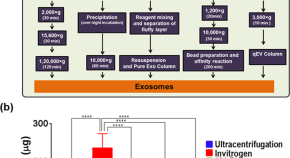
Comparative analysis of exosome isolation methods using culture supernatant for optimum yield, purity and downstream applications
- Girijesh Kumar Patel
- Mohammad Aslam Khan
- Ajay Pratap Singh
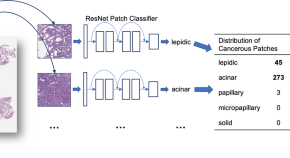
Pathologist-level classification of histologic patterns on resected lung adenocarcinoma slides with deep neural networks
- Jason W. Wei
- Laura J. Tafe
- Saeed Hassanpour
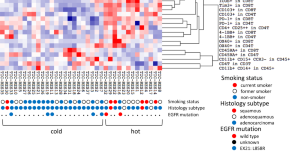
Peripheral T cell cytotoxicity predicts T cell function in the tumor microenvironment
- Kota Iwahori
- Yasushi Shintani
- Hisashi Wada
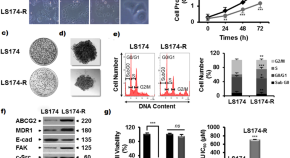
The Phenolic compound Kaempferol overcomes 5-fluorouracil resistance in human resistant LS174 colon cancer cells
- Ichrak Riahi-Chebbi
- Soumaya Souid
- Khadija Essafi-Benkhadir
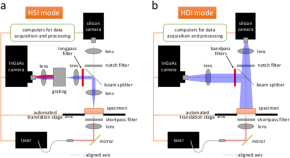
Deep-tissue optical imaging of near cellular-sized features
- Xiangnan Dang
- Neelkanth M. Bardhan
- Angela M. Belcher
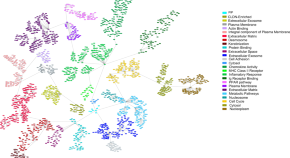
A novel approach to triple-negative breast cancer molecular classification reveals a luminal immune-positive subgroup with good prognoses
- Guillermo Prado-Vázquez
- Angelo Gámez-Pozo
- Juan Ángel Fresno Vara
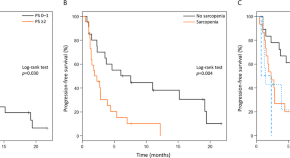
Impact of sarcopenia in patients with advanced non–small cell lung cancer treated with PD-1 inhibitors: A preliminary retrospective study
- Takayuki Shiroyama
- Izumi Nagatomo
- Atsushi Kumanogoh
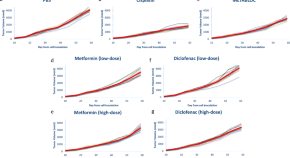
Metabolic therapies inhibit tumor growth in vivo and in silico
- Jorgelindo da Veiga Moreira
- Minoo Hamraz
- Sabine Peres
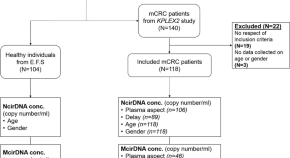
Quantifying circulating cell-free DNA in humans
- Romain Meddeb
- Zahra Al Amir Dache
- Alain R. Thierry
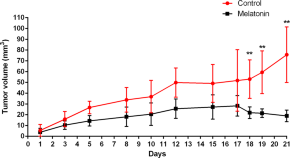
RNA-Seq transcriptome analysis shows anti-tumor actions of melatonin in a breast cancer xenograft model
- Bruna Victorasso Jardim-Perassi
- Pâmela A. Alexandre
- Debora Aparecida Pires de Campos Zuccari
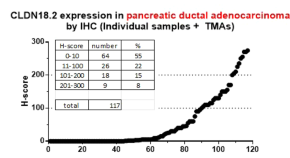
Targeting CLDN18.2 by CD3 Bispecific and ADC Modalities for the Treatments of Gastric and Pancreatic Cancer
- Davide Foletti
- Shu-Hui Liu
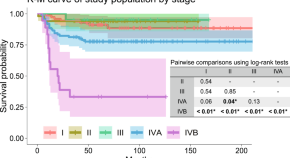
Deep learning-based survival prediction of oral cancer patients
- Dong Wook Kim
- Sanghoon Lee
- Hyung Jun Kim
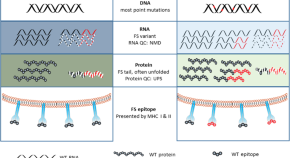
RNA Transcription and Splicing Errors as a Source of Cancer Frameshift Neoantigens for Vaccines
- Stephen Albert Johnston
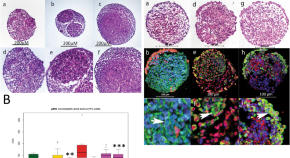
A Human iPSC-derived 3D platform using primary brain cancer cells to study drug development and personalized medicine
- Simon Plummer
- Stephanie Wallace
- David Pamies
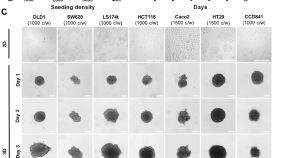
Short-term 3D culture systems of various complexity for treatment optimization of colorectal carcinoma
- Marloes Zoetemelk
- Magdalena Rausch
- Patrycja Nowak-Sliwinska
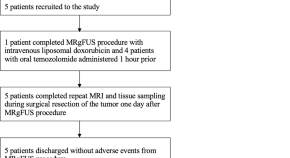
Blood-Brain Barrier Opening in Primary Brain Tumors with Non-invasive MR-Guided Focused Ultrasound: A Clinical Safety and Feasibility Study
- Todd Mainprize
- Nir Lipsman
- Kullervo Hynynen
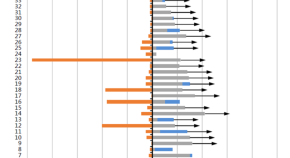
Real-world evidence and clinical observations of the treatment of advanced non-small cell lung cancer with PD-1/PD-L1 inhibitors
- Jingcheng Zhang
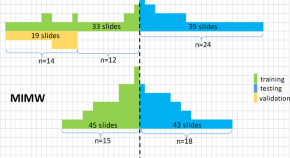
Convolutional neural networks can accurately distinguish four histologic growth patterns of lung adenocarcinoma in digital slides
- Arkadiusz Gertych
- Zaneta Swiderska-Chadaj
- Beatrice S. Knudsen
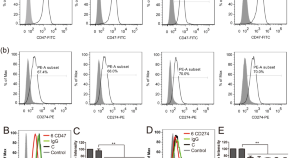
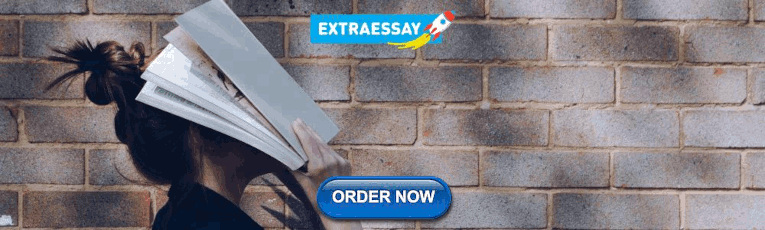
Dual blockage of both PD-L1 and CD47 enhances immunotherapy against circulating tumor cells
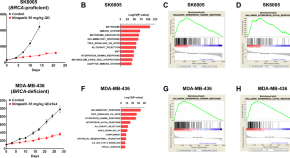
Niraparib activates interferon signaling and potentiates anti-PD-1 antibody efficacy in tumor models
- Kaiming Sun
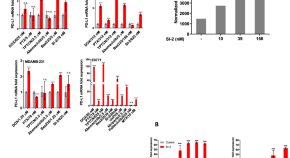
Drug-induced PD-L1 expression and cell stress response in breast cancer cells can be balanced by drug combination
- Yossi Eliaz
- David M. Lonard
Associations between Coffee Products and Breast Cancer Risk: a Case-Control study in Hong Kong Chinese Women
- Priscilla Ming Yi Lee
- Wing Cheong Chan
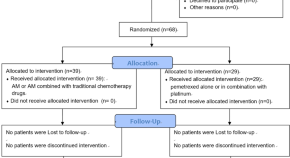
Apatinib Mesylate in the treatment of advanced progressed lung adenocarcinoma patients with EGFR-TKI resistance —A Multicenter Randomized Trial
- Liqin Zhang
- Hongbao Cao
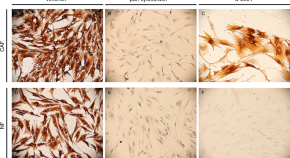
Cancer associated fibroblasts sculpt tumour microenvironment by recruiting monocytes and inducing immunosuppressive PD-1 + TAMs
- Betul Gok Yavuz
- Gurcan Gunaydin
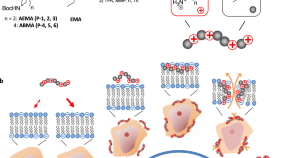
Anticancer polymers designed for killing dormant prostate cancer cells
- Haruko Takahashi
- Kenji Yumoto
- Kenichi Kuroda
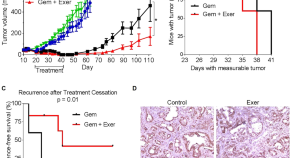
Exercise during preoperative therapy increases tumor vascularity in pancreatic tumor patients
- Claudia Alvarez Florez Bedoya
- Ana Carolina Ferreira Cardoso
- Keri L. Schadler
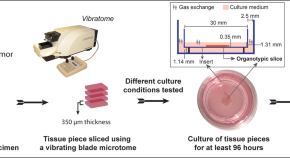
Ex vivo organotypic culture system of precision-cut slices of human pancreatic ductal adenocarcinoma
- Sougat Misra
- Carlos F. Moro
- Caroline S. Verbeke
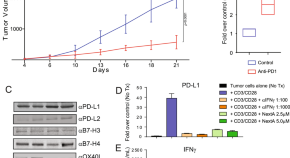
Selective HDAC6 inhibitors improve anti-PD-1 immune checkpoint blockade therapy by decreasing the anti-inflammatory phenotype of macrophages and down-regulation of immunosuppressive proteins in tumor cells
- Eva Sahakian
- Alejandro Villagra
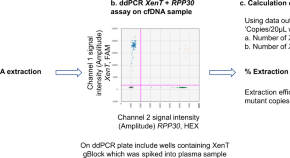
Optimisation of robust singleplex and multiplex droplet digital PCR assays for high confidence mutation detection in circulating tumour DNA
- Vicky Rowlands
- Andrzej J. Rutkowski
- J. Carl Barrett
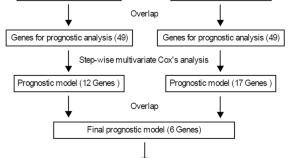
A RNA sequencing-based six-gene signature for survival prediction in patients with glioblastoma
- Shuguang Zuo
- Xinhong Zhang
- Liping Wang
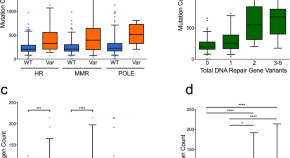
Mutations in DNA repair genes are associated with increased neoantigen burden and a distinct immunophenotype in lung squamous cell carcinoma
- Young Kwang Chae
- Jonathan F. Anker
- Jeffrey H. Chuang
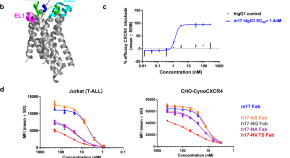
Optimal design, anti-tumour efficacy and tolerability of anti-CXCR4 antibody drug conjugates
- Maria José Costa
- Jyothirmayee Kudaravalli
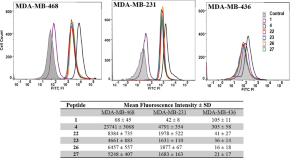
Small Peptide Ligands for Targeting EGFR in Triple Negative Breast Cancer Cells
- Hanieh Hossein-Nejad-Ariani
- Emad Althagafi
- Kamaljit Kaur
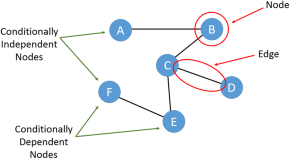
Network Analysis of the Multidimensional Symptom Experience of Oncology
- Nikolaos Papachristou
- Payam Barnaghi
- Christine Miaskowski
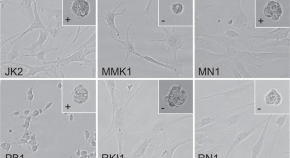
A reference collection of patient-derived cell line and xenograft models of proneural, classical and mesenchymal glioblastoma
- Brett W. Stringer
- Bryan W. Day
- Andrew W. Boyd
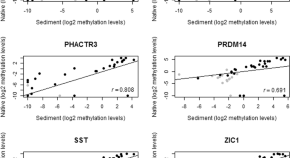
Cervical cancer detection by DNA methylation analysis in urine
- Barbara C. Snoek
- Annina P. van Splunter
- Renske D. M. Steenbergen
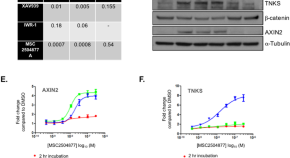
A novel tankyrase inhibitor, MSC2504877, enhances the effects of clinical CDK4/6 inhibitors
- Malini Menon
- Richard Elliott
- Christopher J. Lord
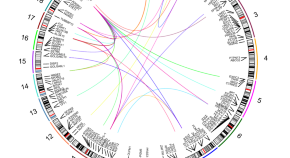
Differentiating between cancer and normal tissue samples using multi-hit combinations of genetic mutations
- Nicholas A. Kinney
- Ramu Anandakrishnan
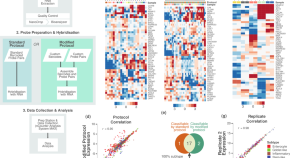
Analytical Validation of Multiplex Biomarker Assay to Stratify Colorectal Cancer into Molecular Subtypes
- Chanthirika Ragulan
- Katherine Eason
- Anguraj Sadanandam
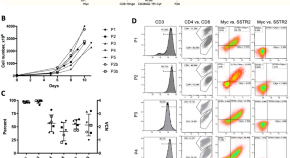
Manufacturing and preclinical validation of CAR T cells targeting ICAM-1 for advanced thyroid cancer therapy
- Yogindra Vedvyas
- Jaclyn E. McCloskey
- Moonsoo M. Jin
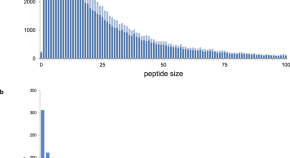
A library of Neo Open Reading Frame peptides (NOPs) as a sustainable resource of common neoantigens in up to 50% of cancer patients
- Ronald H. A. Plasterk
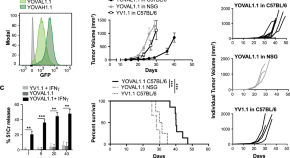
A novel immunogenic mouse model of melanoma for the preclinical assessment of combination targeted and immune-based therapy
- Emily J. Lelliott
- Carleen Cullinane
- Karen E. Sheppard
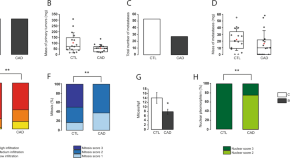
Cadaverine, a metabolite of the microbiome, reduces breast cancer aggressiveness through trace amino acid receptors
- Tünde Kovács
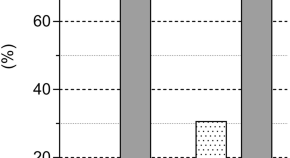
Bovine leukemia virus DNA associated with breast cancer in women from South Brazil
- Daniela Schwingel
- Ana P. Andreolla
- Luiz C. Kreutz
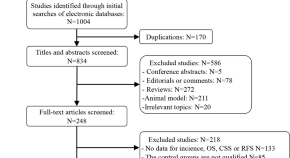
The effect of metformin therapy on incidence and prognosis in prostate cancer: A systematic review and meta-analysis
- Kancheng He
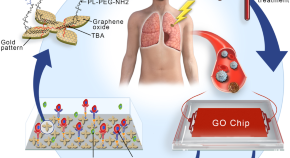
PD-L1 Expression in Circulating Tumor Cells Increases during Radio(chemo)therapy and Indicates Poor Prognosis in Non-small Cell Lung Cancer
- Tae Hyun Kim
- Sunitha Nagrath
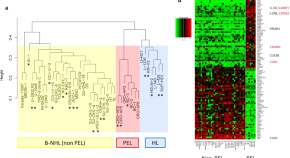
The LL-100 panel: 100 cell lines for blood cancer studies
- Hilmar Quentmeier
- Claudia Pommerenke
- Hans G. Drexler
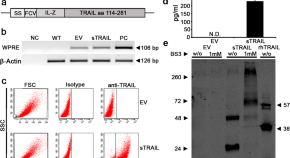
Soluble TRAIL Armed Human MSC As Gene Therapy For Pancreatic Cancer
- Carlotta Spano
- Giulia Grisendi
- Massimo Dominici
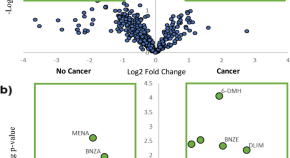
Detection of Volatile Organic Compounds (VOCs) in Urine via Gas Chromatography-Mass Spectrometry QTOF to Differentiate Between Localized and Metastatic Models of Breast Cancer
- Mark Woollam
- Meghana Teli
- Mangilal Agarwal
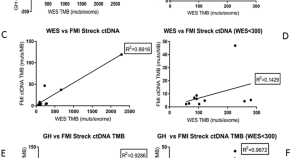
Measuring Tumor Mutational Burden (TMB) in Plasma from mCRPC Patients Using Two Commercial NGS Assays
- Christian H. Poehlein
- Diane Levitan
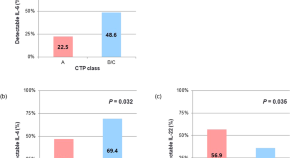
Inflammatory cytokines and change of Th1/Th2 balance as prognostic indicators for hepatocellular carcinoma in patients treated with transarterial chemoembolization
- Hae Lim Lee
- Jeong Won Jang
- Seung Kew Yoon
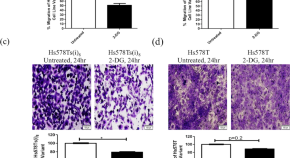
2-Deoxy-D-Glucose inhibits aggressive triple-negative breast cancer cells by targeting glycolysis and the cancer stem cell phenotype
- Sadhbh O’Neill
- Richard K. Porter
- Lorraine O’Driscoll
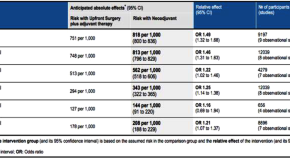
Upfront Surgery versus Neoadjuvant Therapy for Resectable Pancreatic Cancer: Systematic Review and Bayesian Network Meta-analysis
- Alison Bradley
- Robert Van Der Meer
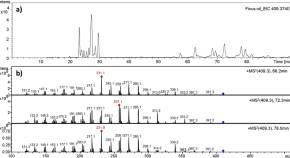
Biological activities of Ficus carica latex for potential therapeutics in Human Papillomavirus (HPV) related cervical cancers
- Arshia Ghanbari
- Adam Le Gresley
- G. Hossein Ashrafi
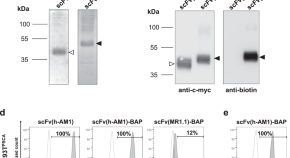
Targeted delivery of TLR3 agonist to tumor cells with single chain antibody fragment-conjugated nanoparticles induces type I-interferon response and apoptosis
- Isabell Schau
- Susanne Michen
- Achim Temme
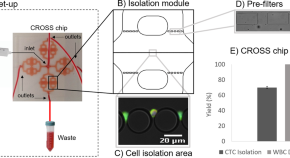
Fast and efficient microfluidic cell filter for isolation of circulating tumor cells from unprocessed whole blood of colorectal cancer patients
- Silvina Ribeiro-Samy
- Marta I. Oliveira
- Lorena Diéguez
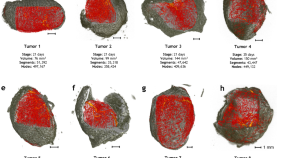
Tumor Ensemble-Based Modeling and Visualization of Emergent Angiogenic Heterogeneity in Breast Cancer
- Spyros K. Stamatelos
- Akanksha Bhargava
- Arvind P. Pathak
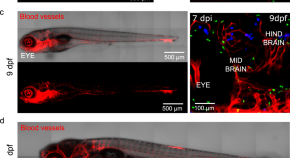
Human macrophages survive and adopt activated genotypes in living zebrafish
- Colin D. Paul
- Alexus Devine
- Kandice Tanner
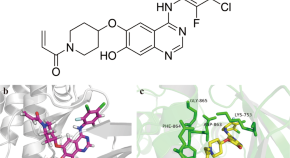
PCC0208027, a novel tyrosine kinase inhibitor, inhibits tumor growth of NSCLC by targeting EGFR and HER2 aberrations
- Hiroshi Kurihara
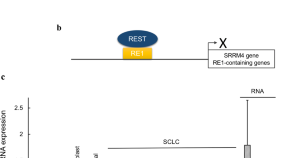
A gapmer antisense oligonucleotide targeting SRRM4 is a novel therapeutic medicine for lung cancer
- Masahito Shimojo
- Yuuya Kasahara
- Satoshi Obika
Risk factors for immune-related adverse events associated with anti-PD-1 pembrolizumab
- Yeonghee Eun
- In Young Kim
- Jaejoon Lee
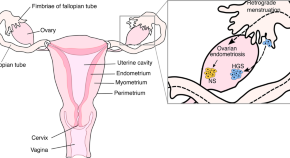
Ovarian cancer cell lines derived from non-serous carcinomas migrate and invade more aggressively than those derived from high-grade serous carcinomas
- Amelia Hallas-Potts
- John C. Dawson
- C. Simon Herrington
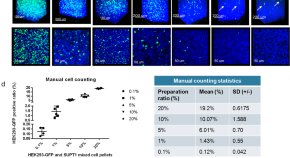
Three-dimensional imaging and quantitative analysis in CLARITY processed breast cancer tissues
- Laurie J. Goodman
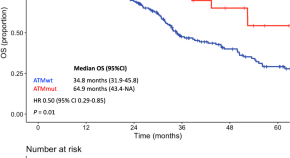
Prognostic impact of ATM mutations in patients with metastatic colorectal cancer
- Giovanni Randon
- Giovanni Fucà
- Filippo Pietrantonio
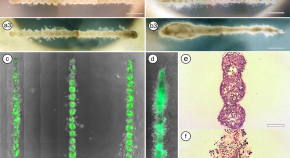
A 3D bioprinter platform for mechanistic analysis of tumoroids and chimeric mammary organoids
- John A. Reid
- Xavier-Lewis Palmer
- Robert D. Bruno
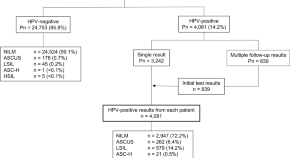
Carcinogenic risk of human papillomavirus (HPV) genotypes and potential effects of HPV vaccines in Korea
- Eunhyang Park
- Young Lyun Oh
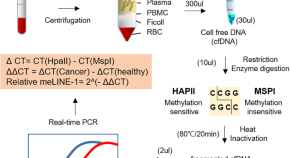
Methylation of LINE-1 in cell-free DNA serves as a liquid biopsy biomarker for human breast cancers and dog mammary tumors
- Kang-Hoon Lee
- Tae-Jin Shin
- Je-Yoel Cho
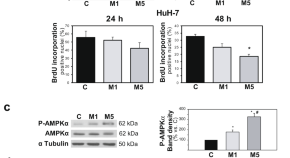
Metformin and glucose starvation decrease the migratory ability of hepatocellular carcinoma cells: targeting AMPK activation to control migration
- Anabela C. Ferretti
- Florencia Hidalgo
- Cristián Favre
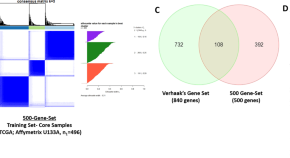
Relevance of a TCGA-derived Glioblastoma Subtype Gene-Classifier among Patient Populations
- Wan-Yee Teo
- Karthik Sekar
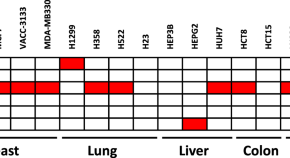
Identification of recurrent fusion genes across multiple cancer types
- Yan-Ping Yu
- Jian-Hua Luo
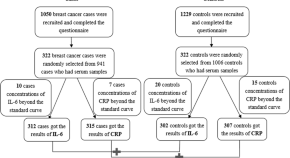
Direct and indirect associations between dietary magnesium intake and breast cancer risk
- Wu-Qing Huang
- Wei-Qing Long
- Cai-Xia Zhang
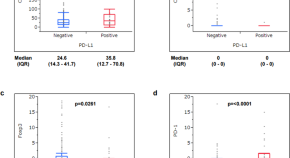
PD-L1 expression combined with microsatellite instability/CD8+ tumor infiltrating lymphocytes as a useful prognostic biomarker in gastric cancer
- Toshiaki Morihiro
- Shinji Kuroda
- Toshiyoshi Fujiwara
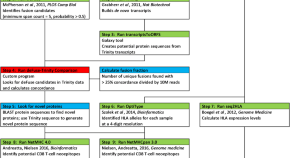
Identification of candidate neoantigens produced by fusion transcripts in human osteosarcomas
- Susan K. Rathe
- Flavia E. Popescu
- David A. Largaespada
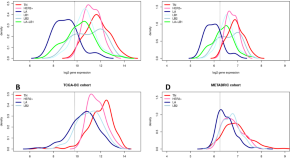
PCA-PAM50 improves consistency between breast cancer intrinsic and clinical subtyping reclassifying a subset of luminal A tumors as luminal B
- Praveen-Kumar Raj-Kumar
- Jianfang Liu
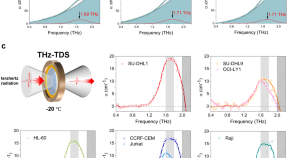
Detection and manipulation of methylation in blood cancer DNA using terahertz radiation
- Hwayeong Cheon
- Jin Ho Paik
- Joo-Hiuk Son
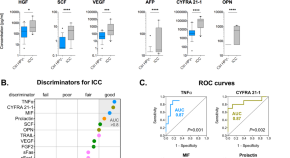
Features of the cervicovaginal microenvironment drive cancer biomarker signatures in patients across cervical carcinogenesis
- Paweł Łaniewski
- Melissa M. Herbst-Kralovetz
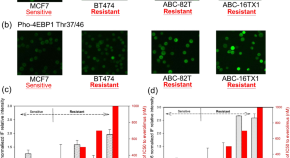
Immunofluorescence can assess the efficacy of mTOR pathway therapeutic agent Everolimus in breast cancer models
- Chun-Ting Kuo
- Chen-Lin Chen
- Andrew M. Wo
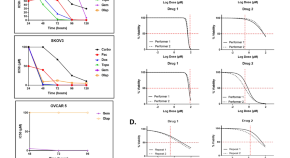
Prospective Validation of an Ex Vivo , Patient-Derived 3D Spheroid Model for Response Predictions in Newly Diagnosed Ovarian Cancer
- Stephen Shuford
- Christine Wilhelm
- Teresa M. DesRochers
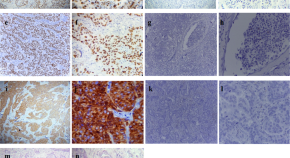
Ki-67, p53 and BCL-2 Expressions and their Association with Clinical Histopathology of Breast Cancer among Women in Tanzania
- Hidaya Mansouri
- Leah F. Mnango
- Emmanuel A. Mpolya
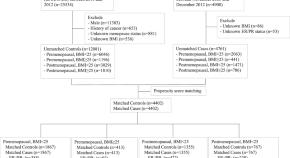
Association of white blood cell count with breast cancer burden varies according to menopausal status, body mass index, and hormone receptor status: a case-control study
- Byoungjin Park
- Hye Sun Lee
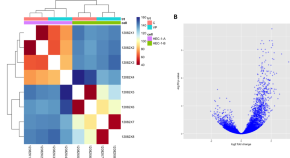
Differential gene expression induced by Verteporfin in endometrial cancer cells
- Lisa Gahyun Bang
- Venkata Ramesh Dasari
- Radhika P. Gogoi
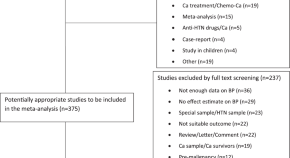
Association between blood pressure and risk of cancer development: a systematic review and meta-analysis of observational studies
- Aristeidis Seretis
- Sofia Cividini
- Konstantinos K. Tsilidis
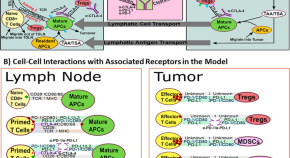
A QSP Model for Predicting Clinical Responses to Monotherapy, Combination and Sequential Therapy Following CTLA-4, PD-1, and PD-L1 Checkpoint Blockade
- Oleg Milberg
- Aleksander S. Popel
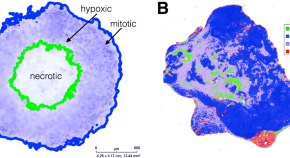
The impact of proliferation-migration tradeoffs on phenotypic evolution in cancer
- Jill A. Gallaher
- Joel S. Brown
- Alexander R. A. Anderson
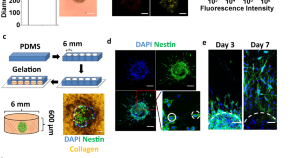
Endothelial cells promote 3D invasion of GBM by IL-8-dependent induction of cancer stem cell properties
- Michael G. McCoy
- Dennis Nyanyo
- Claudia Fischbach
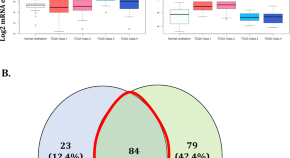
Targeting Epidermal Growth Factor Receptor (EGFR) and Human Epidermal Growth Factor Receptor 2 (HER2) Expressing Bladder Cancer Using Combination Photoimmunotherapy (PIT)
- Mohammad R. Siddiqui
- Reema Railkar
- Piyush K. Agarwal
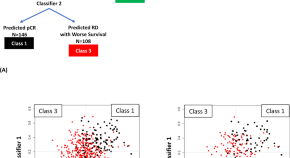
A Predictor of Pathological Complete Response to Neoadjuvant Chemotherapy Stratifies Triple Negative Breast Cancer Patients with High Risk of Recurrence
- Marcia V. Fournier
- Edward C. Goodwin
- Adam M. Brufsky
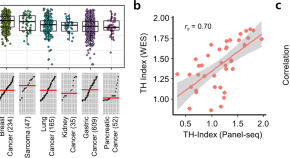
Intratumor heterogeneity inferred from targeted deep sequencing as a prognostic indicator
- Bo Young Oh
- Hyun-Tae Shin
- Woong-Yang Park
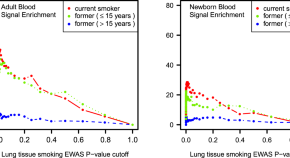
DNA methylation signature of smoking in lung cancer is enriched for exposure signatures in newborn and adult blood
- K. M. Bakulski
- J. A. Colacino
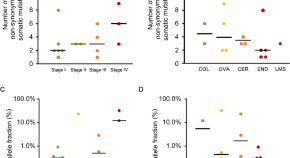
Liquid biopsy-based comprehensive gene mutation profiling for gynecological cancer using CAncer Personalized Profiling by deep Sequencing
- Naoyuki Iwahashi
- Kazuko Sakai
- Kazuhiko Ino
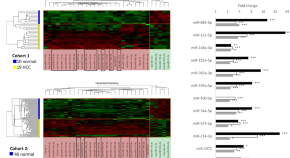
Circulating microRNAs as Potential Diagnostic and Prognostic Biomarkers in Hepatocellular Carcinoma
- Ye Shen Wong
- Caroline G. L. Lee
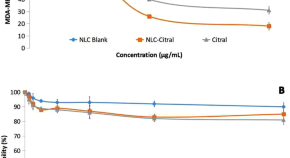
In vitro cytotoxicity and anticancer effects of citral nanostructured lipid carrier on MDA MBA-231 human breast cancer cells
- Noraini Nordin
- Swee Keong Yeap
- Noorjahan Banu Alitheen
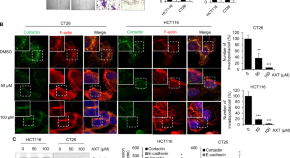
Astaxanthin suppresses the metastasis of colon cancer by inhibiting the MYC-mediated downregulation of microRNA-29a-3p and microRNA-200a
- Hye-Youn Kim
- Young-Mi Kim
- Suntaek Hong
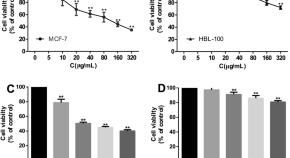
Anti-breast Cancer Activity of SPG-56 from Sweet Potato in MCF-7 Bearing Mice in Situ through Promoting Apoptosis and Inhibiting Metastasis
- Zhaoxing Li
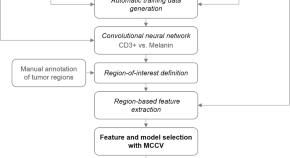
Automatic discovery of image-based signatures for ipilimumab response prediction in malignant melanoma
- Nathalie Harder
- Ralf Schönmeyer
- Günter Schmidt
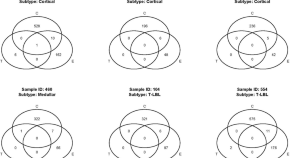
Detection of novel fusion-transcripts by RNA-Seq in T-cell lymphoblastic lymphoma
- Pilar López-Nieva
- Pablo Fernández-Navarro
- José Fernández-Piqueras
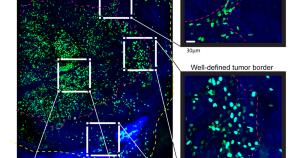
Intravital imaging of glioma border morphology reveals distinctive cellular dynamics and contribution to tumor cell invasion
- Maria Alieva
- Verena Leidgens
- Jacco van Rheenen
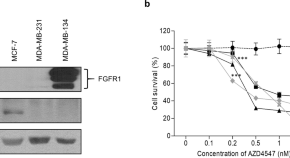
A Selective FGFR inhibitor AZD4547 suppresses RANKL/M-CSF/OPG-dependent ostoclastogenesis and breast cancer growth in the metastatic bone microenvironment
- Yoon Ji Choi
- Kyong Hwa Park
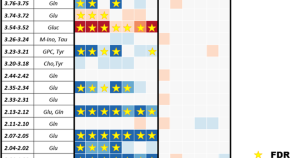
Magnetic Resonance Spectroscopy-based Metabolomic Biomarkers for Typing, Staging, and Survival Estimation of Early-Stage Human Lung Cancer
- Yannick Berker
- Lindsey A. Vandergrift
- Leo L. Cheng
Quick links
- Explore articles by subject
- Guide to authors
- Editorial policies

Journal of Cancer Biology and Research
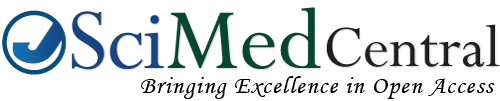
Clinical Journal of Heart Disease
- Open Access :   Creative Commons
- Review Process :   Double Blind
- Online ISSN :   2373-9436
- JsciMed Central Publisher Id (DOI) :   10.47739
- Article Formats Available :   PDF, Fulltext, XML, ePub
- Submit Manuscript :   [email protected]
- Email :   [email protected]
- DOI :   https://doi.org/10.47739/2373-9436/
- Telephone :   302-261-5000
- For Journal related queries :   Journal mail id
- For APC queries :   [email protected]
- For Other queries :   [email protected]
- Telephone (Whatsapp) :   302-261-5000
- + View More + Hide More

Journal Profile
Editor spotlight, special issues, jscimed central, submission - publication process ...

Recent Articles
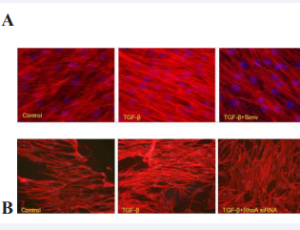
Subscribe to Newsletters
And stay informed about our news and events

An official website of the United States government
The .gov means it’s official. Federal government websites often end in .gov or .mil. Before sharing sensitive information, make sure you’re on a federal government site.
The site is secure. The https:// ensures that you are connecting to the official website and that any information you provide is encrypted and transmitted securely.
- Publications
- Account settings
Preview improvements coming to the PMC website in October 2024. Learn More or Try it out now .
- Advanced Search
- Journal List
- Comput Struct Biotechnol J
Applied machine learning in cancer research: A systematic review for patient diagnosis, classification and prognosis
Konstantina kourou.
a Unit of Medical Technology and Intelligent Information Systems, Dept. of Materials Science and Engineering, University of Ioannina, Ioannina, Greece
g Foundation for Research and Technology-Hellas, Institute of Molecular Biology and Biotechnology, Dept. of Biomedical Research, Ioannina GR45110, Greece
Konstantinos P. Exarchos
b Dept. of Respiratory Medicine, Faculty of Medicine, University of Ioannina, Ioannina, Greece
Costas Papaloukas
c Dept. of Biological Applications and Technology, University of Ioannina, Ioannina, Greece
Prodromos Sakaloglou
d Dept. of Precision and Molecular Medicine, Unit of Liquid Biopsy in Oncology, Ioannina University Hospital, Ioannina, Greece
e Laboratory of Medical Genetics in Clinical Practice, School of Health Sciences, Faculty of Medicine, University of Ioannina, Ioannina, Greece
Themis Exarchos
f Dept. of Informatics, Ionian University, Corfu, Greece
Dimitrios I. Fotiadis
Associated data.
Artificial Intelligence (AI) has recently altered the landscape of cancer research and medical oncology using traditional Machine Learning (ML) algorithms and cutting-edge Deep Learning (DL) architectures. In this review article we focus on the ML aspect of AI applications in cancer research and present the most indicative studies with respect to the ML algorithms and data used. The PubMed and dblp databases were considered to obtain the most relevant research works of the last five years. Based on a comparison of the proposed studies and their research clinical outcomes concerning the medical ML application in cancer research, three main clinical scenarios were identified. We give an overview of the well-known DL and Reinforcement Learning (RL) methodologies, as well as their application in clinical practice, and we briefly discuss Systems Biology in cancer research. We also provide a thorough examination of the clinical scenarios with respect to disease diagnosis, patient classification and cancer prognosis and survival. The most relevant studies identified in the preceding year are presented along with their primary findings. Furthermore, we examine the effective implementation and the main points that need to be addressed in the direction of robustness, explainability and transparency of predictive models. Finally, we summarize the most recent advances in the field of AI/ML applications in cancer research and medical oncology, as well as some of the challenges and open issues that need to be addressed before data-driven models can be implemented in healthcare systems to assist physicians in their daily practice.
1. Introduction
Artificial Intelligence (AI) has recently made eminent progress in many areas, including medicine and biomedical research. AI, a branch of computer science, encompasses mathematical methods that enable the decision making or action, the rational and autonomous reasoning, and the effective adaptation to complex and unseen situations [1] . AI systems regroup several different algorithms originated from the subfield of Machine Learning (ML) to advance the automation of human experts’ tasks leading to real and tangible results in healthcare. Given the digital acquisition of high-dimensional annotated medical data, the improvements in ML methods, the open ML data science and the evolving computational power and storage services, we could anticipate the tremendous progress of AI in the medical practice landscape [2] , [3] .
Recently, the medical applications of AI have expanded to clinical practice, translational medicine and the biomedical research of various diseases, such as cancer [1] , [4] . Current AI systems, based solely on ML methodologies, have been applied to different aspects of clinical practice including: (i) the image-based computer-aided detection and diagnosis within many medical specialties (i.e. pathology, radiology, ophthalmology and dermatology), (ii) the interpretation of genomic data for identifying genetic variants based on high-throughput sequencing technologies, (iii) the prediction of patients prognosis and monitoring, (iv) the discovery of novel biomarkers through the integration of omics and phenotype data, (v) the detection of health status in terms of biological signals collected from wearable devices, and finally (vi) the development and application of autonomous robots in medical interventions [1] .
Using AI/ML technologies in precision oncology and integrating them into clinical practice, however, raises technological challenges in model development [5] , [6] . Data curation and sanitization reduce the bias in collection and management preventing subsequently errors during the training and testing phases. These challenges along with social, economic and legal aspects should be considered before the deployment of AI/ML systems in medical practice to empower clinicians for better prevention, diagnosis, treatment and care in oncology. In addition, improving the performance, reproducibility and reliability of the AI/ML models would augment the work of clinicians by making better diagnostic decisions and tailoring the medical treatment to the patient's unique phenotype.
AI today is dominated by ML techniques capable of extracting patterns from large amounts of data as well as building reasoning systems for patient risk stratification and better decision making. For more accurate patient-level predictions and for modeling disease prognosis and risk prediction, data mining techniques and adaptive ML algorithms have consistently outperformed traditional statistical approaches [7] . ML-based techniques have the advantage of being able to automate the process of hypothesis formulation and evaluation, while assigning parameter weights to predictors based on correlates with the outcome prediction [6] , [8] . Despite this, the enormous promise of AI in cancer research should be carefully addressed alongside answers to the challenges of transparency and reproducibility [9] , [10] , [11] . To ensure the high potential of AI and ML in medicine and clinical trials, we need to adopt a framework for making the scientific research more transparent and reproducible.
In cancer research and oncology, the successful application of Deep Learning (DL) techniques has recently demonstrated fundamental improvements in image-based disease diagnosis and detection [12] , [13] . Generally, DL architectures correspond to artificial neural networks of multiple non-linear layers. Over the last decade, a variety of DL designs have been developed based on the input data types and the study aim (s). Concurrently, the evaluation of the model's performance has shown that DL application on cancer prognosis outperforms other conventional ML techniques [14] . DL frameworks have been also applied towards cancer diagnosis, classification and treatment by exploiting genomic profiles and phenotype data [1] , [7] , [15] .
In this review article, we focus on the ML aspect of AI-based applications in cancer research and medical oncology and present relevant studies that have been published the last five years (2016–2020) concerning the development of robust ML models towards patient diagnosis, classification and prognosis. The selection of the material was based on three clinical scenarios considering both the ML-based techniques and the heterogeneous data sources that were employed. We provide the search criteria of the literature review to obtain the most relevant studies, summarize the successful clinical scenarios towards applying robust and validated ML methods, discuss the state-of-the-art DL and Reinforcement Learning (RL) applications, present the impact of ML models in terms of robustness and explainability, identify the achievements and new challenges of ML-based systems in healthcare and discuss future research investigations along with the unsolved problems of reproducibility and transparency with possible solutions in the field. Systems biology and network-centered methods for computationally analyzing various sources of omics data and better comprehending the complex structures of biological processes and cellular components within cancer cells are also explained.
2. Literature review
The PubMed biomedical repository [16] and the dblp computer science bibliography [17] were selected to perform a literature overview on ML-based studies in cancer towards disease diagnosis, disease outcome prediction and patients’ classification. We searched and selected original research journal papers excluding reviews and technical reports between 2016 (January) and 2020 (December). In the PubMed’s advanced search option, we added the query terms “Cancer AND machine learning”, “Cancer AND artificial intelligence” and “Cancer AND deep learning”, separately, in the title field and not in the abstract to obtain the relevant studies. The same strategy and keywords were followed and applied to the dblp query search. According to our search results a total of 921 and 165 studies were found in PubMed and dblp databases, respectively, for the three queries. Duplicate studies and review or technical reports within the search results were excluded. A total of 734 research studies were further compared to provide a comprehensive overview of the application of ML and DL techniques in oncology research. We systematically reviewed the methods and outcomes of these research works and compared them until we identified the main clinical scenarios where ML methods are widely applied to enhance the automated decision support systems, the selection of appropriate treatments and the explanation of clinical reasoning.
The Tables showing the total number of studies identified in the preceding year for each search query in PubMed [16] and dblp [17] are given as supplementary material (Tables I-III). In the current work, we selected the most representative research from each clinical setting and provided a quick review of their key findings. To summarize the most current computational methods and clinical investigations in connection to early disease diagnosis, prognosis, and clinical outcome prediction for patient monitoring, we examined primarily preliminary studies published in the last year in both archives.
In Fig. 1 , we present the results of our literature overview on cancer diagnosis, prognosis and patients’ risk stratification the last five years on both databases. In Fig. 1 (upper part), a group-based barplot illustrates the number of articles that were identified when considering each search query in the databases. Fig. 1 (bottom part) illustrates the timeline results for each database. The total number of articles as regards to the total sum of the articles within the three queries is depicted per year.

The results of our literature overview on cancer diagnosis, patients’ classification and prognosis. The upper part of the figure presents the literature search results per category when considering each database. The bottom part of the figure depicts the timeline (last five years) results considering the total number of articles for the three search queries.
In the sections that follow we present briefly the popular and rising techniques of DL and RL along with their successful application and impact in oncology research and clinical practice. The clinical scenarios we have identified according to our literature overview are clearly presented along with the relative publications in the next sections. These clinical scenarios are among the most successful domains of biomedical ML-based applications in medical oncology. We provide details on the facets of robustness and explainability when ML-based models are employed in the healthcare systems. A summary and outlook presenting the recent advances and new challenges for the application of ML models for automated decision making in the clinical practice is also given in the last section.
2.1. Deep learning in oncology research
The potential of AI/ML techniques in biomedicine and precision oncology has become apparent with advances in new ML methods for computer-aided diagnosis [7] . These new technologies have been also integrated into the clinical practice for improving patient outcomes and accelerating clinical decision making [14] . DL approaches, a branch of ML, have recently showed great help to physicians in medical oncology with the development of medical-imaging diagnostic systems trying to improve disease diagnosis and the early detection of tumors [18] , [19] . With the availability of huge amounts of data and the parallel and distributed ML frameworks for their analysis, DL architectures have emerged and are categorized into three groups: (i) the deep neural networks (DNNs) [20] , [21] , [22] , (ii) the convolutional neural networks (CNNs) [23] , [24] and (iii) the recurrent neural networks (RNNs) [25] , [26] DL architectures are essentially artificial neural networks with numerous non-linear layers. The key distinguishing aspect of DL is that the feature layers are learnt from data using a general-purpose learning method rather than being created by the user.
ML can be roughly divided into three paradigms: (i) the supervised task that includes a label/class, (ii) the unsupervised task where no label is provided and (iii) the last category of RL techniques where an agent is trained to perform actions sequentially. Supervised techniques are mainly applied in today’s use of ML in automated patient-centered decision making with the decision trees (DTs), support vector machines (SVMs) or linear regression being the most common algorithms [27] . Based on the traditional ML techniques the main descriptors or variables are used to train a model and extract patterns and reasonable representations of feature vectors relevant to the problem under study. Despite this, the ability of conventional machine-learning approaches to analyze natural data in its raw form is limited. On the other hand, nowadays, DL (i.e. the implementation of multi-layered neural networks) has gained a lot of attention for their potential to include multiple levels of representation of features as part of the learning process, increasing thereby the model’s performance, computational feasibility and scalability [28] , [29] . DL approaches can be adapted to new representations of data allowing the different layers of features to be learned from more informative data using a complex learning procedure. DL outperforms in tasks related to perception problems (such as image analysis and sound recognition), while typical ML methods suffer from managing high dimensional datasets.
In cancer research and medical oncology, several DL architectures have been developed and applied for the classification and/or detection of cancer types [30] . The evaluation of DL models’ performances have shown that this type of techniques outperform other conventional ML approaches. DL frameworks have been also developed and further utilized for cancer diagnosis and classification based on gene expression profiles [31] , [32] , [33] . Concerning cancer prognosis and treatment, DL methods have been proposed to tackle the problem of predicting the drug response in certain cancer types [12] .
2.2. Reinforcement learning in oncology research
RL, a distinctive class of ML, has also found applications in cancer research and medical oncology towards finding the optimal treatment policies and computer-aided disease diagnosis [34] , [35] . In an RL model, an agent (i.e. the physician) learns from the interaction with his/her environment to achieve a goal based on the outcome that he/she wants to optimize (reward function). The learning process of an agent in a typical RL cycle is a continuous procedure. The interaction with the environment occurs at discrete time points. Once an environment’s state is received the agent selects a certain action to interact with it. The environment responds then to the action and the reward that the agent will or will not receive is finally determined [36] .
The incorporation of DL and RL systems into clinical practice with reference to available structured and unstructured biomedical and clinical cancer data will improve our understanding of cancer complexity and the role of risk factors and determinants in the development of effective treatment protocols.
2.3. Systems biology in oncology research
Systems biology concerns the integration of different components (i.e. genes, proteins and other cellular components) and how they interact in a dynamic environment. To facilitate our understanding on how cellular components function we need to elucidate in an integrated way how the system is organized with reference to dynamic networks of genes or proteins alongside their interactions with each other [37] . The development of AI models that predict the characteristics of large and interconnected networks found in living organisms would permit the thorough investigation of how signaling molecules produce functional cellular responses. In systems biology, mathematical descriptions of the processes during cancer progression and knowledge from network analysis and ML theories are used to identify the components and their interactions in a network-centered model and integrate them into an interconnected biological pathway. To this end, molecular or cellular associations and causal dependencies can be identified [38] , [39] , [40] .
The last decade, different omics platforms have provided large cancer datasets concerning the biological and cellular processes that can be studied at both the gene and protein levels. Applying AI/ML tools on omics data, based on systems biology and network-based theory, we may be able to expose the intricate structure of biological processes, improving our understanding of cancer onset and progression. Network theory and analysis in oncology research could permit to decipher the organization of biological signals within the cells in terms of nodes (e.g. genes or proteins) and edges which represent the relationships among them allowing to quantify the strength, type and direction of these interactions based primarily on omics data. High-throughput technologies, such as DNA microarrays, facilitate the simultaneous assessment of many gene expression levels as they vary over time. The huge amount of available experimental data may be used to obtain a better understanding of how genes interact with one another, forming a network and allowing for integrative analysis of biological systems [37] , [38] .
In the following sections, we clearly provide the clinical scenarios we found based on our literature review, along with the relevant papers published in the field of cancer research and clinical oncology.
2.4. Cancer detection and diagnosis
Arguably, automated cancer detection and diagnosis is one of the most important and successful domains of biomedical ML applications. According to our search results in PubMed and dblp, the last five years 192 research studies proposed ML-based pipelines based on conventional or state-of-the-art techniques to perform diagnostic tasks in common cancer types such as breast, lung, colon and pancreatic cancers, among others. Most of the studies used imaging data acquired from computed tomography (CT), magnetic resonance imaging (MRI), X-ray radiography and positron-emission tomography (PET) to develop automated diagnostic models based mainly on DL architectures.
In this review article, we present the most recent and indicative studies of the last year using either imaging or clinical, genomic and other relevant medical data to develop ML-based models for disease diagnosis and detection. A large proportion of this comprehensive list corresponds to studies that handle the specific clinical scenario by utilizing solely imaging data as input to DL models (Table I in the supplementary material ).
To this direction, automatic disease diagnosis was studied in terms of CNN models to early detect breast cancer by analyzing histopathological images [41] , [42] , [43] , [44] , [45] , [46] , [47] , [48] , [49] . More specifically, Zheng et al. [42] examined and proposed a CNN-based transfer learning method to early detect breast cancer by efficiently segment the ROIs. In comparison to other machine learning traditional approaches, promising results were obtained with high levels of accuracy (i.e. 97.2%) and a fair balance between sensitivity and specificity metrics (i.e. 98.3%, and 96.5%, respectively). Similar approaches were proposed by Benhammou et al. [43] , Sha et al. [44] , Kumar et al. [45] , Krithiga et al. [46] , Hameed et al. [47] , and Li et al. [48] towards assessing the diagnostic capability of deep CNN architectures by analyzing imaging slides. Based on the models' preprocessing, training, and evaluation procedures, favorable results were suggested with an average percentage of accuracy equal to 90.0%, demonstrating the authors' contribution in assisting clinicians to their diagnostic processes. DL frameworks were also designed and developed in [50] , [51] , [52] , [53] , [54] based on the CNN architecture for the analysis of CT and dermoscopy images in liver and skin cancer, respectively. In the study of Das et al. [53] the Gaussian mixture model (GMM) algorithm was primarily used to effectively segment the lesions and deep neural networks were then employed for the automated diagnostic task. Furthermore, in [54] feature selection and optimization was performed to identify the most important determinants of skin cancer detection while deep CNN was employed for melanoma detection. Promising results were obtained by the studies with high performance in terms of classification accuracy (i.e. ∼ 90.0%).
Conventional ML algorithms, such as DTs, Random Forests (RFs), Naïve Bayes (NB), k Nearest Neighbor (kNN), Artificial Neural Networks (ANNs), Gradient Boosting Machines (GBMs) and SVMs were also applied the last year in medical oncology for the automated detection and diagnosis of cancer. Indicative works include the studies of [55] , [56] , [57] , [58] , [59] , [60] , [61] , [62] where positive results were obtained by employing traditional ML techniques for the analysis of clinical, laboratory, genomic and epidemiological data to effectively diagnose prostate, lung, colorectal, breast and gastric cancers. In a separate work [63] , supervised and unsupervised techniques were applied to transcriptomic data for the identification of potential candidate biomarkers (i.e. genes) with reference to pancreatic cancer onset. Preprocessing steps based on certain bioinformatics workflows were applied to detect the novel gene set that contributes to the extension of prostate cancer to adjacent lymph nodes with Area Under the ROC Curve (AUC) higher than 0.90.
The total number of published studies identified in the previous year based on our literature search results for cancer prognosis and survival prediction is shown in Table II in the supplementary material .
2.5. Cancer patient classification
In medical oncology and cancer research the classification task of disease prediction has been studied thoroughly based on well-established ML algorithms for handling binary or multi-class learning problems. Patient classification into predefined groups would enable the development of ML-based predictive models able to assess risk stratification with generalizable performance. In this regard, numerous research papers were released last year that predicted the identification of key variables for cancer classification using traditional algorithms and DL methods. Most of the studies utilized DL architectures for the analysis of imaging and genomic data with respect to risk prediction and stratification. Indicatively, in [64] , [65] , [66] , [67] , [68] , [69] DL models were trained to classify and detect disease subtypes based on images and genetic data. These data-driven approaches demonstrated the superiority of ML-based frameworks towards exploiting heterogeneous datasets with respect to improved diagnosis and treatment.
Recently, a very interesting study was proposed by Li et al. [70] with regards to the assessment of pan-cancer Ras pathway activation and the identification of hidden key players during disease progression. RNA sequencing, copy number and mutation data were integrated in the DL model to provide insights into the pathway activity. The proposed model achieved superior performance in comparison to relevant studies concerning the classification of abnormal activity of the Ras pathway in tumor samples (i.e. AUC > 0.90) In an alternative study, a colorectal cancer (CRC) cohort [71] was analyzed based on whole-genome sequencing experiments of DNA samples to obtain an ML model with accurate generalization performance towards the early detection of the disease. A comprehensive ML-based pipeline was proposed to investigate the genomic profiles and cancer status and further identify the highly ranked covariates that discriminate control cases and early-stage CRC patients. According to the performance results a mean AUC of 0.92 with a mean sensitivity of 85.0% at 85.0% specificity were achieved.
Furthermore, well-known adaptive ML algorithms have been used widely in the literature for cancer classification by integrating different types of data [72] , [73] , [74] , [75] , [76] . Song et al. [77] proposed a predictive model for long-term prognosis of bladder cancer based on the learning ability of ML algorithms. The validated classification model was developed by utilizing clinical and molecular features able to distinguish cancerous from non-cancerous samples. Positive results were obtained in terms of classification performance with AUC higher than 0.70. Recently, similar works were published [60] , [78] , [79] , [80] , [81] aiming at applying data-driven methodologies to classify cancer data for prediction purposes. These studies correspond to ML-based models that improve the decision making process of physicians during patient monitoring and follow-up. Due to the availability of large amounts of heterogeneous data types in cancer research these studies utilized cancer data coming from patient registries, electronic health records, demographics, sequencing and imaging technologies.
Two distinct research studies [82] , [83] were published currently which use CT data integrated with radiomics features to classify cancer cases for improved prediction of lung cancer and in pulmonary lesions, respectively. The combination of radiomic features with clinical information in terms of ML algorithms empowered the extraction of potential patient characteristics that need to be considered thoroughly for disease screening. The performance metrics of the proposed ML-based methods were high with classification accuracy and AUC higher than 77.0% and 0.80, respectively.
The total number of studies identified in the previous year based on our indicative literature search results for cancer prognosis and survival prediction is shown in Table III in the supplementary material .
2.6. Cancer prognosis and survival prediction
This is another important aspect of cancer research where AI is expected to provide significant insight in the management of patients diagnosed with cancer. Specifically, in this category we have gathered studies aiming to assess the prognosis of patients, i.e. predict approximate survival based on a set of features (clinical, imaging, genomic), evaluate response to treatment and consequently patient prognosis. Due to the volume of data and its complexity, such analyses would be inevitable without the employment of ML algorithms and especially DL techniques. Specifically, during the last year only, approximately 200 studies were published aiming to assess cancer prognosis. Among them, the considerable majority utilized DL techniques, whereas only a small percentage used traditional ML algorithms.
Same as in the previous scenarios as well, and in accordance with the cancer research overall, certain organ cancers are predominantly studied, such as breast, lung, prostate and colorectal. The types of data used vary across the studies, however, we observe propensity towards specific sources of data for each cancer type. Specifically, pathology data are used in prostate cancer, breast and colorectal cancer research utilize genomic data, and lung cancer is largely dependent on imaging data, especially CT scans. Despite those slight propensities per cancer type, we observe that all ML techniques, and especially DL techniques, are primarily used for the analysis of imaging data, indifferent to the type of imaging modality employed.
An interesting approach was recently proposed by [84] where an automated deep learning system was trained to grade prostate biopsies following the Gleason grading system. Similar approaches have been presented in the literature for assessing prostate cancer prognosis [85] , [86] . In the same manner, a DL approach is proposed in [87] to discern between benign and malignant lesions of the skin, resulting in an overall AUC = 0.91. Another commonly used purpose of ML for cancer prognosis, is the assessment of approximate survival of the patient based on a set of features, from the baseline; encompassing information from subsequent follow-up visits achieves higher accuracy. Such studies have been presented in the literature for several types of cancer, e.g. lung cancer [88] , breast cancer [89] , bladder cancer [90] , etc. For a similar end purpose, ML algorithms have also been used for predicting response to treatment and consequently assessing the patient’s overall prognosis and survival [91] .
The total number of relevant studies identified in the preceding year based on our literature search results for cancer prognosis and survival prediction is shown in Tables I-III in the supplementary material .
2.7. Robustness and explainability of AI/ML models
The recent advances in AI/ML raised the issue of vulnerabilities that affect the predictive models and strongly impact their robustness. To this direction, a set of principles for trustworthy and secure use of ML techniques in the digital society have been drawn to augment innovation while protecting fundamental human rights [92] . Although ML techniques could extract complex patterns and correlations from large datasets, there is a severe lack of understanding considering the causal relationships and the explicit rules [93] .
To ensure the right deployment of ML models in the clinical practice in accordance with a sound regulatory framework, three main topics need to be highlighted and addressed. Firstly, the transparency of the models, which relates to the technical requirements and the data used, should be achieved. To obtain a complete view of a ML model the levels of implementation (i.e. technical principles), specifications (i.e. details about the training and testing phase) and interpretability (understanding model’s reasoning) must be fulfilled. The second topic concerns the reliability of the models along with the technical solutions that need to be clarified and adopted to prevent failures of autonomous systems in specific conditions. To assess the reliability of a model its performance and vulnerabilities need to be evaluated. Poor performance and existence of malfunctions indicate that a learned ML model is not reliable. Hence, the approaches of: (i) data sanitization, (ii) robust learning and (iii) extensive testing have been proposed to increase the reliability of ML models. The protection of sensitive data in ML systems is the third point that needs to be encountered for ensuring a good regulatory framework towards building and making use of automated systems. The implementation of data protection principles will guarantee the compliance to the privacy and data protection laws. Nevertheless [94] , the use of anonymization and pseudonymization techniques on sensitive data in accordance with the General Data Protection Regulation (GDPR) in Europe [95] and the guidelines on how the information may be used or shared in accordance with the Health Insurance Portability and Accountability Act (HIPPA) in the United States [96] may increase model’s complexity and impact its explainability.
Understanding the mechanisms and reasoning of a ML system in the digital society could guarantee its reliability. Introducing standardized approaches to assess the robustness of predictive models with respect to the data used for training, promoting model’s transparency through explainability-by-design principle for ML-based systems and designing methodologies to address vulnerabilities ensuring thereby the reliability will promote an effective and secure use of AI/ML systems. Furthermore, the successful establishment of good practices towards the right development and deployment of automated ML-based systems will ensure a regulatory framework for strengthening the trust in AI/ML systems.
Explainable AI (XAI) provides a framework to facilitate the understanding why an AI system or ML model have produced a given result. Interpreting the output of a model and giving the explanations at the local and global levels would benefit the improvement of clinical decision support systems. Model-specific and model-agnostic analysis could be implemented for black-box models’ explanations (such as SVM models) increasing thereby their trustworthiness and transparency in clinical trials [97] . Model-specific explanations are common but not well-adapted for two models with different structures. Once a new architecture for a predictive model is obtained a new method for model exploration and diagnostics should be searched. On the contrary, model-agnostic techniques could enhance models’ exploratory analysis with instance-level exploration methods for better understanding on how a model yields a prediction for a particular single observation. Apart from instance-level explanations, dataset-level-explainers for ML-based predictive models help to understand how the model’s predictions perform for the entire dataset and not for a certain observation [97] . Concerning the explanations of network-based models and tree-based classifiers (e.g. DTs and RFs), XAI techniques related to local and global explanations could benefit more the output interpretation concerning their less complex structure and hyper-parameters tuning. Although, DL techniques have been proved very efficient and effective regarding their performance, explanations on how a DL model has produced a result should be based on more comprehensive techniques with reference to model-specific and model-agnostic analysis.
2.8. Summary and outlook
In the previous review article [98] , we provided a comprehensive overview of ML applications for cancer prognosis and prediction by explaining the main aspects of ML and their clinical implications. On this basis, we analyzed the most representative studies published between 2010 and 2015 which used traditional ML approaches to predict cancer susceptibility, recurrence, and survivability in cancer patients. In this work, we conduct a thorough and complementary literature search on the application of ML-based models in cancer research and oncology the last five years (2015–2020). According to our search results in both the PubMed [16] and dblp [17] databases, a comprehensive list of publications was obtained. In comparison to our previous review article, three different clinical scenarios were identified according to their clinical outcomes regarding disease diagnosis, patient classification and prognosis. To highlight the most recent achievements in the subject, we included the most representative studies from the previous year in each category. Furthermore, we investigated the contrasts between recent research works in terms of data used and cutting-edge ML approaches for addressing each clinical situation in the data-driven era of precision oncology.
AI and ML approaches may be utilized to explore many aspects of cancer biology and extract new insights given that any disruption to the genetic material causes the beginning and development of carcinogenesis. Apparently, a massive quantity of genetic information on neoplasia has now been gathered, and it is rapidly increasing. This knowledge has significantly aided two key goals in cancer research: (i) a better understanding of the processes and mechanisms of oncogenesis, and (ii) their direct use in clinical practice as markers of diagnosis, prognosis, prevention, and cancer treatment. Fig. 2 depicts the major subject themes in cancer biology that have been extensively explored in terms of ML applications during the last decade. We classified the main topics according to the well-established research domains in oncology research and provide indicative paradigms where ML-based methods can be employed.

The most widely researched study topics in cancer biology, where ML-based techniques are frequently used. The six major features are illustrated, as well as the major biological issues they may address. CNVs: Copy Number Variations, DNA: Deoxyribonucleic acid, RNA: Ribonucleic acid, miRNAs: microRNAs
The last decade, AI and its technologies has made tremendous progress helping clinicians to automate tasks, detect the disease earlier while obtaining more real and tangible results for tailoring treatments. In comparison to the traditional statistical approaches, ML-based techniques have the inherent ability to identify patterns from high-dimensional datasets while automating the decision-making process by developing reasoning systems for disease early diagnosis, prognosis and risk stratification.
In the light of the recent advances in DL and RL methods for building ML-oriented systems we herein discuss their main characteristics alongside their major applications in the field of cancer research. Regarding the robustness and explainability of AI/ML systems we provide a brief overview of the standard points that need to be addressed when building ML models towards establishing a trustworthy regulatory framework while ensuring reliability, data protection and transparency as well as understanding of models.
ML methodologies have raised concerns in automated decision making tools and personal data regarding the lack of reasoning and explicit rules in black-box models. Hence, technical solutions need to be adopted for the design of standardized principles to increase the robustness and explainability of AI/ML systems as well as face the challenges of transparency and reproducibility of AI-based solutions. Transparency and reproducibility in AI are paramount for prospectively validate and implement in clinical practice such technologies and models [9] , [10] . Several frameworks and reproducible research practices have been implemented to ensure that the methods and code underpinning a research publication are adequately documented. Transparency is handled in terms of common code, software dependencies, and parameters required to train a model, allowing thereby the research study to be reproducible [99] , [100] . Practical and pragmatic recommendations for the effective documentation of research experiments and results have been proposed in the scientific community towards reproducible research and open science [100] . The degrees of reproducibility that are introduced concern the: (i) experiment reproducible, (ii) data reproducible and (iii) method reproducible. Different set of factors need to be documented within a publication to validate and reproduce the research results. Encouraging the research community to follow the best practices and recommendations for (i) data in publications, (ii) source code implementing AI/ML, (iii) AI methods and (iv) experiments described in scientific publications would be the steppingstone to accelerate transparency and reproducibility in the era of AI research. Several research groups cited replicable and clinically validated results in accordance with the oncology context, as well as transparency and validity in AI/ML-based solutions, concerning the clinical scenarios we addressed in this study and the indicative publications of 2020 described in each case [57] , [67] , [84] .
Furthermore, transparent and reliable predictive models can protect the sensitive data according to anonymization and pseudonymization approaches that have been assessed by the GDPR [94] , [95] . Nowadays, ML-based systems are not yet considered reliable enough to avoid any malfunction without the human supervision. Identifying the vulnerabilities of ML models would foster the predictions of the given input and output in the learning process of a predictive model enhancing its robustness. According to the guidelines for the ethical development of ML-based systems [101] , an ethics by design approach has been proposed for trustworthy AI/ML for GDPR-compliant, ethical and robust systems. Certain ethics and trustworthy aspects are outlined along with possible tools to self-assess an automated decision support system based on cutting-edge ML methodologies.
In addition to the standard technical solutions regarding the trustworthiness of autonomous ML-based systems in clinical practice we should also take into consideration the FAIR (Findable, Accessible, Interoperable and Reusable) data principles [102] . Thinking of the complex nature of cancer and the multistep process of tumorigenesis, one can easily presume that not enough data can be obtained from single centers regarding cancer research. Tailoring treatments to patients according to their status at both the phenotype and genotype levels would accelerate the automated decision process in disease management in the era of precision oncology. Moreover, the rise of omics data and their integration in precision oncology will promote a global and integrative analytical approach. Therefore, the adherence to the FAIR (Findable, Accessible, Interoperable and Reusable) principles when developing computational models leverages the adoption of data quality guidelines, data integration procedures and GDPR-compliance data sharing and access.
Dealing with multiple data modalities, i.e. multimodal frameworks, when building a ML-based framework for cancer prediction and classification, poses a new challenge in the field of cancer research. The development of integrative predictive models by combining the output from different algorithms is an innovation but also a challenge for the interpretation and reliability of the models implications in clinical practice.
To achieve our mission towards precision oncology and better understand the complex mechanisms of cancer, intervention actions should be designed by means of evidence-based decision support tools to prevent what is preventable, optimise diagnostics and treatment and support the quality of life of patients and caregivers. Furthermore, considering the COVID-19 pandemic in the last two years and the situation in the public healthcare systems, we can admit that cancer patients faced a severe and anxious period of follow-up visits trying to avoid a possible COVID-19 diagnosis which resulted in reduced hospitalizations and procedures [103] , [104] . As a result, we can foresee the influence of the COVID-19 pandemic on cancer early detection, on top of worsening prognosis and patient screening.
CRediT authorship contribution statement
Konstantina Kourou: Conceptualization, Writing – original draft, Visualization, Writing - review & editing. Konstantinos P. Exarchos: Conceptualization, Writing - review & editing. Costas Papaloukas: Conceptualization, Writing - review & editing. Prodromos Sakaloglou: Visualization. Themis Exarchos: Conceptualization. Dimitrios I. Fotiadis: Conceptualization, Supervision.
Declaration of Competing Interest
The authors declare that they have no known competing financial interests or personal relationships that could have appeared to influence the work reported in this paper.
Acknowledgement
This work has received funding from the European Union's Horizon 2020 research and innovation programme under grant agreement No 777167.
Appendix A Supplementary data to this article can be found online at https://doi.org/10.1016/j.csbj.2021.10.006 .
Appendix A. Supplementary data
The following are the Supplementary data to this article:

Researchers develop a new way to safely boost immune cells to fight cancer
Cancer is the monster of our society. Last year alone, more than 600,000 people in the United States died from cancer, according to the American Cancer Society. The relentless pursuit of understanding this complex disease has shaped medical progress on developing treatment procedures that are less invasive while still highly effective.
Immunotherapy is on the rise as a possible solution. Immunotherapy involves harnessing the power of the body's immune system to fight against cancer cells. Researchers in the College of Engineering have found a way to revamp a treatment procedure into a groundbreaking practice.
Rong Tong, associate professor in chemical engineering, has teamed up with Wenjun "Rebecca" Cai, associate professor in materials science and engineering, to explore a cancer immunotherapy treatment that has long been of interest to researchers. In their newly published article in the journal Science Advances, Tong and Cai detailed their approach, which involves activating the immune cells in the body and reprogramming them to attack and destroy the cancer cells. This therapeutic method is frequently implemented with the protein cytokine. Cytokines are small protein molecules that act as intercellular biochemical messengers and are released by the body's immune cells to coordinate their response.
"Cytokines are potent and highly effective at stimulating the immune cells to eliminate cancer cells," Tong said. "The problem is they're so potent that if they roam freely throughout the body, they'll activate every immune cell they encounter, which can cause an overactive immune response and potentially fatal side effects."
Tong and Cai, in collaboration with chemical engineering and materials science and engineering graduate students, have developed an innovative approach to employ cytokine proteins as a potential immunotherapy treatment. Unlike previous methods, their technique ensures that the immune cell stimulating cytokines effectively localize within the tumors for weeks while preserving the cytokine's structure and reactivity levels.
Combining forces to take down cancer cells
Current cancer treatments, such as chemotherapy, cannot distinguish between healthy cells and cancer cells. When someone with cancer is treated with chemotherapy, the treatment attacks all of the cells in their body, which can lead to side effects such as hair loss and fatigue. Stimulating the body's immune system to attack tumors is a promising alternative to treat cancer. The delivery of cytokines can jump-start immune cells in the tumor, but overstimulating healthy cells can cause severe side effects.
"Scientists determined a while ago that cytokines can be used to activate and fight against tumors, but they didn't know how to localize them inside the tumor while not exposing toxicity to the rest of the body," said Tong. "Chemical engineers can look at this from an engineering approach and use their knowledge to help refine and elevate the effectiveness of the cytokines so they can work inside the body effectively."
The research team's goal is to find a balance between killing cancer cells in the body while sparing healthy cells.
To accomplish this goal, Tong and his students used their expertise to create specialized particles with distinctive sizes that help determine where the drug is going. These microparticles are designed to stay within the tumor environment after being injected into the body. Cai and her students worked on measuring these particles' surface properties.
"In the field of materials science and engineering, we study the surface chemistry and mechanical behavior of materials, such as the specialized particle created for this project," Cai said. "Surface engineering and characterization, along with particle size, play important roles in controlled drug delivery, ensuring prolonged drug presence and sustained therapeutic effectiveness."
To ensure successful drug delivery, Tong and his chemical engineering students designed a novel strategy that:
- Anchors cytokines to these new microparticles, limiting the harm of cytokines to healthy cells
- Allows the newly particle-anchored cytokines to jump-start immune systems and recruit immune cells to attack cancer cells
"Our strategy not only minimizes cytokine-induced harm to healthy cells, but also prolongs cytokine retention within the tumor," Tong said. "This helps facilitate the recruitment of immune cells for targeted tumor attack."
The next step in the process involves combining the new, localized cytokine therapy method with commercially available, Food and Drug Administration (FDA)-approved checkpoint blockade antibodies, which reactivate the tumor immune cells that have been silenced so they can fight back the cancer cells.
"When there is a tumor inside the body, the body's immune cells are being deactivated by the cancer cells," Tong explained. "The FDA-approved checkpoint blocking antibody helps "take off the brakes" that tumors put on immune cells, while the cytokine molecules "step on the gas" to jump-start the immune system and get an immune cell army to fight cancer cells. These two approaches work together to activate immune cells."
Combining the checkpoint antibodies with the particle-anchored cytokine proved to successfully eliminate many tumors in their study.
Engineering an impact on cancer treatment
Team members hope their impact on immunotherapy treatment is part of a greater movement toward cancer treatment approaches that are harmless to healthy cells. The new approach of attaching cytokines to particles also could be used in the future to deliver other types of immunostimulatory drugs, according to the team.
"Researchers are still looking for safer and more effective cancer treatments," said Tong. "This motivation is what drives us to develop new technologies in the field. The whole class of drugs that are employed to jump-start the immune system to fight cancer cells has largely not yet succeeded. Our goal is to create novel solutions that allow researchers to test these drugs with existing FDA-approved therapeutics, ensuring both safety and enhanced efficacy."
Cai said the nature of cancer treatment research requires expertise across engineering disciplines.
"I view this project as a perfect marriage between chemical engineering and materials science," Cai said. "The former focuses on the synthesis and drug delivery part, the latter on applying advanced materials characterization. This collaboration not only accelerates immunotherapy research, but also has the ability to transform cancer treatment."
- Immune System
- Brain Tumor
- Lung Cancer
- Prostate Cancer
- Colon Cancer
- Skin Cancer
- Immune system
- Chemotherapy
- Monoclonal antibody therapy
- Natural killer cell
- White blood cell
- Prostate cancer
- Endocrine system
Story Source:
Materials provided by Virginia Tech . Original written by Hailey Wade. Note: Content may be edited for style and length.
Journal Reference :
- Liqian Niu, Eungyo Jang, Ai Lin Chin, Ziyu Huo, Wenbo Wang, Wenjun Cai, Rong Tong. Noncovalently particle-anchored cytokines with prolonged tumor retention safely elicit potent antitumor immunity . Science Advances , 2024; 10 (16) DOI: 10.1126/sciadv.adk7695
Cite This Page :
Explore More
- Collisions of Neutron Stars and Black Holes
- Advance in Heart Regenerative Therapy
- Bioluminescence in Animals 540 Million Years Ago
- Profound Link Between Diet and Brain Health
- Loneliness Runs Deep Among Parents
- Food in Sight? The Liver Is Ready!
- Acid Reflux Drugs and Risk of Migraine
- Do Cells Have a Hidden Communication System?
- Mice Given Mouse-Rat Brains Can Smell Again
- How Do Birds Flock? New Aerodynamics
Trending Topics
Strange & offbeat.
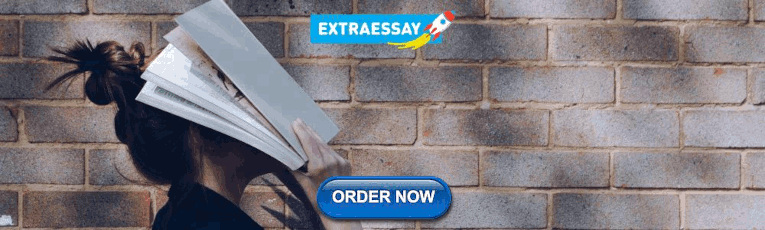
IMAGES
VIDEO
COMMENTS
The Biology of Cancer. Cancer is a disease that begins with genetic and epigenetic alterations occurring in specific cells, some of which can spread and migrate to other tissues. 4 Although the biological processes affected in carcinogenesis and the evolution of neoplasms are many and widely different, we will focus on 4 aspects that are particularly relevant in tumor biology: genomic and ...
Cancer biology is the cornerstone on which much of modern cancer research is based. Continuing to explore the intricacies of this multilayered foundational scientific area is essential.
Nature Cancer aims to publish the most significant advances across the full spectrum of cancer research in the life, physical, applied and social sciences, ...
Abstract. Cancer progression is an evolutionary process. During this process, evolving cancer cell populations encounter restrictive ecological niches within the body, such as the primary tumor, circulatory system, and diverse metastatic sites. Efforts to prevent or delay cancer evolution—and progression—require a deep understanding of the ...
The Cancer Systems Biology Consortium (CSBC), which includes cancer biologists, engineers, mathematicians, physicists, and oncologists, aims to tackle the most perplexing issues in cancer to increase our understanding of tumor biology, treatment options, and patient outcomes.The initiative takes an integrative approach to cancer research, with the goal of improving the lives of people with cancer.
Guida et al. assess seven measures of biological age in SJLIFE Cohort and reveal that survivors of cancer age faster than healthy controls and have increased risk of frailty and death. The aging ...
Cancer systems biology has undoubtedly emerged as an integrative tool to achieve such advances. This Special Issue on "recent developments in cancer systems biology" has compiled several novel approaches that use cutting-edge technologies to build a strong foundation of systems biology in cancer research.
Cancer Research publishes impactful original studies, reviews, and opinion pieces of high significance to the broad cancer research community. Cancer Research seeks manuscripts that offer conceptual or technological advances leading to basic and translational insights into cancer biology. Read More About the Journal.
AIMS AND SCOPE OF JOURNAL: The Annual Review of Cancer Biology reviews a range of subjects in cancer research that represent important and emerging areas in the field. With recent advances in our understanding of the basic mechanisms of cancer development and the translation of an increasing number of these findings into the clinic in the form of targeted treatments for the disease, the Annual ...
Cancer research has been largely focused on the cellular and molecular levels of investigation. Recent data show that not only the cell but also the extracellular matrix plays a major role in the progression of malignancy. ... Therefore, cancer biology involves a specific malignant microenvironment (cells, matrix, messaging) that is able to ...
Cancer is a genetic disease. Most common cancers are caused by acquired mutations in somatic cells. In contrast, specific germline mutations account for rare hereditary cancer syndromes. In general, cancer-associated genes can be divided into two groups: oncogenes and tumour suppressor genes (TSGs). Oncogenes undergo activation and are ...
Advances in Cancer Biology Research. Over the past year, research projects funded by the Division of Cancer Biology (DCB) have led to many new discoveries and advances in basic cancer research that continue to lay the groundwork for future clinical breakthroughs and cancer prevention strategies. Below are examples of important cancer biology ...
In spite of a remarkable progress in cancer genomics, biology, and proteomics during the last several decades, cancer treatment is still not satisfactory and overall survival rate of many of cancer patients stays low [125]. To the date, due to the difficulties in clinical trials, there is no FDA registered topical treatments of melanoma and ...
Cancer Biology & Therapy, Volume 25, Issue 1 (2024) ... Research Paper. Article. Red ginseng polysaccharide promotes ferroptosis in gastric cancer cells by inhibiting PI3K/Akt pathway through down-regulation of AQP3. Yan Wang, Wen-Xian Guan, Yuan Zhou, Xiao-Yu Zhang & Hai-Jian Zhao.
Focus Areas. The mission of the Department of Cancer Biology is to identify and understand the causes of cancer, to develop innovative approaches to reduce cancer incidence, to create and test novel and more effective therapies, and to translate these findings into clinical care for the benefit of patients. Research in our department is highly ...
The Nature Portfolio editors who handle cancer primary research, methods, protocols and reviews bring you the latest articles, covering all aspects from disease mechanisms to therapeutic ...
provides the fundamentals of cancer biology that will enable students of biology and medicine to enter the eld with con dence. It opens with a discussion of global cancer ... 100 research papers and seven books, including the 1st edition of Introduction to Cancer Biology (2012) and Understanding Cancer (2022) for Cambridge University Press.
Organoid biology will further develop with a goal of translating the research into personalized therapy. These research areas may result in the creation of new cancer treatments in the future. Keywords: exosomes, immunotherapy, microbiome, organoid. Cancer research has made remarkable progress and new discoveries are beginning to be made.
cancer gene discovery • tumorigenesis • cancer therapy and resistance • oncogenes • tumor suppressor genes • cancer models • growth control and cell proliferation • metastasis • cell proliferation • cell death • cell-cell and cell-matrix interactions • microenvironment •DNA repair and replication • transcription • chromosome stability • metabolism • immunology ...
Credit: National Cancer Institute. Research in cancer cell biology seeks to define the biological basis underlying the differences between normal cells and cancerous cells. This includes studies of the fundamental mechanisms that drive pre-cancer states, oncogenic transformation, and that support tumor growth and behavior.
Aneuploidy, defined as an abnormal number of chromosomes or chromosome-arms within a cell, is a characteristic trait of human cancer [].Aneuploidy is associated with patient prognosis and with response to anticancer therapies [2, 3], indicating that it can play a driving role in tumorigenesis.It is well established that the fitness advantage conferred by specific aneuploidies depends on the ...
Top 100 in Cancer. This collection highlights our most downloaded* cancer papers published in 2019. Featuring authors from around the world, these papers feature valuable research from an ...
Journal of Cancer Biology & Research maintains experienced world class practicing experts who lay the strong foundation of our journal. They actively participate and closely scrutinize the quality of our incoming articles and make decisions wisely after rigorous peer review processing. Every manuscript is individually assigned to a pool of ...
2. Literature review. The PubMed biomedical repository and the dblp computer science bibliography were selected to perform a literature overview on ML-based studies in cancer towards disease diagnosis, disease outcome prediction and patients' classification. We searched and selected original research journal papers excluding reviews and technical reports between 2016 (January) and 2020 ...
Tel: +91-7834871362, +91-7055064227. 1. Cancer: An Overview. Garima Mathur, Sumitra Nain and Pramod Kumar Sha rma. 12 1. School of Medical and Allied Scien ces, Galgotia university, Greater Noida ...
April 19, 2024. Source: Virginia Tech. Summary: Researchers explore a cancer immunotherapy treatment that involves activating the immune cells in the body and reprogramming them to attack and ...