Module 3: Important Biological Macromolecules
Structure of nucleic acids, learning outcomes.
- Describe the basic structure of nucleic acids
Nucleic acids are the most important macromolecules for the continuity of life. They carry the genetic blueprint of a cell and carry instructions for the functioning of the cell.
The two main types of nucleic acids are deoxyribonucleic acid (DNA) and ribonucleic acid (RNA) . DNA is the genetic material found in all living organisms, ranging from single-celled bacteria to multicellular mammals. It is found in the nucleus of eukaryotes and in the organelles, chloroplasts, and mitochondria. In prokaryotes, the DNA is not enclosed in a membranous envelope.
The entire genetic content of a cell is known as its genome, and the study of genomes is genomics. In eukaryotic cells but not in prokaryotes, DNA forms a complex with histone proteins to form chromatin, the substance of eukaryotic chromosomes. A chromosome may contain tens of thousands of genes. Many genes contain the information to make protein products; other genes code for RNA products. DNA controls all of the cellular activities by turning the genes “on” or “off.”
The other type of nucleic acid, RNA, is mostly involved in protein synthesis. The DNA molecules never leave the nucleus but instead use an intermediary to communicate with the rest of the cell. This intermediary is the messenger RNA (mRNA) . Other types of RNA—like rRNA, tRNA, and microRNA—are involved in protein synthesis and its regulation.
DNA and RNA are made up of monomers known as nucleotides. The nucleotides combine with each other to form a polynucleotide, DNA or RNA. Each nucleotide is made up of three components: a nitrogenous base, a pentose (five-carbon) sugar, and a phosphate group (Figure 1). Each nitrogenous base in a nucleotide is attached to a sugar molecule, which is attached to one or more phosphate groups.
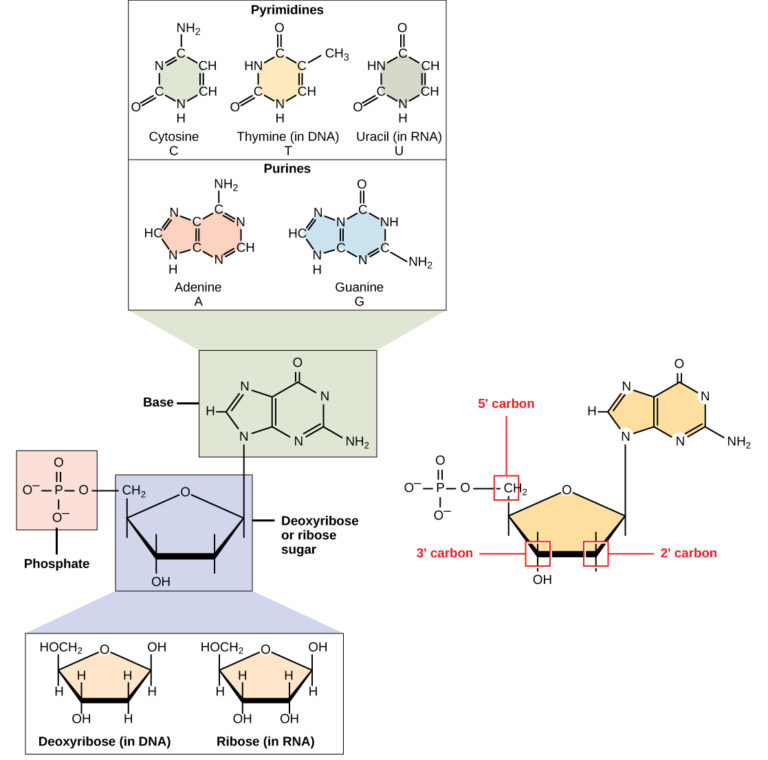
Figure 1. A nucleotide is made up of three components: a nitrogenous base, a pentose sugar, and one or more phosphate groups. Carbon residues in the pentose are numbered 1′ through 5′ (the prime distinguishes these residues from those in the base, which are numbered without using a prime notation). The base is attached to the 1′ position of the ribose, and the phosphate is attached to the 5′ position. When a polynucleotide is formed, the 5′ phosphate of the incoming nucleotide attaches to the 3′ hydroxyl group at the end of the growing chain. Two types of pentose are found in nucleotides, deoxyribose (found in DNA) and ribose (found in RNA). Deoxyribose is similar in structure to ribose, but it has an H instead of an OH at the 2′ position. Bases can be divided into two categories: purines and pyrimidines. Purines have a double ring structure, and pyrimidines have a single ring.
The nitrogenous bases, important components of nucleotides, are organic molecules and are so named because they contain carbon and nitrogen. They are bases because they contain an amino group that has the potential of binding an extra hydrogen, and thus, decreases the hydrogen ion concentration in its environment, making it more basic. Each nucleotide in DNA contains one of four possible nitrogenous bases: adenine (A), guanine (G) cytosine (C), and thymine (T). RNA nucleotides also contain one of four possible bases: adenine, guanine, cytosine, and uracil (U) rather than thymine.
Adenine and guanine are classified as purines . The primary structure of a purine is two carbon-nitrogen rings. Cytosine, thymine, and uracil are classified as pyrimidines which have a single carbon-nitrogen ring as their primary structure (Figure 1). Each of these basic carbon-nitrogen rings has different functional groups attached to it. In molecular biology shorthand, the nitrogenous bases are simply known by their symbols A, T, G, C, and U. DNA contains A, T, G, and C whereas RNA contains A, U, G, and C.
The pentose sugar in DNA is deoxyribose, and in RNA, the sugar is ribose (Figure 1). The difference between the sugars is the presence of the hydroxyl group on the second carbon of the ribose and hydrogen on the second carbon of the deoxyribose. The carbon atoms of the sugar molecule are numbered as 1′, 2′, 3′, 4′, and 5′ (1′ is read as “one prime”). The phosphate residue is attached to the hydroxyl group of the 5′ carbon of one sugar and the hydroxyl group of the 3′ carbon of the sugar of the next nucleotide, which forms a 5′–3′ phosphodiester linkage. The phosphodiester linkage is not formed by simple dehydration reaction like the other linkages connecting monomers in macromolecules: its formation involves the removal of two phosphate groups. A polynucleotide may have thousands of such phosphodiester linkages.
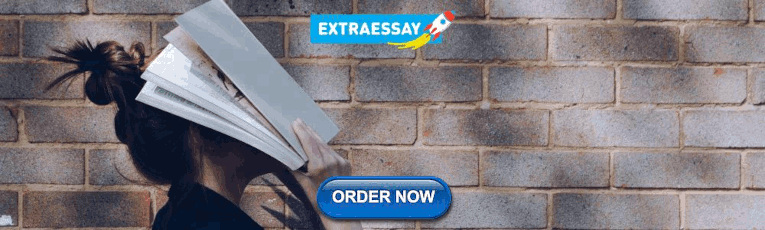
DNA Double-Helix Structure
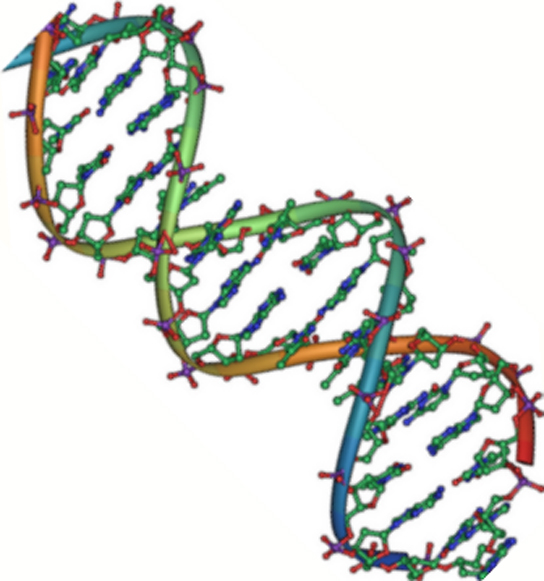
Figure 2. DNA is an antiparallel double helix. The phosphate backbone (the curvy lines) is on the outside, and the bases are on the inside. Each base interacts with a base from the opposing strand. (credit: Jerome Walker/Dennis Myts)
DNA has a double-helix structure (Figure 2). The sugar and phosphate lie on the outside of the helix, forming the backbone of the DNA. The nitrogenous bases are stacked in the interior, like the steps of a staircase, in pairs; the pairs are bound to each other by hydrogen bonds. Every base pair in the double helix is separated from the next base pair by 0.34 nm.
The two strands of the helix run in opposite directions, meaning that the 5′ carbon end of one strand will face the 3′ carbon end of its matching strand. (This is referred to as antiparallel orientation and is important to DNA replication and in many nucleic acid interactions.)
Only certain types of base pairing are allowed. For example, a certain purine can only pair with a certain pyrimidine. This means A can pair with T, and G can pair with C, as shown in Figure 3. This is known as the base complementary rule. In other words, the DNA strands are complementary to each other. If the sequence of one strand is AATTGGCC, the complementary strand would have the sequence TTAACCGG. During DNA replication, each strand is copied, resulting in a daughter DNA double helix containing one parental DNA strand and a newly synthesized strand.
Practice Question
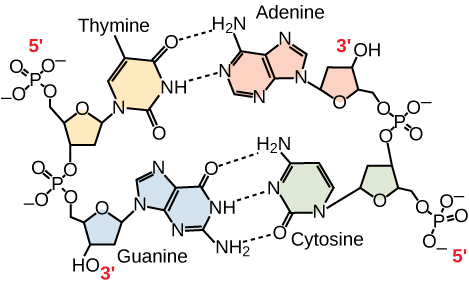
Figure 3. In a double stranded DNA molecule, the two strands run antiparallel to one another so that one strand runs 5′ to 3′ and the other 3′ to 5′. The phosphate backbone is located on the outside, and the bases are in the middle. Adenine forms hydrogen bonds (or base pairs) with thymine, and guanine base pairs with cytosine.
A mutation occurs, and cytosine is replaced with adenine. What impact do you think this will have on the DNA structure?
Ribonucleic acid, or RNA, is mainly involved in the process of protein synthesis under the direction of DNA. RNA is usually single-stranded and is made of ribonucleotides that are linked by phosphodiester bonds. A ribonucleotide in the RNA chain contains ribose (the pentose sugar), one of the four nitrogenous bases (A, U, G, and C), and the phosphate group.
There are four major types of RNA: messenger RNA (mRNA), ribosomal RNA (rRNA), transfer RNA (tRNA), and microRNA (miRNA). The first, mRNA, carries the message from DNA, which controls all of the cellular activities in a cell. If a cell requires a certain protein to be synthesized, the gene for this product is turned “on” and the messenger RNA is synthesized in the nucleus. The RNA base sequence is complementary to the coding sequence of the DNA from which it has been copied. However, in RNA, the base T is absent and U is present instead. If the DNA strand has a sequence AATTGCGC, the sequence of the complementary RNA is UUAACGCG. In the cytoplasm, the mRNA interacts with ribosomes and other cellular machinery (Figure 4).
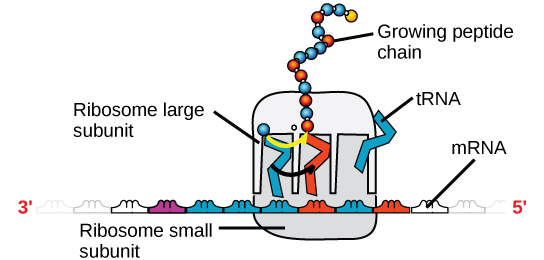
Figure 4. A ribosome has two parts: a large subunit and a small subunit. The mRNA sits in between the two subunits. A tRNA molecule recognizes a codon on the mRNA, binds to it by complementary base pairing, and adds the correct amino acid to the growing peptide chain.
The mRNA is read in sets of three bases known as codons. Each codon codes for a single amino acid. In this way, the mRNA is read and the protein product is made. Ribosomal RNA (rRNA) is a major constituent of ribosomes on which the mRNA binds. The rRNA ensures the proper alignment of the mRNA and the ribosomes; the rRNA of the ribosome also has an enzymatic activity (peptidyl transferase) and catalyzes the formation of the peptide bonds between two aligned amino acids. Transfer RNA (tRNA) is one of the smallest of the four types of RNA, usually 70–90 nucleotides long. It carries the correct amino acid to the site of protein synthesis. It is the base pairing between the tRNA and mRNA that allows for the correct amino acid to be inserted in the polypeptide chain. microRNAs are the smallest RNA molecules and their role involves the regulation of gene expression by interfering with the expression of certain mRNA messages.
Contribute!
Improve this page Learn More
- Biology 2e. Provided by : OpenStax. Located at : http://cnx.org/contents/[email protected] . License : CC BY: Attribution . License Terms : Access for free at https://openstax.org/books/biology-2e/pages/1-introduction
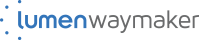
Browse Course Material
Course info, instructors.
- Prof. Barbara Imperiali
- Prof. Adam Martin
- Dr. Diviya Ray
Departments
As taught in.
- Functional Genomics
- Biochemistry
- Cell Biology
- Microbiology
- Molecular Biology
Learning Resource Types
Introductory biology, lecture 6: nucleic acids, description.
In this final lecture of the Biochemistry unit, Professor Imperiali covers nucleotides and nucleic acids, discussing their structures and their importance as fundamental units for information storage and information transfer.
Instructor: Barbara Imperiali
- Download video
- Download transcript

You are leaving MIT OpenCourseWare

- school Campus Bookshelves
- menu_book Bookshelves
- perm_media Learning Objects
- login Login
- how_to_reg Request Instructor Account
- hub Instructor Commons
- Download Page (PDF)
- Download Full Book (PDF)
- Periodic Table
- Physics Constants
- Scientific Calculator
- Reference & Cite
- Tools expand_more
- Readability
selected template will load here
This action is not available.
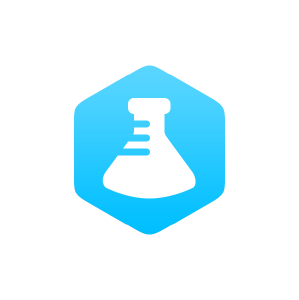
17.E: Nucleic Acids (Exercises)
- Last updated
- Save as PDF
- Page ID 306724
19.1: Nucleotides
Concept review exercises.
- deoxyribose
- nitrogenous base (adenine, guanine, cytosine, and thymine), 2-deoxyribose, and H 3 PO 4
- nitrogenous base (adenine, guanine, cytosine, and uracil), ribose, and H 3 PO 4
- pentose sugar
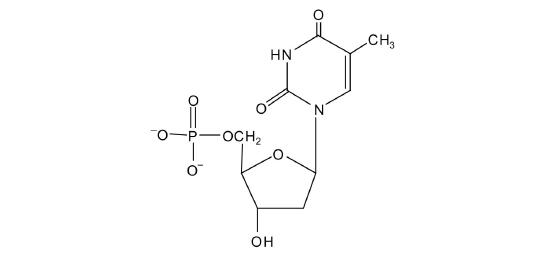
19.2: Nucleic Acid Structure
- Name the two kinds of nucleic acids.
- Which type of nucleic acid stores genetic information in the cell?
- What are complementary bases?
- Why is it structurally important that a purine base always pair with a pyrimidine base in the DNA double helix?
- deoxyribonucleic acid (DNA) and ribonucleic acid (RNA)
- the specific base pairings in the DNA double helix in which guanine is paired with cytosine and adenine is paired with thymine
- The width of the DNA double helix is kept at a constant width, rather than narrowing (if two pyrimidines were across from each other) or widening (if two purines were across from each other).
- identify the 5′ end and the 3′ end of the molecule.
- circle the atoms that comprise the backbone of the nucleic acid chain.
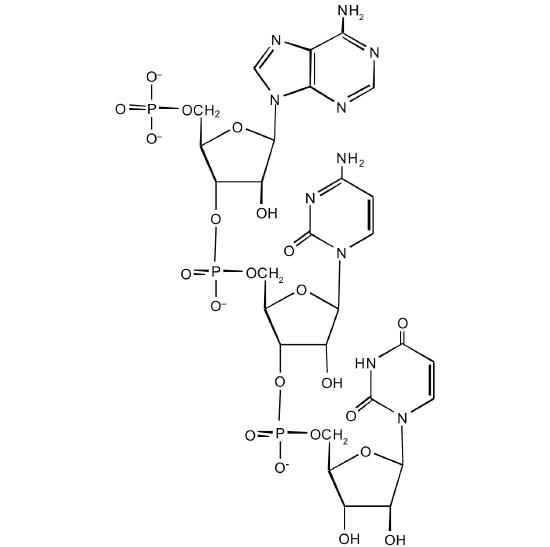
5′ ATGCGACTA 3′ 3′ TACGCTGAT 5′
5′ CGATGAGCC 3′ 3′ GCTACTCGG 5′

- 22 (2 between each AT base pair and 3 between each GC base pair)
19.3: Replication and Expression of Genetic Information
- In DNA replication, a parent DNA molecule produces two daughter molecules. What is the fate of each strand of the parent DNA double helix?
- What is the role of DNA in transcription? What is produced in transcription?
- Which type of RNA contains the codon? Which type of RNA contains the anticodon?
- Each strand of the parent DNA double helix remains associated with the newly synthesized DNA strand.
- DNA serves as a template for the synthesis of an RNA strand (the product of transcription).
- codon: mRNA; anticodon: tRNA
- Describe how replication and transcription are similar.
- Describe how replication and transcription differ.
- What is the sequence of complementary template strand?
- What is the sequence of the mRNA that would be produced during transcription from this segment of DNA?
- Both processes require a template from which a complementary strand is synthesized.
- 3′‑TACTCGCTGAAACGCCCTAAT‑5′
- 5′‑AUGAGCGACUUUGCGGGAUUA‑3′
19.4: Protein Synthesis and the Genetic Code
- What are the roles of mRNA and tRNA in protein synthesis?
- What is the initiation codon?
- What are the termination codons and how are they recognized?
- mRNA provides the code that determines the order of amino acids in the protein; tRNA transports the amino acids to the ribosome to incorporate into the growing protein chain.
- UAA, UAG, and UGA; they are recognized by special proteins called release factors, which signal the end of the translation process.
- 5′‑UUU‑3′
- 5′‑CAU‑3′
- 5′‑AGC‑3′
- 5′‑CCG‑3′
- 5′‑UUG‑3′
- 5′‑GAA‑3′
- 5′‑UCC‑3′
- 5′‑CAC‑3′
- The peptide hormone oxytocin contains 9 amino acid units. What is the minimum number of nucleotides needed to code for this peptide?
- Myoglobin, a protein that stores oxygen in muscle cells, has been purified from a number of organisms. The protein from a sperm whale is composed of 153 amino acid units. What is the minimum number of nucleotides that must be present in the mRNA that codes for this protein?
- Use Figure \(\PageIndex{3}\) to identify the amino acids carried by each tRNA molecule in Exercise 1.
- Use Figure \(\PageIndex{3}\) to identify the amino acids carried by each tRNA molecule in Exercise 2.
- Use Figure \(\PageIndex{3}\) to determine the amino acid sequence produced from this mRNA sequence: 5′‑AUGAGCGACUUUGCGGGAUUA‑3′.
- Use Figure \(\PageIndex{3}\) to determine the amino acid sequence produced from this mRNA sequence: 5′‑AUGGCAAUCCUCAAACGCUGU‑3′
- 3′‑AAA‑5′
- 3′‑GUA‑5′
- 3′‑UCG‑5′
- 3′‑GGC‑5′
- 27 nucleotides (3 nucleotides/codon)
- 1a: phenyalanine; 1b: histidine; 1c: serine; 1d: proline
- met-ser-asp-phe-ala-gly-leu
19.5: Mutations and Genetic Diseases
- What effect can UV radiation have on DNA?
- Is UV radiation an example of a physical mutagen or a chemical mutagen?
- What causes PKU?
- How is PKU detected and treated?
- It can lead to the formation of a covalent bond between two adjacent thymines on a DNA strand, producing a thymine dimer.
- physical mutagen
- the absence of the enzyme phenylalanine hydroxylase
- PKU is diagnosed by assaying a sample of blood or urine for phenylalanine or one of its metabolites; treatment calls for an individual to be placed on a diet containing little or no phenylalanine.
- Identify the mutation as a substitution, an insertion, or a deletion.
- What effect would the mutation have on the amino acid sequence of the protein obtained from this mutated gene (use Figure 19.14)?
- What is a mutagen?
- Give two examples of mutagens.
- Tay-Sachs disease
- substitution
- Phenylalanine (UUU) would be replaced with leucine (CUU).
- a chemical or physical agent that can cause a mutation
- UV radiation and gamma radiation (answers will vary)
19.6: Viruses
- Describe the general structure of a virus.
- How does a DNA virus differ from an RNA virus?
- Why is HIV known as a retrovirus?
- Describe how a DNA virus invades and destroys a cell.
- Describe how an RNA virus invades and destroys a cell.
- How does this differ from a DNA virus?
- What HIV enzyme does AZT inhibit?
- What HIV enzyme does raltegravir inhibit?
- A virus consists of a central core of nucleic acid enclosed in a protective shell of proteins. There may be lipid or carbohydrate molecules on the surface.
- A DNA virus has DNA as its genetic material, while an RNA virus has RNA as its genetic material.
- In a cell, a retrovirus synthesizes a DNA copy of its RNA genetic material.
- The DNA virus enters a host cell and induces the cell to replicate the viral DNA and produce viral proteins. These proteins and DNA assemble into new viruses that are released by the host cell, which may die in the process.
- reverse transcriptase
Additional Exercises
For this nucleic acid segment,

- classify this segment as RNA or DNA and justify your choice.
- determine the sequence of this segment, labeling the 5′ and 3′ ends.
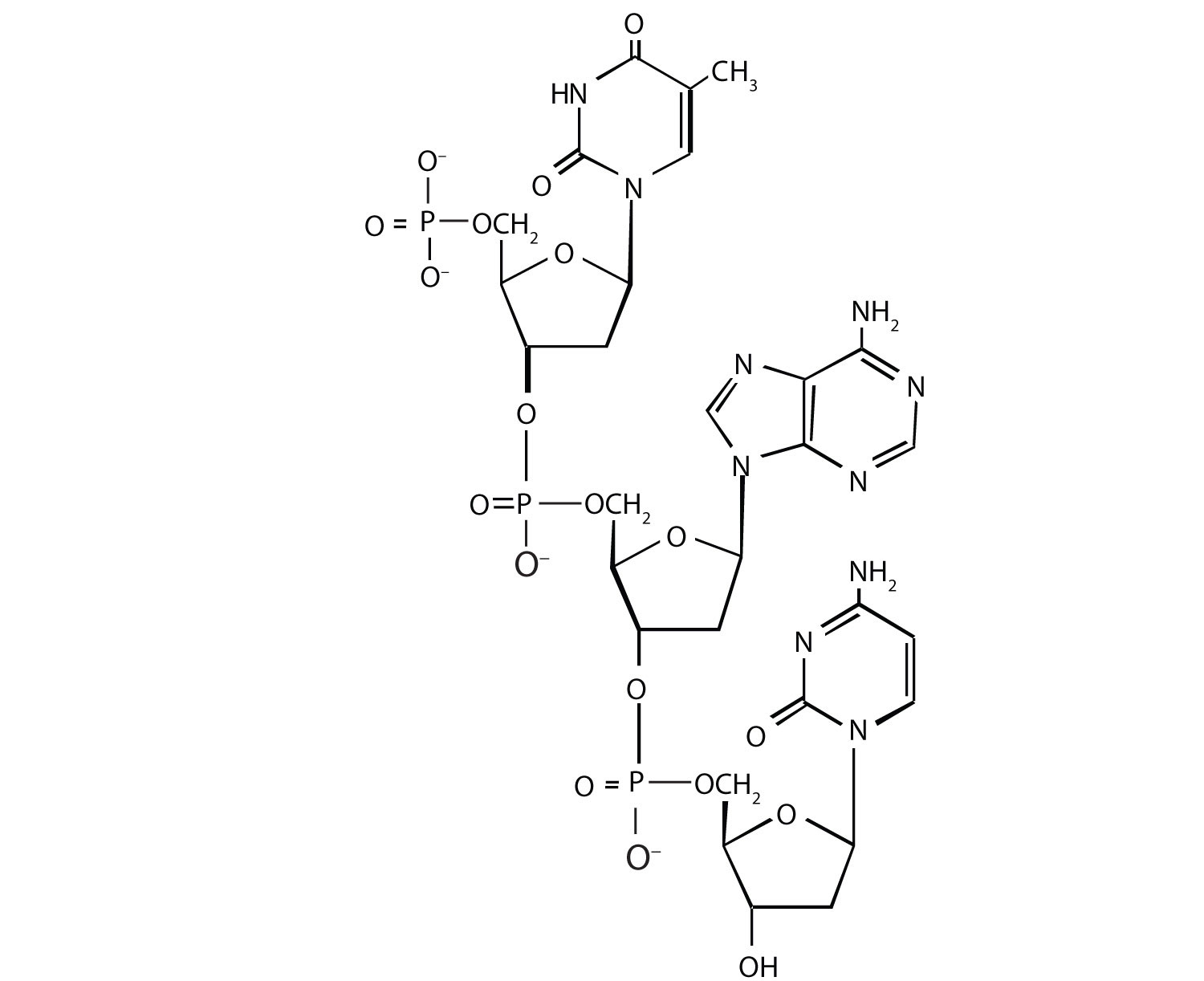
One of the key pieces of information that Watson and Crick used in determining the secondary structure of DNA came from experiments done by E. Chargaff, in which he studied the nucleotide composition of DNA from many different species. Chargaff noted that the molar quantity of A was always approximately equal to the molar quantity of T, and the molar quantity of C was always approximately equal to the molar quantity of G. How were Chargaff’s results explained by the structural model of DNA proposed by Watson and Crick?
Suppose Chargaff (see Exercise 3) had used RNA instead of DNA. Would his results have been the same; that is, would the molar quantity of A approximately equal the molar quantity of T? Explain.
In the DNA segment
5′‑ATGAGGCATGAGACG‑3′ (coding strand) 3′‑TACTCCGTACTCTGC‑5′ (template strand)
- What products would be formed from the segment’s replication?
- Write the mRNA sequence that would be obtained from the segment’s transcription.
- What is the amino acid sequence of the peptide produced from the mRNA in Exercise 5b?
5′‑ATGACGGTTTACTAAGCC‑3′ (coding strand) 3′‑TACTGCCAAATGATTCGG‑5′ (template strand)
- What is the amino acid sequence of the peptide produced from the mRNA in Exercise 6b?
A hypothetical protein has a molar mass of 23,300 Da. Assume that the average molar mass of an amino acid is 120.
- How many amino acids are present in this hypothetical protein?
- What is the minimum number of codons present in the mRNA that codes for this protein?
- What is the minimum number of nucleotides needed to code for this protein?
Bradykinin is a potent peptide hormone composed of nine amino acids that lowers blood pressure.
- The amino acid sequence for bradykinin is arg-pro-pro-gly-phe-ser-pro-phe-arg. Postulate a base sequence in the mRNA that would direct the synthesis of this hormone. Include an initiation codon and a termination codon.
- What is the nucleotide sequence of the DNA that codes for this mRNA?
A particular DNA coding segment is ACGTTA G CCCCAGCT.
- Write the sequence of nucleotides in the corresponding mRNA.
- Determine the amino acid sequence formed from the mRNA in Exercise 9a during translation.
What amino acid sequence results from each of the following mutations?
- replacement of the underlined guanine by adenine
- insertion of thymine immediately after the underlined guanine
- deletion of the underlined guanine
A particular DNA coding segment is TAC G ACGTAAC A AGC.
- Determine the amino acid sequence formed from the mRNA in Exercise 10a during translation.
- replacement of the underlined adenine by thymine
Two possible point mutations are the substitution of lysine for leucine or the substitution of serine for threonine. Which is likely to be more serious and why?
Two possible point mutations are the substitution of valine for leucine or the substitution of glutamic acid for histidine. Which is likely to be more serious and why?
- RNA; the sugar is ribose, rather than deoxyribose
- 5′‑GUA‑3′
In the DNA structure, because guanine (G) is always paired with cytosine (C) and adenine (A) is always paired with thymine (T), you would expect to have equal amounts of each.
- Each strand would be replicated, resulting in two double-stranded segments.
- 5′‑AUGAGGCAUGAGACG‑3′
- met-arg-his-glu-thr
- 5′‑ACGUUAGCCCCAGCU‑3′
- thr-leu-ala-pro-ala
- thr-leu-thr-pro-ala
- thr-leu-val-pro-ser
- thr-leu-pro-gin
substitution of lysine for leucine because you are changing from an amino acid with a nonpolar side chain to one that has a positively charged side chain; both serine and threonine, on the other hand, have polar side chains containing the OH group.
3.5 Nucleic Acids
Learning objectives.
- Describe the structure of nucleic acids and define the two types of nucleic acids
- Explain the structure and role of DNA
- Explain the structure and roles of RNA
Nucleic acids are the most important macromolecules for the continuity of life. They carry the genetic blueprint of a cell and carry instructions for the functioning of the cell.
DNA and RNA
The two main types of nucleic acids are deoxyribonucleic acid (DNA) and ribonucleic acid (RNA) . DNA is the genetic material found in all living organisms, ranging from single-celled bacteria to multicellular mammals. It is found in the nucleus of eukaryotes and in the organelles, chloroplasts, and mitochondria. In prokaryotes, the DNA is not enclosed in a membranous envelope.
The entire genetic content of a cell is known as its genome, and the study of genomes is genomics. In eukaryotic cells but not in prokaryotes, DNA forms a complex with histone proteins to form chromatin, the substance of eukaryotic chromosomes. A chromosome may contain tens of thousands of genes. Many genes contain the information to make protein products; other genes code for RNA products. DNA controls all of the cellular activities by turning the genes “on” or “off.”
The other type of nucleic acid, RNA, is mostly involved in protein synthesis. The DNA molecules never leave the nucleus but instead use an intermediary to communicate with the rest of the cell. This intermediary is the messenger RNA (mRNA) . Other types of RNA—like rRNA, tRNA, and microRNA—are involved in protein synthesis and its regulation.
DNA and RNA are made up of monomers known as nucleotides . The nucleotides combine with each other to form a polynucleotide , DNA or RNA. Each nucleotide is made up of three components: a nitrogenous base, a pentose (five-carbon) sugar, and a phosphate group ( Figure 3.31 ). Each nitrogenous base in a nucleotide is attached to a sugar molecule, which is attached to one or more phosphate groups.
The nitrogenous bases, important components of nucleotides, are organic molecules and are so named because they contain carbon and nitrogen. They are bases because they contain an amino group that has the potential of binding an extra hydrogen, and thus, decreases the hydrogen ion concentration in its environment, making it more basic. Each nucleotide in DNA contains one of four possible nitrogenous bases: adenine (A), guanine (G) cytosine (C), and thymine (T).
Adenine and guanine are classified as purines . The primary structure of a purine is two carbon-nitrogen rings. Cytosine, thymine, and uracil are classified as pyrimidines which have a single carbon-nitrogen ring as their primary structure ( Figure 3.31 ). Each of these basic carbon-nitrogen rings has different functional groups attached to it. In molecular biology shorthand, the nitrogenous bases are simply known by their symbols A, T, G, C, and U. DNA contains A, T, G, and C whereas RNA contains A, U, G, and C.
The pentose sugar in DNA is deoxyribose, and in RNA, the sugar is ribose ( Figure 3.31 ). The difference between the sugars is the presence of the hydroxyl group on the second carbon of the ribose and hydrogen on the second carbon of the deoxyribose. The carbon atoms of the sugar molecule are numbered as 1′, 2′, 3′, 4′, and 5′ (1′ is read as “one prime”). The phosphate residue is attached to the hydroxyl group of the 5′ carbon of one sugar and the hydroxyl group of the 3′ carbon of the sugar of the next nucleotide, which forms a 5′–3′ phosphodiester linkage. The phosphodiester linkage is not formed by simple dehydration reaction like the other linkages connecting monomers in macromolecules: its formation involves the removal of two phosphate groups. A polynucleotide may have thousands of such phosphodiester linkages.
DNA Double-Helix Structure
DNA has a double-helix structure ( Figure 3.32 ). The sugar and phosphate lie on the outside of the helix, forming the backbone of the DNA. The nitrogenous bases are stacked in the interior, like the steps of a staircase, in pairs; the pairs are bound to each other by hydrogen bonds. Every base pair in the double helivx is separated from the next base pair by 0.34 nm. The two strands of the helix run in opposite directions, meaning that the 5′ carbon end of one strand will face the 3′ carbon end of its matching strand. (This is referred to as antiparallel orientation and is important to DNA replication and in many nucleic acid interactions.)
Only certain types of base pairing are allowed. For example, a certain purine can only pair with a certain pyrimidine. This means A can pair with T, and G can pair with C, as shown in Figure 3.33 . This is known as the base complementary rule. In other words, the DNA strands are complementary to each other. If the sequence of one strand is AATTGGCC, the complementary strand would have the sequence TTAACCGG. During DNA replication, each strand is copied, resulting in a daughter DNA double helix containing one parental DNA strand and a newly synthesized strand.
A mutation occurs, and cytosine is replaced with adenine. What impact do you think this will have on the DNA structure?
Ribonucleic acid, or RNA, is mainly involved in the process of protein synthesis under the direction of DNA. RNA is usually single-stranded and is made of ribonucleotides that are linked by phosphodiester bonds. A ribonucleotide in the RNA chain contains ribose (the pentose sugar), one of the four nitrogenous bases (A, U, G, and C), and the phosphate group.
There are four major types of RNA: messenger RNA (mRNA), ribosomal RNA (rRNA), transfer RNA (tRNA), and microRNA (miRNA). The first, mRNA, carries the message from DNA, which controls all of the cellular activities in a cell. If a cell requires a certain protein to be synthesized, the gene for this product is turned “on” and the messenger RNA is synthesized in the nucleus. The RNA base sequence is complementary to the coding sequence of the DNA from which it has been copied. However, in RNA, the base T is absent and U is present instead. If the DNA strand has a sequence AATTGCGC, the sequence of the complementary RNA is UUAACGCG. In the cytoplasm, the mRNA interacts with ribosomes and other cellular machinery ( Figure 3.34 ).
The mRNA is read in sets of three bases known as codons. Each codon codes for a single amino acid. In this way, the mRNA is read and the protein product is made. Ribosomal RNA (rRNA) is a major constituent of ribosomes on which the mRNA binds. The rRNA ensures the proper alignment of the mRNA and the ribosomes; the rRNA of the ribosome also has an enzymatic activity (peptidyl transferase) and catalyzes the formation of the peptide bonds between two aligned amino acids. Transfer RNA (tRNA) is one of the smallest of the four types of RNA, usually 70–90 nucleotides long. It carries the correct amino acid to the site of protein synthesis. It is the base pairing between the tRNA and mRNA that allows for the correct amino acid to be inserted in the polypeptide chain. microRNAs are the smallest RNA molecules and their role involves the regulation of gene expression by interfering with the expression of certain mRNA messages. Table 3.2 summarizes features of DNA and RNA.
Even though the RNA is single stranded, most RNA types show extensive intramolecular base pairing between complementary sequences, creating a predictable three-dimensional structure essential for their function.
As you have learned, information flow in an organism takes place from DNA to RNA to protein. DNA dictates the structure of mRNA in a process known as transcription , and RNA dictates the structure of protein in a process known as translation . This is known as the Central Dogma of Life, which holds true for all organisms; however, exceptions to the rule occur in connection with viral infections.
Link to Learning
To learn more about DNA, explore the Howard Hughes Medical Institute BioInteractive animations on the topic of DNA.
As an Amazon Associate we earn from qualifying purchases.
This book may not be used in the training of large language models or otherwise be ingested into large language models or generative AI offerings without OpenStax's permission.
Want to cite, share, or modify this book? This book uses the Creative Commons Attribution License and you must attribute OpenStax.
Access for free at https://openstax.org/books/biology/pages/1-introduction
- Authors: Connie Rye, Robert Wise, Vladimir Jurukovski, Jean DeSaix, Jung Choi, Yael Avissar
- Publisher/website: OpenStax
- Book title: Biology
- Publication date: Oct 21, 2016
- Location: Houston, Texas
- Book URL: https://openstax.org/books/biology/pages/1-introduction
- Section URL: https://openstax.org/books/biology/pages/3-5-nucleic-acids
© Feb 14, 2022 OpenStax. Textbook content produced by OpenStax is licensed under a Creative Commons Attribution License . The OpenStax name, OpenStax logo, OpenStax book covers, OpenStax CNX name, and OpenStax CNX logo are not subject to the Creative Commons license and may not be reproduced without the prior and express written consent of Rice University.
Nucleic Acid
Nucleic acids are large molecules where genetic information is stored. They are macromolecules that store genetic information and enable protein production. They are composed of individual acid units termed nucleotides. There are two types of nucleic acids: deoxyribonucleic acid, better known as DNA and ribonucleic acid, better known as RNA. They carry the genetic blueprint of a cell and carry instructions for the functioning of the cell.
The nucleic acids are made of nucleotides. DNA and RNA are made up of monomers also known as nucleotides. A nucleotide is made of a nitrogenous base, sugar with five carbon atoms and a phosphate group. This compound was neither a protein nor lipid nor a carbohydrate; therefore, it was a novel type of biological molecule. Each nucleotide is made up of three components: a nitrogenous base, a pentose sugar, and a phosphate group. They are the most important macromolecules for the continuity of life.
Nucleic acids allow organisms to transfer genetic information from one generation to the next. When a cell divides, its DNA is copied and passed from one cell generation to the next generation. DNA is composed of a phosphate-deoxyribose sugar backbone and the nitrogenous bases adenine (A), guanine (G), cytosine (C), and thymine (T). It is found in the nucleus of eukaryotes and in the organelles, chloroplasts, and mitochondria. The order, or sequence, of the nucleotides in DNA allows nucleic acid to encode an organism’s genetic blueprint.
DNA is organized into chromosomes and found within the nucleus of our cells. Its function in any cell is to carry the sequence of bases which will be transcribed into RNA. The function of RNA is much more difficult to explain. RNA has ribose sugar and the nitrogenous bases A, G, C, and uracil (U). One well-known function is for messenger RNA to be translated into proteins, mainly enzymes. But there are other types of RNA, and these are called non-coding RNAs.
Through the Use of Nanoinclusions, Researchers Enhance the Thermoelectric Properties of n-type Transition Metal Selenide
Ph indicator – a halochromic chemical compound, alcohol – an organic compound, production of ethylene by cations, advance wet processing technology, cytokines – a group of signaling molecules made by cells, the raven’s spell, libertarian socialism, credit risk management of ific bank, hypophosphorous acid (properties, uses), latest post, cathodic protection – a technique for controlling corrosion, electromagnetism – a discipline of physics, astronomers measure the heaviest black hole pair ever discovered, even passive smokers are extensively colonized by microbes, webb discovers proof that a neutron star powers the young supernova remnant, flyback transformer (fbt).

An official website of the United States government
The .gov means it’s official. Federal government websites often end in .gov or .mil. Before sharing sensitive information, make sure you’re on a federal government site.
The site is secure. The https:// ensures that you are connecting to the official website and that any information you provide is encrypted and transmitted securely.
- Publications
- Account settings
Preview improvements coming to the PMC website in October 2024. Learn More or Try it out now .
- Advanced Search
- Journal List
- Nucleic Acids Res
- v.51(15); 2023 Aug 25
- PMC10450167
DoubleHelix: nucleic acid sequence identification, assignment and validation tool for cryo-EM and crystal structure models
Grzegorz chojnowski.
European Molecular Biology Laboratory, Hamburg Unit, Notkestraße 85, 22607 Hamburg, Germany
Associated Data
The doubleHelix program source code and installation instructions are available at https://gitlab.com/gchojnowski/doublehelix . The doubleHelix sequence assignment algorithm has been added to the checkMySequence program to enable comprehensive validation of sequence assignment in the models of protein-nucleic acid complexes. The updated method is available at https://gitlab.com/gchojnowski/checkmysequence . Corrected models of mammalian and bacterial ribosome structures presented in this work are available at https://doi.org/10.5281/zenodo.7650444 .
Sequence assignment is a key step of the model building process in both cryogenic electron microscopy (cryo-EM) and macromolecular crystallography (MX). If the assignment fails, it can result in difficult to identify errors affecting the interpretation of a model. There are many model validation strategies that help experimentalists in this step of protein model building, but they are virtually non-existent for nucleic acids. Here, I present doubleHelix—a comprehensive method for assignment, identification, and validation of nucleic acid sequences in structures determined using cryo-EM and MX. The method combines a neural network classifier of nucleobase identities and a sequence-independent secondary structure assignment approach. I show that the presented method can successfully assist sequence-assignment step in nucleic-acid model building at lower resolutions, where visual map interpretation is very difficult. Moreover, I present examples of sequence assignment errors detected using doubleHelix in cryo-EM and MX structures of ribosomes deposited in the Protein Data Bank, which escaped the scrutiny of available model-validation approaches. The doubleHelix program source code is available under BSD-3 license at https://gitlab.com/gchojnowski/doublehelix .
Graphical Abstract

INTRODUCTION
Nucleic acids are key players in many cellular processes ranging from gene expression to the catalysis of chemical reactions. For many nucleic acid molecules tertiary structure determines function, much like for proteins. Nevertheless, our understanding of the structure-function relationship in nucleic acids clearly lags behind proteins, which is reflected by the disproportion of structures deposited in the Protein Data Bank (PDB) ( 1 ). As of January 2023, out of 200 708 available structures only 15 374 (7%) contained a nucleic acid component. The resolution revolution in cryogenic electron microscopy (cryo-EM) seems to be slowly changing this picture as more and more challenging nucleic-acid complexes are being determined using this technique. In 2022, out of 1454 structures with nucleic-acid components deposited in the PDB as many as 804 (55%) were determined using cryo-EM. Many of these cryo-EM structures would be very difficult to solve using other techniques owing to their size and structural heterogeneity ( 2 ).
The release of the Artificial Intelligence (AI) based structure prediction programs AlphaFold2 ( 3 ) and RoseTTAFold ( 4 ) provided means for accurate and widely accessible structure prediction of protein structures. Although they did not solve the problem of protein structure determination the accurate predictions proved useful for solving the phase problem in macromolecular X-ray crystallography and the interpretation of cryo-EM maps ( 5 ). There is no AlphaFold2 or RoseTTAFold equivalent for nucleic acids and most likely won’t be soon as building AI 3D structure prediction tools requires huge and diverse training sets of reliable structural models that are currently not available for nucleic acids. The PDB-deposited models are strongly biased towards ribosomal RNA that is usually highly conserved across all kingdoms of live. Moreover, as will be shown later in this work, available experimental RNA structures contain difficult to identify errors that may reduce generalization properties of structure prediction methods. Although attempts to build such tools are already taken (e.g. RoseTTAFoldNA ( 6 ) and ARES ( 7 )), experimental techniques will continue to be the method of choice for detailed structural studies of nucleic acid complexes, with all their limitations and bottlenecks.
MX and cryo-EM remain the most frequently used experimental approaches for the structure determination of large biomolecules. The main result of both these methods is an atomic model traced into a map - an interpretation of experimental observations given a priori knowledge of biomolecular structure. Although, the main effort of the method developer community is clearly focused on proteins, several techniques facilitating experimental determination of nucleic acid structures in cryo-EM and MX have been developed, e.g. NAUTILUS ( 8 ), ARP/wARP ( 9 ), PHENIX ( 10 ), RCrane ( 11 ), COOT ( 12 ), ISOLDE ( 13 ), DeepTracer ( 14 ) and ModelAngelo ( 15 ). As with proteins, nucleic acid model building in these methods usually starts with tracing into a density map a ribose-phosphate backbone that makes up two-thirds of a polynucleotide mass. The backbone model is subsequently assigned to a target sequence and complemented with base moieties.
Sequence assignment is a crucial step in macromolecular model building. It is required for the identification and completion of missing fragments in initial models. It is also a fundamental prerequisite of a model interpretation. Failure may lead to register-shift errors, where residues are systematically assigned an identity of a residue a few positions before or ahead in sequence. Although register-shifts may bias model interpretation, they remain one of the most difficult problems to identify and correct in macromolecular models ( 16 ). In protein models, register-shifts often result in backbone-geometry outliers when several sidechains are forced into too small density volumes, which can be detected using geometry validation approaches like CaBLAM ( 17 ). Moreover, backbone tracing issues (e.g. deletion or insertion) that caused register shift can be occasionally detected as a geometry outlier ( 18 ). Nevertheless, it has been shown that regardless of the effort made to validate protein models, register-shift errors are relatively common in PDB ( 19 , 20 ). Particularly affected are very large structures, for example ribosomes, where detailed inspection of a model using interactive tools (e.g. COOT or ISOLDE) is rarely feasible.
Sequence assignment errors in nucleic acids are even more difficult to identify than in proteins. They rarely result in severe geometry issues during model refinement as the ribose-phosphate backbone dominates scattering and its geometry is weakly affected by the presence of misassigned nucleobases. Moreover, small differences between different types of purines and pyrimidines makes visual validation of a sequence assignment very challenging unless high-resolution maps are available.
The most prominent issue related to a sequence assignment error in nucleic acid structures are steric clashes arising from the presence of base-paired nucleobases that don’t fit their secondary structure context - non-isostericity ( 21 ). For example, a Watson–Crick pair in cis orientation that erroneously involves two guanines is too large to fit into a double-helical region ( 22 ). At lower resolutions, however, this will be promptly masked by a refinement software and the bases that are weakly restrained by a map shifted to a non-clashing conformation. Nevertheless, these relatively rare issues can be in principle be detected using standard model-validation software, e.g. Molprobity ( 17 ).
Another, rarely recognised, issue related to the sequence assignment in model building are unknown target sequences. Until recently, structural studies of macromolecules of unknown identity, e.g. extracted from natural sources, were attempted predominantly using MX ( 23 ). Recent developments in cryo-EM, however, forged a completely new path to the studies of uncharacterised macromolecules. It has been shown that cryo-EM reconstructions of protein nucleic-acid complexes, at resolutions high enough for de-novo model building, can be determined directly in a cell using subtomogram averaging ( 24 ). High-resolution structural information can be also retrieved from a systematic cryo-EM analysis of cell lysate fractions ( 25 , 26 ). In a recent study Skalidis and co-workers ( 27 ) presented a complete workflow for identifying biomolecules directly from native cell extracts combining cryo-EM with AI structure prediction methods. Although there are in principle no technical limitations to the identification of nucleic-acid sequences directly from cryo-EM reconstruction, to the best of my knowledge there is no computational tool available that could be used for this purpose.
In this work, I present doubleHelix ; a computer program for comprehensive nucleic-acid sequence identification, assignment, and validation in MX and cryo-EM models. Similarly to a previously developed program findMySequence ( 28 ) for protein-sequence identification, doubleHelix uses neural network classifiers for estimating residue-type probabilities given a backbone model and a density map. What makes the doubleHelix program unique is the way it addresses the inherent nucleobase-type ambiguity that makes it impossible to distinguish adenine from guanine and cytosine from uracil or thymine unless a very high-resolution experimental data is available. The program estimates only the probabilities of purines and pyrimidines in a model. This information is complemented with base-pairing restraints obtained using a new approach that relies on a backbone conformation ignoring nucleobase identities that are not known before the sequence assignment. The base-pair identification approach is based on alignment of recurrent nucleic-acid structural motifs of known secondary structure (e.g. A- or B-form double helices) to the target model. I show that despite its simplicity this approach is both highly specific and accurate. Moreover, the secondary structure information it provides readily improves sequence assignment and identification performance at lower resolutions where base-type classification reliability is reduced. I also show an example of a previously unidentified RNA-sequence assignment errors in mammalian and bacterial ribosome structures deposited in the PDB that could have been avoided if doubleHelix had been used for model building and validation.
AN OVERVIEW OF THE DOUBLEHELIX METHOD
The doubleHelix program requires on input a model in PDB or mmCIF format. For the sequence identification and assignment, it also requires a corresponding map, which can be provided in CCP4/MRC format for cryo-EM models or as a MTZ file with structure factor amplitudes and phases for crystal structures. The doubleHelix program provides four basic functionalities:
- Secondary structure restraints generator for nucleic-acid models
Given RNA or DNA model on input the program generates base-pair and -stacking restraints in formats accepted by COOT and popular refinement programs REFMAC5 ( 29 ), PHENIX ( 30 ) and ISOLDE ( 13 ). Additionally, it generates a PYMOL ( 31 ) script that can be used for visualising the restraints (Figure (Figure1C). 1C ). The restraints are also generated for interacting model fragments modelled as separate chains (e.g. DNA duplexes).

Schematic representation of the doubleHelix workflow. Key steps are color-coded and grouped in dashed rectangles; ( A ) input map (cryo-EM or MX), nucleic acid model, and target sequences ( B ) nucleobase-type probability estimation, ( C ) base-pair and refinement restraints assignment based on matched recurrent structural motifs, ( D ) sequence identification and ( E ) assignment based on estimated nucleobase-type probabilities and secondary structure. All steps are integrated in the software and performed automatically.
- Identification of unknown sequences of nucleic-acid models
For a nucleic acid model and a corresponding map (CCP4/MRC or MTZ formats for cryo-EM and MX, respectively), the program identifies the most plausible sequence from a sequence-database in FASTA format given estimated nucleobase-type probabilities and input model secondary structure (Figure (Figure1D). 1D ). By default, the program identifies a sequence that best matches all nucleic-acid chains fragments in the input model.
- Assignment of nucleic acid models to known target sequences
For a nucleic acid model and a corresponding map (CCP4/MRC or MTZ formats for cryo-EM and MX, respectively), the program assigns continuous polynucleotide chain fragments to the target sequence and rebuilds the bases accordingly. Apart from the estimated nucleobase-type probabilities, base-pairs identified within the fragments are used as additional restraints (Figure (Figure1E 1E ).
- Sequence assignment validation in nucleic-acid models
Given a nucleic-acid model, a corresponding map (CCP4/MRC or MTZ formats for EM and MX, respectively) and the set of all model sequences, the program evaluates the plausibility of the model's sequence assignment. This feature is implemented as an extension of the checkMySequence program and uses an algorithm described previously ( 19 ). Users interested in the validation of nucleic acid model sequence assignment should refer to instructions available on the checkMySequence project page.
MATERIALS AND METHODS
Recurrent structural motifs in nucleic acids.
The doubleHelix program identifies base pairs in RNA and DNA models from a local similarity of backbone coordinates with small ‘search-fragments’ of known secondary structure. Model, double helical A-RNA and B-DNA search-fragments were generated using the X3DNA suite ( 32 ). Non-helical search fragments were selected using RNA Bricks ( 33 ) database. Selected sets of recurrent RNA fragments classified as ‘loops’ with at least 500, 100, 25 and 10 occurrences in the database correspond to 83, 532, 1430 and 2664 search-fragments respectively (as of 28 March 2020).
Ribosome crystal structures for secondary structure assignment benchmarks
As a reference for the secondary structure assignment benchmarks, I used crystal structures of ribosomes available in PDB as of 28 March 2020. From all structures determined at a resolution better than 3.0 Å, I selected ones with crystallographic R-free factor below 0.3. To reduce the set redundancy, from each group of similar structures (e.g. originating from the same publication) I selected models with the lowest R-work/R-free difference. The resulting set contained eight structures originating from Haloarcula marismortui , Thermus thermophilus , and Deinococcus radiodurans (PDB entries 1s72, 4ybb, 7rqa, 1hnx, 1fjg, 1k73, 4y4o, 6oxi). For each of the models, secondary structure was determined using the ClaRNA program ( 34 ) and used as a ground-truth.
Reference set of ribosome cryo-EM structures for sequence identification benchmarks
From the PDB, I selected cryo-EM structures of ribosomes determined at a resolution better than 3.5 Å. Among 102 such structures available as of 4 February 2020, I selected 17 with half-maps available for download in the Electron Microscopy Data Bank (EMDB). For each of the half-map pairs local resolution maps were calculated using Resmap version 1.1.4 ( 35 ) with default parameters.
The selected models (PDB entries 3j79, 3j7a, 3j7q, 5iqr, 5mdv, 5mdw, 5mdy, 5ngm, 5umd, 5wdt, 5we4, 5wfs, 6okk, 6p5i, 6p5j, 6p5k, 6p5n) originated from five different organisms: Plasmodium falciparum, Escherichia coli, Staphylococcus aureus, Sus scrofa and Oryctolagus cuniculus . For each of them, nucleotide sequences corresponding to RNA features annotated based on the genome sequence were downloaded from NCBI ( 36 ) and used as references for the sequence identification benchmarks. The reference sets contain tRNA, rRNA and ncRNA sequences, except for those corresponding to eukaryotic organisms that additionally contain mRNA sequences. To ensure that exact matches are available in the reference sets I added target rRNA sequences to each of them.
Structures used for training neural network classifiers
As map features observed in cryo-EM and MX maps differ in fine detail, two separate neural networks were trained for each of these experimental methods.
For training the cryo-EM nucleobase-type classifier from the cryo-EM structures of ribosomes initially selected for sequence identification benchmarks, for which half-maps were not available in EMDB, I randomly selected 10 (PDB entries 5afi, 5mmi, 5u9f, 5wdt, 6eri, 6h4n, 6ogi, 6om0, 6q8y, 6sgc). Additionally, 142 PDB-deposited cryo-EM structures containing a DNA, but not an RNA component determined at resolution 3.5 Å or better with map-to-model correlation coefficient above 0.8 as estimated for complete models (including non-NA components) using phenix.map_model_cc ( 37 ) were added to the training set.
For training a crystal structure nucleobase-type classifier, I selected eight structures and corresponding maps that were also used for benchmarking secondary structure assignment procedure. Moreover, 100 crystal structures randomly selected from PDB that contain a DNA, but not an RNA component, determined at resolution 3.5 Å or better with R-free–R-work below 0.3 were added to the training set.
Ribosome structures for the base-type classifier benchmarks
For benchmarking the residue type neural network classifiers, I selected five the highest resolution cryo-EM and MX structures available in PDB as of 24 April 2023 and released after training the base-type classifiers. The resulting set contained crystal structurers refined between 2.3 and 2.5 Å resolution (PDB entries 8cvl, 6xhv, 8cvj, 7rqe and 7rqa). The resolution of selected cryo-EM structures varied between 1.5 and 1.9 Å (PDB entries 8b0x, 8glp, 8a3d, 8aye and 7k00). All the models contain modified nucleic acids residues as modelled by their authors. Each modified residue in the set was classified as a purine or pyrimidine based on the presence of imidazole rings. Specifically, the classification was based on the presence of both N1 and N9 atoms within a base, which are located approximately 4.1 Å apart from each other.
Ribosome crystal structures for sequence identification and assignment benchmarks
For RNA sequence identification benchmarks, I arbitrarily selected two crystal structure models of Thermus thermophilus 30S ribosomal subunit determined at a resolution 2.8Å (PDB entry 2uub) and 3.3Å (PDB entry 6mpi). For both targets, re-refined structures were downloaded from the PDB_REDO server ( 38 ). Initially, a randomly selected 90% of ribosomal RNA nucleobases were mutated in both models (purines to pyrimidines and vice versa) keeping canonical base-pairing interactions identified using doubleHelix (the procedure is implemented in doubleHelix program and can be enabled with an option “- - randomize = 0.9 "). The model coordinates were subsequently randomised with 0.2Å RMSD ignoring any geometry restraints and automatically refined using the PDB_REDO web server. For both models the randomisation procedure clearly affected the R-work/R-free factor values that increased from 0.19/0.23 to 0.25/0.29 and from 0.20/0.25 to 0.23/0.27 for better and worse resolution structures respectively. The automatically refined randomised models with corresponding maximum likelihood, combined 2mFo-DFc maps were used for sequence identification and assignment benchmarks.
For the sequence identification benchmarks, nucleotide sequences corresponding to RNA features annotated based on the Thermus thermophilus genome were downloaded from NCBI and used for making queries.
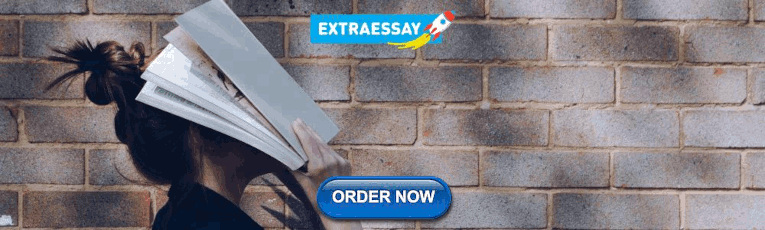
Neural network base-type classifier
To estimate the probability that a given nucleotide fitted into a map corresponds to a purine or pyrimidine two independent neural-network classifiers were prepared. The classifiers have identical architecture but are trained on distinct training sets derived from crystal structures or cryo-EM models and their respective maps.
Nucleotides are described with a vector of map values sampled around a putative base moiety (a residue descriptor). The map is sampled on a regular grid with 1.0 Å spacing. The grid is centred at the N1 or N9 atom for purine and pyrimidine respectively and spanned by orthonormal vectors defined by glycosidic bond (e x ), the normal vector of the ribose best-fitting plane (e y ), and their cross product (e z = e x × e y ). For a given nucleotide the input to the classifier contains a cloud of 403 grid points that are within 1.0 Å distance from any atom of a nucleobase mutated to Guanine in any rotation around the glycosidic bond. In practice a precomputed cloud is aligned to each nucleotide using C2’, C1’ and O4’ ribose or deoxyribose atoms.
The neural-network model input is a vector of length 403 (the residue descriptor described above). The model contains two, fully connected hidden layers. The first layer has a ReLU (Rectified Linear Unit) activation function, which sets all negative neuron inputs to zero, and 403 output features. The second layer has 2 output features and uses the log-softmax normalisation function enabling estimation of output classification probabilities. To avoid overfitting, an additional dropout layer was inserted between the two hidden layers. The dropout layer at each training step disables neuron connections selected at random with probability P . The models were trained for 1000 epochs with P = 0.5, a batch size of 20 residue descriptors in each parameters update cycle, and a 10% validation set. The models were trained using the ADAM optimization algorithm ( 39 ) with a learning rate of 1e–5 that resulted in the best test-set accuracies.
For training the crystal structure classifier I used 84 887 and 9431 nucleobase descriptors for training and test-set respectively. The accuracies of a resulting model were 0.98 and 0.96 for the training and test sets, respectively. Similarly, for training the EM classifier I used 85 092 and 9454 residue descriptors in training and test-sets, respectively. The resulting model estimated accuracies were 0.95 and 0.92 for training and test set, respectively.
Secondary structure assignment procedure
Unlike DNA, which occurs in nature predominantly in a double-helical form, RNA molecules are often single-stranded and fold into complex structures stabilised by stacking and base-pairing interactions ( 40 ). Folded RNA molecules have a modular architecture in which the double-helical regions are intertwined with different types of loops that define the topology of the structure and stabilise it through long-range interactions. Many of these loops are recurrent and can be found in a similar structural context in many, possibly evolutionary unrelated, RNA molecules ( 33 ). Most importantly, it is the overall module geometry and base-pairing pattern rather than the nucleotide sequence that is conserved across different occurrences of the same module ( 41 ). This feature of RNA molecules is used in the doubleHelix program for the inference of base-pairing interactions from the local geometry of sugar-phosphate backbone. This approach ignores both identities and mutual orientation of bases, which is particularly important in the analysis of preliminary, not fully refined models, where base identities are not yet known and their coordinates, unlike relatively heavy backbone, may be inaccurate.
The program superposes small RNA or DNA search-fragments of known secondary structure onto the input model using an algorithm described previously as a part of a model-building program Brickworx ( 2 ). First, all possible triplets of phosphate group P-atoms in a search-fragment are structurally aligned with similar P-atom triplets from the input structure. Resulting rigid body transformation is applied to the complete fragment to identify matching nucleotides in the search fragment and input structure. Finally, the match is refined using all sugar-phosphate backbone atoms. If the resulting root-mean-square deviation (RMSD) is below 1.0 Å (the threshold defined in the Results section), search-fragment base pairs are assigned to the corresponding residues in the input model. If multiple, overlapping matches of search-fragments are identified, the one with the lowest RMSD is selected.
For the sake of computational efficiency, the input model processing is divided into two steps. Firstly, only a double-helical fragment is matched to identify Watson-Crick base-pairs. In this step, A-RNA or B-DNA search fragments are used depending on the target nucleic acid type. Next, all nucleotides within stacked Watson-Crick base-paired regions (except flanking residues) are removed from the input model. In the second step, used only in case of RNA targets, a predefined set of recurrent RNA motifs is matched to the remaining nucleotides in the input model. In case of base-pair assignment conflicts, the ones detected in the second step are given preference.
Sequence identification procedure
For the identification of the most plausible sequence in a database, given input model residue-type probabilities and secondary structure, doubleHelix uses sequence-comparison tools from the INFERNAL suite ( 42 ) (Figure (Figure1D). 1D ). Initially, predicted residue-type probabilities are converted into a multiple sequence alignment (MSA), where fractions of residue types in each column correspond to predicted probabilities. The MSA, combined with base-pairing pattern is encoded in a Stockholm format (STO), which is an input to the cmbuild program. The resulting Covariance Model (CM) is further calibrated with cmalibrate and used to query sequence databases using the cmsearch program with default parameters. The Hidden Markov Model (profile-HMM) queries are enabled by adding the - - hmmonly keyword to cmsearch and skipping the calibration step. Sequence hits with the lowest E-values are returned to the user (3 by default).
Sequence assignment procedure
Analogously to the sequence identification procedure, doubleHelix uses the estimated base-type probabilities to assign RNA or DNA models to a target sequence. For a continuous polynucleotide fragment in the input model a neural network classifier is used to estimate base-type (purine or pyrimidine) probabilities for each residue. The resulting scoring matrix is aligned to the target sequence and the probability of each tentative alignment is approximated by a product of the probability estimates for each residue in the fragment, assuming their independence. If any residue pair in the fragment forms a Watson–Crick base-pair (detected using a procedure described above) an additional, low-probability correction factor (0.1) is used if for a tentative alignment the two bases are either both purines or pyrimidines, which is very unlikely for a Watson–Crick interaction. Otherwise, the correction term is 1.
Although the neural-network classifier has been calibrated and the predicted residue-type probabilities generally reflect expected frequencies, the accuracy of predictions may vary depending on local map resolution and quality of the models ( 9 ). Therefore, for each tentative assignment of a fragment to a target sequence the method estimates a p-value, or probability that a given alignment has been observed by chance. A tentative alignment probability is compared to a background distribution of the fragment alignment probabilities for a long, random sequence. To additionally account for the varying target-sequence lengths an additional extreme-value distribution theorem correction is applied as described before ( 19 ).
Implementation
The doubleHelix program was implemented using Python3 with an extensive use of numpy ( 43 ), scipy ( 44 ), CCTBX ( 45 ) and CCP4 ( 46 ) libraries and utility programs. The neural network classifier used in this work was trained using Pytorch ( 47 ) and deployed to a C code using keras2c ( https://github.com/f0uriest/keras2c ). For making the rRNA sequence database queries, the program uses INFERNAL suite version 1.1.4.
RESULTS AND DISCUSSION
Secondary structure assignment.
The base-pair assignment in the doubleHelix program relies on structural alignment of recurrent motifs with known secondary structure (search-fragments) to the input model. The method uses five different sets of search-fragments. First, A- or B-form double helices, for RNA and DNA target models, respectively, are tried. Additionally, for RNA models, the method uses four sets of the most frequent recurrent RNA motifs from the RNA Bricks database (see Materials and Methods for details). Although the double-helical search fragments can be used for the identification of the canonical Watson–Crick base-pairs only, the other search fragments allow for the identification of any base-pairing interaction type.
The base-pair assignment procedure parameters providing maximum performance are 2-base-pair double-helical search fragments with 1.0 Å RMSD threshold (Figure (Figure2A). 2A ). This can be explained with a relatively high structural heterogeneity observed in double-helical structures ( 48 ), which cannot be represented using longer, idealised search models. Interestingly, an additional search step with recurrent RNA motifs with at least 500 occurrences in the RNA Bricks database further improves precision of the Watson-Crick base-pairs assignment. The resulting Watson-Crick base-pair assignment f 1-score 0.94 is comparable to a value of 0.95 reported for a recently described program CSSR ( 49 ), which also relies on backbone conformations only. With doubleHelix this translates to the recall of 0.91 and precision 0.98 (no corresponding results were reported for CSSR). Unlike doubleHelix, however, CSSR focuses exclusively on pairs of nucleotides compatible with canonical base pairing (A/U, G/C or G/U) that makes the two methods not directly comparable. Another advantage of the doubleHelix approach over CSSR is its ability to identify stackings and non-canonical base-pairs with recall/precision of 0.63/0.89 and 0.47/0.94, respectively (Figure (Figure2B). 2B ). This, however, requires the use of a larger set of recurrent RNA motifs with at least 100 instances in the RNA Bricks database that results in an increased computational cost. For example, for a tRNA model (76 nucleotides, PDB entry 1ehz) processing time on a standard laptop increases from 4 s in default configuration to 13 s. For a complete porcine 28S rRNA (3938 nucleotides, PDB entry 3j7q) these times increase from 1 to almost 8 h. This, however, is needed only for the accurate assignment of non-canonical base-pairs in RNA models (e.g. as refinement restraints). By default, for the identification of Watson–Crick base-pairs the doubleHelix uses the smallest set of RNA recurrent motifs providing maximum performance at a reasonable computational cost.

Performance of RNA secondary structure assignment based on structural alignment of recurrent motifs for ( A ) canonical (Watson–Crick) and ( B ) non-canonical base-pairs. Each data point represents performance for a given stem length (2 or 3 bp) and main-chain atoms RMSD maximising classification f 1-score (harmonic mean of precision and recall). Data-point labels correspond to the number of recurrent RNA motifs used for model interpretation; RNA stem only (0), motifs with at least 500 (1), 100 (2), 25 (3) and 10 (4) occurrences in RNA Bricks database. Precision and recall were estimated using ClaRNA ( 34 ) base-pair classification as ground truth.
Base-type classifier benchmarks
The neural network residue-type classifiers used in this work were trained ignoring any base modifications in the structures. However, these modifications can effectively alter the scattering properties of a base and introduce bias in the classification results. To examine the impact of the base modifications on the classification performance, I selected ten high-resolution models of ribosomes containing modified ribonucleotides, as described in Materials and Methods section.
The crystal structure models of ribosomes contained in total 45 784 standard ribonucleotides out of which 99% have been correctly classified. Among 358 modified bases the most frequent were pseudouridines (81) and all of them were correctly classified as pyrimidines. For other ribonucleotides with modified bases, 222 out of 234 (95%) were correctly classified as purines or pyrimidines. For the remaining modifications that do not affect the base (e.g. O2’-methyluridine), 39 out of 42 (93%) were classified correctly.
In the set of cryo-EM models, 98% out of 28 791 standard ribonucleotides, 297 out of 301 (99%) modifications not affecting bases, and all 294 pseudouridines were classified correctly. Among ribonucleotides with base modifications 89 out of 91 (98%) were classified correctly.
Overall, the performance of base-type classifiers for both cryo-EM and MX agrees with the estimates from the training procedure. This includes modified bases, even though given high-resolution of the maps, the modifications are usually resolved in the density. It can be expected that at lower resolutions the effect of base-modifications will be also negligible as modifications are not resolved in the maps anyway.
Sequence identification in cryo-EM
For the identification of a nucleic acid model the doubleHelix program finds the most plausible sequence in a database given nucleobase-type probabilities (purine or pyrimidine) estimated based on a backbone model and corresponding cryo-EM map. Secondary structure restraints, derived directly from a backbone model, are used as an additional source of information. Both base-type probabilities and base-pairing information are used to query sequence databases using the INFERNAL suite as described in the Materials and Methods section. I observed that this approach allows for a sequence identification up to 4.5Å local resolution for fragments of 50 amino acid residues (Figure (Figure3A) 3A ) when Covariance Models (CMs) and secondary structure information is used. The use of Hidden Markov Models (HMMs), which neglects base-pairing information, clearly reduces the method performance (Figure (Figure3A). 3A ). The use of longer fragments of 100 residues, further increases the resolution limit of the method applicability up to 5.5 Å when the base-pairing information and CMs are used (Figure (Figure3A). 3A ). Overall, the E -value provided by the INFERNAL suite is a reliable measure of the sequence identification reliability. There are, however, a few model fragments for which the identified sequence has a relatively low sequence identity to the target, despite a reliable E -value score (Figure (Figure3B). 3B ). This issue can be attributed to the reduction of the sequence identification problem to purines and pyrimidines only that may occasionally make different sequence fragments practically indistinguishable.

Sequence-identification benchmarks with continuous fragments of 50 and 100 nucleic acid residues selected at random from cryo-EM ribosomal RNA models. Comparison of the method performances for an identification of model sequence with (CM) and without (HMM) the use of base-pairing information. The sequence identification performance is shown as a function of ( A ) local resolution of EM maps and ( B ) E -value of the sequence assignment estimated by the INFERNAL suite. The (B) plot horizontal axis shows -log(E-value); higher values correspond to lower E -values and more reliable sequence identification results. The continuous and dashed curves are logistic regression estimates of a probability that an identified sequence will have at least 90% sequence identity to the target sequence.
Sequence assignment in cryo-EM
It has been shown that a neural network-based assignment of protein model sequence can provide reliable results up to local map resolutions where de novo model building would be very challenging ( 50 ). Moreover, a carefully derived sequence assignment reliability score, the p-value, accurately separates correct from wrong alignments independently of local map resolution. The assignment of polynucleotide sequences, however, presents a particular challenge compared to proteins. First of all, nucleic acid models are often built de novo into lower resolution map regions, as double-helical fragments are excellent and universal models for map interpretation. Moreover, even at moderate resolutions the target sequence is effectively reduced to only two types of nucleobases (purines and pyrimidines) that greatly increases sequence assignment ambiguity.
I observed that similarly to proteins the neural-network classifier implemented in doubleHelix provides a reliable means of assigning polynucleotide sequences to model fragments at local resolutions as low as 5Å, even though the required fragment lengths are clearly longer (Figure (Figure4A). 4A ). For a total number of 18 655 RNA test-fragments of 20 residues (see Materials and Methods for details), the assigned sequence matched the corresponding model in 83% (15 461) of cases. For longer fragments of 40 residues the number of correctly assigned sequences increases to 95% (17 383).

Medians and 90% confidence intervals for sequence assignment p-value as a function of local resolution for RNA chain test-fragments of 20 and 40 nucleic acid residues. Panel ( A ) shows fragments with sequences matching reference. Fragments for which assigned and reference model sequences differ are presented in panel ( B ). The dashed line corresponds to a 99.5% one-sided confidence interval estimated for fragments of 20 nucleic acids with an assigned sequence different from the input-model sequence. Blue circles depict an outlier presented in the text (porcine 28S rRNA, PDB entry 3j7q). The plots' ordinate axes show –log(p-value) for the test-fragments; higher values correspond to lower p-values and more reliable sequence assignments.
The p-value, or the probability of observing a given sequence assignment by chance, provides a reliable estimate of the alignment accuracy that doesn’t depend on local map resolution (Figure (Figure4B). 4B ). Indeed, a one-sided 99.5% confidence interval for fragments with sequence assignment that doesn’t match the reference (dashed line on Figure Figure4) 4 ) corresponds to 62% and 17% of cases with matching sequences for fragments of 20 and 40 residues respectively. Although sequence mismatches with a p-value outside this region are expected to be very rare, I observed several such test-fragments in the benchmark set (blue circles on Figure Figure4B), 4B ), all of them correspond to a single model of porcine 28S rRNA (PDB entry 3j7q). I will discuss this outlier in more detail in the next section.
Sequence assignment outlier: mammalian 28S rRNA
In the cryo-EM benchmark set, I identified several clear outliers, where RNA test-fragments were assigned reliable (low p-value) sequences different from the reference model (Figure (Figure4B). 4B ). All the fragments originate from an expansion segment (ES7a) of a cryo-EM structure of porcine 28S ribosomal subunit determined at 3.4Å resolution (PDB entry 3j7q). Closer inspection of the model revealed several nucleobases poorly fitting the EM reconstruction, which is understandable given limited resolution, but no clearly visible sequence assignment issues. As there is no higher resolution structure for the porcine ribosome available, which could be used to validate the sequence register, I decided to use as a reference the closest homologue from rabbit (98% of sequence identity), for which a structure determined at 3Å resolution has been recently deposited (PDB entry 6r5q). For a detailed comparison I selected a fragment of the ES7a that has a strictly conserved sequence in both organisms according to an alignment generated using R-coffee ( 51 ). Structural alignment of the corresponding model fragments, however, revealed multiple differences between corresponding nucleobase identities, several of them resulting in base-pairing violations (Figure (Figure5C). 5C ). I also observed that several differences in sequence preserving secondary structure are visible as clear density-fit outliers (Figure (Figure5A). 5A ). The problem is easily solved by shifting the sequences of the two chain fragments by one residue, as suggested by the doubleHelix program, which results in a perfect fit between the porcine and rabbit models (Figure (Figure5B 5B ).

Fragments with strictly conserved sequence of porcine ( A ) and rabbit ( B ) expansion segments (ES7a) in 28S rRNA with corresponding cryo-EM reconstructions at 3.4Å and 3.0Å resolution, respectively. Black model on panel (A) and grey on panel (B) represent deposited coordinates whereas porcine structure with sequence re-assigned using doubleHelix is depicted in red. Aligned sequences and secondary structures of the rabbit and porcine models are presented on panel ( C ). Although the register shift in deposited porcine structure preserves most of the base-pairs (green and blue boxes), several of them are visible as clear density-fit outliers (bases in red rectangles and labelled on panel A). There are also multiple secondary structure violations (shown in orange). Secondary structure presented on panel (C) was identified from the corrected model using ClaRNA. The figure was prepared using ChimeraX ( 52 ) and R-chie webserver ( 53 ).
RNA sequence identification and assignment in MX
A crystallographic diffraction experiment provides only amplitudes of complex structure factors required for calculating electron-density maps. Missing phases need to be derived from other sources, for example a tentative model of the unknown crystal structure from Molecular Replacement procedure. The use of model-derived phases for calculating electron-density maps inevitably results in so-called ‘model bias’ - the presence in a map of model features that are absent in a crystal structure. The same issue may be expected when a tentative crystal structure model polynucleotide sequence doesn’t match an unknown crystal structure. Although the model bias is reduced in maximum likelihood maps ( 54 ), routinely used for model building and refinement in MX, the problem is not completely eliminated. To investigate how the model-bias issue affects the sequence identification and assignment procedures I benchmarked them using ribosome crystal structures with randomised rRNA sequences. I used two crystal structure models of Thermus thermophilus 30S ribosomal subunit determined at resolutions 2.8Å (PDB entry 2uub) and 3.3Å (PDB entry 6mpi). Additionally, to remove any effect related to the presence of protein chain models that were refined in the presence of the correct rRNA sequences all atomic coordinates were randomised with 0.2Å RMSD. The resulting, randomized 30S models were refined using REFMAC5 and the PDB_REDO webserver. Interestingly, observed model bias in both randomised structures is moderate and clear sequence mismatches can be noticed for few (but not all) nucleobases (Figure (Figure6B 6B and D ).

Corresponding fragments of Thermus thermophilus 28S ribosomal subunit crystal structures used for the sequence identification and assignment benchmarks. Two models with randomised rRNA sequence were generated based on crystal structures determined at a resolution of 2.8 Å (PDB entry 2uub, panels A and B ) and 3.3Å (PDB entry 6mpi, panels C and D ). The panels depict residue range 1262–1273 with corresponding combined 2mFo-DFc (grey) and difference mFo-DFc (red/green) maximum likelihood maps contoured at 2σ and 3σ levels respectively; as deposited (A, C) and after randomising atomic coordinates and mutating 90% of nucleobases (B, D, see Materials and Methods for details). Both deposited and randomised structures were automatically refined using the PDB_REDO web server.
The sequence identification procedure was very effective for the randomised 2.8Å resolution model. Among 1000 continuous, randomly selected rRNA fragments of 100 residues, doubleHelix identified a correct target sequence in 99% of cases. For shorter fragments of 50 residues this fraction reduces to 76%. At lower resolution (randomised 6mpi model at 3.3Å resolution) the performance clearly reduces. The program provided a correct hit in 86% and 62% and of cases for test-fragments of 100 and 50 residues respectively. Interestingly, I also observed that the use of secondary structure information for the sequence identification clearly improves the method performance. Without base-pairing restraints the fraction of correctly identified sequences for fragments of 100 residues reduces to 90% and 72% for better and worse resolution structures. In all cases the incorrect hits can be easily filtered based on E-value returned by INFERNAL suite where values smaller than 0.1 guarantee a correct solution (Figure (Figure7A 7A ).

Performance of ( A ) sequence-identification and ( B ) sequence-assignment for fragments selected at random from two MX ribosomal RNA models determined at a resolution of 2.8Å (PDB entry 2uub) and 3.3Å (PDB entry 6mpi). The random fragments used for sequence identification and assignment had 50 and 20 nucleic acids, respectively. The continuous curves are logistic regression estimates of a probability that an identified sequence will have at least 90% sequence identity to the target sequence.
For sequence assignment the method correctly identified sequences of 46% and 65% of 1000 continuous rRNA fragments of 20 nucleic acid residues selected at random from randomised models at worse and better resolution respectively. These numbers increase to 82% and 94% for longer fragments of 40 residues. The correct sequence assignments are also clearly separated from incorrect ones by p-value with the 99.5% one-sided confidence interval for wrongly assigned fragments matching a value estimated for EM models (Figures (Figures4B 4B and 7B ).
Case study: sequence register errors in crystal structure of the large ribosomal subunit of S. Aureus
The doubleHelix program has been integrated into a previously developed tool called checkMySequence , which is used for validating sequence assignment in protein models. This integration enables a fully automated validation of sequence assignment for complete protein-nucleic acid complexes. Benchmarks of the updated checkMySequence method revealed a particularly interesting structure of the large ribosomal subunit from S. aureus . The program identified in this model plausible register shift errors in two ribosomal proteins (L18 and L4) and 5S rRNA. The structure was determined at 3.5Å resolution (PDB entry 4wce, ( 55 )) and refined to R-work/R-free factor values of 0.202/0.246 and clashscore 11.
A detailed discussion of the register shift error correction in ribosomal proteins is out of scope of this work and will be presented only briefly. The protein chains were replaced with corresponding predictions from AlphaFold2 database (release 4 for UniProt entries {"type":"entrez-protein","attrs":{"text":"Q2FW07","term_id":"122538859","term_text":"Q2FW07"}} Q2FW07 and {"type":"entrez-protein","attrs":{"text":"Q2FW22","term_id":"115504959","term_text":"Q2FW22"}} Q2FW22 for L4 and L18 respectively) subsequently refined using COOT in real space with self-restrains generated at 5 Å cut-off. Both predicted models were assigned a very high confidence score (pLDDT > 90), which was observed to usually correspond to minor differences in loop and side-chain conformations compared to reliable experimental models ( 56 ). Comparison of the deposited and corrected protein chains of both proteins revealed multiple plausible tracing issues and confirmed register-shifts suggested by checkMySequence .
The target sequence of the 5S rRNA chain consists of 114 residues that were all traced in the map (chain Y in the deposited model). The checkMySequence scan revealed an alternative sequence assignment with p-value of 0.01 (very reliable according to Figure Figure7B) 7B ) for a chain fragment following residue 81. The alternative sequence corresponds to a register shift by one base which suggests that a residue could have been omitted in the deposited model (deletion). Although closer inspection of the crystal structure revealed several clear density outliers for bases (Figure (Figure8A), 8A ), there were no signs of tracing issues. To confirm the chain sequence correctness, I performed sequence identification using doubleHelix with the deposited 5S chain coordinates, corresponding maximum likelihood 2mFo-DFc map, and a set of RNA sequences for S. aureus strain NCTC8325 downloaded from NCBI. At the time of writing (25.11.2022) there were two assemblies available (GCF_900475245 and GCF_900475245) each containing roughly 100 tRNA, rRNA, and ncRNA sequences.

Fragment of 5S rRNA model from a crystal structure of large ribosomal subunit of S. aureus refined at 3.5 Å resolution. High negative (red) and positive (green) difference density map values near Guanine Y/87 and Cytosine Y/86 correspond to excess and missing atoms in the model ( A ). After re-assigning the model to a sequence of different 5S gene variant and subsequent restrained refinement in REFMAC5 and PDB_REDO map-model fit clearly improves ( B ). The new sequence also clearly improves base-pairing pattern of the model ( C ). For clarity, only a sequence fragment including the stem loop depicted in panels (A, B) is shown (highlighted with a red box). Most sequence mismatches between the two 5S sequence variants can be corrected by introducing a gap to the alignment (shown as a grey box) that corresponds to a single base register-shift identified by checkMySequence . Maximum likelihood combined 2mFo-DFc and difference mFo-DFc maps on panels (A, B) are contoured at 2σ and 3σ level, respectively. Secondary structure presented on panel (C) was identified in the corrected model using ClaRNA. The figure was prepared using Pymol ( 31 ) and R-chie webserver ( 53 ).
In the RNA sequence sets doubleHelix identified two, different 5S genes (96% sequence identity), both very reliable hits with E -value below 1e-20 (see Figure Figure7A). 7A ). One of the sequences, however, scored visibly better that the other (7e-25 versus 4e-21 for {"type":"entrez-nucleotide","attrs":{"text":"NC_007795","term_id":"88193823","term_text":"NC_007795"}} NC_007795 .1_rrna_7 and {"type":"entrez-nucleotide","attrs":{"text":"NC_007795","term_id":"88193823","term_text":"NC_007795"}} NC_007795 .1_rrna_6 respectively), which has been previously shown to be a good evidence for a better fit to the data for protein models ( 28 ). The deposited 5S model was assigned the sequence variant with worse E-value, which resulted in the map-model fit outliers mentioned above (Figure (Figure8A). 8A ). The model, after re-assigning the 5S sequence variant identified with better E-value and subsequent restrained refinement with REFMAC5 and PDB_REDO shows much better fit to the map (Figure (Figure8B). 8B ). The new base identities are also more favourable for the formation of canonical base-pairs in the model (Figure (Figure8C). 8C ). The final model of complete ribosome, after correcting tracing issues in the two protein chains (L4 and L18) and the 5S chain sequence refines with clearly better scores; R-work/R-free reduces to 0.195/0.237 (from 0.202/0.247) and clashscore to 5 (from 11). The additional base-pairs, visually better map-to-model fit and reduced global model-quality scores together provide good evidence of improved agreement of the corrected model with the experimental data.
CONCLUSIONS
Sequence assignment is a key step of macromolecular model building that may lead to difficult to identify errors affecting structure interpretation. Nevertheless, it has been shown that protein models deposited in the PDB, despite expensive model validation efforts, contain many register-shift errors ( 16 , 20 , 57 ). Validation and assignment of nucleic acid sequences presents a particular challenge compared to proteins; the models are usually poorly resolved in cryo-EM and MX maps and available sequence-information is in practice limited to two nucleobase-types. Moreover, validation of nucleic acid models addresses predominantly backbone conformations that are rarely affected by nucleobase-sequence assignment issues. In consequence, the reliability of available, experimentally determined nucleic acid models is very difficult to assess. This may result in unintended error propagation, where a newly deposited model contains an error inherited from an earlier one used for model building. Errors can also detrimentally affect efforts of bioinformaticians working on large-scale structural analyses or structure prediction methods.
Here, I presented doubleHelix ; a new program for a comprehensive assignment, identification, and validation of nucleic acid sequences in cryo-EM and MX. I show that the approach, which relies on a neural-network base-type classifier, can successfully identify and assign sequences of cryo-EM model fragments at local resolution as low as 5 Å. I also show that base-pairing information, derived from backbone-geometry, clearly improves the program's performance at lower resolutions but is not essential. This is particularly important for large nucleic acid structures with very low content of paired bases; for example the trypanosomal mitochondrial ribosome ( 58 ).
Nucleic acid model building in cryo-EM and MX usually begins with tracing a ribose-phosphate backbone, which is then assigned to a target sequence. Although the former step can be addressed by several fully automated and interactive tools, the sequence assignment remains an open issue. Popular crystal structure building programs and modern, AI-based EM model building tools (e.g. DeepTracer or ModelAngelo) usually produce intermediate models that need to be completed and partially assigned to the target sequence using interactive software ( 14 , 15 ). The doubleHelix program should prove useful in this model building step. It can be used for assigning nucleic acid model fragments to the target sequence after each round of model rebuilding (and refinement in case of MX). It can help a user identify model connectivity and make decisions about consecutive modelling steps. Particularly important for this purpose is the sequence-assignment score provided by doubleHelix (p-value) that can help assessing local correctness of intermediate models.
The doubleHelix software can be also used for generating base-pairing restraints ready-to-use with the most popular cryo-EM and MX model-building and refinement tools (REFMAC5, PHENIX, COOT, ISOLDE). I have shown that the approach can successfully identify 91% of canonical base-pairs with 98% precision without relying on base conformation or identity. This may be particularly useful in early stages of the model building process as available base-pairing restraints generation approaches are strictly dependent on base-identities and the detection of hydrogen-bonding patterns that requires accurate relative positioning of paired-bases (LibG ( 59 ), Phenix suite ( 60 )). An exception here is a pipeline implemented in PDB_REDO ( 61 ) relying on base-pair assignment by DSSR suite, which is by design less sensitive to the structure distortions ( 62 ).
The presented doubleHelix benchmarks revealed a plausible sequence-register error in an expansion segment ES7a of a mammalian ribosome model deposited in the PDB (PDB entry 3j7q/EMDB entry EMD-2650). Expansion segments (ES) are present only in eukaryotic ribosomes and exhibit a surprising level of variability between different organisms. Nevertheless, the function of ES remains poorly understood, which makes them an important research target ( 63 ). Ribosomes are usually highly conserved across all kingdoms of live and ribosome models already available in the PDB can be often used to greatly simplify model building and refinement process of newly determined structures. The high variability of the ES at a structural and sequence level makes them one of the few rRNA regions that require in-depth modelling. Not surprisingly, this results in sporadic errors, which may hinder efforts aimed at understanding ES function. The problem is even more important for newly determined nucleic-acid complex structures for which at least partial models that could be used for model building are not available in the PDB. This makes the doubleHelix program particularly useful in structure determination using cryo-EM and MX as a reliable tool for nucleic-acid model sequence assignment and validation.
To facilitate the use of doubleHelix for model validation, it has been incorporated into a previously released, open-source sequence-assignment validation tool checkMySequence that can now process complete protein-nucleic acid complexes. The method enables a conceptually simple and fully automated detection of the most common sequence assignment issues in models of proteins, RNA, DNA, and their complexes that include register-shifts, sequence mismatches (single-residue differences between model and target sequence), problems with residue numbering (e.g. continuous residue numbering ignoring parts of a model that were not traced). With an example of bacterial ribosome crystal structure, I show that the checkMySequence can successfully identify errors in both protein and nucleic acid components of complex structures, resulting from model tracing issues and errors in reference sequences that would be otherwise very difficult to identify. Owing to its performance and full automation, checkMySequence is applicable to the analysis of very large models. For example, validation of a complete cryo-EM structures of 80S ribosome at 3.4 Å resolution discussed in this work, with 48 chains and over 11 000 protein and nucleic acid residues takes less than six minutes on a laptop.
ACKNOWLEDGEMENTS
I would like to thank all the microscopists who decided to deposit half-maps to EMDB which was of great help while developing doubleHelix . I would also like to thank Sean Eddy for his help with the configuration of INFERNAL suite. I am very grateful to Daniel J. Rigden and Katherine S. H. Beckham for critical reading of the manuscript and very helpful comments.
Data Availability
Funding for open access charge: European Molecular Biology Laboratory.
Conflict of interest statement . None declared.
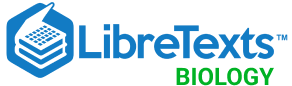
- school Campus Bookshelves
- menu_book Bookshelves
- perm_media Learning Objects
- login Login
- how_to_reg Request Instructor Account
- hub Instructor Commons
- Download Page (PDF)
- Download Full Book (PDF)
- Periodic Table
- Physics Constants
- Scientific Calculator
- Reference & Cite
- Tools expand_more
- Readability
selected template will load here
This action is not available.
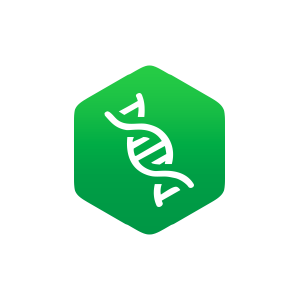
Nucleic Acid Structure and Metabolism
- Last updated
- Save as PDF
- Page ID 72479
- 23S rRNA of the large ribosomal subunit from Deinococcus radiodurans (2O44)
- Adenine mispaired with 8-oxoguanine by MutY adenine DNA glycosylase (1RRQ)
- Androgen Receptor DNA-Binding Domain Bound to a Direct Repeat Response Element (1R4I)
- A Hoogsteen base pair embedded in undistorted B-DNA - MATALPHA2 Homeodomain bound to DNA (1K61)
- Bacterial mRNA cap (Guanine-n7) methyltransferase (1RI1)
- C'2 endo ribose, 1BNA (B-DNA)
- C'3-endo ribose, A-RNA (413D, double stranded)
- C-terminal domain of the human DNA primase large subunit with bound DNA template-RNA primer (5F0Q)
- C2H2-type zinc finger domain (699-729) from zinc finger protein 473 (2YRH)
- Catabolite activator protein CAP-DNA complex with bound cAMP (2CGP)
- Catalytic core of human DNA polymerase alpha in ternary complex with an RNA-primed DNA template and dCTP (4QCL)
- Class II E. coli threonyl-tRNA synthase - tRNA(Thr) complex (1QF6)
- Class I E. Coli Cysteinyl-tRNA synthetase -tRNACys (1U0B)
- Closed ring structure of the E. Coli Rho transcription termination factor in complex with nucleic acid in the motor domains (2HT1)
- Core human replisome (7PFO)
- Core human replisome (7PFO) - short load
- Core structure of U6 small nuclear RNA - protein (ribonucleoprotein) complex (4N0T)
- Cyclid-di-GMP RNA riboswitch (3IRW)
- Divalent cation-sensing regulatory RNA (2QBZ)
- dsRNA with G-U wobble base pairs (6L0Y)
- E.Coli topoisomerase I in complex with ssDNA (4RUL)
- E. Coli DNA Mismatch Repair Protein Muts Binding to a G-T Mismatch (1E3M)
- E. Coli MutS scanning form (EMD-11791, PDB 7AI5)
- E. Coli MutS with MutL in kink clamped form (EMD-11795, PDB 7AIC)
- E. coli replicative DNA polymerase-clamp-exonuclase-theta complex bound to DNA in the editing mode (5M1S)
- E. coli replicative DNA polymerase III (alpha, beta2, epsilon, tau complex) bound to DNA (5FKV)
- E. coli Rho-dependent Transcription Pre-termination Complex (6XAS)
- E. Coli Rho transcription termination factor in complex with ssRNA substrate and ANPPNP (1PVO)
- E. Coli ribosome (7K00)
- E. Coli Trp repressor - operator complex (1TRO)
- Ephydatia fluviatilis PiwiA-piRNA-target complex (7KX9)
- Escherichia Coli Replication Terminator Protein (Tus) Complexed With DNA- Locked form (2I06)
- Escherichia Coli Replication Terminator Protein (Tus) Complexed With TerA DNA in the permissive form (2I05)
- Eukaryotic 80S ribosome with bound mRNA and tRNAs in intermediate translocation state (6GX3)
- Foot-and-mouth disease virus RNA-polymerase in complex with a template- primer RNA, PPi and UTP (2E9Z)
- Full-length Schistosoma manson catalytically active hammerhead ribozyme (3dz5)
- GCN4 basic region leucine zipper binds DNA as a dimer of uninterrupted alpha helices (1YSA)
- GlmS ribozyme bound to glucosamine-6-phosphate (2Z75)
- Glutamine Phosphoribosylpyrophosphate Amidotransferase from Arabidopsis thaliana (6LBP)
- GT Wobble Base-Pairing in Z-DNA form of d(CGCGTG) (1VTT)
- Guanine-responsive riboswitch bound to metabolite hypoxanthine (4FE5)
- Hairpin ribozyme (human) in the catalytically-active conformation (1M5K)
- Human-house-mastadenovirus C RNA polymerase II core pre-initiation complex with open promoter DNA (7NVU)
- Human Argonaute2 Bound to a Guide and Target RNA (4W5O)
- Human C Complex Spliceosome (7A5P)
- Human DNA Ligase I bound to 5'-adenylated, nicked DNA (1X9N)
- human full-length heteromeric alpha1-beta3-gamma 2L GABA(A)R in complex with diazepam (Valium) and GABA (6HUP)
- Human fully-assembled precatalytic spliceosome (pre-B complex) (6QX9)
- Human Hsf1 with Satellite III repeat DNA - HTH Domain (5d5v)
- human nucleosome (3afa)
- Human p53 tetramer bound to the natural CDKN1A(p21) p53-response element (3TS8)
- Human primosome without nucleic acids (5EXR)
- Klf4 zinc finger DNA binding domain in complex with methylated DNA(4m9e)
- Lactose operon repressor and its complexes with DNA (1LBG)
- Lactose operon repressor and its complexes with DNA and inducer (1lbg)
- Minor groove RNA triplex in the crystal structure of a ribosomal frameshifting viral pseudoknot (437D)
- Myc-Max and Mad-Max recognizing DNA(1nkp
- N-terminal fragment of the yeast transcriptional activator GAL4 bound to DNA (1D66)
- NMR solution structure of Dimer of LAC repressor DNA-binding domain complexed to its natural operator O3 (2KEK)
- Parallel quadruplexes from human telomeric DNA (1KF1)
- Pi stacking in B-DNA (5t4w)
- Poliovirus polymerase elongation complex with 2,3-dideoxy-CTP (3OLB)
- POU-HMG-DNA ternary complex - HTM-HMG domain (1gt0)
- POU protein - DNA complex HTH-HD domain (3l1p)
- Predicted AlphaFold structure of E. Coli DNA Polymerase I (P00582)
- Protein toxin (ToxN) - LncRNA antitoxin (ToxI)) complex (2xdb)
- REV Response element RNA complexed with REV peptide (1ETF)
- Ribonucleoprotein with 12 nt guide regions and 13 RNA substrates - small nucleolar RNA (5GIO)
- Saccharomyces cerevisiae Cet1-Ceg1 mRNA Capping Apparatus (3KYH)
- Solution conformation of a ssDNA:DFAME fluorophore complex (8FI0)
- Solution conformation of a parallel DNA triple helix (1BWG)
- Structure of a short oligomer of double-stranded DNA (1BNA)
- Structure of S. pombe Mei2 RRM3 domain bound to ln RNA (6YYM)
- Structure of the Holliday junction intermediate in Cre-loxP site-specific recombination (3CRX)
- T. aquaticus transcription initiation complex containing bubble promoter and RNA (4XLN)
- T. aquaticus transcription initiation complex containing bubble promoter and RNA (4XLN)-optimal
- T. aquaticus transcription initiation complex containing bubble promoter and RNA (4XLN)-V2simple
- T. thermophilus RNAP polymerase elongation complex with the NTP substrate analog (2O5J)
- T4 hairpin loop on a Z-DNA stem (1D16)
- Template for hovering iCn3Ds
- Viral suppressor of RNA silencing protein and small interfering RNAs (6BJV)
- Yeast cleavage and polyadenylation specificity factor (CPF) polymerase module in complex with Mpe1, the yPIM of Cft2 and the pre-cleaved CYC1 RNA (7ZGR)
- Yeast mRNA decapping enzyme Dcp1-Dcp2 complex in the ATP bound closed conformation (2QKM)
- Yeast phenylalanine tRNA (1EHZ)
- Yeast phenylalanine tRNA Domain Coded (1EHZ)
- Yeast Topoisomerase II-DNA-AMPPNP complex (4GFH)
- Z-DNA (4OCB)
Nucleic Acids
Nucleic acids are long-chain polymeric molecules. The monomer or the repeating unit is known as the nucleotides and hence sometimes nucleic acids are referred to as polynucleotides. Nucleic acids can be defined as organic molecules present in living cells. It plays a key factor in transferring genetic information from one generation to the next. Nucleic acids are composed of DNA-deoxyribonucleic acid and RNA-ribonucleic acid that form the polymers of nucleotides.
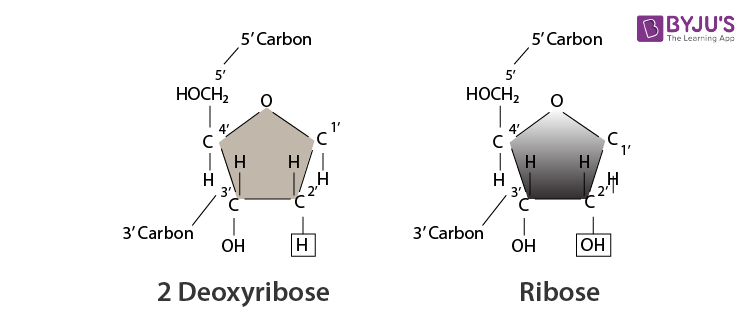
In the nucleus, nucleotide monomers are linked together comprising of distinct components namely a Phosphate Group, Nitrogenous Bases or Ribose and Deoxyribose. Pyrimidines and Purines are two types of nitrogenous bases. Pyrimidines are composed of cytosine and thymine. Purines are composed of guanine and adenine. Thymine is replaced by Uracil in ribonucleic acid whereas deoxyribonucleic acid comprises of all four bases.
Table of Contents
Dna structure, rna structure, functions of nucleic acids.
DNA consists of instructions that monitor the performance of all cell functions. It is a cellular molecule that is organized into chromosomes. They are present in the nucleus of the cells and contain cellular activities.
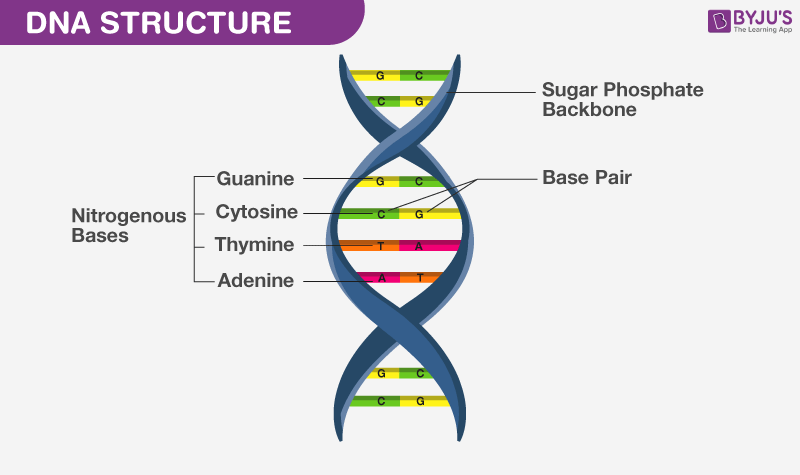
It is a double helix formed by 2 polynucleotide chains that are twisted. There are 2 strands of DNA which are parallel to each other. Hydrogen bond binds two helices and the bases are bundled within the helix. Due to the presence of phosphate groups, DNA is negatively charged.
Chemically, DNA is composed of a pentose sugar, phosphoric acid and some cyclic bases containing nitrogen. The sugar moiety present in DNA molecules is β-D-2-deoxyribose. The cyclic bases that have nitrogen in them are adenine (A), guanine (G), cytosine(C) and thymine (T). These bases and their arrangement in the molecules of DNA play an important role in the storage of information from one generation to the next one.
RNA plays a vital role in the synthesis of proteins that mainly involves decoding and translation of genetic code and transcription to produce proteins.
RNA molecules are also composed of phosphoric acid, a pentose sugar and some cyclic bases containing nitrogen . RNA has β-D-ribose in it as the sugar moiety. The heterocyclic bases present in RNA are adenine (A), guanine (G), cytosine(C) and uracil (U). In RNA the fourth base is different from that of DNA. The RNA generally consists of a single strand which sometimes folds back.
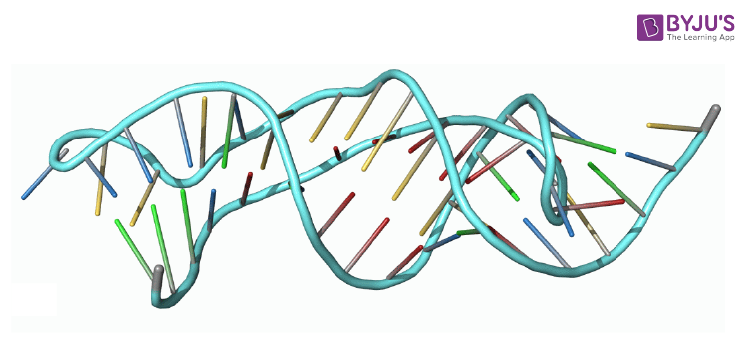
There are several different types of RNA and each has a specific function.
- Ribosomal RNA – It is one of the components of ribosomes that are involved in protein synthesis.
- Transfer RNA – It is essential for the translation of mRNA in protein synthesis.
- Micro RNAs – It is the smallest among all RNA that helps in regulating gene expressions.
- Messenger RNA – It is the RNA transcript that is produced during DNA transcription.
- Nucleic Acid is responsible for the synthesis of protein in our body
- RNA is a vital component of protein synthesis.
- Loss of DNA content is linked to many diseases.
- DNA is an essential component required for transferring genes from parents to offspring.
- All the information of a cell is stored in DNA.
- DNA fingerprinting is a method used by forensic experts to determine paternity. It is also used for the identification of criminals. It has also played a major role in studies regarding biological evolution and genetics.
Recommended Videos
Biomolecules.
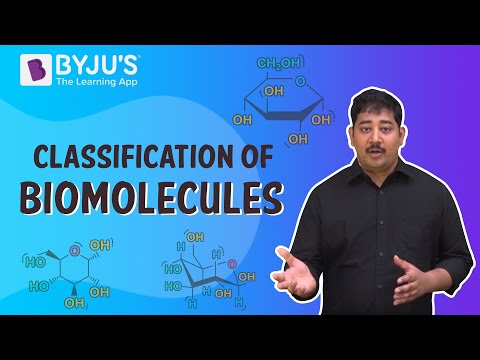
Put your understanding of this concept to test by answering a few MCQs. Click ‘Start Quiz’ to begin!
Select the correct answer and click on the “Finish” button Check your score and answers at the end of the quiz
Visit BYJU’S for all Chemistry related queries and study materials
Your result is as below
Request OTP on Voice Call
Leave a Comment Cancel reply
Your Mobile number and Email id will not be published. Required fields are marked *
Post My Comment

- Share Share
Register with BYJU'S & Download Free PDFs
Register with byju's & watch live videos.

- Search Menu
- Chemical Biology and Nucleic Acid Chemistry
- Computational Biology
- Critical Reviews and Perspectives
- Data Resources and Analyses
- Gene Regulation, Chromatin and Epigenetics
- Genome Integrity, Repair and Replication
- Methods Online
- Molecular Biology
- Nucleic Acid Enzymes
- RNA and RNA-protein complexes
- Structural Biology
- Synthetic Biology and Bioengineering
- Advance Articles
- Breakthrough Articles
- Special Collections
- Scope and Criteria for Consideration
- Author Guidelines
- Data Deposition Policy
- Database Issue Guidelines
- Web Server Issue Guidelines
- Submission Site
- About Nucleic Acids Research
- Editors & Editorial Board
- Information of Referees
- Self-Archiving Policy
- Dispatch Dates
- Advertising and Corporate Services
- Journals Career Network
- Journals on Oxford Academic
- Books on Oxford Academic
Article Contents
Introduction, data availability, interactive tree of life (itol) v6: recent updates to the phylogenetic tree display and annotation tool.

- Article contents
- Figures & tables
- Supplementary Data
Ivica Letunic, Peer Bork, Interactive Tree of Life (iTOL) v6: recent updates to the phylogenetic tree display and annotation tool, Nucleic Acids Research , 2024;, gkae268, https://doi.org/10.1093/nar/gkae268
- Permissions Icon Permissions
The Interactive Tree Of Life ( https://itol.embl.de ) is an online tool for the management, display, annotation and manipulation of phylogenetic and other trees. It is freely available and open to everyone. iTOL version 6 introduces a modernized and completely rewritten user interface, together with numerous new features. A new dataset type has been introduced (colored/labeled ranges), greatly upgrading the functionality of the previous simple colored range annotation function. Additional annotation options have been implemented for several existing dataset types. Dataset template files now support simple assignment of annotations to multiple tree nodes through substring matching, including full regular expression support. Node metadata handling has been greatly extended with novel display and exporting options, and it can now be edited interactively or bulk updated through annotation files. Tree labels can be displayed using multiple simultaneous font styles, with precise positioning, sizing and styling of each individual label part. Various bulk label editing functions have been implemented, simplifying large scale changes of all tree node labels. iTOL’s automatic taxonomy assignment functions now support trees based on the Genome Taxonomy Database (GTDB), in addition to the NCBI taxonomy. The functionality of the optional user account pages has been expanded, simplifying the management, navigation and sharing of projects and trees. iTOL currently handles more than one and a half million trees from >130 000 individual user accounts.

Phylogenetics and phylogenetic trees play pivotal roles in biological and scientific studies, serving as foundational tools for understanding evolutionary relationships and biodiversity.
Numerous tree visualization tools have been developed through the years (e.g. ( 1–4 ), including iTOL ( 5 ), which was one of the first to introduce tree annotation through diverse supplementary data. Alongside iTOL, many software packages and libraries have emerged, such as the ETE toolkit ( 6 ), ggtree ( 7 ), tvBOT ( 8 ), Evolview ( 9 ) or PhyD3 ( 10 ), which offer sophisticated tree annotation capabilities.
As the heterogeneity and complexity of data and supplementary data increases, there's a pressing need for tools to adapt and evolve. With version 6, iTOL has undergone a significant redesign, with expanded and streamlined functionality. This redesign aims to simplify data handling and enhance user experience, aligning with the evolving demands of phylogenetic analysis. Here we provide an overview of iTOL’s current features and recent enhancements, emphasizing its continued relevance and significance in scientific research.
Basic features
iTOL is an online tool, accessible with any modern web browser (Figure 1 ). The tree display engine is implemented in pure Javascript, utilizing the HTML5 Canvas element for visualization. The majority of display computations and features are executed by the user's web browser, allowing fine grained interactive control over various display parameters with immediate visualization updates.

iTOL’s user interface. A phylogenetic tree annotated with several datasets is displayed, highlighting several new features. ( A ) The main control panel has been streamlined, and allows quick access to a larger set of parameters and functions. ( B ) The new ‘Colored/labeled ranges’ dataset type can be visualized as standard color filled shapes, or as various types of brackets. Each range can be separately labeled, with precise positioning of the label relative to the range shape. Gradient color fills and individual border styles can be defined for each range. ( C ) Multiple font styles can be mixed within the tree labels. Styles can be simply defined and applied interactively through the new label style creator. ( D ) Metadata and other branch labels can have custom backgrounds and their position can be fine-tuned relative to the branch. Leaves and internal tree nodes can be marked with custom shapes with user defined colors and borders.
User interface updates
In iTOL version 6, the complete user interface has been rewritten from scratch, utilizing current web technologies and introducing many new convenience functions (Figure 1 ).
The main control panel has been streamlined and compacted, with collapsible subpanels allowing simple access to a larger set of parameters and functions. Most options contain inline help popups, which can be useful to new users.
iTOL’s help pages have been updated as well, with detailed explanation of all novel features. Most of the functionality is also covered in a set of narrated video tutorials, with crosslinks from the help pages directly to the relevant sections in the videos.
Input types and basic functions
iTOL supports most commonly used phylogenetic tree formats such as Newick, Nexus ( 11 ) and phyloXML ( 12 ). Phylogenetic placement files created by EPA ( 13 ) and pplacer ( 14 ), as well as QIIME 2 trees and annotation files ( 15 ) are also supported. With iTOL v6, certain tree branch annotations embedded in the phyloXML files (color and width) will be automatically parsed and applied to the uploaded tree.
All additional data used for various types of tree annotation are provided in plain text files, and visualized by simply dragging and dropping them onto the user's web browser. Annotation files now support simple assignment of annotations to multiple tree nodes through substring matching, including full regular expression support, making them more compact and lowering the amount of work needed.
iTOL provides most common functions available in any phylogenetic tree viewer. Various tree display formats are supported: phylograms or cladograms, rooted or unrooted, rectangular or radial.
iTOL can manipulate the trees in various ways, and basic editing functions allow users to interactively delete or move single nodes or whole clades. Clades can also be pruned or collapsed, either manually or automatically, based on various parameters (such as associated bootstrap values, average branch length distances or any other numeric metadata value associated with tree nodes). iTOL v6 introduces support for the collapsing of clades through a simple text file with a list of nodes to collapse. Such files can be manually created, or exported from trees with interactively collapsed clades, allowing simple transfer of the collapsed clade status to other user trees.
Trees can be re-rooted manually on any node, or automatically using the midpoint rooting method. Tree leaves can be sorted in various ways, either manually or automatically, and their order rearranged in each individual clade.
Performing large scale editing of tree node labels in iTOL has been simplified by the introduction of the bulk label editor. It provides several convenience functions which can be applied to all tree labels with a single button click, from simple removal of quotes to powerful regular expression text replacements.
Automatic taxonomy assignments
iTOL’s automatic taxonomy assignment functionality provides a simple way of deducing the correct labels and classes of all tree nodes based on external taxonomy databases. In addition to the NCBI taxonomy database ( 16 ), iTOL now also supports GTDB (Genome Taxonomy Database) ( 17 ). The taxonomy assignment function requires trees with taxonomy IDs (NCBI or GTDB) at their leaf nodes. It will replace the ID labels with correct taxonomic names, as well as resolve the correct names and classes of all internal tree nodes, based on their child nodes.
A complete overview of changes and functions added since the last publication ( 18 ) are listed on the iTOL’s version history page ( https://itol.embl.de/version_history.cgi ).
Tree annotation
iTOL v6 introduces various new annotation features, extended functionality in the visualization of existing dataset types, as well as a new dataset type, colored/labeled ranges (Figure 1 ). Limited colored leaf ranges were available in iTOL as a separate annotation feature since the initial release. The new dataset type replicates and greatly extends this functionality with many new features. Since colored ranges are now an independent dataset, there can be multiple different versions present on a single tree, allowing users to simply highlight different parts of the tree as required. Ranges can now be filled with color gradients, and highlighted with individually styled borders of varying widths and colors. Each colored range can be labeled separately, with automatic positioning of the label which can be fine-tuned by the user. Colored ranges can automatically cover not only the tree structure itself, but also any external datasets displayed outside the tree, allowing users to easily highlight the relevant sections of the complete annotated tree. In addition to the standard colored shapes, ranges can be visualized as various types of brackets, further extending their possible uses.
Extended support for node metadata
iTOL v6 expands the node metadata handling and visualization options with several new features. In addition to automatic import of bootstrap values and metadata from MRBAYES ( 19 ) and The New Hampshire X (NHX) formatted trees, custom metadata values can now be imported or updated directly from user generated plain text annotation files. In addition to the bulk importing or editing, an interactive metadata editor has been implemented, allowing simple access to each individual node's metadata values. The metadata editor can also be used to define node classes, which can be used in the automatic clade collapsing function.
During metadata visualization on trees where multiple metadata values are available per node, the display can now be filtered via individual thresholds for each metadata category with full support for both ‘AND’ and ‘OR’ Boolean operators.
Tree labels with multiple font styles
The ability to mix different font styles within tree labels is a feature that was often requested by users, and is now available in iTOL v6. Through a simple interactive form directly in the web interface, users can split the labels into different parts as desired, and define their individual font styles. For each part of the label, it is possible to define a separate color, size, font family, bold/italic style and sub or superscript position. Additionally, the position of each part can be fine-tuned vertically and horizontally as needed.
Several filtering options allow the application of styles to a subset of labels only, for example by including or excluding labels which match a particular string, or by requiring a specific number of parts to be present.
User accounts and tree management
In addition to the anonymous direct tree upload and annotation, iTOL provides a personal account system, which currently has >130 000 registered users, managing more than 1 600 000 uploaded trees. The current version brings a complete redesign of the user account pages (Figure 2 ). Users can organize their trees into various workspaces and projects. Trees uploaded into each project are displayed in a table with several advanced features. Tree lists can be sorted on any of the available columns, while the columns can be toggled and reordered as desired. For projects with many trees, users can specify the paging size of the table, making the navigation through the workspace simpler. All these settings can be applied to each project separately, or defined as user defaults for all their workspaces and projects.
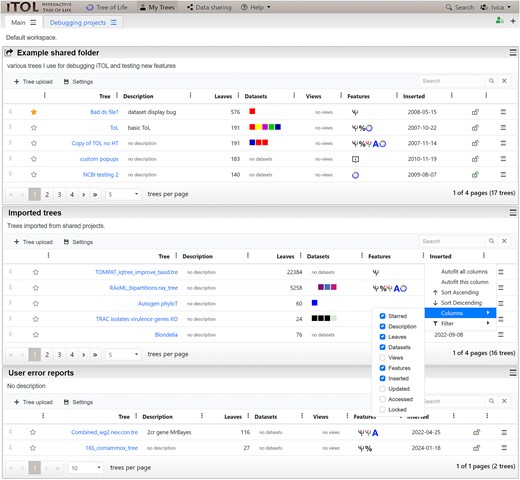
Illustration of the user account page redesign in iTOL v6. Trees can be organized into various workspaces and projects. Projects can be collapsed and rearranged, or moved between workspaces. Tree lists in each project can be customized according to user preferences, where columns can be sorted, reordered or hidden. Trees can be rearranged or moved to other projects by direct drag and drop. All settings can be saved individually for each project, or applied globally.
Several new tree attributes have been introduced, like the ability to mark important trees with a star, or to prevent any public access to a particular tree.
In addition to the standard user accounts created directly, iTOL now supports Single Sign-On Authentication (SSO) via Google or Microsoft services for both login and registration. Users can simply use their existing external accounts in iTOL, without the need to remember new passwords or provide any additional information.
One of iTOL’s primary uses is the creation of high-quality figures for publication or inclusion into other documents. Due to constantly increasing number of active users, the backend server has been extended to make the tree export faster. To prevent the overloading of the export server in peak usage times, we developed a simple queueing system, which processes user exports sequentially. Introduction of the export queuing system made the process more stable for all users, and increased the overall response times of the server.
Taken together, iTOL version 6 provides numerous improvements of both backend and frontend user interfaces, introduces many new annotation features and streamlines the tree annotation process, improving the user experience.
iTOL is free and open to all users without login requirement at https://itol.embl.de/ .
Funding for open access charge: European Molecular Biology Laboratory. This work was supported by the Ministry of Science, Research and the Arts Baden-Württemberg (MWK) within the framework of LIBIS/de.NBI.
Conflict of interest statement . None declared.
Huson D.H. , Scornavacca C. Dendroscope 3: an interactive tool for rooted phylogenetic trees and networks . Syst. Biol. 2012 ; 61 : 1061 – 1067 .
Google Scholar
Chevenet F. , Brun C. , Banuls A.L. , Jacq B. , Christen R. TreeDyn: towards dynamic graphics and annotations for analyses of trees . BMC Bioinf. 2006 ; 7 : 439 .
Zmasek C.M. , Eddy S.R. ATV: display and manipulation of annotated phylogenetic trees . Bioinformatics . 2001 ; 17 : 383 – 384 .
Procter J.B. , Thompson J. , Letunic I. , Creevey C. , Jossinet F. , Barton G.J. Visualization of multiple alignments, phylogenies and gene family evolution . Nat. Methods . 2010 ; 7 : S16 – S25 .
Letunic I. , Bork P. Interactive Tree of Life (iTOL): an online tool for phylogenetic tree display and annotation . Bioinformatics . 2007 ; 23 : 127 – 128 .
Huerta-Cepas J. , Serra F. , Bork P. ETE 3: reconstruction, analysis, and visualization of phylogenomic data . Mol. Biol. Evol. 2016 ; 33 : 1635 – 1638 .
Yu G. Using ggtree to visualize data on tree-like structures . Curr. Protoc. Bioinformatics . 2020 ; 69 : e96 .
Xie J. , Chen Y. , Cai G. , Cai R. , Hu Z. , Wang H. Tree Visualization by one table (tvBOT): a web application for visualizing, modifying and annotating phylogenetic trees . Nucleic Acids Res. 2023 ; 51 : W587 – W592 .
Subramanian B. , Gao S. , Lercher M.J. , Hu S. , Chen W.H. Evolview v3: a webserver for visualization, annotation, and management of phylogenetic trees . Nucleic Acids Res. 2019 ; 47 : W270 – W275 .
Kreft L. , Botzki A. , Coppens F. , Vandepoele K. , Van Bel M. PhyD3: a phylogenetic tree viewer with extended phyloXML support for functional genomics data visualization . Bioinformatics . 2017 ; 33 : 2946 – 2947 .
Wilgenbusch J.C. , Swofford D Inferring evolutionary trees with PAUP* . Curr. Protoc. Bioinformatics . 2003 ; Chapter 6 : Unit 6.4 .
Han M.V. , Zmasek C.M. phyloXML: XML for evolutionary biology and comparative genomics . BMC Bioinf. 2009 ; 10 : 356 .
Berger S.A. , Krompass D. , Stamatakis A. Performance, accuracy, and web server for evolutionary placement of short sequence reads under maximum likelihood . Syst. Biol. 2011 ; 60 : 291 – 302 .
Matsen F.A. , Kodner R.B. , Armbrust E.V. pplacer: linear time maximum-likelihood and bayesian phylogenetic placement of sequences onto a fixed reference tree . BMC Bioinf. 2010 ; 11 : 538 .
Lawley B. , Tannock G.W. Analysis of 16S rRNA gene amplicon sequences using the QIIME software package . Methods Mol. Biol. 2017 ; 1537 : 153 – 163 .
Schoch C.L. , Ciufo S. , Domrachev M. , Hotton C.L. , Kannan S. , Khovanskaya R. , Leipe D. , McVeigh R. , O’Neill K. , Robbertse B. et al. . NCBI Taxonomy: a comprehensive update on curation, resources and tools . Database . 2020 ; 2020 : baaa062 .
Parks D.H. , Chuvochina M. , Rinke C. , Mussig A.J. , Chaumeil P.A. , Hugenholtz P. GTDB: an ongoing census of bacterial and archaeal diversity through a phylogenetically consistent, rank normalized and complete genome-based taxonomy . Nucleic Acids Res. 2022 ; 50 : D785 – D794 .
Letunic I. , Bork P. Interactive Tree of Life (iTOL) v5: an online tool for phylogenetic tree display and annotation . Nucleic Acids Res. 2021 ; 49 : W293 – W296 .
Ling C. , Hamada T. , Gao J. , Zhao G. , Sun D. , Shi W. MrBayes tgMC(3)++: a high performance and resource-efficient GPU-oriented phylogenetic analysis method . IEEE/ACM Trans. Comput. Biol. Bioinf. 2016 ; 13 : 845 – 854 .
Email alerts
Citing articles via.
- Editorial Board
Affiliations
- Online ISSN 1362-4962
- Print ISSN 0305-1048
- Copyright © 2024 Oxford University Press
- About Oxford Academic
- Publish journals with us
- University press partners
- What we publish
- New features
- Open access
- Institutional account management
- Rights and permissions
- Get help with access
- Accessibility
- Advertising
- Media enquiries
- Oxford University Press
- Oxford Languages
- University of Oxford
Oxford University Press is a department of the University of Oxford. It furthers the University's objective of excellence in research, scholarship, and education by publishing worldwide
- Copyright © 2024 Oxford University Press
- Cookie settings
- Cookie policy
- Privacy policy
- Legal notice
This Feature Is Available To Subscribers Only
Sign In or Create an Account
This PDF is available to Subscribers Only
For full access to this pdf, sign in to an existing account, or purchase an annual subscription.
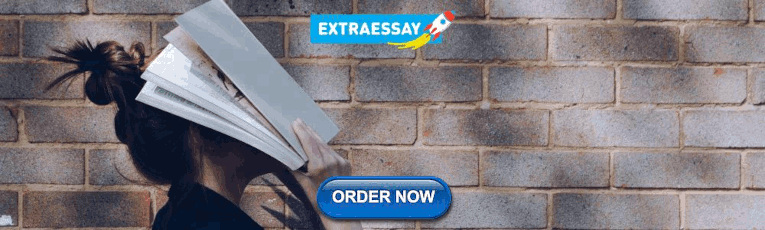
IMAGES
VIDEO
COMMENTS
Nucleic acids, macromolecules made out of units called nucleotides, come in two naturally occurring varieties: deoxyribonucleic acid ( DNA) and ribonucleic acid ( RNA ). DNA is the genetic material found in living organisms, all the way from single-celled bacteria to multicellular mammals like you and me. Some viruses use RNA, not DNA, as their ...
Introduction to Nucleic Acids. Alongside proteins, lipids, and complex carbohydrates (polysaccharides), nucleic acids are one of the four major types of macromolecules that are essential for all known forms of life. The nucleic acids consist of two major macromolecules, Deoxyribonucleic acid (DNA) and ribonucleic acid (RNA) that carry the ...
nucleic acid, naturally occurring chemical compound that is capable of being broken down to yield phosphoric acid, sugars, and a mixture of organic bases (purines and pyrimidines).Nucleic acids are the main information-carrying molecules of the cell, and, by directing the process of protein synthesis, they determine the inherited characteristics of every living thing.
Courses. Nucleic acids (DNA and RNA) comprise the fourth group of biological macromolecules and contain phosphorus (P) in addition to carbon, hydrogen, oxygen, and nitrogen. Conserved through evolution in all organisms, nucleic acids store and transmit hereditary information. As will be explored in more detail in Chapters 14-17, DNA contains ...
Nucleic acids are the most important macromolecules for the continuity of life.They carry the genetic blueprint of a cell and carry instructions for the functioning of the cell. The two main types of nucleic acids are deoxyribonucleic acid (DNA) and ribonucleic acid (RNA).DNA is the genetic material found in all living organisms, ranging from single-celled bacteria to multicellular mammals.
The nucleic acids, DNA and RNA, may be thought of as the information molecules of the cell. In this section, we will examine the structures of DNA and RNA, and how these structures are related to the functions these molecules perform. We will begin with DNA, which is the hereditary information in every cell, that is copied and passed on from ...
DNA structure and function. DNA is the information molecule. It stores instructions for making other large molecules, called proteins. These instructions are stored inside each of your cells, distributed among 46 long structures called chromosomes. These chromosomes are made up of thousands of shorter segments of DNA, called genes.
Both nucleic acids—DNA and RNA—are polymers composed of monomers known as nucleotides, which in turn consist of phosphoric acid (H 3 PO 4), a nitrogenous base, and a pentose sugar. The two types of nitrogenous bases most important in nucleic acids are purines —adenine (A) and guanine (G)—and pyrimidines —cytosine (C), thymine (T), and ...
In this final lecture of the Biochemistry unit, Professor Imperiali covers nucleotides and nucleic acids, discussing their structures and their importance as fundamental units for information storage and information transfer. Instructor: Barbara Imperiali. MIT OpenCourseWare is a web based publication of virtually all MIT course content.
peptide bond. Identify which scientist or group of scientists was responsible for making each of the following discoveries.The double-helix structure of DNA: Mulder Berzelius Miescher Levene Watson, Crick, and Franklin. The chemical composition of proteins: Mulder Berzelius Miescher Levene Watson, Crick, and Franklin. The monomer of nucleic ...
Nucleic acids are organic compounds that contain carbon, hydrogen, oxygen, nitrogen, and phosphorus. They are made of smaller units called nucleotides. Nucleic acids are named for the nucleus of the cell, where some of them are found. Nucleic acids are found not only in all living cells but also in viruses.
A nucleic acid is an organic compound, such as DNA or RNA, that is built of monomers called nucleotides. Many nucleotides bind together to form a chain called a polynucleotide. The nucleic acid DNA (deoxyribonucleic acid) consists of two polynucleotide chains. The nucleic acid RNA (ribonucleic acid) consists of just one polynucleotide chain.
Study with Quizlet and memorize flashcards containing terms like The sugar-phosphate backbones of DNA are connected to one another by sequences of _____ different bases., Nucleic acids carry the _____ codes of life., Adenine pairs with _____, and guanine pairs with _____ in DNA. and more.
Bradykinin is a potent peptide hormone composed of nine amino acids that lowers blood pressure. The amino acid sequence for bradykinin is arg-pro-pro-gly-phe-ser-pro-phe-arg. Postulate a base sequence in the mRNA that would direct the synthesis of this hormone. Include an initiation codon and a termination codon.
Roles of Nucleic Acids. DNA is also known as the hereditary material or genetic information. It is found in genes, and its sequence of bases makes up a code. Between "starts" and "stops," the code carries instructions for the correct sequence of amino acids in a protein (see Figure below).DNA and RNA have different functions relating to the genetic code and proteins.
Nucleic acids are the most important macromolecules for the continuity of life. They carry the genetic blueprint of a cell and carry instructions for the functioning of the cell. DNA and RNA. The two main types of nucleic acids are deoxyribonucleic acid (DNA) and ribonucleic acid (RNA).DNA is the genetic material found in all living organisms, ranging from single-celled bacteria to ...
Structures of Nucleic Acids. CHAPTER 2 STRUCTURES OF NUCLEIC ACIDS. DNA and RNA are both nucleic acids, which are the polymeric acids isolated from the nucleus of cells. DNA and RNA can be represented as simple strings of letters, where each letter corresponds to a particular nucleotide, the monomeric component of the nucleic acid polymers.
Nucleotides are the building blocks of nucleic acids: they are the monomers which, repeated many times, form the polymers DNA and RNA. Nucleotides are composed of a five-carbon sugar covalently attached to a phosphate group and a base containing nitrogen atoms. Figure 1 shows the structure of the nucleotides making up nucleic acids.
Nucleic acids allow organisms to transfer genetic information from one generation to the next. When a cell divides, its DNA is copied and passed from one cell generation to the next generation. DNA is composed of a phosphate-deoxyribose sugar backbone and the nitrogenous bases adenine (A), guanine (G), cytosine (C), and thymine (T).
Nucleic acids are long-chain polymeric molecules, the monomer (the repeating unit) is known as the nucleotides and hence sometimes nucleic acids are referred to as polynucleotides. Deoxyribonucleic acid (DNA) and ribonucleic acid (RNA) are two major types of nucleic acids. DNA and RNA are responsible for the inheritance and transmission of ...
Validation and assignment of nucleic acid sequences presents a particular challenge compared to proteins; the models are usually poorly resolved in cryo-EM and MX maps and available sequence-information is in practice limited to two nucleobase-types. Moreover, validation of nucleic acid models addresses predominantly backbone conformations that ...
Class I E. Coli Cysteinyl-tRNA synthetase -tRNACys (1U0B) Closed ring structure of the E. Coli Rho transcription termination factor in complex with nucleic acid in the motor domains (2HT1) Core human replisome (7PFO) Core human replisome (7PFO) - short load. Core structure of U6 small nuclear RNA - protein (ribonucleoprotein) complex (4N0T)
nucleic acid samples, sophisticated systems are required for biosample search and retrieval to be efficient. Automated systems notwithstanding, the effective utility of these biosample ... biosample is facilitated by the assignment of biochemical identifiers. These identifiers act as an It is made available under a CC-BY-NC-ND 4.0 International ...
Nucleic acids can be defined as organic molecules present in living cells. It plays a key factor in transferring genetic information from one generation to the next. Nucleic acids are composed of DNA-deoxyribonucleic acid and RNA-ribonucleic acid that form the polymers of nucleotides. In the nucleus, nucleotide monomers are linked together ...
Automatic taxonomy assignments. iTOL's automatic taxonomy assignment functionality provides a simple way of deducing the correct labels and classes of all tree nodes based on external taxonomy databases. In addition to the NCBI taxonomy database , iTOL now also supports GTDB (Genome Taxonomy Database) . The taxonomy assignment function ...