Log in using your username and password
- Search More Search for this keyword Advanced search
- Latest content
- Publish with us
- About the journal
- Meet the editors
- Specialist reviews
- BMJ Journals More You are viewing from: Google Indexer
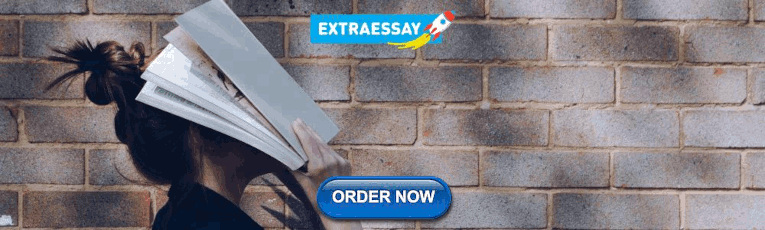
You are here
- Volume 1, Issue 1
- Data linkage in medical research
- Article Text
- Article info
- Citation Tools
- Rapid Responses
- Article metrics

- http://orcid.org/0000-0002-3418-2856 Katie Harron
- UCL Great Ormond Street Institute of Child Health Population Policy and Practice , London , UK
- Correspondence to Dr Katie Harron, UCL Great Ormond Street Institute of Child Health, Population Policy and Practice, London, UK; k.harron{at}ucl.ac.uk
https://doi.org/10.1136/bmjmed-2021-000087
Statistics from Altmetric.com
Request permissions.
If you wish to reuse any or all of this article please use the link below which will take you to the Copyright Clearance Center’s RightsLink service. You will be able to get a quick price and instant permission to reuse the content in many different ways.
Key messages
Data linkage in medical research allows researchers to exploit and enhance existing data sources without the time and cost associated with primary data collection
Methods used to quantify, interpret, and account for errors in the linkage process are needed, alongside guidelines for transparent reporting
Data linkage provides an opportunity to harness existing data for medical research. This article outlines key approaches for data linkage, and describes methods used to quantify, interpret, and account for errors.
Data linkage combines data from different sources that relate to the same person to create a new, enhanced data resource. This technique allows researchers to exploit and enhance existing data sources without the time and cost associated with primary data collection. Linked data can be used to supplement follow-up in conventional cohort studies or trials, or to generate real world evidence by creating population level electronic cohorts that are entirely derived from administrative data ( figure 1 ). 1 2 These longitudinal data sources help us to answer questions that require large sample sizes (eg, for rare diseases) or whole population coverage (eg, for pandemic response planning), which consider a wide range of risk factors and outcomes (including social determinants) and are especially powerful for capturing populations that are hard to reach. 3 4 Figure 1 illustrates two real world examples of how data linkage has been used to inform medical research, to improve clinical trial follow-up and to examine outcomes from birth.
- Download figure
- Open in new tab
- Download powerpoint
Examples of linkage used to support clinical trials and create whole population cohorts. 21 22
Choosing the right approach
A barrier to generating linked data that are fit for purpose is the availability of accurate identifiers that can be used to link the same person across multiple data sources. 5 Recording of unique identifiers such as the NHS number nearly always involves some degree of error or missing data. 6 Therefore, linkage often depends on the use of non-unique identifiers such as name, postcode, and date of birth, or even indirect identifiers such as procedure dates or other clinical variables. 7 In combination, these variables can allow us to identify records that belong to the same person—but errors, changes over time, or missing data can still hamper attempts to find the correct link.
Linkage error
Irrespective of the linkage methods implemented, use of imperfect and dynamic identifiers can lead to linkage error. Linkage errors manifest as false matches (where records belonging to different individuals are linked together) or missed matches (where records belonging to the same individual are not linked). Analogous to false positives and false negatives, these linkage errors can be viewed through a diagnostic accuracy lens ( table 1 ). While carefully designed linkage algorithms and high quality recording of identifying information can facilitate accurate linkage, even small amounts of error can lead to bias. 10 This problem is particularly evident when individuals from certain subgroups are less likely to link accurately. 11 For example, maintaining consistent linkage quality across ethnic groups can be a challenge. 12
- View inline
Linkage accuracy tool
Any linkage strategy will allow, to a certain extent, a trade-off between the two types of errors. 9 In probabilistic linkage, this trade-off depends on the choice of threshold (that is, the weight above which records have been classified as links; figure 2 ). As the threshold is lowered, sensitivity of linkage (that is, the proportion of true matches captured) increases, but the false match rate also increases. A similar diagram could be drawn to represent the trade-off in deterministic linkage as we decide which matching rule or match rank should be used to classify records. Sensitivity analyses can be used to explore the impact of the choice of threshold or matching rule on results. 13
Example of trade-off between false matches and missed matches in probabilistic linkage. In this example, probabilistic match weights are used to classify records as belonging to the same individual or not. A threshold of ≥15 would mean that <1% of linked records were false matches but 40% of the true matches were not captured. Decreasing the threshold to −5 would increase the proportion of true matches captured to 90%, but would also increase the false match rate to 30%
Design of a linkage strategy should be informed by the intended application or research question. For example, when creating a system to support drug administration using linked records, we would need to ensure that treatments are not delivered to the wrong patient: a conservative or specific approach aiming to minimise false matches would be appropriate. Conversely, use of linked data to invite members of the public for screening programmes might prioritise coverage at the expense of sending some invitations in error: a more sensitive approach might be appropriate in this setting. Minimising the difference between error types might also important in some situations. For example, when mortality rates are estimated by linking a cohort to mortality records, the correct rate might still be estimated if the number of false and missed matches cancel out.
Quality control and accounting for linkage error
Several methods can be used to evaluate the quality of linkage. 14 These methods focus on identifying potential sources of bias (that is, which characteristics are associated with errors) by examining the characteristics of records that are linked versus unlinked, or that have high versus low quality identifier data, or that are easily identifiable as having been linked incorrectly (eg, through quality control checks). 15 Accounting for linkage error in analysis is an ongoing area of methodological research, but includes approaches that view uncertainty in linkage as a missing data problem best handled with some form of multiple imputation or weighting, and those that attempt to quantify and adjust for errors using quantitative bias analysis. 16 Reporting guidelines are available that explicitly aim to support transparent reporting of linkage studies. 5 17
Remaining challenges
The biggest barriers to realising the full potential of data linkage as a powerful research tool are gaining and maintaining public trust, and reducing the costs, delays, and inefficiencies in how linked data are made available for research in the public interest. 18 19 For example, proposals to routinely link health records in primary and secondary care in order to support planning and research in England (from care.data in 2012 to General Practice Data for Planning and Research in 2021) have repeatedly raised public concerns about the lack of transparency surrounding how linked data are to be used, processes for opting out, and commercial interests. However, the covid-19 pandemic has highlighted that efficient and secure access to linked data can support agile and responsive research: building on the success of initiatives such as OpenSafely and the British Heart Foundation's CVD-COVID-UK consortium (both of which link primary and secondary care data for the UK) could provide a way forward. 1 20
Data availability statement
No data are available.
- Denholm R ,
- Hollings S , et al
- Fitzpatrick T ,
- Perrier L ,
- Shakik S , et al
- Lebenbaum M ,
- Lam K , et al
- Aldridge RW ,
- Menezes D ,
- Lewer D , et al
- Gilbert R et al
- Ludvigsson JF ,
- Otterblad-Olausson P ,
- Pettersson BU , et al
- Sharples LD ,
- Harron K , et al
- Hagger-Johnson G ,
- Goldstein H , et al
- Doidge JC ,
- Boyd J , et al
- Bohensky MA ,
- Sundararajan V , et al
- Grath-Lone LM ,
- Etoori D , et al
- Harron KL ,
- Knight HE , et al
- Doidge J et al
- Goldstein H
- Benchimol EI ,
- Guttmann A , et al
- Taylor JA ,
- Espuny Pujol F , et al
- Cavallaro F et al
- Rentsch CT ,
- Morton CE , et al
- Verfürden ML ,
- Gilbert R ,
- Lucas A , et al
- Knight HE ,
- Cromwell DA ,
- Gurol-Urganci I , et al
Contributors KH wrote the article.
Competing interests We have read and understood the BMJ policy on declaration of interests and declare the following interests: none.
Patient and public involvement Patients and the public were not involved in the design, or conduct, or reporting, or dissemination plans of this research.
Provenance and peer review Commissioned; not externally peer reviewed.
Read the full text or download the PDF:
Thank you for visiting nature.com. You are using a browser version with limited support for CSS. To obtain the best experience, we recommend you use a more up to date browser (or turn off compatibility mode in Internet Explorer). In the meantime, to ensure continued support, we are displaying the site without styles and JavaScript.
- View all journals
- My Account Login
- Explore content
- About the journal
- Publish with us
- Sign up for alerts
- Open access
- Published: 13 July 2023
What prevents us from reusing medical real-world data in research
- Julia Gehrmann ORCID: orcid.org/0000-0002-4101-5458 1 ,
- Edit Herczog ORCID: orcid.org/0000-0002-2930-5401 2 ,
- Stefan Decker ORCID: orcid.org/0000-0001-6324-7164 3 , 4 &
- Oya Beyan ORCID: orcid.org/0000-0001-7611-3501 1 , 4
Scientific Data volume 10 , Article number: 459 ( 2023 ) Cite this article
4177 Accesses
1 Citations
5 Altmetric
Metrics details
- Epidemiology
- Genetics research
- Outcomes research
- Preclinical research
Medical real-world data stored in clinical systems represents a valuable knowledge source for medical research, but its usage is still challenged by various technical and cultural aspects. Analyzing these challenges and suggesting measures for future improvement are crucial to improve the situation. This comment paper represents such an analysis from the perspective of research.
Introduction
Recent studies show that Medical Data Science (MDS) carries great potential to improve healthcare 1 , 2 , 3 . Thereby, considering data from several medical areas and of different types, i.e. using multimodal data, significantly increases the quality of the research results 4 , 5 . On the other hand, the inclusion of more features in an MDS analysis means that more medical cases are required to represent the full range of possible feature combinations in a quantity that would be sufficient for a meaningful analysis. Historically, data acquisition in medical research applies prospective data collection, e.g. in clinical studies. However, prospectively collecting the amount of data needed for advanced multimodal data analyses is not feasible for two reasons. Firstly, such a data collection process would cost an enormous amount of money. Secondly, it would take decades to generate enough data for longitudinal analyses, while the results are needed now. A worthwhile alternative is using real-world data (RWD) from clinical systems of e.g. university hospitals. This data is immediately accessible in large quantities, providing full flexibility in the choice of the analyzed research questions 6 , 7 . However, when compared to prospectively curated data, medical RWD usually lacks quality due to the specificities of medical RWD outlined in section 2. The reduced quality makes its preparation for analysis more challenging. Table 1 summarizes the advantages and disadvantages of both data curation strategies.
Considering all the above-mentioned aspects, secondary use of RWD is a great opportunity to immediately enable comprehensive and meaningful MDS analyses. These, in turn, promise increased clinical process efficiency, higher patient safety, performant clinical decision support systems, personalized care and improved healthcare system sustainability 1 . Yet MDS reusing RWD is still not established in practice for various reasons 2 . One such reason is the lack of standardized data curation frameworks specifying how to access and combine multimodal clinical data from operational clinical systems 8 , 9 . To maximize the usability of medical RWD for research, such a framework should support data management according to the “FAIR” paradigm, which states that properly managed data should be discoverable, accessible, interoperable, and reusable (FAIR). These are high-level principles, i.e., they do not specify a specific technology, method, or standard, but rather serve as guidance 10 . The extent to which a data set fulfills the four principles is known as its FAIRness. The process of increasing the FAIRness of data is referred to as FAIRification 11 .
To support the scientific reuse of medical RWD with maximal FAIRness, the German Medical Informatics Initiative (MI-I) established Data Integration Centers (DIC) and Medical Data Integration Centers (MeDIC) at German University Hospitals 12 , 13 , 14 , 15 , 16 . The challenges encountered at MeDIC Cologne have compelled us to write this comment paper, which aims to address key issues surrounding the reuse of medical real-world data (RWD) in research. In addition to the technical challenges extensively discussed in existing literature, we also delve into the cultural aspects and uncertainties that scientists, patients, and governing entities confront when reusing medical RWD. As part of our contribution, we propose high-level measures to enhance the reusability of medical RWD for research purposes. Finally, we evaluate the current usability of medical RWD in terms of the FAIR principles. Our insights draw upon personal experiences, as well as relevant findings from recent English and German literature (2016–2022) obtained through Google Scholar. However, it is important to note that the challenges and measures presented in this paper primarily reflect our personal perspectives and may not encompass all possible aspects.
Specificities of medical real-world data
The main difference between medical data and other scientific data is its high level of intrinsic sensitivity requiring thorough preservation of privacy 17 . Medical data can contain a variety of information, including demographics, healthcare provider notes, radiological findings, results of laboratory or genetic tests, presence or absence of biomarkers, administrative information, case summaries for clinical registries, biometric information, patient-reported information and recordings from medical devices or wearable sensors 18 , 19 . This variety is also reflected in the data formats available that range from tabular, time series and natural language data to images and videos 20 . Issues that are typically attributed to the secondary use of medical RWD are their low volume, i.e. small data set sizes, their high sparsity and their tendency towards poor quality 21 . These issues result from the inherent heterogeneity of treatments, outcomes, study design, analytical methods, and approaches for collecting, processing and interpreting data in the medical field 19 . Thus, the availability and quality of features for a patient strongly depend on the conditions present, the treating or examining department, comorbidity as well as current or previous examination results.
Reusing medical real-world data for medical data science
The main tasks in facilitating, or even enabling, the reuse of medical RWD in a research context are to promote interoperability, harmonization, data quality, and ensure privacy, to optimize the retrieval and management of patient consent, and to establish rules for data use and access 12 , 13 . These measures aim to address the various challenges of scientifically reusing routine clinical data described below.
Challenges in balancing benefits and harms
Personal, i.e. non-anonymized medical data, is inherently sensitive 1 , 17 , 22 . As a result, uncertainties in MDS project preparation and execution arise for all roles involved in performing research on medical RWD, i.e. for patients, researchers and governing entities. The patients may lack trust in research using their personal data. Concerns about data misuse, becoming completely transparent and data leakage - especially in the case of long-term storage - can result in the patients overprotecting their own data and not giving their consent for its reuse in research 23 , 24 , 25 . On the other hand, it has also been shown that most EU citizens support secondary use of medical data if it serves further common good 24 . So, convincing patients about the social expediency of MDS can decrease their ambivalence and avoid overprotection. This can be achieved, for example, by reporting on MDS success stories 13 . A second important aspect is patient empowerment by informing patients about the processing and use of their data through open scientific communication and enabling their active engagement in the form of a dynamic consent management 12 , 23 .
However, there are also concerns on the part of the researcher resulting e.g. from a lack of explicit training in a complex landscape of ethical and legal requirements. These could be mitigated by discussions in interdisciplinary team meetings but differences in the daily work routine make it difficult to arrange such meetings 8 , 9 , 18 , 21 . As a consequence of unresolved concerns, researchers could delay or even cancel their MDS projects. Moreover, even governing entities such as data protection officers and ethics committees exhibit a certain level of uncertainty regarding permissible practices in MDS. They tend to overprotect the rights of the patients whose medical data is to be used while underestimating the necessity of reusing medical RWD for research purposes 9 , 23 , 26 , 27 . This leads to restrictive policies hindering scientific progress.
In general, education is a promising approach to address the uncertainties mentioned above. Technical training for medical researchers and governing entities as well as ethical and legal training for technical experts can increase confidence in project-related decision making 1 , 18 , 23 , 24 , 27 , 28 . The same effect can be achieved by developing MDS guidelines and actionable data protection concepts (DPC) 13 , 14 , 15 , 16 . A good example is the DPC of the MI-I that was developed in collaboration with the German working group of medical ethics committees (AK-EK) 12 . Figure 1 summarizes the sources and consequences of the aforementioned uncertainties that lead to significant challenges in the reuse of medical RWD. Each source of uncertainty is associated with the roles it affects and possible measures to mitigate its impact. The challenges posed by these uncertainties are discussed in more detail below.
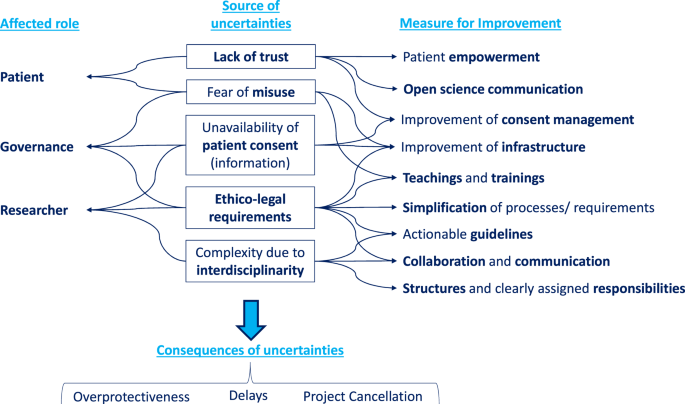
Sources and consequences of uncertainties that lead to significant challenges in the reuse of medical RWD. The sources of uncertainties are individually assigned to the roles they affect and possible measures to counteract them.
Uncertainties due to the legal framework
As mentioned above, the complex legal landscape resulting from various intervening laws contributes significantly to the uncertainty surrounding the reuse of medical RWD. At the European level, the General Data Protection Regulation (GDPR) holds substantial influence over the legal framework. In general, it prohibits the processing of health-related personal data (GDPR Art. 9 (1)) unless the informed consent of every affected person is given (GDPR Art. 9 (2a)) or a scientific exemption is present (GDPR Art. 9 (2j)). The latter is the case if the processing is in the public interest, secured by data protection measures, and adequately justified by a sufficient scientific goal. However, substantiating the presence of such a scientific exemption poses significant challenges 29 , 30 . Similarly, or even more difficult, is obtaining informed consent of patients after they have left the clinics. As such, both GDPR-based possibilities to justify the secondary use of RWD in research are difficult to implement in practice 26 , 29 . If the processing is legally based on the scientific exemption, GDPR Art. 89 further mandates the implementation of appropriate privacy safeguards supported by technical and organizational measures. Additionally, it stipulates that only the data necessary for the project should be utilized (principle of data minimization) 30 , 31 . This ensures the protection of sensitive personal data, but also introduces further challenges for the researchers.
The situation becomes further complicated due to the GDPR allowing for various interpretations by the data protection laws of EU member states 30 , 31 . Moreover, there are country-specific regulations, such as job-specific laws, that impact the legal framework of MDS 31 . This complex scenario poses particular challenges for international MDS projects 29 . As a result, identifying the correct legal basis and implementing appropriate data protection measures becomes exceptionally difficult 29 , 30 . This task, crucial in the preparation of clinical data set compilation, necessitates not only technical and medical expertise but also a comprehensive understanding of legal aspects. Thus, a well-functioning interdisciplinary team or researchers with broad training are essential.
Analyses of the current legal framework for data-driven medical research suggest that this framework is remote from practice and thus inhibits scientific progress 31 , 32 . To address these limitations, certain legal amendments or substantial infrastructure enhancements are necessary. Particularly, the infrastructure should focus on incorporating components and tools that facilitate semi-automated data integration and data anonymization. Although the current legal framework permits physicians to access, integrate, and anonymize data from their own patients, they often lack the technical expertise and time to effectively carry out these tasks. By implementing an infrastructure that enables semi-automated data integration and anonymization, researchers would be able to legally utilize valuable medical RWD without imposing additional workload on physicians 29 , 30 . Attaining a fully automated solution is not feasible since effective data integration and anonymization, leading to meaningful data sets, necessitate manual parameter selection by a domain expert. Nonetheless, by prioritizing maximal automation and specifically assigning domain experts to handle the manual steps in the process, rapid and compliant access to medical RWD, along with reduced uncertainties for researchers, can be achieved.
Ethical considerations and overprotectiveness
Not only the legal framework, but also ethical considerations can cause uncertainties. These can affect the patients and researchers but, in the context of an MDS project, especially the ethics committees as they have to judge whether a project is ethically justifiable. There are a variety of ethical principles to be taken into account for such a decision. These principles encompass patient privacy, data ownership, individual autonomy, confidentiality, necessity of data processing, non-maleficence and beneficence 1 , 33 . Considered jointly, they result in a trade-off to be made between the preservation of ethical rights of treated patients and the beneficence of the scientific project 15 , 18 , 26 . Criticism often arises concerning the prevailing trade-off in favor of patients’ privacy, where ethics committees tend to overprotect patient data 23 , 27 . What is frequently overlooked is the ethical responsibility to share and reuse medical RWD to advance medical progress in diagnoses and treatment. Thus, a consequence of overprotecting data is suboptimal patient care which is, in turn, unethical 1 , 9 , 26 . Measures to prevent overprotection are increasing the awareness of its risks through education, as well as the development of clear ethical regulations and guidelines 28 . To facilitate the latter, the data set compilation process for medical RWD should be simplified, e.g. by standardization of processes and data formats because its current complexity challenges the creation of regulations and guidelines 17 .
Uncertainties in project planning
Many of the mentioned concerns related to legal and ethical requirements occur during project planning and design. Here a variety of decisions are made regarding the composition of the RWD set and its processing. These affect all subsequent project steps, but must be determined at an early stage if the project framework necessitates approvals from governing entities. This is because the governing entities require all planned processing steps to be documented in a study plan, serving as the foundation for their decision-making process. This results in long project planning phases due to uncertainties in a complex multi-player environment 13 , 14 , 15 , 16 , 21 . Additionally, creating a strict study plan usually works for clinical trials, but in data science, meaningful results often require more flexibility. For instance, it might be necessary to redesign the project plan throughout data processing. Therefore, project frameworks that show researchers how to reshape their project in specific cases would be much better suited for secondary use of medical RWD 25 , 34 . Taking it a step further, a general guideline or regulation on how to conduct MDS projects would decrease planning time and the risk of errors, both of which are higher if each project is designed individually 14 . To already now minimize the uncertainties in project planning and, thereby, the duration of the planning phase, research teams should communicate intensely and collaboratively plan their tasks 9 , 18 . Since this is a challenging task in a highly interdisciplinary environment, early definition of structures, binding deadlines, and clear assignment of responsibilities, such as designating a person responsible for timely data provision in each department, are crucial 8 , 14 .
The role of the patient consent
As mentioned in the introduction to this section 3.1, dynamical consent management allowing the patients to effectively give and withdraw their consent at any point in time is a crucial measure to foster patient empowerment. As a result, it also leads to more acceptance of MDS by the affected individuals. Furthermore, in section 3.1.1 the informed patient consent was mentioned as a possible legal justification for processing personal sensitive data. However, the traditional informed consent requires patients to explicitly consent to the specific processing of their data. This means their consent is tied to a specific project 35 , 36 . For retrospective projects such a consent cannot be obtained during the patients’ stay at the hospital because the project idea does not exist at that time. Hence, the researcher would have to retrospectively contact all patients whose data is needed for the project, describe the project objective and methodology to them and then ask for their consent. This requires great effort, is, itself, questionable in terms of data protection and even not feasible if the patients are deceased. Making clinical data truly reusable in a research context, therefore, requires a broad consent in which the patients generally agree to the secondary use of their data in ethically approved research contexts. Furthermore, the retrieval of such a broad consent must be integrated into daily clinical routine and the consent management needs to be digitized. Otherwise, the information about the patient consent status might not be easily retrievable for the researcher 8 , 18 , 21 , 37 .
Previous research has documented that most patients are willing to share their data and even perceive sharing their medical data as a common duty 38 . Therefore, it is highly likely that extensively introducing a broad consent such as the one developed by the MI-I in Germany into clinical practice, combined with a fully digital and dynamic consent management, would have a significant positive impact on the feasibility of MDS projects 39 . It would allow patients to actively determine which future research projects may use their data.
Technical challenges
When describing the challenges resulting from balancing benefits and harms in MDS projects, some measures were suggested that require technical solutions. One example for this is the implementation of data protection measures like data access control, safe data transfer, encryption, or de-identification 20 . However, there are not only technical solutions but also challenges, as shown in Fig. 2 .
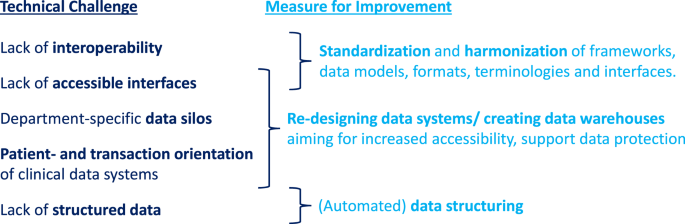
Technical challenges of curating medical RWD sets and possible measures for improvement.
One category of technical challenges results from the specificities of medical data outlined in section 2. Medical RWD is characterized by a higher level of heterogeneity regarding data types and feature availability than data from any other scientific field 18 , 19 , 26 . Thus, compiling usable medical data sets from RWD requires the technical capabilities of skillful data integration, type conversion and data imputation. However, heterogeneity is not restricted to data formats. A common problem is differences in the primary purpose of data acquisition or primary care leading to different data formats and standards being used 8 . This results in different physicians, clinical departments, or clinical sites not necessarily using the same data scales or units, syntax, data models, ontology, or terminology. Hence, it is difficult to decide which standards to use in an MDS project. A subsequent challenge arising from this lack of interoperability is the conversion between standards that potentially leads to information loss 19 , 26 , 40 . Last but not least, heterogeneity is also reflected in different identifiers being used in different sites. This challenges the linkage of related medical records, which may even become impossible once the data is de-identified 41 . Promising and important measures to meet the challenges concerning heterogeneity are the development, standardization, harmonization and, eventually, deployment of conceptual frameworks, data models, formats, terminologies, and interfaces 8 , 13 , 14 , 16 , 42 . An example illustrating the feasibility and effectiveness of these measures is the widely used DICOM standard for Picture Archiving and Communications systems (PACS) 18 . Similar effects are expected from the deployment of the HL7 FHIR standard for general healthcare related data that is currently being developed 43 . However, besides appreciating the benefits of new approaches, the potential of already existing standards like the SNOMED CT terminology should not be neglected. It still has limitations, such as its complexity challenging the identification of respectively fitting codes and its incompleteness partly requiring to add own codes. On the other hand, SNOMED CT is already very comprehensive. Once its practical applicability is improved, SNOMED CT could be introduced as an obligatory standard in medical data systems fostering interoperability 13 , 16 , 42 .
Another significant technical challenge is the fact that a majority of medical RWD is typically available in a semi-structured or unstructured format, while the application of most machine learning algorithms necessitates structured data 8 , 19 , 42 , 44 . Primary care documentation often relies on free text fields or letters because they can capture all real-world contingencies while structured and standardized data models cannot. Additionally documenting the cases in a structured way, is too time-consuming for clinical practice. So, the primary clinical systems mainly contain semi-structured or unstructured RWD 7 , 13 , 23 . To increase the amount of available structured data, automated data structuring using Natural Language Processing (NLP) is a possible solution. However, it is not easy to implement for various reasons. Among them are the already mentioned inconsistent application of terms and abbreviations in medical texts and the requirement to manually structure some free text data sets to get annotated training data 13 , 42 .
Workflows in primary care settings not only lead to predominantly semi-structured or unstructured documentation of medical cases, but also greatly influence the design of clinical data management systems. In primary care and administrative contexts, such as accounting, clinical staff typically need a comprehensive overview of all data pertaining to an individual patient or case. As a result, clinical data management systems have been developed with a case- or patient-centric design that presents data in a transaction-oriented manner. However, this design is at odds with the need for query-driven extract-transform-load (ETL) processes when accessing data for MDS projects. These projects typically require only a subset of the available data features, but for a group of patients 8 , 26 . Developing a functional ETL pipeline is further complicated by the overall lack of accessible interfaces to the data management systems and the fragmented distribution of data across various clinical departments’ systems 8 , 13 .
This means the design of primary clinical systems could be improved significantly if it allowed for more flexibility, i.e. support patient- and case-centricity for primary care as well as data-centricity for secondary use. Moreover, the system design should comply with data specifications and developed standards rather than requiring the data to be created according to system specifications 13 . However, a complete redesign of primary clinical systems is most likely not feasible. An alternative solution is creating clinical data repositories in the form of data lakes or data warehouses that extract and transform medical RWD from primary systems and make it usable for research 45 , 46 . In this context, the use of standardized platforms and frameworks such as OMOP or i2b2 further increases the interoperability of the collected data 47 . In Germany, the MI-I established DIC and MeDIC whose goal is the creation of such data repositories for the medical RWD gathered at German university hospitals. As a common standard they agreed on the HL7 FHIR based MI-I core data set (CDS) 48 . Because this is work in progress and the data repositories are populated with data from primary clinical systems, the DIC and MeDIC still need to address the challenges identified in this comment paper to create FAIR data repositories for research.
Can we enable practical and FAIR research on medical real-world data?
The previous section has shown that compiling medical RWD sets for research carries several cultural and technical challenges. We can see that classical medical research and data science on RWD have not yet reached agreement. At university hospitals, there is still a clear focus on primary care and traditional clinical trials that is at odds with the demands of data science. Besides the technical and regulatory conflicts, there is the conflict between the principle of data minimization in medical research contradicting the explorative big data approach of data science. Thus, it should be assessed by governing entities whether the beneficence of explorative big data outweighs the ethical benefits of data minimization.
Another important measure to enable FAIR MDS is to offer data systems, e.g. data repositories, meeting the needs of data scientists. These systems should enable comprehensive query-driven data exports and increase interoperability by using shared coding systems and terminologies. To simultaneously foster compliance to legal and ethical requirements, the systems should follow the paradigm of Privacy by Design, i.e. enforcing data protection e.g. by authorization, authentication and only allowing de-identified data to be exported. A resulting positive effect would be a decrease in uncertainties for the researchers since they would have to deal with fewer concerns about data protection and security. As long as the data infrastructure does not follow Privacy by Design, the uncertainties about the secondary use of routine clinical data remain for researchers, e.g. when determining the correct legal basis for the processing of medical RWD or designing the project aiming for ethical compliance. A possible measure to decrease these uncertainties is the simplification of project approval processes, e.g. by only requiring a single project application to be sent to an interdisciplinary deciding committee covering ethics, data security and data protection. Further simplification could be achieved by requesting flexible project frameworks rather than strict project plans from the researchers in the design phase. On the part of patients and governing entities, uncertainty regarding the justification of an MDS analysis often manifests itself in the form of overprotection. Section 3.1 described that an important measure to mitigate all such concerns is offering trainings for researchers, governing entities and patients. Moreover, enhanced patient engagement in form of open science communication and dynamic consent management could further decrease the ambivalence of patients. Secondly, a digital and dynamic consent management would increase the availability and reliability of the information whether a patient currently consents to the secondary usage of their data.
Considering FAIRness as the gold standard for scientific usability of data, the current usability level of medical RWD for MDS can be improved significantly:
Findability : The data system infrastructure at university hospitals is so fragmented that most data features are only findable with intense communication or experience, either from previous projects or clinical routine. Systematic investigation on available features in the individual data systems and the creation of data repositories as carried out by the DIC and MeDIC of the MI-I could help to increase findability.
Accessibility : The access to medical data is currently complicated by uncertainties regarding privacy protection, complex ethico-legal requirements and the design of primary clinical systems lacking query orientation and accessible interfaces. Redesigning the systems or creating data repositories aiming for Privacy by Design and technical accessibility of clinical data would significantly ease the compilation of medical RWD sets for research.
Interoperability : The interoperability is currently mainly restricted to the usage of the same patient identifiers within a hospital. Different departments often use different documentation policies, abbreviations, units, or own case IDs while different hospitals use different patient identifiers. Standardization as an agreement on common terminology, data models and coding systems would help to increase interoperability.
Reusability : Given the current legal situation, true reusability is only achievable with anonymized data sets or a broad patient consent allowing the processing of patient data in ethically approved MDS projects. Otherwise, data sets are compiled and used on a project-specific basis. Once the legal basis for creating a reusable data set is established and implemented, metadata documenting data provenance should be created to further promote reusability.
To conclude, reusing medical RWD in MDS is not infeasible, but the current situation still poses a variety of challenges. This comment paper has outlined these challenges from the research perspective with a special focus on the situation in Germany and proposed high-level measures on how to effectively address them. Implementing these measures will itself be a big challenge but significantly increase the usability of medical RWD for MDS and hence promote improvements in future healthcare. Thereby the technical changes will be easier to implement than the cultural ones.
Gruson, D., Helleputte, T., Rousseau, P. & Gruson, D. Data science, artificial intelligence, and machine learning: opportunities for laboratory medicine and the value of positive regulation. Clinical biochemistry 69 , 1–7 (2019).
Article PubMed Google Scholar
Fröhlich, H. et al . From hype to reality: data science enabling personalized medicine. BMC medicine 16 , 1–15 (2018).
Article Google Scholar
Thrall, J. H. et al . Artificial intelligence and machine learning in radiology: opportunities, challenges, pitfalls, and criteria for success. Journal of the American College of Radiology 15 , 504–508 (2018).
Boehm, K. M., Khosravi, P., Vanguri, R., Gao, J. & Shah, S. P. Harnessing multimodal data integration to advance precision oncology. Nature Reviews Cancer 22 , 114–126 (2022).
Article CAS PubMed Google Scholar
Behrad, F. & Abadeh, M. S. An overview of deep learning methods for multimodal medical data mining. Expert Systems with Applications 117006 (2022).
Zakim, D. & Schwab, M. Data collection as a barrier to personalized medicine. Trends in pharmacological sciences 36 , 68–71 (2015).
Khozin, S., Blumenthal, G. M. & Pazdur, R. Real-world data for clinical evidence generation in oncology. JNCI: Journal of the National Cancer Institute 109 , djx187 (2017).
Gehring, S. & Eulenfeld, R. German medical informatics initiative: unlocking data for research and health care. Methods of information in medicine 57 , e46–e49 (2018).
Article PubMed PubMed Central Google Scholar
Krumholz, H. M., Terry, S. F. & Waldstreicher, J. Data acquisition, curation, and use for a continuously learning health system. Jama 316 , 1669–1670 (2016).
Wilkinson, M. D. et al . The fair guiding principles for scientific data management and stewardship. Scientific data 3 , 1–9 (2016).
Sinaci, A. A. et al . From raw data to fair data: the fairification workflow for health research. Methods of information in medicine 59 , e21–e32 (2020).
Semler, S. C., Wissing, F. & Heyder, R. German medical informatics initiative. Methods of information in medicine 57 , e50–e56 (2018).
Haarbrandt, B. et al . Highmed–an open platform approach to enhance care and research across institutional boundaries. Methods of information in medicine 57 , e66–e81 (2018).
Prasser, F., Kohlbacher, O., Mansmann, U., Bauer, B. & Kuhn, K. A. Data integration for future medicine (difuture). Methods of information in medicine 57 , e57–e65 (2018).
Winter, A. et al . Smart medical information technology for healthcare (smith). Methods of information in medicine 57 , e92–e105 (2018).
Prokosch, H.-U. et al . Miracum: medical informatics in research and care in university medicine. Methods of information in medicine 57 , e82–e91 (2018).
Ethikrat, D. Big data und gesundheit–datensouveränität als informationelle freiheitsgestaltung. Stellungnahme, Deutscher Ethikrat. Vorabfassung (2017).
He, J. et al . The practical implementation of artificial intelligence technologies in medicine. Nature medicine 25 , 30–36 (2019).
Article CAS PubMed PubMed Central Google Scholar
Lee, C. H. & Yoon, H.-J. Medical big data: promise and challenges. Kidney research and clinical practice 36 , 3 (2017).
Kubben, P., Dumontier, M. & Dekker, A. Fundamentals Of Clinical Data Science (Springer Nature, 2019).
Chen, D. et al . Deep learning and alternative learning strategies for retrospective real-world clinical data. NPJ digital medicine 2 , 1–5 (2019).
Newaz, A. I., Sikder, A. K., Rahman, M. A. & Uluagac, A. S. A survey on security and privacy issues in modern healthcare systems: Attacks and defenses. ACM Transactions on Computing for Healthcare 2 , 1–44 (2021).
Köngeter, A., Jungkunz, M., Winkler, E. C., Schickhardt, C. & Mehlis, K. Sekundärnutzung klinischer daten aus der patientenversorgung für forschungszwecke–eine qualitative interviewstudie zu nutzen-und risikopotenzialen aus sicht von expertinnen und experten für den deutschen forschungskontext. In Datenreiche Medizin und das Problem der Einwilligung , 185–210 (Springer, Berlin, Heidelberg, 2022).
Skovgaard, L. L., Wadmann, S. & Hoeyer, K. A review of attitudes towards the reuse of health data among people in the european union: The primacy of purpose and the common good. Health policy 123 , 564–571 (2019).
Mannheimer, S., Pienta, A., Kirilova, D., Elman, C. & Wutich, A. Qualitative data sharing: Data repositories and academic libraries as key partners in addressing challenges. American Behavioral Scientist 63 , 643–664 (2019).
Meystre, S. M. et al . Clinical data reuse or secondary use: current status and potential future progress. Yearbook of medical informatics 26 , 38–52 (2017).
Prainsack, B. & Spector, T. Ethics for healthcare data is obsessed with risk–not public benefits. The conversation (2018).
Salerno, J., Knoppers, B. M., Lee, L. M., Hlaing, W. M. & Goodman, K. W. Ethics, big data and computing in epidemiology and public health. Annals of Epidemiology 27 , 297–301 (2017).
McLennan, S. Die ethische aufsicht über die datenwissenschaft im gesundheitswesen. In Datenreiche Medizin und das Problem der Einwilligung , 55–69 (Springer, Berlin, Heidelberg, 2022).
Shabani, M. & Borry, P. Rules for processing genetic data for research purposes in view of the new eu general data protection regulation. European Journal of Human Genetics 26 , 149–156 (2018).
Krawczak, M. & Weichert, T. Vorschlag einer modernen dateninfrastruktur für die medizinische forschung in deutschland (version 1.3). Manuskript, Netzwerk Datenschutzexpertise (2017).
Weichert, T. Datenschutzrechtliche Rahmenbedingungen Medizinischer Forschung (Medizinisch Wissenschaftliche Verlagsgesellschaft, Berlin, 2022).
Rumbold, J. M. & Pierscionek, B. K. A critique of the regulation of data science in healthcare research in the european union. BMC medical ethics 18 , 1–11 (2017).
Natarajan, P., Frenzel, J. C. & Smaltz, D. H. Demystifying Big Data And Machine Learning For Healthcare (CRC Press, 2017).
Vlahou, A. et al . Data sharing under the general data protection regulation: time to harmonize law and research ethics? Hypertension 77 , 1029–1035 (2021).
Hallinan, D. Broad consent under the gdpr: an optimistic perspective on a bright future. Life sciences, society and policy 16 , 1–18 (2020).
Sun, W. et al . Data processing and text mining technologies on electronic medical records: a review. Journal of healthcare engineering 2018 (2018).
Richter, G., Borzikowsky, C., Hoyer, B. F., Laudes, M. & Krawczak, M. Secondary research use of personal medical data: patient attitudes towards data donation. BMC medical ethics 22 , 1–10 (2021).
Zenker, S. et al . Data protection-compliant broad consent for secondary use of health care data and human biosamples for (bio) medical research: towards a new german national standard. Journal of Biomedical Informatics 131 , 104096 (2022).
Huang, M. Z., Gibson, C. J. & Terry, A. L. Measuring electronic health record use in primary care: a scoping review. Applied clinical informatics 9 , 015–033 (2018).
Stammler, S. et al . Mainzelliste secureepilinker (mainsel): privacy-preserving record linkage using secure multi-party computation. Bioinformatics 38 , 1657–1668 (2022).
Vuokko, R., Mäkelä-Bengs, P., Hyppönen, H. & Doupi, P. Secondary use of structured patient data: interim results of a systematic review. In MIE , 291–295 (2015).
Rinaldi, E., Saas, J. & Thun, S. Use of loinc and snomed ct with fhir for microbiology data. Studies in health technology and informatics 278 , 156–162 (2021).
PubMed Google Scholar
Kindermann, A. et al . Preliminary analysis of structured reporting in the highmed use case cardiology: challenges and measures. Stud Health Technol Inform (Forthcoming) (2021).
Hamoud, A., Hashim, A. S. & Awadh, W. A. Clinical data warehouse: a review. Iraqi Journal for Computers and Informatics 44 (2018).
Cappiello, C., Gribaudo, M., Plebani, P., Salnitri, M. & Tanca, L. Enabling real-world medicine with data lake federation: A research perspective. In VLDB Workshop on Data Management and Analytics for Medicine and Healthcare , 39–56 (Springer, 2022).
Rinner, C., Gezgin, D., Wendl, C. & Gall, W. A clinical data warehouse based on omop and i2b2 for austrian health claims data. In eHealth , 94–99 (2018).
Medical Informatics Initiative. The medical informatics initiative’s core data set. https://www.medizininformatik-initiative.de/en/medical-informatics-initiatives-core-data-set . Online; accessed 16-June-2023 (2017).
Download references
Acknowledgements
We acknowledge support for the Article Processing Charge from the DFG (German Research Foundation, 491454339).
Open Access funding enabled and organized by Projekt DEAL.
Author information
Authors and affiliations.
University of Cologne, Faculty of Medicine and University Hospital Cologne, Institute for Biomedical Informatics, Cologne, Germany
Julia Gehrmann & Oya Beyan
Vision & Values, Brussels, Belgium
Edit Herczog
Chair of Computer Science 5, RWTH Aachen University, Aachen, Germany
Stefan Decker
Department of Data Science and Artificial Intelligence, Fraunhofer FIT, Sankt Augustin, Germany
Stefan Decker & Oya Beyan
You can also search for this author in PubMed Google Scholar
Corresponding author
Correspondence to Julia Gehrmann .
Ethics declarations
Competing interests.
The authors declare no competing interests.
Additional information
Publisher’s note Springer Nature remains neutral with regard to jurisdictional claims in published maps and institutional affiliations.
Rights and permissions
Open Access This article is licensed under a Creative Commons Attribution 4.0 International License, which permits use, sharing, adaptation, distribution and reproduction in any medium or format, as long as you give appropriate credit to the original author(s) and the source, provide a link to the Creative Commons license, and indicate if changes were made. The images or other third party material in this article are included in the article’s Creative Commons license, unless indicated otherwise in a credit line to the material. If material is not included in the article’s Creative Commons license and your intended use is not permitted by statutory regulation or exceeds the permitted use, you will need to obtain permission directly from the copyright holder. To view a copy of this license, visit http://creativecommons.org/licenses/by/4.0/ .
Reprints and permissions
About this article
Cite this article.
Gehrmann, J., Herczog, E., Decker, S. et al. What prevents us from reusing medical real-world data in research. Sci Data 10 , 459 (2023). https://doi.org/10.1038/s41597-023-02361-2
Download citation
Received : 17 February 2023
Accepted : 03 July 2023
Published : 13 July 2023
DOI : https://doi.org/10.1038/s41597-023-02361-2
Share this article
Anyone you share the following link with will be able to read this content:
Sorry, a shareable link is not currently available for this article.
Provided by the Springer Nature SharedIt content-sharing initiative
Quick links
- Explore articles by subject
- Guide to authors
- Editorial policies
Sign up for the Nature Briefing: Translational Research newsletter — top stories in biotechnology, drug discovery and pharma.

Data Resources in the Health Sciences
- Clinical Data
Introduction to Clinical Data
Electronic health record, administrative data, claims data, patient / disease registries, health surveys, clinical trials registries and databases, clinical research datasets.
- Scientific Data
- Statistics Sources: Health Sciences
- Preserve/Store Data
- Describe Data
- Analyze/Visualize Data
Defining Clinical Data Repositories
State of the Industry: Seven Characteristics of a Clinical Research Data Repository HIMSS
A Practical Guide to Clinical Data Warehousing Association for Clinical Data Management (ACDM)
Clinical data is a staple resource for most health and medical research. Clinical data is either collected during the course of ongoing patient care or as part of a formal clinical trial program. Clinical data falls into six major types:
- Electronic health records
- Administrative data
- Claims data
- Patient / Disease registries
- Health surveys
- Clinical trials data
See boxes below for examples of each major type.
The purest type of electronic clinical data which is obtained at the point of care at a medical facility, hospital, clinic or practice. Often referred to as the electronic medical record (EMR), the EMR is generally not available to outside researchers. The data collected includes administrative and demographic information, diagnosis, treatment, prescription drugs, laboratory tests, physiologic monitoring data, hospitalization, patient insurance, etc.
Individual organizations such as hospitals or health systems may provide access to internal staff. Larger collaborations, such as the NIH Collaboratory Distributed Research Network provides mediated or collaborative access to clinical data repositories by eligible researchers . Additionally, t he UW De-identified Clinical Data Repository (DCDR) and the Stanford Center for Clinical Informatics allow for initial cohort identification.
Often associated with electronic health records, these are primarily hospital discharge data reported to a government agency like AHRQ.
- Healthcare Cost & Utilization Project (H-CUP) HCUPnet is a free, on-line query system based on data from the Healthcare Cost and Utilization Project (HCUP). It provides access to health statistics and information on hospital inpatient and emergency department utilization. The project includes a number of datasets and sample studies listed under the information icon. Datasets are available for purchase. more... less... Nationwide Inpatient Sample Kids Inpatient Database State Inpatient Databases State Ambulatory Surgery Databases State Emergency Department Databases
Claims data describe the billable interactions (insurance claims) between insured patients and the healthcare delivery system. Claims data falls into four general categories: inpatient, outpatient, pharmacy, and enrollment. The sources of claims data can be obtained from the government (e.g., Medicare) and/or commercial health firms (e.g., United HealthCare).
- Basic Stand Alone (BSA) Medicare Claims Public Use Files (PUFs) This is the Basic Stand Alone (BSA) Public Use Files (PUF) for Medicare claims. This is a claim-level file in which each record is a claim incurred by a 5% sample of Medicare beneficiaries. Claims include inpatient/outpatient care, prescription drugs, DME, SNF, hospice, etc. There are some demographic and claim-related variables provided in every PUF.
- Medicare Provider Utilization and Payment Data Data that summarize utilization and payments for procedures, services, and prescription drugs provided to Medicare beneficiaries by specific inpatient and outpatient hospitals, physicians, and other suppliers.
- Medicaid Data Sources The Medicaid Analytic eXtract data contains state-submitted data on Medicaid eligibility, service utilization and payments. The CMS-64 provides data on Medicaid and SCHIP Budget and Expenditure Systems.
- Medicaid Statistical Information System MSIS is the basic source of state-submitted eligibility and claims data on the Medicaid population, their characteristics, utilization, and payments and is available by clicking on the link on the left-side column.
Disease registries are clinical information systems that track a narrow range of key data for certain chronic conditions such as Alzheimer's Disease, cancer, diabetes, heart disease, and asthma. Registries often provide critical information for managing patient conditions.
- Global Alzheimer's Association Interactive Network (GAAIN) The Global Alzheimer’s Association Interactive Network (GAAIN) is a collaborative project that will provide researchers around the globe with access to a vast repository of Alzheimer’s disease research data and the sophisticated analytical tools and computational power needed to work with that data.
- National Cardiovascular Data Registry (NCDR) The NCDR® is the American College of Cardiology’s worldwide suite of data registries helping hospitals and private practices measure and improve the quality of cardiovascular care they provide. The NCDR encompasses six hospital-based registries and one outpatient registry. There are currently more than 2,400 hospitals and nearly 1,000 outpatient providers participating in NCDR registries.
- National Program of Cancer Registries CDC provides support for states and territories to maintain registries that provide high-quality data. Data collected by local cancer registries enable public health professionals to understand and address the cancer burden more effectively.
- National Trauma Data Bank The National Trauma Data Bank® (NTDB) is the largest aggregation of trauma registry data ever assembled. The goal of the NTDB is to inform the medical community, the public, and decision makers about a wide variety of issues that characterize the current state of care for injured persons.
- Surveillance, Prevention, and Management of Diabetes Mellitus DataLink (SUPREME DM)
In order to provide an accurate evaluation of the population health, national surveys of the most common chronic conditions are generally conducted to provide prevalence estimates. National surveys are one of the few types of data collected specifically for research purposes, thus making it more widely accessible.
- Medicare Current Beneficiary Survey The Medicare Current Beneficiary Survey (MCBS) is a continuous, multipurpose survey of a nationally representative sample of the Medicare population. The central goals of MCBS are to determine expenditures and sources of payment for all services used by Medicare beneficiaries.
- National Health & Nutrition Examination Survey (NHANES) The National Health and Nutrition Examination Survey (NHANES) is a program of studies designed to assess the health and nutritional status of adults and children in the United States. The survey is unique in that it combines interviews and physical examinations.
- National Medical Expenditure Survey The Medical Expenditure Panel Survey (MEPS) is a set of large-scale surveys of families and individuals, their medical providers, and employers across the United States. MEPS is the most complete source of data on the cost and use of health care and health insurance coverage.
- National Center for Health Statistics A rich source of health data and statistics on a variety of topics.
- CMS Data Navigator Center for Medicare & Medicaid Services - Research, Statistics, Data & Systems
- National Health and Aging Trends Study (NHATS) NHATS is a study of Medicare beneficiaries age 65 years and older. The study is being conducted by the Johns Hopkins University Bloomberg School of Public Health, with data collection by Westat, and support from the National Institute on Aging. NHATS is intended to foster research that will guide efforts to reduce disability, maximize health and independent functioning, and enhance quality of life at older ages.
- ClinicalTrials.gov o Registry and results database hosted by the NIH. o Information on publicly and privately supported clinical studies from around the world.
- Cochrane Library o Trials database, CENTRAL, is component of Cochrane Library o Reports of randomized and quasi-randomized clinical trials taken from Medline, Embase, and elsewhere.
- WHO International Clinical Trials Registry Platform (ICTRP) o Clinical trial registration data from over 15 trial registries, including registries from the European Union, Africa, China, Japan, Brazil, and Australia. o Use "standard search" to look for NCT or ISRCTN numbers cited in articles.
- European Union Clinical Trials Database o Protocol and results information on interventional clinical trials conducted in the EU. o Good source of pediatric drug development trials.
- CenterWatch o Portal for actively recruiting pharmaceutical industry-sponsored clinical trials.
Clinical research data may be available through national or discipline-specific organizations. Level of access is likely restricted but available through proper channels.
Proprietary research data may also be available through individual agreements with private companies.
- Biologic Specimen and Data Repository Information Coordinating Center (NHLBI) Listing of studies with resources available for searching and request via BioLINCC.
- Biomedical Translational Research Information System (BTRIS) Research data available to the NIH intramural community only.
- Clinical Data Study Request Clinical trials data. Partners include Pharmaceutical companies.
- NIMH Clinical Trials - Limited Access Datasets Requirements for access at the bottom of the page.
- YODA (Yale Open Data Access) Access to participant-level clinical research data and/or comprehensive reports of clinical research. Partners include Medtronic and Johnson & Johnson.
- << Previous: Find Data
- Next: Scientific Data >>
- Last Updated: Feb 7, 2024 10:58 AM
- URL: https://guides.lib.uw.edu/hsl/data
Be boundless
1959 NE Pacific Street | T334 Health Sciences Building | Box 357155 | Seattle, WA 98195-7155 | 206-543-3390
© 2024 University of Washington | Seattle, WA

- Sponsored Article
Advancing Clinical Research Through Effective Data Delivery
Novel data collection and delivery strategies help usher the clinical research industry into its next era..
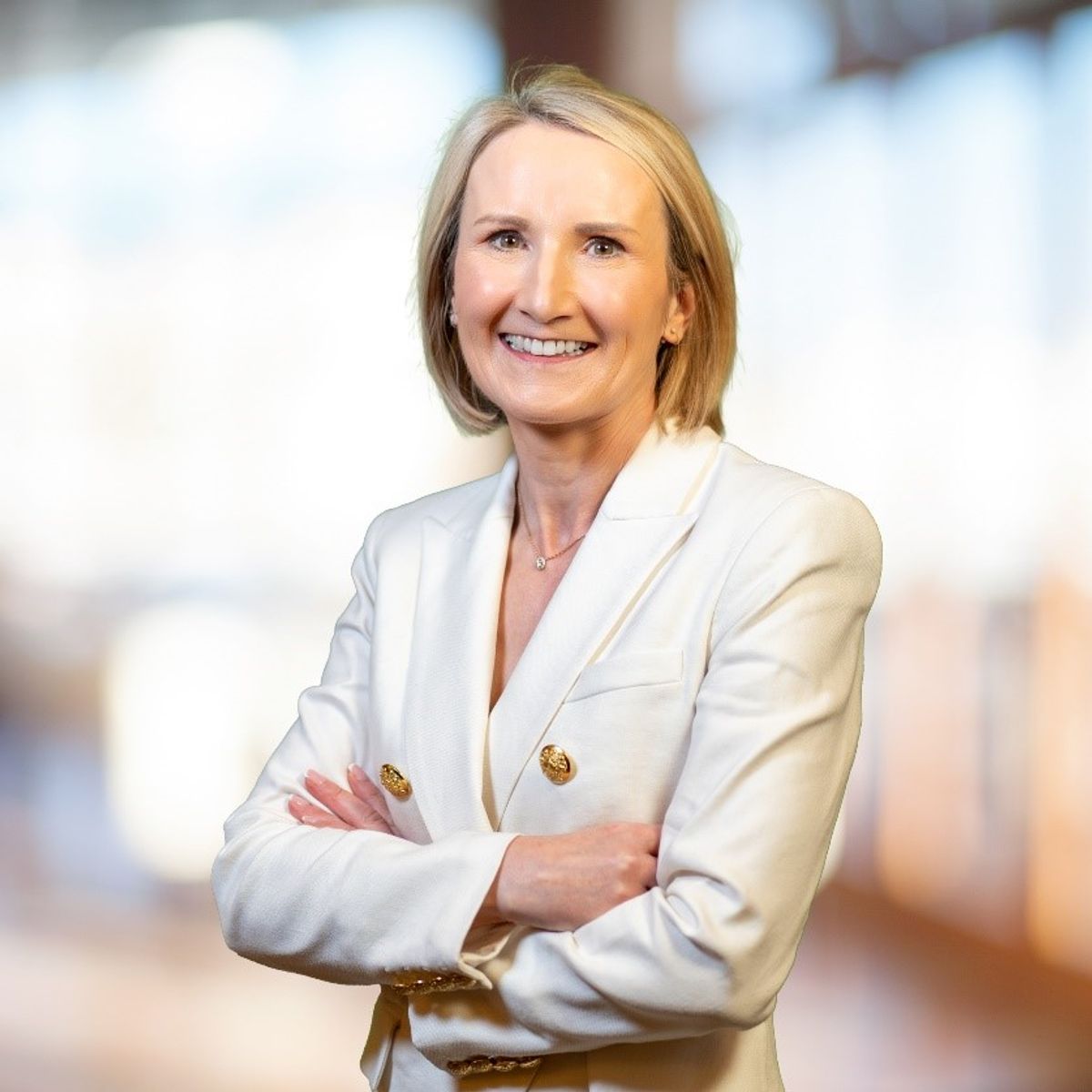
The clinical research landscape is rapidly transforming. Instead of viewing patients as subjects, sponsors now use the patients’ input to help reduce the burden they face during trials. This patient-centric approach is necessary to ensure that the clinical trial staff recruit and retain enough participants and it has led the industry to modify all stages of the clinical trial life cycle, from design to analysis. “What we are seeing is a lot more openness to innovations, digitization, remote visits for the patient, and telemedicine, for example,” said Rose Kidd, the president of Global Operations Delivery at ICON, who oversees a variety of areas including site and patient solutions, study start up, clinical data science, biostatistics, medical writing, and pharmacovigilance. “It is becoming a lot more decentralized in terms of how we collect clinical data, which is really constructive for the industry, and also hugely positive for patients.”
The Increasing Complexity of Clinical Trials
Accurate data is central to the success of a clinical trial. “Research results are only as reliable as the data on which they are based,” Kidd remarked. “If your data is of high quality, the conclusions of that data are trustworthy.” Sponsors are now collecting more data than ever through their trials. 1 This allows them to observe trends and make well-informed decisions about a drug’s or device’s development.
However, these changes in data volume complicate how clinicians design and run their clinical trials. They must capture enough data to fully assess the drug or device without severely disrupting a patient’s lifestyle. Additionally, the investigational sites must ensure that they have enough staff to collect the data in the clinic or through home visits and keep up with their country’s clinical trial regulations. They also must develop efficient data collection and delivery strategies to ensure a trial’s success. While poorly collected data can introduce noise, properly collected data allows clinical trial leads to quickly consolidate and analyze this information. 2 And they often require support with this process.
Innovative Solutions to Improve Data Collection and Delivery
Fortunately, sponsors can find that support with ICON, the healthcare intelligence and clinical research organization. “We essentially advance clinical research [by] providing outsourced services to the pharmaceutical industry, to the medical device industry, and also to government and public health organizations,” Kidd explained. With expertise in numerous therapeutic areas, such as oncology, cell and gene therapies, cardiovascular, biosimilars, vaccines, and rare diseases to mention just a few, ICON helps the pharmaceutical industry efficiently bring devices and drugs to the patients that need them, while ensuring patient safety and meeting local regulations.
One of the areas that Kidd’s team is specifically focused on is providing solutions to advance the collection, delivery, and analysis of clinical data.
The platform that ICON provides to support sponsors in this regard not only stores data directly entered into the system by clinicians during their site or home visits, but also serves as an electronic diary for patients to remotely record their symptoms as they happen. This makes it easier for patients to participate in clinical trials while maintaining their jobs and familial responsibilities. Moreover, this solution provides clinical trial staff with insights into their data as they emerge, such as adverse event profiles and the geographical spread of these events. However, this requires that the data is input into the system in the same manner at every participating site.
To address this problem, ICON’s solutions also include a site-facing web portal that helps to reduce the training burden by standardizing data capture and allowing site teams to learn key information about a drug or device. The portal also offers a visit-by-visit guide to ensure that clinicians are asking the necessary questions for a particular visit and helps them remember how to record the data correctly. “It is training at their fingertips when they need it most,” Kidd said. Solutions like these help sponsors obtain the high-quality clinical data that they need to progress from the trial to the market.
Clinical research is evolving and data strategies that support sites and patients alike must similarly evolve. With the right expertise, experience, and technology solutions, ICON is supporting better decision-making by sponsors.
- Crowley E, et al. Using systematic data categorisation to quantify the types of data collected in clinical trials: The DataCat project . Trials . 2020;21(1):535.
- McGuckin T, et al. Understanding challenges of using routinely collected health data to address clinical care gaps: A case study in Alberta, Canada . BMJ Open Qual . 2022;11(1):e001491.
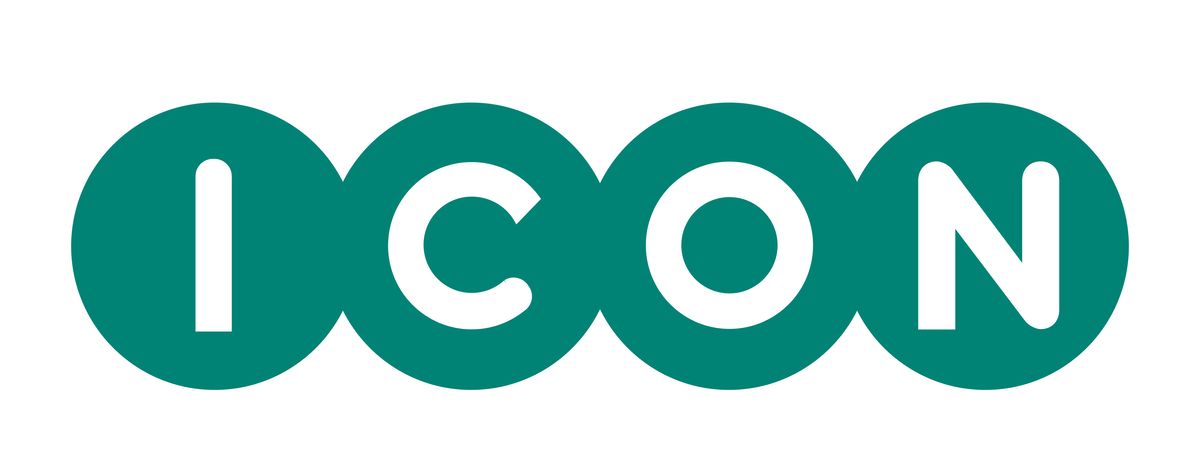
Related drug development Research Resources
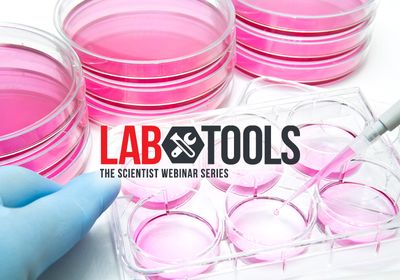
Building Advanced Cell Models for Toxicity Testing

How Cloud Labs and Remote Research Shape Science
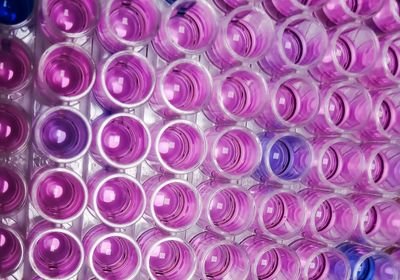
High Throughput Screening Models Ramp up Lead Discovery
Advertisement
Ethical Data Collection for Medical Image Analysis: a Structured Approach
- Original Paper
- Published: 10 April 2023
- Volume 16 , pages 95–108, ( 2024 )
Cite this article
- S. T. Padmapriya 1 &
- Sudhaman Parthasarathy ORCID: orcid.org/0000-0001-7439-6878 1
1806 Accesses
2 Citations
2 Altmetric
Explore all metrics
Due to advancements in technology such as data science and artificial intelligence, healthcare research has gained momentum and is generating new findings and predictions on abnormalities leading to the diagnosis of diseases or disorders in human beings. On one hand, the extensive application of data science to healthcare research is progressing faster, while on the other hand, the ethical concerns and adjoining risks and legal hurdles those data scientists may face in the future slow down the progression of healthcare research. Simply put, the application of data science to ethically guided healthcare research appears to be a dream come true. Hence, in this paper, we discuss the current practices, challenges, and limitations of the data collection process during medical image analysis (MIA) conducted as part of healthcare research and propose an ethical data collection framework to guide data scientists to address the possible ethical concerns before commencing data analytics over a medical dataset.
Similar content being viewed by others
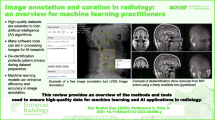
Image annotation and curation in radiology: an overview for machine learning practitioners
Fabio Galbusera & Andrea Cina
Medical Image Data and Datasets in the Era of Machine Learning—Whitepaper from the 2016 C-MIMI Meeting Dataset Session
Marc D. Kohli, Ronald M. Summers & J. Raymond Geis
A Standardised Approach for Preparing Imaging Data for Machine Learning Tasks in Radiology
Avoid common mistakes on your manuscript.
Introduction
Medical image analysis (MIA) has gained significant importance in the recent years due to the advancement in technology specifically in disciplines such as artificial intelligence, machine learning, and data science. Data analytics for MIA has become inevitable for diagnosing diseases or disorders in human beings at the initial stage. MIA is the process of using computer algorithms to examine the medical images that are available, such as X-rays, CT scans, and MRI scans (Chen et al. 2022 ). MIA focuses on identifying specific structures in the images, measuring sizes or positions of structures, and tracing abnormal or affected segments. This is vital for diagnosing diseases or disorders and providing suitable medical solutions to get the patient into a stable state as early as possible (Chakraborthy and Mali 2023 ). The underlying principle of medical image analysis is to facilitate the researchers and the practitioners to diagnose the abnormal state of diseases or disorders by using extensive data analytics based on the dataset, i.e., medical images maintained in the medical or healthcare centers and medical research laboratories. The most widely used techniques in medical image analysis include image segmentation, registration, and visualization (Van et al. 2022 ).
Data ethics and medical image analysis have become inseparable entities as MIA is grounded in the dataset provided by the healthcare centers or research laboratories. The process they have adopted to collect, store, and share data for MIA should necessarily address the data privacy of the patients. To date, there is no structured procedure or universally acceptable approach for carrying out the data collection process for MIA ethically (Martin et al. 2022 ). One of the key concerns in medical image analysis is protecting patient data, which may consist of sensitive information such as their medical history and personal identification. Ensuring that the patient data is secured and protected from unauthorized access is required to maintain the patient’s privacy and maintain trust in the healthcare system. This includes avoiding any bias in the selection of training data, as well as ensuring that the analysis is not discriminatory against certain groups or individuals. Thus, data ethics for medical image analysis should receive attention and need to be studied so as to guide the researchers and practitioners on adopting a structured approach for ethical data collection and analytics (Carter et al. 2015 ).
Gathering research data for medical image analysis is a herculean task for data scientists. To ensure data privacy, medical ethics commissions from participating institutions must approve all data collection. The use, distribution, and reuse of datasets is a distinguishing aspect of today’s medical research field. Several technological advancements, such as artificial intelligence and data science, can only be achieved through the use of medical datasets. While much has been written about the ethics of medical data collection in a number of contexts, there is little guidance on which values are at risk and how we should make decisions in an incredibly challenging health and research environment. Ethical data collection is vital for medical image analysis because it ensures that the data used to train and test models are obtained in a way that respects patient’s privacy and anonymity (Beauchamp and Childress 1994 ). This includes obtaining informed consent from the patients before collecting their medical images and ensuring that their personal information is protected. It is thus observed that the ethical data collection process for medical image analysis must be accurate, reliable, and generalizable to the population they are intended to serve (Beauchamp 2003 ).
If the data used to train models is not representative of the population or biased, the data models developed from those datasets may not contribute to accurate predictions thereby misguiding the patient’s treatment. While the potential of data analytics in many disciplines, including health and research, is widely acknowledged, there are certain inherent issues with the ethics of the data-gathering process for MIA. These difficulties have been identified as being related to the peculiarities of the medical dataset, as well as the difficulty in acquiring, integrating, processing, evaluating, and interpreting data for medical image analysis. In addition, it involves addressing privacy concerns, data security, governance, data sharing, and data compliance as per stipulated laws and regulations (Altman 1980 ; Krutzinna and Floridi 2019 ), as well as operational and ownership issues (Sivarajah et al. 2017 ). We would like to place on record that all these challenges and issues inevitably influence each other.
In recent years, the extensive use of data science has had uncomplimentary effects, such as an increase in privacy invasion, data-driven prejudice, and data-driven decision-making without justification (Martens 2022 ). Data ethics is primarily concerned with right and wrong. When data science is applied to medical images, addressing ethical concerns may result in better datasets and data models, possibly with more accurate predictions or more user acceptance of the data models. The General Data Protection Regulation (GDPR) in Europe addresses numerous data science elements linked to data privacy, including explainability (Martens 2022 ). The theme “data-related problems” focuses on the most important ethical dilemmas that might occur in connection with the gathering and use of data. Data scientists can forecast future events based on historical trends thanks to the large volume of data that is collected, stored, and made available to them (Saltz and Dewar 2019 ). It has been repeatedly observed that data ethics are necessary when analyzing medical images.
Organizations that use data science should also provide ethical training and interactive ethical evaluations to assist employees in resolving ethical problems (Leonelli 2016 ). However, it is uncertain whether these organizations have the breadth and depth of expertise required to provide this training effectively. Previous research (Saltz and Dewar 2019 ) identified three main data-related challenges: data accuracy and validity, data misuse, and privacy and anonymity. The ethical use of data is not necessarily implied by the ability to access or gather it. The ethical issues that can come up when creating and applying analytical models are the main emphasis of the data model. An analytical model is a computational tool for analyzing, interpreting, and forecasting future events based on historical data. An analytical model, in other words, is a series of mathematical operations that provide a forecast of a certain state based on prior knowledge. However, applying an algorithm could bring about or exacerbate a variety of unethical circumstances (Saltz and Dewar 2019 ).
The paper is organized as follows. “ Current Practices, Challenges, and Limitations ” describes the current practices, challenges, and limitations in medical image analysis. “ Ethical Data Collection for MIA—a Structured Approach ” describes our proposed structured approach for ethical data collection for conducting medical image analysis. “ Data Science Ethics Equilibrium ” discusses the application of the proposed approach followed by the conclusion.
Current Practices, Challenges, and Limitations
Related works.
MIA refers to the process of using computational methods to extract meaningful information from the medical images obtained in the form of X-ray, CT, or MRI scans. Performing medical image analysis includes computer-aided diagnosis (CAD), image segmentation, image registration, and image-guided therapy. Recently, all such analyses are supported by deep learning techniques and explainable artificial intelligence (AI) to improve the accuracy of predictions leading to earlier diagnosis of diseases or disorders (Shen et al. 2017 ). The computer-aided diagnosis uses machine learning algorithms to analyze images and identify potential abnormalities.
The image segmentation involves separating an image into different regions or structures, such as tumors or organs. The process of image registration is carried out by aligning or registering multiple images of the same patient taken at different times or using different modalities. The image-guided therapy involves using image analysis techniques to guide and monitor surgical procedures or other forms of therapy (Altaf et al. 2019 ). The deep learning techniques play a significant role in medical image analysis specifically for tasks such as image segmentation and diagnosis. Explainable AI is gaining momentum in developing methods that can provide insights into the decision-making process of a model to improve the precision and interpretability (Weese and Lorenze 2016 ).
Armed with our understanding of medical image analysis (MIA) based on the related previous research works (Kalaiselvi et al. 2020a , b , 2021 , 2022a , b , Kalaiselvi and Padmapriya 2022 , Padmapriya et al. 2021 , 2022 ) on MIA by the first author, we now present the current practices, challenges, and limitations in the data collection process for performing medical image analysis.
In medical image analysis, the tasks such as brain tumor detection, classification (Kalaiselvi et al. 2020a , b , 2021 , 2022a ), and segmentation (Kalaiselvi et al. 2022b , Kalaiselvi and Padmapriya 2022 ) from magnetic resonance imaging (MRI), and COVID-19 detection from X-ray and computed tomography (CT) (Padmapriya et al. 2021 , 2022 ) were carried out using deep learning techniques. Brain tumor classification and segmentation were carried out using the brain tumor segmentation (BraTS) dataset (Menze et al. 2014 ). It is a publicly available dataset of magnetic resonance imaging (MRI) scans of brain tumors. It is used for evaluating the performance of algorithms for brain tumor segmentation, which is the process of identifying and separating the tumor from the surrounding healthy tissue in an MRI scan.
The BraTS dataset consists of multi-modal MRI scans from more than 200 patients with gliomas, which are the most common type of brain tumor. The scans include T1, T1-weighted with contrast, T2, and fluid-attenuated inversion recovery (FLAIR) modalities. The dataset also includes manual segmentations of the tumors, which are used as the ground truth for evaluating algorithm performance. The challenges in collecting and articulating these datasets are data variability and inconsistency, annotation and labeling, limited availability of data, data storage, and management.
Challenges and Limitations
Medical image analysis is a rapidly evolving field, and there are several challenges that researchers and practitioners currently face when collecting the correct dataset (Altaf et al. 2019 ). Some of these challenges are erroneous data in the dataset, noisy data, incomplete dataset, limited domain adaptation, complexity of images, lack of interpretability, data annotation, integration with clinical workflows, and explainable AI (Weese and Lorenze 2016 ). A successful and effective medical image analysis demands a large volume of high-quality data without compromising on privacy and ethical concerns.
Medical images can vary significantly depending on the imaging modality, patient population, and clinical context. This can make it difficult to develop models that can be generalized across different domains. It can be very complex and difficult to interpret, particularly in the case of 3D images or images that contain multiple structures or organs. Most of the existing image analysis techniques are based on deep learning techniques, which require domain knowledge for the practitioners to correctly interpret and understand the results.
The annotation process for medical images is time-consuming and expensive and requires expert knowledge. The integration of computer-aided diagnostic systems in clinical workflows is still a challenge and requires the development of efficient and user-friendly systems. Developing models that can provide insights into their decision-making process is important to improve trust and interpretability, but it can be a challenging task. Medical image analysis is a complex field, and there are several limitations regarding dataset collection and its usage. Some of these limitations include limited accuracy, lack of robustness, limited interpretability, lack of generalizability, limited scalability, privacy and ethical concerns, and limited clinical adoption (Habuza et al. 2021 ). Collecting the right dataset while retaining privacy and handling ethical concerns is a difficult task for MIA.
The existing methods used in MIA are not robust enough to respond to the variations in imaging modalities, acquisition protocols, and patient populations. The models that were trained on a specific dataset may not be sufficient to reach generalized results or observations. This could later become a limitation in terms of clinical adoption as well (Yaffe 2019 ). Medical image analysis may also demand the use of the same dataset for multiple iterations or repurposing. This is impossible if researchers or practitioners are not provided with the required rights for such usage. Failing to address such issues may also lead to constrained scalability in the future during MIA. Medical image analysis often requires access to sensitive personal information which may raise a red flag due to privacy and ethical concerns.
Based on our above observations on the related previous research on the data collection process part of medical image analysis, we now state the most significant challenges and limitations of the current data collection process during MIA as follows:
Data privacy for the medical images, as they hold sensitive information about the patients.
There is no standard procedure or regulations in practice to carry out an ethically guided data collection process.
An extension activity to collecting a dataset of medical images is properly annotating and labeling them. This is mostly left out in practice as it is a time-consuming and expensive process. This may cause ethical issues in the future.
Datasets of medical images can be either from a data repository (stored images) or sometimes collected dynamically in real-time from patients. The ethical procedures would certainly vary depending on the method adopted for data collection and storage.
We deem that once these are addressed with a structured approach for managing the data collection process ethically during MIA, the researchers and the practitioners will be able to perform extensive analysis using cutting-edge techniques involving artificial intelligence, data science, and machine learning.
Ethical Data Collection for Medical Image Analysis—a Structured Approach
While data science can help in so many ways, it can cause harm too. By developing a shared sense of ethical values, we can reap benefits while minimizing harms. Based on the previous research (Kalaiselvi et al. 2020a , b , 2021 , 2022a , b , Kalaiselvi and Padmapriya 2022 , Padmapriya et al. 2021 , 2022 ) on medical image analysis by the first author and related research works involving data science during MIA as discussed in the previous section, we draw the elements for developing a structured approach to facilitating an ethical data collection process during MIA.
The structured approach is grounded specifically in the previous research works on applications of data science in healthcare, namely big data repositories (Xafis and Labude 2019 ), big data and medicines (Schaefer et al. 2019 ), real-time healthcare dataset (Lipworth 2019 ), artificial intelligence, and machine learning on a big dataset from healthcare systems (Lysaght et al. 2019 ; Ballantyne and Stewart 2019 ; Laurie 2019 ). This structured approach is presented below (Fig. 1 ) in the form of a framework. Even though it can be connected to theory with regard to data science research literature and descriptions, the goal of the framework for ethical data collection for carrying out MIA is to have a tool rather than a theory that helps justify actions or a framework that paves the way for better and deeper understanding of complicated issues (Dawson 2010 ).
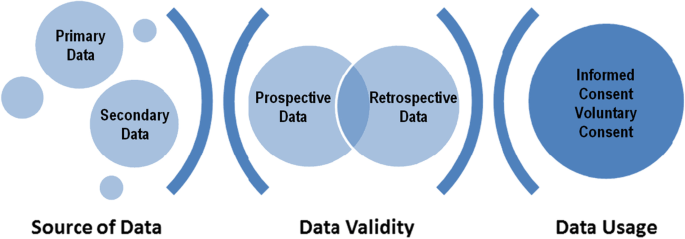
Ethical data collection framework
In a complex world, with many actors, it is often difficult to see where the problem lies and how to define limits on the use of data science. In these circumstances, using an ethical data collection framework will help the data scientists think about the ethical questions to consider as we define these limits. From Fig. 1 , we observe that there are two possible sources of data—a primary dataset and a secondary dataset for conducting the medical image analysis. The medical data such as X-rays, CT scans, and MRI scans that are collected directly from the human beings are considered a primary dataset. The metadata that arises from the original dataset is viewed as a secondary dataset. It is generally considered inappropriate to use this dataset (primary or secondary) without permission. This includes recording metadata during the data collection process, such as who collected the data, what time it took place, and how long it lasted.
Concerns about data validity are not new. Anyone with basic statistics training should be able to think through the concerns we discuss. Many data scientists seem to forget these simple fundamentals. It should be noted that untimely use of data analytics may lead to erroneous inferences and findings. It is trivial that one cannot expect a fair execution of an algorithm during MIA if this algorithm uses an erroneous or invalid dataset as its input. There is no way a computer could have prejudice or stereotypes. The assumptions, model, training data, and other boundary conditions were all specified by human beings, who may reflect their biases in the data analytics outcome, possibly without even realizing it. Despite the fact that the analysis technique itself may be perfectly neutral, stakeholders have just recently started to consider how an algorithm’s dataset can lead to unfair outcomes.
Assume that you have a suggestion to enhance the process for entering patient data into electronic medical records that would reduce errors and enhance the integration of data entry with the workflow for patient care. In this scenario, when you do an experiment to test a hypothesis, the type of data you need is unquestionably prospective data, not retrospective data (i.e., pre-existing information). The prospective data is inevitable for MIA as new patients are cared for and their data recorded. Data scientists should remember that the ethical concerns for the prospective dataset and retrospective dataset would vary and permission or rights to capture and utilize those datasets for the MIA must be obtained prior to building a data model and performing analysis.
It should be noted that the prospective data collection process demands a review of dataset by the Institutional Review Board (IRB). It is also applicable to human subject-based social science research. In the current era, the use of the prospective dataset has become inevitable by data scientists as it contributes to medical research significantly. The past population is not the same as the future population. More so, MIA involving the dataset based on the past will work in the future only to the extent the future resembles the past. In these circumstances, the data scientists may have to watch out for singularities, but should also worry about gradual drift.
When discussing datasets for medical image analysis, data privacy is undoubtedly another pressing issue that comes to mind for data scientists. The roadmap to make use of it through its collection, connection, and analysis while also minimizing the negative effects that can result from the dissemination of data about human beings is a challenging task. The current need is to define rules and regulations that regulatory bodies would agree to. It should also be noted that maintaining anonymity forever is very difficult in practice.
The process of deleting identifying information so that the remaining information can never be utilized to extract the identity of any specific person is known as “anonymization” (also known by the term “anonymity”) (Xafis and Labude 2019 ). Considering both the data by itself and the data when combined with other information to which the organization has or is likely to have access to, as well as the measures and safeguards implemented by the organization to mitigate the risk of identification, data would not be considered anonymous if there is a space to re-identify an individual.
With enough other data, anonymity is practically impossible in practice. The diversity of datasets can be eliminated by combining them with external data. Aggregation only works when the entities being aggregated have unknown structures. In a facial recognition dataset, for instance, faces can be gradually recognized even in difficult situations like partial occlusion. The simplest way to prevent data misuse if anonymity cannot be achieved or is difficult to implement is to avoid publishing the dataset in public forums and to ensure that the data scientists are well informed about the level of consent (informed or voluntary) given by the data owners for its use during MIA.
To protect data privacy during MIA, the validity period for the usage of the collected data should be well defined, either for the purpose of research or to get new insights into the diseases or disorders. Healthcare centers or medical research laboratories legitimately collect data as part of performing MIA. However, it will make an effort to not use the data in egregiously inappropriate ways in order to maintain the consumers’ trust. But after the tests, this dataset becomes an asset that is likely sold to a different party with malicious intentions. Thus, to ensure data privacy, either the collected data must be destroyed, not sold, after a stipulated time period, or the consent for its usage should be explicitly mentioned in the agreement approved by the data owner during the data collection process itself. Modern data science systems must deal with data privacy by design. As there are too many players in the race to exploit the dataset for personal or business gains, it should be noted that the data sharing is contractual and not based on trust.
For utilizing a sensitive dataset extracted from medical records, such as de-identified data used for MIA, data scientists must first go through a straightforward licensing process. This could be carried out by means of signing a contract or agreement with the data owners or other trusted parties who hold the data repository. When it comes to utilization of the collected dataset, before commencing the medical image analysis, the data scientists should verify whether either informed consent or voluntary consent exists for the chosen dataset. By “informed consent,” we mean that the human subject must be made aware of the experiment, must give their permission for it to proceed, and must be given the option to revoke their consent at any time by notifying them. However, consent can be obtained voluntarily, i.e., without coercion for the dataset to perform MIA without any hassle. Ideally, any data science project that involves human subjects should necessarily consider the feedback from the Institutional Review Board (IRB).
The IRB comprises diverse members, including non-scientists, and approves human subject study, balances potential harm to subjects against the benefits to science, and manages necessary violations of informed consent. Regarding voluntary consent, i.e., voluntary disclosure, the stakeholders (data owners) should be informed that anything they disclose voluntarily to others has much less protection than anything they keep completely to themselves. For example, the telephone company must know what number you dialed to be able to place the call. Hence, when you have disclosed this metadata to someone, even if it is not the content of your conversation, there is always a possibility that this may quickly become problematic due to ubiquity.
Data scientists should abide by the laws framed by the medical council in different countries (USA, European Union, India, Australia, and so on) while retaining datasets on human subjects for future reference and usage. After some years, if such medical records are available on the public domain through published research papers, research reports, or design patents, then this would impact the privacy of the data owner and may later lead to legal hurdles as well. Thus, knowing how to remove such records should also be a matter of concern for data scientists during MIA.
Between voluntary consent and informed consent, there will always be a trade-off. It should be emphasized that while “voluntary” consent is given by the stakeholders to carry out a desired action when necessary, “informed” consent is based on information that is concealed in numerous pages of fine print. Facebook explicitly tells users in its agreement that it may collect user data for research purposes. But Facebook got its user’s dissatisfaction for irrelevant usage of their dataset or due to the limitations on the agreement (informed consent). Hence, it is observed that informed consent and the voluntary consent when used wisely would ensure data privacy and facilitate the data scientists in collecting and using the data for effective MIA ethically. However, if it is used otherwise, data science can result in major asymmetries during MIA. In practice, many user agreements are “all-or-nothing.” This amounts to data owners (person who holds accountability for a specific dataset) completely giving up control of shared data to get any service benefits at all. Later, such owners often complain about the loss of privacy. Hence, the ideal solution to deal with this situation would be for data scientists to provide graduated choices, so the data owners could make the tradeoffs they like, for example, incognito browsing.
Another problematic area in the ethical data collection process is “repurposing.” By “repurposing,” we refer to the collection of a dataset for one objective during MIA and later using it for a different objective without obtaining consent from the data owner. It should be noted that “repurposing” can be problematic. Imagine that one gives their dataset to a medical team to obtain a specific service. However, they may not want their dataset (medical records) to be used for other purposes by the data scientists during MIA, nor do they permit them to share the data with others. This means that the data owner’s consent can often be limited to disallow repurposing, thereby indicating that the “context” matters during the data collection and its subsequent usage by the data scientists.
Repurposing is also occasionally inevitable for carrying out medical research using data science or artificial intelligence to generate new insights on certain diseases or disorders. For instance, a patient may willingly allow the repurposing of their medical data with a hospital to receive better care. However, the specific research questions may not be known to the medical team at when this particular patient receives care at the hospital. This is referred to as retrospective data analysis in data science during MIA.
Data Science Ethics Equilibrium
The simplest way to maintain equilibrium between the ethical concerns and utilization of data is to ensure that the data collection process during MIA adheres strictly to data compliance. Data scientists (or their companies) must comply with laws. They must at least do the minimum required to meet the letter of the law. Ideally, compliance follows regulations, which in turn follows the social impact of technology. Data scientists ought to be enthusiastic about the good that data science can accomplish. But if businesses do not self-regulate, there will be backlash from the public that will cost them and impede us from fully utilizing the promise of data science.
Data scientists must therefore conduct themselves morally if they are to continue to be proud of and successful in their data analytics on medical images for improved comprehension and diagnosis. Do not surprise people with data analytics carried out based on their health dataset without their consent. It is unfair to surprise others by utilizing their data for MIA irrespective of the goals and objectives of such analysis. As part of MIA, data scientists should own the result ethically. Even though there is nothing “wrong” with the process, it should be changed if data analytics lead to in unwanted results.
The structured approach (Fig. 1 ) is meant to help data scientists take the ethical concerns that should be considered during the data collection process and its utilization for the purpose of MIA into account. The proposed framework strives to achieve a stable equilibrium between the ethical concerns and the data utilization for MIA by helping data scientists respond to the following questions. They are the following: Who is the data owner? What would be the purpose of utilizing the data? And is there a way to hide certain portions in exposed data? Also, after performing the data analytics over medical dataset on human beings, data scientists should own the outcomes by addressing the following questions. They are the following: Are we doing a valid data analysis? Is the data analysis transparent, fair, and accountable? And is there any societal impact?
Ethical analysis is difficult in the sense that in a complex world, with many actors, it is often challenging to see where the problem lies and how to define limits to the use of data science. Making the right decision does not guarantee success. But surrendering morality invariably ends in disaster. Data is the primary component of all data science. Algorithms that result in specific data science models can be applied to such data. One can debate which of these is unethical, but data can undoubtedly be unethical if it contains a data subject’s private information that they do not want to be known or if there is bias against particularly vulnerable groups. A predictive model can undoubtedly be unethical, most typically as a result of its reliance on unethical data. In such cases, a predictive model is likely to utilize personal data and generate outcomes that negatively discriminate against a group of individuals. When it comes to applying data science, a practice is not simply moral or immoral. Rather, it frequently involves striking a balance between moral considerations and the usefulness of the data.
At one extreme, there is absolutely no interest in or investment in data science ethics, and at the other, the ethical problem is so great that no data is used. Data science approaches are chosen based on how important ethical issues are and how useful the data is. The equilibrium that is reached depends heavily on the context, and it is influenced by the potential effects on people and society, as well as how positive or negative these effects are considered. Medical image analysis using data science will undoubtedly require law enforcement and entail stringent requirements. The data scientists need to adhere to stricter data science ethics procedures in such circumstances.
Conclusions
The ethical data collection framework presented in this paper identified three important components, namely the source of data, data validity, and its usage with due consent from data owners while conducting MIA. Even though this list is not all-inclusive, the articulation of these elements and its underlying idea has the ability to highlight crucial factors to take into account when using data science in healthcare research. The framework also highlighted general issues that cut across all data science applications in healthcare contexts, such as data privacy, data repurposing, prospective dataset, retrospective dataset, and, finally, limited informed consent or open voluntary consent for data usage.
Most data of interest during MIA are provided by human beings, about human beings, or affect human beings. Thus, we must consider this impact as we practice data science. It is crucial that we pay careful attention to the ethical concerns around data collection, its validity, and utility during medical image analysis. Otherwise, the results or the findings of MIA cannot be disseminated among the intended audience as it may invite legal hurdles and privacy concerns or harm the reputation of the medical research laboratories. Each new algorithm, analysis, or system must be considered in terms of how it will affect society. Data scientists are unable to shirk their obligations. In reality, the technology is advancing quickly whereas regulations governing data privacy and its related ethical concerns move slowly. Thus, we will be regulating yesterday’s technologies. This may lead to many abuses because they comply with outdated regulations and, at the same time, disallow possible benefits because they conflict with outdated or needlessly broad regulations.
Altaf, F., S.M. Islam, N. Akhtar, and N.K. Janjua. 2019. Going deep in medical image analysis: concepts, methods, challenges, and future directions. IEEE Access 7: 99540–99572. https://doi.org/10.1109/ACCESS.2019.2929365 .
Article Google Scholar
Altman, D.G. 1980. Statistics and ethics in medical research: V--analysing data. British Medical Journal 281(6253): 1473. https://doi.org/10.1136/bmj.281.6253.1473 .
Ballantyne, A., and C. Stewart. 2019. Big data and public-private partnerships in healthcare and research. Asian Bioethics Review 11 (3): 315–326. https://doi.org/10.1007/s41649-019-00100-7 .
Beauchamp, T.L. 2003. Methods and principles in biomedical ethics. Journal of Medical Ethics 29 (5): 269–274. https://doi.org/10.1136/jme.29.5.269 .
Beauchamp, T.L., and J.F. Childress. 1994. Principles of Biomedical Ethics . New York, NY: Oxford University Press.
Google Scholar
Carter, P., G.T. Laurie, and M. Dixon-Woods. 2015. The social licence for research: why care.data ran into trouble. Journal of Medical Ethics 41 (5): 404–409. https://doi.org/10.1136/medethics-2014-102374 .
Chakraborty, S., and K. Mali. 2023. An overview of biomedical image analysis from the deep learning perspective. In Research Anthology on Improving Medical Imaging Techniques for Analysis and Intervention , edited by Management Association, Information Resources, 43–59. Hershey, PA: IGI Global. https://doi.org/10.4018/978-1-6684-7544-7.ch003 .
Chen, X., X. Wang, K. Zhang, K.M. Fung, T.C. Thai, K. Moore, R.S. Mannel, H. Liu, B. Zheng, and Y. Qiu. 2022. Recent advances and clinical applications of deep learning in medical image analysis. Medical Image Analysis 79: 102444. https://doi.org/10.1016/j.media.2022.102444 .
Dawson, Angus. 2010. Theory and practice in public health ethics: a complex relationship. In Public health ethics and practice , ed. Stephen Peckham, and Alison Hann, 191–209. Bristol: Policy Press. https://doi.org/10.1332/policypress/9781847421029.003.0012 .
Habuza, T., A.N. Navaz, F. Hashim, F. Alnajjar, N. Zaki, M.A. Serhani, and Y. Statsenko. 2021. AI applications in robotics, diagnostic image analysis and precision medicine: current limitations, future trends, guidelines on CAD systems for medicine. Informatics in Medicine Unlocked 24: 100596. https://doi.org/10.1016/j.imu.2021.100596 .
Kalaiselvi, T., and S.T. Padmapriya. 2022. Multimodal MRI brain tumor segmentation—a ResNet-based U-Net approach. In Brain Tumor MRI Image Segmentation Using Deep Learning Techniques , ed. J. Chaki, 123–135. Cambridge, MA: Academic Press (Elsevier). https://doi.org/10.1016/B978-0-323-91171-9.00013-2 .
Kalaiselvi, T., S.T. Padmapriya, P. Sriramakrishnan, and K. Somasundaram. 2020a. Deriving tumor detection models using convolutional neural networks from MRI of human brain scans. International Journal of Information Technology 12 (2): 403–408. https://doi.org/10.1007/s41870-020-00438-4 .
Kalaiselvi, T., T. Padmapriya, P. Sriramakrishnan, and V. Priyadharshini. 2020b. Development of automatic glioma brain tumor detection system using deep convolutional neural networks. International Journal of Imaging Systems and Technology 30 (4): 926–938. https://doi.org/10.1002/ima.22433 .
Kalaiselvi, T., S.T. Padmapriya, P. Sriramakrishnan, and K. Somasundaram. 2021. A deep learning approach for brain tumour detection system using convolutional neural networks. International Journal of Dynamical Systems and Differential Equations 11 (5-6): 514–526. https://doi.org/10.1504/IJDSDE.2021.120046 .
Kalaiselvi, T., S.T. Padmapriya, K. Somasundaram, and S. Praveenkumar. 2022a. E-Tanh: a novel activation function for image processing neural network models. Neural Computing and Applications 34 (19): 16563–16575. https://doi.org/10.1007/s00521-022-07245-x .
Kalaiselvi, T., S.T. Padmapriya, K. Somasundaram, and R. Vasanthi. 2022b. A novel activation function for brain tumor segmentation using V-NET approach. Journal of Scientific Research 66(2): 156-162. https://doi.org/10.37398/JSR.2022.660221 .
Krutzinna, J., and L. Floridi, eds. 2019. The ethics of medical data donation . Cham: Springer. https://doi.org/10.1007/978-3-030-04363-6 .
Laurie, G.T. 2019. Cross-sectoral big data: the Application of an ethics framework for big data in health and research. Asian Bioethics Review 11 (3): 327–339. https://doi.org/10.1007/s41649-019-00093-3 .
Leonelli, S. 2016. Locating ethics in data science: responsibility and accountability in global and distributed knowledge production systems. Philosophical Transactions of the Royal Society A 374 (2083): 20160122. https://doi.org/10.1098/rsta.2016.0122 .
Lipworth, W. 2019. Real-world data to generate evidence about healthcare interventions. Asian Bioethics Review 11 (3): 289–298. https://doi.org/10.1007/s41649-019-00095-1 .
Lysaght, T., H.Y. Lim, V. Xafis, and K.Y. Ngiam. 2019. AI-assisted decision-making in healthcare: the application of an ethics framework for big data in health and research. Asian Bioethics Review 11 (3): 299–314. https://doi.org/10.1007/s41649-019-00096-0 .
Martin, C., K. DeStefano, H. Haran, S. Zink, J. Dai, D. Ahmed, A. Razzak, K. Lin, A. Kogler, J. Waller, and M. Umair. 2022. The ethical considerations including inclusion and biases, data protection, and proper implementation among AI in radiology and potential implications. Intelligence-Based Medicine 6: 100073. https://doi.org/10.1016/j.ibmed.2022.100073 .
Menze, B.H., A. Jakab, S. Bauer, J. Kalpathy-Cramer, K. Farahani, J. Kirby, Y. Burren, N. Porz, J. Slotboom, R. Wiest, and K. Van Leemput. 2014. The multimodal brain tumor image segmentation benchmark (BRATS). IEEE Transactions on Medical Imaging 34 (10): 1993–2024. https://doi.org/10.1109/TMI.2014.2377694 .
Padmapriya, S.T., T. Kalaiselvi, K. Somasundaram, C.N. Kumar, and V. Priyadharshini. 2021. Novel artificial intelligence learning models for COVID-19 detection from X-ray and ct chest images. International Journal of Computational Intelligence in Control 13 (2): 9–17.
Padmapriya, T., T. Kalaiselvi, and V. Priyadharshini. 2022. Multimodal covid network: multimodal bespoke convolutional neural network architectures for COVID-19 detection from chest X-ray’s and computerized tomography scans. International Journal of Imaging Systems and Technology 32 (3): 704–716. https://doi.org/10.1002/ima.22712 .
Martens, David. 2022. Data science ethics: concepts, techniques and cautionary tales . Oxford: Oxford University Press.
Book Google Scholar
Saltz, J.S., and N. Dewar. 2019. Data science ethical considerations: a systematic literature review and proposed project framework. Ethics and Information Technology 21: 197–208. https://doi.org/10.1007/s10676-019-09502-5 .
Schaefer, G.O., E.S. Tai, and S. Sun. 2019. Precision medicine and big data: the application of an ethics framework for big data in health and research. Asian Bioethics Review 11 (3): 275–288. https://doi.org/10.1007/s41649-019-00094-2 .
Shen, D., G. Wu, and H.I. Suk. 2017. Deep learning in medical image analysis. Annual Review of Biomedical Engineering 19: 221. https://doi.org/10.1146/annurev-bioeng-071516-044442 .
Sivarajah, U., M.M. Kamal, Z. Irani, and V. Weerakkody. 2017. Critical analysis of big data challenges and analytical methods. Journal of Business Research 70: 263–286. https://doi.org/10.1016/j.jbusres.2016.08.001 .
Van der Velden, B.H., H.J. Kuijf, K.G. Gilhuijs, and M.A. Viergever. 2022. Explainable artificial intelligence (XAI) in deep learning-based medical image analysis. Medical Image Analysis 79: 102470. https://doi.org/10.1016/j.media.2022.102470 .
Weese, J., and C. Lorenz. 2016. Four challenges in medical image analysis from an industrial perspective. Medical Image Analysis 33: 44–49. https://doi.org/10.1016/j.media.2016.06.023 .
Xafis, V., and M.K. Labude. 2019. Openness in big data and data repositories. Asian Bioethics Review 11 (3): 255–273. https://doi.org/10.1007/s41649-019-00097-z .
Yaffe, M.J. 2019. Emergence of “big data” and its potential and current limitations in medical imaging. Seminars in Nuclear Medicine 49(2): 94–104. https://doi.org/10.1053/j.semnuclmed.2018.11.010 .
Download references
Author information
Authors and affiliations.
Department of Applied Mathematics and Computational Science, Thiagarajar College of Engineering, Madurai, India
S. T. Padmapriya & Sudhaman Parthasarathy
You can also search for this author in PubMed Google Scholar
Corresponding author
Correspondence to Sudhaman Parthasarathy .
Ethics declarations
Ethics approval.
Not applicable
Consent to Participate
Consent for publication, conflict of interest.
The authors declare no competing interests.
Additional information
Publisher’s note.
Springer Nature remains neutral with regard to jurisdictional claims in published maps and institutional affiliations.
Rights and permissions
Springer Nature or its licensor (e.g. a society or other partner) holds exclusive rights to this article under a publishing agreement with the author(s) or other rightsholder(s); author self-archiving of the accepted manuscript version of this article is solely governed by the terms of such publishing agreement and applicable law.
Reprints and permissions
About this article
Padmapriya, S.T., Parthasarathy, S. Ethical Data Collection for Medical Image Analysis: a Structured Approach. ABR 16 , 95–108 (2024). https://doi.org/10.1007/s41649-023-00250-9
Download citation
Received : 24 January 2023
Revised : 24 March 2023
Accepted : 26 March 2023
Published : 10 April 2023
Issue Date : January 2024
DOI : https://doi.org/10.1007/s41649-023-00250-9
Share this article
Anyone you share the following link with will be able to read this content:
Sorry, a shareable link is not currently available for this article.
Provided by the Springer Nature SharedIt content-sharing initiative
- Data ethics
- Medical imaging
- Data collection
- Data science
- Research ethics
- Data privacy
- Data analytics
- Find a journal
- Publish with us
- Track your research
This paper is in the following e-collection/theme issue:
Published on 18.4.2024 in Vol 26 (2024)
The Alzheimer’s Knowledge Base: A Knowledge Graph for Alzheimer Disease Research
Authors of this article:

Original Paper
- Joseph D Romano 1, 2, 3 , MA, MPhil, PhD ;
- Van Truong 1, 4, 5 , MS ;
- Rachit Kumar 1, 4, 5, 6 , BS ;
- Mythreye Venkatesan 7 , BE, MS ;
- Britney E Graham 7 , PhD ;
- Yun Hao 1, 4 , PhD ;
- Nick Matsumoto 7 , BA ;
- Xi Li 7 , MS ;
- Zhiping Wang 7 , MS, PhD ;
- Marylyn D Ritchie 1, 3, 5 , PhD ;
- Li Shen 1, 3 , PhD ;
- Jason H Moore 7 , PhD
1 Institute for Biomedical Informatics, Perelman School of Medicine, University of Pennsylvania, Philadelphia, PA, United States
2 Center of Excellence in Environmental Toxicology, Perelman School of Medicine, University of Pennsylvania, Philadelphia, PA, United States
3 Department of Biostatistics, Epidemiology and Informatics, Perelman School of Medicine, University of Pennsylvania, Philadelphia, PA, United States
4 Graduate Group in Genomics and Computational Biology, Perelman School of Medicine, University of Pennsylvania, Philadelphia, PA, United States
5 Department of Genetics, Perelman School of Medicine, University of Pennsylvania, Philadelphia, PA, United States
6 Medical Scientist Training Program, Perelman School of Medicine, University of Pennsylvania, Philadelphia, PA, United States
7 Department of Computational Biomedicine, Cedars-Sinai Medical Center, Los Angeles, CA, United States
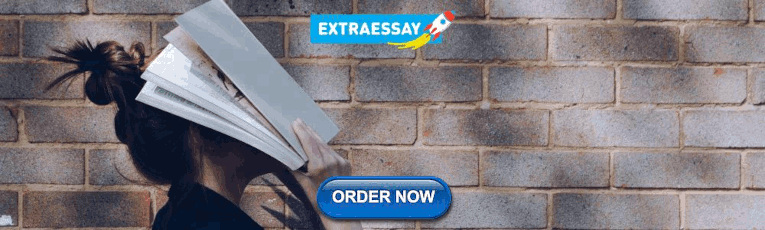
Corresponding Author:
Joseph D Romano, MA, MPhil, PhD
Institute for Biomedical Informatics
Perelman School of Medicine
University of Pennsylvania
403 Blockley Hall
423 Guardian Drive
Philadelphia, PA, 19104
United States
Phone: 1 2155735571
Email: [email protected]
Background: As global populations age and become susceptible to neurodegenerative illnesses, new therapies for Alzheimer disease (AD) are urgently needed. Existing data resources for drug discovery and repurposing fail to capture relationships central to the disease’s etiology and response to drugs.
Objective: We designed the Alzheimer’s Knowledge Base (AlzKB) to alleviate this need by providing a comprehensive knowledge representation of AD etiology and candidate therapeutics.
Methods: We designed the AlzKB as a large, heterogeneous graph knowledge base assembled using 22 diverse external data sources describing biological and pharmaceutical entities at different levels of organization (eg, chemicals, genes, anatomy, and diseases). AlzKB uses a Web Ontology Language 2 ontology to enforce semantic consistency and allow for ontological inference. We provide a public version of AlzKB and allow users to run and modify local versions of the knowledge base.
Results: AlzKB is freely available on the web and currently contains 118,902 entities with 1,309,527 relationships between those entities. To demonstrate its value, we used graph data science and machine learning to (1) propose new therapeutic targets based on similarities of AD to Parkinson disease and (2) repurpose existing drugs that may treat AD. For each use case, AlzKB recovers known therapeutic associations while proposing biologically plausible new ones.
Conclusions: AlzKB is a new, publicly available knowledge resource that enables researchers to discover complex translational associations for AD drug discovery. Through 2 use cases, we show that it is a valuable tool for proposing novel therapeutic hypotheses based on public biomedical knowledge.
Introduction
Alzheimer disease (AD) is a progressive, neurodegenerative disease affecting an estimated 6.5 million Americans aged ≥65 years and represents a significant clinical, economic, and emotional burden worldwide [ 1 ]. AD is often cited as one of the greatest health care problems of the 21st century, particularly in high-income nations with an increasing proportion of older adults. Despite its societal impact, effective pharmaceutical treatments for AD remain notoriously elusive. The US Food and Drug Administration has approved 5 drugs for the treatment of AD, 4 of which (donepezil, rivastigmine, galantamine, and memantine) only temporarily treat symptoms but do not alter the overall progression of the disease [ 2 ], whereas the fifth (aducanumab) is highly controversial in terms of evidence of effectiveness and its safety profile [ 3 ]. AD researchers have prioritized the discovery and approval of new therapies for the disease both in terms of newly discovered compounds and by repurposing drugs that are already approved to treat other (non-AD) human diseases.
AD is associated with substantial changes in pathology, including the presence of neuritic plaques associated with the amyloid-β protein, extracellular deposition of amyloid-β, and neurofibrillary tangles. Previous research has shown that these neuropathological changes begin to occur years before clinical symptoms are apparent [ 4 , 5 ]. Despite decades of research, why this pathology begins to develop remains largely unknown [ 6 ]. Current consensus is that AD risk is multifactorial. The most well-established risk factors include age; family history; and certain genetic factors, especially the presence of the σ4 allele of the apolipoprotein E gene, which is involved in fat metabolism and cholesterol transport. However, the exact mechanism through which these factors—including APOE -σ4 presence—cause or contribute to AD risk is unknown [ 7 ].
Of the many techniques used in AD therapeutics research, there is a wealth of computer-aided approaches that leverage recent advances in bioinformatics, epidemiology, artificial intelligence (AI), and machine learning (ML). For example, Rodriguez et al [ 8 ] developed an ML framework to assess gene lists constructed by differential gene expression data in response to drug treatment to determine whether those drugs would be candidates for repurposing in AD. Tsuji et al [ 9 ] used an autoencoder neural network to perform dimensionality reduction of a high-density protein interaction network to identify new possible drug targets and then found drugs associated with those targets. Genome-wide association studies have long been used for the identification of genes that confer AD risk, particularly for rare genes or genes with small (but statistically significant) contributions to disease risk [ 10 ].
In this paper, we describe the design and deployment of a major new knowledge resource for computational AD research—named The Alzheimer’s Knowledge Base (AlzKB) [ 11 ]—with a particular focus on drug discovery and drug repurposing. The overall structure and contents of AlzKB are summarized in Figure 1 . At its core, AlzKB consists of a large, heterogeneous graph database describing entities related to AD at multiple levels of biological organization, with rich semantic relationships describing how those entities are linked to one another. To demonstrate its value, we present two data-driven analyses involving ML on AlzKB’s knowledge graph: (1) predicting Parkinson disease (PD) genes that may also be associated with AD and (2) generating and explaining drug repurposing hypotheses for treating AD, both of which replicate existing knowledge while proposing entirely novel directions for future experimental validation. AlzKB is free, open source, and publicly available [ 11 ] and consists entirely of publicly sourced knowledge integrated from 22 diverse web-based biomedical databases. We hypothesized that the relationships and entities in AlzKB contain valuable knowledge that cannot be effectively captured in existing data resources, with the additional advantage of improving the explainability of new predictions.

Existing Graph-Based Approaches to AD Research
Due to the increased popularity and success of analyses using integrated knowledge, previous efforts have used knowledge graphs in AD research for a variety of purposes, including drug repurposing [ 12 - 14 ] and gene identification [ 15 ] and as general informational resources [ 16 ]. Similar to AlzKB, these bodies of work draw from a variety of sources to construct the underlying knowledge graphs, including scientific literature and formally structured biomedical databases. Some, including the Alzheimer Disease Knowledge Graph [ 14 ] and the Heterogeneous network-based data set for AD [ 16 ], have been released as publicly accessible resources similar to AlzKB. Other studies have used existing resources not specifically intended for AD research (such as the Semantic MEDLINE Database [ 13 ]) to answer questions related to AD. To our knowledge, AlzKB is the largest graph-based knowledge representation that focuses solely on AD and draws from the greatest number of source databases. For comparison, the next largest AD-specific knowledge graph that we are aware of is AD-KG, which contains 30,729 nodes and 398,544 edges (compared to AlzKB’s 118,902 nodes and 1,309,527 edges). Our emphasis on merging similar nodes or edges and cleaning the graph structure using an underlying biomedical ontology reduces the amount of noise that tends to be associated with many different node or edge types in a single graph, enabling more robust inference about relationships in AD, especially when used with emerging graph ML algorithms. Furthermore, AlzKB offers a public, web interface that allows for easy access and application to new research questions, whereas existing resources have either restricted access or are entirely unavailable for reuse. Given the challenge of identifying new or repurposed drugs for etiologically complex diseases such as AD, AlzKB represents a major step forward by improving both quantitatively and structurally on existing resources.
AlzKB Ontology
Graph databases are renowned for their flexibility in representing data that do not conform to a rigid, tabular structure, but this comes at the expense of implicitly enforcing consistency and semantic standardization [ 17 ]. To mitigate this issue, we designed a Web Ontology Language (OWL) 2 ontology—describing the types of entities relevant to AD and treatment of AD, as well as the types of relationships that link those entities—that serves as a template for nodes and edges in the knowledge graph. Ontologies (including OWL 2 ontologies) are formal representations of knowledge that are frequently used in biomedicine to computationally structure, retrieve, and make inferences about knowledge within a domain of interest [ 18 ]. Briefly, as many of the components of a graph database have a 1-to-1 correspondence with components of an OWL 2 ontology (eg, OWL 2 classes are equivalent to graph database node labels, and OWL 2 object properties are equivalent to edge types in a graph database), it is possible to populate the ontology using biomedical knowledge and translate the contents of the populated ontology into an equivalent graph database. Therefore, enforcing consistency in the ontology becomes equivalent to enforcing consistency in the graph database.
We constructed the ontology manually using the Protégé ontology editor (version 5.5.0; Stanford Center for Biomedical Informatics Research) [ 19 ] following an iterative process guided by expert domain knowledge. First, we prototyped a class hierarchy containing the types of nodes (eg, gene, disease, pathway, and drug) desired in the knowledge base. We then annotated these classes with data properties (eg, drugs can be assigned a property value corresponding to molecular weight) and object properties (relationship types that link 2 entities, such as “drug treats disease”). A thorough description of the components of OWL 2 ontologies is provided by Hitzler et al [ 20 ]. Finally, we placed restrictions on the ontology to reflect biology and clinical practice. For example, we specified restrictions stating that all pathways must contain one or more genes or that all drugs in the knowledge base must have a valid DrugBank ID. We repeated these steps several times, making revisions on previous iterations until several domain experts agreed that the semantic contents of the ontology were consistent with current AD knowledge and systems biology processes involved in AD etiology. After collecting the data sources used to populate the ontology (see the following section), we included additional data properties corresponding to identifiers in those source databases, enabling data provenance and facilitating both interoperability and validation. The final ontology structure consists of entity types involved in AD etiology (modeled as OWL 2 classes), types of semantic relationships that can link those entity types (modeled as OWL 2 object properties), and properties that can be annotated onto entities of specific types (modeled as OWL 2 data properties). Both before and after populating the ontology with individuals (see the Implementing AlzKB section), we validated its contents and structure by running FaCT++—an ontology inference engine that identifies errors by evaluating all assertions in the ontology against the ontology’s class or property hierarchy and other restrictions [ 21 ].
Collecting and Assembling Third-Party Data Sources
Using the AlzKB ontology’s class hierarchy as a starting point, we determined a set of the most important entity types to include in the first release of the knowledge base. For example, we prioritized inclusion of entities representing diseases (specifically AD and its various subtypes), genes, and drugs, among others. Similarly, we identified important relationship types (eg, “DRUG_BINDS_GENE” or “GENE_ASSOCIATED_WITH_DISEASE”) to include in the knowledge base. For each of these entity and relationship types, we identified a third-party, public data source that would serve as a collection of “ground truth knowledge” for that entity or relationship type. In the assembled knowledge base, there is roughly a 1-to-1 correspondence between a data record in the original “ground truth” data source and its corresponding entity or relationship in AlzKB, with some important exceptions. For example, we made the decision to only include neurological diseases in AlzKB rather than all diseases described in the “ground truth” data source (in this case, the Disease Ontology). We also identified instances in which properties from additional data sources could be used to augment the “ground truth” entities. For example, while DrugBank is used to specify the drugs described in AlzKB, we also used fields from Distributed Structure-Searchable Toxicity and PubChem to augment the properties annotated onto drugs (such as molecular weight, chemical fingerprint, and synonyms).
Implementing AlzKB
We populated the ontology by sequentially carrying out the following steps:
- Import distinct entities from each data source corresponding to the corresponding ontology class and define those entities as ontology individuals (ie, instances of that class). For example, the drug memantine is defined as an instance of the ontology class Drug.
- Populate data properties for all instances of each ontology class using data from relevant sources. For example, memantine is annotated with the Chemical Abstracts Service Registry number 19982-08-2.
- Populate object properties as the semantic relationships linking pairs of entities using the appropriate data source. For example, an object property of type “DRUG_TREATS_DISEASE” links memantine to the instance of Disease named Alzheimer’s Disease.
After populating the AlzKB ontology with entities, relationships, and data properties, we serialized the ontology into the Resource Description Framework (RDF) or XML graph data format, which is compatible with modern graph database software as an input format. A complete list of the data sources used in AlzKB at the time of writing is provided in Table 1 . We then populated a Neo4j graph database (version 4.4.5; Neo4j, Inc) [ 22 ] with the contents of the RDF or XML file using the neosemantics library [ 23 ], which parses the RDF data, inserting semantic triples into the graph database corresponding to each entity or relationship. Finally, we stripped the newly populated graph database of unnecessary artifacts that are components of the OWL 2 standard, leaving only nodes, relationships, and properties defined within the hierarchy. For the publicly hosted version of AlzKB, we created a web server that hosts both the static AlzKB website (containing information, documentation, and use details) and the Neo4j graph database, which is available by navigating to a subdomain [ 24 ] of the main website [ 11 ]. For reproducibility, this entire pipeline (including mappings to source databases) is provided as a single Python script available on GitHub (the most recent version) [ 25 ] or Zenodo (an archived version of the code at the time of publication) [ 26 ].
a As source data elements do not correspond in a 1-to-1 manner with entities in the graph (eg, entities may be merged, filtered, or used as edges rather than nodes), actual counts for entities in AlzKB stratified by source are not available. The sizes are the best available estimates at the time of publication. Table 2 and Table S1 in Multimedia Appendix 1 [ 50 - 56 ] provide actual node and edge type counts in AlzKB.
b AOP-DB: Adverse Outcome Pathway Database.
c The derived data are structured in part using Hetionet.
d AD: Alzheimer disease.
e EPA: Environmental Protection Agency.
f DSSTox: Distributed Structure-Searchable Toxicity.
g ACToR: Aggregated Computational Toxicology Resource.
h GWAS: genome-wide association studies.
i LINCS: Library of Integrated Network-Based Cellular Signatures.
j NCBI: National Center for Biotechnology Information.
k MeSH: Medical Subject Headings.
l SIDER: Side Effect Resource.
m Counts not applicable (TISSUES associations map to edges rather than nodes in the graph).
Validating AlzKB Using Real-World Use Cases
After building AlzKB’s knowledge graph, we designed two ML-based use cases that resemble real-world tasks for which AlzKB was originally designed: (1) proposing genetic targets for new drugs based on disease similarity and topological graph features and (2) predicting new edges in the knowledge graph linking AD to repurposed drugs via a graph completion model. These 2 use cases are intended to assess the external validity of AlzKB—for the ML models to perform well on tasks defined using real-world evaluation end points (eg, effective drugs or etiologically important genes), the informative patterns and phenomena underlying those end points need to be adequately captured in the knowledge graph.
In the first use case (identifying genetic targets via graph topology measures), we trained a random forest (RF) classifier (implemented in the scikit-learn library [Python Software Foundation] for the Python programming language) using the following topological graph features, which are computed for every node pair in the graph (regardless of whether an edge does or does not exist between them): common neighbors, total neighbors, preferential attachment, Adamic-Adar, and resource allocation [ 57 - 60 ]. Each feature gives a different measure of network “relatedness” for a pair of nodes, which are then used as predictive features in the RF model. For a given node pair ( n 1 , n 2 ), these metrics are defined as follows:
where N(n 1 ) is the set of neighbor (adjacent) nodes of node i . Our training procedure for the RF model included 3-fold grid search cross-validation to optimize hyperparameters, an 80%/20% train/test split, and repeating the procedure 10 times with random sampling.
To accomplish the second use case (drug repurposing via graph completion models), we implemented and compared the performance of 5 graph completion algorithms applied to the entire AlzKB knowledge graph. These models learn low-dimensional representations of graph nodes as vector embeddings. The embeddings are then combined to propose all possible triples in the graph (source node, edge, and target node), and scores are generated to indicate the plausibility of the triple. The 5 models we evaluated are TransE, RotatE, DistMult, ComplEx, and ConvE [ 60 ].
We implemented the 5 models using PyKEEN—a Python library for knowledge graph embeddings [ 50 ]. We randomly split the data set of all triples into 80/10/10 training/validation/testing sets and used grid search to empirically set embedding dimensions to 256 and the number of epochs to 100 with early stopping allowed. All remaining hyperparameters were set to the PyKEEN defaults. We trained the models on Google Colab using a single Tesla T4 graphics processing unit and evaluated the results using the rank-based evaluation metrics hits@k ( k =1, 3, and 10) and mean reciprocal rank (MRR) [ 61 ]. Ranking-based evaluation sorts the scores of triples in descending order and sets their rank as the index in the sorted list. In the case of multiple “true” triples having an equal score, we used the average of the most optimistic (best) and pessimistic (worst) ranks across the metrics. Briefly, hits@k is the ratio of true triples in the test set that have been ranked within the top k predictions of the model. Higher values indicate better performance. The MRR, also known as inverse harmonic mean rank, is the arithmetic mean of the inverse rank of the true triples. We performed evaluation on both left- and right-side predictions (ie, how well they can predict missing entities in partial triples without either the head [source] or tail [target] entities).
Ethical Considerations
No human participants were involved in this research. All data used to build and evaluate AlzKB were derived from publicly available biomedical knowledge retrieved from open access databases. None of the data included were derived from individual human participants. Similarly, AlzKB is entirely open source and publicly available and complies with the licensing terms of all 22 source databases used to build the knowledge base.
Knowledge Base Description
The first release of AlzKB (version 1.0) [ 26 ] contains 118,902 distinct nodes (representing biomedical entities) and 1,309,527 relationships linking those nodes. A full summary of node and relationship types with counts, respectively, is provided in Table 2 and Table S1 in Multimedia Appendix 1 . Users can interact with AlzKB in their web browser using the built-in Neo4j interface or programmatically by connecting to the graph database over the internet. We also provide instructions for installing a local copy of the graph database as well as how to build the database from its original data sources.
Proposing New Therapeutic Targets for AD
As a proof of concept, we performed an analysis to predict whether known PD genes are also linked to AD etiology. PD is a chronic, progressive neurological disorder characterized by uncontrollable movements and possible mental and behavioral changes. Similar to AD, the precise etiology of PD is not fully understood, but the disease is characterized by the death or dysfunction of basal ganglia neurons. A growing body of work has established physiological and genetic similarities between PD and AD [ 62 ], and it has been proposed that drugs targeting PD genes could potentially treat AD as well. To approach this hypothesis computationally, we defined a binary classification task to predict whether gene nodes in the AlzKB knowledge graph are or are not AD genes [ 63 ]. To assemble the data set, we considered all gene nodes adjacent to AD as positive (n=101) and all gene nodes not adjacent to AD as negative (n=62,306). The negative samples are assumed to contain a mixture of true negatives and false negatives; in link prediction tasks, the goal is to recover the false negatives. We further filtered the negative nodes to omit PD genes (n=73) and orphan gene nodes (n=43,032) and down sampled the remaining genes to 303 (ie, 3 times the number of positive samples). To evaluate the performance, we used accuracy, balanced accuracy, precision, recall, F 1 -score, area under the receiver operating characteristic curve, and area under the precision-recall curve, as shown in Figure 2 .
The RF model predicted gene-disease relationships with an average balanced accuracy of 96.2% (precision=0.88; recall=0.98). We applied the trained models to predict PD genes that are likely to also be AD genes. Of the 73 PD genes in AlzKB, 8 (11%; FYN , DCTN1 , SNCA , SYNJ1 , RSP12 , ATXN2 , KCNIP3 , and CHRNB1 ; described in Table 3 ) were predicted to be AD genes. A total of 10% (7/73) of the genes were predicted to be AD genes in all 10 models, whereas CHRNB1 was predicted in 7 of the 10 models.

Drug Repurposing via Graph Data Science
As a second use case, we considered the task of repurposing existing drugs—currently used to treat other diseases—based on patterns in the knowledge graph that suggest that they may also treat AD. To do this, we trained 5 state-of-the-art knowledge graph completion methods (TransE, RotatE, DistMult, ComplEx, and ConvE) [ 51 ] on AlzKB and selected the highest-performing one to predict links between drugs and AD. Additional details about the differences between these methods are provided in Multimedia Appendix 1 .
The performance of the 5 different knowledge graph completion models is shown in Table 4 . Among them, RotatE performed best, with the highest MRR and hits@k values. Therefore, we used RotateE to make predictions on the test set to obtain missing head entities with the template ([ drug ], DRUG_TREATS_DISEASE, AD). The top 10 predicted drugs are listed in Table 5 along with their current approved use and relevant clinical trial status pertaining to AD efficacy. Of the top 10 predictions, 3 (30%) have been investigated in clinical trials to treat symptoms of AD. To further explore these predictions, we generated visualizations of a minimum spanning tree linking the 10 drugs to AD in AlzKB’s knowledge graph, as shown in Figure 3 . The visualization shows that the shortest paths between the drugs and AD are mediated by a small set of AD-associated genes, each of which is associated with one or more of the proposed drugs. The visualization is suggestive of interpretable biological mechanisms through which the drugs could act on AD etiology and provides hypotheses to further explore their validity.
a MRR: mean reciprocal rank.
b Italicized values indicate maximum scores within a given column.
a No known AD-related clinical trials for the given drug.
b ER+: estrogen-receptor positive.

Principal Findings
AlzKB is a freely available resource for the biomedical research community, with the primary goal of expanding the repertoire of therapies for AD via drug repurposing. In the previous sections, we described the current contents of AlzKB, the process of constructing it, and 2 specific data-driven use cases that illustrate how it can be applied to drug repurposing tasks. These use cases consisted of predicting the shared genetic architecture of AD and PD (potentially allowing for PD therapies to be repurposed for AD) and directly proposing drugs to repurpose for treating AD by predicting new links between drug and disease nodes in the knowledge graph. In both cases, the results are both biologically plausible and supported by quantitative metrics, yielding new hypotheses that merit experimental validation. AlzKB is a flexible resource that is not limited to these analyses, and we encourage other research teams to use it for different and complementary knowledge discovery tasks.
The Role of AlzKB in Biomedical Knowledge Discovery
AD and other neurodegenerative diseases present one of the greatest challenges in modern biomedicine. AD is by and large a disease of old age, and as improvements to health care continue to increase the overall global life expectancy, we can expect the number of people with various forms of dementia to also increase. As the etiology and pathophysiology of AD are highly multifactorial, there is likely no single “cure” for the disease. Instead, researchers and public health officials have shifted much of their focus toward finding therapies that reduce risk, slow the progression of the disease, or reverse neuronal damage. In addition, as there are various subtypes of AD with underlying mechanisms, any therapy might be effective for only some patients with AD. Therefore, an essential step for reducing global disease burden is to propose many new therapeutic agents that target various aspects of AD pathology. This is precisely the motivating use case for AlzKB. As we have demonstrated, AlzKB provides a rich representation of existing knowledge about AD and the biological context in which it acts. The 2 ML-based use cases we presented previously use real-world end points to demonstrate that the knowledge captured in AlzKB is meaningful and representative of the biological processes underlying the disease. AlzKB stands to become a major resource in the AD research community, where pattern analysis and integration with observational data can be used to propose a diverse array of new therapeutic hypotheses along with interpretable mechanistic explanations of how those therapies may act in the human body.
Building the initial release of AlzKB was a highly interdisciplinary effort involving contributions from experts in translational bioinformatics, data science, and clinical informatics as well as medical scientists. Although each of these domains was essential in delivering a knowledge base that reflects important biomedical patterns describing AD etiology and treatment, a key need during the design and implementation phases was data literacy. To support future work in this and related areas, we encourage the inclusion of informatics and data analysis techniques in all types of biomedical curricula. Beyond AlzKB, our approach for building the knowledge graph is generalizable to practically any domain and depends on (1) defining an ontology using expert knowledge that formally describes the domain of interest and (2) identifying source databases that provide the entities and relationships described in the ontology. We are directly involved in the ongoing development of other knowledge bases using this same approach, including ComptoxAI—a knowledge base that supports AI research in toxicology [ 64 ]. As both knowledge bases share many of the same “core” entities (genes, diseases, pathways, and anatomical structures), the knowledge graphs are already semantically harmonized and ready for integration in larger, cross-disciplinary biomedical knowledge applications.
Discovering Putative Therapies Through Graph Data Science
Of the PD genes predicted to also be AD genes (see the Proposing New Therapeutic Targets for AD section; Table 3 ), some are involved in neuronal signaling and structure, and some are known to be involved in a wide range of neurological disorders. FYN has seen recent attention and investigation into its possible link to AD due to its broad expression in brain tissue and known interactions with tau proteins [ 65 , 66 ]. Among the other identified genes, one ( CHRNB1 ) is known to be involved in acetylcholine signaling [ 67 , 68 ], and another ( KCNIP3 ) codes a protein that interacts with presenilin, and mutations in presenilin are causal for hereditary AD [ 69 , 70 ]. Some of these gene hits ( ATXN2 and DCTN1 ) have limited or no current research directly linking them to AD but are biologically plausible. As such, they may represent novel therapeutic targets or targets for further research and investigation [ 71 ]. For example, DCTN1 encodes the dynactin-1 protein, and deficits in dynactin are connected to several neurodegenerative diseases; however, there is limited research linking this gene to AD [ 72 , 73 ].
Among the drug repurposing predictions (see the Drug Repurposing via Graph Data Science section; Table 5 ) are some agents that have previously been proposed for the treatment of AD (risperidone and sertraline) or for symptoms associated with AD (nicotine). Sumatriptan has been the subject of several studies focused on AD [ 74 ] and is connected to a strong comorbidity of migraine headaches and dementia in women [ 75 ]. Pimozide has been shown to reduce the aggregation of tau protein in mice [ 76 ] and is linked to AD in a number of unrelated in silico models [ 77 ]. The inclusion of nicotine is also noteworthy as it has seen recent interest among AD researchers and is the subject of an ongoing clinical trial to improve memory [ 78 ]. Other drugs listed in Table 5 have not yet been identified as AD treatments and represent novel repurposing candidates. Each can be considered a testable hypothesis meriting further investigation, giving credence to the increased detective power of AlzKB’s knowledge graph approach over existing AD data resources. It should be noted that this approach can only propose new indications for existing drugs and is based on existing knowledge and derived from known biological associations with those drugs. Other approaches (including emerging techniques in graph ML) could be used to propose entirely new drugs that could treat AD.
Future Directions With AlzKB
AlzKB is a growing resource, and we have plans for adding new features and data types that are in various stages of implementation. As a central hypothesis of AD pathogenesis revolves around the atypical accumulation of proteins within and around brain cells, an important step will be to adequately distinguish and differentiate genes from the proteins that those genes code for. Existing data resources available for inclusion in AlzKB largely fail to make this distinction in a way that is accepted by the scientific community, so we are currently evaluating options to use either postprocessing of existing knowledge sources or synthesis of new knowledge to achieve a good representation of genes, proteins, and functional or structural variants that are key to understanding AD.
Current ML models often do not generalize well to heterogeneous graphs such as the one that constitutes AlzKB’s knowledge graph. This is largely because traditional models cannot use the network structure and heterogeneous nature of different entity types. Several promising algorithms can be used for prediction on heterogeneous graphs—including GraphSAGE [ 79 ] and metapath2vec [ 80 ]—but most fail to scale effectively when the number of node or edge types increases. As any effective therapy must be accompanied by a mechanistic understanding of how it functions, we also need to ensure that new heterogeneous graph ML models are explainable . With this in mind, we are using AlzKB as a motivating resource for designing new, cutting-edge algorithms that produce interpretable predictions from highly heterogeneous knowledge graphs. Furthermore, the increasing popularity of large language models (LLMs; such as GPT-4) presents a wealth of opportunities for incorporating knowledge graphs such as AlzKB into diverse AI applications [ 81 ]. One application we are considering is using AlzKB to provide LLMs with formalized knowledge about AD that allows them to more effectively produce informative outputs about AD etiology. Currently, LLMs can perform poorly on technically complex or poorly understood domains due to a scarcity of relevant content in their training corpora, and augmenting their performance using domain-specific knowledge graphs is an emerging strategy for fixing that issue. As we do so, these will be released alongside AlzKB with educational resources that facilitate ease of use and adoptability by various stakeholders.
Knowledge graphs—including AlzKB—come with several important limitations that will be crucial to address in coming years. One of these is the subjective nature of determining what does and does not constitute “knowledge,” implying broad acceptance by the scientific community (as opposed to “data,” which consist of individual observations). Currently, we use expert domain knowledge and careful screening of source databases to accomplish this, but with the advent of broadly accessible generative AI tools, there may be emerging strategies that minimize sources of human bias [ 82 ]. Furthermore, new predictions made using knowledge graphs still necessitate costly and time-consuming experimental or observational follow-up studies to validate those predictions. This is due in part to the absence of negative samples for training predictive models. While the presence of an edge between 2 nodes in a knowledge graph is interpreted as a “positive sample” for model training, the absence of an edge simply means that we do not know whether a relationship does or does not exist, and therefore, it may not in fact be a negative sample. New methods, including self-supervised contrastive learning, show promise in alleviating this issue [ 83 ], but further work is needed to determine whether these generalize well to AlzKB and similar highly heterogeneous biomedical knowledge graphs. Nonetheless, these are active areas of research in the AI, informatics, and computer science communities, and in spite of them, our results are still robust enough to provide compelling evidence demonstrating AlzKB’s scientific value.
Ultimately, we aim to provide AlzKB as a robust resource that helps unravel the etiology of AD. It is already a large, high-quality knowledge base from which graph-based AI or ML approaches can be developed for drug repurposing and drug discovery. As we and the rest of the biomedical research community make these discoveries in the coming years, they will be included and publicized on the AlzKB website as a public resource to drive innovation and scientific progress.
Obtaining AlzKB for Local Use and Extending the Knowledge Graph
As it is a public and open-source resource for scientific discovery, we provide AlzKB through a variety of interfaces with distinct advantages for different use cases and user types. Casual users who wish to browse the knowledge base or perform simple analyses can do so directly through the Neo4j browser interface [ 24 ]. However, for more advanced use cases (or when computational needs exceed those available on the public version of the knowledge base), AlzKB can be either downloaded and populated locally into a Neo4j installation or built from the original source data files via the tools included on the AlzKB GitHub repository [ 25 ]. The latter of these options also allows users to extend the knowledge base to include additional data sources, entity types, or relationships beyond those provided in the official knowledge base distribution. We also encourage users who make modifications to the knowledge base to submit their changes for review to be included in the main code distribution. Instructions for how to contribute to AlzKB are also available on the GitHub repository.
As the data sources included in AlzKB are all, themselves, from open-source databases, we urge users to ensure that any new data sources they merge into AlzKB similarly comply with open-source standards. In brief, AlzKB can only be maintained under the most restrictive license terms of its included third-party sources, so restrictive license terms in a database being considered decrease that database’s suitability for inclusion. We hope for AlzKB to be recognized as a community effort for aggregating and democratizing the discovery of new AD therapeutics and, therefore, encourage public discussion of new methods and data sources to be included.
Conclusions
In this work, we introduced AlzKB as a free, publicly available toolkit and data resource for novel discoveries in AD research, with a particular focus on therapeutic approaches to treating AD. AlzKB is both new and continually growing, and we aim to cultivate a community of researchers to collaboratively increase the impact, speed, and throughput of AD research, along with rapid dissemination to health care, academia, and the pharmaceutical industry. In the future, we will develop new AI and data science methods to continually extract knowledge from AlzKB, but in this study, we already demonstrate through graph data science that AlzKB can both replicate existing AD knowledge and generate entirely new, testable hypotheses to drive the future of drug repurposing and drug discovery.
Acknowledgments
The Alzheimer’s Knowledge Base is supported by US National Institutes of Health grants U01-AG066833, R01-LM010098, R01-LM013463 (principal investigator [PI]: JHM), and R00-LM013646 (PI: JDR).
Data Availability
The data sets generated during and analyzed during this study are available in the GitHub and Zenodo repositories [ 25 , 26 ].
Conflicts of Interest
None declared.
Supplemental information providing expanded details on the knowledge graph completion methods used to validate Alzheimer’s Knowledge Base, as well as counts for relationship types in the knowledge graph.
- 2022 Alzheimer's disease facts and figures. Alzheimers Dement. Apr 2022;18(4):700-789. [ CrossRef ] [ Medline ]
- Yiannopoulou KG, Papageorgiou SG. Current and future treatments in Alzheimer disease: an update. J Cent Nerv Syst Dis. Feb 29, 2020;12:1179573520907397. [ FREE Full text ] [ CrossRef ] [ Medline ]
- Rabinovici GD. Controversy and progress in Alzheimer's disease - FDA approval of aducanumab. N Engl J Med. Aug 26, 2021;385(9):771-774. [ CrossRef ] [ Medline ]
- DeTure MA, Dickson DW. The neuropathological diagnosis of Alzheimer's disease. Mol Neurodegener. Aug 02, 2019;14(1):32. [ FREE Full text ] [ CrossRef ] [ Medline ]
- Aisen PS, Cummings J, Jack CRJ, Morris JC, Sperling R, Frölich L, et al. On the path to 2025: understanding the Alzheimer's disease continuum. Alzheimers Res Ther. Aug 09, 2017;9(1):60. [ FREE Full text ] [ CrossRef ] [ Medline ]
- Fan L, Mao C, Hu X, Zhang S, Yang Z, Hu Z, et al. New insights into the pathogenesis of Alzheimer's disease. Front Neurol. 2019;10:1312. [ FREE Full text ] [ CrossRef ] [ Medline ]
- Silva MV, de Mello Gomide Loures C, Alves LC, de Souza LC, Borges KB, Carvalho MD. Alzheimer's disease: risk factors and potentially protective measures. J Biomed Sci. May 09, 2019;26(1):33. [ FREE Full text ] [ CrossRef ] [ Medline ]
- Rodriguez S, Hug C, Todorov P, Moret N, Boswell SA, Evans K, et al. Machine learning identifies candidates for drug repurposing in Alzheimer's disease. Nat Commun. Feb 15, 2021;12(1):1033. [ FREE Full text ] [ CrossRef ] [ Medline ]
- Tsuji S, Hase T, Yachie-Kinoshita A, Nishino T, Ghosh S, Kikuchi M, et al. Artificial intelligence-based computational framework for drug-target prioritization and inference of novel repositionable drugs for Alzheimer's disease. Alzheimers Res Ther. May 03, 2021;13(1):92. [ FREE Full text ] [ CrossRef ] [ Medline ]
- Grupe A, Abraham R, Li Y, Rowland C, Hollingworth P, Morgan A, et al. Evidence for novel susceptibility genes for late-onset Alzheimer's disease from a genome-wide association study of putative functional variants. Hum Mol Genet. Apr 15, 2007;16(8):865-873. [ CrossRef ] [ Medline ]
- The Alzheimer's KnowledgeBase (AlzKB). AlzKB. URL: https://alzkb.ai/ [accessed 2023-02-24]
- Daluwatumulle G, Wijesinghe R, Weerasinghe R. In silico drug repurposing using knowledge graph embeddings for Alzheimer's disease. In: Proceedings of the 9th International Conference on Bioinformatics Research and Applications. 2022. Presented at: ICBRA '22; September 18-20, 2022; Berlin, Germany. [ CrossRef ]
- Nian Y, Hu X, Zhang R, Feng J, Du J, Li F, et al. Mining on Alzheimer's diseases related knowledge graph to identity potential AD-related semantic triples for drug repurposing. BMC Bioinformatics. Sep 30, 2022;23(Suppl 6):407. [ FREE Full text ] [ CrossRef ] [ Medline ]
- Hsieh KL, Plascencia-Villa G, Lin KH, Perry G, Jiang X, Kim Y. Synthesize heterogeneous biological knowledge via representation learning for Alzheimer's disease drug repurposing. iScience. Nov 26, 2022;26(1):105678. [ FREE Full text ] [ CrossRef ] [ Medline ]
- Binder J, Ursu O, Bologa C, Jiang S, Maphis N, Dadras S, et al. Machine learning prediction and tau-based screening identifies potential Alzheimer's disease genes relevant to immunity. Commun Biol. Feb 11, 2022;5(1):125. [ FREE Full text ] [ CrossRef ] [ Medline ]
- Sügis E, Dauvillier J, Leontjeva A, Adler P, Hindie V, Moncion T, et al. HENA, heterogeneous network-based data set for Alzheimer's disease. Sci Data. Aug 14, 2019;6(1):151. [ CrossRef ] [ Medline ]
- Robinson I, Webber J, Eifrem E. Graph Databases: New Opportunities for Connected Data. Sebastopol, CA. O'Reilly Media; 2015.
- Davis R, Shrobe H, Szolovits P. What is a knowledge representation? AI Mag. 1993;14(1):17. [ CrossRef ]
- Musen MA, Protégé Team. The Protégé project: a look back and a look forward. AI Matters. Jun 2015;1(4):4-12. [ FREE Full text ] [ CrossRef ] [ Medline ]
- Hitzler P, Krötzsch M, Parsia B, Patel-Schneider PF, Rudolph S. OWL 2 Web ontology language primer. World Wide Web Consortium. Apr 21, 2009. URL: https://www.w3.org/TR/2009/WD-owl2-primer-20090421/ [accessed 2024-03-25]
- Tsarkov D, Horrocks I. FaCT++ description logic reasoner: system description. In: Proceedings of the International Joint Conference on Automated Reasoning. 2006. Presented at: IJCAR 2006; August 17-20, 2006; Seattle, WA. [ CrossRef ]
- Neo4j. URL: https://neo4j.com/ [accessed 2022-10-25]
- Barrasa J, Cowley A. neosemantics (n10s): Neo4j RDF and semantics toolkit. Neo4j. URL: https://neo4j.com/labs/neosemantics/ [accessed 2022-10-25]
- Neo4j browser. Neo4j. URL: http://neo4j.alzkb.ai/browser/ [accessed 2023-02-24]
- EpistasisLab/AlzKB. GitHub. URL: https://github.com/EpistasisLab/AlzKB [accessed 2023-02-24]
- Romano J, Wang P. EpistasisLab/AlzKB: AlzKB first DOI release. Zenodo. Aug 22, 2022. URL: https://zenodo.org/records/7015728 [accessed 2024-03-27]
- Mortensen HM, Senn J, Levey T, Langley P, Williams AJ. The 2021 update of the EPA's adverse outcome pathway database. Sci Data. Jul 12, 2021;8(1):169. [ FREE Full text ] [ CrossRef ] [ Medline ]
- Bastian F, Parmentier G, Roux J, Moretti S, Laudet V, Robinson-Rechavi M. Bgee: integrating and comparing heterogeneous transcriptome data among species. In: Proceedings of the Data Integration in the Life Sciences. 2008. Presented at: DILS 2008; June 25-27, 2008; Evry, France. [ CrossRef ]
- Schriml LM, Mitraka E, Munro J, Tauber B, Schor M, Nickle L, et al. Human Disease Ontology 2018 update: classification, content and workflow expansion. Nucleic Acids Res. Jan 08, 2019;47(D1):D955-D962. [ FREE Full text ] [ CrossRef ] [ Medline ]
- Piñero J, Queralt-Rosinach N, Bravo A, Deu-Pons J, Bauer-Mehren A, Baron M, et al. DisGeNET: a discovery platform for the dynamical exploration of human diseases and their genes. Database (Oxford). 2015;2015:bav028. [ FREE Full text ] [ CrossRef ] [ Medline ]
- Wishart DS, Knox C, Guo AC, Cheng D, Shrivastava S, Tzur D, et al. DrugBank: a knowledgebase for drugs, drug actions and drug targets. Nucleic Acids Res. Jan 2008;36(Database issue):D901-D906. [ FREE Full text ] [ CrossRef ] [ Medline ]
- Grulke CM, Williams AJ, Thillanadarajah I, Richard AM. EPA's DSSTox database: history of development of a curated chemistry resource supporting computational toxicology research. Comput Toxicol. Nov 01, 2019;12:100096. [ FREE Full text ] [ CrossRef ] [ Medline ]
- Judson R, Richard A, Dix D, Houck K, Elloumi F, Martin M, et al. ACToR--Aggregated computational toxicology resource. Toxicol Appl Pharmacol. Nov 15, 2008;233(1):7-13. [ CrossRef ] [ Medline ]
- Ashburner M, Ball CA, Blake JA, Botstein D, Butler H, Cherry JM, et al. Gene ontology: tool for the unification of biology. The Gene Ontology Consortium. Nat Genet. May 2000;25(1):25-29. [ FREE Full text ] [ CrossRef ] [ Medline ]
- Gene Ontology Consortium. The Gene Ontology resource: enriching a GOld mine. Nucleic Acids Res. Jan 08, 2021;49(D1):D325-D334. [ FREE Full text ] [ CrossRef ] [ Medline ]
- Buniello A, MacArthur JA, Cerezo M, Harris LW, Hayhurst J, Malangone C, et al. The NHGRI-EBI GWAS Catalog of published genome-wide association studies, targeted arrays and summary statistics 2019. Nucleic Acids Res. Jan 08, 2019;47(D1):D1005-D1012. [ FREE Full text ] [ CrossRef ] [ Medline ]
- Himmelstein DS, Lizee A, Hessler C, Brueggeman L, Chen SL, Hadley D, et al. Systematic integration of biomedical knowledge prioritizes drugs for repurposing. Elife. Sep 22, 2017;6:e26726. [ FREE Full text ] [ CrossRef ] [ Medline ]
- The human reference protein interactome mapping project. The Human Reference Interactome. URL: http://www.interactome-atlas.org/ [accessed 2023-02-24]
- Duan Q, Reid SP, Clark NR, Wang Z, Fernandez NF, Rouillard AD, et al. L1000CDS: LINCS L1000 characteristic direction signatures search engine. NPJ Syst Biol Appl. Aug 04, 2016;2(1):16015. [ FREE Full text ] [ CrossRef ] [ Medline ]
- Lipscomb CE. Medical Subject Headings (MeSH). Bull Med Libr Assoc. Jul 2000;88(3):265-266. [ FREE Full text ] [ Medline ]
- Maglott D, Ostell J, Pruitt KD, Tatusova T. Entrez Gene: gene-centered information at NCBI. Nucleic Acids Res. Jan 2011;39(Database issue):D52-D57. [ FREE Full text ] [ CrossRef ] [ Medline ]
- Schaefer C, Anthony K, Krupa S, Buchoff J, Day M, Hannay T, et al. PID: the pathway interaction database. Nat Prec. Aug 29, 2008. [ CrossRef ]
- Himmelstein D, Pouya K, Hessler CS, Green AJ, Baranzini S. PharmacotherapyDB 1.0: the open catalog of drug therapies for disease. Figshare. 2016. URL: https://tinyurl.com/mv8k46em [accessed 2024-03-25]
- Kim S, Chen J, Cheng T, Gindulyte A, He J, He S, et al. PubChem in 2021: new data content and improved web interfaces. Nucleic Acids Res. Jan 08, 2021;49(D1):D1388-D1395. [ FREE Full text ] [ CrossRef ] [ Medline ]
- Wu G, Haw R. Functional interaction network construction and analysis for disease discovery. Methods Mol Biol. 2017;1558:235-253. [ CrossRef ] [ Medline ]
- Kuhn M, Letunic I, Jensen LJ, Bork P. The SIDER database of drugs and side effects. Nucleic Acids Res. Jan 04, 2016;44(D1):D1075-D1079. [ FREE Full text ] [ CrossRef ] [ Medline ]
- Palasca O, Santos A, Stolte C, Gorodkin J, Jensen LJ. TISSUES 2.0: an integrative web resource on mammalian tissue expression. Database (Oxford). Jan 01, 2018;2018:2. [ FREE Full text ] [ CrossRef ] [ Medline ]
- Mungall CJ, Torniai C, Gkoutos GV, Lewis SE, Haendel MA. Uberon, an integrative multi-species anatomy ontology. Genome Biol. Jan 31, 2012;13(1):R5. [ FREE Full text ] [ CrossRef ] [ Medline ]
- Martens M, Ammar A, Riutta A, Waagmeester A, Slenter DN, Hanspers KA, et al. WikiPathways: connecting communities. Nucleic Acids Res. Jan 08, 2021;49(D1):D613-D621. [ FREE Full text ] [ CrossRef ] [ Medline ]
- Ali M, Berrendorf M, Hoyt CT, Vermue L, Sharifzadeh S, Tresp V, et al. PyKEEN 1.0: a python library for training and evaluating knowledge graph embeddings. J Mach Learn Res. 2021;22(82):1-6. [ FREE Full text ]
- Zamini M, Reza H, Rabiei M. A review of knowledge graph completion. Information. Aug 21, 2022;13(8):396. [ CrossRef ]
- Bordes A, Usunier N, Garcia-Duran A, Weston J, Yakhnenko O. Translating embeddings for modeling multi-relational data. In: Proceedings of the 26th International Conference on Neural Information Processing Systems - Volume 2. 2013. Presented at: NIPS'13; December 5-10, 2013; Lake Tahoe, Nevada.
- Sun Z, Deng ZH, Nie JY, Tang J. RotatE: knowledge graph embedding by relational rotation in complex space. arXiv. Preprint posted online February 26, 2019. [ FREE Full text ]
- Yang B, Yih WT, He X, Gao J, Deng L. Embedding entities and relations for learning and inference in knowledge bases. arXiv. Preprint posted online December 20, 2014. [ FREE Full text ]
- Trouillon T, Welbl J, Riedel S, Gaussier E, Bouchard G. Complex embeddings for simple link prediction. arXiv. Preprint posted online June 20, 2016. [ FREE Full text ]
- Dettmers T, Minervini P, Stenetorp P, Riedel S. Convolutional 2D knowledge graph embeddings. arXiv. Preprint posted online July 5, 2017. [ FREE Full text ] [ CrossRef ]
- Newman ME. Clustering and preferential attachment in growing networks. Phys Rev E. Jul 26, 2001;64(2):025102. [ CrossRef ]
- Barabasi AL, Albert R. Emergence of scaling in random networks. Science. Oct 15, 1999;286(5439):509-512. [ CrossRef ] [ Medline ]
- Adamic LA, Adar E. Friends and neighbors on the web. Soc Netw. Jul 2003;25(3):211-230. [ CrossRef ]
- Zhou T, Lü L, Zhang YC. Predicting missing links via local information. Eur Phys J B. Oct 10, 2009;71(4):623-630. [ CrossRef ]
- Gao Z, Ding P, Xu R. KG-Predict: a knowledge graph computational framework for drug repurposing. J Biomed Inform. Aug 2022;132:104133. [ FREE Full text ] [ CrossRef ] [ Medline ]
- Nussbaum RL, Ellis CE. Alzheimer's disease and Parkinson's disease. N Engl J Med. Apr 03, 2003;348(14):1356-1364. [ CrossRef ] [ Medline ]
- Abbas K, Abbasi A, Dong S, Niu L, Yu L, Chen B, et al. Application of network link prediction in drug discovery. BMC Bioinformatics. Apr 12, 2021;22(1):187. [ FREE Full text ] [ CrossRef ] [ Medline ]
- Romano JD, Hao Y, Moore JH, Penning TM. Automating predictive toxicology using ComptoxAI. Chem Res Toxicol. Aug 15, 2022;35(8):1370-1382. [ FREE Full text ] [ CrossRef ] [ Medline ]
- Iannuzzi F, Sirabella R, Canu N, Maier TJ, Annunziato L, Matrone C. Fyn tyrosine kinase elicits amyloid precursor protein Tyr682 phosphorylation in neurons from Alzheimer's disease patients. Cells. Jul 30, 2020;9(8):1807. [ FREE Full text ] [ CrossRef ] [ Medline ]
- Nygaard HB, van Dyck CH, Strittmatter SM. Fyn kinase inhibition as a novel therapy for Alzheimer's disease. Alzheimers Res Ther. Feb 5, 2014;6(1):8. [ FREE Full text ] [ CrossRef ] [ Medline ]
- Lardenoije R, Roubroeks JA, Pishva E, Leber M, Wagner H, Iatrou A, et al. Alzheimer's disease-associated (hydroxy)methylomic changes in the brain and blood. Clin Epigenetics. Nov 27, 2019;11(1):164. [ FREE Full text ] [ CrossRef ] [ Medline ]
- Lombardo S, Maskos U. Role of the nicotinic acetylcholine receptor in Alzheimer's disease pathology and treatment. Neuropharmacology. Sep 2015;96(Pt B):255-262. [ FREE Full text ] [ CrossRef ] [ Medline ]
- Jo DG, Lee JY, Hong YM, Song S, Mook-Jung I, Koh JY, et al. Induction of pro-apoptotic calsenilin/DREAM/KChIP3 in Alzheimer's disease and cultured neurons after amyloid-beta exposure. J Neurochem. Feb 2004;88(3):604-611. [ FREE Full text ] [ CrossRef ] [ Medline ]
- Jin JK, Choi JK, Wasco W, Buxbaum JD, Kozlowski PB, Carp RI, et al. Expression of calsenilin in neurons and astrocytes in the Alzheimer's disease brain. Neuroreport. Apr 04, 2005;16(5):451-455. [ CrossRef ] [ Medline ]
- Rosas I, Martínez C, Clarimón J, Lleó A, Illán-Gala I, Dols-Icardo O, et al. Role for ATXN1, ATXN2, and HTT intermediate repeats in frontotemporal dementia and Alzheimer's disease. Neurobiol Aging. Mar 2020;87:139.e1-139.e7. [ CrossRef ] [ Medline ]
- Aboud O, Parcon PA, DeWall KM, Liu L, Mrak RE, Griffin WS. Aging, Alzheimer's, and APOE genotype influence the expression and neuronal distribution patterns of microtubule motor protein dynactin-P50. Front Cell Neurosci. Mar 25, 2015;9:103. [ FREE Full text ] [ CrossRef ] [ Medline ]
- Caroppo P, Le Ber I, Clot F, Rivaud-Péchoux S, Camuzat A, De Septenville A, et al. DCTN1 mutation analysis in families with progressive supranuclear palsy-like phenotypes. JAMA Neurol. Feb 2014;71(2):208-215. [ FREE Full text ] [ CrossRef ] [ Medline ]
- Zochodne DW, Ho LT. Sumatriptan blocks neurogenic inflammation in the peripheral nerve trunk. Neurology. Jan 1994;44(1):161-163. [ CrossRef ] [ Medline ]
- Liu CT, Wu BY, Hung YC, Wang LY, Lee YY, Lin TK, et al. Decreased risk of dementia in migraine patients with traditional Chinese medicine use: a population-based cohort study. Oncotarget. Oct 03, 2017;8(45):79680-79692. [ FREE Full text ] [ CrossRef ] [ Medline ]
- Kim YD, Jeong EI, Nah J, Yoo SM, Lee WJ, Kim Y, et al. Pimozide reduces toxic forms of tau in TauC3 mice via 5' adenosine monophosphate-activated protein kinase-mediated autophagy. J Neurochem. Sep 11, 2017;142(5):734-746. [ FREE Full text ] [ CrossRef ] [ Medline ]
- Kumar S, Chowdhury S, Kumar S. In silico repurposing of antipsychotic drugs for Alzheimer's disease. BMC Neurosci. Oct 27, 2017;18(1):76. [ FREE Full text ] [ CrossRef ] [ Medline ]
- van Duijn CM, Hofman A. Relation between nicotine intake and Alzheimer's disease. BMJ. Jun 22, 1991;302(6791):1491-1494. [ FREE Full text ] [ CrossRef ] [ Medline ]
- Hamilton WL, Ying R, Leskovec J. Inductive representation learning on large graphs. arXiv. Preprint posted online June 7, 2017. [ FREE Full text ]
- Dong Y, Chawla NV, Swami A. metapath2vec: scalable representation learning for heterogeneous networks. In: Proceedings of the 23rd ACM SIGKDD International Conference on Knowledge Discovery and Data Mining. 2017. Presented at: KDD '17; August 13-17, 2017; Halifax, NS. URL: https://dl.acm.org/doi/10.1145/3097983.3098036
- Pan S, Luo L, Wang Y, Chen C, Wang J, Wu X. Unifying large language models and knowledge graphs: a roadmap. arXiv. Preprint posted online June 14, 2023. [ FREE Full text ] [ CrossRef ]
- Zhu Y, Wang X, Chen J, Qiao S, Ou Y, Yao Y, et al. LLMs for knowledge graph construction and reasoning: recent capabilities and future opportunities. arXiv. Preprint posted online May 22, 2023. [ FREE Full text ]
- Kefato ZT, Girdzijauskas S. Self-supervised Graph Neural Networks without explicit negative sampling. arXiv. Preprint posted online March 27, 2021. [ FREE Full text ]
Abbreviations
Edited by T de Azevedo Cardoso; submitted 24.02.23; peer-reviewed by P Dabas, N Mungoli, B Xie, C Sun; comments to author 21.04.23; revised version received 23.06.23; accepted 07.11.23; published 18.04.24.
©Joseph D Romano, Van Truong, Rachit Kumar, Mythreye Venkatesan, Britney E Graham, Yun Hao, Nick Matsumoto, Xi Li, Zhiping Wang, Marylyn D Ritchie, Li Shen, Jason H Moore. Originally published in the Journal of Medical Internet Research (https://www.jmir.org), 18.04.2024.
This is an open-access article distributed under the terms of the Creative Commons Attribution License (https://creativecommons.org/licenses/by/4.0/), which permits unrestricted use, distribution, and reproduction in any medium, provided the original work, first published in the Journal of Medical Internet Research, is properly cited. The complete bibliographic information, a link to the original publication on https://www.jmir.org/, as well as this copyright and license information must be included.
- Skip to main content
- Skip to FDA Search
- Skip to in this section menu
- Skip to footer links

The .gov means it’s official. Federal government websites often end in .gov or .mil. Before sharing sensitive information, make sure you're on a federal government site.
The site is secure. The https:// ensures that you are connecting to the official website and that any information you provide is encrypted and transmitted securely.
U.S. Food and Drug Administration
- Search
- Menu
- Medical Devices
- Medical Devices News and Events
CDRH Unveils New Dataset to Help Improve Chemical Characterization Methods for Biocompatibility of Medical Devices
FOR IMMEDIATE RELEASE April 16, 2024
The following is attributed to Jeff Shuren, M.D., J.D., director of the FDA’s Center for Devices and Radiological Health (CDRH) and Ed Margerrison, Ph.D., director of the Office of Science and Engineering Laboratories (OSEL), CDRH
Today, the FDA’s Center for Devices and Radiological Health (CDRH) is unveiling a new public dataset designed to help assist chemistry labs in ensuring the robustness of chemical characterization methods used to assess the biocompatibility of medical devices.
This dataset will allow analytical chemistry labs to determine their ability to detect a broad range of potential chemicals. Provided are links to the first set of chemicals along with their physicochemical properties, as well as the CDRH provided results when using gas chromatography (GC) protocols.
The example chemicals can be assessed by laboratories using their own GC protocols to determine whether the chemicals are detectable compared with one of the specific chemicals in the dataset measured by the relative response factor (RRF). If detectability is shown to be similar to the RRFs obtained by CDRH, there can be an increased confidence in the protocols being used by the lab. In the future, CDRH intends to increase the breadth of the dataset of chemicals and RRF information it makes publicly available, and to add other detection methodologies such as liquid chromatography (LC).
This new dataset is part of CDRH’s ongoing commitment to help reduce the burden of premarket processes, while increasing the consistency and transparency of biocompatibility assessment methods.
In 2016, CDRH issued the first version of FDA’s Biocompatibility Guidance based on the international consensus standard ISO 10993 Part 1, which outlines the Center’s approach to the biocompatibility evaluation of medical devices within a risk management process. A central tenet of the CDRH biocompatibility evaluation is for sponsors to have the option, for some biocompatibility endpoints, to undertake extraction studies to identify and quantify chemicals released from a device and then to perform a toxicological risk assessment (TRA) to determine if the chemicals pose safety issues in the use of the device.
A successful TRA determination is dependent on the sensitivity of the analytical chemistry method used to detect and quantify chemicals that may be present following extraction from a medical device. This non-animal-based analytical chemistry testing approach is used as a surrogate to predict the exposure of chemicals that may be released by medical devices in use.
Through this new approach and other actions, CRDH will continue working to make the premarket review process as efficient and seamless as possible for developers and other stakeholders, while enhancing transparency around biocompatibility assessment and prioritizing safety for all devices undergoing the premarket review process.
Additional Resources
- Biocompatibility and Toxicology Program: Research on Medical Devices, Biocompatibility, and Toxicology
- Materials and Chemical Characterization Program: Research on the Materials and Chemical Characterization of Medical Devices
Numbers, Facts and Trends Shaping Your World
Read our research on:
Full Topic List
Regions & Countries
- Publications
- Our Methods
- Short Reads
- Tools & Resources
Read Our Research On:
What the data says about abortion in the U.S.
Pew Research Center has conducted many surveys about abortion over the years, providing a lens into Americans’ views on whether the procedure should be legal, among a host of other questions.
In a Center survey conducted nearly a year after the Supreme Court’s June 2022 decision that ended the constitutional right to abortion , 62% of U.S. adults said the practice should be legal in all or most cases, while 36% said it should be illegal in all or most cases. Another survey conducted a few months before the decision showed that relatively few Americans take an absolutist view on the issue .
Find answers to common questions about abortion in America, based on data from the Centers for Disease Control and Prevention (CDC) and the Guttmacher Institute, which have tracked these patterns for several decades:
How many abortions are there in the U.S. each year?
How has the number of abortions in the u.s. changed over time, what is the abortion rate among women in the u.s. how has it changed over time, what are the most common types of abortion, how many abortion providers are there in the u.s., and how has that number changed, what percentage of abortions are for women who live in a different state from the abortion provider, what are the demographics of women who have had abortions, when during pregnancy do most abortions occur, how often are there medical complications from abortion.
This compilation of data on abortion in the United States draws mainly from two sources: the Centers for Disease Control and Prevention (CDC) and the Guttmacher Institute, both of which have regularly compiled national abortion data for approximately half a century, and which collect their data in different ways.
The CDC data that is highlighted in this post comes from the agency’s “abortion surveillance” reports, which have been published annually since 1974 (and which have included data from 1969). Its figures from 1973 through 1996 include data from all 50 states, the District of Columbia and New York City – 52 “reporting areas” in all. Since 1997, the CDC’s totals have lacked data from some states (most notably California) for the years that those states did not report data to the agency. The four reporting areas that did not submit data to the CDC in 2021 – California, Maryland, New Hampshire and New Jersey – accounted for approximately 25% of all legal induced abortions in the U.S. in 2020, according to Guttmacher’s data. Most states, though, do have data in the reports, and the figures for the vast majority of them came from each state’s central health agency, while for some states, the figures came from hospitals and other medical facilities.
Discussion of CDC abortion data involving women’s state of residence, marital status, race, ethnicity, age, abortion history and the number of previous live births excludes the low share of abortions where that information was not supplied. Read the methodology for the CDC’s latest abortion surveillance report , which includes data from 2021, for more details. Previous reports can be found at stacks.cdc.gov by entering “abortion surveillance” into the search box.
For the numbers of deaths caused by induced abortions in 1963 and 1965, this analysis looks at reports by the then-U.S. Department of Health, Education and Welfare, a precursor to the Department of Health and Human Services. In computing those figures, we excluded abortions listed in the report under the categories “spontaneous or unspecified” or as “other.” (“Spontaneous abortion” is another way of referring to miscarriages.)
Guttmacher data in this post comes from national surveys of abortion providers that Guttmacher has conducted 19 times since 1973. Guttmacher compiles its figures after contacting every known provider of abortions – clinics, hospitals and physicians’ offices – in the country. It uses questionnaires and health department data, and it provides estimates for abortion providers that don’t respond to its inquiries. (In 2020, the last year for which it has released data on the number of abortions in the U.S., it used estimates for 12% of abortions.) For most of the 2000s, Guttmacher has conducted these national surveys every three years, each time getting abortion data for the prior two years. For each interim year, Guttmacher has calculated estimates based on trends from its own figures and from other data.
The latest full summary of Guttmacher data came in the institute’s report titled “Abortion Incidence and Service Availability in the United States, 2020.” It includes figures for 2020 and 2019 and estimates for 2018. The report includes a methods section.
In addition, this post uses data from StatPearls, an online health care resource, on complications from abortion.
An exact answer is hard to come by. The CDC and the Guttmacher Institute have each tried to measure this for around half a century, but they use different methods and publish different figures.
The last year for which the CDC reported a yearly national total for abortions is 2021. It found there were 625,978 abortions in the District of Columbia and the 46 states with available data that year, up from 597,355 in those states and D.C. in 2020. The corresponding figure for 2019 was 607,720.
The last year for which Guttmacher reported a yearly national total was 2020. It said there were 930,160 abortions that year in all 50 states and the District of Columbia, compared with 916,460 in 2019.
- How the CDC gets its data: It compiles figures that are voluntarily reported by states’ central health agencies, including separate figures for New York City and the District of Columbia. Its latest totals do not include figures from California, Maryland, New Hampshire or New Jersey, which did not report data to the CDC. ( Read the methodology from the latest CDC report .)
- How Guttmacher gets its data: It compiles its figures after contacting every known abortion provider – clinics, hospitals and physicians’ offices – in the country. It uses questionnaires and health department data, then provides estimates for abortion providers that don’t respond. Guttmacher’s figures are higher than the CDC’s in part because they include data (and in some instances, estimates) from all 50 states. ( Read the institute’s latest full report and methodology .)
While the Guttmacher Institute supports abortion rights, its empirical data on abortions in the U.S. has been widely cited by groups and publications across the political spectrum, including by a number of those that disagree with its positions .
These estimates from Guttmacher and the CDC are results of multiyear efforts to collect data on abortion across the U.S. Last year, Guttmacher also began publishing less precise estimates every few months , based on a much smaller sample of providers.
The figures reported by these organizations include only legal induced abortions conducted by clinics, hospitals or physicians’ offices, or those that make use of abortion pills dispensed from certified facilities such as clinics or physicians’ offices. They do not account for the use of abortion pills that were obtained outside of clinical settings .
(Back to top)
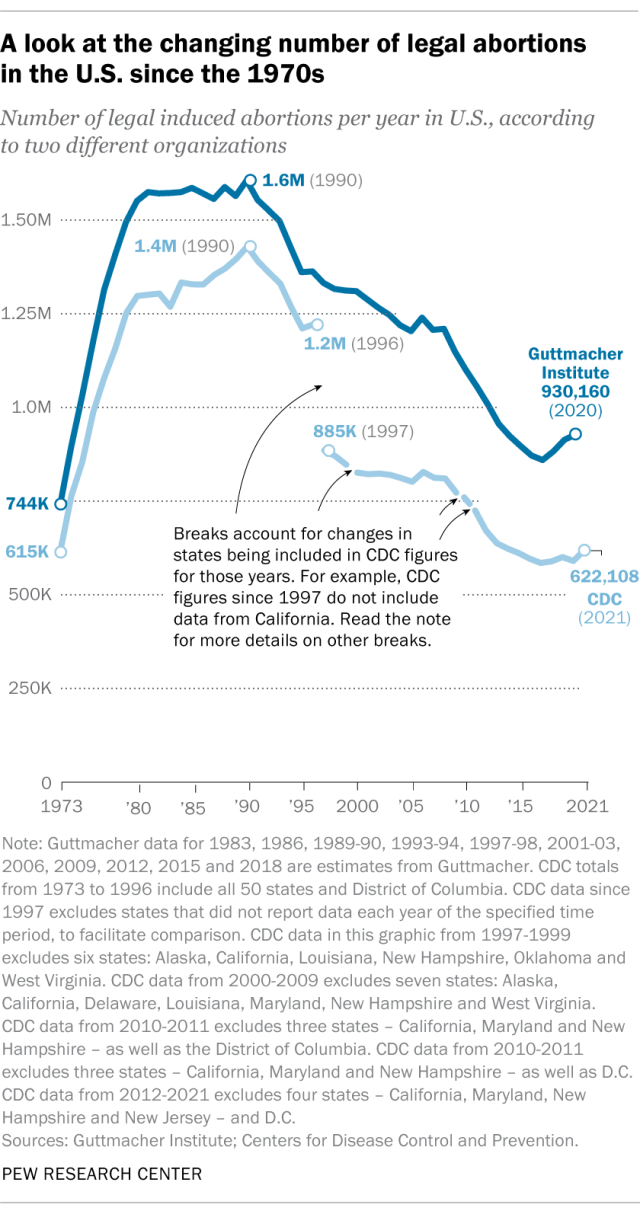
The annual number of U.S. abortions rose for years after Roe v. Wade legalized the procedure in 1973, reaching its highest levels around the late 1980s and early 1990s, according to both the CDC and Guttmacher. Since then, abortions have generally decreased at what a CDC analysis called “a slow yet steady pace.”
Guttmacher says the number of abortions occurring in the U.S. in 2020 was 40% lower than it was in 1991. According to the CDC, the number was 36% lower in 2021 than in 1991, looking just at the District of Columbia and the 46 states that reported both of those years.
(The corresponding line graph shows the long-term trend in the number of legal abortions reported by both organizations. To allow for consistent comparisons over time, the CDC figures in the chart have been adjusted to ensure that the same states are counted from one year to the next. Using that approach, the CDC figure for 2021 is 622,108 legal abortions.)
There have been occasional breaks in this long-term pattern of decline – during the middle of the first decade of the 2000s, and then again in the late 2010s. The CDC reported modest 1% and 2% increases in abortions in 2018 and 2019, and then, after a 2% decrease in 2020, a 5% increase in 2021. Guttmacher reported an 8% increase over the three-year period from 2017 to 2020.
As noted above, these figures do not include abortions that use pills obtained outside of clinical settings.
Guttmacher says that in 2020 there were 14.4 abortions in the U.S. per 1,000 women ages 15 to 44. Its data shows that the rate of abortions among women has generally been declining in the U.S. since 1981, when it reported there were 29.3 abortions per 1,000 women in that age range.
The CDC says that in 2021, there were 11.6 abortions in the U.S. per 1,000 women ages 15 to 44. (That figure excludes data from California, the District of Columbia, Maryland, New Hampshire and New Jersey.) Like Guttmacher’s data, the CDC’s figures also suggest a general decline in the abortion rate over time. In 1980, when the CDC reported on all 50 states and D.C., it said there were 25 abortions per 1,000 women ages 15 to 44.
That said, both Guttmacher and the CDC say there were slight increases in the rate of abortions during the late 2010s and early 2020s. Guttmacher says the abortion rate per 1,000 women ages 15 to 44 rose from 13.5 in 2017 to 14.4 in 2020. The CDC says it rose from 11.2 per 1,000 in 2017 to 11.4 in 2019, before falling back to 11.1 in 2020 and then rising again to 11.6 in 2021. (The CDC’s figures for those years exclude data from California, D.C., Maryland, New Hampshire and New Jersey.)
The CDC broadly divides abortions into two categories: surgical abortions and medication abortions, which involve pills. Since the Food and Drug Administration first approved abortion pills in 2000, their use has increased over time as a share of abortions nationally, according to both the CDC and Guttmacher.
The majority of abortions in the U.S. now involve pills, according to both the CDC and Guttmacher. The CDC says 56% of U.S. abortions in 2021 involved pills, up from 53% in 2020 and 44% in 2019. Its figures for 2021 include the District of Columbia and 44 states that provided this data; its figures for 2020 include D.C. and 44 states (though not all of the same states as in 2021), and its figures for 2019 include D.C. and 45 states.
Guttmacher, which measures this every three years, says 53% of U.S. abortions involved pills in 2020, up from 39% in 2017.
Two pills commonly used together for medication abortions are mifepristone, which, taken first, blocks hormones that support a pregnancy, and misoprostol, which then causes the uterus to empty. According to the FDA, medication abortions are safe until 10 weeks into pregnancy.
Surgical abortions conducted during the first trimester of pregnancy typically use a suction process, while the relatively few surgical abortions that occur during the second trimester of a pregnancy typically use a process called dilation and evacuation, according to the UCLA School of Medicine.
In 2020, there were 1,603 facilities in the U.S. that provided abortions, according to Guttmacher . This included 807 clinics, 530 hospitals and 266 physicians’ offices.
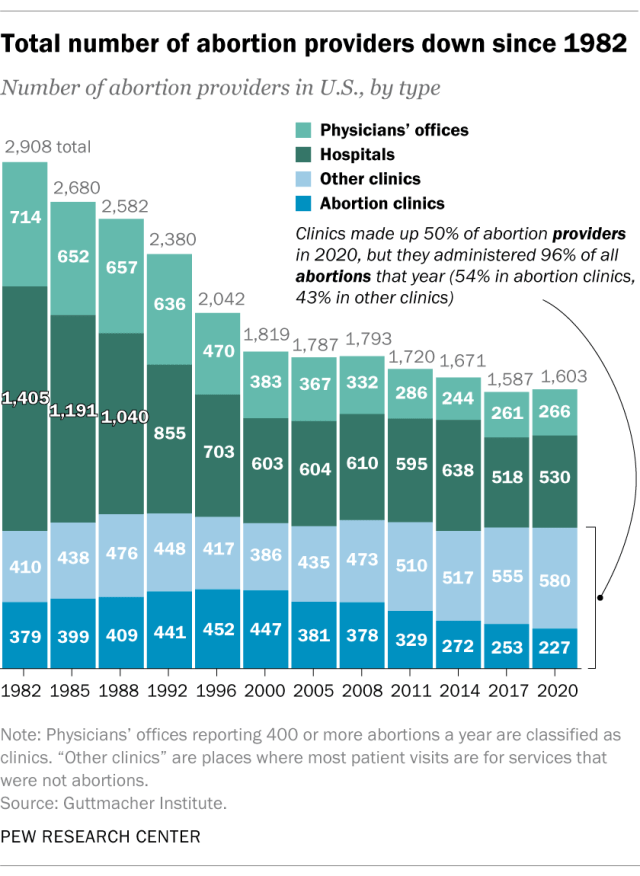
While clinics make up half of the facilities that provide abortions, they are the sites where the vast majority (96%) of abortions are administered, either through procedures or the distribution of pills, according to Guttmacher’s 2020 data. (This includes 54% of abortions that are administered at specialized abortion clinics and 43% at nonspecialized clinics.) Hospitals made up 33% of the facilities that provided abortions in 2020 but accounted for only 3% of abortions that year, while just 1% of abortions were conducted by physicians’ offices.
Looking just at clinics – that is, the total number of specialized abortion clinics and nonspecialized clinics in the U.S. – Guttmacher found the total virtually unchanged between 2017 (808 clinics) and 2020 (807 clinics). However, there were regional differences. In the Midwest, the number of clinics that provide abortions increased by 11% during those years, and in the West by 6%. The number of clinics decreased during those years by 9% in the Northeast and 3% in the South.
The total number of abortion providers has declined dramatically since the 1980s. In 1982, according to Guttmacher, there were 2,908 facilities providing abortions in the U.S., including 789 clinics, 1,405 hospitals and 714 physicians’ offices.
The CDC does not track the number of abortion providers.
In the District of Columbia and the 46 states that provided abortion and residency information to the CDC in 2021, 10.9% of all abortions were performed on women known to live outside the state where the abortion occurred – slightly higher than the percentage in 2020 (9.7%). That year, D.C. and 46 states (though not the same ones as in 2021) reported abortion and residency data. (The total number of abortions used in these calculations included figures for women with both known and unknown residential status.)
The share of reported abortions performed on women outside their state of residence was much higher before the 1973 Roe decision that stopped states from banning abortion. In 1972, 41% of all abortions in D.C. and the 20 states that provided this information to the CDC that year were performed on women outside their state of residence. In 1973, the corresponding figure was 21% in the District of Columbia and the 41 states that provided this information, and in 1974 it was 11% in D.C. and the 43 states that provided data.
In the District of Columbia and the 46 states that reported age data to the CDC in 2021, the majority of women who had abortions (57%) were in their 20s, while about three-in-ten (31%) were in their 30s. Teens ages 13 to 19 accounted for 8% of those who had abortions, while women ages 40 to 44 accounted for about 4%.
The vast majority of women who had abortions in 2021 were unmarried (87%), while married women accounted for 13%, according to the CDC , which had data on this from 37 states.
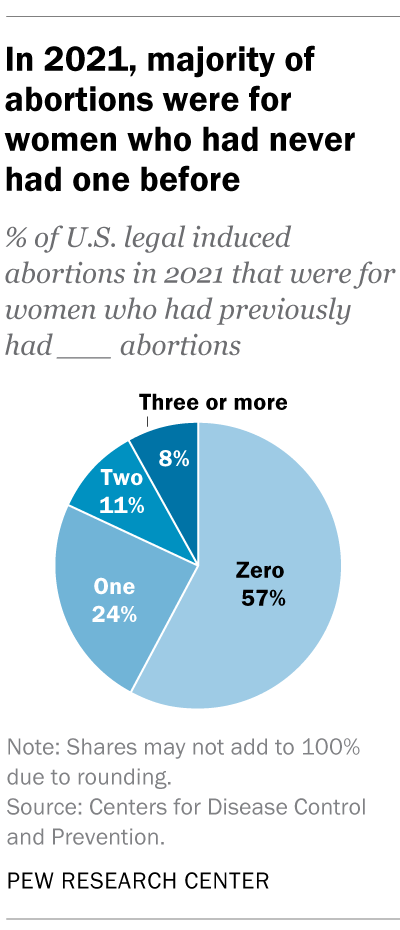
In the District of Columbia, New York City (but not the rest of New York) and the 31 states that reported racial and ethnic data on abortion to the CDC , 42% of all women who had abortions in 2021 were non-Hispanic Black, while 30% were non-Hispanic White, 22% were Hispanic and 6% were of other races.
Looking at abortion rates among those ages 15 to 44, there were 28.6 abortions per 1,000 non-Hispanic Black women in 2021; 12.3 abortions per 1,000 Hispanic women; 6.4 abortions per 1,000 non-Hispanic White women; and 9.2 abortions per 1,000 women of other races, the CDC reported from those same 31 states, D.C. and New York City.
For 57% of U.S. women who had induced abortions in 2021, it was the first time they had ever had one, according to the CDC. For nearly a quarter (24%), it was their second abortion. For 11% of women who had an abortion that year, it was their third, and for 8% it was their fourth or more. These CDC figures include data from 41 states and New York City, but not the rest of New York.
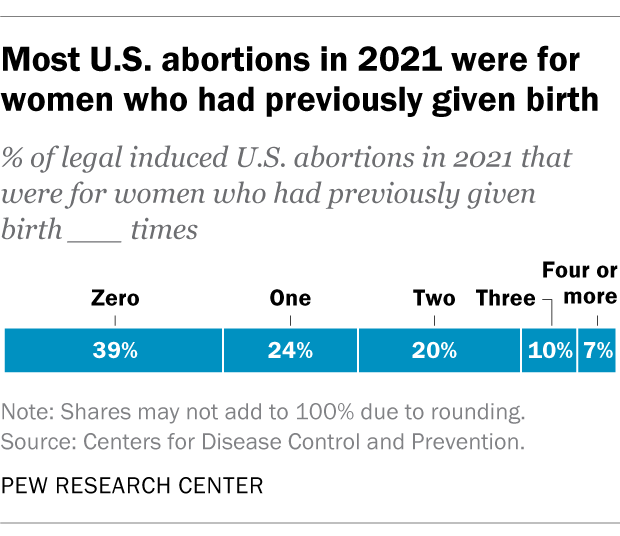
Nearly four-in-ten women who had abortions in 2021 (39%) had no previous live births at the time they had an abortion, according to the CDC . Almost a quarter (24%) of women who had abortions in 2021 had one previous live birth, 20% had two previous live births, 10% had three, and 7% had four or more previous live births. These CDC figures include data from 41 states and New York City, but not the rest of New York.
The vast majority of abortions occur during the first trimester of a pregnancy. In 2021, 93% of abortions occurred during the first trimester – that is, at or before 13 weeks of gestation, according to the CDC . An additional 6% occurred between 14 and 20 weeks of pregnancy, and about 1% were performed at 21 weeks or more of gestation. These CDC figures include data from 40 states and New York City, but not the rest of New York.
About 2% of all abortions in the U.S. involve some type of complication for the woman , according to an article in StatPearls, an online health care resource. “Most complications are considered minor such as pain, bleeding, infection and post-anesthesia complications,” according to the article.
The CDC calculates case-fatality rates for women from induced abortions – that is, how many women die from abortion-related complications, for every 100,000 legal abortions that occur in the U.S . The rate was lowest during the most recent period examined by the agency (2013 to 2020), when there were 0.45 deaths to women per 100,000 legal induced abortions. The case-fatality rate reported by the CDC was highest during the first period examined by the agency (1973 to 1977), when it was 2.09 deaths to women per 100,000 legal induced abortions. During the five-year periods in between, the figure ranged from 0.52 (from 1993 to 1997) to 0.78 (from 1978 to 1982).
The CDC calculates death rates by five-year and seven-year periods because of year-to-year fluctuation in the numbers and due to the relatively low number of women who die from legal induced abortions.
In 2020, the last year for which the CDC has information , six women in the U.S. died due to complications from induced abortions. Four women died in this way in 2019, two in 2018, and three in 2017. (These deaths all followed legal abortions.) Since 1990, the annual number of deaths among women due to legal induced abortion has ranged from two to 12.
The annual number of reported deaths from induced abortions (legal and illegal) tended to be higher in the 1980s, when it ranged from nine to 16, and from 1972 to 1979, when it ranged from 13 to 63. One driver of the decline was the drop in deaths from illegal abortions. There were 39 deaths from illegal abortions in 1972, the last full year before Roe v. Wade. The total fell to 19 in 1973 and to single digits or zero every year after that. (The number of deaths from legal abortions has also declined since then, though with some slight variation over time.)
The number of deaths from induced abortions was considerably higher in the 1960s than afterward. For instance, there were 119 deaths from induced abortions in 1963 and 99 in 1965 , according to reports by the then-U.S. Department of Health, Education and Welfare, a precursor to the Department of Health and Human Services. The CDC is a division of Health and Human Services.
Note: This is an update of a post originally published May 27, 2022, and first updated June 24, 2022.
Support for legal abortion is widespread in many countries, especially in Europe
Nearly a year after roe’s demise, americans’ views of abortion access increasingly vary by where they live, by more than two-to-one, americans say medication abortion should be legal in their state, most latinos say democrats care about them and work hard for their vote, far fewer say so of gop, positive views of supreme court decline sharply following abortion ruling, most popular.
1615 L St. NW, Suite 800 Washington, DC 20036 USA (+1) 202-419-4300 | Main (+1) 202-857-8562 | Fax (+1) 202-419-4372 | Media Inquiries
Research Topics
- Age & Generations
- Coronavirus (COVID-19)
- Economy & Work
- Family & Relationships
- Gender & LGBTQ
- Immigration & Migration
- International Affairs
- Internet & Technology
- Methodological Research
- News Habits & Media
- Non-U.S. Governments
- Other Topics
- Politics & Policy
- Race & Ethnicity
- Email Newsletters
ABOUT PEW RESEARCH CENTER Pew Research Center is a nonpartisan fact tank that informs the public about the issues, attitudes and trends shaping the world. It conducts public opinion polling, demographic research, media content analysis and other empirical social science research. Pew Research Center does not take policy positions. It is a subsidiary of The Pew Charitable Trusts .
Copyright 2024 Pew Research Center
Terms & Conditions
Privacy Policy
Cookie Settings
Reprints, Permissions & Use Policy

An official website of the United States government
The .gov means it’s official. Federal government websites often end in .gov or .mil. Before sharing sensitive information, make sure you’re on a federal government site.
The site is secure. The https:// ensures that you are connecting to the official website and that any information you provide is encrypted and transmitted securely.
- Publications
- Account settings
Preview improvements coming to the PMC website in October 2024. Learn More or Try it out now .
- Advanced Search
- Journal List

Electronic health records to facilitate clinical research
Martin r. cowie.
1 National Heart and Lung Institute, Imperial College London, Royal Brompton Hospital, Sydney Street, London, SW3 6HP UK
Juuso I. Blomster
2 Astra Zeneca R&D, Molndal, Sweden
3 University of Turku, Turku, Finland
Lesley H. Curtis
4 Duke Clinical Research Institute, Durham, NC USA
Sylvie Duclaux
5 Servier, Paris, France
6 Robertson Centre for Biostatistics, University of Glasgow, Glasgow, UK
Fleur Fritz
7 University of Münster, Münster, Germany
Samantha Goldman
8 Daiichi-Sankyo, London, UK
Salim Janmohamed
9 GlaxoSmithKline, Stockley Park, UK
Jörg Kreuzer
10 Boehringer-Ingelheim, Pharma GmbH & Co KG, Ingelheim, Germany
Mark Leenay
11 Optum International, London, UK
Alexander Michel
12 Bayer Pharma, Berlin, Germany
13 Pfizer Ltd., Surrey, UK
Jill P. Pell
14 Institute of Health and Wellbeing, University of Glasgow, Glasgow, UK
Mary Ross Southworth
15 Food and Drug Administration, Silver Spring, MD USA
Wendy Gattis Stough
16 Campbell University College of Pharmacy and Health Sciences, Campbell, NC USA
Martin Thoenes
17 Edwards LifeSciences, Nyon, Switzerland
Faiez Zannad
18 INSERM, Centre d’Investigation Clinique 9501 and Unité 961, Centre Hospitalier Universitaire, Nancy, France
19 Department of Cardiology, Nancy University, Université de Lorraine, Nancy, France
Andrew Zalewski
20 Glaxo Smith Kline, King of Prussia, Pennsylvania, USA
Electronic health records (EHRs) provide opportunities to enhance patient care, embed performance measures in clinical practice, and facilitate clinical research. Concerns have been raised about the increasing recruitment challenges in trials, burdensome and obtrusive data collection, and uncertain generalizability of the results. Leveraging electronic health records to counterbalance these trends is an area of intense interest. The initial applications of electronic health records, as the primary data source is envisioned for observational studies, embedded pragmatic or post-marketing registry-based randomized studies, or comparative effectiveness studies. Advancing this approach to randomized clinical trials, electronic health records may potentially be used to assess study feasibility, to facilitate patient recruitment, and streamline data collection at baseline and follow-up. Ensuring data security and privacy, overcoming the challenges associated with linking diverse systems and maintaining infrastructure for repeat use of high quality data, are some of the challenges associated with using electronic health records in clinical research. Collaboration between academia, industry, regulatory bodies, policy makers, patients, and electronic health record vendors is critical for the greater use of electronic health records in clinical research. This manuscript identifies the key steps required to advance the role of electronic health records in cardiovascular clinical research.
Introduction
Electronic health records (EHRs) provide opportunities to enhance patient care, to embed performance measures in clinical practice, and to improve the identification and recruitment of eligible patients and healthcare providers in clinical research. On a macroeconomic scale, EHRs (by enabling pragmatic clinical trials) may assist in the assessment of whether new treatments or innovation in healthcare delivery result in improved outcomes or healthcare savings.
Concerns have been raised about the current state of cardiovascular clinical research: the increasing recruitment challenges; burdensome data collection; and uncertain generalizability to clinical practice [ 1 ]. These factors add to the increasing costs of clinical research [ 2 ] and are thought to contribute to declining investment in the field [ 1 ].
The Cardiovascular Round Table (CRT) of the European Society of Cardiology (ESC) convened a two-day workshop among international experts in cardiovascular clinical research and health informatics to explore how EHRs could advance cardiovascular clinical research. This paper summarizes the key insights and discussions from the workshop, acknowledges the barriers to EHR implementation in clinical research, and identifies practical solutions for engaging stakeholders (i.e., academia, industry, regulatory bodies, policy makers, patients, and EHR vendors) in the implementation of EHRs in clinical research.
Overview of electronic health records
Broadly defined, EHRs represent longitudinal data (in electronic format) that are collected during routine delivery of health care [ 3 ]. EHRs generally contain demographic, vital statistics, administrative, claims (medical and pharmacy), clinical, and patient-centered (e.g., originating from health-related quality-of-life instruments, home-monitoring devices, and frailty or caregiver assessments) data. The scope of an EHR varies widely across the world. Systems originating primarily as billing systems were not designed to support clinical work flow. Moving forward, EHR should be designed to optimize diagnosis and clinical care, which will enhance their relevance for clinical research. The EHR may reflect single components of care (e.g., primary care, emergency department, and intensive care unit) or data from an integrated hospital-wide or inter-hospital linked system [ 4 ]. EHRs may also change over time, reflecting evolving technology capabilities or external influences (e.g., changes in type of data collected related to coding or reimbursement practices).
EHRs emerged largely as a means to improve healthcare quality [ 5 – 7 ] and to capture billing data. EHRs may potentially be used to assess study feasibility, facilitate patient recruitment, streamline data collection, or conduct entirely EHR-based observational, embedded pragmatic, or post-marketing randomized registry studies, or comparative effectiveness studies. The various applications of EHRs for observational studies, safety surveillance, clinical research, and regulatory purposes are shown in Table 1 [ 3 , 8 – 10 ].
Table 1
Electronic health records in research
a Sentinel is the United States Food and Drug Administration’s national electronic system to proactively monitor medical product safety post-marketing, through rapidly and securely accessing data from large amounts of electronic healthcare records, insurance claims, and registries, from a diverse group of data partners [ 24 ]
PROBE prospective randomized open blinded endpoint, eCRF electronic case report form, SAE serious adverse event
Electronic health records for research applications
Epidemiologic and observational research.
EHR data have been used to support observational studies, either as stand-alone data or following linkage to primary research data or other administrative data sets [ 3 , 11 – 14 ]. For example, the initial Euro Heart Survey [ 15 ] and subsequent Eurobservational Research Program (EORP) [ 16 ], the American College of Cardiology National Cardiovascular Data Registry (ACC-NCDR) [ 14 ], National Registry of Myocardial Infarction (NRMI), and American Heart Association Get With the Guidelines (AHA GWTG) [ 17 ] represent clinical data (collected from health records into an electronic case report form [eCRF] designed for the specific registry) on the management of patients across a spectrum of different cardiovascular diseases. However, modern EHR systems can minimize or eliminate the need for duplicate data collection (i.e., in a separate registry-specific eCRF), are capable of integrating large amounts of medical information accumulated throughout the patient’s life, enabling longitudinal study of diseases using the existing informatics infrastructure [ 18 ]. For example, EHR systems increasingly house imaging data which provide more detailed disease characterization than previously available in most observational data sets. In some countries (e.g., Farr Institute in Scotland [ 19 ]), the EHR can be linked, at an individual level, to other data sets, including general population health and lifestyle surveys, disease registries, and data collected by other sectors (e.g., education, housing, social care, and criminal justice). EHR data support a wide range of epidemiological research on the natural history of disease, drug utilization, and safety, as well as health services research.
Safety surveillance and regulatory uses
Active post-marketing safety surveillance and signal detection are important, emerging applications for EHRs, because they can provide realistic rates of events (unlike spontaneous event reports) and information on real-world use of drugs [ 20 ]. The EU-ADR project linked 8 databases in four European countries (Denmark, Italy, The Netherlands, United Kingdom) to enable analysis of select target adverse drug events [ 21 ]. The European Medicines Agency (EMA) coordinates the European Network of Centres for Pharmacoepidemiology and Pharmacovigilance (ENCePP) which aims to conduct post-marketing risk assessment using various EHR sources [ 22 , 23 ]. In the United States, the Food and Drug Administration (FDA) uses EHR data from several different sources (e.g., Sentinel and Mini-Sentinel System [ 24 ], Centers for Medicare and Medicaid Services [CMS], Veterans Affairs, Department of Defense, Substance Abuse and Mental Health Services Administration) to support post-marketing safety investigations [ 25 ].
Prospective clinical research
National patient registries that contain data extracted from the EHR are an accepted modality to assess guideline adherence and the effectiveness of performance improvement initiatives [ 26 – 33 ]. However, the use of EHRs for prospective clinical research is still limited, despite the fact that data collected for routine medical care overlap considerably with data collected for research. The most straightforward and generally accepted application for EHR is assessing trial feasibility and facilitating patient recruitment, and EHRs are currently used for this purpose in some centers. Using EHR technology to generate lists of patients who might be eligible for research is recognized as an option to meet meaningful use standards for EHR in the United States [ 6 ]. However, incomplete data may prohibit screening for the complete list of eligibility criteria [ 34 ], but EHRs may facilitate pre-screening of patients by age, gender, and diagnosis, particularly for exclusion of ineligible patients, and reduce the overall screening burden in clinical trials [ 35 ]. A second, and more complex, step involves the reuse of information collected in EHRs for routine clinical care as source data for research. Using EHRs as the source for demographic information, co-morbidities, and concomitant medications has several advantages over separately recording these data into an eCRF. Transcription errors may be reduced, since EHR data are entered by providers directly involved in a patient’s care as opposed to secondary eCRF entry by study personnel. The eCRF may be a redundant and costly step in a clinical trial, since local health records (electronic or paper) are used to verify source data entered into the eCRF. Finally, EHRs might enhance patient safety and reduce timelines if real-time EHR systems are used in clinical trials, in contrast to delays encountered with manual data entry into an eCRF. The EHR may facilitate implementation of remote data monitoring, which has the potential to greatly reduce clinical trial costs. The Innovative Medicine Initiative (IMI) Electronic Health Records for Clinical Research (EHR4CR, http://www.ehr4cr.eu ) project is one example, where tools and processes are being developed to facilitate reuse of EHR data for clinical research purposes. Systems to assess protocol feasibility and identify eligible patients for recruitment have been implemented, and efforts to link EHRs with clinical research electronic data collection are ongoing [ 36 ].
A shift towards pragmatic trials has been proposed as a mechanism to improve clinical trial efficiency [ 37 ]. Most of the data in a pragmatic trial are collected in the context of routine clinical care, which reduce trial-specific clinic visits and assessments, and should also reduce costs [ 38 ]. This concept is being applied in the National Institutes of Health (NIH) Health Care Systems Research Collaboratory. Trials conducted within the NIH Collaboratory aim to answer questions related to care delivery and the EHR contains relevant data for this purpose. Studies may have additional data collection modules if variables not routinely captured in the EHR are needed for a specific study. Similarly, the Patient-Centered Outcomes Research Institute (PCORI) has launched PCORnet, a research network that uses a common data platform alongside the existing EHR to conduct observational and interventional comparative effectiveness research [ 9 , 39 , 40 ].
The integration of EHRs in the conventional randomized controlled trials intended to support a new indication is more complex. EHRs may be an alternative to eCRFs when data collection is focused and limited to critical variables that are consistently collected in routine clinical care. Regulatory feedback indicates that while a new indication for a marketed drug might be achieved through EHRs, first marketing authorization using data entirely from EHRs would most likely not be possible with current systems until validation studies are performed and reviewed by regulatory agencies. The EHR could also be used to collect serious adverse events (SAE) that result in hospitalization, or to collect endpoints that do not necessarily require blinded adjudication (e.g., death), although the utility of EHRs for this purpose is dependent on the type of endpoint, whether it can reliably be identified in the EHR, and the timeliness of EHR data availability. Events that are coded for reimbursement (e.g., hospitalizations, MI) or new diagnoses, where disease-specific therapy is initiated (e.g., initiation of glucose lowering drugs to define new onset diabetes) tend to be more reliable. The reliability of endpoint collection varies by region and depends on the extent of linkage between different databases.
Challenges to using electronic health records in clinical trials and steps toward solutions
Challenges to using EHRs in clinical trials have been identified, related to data quality and validation, complete data capture, heterogeneity between systems, and developing a working knowledge across systems (Table 2 ). Ongoing projects, such as those conducted within the NIH Collaboratory and PCORnet [ 39 , 41 ] in the United States or the Farr Institute of Health Informatics Research in Scotland, have demonstrated the feasibility of using EHRs for aspects of clinical research, particularly comparative effectiveness. The success of these endeavors is connected to careful planning by a multi-stakeholder group committed to patient privacy, data security, fair governance, robust data infrastructure, and quality science from the outset. The next hurdle is to adapt the accrued knowledge for application to a broader base of clinical trials.
Table 2
Challenges of using electronic health records in research
EHR electronic health record, SAE serious adverse event
Data quality and validation
Data quality and validation are key factors in determining whether EHRs might be suitable data sources in clinical trials. Concerns about coding inaccuracies or bias introduced by selection of codes driven by billing incentives rather than clinical care may be diminished when healthcare providers enter data directly into the EHRs or when EHRs are used throughout all areas of the health-system, but such systems have not yet been widely implemented [ 42 ]. Excessive or busy workloads may also contribute to errors in clinician data entry [ 43 ]. Indeed, errors in EHRs have been reported [ 43 – 45 ].
Complete data capture is also a critical aspect of using EHRs for clinical research, particularly if EHRs are used for endpoint ascertainment or SAE collection. Complete data capture can be a major barrier in regions, where patients receive care from different providers or hospitals operating in different EHR systems that are not linked.
Consistent, validated methods for assessing data quality and completeness have not yet been adopted [ 46 ], but validation is a critical factor for the regulatory acceptance of EHR data. Proposed validation approaches include using both an eCRF and EHRs in a study in parallel and comparing results using the two data collection methods. This approach will require collaborative efforts to embed EHR substudies in large cardiovascular studies conducted by several sponsors. Assessing selected outcomes of interest from several EHR-based trials to compare different methodologies with an agreed statistical framework will be required to gauge precision of data collection via EHRs. A hybrid approach has also been proposed, where the EHR is used to identify study endpoints (e.g., death, hospitalization, myocardial infarction, and cancer), followed by adjudication and validation of EHR findings using clinical data (e.g., electrocardiogram and laboratory data).
Validity should be defined a priori and should be specific to the endpoints of interest as well as relevant to the country or healthcare system. Validation studies should aim to assess both the consistency between EHR data and standard data collection methods, and also how identified differences influence a study’s results. Proposed uses of EHRs for registration trials and methods for their validation will likely be considered by regulatory agencies on a case-by-case basis, because of the limited experience with EHRs for this purpose at the current time. Collaboration among industry sponsors to share cumulative experiences with EHR validation studies might lead to faster acceptance by regulatory authorities.
The ESC-CRT recommends that initial efforts to integrate EHRs in clinical trials focus on a few efficacy endpoints of interest, preferably objective endpoints (e.g., all-cause or cause-specific mortality) that are less susceptible to bias or subjective interpretation. As noted above, mortality may be incompletely captured in EHRs, particularly if patients die outside of the hospital, or at another institution using a non-integrated EHR. Thus, methods to supplement endpoint ascertainment in the EHR may be necessary if data completeness is uncertain. Standardized endpoint definitions based on the EHR should be included in the study protocol and analysis plan. A narrow set of data elements for auditing should be prospectively defined to ensure the required variables which are contained in the EHR.
Early interaction between sponsors, clinical investigators, and regulators is recommended to enable robust designs for clinical trials aiming to use EHRs for endpoint ascertainment. Plans to translate Good Clinical Practice into an EHR facilitated research environment should be described. Gaps in personnel training and education should be identified and specific actions to address training deficiencies should be communicated to regulators and in place prior to the start of the trial.
Timely access to electronic health record data
The potential for delays in data access is an important consideration when EHRs are used in clinical trials. EHRs may contain data originally collected as free text that was later coded for the EHR. Thus, coded information may not be available for patient identification/recruitment during the admission. Similarly, coding may occur weeks or months after discharge. In nationally integrated systems, data availability may also be delayed. These delays may be critical depending on the purpose of data extracted from the EHR (e.g., SAE reporting, source data, or endpoints in a time-sensitive study).
Heterogeneity between systems
Patients may be treated by multiple healthcare providers who operate independently of one another. Such patients may have more than one EHR, and these EHRs may not be linked. This heterogeneity adds to the complexity of using EHRs for clinical trials, since data coordinating centres have to develop processes for interacting or extracting data from any number of different systems. Differences in quality [ 47 ], non-standardized terminology, incomplete data capture, issues related to data sharing and data privacy, lack of common data fields, and the inability of systems to be configured to communicate with each other may also be problematic. Achieving agreement on a minimum set of common data fields to enable cross communication between systems would be a major step forward towards enabling EHRs to be used in clinical trials across centers and regions [ 48 , 49 ].
Data security and privacy
Privacy issues and information governance are among the most complex aspects of implementing EHRs for clinical research, in part because attitudes and regulations related to data privacy vary markedly around the world. Data security and appropriate use are high priorities, but access should not be restricted to the extent that the data are of limited usefulness. Access to EHR data by regulatory agencies will be necessary for auditing purposes in registration trials. Distributed analyses have the advantage of allowing data to remain with the individual site and under its control [ 39 , 41 ].
Pre-trial planning is critical to anticipate data security issues and to develop optimal standards and infrastructure. For pivotal registration trials, patients should be informed during the consent process about how their EHRs will be used and by whom. Modified approaches to obtaining informed consent for comparative effectiveness research studies of commonly used clinical practices or interventions may be possible [ 50 ]. A general upfront consent stating that EHR data may be used for research is a proactive step that may minimize later barriers to data access, although revision of existing legislation or ethics board rules may be needed to allow this approach. Patients and the public should be recognized as important stakeholders, and they can be advocates for clinical research using EHRs and improve the quality of EHR-based research if they are educated and engaged in the process and the purpose and procedures for EHR use are transparent. Developing optimal procedures for ensuring patients that are informed and protected, balanced with minimizing barriers to research is a major consideration as EHR-based research advances.
System capabilities
EHRs for use in clinical research need a flexible architecture to accommodate studies of different interventions or disease states. EHR systems may be capable of matching eligibility criteria to relevant data fields and flagging potential trial subjects to investigators. Patient questionnaires and surveys can be linked to EHRs to provide additional context to clinical data. Pre-population of eCRFs has been proposed as a potential role for EHRs, but the proportion of fields in an EHR that can be mapped to an eCRF varies substantially across systems.
EHRs may be more suitable for pragmatic trials where data collection mirrors those variables collected in routine clinical care. Whether regulators would require collection of additional elements to support a new drug or new indication depends on the drug, intended indication, patient population, and potential safety concerns.
Sustainability
The sustainability of EHRs in clinical research will largely depend on the materialization of their promised efficiencies. Programs like the NIH Collaboratory [ 41 ] and PCORnet [ 39 , 41 ], and randomized registry trials [ 51 , 52 ] are demonstrating the feasibility of these more efficient approaches to clinical research. The sustainability of using EHRs for pivotal registration clinical trials will depend on regulatory acceptance of the approach and whether the efficiencies support a business case for their use.
Role of stakeholders
To make the vision of EHRs in clinical trials a reality, stakeholders should collaborate and contribute to the advancement of EHRs for research. Professional bodies, such as the ESC, can play a major role in the training and education of researchers and the public about the potential value of EHR. Clinical trialists and industry must be committed to advancing validation methodology [ 53 ]. Investigators should develop, conduct, and promote institutional EHR trials that change clinical practice; such experience may encourage EHR trial adoption by industry and the agencies. Development of core or minimal data sets could streamline the process, reduce redundancy and heterogeneity, and decrease start-up time for future EHR-based clinical trials. These and other stakeholder contributions are outlined in Table 3 .
Table 3
Role and influence of stakeholders in advancing the use of electronic health records in clinical research
CARDS cardiology audit and registration data standards, CRO contract research organization, eCRF electronic case report form, IT information technology, EHR electronic health record, EORP European Observational Research Program
Electronic health records are a promising resource to improve the efficiency of clinical trials and to capitalize on novel research approaches. EHRs are useful data sources to support comparative effectiveness research and new trial designs that may answer relevant clinical questions as well as improve efficiency and reduce the cost of cardiovascular clinical research. Initial experience with EHRs has been encouraging, and accruing knowledge will continue to transform the application of EHRs for clinical research. The pace of technology has produced unprecedented analytic capabilities, but these must be pursued with appropriate measures in place to manage security, privacy, and ensure adequacy of informed consent. Ongoing programs have implemented creative solutions for these issues using distributed analyses to allow organizations to retain data control and by engaging patient stakeholders. Whether EHRs can be successfully applied to the conventional drug development in pivotal, registration trials remains to be seen and will depend on demonstration of data quality and validity, as well as realization of expected efficiencies.
Acknowledgments
This paper was generated from discussions during a cardiovascular round table (CRT) Workshop organized on 23–24 April 2015 by the European Society of Cardiology (ESC). The CRT is a strategic forum for high-level dialogues between academia, regulators, industry, and ESC leadership to identify and discuss key strategic issues for the future of cardiovascular health in Europe and other parts of the world. We acknowledge Colin Freer for his participation in the meeting. This article reflects the views of the authors and should not be construed to represent FDA’s views or policies. The opinions expressed in this paper are those of the authors and cannot be interpreted as the opinion of any of the organizations that employ the authors. MRC’s salary is supported by the National Institute for Health Research (NIHR) Cardiovascular Biomedical Research Unit at the Royal Brompton Hospital, London, UK.
Conflict of interest
Martin R. Cowie: Research grants from ResMed, Boston Scientific, and Bayer; personal fees from ResMed, Boston Scientific, Bayer, Servier, Novartis, St. Jude Medical, and Pfizer. Juuso Blomster: Astra Zeneca employee. Lesley Curtis: Funding from FDA for work with the Mini-Sentinel program and from PCORI for work with the PCORnet program. Sylvie Duclaux: None. Ian Ford: None. Fleur Fritz: None. Samantha Goldman: None. Salim Janmohamed: GSK employee and shareholder. Jörg Kreuzer: Employee of Boehringer-Ingelheim. Mark Leenay: Employee of Optum. Alexander Michel: Bayer employee and shareholder. Seleen Ong: Employee of Pfizer. Jill Pell: None. Mary Ross Southworth: None. Wendy Gattis Stough: Consultant to European Society of Cardiology, Heart Failure Association of the European Society of Cardiology, European Drug Development Hub, Relypsa, CHU Nancy, Heart Failure Society of America, Overcome, Stealth BioTherapeutics, Covis Pharmaceuticals, University of Gottingen, and University of North Carolina. Martin Thoenes: Employee of Edwards Lifesciences. Faiez Zannad: Personal fees from Boston Scientific, Servier, Pfizer, Novartis, Takeda, Janssen, Resmed, Eli Lilly, CVRx, AstraZeneca, Merck, Stealth Peptides, Relypsa, ZS Pharma, Air Liquide, Quantum Genomics, Bayer for Steering Committee, Advisory Board, or DSMB member. Andrew Zalewski: Employee of GSK.
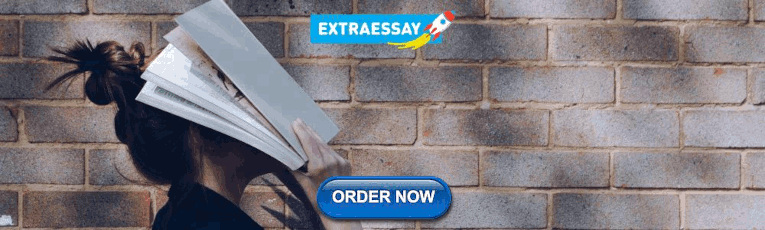
IMAGES
VIDEO
COMMENTS
With increasing attention being paid to patient-reported outcomes in observational, comparative effectiveness, and clinical trials research, data collection approaches that combine medical record abstraction, patient interviews, and administrative data will be more commonly utilized in the future. In the present editorial, we discuss a number ...
Step 1: Data collection. Private and public organizations around the world collect data through surveys, censuses, and other methods. Step 2: Data publication. Organizations publish the data they have collected and share details on the methodology. Depending on the nature of the data, they may be publicly available for anyone to download, or ...
We offer best-practice recommendations for journal reviewers, editors, and authors regarding data collection and preparation. Our recommendations are applicable to research adopting different epistemological and ontological perspectives—including both quantitative and qualitative approaches—as well as research addressing micro (i.e., individuals, teams) and macro (i.e., organizations ...
Easy access to large quantities of accurate health data is required to understand medical and scientific information in real-time; evaluate public health measures before, during, and after times ...
At Johns Hopkins, OMOP has converted more than 1 billion pieces of information in the medical records of 2.6 million patients from the past six years — creating a trove of data that is regularly updated. Like any research project that uses patient information, Institutional Review Board approval is required before researchers can use OMOP for ...
This chapter has set out data collection methods, descriptive statistical methods, and inferential statistical methods. Of particular significance in medical imaging research is the collection of knowledge and attitudes via a combination of quantitative and qualitative methods. Many research studies in medical radiation science require some ...
Data linkage in medical research allows researchers to exploit and enhance existing data sources without the time and cost associated with primary data collection. Methods used to quantify, interpret, and account for errors in the linkage process are needed, alongside guidelines for transparent reporting. Data linkage provides an opportunity to ...
The term "data" in clinical research refers to observations that are structured in such a way as to be "amenable to inspection and/or analysis" [ 3 ]. In other words, they represent the evidence for conclusions drawn in a trial. All data collected in biomedical research studies are either numerical or nonnumerical.
We provide an overview of the different data-collection approaches that are commonly used in carrying out clinical, public health, and translational research. We discuss several of the factors that researchers need to consider in using data collected in questionnaire surveys, from proxy informants, through the review of medical records, and in the collection of biologic samples.
Method. Prospective observational study comparing the completeness of data capture and level of agreement between three data collection methods; manual data collection from ward-based sources, administrative data from an electronic patient management program (i.PM), and inpatient medical record review (gold standard) for hospital length of stay ...
Data collection is an essential component of any research project, particularly in the medical and life sciences fields. It involves gathering information, measurements, and observations that will later be used to answer research questions or test hypotheses. Effective data collection is crucial in ensuring that research findings are accurate, reliable, and valid. In this blog post, we will ...
Recent studies show that Medical Data Science (MDS) carries great potential to improve healthcare 1, 2, 3. Thereby, considering data from several medical areas and of different types, i.e. using ...
Clinical data is a staple resource for most health and medical research. Clinical data is either collected during the course of ongoing patient care or as part of a formal clinical trial program. Clinical data falls into six major types: ... with data collection by Westat, and support from the National Institute on Aging. NHATS is intended to ...
Innovative Solutions to Improve Data Collection and Delivery Fortunately, sponsors can find that support with ICON, the healthcare intelligence and clinical research organization. "We essentially advance clinical research [by] providing outsourced services to the pharmaceutical industry, to the medical device industry, and also to government ...
Based on our above observations on the related previous research on the data collection process part of medical image analysis, we now state the most significant challenges and limitations of the current data collection process during MIA as follows: 1. Data privacy for the medical images, as they hold sensitive information about the patients. 2.
Box 5-4. Successful Collection of Data by a Health Plan: Aetna Aetna was the first national, commercial plan to start collecting race and ethnicity data for all of its members. In 2002, Aetna began directly collecting these data using electronic and paper enrollment forms. Multiple mechanisms are now used to capture race, ethnicity, and ...
Prevention is the most cost-effective activity that will ensure the integrity of data collection. A detailed and comprehensive research manual will standardize data collection. Poorly written manuals are vague and ambiguous. The research manual is based off your protocol. The manual should spell out every step of the data collection process.
The Benefits of Public Health Data Collection. Making data-driven decisions is virtually impossible without relevant, up-to-date information. Whether tracking the spread of disease, informing the public on the latest prevention strategies, or promoting public health throughout the population, professionals must understand the importance of data collection as they collaborate with other public ...
Background: As global populations age and become susceptible to neurodegenerative illnesses, new therapies for Alzheimer disease (AD) are urgently needed. Existing data resources for drug discovery and repurposing fail to capture relationships central to the disease's etiology and response to drugs. Objective: We designed the Alzheimer's Knowledge Base (AlzKB) to alleviate this need by ...
Abstract. We provide an overview of the different data-collection approaches that are commonly used in carrying out clinical, public health, and translational research. We discuss several of the factors that researchers need to consider in using data collected in questionnaire surveys, from proxy informants, through the review of medical ...
FOR IMMEDIATE RELEASE April 16, 2024. The following is attributed to Jeff Shuren, M.D., J.D., director of the FDA's Center for Devices and Radiological Health (CDRH) and Ed Margerrison, Ph.D ...
To explore the motives and experiences of patients with breast cancer who chose Korean medical treatment, we utilized a qualitative research methodology. For data collection, we will conduct in-depth interviews based on semi-structured questionnaires, and for data analysis, we aim to adopt the grounded theory method proposed by Glaser and ...
The CDC data that is highlighted in this post comes from the agency's "abortion surveillance" reports, which have been published annually since 1974 (and which have included data from 1969). Its figures from 1973 through 1996 include data from all 50 states, the District of Columbia and New York City - 52 "reporting areas" in all.
Abstract. Electronic health records (EHRs) provide opportunities to enhance patient care, embed performance measures in clinical practice, and facilitate clinical research. Concerns have been raised about the increasing recruitment challenges in trials, burdensome and obtrusive data collection, and uncertain generalizability of the results.