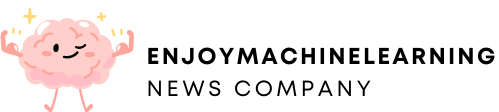
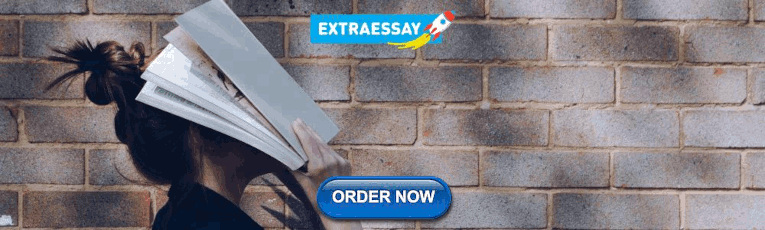
37 Research Topics In Data Science To Stay On Top Of
- February 22, 2024
As a data scientist, staying on top of the latest research in your field is essential.
The data science landscape changes rapidly, and new techniques and tools are constantly being developed.
To keep up with the competition, you need to be aware of the latest trends and topics in data science research.
In this article, we will provide an overview of 37 hot research topics in data science.
We will discuss each topic in detail, including its significance and potential applications.
These topics could be an idea for a thesis or simply topics you can research independently.
Stay tuned – this is one blog post you don’t want to miss!
37 Research Topics in Data Science
1.) predictive modeling.
Predictive modeling is a significant portion of data science and a topic you must be aware of.
Simply put, it is the process of using historical data to build models that can predict future outcomes.
Predictive modeling has many applications, from marketing and sales to financial forecasting and risk management.
As businesses increasingly rely on data to make decisions, predictive modeling is becoming more and more important.
While it can be complex, predictive modeling is a powerful tool that gives businesses a competitive advantage.
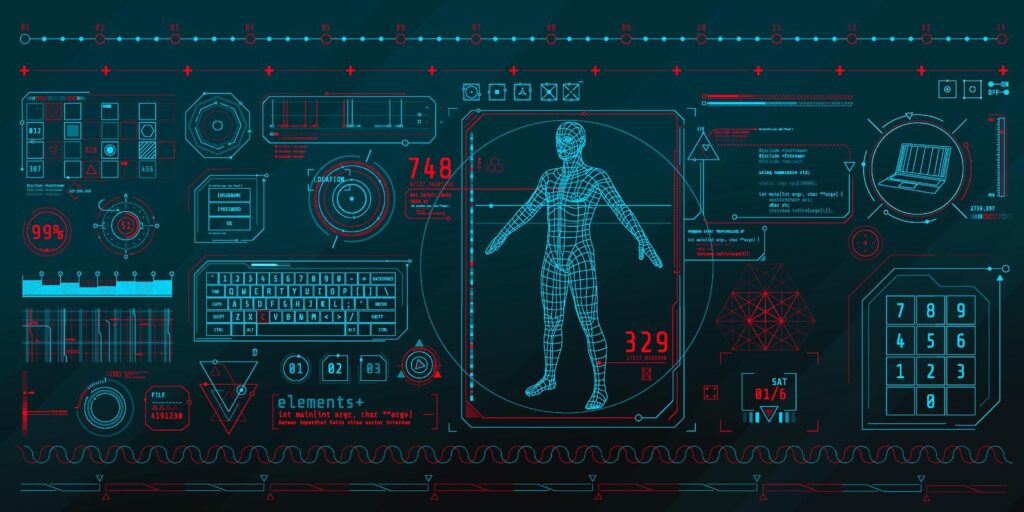
2.) Big Data Analytics
These days, it seems like everyone is talking about big data.
And with good reason – organizations of all sizes are sitting on mountains of data, and they’re increasingly turning to data scientists to help them make sense of it all.
But what exactly is big data? And what does it mean for data science?
Simply put, big data is a term used to describe datasets that are too large and complex for traditional data processing techniques.
Big data typically refers to datasets of a few terabytes or more.
But size isn’t the only defining characteristic – big data is also characterized by its high Velocity (the speed at which data is generated), Variety (the different types of data), and Volume (the amount of the information).
Given the enormity of big data, it’s not surprising that organizations are struggling to make sense of it all.
That’s where data science comes in.
Data scientists use various methods to wrangle big data, including distributed computing and other decentralized technologies.
With the help of data science, organizations are beginning to unlock the hidden value in their big data.
By harnessing the power of big data analytics, they can improve their decision-making, better understand their customers, and develop new products and services.
3.) Auto Machine Learning
Auto machine learning is a research topic in data science concerned with developing algorithms that can automatically learn from data without intervention.
This area of research is vital because it allows data scientists to automate the process of writing code for every dataset.
This allows us to focus on other tasks, such as model selection and validation.
Auto machine learning algorithms can learn from data in a hands-off way for the data scientist – while still providing incredible insights.
This makes them a valuable tool for data scientists who either don’t have the skills to do their own analysis or are struggling.
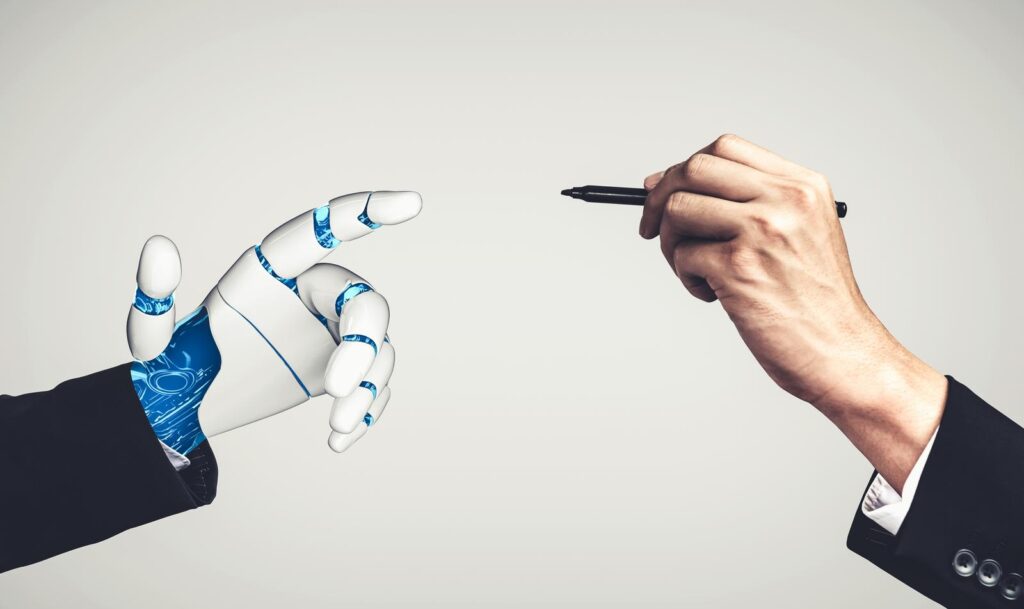
4.) Text Mining
Text mining is a research topic in data science that deals with text data extraction.
This area of research is important because it allows us to get as much information as possible from the vast amount of text data available today.
Text mining techniques can extract information from text data, such as keywords, sentiments, and relationships.
This information can be used for various purposes, such as model building and predictive analytics.
5.) Natural Language Processing
Natural language processing is a data science research topic that analyzes human language data.
This area of research is important because it allows us to understand and make sense of the vast amount of text data available today.
Natural language processing techniques can build predictive and interactive models from any language data.
Natural Language processing is pretty broad, and recent advances like GPT-3 have pushed this topic to the forefront.
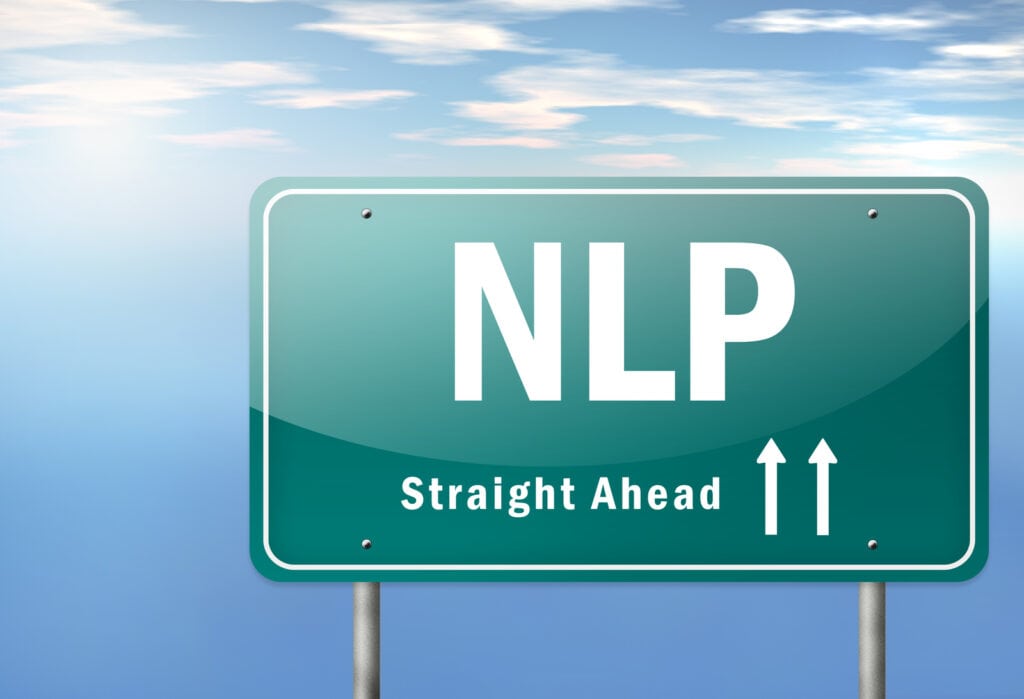
6.) Recommender Systems
Recommender systems are an exciting topic in data science because they allow us to make better products, services, and content recommendations.
Businesses can better understand their customers and their needs by using recommender systems.
This, in turn, allows them to develop better products and services that meet the needs of their customers.
Recommender systems are also used to recommend content to users.
This can be done on an individual level or at a group level.
Think about Netflix, for example, always knowing what you want to watch!
Recommender systems are a valuable tool for businesses and users alike.
7.) Deep Learning
Deep learning is a research topic in data science that deals with artificial neural networks.
These networks are composed of multiple layers, and each layer is formed from various nodes.
Deep learning networks can learn from data similarly to how humans learn, irrespective of the data distribution.
This makes them a valuable tool for data scientists looking to build models that can learn from data independently.
The deep learning network has become very popular in recent years because of its ability to achieve state-of-the-art results on various tasks.
There seems to be a new SOTA deep learning algorithm research paper on https://arxiv.org/ every single day!
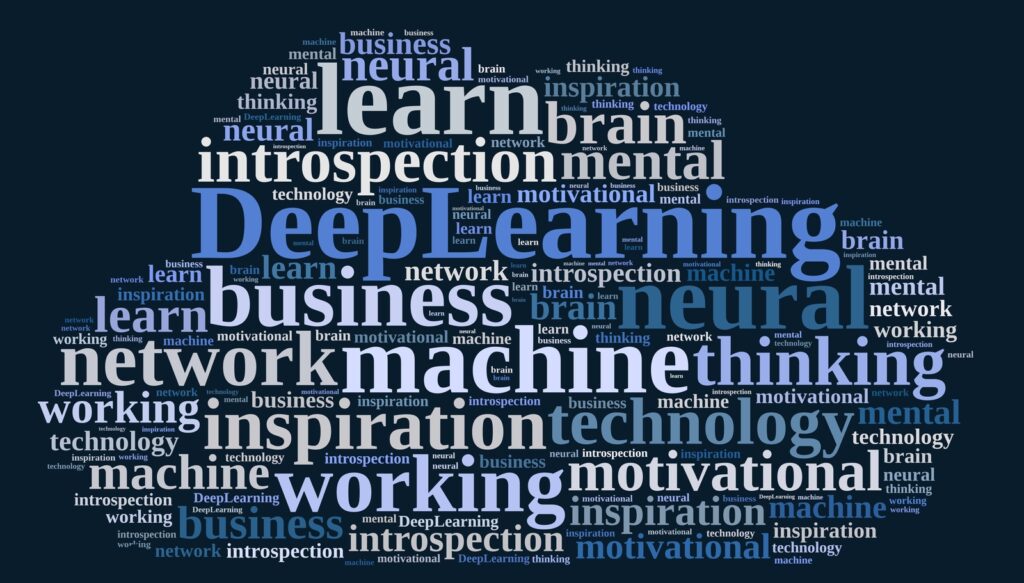
8.) Reinforcement Learning
Reinforcement learning is a research topic in data science that deals with algorithms that can learn on multiple levels from interactions with their environment.
This area of research is essential because it allows us to develop algorithms that can learn non-greedy approaches to decision-making, allowing businesses and companies to win in the long term compared to the short.
9.) Data Visualization
Data visualization is an excellent research topic in data science because it allows us to see our data in a way that is easy to understand.
Data visualization techniques can be used to create charts, graphs, and other visual representations of data.
This allows us to see the patterns and trends hidden in our data.
Data visualization is also used to communicate results to others.
This allows us to share our findings with others in a way that is easy to understand.
There are many ways to contribute to and learn about data visualization.
Some ways include attending conferences, reading papers, and contributing to open-source projects.
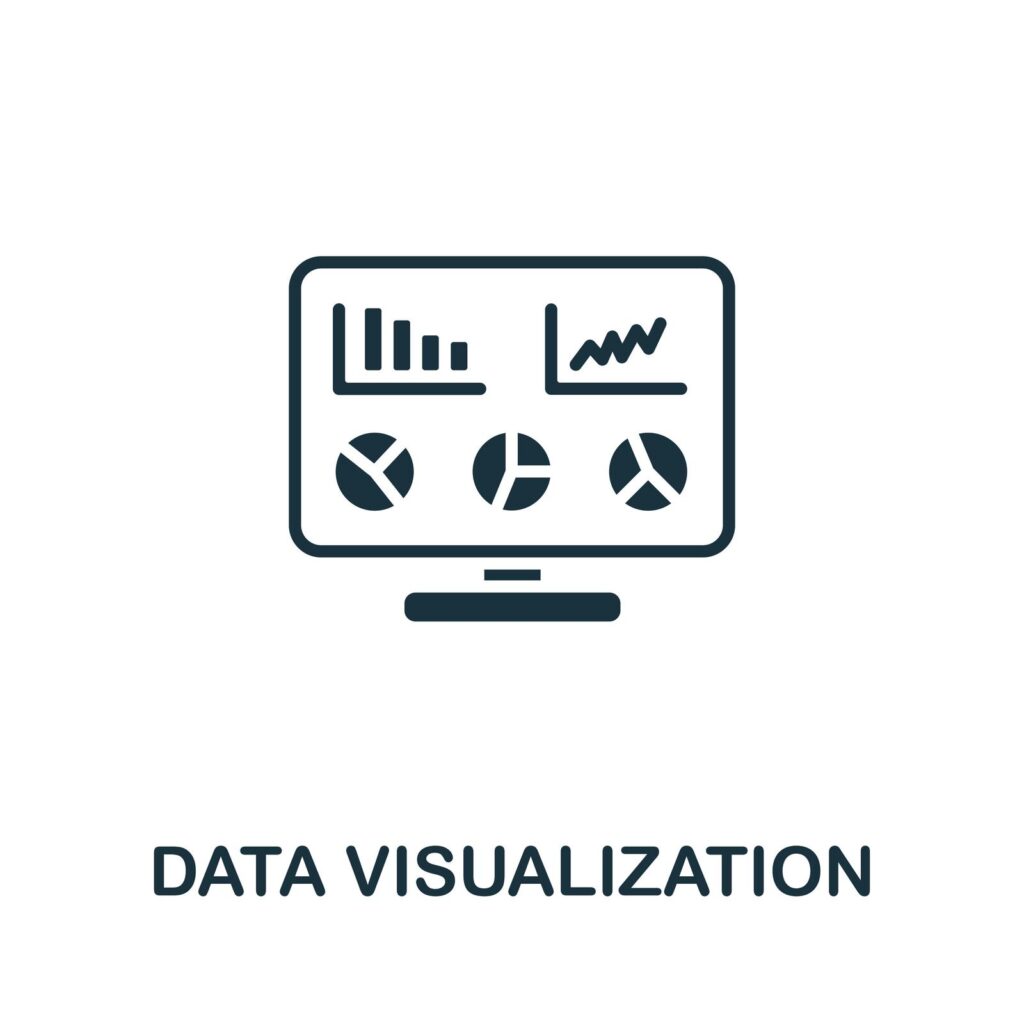
10.) Predictive Maintenance
Predictive maintenance is a hot topic in data science because it allows us to prevent failures before they happen.
This is done using data analytics to predict when a failure will occur.
This allows us to take corrective action before the failure actually happens.
While this sounds simple, avoiding false positives while keeping recall is challenging and an area wide open for advancement.
11.) Financial Analysis
Financial analysis is an older topic that has been around for a while but is still a great field where contributions can be felt.
Current researchers are focused on analyzing macroeconomic data to make better financial decisions.
This is done by analyzing the data to identify trends and patterns.
Financial analysts can use this information to make informed decisions about where to invest their money.
Financial analysis is also used to predict future economic trends.
This allows businesses and individuals to prepare for potential financial hardships and enable companies to be cash-heavy during good economic conditions.
Overall, financial analysis is a valuable tool for anyone looking to make better financial decisions.
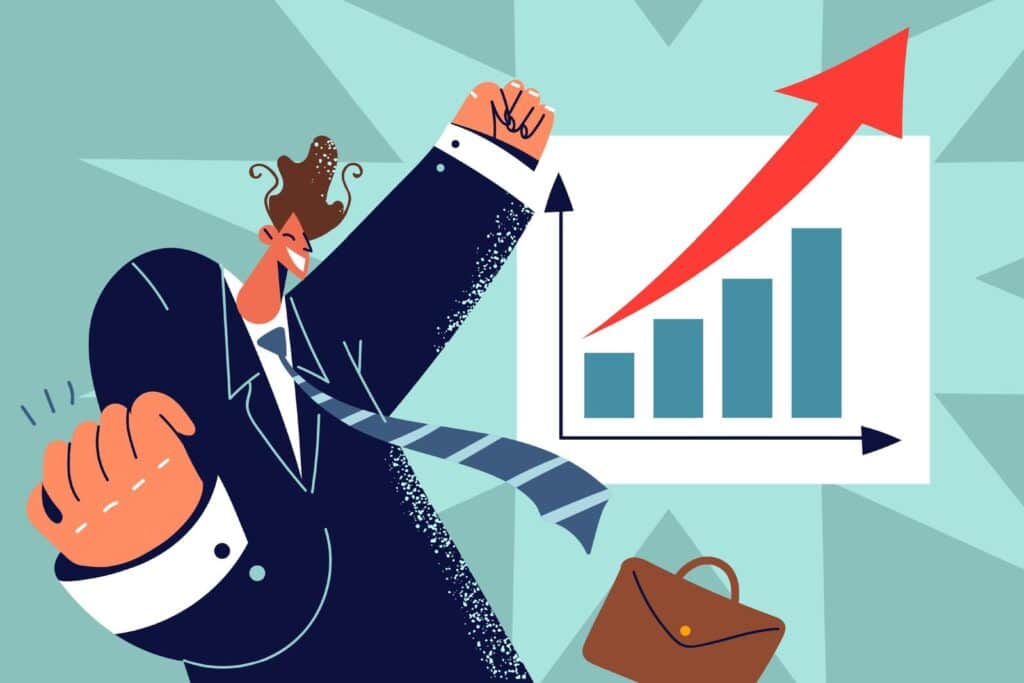
12.) Image Recognition
Image recognition is one of the hottest topics in data science because it allows us to identify objects in images.
This is done using artificial intelligence algorithms that can learn from data and understand what objects you’re looking for.
This allows us to build models that can accurately recognize objects in images and video.
This is a valuable tool for businesses and individuals who want to be able to identify objects in images.
Think about security, identification, routing, traffic, etc.
Image Recognition has gained a ton of momentum recently – for a good reason.
13.) Fraud Detection
Fraud detection is a great topic in data science because it allows us to identify fraudulent activity before it happens.
This is done by analyzing data to look for patterns and trends that may be associated with the fraud.
Once our machine learning model recognizes some of these patterns in real time, it immediately detects fraud.
This allows us to take corrective action before the fraud actually happens.
Fraud detection is a valuable tool for anyone who wants to protect themselves from potential fraudulent activity.
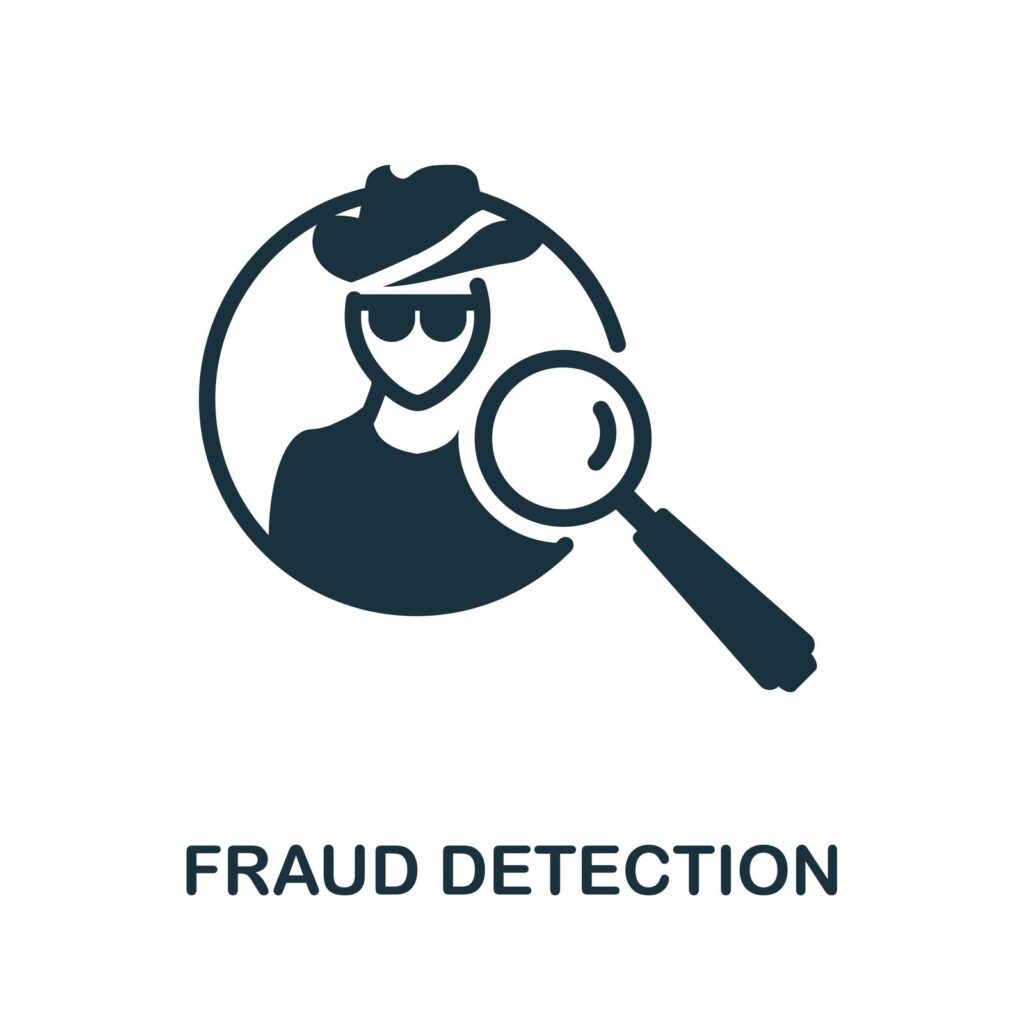
14.) Web Scraping
Web scraping is a controversial topic in data science because it allows us to collect data from the web, which is usually data you do not own.
This is done by extracting data from websites using scraping tools that are usually custom-programmed.
This allows us to collect data that would otherwise be inaccessible.
For obvious reasons, web scraping is a unique tool – giving you data your competitors would have no chance of getting.
I think there is an excellent opportunity to create new and innovative ways to make scraping accessible for everyone, not just those who understand Selenium and Beautiful Soup.
15.) Social Media Analysis
Social media analysis is not new; many people have already created exciting and innovative algorithms to study this.
However, it is still a great data science research topic because it allows us to understand how people interact on social media.
This is done by analyzing data from social media platforms to look for insights, bots, and recent societal trends.
Once we understand these practices, we can use this information to improve our marketing efforts.
For example, if we know that a particular demographic prefers a specific type of content, we can create more content that appeals to them.
Social media analysis is also used to understand how people interact with brands on social media.
This allows businesses to understand better what their customers want and need.
Overall, social media analysis is valuable for anyone who wants to improve their marketing efforts or understand how customers interact with brands.
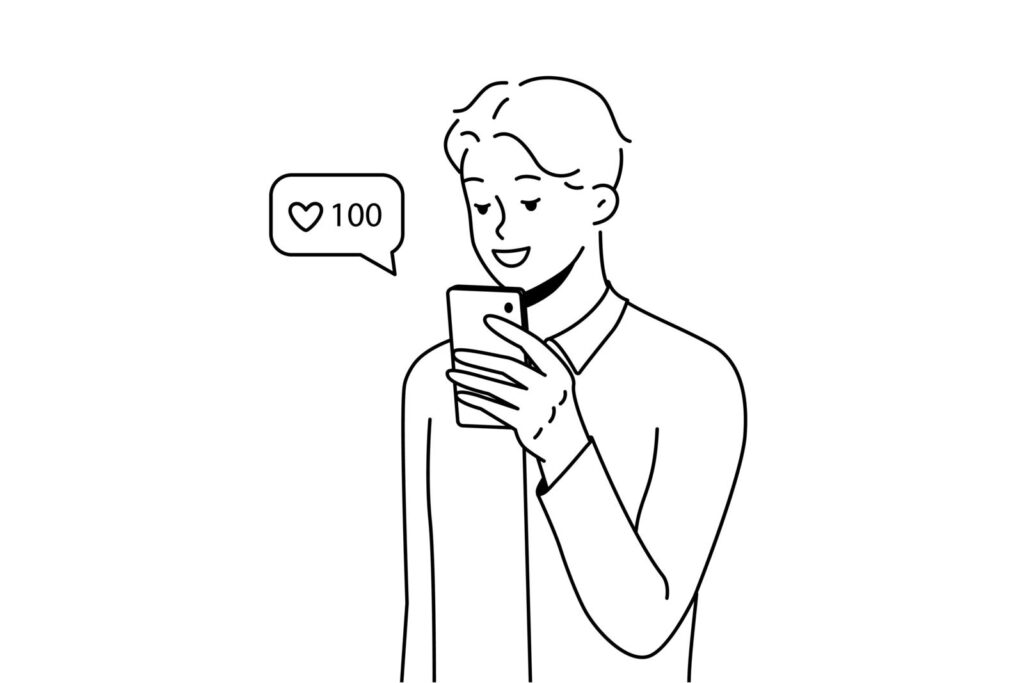
16.) GPU Computing
GPU computing is a fun new research topic in data science because it allows us to process data much faster than traditional CPUs .
Due to how GPUs are made, they’re incredibly proficient at intense matrix operations, outperforming traditional CPUs by very high margins.
While the computation is fast, the coding is still tricky.
There is an excellent research opportunity to bring these innovations to non-traditional modules, allowing data science to take advantage of GPU computing outside of deep learning.
17.) Quantum Computing
Quantum computing is a new research topic in data science and physics because it allows us to process data much faster than traditional computers.
It also opens the door to new types of data.
There are just some problems that can’t be solved utilizing outside of the classical computer.
For example, if you wanted to understand how a single atom moved around, a classical computer couldn’t handle this problem.
You’ll need to utilize a quantum computer to handle quantum mechanics problems.
This may be the “hottest” research topic on the planet right now, with some of the top researchers in computer science and physics worldwide working on it.
You could be too.
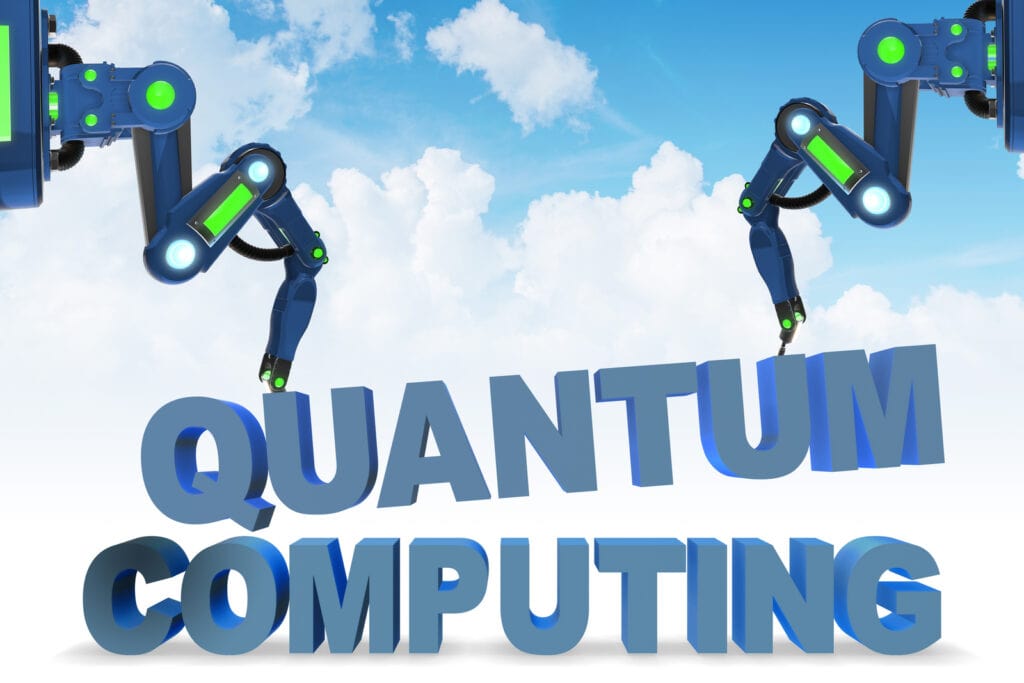
18.) Genomics
Genomics may be the only research topic that can compete with quantum computing regarding the “number of top researchers working on it.”
Genomics is a fantastic intersection of data science because it allows us to understand how genes work.
This is done by sequencing the DNA of different organisms to look for insights into our and other species.
Once we understand these patterns, we can use this information to improve our understanding of diseases and create new and innovative treatments for them.
Genomics is also used to study the evolution of different species.
Genomics is the future and a field begging for new and exciting research professionals to take it to the next step.
19.) Location-based services
Location-based services are an old and time-tested research topic in data science.
Since GPS and 4g cell phone reception became a thing, we’ve been trying to stay informed about how humans interact with their environment.
This is done by analyzing data from GPS tracking devices, cell phone towers, and Wi-Fi routers to look for insights into how humans interact.
Once we understand these practices, we can use this information to improve our geotargeting efforts, improve maps, find faster routes, and improve cohesion throughout a community.
Location-based services are used to understand the user, something every business could always use a little bit more of.
While a seemingly “stale” field, location-based services have seen a revival period with self-driving cars.
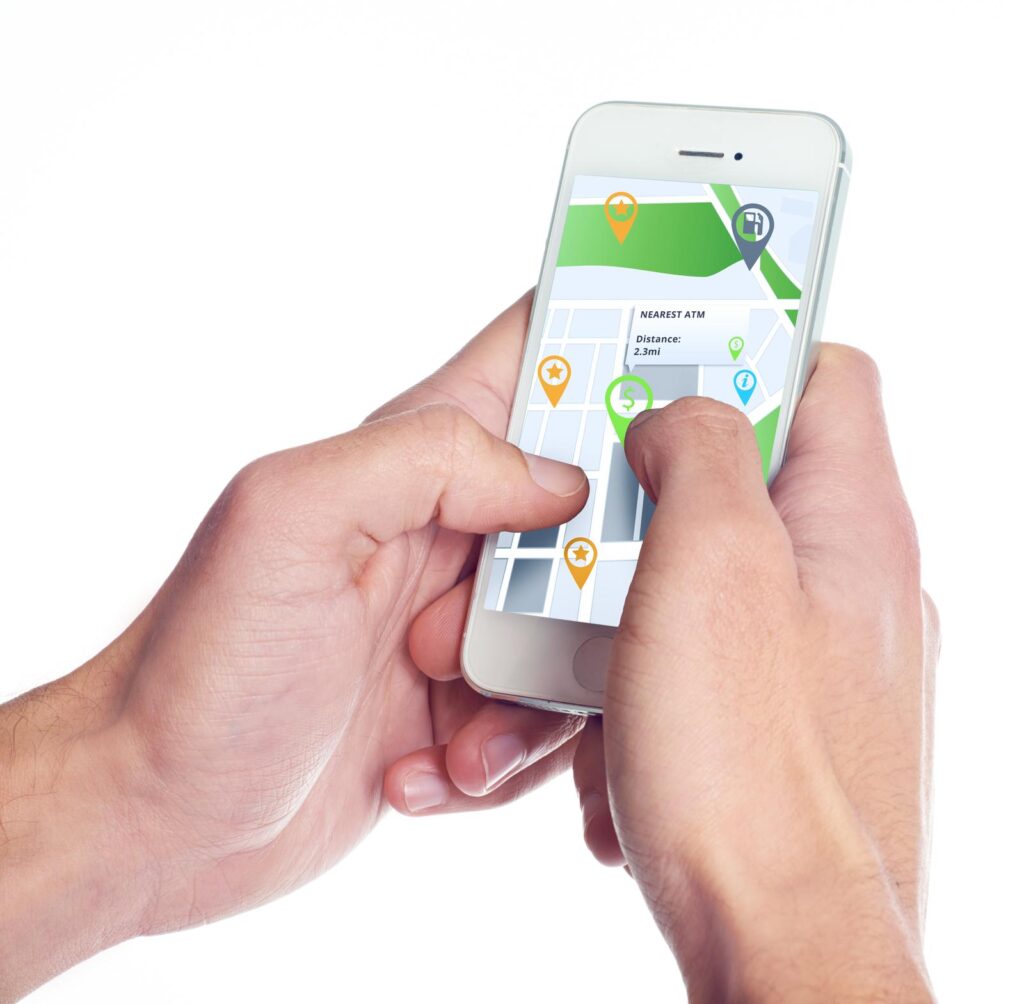
20.) Smart City Applications
Smart city applications are all the rage in data science research right now.
By harnessing the power of data, cities can become more efficient and sustainable.
But what exactly are smart city applications?
In short, they are systems that use data to improve city infrastructure and services.
This can include anything from traffic management and energy use to waste management and public safety.
Data is collected from various sources, including sensors, cameras, and social media.
It is then analyzed to identify tendencies and habits.
This information can make predictions about future needs and optimize city resources.
As more and more cities strive to become “smart,” the demand for data scientists with expertise in smart city applications is only growing.
21.) Internet Of Things (IoT)
The Internet of Things, or IoT, is exciting and new data science and sustainability research topic.
IoT is a network of physical objects embedded with sensors and connected to the internet.
These objects can include everything from alarm clocks to refrigerators; they’re all connected to the internet.
That means that they can share data with computers.
And that’s where data science comes in.
Data scientists are using IoT data to learn everything from how people use energy to how traffic flows through a city.
They’re also using IoT data to predict when an appliance will break down or when a road will be congested.
Really, the possibilities are endless.
With such a wide-open field, it’s easy to see why IoT is being researched by some of the top professionals in the world.
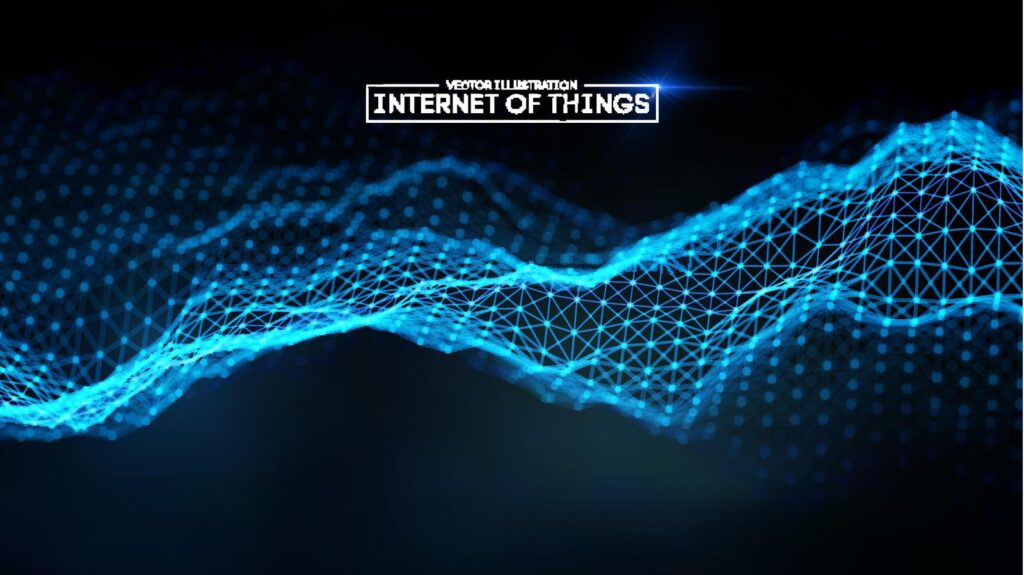
22.) Cybersecurity
Cybersecurity is a relatively new research topic in data science and in general, but it’s already garnering a lot of attention from businesses and organizations.
After all, with the increasing number of cyber attacks in recent years, it’s clear that we need to find better ways to protect our data.
While most of cybersecurity focuses on infrastructure, data scientists can leverage historical events to find potential exploits to protect their companies.
Sometimes, looking at a problem from a different angle helps, and that’s what data science brings to cybersecurity.
Also, data science can help to develop new security technologies and protocols.
As a result, cybersecurity is a crucial data science research area and one that will only become more important in the years to come.
23.) Blockchain
Blockchain is an incredible new research topic in data science for several reasons.
First, it is a distributed database technology that enables secure, transparent, and tamper-proof transactions.
Did someone say transmitting data?
This makes it an ideal platform for tracking data and transactions in various industries.
Second, blockchain is powered by cryptography, which not only makes it highly secure – but is a familiar foe for data scientists.
Finally, blockchain is still in its early stages of development, so there is much room for research and innovation.
As a result, blockchain is a great new research topic in data science that vows to revolutionize how we store, transmit and manage data.
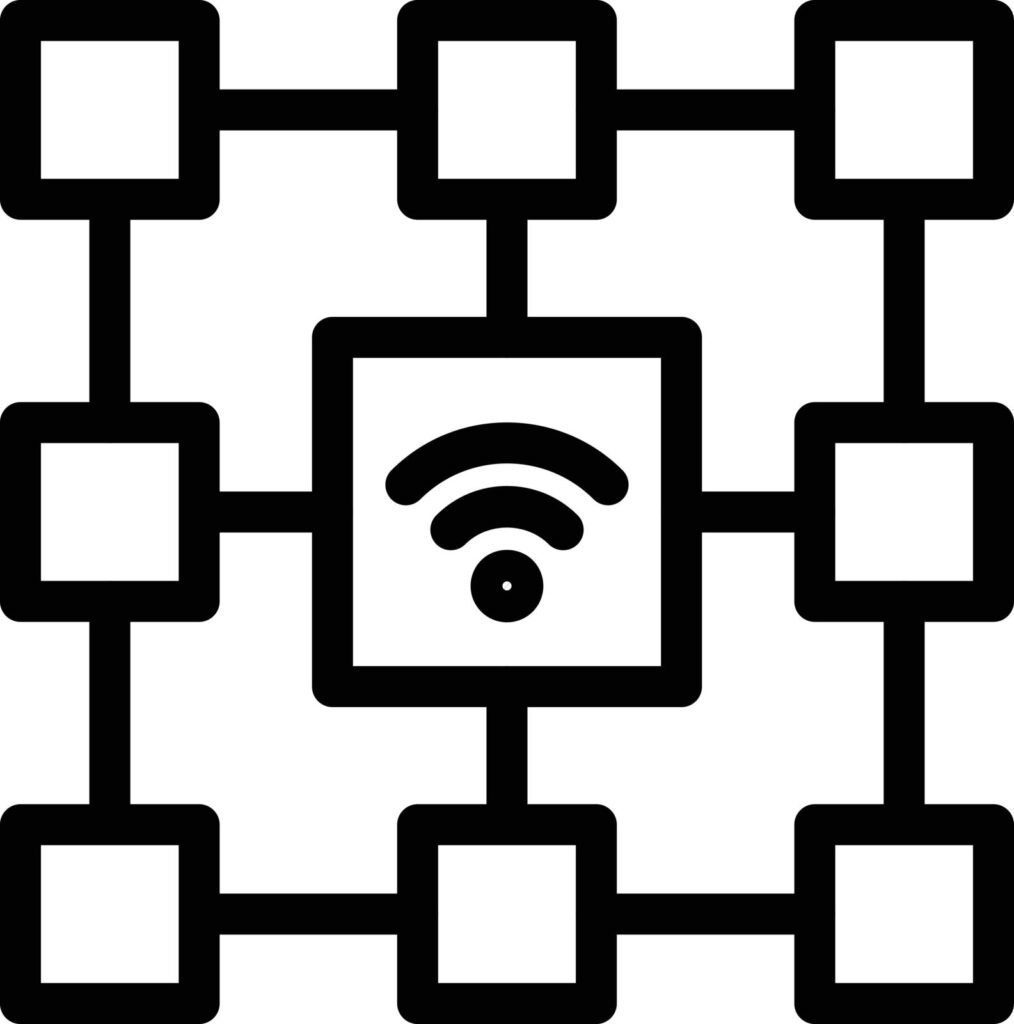
24.) Sustainability
Sustainability is a relatively new research topic in data science, but it is gaining traction quickly.
To keep up with this demand, The Wharton School of the University of Pennsylvania has started to offer an MBA in Sustainability .
This demand isn’t shocking, and some of the reasons include the following:
Sustainability is an important issue that is relevant to everyone.
Datasets on sustainability are constantly growing and changing, making it an exciting challenge for data scientists.
There hasn’t been a “set way” to approach sustainability from a data perspective, making it an excellent opportunity for interdisciplinary research.
As data science grows, sustainability will likely become an increasingly important research topic.
25.) Educational Data
Education has always been a great topic for research, and with the advent of big data, educational data has become an even richer source of information.
By studying educational data, researchers can gain insights into how students learn, what motivates them, and what barriers these students may face.
Besides, data science can be used to develop educational interventions tailored to individual students’ needs.
Imagine being the researcher that helps that high schooler pass mathematics; what an incredible feeling.
With the increasing availability of educational data, data science has enormous potential to improve the quality of education.
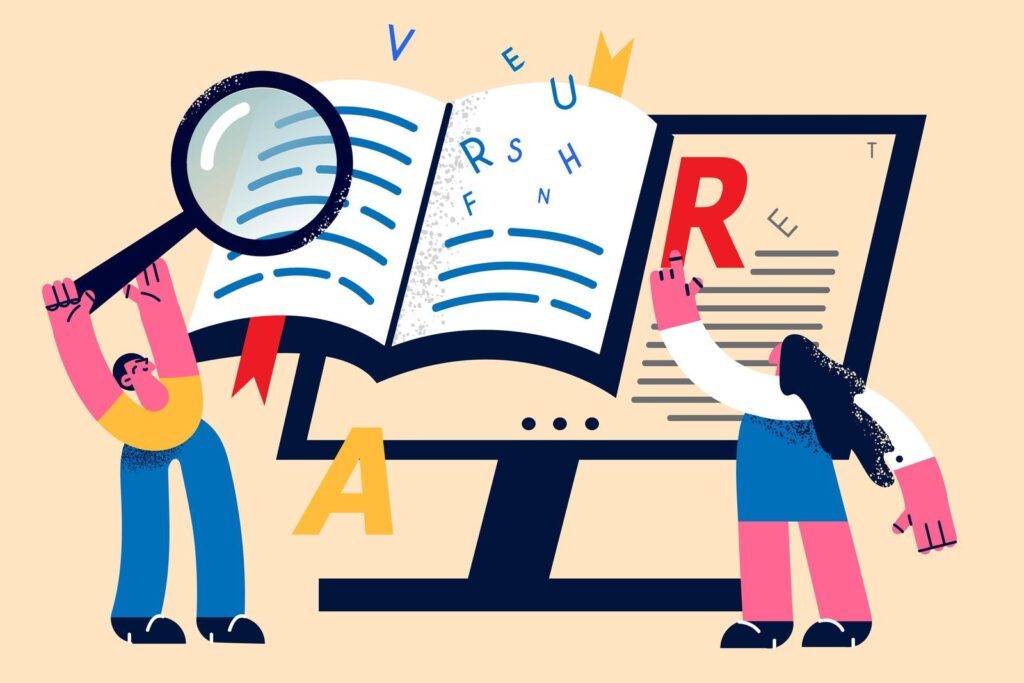
26.) Politics
As data science continues to evolve, so does the scope of its applications.
Originally used primarily for business intelligence and marketing, data science is now applied to various fields, including politics.
By analyzing large data sets, political scientists (data scientists with a cooler name) can gain valuable insights into voting patterns, campaign strategies, and more.
Further, data science can be used to forecast election results and understand the effects of political events on public opinion.
With the wealth of data available, there is no shortage of research opportunities in this field.
As data science evolves, so does our understanding of politics and its role in our world.
27.) Cloud Technologies
Cloud technologies are a great research topic.
It allows for the outsourcing and sharing of computer resources and applications all over the internet.
This lets organizations save money on hardware and maintenance costs while providing employees access to the latest and greatest software and applications.
I believe there is an argument that AWS could be the greatest and most technologically advanced business ever built (Yes, I know it’s only part of the company).
Besides, cloud technologies can help improve team members’ collaboration by allowing them to share files and work on projects together in real-time.
As more businesses adopt cloud technologies, data scientists must stay up-to-date on the latest trends in this area.
By researching cloud technologies, data scientists can help organizations to make the most of this new and exciting technology.
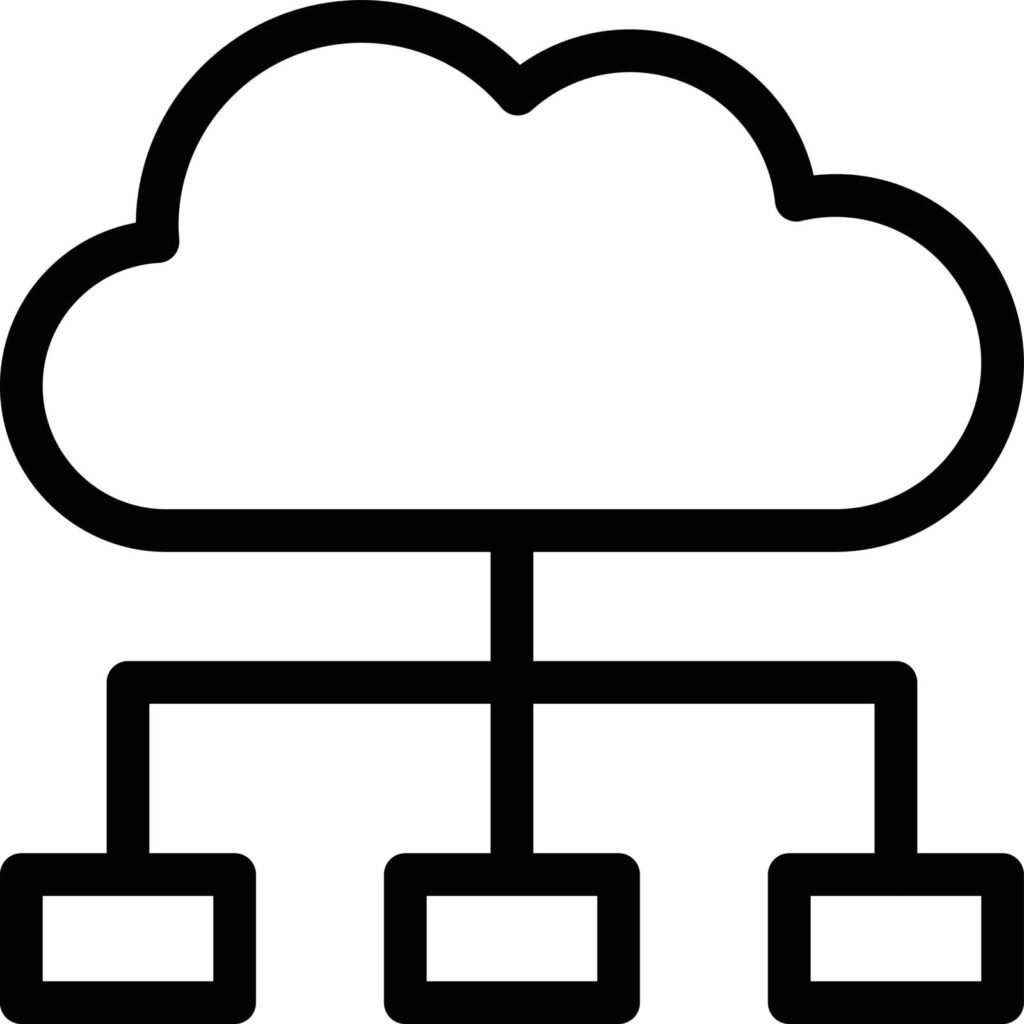
28.) Robotics
Robotics has recently become a household name, and it’s for a good reason.
First, robotics deals with controlling and planning physical systems, an inherently complex problem.
Second, robotics requires various sensors and actuators to interact with the world, making it an ideal application for machine learning techniques.
Finally, robotics is an interdisciplinary field that draws on various disciplines, such as computer science, mechanical engineering, and electrical engineering.
As a result, robotics is a rich source of research problems for data scientists.
29.) HealthCare
Healthcare is an industry that is ripe for data-driven innovation.
Hospitals, clinics, and health insurance companies generate a tremendous amount of data daily.
This data can be used to improve the quality of care and outcomes for patients.
This is perfect timing, as the healthcare industry is undergoing a significant shift towards value-based care, which means there is a greater need than ever for data-driven decision-making.
As a result, healthcare is an exciting new research topic for data scientists.
There are many different ways in which data can be used to improve healthcare, and there is a ton of room for newcomers to make discoveries.
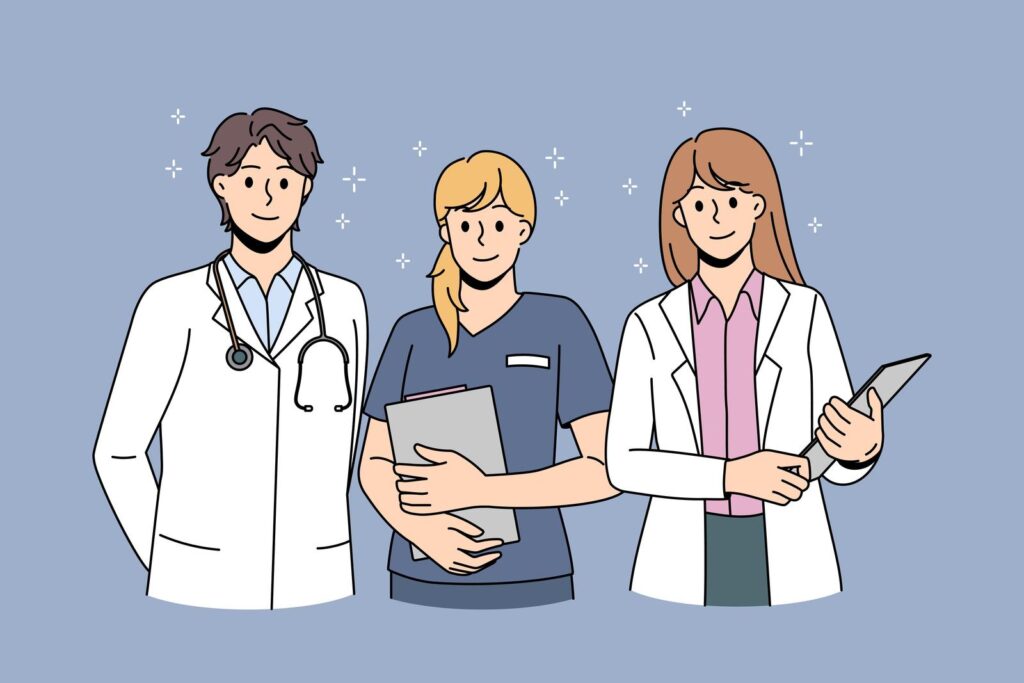
30.) Remote Work
There’s no doubt that remote work is on the rise.
In today’s global economy, more and more businesses are allowing their employees to work from home or anywhere else they can get a stable internet connection.
But what does this mean for data science? Well, for one thing, it opens up a whole new field of research.
For example, how does remote work impact employee productivity?
What are the best ways to manage and collaborate on data science projects when team members are spread across the globe?
And what are the cybersecurity risks associated with working remotely?
These are just a few of the questions that data scientists will be able to answer with further research.
So if you’re looking for a new topic to sink your teeth into, remote work in data science is a great option.
31.) Data-Driven Journalism
Data-driven journalism is an exciting new field of research that combines the best of both worlds: the rigor of data science with the creativity of journalism.
By applying data analytics to large datasets, journalists can uncover stories that would otherwise be hidden.
And telling these stories compellingly can help people better understand the world around them.
Data-driven journalism is still in its infancy, but it has already had a major impact on how news is reported.
In the future, it will only become more important as data becomes increasingly fluid among journalists.
It is an exciting new topic and research field for data scientists to explore.
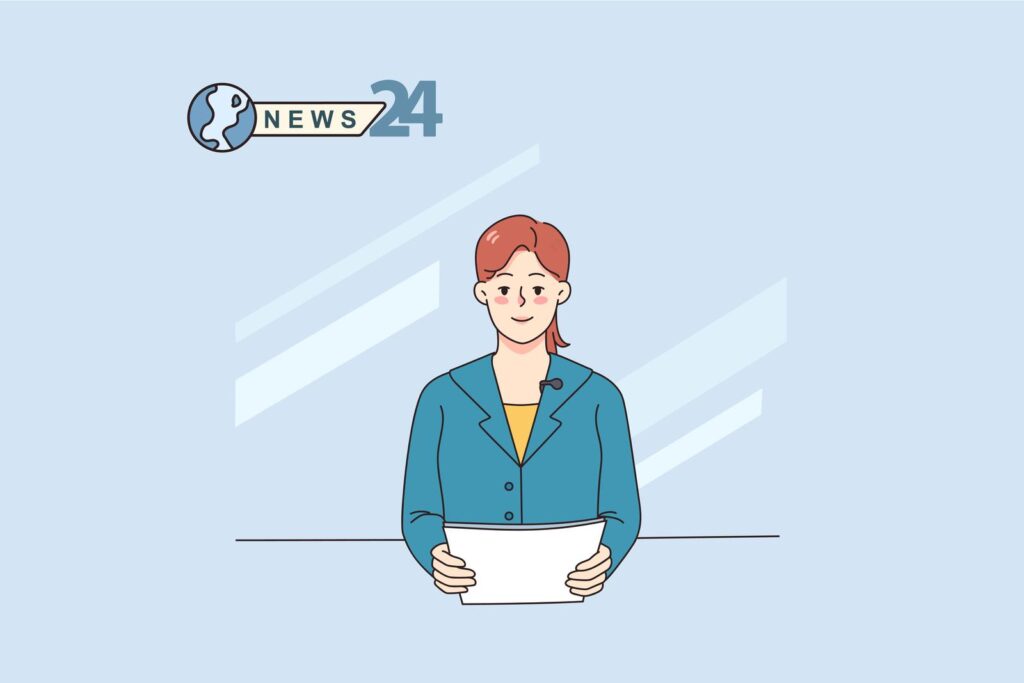
32.) Data Engineering
Data engineering is a staple in data science, focusing on efficiently managing data.
Data engineers are responsible for developing and maintaining the systems that collect, process, and store data.
In recent years, there has been an increasing demand for data engineers as the volume of data generated by businesses and organizations has grown exponentially.
Data engineers must be able to design and implement efficient data-processing pipelines and have the skills to optimize and troubleshoot existing systems.
If you are looking for a challenging research topic that would immediately impact you worldwide, then improving or innovating a new approach in data engineering would be a good start.
33.) Data Curation
Data curation has been a hot topic in the data science community for some time now.
Curating data involves organizing, managing, and preserving data so researchers can use it.
Data curation can help to ensure that data is accurate, reliable, and accessible.
It can also help to prevent research duplication and to facilitate the sharing of data between researchers.
Data curation is a vital part of data science. In recent years, there has been an increasing focus on data curation, as it has become clear that it is essential for ensuring data quality.
As a result, data curation is now a major research topic in data science.
There are numerous books and articles on the subject, and many universities offer courses on data curation.
Data curation is an integral part of data science and will only become more important in the future.
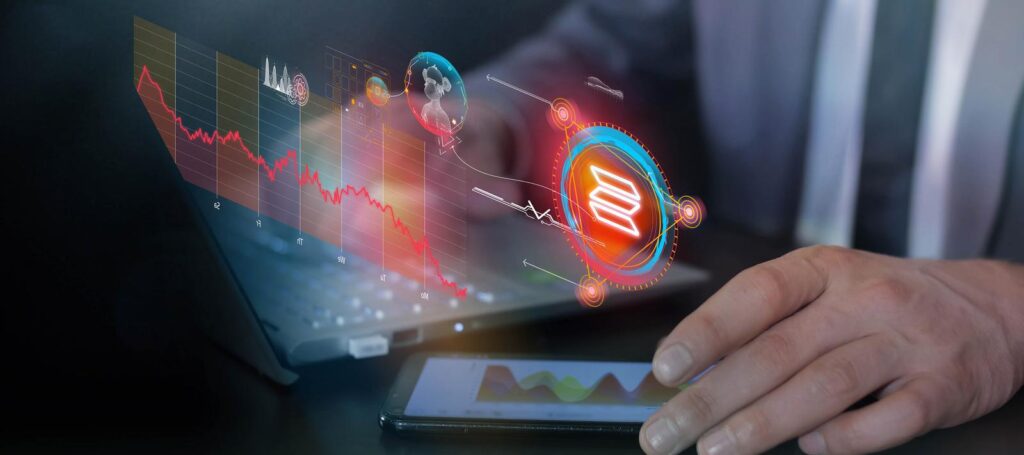
34.) Meta-Learning
Meta-learning is gaining a ton of steam in data science. It’s learning how to learn.
So, if you can learn how to learn, you can learn anything much faster.
Meta-learning is mainly used in deep learning, as applications outside of this are generally pretty hard.
In deep learning, many parameters need to be tuned for a good model, and there’s usually a lot of data.
You can save time and effort if you can automatically and quickly do this tuning.
In machine learning, meta-learning can improve models’ performance by sharing knowledge between different models.
For example, if you have a bunch of different models that all solve the same problem, then you can use meta-learning to share the knowledge between them to improve the cluster (groups) overall performance.
I don’t know how anyone looking for a research topic could stay away from this field; it’s what the Terminator warned us about!
35.) Data Warehousing
A data warehouse is a system used for data analysis and reporting.
It is a central data repository created by combining data from multiple sources.
Data warehouses are often used to store historical data, such as sales data, financial data, and customer data.
This data type can be used to create reports and perform statistical analysis.
Data warehouses also store data that the organization is not currently using.
This type of data can be used for future research projects.
Data warehousing is an incredible research topic in data science because it offers a variety of benefits.
Data warehouses help organizations to save time and money by reducing the need for manual data entry.
They also help to improve the accuracy of reports and provide a complete picture of the organization’s performance.
Data warehousing feels like one of the weakest parts of the Data Science Technology Stack; if you want a research topic that could have a monumental impact – data warehousing is an excellent place to look.
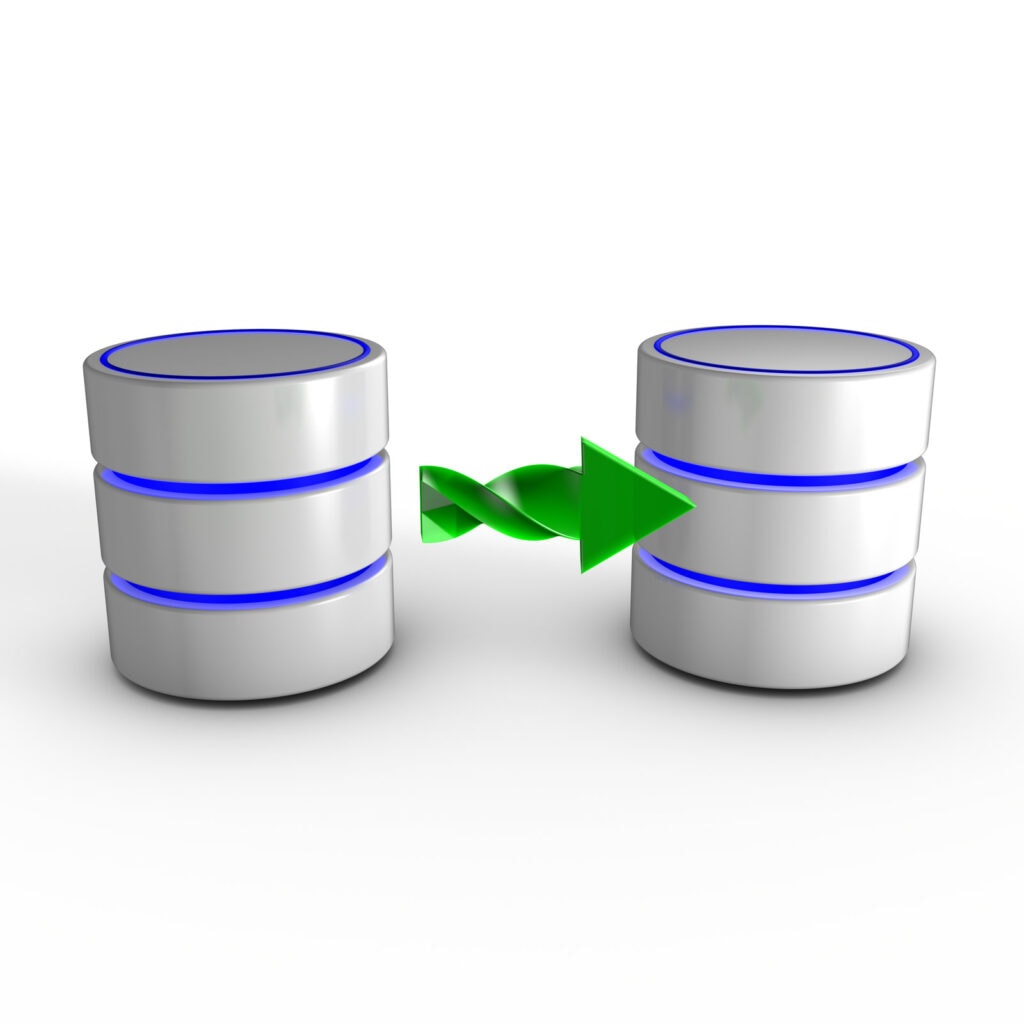
36.) Business Intelligence
Business intelligence aims to collect, process, and analyze data to help businesses make better decisions.
Business intelligence can improve marketing, sales, customer service, and operations.
It can also be used to identify new business opportunities and track competition.
BI is business and another tool in your company’s toolbox to continue dominating your area.
Data science is the perfect tool for business intelligence because it combines statistics, computer science, and machine learning.
Data scientists can use business intelligence to answer questions like, “What are our customers buying?” or “What are our competitors doing?” or “How can we increase sales?”
Business intelligence is a great way to improve your business’s bottom line and an excellent opportunity to dive deep into a well-respected research topic.
37.) Crowdsourcing
One of the newest areas of research in data science is crowdsourcing.
Crowdsourcing is a process of sourcing tasks or projects to a large group of people, typically via the internet.
This can be done for various purposes, such as gathering data, developing new algorithms, or even just for fun (think: online quizzes and surveys).
But what makes crowdsourcing so powerful is that it allows businesses and organizations to tap into a vast pool of talent and resources they wouldn’t otherwise have access to.
And with the rise of social media, it’s easier than ever to connect with potential crowdsource workers worldwide.
Imagine if you could effect that, finding innovative ways to improve how people work together.
That would have a huge effect.
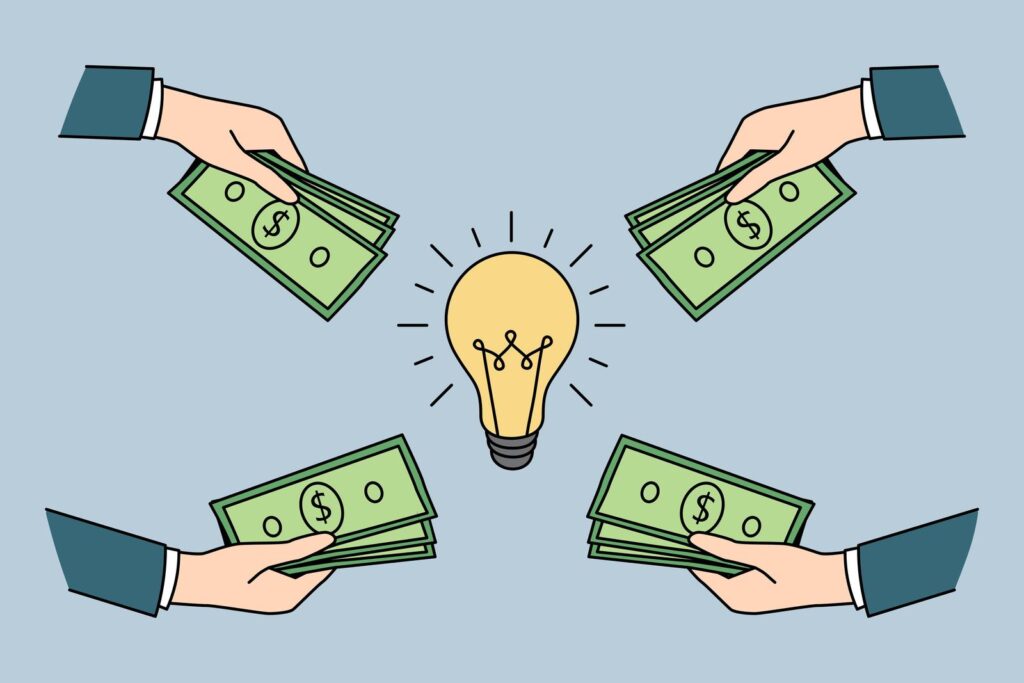
Final Thoughts, Are These Research Topics In Data Science For You?
Thirty-seven different research topics in data science are a lot to take in, but we hope you found a research topic that interests you.
If not, don’t worry – there are plenty of other great topics to explore.
The important thing is to get started with your research and find ways to apply what you learn to real-world problems.
We wish you the best of luck as you begin your data science journey!
Other Data Science Articles
We love talking about data science; here are a couple of our favorite articles:
- Why Are You Interested In Data Science?
- Recent Posts
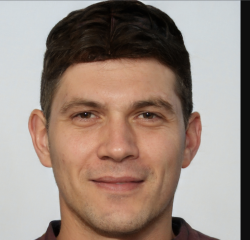
- Effective Strategies for How to Collect Data for Statistical Analysis [Boost Your Analysis Now] - May 15, 2024
- Is Lean Six Sigma Used in Software Development? [Discover the Impact Now] - May 14, 2024
- How many software engineers are employed by Google? [Discover the Surprising Answer Here] - May 14, 2024
Trending now
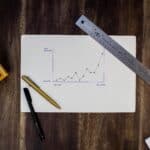

Research Topics & Ideas: Data Science
50 Topic Ideas To Kickstart Your Research Project
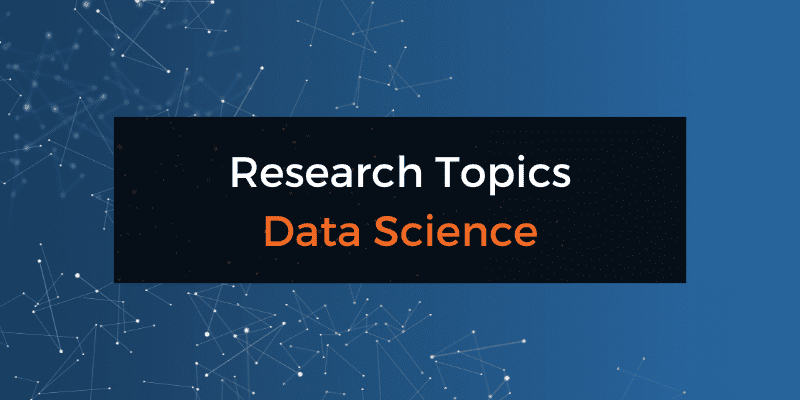
If you’re just starting out exploring data science-related topics for your dissertation, thesis or research project, you’ve come to the right place. In this post, we’ll help kickstart your research by providing a hearty list of data science and analytics-related research ideas , including examples from recent studies.
PS – This is just the start…
We know it’s exciting to run through a list of research topics, but please keep in mind that this list is just a starting point . These topic ideas provided here are intentionally broad and generic , so keep in mind that you will need to develop them further. Nevertheless, they should inspire some ideas for your project.
To develop a suitable research topic, you’ll need to identify a clear and convincing research gap , and a viable plan to fill that gap. If this sounds foreign to you, check out our free research topic webinar that explores how to find and refine a high-quality research topic, from scratch. Alternatively, consider our 1-on-1 coaching service .
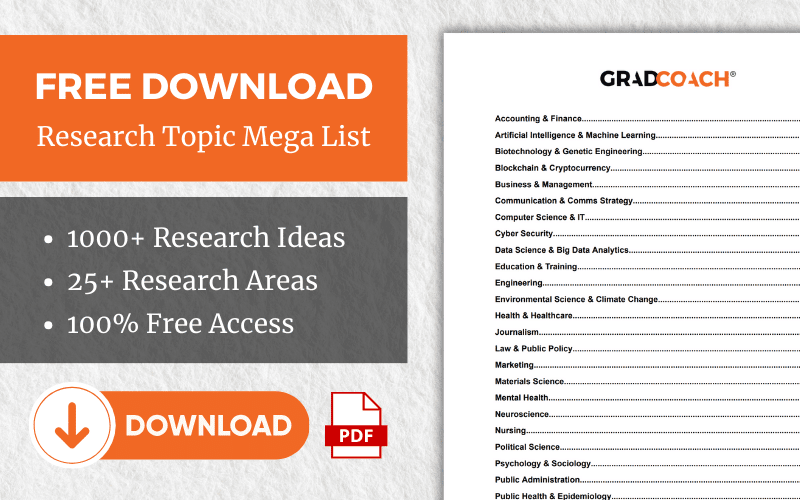
Data Science-Related Research Topics
- Developing machine learning models for real-time fraud detection in online transactions.
- The use of big data analytics in predicting and managing urban traffic flow.
- Investigating the effectiveness of data mining techniques in identifying early signs of mental health issues from social media usage.
- The application of predictive analytics in personalizing cancer treatment plans.
- Analyzing consumer behavior through big data to enhance retail marketing strategies.
- The role of data science in optimizing renewable energy generation from wind farms.
- Developing natural language processing algorithms for real-time news aggregation and summarization.
- The application of big data in monitoring and predicting epidemic outbreaks.
- Investigating the use of machine learning in automating credit scoring for microfinance.
- The role of data analytics in improving patient care in telemedicine.
- Developing AI-driven models for predictive maintenance in the manufacturing industry.
- The use of big data analytics in enhancing cybersecurity threat intelligence.
- Investigating the impact of sentiment analysis on brand reputation management.
- The application of data science in optimizing logistics and supply chain operations.
- Developing deep learning techniques for image recognition in medical diagnostics.
- The role of big data in analyzing climate change impacts on agricultural productivity.
- Investigating the use of data analytics in optimizing energy consumption in smart buildings.
- The application of machine learning in detecting plagiarism in academic works.
- Analyzing social media data for trends in political opinion and electoral predictions.
- The role of big data in enhancing sports performance analytics.
- Developing data-driven strategies for effective water resource management.
- The use of big data in improving customer experience in the banking sector.
- Investigating the application of data science in fraud detection in insurance claims.
- The role of predictive analytics in financial market risk assessment.
- Developing AI models for early detection of network vulnerabilities.
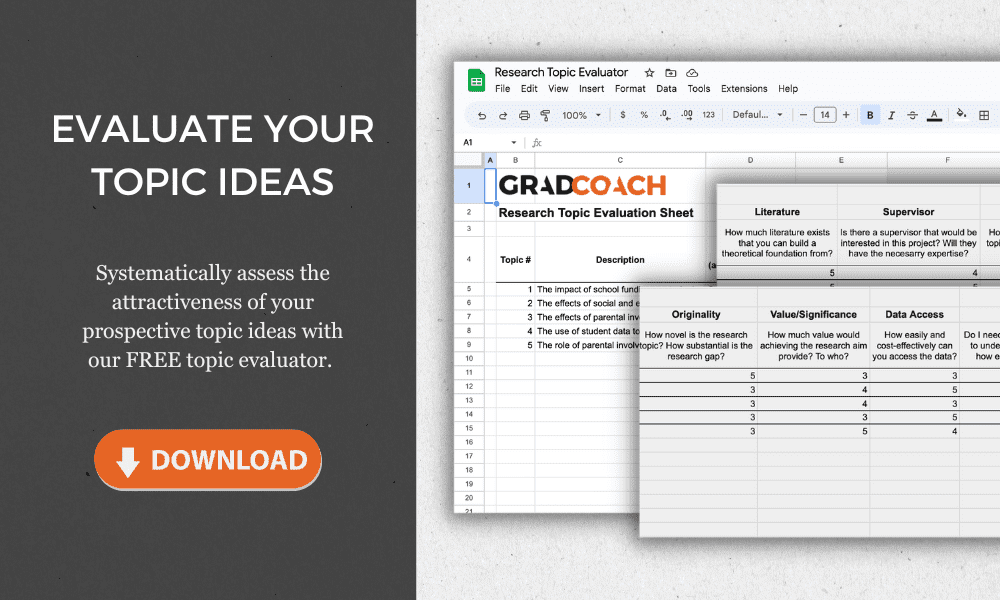
Data Science Research Ideas (Continued)
- The application of big data in public transportation systems for route optimization.
- Investigating the impact of big data analytics on e-commerce recommendation systems.
- The use of data mining techniques in understanding consumer preferences in the entertainment industry.
- Developing predictive models for real estate pricing and market trends.
- The role of big data in tracking and managing environmental pollution.
- Investigating the use of data analytics in improving airline operational efficiency.
- The application of machine learning in optimizing pharmaceutical drug discovery.
- Analyzing online customer reviews to inform product development in the tech industry.
- The role of data science in crime prediction and prevention strategies.
- Developing models for analyzing financial time series data for investment strategies.
- The use of big data in assessing the impact of educational policies on student performance.
- Investigating the effectiveness of data visualization techniques in business reporting.
- The application of data analytics in human resource management and talent acquisition.
- Developing algorithms for anomaly detection in network traffic data.
- The role of machine learning in enhancing personalized online learning experiences.
- Investigating the use of big data in urban planning and smart city development.
- The application of predictive analytics in weather forecasting and disaster management.
- Analyzing consumer data to drive innovations in the automotive industry.
- The role of data science in optimizing content delivery networks for streaming services.
- Developing machine learning models for automated text classification in legal documents.
- The use of big data in tracking global supply chain disruptions.
- Investigating the application of data analytics in personalized nutrition and fitness.
- The role of big data in enhancing the accuracy of geological surveying for natural resource exploration.
- Developing predictive models for customer churn in the telecommunications industry.
- The application of data science in optimizing advertisement placement and reach.
Recent Data Science-Related Studies
While the ideas we’ve presented above are a decent starting point for finding a research topic, they are fairly generic and non-specific. So, it helps to look at actual studies in the data science and analytics space to see how this all comes together in practice.
Below, we’ve included a selection of recent studies to help refine your thinking. These are actual studies, so they can provide some useful insight as to what a research topic looks like in practice.
- Data Science in Healthcare: COVID-19 and Beyond (Hulsen, 2022)
- Auto-ML Web-application for Automated Machine Learning Algorithm Training and evaluation (Mukherjee & Rao, 2022)
- Survey on Statistics and ML in Data Science and Effect in Businesses (Reddy et al., 2022)
- Visualization in Data Science VDS @ KDD 2022 (Plant et al., 2022)
- An Essay on How Data Science Can Strengthen Business (Santos, 2023)
- A Deep study of Data science related problems, application and machine learning algorithms utilized in Data science (Ranjani et al., 2022)
- You Teach WHAT in Your Data Science Course?!? (Posner & Kerby-Helm, 2022)
- Statistical Analysis for the Traffic Police Activity: Nashville, Tennessee, USA (Tufail & Gul, 2022)
- Data Management and Visual Information Processing in Financial Organization using Machine Learning (Balamurugan et al., 2022)
- A Proposal of an Interactive Web Application Tool QuickViz: To Automate Exploratory Data Analysis (Pitroda, 2022)
- Applications of Data Science in Respective Engineering Domains (Rasool & Chaudhary, 2022)
- Jupyter Notebooks for Introducing Data Science to Novice Users (Fruchart et al., 2022)
- Towards a Systematic Review of Data Science Programs: Themes, Courses, and Ethics (Nellore & Zimmer, 2022)
- Application of data science and bioinformatics in healthcare technologies (Veeranki & Varshney, 2022)
- TAPS Responsibility Matrix: A tool for responsible data science by design (Urovi et al., 2023)
- Data Detectives: A Data Science Program for Middle Grade Learners (Thompson & Irgens, 2022)
- MACHINE LEARNING FOR NON-MAJORS: A WHITE BOX APPROACH (Mike & Hazzan, 2022)
- COMPONENTS OF DATA SCIENCE AND ITS APPLICATIONS (Paul et al., 2022)
- Analysis on the Application of Data Science in Business Analytics (Wang, 2022)
As you can see, these research topics are a lot more focused than the generic topic ideas we presented earlier. So, for you to develop a high-quality research topic, you’ll need to get specific and laser-focused on a specific context with specific variables of interest. In the video below, we explore some other important things you’ll need to consider when crafting your research topic.
Get 1-On-1 Help
If you’re still unsure about how to find a quality research topic, check out our Research Topic Kickstarter service, which is the perfect starting point for developing a unique, well-justified research topic.
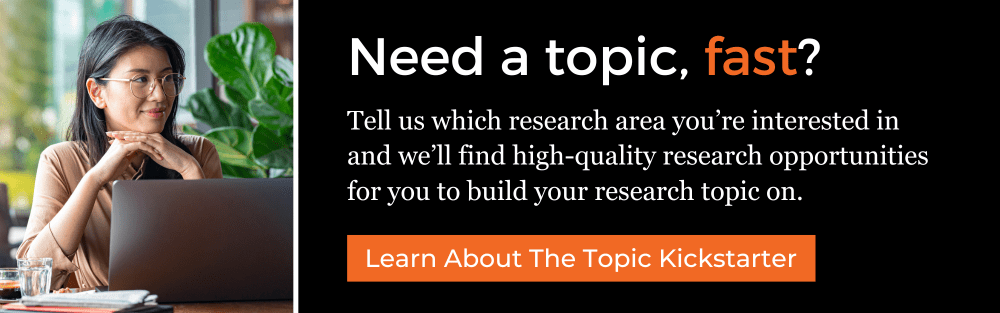
You Might Also Like:
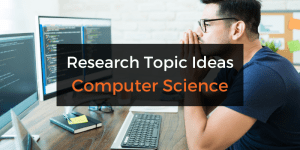
Submit a Comment Cancel reply
Your email address will not be published. Required fields are marked *
Save my name, email, and website in this browser for the next time I comment.
- Print Friendly
17 Most Important Data Science Trends of 2023
There’s nothing constant in our lives but change. Over the years, we’ve seen how businesses have become more modern, adopting the latest technology to boost productivity and increase the return on investment.
Data analytics, big data , artificial intelligence , and data science are the trending keywords in the current scenario. Enterprises want to adopt data-driven models to streamline their business processes and make better decisions based on data analytical insights.
With the pandemic disrupting industries around the world, SMEs and large enterprises had no option but to adapt to the changes in less time. This led to increasing investments in data analytics and data science. Data has become the center point for almost every organization.
As businesses rely on data analytics to avoid and overcome several challenges, we see new trends emerging in the industries. AI trends 2023 by Gartner are an example of development. The trends have been divided into three major heads- accelerating change, operationalizing business value, and distribution of everything (data and insights).
In this blog, we’ll look at the most important data science trends in 2023 and understand how big data and data analytics are becoming an inherent part of every enterprise, irrespective of the industry.
Top Data Science Trends of 2023
1. big data on the cloud .
Data is already being generated in abundance. The problem lies with collecting, tagging, cleaning, structuring, formatting, and analyzing this huge volume of data in one place. How to collect data? Where to store and process it? How should we share the insights with others?
Data science models and artificial intelligence come to the rescue. However, storage of data is still a concern. It has been found that around 45% of enterprises have moved their big data to cloud platforms. Businesses are increasingly turning towards cloud services for data storage, processing, and distribution. One of the major data management trends in 202 3 is the use of public and private cloud services for big data and data analytics .
2. Emphasis on Actionable Data
What use is data in its raw, unstructured, and complex format if you don’t know what to do with it? The emphasis is on actionable data that brings together big data and business processes to help you make the right decisions.
Investing in expensive data software will not give any results unless the data is analyzed to derive actionable insights . It is these insights that help you in understanding the current position of your business, the trends in the market, the challenges and opportunities, etc. Actionable data empowers you to become a better decision-maker and do what’s right for the business. From arranging activities/ jobs in the enterprise , streamlining the workflows, and distributing projects between teams, insights from actionable data help you increase the overall efficiency of the business .
3. Data as a Service- Data Exchange in Marketplaces
Data is now being offered as a service as well. How is that possible?
You must have seen websites embedding Covid-19 data to show the number of cases in a region or the number of deaths, etc. This data is provided by other companies that offer data as a service . This data can be used by enterprises as a part of their business processes.
Since it might lead to data privacy issues and complications, companies are coming with procedures that minimize the data risk of a data breach or attract a lawsuit. Data can be moved from the vendor’s platform to the buyer’s platforms with little or no disturbance and data breach of any kind. Data exchange in marketplaces for analytics and insights is one of the prominent data analytics trends in 2023. It is referred to as DaaS in short.
4. Use of Augmented Analytics
What is augmented analytics? AA is a concept of data analytics that uses AI, machine learning, and natural language processing to automate the analysis of massive data. What is normally handled by a data scientist is now being automated in delivering insights in real-time.
It takes less time for enterprises to process the data and derives insights from it. The result is also more accurate, thus leading to better decisions. From assisting with data preparation to data processing , analytics, and visualization, AI, ML , and NLP help experts explore data and generate in-depth reports and predictions. Data from within the enterprise and outside the enterprise can be combined through augmented analytics.
Actionable Advice for Data-Driven Leaders
Struggling to reap the right kind of insights from your business data? Get expert tips, latest trends, insights, case studies, recommendations and more in your inbox.
5. Cloud Automation and Hybrid Cloud Services
The automation of cloud computing services for public and private clouds is achieved using artificial intelligence and machine learning . AIOps is artificial intelligence for IT operations. This is bringing a change in the way enterprises look at big data and cloud services by offering more data security , scalability, centralized database and governance system, and ownership of data at low cost.
One of the big data predictions for 2023 is the increase in the use of hybrid cloud services. A hybrid cloud is an amalgamation of a public cloud and a private cloud platform.
Public clouds are cost-effective but do not provide high data security. A private cloud is more secure but expensive and not a feasible option for all SMEs . The feasible solution is a combination of both where cost and security are balanced to offer more agility. A hybrid cloud helps optimize the resources and performance of the enterprise.
6. Focus on Edge Intelligence
Gartner and Forrester have predicted that edge computing will become a mainstream process in 2023. Edge computing or edge intelligence is where data analysis and data aggregation are done close to the network. Industries wish to take advantage of the internet of things (IoT) and data transformation services to incorporate edge computing into business systems.
This results in greater flexibility, scalability, and reliability, leading to a better performance of the enterprise. It also reduces latency and increases the processing speed. When combined with cloud computing services, edge intelligence allows employees to work remotely while improving the quality and speed of productivity.
7. Hyperautomation
Another dominant trend in data science in 202 3 is hyper-automation, which began in 2020. Brian Burke, Research Vice President of Gartner, has once said that hyper-automation is inevitable and irreversible, and anything and everything that can be automated should be automated to improve efficiency.
By combining automation with artificial intelligence , machine learning, and smart business processes , you can unlock a higher level of digital transformation in your enterprise. Advanced analytics, business process management, and robotic process automation are considered the core concepts of hyper-automation. The trend is all set to grow in the next few years, with more emphasis on robotic process automation (RPA).
8. Use of Big Data in the Internet of Things (IoT)
Internet of Things (IoT) is a network of physical things embedded with software, sensors, and the latest technology. This allows different devices across the network to connect with each other and exchange information over the internet. By integrating the Internet of Things with machine learning and data analytics , you can increase the flexibility of the system and improve the accuracy of the responses provided by the machine learning algorithm.
While many large-scale enterprises are already using IoT in their business, SMEs are starting to follow the trend and become better equipped to handle data. When this occurs in full swing, it is bound to disrupt the traditional business systems and result in tremendous changes in how business systems and processes are developed and used.
9. Automation of Data Cleaning
For advanced analytics in 2023, having data is not sufficient. We already mentioned in the previous points how big data is of no use if it’s not clean enough for analytics. It also refers to incorrect data, data redundancy, and duplicate data with no structure or format.
This causes the data retrieval process to slow down. That directly leads to the loss of time and money for enterprises. On a large scale, this loss could be counted in millions. Many researchers and enterprises are looking for ways to automate data cleaning or scrubbing to speed up data analytics and gain accurate insights from big data. Artificial intelligence and machine learning will play a major role in data cleaning automation.
10. Increase in Use of Natural Language Processing
Famously known as NLP, it started as a subset of artificial intelligence. It is now considered a part of the business processes used to study data to find patterns and trends. It is said that NLP will be used for the immediate retrieval of information from data repositories in 2023. Natural Language Processing will have access to quality information that will result in quality insights.
Not just that, NLP also provides access to sentiment analysis. This way, you will have a clear picture of what your customers think and feel about your business and your competitors. When you know what your customers and target audience expect, it becomes easier to provide them with the required products/ services and enhance customer satisfaction .
11. Quantum Computing for Faster Analysis
One of the trending research topics in data science is Quantum computing. Google is already working on this, where decisions are not taken by the binary digits 0 and 1. The decisions are made using quantum bits of a processor called Sycamore. This processor is said to solve a problem in just 200 seconds.
However, Quantum computing is very much in its early stages and needs a lot of fine-tuning before it can be adopted by a range of enterprises in different industries. Nevertheless, it has started to make its presence felt and will soon become an integral part of business processes. The aim of using Quantum computing is to integrate data by comparing data sets for faster analysis. It also helps in understanding the relationship between two or more models.
12. Democratizing AI and Data Science
We have already seen how DaaS is becoming famous. The same is now being applied to machine learning models as well. Thanks to the increase in demand for cloud services, AI and ML models are easier to be offered as a part of cloud computing services and tools.
You can contact a data science company in India to use MLaaS (Machine Learning as a Service) for data visualization, NLP, and deep learning . MLaaS would be a perfect tool for predictive analytics. When you invest in DaaS and MLaaS, you don’t need to build an exclusive data science team in your enterprise. The services are provided by offshore companies.
13. Automation of Machine Learning (AutoML)
Automated machine learning can automate various data science processes such as cleaning data, training models, predicting results and insights, interpreting the results, and much more. These tasks are usually performed by data science teams. We’ve mentioned how data cleaning will be automated for faster analytics. The other manual processes will also follow suit when enterprises adopt AutoML in their business. This is yet in the early stages of development.
14. Computer Vision for High Dimensional Data Analytics
Forrester has predicted that more than 1/3rd of the enterprises will depend on artificial intelligence to reduce workplace disruptions. The advent of the covid-19 pandemic has forced organizations to make some drastic changes to their business processes. The remote working facility has become necessary for most businesses. Similarly, automation is being considered a better option than relying on workers and the human touch.
Using computer vision for high-dimensional data analytics is one of the data science trends in 202 3 that helps enterprises detect inconsistencies, perform quality checks, assure safe practices, speed up the processes, and perform more such actions. Especially seen in the manufacturing industry , CV is making it possible to automate production monitoring and quality assurance.
15. Generative AI for Deepfake and Synthetic Data
Remember the Tiktok videos that were supposedly by Tom Cruise? The videos were created using generative AI, where new content is created using existing data. This trend is set to enter other industries and help train the ML algorithms using synthetic data.
Synthetic data is artificially manufactured instead of being taken from real-life events. There is a surge in privacy concerns for using the images of real people to train facial recognition apps. The challenge can be overcome by using synthetic images of people who don’t exist. Generative AI and synthetic data will become a part of more industries and impact how the AI software works.
16. Blockchain in Data Science
While blockchain has become a part of FinTech and healthcare industries, it’s now entering the IT industry. So how does blockchain help with data science?
- The decentralized ledgers make it easier to manage big data.
- The blockchain’s decentralized structure allows data scientists to run analytics directly from their individual devices.
- Given how blockchain already tracks the origin of data, it becomes easier to validate the information.
Data scientists have to structure the information in a centralized manner to make it ready for data analytics. This process is still time-consuming and requires effort from data scientists. Blockchain can solve the issue effectively.
17. Python is Still the Top Programming Language
Many data scientists feel that Python is an integral part of data science and will continue to be. It shouldn’t be surprising that Python will continue to rule the data science and ML world even in 2023. It’s agile, allows collaborations, and simplifies integrations for other programming languages and libraries. Wannabe data scientists will find that mastering the Python programming language will give them better opportunities in the field.
Conclusion
Data science will continue to be in the limelight in the coming years. We will see more such developments and innovations. The demand for data scientists , data analysts, and AI engineers is set to increase. The easiest way to adopt the latest changes in the business is by hiring a data analytics company .
Stay relevant in this competitive market by adopting the data-driven model in your enterprise. Be prepared to tackle the changing trends and make the right decisions to increase returns.
Originally published on Datasciencecentral.com
Kavika is Head of Information Management at DataToBiz. She is responsible for identification, acquisition, distribution & organization of technical oversight.
- Pingback: Pranjal
- Pingback: John
Leave a Reply Cancel reply
You must be logged in to post a comment.
Data Engineering
AI & Machine Learning
By Use Cases
Business Intelligence & Tableau
+91 70099 35623
[email protected], f-429, industrial area, phase 8b, mohali, pb 160059 punjab, india, ©2024 datatobiz r all rights reserved.
- Privacy Policy
Subscribe To Our Newsletter
Get amazing insights and updates on the latest trends in AI, BI and Data Science technologies

99+ Interesting Data Science Research Topics For Students In 2024
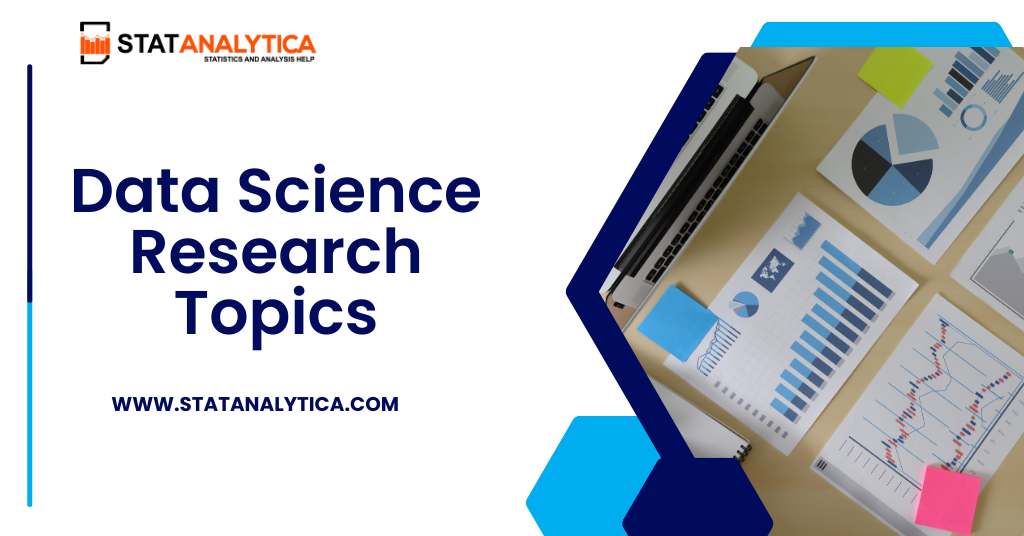
In today’s information-driven world, data science research stands as a pivotal domain shaping our understanding and application of vast data sets. It amalgamates statistics, computer science, and domain knowledge to extract valuable insights from data. Understanding ‘What Is Data Science?’ is fundamental—a field exploring patterns, trends, and solutions embedded within data.
However, the significance of data science research papers in a student’s life cannot be overstated. They foster critical thinking, analytical skills, and a deeper comprehension of the subject matter. To aid students in navigating this realm effectively, this blog dives into essential elements integral to a data science research paper, while also offering a goldmine of 99+ engaging and timely data science research topics for 2024.
Unraveling tips for crafting an impactful research paper and insights on choosing the right topic, this blog is a compass for students exploring data science research topics. Stay tuned to unearth more about ‘data science research topics’ and refine your academic journey.
What Is Data Science?
Table of Contents
Data Science is like a detective for information! It’s all about uncovering secrets and finding valuable stuff in heaps of data. Imagine you have a giant puzzle with tons of pieces scattered around. Data Science helps in sorting these pieces and figuring out the picture they create. It uses tools and skills from math, computer science, and knowledge about different fields to solve real-world problems.
In simpler terms, Data Science is like a chef in a kitchen, blending ingredients to create a perfect dish. Instead of food, it combines data—numbers, words, pictures—to cook up solutions. It helps in understanding patterns, making predictions, and answering tricky questions by exploring data from various sources. In essence, Data Science is the magic that turns data chaos into meaningful insights that can guide decisions and make life better.
Importance Of Data Science Research Paper In Student’s Life
Data Science research papers are like treasure maps for students! They’re super important because they teach students how to explore and understand the world of data. Writing these papers helps students develop problem-solving skills, think critically, and become better at analyzing information. It’s like a fun adventure where they learn how to dig into data and uncover valuable insights that can solve real-world problems.
- Enhances critical thinking: Research papers challenge students to analyze and interpret data critically, honing their thinking skills.
- Fosters analytical abilities: Students learn to sift through vast amounts of data, extracting meaningful patterns and information.
- Encourages exploration: Engaging in research encourages students to explore diverse data sources, broadening their knowledge horizon.
- Develops communication skills: Writing research papers hones students’ ability to articulate complex findings and ideas clearly.
- Prepares for real-world challenges: Through research, students learn to apply theoretical knowledge to practical problems, preparing them for future endeavors.
Elements That Must Be Present In Data Science Research Paper
Here are some elements that must be present in data science research paper:
1. Clear Objective
A data science research paper should start with a clear goal, stating what the study aims to investigate or achieve. This objective guides the entire paper, helping readers understand the purpose and direction of the research.
2. Detailed Methodology
Explaining how the research was conducted is crucial. The paper should outline the tools, techniques, and steps used to collect, analyze, and interpret data. This section allows others to replicate the study and validate its findings.
3. Accurate Data Presentation
Presenting data in an organized and understandable manner is key. Graphs, charts, and tables should be used to illustrate findings clearly, aiding readers’ comprehension of the results.
4. Thorough Analysis and Interpretation
Simply presenting data isn’t enough; the paper should delve into a deep analysis, explaining the meaning behind the numbers. Interpretation helps draw conclusions and insights from the data.
5. Conclusive Findings and Recommendations
A strong conclusion summarizes the key findings of the research. It should also offer suggestions or recommendations based on the study’s outcomes, indicating potential avenues for future exploration.
Here are some interesting data science research topics for students in 2024:
Natural Language Processing (NLP)
- Multi-modal Contextual Understanding: Integrating text, images, and audio to enhance NLP models’ comprehension abilities.
- Cross-lingual Transfer Learning: Investigating methods to transfer knowledge from one language to another for improved translation and understanding.
- Emotion Detection in Text: Developing models to accurately detect and interpret emotions conveyed in textual content.
- Sarcasm Detection in Social Media: Building algorithms that can identify and understand sarcastic remarks in online conversations.
- Language Generation for Code: Generating code snippets and scripts from natural language descriptions using NLP techniques.
- Bias Mitigation in Language Models: Developing strategies to mitigate biases present in large language models and ensure fairness in generated content.
- Dialogue Systems for Personalized Assistance: Creating intelligent conversational agents that provide personalized assistance based on user preferences and history.
- Summarization of Legal Documents: Developing NLP models capable of summarizing lengthy legal documents for quick understanding and analysis.
- Understanding Contextual Nuances in Sentiment Analysis: Enhancing sentiment analysis models to better comprehend contextual nuances and sarcasm in text.
- Hate Speech Detection and Moderation: Building systems to detect and moderate hate speech and offensive language in online content.
Computer Vision
- Weakly Supervised Object Detection: Exploring methods to train object detection models with limited annotated data.
- Video Action Recognition in Uncontrolled Environments: Developing models that can recognize human actions in videos captured in uncontrolled settings.
- Image Generation and Translation: Investigating techniques to generate realistic images from textual descriptions and translate images across different domains.
- Scene Understanding in Autonomous Systems: Enhancing computer vision algorithms for better scene understanding in autonomous vehicles and robotics.
- Fine-grained Visual Classification: Improving models to classify objects at a more granular level, distinguishing subtle differences within similar categories.
- Visual Question Answering (VQA): Creating systems capable of answering questions based on visual input, requiring reasoning and comprehension abilities.
- Medical Image Analysis for Disease Diagnosis: Developing computer vision models for accurate and early diagnosis of diseases from medical images.
- Action Localization in Videos: Building models to precisely localize and recognize specific actions within video sequences.
- Image Captioning with Contextual Understanding: Generating captions for images considering the context and relationships between objects.
- Human Pose Estimation in Real-time: Improving algorithms for real-time estimation of human poses in videos for applications like motion analysis and gaming.
Machine Learning Algorithms
- Self-supervised Learning Techniques: Exploring novel methods for training machine learning models without explicit supervision.
- Continual Learning in Dynamic Environments: Investigating algorithms that can continuously learn and adapt to changing data distributions and tasks.
- Explainable AI for Model Interpretability: Developing techniques to explain the decisions and predictions made by complex machine learning models.
- Transfer Learning for Small Datasets: Techniques to effectively transfer knowledge from large datasets to small or domain-specific datasets.
- Adaptive Learning Rate Optimization: Enhancing optimization algorithms to dynamically adjust learning rates based on data characteristics.
- Robustness to Adversarial Attacks: Building models resistant to adversarial attacks, ensuring stability and security in machine learning applications.
- Active Learning Strategies: Investigating methods to select and label the most informative data points for model training to minimize labeling efforts.
- Privacy-preserving Machine Learning: Developing algorithms that can train models on sensitive data while preserving individual privacy.
- Fairness-aware Machine Learning: Techniques to ensure fairness and mitigate biases in machine learning models across different demographics.
- Multi-task Learning for Jointly Learning Tasks: Exploring approaches to jointly train models on multiple related tasks to improve overall performance.
Deep Learning
- Graph Neural Networks for Representation Learning: Using deep learning techniques to learn representations from graph-structured data.
- Transformer Models for Image Processing: Adapting transformer architectures for image-related tasks, such as image classification and generation.
- Few-shot Learning Strategies: Investigating methods to enable deep learning models to learn from a few examples in new categories.
- Memory-Augmented Neural Networks: Enhancing neural networks with external memory for improved learning and reasoning capabilities.
- Neural Architecture Search (NAS): Automating the design of neural network architectures for specific tasks or constraints.
- Meta-learning for Fast Adaptation: Developing models capable of quickly adapting to new tasks or domains with minimal data.
- Deep Reinforcement Learning for Robotics: Utilizing deep RL techniques for training robots to perform complex tasks in real-world environments.
- Generative Adversarial Networks (GANs) for Data Augmentation: Using GANs to generate synthetic data for enhancing training datasets.
- Variational Autoencoders for Unsupervised Learning: Exploring VAEs for learning latent representations of data without explicit supervision.
- Lifelong Learning in Deep Networks: Strategies to enable deep networks to continually learn from new data while retaining past knowledge.
Big Data Analytics
- Streaming Data Analysis for Real-time Insights: Techniques to analyze and derive insights from continuous streams of data in real-time.
- Scalable Algorithms for Massive Graphs: Developing algorithms that can efficiently process and analyze large-scale graph-structured data.
- Anomaly Detection in High-dimensional Data: Detecting anomalies and outliers in high-dimensional datasets using advanced statistical methods and machine learning.
- Personalization and Recommendation Systems: Enhancing recommendation algorithms for providing personalized and relevant suggestions to users.
- Data Quality Assessment and Improvement: Methods to assess, clean, and enhance the quality of big data to improve analysis and decision-making.
- Time-to-Event Prediction in Time-series Data: Predicting future events or occurrences based on historical time-series data.
- Geospatial Data Analysis and Visualization: Analyzing and visualizing large-scale geospatial data for various applications such as urban planning, disaster management, etc.
- Privacy-preserving Big Data Analytics: Ensuring data privacy while performing analytics on large-scale datasets in distributed environments.
- Graph-based Deep Learning for Network Analysis: Leveraging deep learning techniques for network analysis and community detection in large-scale networks.
- Dynamic Data Compression Techniques: Developing methods to compress and store large volumes of data efficiently without losing critical information.
Healthcare Analytics
- Predictive Modeling for Patient Outcomes: Using machine learning to predict patient outcomes and personalize treatments based on individual health data.
- Clinical Natural Language Processing for Electronic Health Records (EHR): Extracting valuable information from unstructured EHR data to improve healthcare delivery.
- Wearable Devices and Health Monitoring: Analyzing data from wearable devices to monitor and predict health conditions in real-time.
- Drug Discovery and Development using AI: Utilizing machine learning and AI for efficient drug discovery and development processes.
- Predictive Maintenance in Healthcare Equipment: Developing models to predict and prevent equipment failures in healthcare settings.
- Disease Clustering and Stratification: Grouping diseases based on similarities in symptoms, genetic markers, and response to treatments.
- Telemedicine Analytics: Analyzing data from telemedicine platforms to improve remote healthcare delivery and patient outcomes.
- AI-driven Radiomics for Medical Imaging: Using AI to extract quantitative features from medical images for improved diagnosis and treatment planning.
- Healthcare Resource Optimization: Optimizing resource allocation in healthcare facilities using predictive analytics and operational research techniques.
- Patient Journey Analysis and Personalized Care Pathways: Analyzing patient trajectories to create personalized care pathways and improve healthcare outcomes.
Time Series Analysis
- Forecasting Volatility in Financial Markets: Predicting and modeling volatility in stock prices and financial markets using time series analysis.
- Dynamic Time Warping for Similarity Analysis: Utilizing DTW to measure similarities between time series data, especially in scenarios with temporal distortions.
- Seasonal Pattern Detection and Analysis: Identifying and modeling seasonal patterns in time series data for better forecasting.
- Time Series Anomaly Detection in Industrial IoT: Detecting anomalies in industrial sensor data streams to prevent equipment failures and improve maintenance.
- Multivariate Time Series Forecasting: Developing models to forecast multiple related time series simultaneously, considering interdependencies.
- Non-linear Time Series Analysis Techniques: Exploring non-linear models and methods for analyzing complex time series data.
- Time Series Data Compression for Efficient Storage: Techniques to compress and store time series data efficiently without losing crucial information.
- Event Detection and Classification in Time Series: Identifying and categorizing specific events or patterns within time series data.
- Time Series Forecasting with Uncertainty Estimation: Incorporating uncertainty estimation into time series forecasting models for better decision-making.
- Dynamic Time Series Graphs for Network Analysis: Representing and analyzing dynamic relationships between entities over time using time series graphs.
Reinforcement Learning
- Multi-agent Reinforcement Learning for Collaboration: Developing strategies for multiple agents to collaborate and solve complex tasks together.
- Hierarchical Reinforcement Learning: Utilizing hierarchical structures in RL for solving tasks with varying levels of abstraction and complexity.
- Model-based Reinforcement Learning for Sample Efficiency: Incorporating learned models into RL for efficient exploration and planning.
- Robotic Manipulation with Reinforcement Learning: Training robots to perform dexterous manipulation tasks using RL algorithms.
- Safe Reinforcement Learning: Ensuring that RL agents operate safely and ethically in real-world environments, minimizing risks.
- Transfer Learning in Reinforcement Learning: Transferring knowledge from previously learned tasks to expedite learning in new but related tasks.
- Curriculum Learning Strategies in RL: Designing learning curricula to gradually expose RL agents to increasingly complex tasks.
- Continuous Control in Reinforcement Learning: Exploring techniques for continuous control tasks that require precise actions in a continuous action space.
- Reinforcement Learning for Adaptive Personalization: Utilizing RL to personalize experiences or recommendations for individuals in dynamic environments.
- Reinforcement Learning in Healthcare Decision-making: Using RL to optimize treatment strategies and decision-making in healthcare settings.
Data Mining
- Graph Mining for Social Network Analysis: Extracting valuable insights from social network data using graph mining techniques.
- Sequential Pattern Mining for Market Basket Analysis: Discovering sequential patterns in customer purchase behaviors for market basket analysis.
- Clustering Algorithms for High-dimensional Data: Developing clustering techniques suitable for high-dimensional datasets.
- Frequent Pattern Mining in Healthcare Datasets: Identifying frequent patterns in healthcare data for actionable insights and decision support.
- Outlier Detection and Fraud Analysis: Detecting anomalies and fraudulent activities in various domains using data mining approaches.
- Opinion Mining and Sentiment Analysis in Reviews: Analyzing opinions and sentiments expressed in product or service reviews to derive insights.
- Data Mining for Personalized Learning: Mining educational data to personalize learning experiences and adapt teaching methods.
- Association Rule Mining in Internet of Things (IoT) Data: Discovering meaningful associations and patterns in IoT-generated data streams.
- Multi-modal Data Fusion for Comprehensive Analysis: Integrating information from multiple data modalities for a holistic understanding and analysis.
- Data Mining for Energy Consumption Patterns: Analyzing energy usage data to identify patterns and optimize energy consumption in various sectors.
Ethical AI and Bias Mitigation
- Fairness Metrics and Evaluation in AI Systems: Developing metrics and evaluation frameworks to assess the fairness of AI models.
- Bias Detection and Mitigation in Facial Recognition: Addressing biases present in facial recognition systems to ensure equitable performance across demographics.
- Algorithmic Transparency and Explainability: Designing methods to make AI algorithms more transparent and understandable to stakeholders.
- Fair Representation Learning in Unbalanced Datasets: Learning fair representations from imbalanced data to reduce biases in downstream tasks.
- Fairness-aware Recommender Systems: Ensuring fairness and reducing biases in recommendation algorithms across diverse user groups.
- Ethical Considerations in AI for Criminal Justice: Investigating the ethical implications of AI-based decision-making in criminal justice systems.
- Debiasing Techniques in Natural Language Processing: Developing methods to mitigate biases in language models and text generation.
- Diversity and Fairness in Hiring Algorithms: Ensuring diversity and fairness in AI-based hiring systems to minimize biases in candidate selection.
- Ethical AI Governance and Policy: Examining the role of governance and policy frameworks in regulating the development and deployment of AI systems.
- AI Accountability and Responsibility: Addressing ethical dilemmas and defining responsibilities concerning AI system behaviors and decision-making processes.
Tips For Writing An Effective Data Science Research Paper
Here are some tips for writing an effective data science research paper:
Tip 1: Thorough Planning and Organization
Begin by planning your research paper carefully. Outline the sections and information you’ll include, ensuring a logical flow from introduction to conclusion. This organized approach makes writing easier and helps maintain coherence in your paper.
Tip 2: Clarity in Writing Style
Use clear and simple language to communicate your ideas. Avoid jargon or complex terms that might confuse readers. Write in a way that is easy to understand, ensuring your message is effectively conveyed.
Tip 3: Precise and Relevant Information
Include only information directly related to your research topic. Ensure the data, explanations, and examples you use are precise and contribute directly to supporting your arguments or findings.
Tip 4: Effective Data Visualization
Utilize graphs, charts, and tables to present your data visually. Visual aids make complex information easier to comprehend and can enhance the overall presentation of your research findings.
Tip 5: Review and Revise
Before submitting your paper, review it thoroughly. Check for any errors in grammar, spelling, or formatting. Revise sections if necessary to ensure clarity and coherence in your writing. Asking someone else to review it can also provide valuable feedback.
- Hospitality Management Research Topics
Things To Remember While Choosing The Data Science Research Topic
When selecting a data science research topic, consider your interests and its relevance to the field. Ensure the topic is neither too broad nor too narrow, striking a balance that allows for in-depth exploration while staying manageable.
- Relevance and Significance: Choose a topic that aligns with current trends or addresses a significant issue in the field of data science.
- Feasibility : Ensure the topic is researchable within the resources and time available. It should be practical and manageable for the scope of your study.
- Your Interest and Passion: Select a topic that genuinely interests you. Your enthusiasm will drive your motivation and engagement throughout the research process.
- Availability of Data: Check if there’s sufficient data available for analysis related to your chosen topic. Accessible and reliable data sources are vital for thorough research.
- Potential Contribution: Consider how your chosen topic can contribute to existing knowledge or fill a gap in the field. Aim for a topic that adds value and insights to the data science domain.
In wrapping up our exploration of data science research topics, we’ve uncovered a world of importance and guidance for students. From defining data science to understanding its impact on student life, identifying essential elements in research papers, offering a multitude of intriguing topics for 2024, to providing tips for crafting effective papers—the journey has been insightful.
Remembering the significance of topic selection and the key components of a well-structured paper, this voyage emphasizes how data science opens doors to endless opportunities. It’s not just a subject; it’s the compass guiding tomorrow’s discoveries and innovations in our digital landscape.
Related Posts
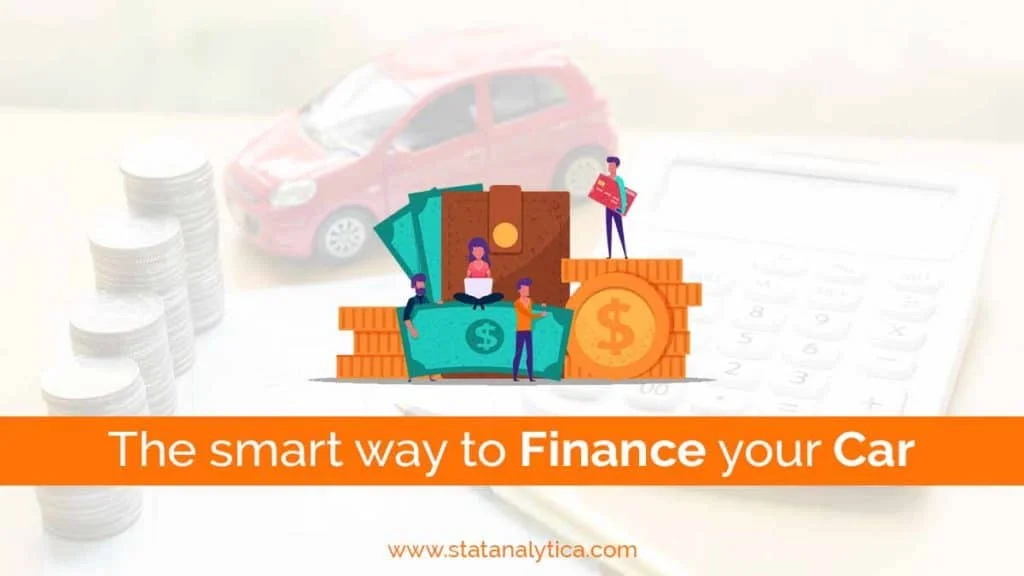
Step by Step Guide on The Best Way to Finance Car
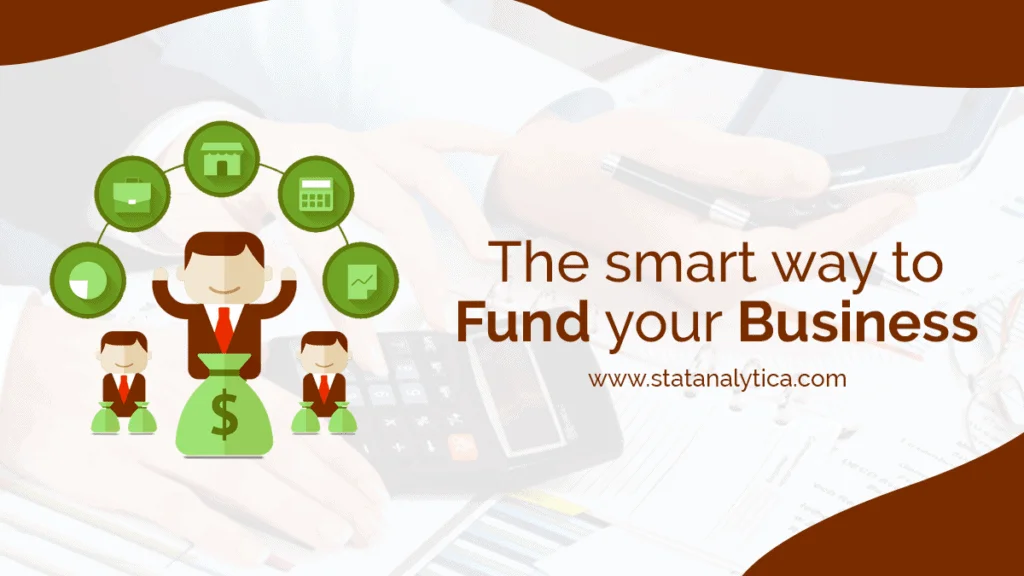
The Best Way on How to Get Fund For Business to Grow it Efficiently
DiscoverDataScience.org
Data Science Trends 2023
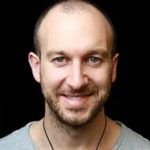
Created by aasif.faizal
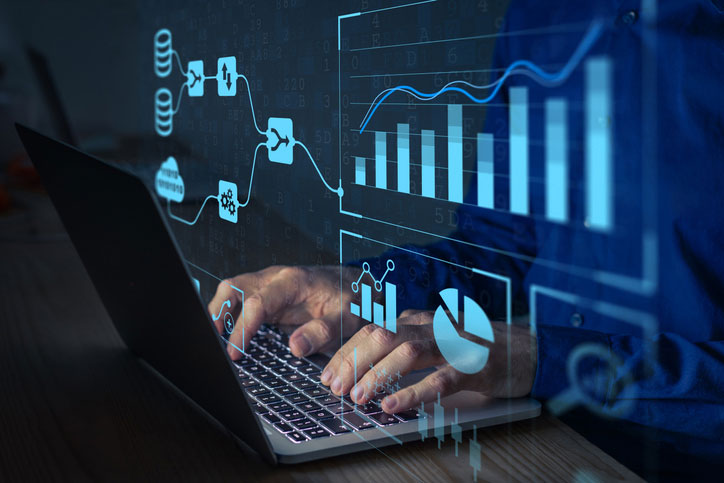
Indeed, based on the exponential rate at which technology is currently advancing, each year is likely to bring even more change than the previous. 2023 is likely to be no different, with several pieces of significant data science news that any big data professional should take note of.
This article will survey some of the biggest pieces of data science news headed our way in the year to come.
Included in this Article:
- Key Themes in Data Science
Data Analytics
Artificial intelligence, data science jobs.
- Cloud Based Operations
Data Visualization Advancements
- Deepfake Video and Audio
Python Growth
Cybersecurity.
- Additional Resources
2023 Trends in Data Science: An Overview of Key Themes
If the 21st century has shown us anything, it’s that big data is only going to continue to get… well, bigger. In fact, even the name “big data” makes reference to the ever-expanding nature of information technology that allows for more and more valuable data to be captured and interpreted. Over the last thirty years especially, we’ve witnessed the way that these insights can transform how entire industries operate, from enhancing marketing research and development to identifying areas for improvement in a company’s production model.
But if big data has been trending for so long already, what developments is it likely to undergo in the year to come? Experts have identified a few of the following as key concerns for big data professionals in 2023:
- Advancing tools. Developments in A.I. and machine learning are certain to continue to make leaps in the coming year, which in turn will greatly impact the instruments data scientists have at their disposal to perform their research and analysis. This has already significantly impacted most branches of data science, all of which employ machine learning tools to function. In part, this is because of the next factor on the list…
- Higher volumes of data. As a direct result of the advances in A.I. and M.L. listed above, businesses are receiving a significantly larger amount of data of all sorts. Some of this expands upon previously existing datasets while other advances have brought in new forms of data altogether. In both cases, adaptations are required to allow businesses to make use of this data as well as finding safe and affordable ways to store it. As you will see, this will be critical in businesses across industries.
- Security threats. For better or worse, these are a perennial trend in the world of data science, with new forms of cyberattack emerging constantly. For those working in the field of cybersecurity , this means strategic planning and rigorous research to identify data breaches as well as preventing new ones. For everyone else working in big data, it means that vigilance is always imperative, as certain types of cyberattacks like phishing and other forms of data related fraud involve deceptive tactics that could target them.
Read on to discover our list of the specific data science trends of 2023 for insight into where we are and where we’re headed.
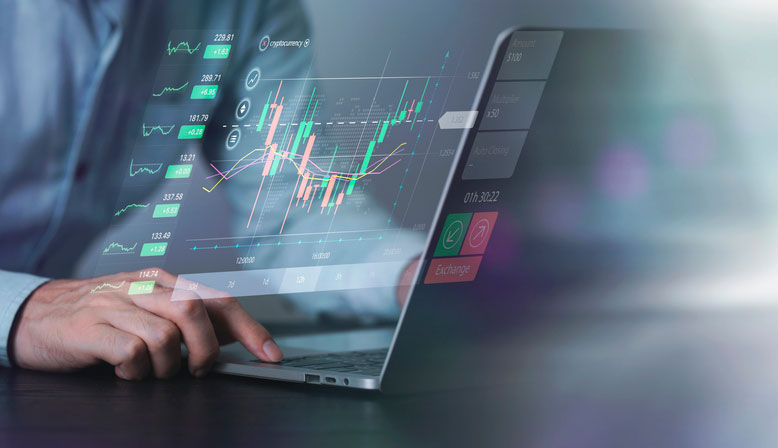
Analytics is one of the key fields of data science likely to undergo major transformations in the year ahead. Below are a few of the new data analytics trends to look out for.
Real-Time Analytics
One of the top rising data trends of 2023 is real-time analytics. Data capturing tools have improved in speed and scope, meaning we have access to an even greater wealth of real-time information that can illuminate our understanding of all sorts of processes. Companies are only just at the beginning of learning how these data sets can be used to guide important business decisions. If you are a data analyst or work in a related field, it will be of great use to follow any news and updates about real-time analytics that arise over the course of this year.
Mobile Analytics
Though it might seem like it couldn’t possibly continue to grow, the presence of mobile devices is ever-expanding in communities all over the globe. This means that there is significantly more mobile data to be captured. For certain industries, mobile analytics are the core of their strategy, providing the most revealing and useful information to guide marketing and advertising tactics. This includes registering user engagement, customer satisfaction, monitoring in-app traffic, and identifying security threats.
Recent years have made clear that artificial intelligence has made monumental leaps that are likely to change not only the way we do business but the way we live. Outside of data science, this has already risen to popular consciousness: A.I. programs that augment or generate images and texts have already begun to trend even among those who don’t know much about information technology.
Below are a few of the ways that artificial intelligence is likely to continue to trend in 2023.
Augmented Analytics
Within the field of big data, one of the main ways artificial intelligence is being employed is to help collect the ever-increasing amounts of data being captured and stored by new devices. This is in step with the global population’s increased dependence on technology to go about their everyday lives.
In order to keep up with the huge amounts of information that are continuously coming in, machine learning and AI tools are improving their processing functions to expedite this process, preparing and meaningfully analyzing a tremendous amount of new data. This is known as Augmented Analytics, and it can help businesses vastly.
Augmented analytics also fits into the category of Business Intelligence. The field is expected to grow at a breathtaking speed in the years to come.
Deep Learning
When people think of A.I., what they imagine is most comparable to deep learning, the branch of artificial intelligence devoted to training computers to behave like humans. These respond to neural network architectures and large sets of data that so far have been shown to be demonstrably effective. Indeed, in 2023 data scientists and casual observers alike are likely to take note of the increasing ability of computers to mirror human interactions.
For businesses, deep learning can be used to anticipate human behaviors, which in turn can impact areas including marketing and overall business strategies. As this technology grows more sophisticated, it will only continue to transform our approach to customer service and impact how businesses understand their customers’ needs.
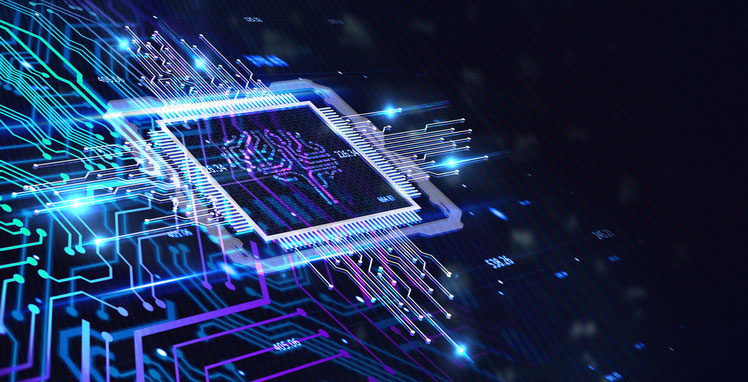
For those who are considering breaking into the field of big data, here’s some exciting data science news: according to the Bureau of Labor Statistics, careers in big data are likely to be trending for years to come. This translates to more job openings and higher overall salaries, as data scientists are becoming ever-more valued by employers in all different sorts of industries.
Indeed, the numbers for data scientists are impressive by any metric: the Bureau of Labor Statistics reports an estimated job growth rate of an astonishing 36% by 2031, which is significantly higher than estimates for other professions. Over the years, different regions across the United States have become hubs for the tech industry, meaning an especially high concentration of data science job opportunities are available. Statistics from May 2021 reveal that the states with the highest employment levels for data scientists are California, New York, Texas, North Carolina, and Illinois.
In step with job growth projections, average data science salaries are exceptionally high relative to other professions. The Bureau of Labor Statistics reports a median annual salary of $100,910, with those in the highest-earning industry of scientific research and development earning a median annual salary of $102,750.
Businesses And Enterprises Moving To Cloud-Based Operations
In step with the increased amounts of data being captured by advanced tools, there is a growing need for enhanced storage solutions. Cloud computing is quickly being embraced as the solution to this problem, offering vastly improved storage opportunities that can keep up pace with the changing state of data capturing. In fact, most are beginning to consider it the very environment in which data-based business will be stored moving forward, though other storage options are technically available.
Of cloud-based computing service options available, the current game-changer is hybrid cloud, which makes use of machine learning and A.I. technology to offer a centralized database that is more cost-efficient than private cloud solutions (which can be all but completely out of reach in terms of cost for smaller businesses) and more secure than public cloud options. The hybrid cloud option is likely to become even more popular in 2023.
Cloud computing impacts the field of big tech in an enormous variety of ways, touching the fields of data science, customer interactions, Artificial Intelligence, transactional systems, DevOps, and more. If you are interested in or employed in any of those fields, staying abreast of developments in cloud-based technology will be crucial to managing your database efficiently and preserving it for the long term.
Several advancements in the field of data visualization are likely to be notable in 2023. Below are a few of the most important pieces of data science news to follow.
Data Visualization Videos
Those who are interested in pursuing careers in data visualization will be interested to learn about the trend toward using video for data visualization. Opting to use video instead of photos or text has been shown to significantly increase not only viewers’ engagement while watching but also increase their levels of fact retention in the days and even weeks after reviewing the data.
The central goal of data visualization is to help translate highly inaccessible information to be not comprehensible for business leaders who will make key decisions based on their findings. Because of this, sharing data sets through an entirely new medium that has been proven to be effective could be game changing for the field, expanding our capacity to learn at the same time that we expand our access to data.
Mobile-Optimized Visualization
Those who work in the arena of data visualization must be mindful not only of not only the medium in which they are working but the interface on which it will be seen. For those who provide data visualizations for remote clients, it’s important to remember that they may often review data visualizations on their mobile devices, meaning your visualization methods must be optimized to suit phones and tablets in addition to computers. Though it may sound simple, ease of access is a crucial factor in establishing long-term relationships with your clients, which means it’s imperative to confirm that your visualization products are reaching them quickly and straightforwardly. This is another data science trend to look out for in 2023.
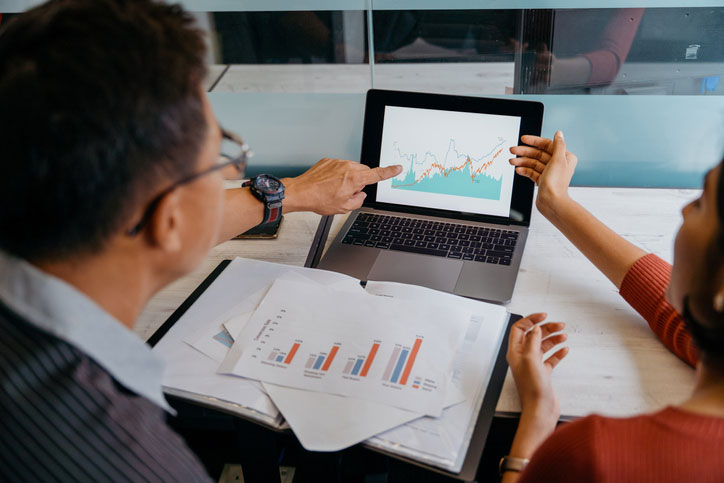
Data as a Service (DaaS)
Having already begun to blossom, the field of DaaS is likely to continue to expand. For those not familiar with the term, Data as a Service is the industry in which organizations that have worked with data for decades share their expertise as well as their intellectual property with clients. This is because they possess uncommon insight into the workings of data that many data scientists seek to cultivate.
Because of the data industry’s ever-growing boom, Data as a Service is all but guaranteed to become an even more widespread industry, offering a great number of job opportunities to those who wish to specialize in the field. This is yet another piece of data science news to stay on the lookout for, as it’s likely to become a crucial part of data management.
Deepfake Video And Audio
Deepfake technology is another example of a tech-world development that has captured attention far outside of the field of big data. This is technology that can vividly create audio or video content by manipulating existing documents. Already, convincing video and audio clips have surfaced featuring public figures saying or doing things that they did not actually do, with highly destructive consequences. Indeed, the implications of this are vast, posing threats not only to the reputations of individuals but also causing destructive political misunderstandings.
Beyond these public examples of deepfake technology being weaponized, businesses in particular should be on the lookout for deepfake scams. Amazing though it may seem, the speech patterns and voices of individuals can be learned and mimicked by machines and used in automated cyberattacks. One famous example of this was in 2019, when a U.K.-based energy company was scammed out of close to a quarter million euros by a fraudulent phone call made using deepfake software to imitate the voice of a top-ranking executive.
Unfortunately, concern about deepfake technology will only continue to grow as machines continue to improve, becoming more convincing in what they are able to represent, quicker in their execution, and accurate in their responsiveness when interacting with real individuals. For those interested in the field of cybersecurity , this is an important new type of cyberattack to consider.
Python has long been one of the leading programming languages in data science, and in recent years has become the standard programming language for those in the field of data analysis. This is only likely to increase in the coming year, thanks to its relative ease of use (it is many beginners’ first programming language), its large number of data science libraries and machine learning libraries, and its availability for use in designing blockchain applications. Because of this, experts suggest that we are likely to see Python become the #1 programming language overall for data scientists , outpacing the other leading programming languages (javascript, java, and r).
If you are interested in pursuing a career in the data sciences, the message here is clear: if you have a choice of which programming language to learn first, Python is your strongest choice. Because of its rising popularity, it is possible that your degree program will also make teaching Python a priority for new students.
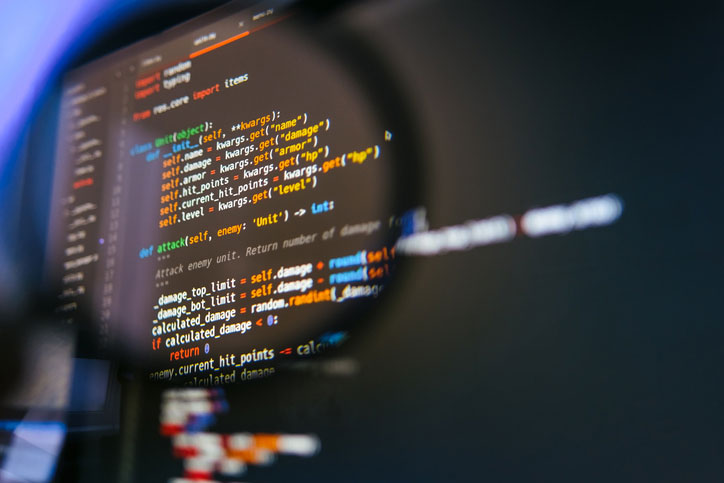
Below are a few of the key issues in cybersecurity that data scientists will face in the year ahead.
Adversarial Machine Learning Combating
Among the key targets of recent cyberattacks have been machine learning algorithms – the same system that has been radicalizing the world of big data in a whole variety of ways mentioned above. To prevent this, cybersecurity experts are working overtime to study adversarial machine learning to understand how these attacks work and what they can do to help prevent them in the future.
Indeed, there is reason to fear that these systems are under threat. A recent study by the Institute of Electrical and Electronic Engineers (IEEE) speaks in no uncertain terms, stating, “industry practitioners are not equipped with tactical and strategic tools to protect, detect and respond to attacks on their Machine Learning (M.L.) systems.” They argue that this is because data science research has so far been out of step with the rapidly growing capacities of M.L., which, being so instrumental to so many big data functions, is a major target for cyberattacks. Their study reveals that these systems themselves are not just attractive to cyberattackers but highly vulnerable, as they are insufficiently understood by data scientists.
For those interested in pursuing careers in cybersecurity , advances in adversarial machine learning is a piece of data science news to follow in 2023, as it is likely to make a lasting impact on many parts of the cybersecurity profession.
Consumer Data Protection
Security scandals have been a huge part of data science news over the years, in particular the Cambridge Analytica scandal , which exposed the illegal harvesting of private data accessed through individuals’ Facebook accounts to inform political campaigning. Indeed, data breaches of this nature are a huge source of anxiety and outrage among the public. This expands the job of cybersecurity experts whose efforts will not only help protect the companies they work for but will also contribute to the overall data safety of the public.
While cybersecurity experts will help figure out best practices for creating security programs as well as strategies to prevent increasingly sophisticated cyberattacks, there will also be significant efforts made in the realm of data science law to curb organizations’ and individuals’ ease of accessing data that is meant to be private.
Additional Resources for a Career in Data Science
If reading our Data Science Trends 2023 list is making you excited about the many developments in big data that are already transforming our world, you may be a perfect candidate to pursue a data science degree. As the article above may have made clear, there is a huge variety of career paths available in the world of data science, and choosing one early will help you gain the education and specialized skills you need to build a thriving career.
To learn more about the many career paths you can take in the world of big data, take a look at our comprehensive guide here .
One of the most popular specializations for data scientists is the field of data analytics, which itself can lead to a huge number of more specific focus areas. To learn more about the world of analytics and discover if it may be the right path for you, visit our guide to a career in analytics here .
After determining your area of focus, the next step is to find the data science program that is right for you. Data scientists can hold a huge variety of degrees and certifications, though the most common route to a high-earning data science career is through a master’s degree program. To learn more about educational opportunities and find the one that is right for you, visit our data science program guide here .
Finally, if you have great abilities with numbers but aren’t certain which industry is the right one to plot your career, we can help you survey the many options available to you. Our guide to exploring a career with numbers will give you the lay of the land so you can make a decision that will make the most of your interests and skills.
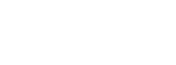
- Related Programs

data science Recently Published Documents
Total documents.
- Latest Documents
- Most Cited Documents
- Contributed Authors
- Related Sources
- Related Keywords
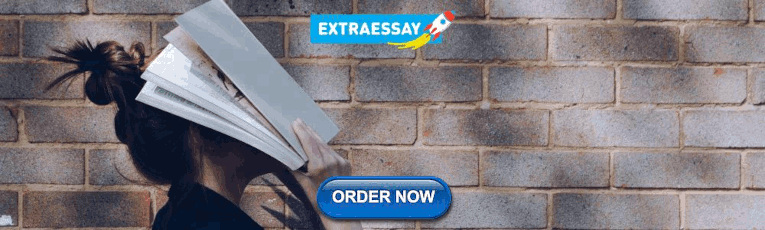
Assessing the effects of fuel energy consumption, foreign direct investment and GDP on CO2 emission: New data science evidence from Europe & Central Asia
Documentation matters: human-centered ai system to assist data science code documentation in computational notebooks.
Computational notebooks allow data scientists to express their ideas through a combination of code and documentation. However, data scientists often pay attention only to the code, and neglect creating or updating their documentation during quick iterations. Inspired by human documentation practices learned from 80 highly-voted Kaggle notebooks, we design and implement Themisto, an automated documentation generation system to explore how human-centered AI systems can support human data scientists in the machine learning code documentation scenario. Themisto facilitates the creation of documentation via three approaches: a deep-learning-based approach to generate documentation for source code, a query-based approach to retrieve online API documentation for source code, and a user prompt approach to nudge users to write documentation. We evaluated Themisto in a within-subjects experiment with 24 data science practitioners, and found that automated documentation generation techniques reduced the time for writing documentation, reminded participants to document code they would have ignored, and improved participants’ satisfaction with their computational notebook.
Data science in the business environment: Insight management for an Executive MBA
Adventures in financial data science, gecoagent: a conversational agent for empowering genomic data extraction and analysis.
With the availability of reliable and low-cost DNA sequencing, human genomics is relevant to a growing number of end-users, including biologists and clinicians. Typical interactions require applying comparative data analysis to huge repositories of genomic information for building new knowledge, taking advantage of the latest findings in applied genomics for healthcare. Powerful technology for data extraction and analysis is available, but broad use of the technology is hampered by the complexity of accessing such methods and tools. This work presents GeCoAgent, a big-data service for clinicians and biologists. GeCoAgent uses a dialogic interface, animated by a chatbot, for supporting the end-users’ interaction with computational tools accompanied by multi-modal support. While the dialogue progresses, the user is accompanied in extracting the relevant data from repositories and then performing data analysis, which often requires the use of statistical methods or machine learning. Results are returned using simple representations (spreadsheets and graphics), while at the end of a session the dialogue is summarized in textual format. The innovation presented in this article is concerned with not only the delivery of a new tool but also our novel approach to conversational technologies, potentially extensible to other healthcare domains or to general data science.
Differentially Private Medical Texts Generation Using Generative Neural Networks
Technological advancements in data science have offered us affordable storage and efficient algorithms to query a large volume of data. Our health records are a significant part of this data, which is pivotal for healthcare providers and can be utilized in our well-being. The clinical note in electronic health records is one such category that collects a patient’s complete medical information during different timesteps of patient care available in the form of free-texts. Thus, these unstructured textual notes contain events from a patient’s admission to discharge, which can prove to be significant for future medical decisions. However, since these texts also contain sensitive information about the patient and the attending medical professionals, such notes cannot be shared publicly. This privacy issue has thwarted timely discoveries on this plethora of untapped information. Therefore, in this work, we intend to generate synthetic medical texts from a private or sanitized (de-identified) clinical text corpus and analyze their utility rigorously in different metrics and levels. Experimental results promote the applicability of our generated data as it achieves more than 80\% accuracy in different pragmatic classification problems and matches (or outperforms) the original text data.
Impact on Stock Market across Covid-19 Outbreak
Abstract: This paper analysis the impact of pandemic over the global stock exchange. The stock listing values are determined by variety of factors including the seasonal changes, catastrophic calamities, pandemic, fiscal year change and many more. This paper significantly provides analysis on the variation of listing price over the world-wide outbreak of novel corona virus. The key reason to imply upon this outbreak was to provide notion on underlying regulation of stock exchanges. Daily closing prices of the stock indices from January 2017 to January 2022 has been utilized for the analysis. The predominant feature of the research is to analyse the fact that does global economy downfall impacts the financial stock exchange. Keywords: Stock Exchange, Matplotlib, Streamlit, Data Science, Web scrapping.
Information Resilience: the nexus of responsible and agile approaches to information use
AbstractThe appetite for effective use of information assets has been steadily rising in both public and private sector organisations. However, whether the information is used for social good or commercial gain, there is a growing recognition of the complex socio-technical challenges associated with balancing the diverse demands of regulatory compliance and data privacy, social expectations and ethical use, business process agility and value creation, and scarcity of data science talent. In this vision paper, we present a series of case studies that highlight these interconnected challenges, across a range of application areas. We use the insights from the case studies to introduce Information Resilience, as a scaffold within which the competing requirements of responsible and agile approaches to information use can be positioned. The aim of this paper is to develop and present a manifesto for Information Resilience that can serve as a reference for future research and development in relevant areas of responsible data management.
qEEG Analysis in the Diagnosis of Alzheimers Disease; a Comparison of Functional Connectivity and Spectral Analysis
Alzheimers disease (AD) is a brain disorder that is mainly characterized by a progressive degeneration of neurons in the brain, causing a decline in cognitive abilities and difficulties in engaging in day-to-day activities. This study compares an FFT-based spectral analysis against a functional connectivity analysis based on phase synchronization, for finding known differences between AD patients and Healthy Control (HC) subjects. Both of these quantitative analysis methods were applied on a dataset comprising bipolar EEG montages values from 20 diagnosed AD patients and 20 age-matched HC subjects. Additionally, an attempt was made to localize the identified AD-induced brain activity effects in AD patients. The obtained results showed the advantage of the functional connectivity analysis method compared to a simple spectral analysis. Specifically, while spectral analysis could not find any significant differences between the AD and HC groups, the functional connectivity analysis showed statistically higher synchronization levels in the AD group in the lower frequency bands (delta and theta), suggesting that the AD patients brains are in a phase-locked state. Further comparison of functional connectivity between the homotopic regions confirmed that the traits of AD were localized in the centro-parietal and centro-temporal areas in the theta frequency band (4-8 Hz). The contribution of this study is that it applies a neural metric for Alzheimers detection from a data science perspective rather than from a neuroscience one. The study shows that the combination of bipolar derivations with phase synchronization yields similar results to comparable studies employing alternative analysis methods.
Big Data Analytics for Long-Term Meteorological Observations at Hanford Site
A growing number of physical objects with embedded sensors with typically high volume and frequently updated data sets has accentuated the need to develop methodologies to extract useful information from big data for supporting decision making. This study applies a suite of data analytics and core principles of data science to characterize near real-time meteorological data with a focus on extreme weather events. To highlight the applicability of this work and make it more accessible from a risk management perspective, a foundation for a software platform with an intuitive Graphical User Interface (GUI) was developed to access and analyze data from a decommissioned nuclear production complex operated by the U.S. Department of Energy (DOE, Richland, USA). Exploratory data analysis (EDA), involving classical non-parametric statistics, and machine learning (ML) techniques, were used to develop statistical summaries and learn characteristic features of key weather patterns and signatures. The new approach and GUI provide key insights into using big data and ML to assist site operation related to safety management strategies for extreme weather events. Specifically, this work offers a practical guide to analyzing long-term meteorological data and highlights the integration of ML and classical statistics to applied risk and decision science.
Export Citation Format
Share document.
Ten Research Challenge Areas in Data Science
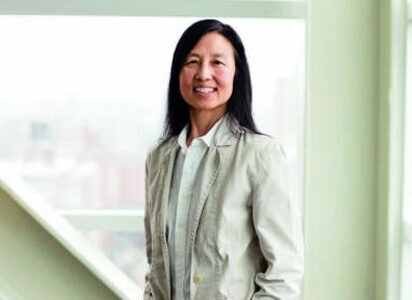
Although data science builds on knowledge from computer science, mathematics, statistics, and other disciplines, data science is a unique field with many mysteries to unlock: challenging scientific questions and pressing questions of societal importance.
Is data science a discipline?
Data science is a field of study: one can get a degree in data science, get a job as a data scientist, and get funded to do data science research. But is data science a discipline, or will it evolve to be one, distinct from other disciplines? Here are a few meta-questions about data science as a discipline.
- What is/are the driving deep question(s) of data science? Each scientific discipline (usually) has one or more “deep” questions that drive its research agenda: What is the origin of the universe (astrophysics)? What is the origin of life (biology)? What is computable (computer science)? Does data science inherit its deep questions from all its constituency disciplines or does it have its own unique ones?
- What is the role of the domain in the field of data science? People (including this author) (Wing, J.M., Janeia, V.P., Kloefkorn, T., & Erickson, L.C. (2018)) have argued that data science is unique in that it is not just about methods, but about the use of those methods in the context of a domain—the domain of the data being collected and analyzed; the domain for which a question to be answered comes from collecting and analyzing the data. Is the inclusion of a domain inherent in defining the field of data science? If so, is the way it is included unique to data science?
- What makes data science data science? Is there a problem unique to data science that one can convincingly argue would not be addressed or asked by any of its constituent disciplines, e.g., computer science and statistics?
Ten research areas
While answering the above meta-questions is still under lively debate, including within the pages of this journal, we can ask an easier question, one that also underlies any field of study: What are the research challenge areas that drive the study of data science? Here is a list of ten. They are not in any priority order, and some of them are related to each other. They are phrased as challenge areas, not challenge questions. They are not necessarily the “top ten” but they are a good ten to start the community discussing what a broad research agenda for data science might look like. 1
- Scientific understanding of learning, especially deep learning algorithms. As much as we admire the astonishing successes of deep learning, we still lack a scientific understanding of why deep learning works so well. We do not understand the mathematical properties of deep learning models. We do not know how to explain why a deep learning model produces one result and not another. We do not understand how robust or fragile they are to perturbations to input data distributions. We do not understand how to verify that deep learning will perform the intended task well on new input data. Deep learning is an example of where experimentation in a field is far ahead of any kind of theoretical understanding.
- Causal reasoning. Machine learning is a powerful tool to find patterns and examine correlations, particularly in large data sets. While the adoption of machine learning has opened many fruitful areas of research in economics, social science, and medicine, these fields require methods that move beyond correlational analyses and can tackle causal questions. A rich and growing area of current study is revisiting causal inference in the presence of large amounts of data. Economists are already revisiting causal reasoning by devising new methods at the intersection of economics and machine learning that make causal inference estimation more efficient and flexible (Athey, 2016), (Taddy, 2019). Data scientists are just beginning to explore multiple causal inference, not just to overcome some of the strong assumptions of univariate causal inference, but because most real-world observations are due to multiple factors that interact with each other (Wang & Blei, 2018).
- Precious data. Data can be precious for one of three reasons: the dataset is expensive to collect; the dataset contains a rare event (low signal-to-noise ratio ); or the dataset is artisanal—small and task-specific. A good example of expensive data comes from large, one-of, expensive scientific instruments, e.g., the Large Synoptic Survey Telescope, the Large Hadron Collider, the IceCube Neutrino Detector at the South Pole. A good example of rare event data is data from sensors on physical infrastructure, such as bridges and tunnels; sensors produce a lot of raw data, but the disastrous event they are used to predict is (thankfully) rare. Rare data can also be expensive to collect. A good example of artisanal data is the tens of millions of court judgments that China has released online to the public since 2014 (Liebman, Roberts, Stern, & Wang, 2017) or the 2+ million US government declassified documents collected by Columbia’s History Lab (Connelly, Madigan, Jervis, Spirling, & Hicks, 2019). For each of these different kinds of precious data, we need new data science methods and algorithms, taking into consideration the domain and intended uses of the data.
- Multiple, heterogeneous data sources. For some problems, we can collect lots of data from different data sources to improve our models. For example, to predict the effectiveness of a specific cancer treatment for a human, we might build a model based on 2-D cell lines from mice, more expensive 3-D cell lines from mice, and the costly DNA sequence of the cancer cells extracted from the human. State-of-the-art data science methods cannot as yet handle combining multiple, heterogeneous sources of data to build a single, accurate model. Since many of these data sources might be precious data, this challenge is related to the third challenge. Focused research in combining multiple sources of data will provide extraordinary impact.
- Inferring from noisy and/or incomplete data. The real world is messy and we often do not have complete information about every data point. Yet, data scientists want to build models from such data to do prediction and inference. A great example of a novel formulation of this problem is the planned use of differential privacy for Census 2020 data (Garfinkel, 2019), where noise is deliberately added to a query result, to maintain the privacy of individuals participating in the census. Handling “deliberate” noise is particularly important for researchers working with small geographic areas such as census blocks, since the added noise can make the data uninformative at those levels of aggregation. How then can social scientists, who for decades have been drawing inferences from census data, make inferences on this “noisy” data and how do they combine their past inferences with these new ones? Machine learning’s ability to better separate noise from signal can improve the efficiency and accuracy of those inferences.
- Trustworthy AI. We have seen rapid deployment of systems using artificial intelligence (AI) and machine learning in critical domains such as autonomous vehicles, criminal justice, healthcare, hiring, housing, human resource management, law enforcement, and public safety, where decisions taken by AI agents directly impact human lives. Consequently, there is an increasing concern if these decisions can be trusted to be correct, reliable, robust, safe, secure, and fair, especially under adversarial attacks. One approach to building trust is through providing explanations of the outcomes of a machine learned model. If we can interpret the outcome in a meaningful way, then the end user can better trust the model. Another approach is through formal methods, where one strives to prove once and for all a model satisfies a certain property. New trust properties yield new tradeoffs for machine learned models, e.g., privacy versus accuracy; robustness versus efficiency. There are actually multiple audiences for trustworthy models: the model developer, the model user, and the model customer. Ultimately, for widespread adoption of the technology, it is the public who must trust these automated decision systems.
- Computing systems for data-intensive applications. Traditional designs of computing systems have focused on computational speed and power: the more cycles, the faster the application can run. Today, the primary focus of applications, especially in the sciences (e.g., astronomy, biology, climate science, materials science), is data. Also, novel special-purpose processors, e.g., GPUs, FPGAs, TPUs, are now commonly found in large data centers. Even with all these data and all this fast and flexible computational power, it can still take weeks to build accurate predictive models; however, applications, whether from science or industry, want real-time predictions. Also, data-hungry and compute-hungry algorithms, e.g., deep learning, are energy hogs (Strubell, Ganesh, & McCallum, 2019). We should consider not only space and time, but also energy consumption, in our performance metrics. In short, we need to rethink computer systems design from first principles, with data (not compute) the focus. New computing systems designs need to consider: heterogeneous processing; efficient layout of massive amounts of data for fast access; the target domain, application, or even task; and energy efficiency.
- Automating front-end stages of the data life cycle. While the excitement in data science is due largely to the successes of machine learning, and more specifically deep learning, before we get to use machine learning methods, we need to prepare the data for analysis. The early stages in the data life cycle (Wing, 2019) are still labor intensive and tedious. Data scientists, drawing on both computational and statistical methods, need to devise automated methods that address data cleaning and data wrangling, without losing other desired properties, e.g., accuracy, precision, and robustness, of the end model.One example of emerging work in this area is the Data Analysis Baseline Library (Mueller, 2019), which provides a framework to simplify and automate data cleaning, visualization, model building, and model interpretation. The Snorkel project addresses the tedious task of data labeling (Ratner et al., 2018).
- Privacy. Today, the more data we have, the better the model we can build. One way to get more data is to share data, e.g., multiple parties pool their individual datasets to build collectively a better model than any one party can build. However, in many cases, due to regulation or privacy concerns, we need to preserve the confidentiality of each party’s dataset. An example of this scenario is in building a model to predict whether someone has a disease or not. If multiple hospitals could share their patient records, we could build a better predictive model; but due to Health Insurance Portability and Accountability Act (HIPAA) privacy regulations, hospitals cannot share these records. We are only now exploring practical and scalable ways, using cryptographic and statistical methods, for multiple parties to share data and/or share models to preserve the privacy of each party’s dataset. Industry and government are exploring and exploiting methods and concepts, such as secure multi-party computation, homomorphic encryption, zero-knowledge proofs, and differential privacy, as part of a point solution to a point problem.
- Ethics. Data science raises new ethical issues. They can be framed along three axes: (1) the ethics of data: how data are generated, recorded, and shared; (2) the ethics of algorithms: how artificial intelligence, machine learning, and robots interpret data; and (3) the ethics of practices: devising responsible innovation and professional codes to guide this emerging science (Floridi & Taddeo, 2016) and for defining Institutional Review Board (IRB) criteria and processes specific for data (Wing, Janeia, Kloefkorn, & Erickson 2018). Example ethical questions include how to detect and eliminate racial, gender, socio-economic, or other biases in machine learning models.
Closing remarks
As many universities and colleges are creating new data science schools, institutes, centers, etc. (Wing, Janeia, Kloefkorn, & Erickson 2018), it is worth reflecting on data science as a field. Will data science as an area of research and education evolve into being its own discipline or be a field that cuts across all other disciplines? One could argue that computer science, mathematics, and statistics share this commonality: they are each their own discipline, but they each can be applied to (almost) every other discipline. What will data science be in 10 or 50 years?
Acknowledgements
I would like to thank Cliff Stein, Gerad Torats-Espinosa, Max Topaz, and Richard Witten for their feedback on earlier renditions of this article. Many thanks to all Columbia Data Science faculty who have helped me formulate and discuss these ten (and other) challenges during our Fall 2019 retreat.
Athey, S. (2016). “Susan Athey on how economists can use machine learning to improve policy,” Retrieved from https://siepr.stanford.edu/news/susan-athey-how-economists-can-use-machine-learning-improve-policy
Berger, J., He, X., Madigan, C., Murphy, S., Yu, B., & Wellner, J. (2019), Statistics at a Crossroad: Who is for the Challenge? NSF workshop report. Retrieved from https://hub.ki/groups/statscrossroad
Connelly, M., Madigan, D., Jervis, R., Spirling, A., & Hicks, R. (2019). The History Lab. Retrieved from http://history-lab.org/
Floridi , L. & Taddeo , M. (2016). What is Data Ethics? Philosophical Transactions of the Royal Society A , vol. 374, issue 2083, December 2016.
Garfinkel, S. (2019). Deploying Differential Privacy for the 2020 Census of Population and Housing. Privacy Enhancing Technologies Symposium, Stockholm, Sweden. Retrieved from http://simson.net/ref/2019/2019-07-16%20Deploying%20Differential%20Privacy%20for%20the%202020%20Census.pdf
Liebman, B.L., Roberts, M., Stern, R.E., & Wang, A. (2017). Mass Digitization of Chinese Court Decisions: How to Use Text as Data in the Field of Chinese Law. UC San Diego School of Global Policy and Strategy, 21 st Century China Center Research Paper No. 2017-01; Columbia Public Law Research Paper No. 14-551. Retrieved from https://scholarship.law.columbia.edu/faculty_scholarship/2039
Mueller, A. (2019). Data Analysis Baseline Library. Retrieved from https://libraries.io/github/amueller/dabl
Ratner, A., Bach, S., Ehrenberg, H., Fries, J., Wu, S, & Ré, C. (2018). Snorkel: Rapid Training Data Creation with Weak Supervision . Proceedings of the 44 th International Conference on Very Large Data Bases.
Strubell E., Ganesh, A., & McCallum, A. (2019),”Energy and Policy Considerations for Deep Learning in NLP. Proceedings of the 57th Annual Meeting of the Association for Computational Linguistics (ACL).
Taddy, M. (2019). Business Data Science: Combining Machine Learning and Economics to Optimize, Automate, and Accelerate Business Decisions , Mc-Graw Hill.
Wang, Y. & Blei, D.M. (2018). The Blessings of Multiple Causes, Retrieved from https://arxiv.org/abs/1805.06826
Wing, J.M. (2019), The Data Life Cycle, Harvard Data Science Review , vol. 1, no. 1.
Wing, J.M., Janeia, V.P., Kloefkorn, T., & Erickson, L.C. (2018). Data Science Leadership Summit, Workshop Report, National Science Foundation. Retrieved from https://dl.acm.org/citation.cfm?id=3293458
J.M. Wing, “ Ten Research Challenge Areas in Data Science ,” Voices, Data Science Institute, Columbia University, January 2, 2020. arXiv:2002.05658 .
Jeannette M. Wing is Avanessians Director of the Data Science Institute and professor of computer science at Columbia University.

Information Age
Insight and Analysis for the CTO
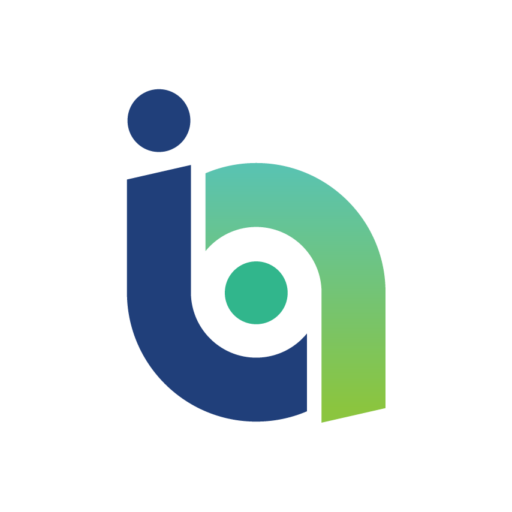
Hot topics and emerging trends in data science
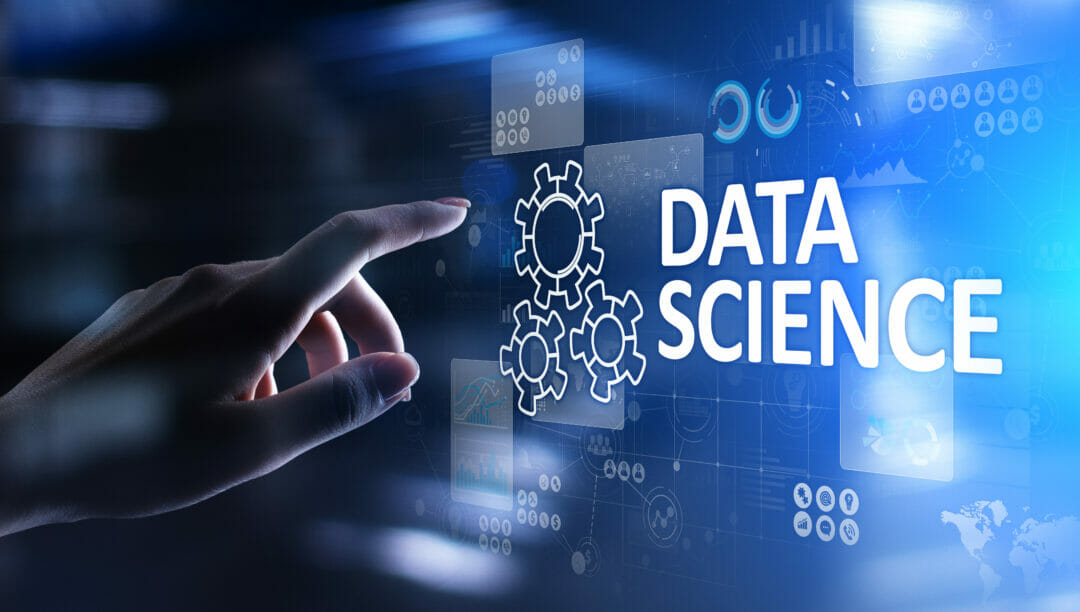
We gauged the perspectives of experts in data science, asking them about the biggest emerging trends in data science.
As one of the fastest evolving areas of tech, data science has seen a rise up the corporate agenda as less and less leaders base business decisions on guess work. With added capabilities such as artificial intelligence (AI) and the edge complementing the work of data scientists , the field is becoming more accessible to employees, but this still requires training of data skills, on the most part. In this article, we explore some key emerging trends in data science, as believed by experts in the field.
Increased involvement of AI and ML
Firstly, it’s believed that the involvement of AI and machine learning (ML) will increase further, and enable more industries to become truly data-centric.
“As businesses start to see the benefits of artificial intelligence and machine learning enabled platforms, they will invest in these technologies further,” said Douggie Melville-Clarke , head of data science at Duco .
“In fact, the Duco State of Reconciliation report – which surveyed 300 heads of global reconciliation utilities, including chief operating officers, heads of financial control and heads of finance transformation – found that 42% of those surveyed will investigate the use of more machine learning in 2021 for the purposes of intelligent data automation.”
Data science in insurance
Melville-Clarke went on to cite the insurance industry, often perceived as a sector that’s had difficulty innovating due to high levels of regulation, as an example for future success when it comes to data science.
He explained: “The insurance industry, for example, has already embraced automation for processes such as underwriting and quote generation. But the more valuable use of artificial intelligence and machine learning is to increase your service and market share through uses like constrained customisation.
“Personalisation is one of the key ways that banks and insurance companies can differentiate themselves, but without machine learning this can be a lengthy and expensive process.
“Machine learning can help these industries tailor their products to meet the individual consumers’ needs in a much more cost-effective way, bettering the customer experience and increasing customisation.”
Digital transformation in the insurance sector: cultural and organisational Johanna Von Geyr, partner and EMEA lead banking, financial services & insurance at ISG, explores digital transformation in the insurance sector. Read here
The evolution of hyperautomation
Along with rising use of AI and ML models, organisations have been combining AI with robotic process automation (RPA), to reduce operational costs through automating decision making. This trend, known as hyperautomation , is predicted to help companies to continue innovating fast in a post-COVID environment in the next few years.
“In many ways, this isn’t a new concept — the key goal of enterprise investment in data science for the past decade has been to automate decision-making processes based on AI and ML,” explained Rich Pugh , co-founder and chief data scientist at Mango Solutions , an Ascent company.
“What is new here is that hyperautomation is underpinned by an ‘RPA-first’ approach that can turbocharge process automation and drive increased collaboration across analytic and IT functions.
“Business leaders need to focus on how to harness enterprise automation and continuous intelligence to elevate the customer experience. Whether that is embedding intelligent thinking into the processes that will drive more informed decision making, such as deploying automation around pricing decisions to deliver a more efficient and personalised service, or leveraging richer real-time customer insights in conjunction with automation to execute highly relevant offers and new services at speed.
“Embarking on the hyperautomation journey begins with achieving some realistic and measurable future outcomes. Specifically, this should include aiming for high-value processes, focusing on automation and change, and initiating a structure to gather the data that will enable future success.”
SaaS and self-service
Dan Sommer , senior director at Qlik , identified software-as-a-service (SaaS) and a self-service approach among users, along with a shift in advanced analytics , as a notable emerging trend in data science.
“To those in the industry, it’s clear that SaaS will be everyone’s new best friend – with a greater migration of databases and applications from on premise to cloud environments,” said Sommer.
“Cloud computing has helped many businesses, organisations, and schools to keep the lights on in virtual environments – and we’re now going to see an enhanced focus on SaaS as hybrid operations look set to remain.
“In addition, we’ll see self-service evolving to self-sufficiency when it comes to effectively using data and analytics. Empowering users to access data, insights and business logic earlier and more intuitively will enable the move from visualisation self-service to data self-sufficiency in the near future.
“Finally, advanced analytics need to look different. In uncertain times, we can no longer count on backward-looking data to build a comprehensive model of the future. Instead, we need to give particular focus to, rather than exclude outliers – and this will define how we tackle threats going forward too.”
The value of SaaS offerings in a post-Covid business environment Jonathan Bowl, AVP & general manager, UK, Ireland & Nordics at Commvault, explores the value of SaaS offerings in a post-COVID business environment. Read here
Data fabric
With employees gradually becoming more comfortable with using data science tools to make decisions, while aided by automation and machine intelligence, a concept that’s materialised as a hot topic for the next stage of development is the concept of ‘data fabric’.
Trevor Morgan , product manager at comforte AG , explained: “A data fabric is more of an architectural overlay on top of massive enterprise data ecosystems. The data fabric unifies disparate data sources and streams across many different topologies (both on-premise and in the cloud), and provides multiple ways of accessing and working with that data for organisational personnel, and with the larger fabric as a contextual backdrop.
“For large enterprises that are moving with hyper-agility while working with multiple or many Big Data environments, data fabric technology will provide the means to harness all this information and make it workable throughout the enterprise.”
New career paths and roles
Another important trend to consider regarding the future of data science is the new career paths and jobs that are set to emerge in the coming years.
“According to the World Economic Forum ( WEF )’s Future of Job’s Report 2020 , 94% of UK employers plan to hire new permanent staff with skills relevant to new technologies and expect existing employees to pick up new skills on the job,” said Anthony Tattersall , vice-president, enterprise, EMEA at Coursera .
“What’s more, WEF’s top emerging jobs in the UK — data scientists, AI and machine learning specialists, big data and Internet of Things — all call for skills of this nature.
“We therefore envision access to a variety of job-relevant credentials, including a path to entry-level digital jobs, will be key to reskilling at scale and accelerating economic recovery in the years ahead.”
The ‘Industrial Data Scientist’
In regards to new roles to emerge in data science, Adi Pendyala , senior director at Aspen Technology , predicts the emergence of the ‘Industrial Data Scientist’: “These scientists will be a new breed of tech-driven, data-empowered domain experts with access to more industrial data than ever before, as well as the accessible AI/ML and analytics tools needed to translate that information into actionable intelligence across the enterprise.
“Industrial data scientists will represent a new kind of crossroads between our traditional understanding of citizen data scientists and industrial domain experts: workers who possess the domain expertise of the latter but are increasingly shifting over to the data realm occupied by the former.”
How to embark on a data science career To kick off our Data Science month, this article will explore how you can embark on a career in data science, and the key factors to consider. Read here
Many organisations are being impacted by a shortage of data scientists in proportion to demand, but Julien Alteirac , regional vice-president, UK&I at Snowflake , believes that new tools, powered by ML, could help to mitigate this skills gap in the near future.
“When it comes to analysing data, most organisations employ an abundance of data analysts and a limited number of data scientists, due in large part to the limited supply and high costs associated with data scientists,” said Alteirac.
“Since analysts lack the data science expertise required to build ML models, data scientists have become a potential bottleneck for broadening the use of ML. However, new and improved ML tools which are more user-friendly are helping organisations realise the power of data science.
“Data analysts are empowered with access to powerful models without needing to manually build them. Specifically, automated machine learning ( AutoML ) and AI services via APIs are removing the need to manually prepare data and then build and train models. AutoML tools and AI services lower the barrier to entry for ML, so almost anyone will now be able to access and use data science without requiring an academic background.”

Aaron Hurst
Aaron Hurst is Information Age's senior reporter, providing news and features around the hottest trends across the tech industry. More by Aaron Hurst
Related Topics
Related stories.
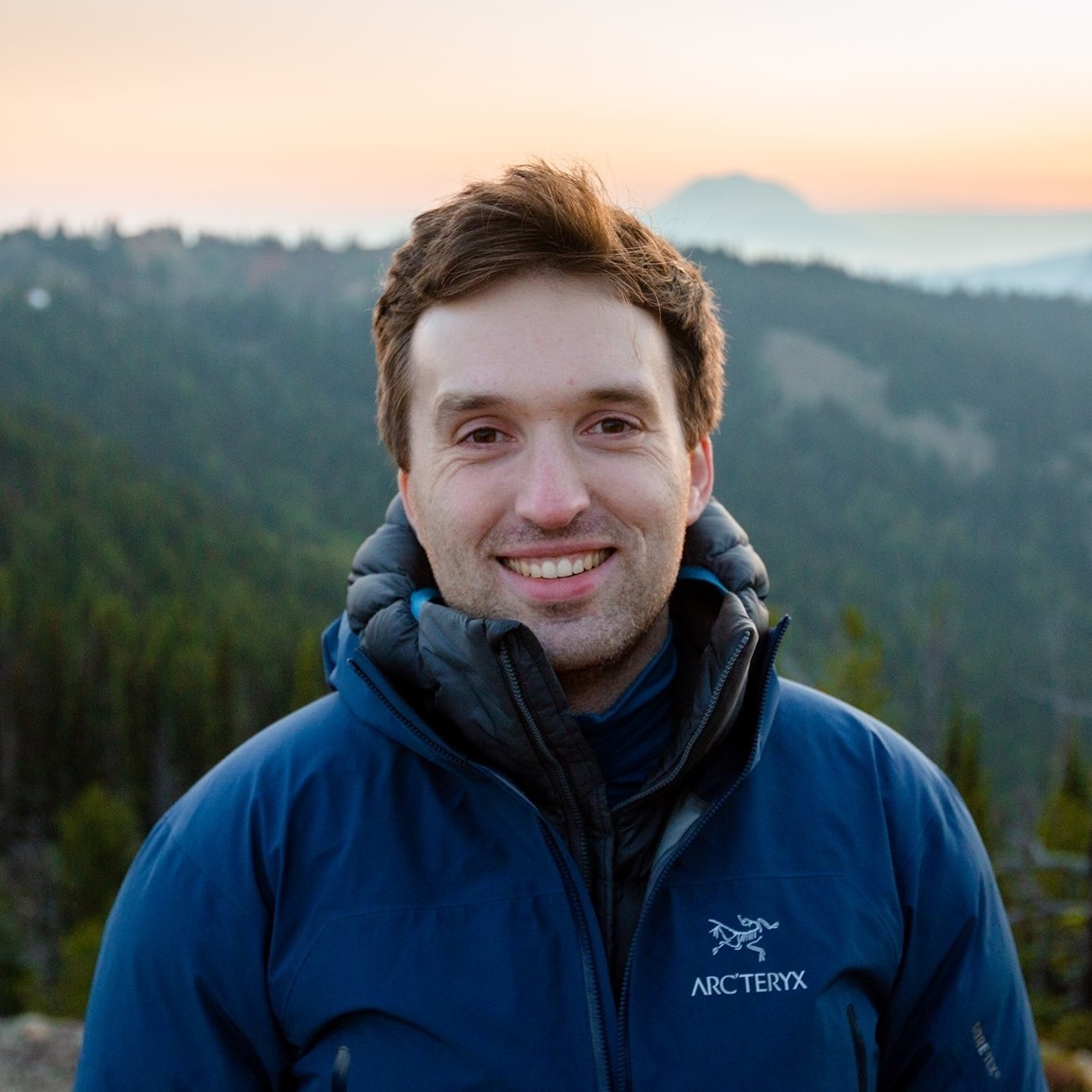
Data Analytics & Data Science
Observability – everything you need to know
Morgan Mclean, Director of Product Management at cybersecurity leader, Splunk, tells Information Age about all things observability
Partner Content
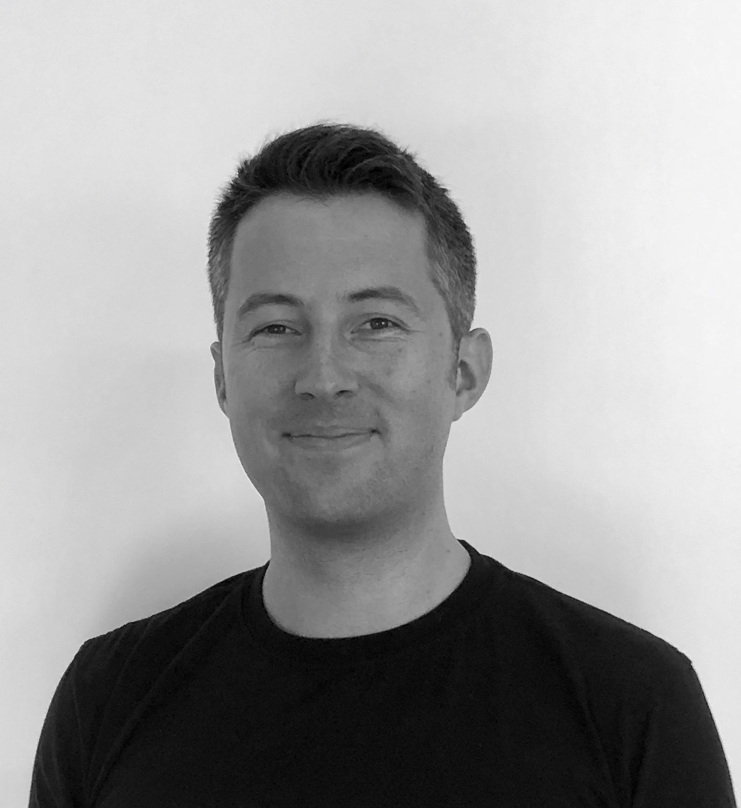
Why data isn’t the answer to everything
Splunk's James Hodge explains the problem with using data (and AI) in helping you make key business decisions
Anna Jordan
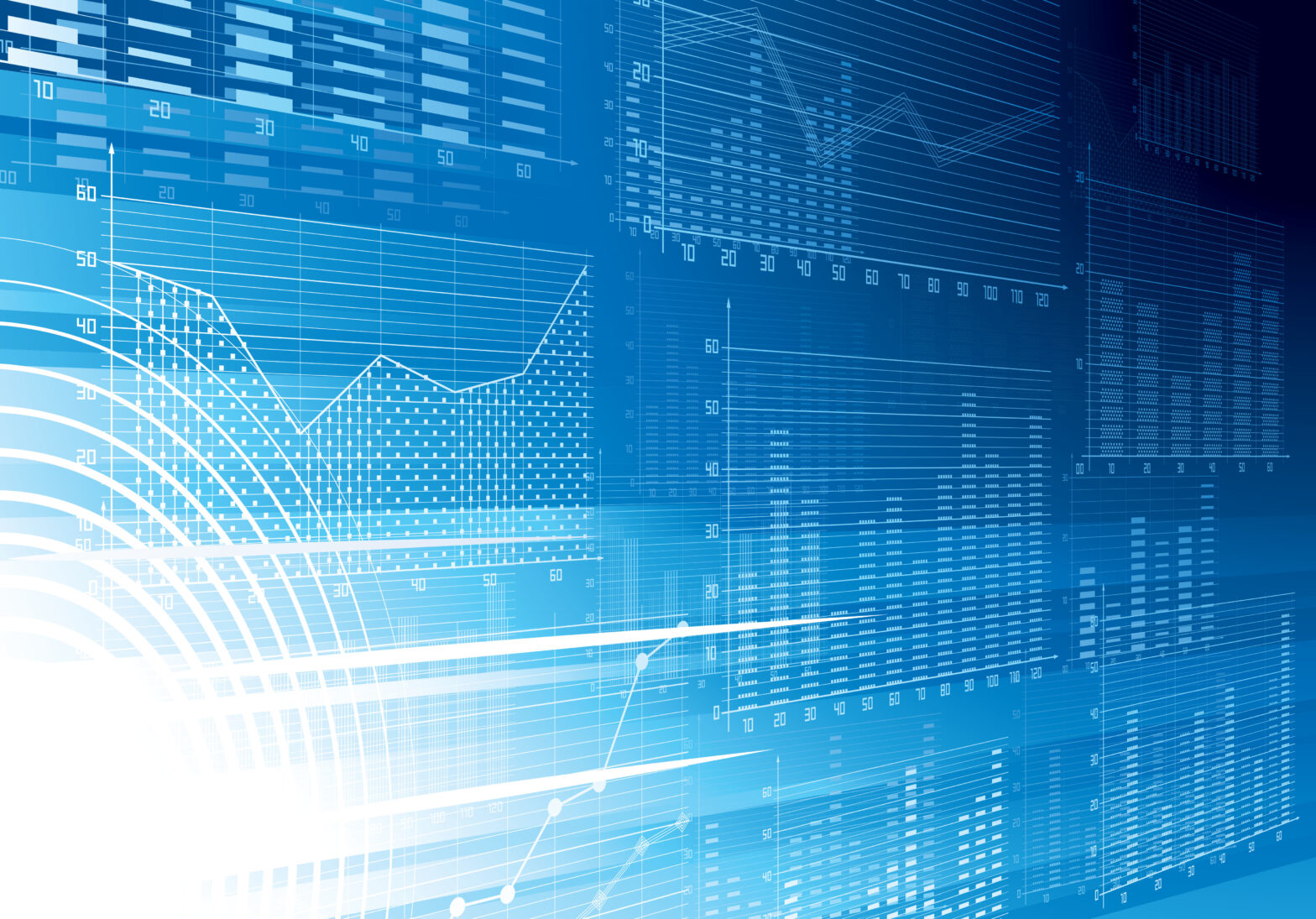
Cloud & Edge Computing
Two-thirds of UKI firms struggling with data insight costs
Research from SAS has revealed widespread struggles faced by businesses in the UK and Ireland to drive value from data insights
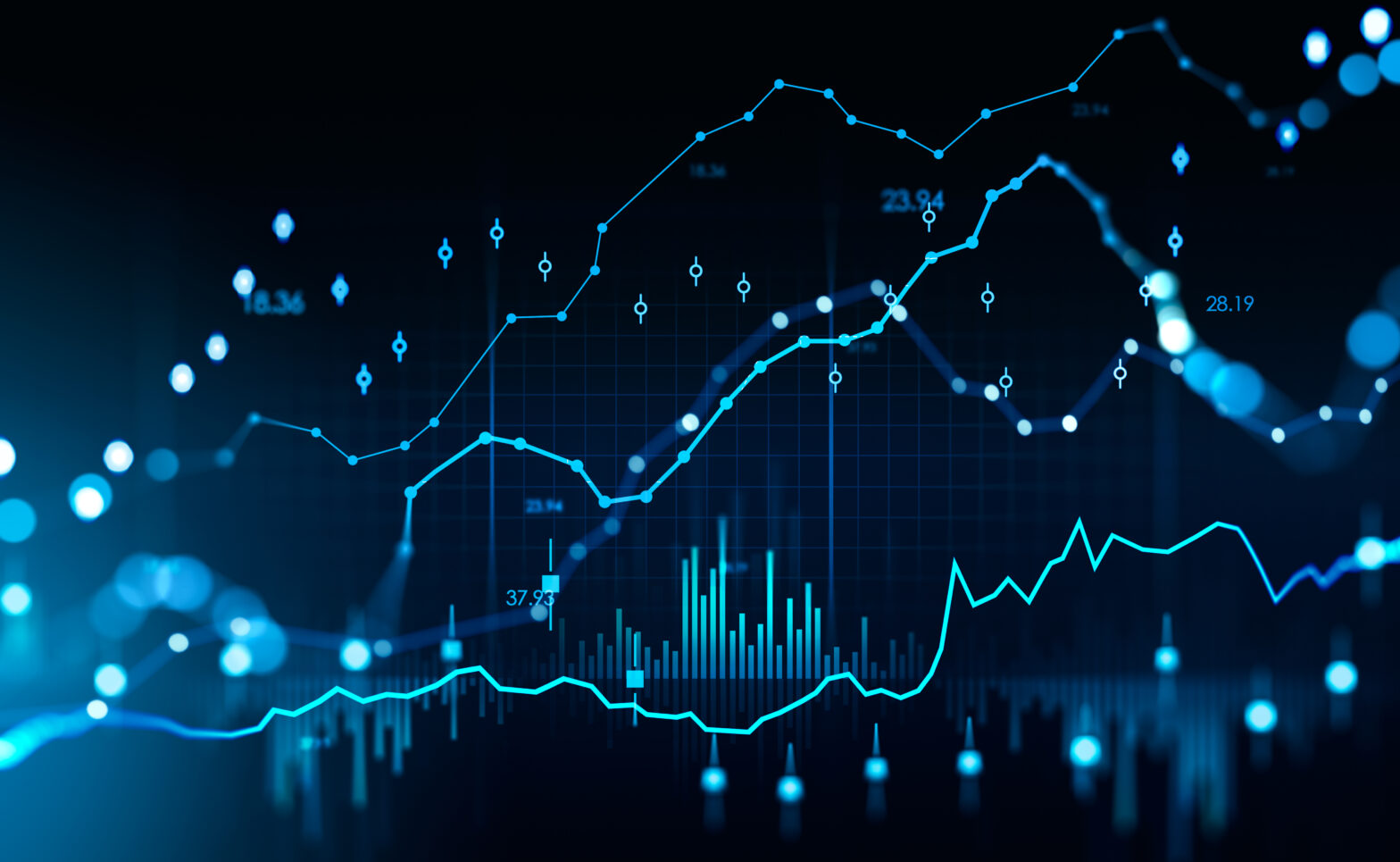
Qlik completes acquisition of Talend
Global data integration and analytics provider Qlik has acquired data management vendor Talend, to bolster automated delivery of business insights
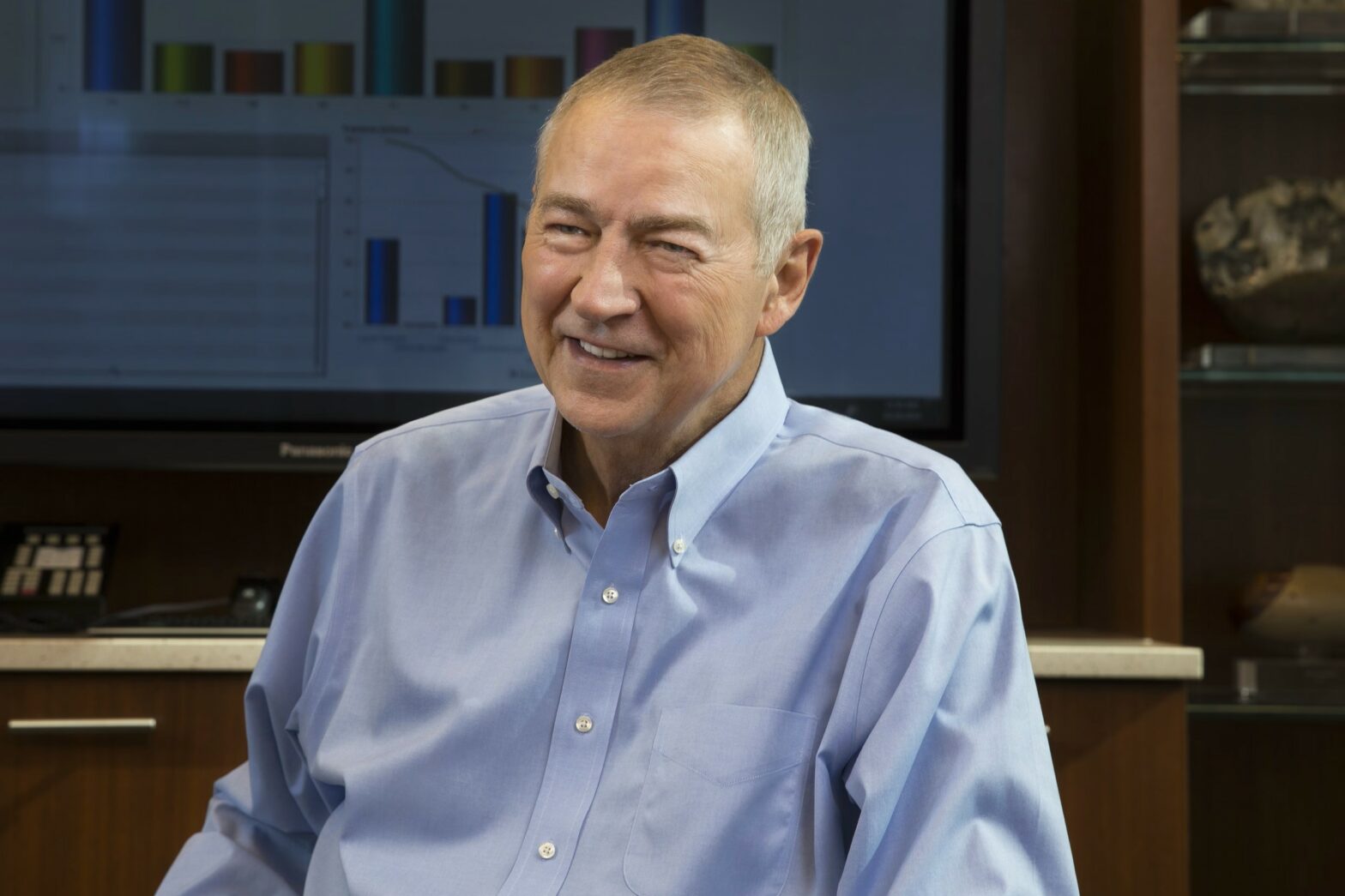
What generative AI means for business analytics
Jim Goodnight, founder and CEO of SAS Institute, tells Information Age his thoughts on the impact generative AI will have on business analytics
Dom Walbanke
- Data, AI, & Machine Learning
- Managing Technology
- Social Responsibility
- Workplace, Teams, & Culture
- AI & Machine Learning
- Diversity & Inclusion
- Big ideas Research Projects
- Artificial Intelligence and Business Strategy
- Responsible AI
- Future of the Workforce
- Future of Leadership
- All Research Projects
AI in Action
- Most Popular
- The Truth Behind the Nursing Crisis
- Work/23: The Big Shift
- Coaching for the Future-Forward Leader
- Measuring Culture
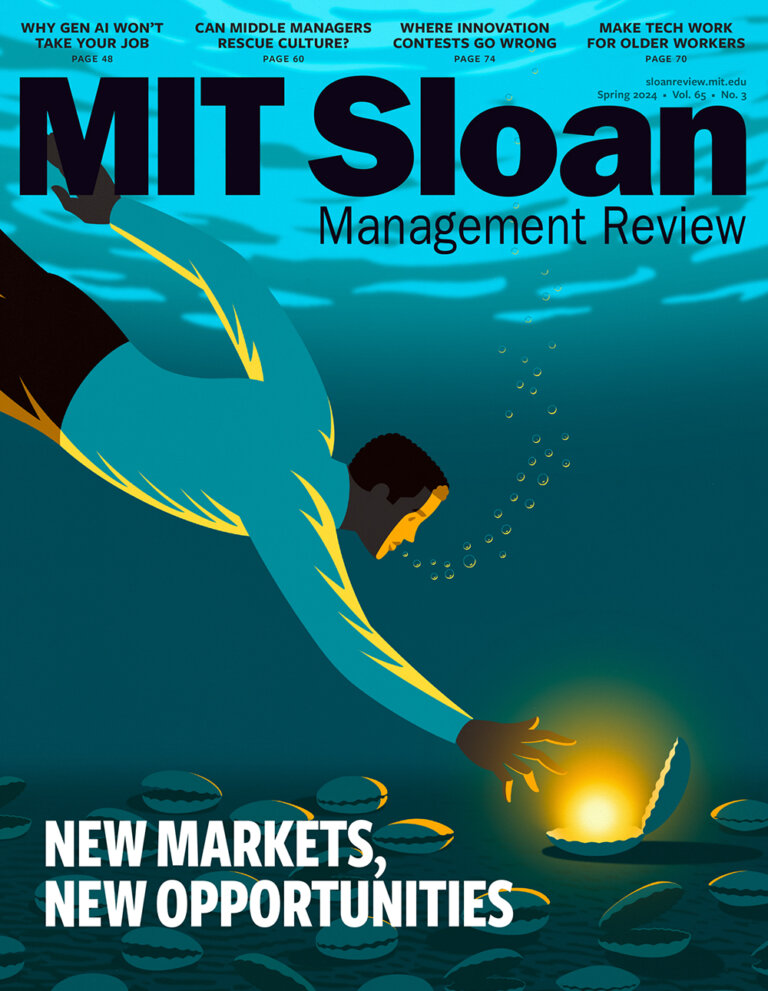
The spring 2024 issue’s special report looks at how to take advantage of market opportunities in the digital space, and provides advice on building culture and friendships at work; maximizing the benefits of LLMs, corporate venture capital initiatives, and innovation contests; and scaling automation and digital health platform.
- Past Issues
- Upcoming Events
- Video Archive
- Me, Myself, and AI
- Three Big Points
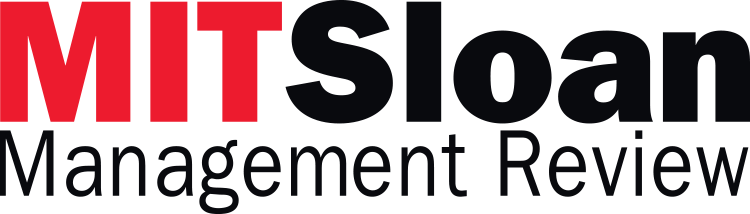
Five Key Trends in AI and Data Science for 2024
These developing issues should be on every leader’s radar screen, data executives say.
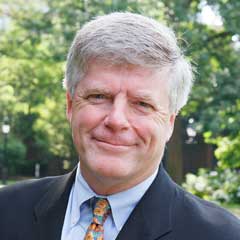
- Data, AI, & Machine Learning
- AI & Machine Learning
- Data & Data Culture
- Technology Implementation
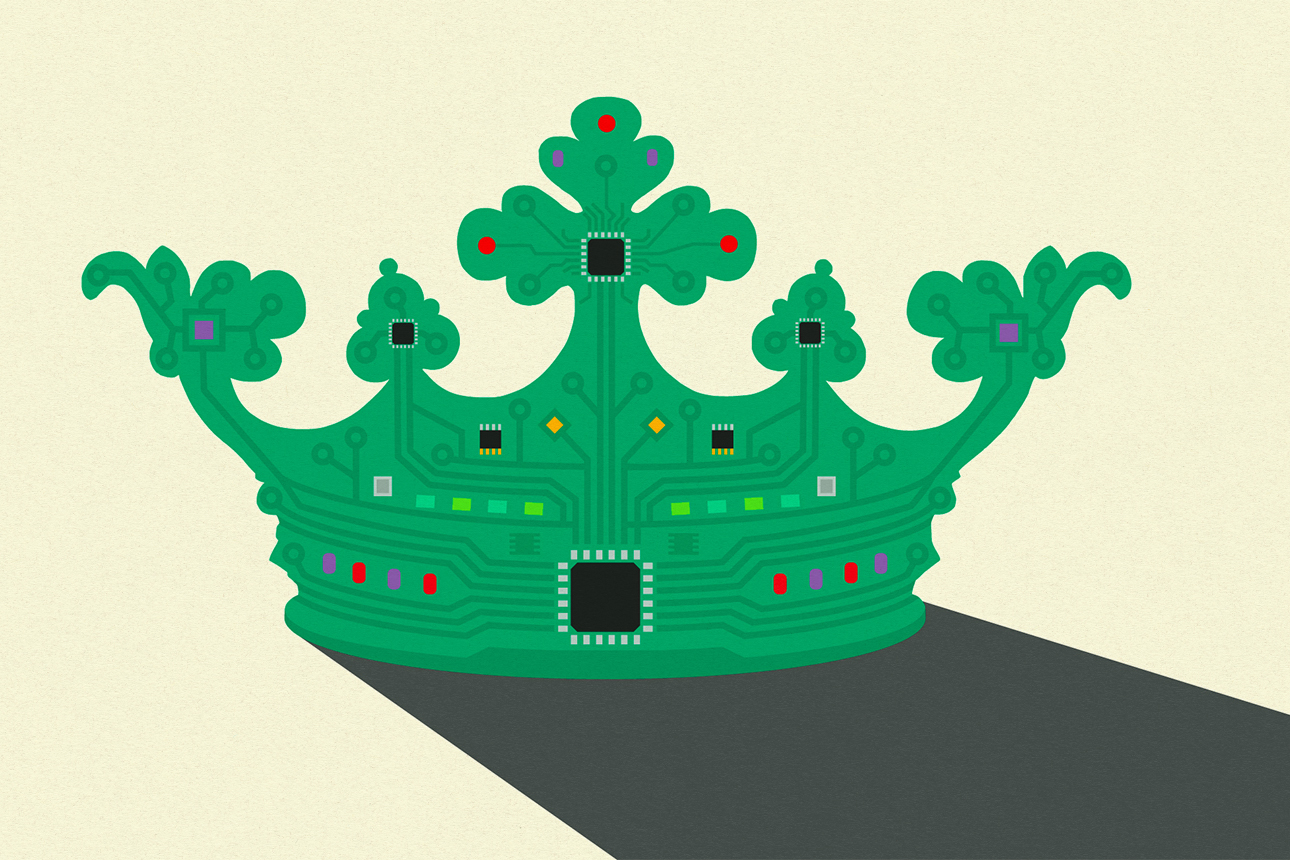
Carolyn Geason-Beissel/MIT SMR | Getty Images
Artificial intelligence and data science became front-page news in 2023. The rise of generative AI, of course, drove this dramatic surge in visibility. So, what might happen in the field in 2024 that will keep it on the front page? And how will these trends really affect businesses?
During the past several months, we’ve conducted three surveys of data and technology executives. Two involved MIT’s Chief Data Officer and Information Quality Symposium attendees — one sponsored by Amazon Web Services (AWS) and another by Thoughtworks . The third survey was conducted by Wavestone , formerly NewVantage Partners, whose annual surveys we’ve written about in the past . In total, the new surveys involved more than 500 senior executives, perhaps with some overlap in participation.
Get Updates on Leading With AI and Data
Get monthly insights on how artificial intelligence impacts your organization and what it means for your company and customers.
Please enter a valid email address
Thank you for signing up
Privacy Policy
Surveys don’t predict the future, but they do suggest what those people closest to companies’ data science and AI strategies and projects are thinking and doing. According to those data executives, here are the top five developing issues that deserve your close attention:
1. Generative AI sparkles but needs to deliver value.
As we noted, generative AI has captured a massive amount of business and consumer attention. But is it really delivering economic value to the organizations that adopt it? The survey results suggest that although excitement about the technology is very high , value has largely not yet been delivered. Large percentages of respondents believe that generative AI has the potential to be transformational; 80% of respondents to the AWS survey said they believe it will transform their organizations, and 64% in the Wavestone survey said it is the most transformational technology in a generation. A large majority of survey takers are also increasing investment in the technology. However, most companies are still just experimenting, either at the individual or departmental level. Only 6% of companies in the AWS survey had any production application of generative AI, and only 5% in the Wavestone survey had any production deployment at scale.
Surveys suggest that though excitement about generative AI is very high, value has largely not yet been delivered.
Production deployments of generative AI will, of course, require more investment and organizational change, not just experiments. Business processes will need to be redesigned, and employees will need to be reskilled (or, probably in only a few cases, replaced by generative AI systems). The new AI capabilities will need to be integrated into the existing technology infrastructure.
Perhaps the most important change will involve data — curating unstructured content, improving data quality, and integrating diverse sources. In the AWS survey, 93% of respondents agreed that data strategy is critical to getting value from generative AI, but 57% had made no changes to their data thus far.
2. Data science is shifting from artisanal to industrial.
Companies feel the need to accelerate the production of data science models . What was once an artisanal activity is becoming more industrialized. Companies are investing in platforms, processes and methodologies, feature stores, machine learning operations (MLOps) systems, and other tools to increase productivity and deployment rates. MLOps systems monitor the status of machine learning models and detect whether they are still predicting accurately. If they’re not, the models might need to be retrained with new data.
Producing data models — once an artisanal activity — is becoming more industrialized.
Most of these capabilities come from external vendors, but some organizations are now developing their own platforms. Although automation (including automated machine learning tools, which we discuss below) is helping to increase productivity and enable broader data science participation, the greatest boon to data science productivity is probably the reuse of existing data sets, features or variables, and even entire models.
3. Two versions of data products will dominate.
In the Thoughtworks survey, 80% of data and technology leaders said that their organizations were using or considering the use of data products and data product management. By data product , we mean packaging data, analytics, and AI in a software product offering, for internal or external customers. It’s managed from conception to deployment (and ongoing improvement) by data product managers. Examples of data products include recommendation systems that guide customers on what products to buy next and pricing optimization systems for sales teams.
But organizations view data products in two different ways. Just under half (48%) of respondents said that they include analytics and AI capabilities in the concept of data products. Some 30% view analytics and AI as separate from data products and presumably reserve that term for reusable data assets alone. Just 16% say they don’t think of analytics and AI in a product context at all.
We have a slight preference for a definition of data products that includes analytics and AI, since that is the way data is made useful. But all that really matters is that an organization is consistent in how it defines and discusses data products. If an organization prefers a combination of “data products” and “analytics and AI products,” that can work well too, and that definition preserves many of the positive aspects of product management. But without clarity on the definition, organizations could become confused about just what product developers are supposed to deliver.
4. Data scientists will become less sexy.
Data scientists, who have been called “ unicorns ” and the holders of the “ sexiest job of the 21st century ” because of their ability to make all aspects of data science projects successful, have seen their star power recede. A number of changes in data science are producing alternative approaches to managing important pieces of the work. One such change is the proliferation of related roles that can address pieces of the data science problem. This expanding set of professionals includes data engineers to wrangle data, machine learning engineers to scale and integrate the models, translators and connectors to work with business stakeholders, and data product managers to oversee the entire initiative.
Another factor reducing the demand for professional data scientists is the rise of citizen data science , wherein quantitatively savvy businesspeople create models or algorithms themselves. These individuals can use AutoML, or automated machine learning tools, to do much of the heavy lifting. Even more helpful to citizens is the modeling capability available in ChatGPT called Advanced Data Analysis . With a very short prompt and an uploaded data set, it can handle virtually every stage of the model creation process and explain its actions.
Of course, there are still many aspects of data science that do require professional data scientists. Developing entirely new algorithms or interpreting how complex models work, for example, are tasks that haven’t gone away. The role will still be necessary but perhaps not as much as it was previously — and without the same degree of power and shimmer.
5. Data, analytics, and AI leaders are becoming less independent.
This past year, we began to notice that increasing numbers of organizations were cutting back on the proliferation of technology and data “chiefs,” including chief data and analytics officers (and sometimes chief AI officers). That CDO/CDAO role, while becoming more common in companies, has long been characterized by short tenures and confusion about the responsibilities. We’re not seeing the functions performed by data and analytics executives go away; rather, they’re increasingly being subsumed within a broader set of technology, data, and digital transformation functions managed by a “supertech leader” who usually reports to the CEO. Titles for this role include chief information officer, chief information and technology officer, and chief digital and technology officer; real-world examples include Sastry Durvasula at TIAA, Sean McCormack at First Group, and Mojgan Lefebvre at Travelers.
Related Articles
This evolution in C-suite roles was a primary focus of the Thoughtworks survey, and 87% of respondents (primarily data leaders but some technology executives as well) agreed that people in their organizations are either completely, to a large degree, or somewhat confused about where to turn for data- and technology-oriented services and issues. Many C-level executives said that collaboration with other tech-oriented leaders within their own organizations is relatively low, and 79% agreed that their organization had been hindered in the past by a lack of collaboration.
We believe that in 2024, we’ll see more of these overarching tech leaders who have all the capabilities to create value from the data and technology professionals reporting to them. They’ll still have to emphasize analytics and AI because that’s how organizations make sense of data and create value with it for employees and customers. Most importantly, these leaders will need to be highly business-oriented, able to debate strategy with their senior management colleagues, and able to translate it into systems and insights that make that strategy a reality.
About the Authors
Thomas H. Davenport ( @tdav ) is the President’s Distinguished Professor of Information Technology and Management at Babson College, a fellow of the MIT Initiative on the Digital Economy, and senior adviser to the Deloitte Chief Data and Analytics Officer Program. He is coauthor of All in on AI: How Smart Companies Win Big With Artificial Intelligence (HBR Press, 2023) and Working With AI: Real Stories of Human-Machine Collaboration (MIT Press, 2022). Randy Bean ( @randybeannvp ) is an industry thought leader, author, founder, and CEO and currently serves as innovation fellow, data strategy, for global consultancy Wavestone. He is the author of Fail Fast, Learn Faster: Lessons in Data-Driven Leadership in an Age of Disruption, Big Data, and AI (Wiley, 2021).
More Like This
Add a comment cancel reply.
You must sign in to post a comment. First time here? Sign up for a free account : Comment on articles and get access to many more articles.
Comment (1)
Nicolas corzo.
More From Forbes
The Top 5 Data Science And Analytics Trends In 2023
- Share to Facebook
- Share to Twitter
- Share to Linkedin
Data is increasingly the differentiator between winners and also-rans in business. Today, information can be captured from many different sources, and technology to extract insights is becoming increasingly accessible.
Moving to a data-driven business model – where decisions are made based on what we know to be true rather than “gut feeling” – is core to the wave of digital transformation sweeping through every industry in 2023 and beyond. It helps us to react with certainty in the face of uncertainty – especially when wars and pandemics upset the established order of things.
But the world of data and analytics never stands still. New technologies are constantly emerging that offer faster and more accurate access to insights. And new trends emerge, bringing us new thinking on the best ways to put it to work across business and society at large. So, here’s my rundown of what I believe are the most important trends that will affect the way we use data and analytics to drive business growth in 2023.
Data Democratization
One of the most important trends will be the continued empowerment of entire workforces – rather than data engineers and data scientists – to put analytics to work. This is giving rise to new forms of augmented working, where tools, applications, and devices push intelligent insights into the hands of everybody in order to allow them to do their jobs more effectively and efficiently.
Best Travel Insurance Companies
Best covid-19 travel insurance plans.
In 2023, businesses will understand that data is the key to understanding customers, developing better products and services, and streamlining their internal operations to reduce costs and waste. However, it’s becoming increasingly clear that this won’t fully happen until the power to act on data-driven insights is available to frontline, shop floor, and non-technical staff, as well as functions such as marketing and finance.
Some great examples of data democracy in practice include lawyers using natural language processing (NLP) tools to scan pages of documents of case law, or retail sales assistants using hand terminals that can access customer purchase history in real time and recommend products to up-sell and cross-sell. Research by McKinsey has found that companies that make data accessible to their entire workforce are 40 times more likely to say analytics has a positive impact on revenue.
Artificial Intelligence
Artificial intelligence (AI) is perhaps the one technology trend that will have the biggest impact on how we live, work and do business in the future. Its effect on business analytics will be to enable more accurate predictions, reduce the amount of time we spend on mundane and repetitive work like data gathering and data cleansing, and to empower workforces to act on data-driven insights, whatever their role and level of technical expertise (see Data Democratization, above).
Put simply; AI allows businesses to analyze data and draw out insights far more quickly than would ever be possible manually, using software algorithms that get better and better at their job as they are fed more data. This is the basic principle of machine learning (ML), which is the form of AI used in business today. AI and ML technologies include NLP, which enables computers to understand and communicate with us in human languages, computer vision which enables computers to understand and process visual information using cameras, just as we do with our eyes; and generative AI, which can create text, images, sounds and video from scratch.
Cloud and Data-as-a-Service
I’ve put these two together because cloud is the platform that enables data-as-a-service technology to work. Basically, it means that companies can access data sources that have been collected and curated by third parties via cloud services on a pay-as-you-go or subscription-based billing model. This reduces the need for companies to build their own expensive, proprietary data collection and storage systems for many types of applications.
As well as raw data, DaaS companies offer analytics tools as-a-service. Data accessed through DaaS is typically used to augment a company’s proprietary data that it collects and processes itself in order to create richer and more valuable insights. It plays a big part in the democratization of data mentioned previously, as it allows businesses to work with data without needing to set up and maintain expensive and specialized data science operations. In 2023, it’s estimated that the value of the market for these services will grow to $10.7 billion .
Real-Time Data
When digging into data in search of insights, it's better to know what's going on right now – rather than yesterday, last week, or last month. This is why real-time data is increasingly becoming the most valuable source of information for businesses.
Working with real-time data often requires more sophisticated data and analytics infrastructure, which means more expense, but the benefit is that we’re able to act on information as it happens. This could involve analyzing clickstream data from visitors to our website to work out what offers and promotions to put in front of them, or in financial services, it could mean monitoring transactions as they take place around the world to watch out for warning signs of fraud. Social media sites like Facebook analyze hundreds of gigabytes of data per second for various use cases, including serving up advertising and preventing the spread of fake news. And in South Africa’s Kruger National Park, a joint initiative between the WWF and ZSL analyzes video footage in real-time to alert law enforcement to the presence of poachers .
As more organizations look to data to provide them with a competitive edge, those with the most advanced data strategies will increasingly look towards the most valuable and up-to-date data. This is why real-time data and analytics will be the most valuable big data tools for businesses in 2023.
Data Governance and Regulation
Data governance will also be big news in 2023 as more governments introduce laws designed to regulate the use of personal and other types of data. In the wake of the likes of European GDPR, Canadian PIPEDA, and Chinese PIPL, other countries are likely to follow suit and introduce legislation protecting the data of their citizens. In fact, analysts at Gartner have predicted that by 2023, 65% of the world’s population will be covered by regulations similar to GDPR.
This means that governance will be an important task for businesses over the next 12 months, wherever they are located in the world, as they move to ensure that their internal data processing and handling procedures are adequately documented and understood. For many businesses, this will mean auditing exactly what information they have, how it is collected, where it is stored, and what is done with it. While this may sound like extra work, in the long term, the idea is that everyone will benefit as consumers will be more willing to trust organizations with their data if they are sure it will be well looked after. Those organizations will then be able to use this data to develop products and services that align more closely with what we need at prices we can afford.
To stay on top of the latest on the latest trends, make sure to subscribe to my newsletter , follow me on Twitter , LinkedIn , and YouTube , and check out my books ‘Data Strategy: How To Profit From A World Of Big Data, Analytics And Artificial Intelligence’ and ‘ Business Trends in Practice ’.

- Editorial Standards
- Reprints & Permissions
Join The Conversation
One Community. Many Voices. Create a free account to share your thoughts.
Forbes Community Guidelines
Our community is about connecting people through open and thoughtful conversations. We want our readers to share their views and exchange ideas and facts in a safe space.
In order to do so, please follow the posting rules in our site's Terms of Service. We've summarized some of those key rules below. Simply put, keep it civil.
Your post will be rejected if we notice that it seems to contain:
- False or intentionally out-of-context or misleading information
- Insults, profanity, incoherent, obscene or inflammatory language or threats of any kind
- Attacks on the identity of other commenters or the article's author
- Content that otherwise violates our site's terms.
User accounts will be blocked if we notice or believe that users are engaged in:
- Continuous attempts to re-post comments that have been previously moderated/rejected
- Racist, sexist, homophobic or other discriminatory comments
- Attempts or tactics that put the site security at risk
- Actions that otherwise violate our site's terms.
So, how can you be a power user?
- Stay on topic and share your insights
- Feel free to be clear and thoughtful to get your point across
- ‘Like’ or ‘Dislike’ to show your point of view.
- Protect your community.
- Use the report tool to alert us when someone breaks the rules.
Thanks for reading our community guidelines. Please read the full list of posting rules found in our site's Terms of Service.
20 Data Science Topics and Areas
It is no doubt that data science topics and areas are some of the hottest business points today.
We collected some basic and advanced topics in data science to give you ideas on where to master your skills.
In today’s landscape, businesses are investing in corporate data science training to enhance their employees’ data science capabilities.
Data science topics also are hot subjects you can use as directions to prepare yourself for data science job interview questions.
1. The core of data mining process
This is an example of a wide data science topic.
What is it?
Data mining is an iterative process that involves discovering patterns in large data sets. It includes methods and techniques such as machine learning, statistics, database systems and etc.
The two main data mining objectives are to find out patterns and establish trends and relationship in a dataset in order to solve problems.
The general stages of the data mining process are: problem definition, data exploration, data preparation, modeling, evaluation, and deployment.
Core terms related to data mining are classification, predictions, association rules, data reduction, data exploration, supervised and unsupervised learning, datasets organization, sampling from datasets, building a model and etc.
2. Data visualization
Data visualization is the presentation of data in a graphical format.
It enables decision-makers of all levels to see data and analytics presented visually, so they can identify valuable patterns or trends.
Data visualization is another broad subject that covers the understanding and use of basic types of graphs (such as line graphs, bar graphs, scatter plots , histograms, box and whisker plots , heatmaps.
You cannot go without these graphs. In addition, here you need to learn about multidimensional variables with adding variables and using colors, size, shapes, animations.
Manipulation also plays a role here. You should be able to rascal, zoom, filter, aggregate data.
Using some specialized visualizations such as map charts and tree maps is a hot skill too.
3. Dimension reduction methods and techniques
Dimension Reduction process involves converting a data set with vast dimensions into a dataset with lesser dimensions ensuring that it provides similar information in short.
In other words, dimensionality reduction consists of series of techniques and methods in machine learning and statistics to decrease the number of random variables.
There are so many methods and techniques to perform dimension reduction.
The most popular of them are Missing Values, Low Variance, Decision Trees, Random Forest, High Correlation, Factor Analysis, Principal Component Analysis, Backward Feature Elimination.
4. Classification
Classification is a core data mining technique for assigning categories to a set of data.
The purpose is to support gathering accurate analysis and predictions from the data.
Classification is one of the key methods for making the analysis of a large amount of datasets effective.
Classification is one of the hottest data science topics too. A data scientist should know how to use classification algorithms to solve different business problems.
This includes knowing how to define a classification problem, explore data with univariate and bivariate visualization, extract and prepare data, build classification models, evaluate models, and etc. Linear and non-linear classifiers are some of the key terms here.
5. Simple and multiple linear regression
Linear regression models are among the basic statistical models for studying relationships between an independent variable X and Y dependent variable.
It is a mathematical modeling which allows you to make predictions and prognosis for the value of Y depending on the different values of X.
There are two main types of linear regression: simple linear regression models and multiple linear regression models.
Key points here are terms such as correlation coefficient, regression line, residual plot, linear regression equation and etc. For the beginning, see some simple linear regression examples .
6. K-nearest neighbor (k-NN)
N-nearest-neighbor is a data classification algorithm that evaluates the likelihood a data point to be a member of one group. It depends on how near the data point is to that group.
As one of the key non-parametric method used for regression and classification, k-NN can be classified as one of the best data science topics ever.
Determining neighbors, using classification rules, choosing k are a few of the skills a data scientist should have. K-nearest neighbor is also one of the key text mining and anomaly detection algorithms .
7. Naive Bayes
Naive Bayes is a collection of classification algorithms which are based on the so-called Bayes Theorem .
Widely used in Machine Learning, Naive Bayes has some crucial applications such as spam detection and document classification.
There are different Naive Bayes variations. The most popular of them are the Multinomial Naive Bayes, Bernoulli Naive Bayes, and Binarized Multinomial Naive Bayes.
8. Classification and regression trees (CART)
When it comes to algorithms for predictive modeling machine learning, decision trees algorithms have a vital role.
The decision tree is one of the most popular predictive modeling approaches used in data mining, statistics and machine learning that builds classification or regression models in the shape of a tree (that’s why they are also known as regression and classification trees).
They work for both categorical data and continuous data.
Some terms and topics you should master in this field involve CART decision tree methodology, classification trees, regression trees, interactive dihotomiser, C4.5, C5.5, decision stump, conditional decision tree, M5, and etc.
9. Logistic regression
Logistic regression is one of the oldest data science topics and areas and as the linear regression, it studies the relationship between dependable and independent variable.
However, we use logistic regression analysis where the dependent variable is dichotomous (binary).
You will face terms such as sigmoid function, S-shaped curve, multiple logistic regression with categorical explanatory variables, multiple binary logistic regression with a combination of categorical and continuous predictors and etc.
10. Neural Networks
Neural Networks act as a total hit in the machine learning nowadays. Neural networks (also known as artificial neural networks) are systems of hardware and/or software that mimic the human brain neurons operation.
The above were some of the basic data science topics. Here is a list of more interesting and advanced topics:
11. Discriminant analysis
12. Association rules
13. Cluster analysis
14. Time series
15. Regression-based forecasting
16. Smoothing methods
17. Time stamps and financial modeling
18. Fraud detection
19. Data engineering – Hadoop, MapReduce, Pregel.
20. GIS and spatial data
For continuous learning, explore online data science courses for mastering these topics.
What are your favorite data science topics? Share your thoughts in the comment field above.
About The Author

Silvia Valcheva
Silvia Valcheva is a digital marketer with over a decade of experience creating content for the tech industry. She has a strong passion for writing about emerging software and technologies such as big data, AI (Artificial Intelligence), IoT (Internet of Things), process automation, etc.
Leave a Reply Cancel Reply
This site uses Akismet to reduce spam. Learn how your comment data is processed .
TechRepublic
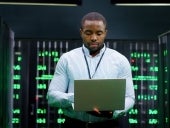
8 Best Data Science Tools and Software
Apache Spark and Hadoop, Microsoft Power BI, Jupyter Notebook and Alteryx are among the top data science tools for finding business insights. Compare their features, pros and cons.
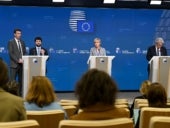
EU’s AI Act: Europe’s New Rules for Artificial Intelligence
Europe's AI legislation, adopted March 13, attempts to strike a tricky balance between promoting innovation and protecting citizens' rights.
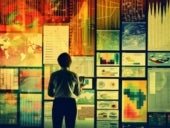
10 Best Predictive Analytics Tools and Software for 2024
Tableau, TIBCO Data Science, IBM and Sisense are among the best software for predictive analytics. Explore their features, pricing, pros and cons to find the best option for your organization.
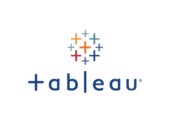
Tableau Review: Features, Pricing, Pros and Cons
Tableau has three pricing tiers that cater to all kinds of data teams, with capabilities like accelerators and real-time analytics. And if Tableau doesn’t meet your needs, it has a few alternatives worth noting.
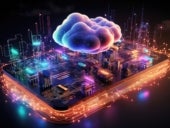
Top 6 Enterprise Data Storage Solutions for 2024
Amazon, IDrive, IBM, Google, NetApp and Wasabi offer some of the top enterprise data storage solutions. Explore their features and benefits, and find the right solution for your organization's needs.
Latest Articles
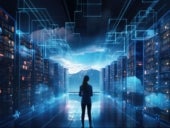
8 Best B2B Database Providers for 2024
In this guide, we explore the top B2B database providers that collect and manage reliable and accurate data for your business’ marketing and sales needs.
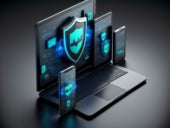
Report: Organisations Have Endpoint Security Tools But Are Still Falling Short on the Basics
AI PCs could soon see organisations invest in whole fleets of new managed devices, but Absolute Security data shows they are failing to maintain endpoint protection and patching the devices they have.
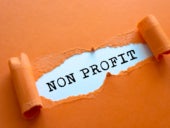
6 Best Nonprofit CRM Software for 2024
Find the perfect CRM solution for your nonprofit organization. Explore the features, pricing and more of the top-rated CRM options tailored for nonprofits.
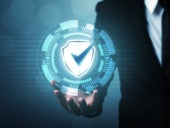
How Can Businesses Defend Themselves Against Common Cyberthreats?
TechRepublic consolidated expert advice on how businesses can defend themselves against the most common cyberthreats, including zero-days, ransomware and deepfakes.
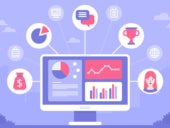
Top 10 CRM Features and Functionalities
Discover the top CRM features for business success. Explore a curated list of key capabilities to consider when choosing the right CRM solution, including marketing tools, activity tracking and more.
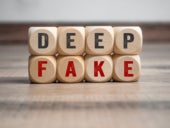
Combatting Deepfakes in Australia: Content Credentials is the Start
The production of deepfakes is accelerating at more than 1,500% in Australia, forcing organisations to create and adopt standards like Content Credentials.
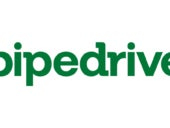
The Top 5 Pipedrive Alternatives for 2024
Discover the top alternatives to Pipedrive. Explore a curated list of CRM platforms with similar features, pricing and pros and cons to find the best fit for your business.
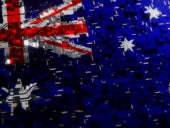
The Australian Government’s Manufacturing Objectives Rely on IT Capabilities
The intent of the Future Made in Australia Act is to build manufacturing capabilities across all sectors, which will likely lead to more demand for IT skills and services.
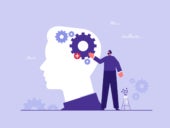
Udemy Report: Which IT Skills Are Most in Demand in Q1 2024?
Informatica PowerCenter, Microsoft Playwright and Oracle Database SQL top Udemy’s list of most popular tech courses.
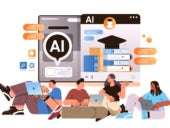
The 10 Best AI Courses in 2024
Today’s options for best AI courses offer a wide variety of hands-on experience with generative AI, machine learning and AI algorithms.
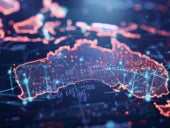
Gartner: 4 Bleeding-Edge Technologies in Australia
Gartner recently identified emerging tech that will impact enterprise leaders in APAC. Here’s what IT leaders in Australia need to know about these innovative technologies.
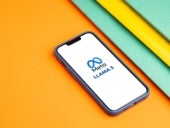
Llama 3 Cheat Sheet: A Complete Guide for 2024
Learn how to access Meta’s new AI model Llama 3, which sets itself apart by being open to use under a license agreement.
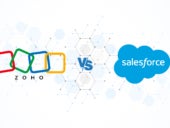
Zoho vs Salesforce (2024): Which CRM Is Better?
Look at Zoho CRM and Salesforce side-by-side to compare the cost per functionality and top pros and of each provider to determine which is better for your business needs.
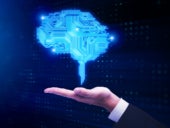
9 Innovative Use Cases of AI in Australian Businesses in 2024
Australian businesses are beginning to effectively grapple with AI and build solutions specific to their needs. Here are notable use cases of businesses using AI.
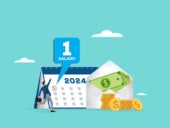
How Are APAC Tech Salaries Faring in 2024?
The year 2024 is bringing a return to stable tech salary growth in APAC, with AI and data jobs leading the way. This follows downward salary pressure in 2023, after steep increases in previous years.
Create a TechRepublic Account
Get the web's best business technology news, tutorials, reviews, trends, and analysis—in your inbox. Let's start with the basics.
* - indicates required fields
Sign in to TechRepublic
Lost your password? Request a new password
Reset Password
Please enter your email adress. You will receive an email message with instructions on how to reset your password.
Check your email for a password reset link. If you didn't receive an email don't forgot to check your spam folder, otherwise contact support .
Welcome. Tell us a little bit about you.
This will help us provide you with customized content.
Want to receive more TechRepublic news?
You're all set.
Thanks for signing up! Keep an eye out for a confirmation email from our team. To ensure any newsletters you subscribed to hit your inbox, make sure to add [email protected] to your contacts list.
Does Granger causality exist between article usage and publication counts? A topic-level time-series evidence from IEEE Xplore
- Published: 10 May 2024
Cite this article
- Wencan Tian 1 ,
- Yongzhen Wang 1 ,
- Zhigang Hu 2 ,
- Ruonan Cai 3 ,
- Guangyao Zhang 1 , 4 &
- Xianwen Wang ORCID: orcid.org/0000-0002-7236-9267 1
42 Accesses
Explore all metrics
In this study, employing the IEEE Xplore database as the data source, articles on different topics (keywords) and their usage data generated from January 2011 to December 2020 were collected and analyzed. The study examined the temporal relationships between these usage data and publication counts at the topic level via Granger causality analysis. The study found that almost 80% of the topics exhibit significant usage-publication interactions from a time-series perspective, with varying time lag lengths depending on the direction of the Granger causality results. Topics that present bidirectional Granger causality show longer time lag lengths than those exhibiting unidirectional causality. Additionally, the study found that the direction of the unidirectional Granger causality was influenced by the significance of a topic. Topics with a greater preference for article usage as the Granger cause of publication counts were deemed more important. The findings’ reliability was confirmed by varying the maximum lag period. This study provides strong support for using usage data to identify hot topics of research.
This is a preview of subscription content, log in via an institution to check access.
Access this article
Price includes VAT (Russian Federation)
Instant access to the full article PDF.
Rent this article via DeepDyve
Institutional subscriptions
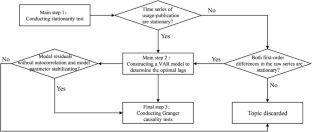
https://www.nltk.org/
Degree centrality refers to the number of direct connections a node has with other nodes (in a topic co-occurrence network, the nodes represent topics). Betweenness centrality measures the frequency of a node’s appearance on all shortest paths within the network, quantifying its role and importance as a mediator or “bridge” within the network. Closeness centrality is defined by calculating the reciprocal of the shortest path lengths from a certain node to all other nodes in the network, which is used to measure the average proximity of a node to all other nodes within the network. Eigenvector centrality involves the adjacency matrix of the network and the eigenvector corresponding to its largest eigenvalue, with the underlying idea that one’s own importance depends on the importance of the nodes to which one is connected.
https://networkx.org/
Akaike, H. (1970). Statistical predictor identification. Annals of the Institute of Statistical Mathematics, 22 (2), 203. https://doi.org/10.1007/BF02506337
Article MathSciNet Google Scholar
Bai, R., Liu, B., & Leng, F. (2020). Frontier identification of emerging scientific research based on multi-indicators. Journal of the China Society for Scientific and Technical Information, 39 (7), 747–760. https://doi.org/10.3772/j.issn.1000-0135.2020.07.007
Article Google Scholar
Baker, K. S., & Mayernik, M. S. (2020). Disentangling knowledge production and data production. Ecosphere, 11 (7), e03191. https://doi.org/10.1002/ecs2.3191
Blei, D. M., Ng, A. Y., & Jordan, M. I. (2003). Latent dirichlet allocation. Journal of Machine Learning Research, 3 (4–5), 993–1022.
Google Scholar
Bollen, J., & Van De Sompel, H. (2006). Mapping the structure of science through usage. Scientometrics, 69 (2), 227–258. https://doi.org/10.1007/s11192-006-0151-8
Bollen, J., & Van De Sompel, H. (2008). Usage impact factor: The effects of sample characteristics on usage-based impact metrics. Journal of the American Society for Information Science and Technology, 59 (1), 136–149. https://doi.org/10.1002/asi.20746
Borner, K., Penumarthy, S., Meiss, M., & Ke, W. (2006). Mapping the diffusion of scholarly knowledge among major US research institutions. Scientometrics, 68 (3), 415–426. https://doi.org/10.1007/s11192-006-0120-2
Boyack, K. W., & Klavans, R. (2010). Co-citation analysis, bibliographic coupling, and direct citation: Which citation approach represents the research front most accurately? Journal of the American Society for Information Science and Technology, 61 (12), 2389–2404. https://doi.org/10.1002/asi.21419
Breitzman, A. (2021). The relationship between web usage and citation statistics for electronics and information technology articles. Scientometrics, 126 (3), 2085–2105. https://doi.org/10.1007/s11192-020-03851-5
Brody, T., Harnad, S., & Carr, L. (2006). Earlier web usage statistics as predictors of later citation impact. Journal of the American Society for Information Science and Technology, 57 (8), 1060–1072. https://doi.org/10.1002/asi.20373
Chen, C. M. (2006). CiteSpace II: Detecting and visualizing emerging trends and transient patterns in scientific literature. Journal of the American Society for Information Science and Technology, 57 (3), 359–377. https://doi.org/10.1002/asi.20317
Chen, W. M. Y., Bukhari, M., Cockshull, F., & Galloway, J. (2020). The relationship between citations, downloads and alternative metrics in rheumatology publications: A bibliometric study. Rheumatology, 59 (2), 277–280. https://doi.org/10.1093/rheumatology/kez163
Chen, W., & Chen, W. (2022). Predicting popularity of emerging topics with multivariable LSTM and bibliometric indicators. Data Analysis and Knowledge Discovery, 6 (10), 35–45. https://doi.org/10.11925/infotech.2096-3467.2022.0075
Chi, P.-S. (2020). The field-specific citation and usage patterns of book literature in the book citation index. Research Evaluation, 29 (2), 203–214. https://doi.org/10.1093/reseval/rvz037
Chi, P.-S., & Glänzel, W. (2018). Comparison of citation and usage indicators in research assessment in scientific disciplines and journals. Scientometrics, 116 (1), 537–554. https://doi.org/10.1007/s11192-018-2708-8
Chi, P.-S., Gorraiz, J., & Glanzel, W. (2019). Comparing capture, usage and citation indicators: An altmetric analysis of journal papers in chemistry disciplines. Scientometrics, 120 (3), 1461–1473. https://doi.org/10.1007/s11192-019-03168-y
Clarkson, J. J., Janiszewski, C., & Cinelli, M. D. (2013). The desire for consumption knowledge. Journal of Consumer Research, 39 (6), 1313–1329. https://doi.org/10.1086/668535
Dickey, D., & Fuller, W. (1979). Distribution of the estimators for autoregressive time-series with a unit root. Journal of the American Statistical Association, 74 (366), 427–431. https://doi.org/10.2307/2286348
Ding, W., & Chen, C. (2014). Dynamic topic detection and tracking: A comparison of HDP, C-word, and cocitation methods. Journal of the Association for Information Science and Technology, 65 (10), 2084–2097. https://doi.org/10.1002/asi.23134
Ding, Y., Dong, X., Bu, Y., Zhang, B., Lin, K., & Hu, B. (2021). Revisiting the relationship between downloads and citations: A perspective from papers with different citation patterns in the case of the Lancet. Scientometrics, 126 (9), 7609–7621. https://doi.org/10.1007/s11192-021-04099-3
Dorta-González, P., & Dorta-González, M. I. (2023). The funding effect on citation and social attention: The UN sustainable development goals (SDGs) as a case study. Online Information Review, 47 (7), 1358–1376. https://doi.org/10.1108/OIR-05-2022-0300
Fang, Z., Costas, R., Tian, W., Wang, X., & Wouters, P. (2020). An extensive analysis of the presence of altmetric data for web of science publications across subject fields and research topics. Scientometrics, 124 (3), 2519–2549. https://doi.org/10.1007/s11192-020-03564-9
Glaenzel, W., & Gorraiz, J. (2015). Usage metrics versus altmetrics: Confusing terminology? Scientometrics, 102 (3), 2161–2164. https://doi.org/10.1007/s11192-014-1472-7
Glänzel, W., & Thijs, B. (2012). Using’ core documents’ for detecting and labelling new emerging topics. Scientometrics, 91 (2), 399–416. https://doi.org/10.1007/s11192-011-0591-7
Gorraiz, J., Gumpenberger, C., & Schloegl, C. (2014). Usage versus citation behaviours in four subject areas. Scientometrics, 101 (2), 1077–1095. https://doi.org/10.1007/s11192-014-1271-1
Granger, C. (1969). Investigating causal relations by econometric models and cross-spectral methods. Econometrica, 37 (3), 424–438. https://doi.org/10.2307/1912791
Guerrero-Bote, V. P., & Moya-Anegón, F. (2014). Relationship between downloads and citations at journal and paper levels, and the influence of language. Scientometrics, 101 (2), 1043–1065. https://doi.org/10.1007/s11192-014-1243-5
Hu, B., Ding, Y., Dong, X., Bu, Y., & Ding, Y. (2021). On the relationship between download and citation counts: An introduction of granger-causality inference. Journal of Informetrics, 15 (2), 101125. https://doi.org/10.1016/j.joi.2020.101125
Jeong, D. H., & Song, M. (2014). Time gap analysis by the topic model-based temporal technique. Journal of Informetrics, 8 (3), 776–790. https://doi.org/10.1016/j.joi.2014.07.005
Khan, M. S., & Younas, M. (2017). Analyzing readers behavior in downloading articles from IEEE digital library: A study of two selected journals in the field of education. Scientometrics, 110 (3), 1523–1537. https://doi.org/10.1007/s11192-016-2232-7
Kurtz, M. J., & Henneken, E. A. (2017). Measuring metrics—a 40-year longitudinal cross-validation of citations, downloads, and peer review in astrophysics. Journal of the Association for Information Science and Technology, 68 (3), 695–708. https://doi.org/10.1002/asi.23689
Lanham, R. A. (2007). The economics of attention: Style and substance in the age of information. University of Chicago Press. https://press.uchicago.edu/ucp/books/book/chicago/E/bo3680280.html
Lee, L. C., Lin, P. H., Chuang, Y. W., & Lee, Y. Y. (2011). Research output and economic productivity: A Granger causality test. Scientometrics, 89 (2), 465. https://doi.org/10.1007/s11192-011-0476-9
Lee, W. H. (2008). How to identify emerging research fields using scientometrics: An example in the field of information security. Scientometrics, 76 (3), 503–525. https://doi.org/10.1007/s11192-007-1898-2
Liang, Z., Mao, J., Lu, K., Ba, Z., & Li, G. (2021). Combining deep neural network and bibliometric indicator for emerging research topic prediction. Information Processing & Management, 58 (5), 102611. https://doi.org/10.1016/j.ipm.2021.102611
Lippi, G., & Favaloro, E. J. (2013). Article downloads and citations: Is there any relationship? Clinica Chimica Acta, 415 , 195–195. https://doi.org/10.1016/j.cca.2012.10.037
Luan, C., Deng, S., & Allison, J. R. (2022). Mutual granger “causality” between scientific instruments and scientific publications. Scientometrics, 127 (11), 6209–6229. https://doi.org/10.1007/s11192-022-04516-1
Markusova, V., Bogorov, V., & Libkind, A. (2018). Usage metrics vs classical metrics: Analysis of Russia’s research output. Scientometrics, 114 (2), 593–603. https://doi.org/10.1007/s11192-017-2597-2
Masoumi, N., & Khajavi, R. (2023). A fuzzy classifier for evaluation of research topics by using keyword co-occurrence network and sponsors information. Scientometrics, 128 (3), 1485–1512. https://doi.org/10.1007/s11192-022-04618-w
McGillivray, B., & Astell, M. (2019). The relationship between usage and citations in an open access mega-journal. Scientometrics, 121 (2), 817–838. https://doi.org/10.1007/s11192-019-03228-3
Miao, Z., Du, J., Dong, F., Liu, Y., & Wang, X. (2020). Identifying technology evolution pathways using topic variation detection based on patent data: A case study of 3D printing. Futures, 118 , 102530. https://doi.org/10.1016/j.futures.2020.102530
Park, I., Lee, K., & Yoon, B. (2015). Exploring promising research frontiers based on knowledge maps in the solar cell technology field. Sustainability, 7 (10), 13660–13689. https://doi.org/10.3390/su71013660
Porter, A. L., Garner, J., Carley, S. F., & Newman, N. C. (2019). Emergence scoring to identify frontier R&D topics and key players. Technological Forecasting and Social Change, 146 , 628–643. https://doi.org/10.1016/j.techfore.2018.04.016
Rowlands, I., & Nicholas, D. (2007). The missing link: Journal usage metrics. Aslib Proceedings, 59 (3), 222–228. https://doi.org/10.1108/00012530710752025
Schloegl, C., & Gorraiz, J. (2010). Comparison of citation and usage indicators: The case of oncology journals. Scientometrics, 82 (3), 567–580. https://doi.org/10.1007/s11192-010-0172-1
Schloegl, C., Gorraiz, J., Gumpenberger, C., Jack, K., & Kraker, P. (2014). Comparison of downloads, citations and readership data for two information systems journals. Scientometrics, 101 (2), 1113–1128. https://doi.org/10.1007/s11192-014-1365-9
Small, H., Boyack, K. W., & Klavans, R. (2014). Identifying emerging topics in science and technology. Research Policy, 43 (8), 1450–1467. https://doi.org/10.1016/j.respol.2014.02.005
Tahamtan, I., & Bornmann, L. (2019). What do citation counts measure? An updated review of studies on citations in scientific documents published between 2006 and 2018. Scientometrics, 121 (3), 1635–1684. https://doi.org/10.1007/s11192-019-03243-4
Thelwall, M., & Maflahi, N. (2015). Are scholarly articles disproportionately read in their own country? An analysis of mendeley readers. Journal of the Association for Information Science and Technology, 66 (6), 1124–1135. https://doi.org/10.1002/asi.23252
Tian, W., Fang, Z., Wang, X., & Costas, R. (2024). A multi-dimensional analysis of usage counts, mendeley readership, and citations for journal and conference papers. Scientometrics, 129 (2), 985–1013. https://doi.org/10.1007/s11192-023-04909-w
Tian, W., Wang, Y., & Wang, X. (2023). Granger causality between usage counts and publication numbers. In Proceedings of the 19th international conference on scientometrics and informetrics - (ISSI 2023) 2-5 July 2023, Bloomington, Indiana, USA.
Tian, W., Hu, Z., & Wang, X. (2019). Upgrading from 3G to 5G: Topic evolution and persistence among scientists . In Proceedings of the 17th international conference on scientometrics and informetrics (pp. 1156–1165)
Uddin, S., & Khan, A. (2016). The impact of author-selected keywords on citation counts. Journal of Informetrics, 10 (4), 1166–1177. https://doi.org/10.1016/j.joi.2016.10.004
Vaughan, L., Tang, J., & Yang, R. (2017). Investigating disciplinary differences in the relationships between citations and downloads. Scientometrics, 111 (3), 1533–1545. https://doi.org/10.1007/s11192-017-2308-z
Waltman, L. (2016). A review of the literature on citation impact indicators. Journal of Informetrics, 10 (2), 365–391. https://doi.org/10.1016/j.joi.2016.02.007
Wan, J., Hua, P., Rousseau, R., & Sun, X. (2010). The journal download immediacy index (DII): Experiences using a Chinese full-text database. Scientometrics, 82 (3), 555–566. https://doi.org/10.1007/s11192-010-0171-2
Wang, X., & Fang, Z. (2016). Detecting and tracking the real-time hot topics: A study on computational neuroscience. arXiv: 1608.05517
Wang, X., Liu, C., Mao, W., & Fang, Z. (2015). The open access advantage considering citation, article usage and social media attention. Scientometrics, 103 (3), 1149–1149. https://doi.org/10.1007/s11192-015-1589-3
Wang, X., Mao, W., Xu, S., & Zhang, C. (2014). Usage history of scientific literature: Nature metrics and metrics of nature publications. Scientometrics, 98 (3), 1923–1933. https://doi.org/10.1007/s11192-013-1167-5
Wang, X., Wang, Z., & Xu, S. (2013). Tracing scientist’s research trends realtimely. Scientometrics, 95 (2), 717–729. https://doi.org/10.1007/s11192-012-0884-5
Wu, H., Yi, H., & Li, C. (2021). An integrated approach for detecting and quantifying the topic evolutions of patent technology: A case study on graphene field. Scientometrics, 126 (8), 6301–6321. https://doi.org/10.1007/s11192-021-04000-2
Xu, H., Winnink, J., Yue, Z., Zhang, H., & Pang, H. (2021). Multidimensional scientometric indicators for the detection of emerging research topics. Technological Forecasting and Social Change, 163 , 120490. https://doi.org/10.1016/j.techfore.2020.120490
Ye, G., Wang, C., Wu, C., Peng, Z., Wei, J., Song, X., Tan, Q., & Wu, L. (2023). Research frontier detection and analysis based on research grants information: A case study on health informatics in the US. Journal of Informetrics, 17 (3), 101421. https://doi.org/10.1016/j.joi.2023.101421
Zahedi, Z., & Haustein, S. (2018). On the relationships between bibliographic characteristics of scientific documents and citation and mendeley readership counts: A large-scale analysis of web of science publications. Journal of Informetrics, 12 (1), 191–202. https://doi.org/10.1016/j.joi.2017.12.005
Zhang, C., Bu, Y., Ding, Y., & Xu, J. (2018). Understanding scientific collaboration: Homophily, transitivity, and preferential attachment. Journal of the Association for Information Science and Technology, 69 (1), 72–86. https://doi.org/10.1002/asi.23916
Zhang, G., Shang, F., Wang, L., Xie, W., Jia, P., Jiang, C., & Wang, X. (2023). Is peer review duration shorter for attractive manuscripts? Journal of Information Science . https://doi.org/10.1177/01655515231174382
Zhang, G., Wang, Y., Xie, W., Du, H., Jiang, C., & Wang, X. (2021). The open access usage advantage: A temporal and spatial analysis. Scientometrics, 126 (7), 6187–6199. https://doi.org/10.1007/s11192-020-03836-4
Zhao, S. X., Lou, W., Tan, A. M., & Yu, S. (2018). Do funded papers attract more usage? Scientometrics, 115 (1), 153–168. https://doi.org/10.1007/s11192-018-2662-5
Zong, Q., Fan, L., Xie, Y., & Huang, J. (2020). The relationship of polarity of post-publication peer review to citation count evidence from publons. Online Information Review, 44 (3), 583–602. https://doi.org/10.1108/OIR-01-2019-0027
Download references
Acknowledgements
The present study is an extended version of a paper presented at the 19th International Conference on Scientometrics and Informetrics 2023 (ISSI 2023), Bloomington, Indiana (USA), 2-5 July 2023 (Tian et al., 2023 ). This study is partially supported by the National Natural Science Foundation of China (71974029, 71974030) and LiaoNing Revitalization Talents Program (XLYC2007149). Wencan Tian is financially supported by the China Scholarship Council (202106060134). The authors are grateful to the anonymous reviewers for their helpful comments and suggestions.
Author information
Authors and affiliations.
WISE Lab, Institute of Science of Science and S&T Management, Dalian University of Technology, Dalian, China
Wencan Tian, Yongzhen Wang, Guangyao Zhang & Xianwen Wang
Institute for Science Technology and Society, South China Normal University, Guangzhou, China
School of Business, Shandong University, Weihai, China
UNU-MERIT, Maastricht University, Maastricht, The Netherlands
Guangyao Zhang
You can also search for this author in PubMed Google Scholar
Corresponding author
Correspondence to Xianwen Wang .
Ethics declarations
Conflict of interest.
The authors declare that they have no conflicts of interest.
Additional information
Publisher's note.
Springer Nature remains neutral with regard to jurisdictional claims in published maps and institutional affiliations.
See Fig. 7 .
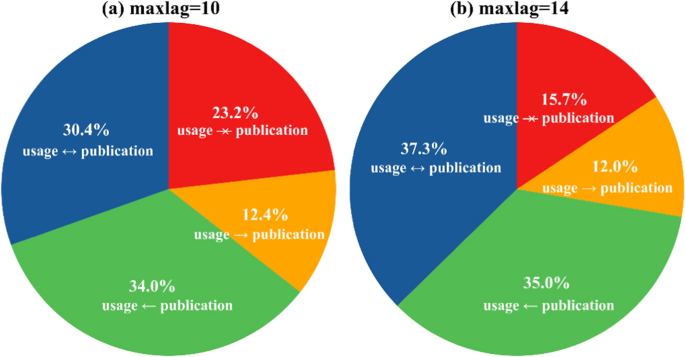
Results of robustness test. Both reducing and raising the maximum lag time demonstrate a strong statistically significant causal association between article usage and publication counts, demonstrating that the results from this study are robust. Specifically, when the maximum lag was set to 10, 76.8% of the topics exhibited an inherent logical link between article usage and publication counts; when the maximum lag was set to 14, this proportion was 84.3%
Rights and permissions
Springer Nature or its licensor (e.g. a society or other partner) holds exclusive rights to this article under a publishing agreement with the author(s) or other rightsholder(s); author self-archiving of the accepted manuscript version of this article is solely governed by the terms of such publishing agreement and applicable law.
Reprints and permissions
About this article
Tian, W., Wang, Y., Hu, Z. et al. Does Granger causality exist between article usage and publication counts? A topic-level time-series evidence from IEEE Xplore. Scientometrics (2024). https://doi.org/10.1007/s11192-024-05038-8
Download citation
Received : 07 August 2023
Accepted : 19 April 2024
Published : 10 May 2024
DOI : https://doi.org/10.1007/s11192-024-05038-8
Share this article
Anyone you share the following link with will be able to read this content:
Sorry, a shareable link is not currently available for this article.
Provided by the Springer Nature SharedIt content-sharing initiative
- Article usage data
- Publication counts
- IEEE Xplore
- Time-series
- Granger causality test
- Find a journal
- Publish with us
- Track your research
Featured Topics
Featured series.
A series of random questions answered by Harvard experts.
Explore the Gazette
Read the latest.
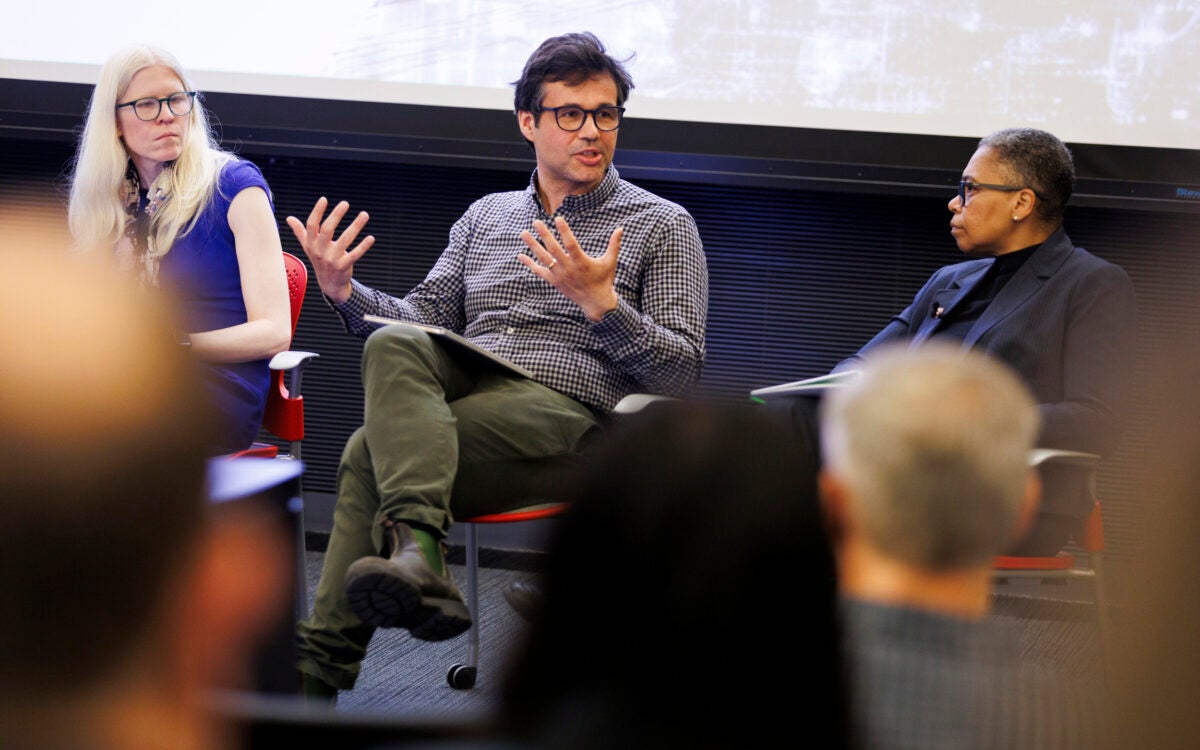
What is ‘original scholarship’ in the age of AI?
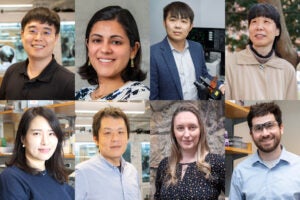
Complex questions, innovative approaches
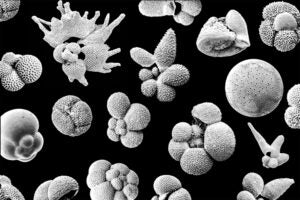
Early warning sign of extinction?
Epic science inside a cubic millimeter of brain.
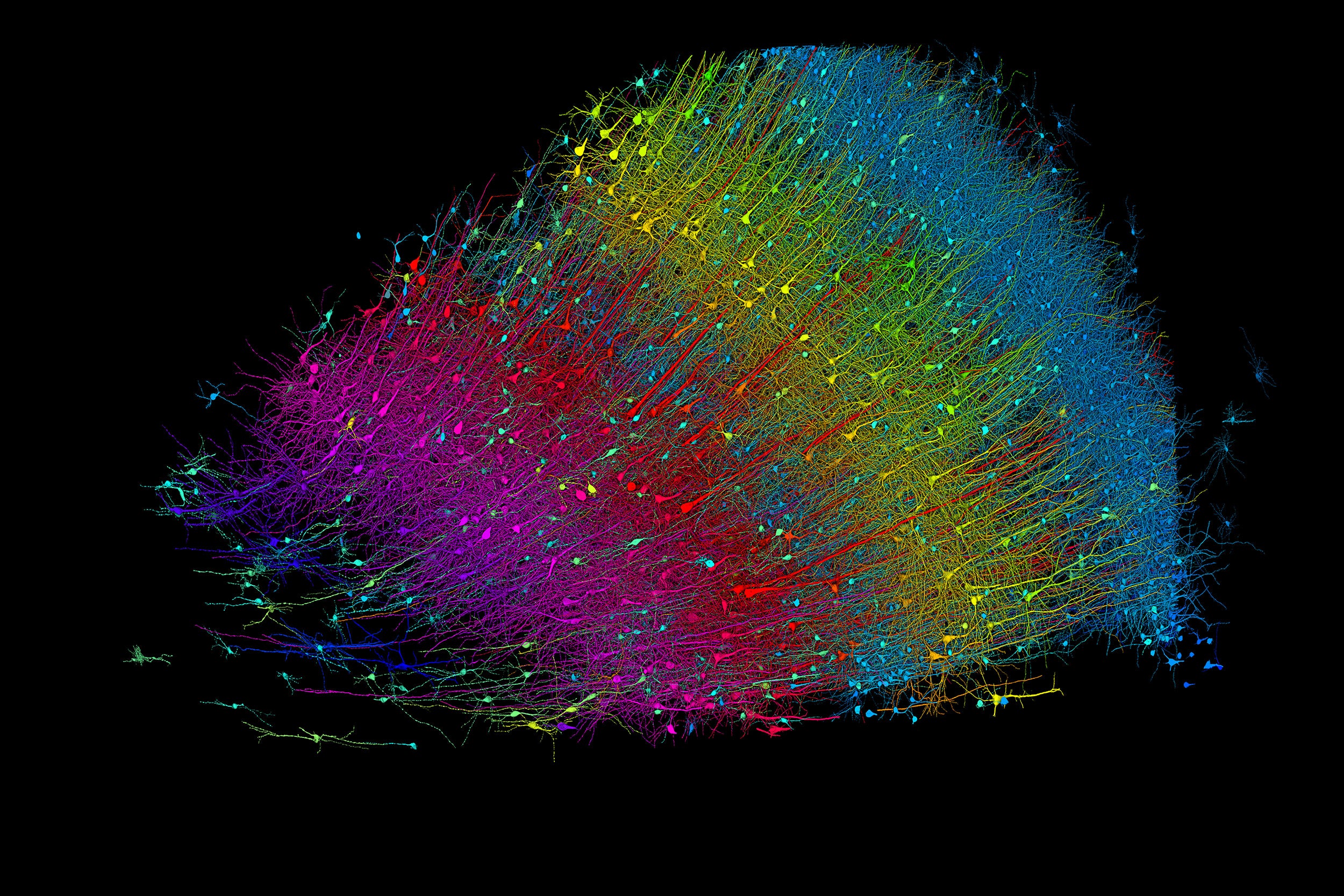
Six layers of excitatory neurons color-coded by depth.
Credit: Google Research and Lichtman Lab
Anne J. Manning
Harvard Staff Writer
Researchers publish largest-ever dataset of neural connections
A cubic millimeter of brain tissue may not sound like much. But considering that that tiny square contains 57,000 cells, 230 millimeters of blood vessels, and 150 million synapses, all amounting to 1,400 terabytes of data, Harvard and Google researchers have just accomplished something stupendous.
Led by Jeff Lichtman, the Jeremy R. Knowles Professor of Molecular and Cellular Biology and newly appointed dean of science , the Harvard team helped create the largest 3D brain reconstruction to date, showing in vivid detail each cell and its web of connections in a piece of temporal cortex about half the size of a rice grain.
Published in Science, the study is the latest development in a nearly 10-year collaboration with scientists at Google Research, combining Lichtman’s electron microscopy imaging with AI algorithms to color-code and reconstruct the extremely complex wiring of mammal brains. The paper’s three first co-authors are former Harvard postdoc Alexander Shapson-Coe, Michał Januszewski of Google Research, and Harvard postdoc Daniel Berger.
The ultimate goal, supported by the National Institutes of Health BRAIN Initiative , is to create a comprehensive, high-resolution map of a mouse’s neural wiring, which would entail about 1,000 times the amount of data the group just produced from the 1-cubic-millimeter fragment of human cortex.
“The word ‘fragment’ is ironic,” Lichtman said. “A terabyte is, for most people, gigantic, yet a fragment of a human brain — just a minuscule, teeny-weeny little bit of human brain — is still thousands of terabytes.”
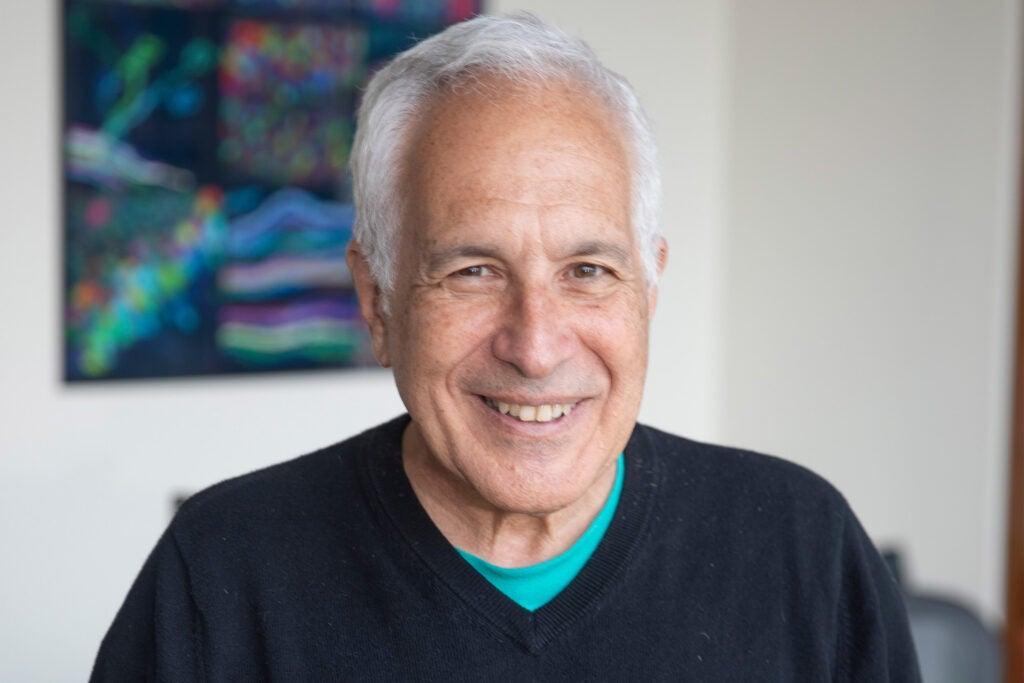
Jeff Lichtman.
Kris Snibbe/Harvard Staff Photographer
The latest map contains never-before-seen details of brain structure, including a rare but powerful set of axons connected by up to 50 synapses. The team also noted oddities in the tissue, such as a small number of axons that formed extensive whorls. Because the sample was taken from a patient with epilepsy, the researchers don’t know whether such formations are pathological or simply rare.
Lichtman’s field is connectomics, which seeks to create comprehensive catalogs of brain structure, down to individual cells. Such completed maps would unlock insights into brain function and disease, about which scientists still know very little.
Google’s state-of-the-art AI algorithms allow for reconstruction and mapping of brain tissue in three dimensions. The team has also developed a suite of publicly available tools researchers can use to examine and annotate the connectome.
“Given the enormous investment put into this project, it was important to present the results in a way that anybody else can now go and benefit from them,” said Google collaborator Viren Jain.
Next the team will tackle the mouse hippocampal formation, which is important to neuroscience for its role in memory and neurological disease.
Share this article
You might like.
Symposium considers how technology is changing academia
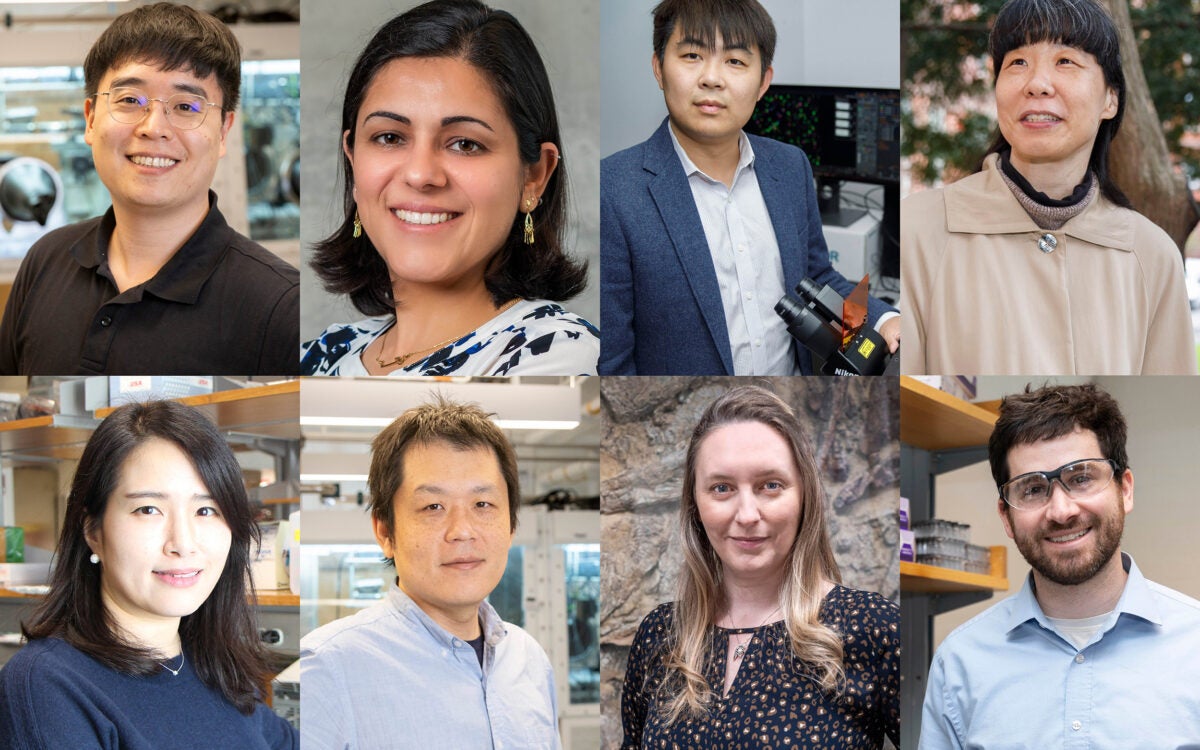
Seven projects awarded Star-Friedman Challenge grants
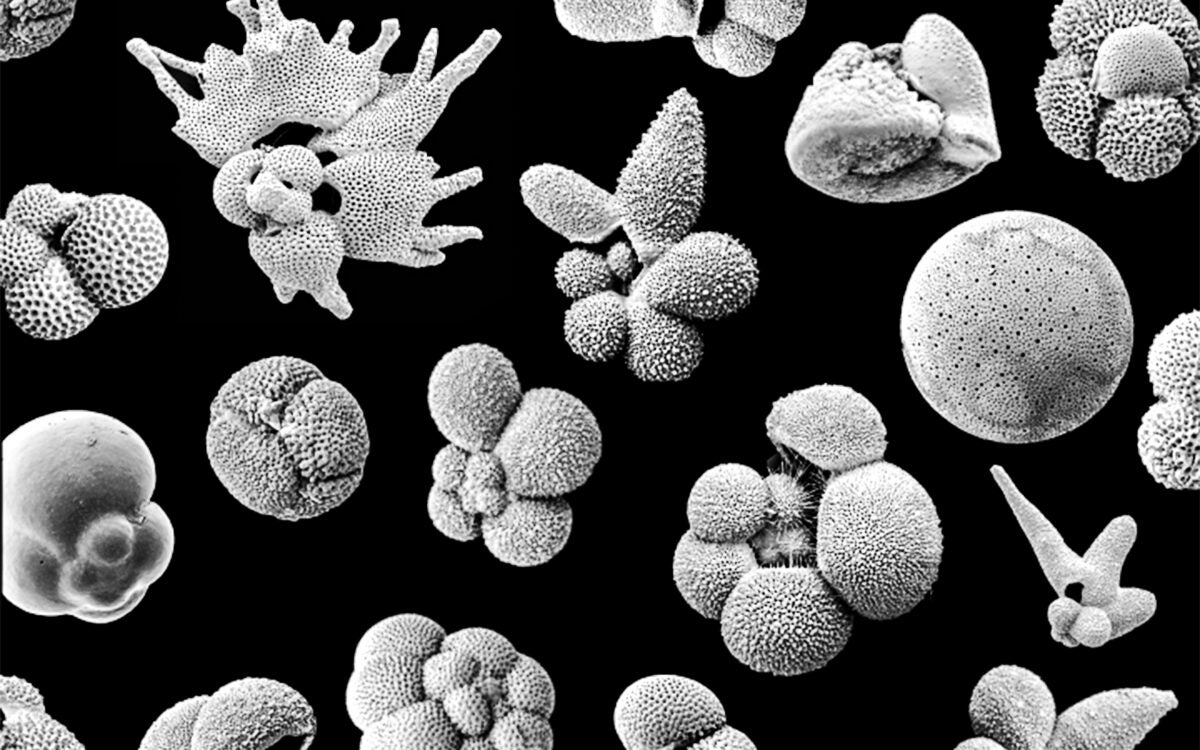
Fossil record stretching millions of years shows tiny ocean creatures on the move before Earth heats up
How far has COVID set back students?
An economist, a policy expert, and a teacher explain why learning losses are worse than many parents realize
Excited about new diet drug? This procedure seems better choice.
Study finds minimally invasive treatment more cost-effective over time, brings greater weight loss
News, Views & Insights
- Best of Blog (70)
- Astronomy (28)
- Computational Thinking (64)
- Current Events (36)
- Data Analysis and Visualization (138)
- Data Repository (11)
- Design (24)
- Developer Insights (76)
- Digital Humanities (7)
- Education (193)
- Events (44)
- Finance (24)
- Function Repository (12)
- Geosciences (12)
- High-Performance Computing (12)
- History (18)
- Image Processing (48)
- Machine Learning (16)
- Mathematica News (112)
- Mathematica Q&A (13)
- Mathematics (129)
- New Technology (41)
- Other Application Areas (135)
- Raspberry Pi (18)
- Recreational Computation (163)
- Software Development (35)
- System Modeler (49)
- Uncategorized (1)
- Wolfram Cloud (24)
- Wolfram Community (19)
- Wolfram Demonstrations Project (31)
- Wolfram Language (297)
- Wolfram News (278)
- Wolfram Notebooks (37)
- Wolfram U (28)
- Wolfram|Alpha (48)
- Wolfram|One (7)
From Data to Discovery: Studying Computational Biology with Wolfram
Navigating quantum computing: accelerating next-generation innovation, food and sun: wolfram language recipe graphs for the solar eclipse, marking a milestone: four years of daily study groups.
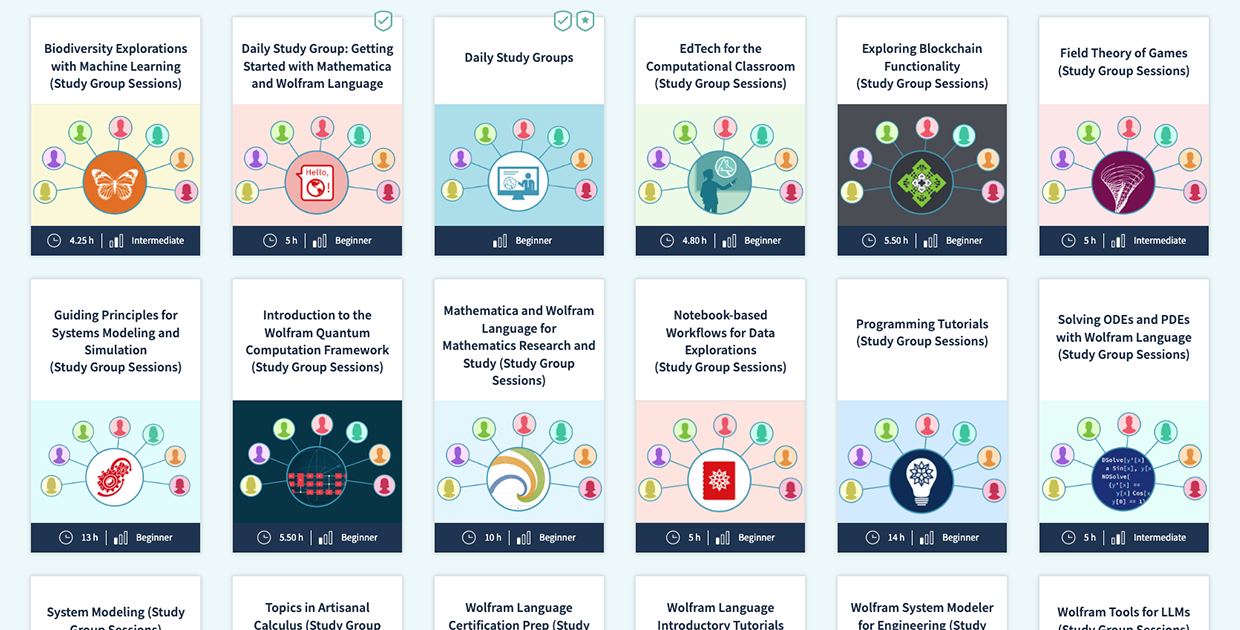
Four years ago, as the COVID pandemic wreaked havoc to class and event schedules, instructors and organizations were scrambling to create meaningful learning opportunities for students. In April 2020, Stephen Wolfram challenged the Wolfram U team to establish a unique online program for building computational skills with Daily Study Groups . The program was enthusiastically received by learners of all ages, and, after recently completing our 50th Daily Study Group, this is the perfect time to reflect on the program, celebrate a milestone and look ahead to future developments.
What Are Wolfram Daily Study Groups?
The mission behind Daily Study Groups was pretty simple. They were to facilitate learning cohorts that met together online for one hour daily, Monday through Friday, for one or more weeks. They were to offer interesting, timely and fun computational topics that provided hands-on access to the latest Wolfram technology and a Study Group instructor who was knowledgeable in the field. They were to provide support to online sessions with helpful staff who assisted in polling the group to review key concepts, introducing practice problems and answering questions. Finally, the Daily Study Groups would offer certifications to those who went the extra mile and successfully completed quizzes, practice problems and exams. After running almost five hundred daily sessions for thousands of participants, we can call the program a huge success!
What Do You Study?
Our first Daily Study Group was a primer on learning Wolfram Language . The Study Groups that have proven to be the most popular are based on programming topics (such as Wolfram Language Basics , Programming Proficiency and Creating Custom User Interfaces) and college-level mathematics courses such as calculus, differential equations, linear algebra and statistics. Computational topics are also well-represented in Daily Study Groups in areas of data science , cryptography , machine learning , signal processing and game theory .
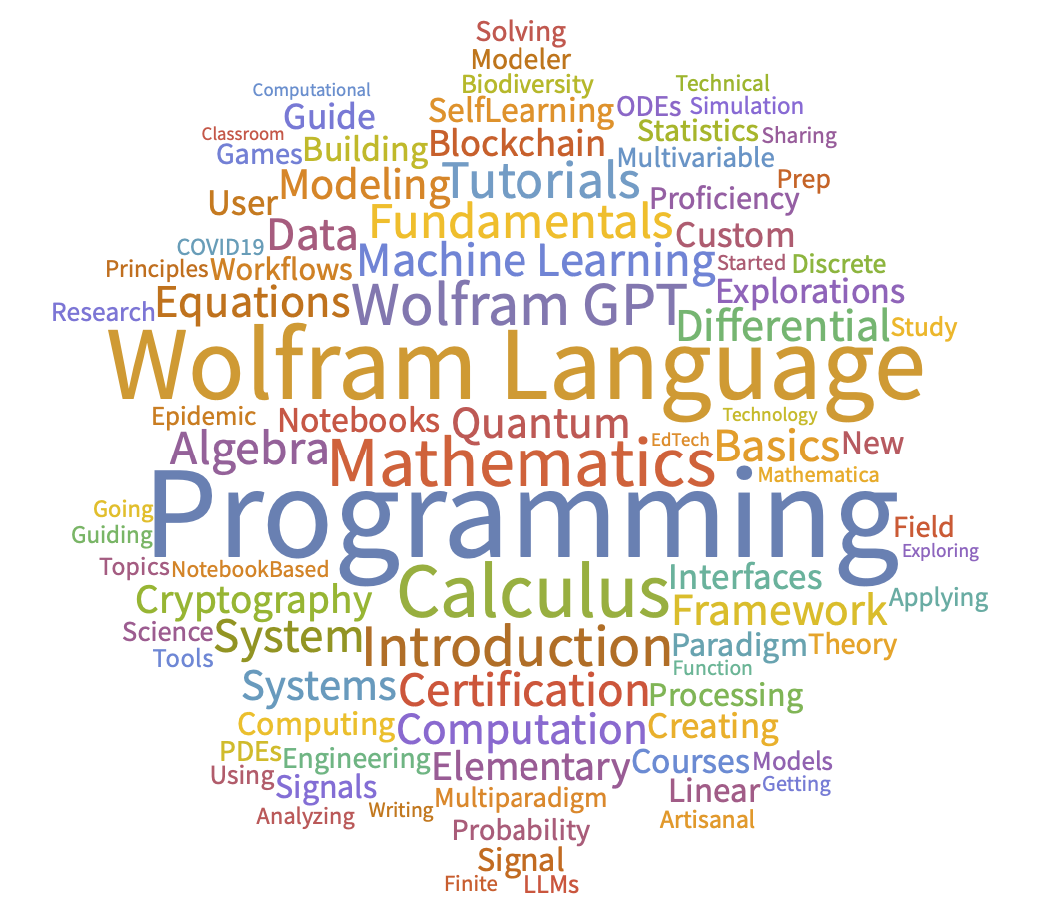
Are Trending Hot Topics Covered?
Daily Study Groups are a great way to learn more about trending topics and technology, and Wolfram users are always curious to explore the latest. During the pandemic, we hosted the Study Groups COVID-19 Data Analysis and Visualization , Biodiversity Explorations with Machine Learning and Building and Applying Epidemiological Models . Daily Study Groups have helped participants learn about cutting-edge topics like quantum computing, blockchain and Wolfram GPT; our 50th Daily Study Group was all about LLM functionality. The following poll shows the range of interest in different tools at this Study Group:
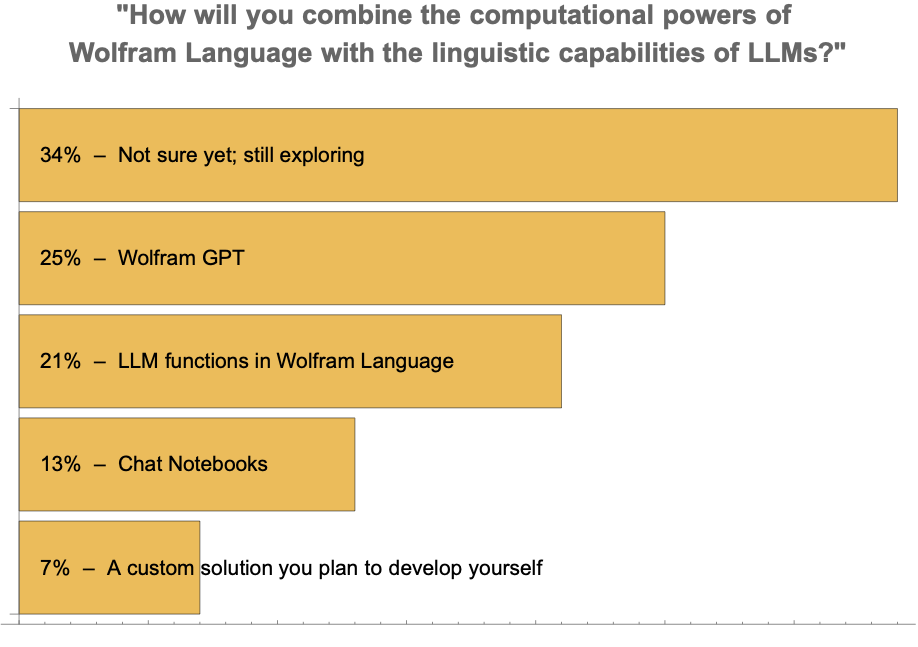
Early Access to Wolfram Interactive Courses
Joining the Daily Study Groups can also sometimes provide access to pre-released course content, giving participants a sneak peak at upcoming courses and helping us to collect valuable feedback before a full public release. Our interactive courses cover a wide range of computational topics, and we discovered that running Daily Study Groups based on these courses was a great way to further engage students and encourage them to complete coursework and earn Wolfram certifications. A recent Study Group followed this model for Introduction to Finite Mathematics . The Study Group followed lessons from the interactive course and participants were the first to have access to course quizzes and exercises and even prepare for the final exam. We’re pleased that many from the Study Group went on to pass the exam and earn a Level 1 certification for proficiency in finite mathematics.
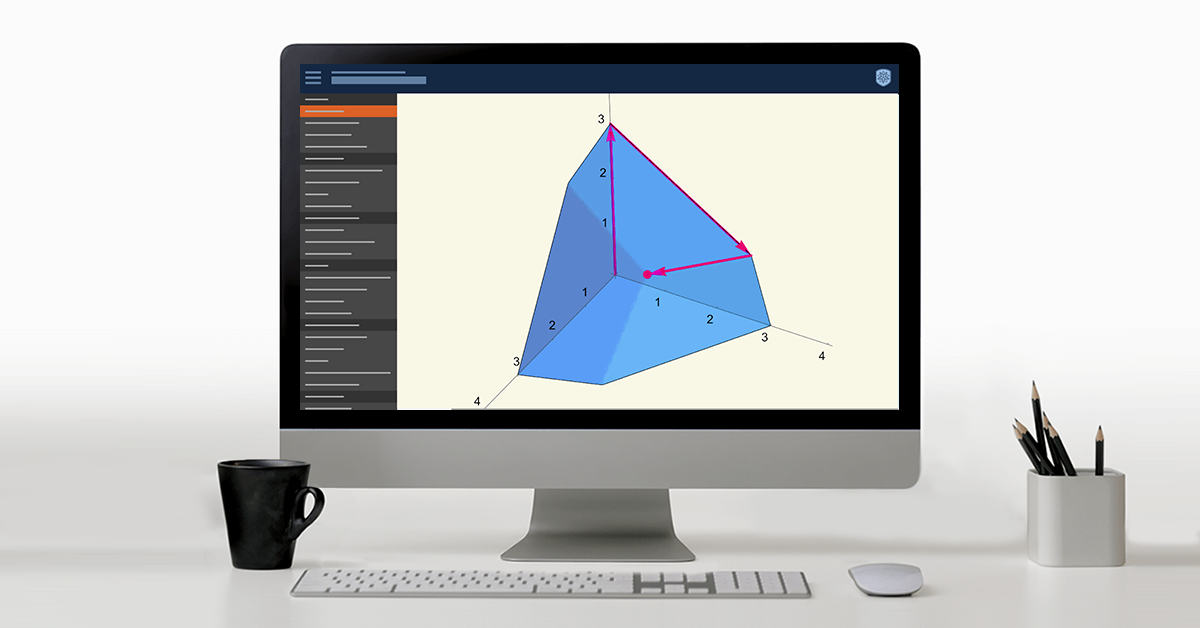
Community Engagement
Each Daily Study Group establishes a Wolfram U group discussion on Wolfram Community . Many of these discussions have grown to be incredibly active and useful to Community members. Check out the recent discussion threads, and keep in mind that you only get full access to Study Group materials, including lesson notebooks, videos, quizzes, certification opportunities and more, when you sign up for a Wolfram Daily Study Group.
Learning and Certifications
More than 2,300 Wolfram certifications have been granted through Daily Study Group programs so far, and we look forward to awarding many more. Level 2 certification for applied expertise in Wolfram Language programming is a brand-new certification level offered by Wolfram U, and we were pleased to introduce it in a Daily Study Group earlier this year. Congratulations to Michael Ulrey, who is the very first to be recognized with the Level 2 certificate for his project work with Bell’s theorem, visualizing pertinent sets of correlations. We know there are many Wolfram Language users out there with Level 2–caliber project work. I hope you’ll be ready to promote your skills and knowledge by applying for Wolfram certifications, which are easily sharable to professional profile pages and applications.
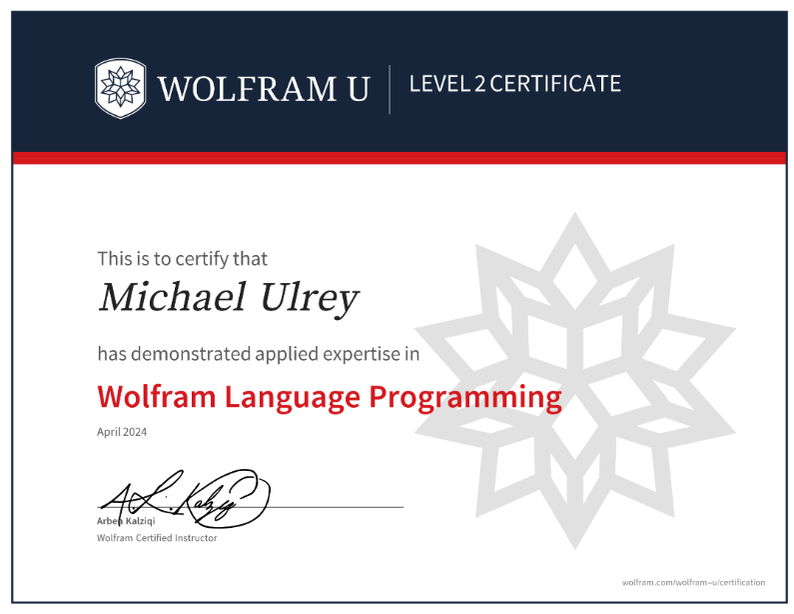
A wide variety of certifications is available. Participating in a Daily Study Group is an enjoyable way to complete coursework and earn certifications, but many certifications are obtainable through independent completion of courses at Wolfram U, allowing you to manage learning time at your own pace and schedule. I encourage you to browse the full catalog and find topics of interest to you. The following is a sample of available Wolfram certifications:
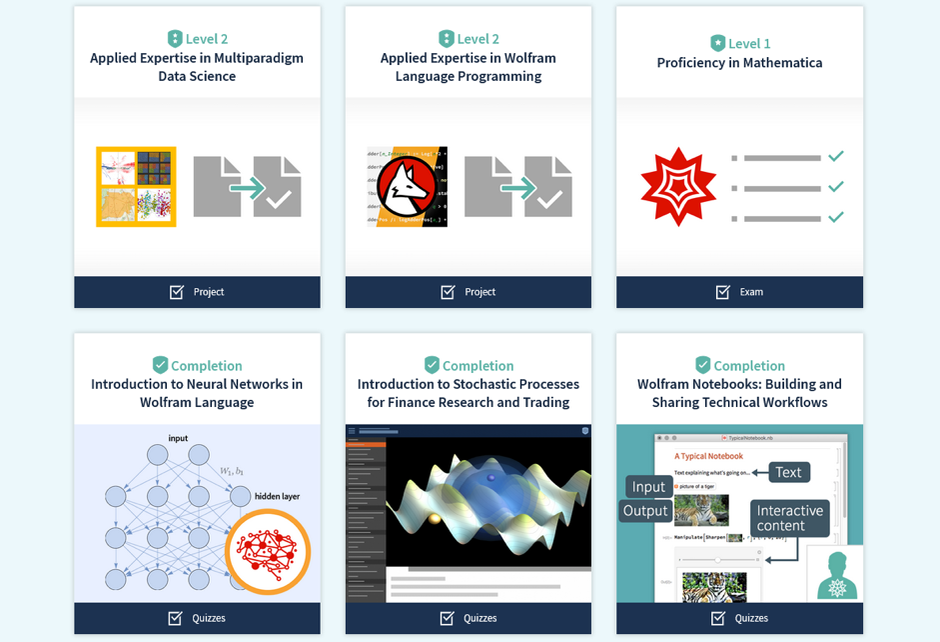
What Participants Are Saying
One of the best things about being part of a Daily Study Group is hearing how helpful they are to so many people. We read all our survey comments, and it’s a pleasure to receive this kind of feedback:
- “As someone who has teaching experience about 20+ years, these sessions provided new insights, introduced some new topics and inspired me to explore more.”
- “I am a student who has benefited greatly from your instruction since the Daily Study Group: Introduction to Multivariable Calculus. I want to express my sincere gratitude for your dedication to our education. Your willingness to answer our questions during class and carefully consider our survey responses has been invaluable.”
- “That was a wonderful study group idea… I really loved it and I hope you will have more of this kind of study group. It was clear that the presenters had spent many hours preparing their notebooks, and prior to that researching the topics… which made a wonderful opportunity for the listener to brush up on a topic, or learn a new topic, and see how it is implemented in Wolfram Language. You had me mesmerized. More, more, more… Thank you.”
What’s Next?
More courses, more computational explorations and more learning! You can count on Wolfram U and Daily Study Groups to keep up with expanding technologies and the latest content from Wolfram. Watch for upcoming Study Groups in complex analysis, electric circuits, computational physics, machine learning, generative AI and, of course, opportunities for getting started and building skills with Wolfram Language. Consult our current Study Group schedule any time to see the latest.
Thanks to a Fantastic Team
At Wolfram, we’re fortunate to be surrounded by colleagues with specialized fields of interests and experience in academia and teaching. I want to take this opportunity to thank all the Study Group instructors, teaching assistants and Wolfram U staff who have helped to provide such a rich resource to so many over the past four years. Running a daily online program is a big task and requires much coordination and teamwork. Thanks also to the folks from all sorts of backgrounds, from all around the world, who have participated in Daily Study Groups. The secret to the success of Wolfram Daily Study Groups comes down to a combination of talented instructors and staff, reliable technology, motivated students and the power of Wolfram Language.
! Please enter your comment (at least 5 characters).
! Please enter your name.
! Please enter a valid email address.
Related Posts
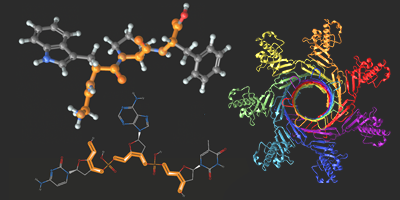
Speedy, secure, sustainable -- that's the future of telecom
New study uncovers technologies that could unveil energy-efficient information processing and sophisticated data security.
Advanced information processing technologies offer greener telecommunications and strong data security for millions, a study led by University of Maryland (UMD) researchers revealed.
A new device that can process information using a small amount of light could enable energy-efficient and secure communications. Work led by You Zhou, an assistant professor in UMD's Department of Materials Science and Engineering (MSE), in collaboration with researchers at the U.S. Department of Energy's (DOE) Brookhaven National Laboratory, was published today in the journal Nature Photonics.
Optical switches, the devices responsible for sending information via telephone signals, rely on light as a transmission medium and on electricity as a processing tool, requiring an extra set of energy to interpret the data. A new alternative engineered by Zhou uses only light to power a full transmission, which could improve speed and energy efficiency for telecommunications and computation platforms.
Early tests of this technology have shown significant energy improvements. While conventional optical switches require between 10 to 100 femtojoules to enable a communication transmission, Zhou's device consumes one hundred times less energy, which is only one tenth to one femtojoule. Building a prototype that enables information processing using small amounts of light, via a material's property known as "non-linear response," paved the way for new opportunities in his research group.
"Achieving strong non-linearity was unexpected, which opened a new direction that we were not previously exploring: quantum communications," said Zhou.
To build the device, Zhou used the Quantum Material Press (QPress) at the Center for Functional Nanomaterials (CFN), a DOE Office of Science user facility at Brookhaven Lab that offers free access to world-class equipment for scientists conducting open research. The QPress is an automated tool for synthesizing quantum materials with layers as thin as a single atom.
"We have been collaborating with Zhou's group for several years. They are one of the earliest adopters of our QPress modules, which include an exfoliator, cataloger, and stacker," said co-author Suji Park, a staff scientist in the Electronic Nanomaterials Group at CFN. "Specifically, we have provided high-quality exfoliated flakes tailored to their requests, and we worked together closely to optimize the exfoliation conditions for their materials. This partnership has significantly enhanced their sample fabrication process."
Next up, Zhou's research team aims to increase energy efficiency down to the smallest amount of electromagnetic energy, a main challenge in enabling the so-called quantum communications, which offer a promising alternative for data security.
In the wake of rising cyberattacks, building sophisticated protection against hackers has grown scientific interest. Data transmitted over conventional communication channels can be read and copied without leaving a trace, which cost thousands of breaches for 350 million users last year, according to a recent Statista report.
Quantum communications, on the other hand, offer a promising alternative as they encode the information using light, which cannot be intercepted without altering its quantum state. Zhou's method to improve materials' nonlinearity is a step closer to enabling those technologies.
This study was supported by the DOE Office of Science and the National Science Foundation.
Editor's Note: This news release is being jointly issued by the University of Maryland and Brookhaven National Laboratory.
- Telecommunications
- Quantum Computing
- Information Technology
- Computers and Internet
- Information and communication technologies
- Electric power
- Nuclear fusion
- Quantum entanglement
- Cryptography
- Photography
Story Source:
Materials provided by DOE/Brookhaven National Laboratory . Original written by Daniela Benites, University of Maryland. Note: Content may be edited for style and length.
Journal Reference :
- Liuxin Gu, Lifu Zhang, Ruihao Ni, Ming Xie, Dominik S. Wild, Suji Park, Houk Jang, Takashi Taniguchi, Kenji Watanabe, Mohammad Hafezi, You Zhou. Giant optical nonlinearity of Fermi polarons in atomically thin semiconductors . Nature Photonics , 2024; DOI: 10.1038/s41566-024-01434-x
Cite This Page :
Explore More
- Nature's 3D Printer: Bristle Worms
- Giant ' Cotton Candy' Planet
- A Young Whale's Journey
- No Inner Voice Linked to Poorer Verbal Memory
- Bird Flu A(H5N1) Transmitted from Cow to Human
- Universe's Oldest Stars in Our Galactic Backyard
- Polygenic Embryo Screening for IVF: Opinions
- VR With Cinematoghraphics More Engaging
- 2023 Was the Hottest Summer in 2000 Years
- Fastest Rate of CO2 Rise Over Last 50,000 Years
Trending Topics
Strange & offbeat.
- Frontiers in Applied Mathematics and Statistics
- Mathematics of Computation and Data Science
- Research Topics
Fundamental Mathematical Topics in Data Science
Total Downloads
Total Views and Downloads
About this Research Topic
Since the turn of the century, there has been a surge of interest in research on data science. Techniques related to data science have become the main driving force behind numerous areas of industry and many new research directions have been developed, with new scientific questions raised from the study of ...
Keywords : sparse representation, reproducing kernels, machine learning, image processing, non-convex optimization
Important Note : All contributions to this Research Topic must be within the scope of the section and journal to which they are submitted, as defined in their mission statements. Frontiers reserves the right to guide an out-of-scope manuscript to a more suitable section or journal at any stage of peer review.
Topic Editors
Topic coordinators, recent articles, submission deadlines.
Submission closed.
Participating Journals
Total views.
- Demographics
No records found
total views article views downloads topic views
Top countries
Top referring sites, about frontiers research topics.
With their unique mixes of varied contributions from Original Research to Review Articles, Research Topics unify the most influential researchers, the latest key findings and historical advances in a hot research area! Find out more on how to host your own Frontiers Research Topic or contribute to one as an author.
Department of Physics
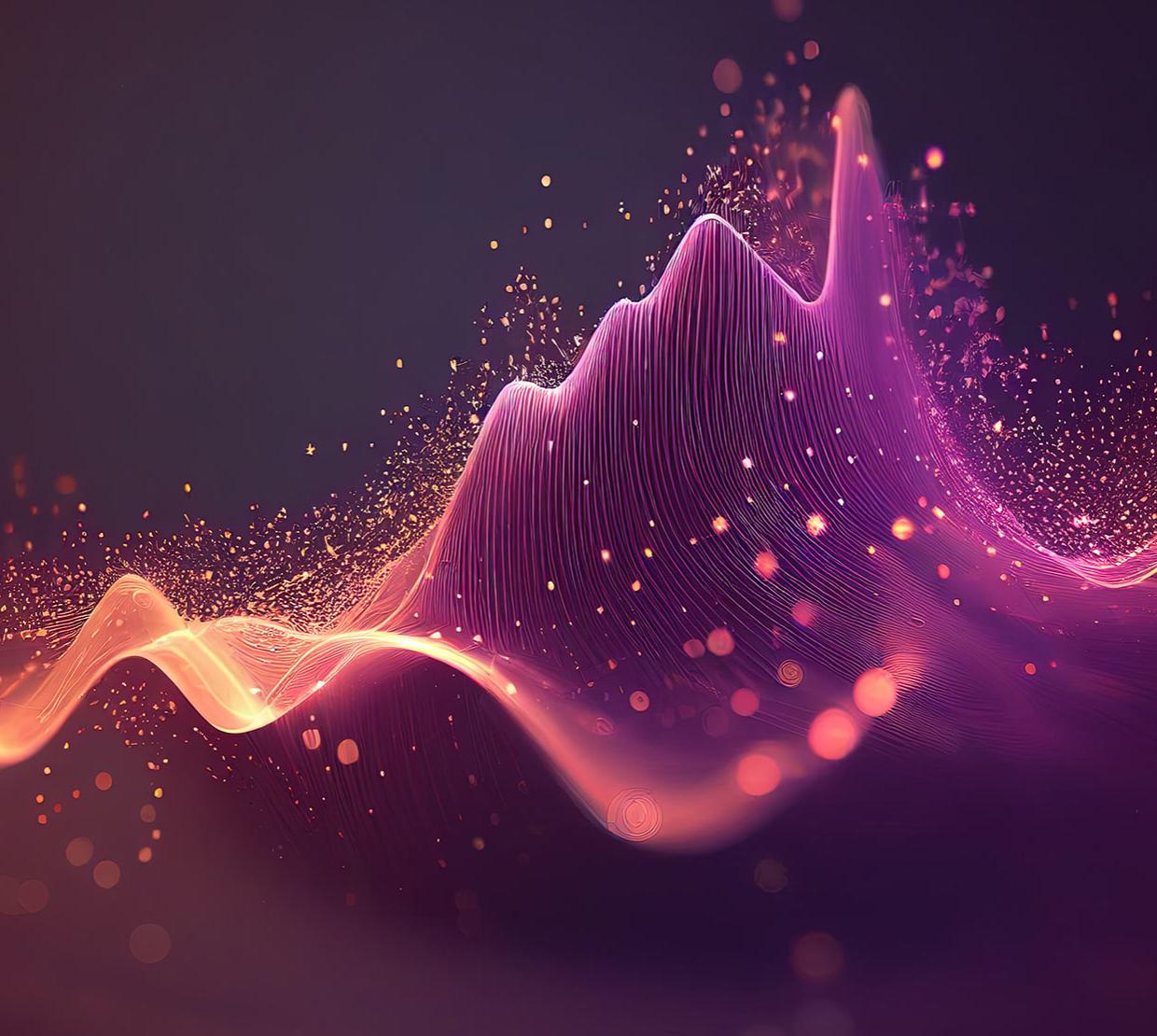
College of Science hosts Inaugural Research Showcase
Extending the reach and impact of science.
Tuesday, May 21, 2024 11 a.m. – 2 p.m. Memorial Union Multipurpose Room 13
This event will feature SciRIS awardee presentations, panel discussion on artificial intelligence in the College of Science, and posters and science education demonstrations by Oregon Museum of Science and Industry (OMSI) Fellows.
Schedule of Events
11 – 11:10 a.m..
Welcome and introduction from Vrushali Bokil , Associate Dean of Research and Graduate Studies
11:10 – noon
SciRIS Awards Showcase
The College of Science Research and Innovation Seed (SciRIS) program funds projects based on collaborative research within our community and beyond. The program awards seed funding for high-impact collaborative proposals that build teams, pursue fundamental discoveries and create societal impact. Founded in 2018 , SciRIS accelerates the pace of research, discovery and innovation in the College of Science by enabling scientists to work across an array of disciplines in a mentored environment. We showcase some of the recent awards made under this program.
Francis Chan : “The Hypoxic Barrier Hypothesis: have we missed a fundamental dynamic of oxygen use in microbes and ecosystems?”
Kim Halsey : “Leveraging volatile organic compounds to detect cyanotoxin contamination in Oregon lakes”
Maude David : “Leveraging organ-on-a-chip systems to mimic the gut sensory system: toward screening microbiota-vagal interactions”
Yuan Jiang : “Harnesses longitudinal microbiome data to define the ecological roles of host-associated microbes”
Alysia Vrailas-Mortimer : “A New Model to Study the role of Iron in Parkinson’s Disease”
Noon – 1 p.m.
Lunch & Networking: OMSI Communication Fellows demonstration and poster session
Oregon State University and the Oregon Museum of Science and Industry (OMSI), one of the nation's leading science centers, have enjoyed a close partnership since 2016. OMSI hosts its popular Science Communication Fellowship cohort program on OSU’s Corvallis campus every spring. More than 70 students, faculty and staff from across science at OSU have completed the training program, including the Colleges of Science; Engineering; Earth, Ocean, and Atmospheric Science; Agricultural Sciences; Forestry; and Public Health and Human Sciences. The COS partners with OMSI in offering this fellowship to our students. Here we showcase some of our COS OMSI Science Communication Fellows.
Akasit Visootsat & Yuan Gao (Physics): “What & How to see motor proteins?”
Sunni Patton (Microbiology): “Exploring the Coral Microbiome”
Austin Vick (Integrative Biology): “What can the common fruit fly tell us about our health”
Panel Session: AI in Research Moderators: Vrushali Bokil, Bettye Maddux and Jeff Hare
The panel will discuss ideas for incorporating AI and data science across four priority research areas: clean energy, integrated health and biotechnology, climate solutions and robotics.
Tim Zuehlsdorff , Assistant Professor, Department of Chemistry
Jeff Hazboun , Assistant Professor, Department of Physics
Ryan Mehl , Professor, Director of GCE4All Research Center, Department of Biochemistry & Biophysics
Marilyn Rampersad Mackiewicz , Assistant Professor, Department of Chemistry
Francis Chan , Associate Professor, Director, Cooperative Institute for Marine Ecosystem and Resources Studies, Department of Integrative Biology
Read more stories about: events , biochemistry & biophysics , chemistry , integrative biology , mathematics , microbiology , physics , statistics , research
Related Stories
Across the department, explore related stories.
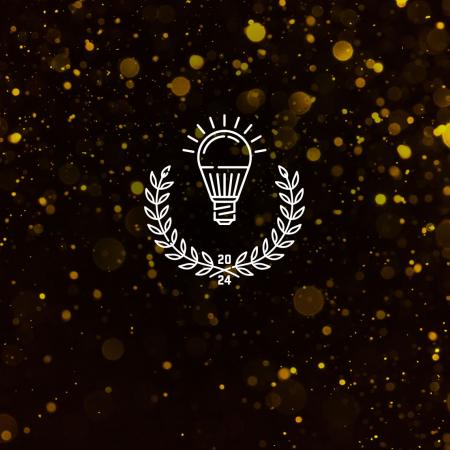
Celebrating inclusive excellence, administration, service and performance: 2024 College of Science Awards
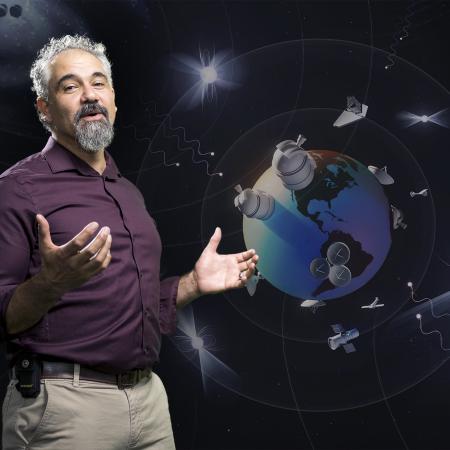
Gravitational waves discovery topic of Dec. 6 Oregon State Science Pub
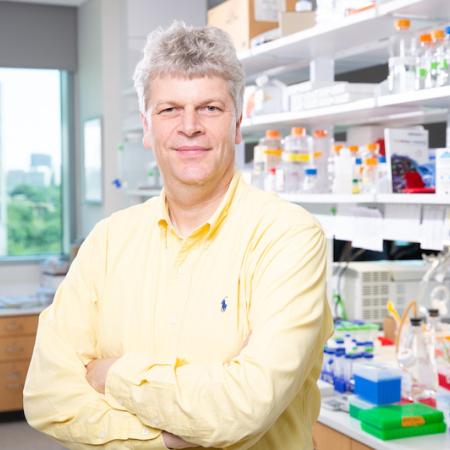
Distinguished biophysicist to discuss mechanisms of cancer cell adaptation at annual Yunker Lecture
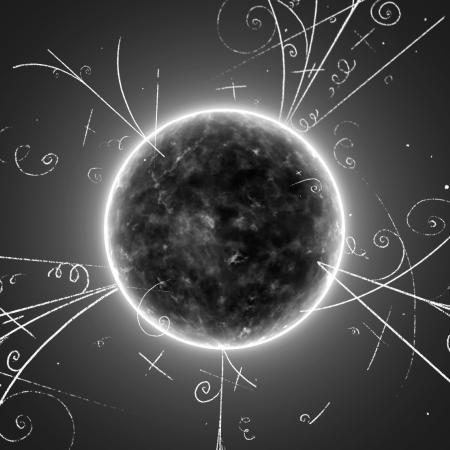
Gilfillan Lecture: "Dealing with big data: What to do when your neutrino detector is the size of a building"
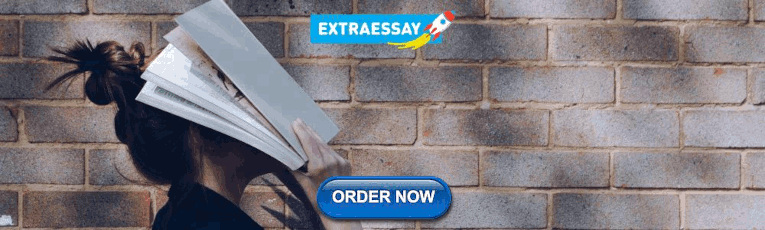
IMAGES
VIDEO
COMMENTS
As a result, cybersecurity is a crucial data science research area and one that will only become more important in the years to come. 23.) Blockchain. Blockchain is an incredible new research topic in data science for several reasons. First, it is a distributed database technology that enables secure, transparent, and tamper-proof transactions.
If you're just starting out exploring data science-related topics for your dissertation, thesis or research project, you've come to the right place. In this post, we'll help kickstart your research by providing a hearty list of data science and analytics-related research ideas, including examples from recent studies.. PS - This is just the start…
99+ Data Science Research Topics: A Path to Innovation. In today's rapidly advancing digital age, data science research plays a pivotal role in driving innovation, solving complex problems, and shaping the future of technology. Choosing the right data science research topics is paramount to making a meaningful impact in this field.
Data analytics, big data, artificial intelligence, and data science are the trending keywords in the current scenario. Enterprises want to adopt data-driven models to streamline their business processes and make better decisions based on data analytical insights. 3. 3. 3. Remember the Tiktok videos that were supposedly by Tom Cruise?
In this article, we have listed 10 such research and thesis topic ideas to take up as data science projects in 2022. Handling practical video analytics in a distributed cloud: With increased dependency on the internet, sharing videos has become a mode of data and information exchange. The role of the implementation of the Internet of Things ...
A data science research paper should start with a clear goal, stating what the study aims to investigate or achieve. This objective guides the entire paper, helping readers understand the purpose and direction of the research. 2. Detailed Methodology. Explaining how the research was conducted is crucial.
1. Introduction. Statistics and data science are more popular than ever in this era of data explosion and technological advances. Decades ago, John Tukey (Brillinger, 2014) said, "The best thing about being a statistician is that you get to play in everyone's backyard."More recently, Xiao-Li Meng (2009) said, "We no longer simply enjoy the privilege of playing in or cleaning up everyone ...
Learn about the latest research topics and trends in data science, such as deep learning, explainable AI, data privacy, data visualization, natural language processing, and data engineering.
Real-Time Analytics. One of the top rising data trends of 2023 is real-time analytics. Data capturing tools have improved in speed and scope, meaning we have access to an even greater wealth of real-time information that can illuminate our understanding of all sorts of processes.
E ven though Big data is in the mainstream of operations as of 2020, there are still potential issues or challenges the researchers can address. Some of these issues overlap with the data science field. In this article, the top 20 interesting latest research problems in the combination of big data and data science are covered based on my personal experience (with due respect to the ...
The following are the hottest data science topics and areas that any aspiring data. scientist should know whether they are data analysts or just business intelligence specialists who aim to ...
Find the latest published documents for data science, Related hot topics, top authors, the most cited documents, and related journals. ScienceGate; Advanced Search; Author Search ... of this paper is to develop and present a manifesto for Information Resilience that can serve as a reference for future research and development in relevant areas ...
J.M. Wing, " Ten Research Challenge Areas in Data Science ," Voices, Data Science Institute, Columbia University, January 2, 2020. arXiv:2002.05658. Jeannette M. Wing is Avanessians Director of the Data Science Institute and professor of computer science at Columbia University. December 30, 2019.
We'll also outline how these trends will impact both data scientists' work and everyday life. Whether you're actively involved in the data science community, or just concerned about your data privacy, these are the top trends to monitor. 1. Explosion in deepfake video and audio. "Deep fake" searches have increased by 900% in 5 years.
In this article we will discuss some of the hot subtopics in the AI research, many of these topics are interlinked and come under broad umbrella of artificial intelligence: Large scale Machine Learning. Machine Learning (ML) is concerned about developing systems that improve their performance with experience.
Examples are sharing the data concerning the Ebola virus and, more recently, the first genome sequence of the SARS-CoV-2 virus. These examples provide an inspiring model for how global research collaborations can help address societal challenges.This Research Topic addresses a holistic view of Data science; a view that has implications for the ...
The Data Science Life Cycle. End-to-end projects involve real-world problems which you solve using the 6 stages of the data science life cycle: Business understanding. Data understanding. Data preparation. Modeling. Validation. Deployment. Here's how to execute a data science project from end to end in more detail.
by The 365 Team 6 min read. Trending Topics. Data Analytics for SaaS Companies: A Case Study of Sage. by The 365 Team 6 min read. Trending Topics. Data Stories at Jayride: Data Science in the Travel Industry. 5 min read. Trending Topics. A Guide to Data-Driven Recruitment: Using Workwolf to Reduce Bias and Increase Efficiency.
Data fabric. With employees gradually becoming more comfortable with using data science tools to make decisions, while aided by automation and machine intelligence, a concept that's materialised as a hot topic for the next stage of development is the concept of 'data fabric'. Trevor Morgan, product manager at comforte AG, explained: "A ...
5. Data, analytics, and AI leaders are becoming less independent. This past year, we began to notice that increasing numbers of organizations were cutting back on the proliferation of technology and data "chiefs," including chief data and analytics officers (and sometimes chief AI officers).
Today, information can be captured from many different sources, and technology to extract insights is becoming increasingly accessible. The Top 5 Data Science And Analytics Trends In 2023. Adobe ...
There are so many methods and techniques to perform dimension reduction. The most popular of them are Missing Values, Low Variance, Decision Trees, Random Forest, High Correlation, Factor Analysis, Principal Component Analysis, Backward Feature Elimination. 4. Classification.
8 Best Data Science Tools and Software. Apache Spark and Hadoop, Microsoft Power BI, Jupyter Notebook and Alteryx are among the top data science tools for finding business insights. Compare their ...
This study provides strong support for using usage data to identify hot topics of research. In this study, employing the IEEE Xplore database as the data source, articles on different topics (keywords) and their usage data generated from January 2 ... An extensive analysis of the presence of altmetric data for web of science publications across ...
The ultimate goal, supported by the National Institutes of Health BRAIN Initiative, is to create a comprehensive, high-resolution map of a mouse's neural wiring, which would entail about 1,000 times the amount of data the group just produced from the 1-cubic-millimeter fragment of human cortex. "The word 'fragment' is ironic ...
Computational topics are also well-represented in Daily Study Groups in areas of data science, cryptography, machine learning, signal processing and game theory. Are Trending Hot Topics Covered? Daily Study Groups are a great way to learn more about trending topics and technology, and Wolfram users are always curious to explore the latest.
DOE/Brookhaven National Laboratory. "Speedy, secure, sustainable -- that's the future of telecom." ScienceDaily. ScienceDaily, 14 May 2024. <www.sciencedaily.com / releases / 2024 / 05 ...
This Research Topic will cover mathematical topics crucial to the advancement of data science including, but not limited to: • applications of data science. • functional spaces suitable for big data analysis. • mathematical foundation of machine learning. • non-smooth convex or non-convex sparse optimization for data analysis.
HOT TOPICS IN BEHAVIORAL SCIENCE AND HEALTH SERVICES RESEARCH. ... American Thoracic Society International Conference Abstracts > D102. HOT TOPICS IN BEHAVIORAL SCIENCE AND HEALTH SERVICES RESEARCH ... Send to Citation Mgr. Add to Favorites. Email to a Friend. Track Citations. Rethinking Missing Data Handling in Crisis Standards of Care ...
Read more stories about: events, biochemistry & biophysics, chemistry, integrative biology, mathematics, microbiology, physics, statistics, research. Events All Stories. The College of Science is hosting the inaugural research showcase on Tuesday, May 21, 11 a.m. to 2 p.m. at Oregon State Memorial Union Room 13.