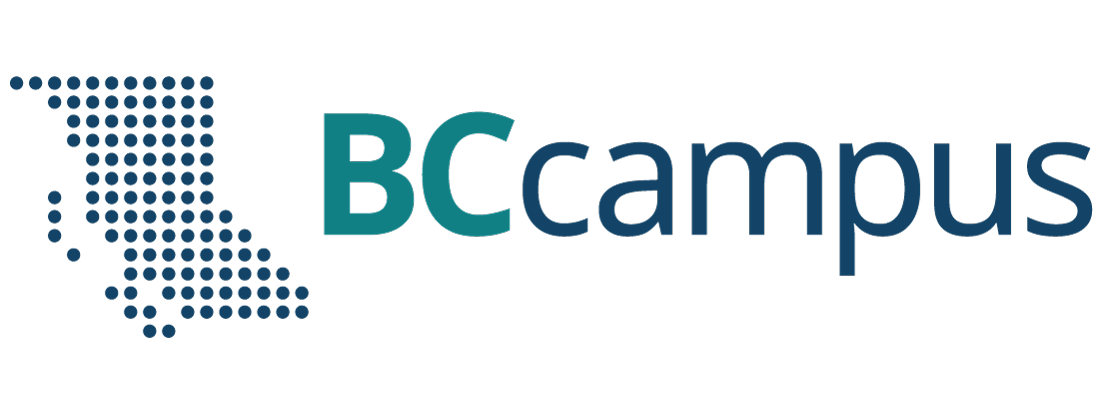
Want to create or adapt books like this? Learn more about how Pressbooks supports open publishing practices.
Chapter 13: Inferential Statistics
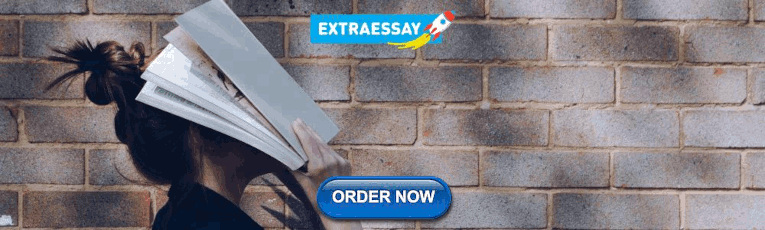
Understanding Null Hypothesis Testing
Learning Objectives
- Explain the purpose of null hypothesis testing, including the role of sampling error.
- Describe the basic logic of null hypothesis testing.
- Describe the role of relationship strength and sample size in determining statistical significance and make reasonable judgments about statistical significance based on these two factors.
The Purpose of Null Hypothesis Testing
As we have seen, psychological research typically involves measuring one or more variables for a sample and computing descriptive statistics for that sample. In general, however, the researcher’s goal is not to draw conclusions about that sample but to draw conclusions about the population that the sample was selected from. Thus researchers must use sample statistics to draw conclusions about the corresponding values in the population. These corresponding values in the population are called parameters . Imagine, for example, that a researcher measures the number of depressive symptoms exhibited by each of 50 clinically depressed adults and computes the mean number of symptoms. The researcher probably wants to use this sample statistic (the mean number of symptoms for the sample) to draw conclusions about the corresponding population parameter (the mean number of symptoms for clinically depressed adults).
Unfortunately, sample statistics are not perfect estimates of their corresponding population parameters. This is because there is a certain amount of random variability in any statistic from sample to sample. The mean number of depressive symptoms might be 8.73 in one sample of clinically depressed adults, 6.45 in a second sample, and 9.44 in a third—even though these samples are selected randomly from the same population. Similarly, the correlation (Pearson’s r ) between two variables might be +.24 in one sample, −.04 in a second sample, and +.15 in a third—again, even though these samples are selected randomly from the same population. This random variability in a statistic from sample to sample is called sampling error . (Note that the term error here refers to random variability and does not imply that anyone has made a mistake. No one “commits a sampling error.”)
One implication of this is that when there is a statistical relationship in a sample, it is not always clear that there is a statistical relationship in the population. A small difference between two group means in a sample might indicate that there is a small difference between the two group means in the population. But it could also be that there is no difference between the means in the population and that the difference in the sample is just a matter of sampling error. Similarly, a Pearson’s r value of −.29 in a sample might mean that there is a negative relationship in the population. But it could also be that there is no relationship in the population and that the relationship in the sample is just a matter of sampling error.
In fact, any statistical relationship in a sample can be interpreted in two ways:
- There is a relationship in the population, and the relationship in the sample reflects this.
- There is no relationship in the population, and the relationship in the sample reflects only sampling error.
The purpose of null hypothesis testing is simply to help researchers decide between these two interpretations.
The Logic of Null Hypothesis Testing
Null hypothesis testing is a formal approach to deciding between two interpretations of a statistical relationship in a sample. One interpretation is called the null hypothesis (often symbolized H 0 and read as “H-naught”). This is the idea that there is no relationship in the population and that the relationship in the sample reflects only sampling error. Informally, the null hypothesis is that the sample relationship “occurred by chance.” The other interpretation is called the alternative hypothesis (often symbolized as H 1 ). This is the idea that there is a relationship in the population and that the relationship in the sample reflects this relationship in the population.
Again, every statistical relationship in a sample can be interpreted in either of these two ways: It might have occurred by chance, or it might reflect a relationship in the population. So researchers need a way to decide between them. Although there are many specific null hypothesis testing techniques, they are all based on the same general logic. The steps are as follows:
- Assume for the moment that the null hypothesis is true. There is no relationship between the variables in the population.
- Determine how likely the sample relationship would be if the null hypothesis were true.
- If the sample relationship would be extremely unlikely, then reject the null hypothesis in favour of the alternative hypothesis. If it would not be extremely unlikely, then retain the null hypothesis .
Following this logic, we can begin to understand why Mehl and his colleagues concluded that there is no difference in talkativeness between women and men in the population. In essence, they asked the following question: “If there were no difference in the population, how likely is it that we would find a small difference of d = 0.06 in our sample?” Their answer to this question was that this sample relationship would be fairly likely if the null hypothesis were true. Therefore, they retained the null hypothesis—concluding that there is no evidence of a sex difference in the population. We can also see why Kanner and his colleagues concluded that there is a correlation between hassles and symptoms in the population. They asked, “If the null hypothesis were true, how likely is it that we would find a strong correlation of +.60 in our sample?” Their answer to this question was that this sample relationship would be fairly unlikely if the null hypothesis were true. Therefore, they rejected the null hypothesis in favour of the alternative hypothesis—concluding that there is a positive correlation between these variables in the population.
A crucial step in null hypothesis testing is finding the likelihood of the sample result if the null hypothesis were true. This probability is called the p value . A low p value means that the sample result would be unlikely if the null hypothesis were true and leads to the rejection of the null hypothesis. A high p value means that the sample result would be likely if the null hypothesis were true and leads to the retention of the null hypothesis. But how low must the p value be before the sample result is considered unlikely enough to reject the null hypothesis? In null hypothesis testing, this criterion is called α (alpha) and is almost always set to .05. If there is less than a 5% chance of a result as extreme as the sample result if the null hypothesis were true, then the null hypothesis is rejected. When this happens, the result is said to be statistically significant . If there is greater than a 5% chance of a result as extreme as the sample result when the null hypothesis is true, then the null hypothesis is retained. This does not necessarily mean that the researcher accepts the null hypothesis as true—only that there is not currently enough evidence to conclude that it is true. Researchers often use the expression “fail to reject the null hypothesis” rather than “retain the null hypothesis,” but they never use the expression “accept the null hypothesis.”
The Misunderstood p Value
The p value is one of the most misunderstood quantities in psychological research (Cohen, 1994) [1] . Even professional researchers misinterpret it, and it is not unusual for such misinterpretations to appear in statistics textbooks!
The most common misinterpretation is that the p value is the probability that the null hypothesis is true—that the sample result occurred by chance. For example, a misguided researcher might say that because the p value is .02, there is only a 2% chance that the result is due to chance and a 98% chance that it reflects a real relationship in the population. But this is incorrect . The p value is really the probability of a result at least as extreme as the sample result if the null hypothesis were true. So a p value of .02 means that if the null hypothesis were true, a sample result this extreme would occur only 2% of the time.
You can avoid this misunderstanding by remembering that the p value is not the probability that any particular hypothesis is true or false. Instead, it is the probability of obtaining the sample result if the null hypothesis were true.
Role of Sample Size and Relationship Strength
Recall that null hypothesis testing involves answering the question, “If the null hypothesis were true, what is the probability of a sample result as extreme as this one?” In other words, “What is the p value?” It can be helpful to see that the answer to this question depends on just two considerations: the strength of the relationship and the size of the sample. Specifically, the stronger the sample relationship and the larger the sample, the less likely the result would be if the null hypothesis were true. That is, the lower the p value. This should make sense. Imagine a study in which a sample of 500 women is compared with a sample of 500 men in terms of some psychological characteristic, and Cohen’s d is a strong 0.50. If there were really no sex difference in the population, then a result this strong based on such a large sample should seem highly unlikely. Now imagine a similar study in which a sample of three women is compared with a sample of three men, and Cohen’s d is a weak 0.10. If there were no sex difference in the population, then a relationship this weak based on such a small sample should seem likely. And this is precisely why the null hypothesis would be rejected in the first example and retained in the second.
Of course, sometimes the result can be weak and the sample large, or the result can be strong and the sample small. In these cases, the two considerations trade off against each other so that a weak result can be statistically significant if the sample is large enough and a strong relationship can be statistically significant even if the sample is small. Table 13.1 shows roughly how relationship strength and sample size combine to determine whether a sample result is statistically significant. The columns of the table represent the three levels of relationship strength: weak, medium, and strong. The rows represent four sample sizes that can be considered small, medium, large, and extra large in the context of psychological research. Thus each cell in the table represents a combination of relationship strength and sample size. If a cell contains the word Yes , then this combination would be statistically significant for both Cohen’s d and Pearson’s r . If it contains the word No , then it would not be statistically significant for either. There is one cell where the decision for d and r would be different and another where it might be different depending on some additional considerations, which are discussed in Section 13.2 “Some Basic Null Hypothesis Tests”
Although Table 13.1 provides only a rough guideline, it shows very clearly that weak relationships based on medium or small samples are never statistically significant and that strong relationships based on medium or larger samples are always statistically significant. If you keep this lesson in mind, you will often know whether a result is statistically significant based on the descriptive statistics alone. It is extremely useful to be able to develop this kind of intuitive judgment. One reason is that it allows you to develop expectations about how your formal null hypothesis tests are going to come out, which in turn allows you to detect problems in your analyses. For example, if your sample relationship is strong and your sample is medium, then you would expect to reject the null hypothesis. If for some reason your formal null hypothesis test indicates otherwise, then you need to double-check your computations and interpretations. A second reason is that the ability to make this kind of intuitive judgment is an indication that you understand the basic logic of this approach in addition to being able to do the computations.
Statistical Significance Versus Practical Significance
Table 13.1 illustrates another extremely important point. A statistically significant result is not necessarily a strong one. Even a very weak result can be statistically significant if it is based on a large enough sample. This is closely related to Janet Shibley Hyde’s argument about sex differences (Hyde, 2007) [2] . The differences between women and men in mathematical problem solving and leadership ability are statistically significant. But the word significant can cause people to interpret these differences as strong and important—perhaps even important enough to influence the college courses they take or even who they vote for. As we have seen, however, these statistically significant differences are actually quite weak—perhaps even “trivial.”
This is why it is important to distinguish between the statistical significance of a result and the practical significance of that result. Practical significance refers to the importance or usefulness of the result in some real-world context. Many sex differences are statistically significant—and may even be interesting for purely scientific reasons—but they are not practically significant. In clinical practice, this same concept is often referred to as “clinical significance.” For example, a study on a new treatment for social phobia might show that it produces a statistically significant positive effect. Yet this effect still might not be strong enough to justify the time, effort, and other costs of putting it into practice—especially if easier and cheaper treatments that work almost as well already exist. Although statistically significant, this result would be said to lack practical or clinical significance.
Key Takeaways
- Null hypothesis testing is a formal approach to deciding whether a statistical relationship in a sample reflects a real relationship in the population or is just due to chance.
- The logic of null hypothesis testing involves assuming that the null hypothesis is true, finding how likely the sample result would be if this assumption were correct, and then making a decision. If the sample result would be unlikely if the null hypothesis were true, then it is rejected in favour of the alternative hypothesis. If it would not be unlikely, then the null hypothesis is retained.
- The probability of obtaining the sample result if the null hypothesis were true (the p value) is based on two considerations: relationship strength and sample size. Reasonable judgments about whether a sample relationship is statistically significant can often be made by quickly considering these two factors.
- Statistical significance is not the same as relationship strength or importance. Even weak relationships can be statistically significant if the sample size is large enough. It is important to consider relationship strength and the practical significance of a result in addition to its statistical significance.
- Discussion: Imagine a study showing that people who eat more broccoli tend to be happier. Explain for someone who knows nothing about statistics why the researchers would conduct a null hypothesis test.
- The correlation between two variables is r = −.78 based on a sample size of 137.
- The mean score on a psychological characteristic for women is 25 ( SD = 5) and the mean score for men is 24 ( SD = 5). There were 12 women and 10 men in this study.
- In a memory experiment, the mean number of items recalled by the 40 participants in Condition A was 0.50 standard deviations greater than the mean number recalled by the 40 participants in Condition B.
- In another memory experiment, the mean scores for participants in Condition A and Condition B came out exactly the same!
- A student finds a correlation of r = .04 between the number of units the students in his research methods class are taking and the students’ level of stress.
Long Descriptions
“Null Hypothesis” long description: A comic depicting a man and a woman talking in the foreground. In the background is a child working at a desk. The man says to the woman, “I can’t believe schools are still teaching kids about the null hypothesis. I remember reading a big study that conclusively disproved it years ago.” [Return to “Null Hypothesis”]
“Conditional Risk” long description: A comic depicting two hikers beside a tree during a thunderstorm. A bolt of lightning goes “crack” in the dark sky as thunder booms. One of the hikers says, “Whoa! We should get inside!” The other hiker says, “It’s okay! Lightning only kills about 45 Americans a year, so the chances of dying are only one in 7,000,000. Let’s go on!” The comic’s caption says, “The annual death rate among people who know that statistic is one in six.” [Return to “Conditional Risk”]
Media Attributions
- Null Hypothesis by XKCD CC BY-NC (Attribution NonCommercial)
- Conditional Risk by XKCD CC BY-NC (Attribution NonCommercial)
- Cohen, J. (1994). The world is round: p < .05. American Psychologist, 49 , 997–1003. ↵
- Hyde, J. S. (2007). New directions in the study of gender similarities and differences. Current Directions in Psychological Science, 16 , 259–263. ↵
Values in a population that correspond to variables measured in a study.
The random variability in a statistic from sample to sample.
A formal approach to deciding between two interpretations of a statistical relationship in a sample.
The idea that there is no relationship in the population and that the relationship in the sample reflects only sampling error.
The idea that there is a relationship in the population and that the relationship in the sample reflects this relationship in the population.
When the relationship found in the sample would be extremely unlikely, the idea that the relationship occurred “by chance” is rejected.
When the relationship found in the sample is likely to have occurred by chance, the null hypothesis is not rejected.
The probability that, if the null hypothesis were true, the result found in the sample would occur.
How low the p value must be before the sample result is considered unlikely in null hypothesis testing.
When there is less than a 5% chance of a result as extreme as the sample result occurring and the null hypothesis is rejected.
Research Methods in Psychology - 2nd Canadian Edition Copyright © 2015 by Paul C. Price, Rajiv Jhangiani, & I-Chant A. Chiang is licensed under a Creative Commons Attribution-NonCommercial-ShareAlike 4.0 International License , except where otherwise noted.
Share This Book
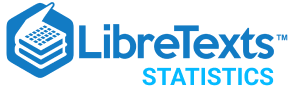
- school Campus Bookshelves
- menu_book Bookshelves
- perm_media Learning Objects
- login Login
- how_to_reg Request Instructor Account
- hub Instructor Commons
- Download Page (PDF)
- Download Full Book (PDF)
- Periodic Table
- Physics Constants
- Scientific Calculator
- Reference & Cite
- Tools expand_more
- Readability
selected template will load here
This action is not available.
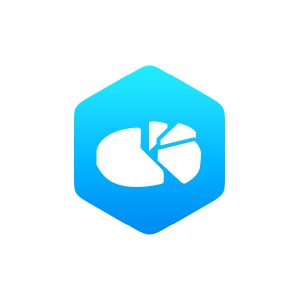
7.4: Null Hypothesis Significance Testing
- Last updated
- Save as PDF
- Page ID 22069
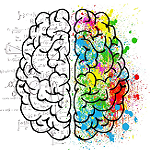
- Michelle Oja
- Taft College
Null Hypotheses and Research Hypotheses
So far, so good? We develop a directional research hypothesis that names our groups, the DV (the outcome that was measured), and indicates a direction (which group will be higher). And we have a null hypothesis that says that the groups will have similar means on the DV. It’s at this point that things get somewhat counterintuitive. Because the null hypothesis seems to correspond to the opposite of what I want to believe, and then we focus exclusively on that, almost to the neglect of the thing I’m actually interested in (the research hypothesis). In our growth mindset example, the null hypothesis is that the sample of junior high students with high beliefs in growth mindset will have similar average study times compared to the population of all junior high students. But for Blackwell, Trzseniewski, and Dweck (2007), and, really, any teacher ever, we actually want to believe that the understanding that your intelligence and abilities can always improve (high belief in growth mindset) will result in working harder and spending more time on homework. So the alternative to this null hypothesis is that those junior high students with higher growth mindset scores will spend more time on their math homework than those from the population of junior high students. The important thing to recognize is that the goal of a hypothesis test is not to show that the research hypothesis is (probably) true; the goal is to show that the null hypothesis is (probably) false. Most people find this pretty weird.
The best way to think about it, in my experience, is to imagine that a hypothesis test is a criminal trial… the trial of the null hypothesis . The null hypothesis is the defendant, the researcher is the prosecutor, and the statistical test itself is the judge. Just like a criminal trial, there is a presumption of innocence: the null hypothesis is deemed to be true unless you, the researcher, can prove beyond a reasonable doubt that it is false. You are free to design your experiment however you like, and your goal when doing so is to maximize the chance that the data will yield a conviction… for the crime of being false. The catch is that the statistical test sets the rules of the trial, and those rules are designed to protect the null hypothesis – specifically to ensure that if the null hypothesis is actually true, the chances of a false conviction are guaranteed to be low. This is pretty important: after all, the null hypothesis doesn’t get a lawyer. And given that the researcher is trying desperately to prove it to be false, someone has to protect it.
Okay, so the null hypothesis always states that there's no difference. In our examples so far, we've been saying that there's no difference between the sample mean and population mean. But we don’t really expect that, or why would we be comparing the means? The purpose of null hypothesis significance testing is to be able to reject the expectation that the means of the two groups are the same .
- Rejecting the null hypothesis means that \( \bar{X} \neq \mu \).
- Rejecting the null hypothesis doesn't automatically mean that the research hypothesis is supported.
- Retaining the null hypothesis means that \( \bar{X} = \mu \).
- This means that our research hypothesis cannot be true.
We only reject or retain the null hypothesis. If we reject the null hypothesis (which says that everything is similar), we are saying that some means are statistically different from some other means. We only support the research hypothesis if the means are in the direction that we said. For example, if we rejected the null hypothesis that junior highers with high growth mindset spend as much time on homework as all junior highers, we can't automatically say that junior high students with high growth mindset study more than the population of junior high students. Instead, we'd have to look at the actual means of each group, and then decide if the research hypothesis was supported or not.
I hope that it's obvious that you don't have to look at the group means if the null hypothesis is retained?
In sum, you reject or retain the null hypothesis, and your support or or don’t support the research hypothesis.
Why predict that two things are similar?
Because each sample’s mean will vary around the population mean (see the first few sections of this chapter to remind yourself of this), we can’t tell if our sample’s mean is within a “normal” variance. But we can gather data to show that this sample’s mean is different (enough) from the population’s mean. This is rejecting the null hypothesis .
We use statistics to determine the probability of the null hypothesis being true.
Exercise \(\PageIndex{1}\)
Does a true null hypothesis say the sample mean and the population mean are similar or different?
A null hypothesis always says that the means are similar (or that there is no relationship between the variables).
Why can’t we prove that the mean of our sample is different from the mean of the population? Remember the first few sections of this chapter, that showed how different samples from the same population have different means and standard deviations. Researchers are a conservative bunch; we don't want to stake our reputation on a sample mean that could be fluke, one of the extreme handfuls of green gumballs even when the mean difference between hands was zero.
But what we can show is that our sample is so extreme that it is statistically unlikely to be similar to the population.
Null hypothesis significant testing is like how courts decide if defendants are Guilty or Not Guilty, not their Guilt v. Innocent. Similarly, we decide if the sample is similar to the population or not.
This is a tough concept to grasp, so we'll keep working on it. And if you never get it, that's okay, too, as long as you remember the pattern of rejecting or retaining the null hypothesis, and supporting or not supporting the research hypothesis.
Hypothesis Testing (cont...)
Hypothesis testing, the null and alternative hypothesis.
In order to undertake hypothesis testing you need to express your research hypothesis as a null and alternative hypothesis. The null hypothesis and alternative hypothesis are statements regarding the differences or effects that occur in the population. You will use your sample to test which statement (i.e., the null hypothesis or alternative hypothesis) is most likely (although technically, you test the evidence against the null hypothesis). So, with respect to our teaching example, the null and alternative hypothesis will reflect statements about all statistics students on graduate management courses.
The null hypothesis is essentially the "devil's advocate" position. That is, it assumes that whatever you are trying to prove did not happen ( hint: it usually states that something equals zero). For example, the two different teaching methods did not result in different exam performances (i.e., zero difference). Another example might be that there is no relationship between anxiety and athletic performance (i.e., the slope is zero). The alternative hypothesis states the opposite and is usually the hypothesis you are trying to prove (e.g., the two different teaching methods did result in different exam performances). Initially, you can state these hypotheses in more general terms (e.g., using terms like "effect", "relationship", etc.), as shown below for the teaching methods example:
Depending on how you want to "summarize" the exam performances will determine how you might want to write a more specific null and alternative hypothesis. For example, you could compare the mean exam performance of each group (i.e., the "seminar" group and the "lectures-only" group). This is what we will demonstrate here, but other options include comparing the distributions , medians , amongst other things. As such, we can state:
Now that you have identified the null and alternative hypotheses, you need to find evidence and develop a strategy for declaring your "support" for either the null or alternative hypothesis. We can do this using some statistical theory and some arbitrary cut-off points. Both these issues are dealt with next.
Significance levels
The level of statistical significance is often expressed as the so-called p -value . Depending on the statistical test you have chosen, you will calculate a probability (i.e., the p -value) of observing your sample results (or more extreme) given that the null hypothesis is true . Another way of phrasing this is to consider the probability that a difference in a mean score (or other statistic) could have arisen based on the assumption that there really is no difference. Let us consider this statement with respect to our example where we are interested in the difference in mean exam performance between two different teaching methods. If there really is no difference between the two teaching methods in the population (i.e., given that the null hypothesis is true), how likely would it be to see a difference in the mean exam performance between the two teaching methods as large as (or larger than) that which has been observed in your sample?
So, you might get a p -value such as 0.03 (i.e., p = .03). This means that there is a 3% chance of finding a difference as large as (or larger than) the one in your study given that the null hypothesis is true. However, you want to know whether this is "statistically significant". Typically, if there was a 5% or less chance (5 times in 100 or less) that the difference in the mean exam performance between the two teaching methods (or whatever statistic you are using) is as different as observed given the null hypothesis is true, you would reject the null hypothesis and accept the alternative hypothesis. Alternately, if the chance was greater than 5% (5 times in 100 or more), you would fail to reject the null hypothesis and would not accept the alternative hypothesis. As such, in this example where p = .03, we would reject the null hypothesis and accept the alternative hypothesis. We reject it because at a significance level of 0.03 (i.e., less than a 5% chance), the result we obtained could happen too frequently for us to be confident that it was the two teaching methods that had an effect on exam performance.
Whilst there is relatively little justification why a significance level of 0.05 is used rather than 0.01 or 0.10, for example, it is widely used in academic research. However, if you want to be particularly confident in your results, you can set a more stringent level of 0.01 (a 1% chance or less; 1 in 100 chance or less).
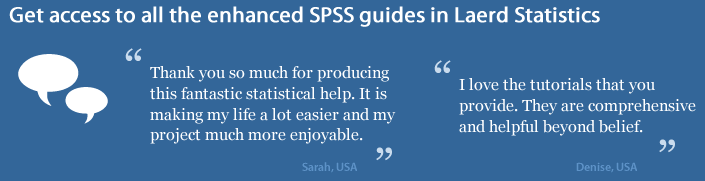
One- and two-tailed predictions
When considering whether we reject the null hypothesis and accept the alternative hypothesis, we need to consider the direction of the alternative hypothesis statement. For example, the alternative hypothesis that was stated earlier is:
The alternative hypothesis tells us two things. First, what predictions did we make about the effect of the independent variable(s) on the dependent variable(s)? Second, what was the predicted direction of this effect? Let's use our example to highlight these two points.
Sarah predicted that her teaching method (independent variable: teaching method), whereby she not only required her students to attend lectures, but also seminars, would have a positive effect (that is, increased) students' performance (dependent variable: exam marks). If an alternative hypothesis has a direction (and this is how you want to test it), the hypothesis is one-tailed. That is, it predicts direction of the effect. If the alternative hypothesis has stated that the effect was expected to be negative, this is also a one-tailed hypothesis.
Alternatively, a two-tailed prediction means that we do not make a choice over the direction that the effect of the experiment takes. Rather, it simply implies that the effect could be negative or positive. If Sarah had made a two-tailed prediction, the alternative hypothesis might have been:
In other words, we simply take out the word "positive", which implies the direction of our effect. In our example, making a two-tailed prediction may seem strange. After all, it would be logical to expect that "extra" tuition (going to seminar classes as well as lectures) would either have a positive effect on students' performance or no effect at all, but certainly not a negative effect. However, this is just our opinion (and hope) and certainly does not mean that we will get the effect we expect. Generally speaking, making a one-tail prediction (i.e., and testing for it this way) is frowned upon as it usually reflects the hope of a researcher rather than any certainty that it will happen. Notable exceptions to this rule are when there is only one possible way in which a change could occur. This can happen, for example, when biological activity/presence in measured. That is, a protein might be "dormant" and the stimulus you are using can only possibly "wake it up" (i.e., it cannot possibly reduce the activity of a "dormant" protein). In addition, for some statistical tests, one-tailed tests are not possible.
Rejecting or failing to reject the null hypothesis
Let's return finally to the question of whether we reject or fail to reject the null hypothesis.
If our statistical analysis shows that the significance level is below the cut-off value we have set (e.g., either 0.05 or 0.01), we reject the null hypothesis and accept the alternative hypothesis. Alternatively, if the significance level is above the cut-off value, we fail to reject the null hypothesis and cannot accept the alternative hypothesis. You should note that you cannot accept the null hypothesis, but only find evidence against it.
Arcus Education Portal
Data Education for the CHOP Researcher
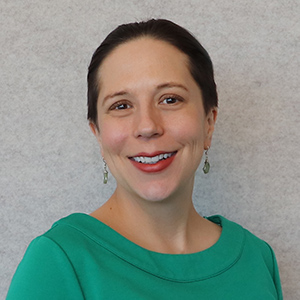
Null Hypothesis Statistical Testing (NHST)
Null Hypothesis Significance Testing (NHST) is a common statistical test to see if your research findings are statistically interesting. Its usefulness is sometimes challenged, particularly because NHST relies on p values, which are sporadically under fire from statisticians. The important thing to remember is not the latest p-value-related salvo in the statistical press, but rather that NHST is often best used alongside other measures that strengthen your claims, like effect size.
So what is null hypothesis statistical testing?
NHST is the statistical comparison of two means:
- an expected mean (like an IQ mean of 100) and observed mean (like mean IQ of children exposed to industrial pesticides in utero )
- group 1 and group 2, like the mean number of headaches in a placebo group and drug group
- the same group with two conditions (like mean face recognition accuracy before and after playing a violent video game)
As we know, any time you measure the mean of a sample, that mean could be larger or smaller than what you’re comparing it to just because of random chance. Or, the mean could be different because there’s a true difference between the two things you’re comparing. NHST helps us decide which possibility seems to be most supported by the evidence.
For example, let’s say I suspect that CHOP tends to have short employees more often than tall employees, because short people have a gene (CHOP1) that causes both shortness and an affinity for healthcare employment. Since men and women have height differences, we’ll concentrate our focus only on CHOP’s women employees, in order to simplify our investigation. I suspect their average height is lower than the average height for women generally.
One of these suspicions is empirically testable with NHST – does CHOP indeed have women employees that tend to be shorter than women generally? It is important to remember that null hypothesis testing cannot provide evidence to back up a claim of what caused a difference, but only evidence to back up the assertion that there is a difference. We can consider ourselves to have two hypotheses: a statistical hypothesis (we’ll find that CHOP-employed women are shorter than women generally), and a scientific or explanatory hypothesis (CHOP has shorter women because there’s a short-and-likes-healthcare gene that attracts short women to work at CHOP). Null hypothesis testing relates only to the statistical hypothesis.
I want to check my claim that CHOP’s female workforce really is different than women generally as far as height. I take a sample of 20 women employed at CHOP and discover that while the average height for women in the USA is 163.2 cm, the average height of our 20 female employees is 160.5 cm. The standard deviation of the sample is 10 cm. Now, the fact that the average height is lower than expected could just be random chance. After all, 20 is a small sample size, and I could have had weird luck and randomly picked 20 of the shorter women at CHOP.
Null hypothesis testing is like criminal prosecution. In criminal prosecution, the jury assumes that the defendant is innocent until proven guilty beyond a reasonable doubt. In our case, we assume that there’s no real difference happening, no real disparity between the height of women at CHOP and women generally, until evidence strongly indicates the contrary. Assuming “innocence” here is our null hypothesis .
The null hypothesis (H 0 ) is always “nothing new or different is happening here, the means are the same.” In our case, the null hypothesis is that CHOP women actually have the same height, on average, as women generally. Sure, we might pick an unusually short or tall sample of CHOP women by happenstance, but the collection of all CHOP women is no different in height than women generally.
The “alternative hypothesis” (H a ) is that there really is a group difference, that the means are different. Your alternative hypothesis could be that the mean of the group you’re studying (CHOP-employed women, in our case) is larger than, smaller than, or different from (you don’t know which direction) the other mean. This “other mean” could be a different group you’re studying, or an expected mean, which in our case is the mean height of American women, which we believe is 163.2 cm. In our case, the alternative hypothesis is that the mean of women employed at CHOP is less than 163.2 cm.
The data we observed, the sample of 20 CHOP women with an average height of 160.5 and standard deviation of 10 cm, could conceivably occur under either hypothesis. So, did we just happen to get an unusually short group of 20 women from an otherwise totally average population? Or did we get a measurement that points to a real difference in the height of women at CHOP?
This is where we have to define our version of “reasonable doubt”. We’ll measure the probability that our sample would occur under the null hypothesis, and make the call based on that. What level of probability do we choose? Typically, we say 5% or 0.05. If the sample we observed has less than a 5% probability of occurring under the null hypothesis, we say we can reject the null hypothesis. That’s our “reasonable doubt”. Keep in mind that we’re admitting that on average, given a lot of “innocent” data, we’re going to get “false positives” around 5% of the time – we’ll reject the null hypothesis when we shouldn’t. This is why statistical significance is evidence , not proof . To make a strong claim, we rely on multiple measurements carried out at different times by different people that all demonstrate similar evidence. This is why replication is so crucial in science.
If you want to see the math of hypothesis testing illustrated and described simply yet in detail, you should definitely check out the Khan Academy videos on hypothesis testing .
In our case, we can do a T test which considers our sample measurements in cm (156, 155.8, 159.5, 174.6, 147.3, 163, 172.5, 156.1, 164.7, 170.1, 170.6, 161, 166.6, 149.8, 163.5, 145.8, 156.7, 158.2, 153.3, 164.1) and considers the likelihood of this sample coming from all CHOP women having an average height of 163.2 cm (the same as women in general). In R, I can use t.test:
My p value is 0.07437, so there’s a 7.437% chance that this sample belongs under the null hypothesis. That’s bigger than 5%, so we can’t reject the null hypothesis. We have to assume that CHOP women are no different than women generally, where height is concerned. Sure, we have kind of a short sample, but we can’t reject the possibility that we just had a sample that tended to be a little short, by random luck.
Now, what if I had a much larger sample, say, 2000 women at CHOP, with the same mean and standard deviation?
Consider what’s weirder: rolling 3 ones in a row with a die, or rolling 30 ones in a row with a die. We instinctively know that an unexpected outcome with just a few elements can happen pretty easily, and while it might draw our attention, we don’t jump to conclusions. But when the repetition of the unusual outcome gets bigger, we start to wonder what’s up. The T test is the same way – it is sensitive to changes in sample size. In the case of our 2000 woman sample, this could be our T test output:
In this case, our p-value is so low it can’t be accurately calculated by my computer. Definitely way, way less than 0.05. We would therefore reject the null hypothesis and assert that we have some evidence to support the fact that CHOP women are shorter, on average, than women in general.
Our observed height difference is statistically significant, but is it really important or interesting? Are we noticing something that, while real, isn’t a big difference? In our case, the difference in means is less than 3 cm, which is pretty small. When we compare the difference between our groups with the difference within our groups (the natural variation in height, described as the standard deviation of 10 cm), we see that the effect size of “works at CHOP” is dwarfed by the natural variance of heights in either sample.
Cohen’s d is a commonly used effect size, and we can calculate it very simply. We take our sample mean (to be precise, it’s 160.45), subtract our H 0 mean (163.2), and divide that difference by the standard deviation (we assume it’s 10 cm across the board). The figure we end up with, -0.275, shows that it’s a small effect size in the negative direction. Cohen suggested that d=0.2 be considered a ‘small’ effect size, 0.5 a ‘medium’ effect size, and 0.8 a ‘large’ effect size, but effect size is somewhat subjective and depends on the domain. For a company manufacturing machine screws for the space station, an effect size of ± 0.275 might be huge, while a chemotherapeutic drug trial might consider even an effect size of ± 0.8 to be small.
The use of effect size is a great way to contextualize findings. We had an extremely significant p value in our 2000-person sample, so we can be pretty confident in our assertion that CHOP women are clearly shorter than women generally (as long as we practiced good science in our sampling, etc.). But the difference? Not a big one, and probably not one anyone would ever notice or care about. When we plot our 2000 CHOP sample along with a 2000-woman sample from the general population, we see that there’s a difference, but not one that stands out a lot:
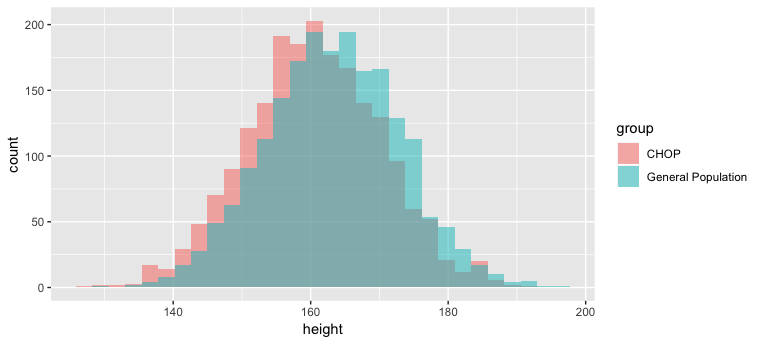
Want to learn more about hypothesis testing, including two-tailed tests (here I just covered a one-tailed test)? I recommend the charming and useful Handbook of Biological Statistics , particularly the section on hypothesis testing .
Like this article? Click "Like" to let us know. Like
13.1 Understanding Null Hypothesis Testing
Learning objectives.
- Explain the purpose of null hypothesis testing, including the role of sampling error.
- Describe the basic logic of null hypothesis testing.
- Describe the role of relationship strength and sample size in determining statistical significance and make reasonable judgments about statistical significance based on these two factors.
The Purpose of Null Hypothesis Testing
As we have seen, psychological research typically involves measuring one or more variables in a sample and computing descriptive statistics for that sample. In general, however, the researcher’s goal is not to draw conclusions about that sample but to draw conclusions about the population that the sample was selected from. Thus researchers must use sample statistics to draw conclusions about the corresponding values in the population. These corresponding values in the population are called parameters . Imagine, for example, that a researcher measures the number of depressive symptoms exhibited by each of 50 adults with clinical depression and computes the mean number of symptoms. The researcher probably wants to use this sample statistic (the mean number of symptoms for the sample) to draw conclusions about the corresponding population parameter (the mean number of symptoms for adults with clinical depression).
Unfortunately, sample statistics are not perfect estimates of their corresponding population parameters. This is because there is a certain amount of random variability in any statistic from sample to sample. The mean number of depressive symptoms might be 8.73 in one sample of adults with clinical depression, 6.45 in a second sample, and 9.44 in a third—even though these samples are selected randomly from the same population. Similarly, the correlation (Pearson’s r ) between two variables might be +.24 in one sample, −.04 in a second sample, and +.15 in a third—again, even though these samples are selected randomly from the same population. This random variability in a statistic from sample to sample is called sampling error . (Note that the term error here refers to random variability and does not imply that anyone has made a mistake. No one “commits a sampling error.”)
One implication of this is that when there is a statistical relationship in a sample, it is not always clear that there is a statistical relationship in the population. A small difference between two group means in a sample might indicate that there is a small difference between the two group means in the population. But it could also be that there is no difference between the means in the population and that the difference in the sample is just a matter of sampling error. Similarly, a Pearson’s r value of −.29 in a sample might mean that there is a negative relationship in the population. But it could also be that there is no relationship in the population and that the relationship in the sample is just a matter of sampling error.
In fact, any statistical relationship in a sample can be interpreted in two ways:
- There is a relationship in the population, and the relationship in the sample reflects this.
- There is no relationship in the population, and the relationship in the sample reflects only sampling error.
The purpose of null hypothesis testing is simply to help researchers decide between these two interpretations.
The Logic of Null Hypothesis Testing
Null hypothesis testing is a formal approach to deciding between two interpretations of a statistical relationship in a sample. One interpretation is called the null hypothesis (often symbolized H 0 and read as “H-naught”). This is the idea that there is no relationship in the population and that the relationship in the sample reflects only sampling error. Informally, the null hypothesis is that the sample relationship “occurred by chance.” The other interpretation is called the alternative hypothesis (often symbolized as H 1 ). This is the idea that there is a relationship in the population and that the relationship in the sample reflects this relationship in the population.
Again, every statistical relationship in a sample can be interpreted in either of these two ways: It might have occurred by chance, or it might reflect a relationship in the population. So researchers need a way to decide between them. Although there are many specific null hypothesis testing techniques, they are all based on the same general logic. The steps are as follows:
- Assume for the moment that the null hypothesis is true. There is no relationship between the variables in the population.
- Determine how likely the sample relationship would be if the null hypothesis were true.
- If the sample relationship would be extremely unlikely, then reject the null hypothesis in favor of the alternative hypothesis. If it would not be extremely unlikely, then retain the null hypothesis .
Following this logic, we can begin to understand why Mehl and his colleagues concluded that there is no difference in talkativeness between women and men in the population. In essence, they asked the following question: “If there were no difference in the population, how likely is it that we would find a small difference of d = 0.06 in our sample?” Their answer to this question was that this sample relationship would be fairly likely if the null hypothesis were true. Therefore, they retained the null hypothesis—concluding that there is no evidence of a sex difference in the population. We can also see why Kanner and his colleagues concluded that there is a correlation between hassles and symptoms in the population. They asked, “If the null hypothesis were true, how likely is it that we would find a strong correlation of +.60 in our sample?” Their answer to this question was that this sample relationship would be fairly unlikely if the null hypothesis were true. Therefore, they rejected the null hypothesis in favor of the alternative hypothesis—concluding that there is a positive correlation between these variables in the population.
A crucial step in null hypothesis testing is finding the likelihood of the sample result if the null hypothesis were true. This probability is called the p value . A low p value means that the sample result would be unlikely if the null hypothesis were true and leads to the rejection of the null hypothesis. A p value that is not low means that the sample result would be likely if the null hypothesis were true and leads to the retention of the null hypothesis. But how low must the p value be before the sample result is considered unlikely enough to reject the null hypothesis? In null hypothesis testing, this criterion is called α (alpha) and is almost always set to .05. If there is a 5% chance or less of a result as extreme as the sample result if the null hypothesis were true, then the null hypothesis is rejected. When this happens, the result is said to be statistically significant . If there is greater than a 5% chance of a result as extreme as the sample result when the null hypothesis is true, then the null hypothesis is retained. This does not necessarily mean that the researcher accepts the null hypothesis as true—only that there is not currently enough evidence to reject it. Researchers often use the expression “fail to reject the null hypothesis” rather than “retain the null hypothesis,” but they never use the expression “accept the null hypothesis.”
The Misunderstood p Value
The p value is one of the most misunderstood quantities in psychological research (Cohen, 1994) [1] . Even professional researchers misinterpret it, and it is not unusual for such misinterpretations to appear in statistics textbooks!
The most common misinterpretation is that the p value is the probability that the null hypothesis is true—that the sample result occurred by chance. For example, a misguided researcher might say that because the p value is .02, there is only a 2% chance that the result is due to chance and a 98% chance that it reflects a real relationship in the population. But this is incorrect . The p value is really the probability of a result at least as extreme as the sample result if the null hypothesis were true. So a p value of .02 means that if the null hypothesis were true, a sample result this extreme would occur only 2% of the time.
You can avoid this misunderstanding by remembering that the p value is not the probability that any particular hypothesis is true or false. Instead, it is the probability of obtaining the sample result if the null hypothesis were true.

“Null Hypothesis” retrieved from http://imgs.xkcd.com/comics/null_hypothesis.png (CC-BY-NC 2.5)
Role of Sample Size and Relationship Strength
Recall that null hypothesis testing involves answering the question, “If the null hypothesis were true, what is the probability of a sample result as extreme as this one?” In other words, “What is the p value?” It can be helpful to see that the answer to this question depends on just two considerations: the strength of the relationship and the size of the sample. Specifically, the stronger the sample relationship and the larger the sample, the less likely the result would be if the null hypothesis were true. That is, the lower the p value. This should make sense. Imagine a study in which a sample of 500 women is compared with a sample of 500 men in terms of some psychological characteristic, and Cohen’s d is a strong 0.50. If there were really no sex difference in the population, then a result this strong based on such a large sample should seem highly unlikely. Now imagine a similar study in which a sample of three women is compared with a sample of three men, and Cohen’s d is a weak 0.10. If there were no sex difference in the population, then a relationship this weak based on such a small sample should seem likely. And this is precisely why the null hypothesis would be rejected in the first example and retained in the second.
Of course, sometimes the result can be weak and the sample large, or the result can be strong and the sample small. In these cases, the two considerations trade off against each other so that a weak result can be statistically significant if the sample is large enough and a strong relationship can be statistically significant even if the sample is small. Table 13.1 shows roughly how relationship strength and sample size combine to determine whether a sample result is statistically significant. The columns of the table represent the three levels of relationship strength: weak, medium, and strong. The rows represent four sample sizes that can be considered small, medium, large, and extra large in the context of psychological research. Thus each cell in the table represents a combination of relationship strength and sample size. If a cell contains the word Yes , then this combination would be statistically significant for both Cohen’s d and Pearson’s r . If it contains the word No , then it would not be statistically significant for either. There is one cell where the decision for d and r would be different and another where it might be different depending on some additional considerations, which are discussed in Section 13.2 “Some Basic Null Hypothesis Tests”
Although Table 13.1 provides only a rough guideline, it shows very clearly that weak relationships based on medium or small samples are never statistically significant and that strong relationships based on medium or larger samples are always statistically significant. If you keep this lesson in mind, you will often know whether a result is statistically significant based on the descriptive statistics alone. It is extremely useful to be able to develop this kind of intuitive judgment. One reason is that it allows you to develop expectations about how your formal null hypothesis tests are going to come out, which in turn allows you to detect problems in your analyses. For example, if your sample relationship is strong and your sample is medium, then you would expect to reject the null hypothesis. If for some reason your formal null hypothesis test indicates otherwise, then you need to double-check your computations and interpretations. A second reason is that the ability to make this kind of intuitive judgment is an indication that you understand the basic logic of this approach in addition to being able to do the computations.
Statistical Significance Versus Practical Significance
Table 13.1 illustrates another extremely important point. A statistically significant result is not necessarily a strong one. Even a very weak result can be statistically significant if it is based on a large enough sample. This is closely related to Janet Shibley Hyde’s argument about sex differences (Hyde, 2007) [2] . The differences between women and men in mathematical problem solving and leadership ability are statistically significant. But the word significant can cause people to interpret these differences as strong and important—perhaps even important enough to influence the college courses they take or even who they vote for. As we have seen, however, these statistically significant differences are actually quite weak—perhaps even “trivial.”
This is why it is important to distinguish between the statistical significance of a result and the practical significance of that result. Practical significance refers to the importance or usefulness of the result in some real-world context. Many sex differences are statistically significant—and may even be interesting for purely scientific reasons—but they are not practically significant. In clinical practice, this same concept is often referred to as “clinical significance.” For example, a study on a new treatment for social phobia might show that it produces a statistically significant positive effect. Yet this effect still might not be strong enough to justify the time, effort, and other costs of putting it into practice—especially if easier and cheaper treatments that work almost as well already exist. Although statistically significant, this result would be said to lack practical or clinical significance.

“Conditional Risk” retrieved from http://imgs.xkcd.com/comics/conditional_risk.png (CC-BY-NC 2.5)
Key Takeaways
- Null hypothesis testing is a formal approach to deciding whether a statistical relationship in a sample reflects a real relationship in the population or is just due to chance.
- The logic of null hypothesis testing involves assuming that the null hypothesis is true, finding how likely the sample result would be if this assumption were correct, and then making a decision. If the sample result would be unlikely if the null hypothesis were true, then it is rejected in favor of the alternative hypothesis. If it would not be unlikely, then the null hypothesis is retained.
- The probability of obtaining the sample result if the null hypothesis were true (the p value) is based on two considerations: relationship strength and sample size. Reasonable judgments about whether a sample relationship is statistically significant can often be made by quickly considering these two factors.
- Statistical significance is not the same as relationship strength or importance. Even weak relationships can be statistically significant if the sample size is large enough. It is important to consider relationship strength and the practical significance of a result in addition to its statistical significance.
- Discussion: Imagine a study showing that people who eat more broccoli tend to be happier. Explain for someone who knows nothing about statistics why the researchers would conduct a null hypothesis test.
- The correlation between two variables is r = −.78 based on a sample size of 137.
- The mean score on a psychological characteristic for women is 25 ( SD = 5) and the mean score for men is 24 ( SD = 5). There were 12 women and 10 men in this study.
- In a memory experiment, the mean number of items recalled by the 40 participants in Condition A was 0.50 standard deviations greater than the mean number recalled by the 40 participants in Condition B.
- In another memory experiment, the mean scores for participants in Condition A and Condition B came out exactly the same!
- A student finds a correlation of r = .04 between the number of units the students in his research methods class are taking and the students’ level of stress.
- Cohen, J. (1994). The world is round: p < .05. American Psychologist, 49 , 997–1003. ↵
- Hyde, J. S. (2007). New directions in the study of gender similarities and differences. Current Directions in Psychological Science, 16 , 259–263. ↵

Share This Book
- Increase Font Size
If you're seeing this message, it means we're having trouble loading external resources on our website.
If you're behind a web filter, please make sure that the domains *.kastatic.org and *.kasandbox.org are unblocked.
To log in and use all the features of Khan Academy, please enable JavaScript in your browser.
AP®︎/College Statistics
Course: ap®︎/college statistics > unit 10.
- Idea behind hypothesis testing
Examples of null and alternative hypotheses
- Writing null and alternative hypotheses
- P-values and significance tests
- Comparing P-values to different significance levels
- Estimating a P-value from a simulation
- Estimating P-values from simulations
- Using P-values to make conclusions
Want to join the conversation?
- Upvote Button navigates to signup page
- Downvote Button navigates to signup page
- Flag Button navigates to signup page

Video transcript
Null hypothesis significance tests. A mix-up of two different theories: the basis for widespread confusion and numerous misinterpretations
- Published: 25 February 2014
- Volume 102 , pages 411–432, ( 2015 )
Cite this article
- Jesper W. Schneider 1
3233 Accesses
71 Citations
7 Altmetric
Explore all metrics
The statistician cannot excuse himself from the duty of getting his head clear on the principles of scientific inference, but equally no other thinking man can avoid a like obligation (Fisher 1951 , p. 2)
Null hypothesis statistical significance tests (NHST) are widely used in quantitative research in the empirical sciences including scientometrics. Nevertheless, since their introduction nearly a century ago significance tests have been controversial. Many researchers are not aware of the numerous criticisms raised against NHST. As practiced, NHST has been characterized as a ‘null ritual’ that is overused and too often misapplied and misinterpreted. NHST is in fact a patchwork of two fundamentally different classical statistical testing models, often blended with some wishful quasi-Bayesian interpretations. This is undoubtedly a major reason why NHST is very often misunderstood. But NHST also has intrinsic logical problems and the epistemic range of the information provided by such tests is much more limited than most researchers recognize. In this article we introduce to the scientometric community the theoretical origins of NHST, which is mostly absent from standard statistical textbooks, and we discuss some of the most prevalent problems relating to the practice of NHST and trace these problems back to the mix-up of the two different theoretical origins. Finally, we illustrate some of the misunderstandings with examples from the scientometric literature and bring forward some modest recommendations for a more sound practice in quantitative data analysis.
This is a preview of subscription content, log in via an institution to check access.
Access this article
Price includes VAT (Russian Federation)
Instant access to the full article PDF.
Rent this article via DeepDyve
Institutional subscriptions
Similar content being viewed by others
A Holistic Approach to Empirical Analysis: The Insignificance of P, Hypothesis Testing and Statistical Significance*
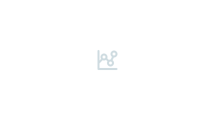
Statistical significance and its critics: practicing damaging science, or damaging scientific practice?
The e-value: a fully bayesian significance measure for precise statistical hypotheses and its research program.
Notice, other hypotheses to be nullified, such as a directional, non-zero or interval estimates are possible but seldom used, hence the ‘null ritual’.
Statistical power is the probability of rejecting H 0 when it is false (Cohen 1988 ). Statistical power is affected by α and β levels, the size of the effect and the size of the sample used to detect it. These elements make it possible to define the probability density function for the alternative hypothesis.
E.g., a sampling design where one either chooses to toss a coin until it produces a pre-specified pattern, or instead doing a pre-specified number of tosses. The results can be identical, but the p values will be different.
For example, the instructions to authors in the journal Epidemiology reads “We strongly discourage the use of p values and language referring to statistical significance” ( http://edmgr.ovid.com/epid/accounts/ifauth.htm ).
Abelson, R. P. (1997). On the surprising longevity of flogged horses: Why there is a case for the significance test. Psychological Science, 8 (1), 12–15.
Google Scholar
American Psychological Association. (2010). Publication Manual of the APA (6th ed.). Washington, DC: APA.
Anderson, D. R. (2008). Model based inference in the life sciences: A primer on evidence . New York: Springer.
Anderson, D. R., Burnham, K. P., & Thompson, W. L. (2000). Null hypothesis testing: Problems, prevalence, and an alternative. Journal of Wildlife Management, 64 , 912–923.
Armstrong, J. S. (2007). Significance tests harm progress in forecasting. International Journal of Forecasting, 23 (2), 321–327.
Armstrong, J. S. (2012). Illusions in regression analysis. International Journal of Forecasting, 28 (3), 689–694.
Beninger, P. G., Boldina, I., & Katsanevakis, S. (2012). Strengthening statistical usage in marine ecology. Journal of Experimental Marine Biology and Ecology, 426 , 97–108.
Berger, J. O., & Berry, D. A. (1988). Statistical analysis and the illusion of objectivity. American Scientist, 76 (2), 159–165.
Berger, J. O., & Sellke, T. (1987). Testing a point null hypothesis—The irreconcilability of p -values and evidence. Journal of the American Statistical Association, 82 (397), 112–122.
MathSciNet MATH Google Scholar
Berk, R. A., & Freedman, D. A. (2003). Statistical assumptions as empirical commitments. In T. G. Blomberg & S. Cohen (Eds.), Law, punishment, and social control: Essays in honor of Sheldon Messinger (pp. 235–254). New York: Aldine.
Berk, R. A., Western, B., & Weiss, R. E. (1995). Statistical inference for apparent populations. Sociological Methodology, 25 , 421–458.
Berkson, J. (1938). Some difficulties of interpretation encountered in the application of the Chi square test. Journal of the American Statistical Association, 33 (203), 526–536.
MATH Google Scholar
Berkson, J. (1942). Tests of significance considered as evidence. Journal of the American Statistical Association, 37 (219), 325–335.
Boring, E. G. (1919). Mathematical versus scientific significance. Psychological Bulletin, 16 , 335–338.
Bornmann, L., & Leydesdorff, L. (2013). Statistical tests and research assessments: A comment on Schneider (2012). Journal of the American Society for Information Science and Technology, 64 (6), 1306–1308.
Carver, R. P. (1978). The case against statistical significance testing. Harvard Educational Review, 48 (3), 378–399.
MathSciNet Google Scholar
Chow, S. L. (1998). Précis of Statistical significance: Rationale, validity, and utility. Behavioral and Brain Sciences, 2 , 169–239.
Clark, C. A. (1963). Hypothesis testing in relation to statistical methodology. Review of Educational Research, 33 , 455–473.
Cohen, J. (1988). Statistical power analysis for the behavioral sciences (2nd ed.). Hillsdale, NJ: Lawrence Erlbaum.
Cohen, J. (1990). Things I have learned (so far). American Psychologist, 45 (12), 1304–1312.
Cohen, J. (1994). The earth is round ( p < 0.05). American Psychologist, 49 (12), 1003–1007.
Cortina, J. M., & Dunlap, W. P. (1997). On the logic and purpose of significance testing. Psychological Methods, 2 (2), 161–172.
Cumming, G. (2012). Understanding the new statistics. Effect sizes, confidence intervals, and meta-analysis . New York: Routledge.
Dixon, P., & O’Reilly, T. (1999). Scientific versus statistical inference. Canadian Journal of Experimental Psychology-Revue Canadienne De Psychologie Experimentale, 53 (2), 133–149.
Ellis, P. D. (2010). The essential guide to effect sizes: Statistical power, meta-analysis, and the interpretation of research results . Cambridge: Cambridge University Press.
Falk, R., & Greenbaum, C. W. (1995). Significance tests die hard. Theory and Psychology, 5 , 396–400.
Fisher, R. A. (1925). Statistical methods for research workers (1st ed.). London: Oliver & Boyd.
Fisher, R. A. (1935a). The design of experiments (1st ed.). Edinburgh: Oliver & Boyd.
Fisher, R. A. (1935b). Statistical tests. Nature, 136 , 474.
Fisher, R. A. (1935c). The logic of inductive inference. Journal of the Royal Statistical Society, 98 , 71–76.
Fisher, R. A. (1951). The design of experiments (6th ed.). Edinburgh: Oliver & Boyd.
Fisher, R. A. (1955). Statistical methods and scientific induction. Journal of the Royal Statistical Society B, 17 , 69–78.
Fisher, R. A. (1956). Statistical methods and scientific inference . London: Oliver & Boyd.
Frick, R. W. (1996). The appropriate use of null hypothesis testing. Psychological Methods, 1 (4), 379–390.
Gelman, A., Carlin, J. B., Stern, H. S., & Rubin, D. B. (2004). Bayesian data analysis . Boca Raton: Chapman & Hall/CRC.
Gelman, A., & Stern, H. (2006). The difference between “significant” and “not significant” is not itself statistically significant. The American Statistician, 60 (4), 328–331.
Gigerenzer, G. (1993). The superego, the ego, and the id in statistical reasoning. In G. Keren & C. Lewis (Eds.), A handbook for data analysis in the behavioral sciences: methodological issues (pp. 311–339). Hillsdale: Erlbaum.
Gigerenzer, G. (2004). Mindless statistics. The Journal of Socio-Economics, 33 (5), 587–606.
Gigerenzer, G., Swijtink, Z., Porter, T., Daston, L., Beatty, J., & Kruger, L. (1989). The empire of chance: How probability changed science and everyday life . New York: Cambridge University Press.
Gill, J. (2007). Bayesian methods: A social and behavioral sciences approach (2nd ed.). Boca Raton: Chapman & Hall/CRC.
Glass, G. (2006). Meta-analysis: The quantitative synthesis of research findings. In J. L. Green, G. Camilli, & P. B. Elmore (Eds.), Handbook of Complementary Methods in Education Research . Mahwah, NJ: Lawrence Erlbaum.
Good, I. J. (1950). Probability and the weighing of evidence . London: Griffin.
Goodman, S. N. (1993). P values, hypothesis tests, and likelihood: Implications for epidemiology of a neglected historical debate. American Journal of Epidemiology, 137 (5), 485–496.
Goodman, S. N. (1999a). Toward evidence-based medical statistics. 1: The P value fallacy. Annals of Internal Medicine, 130 (12), 995–1004.
Goodman, S. N. (1999b). Toward evidence-based medical statistics. 2: The Bayes factor. Annals of Internal Medicine, 130 (12), 1005–1013.
Goodman, S. N. (2003). Commentary: The P -value, devalued. International Journal of Epidemiology, 32 (5), 699–702.
Goodman, S. N. (2008). A dirty dozen: Twelve P -value misconceptions. Seminars in Hematology, 45 (3), 135–140.
Goodman, S. N., & Greenland, S. (2007). Why most published research findings are false: Problems in the analysis. PLoS Medicine, 4 (4), e168.
Greenland, S. (1990). Randomization, statistics, and causal Inference. Epidemiology, 1 (6), 421–429.
Greenland, S., & Poole, C. (2013). Living with statistics in observational research. Epidemiology, 24 (1), 73–78.
Hacking, I. (1965). Logic of statistical inference . Cambridge: Cambridge University Press.
Haller, H., & Krauss, S. (2002). Misinterpretations of significance: A problem students share with their teachers. Methods of Psychological Research, 7 (1), 1–20.
Harlow, L. L., Muliak, S. A., & Steiger, J. H. (Eds.). (1997). What if there were no significance tests? . Mahwah: Lawrence Erlbaum.
Hubbard, R. (2004). Alphabet soup: Blurring the distinctions between p’s and a’s in psychological research. Theory and Psychology, 14 (3), 295–327.
Hubbard, R., & Armstrong, J. S. (2006). Why we don’t really know what statistical significance means: Implications for educators. Journal of Marketing Education, 28 (2), 114–120.
Hubbard, R., & Bayarri, M. J. (2003). Confusion over measures of evidence (p’s) versus errors (α’s) in classical statistical testing. American Statistician, 57 (3), 171–178.
Hubbard, R., & Lindsay, R. M. (2008). Why P values are not a useful measure of evidence in statistical significance testing. Theory and Psychology, 18 (1), 69–88.
Hubbard, R., & Ryan, P. A. (2000). The historical growth of statistical significance testing in psychology and its future prospects. Educational and Psychological Measurement, 60 , 661–681.
Hunter, J. E. (1997). Needed: A ban on the significance test. Psychological Science, 8 , 3–7.
Hurlbert, S. H., & Lombardi, C. M. (2009). Final collapse of the Neyman–Pearson decision theoretic framework and rise of the neoFisherian. Annales Zoologici Fennici, 46 (5), 311–349.
Ioannidis, J. P. A. (2005). Why most published research findings are false. PLoS Medicine, 2 (8), 696–701.
Jeffreys, H. (1939). The theory of probability (1st ed.). Oxford: Oxford University Press.
Jeffreys, H. (1961). The theory of probability (3rd ed.). Oxford: Oxford University Press.
Kirk, R. E. (1996). Practical significance: a concept whose time has come. Educational and Psychological Measurement, 61 (5), 246–759.
Kline, R. B. (2004). Beyond significance testing: reforming data analysis methods in behavioral research . Washington, DC: American Psychological Association.
Kline, R. B. (2013). Beyond significance testing: reforming data analysis methods in behavioral research (2nd ed.). Washington, DC: American Psychological Association.
Krämer, W., & Gigerenzer, G. (2005). How to confuse with statistics or: The use and misuse of conditional probabilities. Statistical Science, 20 (3), 223–230.
Kruschke, J. K. (2010). What to believe: Bayesian methods for data analysis. Trends in Cognitive Sciences, 14 (7), 293–300.
Lehmann, E. L. (1993). The Fisher, Neyman–Pearson theories of testing hypotheses: One theory or two? Journal of the American Statistical Association, 88 (424), 1242–1249.
Leydesdorff, L. (2013). Does the specification of uncertainty hurt the progress of scientometrics? Journal of Informetrics, 7 (2), 292–293.
Lindley, D. (1957). A statistical paradox. Biometrika, 44 , 187–192.
Ludwig, D. A. (2005). Use and misuse of p -values in designed and observational studies: Guide for researchers and reviewers. Aviation, Space and Environmental Medicine, 76 (7), 675–680.
Lykken, D. T. (1968). Statistical significance in psychological research. Psychological Bulletin, 70 (3, Part 1), 151–159.
Mayo, D. (1996). Error and the growth of experimental knowledge . Chicago University Press: Chicago, IL.
Mayo, D. (2006). Philosophy of Statistics. In S. Sarkar & J. Pfeifer (Eds.), The philosophy of science: An encyclopedia (pp. 802–815). London: Routledge.
Meehl, P. E. (1978). Theoretical risks and tabular asterisk: Sir Karl, Sir Ronald, and the slow progress of soft psychology. Journal of Counseling and Clinical Psychology, 46 , 806–834.
Meehl, P. E. (1990). Appraising and amending theories: the strategy of Lakatosian defense and two principles that warrant it. Psychological Inquiry, 1 , 108–141.
Morrison, D. E., & Henkel, R. E. (Eds.). (1970). The significance test controversy . Chicago: Aldine.
Neyman, J. (1937). Outline of a theory of statistical estimation based on the classical theory of probability. Philosophical Transactions of the Royal Society A, 236 , 333–380.
Neyman, J., & Pearson, E. S. (1928). On the use and interpretation of certain test criteria of statistical inference, part I. Biometrika, 20A , 175–240.
Neyman, J., & Pearson, E. S. (1933a). On the problem of the most efficient test of statistical hypotheses. Philosophical Transactions of the Royal Society of London A, 231 , 289–337.
Neyman, J., & Pearson, E. S. (1933b). The testing of statistical hypotheses in relation to probabilituies a priori. Proceedings of the Cambridge Philosophical Society, 29 , 492–510.
Nickerson, R. S. (2000). Null hypothesis significance testing: a review of an old and continuing controversy. Psychological Methods, 5 (2), 241–301.
Oakes, M. (1986). Statistical inference: A commentary for the social and behavioral sciences . New York: Wiley.
Pollard, P., & Richardson, J. T. E. (1987). On the probability of making Type I errors. Psychological Bulletin, 102 , 159–163.
Rosnow, R. L., & Rosenthal, R. (1989). Statistical procedures and the justification of knowledge in psychological science. American Psychologist, 44 (10), 1276–1284.
Royall, R. M. (1997). Statistical evidence: A likelihood paradigm . London: Chapman & Hall.
Rozeboom, W. W. (1960). The fallacy of the null-hypothesis significance test. Psychological Bulletin, 57 (5), 416–428.
Scarr, S. (1997). Rules of evidence: A larger context for the statistical debate. Psychological Science, 8 , 16–17.
Schneider, J. W. (2012). Testing university rankings statistically: Why this perhaps is not such a good idea after all. Some reflections on statistical power, effect size, random sampling and imaginary populations. In É. Archambault, Y. Gingras, & V. Larivière (Eds.), Proceedings of the 17th international conference on science and technology indicators, Montreal . Retrieved, from http://2012.sticonference.org/Proceedings/vol2/Schneider_Testing_719.pdf .
Schneider, J. W. (2013). Caveats for using statistical significance tests in research assessments. Journal of Informetrics, 7 (1), 50–62.
Schneider, A. L., & Darcy, R. E. (1984). Policy implications of using significance tests in evaluation research. Evaluation Review, 8 (4), 573–582.
Schrodt, P. A. (2006). Beyond the linear frequentist orthodoxy. Political Analysis, 14 (3), 335–339.
Schwab, A., Abrahamson, E., Starbuck, W. H., & Fidler, F. (2011). Researchers should make thoughtful assessments instead of null-hypothesis significance tests. Organization Science, 22 (4), 1105–1120.
Sellke, T., Bayarri, M. J., & Berger, J. O. (2001). Calibration of rho values for testing precise null hypotheses. The American Statistician, 55 , 62–71.
Simmons, J. P., Nelson, L. D., & Simonsohn, U. (2011). False-positive psychology: undisclosed flexibility in data collection and analysis allows presenting anything as significant. Psychological Science, 22 (11), 1359–1366.
Spielman, S. (1974). The logic of tests of significance. Philosophy of Science, 41 , 211–226.
Starbuck, W. H. (2006). The production of knowledge: The challenge of social science research . Oxford: Oxford University Press.
Taagepera, R. (2008). Making social sciences more scientific: The need for predictive models . Oxford: Oxford University Press.
Tukey, J. W. (1977). Exploratory data analysis . Reading: Addison-Wesley.
Tukey, J. W. (1991). The philosophy of multiple comparisons. Statistical Science, 6 (1), 100–116.
Wagenmakers, E. J. (2007). A practical solution to the pervasive problem of p values. Psychonomic Bulletin & Review, 14 (5), 779–804.
Webster, E. J., & Starbuck, W. H. (1988). Theory building in industrial and organizational psychology. In C. L. Cooper & I. Robertson (Eds.), International review of industrial and organizational psychology (pp. 93–138). London: Wiley.
Wetzels, R., Matzke, D., Lee, M. D., Rouder, J. N., Iverson, G. J., & Wagenmakers, E.-J. (2011). Statistical evidence in experimental psychology: An empirical comparison using 855 t tests. Perspectives on Psychological Science, 6 (3), 291–298.
Wilkinson, L., & Task Force on Statistical Inference, APA Board on Scientific Affairs (1999). Statistical methods in psychology journals - Guidelines and explanations. American Psychologist, 54 (8), 594–604.
Ziliak, S. T., & McCloskey, D. N. (2008). The cult of statistical significance: How the standard error costs us jobs, justice, and lives . Ann Arbor: The University of Michigan Press.
Download references
Author information
Authors and affiliations.
Department of Political Science & Government, Danish Centre for Studies in Research and Research Policy, Aarhus University, Bartholins Allé 7, 8000, Aarhus C, Denmark
Jesper W. Schneider
You can also search for this author in PubMed Google Scholar
Corresponding author
Correspondence to Jesper W. Schneider .
Rights and permissions
Reprints and permissions
About this article
Schneider, J.W. Null hypothesis significance tests. A mix-up of two different theories: the basis for widespread confusion and numerous misinterpretations. Scientometrics 102 , 411–432 (2015). https://doi.org/10.1007/s11192-014-1251-5
Download citation
Received : 03 February 2014
Published : 25 February 2014
Issue Date : January 2015
DOI : https://doi.org/10.1007/s11192-014-1251-5
Share this article
Anyone you share the following link with will be able to read this content:
Sorry, a shareable link is not currently available for this article.
Provided by the Springer Nature SharedIt content-sharing initiative
- Null hypothesis significance test
- Fisher’s significance test
- Neyman–Pearson’s hypothesis test
- Statistical inference
- Scientometrics
Mathematic Subject Classification
Jel classification.
- Find a journal
- Publish with us
- Track your research
- Open supplemental data
- Reference Manager
- Simple TEXT file
People also looked at
Review article, when null hypothesis significance testing is unsuitable for research: a reassessment.
- 1 Department of Psychology, University of Cambridge, Cambridge, United Kingdom
- 2 Meta-Research Innovation Center at Stanford and Department of Medicine, Department of Health Research and Policy, and Department of Statistics, Stanford University, Stanford, CA, United States
Null hypothesis significance testing (NHST) has several shortcomings that are likely contributing factors behind the widely debated replication crisis of (cognitive) neuroscience, psychology, and biomedical science in general. We review these shortcomings and suggest that, after sustained negative experience, NHST should no longer be the default, dominant statistical practice of all biomedical and psychological research. If theoretical predictions are weak we should not rely on all or nothing hypothesis tests. Different inferential methods may be most suitable for different types of research questions. Whenever researchers use NHST they should justify its use, and publish pre-study power calculations and effect sizes, including negative findings. Hypothesis-testing studies should be pre-registered and optimally raw data published. The current statistics lite educational approach for students that has sustained the widespread, spurious use of NHST should be phased out.
“What used to be called judgment is now called prejudice and what used to be called prejudice is now called a null hypothesis. In the social sciences, particularly, it is dangerous nonsense (dressed up as the “scientific method”) and will cause much trouble before it is widely appreciated as such.”
( Edwards, 1972 ; p.180.)
“…the mathematical rules of probability theory are not merely rules for calculating frequencies of random variables; they are also the unique consistent rules for conducting inference (i.e., plausible reasoning)”
( Jaynes, 2003 ; p. xxii).
The Replication Crisis and Null Hypothesis Significance Testing (NHST)
There is increasing discontent that many areas of psychological science, cognitive neuroscience, and biomedical research ( Ioannidis, 2005 ; Ioannidis et al., 2014 ) are in a crisis of producing too many false positive non-replicable results ( Begley and Ellis, 2012 ; Aarts et al., 2015 ). This wastes research funding, erodes credibility and slows down scientific progress. Since more than half a century many methodologists have claimed repeatedly that this crisis may at least in part be related to problems with Null Hypothesis Significance Testing (NHST; Rozeboom, 1960 ; Bakan, 1966 ; Meehl, 1978 ; Gigerenzer, 1998 ; Nickerson, 2000 ). However, most scientists (and in particular psychologists, biomedical scientists, social scientists, cognitive scientists, and neuroscientists) are still near exclusively educated in NHST, they tend to misunderstand and abuse NHST and the method is near fully dominant in scientific papers ( Chavalarias et al., 1990-2015 ). Here we provide an accessible critical reassessment of NHST and suggest that while it may have legitimate uses when there are precise quantitative predictions and/or as a heuristic, it should be abandoned as the cornerstone of research.
Our paper does not concern specifically the details of neuro-imaging methodology, many papers dealt with such details recently ( Pernet and Poline, 2015 ; Nichols et al., 2016 , 2017 ). Rather, we take a more general view in discussing fundamental problems that can affect any scientific field, including neuroscience and neuro-imaging. In relation to this it is important to see that non-invasive neuroscience data related to behavioral tasks cannot be interpreted if task manipulations did not work and/or behavior is unclear. This is because most measured brain activity changes can be interpreted in many different ways on their own ( Poldrack, 2006 ; see Section 2.7 in Nichols et al., 2016 ). So, as most behavioral data are analyzed by NHST statistics NHST based inference from behavioral data also plays a crucial role in interpreting brain data.
The Origins of NHST as a Weak Heuristic and a Decision Rule
Nhst as a weak heuristic based on the p -value: fisher.
p -values were popularized by Fisher (1925) . In the context of the current NHST approach Fisher only relied on the concepts of the null hypothesis (H 0 ) and the exact p-value (hereafter p will refer to the p -value and “pr” to probability; see Appendix 1 in Supplementary Material for terms). He thought that experiments should aim to reject (or “nullify”; henceforth the name “null hypothesis”) H 0 which assumes that the data demonstrates random variability according to some distribution around a certain value. Discrepancy from H 0 is measured by a test statistic whose values can be paired with one or two-tailed p -values which tell us how likely it is that we would have found our data or more extreme data if H 0 was really correct. Formally we will refer to the p -value as: pr(data or more extreme data|H 0 ). It is important to realize that the p -value represents the “extremeness” of the data according to an imaginary data distribution assuming there is no bias in data sampling.
The late Fisher viewed the exact p -value as a heuristic piece of inductive evidence which gives an indication of the plausibility of H 0 together with other available evidence, like effect sizes (see Hubbard and Bayarri, 2003 ; Gigerenzer et al., 2004 ). Fisher recommended that H 0 can usually be rejected if p ≤ 0.05 but in his system there is no mathematical justification for selecting a particular p -value for the rejection of H 0 . Rather, this is up to the substantively informed judgment of the experimenter. Fisher thought that a hypothesis is demonstrable only when properly designed experiments “ rarely fail” to give us statistically significant results ( Gigerenzer et al., 1989 , p. 96; Goodman, 2008 ). Hence, a single significant result should not represent a “scientific fact” but should merely draw attention to a phenomenon which seems worthy of further investigation including replication ( Goodman, 2008 ). In contrast to the above, until recently replication studies have been very rare in many scientific fields; lack of replication efforts has been a particular problem in the psychological sciences ( Makel et al., 2012 ), but this may hopefully change now with the wide attention that replication has received ( Aarts et al., 2015 ).
Neyman and Pearson: A Decision Mechanism Optimized for the Long-Run
The concepts of the alternative hypothesis (H 1 ), α, power, β, Type I, and Type II errors were introduced by Neyman and Pearson ( Neyman and Pearson, 1933 ; Neyman, 1950 ) who set up a formal decision procedure motivated by industrial quality control problems ( Gigerenzer et al., 1989 ). Their approach aimed to minimize the false negative (Type II) error rate to an acceptable level (β) and consequently to maximize power (1-β) subject to a bound (α) on false positive (Type I) errors ( Hubbard and Bayarri, 2003 ). α can be set by the experimenter to an arbitrary value and Type-II error can be controlled by setting the sample size so that the required effect size can be detected (see Figure 1 for illustration). In contrast to Fisher, this framework does not use the p -value as a measure of evidence. We merely determine the critical value of the test statistic associated with α and reject H 0 whenever the test statistic is larger than the critical value. The exact p -value is irrelevant because the sole objective of the decision framework is long-run error minimization and only the critical threshold but not the exact p -value plays any role in achieving this goal ( Hubbard and Bayarri, 2003 ). Neyman and Pearson rejected the idea of inductive reasoning and offered a reasoning - free inductive behavioral rule to choose between two behaviors, accepting or rejecting H 0 , irrespective of the researcher's belief about whether H 0 and H 1 are true or not ( Neyman and Pearson, 1933 ).
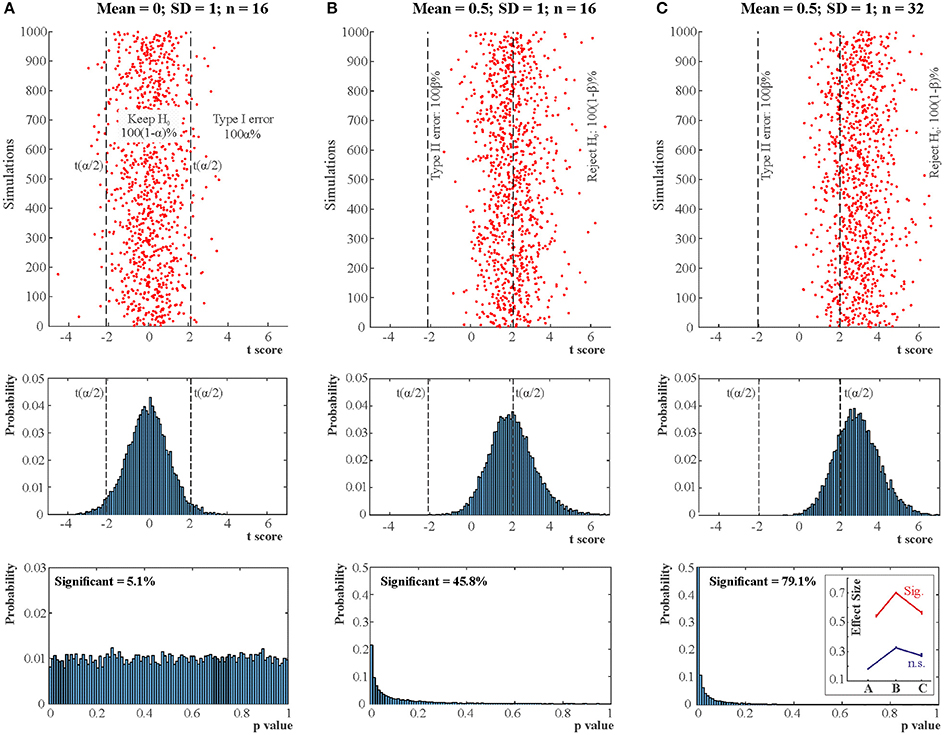
Figure 1 . NHST concepts make sense in the context of a long run of studies. 3 × 10,000 studies with normally distributed data were simulated for 3 situations ( A: True H 0 situation: Mean = 0; SD = 1; n = 16. B: Mean = 0.5; SD = 1; n = 16; Power = 0.46. C: Mean = 0.5; SD = 1; n = 32; Power = 0.78.). One sample two-tailed t- tests determined whether the sample means were zero. The red dots in the top panels show t scores for 3 × 1,000 studies (not all studies are shown for better visibility). The vertical dashed lines mark the critical rejection thresholds for H 0 , t(α/2) for the two-tailed test. The studies producing a t statistic more extreme than these thresholds are declared statistically significant. The middle panels show the distribution of t scores for all 3 × 10,000 studies (bins = 0.1). The bottom panels show the distribution of p -values for all 3 × 10,000 studies (bins = 0.01) and state the proportion of significant studies. The inset in the bottom right panel shows the mean absolute effect sizes in standard deviation units for situations A-C from all significant (Sig.) and non-significant (n.s.) studies with 95% bias corrected and accelerated bootstrap confidence intervals (10,000 permutations). The real effect size was 0 in situation (A) and 0.5 in situations (B,C) . Note that the less is the power the more statistically significant studies overstate the effect size. Also note that p -values are randomly distributed and the larger is power the more right skewed is the distribution of p -values. In the true H 0 situation the distribution of p -values is uniform between 0 and 1. See further explanation of this figure in Appendix 2 in Supplementary Material.
Crucially, the Neyman–Pearson approach is designed to work efficiently ( Neyman and Pearson, 1933 ) in the context of long-run repeated testing (exact replication). Hence, there is a major difference between the p -value which is computed for a single data set and α, β, power, Type I, and Type II error which are so called “ frequentist” concepts and they make sense in the context of a long-run of many repeated experiments . If we only run a single experiment all we can claim is that if we had run a long series of experiments we would have had 100α% false positives (Type I error) had H 0 been true and 100β% false negatives (Type II error) had H 1 been true provided we got the power calculations right. Note the conditionals.
In the Neyman–Pearson framework optimally setting α and β assures long-term decision-making efficiency in light of our costs and benefits by committing Type I and Type II errors. However, optimizing α and β is much easier in industrial quality control than in research where often there is no reason to expect a specific effect size associated with H 1 ( Gigerenzer et al., 1989 ). For example, if a factory has to produce screw heads with a diameter of 1 ± 0.01 cm than we know that we have to be able to detect a deviation of 0.01 cm to produce acceptable quality output. In this setting we know exactly the smallest effect size we are interested in (0.01 cm) and we can also control the sample size very efficiently because we can easily take a sample of a large number of screws from a factory producing them by the million assuring ample power. On the one hand, failing to detect too large or too small screws (Type II error) will result in our customers canceling their orders (or, in other industrial settings companies may deliver faulty cars or exploding laptops to customers exposing themselves to substantial litigation and compensation costs). On the other hand, throwing away false positives (Type I error), i.e., completely good batches of screws which we think are too small or too large, will also cost us a certain amount of money. Hence, we have a very clear scale (monetary value) to weigh the costs and benefits of both types of errors and we can settle on some rationally justified values of α and β so as to minimize our expenses and maximize our profit.
In contrast to such industrial settings, controlling the sample size and effect size and setting rational α and β levels is not that straightforward in most research settings where the true effect sizes being pursued are largely unknown and deciding about the requested size of a good enough effect can be very subjective. For example, what is the smallest difference of interest between two participant groups in a measure of “fMRI activity”? Or, what is the smallest difference of interest between two groups of participants when we measure their IQ or reaction time? And, even if we have some expectations about the “true effect size,” can we test enough participants to ensure a small enough β? Further, what is the cost of falsely claiming that a vaccine causes autism thereby generating press coverage that grossly misleads the public ( Deer, 2011 ; Godlee, 2011 )? What is the cost of running too many underpowered studies thereby wasting perhaps most research funding, boosting the number of false positive papers and complicating interpretation ( Schmidt, 1992 ; Ioannidis, 2005 ; Button et al., 2013 )? More often than not researchers do not know the “true” size of an effect they are interested in, so they cannot assure adequate sample size and it is also hard to estimate general costs and benefits of having particular α and β values. While some “rules of thumb” exist about what are small, modest, and large effects (e.g., Cohen, 1962 , 1988 ; Jaeschke et al., 1989 ; Sedlmeier and Gigerenzer, 1989 ), some large effects may not be actionable (e.g., a change in some biomarker that is a poor surrogate and thus bears little relationship to major, clinical outcomes), while some small effects may be important and may change our decision (e.g., most survival benefits with effective drugs are likely to be small, but still actionable).
Given the above ambiguity, researchers fall back to the default α = 0.05 level with usually undefined power. So, the unjustified α and β levels completely discredit the originally intended “ efficiency” rationale of the creators of the Neyman–Pearson decision mechanism ( Neyman and Pearson, 1933 ).
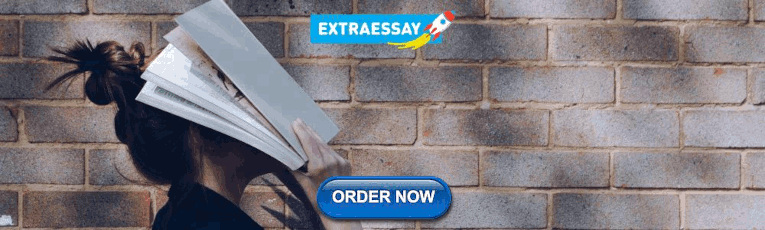
P -Values Are Random Variables and They Correspond to Standardized Effect Size Measures
Contrary to the fact that in Figure 1 all 10,000 true H 0 and 10,000 true H 1 samples were simulated from identical H 0 and H 1 distributions, the t scores and the associated p -values reflect a dramatic spread. That is, p -values are best viewed as random variables which can take on a range of values depending on the actual data ( Sterling, 1959 ; Murdoch et al., 2008 ). Consequently, it is impossible to tell from the outcome of a single (published) experiment delivering a statistically significant result whether a true effect exist. The only difference between the true H 0 and true H 1 situations is that when H 0 is true in all experiments, the distribution of p -values is uniform between 0 and 1 whereas when H 1 is true in all experiments p -values are more likely to fall on the left of the 0–1 interval, that is, their distribution becomes right skewed. The larger is the effect size and power the stronger is this right skew (Figure 2 ). This fact led to the suggestion that comparing this skew allows us to determine the robustness of findings in some fields by studying “p curves” ( Hung et al., 1997 ; Simonsohn et al., 2014a , b ). Hence, from this perspective, replication, and unbiased publication of all results (“positive” and “negative”) is again crucial if we rely on NHST because only then can they inform us about the distribution of p -values.
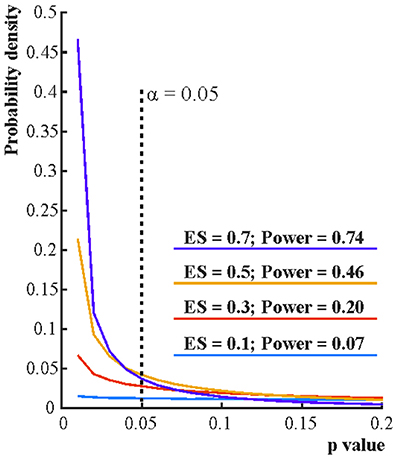
Figure 2 . The distribution of p -values if the alternative hypothesis (H 1 ) is true. Each line depicts the distribution of p -values resulting from one-sample two-tailed t -tests testing whether the sample mean was zero. Effect sizes (ES) indicate the true sample means for normally distributed data with standard deviation 1. For each effect size one million simulations were run with 16 cases in each simulation. The distribution of the p -value is becoming increasingly right skewed with increasing effect size and power. Note that α, the Type I error rate, is fix irrespective of what p -value is found in an experiment.
Another point to notice is that both p -values and usual standardized effect size measures (Cohen's D, correlation values, etc.) are direct functions of NHST test statistics. Hence, for given degrees of freedom NHST test statistics, effect size measures and p -values will have non-linear correspondence as illustrated in Figure 3 .
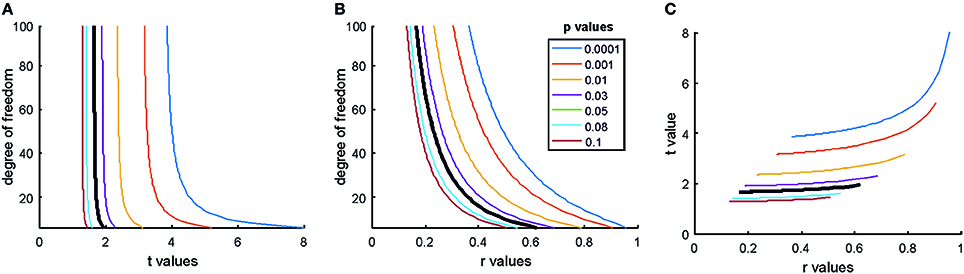
Figure 3 . The relationship of the p -value, test statistic and effect sizes. (A) The relationship of t values, degrees of freedoms and p -values for Pearson correlation studies (df = n–2). (B) The relationship of Pearson correlation (r) values, degrees of freedoms, and p -values [r = t/sqrt(df + t 2 )]. (C) The relationship of r - and t -value pairs for each degree of freedom at various p -values. The bold black lines mark the usual significance level of α = 0.05. Note that typically only results which exceed the α = 0.05 threshold are reported in papers. Hence, papers mostly report exaggerated effect sizes.
NHST in Its Current Form
The current NHST merged the approaches of Fisher and Neyman and Pearson and is often applied stereotypically as a “mindless null ritual” ( Gigerenzer, 2004 ). Researchers set H 0 nearly always “predicting” zero effect but do not quantitatively define H 1 . Hence, pre-experimental power cannot be calculated for most tests which is a crucial omission in the Neyman–Pearson framework. Researchers compute the exact p -value as Fisher did but also mechanistically reject H 0 and accept the undefined H 1 if p ≤ (α = 0.05) without flexibility following the behavioral decision rule of Neyman and Pearson. As soon as p ≤ α, findings have the supposed right to become a scientific fact defying the exact replication demands of Fisher and the belief neutral approach of Neyman and Pearson. Researchers also interpret the exact p -value and use it as a relative measure of evidence against H 0 , as Fisher did. A “ highly significant” result with a small p -value is perceived as much stronger evidence than a weakly significant one. However, while Fisher was conscious of the weak nature of the evidence provided by the p -value ( Wasserstein and Lazar, 2016 ), generations of scientists encouraged by incorrect editorial interpretations ( Bakan, 1966 ) started to exclusively rely on the p -value in their decisions even if this meant neglecting their substantive knowledge: scientific conclusions merged with reading the p -value ( Goodman, 1999 ).
Neglecting the Full Context of NHST Leads to Confusions About the p- value
Most textbooks illustrate NHST by partial 2 × 2 tables (see Table 1 ) which fail to contextualize long-run conditional probabilities and fail to clearly distinguish between long-run probabilities and the p -value which is computed for a single data set ( Pollard and Richardson, 1987 ). This leads to major confusions about the meaning of the p -value (see Appendix 2 in Supplementary Material).
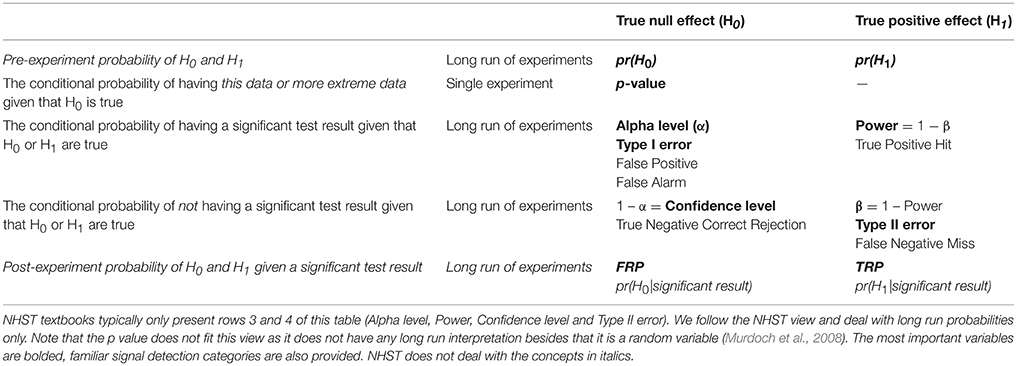
Table 1 . “pr” stands for probability.
First, both H 0 and H 1 have some usually unknown pre-study or “prior” probabilities, pr(H 0 ) and pr(H 1 ). Nevertheless, these probabilities may be approximated through extensive substantive knowledge. For example, we may know about a single published study claiming to demonstrate H 1 by showing a difference between appropriate experimental conditions. However, in conferences we may have also heard about 9 highly powered but failed replication attempts very similar to the original study. In this case we may assume that the odds of H 0 :H 1 are 9:1, that is, pr(H 1 ) is 1/10. Of course, these pre-study odds are usually hard to judge unless we demand to see our colleagues' “null results” hidden in their drawers because of the practice of not publishing negative findings. Current scientific practices appreciate the single published “positive” study more than the 9 unpublished negative ones perhaps because NHST logic only allows for rejecting H 0 but does not allow for accepting it and because researchers erroneously often think that the single published positive study has a very small, acceptable error rate of providing false positive statistically significant results which equals α, or the p -value. So, they often spuriously assume that the negative studies somehow lacked the sensitivity to show an effect while the single positive study is perceived as a well-executed sensitive experiment delivering a “conclusive” verdict rather than being a “lucky” false positive ( Bakan, 1966 ). (See a note on pilot studies in Serious Underestimation of the Proportion of False Positive Findings in NHST).
NHST completely neglects the above mentioned pre-study information and exclusively deals with rows 2–4 of Table 1 . NHST computes the one or two-tailed p -value for a particular data set assuming that H 0 is true. Additionally, NHST logic takes long-run error probabilities (α and β) into account conditional on H 0 and H 1 . These long-run probabilities are represented in typical 2 × 2 NHST contingency tables but note that β is usually unknown in real studies.
As we have seen, NHST never computes the probability of H 0 and H 1 being true or false, all we have is a decision mechanism hoping for the best individual decision in view of long-run Type I and Type II error expectations. Nevertheless, following the repeated testing logic of the NHST framework, for many experiments we can denote the long-run probability of H 0 being true given a statistically significant result as False Report Probability (FRP), and the long-run probability of H 1 being true given a statistically significant result as True Report Probability (TRP). FRP and TRP are represented in row 5 of Table 1 and it is important to see that they refer to completely different conditional probabilities than the p -value.
Simply put, the p -value is pretty much the only thing that NHST computes but scientists usually would like to know the probability of their theory being true or false in light of their data ( Pollard and Richardson, 1987 ; Goodman, 1993 ; Jaynes, 2003 ; Wagenmakers, 2007 ). That is, researchers are interested in the post-experimental probability of H 0 and H 1 . Most probably, for the reason that researchers do not get what they really want to see and the only parameter NHST computes is the p -value it is well-documented ( Oakes, 1986 ; Gliner et al., 2002 ; Castro Sotos et al., 2007 , 2009 ; Wilkerson and Olson, 2010 ; Hoekstra et al., 2014 ) that many, if not most researchers confuse FRP with the p -value or α and they also confuse the complement of p -value (1-p) or α (1-α) with TRP ( Pollard and Richardson, 1987 ; Cohen, 1994 ). These confusions are of major portend because the difference between these completely different parameters is not minor, they can differ by orders of magnitude, the long-run FRP being much larger than the p -value under realistic conditions ( Sellke et al., 2001 ; Ioannidis, 2005 ). The complete misunderstanding of the probability of producing false positive findings is most probably a key factor behind vastly inflated confidence in research findings and we suggest that this inflated confidence is an important contributor to the current replication crisis in biomedical science and psychology.
Serious Underestimation of the Proportion of False Positive Findings in NHST
Ioannidis (2005) has shown that most published research findings relying on NHST are likely to be false. The modeling supporting this claim refers to the long-run FRP and TRP which we can compute by applying Bayes' theorem (see Figure 4 for illustration, see computational details and further illustration in Appendix 3 in Supplementary Material). The calculations must consider α, the power (1-β) of the statistical test used, the pre-study probabilities of H 0 and H 1 , and it is also insightful to consider bias ( Berger, 1985 ; Berger and Delampady, 1987 ; Berger and Sellke, 1987 ; Pollard and Richardson, 1987 ; Lindley, 1993 ; Sellke et al., 2001 ; Sterne and Smith, 2001 ; Ioannidis, 2005 ).
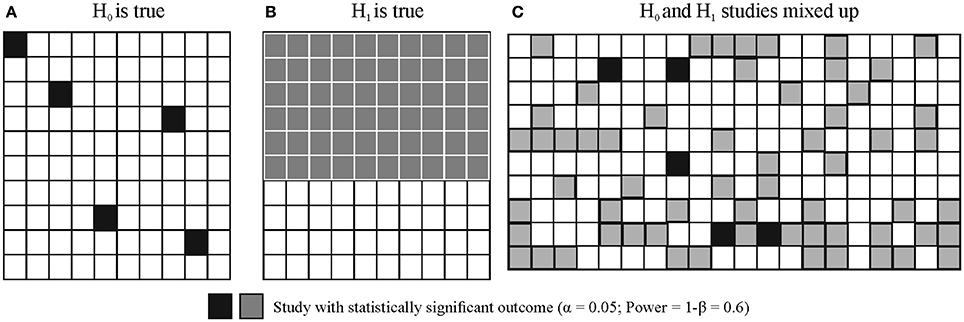
Figure 4 . Illustration of long run False Positive Probability (FRP) and True Positive Probability (TRP) of studies. Let's assume that we run 2 × 100 studies, H 0 is true in 100 studies and H 1 is true in 100 studies with α = 0.05 and Power = 1−β = 0.6. (A) Shows the outcome of true H 0 studies, 5 of the 100 studies coming up statistically significant. (B) Shows the outcome of true H 1 studies, 60 of the 100 studies coming up statistically significant [note that realistically the 60 studies would be scattered around just as in panel (A) but for better visibility they are represented in a block]. (C) Illustrates that true H 0 and true H 1 studies would be indistinguishable. That is, researchers do not know which study tested a true H 0 or true H 1 situation (i.e., they could not distinguish studies represented by black and gray squares). All they know is whether the outcome of a particular study out of the 200 studies run was statistically significant or not. FRP is the ratio of false positive (H 0 is true) statistically significant studies to all statistically significant studies: 5/65 = 0.0769. TRP is the ratio of truly positive (H 1 is true) statistically significant studies to all statistically significant studies: 60/65 = 0.9231 = 1 − FRP = 1 − 0.0769.
While NHST neglects the pre-study odds of H 0 and H 1 , these are crucial to take into account when calculating FRP and TRP. For example, let's assume that we run 200 experiments and in 100 studies our experimental ideas are wrong (that is, we test true H 0 situations) while in 100 studies our ideas are correct (that is, we test true H 1 situations). Let's also assume that the power (1-β) of our statistical test is 0.6 and α = 0.05. In this case in 100 studies (true H 0 ) we will have 5% of results significant by chance alone and in the other 100 studies (true H 1 ) 60% of studies will come up significant. FRP is the ratio of false positive studies to all studies which come up significant:
That is, we will have 5 false positives out of a total of 65 statistically significant outcomes which means that the proportion of false positive studies amongst all statistically significant results is 7.69%, higher than the usually assumed 5%. However, this example still assumes that we get every second hypothesis right. If we are not as lucky and only get every sixth hypothesis right then if we run 600 studies, 500 of them will have true H 0 true situations and 100 of them will have true H 1 situations. Hence, the computation will look like:
Hence, nearly 1/3 of all statistically significant findings will be false positives irrespective of the p -value. Note that this issue is basically the consequence of running multiple NHST tests throughout the whole literature and FRP can be considered the uncontrolled false discovery rate (FDR) across all studies run (see Section Family-Wise Error Rate (FWER) and FDR Correction in NHST).
Crucially, estimating pre-study odds is difficult, primarily due to the lack of publishing negative findings and to the lack of proper documentation of experimenter intentions before an experiment is run: We do not know what percent of the published statistically significant findings are lucky false positives explained post-hoc ( Kerr, 1998 ) when in fact researchers could not detect the originally hypothesized effect and/or worked out analyses depending on the data ( Gelman and Loken, 2014 ). However, it is reasonable to assume that only the most risk avoidant studies have lower H 0 :H 1 odds than 1, relatively conservative studies have low to moderate H 0 :H 1 odds (1–10) while H 0 :H 1 odds can be much higher in explorative research (50–100 or even higher; Ioannidis, 2005 ).
The above H 0 :H 1 assumptions are reasonable, as they are supported by empirical data in many different fields. For example, half or more of the drugs tested in large, late phase III trials show higher effectiveness against older comparators (H 0 :H 1 = <1; Soares et al., 2005 ). Conversely, the vast majority of tested hypotheses in large-scale exploratory research reflect null effects, e.g., in the search of genetic variants associated with various diseases in the candidate gene era where investigators were asking hypotheses one or a few at a time (the same way that investigators continue to test hypotheses in most other biomedical and social science fields) yielded thousands of putative discovered associations, but only 1.2% of them were subsequently validated to be non-null when large-scale consortia with accurate measurements and rigorous analyses plans assessed them ( Chanock et al., 2007 ; Ioannidis et al., 2011 ). Of the hundreds of thousands to many millions of variables assessed in current agnostic–omics testing, much less than 1% are likely to reflect non-null effects (H 0 :H 1 >>100). Lower rates of H 0 :H 1 would be incompatible with logical considerations of how many variables are needed to explain all the variance of a disease or outcome risk.
Besides H 0 :H 1 odds bias is another important determinant of FRP and TRP ( Ioannidis, 2005 ). Whenever, H 0 is not rejected findings have far more difficulty to be published and the researcher may feel that she wasted her efforts. Further, positive findings are more likely to get cited than negative findings ( Kjaergard and Gluud, 2002 ; Jannot et al., 2013 ; Kivimäki et al., 2014 ). Consequently, researchers may often be highly biased to reject H 0 and publish positive findings. Researcher bias affects FRP even if our NHST decision criteria, α and β, are formally unchanged. Ioannidis (2005) introduced the u bias parameter. The impact of u is that after some data tweaking and selective reporting (see Section NHST May Foster Selective Reporting and Subjectivity) u fraction of otherwise non-significant true H 0 results will be reported as significant and u faction of otherwise non-significant true H 1 results will be reported as significant. If u increases, FRP increases and TRP decreases. For example, if α = 0.05, power = 0.6, and H 0 :H 1 odds = 1 then a 10% bias (u = 0.1) will raise FRP to 18.47%. A 20% bias will raise FRP to 26.09%. If H 0 :H 1 odds = 6 then FRP will be 67.92%. Looking at these numbers the replication crisis does not seem surprising: using NHST very high FRP can be expected even with modestly high H 0 :H 1 odds and moderate bias ( Etz and Vandekerckhove, 2016 ). Hence, under realistic conditions FRP not only extremely rarely equals α or the p -value (and TRP extremely rarely equals 1-α and/or 1- p -value) but also, FRP is much larger than the generally assumed 5% and TRP is much lower than the generally assumed 95%. Overall, α or the p -value practically says nothing about the likelihood of our research findings being true or false.
At this point it is worth noting that it could be argued that unpublished pilot experiments may prompt us to run studies and hence, often H 0 :H 1 odds would be lower than 1. However, unpublished pilot data often comes from small scale underpowered studies with high FRP, undocumented initial hypotheses and analysis paths. Hence, we doubt that statistically significant pilot results inevitably mean low H 0 :H 1 odds.
The Neglect of Power Reinterpreted
In contrast to the importance of power in determining FRP and TRP, NHST studies tend to ignore power and β and emphasize α and low p -values. Often, finding a statistically significant effect erroneously seems to override the importance of power. However, statistical significance does not protect us from false positives. FRP can only be minimized by keeping H 0 :H 1 odds and bias low and power high ( Pollard and Richardson, 1987 ; Button et al., 2013 ; Bayarri et al., 2016 ). Hence, power is not only important so that we increase our chances to detect true effects but it is also crucial in keeping FRP low. While power in principle can be adjusted easily by increasing sample size, power in many/most fields of biomedical science and psychology has been notoriously low and the situation has not improved much during the past 50 years ( Cohen, 1962 ; Sedlmeier and Gigerenzer, 1989 ; Rossi, 1990 ; Hallahan and Rosenthal, 1996 ; Button et al., 2013 ; Szucs and Ioannidis, 2017 ). Clearly, besides making sure that research funding is not wasted, minimizing FRP also provides very strong rationale for increasing the typically used sample sizes in studies.
NHST Logic is Incomplete
Nhst misleads because it neglects pre-data probabilities.
Besides often being subject to conceptual confusion and generating misleading inferences especially in the setting of weak power, NHST has further serious problems. NHST logic is based on the so-called modus tollens (denying the consequent) argumentation (see footnote in Appendix 4 in Supplementary Material): It sets up a H 0 model and assumes that if the data fits this model than the test statistic associated with the data should not take more extreme values than a certain threshold ( Meehl, 1967 ; Pollard and Richardson, 1987 ). If the test statistic contradicts this expectation then NHST assumes that H 0 can be rejected and consequently its complement, H 1 can be accepted. While this logic may be able to minimize Type I error in well-powered high-quality well-controlled tests (Section Neyman and Pearson: A decision Mechanism Optimized for the Long-Run), it is inadequate if we use it to decide about the truth of H 1 in a single experiment, because there is always space for Type I and Type II error ( Falk and Greenbaum, 1995 ). So, our conclusion is never certain and the only way to see how much error we have is to calculate the long-run FRP and TRP using appropriate α and power levels and prior H 0 :H 1 odds. The outcome of the calculation can easily conflict with NHST decisions (see Appendix 4 in Supplementary Material).
NHST Neglects Predictions under H 1 Facilitating Sloppy Research
NHST does not require us to specify exactly what data H 1 would predict. Whereas, the Neyman–Pearson approach requires researchers to specify an effect size associated with H 1 and compute power (1-β), in practice this is easy to neglect because the NHST machinery only computes the p -value conditioned on H 0 and it is able to provide this result even if H 1 is not specified at all. A widespread misconception flowing from the fuzzy attitude of NHST to H 1 is that rejecting H 0 allows for accepting a specific H 1 ( Nickerson, 2000 ). This is what most practicing researchers do in practice when they reject H 0 and argue for their specific H 1 in turn. However, NHST only computes probabilities conditional on H 0 and it does not allow for the acceptance of either H 0 , a specific H 1 or a generic H 1 . Rather, it only allows for the rejection of H 0 . Hence, if we reject H 0 we will have no idea about how well our data fits a specific H 1 . This cavalier attitude to H 1 can easily lead us astray even when contrasting H 0 just with a single alternative hypothesis as illustrated by the invalid inference based on NHST logic in Table 2 ( Pollard and Richardson, 1987 ).
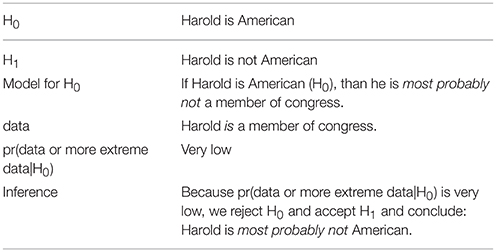
Table 2 . Potential NHST style argument (based on Pollard and Richardson, 1987 ).
Our model says that if H 0 is true, it is a very rare event that Harold is a member of congress. This rare event then happens which is equivalent to finding a small p -value. Hence, we conclude that H 0 can be rejected and H 1 is accepted (i.e., Harold is a member of congress and therefore he is not American.). However, if we carefully explicate all probabilities it is easy to see that we are being mislead by NHST logic. First, because we have absolutely no idea about Harold's nationality we can set pre-data probabilities of both H 1 and H 0 to 1/2, which means that H 0 :H 1 odds are uninformative, 1:1. Then we can explicate the important conditional probabilities of the data (Harold is a member of congress) given the possible hypotheses. We can assign arbitrary but plausible probabilities:
That is, while the data is indeed rare under H 0 , its probability is actually zero under H 1 (in other words, the data is very unlikely under both the null and the alternative models). So, even if p ≈ 0.0000001, it does not make sense to reject H 0 and accept H 1 because this data just cannot happen if H 1 is true. If we only have these two hypotheses to choose from then it only makes sense to accept H 0 because the data is still possible under H 0 ( Jaynes, 2003 ). In fact, using Bayes' theorem we can formally show that the probability of H 0 is actually 1 (Appendix 5 in Supplementary Material).
In most real world problems multiple alternative hypotheses compete to explain the data. However, by using NHST we can only reject H 0 and argue for some H 1 without any formal justification of why we prefer a particular hypothesis whereas it can be argued that it only makes sense to reject any hypothesis if another one better fits the data ( Jaynes, 2003 ). We only have qualitative arguments to accept a specific H 1 and the exclusive focus on H 0 makes unjustified inference too easy. For example, if we assume that H 0 predicts normally distributed data with mean 0 and standard deviation 1 then we have endless options to pick H 1 ( Hubbard and Bayarri, 2003 ): Does H 1 imply that the data have a mean other than zero, the standard deviation other than 1 and/or does it represent non-normally distributed data? NHST allows us to consider any of these options implicitly and then accept one of them post-hoc without any quantitative justification of why we chose that particular option. Further, merging all alternative hypotheses into a single H 1 is not only too simplistic for most real world problems but it also poses an “inferential double standard” ( Rozeboom, 1960 ): The procedure pits the well-defined H 0 against a potentially infinite number of alternatives.
Vague H 1 definitions (the lack of quantitative predictions) enable researchers to avoid the falsification of their favorite hypotheses by intricately redefining them (especially in fields such as psychology and cognitive neuroscience where theoretical constructs are often vaguely defined) and never providing any definitive assessment of the plausibility of a favorite hypothesis in light of credible alternatives ( Meehl, 1967 ). This problem is reflected in papers aiming at the mere demonstration of often little motivated significant differences between conditions ( Giere, 1972 ) and post-hoc explanations of likely unexpected but statistically significant findings. For example, neuroimaging studies often attempt to explain why an fMRI BOLD signal “deactivation” happened instead of a potentially more reasonable looking “activation” (or, vice versa). Most such findings may be the consequence of the data randomly deviating into the wrong direction relative to zero between-condition difference. Even multiple testing correction will not help such studies as they still rely on standard NHST just with adjusted α thresholds. Similarly, patient studies often try to explain an unexpected difference between patient and control groups (e.g., the patient group is “better” on a measure or shows “more” or “less” brain activation) by some kind of “compensatory mechanism.” In such cases what happens is that “ the burden of inference has been delegated to the statistical test ,” indeed, and simply because p ≤ α odd looking observations and claims are to be trusted as scientific facts ( Bakan, 1966 , p. 423; Lykken, 1968 ).
Finally, paradoxically, when real life practicing researchers achieve their “goal” and successfully reject H 0 they may be left in complete existential vacuum because during the rejection of H 0 NHST “ saws off its own limb” ( Jaynes, 2003 ; p. 524): If we manage to reject H 0 then it follows that pr(data or more extreme data|H 0 ) is useless because H 0 is not true. Thus, we are left with nothing to characterize the probability of our data in the real world; we will not know pr(data|H 1 ) for example, because H 1 is formally undefined and NHST never tells us anything about it. In light of these problems Jaynes (2003) suggested that the NHST framework addresses an ill-posed problem and provides invalid responses to questions of statistical inference.
It is noteworthy that some may argue that Jaynes's argument is formally invalid as the NHST approach can be used to reject a low probability H 0 in theory . However, recall that (1) NHST does not deliver final objective theoretical decisions, there is no theoretical justification for any α thresholds marking a boundary of informal surprise and NHST merely aims to minimize Type I error on the long run (and in fact, Neyman and Pearson (1933) considered their procedure a theory-free decision mechanism and Fisher considered it a heuristic). (2) NHST can only reject H 0 (heuristically or in a theory-free manner) and (3) cannot provide support for any H 1 . We could also add that many practicing biomedical and social scientists may not have clear quantitative predictions under H 0 besides expecting to reject a vague null effect (see Section NHST in Sciences with and without Exact Quantitative Predictions for the difference between sciences with and without exact predictions). Hence, their main (ultimate) objective of using NHST is often actually not the falsification of the exact theoretical predictions of a well-defined theory (H 0 ). Rather, they are more interested in arguing in favor of an alternative theory. For example, with a bit of creativity fMRI “activation” in many different (perhaps post-hoc defined) ROIs can easily be “explained” by some theory when H 0 (“no activation”) is rejected in any of the ROIs. However, supporting a specific alternative theory is just not possible in the NHST framework and in this context Jaynes' comment is perfectly valid: NHST provides an ill-defined framework, after rejecting H 0 real-world researchers have no formal hypothesis test outcomes to support their “positive” arguments.
NHST Is Unsuitable for Large Datasets
In consequence of the recent ‘big data’ revolution access to large databases has increased dramatically potentially increasing power tremendously (though, large data sets with many variables are still relatively rare in neuroscience research). However, NHST leads to worse inference with large databases than with smaller ones ( Meehl, 1967 ; Khoury and Ioannidis, 2014 ). This is due to how NHST tests statistics are computed, the properties of real data and to the lack of specifying data predicted by H 1 ( Bruns and Ioannidis, 2016 ).
Most NHST studies rely on nil null hypothesis testing ( Nickerson, 2000 ) which means that H 0 expects a true mean difference of exactly zero between conditions with some variation around this true zero mean. Further, NHST machinery guarantees that we can detect any tiny irrelevant effect sizes if sample size is large enough. This is because test statistics are typically computed as the ratio of the relevant between condition differences and associated variability of the data weighted by some function of the sample size [difference/variability × f(sample size)]. The p -value is smaller if the test statistic is larger. Thus, the larger is the difference between conditions and/or the smaller is variability and/or the larger is the sample size the larger is the test statistic and the smaller is the p -value (see Figure 3 for examples). Consequently, by increasing sample size enough it is guaranteed that H 0 can be rejected even with miniature effect sizes ( Ziliak and McCloskey, 2008 ).
Parameters of many real data sets are much more likely to differ than to be the same for reasons completely unrelated to our hypotheses ( Meehl, 1967 , 1990 ; Edwards, 1972 ). First, many psychological, social and biomedical phenomena are extremely complex reflecting the contribution of very large numbers of interacting (latent) factors, let it be at the level of society, personality or heavily networked brain function or other biological networks ( Lykken, 1968 ; Gelman, 2015 ). Hence, if we select any two variables related to these complex networks most probably there will be some kind of at least remote connection between them. This phenomenon is called “crud factor” Meehl (1990) or “ambient correlational noise” ( Lykken, 1968 ) and it is unlikely to reflect a causal relationship. In fact some types of variables, such as intake of various nutrients and other environmental exposures are very frequently correlated among themselves and with various disease outcomes without this meaning that they have anything to do with causing disease outcomes ( Patel and Ioannidis, 2014a , b ). Second, unlike in physical sciences it is near impossible to control for the relationship of all irrelevant variables which are correlated with the variable(s) of interest ( Rozeboom, 1960 ; Lykken, 1968 ). Consequently, there can easily be a small effect linking two randomly picked variables even if their statistical connection merely communicates that they are part of a vast complex interconnected network of variables. Only a few of these tiny effects are likely to be causal and of any portend ( Siontis and Ioannidis, 2011 ).
The above issues have been demonstrated empirically and by simulations. For example, Bakan (1966 ; see also Berkson, 1938 ; Nunnally, 1960 ; Meehl, 1967 ) subdivided the data of 60,000 persons according to completely arbitrary criteria, like living east or west of the Mississippi river, living in the north or south of the USA, etc. and found all tests coming up statistically significant. Waller (2004) examined the personality questionnaire data of 81,000 individuals to see how many randomly chosen directional null hypotheses can be rejected. If sample size is large enough, 50% of directional hypothesis tests should be significant irrespective of the hypothesis. As expected, nearly half (46%) of Waller's (2004) results were significant. Simulations suggest that in the presence of even tiny residual confounding (e.g., some omitted variable bias) or other bias, large observational studies of null effects will generate results that may be mistaken as revealing thousands of true relationships ( Bruns and Ioannidis, 2016 ). Experimental studies may also suffer the same problem, if they have even minimal biases.
NHST in Sciences with and without Exact Quantitative Predictions
Due to the combination of the above properties of real-world data sets and statistical machinery theory testing radically differs in sciences with exact and non-exact quantitative predictions ( Meehl, 1967 ). In physical sciences increased measurement precision and increased amounts of data increase the difficulties a theory must pass before it is accepted. This is because theoretical predictions are well-defined, numerically precise and it is also easier to control measurements ( Lykken, 1968 ). Hence, NHST may be used to aim to falsify exact theoretical predictions. For example, a theory may predict that a quantity should be let's say 8 and the experimental setup can assure that really only very few factors influence measurements—these factors can then be taken into account during analysis. Hence, increased measurement precision will make it easier to demonstrate a departure from numerically exact predictions. So, a “five sigma” deviation rule may make good sense in physics where precise models are giving precise predictions about variables.
In sciences using NHST without clear numerical predictions the situation is the opposite of the above, because NHST does not demand the exact specification of H 1 , so theories typically only predict a fairly vague “ difference” between groups or experimental conditions rather than an exact numerical discrepancy between measures of groups or conditions. However, as noted, groups are actually likely to differ and if sample size increases and variability in data decreases it will become easier and easier to reject any kind of H 0 when following the NHST approach. In fact, with precise enough measurements, large enough sample size and repeated “falsification” attempts H 0 is guaranteed to be rejected on the long run (see Section The Rejection of H 0 is Guaranteed on the Long-Run) even if the underlying processes generating the data in two experimental conditions are exactly the same. Hence, ultimately any H 1 can be accepted, claiming support for any kind of theory. For example, in an amusing demonstration Carver (1993) used Analysis of Variance to re-analyze the data of Michaelson and Morley (1887) who came up with a “dreaded” null finding and based on this they suggested that the speed of light was constant (H 0 ) thereby providing empirical support for Einstein's theory of relativity. Carver (1993) found that that the speed of light was actually not constant at p < 0.001. The catch? The effect size as measured by Eta 2 was 0.005. While some may feel that Einstein's theory has now been falsified, perhaps it is also worth considering that here the statistically significant result is essentially insignificant. This example also highlights the fact we are not arguing against the Popperian view of scientific progress by falsifying theories. Rather, we discuss why NHST is a very imperfect method for this falsification (see further arguments as well).
A typical defense of NHST may be that we actually may not want to increase power endlessly, just as much as we still think that it allows us to detect reasonable effect sizes ( Giere, 1972 ). For example, equivalence testing may be used to reject the hypothesis that a meaningfully large effect exist (e.g., Wellek, 2010 ) or researchers may check for the sign of expected effects ( Gelman and Tuerlinckx, 2000 ). However, because typically only statistically significant data is published, published studies most probably exaggerate effect sizes. So, estimating true (expected) effect sizes is very difficult. A more reasoned approach may be to consider explicitly what the consequences (“costs”) are of a false-positive, true-positive, false-negative, and true-negative result. Explicit modeling can suggest that the optimal combination of Type 1 error and power may need to be different depending on what these assumed costs are ( Djulbegovic et al., 2014 ). Different fields may need to operate at different optimal ratios of false-positives to false-negatives ( Ioannidis et al., 2011 ).
NHST May Foster Selective Reporting and Subjectivity
Because NHST never evaluates H 1 formally and it is fairly biased toward the rejection of H 0 , reporting bias against H 0 can easily infiltrate the literature even if formal NHST parameters are fixed (see Section Serious Underestimation of the Proportion of False Positive Findings in NHST about the “u” bias parameter). Overall, a long series of exploratory tools and questionable research practices are utilized in search for statistical significance ( Ioannidis and Trikalinos, 2007 ; John et al., 2012 ). Researchers can influence their data during undocumented analysis and pre-processing steps and by the mere choice of structuring the data (constituting researcher degrees of freedom ; Simmons et al., 2011 ). This is particularly a problem in neuroimaging where the complexity and idiosyncrasy of analyses is such that it is usually impossible to replicate exactly what happened and why during data analysis ( Kriegeskorte et al., 2009 ; Vul et al., 2009 ; Carp, 2012 ). Another term that has been used to describe the impact of diverse analytical choices is “vibration of effects” ( Ioannidis, 2008 ). Different analytical options, e.g., choice of adjusting covariates in a regression model can result in a cloud of results, instead of a single result, and this may entice investigators to select a specific result that is formally significant, while most analytical options would give non-significant results or even results with effects in the opposite direction (“Janus effect”; Patel et al., 2015 ). Another common mechanism that may generate biased results with NHST is when investigators continue data collection and re-analyse the accumulated data sequentially without accounting for the penalty induced by this repeated testing ( DeMets and Lan, 1994 ; Goodman, 1999 ; Szucs, 2016 ). The unplanned testing is usually undocumented and researchers may not even be conscious that it exposes them to Type I error accumulation. Bias may be the key explanation why in most biomedical and social science disciplines, the vast majority of published papers with empirical data report statistically significant results ( Kavvoura et al., 2007 ; Fanelli, 2010 ; Chavalarias et al., 1990-2015 ). Overall, it is important to see that NHST can easily be infiltrated by several undocumented subjective decisions. (Bayesian methods are often blamed such subjectivity, see Section Teach Alternative Approaches Seriously.)
The Rejection of H 0 Is Guaranteed on the Long-Run
If H 0 is true, with α = 0.05, 5% of our tests will be statistically significant on the long-run. The riskier experiments we run, the larger are H 0 :H 1 odds and bias and the larger is the long-run FRP. For example, in a large laboratory with 20 post-docs and PhD students, each person running 5 experiments a year implementing 10 significance tests in each experiment we can expect 20 × 5 × 10 × 0.05 = 50 [usually publishable] false results a year at α = 0.05 if H 0 is true. Coupled with the fact that a large number of unplanned tests may be run in each study ( Simmons et al., 2011 ; Gelman and Loken, 2014 ) and that negative results and failed replications are often not published, this leads to “ unchallenged fallacies” clogging up the research literature ( Ioannidis, 2012 ; p1; Sterling, 1959 ; Bakan, 1966 ; Sterling et al., 1995 ). Moreover, such published false positive true H 0 studies will also inevitably overestimate the effect size of the non-existent effects or of existent, but unimportantly tiny, effects ( Schmidt, 1992 , 1996 ; Sterling et al., 1995 ; Ioannidis, 2008 ). These effects may even be confirmed by meta-analyses, because meta-analyses typically are not able to incorporate unpublished negative results ( Sterling et al., 1995 ) and they cannot correct many of the biases that have infiltrated the primary studies. For example, such biases may result in substantial exaggeration of measured effect sizes in meta-analyses (see e.g., Szucs and Ioannidis, 2017 ).
Given that the predictions of H 1 are rarely precise and that theoretical constructs in many scientific fields (including psychology and cognitive neuroscience) are often poorly defined ( Pashler and Harris, 2012 ), it is easy to claim support for a popular theory with many kinds of data falsifying H 0 even if the constructs measured in many papers are just very weakly linked to the original paper, or not linked at all. Overall, the literature may soon give the impression of a steady stream of replications throughout many years. Even when “negative” results appear, citation bias may still continue to distort the literature and the prevailing theory may continue to be based on the “positive” results. Hence, citation bias may maintain prevailing theories even when they are clearly false and unfounded ( Greenberg, 2009 ).
NHST Does Not Facilitate Systematic Knowledge Integration
Due to high FRP the contemporary research literature provides statistically significant “evidence” for nearly everything ( Schoenfeld and Ioannidis, 2012 ). Because NHST emphasizes all or none p -value based decisions rather than the magnitude of effects, often only p -values are reported for critical tests, effect size reports are often missing and interval estimates and confidence intervals are not reported. In an assessment of the entire biomedical literature in 1990–2015, 96% of the papers that used abstracts reported at least some p -value below 0.05, while only 4% of a random sample of papers presented consistently effect sizes with confidence intervals ( Chavalarias et al., 1990-2015 ). However, oddly enough, the main NHST “measure of evidence,” the p -value cannot be compared across studies. It is a frequent misconception that a lower p -value always means stronger evidence irrespective of the sample size and effect size ( Oakes, 1986 ; Schmidt, 1996 ; Nickerson, 2000 ). Besides the non-comparable p -values, NHST does not offer any formal mechanism for systematic knowledge accumulation and integration ( Schmidt, 1996 ) unlike Bayesian methods which can take such pre-study information into account. Hence, we end up with many fragmented studies which are most often unable to say anything formal about their favorite H 1 s (accepted in a qualitative manner). Methods do exist for the meta-analysis of p -values (see e.g., Cooper et al., 2009 ) and these are still used in some fields. However, practically such meta-analyses still say nothing about the magnitude of the effect size of the phenomenon being addressed. These methods are potentially acceptable when the question is whether there is any non-null signal among multiple studies that have been performed, e.g., in some types of genetic associations where it is taken for granted that the effect sizes are likely to be small anyhow ( Evangelou and Ioannidis, 2013 ).
Family-Wise Error Rate (FWER) and FDR Correction in NHST
An increasingly important problem is that with the advent of large data sets researchers can use NHST to test multiple, related hypotheses. For example, this problem routinely appears in neuro-imaging where a large amount of non-independent data points are collected and then the same hypothesis test may be run on tens of thousands of observations, for example, from a brain volume, or from 256 electrodes placed on the scalp, each electrode recording voltage 500 times a second. Analysis procedures that generate different views of data (e.g., time-frequency or independent component analyses) may further boost the amount of tests to be run.
Regarding these multiple testing situations, a group of statistical tests which are somehow related to each other can be defined as a “family of comparisons.” The probability that a family of comparisons contains at least one false positive error is called the family wise error rate (FWER). If the repeated tests concern independent data sets where H 0 is true than the probability of having at least one Type I error in k independent tests, each with significance level α, is α TOTAL = 1 - (1 - α) k . For example if k = 1, 2, 3, 4, 5, and 10 than α TOTAL is 5, 9.75, 14.26, 18.55, 22.62, and 40.13%, respectively (see Curran-Everett, 2000 ; Szucs, 2016 for graphical illustrations and simulations for non-independent data).
There are numerous procedures which can take multiple testing into account by correcting p -values. The simplest of these procedures is Bonferroni correction which computes an adjusted p -value threshold as α/n where α is the statistical significance threshold for a single test and n is the number of tests run. Hence, if we run 5 tests which can be defined as a family of tests and our original α is 0.05 then the Bonferroni corrected adjusted α level is 0.05/5 = 0.01. Any p -values above this threshold should not be considered to demonstrate statistically significant effects. Besides the Bonferroni correction there are other alternative methods of FWER correction, like the Tukey Honestly Significant Difference test, the Scheffe test, Holm's method, Sidak's method; Hochberg's method, etc. Some of these corrections also take the dependency (non-independence) of tests into account (see e.g., Shaffer, 1995 ; Nichols and Hayasaka, 2003 for review).
FWER control is a conservative procedure in keeping Type I error rate low but it also sacrifices power increasing Type II error. An alternative to FWER control is False Discovery Rate (FDR) control which allows more Type I errors but assures higher power. Using the same logic as the computation of FRP discussed before, FDR control considers the estimated proportion of false positive statistically significant findings amongst all statistically significant findings (i.e., the proportion of erroneously rejected null hypotheses out of all rejected null hypotheses; Benjamini and Hochberg, 1995 ). FDR computation is illustrated by Table 3 . If we run M hypothesis tests then a certain number of them are likely to test true null effects (M 0 in Table 3 ) and some other number of them are likely to test non-null effects with true alternative hypotheses (M 1 in Table 3 ). Depending on our α level and power (1-β), a certain number of the M 0 and M 1 tests will reject the null hypothesis (FP and TN, respectively, see Table 3 for abbreviations) while some other number of them will not reject the null hypothesis (TN and FN, respectively). If we know the exact numbers in Table 3 then the proportion of false positive statistically significant findings can be computed as the ratio of false positive results to all statistically significant results: Q = FP/(FP + TP) = FP/R (assuming that R≠ 0).
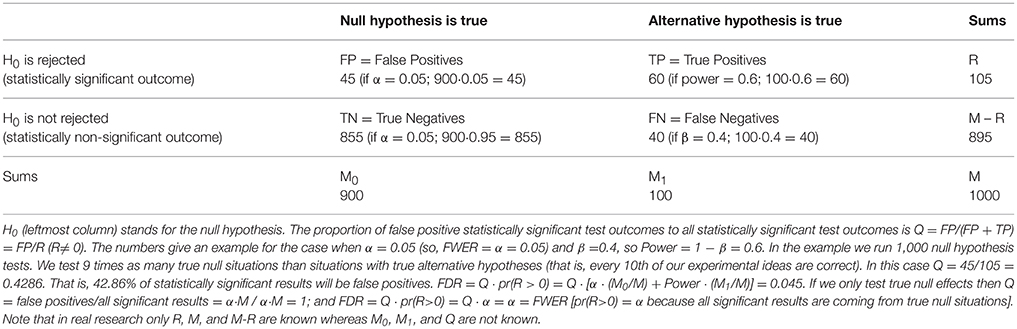
Table 3 . Illustrating the logic behind FDR computation.
Of course, in real research settings we do not know how many of our tests test true null effects and we only know how many tests we run and how many of them return statistically significant and non-significant results. So Q can be considered a random variable. However, as Q cannot be controlled directly FDR is defined as the expected value of the proportion of false positive errors: FDR = E[FP/R|R > 0] · pr(R > 0), a variable which can be controlled (see Benjamini and Hochberg, 1995 ; Curran-Everett, 2000 ; Nichols and Hayasaka, 2003 ; Bennett et al., 2009 ; Benjamini, 2010 ; Goeman and Solari, 2014 ). Some FDR estimation procedures can also factor in dependency between tests ( Benjamini and Yekutieli, 2001 ).
In contrast to FDR, using the notation in Table 3 , FWER can be expressed as FWER = pr(FP≥1) = 1 − pr(FP = 0) that is, the probability that there is at least one false positive Type I error in a family of observations. If the null hypothesis is true in all tests we run then FDR = FWER while if there are situations with true alternative hypotheses then FDR < FWER (see Table 3 for example). Also, various other FDR and FWER measures can be derived (see the above cited reviews). It can be argued that controlling FDR is more useful in research where a very large number of tests are carried out routinely, like neuro-imaging or genetics but less useful in behavioral psychological and social science research where fewer hypotheses may be tested at any one time and accepting any single hypothesis as statistically significant may have large impact on inferences ( Gelman et al., 2012 ). This last statement is also true for behavioral data used to support the interpretation of neuro-imaging findings.
Most relevant to our paper, both FWER and FDR error rate corrections are based on the same NHST procedure. That is, they do not modify the procedure in any ways other than aiming to decrease Type I error toward initially expected levels when multiple NHST tests are run. That is, these methods can help in constraining the number of random findings when a single hypothesis is tested simultaneously in many data points (e.g., voxels) but do nothing to protect against many of the other problems discussed in this paper (e.g., generating a high amount of false positives across the literature; being sensitive to undocumented biasing procedures; neglecting predictions under H 1 ; not providing probability statements for H 1 ; neglecting pre-data probabilities; being unable to effectively integrate study results). These problems are valid even when just one single NHST test is run. In addition, empirical analyses of large fMRI data sets found that the most popular fMRI analysis software packages implemented erroneous multiple testing corrections and hence, generate much higher levels of false positive results than expected ( Eklund et al., 2012 , 2016 ). This casts doubts on a substantial part of the published fMRI literature. Further, Carp (2012) reported that about 40% of 241 relatively recent fMRI papers actually did not report having used multiple testing correction. So, a very high percentage of fMRI literature may have been exposed to high false positive rates either multiple correction was used or not (see also ( Szucs and Ioannidis, 2017 ) on statistical power).
In the NHST framework the multiple comparison problem is exacerbated by the fact that we may test a very large number of precise null hypotheses ( Neath and Cavanaugh, 2006 ), often without much theoretical justification (e.g., in many explorative whole brain analyses). However, as the H 0 :H 1 odds may be high the NHST mechanism may produce a very large number of falsely significant results. Similarly high numbers of false alarms are produced under realistic conditions even if some rudimentary model is used for the data (e.g., expecting positive or negative difference between conditions; Gelman and Tuerlinckx, 2000 ). In contrast, while currently there is no standard way to correct for multiple comparisons with Bayesian methods, Bayesian methods have been shown to be more conservative than NHST in some situations ( Gelman and Tuerlinckx, 2000 ) and they offer various methods for correcting for multiple comparisons (e.g., Westfall et al., 1997 ; Gelman et al., 2014 ). In addition, Bayesian methods are strongly intertwined with explicit model specifications. These models can then be used to generate simulated data and to study model response behavior. This may offer a way to judge the reasonableness of analyses offering richer information than NHST accept/reject decisions ( Gelman et al., 2014 ). The challenge of course is the development of models. However, below we argue that efficient model development can only happen if we refocus our efforts on understanding data patters from the testing of often very vaguely defined hypotheses. In addition, Bayesian methods are also able to formally aggregate data from many experiments (e.g., adding data serially and by hierarchical models; Gelman et al., 2014 ). This can further maximize large-scale joint efforts for better model specifications.
The State of the Art Must Change
Nhst is unsuitable as the cornerstone of scientific inquiry in most fields.
In summary, NHST provides the illusion of certainty through supposedly ‘objective’ binary accept/reject decisions ( Cohen, 1994 ; Ioannidis, 2012 ) based on practically not very useful p -values ( Bakan, 1966 ). However, researchers usually never give any formal assessment of how well their theory (a specific H 1 ) fits the facts and, instead of gradual model building ( Gigerenzer, 1998 ) and comparing the plausibility of theories, they can get away with destroying a strawman: they disprove an H 0 (which happens inevitably sooner or later) with a machinery biased to disproving it without ever going into much detail about the exact behavior of variables under exactly specified hypotheses ( Kranz, 1999 ; Jaynes, 2003 ). NHST also does not allow for systematic knowledge accumulation. In addition, both because of its shortcomings and because it is subject to major misunderstandings it facilitates the production of non-replicable false positive reports. Such reports ultimately erode scientific credibility and result in wasting perhaps most of the research funding in some areas ( Ioannidis, 2005 ; Macleod et al., 2014 ; Kaplan and Irvin, 2015 ; Nosek et al., 2015 ).
NHST seems to dominate biomedical research for various reasons. First, it allows for the easy production of a large number of publishable papers (irrespective of their truth value) providing a response to publication pressure. Second, NHST seems deceptively simple: because the burden of inference ( Bakan, 1966 ) has been delegated to the significance test all too often researchers' statistical world view is narrowed to checking an inequality: is p ≤ 0.05 ( Cohen, 1994 )? After passing this test, an observation can become a “scientific fact” contradicting the random nature of statistical inference ( Gelman, 2015 ). Third, in biomedical and social science NHST is often falsely perceived as the single objective approach to scientific inference ( Gigerenzer et al., 1989 ) and alternatives are simply not taught and/or understood.
We have now decades of negative experience with NHST which gradually achieved dominance in biomedical and social science since the 1930s ( Gigerenzer et al., 1989 ). Critique of NHST started not much later ( Jeffreys, 1939, 1948, 1961 ) and has been forcefully present since then ( Jeffreys, 1939, 1948, 1961 ; Eysenck, 1960 ; Nunnally, 1960 ; Rozeboom, 1960 ; Clark, 1963 ; Bakan, 1966 ; Meehl, 1967 ; Lykken, 1968 ) and continues to-date ( Wasserstein and Lazar, 2016 ). The problems are numerous, and as Edwards (1972 , p. 179) concluded 44 years ago: “ any method which invites the contemplation of a null hypothesis is open to grave misuse, or even abuse .” Time has proven this statement and that problems are unlikely to go away. We suggest that that it is really time for change now.
When and How to Use NHST
Importantly, we do not want to ban NHST ( Hunter, 1997 ), we realize that it may be reasonable to use it in some well-justified cases. In all cases when NHST is used its use must be justified clearly rather than used as an automatic default and single cornerstone procedure. On the one hand, NHST can be used when very precise quantitative theoretical predictions can be tested, hence, both power and effect size can be estimated well as intended by Neyman and Pearson (1933) . On the other hand, when theoretical predictions are not precise, reasonably powered NHST tests may be used as an initial heuristic look at the data as Fisher (1925) intended. However, in these cases (when well-justified theoretical predictions are lacking) if studies are not pre-registered (see below) NHST tests can only be considered preliminary (exploratory) heuristics. Hence, their findings should only be taken seriously if they are replicated, optimally within the same paper ( Nosek et al., 2013 ). These replications must be well powered to keep FRP low. As discussed, NHST can only reject H 0 and can accept neither a generic or specific H 1 . So, on its own NHST cannot provide evidence “for” something even if findings are replicated.
For example, if initially researches do not know where to expect experimental effects in a particular experimental task, they could run a whole brain, multiple-testing corrected search for statistical significance in a group of participants. Such a search would provide heuristic evidence if they identify some brain areas reacting to manipulations. In order to confirm these effects they would need to carefully study for example the BOLD signal or EEG amplitude changes in areas or over electrodes of interest, make predictions about the behavior of these variables, replicate measurements and minimally confirm the previous NHST results before the findings can be taken seriously. Much better, if researchers can also provide some model for the behavior of their variables, make model predictions and then confirm these with likelihood-based and/or Bayesian methods. Making such predictions would probably require intimate familiarity with a lot of raw data.
Ways to Change
In most biomedical, neuroscience, psychology, and social science fields currently popular analysis methods are based on NHST. It is clear that analysis software and researcher knowledge cannot be changed overnight. Below we summarize some further recommendations which we think can minimize the negative features of NHST even if it continues to be dominant for a while. A very important practical goal would be to change the incentive structure of biomedical and social science to bring it in line with these and similar other recommendations ( Wagenmakers et al., 2011 ; Begley and Ellis, 2012 ; Nosek et al., 2013 ; Stodden et al., 2016 ). Also note that we are not arguing against statistical inference which we consider the “logic of science” ( Jaynes, 2003 ; p. xxii.), quantitative and well justified statistical inference should be at the core of the scientific enterprise.
If Theory Is Weak, Focus on Raw Data, Estimating Effect Sizes, and Their Uncertainty
The currently dominant, NHST influenced approach is that instead of understanding raw data researchers often just focus on the all or nothing rejection of a vaguely defined H 0 and shift their attention to interpreting brain “activations” revealed by potentially highly misleading statistical parameter maps. Based on these maps then strong (qualitative) claims may be made about alternative theories whose support may in fact never be tested. So, current approaches seem to reward exuberant theory building based on small and underpowered studies ( Szucs and Ioannidis, 2017 ) much more than meticulous data collection and understanding and modeling extensive raw data patterns. For analogy, in astronomy theories typically built on thousands of years of sky observation data open to everyone. For example, Kepler could identify the correct laws of planetary motion because he had access to the large volume of observational data accumulated by Tycho Brache who devoted decades of his life to much more precise data collection than previously done. Similarly, the crucial tests of Einstein's theories were precise predictions about data which could be verified or falsified ( Smolin, 2006 ; Chaisson and McMillan, 2017 ).
Overall, if we just consider competing “theories” without ever deeply considering extensive raw data patterns it is unlikely that major robust scientific breakthroughs will be done whereas many different plausible looking theories can be promoted. Imagine, for example, a situation where astronomers would have only published the outcomes of their NHST tests, some rejecting that the sun is in the middle of the universe while others rejecting that the earth is in the middle of the universe while publishing no actual raw data. Meta-analyses of published effect sizes would have confirmed both positions as both camps would have only published test statistics which passed the statistical significance threshold. Luckily, real astronomers recorded a lot of data and derived testable theories with precise predictions.
In basic biomedical and psychology research we often cannot provide very well worked out hypotheses and even a simple directional hypothesis may seem particularly enlightening. Such rudimentary state of knowledge can be respected. However, in such pre-hypothesis stage substantively blind all or nothing accept/reject decisions may be unhelpful and may maintain our ignorance rather than facilitate organizing new information into proper quantitative scientific models. It is much more meaningful to focus on assessing the magnitude of effects along with estimates of uncertainty, let these be error terms, confidence intervals or Bayesian credible intervals ( Edwards, 1972 ; Luce, 1988 ; Schmidt, 1996 ; Jaynes, 2003 ; Gelman, 2013a , b ; see Morey et al., 2016 on the difference between classical confidence intervals and Bayesian credible intervals). These provide more direct information on the actual “empirical” behavior of our variables and/or the precision of interval estimation. Gaining enough experience with interval estimates and assuring their robustness by building replication into design ( Nosek et al., 2013 ) may then allow us to describe the behavior of variables by more and more precise scientific models which may provide more clear predictions ( Schmidt, 1996 ; Jaynes, 2003 ; Gelman, 2013a , b ).
The above problem does not only concern perceived “soft areas” of science where measurement, predictions, control and quantification are thought to be less rigorous than in “hard” areas ( Meehl, 1978 ). In many fields, for example, in cognitive neuroscience, the measurement methods may be “hard” but theoretical predictions and analysis often may be just as “soft” as in any area of “soft” psychology: Using a state of the art fMRI scanner for data collection and novel but extremely complicated and often not well understood analysis paths will not make a badly defined theory well-defined.
The change of emphasis suggested here would require that instead of p -values and reporting the outcomes of all/nothing hypothesis tests studies should focus on reporting data in original units of measurement as well as providing derived effect sizes. It is important to publish data summaries (means, standard errors, nowadays extremely rarely plotted empirical data distributions) in original units of measurement as derived measures may be highly biased by some (undocumented) analysis techniques. If we have clear and pre-registered hypotheses then it is relatively straightforward to publish raw data summaries (e.g., mean BOLD signal or ERP amplitude change with standard errors) related to those hypotheses. Clear data presentation usually gets difficult when there are lots of incidental findings. Usually unlimited amount of data summaries can now be published cost free in Supplementary Materials.
Pre-registration
In our view one of the most important and virtually cost-free (to researchers) improvement would be to pre-register hypotheses and analysis parameters and approaches (in line with Section 5.2 in Nichols et al., 2016 ; p11; Gelman and Loken, 2014 ). Pre-registration can easily be done for example, at the website of the Open Science Foundation (osf.org), also in a manner that it does not immediately become public. Hence, competitors will not be able to scoop good ideas before the study is published. Considering the extreme analysis flexibility offered by high-dimensional neuroscience data ( Kriegeskorte et al., 2009 ; Vul et al., 2009 ; Carp, 2012 ) pre-registration seems a necessary pre-condition of robust hypothesis driven neuroscience research. Pre-registration would likely help to cleanse non-replicable “unchallenged fallacies” ( Ioannidis, 2012 ) from the literature. For example, Kaplan and Irvin (2015) found that pre-registering the primary hypotheses of clinical studies decreased the proportion of positive findings from 57% (17 of 30 studies) to 8% (2 out of 25 studies). Hence, another benefit of pre-registration would be to decrease publication volumes. This would require changing the incentive system motivating scientists ( Nosek et al., 2013 ).
It is to note that honesty regarding pre-registration and challenging questionable research practices ( Simmons et al., 2011 ) is the shared responsibility of all co-authors. Some fields in medical research have already over 10 years of experience with pre-registration. This experience shows that pre-registration needs to be thorough to be reliable. For example, many observational studies claimed to have been registered but closer scrutiny shows that registration has actually happened after the study/analysis was done ( Boccia et al., 2016 ). In other cases, clinical trials may be seemingly properly pre-registered before they start recruiting patients, but analyses and outcomes were still manipulated after registration ( Ioannidis et al., 2017 ). Hence, proper safeguards should be put in place to ensure that scientists are accountable for any misconduct regarding breaching pre-registration rules.
Publish All Analysis Scripts with Analysis Settings
Another cost-free improvement is to publish all analysis scripts with the ability to regenerate all figures and tables ( Laine et al., 2007 ; Peng, 2009 , 2011 ; Diggle and Zeger, 2010 ; Keiding, 2010 ; Doshi et al., 2013 ). This does not require large storage space and can also be done in Supplementary Material. If researchers keep this expectation in mind from the start of a project then implementing it becomes relatively straightforward. Program code will often provide information which is missing from papers. With regard to missing analysis information it is important to be conscious of the fact that seemingly innocuous and irrelevant analysis settings (e.g., slightly changing initial filtering parameters) can have major impact on final statistical outcomes at the end of a complicated processing pipeline. For example, modified initial settings may change the statistically significant/non-significant status of final important test statistics. This can be an issue if multiple settings can be justified and/or if some settings leading to significant outcomes are actually less justified than alternative settings. Publishing scripts will also provide more information on potential statistical errors ( Bakker and Wicherts, 2001 ; Nuijten et al., 2016 ).
It is to note that in-house analysis scripts may provide substantial competitive advantage to researchers who are able to programme these. Hence, the unconditional release of these scripts may deprive researchers from an important competitive asset. In such cases in house scripts could be documented in brief methods papers which could be cited when the relevant scripts are used so that researchers benefit from citations. Perhaps specialized methods repository journals could be set up for this purpose. For some recent recommendations for improving computational reproducibility practices see Stodden et al. (2016) .
Publish Raw Data
In an ideal world researchers should publish all raw data. This is easy with small volumes of behavioral data but it has serious monetary and time investment costs with large neural data volumes (see also Nichols et al., 2017 ). Some repositories have already been set up and it is important that funders cover these costs and optimally provide infrastructure (see Pernet and Poline, 2015 ; Nichols et al., 2017 ). Incentives such as a badge system may help promote availability of more raw data ( Nosek et al., 2015 ). In our opinion it is important to publish unprocessed raw data because processed data may already have been distorted/biased in undocumented ways. In general, it is more and more usual to reanalyse data from large repositories, so much further development can be expected in this area (e.g., Eklund et al., 2012 ).
Publish Data (Summaries) Irrespective of Statistical Significance, Promote Building Good Quality Datasets Including Large Replication Studies
It is important to publish data summaries and/or data sets, including the ones not resulting in statistically significant findings. Without these datasets true effect sizes simply cannot be determined. This will require that these datasets become citeable so that their authors can be rewarded if data is used for secondary analyses. Considering for example the above mentioned case of Tycho Brache it is clear that his data collection exercise was a necessary precondition of crowning the Copernican/Newtonian revolution of astronomy ( Chaisson and McMillan, 2017 ). Hence, we should be able to reward the mere collection of large volumes of good quality data: such activity can prove to be an immense service to the whole profession. Initiatives, like registered multi-lab replication studies should also be prioritized when the validity of important proposals is at stake. Funders are currently often reluctant to fund such studies. However, they should realize that the continuous seeking of new results and theories may just waste most of their resources ( Ioannidis et al., 2014 ; Kaplan and Irvin, 2015 ).
Increase Statistical Power and Publish Pre-study Power Calculations
In real world research it is usually impossible to determine the statistical power of NHST tests exactly. However, if raw data summaries and/or raw data is published irrespective of statistical significance (Section Pre-registration, Publish Raw Data, and Publish Data (Summaries) Irrespective of Statistical Significance, Promote Building Good Quality Datasets Including Large Replication Studies) then we have a much better chance of trying to determine power. Another option is to determine power to detect pre-defined standardized effect sizes. In any case, power in psychology and neuroscience should be much higher than what it is nowadays ( Szucs and Ioannidis, 2017 ). We hypothesize that pre-registration would facilitate increasing power because researchers could less expect to rely on incidentally finding something statistically significant to report from their studies. Hence, they would have more interest in assuring that they are able to respond their primary, registered hypotheses.
Better Training and Better Use of More Statistical Methods: from Believers to Thinkers
A core problem seems to be that the statistical subject knowledge of many researchers in biomedical and social science has been shown to be poor ( Oakes, 1986 ; Gliner et al., 2002 ; Castro Sotos et al., 2007 , 2009 ; Wilkerson and Olson, 2010 ; Hoekstra et al., 2014 ). NHST perfectly fits with poor understanding because of the perceived simplicity of interpreting its outcome: is p ≤ 0.05 ( Cohen, 1994 )?
We suggest that the weak statistical understanding is probably due to inadequate “statistics lite” education. This approach does not build up appropriate mathematical fundamentals and does not provide scientifically rigorous introduction into statistics. Hence, students' knowledge may remain imprecise, patchy, and prone to serious misunderstandings. What this approach achieves, however, is providing students with false confidence of being able to use inferential tools whereas they usually only interpret the p-value provided by black box statistical software . While this educational problem remains unaddressed, poor statistical practices will prevail regardless of what procedures and measures may be favored and/or banned by editorials.
All too often statistical understanding is perceived as something external to the subject matter of substantive research. However, it is important to see that statistical understanding influences most decisions about substantive questions, because it underlies the thinking of researchers even if this remains implicit . While common sense “statistics” may be able to cope with simple situations, common sense is not enough to decipher scientific puzzles involving dozens, hundreds, or even thousands of interrelated variables. In such cases well justified applications of probability theory are necessary ( Jaynes, 2003 ). Hence, instead of delegating their judgment to “automatized” but ultimately spurious decision mechanisms, researchers should have confidence in their own informed judgment when they make an inference. Such confidence requires deep study.
Understanding probability is difficult. Common sense is notoriously weak in understanding phenomena based on probabilities ( Gigerenzer et al., 2005 ). We cannot assume that without proper training biomedical and social science graduates would get miraculously enlightened about probability. Some of the best symbolic thinking minds of humanity devoted hundreds of years to the proper understanding of probability and statisticians still do not agree on how best to draw statistical inference ( Stigler, 1986 ; Gigerenzer et al., 1989 ), e.g., the recent American Statistical Association statement on p -values ( Wasserstein and Lazar, 2016 ) was accompanied by 21 editorials from the statisticians and methodologists who participated in crafting it and who disagreed in different aspects among themselves.
There is no reason to assume that understanding twenty first and twenty second century science will require less mathematical and statistical understanding than before. Such as there is no royal road to mathematics, there is no royal road to statistics which is heavily based on mathematics. If statistical understanding does not improve it will not matter whether editorials enforce bootstrapping, likelihood estimation or Bayesian approaches, they will all remain opaque to the untrained mind and open to abuse such as the NHST of the twentieth century.
One approach would be to phase out the ‘statistics lite’ education approach for all research stream students and teach statistics rigorously. A typical research stream undergraduate training could include, for example, 3–4 semesters of calculus, one semester of introductory statistics, three more semesters of calculus based statistics, and then finally two semesters of more specialized statistics. An alternative and/or complementary approach would be to enhance the training of professional applied statisticians and to ensure that all research involves knowledgeable statisticians or equivalent methodologists. At a minimum, all scientists should be well trained in understanding evidence and statistics and being in a position to recognize that they may need help from a methodologist expert ( Marusic and Marusic, 2003 ; Moharari et al., 2009 ; Vujaklija et al., 2010 ).
Teach Alternative Approaches Seriously
It is important that researchers are conscious that NHST only represents a small segment of available statistical techniques. Besides NHST, Bayesian and likelihood based approaches should also be taught, with explanation of the strengths and weaknesses of each inferential method. Hypotheses could be tested by either likelihood ratio testing, and/or Bayesian methods which usually view probability as characterizing the state of our beliefs about the world ( Pearl, 1988 ; Jaynes, 2003 ; MacKay, 2003 ; Sivia and Skilling, 2006 ; Gelman et al., 2014 ; for neuroscience data see e.g., Lorenz et al., 2017 ). The above alternative approaches typically require model specifications about alternative hypotheses, they can give probability statements about H 0 and alternative hypotheses, they allow for clear model comparison, are insensitive to data collection procedures and do not suffer from problems with large samples. In addition, Bayesian methods can also factor in pre-study (prior) information into model evaluations which may be important for integrating current and previous research findings. Hence, the above alternative approaches seem more suitable for the purpose of scientific inquiry than NHST and ample literature is available on both. The problem is that usually none of these alternative approaches are taught properly in statistics courses for students in psychology, neuroscience, biomedical science and social science. For example, across 1,000 abstracts randomly selected from the biomedical literature of 1990–2015, none reported results in a Bayesian framework ( Chavalarias et al., 1990-2015 ).
It is important to note that Bayesian methods are often accused of subjectivity because they can take prior information into account. However, Bayesian methods are able to consider prior expectations formally and explicitly in their models provided that necessary information (e.g., raw data and/or extensive reporting of data parameters) from previous studies is available. In contrast, as we have discussed NHST can be latently biased by subjectivity at many points without ever revealing any of the biases. In contrast, different reasonable Bayesian priors can be implemented and their impact on outcomes can be debated explicitly and ultimately, the goodness of model predictions can be tested. Hence, we do not see the use of Bayesian priors as a drawback. Rather, explicit priors can represent a strength as they allow for formal knowledge integration from previous studies.
There Is No Automatic Inference: New-Old Dangers Ahead?
Perhaps the most worrisome false belief about statistics is the belief in automatic statistical inference ( Bakan, 1966 ; Gigerenzer and Marewski, 1998 ), the illusion that plugging in some numbers into some black box algorithm will give a number (perhaps the p -value or some other metric) that conclusively proves or disproves hypotheses ( Bakan, 1966 ). There is no reason to assume that any kind of “new statistics” ( Cumming, 2014 ) will not suffer the fate of NHST if statistical understanding is inadequate. For example, it has been shown that confidence intervals are misinterpreted just as badly as p -values by undergraduates, graduates, and researchers alike and self-declared statistical experience even slightly positively correlates with the number of errors ( Hoekstra et al., 2014 ). Or, many times black box machine learning algorithms may be run uncritically and/or on relatively small data volumes. However, the more complex is a dataset the more chance such substantively blind search algorithms have to find some relationships where nothing worthy of mention exist. So, uncritical applications are likely to further boost the proportion of false positive findings irrespective of the sophistication of the algorithms ( Skokic et al., 2016 ). Similarly, the proper use of Bayesian methods may require use of advanced simulation methods and a clear understanding and justification of probability distribution models. In contrast to this, it is frequent to see a kind of “automatic” determination of Bayes factors or posterior estimates, again, provided by black box statistical packages which again, promise to take the load of thinking off the shoulders of researchers.
Author Note
A previous version of this manuscript was available as a pre-print ( http://biorxiv.org/content/early/2016/12/20/095570 ). The copyright holder for this preprint is the author/funder. It is made available under a CC-BY-NC-ND 4.0 International license.
Author Contributions
DS wrote the first draft of the manuscript. DS and JI revised successive drafts.
Conflict of Interest Statement
The authors declare that the research was conducted in the absence of any commercial or financial relationships that could be construed as a potential conflict of interest.
Acknowledgments
DS is supported by a twenty-first Century Science Initiative in Understanding Human Cognition Scholar Award (220020370) from the James S. McDonnell Foundation. METRICS is supported from a grant from the Laura and John Arnold Foundation.
Supplementary Material
The Supplementary Material for this article can be found online at: https://www.frontiersin.org/article/10.3389/fnhum.2017.00390/full#supplementary-material
Aarts, A. A., Anderson, J. E., Anderson, C. J., Attridge, P. R., Attwood, A., Axt, J., et al. (2015). Estimating the reproducibility of psychological science. Science 349, 943. doi: 10.1126/science.aac4716
CrossRef Full Text | Google Scholar
Bakan, D. (1966). The test of significance in psychological research. Psychol. Bull. 66, 423–437. doi: 10.1037/h0020412
PubMed Abstract | CrossRef Full Text | Google Scholar
Bakker, M., and Wicherts, J. M. (2001). The misreporting of statistical results in psychology journals. Behav. Res. Methods 43, 666–678. doi: 10.3758/s13428-011-0089-5
Bayarri, M. J., Benjamin, D. J., Berger, J. O., and Sellke, T. M. (2016). Rejection odds and rejection ratios: a proposal for statistical practice in testing hypotheses. J. Math. Psychol. 72, 90–103. doi: 10.1016/j.jmp.2015.12.007
Begley, C. G., and Ellis, L. M. (2012). Raise standards for preclinical cancer research. Nature 483, 531–533. doi: 10.1038/483531a
Benjamini, Y. (2010). Simulataneous and selective inference: current successes and future challenges. Biometr. J. 52, 708–721. doi: 10.1002/bimj.200900299
Benjamini, Y., and Hochberg, Y. (1995). Controlling the false discovery rate: a practical and powerful approach to multiple testing. R. Statist. Soc. B 57, 89–300.
Benjamini, Y., and Yekutieli, D. (2001). The control of false discovery rate in multiple testing under dependency. Ann. Stat. 29, 1165–1188. Available online at: http://www.jstor.org/stable/2674075
Google Scholar
Bennett, C. M., Wolford, G. L., and Miller, M. B. (2009). The principled control of false positives in neuroimaging. Soc. Cogn. Affect. Neurosci. 4, 417–442. doi: 10.1093/scan/nsp053
Berger, J. O. (1985). Statistical Decision Theory and Bayesian Analysis, 2nd Edition . New York, NY: Springer. doi: 10.1007/978-1-4757-4286-2
Berger, J. O., and Delampady, M. (1987). Testing precise hypothesis. Stat. Sci . 2, 317–352. doi: 10.1214/ss/1177013238
Berger, J. O., and Sellke, T. (1987). Testing a point null hypothesis: the irreconcilability of p -values and evidence. J. Am. Stat. Assoc. 82, 112–122. doi: 10.2307/2289139
Berkson, J. (1938). Some difficulties of interpretation encountered in the application of the chi-square test. J. Am. Stat. Assoc . 33, 526–542. doi: 10.1080/01621459.1938.10502329
Boccia, S., Rothman, K. J., Panic, N., Flacco, M. E., Rosso, A., Pastorino, R., et al. (2016). Registration practices for observational studies on ClinicalTrials.gov indicated low adherence. J Clin Epidemiol. 70, 176–182. doi: 10.1016/j.jclinepi.2015.09.009
Bruns, S., and Ioannidis, J. P. (2016). p-Curve and p-Hacking in observational research. PLoS ONE 112:e0149144. doi: 10.1371/journal.pone.0149144
Button, K. S., Ioannidis, J., Mokrysz, C., and Nosek, B. A. (2013). Power failure: why small sample size undermines the reliability of neuroscience. Nat. Rev. Neurosci . 14, 365–376. doi: 10.1038/nrn3475
Carp, J. (2012). The secret lives of experiments: methods reporting in the fMRI literature. Neuroimage 63, 289–300. doi: 10.1016/j.neuroimage.2012.07.004
Carver, R. P. (1993). The case against statistical significance testing, revisited. J. Exp. Educ. 61, 287–292. doi: 10.1080/00220973.1993.10806591
Castro Sotos, A. E., Vanhoof, S., Van den Noortage, W., and Onghena, P. (2007). Students' misconceptions of statistical inference: a review of the empirical evidence from research on statistics education. Educ. Res. Rev . 2, 98–113. doi: 10.1016/j.edurev.2007.04.001
Castro Sotos, A. E., Vanhoof, S., Van den Noortage, W., and Onghena, P. (2009). How confident are students in their misconceptions about hypothesis tests? J. Stat. Educ. 17. Available online at: https://ww2.amstat.org/publications/jse/v17n2/castrosotos.pdf
Chaisson, E., and McMillan, S. (2017). Astronomy Today . Pearson.
Chanock, S. J., Manolio, T., Boehnke, M., Boerwinkle, E., Hunter, D. J., Thomas, G., et al. (2007). Replicating genotype-phenotype associations. Nature 447, 655–660. doi: 10.1038/447655a
Chavalarias, D., Wallach, J., Li, A., and Ioannidis, J. P. (1990-2015). Evolution of reporting P-values in the biomedical literature. JAMA 315, 1141–1148. doi: 10.1001/jama.2016.1952
Clark, C. A. (1963). Hypothesis testing in relation to statistical methodology. Rev. Educ. Res . 33, 455–473. doi: 10.2307/1169648
Cohen, J. (1962). The statistical power of abnormal - social psychological research: a review. J. Abnorm. Soc. Psychol. 65, 145–153. doi: 10.1037/h0045186
Cohen, J. (1988). Statistical Power Analysis for the Behavioural Sciences . Academic Press.
Cohen, J. (1994). The earth is round p < 0.05. Am. Psychol . 49, 997–1003. doi: 10.1037/0003-066X.49.12.997
CrossRef Full Text
Cooper, H., Hedges, L. V., and Valentine, J. C. (2009). The Handbook of Research Synthesis and Meta-analysis . New York, NY: Sage.
Cumming, G. (2014). The new statistics: why and how? Psychol. Sci. 25, 7–28. doi: 10.1177/0956797613504966
Curran-Everett, D. (2000). Multiple comparisons: philosophies and illustrations. Am. J. Physiol. Regul. Integr. Comp. Physiol. 279, R1–R8. Available online at: http://ajpregu.physiology.org/content/279/1/R1
PubMed Abstract | Google Scholar
Deer, B. (2011). How the case against the MMR vaccine was fixed. Br. Med. J . 342:c5347. doi: 10.1136/bmj.c5347
DeMets, D., and Lan, K. K. G. (1994). Interim analysis: the alpha spending function approach. Stat. Med. 13, 1341–1352. doi: 10.1002/sim.4780131308
Diggle, P. J., and Zeger, S. L. (2010). Embracing the concept of reproducible research. Biostatistics 11:375. doi: 10.1093/biostatistics/kxq029
Djulbegovic, D., Hozo, I., and Ioannidis, J. P. (2014). Improving the drug development process: more not less random trials. JAMA 311, 355–356. doi: 10.1001/jama.2013.283742
Doshi, P., Goodman, S. N., and Ioannidis, J. P. (2013). Raw data from clinical trials: within reach? Trends Pharmacol. Sci . 34, 645–647. doi: 10.1016/j.tips.2013.10.006
Edwards, A. W. F. (1972). Likelihood: An Account of the Statistical Concept of Likelihood and Its Application to Scientific Inference . Cambridge, UK: Cambridge University Press.
Eklund, A., Andersson, M., Josephson, C., Johannesson, M., and Knutsson, H. (2012). Does parametric fMRI analysis with SPM yield valid results? - An empirical study of 1484 datasets. Neuroimage 61, 565–578. doi: 10.1016/j.neuroimage.2012.03.093
Eklund, A., Nichols, T. E., and Knutson, H. (2016). Cluster failure: why fMRI inferences for spatial extent have inflated false-positives. Proc. Natl. Acad. Sci . U.S.A. 113, 7900–7905. doi: 10.1073/pnas.1602413113
Etz, A., and Vandekerckhove, J. (2016). A Bayesian perspective on the reproducibility project: psychology. PLoS ONE 112:e0149794. doi: 10.1371/journal/pone.0149794
Evangelou, E., and Ioannidis, J. (2013). Meta-analysis methods for genome-wide association studies and beyond. Nat. Rev. Genet. 14, 379–389. doi: 10.1038/nrg3472
Eysenck, H. J. (1960). The concept of statistical significance and the controversy about one tailed tests. Psychol. Rev . 67, 269–271. doi: 10.1037/h0048412
Falk, R., and Greenbaum, C. W. (1995). Significance tests die hard: the Amazing persistence of a probabilistic misconception. Theory Psychol . 5, 75–98. doi: 10.1177/0959354395051004
Fanelli, D. (2010). Do pressures to publish increase scientists' bias? An empirical support from US states data. PLoS ONE 5:e10271. doi: 10.1371/journal.pone.0010271
Fisher, R. (1925). Statistical Methods for Research Workers, First Edition. Edinburgh: Oliver and Boyd.
Gelman, A. (2013a). Commentary: p-values and statistical practice. Epidemiology 24, 69–72. doi: 10.1097/EDE.0b013e31827886f7
Gelman, A. (2013b). Interrogating p values. J. Math. Psychol. 57, 188–189. doi: 10.1016/j.jmp.2013.03.005
Gelman, A. (2015). The connection between varying treatment effects and the crisis of unreplicable research: a bayesian perspective. J. Manage. 41, 632–643. doi: 10.1177/0149206314525208
Gelman, A., Carlin, J. B., Stern, H. S., Dunson, D. B., Vehtari, A., and Rubin, D. (2014). Bayesian Data Analysis . CRC Press.
Gelman, A., Hill, J., and Yajima, M. (2012). Why we (usually) do not have to worry about multiple comparisons. J. Res. Educ. Effect. 5, 189–211. doi: 10.1080/19345747.2011.618213
Gelman, A., and Loken, E. (2014). The statistical crisis in science. Data dependent analysis – A ‘garden of forking paths’ explains why many statistically significant comparisons don't hold up. Am. Sci . 102, 460–465. doi: 10.1511/2014.111.460
Gelman, A., and Tuerlinckx, F. (2000). Type S error rates for classical and Bayesian single and multiple comparison procedures. Comput. Stat. 15, 373–390. doi: 10.1007/s001800000040
Giere, R. N. (1972). The significance test controversy. Br. J. Philos. Sci. 23, 170–181. doi: 10.1093/bjps/23.2.170
Gigerenzer, G. (1998). We need statistical thinking, not statistical rituals. Behav. Brain Sci. 21, 199–200. doi: 10.1017/S0140525X98281167
Gigerenzer, G. (2004). Mindless statistics. J. Socio Econ. 33, 587–606. doi: 10.1016/j.socec.2004.09.033
Gigerenzer, G., Hertwig, R., van den Broek, E., Fasolo, B., and Katsikopoulos, K. V. (2005). ‘A 30% chance tomorrow’: how does the public understand probabilistic weather forecasts? Risk Analysis 25, 623–629. doi: 10.1111/j.1539-6924.2005.00608.x
Gigerenzer, G., Krauss, S., and Vitouch, O. (2004). “The null ritual: what you always wanted to know about significance testing but were afraid to ask,” in The Sage Handbook of Quantitative Methodology for the Social Sciences , ed D. Kaplan (Thousand Oaks, CA: Sage), 391–408. doi: 10.4135/9781412986311.n21
Gigerenzer, G., and Marewski, J. N. (1998). Surrogate science: the idol of a universal method for scientific inference. J. Manage . 41, 421–400. doi: 10.1177/0149206314547522
Gigerenzer, G., Swijtnik, Z., Porter, T., Daston, L., Beatty, J., and Kruger, L. (1989). The Empire of Chance. Camridge, UK: Cambridge University Press. doi: 10.1017/CBO9780511720482
Gliner, J. A., Leech, N. L., and Morgan, G. A. (2002). Problems with null hypothesis significance testing NHST: what do the textbooks say? J. Exp. Educ . 7, 83–92. doi: 10.1080/00220970209602058
Godlee, F. (2011). Wakefield's article linking MMR vaccine and autism was fraudulent. Br. Med. J. 342:c7452. doi: 10.1136/bmj.c7452
Goeman, J. J., and Solari, A. (2014). Multiple hypothesis testing in genomics. Stat. Med. 20, 1946–1978. doi: 10.1002/sim.6082
Goodman, S. N. (1993). p values, hypothesis tests and likelihood: implications for epidemiology of a neglected historical debate. Epidemiology 5, 485–496. doi: 10.1093/oxfordjournals.aje.a116700
Goodman, S. N. (1999). Toward evidence-based medical statistics 1: the p value fallacy. Ann. Intern. Med. 130, 995–1004. doi: 10.7326/0003-4819-130-12-199906150-00008
Goodman, S. N. (2008). A dirty dozen: twelve p value misconceptions. Semin. Hematol. 45, 135–140. doi: 10.1053/j.seminhematol.2008.04.003
Greenberg, S. A. (2009). How citation distortions create unfounded authority: analysis of a citation network. BMJ . 339:b2680. doi: 10.1136/bmj.b2680
Hallahan, M., and Rosenthal, R. (1996). Statistical power: concepts, procedures and applications. Behav. Res. Theory 34, 489–499.
Hoekstra, R., Morey, R. D., Rouder, J. N., and Wagenmakers, E. J. (2014). Robust misinterpretation of confidence intervals. Psychon. Bull. Rev . 21, 1157–1164. doi: 10.3758/s13423-013-0572-3
Hubbard, R., and Bayarri, M. J. (2003). Confusion over measures of evidence p's versus errors α's in classical statistical testing. Am. Stat . 57, 171–182. doi: 10.1198/0003130031856
Hung, H. M. J., O'Neill, T., Bauer, P., and Kohne, K. (1997). The behavior of the p value when the alternative hypothesis is true. Biometrics 53, 11–22. doi: 10.2307/2533093
Hunter, J. E. (1997). Needed: a ban on the significance test. Psychol. Sci. 8, 3–7. doi: 10.1111/j.1467-9280.1997.tb00534.x
Ioannidis, J. P. (2008). Why most true discovered associations are inflated. Epidemiology 19, 640–648. doi: 10.1097/EDE.0b013e31818131e7
Ioannidis, J. P., Caplan, A. L., and Dal-Ré, R. (2017). Outcome reporting bias in clinical trials: why monitoring matters. BMJ 356:j408. doi: 10.1136/bmj.j408
Ioannidis, J. P., Tarone, R., and McLaughlin, J. (2011). The false-positive to false-negative ratio in epidemiological studies. Epidemiology 22, 450–456. doi: 10.1097/EDE.0b013e31821b506e
Ioannidis, J. P., and Trikalinos, T. A. (2007). An exploratory test for an excess of significant findings. Clin. Trials 4, 245–253. doi: 10.1177/1740774507079441
Ioannidis, J. P. A. (2012). Why science is not necessarily self-correcting. Perspect. Psychol. Sci . 7, 645–654. doi: 10.1177/1745691612464056
Ioannidis, J. P. A. (2005). Why most published research findings are false. PLoS Med. 2:e124. doi: 10.1371/journal.pmed.0020124
Ioannidis, J. P. A., Greenland, S., Hlatky, M. A., Khoury, M. J., MacLeod, M. R., Moher, D., et al. (2014). Increasing value and reducing waste and research design, conduct and analysis. Lancet 383, 166–175. doi: 10.1016/S0140-6736(13)62227-8
Jaeschke, R., Singer, J., and Guyatt, G. H. (1989). Measurement of health status: ascertaining the minimal clinically important difference. Controlled Clin. Trials 104, 407–415. doi: 10.1016/0197-2456(89)90005-6
Jannot, A. S., Agoritsas, T., Gayet-Ageron, A., and Perneger, T. V. (2013). Citation bias favoring statistically significant studies was present in medical research. J. Clin. Epidemiol. 66, 296–301. doi: 10.1016/j.jclinepi.2012.09.015
Jaynes, E. T. (2003). Probability Theory: The Logic of Science . Cambridge, UK: Cambridge University Press. doi: 10.1017/CBO9780511790423
Jeffreys, H. (1939, 1948, 1961). The Theory of Probability. Oxford: Oxford University Press.
John, L. K., Loewenstein, G., and Prelec, D. (2012). Measuring the prevalence of questionable research practices with incentives for truth-telling. Psychol. Sci. 23, 524–532. doi: 10.1177/0956797611430953
Kaplan, R. M., and Irvin, V. L. (2015). Likelihood of null effects of large NHLBI clinical trials has increased over time. PLoS ONE 10:e0132382. doi: 10.1371/journal.pone.0132382
Kavvoura, F. K., Liberopoulos, G., and Ioannidis, J. P. (2007). Selection in reported epidemiological risks: an empirical assessment. PLoS Med. 3:e79. doi: 10.1371/journal.pmed.0040079
Keiding, N. (2010). Reproducible research and the substantive context. Biostatistics 11, 376–378. doi: 10.1093/biostatistics/kxq033
Kerr, N. L. (1998). HARKing: hypothesizing after the results are known. Pers. Soc. Psychol. Rev. 2, 196–217. doi: 10.1207/s15327957pspr0203_4
Khoury, M. J., and Ioannidis, J. P. (2014). Big data meets public health: human well-being could benefit from large-scale data if large-scale noise is minimized. Science 346, 1054–1055. doi: 10.1126/science.aaa2709
Kivimäki, M., Batty, G. D., Kawachi, I., Virtanen, M., Singh-Manoux, A., and Brunner, E. J. (2014). Don't let the truth get in the way of a good story: an illustration of citation bias in epidemiologic research. Am. J. Epidemiol. 180, 446–448. doi: 10.1093/aje/kwu164
Kjaergard, L. L., and Gluud, C. (2002). Citation bias of hepato-biliary randomized clinical trials. J. Clin. Epidemiol. 55, 407–410. doi: 10.1016/S0895-4356(01)00513-3
Kranz, D. H. (1999). The null hypothesis testing controversy in psychology. J. Am. Stat. Assoc . 94, 1372–1381. doi: 10.1080/01621459.1999.10473888
Kriegeskorte, N., Simmons, W. K., Bellgowan, P. S. F., and Baker, C. I. (2009). Circular analysis in systems neuroscience – the dangers of double dipping. Nat. Neurosci. 12, 535–540. doi: 10.1038/nn.2303
Laine, C., Goodman, S. N., Griswold, M. E., and Sox, H. C. (2007). Reproducible research: moving toward research the public can really trust. Ann. Intern. Med. 146, 450–453. doi: 10.7326/0003-4819-146-6-200703200-00154
Lindley, D. V. (1993). The analysis of experimental data: the appreciation of tea and wine. Teach. Stat. 15, 22–25. doi: 10.1111/j.1467-9639.1993.tb00252.x
Lorenz, R., Hampshire, A., and Leech, R. (2017). Neuroadaptive bayeisan optmizaiton and hypothesis testing. Trends Cogn. Sci. 21, 155–167. doi: 10.1016/j.tics.2017.01.006
Luce, R. D. (1988). The tools to theory hypothesis. Review of G. Gigerenzer and D.J. Murray, ‘Cognition as intuitive statistics’. Contemp. Psychol. 33, 582–583. doi: 10.1037/030460
Lykken, D. T. (1968). Statistical significance in psychological research. Psychol. Bull . 70, 151–159. doi: 10.1037/h0026141
MacKay, D. J. C. (2003). Information Theory, Inference and Learning Algorithms. Cambridge, UK: Cambridge University Press.
Macleod, M. R., Michie, S., Roberts, I., Dirnagl, U., Chalmers, I., Ioannidis, J. P., et al. (2014). Biomedical research: increasing value, reducing waste. Lancet 383, 101–104. doi: 10.1016/S0140-6736(13)62329-6
Makel, M., Plucker, J., and Hegarty, B. (2012). Replications in psychology research: how often do they really occur? Perspect. Psychol. Sci. 7, 537–542. doi: 10.1177/1745691612460688
Marusic, A., and Marusic, M. (2003). Teaching students how to read and write science: a mandatory course on scientific research and communication in medicine. Acad. Med. 78, 1235–1239. doi: 10.1097/00001888-200312000-00007
Meehl, P. E. (1967). Theory testing in psychology and physics: a methodological paradox. Philos. Sci. 34, 103–115. doi: 10.1086/288135
Meehl, P. E. (1978). Theoretical risks and tabular asterisks: Sir Karl, Sir Ronald, and the slow progress of soft psychology. J. Consult. Clin. Psychol. 46, 806–834. doi: 10.1037/0022-006X.46.4.806
Meehl, P. E. (1990). Why summaries of research on psychological theories are often uninterpretable. Psychol. Rep . 66, 195–244. doi: 10.2466/pr0.1990.66.1.195
Michaelson, A. M., and Morley, E. W. (1887). On the relative motion of the earth and the luminiferous ether. American Journal of Science . 34, 333–345. doi: 10.2475/ajs.s3-34.203.333
Moharari, R. S., Rahimi, E. R., Najafi, A., et al. (2009). Teaching critical appraisal and statistics in anesthesia journal club. Q. J. Med. 102, 139–141. doi: 10.1093/qjmed/hcn131
Morey, R. D., Hoekstra, R., Rouder, J. N., Lee, M. D., and Wagenmakers, E.-J. (2016). The fallacy of placing confidence in confidence intervals. Psychon. Bull. Rev. 23, 103–123. doi: 10.3758/s13423-015-0947-8
Murdoch, D. J., Tsai, Y. L., and Adcock, J. (2008). P values are random variables. Am. Stat. 62, 242–245. doi: 10.1198/000313008X332421
Neath, A. A., and Cavanaugh, J. E. (2006). A Bayesian approach to the multiple comparison problem. J. Data Sci. 4, 131–146.
Neyman, J. (1950). Probability and Statistics . New York, NY: Holt.
Neyman, J., and Pearson, E. S. (1933). On the problem of the most efficient tests of statistical hypotheses. Philos. Trans. R. Soc. Lond. Ser. A 231, 289–337.
Nichols, T., and Hayasaka, S. (2003). Controlling the familywise error rate in neuroimaging: a comparative review. Stat. Methods Med. Res. 12, 419–446. doi: 10.1191/0962280203sm341ra
Nichols, T. E., Das, S., Eickhoff, S. B., Evans, A. C., Glatard, T., Hanke, M., et al. (2016). Best practices in data analysis and sharing in neuroimaging using MRI. bioRxiv. doi: 10.1101/054262
Nichols, T. E., Das, S., Eickhoff, S. B., Evans, A. C., Glatard, T., Hanke, M., et al. (2017). Best practices in data analysis and sharing in neuroimaging using MRI. Nat. Neurosci. 20, 299–303. doi: 10.1038/nn.4500
Nickerson, R. S. (2000). Null hypothesis significance testing: a review of an old and continuing controversy. Psychol. Methods 5, 241–301. doi: 10.1037/1082-989X.5.2.241
Nosek, B. A., Alter, G., Banks, G. C., Borsboom, D., Bowman, S. D., Breckler, S. J., et al. (2015). Promoting an open research culture. Science 348, 1422–1425 doi: 10.1016/j.jmp.2015.12.007
Nosek, B. A., Spies, J. R., and Motyl, M. (2013). Scientific utopia II: restructuring incentives and practices to promote truth over publishability. Perspect. Psychol. Sci . 7, 615–631. doi: 10.1177/1745691612459058
Nuijten, M. B., Hartgerink, C. H., van Assen, M. A., Epskamp, S., and Wicherts, J. M. (2016). The prevalence of statistical reporting errors in psychology 1985-2013. Behav. Res. Methods . 48, 1205–1226. Available online at: https://link.springer.com/article/10.3758%2Fs13428-015-0664-2
Nunnally, J. (1960). The place of statistics in psychology. Educ. Psychol. Measur . 20, 641–650. doi: 10.1177/001316446002000401
Oakes, M. L. (1986). Statistical Inference: A Commentary for the Social and Behavioural Sciences . New York, NY: Wiley.
Pashler, H., and Harris, C. R. (2012). Is the replicability crisis overblown? Three arguments examined. Perspect. Psychol. Sci. 7, 531–536. doi: 10.1177/1745691612463401
Patel, C. J., and Ioannidis, J. P. (2014a). Placing epidemiological results in the context of multiplicity and typical correlations of exposures. J. Epidemiol. Community Health 68, 1096–1100. doi: 10.1136/jech-2014-204195
Patel, C. J., and Ioannidis, J. P. (2014b). Studying the elusive environment in large scale. JAMA 311, 2173–2174. doi: 10.1136/jech-2014-204195
Patel, C. J., Burford, B., and Ioannidis, J. P. (2015). Assessment of vibration of effects due to model specification can demonstrate the instability of observational associations. J. Clin. Epidemiol. 68, 1046–1058. doi: 10.1016/j.jclinepi.2015.05.029
Pearl, J. (1988). Probabilistic Reasoning in Intelligent Systems. San Francisco, CA: Morgan.
Peng, R. D. (2009). Reproducible research and biostatistics. Biostatistics 10, 405–408. doi: 10.1093/biostatistics/kxp014
Peng, R. D. (2011). Reproducible research in computational science. Science 334, 1226–1227. doi: 10.1126/science.1213847
Pernet, C., and Poline, J.-B. (2015). Improving functional magnetic imaging reproducibility. Gigascience 4:15. doi: 10.1186/s13742-015-0055-8
Poldrack, R. A. (2006). Can cognitive processes be inferred from neuroimaging data? Trends Cogn. Sci. 10, 59–63. doi: 10.1016/j.tics.2005.12.004
Pollard, P., and Richardson, J. T. E. (1987). On the probability of making Type-I errors. Psychol. Bull. 102, 159–163. doi: 10.1037/0033-2909.102.1.159
Rossi, J. S. (1990). Statistical power of psychological research: what have we gained in 20 years? J. Consult. Clin. Psychol . 58, 646–656. doi: 10.1037/0022-006X.58.5.646
Rozeboom, W. W. (1960). The fallacy of the null hypothesis significance test. Psychol. Bull . 57, 416–428. doi: 10.1037/h0042040
Schmidt, F. L. (1992). What do data really mean? Research findings, meta-analysis and cumulative knowledge in psychology. Am. Psychol. 47, 1173–1181. doi: 10.1037/0003-066X.47.10.1173
Schmidt, F. L. (1996). Statistical significance testing and cumulative knowledge in psychology: implications for training of researchers. Psychol. Methods 1, 115–129. doi: 10.1037/1082-989X.1.2.115
Schoenfeld, J. D., and Ioannidis, J. P. A. (2012). Is everything we eat is associated with cancer? A systematic cookbook review. Am. J. Clin. Nutri. 97, 127–134. doi: 10.3945/ajcn.112.047142
Sedlmeier, P., and Gigerenzer, G. (1989). Do studies of statistical power have an effect on the power of the studies? Psychol. Bull. 105, 309–316. doi: 10.1037/0033-2909.105.2.309
Sellke, T., Bayarri, M. J., and Berger, J. O. (2001). Calibration of p values for testing precise null hypotheses. Am. Stat. 55, 62–71. doi: 10.1198/000313001300339950
Shaffer, J. P. (1995). Multiple hypothesis testing. Annu. Rev. Psychol. 46, 561–584 doi: 10.1146/annurev.ps.46.020195.003021
Simmons, J., Nelson, L., and Simonsohn, U. (2011). False-positive psychology: undisclosed flexibility in data collection and analysis allow presenting anything as significant. Psychol. Sci. 22, 1359–1366. doi: 10.1177/0956797611417632
Simonsohn, U., Nelson, L. D., and Simmons, J. P. (2014a). P-Curve: a key to the file drawer. J. Exp. Psychol. 1432, 534–547. doi: 10.1037/a0033242
Simonsohn, U., Nelson, L. D., and Simmons, J. P. (2014b). p -Curve and effect size: correcting for publication bias using only significant results. Psychol. Sci. 96, 666–681. doi: 10.1177/1745691614553988
Siontis, G. C., and Ioannidis, J. P. (2011). Risk factors and interventions with statistically significant tiny effects. Int. J. Epidemiol. 40, 1292–1307. doi: 10.1093/ije/dyr099
Sivia, D. S., and Skilling, J. (2006). Data Analysis: A Bayesian Tutorial . Oxford University Press.
Skokic, M., Collins, J., Callahan-Flintoft, C., Bowman, H., and Wyble, B. (2016). I tried a bunch of things: the dangers of unexpected overfitting in classification. BioRxiv .
Smolin, L. (2006). The Trouble with Physics . London: Penguin.
Soares, H. P., Kumar, A., Daniels, S., Swann, S., Cantor, A., Hozo, I., et al. (2005). Evaluation of new treatments in radiation oncology: are they better than standard treatments? JAMA 293, 970–978. doi: 10.1001/jama.293.8.970
Sterling, T. D. (1959). Publication decisions and their possible effects on inferences drawn from tests of significance—or vice versa. J. Am. Stat. Assoc. 54, 30–34. doi: 10.1080/01621459.1959.10501497
Sterling, T. D., Rosenbaum, W. L., and Weinkam, J. J. (1995). Publication decisions revisited: The effect of the outcome of statistical tests on the decision to publish and vice versa. Am. Stat. 49, 108–112. doi: 10.1080/00031305.1995.10476125
Sterne, J. A. C., and Smith, G. D. (2001). Sifting the evidence - what's wrong with significance tests? Br. Med. J. 322, 226–231. doi: 10.1136/bmj.322.7280.226
Stigler, S. M. (1986). The History of Statistics . Cambridge, MA; London: Harvard University Press.
Stodden, V., McNutt, M., Bailey, D. H., Deelman, E., Gil, Y., Hanson, B., et al. (2016). Enhancing reproducibility for computational methods. Science 354, 1240–1241. doi: 10.1126/science.aah6168
Szucs, D. (2016). A tutorial on hunting statistical significance by chasing N. Front. Psychol. 7:1444. doi: 10.3389/fpsyg.2016.01444
Szucs, D., and Ioannidis, J. P. A. (2017). Empirical assessment of published effect sizes and power in the recent cognitive neuroscience and psychology literature. PLoS Biol. 15:e2000797. doi: 10.1371/journal.pbio.2000797
Vujaklija, A., Hren, D., Sambunjak, D., Vodopivec, I., Ivanis, A., Marusic, A., et al. (2010). Can teaching research methodology influence students' attitude toward science? Cohort study and nonrandomized trial in a single medical school. J. Investig. Med. 58, 282–286. doi: 10.2310/JIM.0b013e3181cb42d9
Vul, E., Harris, C., Winkielman, P., and Pashler, H. (2009). Puzzlingly high correlations in fMRI studies of emotion, personality and social cognition. Perspect. Psychol. Sci . 4, 274–324. doi: 10.1111/j.1745-6924.2009.01125.x
Wagenmakers, E.-J. (2007). A practical solution to the pervasive problem of p values. Psychon. Bull. Rev. 14, 779–804. doi: 10.3758/BF03194105
Wagenmakers, E. J., Wetzels, R., Borsboom, D., and van der Maas, H. L. J. (2011). Why psychologists must change the way they analyse their data: the case of psi: comment on Bem (2011). J. Pers. Soc. Psychol. 100, 426–432. doi: 10.1037/a0022790
Waller, N. G. (2004). The fallacy of the null hypothesis in soft psychology. Appl. Prevent. Psychol . 11, 83–86. doi: 10.1016/j.appsy.2004.02.015
Wasserstein, R. L., and Lazar, N. A. (2016). The ASA statement on p values: context, process, and purpose. Am. Stat. 70, 129–133. doi: 10.1080/00031305.2016.1154108
Wellek, S. (2010). Testing Statistical Hypotheses of Equivalence and Noninferiority, 2nd Edition. Chapman and Hall/CRC. doi: 10.1201/EBK1439808184
Westfall, P. H., Johnson, W. O., and Utts, J. M. (1997). A Bayesian perspective on the Bonferroni adjustment. Biometrika 84, 419–427. doi: 10.1093/biomet/84.2.419
Wilkerson, M., and Olson, M. R. (2010). Misconceptions about sample size, statistical significance and treatment effect. J. Psychol . 131, 627–631. doi: 10.1080/00223989709603844
Ziliak, T., and McCloskey, N. (2008). The Cult of Statistical Significance . Ann Arbor, MI: The University of Michigan Press.
Keywords: replication crisis, false positive findings, research methodology, null hypothesis significance testing, Bayesian methods
Citation: Szucs D and Ioannidis JPA (2017) When Null Hypothesis Significance Testing Is Unsuitable for Research: A Reassessment. Front. Hum. Neurosci . 11:390. doi: 10.3389/fnhum.2017.00390
Received: 03 February 2017; Accepted: 13 July 2017; Published: 03 August 2017.
Reviewed by:
Copyright © 2017 Szucs and Ioannidis. This is an open-access article distributed under the terms of the Creative Commons Attribution License (CC BY) . The use, distribution or reproduction in other forums is permitted, provided the original author(s) or licensor are credited and that the original publication in this journal is cited, in accordance with accepted academic practice. No use, distribution or reproduction is permitted which does not comply with these terms.
*Correspondence: Denes Szucs, [email protected]
What is The Null Hypothesis & When Do You Reject The Null Hypothesis
Julia Simkus
Editor at Simply Psychology
BA (Hons) Psychology, Princeton University
Julia Simkus is a graduate of Princeton University with a Bachelor of Arts in Psychology. She is currently studying for a Master's Degree in Counseling for Mental Health and Wellness in September 2023. Julia's research has been published in peer reviewed journals.
Learn about our Editorial Process
Saul Mcleod, PhD
Editor-in-Chief for Simply Psychology
BSc (Hons) Psychology, MRes, PhD, University of Manchester
Saul Mcleod, PhD., is a qualified psychology teacher with over 18 years of experience in further and higher education. He has been published in peer-reviewed journals, including the Journal of Clinical Psychology.
Olivia Guy-Evans, MSc
Associate Editor for Simply Psychology
BSc (Hons) Psychology, MSc Psychology of Education
Olivia Guy-Evans is a writer and associate editor for Simply Psychology. She has previously worked in healthcare and educational sectors.
On This Page:
A null hypothesis is a statistical concept suggesting no significant difference or relationship between measured variables. It’s the default assumption unless empirical evidence proves otherwise.
The null hypothesis states no relationship exists between the two variables being studied (i.e., one variable does not affect the other).
The null hypothesis is the statement that a researcher or an investigator wants to disprove.
Testing the null hypothesis can tell you whether your results are due to the effects of manipulating the dependent variable or due to random chance.
How to Write a Null Hypothesis
Null hypotheses (H0) start as research questions that the investigator rephrases as statements indicating no effect or relationship between the independent and dependent variables.
It is a default position that your research aims to challenge or confirm.
For example, if studying the impact of exercise on weight loss, your null hypothesis might be:
There is no significant difference in weight loss between individuals who exercise daily and those who do not.
Examples of Null Hypotheses
When do we reject the null hypothesis .
We reject the null hypothesis when the data provide strong enough evidence to conclude that it is likely incorrect. This often occurs when the p-value (probability of observing the data given the null hypothesis is true) is below a predetermined significance level.
If the collected data does not meet the expectation of the null hypothesis, a researcher can conclude that the data lacks sufficient evidence to back up the null hypothesis, and thus the null hypothesis is rejected.
Rejecting the null hypothesis means that a relationship does exist between a set of variables and the effect is statistically significant ( p > 0.05).
If the data collected from the random sample is not statistically significance , then the null hypothesis will be accepted, and the researchers can conclude that there is no relationship between the variables.
You need to perform a statistical test on your data in order to evaluate how consistent it is with the null hypothesis. A p-value is one statistical measurement used to validate a hypothesis against observed data.
Calculating the p-value is a critical part of null-hypothesis significance testing because it quantifies how strongly the sample data contradicts the null hypothesis.
The level of statistical significance is often expressed as a p -value between 0 and 1. The smaller the p-value, the stronger the evidence that you should reject the null hypothesis.
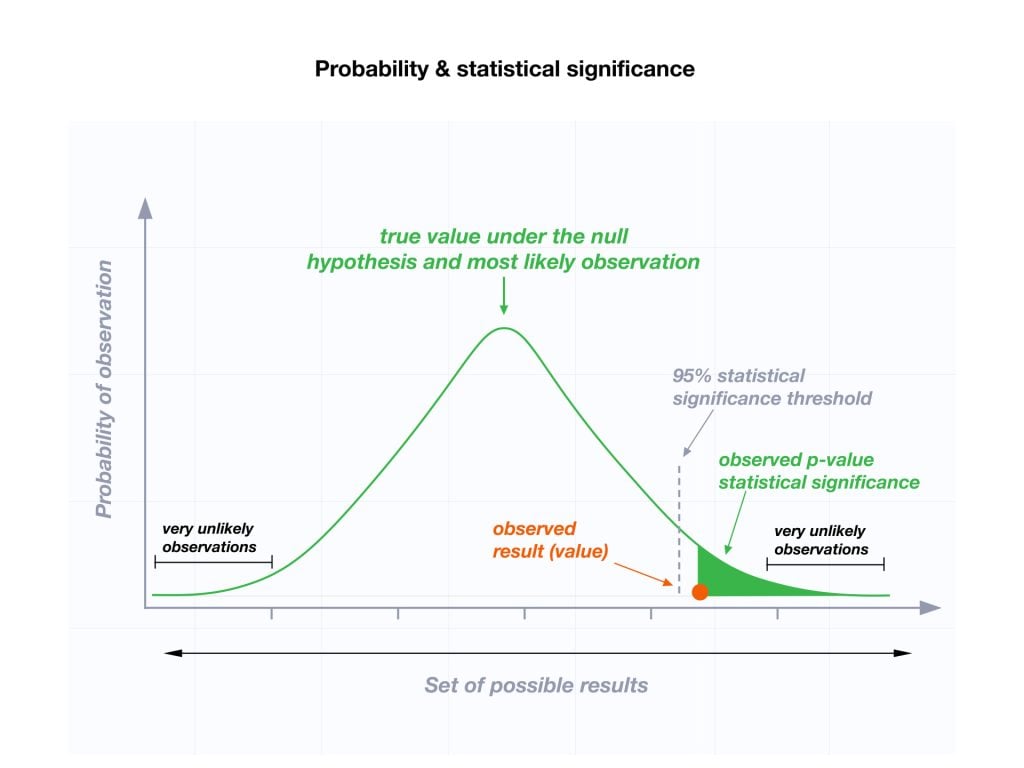
Usually, a researcher uses a confidence level of 95% or 99% (p-value of 0.05 or 0.01) as general guidelines to decide if you should reject or keep the null.
When your p-value is less than or equal to your significance level, you reject the null hypothesis.
In other words, smaller p-values are taken as stronger evidence against the null hypothesis. Conversely, when the p-value is greater than your significance level, you fail to reject the null hypothesis.
In this case, the sample data provides insufficient data to conclude that the effect exists in the population.
Because you can never know with complete certainty whether there is an effect in the population, your inferences about a population will sometimes be incorrect.
When you incorrectly reject the null hypothesis, it’s called a type I error. When you incorrectly fail to reject it, it’s called a type II error.
Why Do We Never Accept The Null Hypothesis?
The reason we do not say “accept the null” is because we are always assuming the null hypothesis is true and then conducting a study to see if there is evidence against it. And, even if we don’t find evidence against it, a null hypothesis is not accepted.
A lack of evidence only means that you haven’t proven that something exists. It does not prove that something doesn’t exist.
It is risky to conclude that the null hypothesis is true merely because we did not find evidence to reject it. It is always possible that researchers elsewhere have disproved the null hypothesis, so we cannot accept it as true, but instead, we state that we failed to reject the null.
One can either reject the null hypothesis, or fail to reject it, but can never accept it.
Why Do We Use The Null Hypothesis?
We can never prove with 100% certainty that a hypothesis is true; We can only collect evidence that supports a theory. However, testing a hypothesis can set the stage for rejecting or accepting this hypothesis within a certain confidence level.
The null hypothesis is useful because it can tell us whether the results of our study are due to random chance or the manipulation of a variable (with a certain level of confidence).
A null hypothesis is rejected if the measured data is significantly unlikely to have occurred and a null hypothesis is accepted if the observed outcome is consistent with the position held by the null hypothesis.
Rejecting the null hypothesis sets the stage for further experimentation to see if a relationship between two variables exists.
Hypothesis testing is a critical part of the scientific method as it helps decide whether the results of a research study support a particular theory about a given population. Hypothesis testing is a systematic way of backing up researchers’ predictions with statistical analysis.
It helps provide sufficient statistical evidence that either favors or rejects a certain hypothesis about the population parameter.
Purpose of a Null Hypothesis
- The primary purpose of the null hypothesis is to disprove an assumption.
- Whether rejected or accepted, the null hypothesis can help further progress a theory in many scientific cases.
- A null hypothesis can be used to ascertain how consistent the outcomes of multiple studies are.
Do you always need both a Null Hypothesis and an Alternative Hypothesis?
The null (H0) and alternative (Ha or H1) hypotheses are two competing claims that describe the effect of the independent variable on the dependent variable. They are mutually exclusive, which means that only one of the two hypotheses can be true.
While the null hypothesis states that there is no effect in the population, an alternative hypothesis states that there is statistical significance between two variables.
The goal of hypothesis testing is to make inferences about a population based on a sample. In order to undertake hypothesis testing, you must express your research hypothesis as a null and alternative hypothesis. Both hypotheses are required to cover every possible outcome of the study.
What is the difference between a null hypothesis and an alternative hypothesis?
The alternative hypothesis is the complement to the null hypothesis. The null hypothesis states that there is no effect or no relationship between variables, while the alternative hypothesis claims that there is an effect or relationship in the population.
It is the claim that you expect or hope will be true. The null hypothesis and the alternative hypothesis are always mutually exclusive, meaning that only one can be true at a time.
What are some problems with the null hypothesis?
One major problem with the null hypothesis is that researchers typically will assume that accepting the null is a failure of the experiment. However, accepting or rejecting any hypothesis is a positive result. Even if the null is not refuted, the researchers will still learn something new.
Why can a null hypothesis not be accepted?
We can either reject or fail to reject a null hypothesis, but never accept it. If your test fails to detect an effect, this is not proof that the effect doesn’t exist. It just means that your sample did not have enough evidence to conclude that it exists.
We can’t accept a null hypothesis because a lack of evidence does not prove something that does not exist. Instead, we fail to reject it.
Failing to reject the null indicates that the sample did not provide sufficient enough evidence to conclude that an effect exists.
If the p-value is greater than the significance level, then you fail to reject the null hypothesis.
Is a null hypothesis directional or non-directional?
A hypothesis test can either contain an alternative directional hypothesis or a non-directional alternative hypothesis. A directional hypothesis is one that contains the less than (“<“) or greater than (“>”) sign.
A nondirectional hypothesis contains the not equal sign (“≠”). However, a null hypothesis is neither directional nor non-directional.
A null hypothesis is a prediction that there will be no change, relationship, or difference between two variables.
The directional hypothesis or nondirectional hypothesis would then be considered alternative hypotheses to the null hypothesis.
Gill, J. (1999). The insignificance of null hypothesis significance testing. Political research quarterly , 52 (3), 647-674.
Krueger, J. (2001). Null hypothesis significance testing: On the survival of a flawed method. American Psychologist , 56 (1), 16.
Masson, M. E. (2011). A tutorial on a practical Bayesian alternative to null-hypothesis significance testing. Behavior research methods , 43 , 679-690.
Nickerson, R. S. (2000). Null hypothesis significance testing: a review of an old and continuing controversy. Psychological methods , 5 (2), 241.
Rozeboom, W. W. (1960). The fallacy of the null-hypothesis significance test. Psychological bulletin , 57 (5), 416.


Null Hypothesis Significance Testing in Behavior Analysis: Problems and Recommendations
##article.authors##.
- PDF (Português (Brasil))
How to Cite
- Endnote/Zotero/Mendeley (RIS)
Copyright (c) 2024 Bruna Rodrigues Lins, Bruno Strapasson

This work is licensed under a Creative Commons Attribution 4.0 International License .

Data statement
- It is a theoretical paper, there is no associated data.
Preprints disclaimer
Announcements, request your next prereview on scielo preprints via the coar notify protocol.
Next time you post a preprint on SciELO Preprints , make sure to request a review from the PREreview community!
FAQ Updated
All authors are requested to consult the FAQ before submitting their manuscript.
Annals of ANPOCS Annual Meetings at SciELO Preprints
Starting this year, when your article is approved at the ANPOCS meetings, you can choose to publish it on the SciELO Preprints server.
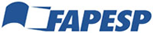
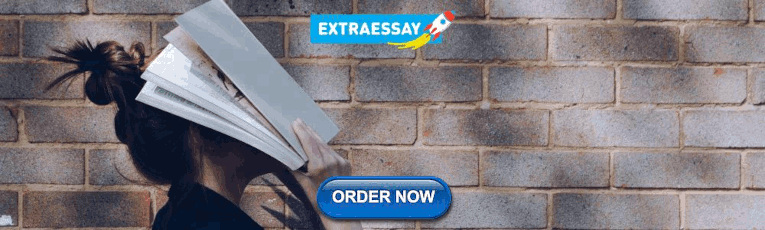
IMAGES
VIDEO
COMMENTS
Fisher, significance testing, and the p-value. The method developed by ( Fisher, 1934; Fisher, 1955; Fisher, 1959) allows to compute the probability of observing a result at least as extreme as a test statistic (e.g. t value), assuming the null hypothesis of no effect is true.This probability or p-value reflects (1) the conditional probability of achieving the observed outcome or larger: p(Obs ...
The null hypothesis in statistics states that there is no difference between groups or no relationship between variables. It is one of two mutually exclusive hypotheses about a population in a hypothesis test. When your sample contains sufficient evidence, you can reject the null and conclude that the effect is statistically significant.
A crucial step in null hypothesis testing is finding the likelihood of the sample result if the null hypothesis were true. This probability is called the p value. A low p value means that the sample result would be unlikely if the null hypothesis were true and leads to the rejection of the null hypothesis. A high p value means that the sample ...
Page 6.1 (hyp-test.docx, 5/8/2016) 6: Introduction to Null Hypothesis Significance Testing . Acronyms and symbols . P . P value . p . binomial parameter "probability of success" n . sample size . H. 0. the null hypothesis . H. a. the alternative hypothesis . P. value . Statistical inference is the act of generalizing from sample (the data ...
The purpose of null hypothesis significance testing is to be able to reject the expectation that the means of the two groups are the same. Reject the null hypothesis: The sample mean is different from the population mean. Rejecting the null hypothesis means that X¯ ≠ μ X ¯ ≠ μ. Rejecting the null hypothesis doesn't automatically mean ...
There are 5 main steps in hypothesis testing: State your research hypothesis as a null hypothesis and alternate hypothesis (H o) and (H a or H 1 ). Collect data in a way designed to test the hypothesis. Perform an appropriate statistical test. Decide whether to reject or fail to reject your null hypothesis. Present the findings in your results ...
Let's return finally to the question of whether we reject or fail to reject the null hypothesis. If our statistical analysis shows that the significance level is below the cut-off value we have set (e.g., either 0.05 or 0.01), we reject the null hypothesis and accept the alternative hypothesis. Alternatively, if the significance level is above ...
Null Hypothesis Significance Testing (NHST) is a common statistical test to see if your research findings are statistically interesting. Its usefulness is sometimes challenged, particularly because NHST relies on p values, which are sporadically under fire from statisticians. The important thing to remember is not the latest p-value-related salvo in the statistical press, but rather that NHST ...
Unit test. Significance tests give us a formal process for using sample data to evaluate the likelihood of some claim about a population value. Learn how to conduct significance tests and calculate p-values to see how likely a sample result is to occur by random chance. You'll also see how we use p-values to make conclusions about hypotheses.
Null hypothesis testing is a formal approach to deciding between two interpretations of a statistical relationship in a sample. ... When this happens, the result is said to be statistically significant. If there is greater than a 5% chance of a result as extreme as the sample result when the null hypothesis is true, then the null hypothesis is ...
Basic definitions. The null hypothesis and the alternative hypothesis are types of conjectures used in statistical tests to make statistical inferences, which are formal methods of reaching conclusions and separating scientific claims from statistical noise.. The statement being tested in a test of statistical significance is called the null hypothesis. . The test of significance is designed ...
Obviously, when the null hypothesis is correct, we want a low probability that hypothesis tests will produce statistically significant results. For example, if alpha is 0.05, your analysis has a 5% chance of producing a significant result when the null hypothesis is correct.
The p value determines statistical significance. An extremely low p value indicates high statistical significance, while a high p value means low or no statistical significance. Example: Hypothesis testing. To test your hypothesis, you first collect data from two groups. The experimental group actively smiles, while the control group does not.
It is the opposite of your research hypothesis. The alternative hypothesis--that is, the research hypothesis--is the idea, phenomenon, observation that you want to prove. If you suspect that girls take longer to get ready for school than boys, then: Alternative: girls time > boys time. Null: girls time <= boys time.
Null hypothesis statistical significance tests (NHST) are widely used in quantitative research in the empirical sciences including scientometrics. Nevertheless, since their introduction nearly a century ago significance tests have been controversial. Many researchers are not aware of the numerous criticisms raised against NHST. As practiced, NHST has been characterized as a 'null ritual ...
Using P values and Significance Levels Together. If your P value is less than or equal to your alpha level, reject the null hypothesis. The P value results are consistent with our graphical representation. The P value of 0.03112 is significant at the alpha level of 0.05 but not 0.01.
Null hypothesis significance testing (NHST) has several shortcomings that are likely contributing factors behind the widely debated replication crisis of (cognitive) neuroscience, psychology, and biomedical science in general. We review these shortcomings and suggest that, after sustained negative experience, NHST should no longer be the default, dominant statistical practice of all biomedical ...
Research question: Null hypothesis (H 0): General: Test-specific: Does tooth flossing affect the number of cavities? Tooth flossing has no effect on the number of cavities.: t test:. The mean number of cavities per person does not differ between the flossing group (µ 1) and the non-flossing group (µ 2) in the population; µ 1 = µ 2.: Does the amount of text highlighted in the textbook ...
Calculating the p-value is a critical part of null-hypothesis significance testing because it quantifies how strongly the sample data contradicts the null hypothesis. The level of statistical significance is often expressed as a p -value between 0 and 1. The smaller the p-value, the stronger the evidence that you should reject the null hypothesis.
The dissemination of research with groups using null hypothesis significance testing (NHST) in data analysis has grown in this area. It brings with it related problems (intrinsic and due to misuse). Such problems often go unnoticed in the current peer review system, compromising the reliability of some conclusions available in the scientific ...
Statistical tests are used in hypothesis testing. They can be used to: determine whether a predictor variable has a statistically significant relationship with an outcome variable. estimate the difference between two or more groups. Statistical tests assume a null hypothesis of no relationship or no difference between groups. Then they ...
To start this test, you write the null hypothesis, Ho, and the alternative hypothesis, H₁, as follows. Ho: μ=7 H₁ = 7 Suppose you also know the following information. ... O Since the p-value is greater than the level of significance, the null hypothesis is not rejected. AI-Generated Solution. AI-generated content may present inaccurate or ...